
An official website of the United States government
The .gov means it’s official. Federal government websites often end in .gov or .mil. Before sharing sensitive information, make sure you’re on a federal government site.
The site is secure. The https:// ensures that you are connecting to the official website and that any information you provide is encrypted and transmitted securely.
- Publications
- Account settings
Preview improvements coming to the PMC website in October 2024. Learn More or Try it out now .
- Advanced Search
- Journal List
- v.12(6); 2020 Jun

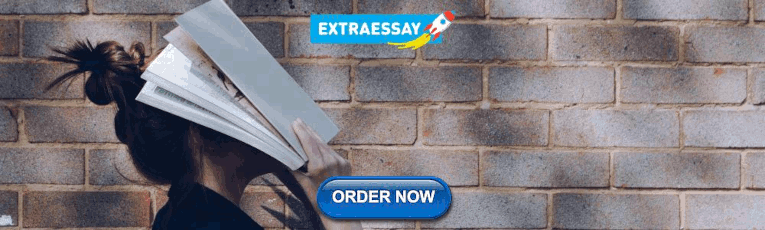
Social Media Use and Its Connection to Mental Health: A Systematic Review
Fazida karim.
1 Psychology, California Institute of Behavioral Neurosciences and Psychology, Fairfield, USA
2 Business & Management, University Sultan Zainal Abidin, Terengganu, MYS
Azeezat A Oyewande
3 Family Medicine, California Institute of Behavioral Neurosciences and Psychology, Fairfield, USA
4 Family Medicine, Lagos State Health Service Commission/Alimosho General Hospital, Lagos, NGA
Lamis F Abdalla
5 Internal Medicine, California Institute of Behavioral Neurosciences and Psychology, Fairfield, USA
Reem Chaudhry Ehsanullah
Safeera khan.
Social media are responsible for aggravating mental health problems. This systematic study summarizes the effects of social network usage on mental health. Fifty papers were shortlisted from google scholar databases, and after the application of various inclusion and exclusion criteria, 16 papers were chosen and all papers were evaluated for quality. Eight papers were cross-sectional studies, three were longitudinal studies, two were qualitative studies, and others were systematic reviews. Findings were classified into two outcomes of mental health: anxiety and depression. Social media activity such as time spent to have a positive effect on the mental health domain. However, due to the cross-sectional design and methodological limitations of sampling, there are considerable differences. The structure of social media influences on mental health needs to be further analyzed through qualitative research and vertical cohort studies.
Introduction and background
Human beings are social creatures that require the companionship of others to make progress in life. Thus, being socially connected with other people can relieve stress, anxiety, and sadness, but lack of social connection can pose serious risks to mental health [ 1 ].
Social media
Social media has recently become part of people's daily activities; many of them spend hours each day on Messenger, Instagram, Facebook, and other popular social media. Thus, many researchers and scholars study the impact of social media and applications on various aspects of people’s lives [ 2 ]. Moreover, the number of social media users worldwide in 2019 is 3.484 billion, up 9% year-on-year [ 3 - 5 ]. A statistic in Figure 1 shows the gender distribution of social media audiences worldwide as of January 2020, sorted by platform. It was found that only 38% of Twitter users were male but 61% were using Snapchat. In contrast, females were more likely to use LinkedIn and Facebook. There is no denying that social media has now become an important part of many people's lives. Social media has many positive and enjoyable benefits, but it can also lead to mental health problems. Previous research found that age did not have an effect but gender did; females were much more likely to experience mental health than males [ 6 , 7 ].

Impact on mental health
Mental health is defined as a state of well-being in which people understand their abilities, solve everyday life problems, work well, and make a significant contribution to the lives of their communities [ 8 ]. There is debated presently going on regarding the benefits and negative impacts of social media on mental health [ 9 , 10 ]. Social networking is a crucial element in protecting our mental health. Both the quantity and quality of social relationships affect mental health, health behavior, physical health, and mortality risk [ 9 ]. The Displaced Behavior Theory may help explain why social media shows a connection with mental health. According to the theory, people who spend more time in sedentary behaviors such as social media use have less time for face-to-face social interaction, both of which have been proven to be protective against mental disorders [ 11 , 12 ]. On the other hand, social theories found how social media use affects mental health by influencing how people view, maintain, and interact with their social network [ 13 ]. A number of studies have been conducted on the impacts of social media, and it has been indicated that the prolonged use of social media platforms such as Facebook may be related to negative signs and symptoms of depression, anxiety, and stress [ 10 - 15 ]. Furthermore, social media can create a lot of pressure to create the stereotype that others want to see and also being as popular as others.
The need for a systematic review
Systematic studies can quantitatively and qualitatively identify, aggregate, and evaluate all accessible data to generate a warm and accurate response to the research questions involved [ 4 ]. In addition, many existing systematic studies related to mental health studies have been conducted worldwide. However, only a limited number of studies are integrated with social media and conducted in the context of social science because the available literature heavily focused on medical science [ 6 ]. Because social media is a relatively new phenomenon, the potential links between their use and mental health have not been widely investigated.
This paper attempt to systematically review all the relevant literature with the aim of filling the gap by examining social media impact on mental health, which is sedentary behavior, which, if in excess, raises the risk of health problems [ 7 , 9 , 12 ]. This study is important because it provides information on the extent of the focus of peer review literature, which can assist the researchers in delivering a prospect with the aim of understanding the future attention related to climate change strategies that require scholarly attention. This study is very useful because it provides information on the extent to which peer review literature can assist researchers in presenting prospects with a view to understanding future concerns related to mental health strategies that require scientific attention. The development of the current systematic review is based on the main research question: how does social media affect mental health?
Research strategy
The research was conducted to identify studies analyzing the role of social media on mental health. Google Scholar was used as our main database to find the relevant articles. Keywords that were used for the search were: (1) “social media”, (2) “mental health”, (3) “social media” AND “mental health”, (4) “social networking” AND “mental health”, and (5) “social networking” OR “social media” AND “mental health” (Table 1 ).
Out of the results in Table 1 , a total of 50 articles relevant to the research question were selected. After applying the inclusion and exclusion criteria, duplicate papers were removed, and, finally, a total of 28 articles were selected for review (Figure 2 ).

PRISMA, Preferred Reporting Items for Systematic Reviews and Meta-Analyses
Inclusion and exclusion criteria
Peer-reviewed, full-text research papers from the past five years were included in the review. All selected articles were in English language and any non-peer-reviewed and duplicate papers were excluded from finally selected articles.
Of the 16 selected research papers, there were a research focus on adults, gender, and preadolescents [ 10 - 19 ]. In the design, there were qualitative and quantitative studies [ 15 , 16 ]. There were three systematic reviews and one thematic analysis that explored the better or worse of using social media among adolescents [ 20 - 23 ]. In addition, eight were cross-sectional studies and only three were longitudinal studies [ 24 - 29 ].The meta-analyses included studies published beyond the last five years in this population. Table 2 presents a selection of studies from the review.
IGU, internet gaming disorder; PSMU, problematic social media use
This study has attempted to systematically analyze the existing literature on the effect of social media use on mental health. Although the results of the study were not completely consistent, this review found a general association between social media use and mental health issues. Although there is positive evidence for a link between social media and mental health, the opposite has been reported.
For example, a previous study found no relationship between the amount of time spent on social media and depression or between social media-related activities, such as the number of online friends and the number of “selfies”, and depression [ 29 ]. Similarly, Neira and Barber found that while higher investment in social media (e.g. active social media use) predicted adolescents’ depressive symptoms, no relationship was found between the frequency of social media use and depressed mood [ 28 ].
In the 16 studies, anxiety and depression were the most commonly measured outcome. The prominent risk factors for anxiety and depression emerging from this study comprised time spent, activity, and addiction to social media. In today's world, anxiety is one of the basic mental health problems. People liked and commented on their uploaded photos and videos. In today's age, everyone is immune to the social media context. Some teens experience anxiety from social media related to fear of loss, which causes teens to try to respond and check all their friends' messages and messages on a regular basis.
On the contrary, depression is one of the unintended significances of unnecessary use of social media. In detail, depression is limited not only to Facebooks but also to other social networking sites, which causes psychological problems. A new study found that individuals who are involved in social media, games, texts, mobile phones, etc. are more likely to experience depression.
The previous study found a 70% increase in self-reported depressive symptoms among the group using social media. The other social media influence that causes depression is sexual fun [ 12 ]. The intimacy fun happens when social media promotes putting on a facade that highlights the fun and excitement but does not tell us much about where we are struggling in our daily lives at a deeper level [ 28 ]. Another study revealed that depression and time spent on Facebook by adolescents are positively correlated [ 22 ]. More importantly, symptoms of major depression have been found among the individuals who spent most of their time in online activities and performing image management on social networking sites [ 14 ].
Another study assessed gender differences in associations between social media use and mental health. Females were found to be more addicted to social media as compared with males [ 26 ]. Passive activity in social media use such as reading posts is more strongly associated with depression than doing active use like making posts [ 23 ]. Other important findings of this review suggest that other factors such as interpersonal trust and family functioning may have a greater influence on the symptoms of depression than the frequency of social media use [ 28 , 29 ].
Limitation and suggestion
The limitations and suggestions were identified by the evidence involved in the study and review process. Previously, 7 of the 16 studies were cross-sectional and slightly failed to determine the causal relationship between the variables of interest. Given the evidence from cross-sectional studies, it is not possible to conclude that the use of social networks causes mental health problems. Only three longitudinal studies examined the causal relationship between social media and mental health, which is hard to examine if the mental health problem appeared more pronounced in those who use social media more compared with those who use it less or do not use at all [ 19 , 20 , 24 ]. Next, despite the fact that the proposed relationship between social media and mental health is complex, a few studies investigated mediating factors that may contribute or exacerbate this relationship. Further investigations are required to clarify the underlying factors that help examine why social media has a negative impact on some peoples’ mental health, whereas it has no or positive effect on others’ mental health.
Conclusions
Social media is a new study that is rapidly growing and gaining popularity. Thus, there are many unexplored and unexpected constructive answers associated with it. Lately, studies have found that using social media platforms can have a detrimental effect on the psychological health of its users. However, the extent to which the use of social media impacts the public is yet to be determined. This systematic review has found that social media envy can affect the level of anxiety and depression in individuals. In addition, other potential causes of anxiety and depression have been identified, which require further exploration.
The importance of such findings is to facilitate further research on social media and mental health. In addition, the information obtained from this study can be helpful not only to medical professionals but also to social science research. The findings of this study suggest that potential causal factors from social media can be considered when cooperating with patients who have been diagnosed with anxiety or depression. Also, if the results from this study were used to explore more relationships with another construct, this could potentially enhance the findings to reduce anxiety and depression rates and prevent suicide rates from occurring.
The content published in Cureus is the result of clinical experience and/or research by independent individuals or organizations. Cureus is not responsible for the scientific accuracy or reliability of data or conclusions published herein. All content published within Cureus is intended only for educational, research and reference purposes. Additionally, articles published within Cureus should not be deemed a suitable substitute for the advice of a qualified health care professional. Do not disregard or avoid professional medical advice due to content published within Cureus.
The authors have declared that no competing interests exist.
Advertisement
Social Media and Mental Health: Benefits, Risks, and Opportunities for Research and Practice
- Published: 20 April 2020
- Volume 5 , pages 245–257, ( 2020 )
Cite this article
- John A. Naslund 1 ,
- Ameya Bondre 2 ,
- John Torous 3 &
- Kelly A. Aschbrenner 4
371k Accesses
184 Citations
148 Altmetric
12 Mentions
Explore all metrics
Avoid common mistakes on your manuscript.
Introduction
Social media has become a prominent fixture in the lives of many individuals facing the challenges of mental illness. Social media refers broadly to web and mobile platforms that allow individuals to connect with others within a virtual network (such as Facebook, Twitter, Instagram, Snapchat, or LinkedIn), where they can share, co-create, or exchange various forms of digital content, including information, messages, photos, or videos (Ahmed et al. 2019 ). Studies have reported that individuals living with a range of mental disorders, including depression, psychotic disorders, or other severe mental illnesses, use social media platforms at comparable rates as the general population, with use ranging from about 70% among middle-age and older individuals to upwards of 97% among younger individuals (Aschbrenner et al. 2018b ; Birnbaum et al. 2017b ; Brunette et al. 2019 ; Naslund et al. 2016 ). Other exploratory studies have found that many of these individuals with mental illness appear to turn to social media to share their personal experiences, seek information about their mental health and treatment options, and give and receive support from others facing similar mental health challenges (Bucci et al. 2019 ; Naslund et al. 2016b ).
Across the USA and globally, very few people living with mental illness have access to adequate mental health services (Patel et al. 2018 ). The wide reach and near ubiquitous use of social media platforms may afford novel opportunities to address these shortfalls in existing mental health care, by enhancing the quality, availability, and reach of services. Recent studies have explored patterns of social media use, impact of social media use on mental health and wellbeing, and the potential to leverage the popularity and interactive features of social media to enhance the delivery of interventions. However, there remains uncertainty regarding the risks and potential harms of social media for mental health (Orben and Przybylski 2019 ) and how best to weigh these concerns against potential benefits.
In this commentary, we summarized current research on the use of social media among individuals with mental illness, with consideration of the impact of social media on mental wellbeing, as well as early efforts using social media for delivery of evidence-based programs for addressing mental health problems. We searched for recent peer reviewed publications in Medline and Google Scholar using the search terms “mental health” or “mental illness” and “social media,” and searched the reference lists of recent reviews and other relevant studies. We reviewed the risks, potential harms, and necessary safety precautions with using social media for mental health. Overall, our goal was to consider the role of social media as a potentially viable intervention platform for offering support to persons with mental disorders, promoting engagement and retention in care, and enhancing existing mental health services, while balancing the need for safety. Given this broad objective, we did not perform a systematic search of the literature and we did not apply specific inclusion criteria based on study design or type of mental disorder.
Social Media Use and Mental Health
In 2020, there are an estimated 3.8 billion social media users worldwide, representing half the global population (We Are Social 2020 ). Recent studies have shown that individuals with mental disorders are increasingly gaining access to and using mobile devices, such as smartphones (Firth et al. 2015 ; Glick et al. 2016 ; Torous et al. 2014a , b ). Similarly, there is mounting evidence showing high rates of social media use among individuals with mental disorders, including studies looking at engagement with these popular platforms across diverse settings and disorder types. Initial studies from 2015 found that nearly half of a sample of psychiatric patients were social media users, with greater use among younger individuals (Trefflich et al. 2015 ), while 47% of inpatients and outpatients with schizophrenia reported using social media, of which 79% reported at least once-a-week usage of social media websites (Miller et al. 2015 ). Rates of social media use among psychiatric populations have increased in recent years, as reflected in a study with data from 2017 showing comparable rates of social media use (approximately 70%) among individuals with serious mental illness in treatment as compared with low-income groups from the general population (Brunette et al. 2019 ).
Similarly, among individuals with serious mental illness receiving community-based mental health services, a recent study found equivalent rates of social media use as the general population, even exceeding 70% of participants (Naslund et al. 2016 ). Comparable findings were demonstrated among middle-age and older individuals with mental illness accessing services at peer support agencies, where 72% of respondents reported using social media (Aschbrenner et al. 2018b ). Similar results, with 68% of those with first episode psychosis using social media daily were reported in another study (Abdel-Baki et al. 2017 ).
Individuals who self-identified as having a schizophrenia spectrum disorder responded to a survey shared through the National Alliance of Mental Illness (NAMI) and reported that visiting social media sites was one of their most common activities when using digital devices, taking up roughly 2 h each day (Gay et al. 2016 ). For adolescents and young adults ages 12 to 21 with psychotic disorders and mood disorders, over 97% reported using social media, with average use exceeding 2.5 h per day (Birnbaum et al. 2017b ). Similarly, in a sample of adolescents ages 13–18 recruited from community mental health centers, 98% reported using social media, with YouTube as the most popular platform, followed by Instagram and Snapchat (Aschbrenner et al. 2019 ).
Research has also explored the motivations for using social media as well as the perceived benefits of interacting on these platforms among individuals with mental illness. In the sections that follow (see Table 1 for a summary), we consider three potentially unique features of interacting and connecting with others on social media that may offer benefits for individuals living with mental illness. These include: (1) Facilitate social interaction; (2) Access to a peer support network; and (3) Promote engagement and retention in services.
Facilitate Social Interaction
Social media platforms offer near continuous opportunities to connect and interact with others, regardless of time of day or geographic location. This on demand ease of communication may be especially important for facilitating social interaction among individuals with mental disorders experiencing difficulties interacting in face-to-face settings. For example, impaired social functioning is a common deficit in schizophrenia spectrum disorders, and social media may facilitate communication and interacting with others for these individuals (Torous and Keshavan 2016 ). This was suggested in one study where participants with schizophrenia indicated that social media helped them to interact and socialize more easily (Miller et al. 2015 ). Like other online communication, the ability to connect with others anonymously may be an important feature of social media, especially for individuals living with highly stigmatizing health conditions (Berger et al. 2005 ), such as serious mental disorders (Highton-Williamson et al. 2015 ).
Studies have found that individuals with serious mental disorders (Spinzy et al. 2012 ) as well as young adults with mental illness (Gowen et al. 2012 ) appear to form online relationships and connect with others on social media as often as social media users from the general population. This is an important observation because individuals living with serious mental disorders typically have few social contacts in the offline world and also experience high rates of loneliness (Badcock et al. 2015 ; Giacco et al. 2016 ). Among individuals receiving publicly funded mental health services who use social media, nearly half (47%) reported using these platforms at least weekly to feel less alone (Brusilovskiy et al. 2016 ). In another study of young adults with serious mental illness, most indicated that they used social media to help feel less isolated (Gowen et al. 2012 ). Interestingly, more frequent use of social media among a sample of individuals with serious mental illness was associated with greater community participation, measured as participation in shopping, work, religious activities, or visiting friends and family, as well as greater civic engagement, reflected as voting in local elections (Brusilovskiy et al. 2016 ).
Emerging research also shows that young people with moderate to severe depressive symptoms appear to prefer communicating on social media rather than in-person (Rideout and Fox 2018 ), while other studies have found that some individuals may prefer to seek help for mental health concerns online rather than through in-person encounters (Batterham and Calear 2017 ). In a qualitative study, participants with schizophrenia described greater anonymity, the ability to discover that other people have experienced similar health challenges and reducing fears through greater access to information as important motivations for using the Internet to seek mental health information (Schrank et al. 2010 ). Because social media does not require the immediate responses necessary in face-to-face communication, it may overcome deficits with social interaction due to psychotic symptoms that typically adversely affect face-to-face conversations (Docherty et al. 1996 ). Online social interactions may not require the use of non-verbal cues, particularly in the initial stages of interaction (Kiesler et al. 1984 ), with interactions being more fluid and within the control of users, thereby overcoming possible social anxieties linked to in-person interaction (Indian and Grieve 2014 ). Furthermore, many individuals with serious mental disorders can experience symptoms including passive social withdrawal, blunted affect, and attentional impairment, as well as active social avoidance due to hallucinations or other concerns (Hansen et al. 2009 ), thus potentially reinforcing the relative advantage, as perceived by users, of using social media over in person conversations.
Access to a Peer Support Network
There is growing recognition about the role that social media channels could play in enabling peer support (Bucci et al. 2019 ; Naslund et al. 2016b ), referred to as a system of mutual giving and receiving where individuals who have endured the difficulties of mental illness can offer hope, friendship, and support to others facing similar challenges (Davidson et al. 2006 ; Mead et al. 2001 ). Initial studies exploring use of online self-help forums among individuals with serious mental illnesses have found that individuals with schizophrenia appeared to use these forums for self-disclosure and sharing personal experiences, in addition to providing or requesting information, describing symptoms, or discussing medication (Haker et al. 2005 ), while users with bipolar disorder reported using these forums to ask for help from others about their illness (Vayreda and Antaki 2009 ). More recently, in a review of online social networking in people with psychosis, Highton-Williamson et al. ( 2015 ) highlight that an important purpose of such online connections was to establish new friendships, pursue romantic relationships, maintain existing relationships or reconnect with people, and seek online peer support from others with lived experience (Highton-Williamson et al. 2015 ).
Online peer support among individuals with mental illness has been further elaborated in various studies. In a content analysis of comments posted to YouTube by individuals who self-identified as having a serious mental illness, there appeared to be opportunities to feel less alone, provide hope, find support and learn through mutual reciprocity, and share coping strategies for day-to-day challenges of living with a mental illness (Naslund et al. 2014 ). In another study, Chang ( 2009 ) delineated various communication patterns in an online psychosis peer-support group (Chang 2009 ). Specifically, different forms of support emerged, including “informational support” about medication use or contacting mental health providers, “esteem support” involving positive comments for encouragement, “network support” for sharing similar experiences, and “emotional support” to express understanding of a peer’s situation and offer hope or confidence (Chang 2009 ). Bauer et al. ( 2013 ) reported that the main interest in online self-help forums for patients with bipolar disorder was to share emotions with others, allow exchange of information, and benefit by being part of an online social group (Bauer et al. 2013 ).
For individuals who openly discuss mental health problems on Twitter, a study by Berry et al. ( 2017 ) found that this served as an important opportunity to seek support and to hear about the experiences of others (Berry et al. 2017 ). In a survey of social media users with mental illness, respondents reported that sharing personal experiences about living with mental illness and opportunities to learn about strategies for coping with mental illness from others were important reasons for using social media (Naslund et al. 2017 ). A computational study of mental health awareness campaigns on Twitter provides further support with inspirational posts and tips being the most shared (Saha et al. 2019 ). Taken together, these studies offer insights about the potential for social media to facilitate access to an informal peer support network, though more research is necessary to examine how these online interactions may impact intentions to seek care, illness self-management, and clinically meaningful outcomes in offline contexts.
Promote Engagement and Retention in Services
Many individuals living with mental disorders have expressed interest in using social media platforms for seeking mental health information (Lal et al. 2018 ), connecting with mental health providers (Birnbaum et al. 2017b ), and accessing evidence-based mental health services delivered over social media specifically for coping with mental health symptoms or for promoting overall health and wellbeing (Naslund et al. 2017 ). With the widespread use of social media among individuals living with mental illness combined with the potential to facilitate social interaction and connect with supportive peers, as summarized above, it may be possible to leverage the popular features of social media to enhance existing mental health programs and services. A recent review by Biagianti et al. ( 2018 ) found that peer-to-peer support appeared to offer feasible and acceptable ways to augment digital mental health interventions for individuals with psychotic disorders by specifically improving engagement, compliance, and adherence to the interventions and may also improve perceived social support (Biagianti et al. 2018 ).
Among digital programs that have incorporated peer-to-peer social networking consistent with popular features on social media platforms, a pilot study of the HORYZONS online psychosocial intervention demonstrated significant reductions in depression among patients with first episode psychosis (Alvarez-Jimenez et al. 2013 ). Importantly, the majority of participants (95%) in this study engaged with the peer-to-peer networking feature of the program, with many reporting increases in perceived social connectedness and empowerment in their recovery process (Alvarez-Jimenez et al. 2013 ). This moderated online social therapy program is now being evaluated as part of a large randomized controlled trial for maintaining treatment effects from first episode psychosis services (Alvarez-Jimenez et al. 2019 ).
Other early efforts have demonstrated that use of digital environments with the interactive peer-to-peer features of social media can enhance social functioning and wellbeing in young people at high risk of psychosis (Alvarez-Jimenez et al. 2018 ). There has also been a recent emergence of several mobile apps to support symptom monitoring and relapse prevention in psychotic disorders. Among these apps, the development of PRIME (Personalized Real-time Intervention for Motivational Enhancement) has involved working closely with young people with schizophrenia to ensure that the design of the app has the look and feel of mainstream social media platforms, as opposed to existing clinical tools (Schlosser et al. 2016 ). This unique approach to the design of the app is aimed at promoting engagement and ensuring that the app can effectively improve motivation and functioning through goal setting and promoting better quality of life of users with schizophrenia (Schlosser et al. 2018 ).
Social media platforms could also be used to promote engagement and participation in in-person services delivered through community mental health settings. For example, the peer-based lifestyle intervention called PeerFIT targets weight loss and improved fitness among individuals living with serious mental illness through a combination of in-person lifestyle classes, exercise groups, and use of digital technologies (Aschbrenner et al. 2016b , c ). The intervention holds tremendous promise as lack of support is one of the largest barriers towards exercise in patients with serious mental illness (Firth et al. 2016 ), and it is now possible to use social media to counter such. Specifically, in PeerFIT, a private Facebook group is closely integrated into the program to offer a closed platform where participants can connect with the lifestyle coaches, access intervention content, and support or encourage each other as they work towards their lifestyle goals (Aschbrenner et al. 2016a ; Naslund et al. 2016a ). To date, this program has demonstrated preliminary effectiveness for meaningfully reducing cardiovascular risk factors that contribute to early mortality in this patient group (Aschbrenner, Naslund, Shevenell, Kinney, et al., 2016), while the Facebook component appears to have increased engagement in the program, while allowing participants who were unable to attend in-person sessions due to other health concerns or competing demands to remain connected with the program (Naslund et al. 2018 ). This lifestyle intervention is currently being evaluated in a randomized controlled trial enrolling young adults with serious mental illness from real world community mental health services settings (Aschbrenner et al. 2018a ).
These examples highlight the promise of incorporating the features of popular social media into existing programs, which may offer opportunities to safely promote engagement and program retention, while achieving improved clinical outcomes. This is an emerging area of research, as evidenced by several important effectiveness trials underway (Alvarez-Jimenez et al. 2019 ; Aschbrenner et al. 2018a ), including efforts to leverage online social networking to support family caregivers of individuals receiving first episode psychosis services (Gleeson et al. 2017 ).
Challenges with Social Media for Mental Health
The science on the role of social media for engaging persons with mental disorders needs a cautionary note on the effects of social media usage on mental health and wellbeing, particularly in adolescents and young adults. While the risks and harms of social media are frequently covered in the popular press and mainstream news reports, careful consideration of the research in this area is necessary. In a review of 43 studies in young people, many benefits of social media were cited, including increased self-esteem and opportunities for self-disclosure (Best et al. 2014 ). Yet, reported negative effects were an increased exposure to harm, social isolation, depressive symptoms, and bullying (Best et al. 2014 ). In the sections that follow (see Table 1 for a summary), we consider three major categories of risk related to use of social media and mental health. These include: (1) Impact on symptoms; (2) Facing hostile interactions; and (3) Consequences for daily life.
Impact on Symptoms
Studies consistently highlight that use of social media, especially heavy use and prolonged time spent on social media platforms, appears to contribute to increased risk for a variety of mental health symptoms and poor wellbeing, especially among young people (Andreassen et al. 2016 ; Kross et al. 2013 ; Woods and Scott 2016 ). This may partly be driven by the detrimental effects of screen time on mental health, including increased severity of anxiety and depressive symptoms, which have been well documented (Stiglic and Viner 2019 ). Recent studies have reported negative effects of social media use on mental health of young people, including social comparison pressure with others and greater feeling of social isolation after being rejected by others on social media (Rideout and Fox 2018 ). In a study of young adults, it was found that negative comparisons with others on Facebook contributed to risk of rumination and subsequent increases in depression symptoms (Feinstein et al. 2013 ). Still, the cross-sectional nature of many screen time and mental health studies makes it challenging to reach causal inferences (Orben and Przybylski 2019 ).
Quantity of social media use is also an important factor, as highlighted in a survey of young adults ages 19 to 32, where more frequent visits to social media platforms each week were correlated with greater depressive symptoms (Lin et al. 2016 ). More time spent using social media is also associated with greater symptoms of anxiety (Vannucci et al. 2017 ). The actual number of platforms accessed also appears to contribute to risk as reflected in another national survey of young adults where use of a large number of social media platforms was associated with negative impact on mental health (Primack et al. 2017 ). Among survey respondents using between 7 and 11 different social media platforms compared with respondents using only 2 or fewer platforms, there were 3 times greater odds of having high levels of depressive symptoms and a 3.2 times greater odds of having high levels of anxiety symptoms (Primack et al. 2017 ).
Many researchers have postulated that worsening mental health attributed to social media use may be because social media replaces face-to-face interactions for young people (Twenge and Campbell 2018 ) and may contribute to greater loneliness (Bucci et al. 2019 ) and negative effects on other aspects of health and wellbeing (Woods and Scott 2016 ). One nationally representative survey of US adolescents found that among respondents who reported more time accessing media such as social media platforms or smartphone devices, there were significantly greater depressive symptoms and increased risk of suicide when compared with adolescents who reported spending more time on non-screen activities, such as in-person social interaction or sports and recreation activities (Twenge et al. 2018 ). For individuals living with more severe mental illnesses, the effects of social media on psychiatric symptoms have received less attention. One study found that participation in chat rooms may contribute to worsening symptoms in young people with psychotic disorders (Mittal et al. 2007 ), while another study of patients with psychosis found that social media use appeared to predict low mood (Berry et al. 2018 ). These studies highlight a clear relationship between social media use and mental health that may not be present in general population studies (Orben and Przybylski 2019 ) and emphasize the need to explore how social media may contribute to symptom severity and whether protective factors may be identified to mitigate these risks.
Facing Hostile Interactions
Popular social media platforms can create potential situations where individuals may be victimized by negative comments or posts. Cyberbullying represents a form of online aggression directed towards specific individuals, such as peers or acquaintances, which is perceived to be most harmful when compared with random hostile comments posted online (Hamm et al. 2015 ). Importantly, cyberbullying on social media consistently shows harmful impact on mental health in the form of increased depressive symptoms as well as worsening of anxiety symptoms, as evidenced in a review of 36 studies among children and young people (Hamm et al. 2015 ). Furthermore, cyberbullying disproportionately impacts females as reflected in a national survey of adolescents in the USA, where females were twice as likely to be victims of cyberbullying compared with males (Alhajji et al. 2019 ). Most studies report cross-sectional associations between cyberbullying and symptoms of depression or anxiety (Hamm et al. 2015 ), though one longitudinal study in Switzerland found that cyberbullying contributed to significantly greater depression over time (Machmutow et al. 2012 ).
For youth ages 10 to 17 who reported major depressive symptomatology, there were over 3 times greater odds of facing online harassment in the last year compared with youth who reported mild or no depressive symptoms (Ybarra 2004 ). Similarly, in a 2018 national survey of young people, respondents ages 14 to 22 with moderate to severe depressive symptoms were more likely to have had negative experiences when using social media and, in particular, were more likely to report having faced hostile comments or being “trolled” from others when compared with respondents without depressive symptoms (31% vs. 14%) (Rideout and Fox 2018 ). As these studies depict risks for victimization on social media and the correlation with poor mental health, it is possible that individuals living with mental illness may also experience greater hostility online compared to individuals without mental illness. This would be consistent with research showing greater risk of hostility, including increased violence and discrimination, directed towards individuals living with mental illness in in-person contexts, especially targeted at those with severe mental illnesses (Goodman et al. 1999 ).
A computational study of mental health awareness campaigns on Twitter reported that while stigmatizing content was rare, it was actually the most spread (re-tweeted) demonstrating that harmful content can travel quickly on social media (Saha et al. 2019 ). Another study was able to map the spread of social media posts about the Blue Whale Challenge, an alleged game promoting suicide, over Twitter, YouTube, Reddit, Tumblr, and other forums across 127 countries (Sumner et al. 2019 ). These findings show that it is critical to monitor the actual content of social media posts, such as determining whether content is hostile or promotes harm to self or others. This is pertinent because existing research looking at duration of exposure cannot account for the impact of specific types of content on mental health and is insufficient to fully understand the effects of using these platforms on mental health.
Consequences for Daily Life
The ways in which individuals use social media can also impact their offline relationships and everyday activities. To date, reports have described risks of social media use pertaining to privacy, confidentiality, and unintended consequences of disclosing personal health information online (Torous and Keshavan 2016 ). Additionally, concerns have been raised about poor quality or misleading health information shared on social media and that social media users may not be aware of misleading information or conflicts of interest especially when the platforms promote popular content regardless of whether it is from a trustworthy source (Moorhead et al. 2013 ; Ventola 2014 ). For persons living with mental illness, there may be additional risks from using social media. A recent study that specifically explored the perspectives of social media users with serious mental illnesses, including participants with schizophrenia spectrum disorders, bipolar disorder, or major depression, found that over one third of participants expressed concerns about privacy when using social media (Naslund and Aschbrenner 2019 ). The reported risks of social media use were directly related to many aspects of everyday life, including concerns about threats to employment, fear of stigma and being judged, impact on personal relationships, and facing hostility or being hurt (Naslund and Aschbrenner 2019 ). While few studies have specifically explored the dangers of social media use from the perspectives of individuals living with mental illness, it is important to recognize that use of these platforms may contribute to risks that extend beyond worsening symptoms and that can affect different aspects of daily life.
