- I Tried Both: Apple Watch 9 vs Fitbit Charge 6
- Best Places to Print Photos Online
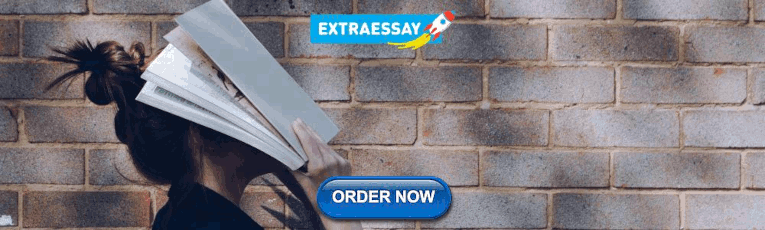
What Is a Smartwatch and What Do They Do?
Everything you need to know about smartwatches
- Bard College
- Sarah Lawrence College
:max_bytes(150000):strip_icc():format(webp)/ryanperiansquare-de5f69cde760457facb17deac949263e-180a645bf10845498a859fbbcda36d46.jpg)
- Western Governors University
In This Article
Jump to a Section
- A Brief History
- Standard Features
- Main Categories
Smartwatch Market Growth
- Frequently Asked Questions
A smartwatch is a portable device that's designed to be worn on a wrist. Like smartphones, they use touchscreens, offer apps, and often record your heart rate and other vital signs.
The Apple Watch and Wear (formerly Android Wear) models prompted more consumers to appreciate the usefulness of wearing a mini computer on their wrists. In addition, specialty smartwatches for outdoor activities often supplement other, bulkier devices in an adventurer's tool kit.
A Short History of the Smartwatch
While digital watches have been around for decades—some with abilities like calculators and unit converters—only in the 2010s did tech companies begin releasing watches with smartphone-like abilities.
Apple, Samsung, Sony, and other major players offer smartwatches on the consumer market, but a small startup actually deserves credit for popularizing the modern-day smartwatch. When Pebble announced its first smartwatch in 2013, it raised a record amount of funding on Kickstarter and went on to sell more than one million units.
The Pebble smartwatch was discontinued when the company shut down in 2016, but still has a number of fans and enthusiasts who continue to use and develop for it .
At the same time, advances in silicon miniaturization opened the door to other kinds of dedicated-purpose smartwatches. Companies like Garmin, for example, support smartwatches like the Fenix, which are more rugged and optimized with sensors and trackers to support back-country expeditions. Likewise, companies like Suunto released smartwatches optimized for scuba diving that withstand extended time at significant depths.
What Do Smartwatches Do?
Most smartwatches—whether they're intended for daily use (as with the Apple Watch) or for specific purposes (as with the Garmin Fenix)—offer a suite of standard features:
- Notifications : Smartphones display notifications to alert you of important events or activities. The types of notifications differ; devices connected to a smartphone may simply mirror the phone's notifications on your wrist, but other smartwatches display notifications that only a wearable could provide. For example, newer Apple Watches includes a fall sensor. If you fall while wearing the watch, it senses your subsequent movement. If it doesn't detect any movement, it sends a series of escalating notifications. Fail to respond to the notification, and the watch assumes you're injured and alerts authorities on your behalf.
- Apps : Beyond displaying notifications from your phone, a smartwatch is only as good as the apps it supports. App ecosystems vary, and they're tied to either Apple's or Google's environments. Smartwatches with a dedicated purpose, such as hiking or diving, generally support the apps they need to accomplish that purpose without the opportunity to add other kinds of apps.
- Media management : Most smartwatches paired with smartphones can manage media playback for you. For example, when you're listening to music on an iPhone using Apple's AirPods, you can use your Apple Watch to change volume and tracks.
- Answer messages by voice : Remember the old Dick Tracy comics, where the hero detective used a watch as a phone? Modern smartwatches running either the watchOS or Wear operating systems support voice dictation.
- Fitness tracking : If you’re a hard-core athlete, a dedicated fitness band is likely a better choice than a smartwatch. Still, many smartwatches include a heart rate monitor and a pedometer to help track your workouts.
- GPS : Most smartwatches include a GPS for tracking your location or receiving location-specific alerts.
- Good battery life : Modern smartwatches feature batteries that get you through the day, with normal use, with a bit of juice still left to go. Battery use varies; the Apple Watch typically gets 18 hours of normal use on a single charge, while the Pebble gets two or three days.
Types of Smartwatches
Broadly speaking, smartwatches occupy two niches in the wearables market. First, a general-purpose smartwatch—like the Apple Watch and most Google-powered Wear devices—blend form and function. They're designed to replace mechanical wristwatches and are heavily smartphone-dependent. Think of them as a support device for your phone that you happen to keep on your wrist.
You also see vendor-specific classes of general-purpose smartwatches in the consumer market:
- Apple Watch : Designed and sold by Apple.
- Pixel Watch : Designed and sold by Google, compatible with Android phones but not currently with Apple devices.
- Wear watches : Designed and sold by many vendors, using Google's Wear operating system.
- Tizen watches : Proprietary operating system designed by Samsung for its popular Galaxy line of smartwatches.
The other niche includes specialty devices intended for specific-use cases. These devices often offer a more robust version of a fitness tracker, insofar as they bleed between a phone-dependent smartwatch and a stand-alone fitness tracker like a Fitbit.
Examples of these specialized devices include:
- Hiking watches : Intended for remote travel and featuring solid battery life, GPS tracking and navigation, basic vitals, and weather forecasting. Often engineered for advanced durability to protect against bumps, drops, dust, and water. Examples include the Garmin Fenix 5 Plus, the Suunto 9 Baro, and the 2022 Apple Watch Ultra .
- Diving watches : Connect your first-stage regulator to a Bluetooth transmitter to use a diving watch. Garmin's Descent Mk2i and Suunto's DX offer depth, time-remaining, temperature, and other important indicators. And the Apple Watch Ultra can make use of the Oceanic+ Dive Computer App to calculate dive times, display a number of different stats, and can handle depths of up to 130 feet (40 meters).
- Flying watches : A niche market, but Garmin's MARQ Aviator Gen. 2 offers a jet-lag advisor, GPS-powered moving map, NEXRAD weather reports (using METARs, TAFs and MOS2), flight logging, a barometric altimeter, and more.
Smartwatches settled into a steep growth curve in the late 2010s in terms of global market adoption. Data from Statista shows that sales rose from five million units worldwide in 2014 to an estimated 173 million in 2022. Apple's market share rose from 13- to 30-percent from the second fiscal quarter of 2017 to the same period in 2021. With Samsung in the second-place spot with a 10-percent market share.
During the same period, specialty vendors like Garmin saw a 4.1-percent increase in year-over-year growth, while fitness-tracker-only vendors like Fitbit saw a nearly 22-percent market plunge.
Statista predicts that over 253 million smartwatches will ship worldwide by 2025.
Hybrid smartwatches are watches with the traditional looks and feels of a watch, but they also come with smartwatch functionality.
Fitbits are fitness trackers, which do have functionality similar to smartwatches, but they focus on fitness-oriented features and don't often come with the advanced features of smartwatches.
Get the Latest Tech News Delivered Every Day
- I Tried Both: Apple Watch Series 9 vs Fitbit Charge 6
- The First Steps for Wearable Activity Trackers
- Is the Oura Ring Worth It? 4 Reasons Why You Should Buy One
- All the Things You Can Track With Wearables
- Should You Buy an Apple Watch?
- Apple Watch vs. Fitbit Blaze
- Apple Watch X: News and Expected Price, Release Date, Specs; and More Rumors
- Apple Watch Series 9 Review
- How to Wear Your Fitbit
- Wear OS vs. watchOS: Which Is the Better Software?
- Meta Smartwatch: News, Rumors, and Estimated Price, Release Date, and Specs
- The Best-Looking Smartwatches
- Getting Started With Your Smartwatch
- The 10 Best Tips and Tricks for Wear OS
- The Complete Guide to the Wear Operating System
- What Is the Fitbit Ace?
The unexpected health impacts of wearable tech
We didn’t realize how much doctors would be seeing of our health data.
By Nicole Wetsman
Illustration by Micha Huigen
Share this story
:format(webp)/cdn.vox-cdn.com/uploads/chorus_asset/file/22968887/VRG_ILLO_ANI_4841_10_Year_health_tech.jpg)
When Tim Cook took the stage at a September 2014 event to announce the hotly anticipated, long-rumored Apple Watch, he described it as a “comprehensive health and fitness device.” The announcement set the foundation for what the company thought smartwatches and wearable devices would be — not just for fun, not just for notifications, but for your health.
It was a big promise for a new, unproven product, made at a time when no one was quite sure what smartwatches were for or what they might be able to do. In the years before the Apple Watch launched, similar devices were steadily flooding the market. “It looked like the world would soon be awash in smartwatches. Samsung, Sony, LG, Asus, Motorola, Pebble, Meta — companies big and small attempted to find out what people want their wrists to do,” wrote David Pierce , then the deputy editor at The Verge . There didn’t seem to be a clear answer. Dan Seifert, who runs The Verge ’s reviews desk, wondered in 2014 if there even needed to be an answer: maybe there could just be a range of watches, each with a different focus.
People were using the devices to monitor their health — and gradually, more started to bring that to their doctors
At first, Apple didn’t appear to have a good answer, either. The company over-indexed on apps and communication, while its health features remained basic. The watch wasn’t smart enough yet and didn’t feel essential to a daily routine, Walt Mossberg said in one 2016 column. “It needs a capability more compelling than what’s out there so far,” he wrote .
But Apple continued to sharpen its focus on fitness, and smartwatches became more and more popular. Sales for all smartwatches, not just Apple Watches, went up 61 percent in 2018. And their features started to fit more closely into the framework laid out by Cook in 2014. People were using the devices to monitor their health — tracking their heart rate, their steps, and their sleep. And gradually, more and more of them started to bring that information along to their doctors appointments.
The permeation through healthcare is particularly noticeable in three areas: cardiology, sleep medicine, and sports medicine. Smartwatches made by Apple, Fitbit, Withings, Samsung, and other companies have features specific to each of those fields, and over the past decade, the devices became impossible for doctors to ignore.
“People come in and they have their device, and they’ve downloaded that data, and they want to show it to you,” says Matthew Ebben, a sleep specialist at Weill Cornell Medicine in New York. “It happens all the time.”
The Verge asked cardiologists, sleep doctors, and sports physicians how all of that data influences their interactions with patients. For better or for worse, they know it’s here to stay.
Four years after Cook called Apple’s smartwatch a health device, he was back to tout bigger claims: Apple Watches could be lifesaving . The company announced that it had Food and Drug Administration clearance for an app that could detect irregular heart rhythms. The Apple Watch was now officially a medical device and was allowed to tell people that they might have a medical problem.
The app was a big step for Apple, and a big step for smartwatches generally — soon after, Fitbit, Withings, and Samsung all added programs to detect irregular heart rhythms. Early on, though, many doctors were hesitant to embrace the tech. They told The Verge they were worried that there could be false positive readings that could push people to seek out unnecessary medical care, straining the system.
Five or six years ago, cardiologists started to see a trickle of patients bring in heart rate data from smartwatches. But a few months after the FDA cleared the Apple Watch’s app in 2018, things changed. “People were watching ads and hearing Tim Cook say that it’s a lifesaving device. So we started getting a lot more of those patients,” says Mohamed Elshazly, a cardiac electrophysiologist at the Cleveland Clinic. “We have definitely seen an uptick in the last two to three years.”
:format(webp)/cdn.vox-cdn.com/uploads/chorus_asset/file/22967879/dseifert_200930_4218_0007.0.jpg)
Elshazly remembers the first time he talked with a patient about their Apple Watch. Around four years ago, a man in his 60s — an active runner — came to see him after having occasional heart palpitations for a year. Other doctors hadn’t been able to offer a diagnosis because he hadn’t been wearing a heart monitor during any of those incidents.
“Then I looked at talking to him, and I’m looking at his hand, and he’s wearing an Apple Watch,” Elshazly says. When the Apple Watch detects an abnormal heart rhythm, it prompts the user to use their index finger to take an EKG reading on the watch. The patient had followed those directions during one of the palpitations. Elshazly asked to see the patient’s phone, pulled up the health app, and looked at the reading. “It was atrial fibrillation,” he says. “So I was able to tell the patient, we have a diagnosis.”
“They also let patients be more engaged in their own care.”
Four years later, wearables are a regular part of patient visits. Sometimes, patients come into the office with readouts from their device that they want to show the doctor. Other visits are more like Elshazly’s first encounter with an Apple Watch — he spots a wearable on their wrist and asks about it.
In many ways, they’ve been helpful to cardiologists and their patients. Before, it could be challenging to diagnose people with heart conditions that cause abnormal heart rhythms, like atrial fibrillation. The conditions don’t mean someone’s heart is having problems all the time — it may flutter or feel strange for a moment before returning to normal. Doctors would send a patient home for a week or so with a heart monitor to try to catch one of the incidents. But if a flutter didn’t happen in that window, they sometimes resorted to surgically implanting a device in the patient’s chest to track heart rhythms.
“It’s an invasive procedure and quite a bit more expensive than an Apple Watch,” says Byron Allen, a cardiologist and clinical professor at the University of California at Irvine. Wearable devices like the Apple Watch and Fitbits, which also have an electrocardiogram feature, make it easier for doctors to monitor patients at home. “They also let patients be more engaged in their own care,” he says.
False positive Apple Watch readings “cause anxiety for the patient”
Most doctors still wouldn’t actively recommend a cardiac patient go out and buy a wearable device as part of their treatment, Elshazly says — they’re still fairly new, and while most people have stories of times when they were effective, there isn’t good comprehensive data on how much of a difference they make in public health overall. But if someone already has a device, doctors should make use of the data they offer, he says.
But there are downsides to the influx of wearable data, Allen says — those early worries about false positives proved valid. He estimates around a third of patients who come in worried about an Apple Watch notification have a false positive reading. “Those cause anxiety for the patient, and it takes quite a bit of time to sort through,” he says.
Doctors can also struggle to manage the sheer volume of heart rate and heart rhythm information wearable devices can collect. It’s not high enough yet to be entirely overwhelming, but it’s enough that cardiologists know they need to start figuring out how to sort through and manage it. “The engineers have been able to give us all this data, and create all these sensors for us,” Elshazly says. “Now it’s up to us to analyze that data and figure out how to make clinical sense of it.”
Sports and fitness
Liz Joy knows that more and more people are using smartwatches and other wearable devices as part of their health and fitness routines — tracking steps, monitoring their heart rate, and logging runs. But they don’t often bring them up at appointments, says Joy, a sports medicine specialist and senior medical director of wellness and nutrition at Intermountain Healthcare in Utah. “It doesn’t happen as often as you would think. Maybe 10 percent of the time,” she told The Verge .
Joy thinks that’s because most people don’t think about the information collected by the devices as something they should bring up at a doctor’s appointment. “If you don’t ask, they’re not going to tell,” she says.
Most of the time, when the devices do come up, Joy usually uses it as an opening to talk about a range of topics: the amount of activity they do each day, any strength training they might want to incorporate into their routine, and so on. They can be helpful for people to keep tabs on the amount they’re moving around, even though they might not play a big role in someone’s nutrition or exercise programs. Many conversations aim to refocus people using activity trackers from the raw stats to how activity makes them feel and their overall goals, Joy says.
:format(webp)/cdn.vox-cdn.com/uploads/chorus_asset/file/22967886/akrales_190328_3319_0023.jpg)
Fitness and fitness-adjacent information, like step counts, was some of the earliest wearable data available. But it was often divorced from context — the main goal was hitting a target , and that target could often be arbitrary.
“They say, ‘I try to get 10,000 steps a day.’ And I’ll ask them where they think that recommendation came from, and nine times out of 10, they have no idea,” she says. “There’s nothing wrong with getting 10,000 steps, it’s just that they don’t need that many to get health benefits.”
In some situations, though, the widespread use of wearable devices as fitness trackers can be dangerous — like for patients with eating disorders or obsessive-compulsive behaviors, who Joy also sees. “I’d say it’s more of a curse than a blessing with that group,” she says.
“We talk about how to avoid tracking, because it’s not healthy for them. We want them to be more mindful.”
Dusty Marie Narducci, a sports medicine and eating disorder specialist at the University of South Florida, discourages trackers for those patients. “We talk about how to avoid tracking, because it’s not healthy for them. We want them to be more mindful, and try different activities — like, say, kayaking — rather than actively tracking their running,” she says.
On the opposite end of the spectrum are intense athletes, who tend to have the most intensive use of their smartwatches. Triathletes and ultramarathoners often keep close tabs on every possible metric, Narducci says. “There’s so much data that they come in with.”
But it’s hard to figure out what to do with that data. There’s no clear sign that the training and fitness information collected on a smartwatch or wearable devices can help people reach their goals faster or more effectively. Focusing on that information might actually make things worse, Narducci says.
“They’re always looking at their watch and trying to fix something. They spend so much time setting everything up or tracking it after, rather than focusing on why they’re exercising, or other things that might make them better performers,” she says. “You always have to ask yourself what you’re going to do with this data, and what time you’re taking away from other things to focus on it.”
Sleep medicine
When patients first started showing up to Seema Khosla’s office around 10 years ago with digital devices tracking their sleep, she wasn’t really sure what to make of it. “They would come in with their Fitbit, or I had one lady bring in her laptop,” says Khosla, medical director of the North Dakota Center for Sleep. “That was the reason they made their appointment, because their tracker said there’s something not quite right.”
Fitbit has monitored sleep since the earliest iterations of its devices — the clip-on Fitbit Classic, released in 2009, told wearers how much time they spent asleep during the night (or at least tried to ). Garmin had early sleep features starting in 2014 , as well. When the devices first started to come on the market, Khosla says she probably had around one patient a month bring them in. “Now it’s easily ten a week,” she says.
“They say, ‘so, what am I looking at?’”
The trouble with the information offered by sleep tracking devices is that it’s hard for most people to know what to do with it. That’s just as true now as it was when the features were first introduced. The sleep features on the Samsung Galaxy Watch 3, which came out in 2020, aren’t that helpful, Dan Seifert wrote in The Verge ’s review . “Wearable devices like this are missing a lot of the necessary context for what impacts the quality of your sleep,” he said.
That’s why people go see sleep doctors about their data, says Ebben, the specialist from Weill Cornell Medicine. “They say, ‘so, what am I looking at?’”
:format(webp)/cdn.vox-cdn.com/uploads/chorus_asset/file/22967893/jawbone_up_3_5274_2.0.jpg)
At first, Khosla says she wasn’t quite sure what she was looking at, either. She rarely initiated conversations with patients about their wearable devices, and only discussed it when the patients brought it up themselves. “Now, if I spy a Fitbit on their wrist, I’ll ask about it,” she says. It functions as a patient engagement tool: she asks them why they track their sleep, what they think about the information it gives them, and how it makes them feel. It gives her a way to point out the importance of things like a consistent bedtime — if someone’s data shows that they go to sleep at a range of times, it’s a clear opening to raise the issue.
Devices offer more objective information than a sleep log, which doctors often ask patients to keep before a visit to capture their sleep patterns. “Inevitably they end up filling that out in the parking lot before the appointment,” Khosla says.
Ebben has started to notice a drop off in patients bringing in data
But while sleep tracking features on wearables have improved over the years, they’re still not accurate enough to rely on entirely, Ebben says. They’re pretty good at figuring out when people are actually asleep during the night but aren’t as good at flagging when someone is awake. The various charts showing when someone is in deep sleep tend to be inaccurate, as well. It can be hard to treat someone with sleep issues if they’re fixated on data from a device, he says.
“They want to talk about what happened at 2:30AM on Thursday. We try to refocus on how they feel, if they feel like they’re waking up during the night — those are the things that are actually important to the person,” Ebben says.
Khosla says she’ll look at the information on deep sleep, particularly if the wearable has flagged something concerning. The varying accuracy, though, can make patients unnecessarily anxious, she says. She tries to keep tabs on the various devices so she can tell them what to expect. “You can tell them that theirs tends to underestimate wakefulness, or overestimate something else.”
People who use the devices seem to be starting to understand their limitations, Ebben says. After a surge in patients bringing in data, he’s started to notice a drop-off. “I think people are more aware that this is more entertainment than data.”
Google scrambles to manually remove weird AI answers in search
Apple’s wwdc may include ai-generated emoji and an openai partnership, hbo’s moviepass doc is a snapshot of how c-suites kill companies, lego’s first legend of zelda set is a 2,500-piece great deku tree, microsoft confirms call of duty: black ops 6 is coming to xbox game pass.

More from Science
:format(webp)/cdn.vox-cdn.com/uploads/chorus_asset/file/23935561/acastro_STK103__04.jpg)
Amazon — like SpaceX — claims the labor board is unconstitutional
:format(webp)/cdn.vox-cdn.com/uploads/chorus_asset/file/25288452/246992_AI_at_Work_REAL_COST_ECarter.png)
How much electricity does AI consume?
:format(webp)/cdn.vox-cdn.com/uploads/chorus_asset/file/25287681/1371856480.jpg)
A Big Tech-backed campaign to plant trees might have taken a wrong turn
:format(webp)/cdn.vox-cdn.com/uploads/chorus_asset/file/25287408/2003731596.jpg)
SpaceX successfully launches Odysseus in bid to return US to the lunar surface
- Share full article
Advertisement
Supported by
Lindsay Crouse
I Ditched My Smart Watch, and I Don’t Regret It

By Lindsay Crouse
Ms. Crouse is a writer and producer in Opinion who writes on gender, ambition and power. Her best marathon time is a 2:53.
My rock bottom was when I caught myself at a nice dinner, transfixed by my phone under the table. I was opening the app for my smart watch, checking whether the numbers it assigned to my “training status” had improved since I finished my run an hour ago. The numbers hadn’t budged, so I closed the app and refreshed it, frowning a little. Was it broken? My companion asked what I was doing. “Nothing,” I lied.
At first, I loved that smart watch, which I used to get faster at racing marathons. Suddenly, I had metrics on things I didn’t even realize my body did: lactate thresholds, VO₂ max, heart rate variability. Each evening I had a full report, telling me what this device thought of my performance.
Soon I couldn’t stop thinking about the numbers on the watch. I was addicted.
At the dawn of the smart watch era, the introduction of the Apple Watch in 2015, Tim Cook pitched the new device as aspirational tech, the next must-have gadget, loaded with apps and features. After a choppy start, smart watches have exploded in popularity in recent years and are expected to reach shipments of 230 million units by 2026. Lately, companies have been marketing these devices less as luxury products and more as essential medical devices , necessities for anyone concerned about health. Amid the pandemic, this pitch seems to be working .
Some brands now have ECG monitors , which let you check for atrial fibrillation, and pulse oximeters , a useful feature during a coronavirus infection, when low blood oxygen can be a useful signal. Others can track your skin’s exposure to the sun. If you fall down in the woods, Apple assures us in some rather alarming advertising, its watch can call for help. And this kind of monitoring isn’t confined to watches: Smart beds that will report via an app on how you slept last night are among an arsenal of health-monitoring home technologies. There are light bulbs in development that will measure your heart rate and body temperature. A breathalyzing product called Lumen invites users to breathe into a tube that it claims can read your metabolism “to see if you have enough energy for your workout or if you should fuel up.”
The message is clear. Self-quantification isn’t just aspirational anymore — it’s essential. A roster of famously healthy-seeming people endorse these claims. Coros makes a watch for the marathon world-record holder Eliud Kipchoge. Jennifer Aniston says she’s “addicted” to her Oura ring , which tracks everything from breathing patterns to blood oxygen to sleep. In 2017 Gwyneth Paltrow promoted the Frédérique Constant Horological smart watch and its pointed command that women “Move More, Sleep Better, Improve.”
But does this constant monitoring of our vital signs truly yield better health? There’s no clear answer yet. One study found that people trying to lose weight who used wearable technology to help actually lost less weight than their watch-free counterparts. A review in the American Journal of Medicine found “little indication that wearable devices provide a benefit for health outcomes.” Another issue is that the measuring abilities of wearables are imperfect for some metrics.
I also worry that the safety-net sales pitch ignores one major downside to all this quantification: It can interfere with our ability to know our own bodies. Once you outsource your well-being to a device and convert it into a number, it stops being yours. The data stands in for self-awareness. We let a gadget tell us when and how to move, when we’re tired, when we’re hungry.
With my smart watch, sometimes I would wake up in the morning and check my app to see how I slept — instead of just taking a moment to notice that I was still tired. When I discovered that my watch could measure my stress levels, it was as if I’d started carrying around an expensive psychological pyramid scheme on my wrist. The more I used my watch to monitor my stress, the higher my stress levels rose.
It’s an extension of our hustle-oriented culture, said the executive coach and performance expert Brad Stulberg, author of “The Practice of Groundedness.” “Our culture promotes the limiting belief that measurable achievement is the predominant arbiter of success, and these devices play right into that,” he told me. “It’s like you’re trying to win at this game instead of living your life. Instead of learning what your body feels like, you have a number.”
