Press release
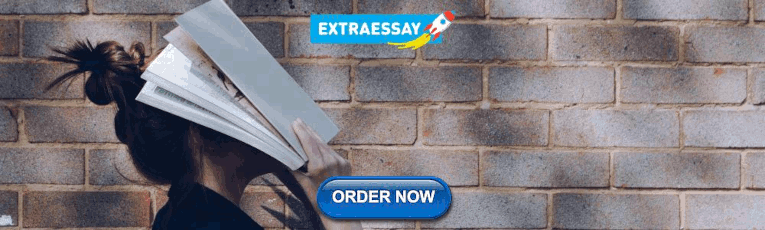
Global Education Monitoring (GEM) Report 2020

Fewer than 10% of countries have laws that help ensure full inclusion in education, according to UNESCO’s 2020 Global Education Monitoring Report: Inclusion and education – All means all.
The report provides an in-depth analysis of key factors for exclusion of learners in education systems worldwide including background, identity and ability (i.e. gender, age, location, poverty, disability, ethnicity, indigeneity, language, religion, migration or displacement status, sexual orientation or gender identity expression, incarceration, beliefs and attitudes). It identifies an exacerbation of exclusion during the COVID-19 pandemic and estimates that about 40% of low and lower-middle-income countries have not supported disadvantaged learners during temporary school shutdown. The 2020 Global Education Monitoring (GEM) Report urges countries to focus on those left behind as schools reopen so as to foster more resilient and equal societies.
Persistence of exclusion: This year’s report is the fourth annual UNESCO GEM Report to monitor progress across 209 countries in achieving the education targets adopted by UN Member States in the 2030 Agenda for Sustainable Development. It notes that 258 million children and youth were entirely excluded from education, with poverty as the main obstacle to access. In low- and middle-income countries, adolescents from the richest 20% of all households were three times as likely to complete lower secondary school as were as those from the poorest homes. Among those who did complete lower secondary education, students from the richest households were twice as likely to have basic reading and mathematics skills as those from the poorest households. Despite the proclaimed target of universal upper secondary completion by 2030, hardly any poor rural young women complete secondary school in at least 20 countries, most of them in sub-Saharan Africa.
Also according to the report, 10-year old students in middle and high-income countries who were taught in a language other than their mother tongue typically scored 34% below native speakers in reading tests. In ten low- and middle-income countries, children with disabilities were found to be 19% less likely to achieve minimum proficiency in reading than those without disabilities. In the United States, for example, LGBTI students were almost three times more likely to say that they had stayed home from school because of feeling unsafe.
Inequitable foundations: Alongside today’s publication, UNESCO GEM Report team launched a new website, PEER, with information on laws and policies concerning inclusion in education for every country in the world. PEER shows that many countries still practice education segregation, which reinforces stereotyping, discrimination and alienation. Laws in a quarter of all countries require children with disabilities to be educated in separate settings, rising to over 40% in Latin America and the Caribbean, as well as in Asia.
Blatant exclusion: Two countries in Africa still ban pregnant girls from school, 117 allowed child marriages, while 20 had yet to ratify the Convention 138 of the International Labour Organization which bans child labour. In several central and eastern European countries, Roma children were segregated in mainstream schools. In Asia, displaced people, such as the Rohingya were taught in parallel education systems. In OECD countries, more than two-thirds of students from immigrant backgrounds attended schools where they made up at least 50% of the student population, which reduced their chance of academic success.
Parents’ discriminatory beliefs were found to form one barrier to inclusion: Some 15% of parents in Germany and 59% in Hong Kong, China, feared that children with disabilities disturbed others’ learning. Parents with vulnerable children also wished to send them to schools that ensure their well-being and respond to their needs. In Queensland, Australia, 37% of students in special schools had moved away from mainstream establishments.
The Report shows that education systems often fail to take learners’ special needs into account. Just 41 countries worldwide officially recognized sign language and, globally, schools were more eager to get internet access than to cater for learners with disabilities. Some 335 million girls attended schools that did not provide them with the water, sanitation and hygiene services they required to continue attending class during menstruation.
Alienating learners: When learners are inadequately represented in curricula and textbooks they can feel alienated. Girls and women only made up 44% of references in secondary school English-language textbooks in Malaysia and Indonesia, 37% in Bangladesh and 24% in the province of Punjab in Pakistan. The curricula of 23 out of 49 European countries do not address issues of sexual orientation, gender identity, or expression.
Teachers need and want training on inclusion, which fewer than 1 in 10 primary school teachers in ten Francophone countries in sub-Saharan Africa said they had received. A quarter of teachers across 48 countries reported they wanted more training on teaching students with special needs.
Chronic lack of quality data on those left behind: Almost half of low- and middle-income countries do not collect enough education data about children with disabilities. Household surveys are key for breaking education data down by individual characteristics. But 41% of countries – home to 13% of the world’s population – did not conduct surveys or make available data from such surveys. Figures on learning are mostly taken from school, failing to take into account those not attending.
Signs of progress towards inclusion: The Report and its PEER website note that many countries were using positive, innovative approaches to transition towards inclusion. Many were setting up resource centres for multiple schools and enabling mainstream establishments to accommodate children from special schools, as was the case in Malawi, Cuba and Ukraine. The Gambia, New Zealand and Samoa were using itinerant teachers to reach underserved populations.
Many countries were also seen to go out of their way to accommodate different learners’ needs: Odisha state in India, for example, used 21 tribal languages in its classrooms, Kenya adjusted its curriculum to the nomadic calendar and, in Australia, the curricula of 19% of students were adjusted by teachers so that their expected outcomes could match students’ needs.
The report includes material for a digital campaign, All means All, which promotes a set of key recommendations for the next ten years.
Related items
- Country page: Pakistan
- UNESCO Office in Islamabad
- SDG: SDG 4 - Ensure inclusive and equitable quality education and promote lifelong learning opportunities for all
This article is related to the United Nation’s Sustainable Development Goals .

Other recent press releases


- High contrast
- Press Centre
Search UNICEF
Education sector analysis, methodological guidelines - volume3.

This present volume is the third in a series of education sector analysis (ESA) guidelines following two volumes published in 2014. The series provides methodologies and applied examples for diagnosing education systems and informing national education policies and plans. This volume proposes guidelines to strengthen national capacities in analyzing education systems in four areas: inclusive education system for children with disabilities, risk analysis for resilient education systems, functioning and effectiveness of the educational administration, and stakeholder mapping and problem-driven analysis (governance and political economy).
The present volume was prepared by experts from various backgrounds (including education, economics, sociology, political science and other social sciences) from UNESCO‘s International Institute for Educational Planning, UNICEF, the United Kingdom’s Foreign, Commonwealth & Development Office and the Global Partnership for Education.

Files available for download
Related topics, more to explore.
Methodological guidelines - Volume1
Methodological guidelines - Volume2
UNICEF Goodwill Ambassador Millie Bobby Brown calls for ‘a world where periods don’t hold us back’ in new video for Menstrual Hygiene Day
10 Fast Facts: Menstrual health in schools
This site belongs to UNESCO's International Institute for Educational Planning
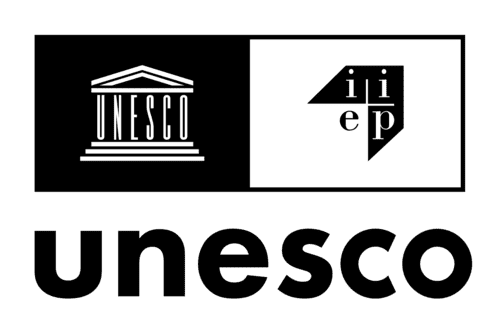
IIEP Learning Portal

Search form
- issue briefs
- Plan for learning
Education sector analysis
An education sector analysis (ESA) is an in-depth, holistic diagnosis of an education system. It assists with understanding how an education system (and its subsectors) works, why it works that way, and how to improve it. An ESA provides the evidence base for decision-making and is the first step in preparing an education sector plan.
An ESA is a nationally driven process, involving collaboration and dialogue among different actors and institutions in a system. Empowering and consulting the different stakeholders throughout the process are essential, as ‘sustainable changes that lead to improved learning outcomes cannot be brought about in the absence of involvement of the individuals and groups who will implement the change’ (Faul and Martinez, 2019: 31).
The ESA process must therefore be participative and aim to create an understanding of the key stakeholders in the education system, their incentives, relationships and accountability, as well as how these dynamics shape education systems (IIEP-UNESCO et al., 2021).
What does an ESA cover?
An ESA includes context analysis, existing policy analysis, cost and finance analysis, education performance analysis, and system capacity analysis, including stakeholder analysis (IIEP-UNESCO and GPE, 2015). Any challenges identified through the ESA should be analysed through the lens of Sustainable Development Goal 4 (UNESCO, 2016). Quality of learning is one factor analysed in the performance of the education system along with issues related to access and coverage, equity and inclusion, and internal and external efficiency of the system. Quality of learning involves analysing the range of inputs and processes including teachers, learning and teaching materials, school facilities, and learning outcomes (IIEP-UNESCO and GPE, 2015; IIEP-UNESCO, World Bank, and UNICEF, 2014).
Teachers play a decisive role in ensuring learning quality. Teacher management features – ranging from recruitment and deployment to pre- and in-service training, career pathways, motivation and job satisfaction, absenteeism and effective teaching time – also need to be analysed. Typical indicators include (IIEP-UNESCO, World Bank, and UNICEF, 2014):
- Pupil/teacher ratio by level for primary education
- Pupil/trained teacher ratio
- Teacher utilization rate
- The consistency in teacher allocation (R2 coefficient)
- Theoretical teaching time in relation to theoretical instruction time for secondary teachers
- The percentage of pre- and in-service teachers trained by level
- The number of teachers disaggregated by status (civil servants, contract, or community teachers)
- Qualifications and teaching experience
Learning and teaching materials
An ESA should analyse the equitable allocation of learning and teaching materials and other inputs among different schools and regions. An ESA should include indicators such as the proportion of teachers with teacher guides, pupil/textbook ratios, and the notion of useful pupil/textbook ratio (IIEP-UNESCO, World Bank, and UNICEF, 2014). Qualitative information gathered through teacher interviews, for example, can also be integrated into the analysis to complement quantitative data. For instance, in crisis-affected areas, quantitative data may be weak regarding the actual distribution and use of textbooks throughout the country (IIEP UNESCO and GPE, 2016).
School facilities
School facilities (school buildings and infrastructure such as electricity or school landscaping) can have a significant impact on students’ learning achievements. Proper water, sanitation and hygiene (WASH) facilities in schools can improve access to education and learning outcomes, particularly for girls (UNICEF and WHO, 2018). Relevant indicators include classroom utilization rate and, when applicable, type of classroom (such as temporary, open air, permanent, or home-based classrooms); the percentage of schools with functioning WASH facilities; the percentage of schools with electricity; the percentage of schools with boundary walls for security reasons; and the percentage of classrooms that need to be rehabilitated (IIEP-UNESCO, World Bank, and UNICEF, 2014).
Learning outcomes
Student assessments include national examinations and admission tests, national large-scale learning assessments, regional or international standardized assessments, citizen-led assessments, and household surveys. The analysis of learning assessments enables education planners and decision makers to understand whether the education system is transferring knowledge to students as expected, as well as whether this transfer is equitable or is leaving certain population groups or geographic areas behind. Learning assessments can further help countries track the progress of learning achievements over time, compare results with comparable countries, and identify plausible causes for weak learning outcomes (IIEP UNESCO, World Bank, and UNICEF, 2014).
However, there are several risks when using learning data, such as the accuracy of data and their interpretation; the use of a single test score for decision-making; the use of learning assessment data to legitimize predefined agendas; and narrowing educational measurements to simplified indicators (Raudonyte, 2019).
Changes in learning assessment results over time should be interpreted with caution and cross-checked with other evidence. For instance, a sharp increase in enrolments may affect learning outcomes (IIEP-UNESCO, World Bank, and UNICEF, 2014).
ESA data sources
An effective ESA relies on both qualitative and quantitative rigorous data. Relevant data sources include (IIEP-UNESCO and GPE, 2015; IIEP-UNESCO et al., 2021; IIEP-UNESCO, World Bank, and UNICEF, 2014):
- National, regional and international learning assessments: provide information on whether the education system is transferring knowledge as expected; track progress on learning achievements over time; allow comparisons with comparable countries; and identify plausible reasons behind weak learning outcomes.
- School data on students, textbooks, teachers, and subsidies: provide information on resource distribution and learning time, among others.
- Administrative manuals: provide information on teacher management, teaching time, and other resources.
- Teacher training institute data: provide information on whether the capacities of teacher training institutes meet current and projected needs.
- Human resources data: provide information about teacher recruitment, deployment and utilization, among others.
- Sample surveys: can be used to assess teaching and learning time.
- Household surveys: provide information on the relationship between the level of literacy and the number of years of schooling.
- Specific research exercises: provide valuable information on relevant issues faced by education systems.
- Interviews and questionnaires of stakeholders: provide relevant qualitative information, for instance related to institutional capacity.
An ESA should further assess information gaps and whether primary data collection will need to be undertaken to obtain missing information (IIEP-UNESCO and GPE, 2015).
Plans and policies
- Liberia: Education Sector Analysis
- Somalia: Education Sector Analysis
- IIEP-UNESCO; Global Partnership for Education. 2015. Guidelines for Education Sector Plan Preparation
- IIEP-UNESCO; Global Partnership for Education; UNICEF; Foreign, Commonwealth and Development Office. 2021. Education Sector Analysis Methodological Guidelines: Vol. 3: Thematic Analyses
- IIEP-UNESCO; World Bank; UNICEF. 2014. Education Sector Analysis Methodological Guidelines: Vol 1: Sector-wide Analysis, With Emphasis on Primary and Secondary Education
- IIEP-UNESCO; World Bank; UNICEF. 2014. Education Sector Analysis Methodological Guidelines: Vol. 2: Sub-sector Specific Analysis
- UNESCO-UIS. 2009. Education Indicators: Technical Guidelines
Faul, M.; Martinez, R. 2019. Education System Diagnostics. What is an 'Education System Diagnostic', Why Might it be Useful, and What Currently Exists?
IIEP-UNESCO; GPE (Global Partnership for Education). 2015. Guidelines for Education Sector Plan Preparation. Paris: IIEP-UNESCO.
––––. 2016. Guidelines for Transitional Education Plan Preparation. Washington, DC: GPE.
IIEP-UNESCO; GPE (Global Partnership for Education); UNICEF; FCDO (Foreign, Commonwealth and Development Office). 2021. Education Sector Analysis Methodological Guidelines: Vol. 3: Thematic Analyses . Dakar: IIEP-UNESCO.
IIEP-UNESCO; World Bank; UNICEF. 2014. Education Sector Analysis Methodological Guidelines: Vol 1: Sector-wide Analysis, with Emphasis on Primary and Secondary Education. Dakar: IIEP-UNESCO.
Raudonyte, I. 2019. Use of Learning Assessment Data in Education Policy-making. Paris: IIEP UNESCO.
UNESCO. 2016. Mainstreaming SDG4-Education 2030 in Sector-wide Policy and Planning: Technical Guidelines for UNESCO Field Offices. Paris: UNESCO.
UNICEF; WHO (World Health Organization). 2018. Drinking Water, Sanitation and Hygiene in Schools: Global Baseline Report 2018. New York, NY: UNICEF and WHO.
Related information
- Supporting education sector analyses [IIEP-UNESCO Dakar]
How higher-education institutions can transform themselves using advanced analytics
Leaders in most higher-education institutions generally understand that using advanced analytics can significantly transform the way they work by enabling new ways to engage current and prospective students, increase student enrollment, improve student retention and completion rates , and even boost faculty productivity and research. However, many leaders of colleges and universities remain unsure of how to incorporate analytics into their operations and achieve intended outcomes and improvements. What really works? Is it a commitment to new talent, technologies, or operating models? Or all of the above?
To answer these questions, we interviewed more than a dozen senior leaders at colleges and universities known for their transformations through analytics. We also conducted in-depth, on-campus visits at the University of Maryland University College (UMUC), a public institution serving primarily working adults through distance learning, and Northeastern University, a private nonprofit institution in Boston, to understand how their transformations went. 1 Our research base included presidents, vice presidents of enrollment management, chief data officers, provosts, and chief financial officers. In September 2017, we conducted on-campus visits to meet with leaders at several levels at both the University of Maryland University College (UMUC) and Northeastern University. We thank these leaders for generously agreeing to have their observations and experiences included in this article. We combined insights from these interviews and site visits with those gleaned from our work with more than 100 higher-education engagements across North America over the past five years, and we tapped McKinsey’s wide-ranging expertise in analytics-enabled transformations in both the public and private sectors.
Our conversations and engagements revealed several potential pitfalls that organizations may face when building their analytics capabilities—as well as several practical steps education leaders can take to avoid these traps.
Understanding the challenges
Advanced analytics use cases.
Northeastern used advanced analytics to help grow its U.S. News & World Report ranking among national universities from 115 in 2006 to 40 in 2017.
UMUC used advanced analytics to achieve a 20 percent increase in new student enrollment while spending 20 percent less on marketing.
Transformation through advanced analytics can be difficult for any organization; in higher education, the challenges are compounded by sector-specific factors related to governance and talent. Leaders in higher education cannot simply pay lip service to the power of analytics; they must first address some or all of the most common obstacles.
Being overly focused on external compliance . Many higher-education institutions’ data analytics teams focus most of their efforts on generating reports to satisfy operational, regulatory, or statutory compliance. The primary goal of these teams is to churn out university statistics that accrediting bodies and other third parties can use to assess each institution’s performance. Any requests outside the bounds of these activities are considered emergencies rather than standard, necessary assignments. Analytics teams in this scenario have very limited time to support strategic, data-driven decision making.
Isolating the analytics program in an existing department . In our experience, analytics teams in higher-education institutions usually report to the head of an existing function or department—typically the institutional research team or the enrollment-management group. As a result, the analytics function becomes associated with the agenda of that department rather than a central resource for all, with little to no contact with executive leadership. Under this common scenario, the impact of analytics remains limited, and analytics insights are not embedded into day-to-day decision making of the institution as a whole.
Failing to establish a culture of data sharing and hygiene . In many higher-education institutions, there is little incentive (and much reluctance) to share data. As a result, most higher-education institutions lack good data hygiene —that is, established rules for who can access various forms of data, as well as formal policies for how they can share those data across departments. For example, analytics groups in various university functions may use their own data sets to determine retention rates for different student segments—and when they get together, they often disagree on which set of numbers is right.
