- Search Menu
- Sign in through your institution
- Browse content in Arts and Humanities
- Browse content in Archaeology
- Anglo-Saxon and Medieval Archaeology
- Archaeological Methodology and Techniques
- Archaeology by Region
- Archaeology of Religion
- Archaeology of Trade and Exchange
- Biblical Archaeology
- Contemporary and Public Archaeology
- Environmental Archaeology
- Historical Archaeology
- History and Theory of Archaeology
- Industrial Archaeology
- Landscape Archaeology
- Mortuary Archaeology
- Prehistoric Archaeology
- Underwater Archaeology
- Urban Archaeology
- Zooarchaeology
- Browse content in Architecture
- Architectural Structure and Design
- History of Architecture
- Residential and Domestic Buildings
- Theory of Architecture
- Browse content in Art
- Art Subjects and Themes
- History of Art
- Industrial and Commercial Art
- Theory of Art
- Biographical Studies
- Byzantine Studies
- Browse content in Classical Studies
- Classical Literature
- Classical Reception
- Classical History
- Classical Philosophy
- Classical Mythology
- Classical Art and Architecture
- Classical Oratory and Rhetoric
- Greek and Roman Papyrology
- Greek and Roman Archaeology
- Greek and Roman Epigraphy
- Greek and Roman Law
- Late Antiquity
- Religion in the Ancient World
- Digital Humanities
- Browse content in History
- Colonialism and Imperialism
- Diplomatic History
- Environmental History
- Genealogy, Heraldry, Names, and Honours
- Genocide and Ethnic Cleansing
- Historical Geography
- History by Period
- History of Emotions
- History of Agriculture
- History of Education
- History of Gender and Sexuality
- Industrial History
- Intellectual History
- International History
- Labour History
- Legal and Constitutional History
- Local and Family History
- Maritime History
- Military History
- National Liberation and Post-Colonialism
- Oral History
- Political History
- Public History
- Regional and National History
- Revolutions and Rebellions
- Slavery and Abolition of Slavery
- Social and Cultural History
- Theory, Methods, and Historiography
- Urban History
- World History
- Browse content in Language Teaching and Learning
- Language Learning (Specific Skills)
- Language Teaching Theory and Methods
- Browse content in Linguistics
- Applied Linguistics
- Cognitive Linguistics
- Computational Linguistics
- Forensic Linguistics
- Grammar, Syntax and Morphology
- Historical and Diachronic Linguistics
- History of English
- Language Evolution
- Language Reference
- Language Variation
- Language Families
- Language Acquisition
- Lexicography
- Linguistic Anthropology
- Linguistic Theories
- Linguistic Typology
- Phonetics and Phonology
- Psycholinguistics
- Sociolinguistics
- Translation and Interpretation
- Writing Systems
- Browse content in Literature
- Bibliography
- Children's Literature Studies
- Literary Studies (Romanticism)
- Literary Studies (American)
- Literary Studies (Modernism)
- Literary Studies (Asian)
- Literary Studies (European)
- Literary Studies (Eco-criticism)
- Literary Studies - World
- Literary Studies (1500 to 1800)
- Literary Studies (19th Century)
- Literary Studies (20th Century onwards)
- Literary Studies (African American Literature)
- Literary Studies (British and Irish)
- Literary Studies (Early and Medieval)
- Literary Studies (Fiction, Novelists, and Prose Writers)
- Literary Studies (Gender Studies)
- Literary Studies (Graphic Novels)
- Literary Studies (History of the Book)
- Literary Studies (Plays and Playwrights)
- Literary Studies (Poetry and Poets)
- Literary Studies (Postcolonial Literature)
- Literary Studies (Queer Studies)
- Literary Studies (Science Fiction)
- Literary Studies (Travel Literature)
- Literary Studies (War Literature)
- Literary Studies (Women's Writing)
- Literary Theory and Cultural Studies
- Mythology and Folklore
- Shakespeare Studies and Criticism
- Browse content in Media Studies
- Browse content in Music
- Applied Music
- Dance and Music
- Ethics in Music
- Ethnomusicology
- Gender and Sexuality in Music
- Medicine and Music
- Music Cultures
- Music and Media
- Music and Culture
- Music and Religion
- Music Education and Pedagogy
- Music Theory and Analysis
- Musical Scores, Lyrics, and Libretti
- Musical Structures, Styles, and Techniques
- Musicology and Music History
- Performance Practice and Studies
- Race and Ethnicity in Music
- Sound Studies
- Browse content in Performing Arts
- Browse content in Philosophy
- Aesthetics and Philosophy of Art
- Epistemology
- Feminist Philosophy
- History of Western Philosophy
- Metaphysics
- Moral Philosophy
- Non-Western Philosophy
- Philosophy of Language
- Philosophy of Mind
- Philosophy of Perception
- Philosophy of Action
- Philosophy of Law
- Philosophy of Religion
- Philosophy of Science
- Philosophy of Mathematics and Logic
- Practical Ethics
- Social and Political Philosophy
- Browse content in Religion
- Biblical Studies
- Christianity
- East Asian Religions
- History of Religion
- Judaism and Jewish Studies
- Qumran Studies
- Religion and Education
- Religion and Health
- Religion and Politics
- Religion and Science
- Religion and Law
- Religion and Art, Literature, and Music
- Religious Studies
- Browse content in Society and Culture
- Cookery, Food, and Drink
- Cultural Studies
- Customs and Traditions
- Ethical Issues and Debates
- Hobbies, Games, Arts and Crafts
- Natural world, Country Life, and Pets
- Popular Beliefs and Controversial Knowledge
- Sports and Outdoor Recreation
- Technology and Society
- Travel and Holiday
- Visual Culture
- Browse content in Law
- Arbitration
- Browse content in Company and Commercial Law
- Commercial Law
- Company Law
- Browse content in Comparative Law
- Systems of Law
- Competition Law
- Browse content in Constitutional and Administrative Law
- Government Powers
- Judicial Review
- Local Government Law
- Military and Defence Law
- Parliamentary and Legislative Practice
- Construction Law
- Contract Law
- Browse content in Criminal Law
- Criminal Procedure
- Criminal Evidence Law
- Sentencing and Punishment
- Employment and Labour Law
- Environment and Energy Law
- Browse content in Financial Law
- Banking Law
- Insolvency Law
- History of Law
- Human Rights and Immigration
- Intellectual Property Law
- Browse content in International Law
- Private International Law and Conflict of Laws
- Public International Law
- IT and Communications Law
- Jurisprudence and Philosophy of Law
- Law and Society
- Law and Politics
- Browse content in Legal System and Practice
- Courts and Procedure
- Legal Skills and Practice
- Primary Sources of Law
- Regulation of Legal Profession
- Medical and Healthcare Law
- Browse content in Policing
- Criminal Investigation and Detection
- Police and Security Services
- Police Procedure and Law
- Police Regional Planning
- Browse content in Property Law
- Personal Property Law
- Study and Revision
- Terrorism and National Security Law
- Browse content in Trusts Law
- Wills and Probate or Succession
- Browse content in Medicine and Health
- Browse content in Allied Health Professions
- Arts Therapies
- Clinical Science
- Dietetics and Nutrition
- Occupational Therapy
- Operating Department Practice
- Physiotherapy
- Radiography
- Speech and Language Therapy
- Browse content in Anaesthetics
- General Anaesthesia
- Neuroanaesthesia
- Clinical Neuroscience
- Browse content in Clinical Medicine
- Acute Medicine
- Cardiovascular Medicine
- Clinical Genetics
- Clinical Pharmacology and Therapeutics
- Dermatology
- Endocrinology and Diabetes
- Gastroenterology
- Genito-urinary Medicine
- Geriatric Medicine
- Infectious Diseases
- Medical Toxicology
- Medical Oncology
- Pain Medicine
- Palliative Medicine
- Rehabilitation Medicine
- Respiratory Medicine and Pulmonology
- Rheumatology
- Sleep Medicine
- Sports and Exercise Medicine
- Community Medical Services
- Critical Care
- Emergency Medicine
- Forensic Medicine
- Haematology
- History of Medicine
- Browse content in Medical Skills
- Clinical Skills
- Communication Skills
- Nursing Skills
- Surgical Skills
- Medical Ethics
- Browse content in Medical Dentistry
- Oral and Maxillofacial Surgery
- Paediatric Dentistry
- Restorative Dentistry and Orthodontics
- Surgical Dentistry
- Medical Statistics and Methodology
- Browse content in Neurology
- Clinical Neurophysiology
- Neuropathology
- Nursing Studies
- Browse content in Obstetrics and Gynaecology
- Gynaecology
- Occupational Medicine
- Ophthalmology
- Otolaryngology (ENT)
- Browse content in Paediatrics
- Neonatology
- Browse content in Pathology
- Chemical Pathology
- Clinical Cytogenetics and Molecular Genetics
- Histopathology
- Medical Microbiology and Virology
- Patient Education and Information
- Browse content in Pharmacology
- Psychopharmacology
- Browse content in Popular Health
- Caring for Others
- Complementary and Alternative Medicine
- Self-help and Personal Development
- Browse content in Preclinical Medicine
- Cell Biology
- Molecular Biology and Genetics
- Reproduction, Growth and Development
- Primary Care
- Professional Development in Medicine
- Browse content in Psychiatry
- Addiction Medicine
- Child and Adolescent Psychiatry
- Forensic Psychiatry
- Learning Disabilities
- Old Age Psychiatry
- Psychotherapy
- Browse content in Public Health and Epidemiology
- Epidemiology
- Public Health
- Browse content in Radiology
- Clinical Radiology
- Interventional Radiology
- Nuclear Medicine
- Radiation Oncology
- Reproductive Medicine
- Browse content in Surgery
- Cardiothoracic Surgery
- Gastro-intestinal and Colorectal Surgery
- General Surgery
- Neurosurgery
- Paediatric Surgery
- Peri-operative Care
- Plastic and Reconstructive Surgery
- Surgical Oncology
- Transplant Surgery
- Trauma and Orthopaedic Surgery
- Vascular Surgery
- Browse content in Science and Mathematics
- Browse content in Biological Sciences
- Aquatic Biology
- Biochemistry
- Bioinformatics and Computational Biology
- Developmental Biology
- Ecology and Conservation
- Evolutionary Biology
- Genetics and Genomics
- Microbiology
- Molecular and Cell Biology
- Natural History
- Plant Sciences and Forestry
- Research Methods in Life Sciences
- Structural Biology
- Systems Biology
- Zoology and Animal Sciences
- Browse content in Chemistry
- Analytical Chemistry
- Computational Chemistry
- Crystallography
- Environmental Chemistry
- Industrial Chemistry
- Inorganic Chemistry
- Materials Chemistry
- Medicinal Chemistry
- Mineralogy and Gems
- Organic Chemistry
- Physical Chemistry
- Polymer Chemistry
- Study and Communication Skills in Chemistry
- Theoretical Chemistry
- Browse content in Computer Science
- Artificial Intelligence
- Computer Architecture and Logic Design
- Game Studies
- Human-Computer Interaction
- Mathematical Theory of Computation
- Programming Languages
- Software Engineering
- Systems Analysis and Design
- Virtual Reality
- Browse content in Computing
- Business Applications
- Computer Games
- Computer Security
- Computer Networking and Communications
- Digital Lifestyle
- Graphical and Digital Media Applications
- Operating Systems
- Browse content in Earth Sciences and Geography
- Atmospheric Sciences
- Environmental Geography
- Geology and the Lithosphere
- Maps and Map-making
- Meteorology and Climatology
- Oceanography and Hydrology
- Palaeontology
- Physical Geography and Topography
- Regional Geography
- Soil Science
- Urban Geography
- Browse content in Engineering and Technology
- Agriculture and Farming
- Biological Engineering
- Civil Engineering, Surveying, and Building
- Electronics and Communications Engineering
- Energy Technology
- Engineering (General)
- Environmental Science, Engineering, and Technology
- History of Engineering and Technology
- Mechanical Engineering and Materials
- Technology of Industrial Chemistry
- Transport Technology and Trades
- Browse content in Environmental Science
- Applied Ecology (Environmental Science)
- Conservation of the Environment (Environmental Science)
- Environmental Sustainability
- Environmentalist Thought and Ideology (Environmental Science)
- Management of Land and Natural Resources (Environmental Science)
- Natural Disasters (Environmental Science)
- Nuclear Issues (Environmental Science)
- Pollution and Threats to the Environment (Environmental Science)
- Social Impact of Environmental Issues (Environmental Science)
- History of Science and Technology
- Browse content in Materials Science
- Ceramics and Glasses
- Composite Materials
- Metals, Alloying, and Corrosion
- Nanotechnology
- Browse content in Mathematics
- Applied Mathematics
- Biomathematics and Statistics
- History of Mathematics
- Mathematical Education
- Mathematical Finance
- Mathematical Analysis
- Numerical and Computational Mathematics
- Probability and Statistics
- Pure Mathematics
- Browse content in Neuroscience
- Cognition and Behavioural Neuroscience
- Development of the Nervous System
- Disorders of the Nervous System
- History of Neuroscience
- Invertebrate Neurobiology
- Molecular and Cellular Systems
- Neuroendocrinology and Autonomic Nervous System
- Neuroscientific Techniques
- Sensory and Motor Systems
- Browse content in Physics
- Astronomy and Astrophysics
- Atomic, Molecular, and Optical Physics
- Biological and Medical Physics
- Classical Mechanics
- Computational Physics
- Condensed Matter Physics
- Electromagnetism, Optics, and Acoustics
- History of Physics
- Mathematical and Statistical Physics
- Measurement Science
- Nuclear Physics
- Particles and Fields
- Plasma Physics
- Quantum Physics
- Relativity and Gravitation
- Semiconductor and Mesoscopic Physics
- Browse content in Psychology
- Affective Sciences
- Clinical Psychology
- Cognitive Psychology
- Cognitive Neuroscience
- Criminal and Forensic Psychology
- Developmental Psychology
- Educational Psychology
- Evolutionary Psychology
- Health Psychology
- History and Systems in Psychology
- Music Psychology
- Neuropsychology
- Organizational Psychology
- Psychological Assessment and Testing
- Psychology of Human-Technology Interaction
- Psychology Professional Development and Training
- Research Methods in Psychology
- Social Psychology
- Browse content in Social Sciences
- Browse content in Anthropology
- Anthropology of Religion
- Human Evolution
- Medical Anthropology
- Physical Anthropology
- Regional Anthropology
- Social and Cultural Anthropology
- Theory and Practice of Anthropology
- Browse content in Business and Management
- Business Ethics
- Business History
- Business Strategy
- Business and Technology
- Business and Government
- Business and the Environment
- Comparative Management
- Corporate Governance
- Corporate Social Responsibility
- Entrepreneurship
- Health Management
- Human Resource Management
- Industrial and Employment Relations
- Industry Studies
- Information and Communication Technologies
- International Business
- Knowledge Management
- Management and Management Techniques
- Operations Management
- Organizational Theory and Behaviour
- Pensions and Pension Management
- Public and Nonprofit Management
- Strategic Management
- Supply Chain Management
- Browse content in Criminology and Criminal Justice
- Criminal Justice
- Criminology
- Forms of Crime
- International and Comparative Criminology
- Youth Violence and Juvenile Justice
- Development Studies
- Browse content in Economics
- Agricultural, Environmental, and Natural Resource Economics
- Asian Economics
- Behavioural Finance
- Behavioural Economics and Neuroeconomics
- Econometrics and Mathematical Economics
- Economic History
- Economic Methodology
- Economic Systems
- Economic Development and Growth
- Financial Markets
- Financial Institutions and Services
- General Economics and Teaching
- Health, Education, and Welfare
- History of Economic Thought
- International Economics
- Labour and Demographic Economics
- Law and Economics
- Macroeconomics and Monetary Economics
- Microeconomics
- Public Economics
- Urban, Rural, and Regional Economics
- Welfare Economics
- Browse content in Education
- Adult Education and Continuous Learning
- Care and Counselling of Students
- Early Childhood and Elementary Education
- Educational Equipment and Technology
- Educational Strategies and Policy
- Higher and Further Education
- Organization and Management of Education
- Philosophy and Theory of Education
- Schools Studies
- Secondary Education
- Teaching of a Specific Subject
- Teaching of Specific Groups and Special Educational Needs
- Teaching Skills and Techniques
- Browse content in Environment
- Applied Ecology (Social Science)
- Climate Change
- Conservation of the Environment (Social Science)
- Environmentalist Thought and Ideology (Social Science)
- Natural Disasters (Environment)
- Social Impact of Environmental Issues (Social Science)
- Browse content in Human Geography
- Cultural Geography
- Economic Geography
- Political Geography
- Browse content in Interdisciplinary Studies
- Communication Studies
- Museums, Libraries, and Information Sciences
- Browse content in Politics
- African Politics
- Asian Politics
- Chinese Politics
- Comparative Politics
- Conflict Politics
- Elections and Electoral Studies
- Environmental Politics
- European Union
- Foreign Policy
- Gender and Politics
- Human Rights and Politics
- Indian Politics
- International Relations
- International Organization (Politics)
- International Political Economy
- Irish Politics
- Latin American Politics
- Middle Eastern Politics
- Political Behaviour
- Political Economy
- Political Institutions
- Political Theory
- Political Methodology
- Political Communication
- Political Philosophy
- Political Sociology
- Politics and Law
- Politics of Development
- Public Policy
- Public Administration
- Quantitative Political Methodology
- Regional Political Studies
- Russian Politics
- Security Studies
- State and Local Government
- UK Politics
- US Politics
- Browse content in Regional and Area Studies
- African Studies
- Asian Studies
- East Asian Studies
- Japanese Studies
- Latin American Studies
- Middle Eastern Studies
- Native American Studies
- Scottish Studies
- Browse content in Research and Information
- Research Methods
- Browse content in Social Work
- Addictions and Substance Misuse
- Adoption and Fostering
- Care of the Elderly
- Child and Adolescent Social Work
- Couple and Family Social Work
- Direct Practice and Clinical Social Work
- Emergency Services
- Human Behaviour and the Social Environment
- International and Global Issues in Social Work
- Mental and Behavioural Health
- Social Justice and Human Rights
- Social Policy and Advocacy
- Social Work and Crime and Justice
- Social Work Macro Practice
- Social Work Practice Settings
- Social Work Research and Evidence-based Practice
- Welfare and Benefit Systems
- Browse content in Sociology
- Childhood Studies
- Community Development
- Comparative and Historical Sociology
- Economic Sociology
- Gender and Sexuality
- Gerontology and Ageing
- Health, Illness, and Medicine
- Marriage and the Family
- Migration Studies
- Occupations, Professions, and Work
- Organizations
- Population and Demography
- Race and Ethnicity
- Social Theory
- Social Movements and Social Change
- Social Research and Statistics
- Social Stratification, Inequality, and Mobility
- Sociology of Religion
- Sociology of Education
- Sport and Leisure
- Urban and Rural Studies
- Browse content in Warfare and Defence
- Defence Strategy, Planning, and Research
- Land Forces and Warfare
- Military Administration
- Military Life and Institutions
- Naval Forces and Warfare
- Other Warfare and Defence Issues
- Peace Studies and Conflict Resolution
- Weapons and Equipment

- < Previous chapter
- Next chapter >

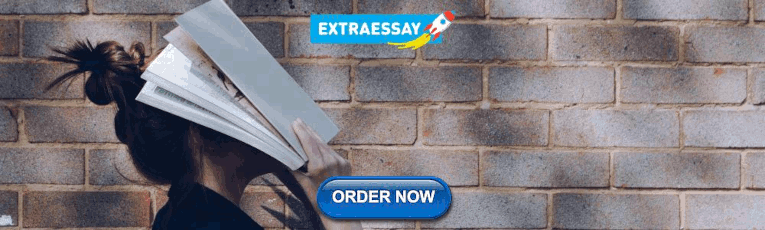
28 Secondary Data Analysis
Department of Psychology, Michigan State University
Richard E. Lucas, Department of Psychology, Michigan State University, East Lansing, MI
- Published: 01 October 2013
- Cite Icon Cite
- Permissions Icon Permissions
Secondary data analysis refers to the analysis of existing data collected by others. Secondary analysis affords researchers the opportunity to investigate research questions using large-scale data sets that are often inclusive of under-represented groups, while saving time and resources. Despite the immense potential for secondary analysis as a tool for researchers in the social sciences, it is not widely used by psychologists and is sometimes met with sharp criticism among those who favor primary research. The goal of this chapter is to summarize the promises and pitfalls associated with secondary data analysis and to highlight the importance of archival resources for advancing psychological science. In addition to describing areas of convergence and divergence between primary and secondary data analysis, we outline basic steps for getting started and finding data sets. We also provide general guidance on issues related to measurement, handling missing data, and the use of survey weights.
The goal of research in the social science is to gain a better understanding of the world and how well theoretical predictions match empirical realities. Secondary data analysis contributes to these objectives through the application of “creative analytical techniques to data that have been amassed by others” ( Kiecolt & Nathan, 1985 , p. 10). Primary researchers design new studies to answer research questions, whereas the secondary data analyst uses existing resources. There is a deliberate coupling of research design and data analysis in primary research; however, the secondary data analyst rarely has had input into the design of the original studies in terms of the sampling strategy and measures selected for the investigation. For better or worse, the secondary data analyst simply has access to the final products of the data collection process in the form of a codebook or set of codebooks and a cleaned data set.
The analysis of existing data sets is routine in disciplines such as economics, political science, and sociology, but it is less well established in psychology ( but see Brooks-Gunn & Chase-Lansdale, 1991 ; Brooks-Gunn, Berlin, Leventhal, & Fuligini, 2000 ). Moreover, biases against secondary data analysis in favor of primary research may be present in psychology ( see McCall & Appelbaum, 1991 ). One possible explanation for this bias is that psychology has a rich and vibrant experimental tradition, and the training of many psychologists has likely emphasized this approach as the “gold standard” for addressing research questions and establishing causality ( see , e.g., Cronbach, 1957 ). As a result, the nonexperimental methods that are typically used in secondary analyses may be viewed by some as inferior. Psychological scientists trained in the experimental tradition may not fully appreciate the unique strengths that nonexperimental techniques have to offer and may underestimate the time, effort, and skills required for conducting secondary data analyses in a competent and professional manner. Finally, biases against secondary data analysis might stem from lingering concerns over the validity of the self-report methods that are typically used in secondary data analysis. These can include concerns about the possibility that placement of items in a survey can influence responses (e.g., differences in the average levels of reported marital and life satisfaction when questions occur back to back as opposed to having the questions separated in the survey; see Schwarz, 1999 ; Schwarz & Strack, 1999 ) and concerns with biased reporting of sensitive behaviors ( but see Akers, Massey, & Clarke, 1983 ).
Despite the initial reluctance to widely embrace secondary data analysis as a tool for psychological research, there are promising signs that the skepticism toward secondary analyses will diminish as psychology seeks to position itself as a hub science that plays a key role in interdisciplinary inquiry ( see Mroczek, Pitzer, Miller, Turiano, & Fingerman, 2011 ). Accordingly, there is a compelling argument for including secondary data analysis into the suite of methodological approaches used by psychologists ( see Trzesniewski, Donnellan, & Lucas, 2011 ).
The goal of this chapter is to summarize the promises and pitfalls associated with secondary data analysis and to highlight the importance of archival resources for advancing psychological science. We limit our discussion to analyses based on large-scale and often longitudinal national data sets such as the National Longitudinal Study of Adolescent Health (Add Health), the British Household Panel Study (BHPS), the German Socioeconomic Panel Study (GSOEP), and the National Institute of Child Health and Human Development (NICHD) Study of Early Child Care and Youth Development (SEC-CYD). However, much of our discussion applies to all secondary analyses. The perspective and specific recommendations found in this chapter draw on the edited volume by Trzesniewski et al. (2011 ). Following a general introduction to secondary data analysis, we will outline the necessary steps for getting started and finding data sets. Finally, we provide some general guidance on issues related to measurement, approaches to handling missing data, and survey weighting. Our treatment of these important topics is intended to draw attention to the relevant issues rather than to provide extensive coverage. Throughout, we take a practical approach to the issues and offer tips and guidance rooted in our experiences as data analysts and researchers with substantive interests in personality and life span developmental psychology.
Comparing Primary Research and Secondary Research
As noted in the opening section, it is possible that biases against secondary data analysis exist in the minds of some psychological scientists. To address these concerns, we have found it can be helpful to explicitly compare the processes of secondary analyses with primary research ( see also McCall & Appelbaum, 1991 ). An idealized and simplified list of steps is provided in Table 28.1 . As is evident from this table, both techniques start with a research question that is ideally rooted in existing theory and previous empirical results. The areas of biggest divergence between primary and secondary approaches occur after researchers have identified their questions (i.e., Steps 2 through 5 in Table 28.1 ). At this point, the primary researcher develops a set of procedures and then engages in pilot testing to refine procedures and methods, whereas the secondary analyst searches for data sets and evaluates codebooks. The primary researcher attempts to refine her or his procedures, whereas the secondary analyst determines whether a particular resource is appropriate for addressing the question at hand. In the next stages, the primary researcher collects new data, whereas the secondary data analyst constructs a working data set from a much larger data archive. At these stages, both types of researchers must grapple with the practical considerations imposed by real world constraints. There is no such thing as a perfect single study ( see Hunter & Schmidt, 2004 ), as all data sets are subject to limitations stemming from design and implementation. For example, the primary researcher may not have enough subjects to generate adequate levels of statistical power (because of a failure to take power calculations into account during the design phase, time or other resource constraints during the data collection phase, or because of problems with sample retention), whereas the secondary data analyst may have to cope with impoverished measurement of core constructs. Both sets of considerations will affect the ability of a given study to detect effects and provide unbiased estimates of effect sizes.
Table 28.1 also illustrates the fact that there are considerable areas of overlap between the two techniques. Researchers stemming from both traditions analyze data, interpret results, and write reports for dissemination to the wider scientific community. Both kinds of research require a significant investment of time and intellectual resources. Many skills required in conducting high-quality primary research are also required in conducting high-quality secondary data analysis including sound scientific judgment, attention to detail, and a firm grasp of statistical methodology.
Note: Steps modified and expanded from McCall and Appelbaum (1991 ).
We argue that both primary research and secondary data analysis have the potential to provide meaningful and scientifically valid research findings for psychology. Both approaches can generate new knowledge and are therefore reasonable ways of evaluating research questions. Blanket pronouncements that one approach is inherently superior to the other are usually difficult to justify. Many of the concerns about secondary data analysis are raised in the context of an unfair comparison—a contrast between the idealized conceptualization of primary research with the actual process of a secondary data analysis. Our point is that both approaches can be conducted in a thoughtful and rigorous manner, yet both approaches involve concessions to real-world constraints. Accordingly, we encourage all researchers and reviewers of papers to keep an open mind about the importance of both types of research.