In this commentary, we considered ways in which social media may yield benefits for individuals living with mental illness, while contrasting these with the possible harms. Studies reporting on the threats of social media for individuals with mental illness are mostly cross-sectional, making it difficult to draw conclusions about direction of causation. However, the risks are potentially serious. These risks should be carefully considered in discussions pertaining to use of social media and the broader use of digital mental health technologies, as avenues for mental health promotion or for supporting access to evidence-based programs or mental health services. At this point, it would be premature to view the benefits of social media as outweighing the possible harms, when it is clear from the studies summarized here that social media use can have negative effects on mental health symptoms, can potentially expose individuals to hurtful content and hostile interactions, and can result in serious consequences for daily life, including threats to employment and personal relationships. Despite these risks, it is also necessary to recognize that individuals with mental illness will continue to use social media given the ease of accessing these platforms and the immense popularity of online social networking. With this in mind, it may be ideal to raise awareness about these possible risks so that individuals can implement necessary safeguards, while highlighting that there could also be benefits. Being aware of the risks is an essential first step, before then recognizing that use of these popular platforms could contribute to some benefits like finding meaningful interactions with others, engaging with peer support networks, and accessing information and services.
To capitalize on the widespread use of social media and to achieve the promise that these platforms may hold for supporting the delivery of targeted mental health interventions, there is need for continued research to better understand how individuals living with mental illness use social media. Such efforts could inform safety measures and also encourage use of social media in ways that maximize potential benefits while minimizing risk of harm. It will be important to recognize how gender and race contribute to differences in use of social media for seeking mental health information or accessing interventions, as well as differences in how social media might impact mental wellbeing. For example, a national survey of 14- to 22-year olds in the USA found that female respondents were more likely to search online for information about depression or anxiety and to try to connect with other people online who share similar mental health concerns when compared with male respondents (Rideout and Fox 2018 ). In the same survey, there did not appear to be any differences between racial or ethnic groups in social media use for seeking mental health information (Rideout and Fox 2018 ). Social media use also appears to have a differential impact on mental health and emotional wellbeing between females and males (Booker et al. 2018 ), highlighting the need to explore unique experiences between gender groups to inform tailored programs and services. Research shows that lesbian, gay, bisexual, or transgender individuals frequently use social media for searching for health information and may be more likely compared with heterosexual individuals to share their own personal health experiences with others online (Rideout and Fox 2018 ). Less is known about use of social media for seeking support for mental health concerns among gender minorities, though this is an important area for further investigation as these individuals are more likely to experience mental health problems and online victimization when compared with heterosexual individuals (Mereish et al. 2019 ).
Similarly, efforts are needed to explore the relationship between social media use and mental health among ethnic and racial minorities. A recent study found that exposure to traumatic online content on social media showing violence or hateful posts directed at racial minorities contributed to increases in psychological distress, PTSD symptoms, and depression among African American and Latinx adolescents in the USA (Tynes et al. 2019 ). These concerns are contrasted by growing interest in the potential for new technologies including social media to expand the reach of services to underrepresented minority groups (Schueller et al. 2019 ). Therefore, greater attention is needed to understanding the perspectives of ethnic and racial minorities to inform effective and safe use of social media for mental health promotion efforts.
Research has found that individuals living with mental illness have expressed interest in accessing mental health services through social media platforms. A survey of social media users with mental illness found that most respondents were interested in accessing programs for mental health on social media targeting symptom management, health promotion, and support for communicating with health care providers and interacting with the health system (Naslund et al. 2017 ). Importantly, individuals with serious mental illness have also emphasized that any mental health intervention on social media would need to be moderated by someone with adequate training and credentials, would need to have ground rules and ways to promote safety and minimize risks, and importantly, would need to be free and easy to access.
An important strength with this commentary is that it combines a range of studies broadly covering the topic of social media and mental health. We have provided a summary of recent evidence in a rapidly advancing field with the goal of presenting unique ways that social media could offer benefits for individuals with mental illness, while also acknowledging the potentially serious risks and the need for further investigation. There are also several limitations with this commentary that warrant consideration. Importantly, as we aimed to address this broad objective, we did not conduct a systematic review of the literature. Therefore, the studies reported here are not exhaustive, and there may be additional relevant studies that were not included. Additionally, we only summarized published studies, and as a result, any reports from the private sector or websites from different organizations using social media or other apps containing social media–like features would have been omitted. Although, it is difficult to rigorously summarize work from the private sector, sometimes referred to as “gray literature,” because many of these projects are unpublished and are likely selective in their reporting of findings given the target audience may be shareholders or consumers.
Another notable limitation is that we did not assess risk of bias in the studies summarized in this commentary. We found many studies that highlighted risks associated with social media use for individuals living with mental illness; however, few studies of programs or interventions reported negative findings, suggesting the possibility that negative findings may go unpublished. This concern highlights the need for a future more rigorous review of the literature with careful consideration of bias and an accompanying quality assessment. Most of the studies that we described were from the USA, as well as from other higher income settings such as Australia or the UK. Despite the global reach of social media platforms, there is a dearth of research on the impact of these platforms on the mental health of individuals in diverse settings, as well as the ways in which social media could support mental health services in lower income countries where there is virtually no access to mental health providers. Future research is necessary to explore the opportunities and risks for social media to support mental health promotion in low-income and middle-income countries, especially as these countries face a disproportionate share of the global burden of mental disorders, yet account for the majority of social media users worldwide (Naslund et al. 2019 ).
Future Directions for Social Media and Mental Health
As we consider future research directions, the near ubiquitous social media use also yields new opportunities to study the onset and manifestation of mental health symptoms and illness severity earlier than traditional clinical assessments. There is an emerging field of research referred to as “digital phenotyping” aimed at capturing how individuals interact with their digital devices, including social media platforms, in order to study patterns of illness and identify optimal time points for intervention (Jain et al. 2015 ; Onnela and Rauch 2016 ). Given that most people access social media via mobile devices, digital phenotyping and social media are closely related (Torous et al. 2019 ). To date, the emergence of machine learning, a powerful computational method involving statistical and mathematical algorithms (Shatte et al. 2019 ), has made it possible to study large quantities of data captured from popular social media platforms such as Twitter or Instagram to illuminate various features of mental health (Manikonda and De Choudhury 2017 ; Reece et al. 2017 ). Specifically, conversations on Twitter have been analyzed to characterize the onset of depression (De Choudhury et al. 2013 ) as well as detecting users’ mood and affective states (De Choudhury et al. 2012 ), while photos posted to Instagram can yield insights for predicting depression (Reece and Danforth 2017 ). The intersection of social media and digital phenotyping will likely add new levels of context to social media use in the near future.
Several studies have also demonstrated that when compared with a control group, Twitter users with a self-disclosed diagnosis of schizophrenia show unique online communication patterns (Birnbaum et al. 2017a ), including more frequent discussion of tobacco use (Hswen et al. 2017 ), symptoms of depression and anxiety (Hswen et al. 2018b ), and suicide (Hswen et al. 2018a ). Another study found that online disclosures about mental illness appeared beneficial as reflected by fewer posts about symptoms following self-disclosure (Ernala et al. 2017 ). Each of these examples offers early insights into the potential to leverage widely available online data for better understanding the onset and course of mental illness. It is possible that social media data could be used to supplement additional digital data, such as continuous monitoring using smartphone apps or smart watches, to generate a more comprehensive “digital phenotype” to predict relapse and identify high-risk health behaviors among individuals living with mental illness (Torous et al. 2019 ).
With research increasingly showing the valuable insights that social media data can yield about mental health states, greater attention to the ethical concerns with using individual data in this way is necessary (Chancellor et al. 2019 ). For instance, data is typically captured from social media platforms without the consent or awareness of users (Bidargaddi et al. 2017 ), which is especially crucial when the data relates to a socially stigmatizing health condition such as mental illness (Guntuku et al. 2017 ). Precautions are needed to ensure that data is not made identifiable in ways that were not originally intended by the user who posted the content as this could place an individual at risk of harm or divulge sensitive health information (Webb et al. 2017 ; Williams et al. 2017 ). Promising approaches for minimizing these risks include supporting the participation of individuals with expertise in privacy, clinicians, and the target individuals with mental illness throughout the collection of data, development of predictive algorithms, and interpretation of findings (Chancellor et al. 2019 ).
In recognizing that many individuals living with mental illness use social media to search for information about their mental health, it is possible that they may also want to ask their clinicians about what they find online to check if the information is reliable and trustworthy. Alternatively, many individuals may feel embarrassed or reluctant to talk to their clinicians about using social media to find mental health information out of concerns of being judged or dismissed. Therefore, mental health clinicians may be ideally positioned to talk with their patients about using social media and offer recommendations to promote safe use of these sites while also respecting their patients’ autonomy and personal motivations for using these popular platforms. Given the gap in clinical knowledge about the impact of social media on mental health, clinicians should be aware of the many potential risks so that they can inform their patients while remaining open to the possibility that their patients may also experience benefits through use of these platforms. As awareness of these risks grows, it may be possible that new protections will be put in place by industry or through new policies that will make the social media environment safer. It is hard to estimate a number needed to treat or harm today given the nascent state of research, which means the patient and clinician need to weigh the choice on a personal level. Thus, offering education and information is an important first step in that process. As patients increasingly show interest in accessing mental health information or services through social media, it will be necessary for health systems to recognize social media as a potential avenue for reaching or offering support to patients. This aligns with growing emphasis on the need for greater integration of digital psychiatry, including apps, smartphones, or wearable devices, into patient care and clinical services through institution-wide initiatives and training clinical providers (Hilty et al. 2019 ). Within a learning healthcare environment where research and care are tightly intertwined and feedback between both is rapid, the integration of digital technologies into services may create new opportunities for advancing use of social media for mental health.
As highlighted in this commentary, social media has become an important part of the lives of many individuals living with mental disorders. Many of these individuals use social media to share their lived experiences with mental illness, to seek support from others, and to search for information about treatment recommendations, accessing mental health services and coping with symptoms (Bucci et al. 2019 ; Highton-Williamson et al. 2015 ; Naslund et al. 2016b ). As the field of digital mental health advances, the wide reach, ease of access, and popularity of social media platforms could be used to allow individuals in need of mental health services or facing challenges of mental illness to access evidence-based treatment and support. To achieve this end and to explore whether social media platforms can advance efforts to close the gap in available mental health services in the USA and globally, it will be essential for researchers to work closely with clinicians and with those affected by mental illness to ensure that possible benefits of using social media are carefully weighed against anticipated risks.
Abdel-Baki, A., Lal, S., Charron, D.-C., Stip, E., & Kara, N. (2017). Understanding access and use of technology among youth with first-episode psychosis to inform the development of technology-enabled therapeutic interventions. Early Intervention in Psychiatry, 11 (1), 72–76.
PubMed Google Scholar
Ahmed, Y. A., Ahmad, M. N., Ahmad, N., & Zakaria, N. H. (2019). Social media for knowledge-sharing: a systematic literature review. Telematics and Informatics, 37 , 72–112.
Google Scholar
Alhajji, M., Bass, S., & Dai, T. (2019). Cyberbullying, mental health, and violence in adolescents and associations with sex and race: data from the 2015 youth risk behavior survey. Global Pediatric Health, 6 , 2333794X19868887.
PubMed PubMed Central Google Scholar
Alvarez-Jimenez, M., Bendall, S., Lederman, R., Wadley, G., Chinnery, G., Vargas, S., Larkin, M., Killackey, E., McGorry, P., & Gleeson, J. F. (2013). On the HORYZON: moderated online social therapy for long-term recovery in first episode psychosis. Schizophrenia Research, 143 (1), 143–149.
Alvarez-Jimenez, M., Gleeson, J., Bendall, S., Penn, D., Yung, A., Ryan, R., et al. (2018). Enhancing social functioning in young people at ultra high risk (UHR) for psychosis: a pilot study of a novel strengths and mindfulness-based online social therapy. Schizophrenia Research, 202 , 369–377.
Alvarez-Jimenez, M., Bendall, S., Koval, P., Rice, S., Cagliarini, D., Valentine, L., et al. (2019). HORYZONS trial: protocol for a randomised controlled trial of a moderated online social therapy to maintain treatment effects from first-episode psychosis services. BMJ Open, 9 (2), e024104.
Andreassen, C. S., Billieux, J., Griffiths, M. D., Kuss, D. J., Demetrovics, Z., Mazzoni, E., & Pallesen, S. (2016). The relationship between addictive use of social media and video games and symptoms of psychiatric disorders: a large-scale cross-sectional study. Psychology of Addictive Behaviors, 30 (2), 252.
Aschbrenner, K. A., Naslund, J. A., & Bartels, S. J. (2016a). A mixed methods study of peer-to-peer support in a group-based lifestyle intervention for adults with serious mental illness. Psychiatric Rehabilitation Journal, 39 (4), 328–334.
Aschbrenner, K. A., Naslund, J. A., Shevenell, M., Kinney, E., & Bartels, S. J. (2016b). A pilot study of a peer-group lifestyle intervention enhanced with mHealth technology and social media for adults with serious mental illness. The Journal of Nervous and Mental Disease, 204 (6), 483–486.
Aschbrenner, K. A., Naslund, J. A., Shevenell, M., Mueser, K. T., & Bartels, S. J. (2016c). Feasibility of behavioral weight loss treatment enhanced with peer support and mobile health technology for individuals with serious mental illness. Psychiatric Quarterly, 87 (3), 401–415.
Aschbrenner, K. A., Naslund, J. A., Gorin, A. A., Mueser, K. T., Scherer, E. A., Viron, M., et al. (2018a). Peer support and mobile health technology targeting obesity-related cardiovascular risk in young adults with serious mental illness: protocol for a randomized controlled trial. Contemporary Clinical Trials, 74 , 97–106.
Aschbrenner, K. A., Naslund, J. A., Grinley, T., Bienvenida, J. C. M., Bartels, S. J., & Brunette, M. (2018b). A survey of online and mobile technology use at peer support agencies. Psychiatric Quarterly , 1–10.
Aschbrenner, K. A., Naslund, J. A., Tomlinson, E. F., Kinney, A., Pratt, S. I., & Brunette, M. F. (2019). Adolescents’ use of digital technologies and preferences for mobile health coaching in mental health settings. Frontiers in Public Health. 7 , 178.
Badcock, J. C., Shah, S., Mackinnon, A., Stain, H. J., Galletly, C., Jablensky, A., & Morgan, V. A. (2015). Loneliness in psychotic disorders and its association with cognitive function and symptom profile. Schizophrenia Research, 169 (1–3), 268–273.
Batterham, P. J., & Calear, A. J. (2017). Preferences for internet-based mental health interventions in an adult online sample: findings from ann online community survey. JMIR Mental Health, 4 (2), e26.
Bauer, R., Bauer, M., Spiessl, H., & Kagerbauer, T. (2013). Cyber-support: an analysis of online self-help forums (online self-help forums in bipolar disorder). Nordic Journal of Psychiatry, 67 (3), 185–190.
Berger, M., Wagner, T. H., & Baker, L. C. (2005). Internet use and stigmatized illness. Social Science & Medicine, 61 (8), 1821–1827.
Berry, N., Lobban, F., Belousov, M., Emsley, R., Nenadic, G., & Bucci, S. (2017). # WhyWeTweetMH: understanding why people use Twitter to discuss mental health problems. Journal of Medical Internet Research, 19 (4), e107.
Berry, N., Emsley, R., Lobban, F., & Bucci, S. (2018). Social media and its relationship with mood, self-esteem and paranoia in psychosis. Acta Psychiatrica Scandinavica, 138 , 558–570.
Best, P., Manktelow, R., & Taylor, B. (2014). Online communication, social media and adolescent wellbeing: a systematic narrative review. Children and Youth Services Review, 41 , 27–36.
Biagianti, B., Quraishi, S. H., & Schlosser, D. A. (2018). Potential benefits of incorporating peer-to-peer interactions into digital interventions for psychotic disorders: a systematic review. Psychiatric Services, 69 (4), 377–388.
Bidargaddi, N., Musiat, P., Makinen, V.-P., Ermes, M., Schrader, G., & Licinio, J. (2017). Digital footprints: facilitating large-scale environmental psychiatric research in naturalistic settings through data from everyday technologies. Molecular Psychiatry, 22 (2), 164.
Birnbaum, M. L., Ernala, S. K., Rizvi, A. F., De Choudhury, M., & Kane, J. M. (2017a). A collaborative approach to identifying social media markers of schizophrenia by employing machine learning and clinical appraisals. Journal of Medical Internet Research, 19 (8), e289.
Birnbaum, M. L., Rizvi, A. F., Correll, C. U., Kane, J. M., & Confino, J. (2017b). Role of social media and the Internet in pathways to care for adolescents and young adults with psychotic disorders and non-psychotic mood disorders. Early Intervention in Psychiatry, 11 (4), 290–295.
Booker, C. L., Kelly, Y. J., & Sacker, A. (2018). Gender differences in the associations between age trends of social media interaction and well-being among 10-15 year olds in the UK. BMC Public Health, 18 (1), 321.
Brunette, M., Achtyes, E., Pratt, S., Stilwell, K., Opperman, M., Guarino, S., & Kay-Lambkin, F. (2019). Use of smartphones, computers and social media among people with SMI: opportunity for intervention. Community Mental Health Journal , 1–6.
Brusilovskiy, E., Townley, G., Snethen, G., & Salzer, M. S. (2016). Social media use, community participation and psychological well-being among individuals with serious mental illnesses. Computers in Human Behavior, 65 , 232–240.
Bucci, S., Schwannauer, M., & Berry, N. (2019). The digital revolution and its impact on mental health care. Psychology and Psychotherapy: Theory, Research and Practice, 92 (2), 277–297.
Chancellor, S., Birnbaum, M. L., Caine, E. D., Silenzio, V. M., & De Choudhury, M. (2019). A taxonomy of ethical tensions in inferring mental health states from social media. In Proceedings of the Conference on Fairness, Accountability, and Transparency, 79–88.
Chang, H. J. (2009). Online supportive interactions: using a network approach to examine communication patterns within a psychosis social support group in Taiwan. Journal of the American Society for Information Science and Technology, 60 (7), 1504–1517.
Davidson, L., Chinman, M., Sells, D., & Rowe, M. (2006). Peer support among adults with serious mental illness: a report from the field. Schizophrenia Bulletin, 32 (3), 443–450.
De Choudhury, M., Gamon, M., & Counts, S. (2012). Happy, nervous or surprised? classification of human affective states in social media. Paper presented at the sixth international Association for Advancement of Artificial Intelligence (AAAI) Conference on Weblogs and Social Meedia, 435–438.
De Choudhury, M., Gamon, M., Counts, S., & Horvitz, E. (2013). Predicting depression via social media. Paper presented at the seventh international Association for Advancement of Artificial Intelligence (AAAI) Conference on Weblogs and Social Media, 128–137.
Docherty, N. M., Hawkins, K. A., Hoffman, R. E., Quinlan, D. M., Rakfeldt, J., & Sledge, W. H. (1996). Working memory, attention, and communication disturbances in schizophrenia. Journal of Abnormal Psychology, 105 (2), 212–219.
Ernala, S. K., Rizvi, A. F., Birnbaum, M. L., Kane, J. M., & De Choudhury, M. (2017). Linguistic markers indicating therapeutic outcomes of social media disclosures of schizophrenia. Proceedings of the ACM on Human-Computer Interaction, 1 (1), 43.
Feinstein, B. A., Hershenberg, R., Bhatia, V., Latack, J. A., Meuwly, N., & Davila, J. (2013). Negative social comparison on Facebook and depressive symptoms: rumination as a mechanism. Psychology of Popular Media Culture, 2 (3), 161.
Firth, J., Cotter, J., Torous, J., Bucci, S., Firth, J. A., & Yung, A. R. (2015). Mobile phone ownership and endorsement of “mHealth” among people with psychosis: a meta-analysis of cross-sectional studies. Schizophrenia Bulletin, 42 (2), 448–455.
Firth, J., Rosenbaum, S., Stubbs, B., Gorczynski, P., Yung, A. R., & Vancampfort, D. (2016). Motivating factors and barriers towards exercise in severe mental illness: a systematic review and meta-analysis. Psychological Medicine, 46 (14), 2869–2881.
Gay, K., Torous, J., Joseph, A., Pandya, A., & Duckworth, K. (2016). Digital technology use among individuals with schizophrenia: results of an online survey. JMIR Mental Health, 3 (2), e15.
Giacco, D., Palumbo, C., Strappelli, N., Catapano, F., & Priebe, S. (2016). Social contacts and loneliness in people with psychotic and mood disorders. Comprehensive Psychiatry, 66 , 59–66.
Gleeson, J., Lederman, R., Herrman, H., Koval, P., Eleftheriadis, D., Bendall, S., Cotton, S. M., & Alvarez-Jimenez, M. (2017). Moderated online social therapy for carers of young people recovering from first-episode psychosis: study protocol for a randomised controlled trial. Trials, 18 (1), 27.
Glick, G., Druss, B., Pina, J., Lally, C., & Conde, M. (2016). Use of mobile technology in a community mental health setting. Journal of Telemedicine and Telecare, 22 (7), 430–435.
Goodman, L. A., Thompson, K. M., Weinfurt, K., Corl, S., Acker, P., Mueser, K. T., & Rosenberg, S. D. (1999). Reliability of reports of violent victimization and posttraumatic stress disorder among men and women with serious mental illness. Journal of Traumatic Stress: Official Publication of the International Society for Traumatic Stress Studies, 12 (4), 587–599.
Gowen, K., Deschaine, M., Gruttadara, D., & Markey, D. (2012). Young adults with mental health conditions and social networking websites: seeking tools to build community. Psychiatric Rehabilitation Journal, 35 (3), 245–250.
Guntuku, S. C., Yaden, D. B., Kern, M. L., Ungar, L. H., & Eichstaedt, J. C. (2017). Detecting depression and mental illness on social media: an integrative review. Current Opinion in Behavioral Sciences, 18 , 43–49.
Haker, H., Lauber, C., & Rössler, W. (2005). Internet forums: a self-help approach for individuals with schizophrenia? Acta Psychiatrica Scandinavica, 112 (6), 474–477.
Hamm, M. P., Newton, A. S., Chisholm, A., Shulhan, J., Milne, A., Sundar, P., Ennis, H., Scott, S. D., & Hartling, L. (2015). Prevalence and effect of cyberbullying on children and young people: a scoping review of social media studies. JAMA Pediatrics, 169 (8), 770–777.
Hansen, C. F., Torgalsbøen, A.-K., Melle, I., & Bell, M. D. (2009). Passive/apathetic social withdrawal and active social avoidance in schizophrenia: difference in underlying psychological processes. The Journal of Nervous and Mental Disease, 197 (4), 274–277.
Highton-Williamson, E., Priebe, S., & Giacco, D. (2015). Online social networking in people with psychosis: a systematic review. International Journal of Social Psychiatry, 61 (1), 92–101.
Hilty, D. M., Chan, S., Torous, J., Luo, J., & Boland, R. J. (2019). Mobile health, smartphone/device, and apps for psychiatry and medicine: competencies, training, and faculty development issues. Psychiatric Clinics, 42 (3), 513–534.
Hswen, Y., Naslund, J. A., Chandrashekar, P., Siegel, R., Brownstein, J. S., & Hawkins, J. B. (2017). Exploring online communication about cigarette smoking among Twitter users who self-identify as having schizophrenia. Psychiatry Research, 257 , 479–484.
Hswen, Y., Naslund, J. A., Brownstein, J. S., & Hawkins, J. B. (2018a). Monitoring online discussions about suicide among Twitter users with schizophrenia: exploratory study. JMIR Mental Health, 5 (4), e11483.
Hswen, Y., Naslund, J. A., Brownstein, J. S., & Hawkins, J. B. (2018b). Online communication about depression and anxiety among twitter users with schizophrenia: preliminary findings to inform a digital phenotype using social media. Psychiatric Quarterly, 89 (3), 569–580.
Indian, M., & Grieve, R. (2014). When Facebook is easier than face-to-face: social support derived from Facebook in socially anxious individuals. Personality and Individual Differences, 59 , 102–106.
Jain, S. H., Powers, B. W., Hawkins, J. B., & Brownstein, J. S. (2015). The digital phenotype. Nature Biotechnology, 33 (5), 462–463.
Kiesler, S., Siegel, J., & McGuire, T. W. (1984). Social psychological aspects of computer-mediated communication. American Psychologist, 39 , 1123–1134.
Kross, E., Verduyn, P., Demiralp, E., Park, J., Lee, D. S., Lin, N., Shablack, H., Jonides, J., & Ybarra, O. (2013). Facebook use predicts declines in subjective well-being in young adults. PLoS One, 8 (8), e69841.
Lal, S., Nguyen, V., & Theriault, J. (2018). Seeking mental health information and support online: experiences and perspectives of young people receiving treatment for first-episode psychosis. Early Intervention in Psychiatry, 12 (3), 324–330.
Lin, L. Y., Sidani, J. E., Shensa, A., Radovic, A., Miller, E., Colditz, J. B., Hoffman, B. L., Giles, L. M., & Primack, B. A. (2016). Association between social media use and depression among US young adults. Depression and Anxiety, 33 (4), 323–331.
Machmutow, K., Perren, S., Sticca, F., & Alsaker, F. D. (2012). Peer victimisation and depressive symptoms: can specific coping strategies buffer the negative impact of cybervictimisation? Emotional and Behavioural Difficulties, 17 (3–4), 403–420.
Manikonda, L., & De Choudhury, M. (2017). Modeling and understanding visual attributes of mental health disclosures in social media. In Proceedings of the 2017 CHI Conference on Human Factors in Computing Systems, 170–181.
Mead, S., Hilton, D., & Curtis, L. (2001). Peer support: a theoretical perspective. Psychiatric Rehabilitation Journal, 25 (2), 134–141.
Mereish, E. H., Sheskier, M., Hawthorne, D. J., & Goldbach, J. T. (2019). Sexual orientation disparities in mental health and substance use among Black American young people in the USA: effects of cyber and bias-based victimisation. Culture, Health & Sexuality, 21 (9), 985–998.
Miller, B. J., Stewart, A., Schrimsher, J., Peeples, D., & Buckley, P. F. (2015). How connected are people with schizophrenia? Cell phone, computer, email, and social media use. Psychiatry Research, 225 (3), 458–463.
Mittal, V. A., Tessner, K. D., & Walker, E. F. (2007). Elevated social Internet use and schizotypal personality disorder in adolescents. Schizophrenia Research, 94 (1–3), 50–57.
Moorhead, S. A., Hazlett, D. E., Harrison, L., Carroll, J. K., Irwin, A., & Hoving, C. (2013). A new dimension of health care: systematic review of the uses, benefits, and limitations of social media for health communication. Journal of Medical Internet Research, 15 (4), e85.
Naslund, J. A., & Aschbrenner, K. A. (2019). Risks to privacy with use of social media: understanding the views of social media users with serious mental illness. Psychiatric Services, 70 (7), 561–568.
Naslund, J. A., Grande, S. W., Aschbrenner, K. A., & Elwyn, G. (2014). Naturally occurring peer support through social media: the experiences of individuals with severe mental illness using YouTube. PLoS One, 9 (10), e110171.
Naslund, J. A., Aschbrenner, K. A., & Bartels, S. J. (2016). How people living with serious mental illness use smartphones, mobile apps, and social media. Psychiatric Rehabilitation Journal, 39 (4), 364–367.
Naslund, J. A., Aschbrenner, K. A., Marsch, L. A., & Bartels, S. J. (2016a). Feasibility and acceptability of Facebook for health promotion among people with serious mental illness. Digital Health, 2 , 2055207616654822.
PubMed Central Google Scholar
Naslund, J. A., Aschbrenner, K. A., Marsch, L. A., & Bartels, S. J. (2016b). The future of mental health care: peer-to-peer support and social media. Epidemiology and Psychiatric Sciences, 25 (2), 113–122.
Naslund, J. A., Aschbrenner, K. A., McHugo, G. J., Unützer, J., Marsch, L. A., & Bartels, S. J. (2019). Exploring opportunities to support mental health care using social media: A survey of social media users with mental illness. Early Intervention in Psychiatry, 13 (3), 405–413.
Naslund, J. A., Aschbrenner, K. A., Marsch, L. A., McHugo, G. J., & Bartels, S. J. (2018). Facebook for supporting a lifestyle intervention for people with major depressive disorder, bipolar disorder, and schizophrenia: an exploratory study. Psychiatric Quarterly, 89 (1), 81–94.
Naslund, J. A., Gonsalves, P. P., Gruebner, O., Pendse, S. R., Smith, S. L., Sharma, A., & Raviola, G. (2019). Digital innovations for global mental health: opportunities for data science, task sharing, and early intervention. Current Treatment Options in Psychiatry , 1–15.
Onnela, J.-P., & Rauch, S. L. (2016). Harnessing smartphone-based digital phenotyping to enhance behavioral and mental health. Neuropsychopharmacology, 41 (7), 1691–1696.
Orben, A., & Przybylski, A. K. (2019). The association between adolescent well-being and digital technology use. Nature Human Behaviour, 3 (2), 173–182.
Patel, V., Saxena, S., Lund, C., Thornicroft, G., Baingana, F., Bolton, P., et al. (2018). The Lancet Commission on global mental health and sustainable development. The Lancet, 392 (10157), 1553–1598.
Primack, B. A., Shensa, A., Escobar-Viera, C. G., Barrett, E. L., Sidani, J. E., Colditz, J. B., & James, A. E. (2017). Use of multiple social media platforms and symptoms of depression and anxiety: a nationally-representative study among US young adults. Computers in Human Behavior, 69 , 1–9.
Reece, A. G., & Danforth, C. M. (2017). Instagram photos reveal predictive markers of depression. EPJ Data Science, 6 (1), 15.
Reece, A. G., Reagan, A. J., Lix, K. L., Dodds, P. S., Danforth, C. M., & Langer, E. J. (2017). Forecasting the onset and course of mental illness with Twitter data. Scientific Reports, 7 (1), 13006.
Rideout, V., & Fox, S. (2018). Digital health practices, social media use, and mental well-being among teens and young adults in the U.S. Retrieved from San Francisco, CA: https://www.hopelab.org/reports/pdf/a-national-survey-by-hopelab-and-well-being-trust-2018.pdf . Accessed 10 Jan 2020.
Saha, K., Torous, J., Ernala, S. K., Rizuto, C., Stafford, A., & De Choudhury, M. (2019). A computational study of mental health awareness campaigns on social media. Translational behavioral medicine, 9 (6), 1197–1207.
Schlosser, D. A., Campellone, T., Kim, D., Truong, B., Vergani, S., Ward, C., & Vinogradov, S. (2016). Feasibility of PRIME: a cognitive neuroscience-informed mobile app intervention to enhance motivated behavior and improve quality of life in recent onset schizophrenia. JMIR Research Protocols, 5 (2).
Schlosser, D. A., Campellone, T. R., Truong, B., Etter, K., Vergani, S., Komaiko, K., & Vinogradov, S. (2018). Efficacy of PRIME, a mobile app intervention designed to improve motivation in young people with schizophrenia. Schizophrenia Bulletin, 44 (5), 1010–1020.
Schrank, B., Sibitz, I., Unger, A., & Amering, M. (2010). How patients with schizophrenia use the internet: qualitative study. Journal of Medical Internet Research, 12 (5), e70.
Schueller, S. M., Hunter, J. F., Figueroa, C., & Aguilera, A. (2019). Use of digital mental health for marginalized and underserved populations. Current Treatment Options in Psychiatry, 6 (3), 243–255.
Shatte, A. B., Hutchinson, D. M., & Teague, S. J. (2019). Machine learning in mental health: a scoping review of methods and applications. Psychological Medicine, 49 (9), 1426–1448.
Spinzy, Y., Nitzan, U., Becker, G., Bloch, Y., & Fennig, S. (2012). Does the Internet offer social opportunities for individuals with schizophrenia? A cross-sectional pilot study. Psychiatry Research, 198 (2), 319–320.
Stiglic, N., & Viner, R. M. (2019). Effects of screentime on the health and well-being of children and adolescents: a systematic review of reviews. BMJ Open, 9 (1), e023191.
Sumner, S. A., Galik, S., Mathieu, J., Ward, M., Kiley, T., Bartholow, B., et al. (2019). Temporal and geographic patterns of social media posts about an emerging suicide game. Journal of Adolescent Health, 65 (1), 94–100.
Torous, J., & Keshavan, M. (2016). The role of social media in schizophrenia: evaluating risks, benefits, and potential. Current Opinion in Psychiatry, 29 (3), 190–195.
Torous, J., Chan, S. R., Tan, S. Y.-M., Behrens, J., Mathew, I., Conrad, E. J., et al. (2014a). Patient smartphone ownership and interest in mobile apps to monitor symptoms of mental health conditions: a survey in four geographically distinct psychiatric clinics. JMIR Mental Health, 1 (1), e5.
Torous, J., Friedman, R., & Keshavan, M. (2014b). Smartphone ownership and interest in mobile applications to monitor symptoms of mental health conditions. JMIR mHealth and uHealth, 2 (1), e2.
Torous, J., Wisniewski, H., Bird, B., Carpenter, E., David, G., Elejalde, E., et al. (2019). Creating a digital health smartphone app and digital phenotyping platform for mental health and diverse healthcare needs: an interdisciplinary and collaborative approach. Journal of Technology in Behavioral Science, 4 (2), 73–85.
Trefflich, F., Kalckreuth, S., Mergl, R., & Rummel-Kluge, C. (2015). Psychiatric patients' internet use corresponds to the internet use of the general public. Psychiatry Research, 226 , 136–141.