Compounding this challenge, many higher-education institutions struggle to link the myriad legacy data systems teams use in different functions or working groups. Even with the help of a software platform vendor, the lead time to install, train, and win buy-in for these technical changes can take time, perhaps two to three years, before institutions see tangible outcomes from their analytics programs. In the meantime, institutions struggle to instill a culture and processes built around the possibilities of data-driven decision making.
Lacking the appropriate talent. Budgets and other constraints can make it difficult for higher-education institutions to meet market rates for analytics talent. Colleges and universities could potentially benefit from sourcing analytics talent among their graduate students and faculty, but it can be a struggle to attract and retain them. Furthermore, to successfully pursue transformation through analytics, higher-education institutions need leaders who are fluent in not only management but also data analytics and can solve problems in both areas.
Would you like to learn more about the Social Sector Practice ?
Deploying best practices.
These challenges can seem overwhelming, but transformation through analytics is possible when senior leaders in higher-education institutions endeavour to change both operations and mind-sets.
Leaders point to five action steps to foster success:
Articulate an analytics mandate that goes beyond compliance . Senior leaders in higher education must signal that analytics is a strategic priority. Indeed, to realize the potential of analytics, the function cannot be considered solely as a cost center for compliance. Instead, this team must be seen as a source of innovation and an economic engine for the institution. As such, leaders must articulate the team’s broader mandate. According to the leaders we interviewed, the transformation narrative must focus on how analytics can help the institution facilitate the student journey from applicant to alumnus while providing unparalleled learning, research, and teaching opportunities, as well as foster a strong, financially sustainable institution.
Establish a central analytics team with direct reporting lines to executive leaders . To mitigate the downsides of analytics teams couched in existing departments or decentralized across several functions, higher-education leaders must explicitly allocate the requisite financial and human resources to establish a central department or function to oversee and manage the use of analytics across the institution. This team can be charged with managing a central, integrated platform for collecting, analyzing, and modeling data sets and producing insights quickly.
For example, UMUC has a designated “data czar” to help define standards for how information is captured, managed, shared, and stored online. When conflicts arise, the data czar weighs in and helps de-escalate problems. Having a central point of contact has improved the consistency and quality of the university’s data: there is now a central source of truth, and all analysts have access to the data. Most important, the university now has a data evangelist who can help cultivate an insights-driven culture at the institution.
In another example, leaders at Northeastern created an analytics center of excellence structured as a “virtual” entity. The center is its own entity and is governed by a series of rotating chairs to ensure the analytics team is aware of and paying equal attention to priorities from across the university.
In addition to enjoying autonomous status outside a subfunction or single department, the analytics team should report to the most-senior leaders in the institution—in some cases, the provost. When given a more substantial opportunity to influence decisions, analytics leaders gain a greater understanding of the issues facing the university and how they affect the institution’s overall strategy. Leaders can more easily identify the data sets that might provide relevant insights to university officials—not just in one area, but across the entire organization—and they can get a jump-start on identifying possible solutions.
Analysts at Northeastern, for instance, were able to quantify the impact of service-learning programs on student retention, graduation, and other factors, thereby providing support for key decisions about these programs.
Win analytics buy-in from the front line and create a culture of data-driven decision making . To overcome the cultural resistance to data sharing, the analytics team must take the lead on engendering meaningful communications about analytics across the institution. To this end, it helps to have members of the centralized analytics function interact formally and frequently with different departments across the university. A hub-and-spoke model can be particularly effective: analysts sit alongside staffers in the operating units to facilitate sharing and directly aid their decision making. These analysts can serve as translators, helping working groups understand how to apply analytics to tackle specific problems, while also taking advantage of data sets provided by other departments. The university leaders we spoke with noted that their analysts may rotate into different functional areas to learn more about the university’s departments and to ensure that the department leaders have a link back to the analytics function.

How to improve student educational outcomes: New insights from data analytics
Of course, having standardized, unified systems for processing all university data can help enable robust analysis. However, universities seeking to create a culture of data-driven decision making need not wait two years until a new data platform is up and running. Instead, analysts can define use cases—that is, places where data already exist and where analysis can be conducted relatively quickly to yield meaningful insights. Teams can then share success stories and evangelize the impact of shared data analytics, thereby prompting others to take up their own analytics-driven initiatives.
The analysts from UMUC’s decision-support unit sometimes push relevant data and analyses to the relevant departments to kick-start reflection and action, rather than waiting for the departments to request the information. However, the central unit avoids producing canned reports; analysts tend to be successful only when they engage departments in an honest and objective exploration of the data without preexisting biases.
Strengthen in-house analytical capabilities . The skills gap is an obvious impediment to colleges’ and universities’ attempts to transform operations through advanced analytics—thus, it is perfectly acceptable to contract out work in the short term. However, while supplementing a skills gap with external expertise may help accelerate transformations, it can never fully replace the need for in-house capacity; the effort to push change across the institution must be owned and led internally.
To do so, institutions will need to change their approaches to talent acquisition and development . They may need to look outside usual sources to find professionals who understand core analytics technologies (cloud computing, data science, machine learning, and statistics, for instance) as well as design thinking and operations. Institutions may also need to appeal to new hires with competitive financial compensation and by emphasizing the opportunity to work autonomously on intellectually challenging projects that will make an impact on generations of students and contribute to an overarching mission.
Do not let great be the enemy of good . It takes time to launch a successful analytics program. At the outset, institutions may lack certain types of data, and not every assessment will yield insightful results—but that is no reason to pull back on experimentation. Colleges and universities can instead deploy a test-and-learn approach: identify areas with clear problems and good data, conduct analyses, launch necessary changes, collect feedback, and iterate as needed. These cases can help demonstrate the impact of analytics to other parts of the organization and generate greater interest and buy-in.

Subscribe to the Shortlist
McKinsey’s new weekly newsletter, featuring must-read content on a range of topics, every Friday
Realizing impact from analytics
It is easy to forget that analytics is a beginning, not an end . Analytics is a critical enabler to help colleges and universities solve tough problems—but leaders in higher-education institutions must devote just as much energy to acting on the insights from the data as they do on enabling analysis of the data. Implementation requires significant changes in culture, policy, and processes. When outcomes improve because a university successfully implemented change—even in a limited environment—the rest of the institution takes notice. This can strengthen the institutional will to push further and start tackling other areas of the organization that need improvement.
Some higher-education institutions have already overcome these implementation challenges and are realizing significant impact from their use of analytics. Northeastern University, for example, is using a predictive model to determine which applicants are most likely to be the best fit for the school if admitted. Its analytics team relies on a range of data to make forecasts, including students’ high school backgrounds, previous postsecondary enrollments, campus visit activity, and email response rates. According to the analytics team, an examination of the open rate for emails was particularly insightful as it was more predictive of whether students actually enrolled at Northeastern than what the students said or whether they visited campus.
Meanwhile, the university also looked at National Student Clearinghouse data, which tracks where applicants land at the end of the enrollment process, and learned that the institutions it had considered core competitors were not. Instead, competition was coming from sources it had not even considered. It also learned that half of its enrollees were coming from schools that the institution’s admissions office did not visit. The team’s overall analysis prompted Northeastern to introduce a number of changes to appeal to those individuals most likely to enroll once admitted, including offering combined majors. The leadership team also shifted some spending from little-used programs to bolster programs and features that were more likely to attract targeted students. Due in part to these changes, Northeastern improved its U.S. News & World Report ranking among national universities from 115 in 2006 to 40 in 2017.
In another example, in 2013 UMUC was trying to pinpoint the source of a decline in enrollment. It was investing significant dollars in advertising and was generating a healthy number of leads—however, conversion rates were low. Data analysts at the institution assessed the university’s returns on investment for various marketing efforts and discovered a bottleneck—UMUC’s call centers were overused and underresourced. The university invested in new call-center capabilities and within a year realized a 20 percent increase in new student enrollment while spending 20 percent less on advertising.
The benefits we discussed barely scratch the surface; the next wave of advanced analytics will, among other things, enable bespoke, personalized student experiences, with teaching catered to students’ individual learning styles and competency levels. To realize the great promise of analytics in the years to come, senior leaders must focus on more than just making incremental improvements in business processes or transactions. Our conversations with leaders in higher education point to the need for colleges and universities to establish a strong analytics function as well as a culture of data-driven decision making and a focus on delivering measurable outcomes. In doing so, institutions can create significant value for students—and sustainable operations for themselves.
Marc Krawitz is an associate partner in McKinsey’s New Jersey office. Jonathan Law is a partner in the New York office and leads the Higher-Education Practice. Sacha Litman is an associate partner in the Washington, DC, office and leads public and social sector analytics.
The authors would like to thank business and technology leaders at the University of Maryland University College and Northeastern University for their contributions to this article.
Explore a career with us
Related articles.

Shaking up the leadership model in higher education

Three more reasons why US education is ready for investment

Boosting productivity in US higher education
- Review Article
- Open access
- Published: 22 June 2020
Teaching analytics, value and tools for teacher data literacy: a systematic and tripartite approach
- Ifeanyi Glory Ndukwe 1 &
- Ben Kei Daniel 1
International Journal of Educational Technology in Higher Education volume 17 , Article number: 22 ( 2020 ) Cite this article
27k Accesses
41 Citations
14 Altmetric
Metrics details
Teaching Analytics (TA) is a new theoretical approach, which combines teaching expertise, visual analytics and design-based research to support teacher’s diagnostic pedagogical ability to use data and evidence to improve the quality of teaching. TA is now gaining prominence because it offers enormous opportunities to the teachers. It also identifies optimal ways in which teaching performance can be enhanced. Further, TA provides a platform for teachers to use data to reflect on teaching outcome. The outcome of TA can be used to engage teachers in a meaningful dialogue to improve the quality of teaching. Arguably, teachers need to develop their teacher data literacy and data inquiry skills to learn about teaching challenges. These skills are dependent on understanding the connection between TA, LA and Learning Design (LD). Additionally, they need to understand how choices in particular pedagogues and the LD can enhance their teaching experience. In other words, teachers need to equip themselves with the knowledge necessary to understand the complexity of teaching and the learning environment. Providing teachers access to analytics associated with their teaching practice and learning outcome can improve the quality of teaching practice. This research aims to explore current TA related discussions in the literature, to provide a generic conception of the meaning and value of TA. The review was intended to inform the establishment of a framework describing the various aspects of TA and to develop a model that can enable us to gain more insights into how TA can help teachers improve teaching practices and learning outcome. The Tripartite model was adopted to carry out a comprehensive, systematic and critical analysis of the literature of TA. To understand the current state-of-the-art relating to TA, and the implications to the future, we reviewed published articles from the year 2012 to 2019. The results of this review have led to the development of a conceptual framework for TA and established the boundaries between TA and LA. From the analysis the literature, we proposed a Teaching Outcome Model (TOM) as a theoretical lens to guide teachers and researchers to engage with data relating to teaching activities, to improve the quality of teaching.
Introduction
Educational institutions today are operating in an information era, where machines automatically generate data rather than manually; hence, the emergence of big data in education ( Daniel 2015 ). The phenomenon of analytics seeks to acquire insightful information from data that ordinarily would not be visible by the ordinary eyes, except with the application of state-of-the-art models and methods to reveal hidden patterns and relationships in data. Analytics plays a vital role in reforming the educational sector to catch up with the fast pace at which data is generated, and the extent to which such data can be used to transform our institutions effectively. For example, with the extensive use of online and blended learning platforms, the application of analytics will enable educators at all levels to gain new insights into how people learn and how teachers can teach better. However, the current discourses on the use of analytics in Higher Education (HE) are focused on the enormous opportunities analytics offer to various stakeholders; including learners, teachers, researchers and administrators.
In the last decade, extensive literature has proposed two weaves of analytics to support learning and improve educational outcomes, operations and processes. The first form of Business Intelligence introduced in the educational industry is Academic Analytics (AA). AA describes data collected on the performance of academic programmes to inform policy. Then, Learning Analytics (LA), emerged as the second weave of analytics, and it is one of the fastest-growing areas of research within the broader use of analytics in the context of education. LA is defined as the "measurement, collection, analysis and reporting of data about the learner and their learning contexts for understanding and optimising learning and the environments in which it occurs" ( Elias 2011 ). LA was introduced to attend to teaching performance and learning outcome ( Anderson 2003 ; Macfadyen and Dawson 2012 ). Typical research areas in LA, include student retention, predicting students at-risk, personalised learning which in turn are highly student-driven ( Beer et al. 2009 ; Leitner et al. 2017 ; Pascual-Miguel et al. 2011 ; Ramos and Yudko 2008 ). For instance, Griffiths ( Griffiths 2017 ), employed LA to monitor students’ engagements and behavioural patterns on a computer-supported collaborative learning environment to predict at-risk students. Similarly, Rienties et al. ( Rienties et al. 2016 ) looked at LA approaches in their capacity to enhance the learner’s retention, engagement and satisfaction. However, in the last decade, LA research has focused mostly on the learner and data collections, based on digital data traces from Learning Management Systems (LMS) ( Ferguson 2012 ), not the physical classroom.
Teaching Analytics (TA) is a new theoretical approach that combines teaching expertise, visual analytics and design-based research, to support the teacher with diagnostic and analytic pedagogical ability to improve the quality of teaching. Though it is a new phenomenon, TA is now gaining prominence because it offers enormous opportunities to the teachers.
Research on TA pays special attention to teacher professional practice, offering data literacy and visual analytics tools and methods ( Sergis et al. 2017 ). Hence, TA is the collection and use of data related to teaching and learning activities and environments to inform teaching practice and to attain specific learning outcomes. Some authors have combined the LA, and TA approaches into Teaching and Learning Analytics (TLA) ( Sergis and Sampson 2017 ; Sergis and Sampson 2016 ). All these demonstrate the rising interest in collecting evidence from educational settings for awareness, reflection, or decision making, among other purposes. However, the most frequent data that have been collected and analysed about TA focus on the students (e.g., different discussion and learning activities and some sensor data such as eye-tracking, position or physical actions) ( Sergis and Sampson 2017 ), rather than monitoring teacher activities. Providing teachers access to analytics of their teaching, and how they can effectively use such analytics to improve their teaching process is a critical endeavour. Also, other human-mediated data gathering in the form of student feedback, self and peer observations or teacher diaries can be employed to enrich TA further. For instance, visual representations such as dashboards can be used to present teaching data to help teachers reflect and make appropriate decisions to inform the quality of teaching. In other words, TA can be regarded as a reconceptualisation of LA for teachers to improve teaching performance and learning outcome. The concept of TA is central to the growing data-rich technology-enhanced learning and teaching environment ( Flavin 2017 ; Saye and Brush 2007 ). Further, it provides teachers with the opportunity to engage in data-informed pedagogical improvement.
While LA is undeniably an essential area of research in educational technology and the learning sciences, automatically extracted data from an educational platform mainly provide an overview of student activities, and participation. Nevertheless, it hardly indicates the role of the teacher in these activities, or may not otherwise be relevant to teachers’ individual needs (for Teaching Professional Development (TPD) or improvement of their classroom practice). Many teachers generally lack adequate data literacy skills ( Sun et al. 2016 ). Teacher data literacy skill and teacher inquiry skill using data are the foundational concepts underpinning TA ( Kaser and Halbert 2014 ). The development of these two skills is dependent on understanding the connection between TA, LA and Learning Design (LD). In other words, teachers need to equip themselves with knowledge through interaction with sophisticated data structures and analytics. Hence, TA is critical to improving teachers’ low efficacy towards educational data.
Additionally, technology has expanded the horizon of analytics to various forms of educational settings. As such, the educational research landscape needs efficient tools for collecting data and analyzing data, which in turn requires explicit guidance on how to use the findings to inform teaching and learning ( McKenney and Mor 2015 ). Increasing the possibilities for teachers to engage with data to assess what works for the students and courses they teach is instrumental to quality ( Van Harmelen and Workman 2012 ). TA provides optimal ways of performing the analysis of data obtained from teaching activities and the environment in which instruction occurs. Hence, more research is required to explore how teachers can engage with data associated with teaching to encourage teacher reflection, improve the quality of teaching, and provide useful insights into ways teachers could be supported to interact with teaching data effectively. However, it is also essential to be aware that there are critical challenges associated with data collection. Moreover, designing the information flow that facilitates evidence-based decision-making requires addressing issues such as the potential risk of bias; ethical and privacy concerns; inadequate knowledge of how to engage with analytics effectively.
To ensure that instructional design and learning support is evidence-based, it is essential to empower teachers with the necessary knowledge of analytics and data literacy. The lack of such knowledge can lead to poor interpretation of analytics, which in turn can lead to ill-informed decisions that can significantly affect students; creating more inequalities in access to learning opportunities and support regimes. Teacher data literacy refers to a teachers’ ability to effectively engage with data and analytics to make better pedagogical decisions.
The primary outcome of TA is to guide educational researchers to develop better strategies to support the development of teachers’ data literacy skills and knowledge. However, for teachers to embrace data-driven approaches to learning design, there is a need to implement bottom-up approaches that include teachers as main stakeholders of a data literacy project, rather than end-users of data.
The purpose of this research is to explore the current discusses in the literature relating to TA. A vital goal of the review was to extend our understanding of conceptions and value of TA. Secondly, we want to contextualise the notion of TA and develop various concepts around TA to establish a framework that describes multiple aspects of TA. Thirdly, to examine different data collections/sources, machine learning algorithms, visualisations and actions associated with TA. The intended outcome is to develop a model that would provide a guide for the teacher to improve teaching practice and ultimately enhance learning outcomes.
The research employed a systematic and critical analysis of articles published from the year 2012 to 2019. A total of 58 publications were initially identified and compiled from the Scopus database. After analysing the search results, 31 papers were selected for review. This review examined research relating to the utilisation of analytics associated with teaching and teacher activities and provided conceptual clarity on TA. We found that the literature relating to conception, and optimisation of TA is sporadic and scare, as such the notion of TA is theoretically underdeveloped.
Methods and procedures
This research used the Tripartite model ( Daniel and Harland 2017 ), illustrated in Fig. 1 , to guide the systematic literature review. The Tripartite model draws from systematic review approaches such as the Cochrane, widely used in the analyses of rigorous studies, to provide the best evidence. Moreover, the Tripartite model offers a comprehensive view and presentation of the reports. The model composes of three fundamental components; descriptive (providing a summary of the literature), synthesis (logically categorising the research based on related ideas, connections and rationales), and critique (criticising the novel, providing evidence to support, discard or offer new ideas about the literature). Each of these phases is detailed fully in the following sections.