Advantages and Disadvantages of Secondary Data Analysis
The foremost reason why psychologists should learn about secondary data analysis is that there are many existing data sets that can be used to answer interesting and important questions. Individuals who are unaware of these resources are likely to miss crucial opportunities to contribute new knowledge to the discipline and even risk reinventing the proverbial wheel by collecting new data. Regrettably, new data collection efforts may occur on a smaller scale than what is available in large national datasets. Researchers who are unaware of the potential treasure trove of variables in existing data sets risk unnecessarily duplicating considerable amounts of time and effort. At the very least, researchers may wish to familiarize themselves with publicly available data to truly address gaps in the literature when they undertake projects that involve new data collection.
The biggest advantage of secondary analyses is that the data have already been collected and are ready to be analyzed ( see Hofferth, 2005 ), thus conserving time and resources. Existing data sources are often of much larger and higher quality than could be feasibly collected by a single investigator. This advantage is especially pronounced when considering the investments of time and money necessary to collect longitudinal data. Some data sets were collected with scientific sampling plans (such as the GSOEP), which make it possible to generalize the findings to a specific population. Further, many publicly available data sets are quite large, and therefore provide adequate statistical power for conducting many analyses, including hypotheses about statistical interactions. Investigations of interactions often require a surprisingly high number of participants to achieve respectable levels of statistical power in the face of measurement error ( see Aiken & West, 1991 ). 1 Large-scale data sets are also well suited for subgroup analyses of populations that are often under-represented in smaller research studies.
Another advantage of secondary data analysis is that it forces researchers to adopt an open and transparent approach to their craft. Because data are publicly available, other investigators may attempt to replicate findings and specify alternative models for a given research question. This reality encourages transparency and detailed record keeping on the part of the researcher, including careful reporting of analysis and a reasoned justification for all analytic decisions. Freese (2007 ) has provided a useful discussion about policies for archiving material necessary for replicating results, and his treatment of the issues provides guidance to researchers interested in maintaining good records.
Despite the many advantages of secondary data analysis, it is not without its disadvantages. The most significant challenge is simply the flipside of the primary advantage—the data have already been collected by somebody else! Analysts must take advantage of what has been collected without input into design and measurement issues. In some cases, an existing data set may not be available to address the particular research questions of a given investigator without some limitations in terms of sampling, measurement, or other design feature. For example, data sets commonly used for secondary analysis often have a great deal of breadth in terms of the range of constructs assessed (e.g., finances, attitudes, personality, life satisfaction, physical health), but these constructs are often measured with a limited number of survey items. Issues of measurement reliability and validity are usually a major concern. Therefore, a strong grounding in basic and advanced psychometrics is extremely helpful for responding to criticisms and concerns about measurement issues that arise during the peer-review process.
A second consequence of the fact that the data have been collected by somebody else is that analysts may not have access to all of the information about data collection procedures and issues. The analyst simply receives a cleaned data set to use for subsequent analyses. Perhaps not obvious to the user is the amount of actual cleaning that occurred behind the scenes. Similarly, the complicated sampling procedures used in a given study may not be readily apparent to users, and this issue can prevent the appropriate use of survey weights ( Shrout & Napier, 2011 ).
Another significant disadvantage for secondary data analysis is the large amount of time and energy initially required to review data documentation. It can take hours and even weeks to become familiar with the codebooks and to discover which research questions have already been addressed by investigators using the existing data sets. It is very easy to underestimate how long it will take to move from an initial research idea to a competent final analysis. There is a risk that, unbeknownst to one another, researchers in different locations will pursue answers to the same research questions. On the other hand, once a researcher has become familiar with a data set and developed skills to work with the resource, they are able to pursue additional research questions resulting in multiple publications from the same data set. It is our experience that the process of learning about a data set can help generate new research ideas as it becomes clearer how the resource can be used to contribute to psychological science. Thus, the initial time and energy expended to learn about a resource can be viewed as initial investment that holds the potential to pay larger dividends over time.
Finally, a possible disadvantage concerns how secondary data analyses are viewed within particular subdisciplines of psychology and by referees during the peer-review process. Some journals and some academic departments may not value secondary data analyses as highly as primary research. Such preferences might break along Cronbach’s two disciplines or two streams of psychology—correlational versus experimental ( Cronbach, 1957 ; Tracy, Robins, & Sherman, 2009 ). The reality is that if original data collection is more highly valued in a given setting, then new investigators looking to build a strong case for getting hired or getting promoted might face obstacles if they base a career exclusively on secondary data analysis. Similarly, if experimental methods are highly valued and correlational methods are denigrated in a particular subfield, then results of secondary data analyses will face difficulties getting attention (and even getting published). The best advice is to be aware of local norms and to act accordingly.
Steps for Beginning a Secondary Data Analysis
Step 1: Find Existing Data Sets . After generating a substantive question, the first task is to find relevant data sets ( see Pienta, O’Rouke, & Franks, 2011 ). In some cases researchers will be aware of existing data sets through familiarity with the literature given that many well-cited papers have used such resources. For example, the GSOEP has now been widely used to address questions about correlates and developmental course of subjective well-being (e.g., Baird, Lucas, & Donnellan, 2010 ; Gerstorf, Ram, Estabrook, Schupp, Wagner, & Lindenberger, 2008 ; Gerstorf, Ram, Goebel, Schupp, Lindenberger, & Wagner, 2010 ; Lucas, 2005 ; 2007 ), and thus, researchers in this area know to turn to this resource if a new question arises. In other cases, however, researchers will attempt to find data sets using established archives such as the University of Michigan’s Interuniversity Consortium for Political and Social Research (ICPSR; http://www.icpsr.umich.edu/icpsrweb/ICPSR/ ). In addition to ICPSR, there are a number of other major archives ( see Pienta et al., 2011 ) that house potentially relevant data sets. Here are just a few starting points:
The Henry A. Murray Research Archive ( http://www.murray.harvard.edu/ )
The Howard W Odum Institute for Research in Social Science ( http://www.irss.unc.edu/odum/jsp/home2.jsp )
The National Opinion Research Center ( http://norc.org/homepage.htm )
The Roper Center of Public Opinion Research ( http://ropercenter.uconn.edu/ )
The United Kingdom Data Archive ( http://www.data-archive.ac.uk/ )
Individuals in charge of these archives and data depositories often catalog metadata, which is the technical term for information about the constituent data sets. Typical kinds of metadata include information about the original investigators, a description of the design and process of data collection, a list of the variables assessed, and notes about sampling weights and missing data. Searching through this information is an efficient way of gaining familiarity with data sets. In particular, the ICPSR has an impressive infrastructure for allowing researchers to search for data sets through a cataloguing of study metadata. The ICPSR is thus a useful starting point for finding the raw material for a secondary data analysis. The ICPSR also provides a new user tutorial for searching their holdings ( http://www.icpsr.umich.edu/icpsrweb/ICPSR/help/newuser.jsp ). We recommend that researchers search through their holdings to make a list of potential data sets. At that point, the next task is to obtain relevant codebooks to learn more about each resource.
Step 2: Read Codebooks . Researchers interesting in using an existing data set are strongly advised to thoroughly read the accompanying codebook ( Pienta et al., 2011 ). There are several reasons why a comprehensive understanding of the codebook is a critical first step when conducting a secondary data analysis. First, the codebook will detail the procedures and methods used to acquire the data and provide a list of all of the questions and assessments collected. A thorough reading of the codebook can provide insights into important covariates that can be included in subsequent models, and a careful reading will draw the analyst’s attention to key variables that will be missing because no such information was collected. Reading through a codebook can also help to generate new research questions.
Second, high-quality codebooks often report basic descriptive information for each variable such as raw frequency distributions and information about the extent of missing values. The descriptive information in the codebook can give investigators a baseline expectation for variables under consideration, including the expected distributions of the variables and the frequencies of under-represented groups (such as ethnic minority participants). Because it is important to verify that the descriptive statistics in the published codebook match those in the file analyzed by the secondary analyst, a familiarity with the codebook is essential. In addition to codebooks, many existing resources provide copies of the actual surveys completed by participants ( Pienta et al., 2011 ). However, the use of actual pencil-and-paper surveys is becoming less common with the advent of computer assisted interview techniques and Internet surveys. It is often the case that survey methods involve skip patterns (e.g., a participant is not asked about the consequences of her drinking if she responds that she doesn’t drink alcohol) that make it more difficult to assume the perspective of the “typical” respondent in a given study ( Pienta et al., 2011 ). Nonetheless, we recommend that analysts try to develop an understanding for the experiences of the participant in a given study. This perspective can help secondary analysts develop an intuitive understanding of certain patterns of missing data and anticipate concerns about question ordering effects ( see , e.g., Schwarz, 1999 ).
Step 3: Acquire Datasets and Construct a Working Datafile . Although there is a growing availability of Web-based resources for conducting basic analyses using selected data sets (e.g., the Survey Documentation Analysis software used by ICPSR), we are convinced that there is no substitute for the analysis of the raw data using the software packages of preference for a given investigator. This means that the analysts will need to acquire the data sets that they consider most relevant. This is typically a very straightforward process that involves acknowledging researcher responsibilities before downloading the entire data set from a website. In some cases, data are classified as restricted-use, and there are more extensive procedures for obtaining access that may involve submitting a detailed security plan and accompanying legal paperwork before becoming an authorized data user. When data involve children and other sensitive groups, Institutional Review Board approval is often required.
Each data set has different usage requirements, so it is difficult to provide blanket guidance. Researchers should be aware of the policies for using each data set and recognize their ethical responsibility for adhering to those regulations. A central issue is that the researcher must avoid deductive disclosure whereby otherwise anonymous participants are identified because of prior knowledge in conjunction with the personal characteristics coded in the dataset (e.g., gender, racial/ethnic group, geographic location, birth date). Such a practice violates the major ethical principles followed by responsible social scientists and has the potential to harm research participants.
Once the entire set of raw data is acquired, it is usually straightforward to import the files into the kinds of statistical packages used by researchers (e.g., R, SAS, SPSS, and STATA). At this point, it is likely that researchers will want to create smaller “working” file by pulling only relevant variables from the larger master files. It is often too cumbersome to work with a computer file that may have more than a thousand columns of information. The solution is to construct a working data file that has all of the needed variables tied to a particular research project. Researchers may also need to link multiple files by matching longitudinal data sets and linking to contextual variables such as information about schools or neighborhoods for data sets with a multilevel structure (e.g., individuals nested in schools or neighborhoods).
Explicit guidance about managing a working data file can be found in Willms (2011 ). Here, we simply highlight some particularly useful advice: (1) keep exquisite notes about what variables were selected and why; (2) keep detailed notes regarding changes to each variable and reasons why; and (3) keep track of sample sizes throughout this entire process. The guiding philosophy is to create documentation that is clear enough for an outside user to follow the logic and procedures used by the researcher. It is far too easy to overestimate the power of memory only to be disappointed when it comes time to revisit a particular analysis. Careful documentation can save time and prevent frustration. Willms (2011 ) noted that “keeping good notes is the sine qua non of the trade” (p. 33).
Step 4: Conduct Analyses . After assembling the working data file, the researcher will likely construct major study variables by creating scale composites (e.g., the mean of the responses to the items assessing the same construct) and conduct initial analyses. As previously noted, a comparison of the distributions and sample sizes with those in the study codebook is essential at this stage. Any deviations for the variables in the working data file and the codebook should be understood and documented. It is particularly useful to keep track of missing values to make sure that they have been properly coded. It should go without saying that an observed value of-9999 will typically require recoding to a missing value in the working file. Similarly, errors in reverse scoring items can be particularly common (and troubling) so researchers are well advised to conduct through item-level and scale analyses and check to make sure that reverse scoring was done correctly (e.g., examine the inter-item correlation matrix when calculating internal consistency estimates to screen for negative correlations). Willms (2011 ) provides some very savvy advice for the initial stages of actual data analysis: “Be wary of surprise findings” (p. 35). He noted that “too many times I have been excited by results only to find that I have made some mistake” (p. 35). Caution, skepticism, and a good sense of the underlying data set are essential for detecting mistakes.
An important comment about the nature of secondary data analysis is again worth emphasizing: These data sets are available to others in the scholarly community. This means that others should be able to replicate your results! It is also very useful to adopt a self-critical perspective because others will be able to subject findings to their own empirical scrutiny. Contemplate alternative explanations and attempt to conduct analyses to evaluate the plausibility of these explanations. Accordingly, we recommend that researchers strive to think of theoretically relevant control variables and include them in the analytic models when appropriate. Such an approach is useful both from the perspective of scientific progress (i.e., attempting to curb confirmation biases) and in terms of surviving the peer-review process.
Special Issue: Measurement Concerns in Existing Datasets
One issue with secondary data analyses that is likely to perplex psychologists are concerns regarding the measurement of core constructs. The reality is that many of the measures available in large-scale data sets consist of a subset of items derived from instruments commonly used by psychologists ( see Russell & Matthews, 2011 ). For example, the 10-item Rosenberg Self-Esteem scale ( Rosenberg, 1965 ) is the most commonly used measure of global self-esteem in the literature ( Donnellan, Trzesniewski, & Robins, 2011 ). Measures of self-esteem are available in many data sets like Monitoring the Future ( see Trzesniewski & Donnellan, 2010 ) but these measures are typically shorter than the original Rosenberg scale. Similarly, the GSOEP has a single-item rating of subjective well-being in the form of happiness, whereas psychologists might be more accustomed to measuring this construct with at least five items (e.g., Diener, Emmons, Larsen, & Griffin, 1985 ). Researchers using existing data sets will have to grapple with the consequences of having relatively short assessments in terms of the impact on reliability and validity.
For purposes of this chapter, we will make use of a conventional distinction between reliability and validity. Reliability will refer to the degree of measurement error present in a given set of scores (or alternatively the degree of consistency or precision in scores), whereas validity will refer to the degree to which measures capture the construct of interest and predict other variables in ways that are consistent with theory. More detailed but accessible discussions of reliability and validity can be found in Briggs and Cheek (1986 ), Clark and Watson (1995 ), John and Soto (2007 ), Messick (1995 ), Simms (2008 ), and Simms and Watson (2007 ). Widaman, Little, Preacher, and Sawalani (2011 ) have provided a discussion of these issues in the context of the shortened assessments available in existing data sets.
Short Measures and Reliability . Classical Test Theory (e.g., Lord & Novick, 1968 ) is the measurement perspective most commonly used among psychologists. According to this measurement philosophy, any observed score is a function of the underlying attribute (the so-called “true score”) and measurement error. Reliability is conceptualized as any deviation or inconsistency in observed scores for the same attribute across multiple assessments of that attribute. A thought experiment may help crystallize insights about reliability (e.g., Lord & Novick, 1968 ): Imagine a thousand identical clones each completing the same self-esteem instrument simultaneously. The underlying self-esteem attribute (i.e., the true scores) should be the same for each clone (by definition), whereas the observed scores may fluctuate across clones because of random measurement errors (e.g., a single clone misreading an item vs. another clone being frustrated by an extremely hot testing room). The extent of the observed fluctuations in reported scores across clones offers insight into how much measurement error is present in this instrument. If scores are tightly clustered around a single value, then measurement error is minimal; however, if scores are dramatically different across clones, then there is a clear indication of problems with reliability. The measure is imprecise because it yields inconsistent values across the same true scores.
These ideas about reliability can be applied to observed samples of scores such that the total observed variance is attributable to true score variance (i.e., true individual differences in underlying attributes) and variance stemming from random measurement errors. The assumption that measurement error is random means that it has an expected value of zero across observations. Using this framework, reliability can then be defined as the ratio of true score variance to the total observed variance. An assessment that is perfectly reliable (i.e., has no measurement error) will have a ratio of 1.0, whereas an assessment that is completely unreliable will yield a ratio of 0.0 ( see John & Soto, 2007 , for an expanded discussion). This perspective provides a formal definition of a reliability coefficient.
Psychologists have developed several tools to estimate the reliability of their measures, but the approach that is most commonly used is coefficient a ( Cronbach, 1951 ; see Schmitt, 1996 , for an accessible review). This approach considers reliability from the perspective of internal consistency. The basic idea is that fluctuations across items assessing the same construct reflect the presence of measurement error. The formula for the standardized α is a fairly simple function of the average inter-item correlation (a measure of inter-item homogeneity) and the total number of items in a scale. The α coefficient is typically judged acceptable if it is above 0.70, but the justification for this particular cutoff is somewhat arbitrary ( see Lance, Butts, & Michels, 2006 ). Researchers are therefore advised to take a more critical perspective on this statistic. A relevant concern is that α is negatively impacted when the measure is short.
Given concerns with scale length and α, many methodologically oriented researchers recommend evaluating and reporting the average inter-item correlation because it can be interpreted independently of length and thus represents a “more straightforward indicator of internal consistency” ( Clark & Watson, 1995 , p. 316). Consider that it is common to observe an average inter-item correlation for the 10-item Rosenberg Self-Esteem ( Rosenberg, 1965 ) scale around 0.40 (this is based on typically reported a coefficients; see Donnellan et al., 2011 ). This same level of internal homogeneity (i.e., an inter-item correlation of 0.40) yields an α of around 0.67 with a 3-item scale but an α of around 0.87 with 10 items. A measure of a broader construct like Extraversion may generate an average inter-item correlation of 0.20 ( Clark & Watson, 1995 , p. 316), which would translate to an α of 0.43 for a 3-item scale and 0.71 for a 10-item scale. The point is that α coefficients will fluctuate with scale length and the breadth of the construct. Because most scales in existing resources are short, the α coefficients might fall below the 0.70 convention despite having a respectable level of inter-item correlation.
Given these considerations, we recommend that researchers consider the average inter-item correlation more explicitly when working with secondary data sets. It is also important to consider the breadth of the underlying construct to generate expectations for reasonable levels of item homogeneity as indexed by the average inter-item correlation. Clark and Watson (1995 ; see also Briggs & Cheek, 1986 ) recommend values of around 0.40 to 0.50 for measures of fairly narrow constructs (e.g., self-esteem) and values of around 0.15 to 0.20 for measures of broader constructs (e.g., neuroticism). It is our experience that considerations about internal consistency often need to be made explicit in manuscripts so that reviewers will not take an unnecessarily harsh perspective on α’s that fall below their expectations. Finally, we want to emphasize that internal consistency is but one kind of reliability. In some cases, it might be that test—retest reliability is more informative and diagnostic of the quality of a measure ( McCrae, Kurtz, Yamagata, & Terracciano, 2011 ). Fortunately, many secondary data sets are longitudinal so it possible to get an estimate of longer term test-retest reliability from the existing data.
Beyond simply reporting estimates of reliability, it is worth considering why measurement reliability is such an important issue in the first place. One consequence of reliability for substantive research is that measurement imprecision tends to depress observed correlations with other variables. This notion of attenuation resulting from measurement error and a solution were discussed by Spearman as far back as 1904 ( see , e.g., pp. 88–94). Unreliable measures can affect the conclusions drawn from substantive research by imposing a downward bias on effect size estimation. This is perhaps why Widaman et al. (2011 ) advocate using latent variable structural modeling methods to combat this important consequence of measurement error. Their recommendation is well worth considering for those with experience with this technique ( see Kline, 2011 , for an introduction). Regardless of whether researchers use observed variables or latent variables for their analyses, it is important to recognize and appreciate the consequences of reliability.
Short Measures and Validity . Validity, for our purposes, reflects how well a measure captures the underlying conceptual attribute of interest. All discussions of validity are based, in part, on agreement in a field as to how to understand the construct in question. Validity, like reliability, is assessed as a matter of degree rather than a categorical distinction between valid or invalid measures. Cronbach and Meehl (1955 ) have provided a classic discussion of construct validity, perhaps the most overarching and fundamental form of validity considered in psychological research ( see also Smith, 2005 ). However, we restrict our discussion to content validity and criterion-related validity because these two types of validity are particularly relevant for secondary data analysis and they are more immediately addressable.
Content validity describes how well a measure captures the entire domain of the construct in question. Judgments regarding content validity are ideally made by panels of experts familiar with the focal construct. A measure is considered construct deficient if it fails to assess important elements of the construct. For example, if thoughts of suicide are an integral aspect of the concept depression and a given self-report measure is missing items that tap this content, then the measure would be deemed construct-deficient. A measure can also suffer from construct contamination if it includes extraneous items that are irrelevant to the focal construct. For example, if somatic symptoms like a rapid heartbeat are considered to reflect the construct of anxiety and not part of depression, then a depression inventory that has such an item would suffer from construct contamination. Given the reduced length of many assessments, concerns over construct deficiency are likely to be especially pressing. A short assessment may not include enough items to capture the full breadth of a broad construct. This limitation is not readily addressed and should be acknowledged ( see Widaman et al., 2011 ). In particular, researchers may need to clearly specify that their findings are based on a narrower content domain than is normally associated with the focal construct of interest.
A subtle but important point can arise when considering the content of measures with particularly narrow content. Internal consistency will increase when there is redundancy among items in the scale; however, the presence of similar items may decrease predictive power. This is known as the attenuation paradox in psycho metrics ( see Clark & Watson, 1995 ). When items are nearly identical, they contribute redundant information about a very specific aspect of the construct. However, the very specific attribute may not have predictive power. In essence, reliability can be maximized at the expense of creating a measure that is not very useful from the point of view of prediction (and likely explanation). Indeed, Clark and Watson (1995 ) have argued that the “goal of scale construction is to maximize validity rather than reliability” (p. 316). In short, an evaluation of content validity is also important when considering the predictive power of a given measure.
Whereas content validity is focused on the internal attributes of a measure, criterion-related validity is based on the empirical relations between measures and other variables. Using previous research and theory surrounding the focal construct, the researcher should develop an expectation regarding the magnitude and direction of observed associations (i.e., correlations) with other variables. A good supporting theory of a construct should stipulate a pattern of association, or nomological network, concerning those other variables that should be related and unrelated to the focal construct. This latter requirement is often more difficult to specify from existing theories, which tend to provide a more elaborate discussion of convergent associations rather than discriminant validity ( Widaman et al., 2011 ). For example, consider a very truncated nomological network for Agreeableness (dispositional kindness and empathy). Measures of this construct should be positively associated with romantic relationship quality, negatively related to crime (especially violent crime), and distinct from measures of cognitive ability such as tests of general intelligence.
Evaluations of criterion-related validity can be conducted within a data set as researchers document that a measure has an expected pattern of associations with existing criterion-related variables. Investigators using secondary data sets may want to conduct additional research to document the criterion-related validity of short measures with additional convenience samples (e.g., the ubiquitous college student samples used by many psychologists; Sears, 1986 ). For example, there are six items in the Add Health data set that appear to measure self-esteem (e.g., “I have a lot of good qualities” and “I like myself just the way I am”) ( see Russell, Crockett, Shen, &Lee, 2008 ). Although many of the items bear a strong resemblance to the items on the Rosenberg Self-Esteem scale ( Rosenberg, 1965 ), they are not exactly the same items. To obtain some additional data on the usefulness of this measure, we administered the Add Health items to a sample of 387 college students at our university along with the Rosenberg Self-Esteem scale and an omnibus measure of personality based on the Five-Factor model ( Goldberg, 1999 ). The six Add Health items were strongly correlated with the Rosenberg ( r = 0.79), and both self-esteem measures had a similar pattern of convergent and divergent associations with the facets of the Five-Factor model (the two profiles were very strongly associated: r > 0.95). This additional information can help bolster the case for the validity of the short Add Health self-esteem measure.
Special Issue: Missing Data in Existing Data Sets
Missing data is a fact of life in research— individuals may drop out of longitudinal studies or refuse to answer particular questions. These behaviors can affect the generalizability of findings because results may only apply to those individuals who choose to complete a study or a measure. Missing data can also diminish statistical power when common techniques like listwise deletion are used (e.g., only using cases with complete information, thereby reducing the sample size) and even lead to biased effect size estimates (e.g., McKnight & McKnight, 2011 ; McKnight, McKnight, Sidani, & Figuredo, 2007 ; Widaman, 2006 ). Thus, concerns about missing data are important for all aspects of research, including secondary data analysis. The development of specific techniques for appropriately handling missing data is an active area of research in quantitative methods ( Schafer & Graham, 2002 ).
Unfortunately, the literature surrounding missing data techniques is often technical and steeped in jargon, as noted by McKnight et al. (2007 ). The reality is that researchers attempting to understand issues of missing data need to pay careful attention to terminology. For example, a novice researcher may not immediately grasp the classification of missing data used in the literature ( see Schafer & Graham, 2002 , for a clear description). Consider the confusion that may stem from learning that data are missing at random (MAR) versus data are missing completely at random (MCAR). The term MAR does not mean that missing values only occurred because of chance factors. This is the case when data are missing completely at random (MCAR). Data that are MCAR are absent because of truly random factors. Data that are MAR refers to the situation in which the probability that the observations are missing depends only on other available information in the data set. Data that are MAR can be essentially “ignored” when the other factors are included in a statistical model. The last type of missing data, data missing not at random (MNAR), is likely to characterize the variables in many real-life data sets. As it stands, methods for handing data that are MAR and MCAR are better developed and more easily implemented than methods for handling data MNAR. Thus, many applied researchers will assume data are MAR for purposes of statistical modeling (and the ability to sleep comfortably at night). Fortunately, such an assumption might not create major problems for many analyses and may in fact represent the “practical state of the art” ( Schafer & Graham, 2002 , p. 173).
The literature on missing data techniques is growing, so we simply recommend that researchers keep current on developments in this area. McKnight et al. (2007 ) and Widaman (2006 ) both provide an accessible primer on missing data techniques. In keeping with the largely practical bent to the chapter, we suggest that researchers keep careful track of the amount of missing data present in their analyses and report such information clearly in research papers ( see McKnight & McKnight, 2011 ). Similarly, we recommend that researchers thoroughly screen their data sets for evidence that missing values depend on other measured variables (e.g., scores at Time 1 might be associated with Time 2 dropout). In general, we suggest that researchers avoid listwise and pairwise deletion methods because there is very little evidence that these are good practices ( see Jeličić, Phelps, & Lerner, 2009 ; Widaman, 2006 ). Rather, it might be easiest to use direct fitting methods such as the estimation procedures used in conventional structural equation modeling packages (e.g., Full Information Maximum Likelihood; see Allison, 2003 ). At the very least, it is usually instructive to compare results using listwise deletion with results obtained with direct model fitting in terms of the effect size estimates and basic conclusions regarding the statistical significance of focal coefficients.
Special Issue: Sample Weighting in Existing Data Sets
One of the advantages of many existing data sets is that they were collected using probabilistic sampling methods so that researchers can obtain unbiased population estimates. Such estimates, however, are only obtained when complex survey weights are formally incorporated into the statistical modeling procedures. Such weighting schemes can affect the correlations between variables, and therefore all users of secondary data sets should become familiar with sampling design when they begin working with a new data set. A considerable amount of time and effort is dedicated toward generating complex weighting schemes that account for the precise sampling strategies used in the given study, and users of secondary data sets should give careful consideration to using these weights appropriately.