Twenge, J. M., & Campbell, W. K. (2018). Associations between screen time and lower psychological well-being among children and adolescents: evidence from a population-based study. Preventive Medicine Reports, 12 , 271–283.
Twenge, J. M., Joiner, T. E., Rogers, M. L., & Martin, G. N. (2018). Increases in depressive symptoms, suicide-related outcomes, and suicide rates among US adolescents after 2010 and links to increased new media screen time. Clinical Psychological Science, 6 (1), 3–17.
Tynes, B. M., Willis, H. A., Stewart, A. M., & Hamilton, M. W. (2019). Race-related traumatic events online and mental health among adolescents of color. Journal of Adolescent Health, 65 (3), 371–377.
Vannucci, A., Flannery, K. M., & Ohannessian, C. M. (2017). Social media use and anxiety in emerging adults. Journal of Affective Disorders, 207 , 163–166.
Vayreda, A., & Antaki, C. (2009). Social support and unsolicited advice in a bipolar disorder online forum. Qualitative Health Research, 19 (7), 931–942.
Ventola, C. L. (2014). Social media and health care professionals: benefits, risks, and best practices. Pharmacy and Therapeutics, 39 (7), 491–520.
We Are Social. (2020). Digital in 2020. Retrieved from https://wearesocial.com/global-digital-report-2019 . Accessed 10 Jan 2020.
Webb, H., Jirotka, M., Stahl, B. C., Housley, W., Edwards, A., Williams, M., ... & Burnap, P. (2017). The ethical challenges of publishing Twitter data for research dissemination . Paper presented at the proceedings of the 2017 ACM on Web Science Conference, 339–348.
Williams, M. L., Burnap, P., & Sloan, L. (2017). Towards an ethical framework for publishing twitter data in social research: taking into account users’ views, online context and algorithmic estimation. Sociology, 51 (6), 1149–1168.
Woods, H. C., & Scott, H. (2016). # Sleepyteens: social media use in adolescence is associated with poor sleep quality, anxiety, depression and low self-esteem. Journal of Adolescence, 51 , 41–49.
Ybarra, M. L. (2004). Linkages between depressive symptomatology and internet harassment among young regular Internet users. Cyberpsychology & Behavior, 7 (2), 247–257.
Download references
Dr. Naslund is supported by a grant from the National Institute of Mental Health (U19MH113211). Dr. Aschbrenner is supported by a grant from the National Institute of Mental Health (1R01MH110965-01).
Author information
Authors and affiliations.
Department of Global Health and Social Medicine, Harvard Medical School, 641 Huntington Avenue, Boston, MA, 02115, USA
John A. Naslund
Digital Mental Health Research Consultant, Mumbai, India
Ameya Bondre
Division of Digital Psychiatry, Beth Israel Deaconess Medical Center, Harvard Medical School, Boston, MA, USA
John Torous
Department of Psychiatry, Geisel School of Medicine at Dartmouth, Lebanon, NH, USA
Kelly A. Aschbrenner
You can also search for this author in PubMed Google Scholar
Corresponding author
Correspondence to John A. Naslund .
Ethics declarations
Conflict of interest.
The authors declare that they have no conflict of interest.
Additional information
Publisher’s note.
Springer Nature remains neutral with regard to jurisdictional claims in published maps and institutional affiliations.
Rights and permissions
Reprints and permissions
About this article
Naslund, J.A., Bondre, A., Torous, J. et al. Social Media and Mental Health: Benefits, Risks, and Opportunities for Research and Practice. J. technol. behav. sci. 5 , 245–257 (2020). https://doi.org/10.1007/s41347-020-00134-x
Download citation
Received : 19 October 2019
Revised : 24 February 2020
Accepted : 17 March 2020
Published : 20 April 2020
Issue Date : September 2020
DOI : https://doi.org/10.1007/s41347-020-00134-x
Share this article
Anyone you share the following link with will be able to read this content:
Sorry, a shareable link is not currently available for this article.
Provided by the Springer Nature SharedIt content-sharing initiative
- Find a journal
- Publish with us
- Track your research
Thank you for visiting nature.com. You are using a browser version with limited support for CSS. To obtain the best experience, we recommend you use a more up to date browser (or turn off compatibility mode in Internet Explorer). In the meantime, to ensure continued support, we are displaying the site without styles and JavaScript.
- View all journals
- Explore content
- About the journal
- Publish with us
- Sign up for alerts
- Review Article
- Published: 07 May 2024
Mechanisms linking social media use to adolescent mental health vulnerability
- Amy Orben ORCID: orcid.org/0000-0002-2937-4183 1 ,
- Adrian Meier ORCID: orcid.org/0000-0002-8191-2962 2 ,
- Tim Dalgleish ORCID: orcid.org/0000-0002-7304-2231 1 &
- Sarah-Jayne Blakemore 3 , 4
Nature Reviews Psychology ( 2024 ) Cite this article
5028 Accesses
168 Altmetric
Metrics details
- Psychiatric disorders
- Science, technology and society
Research linking social media use and adolescent mental health has produced mixed and inconsistent findings and little translational evidence, despite pressure to deliver concrete recommendations for families, schools and policymakers. At the same time, it is widely recognized that developmental changes in behaviour, cognition and neurobiology predispose adolescents to developing socio-emotional disorders. In this Review, we argue that such developmental changes would be a fruitful focus for social media research. Specifically, we review mechanisms by which social media could amplify the developmental changes that increase adolescents’ mental health vulnerability. These mechanisms include changes to behaviour, such as sharing risky content and self-presentation, and changes to cognition, such as modifications in self-concept, social comparison, responsiveness to social feedback and experiences of social exclusion. We also consider neurobiological mechanisms that heighten stress sensitivity and modify reward processing. By focusing on mechanisms by which social media might interact with developmental changes to increase mental health risks, our Review equips researchers with a toolkit of key digital affordances that enables theorizing and studying technology effects despite an ever-changing social media landscape.
You have full access to this article via your institution.
Similar content being viewed by others
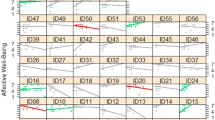
The effect of social media on well-being differs from adolescent to adolescent
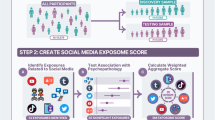
Probing the digital exposome: associations of social media use patterns with youth mental health
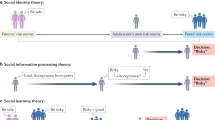
Social contextual risk taking in adolescence
Introduction.
Adolescence is a period marked by profound neurobiological, behavioural and environmental changes that facilitate the transition from familial dependence to independent membership in society 1 , 2 . This critical developmental stage is also characterized by diminished well-being and increased vulnerability to the onset of mental health conditions 3 , 4 , 5 , particularly socio-emotional disorders such as depression, and eating disorders 4 , 6 (Fig. 1 ). Notable symptoms of socio-emotional disorders include heightened negative affect, mood dysregulation and an increased focus on distress or challenges concerning interpersonal relationships, including heightened sensitivity to peers or perceptions of others 6 . Although some risk factors for socio-emotional disorders do not necessarily occur in adolescence (including genetic predispositions, adverse childhood experiences and poverty 7 , 8 , 9 ), the unique developmental characteristics of this period of life can interact with pre-existing vulnerabilities, increasing the risk of disorder onset 10 .
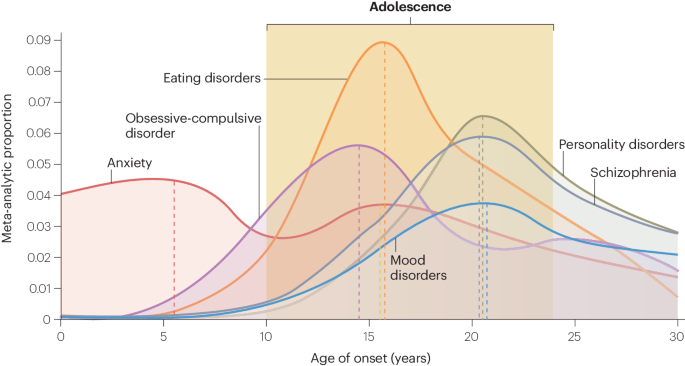
Meta-analytic proportion of age of onset of anxiety (red), obsessive-compulsive disorder (purple), eating disorders (orange), personality disorders (green), schizophrenia (grey) and mood disorders (blue). The peak age of onset (dotted lines) is 5.5 and 15.5 years for anxiety, 14.5 years for obsessive-compulsive disorder, 15.5 years for eating disorders and 20.5 years for personality disorders, schizophrenia and mood disorders. Adapted from ref. 258 , CC BY 4.0 ( https://creativecommons.org/licenses/by/4.0/ ).
Over the past decade, declines in adolescent mental health have become a great concern 11 , 12 . The prevalence of socio-emotional disorders has increased in the adolescent age range (10–24 years 2 ) 13 , 14 , 15 , 16 , 17 , 18 , 19 , 20 , 21 , leading to mounting pressures on child and adolescent mental health services 16 , 21 , 22 . This increase has not been as pronounced among other age groups when compared with adolescents 20 , 22 , 23 (measured in ref. 20 , ref. 22 and ref. 23 as age 12–25 years, 12–20 years and 18–25 years, respectively), even if some studies have found increases across the entire lifespan 24 , 25 . Although these trends might not be generalizable across the world 26 or to subclinical indicators of distress 15 , similar trends have been found in a range of countries 27 . Declines in adolescent mental health, especially socio-emotional problems, are consistent across datasets and researchers have argued that they are not solely driven by changes in social attitudes, stigma or reporting of distress 28 , 29 .
Concurrently, adolescents’ lives have become increasingly digital, with most young people using social media platforms throughout the day 30 . Ninety-five per cent of UK adolescents aged 15 years use social media 31 , and 50% of US adolescents aged 13–17 years report being almost constantly online 32 . The social media environment impacts adolescent and adult life across many domains (for example, by enabling social communication or changing the way news is accessed) and influences individuals, dyads and larger social systems 33 , 34 , 35 , 36 . Because social media is inherently social and relational 37 , it potentially overlaps and interacts with the developmental changes that make adolescents vulnerable to the onset of mental health problems 38 , 39 (Fig. 2 ). Thus, it has been intensely debated whether the increase in social media use during the past decade has a causal role in the decline of adolescent mental health 40 . Indeed, rapid changes to the environment experienced before and during adolescence might be a fruitful area to explore when examining current mental health trends 41 .
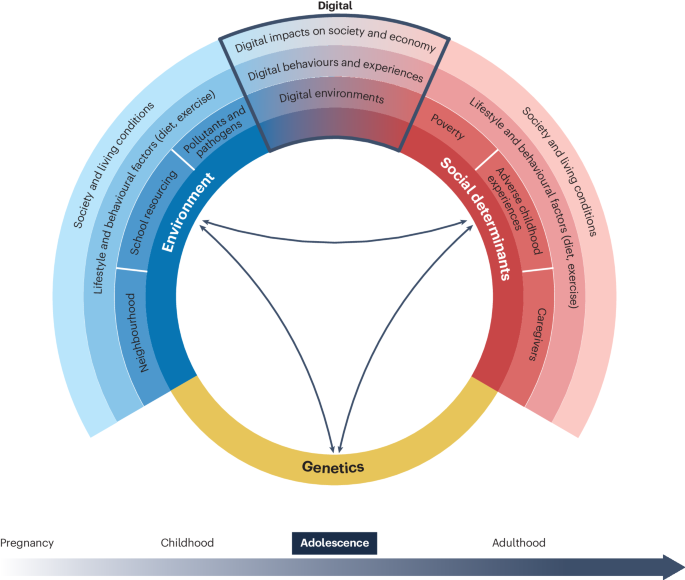
During adolescence, the interaction between genetic programming (yellow), social determinants (red) and environmental factors (blue), as well as the developmental changes discussed in this Review, increases the risk for onset of mental health conditions. Digital environments, mediated behaviours and experiences, and the impact that this technology has on society and economy more generally, are one aspect of the complex forces that might lead to the declines in adolescent mental health observed in the last decade. Adapted from ref. 259 , Springer Nature Limited.
Although there are many environmental changes that could be relevant, a substantial body of research has emerged to investigate the potential link between social media use and declines in adolescent mental health 42 , 43 using various research approaches, including cross-sectional studies 44 , longitudinal observational data analyses 45 , 46 , 47 and experimental studies 48 , 49 . However, the scientific results have been mixed and inconclusive (for reviews, see refs. 43 , 50 , 51 , 52 , 53 ), which has made it difficult to establish evidence-based recommendations, regulations and interventions aimed at ensuring that social media use is not harmful to adolescents 54 , 55 , 56 , 57 .
Many researchers attribute the mixed results to insufficient study specificity. For instance, the relationship between social media use and mental health varies notably across individuals 45 , 58 and developmental time windows 59 . Yet studies often examine adolescents without differentiating them based on age or developmental stage 60 , which prevents systematic accounts of individual and subgroup differences. Additionally, most studies only rely on self-reported measures of time spent on social media 61 , 62 , and overlook more nuanced aspects of social media use such as the nature of the activities 63 and the content or features that users engage with 52 . These factors need to be considered to unpack any broader relationships 35 , 64 , 65 , 66 . Furthermore, the measurement of mental health often conflates positive and negative mental health outcomes as well as various mental health conditions, which could all be differentially related to social media use 52 , 67 .
This research space presents substantial complexity 68 . There is an ever-increasing range of potential combinations of social media predictors, well-being and mental health outcomes and participant groups of varying backgrounds and demographics that can become the target of scientific investigation. However, the pressure to deliver policy and public-facing recommendations and interventions leaves little time to investigate comprehensively each of these combinations. Researchers need to be able to pinpoint quickly the research programmes with the maximum potential to create translational and real-world impact for adolescent mental health.
In this Review, we aim to delineate potential avenues for future research that could lead to concrete interventions to improve adolescent mental health by considering mechanisms at the nexus between pre-existing processes known to increase adolescent mental health vulnerability and digital affordances introduced by social media. First, we describe the affordance approach to understanding the effects of social media. We then draw upon research on adolescent development, mental health and social media to describe behavioural, cognitive and neurobiological mechanisms by which social media use might amplify changes during adolescent development to increase mental health vulnerability during this period of life. The specific mechanisms within each category were chosen because they have a strong evidence base showing that they undergo substantive changes during adolescent development, are implicated in mental health risk and can be modulated by social media affordances. Although the ways in which social media can also improve mental health resilience are not the focus of our Review and therefore are not reviewed fully here, they are briefly discussed in relation to each mechanism. Finally, we discuss future research focused on how to systematically test the intersection between social media and adolescent mental health.
Social media affordances
To study the impact of social media on adolescent mental health, its diverse design elements and highly individualized uses must be conceptualized. Initial research predominately related access to or time spent on social media to mental health outcomes 46 , 69 , 70 . However, social media is not similar to a toxin or nutrient for which each exposure dose has a defined link to a health-related outcome (dose–response relationship) 56 . Social media is a diverse environment that cannot be summarized by the amount of time one spends interacting with it 71 , 72 , and individual experiences are highly varied 45 .
Previous psychological reviews often focused on social media ‘features’ 73 and ‘affordances’ 74 interchangeably. However, these terms have distinct definitions in communication science and information systems research. Social media features are components of the technology intentionally designed to enable users to perform specific actions, such as liking, reposting or uploading a story 75 , 76 . By contrast, affordances describe the perceptions of action possibilities users have when engaging with social media and its features, such as anonymity (the difficulty with which social media users can identify the source of a message) and quantifiability (how countable information is).
The term ‘affordance’ came from ecological psychology and visuomotor research, and was described as mainly determined by human perception 77 . ‘Affordance’ was later adopted for design and human–computer interaction contexts to refer to the action possibilities that are suggested to the user by the technology design 78 . Communication research synthesizes both views. Affordances are now typically understood as the perceived — and therefore flexible — action possibilities of digital environments, which are jointly shaped by the technology’s features and users’ idiosyncratic perceptions of those features 79 .
Latent action possibilities can vary across different users, uses and technologies 79 . For example, ‘stories’ are a feature of Instagram designed to share content between users. Stories can also be described in terms of affordances when users perceive them as a way to determine how long their content remains available on the platform (persistence) or who can see that content (visibility) 80 , 81 , 82 , 83 , 84 . Low persistence (also termed ephemerality) and comparatively low visibility can be achieved through a technology feature (Instagram stories), but are not an outcome of technology use itself; they are instead perceived action possibilities that can vary across different technologies, users and designs 79 .
The affordances approach is particularly valuable for theorizing at a level above individual social media apps or specific features, which makes this approach more resilient to technological changes or shifts in platform popularity 79 , 85 . However, the affordances approach can also be related back to specific types of social media by assessing the extent to which certain affordances are ‘built into’ a particular platform through feature design 35 . Furthermore, because affordances depend on individuals’ perceptions and actions, they are more aligned than features with a neurocognitive and behavioural perspective to social media use. Affordances, similar to neurocognitive and behavioural research, emphasize the role of the user (how the technology is perceived, interpreted and used) rather than technology design per se. In this sense, the affordances approach is essential to overcome technological determinism of mental health outcomes, which overly emphasizes the role of technology as the driver of outcomes but overlooks the agency and impact of the people in question 86 . This flexibility and alignment with psychological theory has contributed to the increasing popularity of the affordance approach 35 , 73 , 74 , 85 , 87 and previous reviews have explored relevant social media affordances in the context of interpersonal communication among adults and adolescents 35 , 88 , 89 , adolescent body image concerns 73 and work contexts 33 . Here, we focus on the affordances of social media that are relevant for adolescent development and its intersection with mental health (Table 1 ).
Behavioural mechanisms
Adolescents often use social media differently to adults, engaging with different platforms and features and, potentially, perceiving or making use of affordances in distinctive ways 35 . These usage differences might interact with developmental characteristics and changes to amplify mental health vulnerability (Fig. 3 ). We examine two behavioural mechanisms that might govern the impact of social media use on mental health: risky posting behaviours and self-presentation.
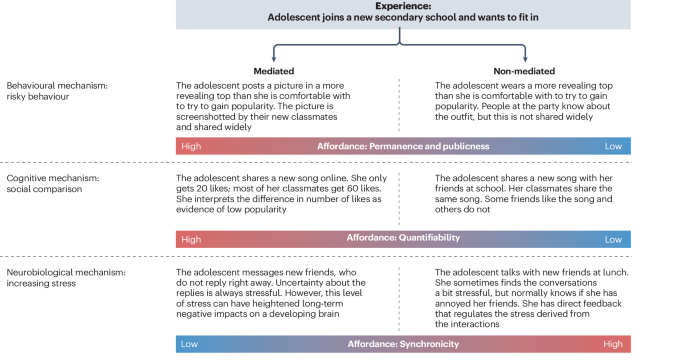
Social media affordances can amplify the impact that common adolescent developmental mechanisms (behavioural, cognitive and neurobiological) have on mental health. At the behavioural level (top), affordances such as permanence and publicness lead to an increased impact of risk-taking behaviour on mental health compared with similar behaviours in non-mediated environments. At the cognitive level (middle), high quantifiability influences the effects of social comparison. At the neurobiological level (bottom), low synchronicity can amplify the effects of stress on the developing brain.
Risky posting behaviour
Sensation-seeking peaks in adolescence and self-regulation abilities are still not fully developed in this period of life 90 . Thus, adolescents often engage in more risky behaviours than other age groups 91 . Adolescents are more likely to take risks in situations involving peers 92 , 93 , perhaps because they are motivated to avoid social exclusion 94 , 95 . Whether adolescent risk-taking behaviour is inherently adaptive or maladaptive is debated. Although some risk-taking behaviours can be adaptive and part of typical development, others can increase mental health vulnerability. For example, data from a prospective UK panel study of more than 5,500 young people showed that engaging in more risky behaviours (including social and health risks) at age 16 years increases the odds of a range of adverse outcomes at age 18 years, such as depression, anxiety and substance abuse 96 .
Social media can increase adolescents’ engagement in risky behaviours both in non-mediated and mediated environments (environments in which the behaviour is executed in or through a technology, such as a mobile phone and social media). First, affordances such as quantifiability in conjunction with visibility and association (the degree with which links between people, between people and content or between a presenter and their audience can be articulated) can promote more risky behaviours in non-mediated environments and in-person social interactions. For example, posts from university students containing references to alcohol gain more likes than posts not referencing alcohol and liking such posts predicts an individual’s subsequent drinking habits 97 . Users expecting likes from their audience are incentivized to engage in riskier posting behaviour (such as more frequent or more extreme posts containing references to alcohol). The relationship between risky online behaviour and offline behaviour is supported by meta-analyses that found a positive correlation between adolescents’ social media use and their engagement in behaviours that might expose them to harm or risk of injury (for example, substance use or risky sexual behaviours) 98 . Further, affordances such as persistence and visibility can mean that risky behaviours in mediated and non-mediated environments remain public for long periods of time, potentially influencing how an adolescent is perceived by peers over the longer term 39 , 99 .
Adolescence can also be a time of more risky social media use. For most forms of semi-public and public social media use, users typically do not know who exactly will be able to see their posts. Thus, adolescents need to self-present to an ‘imagined audience’ 100 and avoid posting the wrong kind of content as the boundaries between different social spheres collapse (context collapse 101 ). However, young people can underestimate the risks of disclosing revealing information in a social media environment 102 . Affordances such as visibility, replicability (social media posts remain in the system and can be screenshotted and shared even if they are later deleted 39 ), association and persistence could heighten the risk of experiencing cyberbullying, victimization and online harassment 103 . For example, adolescents can forward privately received sexual images to larger friendship groups, increasing the risk of online harassment over the subject of the sexual images 104 . Further, low bandwidth (a relative lack of socio-emotional cues) and high anonymity have the potential to disinhibit interactions between users and make behaviours and reactions more extreme 105 , 106 . For example, anonymity was associated with more trolling behaviours during an online group discussion in an experiment with 242 undergraduate students 107 .
Thus, social media might drive more risky behaviours in both mediated and non-mediated contexts, increasing mental health vulnerability. However, the evidence is still not clear cut and often discounts adolescent agency and understanding. For example, mixed-methods research has shown that young people often understand the risks of posting private or sexual content and use social media apps that ensure that posts are deleted and inaccessible after short periods of time to counteract them 39 (even though posts can still be captured in the meantime). Future work will therefore need to investigate how adolescents understand and balance such risks and how such processes relate to social media’s impact on mental health.
Self-presentation and identity
The adolescent period is characterized by an abundance of self-presentation activities on social media 74 , where the drive to present oneself becomes a fundamental motivation for engagement 108 . These activities include disclosing, concealing and modifying one’s true self, and might involve deception, to convey a desired impression to an audience 109 . Compared with adults, adolescents more frequently take part in self-presentation 102 , which can encompass both realistic and idealized portrayals of themselves 110 . In adults, authentic self-presentation has been associated with increased well-being, and inauthentic presentation (such as when a person describes themselves in ways not aligned with their true self) has been associated with decreased well-being 111 , 112 , 113 .
Several social media affordances shape the self-presentation behaviours of adolescents. For example, the editability of social media profiles enables users to curate their online identity 84 , 114 . Editability is further enhanced by highly visible (public) self-presentations. Additionally, the constant availability of social media platforms enables adolescents to access and engage with their profiles at any time, and provides them with rapid quantitative feedback about their popularity among peers 89 , 115 . People receive more direct and public feedback on their self-presentation on social media than in other types of environment 116 , 117 . The affordances associated with self-presentation can have a particular impact during adolescence, a period characterized by identity development and exploration.
Social media environments might provide more opportunities than offline environments for shaping one’s identity. Indeed, public self-presentation has been found to invoke more prominent identity shifts (substantial changes in identity) compared with private self-presentation 118 , 119 . Concerns have been raised that higher Internet use is associated with decreased self-concept clarity. Only one study of 101 adolescents as well as adults reviewed in a 2021 meta-analysis 120 showed that the intensity of Facebook use (measured by the Facebook Intensity Scale) predicted a longitudinal decline in self-concept clarity 3 months later, but the converse was not the case and changes in self-concept clarity did not predict Facebook use 121 . This result is still not enough to show a causal relationship 121 . Further, the affordances of persistence and replicability could also curtail adolescents’ ability to explore their identity freely 122 .
By contrast, qualitative research has highlighted that social media enables adolescents to broaden their horizons, explore their identity and identify and reaffirm their values 123 . Social media can help self-presentation by enabling adolescents to elaborate on various aspects of their identity, such as ethnicity and race 124 or sexuality 125 . Social media affordances such as editability and visibility can also facilitate this process. Adolescents can modify and curate self-presentations online, try out new identities or express previously undisclosed aspects of their identity 126 , 127 . They can leverage social media affordances to present different facets of themselves to various social groups by using different profiles, platforms and self-censorship and curation of posts 128 , 129 . Presenting and exploring different aspects of one’s identity can have mental health implications for minority teens. Emerging research shows a positive correlation between well-being and problematic Internet use in transgender, non-binary and gender-diverse adolescents (age 13–18 years), and positive sentiment has been associated with online identity disclosures in transgender individuals with supportive networks (both adolescent and adult) 130 , 131 .
Cognitive mechanisms
Adolescents and adults might experience different socio-cognitive impacts from the same social media activity. In this section, we review four cognitive mechanisms via which social media and its affordances might influence the link between adolescent development and mental health vulnerabilities (Fig. 3 ). These mechanisms (self-concept development, social comparison, social feedback and exclusion) roughly align with a previous review that examined self-esteem and social media use 115 .
Self-concept development
Self-concept refers to a person’s beliefs and evaluations about their own qualities and traits 132 , which first develops and becomes more complex throughout childhood and then accelerates its development during adolescence 133 , 134 , 135 . Self-concept is shaped by socio-emotional processes such as self-appraisal and social feedback 134 . A negative and unstable self-concept has been associated with negative mental health outcomes 136 , 137 .
Perspective-taking abilities also develop during adolescence 133 , 138 , 139 , as does the processing of self-relevant stimuli (measured by self-referential memory tasks, which assess memory for self-referential trait adjectives 140 , 141 ). During adolescence, direct self-evaluations and reflected self-evaluations (how someone thinks others evaluate them) become more similar. Further, self-evaluations have a distinct positive bias during childhood, but this positivity bias decreases in adolescence as evaluations of the self are integrated with judgements of other people’s perspectives 142 . Indeed, negative self-evaluations peak in late adolescence (around age 19 years) 140 .
The impact of social media on the development of self-concept could be heightened during adolescence because of affordances such as personalization of content 143 (the degree to which content can be tailored to fit the identity, preferences or expectations of the receiver), which adapts the information young people are exposed to. Other affordances with similar impacts are quantifiability, availability (the accessibility of the technology as well as the user’s accessibility through the technology) and public visibility of interactions 89 , which render the evaluations of others more prominent and omnipresent. The prominence of social evaluation can pose long-term risks to mental health under certain conditions and for some users 144 , 145 . For example, receiving negative evaluations from others or being exposed to cyberbullying behaviours 146 , 147 can, potentially, have heightened impact at times of self-concept development.
A pioneering cross-sectional study of 150 adolescents showed that direct self-evaluations are more similar to reflected self-evaluations, and self-evaluations are more negative, in adolescents aged 11–21 years who estimate spending more time on social media 148 . Further, longitudinal data have shown bidirectional negative links between social media use and satisfaction with domains of the self (such as satisfaction with family, friends or schoolwork) 47 .
Although large-scale evidence is still unavailable, these findings raise the interesting prospect that social media might have a negative influence on perspective-taking and self-concept. There is less evidence for the potential positive influence of social media on these aspects of adolescent development, demonstrating an important research gap. Some researchers hypothesize that social media enables self-concept unification because it provides ample opportunity to find validation 89 . Research has also discussed how algorithmic curation of personalized social media feeds (for example, TikTok algorithms tailoring videos viewed to the user’s interests) enables users to reflect on their self-concept by being exposed to others’ experiences and perspectives 143 , an area where future research can provide important insights.
Social comparison
Social comparison (thinking about information about other people in relation to the self 149 ) also influences self-concept development and becomes particularly important during adolescence 133 , 150 . There are a range of social media affordances that can amplify the impact of social comparison on mental health. For example, quantifiability enables like or follower counts to be easily compared with others as a sign of status, which facilitates social ranking 151 , 152 , 153 , 154 . Studies of older adolescents and adults aged, on average, 20 years have also found that the number of likes or reactions received predict, in part, how successful users judge their self-presentation posts on Facebook 155 . Furthermore, personalization enables the content that users see on social media to be curated so as to be highly relevant and interesting for them, which should intensify comparisons. For example, an adolescent interested in sports and fitness content will receive personalized recommendations fitting those interests, which should increase the likelihood of comparisons with people portrayed in this content. In turn, the affordance of association can help adolescents surround themselves with similar peers and public personae online, enhancing social comparison effects 63 , 156 . Being able to edit posts (via the affordance of editability) has been argued to contribute to the positivity bias on social media: what is portrayed online is often more positive than the offline experience. Thus, upward comparisons are more likely to happen in online spaces than downward or lateral comparisons 157 . Lastly, the verifiability of others’ idealized self-presentations is often low, meaning that users have insufficient cues to gauge their authenticity 158 .
Engaging in comparisons on social media has been associated with depression in correlational studies 159 . Furthermore, qualitative research has shown that not receiving as many positive evaluations as expected (or if positive evaluations are not provided quickly enough) increases negative emotions in children and adolescents aged between age 9 and 19 years 39 . This result aligns with a reinforcement learning modelling study of Instagram data, which found that the likes a user receives on their own posts become less valuable and less predictive of future posting behaviour if others in their network receive more likes on their posts 160 . Although this study did not measure mood or mental health, it shows that the value of the likes are not static but inherently social; their impact depends on how many are typically received by other people in the same network.
Among the different types of social comparison that adolescents engage in (comparing one’s achievements, social status or lifestyle), the most substantial concerns have been raised about body-related comparisons. One review suggested that social media affordances create a ‘perfect storm’ for body image concerns that can contribute to both socio-emotional and eating disorders 73 . Social media affordances might increase young people’s focus on other people’s appearances as well as on their own appearance by showing idealized, highly edited images, providing quantified feedback and making the ability to associate and compare oneself with peers constantly available 161 , 162 . The latter puts adolescents who are less popular or receive less social support at particular risk of low self-image and social distress 35 .
Affordances enable more prominent and explicit social comparisons in social media environments relative to offline environments 158 , 159 , 163 , 164 , 165 . However, this association could have a positive impact on mental health 164 , 166 . Initial evidence suggests beneficial outcomes of upward comparisons on social media, which can motivate behaviour change and yield positive downstream effects on mental health 164 , 166 . Positive motivational effects (inspiration) have been observed among young adults for topics such as travelling and exploring nature, as well as fitness and other health behaviours, which can all improve mental health 167 . Importantly, inspiration experiences are not a niche phenomenon on social media: an experience sampling study of 353 Dutch adolescents (mean age 13–15 years) found that participants reported some level of social media-induced inspiration in 33% of the times they were asked to report on this over the course of 3 weeks 168 . Several experimental and longitudinal studies show that inspiration is linked to upward comparison on social media 157 , 164 , 166 . However, the positive, motivating side of social comparison on social media has only been examined in a few studies and requires additional investigation.
Social feedback
Adolescence is also a period of social reorientation, when peers tend to become more important than family 169 , peer acceptance becomes increasingly relevant 170 , 171 , 172 and young people spend increasing amounts of time with peers 173 . In parallel, there is a heightened sensitivity to negative socio-emotional or self-referential cues 140 , 174 , higher expectation of being rejected by others 175 and internalization of such rejection 142 , 176 compared with other phases in life development. A meta-analysis of both adolescents and adults found that oversensitivity to social rejection is moderately associated with both depression and anxiety 177 .
Social media affordances might amplify the potential impact of social feedback on mental health. Wanting to be accepted by peers and increased susceptibility to social rewards could be a motivator for using social media in the first place 178 . Indeed, receiving likes as social reward activated areas of the brain (such as the nucleus accumbens) that are also activated by monetary reward 179 . Quantifiability amplifies peer acceptance and rejection (via like counts), and social rejection has been linked to adverse mental health outcomes 170 , 180 , 181 , 182 . Social media can also increase feelings of being evaluated, the risk of social rejection and rumination about potential rejection due to affordances such as quantifiability, synchronicity (the degree to which an interaction happens in real time) and variability of social rewards (the degree to which social interaction and feedback occur on variable time schedules). For example, one study of undergraduate students found that active communication such as messaging was associated with feeling better after Facebook use; however, this was not the case if the communication led to negative feelings such as rumination (for example, after no responses to the messages) 183 .
In a study assessing threatened social evaluation online 184 , participants were asked to record a statement about themselves and were told their statements would be rated by others. To increase the authenticity of the threat, participants were asked to rate other people’s recordings. Threatened social evaluation online in this study decreased mood, most prominently in people with high sensitivity to social rejection. Adolescents who are more sensitive to social rejection report more severe depressive symptoms and maladaptive ruminative brooding in both mediated and non-mediated social environments, and this association is most prominent in early adolescence 185 . Not receiving as much online social approval as peers led to more severe depressive symptoms in a study of American ninth-grade adolescents (between age 14 and 15 years), especially those who were already experiencing peer victimization 153 . Furthermore, individuals with lower self-esteem post more negative and less positive content than individuals with higher self-esteem. Posted negative content receives less social reward and recognition from others than positive content, possibly creating a vicious cycle 186 . Negative experiences pertaining to social exclusion and status are also risk factors for socio-emotional disorders 180 .