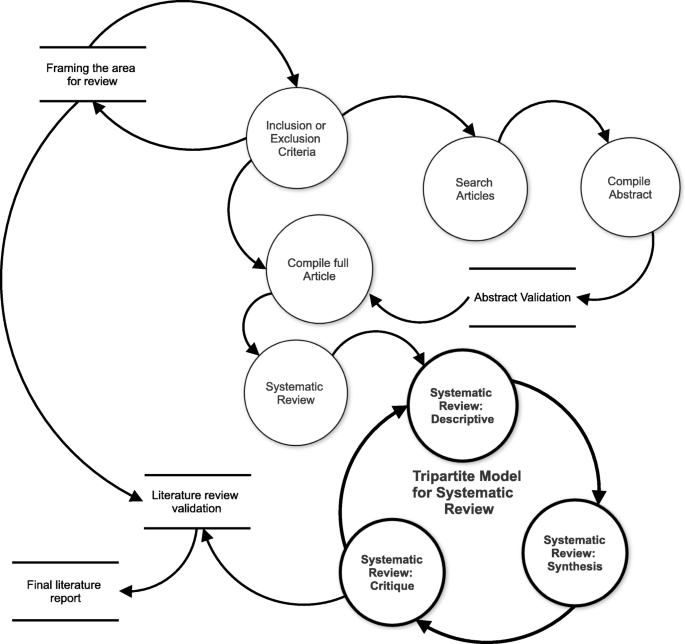
Tripartite Model. The Tripartite Model: A Systematic Literature Review Process ( Daniel and Harland 2017 )
To provide clarity; the review first focused on describing how TA is conceptualised and utilised. Followed by the synthesis of the literature on the various tools used to harvest, analyse and present teaching-related data to the teachers. Then the critique of the research which led to the development of a conceptual framework describing various aspects of TA. Finally, this paper proposes a Teaching Outcome Model (TOM). TOM is intended to offer teachers help on how to engage and reflect on teaching data.
TOM is a TA life cycle which starts with the data collection stage; where the focus is on teaching data. Then the data analysis stage; the application of different Machine Learning (ML) techniques to the data to discover hidden patterns. Subsequently, the data visualisation stage, where data presentation is carried out in the form of a Teaching Analytics Dashboard (TAD) for the teacher. This phase is where the insight generation, critical thinking and teacher reflection are carried out. Finally, the action phase, this is where actions are implemented by teachers to improve teaching practice. Some of these actions include improving the LD, changing teaching method, providing appropriate feedback and assessment or even carrying out more research. This research aims to inform the future work in the advancement of TA research field.
Framing research area for review
As stated in the introduction, understanding current research on TA can be used to provide teachers with strategies that can help them utilise various forms of data to optimise teaching performance and outcome. Framing the review was guided by some questions and proposed answers to address those questions (see Table 1 )
Inclusion and exclusion criteria
The current review started with searching through the Scopus database using the SciVal visualisation and analytical tool. The rationale for choosing the Scopus database is that it contains the largest abstract and citation database of peer-reviewed research literature with diverse titles from publishers worldwide. Hence, it is only conceivable to search for and find a meaningful balance of the published content in the area of TA. Also, the review included peer-reviewed journals and conference proceedings. We excluded other documents and source types, such as book series, books, editorials, trade publications on the understanding that such sources might lack research on TA. Also, this review excluded articles published in other languages other than English.
Search strategy
This review used several keywords and combinations to search on terms related to TA. For instance: ’Teaching Analytics’ AND ’Learning Analytics’ OR ’Teacher Inquiry’ OR ’Data Literacy’ OR ’Learning Design’ OR ’Computer-Supported Collaborative Learning’ OR ’Open Learner Model’ OR ’Visualisation’ OR ’Learning Management System’ OR ’Intelligent Tutoring System’ OR ’Student Evaluation on Teaching’ OR ’Student Ratings’.
This review searched articles published between 2012 to 2019. The initial stage of the literature search yielded 58 papers. After the subsequent screening of previous works and removing duplicates and titles that did not relate to the area of research, 47 articles remained. As such, a total of 36 studies continued for full-text review. Figure 2 , shows the process of finalising the previous studies of this review.
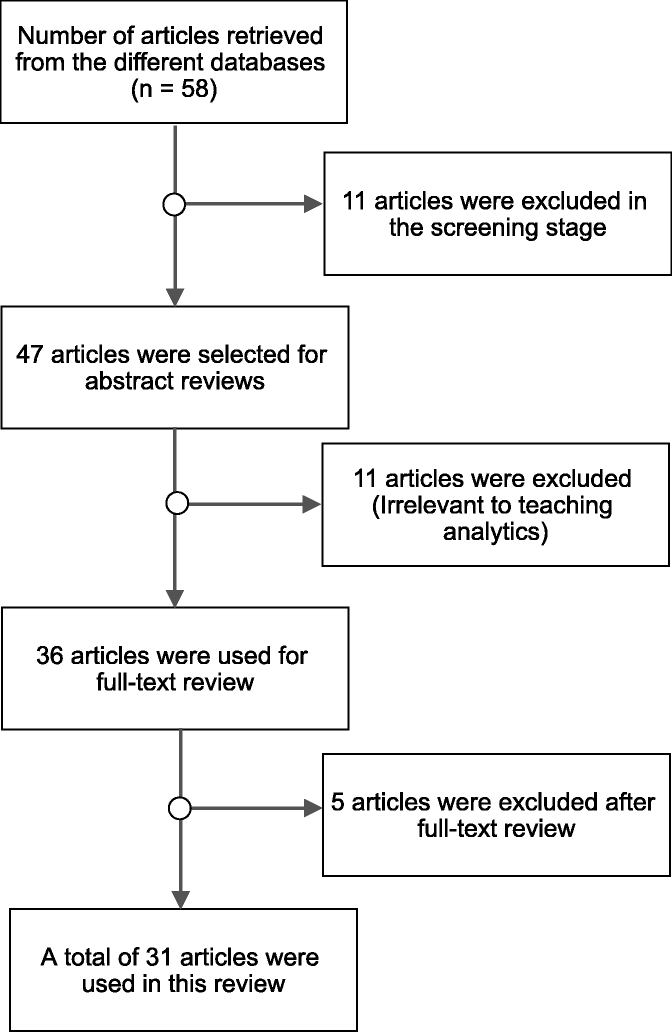
Inclusion Exclusion Criteria Flowchart. The selection of previous studies
Compiling the abstracts and the full articles
The review ensured that the articles identified for review were both empirical and conceptual papers. The relevance of each article was affirmed by requiring that chosen papers contained various vital phrases all through the paper, as well as, title, abstract, keywords and, afterwards, the entire essay. In essence, were reviewed giving particular cognisance and specific consideration to those section(s) that expressly related to the field of TA. In doing as such, to extract essential points of view on definitions, data sources, tools and technologies associated with analytics for the teachers. Also, this review disregarded papers that did not, in any way, relate to analytics in the context of the teachers. Finally, 31 articles sufficed for this review.
Systematic review: descriptive
Several studies have demonstrated that TA is an important area of inquiry ( Flanders 1970 ; Gorham 1988 ; Pennings et al. 2014 ; Schempp et al. 2004 ), that enables researchers to explore analytics associated with teaching process systematically. Such analytics focus on data related to the teachers, students, subjects taught and teaching outcomes. The ultimate goal of TA is to improve professional teaching practice ( Huang 2001 ; Sergis et al. 2017 ). However, there is no consensus on what constitutes TA. Several studies suggest that TA is an approach used to analyse teaching activities ( Barmaki and Hughes 2015 ; Gauthier 2013 ; KU et al. 2018 ; Saar et al. 2017 ), including how teachers deliver lectures to students, tools usage pattern, or dialogue. While various other studies recognise TA as the ability to applying analytical methods to improve teacher awareness of student activities for appropriate intervention ( Ginon et al. 2016 ; Michos and Hernández Leo 2016 ; Pantazos et al. 2013 ; Taniguchi et al. 2017 ; Vatrapu et al. 2013 ). A hand full of others indicate TA as analytics that combines both teachers and students activities ( Chounta et al. 2016 ; Pantazos and Vatrapu 2016 ; Prieto et al. 2016 ; Suehiro et al. 2017 ). Hence, it is particularly problematic and challenging to carry out a systematic study in the area of analytics for the teachers to improve teaching practice, since there is no shared understanding of what constitutes analytics and how best to approach TA.
Researchers have used various tools to automatically harvest important episodes of interactive teacher and student behaviour during teaching, for teacher reflection. For instance, KU et al. ( 2018 ), utilised instruments such as; Interactive Whiteboard (IWB), Document Camera (DC), and Interactive Response System (IRS) to collect classroom instructional data during instruction. Similarly, Vatrapu et al. ( 2013 ) employed eye-tracking tools to capture eye-gaze data on various visual representations. Thomas ( 2018 ) also extracted multimodal features from both the speaker and the students’ audio-video data, using digital devices such as cameras and high-definition cameras. Data collected from some of these tools not only provide academics with real-time data but also attract more details about teaching and learning than the teacher may realise. However, the cost of using such digital tools for large-scale verification is high, and cheaper alternatives are sort after. For instance, Suehiro et al. ( 2017 ) proposed a novel approach of using e-books to extract teaching activity logs in a face-to-face class efficiently.
Vatrapu ( 2012 ) considers TA as a subset of LA dedicated to supporting teachers to understand the learning and teaching process. However, this definition does not recognise that both the learning and teaching processes are intertwined. Also, most of the research in LA collects data about the student learning or behaviour, to provide feedback to the teacher ( Vatrapu et al. 2013 ; Ginon et al. 2016 ; Goggins et al. 2016 ; Shen et al. 2018 ; Suehiro et al. 2017 ), see, for example, the iKlassroom conceptual proposal by Vatrapu et al. ( 2013 ), which highlights a map of the classroom to help contextualise real-time data about the learners in a lecture. Although, a few research draw attention to the analysis of teacher-gathering and teaching practice artefacts, such as lesson plans. Xu and Recker ( 2012 ) examined teachers tool usage patterns. Similarly, Gauthier ( 2013 ) extracted the analysis of the reasoning behind the expert teacher and used such data to improve the quality of teaching.
Multimodal analytics is an emergent trend used to complement available digital trace with data captured from the physical world ( Prieto et al. 2017 ). Isolated examples include the smart school multimodal dataset conceptual future proposal by Prieto et al. ( 2017 ), which features a plan of implementing a smart classroom to help contextualise real-time data about both the teachers and learners in a lecture. Another example, Prieto et al. ( 2016 ), explored the automatic extraction of orchestration graphs from a multimodal dataset gathered from only one teacher, classroom space, and a single instructional design. Results showed that ML techniques could achieve reasonable accuracy towards automated characterisation in teaching activities. Furthermore, Prieto et al. ( 2018 ) applied more advanced ML techniques to an extended version of the previous dataset to explore the different relationships that exist between datasets captured by multiple sources.
Previous studies have shown that teachers want to address common issues such as improving their TPD and making students learn effectively ( Charleer et al. 2013 ; Dana and Yendol-Hoppey 2019 ; Pennings et al. 2014 ). Reflection on teaching practice plays an essential role in helping teachers address these issues during the process of TPD ( Saric and Steh 2017 ; Verbert et al. 2013 ). More specifically, reflecting on personal teaching practice provides opportunities for teachers to re-examine what they have performed in their classes ( Loughran 2002 ; Mansfield 2019 ; Osterman and Kottkamp 1993 ). Which, in turn, helps them gain an in-depth understanding of their teaching practice, and thus improve their TPD. For instance, Gauthier ( 2013 ), used a visual teach-aloud method to help teaching practitioners reflect and gain insight into their teaching practices. Similarly, Saar et al. ( 2017 ) talked about a self-reflection as a way to improve teaching practice. Lecturers can record and observe their classroom activities, analyse their teaching and make informed decisions about any necessary changes in their teaching method.
The network analysis approach is another promising field of teacher inquiry, especially if combined with systematic, effective qualitative research methods ( Goggins et al. 2016 ). However, researchers and teacher who wish to utilise social network analysis must be specific about what inquiry they want to achieve. Such queries must then be checked and validated against a particular ontology for analytics ( Goggins 2012 ). Goggins et al. ( 2016 ), for example, aimed at developing an awareness of the types of analytics that could help teachers in Massive Open Online Courses (MOOCs) participate and collaborate with student groups, through making more informed decisions about which groups need help, and which do not. Network theory offers a particularly useful framework for understanding how individuals and groups respond to each other as they evolve. Study of the Social Network (SNA) is the approach used by researchers to direct analytical studies informed by network theory. SNA has many specific forms, each told by graph theory, probability theory, and algebraic modelling to various degrees. There are gaps in our understanding of the link between analytics and pedagogy. For example, which unique approaches to incorporating research methods for qualitative and network analysis would produce useful information for teachers in MOOCs? A host of previous work suggests a reasonable path to scaling analytics for MOOCs will involve providing helpful TA perspectives ( Goggins 2012 ; Goggins et al. 2016 ; Vatrapu et al. 2012 ).
Teacher facilitation is considered a challenging and critical aspect of active learning ( Fischer et al. 2014 ). Both educational researchers and practitioners have paid particular attention to this process, using different data gathering and visualisation methods, such as classroom observation, student feedback, audio and video recordings, or teacher self-reflection. TA enables teachers to perform analytics through visual representations to enhance teachers’ experience ( Vatrapu et al. 2011 ). As in a pedagogical environment, professionals have to monitor several data such as questions, mood, ratings, or progress. Hence, dashboards have become an essential factor in improving and conducting successful teaching. Dashboards are visualisation tools enable teachers to monitor and observe teaching practice to enhance teacher self-reflection ( Yigitbasioglu and Velcu 2012 ). While a TAD is a category of dashboard meant for teachers and holds a unique role and value [62]. First, TAD could allow teachers to access students learning in an almost real-time and scalable manner ( Mor et al. 2015 ), consequently, enabling teachers to improve their self-knowledge by monitoring and observing students activities. TAD assists the teachers in obtaining an overview of the whole classroom as well as drill down into details about individual and groups of students to identify student competencies, strengths and weaknesses. For instance, Pantazos and Vatrapu ( 2016 ) described TAD for repertory grid data to enable teachers to conduct systematic visual analytics of classroom learning data for formative assessment purposes. Second, TAD also allows for tracking on teacher self-activities ( van Leeuwen et al. 2019 ), as well as students feedback about their teaching practice. For example,Barmaki and Hughes ( 2015 ) explored a TAD that provides automated real-time feedback based on speakers posture, to support teachers practice classroom management and content delivery skills. It is a pedagogical point that dashboards can motivate teachers to reflect on teaching activities, help them improve teaching practice and learning outcome ( 2016 ). The literature has extensively described extensively, different teaching dashboards. For instance, Dix and Leavesley ( 2015 ), broadly discussed the idea of TAD and how they can represent visual tools for academics to interface with learning analytics and other academic life. Some of these academic lives may include schedules such as when preparing for class or updating materials, or meeting times such as meeting appointments with individual or collective group of students. Similarly, Vatrapu et al. ( 2013 ) explored TAD using visual analytics techniques to allow teachers to conduct a joint analysis of students personal constructs and ratings of domain concepts from the repertory grids for formative assessment application.
Systematic review: synthesis
In this second part of the review process, we extracted selected ideas from previous studies. Then group them based on data sources, analytical methods used, types of visualisations performed and actions.
Data sources and tools
Several studies have used custom software and online applications such as employing LMS and MOOCs to collect online classroom activities ( Goggins et al. 2016 ; KU et al. 2018 ; Libbrecht et al. 2013 ; Müller et al. 2016 ; Shen et al. 2018 ; Suehiro et al. 2017 ; Vatrapu et al. 2013 ; Xu and Recker 2012 ). Others have used modern devices including eye-tracker, portable electroencephalogram (EEG), gyroscope, accelerometer and smartphones ( Prieto et al. 2016 ; Prieto et al. 2018 ; Saar et al. 2017 ; Saar et al. 2018 ; Vatrapu et al. 2013 ), and conventional instruments such as video and voice recorders ( Barmaki and Hughes 2015 ; Gauthier 2013 ; Thomas 2018 ), to record classroom activities. However, some authors have pointed out several issues with modern devices such as expensive equipment, high human resource and ethical concerns ( KU et al. 2018 ; Prieto et al. 2017 ; Prieto et al. 2016 ; Suehiro et al. 2017 ).
In particular, one study by Chounta et al. ( 2016 ) recorded classroom activities using humans to code tutor-student dialogue manually. However, they acknowledged that manual coding of lecture activities is complicated and cumbersome. Some authors also subscribe to this school of thought and have attempted to address this issue by applying Artificial Intelligence (AI) techniques to automate and scale the coding process to ensure quality in all platforms ( Prieto et al. 2018 ; Saar et al. 2017 ; Thomas 2018 ). Others have proposed re-designing TA process to automate the process of data collection as well as making the teacher autonomous in collecting data about their teaching ( Saar et al. 2018 ; Shen et al. 2018 ). Including using technology that is easy to set up, effortless to use, does not require much preparation and at the same time, not interrupting the flow of the class. In this way, they would not require researcher assistance or outside human observers. Table 2 , summarises the various data sources as well as tools that are used to harvest teaching data with regards to TA.
The collection of evidence from both online and real classroom practice is significant both for educational research and TPD. LA deals mostly with data captured from online and blended learning platforms (e.g., log data, social network and text data). Hence, LA provides teachers with data to monitor and observe students online class activities (e.g., discussion boards, assignment submission, email communications, wiki activities and progress). However, LA neglects to capture physical occurrences of the classroom and do not always address individual teachers’ needs. TA requires more adaptable forms of classroom data collection (e.g., through video- recordings, sensor recording or by human observers) which are tedious, human capital intensive and costly. Other methods have been explored to balance the trade-off between data collected online, and data gathered from physical classroom settings by implementing alternative designs approach ( Saar et al. 2018 ; Suehiro et al. 2017 ).