In some cases, the addition of sampling weights will have little substantive implication on findings, so extensive concern over weighting might be overstated. On the other hand, any potential difference is ultimately an empirical question, so researchers are well advised to consider the importance of sampling weights ( Shrout & Napier, 2011 ). The problem is that many psychologists are not well versed in the use of sampling weights ( Shrout & Napier, 2011 ). Thus, psychologists may not be in a strong position to evaluate whether sample weighting concerns are relevant. In addition, it is sometimes necessary to use specialized software packages or add-ons to adjust analytic models appropriately for sampling weights. Programs such as STATA and SAS have such capabilities in the base package, whereas packages like SPSS sometimes require a complex survey model add-on that integrates with its existing capabilities. Whereas the graduate training of the modal sociologist or demographer is likely to emphasize survey research and thus presumably cover sampling, this is not the case with the methodological training of many psychologists ( Aiken, West, & Millsap, 2008 ). Psychologists who are unfamiliar with sample weighting procedures are well advised to seek the counsel of a survey methodologist before undertaking data analysis.
In terms of practical recommendations, it is important for the user of the secondary data set to develop a clear understanding of how the data were collected by reading documentation about the design and sampling procedure ( Shrout & Napier, 2011 ). This insight will provide a conceptual framework for understanding weighting schemes and for deciding how to appropriately weight the data. Once researchers have a clear idea of the sampling scheme and potential weights, actually incorporating available weights into analyses is not terribly difficult, provided researchers have the appropriate software ( Shrout & Napier, 2011 ). Weighting tutorials are often available for specific data sets. For example, the Add Health project has a document describing weighting ( http://www.cpc.unc.edu/projects/addhealth/faqs/aboutdata/weight1.pdf ) as does the Centers for Disease Control and Prevention for use with their Youth Risk Behavior Surveys ( http://www.cdc.gov/HealthyYouth/yrbs/pdf/YRBS_analysis_software.pdf ). These free documents may also provide useful and accessible background even for those who may not use the data from these projects.
Secondary data analysis refers to the analysis of existing data that may not have been explicitly collected to address a particular research question. Many of the quantitative techniques described in this volume can be applied using existing resources. To be sure, strong data analytic skills are important for fully realizing the potential benefits of secondary data sets, and such skills can help researchers recognize the limits of a data set for any given analysis.
In particular, measurement issues are likely to create the biggest hurdles for psychologists conducting secondary analyses in terms of the challenges associated with offering a reasonable interpretation of the results and in surviving the peer-review process. Accordingly, a familiarity with basic issues in psychometrics is very helpful. Beyond such skills, the effective use of these existing resources requires patience and strong attention to detail. Effective secondary data analysis also requires a fair bit of curiosity to seek out those resources that might be used to make important contribution to psychological science.
Ultimately, we hope that the field of psychology becomes more and more accepting of secondary data analysis. As psychologists use this approach with increasing frequency, it is likely that the organizers of major ongoing data collection efforts will be increasingly open to including measures of prime interest to psychologists. The individuals in charge of projects like the BHPS, the GSOEP, and the National Center for Education Statistics ( http://nces.ed.gov/ ) want their data to be used by the widest possible audiences and will respond to researcher demands. We believe that it is time that psychologists join their colleagues in economics, sociology, and political science in taking advantage of these existing resources. It is also time to move beyond divisive discussions surrounding the presumed superiority of primary data collection over secondary analysis. There is no reason to choose one over the other when the field of psychology can profit from both. We believe that the relevant topics of debate are not about the method of initial data collection but, rather, about the importance and intrinsic interest of the underlying research questions. If the question is important and the research design and measures are suitable, then there is little doubt in our minds that secondary data analysis can make a contribution to psychological science.
Author Note
M. Brent Donnellan, Department of Psychology, Michigan State University, East Lansing, MI 48824.
Richard E. Lucas, Department of Psychology, Michigan State University, East Lansing, MI 48824.
One consequence of large sample sizes, however, is that issues of effect size interpretation become paramount given that very small correlations or very small mean differences between groups are likely to be statistically significant using conventional null hypothesis significance tests (e.g., Trzesniewski & Donnellan, 2009 ). Researchers will therefore need to grapple with issues related to null hypothesis significance testing ( see Kline, 2004 ).
Aiken, L. S. , & West, S. G. ( 1991 ). Multiple regression: Testing and interpreting interactions . Newbury Park, CA: Sage.
Google Scholar
Google Preview
Aiken, L. S. , West, S. G. , & Millsap, R. E. ( 2008 ). Doctoral training in statistics, measurement, and methodology in psychology: Replication and extension of Aiken, West, Sechrest, and Reno’s (1990) survey of Ph.D. programs in North America. American Psychologist, 63, 32–50.
Akers, R. L. , Massey, J. , & Clarke, W ( 1983 ). Are self-reports of adolescent deviance valid? Biochemical measures, randomized response, and the bogus pipeline in smoking behavior. Social Forces, 62, 234–251.
Allison, P. D. ( 2003 ). Missing data techniques for structural equation modeling. Journal of Abnormal Psychology, 112, 545–557.
Baird, B. M. , Lucas, R. E. , & Donnellan, M. B. ( 2010 ). Life Satisfaction across the lifespan: Findings from two nationally representative panel studies. Social Indicators Research, 99, 183–203.
Briggs, S. R. , & Cheek, J. M. ( 1986 ). The role of factor analysis in the development and evaluation of personality scales. Journal of Personality 54, 106–148.
Brooks-Gunn, J. , Berlin, L. J. , Leventhal, T. , & Fuligini, A. S. ( 2000 ). Depending on the kindness of strangers: Current national data initiatives and developmental research. Child Development, 71, 257–268.
Brooks-Gunn, J. , & Chase-Lansdale, P. L. ( 1991 ) (Eds.). Secondary data analyses in developmental psychology [Special section]. Developmental Psychology, 27, 899–951.
Clark, L. A. , & Watson, D. ( 1995 ). Constructing validity: Basic issues in objective scale development. Psychological Assessment, 7, 309–319.
Cronbach, L. J. ( 1951 ). Coefficient alpha and the internal structure of tests. Psychometrika, 16, 297–234.
Cronbach, L. J. ( 1957 ). The two disciplines of scientific psychology. American Psychologist, 12, 671–684.
Cronbach, L. J. , & Meehl, P. ( 1955 ). Construct validity in psychological tests. Psychological Bulletin, 52, 281–302.
Diener, E. , Emmons, R. A. , Larsen, R. J. , & Griffin, S. ( 1985 ). The Satisfaction with Life Scale. Journal of Personality Assessment, 49, 71–75.
Donnellan, M. B. , Trzesniewski, K. H. , & Robins, R. W. ( 2011 ). Self-esteem: Enduring issues and controversies. In T Chamorro-Premuzic , S. von Stumm , and A. Furnham (Eds). The Wiley-Blackwell Handbook of Individual Differences (pp. 710–746). New York: Wiley-Blackwell.
Freese, J. ( 2007 ). Replication standards for quantitative social science: Why not sociology? Sociological Methods & Research, 36, 153–172.
Gerstorf, D. , Ram, N. , Estabrook, R. , Schupp, J. , Wagner, G. G. , & Lindenberger, U. ( 2008 ). Life satisfaction shows terminal decline in old age: Longitudinal evidence from the German Socio-Economic Panel Study (SOEP). Developmental Psychology, 44, 1148–1159.
Gerstorf, D. , Ram, N. , Goebel, J. , Schupp, J. , Lindenberger, U. , & Wagner, G. G. ( 2010 ). Where people live and die makes a difference: Individual and geographic disparities in well-being progression at the end of life. Psychology and Aging, 25, 661–676.
Goldberg, L. R. ( 1999 ). A broad-bandwidth, public domain, personality inventory measuring the lower-level facets of several five-factor models. In I Mervielde , I. Deary , F. De Fruyt , & F. Ostendorf (Eds.), Personality psychology in Europe (Vol. 7, pp. 7–28). Tilburg, The Netherlands: Tilburg University Press.
Hofferth, S. L. , ( 2005 ). Secondary data analysis in family research. Journal of Marriage and the Family, 67, 891–907.
Hunter, J. E. , & Schmidt, F. L. ( 2004 ). Methods of meta-analysis: Correcting error and bias in research findings (2nd ed.). Newbury Park, CA: Sage.
Jeličić, H. , Phelps, E. , & Lerner, R. M. ( 2009 ). Use of missing data methods in longitudinal studies: The persistence of bad practices in developmental psychology. Developmental Psychology, 45, 1195–1199.
John, O. P. , & Soto, C. J. ( 2007 ). The importance of being valid. In R. W Robins , R. C. Fraley , and R. F. Krueger (Eds). Handbook of Research Methods in Personality Psychology (pp. 461–494). New York: Guilford Press.
Kiecolt, K. J. & Nathan, L. E. ( 1985 ). Secondary analysis of survey data . Sage University Paper series on Quantitative Applications in the Social Sciences, No. 53). Newbury Park, CA: Sage.
Kline, R. B. ( 2004 ). Beyond significance testing: Reforming data analysis methods in behavioral research . Washington, DC: American Psychological Association.
Kline, R. B. ( 2011 ). Principles and practice of structural equation modeling (3rd ed.). New York: Guildford Press.
Lance, C. E. , Butts, M. M. , & Michels, L. C. ( 2006 ). The sources of four commonly reported cutoff criteria: What did they really say? Organizational Research Methods, 9, 202–220.
Lord, F. , & Novick, M. R. ( 1968 ). Statistical theories of mental test scores . Reading, MA: Addison-Wesley.
Lucas, R. E. ( 2005 ). Time does not heal all wounds. Psychological Science, 16, 945–950.
Lucas, R. E. ( 2007 ). Adaptation and the set-point model of subjective well-being: Does happiness change after major life events? Current Directions in Psychological Science, 16, 75–79.
McCall, R. B. , & Appelbaum, M. I. ( 1991 ). Some issues of conducting secondary analyses. Developmental Psychology, 27, 911–917.
McCrae, R. R. , Kurtz, J. E. , Yamagata, S. , & Terracciano, A. ( 2011 ). Internal consistency, retest reliability, and their implications for personality scale validity. Personality and Social Psychology Review, 15, 28–50.
Messick, S. ( 1995 ). Validity of psychological assessment: Validation of inferences from persons’ responses and performances as scientific inquiry into score meaning. American Psychologist, 50, 741–749.
McKnight, P. E. , & McKnight, K. M. ( 2011 ). Missing data in secondary data analysis. In K. H. Trzesniewski , M. B. Donnellan , & R. E. Lucas (Eds). Secondary data analysis: An introduction for psychologists (pp. 83–101). Washington, DC: American Psychological Association.
McKnight, P. E. , McKnight, K. M. , Sidani, S. , & Figuredo, A. ( 2007 ). Missing data: A gentle introduction . New York: Guilford Press.
Mroczek, D. K. , Pitzer, L. , Miller, L. , Turiano, N. , & Fingerman, K. ( 2011 ). The use of secondary data in adult development and aging research. In K. H. Trzesniewski , M. B. Donnellan , and R. E. Lucas (Eds). Secondary data analysis: An introduction for psychologists (pp. 121–132). Washington, DC: American Psychological Association.
Pienta, A. M. , O’Rourke, J. M. , & Franks, M. M. ( 2011 ). Getting started: Working with secondary data. In K. H. Trzesniewski , M. B. Donnellan , and R. E. Lucas (Eds). Secondary data analysis: An introduction for psychologists (pp. 13–25). Washington, DC: American Psychological Association.
Rosenberg, M. ( 1965 ). Society and adolescent self image , Princeton, NJ: Princeton University.
Russell, S. T. , Crockett, L. J. , Shen, Y-L , & Lee, S-A. ( 2008 ). Cross-ethnic invariance of self-esteem and depression measures for Chinese, Filipino, and European American adolescents. Journal of Youth and Adolescence, 37, 50–61.
Russell, S. T. , & Matthews, E. ( 2011 ). Using secondary data to study adolescence and adolescent development. In K. H. Trzesniewski , M. B. Donnellan , & R. E. Lucas (Eds). Secondary data analysis: An introduction for psychologists (pp. 163–176). Washington, DC: American Psychological Association.
Schafer, J. L. & Graham, J. W ( 2002 ). Missing data: Our view of the state of the art. Psychological Methods, 7, 147–177.
Schmitt, N. ( 1996 ). Uses and abuses of coefficient alpha. Psychological Assessment, 8, 350–353.
Schwarz, N. ( 1999 ). Self-reports: How the questions shape the answers. American Psychologist, 54, 93–105.
Schwarz, N. & Strack, F. ( 1999 ). Reports of subjective well-being: Judgmental processes and their methodological implications. In D. Kahneman , E. Diener , & N. Schwarz (Eds.). Well-being: The foundations of hedonic psychology (pp.61–84). New York: Russell Sage Foundation.
Sears, D. O. ( 1986 ). College sophomores in the lab: Influences of a narrow data base on social psychology’s view of human nature. Journal of Personality and Social Psychology, 51, 515–530.
Shrout, P. E. , & Napier, J. L. ( 2011 ). Analyzing survey data with complex sampling designs. In K. H. Trzesniewski , M. B. Donnellan , & R. E. Lucas (Eds). Secondary data analysis: An introduction for psychologists (pp. 63–81). Washington, DC: American Psychological Association.
Simms, L. J. ( 2008 ). Classical and modern methods of psychological scale construction. Social and Personality Psychology Compass, 2/1, 414–433.
Simms, L. J. , & Watson, D. ( 2007 ). The construct validation approach to personality scale creation. In R. W Robins , R. C. Fraley , & R. F. Krueger (Eds). Handbook of Research Methods in Personality Psychology (pp. 240–258). New York: Guilford Press.
Smith, G. X ( 2005 ). On construct validity: Issues of method and measurement. Psychological Assessment, 17, 396–408.
Tracy, J. L. , Robins, R. W. , & Sherman, J. W. ( 2009 ). The practice of psychological science: Searching for Cronbach’s two streams in social-personality psychology. Journal of Personality and Social Psychology, 96, 1206–1225.
Trzesniewski, K.H. & Donnellan, M. B. ( 2009 ). Re-evaluating the evidence for increasing self-views among high school students: More evidence for consistency across generations (1976–2006). Psychological Science, 20, 920–922.
Trzesniewski, K. H. & Donnellan, M. B. ( 2010 ). Rethinking “Generation Me”: A study of cohort effects from 1976–2006. Perspectives in Psychological Science , 5, 58–75.
Trzesniewski, K. H. , Donnellan, M. B. , & Lucas, R. E. ( 2011 ) (Eds). Secondary data analysis: An introduction for psychologists . Washington, DC: American Psychological Association.
Widaman, K. F. ( 2006 ). Missing data: What to do with or without them. Monographs of the Society for Research in Child Development, 71, 42–64.
Widaman, K. F. , Little, T. D. , Preacher, K. K. , & Sawalani, G. M. ( 2011 ). On creating and using short forms of scales in secondary research. In K. H. Trzesniewski , M. B. Donnellan , & R. E. Lucas (Eds). Secondary data analysis: An introduction for psychologists (pp. 39–61). Washington, DC: American Psychological Association.
Willms, J. D. ( 2011 ). Managing and using secondary data sets with multidisciplinary research teams. In K. H. Trzesniewski , M. B. Donnellan , & R. E. Lucas (Eds). Secondary data analysis: An introduction for psychologists (pp. 27–38). Washington, DC: American Psychological Association.
- About Oxford Academic
- Publish journals with us
- University press partners
- What we publish
- New features
- Open access
- Institutional account management
- Rights and permissions
- Get help with access
- Accessibility
- Advertising
- Media enquiries
- Oxford University Press
- Oxford Languages
- University of Oxford
Oxford University Press is a department of the University of Oxford. It furthers the University's objective of excellence in research, scholarship, and education by publishing worldwide
- Copyright © 2024 Oxford University Press
- Cookie settings
- Cookie policy
- Privacy policy
- Legal notice
This Feature Is Available To Subscribers Only
Sign In or Create an Account
This PDF is available to Subscribers Only
For full access to this pdf, sign in to an existing account, or purchase an annual subscription.
A Guide To Secondary Data Analysis
What is secondary data analysis? How do you carry it out? Find out in this post.
Historically, the only way data analysts could obtain data was to collect it themselves. This type of data is often referred to as primary data and is still a vital resource for data analysts.
However, technological advances over the last few decades mean that much past data is now readily available online for data analysts and researchers to access and utilize. This type of data—known as secondary data—is driving a revolution in data analytics and data science.
Primary and secondary data share many characteristics. However, there are some fundamental differences in how you prepare and analyze secondary data. This post explores the unique aspects of secondary data analysis. We’ll briefly review what secondary data is before outlining how to source, collect and validate them. We’ll cover:
- What is secondary data analysis?
- How to carry out secondary data analysis (5 steps)
- Summary and further reading
Ready for a crash course in secondary data analysis? Let’s go!
1. What is secondary data analysis?
Secondary data analysis uses data collected by somebody else. This contrasts with primary data analysis, which involves a researcher collecting predefined data to answer a specific question. Secondary data analysis has numerous benefits, not least that it is a time and cost-effective way of obtaining data without doing the research yourself.
It’s worth noting here that secondary data may be primary data for the original researcher. It only becomes secondary data when it’s repurposed for a new task. As a result, a dataset can simultaneously be a primary data source for one researcher and a secondary data source for another. So don’t panic if you get confused! We explain exactly what secondary data is in this guide .
In reality, the statistical techniques used to carry out secondary data analysis are no different from those used to analyze other kinds of data. The main differences lie in collection and preparation. Once the data have been reviewed and prepared, the analytics process continues more or less as it usually does. For a recap on what the data analysis process involves, read this post .
In the following sections, we’ll focus specifically on the preparation of secondary data for analysis. Where appropriate, we’ll refer to primary data analysis for comparison.
2. How to carry out secondary data analysis
Step 1: define a research topic.
The first step in any data analytics project is defining your goal. This is true regardless of the data you’re working with, or the type of analysis you want to carry out. In data analytics lingo, this typically involves defining:
- A statement of purpose
- Research design
Defining a statement of purpose and a research approach are both fundamental building blocks for any project. However, for secondary data analysis, the process of defining these differs slightly. Let’s find out how.
Step 2: Establish your statement of purpose
Before beginning any data analytics project, you should always have a clearly defined intent. This is called a ‘statement of purpose.’ A healthcare analyst’s statement of purpose, for example, might be: ‘Reduce admissions for mental health issues relating to Covid-19′. The more specific the statement of purpose, the easier it is to determine which data to collect, analyze, and draw insights from.
A statement of purpose is helpful for both primary and secondary data analysis. It’s especially relevant for secondary data analysis, though. This is because there are vast amounts of secondary data available. Having a clear direction will keep you focused on the task at hand, saving you from becoming overwhelmed. Being selective with your data sources is key.
Step 3: Design your research process
After defining your statement of purpose, the next step is to design the research process. For primary data, this involves determining the types of data you want to collect (e.g. quantitative, qualitative, or both ) and a methodology for gathering them.
For secondary data analysis, however, your research process will more likely be a step-by-step guide outlining the types of data you require and a list of potential sources for gathering them. It may also include (realistic) expectations of the output of the final analysis. This should be based on a preliminary review of the data sources and their quality.
Once you have both your statement of purpose and research design, you’re in a far better position to narrow down potential sources of secondary data. You can then start with the next step of the process: data collection.
Step 4: Locate and collect your secondary data
Collecting primary data involves devising and executing a complex strategy that can be very time-consuming to manage. The data you collect, though, will be highly relevant to your research problem.
Secondary data collection, meanwhile, avoids the complexity of defining a research methodology. However, it comes with additional challenges. One of these is identifying where to find the data. This is no small task because there are a great many repositories of secondary data available. Your job, then, is to narrow down potential sources. As already mentioned, it’s necessary to be selective, or else you risk becoming overloaded.
Some popular sources of secondary data include:
- Government statistics , e.g. demographic data, censuses, or surveys, collected by government agencies/departments (like the US Bureau of Labor Statistics).
- Technical reports summarizing completed or ongoing research from educational or public institutions (colleges or government).
- Scientific journals that outline research methodologies and data analysis by experts in fields like the sciences, medicine, etc.
- Literature reviews of research articles, books, and reports, for a given area of study (once again, carried out by experts in the field).
- Trade/industry publications , e.g. articles and data shared in trade publications, covering topics relating to specific industry sectors, such as tech or manufacturing.
- Online resources: Repositories, databases, and other reference libraries with public or paid access to secondary data sources.
Once you’ve identified appropriate sources, you can go about collecting the necessary data. This may involve contacting other researchers, paying a fee to an organization in exchange for a dataset, or simply downloading a dataset for free online .
Step 5: Evaluate your secondary data
Secondary data is usually well-structured, so you might assume that once you have your hands on a dataset, you’re ready to dive in with a detailed analysis. Unfortunately, that’s not the case!
First, you must carry out a careful review of the data. Why? To ensure that they’re appropriate for your needs. This involves two main tasks:
Evaluating the secondary dataset’s relevance
- Assessing its broader credibility
Both these tasks require critical thinking skills. However, they aren’t heavily technical. This means anybody can learn to carry them out.
Let’s now take a look at each in a bit more detail.
The main point of evaluating a secondary dataset is to see if it is suitable for your needs. This involves asking some probing questions about the data, including:
What was the data’s original purpose?
Understanding why the data were originally collected will tell you a lot about their suitability for your current project. For instance, was the project carried out by a government agency or a private company for marketing purposes? The answer may provide useful information about the population sample, the data demographics, and even the wording of specific survey questions. All this can help you determine if the data are right for you, or if they are biased in any way.
When and where were the data collected?
Over time, populations and demographics change. Identifying when the data were first collected can provide invaluable insights. For instance, a dataset that initially seems suited to your needs may be out of date.
On the flip side, you might want past data so you can draw a comparison with a present dataset. In this case, you’ll need to ensure the data were collected during the appropriate time frame. It’s worth mentioning that secondary data are the sole source of past data. You cannot collect historical data using primary data collection techniques.
Similarly, you should ask where the data were collected. Do they represent the geographical region you require? Does geography even have an impact on the problem you are trying to solve?
What data were collected and how?
A final report for past data analytics is great for summarizing key characteristics or findings. However, if you’re planning to use those data for a new project, you’ll need the original documentation. At the very least, this should include access to the raw data and an outline of the methodology used to gather them. This can be helpful for many reasons. For instance, you may find raw data that wasn’t relevant to the original analysis, but which might benefit your current task.
What questions were participants asked?
We’ve already touched on this, but the wording of survey questions—especially for qualitative datasets—is significant. Questions may deliberately be phrased to preclude certain answers. A question’s context may also impact the findings in a way that’s not immediately obvious. Understanding these issues will shape how you perceive the data.
What is the form/shape/structure of the data?
Finally, to practical issues. Is the structure of the data suitable for your needs? Is it compatible with other sources or with your preferred analytics approach? This is purely a structural issue. For instance, if a dataset of people’s ages is saved as numerical rather than continuous variables, this could potentially impact your analysis. In general, reviewing a dataset’s structure helps better understand how they are categorized, allowing you to account for any discrepancies. You may also need to tidy the data to ensure they are consistent with any other sources you’re using.
This is just a sample of the types of questions you need to consider when reviewing a secondary data source. The answers will have a clear impact on whether the dataset—no matter how well presented or structured it seems—is suitable for your needs.
Assessing secondary data’s credibility
After identifying a potentially suitable dataset, you must double-check the credibility of the data. Namely, are the data accurate and unbiased? To figure this out, here are some key questions you might want to include:
What are the credentials of those who carried out the original research?
Do you have access to the details of the original researchers? What are their credentials? Where did they study? Are they an expert in the field or a newcomer? Data collection by an undergraduate student, for example, may not be as rigorous as that of a seasoned professor.
And did the original researcher work for a reputable organization? What other affiliations do they have? For instance, if a researcher who works for a tobacco company gathers data on the effects of vaping, this represents an obvious conflict of interest! Questions like this help determine how thorough or qualified the researchers are and if they have any potential biases.
Do you have access to the full methodology?
Does the dataset include a clear methodology, explaining in detail how the data were collected? This should be more than a simple overview; it must be a clear breakdown of the process, including justifications for the approach taken. This allows you to determine if the methodology was sound. If you find flaws (or no methodology at all) it throws the quality of the data into question.
How consistent are the data with other sources?
Do the secondary data match with any similar findings? If not, that doesn’t necessarily mean the data are wrong, but it does warrant closer inspection. Perhaps the collection methodology differed between sources, or maybe the data were analyzed using different statistical techniques. Or perhaps unaccounted-for outliers are skewing the analysis. Identifying all these potential problems is essential. A flawed or biased dataset can still be useful but only if you know where its shortcomings lie.
Have the data been published in any credible research journals?
Finally, have the data been used in well-known studies or published in any journals? If so, how reputable are the journals? In general, you can judge a dataset’s quality based on where it has been published. If in doubt, check out the publication in question on the Directory of Open Access Journals . The directory has a rigorous vetting process, only permitting journals of the highest quality. Meanwhile, if you found the data via a blurry image on social media without cited sources, then you can justifiably question its quality!
Again, these are just a few of the questions you might ask when determining the quality of a secondary dataset. Consider them as scaffolding for cultivating a critical thinking mindset; a necessary trait for any data analyst!
Presuming your secondary data holds up to scrutiny, you should be ready to carry out your detailed statistical analysis. As we explained at the beginning of this post, the analytical techniques used for secondary data analysis are no different than those for any other kind of data. Rather than go into detail here, check out the different types of data analysis in this post.
3. Secondary data analysis: Key takeaways
In this post, we’ve looked at the nuances of secondary data analysis, including how to source, collect and review secondary data. As discussed, much of the process is the same as it is for primary data analysis. The main difference lies in how secondary data are prepared.
Carrying out a meaningful secondary data analysis involves spending time and effort exploring, collecting, and reviewing the original data. This will help you determine whether the data are suitable for your needs and if they are of good quality.
Why not get to know more about what data analytics involves with this free, five-day introductory data analytics short course ? And, for more data insights, check out these posts:
- Discrete vs continuous data variables: What’s the difference?
- What are the four levels of measurement? Nominal, ordinal, interval, and ratio data explained
- What are the best tools for data mining?
Root out friction in every digital experience, super-charge conversion rates, and optimize digital self-service
Uncover insights from any interaction, deliver AI-powered agent coaching, and reduce cost to serve
Increase revenue and loyalty with real-time insights and recommendations delivered to teams on the ground
Know how your people feel and empower managers to improve employee engagement, productivity, and retention
Take action in the moments that matter most along the employee journey and drive bottom line growth
Whatever they’re are saying, wherever they’re saying it, know exactly what’s going on with your people
Get faster, richer insights with qual and quant tools that make powerful market research available to everyone
Run concept tests, pricing studies, prototyping + more with fast, powerful studies designed by UX research experts
Track your brand performance 24/7 and act quickly to respond to opportunities and challenges in your market
Explore the platform powering Experience Management
- Free Account
- For Digital
- For Customer Care
- For Human Resources
- For Researchers
- Financial Services
- All Industries
Popular Use Cases
- Customer Experience
- Employee Experience
- Net Promoter Score
- Voice of Customer
- Customer Success Hub
- Product Documentation
- Training & Certification
- XM Institute
- Popular Resources
- Customer Stories
- Artificial Intelligence
Market Research
- Partnerships
- Marketplace
The annual gathering of the experience leaders at the world’s iconic brands building breakthrough business results, live in Salt Lake City.