Add a social or competitive component, as in the fitness app Strava or the community features on Peloton, and the feelings of control and empowerment that fitness can foster can morph quickly into the opposite. Halfway through one marathon training cycle, I discovered a new trick: My watch could measure my overall fitness level, assigning it a number, plotting its change over time and telling me how my levels compared with others’, sorted by gender and age. I craved its approval.
If it feels like an addiction, that’s because it can work similarly to smartphone and other digital addictions. Dependency is what these devices are designed to foster.
“These technologies have, in essence, druggified even exercise,” said Anna Lembke, a professor of psychiatry and behavioral sciences at Stanford University, and the author of “Dopamine Nation: Finding Balance in the Age of Indulgence,” which explores addictive behavior. “You may think, ‘A wearable device that keeps track of my actions couldn’t possibly be bad because it’s just a watch, and I’m just, for example, monitoring my heart rate, which is about my physical wellness.’ But in fact, we very much can become compulsively fixated on these wearable devices — in a way that is akin to addiction.”
These devices don’t just record your behavior — they influence it and keep you coming back. You become dependent on external validation. This in itself is nothing new: As with weighing yourself on a scale, or calculating your body mass index, or measuring your step goals, it’s easier to read a number than it is to know instinctively whether you are healthy. But you can’t quantify your way to good health. The reality is much harder.
For a while, my smart watch probably did help me get healthier. I know I got fitter. But I started to feel that my health wasn’t grounded in my own body anymore, or even in my mind. I didn’t know how my workout had gone until I opened the app.
I had been using numbers — and the success they validated — as a shortcut to feeling good enough in general. Exercise wasn’t helping me rebound from pressure anymore; it was adding to it.
Of course these watches can be useful: for health data, reminding you to move more or maybe even that emergency call if you wind up falling in the woods. Many of us make better choices when we know we’re being watched.
But if you’re thinking it might be time for a break from the numbers, I propose a challenge for the year ahead: Try taking your cues from your body instead of a device.
That’s what I did. At some point in the pandemic, I took off my watch. It left a stripe of skin on my wrist where it had spent years blocking the sun. Then I lost it and never bothered to find it.
The adjustment didn’t always feel natural. Once you outsource your confidence to something else, it takes a while to come back. But finally, I stopped counting, stopped tracking.
Now sometimes I come back from running in the dreariness of the pandemic and feel great, like the grown-up Peter Pan in “Hook,” out there relearning how to fly. The miles disappear when they’re done. And the only one who knows they happened is me.
Lindsay Crouse ( @lindsaycrouse ) is an editor and producer in Opinion who writes on gender, ambition and power. She produced the Emmy-nominated Opinion Video series “Equal Play,” which brought widespread reform to women’s sports.
The Times is committed to publishing a diversity of letters to the editor. We’d like to hear what you think about this or any of our articles. Here are some tips . And here’s our email: [email protected] .
Follow The New York Times Opinion section on Facebook , Twitter (@NYTopinion) and Instagram .
Understanding continued smartwatch usage: the role of emotional as well as health and fitness factors
- Research Paper
- Open access
- Published: 18 February 2021
- Volume 31 , pages 795–809, ( 2021 )
Cite this article
You have full access to this open access article
- Carolin Siepmann ORCID: orcid.org/0000-0001-5259-2704 1 &
- Pascal Kowalczuk 1
26k Accesses
30 Citations
9 Altmetric
Explore all metrics
Smartwatches are the most popular wearable device and increasingly subject to empirical research. In recent years, the focus has shifted from revealing determinants of smartwatch adoption to understanding factors that cause long-term usage. Despite their importance for personal fitness, health monitoring, and for achieving health and fitness goals, extant research on the continuous use intention of smartwatches mostly disregards health and fitness factors. Grounding on self-determination theory, this study addresses this gap and investigates the impact of health and fitness as well as positive and negative emotional factors encouraging or impeding consumers to continuously use smartwatches. We build upon the expectation-confirmation model (ECM) and extend it with emotional (device annoyance and enjoyment) as well as health and fitness factors (goal pursuit motivation and self-quantification behavior). We use structural equation modeling to validate our model based on 335 responses from actual smartwatch users. Results prove the applicability of the ECM to the smartwatch context and highlight the importance of self-quantification as a focal construct for explaining goal pursuit motivation, perceived usefulness, confirmation and device annoyance. Further, we identify device annoyance as an important barrier to continuous smartwatch use. Based on our results, we finally derive implications for researchers and practitioners alike.
Similar content being viewed by others
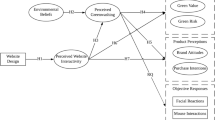
Perceived Greenwashing: The Effects of Green Marketing on Environmental and Product Perceptions
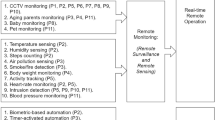
Usage and impact of the internet-of-things-based smart home technology: a quality-of-life perspective
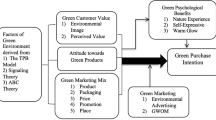
Effect of green marketing mix, green customer value, and attitude on green purchase intention: evidence from the USA
Avoid common mistakes on your manuscript.
Introduction
Smartwatches are wearable devices which are equipped with a screen and sensors (e.g., accelerometers, IR sensors). A wireless connection to the internet on its own or through a smartphone allows to run proprietary as well as third-party apps (Nascimento et al. 2018 ). By increasingly meeting aesthetic demands, smartwatches straddle the line between technological device and fashion accessory. As smartwatches integrate functions of other wearables such as fitness trackers, they have the unique potential to address all three basic psychological needs postulated in self-determination theory (SDT) (Deci and Ryan 2000 ): autonomy, competence, and relatedness. Thus, it is not surprising that with 42% they take the lead in terms of end-user spendings on wearable devices in 2019 (Goasduff 2019 ). Projected 109.2 million global unit shipments of smartwatches in 2023 reveal the continued end-user demand and underline the economic importance of these devices. To date, Apple holds the largest share of the smartwatch market, followed by Samsung and Garmin (Ubrani et al. 2019 ).
For companies it is beneficial when their customers decide to continuously use their smartwatches. Not only will they be able to sell accessories (i.e., bands) and services (i.e., apps, abonnements, in-app purchases), but it is also guaranteed that follow-up models will be sold. Sustained smartwatch use, however, is not only desired by companies selling them, but there are indeed multiple parties which profit from long-term use. By continuously wearing smartwatches, users benefit from the available functionalities, many of which are only possible because of the physical proximity and even skin contact. Smartwatches continuously monitor physiological parameters and daily activities, thereby encouraging individuals to live a healthier lifestyle, which will ultimately result in reduced health care expenses. Thus, long-term smartwatch usage for personal fitness and health monitoring is highly desirable on the individual level for end-users as well as on the macro-level for government and health insurance funds.
The importance of establishing prolonged smartwatch use calls for a deeper understanding of which mechanisms drive the continued use of smartwatches. We aim at furthering our knowledge in this domain by answering the following research questions:
RQ1: In how far and through which mechanisms do health- and fitness-related factors drive the continuous smartwatch usage?
RQ2: How do positive and negative emotional reactions affect the continuous smartwatch usage?
To answer these questions, we build upon the expectation-confirmation model (ECM) and develop an integrated framework explaining the continuance intention through positive and negative emotional as well as health- and fitness-related variables. Thereby, our study contributes to literature in the following ways. First, prior studies have mostly concentrated on investigating the adoption (e.g., Krey et al. 2019 ), purchase (e.g., Hsiao and Chen 2018 ), and behavioral intention to use (e.g., Choi and Kim 2016 ) wearables and smartwatches. Due to the benefits associated with long-term smartwatch use, in recent years, the focus has shifted towards understanding reasons for either abandoning fitness trackers (Attig and Franke 2020 ) and smartwatches (Shen et al. 2018 ) or continuously using them after initial adoption (e.g., Chuah 2019 ). We add to the last stream of literature by applying the ECM to the smartwatch context.
Second, despite their significant role in ongoing health monitoring, there is little academic research exploring how health- and fitness-related factors drive the continuance intention of smartwatches. Up to now, only Dehghani ( 2018 ) unveiled the importance of health factors for continuously using smartwatches by identifying healthtology, the importance of health factors for continuous smartwatch use, in his qualitative study.
By drawing on SDT, we argue that health- and fitness-related factors are particularly important for explaining why consumers continuously use smartwatches. Specifically, we introduce self-quantification and the new construct health and fitness goal pursuit motivation as important factors associated with continuous smartwatch use. Self-quantification, or self-tracking, belongs to the growing trend of self-optimization and refers to regularly gathering and subsequently analyzing health and fitness data (Lupton 2014 ). Thus, self-quantifiers draw on the functionalities of self-tracking devices such as smartwatches to generate insights about themselves. It becomes obvious that the self-quantification movement was only made possible by technological developments, which initiated the shift from niche to mainstream product (Day 2016 ) and which allow to precisely measure and track physiological data in everyday life.
In addition, psychologists emphasize the importance of goals and goal pursuit for understanding consumer choices (e.g., Kopetz et al. 2012 ). Research substantiated the importance of goal pursuit for health-related behavior (Benning et al. 2020 ). We argue that investigating the interplay of self-quantification and health and fitness goal pursuit motivation furthers our understanding of lasting smartwatch use.
Third, we contribute to literature by integrating emotional drivers of and barriers to continuous smartwatch usage. Prior research in this field considered positive emotional reactions towards smartwatches in terms of hedonic value (Hong et al. 2017 ), benefits (Chuah 2019 ), motivation (Dehghani et al. 2018 ), or as perceived enjoyment (Nascimento et al. 2018 ). So far, no research has incorporated negative emotional reactions elicited by smartwatch usage. We not only consider the positive emotion enjoyment but also introduce the novel construct device annoyance, which captures the feeling of being bothered by notifications of the smartwatch. By integrating annoyance elicited by smartwatch use, we address the discussed research gap and investigate how positive and negative emotional reactions affect the continuance intention.
The remainder of this paper is organized as follows. In the next section, we give an overview of the theoretical foundation and related research. Afterwards, we derive hypotheses and present the empirical validation of our model using covariance-based structural equation modeling (CB-SEM) on data from 335 actual smartwatch users. In the last section, we discuss our findings and implications and ultimately name potential limitations and future research directions.
- Expectation-confirmation model
Theoretical background
Research has extensively used the technology acceptance model (TAM) to explain consumer intentions to adopt innovative technologies (Davis 1989 ). Due to the importance of repeated usage for the success of smartwatches, we draw upon the ECM, which has been established by Bhattacherjee ( 2001 ). Prior research proved its applicability to the contexts of social networks (Jin et al. 2009 ), personal IT devices (Chen 2014 ), and wearables (Nascimento et al. 2018 ). The ECM grounds on the TAM as well as the expectation-confirmation theory (ECT) as proposed by Oliver ( 1980 ). In contrast to the TAM, the ECM focuses on post-usage rather than pre-usage expectations and posits that users’ continuance intention is driven by satisfaction and perceived usefulness (Bhattacherjee 2001 ). While perceived usefulness captures pre-usage expectations in the TAM, in the ECM, it reflects the aggregation of long-term post-usage beliefs about the extent to which using a technology leads to higher performance, productivity, and effectiveness (Bhattacherjee 2001 ; Davis 1989 ). Satisfaction, defined as the user’s assessment of feelings resulting from technology use, is driven by usefulness and confirmation. Confirmation describes the perceived congruence between expected use and actual performance and is hypothesized to also influence perceived usefulness (Bhattacherjee 2001 ). While the ECM has been widely used to explain the continuance intention for different technologies, research has also leveled criticism against it (Nascimento et al. 2018 ). One aspect which has been raised in prior literature is related to the fact that the ECM disregards the impact of motivational factors on continued IT usage (Sørebø et al. 2009 ). Another point of criticism regards the fact that emotional factors such as enjoyment might not only play an important role for the initial but also for the continued technology use (Thong et al. 2006 ). As these aspects provide fruitful avenues for extending and applying the expectation confirmation model to the context of smartwatches, we address these limitations by integrating positive and negative emotional factors as well as the goal pursuit motivation and self-quantification, two health- and fitness-related factors.
- Self-determination theory
Deci and Ryan’s SDT (Deci and Ryan 1985a ; Deci and Ryan 2000 ) is a basic theory of human motivation. The theory posits that the intrinsic motivation to engage in a certain activity is determined by the degree to which this activity fulfils the three basic psychological needs: autonomy, competence, and relatedness (Deci and Ryan 2000 ). SDT has been previously used as a framework to explain the motivational impact of digital technologies on physical activities (Kerner and Goodyear 2017 ). Since smartwatches have the potential to fulfil all three basic psychological needs, we argue that SDT can be also consulted as an explanation for continued smartwatch use.
Smartwatch functions allow users to record their workouts and activity progress (e.g., through monitoring step count and calories burnt), to connect and share their achievements with others, to engage in competitions to earn awards, and to tailor their goals according to their needs. Thereby, smartwatches address the three basic psychological needs proposed in SDT. The need for competence is enhanced by feedback on physical activities. Smartwatches provide users with an increased sense of autonomy and empowerment by providing transparent health data which can be accessed and analyzed without having to consult a professional. Ultimately, smartwatches satisfy the need for relatedness through the embedded social features, which allow users to engage with others by for example sending them motivational or appreciating messages. As users experience a fulfillment of their basic psychological needs, they may develop self-determined motivations, associated with continuous smartwatch use, self-tracking, and engagement in physical activities (Teixeira et al. 2012 ).
Research on continuous use of smartwatches
To date, research on wearables mainly concentrated on the adoption intention of smartwatches (e.g., Chuah et al. 2016 ; Krey et al. 2019 ; Wu et al. 2016 ) and specific health and fitness devices (e.g., Gao et al. 2015 ). In contrast, we place the focus of our research on the continuous use intention of smartwatches. In Table 1 , we provide a detailed analysis of this stream of research and outline how we contribute to current literature.
Hong et al. ( 2017 ) analyzed the impact of utilitarian and hedonic values based on a synthesis of theories. They showed that consumer innovativeness is positively associated with continuance use intention of smartwatches. By grounding on uses and gratification theory (U>), Cho and Lee ( 2017 ) used focus group and regression analysis to study the impact of practical and social factors on the continuance intention. Ensuing from theory of planned behavior (TPB), Song et al. ( 2018 ) showed that technology- and fashion-related factors enhance the attitude towards smartwatches. Their results further indicate that control-related factors indirectly affect the continuance intention through perceived behavioural control. Dehghani et al. ( 2018 ) demonstrated that the continuance intention is driven by operational imperfection, hedonic motivation, and aesthetic appeal. Further, Chuah ( 2019 ) established benefits and lifestyle incongruence as antecedents of continuance intention through inspiration and well-being.
In literature on continuous smartwatch use, only few studies based their research on the ECM despite its popularity in other realms. Among these, Ogbanufe and Gerhart ( 2018 ) investigated the indirect effects of utilitarian aspects (i.e., information and system quality) on continued use of smartphone features. While Pal et al. ( 2018 ) also addressed the utilitarian side of smartwatches by integrating perceived accuracy and functional limitations, they additionally studied the effect of hedonic motivation on continuous usage. They demonstrated a positive effect of perceived usefulness, hedonic motivation, perceived comfort, and self-socio motivation on continuous usage. Perceived privacy, battery-life concern as well as perceived accuracy and functional limitations were in contrast shown to negatively affect the continuance use of smartwatches. Nascimento et al. ( 2018 ) acknowledged both the hedonic and utilitarian side of smartwatches. Apart from habit, they introduced perceived usability, capturing the ease of use, and perceived enjoyment as antecedents of continuance intention. Very recently, Bölen ( 2020 ) integrated individual mobility, habit, and perceived aesthetics to the ECM. Moreover, Gupta et al. ( 2020 ) investigated the indirect and direct impact of perceived health outcomes, the perceived benefits fitness wearables had on the own health, on the continuous intention to use smartwatches. The authors show that the backward-looking assessment of perceived health benefits increases satisfaction and continuance intention (Gupta et al. 2020 ).
Overall, previous literature on continuance intention disregards health and fitness factors as motivational variables. Drawing on SDT and the evermore emerging health and fitness trend, we, however, argue that these factors are required to satisfy the basic psychological needs and are thus important for explaining prolonged smartwatch usage.
Model development
To explain the continuance intention of smartwatches, we develop a new model by extending the ECM (Bhattacherjee 2001 ) with emotional as well as health- and fitness-related factors. Thereby, we are the first to integrate self-quantification behavior, goal pursuit motivation, and device annoyance into the ECM (Fig. 1 ).
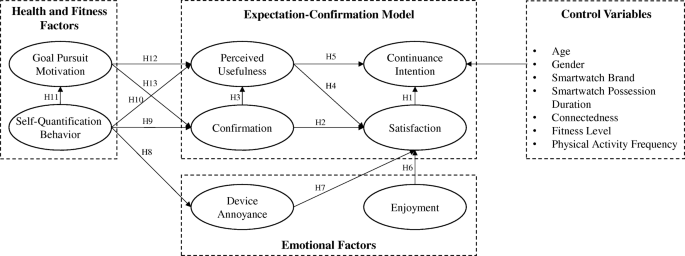
Proposed model and hypotheses
The expectation-confirmation model
In line with the assumptions of the ECM and prior research building upon the ECM to explain continuous smartwatch usage (e.g., Nascimento et al. 2018 ; Pal et al. 2018 ; Gupta et al. 2020 ), we derive our first five hypotheses:
H1: Satisfaction positively affects continuance intention.
H2–3: Confirmation positively affects satisfaction (H2) and perceived usefulness (H3).
H4–5: Perceived usefulness positively affects satisfaction (H4) and continuance intention (H5).
The influence of emotional factors
According to SDT, extrinsic motivation describes the behavior driven by external achievements and rewards (Deci and Ryan 1985b ), whereas intrinsic motivation refers to internal rewards, such as pleasure and satisfaction of an activity (Deci 1971 ). In accordance with Venkatesh ( 2000 ), we define enjoyment as the degree to which the use of smartwatches is perceived as enjoyable in its own right. Enjoyment belongs to intrinsic motivations and arises when all three basic psychological needs are satisfied by the activity performed (Ryan et al. 2006 ). Within the research field of smartwatch adoption, Choi and Kim ( 2016 ) found a strong positive effect of perceived enjoyment on the attitude towards using a smartwatch. Krey et al. ( 2019 ) demonstrated that expected enjoyment indirectly over attitude enhances the smartwatch adoption intention. In terms of prior research on continued smartwatch usage, Pal et al. ( 2018 ) found enjoyment to significantly affect satisfaction and continuance intention, whereas in the study conducted by Nascimento et al. ( 2018 ) only the effect on satisfaction turned out significant. Based on these validated findings and the fact that satisfaction encompasses an assessment of feelings resulting from wearable usage, we hypothesize that enjoyment enhances smartwatch satisfaction.
H6: Enjoyment positively affects satisfaction.
Since smartwatches allow to quickly and easily view incoming messages with a glance at the small display, they are perceived as true wearables (Beh et al. 2019 ) and less intriguing than the larger smartphones. If the functionalities of the smartwatch are fully exhausted, they will not only give immediate feedback to trainings, but these electronic devices will use either tones or vibration as a signal for incoming calls or messages, app notifications, reminders to move, and training sessions completed by friends and family if connected. As users receive important and transparent information about their health and fitness, smartwatches satisfy the need for autonomy. Despite the benefits offered by smartwatches, the many interruptions through the course of a day might be perceived as bothering or irritating and will probably lead to device annoyance.
In an advertising context, Hutter et al. ( 2013 ) defined annoyance as an unpleasant emotional reaction to subjective overexposure to a certain kind of media. We argue that overexposure in a smartwatch context can result from the many interruptions and notifications of the smartwatch. Annoyance may arise only after some time of usage and potentially even mitigates the feeling of autonomy. Thus, if present, device annoyance leads to decreased satisfaction, resulting from the assessment of negative feelings arising from smartwatch use.
H7: Device annoyance negatively affects satisfaction.
The influence of health and fitness factors
Consumers in the pursuit of fitness, health, and longevity increasingly engage themselves in self-quantification. The quantified-self movement describes the growing popularity of generating self-knowledge through self-tracking with “any kind of biological, physical, behavioral, or environmental information” (Swan 2013 , p. 85). Following Maltseva and Lutz ( 2018 ), we define self-quantification as the process of collecting and reflecting on personal data by using smartwatches and self-tracking apps. Self-quantification mirrors a high involvement in physiological data collection and analysis. We posit that high self-quantifiers will be much more attached, probably even emotionally connected, to the technological device on their wrist and appreciate its notifications. Thus, they will be less annoyed by its functionalities and notifications about sport activities.
H8: Self-quantification behavior negatively affects device annoyance.
By quantifying themselves, users receive tailored analyses about their health conditions and physical activities. Research showed that health and fitness interest increases the adoption intention of wearable fitness trackers (Lee and Lee 2018 ). Individuals who are more physically active will more likely use fitness trackers as they perceive them as motivating (Rupp et al. 2018 ). Furthermore, Li et al. ( 2018 ) substantiated that the activity amount and frequency positively affect confirmation in the context of fitness-tracking apps. Since self-tracking is a core function of smartwatches, consumers who are interested in their health and fitness can use these devices for quantifying themselves. When doing so, they will experience smartwatches as precise and comprehensive measures of physiological and biological data. We conclude that self-quantification behavior increases confirmation as smartwatches allow these individuals to monitor their health as initially expected. Thus, we argue that individuals with a desire to quantify themselves will perceive a higher congruence between the expected and actual smartwatch performance (Bhattacherjee 2001 ).
H9: Self-quantification behavior positively affects confirmation.
Users who engage in self-quantification behavior habitually use smartwatches for quantifying themselves. Thereby, these users become increasingly familiar with the various functionalities provided by the smartwatches (Alsharo et al. 2020 ; Gefen 2003 ). As self-quantifiers are driven by the aim of experiencing greater self-understanding and self-improvement (DuFault and Schouten 2020 ), they will gain more advantages of the self-tracking functionalities of smartwatches. Consequently, they will regard them as highly useful technologies which help to increase their personal efficiency (Chuah et al. 2016 ).
H10: Self-quantification behavior positively affects perceived usefulness.
Furthermore, by automatically tracking and thus quantifying daily activities, smartwatches create an awareness of one’s goals by making them and the individual progress towards these goals measurable. Drawing on qualitative findings, Pettinico and Milne ( 2017 ) argued that quantified results help users to focus on their goals and daily activities in pursuit of those goals. Also, Jarrahi et al. ( 2018 ) demonstrated that using fitness trackers could increase goal-directed behavior. In an empirical study, Zhang et al. ( 2019 ) showed that quantification induces greater goal pursuit motivation. Further, research has established that individuals who perceive themselves as closer to achieving a goal have a higher motivation to pursue the goal (Laran 2016 ). Therefore, we suppose that self-quantification leads to more transparent health data, which will enhance perceptions of health and fitness goal progress, and ultimately results in increased goal pursuit motivation.
H11: Self-quantification behavior positively affects goal pursuit motivation.
We define goals as “internal representations of desired states” (Austin and Vancouver 1996 , p. 338) and goal pursuit motivation as the extent to which an individual engages in a certain behavior to reach this desired end state (Laran 2016 ). Bagozzi and Edwards ( 1998 ) showed that goal intention increases activities of trying to reach one’s goals and initiates goal-directed behaviors, which result in enhanced degree of goal attainment. Thus, we suppose that individuals with high health and fitness goal pursuit motivations will try harder to reach their goals by exercising regularly and living healthy to satisfy their need for competence. Furthermore, Laran ( 2016 ) substantiated that, in situations of conscious goal pursuit, individuals are aware of their goals and actively use feedback on their performance to plan subsequent behavior. Thus, when pursuing a healthy and active lifestyle, individuals use their smartwatches to attain feedback based on transparent and objective information provided by these wearable devices. Therefore, high goal pursuit motivation will enhance the perceived usefulness of the smartwatch for attaining health and fitness goals.
H12: Goal pursuit motivation positively affects perceived usefulness.
We further hypothesize that goal pursuit motivation, which is driven by self-quantification behavior, also increases confirmation. We argue that by providing goal-related information, smartwatches provide individuals with information about their goal progress (Zhang et al. 2019 ), thereby supporting them in attaining their goals. Thus, goal pursuit motivation has a positive impact on the perceived congruence between the expected and actual performance of the smartwatch.
H13: Goal pursuit motivation positively affects confirmation.
Research method
Instrument development and data collection.