Analysis methods
Multimodal analytics is the emergent trend that will complement readily available digital traces, with data captured from the physical world. Several articles in the literature have used multimodal approaches to analyse teaching processes in the physical world ( Prieto et al. 2016 ; Prieto et al. 2017 ; Prieto et al. 2018 ; Saar et al. 2017 ; Thomas 2018 ). In university settings, unobtrusive computer vision approaches to assess student attention from their facial features, and other behavioural signs have been applied ( Thomas 2018 ). Most of the studies that have ventured into multimodal analytics applied ML algorithms to their captured datasets to build models of the phenomena under investigation ( Prieto et al. 2016 ; Prieto et al. 2018 ). Apart from research areas that involve multimodal analytics, other areas of TA research have also applied in ML techniques such as teachers tool usage patterns ( Xu and Recker 2012 ), online e-books ( Suehiro et al. 2017 ), students written-notes ( Taniguchi et al. 2017 ). Table 3 outlines some of the ML techniques applied from previous literature in TA.
Visualisation methods
TA allows teachers to apply visual analytics and visualisation techniques to improve TPD. The most commonly used visualisation techniques in TA are statistical graphs such as line charts, bar charts, box plots, or scatter plots. Other visualisation techniques include SNA, spatial, timeline, static and real-time visualisations. An essential visualisation factor for TA is the number of users represented in a visualisation technique. Serving single or individual users allows the analyst to inspect the viewing behaviour of one participant. Visualising multiple or group users at the same time can allow one to find strategies of groups. However, these representations might suffer from visual clutter if too much data displays at the same time. Here, optimisation strategies, such as averaging or bundling of lines might be used, to achieve better results. Table 4 represents the visualisation techniques mostly used in TA.
Systematic review: critique
Student evaluation on teaching (set) data.
Although the literature has extensively reported various data sources used for TA, this study also draws attention to student feedback on teaching, as another form of data that originates from the classroom. The analytics of student feedback on teaching could support teacher reflection on teaching practice and add value to TA. Student feedback on teaching is also known as student ratings, or SET is a form of textual data. It can be described as a combination of both quantitative and qualitative data that express students opinions about particular areas of teaching performance. It has existed since the 1920s ( Marsh 1987 ; Remmers and Brandenburg 1927 ), and used as a form of teacher feedback. In addition to serving as a source of input for academic improvement ( Linse 2017 ), many universities also rely profoundly on SET for hiring, promoting and firing instructors ( Boring et al. 2016 ; Harland and Wald 2018 ).
Technological advancement has enabled institutions of Higher Education (HE) to administer course evaluations online, forgoing the traditional paper-and-pencil ( Adams and Umbach 2012 ). There has been much research around online teaching evaluations. Asare and Daniel ( 2017 ) investigated the factors influencing the rate at which students respond to online SET. While there is a verity of opinions as to the validity of SET as a measure of teaching performance, many teaching academics and administrators perceive that SET is still the primary measure that fills this gap ( Ducheva et al. 2013 ; Marlin Jr and Niss 1980 ). After all, who experiences teaching more directly than students? These evaluations generally consist of questions addressing the instructor’s teaching, the content and activities of the paper, and the students’ own learning experience, including assessment. However, it appears these schemes gather evaluation data and pass on the raw data to the instructors and administrators, stopping short of deriving value from the data to facilitate improvements in the instruction and the learning experiences. This measure is especially critical as some teachers might have the appropriate data literacy skills to interpret and use such data.
Further, there are countless debates over the validity of SET data ( Benton and Cashin 2014 ; MacNell et al. 2015 ). These debates have highlighted some shortcomings of student ratings of teaching in light of the quality of instruction rated ( Boring 2015 ; Braga et al. 2014 ). For Edström, what matters is how the individual teacher perceives an evaluation. It could be sufficient to undermine TPD, especially if the teachers think they are the subjects of audit ( Edström 2008 ). However, SET is today an integral part of the universities evaluation process ( Ducheva et al. 2013 ). Research has also shown that there is substantial room for utilising student ratings for improving teaching practice, including, improving the quality of instruction, learning outcomes, and teaching and learning experience ( Linse 2017 ; Subramanya 2014 ). This research aligns to the side of the argument that supports using SET for instructional improvements, to the enhancement of teaching experience.
Systematically, analytics of SET could provide valuable insights, which can lead to improving teaching performance. For instance, visualising SET can provide some way, a teacher can benchmark his performance over a while. Also, SET could provide evidence to claim for some level of data fusion in TA, as argued in the conceptualisation subsection of TA.
Transformational TA
The growing research into big data in education has led to renewed interests in the use of various forms of analytics ( Borgman et al. 2008 ; Butson and Daniel 2017 ; Choudhury et al. 2002 ). Analytics seeks to acquire insightful information from hidden patterns and relationships in data that ordinarily would not be visible by the natural eyes, except with the application of state-of-the-art models and methods. Big data analytics in HE provides lenses on students, teachers, administrators, programs, curriculum, procedures, and budgets ( Daniel 2015 ). Figure 3 illustrates the types of analytics that applies to TA to transform HE.
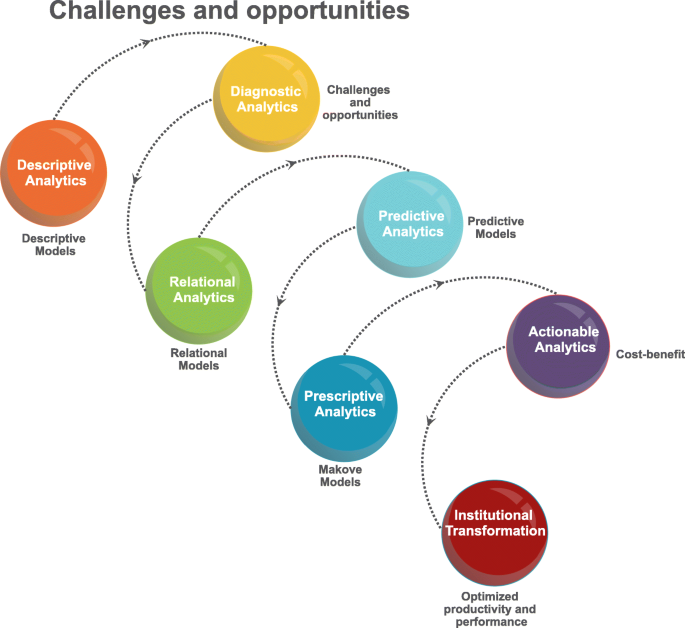
Types of analytics in higher education ( Daniel 2019 )
Descriptive Analytics Descriptive analytics aims to interpret historical data to understand better organisational changes that have occurred. They are used to answer the "What happened?" information regarding a regulatory process such as what are the failure rates in a particular program ( Olson and Lauhoff 2019 ). It applies simple statistical techniques such as mean, median, mode, standard deviation, variance, and frequency to model past behaviour ( Assunção et al. 2015 ; ur Rehman et al. 2016 ). Barmaki and Hughes ( 2015 ) carried out some descriptive analytics to know the mean view time, mean emotional activation, and area of interest analysis on the data generated from 27 stimulus images to investigate the notational, informational and emotional aspect of TA. Similarly, Michos and Hernández-Leo ( 2016 ) demonstrated how descriptive analytics could support teachers’ reflection and re-design their learning scenarios.
Diagnostic Analytics Diagnostic analytics is higher-level analytics that further diagnoses descriptive analytics ( Olson and Lauhoff 2019 ). They are used to answer the "Why it happened?". For example, a teacher may need to carry out diagnostic analytics to know why there is a high failure rate in a particular programme or why students rated a course so low for a specific year compared to the previous year. Diagnostic analytics uses some data mining techniques such as; data discovery, drill-down and correlations to further explore trends, patterns and behaviours ( Banerjee et al. 2013 ). Previous research has applied the repertory grid technique as a pedagogical method to support the teachers perform knowledge diagnostics of students about a specific topic of study ( Pantazos and Vatrapu 2016 ; Vatrapu et al. 2013 ).
Relational Analytics Relational analytics is the measure of relationships that exists between two or more variables. Correlation analysis is a typical example of relational analytics that measures the linear relationship between two variables ( Rayward-Smith 2007 ). For instance, Thomas ( 2018 ) applied correlation analysis to select the best features from the speaker and audience measurements. Some researchers have also referred to other forms of relational analytics, such as co-occurrence analysis to reveal students hidden abstract impressions from students written notes ( Taniguchi et al. 2017 ). Others have used relational analytics to differentiate critical formative assessment futures of an individual student to assist teachers in the understanding of the primary components that affect student performance ( Pantazos et al. 2013 ; Michos and Hernández Leo 2016 ). A few others have applied it to distinguish elements or term used to express similarities or differences as they relate to their contexts ( Vatrapu et al. 2013 ). Insights generated from this kind of analysis can be considered to help improve teaching in future lectures and also compare different teaching styles. Sequential pattern mining is also another type of relational analytics used to determine the relationship that exists between subsequent events ( Romero and Ventura 2010 ). It can be applied in multimodal analytics to cite the relationship between the physical aspect of the learning and teaching process such as the relationship between ambient factors and learning; or the investigation of robust multimodal indicators of learning, to help in teacher decision-making ( Prieto et al. 2017 ).
Predictive Analytics Predictive analytics aims to predict future outcomes based on historical and current data ( Gandomi and Haider 2015 ). Just as the name infers, predictive analytics attempts to predict future occurrences, patterns and trends under varying conditions ( Joseph and Johnson 2013 ). It makes use of different techniques such as regression analysis, forecasting, pattern matching, predictive modelling and multi-variant statistics ( Gandomi and Haider 2015 ; Waller and Fawcett 2013 ). In prediction, the goal is to predict students and teachers activities to generate information that can support decision-making by the teacher ( Chatti et al. 2013 ). Predictive analytics is used to answer the "What will happen". For instance, what are the interventions and preventive measures a teacher can take to minimise the failure rate? Herodotou et al. ( Herodotou et al. 2019 ) provided evidence on how predictive analytics can be used by teachers to support active learning. An extensive body of literature suggests that predictive analytics can help teachers improve teaching practice ( Barmaki and Hughes 2015 ; Prieto et al. 2016 ; Prieto et al. 2018 ; Suehiro et al. 2017 ) and also to identify group of students that might need extra support to reach desired learning outcomes ( Goggins et al. 2016 ; Thomas 2018 ).
Prescriptive Analytics Prescriptive analytics provides recommendations or can automate actions in a feedback loop that might modify, optimise or pre-empt outcomes ( Williamson 2016 ). It is used to answer the "How will it best happen?". For instance, how will teachers make the right interventions for students that have been perceived to be at risk to minimise the student dropout rate or what kinds of resources are needed to support students who might need them to succeed? It determines the optimal action that enhances the business processes by providing the cause-effect relationship and applying techniques such as; graph analysis, recommendation engine, heuristics, neural networks, machine learning and Markov process ( Bihani and Patil 2014 ; ur Rehman et al. 2016 ). For example, applying curriculum Knowledge graph and learning Path recommendation to support teaching and learners learning process ( Shen et al. 2018 ).
Actionable Analytics Actionable analytics refers to analytics that prompt action ( Gudivada et al. 2016 ; Gudivada et al. 2018 ; Winkler and Söllner 2018 ). Norris et al. ( 2008 ) used the term action analytics to describe "the emergence of a new generation of tools, solutions, and behaviours that are giving rise to more powerful and effective utilities through which colleges and universities can measure performance and provoke pervasive actions to improve it". The educational sector can leverage some of these innovative, new and cutting edge technologies and techniques such as Natural Language Processing (NLP) ( Sergis and Sampson 2016 ; Taniguchi et al. 2017 ), big data analytics ( Goggins et al. 2016 ) and deep learning ( Prieto et al. 2018 ) to support teacher in both the teaching and learning processes.
Institutional Transformation Data in themselves are not useful; they only become valuable if they can be used to generate insight. In other words, analytics can be applied to institutional data to optimise productivity and performance of the institutional operations, thereby providing value that can transform the institutional practices. In education, there are various purposes of analytics, ranging from those that provide institutions with an overview or deep-down microscopic view of individual students, faculty, curriculum, programs, operations and budgets, to those capable of predicting future trends. Unveiling the value of TA empowers the teachers to identify issues and transform difficulties into opportunities. These opportunities can be employed to optimises the institutional processes, enhance learner experiences and improve teaching performance. TA and LA both play a vital role in effectively reforming and transforming the educational sector to catch up with the fast pace at which data generates. For example, with the extensive use of online and blended learning platforms, the application of analytics will enable institutional stakeholders at all levels to gain new insights into educational data. Today, the HE sector is at crossroads, where there is a need for synergies in learning research and data analytics to transform the way teaching and learning are fundamentally carried out.
The link between TA, LA and LD
Primarily, TA aims to link the centrepiece of LA and remodel them to address teaching challenges. More specifically, TA argues that connecting and analysing insights generated from LA methods and tools with those generated from in-class methods and tools, through TA tools could support teacher reflection and improve TPD based on evidence. Hence, this concept is presented further in the next subsection.
Conceptual framework of TA
Based on the different perceptions of TA described in previous reviews, this study proposes a conceptual framework for TA to model the complex interaction existing around TA. Three nodes (LA, TA and LD) are interconnected to each other forming a triadic network with the teacher at the centre, performing value-added interactions to make informed based decisions. Each part of this interconnection forms a triangle, totalling three triangles (A, B and C) (see Fig. 4 ).
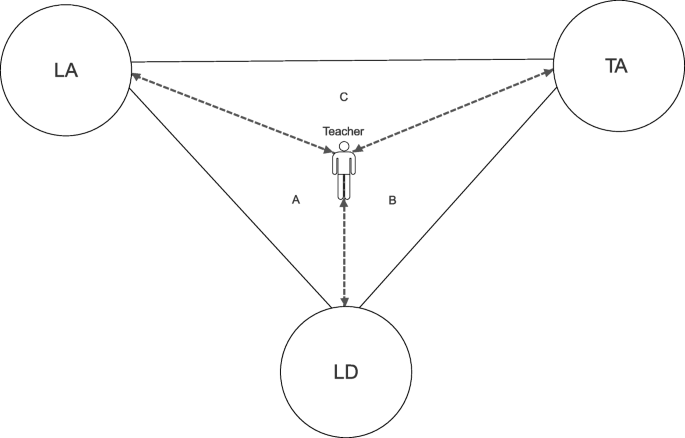
Conceptualisation of TA. Triadic TA Conceptual Framework
The proposed framework is not bound to any particular implementation of learning or design technology. Instead, the point is to describe the elements of analytics and data sources that are key for each domain to guide the use of analytical methods, tools and technology to support the multiple dimensions of learning design successfully.
This triad illustrates the interaction occurring between the teacher, the LA and the LD, to inform TPD. Hernández-Leo et al. ( 2019 ) argued that LD could contribute to structuring and orchestrating the design intent with learners digital trace patterns, advancing the knowledge and interpretation of LA. LA tailored to fit the design intent could be considered by teachers as contributing to the enhancement of the LD in subsequent design interactions. For example, LA could be an information tool to inform the tutors or designers of pedagogical decision making ( Persico and Pozzi 2015 ). Hence, a teacher may want to utilise LA to make just-in-time pedagogical decisions, such as grouping students based on their performance.
Similarly, a teacher may want to investigate if the estimated time taken for students to carry out learning tasks is reasonable or whether adjustments need to be made to the course design ( Hernández-Leo et al. 2019 ; Pozzi and Persico 2013 ). This domain can also provide teachers with analytics regarding the challenges and difficulties students face in the problem-solving phase while performing a task. In return, they give the teacher information in the form of TAD summarising the various challenges students encountered with that activity. They may also provide solutions on how to address them. For example, an early alert system that instantiates a dashboard for instructors using some metrics calculations such as login counts and page views ( Thille and Zimmaro 2017 ). The data sources in the LA node can improve teachers’ awareness, which could also lead to the improvement of LD and help to distinguish design elements that could modify future designs. Data collection in this domain is mostly automatic through virtual learning environments (e.g., LMS, MOOCs). Other forms of data collection may include social media platforms (e.g., Facebook, Tweeter), wearable sensors (e.g., eye-trackers, EEG), software tools that support and collect data related to specific student activities and attendance ( Bakharia et al. 2016 ; Bos and Brand-Gruwel 2016 ).
This triangle represents the relationship between the teacher, the LD and TA. While experiencing LD, TA endeavours to handle continues teachers’ engagement, progression, achievement and learners satisfaction ( Bakharia et al. 2016 ; Sergis and Sampson 2017 ). For example, exploring the impact of video shot on instructor performance and student learning. Using MOOC AB testing, teachers could experiment whether a difference in video production setting would have any impact on the instructors acting performance, or whether any changes in format and instructors performance will result in detectable differences in student viewing behaviour ( Chen et al. 2016 ).
Further, data sources in TA could assist teacher reflection on the impacts of their LD. Data collection could also be automatic by the use of wearable sensors on the teachers while performing teaching activities, also known as in-class analytics. Several institutions now record video contents of their face-to-face classes. Some others even go a step further by collecting their physiological data. These datasets, as mentioned earlier, have a way of exemplifying and illustrating things that ordinarily, a book of pedagogy cannot convey, in providing systematic feedback for the teachers. It involves capturing data during a traditional in-class, face-to-face teacher-centric instruction or teacher-student interaction (where students learn by directly or indirectly interacting with instructors in a lab or lecture hall) and analysing data to identify areas of possible improvements. The kind of data usually captured in this setting are audio, video, body movement, brain activity, cortex activity, to mention just a few. For example, a teacher can perform diagnostic analysis on class recorded videos to expose what is intrinsic during his lecture. This kind of diagnostic analysis could help teachers understand more about their teaching and discover areas of further improvement. SET is another form of data about the teachers; they are collected via the institutional application platforms ( Hernández-Leo et al. 2019 ) and can be visualised to improve teaching performance..
Analytics that happens in the LD involves the visualisation of teaching design to facilitate teacher reflection on the lesson plan, visualisation of the extent to which the lesson plan aligns with the educational objectives, and finally, validation of the lesson plan to highlight potential inconsistencies in the teaching design. For example, a teacher can visualise the number of assessment activities of the lesson plan or the various types of educational resources used in the lesson plan, to know if they are still valid or obsolete. Similarly, a teacher could analyse the time allocated for each lesson activity, to find out if the time allocated for each activity is good enough, or visualise the level of inconsistencies of time misappropriations and imbalances between the overall lesson plan and the individual lesson activities.