- English/AU & NZ
- Español/Europa
- Español/América Latina
- Português Brasileiro
- REQUEST DEMO
- Experience Management
- Secondary Research
Try Qualtrics for free
Secondary research: definition, methods, & examples.
19 min read This ultimate guide to secondary research helps you understand changes in market trends, customers buying patterns and your competition using existing data sources.
In situations where you’re not involved in the data gathering process ( primary research ), you have to rely on existing information and data to arrive at specific research conclusions or outcomes. This approach is known as secondary research.
In this article, we’re going to explain what secondary research is, how it works, and share some examples of it in practice.
Free eBook: The ultimate guide to conducting market research
What is secondary research?
Secondary research, also known as desk research, is a research method that involves compiling existing data sourced from a variety of channels . This includes internal sources (e.g.in-house research) or, more commonly, external sources (such as government statistics, organizational bodies, and the internet).
Secondary research comes in several formats, such as published datasets, reports, and survey responses , and can also be sourced from websites, libraries, and museums.
The information is usually free — or available at a limited access cost — and gathered using surveys , telephone interviews, observation, face-to-face interviews, and more.
When using secondary research, researchers collect, verify, analyze and incorporate it to help them confirm research goals for the research period.
As well as the above, it can be used to review previous research into an area of interest. Researchers can look for patterns across data spanning several years and identify trends — or use it to verify early hypothesis statements and establish whether it’s worth continuing research into a prospective area.
How to conduct secondary research
There are five key steps to conducting secondary research effectively and efficiently:
1. Identify and define the research topic
First, understand what you will be researching and define the topic by thinking about the research questions you want to be answered.
Ask yourself: What is the point of conducting this research? Then, ask: What do we want to achieve?
This may indicate an exploratory reason (why something happened) or confirm a hypothesis. The answers may indicate ideas that need primary or secondary research (or a combination) to investigate them.
2. Find research and existing data sources
If secondary research is needed, think about where you might find the information. This helps you narrow down your secondary sources to those that help you answer your questions. What keywords do you need to use?
Which organizations are closely working on this topic already? Are there any competitors that you need to be aware of?
Create a list of the data sources, information, and people that could help you with your work.
3. Begin searching and collecting the existing data
Now that you have the list of data sources, start accessing the data and collect the information into an organized system. This may mean you start setting up research journal accounts or making telephone calls to book meetings with third-party research teams to verify the details around data results.
As you search and access information, remember to check the data’s date, the credibility of the source, the relevance of the material to your research topic, and the methodology used by the third-party researchers. Start small and as you gain results, investigate further in the areas that help your research’s aims.
4. Combine the data and compare the results
When you have your data in one place, you need to understand, filter, order, and combine it intelligently. Data may come in different formats where some data could be unusable, while other information may need to be deleted.
After this, you can start to look at different data sets to see what they tell you. You may find that you need to compare the same datasets over different periods for changes over time or compare different datasets to notice overlaps or trends. Ask yourself: What does this data mean to my research? Does it help or hinder my research?
5. Analyze your data and explore further
In this last stage of the process, look at the information you have and ask yourself if this answers your original questions for your research. Are there any gaps? Do you understand the information you’ve found? If you feel there is more to cover, repeat the steps and delve deeper into the topic so that you can get all the information you need.
If secondary research can’t provide these answers, consider supplementing your results with data gained from primary research. As you explore further, add to your knowledge and update your findings. This will help you present clear, credible information.
Primary vs secondary research
Unlike secondary research, primary research involves creating data first-hand by directly working with interviewees, target users, or a target market. Primary research focuses on the method for carrying out research, asking questions, and collecting data using approaches such as:
- Interviews (panel, face-to-face or over the phone)
- Questionnaires or surveys
- Focus groups
Using these methods, researchers can get in-depth, targeted responses to questions, making results more accurate and specific to their research goals. However, it does take time to do and administer.
Unlike primary research, secondary research uses existing data, which also includes published results from primary research. Researchers summarize the existing research and use the results to support their research goals.
Both primary and secondary research have their places. Primary research can support the findings found through secondary research (and fill knowledge gaps), while secondary research can be a starting point for further primary research. Because of this, these research methods are often combined for optimal research results that are accurate at both the micro and macro level.
Sources of Secondary Research
There are two types of secondary research sources: internal and external. Internal data refers to in-house data that can be gathered from the researcher’s organization. External data refers to data published outside of and not owned by the researcher’s organization.
Internal data
Internal data is a good first port of call for insights and knowledge, as you may already have relevant information stored in your systems. Because you own this information — and it won’t be available to other researchers — it can give you a competitive edge . Examples of internal data include:
- Database information on sales history and business goal conversions
- Information from website applications and mobile site data
- Customer-generated data on product and service efficiency and use
- Previous research results or supplemental research areas
- Previous campaign results
External data
External data is useful when you: 1) need information on a new topic, 2) want to fill in gaps in your knowledge, or 3) want data that breaks down a population or market for trend and pattern analysis. Examples of external data include:
- Government, non-government agencies, and trade body statistics
- Company reports and research
- Competitor research
- Public library collections
- Textbooks and research journals
- Media stories in newspapers
- Online journals and research sites
Three examples of secondary research methods in action
How and why might you conduct secondary research? Let’s look at a few examples:
1. Collecting factual information from the internet on a specific topic or market
There are plenty of sites that hold data for people to view and use in their research. For example, Google Scholar, ResearchGate, or Wiley Online Library all provide previous research on a particular topic. Researchers can create free accounts and use the search facilities to look into a topic by keyword, before following the instructions to download or export results for further analysis.
This can be useful for exploring a new market that your organization wants to consider entering. For instance, by viewing the U.S Census Bureau demographic data for that area, you can see what the demographics of your target audience are , and create compelling marketing campaigns accordingly.
2. Finding out the views of your target audience on a particular topic
If you’re interested in seeing the historical views on a particular topic, for example, attitudes to women’s rights in the US, you can turn to secondary sources.
Textbooks, news articles, reviews, and journal entries can all provide qualitative reports and interviews covering how people discussed women’s rights. There may be multimedia elements like video or documented posters of propaganda showing biased language usage.
By gathering this information, synthesizing it, and evaluating the language, who created it and when it was shared, you can create a timeline of how a topic was discussed over time.
3. When you want to know the latest thinking on a topic
Educational institutions, such as schools and colleges, create a lot of research-based reports on younger audiences or their academic specialisms. Dissertations from students also can be submitted to research journals, making these places useful places to see the latest insights from a new generation of academics.
Information can be requested — and sometimes academic institutions may want to collaborate and conduct research on your behalf. This can provide key primary data in areas that you want to research, as well as secondary data sources for your research.
Advantages of secondary research
There are several benefits of using secondary research, which we’ve outlined below:
- Easily and readily available data – There is an abundance of readily accessible data sources that have been pre-collected for use, in person at local libraries and online using the internet. This data is usually sorted by filters or can be exported into spreadsheet format, meaning that little technical expertise is needed to access and use the data.
- Faster research speeds – Since the data is already published and in the public arena, you don’t need to collect this information through primary research. This can make the research easier to do and faster, as you can get started with the data quickly.
- Low financial and time costs – Most secondary data sources can be accessed for free or at a small cost to the researcher, so the overall research costs are kept low. In addition, by saving on preliminary research, the time costs for the researcher are kept down as well.
- Secondary data can drive additional research actions – The insights gained can support future research activities (like conducting a follow-up survey or specifying future detailed research topics) or help add value to these activities.
- Secondary data can be useful pre-research insights – Secondary source data can provide pre-research insights and information on effects that can help resolve whether research should be conducted. It can also help highlight knowledge gaps, so subsequent research can consider this.
- Ability to scale up results – Secondary sources can include large datasets (like Census data results across several states) so research results can be scaled up quickly using large secondary data sources.
Disadvantages of secondary research
The disadvantages of secondary research are worth considering in advance of conducting research :
- Secondary research data can be out of date – Secondary sources can be updated regularly, but if you’re exploring the data between two updates, the data can be out of date. Researchers will need to consider whether the data available provides the right research coverage dates, so that insights are accurate and timely, or if the data needs to be updated. Also, fast-moving markets may find secondary data expires very quickly.
- Secondary research needs to be verified and interpreted – Where there’s a lot of data from one source, a researcher needs to review and analyze it. The data may need to be verified against other data sets or your hypotheses for accuracy and to ensure you’re using the right data for your research.
- The researcher has had no control over the secondary research – As the researcher has not been involved in the secondary research, invalid data can affect the results. It’s therefore vital that the methodology and controls are closely reviewed so that the data is collected in a systematic and error-free way.
- Secondary research data is not exclusive – As data sets are commonly available, there is no exclusivity and many researchers can use the same data. This can be problematic where researchers want to have exclusive rights over the research results and risk duplication of research in the future.
When do we conduct secondary research?
Now that you know the basics of secondary research, when do researchers normally conduct secondary research?
It’s often used at the beginning of research, when the researcher is trying to understand the current landscape . In addition, if the research area is new to the researcher, it can form crucial background context to help them understand what information exists already. This can plug knowledge gaps, supplement the researcher’s own learning or add to the research.
Secondary research can also be used in conjunction with primary research. Secondary research can become the formative research that helps pinpoint where further primary research is needed to find out specific information. It can also support or verify the findings from primary research.
You can use secondary research where high levels of control aren’t needed by the researcher, but a lot of knowledge on a topic is required from different angles.
Secondary research should not be used in place of primary research as both are very different and are used for various circumstances.
Questions to ask before conducting secondary research
Before you start your secondary research, ask yourself these questions:
- Is there similar internal data that we have created for a similar area in the past?
If your organization has past research, it’s best to review this work before starting a new project. The older work may provide you with the answers, and give you a starting dataset and context of how your organization approached the research before. However, be mindful that the work is probably out of date and view it with that note in mind. Read through and look for where this helps your research goals or where more work is needed.
- What am I trying to achieve with this research?
When you have clear goals, and understand what you need to achieve, you can look for the perfect type of secondary or primary research to support the aims. Different secondary research data will provide you with different information – for example, looking at news stories to tell you a breakdown of your market’s buying patterns won’t be as useful as internal or external data e-commerce and sales data sources.
- How credible will my research be?
If you are looking for credibility, you want to consider how accurate the research results will need to be, and if you can sacrifice credibility for speed by using secondary sources to get you started. Bear in mind which sources you choose — low-credibility data sites, like political party websites that are highly biased to favor their own party, would skew your results.
- What is the date of the secondary research?
When you’re looking to conduct research, you want the results to be as useful as possible , so using data that is 10 years old won’t be as accurate as using data that was created a year ago. Since a lot can change in a few years, note the date of your research and look for earlier data sets that can tell you a more recent picture of results. One caveat to this is using data collected over a long-term period for comparisons with earlier periods, which can tell you about the rate and direction of change.
- Can the data sources be verified? Does the information you have check out?
If you can’t verify the data by looking at the research methodology, speaking to the original team or cross-checking the facts with other research, it could be hard to be sure that the data is accurate. Think about whether you can use another source, or if it’s worth doing some supplementary primary research to replicate and verify results to help with this issue.
We created a front-to-back guide on conducting market research, The ultimate guide to conducting market research , so you can understand the research journey with confidence.
In it, you’ll learn more about:
- What effective market research looks like
- The use cases for market research
- The most important steps to conducting market research
- And how to take action on your research findings
Download the free guide for a clearer view on secondary research and other key research types for your business.
Related resources
Market intelligence 10 min read, marketing insights 11 min read, ethnographic research 11 min read, qualitative vs quantitative research 13 min read, qualitative research questions 11 min read, qualitative research design 12 min read, primary vs secondary research 14 min read, request demo.
Ready to learn more about Qualtrics?
- Privacy Policy

Home » Secondary Data – Types, Methods and Examples
Secondary Data – Types, Methods and Examples
Table of Contents

Secondary Data
Definition:
Secondary data refers to information that has been collected, processed, and published by someone else, rather than the researcher gathering the data firsthand. This can include data from sources such as government publications, academic journals, market research reports, and other existing datasets.
Secondary Data Types
Types of secondary data are as follows:
- Published data: Published data refers to data that has been published in books, magazines, newspapers, and other print media. Examples include statistical reports, market research reports, and scholarly articles.
- Government data: Government data refers to data collected by government agencies and departments. This can include data on demographics, economic trends, crime rates, and health statistics.
- Commercial data: Commercial data is data collected by businesses for their own purposes. This can include sales data, customer feedback, and market research data.
- Academic data: Academic data refers to data collected by researchers for academic purposes. This can include data from experiments, surveys, and observational studies.
- Online data: Online data refers to data that is available on the internet. This can include social media posts, website analytics, and online customer reviews.
- Organizational data: Organizational data is data collected by businesses or organizations for their own purposes. This can include data on employee performance, financial records, and customer satisfaction.
- Historical data : Historical data refers to data that was collected in the past and is still available for research purposes. This can include census data, historical documents, and archival records.
- International data: International data refers to data collected from other countries for research purposes. This can include data on international trade, health statistics, and demographic trends.
- Public data : Public data refers to data that is available to the general public. This can include data from government agencies, non-profit organizations, and other sources.
- Private data: Private data refers to data that is not available to the general public. This can include confidential business data, personal medical records, and financial data.
- Big data: Big data refers to large, complex datasets that are difficult to manage and analyze using traditional data processing methods. This can include social media data, sensor data, and other types of data generated by digital devices.
Secondary Data Collection Methods
Secondary Data Collection Methods are as follows:
- Published sources: Researchers can gather secondary data from published sources such as books, journals, reports, and newspapers. These sources often provide comprehensive information on a variety of topics.
- Online sources: With the growth of the internet, researchers can now access a vast amount of secondary data online. This includes websites, databases, and online archives.
- Government sources : Government agencies often collect and publish a wide range of secondary data on topics such as demographics, crime rates, and health statistics. Researchers can obtain this data through government websites, publications, or data portals.
- Commercial sources: Businesses often collect and analyze data for marketing research or customer profiling. Researchers can obtain this data through commercial data providers or by purchasing market research reports.
- Academic sources: Researchers can also obtain secondary data from academic sources such as published research studies, academic journals, and dissertations.
- Personal contacts: Researchers can also obtain secondary data from personal contacts, such as experts in a particular field or individuals with specialized knowledge.
Secondary Data Formats
Secondary data can come in various formats depending on the source from which it is obtained. Here are some common formats of secondary data:
- Numeric Data: Numeric data is often in the form of statistics and numerical figures that have been compiled and reported by organizations such as government agencies, research institutions, and commercial enterprises. This can include data such as population figures, GDP, sales figures, and market share.
- Textual Data: Textual data is often in the form of written documents, such as reports, articles, and books. This can include qualitative data such as descriptions, opinions, and narratives.
- Audiovisual Data : Audiovisual data is often in the form of recordings, videos, and photographs. This can include data such as interviews, focus group discussions, and other types of qualitative data.
- Geospatial Data: Geospatial data is often in the form of maps, satellite images, and geographic information systems (GIS) data. This can include data such as demographic information, land use patterns, and transportation networks.
- Transactional Data : Transactional data is often in the form of digital records of financial and business transactions. This can include data such as purchase histories, customer behavior, and financial transactions.
- Social Media Data: Social media data is often in the form of user-generated content from social media platforms such as Facebook, Twitter, and Instagram. This can include data such as user demographics, content trends, and sentiment analysis.
Secondary Data Analysis Methods
Secondary data analysis involves the use of pre-existing data for research purposes. Here are some common methods of secondary data analysis:
- Descriptive Analysis: This method involves describing the characteristics of a dataset, such as the mean, standard deviation, and range of the data. Descriptive analysis can be used to summarize data and provide an overview of trends.
- Inferential Analysis: This method involves making inferences and drawing conclusions about a population based on a sample of data. Inferential analysis can be used to test hypotheses and determine the statistical significance of relationships between variables.
- Content Analysis: This method involves analyzing textual or visual data to identify patterns and themes. Content analysis can be used to study the content of documents, media coverage, and social media posts.
- Time-Series Analysis : This method involves analyzing data over time to identify trends and patterns. Time-series analysis can be used to study economic trends, climate change, and other phenomena that change over time.
- Spatial Analysis : This method involves analyzing data in relation to geographic location. Spatial analysis can be used to study patterns of disease spread, land use patterns, and the effects of environmental factors on health outcomes.
- Meta-Analysis: This method involves combining data from multiple studies to draw conclusions about a particular phenomenon. Meta-analysis can be used to synthesize the results of previous research and provide a more comprehensive understanding of a particular topic.
Secondary Data Gathering Guide
Here are some steps to follow when gathering secondary data:
- Define your research question: Start by defining your research question and identifying the specific information you need to answer it. This will help you identify the type of secondary data you need and where to find it.
- Identify relevant sources: Identify potential sources of secondary data, including published sources, online databases, government sources, and commercial data providers. Consider the reliability and validity of each source.
- Evaluate the quality of the data: Evaluate the quality and reliability of the data you plan to use. Consider the data collection methods, sample size, and potential biases. Make sure the data is relevant to your research question and is suitable for the type of analysis you plan to conduct.
- Collect the data: Collect the relevant data from the identified sources. Use a consistent method to record and organize the data to make analysis easier.
- Validate the data: Validate the data to ensure that it is accurate and reliable. Check for inconsistencies, missing data, and errors. Address any issues before analyzing the data.
- Analyze the data: Analyze the data using appropriate statistical and analytical methods. Use descriptive and inferential statistics to summarize and draw conclusions from the data.
- Interpret the results: Interpret the results of your analysis and draw conclusions based on the data. Make sure your conclusions are supported by the data and are relevant to your research question.
- Communicate the findings : Communicate your findings clearly and concisely. Use appropriate visual aids such as graphs and charts to help explain your results.
Examples of Secondary Data
Here are some examples of secondary data from different fields:
- Healthcare : Hospital records, medical journals, clinical trial data, and disease registries are examples of secondary data sources in healthcare. These sources can provide researchers with information on patient demographics, disease prevalence, and treatment outcomes.
- Marketing : Market research reports, customer surveys, and sales data are examples of secondary data sources in marketing. These sources can provide marketers with information on consumer preferences, market trends, and competitor activity.
- Education : Student test scores, graduation rates, and enrollment statistics are examples of secondary data sources in education. These sources can provide researchers with information on student achievement, teacher effectiveness, and educational disparities.
- Finance : Stock market data, financial statements, and credit reports are examples of secondary data sources in finance. These sources can provide investors with information on market trends, company performance, and creditworthiness.
- Social Science : Government statistics, census data, and survey data are examples of secondary data sources in social science. These sources can provide researchers with information on population demographics, social trends, and political attitudes.
- Environmental Science : Climate data, remote sensing data, and ecological monitoring data are examples of secondary data sources in environmental science. These sources can provide researchers with information on weather patterns, land use, and biodiversity.
Purpose of Secondary Data
The purpose of secondary data is to provide researchers with information that has already been collected by others for other purposes. Secondary data can be used to support research questions, test hypotheses, and answer research objectives. Some of the key purposes of secondary data are:
- To gain a better understanding of the research topic : Secondary data can be used to provide context and background information on a research topic. This can help researchers understand the historical and social context of their research and gain insights into relevant variables and relationships.
- To save time and resources: Collecting new primary data can be time-consuming and expensive. Using existing secondary data sources can save researchers time and resources by providing access to pre-existing data that has already been collected and organized.
- To provide comparative data : Secondary data can be used to compare and contrast findings across different studies or datasets. This can help researchers identify trends, patterns, and relationships that may not have been apparent from individual studies.
- To support triangulation: Triangulation is the process of using multiple sources of data to confirm or refute research findings. Secondary data can be used to support triangulation by providing additional sources of data to support or refute primary research findings.
- To supplement primary data : Secondary data can be used to supplement primary data by providing additional information or insights that were not captured by the primary research. This can help researchers gain a more complete understanding of the research topic and draw more robust conclusions.
When to use Secondary Data
Secondary data can be useful in a variety of research contexts, and there are several situations in which it may be appropriate to use secondary data. Some common situations in which secondary data may be used include:
- When primary data collection is not feasible : Collecting primary data can be time-consuming and expensive, and in some cases, it may not be feasible to collect primary data. In these situations, secondary data can provide valuable insights and information.
- When exploring a new research area : Secondary data can be a useful starting point for researchers who are exploring a new research area. Secondary data can provide context and background information on a research topic, and can help researchers identify key variables and relationships to explore further.
- When comparing and contrasting research findings: Secondary data can be used to compare and contrast findings across different studies or datasets. This can help researchers identify trends, patterns, and relationships that may not have been apparent from individual studies.
- When triangulating research findings: Triangulation is the process of using multiple sources of data to confirm or refute research findings. Secondary data can be used to support triangulation by providing additional sources of data to support or refute primary research findings.
- When validating research findings : Secondary data can be used to validate primary research findings by providing additional sources of data that support or refute the primary findings.
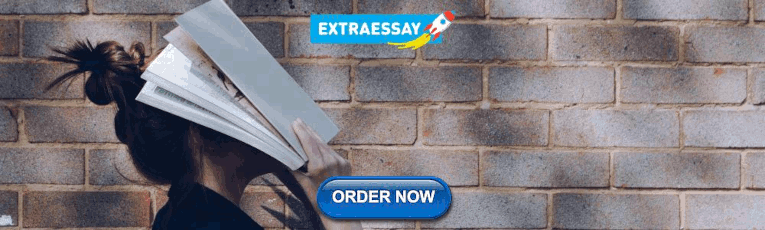
Characteristics of Secondary Data
Secondary data have several characteristics that distinguish them from primary data. Here are some of the key characteristics of secondary data:
- Non-reactive: Secondary data are non-reactive, meaning that they are not collected for the specific purpose of the research study. This means that the researcher has no control over the data collection process, and cannot influence how the data were collected.
- Time-saving: Secondary data are pre-existing, meaning that they have already been collected and organized by someone else. This can save the researcher time and resources, as they do not need to collect the data themselves.
- Wide-ranging : Secondary data sources can provide a wide range of information on a variety of topics. This can be useful for researchers who are exploring a new research area or seeking to compare and contrast research findings.
- Less expensive: Secondary data are generally less expensive than primary data, as they do not require the researcher to incur the costs associated with data collection.
- Potential for bias : Secondary data may be subject to biases that were present in the original data collection process. For example, data may have been collected using a biased sampling method or the data may be incomplete or inaccurate.
- Lack of control: The researcher has no control over the data collection process and cannot ensure that the data were collected using appropriate methods or measures.
- Requires careful evaluation : Secondary data sources must be evaluated carefully to ensure that they are appropriate for the research question and analysis. This includes assessing the quality, reliability, and validity of the data sources.
Advantages of Secondary Data
There are several advantages to using secondary data in research, including:
- Time-saving : Collecting primary data can be time-consuming and expensive. Secondary data can be accessed quickly and easily, which can save researchers time and resources.
- Cost-effective: Secondary data are generally less expensive than primary data, as they do not require the researcher to incur the costs associated with data collection.
- Large sample size : Secondary data sources often have larger sample sizes than primary data sources, which can increase the statistical power of the research.
- Access to historical data : Secondary data sources can provide access to historical data, which can be useful for researchers who are studying trends over time.
- No ethical concerns: Secondary data are already in existence, so there are no ethical concerns related to collecting data from human subjects.
- May be more objective : Secondary data may be more objective than primary data, as the data were not collected for the specific purpose of the research study.
Limitations of Secondary Data
While there are many advantages to using secondary data in research, there are also some limitations that should be considered. Some of the main limitations of secondary data include:
- Lack of control over data quality : Researchers do not have control over the data collection process, which means they cannot ensure the accuracy or completeness of the data.
- Limited availability: Secondary data may not be available for the specific research question or study design.
- Lack of information on sampling and data collection methods: Researchers may not have access to information on the sampling and data collection methods used to gather the secondary data. This can make it difficult to evaluate the quality of the data.
- Data may not be up-to-date: Secondary data may not be up-to-date or relevant to the current research question.
- Data may be incomplete or inaccurate : Secondary data may be incomplete or inaccurate due to missing or incorrect data points, data entry errors, or other factors.
- Biases in data collection: The data may have been collected using biased sampling or data collection methods, which can limit the validity of the data.
- Lack of control over variables: Researchers have limited control over the variables that were measured in the original data collection process, which can limit the ability to draw conclusions about causality.
About the author
Muhammad Hassan
Researcher, Academic Writer, Web developer
You may also like

Primary Data – Types, Methods and Examples

Qualitative Data – Types, Methods and Examples

Research Data – Types Methods and Examples

Quantitative Data – Types, Methods and Examples

Information in Research – Types and Examples

15 Secondary Research Examples

Secondary research is the analysis, summary or synthesis of already existing published research. Instead of collecting original data, as in primary research , secondary research involves data or the results of data analyses already collected.
It is generally published in books, handbooks, textbooks, articles, encyclopedias, websites, magazines, literature reviews and meta-analyses. These are usually referred to as secondary sources .
Secondary research is a good place to start when wanting to acquire a broad view of a research area. It is usually easier to understand and may not require advanced training in research design and statistics.
Secondary Research Examples
1. literature review.
A literature review summarizes, reviews, and critiques the existing published literature on a topic.
Literature reviews are considered secondary research because it is a collection and analysis of the existing literature rather than generating new data for the study.
They hold value for academic studies because they enable us to take stock of the existing knowledge in a field, evaluate it, and identify flaws or gaps in the existing literature. As a result, they’re almost universally used by academics prior to conducting primary research.
Example 1: Workplace stress in nursing: a literature review
Citation: McVicar, A. (2003). Workplace stress in nursing: a literature review. Journal of advanced nursing , 44 (6), 633-642. Source: https://doi.org/10.1046/j.0309-2402.2003.02853.x
Summary: This study conducted a systematic analysis of literature on the causes of stress for nurses in the workplace. The study explored the literature published between 2000 and 2014. The authors found that the literature identifies several main causes of stress for nurses: professional relationships with doctors and staff, communication difficulties with patients and their families, the stress of emergency cases, overwork, lack of staff, and lack of support from the institutions. They conclude that understanding these stress factors can help improve the healthcare system and make it better for both nurses and patients.
Example 2: The impact of shiftwork on health: a literature review
Citation: Matheson, A., O’Brien, L., & Reid, J. A. (2014). The impact of shiftwork on health: a literature review. Journal of Clinical Nursing , 23 (23-24), 3309-3320. Source: https://doi.org/10.1111/jocn.12524
In this literature review, 118 studies were analyzed to examine the impact of shift work on nurses’ health. The findings were organized into three main themes: physical health, psychosocial health, and sleep. The majority of shift work research has primarily focused on these themes, but there is a lack of studies that explore the personal experiences of shift workers and how they navigate the effects of shift work on their daily lives. Consequently, it remains challenging to determine how individuals manage their shift work schedules. They found that, while shift work is an inevitable aspect of the nursing profession, there is limited research specifically targeting nurses and the implications for their self-care.