We mostly relied on established reflective multi-item measures to assess the latent constructs in our model. Specifically, scales for the constructs of the ECM (Bhattacherjee 2001 ; Bhattacherjee and Lin 2015 ; Davis 1989 ), enjoyment (Venkatesh and Bala 2008 ), and self-quantification behavior (Maltseva and Lutz 2018 ) were adapted to the smartwatch context. Based on Hutter et al.’s ( 2013 ) annoyance scale, we developed a measure for device annoyance. Further, we extended the scale for goal pursuit motivation (Zhang et al. 2019 ) to capture both the motivation to exercise and to live a healthier life. Lastly, we included several control variables to account for individual differences. Besides age and sex, our participants were asked to indicate their smartwatch brand, the duration of smartwatch possession, and if they are connected with friends or family members via the smartwatch (dichotomous question). Additionally, we measured their fitness level with three items from Zhang et al. ( 2019 ), and asked how often they engage in physical activities (1 = daily; 5 = once a month or less). We measured all latent constructs on seven-point Likert scales ranging from 1 (strongly disagree) to 7 (strongly agree), except for satisfaction, which we assessed on a five-point semantic differential scale.
Data were collected through a questionnaire, which we administered online in November and December 2019. As respondents, we recruited 335 German smartwatch users (M age = 34.45, SD = 12.42) of which 90.7% had owned a smartwatch for at least two months. As our sample varied in terms of smartwatch use duration and intensity, we do not expect that sample bias alters our results. Table 2 provides a detailed overview of the study’s respondents.
Measurement model assessment
Prior to testing the hypothesized relationships, we assessed the adequacy of the measurement model. Given the substantial changes we made to the established scale of goal pursuit motivation to capture both health and fitness goal pursuit, we conducted an exploratory factor analysis (EFA) on this scale. The EFA indicated a Kaiser-Meyer-Olkin (KMO) measure of sampling adequacy of .89, exceeding the critical value of .60 (Kaiser 1970 ; Kaiser and Rice 1974 ). In addition, the results of Bartlett’s test of sphericity (Bartlett 1951 ) verified the sampling adequacy of the data set for factorability ( p < .001). A scree plot of eigenvalues implied a one-factor solution to be most appropriate for measuring goal pursuit motivation. Furthermore, each measurement item achieved a factor loading above .84 and a communality value above .71, exceeding the recommended value of .40 (Field 2005 ). Finally, the corrected item-total correlation coefficients ranged from .82 to .88, indicating high homogeneity of the items. Summarizing, the results of the EFA show that we can include goal pursuit motivation in our further analysis.
Subsequently, we employed CB-SEM through R and the package lavaan (Rosseel 2012 ) to test the measurement and structural model. Since prior analysis showed that multivariate normality of the variables is not given, we applied a maximum likelihood estimation with robust standard errors and a mean-adjusted chi-square test statistic (MLM; Rosseel 2012 ). This procedure, which is also referred to as Satorra-Bentler chi-square test, introduces a scaling factor to the test statistics (Satorra and Bentler 1994 ). Simulation studies have shown that Satorra-Bentler scaled test statistics yield more accurate results than standard maximum likelihood estimators when the distribution of scores deviates from a normal distribution (Boomsma and Hoogland 2001 ).
In CB-SEM, no absolute standard regarding adequate sample size exists. However, our sample fulfils some general recommendations. The sample size of 335 is larger than the often-recommended size of N = 200 (Boomsma and Hoogland 2001 ). Further, the general rule of thumb to have 10 times the number of respondents as items (Kline 2011 ) is fulfilled because the ratio of cases to indicators is 10.8:1. Thus, we reason that the current sample is deemed suitable for analyzing the proposed model. Table 3 provides an overview of the measurement model assessment.
We achieved construct reliability and validity since, for each scale, Cronbach’s alpha is above .70 (Nunnally 1978 ), composite reliability (C.R.) is greater than .70, and average variance extracted (AVE) is above .50 (Hair et al. 2014 ). Overall high factor loadings prove the applicability of the scales. The results of the confirmatory factor analysis (CFA) provide evidence for construct validity and goodness of fit in the data Footnote 1 : χ 2 /df = 1.88, Comparative Fit Index (CFI) = .946, Tucker-Lewis Index (TLI) = .939, Root Mean Square Error of Approximation (RMSEA) = .058, Standardized Root Mean Square Residual (SRMR) = .048.
Following the CFA, we conducted further analyses to establish discriminant validity. As shown in Table 4 , discriminant validity is achieved since the AVE values, which are shown below the diagonal, exceed the squared correlations between the relevant factors (Fornell and Larcker 1981 ). Further, the values for the heterotrait-monotrait ratio (HTMT), which are shown above the diagonal, are well below the threshold value of .85 (Henseler et al. 2015 ).
Common method bias
As the data of our study is self-reported, we used procedural as well as statistical remedies to control for common method bias (CMB). First, we randomized the order of the questions in the online questionnaire. Second, we conducted Harman’s single factor test with an unrotated factor solution (Podsakoff et al. 2003 ), which showed that the single factor explained <50% of variance (38%). Third, we followed Eichhorn ( 2014 ) and included a common latent factor in the CFA. The model indicates that the factor explains 27% (R = .54 2 ) of variance. Lastly, we conducted the common marker variable approach. To do so, we included social desirability, which we measured with a short version of the Marlowe-Crowne Social Desirability Scale (Crowne and Marlowe 1960 ; Fischer and Fick 1993 ), as the common marker variable and also included a latent factor, as explained above. Results of the common marker variable approach imply that the common variance amounts to 5.29% (R = .23 2 ). Based on the results of the three statistical tests, we conclude that common method bias is no serious threat in this study.
As for the CFA, we employed a maximum likelihood estimation with robust standard errors to estimate the full model (Satorra and Bentler 1994 ). Since all robust indices for the overall fit have appropriate values (χ 2 /df = 2.03, CFI = .935, TLI = .928, RMSEA = .063, SRMR = .088), the hypothesized model is considered acceptable.
As outlined in Fig. 2 , all hypotheses are supported. Regarding the ECM, results imply that satisfaction is a strong driver of continuance intention (H1, β = .500, p ≤ .001). Further, confirmation was found to be a significant driver of both satisfaction (H2, β = .507, p ≤ .001) and perceived usefulness (H3, β = .255, p ≤ .001). Perceived usefulness in turn shows a significant positive effect on satisfaction (H4, β = .161, p ≤ .01) and continuance intention (H5, β = .160, p ≤ .01). Results for the integrated emotional factors imply that perceived enjoyment has a significant positive (H6; β = .351, p ≤ .001) and device annoyance a significant negative impact (H7; β = −.100, p ≤ .05) on satisfaction. In terms of the health and fitness factors, self-quantification behavior was found to significantly reduce device annoyance (H8, β = −.243, p ≤ .001) and to increase confirmation (H9, β = .192, p ≤ .05), perceived usefulness (H10, β = .227, p ≤ .001), and goal pursuit motivation (H11, β = .544, p ≤ .001). The latter exerts a positive effect on perceived usefulness (H12, β = .464, p ≤ .001) and confirmation (H13, β = .243, p ≤ .01). Overall, the model has an appropriate predictive power for perceived usefulness (R 2 = .57), satisfaction (R 2 = .58), and continuance intention (R 2 = .35), emphasizing the relevance of the proposed emotional as well as health and fitness factors.

Structural equation modeling results
To assess the robustness of our results, we integrated the control variables age, sex, smartwatch brand, duration of smartwatch possession, connectedness with friends and family members, and fitness level. After including these control variables into the model, the results remained stable. Only the effect of age on continuance intention was positive and significant (β = .111, p ≤ .01), indicating that older participants have a higher intention to continuously use smartwatches.
Discussion of results
The primary focus of the present paper is to further our knowledge in the domain of continued smartwatch usage. Specifically, we aimed at a) identifying the mechanisms through which health- and fitness-related factors drive the continuous smartwatch usage and b) understanding how positive and negative emotional reactions affect the continuous smartwatch usage.
The results confirm all relationships proposed in the ECM and thereby provide support for previous research on continuance intention of smartwatches (Nascimento et al. 2018 ; Ogbanufe and Gerhart 2018 ; Pal et al. 2018 ). Thus, we once more show the applicability of the ECM to the smartwatch context. In contrast to the results obtained by Bölen ( 2020 ) and Gupta et al. ( 2020 ), but in line with the ECM, we provide evidence that perceived usefulness significantly increases continuance intention. These results underline that continuous smartwatch use is not only driven by hedonic (Dehghani et al. 2018 ), but also by utilitarian aspects. This is especially true for individuals who aim at quantifying themselves with the goal of reaching health- and fitness-related goals.
Regarding the impact of health and fitness factors, the results show a highly significant impact of self-quantification on usefulness. This corroborates research on health information systems showing that habitually using a technology increases familiarity with its functionalities and thus usefulness (Alsharo et al. 2020 ). Moreover, the results indicate that self-quantification positively affects confirmation. Since those who are physically active will more likely use fitness trackers (Rupp et al. 2018 ), our research extends previous findings that the activity amount and frequency positively affect confirmation (Li et al. 2018 ). Furthermore, in line with previous qualitative (Pettinico and Milne 2017 ) and quantitative research (Zhang et al. 2019 ), we demonstrate that self-quantification itself has a strong impact on goal pursuit motivation. This underlines that self-tracking produces performance feedback and thus enhances the users’ motivation to pursue their goals (Zhang et al. 2019 ). Thus, self-quantification enhances the perception that goals are attainable. These results provide support for the notion that smartwatches should not only be regarded as a technological gimmick. Instead, they can intrinsically motivate users to increase their physical activity and help boosting their health conditions.
We observe a strong and significant effect of goal pursuit motivation on perceived usefulness. This effect implies that individuals with high health and fitness goal pursuit motivations value the smartwatch as a useful technology that helps them achieving their goals by providing feedback (Laran 2016 ). The significant impact of goal pursuit motivation on confirmation further proves that individuals who are motivated by smartwatches to exercise regularly and live healthy perceive a higher congruence between the expected and actual performance of the smartwatch. Thereby, we extend current literature on fitness tracking devices which is limited to analyzing the antecedents of goal pursuit motivation (Zhang et al. 2019 ).
Concerning the effects of emotional factors, the results provide evidence for a strong and highly significant impact of enjoyment on satisfaction. Thus, we corroborate prior smartwatch research. Previous studies demonstrated that users who perceive smartwatches as enjoyable have more positive attitudes towards (Choi and Kim 2016 ; Krey et al. 2019 ) and are more satisfied with these devices (Nascimento et al. 2018 ; Pal et al. 2018 ). In contrast, device annoyance has not been studied so far. The effect of device annoyance on satisfaction is significant ( p ≤ .05) but not as strong and highly significant as the effect of enjoyment. As we observe that self-quantification exerts a strong negative impact on device annoyance, we conclude that those individuals who regularly quantify themselves appreciate the notifications of the smartwatch and device annoyance is thus less likely to arise. While the observed results reduce the potential negative effects of device annoyance, it is still important to note that annoyance may arise from the many interruptions and notifications of the smartwatch and that individuals who perceive device annoyance potentially perceive using the smartwatch as less satisfying. In summary, we confirm previous results on enjoyment and additionally identify device annoyance as an important barrier to smartwatch satisfaction and thus relevant for sustained smartwatch use.
Theoretical contribution
Smartwatches belong to the most popular wearable devices and have thus received increasing academic interest over the last couple of years. Lately, the focus has shifted from studying determinants of smartwatch adoption to understanding the drivers and barriers of continued smartwatch usage. Research in this field, however, is still in its infancy and more knowledge regarding the role of health- and fitness-related factors in explaining long-term usage is required. We contribute to literature by (1) providing support for the applicability of the ECM to smart connected devices and especially smartwatches, (2) integrating two relevant health- and fitness-related constructs which have not been studied in the context of continuous smartwatch use before, and (3) revealing emotional drivers of and barriers to continuous smartwatch usage.
First, we add to the development of research on continued use of smartwatches. Current literature on wearables mainly focused on the adoption (e.g., Krey et al. 2019 ) or behavioral intention (e.g., Choi and Kim 2016 ) to use smartwatches or health and fitness wearables rather than on the continuance intention of smartwatches (e.g., Hong et al. 2017 ). By concentrating on the continued use of smartwatches, we address the increasing demand for establishing long-term usage to ensure revenues and to increase positive health benefits for users.
Second, we generate interesting novel insights from the interplay of the two health- and fitness-related constructs self-quantification behavior and goal pursuit motivation and their impact on long-term smartwatch use. Results confirm that self-quantification and goal pursuit motivation are both important antecedents of perceived usefulness and satisfaction. Further, we can determine self-quantification as a driver of goal pursuit motivation.
Third, we show that apart from usefulness and confirmation, resulting from a rational assessment of a smartwatch, also emotional factors significantly affect smartwatch satisfaction. By integrating enjoyment and device annoyance into our model, we equally address the impact of positive and negative emotional variables on smartwatch satisfaction. While we identify enjoyment as a driver of satisfaction, device annoyance is established as a barrier to satisfaction.
In addition to the points discussed above, this study contributes to IS research by bridging the gap between behavioral and design science research. Typically, design science and behavioral science are viewed as distinct research paradigms (Wimmer and Yoon 2017 ). Design science understands research as building and evaluating artefacts which target certain real-world problems, whereas behavioral science rather concentrates on developing and testing theories (Hevner et al. 2004 ). Both approaches, however, should be viewed as complementary (Wimmer and Yoon 2017 ). We combine design and behavioral science research as we test established theories which explore the underlying mechanisms constituting continuance intention. Further, we extend traditional theories with elements that help developers to design solutions (Hevner et al. 2004 ) to further increase the continued usage.
In summary, by developing an integrative framework containing established and novel emotional as well as health and fitness factors, we contribute to current literature and provide insights into the psychological mechanisms translating perceived benefits into outcomes relevant for government, healthcare, academics, and industry.
Managerial implications
Based on our results, we can derive implications for several recipients. Since government and health insurance funds benefit from a healthy population, they could support smartwatch purchases to help users achieve their fitness goals and in turn save treatment costs. Our research shows that individuals who engage in self-quantification have an increased motivation to pursue their health and fitness goals, indicating a higher intention to engage in physical activities. As smartwatch adoption and initial use does not automatically imply that users engage in self-quantification, they should be shown and taught how they can use the functionalities provided by the watch and the possibility to track data. Moreover, we recommend that marketers highlight the benefits for health and fitness associated with smartwatches in their advertising campaigns. The importance of engaging users in self-quantification is further established by our research which shows that self-quantification significantly reduces device annoyance, the negative emotional reaction elicited by receiving bothering messages. Since device annoyance is a strong inhibitor of satisfaction, companies should concentrate on reducing or even preventing this negative emotional reaction towards smartwatches to increase satisfaction and in turn continuance intention. Apart from engaging users in self-quantification, another possibility to mitigate annoyance is related to the functionalities of the smartwatch itself. Given the fact that annoyance may arise from interruptions of the smartwatch, companies should include easily accessible menus allowing users to customize their smartwatch notifications according to their needs. Furthermore, based on the fact that enjoyment significantly enhances satisfaction, we suggest that marketers should emphasize the hedonic side of smartwatches along their utilitarian aspects.
Limitations and future research
Our research has some limitations that warrant future research. In our study, we explicitly considered smartwatch characteristics addressing the two basic psychological needs of autonomy and competence. Smartwatches also touch upon the need for relatedness as they enable users to connect and interact with friends and family members. Due to the importance of fulfilling the three basic psychological needs, we suggest placing special emphasis on the social component of smartwatches in future research. Given the fact that age evokes a significant effect on continuous intention, it might be interesting to assess age-related differences more deeply. To further evaluate the generalizability of our model, we propose replicating our study with a culturally more diverse sample. Also, we suggest applying our model in the context of other wearable devices such as activity trackers. There, special emphasis should be placed on the newly established construct device annoyance, which might also be a barrier to the success of other smart devices.
Wearables are associated with data security and privacy concerns owing to the sensitive information which they continuously collect and store (Motti and Caine 2015 ). Qualitative research identified health information privacy concerns as barrier to wearable health technology growth (Becker 2018 ). While one would generally assume that these concerns impose greater threat to initial wearable adoption, research has demonstrated that data vulnerability grows over time as userbase increases (Biswas and Mukhopadhyay 2018 ). If software becomes more vulnerable to cyber-attacks over time, it might be a fruitful avenue for future research to assess the impact of privacy concerns on continuous smartwatch usage.
Noting that smartwatches can elicit status through aesthetic design and better athletic appearance, future research should further examine internal and external motives for continued use. Despite the discussed limitations, we provide a first systematic understanding of the impact of positive and negative emotional as well as health- and fitness-related factors on continuous smartwatch use.
Following Brosseau-Liard et al. ( 2012 ), Brosseau-Liard and Savalei ( 2014 ), and Savalei ( 2018 ), we report the new robust goodness of fit values.
Alsharo, M., Alnsour, Y., & Alabdallah, M. (2020). How habit affects continuous use: evidence from Jordan’s national health information system. Informatics for Health and Social Care, 45 (1), 43–56. https://doi.org/10.1080/17538157.2018.1540423 .
Article Google Scholar
Attig, C., & Franke, T. (2020). Abandonment of personal quantification: a review and empirical study investigating reasons for wearable activity tracking attrition. Computers in Human Behavior, 102 , 223–237. https://doi.org/10.1016/j.chb.2019.08.025 .
Austin, J. T., & Vancouver, J. B. (1996). Goal constructs in psychology: structure, process, and content. Psychological Bulletin, 120 (3), 338–375. https://doi.org/10.1037/0033-2909.120.3.338 .
Bagozzi, R. P., & Edwards, E. A. (1998). Goal setting and goal pursuit in the regulation of body weight. Psychology and Health, 13 (4), 593–621. https://doi.org/10.1080/08870449808407421 .
Bartlett, M. S. (1951). The effect of standardization on a χ 2 approximation in factor analysis. Biometrika, 38 (3/4), 337–344. https://doi.org/10.2307/2332580 .
Becker, M. (2018). Understanding Users’ Health Information Privacy Concerns for Health Wearables. 51st Hawaii International Conference on System Sciences , Big Island, Hawaii, 2018.
Beh, P. K., Ganesan, Y., Iranmanesh, M., & Foroughi, B. (2019). Using smartwatches for fitness and health monitoring: the UTAUT2 combined with threat appraisal as moderators. Behaviour & Information Technology , 1-18. https://doi.org/10.1080/0144929X.2019.1685597 .
Benning, T. M., Dellaert, B. G., & Arentze, T. A. (2020). The impact of health vs. non-health goals on individuals’ lifestyle program choices: a discrete choice experiment approach. BMC Public Health, 20 , 1–9. https://doi.org/10.1186/s12889-020-8416-3 .
Bhattacherjee, A. (2001). Understanding information systems continuance: an expectation-confirmation model. MIS Quarterly, 25 , 351–370. https://doi.org/10.2307/3250921 .
Bhattacherjee, A., & Lin, C. P. (2015). A unified model of IT continuance: three complementary perspectives and crossover effects. European Journal of Information Systems, 24 (4), 364–373. https://doi.org/10.1057/ejis.2013.36 .
Biswas, B., & Mukhopadhyay, A. (2018). G-RAM framework for software risk assessment and mitigation strategies in organisations. Journal of Enterprise Information Management, 31 (2), 276–299. https://doi.org/10.1108/JEIM-05-2017-0069 .
Bölen, M. C. (2020). Exploring the determinants of users’ continuance intention in smartwatches. Technology in Society, 60 , 101209. https://doi.org/10.1016/j.techsoc.2019.101209 .
Boomsma, A., & Hoogland, J. J. (2001). The robustness of LISREL modeling revisited. Structural equation models: present and future. A Festschrift in honor of Karl Jöreskog, 2 (3), 139–168.
Google Scholar
Brosseau-Liard, P. E., & Savalei, V. (2014). Adjusting relative fit indices for nonnormality. Multivariate Behavioral Research, 49 (5), 460–470. https://doi.org/10.1080/00273171.2014.933697 .
Brosseau-Liard, P., Savalei, V., & Li, L. (2012). An investigation of the sample performance of two non-normality corrections for RMSEA. Multivariate Behavioral Research, 47 (6), 904–930. https://doi.org/10.1080/00273171.2012.715252 .
Chen, C.-W. (2014). “BYOD flexibility: The effects of flexibility of multiple IT device use on users’ attitudes and continuance intention. Proceedings of the 20th Americas Conference on Information Systems , 1-9.
Cho, J., & Lee, H. E. (2017). Contextualization of motivations determining the continuance intention to use smart devices among people with physical disabilities. Telematics and Informatics, 34 (1), 338–350. https://doi.org/10.1016/j.tele.2016.05.011 .
Cho, W. C., Lee, K. Y., & Yang, S. B. (2019). What makes you feel attached to smartwatches? The stimulus–organism–response (S–O–R) perspectives. Information Technology & People, 32 (2), 319–343. https://doi.org/10.1108/ITP-05-2017-0152 .
Choi, J., & Kim, S. (2016). Is the smartwatch an IT product or a fashion product? A study on factors affecting the intention to use smartwatches. Computers in Human Behavior, 63 , 777–786. https://doi.org/10.1016/j.chb.2016.06.007 .
Chuah, S. H. W. (2019). You inspire me and make my life better: investigating a multiple sequential mediation model of smartwatch continuance intention. Telematics and Informatics, 43 , 101245. https://doi.org/10.1016/j.tele.2019.101245 .
Chuah, S. H. W., Rauschnabel, P. A., Krey, N., Nguyen, B., Ramayah, T., & Lade, S. (2016). Wearable technologies: the role of usefulness and visibility in smartwatch adoption. Computers in Human Behavior, 65 , 276–284. https://doi.org/10.1016/j.chb.2016.07.047 .
Crowne, D. P., & Marlowe, D. (1960). A scale of social desirability independent of psychopathology. Journal of Consulting Psychology, 24 , 349–354. https://doi.org/10.1037/h0047358 .
Davis, F. D. (1989). Perceived usefulness, perceived ease of use, and user acceptance of information technology. MIS Quarterly, 13 (3), 319–340. https://doi.org/10.2307/249008 .
Day, S. (2016). Self-tracking over time: The FITBIT® phenomenon. The 7th Annual Conference of Computing and Information Technology Research and Education New Zealand (CITRENZ2016) and the 29th Annual Conference of the National Advisory Committee on Computing Qualifications , Wellington, New Zealand, 1–6.
Deci, E. L. (1971). Effects of externally mediated rewards on intrinsic motivation. Journal of Personality and Social Psychology, 18 (1), 105–115. https://doi.org/10.1037/h0030644 .
Deci, E. L., & Ryan, R. M. (1985a). Intrinsic motivation and self-determination in human behaviour . NY: Plenum.
Deci, E. L., & Ryan, R. M. (1985b). The general causality orientations scale: self-determination in personality. Journal of Research in Personality, 19 (2), 109–134. https://doi.org/10.1016/0092-6566(85)90023-6 .
Deci, E. L., & Ryan, R. M. (2000). The “what” and “why” of goal pursuits: human needs and the self-determination of behavior. Psychological Inquiry, 11 (4), 227–268. https://doi.org/10.1207/S15327965PLI1104_01 .
Dehghani, M. (2018). Exploring the motivational factors on continuous usage intention of smartwatches among actual users. Behaviour & Information Technology, 37 (2), 145–158. https://doi.org/10.1080/0144929X.2018.1424246 .
Dehghani, M., Kim, K. J., & Dangelico, R. M. (2018). Will smartwatches last? Factors contributing to intention to keep using smart wearable technology. Telematics and Informatics, 35 (2), 480–490. https://doi.org/10.1016/j.tele.2018.01.007 .
DuFault, B. L., & Schouten, J. W. (2020). Self-quantification and the datapreneurial consumer identity. Consumption Markets & Culture, 23 (3), 290–316. https://doi.org/10.1080/10253866.2018.1519489 .
Eichhorn, B. R. (2014). Common method variance techniques. In Cleveland State University, Department of Operations & Supply Chain Management . Cleveland: SAS Institute Inc..
Field, A. (2005). Discovering statistics using SPSS . Thousand Oaks: SAGE.
Fischer, D. G., & Fick, C. (1993). Measuring social desirability: short forms of the Marlowe-Crowne social desirability scale. Educational and Psychological Measurement, 53 (2), 417–424. https://doi.org/10.1177/0013164493053002011 .
Fornell, C., & Larcker, D. F. (1981). Evaluating structural equation models with unobservable variables and measurement error. Journal of Marketing Research, 18 (1), 39–50. https://doi.org/10.1177/002224378101800104 .
Gao, Y., Li, H., & Luo, Y. (2015). An empirical study of wearable technology acceptance in healthcare. Industrial Management & Data Systems, 115 (9), 1704–1723. https://doi.org/10.1108/IMDS-03-2015-0087 .
Gefen, D. (2003). TAM or just plain habit: a look at experienced online shoppers. Journal of Organizational and End User Computing (JOEUC), 15 (3), 1–13. https://doi.org/10.4018/joeuc.2003070101 .
Goasduff, L. (2019). Gartner says global end-user spending on wearable devices to total $52 billion in 2020 . Gartner. https://www.gartner.com/en/newsroom/press-releases/2019-10-30-gartner-says-global-end-user-spending-on-wearable-dev . Accessed 27 Nov 2019.