This area presents the communication between the teacher, the LA and the TA. Chinchu Thomas ( 2018 ) explored the correlation between student ratings on teaching and student physiological data. Similarly, Schmidlin ( 2015 ) established how to analyse and cross-reference data without decrypting the data sources. Hence, we argue that SET could be linked with LA such as student digital traces from LMS ( Stier et al. 2019 ) and other forms of data (such as attendance data), without compromising privacy. This claim for data fusion could support the teachers to make informed-decisions in new ways. For example, analytics performed on linked datasets could quickly reveal those student opinions that may not count at the end of the semester courses.
Visualisations that could quickly realise students with low participation rates and link it to their opinions, without revealing any identity. Additionally, teachers may be interested in comparing the view of students with low participation rate with those of high participation rate. This kind of information may lead teachers towards making explicit judgements with evidence. A tutor may choose to disregard the opinions of those students that participated less than 20 per cent in-class activities and assignments, as well as had a low attendance rate. Hence, narrowing concentration more on the opinions of students that participated in improving teaching practice.
However, considering ethical concerns, data fusion at the individual level still requires explicit and informed consent from the students whose data are collected ( Menchen-Trevino 2016 ). Other issues such as privacy concerns, data fusion can be problematic as this usually requires that the teachers know student identities. However, from a programmatic perspective, extra measures can be put in place to address this concern. Algorithms can be interfaced to mask student identities to some other unique identities to make them anonymous but linked ( Schmidlin et al. 2015 ) to provide a richer set of data for the teacher to make informed decisions.
Teachers can get a better picture towards improving the context in which learning happens, only if they can be informed about both how they teach and how students learn. Hence, this framework aims to continually provide teachers with interesting information from intelligent feedback based on data generated from users and learning context to improve their learning design and teaching outcome continuously.
Teaching Outcome Model (TOM)
Design-based research advances instructional design work, theory, and implementation as iterative, participatory, and located rather than processes "owned and operated" by designers of instructions ( Wang and Hannafin 2005 ). TOM is an iterative process that follows a design-based research approach to guide teachers, researchers, faculty and administrators on how to utilise data to improve the quality of teaching and learning outcome. This model enables teachers to investigate and evaluate their work using data. Consequently, improving the teacher use of data to inform teaching practice. To build more awareness with regards to teaching data, TOM models TA through iterative cycles of data collection, data analysis, data visualisation and action stages which are interdependent of each other (see Fig. 5 ). Design-based research, as a pragmatic methodology, can guide TOM while generating insights that can support teacher reflections on teaching and student learning. Conversely, TOM ensures that design-based research methodologies can be operational and systemised. Following the various stages outlined in the model, teachers can regularly identify, match and adjust teaching practice, and learning design to all the learners need.
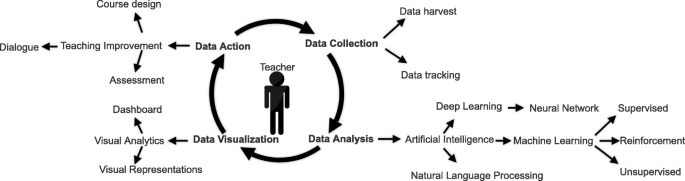
Teaching Outcome Model. TA Life cycle
In the data collection stage, a constant stream of data accumulates from the digital traces relating to teaching daily activities and engagements, including structured and unstructured data, visual and non-visual data, historical and real-time data. It is also important to note that the rate at which diverse data accumulates in our educational system will keep growing. According to Voithofer and Golan ( 2018 ), there are several ways to mine teaching and learning data without professional knowledge that is beyond the necessary teacher training experience in data literacy, administering learning design and class orchestration. Subscribing to this school of thought, adopting Big data infrastructure in our institutions will guarantee easy access to data by the various stakeholders, this will also mitigate the bottleneck of disparate data points existing in our educational sector. Therefore, enabling educators to focus more attention on instruction, setting up interactive class activities, and participating more on discussions that will create more data for evidence-based decision making. Also, the misuse of data is a broad primary concern ( Roberts et al. 2017 ). One critical matter is identifying the types of data that can be collected, analysed and visualized; to ensure that the right people have access to the data for the right purpose. As such, implementing data governance policies around institutional data such as; ’open definition of purpose, scope and boundaries, even if that is broad and in some respects, open-ended’ is critical ( Kay et al. 2012, p 6 ). This sort of measure will introduce clarity and address issues around who controls what data as well as security and privacy issues around data.
Analysis stage
This step involves the different ways of working with data to ensure data quality. Professionals such as data scientists, programmers, engineers and researchers need to work together with the teachers at this level. They can apply data mining techniques, statistical methods, complex algorithms, and AI techniques (such as NLP, AI, ML, deep learning) to adequately transform data into the useful analytical process. Analytics in the education space presents in diverse forms including, descriptive, diagnostic, predictive and prescriptive. These different forms of analytics can be utilised to offer a high-level view or fine-grained view of individual learners, teacher, faculty and their various activities, engagements and behaviours. Unravelling the value of data analytics empowers teachers and researchers to identify problems and transform challenges into opportunities that can be utilised to support teacher reflection and enrich teacher data-literacy experiences. For example, teachers can apply NLP on text data to gather topics from discussion posts, contributions participants have made within collaborative projects and their sentiments.
Furthermore, ML techniques could be combined with TA to enhance teaching outcome. For instance, chatbots could support the teacher by acting as a teacher assistant in large classes. An essential consideration in analytics, however, is that data can be easily de-identified ( Roberts et al. 2017 ; Cumbley and Church 2013 ), especially when data sets increase in size and scope and are combined to generate big data. To resolve these concerns, a particular university introduced a two-stage method of data de-identification coupled with data governance to restrict data access ( De Freitas et al. 2015 ).
Visualisation stage
This stage ensures data presentation in useful and meaningful ways to teachers. Empowering teachers with interactive visual interfaces and dashboards that facilitate teacher cognition and promote reflection about pre-processed and fine-grained teaching and learning activities. Through TAD, can project real-time and historical information from different data sources that might not be necessarily interoperable, and results summarised ( Moore 2018 ). However, visualisation is "what you see is what you get"; meaning that information presentation method may affect its interpretation, and consequently, may influence decision-making. Hence, it is necessary to address issues around visualisations in diverse forms such as; visual analytics and exploratory data analysis to create room for visual interactivity, exploratory visualisation to discover trends, patterns, relationships and behaviours. For example, a teacher can use a TAD to monitor student engagement. When the student engagement is poor, it may prompt the teacher to take necessary actions such as; changing teaching material and making it more interactive. Additionally, there are also questions around privacy, such as who has access to visualisations relevant to an instructor, such as other faculty members participating in the course, directly or indirectly, administrators, researchers, potential employees of other institutions.
Action stage
At this stage, informed-decision leads to action and actions unavoidably reshape our environment; subsequently, regenerate new data. Additionally, there is a to create tools that will be useful to the teacher to understand and make meaning of data quickly. Actions taken by teachers can be used to improve the course design and assessment (value-added formative assessment). In any case, predictive analytics prompts an epistemological question; how should we ensure effective action by the teacher based on flawed predictions such that the system does not collapse?
Discussion and conclusion
This article presents the result of a systematic literature review aimed at describing the conception, and synthesis of the current research on the notion of TA, to provide insight into how TA can be used to improve the quality of teaching. The first part of the article described what is meant by TA to consolidate the divergent discourse on TA. The review showed that TA applies to analytics on teaching activities as well as methods of improving teachers’ awareness on students’ activities, including supporting the teachers to understand student learning behaviours to provide adequate feedback to teachers. In essence, the primary goal of TA is to improve teaching performance. The literature also revealed the several tools and methods are available for extracting digital traces associated with teaching in addition to traditional student evaluation tools. However, one of the main challenges recognised was the cost associated with some devices used to capture in-class activities, and ML techniques have been proposed to minimise this challenge.
The literature has also recognised teacher inquiry as a promising area of research in TA and came to a consensus that methods, like multimodal analytics and SNA, could help promote teacher inquiry and teacher reflection. Visualisations and visual analytics techniques are very significant in TA and also encourage teacher inquiry. The use of visualisation dashboards and TAD are essential tools that the modern-day teachers require to carry out a continuous and efficient reflection on teaching practice.
The emphasis of the synthesis of TA was clearly on data collection, analysis and visualisation, as illustrated in Fig. 6 . In the literature, the various kinds of data collected and used to improve teaching practice, include:
Digital trace data; "records of activity (trace data) undertaken through an online information system (thus, digital)" [119]. They incorporate various activities generated from custom applications and learning environments that leave digital footprints.
Image data are photographic or trace objects that represent the underlying pixel data of an area of an image element.
Physiological data are body measurement based on body-mounted sensors ( Lazar et al. 2017 ), used to extract data from teachers while performing classroom teaching activities.
Audio-video stream data or recorded lecturer data with captured physical teaching activities and students learning activities. Hence, attainable with mounted cameras, computer or mobile cameras connected to applications like Zoom and Skype, eye tracks with recording capabilities and digital cameras connected to learning environments such as Eco365.
Social data are data with online social activities, including utilising the repertory grid technique to collect students’ assessment data from social media sites.
Text data, including quantitative and qualitative data, data generated from text documents such as discussion forums, students essay or articles, emails and chat messages.
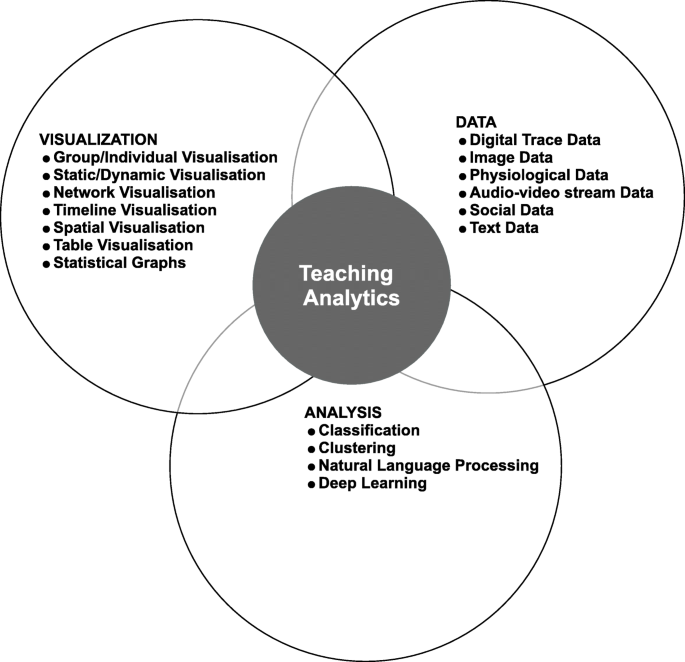
Dimensions of TA. Illustration of TA based on the literature
Analysis in this context refers to the application of Educational Data Mining (EDM) and deep learning techniques mostly used to process data. EDM approaches is a complicated process that requires an interweaving of various specialised knowledge and ML algorithms, especially to improve teaching and learning ( Chen 2019 ). NLP and classification are the two main EDM techniques applied in TA. However, the review also recognised the use of other methods such as clustering and deep learning techniques, to support teachers.
As commonly said, a picture is worth more than a thousand words; visualisation can effectively communicate and reveal structures, patterns and trends in variables and their interconnections. Research in TA has applied several visualisation techniques including Network, Timeline, Spatial, Table and Statistical Graphs. For instance, SNA is a form of visual analytics that is used to support teachers to determine how different groups interact and engage with course resources. Identifying differences in interaction patterns for different groups of students may result in different learning outcomes, such as, how access patterns of successful groups of students differ from that of unsuccessful students. Applying visualisation techniques can support teachers in areas such as advising underperforming students about effective ways to approach study. Visualisation can enable teachers to identify groups of students that might need assistance and discover new and efficient means of using collaborative systems to achieve group work that can be taught explicitly to students.
However, while acknowledging the incomplete nature of data and complexities associated with data collection, analysis and use, teachers should take caution to avoid bais. Data collected in one context may not be directly applicable to another or have both benefits and cost for individuals or groups from which data was harvested. Therefore, key stakeholders, including teachers, course directors, unit coordinators and researchers must pay proper attention to predictive models and algorithms and take extra care to ensure that the contexts of data analysed are carefully considered. There are also privacy concerns, such as who has access to view analytics relating to a teacher, including other faculty members both directly or indirectly involved in the course, administrators, researchers, future employees of other institutions. It will be useful for institutions to have clear guidelines as to who has access to what and who views what. Other issues around data include how long should data remain accessible ( Siemens 2013 ), with big data technology and infrastructure, data should be kept for as long as it can exist. Pardo and Siemens ( 2014 ) acknowledged that the use of analytics in higher education research has no clear interpretation of the right to privacy. They seem opposed to the need for absolute privacy, on the basis that the use of historical data enhances research with potential rewards for the future of teaching professional development and student outcome.
The review provided in the current article highlighted the significant limitations in the existing literature on teaching analytics. The TAD is proposed to guide teachers, developers, and researchers to understand and optimise teaching and the learning environments. The critical aspect of this review is establishing the link between LA, TA and LD and its value in informing teachers’ inquiry process. Also, the review describes the relationship between LA, TA and LD. Finally, the article proposes TOM, which draws from a research-based approach to guide teachers on how to utilise data to improve teaching. The outcome of this model is a TAD that provides actionable insights for teacher reflection and informed decision-making. Therefore, showing the value that TA brings to pedagogic interventions and teacher reflection.
Theoretical implications
The analysis of data collected from the interaction of teachers with technology and students is a promising approach for advancing our understanding of the teaching process and how it can be supported. Teachers can use data obtained from their teaching to reflect on their pedagogical design and optimise the learning environment to meet students’ diverse needs and expectations.
Teacher-centric learning design can improve the utility of new technologies and subsequent acceptance of the use of these technologies to improve the quality of teaching and enhance students learning experience. TAD is one class of tools that can be designed in such a way that will improve teaching practice.
Research on learning analytics has revealed useful insights about students’ learning and the context in which they learn. While the ability to track, harvest and analyse various forms of learning analytics can reveal useful insights about learners’ engagement with learning environments, our review suggests that there is limited focus on analytics relating to the teacher, their teaching approaches and activities. Also, there has been increasing advances in the design of learner and teaching dashboards. However, many teachers still struggle with understanding and interpreting dashboards partly because they lack data literacy skills, and mostly because most the design of many of the tools does not include teachers as partners.
Although, TAD enable teachers to inspect, and understand the processes and progress relating to their teaching, the current implementations of TAD in general, does not adequately provide teachers with the details they need or want in a readily usable format. Educational technology developers can utilise our proposed model to design better tools for improving teaching practice. For example, a TAD can be designed to perform text analytics on students qualitative comments about a course taught, and results presented to the teacher in the form of themes, sentiments and classification; such that it will support the instructor’s needs and preferences for insight generation and reflection.
Teachers monitor, observe and track both teaching and learning activities to make appropriate decisions. Moreover, it is also important to note that visualisations can be misrepresented, misinterpreted or misused by the viewer [122]. Hence, perception and cognition remain a significant challenge in TAD. Consequently, it becomes necessary to design and write algorithms that extract information visualisation, in such a way that allows adequate understanding by teachers. It is also crucial for dashboards to integrate multiple sources such as combining both the learning and teaching activities into a TAD, to create room for teachers to comprehend, reflect on and act upon the presented information quickly.
Also, the current state of technology shows little progress in taking TA, raising concerns about the accurate validity and scalability of innovations such as predictive analytics and TAD. Furthermore, the ethical issues of data use are not considered sufficient to establish institutional policies which incorporate TA as part of quality education models.
Finally, consideration of the framework’s three layers as a whole raises new questions and opportunities. For example, linking educational performance and satisfaction to specific learning design involves consideration of elements of all three layers. This review has shown that TA is a new and essential area of analytics in education. The study also suggests that the conceptualisation of teaching analytics is still at its infancy. However, the practical and successful use of teaching analytics is highly dependent on the development of conceptual and theoretical foundations into consideration.
Implications for practice
This review has uncovered the value of TA and its role in fostering data literacy skills in teachers to support evidence-based teaching. The purpose of TOM is to guide the development of teaching dashboard, and for researchers to develop strategies that help meaningful ways in which data can be presented to teachers. Teacher dashboards can empower the teachers with tools that create new opportunities to make data-informed strategic decisions, utilising the power of analytics and visualisation techniques. Consequently, increasing the efficiency and effectiveness of the institution, including, improving teaching practice, curriculum development and improvement, active learning engagement and improved students’ success. TOM also presents a platform for teaching academics who may have the best understanding of their course contexts, to provide a significant contribution to a culture of data-informed teaching practice within an institution.
The responsibility for managing the systems that provide the analytics usually falls within the control and supervision of the institution’s information technology (IT) department, and often, they have little to no knowledge of their pedagogical applications to teaching and learning. Likewise, academics and their fields of learning support are often deprived of IT skills and have little to no professional understanding of how software systems work. TOM provides opportunities for the teachers to be involved in the design of TA by providing significant interaction and collaboration between the IT and the other sectors that interpret and act upon the information flow.
Additionally, institutions need to provide teaching staff with the necessary training that fosters the development of data literacy skills, and in the use of data and analytical or visualisation dashboards to monitor their teaching practice. Based on some of the challenges identified in the present review, it is imperative institutions ensure that data is collected transparently, with the awareness of all the stakeholders involved, and informed consent of individuals where appropriate. With the advancements in computing technology, data collection, analysis and use have significantly increased, large amounts of data can be continually pulled from different sources and processed at fast speeds. Big data offers institutions the opportunity to implement big data infrastructures and utilise the full potential of data analytics and visualisation. However, institutions also need to consider implementing a data governance framework to guide the implementation and practice of analytics.
The conceptual framework of TA was established to demonstrate the relationship between LA, TA and LD, which can be useful knowledge to various institutional stakeholders, including the learners, teachers, researchers and administrators. However, there are also issues around data ownership, intellectual property rights, and licensing for data re-use (the students, the instructor, the researcher or the institution). For instance, the same data sources can be shared amongst the various stakeholders, but with different level of access, as such data sharing agreement would be needed to guide sharability without infringing on rights, violating privacy or disadvantaging individuals. The implementation of data sharing agreement would require the building of institutional, group as well as individual trust, which would include guidelines on sharing data within the institution and between third parties, such as external organisations and other institutions. In general, stricter data management policies that guide data collection, analysis and use is essential for every institution.
Limitations and future research
Teaching analytics is an emergent phenomenon in the learning analytics and data science literature, with a limited body of published work in the area, as such conclusions drawn from the review are limited to the databases interrogated and articles reviewed. Further, findings in the review are likely to be influenced by our interpretation of the literature and untestable assumptions. For example, linking LA, TA and LD and their underlying assumptions is not grounded in empirical work. The review serves as an advocacy for teacher data literacy and the ability to work with various forms of data. However, working with a single data point may not be publicly accessible to teachers.