Example 3: Social media and entrepreneurship research: A literature review
Citation: Olanrewaju, A. S. T., Hossain, M. A., Whiteside, N., & Mercieca, P. (2020). Social media and entrepreneurship research: A literature review. International Journal of Information Management , 50 , 90-110. Source: https://doi.org/10.1016/j.ijinfomgt.2019.05.011
In this literature review, 118 studies were analyzed to examine the impact of shift work on nurses’ health. The findings were organized into three main themes: physical health, social health , and sleep. The majority of shift work research has primarily focused on these themes, but there is a lack of studies that explore the personal experiences of shift workers and how they navigate the effects of shift work on their daily lives. Consequently, it remains challenging to determine how individuals manage their shift work schedules. They found that, while shift work is an inevitable aspect of the nursing profession, there is limited research specifically targeting nurses and the implications for their self-care.
Example 4: Adoption of electric vehicle: A literature review and prospects for sustainability
Citation: Kumar, R. R., & Alok, K. (2020). Adoption of electric vehicle: A literature review and prospects for sustainability. Journal of Cleaner Production , 253 , 119911. Source: https://doi.org/10.1016/j.jclepro.2019.119911
This study is a literature review that aims to synthesize and integrate findings from existing research on electric vehicles. By reviewing 239 articles from top journals, the study identifies key factors that influence electric vehicle adoption. Themes identified included: availability of charging infrastructure and total cost of ownership. The authors propose that this analysis can provide valuable insights for future improvements in electric mobility.
Example 5: Towards an understanding of social media use in the classroom: a literature review
Citation: Van Den Beemt, A., Thurlings, M., & Willems, M. (2020). Towards an understanding of social media use in the classroom: a literature review. Technology, Pedagogy and Education , 29 (1), 35-55. Source: https://doi.org/10.1080/1475939X.2019.1695657
This study examines how social media can be used in education and the challenges teachers face in balancing its potential benefits with potential distractions. The review analyzes 271 research papers. They find that ambiguous results and poor study quality plague the literature. However, they identify several factors affecting the success of social media in the classroom, including: school culture, attitudes towards social media, and learning goals. The study’s value is that it organizes findings from a large corpus of existing research to help understand the topic more comprehensively.
2. Meta-Analyses
Meta-analyses are similar to literature reviews, but are at a larger scale and tend to involve the quantitative synthesis of data from multiple studies to identify trends and derive estimates of overall effect sizes.
For example, while a literature review might be a qualitative assessment of trends in the literature, a meta analysis would be a quantitative assessment, using statistical methods, of studies that meet specific inclusion criteria that can be directly compared and contrasted.
Often, meta-analysis aim to identify whether the existing data can provide an authoritative account for a hypothesis and whether it’s confirmed across the body of literature.
Example 6: Cholesterol and Alzheimer’s Disease Risk: A Meta-Meta-Analysis
Citation: Sáiz-Vazquez, O., Puente-Martínez, A., Ubillos-Landa, S., Pacheco-Bonrostro, J., & Santabárbara, J. (2020). Cholesterol and Alzheimer’s disease risk: a meta-meta-analysis. Brain sciences , 10 (6), 386. Source: https://doi.org/10.3390/brainsci10060386
This study examines the relationship between cholesterol and Alzheimer’s disease (AD). Researchers conducted a systematic search of meta-analyses and reviewed several databases, collecting 100 primary studies and five meta-analyses to analyze the connection between cholesterol and Alzheimer’s disease. They find that the literature compellingly demonstrates that low-density lipoprotein cholesterol (LDL-C) levels significantly influence the development of Alzheimer’s disease, but high-density lipoprotein cholesterol (HDL-C), total cholesterol (TC), and triglycerides (TG) levels do not show significant effects. This is an example of secondary research because it compiles and analyzes data from multiple existing studies and meta-analyses rather than collecting new, original data.
Example 7: The power of feedback revisited: A meta-analysis of educational feedback research
Citation: Wisniewski, B., Zierer, K., & Hattie, J. (2020). The power of feedback revisited: A meta-analysis of educational feedback research. Frontiers in Psychology , 10 , 3087. Source: https://doi.org/10.3389/fpsyg.2019.03087
This meta-analysis examines 435 empirical studies research on the effects of feedback on student learning. They use a random-effects model to ascertain whether there is a clear effect size across the literature. The authors find that feedback tends to impact cognitive and motor skill outcomes but has less of an effect on motivational and behavioral outcomes. A key (albeit somewhat obvious) finding was that the manner in which the feedback is provided is a key factor in whether the feedback is effective.
Example 8: How Much Does Education Improve Intelligence? A Meta-Analysis
Citation: Ritchie, S. J., & Tucker-Drob, E. M. (2018). How much does education improve intelligence? A meta-analysis. Psychological science , 29 (8), 1358-1369. Source: https://doi.org/10.1177/0956797618774253
This study investigates the relationship between years of education and intelligence test scores. The researchers analyzed three types of quasiexperimental studies involving over 600,000 participants to understand if longer education increases intelligence or if more intelligent students simply complete more education. They found that an additional year of education consistently increased cognitive abilities by 1 to 5 IQ points across all broad categories of cognitive ability. The effects persisted throughout the participants’ lives, suggesting that education is an effective way to raise intelligence. This study is an example of secondary research because it compiles and analyzes data from multiple existing studies rather than gathering new, original data.
Example 9: A meta-analysis of factors related to recycling
Citation: Geiger, J. L., Steg, L., Van Der Werff, E., & Ünal, A. B. (2019). A meta-analysis of factors related to recycling. Journal of environmental psychology , 64 , 78-97. Source: https://doi.org/10.1016/j.jenvp.2019.05.004
This study aims to identify key factors influencing recycling behavior across different studies. The researchers conducted a random-effects meta-analysis on 91 studies focusing on individual and household recycling. They found that both individual factors (such as recycling self-identity and personal norms) and contextual factors (like having a bin at home and owning a house) impacted recycling behavior. The analysis also revealed that individual and contextual factors better predicted the intention to recycle rather than the actual recycling behavior. The study offers theoretical and practical implications and suggests that future research should examine the effects of contextual factors and the interplay between individual and contextual factors.
Example 10: Stress management interventions for police officers and recruits
Citation: Patterson, G. T., Chung, I. W., & Swan, P. W. (2014). Stress management interventions for police officers and recruits: A meta-analysis. Journal of experimental criminology , 10 , 487-513. Source: https://doi.org/10.1007/s11292-014-9214-7
The meta-analysis systematically reviews randomized controlled trials and quasi-experimental studies that explore the effects of stress management interventions on outcomes among police officers. It looked at 12 primary studies published between 1984 and 2008. Across the studies, there were a total of 906 participants. Interestingly, it found that the interventions were not effective. Here, we can see how secondary research is valuable sometimes for showing there is no clear trend or consensus in existing literature. The conclusions suggest a need for further research to develop and implement more effective interventions addressing specific stressors and using randomized controlled trials.
3. Textbooks
Academic textbooks tend not to present new research. Rather, they present key academic information in ways that are accessible to university students and academics.
As a result, we can consider textbooks to be secondary rather than primary research. They’re collections of information and research produced by other people, then re-packaged for a specific audience.
Textbooks tend to be written by experts in a topic. However, unlike literature reviews and meta-analyses, they are not necessarily systematic in nature and are not designed to progress current knowledge through identifying gaps, weaknesses, and strengths in the existing literature.
Example 11: Psychology for the Third Millennium: Integrating Cultural and Neuroscience Perspectives
This textbook aims to bridge the gap between two distinct domains in psychology: Qualitative and Cultural Psychology , which focuses on managing meaning and norms, and Neuropsychology and Neuroscience, which studies brain processes. The authors believe that by combining these areas, a more comprehensive general psychology can be achieved, which unites the biological and cultural aspects of human life. This textbook is considered a secondary source because it synthesizes and integrates information from various primary research studies, theories, and perspectives in the field of psychology.
Example 12: Cultural Sociology: An Introduction
Citation: Bennett, A., Back, L., Edles, L. D., Gibson, M., Inglis, D., Jacobs, R., & Woodward, I. (2012). Cultural sociology: an introduction . New York: John Wiley & Sons.
This student textbook introduces cultural sociology and proposes that it is a valid model for sociological thinking and research. It gathers together existing knowledge within the field to prevent an overview of major sociological themes and empirical approaches utilized within cultural sociological research. It does not present new research, but rather packages existing knowledge in sociology and makes it understandable for undergraduate students.
Example 13: A Textbook of Community Nursing
Citation: Chilton, S., & Bain, H. (Eds.). (2017). A textbook of community nursing . New York: Routledge.
This textbook presents an evidence-based introduction to professional topics in nursing. In other words, it gathers evidence from other research and presents it to students. It covers areas such as care approaches, public health, eHealth, therapeutic relationships, and mental health. Like many textbooks, it brings together its own secondary research with user-friendly elements like exercises, activities, and hypothetical case studies in each chapter.
4. White Papers
White papers are typically produced within businesses and government departments rather than academic research environments.
Generally, a white paper will focus on a specific topic of concern to the institution in order to present a state of the current situation as well as opportunities that could be pursued for change, improvement, or profit generation in the future.
Unlike a literature review, a white paper generally doesn’t follow standards of academic rigor and may be presented with a bias toward, or focus on, a company or institution’s mission and values.
Example 14: Future of Mobility White Paper
Citation: Shaheen, S., Totte, H., & Stocker, A. (2018). Future of Mobility White Paper. UC Berkeley: Institute of Transportation Studies at UC Berkeley Source: https://doi.org/10.7922/G2WH2N5D
This white paper explores the how transportation is changing due to concerns over climate change, equity of access to transit, and rapid technological advances (such as shared mobility and automation). The authors aggregate current information and research on key trends, emerging technologies/services, impacts on California’s transportation ecosystem, and future growth projections by reviewing state agency publications, peer-reviewed articles, and forecast reports from various sources. This white paper is an example of secondary research because it synthesizes and integrates information from multiple primary research sources, expert interviews, and input from an advisory committee of local and state transportation agencies.
Example 15: White Paper Concerning Philosophy of Education and Environment
Citation: Humphreys, C., Blenkinsop, S. White Paper Concerning Philosophy of Education and Environment. Stud Philos Educ 36 (1): 243–264. Source: https://doi.org/10.1007/s11217-017-9567-2
This white paper acknowledges the increasing significance of climate change, environmental degradation, and our relationship with nature, and the need for philosophers of education and global citizens to respond. The paper examines five key journals in the philosophy of education to identify the scope and content of current environmental discussions. By organizing and summarizing the located articles, it assesses the possibilities and limitations of these discussions within the philosophy of education community. This white paper is an example of secondary research because it synthesizes and integrates information from multiple primary research sources, specifically articles from the key journals in the field, to analyze the current state of environmental discussions.
5. Academic Essays
Students’ academic essays tend to present secondary rather than primary research. The student is expected to study current literature on a topic and use it to present a thesis statement.
Academic essays tend to require rigorous standards of analysis, critique, and evaluation, but do not require systematic investigation of a topic like you would expect in a literature review.
In an essay, a student may identify the most relevant or important data from a field of research in order to demonstrate their knowledge of a field of study. They may also, after demonstrating sufficient knowledge and understanding, present a thesis statement about the issue.
Secondary research involves data that has already been collected. The published research might be reviewed, included in a meta-analysis, or subjected to a re-analysis.
These findings might be published in a peer-reviewed journal or handbook, become the foundation of a book for public consumption, or presented in a more narrative form for a popular website or magazine.
Sources for secondary research can range from scientific journals to government databases and archived data accumulated by research institutes.
University students might engage in secondary research to become familiar with an area of research. That might help spark an intriguing hypothesis for a research project of master’s thesis.
Secondary research can yield new insights into human behavior , or confirm existing conceptualizations of psychological constructs.
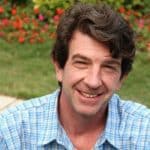
Dave Cornell (PhD)
Dr. Cornell has worked in education for more than 20 years. His work has involved designing teacher certification for Trinity College in London and in-service training for state governments in the United States. He has trained kindergarten teachers in 8 countries and helped businessmen and women open baby centers and kindergartens in 3 countries.
- Dave Cornell (PhD) https://helpfulprofessor.com/author/dave-cornell-phd/ 10 Sensorimotor Stage Examples
- Dave Cornell (PhD) https://helpfulprofessor.com/author/dave-cornell-phd/ 11 Unconditioned Stimulus Examples
- Dave Cornell (PhD) https://helpfulprofessor.com/author/dave-cornell-phd/ 10 Conditioned Stimulus Examples (With Pictures)
- Dave Cornell (PhD) https://helpfulprofessor.com/author/dave-cornell-phd/ 25 Positive Punishment Examples
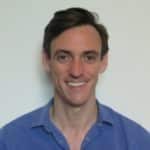
Chris Drew (PhD)
This article was peer-reviewed and edited by Chris Drew (PhD). The review process on Helpful Professor involves having a PhD level expert fact check, edit, and contribute to articles. Reviewers ensure all content reflects expert academic consensus and is backed up with reference to academic studies. Dr. Drew has published over 20 academic articles in scholarly journals. He is the former editor of the Journal of Learning Development in Higher Education and holds a PhD in Education from ACU.
- Chris Drew (PhD) #molongui-disabled-link 10 Sensorimotor Stage Examples
- Chris Drew (PhD) #molongui-disabled-link 11 Unconditioned Stimulus Examples
- Chris Drew (PhD) #molongui-disabled-link 10 Conditioned Stimulus Examples (With Pictures)
- Chris Drew (PhD) #molongui-disabled-link 25 Positive Punishment Examples
Leave a Comment Cancel Reply
Your email address will not be published. Required fields are marked *

An official website of the United States government
The .gov means it’s official. Federal government websites often end in .gov or .mil. Before sharing sensitive information, make sure you’re on a federal government site.
The site is secure. The https:// ensures that you are connecting to the official website and that any information you provide is encrypted and transmitted securely.
- Publications
- Account settings
Preview improvements coming to the PMC website in October 2024. Learn More or Try it out now .
- Advanced Search
- Journal List
- Shanghai Arch Psychiatry
- v.26(6); 2014 Dec
Language: English | Chinese
Secondary analysis of existing data: opportunities and implementation
现有数据的分析 : 机遇与实施, hui g cheng.
1 Shanghai Mental Health Center, Shanghai Jiao Tong University School of Medicine, Shanghai, China
Michael R. PHILLIPS
2 Departments of Psychiatry and Global Health, Emory University, Georgia, United States
The secondary analysis of existing data has become an increasingly popular method of enhancing the overall efficiency of the health research enterprise. But this effort depends on governments, funding agencies, and researchers making the data collected in primary research studies and in health-related registry systems available to qualified researchers who were not involved in the original research or in the creation and maintenance of the registry systems. The benefits of doing this are clear but the barriers are many, so the effort of increasing access to such material has been slow, particularly in low- and middleincome countries. This article introduces the rationale and concept of the secondary analysis of existing data, describes several sources of publicly available datasets, provides general guidelines for conducting secondary analyses of existing data, and discusses the advantages and disadvantages of analyzing existing data.
概述
现有数据的二次分析已成为提升卫生研究机构 整体效率的一种日益流行的方法。该工作取决于政府、 资助机构以及研究者,取决于他们能不能让没有参与 原始研究、没有参与创建和维护登记系统的其他合格 研究人员获得原始研究数据或登记系统的数据。二次 分析的好处是显而易见的,但面临的障碍很多。因此 提高这些数据可获得性的工作进展缓慢,在低收入和 中等收入国家尤为如此。本文介绍了现有数据二次分 析的基本原理和概念,描述了若干个可公开获得的数 据库,为现有数据的二次分析提供一般准则,并讨论 了现有数据分析的优势和不足。
1. Background
A typical mental health research project begins with the development of a comprehensive research proposal and is (hopefully) followed by the successful acquisition of funding; the researcher then collects data, analyzes the results, and writes-up one or more research reports. Another less common, but no less important, research method is the analysis of existing data. The analysis of existing data is a cost-efficient way to make full use of data that are already collected to address potentially important new research questions or to provide a more nuanced assessment of the primary results from the original study. In this article we discuss the distinction between primary and secondary data, provide information about existing mental health-related data that are publically available for further analysis, list the steps of conducting analyzes of existing data, and discuss the pros and cons of analyzing existing data.
2. Data sources
2.1. ‘primary data’, ‘secondary data’, or ‘existing data’.
There is frequently confusion about the use of the terms ‘primary data’, ‘primary data analysis’, ‘secondary data’, and ‘secondary data analysis’. This confusion arises because it is never completely clear whether data employed in an analysis should be considered ‘primary data’ or ‘secondary data’. Based on the usage of the National Institute of Health (NIH) in the United States, ‘primary data analysis’ is limited to the analysis of data by members of the research team that collected the data, which are conducted to answer the original hypotheses proposed in the study. All other analyses of data collected for specific research studies or analyses of data collected for other purposes (including registry data) are considered ‘secondary analyses of existing data’, whether or not the persons conducting the analyses participated in the collection of the data. This replacement of the traditional term ‘secondary data analysis’ with the term ‘secondary analysis of existing data’ is a much clearer categorization because it avoids the confusion of trying to decide whether the data employed in an analysis is ‘primary data’ or ‘secondary data’.
Of course, there are cases where the distinction is less clear. One example would be the analysis of data by a researcher who has no connection with the data collection team to address a research question that overlaps with the hypotheses considered in the original study. Another example would be when a member of the original research team subsequently revisits the original hypothesis in an analysis that uses different statistical methods. These situations commonly occur in the analyses of large-scale population surveys where the research questions are generally broad (e.g., sociodemographic correlates of depression) and when the participating researchers share the cleaned data with the broader research community. In both of these situations, based on a strict application of the NIH usage, the analyses would be considered ‘secondary analysis of existing data’ NOT ‘primary data analysis’ and NOT ‘secondary data analysis’. In fact, we recommend avoiding the ambiguous term ‘secondary data analysis’ entirely.
2.2 . Sources of existing data
Existing data can be private or public. To maximize the output of data collection efforts, researchers often assess many more variables than those strictly needed to answer their original hypotheses. Often times, these data are not fully used or explored by the original research team due to restrictions in time, resources, or interest. Unfortunately, the vast majority of these completed datasets are not made available, and in many countries (including China), there isn’t even a registry or other means of determining what data have been previously collected about a specific research topic (so there are many unnecessarily duplicated studies). However, if the research team is willing to share their data with other researchers who have the interest, skills, and resources to conduct additional analyses, this can greatly increase the productivity of the research team that conducted the original study. This type of exchange usually involves an agreement between the data collection team and the data analysis team to clarify details about data sharing protocols and how the data should be used.
There are several publically available health-related electronic databases that can be used to address a variety of research topics. A few examples follow. (a) The World Health Organization (WHO) Global Health Observatory Data Repository ( http://apps.who.int/ gho/data/?theme=main ) provides statistics on an array of health-related topics for countries around the world. However, these statistics are generally at the country-level so regional or population subgroup-specific data are not usually available. Another similar source is data available on the website of the Institute of Health Metrics and Evaluation at the University of Washington in the United States ( http://www.healthdata.org/ ). This website includes the Global Burden of Disease (GBD) estimates which quantify country-level healthrelated burden (i.e., cause-specific mortality and disability) from 1990 to 2010 and data visualization tools which make it possible to compare the relative importance of different health conditions (including mental disorders) between countries and between different population groups within countries ( http:// www.healthdata.org/gbd/data-visualizations ).
(b) Established in 1962, the Inter-university Consortium for Political and Social Research (ICPSR, http://www.icpsr.umich.edu/icpsrweb/landing.jsps ) is a major data source for scholars in the social sciences. Located at the University of Michigan in the United States, ICPSR is a membership-based network that includes 65, 000 datasets from over 8, 000 discrete studies or surveys, including a number of largescale population surveys conducted in the United States and other countries. The website provides online analysis tools to generate simple descriptive statistics including frequencies and cross-tabulations. In addition to ASCII and .txt format, the website also provides options for downloading data in formats that are compatible with popular statistical software packages such as SAS, Stata, SPSS, and R. The website also provides technical support in data analysis and in the identification of potential data sources. In order to download data, users need to register with the system.
(c) A variety of government agencies in the United States regularly collect data on different health-related topics and post them online for free download once data cleaning is completed. For example, the United States Census Bureau ( http://www.census.gov/data.html ) provides basic demographic data and the Centers for Disease Control and Prevention ( http://www.cdc.gov ) provides access to data on causespecific disability, mortality, and an array of health conditions including injuries and violence, alcohol use, and tobacco smoking. The Substance Abuse and Mental Health Services Administration have a range of datasets posted on their website ( http://www.samhsa.gov/data/ ) about various mental and substance use disorders. Users interested in more information about publicly available health-related data can refer to Secondary data sources for public health: A practical guide by Boslaugh. [1]
3. Conducting a secondary analysis of existing data
There are two general approaches for analyzing existing data: the ‘research question-driven’ approach and the ‘data-driven’ approach. In the research question approach, researchers have an a priori hypothesis or a question in mind and then look for suitable datasets to address the question. In the data-driven approach researchers glance through variables in a particular dataset and decide what kind of questions can be answered by the available data. In practice, the two approaches are often used jointly and iteratively. Researchers typically start with a general idea about the question or hypothesis and then look for available datasets which contain the variables needed to address the research questions of interest. If they do not find datasets that contain all variables needed, they usually modify the research question(s) or the analysis plan based on the best available data.
When conducting either research question-driven or data-driven approaches to the analysis of existing data, researchers need to follow the same basic steps.
(a) There needs to be an analytic plan that includes the specific variables to be considered and the types of analyses that will be conducted. (In the research question-driven approach this is determined before the researchers look at the actual data available in the dataset; in the data-driven approach this is determined after the researchers look through the dataset.)
(b) Researchers must have a comprehensive understanding of the strengths and weaknesses of the dataset. This involves obtaining detailed descriptions of the population under study, sampling scheme and strategy, time frame of data collection, assessment tools, response levels, and quality control measures. To the extent possible, researchers need to obtain and study in detail all survey instruments, codebooks, guidebooks and any other documentation provided for users of the databases. These documents should provide sufficient information to assess the internal and external validity of the data and allow researchers to determine whether or not there are enough cases in the dataset to generate meaningful estimates about the topic(s) of interest.
(c) Before conducting the analysis, researchers need to generate operational definitions of the exposure variable(s), outcome variable(s), covariates, and confounding variables that will be considered in the analysis.
(d) The first step in the analysis is to run frequency tables and cross-tabulations of all variables that will be included in the main analysis. This provides information about the use of the coding pattern for each variable and about the profile of missing data for each variable. Due attention should be paid to skip patterns, which can result in large numbers of missing values for certain variables. In comprehensive surveys that take a long time to complete, skipping a group of questions that are not relevant for a particular respondent (i.e., ‘skips’) is a common method used to reduce interviewee burden and to avoid interviewee burn-out. For example, in a survey about alcohol-related problems, the survey module typically starts with questions about whether the interviewee has ever drunk alcohol. If the answer is negative, all questions about drinking behaviors and related problems are skipped because it is safe to assume that this interviewee does not have any such problems. Prior to conducting the full analysis, these types of missing values (which indicate that a particular condition is not relevant for the respondent) need to be distinguished from missing values for which the data is, in fact, missing (which indicate that the status of the individual related to the variable is unknown). Researchers should be aware of these skips in order to make a strategic judgment about the coding of these variables.
(e) Finally, the researcher should recode the original variables in order to properly handle missing values and, if necessary, to transform the distribution of the variables so that they meet the assumptions of the statistical model to be used in the intended analysis. The recoded variables should be stored in a new dataset and all syntax for the recoding of variables (and for the analysis itself) should be documented. The original dataset should NEVER be altered in any way.
(f) When using data from longitudinal surveys or when using data stored in different datasets, it is critical to check the accuracy of the identifier variable(s) to ensure that the data from different time periods or from different datasets is matched correctly when merging the datasets.
(g) For longitudinal studies, the assessment methods and the coding methods for key variables can change over time. Thus, close examination of the survey questionnaires and codebooks are essential to ensure that each variable in the combined dataset has a uniform interpretation throughout the study. This may require the creation of separate uniform variables that are constructed in different ways at different points in time throughout the study, such as the crosswalks to convert diagnostic categories between DSM-III, DSM-IV, and DSM-5.
(h) Many population-based surveys, particularly those focused on assessing the prevalence of relatively uncommon conditions such as schizophrenia, employ multi-stage sampling strategies to enrich the sample. In this case, the data set usually includes design variables for each case (including sampling weight, strata, and primary sampling unit) that are needed to adjust the analysis of interest (such as the prevalence of a condition, odds ratios, mean differences, etc.). Researchers who conduct secondary analysis of existing data should consider the design variables used in the original study and apply these variables appropriately in their own analyses in order to generate less biased estimates. [2] , [3]
4. Pros and cons of the secondary analysis of existing data
4.1 . advantages.
The most obvious advantage of the secondary analysis of existing data is the low cost. There is sometimes a fee required to obtain access to such datasets, but this is almost always a tiny proportion of what it would cost to conduct an original study. Also, the data posted online are usually cleaned by professional staff members who often provide detailed documentation about the data collection and data cleaning process. Moreover, teams conducting large-scale population-based surveys that are made available to others usually employ statisticians to generate ready-to-use survey weights and design variables - something that most users of the data are unable to do - so this helps users make necessary adjustments to their estimates. This is a great boon to graduate students and others who have lots of good ideas but no money to conduct the studies that could test their ideas.
Researchers who would rather spend their time testing hypotheses and thinking about different research approaches rather than collecting primary data can find a large amount of data online. The increasing availability of such data online encourages the creative use and cross-linking of information from different data sources. For example, experts in hierarchical models can combine data from individual surveys with aggregate data from different administrative levels of a community (e.g., village, township, county, province, etc.) to examine the factors associated with healthrelated outcomes at each level. The availability of such databases also provides statisticians with real-life data to test new statistical models. Such analyses could identify potential new interventions to existing problems that can subsequently be tested in prospective studies.
4.2 . Disadvantages
Inherent to the nature of the secondary analysis of existing data, the available data are not collected to address the particular research question or to test the particular hypothesis. It is not uncommon that some important third variables were not available for the analysis. Similarly, the data may not be collected for all population subgroups of interest or for all geographic regions of interest. Another problem is that to protect the confidentiality of respondents, publicly available datasets usually delete identifying variables about respondents, variables that may be important in the intended analysis such as zip codes, the names of the primary sampling units, and the race, ethnicity, and specific age of respondents. This can create residual confounding when the omitted variables are crucial covariates to control for in the secondary analysis.
Another major limitation of the analysis of existing data is that the researchers who are analyzing the data are not usually the same individuals as those involved in the data collection process. Therefore, they are probably unaware of study-specific nuances or glitches in the data collection process that may be important to the interpretation of specific variables in the dataset. Sometimes, the amount of documentation is daunting (particularly for complex, large-scale surveys conducted by government agencies), so users may miss important details unless they are prominently presented in the documents. Succinct documentation of important information about the validity of the data (by the provider) and careful examination of all relevant documents (by the user) can mitigate this problem.