Gupta, A., Dhiman, N., Yousaf, A., & Arora, N. (2020). Social comparison and continuance intention of smart fitness wearables: an extended expectation confirmation theory perspective. Behaviour & Information Technology , 1-14. https://doi.org/10.1080/0144929X.2020.1748715 .
Hair Jr., J. F., Babin, B. J., Anderson, R. E., & Black, W. C. (2014). Multivariate data analysis, Pearson custom library (7th ed.). Harlow, Essex: Pearson.
Henseler, J., Ringle, C. M., & Sarstedt, M. (2015). A new criterion for assessing discriminant validity in variance-based structural equation modelling. Journal of the Academy of Marketing Science, 43 (1), 115–135. https://doi.org/10.1007/s11747-014-0403-8 .
Hevner, A., March, S. T., Park, J., & Ram, S. (2004). Design science research in information systems. MIS Quarterly, 28 (1), 75–105. https://doi.org/10.2307/25148625 .
Hong, J. C., Lin, P. H., & Hsieh, P. C. (2017). The effect of consumer innovativeness on perceived value and continuance intention to use smartwatch. Computers in Human Behavior, 67 , 264–272. https://doi.org/10.1016/j.chb.2016.11.001 .
Hsiao, K. L., & Chen, C. C. (2018). What drives smartwatch purchase intention? Perspectives from hardware, software, design, and value. Telematics and Informatics, 35 (1), 103–113. https://doi.org/10.1016/j.tele.2017.10.002 .
Hutter, K., Hautz, J., Dennhardt, S., & Füller, J. (2013). The impact of user interactions in social media on brand awareness and purchase intention: the case of MINI on Facebook. Journal of Product & Brand Management, 22 (5/6), 342–351. https://doi.org/10.1108/JPBM-05-2013-0299 .
Jarrahi, M. H., Gafinowitz, N., & Shin, G. (2018). Activity trackers, prior motivation, and perceived informational and motivational affordances. Personal and Ubiquitous Computing, 22 (2), 433–448. https://doi.org/10.1007/s00779-017-1099-9 .
Jin, X. L., Cheung, C. M. K., Lee, M. K. O., & Chen, H.-P. (2009). How to keep members using the information in a computer-supported social network. Computers in Human Behavior, 25 (5), 1172–1181. https://doi.org/10.1016/j.chb.2009.04.008 .
Kaiser, H. F. (1970). A second generation little jiffy. Psychometrika, 35 (4), 401–415.
Kaiser, H. F., & Rice, J. (1974). Little jiffy, mark IV. Educational and Psychological Measurement, 34 (1), 111–117.
Kerner, C., & Goodyear, V. A. (2017). The motivational impact of wearable healthy lifestyle technologies: a self-determination perspective on Fitbits with adolescents. American Journal of Health Education, 48 (5), 287–297. https://doi.org/10.1080/19325037.2017.1343161 .
Kline, R. B. (2011). Principles and practice of structural equation modeling (3rd ed.). New York: The Guilford Press.
Kopetz, C. E., Kruglanski, A. W., Arens, Z. G., Etkin, J., & Johnson, H. M. (2012). The dynamics of consumer behavior: a goal systemic perspective. Journal of Consumer Psychology, 22 (2), 208–223. https://doi.org/10.1016/j.jcps.2011.03.001 .
Krey, N., Chuah, S., Ramayah, T., & Rauschnabel, P. (2019). How functional and emotional ads drive smartwatch adoption: the moderating role of consumer innovativeness and extraversion. Internet Research, 29 (3), 578–602. https://doi.org/10.1108/IntR-12-2017-0534 .
Laran, J. (2016). Consumer goal pursuit. Current Opinion in Psychology, 10 , 22–26. https://doi.org/10.1016/j.copsyc.2015.10.015 .
Lee, S. Y., & Lee, K. (2018). Factors that influence an individual’s intention to adopt a wearable healthcare device: the case of a wearable fitness tracker. Technological Forecasting and Social Change, 129 , 154–163. https://doi.org/10.1016/j.techfore.2018.01.002 .
Li, J., Liu, X., Ma, L., & Zhang, W. (2018). Users’ intention to continue using social fitness-tracking apps: expectation confirmation theory and social comparison theory perspective. Informatics for Health and Social Care, 44 (3), 298–312. https://doi.org/10.1080/17538157.2018.1434179 .
Lupton, D. (2014). Self-tracking cultures: Towards a sociology of personal informatics. Proceedings of the 26th Australian computer-human interaction conference on designing futures : the future of design, USA , 77–86. https://doi.org/10.1145/2686612.2686623 .
Maltseva, K., & Lutz, C. (2018). A quantum of self: a study of self-quantification and self-disclosure. Computers in Human Behavior, 81 , 102–114. https://doi.org/10.1016/j.chb.2017.12.006 .
Motti, V. G., & Caine, K. (2015). Users’ privacy concerns about wearables. In Brenner M., Christin N., Johnson B., Rohloff K. (eds). Financial Cryptography and Data Security. FC 2015. Lecture Notes in Computer Science, vol 8976 . Berlin, Heidelberg: Springer. https://doi.org/10.1007/978-3-662-48051-9_17
Nascimento, B., Oliveira, T., & Tam, C. (2018). Wearable technology: what explains continuance intention in smartwatches? Journal of Retailing and Consumer Services, 43 , 157–169. https://doi.org/10.1016/j.jretconser.2018.03.017 .
Nunnally, J. C. (1978). Psychometric theory . New York: McGraw-Hill.
Ogbanufe, O., & Gerhart, N. (2018). Watch it! Factors driving continued feature use of the smartwatch. International Journal of Human-Computer Interaction, 34 (11), 999–1014. https://doi.org/10.1080/10447318.2017.1404779 .
Oliver, R. L. (1980). A cognitive model of the antecedents and consequences of satisfaction decisions. Journal of Marketing Research, 17 (4), 460–469. https://doi.org/10.1177/002224378001700405 .
Pal, D., Funilkul, S., & Vanijja, V. (2018). The future of smartwatches: assessing the end-users’ continuous usage using an extended expectation-confirmation model. Universal Access in the Information Society, 1-21. https://doi.org/10.1007/s10209-018-0639-z .
Pettinico, G., & Milne, G. R. (2017). Living by the numbers: understanding the “quantification effect”. Journal of Consumer Marketing, 34 (4), 281–291. https://doi.org/10.1108/JCM-06-2016-1839 .
Podsakoff, P. M., MacKenzie, S. B., Lee, J. Y., & Podsakoff, N. P. (2003). Common method biases in behavioral research: a critical review of the literature and recommended remedies. Journal of Applied Psychology, 88 (5), 879–903. https://doi.org/10.1037/0021-9010.88.5.879 .
Rosseel Y. (2012). Lavaan: an R package for structural equation modeling. Journal of Statistical Software, 48 (2), 1–36. https://doi.org/10.18637/jss.v048.i02 .
Rupp, M. A., Michaelis, J. R., McConnell, D. S., & Smither, J. A. (2018). The role of individual differences on perceptions of wearable fitness device trust, usability, and motivational impact. Applied Ergonomics, 70 , 77–87. https://doi.org/10.1016/j.apergo.2018.02.005 .
Ryan, R. M., Rigby, C. S., & Przybylski, A. (2006). The motivational pull of video games: a self-determination theory approach. Motivation and Emotion, 30 , 347–363. https://doi.org/10.1007/s11031-006-9051-8 .
Satorra, A., & Bentler, P. M. (1994). Corrections to test statistics and standard errors in covariance structure analysis. In A. von Eye & C. C. Clogg (Eds.), Latent variable analysis: Applications to development research (pp. 399–419). Newbury Park: Sage.
Savalei, V. (2018). On the computation of the RMSEA and CFI from the mean-and-variance corrected test statistic with nonnormal data in SEM. Multivariate Behavioral Research, 53 (3), 419–429. https://doi.org/10.1080/00273171.2018.1455142 .
Shen, X. L., Li, Y. J., & Sun, Y. (2018). Wearable health information systems intermittent discontinuance. Industrial Management & Data Systems, 118 (3), 506–523. https://doi.org/10.1108/IMDS-05-2017-0222 .
Song, J., Kim, J., & Cho, K. (2018). Understanding users’ continuance intentions to use smart-connected sports products. Sport Management Review, 21 (5), 477–490. https://doi.org/10.1016/j.smr.2017.10.004 .
Sørebø, Ø., Halvari, H., Gulli, V. F., & Kristiansen, R. (2009). The role of self-determination theory in explaining teachers’ motivation to continue to use e-learning technology. Computers & Education, 53 (4), 1177–1187. https://doi.org/10.1016/j.compedu.2009.06.001 .
Swan, M. (2013). The quantified self: fundamental disruption in big data science and biological discovery. Big Data, 1 (2), 85–99. https://doi.org/10.1089/big.2012.0002 .
Teixeira, P. J., Carraça, E. V., Markland, D., Silva, M. N., & Ryan, R. M. (2012). Exercise, physical activity, and self-determination theory: a systematic review. International Journal of Behavioral Nutrition and Physical Activity, 9 (78), 1–30. https://doi.org/10.1186/1479-5868-9-78 .
Thong, J. Y., Hong, S. J., & Tam, K. Y. (2006). The effects of post-adoption beliefs on the expectation-confirmation model for information technology continuance. International Journal of Human-Computer Studies, 64 (9), 799–810. https://doi.org/10.1016/j.ijhcs.2006.05.001 .
Ubrani, J., Llamas, R., & Shirer, M. (2019). Worldwide Wearables market to top 300 million units in 2019 and nearly 500 million units in 2023 , IDC. Retrieved from https://www.idc.com/getdoc.jsp?containerId=prUS45737919 .
Venkatesh, V. (2000). Determinants of perceived ease of use: integrating perceived behavioral control, computer anxiety and enjoyment into the technology acceptance model. Information Systems Research, 11 (4), 342–365. https://doi.org/10.1287/isre.11.4.342.11872 .
Venkatesh, V., & Bala, H. (2008). Technology acceptance model 3 and a research agenda on interventions. Decision Sciences, 39 (2), 273–315. https://doi.org/10.1111/j.1540-5915.2008.00192.x .
Wimmer, H., & Yoon, V. Y. (2017). Counterfeit product detection: bridging the gap between design science and behavioral science in information systems research. Decision Support Systems, 104 , 1–12. https://doi.org/10.1016/j.dss.2017.09.005 .
Wu, L. H., Wu, L. C., & Chang, S. C. (2016). Exploring consumers’ intention to accept smartwatch. Computers in Human Behavior, 64 , 383–392. https://doi.org/10.1016/j.chb.2016.07.005 .
Zhang, Y. D., Li, D. J., Zhang, C. B., & Zhang, H. L. (2019). Quantified or nonquantified: how quantification affects consumers’ motivation in goal pursuit. Journal of Consumer Behaviour, 18 (2), 120–134. https://doi.org/10.1002/cb.1752 .
Download references
Open Access funding enabled and organized by Projekt DEAL.
Author information
Authors and affiliations.
Chair of Marketing, University of Duisburg-Essen, 47057, Duisburg, Germany
Carolin Siepmann & Pascal Kowalczuk
You can also search for this author in PubMed Google Scholar
Corresponding author
Correspondence to Carolin Siepmann .
Additional information
Responsible Editor: Edith Maier
Publisher’s note
Springer Nature remains neutral with regard to jurisdictional claims in published maps and institutional affiliations.
This article is part of the Topical Collection on Digital Healthcare Services
Rights and permissions
Open Access This article is licensed under a Creative Commons Attribution 4.0 International License, which permits use, sharing, adaptation, distribution and reproduction in any medium or format, as long as you give appropriate credit to the original author(s) and the source, provide a link to the Creative Commons licence, and indicate if changes were made. The images or other third party material in this article are included in the article's Creative Commons licence, unless indicated otherwise in a credit line to the material. If material is not included in the article's Creative Commons licence and your intended use is not permitted by statutory regulation or exceeds the permitted use, you will need to obtain permission directly from the copyright holder. To view a copy of this licence, visit http://creativecommons.org/licenses/by/4.0/ .
Reprints and permissions
About this article
Siepmann, C., Kowalczuk, P. Understanding continued smartwatch usage: the role of emotional as well as health and fitness factors. Electron Markets 31 , 795–809 (2021). https://doi.org/10.1007/s12525-021-00458-3
Download citation
Received : 31 July 2020
Accepted : 05 January 2021
Published : 18 February 2021
Issue Date : December 2021
DOI : https://doi.org/10.1007/s12525-021-00458-3
Share this article
Anyone you share the following link with will be able to read this content:
Sorry, a shareable link is not currently available for this article.
Provided by the Springer Nature SharedIt content-sharing initiative
- Smartwatches
- Continuance intention
- Self-quantification
- Device annoyance
JEL classification
Advertisement
- Find a journal
- Publish with us
- Track your research
Smart Watches: Minimizing Ageing Issues through the Technology Report
Introduction, problem statement, high-level requirements: assumptions, constraints, stakeholders, interaction mechanisms.
There is need for radical transformation on how ageism has been perceived and handled over the years. Old age and the associated impacts of aging have led to myriads of health complications, loss of memory, high dependency level, and loss of social touch with events taking place in the immediate world. This essay briefly explores how technology can be embraced in order to minimize the effects of old age.
The old generation faces a lot of social discrimination especially in the modern societies. Old age seems to be a bother to the young generation bearing in mind that old people often experience higher levels of dependency than the young generation. They are also faced with additional health complications that demand regular attention (Mann 2005, p.26).
Moreover, old people are relatively slow in terms of responding to stimuli and therefore, they require assistance and guidance from time to time. However, there are ways through which modern technology can be adopted in order to combat the negative effects of old age.
Smart watches are wrist watches that have been invented so that they can improve the overall functioning of watches since most users have been unable to properly utilize the watch technology (Morley & Charles 2008, p.62). They have been used to improve technology in connecting several people. In other words, this type of wrist watch is meant to offer users with additional features that can improve their social lives.
Smart watches came into demand since they can be used for multiple purposes. For instance, they can be used to access the internet as any other computer. In addition, they can indicate updates on weather, sports, and traffic movement. Needless to say, electronic mails and several other sites connected to the internet can be accessed using a smart watch. Therefore, smart watches are specially designed to meet the needs of all types of people although individuals who are in old age can immensely benefit from this type of technology.
The main constraint that faces this modern application is that most people are not ready and willing to embrace the new technology installed in smart watches. Google glasses are also able to interface most of the applications at ago to enhance usability. It also improves the performance of the various individuals who are using it. The technology will also be enhancing the reliability and the way people interact.
Since Smart watches are worn on the wrists, the technology will enhance them to use it at any moment and at any place wherever they are. For example the smart watches can be used when cycling, swimming and also when jogging and at the various working places. They are light, consume less energy and are relatively cheap.
Another challenge is the issue of size. Quite a number of users have argued that the size is not appealing as the display of the information is not as large as expected by most users.
Smart watches are a type of wrist watches that have been designed with high level of interaction in order to offer multiple services to users especially those who are in old age (Stair & George 2010, p.12). Users are able to maintain their social lives in spite of old age. Some of the core features and functions of this type of watch include data storage, sending messages through speaking, monitoring sugar level, blood pressure, and heart beat as well as taking and sharing both audio and video files.
Smart watches use technology known as SPOT (Smart Personal Object Technology) that is aimed at bringing the power of technology to users.NET. They are computerized and they enhance low power consumption and low energy sources as compared to the other devices such as laptops and other technology related products. The main aim of building the smart watches is to improve communication and also make the gadgets more useful in the day to day lives.
SPOT is a technology that is used to deliver the necessary information and domains that aid in improving the welfare of the technology used. SPOT uses the main mode of technology has the FM broadcasting does. IT delivers the web-based data that is related to the smart appliances such as the smart watches.
The application and technology will be used by old people from all types of backgrounds. It will be modified by adding several vital applications so that old people will find it easy to use even in an environment with several communication barriers.
Easier and faster mode of communications will be applied to increase the rate at which the information is passed from one person to another. Some of the Input output devices that will be used in the project are the LCD displays, microprocessors and the numeric displays that are able to display to the users. The input and the output devices also enable users to boost their performance when interacting with the device.
The private life and work environment of an individual using a smart watch will be improved due to the hardware used to manufacture the gadget. The vibration feedback integrated acceleration sensors installed in this type of watch will indeed boost the user’s experience (Heckman 2008, p.72).
It is definite that the user will be offered with a non-obtrusive interaction with the computerized watch. Since the watch will be able to recognize physical activities, an aged user will be able to complete his or her social life without relying on external assistance.
Heckman, D 2008, A Small World: Smart Houses and the Dream of the Perfect Day , Duke University Press, Durham.
Mann, WC 2005, Smart Technology for Aging, Disability and Independence: The State of the Science , John Wiley & Sons, Hoboken.
Morley, D & Charles, S.P 2008, Understanding Computers: Today and Tomorrow , Course Technology, Boston.
Stair, R M & George W. R 2010, Fundamentals of Information Systems , Course Technology Boston.
- Smartwatches: Computer on the Wrist
- Effects of Ageing Population as Driving Force
- Individuals and Families in a Diverse Society: Ageing Population
- Youth Issues: The State of Children’s Rights in UAE
- Doing the Right Thing
- Physical Appearance in Old Age and Social Degradation
- Adult Education for Social Change: The Role of a Grassroots Organization in Canada
- “Savage Inequality” a Book by Jonathan Kozol
- Chicago (A-D)
- Chicago (N-B)
IvyPanda. (2019, May 28). Smart Watches: Minimizing Ageing Issues through the Technology. https://ivypanda.com/essays/smart-watches-report/
"Smart Watches: Minimizing Ageing Issues through the Technology." IvyPanda , 28 May 2019, ivypanda.com/essays/smart-watches-report/.
IvyPanda . (2019) 'Smart Watches: Minimizing Ageing Issues through the Technology'. 28 May.
IvyPanda . 2019. "Smart Watches: Minimizing Ageing Issues through the Technology." May 28, 2019. https://ivypanda.com/essays/smart-watches-report/.
1. IvyPanda . "Smart Watches: Minimizing Ageing Issues through the Technology." May 28, 2019. https://ivypanda.com/essays/smart-watches-report/.
Bibliography
IvyPanda . "Smart Watches: Minimizing Ageing Issues through the Technology." May 28, 2019. https://ivypanda.com/essays/smart-watches-report/.
Articles on Smartwatches
Displaying all articles.
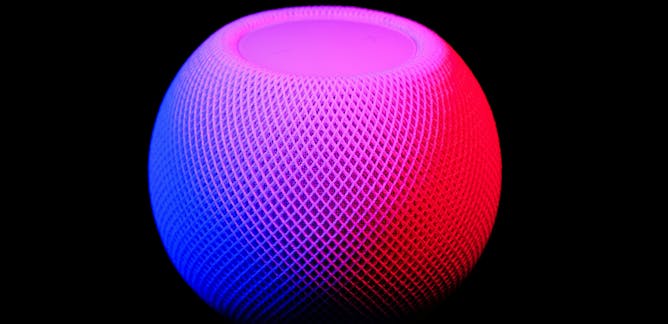
AI is making smart devices – watches, speakers, doorbells – easier to hack. Here’s how to stay safe
Chao Chen , RMIT University ; Kok-Leong Ong , RMIT University , and Lin Li , RMIT University
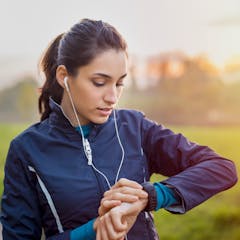
Why some amateur athletes are giving up on smartwatches
Matthieu Quidu , Université Claude Bernard Lyon 1 and Brice Favier-Ambrosini , Université du Québec à Chicoutimi (UQAC)
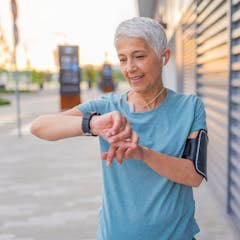
Should you really use your smartwatch or fitness wearable to monitor your heart?
Jessica Orchard , University of Sydney and Ben Freedman , University of Sydney
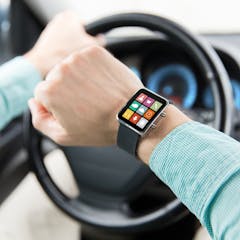
Smartwatches are a bigger distraction to drivers than mobile phones
Pierre-Majorique Léger , HEC Montréal and Sylvain Senecal , HEC Montréal
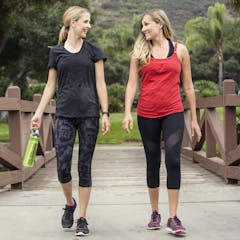
Do we really need to walk 10,000 steps a day?
Lindsay Bottoms , University of Hertfordshire
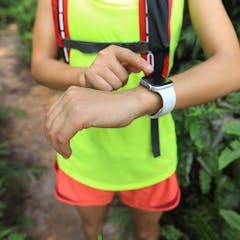
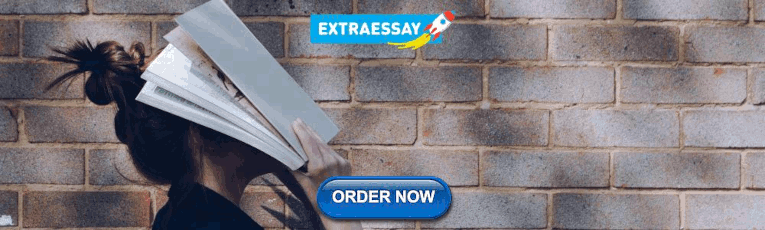
The risks of algorithmic (il)literacy on healthcare platforms
Handan Vicdan , EM Lyon Business School
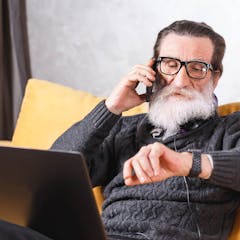
Better design could make mobile devices easier for seniors to use
Edward Henry Steinfeld , University at Buffalo
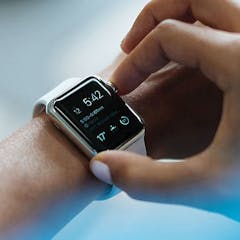
The social factors that influence whether you’ll use your wearable device
Deborah Lupton , University of Canberra
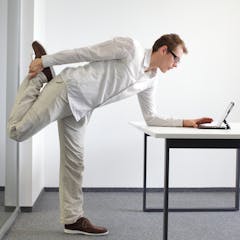
How to stop sitting yourself to death
David Alter , University of Toronto
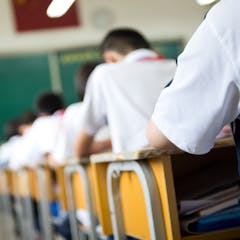
Students are using ‘smart’ spy technology to cheat in exams
Ritesh Chugh , CQUniversity Australia
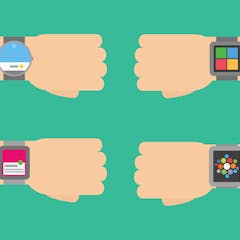
Soon smartwatches will listen to your body to work out how you’re feeling
Andrew McStay , Bangor University
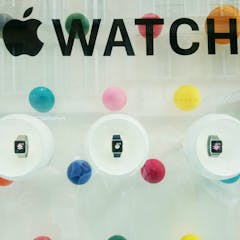
Apple Watch: phantom menace of smartwatches could make us even more self-obsessed
Andre Spicer , City, University of London
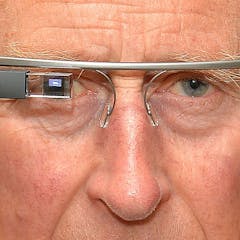
Google Glass finally cracks: it was a product looking for a market
Paul Levy , University of Brighton
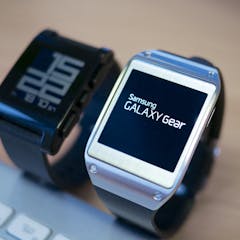
Samsung heats up wearable tech race with graphene wafers
Andrew Bissette , University of Oxford
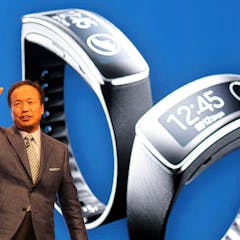
Power up! Samsung Galaxy S5’s battery boost … and more
Ara Sarafian, The Conversation
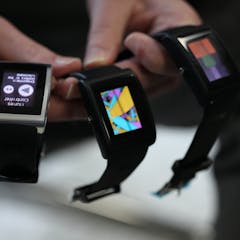
Wearable tech sees all, so choose what you want to share
Christopher Baber , University of Birmingham
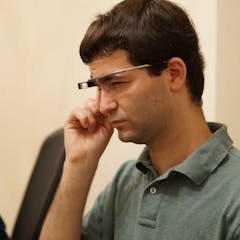
Not long now before on-body gadgets get under your skin
Tim Dant , Lancaster University
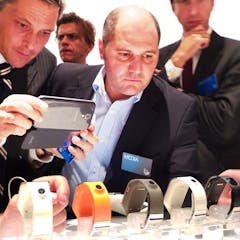
Scoff now, but you’re probably getting a smartwatch
Matthew Higgs , UCL
Related Topics
- Artificial intelligence (AI)
- Digital economy
- Google Glass
- Physical activity
- Wearable technology
Top contributors
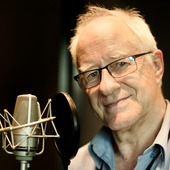
Professor Emeritus, The University of Queensland
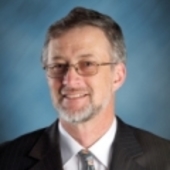
Fellow of the Faculty of Engineering and Information Technology, University of Technology Sydney
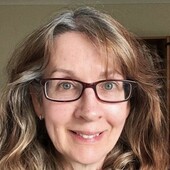
SHARP Professor, Vitalities Lab, Centre for Social Research in Health and Social Policy Centre, and the ARC Centre of Excellence for Automated Decision-Making and Society, UNSW Sydney
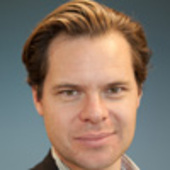
Professor of Organisational Behaviour, Bayes Business School, City, University of London
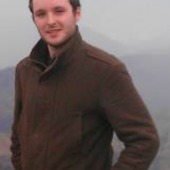
Postdoctoral research associate, University of Oxford
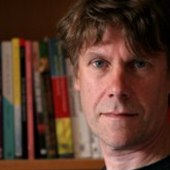
Professor of Sociology (retired), Lancaster University
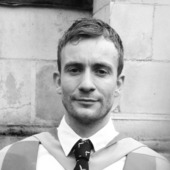
Postdoctoral Research Associate, UCL
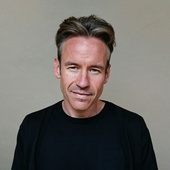
Professor of Technology & Society, Bangor University
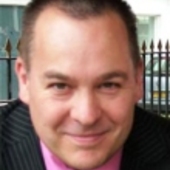
Chair of Pervasive and Ubiquitous Computing, University of Birmingham
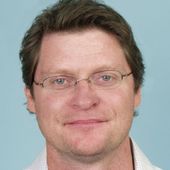
Senior Research Fellow, Deakin University
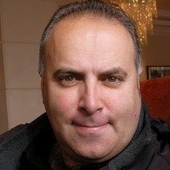
Senior Lecturer and Researcher in Innovation and Digital Leadership, University of Brighton
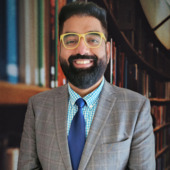
Associate Professor - Information and Communications Technology, CQUniversity Australia
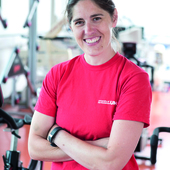
Reader in Exercise and Health Physiology, University of Hertfordshire
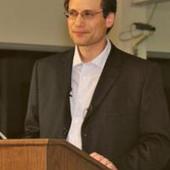
Associate Professor of Medicine and Senior Scientist, Toronto Rehabilitation Institute, University of Toronto
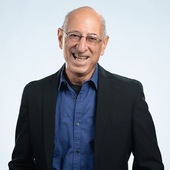
SUNY Distinguished Professor of Architecture, University at Buffalo
- X (Twitter)
- Unfollow topic Follow topic

An official website of the United States government
The .gov means it’s official. Federal government websites often end in .gov or .mil. Before sharing sensitive information, make sure you’re on a federal government site.