Moreover, the combination of analytics on the several data points may lead to some level of identification, and this would require navigating issues around access, protecting privacy, and obtaining appropriate consents. Therefore, it is almost impossible for individual teachers to comprehend not only the scope of data collected, analysed and used but also the consequences of the different layers of collection, analysis and use. Consequently, making it challenging for teachers to make use of the full potentials of data to make informed choices in learning design. No matter how straightforward or transparent institutional policies around data are, the sheer complexity of the collection, analysis and use has made it impossible, posing a fundamental issue for the stakeholders trying to use analytics to enhance teaching practice and learning outcome across an institution.
In future research, we hope to carry out more extensive empirical research on how TOM could be applied to address issues with regards to ethical and privacy concerns about the utilization of TA. We are currently exploring how teaching analytics dashboards can be used to support teacher data literacy and use analytics to improve teaching practice and learning outcome.
Availability of data and materials
Not applicable.
Abbreviations
Academic analytics
Artificial intelligence
Educational data mining
Higher education
Interactive whiteboard
- Learning analytics
Learning design
Learning management system
Machine learning
Massive open online courses
Natural language processing
Open learners model
Student evaluation of teaching
Social network analysis
- Teaching analytics
Teaching analytics dashboard
Term frequency inverse document frequency
- Teaching and learning analytics
- Teaching outcome model
Technology, pedagogy, and content knowledge
Teacher professional development
Adams, M.J., & Umbach, P.D. (2012). Nonresponse and online student evaluations of teaching: Understanding the influence of salience, fatigue, and academic environments. Research in Higher Education , 53 (5), 576–591.
Article Google Scholar
Anderson, T. (2003). Getting the mix right again: An updated and theoretical rationale for interaction. The International Review of Research in Open Distributed Learning , 4 (2).
Asare, S., & Daniel, B.K. (2017). Factors influencing response rates in online student evaluation systems: A systematic review approach. In E-Learn: World Conference on E-Learning in Corporate, Government, Healthcare, and Higher Education . Association for the Advancement of Computing in Education (AACE), (pp. 537–541).
Assunção, M.D., Calheiros, R.N., Bianchi, S., Netto, M.A., Buyya, R. (2015). Big data computing and clouds: Trends and future directions. Journal of Parallel and Distributed Computing , 79 , 3–15.
Bakharia, A., Corrin, L., De Barba, P., Kennedy, G., Gašević, D., Mulder, R., Williams, D., Dawson, S., Lockyer, L. (2016). A conceptual framework linking learning design with learning analytics. In Proceedings of the Sixth International Conference on Learning Analytics & Knowledge . ACM, (pp. 329–338).
Banerjee, A., Bandyopadhyay, T., Acharya, P. (2013). Data analytics: Hyped up aspirations or true potential? Vikalpa , 38 (4), 1–12.
Barmaki, R., & Hughes, C.E. (2015). Providing real-time feedback for student teachers in a virtual rehearsal environment. In Proceedings of the 2015 ACM on International Conference on Multimodal Interaction . ACM, (pp. 531–537).
Beer, C., Jones, D., Clark, K. (2009). The indicators project identifying effective learning: Adoption, activity, grades and external factors. In Ascilite . Citeseer.
Benton, S.L., & Cashin, W.E. (2014). Student ratings of instruction in college and university courses , (pp. 279–326): Springer.
Bihani, P., & Patil, S. (2014). A comparative study of data analysis techniques. International Journal of Emerging Trends & Technology in Computer Science , 3 (2), 95–101.
Google Scholar
Borgman, C.L., Abelson, H., Dirks, L., Johnson, R., Koedinger, K.R., Linn, M.C., Lynch, C.A., Oblinger, D.G., Pea, R.D., Salen, K. (2008). Fostering learning in the networked world: The cyberlearning opportunity and challenge. a 21st century agenda for the national science foundation. https://doi.org/10.1037/e532532011-001 .
Boring, A. (2015). Gender biases in student evaluation of teachers . Paris. https://doi.org/10.1016/j.jpubeco.2016.11.006 .
Boring, A., Ottoboni, K., Stark, P.B. (2016). Student evaluations of teaching are not only unreliable, they are significantly biased against female instructors. Impact of Social Sciences Blog . The London School of Economics and Political Science.
Bos, N., & Brand-Gruwel, S. (2016). Student differences in regulation strategies and their use of learning resources: implications for educational design. In Proceedings of the Sixth International Conference on Learning Analytics & Knowledge . ACM, (pp. 344–353).
Braga, M., Paccagnella, M., Pellizzari, M. (2014). Evaluating students’ evaluations of professors. Economics of Education Review , 41 , 71–88.
Butson, R., & Daniel, B. (2017). The Rise of Big Data and Analytics in Higher Education , (pp. 127–140): Auerbach Publications.
Charleer, S., Klerkx, J., Odriozola, S., Luis, J., Duval, E. (2013). Improving awareness and reflection through collaborative, interctive visualizations of badges. In ARTEL13: Proceedings of the 3rd Workshop on Awareness and Reflection in Technology-enhanced Learning, vol. 1103 . CEUR Workshop Proceedings, (pp. 69–81).
Chatti, M.A., Dyckhoff, A.L., Schroeder, U., Thüs, H. (2013). A reference model for learning analytics. 5-6 , 4 , 318–331.
Chen, L.-L. (2019). Enhancing teaching with effective data mining protocols. Journal of Educational Technology Systems , 47 (4), 500–512.
Chen, Z., Chudzicki, C., Palumbo, D., Alexandron, G., Choi, Y.-J., Zhou, Q., Pritchard, D.E. (2016). Researching for better instructional methods using ab experiments in moocs: results and challenges. Research and Practice in Technology Enhanced Learning , 11 (1), 9.
Choudhury, S., Hobbs, B., Lorie, M., Flores, N. (2002). A framework for evaluating digital library services. D-Lib magazine , 8 (7/8), 1082–9873.
Chounta, I.-A., McLaren, B.M., Albacete, P.L., Jordan, P.W., Katz, S. (2016). Analysis of human-to-human tutorial dialogues: Insights for teaching analytics. In IWTA@ EC-TEL , (pp. 9–17).
Cumbley, R., & Church, P. (2013). Is “big data” creepy? Computer Law & Security Review , 29 (5), 601–609.
Dana, N.F., & Yendol-Hoppey, D. (2019). The Reflective Educator’s Guide to Classroom Research: Learning to Teach and Teaching to Learn Through Practitioner Inquiry : Corwin.
Daniel, B. (2015). Big data and analytics in higher education: Opportunities and challenges. British Journal of Educational Technology , 46 (5), 904–920. https://doi.org/10.1111/bjet.12230 .
Daniel, B., & Harland, T. (2017). Higher Education Research Methodology: A Step-by-Step Guide to the Research Process . Routledge London. https://doi.org/10.4324/9781315149783 .
Daniel, B.K. (2019). Artificial reality: The practice of analytics and big data in educational research. In: Pedersen, J.S., & Wilkinson, A. (Eds.) In Big data: Promise, application and pitfalls . https://doi.org/10.4337/9781788112352.00018 . Edward Elgar, Cheltenham, (pp. 287–300).
Chapter Google Scholar
De Freitas, S., Gibson, D., Du Plessis, C., Halloran, P., Williams, E., Ambrose, M., Dunwell, I., Arnab, S. (2015). Foundations of dynamic learning analytics: Using university student data to increase retention. British Journal of Educational Technology , 46 (6), 1175–1188.
Dix, A.J., & Leavesley, J. (2015). Learning analytics for the academic: An action perspective. J. UCS , 21 (1), 48–65.
Ducheva, Z., Pehlivanova, M., Dineva, S. (2013). Possibilities for students to evaluate and improve electronic courses. In The 8th International Conferemnce on Virtual Learning ICVL .
Edström, K. (2008). Doing course evaluation as if learning matters most. Higher Education Research & Development , 27 (2), 95–106.
Elias, T. (2011). Learning analytics. Learning , 1–22.
Ferguson, R. (2012). Learning analytics: drivers, developments and challenges. International Journal of Technology Enhanced Learning , 4 (5/6), 304–317.
Fischer, F., Wild, F., Sutherland, R., Zirn, L. (2014). Grand Challenge Problems from the Alpine Rendez-Vous , (pp. 3–71): Springer.
Flanders, N.A. (1970). Analyzing Teacher Behavior . Addison-Wesley P.C.
Flavin, M. (2017). Disruptive Technology Enhanced Learning: The Use and Misuse of Digital Technologies in Higher Education : Springer. https://doi.org/10.1057/978-1-137-57284-4 .
Gandomi, A., & Haider, M. (2015). Beyond the hype: Big data concepts, methods, and analytics. International Journal of Information Management , 35 (2), 137–144.
Gauthier, G. (2013). Using teaching analytics to inform assessment practices in technology mediated problem solving tasks. In IWTA@ LAK .
Ginon, B., Johnson, M.D., Turker, A., Kickmeier-Rust, M. (2016). Helping Teachers to Help Students by Using an Open Learner Model. https://doi.org/10.1007/978-3-319-45153-4_69 .
Goggins, S.P. (2012). Group informatics: A multi-domain perspective on the development of teaching analytics. In Proceedings of the TaPTA Workshop at EC-TEL .
Goggins, S.P., Galyen, K., Petakovic, E., Laffey, J.M. (2016). Connecting performance to social structure and pedagogy as a pathway to scaling learning analytics in moocs: an exploratory study. Journal of Computer Assisted Learning , 32 (3), 244–266.
Gorham, J. (1988). The relationship between verbal teacher immediacy behaviors and student learning. Communication Education , 37 (1), 40–53.
Griffiths, D. (2017). The use of models in learning design and learning analytics. Interaction Design and Architecture(s) Journal , 33 , 113–133.
Gudivada, V.N., Irfan, M., Fathi, E., Rao, D. (2016). Cognitive analytics: Going beyond big data analytics and machine learning (Vol. 35, pp. 169–205).
Gudivada, V.N., Rao, D.L., Ding, J. (2018). 2. Evolution and Facets of Data Analytics for Educational Data Mining and Learning Analytics , (pp. 16–42). New York. https://doi.org/10.4324/9780203728703-3 .
Harland, T., & Wald, N. (2018). Vanilla teaching as a rational choice: the impact of research and compliance on teacher development. Teaching in Higher Education , 23 (4), 419–434.
Hernández-Leo, D., Martinez-Maldonado, R., Pardo, A., Muñoz-Cristóbal, J.A., Rodríguez-Triana, M.J. (2019). Analytics for learning design: A layered framework and tools. British Journal of Educational Technology , 50 (1), 139–152.
Herodotou, C., Hlosta, M., Boroowa, A., Rienties, B., Zdrahal, Z., Mangafa, C. (2019). Empowering online teachers through predictive learning analytics. British Journal of Educational Technology . https://doi.org/10.1111/bjet.12853 .
Huang, C.-W. (2001). Educlick: A computer-supported formative evaluation system with wireless devices in ordinary classroom. In Proceedings of Int. Conference on Computers in Education, 2010 , (pp. 1462–1469).
Joseph, R.C., & Johnson, N.A. (2013). Big data and transformational government. IT Professional , 15 (6), 43–48.
Kaser, L., & Halbert, J. (2014). Creating and sustaining inquiry spaces for teacher learning and system transformation. European Journal of Education , 49 (2), 206–217.
Kay, D., Korn, N., Oppenheim, C. (2012). Legal, risk and ethical aspects of analytics in higher education. Analytics Series . JISC Cetis (Centre for educational technology and interoperability standards).
KU, O., LIANG, J.-K., CHANG, S.-B., WU, M. (2018). Sokrates teaching analytics system (stas): An automatic teaching behavior analysis system for facilitating teacher professional development. In Proceedings of the 26th International Conference on Computers in Education. Philippines: Asia-Pacific Society for Computers in Education .
Laney, D. (2001). 3d data management: Controlling data volume, velocity and variety. META Group Research Note. META group research note , 6 (70), 1.
Lazar, J., Feng, J.H., Hochheiser, H. (2017). Research Methods in Human-computer Interaction : Morgan Kaufmann.
Leitner, P., Khalil, M., Ebner, M. (2017). Learning analytics in higher education—a literature review , (pp. 1–23): Springer.
Libbrecht, P., Kortenkamp, U., Rebholz, S., Müller, W. (2013). Tales of a companion teacher analytics. In IWTA@ LAK .
Linse, A.R. (2017). Interpreting and using student ratings data: Guidance for faculty serving as administrators and on evaluation committees. Studies in Educational Evaluation , 54 , 94–106.
Loughran, J.J. (2002). Effective reflective practice: In search of meaning in learning about teaching. Journal of teacher education , 53 (1), 33–43.
Macfadyen, L.P., & Dawson, S. (2012). Numbers are not enough. why e-learning analytics failed to inform an institutional strategic plan. Journal of Educational Technology Society , 15 (3).
MacNell, L., Driscoll, A., Hunt, A.N. (2015). What’s in a name: Exposing gender bias in student ratings of teaching. Innovative Higher Education , 40 (4), 291–303.
Mansfield, J. (2019). Pedagogical Equilibrium: The Development of Teachers’ Professional Knowledge : Routledge.
Marlin Jr, J.W., & Niss, J.F. (1980). End-of-course evaluations as indicators of student learning and instructor effectiveness. The Journal of Economic Education , 11 (2), 16–27.
Marsh, H.W. (1987). Students’ evaluations of university teaching: Research findings, methodological issues, and directions for future research. International Journal of Educational Research , 11 (3), 253–388.
McKenney, S., & Mor, Y. (2015). Supporting teachers in data–informed educational design. British journal of educational technology , 46 (2), 265–279.
Menchen-Trevino, E. (2016). Web historian: Enabling multi-method and independent research with real-world web browsing history data. In IConference 2016 Proceedings . https://doi.org/10.9776/16611 .
Michos, K., & Hernández Leo, D. (2016). Towards understanding the potential of teaching analytics within educational communities. In: Vatrapu, R.G.B.B.S., & Kickmeier-Rust, M. (Eds.) In Vatrapu R, Kickmeier-Rust M, Ginon B, Bull S. IWTA 2016 International Workshop on Teaching Analytics. Proceedings of the Fourth International Workshop on Teaching Analytics, in Conjunction with EC-TEL 2016; 2016 Sept 16; Lyon, France.[place Unknown]: CEUR Workshop Proceedings , (pp. 1–8).
Moore, B.L. (2018). 6. The Role of Data Analytics in Education Possibilities and Limitations, 1st edn.https://doi.org/10.4324/9780203728703-8.
Mor, Y., Ferguson, R., Wasson, B. (2015). Learning design, teacher inquiry into student learning and learning analytics: A call for action. British Journal of Educational Technology , 46 (2), 221–229.
Müller, W., Rebholz, S., Libbrecht, P. (2016). Automatic inspection of e-portfolios for improving formative and summative assessment. In International Symposium on Emerging Technologies for Education . Springer, (pp. 480–489).
Norris, D., Baer, L., Leonard, J., Pugliese, L., Lefrere, P. (2008). Action analytics: Measuring and improving performance that matters in higher education. EDUCAUSE Review , 43 (1), 42.
Olson, D.L., & Lauhoff, G. (2019). Descriptive data mining , (pp. 129–130): Springer.
Osterman, K.F., & Kottkamp, R.B. (1993). Reflective Practice for Educators: Improving Schooling Through Professional Development : ERIC.
Pantazos, K., & Vatrapu, R. (2016). Enhancing the professional vision of teachers: A physiological study of teaching analytics dashboards of students’ repertory grid exercises in business education. In System Sciences (HICSS), 2016 49th Hawaii International Conference On . IEEE, (pp. 41–50).
Pantazos, K., Vatrapu, R.K., Hussain, A. (2013). Visualizing repertory grid data for formative assessment. In IWTA@ LAK .
Papamitsiou, Z., & Economides, A.A. (2016). Learning analytics for smart learning environments: A meta-analysis of empirical research results from 2009 to 2015. Learning, Design, and Technology: An International Compendium of Theory, Research, Practice, and Policy , 1–23. https://doi.org/10.1007/978-3-319-17727-4_15-1 .
Pardo, A., & Siemens, G. (2014). Ethical and privacy principles for learning analytics. British Journal of Educational Technology , 45 (3), 438–450.
Pascual-Miguel, F., Chaparro-Pelaez, J., Hernandez-Garcia, A., Iglesias-Pradas, S. (2011). A characterisation of passive and active interactions and their influence on students’ achievement using moodle lms logs. International Journal of Technology Enhanced Learning , 3 (4), 403–414.
Pennings, H.J., Brekelmans, M., Wubbels, T., van der Want, A.C., Claessens, L.C., van Tartwijk, J. (2014). A nonlinear dynamical systems approach to real-time teacher behavior: Differences between teachers. Nonlinear Dynamics, Psychology, and Life Sciences , 18 (1), 23–45.
Persico, D., & Pozzi, F. (2015). Informing learning design with learning analytics to improve teacher inquiry. British Journal of Educational Technology , 46 (2), 230–248.
Pozzi, F., & Persico, D. (2013). Sustaining learning design and pedagogical planning in cscl. Research in Learning Technology , 21 . https://doi.org/10.3402/rlt.v21i0.17585 .
Prieto, L.P., Magnuson, P., Dillenbourg, P., Saar, M. (2017). Reflection for action: Designing tools to support teacher reflection on everyday evidence. https://doi.org/10.31219/osf.io/bj2rp .
Prieto, L.P., Rodriguez-Triana, M.J., Kusmin, M., Laanpere, M. (2017). Smart school multimodal dataset and challenges. In Joint Proceedings of the Sixth Multimodal Learning Analytics (MMLA) Workshop and the Second Cross-LAK Workshop Co-located with 7th International Learning Analytics and Knowledge Conference, vol. 1828 . CEUR, (pp. 53–59).
Prieto, L.P., Sharma, K., Dillenbourg, P., Jesús, M. (2016). Teaching analytics: towards automatic extraction of orchestration graphs using wearable sensors. In Proceedings of the Sixth International Conference on Learning Analytics & Knowledge . ACM, (pp. 148–157).
Prieto, L.P., Sharma, K., Kidzinski, Ł., Rodríguez-Triana, M.J., Dillenbourg, P. (2018). Multimodal teaching analytics: Automated extraction of orchestration graphs from wearable sensor data. Journal of Computer Assisted Learning , 34 (2), 193–203.