5. Government support for secondary analysis of existing data
This paper discusses several issues related to the secondary analysis of existing data. There are definitely limitations to such analyses, but the great advantage is that secondary analyses can dramatically increase the overall efficiency of the research effort and - a secondary advantage - give young researchers with good ideas but little access to research funds the opportunity to test their ideas. Recognizing the importance of making the most of high-quality research data and of rapidly translating research findings into actionable knowledge, starting in 2003 the United States National Institute of Health, the largest funding agency for biomedical research in the world, required all projects with annual direct costs of 500, 000 US dollars or more to include data-sharing plans in their proposals. Moreover, NIH has released several program announcements specifically designed to promote secondary analysis of existing datasets. Other countries and some large health care providers also make registry data available to qualified researchers. These practices ensure that other researchers not involved in the studies or in the creation and maintenance of the registries will be able to use the data generated by these big projects or by the registries to test a wide range of hypotheses. Other governments (including the Chinese government), health-related non-government organizations, and other funders of biomedical research need to follow these examples. Failure to provide qualified researchers access to government-generated registry data or to government-supported research data results in a huge but unnecessary wastage of economic and intellectual resources that could be better employed to improve the health of the nation.
Dr. Hui Cheng is an epidemiologist by training. She is currently a post-doctoral research associate at Shanghai Mental Health Center, Shanghai Jiao Tong University School of Medicine. She has published findings from studies on mental health related topics using public data. Her main interest is substance use and related problems, and public mental health.
Funding Statement
This work was supported by a grant from the China Medical Board (13-165) to HGC.
Conflict of interest: The authors declare no conflict of interest related to this article.
- USC Libraries
- Research Guides
Organizing Your Social Sciences Research Paper
- Secondary Sources
- Purpose of Guide
- Design Flaws to Avoid
- Independent and Dependent Variables
- Glossary of Research Terms
- Reading Research Effectively
- Narrowing a Topic Idea
- Broadening a Topic Idea
- Extending the Timeliness of a Topic Idea
- Academic Writing Style
- Applying Critical Thinking
- Choosing a Title
- Making an Outline
- Paragraph Development
- Research Process Video Series
- Executive Summary
- The C.A.R.S. Model
- Background Information
- The Research Problem/Question
- Theoretical Framework
- Citation Tracking
- Content Alert Services
- Evaluating Sources
- Primary Sources
- Tiertiary Sources
- Scholarly vs. Popular Publications
- Qualitative Methods
- Quantitative Methods
- Insiderness
- Using Non-Textual Elements
- Limitations of the Study
- Common Grammar Mistakes
- Writing Concisely
- Avoiding Plagiarism
- Footnotes or Endnotes?
- Further Readings
- Generative AI and Writing
- USC Libraries Tutorials and Other Guides
- Bibliography
In the social sciences, a secondary source is usually a scholar book, journal article, or digital or print document that was created by someone who did not directly experience or participate in the events or conditions under investigation. Secondary sources are not evidence per se, but rather, provide an interpretation, analysis, or commentary derived from the content of primary source materials and/or other secondary sources.
Value of Secondary Sources
To do research, you must cite research. Primary sources do not represent research per se, but only the artifacts from which most research is derived. Therefore, the majority of sources in a literature review are secondary sources that present research findings, analysis, and the evaluation of other researcher's works.
Reviewing secondary source material can be of valu e in improving your overall research paper because secondary sources facilitate the communication of what is known about a topic. This literature also helps you understand the level of uncertainty about what is currently known and what additional information is needed from further research. It is important to note, however, that secondary sources are not the subject of your analysis. Instead, they represent various opinions, interpretations, and arguments about the research problem you are investigating--opinions, interpretations, and arguments with which you may either agree or disagree with as part of your own analysis of the literature.
Examples of secondary sources you could review as part of your overall study include: * Bibliographies [also considered tertiary] * Biographical works * Books, other than fiction and autobiography * Commentaries, criticisms * Dictionaries, Encyclopedias [also considered tertiary] * Histories * Journal articles [depending on the discipline, they can be primary] * Magazine and newspaper articles [this distinction varies by discipline] * Textbooks [also considered tertiary] * Web site [also considered primary]
- << Previous: Primary Sources
- Next: Tiertiary Sources >>
- Last Updated: May 25, 2024 4:09 PM
- URL: https://libguides.usc.edu/writingguide
Thank you for visiting nature.com. You are using a browser version with limited support for CSS. To obtain the best experience, we recommend you use a more up to date browser (or turn off compatibility mode in Internet Explorer). In the meantime, to ensure continued support, we are displaying the site without styles and JavaScript.
- View all journals
- Explore content
- About the journal
- Publish with us
- Sign up for alerts
- Perspective
- Published: 21 May 2024
The state of the art in secondary pharmacology and its impact on the safety of new medicines
- Richard J. Brennan ORCID: orcid.org/0000-0002-0449-2730 1 na1 ,
- Stephen Jenkinson 2 na1 nAff16 ,
- Andrew Brown ORCID: orcid.org/0000-0002-5616-3664 3 ,
- Annie Delaunois ORCID: orcid.org/0000-0001-6345-7989 4 ,
- Bérengère Dumotier ORCID: orcid.org/0000-0002-3360-1510 5 ,
- Malar Pannirselvam 6 nAff17 ,
- Mohan Rao 7 nAff18 ,
- Lyn Rosenbrier Ribeiro 4 , 8 nAff19 ,
- Friedemann Schmidt ORCID: orcid.org/0000-0003-0265-0974 9 ,
- Alicia Sibony 4 ,
- Yoav Timsit 10 ,
- Vicencia Toledo Sales 11 ,
- Duncan Armstrong 10 nAff20 ,
- Armando Lagrutta ORCID: orcid.org/0000-0002-0136-6210 12 ,
- Scott W. Mittlestadt 13 ,
- Russell Naven 11 nAff21 ,
- Ravikumar Peri 11 nAff22 ,
- Sonia Roberts 14 ,
- James M. Vergis ORCID: orcid.org/0009-0000-1362-2368 15 &
- Jean-Pierre Valentin ORCID: orcid.org/0000-0002-3599-9155 4 na1
Nature Reviews Drug Discovery ( 2024 ) Cite this article
1853 Accesses
14 Altmetric
Metrics details
- Pharmacology
Secondary pharmacology screening of investigational small-molecule drugs for potentially adverse off-target activities has become standard practice in pharmaceutical research and development, and regulatory agencies are increasingly requesting data on activity against targets with recognized adverse effect relationships. However, the screening strategies and target panels used by pharmaceutical companies may vary substantially. To help identify commonalities and differences, as well as to highlight opportunities for further optimization of secondary pharmacology assessment, we conducted a broad-ranging survey across 18 companies under the auspices of the DruSafe leadership group of the International Consortium for Innovation and Quality in Pharmaceutical Development. Based on our analysis of this survey and discussions and additional research within the group, we present here an overview of the current state of the art in secondary pharmacology screening. We discuss best practices, including additional safety-associated targets not covered by most current screening panels, and present approaches for interpreting and reporting off-target activities. We also provide an assessment of the safety impact of secondary pharmacology screening, and a perspective on opportunities and challenges in this rapidly developing field.
This is a preview of subscription content, access via your institution
Access options
Access Nature and 54 other Nature Portfolio journals
Get Nature+, our best-value online-access subscription
24,99 € / 30 days
cancel any time
Subscribe to this journal
Receive 12 print issues and online access
195,33 € per year
only 16,28 € per issue
Buy this article
- Purchase on Springer Link
- Instant access to full article PDF
Prices may be subject to local taxes which are calculated during checkout
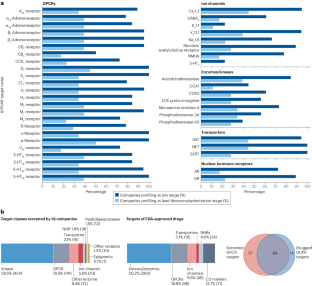
Similar content being viewed by others
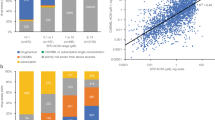
A preclinical secondary pharmacology resource illuminates target-adverse drug reaction associations of marketed drugs
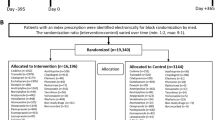
The INGENIOUS trial: Impact of pharmacogenetic testing on adverse events in a pragmatic clinical trial
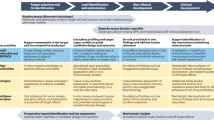
The evolving role of investigative toxicology in the pharmaceutical industry
Jalencas, X. & Mestres, J. On the origins of drug polypharmacology. MedChemComm 4 , 80–87 (2013).
Article CAS Google Scholar
Finan, C. et al. The druggable genome and support for target identification and validation in drug development. Sci. Transl Med. 9 , eaag1166 (2017).
Article PubMed PubMed Central Google Scholar
Dai, S. X., Li, W. X., Li, G. H. & Huang, J. F. Proteome-wide prediction of targets for aspirin: new insight into the molecular mechanism of aspirin. PeerJ 4 , e1791 (2016).
Shapiro, P. A promiscuous kinase inhibitor reveals secrets to cancer cell survival. J. Biol. Chem. 294 , 8674–8675 (2019).
Article CAS PubMed PubMed Central Google Scholar
Bowes, J. et al. Reducing safety-related drug attrition: the use of in vitro pharmacological profiling. Nat. Rev. Drug Discov. 11 , 909–922 (2012).
Article CAS PubMed Google Scholar
Lynch, J. J. III, Van Vleet, T. R., Mittelstadt, S. W. & Blomme, E. A. G. Potential functional and pathological side effects related to off-target pharmacological activity. J. Pharmacol. Toxicol. Methods 87 , 108–126 (2017).
Bendels, S. et al. Safety screening in early drug discovery: an optimized assay panel. J. Pharmacol. Toxicol. Methods 99 , 106609 (2019).
Cook, D. et al. Lessons learned from the fate of AstraZeneca’s drug pipeline: a five-dimensional framework. Nat. Rev. Drug Discov. 13 , 419–431 (2014).
Guengerich, F. P. Mechanisms of drug toxicity and relevance to pharmaceutical development. Drug Metab. Pharmacokinet. 26 , 3–14 (2011).
Weaver, R. J. & Valentin, J. P. Today’s challenges to de-risk and predict drug safety in human “Mind-the-Gap. Toxicol. Sci. 167 , 307–321 (2019).
Hishigaki, H. & Kuhara, S. hERGAPDbase: a database documenting hERG channel inhibitory potentials and APD-prolongation activities of chemical compounds. Database 2011 , bar017 (2011).
Gintant, G., Sager, P. T. & Stockbridge, N. Evolution of strategies to improve preclinical cardiac safety testing. Nat. Rev. Drug Discov. 15 , 457–471 (2016).
Cavero, I. & Guillon, J. M. Safety pharmacology assessment of drugs with biased 5-HT(2B) receptor agonism mediating cardiac valvulopathy. J. Pharmacol. Toxicol. Methods 69 , 150–161 (2014).
Keller, D. A., Brennan, R. J. & Leach, K. L. in Antitargets and Drug Safety (eds Urbán, L., Patel, V. F. & Vaz, R. J.) 365–400 (Wiley, 2015).
Hasinoff, B. B. & Patel, D. The lack of target specificity of small molecule anticancer kinase inhibitors is correlated with their ability to damage myocytes in vitro. Toxicol. Appl. Pharmacol. 249 , 132–139 (2010).
Hasinoff, B. B. The cardiotoxicity and myocyte damage caused by small molecule anticancer tyrosine kinase inhibitors is correlated with lack of target specificity. Toxicol. Appl. Pharmacol. 244 , 190–195 (2010).
Force, T. & Kolaja, K. L. Cardiotoxicity of kinase inhibitors: the prediction and translation of preclinical models to clinical outcomes. Nat. Rev. Drug Discov. 10 , 111–126 (2011).
Urbán, L., Patel, V. F. & Vaz, R. J. (eds) Antitargets and Drug Safety (Wiley, 2015).
Deaton, A. M. et al. Rationalizing secondary pharmacology screening using human genetic and pharmacological evidence. Toxicol. Sci. 167 , 593–603 (2019).
Dodson, A. et al. Aggregation and analysis of secondary pharmacology data from investigational new drug submissions at the US Food and Drug Administration. J. Pharmacol. Toxicol. Methods 111 , 107098 (2021).
Papoian, T. et al. Secondary pharmacology data to assess potential off-target activity of new drugs: a regulatory perspective. Nat. Rev. Drug Discov. 14 , 294 (2015).
Papoian, T. et al. Regulatory forum review*: utility of in vitro secondary pharmacology data to assess risk of drug-induced valvular heart disease in humans: regulatory considerations. Toxicol. Pathol. 45 , 381–388 (2017).
Safety Testing of Drug Metabolites: Guidance for Industry . (FDA, 2020).
Valentin, J. P. et al. In vitro secondary pharmacological profiling: an IQ-DruSafe industry survey on current practices. J. Pharmacol. Toxicol. Methods 93 , 7–14 (2018).
Santos, R. et al. A comprehensive map of molecular drug targets. Nat. Rev. Drug Discov. 16 , 19–34 (2017).
Strauss, D. G., Wu, W. W., Li, Z., Koerner, J. & Garnett, C. Translational models and tools to reduce clinical trials and improve regulatory decision making for QTc and proarrhythmia risk (ICH E14/S7B Updates). Clin. Pharmacol. Ther. 109 , 319–333 (2021).
Vargas, H. M. et al. Time for a fully integrated nonclinical-clinical risk assessment to streamline QT prolongation liability determinations: a pharma industry perspective. Clin. Pharmacol. Ther. 109 , 310–318 (2021).
Article PubMed Google Scholar
Harvey, R. D. in Muscarinic Receptors. Handbook of Experimental Pharmacology , Vol. 208 (eds Fryer, A., Christopoulos, A. & Nathanson, N.) 299–316 (Springer, 2012).
Nguyen, T., Thomas, B. F. & Zhang, Y. Overcoming the psychiatric side effects of the cannabinoid CB1 receptor antagonists: current approaches for therapeutics development. Curr. Top. Med. Chem. 19 , 1418–1435 (2019).
De Vries, T. J. & Shippenberg, T. S. Neural systems underlying opiate addiction. J. Neurosci. 22 , 3321–3325 (2002).
Lucas, J. A., Miller, A. T., Atherly, L. O. & Berg, L. J. The role of Tec family kinases in T cell development and function. Immunol. Rev. 191 , 119–138 (2003).
Popa-Nita, O., Marois, L., Paré, G. & Naccache, P. H. Crystal-induced neutrophil activation: X. proinflammatory role of the tyrosine kinase Tec. Arthritis Rheum. 58 , 1866–1876 (2008).
Greenwell, I. B., Ip, A. & Cohen, J. B. PI3K inhibitors: understanding toxicity mechanisms and management. Oncology 31 , 821–828 (2017).
PubMed Google Scholar
James, M. O. et al. Therapeutic applications of dichloroacetate and the role of glutathione transferase zeta-1. Pharmacol. Ther. 170 , 166–180 (2017).
Freeman-Cook, K. et al. Expanding control of the tumor cell cycle with a CDK2/4/6 inhibitor. Cancer Cell 39 , 1404–1421.e11 (2021).
Howell, K. R., Floyd, K. & Law, A. J. PKBγ/AKT3 loss-of-function causes learning and memory deficits and deregulation of AKT/mTORC2 signaling: relevance for schizophrenia. PLoS ONE 12 , e0175993 (2017).
Bavetsias, V. & Linardopoulos, S. Aurora kinase inhibitors: current status and outlook. Front. Oncol. 5 , 278 (2015).
Brianso, F., Carrascosa, M. C., Oprea, T. I. & Mestres, J. Cross-pharmacology analysis of G protein-coupled receptors. Curr. Top. Med. Chem. 11 , 1956–1963 (2011).
Olney, J. W. et al. NMDA antagonist neurotoxicity: mechanism and prevention. Science 254 , 1515–1518 (1991).
Kenna, J. G. et al. Can bile salt export pump inhibition testing in drug discovery and development reduce liver injury risk? An international transporter consortium perspective. Clin. Pharmacol. Ther. 104 , 916–932 (2018).
Fabian, M. A. et al. A small molecule–kinase interaction map for clinical kinase inhibitors. Nat. Biotechnol. 23 , 329–336 (2005).
Hanson, S. M. et al. What makes a kinase promiscuous for inhibitors? Cell Chem. Biol. 26 , 390–399 (2019).
Dy, G. K. & Adjei, A. A. Understanding, recognizing, and managing toxicities of targeted anticancer therapies. CA Cancer J. Clin. 63 , 249–279 (2013).
Hartmann, J. T., Haap, M., Kopp, H. G. & Lipp, H. P. Tyrosine kinase inhibitors - a review on pharmacology, metabolism and side effects. Curr. Drug Metab. 10 , 470–481 (2009).
Grossman, M. & Adler, E. in Protein Kinases — Promising Targets for Anticancer Drug Research (ed. Singh R. K.) Ch. 2 (IntechOpen, 2021).
Shah, D. R., Shah, R. R. & Morganroth, J. Tyrosine kinase inhibitors: their on-target toxicities as potential indicators of efficacy. Drug Saf. 36 , 413–426 (2013).
Chen, J. et al. Expression and function of the epidermal growth factor receptor in physiology and disease. Physiol. Rev. 96 , 1025–1069 (2016).
Gurule, N. J. & Heasley, L. E. Linking tyrosine kinase inhibitor-mediated inflammation with normal epithelial cell homeostasis and tumor therapeutic responses. Cancer Drug Resist. 1 , 118–125 (2018).
Solassol, I., Pinguet, F. & Quantin, X. FDA- and EMA-approved tyrosine kinase inhibitors in advanced EGFR-mutated non-small cell lung cancer: safety, tolerability, plasma concentration monitoring, and management. Biomolecules 9 , 668 (2019).
Galanis, A. & Levis, M. Inhibition of c-Kit by tyrosine kinase inhibitors. Haematologica 100 , e77–e79 (2015).
Livingstone, E., Zimmer, L., Vaubel, J. & Schadendorf, D. BRAF, MEK and KIT inhibitors for melanoma: adverse events and their management. Chin. Clin. Oncol. 3 , 29 (2014).
Schmidinger, M. Understanding and managing toxicities of vascular endothelial growth factor (VEGF) inhibitors. EJC Suppl. 11 , 172–191 (2013).
Jonker, D. J. et al. A phase I study to determine the safety, pharmacokinetics and pharmacodynamics of a dual VEGFR and FGFR inhibitor, brivanib, in patients with advanced or metastatic solid tumors. Ann. Oncol. 22 , 1413–1419 (2011).
Park, S. et al. Biomarker-driven phase 2 umbrella trial study for patients with recurrent small cell lung cancer failing platinum-based chemotherapy. Cancer 126 , 4002–4012 (2020).
Tirronen, A. et al. The ablation of VEGFR-1 signaling promotes pressure overload-induced cardiac dysfunction and sudden death. Biomolecules 11 , 452 (2021).
Lamore, S. D. et al. Deconvoluting kinase inhibitor induced cardiotoxicity. Toxicol. Sci. 158 , 213–226 (2017).
Jagasia, M. et al. ROCK2 inhibition with belumosudil (KD025) for the treatment of chronic graft-versus-host disease. J. Clin. Oncol. 39 , 1888–1898 (2021).
Hartmann, S., Ridley, A. J. & Lutz, S. The function of Rho-associated kinases ROCK1 and ROCK2 in the pathogenesis of cardiovascular disease. Front. Pharmacol. 6 , 276 (2015).
Zuhl, A. M. et al. Chemoproteomic profiling reveals that cathepsin D off-target activity drives ocular toxicity of β-secretase inhibitors. Nat. Commun. 7 , 13042 (2016).
Martin, K. et al. Pharmacological inhibition of MALT1 protease leads to a progressive IPEX-like pathology. Front. Immunol. 11 , 745 (2020).
Artero, A., Tarín, J. J. & Cano, A. The adverse effects of estrogen and selective estrogen receptor modulators on hemostasis and thrombosis. Semin. Thromb. Hemost. 38 , 797–807 (2012).
Delgado, B. J. & Lopez-Ojeda, W. Estrogen (StatPearls, 2024).
Jia, M., Dahlman-Wright, K. & Gustafsson, J. A. Estrogen receptor alpha and beta in health and disease. Best Pract. Res. Clin. Endocrinol. Metab. 29 , 557–568 (2015).
DIRECTIVE 2010/63/EU OF THE EUROPEAN PARLIAMENT AND OF THE COUNCIL of 22 September 2010 on the protection of animals used for scientific purposes. OJEU https://eur-lex.europa.eu/LexUriServ/LexUriServ.do?uri=OJ:L:2010:276:0033:0079:en:PDF (2010).
FDA Modernization Act of 2021. https://www.congress.gov/bill/117th-congress/house-bill/2565?s=3&r=1 (2021).
Jenkinson, S., Schmidt, F., Rosenbrier Ribeiro, L., Delaunois, A. & Valentin, J. P. A practical guide to secondary pharmacology in drug discovery. J. Pharmacol. Toxicol. Methods 105 , 106869 (2020).
Armstrong, D. et al. in Pharmaceutical Sciences Encyclopedia (eds Gad S. C. et al.) 1–29 (Wiley, 2010).
Redfern, W. et al. Relationships between preclinical cardiac electrophysiology, clinical QT interval prolongation and torsade de pointes for a broad range of drugs: evidence for a provisional safety margin in drug development. Cardiovasc. Res. 58 , 32–45 (2003).
Rosenbrier Ribeiro, L. & Ian Storer, R. A semi-quantitative translational pharmacology analysis to understand the relationship between in vitro ENT1 inhibition and the clinical incidence of dyspnoea and bronchospasm. Toxicol. Appl. Pharmacol. 317 , 41–50 (2017).
Redfern, W. S. et al. Safety pharmacology–a progressive approach. Fundam. Clin. Pharmacol. 16 , 161–173 (2002).
European Medicines Agency. ICH Topic S 7 A: Safety pharmacology studies for human pharmaceuticals. European Medicines Agency ema.europa.eu/en/documents/scientific-guideline/ich-s-7-safety-pharmacology-studies-human-pharmaceuticals-step-5_en.pdf (2001).
ICH Expert Working Group. ICH harmonised tripartite guideline: The non-clinical evaluation of the potential for delayed ventricular repolarization (QT interval prolongation) by human pharmaceuticals. ICH S7B guideline https://database.ich.org/sites/default/files/S7B_Guideline.pdf (2005).
Committee for Medicinal Products for Human Use. ICH guideline M4 (R4) on common technical document (CTD) for the registration of pharmaceuticals for human use - organisation of CTD. European Medicines Agency https://www.ema.europa.eu/documents/scientific-guideline/ich-guideline-m4-r4-common-technical-document-ctd-registration-pharmaceuticals-human-use_en.pdf (2021).
International Council for Harmonisation of Technical Requirements for Pharmaceuticals for Human Use. ICH E14/S7B Implementation Working Group: Clinical and nonclinical evaluation of QT/QTc interval prolongation and proarrhythmic potential — Questions and Answers. ICH https://database.ich.org/sites/default/files/E14-S7B_QAs_Step4_2022_0221.pdf (2022).
U.S. Department of Health and Human Services, Food and Drug Administration & Center for Drug Evaluation and Research. Assessment of Abuse Potential of Drugs: Guidance for Industry. FDA https://www.fda.gov/media/116739/download (2017).
Harding, S. D. et al. The IUPHAR/BPS guide to PHARMACOLOGY in 2022: curating pharmacology for COVID-19, malaria and antibacterials. Nucleic Acids Res. 50 , D1282–D1294 (2022).
Harmer, A. R., Valentin, J. P. & Pollard, C. E. On the relationship between block of the cardiac Na + channel and drug-induced prolongation of the QRS complex. Br. J. Pharmacol. 164 , 260–273 (2011).
Mellor, H. R., Bell, A. R., Valentin, J. P. & Roberts, R. R. Cardiotoxicity associated with targeting kinase pathways in cancer. Toxicol. Sci. 120 , 14–32 (2011).
Sameshima, T. et al. Small-scale panel comprising diverse gene family targets to evaluate compound promiscuity. Chem. Res. Toxicol. 33 , 154–161 (2020).
Simon, I. A. et al. Ligand selectivity hotspots in serotonin GPCRs. Trends Pharmacol. Sci. 44 , 978–990 (2023).
Center for Drug Evaluation and Research. Guidance for Industry: Suicidal Ideation and Behavior: prospective assessment of occurrence in clinical trials. FDA fda.gov/regulatory-information/search-fda-guidance-documents/guidance-industry-suicidal-ideation-and-behavior-prospective-assessment-occurrence-clinical-trials (2012).
Urban, L. et al. Translation of off-target effects: prediction of ADRs by integrated experimental and computational approach. Toxicol. Res. 3 , 433–444 (2014).
Center for Drug Evaluation and Research. Assessment of Pressor Effects of Drugs Guidance for Industry. FDA fda.gov/regulatory-information/search-fda-guidance-documents/assessment-pressor-effects-drugs-guidance-industry (2022).
International Council for Harmonisation of Technical Requirements for Pharmaceuticals for Human Use. Testing for Carcinogenicity of Pharmaceuticals S1B(R1). ICH Database database.ich.org/sites/default/files/ICHS1B%28R1%29_Step4_Presentation_2022_0809.pdf (2022).
Carss, K. J. et al. Using human genetics to improve safety assessment of therapeutics. Nat. Rev. Drug Discov. 22 , 145–162 (2023).
Whitebread, S. et al. Secondary pharmacology: screening and interpretation of off-target activities – focus on translation. Drug Discov. Today 21 , 1232–1242 (2016).
Paolini, G. V., Shapland, R. H., van Hoorn, W. P., Mason, J. S. & Hopkins, A. L. Global mapping of pharmacological space. Nat. Biotechnol. 24 , 805–815 (2006).
Hresko, R. C. & Hruz, P. W. HIV protease inhibitors act as competitive inhibitors of the cytoplasmic glucose binding site of GLUTs with differing affinities for GLUT1 and GLUT4. PLoS ONE 6 , e25237 (2011).
Conn, P. J., Christopoulos, A. & Lindsley, C. W. Allosteric modulators of GPCRs: a novel approach for the treatment of CNS disorders. Nat. Rev. Drug Discov. 8 , 41–54 (2009).
Fischer, G., Rossmann, M. & Hyvönen, M. Alternative modulation of protein-protein interactions by small molecules. Curr. Opin Biotechnol. 35 , 78–85 (2015).
Jones, L. H. et al. Targeted protein degraders: a call for collective action to advance safety assessment. Nat. Rev. Drug Discov. 21 , 401–402 (2022).
Valeur, E. et al. New modalities for challenging targets in drug discovery. Angew. Chem. Int. Ed. 56 , 10294–10323 (2017).
Prachayasittikul, V. et al. Exploring the epigenetic drug discovery landscape. Expert Opin Drug Discov. 12 , 345–362 (2017).
Blanco, M. J. & Gardinier, K. M. New chemical modalities and strategic thinking in early drug discovery. ACS Med. Chem. Lett. 11 , 228–231 (2020).