The site is secure. The https:// ensures that you are connecting to the official website and that any information you provide is encrypted and transmitted securely.
- Publications
- Account settings
Preview improvements coming to the PMC website in October 2024. Learn More or Try it out now .
- Advanced Search
- Journal List
- BMC Med Inform Decis Mak
- PMC10625201

Smartwatches in healthcare medicine: assistance and monitoring; a scoping review
Mohsen masoumian hosseini.
1 Department of E-Learning in Medical Sciences, Tehran University of Medical Sciences, Tehran, Iran
2 CyberPatient Research Affiliate, Interactive Health International, Department of the surgery, University of British Columbia, Vancouver, Canada
Seyedeh Toktam Masoumian Hosseini
3 Department of Nursing, School of Nursing and Midwifery, Torbat Heydariyeh University of Medical Sciences, Torbat Heydariyeh, Iran
Karim Qayumi
4 Professor at Department of Surgery, University of British Columbia, Vancouver, Canada
Shahriar Hosseinzadeh
5 CyberPatient Research Coordinator, Interactive Health International, Department of Surgery, University of British Columbia, Vancouver, Canada
Seyedeh Saba Sajadi Tabar
6 Universal Scientific Education and Research Network (USERN), Tehran, Iran
Associated Data
Considering that the study is a review, no data are available.
Smartwatches have become increasingly popular in recent times because of their capacity to track different health indicators, including heart rate, patterns of sleep, and physical movements. This scoping review aims to explore the utilisation of smartwatches within the healthcare sector. According to Arksey and O'Malley's methodology, an organised search was performed in PubMed/Medline, Scopus, Embase, Web of Science, ERIC and Google Scholar. In our search strategy, 761 articles were returned. The exclusion/inclusion criteria were applied. Finally, 35 articles were selected for extracting data. These included six studies on stress monitoring, six on movement disorders, three on sleep tracking, three on blood pressure, two on heart disease, six on covid pandemic, three on safety and six on validation. The use of smartwatches has been found to be effective in diagnosing the symptoms of various diseases. In particular, smartwatches have shown promise in detecting heart diseases, movement disorders, and even early signs of COVID-19. Nevertheless, it should be emphasised that there is an ongoing discussion concerning the reliability of smartwatch diagnoses within healthcare systems. Despite the potential advantages offered by utilising smartwatches for disease detection, it is imperative to approach their data interpretation with prudence. The discrepancies in detection between smartwatches and their algorithms have important implications for healthcare use. The accuracy and reliability of the algorithms used are crucial, as well as high accuracy in detecting changes in health status by the smartwatches themselves. This calls for the development of medical watches and the creation of AI-hospital assistants. These assistants will be designed to help with patient monitoring, appointment scheduling, and medication management tasks. They can educate patients and answer common questions, freeing healthcare providers to focus on more complex tasks.
Introduction
In the mobile technology market, smartwatches are experiencing tremendous growth as wearables and medical devices converge to monitor personal health in real-time, including cardiovascular health measures [ 1 , 2 ]. Consumers can now access a personalised medical data report through these devices, which could prove useful in preventing and treating diseases [ 3 ]. The rise of smartwatches has empowered numerous patients to actively engage in self-care and advocate for the health of others. With the increasing prevalence of these individuals, known as 'e-patients', it is imperative for medical institutions to adequately educate their students on effectively collaborating with and providing healthcare services to them. Failing to do so could potentially jeopardise patients' lives [ 4 ]. Similar to the access to online tools that smartwatches provide for medical schools and students, access to e-patients is also increasing. Mobile decision support tools, for example, use simple graphics to communicate calculator results (such as the Framingham Risk Score) in a way that is relevant to both the learner and the patient [ 5 ].
The Framingham Risk Score is an algorithm designed to assess the probability of developing cardiovascular disease over a period of 10 years [ 6 ]. Initially focused on coronary heart disease, it was expanded in 2008 to encompass cerebrovascular events, peripheral artery disease, and heart failure. Considering variables such as age, sex, blood pressure levels, cholesterol levels, smoking status, and diabetes status, this risk score applies specifically to individuals aged between 30–79 without any previous history of CVD [ 7 ]. There are many apps explicitly aimed at patients, including simple medical calculators, e.g. BMI calculators, risk calculators, medication reminders, pharmacy finders and even diagnostic tools [ 8 ]. As many patients are unable to communicate their condition to their companions or medical staff, these smart tools will help caregivers be more alert and apply more appropriate comfort measures. The notion of comfort measures has gained significant attention in both nursing and medical literature, reflecting a systematic approach that entails proactive engagement as well as thoughtful restraint [ 9 ]. These measures can range from the simplest to more intricate interventions, all serving to provide supportive care. Importantly, they are applicable across various healthcare settings and warrant careful consideration within the clinical management of patients burdened with multiple chronic comorbidities [ 9 ].
The FDA has approved clinical smartwatches for detecting medically significant events and recording and processing data [ 10 ]. There are no approved directly addressed smartwatches in the European Union (EU). However, to comply with EU regulations, smartwatch manufacturers must navigate through various frameworks such as the Radio Equipment Directive 2014/53/EU and Medical Device Regulation 2017/745. These regulations emphasize safety standards and touch upon essential aspects like electromagnetic compatibility and efficient radio spectrum utilization [ 11 , 12 ].
Smartwatches often come with advanced features like a heart rate monitor. These high-tech devices utilize a technique called photoplethysmography to accurately measure the user's heart rate. By utilizing light beams and specialized sensors on the smartwatch, changes in blood volume flowing through the wrist can be precisely quantified. This process generates a PPG waveform that provides valuable data for determining an individual's heart rate [ 13 , 14 ]. One instance where this technology proves beneficial is when an epilepsy patient wears a smartwatch equipped with an artificial intelligence (AI) algorithm capable of identifying high-risk seizures and initiating human assistance promptly [ 15 ]. This particular smartwatch provides physiological data to monitor vital signs and issues alerts that help save lives, as attending a seizure is associated with a lower rate of severe injury and death [ 15 ]. Other wearable devices run algorithms that detect conditions such as atrial fibrillation or medically necessary conditions where early intervention can positively impact the quality of life. Researchers have shown that wearable biomarkers are more accurate at predicting blood and urine measurements than two vital signs in the clinic. They can provide clinicians with predictions from thousands of measurements in a fraction of the time it takes to measure a single vital sign [ 13 ]. It is important to note that these smartwatches are based on algorithmic interpretations of clinical outcomes, and their predictive power ultimately depends on the support and strength of their algorithms [ 16 , 17 ]. In this review, we discuss the applications of these wearable devices to patient health and HealthWorks' educational initiatives. This article seeks to answer several questions, including whether smartwatches can be trusted for clinical measurements and what might facilitate the development of trust in these devices.
Reason for scoping review
A scoping review was conducted since AI and machine learning algorithms are emerging technologies applied to smartwatches. Additionally, scoping reviews are helpful when attempting to evaluate the effectiveness of large-scale or emerging research [ 18 ].
Scoping reviews aim to identify and map relevant evidence on a topic, field, context or question that meets predetermined inclusion criteria [ 19 , 20 ]. They provide important insights into the characteristics of a body of evidence and can highlight knowledge gaps for subsequent syntheses. Unlike traditional systematic reviews, scoping reviews have broader questions and may include multiple types of evidence. Scoping reviews are useful for obtaining a comprehensive overview of the evidence and identifying gaps in the existing literature without the need for methodological judgement or risk of bias assessment [ 21 ]. They differ from evidence maps, which present the results of a systematic search in searchable databases to identify knowledge gaps and future research needs. Scoping reviews also differ from other forms of evidence synthesis. Scoping reviews do not make recommendations for clinical practise and often do not assess methodological quality or risk of bias in studies. The PRISMA ScR is a new approach to reporting scoping reviews based on the popular PRISMA statement and checklist [ 22 ].
A scoping review can collect different types of evidence from different areas, including both empirical and non-empirical sources [ 23 ]. This type of review is suitable for investigating, identifying, presenting, reporting, or discussing features or concepts across a wide range of evidence sources [ 24 ]. Scoping reviews are particularly useful when comparing measures is neither practical nor feasible due to cost or time constraints. Although they often involve reviews of numerous sources, these reviews do not expect or allow for statistical pooling, formal risk assessment, or quality assessment [ 25 ].
Search strategy
This study's methodology was based on the scoping review methodology that was developed by Arksey and O'Malley [ 20 ] and used the methodological enhancement suggested by Levac et al. (2010) [ 26 ]. As outlined in this framework, scoping reviews have six stages: (1) identifying a research question; (2) identifying relevant studies; (3) selecting studies; (4) charting the data; (5) collating, summarizing and reporting the results and (6) consulting with stakeholders.
Stage 1: Identifying the research question
In consultation with the research team and key stakeholders, the overall main research question developed is: ‘Whether all smartwatches can be trusted for clinical measurements and what might facilitate the development of trust in these devices?’.
Stage 2: Identifying relevant studies
Search strategy and information sources.
First step in identifying articles related to this topic was to conduct a limited search of PubMed/Medline, SCOPUS, Embase, Web of Sciences, and ERIC. For the development of a comprehensive search strategy, the text words contained in the titles of relevant articles, as well as the index terms utilized to describe the articles, were analyzed (see Table Table1). 1 ). Depending on the database and/or information source involved, the search strategy was tailored to include all keywords and index terms identified. Additional studies were screened from the reference list of all included sources of evidence. We also searched a variety of grey literature sources in order to ensure that all relevant information was obtained. The review team searched relevant grey literature databases (such as Grey Literature Report, Google Scholar, OpenGrey, and Web of Science Conference Proceedings) for studies, reports, and conference abstracts of interest to the topic. A Research librarian developed the search strategy and revised it following input from stakeholders. In order to prevent bias, the research team blinded the stakeholders to the original search strategy that was developed. The search papers focused only on English-language studies. Considering that smartwatch medical applications have only been introduced to the market recently, the search period was restricted to the period following 2017. After conducting the search, all citations identified were gathered into an EndNote 8 database and duplicates were removed.
Database search strategy
This table presents the search strategy for specific keywords in different databases. This column provides an overview of the steps and techniques used to retrieve relevant information from each database based on the keywords organised
Stage 3: Study selection
Inclusion/exclusion criteria.
- Types of research: any type of study design, in addition to randomized controlled trials (RCT and CT), controlled clinical trials, case studies, correlational studies, longitudinal studies, experimental studies, and quasi-experimental studies. There were no limitations regarding the study design or geographic location.
- Types of Participants: All Participants that deal with the healthcare system
- Types of interventions: The study focused on smartwatches, wristband, fitness-bound, wireless watch, Wearable movement sensors
- The studies included focused on any of the following areas: (a) development; (b) implementation; (c) evaluation; or (d) comparative validation of such measures.
- Types of outcomes: satisfaction, knowledge, skills, attitudes, and behaviours were the outcomes of interest.
Two screening stages took place in the review process: a review of the title and abstract, followed by an assessment of the complete text. The initial phase involved M.M.H and T.M.H, who independently scrutinized all obtained citations to determine if they met a set of minimum inclusion criteria. In order to ensure that the criteria were robust enough to capture any articles relating to smartwatches, a sample of abstracts was tested prior to undertaking the abstract review. The full-text review included any articles that either or both reviewers considered to be relevant. The full-text articles were then independently assessed by both investigators to determine if they fulfilled the inclusion and exclusion criteria. A Cohen's κ coefficient of the agreement was calculated both at the title and abstract review phase and at the full article review phase in order to determine the inter-rater agreement. In case of disagreements regarding study eligibility at the full-text review stage, any further disagreements were resolved through discussion with a third investigator until consensus was achieved. In the final scoping review, the search results and the inclusion process were comprehensively reported along with a flow diagram reflecting Preferred Reporting Items for Systematic Reviews and Meta-analyses extension for scoping reviews (PRISMA-ScR) (Fig. 1 ).

An overview of the article selection process according to ScR-PRISMA
Stage 4: Data collection
Data extraction was performed using the PRISMA-ScR Checklist, which consists of 22 items developed by IBJ [ 27 ]. To ensure that the form captured the information accurately, the form was reviewed by the research team and pretested by all reviewers before implementation. Studies characteristics that were extracted included to publication year, publication type (eg, original research or review type), study design, country, participants' population characteristics, intervention setting, description of quality indicators including definition, numerator, dominator, psychometrics of the indicators (face validity, reliability, construct validity, risk adjustment). The data abstraction process was conducted in duplicate by two reviewers who independently extracted the data from all included studies.
Stage 5: Data summary and synthesis of results
Quality assessment is not an integral part of scoping reviews, but in this review, the quality checklist developed in BEME guide 11 was used to assess quality [ 28 ]. Based on the provided checklist, excellent quality studies met eight or more of the established criteria. Studies deemed moderate quality fulfilled six or seven indicators, while studies with low quality met five or fewer criteria. Each study was assigned either a 0 or 1 for each index measurement. Studies that met the index received a positive score, whereas studies that did not meet the index or were vaguely described received a zero score. Using the IBJ checklist as a guide, selected articles were analyzed and themes were extracted.
Stage 6: Consultation
There is a suggestion by Levac et al. that the consultation stage provides opportunities for stakeholder involvement, which may provide insights beyond those that have been described in the literature. An integral part of the study's healthcare-centred approach was the involvement of stakeholders, including a patient partner who served as both a consultant and knowledge user throughout the study.
Descriptive
Following the articles' quality assessment, 32 studies were analysed for data extraction. The majority of studies were conducted in the United State America (USA) (N = 10), three in China, three in Taiwan, three in United Kingdom (UK), in countries including Finland, Germany, Norway and Spain, each one two studies, and one study in the rest (Greece, Switzerland, Slovenia, Canada, Australia, Belgium, Brazil and France). Thirteen of the studies were experimental, four were cohort studies, and one study of each of the designs (longitudinal cohort study design, longitudinal observational study, multicenter observational study, randomized controlled trial (RCT), randomized, accelerometer data, combined method, feasibility study, observational study, prospective, nonrandomized, and adjudicator-blinded study, Prospective, single-arm, cross-sectional study, prospective comparative and prospective study). Three studies did not have a specific design due to the nature of the research, and one study was a systematic review (Table 2 ). As shown in Table 2 , the geographical distribution of the studies, the purpose, the outcome and the (Table (Table2) 2 ) number of participants are listed with a description of the type of study. In this review, the text was categorised according to the themes addressed in the studies, e.g. methodology, results and impact. We also identified the main themes and patterns that emerged from the analysis. This helped researchers understand the study's impact. These included six studies on stress monitoring, six on movement disorders, three on sleep tracking, three on blood pressure, two on heart disease, six on covid pandemic, three on safety and six on Validations. Figure 2 shows the distribution of these studies graphically. An analysis of the keywords used in these studies indicates that most were related to smartwatches, Covid-19, and digital health. A visual representation of their distribution can be found in Fig. 3 .
Characteristics of studies included in the scoping review

A graphical representation of the distribution and classification of studies based on the use of smartwatches

Keyword analysis of studies
Stress level
As one of the leading branches of human–computer interaction, affective computing uses technology to detect a person's emotional state [ 29 ]. A stress detection system can be used for various purposes, such as monitoring the stress of drivers, detecting and alleviating the stress of passengers, monitoring the stress levels of employees, and assisting psychologists with online therapy sessions [ 30 ]. Stress detection occurs in various environments, including laboratories, hospitals, clinics, offices, schools, cars and everyday situations. When the brain receives sensory signals from the eye, nose and ear, it triggers a stress response. As a result of the stress response, heart rate increases, muscles tense, blood pressure rises, frequency of inhalation increases, blood sugar levels rise, and senses become heightened. Perceived stress is the way a person interprets and analyses stressful situations [ 31 ]. It can be assessed through regular self-reporting by individuals. Self-reported perceptions of stress can misrepresent stressful episodes, and people tend to forget stressful events, contributing to discrepancies between physiological and perceived stress levels [ 32 ]. Instruments to measure stress should be unobtrusive and non-invasive to collect data accurately. The latest technologies provide us with non-invasive and completely transparent devices to monitor stress. Heart rate variability (HRV) is one of the best-known signals for stress detection. Several features have been used in the literature to distinguish between stress and relaxation, including mean RR, mean heart rate, normalised low frequency, sympathovagal balance index (SVI) and morphological variability (MV) [ 33 ]. Combining features from smartphones and wearable devices (EDA, HR sensors) would be necessary to develop a successful stress detection system. Collecting contextual information (activity, social interaction, GPS and ambient light) about a user will also help researchers to anticipate their condition [ 34 , 35 ].
Photoplethysmography (PPG) is a low-cost optical technique for measuring blood volume pulse by light absorption by blood. BVP features can be used directly or to extract heart rate variability or IBI features. A wearable physiological measurement device must provide high-quality data that is complete, relevant, timely, sufficiently detailed, appropriately presented and contains enough contextual information to facilitate decision-making and provide accurate results. Muhammad Ali Fauzi's study [ 36 ] compared three learning strategies for stress detection tasks. All three strategies used logistic regression (LR) as a machine learning model. Unlike individual learning, this learning strategy is based on a central server that combines the data and trains the integrated model. The user devices only need to perform the task of detecting stress and inferring its cause, while the server performs the tasks of feature extraction and training the model. In this study, a comparison is made between individual, centralised and federated learning for smartwatch-based stress detection. Individual learning provides higher accuracy and privacy than centralised and federated learning. The results of this study show that federated learning performs relatively mediocre in stress detection. The average accuracy was 0.8575, the average precision was 0.9892, the average recall was 0.5208, and the average F1 -measure was 0.6339. In his study, Fauzi proposed an application that could monitor the physiological signals of health professionals to detect occupational stress. The smartwatches would collect data from individual sensors, such as heart rate and skin temperature, to detect changes in physiological signals. The data would then be used to create individual classifiers and sets of classifiers to detect stress levels. In addition, the experiment found that these classifiers could be used in real-time to monitor the stress levels of hospital staff effectively.
In one interesting review study, a wearable platform on the wrist was used to detect and analyse cortisol from small amounts of sweat to study stress [ 35 ]. In some studies, the Empatica E4 wristband includes biomarkers that measure skin temperature, movement-based activity (accelerometer), electrodermal fluctuation and blood volume pulse [ 37 – 40 ]. Studies have used various wearable technologies, including Microsoft Band (10%), Cortiwatch (10%), ARM -Cortex4 smart wristband with DSP functionality (10%) and Empatica E4 (10%), which detects and analyses cortisol in small amounts of sweat on the wrist to detect stress [ 41 ]. A wearable device, such as a smartwatch sensor, and machine learning techniques can be used to detect stress in hospitals. However, some wearable devices are not user-friendly and uncomfortable to wear at work (e.g. devices worn on the chest, GSR sensors positioned with the finger, etc.). A study by Fauzi proposed a method for detecting continuous stress using single classifiers and classifier ensembles. This study used seven machine learning methods for stress detection, including Naive Bayes, Support Vector Machines, Neural Networks and an ensemble approach. It was found that single classifiers had the best accuracy, LR had the best precision, and NN had the best recall for stress detection. In addition, the ensemble approach performed better than all individual classifiers [ 37 ].
Movement disorders
Several studies have shown that smartphones can track symptoms of various diseases over the long term, including chronic pain, rheumatoid arthritis, heart failure and COVID-19. It allows researchers to understand better how symptoms change over time, which can help inform treatment and management plans. The study by Syed Mustafa Ali [ 42 ] examined the longitudinal engagement of users of a smartwatch app in people living with MLTC-M, stratifying engagement patterns by age, gender, number of disease domains and question type. In the 'Watch Your Steps' study, people living with MLTC-M were asked to complete several daily and weekly questions and active tasks over 90 days. The Google Fit Research team developed the "Watch Your Steps" study app, which asked participants to complete three types of tasks: Core symptom questions, organ-specific questions and active tasks, including a sit-stand test, a walk test and a tap test. Fossil Sport smartwatches were preloaded with the study app and loaned to the participants. They were instructed to dock their watch every night for charging, and contact details for support in case of problems were included in the instruction manual. The engagement was recorded over time using longitudinal charts of daily completion rates. Fifty-three individuals with MLTC-M participated in the study. The majority were white, and the average completion rate was 45%. Most participants did not find the data collection tasks distracting, and almost all reported that the smartwatch did not interfere with their normal daily activities. They showed that using a smartwatch to collect health data is feasible and acceptable for people with MLTC-M over 90 days. This study suggests that people living with MLTC-M can use smartwatches to report multiple symptoms per day and that this data could be integrated into electronic health records to support clinical care.
Autism is a disorder with three characteristic symptoms: social development, communication and repetitive behaviours. These behaviours occur when a child tries to regulate sensory input from their environment. The study by Amiri et al. (2017) [ 43 ] developed an Internet-of-Things (IoT) framework called WearSense that uses the sensory capabilities of modern smartwatches to detect stereotypic behaviours in children with autism. They recruited 12 healthy subjects aged 23 to 33 years, from whom 165 samples were collected. They also recruited two subjects aged 15 and 16 who had been diagnosed with autism and recorded sensor data from them as they went about their regular daily routines. The subjects were asked to perform three tasks: hitting their hand in front of their face, drawing on a piece of paper and bumping their head. Participants wore a Moto360 SmartWatch protected by a 3D-printed shield that sent and stored data to a smartphone via Bluetooth. The study employed the built-in accelerometer of a smartwatch to accurately detect three distinct behaviors commonly observed in children with autism: hand flapping, painting, and sobbing. The processing component extracted 34 distinct features in each dimension of the tri-axis accelerometer, yielding a total of 102 discernible characteristics. Subsequently, several classification techniques were evaluated and compared for efficacy; ultimately demonstrating that an ensemble comprising 40 decision trees K-fold cross-validation rendered the highest level of accuracy at approximately 94.6%. This impressive degree of accuracy effectively underscores both the high caliber data captured from the smartwatch as well as commendable feature extraction methodologies implemented throughout this inquiry. Furthermore, employing a smartwatch for identifying these targeted behaviors holds significant promise in facilitating ongoing monitoring efforts pertaining to individuals who manifest autistic tendencies—thereby enabling comprehensive analysis and informed decision-making amongst parents, caregivers, and clinicians alike.