The impact of social media experiences on self-esteem can be very heterogeneous, varying substantially across individuals. As a benefit, positive social feedback obtained via social media can increase users’ self-esteem 115 , an association also found among adolescents 187 . For instance, receiving likes on one’s profile or posted photographs can bolster self-esteem in the short term 144 , 188 . A study linking behavioural data and self-reports from Facebook users found that receiving quick responses on public posts increased a sense of social support and decreased loneliness 189 . Furthermore, a review of reviews consistently documented that users who report more social media use also perceive themselves to have more social resources and support online 52 , although this association has mostly been studied among young adults using social network sites such as Facebook. Whether such social feedback benefits extend to adolescents’ use of platforms centred on content consumption (such as TikTok or Instagram) is an open question.
Social inclusion and exclusion
Adolescents are more sensitive to the negative emotional impacts of being excluded than are adults 170 , 190 . It has been proposed that, as the importance of social affiliation increases during this period of life 134 , 191 , 192 , adolescents are more sensitive to a range of social stimuli, regardless of valence 193 . These include social feedback (such as compliments or likes) 95 , 194 , negative socio-emotional cues (such as negative facial expressions or social exclusion) 174 and social rejection 172 , 185 . By contrast, social inclusion (via friendships in adolescence) is protective against emotional disorders 195 and more social support is related to higher adolescent well-being 196 .
Experiencing ostracism and exclusion online decreases self-esteem and positive emotion 197 . This association has been found in vignette experiments where participants received no, only a few or a lot of likes 198 , or experiments that used mock-ups of social media sites where others received more likes than participants 153 . Being ostracized (not receiving attention or feedback) or rejected through social media features (receiving dislikes and no likes) is also associated with a reduced sense of belonging, meaningfulness, self-esteem and control 199 . Similar results were found when ostracism was experienced over messaging apps, such as not receiving a reply via WhatsApp 200 .
Evidence on whether social media also enables adolescents to experience positive social inclusion is mostly indirect and mixed. Some longitudinal surveys have found that prosocial feedback received on social media during major life events (such as university admissions) helps to buffer against stress 201 . Adult participants of a longitudinal study reported that social media offered more informational support than offline contexts, but offline contexts more often offered emotional or instrumental support 202 . Higher social network site use is, on average, associated with a perception of having more social resources and support in adults (for an overview of meta-analyses, see ref. 52 ). However, most of these studies have not investigated social support among adolescents, and it is unclear whether early findings (for example, on Facebook or Twitter) generalize to a social media landscape more strongly characterized by content consumption than social interaction (such as Instagram or TikTok).
Still, a review of social media use and offline interpersonal outcomes among adolescents documents both positive (sense of belonging and social capital) and negative (alienation from peers and perceived isolation) correlates 203 . Experience sampling research on emotional support among young adults has further shown that online social support is received and perceived as effective, and its perceived effectiveness is similar to in-person social support 204 . Social media use also has complex associations with friendship closeness among adolescents. For example, one experience sampling study found that greater use of WhatsApp or Instagram is associated with higher friendship closeness among adolescents; however, within-person examinations over time showed small negative associations 205 .
Neurobiological mechanisms
The long-term impact of environmental changes such as social media use on mental health might be amplified because adolescence is a period of considerable neurobiological development 95 (Fig. 3 ). During adolescence, overall cortical grey matter declines and white matter increases 206 , 207 . Development is particularly protracted in brain regions associated with social cognition and executive functions such as planning, decision-making and inhibiting prepotent responses. The changes in grey and white matter are thought to reflect axonal growth, myelination and synaptic reorganization, which are mechanisms of neuroplasticity influenced by the environment 208 . For example, research in rodents has demonstrated that adolescence is a sensitive period for social input, and that social isolation in adolescence has unique and more deleterious consequences for neural, behavioural and mental health development than social isolation before puberty or in adulthood 206 , 209 . There is evidence that brain regions involved in motivation and reward show greater activation to rewarding and motivational stimuli (such as appetitive stimuli and the presence of peers) in early and/or mid adolescence compared with other age groups 210 , 211 , 212 , 213 , 214 .
Little is known about the potential links between social media and neurodevelopment due to the paucity of research investigating these associations. Furthermore, causal chains (for example, social media increasing stress, which in turn influences the brain) have not yet been accurately delineated. However, it would be amiss not to recognize that brain development during adolescence forms part of the biological basis of mental health vulnerability and should therefore be considered. Indeed, the brain is proposed to be particularly plastic in adolescence and susceptible to environmental stimuli, both positive and negative 208 . Thus, even if adults and adolescents experienced the same affective consequences from social media use (such as increases in peer comparison or stress), these consequences might have a greater impact in adolescence.
A cross-sectional study (with some longitudinal elements) suggested that habitual checking of social media (for example, checking for rewards such as likes) might exacerbate reward sensitivity processes, leading to long-term hypersensitization of the reward system 215 . Specifically, frequently checking social media was associated with reduced activation in brain regions such as the dorsolateral prefrontal cortex and the amygdala in response to anticipated social feedback in young people. Brain activation during the same social feedback task was measured over subsequent years. Upon follow-up, anticipating feedback was associated with increased activation of the same brain regions among the individuals who checked social media frequently initially 215 . Although longitudinal brain imaging measurements enabled trajectories of brain development to be specified, the measures of social media use were only acquired once in the first wave of data collection. The study therefore cannot account for confounds such as personality traits, which might influence both social media checking behaviours and brain development. Other studies of digital screen use and brain development have found no impact on adolescent functional brain organization 216 .
Brain development and heightened neuroplasticity 208 render adolescence a particularly sensitive period with potentially long-term impacts into adulthood. It is possible that social media affordances that underpin increased checking and reward-seeking behaviours (such as quantifiability, variability of social rewards and permanent availability of peers) might have long-term consequences on reward processing when experienced during adolescence. However, this suggestion is still speculative and not backed up by evidence 217 .
Stress is another example of the potential amplifying effect of social media on adolescent mental health vulnerability due to neural development. Adolescents show higher stress reactivity because of maturational changes to, and increased reactivity in, the hypothalamic–pituitary–adrenal axis 218 , 219 . Compared with children and adults, adolescents experience an increase in self-consciousness and associated emotional states such as self-reported embarrassment and related physiological measures of arousal (such as skin conductance), and heightened neural response patterns compared with adults, when being evaluated or observed by peers 220 . Similarly, adolescents (age 13–17 years) show higher stress responses (higher levels of cortisol or blood pressure) compared with children (age 7–12 years) when they perform in front of others or experience social rejection 221 .
Such changes in adolescence might confer heightened risk for the onset of mental health conditions, especially socio-emotional disorders 6 . Both adolescent rodents and humans show prolonged hypothalamic–pituitary–adrenal activation after experiencing stress compared with conspecifics of different ages 218 , 219 . In animal models, stress during adolescence has been shown to result in increased anxiety levels in adulthood 222 and alterations in emotional and cognitive development 223 . Furthermore, human studies have linked stress in adolescence to a higher risk of mental health disorder onset 218 and reviews of cross-species work have illustrated a range of brain changes due to adolescent stress 224 , 225 .
There is still little conclusive neurobiological evidence about social media use and stress, and a lack of understanding about which affordances might be involved (although there has been a range of work studying digital stress; Box 1 ). Studies of changes in cortisol levels or hypothalamic–pituitary–adrenal functioning and their relation to social media use have been mixed and inconclusive 226 , 227 . These results could be due to the challenge of studying stress responses in adolescents, particularly as cortisol fluctuates across the day and one-point readings can be unreliable. However, the increased stress sensitivity during the adolescent developmental period might mean that social media use can have a long-term influence on mental health due to neurobiological mechanisms. These processes are therefore important to understand in future research.
Box 1 Digital stress
Digital stress is not a unified construct. Thematic content analyses have categorized digital stress into type I stressors (for example, mean attacks, cyberbullying or shaming) and type II stressors (for example, interpersonal stress due to pressure to stay available) 260 . Other reviews have noted its complexity, and categorized digital stress into availability stress (stress that results from having to be constantly available), approval anxiety (anxiety regarding others’ reaction to their own profile, posts or activities online), fear of missing out (stress about being absent from or not experiencing others’ rewarding experiences) and communication overload (stress due to the scale, intensity and frequency of online communication) 261 .
Digital stress has been systematically linked to negative mental health outcomes. Higher digital stress was longitudinally associated with higher depressive symptoms in a questionnaire study 262 . Higher social media stress was also longitudinally related to poorer sleep outcomes in girls (but not boys) 263 . Studies and reviews have linked cyberbullying victimization (a highly stressful experience) to decreased mental health outcomes such as depression, and psychosocial outcomes such as self-esteem 103 , 146 , 147 , 264 , 265 . A systematic review of both adolescents and adults found a medium association ( r = 0.26–0.34) between different components of digital stress and psychological distress outcomes such as anxiety, depression or loneliness, which was not moderated by age or sex (except for connection overload) 266 . However, the causal structure giving rise to such results is still far from clear. For example, surveys have linked higher stress levels to more problematic social media use and fear of missing out 267 , 268 .
Thus, the impact of digital stress on mental health is probably complex and influenced by the type of digital stressor and various affordances. For example, visibility and availability increase fear of negative public evaluation 269 and high availability and a social norm of responding quickly to messages drive constant monitoring in adolescents due to a persistent fear of upsetting friends 270 .
A range of relevant evidence from qualitative and quantitative studies documents that adolescents often ruminate about online interactions and messages. For example, online salience (constantly thinking about communication, content or events happening online) was positively associated with stress on both between-person and within-person levels in a cross-sectional quota sample of adults and three diary studies of young adults 271 , 272 . Online salience has also been associated with lower well-being in a pre-registered study of momentary self-reports from young adults with logged online behaviours. However, this study also noted that positive thoughts were related to higher well-being 273 . Furthermore, although some studies found no associations between the amount of communication and digital stress 272 , a cross-sectional study found that younger users’ (age 14–34 years and 35–49 years) perception of social pressure to be constantly available was related to communication load (measured by questions about the amount of use, as well as the urge to check email and social media) and Internet multitasking, whereas this was not the case for older users aged 50–85 years 274 . By contrast, communication load and perceived stress were associated only among older users.
Summary and future directions
To help to understand the potential role of social media in the decline of adolescent mental health over the past decade, researchers should study the mechanisms linking social media, adolescent development and mental health. Specifically, social media environments might amplify the socio-cognitive processes that render adolescents more vulnerable to mental health conditions in the first place. We outline various mechanisms at three levels of adolescent development — behavioural, cognitive and neurobiological — that could be involved in the decline of adolescent mental health as a function of social media engagement. To do so, we delineate specific social media affordances, such as quantification of social feedback or anonymity, which can also have positive impacts on mental health.
Our Review sets out clear recommendations for future research on the intersection of social media and adolescent mental health. The foundation of this research lies in the existing literature investigating the underlying processes that heighten adolescents’ risk of developing socio-emotional disorders. Zooming in on the potential mechanistic targets impacted by social media uses and affordances will produce specific research questions to facilitate controlled and systematic scientific inquiry relevant for intervention and translation. This approach encourages researchers to pinpoint the mechanisms and levels of explanation they want to include and will enable them to identify what factors to additionally consider, such as participants’ age 60 , the specific mental health outcomes being measured, the types of social media being examined and the populations under study 52 , 228 . Targeted and effective research should prioritize the most promising areas of study and acknowledge that all research approaches have inherent limitations 229 . Researchers must embrace methodological diversity, which in turn will facilitate triangulation. Surveys, experience sampling designs in conjunction with digital trace data, as well as experimental or neuroimaging paradigms and computational modelling (such as reinforcement learning) can all be used to address research questions comprehensively 230 . Employing such a multi-method approach enables the convergence of evidence and strengthens the reliability of findings 231 .
Mental health and developmental research can also become more applicable to the study of social media by considering how studies might already be exploring features of the digital environment, such as its design features and perceived affordances. Many cognitive neuroscience studies that investigate social processes and mental health during adolescence necessarily design tasks that can be completed in controlled experimental or brain scanning environments. Consequently, they tend to focus on digitally mediated interactions. However, researchers conceptualize and generalize their results to face-to-face interactions. For example, it is common across the discipline to not explicitly describe the interactions under study as being about social processes in digital environments (such as studies that assess social feedback based on the number of ‘thumbs up’ or ‘thumbs down’ received in social media 232 ). Considering whether cognitive neuroscience studies include key affordances of mediated (or non-mediated) environments, and discussing these in published papers, will make studies searchable within the field of social media research, enabling researchers to broaden the impact of their work and systematically specify generalizations to offline environments 233 .
To bridge the gap between knowledge about mediated and non-mediated social environments, it is essential to directly compare the two 233 . It is often assumed that negative experiences online have a detrimental impact on mental health. However, it remains unclear whether this mechanism is present in both mediated and non-mediated spaces or whether it is specific to the mediated context. For instance, our Review highlights that the quantification of social feedback through likes is an important affordance of social media 160 . Feedback on social media platforms might therefore elicit a greater sense of certainty because it is quantified compared with the more subjective and open-to-interpretation feedback received face to face 151 . Conducting experiments in which participants receive feedback that is more or less quantified and uncertain, specifically designed to compare mediated and non-mediated environments, would provide valuable insights. Such research efforts could also establish connections with computational neuroscience studies demonstrating that people tend to learn faster from stimuli that are less uncertain 234 .
We have chosen not to make recommendations concerning interventions targeting social media use to improve adolescent mental health for several reasons. First, we did not fully consider the bidirectional interactions between environment and development 35 , 235 , or the factors modulating adolescents’ differential susceptibility to the effects of social media 45 , 58 . For example, mental health status also influences how social media is used 47 , 58 , 59 , 236 , 237 (Box 2 ). These bidirectional interactions could be addressed using network or complexity science approaches 238 . Second, we do not yet know how the potential mechanisms by which social media might increase mental health vulnerability compare in magnitude, importance, scale and ease and/or cost of intervention with other factors and mechanisms that are already well known to influence mental health, such as poverty or loneliness. Last, social media use will probably interact with these predictors in ways that have not been delineated and can also support mental health resilience (for example, through social support or online self-help programmes). These complexities should be considered in future research, which will need to pinpoint not just the existence of mechanisms but their relative importance, to identify policy and intervention priorities.
Our Review has used a broad definition of mental health. Focusing on specific diagnostic or transdiagnostic symptomatology might reveal different mechanisms of interest. Furthermore, our Review is limited to mechanisms related to behaviour and neurocognitive development, disregarding other levels of explanation (such as genetics and culture) 34 , and also studying predominately Western-centric samples 239 . Mechanisms do not operate solely in linear pathways but exist within networks of interacting risk and resilience factors, characterized by non-linear and complex dynamics across diverse timescales 9 . Mechanisms and predisposing factors can interact and combine, amplifying mental health vulnerability. Mental health can be considered a dynamic system in which gradual changes to external conditions can have substantial downstream consequences due to system properties such as feedback loops 240 , 241 , 242 . These consequences are especially prominent in times of change and pre-existing vulnerability, such as adolescence 10 .
Indeed, if social media is a contributing factor to the current decline in adolescent mental health, as is commonly assumed, then it is important to identify and investigate mechanisms that are specifically tailored to the adolescent age range and make the case for why they matter. Without a thorough examination of these mechanisms and policy analysis to indicate whether they should be a priority to address, there is insufficient evidence to support the hypothesis that social media is the primary — or even just an influential and important — driver of mental health declines. Researchers need to stop studying social media as monolithic and uniform, and instead study its features, affordances and outcomes by leveraging a range of methods including experiments, questionnaires, qualitative research and industry data. Ultimately, this comprehensive approach will enhance researchers’ ability to address the potential challenges that the digital era poses on adolescent mental health.
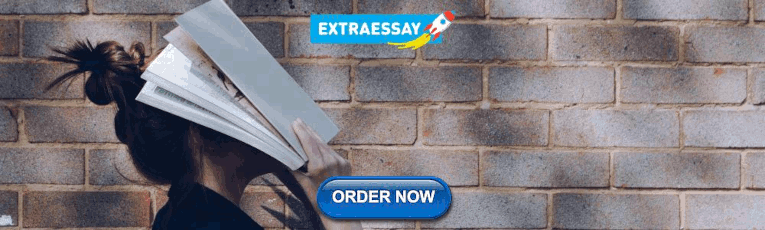
Box 2 Effects of mental health on social media use
Although a lot of scientific discussion has focused on the impact of social media use on mental health, cross-sectional studies cannot differentiate between whether social media use is influencing mental health or mental health is influencing social media use, or a third factor is influencing both 51 . It is likely that mental health status influences social media use creating reinforcing cycles of behaviour, something that has been considered in the communication sciences literature under the term ‘transactional media effects’ 58 , 236 , 237 . According to communication science models, media use and its consequences are components of reciprocal processes 275 .
There are similar models in mental health research. For example, people’s moods influence their judgements of events, which can lead to self-perpetuating cycles of negativity (or positivity); a mechanism called ‘mood congruency’ 276 . Behavioural studies have also shown that people experiencing poor mental health behave in ways that decrease their opportunity to experience environmental reward such as social activities, maintaining poor mental health 277 , 278 . Although for many people these behaviours are a form of coping (for example, by avoiding stressful circumstances), they often worsen symptoms of mental health conditions 279 .
Some longitudinal studies found that a decrease in adolescent well-being predicted an increase in social media use 1 year later 47 , 59 . However, other studies have found no relationships between well-being and social media use over long-term or daily time windows 45 , 46 . One reason behind the heterogeneity of the results could be that how mental health impacts social media use is highly individual 45 , 280 .
Knowledge on the impact of mental health on social media use is still in its infancy and studies struggle to reach coherent conclusions. However, findings from the mental health literature can be used to generate hypotheses about how aspects of mental health might impact social media use. For example, it has been repeatedly found that young people with anxiety or eating disorders engage in more social comparisons than individuals without these disorders 281 , 282 , and adolescents with depression report more unfavourable social comparisons on social media than adolescents without depression 283 . Similar results have been found for social feedback seeking (for example, reassurance), including in social media environments 159 . Specifically, depressive symptoms were more associated with social comparison and feedback seeking, and these associations were stronger in women and in adolescents who were less popular. Individuals from the general population with lower self-esteem post more negative and less positive content than individuals with higher self-esteem, which in turn is associated with receiving less positive feedback from others 185 . There are therefore a wide range of possible ways in which diverse aspects of mental health might influence specific facets of how social media is used — and, in turn, how it ends up impacting the user.
Savin-Williams, R. Adolescence: An Ethological Perspective (Springer, 1987).
Sawyer, S. M., Azzopardi, P. S., Wickremarathne, D. & Patton, G. C. The age of adolescence. Lancet Child. Adolesc. Health 2 , 223–228 (2018).
Article PubMed Google Scholar
Paus, T., Keshavan, M. & Giedd, J. N. Why do many psychiatric disorders emerge during adolescence? Nat. Rev. Neurosci. 9 , 947–957 (2008).
Article PubMed PubMed Central Google Scholar
Solmi, M. et al. Age at onset of mental disorders worldwide: large-scale meta-analysis of 192 epidemiological studies. Mol. Psychiatry 27 , 281–295 (2022).
Orben, A., Lucas, R. E., Fuhrmann, D. & Kievit, R. A. Trajectories of adolescent life satisfaction. R. Soc. Open. Sci. 9 , 211808 (2022).
Rapee, R. M. et al. Adolescent development and risk for the onset of social-emotional disorders: a review and conceptual model. Behav. Res. Ther. 123 , 103501 (2019). This review describes why adolescence is a sensitive period for mental health vulnerability.
Arango, C. et al. Risk and protective factors for mental disorders beyond genetics: an evidence‐based atlas. World Psychiatry 20 , 417–436 (2021).
Ioannidis, K., Askelund, A. D., Kievit, R. A. & van Harmelen, A.-L. The complex neurobiology of resilient functioning after childhood maltreatment. BMC Med. 18 , 32 (2020).
Kraemer, H. C., Stice, E., Kazdin, A., Offord, D. & Kupfer, D. How do risk factors work together? Mediators, moderators, and independent, overlapping, and proxy risk factors. AJP 158 , 848–856 (2001).
Article Google Scholar
Hankin, B. L. & Abramson, L. Y. Development of gender differences in depression: an elaborated cognitive vulnerability–transactional stress theory. Psychol. Bull. 127 , 773–796 (2001).
Collishaw, S., Maughan, B., Natarajan, L. & Pickles, A. Trends in adolescent emotional problems in England: a comparison of two national cohorts twenty years apart: twenty-year trends in emotional problems. J. Child. Psychol. Psychiatry 51 , 885–894 (2010).
Pitchforth, J. M., Viner, R. M. & Hargreaves, D. S. Trends in mental health and wellbeing among children and young people in the UK: a repeated cross-sectional study, 2000–14. Lancet 388 , S93 (2016).
Coley, R. L., O’Brien, M. & Spielvogel, B. Secular trends in adolescent depressive symptoms: growing disparities between advantaged and disadvantaged schools. J. Youth Adolescence 48 , 2087–2098 (2019).
Patalay, P. & Gage, S. H. Changes in millennial adolescent mental health and health-related behaviours over 10 years: a population cohort comparison study. Int. J. Epidemiol. 48 , 1650–1664 (2019).
Pitchforth, J. M. et al. Mental health and well-being trends among children and young people in the UK, 1995–2014: analysis of repeated cross-sectional national health surveys. Psychol. Med. 49 , 1275–1285 (2019).
Plana‐Ripoll, O. et al. Temporal changes in sex‐ and age‐specific incidence profiles of mental disorders—a nationwide study from 1970 to 2016. Acta Psychiatr. Scand. 145 , 604–614 (2022).
Twenge, J. M., Cooper, A. B., Joiner, T. E., Duffy, M. E. & Binau, S. G. Age, period, and cohort trends in mood disorder indicators and suicide-related outcomes in a nationally representative dataset, 2005–2017. J. Abnorm. Psychol. 128 , 185–199 (2019).
van Vuuren, C. L., Uitenbroek, D. G., van der Wal, M. F. & Chinapaw, M. J. M. Sociodemographic differences in 10-year time trends of emotional and behavioural problems among adolescents attending secondary schools in Amsterdam, The Netherlands. Eur. Child. Adolesc. Psychiatry 27 , 1621–1631 (2018).
Collishaw, S. Annual research review: secular trends in child and adolescent mental health. J. Child. Psychol. Psychiatry 56 , 370–393 (2015).
Goodwin, R. D. et al. Trends in U.S. depression prevalence from 2015 to 2020: the widening treatment gap. Am. J. Prev. Med. 63 , 726–733 (2022).
Mojtabai, R. & Olfson, M. National trends in mental health care for US adolescents. JAMA Psychiatry 77 , 703 (2020).
Mojtabai, R., Olfson, M. & Han, B. National trends in the prevalence and treatment of depression in adolescents and young adults. Pediatrics 138 , e20161878 (2016).
Goodwin, R. D., Weinberger, A. H., Kim, J. H., Wu, M. & Galea, S. Trends in anxiety among adults in the United States, 2008–2018: rapid increases among young adults. J. Psychiatr. Res. 130 , 441–446 (2020).
Beerten, S. G. et al. Trends in the registration of anxiety in Belgian primary care from 2000 to 2021: a registry-based study. Br. J. Gen. Pract. 73 , e460–e467 (2022).
Walrave, R. et al. Trends in the epidemiology of depression and comorbidities from 2000 to 2019 in Belgium. BMC Prim. Care 23 , 163 (2022).
Vuorre, M. & Przybylski, A. K. Global well-being and mental health in the internet age. Clin. Psychol. Sci . https://doi.org/10.1177/21677026231207791 (2023).
Steffen, A., Thom, J., Jacobi, F., Holstiege, J. & Bätzing, J. Trends in prevalence of depression in Germany between 2009 and 2017 based on nationwide ambulatory claims data. J. Affect. Disord. 271 , 239–247 (2020).
Ford, T. Editorial Perspective: why I am now convinced that emotional disorders are increasingly common among young people in many countries. J. Child. Psychol. Psychiatr. 61 , 1275–1277 (2020).
McElroy, E., Tibber, M., Fearon, P., Patalay, P. & Ploubidis, G. B. Socioeconomic and sex inequalities in parent‐reported adolescent mental ill‐health: time trends in four British birth cohorts. J. Child Psychol. Psychiatry 64 , 758–767 (2022).
OECD. Society at a Glance 2019: OECD Social Indicators (Organisation for Economic Co-operation and Development, 2019).
Ofcom. Online Nation (2021). Ofcom.org.uk https://www.ofcom.org.uk/research-and-data/online-research/online-nation (2022).
Anderson, M. & Jiang, J. Teens’ Social Media Habits and Experiences (Pew Research Center, 2018).
McFarland, L. A. & Ployhart, R. E. Social media: a contextual framework to guide research and practice. J. Appl. Psychol. 100 , 1653–1677 (2015).
Büchi, M. Digital well-being theory and research. N. Media Soc. 26 , 172–189 (2024).
Nesi, J., Choukas-Bradley, S. & Prinstein, M. J. Transformation of adolescent peer relations in the social media context: part 1—a theoretical framework and application to dyadic peer relationships. Clin. Child. Fam. Psychol. Rev. 21 , 267–294 (2018). This landmark paper applies the idea of affordances to understanding the impact of social media on adolescent social relationships.
Taffel, S. Perspectives on the postdigital: beyond rhetorics of progress and novelty. Convergence 22 , 324–338 (2016).
Papacharissi, Z. We have always been social. Soc. Media + Society 1 , 205630511558118 (2015).
Google Scholar
Crone, E. A. & Konijn, E. A. Media use and brain development during adolescence. Nat. Commun. 9 , 1–10 (2018). This article describes adolescent cognitive and neural development and its intersection with new types of technology.
Weinstein, E. & James, C. Behind Their Screens: What Teens Are Facing (and Adults Are Missing) (MIT Press, 2022).
Twenge, J. M., Joiner, T. E., Rogers, M. L. & Martin, G. N. Increases in depressive symptoms, suicide-related outcomes, and suicide rates among U.S. adolescents after 2010 and links to increased new media screen time. Clin. Psychol. Sci. 6 , 3–17 (2017).
Gunnell, D., Kidger, J. & Elvidge, H. Adolescent mental health in crisis. BMJ 361 , k2608 (2018).
Odgers, C. L., Schueller, S. M. & Ito, M. Screen time, social media use, and adolescent development. Annu. Rev. Dev. Psychol. 2 , 485–502 (2020).
Valkenburg, P. M., Meier, A. & Beyens, I. Social media use and its impact on adolescent mental health: an umbrella review of the evidence. Curr. Opin. Psychol. 44 , 58–68 (2022).
Kreski, N. et al. Social media use and depressive symptoms among United States adolescents. J. Adolesc. Health 68 , 572–579 (2020).
Beyens, I., Pouwels, J. L., van Driel, I. I., Keijsers, L. & Valkenburg, P. M. The effect of social media on well-being differs from adolescent to adolescent. Sci. Rep. 10 , 10763 (2020). This landmark paper highlights that the impacts of social media on well-being are highly individual.
Jensen, M., George, M. J., Russell, M. R. & Odgers, C. L. Young adolescents’ digital technology use and mental health symptoms: little evidence of longitudinal or daily linkages. Clin. Psychol. Sci. 7 , 1416–1433 (2019).
Orben, A., Dienlin, T. & Przybylski, A. K. Social media’s enduring effect on adolescent life satisfaction. Proc. Natl Acad. Sci. USA 116 , 10226–10228 (2019).
Allcott, H., Braghieri, L., Eichmeyer, S. & Gentzkow, M. The welfare effects of social media. Am. Economic Rev. 110 , 629–676 (2020).
Nassen, L.-M., Vandebosch, H., Poels, K. & Karsay, K. Opt-out, abstain, unplug. A systematic review of the voluntary digital disconnection literature. Telemat. Inform. 81 , 101980 (2023).
Dienlin, T. & Johannes, N. The impact of digital technology use on adolescent well-being. Dialogues Clin. Neurosci. 22 , 135–142 (2020).
Odgers, C. L. & Jensen, M. R. Annual research review: adolescent mental health in the digital age: facts, fears, and future directions. J. Child. Psychol. Psychiatry 61 , 336–348 (2020).
Meier, A. & Reinecke, L. Computer-mediated communication, social media, and mental health: a conceptual and empirical meta-review. Commun. Res. 48 , 1182–1209 (2021). This review provides a hierarchical taxonomy of the levels of analysis at which social media can be conceptualized and measured.
Orben, A. Teenagers, screens and social media: a narrative review of reviews and key studies. Soc. Psychiatry Psychiatr. Epidemiol. 55 , 407–414 (2020).
Bell, V., Bishop, D. V. M. & Przybylski, A. K. The debate over digital technology and young people. BMJ 351 , h3064 (2015).
Online Safety Act 2023. legislation.gov.uk , https://www.legislation.gov.uk/ukpga/2023/50/enacted (2023).
Hawkes, N. CMO report is unable to shed light on impact of screen time and social media on children’s health. BMJ 364 , l643 (2019).
US Department of Health and Human Services. Social Media and Youth Mental Health: The U.S. Surgeon General’s Advisory (2023).
Valkenburg, P. M. & Peter, J. The differential susceptibility to media effects model: differential susceptibility to media effects model. J. Commun. 63 , 221–243 (2013). This landmark paper examines how the impact of media is influenced by individual differences.
Orben, A., Przybylski, A. K., Blakemore, S.-J. & Kievit, R. A. Windows of developmental sensitivity to social media. Nat. Commun. 13 , 1649 (2022). This large-scale data analysis shows that adolescent development potentially influences how social media impacts well-being.
Orben, A. & Blakemore, S.-J. How social media affects teen mental health: a missing link. Nature 614 , 410–412 (2023).
Shaw, H. et al. Quantifying smartphone “use”: choice of measurement impacts relationships between “usage” and health. Technol. Mind Behav . 1 , https://doi.org/10.1037/tmb0000022 (2020).
Parry, D. A. et al. A systematic review and meta-analysis of discrepancies between logged and self-reported digital media use. Nat. Hum. Behav. 5 , 1535–1547 (2021).
Verduyn, P., Gugushvili, N. & Kross, E. Do social networking sites influence well-being? The extended active-passive model. Curr. Dir. Psychol. Sci. 31 , 62–68 (2022).
Davidson, B. I., Shaw, H. & Ellis, D. A. Fuzzy constructs in technology usage scales. Comput. Hum. Behav. 133 , 107206 (2022).
Shaw, D. J., Kaye, L. K., Ngombe, N., Kessler, K. & Pennington, C. R. It’s not what you do, it’s the way that you do it: an experimental task delineates among passive, reactive and interactive styles of behaviour on social networking sites. PLoS ONE 17 , e0276765 (2022).
Griffioen, N., Van Rooij, M., Lichtwarck-Aschoff, A. & Granic, I. Toward improved methods in social media research. Technol. Mind Behav . 1 , https://doi.org/10.1037/tmb0000005 (2020).
Valkenburg, P. M. Social media use and well-being: what we know and what we need to know. Curr. Opin. Psychol. 45 , 101294 (2022).
Yang, C., Holden, S. M. & Ariati, J. Social media and psychological well-being among youth: the multidimensional model of social media use. Clin. Child. Fam. Psychol. Rev. 24 , 631–650 (2021).
Kelly, Y., Zilanawala, A., Booker, C. & Sacker, A. Social media use and adolescent mental health: findings from the UK Millennium Cohort Study. EClinicalMedicine 6 , 59–68 (2019).
Orben, A. & Przybylski, A. K. The association between adolescent well-being and digital technology use. Nat. Hum. Behav. 3 , 173–182 (2019).
Sultan, M., Scholz, C. & van den Bos, W. Leaving traces behind: using social media digital trace data to study adolescent wellbeing. Comput. Hum. Behav. Rep. 10 , 100281 (2023).
Kaye, L., Orben, A., Ellis, D., Hunter, S. & Houghton, S. The conceptual and methodological mayhem of “screen time”. IJERPH 17 , 3661 (2020).
Choukas-Bradley, S., Roberts, S. R., Maheux, A. J. & Nesi, J. The perfect storm: a developmental–sociocultural framework for the role of social media in adolescent girls’ body image concerns and mental health. Clin. Child. Fam. Psychol. Rev. 25 , 681–701 (2022). This review focuses on how social media can influence adolescent development of body image.
Moreno, M. A. & Uhls, Y. T. Applying an affordances approach and a developmental lens to approach adolescent social media use. Digital Health 5 , 205520761982667 (2019).
Smock, A. D., Ellison, N. B., Lampe, C. & Wohn, D. Y. Facebook as a toolkit: a uses and gratification approach to unbundling feature use. Comput. Hum. Behav. 27 , 2322–2329 (2011).
Bayer, J. B., Triêu, P. & Ellison, N. B. Social media elements, ecologies, and effects. Annu. Rev. Psychol. 71 , 471–497 (2020).