Sutton, C. W. The role of targeted chemical proteomics in pharmacology. Br. J. Pharmacol. 166 , 457–475 (2012).
van Esbroeck, A. C. M. et al. Activity-based protein profiling reveals off-target proteins of the FAAH inhibitor BIA 10-2474. Science 356 , 1084–1087 (2017).
Freeth, J. & Soden, J. New advances in cell microarray technology to expand applications in target deconvolution and off-target screening. SLAS Discov. 25 , 223–230 (2020).
Hasselgren, C. et al. in Chemoinformatics for Drug Discovery (ed. Bajorath, J.) 267–290 (Wiley, 2013).
Raies, A. B. & Bajic, V. B. In silico toxicology: computational methods for the prediction of chemical toxicity. Wiley Interdiscip. Rev. Comput. Mol. Sci. 6 , 147–172 (2016).
Ietswaart, R. et al. Machine learning guided association of adverse drug reactions with in vitro target-based pharmacology. EBioMedicine 57 , 102837 (2020).
Vamathevan, J. et al. Applications of machine learning in drug discovery and development. Nat. Rev. Drug Discov. 18 , 463–477 (2019).
Scott, C., Dodson, A., Saulnier, M., Snyder, K. & Racz, R. Analysis of secondary pharmacology assays received by the US Food and Drug Administration. J. Pharmacol. Toxicol. Methods 117 , 107205 (2022).
Valentin, J. P. & Leishman, D. 2000–2023 over two decades of ICH S7A: has the time come for a revamp? Regul. Toxicol. Pharmacol. 139 , 105368 (2023).
Valentin, J. P., Sibony, A., Rosseels, M. L. & Delaunois, A. “Appraisal of state-of-the-art” the 2021 distinguished service award of the safety pharmacology society: reflecting on the past to tackle challenges ahead. J. Pharmacol. Toxicol. Methods 123 , 107269 (2023).
Pottel, J. et al. The activities of drug inactive ingredients on biological targets. Science 369 , 403–413 (2020).
Sipes, N. S. et al. Profiling 976 ToxCast chemicals across 331 enzymatic and receptor signaling assays. Chem. Res. Toxicol. 26 , 878–895 (2013).
Bolden, J. E. et al. Inducible in vivo silencing of Brd4 identifies potential toxicities of sustained BET protein inhibition. Cell Rep. 8 , 1919–1929 (2014).
Wagoner, M. et al. Bromodomain and extraterminal (BET) domain inhibitors induce a loss of intestinal stem cells and villous atrophy. Toxicol. Lett. 229 , S75–S76 (2014).
Article Google Scholar
Abbruzzese, G. et al. A European observational study to evaluate the safety and the effectiveness of safinamide in routine clinical practice: the SYNAPSES trial. J. Parkinsons Dis. 11 , 187–198 (2021).
Blackwell, B. & Mabbitt, L. A. Tyramine in cheese related to hypertensive crises after monoamine-oxidase inhibition. Lancet 1 , 938–940 (1965).
Finberg, J. P. & Rabey, J. M. Inhibitors of MAO-A and MAO-B in psychiatry and neurology. Front. Pharmacol. 7 , 340 (2016).
Gross, M. E. et al. Phase 2 trial of monoamine oxidase inhibitor phenelzine in biochemical recurrent prostate cancer. Prostate Cancer Prostatic Dis. 24 , 61–68 (2021).
Woolley, M. L., Marsden, C. A. & Fone, K. C. 5-HT6 receptors. Curr. Drug. Targets CNS Neurol. Disord. 3 , 59–79 (2004).
Boyce, M. et al. Effect of netazepide, a gastrin/CCK2 receptor antagonist, on gastric acid secretion and rabeprazole-induced hypergastrinaemia in healthy subjects. Br. J. Clin. Pharmacol. 79 , 744–755 (2015).
Boyce, M., Warrington, S. & Black, J. Netazepide, a gastrin/CCK2 receptor antagonist, causes dose-dependent, persistent inhibition of the responses to pentagastrin in healthy subjects. Br. J. Clin. Pharmacol. 76 , 689–698 (2013).
Dufresne, M., Seva, C. & Fourmy, D. Cholecystokinin and gastrin receptors. Physiol. Rev. 86 , 805–847 (2006).
Horinouchi, Y. et al. Reduced anxious behavior in mice lacking the CCK2 receptor gene. Eur. Neuropsychopharmacol. 14 , 157–161 (2004).
Moore, A. R. et al. Netazepide, a gastrin receptor antagonist, normalises tumour biomarkers and causes regression of type 1 gastric neuroendocrine tumours in a nonrandomised trial of patients with chronic atrophic gastritis. PLoS ONE 8 , e76462 (2013).
Wang, H., Wong, P. T., Spiess, J. & Zhu, Y. Z. Cholecystokinin-2 (CCK2) receptor-mediated anxiety-like behaviors in rats. Neurosci. Biobehav. Rev. 29 , 1361–1373 (2005).
Zanoveli, J. M., Netto, C. F., Guimarães, F. S. & Zangrossi, H. Jr Systemic and intra-dorsal periaqueductal gray injections of cholecystokinin sulfated octapeptide (CCK-8s) induce a panic-like response in rats submitted to the elevated T-maze. Peptides 25 , 1935–1941 (2004).
Falkai, P. et al. The efficacy and safety of cariprazine in the early and late stage of schizophrenia: a post hoc analysis of three randomized, placebo-controlled trials. CNS Spectr. 28 , 104–111 (2021).
Guma, E. et al. Role of D3 dopamine receptors in modulating neuroanatomical changes in response to antipsychotic administration. Sci. Rep. 9 , 7850 (2019).
Guo, K. et al. Safety profile of antipsychotic drugs: analysis based on a provincial spontaneous reporting systems database. Front. Pharmacol. 13 , 848472 (2022).
Heidbreder, C. A. et al. The role of central dopamine D3 receptors in drug addiction: a review of pharmacological evidence. Brain Res. Rev. 49 , 77–105 (2005).
Periclou, A. et al. Relationship between plasma concentrations and clinical effects of cariprazine in patients with schizophrenia or bipolar mania. Clin. Transl Sci. 13 , 362–371 (2020).
Hodge, R. J. & Nunez, D. J. Therapeutic potential of Takeda-G-protein-receptor-5 (TGR5) agonists. Hope or hype? Diabetes Obes. Metab. 18 , 439–443 (2016).
McNeil, B. D. et al. Identification of a mast-cell-specific receptor crucial for pseudo-allergic drug reactions. Nature 519 , 237–241 (2015).
Grimes, J. et al. MrgX2 is a promiscuous receptor for basic peptides causing mast cell pseudo-allergic and anaphylactoid reactions. Pharmacol. Res. Perspect. 7 , e00547 (2019).
Barrett, J. et al. Tachykinin receptors (version 2019.4). in IUPHAR/BPS Guide to Pharmacology CITE . https://doi.org/10.2218/gtopdb/F62/2019.4 (2019).
Smits, G. J. & Lefebvre, R. A. Tachykinin receptors involved in the contractile effect of the natural tachykinins in the rat gastric fundus. J. Auton. Pharmacol. 14 , 383–392 (1994).
Valero, M. S. et al. Contractile effect of tachykinins on rabbit small intestine. Acta Pharmacol. Sin. 32 , 487–494 (2011).
Vilain, P., Emonds-Alt, X., Le Fur, G. & Brelière, J. C. Tachykinin-induced contractions of the guinea pig ileum longitudinal smooth muscle: tonic and phasic muscular activities. Can. J. Physiol. Pharmacol. 75 , 587–590 (1997).
Amenyogbe, E. et al. A review on sex steroid hormone estrogen receptors in mammals and fish. Int. J. Endocrinol. 2020 , 5386193 (2020).
Scarpin, K. M., Graham, J. D., Mote, P. A. & Clarke, C. L. Progesterone action in human tissues: regulation by progesterone receptor (PR) isoform expression, nuclear positioning and coregulator expression. Nucl. Recept. Signal. 7 , e009 (2009).
Spitz, I. M. Progesterone receptor antagonists. Curr. Opin Investig. Drugs 7 , 882–890 (2006).
CAS PubMed Google Scholar
Chen, J. Y. et al. Two distinct actions of retinoid-receptor ligands. Nature 382 , 819–822 (1996).
Chung, S. S. et al. Pharmacological activity of retinoic acid receptor alpha-selective antagonists in vitro and in vivo. ACS Med. Chem. Lett. 4 , 446–450 (2013).
Scimemi, A. Structure, function, and plasticity of GABA transporters. Front. Cell Neurosci. 8 , 161 (2014).
Zafar, S. & Jabeen, I. Structure, function, and modulation of γ-aminobutyric acid transporter 1 (GAT1) in neurological disorders: a pharmacoinformatic prospective. Front. Chem. 6 , 397 (2018).
Frosina, G., Marubbi, D., Marcello, D., Vecchio, D. & Daga, A. The efficacy and toxicity of ATM inhibition in glioblastoma initiating cells-driven tumor models. Crit. Rev. Oncol. Hematol. 138 , 214–222 (2019).
Majd, N. K. et al. The promise of DNA damage response inhibitors for the treatment of glioblastoma. Neuro-Oncol. Adv. 3 , vdab015 (2021).
Pizzamiglio, L. et al. New role of ATM in controlling GABAergic tone during development. Cereb. Cortex 26 , 3879–3888 (2016).
Tassinari, V. et al. Atrophy, oxidative switching and ultrastructural defects in skeletal muscle of the ataxia telangiectasia mouse model. J. Cell Sci. 132 , jcs223008 (2019).
Bhushan, B. et al. Dual role of epidermal growth factor receptor in liver injury and regeneration after acetaminophen overdose in mice. Toxicol. Sci. 155 , 363–378 (2017).
Kirchner, S. in Polypharmacology in Drug Discovery (ed. Peters, J.-U.) Ch. 4 (Wiley, 2012).
Horta, E., Bongiorno, C., Ezzeddine, M. & Neil, E. C. Neurotoxicity of antibodies in cancer therapy: a review. Clin. Neurol. Neurosurg. 188 , 105566 (2020).
Huang, J. et al. Safety profile of epidermal growth factor receptor tyrosine kinase inhibitors: a disproportionality analysis of FDA adverse event reporting system. Sci. Rep. 10 , 4803 (2020).
Miroddi, M. et al. Systematic review and meta-analysis of the risk of severe and life-threatening thromboembolism in cancer patients receiving anti-EGFR monoclonal antibodies (cetuximab or panitumumab). Int. J. Cancer 139 , 2370–2380 (2016).
Ohmori, T. et al. Molecular and clinical features of EGFR-TKI-associated lung injury. Int. J. Mol. Sci. 22 , 792 (2021).
Rizzo, A. et al. Anti-EGFR monoclonal antibodies in advanced biliary tract cancer: a systematic review and meta-analysis. In Vivo 34 , 479–488 (2020).
Shah, R. R. & Shah, D. R. Safety and tolerability of epidermal growth factor receptor (EGFR) tyrosine kinase inhibitors in oncology. Drug Saf. 42 , 181–198 (2019).
Tischer, B., Huber, R., Kraemer, M. & Lacouture, M. E. Dermatologic events from EGFR inhibitors: the issue of the missing patient voice. Support. Care Cancer 25 , 651–660 (2017).
Baruch, A. et al. Antibody-mediated activation of the FGFR1/Klothoβ complex corrects metabolic dysfunction and alters food preference in obese humans. Proc. Natl Acad. Sci. USA 117 , 28992–29000 (2020).
Chae, Y. K. et al. Inhibition of the fibroblast growth factor receptor (FGFR) pathway: the current landscape and barriers to clinical application. Oncotarget 8 , 16052–16074 (2017).
Gile, J. J. et al. FGFR inhibitor toxicity and efficacy in cholangiocarcinoma: multicenter single-institution cohort experience. JCO Precis. Oncol . https://doi.org/10.1200/PO.21.00064 (2021).
Kommalapati, A., Tella, S. H., Borad, M., Javle, M. & Mahipal, A. FGFR inhibitors in oncology: insight on the management of toxicities in clinical practice. Cancers 13 , 2968 (2021).
Mahipal, A., Tella, S. H., Kommalapati, A., Yu, J. & Kim, R. Prevention and treatment of FGFR inhibitor-associated toxicities. Crit. Rev. Oncol. Hematol. 155 , 103091 (2020).
Sonoda, J., Chen, M. Z. & Baruch, A. FGF21-receptor agonists: an emerging therapeutic class for obesity-related diseases. Horm. Mol. Biol. Clin. Investig. 30 , 20170002 (2017).
Tassi, E. et al. Blood pressure control by a secreted FGFBP1 (fibroblast growth factor-binding protein). Hypertension 71 , 160–167 (2018).
Wu, A.-L. et al. Antibody-mediated activation of FGFR1 induces FGF23 production and hypophosphatemia. PLoS ONE 8 , e57322 (2013).
Xie, Y. et al. FGF/FGFR signaling in health and disease. Signal Transduct. Target. Ther. 5 , 181 (2020).
Gómez-Sintes, R. et al. Neuronal apoptosis and reversible motor deficit in dominant-negative GSK-3 conditional transgenic mice. EMBO J. 26 , 2743–2754 (2007).
Hurcombe, J. A. et al. Podocyte GSK3 is an evolutionarily conserved critical regulator of kidney function. Nat. Commun. 10 , 403 (2019).
Boucher, J. et al. Differential roles of insulin and IGF-1 receptors in adipose tissue development and function. Diabetes 65 , 2201–2213 (2016).
Cai, W. et al. Insulin regulates astrocyte gliotransmission and modulates behavior. J. Clin. Investig. 128 , 2914–2926 (2018).
Srivastava, S. P. & Goodwin, J. E. Cancer biology and prevention in diabetes. Cells 9 , 1380 (2020).
Bharate, J. B. et al. Rational design, synthesis and biological evaluation of pyrimidine-4,6-diamine derivatives as type-II inhibitors of FLT3 selective against c-KIT. Sci. Rep. 8 , 3722 (2018).
Omdal, R., Skoie, I. M. & Grimstad, T. Fatigue is common and severe in patients with mastocytosis. Int. J. Immunopathol. Pharmacol. 32 , 2058738418803252 (2018).
Openshaw, R. L. et al. Map2k7 haploinsufficiency induces brain imaging endophenotypes and behavioral phenotypes relevant to schizophrenia. Schizophr. Bull. 46 , 211–223 (2020).
Cocco, E., Scaltriti, M. & Drilon, A. NTRK fusion-positive cancers and TRK inhibitor therapy. Nat. Rev. Clin. Oncol. 15 , 731–747 (2018).
Drilon, A. TRK inhibitors in TRK fusion-positive cancers. Ann. Oncol. 30 , viii23–viii30 (2019).
Gambella, A. et al. NTRK fusions in central nervous system tumors: a rare, but worthy target. Int. J. Mol. Sci. 21 , 753 (2020).
Han, S.-Y. TRK inhibitors: tissue-agnostic anti-cancer drugs. Pharmaceuticals 14 , 632 (2021).
Rohrberg, K. S. & Lassen, U. Detecting and targeting NTRK fusions in cancer in the era of tumor agnostic oncology. Drugs 81 , 445–452 (2021).
Sanchez-Ortiz, E. et al. TrkA gene ablation in basal forebrain results in dysfunction of the cholinergic circuitry. J. Neurosci. 32 , 4065–4079 (2012).
Chong, C. R., Ong, G. J. & Horowitz, J. D. Emerging drugs for the treatment of angina pectoris. Expert Opin Emerg. Drugs 21 , 365–376 (2016).
Heinemann-Yerushalmi, L. et al. BCKDK regulates the TCA cycle through PDC in the absence of PDK family during embryonic development. Dev. Cell 56 , 1182–1194 (2021).
Stakišaitis, D. et al. The importance of gender-related anticancer research on mitochondrial regulator sodium dichloroacetate in preclinical studies in vivo. Cancers 11 , 1210 (2019).
Wang, H. et al. Deletion of PDK1 in oligodendrocyte lineage cells causes white matter abnormality and myelination defect in the central nervous system. Neurobiol. Dis. 148 , 105212 (2021).
Drullinsky, P. R. & Hurvitz, S. A. Mechanistic basis for PI3K inhibitor antitumor activity and adverse reactions in advanced breast cancer. Breast Cancer Res. Treat. 181 , 233–248 (2020).
Gustafson, D., Fish, J. E., Lipton, J. H. & Aghel, N. Mechanisms of cardiovascular toxicity of BCR-ABL1 tyrosine kinase inhibitors in chronic myelogenous leukemia. Curr. Hematol. Malig. Rep. 15 , 20–30 (2020).
Nunnery, S. E. & Mayer, I. A. Management of toxicity to isoform α-specific PI3K inhibitors. Ann. Oncol. 30 , x21–x26 (2019).
Yap, T. A., Bjerke, L., Clarke, P. A. & Workman, P. Drugging PI3K in cancer: refining targets and therapeutic strategies. Curr. Opin Pharmacol. 23 , 98–107 (2015).
Chen, Y. et al. Focal adhesion kinase promotes hepatic stellate cell activation by regulating plasma membrane localization of TGFβ receptor 2. Hepatol. Commun. 4 , 268–283 (2020).
Dawson, J. C., Serrels, A., Stupack, D. G., Schlaepfer, D. D. & Frame, M. C. Targeting FAK in anticancer combination therapies. Nat. Rev. Cancer 21 , 313–324 (2021).
Guidetti, G. F., Torti, M. & Canobbio, I. Focal adhesion kinases in platelet function and thrombosis. Arterioscler. Thromb. Vasc. Biol. 39 , 857–868 (2019).
Lassiter, D. G. et al. FAK tyrosine phosphorylation is regulated by AMPK and controls metabolism in human skeletal muscle. Diabetologia 61 , 424–432 (2018).
Peng, X. et al. Cardiac developmental defects and eccentric right ventricular hypertrophy in cardiomyocyte focal adhesion kinase (FAK) conditional knockout mice. Proc. Natl Acad. Sci. USA 105 , 6638–6643 (2008).
Sorkin, M. et al. Novel strategies to attenuate skin fibrosis: targeted inhibition of focal adhesion kinase in dermal fibroblasts. J. Am. Coll. Surg. 211 , S127 (2010).
Weng, Y. et al. Liver epithelial focal adhesion kinase modulates fibrogenesis and hedgehog signaling. JCI Insight 5 , e141217 (2020).
Zhang, J. & Hochwald, S. N. The role of FAK in tumor metabolism and therapy. Pharmacol. Ther. 142 , 154–163 (2014).
Zhao, X.-K. et al. Focal adhesion kinase regulates hepatic stellate cell activation and liver fibrosis. Sci. Rep. 7 , 4032 (2017).
Greathouse, K. M., Henderson, B. W., Gentry, E. G. & Herskowitz, J. H. Fasudil or genetic depletion of ROCK1 or ROCK2 induces anxiety-like behaviors. Behav. Brain Res. 373 , 112083 (2019).
Kusuhara, S. & Nakamura, M. Ripasudil hydrochloride hydrate in the treatment of glaucoma: safety, efficacy, and patient selection. Clin. Ophthalmol. 14 , 1229–1236 (2020).
Li, J. et al. Renal protective effects of empagliflozin via inhibition of EMT and aberrant glycolysis in proximal tubules. JCI Insight 5 , e129034 (2020).
McLeod, R. et al. First-in-human study of AT13148, a dual ROCK-AKT inhibitor in patients with solid tumors. Clin. Cancer Res. 26 , 4777–4784 (2020).
Sunamura, S. et al. Different roles of myocardial ROCK1 and ROCK2 in cardiac dysfunction and postcapillary pulmonary hypertension in mice. Proc. Natl Acad. Sci. USA 115 , E7129–E7138 (2018).
Pappu, R. Essential tole for the RHO-KINASES in intestinal stem cell viability and maintenance of organ homeostasis [abstract T.126]. Federation of Clinical Immunology Societies Meeting 2019 (2019).
Zheng, K. et al. miR-135a-5p mediates memory and synaptic impairments via the Rock2/Adducin1 signaling pathway in a mouse model of Alzheimer’s disease. Nat. Commun. 12 , 1903 (2021).
De Kock, L. et al. De novo variant in tyrosine kinase SRC causes thrombocytopenia: case report of a second family. Platelets 30 , 931–934 (2019).
Li, J. et al. Heat-induced epithelial barrier dysfunction occurs via C-Src kinase and P120ctn expression regulation in the lungs. Cell. Physiol. Biochem. 48 , 237–250 (2018).
Revilla, N. et al. Clinical and biological assessment of the largest family with SRC‐RT due to p. E527K gain‐of‐function variant [abstract]. Res. Pract. Thromb. Haemost. 5 (suppl. 2), 145–146 (2021).
Google Scholar
Yo, S., Thenganatt, J., Lipton, J. & Granton, J. Incident pulmonary arterial hypertension associated with Bosutinib. Pulm. Circ. 10 , 1–4 (2020).
Yurttas, N. O. & Eskazan, A. E. Tyrosine kinase inhibitor-associated platelet dysfunction: does this need to have a significant clinical impact? Clin. Appl. Thromb. Hemost. 25 , https://doi.org/10.1177/1076029619866925 (2019).
Garcia-Serna, R., Vidal, D., Remez, N. & Mestres, J. Large-scale predictive drug safety: from structural alerts to biological mechanisms. Chem. Res. Toxicol. 28 , 1875–1887 (2015).
Compilation of CDER NME and new biologic approvals 1985–2022. FDA fda.gov/media/135307/download (2022).
Download references
Acknowledgements
The authors thank the following individuals for their valuable contributions: K. A. Henderson from Amgen, C. J. B. Larner from AstraZeneca, A. Fekete from Novartis, E. Pawluk from UCB Biopharma and the entire membership of the IQ-DruSafe In vitro Secondary Pharmacology Working Group. J.-P.V., R.J.B. and S.J. are the Chair and Co-Chairs of the IQ-DruSafe In vitro Secondary Pharmacology Working Group, respectively.
Author information
Stephen Jenkinson
Present address: Metrion Biosciences, San Diego, CA, USA
Malar Pannirselvam
Present address: GSK, Waltham, MA, USA
Present address: Neurocrine Biosciences, San Diego, CA, USA
Lyn Rosenbrier Ribeiro
Present address: Grunenthal, Berkshire, UK
Duncan Armstrong
Present address: Armstrong Pharmacology, Macclesfield, UK
Russell Naven
Present address: Novartis Biomedical Research, Cambridge, MA, USA
Ravikumar Peri
Present address: Alexion Pharmaceuticals, Wilmington, DE, USA
These authors contributed equally: Richard J. Brennan, Stephen Jenkinson, Jean-Pierre Valentin.
Authors and Affiliations
Sanofi, Cambridge, MA, USA
Richard J. Brennan
Pfizer, La Jolla, CA, USA
GSK, Stevenage, UK
Andrew Brown
UCB Biopharma, Braine-l’Alleud, Belgium
Annie Delaunois, Lyn Rosenbrier Ribeiro, Alicia Sibony & Jean-Pierre Valentin
Novartis Biomedical Research, Basel, Switzerland
Bérengère Dumotier
Vertex Pharmaceuticals, Boston, MA, USA
Janssen Research & Development, San Diego, CA, USA
AstraZeneca, Cambridge, UK
Sanofi, Frankfurt, Germany
Friedemann Schmidt
Novartis Biomedical Research, Cambridge, MA, USA
Yoav Timsit & Duncan Armstrong
Takeda Pharmaceuticals, Cambridge, MA, USA
Vicencia Toledo Sales, Russell Naven & Ravikumar Peri
Merck, North Wales, PA, USA
Armando Lagrutta
AbbVie, North Chicago, IL, USA
Scott W. Mittlestadt
Roche Pharma Research and Early Development, Roche Innovation Center, Basel, Switzerland
Sonia Roberts
Faegre Drinker Biddle and Reath, LLP, Washington, DC, USA
James M. Vergis
You can also search for this author in PubMed Google Scholar
Contributions
R.J.B., S.J., A.B., A.D., B.D., M.P., M.R., L.R.R., F.S., A.S., Y.T., V.T.S., D.A., A.L., S.W.M., R.N., R.P., S.R., J.M.V. and J.-P.V. researched data for the article. R.J.B., S.J., A.B., A.D., B.D., M.P., M.R., L.R.R., F.S., A.S., Y.T., V.T.S., A.L., S.W.M., R.P., S.R., J.M.V. and J.-P.V. reviewed and/or edited the manuscript before submission. R.J.B., S.J., A.B., A.D., B.D., M.P., M.R., L.R.R., F.S., A.S., Y.T., V.T.S., D.A., A.L., S.W.M., R.P., S.R. and J.-P.V. made a substantial contribution to discussion of content. R.J.B., S.J., A.B., A.D., B.D, M.P., L.R.R., F.S., A.S., Y.T., V.T.S., S.W.M., S.R. and J.-P.V. wrote the article.
Corresponding author
Correspondence to Richard J. Brennan .
Ethics declarations
Competing interests.
A.B., A.D., B.D., M.P., M.R., L.R.R., F.S., A.S., Y.T., V.T.S., A.L., S.W.M., R.N., R.P., S.R. and J.-P.V. are employees of pharmaceutical companies. R.J.B. is a Director/Trustee and Scientific Advisory Board member of Lhasa Ltd., which manages the Effiris consortium. S.J. is an employee of a CRO providing screening services to pharmaceutical companies. D.A., R.J.B., S.J., A.B., A.D., M.P., M.R., L.R.R., F.S., A.S., Y.T., V.T.S., A.L., S.W.M., R.N., R.P., S.R. and J.-P.V. hold shares, share rights and/or stock options in pharmaceutical companies. J.M.V. declares no competing interests.
Peer review
Peer review information.
Nature Reviews Drug Discovery thanks Wolfgang Jarolimek and the other, anonymous, reviewer(s) for their contribution to the peer review of this work.
Additional information
Publisher’s note Springer Nature remains neutral with regard to jurisdictional claims in published maps and institutional affiliations.
Related links
CiPA Initiative: https://cipaproject.org/
CredibleMeds QTDrugs Lists: https://www.crediblemeds.org/druglist
Effiris Consortium: https://www.lhasalimited.org/effiris-a-secondary-pharmacology-model-suite-powered-by-privacy-preserving-data-sharing/
Elsevier PharmaPendium: https://www.pharmapendium.com
International Consortium for Innovation and Quality in Pharmaceutical Development (IQ): https://iqconsortium.org
International Council for Harmonisation of Technical Requirements for Pharmaceuticals for Human Use (ICH): https://www.ich.org
NC3Rs: https://www.nc3rs.org.uk/who-we-are/3rs
Pistoia Alliance: https://www.pistoiaalliance.org/
The International Union of Basic and Clinical Pharmacology (IUPHAR): https://www.guidetopharmacology.org/
Who are the top 10 pharmaceutical companies in the world? (2023): https://www.proclinical.com/blogs/2023-7/the-top-10-pharmaceutical-companies-in-the-world-2023
Supplementary information
Supplementary information, supplementary table 1, supplementary table 2, supplementary table 3.
Harmful, unintended results caused by the act of taking a medication. An adverse drug reaction is a special type of adverse event in which a causative relationship can be demonstrated.