The system had an accuracy of 96.7% in detecting three autistic actions. Juan C. Torrado's [ 44 ] study focused on using smartwatches to help people with autism spectrum disorders with emotion regulation problems. It also presents and evaluates a smartwatch and smartphone system designed to accomplish these tasks. The smartwatch in this system detects anger outbursts and displays self-regulation activities previously obtained from the caregiver (smartphone). The detection is done through the smartwatch's sensor technology and involves a process of data collection, training, and evaluation. Smartwatches have a small screen and a tactile surface, so interaction is mainly through touch and sliding. There are some recent studies on text input approaches, but they limit ourselves to the simplest known interaction options: short touches and horizontal sliding. The smartwatch authoring tool has been implemented on the Android platform to make it as easy as possible for family members, caregivers, or others who are responsible for the person to use it. They used LG Watch Urbane smartwatches, a Nexus 5 smartphone, and an Android Wear interface to experiment with self-regulation strategies. They designed a simple assistance system that included linear sequences of "screens" with images, text, animations (GIFs), and positive reinforcement at the end with personalized content and a question format. They showed the results for each day and each user and enumerated the events when the self-regulation strategies were triggered. The results showed that the smartwatch helped users regain a state of calm. They found that the smartwatch can help individuals with alexithymia and emotional dysregulation control their stress episodes triggered by various stimuli, except for the learning phase of the experiment.
Clinicians and researchers rely on patient self-reports to understand the mechanisms of falls, but objective, real-world fall data are lacking. Providing clinically useful, objective measures of adherence to assistive devices could help reduce fall risk. Advances in machine learning have enabled activity recognition systems to monitor mobility in the elderly. Such systems work best when the information contains patterns that differ significantly between activities. In the study by Stephen A et al. (2019) [ 45 ], they tested whether a smartphone or smartwatch could detect whether an older adult was walking with or without an assistive device. They hypothesized that smartwatches would perform better than smartphones. They recruited 20 older adults from an adult day centre in Evanston, IL. They completed the Berg Balance Scale and Mini-Mental State Exam and provided written consent witnessed by a third person. They collected sensor data from participants who walked with and without assistive devices while completing the six-minute walk test, the 10-m walk test, and the standing and walking time tests. A physical therapist monitored participants' vital signs and provided on-call assistance to prevent falls. Participants wore an Android smartphone with a custom app that recorded triaxial accelerometer and gyroscope data at a frequency of approximately 50 Hz. Participants wore a phone and watched with a triaxial accelerometer, and data were collected throughout the session, including rest breaks. They collected enough data to train our classifiers with hundreds of samples and compared the classification accuracy of the smartphone with the smartwatch for all types of cross-validation. Fourteen older adults conducted a study in which they wore a smartphone and a smartwatch. Using accelerometer data, they trained machine learning classifiers that could predict whether a participant walked with or without an assistive device. They found that smartwatches provided much higher quality data for detecting walkers and canes compared to smartphones and that a second sensor on the hip was required for the user-generated classifiers to make the most accurate predictions.
Aggressive behavior is a prevalent issue among individuals diagnosed with dementia. Although caregivers have traditionally relied on direct observation to identify such behavior, this approach has limitations. However, advancements in technology present promising opportunities for addressing this challenge. Specifically, computer vision and human activity recognition technology, coupled with wrist-mounted inertial measurement units like smartwatches, holds great potential in accurately detecting aggressive events within healthcare and elderly-care facilities. To explore these possibilities further, F Tchuente et al. (2020) [ 46 ] conducted a study involving wearable smartwatch technology combined with machine learning techniques to classify human aggressive behavior. The research team collected data from accelerometer and gyroscope sensors embedded within Microsoft Band 2 devices. These recordings were subsequently analyzed using the Waikato environment for knowledge analysis, leveraging six distinct machine-learning classifiers along with three feature selectors. The selection process for classification models and feature selectors was based on various performance metrics, including accuracy, sensitivity, specificity, F-score, and Matthews correlation coefficient—an established measure used to evaluate predictive models' quality across imbalanced data sets. The study analyzed various classification methods and found that the k-nearest neighbors algorithm combined with the ReliefF feature selector exhibited exceptional effectiveness in differentiating aggressive and non-aggressive actions, boasting a remarkable accuracy rate of 99.6%. Additionally, this method demonstrated high sensitivity (98.4%), specificity (99.8%), precision (98.9%), F-score (0.987), and Matthews correlation coefficient (0.984). Conversely, models utilizing naïve Bayes or support vector machines performed poorly in this context. Furthermore, their findings revealed that wearing a smartwatch on the dominant wrist was the optimal approach for single-watch classification. This research successfully showcased how accelerometer and gyroscope data from smartwatches can be harnessed to identify aggressive movements with great precision effectively.
Sleep tracking
Sleep apnoea is a sleep disorder in which breathing is interrupted and is associated with various health problems. The current diagnostic system for this disorder is costly, resulting in limited accessibility. Several methods have been proposed to detect sleep apnoea, but none focus on smartwatch technology. ApneaDetector is a smartwatch-based system developed by CHEN et al. (2021) [ 47 ] that uses built-in sensors to detect sleep apnoea. The system processes raw accelerometer data using signal denoising and calibration techniques to detect breathing cycles and possible apnoea events. According to their clinical study, 92% of OSA and 70% of hypopnoea events produce signal spikes that the system can detect. By removing linear trends in the accelerometer data for apnoea events using the first-order differentiation technique, spikes in normal sleep can also be identified from the calibrated data. A clinical sleep study was then conducted at Penn State Milton S. Hershey Medical Centre with 20 subjects using the ApneaDetector smartwatch to collect sensor data and evaluate different classification algorithms for sleep events. The study found that while the ApneaDetector accurately classified normal and sleep apnoea events, it had problems with more specific categorisations such as OSA, CSA and hypopnoea. However, the estimated total sleep time is acceptable for calculating the AHI value for diagnosing sleep apnoea. This work used the sensor data to calculate AHI, a standard metric for diagnosing sleep apnoea.
A pilot study used a smartwatch with seven sensors to screen for OSA by generating respiratory waveforms and detecting sleep–wake states using PPG signals. A machine learning algorithm established an initial screening model, evaluated the risk of sleep apnea, and utilized acceleration signal from the wrist for effective signal screening and abnormal scene discrimination. Participants were recruited from the Outpatient Department of Chinese PLA General Hospital. Physicians conducted a full examination on patients before testing for sleep monitoring. A detailed assessment of their sleep habits, physical condition, symptoms and complications was carried out. The study used polysomnography to compare the smartwatch against medical devices in diagnosing OSA among 20 patients. Results showed that the screening algorithm from the smartwatch is consistent with those from medical tests and had similar predictive ability compared to HSAT or PSG. In summary, PPG-based smartwatches were more effective than simultaneous in-lab PSG or HSAT devices when screening suspected cases of OSA [ 48 ].
Consumer wearables like activity trackers, smartwatches and rings can accurately measure sleep parameters with valid data collection and analysis. A study compared the Oura ring's sleep data to a medically approved actigraphy device in 45 healthy individuals aged between 18–55 years old who wore Gear Sport smartwatch, ActiGraph wristband, and Oura ring for a week. The Oura ring uses various sensors to estimate heart rate variability, respiratory rate, physical activity intensity along with acceleration and gyroscope data while wGT3X-BT by ActiGraph measures wrist acceleration in three orthogonal axes at 80 Hz for estimating sleep parameters. The study examined 4 sleep attributes using the Cole-Kripke algorithm and Troiano wear time validation. Results revealed that the Oura ring was more accurate in detecting these attributes than Samsung Gear Sport smartwatch [ 49 ].
Blood pressures
Hypertension is a leading risk factor for heart disease, and unvalidated devices cannot be used in clinical practice. To increase the reliability of smartwatch-based BP measurements, more research is needed on both normotensive and hypertensive subjects. In Falter's study [ 50 ], consecutive patients scheduled for 24-h ambulatory blood pressure monitoring were recruited from the cardiology outpatient clinic. Measurements were taken using validated devices including an automatic cuff-based upper-arm sphygmomanometer and a Samsung Galaxy Watch Active 2 smartwatch calibrated at inclusion. Patients performed multiple measurements with both conventional BP monitors and smartwatches over a minimum of 24 h to ensure accuracy. A total of 40 patients participated in the study, The smartwatch overestimates BP up to 140 mmHg, after which it underestimates BP, illustrating the presence of proportional and differential bias. The precision of the smartwatch measurements is higher at higher BP values, while the precision of the gold standard method is higher at lower BP values. Daytime smartwatch measures were accurate for measuring blood pressure at 135/85 mmHg. The sensitivity and specificity were 84.6 and 88.9%, respectively. Blood pressure variability was higher in the ABPM measurements as compared to the smartwatch measurements, and the CV was significantly lower in the smartwatch measurements. The results of this study indicate that the smartwatch currently suffers from an anchoring point that is set when calibrating the device, resulting in an overestimation of lower BPs and an underestimation of higher BPs. The Samsung Galaxy Watch Active 2 shows a systematic bias toward a calibration point, overestimating low BPs and underestimating high BPs, and is not ready for clinical usage.
The study by Mark Tsou [ 51 ] evaluated the applicability of smartwatches in PM2.5 health assessment by evaluating whether smartwatches are good complements to certified medical devices for PM2.5 health studies, especially for developing countries. A total of 49 subjects were recruited. Each subject carried a small low-cost sensing device for personal PM2.5 and temperature monitoring for 7 consecutive days. The smartwatch employs optical HR measurement technology, and the data can be downloaded from Garmin Connect. During the same monitoring period, subjects wore smartwatches and ECG monitor devices (RootiRx, Rooti Labs Ltd.,Taipei, Taiwan) for 2 consecutive days. The activities recorded were categorized as follows: resting, commuting, working, cooking, worshipping, shopping, exercising, eating, bathing/showering, sedentary activities, and other activities. PM2.5 levels were significantly associated with heart rate (HR) in males and females aged 40 to 64 years and 65 to 75 years, respectively. The effects of PM2.5 on HR were presented as percentage changes per interquartile range (IQR) increase, and 95% confidence intervals (CI) were included. Heart rate was associated with personal PM2.5 exposure in models adjusted for subject, age, gender, body mass index, temperature, activity, and time of day. The results indicated that the elevated PM2.5 concentration was significantly associated with G-HR for low-intensity activities and marginally associated with G-HR for moderate- to high-intensity activities.
Commercial smartwatches offer a potential strategy for healthy behaviour modifications through 24-h BP, dynamic BP variability and heart rate monitoring. However, there is currently insufficient evidence to support their use in improving hypertension management. Yen [ 52 ] conducted a single-blinded, two-arm study to test the effectiveness of a commercial smartwatch with BP-monitoring feature. The study included adults aged 20–65 living in Taipei City and had an experimental group (wearing ASUS VivoWatch BP) and control group (using Mi Smart Band 3 without BP monitoring). Both groups had similar characteristics at baseline, but the experimental group showed significant improvements in DBP, SBP, resting HR, body weight, BMI, body fat, and skeletal muscle index compared to the control group. Participants' blood pressure and resting heart rate improved after 3 months of using a smartwatch with BP-monitoring feature. Their body weight, BMI, body fat and skeletal muscle index also decreased. The smartwatch increased awareness of high BP and helped modify related risk factors. However, only DBP had a significant correlation between the data from the smartwatch and sphygmomanometer.
Heart disease
The Kardia Band is an Apple Watch accessory that can diagnose atrial fibrillation (AF) through an automatic algorithm. In a non-randomized study [ 53 ], patients with AF wore the KB before and after scheduled elective CV procedures. The accuracy of the KB automated algorithm was evaluated by comparing its results to physician interpreted KB rhythm strips and simultaneous ECGs. The study found that KB automated interpretation had high sensitivity and specificity in diagnosing AF compared to physician interpreted 12-lead ECG and KB rhythm strip. The algorithm correctly diagnosed AF with 93% sensitivity, 84% specificity, and a K coefficient of 0.77 when compared to electrophysiologist-interpreted ECGs. Physician interpretation showed similar results with 99% sensitivity, 83% specificity, and a K coefficient of 0.83 for assessing the quality of KB tracings produced by smartwatches.
PPG technology is utilized for passive and continuous monitoring of AF detection algorithms through modern wearable devices. The algorithm should have low computational cost and memory requirements to ensure superior diagnosis. A study evaluated the accuracy of the AF detection algorithm by obtaining ECG waveforms and PPG signals from patients undergoing AF catheter ablation while considering other arrhythmias' impact on it. The study included 116 patients with paroxysmal AF and 40 patients with persistent AF. Various PPG features were analyzed, including time domain and frequency domain analyses of PPI, peak height analysis of PPG, and ACF features of PPI. The majority of these features showed significant differences between the AF and SR signals in univariate analysis. Results suggest that using a longer length (25-beat) for analyzing PPG data leads to higher accuracy in discriminating AF from SR compared to using only 10 beats. Additionally, frequent PVCs/PACs can reduce the accuracy of the algorithm used for detecting AF [ 54 ].
Covid pandemic
A recent study conducted at Scripps Research Translational Institute explored the potential of using wearable sensor data to forecast the transmission patterns of Coronavirus disease in 2019. The research team analyzed a dataset consisting of information from 333 participants who actively used the DETECT smartphone application. This application allowed individuals to input their symptoms and test results while simultaneously collecting additional biometric data such as heart rate and sleep patterns through commercially available wearable devices. By incorporating symptom-based indicators and sensor-generated data into their analytical model, researchers achieved significantly higher accuracy levels in distinguishing between positive and negative cases compared to models solely relying on symptoms alone [ 55 ].
Technology has enabled continuous monitoring of personal health parameters, such as stress, physical activity, and sleep, during pregnancy. In order to address issues of this nature, the use of an Internet of Things (IoT)-based system and smartwatch technology for the monitoring of pregnant women was investigated in a longitudinal cohort study design. The study involved participants wearing smartwatches continuously from early pregnancy through three months after the birth of the child. Participation in the study was restricted to Finnish-speaking women carrying singletons during the 12th—15th week of pregnancy. A total of 38 pregnant women were monitored during the COVID-19 outbreak in Finland for a period of eight weeks. Monitoring system based on IoT was developed to collect signals from Samsung Gear Sport smartwatches every two hours for 12 min. PPG signals were used to extract parameters related to heart rate and heart rate variability. During the study, the Samsung watch was used to measure physical activity and sleep, and TST and WASO were calculated for each night. To analyze the data, the Statsmodel Python package was used, and the dependent variables were measurements of HRV, physical activity, and sleep. The findings of this study showed that the pandemic-related restrictions were associated with increased heart rate variability, stress levels, decreased physical activity, and decreased sleep duration. Pregnant women can benefit from the use of Internet of Things (IoT) technologies in monitoring their daily patterns of well-being [ 56 ].
The occurrence of lung damage and potential post-treatment injuries is a significant concern in the context of the widespread covid-19 outbreak. Consequently, both patients affected by this disease and medical personnel involved in their care may benefit from monitoring potential complications even after recovery. A valuable instrument that can assist with such surveillance is the utilization of smartwatches. In the study conducted by Hunter [ 57 ], Fitbit Charge 3 watches were given to each participant along with their anonymized study reference ID numbers. The data from a smartwatch was extracted, including the daily step count and the daily resting heart rate. They defined smartwatch use as wearing the watch for a minimum of one month. Participants were recruited across sites in South East England, with a mean age of 57 years, 74% being White, and 54% having at least one comorbid condition. Within three months of discharge, the mean step count of the entire cohort increased by 37%. At 3 months and 12 months following discharge, the participants' mean heart rates were reduced by approximately 7% and 13%, respectively. While a considerable number of participants did not use smartwatches on a regular basis, this study demonstrated that smartwatches are capable of monitoring physical activity remotely.
Early detection of infectious diseases is crucial in order to reduce disease spread by enhancing self-isolation and enabling early treatment. Despite this, current diagnostic methods involve sampling and nucleic acid-based tests require a substantial amount of time and money. Testing methods currently available are unlikely to identify presymptomatic carriers. Therefore, real-time detection of cases is imperative. It is possible to detect COVID-19 infection well before symptoms appear, using smartwatches and other wearable devices. In order to accomplish this, a real-time heart rate monitoring algorithm was developed to detect early stages of infection. In this study, they explored whether wearable devices could detect COVID-19 at an early, pre-symptomatic stage. In this manner, a method for potentially detecting the onset of illness in real time, in the early stages, was developed. In this study, two methods were developed with the objective of detecting aberrant physiology in humans: the RHR difference method (RHR-Diff) and the heart rate over steps anomaly detection method (HROS-AD). The study enrolled 5,262 participants who responded to surveys regarding their illness, diagnosis and symptom dates, illness severity, and type of symptoms. Among these, 4,642 reported wearing a smartwatch, of which 3,325 wore Fitbits, 984 wore Apple watches, 428 wore Garmin devices, and the remaining wore other devices. Upon enrollment on REDCap, participants were directed to download the MyPHD app, which collects wearable device data in a de-identified and encrypted manner. The data collected by wearable devices included heart rate, steps, and sleep. The data were retrieved at intervals of 15 s, 1 min, and sleep stage. Metadata and symptom surveys were downloaded from the participants and processed using a custom-written R and Python script. Accordingly, 88% and 100% of individuals with symptom onset or diagnosis dates showed elevated signals prior to or at the time of onset or diagnosis, respectively. It was determined that the increased RHR signal had a specific relationship to COVID-19 by analyzing 15 cases of non-COVID-19 illness. According to the findings, elevated heart rates that occur before illness can be used as a general indicator of respiratory illness. Infection with COVID-19 alters sleep and activity patterns, which can be monitored using a wearable device. The duration of sleep and the number of steps decrease at the onset of the outlying RHR-Diff signal that is associated with COVID-19 illness. With this prototype, 63% of COVID-19 infections were detectable with an alarming frequency of 0.66 per month in healthy individuals. It was determined that abnormal physiological events, such as elevated resting heart rate and increased heart rate relative to number of steps, can be detected using a smartwatch at or near the time of infection [ 58 ].
Three vaccines are currently authorized and distributed in the United States to prevent the spread of COVID-19. Although there is substantial variability in individuals' immune response to vaccines, the CDC V-safe program found that the majority of individuals reported some systemic side effects after the second dose. A recent study found a relationship between reactogenicity symptoms after vaccination and a humoral immune response. In the study conducted by Quer et al. (2022) [ 59 ] collected daily wearable sensor data from 7298 volunteers who received at least one dose of the COVID-19 vaccine. They hypothesized that there are digital, objective biomarkers of reactogenicity that could be identified by detecting subtle deviations from an individual's normal resting heart rate. In the DETECT study, 7,298 participants received at least one mRNA vaccination. Of these, 5674 (78%) participants contributed adequate data to evaluate changes in activity and sleep, respectively. They observed that the average RHR increased the day following vaccination, reaching a peak on day 2 and not returning to baseline until day 4 and 6, respectively. The majority of participants experienced an increase from their normal RHR. They explored several participant and vaccine characteristics that could impact immune response, and found that women experienced higher RHR changes with respect to baseline in the 5 days following vaccination after the first dose only. In contrast, RHR responses vary by age, with individuals age 40 having the greatest increase in RHR. Although a direct comparison is not possible, changes comparable to the ones observed after the second dose of the Johnson & Johnson vaccine were detected in their cohort. After adjusting for potential confounding factors, prior COVID-19 infection was independently associated with a higher RHR increase after the first dose, and female sex was independently associated with a higher RHR increase after the first dose. After adjusting for age, device, vaccine type, and prior COVID-19 infection, they observed higher RHR increases from Apple devices on average, but not after the first dose. The first dose of the vaccine had minimal effect on activity and sleep, but the second dose caused a significant decrease in activity and an increase in sleep, which returned to baseline by day 2. They demonstrated that it is possible to detect physiologic manifestations of reactogenicity to COVID-19 vaccination through individual changes in RHR. This provides a potential novel mechanism to identify individuals with either a suboptimal or exaggerated immune response to a vaccine. Similarly, a study using the Garmin Vivosmart 4 smartwatch to measure heart rate and heart rate variability showed that smartwatches are more accurate than patients' self-reports in predicting post-vaccination physiological conditions [ 60 ].
The safety of wearable technologies in clinical settings is a critical factor influencing the advancement and growth of these tools. The integrity and accuracy of patient clinical measurements obtained through these devices must be established to avoid any potential risks that could compromise the health conditions of individuals under medical care. Since smartwatches are primarily designed for commercial purposes, examining the safety considerations associated with utilizing such wearable tools for patients becomes an essential aspect explored within this study.
High-frequency electromagnetic fields are produced by mobile phones and smartwatches. As such, these devices have the potential to affect human health, although the issue is still under debate. Hence, in order to examine the safety of these devices, a prospective observational study was conducted in order to evaluate the potential for electromagnetic interference with CIEDs. It was conducted using a popular smartphone and smartwatch, which provided real-time monitoring and printing of intracardiac electrograms, marker channels and a 3-lead electrocardiogram. In total, 1,352 testing procedures were conducted on 148 patients for cardiac implantable electronic devices, which included 51 pacemakers, 5 cardiac resynchronization therapy pacemakers, 46 inverter defibrillators, 43 cardiac resynchronization therapy defibrillators, and 3 implantable loop recorders. EMIs were observed between the iPhone and an implanted dual-chamber pacemaker in 1 patient, but not between the Apple Watch and the CIED. In connecting mode, an iPhone placed directly over the generator was observed to cause EMI, which resulted in marker channel assignment being lost, as well as EGM loss and noise in the ventricular marker channels. Moreover, this study shows that there is no risk of EMIs between the iPhone and CIEDs, but relatively frequent telemetry interferences do occur between the iPhone and the CIEDs [ 61 ].
There is the potential for electromagnetic interference to adversely affect cardiac implantable electronic devices (CIEDs), such as pacemakers, implantable cardioverter-defibrillators, cardioversion-defibrillators, and cardiac resynchronization therapy pacemakers and defibrillators. Considering their inductive charging functionality, smartwatches could be a significant source of electromagnetic interference (EMI) due to their ability to transfer power wirelessly over distances up to four centimeters (QiTM). The magnetic components in smartwatches emit electromagnetic fields that can interfere with patient monitoring systems and defibrillators. A prospective, multicenter study was conducted to investigate whether the use of the latest generation smartwatches might interfere with the proper functioning of the CIED. The study participants were 171 patients who received CIEDs and presented to two centers in Athens, Greece, for routine follow-up between March and November 2019. Their tests were conducted on two smartwatches of the latest generation for potential EMI as well as on their magnetic chargers. The ECG recording was meticulously analyzed to identify atrial and ventricular pacing inhibition, asynchronous ventricular pacing, rapid ventricular pacing, and asynchronous pacing. The emission levels of the tested smartwatches and their magnetic chargers were evaluated by measuring low-frequency magnetic fields between 110 and 400 kHz. Each smartwatch was activated and measured separately, first directly in contact with the probe, then at a distance of 10 cm and 20 cm [ 62 ].
Hearing loss is caused by repeated exposure to loud noises, as well as metabolic disorders, hypertension, abnormal sleep patterns, occupational accidents, tinnitus, and a reduction in cognitive abilities. In situations in which noise levels are high, people should move away from the source or wear hearing protection. However, noise-induced hearing loss (NIHL) may occur slowly and remain undetected for a considerable period of time. Smartphones can be used to measure noise levels, but there are several limitations. Wearable devices such as smartwatches can overcome smartphone limitations because they are worn on the wrist. In the study conducted by Fischer [ 63 ], a popular smartwatch was evaluated for its ability to accurately monitor noise levels in 13 occupational and recreational settings. The results showed that the smartwatch and a sound level meter used as a reference were in excellent agreement. It was found that the music club (52% of the measurement) emitted the most hazardous noise levels, followed by construction sites (24%), housekeeping (20%), and streets (4%). The sound level meter and smartwatch measurements are offset by an average of 0.5 dBA (SD of 1.8 dBA), which indicates the smartwatch underestimates the sound level. This study compared noise levels measured by a smartwatch and sound level meter. Smartwatch accuracy was lower in settings with rapid acoustic fluctuations, but comparable to the sound level meter across different pressure levels based on SD of LAeq differences and ICC results.