Gibson, J. J. The Scological Approach to Visual Perception (Houghton Mifflin, 1979).
Norman, D. A. The Psychology of Everyday Things (Basic Books, 1988).
Evans, S. K., Pearce, K. E., Vitak, J. & Treem, J. W. Explicating affordances: a conceptual framework for understanding affordances in communication research. J. Comput. Mediat. Commun. 22 , 35–52 (2017).
Bayer, J. B., Ellison, N. B., Schoenebeck, S. Y. & Falk, E. B. Sharing the small moments: ephemeral social interaction on Snapchat. Information . Commun. Soc. 19 , 956–977 (2016).
Fox, J. & McEwan, B. Distinguishing technologies for social interaction: the perceived social affordances of communication channels scale. Commun. Monogr. 84 , 298–318 (2017).
Kreling, R., Meier, A. & Reinecke, L. Feeling authentic on social media: subjective authenticity across instagram stories and posts. Soc. Media + Society 8 , 205630512210862 (2022).
Leonardi, P. M. Social media, knowledge sharing, and innovation: toward a theory of communication visibility. Inf. Syst. Res. 25 , 796–816 (2014).
Treem, J. W. & Leonardi, P. M. Social media use in organizations: exploring the affordances of visibility, editability, persistence, and association. Ann. Int. Commun. Assoc. 36 , 143–189 (2013).
Ellison, N. B., Pyle, C. & Vitak, J. Scholarship on well-being and social media: a sociotechnical perspective. Curr. Opin. Psychol. 46 , 101340 (2022).
Orben, A. The Sisyphean cycle of technology panics. Perspect. Psychol. Sci. 15 , 1143–1157 (2020).
Granic, I., Morita, H. & Scholten, H. Beyond screen time: identity development in the digital age. Psychol. Inq. 31 , 195–223 (2020). This perspective discusses how adolescent identity development might be impacted by digital platforms including social media and video games.
Lieberman, A. & Schroeder, J. Two social lives: how differences between online and offline interaction influence social outcomes. Curr. Opin. Psychol. 31 , 16–21 (2020).
Valkenburg, P. M. & Peter, J. Online communication among adolescents: an integrated model of its attraction, opportunities, and risks. J. Adolesc. Health 48 , 121–127 (2011).
Steinberg, L. et al. Around the world, adolescence is a time of heightened sensation seeking and immature self-regulation. Dev. Sci. 21 , e12532 (2018).
Blakemore, S.-J. & Robbins, T. W. Decision-making in the adolescent brain. Nat. Neurosci. 15 , 1184–1191 (2012).
Steinberg, L. A social neuroscience perspective on adolescent risk-taking. Dev. Rev. 28 , 78–106 (2008).
Chein, J., Albert, D., O’Brien, L., Uckert, K. & Steinberg, L. Peers increase adolescent risk taking by enhancing activity in the brain’s reward circuitry: peer influence on risk taking. Dev. Sci. 14 , F1–F10 (2011).
Blakemore, S.-J. Avoiding social risk in adolescence. Curr. Dir. Psychol. Sci. 27 , 116–122 (2018).
Blakemore, S.-J. & Mills, K. L. Is adolescence a sensitive period for sociocultural processing? Annu. Rev. Psychol. 65 , 187–207 (2014). This review presents adolescence as an important stage of development characterized by changes to social cognition.
Campbell, R. et al. Multiple risk behaviour in adolescence is associated with substantial adverse health and social outcomes in early adulthood: findings from a prospective birth cohort study. Prev. Med. 138 , 106157 (2020).
Kurten, S. et al. Like to drink: dynamics of liking alcohol posts and effects on alcohol use. Comput. Hum. Behav. 129 , 107145 (2022).
Vannucci, A., Simpson, E. G., Gagnon, S. & Ohannessian, C. M. Social media use and risky behaviors in adolescents: a meta‐analysis. J. Adolesc. 79 , 258–274 (2020).
Eichhorn, K. The End of Forgetting: Growing up with Social Media (Harvard Univ. Press, 2019).
Litt, E. & Hargittai, E. The imagined audience on social network sites. Soc. Media + Society 2 , 205630511663348 (2016).
Vitak, J. The impact of context collapse and privacy on social network site disclosures. J. Broadcast. Electron. Media 56 , 451–470 (2012).
Livingstone, S. Taking risky opportunities in youthful content creation: teenagers’ use of social networking sites for intimacy, privacy and self-expression. N. Media Soc. 10 , 393–411 (2008).
Marciano, L., Schulz, P. J. & Camerini, A.-L. Cyberbullying perpetration and victimization in youth: a meta-analysis of longitudinal studies. J. Comput.-Mediat. Commun. 25 , 163–181 (2020).
Mori, C., Temple, J. R., Browne, D. & Madigan, S. Association of sexting with sexual behaviors and mental health among adolescents: a systematic review and meta-analysis. JAMA Pediatr. 173 , 770 (2019).
Suler, J. The online disinhibition effect. Cyberpsychol. Behav. 7 , 321–326 (2004).
Wright, M. F., Harper, B. D. & Wachs, S. The associations between cyberbullying and callous-unemotional traits among adolescents: the moderating effect of online disinhibition. Pers. Individ. Differ. 140 , 41–45 (2019).
Nitschinsk, L., Tobin, S. J. & Vanman, E. J. The disinhibiting effects of anonymity increase online trolling. Cyberpsychol. Behav. Soc. Netw. 25 , 377–383 (2022).
Nadkarni, A. & Hofmann, S. G. Why do people use Facebook? Pers. Individ. Differ. 52 , 243–249 (2012).
Leary, M. R. & Kowalski, R. M. Impression management: a literature review and two-component model. Psychol. Bull. 107 , 34–47 (1990).
Zhao, S., Grasmuck, S. & Martin, J. Identity construction on Facebook: digital empowerment in anchored relationships. Comput. Hum. Behav. 24 , 1816–1836 (2008).
Bij de Vaate, N. A. J. D., Veldhuis, J. & Konijn, E. A. How online self-presentation affects well-being and body image: a systematic review. Telemat. Inform. 47 , 101316 (2020).
Reinecke, L. & Trepte, S. Authenticity and well-being on social network sites: a two-wave longitudinal study on the effects of online authenticity and the positivity bias in SNS communication. Comput. Hum. Behav. 30 , 95–102 (2014).
Twomey, C. & O’Reilly, G. Associations of self-presentation on Facebook with mental health and personality variables: a systematic review. Cyberpsychol. Behav. Soc. Netw. 20 , 587–595 (2017).
Vanden Abeele, M., Schouten, A. P. & Antheunis, M. L. Personal, editable, and always accessible: an affordance approach to the relationship between adolescents’ mobile messaging behavior and their friendship quality. J. Soc. Personal. Relatsh. 34 , 875–893 (2017).
Krause, H.-V., Baum, K., Baumann, A. & Krasnova, H. Unifying the detrimental and beneficial effects of social network site use on self-esteem: a systematic literature review. Media Psychol. 24 , 10–47 (2021).
Carr, C. T. & Foreman, A. C. Identity shift III: effects of publicness of feedback and relational closeness in computer-mediated communication. Media Psychol. 19 , 334–358 (2016).
Walther, J. B. et al. The effect of feedback on identity shift in computer-mediated communication. Media Psychol. 14 , 1–26 (2011).
Gonzales, A. L. & Hancock, J. T. Identity shift in computer-mediated environments. Media Psychol. 11 , 167–185 (2008).
Kelly, A. E. & Rodriguez, R. R. Publicly committing oneself to an identity. Basic. Appl. Soc. Psychol. 28 , 185–191 (2006).
Petre, C. E. The relationship between Internet use and self-concept clarity: a systematic review and meta-analysis. Cyberpsychology 15 , https://doi.org/10.5817/CP2021-2-4 (2021).
Appel, M., Schreiner, C., Weber, S., Mara, M. & Gnambs, T. Intensity of Facebook use is associated with lower self-concept clarity: cross-sectional and longitudinal evidence. J. Media Psychol. 30 , 160–172 (2018).
Talaifar, S. & Lowery, B. S. Freedom and constraint in digital environments: implications for the self. Perspect. Psychol. Sci. 18 , 544–575 (2022).
West, M., Rice, S. & Vella-Brodrick, D. Mid-adolescents’ social media use: supporting and suppressing autonomy. J. Adolesc. Res . https://doi.org/10.1177/07435584231168402 (2023).
Grasmuck, S., Martin, J. & Zhao, S. Ethno-racial identity displays on Facebook. J. Comput.-Mediat. Commun. 15 , 158–188 (2009).
DeVito, M. A., Walker, A. M. & Birnholtz, J. ‘Too Gay for Facebook’: presenting LGBTQ+ identity throughout the personal social media ecosystem. Proc. ACM Hum.–Comput. Interact. 2 , 1–23 (2018).
Ellison, N., Heino, R. & Gibbs, E. Managing impressions online: self-presentation processes in the online dating environment. J. Comput.-Mediat. Commun . 11 , https://doi.org/10.1111/j.1083-6101.2006.00020.x (2006).
Hancock, J. T. in Oxford Handbook of Internet Psychology (eds Joinson, A. et al.) 287–301 (Oxford Univ. Press, 2009).
Davidson, B. I. & Joinson, A. N. Shape shifting across social media. Soc. Media + Society 7 , 205630512199063 (2021).
Davis, J. L. Triangulating the self: identity processes in a connected era: triangulating the self. Symbolic Interaction 37 , 500–523 (2014).
Allen, B. J., Stratman, Z. E., Kerr, B. R., Zhao, Q. & Moreno, M. A. Associations between psychosocial measures and digital media use among transgender youth: cross-sectional study. JMIR Pediatr. Parent. 4 , e25801 (2021).
Haimson, O. L. Mapping gender transition sentiment patterns via social media data: toward decreasing transgender mental health disparities. J. Am. Med. Inform. Assoc. 26 , 749–758 (2019).
Harter, S. The Construction of the Self: Developmental and Sociocultural Foundations (Guilford Press, 2012).
Crone, E. A., Green, K. H., van de Groep, I. H. & van der Cruijsen, R. A neurocognitive model of self-concept development in adolescence. Annu. Rev. Dev. Psychol. 4 , 273–295 (2022). This extensive review discusses how adolescence is an important time for self-concept development.
Pfeifer, J. H. & Peake, S. J. Self-development: integrating cognitive, socioemotional, and neuroimaging perspectives. Deve. Cognit. Neurosci. 2 , 55–69 (2012).
Sebastian, C., Burnett, S. & Blakemore, S.-J. Development of the self-concept during adolescence. Trends Cognit. Sci. 12 , 441–446 (2008).
Crocetti, E., Rubini, M., Luyckx, K. & Meeus, W. Identity formation in early and middle adolescents from various ethnic groups: from three dimensions to five statuses. J. Youth Adolesc. 37 , 983–996 (2008).
Morita, H., Griffioen, N. & Granic, I. in Handbook of Adolescent Digital Media Use and Mental Health (eds Nesi, J., Telzer, E. H. & Prinstein, M. J.) 63–84 (Cambridge Univ. Press, 2022).
Dumontheil, I., Apperly, I. A. & Blakemore, S.-J. Online usage of theory of mind continues to develop in late adolescence. Dev. Sci. 13 , 331–338 (2010).
Weil, L. G. et al. The development of metacognitive ability in adolescence. Conscious. Cogn. 22 , 264–271 (2013).
Moses-Payne, M. E., Chierchia, G. & Blakemore, S.-J. Age-related changes in the impact of valence on self-referential processing in female adolescents and young adults. Cognit. Dev. 61 , 101128 (2022).
Scheuplein, M. et al. Perspective taking and memory for self- and town-related information in male adolescents and young adults. Cognit. Dev. 67 , 101356 (2023).
Rodman, A. M., Powers, K. E. & Somerville, L. H. Development of self-protective biases in response to social evaluative feedback. Proc. Natl Acad. Sci. USA 114 , 13158–13163 (2017).
Lee, A. Y., Mieczkowski, H., Ellison, N. B. & Hancock, J. T. The algorithmic crystal: conceptualizing the self through algorithmic personalization on TikTok. Proc. ACM Hum.–Comput. Interact. 6 , 1–22 (2022).
Thomaes, S. et al. I like me if you like me: on the interpersonal modulation and regulation of preadolescents’ state self-esteem. Child. Dev. 81 , 811–825 (2010).
Valkenburg, P. M., Peter, J. & Schouten, A. P. Friend networking sites and their relationship to adolescents’ well-being and social self-esteem. CyberPsychol. Behav. 9 , 584–590 (2006).
Kwan, I. et al. Cyberbullying and children and young people’s mental health: a systematic map of systematic reviews. Cyberpsychol. Behav. Soc. Netw. 23 , 72–82 (2020).
Przybylski, A. K. & Bowes, L. Cyberbullying and adolescent well-being in England: a population-based cross-sectional study. Lancet Child. Adolesc. Health 1 , 19–26 (2017).
Peters, S. et al. Social media use and the not-so-imaginary audience: behavioral and neural mechanisms underlying the influence on self-concept. Dev. Cognit. Neurosci. 48 , 100921 (2021).
Wood, J. V. What is social comparison and how should we study it? Pers. Soc. Psychol. Bull. 22 , 520–537 (1996).
Dahl, R. E., Allen, N. B., Wilbrecht, L. & Suleiman, A. B. Importance of investing in adolescence from a developmental science perspective. Nature 554 , 441–450 (2018).
Ferguson, A. M., Turner, G. & Orben, A. Social uncertainty in the digital world. Trends Cognit. Sci. 28 , 286–289 (2024).
Blease, C. R. Too many ‘friends,’ too few ‘likes’? Evolutionary psychology and ‘Facebook depression’. Rev. Gen. Psychol. 19 , 1–13 (2015).
Lee, H. Y. et al. Getting fewer “likes” than others on social media elicits emotional distress among victimized adolescents. Child. Dev. 91 , 2141–2159 (2020).
Nesi, J. & Prinstein, M. J. In search of likes: longitudinal associations between adolescents’ digital status seeking and health-risk behaviors. J. Clin. Child. Adolesc. Psychol. 48 , 740–748 (2019).
Carr, C. T., Hayes, R. A. & Sumner, E. M. Predicting a threshold of perceived Facebook post success via likes and reactions: a test of explanatory mechanisms. Commun. Res. Rep. 35 , 141–151 (2018).
Noon, E. J. & Meier, A. Inspired by friends: adolescents’ network homophily moderates the relationship between social comparison, envy, and inspiration on instagram. Cyberpsychol. Behav. Soc. Netw. 22 , 787–793 (2019).
Schreurs, L., Meier, A. & Vandenbosch, L. Exposure to the positivity bias and adolescents’ differential longitudinal links with social comparison, inspiration and envy depending on social media literacy. Curr. Psychol . https://doi.org/10.1007/s12144-022-03893-3 (2022).
Meier, A. & Krause, H.-V. Does passive social media use harm well-being? An adversarial review. J. Media Psychol. 35 , 169–180 (2023).
Nesi, J. & Prinstein, M. J. Using social media for social comparison and feedback-seeking: gender and popularity moderate associations with depressive symptoms. J. Abnorm. Child. Psychol. 43 , 1427–1438 (2015).
Lindström, B. et al. A computational reward learning account of social media engagement. Nat. Commun. 12 , 1311 (2021).
Fardouly, J., Diedrichs, P. C., Vartanian, L. R. & Halliwell, E. Social comparisons on social media: the impact of Facebook on young women’s body image concerns and mood. Body Image 13 , 38–45 (2015).
Scully, M., Swords, L. & Nixon, E. Social comparisons on social media: online appearance-related activity and body dissatisfaction in adolescent girls. Ir. J. Psychol. Med. 40 , 31–42 (2023).
Appel, H., Gerlach, A. L. & Crusius, J. The interplay between Facebook use, social comparison, envy, and depression. Curr. Opin. Psychol. 9 , 44–49 (2016).
Meier, A. & Johnson, B. K. Social comparison and envy on social media: a critical review. Curr. Opin. Psychol. 45 , 101302 (2022).
Verduyn, P., Gugushvili, N., Massar, K., Täht, K. & Kross, E. Social comparison on social networking sites. Curr. Opin. Psychol. 36 , 32–37 (2020).
Meier, A., Gilbert, A., Börner, S. & Possler, D. Instagram inspiration: how upward comparison on social network sites can contribute to well-being. J. Commun. 70 , 721–743 (2020).
Vaterlaus, J. M., Patten, E. V., Roche, C. & Young, J. A. #Gettinghealthy: the perceived influence of social media on young adult health behaviors. Comput. Hum. Behav. 45 , 151–157 (2015).
Valkenburg, P. M., Beyens, I., Pouwels, J. L., Van Driel, I. I. & Keijsers, L. Social media browsing and adolescent well-being: challenging the “passive social media use hypothesis”. J. Comput.-Mediat. Commun. https://doi.org/10.1093/jcmc/zmab015 (2022).
Larson, R. W., Richards, M. H., Moneta, G., Holmbeck, G. & Duckett, E. Changes in adolescents’ daily interactions with their families from ages 10 to 18: disengagement and transformation. Dev. Psychol. 32 , 744–754 (1996).
Sebastian, C., Viding, E., Williams, K. D. & Blakemore, S.-J. Social brain development and the affective consequences of ostracism in adolescence. Brain Cogn. 72 , 134–145 (2010).
Sebastian, C. et al. Developmental influences on the neural bases of responses to social rejection: implications of social neuroscience for education. NeuroImage 57 , 686–694 (2011).
Somerville, L. H. The teenage brain: sensitivity to social evaluation. Curr. Dir. Psychol. Sci. 22 , 121–127 (2013).
Larson, R. W. & How, U. S. Children and adolescents spend time: what it does (and doesn’t) tell us about their development. Curr. Dir. Psychol. Sci. 10 , 160–164 (2001).
Thomas, L. A., De Bellis, M. D., Graham, R. & LaBar, K. S. Development of emotional facial recognition in late childhood and adolescence. Dev. Sci. 10 , 547–558 (2007).
Gunther Moor, B., van Leijenhorst, L., Rombouts, S. A. R. B., Crone, E. A. & Van der Molen, M. W. Do you like me? Neural correlates of social evaluation and developmental trajectories. Soc. Neurosci. 5 , 461–482 (2010).
Silk, J. S. et al. Peer acceptance and rejection through the eyes of youth: pupillary, eyetracking and ecological data from the Chatroom Interact task. Soc. Cognit. Affect. Neurosci. 7 , 93–105 (2012).
Gao, S., Assink, M., Cipriani, A. & Lin, K. Associations between rejection sensitivity and mental health outcomes: a meta-analytic review. Clin. Psychol. Rev. 57 , 59–74 (2017).
Prinstein, M. J., Nesi, J. & Telzer, E. H. Commentary: an updated agenda for the study of digital media use and adolescent development—future directions following Odgers & Jensen (2020). J. Child. Psychol. Psychiatr. 61 , 349–352 (2020).
Meshi, D., Morawetz, C. & Heekeren, H. R. Nucleus accumbens response to gains in reputation for the self relative to gains for others predicts social media use. Front. Hum. Neurosci. 7 , 1–11 (2013).
Crone, E. A. & Dahl, R. E. Understanding adolescence as a period of social–affective engagement and goal flexibility. Nat. Rev. Neurosci. 13 , 636–650 (2012).
Platt, B., Kadosh, K. C. & Lau, J. Y. F. The role of peer rejection in adolescent depression. Depress. Anxiety 30 , 809–821 (2013).
Will, G.-J., Rutledge, R. B., Moutoussis, M. & Dolan, R. J. Neural and computational processes underlying dynamic changes in self-esteem. eLife 6 , e28098 (2017).
Macrynikola, N. & Miranda, R. Active Facebook use and mood: when digital interaction turns maladaptive. Comput. Hum. Behav. 97 , 271–279 (2019).
Grunewald, K., Deng, J., Wertz, J. & Schweizer, S. The effect of online social evaluation on mood and cognition in young people. Sci. Rep. 12 , 20999 (2022).
Andrews, J. L., Khin, A. C., Crayn, T., Humphreys, K. & Schweizer, S. Measuring online and offline social rejection sensitivity in the digital age. Psychol. Assess. 34 , 742–751 (2022).
Forest, A. L. & Wood, J. V. When social networking is not working: individuals with low self-esteem recognize but do not reap the benefits of self-disclosure on Facebook. Psychol. Sci. 23 , 295–302 (2012).
Valkenburg, P. M., Koutamanis, M. & Vossen, H. G. M. The concurrent and longitudinal relationships between adolescents’ use of social network sites and their social self-esteem. Comput. Hum. Behav. 76 , 35–41 (2017).
Burrow, A. L. & Rainone, N. How many likes did I get? purpose moderates links between positive social media feedback and self-esteem. J. Exp. Soc. Psychol. 69 , 232–236 (2017).
Seo, M., Kim, J. & Yang, H. Frequent interaction and fast feedback predict perceived social support: using crawled and self-reported data of Facebook users. J. Comput.-Mediat. Comm. 21 , 282–297 (2016).
Fuhrmann, D., Casey, C. S., Speekenbrink, M. & Blakemore, S.-J. Social exclusion affects working memory performance in young adolescent girls. Dev. Cognit. Neurosci. 40 , 100718 (2019).
Blakemore, S.-J. & Choudhury, S. Development of the adolescent brain: implications for executive function and social cognition. J. Child. Psychol. Psychiat 47 , 296–312 (2006).
Dreyfuss, M. et al. Teens impulsively react rather than retreat from threat. Dev. Neurosci. 36 , 220–227 (2014).
Guyer, A. E., Choate, V. R., Pine, D. S. & Nelson, E. E. Neural circuitry underlying affective response to peer feedback in adolescence. Soc. Cognit. Affect. Neurosci. 7 , 81–92 (2012).
Sherman, L. E., Payton, A. A., Hernandez, L. M., Greenfield, P. M. & Dapretto, M. The power of the like in adolescence: effects of peer influence on neural and behavioral responses to social media. Psychol. Sci. 27 , 1027–1035 (2016).
van Harmelen, A.-L. et al. Adolescent friendships predict later resilient functioning across psychosocial domains in a healthy community cohort. Psychol. Med. 47 , 2312–2322 (2017).
Chu, P. S., Saucier, D. A. & Hafner, E. Meta-analysis of the relationships between social support and well-being in children and adolescents. J. Soc. Clin. Psychol. 29 , 624–645 (2010).
Schneider, F. M. et al. Social media ostracism: the effects of being excluded online. Comput. Hum. Behav. 73 , 385–393 (2017).
Reich, S., Schneider, F. M. & Heling, L. Zero likes—symbolic interactions and need satisfaction online. Comput. Hum. Behav. 80 , 97–102 (2018).
Lutz, S. & Schneider, F. M. Is receiving dislikes in social media still better than being ignored? The effects of ostracism and rejection on need threat and coping responses online. Media Psychol. 24 , 741–765 (2021).
Lutz, S. Why don’t you answer me? Exploring the effects of (repeated exposure to) ostracism via messengers on users’ fundamental needs, well-being, and coping motivation. Media Psychol. 26 , 113–140 (2023).
Rodríguez-Hidalgo, C. T., Tan, E. S. H., Verlegh, P. W. J., Beyens, I. & Kühne, R. Don’t stress me now: assessing the regulatory impact of face-to-face and online feedback prosociality on stress during an important life event. J. Comput.-Mediat. Commun. 25 , 307–327 (2020).
Trepte, S., Dienlin, T. & Reinecke, L. Influence of social support received in online and offline contexts on satisfaction with social support and satisfaction with life: a longitudinal study. Media Psychol. 18 , 74–105 (2015).
Dredge, R. & Schreurs, L. Social media use and offline interpersonal outcomes during youth: a systematic literature review. Mass. Commun. Soc. 23 , 885–911 (2020).
Colasante, T., Lin, L., De France, K. & Hollenstein, T. Any time and place? Digital emotional support for digital natives. Am. Psychol. 77 , 186–195 (2022).
Pouwels, J. L., Valkenburg, P. M., Beyens, I., Van Driel, I. I. & Keijsers, L. Social media use and friendship closeness in adolescents’ daily lives: an experience sampling study. Dev. Psychol. 57 , 309–323 (2021).
Mills, K. L. et al. Structural brain development between childhood and adulthood: convergence across four longitudinal samples. NeuroImage 141 , 273–281 (2016).
Tamnes, C. K. et al. Development of the cerebral cortex across adolescence: a multisample study of inter-related longitudinal changes in cortical volume, surface area, and thickness. J. Neurosci. 37 , 3402–3412 (2017).
Larsen, B. & Luna, B. Adolescence as a neurobiological critical period for the development of higher-order cognition. Neurosci. Biobehav. Rev. 94 , 179–195 (2018).
Petanjek, Z. et al. Extraordinary neoteny of synaptic spines in the human prefrontal cortex. Proc. Natl Acad. Sci. USA 108 , 13281–13286 (2011).
Cohen, J. R. et al. A unique adolescent response to reward prediction errors. Nat. Neurosci. 13 , 669–671 (2010).
Ernst, M. et al. Amygdala and nucleus accumbens in responses to receipt and omission of gains in adults and adolescents. NeuroImage 25 , 1279–1291 (2005).
Galván, A. & McGlennen, K. M. Enhanced striatal sensitivity to aversive reinforcement in adolescents versus adults. J. Cognit. Neurosci. 25 , 284–296 (2013).
Braams, B. R., Van Duijvenvoorde, A. C. K., Peper, J. S. & Crone, E. A. Longitudinal changes in adolescent risk-taking: a comprehensive study of neural responses to rewards, pubertal development, and risk-taking behavior. J. Neurosci. 35 , 7226–7238 (2015).
Schreuders, E. et al. Contributions of reward sensitivity to ventral striatum activity across adolescence and early adulthood. Child. Dev. 89 , 797–810 (2018).
Maza, M. T. et al. Association of habitual checking behaviors on social media with longitudinal functional brain development. JAMA Pediatr. 177 , 160–167 (2023).
Miller, J., Mills, K. L., Vuorre, M., Orben, A. & Przybylski, A. K. Impact of digital screen media activity on functional brain organization in late childhood: evidence from the ABCD study. Cortex 169 , 290–308 (2023).
Flayelle, M. et al. A taxonomy of technology design features that promote potentially addictive online behaviours. Nat. Rev. Psychol. 2 , 136–150 (2023).
Lupien, S. J., McEwen, B. S., Gunnar, M. R. & Heim, C. Effects of stress throughout the lifespan on the brain, behaviour and cognition. Nat. Rev. Neurosci. 10 , 434–445 (2009).
Gunnar, M. R., Wewerka, S., Frenn, K., Long, J. D. & Griggs, C. Developmental changes in hypothalamus–pituitary–adrenal activity over the transition to adolescence: normative changes and associations with puberty. Dev. Psychopathol. 21 , 69–85 (2009).
Somerville, L. H. et al. The medial prefrontal cortex and the emergence of self-conscious emotion in adolescence. Psychol. Sci. 24 , 1554–1562 (2013).
Stroud, L. R. et al. Stress response and the adolescent transition: performance versus peer rejection stressors. Dev. Psychopathol. 21 , 47–68 (2009).
Avital, A. & Richter-Levin, G. Exposure to juvenile stress exacerbates the behavioural consequences of exposure to stress in the adult rat. Int. J. Neuropsychopharm. 8 , 163–173 (2005).
McCormick, C. M., Mathews, I. Z., Thomas, C. & Waters, P. Investigations of HPA function and the enduring consequences of stressors in adolescence in animal models. Brain Cogn. 72 , 73–85 (2010).
Eiland, L. & Romeo, R. D. Stress and the developing adolescent brain. Neuroscience 249 , 162–171 (2013).
Romeo, R. D. The teenage brain. Curr. Direc. Psychol. Sci. 22 , 140–145 (2013).
Afifi, T. D., Zamanzadeh, N., Harrison, K. & Acevedo Callejas, M. WIRED: the impact of media and technology use on stress (cortisol) and inflammation (interleukin IL-6) in fast paced families. Comput. Hum. Behav. 81 , 265–273 (2018).
Morin-Major, J. K. et al. Facebook behaviors associated with diurnal cortisol in adolescents: is befriending stressful? Psychoneuroendocrinology 63 , 238–46 (2016).
Ghai, S. It’s time to reimagine sample diversity and retire the WEIRD dichotomy. Nat. Hum. Behav. 5 , 971–972 (2021).
Munafò, M. R. & Davey Smith, G. Robust research needs many lines of evidence. Nature 553 , 399–401 (2018).
Dale, R., Warlaumont, A. S. & Johnson, K. L. The fundamental importance of method to theory. Nat. Rev. Psychol. 2 , 55–66 (2022).
Parry, D. A., Fisher, J. T., Mieczkowski, H., Sewall, C. J. R. & Davidson, B. I. Social media and well-being: a methodological perspective. Curr. Opin. Psychol. 45 , 101285 (2022).
Will, G.-J. et al. Neurocomputational mechanisms underpinning aberrant social learning in young adults with low self-esteem. Transl. Psychiatry 10 , 96 (2020).
Walther, J. B. Affordances, effects, and technology errors. Ann. Int. Commun. Assoc. 36 , 190–193 (2013).
Piray, P. & Daw, N. D. A model for learning based on the joint estimation of stochasticity and volatility. Nat. Commun. 12 , 6587 (2021).
Bronfenbrenner, U. The Ecology of Human Development: Experiments by Nature and Design (Harvard Univ. Press, 1979).
Slater, M. D. Reinforcing spirals: the mutual influence of media selectivity and media effects and their impact on individual behavior and social identity. Commun. Theory 17 , 281–303 (2007).
Valkenburg, P. M., Peter, J. & Walther, J. B. Media effects: theory and research. Annu. Rev. Psychol. 67 , 315–338 (2016).
Aalbers, G., McNally, R. J., Heeren, A., De Wit, S. & Fried, E. I. Social media and depression symptoms: a network perspective. J. Exp. Psychol. Gen. 148 , 1454–1462 (2019).
Ghai, S., Fassi, L., Awadh, F. & Orben, A. Lack of sample diversity in research on adolescent depression and social media use: a scoping review and meta-analysis. Clin. Psychol. Sci. 11 , 759–772 (2023).
Cramer, A. O. J. et al. Major depression as a complex dynamic system. PLoS ONE 11 , e0167490 (2016).
Kendler, K. S., Zachar, P. & Craver, C. What kinds of things are psychiatric disorders? Psychol. Med. 41 , 1143–1150 (2011).
van de Leemput, I. A. et al. Critical slowing down as early warning for the onset and termination of depression. Proc. Natl Acad. Sci. USA. 111 , 87–92 (2014).
Trepte, S. The social media privacy model: privacy and communication in the light of social media affordances. Commun. Theory 31 , 549–570 (2021).
Reinecke, L. et al. Permanently online and permanently connected: development and validation of the Online Vigilance Scale. PLoS ONE 13 , e0205384 (2018).
Trieu, P., Bayer, J. B., Ellison, N. B., Schoenebeck, S. & Falk, E. Who likes to be reachable? Availability preferences, weak ties, and bridging social capital. Inform. Commun. Soc. 22 , 1096–1111 (2019).
Daft, R. L. & Lengel, R. H. Organizational information requirements, media richness and structural design. Manag. Sci. 32 , 554–571 (1986).
Rhee, L., Bayer, J. B., Lee, D. S. & Kuru, O. Social by definition: how users define social platforms and why it matters. Telemat. Inform. 59 , 101538 (2021).
Valkenburg, P. M. Understanding self-effects in social media: self-effects in social media. Hum. Commun. Res. 43 , 477–490 (2017).
Thorson, K. & Wells, C. Curated flows: a framework for mapping media exposure in the digital age: curated flows. Commun. Theor. 26 , 309–328 (2016).
Zhao, H. & Wagner, C. How TikTok leads users to flow experience: investigating the effects of technology affordances with user experience level and video length as moderators. INTR 33 , 820–849 (2023).
Carr, C. T., Wohn, D. Y. & Hayes, R. A. As social support: relational closeness, automaticity, and interpreting social support from paralinguistic digital affordances in social media. Comput. Hum. Behav. 62 , 385–393 (2016).
Rice, R. E. et al. Organizational media affordances: operationalization and associations with media use: organizational media affordances. J. Commun. 67 , 106–130 (2017).
Scissors, L., Burke, M. & Wengrovitz, S. in Proc. 19th ACM Conf. Computer-Supported Cooperative Work & Social Computing—CSCW ’16 1499–1508 (ACM Press, 2016).
Boyd, D. M. in A Networked Self: Identity, Community and Culture in Social Networking Sites (ed. Papacharissi, Z.) 35–58 (Routledge, 2011).
Valkenburg, P. M. in Handbook of Adolescent Digital Media Use and Mental Health (eds Nesi, J., Telzer, E. H. & Prinstein, M. J.) 39–60 (Cambridge Univ. Press, 2022).
Dennis, Fuller & Valacich, Media Tasks, and communication processes: a theory of media synchronicity. MIS Q. 32 , 575 (2008).
DeAndrea, D. C. Advancing warranting theory: advancing warranting theory. Commun. Theor. 24 , 186–204 (2014).