Ramos, C., & Yudko, E. (2008). "hits"(not "discussion posts") predict student success in online courses: a double cross-validation study. Computers & Education , 50 (4), 1174–1182.
Rayward-Smith, V.J. (2007). Statistics to measure correlation for data mining applications. Computational Statistics & Data Analysis , 51 (8), 3968–3982.
Article MathSciNet MATH Google Scholar
Remmers, H.H., & Brandenburg, G. (1927). Experimental data on the purdue rating scale for instructors. Educational Administration and Supervision , 13 (6), 399–406.
Rienties, B., Boroowa, A., Cross, S., Farrington-Flint, L., Herodotou, C., Prescott, L., Mayles, K., Olney, T., Toetenel, L., Woodthorpe, J. (2016). Reviewing three case-studies of learning analytics interventions at the open university uk. In Proceedings of the Sixth International Conference on Learning Analytics & Knowledge . ACM, (pp. 534–535).
Roberts, L.D., Chang, V., Gibson, D. (2017). Ethical considerations in adopting a university-and system-wide approach to data and learning analytics , (pp. 89–108): Springer. https://doi.org/10.1007/978-3-319-06520-5_7 .
Romero, C., & Ventura, S. (2010). Educational data mining: a review of the state of the art. IEEE Transactions on Systems, Man, and Cybernetics, Part C (Applications and Reviews) , 40 (6), 601–618.
Saar, M., Kusmin, M., Laanpere, M., Prieto, L.P., Rüütmann, T. (2017). Work in progress–semantic annotations and teaching analytics on lecture videos in engineering education. In Global Engineering Education Conference (EDUCON), 2017 IEEE . IEEE, (pp. 1548–1551).
Saar, M., Prieto, L.P., Rodríguez-Triana, M.J., Kusmin, M. (2018). Personalized, teacher-driven in-action data collection: technology design principles. In 2018 IEEE 18th International Conference on Advanced Learning Technologies (ICALT) . IEEE, (pp. 58–62).
Saric, M., & Steh, B. (2017). Critical reflection in the professional development of teachers: Challenges and possibilities. CEPS Journal , 7 (3), 67–85.
Saye, J.W., & Brush, T. (2007). Using technology-enhanced learning environments to support problem-based historical inquiry in secondary school classrooms. Theory Research in Social Education , 35 (2), 196–230.
Schempp, P., McCullick, B., Pierre, P.S., Woorons, S., You, J., Clark, B. (2004). Expert golf instructors’ student-teacher interaction patterns. Research Quarterly for Exercise and Sport , 75 (1), 60–70.
Schmidlin, K., Clough-Gorr, K.M., Spoerri, A. (2015). Privacy preserving probabilistic record linkage (p3rl): a novel method for linking existing health-related data and maintaining participant confidentiality. BMC Medical Research Methodology , 15 (1), 46.
Sergis, S., & Sampson, D.G. (2016). Towards a teaching analytics tool for supporting reflective educational (re) design in inquiry-based stem education. In Advanced Learning Technologies (ICALT), 2016 IEEE 16th International Conference On . https://doi.org/10.1109/icalt.2016.134 . IEEE, (pp. 314–318).
Sergis, S., & Sampson, D.G. (2017). Teaching and learning analytics to support teacher inquiry: A systematic literature review , (pp. 25–63): Springer. https://doi.org/10.1007/978-3-319-52977-6_2 .
Sergis, S., Sampson, D.G., Rodríguez-Triana, M.J., Gillet, D., Pelliccione, L., de Jong, T. (2017). Using educational data from teaching and learning to inform teachers’ reflective educational design in inquiry-based stem education. Computers in Human Behavior . https://doi.org/10.1016/j.chb.2017.12.014 .
Shen, J., Chen, H., Jiang, J. (2018). A research on techniques for data fusion and analysis of cross-platform mooc data. In 2018 17th International Conference on Information Technology Based Higher Education and Training (ITHET) . IEEE, (pp. 1–8).
Siemens, G. (2013). Learning analytics: The emergence of a discipline. American Behavioral Scientist , 57 (10), 1380–1400.
Stier, S., Breuer, J., Siegers, P., Thorson, K. (2019). Integrating Survey Data and Digital Trace Data: Key Issues in Developing an Emerging Field. https://doi.org/10.1177/0894439319843669 .
Subramanya, S. (2014). Toward a more effective and useful end-of-course evaluation scheme. Journal of Research in Innovative Teaching , 7 (1).
Suehiro, D., Taniguchi, Y., Shimada, A., Ogata, H. (2017). Face-to-face teaching analytics: Extracting teaching activities from e-book logs via time-series analysis. In Advanced Learning Technologies (ICALT), 2017 IEEE 17th International Conference On . IEEE, (pp. 267–268).
Sun, J., Przybylski, R., Johnson, B.J. (2016). A review of research on teachers’ use of student data: From the perspective of school leadership. Educational Assessment, Evaluation and Accountability , 28 (1), 5–33.
Taniguchi, Y., Suehiro, D., Shimada, A., Ogata, H. (2017). Revealing hidden impression topics in students’journals based on nonnegative matrix factorization. In Advanced Learning Technologies (ICALT), 2017 IEEE 17th International Conference On . IEEE, (pp. 298–300).
Thille, C., & Zimmaro, D. (2017). Incorporating learning analytics in the classroom. New Directions for Higher Education , 2017 (179), 19–31.
Thomas, C. (2018). Multimodal teaching and learning analytics for classroom and online educational settings. In Proceedings of the 2018 on International Conference on Multimodal Interaction . ACM, (pp. 542–545).
ur Rehman, M.H., Chang, V., Batool, A., Wah, T.Y. (2016). Big data reduction framework for value creation in sustainable enterprises. International Journal of Information Management , 36 (6), 917–928.
Van Harmelen, M., & Workman, D. (2012). Analytics for learning and teaching. CETIS Analytics Series , 1 (3), 1–40.
van Leeuwen, A., Rummel, N., van Gog, T. (2019). What information should cscl teacher dashboards provide to help teachers interpret cscl situations? International Journal of Computer-Supported Collaborative Learning , 1–29. https://doi.org/10.1007/s11412-019-09299-x .
Vatrapu, R.K. (2012). Towards semiology of teaching analytics. In Workshop Towards Theory and Practice of Teaching Analytics, at the European Conference on Technology Enhanced Learning, TAPTA, vol. 12 .
Vatrapu, R.K., Kocherla, K., Pantazos, K. (2013). iklassroom: Real-time, real-place teaching analytics. In IWTA@ LAK .
Vatrapu, R., Reimann, P., Bull, S., Johnson, M. (2013). An eye-tracking study of notational, informational, and emotional aspects of learning analytics representations. In ACM International Conference Proceeding Series . https://doi.org/10.1145/2460296.2460321 . https://www.scopus.com/inward/record.uri?eid=2-s2.0-84876499638&doi=10.1145%2f2460296.2460321&partnerID=40&md5=e7b4d83a3e33e7a1c3c5b5f56d5ebe7d , (pp. 125–134).
Vatrapu, R., Reimann, P., Hussain, A., Kocherla, K. (2013). Towards teaching analytics: Repertory grids for formative assessment (rgfa). In CSCL 2013 Conference Proceedings, vol 2 , (pp. 422–426).
Vatrapu, R., Tanveer, U., Hussain, A. (2012). Towards teaching analytics: communication and negotiation tool (coneto). In Proceedings of the 7th Nordic Conference on Human-Computer Interaction: Making Sense Through Design . ACM, (pp. 775–776).
Vatrapu, R., Teplovs, C., Fujita, N., Bull, S. (2011). Towards visual analytics for teachers’ dynamic diagnostic pedagogical decision-making. In Proceedings of the 1st International Conference on Learning Analytics and Knowledge . ACM, (pp. 93–98).
Verbert, K., Duval, E., Klerkx, J., Govaerts, S., Santos, J.L. (2013). Learning analytics dashboard applications. American Behavioral Scientist , 57 (10), 1500–1509.
Voithofer, R., & Golan, A.M. (2018). 5. Data Sources for Educators, 1st edn.https://doi.org/10.4324/9780203728703-7, (p. 18).
Waller, M.A., & Fawcett, S.E. (2013). Data science, predictive analytics, and big data: a revolution that will transform supply chain design and management. Journal of Business Logistics , 34 (2), 77–84.
Wang, F., & Hannafin, M.J. (2005). Design-based research and technology-enhanced learning environments. Educational Technology Research and Development , 53 (4), 5–23.
Williamson, B. (2016). Digital education governance: data visualization, predictive analytics, and ’real-time’ policy instruments. Journal of Education Policy , 31 (2), 123–141.
Winkler, R., & Söllner, M. (2018). Unleashing the potential of chatbots in education: A state-of-the-art analysis. In Academy of Management Annual Meeting (AOM) . https://doi.org/10.5465/ambpp.2018.15903abstract .
Xu, B., & Recker, M. (2012). Teaching analytics: A clustering and triangulation study of digital library user data. Educational Technology & Society , 15 (3), 103–115.
Yigitbasioglu, O.M., & Velcu, O. (2012). A review of dashboards in performance management: Implications for design and research. International Journal of Accounting Information Systems , 13 (1), 41–59.
Download references
Acknowledgements
The research reported is part of an ongoing PhD research study in the area of Big Data Analytics in Higher Education. We also want to thank members of the Technology Enhanced Learning and Teaching (TELT) Committee of the University of Otago, New Zealand for support and for providing constructive feedback.
This research project was fully sponsored by Higher Education Development Centre, University of Otago, New Zealand.
Author information
Authors and affiliations.
Higher Education Development Centre, University of Otago, Dunedin, New Zealand
Ifeanyi Glory Ndukwe & Ben Kei Daniel
You can also search for this author in PubMed Google Scholar
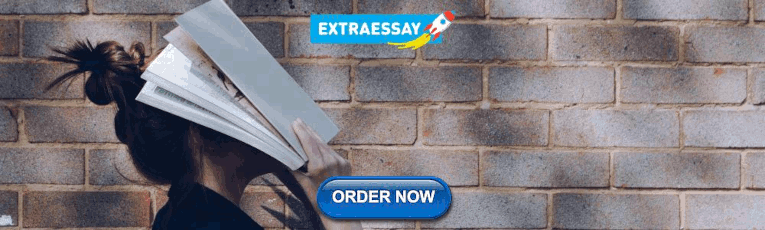
Contributions
IGN conceived and presented the Conceptualisation of Teaching Analytics and Teachingv Outcome Model. BKD developed the Tripartite Approach that was utilised in this research. BKD encouraged IGN to perform a systematic review of teaching analytics that was guided by the Tripartite Approach. BKD supervised the findings of this work. IGN took the lead in writing the manuscript. All authors discussed the results, provided critical feedback and contributed to the final manuscript.
Corresponding author
Correspondence to Ifeanyi Glory Ndukwe .
Ethics declarations
Competing interests.
The authors declare that they have no competing interests. All authors have approved the manuscript and agree with its submission to the International Journal of Education Technology in Higher Education. This manuscript has not been published and is not under consideration for publication elsewhere.
Additional information
Publisher’s note.
Springer Nature remains neutral with regard to jurisdictional claims in published maps and institutional affiliations.
Rights and permissions
Open Access This article is licensed under a Creative Commons Attribution 4.0 International License, which permits use, sharing, adaptation, distribution and reproduction in any medium or format, as long as you give appropriate credit to the original author(s) and the source, provide a link to the Creative Commons licence, and indicate if changes were made. The images or other third party material in this article are included in the article’s Creative Commons licence, unless indicated otherwise in a credit line to the material. If material is not included in the article’s Creative Commons licence and your intended use is not permitted by statutory regulation or exceeds the permitted use, you will need to obtain permission directly from the copyright holder. To view a copy of this licence, visit http://creativecommons.org/licenses/by/4.0/ .
Reprints and permissions
About this article
Cite this article.
Ndukwe, I.G., Daniel, B.K. Teaching analytics, value and tools for teacher data literacy: a systematic and tripartite approach. Int J Educ Technol High Educ 17 , 22 (2020). https://doi.org/10.1186/s41239-020-00201-6
Download citation
Received : 29 October 2019
Accepted : 01 April 2020
Published : 22 June 2020
DOI : https://doi.org/10.1186/s41239-020-00201-6
Share this article
Anyone you share the following link with will be able to read this content:
Sorry, a shareable link is not currently available for this article.
Provided by the Springer Nature SharedIt content-sharing initiative
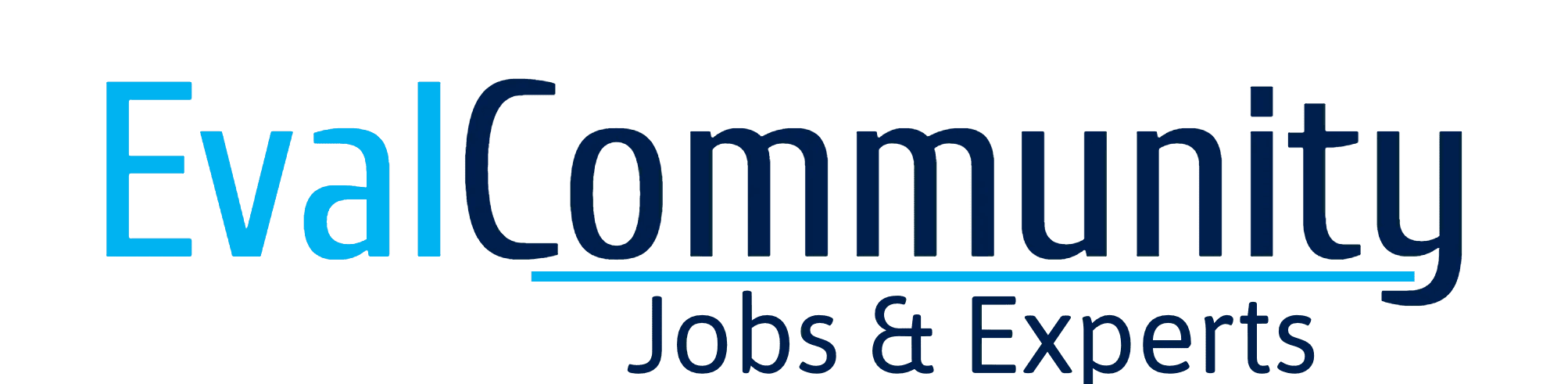
- How to Write Evaluation Reports: Purpose, Structure, Content, Challenges, Tips, and Examples
- Learning Center
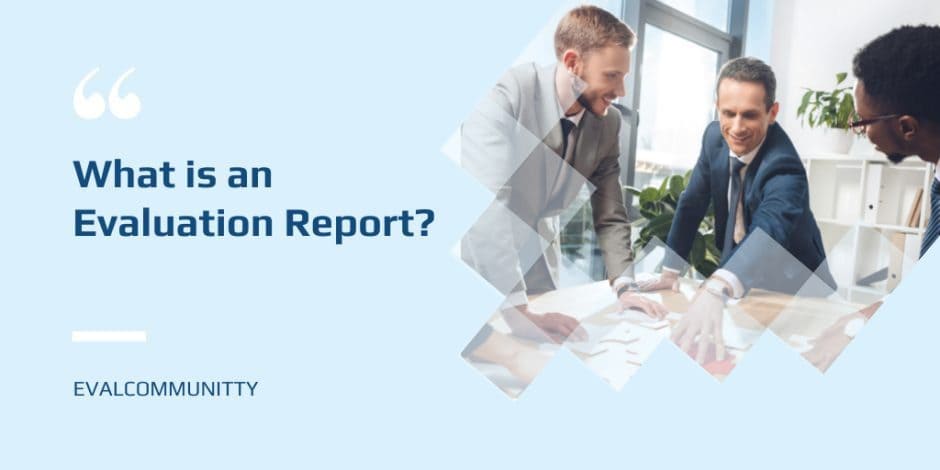
This article explores how to write effective evaluation reports, covering their purpose, structure, content, and common challenges. It provides tips for presenting evaluation findings effectively and using evaluation reports to improve programs and policies. Examples of well-written evaluation reports and templates are also included.
Table of Contents
What is an Evaluation Report?
What is the purpose of an evaluation report, importance of evaluation reports in program management, structure of evaluation report, best practices for writing an evaluation report, common challenges in writing an evaluation report, tips for presenting evaluation findings effectively, using evaluation reports to improve programs and policies, example of evaluation report templates, conclusion: making evaluation reports work for you.
An evaluatio n report is a document that presents the findings, conclusions, and recommendations of an evaluation, which is a systematic and objective assessment of the performance, impact, and effectiveness of a program, project, policy, or intervention. The report typically includes a description of the evaluation’s purpose, scope, methodology, and data sources, as well as an analysis of the evaluation findings and conclusions, and specific recommendations for program or project improvement.
Evaluation reports can help to build capacity for monitoring and evaluation within organizations and communities, by promoting a culture of learning and continuous improvement. By providing a structured approach to evaluation and reporting, evaluation reports can help to ensure that evaluations are conducted consistently and rigorously, and that the results are communicated effectively to stakeholders.
Evaluation reports may be read by a wide variety of audiences, including persons working in government agencies, staff members working for donors and partners, students and community organisations, and development professionals working on projects or programmes that are comparable to the ones evaluated.
Related: Difference Between Evaluation Report and M&E Reports .
The purpose of an evaluation report is to provide stakeholders with a comprehensive and objective assessment of a program or project’s performance, achievements, and challenges. The report serves as a tool for decision-making, as it provides evidence-based information on the program or project’s strengths and weaknesses, and recommendations for improvement.
The main objectives of an evaluation report are:
- Accountability: To assess whether the program or project has met its objectives and delivered the intended results, and to hold stakeholders accountable for their actions and decisions.
- Learning : To identify the key lessons learned from the program or project, including best practices, challenges, and opportunities for improvement, and to apply these lessons to future programs or projects.
- Improvement : To provide recommendations for program or project improvement based on the evaluation findings and conclusions, and to support evidence-based decision-making.
- Communication : To communicate the evaluation findings and conclusions to stakeholders , including program staff, funders, policymakers, and the general public, and to promote transparency and stakeholder engagement.
An evaluation report should be clear, concise, and well-organized, and should provide stakeholders with a balanced and objective assessment of the program or project’s performance. The report should also be timely, with recommendations that are actionable and relevant to the current context. Overall, the purpose of an evaluation report is to promote accountability, learning, and improvement in program and project design and implementation.