Validation or evaluation
Despite the fact that smartwatches' health metrics have generally demonstrated high levels of moderate-to-strong validity, limitations in the current literature exist, including that EE and HR data validity of smartwatches has been found to be less valid in free-living PA assessments, and that most previous validation studies have utilized various Fitbit or Jawbone models. Accordingly, Pope's [ 64 ] study examined the validity, measurement bias, and precision of four popular smartwatches in assessing EE, average HR, and peak HR during active play. The participants for this study were 21 healthy college students from a large metropolitan Midwest U.S. university. They were ages 18 to 35, had a body mass index of 18.5 and performed high-intensity exercise that elicited EE > 300 kcalories for each session. ActiGraph GT3X + -BT accelerometers were used in conjunction with an ActiGraph HR strap attached to the chest for the measurement. For EE data collection, a 1-s epoch was employed, with the following empirically derived cut-points (in counts/minute): light PA: 0—2690; moderate PA: 2691—6166; vigorous PA: 6167—9642; and very VPA: q9643. ActiGraph HR straps mounted on the chest were moistened with water 2 cm below the nipple, and smartwatches were preprogrammed for a 20-min exercise session. They downloaded EE and HR data from the ActiGraph using ActiLife 6.13, and identical data was collected for each smartwatch. Smartwatches demonstrated moderate-to-good precision for average HR and peak HR measurements with approximately the same accuracy as an ActiGraph. EE assessment with the ActiGraph GT3X was found to be valid in comparison to doubly labelled water. However, this tool is not considered the gold standard for EE assessment. In spite of the fact that smartwatches can provide moderate validity when assessing average and peak HR, smartwatch EE assessments are less reliable. Hence, this study suggested that smartwatches should not be used as part of a systems medicine approach to health care.
There has been a growing trend for fitness toward the use of wearable technology over the past few years. A variation in EE estimation accuracy may occur depending on the type and intensity of activities, and algorithms may not take into account the type and intensity of physical activity or the posture of the body. Validating EE estimations requires consideration of the intended use of the device, as well as an assessment of the accuracy of EE estimations made by young adults while walking and running outdoors. Accordingly, Shenglong et al. (2022) [ 65 ] recruited twenty healthy Chinese participants from the campus of the local university for their study. They measured individual VE, VO2 and VCO2 using the Cosmed K5 system for a wide range of metabolic rates. EE was calculated using the ratio between inhaled oxygen and exhaled carbon dioxide. Three smartwatches were examined for validity: Apple Watch Series 6, Garmin Fenix 6, and Huawei GT 2e. Photoplethysmography was used to determine heart rate and GPS was used to determine distance and speed while walking or running outdoors. Data for this study was collected during one visit. Subjects had not consumed food, coffee, tea, or other stimulants, did any vigorous physical activity, or consumed alcohol during 24 h prior to measurements. EE data were taken from the K5 breath-by-breath and summed for each exercise session separately. The EE estimates were obtained directly from the watches. The validity of the smartwatches was determined by several statistical tests, including paired sample t-tests, mean absolute percentage errors (MAPE), and Intraclass Correlation Coefficient (ICC). Excellent, good, moderate, and low agreement thresholds were defined as ICC values of ont, goo). The Apple Watch Series 6 had the highest energy expenditure, followed by the Garmin FENIX 6, and the Huawei Watch GT 2e. GF could likely provide better EE estimates for the outdoor walking than running, and EE tends to be overestimated at lower pace and underestimated at higher pace. Smartwatch EE overestimated EE versus the K5 during outdoor walking and running, and the energy cost of running was overestimated by 24.4% and 21.8%, respectively. This may result in the ineffectiveness of a weight loss program. The findings of this study indicate that smartwatches may have moderate validity in estimating energy expenditure for outdoor walking and running.
Physiological parameters such as heart rate and heart rate variability provide insight into cardiovascular and autonomic nervous system dysfunction as well as mental, physiological, and sleep-related stress. Noninvasive methods for HR and HRV monitoring include Electrocardiography (ECG) and Photoplethysmography (PPG). Studies evaluated the accuracy of HRV parameters extracted from wristbands and smart-watches including Apple Watch, Empatica E4, Microsoft band 2, and the Wavelet wristband against medical-grade ECG devices. They showed that motion artifacts highly affect the reliability of HRV parameters. In the study conducted by Sarhaddi et al. (2022) [ 66 ] evaluated the validity of the Samsung Gear Sport smartwatch in terms of HR and HRV parameters compared with a medical-grade chest ECG monitor in a 24-h continuous free-live setting monitoring. The study included 28 healthy individuals. They collected data using two wearable devices and self-report and background questionnaires. The participants wore a Samsung Gear Sport smartwatch and a Shimmer3 ECG device, and logged their sleep and non-wear time. The Shimmer3 ECG device was used to measure ECG as the gold standard method in their assessment. Also, they developed a customized data collection application for the Samsung Gear Sport watch to collect 16 min of PPG signals every 30 min continuously. They used a deep-learning-based method for PPG peak detection, which is enabled by a dilated Convolutional Neural Networks (CNN) architecture. Also, they developed a two-round peak detection algorithm to locate peaks in ECG signals that obtains higher accuracy in comparison with Pan-Tompkins and Hamilton algorithms. The correlation between the HR and HRV parameters of the smartwatch and Shimmer3 was high (positive), and the LF/HF ratio value showed a moderately positive relationship. The regression analysis was used to compare the accuracy of the extracted parameters from the Samsung smartwatch against the reference ECG. The HR, AVNN, and pNN50 parameters showed good agreement with the ideal lines, but the other HRV parameters showed relatively diverging lines. The Samsung smartwatch underestimates AVNN values, but overestimates other parameters. The Samsung smartwatch and reference ECG showed a high positive correlation between the AVNN and HR values, a moderate positive correlation between the HR and the other HRV parameters, and low positive correlations between the LF, HF, and LF/HF ratio. The results indicate that the accuracy is highest when the watch's parameters are equal to the golden standard values. They validated the accuracy of HR and HRV parameters extracted from PPG signals collected by the Samsung smartwatch during sleep and awake time using short-term HRV analysis. The Samsung smartwatch underestimates HR, SDNN, LF, and LF/HF ratio but overestimates AVNN during sleep time and awake time. Moreover, the watch underestimates RMSSD, pNN50, and HF during sleep time, although it overestimates these parameters during awake time. In concluded this smartwatch can accurately measure HR and HRV parameters during sleep and awake time, and provide acceptable RMSSD, SDNN, LF, and HF.
Falling occurs more frequently with age, and staying on the floor for a prolonged period of time after a fall can have serious consequences, such as hospitalization, a decline in activities of daily living, or a placement in a long-term care facility. There is an increasing availability of assistive technologies, such as call alarm systems and personal emergency response systems. However, consumers are not always able to use these technologies due to difficulties activating these systems. Fall detection can be performed using an app on a smartwatch. The study conducted by Brew B et al. (2022) [ 17 ] aimed to address these issues. This study used a threshold-based algorithm programmed for different smartwatches to automatically detect a fall on 22 volunteer participants. 12 participants were wearing two smartwatches, model A and B, and 10 participants were wearing only one smartwatch, model C, on one wrist. In three phases, the algorithm collected acceleration data and the time of the fall: "prefall", "induced fall" (8 falls around five minutes), and "postfall" (walking back from the crash mat to the area to remove the smartwatches). This study found that an algorithm programmed in commercially available smartwatches to detect induced falls had an overall sensitivity of 77% and specificity of 99%. The fall detection performance depends on the algorithm used, and the sensitivity ranges from 70 to 100% and the specificity from 80 to 100% depending on the type of fall. In addition, they showed the performance of a fall detection algorithm could be strongly dependent on the smartwatch model [ 17 ].
Sensors that measure movement in the body have the potential to revolutionize how clinical status and wellbeing are measured in daily healthcare. Physical disabilities can be described, quantified, and monitored in these instruments, deteriorations can be detected, and treatment responses can be monitored. In a research or clinical setting, consumer smartwatches cannot be used due to their unknown data quality. A smartwatch IMU's accuracy and precision can be evaluated by measuring both absolute errors and correlations with ground truth values. In the study conducted by Auepanwiriyakul [ 67 ], raw IMU sensor data quality was evaluated, followed by a trial of the feasibility of wearable health devices in a clinical environment. Using the Apple Watch Series 3 and 5 IMUs, they compared the inertial accuracy of the consumer smartwatches with two well-known research- and clinical-grade IMU sensors and a gold-standard test for human movement assessment utilizing optical motion tracking. The researchers recruited 15 healthy volunteers and developed a WatchOS App that collected triaxial acceleration and triaxial angular velocity data in real time on Apple Watches at a frequency of 100 Hz in order to record and extract inertial data from Apple Watches. As the base for the experiment, they used an Apple Watch Series 3 and adhered the OptiTrack markers pad to the base with the aid of double-sided tape. Apple Watches demonstrated weak to moderate R2 agreement with OptiTrack. Apple Watch Series 3 and 5 had strong R2 agreement with each other for acceleration and angular velocity. They also had strong agreement with Xsens MTw Awinda. In this study the consumer smartwatches (Apple Watch Series 3 and 5) and research-grade IMU Xsens achieved cleaner linear acceleration signals and lower errors than Axivity, perhaps due to the additional magnetometer and strap down integration (SDI) technology. According to their study on the acceptability of wearable IMU technology by hospital patients and healthcare professionals, this technology is viewed favourably.
Consumer wearables equipped with multisensor technology are an effective means of monitoring objective movement patterns. Various systems have potential for diagnosing PD by analyzing voice, hand movements, gait, facial expressions, eye movements and balance. However, caution should be exercised when interpreting reported accuracies as the models were trained on low sample sizes (n < 100) regarding PD. Varghese et al. (2021) [ 68 ] conducted a prospective study from 2018 to the end of 2021, in which they recruited and measured hand movements of 400 participants using Apple smartwatches and smartphones. The aim was to distinguish PD from other movement disorders and healthy individuals. They compared acceleration amplitudes and tremor frequencies utilizing a seismometer and high-precision shaker with different machine learning models trained for classification performance assessment. Participants wore two smartwatches during a 15-min neurological examination, designed by movement disorder experts. A Trillium Compact seismometer measured ground velocity as part of the study. A shaker table experiment was conducted to validate the method using two Apple watches and a Trillium Compact seismometer. The z-axis accuracy of the method was tested with tremor-typical frequencies and amplitudes, resulting in 43 measurements performed on different days. The watches were connected to iPhones via Bluetooth while data from the seismometer were collected on a digitizer that it was attached to. Each measurement lasted for 20 s for the watches, which were mounted on the shaker table along with a decoupled platform for placing the seismometer. The study utilized various models including SVM, CatBoost, MLP and DL to extract features from acceleration data using Keras and Tensorflow. Results showed that smartwatches had high agreement with seismological sensor validation in measuring movement subtleties or hand-tremor amplitudes and frequencies more accurately than clinical documentation or human vision.
The fast-paced advancements in technology have given rise to a new category of patients called e-patients. These individuals rely on various technological tools to monitor and track the progression of their diseases [ 4 ]. Scholarly works, such as those by Asad et al. (2019) [ 69 ], Masters et al. (2017) [ 4 ], Loda et al. (2019) [ 70 ], and Herrmann-Werner et al. (2019) [ 71 ] highlight the need for medical curriculum integration that enhances communication skills and resolves the challenges associated with engaging e-patients. The studies suggest training medical students on utilizing e-patient knowledge in healthcare delivery, advising patients regarding credible online sources, assessing website credibility strategies, and employing blended-learning teaching methods to improve students' competence when dealing with this unique patient population. Therefore, it becomes crucial for clinical students to possess the necessary skills required for effective engagement with these technologically-savvy individuals. This can only be achieved through comprehensive training provided by educational institutions. Wearable technologies like smartwatches are increasingly popular in healthcare due to their potential to optimize practices and promote healthier habits [ 72 ]. However, integrating these devices presents challenges like seamless integration with clinical workflows and efficient data management [ 73 , 74 ]. Medical students, who use smartwatches, serve as role models for patients, making it crucial for future healthcare professionals to possess technical proficiency and a thorough understanding of these technologies.
Thanks to technological advancements, smartwatches and wearable technology are rising in the diagnosis and symptom reporting field [ 67 , 75 ]. Researchers have conducted studies to assess these technologies' effectiveness, with encouraging outcomes. An investigation revealed that smartwatches and wearables are accurate when identifying initial signs of health issues like irregularities in heart rate [ 64 ]. Moreover, users can receive valuable feedback through this technology. Additionally, researchers discovered that such devices play a crucial role in detecting potential health hazards at an early stage before they escalate into critical conditions [ 52 ]. Another study examined how wearables can monitor patients with chronic conditions. The study discovered that these devices have the capability to perceive variations in essential indicators such as pulse rate and blood pressure, notifying medical professionals of possible issues [ 64 ]. This could prove especially advantageous for individuals unable to communicate effectively with their healthcare providers or are susceptible to abrupt fluctuations in health.
Recent studies have shown that smartwatches can be incredibly useful for various purposes, including motion sickness, heart health, work stress and timely diagnosis of COVID-19 [ 55 , 57 ]. The watch has the capability to assess respiratory rate, levels of oxygen saturation, and body temperature. This functionality provides the potential to detect the virus early on [ 55 ]. However, it should be noted that this method does not possess the ability to differentiate between infections caused specifically by SARS-COV-2 versus other viruses. Nevertheless, its use could lead to identifying diseases arising from diverse infectious agents and potentially forecasting disease severity as well as symptom manifestation [ 76 ]. Disease detection using wearable devices offers many advantages over traditional testing methods, such as no testing infrastructure, materials or personnel, passive testing and high-resolution continuous screening to allow follow-up testing and self-isolation. Although smartwatches are increasing and digital technology was widespread during the COVID-19 pandemic, few studies have used smartwatches for rehabilitation, empowerment or patient engagement in rehabilitation [ 57 , 77 ]. Moreover, the smartwatch can track heart rate and identify any abnormalities, which could serve as an initial indication of potential cardiovascular issues [ 10 , 66 ]. Additionally, employers can utilise smartwatches to monitor employee stress levels in work settings and access valuable information that aids in providing necessary support for their workforce.
Pairing the smartwatches utilized by the patient with additional smart wearable devices that can be employed by both the patient's companions and even healthcare professionals in the intensive care unit holds immense potential to transform it into an invaluable information tool. This confluence of technological advancements plays a pivotal role in diligently monitoring and tracking crucial symptoms exhibited by the patient [ 75 ]. Smartwatches incorporate advanced technology to deliver precise and up-to-date data, enabling medical professionals to comprehend their patients' health conditions. Significantly, individuals have the convenience of monitoring their vital signs and other pertinent health information. They can securely send this information to their caregivers or healthcare professionals to improve the effectiveness of monitoring their state [ 75 ]. This ultimately improves the quality of patient care and enhances understanding of the patient's overall wellness. Furthermore, secure transmission of this data helps expedite diagnosis and treatment processes by reducing time requirements [ 78 , 79 ]. Consequently, patients receive faster and more efficient treatment as a result. Additionally, these smartwatches serve as reminders for patients to take medication promptly, ensuring an added level of comfort and safety in managing their health conditions. It is important to note that smartwatches' functionality heavily relies on their algorithmic operations and program design alongside the specific type utilized in healthcare settings [ 53 ].
Examining the viability of smartwatches as dependable tools necessitates thoroughly considering safety. Previous studies, such as Elvis, have emphasized the significance of user safety in determining the sustained intention to utilize smartwatch applications [ 80 ]. In line with this, Lee has developed an effective tool to assess smartwatch quality from users' perspectives by evaluating factors like usability, functionality, and, most notably, safety concerns [ 81 ]. Moreover, Hong's research in 2022 reveals the positive impact that both safety and convenience can have on individuals' motivation to incorporate smartwatches into their lifestyles [ 82 ]. Alongside assessing their ability to measure clinical data accurately, this research also delves into the Safety concerns surrounding patients' usage of these devices. The findings from multiple studies highlight that smartwatches demonstrate satisfactory levels of safety when used by individuals [ 61 – 63 ].
The world of smartwatches has significantly advanced in recent years. However, most of these watches have been developed for convenience rather than to help with medical diagnosis [ 75 ]. Recent studies indicate that smartwatches' potential in detecting medical symptoms may be limited due to issues with precision and accuracy. The study conducted by Varghese revealed that although smartwatches were capable of capturing subtle tremor signs in Parkinson's disease, there were noticeable differences in amplitude and frequency when compared to a seismometer [ 68 ]. Similarly, the research carried out by Falter showcased insufficient accuracy in smartwatch-based blood pressure measurements, showing a systematic bias towards a calibration point [ 50 ]. Antognoli, on the other hand, emphasized the necessity of metrological validation for wearable devices and determined that while commercial smartwatches offered certain precision and accuracy for heart rate and blood pressure measurements, some degree of bias was present [ 83 ]. Moreover, Poli's investigation from 2022 also examined the precise nature of blood pressure tracking using smartwatches; however, it suggested lower data quality as compared to medical-grade instruments despite achieving acceptable levels of precision and accuracy [ 84 ]. Hence, the research indicates that currently available smartwatches may not meet the necessary standards for precise and accurate detection of medical symptoms. These findings raise concerns about the effectiveness of smartwatches in accurately monitoring and detecting health conditions.
The trustworthiness of smartwatches is a subject of concern due to the opaqueness surrounding the algorithms used for detecting and analyzing users' health information. These algorithms are kept from the general public, which introduces a level of uncertainty regarding their accuracy and reliability [ 79 ]. Furthermore, research studies have pointed out that different brands of smartwatches yield varying results when diagnosing clinical symptoms, sometimes even diverging from those provided by traditional medical devices [ 84 – 86 ]. Recent studies have examined the accuracy and precision of various smartwatch models compared to medical-grade pulse oximeters. Windisch discovered that although the Apple Watch Series 6 generally demonstrated good agreement with medical-grade devices, outliers were still reported, reaching up to a 15% difference [ 86 ]. Jiang, on the other hand, conducted a comparative analysis of different smartwatches and found that the Apple Watch Series 7 performed closest to the reference standard while the Garmin Venu 2 s deviated furthest from it [ 85 ]. These findings suggest that while some smartwatches showcase favorable alignment with medical-grade pulse oximeters regarding accuracy assessments, occasional outliers as well as discrepancies remain present due to variations among different models and contexts considered during evaluations.
Given the crucial nature of healthcare decisions, relying entirely on these watches' information becomes increasingly challenging. This could pose a notable challenge in certain situations where precise diagnosis and treatment are crucial. This study underscores both the significance and pressing need for incorporating smartwatch technologies into health services. It is essential to acknowledge that although smartwatches can serve as valuable tools for monitoring health and overall wellness, they should not be solely relied upon for diagnosing illnesses in most instances [ 87 ]. Hence, we recommend that leading companies in healthcare technology venture into this domain, ensuring that they provide smartwatches equipped with dependable and efficient mechanisms for monitoring individuals' well-being. This calls for the development of medical watches and the creation of AI-hospital assistants.
Smartwatches have the potential to revolutionise healthcare by providing patients with easier access to their health data. They also increase the acceptance of long-term home monitoring [ 88 ]. However, smartwatches in healthcare also raise problems. One problem is that smartwatches focus on aggregating biomedical data and do not take a holistic view of the patient [ 78 ]. The over-reliance on smartwatches and the fewer face-to-face doctor visits lead to a violation of the principle of non-maleficence [ 78 ].
With the increasing availability of 6G technology, AI and medical data, the potential of predictive analytics is becoming more real. This opportunity allows us to develop algorithms that have incredibly high diagnostic power. Using these datasets, we can identify patterns and trends that can be used to predict future health conditions and make informed medical decisions [ 79 ]. Smartwatches has a broad reach and can be significantly improved, but its security and credit ratio still needs to be assessed. This review has attempted to highlight the strengths and weaknesses of some smartwatches in the context of diagnosis and validation; however, further research is needed.
Advancements in technology have led to the rise of e-patients who rely on technology to monitor and track their diseases. Medical curriculum integration is crucial for enhancing communication skills and addressing challenges associated with engaging e-patients. Wearable technologies like smartwatches are increasingly popular in healthcare, but integrating them presents challenges. The review highlights the relationship between smartwatches and their efficiency in health systems. Smartwatches can be effective in diagnosing and reporting symptoms, detecting potential health hazards early, and monitoring patients with chronic conditions. However, safety concerns need to be considered, as most smartwatches have been developed for convenience rather than medical diagnosis. The trustworthiness of smartwatches in healthcare is also a concern due to the opaqueness of their algorithms and varying results in diagnosing clinical symptoms. Instead, there is an increasing demand for a clinical assistant that relies on AI within the health network. This type of assistant would be able to offer more precise and dependable data while also being better equipped to handle the intricacies involved in healthcare. Given these findings, additional investigation will be imperative to fully comprehend and exploit the potential of smartwatches in healthcare and develop more sophisticated and efficient clinical assistants that utilise AI technology.
Authors’ contributions
M.M.H. and S.T.M.H. were responsible for the search strategy and thematic analysis. The manuscript was critically reviewed by K.Q. S.S.S.T. and M.M.H. writing of the manuscript. Sh.H. has enhanced the manuscript by helping to attentively address the reviewers' comments and implementing necessary revisions. All authors not only read and approved the content of the manuscript but also participated in its preparation.
The study was self-founded.
Availability of data and materials
Declarations.
In this study, no ethics approval was required as the scoping review methodology was based on reviewing and collecting data from publicly available materials.
Not applicable.
The authors declare no competing interests.
Publisher’s Note
Springer Nature remains neutral with regard to jurisdictional claims in published maps and institutional affiliations.
- Search Menu
- Sign in through your institution
- Volume 2024, Issue 5, May 2024 (In Progress)
- Volume 2024, Issue 4, April 2024
- Case of the Year
- MSF Case Reports
- Audiovestibular medicine
- Cardiology and cardiovascular systems
- Critical care medicine
- Dermatology
- Emergency medicine
- Endocrinology and metabolism
- Gastroenterology and hepatology
- Geriatrics and gerontology
- Haematology
- Infectious diseases and tropical medicine
- Medical ophthalmology
- Medical disorders in pregnancy
- Paediatrics
- Palliative medicine
- Pharmacology and pharmacy
- Radiology, nuclear medicine, and medical imaging
- Respiratory disorders
- Rheumatology
- Sexual and reproductive health
- Sports medicine
- Substance abuse
- Author Guidelines
- Submission Site
- Open Access
- Editorial Board
- Advertising and Corporate Services
- Journals Career Network
- Self-Archiving Policy
- Journals on Oxford Academic
- Books on Oxford Academic

Article Contents
Wearable devices: monitoring the future, conflict of interest, ethical considerations.
DCI and EMK contributed equally
- Article contents
- Figures & tables
- Supplementary Data
Diomidis C Ioannidis, Efthymia Maria Kapasouri, Vassilios S Vassiliou, Wearable devices: monitoring the future?, Oxford Medical Case Reports , Volume 2019, Issue 12, December 2019, Pages 492–494, https://doi.org/10.1093/omcr/omz143
- Permissions Icon Permissions
Wearable devices are becoming increasingly utilized in our contemporary world, and the Apple Watch is a popular tool for monitoring the heart rate and more recently, heart rhythm. The Apple Watch Series 4 was the first one granted clearance by the Food and Drug Administration (FDA), allowing rhythm detection and identification of Atrial Fibrillation (AF), on 11 September 2018; outlying however that the definitive diagnosis should not be made before a doctor confirms the validity of the possible alarm signal transmitted by the watch [ 1 ]. Nonetheless, Apple watches have been used to inform patients about other rhythm abnormalities as well. In this issue of Oxford Medical Case Reports, Goldstein and Wells present the case of a previously asymptomatic 56-year-old man diagnosed with Atrial Flutter after he noticed a high rate on his watch, which coincided with palpitations. He contacted the medical services 4 days later and an electrocardiogram (ECG) confirmed Atrial Flutter with a 2:1 AV block ( Fig. 1 ). He proceeded to receive anticoagulation and a transoesophageal echocardiogram-guided electrical cardioversion [ 2 ]. In this case of an individual with a high thromboembolic risk score, it was clear that the Apple Watch had an instrumental role in identifying the abnormal rhythm and subsequent confirmation of the Atrial Flutter thus allowing further appropriate medical intervention and pharmacotherapy.

ECG undertaken following the Apple watch notifications demonstrating atrial flutter.
However, concerns have been raised about the accuracy of the watch with regards to rhythm abnormality detection, the need for perhaps unnecessary downstream testing in some individuals and ultimately whether it will benefit patients or simply increase the level of anxiety in those noticing abnormal heart rates or receiving notifications for abnormal findings. Only large population-based registries will allow us to place the exact role of the Apple Watch.
The Apple Watch is a commercially available device, which can be found in stores and online, thus being more commonly used by healthy individuals. It is effective for measuring heart rate [ 3 ], and moreover, as it can detect abnormal cardiac rhythm, it may prove helpful to people who occasionally have symptoms such as palpitations. Newer versions of the device can record the ECG for subsequent reading by a physician, but this function was not available in the country where the patient was present [ 4 ].