Uhlhaas, P. J. et al. Towards a youth mental health paradigm: a perspective and roadmap. Mol. Psychiatry 28 , 3171–3181 (2023).
Kachuri, L. et al. Principles and methods for transferring polygenic risk scores across global populations. Nat. Rev. Genet. 25 , 8–25 (2024).
Weinstein, E. C. & Selman, R. L. Digital stress: adolescents’ personal accounts. N. Media Soc. 18 , 391–409 (2016).
Steele, R. G., Hall, J. A. & Christofferson, J. L. Conceptualizing digital stress in adolescents and young adults: toward the development of an empirically based model. Clin. Child. Fam. Psychol. Rev. 23 , 15–26 (2020).
Nick, E. A. et al. Adolescent digital stress: frequencies, correlates, and longitudinal association with depressive symptoms. J. Adolesc. Health 70 , 336–339 (2022).
Van Der Schuur, W. A., Baumgartner, S. E. & Sumter, S. R. Social media use, social media stress, and sleep: examining cross-sectional and longitudinal relationships in adolescents. Health Commun. 34 , 552–559 (2019).
Fabio, S. & Sonja, P. Is cyberbullying worse than traditional bullying? Examining the differential roles of medium, publicity, and anonymity for the perceived severity of bullying. J. Youth Adolesc. 42 , 739–750 (2013).
Tokunaga, R. S. Following you home from school: a critical review and synthesis of research on cyberbullying victimization. Comput. Hum. Behav. 26 , 277–287 (2010).
Khetawat, D. & Steele, R. G. Examining the association between digital stress components and psychological wellbeing: a meta-analysis. Clin. Child. Fam. Psychol. Rev. 26 , 957–974 (2023).
Beyens, I., Frison, E. & Eggermont, S. “I don’t want to miss a thing”: adolescents’ fear of missing out and its relationship to adolescents’ social needs, Facebook use, and Facebook related stress. Comput. Hum. Behav. 64 , 1–8 (2016).
Wartberg, L., Thomasius, R. & Paschke, K. The relevance of emotion regulation, procrastination, and perceived stress for problematic social media use in a representative sample of children and adolescents. Comput. Hum. Behav. 121 , 106788 (2021).
Winstone, L., Mars, B., Haworth, C. M. A. & Kidger, J. Types of social media use and digital stress in early adolescence. J. Early Adolescence 43 , 294–319 (2023).
West, M., Rice, S. & Vella-Brodrick, D. Exploring the “social” in social media: adolescent relatedness—thwarted and supported. J. Adolesc. Res . https://doi.org/10.1177/07435584211062158 (2021).
Gilbert, A., Baumgartner, S. E. & Reinecke, L. Situational boundary conditions of digital stress: goal conflict and autonomy frustration make smartphone use more stressful. Mob. Media Commun . https://doi.org/10.1177/20501579221138017 (2022).
Freytag, A. et al. Permanently online—always stressed out? The effects of permanent connectedness on stress experiences. Hum. Commun. Res. 47 , 132–165 (2021).
Johannes, N. et al. The relationship between online vigilance and affective well-being in everyday life: combining smartphone logging with experience sampling. Media Psychol. 24 , 581–605 (2021).
Reinecke, L. et al. Digital stress over the life span: the effects of communication load and internet multitasking on perceived stress and psychological health impairments in a german probability sample. Media Psychol. 20 , 90–115 (2017).
Schönbach, K. in The International Encyclopedia of Media Effects (eds Rössler, P., Hoffner, C. A. & Zoonen, L.) 1–11 (Wiley, 2017).
Mayer, J. D., Gaschke, Y. N., Braverman, D. L. & Evans, T. W. Mood-congruent judgment is a general effect. J. Pers. Soc. Psychol. 63 , 119–132 (1992).
Ferster, C. B. A functional analysis of depression. Am. Psychol. 28 , 857–870 (1973).
Carvalho, J. P. & Hopko, D. R. Behavioral theory of depression: reinforcement as a mediating variable between avoidance and depression. J. Behav. Ther. Exp. Psychiatry 42 , 154–162 (2011).
Helbig-Lang, S. & Petermann, F. Tolerate or eliminate? A systematic review on the effects of safety behavior across anxiety disorders. Clin. Psychol. Sci. Pract. 17 , 218–233 (2010).
Marciano, L., Driver, C. C., Schulz, P. J. & Camerini, A.-L. Dynamics of adolescents’ smartphone use and well-being are positive but ephemeral. Sci. Rep. 12 , 1316 (2022).
Rao, P. A. et al. Social anxiety disorder in childhood and adolescence: descriptive psychopathology. Behav. Res. Ther. 45 , 1181–1191 (2007).
Corning, A. F., Krumm, A. J. & Smitham, L. A. Differential social comparison processes in women with and without eating disorder symptoms. J. Couns. Psychol. 53 , 338–349 (2006).
Radovic, A., Gmelin, T., Stein, B. D. & Miller, E. Depressed adolescents’ positive and negative use of social media. J. Adolesc. 55 , 5–15 (2017).
Download references
Acknowledgements
A.O. and T.D. were funded by the Medical Research Council (MC_UU_00030/13). A.O. was funded by the Jacobs Foundation and a UKRI Future Leaders Fellowship (MR/X034925/1). S.-J.B. is funded by Wellcome (grant numbers WT107496/Z/15/Z and WT227882/Z/23/Z), the MRC, the Jacobs Foundation, the Wellspring Foundation and the University of Cambridge.
Author information
Authors and affiliations.
Medical Research Council Cognition and Brain Sciences Unit, University of Cambridge, Cambridge, UK
Amy Orben & Tim Dalgleish
School of Business, Economics and Society, Friedrich-Alexander-Universität Erlangen–Nürnberg, Nürnberg, Germany
Adrian Meier
Department of Psychology, University of Cambridge, Cambridge, UK
Sarah-Jayne Blakemore
Institute for Cognitive Neuroscience, University College London, London, UK
You can also search for this author in PubMed Google Scholar
Contributions
A.O. conceptualized the manuscript; A.O and A.M wrote the original draft; A.O., A.M., T.D. and S.-J.B. reviewed and edited the manuscript. All authors contributed substantially to discussion of the content, and reviewed and/or edited the manuscript before submission.
Corresponding author
Correspondence to Amy Orben .
Ethics declarations
Competing interests.
The authors declare no competing interests.
Peer review
Peer review information.
Nature Reviews Psychology thanks Emily Weinstein, who co-reviewed with Beck Tench; Nastasia Griffioen; and Margarita Panayiotou for their contribution to the peer review of this work.
Additional information
Publisher’s note Springer Nature remains neutral with regard to jurisdictional claims in published maps and institutional affiliations.
Rights and permissions
Springer Nature or its licensor (e.g. a society or other partner) holds exclusive rights to this article under a publishing agreement with the author(s) or other rightsholder(s); author self-archiving of the accepted manuscript version of this article is solely governed by the terms of such publishing agreement and applicable law.
Reprints and permissions
About this article
Cite this article.
Orben, A., Meier, A., Dalgleish, T. et al. Mechanisms linking social media use to adolescent mental health vulnerability. Nat Rev Psychol (2024). https://doi.org/10.1038/s44159-024-00307-y
Download citation
Accepted : 02 April 2024
Published : 07 May 2024
DOI : https://doi.org/10.1038/s44159-024-00307-y
Share this article
Anyone you share the following link with will be able to read this content:
Sorry, a shareable link is not currently available for this article.
Provided by the Springer Nature SharedIt content-sharing initiative
Quick links
- Explore articles by subject
- Guide to authors
- Editorial policies
Sign up for the Nature Briefing newsletter — what matters in science, free to your inbox daily.

Click through the PLOS taxonomy to find articles in your field.
For more information about PLOS Subject Areas, click here .
Loading metrics
Open Access
Peer-reviewed
Research Article
Social impact in social media: A new method to evaluate the social impact of research
Roles Investigation, Writing – original draft
* E-mail: [email protected]
Affiliation Department of Journalism and Communication Studies, Universitat Autonoma de Barcelona, Barcelona, Spain

Affiliation Department of Psychology and Sociology, Universidad de Zaragoza, Zaragoza, Spain
Roles Conceptualization, Investigation, Methodology, Supervision, Writing – review & editing
Affiliation Department of Sociology, Universitat Autonoma de Barcelona, Barcelona, Spain
Affiliation Department of Sociology, Universitat de Barcelona (UB), Barcelona, Spain
- Cristina M. Pulido,
- Gisela Redondo-Sama,
- Teresa Sordé-Martí,
- Ramon Flecha
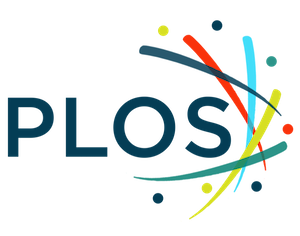
- Published: August 29, 2018
- https://doi.org/10.1371/journal.pone.0203117
- Reader Comments
The social impact of research has usually been analysed through the scientific outcomes produced under the auspices of the research. The growth of scholarly content in social media and the use of altmetrics by researchers to track their work facilitate the advancement in evaluating the impact of research. However, there is a gap in the identification of evidence of the social impact in terms of what citizens are sharing on their social media platforms. This article applies a social impact in social media methodology (SISM) to identify quantitative and qualitative evidence of the potential or real social impact of research shared on social media, specifically on Twitter and Facebook. We define the social impact coverage ratio (SICOR) to identify the percentage of tweets and Facebook posts providing information about potential or actual social impact in relation to the total amount of social media data found related to specific research projects. We selected 10 projects in different fields of knowledge to calculate the SICOR, and the results indicate that 0.43% of the tweets and Facebook posts collected provide linkages with information about social impact. However, our analysis indicates that some projects have a high percentage (4.98%) and others have no evidence of social impact shared in social media. Examples of quantitative and qualitative evidence of social impact are provided to illustrate these results. A general finding is that novel evidences of social impact of research can be found in social media, becoming relevant platforms for scientists to spread quantitative and qualitative evidence of social impact in social media to capture the interest of citizens. Thus, social media users are showed to be intermediaries making visible and assessing evidence of social impact.
Citation: Pulido CM, Redondo-Sama G, Sordé-Martí T, Flecha R (2018) Social impact in social media: A new method to evaluate the social impact of research. PLoS ONE 13(8): e0203117. https://doi.org/10.1371/journal.pone.0203117
Editor: Sergi Lozano, Institut Català de Paleoecologia Humana i Evolució Social (IPHES), SPAIN
Received: November 8, 2017; Accepted: August 15, 2018; Published: August 29, 2018
Copyright: © 2018 Pulido et al. This is an open access article distributed under the terms of the Creative Commons Attribution License , which permits unrestricted use, distribution, and reproduction in any medium, provided the original author and source are credited.
Data Availability: All relevant data are within the paper and its Supporting Information files.
Funding: The research leading to these results has received funding from the 7th Framework Programme of the European Commission under the Grant Agreement n° 613202 P.I. Ramon Flecha, https://ec.europa.eu/research/fp7/index_en.cfm . The funders had no role in study design, data collection and analysis, decision to publish, or preparation of the manuscript.
Competing interests: The authors have declared that no competing interests exist.
Introduction
The social impact of research is at the core of some of the debates influencing how scientists develop their studies and how useful results for citizens and societies may be obtained. Concrete strategies to achieve social impact in particular research projects are related to a broader understanding of the role of science in contemporary society. There is a need to explore dialogues between science and society not only to communicate and disseminate science but also to achieve social improvements generated by science. Thus, the social impact of research emerges as an increasing concern within the scientific community [ 1 ]. As Bornmann [ 2 ] said, the assessment of this type of impact is badly needed and is more difficult than the measurement of scientific impact; for this reason, it is urgent to advance in the methodologies and approaches to measuring the social impact of research.
Several authors have approached the conceptualization of social impact, observing a lack of generally accepted conceptual and instrumental frameworks [ 3 ]. It is common to find a wide range of topics included in the contributions about social impact. In their analysis of the policies affecting land use, Hemling et al. [ 4 ] considered various domains in social impact, for instance, agricultural employment or health risk. Moving to the field of flora and fauna, Wilder and Walpole [ 5 ] studied the social impact of conservation projects, focusing on qualitative stories that provided information about changes in attitudes, behaviour, wellbeing and livelihoods. In an extensive study by Godin and Dore [ 6 ], the authors provided an overview and framework for the assessment of the contribution of science to society. They identified indicators of the impact of science, mentioning some of the most relevant weaknesses and developing a typology of impact that includes eleven dimensions, with one of them being the impact on society. The subdimensions of the impact of science on society focus on individuals (wellbeing and quality of life, social implication and practices) and organizations (speeches, interventions and actions). For the authors, social impact “refers to the impact knowledge has on welfare, and on the behaviours, practices and activities of people and groups” (p. 7).
In addition, the terms “social impact” and “societal impact” are sometimes used interchangeably. For instance, Bornmann [ 2 ] said that due to the difficulty of distinguishing social benefits from the superior term of societal benefits, “in much literature the term ‘social impact’ is used instead of ‘societal impact’”(p. 218). However, in other cases, the distinction is made [ 3 ], as in the present research. Similar to the definition used by the European Commission [ 7 ], social impact is used to refer to economic impact, societal impact, environmental impact and, additionally, human rights impact. Therefore, we use the term social impact as the broader concept that includes social improvements in all the above mentioned areas obtained from the transference of research results and representing positive steps towards the fulfilment of those officially defined social goals, including the UN Sustainable Development Goals, the EU 2020 Agenda, or similar official targets. For instance, the Europe 2020 strategy defines five priority targets with concrete indicators (employment, research and development, climate change and energy, education and poverty and social exclusion) [ 8 ], and we consider the targets addressed by objectives defined in the specific call that funds the research project.
This understanding of the social impact of research is connected to the creation of the Social Impact Open Repository (SIOR), which constitutes the first open repository worldwide that displays, cites and stores the social impact of research results [ 9 ]. The SIOR has linked to ORCID and Wikipedia to allow the synergies of spreading information about the social impact of research through diverse channels and audiences. It is relevant to mention that currently, SIOR includes evidence of real social impact, which implies that the research results have led to actual improvements in society. However, it is common to find evidence of potential social impact in research projects. The potential social impact implies that in the development of the research, there has been some evidence of the effectiveness of the research results in terms of social impact, but the results have not yet been transferred.
Additionally, a common confusion is found among the uses of dissemination, transference (policy impact) and social impact. While dissemination means to disseminate the knowledge created by research to citizens, companies and institutions, transference refers to the use of this knowledge by these different actors (or others), and finally, as already mentioned, social impact refers to the actual improvements resulting from the use of this knowledge in relation to the goals motivating the research project (such as the United Nations Sustainable Development Goals). In the present research [ 3 ], it is argued that “social impact can be understood as the culmination of the prior three stages of the research” (p.3). Therefore, this study builds on previous contributions measuring the dissemination and transference of research and goes beyond to propose a novel methodological approach to track social impact evidences.
In fact, the contribution that we develop in this article is based on the creation of a new method to evaluate the evidence of social impact shared in social media. The evaluation proposed is to measure the social impact coverage ratio (SICOR), focusing on the presence of evidence of social impact shared in social media. Then, the article first presents some of the contributions from the literature review focused on the research on social media as a source for obtaining key data for monitoring or evaluating different research purposes. Second, the SISM (social impact through social media) methodology[ 10 ] developed is introduced in detail. This methodology identifies quantitative and qualitative evidence of the social impact of the research shared on social media, specifically on Twitter and Facebook, and defines the SICOR, the social impact coverage ratio. Next, the results are discussed, and lastly, the main conclusions and further steps are presented.
Literature review
Social media research includes the analysis of citizens’ voices on a wide range of topics [ 11 ]. According to quantitative data from April 2017 published by Statista [ 12 ], Twitter and Facebook are included in the top ten leading social networks worldwide, as ranked by the number of active users. Facebook is at the top of the list, with 1,968 million active users, and Twitter ranks 10 th , with 319 million active users. Between them are the following social networks: WhatsApp, YouTube, Facebook Messenger, WeChat, QQ, Instagram,Qzone and Tumblr. If we look at altmetrics, the tracking of social networks for mentions of research outputs includes Facebook, Twitter, Google+,LinkedIn, Sina Weibo and Pinterest. The social networks common to both sources are Facebook and Twitter. These are also popular platforms that have a relevant coverage of scientific content and easy access to data, and therefore, the research projects selected here for application of the SISM methodology were chosen on these platforms.
Chew and Eysenbach [ 13 ] studied the presence of selected keywords in Twitter related to public health issues, particularly during the 2009 H1N1 pandemic, identifying the potential for health authorities to use social media to respond to the concerns and needs of society. Crooks et al.[ 14 ] investigated Twitter activity in the context of a 5.8 magnitude earthquake in 2011 on the East Coast of the United States, concluding that social media content can be useful for event monitoring and can complement other sources of data to improve the understanding of people’s responses to such events. Conversations among young Canadians posted on Facebook and analysed by Martinello and Donelle [ 15 ] revealed housing and transportation as main environmental concerns, and the project FoodRisc examined the role of social media to illustrate consumers’ quick responses during food crisis situations [ 16 ]. These types of contributions illustrate that social media research implies the understanding of citizens’ concerns in different fields, including in relation to science.
Research on the synergies between science and citizens has increased over the years, according to Fresco [ 17 ], and there is a growing interest among researchers and funding agencies in how to facilitate communication channels to spread scientific results. For instance, in 1998, Lubchenco [ 18 ] advocated for a social contract that “represents a commitment on the part of all scientists to devote their energies and talents to the most pressing problems of the day, in proportion to their importance, in exchange for public funding”(p.491).
In this framework, the recent debates on how to increase the impact of research have acquired relevance in all fields of knowledge, and major developments address the methods for measuring it. As highlighted by Feng Xia et al. [ 19 ], social media constitute an emerging approach to evaluating the impact of scholarly publications, and it is relevant to consider the influence of the journal, discipline, publication year and user type. The authors revealed that people’s concerns differ by discipline and observed more interest in papers related to everyday life, biology, and earth and environmental sciences. In the field of biomedical sciences, Haustein et al. [ 20 ] analysed the dissemination of journal articles on Twitter to explore the correlations between tweets and citations and proposed a framework to evaluate social media-based metrics. In fact, different studies address the relationship between the presence of articles on social networks and citations [ 21 ]. Bornmann [ 22 ] conducted a case study using a sample of 1,082 PLOS journal articles recommended in F1000 to explore the usefulness of altmetrics for measuring the broader impact of research. The author presents evidence about Facebook and Twitter as social networks that may indicate which papers in the biomedical sciences can be of interest to broader audiences, not just to specialists in the area. One aspect of particular interest resulting from this contribution is the potential to use altmetrics to measure the broader impacts of research, including the societal impact. However, most of the studies investigating social or societal impact lack a conceptualization underlying its measurement.
To the best of our knowledge, the assessment of social impact in social media (SISM) has developed according to this gap. At the core of this study, we present and discuss the results obtained through the application of the SICOR (social impact coverage ratio) with examples of evidence of social impact shared in social media, particularly on Twitter and Facebook, and the implications for further research.
Following these previous contributions, our research questions were as follows: Is there evidence of social impact of research shared by citizens in social media? If so, is there quantitative or qualitative evidence? How can social media contribute to identifying the social impact of research?
Methods and data presentation
A group of new methodologies related to the analysis of online data has recently emerged. One of these emerging methodologies is social media analytics [ 23 ], which was initially used most in the marketing research field but also came to be used in other domains due to the multiple possibilities opened up by the availability and richness of the data for different research purposes. Likewise, the concern of how to evaluate the social impact of research as well as the development of methodologies for addressing this concern has occupied central attention. The development of SISM (Social Impact in Social Media) and the application of the SICOR (Social Impact Coverage Ratio) is a contribution to advancement in the evaluation of the social impact of research through the analysis of the social media selected (in this case, Twitter and Facebook). Thus, SISM is novel in both social media analytics and among the methodologies used to evaluate the social impact of research. This development has been made under IMPACT-EV, a research project funded under the Framework Program FP7 of the Directorate-General for Research and Innovation of the European Commission. The main difference from other methodologies for measuring the social impact of research is the disentanglement between dissemination and social impact. While altmetrics is aimed at measuring research results disseminated beyond academic and specialized spheres, SISM contribute to advancing this measurement by shedding light on to what extent evidence of the social impact of research is found in social media data. This involves the need to differentiate between tweets or Facebook posts (Fb/posts) used to disseminate research findings from those used to share the social impact of research. We focus on the latter, investigating whether there is evidence of social impact, including both potential and real social impact. In fact, the question is whether research contributes and/or has the potential to contribute to improve the society or living conditions considering one of these goals defined. What is the evidence? Next, we detail the application of the methodology.
Data collection
To develop this study, the first step was to select research projects with social media data to be analysed. The selection of research projects for application of the SISM methodology was performed according to three criteria.
Criteria 1. Selection of success projects in FP7. The projects were success stories of the 7 th Framework Programme (FP7) highlighted by the European Commission [ 24 ] in the fields of knowledge of medicine, public health, biology and genomics. The FP7 published calls for project proposals from 2007 to 2013. This implies that most of the projects funded in the last period of the FP7 (2012 and 2013) are finalized or in the last phase of implementation.
Criteria 2. Period of implementation. We selected projects in the 2012–2013 period because they combine recent research results with higher possibilities of having Twitter and Facebook accounts compared with projects of previous years, as the presence of social accounts in research increased over this period.
Criteria 3. Twitter and Facebook accounts. It was crucial that the selected projects had active Twitter and Facebook accounts.
Table 1 summarizes the criteria and the final number of projects identified. As shown, 10 projects met the defined criteria. Projects in medical research and public health had higher presence.
- PPT PowerPoint slide
- PNG larger image
- TIFF original image
https://doi.org/10.1371/journal.pone.0203117.t001
After the selection of projects, we defined the timeframe of social media data extraction on Twitter and Facebook from the starting date of the project until the day of the search, as presented in Table 2 .
https://doi.org/10.1371/journal.pone.0203117.t002
The second step was to define the search strategies for extracting social media data related to the research projects selected. In this line, we defined three search strategies.
Strategy 1. To extract messages published on the Twitter account and the Facebook page of the selected projects. We listed the Twitter accounts and Facebook pages related to each project in order to look at the available information. In this case, it is important to clarify that the tweets published under the corresponding Twitter project account are original tweets or retweets made from this account. It is relevant to mention that in one case, the Twitter account and Facebook page were linked to the website of the research group leading the project. In this case, we selected tweets and Facebook posts related to the project. For instance, in the case of the Twitter account, the research group created a specific hashtag to publish messages related to the project; therefore, we selected only the tweets published under this hashtag. In the analysis, we prioritized the analysis of the tweets and Facebook posts that received some type of interaction (likes, retweets or shares) because such interaction is a proxy for citizens’ interest. In doing so, we used the R program and NVivoto extract the data and proceed with the analysis. Once we obtained the data from Twitter and Facebook, we were able to have an overview of the information to be further analysed, as shown in Table 3 .
https://doi.org/10.1371/journal.pone.0203117.t003
We focused the second and third strategies on Twitter data. In both strategies, we extracted Twitter data directly from the Twitter Advanced Search tool, as the API connected to NVivo and the R program covers only a specific period of time limited to 7/9 days. Therefore, the use of the Twitter Advanced Search tool made it possible to obtain historic data without a period limitation. We downloaded the results in PDF and then uploaded them to NVivo.
Strategy 2. To use the project acronym combined with other keywords, such as FP7 or EU. This strategy made it possible to obtain tweets mentioning the project. Table 4 presents the number of tweets obtained with this strategy.
https://doi.org/10.1371/journal.pone.0203117.t004
Strategy 3. To use searchable research results of projects to obtain Twitter data. We defined a list of research results, one for each project, and converted them into keywords. We selected one searchable keyword for each project from its website or other relevant sources, for instance, the brief presentations prepared by the European Commission and published in CORDIS. Once we had the searchable research results, we used the Twitter Advanced Search tool to obtain tweets, as presented in Table 5 .
https://doi.org/10.1371/journal.pone.0203117.t005
The sum of the data obtained from these three strategies allowed us to obtain a total of 3,425 tweets and 1,925 posts on public Facebook pages. Table 6 presents a summary of the results.
https://doi.org/10.1371/journal.pone.0203117.t006
We imported the data obtained from the three search strategies into NVivo to analyse. Next, we select tweets and Facebook posts providing linkages with quantitative or qualitative evidence of social impact, and we complied with the terms of service for the social media from which the data were collected. By quantitative and qualitative evidence, we mean data or information that shows how the implementation of research results has led to improvements towards the fulfilment of the objectives defined in the EU2020 strategy of the European Commission or other official targets. For instance, in the case of quantitative evidence, we searched tweets and Facebook posts providing linkages with quantitative information about improvements obtained through the implementation of the research results of the project. In relation to qualitative evidence, for example, we searched for testimonies that show a positive evaluation of the improvement due to the implementation of research results. In relation to this step, it is important to highlight that social media users are intermediaries making visible evidence of social impact. Users often share evidence, sometimes sharing a link to an external resource (e.g., a video, an official report, a scientific article, news published on media). We identified evidence of social impact in these sources.
Data analysis
γ i is the total number of messages obtained about project i with evidence of social impact on social media platforms (Twitter, Facebook, Instagram, etc.);
T i is the total number of messages from project i on social media platforms (Twitter, Facebook, Instagram, etc.); and
n is the number of projects selected.
Analytical categories and codebook
The researchers who carried out the analysis of the social media data collected are specialists in the social impact of research and research on social media. Before conducting the full analysis, two aspects were guaranteed. First, how to identify evidence of social impact relating to the targets defined by the EU2020 strategy or to specific goals defined by the call addressed was clarified. Second, we held a pilot to test the methodology with one research project that we know has led to considerable social impact, which allowed us to clarify whether or not it was possible to detect evidence of social impact shared in social media. Once the pilot showed positive results, the next step was to extend the analysis to another set of projects and finally to the whole sample. The construction of the analytical categories was defined a priori, revised accordingly and lastly applied to the full sample.
Different observations should be made. First, in this previous analysis, we found that the tweets and Facebook users play a key role as “intermediaries,” serving as bridges between the larger public and the evidence of social impact. Social media users usually share a quote or paragraph introducing evidence of social impact and/or link to an external resource, for instance, a video, official report, scientific article, news story published on media, etc., where evidence of the social impact is available. This fact has implications for our study, as our unit of analysis is all the information included in the tweets or Facebook posts. This means that our analysis reaches the external resources linked to find evidence of social impact, and for this reason, we defined tweets or Facebook posts providing linkages with information about social impact.
Second, the other important aspect is the analysis of the users’ profile descriptions, which requires much more development in future research given the existing limitations. For instance, some profiles are users’ restricted due to privacy reasons, so the information is not available; other accounts have only the name of the user with no description of their profile available. Therefore, we gave priority to the identification of evidence of social impact including whether a post obtained interaction (retweets, likes or shares) or was published on accounts other than that of the research project itself. In the case of the profile analysis, we added only an exploratory preliminary result because this requires further development. Considering all these previous details, the codebook (see Table 7 ) that we present as follows is a result of this previous research.
https://doi.org/10.1371/journal.pone.0203117.t007
How to analyse Twitter and Facebook data
To illustrate how we analysed data from Twitter and Facebook, we provide one example of each type of evidence of social impact defined, considering both real and potential social impact, with the type of interaction obtained and the profiles of those who have interacted.
QUANESISM. Tweet by ZeroHunger Challenge @ZeroHunger published on 3 May 2016. Text: How re-using food waste for animal feed cuts carbon emissions.-NOSHAN project hubs.ly/H02SmrP0. 7 retweets and 5 likes.
The unit of analysis is all the content of the tweet, including the external link. If we limited our analysis to the tweet itself, it would not be evidence. Examining the external link is necessary to find whether there is evidence of social impact. The aim of this project was to investigate the process and technologies needed to use food waste for feed production at low cost, with low energy consumption and with a maximal evaluation of the starting wastes. This tweet provides a link to news published in the PHYS.org portal [ 25 ], which specializes in science news. The news story includes an interview with the main researcher that provides the following quotation with quantitative evidence:
'Our results demonstrated that with a NOSHAN 10 percent mix diet, for every kilogram of broiler chicken feed, carbon dioxide emissions were reduced by 0.3 kg compared to a non-food waste diet,' explains Montse Jorba, NOSHAN project coordinator. 'If 1 percent of total chicken broiler feed in Europe was switched to the 10 percent NOSHAN mix diet, the total amount of CO2 emissions avoided would be 0.62 million tons each year.'[ 25 ]
This quantitative evidence “a NOSHAN 10 percent mix diet, for every kilogram of broiler chicken feed, carbon dioxide emissions carbon dioxide emissions were reduced by 0.3 kg to a non-food waste diet” is linked directly with the Europe 2020 target of Climate Change & Energy, specifically with the target of reducing greenhouse gas emissions by 20% compared to the levels in 1990 [ 8 ]. The illustrative extrapolation the coordinator mentioned in the news is also an example of quantitative evidence, although is an extrapolation based on the specific research result.
This tweet was captured by the Acronym search strategy. It is a message tweeted by an account that is not related to the research project. The twitter account is that of the Zero Hunger Challenge movement, which supports the goals of the UN. The interaction obtained is 7 retweets and 5 likes. Regarding the profiles of those who retweeted and clicked “like”, there were activists, a journalist, an eco-friendly citizen, a global news service, restricted profiles (no information is available on those who have retweeted) and one account with no information in its profile.
The following example illustrates the analysis of QUALESISM: Tweet by @eurofitFP7 published on4 October 2016. Text: See our great new EuroFIT video on youtube! https://t.co/TocQwMiW3c 9 retweets and 5 likes.
The aim of this project is to improve health through the implementation of two novel technologies to achieve a healthier lifestyle. The tweet provides a link to a video on YouTube on the project’s results. In this video, we found qualitative evidence from people who tested the EuroFit programme; there are quotes from men who said that they have experienced improved health results using this method and that they are more aware of how to manage their health:
One end-user said: I have really amazing results from the start, because I managed to change a lot of things in my life. And other one: I was more conscious of what I ate, I was more conscious of taking more steps throughout the day and also standing up a little more. [ 26 ]
The research applies the well researched scientific evidence to the management of health issues in daily life. The video presents the research but also includes a section where end-users talk about the health improvements they experienced. The quotes extracted are some examples of the testimonies collected. All agree that they have improved their health and learned healthy habits for their daily lives. These are examples of qualitative evidence linked with the target of the call HEALTH.2013.3.3–1—Social innovation for health promotion [ 27 ] that has the objectives of reducing sedentary habits in the population and promoting healthy habits. This research contributes to this target, as we see in the video testimonies. Regarding the interaction obtained, this tweet achieved 9 retweets and 5 likes. In this case, the profiles of the interacting citizens show involvement in sport issues, including sport trainers, sport enthusiasts and some researchers.
To summarize the analysis, in Table 8 below, we provide a summary with examples illustrating the evidence found.
https://doi.org/10.1371/journal.pone.0203117.t008
Quantitative evidence of social impact in social media
There is a greater presence of tweets/Fb posts with quantitative evidence (14) than with qualitative evidence (9) in the total number of tweets/Fb posts identified with evidence of social impact. Most of the tweets/Fb posts with quantitative evidence of social impact are from scientific articles published in peer-reviewed international journals and show potential social impact. In Table 8 , we introduce 3 examples of this type of tweets/Fb posts with quantitative evidence:
The first tweet with quantitative social impact selected is from project 7. The aim of this project was to provide high-quality scientific evidence for preventing vitamin D deficiency in European citizens. The tweet highlighted the main contribution of the published study, that is, “Weekly consumption of 7 vitamin D-enhanced eggs has an important impact on winter vitamin D status in adults” [ 28 ]. The quantitative evidence shared in social media was extracted from a news publication in a blog on health news. This blog collects scientific articles of research results. In this case, the blog disseminated the research result focused on how vitamin D-enhanced eggs improve vitamin D deficiency in wintertime, with the published results obtained by the research team of the project selected. The quantitative evidence illustrates that the group of adults who consumed vitamin D-enhanced eggs did not suffer from vitamin D deficiency, as opposed to the control group, which showed a significant decrease in vitamin D over the winter. The specific evidence is the following extracted from the article [ 28 ]:
With the use of a within-group analysis, it was shown that, although serum 25(OH) D in the control group significantly decreased over winter (mean ± SD: -6.4 ± 6.7 nmol/L; P = 0.001), there was no change in the 2 groups who consumed vitamin D-enhanced eggs (P>0.1 for both. (p. 629)
This evidence contributes to achievement of the target defined in the call addressed that is KBBE.2013.2.2–03—Food-based solutions for the eradication of vitamin D deficiency and health promotion throughout the life cycle [ 29 ]. The quantitative evidence shows how the consumption of vitamin D-enhanced eggs reduces vitamin D deficiency.
The second example of this table corresponds to the example of quantitative evidence of social impact provided in the previous section.