(AE). Any untoward medical occurrence in a patient or clinical investigation subject administered a pharmaceutical product, which does not necessarily have a causal relationship with this treatment.
The number of compounds showing activity in a specific assay as a proportion of the total number of compounds evaluated in the assay.
Promiscuity is defined as the specific interaction of a small molecule with multiple biological targets (as opposed to nonspecific binding events) and represents the molecular basis of polypharmacology. The promiscuity rate of a compound refers to the number of off-target hits as a proportion of the total number of targets assayed.
A leadership group of the International Consortium for Innovation and Quality in Pharmaceutical Development with the mission to advance nonclinical safety sciences and impact the global regulatory environment.
(ICH). An initiative that brings together regulatory authorities and the pharmaceutical industry to discuss scientific and technical aspects of pharmaceutical product development and registration. The mission of the ICH is to promote public health by achieving greater harmonization through the development of technical guidelines and requirements for pharmaceutical product registration.
A document through which drug sponsors formally propose that the US Food and Drug Administration (FDA) approve a new pharmaceutical for sale and marketing in the USA.
New drugs containing active ingredients not previously approved by the FDA or marketed as drugs in the USA.
Pharmacological activity of a drug against a target other than its intended therapeutic target.
These studies aim to investigate the mode of action and/or effects of a substance in relation to its desired therapeutic target.
These studies aim to investigate the potential undesirable pharmacodynamic effects of a substance on physiological functions in relation to exposure in the therapeutic range and above. Safety pharmacodynamic effects may result from activity at the primary molecular target, secondary targets or nonspecific interactions.
These studies aim to investigate the mode of action and/or effects of a substance not related to its desired therapeutic target.
Unintended pharmacological effects of a drug, frequently used to describe adverse effects, but this term may also apply to additional beneficial consequences.
(IQ). A not-for-profit organization of pharmaceutical and biotechnology companies with a mission of advancing science and technology to augment the capability of member companies to develop transformational solutions that benefit patients, regulators and the broader research and development community.
Rights and permissions
Springer Nature or its licensor (e.g. a society or other partner) holds exclusive rights to this article under a publishing agreement with the author(s) or other rightsholder(s); author self-archiving of the accepted manuscript version of this article is solely governed by the terms of such publishing agreement and applicable law.
Reprints and permissions
About this article
Cite this article.
Brennan, R.J., Jenkinson, S., Brown, A. et al. The state of the art in secondary pharmacology and its impact on the safety of new medicines. Nat Rev Drug Discov (2024). https://doi.org/10.1038/s41573-024-00942-3
Download citation
Accepted : 05 April 2024
Published : 21 May 2024
DOI : https://doi.org/10.1038/s41573-024-00942-3
Share this article
Anyone you share the following link with will be able to read this content:
Sorry, a shareable link is not currently available for this article.
Provided by the Springer Nature SharedIt content-sharing initiative
Quick links
- Explore articles by subject
- Guide to authors
- Editorial policies
Sign up for the Nature Briefing: Translational Research newsletter — top stories in biotechnology, drug discovery and pharma.

Improving economic policy
Suggested keywords:
- decarbonisation
- climate change
- monetary policy
- / Publications
Research, innovation and data: a fifth freedom in the EU single market?
Research and innovation should be at the top of the EU economic policy agenda, but cannot over-rely on public investment
Share this page:
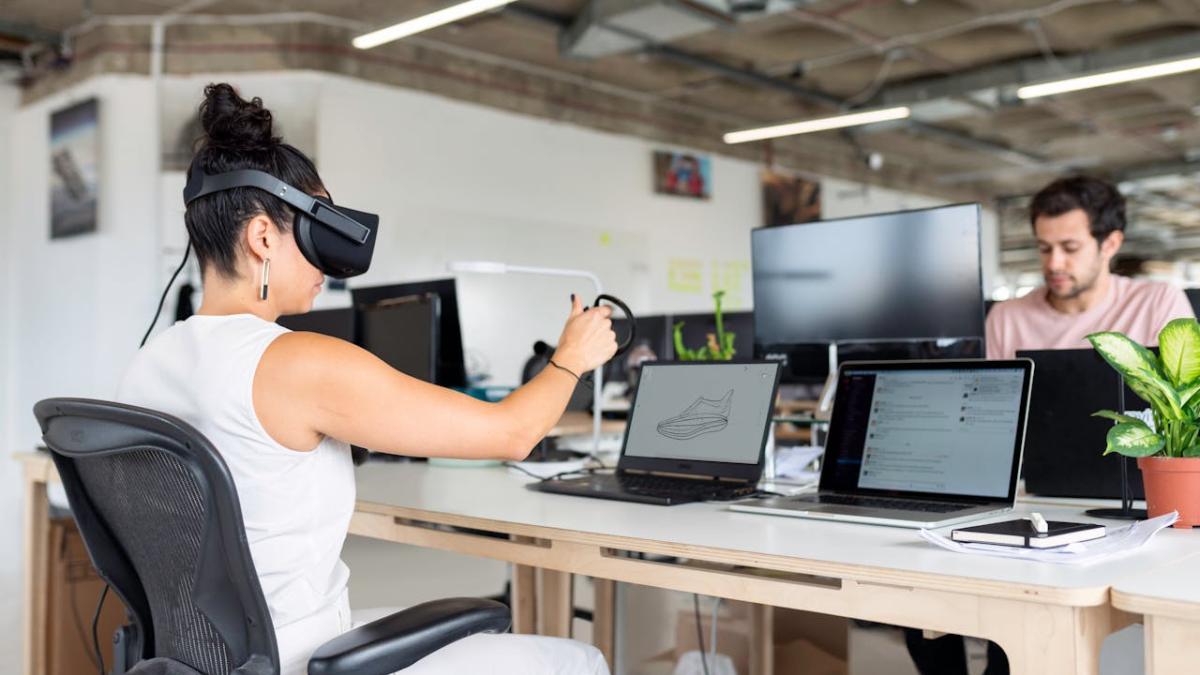
The European Union’s single market famously enables four freedoms: the movement of goods, services, capital and labour. Former Italian prime minister Enrico Letta, in his report on the single market issued and discussed by EU leaders in April, proposed a fifth freedom as a top priority, to encompass research, innovation, data and knowledge that have become indispensable drivers of innovation in modern economies (Letta, 2024) 1 The idea of a fifth freedom is not new. It was mentioned in 1989 by then European Commission president Jacques Delors (Delors, 1989), and by former science and research commissioner Janez Potocnik in 2007. See European Commission archived content, ‘Make “knowledge” a fifth Community freedom, says Potocnik at Green Paper launch’, https://cordis.europa.eu/article/id/27454-make-knowledge-a-fifth-commun… . .
Letta argues that the EU has under-utilised its pools of data, expertise and startups. This wealth of resources benefits global tech giants that are better positioned to capitalise on it and hampers the EU’s strategic autonomy and economic security. He claims that it is a necessary extension of the single market for the EU to become a creator of new technologies and foster the development of leading industrial ecosystems of global importance, with a strong European technological infrastructure in areas including data utilisation, artificial intelligence, quantum computing, biotech, bio-robotics and space.
The Letta report contains a number of constructive and innovative ideas. Most importantly, he does not just attempt to put the fifth freedom on par with other single market freedoms. Instead, he puts it squarely at the top of all single market freedoms: innovation as the necessary condition for the success of all other freedoms, indeed for the success of the EU as an economic project.
Should this be accepted and effectively implemented by the next European Commission, it could herald a major shift in the EU policy environment, which often prioritises precaution over innovation. It would also be a recognition that the empirical evidence of a slow-down in EU productivity growth, and thus in innovation, should be taken seriously (see for example Pinkus et al , 2024). EU productivity growth since the 2009 financial crisis has lagged about a third behind the US. That undermines the EU’s long-term economic welfare.
On the other hand, the fifth freedom sits somewhat uncomfortably in a report on the single market because it has little to do with geographical obstacles or borders. Insisting on the freedom to investigate, explore and create in a borderless single market feels like pushing at an open door. There are hardly any EU internal borders to the mobility of research projects, knowledge and researchers.
Helping data flow
Another positive message from the report is that digital data assumes a central role in Letta’s view of the knowledge economy. Data is a new production factor in modern economies. Eliminating barriers to data access is a powerful catalyst for innovation. Access to computing power and AI technologies are also a necessary ingredient. Letta acknowledges that considerable progress has already been made with several EU digital laws, including the Digital Markets Act, the Digital Services Act, the Data Act and the Data Governance Act. But these are considered insufficient to nurture the necessary level of innovation.
Letta supports the development of European data spaces in key sectors, in line with the European Commission’s (2020) data strategy. Opening access to data and creating data pooling spaces can leverage the value of digital data as a new production factor. Data portability is beneficial because data, once collected by one party for a particular purpose, can often be re-used by other parties for competing or complementary purposes. Data portability thus stimulates competition and innovation in data-driven services markets. Data pooling generates other types of benefits. The valuable insights that can be extracted from a large data pool often exceed the insights that can be extracted from fragmented and smaller datasets.
Letta refers to healthcare as an example of the implementation of the fifth freedom. He cites the European Health Data Space (EHDS) regulation that facilitates the portability of personal health data between medical service providers to stimulate competition between services, and will also create an EU-wide health data pool for research purposes to stimulate medical innovation 2 At time of writing, EHDS has been agreed but not fully ratified; see European Commission press release of 24 April 2024, ‘Commission welcomes European Parliament . EHDS would have been a good template for other data pooling initiatives. Unfortunately, other sectoral data pools may not be as generous to researchers and innovation. Preliminary ideas for a Common European Agricultural Data Space emphasise exclusive data rights for farms, at the expense of data pooling for innovation purposes 3 See the AgriDataSpace project, https://agridataspace-csa.eu/ . .
Typically, Letta’s report recommends removing barriers to cross-border data flows by means of interoperability and data regulations. But there are few restrictions on cross-border data flows inside the EU 4 Annex 5 in European Commission (2017) detected some restrictions, mostly on administrative and tax data, that represent only a tiny part of total data flows. . The real obstacles to access to data are located inside firms that collect and store data in their proprietary silos. They are reluctant to share data with users who generated the data or third parties selected by users, let alone in a common data pool accessible to many users.
The EU Data Act (Regulation (EU) 2023/2854) also makes data pooling difficult. It attributes exclusive data licensing and monopolistic data-pricing rights to device manufacturers, restricting data access for users to very narrowly defined datasets and limiting the use of this data for competitive purposes. That slows down data-driven innovation. The EU Data Governance Act (Regulation (EU) 2022/868) meanwhile does not drive innovation because it excludes precisely those platforms that produce data-driven innovation services, including data analytics, transformation and extraction of value-added from data pools. Over the last couple of years, EU data policies have moved back and forth between the debunked concept of private data ownership and the recognition that data sharing is beneficial for innovation and competition. If the European Commission is to take Letta seriously, is should move away decisively from exclusive data rights and start to see data as a collectively generated production factor that should be leveraged as an major driver of innovation in the digital economy.
Redistributing rents
The EU Digital Markets Act (DMA, Regulation (EU) 2022/1925) is a pioneering attempt to weaken the monopolistic market power of mostly US-based ‘gatekeeper’ platforms. If implemented correctly, it could redistribute some of these monopoly rents to EU consumers and small businesses. But will this redistribution evaporate in non-investable consumer surplus and fragmented financial resources? The EU will need financial instruments to channel these resources back into digital R&D and innovative start-up capital. Even after implementation of the DMA, Europe’s advanced digital technologies may still rely on US platforms to bring their services to consumers and businesses.
It is unlikely that EU publicly financed R&D can compete with these platforms, which are all privately financed for-profit companies. Letta recognises the need to mobilise more private investment as a complement to public-sector investment. One of his most interesting proposals is the creation of an “EU Stock Exchange for Deep Tech” companies that use cutting-edge science and technology, including AI, quantum and biotechnology. Start-ups are high-risk undertakings but offer high gains, if successful. In the EU, because of banking regulations, these types of risky asset are downgraded. The EU should facilitate the creation of a deep-tech stock exchange with specific rules adapted to this risk class.
Letta also argues in favour of creating a strong digital infrastructure layer through consolidation in the telecom sector, allowing many small national telecom providers to merge cross-border into a dozen or so large providers that can invest in advanced infrastructures, including 5G and 6G mobile networks. Among his more provocative and promising ideas, Letta suggests that the time may have come to re-evaluate rules on net neutrality, or non-discriminatory treatment of online traffic. Different treatment of different types of data flows allows optimisation of connectivity, which is important for robotics, the internet of things and AI. It would allow the introduction of innovative use cases that are currently non-compliant with net neutrality. In the US, the FTC abandoned net neutrality in 2018, without major upheaval in the sector.
Another important infrastructure component is cloud-computing capacity for the development of AI models, in which Letta endorses EU public investment. The EU is running far behind the US platforms, which invest massively in cloud computing. Letta suggests that the EU should prioritise shared networks of computational resources and supercomputers, such as the EU’s High-Performance Computing (HPC) initiative 5 See https://eurohpc-ju.europa.eu/index_en . . Unfortunately, HPC’s centralised and public sector governance model is more adapted to academic research than to the requirements of AI start-ups, which require flexible and scalable computing capacity and dedicated AI processors. The public sector will not be able to match the financial resources of the big platforms to invest in hyperscale computing capacity for AI models.
Unfortunately, some of Letta’s more concrete implementation proposals boil down to old European wines in barely new bottles. Letta proposes the creation of a European Knowledge Commons, a centralised digital platform to provide access to publicly funded research, datasets and educational resources, allowing citizens and businesses to tap into a wealth of knowledge for innovation. This Commons should be accompanied by facilitation of cross-border data flows, development of European data spaces, creation of data regulation sandboxes and promotion of researcher mobility within the European Research Area and efforts to retain talent in Europe. There is nothing new in these proposals. Access to EU research findings and data has already opened up considerably over the last decade. But Letta does not mention how sharing knowledge in a commons can be squared with incentives to invest in patentable and commercially exploitable research. Public-private partnerships in strategic areas focused on knowledge exchange and innovation uptake may be important at the research stage, but often become more problematic at the commercial stage, when the public sector may need to spin off successful projects to the private sector.
Almost inevitably, Europe’s old ideas about selecting industrial champions pop up again. Letta clearly favours a centralist, public-sector-driven approach to innovation, in order to be able to draw in substantial private investments. He emphasises public sector financing and commons-based approaches to knowledge accumulation and innovation. He claims that establishing European technological infrastructure involves granting authority to a collective industrial policy at European scale, moving beyond national confines. This is unfortunate. One would have expected the Letta report to acknowledge the enormous private-sector contribution to innovation and productivity growth.
AI and cybersecurity
Letta underscores the importance of the development and deployment of AI technologies, including ethical guidelines and regulatory compliance standards. He argues that even if the most powerful AI models have been developed outside the EU, it can still win the race to make the most of AI applications. He expresses belief in the EU’s position as a leading hub for AI innovation.
This optimistic view may be difficult to square with the realities of the EU AI Act that will impose considerable compliance costs on smaller EU AI developers and may complicate access to model training data 6 The Act has been approved but not published in its final form. See Council of the EU press release of 21 May 2024, ‘Artificial intelligence (AI) act: Council gives final green light to the first worldwide rules on AI’, https://www.consilium.europa.eu/en/press/press-releases/2024/05/21/arti… . . Combined with the lack of adequate computing capacity and finance in the EU, it is easy to understand why EU AI start-ups have opted for collaboration with US platforms. That collaboration gives them access to computing power and inputs data, and also to commercial outlets for their AI model applications, a prerequisite for generating revenue. It is difficult for a start-up to launch a new business model from scratch. Smaller models and applications on top of existing foundation models may have some commercial future but they are vulnerable to competition and high intermediation fees from Big Tech AI services.
Letta’s report rightly observes that the current fragmentation in cybersecurity standards hampers the development of robust security capabilities, by preventing network operators from leveraging centralised network architectures that could benefit from economies of scale. Fragmented national cybersecurity standards and reporting requirements undermine the efficiency of cybersecurity strategies at EU level. Much cybersecurity work is done by giants such as Google and Microsoft because they have a global overview of threats through their sprawling consumer- and business-facing networks. In the absence of EU players of this size, and further handicapped by fragmented national regulation, this remains a source of concern, especially at a time when cyberwarfare is increasingly important.
In sum, the main merit of Letta’s fifth freedom idea is that it puts research and innovation back at the top of the EU economic policy agenda, to counter the slow-down in EU productivity growth. Wrapping it up in a single market freedom anchors it very well in the EU’s institutional and policy foundations. Bringing in data and AI policies to leverage productivity growth chimes with current frontier technologies. Several of his policy proposals challenge the current status quo. However, his reliance on public sector-led industrial policies and investment ignores the fact that private sector R&D and investment now vastly exceed public-sector financing capacities. A Knowledge Commons overlooks the fact that private appropriation of innovation rents has become the main driver of R&D financing. Letta’s ideas will require considerable polishing and fine-tuning to make them fit in the realities of today’s innovation economics.
Delors, J. (1989) ‘Address given by Jacques Delors to the European Parliament (17 January 1989)’, available at https://www.cvce.eu/obj/address_given_by_jacques_delors_to_the_european_parliament_17_january_1989-en-b9c06b95-db97-4774-a700-e8aea5172233.html
European Commission (2017) ‘Impact assessment accompanying proposal for a Regulation on a framework for the free flow of non-personal data in the European Union’, SWD(2017) 304 final, available at https://eur-lex.europa.eu/legal-content/EN/TXT/?uri=SWD:2017:304:FIN
European Commission (2020) ‘A European strategy for data’, COM(2020) 66 final, available at https://eur-lex.europa.eu/legal-content/EN/TXT/?uri=CELEX%3A52020DC0066
Letta, E. (2024) Much More Than a Market , report to the European Council, available at https://www.consilium.europa.eu/media/ny3j24sm/much-more-than-a-market-report-by-enrico-letta.pdf
Pinkus, D., J. Pisani-Ferry, S. Tagliapietra, R. Veugelers, G. Zachmann and J. Zettelmeyer (2024) Coordination for competitiveness , study requested by the ECON committee, European Parliament, available at https://www.europarl.europa.eu/RegData/etudes/STUD/2024/747838/IPOL_STU(2024)747838_EN.pdf
About the authors
Bertin martens.
Bertin Martens is a Senior fellow at Bruegel. He has been working on digital economy issues, including e-commerce, geo-blocking, digital copyright and media, online platforms and data markets and regulation, as senior economist at the Joint Research Centre (Seville) of the European Commission, for more than a decade until April 2022. Prior to that, he was deputy chief economist for trade policy at the European Commission, and held various other assignments in the international economic policy domain. He is currently a non-resident research fellow at the Tilburg Law & Economics Centre (TILEC) at Tilburg University (Netherlands).
His current research interests focus on economic and regulatory issues in digital data markets and online platforms, the impact of digital technology on institutions in society and, more broadly, the long-term evolution of knowledge accumulation and transmission systems in human societies. Institutions are tools to organise information flows. When digital technologies change information costs and distribution channels, institutional and organisational borderlines will shift.
He holds a PhD in economics from the Free University of Brussels.
Disclosure of external interests
Declaration of interests 2023
Related content
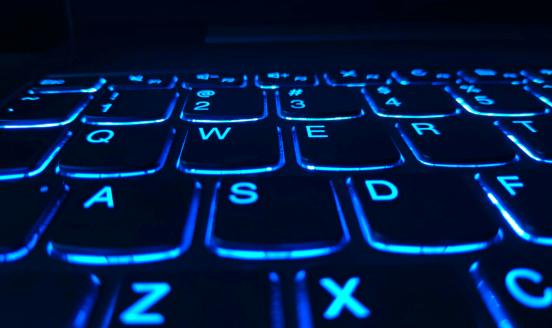
Economic arguments in favour of reducing copyright protection for generative AI inputs and outputs
The licensing of training inputs slows down economic growth compared to what it could be with competitive and high-quality GenAI
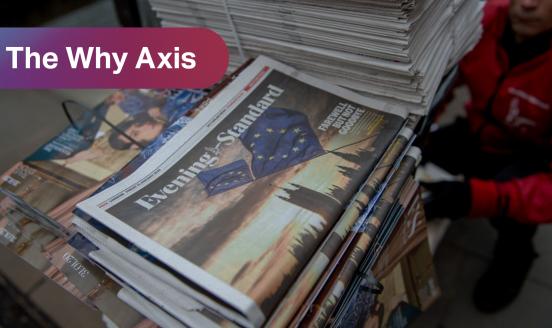
Why should EU copyright protection be reduced to realise the innovation benefits of Generative AI?
The eu artificial intelligence act: premature or precocious regulation.
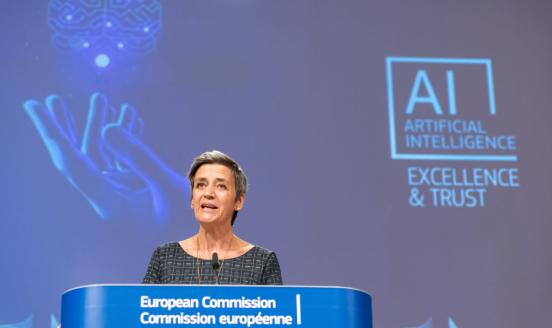
The European Union AI Act: premature or precocious regulation?
As it stands, it is unknown whether the Act will stimulate responsible AI use or smother innovation.
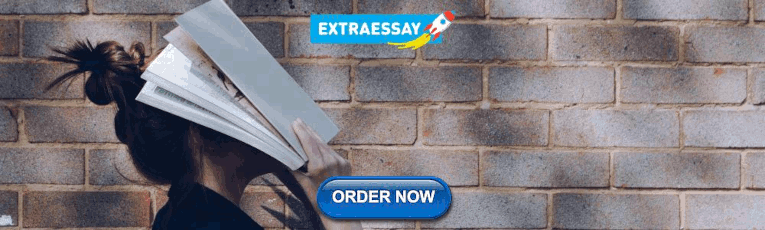
IMAGES
VIDEO
COMMENTS
Secondary analysis of data collected by another researcher for a different purpose, or SDA, is increasing in the medical and social sciences. This is not surprising, given the immense body of health care-related research performed worldwide and the potential beneficial clinical implications of the timely expansion of primary research (Johnston, 2014; Tripathy, 2013).
Secondary research is a research method that uses data that was collected by someone else. In other words, whenever you conduct research using data that already exists, you are conducting secondary research. On the other hand, any type of research that you undertake yourself is called primary research. Example: Secondary research.
Introduction. Secondary data analysis is a valuable research approach that can be used to advance knowledge across many disciplines through the use of quantitative, qualitative, or mixed methods data to answer new research questions (Polit & Beck, 2021).This research method dates to the 1960s and involves the utilization of existing or primary data, originally collected for a variety, diverse ...
SDA involves investigations where data collected for a previous study is analyzed - either by the same researcher(s) or different researcher(s) - to explore new questions or use different analysis strategies that were not a part of the primary analysis (Szabo and Strang, 1997).For research involving quantitative data, SDA, and the process of sharing data for the purpose of SDA, has become ...
In addition to the challenges of secondary research as mentioned in subsection Secondary Data and Analysis, in current research realm of secondary analysis, there is a lack of rigor in the analysis and overall methodology (Ruggiano & Perry, 2019). This has the pitfall of possibly exaggerating the effects of researcher bias (Thorne, 1994, 1998 ...
Abstract. Secondary data analysis refers to the analysis of existing data collected by others. Secondary analysis affords researchers the opportunity to investigate research questions using large-scale data sets that are often inclusive of under-represented groups, while saving time and resources.
Secondary analysis of qualitative data is a topic unto itself and is not discussed in this volume. The interested reader is referred to references such as James and Sorenson (2000) and Heaton (2004). The choice of primary or secondary data need not be an either/or ques-tion. Most researchers in epidemiology and public health will work with both ...
Step 3: Design your research process. After defining your statement of purpose, the next step is to design the research process. For primary data, this involves determining the types of data you want to collect (e.g. quantitative, qualitative, or both) and a methodology for gathering them. For secondary data analysis, however, your research ...
In this paper I offer an overview of secondary qualitative analysis processes and provide general recommendations for researchers to consider in planning and conducting qualitative secondary analysis. I also include a select ... Qualitative Research, Secondary Analysis, Online Research Data .
Secondary analysis is a research methodology by which researchers use pre-existing data in order to investigate new questions or for the verification of the findings of previous works (Heaton ...
Research using secondary analysis of large quantitative datasets can also contribute significantly to policy refinement or change such as when the impact of specific polices is shown to be trending away from the desired direction. ... The 11 papers in this Special Issue demonstrate that researchers have moved forward quickly to leverage the ...
This article covers all the basics of secondary research. ... Meta-analysis Meta-analysis is a type of systematic review, but a systematic review in which statistical analysis is carried out to compare previously published studies and derive new interpretations or new findings. ... 3 Basic tips to write a great research paper title. One of the ...
Secondary analysis is a form of research that uses existing data, or secondary data, collected previously to perform a new study. Researchers might use quantitative or qualitative data another research team or agency gathered or produced for different reasons and analyze it in a new way. That data might be publicly available or the researcher ...
This paper asserts that secondary data analysis is a viable method to utilize in the process of inquiry when a systematic procedure is followed and presents an illustrative research application ...
This includes internal sources (e.g.in-house research) or, more commonly, external sources (such as government statistics, organizational bodies, and the internet). Secondary research comes in several formats, such as published datasets, reports, and survey responses, and can also be sourced from websites, libraries, and museums.
Types of secondary data are as follows: Published data: Published data refers to data that has been published in books, magazines, newspapers, and other print media. Examples include statistical reports, market research reports, and scholarly articles. Government data: Government data refers to data collected by government agencies and departments.
Secondary Research Examples. 1. Literature Review. A literature review summarizes, reviews, and critiques the existing published literature on a topic. Literature reviews are considered secondary research because it is a collection and analysis of the existing literature rather than generating new data for the study.
This paper discusses several issues related to the secondary analysis of existing data. There are definitely limitations to such analyses, but the great advantage is that secondary analyses can dramatically increase the overall efficiency of the research effort and - a secondary advantage - give young researchers with good ideas but little ...
Therefore, the majority of sources in a literature review are secondary sources that present research findings, analysis, and the evaluation of other researcher's works. Reviewing secondary source material can be of value in improving your overall research paper because secondary sources facilitate the communication of what is known about a ...
a, Target composition of the in vitro secondary pharmacology panel of 44 targets suggested by Bowes et al. 5, and its implementation by research phase by the companies that participated in a ...
The European Union's single market famously enables four freedoms: the movement of goods, services, capital and labour. Former Italian prime minister Enrico Letta, in his report on the single market issued and discussed by EU leaders in April, proposed a fifth freedom as a top priority, to encompass research, innovation, data and knowledge ...
A secondary analysis of a gait-analysis data set" by M. Etoom et al. ... Showing 1 through 3 of 0 Related Papers. Related Papers; ... Semantic Scholar is a free, AI-powered research tool for scientific literature, based at the Allen Institute for AI. Learn More. About About Us Meet the Team Publishers Blog (opens in a new tab) AI2 Careers ...