To support the potentially beneficial role of the Apple Watch, the large Apple Heart observational study of 419 297 participants using an Apple Watch over a period of 8 months has been recently published, the ‘Large-Scale Assessment of a Smartwatch to Identify Atrial Fibrillation’. This showed that detection of possible AF for more than 30 seconds was seen in 0.5% of the participants (who were generally younger) but for those over the age of 65, this rose to 3.2%. When the device recording was compared to an ECG patch, the positive predictive value was 0.84, which is very encouraging for potential clinical use [ 5 ].
However, there are certain limitations, which need to be considered. Firstly, there is a concern over the possible overdiagnosis of AF and the unnecessary anxiety that this could bring. Smart watches demonstrate high sensitivity but generally speaking, lower specificity, although with improving technology this is likely to also increase. Therefore, episodes of an otherwise benign dysrhythmia, may be misinterpreted as abnormal, resulting in patient stress, downward testing and even unnecessary anticoagulation treatment [ 6 ].
Linked with this is the fact that such wearable devices are utilized mainly by young healthy individuals who have a very low risk of AF, and therefore, the overall risk of a false positive notification of AF will be higher than the elderly population, who might be less likely to use an Apple Watch [ 7 , 8 ]. Even then, anticoagulation is unlikely to be indicated or beneficial in young; otherwise healthy individuals with very low thromboembolic risk.
Furthermore, another concern stems from the way the device operates. The watch, which is placed on the wrist, has an optical sensor detecting pulse waveform to passively measure heart rate [ 9 ]. Then, using an algorithm, each pulse peak is translated into an R wave in the ECG. Detection of this pulse irregularity is used to identify atrial fibrillation. It is evident that potential skin sensitivities could possibly interrupt this procedure and lead to inaccurate measurements [ 10 ].
However, despite its any possible disadvantages, the Apple Watch is here to stay. It is likely to help patients identify AF and also any other potential rhythm abnormalities in the future, but at the risk of necessitating significant downward testing in healthy individuals. In addition, many more companies are expected to produce similar wearables, thus leading to more people having access to home monitoring and then referring to physicians for further investigations [ 11 ]. Given that these devices can be easily purchased by healthy individuals, as physicians we need to expect and prepare for reviewing patients following Smart Watch notifications as they will become a more common feature of the daily clinical practice.
No conflicts declared.
Dr Vassiliou is the guarantor of this manuscript.
FDA . ( 2018 ). Apple Watch ECG and AF Approval . Retrieved from https://www.accessdata.fda.gov/cdrh_docs/pdf18/DEN180044.pdf
Goldstein L , Wells M . Oxf Med Case Reports OMCR 2019-258 (in press) .
Koshy AN , Sajeev JK , Nerlekar N , Brown AJ , Rajakariar K , Zureik M , et al. Smart watches for heart rate assessment in atrial arrhythmias . Int J Cardiol 2018 ; 266 : 124 – 7 . doi: 10.1016/j.ijcard.2018.02.073 .
Google Scholar
Sanders D , Ungar L , Eskander MA , Seto AH . Ambulatory ECG monitoring in the age of smartphones . Cleveland Clin J Med 2019 ; 86 : 483 – 92 . doi: 10.3949/ccjm.86a.18123 .
Perez M , Mahaffey K , Hedlin H , et al. Large-scale assessment of a smartwatch to identify atrial fibrillation . New Eng J Med 2019 ; 381 : 1909 – 17 .
Cheung CC , Krahn AD , Andrade JG . The emerging role of wearable technologies in detection of arrhythmia . Can J Cardiolo 2018 ; 34 : 1083 – 7 . doi: 10.1016/j.cjca.2018.05.003 .
Foster KR , Torous J . The opportunity and obstacles for smartwatches and wearable sensors . IEEE Pulse 2019 ; 10 : 22 – 5 . doi: 10.1109/MPULS.2018.2885832 .
Turakhia MP , Desai M , Hedlin H , Rajmane A , Talati N , Ferris T , et al. Rationale and design of a large-scale, app-based study to identify cardiac arrhythmias using a smartwatch: the apple heart study . American Heart Journal 2019 ; 207 : 66 – 75 . doi: 10.1016/j.ahj.2018.09.002 .
Apple Inc. Using Apple Watch for Arrhythmia Detection, (December). Retrieved from Apple Inc. (2018). Using Apple Watch for Arrhythmia Detection, (December) . 2018
Carpenter A , Frontera A . Smart-watches: a potential challenger to the implantable loop recorder? Europace 2016 ; 18 : 791 – 3 . doi: 10.1093/europace/euv427 .
Guo Y , Wang H , Zhang H , Liu T , Liang Z , Xia Y , et al. Mobile health technology for atrial fibrillation screening using photoplethysmography-based smart devices: the HUAWEI heart study . J Am College Cardiol 2019 . doi: 10.1016/j.jacc.2019.08.019 .
Author notes
Email alerts, citing articles via, affiliations.
- Online ISSN 2053-8855
- Copyright © 2024 Oxford University Press
- About Oxford Academic
- Publish journals with us
- University press partners
- What we publish
- New features
- Open access
- Institutional account management
- Rights and permissions
- Get help with access
- Accessibility
- Advertising
- Media enquiries
- Oxford University Press
- Oxford Languages
- University of Oxford
Oxford University Press is a department of the University of Oxford. It furthers the University's objective of excellence in research, scholarship, and education by publishing worldwide
- Copyright © 2024 Oxford University Press
- Cookie settings
- Cookie policy
- Privacy policy
- Legal notice
This Feature Is Available To Subscribers Only
Sign In or Create an Account
This PDF is available to Subscribers Only
For full access to this pdf, sign in to an existing account, or purchase an annual subscription.
Watch Your Smartwatch
Ieee account.
- Change Username/Password
- Update Address
Purchase Details
- Payment Options
- Order History
- View Purchased Documents
Profile Information
- Communications Preferences
- Profession and Education
- Technical Interests
- US & Canada: +1 800 678 4333
- Worldwide: +1 732 981 0060
- Contact & Support
- About IEEE Xplore
- Accessibility
- Terms of Use
- Nondiscrimination Policy
- Privacy & Opting Out of Cookies
A not-for-profit organization, IEEE is the world's largest technical professional organization dedicated to advancing technology for the benefit of humanity. © Copyright 2024 IEEE - All rights reserved. Use of this web site signifies your agreement to the terms and conditions.
How-To Geek
Forget the apple watch: here's why i love cheap smartwatches.
I don't need a smartphone on my wrist; I just want a smartwatch!
Quick Links
Cheaper smartwatches have better battery life, you still get most of the features and functionality, heart rate monitoring and step counting are almost as accurate, key takeaways.
- Cheaper smartwatches sometimes offer better battery life.
- Budget smartwatches still offer all essential features like notifications, music, and calls that fulfill most user needs.
- Inexpensive smartwatches provide adequate heart rate monitoring and step-counting accuracy for general fitness tracking.
If you never tried out a smartwatch because they're far too expensive, maybe it's time to check out one of the budget offerings. In fact, not only are they more affordable, but I firmly believe that cheap smartwatches can be superior to more expensive models. Here's why.
The feature set and build quality of smartwatches only get better as you go up in price. Some smartwatches are so advanced that they let you answer calls, track all kinds of health data, and use NFC to pay for groceries. What's strange is that battery life seems to be inversely proportional—the cheaper the smartwatch, the better the battery life.
Of course, battery life highly depends on the exact model in question, but it seems that cheaper smartwatches tend to do a better job on that end. The hardware is more rudimentary, with less bright and sharp screens, slower onboard processors, limited apps, and fewer sensors, yet most smartwatches at any price point use similar battery sizes of about 300–500 mAh. I had a cheap fitness tracker, followed by a cheap smartwatch—the Amazfit GTR 2 —and both devices had batteries that could last 10+ days of everyday use, even with sleep and heart rate monitoring.
Now compare that to the Apple Watch, which boasts a meager 18 hours of battery life . Apple thinks that an "all-day battery" is a thing to be proud of. While I must commend their excellent quality and best health and fitness tracking , their watches are not for me. WearOS smartwatches aren't much better, as they are more resource-heavy than proprietary operating systems from brands like Garmin, Huawei, and Amazfit.
I can't be bothered to charge my watch every evening. Also, I travel frequently and don't want to think about having to charge my watch away from home. I have been to Istanbul twice in the past couple of years, and I didn't bring my smartwatch charger with me. I still came back home after a week with battery life to spare both times.
Although cheap smartwatches don't come with a ridiculous 64GB of storage and fancy neural processing cores like the Apple Watch Ultra 2 , they do almost everything most people want. For example, I can't run Google Keep natively on my smartwatch because I don't have Wear OS, but I still get reminders from my phone on the smartwatch as standard notifications. I don't have AccuWeather to check the weather, but the built-in weather app is just as good.
I can't run games on my smartwatch, but I can't imagine ever doing that anyway. If I need to do something that requires precise input, like using a calculator, and I can't rely on my voice, I won't bother doing it on a tiny smartwatch screen. That's what smartphones are for. My watch tells the time and date, feels responsive, and doesn't miss a single notification, which is all I really need. Everything else is a bonus.
A few other things that my cheap smartwatch can do include sideloading custom watch faces, playing music directly from the smartwatch through the built-in speaker or Bluetooth earbuds, answering calls, setting alarms, responding to voice commands, and running Amazon Alexa. The only app that I really like but can't get is Google Wallet.
I'm sure that pricey smartwatches from brands like Apple, Samsung, and Google do a better job at heart rate monitoring, but I don't care whether my heart rate is 137 or 139 when I run; what matters is that I stay within the aerobic range for the duration of my goal. As long as it's within a few percent of my actual heart rate, I'm okay with it. My cheap smartwatch also has a 5 ATM water resistance rating , so I can take it with me to the pool and beach to track my swimming data.
The same is true for steps—it doesn't matter if your watch tells you that you took 9,000 steps in a day, but you "only" did 8,961. Cheap smartwatches are probably more accurate than smartphone apps , and many people rely on those because they're reasonably accurate. If you ask me, improvements in this area are completely superfluous.
Stop ignoring good deals on cheap smartwatches and fitness trackers—they might surprise you with their features and quality. If you end up liking the overall smartwatch experience and find yourself using it often, you can always upgrade to a nicer model.
Tapping to Agree I hereby consent to the use of cookies, confirm that I have read Cookie Policy and allow this website to collect and process my personal data in a safe and secure way
- Essay Writers for Hire
- Pay For Essays
- Plagiarism Free Essays
- Business Essay Writing Service
- Scholarship Essay Writing
- Masters Essay Writing Service
- Essay Editing
- Persuasive Essay Writing Service
- Personal Statement Writing Service
- College Paper Writing
- Coursework Writing
- Dissertation Editing
- Write My Dissertation
- Essays for Sale
- Buy Research Paper
- Research Paper Help
- Pay For Research Paper
- Write My Research Paper
- Research Paper Editing
- Write My Philosophy Research Paper
- Write My Assignment
- Conclusion Generator
- Paraphrasing Tool
- Essay Maker
- Testimonials
- How It Works
- Engineering
Smart Watches
Smartwatches are critical for improving the efficiency of electricians at work. Productivity is enhanced by allowing individual employees to access all the knowledge they need while dealing with various issues. The knowledge-accessed ranges from procedural information, protection information, mission or job distribution to the technical information required to carry out various electrical and plumbing activities. Efficient efficiency is a hybrid between a healthy working atmosphere and good time control. The task of technology is slowly taking center stage in bridging the holes that remain in maximizing the feedback of employees. The gap that exists in most organizations is access to information and the advent of smart technology is perceived to be a perfect opportunity to make up for periodic trainings (Bertin, Emmanuel, Crespi, & Magedanz, p 175). By letting the employees to have the information by their wrist, is expected to transform the transform the workforce and improve their productivity. The Smart watches will have a picture application that will enable the employees to read-through the information that is captured in a picture format. In addition, the smart watches will have document reading application to support reading document files, and video support to facilitate demonstration via video graphics. The overall objective is therefore to save time and subsequently cost for the organization.
Task allocation: The smart watches are programmable and can be customized to allow supervisors to create teams and allocate them specific task. Supervisors will create accounts for teams and link up the same with the smart watches allowing the employees to access their work schedule ahead of time. The employees will then plan their time based on the task allocated and gather all the resources needed before commencing their work. Smart watches make communication between employees and their managers in relations to the resource allocation for completion of assigned tasks efficient. The information provided in the captured images will be shared among the target employees and that would make it easy to trace any breakdown in communication if the employees feel unsatisfied with the provided information. The smart watches will therefore enable the employee to know the tasks due for completion and plan their time adequately to allow them address all the work (Cho, Namjae, p3). The employees in a team will know their suitability to handle a task based on their skills combination as a team.
Information hub: The new gadgets are equipped with the latest information on plumbing and steam fitting and that means employees can learn the new techniques progressively and become acquainted. Employees handling a task as a team are able to consult with the available information and reduce the time it would take to have the interpretation from senior staff of supervisors on duty before handling a task.
The constant contact and repeated use of work related information is the magic bullet that helps the employees to improve their performance. Unlike periodic trainings on safety, having and using the information to complete task and minimize workplace accidents on a daily basis helps to improve employee consciousness to workplace procedures and safety content (Osman, Ibrahim, Abdel, Anouze, & Ali, p473). Unlike traditional methods of improving employee skills and performances, the smart watches enables the organization and staff to access latest updates relating to different approach to solving construction work challenges. Smart watch will act as an interface for the employees to access and use the latest information to increase efficiency and productivity.
The different codes used in construction and electrical work keep changing and that could mean addition and or removal of older codes. By accessing these information on codes, employees will become more efficient when interpreting diagrams, and or conducting troubleshooting in the course of their duty. Keeping the team updated makes it easy to communicate technically and through reports are addressed directly to the employees. In addition, learning new codes makes it easy for the employees to pick up and work on tasks in future dates when their services are needed (Provost, Fawcett, & Foster, p 317). This aspect of the smart watches has the effect of increasing employee productivity by ensuring that the workforce transform their knowledge and work with the latest and efficient work related content.
Employee autonomy: employees in a work place appreciate all the efforts that enable them to take charge of their tasks in terms of planning and finding solutions. If an employee wants to learn and apply new technology in handling a task, they will likely deliver once they have all the access to information, and tools. Employees who thrive working in a team will become efficient when they access information and freedom to execute a task on their own.
Most employees in the past learned from their supervisors and that explains why it takes longer for one to improve their performances. When a team consults among themselves while getting guidance from documented materials, they internalize the principles and operation procedures better compared to when they get instructions from a supervisor whose knowledge is limited. Pictures and short videos of complete tasks enable the employees to have a pictorial idea of the expected work before commencing their work (Mankins, Michael, & Eric, p67). This will enable them to assess the sufficiency of the tools and materials provided to plate the task. The smart watches in this case provide the employee with a sense of recognition and that is important as a social incentive to inspire positive attitude towards work.
Improved safety: the safety of the staff is important when handling any task that involves the use of heat, electricity, cutting tools among other equipments at the workplace. Every organization understand the importance of prioritizing the safety of the employee and the organization in the course of undertaking any operations by the employees. Smart watches provide a good platform for sensitizing the employees on the safety procedures when handling tasks using different equipments and applications. In this case, the smart watches will have all the safety aspects that are specific to different plumbing and steam heating situations at the work place (Miller, Michael, p248). Different safety gear and procedure of operations are the most important aspects of minimizing workplace accidents. Providing employees with safety information increase their adherence to safety requirements when handling different tasks and that will increase optimum use of labor. The video application in the smart watch will have access to exclusive video graphics that demonstrate the right approach to safe working against the wrong way (Arezes, Pedro, p391). This approach will reinforce the employee commitment to improve on their adherence to safety procedures.
Inspection procedures: The success of every task in the workplace is based on the way in which the operation manual and the inspection manual are drafted. A smart watch give the employees an opportunity to establish the closeness between the operation and the inspection manuals. This information gives the employee a head start in ensuring that they address all the steps in the operations manual satisfactorily (Duhigg, p 231). Giving the employees access to critical information on past inspection findings will ensure that each employee appreciates adherence to operations procedures, hence minimize time needed to conduct a rework. The documented procedure is important in the making of a discipline employee by enabling them to own the process and the results of their work. Improvement and overall productivity of the employee will be realized by making the smart watches employee’s closest and reliable reference material. The time it takes to consult experts is utilized otherwise in a productive way.
Works Cited
Arezes, Pedro M. Occupational Safety and Hygiene Iv: Selected, Extended and Revised Contributions from the International Symposium Occupational Safety and Hygiene, Guimarães, Portugal, 23-24 March 2016. , 2016. Internet resource.
Bertin, Emmanuel, Noel Crespi, and Thomas Magedanz. Evolution of Telecommunication Services [recurso Electrónico]: The Convergence of Telecom and Internet: Technologies and Ecosystems. , n.d. 2013. Internet resource.
Cho, Namjae. The Use of Smart Mobile Equipment for the Innovation in Organizational Coordination [recurso Electrónico]. , n.d. 2012. Internet resource.
Duhigg, Charles. Smarter Faster Better: The Transformative Power of Real Productivity. New York: Random House, 2017. Print.
Mankins, Michael C, and Eric Garton. Time, Talent, Energy: Overcome Organizational Drag and Unleash Your Team's Productive Power. 2017. Internet resource.
Miller, Michael. The Internet of Things: How Smart Tvs, Smart Cars, Smart Homes, and Smart Cities Are Changing the World. , 2015. Internet resource.
Osman, Ibrahim H, Abdel L. Anouze, and Ali Emrouznejad. Handbook of Research on Strategic Performance Management and Measurement Using Data Envelopment Analysis. , 2014. Internet resource.
Provost, Foster, and Tom Fawcett. Data Science for Business: What You Need to Know About Data Mining and Data-Analytic Thinking. Sebastopol, CA: O'Reilly Media, 2013. Internet resource.
- The founder of Kodak - George Eastman
- Investment Casting
- Information technology and architecture creativ...
- Effects of Technology in Our Lives
- War and Engineering
- Lean Manufacturing
Academic levels
Paper formats, urgency types, assignment types, prices that are easy on your wallet.
Our experts are ready to do an excellent job starting at $14.99 per page
We at GrabMyEssay.com
work according to the General Data Protection Regulation (GDPR), which means you have the control over your personal data. All payment transactions go through a secure online payment system, thus your Billing information is not stored, saved or available to the Company in any way. Additionally, we guarantee confidentiality and anonymity all throughout your cooperation with our Company.
Try our service with 15% Discount for your first order! Try our service with 15% Discount for your first order! Try our service with 15% Discount for your first order!
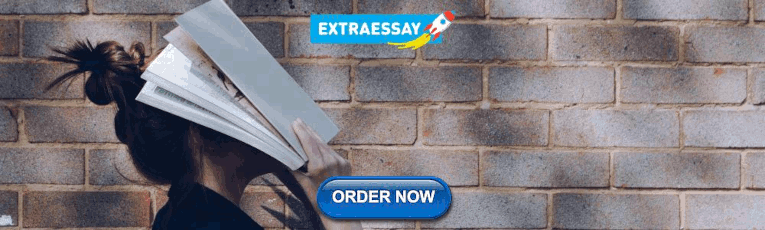
IMAGES
VIDEO
COMMENTS
Second, smart watch research should be reported according to standardized criteria such as the STARE-HI (Statement on reporting of evaluation studies in Health Informatics) guidelines [33] or mini STARE-HI for conference papers [34]. 5. Conclusion. Consumer-grade smart watches have penetrated the health research space rapidly since 2014.
Short Essay On Smart Watch. 887 Words4 Pages. High-tech Wrist Watches From ancient times there are different tools used to measure time. With the advancement of technology the watches just move towards more sophisticated and advanced style. Now watch not only measure time, but also perform multiple tasks. 21st century brings the uniqueness and ...
Frequently Asked Questions. A smartwatch is a portable device that's designed to be worn on a wrist. Like smartphones, they use touchscreens, offer apps, and often record your heart rate and other vital signs. The Apple Watch and Wear (formerly Android Wear) models prompted more consumers to appreciate the usefulness of wearing a mini computer ...
The company over-indexed on apps and communication, while its health features remained basic. The watch wasn't smart enough yet and didn't feel essential to a daily routine, Walt Mossberg said ...
There's no clear answer yet. One study found that people trying to lose weight who used wearable technology to help actually lost less weight than their watch-free counterparts. A review in the ...
Smartwatches are the most popular wearable device and increasingly subject to empirical research. In recent years, the focus has shifted from revealing determinants of smartwatch adoption to understanding factors that cause long-term usage. Despite their importance for personal fitness, health monitoring, and for achieving health and fitness goals, extant research on the continuous use ...
Abstract. Wearable computers are expected to become the next big thing but popular press is divided on whether they will be successful. In this paper we review the existing literature on one type ...
Introduction. In recent times, the niche of wearable devices has grown tremendously, and it will continue to grow until there is a demand for such technologies. One such wearable device is a smartwatch - a portable gadget intended to be worn on a wrist and aimed to record heart rate and other vital signs. The Pebble watch designers created ...
Abstract and Figures. This review paper focuses on analyzing research work related to the utilization of smart watches in health informatics. In recent years, we have seen an ascent in life ...
The main aim of building the smart watches is to improve communication and also make the gadgets more useful in the day to day lives. SPOT is a technology that is used to deliver the necessary information and domains that aid in improving the welfare of the technology used. SPOT uses the main mode of technology has the FM broadcasting does.
Essay On Smart Watch. 2347 Words10 Pages. What is a Smart watch? Although there is not a well-structured definition for a smart watch, but apart from the basic feature of showing time there must be many other features should be present that actually makes the watch smart.
Abstract. This study aims to explore usability issues of watch-type wearable devices and to suggest guidelines for improved operation of smartwatches. To do so, we conducted a series of surveys ...
We conducted a narrative review of the use of wearable devices in health care settings by searching papers in PubMed, EMBASE, Scopus, and the Cochrane Library published since October 2015. ... Some of these technologies are becoming part of people's daily life in the form of accessories such as smart watches, smart bracelets, armbands, ...
Apple Watch: phantom menace of smartwatches could make us even more self-obsessed. Andre Spicer, City, University of London. Smartwatches will bombard us with data about our internal lives. We ...
The search papers focused only on English-language studies. Considering that smartwatch medical applications have only been introduced to the market recently, the search period was restricted to the period following 2017. ... Studies evaluated the accuracy of HRV parameters extracted from wristbands and smart-watches including Apple Watch ...
An Apple Watch browsing French Wikipedia. A smartwatch is a portable and wearable computer device in a form of a watch; modern smartwatches provide a local touchscreen interface for daily use, while an associated smartphone app provides management and telemetry, such as long-term biomonitoring.While early models could perform basic tasks such as calculations, digital time telling, translations ...
Smart watches demonstrate high sensitivity but generally speaking, lower specificity, although with improving technology this is likely to also increase. Therefore, episodes of an otherwise benign dysrhythmia, may be misinterpreted as abnormal, resulting in patient stress, downward testing and even unnecessary anticoagulation treatment [ 6 ].
Heart & Health Monitoring. Heart Rate (constant monitoring by wrist) Daily Resting Heart Rate. Abnormal Heart Rate Detection Alerts. Respiration rate (Know your breathing rate 24×7) Blood Oxygen Saturation (Usually measured by a Pulse Oximeter) Stress Tracking. Relaxation reminders and breathing strategies.
1313 Words6 Pages. A Beginners Guide to Smart Watch Testing. As technology has evolved, people wish to live "smarter". This lead to the evolution of smart devices. We started to upgrade the traditional devices we use in our everyday life to smart devices. Wanting to carry computers with us started the evolution and led to the invention of ...
We followed the proposed framework using Apple Watch. We found that the watch stores a lot of personal information such as contacts details, text messages, calendar details, Emails, pictures, and wallet data including: stored payment cards, gate passes, and event tickets, if any. In addition, the logical acquisition of the backup files revealed ...
This watch has 240 x 240 resolution IPS screen, 2.5D Gorilla glass, to give you ultimate touch technology and viewing experience. This can be easily …show more content…. The smart watch has 1.5inch, touch enabled display set within a metal, silicon plastic shell that is completely waterproof. The display is designed to recognize your ...
Eric built the Smart watch in his dorm room; what he came up …show more content… To name a few we have Apple, Motorola motor, and Sony. A smart watch allows you to stay connected and informed without constantly interrupting your life while on the go. Now you can upgrade and customize your watch...
Now compare that to the Apple Watch, which boasts a meager 18 hours of battery life.Apple thinks that an "all-day battery" is a thing to be proud of. While I must commend their excellent quality and best health and fitness tracking, their watches are not for me.WearOS smartwatches aren't much better, as they are more resource-heavy than proprietary operating systems from brands like Garmin ...
Smart watches provide a good platform for sensitizing the employees on the safety procedures when handling tasks using different equipments and applications. In this case, the smart watches will have all the safety aspects that are specific to different plumbing and steam heating situations at the work place (Miller, Michael, p248).