The third example is a Facebook post from project 3 that is also tweeted. Therefore, this evidence was published in both social media sources analysed. The aim of this project was to measure a range of chemical and physical environmental hazards in food, consumer products, water, air, noise, and the built environment in the pre- and postnatal early-life periods. This Facebook post and tweet links directly to a scientific article [ 30 ] that shows the precision of the spectroscopic platform:
Using 1H NMR spectroscopy we characterized short-term variability in urinary metabolites measured from 20 children aged 8–9 years old. Daily spot morning, night-time and pooled (50:50 morning and night-time) urine samples across six days (18 samples per child) were analysed, and 44 metabolites quantified. Intraclass correlation coefficients (ICC) and mixed effect models were applied to assess the reproducibility and biological variance of metabolic phenotypes. Excellent analytical reproducibility and precision was demonstrated for the 1H NMR spectroscopic platform (median CV 7.2%) . (p.1)
This evidence is linked to the target defined in the call “ENV.2012.6.4–3—Integrating environmental and health data to advance knowledge of the role of environment in human health and well-being in support of a European exposome initiative” [ 31 ]. The evidence provided shows how the project’s results have contributed to building technology for improving the data collection to advance in the knowledge of the role of the environment in human health, especially in early life. The interaction obtained is one retweet from a citizen from Nigeria interested in health issues, according to the information available in his profile.
Qualitative evidence of social impact in social media
We found qualitative evidence of the social impact of different projects, as shown in Table 9 . Similarly to the quantitative evidence, the qualitative cases also demonstrate potential social impact. The three examples provided have in common that they are tweets or Facebook posts that link to videos where the end users of the research project explain their improvements once they have implemented the research results.
https://doi.org/10.1371/journal.pone.0203117.t009
The first tweet with qualitative evidence selected is from project 4. The aim of this project is to produce a system that helps in the prevention of obesity and eating disorders, targeting young people and adults [ 32 ]. The twitter account that published this tweet is that of the Future and Emerging Technologies Programme of the European Commission, and a link to a Euronews video is provided. This video shows how the patients using the technology developed in the research achieved control of their eating disorders, through the testimonies of patients commenting on the positive results they have obtained. These testimonies are included in the news article that complements the video. An example of these testimonies is as follows:
Pierre Vial has lost 43 kilos over the past nine and a half months. He and other patients at the eating disorder clinic explain the effects obesity and anorexia have had on their lives. Another patient, Karin Borell, still has some months to go at the clinic but, after decades of battling anorexia, is beginning to be able to visualise life without the illness: “On a good day I see myself living a normal life without an eating disorder, without problems with food. That’s really all I wish right now”.[ 32 ]
This qualitative evidence shows how the research results contribute to the achievement of the target goals of the call addressed:“ICT-2013.5.1—Personalised health, active ageing, and independent living”. [ 33 ] In this case, the results are robust, particularly for people suffering chronic diseases and desiring to improve their health; people who have applied the research findings are improving their eating disorders and better managing their health. The value of this evidence is the inclusion of the patients’ voices stating the impact of the research results on their health.
The second example is a Facebook post from project 9, which provides a link to a Euronews video. The aim of this project is to bring some tools from the lab to the farm in order to guarantee a better management of the farm and animal welfare. In this video [ 34 ], there are quotes from farmers using the new system developed through the research results of the project. These quotes show how use of the new system is improving the management of the farm and the health of the animals; some examples are provided:
Cameras and microphones help me detect in real time when the animals are stressed for whatever reason,” explained farmer Twan Colberts. “So I can find solutions faster and in more efficient ways, without me being constantly here, checking each animal.”
This evidence shows how the research results contribute to addressing the objectives specified in the call “KBBE.2012.1.1–02—Animal and farm-centric approach to precision livestock farming in Europe” [ 29 ], particularly, to improve the precision of livestock farming in Europe. The interaction obtained is composed of6 likes and 1 share. The profiles are diverse, but some of them do not disclose personal information; others have not added a profile description, and only their name and photo are available.
Interrater reliability (kappa)
The analysis of tweets and Facebook posts providing linkages with information about social impact was conducted following a content analysis method in which reliability was based on a peer review process. This sample is composed of 3,425 tweets and 1,925 Fb/posts. Each tweet and Facebook post was analysed to identify whether or not it contains evidence of social impact. Each researcher has the codebook a priori. We used interrater reliability in examining the agreement between the two raters on the assignment of the categories defined through Cohen’s kappa. We used SPSS to calculate this coefficient. We exported an excel sheet with the sample coded by the two researchers being 1 (is evidence of social impact, either potential or real) and 0 (is not evidence of social impact) to SPSS. The cases where agreement was not achieved were not considered as containing evidence of social impact. The result obtained is 0.979; considering the interpretation of this number according to Landis & Koch [ 35 ], our level of agreement is almost perfect, and thus, our analysis is reliable. To sum up the data analysis, the description of the steps followed is explained:
Step 1. Data analysis I. We included all data collected in an excel sheet to proceed with the analysis. Prior to the analysis, researchers read the codebook to keep in mind the information that should be identified.
Step 2. Each researcher involved reviewed case by case the tweets and Facebook posts to identify whether they provide links with evidence of social impact or not. If the researcher considers there to be evidence of social impact, he or she introduces the value of 1into the column, and if not, the value of 0.
Step 3. Once all the researchers have finished this step, the next step is to export the excel sheet to SPSS to extract the kappa coefficient.
Step 4. Data Analysis II. The following step was to analyse case by case the tweets and Facebook posts identified as providing linkages with information of social impact and classify them as quantitative or qualitative evidence of social impact.
Step 5. The interaction received was analysed because this determines to which extent this evidence of social impact has captured the attention of citizens (in the form of how many likes, shares, or retweets the post has).
Step 6. Finally, if available, the profile descriptions of the citizens interacting through retweeting or sharing the Facebook post were considered.
Step 7. SICOR was calculated. It could be applied to the complete sample (all data projects) or to each project, as we will see in the next section.
The total number of tweets and Fb/posts collected from the 10 projects is 5,350. After the content analysis, we identified 23 tweets and Facebook posts providing linkages to information about social impact. To respond to the research question, which considered whether there is evidence of social impact shared by citizens in social media, the answer was affirmative, although the coverage ratio is low. Both Twitter and Facebook users retweeted or shared evidence of social impact, and therefore, these two social media networks are valid sources for expanding knowledge on the assessment of social impact. Table 10 shows the social impact coverage ratio in relation to the total number of messages analysed.
https://doi.org/10.1371/journal.pone.0203117.t010
The analysis of each of the projects selected revealed some results to consider. Of the 10 projects, 7 had evidence, but those projects did not necessarily have more Tweets and Facebook posts. In fact, some projects with fewer than 70 tweets and 50 Facebook posts have more evidence of social impact than other projects with more than 400 tweets and 400 Facebook posts. This result indicates that the number of tweets and Facebook posts does not determine the existence of evidence of social impact in social media. For example, project 2 has 403 tweets and 423 Facebooks posts, but it has no evidence of social impact on social media. In contrast, project 9 has 62 tweets, 43 Facebook posts, and 2 pieces of evidence of social impact in social media, as shown in Table 11 .
https://doi.org/10.1371/journal.pone.0203117.t011
The ratio of tweets/Fb posts to evidence is 0.43%, and it differs depending on the project, as shown below in Table 12 . There is one project (P7) with a ratio of 4.98%, which is a social impact coverage ratio higher than that of the other projects. Next, a group of projects (P3, P9, P10) has a social impact coverage ratio between 1.41% and 2,99%.The next slot has three projects (P1, P4, P5), with a ratio between 0.13% and 0.46%. Finally, there are three projects (P2, P6, P8) without any tweets/Fb posts evidence of social impact.
https://doi.org/10.1371/journal.pone.0203117.t012
Considering the three strategies for obtaining data, each is related differently to the evidence of social impact. In terms of the social impact coverage ratio, as shown in Table 13 , the most successful strategy is number 3 (searchable research results), as it has a relation of 17.86%, which is much higher than the ratios for the other 2 strategies. The second strategy (acronym search) is more effective than the first (profile accounts),with 1.77% for the former as opposed to 0.27% for the latter.
https://doi.org/10.1371/journal.pone.0203117.t013
Once tweets and Facebook posts providing linkages with information about social impact(ESISM)were identified, we classified them in terms of quantitative (QUANESISM) or qualitative evidence (QUALESISM)to determine which type of evidence was shared in social media. Table 14 indicates the amount of quantitative and qualitative evidence identified for each search strategy.
https://doi.org/10.1371/journal.pone.0203117.t014
First, the results obtained indicated that the SISM methodology aids in calculating the social impact coverage ratio of the research projects selected and evaluating whether the social impact of the corresponding research is shared by citizens in social media. The social impact coverage ratio applied to the sample selected is low, but when we analyse the SICOR of each project separately, we can observe that some projects have a higher social impact coverage ratio than others. Complementary to altmetrics measuring the extent to which research results reach out society, the SICOR considers the question whether this process includes evidence of potential or real social impact. In this sense, the overall methodology of SISM contributes to advancement in the evaluation of the social impact of research by providing a more precise approach to what we are evaluating.
This contribution complements current evaluation methodologies of social impact that consider which improvements are shared by citizens in social media. Exploring the results in more depth, it is relevant to highlight that of the ten projects selected, there is one research project with a social impact coverage ratio higher than those of the others, which include projects without any tweets or Facebook posts with evidence of social impact. This project has a higher ratio of evidence than the others because evidence of its social impact is shared more than is that of other projects. This also means that the researchers produced evidence of social impact and shared it during the project. Another relevant result is that the quantity of tweets and Fb/posts collected did not determine the number of tweets and Fb/posts found with evidence of social impact. Moreover, the analysis of the research projects selected showed that there are projects with less social media interaction but with more tweets and Fb/posts containing evidence of social media impact. Thus, the number of tweets and Fb/posts with evidence of social impact is not determined by the number of publication messages collected; it is determined by the type of messages published and shared, that is, whether they contain evidence of social impact or not.
The second main finding is related to the effectiveness of the search strategies defined. Related to the strategies carried out under this methodology, one of the results found is that the most effective search strategy is the searchable research results, which reveals a higher percentage of evidence of social impact than the own account and acronym search strategies. However, the use of these three search strategies is highly recommended because the combination of all of them makes it possible to identify more tweets and Facebook posts with evidence of social impact.
Another result is related to the type of evidence of social impact found. There is both quantitative and qualitative evidence. Both types are useful for understanding the type of social impact achieved by the corresponding research project. In this sense, quantitative evidence allows us to understand the improvements obtained by the implementation of the research results and capture their impact. In contrast, qualitative evidence allows us to deeply understand how the resultant improvements obtained from the implementation of the research results are evaluated by the end users by capturing their corresponding direct quotes. The social impact includes the identification of both real and potential social impact.
Conclusions
After discussing the main results obtained, we conclude with the following points. Our study indicates that there is incipient evidence of social impact, both potential and real, in social media. This demonstrates that researchers from different fields, in the present case involved in medical research, public health, animal welfare and genomics, are sharing the improvements generated by their research and opening up new venues for citizens to interact with their work. This would imply that scientists are promoting not only the dissemination of their research results but also the evidence on how their results may lead to the improvement of societies. Considering the increasing relevance and presence of the dissemination of research, the results indicate that scientists still need to include in their dissemination and communication strategies the aim of sharing the social impact of their results. This implies the publication of concrete qualitative or quantitative evidence of the social impact obtained. Because of the inclusion of this strategy, citizens will pay more attention to the content published in social media because they are interested in knowing how science can contribute to improving their living conditions and in accessing crucial information. Sharing social impact in social media facilitates access to citizens of different ages, genders, cultural backgrounds and education levels. However, what is most relevant for our argument here is how citizens should also be able to participate in the evaluation of the social impact of research, with social media a great source to reinforce this democratization process. This contributes not only to greatly improving the social impact assessment, as in addition to experts, policy makers and scientific publications, citizens through social media contribute to making this assessment much more accurate. Thus, citizens’ contribution to the dissemination of evidence of the social impact of research yields access to more diverse sectors of society and information that might be unknown by the research or political community. Two future steps are opened here. On the one hand, it is necessary to further examine the profiles of users who interact with this evidence of social impact considering the limitations of the privacy and availability of profile information. A second future task is to advance in the articulation of the role played by citizens’ participation in social impact assessment, as citizens can contribute to current worldwide efforts by shedding new light on this process of social impact assessment and contributing to making science more relevant and useful for the most urgent and poignant social needs.
Supporting information
S1 file. interrater reliability (kappa) result..
This file contains the SPSS file with the result of the calculation of Cohen’s Kappa regards the interrater reliability. The word document exported with the obtained result is also included.
https://doi.org/10.1371/journal.pone.0203117.s001
S2 File. Data collected and SICOR calculation.
This excel contains four sheets, the first one titled “data collected” contains the number of tweets and Facebook posts collected through the three defined search strategies; the second sheet titled “sample” contains the sample classified by project indicating the ID of the message or code assigned, the type of message (tweet or Facebook post) and the codification done by researchers being 1 (is evidence of social impact, either potential or real) and 0 (is not evidence of social impact); the third sheet titled “evidence found” contains the number of type of evidences of social impact founded by project (ESISM-QUANESIM or ESISM-QUALESIM), search strategy and type of message (tweet or Facebook posts); and the last sheet titled “SICOR” contains the Social Impact Coverage Ratio calculation by projects in one table and type of search strategy done in another one.
https://doi.org/10.1371/journal.pone.0203117.s002
Acknowledgments
The research leading to these results received funding from the 7 th Framework Programme of the European Commission under Grant Agreement n° 613202. The extraction of available data using the list of searchable keywords on Twitter and Facebook followed the ethical guidelines for social media research supported by the Economic and Social Research Council (UK) [ 36 ] and the University of Aberdeen [ 37 ]. Furthermore, the research results have already been published and made public, and hence, there are no ethical issues.
- View Article
- PubMed/NCBI
- Google Scholar
- 6. Godin B, Dore C. Measuring the impacts of science; beyond the economic dimension. INRS Urbanisation, Culture et Sociult, HSIT Lecture. 2005. Helsinki, Finland: Helsinki Institute for Science and Technology Studies.
- 7. European Commission. Better regulation Toolbox. 2017: 129–130. Available from https://ec.europa.eu/info/sites/info/files/better-regulation-toolbox_0.pdf
- 8. European 2020 Strategy. 2010. Available from https://ec.europa.eu/info/business-economy-euro/economic-and-fiscal-policy-coordination/eu-economic-governance-monitoring-prevention-correction/european-semester/framework/europe-2020-strategy_en
- 10. Flecha R, Sordé-Martí T. SISM Methodology (Social Impact through Social Media).2016. Available from https://archive.org/details/SISMMethodology
- 12. Statista. Statistics and facts about social media usage. 2017. Available from https://www.statista.com/topics/1164/social-networks/
- 16. FoodRisc Consortium, European Commission, Main researcher: Patrick Wall. FoodRisc—Result in Brief (Seventh Framework Programme). 2017. Available from http://cordis.europa.eu/result/rcn/90678_en.html
- 23. Murphy, L. Grit Report. Greenbook research industry trends report. Grit Q3-Q4 2016; (Vol. 3–4). New York. Retrieved from https://www.greenbook.org/grit
- 24. EC Research & Innovation. Success Stories [Internet]. 2017 [cited 2017 Apr 25]. Available from: http://ec.europa.eu/research/infocentre/success_stories_en.cfm
- 25. PhysOrg. How re-using food waste for animal feed cuts carbon emissions. 2016. Available from: https://phys.org/news/2016-04-re-using-food-animal-carbon-emissions.html
- 26. EuroFIT. EuroFIT International PP Youtube. 2016; minute 2.05–2.20. Available from: https://www.youtube.com/watch?time_continue=155&v=CHkbnD8IgZw
- 27. European Commission<. Work Programme 2013. Cooperation Theme 1 Health. (2013). Available from: https://ec.europa.eu/research/participants/portal/doc/call/fp7/common/1567645-1._health_upd_2013_wp_27_june_2013_en.pdf
- 29. European Commission. Work Programme 2013. Cooperation Theme 2. Food, agriculture, and fisheries, and biotechnology. (2013). Available from: http://ec.europa.eu/research/participants/data/ref/fp7/192042/b-wp-201302_en.pdf
- 31. European Commission. Work Programme 2012. Cooperation Theme 6 Environment (including climate change). (2011). Available from: http://ec.europa.eu/research/participants/data/ref/fp7/89467/f-wp-201201_en.pdf
- 32. Research Information Center. Technology trialled in fight against ticking timebomb of obesity. 2016. Available from: http://www.euronews.com/2016/08/10/technology-trialled-in-fight-against-ticking-timebomb-of-obesity
- 33. European Commission. Work Programme 2013. Cooperation Theme 3. ICT (Information and Communication Technologies) (2012). Available from: http://ec.europa.eu/research/participants/data/ref/fp7/132099/c-wp-201301_en.pdf
- 34. Euronews. Big farmer is watching! Surveillance technology monitors animal wellbeing. 2016. Available from http://www.euronews.com/2016/05/09/big-farmer-is-watching-surveillance-technology-monitors-animal-wellbeing
- 36. Economic and Social Research Council. Social Media Best Practice and Guidance. Using Social Media. 2017. Available from: http://www.esrc.ac.uk/research/impact-toolkit/social-media/using-social-media/
- 37. Townsend, L. & Wallace, C. Social Media Research: A Guide to Ethics. 2016. Available from http://www.gla.ac.uk/media/media_487729_en.pdf
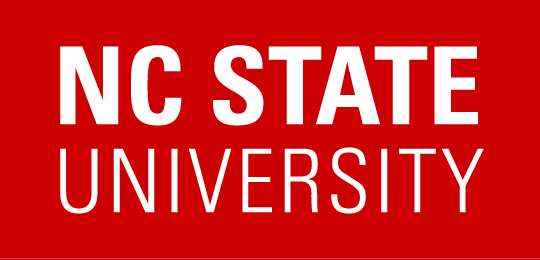
BioResources
- About the Journal
- Authors & Reviewers
- How to Self-Register
- Full Site Navigation
- Editorial Board
- Meet the Staff
- Editorial Policies
- General Instructions
- Ethics & Responsibilities
- Article Preparation
- Submission Instructions
- Acknowledgment of your Peer-Reviewing
- Writing Style Suggestions
- Reviewer Guidelines
- Back and Current Issues
- Scholarly Reviews
- Special Conference Collection Issues
- Competition Print Edition
- FRC: Pulp and Paper Fundamental Research Symposia Proceedings
- Paper Manufacturing Chemistry
- BioResources Early Career Investigator Award
- Distance Education: Online Masters Degree & Individual Courses
- Upcoming Conferences
- Hands-On Courses
- Affiliate Journal
The use of social media and its impact for research
Social media is an omnipresent part of everyday life. It provides users with an easy way to engage and connect with others without meeting face-to-face. This form of communication provides a lot of opportunity for companies and individuals to reach a massive audience. What is the purpose of social media, and how does it tie into science? Well, you see, it all depends on who you know and how active your social media presence is. Is there a benefit for sharing research across social media? The benefits of social media stem from active participation and the generation of new attractive content from an individual. Research is about producing new information, and social media offers unique opportunities to present new content.
Full Article
The Use of Social Media and its Impact for Research
Jessica Rogers
Keywords: Social media; Research; Engagement
Contact information: BioResources Process Editor, Department of Forest Biomaterials, North Carolina State University, Campus Box 8005, Raleigh, NC 27606, USA; e-mail: [email protected]
What is Social Media?
In today’s world social media is an ever-present facet of life that surrounds us. Almost every advertisement, whether television, radio, magazine, movie preview, podcast, newspaper, or elsewhere, will mention its social media presence in some way. ‘You can like us on Facebook, Check us out on Instagram,’ or perhaps ‘Watch our channel on YouTube’, are just some of the hooks that companies will provide to further build their brand and increase their visibility. As of January 2019, there were around 7.7 billion people in the world, of which 3.397 billion were active social media users (Smith 2019). Moreover, there are almost one million new users to some form of social media each day, or a new user every 10 seconds; 300 hours of video are uploaded to YouTube alone every minute (Smith 2019). To summarize, if you have found yourself boycotting the idea of social media, I hate to break it to you but it is here to stay.
The Underlying Purpose of Social Media
For those who do not know, a key theme of social media is ‘engagement’. Have you ever reached out to a company on their social media for any reason? The different social media outlets are simply interactive pathways on the internet that companies and businesses use to form relationships and network with others without leaving one’s desk. As a scientist, it is essential to attend conferences, give lectures, and lead panel discussions to network with others about common science interests. Today, there are an endless amount of resources accessible on the internet at your fingertips that allow you to do the same thing. Twitter first surfaced as a news and social networking site in which users post content and interact with each other through messages called ‘tweets’. The use of hashtags (a type of metadata tag) across all social media platforms allows people to search for certain interests and see all content related to that particular hashtag. This is a quick way to find and engage with people through common interests. Of course, you should still actively participate in your community by attending conventions and conferences, but if you truly seek to engage with more people, then you should not simply ignore the outlet of social media until you try it, as it can connect you with an even larger audience. Think of it this way, your lecture or discussion is most likely already being recorded, so what will you do with that recording?
Social media has a clear and direct purpose for businesses that sell a product or service and are searching for ways to advertise their brand. Of course, there other ways to use social media. Most people use social media to be, well, social, and communicate with family, former colleagues, or keep in touch with old classmates. The idea of a technological way of staying in touch with people is how Facebook was created. Facebook adds 500,000 new accounts each day, which equates to 6 new profiles every second (Smith 2019). So who exactly is in your friends list on Facebook? Who is subscribing to your channel on YouTube? Who is retweeting your tweets? If you want to broaden your impact beyond your discipline, you need to have a strong base of connections in your network.
The average person has 5.54 social media accounts (Smith 2019). Of those accounts, whatever one’s goal is, is it being projected across multiple platforms? Exactly who is engaged? These are all important questions that deal with your potential reach as an individual. The bottom line is if you seek community engagement in what you are doing, you must first be active in that particular community.
Social Media and Research
Now let us change course and focus on a different path of social media, that is where scientists use it to promote their research. The same rule applies. While all social media outlets have the potential for massive reach, it all comes back to a matter of whom you connect with or engage. However, the fast-paced and live aspect of social media can drive skeptical researchers not to publish, but successful reactions and quick responses can increase a researcher’s credibility. Research is about producing new information, and social media offers unique opportunities to present new content.
As a scientist, once you publish your research, you want to share it with as many colleagues and people so that they may read your novel findings. You want to share your hard work with many individuals. Almost all researchers send an email to their colleagues and individuals within their institution, which essentially is the first step in promoting their work. What if you took that one-step further and reached out to the scientific community on social media? You probably already have some form of a social media account and possibly one that relates to the scientific community; ResearchGate is a popular academic social media outlet. ResearchGate is a website that provides scientists with a forum to share and discuss their research as well as find collaborators. If you share your research on your personal account, then the only people that will see it are those whom you connect with. However, if you were active on different community or special interest pages that relate to your area of study and participate in regular discussions with other researchers on these sites, then you may find yourself having a much wider reach. Again, it all stems back to what you wish to accomplish with your research.
What Does that Mean for you?
Before getting started, you must ask yourself what exactly you want to gain from social media. If an increased reach is primarily what you seek, then you must be active in multiple communities related to your specialty. You already stay current on industry news and new research on your own, which is what others may be doing when they discover your research. However, if engagement and stimulated discussions are what you seek, then your active presence is required. Participating and driving discussions and posting content is what ultimately increases your visibility. Sharing and reposting others’ work, and being an active member on social media brings more attention to your profile and can enhance your reputation. A good place to start is with the professional social networking site LinkedIn that allows you to make connections with people based on job interests.
You can always go the old fashioned, tried and true route and send an email about your research, but how many new people reach out to you regarding your work? Maybe next time, try posting your research on a couple of industry pages, tag a few people in the community, and see if you make any new connections or spark any intriguing conversations. Because social media allows you to interact instantly with people across the globe, you may be surprised at who or how many people engage with you.
References Cited
Jaring, P., and Bäck, A. (2017). “How researchers use social media to promote their research and network with industry,” Technology Information Management Review 7(8), 32-39. DOI: 10.22215/timreview/1098
Smith, K. (2019). “123 Amazing social media statistics and facts,” brandwatch , (https://www.brandwatch.com/blog/amazing-social-media-statistics-and-facts/), Accessed 26 March 2019.
Facebook went away. Political divides didn't budge.
In the weeks before and after the 2020 presidential election, researchers at Stanford and elsewhere ran a number of tests to try to understand how much Facebook and its corporate cousin, Instagram, may be contributing to the nation's political divide.
One of those experiments — led by Matthew Gentzkow and Hunt Allcott , senior fellows at the Stanford Institute for Economic Policy Research (SIEPR) — centered on more than 35,000 Facebook and Instagram users who were paid to stay off the platforms in the run-up to Election Day. There’s a lot that researchers could glean from the social media hiatus, including whether people’s political attitudes shifted and in what ways. If views changed dramatically, that would support the argument that Facebook and Instagram, and social media generally, are helping to drive Americans apart.
The results of that deactivation exercise — the largest ever involving social media users and the first to include Instagram — are in: Staying off Facebook and Instagram in the final stretch of the November vote had little or no effect on people’s political views, their negative opinions of opposing parties, or beliefs around claims of election fraud.
But when it comes to Facebook’s impact on what people believed about current events, the researchers reached two conclusions. Those who were off Facebook were worse at answering news quiz questions, but they were also less likely to fall for widely circulated misinformation, suggesting that the platform can be an important conduit for both real and false news.
These findings, newly published by the Proceedings of the National Academy of Sciences, are in line with the main takeaways of the other experiments into Facebook and Instagram’s potential influence around the 2020 election, in which changing news feeds and limiting re-sharing of posts didn’t reduce polarization or change beliefs about whether the voting process was tainted. Those tests were detailed in four papers published in July 2023 in Science and Nature .
Taken together, the papers suggest that, when it comes to U.S. politics, Facebook and Instagram may not have as much ability to shape political attitudes during an election season as the popular narrative suggests.
And like the previous studies, the Gentzkow and Allcott-led study doesn’t absolve Meta Platforms, which owns Facebook and Instagram, from the messy state of U.S. politics. For one thing, the results support the view that Facebook may create harm by distributing misinformation. Gentzkow says it’s also possible that the platforms contributed to polarization in the past, even if people’s use of them in the run-up to the election had limited impact.

“We are not ruling out the possibility that Facebook and Instagram contribute to polarization in other ways over time,” says Gentzkow, who is the Landau Professor of Technology and the Economy in the Stanford School of Humanities and Sciences.
He also notes another finding suggesting that using Facebook in the weeks before the 2020 presidential election may have made people somewhat more likely to vote for Donald Trump and somewhat less likely to vote for Joe Biden. This could suggest that, for Facebook users who still were on the site, Trump’s campaign was savvier at building support than Biden’s team was.
“This effect was not quite statistically significant, so we need to take it with a grain of salt,” Gentzkow says. “But if it’s real, it’s big enough that it could impact the outcome of a close election.”
Meta opens up a trove of data
The study led by Gentzkow and Allcott — and the four that preceded it — are part of a massive research project that has been billed as the most comprehensive, evidence-based investigation yet into the role of social media in American democracy.
The project came together following critiques of Meta’s role in the spread of fake news, Russian influence, and the Cambridge Analytica data breach. The collaboration between academics and Meta researchers involved a series of steps to protect the integrity of the research , which builds on work by Stanford Law School Professor Nathaniel Persily on how to structure partnerships between academia and social media companies. Meta, for example, agreed not to prohibit any findings from being published.
In all, nearly 20 independent social scientists — including Gentzkow; Allcott, a professor at the Stanford Doerr School of Sustainability; Neil Malhotra, a political economist at the Stanford Graduate School of Business; and, Shanto Iyengar and Jennifer Pan, both political scientists in the Stanford School of Humanities and Sciences — are part of the project.
“Access to Meta’s proprietary data has allowed us to jump over big barriers to research on extremely important issues involving social media and politics,” Gentzkow says.
Gentzkow and Allcott’s study — whose 31 co-authors include five current and former SIEPR predocs and one former SIEPR undergraduate research fellow — involved roughly 19,900 Facebook users and 15,600 Instagram users who agreed to stop using the platforms ahead of the 2020 election. About a quarter of them agreed to deactivate their accounts for six weeks before the November vote. The rest comprised a control group that logged off for just one week.
The study’s analysis relies on a number of measures, among them participant surveys, state voting records, campaign donations, and Meta platform data. Some participants also allowed the researchers to track how they used other news and social media services when they were off Facebook or Instagram.
On top of the findings on polarization, knowledge, and Republican support, the authors conclude that Facebook and Instagram help people engage in the political process — mostly through posting about politics and signing petitions online (voter turnout didn’t change).
Takeaways for 2024 and beyond
Gentzkow says that the study’s finding that Facebook and Instagram didn’t change people’s political attitudes or beliefs in claims of electoral fraud in 2020 is especially interesting in light of his previous research with Allcott. In an earlier smaller-scale study of Facebook users who stayed off the platform for a month ahead of the 2018 midterms, the authors did find evidence that it contributes to polarization.
The distinction, Gentzkow says, could be that people are aware enough of political issues during a presidential election, so Facebook and Instagram have little or no effect on their beliefs or attitudes. But during other elections, when information about candidates or issues are not so front and center, social media may have more influence over what people think.
“Even though Facebook and Instagram did not contribute to polarization in the runup to the 2020 election, it’s possible that they are helping to widen political divides in other contexts where people’s views are less entrenched,” Gentzkow says.
And though the study was limited to the six weeks leading up to the presidential vote, it’s still a critical time in U.S. politics — hence the phenomenon known as the “ October surprise .”
“Things happen in the home stretch of a presidential election that can change poll numbers,” he says. “We’ve learned from this study that altering how much time people spend on Facebook and Instagram during that period isn’t likely to make a huge difference.”
More News Topics
When the export-import bank closed up, us companies saw global sales plummet.
- Global Development and Trade
- Money and Finance
- Research Highlight
Adrien Auclert appointed as an economic advisor to French government
- Awards & Appointments
- Taxes and Public Spending
IMF's Gita Gopinath: Geopolitics and its impact on global trade and the dollar
- Copy/Paste Link Link Copied
Understanding How Digital Media Affects Child Development
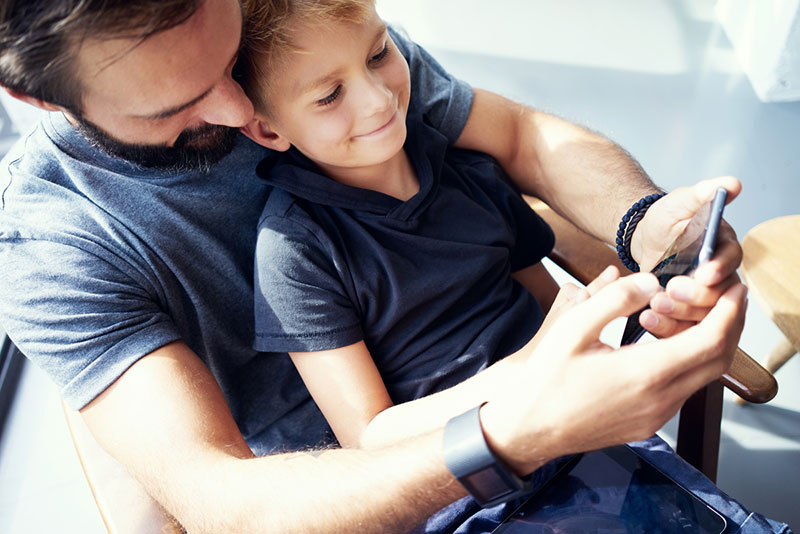
Technology and digital media have become ubiquitous parts of our daily lives. Screen time among children and adolescents was high before COVID-19 emerged, and it has further risen during the pandemic, thanks in part to the lack of in-person interactions.
In this increasingly digital world, we must strive to better understand how technology and media affect development, health outcomes, and interpersonal relationships. In fact, the fiscal year 2023 federal budget sets aside no less than $15 million within NICHD’s appropriation to investigate the effects of technology use and media consumption on infant, child, and adolescent development.
Parents may not closely oversee their children’s media use, especially as children gain independence. However, many scientific studies of child and adolescent media use have relied on parents’ recollections of how much time the children spent in front of a screen. By using software embedded within mobile devices to calculate children’s actual use, NICHD-supported researchers found that parent reports were inaccurate more often than they were on target. A little more than one-third of parents in the study underestimated their children’s usage, and nearly the same proportion overestimated it. With a recent grant award from NICHD, researchers at Baylor College of Medicine plan to overcome the limitation of relying on parental reports by using a novel technology to objectively monitor preschool-age children’s digital media use. They ultimately aim to identify the short- and long-term influences of technology and digital media use on children’s executive functioning, sleep patterns, and weight. This is one of three multi-project program grants awarded in response to NICHD’s recent funding opportunity announcement inviting proposals to examine how digital media exposure and use impact developmental trajectories and health outcomes in early childhood or adolescence. Another grant supports research to characterize the context, content, and use of digital media among children ages 1 to 8 years and to examine associations with the development of emotional regulation and social competence. A third research program seeks to better characterize the complex relationships between social media content, behaviors, brain activity, health, and well-being during adolescence.
I look forward to the findings from these ongoing projects and other studies that promise to inform guidance for technology and media use among children and adolescents. Additionally, the set-aside funding for the current fiscal year will allow us to further expand research in this area. These efforts will help us advance toward our aspirational goal to discover how technology exposure and media use affect developmental trajectories, health outcomes, and parent-child interactions.
Do Facebook and Instagram influence your politics? 35,000 took a break to find out
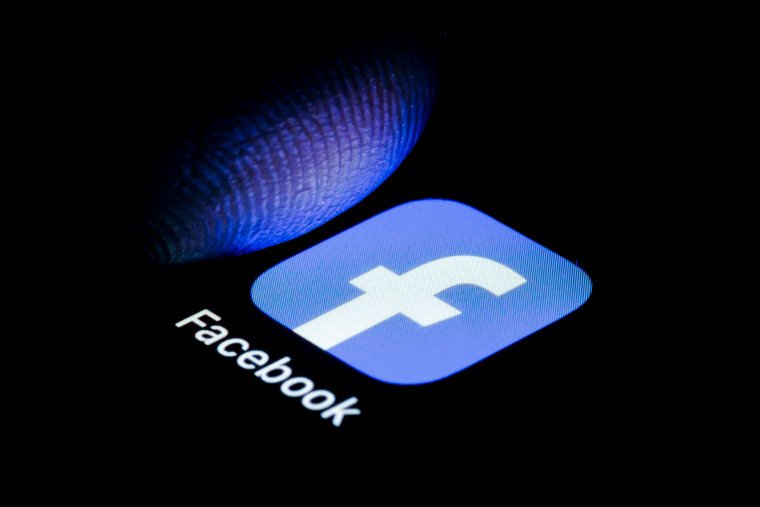
Facebook and Instagram may not have quite the impact on people’s politics as some critics have feared.
A study published Monday by researchers at Stanford University, Meta and other institutions found that a subset of 35,000 people who took breaks from Facebook and Instagram for six weeks before the 2020 presidential election didn’t significantly change their politics by the time the election came around.
The researchers found that staying off the two social media apps, both part of parent company Meta, had nearly zero effect — at least in the short term — on how people viewed the candidates, whether they turned out to vote and how they perceived the legitimacy of the election.
But the researchers also found that staying off Facebook, in particular, was a double-edged sword for understanding the world: Those who deactivated the app appear to be less likely to believe misinformation about the election but also less likely to be knowledgeable about general news.
The research, published in the Proceedings of the National Academy of Sciences, is part of a broader study trying to understand how social media affects democracy. Under fire for years for upending how campaigns are run and how voters consume information, Meta formed a partnership with academics to grant them access to company-held data. University professors retain control over what they report. In the latest study, the authors said that Meta paid for the costs of the research but that it didn’t pay the researchers or their institutions.
Meta said in a statement Monday: “These findings are consistent with previous publications in this study in showing little impact on key political attitudes, beliefs or behaviors.” An earlier set of findings published in July concluded that Facebook had “significant ideological segregation” and that conservative sources dominated its news ecosystem.
Academic researchers and social media users alike have been eager to understand, for example, whether so-called filter bubbles exist and if so what the practical effects are. Social media has been repeatedly blamed for “ ruining political discourse ,” spreading falsehoods and making polarization worse , and research into such questions is relatively young.
A co-author of the latest study, Matthew Gentzkow, a Stanford economist, said one of the study’s findings was how little impact Facebook and Instagram seemed to have on polarization and divisiveness.
“If we’re worried about those things, trying to control what people see on social media and whether they’re on social media may not be the most important lever,” he said in an interview.
On issues such as immigration, mask requirements and policing, those who deactivated Facebook and Instagram remained polarized.
But Gentzkow also said the study isn’t nearly the final word on the subject, because it was limited to the impact of Facebook and Instagram in a relatively narrow six-week time frame.
“This study cannot say one way or the other — in a decadelong sense — whether social media is causing polarization or not,” he said.
Researchers did find a small, unverified impact of Facebook use favoring Donald Trump, in which people who deactivated from Facebook were slightly more likely than others to vote for Joe Biden — the equivalent of 1.3% of Trump voters’ swinging to Biden. That could be because the Trump campaign was using Facebook more effectively or possibly because of other factors, the researchers wrote. Either way, they wrote, the difference “applies to the specific population that selected into our experiment” and “cannot be extrapolated to the broader population without strong assumptions.”
The study bills itself as the “largest-scale evidence available to date on the effect of Facebook and Instagram access on political knowledge, attitudes, and behavior in a presidential election season.” It lists 32 co-authors from 14 institutions.
Researchers didn’t look at the role of Facebook and Instagram after Election Day, when some supporters of Trump used them to promote allegations about election fraud and the company battled to take the allegations down .
Meta worked with the researchers to find participants, placing invitations in people’s feeds in August and September 2020. And participation came with a financial incentive: $25 apiece for people who agreed to deactivate for one week and $150 for people to deactivate for six weeks.
It’s not the first study to wonder what life is like for people not on social media. A study published in 2018, amid an online boycott called #DeleteFacebook, said that “the average Facebook user would require more than $1,000 to deactivate their account for one year.”
And in a study from 2019 , Gentzkow and other researchers found that deactivating Facebook for four weeks before the 2018 midterm elections had a variety of real-world implications, including increased self-reported happiness, reduced factual news knowledge and reduced political polarization. Gentzkow said more research would be needed to explore why that study showed reduced polarization but the most recent one did not.
David Ingram covers tech for NBC News.
Numbers, Facts and Trends Shaping Your World
Read our research on:
Full Topic List
Regions & Countries
- Publications
- Our Methods
- Short Reads
- Tools & Resources
Read Our Research On:
Teens and social media: Key findings from Pew Research Center surveys
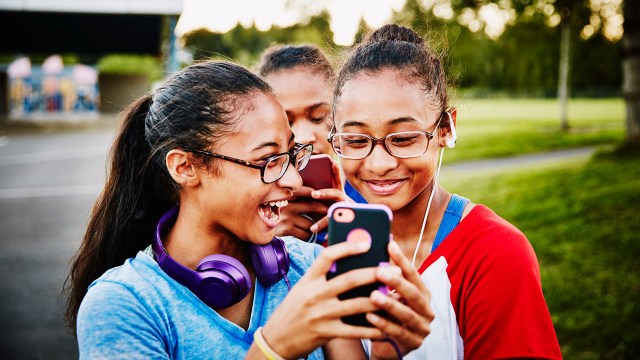
For the latest survey data on social media and tech use among teens, see “ Teens, Social Media, and Technology 2023 .”
Today’s teens are navigating a digital landscape unlike the one experienced by their predecessors, particularly when it comes to the pervasive presence of social media. In 2022, Pew Research Center fielded an in-depth survey asking American teens – and their parents – about their experiences with and views toward social media . Here are key findings from the survey:
Pew Research Center conducted this study to better understand American teens’ experiences with social media and their parents’ perception of these experiences. For this analysis, we surveyed 1,316 U.S. teens ages 13 to 17, along with one parent from each teen’s household. The survey was conducted online by Ipsos from April 14 to May 4, 2022.
This research was reviewed and approved by an external institutional review board (IRB), Advarra, which is an independent committee of experts that specializes in helping to protect the rights of research participants.
Ipsos invited panelists who were a parent of at least one teen ages 13 to 17 from its KnowledgePanel , a probability-based web panel recruited primarily through national, random sampling of residential addresses, to take this survey. For some of these questions, parents were asked to think about one teen in their household. (If they had multiple teenage children ages 13 to 17 in the household, one was randomly chosen.) This teen was then asked to answer questions as well. The parent portion of the survey is weighted to be representative of U.S. parents of teens ages 13 to 17 by age, gender, race, ethnicity, household income and other categories. The teen portion of the survey is weighted to be representative of U.S. teens ages 13 to 17 who live with parents by age, gender, race, ethnicity, household income and other categories.
Here are the questions used for this report, along with responses, and its methodology .
Majorities of teens report ever using YouTube, TikTok, Instagram and Snapchat. YouTube is the platform most commonly used by teens, with 95% of those ages 13 to 17 saying they have ever used it, according to a Center survey conducted April 14-May 4, 2022, that asked about 10 online platforms. Two-thirds of teens report using TikTok, followed by roughly six-in-ten who say they use Instagram (62%) and Snapchat (59%). Much smaller shares of teens say they have ever used Twitter (23%), Twitch (20%), WhatsApp (17%), Reddit (14%) and Tumblr (5%).
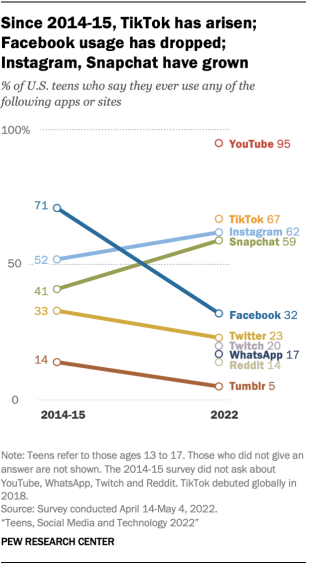
Facebook use among teens dropped from 71% in 2014-15 to 32% in 2022. Twitter and Tumblr also experienced declines in teen users during that span, but Instagram and Snapchat saw notable increases.
TikTok use is more common among Black teens and among teen girls. For example, roughly eight-in-ten Black teens (81%) say they use TikTok, compared with 71% of Hispanic teens and 62% of White teens. And Hispanic teens (29%) are more likely than Black (19%) or White teens (10%) to report using WhatsApp. (There were not enough Asian teens in the sample to analyze separately.)
Teens’ use of certain social media platforms also varies by gender. Teen girls are more likely than teen boys to report using TikTok (73% vs. 60%), Instagram (69% vs. 55%) and Snapchat (64% vs. 54%). Boys are more likely than girls to report using YouTube (97% vs. 92%), Twitch (26% vs. 13%) and Reddit (20% vs. 8%).
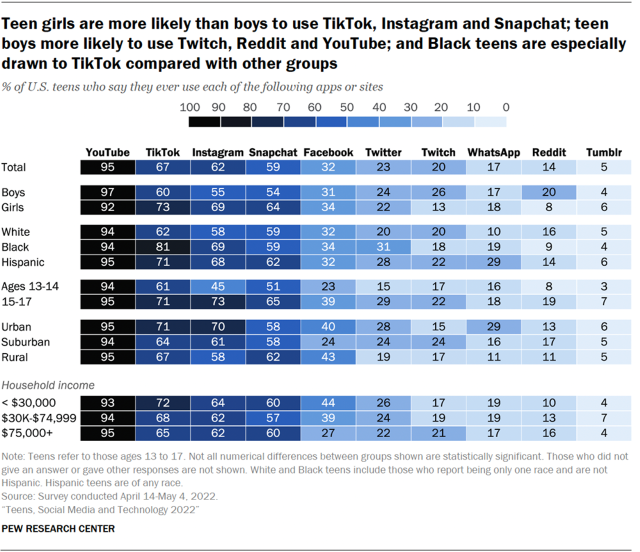
Majorities of teens use YouTube and TikTok every day, and some report using these sites almost constantly. About three-quarters of teens (77%) say they use YouTube daily, while a smaller majority of teens (58%) say the same about TikTok. About half of teens use Instagram (50%) or Snapchat (51%) at least once a day, while 19% report daily use of Facebook.
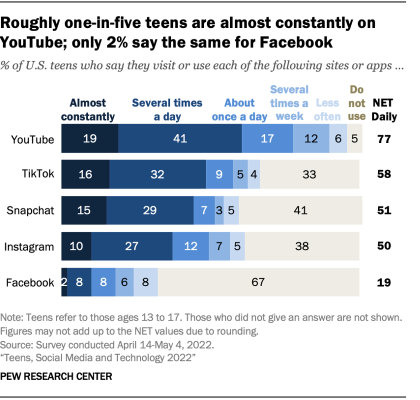
Some teens report using these platforms almost constantly. For example, 19% say they use YouTube almost constantly, while 16% and 15% say the same about TikTok and Snapchat, respectively.
More than half of teens say it would be difficult for them to give up social media. About a third of teens (36%) say they spend too much time on social media, while 55% say they spend about the right amount of time there and just 8% say they spend too little time. Girls are more likely than boys to say they spend too much time on social media (41% vs. 31%).
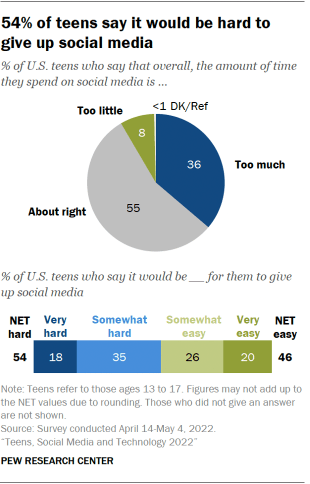
Teens are relatively divided over whether it would be hard or easy for them to give up social media. Some 54% say it would be very or somewhat hard, while 46% say it would be very or somewhat easy.
Girls are more likely than boys to say it would be difficult for them to give up social media (58% vs. 49%). Older teens are also more likely than younger teens to say this: 58% of those ages 15 to 17 say it would be very or somewhat hard to give up social media, compared with 48% of those ages 13 to 14.
Teens are more likely to say social media has had a negative effect on others than on themselves. Some 32% say social media has had a mostly negative effect on people their age, while 9% say this about social media’s effect on themselves.
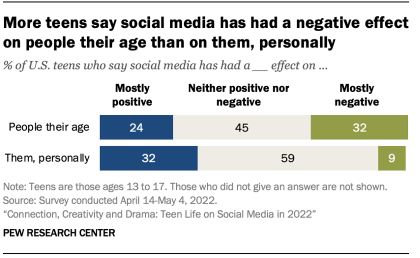
Conversely, teens are more likely to say these platforms have had a mostly positive impact on their own life than on those of their peers. About a third of teens (32%) say social media has had a mostly positive effect on them personally, while roughly a quarter (24%) say it has been positive for other people their age.
Still, the largest shares of teens say social media has had neither a positive nor negative effect on themselves (59%) or on other teens (45%). These patterns are consistent across demographic groups.
Teens are more likely to report positive than negative experiences in their social media use. Majorities of teens report experiencing each of the four positive experiences asked about: feeling more connected to what is going on in their friends’ lives (80%), like they have a place where they can show their creative side (71%), like they have people who can support them through tough times (67%), and that they are more accepted (58%).
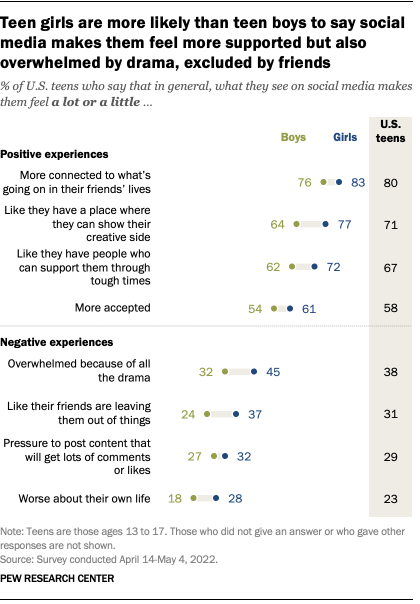
When it comes to negative experiences, 38% of teens say that what they see on social media makes them feel overwhelmed because of all the drama. Roughly three-in-ten say it makes them feel like their friends are leaving them out of things (31%) or feel pressure to post content that will get lots of comments or likes (29%). And 23% say that what they see on social media makes them feel worse about their own life.
There are several gender differences in the experiences teens report having while on social media. Teen girls are more likely than teen boys to say that what they see on social media makes them feel a lot like they have a place to express their creativity or like they have people who can support them. However, girls also report encountering some of the pressures at higher rates than boys. Some 45% of girls say they feel overwhelmed because of all the drama on social media, compared with 32% of boys. Girls are also more likely than boys to say social media has made them feel like their friends are leaving them out of things (37% vs. 24%) or feel worse about their own life (28% vs. 18%).
When it comes to abuse on social media platforms, many teens think criminal charges or permanent bans would help a lot. Half of teens think criminal charges or permanent bans for users who bully or harass others on social media would help a lot to reduce harassment and bullying on these platforms.
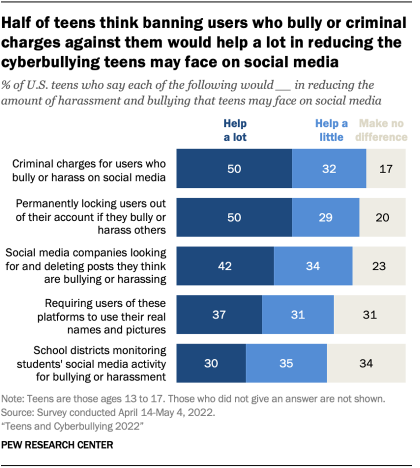
About four-in-ten teens say it would help a lot if social media companies proactively deleted abusive posts or required social media users to use their real names and pictures. Three-in-ten teens say it would help a lot if school districts monitored students’ social media activity for bullying or harassment.
Some teens – especially older girls – avoid posting certain things on social media because of fear of embarrassment or other reasons. Roughly four-in-ten teens say they often or sometimes decide not to post something on social media because they worry people might use it to embarrass them (40%) or because it does not align with how they like to represent themselves on these platforms (38%). A third of teens say they avoid posting certain things out of concern for offending others by what they say, while 27% say they avoid posting things because it could hurt their chances when applying for schools or jobs.
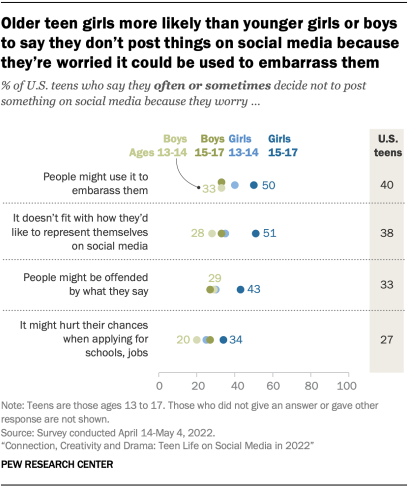
These concerns are more prevalent among older teen girls. For example, roughly half of girls ages 15 to 17 say they often or sometimes decide not to post something on social media because they worry people might use it to embarrass them (50%) or because it doesn’t fit with how they’d like to represent themselves on these sites (51%), compared with smaller shares among younger girls and among boys overall.
Many teens do not feel like they are in the driver’s seat when it comes to controlling what information social media companies collect about them. Six-in-ten teens say they think they have little (40%) or no control (20%) over the personal information that social media companies collect about them. Another 26% aren’t sure how much control they have. Just 14% of teens think they have a lot of control.
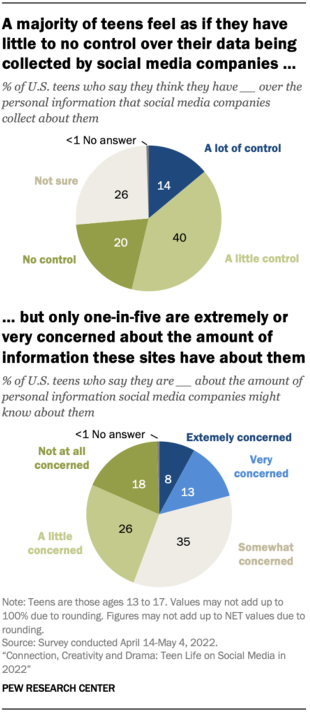
Despite many feeling a lack of control, teens are largely unconcerned about companies collecting their information. Only 8% are extremely concerned about the amount of personal information that social media companies might have and 13% are very concerned. Still, 44% of teens say they have little or no concern about how much these companies might know about them.
Only around one-in-five teens think their parents are highly worried about their use of social media. Some 22% of teens think their parents are extremely or very worried about them using social media. But a larger share of teens (41%) think their parents are either not at all (16%) or a little worried (25%) about them using social media. About a quarter of teens (27%) fall more in the middle, saying they think their parents are somewhat worried.
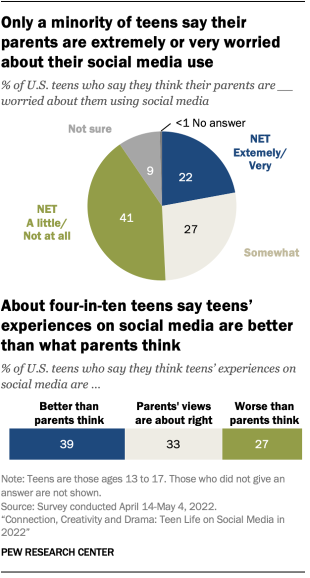
Many teens also believe there is a disconnect between parental perceptions of social media and teens’ lived realities. Some 39% of teens say their experiences on social media are better than parents think, and 27% say their experiences are worse. A third of teens say parents’ views are about right.
Nearly half of parents with teens (46%) are highly worried that their child could be exposed to explicit content on social media. Parents of teens are more likely to be extremely or very concerned about this than about social media causing mental health issues like anxiety, depression or lower self-esteem. Some parents also fret about time management problems for their teen stemming from social media use, such as wasting time on these sites (42%) and being distracted from completing homework (38%).
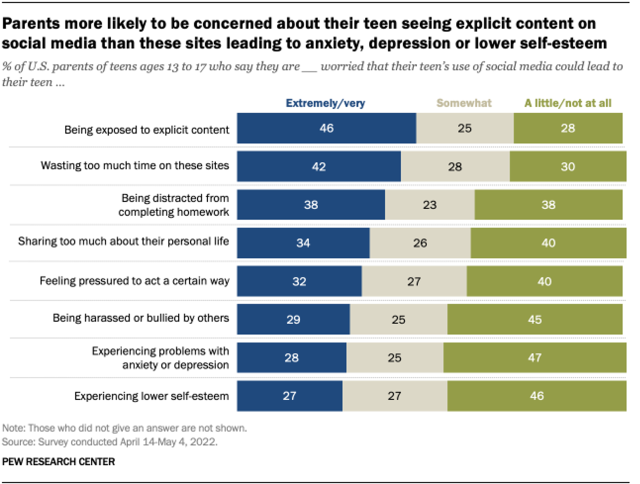
Note: Here are the questions used for this report, along with responses, and its methodology .
CORRECTION (May 17, 2023): In a previous version of this post, the percentages of teens using Instagram and Snapchat daily were transposed in the text. The original chart was correct. This change does not substantively affect the analysis.
- Age & Generations
- Age, Generations & Tech
- Internet & Technology
- Platforms & Services
- Social Media
- Teens & Tech
- Teens & Youth
Emily A. Vogels is a former research associate focusing on internet and technology at Pew Research Center .
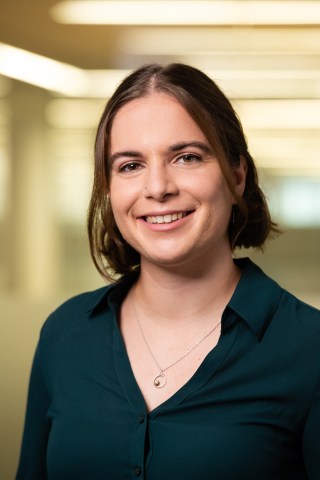
Risa Gelles-Watnick is a former research analyst focusing on internet and technology research at Pew Research Center .
Teens and Video Games Today
As biden and trump seek reelection, who are the oldest – and youngest – current world leaders, how teens and parents approach screen time, who are you the art and science of measuring identity, u.s. centenarian population is projected to quadruple over the next 30 years, most popular.
1615 L St. NW, Suite 800 Washington, DC 20036 USA (+1) 202-419-4300 | Main (+1) 202-857-8562 | Fax (+1) 202-419-4372 | Media Inquiries
Research Topics
- Coronavirus (COVID-19)
- Economy & Work
- Family & Relationships
- Gender & LGBTQ
- Immigration & Migration
- International Affairs
- Methodological Research
- News Habits & Media
- Non-U.S. Governments
- Other Topics
- Politics & Policy
- Race & Ethnicity
- Email Newsletters
ABOUT PEW RESEARCH CENTER Pew Research Center is a nonpartisan fact tank that informs the public about the issues, attitudes and trends shaping the world. It conducts public opinion polling, demographic research, media content analysis and other empirical social science research. Pew Research Center does not take policy positions. It is a subsidiary of The Pew Charitable Trusts .
Copyright 2024 Pew Research Center
Social media use linked to higher risk of vaping, smoking
K ids and young adults who use social media for seven or more hours per day have double the risk of taking up vaping or smoking or both, new research shows.
The study is based on a survey of almost 11,000 young British people ages 10 to 25 who were tracked from 2015 through 2021.
Overall, 8.5% said they currently smoked, 2.5% said they vaped and about 1% did both.
How much time they spent on TikTok, Instagram and other social media platforms tracked well with their odds for smoking or vaping , the study found.
Just 2% of people who reported no social media use engaged in cigarette smoking, but that rose to 17% among those who said they were on social media seven-plus hours per day.
Similarly, less than 1% of those who stayed away from social media vaped, compared to 2.5% of those who were using it seven or more hours daily.
Folks glued to social media for seven or more hours a day were also seven times more prone to both smoke and vape, compared to those who spent no time on social media.
The study was led by Dr Anthony Laverty , of Imperial College London School of Public Health, and published Thursday in the BMJ .
Even a little social media time appeared to raise the odds of smoking or vaping. For example, folks who spent 1 to 3 hours on social media daily were 92% more prone to vaping, versus those who eschewed such platforms, the study found.
Laverty's team stressed, however, that the study wasn't designed to prove cause-and-effect. However, they noted that vaping and smoking are both promoted heavily on these platforms.
"First, and most straightforwardly, there is evidence that the corporations behind cigarette smoking and vaping make use of social media to advertise and promote their products," the researchers said in a journal news release.
"This includes direct advertising which is algorithmically targeted and the use of paid social media influencers who present smoking and vaping as a fashionable and desirable activity," they added. "Greater time spent on social media is likely to increase exposure to these forms of influence."
There's also the "reward-seeking" theory: It's well known that social media can be addictive, in that users constantly chase "likes" and other incentives to stay online. Vaping and smoking, as well, rely on reward-seeking to keep users hooked, the team said.
Then there's the 'forbidden fruit' angle: "As a space that is largely unsupervised by parents/caregivers, social media use may encourage behaviours that are transgressive, including cigarette smoking and vaping," Laverty's group said.
They believe it's time to get tough on social media when it comes to vaping and smoking influencers and the like.
"The companies that own social media platforms have substantial power to modify exposure to material that promotes smoking and vaping if they choose to or are compelled to," the London team wrote. "Voluntary codes seem unlikely to achieve this, and the introduction and enforcement on bans on material that promote this should be considered."
More information
Want to quit vaping? The American Heart Association offers tips that could help.
Copyright © 2024 HealthDay. All rights reserved.
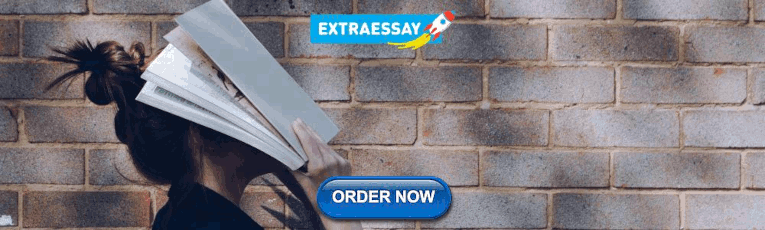
IMAGES
VIDEO
COMMENTS
Impact on mental health. Mental health is defined as a state of well-being in which people understand their abilities, solve everyday life problems, work well, and make a significant contribution to the lives of their communities [].There is debated presently going on regarding the benefits and negative impacts of social media on mental health [9,10].
The relationship between mental health and social media has received significant research and policy attention. However, there is little population-representative data about who social media users ...
Abstract. Research into the impact of social media use (SMU) on well-being (e.g., happiness) and ill-being (e.g., depression) has exploded over the past few years. From 2019 to August 2021, 27 reviews have been published: nine meta-analyses, nine systematic reviews, and nine narrative reviews, which together included hundreds of empirical studies.
Social media has become a prominent fixture in the lives of many individuals facing the challenges of mental illness. Social media refers broadly to web and mobile platforms that allow individuals to connect with others within a virtual network (such as Facebook, Twitter, Instagram, Snapchat, or LinkedIn), where they can share, co-create, or exchange various forms of digital content, including ...
Research linking social media use and adolescent mental health has produced mixed and inconsistent findings and little translational evidence, despite pressure to deliver concrete recommendations ...
Introduction. The past years have witnessed a staggering increase in empirical studies into the effects of social media use (SMU) on adolescents' mental health (e.g. [1∗∗, 2∗, 3]), defined as the absence of mental illness and the presence of well-being [4].This rapid increase may be due to at least two reasons.
This research highlights that social media groups can act as platforms for knowledge exchange and emotional support. ... Findings indicated an overall positive impact of Social Media on the social ...
A 2018 Common Sense Media report found that 81 percent of teens use social media, and more than a third report using social media sites multiple times an hour. These statistics have risen dramatically over the past six years, likely driven by increased access to mobile devices. Rising along with these stats is a growing interest in the impact ...
Those ages 18 to 29 are especially likely to see social media as effective for raising public awareness. For example, in France, 70% of those ages 18 to 29 see social media as an effective way of raising public awareness. Only 48% of those 50 and older share this view, a difference of 22 percentage points.
Follow these links for more in-depth analysis of the impact of social media on American life. Americans' Social Media Use Jan. 31, 2024; Americans' Use of Mobile Technology and Home Broadband Jan. 31 2024; Q&A: How and why we're changing the way we study tech adoption Jan. 31, 2024
This body of social media research also highlights the importance of health care providers taking an active approach in disseminating high-quality content to their patients and the general public. ... The impact of social media information on health disparities is also an important area for future study. Studies are needed to determine whether ...
Social media research is a relatively new field of study that has emerged in conjunction with the development of social media technologies and the upsurge in ... The impact of social media on medical professionalism: A systematic qualitative review of challenges and opportunities. Journal of Medical Internet Research, 15, e184. doi:10.2196/jmir ...
The potential impact of social media on psychosocial development is complex and is an emerging field of research. A systematic review was conducted to investigate existing research relating to social media's effects on psychosocial development. Good practice in systematic review reporting was followed, aligned to the Preferred Reporting Items ...
In a pattern consistent with past Center studies on social media use, there are some stark age differences. Some 84% of adults ages 18 to 29 say they ever use any social media sites, which is similar to the share of those ages 30 to 49 who say this (81%). By comparison, a somewhat smaller share of those ages 50 to 64 (73%) say they use social ...
Social media brings benefits and risks to teens. Psychology can help identify a path forward. New psychological research exposes the harms and positive outcomes of social media. APA's recommendations aim to add science-backed balance to the discussion. By Kirsten Weir Date created: September 1, 2023 15 min read.
The social impact of research has usually been analysed through the scientific outcomes produced under the auspices of the research. The growth of scholarly content in social media and the use of altmetrics by researchers to track their work facilitate the advancement in evaluating the impact of research. However, there is a gap in the identification of evidence of the social impact in terms ...
The Use of Social Media and its Impact for Research. Jessica Rogers. Social media is an omnipresent part of everyday life. It provides users with an easy way to engage and connect with others without meeting face-to-face. This form of communication provides a lot of opportunity for companies and individuals to reach a massive audience.
Social media use may increase feelings of anxiety and depression, specifically in teens and young adults. The addictive nature of social media activates the brain's reward center by releasing dopamine. This is a "feel-good chemical" linked to pleasurable activities. When we post something, our friends and family can "like" it, giving ...
There's a lot that researchers could glean from the social media hiatus, including whether people's political attitudes shifted and in what ways. If views changed dramatically, that would support the argument that Facebook and Instagram, and social media generally, are helping to drive Americans apart.
Pew Research Center's research on the internet, social media and technology in the U.S. and around the world. Many of the topics explored in this report have been studied in depth in the U.S. by Pew Research Center's internet and technology team, which for more than two decades has conducted survey research on the social impact of digital technologies, such as internet and broadband ...
A third research program seeks to better characterize the complex relationships between social media content, behaviors, brain activity, health, and well-being during adolescence. I look forward to the findings from these ongoing projects and other studies that promise to inform guidance for technology and media use among children and adolescents.
Facebook and Instagram may not have quite the impact on people's politics as some critics have feared. A study published Monday said staying off social media apps had nearly zero effect on how ...
Roughly eight-in-ten U.S. adults (83%) report ever using the video-based platform. While a somewhat lower share reports using it, Facebook is also a dominant player in the online landscape. Most Americans (68%) report using the social media platform. Additionally, roughly half of U.S. adults (47%) say they use Instagram.
Through social networks, companies can increase brand awareness, positive. word- of-mouth, sales, and generate greater support. According to Nolcheska (2017) these social media platforms have ...
Girls are more likely than boys to say it would be difficult for them to give up social media (58% vs. 49%). Older teens are also more likely than younger teens to say this: 58% of those ages 15 to 17 say it would be very or somewhat hard to give up social media, compared with 48% of those ages 13 to 14. Teens are more likely to say social ...
Kids and young adults who use social media for seven or more hours per day have double the risk of taking up vaping or smoking or both, new research shows. The study is based on a survey of almost ...