Evaluation reports play a critical role in program management by providing valuable information about program effectiveness and efficiency. They offer insights into the extent to which programs have achieved their objectives, as well as identifying areas for improvement.
Evaluation reports help program managers and stakeholders to make informed decisions about program design, implementation, and funding. They provide evidence-based information that can be used to improve program outcomes and address challenges.
Moreover, evaluation reports are essential in demonstrating program accountability and transparency to funders, policymakers, and other stakeholders. They serve as a record of program activities and outcomes, allowing stakeholders to assess the program’s impact and sustainability.
In short, evaluation reports are a vital tool for program managers and evaluators. They provide a comprehensive picture of program performance, including strengths, weaknesses, and areas for improvement. By utilizing evaluation reports, program managers can make informed decisions to improve program outcomes and ensure that their programs are effective, efficient, and sustainable over time.
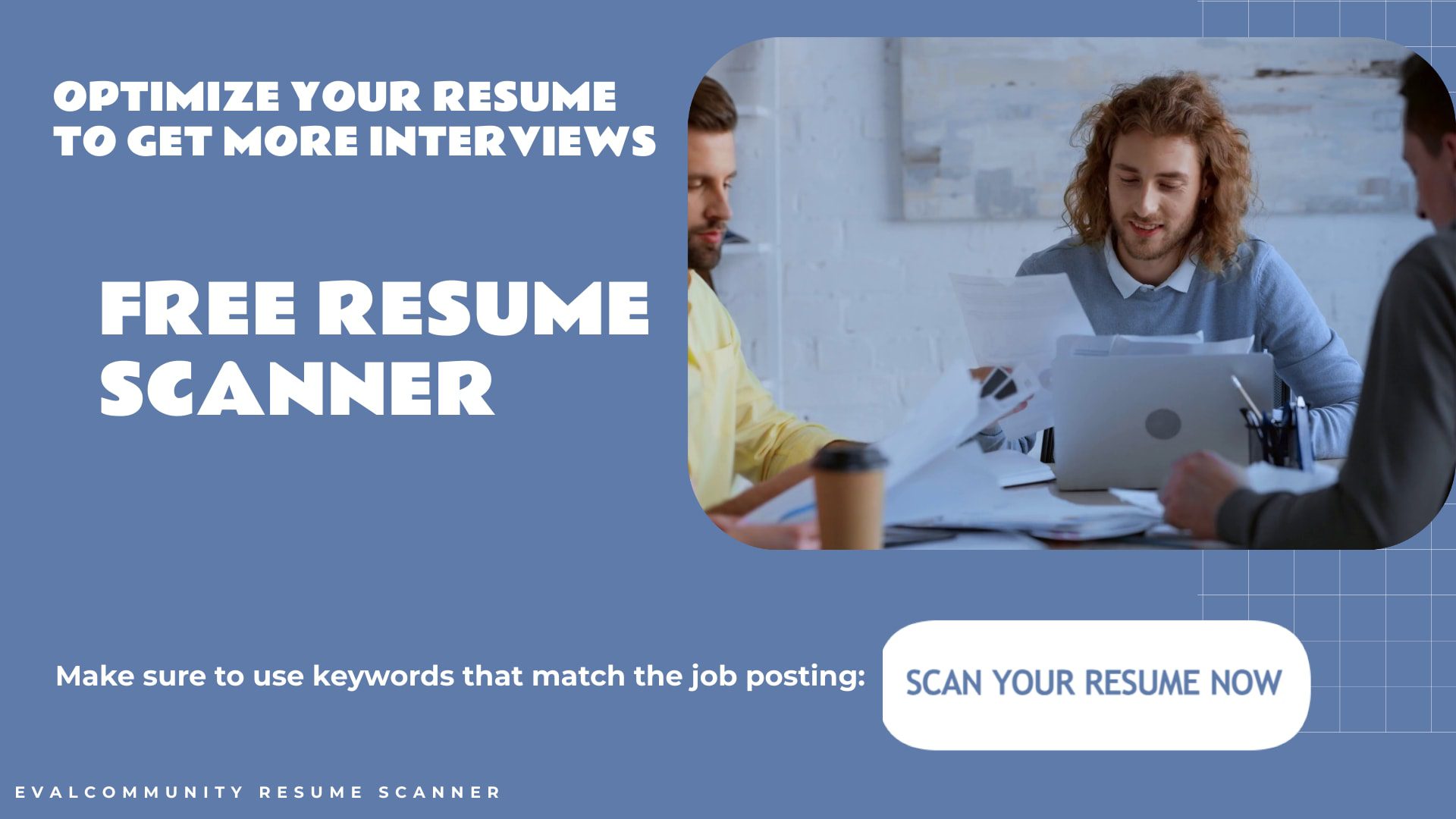
The structure of an evaluation report can vary depending on the requirements and preferences of the stakeholders, but typically it includes the following sections:
- Executive Summary : A brief summary of the evaluation findings, conclusions, and recommendations.
- Introduction: An overview of the evaluation context, scope, purpose, and methodology.
- Background: A summary of the programme or initiative that is being assessed, including its goals, activities, and intended audience(s).
- Evaluation Questions : A list of the evaluation questions that guided the data collection and analysis.
- Methodology: A description of the data collection methods used in the evaluation, including the sampling strategy, data sources, and data analysis techniques.
- Findings: A presentation of the evaluation findings, organized according to the evaluation questions.
- Conclusions : A summary of the main evaluation findings and conclusions, including an assessment of the program or project’s effectiveness, efficiency, and sustainability.
- Recommendations : A list of specific recommendations for program or project improvements based on the evaluation findings and conclusions.
- Lessons Learned : A discussion of the key lessons learned from the evaluation that could be applied to similar programs or projects in the future.
- Limitations : A discussion of the limitations of the evaluation, including any challenges or constraints encountered during the data collection and analysis.
- References: A list of references cited in the evaluation report.
- Appendices : Additional information, such as detailed data tables, graphs, or maps, that support the evaluation findings and conclusions.
The structure of the evaluation report should be clear, logical, and easy to follow, with headings and subheadings used to organize the content and facilitate navigation.
In addition, the presentation of data may be made more engaging and understandable by the use of visual aids such as graphs and charts.
Writing an effective evaluation report requires careful planning and attention to detail. Here are some best practices to consider when writing an evaluation report:
Begin by establishing the report’s purpose, objectives, and target audience. A clear understanding of these elements will help guide the report’s structure and content.
Use clear and concise language throughout the report. Avoid jargon and technical terms that may be difficult for readers to understand.
Use evidence-based findings to support your conclusions and recommendations. Ensure that the findings are clearly presented using data tables, graphs, and charts.
Provide context for the evaluation by including a brief summary of the program being evaluated, its objectives, and intended impact. This will help readers understand the report’s purpose and the findings.
Include limitations and caveats in the report to provide a balanced assessment of the program’s effectiveness. Acknowledge any data limitations or other factors that may have influenced the evaluation’s results.
Organize the report in a logical manner, using headings and subheadings to break up the content. This will make the report easier to read and understand.
Ensure that the report is well-structured and easy to navigate. Use a clear and consistent formatting style throughout the report.
Finally, use the report to make actionable recommendations that will help improve program effectiveness and efficiency. Be specific about the steps that should be taken and the resources required to implement the recommendations.
By following these best practices, you can write an evaluation report that is clear, concise, and actionable, helping program managers and stakeholders to make informed decisions that improve program outcomes.
Catch HR’s eye instantly?
- Resume Review
- Resume Writing
- Resume Optimization
Premier global development resume service since 2012
Stand Out with a Pro Resume
Writing an evaluation report can be a challenging task, even for experienced evaluators. Here are some common challenges that evaluators may encounter when writing an evaluation report:
- Data limitations: One of the biggest challenges in writing an evaluation report is dealing with data limitations. Evaluators may find that the data they collected is incomplete, inaccurate, or difficult to interpret, making it challenging to draw meaningful conclusions.
- Stakeholder disagreements: Another common challenge is stakeholder disagreements over the evaluation’s findings and recommendations. Stakeholders may have different opinions about the program’s effectiveness or the best course of action to improve program outcomes.
- Technical writing skills: Evaluators may struggle with technical writing skills, which are essential for presenting complex evaluation findings in a clear and concise manner. Writing skills are particularly important when presenting statistical data or other technical information.
- Time constraints: Evaluators may face time constraints when writing evaluation reports, particularly if the report is needed quickly or the evaluation involved a large amount of data collection and analysis.
- Communication barriers: Evaluators may encounter communication barriers when working with stakeholders who speak different languages or have different cultural backgrounds. Effective communication is essential for ensuring that the evaluation’s findings are understood and acted upon.
By being aware of these common challenges, evaluators can take steps to address them and produce evaluation reports that are clear, accurate, and actionable. This may involve developing data collection and analysis plans that account for potential data limitations, engaging stakeholders early in the evaluation process to build consensus, and investing time in developing technical writing skills.
Presenting evaluation findings effectively is essential for ensuring that program managers and stakeholders understand the evaluation’s purpose, objectives, and conclusions. Here are some tips for presenting evaluation findings effectively:
- Know your audience: Before presenting evaluation findings, ensure that you have a clear understanding of your audience’s background, interests, and expertise. This will help you tailor your presentation to their needs and interests.
- Use visuals: Visual aids such as graphs, charts, and tables can help convey evaluation findings more effectively than written reports. Use visuals to highlight key data points and trends.
- Be concise: Keep your presentation concise and to the point. Focus on the key findings and conclusions, and avoid getting bogged down in technical details.
- Tell a story: Use the evaluation findings to tell a story about the program’s impact and effectiveness. This can help engage stakeholders and make the findings more memorable.
- Provide context: Provide context for the evaluation findings by explaining the program’s objectives and intended impact. This will help stakeholders understand the significance of the findings.
- Use plain language: Use plain language that is easily understandable by your target audience. Avoid jargon and technical terms that may confuse or alienate stakeholders.
- Engage stakeholders: Engage stakeholders in the presentation by asking for their input and feedback. This can help build consensus and ensure that the evaluation findings are acted upon.
By following these tips, you can present evaluation findings in a way that engages stakeholders, highlights key findings, and ensures that the evaluation’s conclusions are acted upon to improve program outcomes.
Evaluation reports are crucial tools for program managers and policymakers to assess program effectiveness and make informed decisions about program design, implementation, and funding. By analyzing data collected during the evaluation process, evaluation reports provide evidence-based information that can be used to improve program outcomes and impact.
One of the primary ways that evaluation reports can be used to improve programs and policies is by identifying program strengths and weaknesses. By assessing program effectiveness and efficiency, evaluation reports can help identify areas where programs are succeeding and areas where improvements are needed. This information can inform program redesign and improvement efforts, leading to better program outcomes and impact.
Evaluation reports can also be used to make data-driven decisions about program design, implementation, and funding. By providing decision-makers with data-driven information, evaluation reports can help ensure that programs are designed and implemented in a way that maximizes their impact and effectiveness. This information can also be used to allocate resources more effectively, directing funding towards programs that are most effective and efficient.
Another way that evaluation reports can be used to improve programs and policies is by disseminating best practices in program design and implementation. By sharing information about what works and what doesn’t work, evaluation reports can help program managers and policymakers make informed decisions about program design and implementation, leading to better outcomes and impact.
Finally, evaluation reports can inform policy development and improvement efforts by providing evidence about the effectiveness and impact of existing policies. This information can be used to make data-driven decisions about policy development and improvement efforts, ensuring that policies are designed and implemented in a way that maximizes their impact and effectiveness.
In summary, evaluation reports are critical tools for improving programs and policies. By providing evidence-based information about program effectiveness and efficiency, evaluation reports can help program managers and policymakers make informed decisions, allocate resources more effectively, disseminate best practices, and inform policy development and improvement efforts.
There are many different templates available for creating evaluation reports. Here are some examples of template evaluation reports that can be used as a starting point for creating your own report:
- The National Science Foundation Evaluation Report Template – This template provides a structure for evaluating research projects funded by the National Science Foundation. It includes sections on project background, research questions, evaluation methodology, data analysis, and conclusions and recommendations.
- The CDC Program Evaluation Template – This template, created by the Centers for Disease Control and Prevention, provides a framework for evaluating public health programs. It includes sections on program description, evaluation questions, data sources, data analysis, and conclusions and recommendations.
- The World Bank Evaluation Report Template – This template, created by the World Bank, provides a structure for evaluating development projects. It includes sections on project background, evaluation methodology, data analysis, findings and conclusions, and recommendations.
- The European Commission Evaluation Report Template – This template provides a structure for evaluating European Union projects and programs. It includes sections on project description, evaluation objectives, evaluation methodology, findings, conclusions, and recommendations.
- The UNICEF Evaluation Report Template – This template provides a framework for evaluating UNICEF programs and projects. It includes sections on program description, evaluation questions, evaluation methodology, findings, conclusions, and recommendations.
These templates provide a structure for creating evaluation reports that are well-organized and easy to read. They can be customized to meet the specific needs of your program or project and help ensure that your evaluation report is comprehensive and includes all of the necessary components.
- World Health Organisations Reports
- Checkl ist for Assessing USAID Evaluation Reports
In conclusion, evaluation reports are essential tools for program managers and policymakers to assess program effectiveness and make informed decisions about program design, implementation, and funding. By analyzing data collected during the evaluation process, evaluation reports provide evidence-based information that can be used to improve program outcomes and impact.
To make evaluation reports work for you, it is important to plan ahead and establish clear objectives and target audiences. This will help guide the report’s structure and content and ensure that the report is tailored to the needs of its intended audience.
When writing an evaluation report, it is important to use clear and concise language, provide evidence-based findings, and offer actionable recommendations that can be used to improve program outcomes. Including context for the evaluation findings and acknowledging limitations and caveats will provide a balanced assessment of the program’s effectiveness and help build trust with stakeholders.
Presenting evaluation findings effectively requires knowing your audience, using visuals, being concise, telling a story, providing context, using plain language, and engaging stakeholders. By following these tips, you can present evaluation findings in a way that engages stakeholders, highlights key findings, and ensures that the evaluation’s conclusions are acted upon to improve program outcomes.
Finally, using evaluation reports to improve programs and policies requires identifying program strengths and weaknesses, making data-driven decisions, disseminating best practices, allocating resources effectively, and informing policy development and improvement efforts. By using evaluation reports in these ways, program managers and policymakers can ensure that their programs are effective, efficient, and sustainable over time.
Well understanding, the description of the general evaluation of report are clear with good arrangement and it help students to learn and make practices
Patrick Kapuot
Thankyou for very much for such detail information. Very comprehensively said.
hailemichael
very good explanation, thanks
Leave a Comment Cancel Reply
Your email address will not be published.
How strong is my Resume?
Only 2% of resumes land interviews.
Land a better, higher-paying career
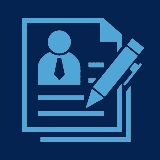
Jobs for You
Water, sanitation and hygiene advisor (wash) – usaid/drc.
- Democratic Republic of the Congo
Health Supply Chain Specialist – USAID/DRC
Chief of party – bosnia and herzegovina.
- Bosnia and Herzegovina
Project Manager I
- United States
Business Development Associate
Director of finance and administration, request for information – collecting information on potential partners for local works evaluation.
- Washington, USA
Principal Field Monitors
Technical expert (health, wash, nutrition, education, child protection, hiv/aids, supplies), survey expert, data analyst, team leader, usaid-bha performance evaluation consultant.
- International Rescue Committee
Manager II, Institutional Support Program Implementation
Senior human resources associate, services you might be interested in, useful guides ....
How to Create a Strong Resume
Monitoring And Evaluation Specialist Resume
Resume Length for the International Development Sector
Types of Evaluation
Monitoring, Evaluation, Accountability, and Learning (MEAL)
LAND A JOB REFERRAL IN 2 WEEKS (NO ONLINE APPS!)
Sign Up & To Get My Free Referral Toolkit Now:
Numbers, Facts and Trends Shaping Your World
Read our research on:
Full Topic List
Regions & Countries
- Publications
- Our Methods
- Short Reads
- Tools & Resources
Read Our Research On:
Is College Worth It?
As economic outcomes for young adults with and without degrees have improved, americans hold mixed views on the value of college, table of contents.
- Labor force trends and economic outcomes for young adults
- Economic outcomes for young men
- Economic outcomes for young women
- Wealth trends for households headed by a young adult
- The importance of a four-year college degree
- Getting a high-paying job without a college degree
- Do Americans think their education prepared them for the workplace?
- Is college worth the cost?
- Acknowledgments
- The American Trends Panel survey methodology
- Current Population Survey methodology
- Survey of Consumer Finances methodology
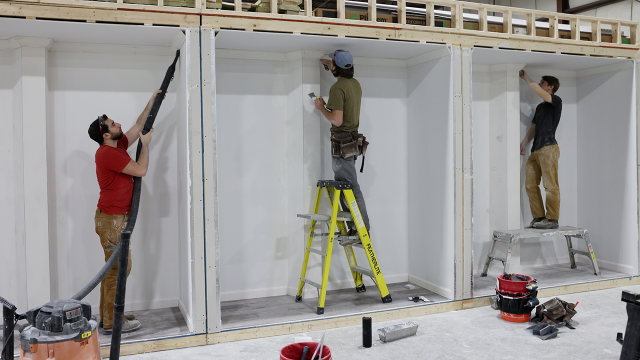
Pew Research Center conducted this study to better understand public views on the importance of a four-year college degree. The study also explores key trends in the economic outcomes of young adults among those who have and have not completed a four-year college degree.
The analysis in this report is based on three data sources. The labor force, earnings, hours, household income and poverty characteristics come from the U.S. Census Bureau’s Annual Social and Economic Supplement of the Current Population Survey. The findings on net worth are based on the Federal Reserve’s Survey of Consumer Finances.
The data on public views on the value of a college degree was collected as part of a Center survey of 5,203 U.S. adults conducted Nov. 27 to Dec. 3, 2023. Everyone who took part in the survey is a member of Pew Research Center’s American Trends Panel (ATP), an online survey panel that is recruited through national, random sampling of residential addresses. Address-based sampling ensures that nearly all U.S. adults have a chance of selection. The survey is weighted to be representative of the U.S. adult population by gender, race, ethnicity, partisan affiliation, education and other categories. Read more about the ATP’s methodology .
Here are the questions used for this report , along with responses, and the survey’s methodology .
Young adults refers to Americans ages 25 to 34.
Noncollege adults include those who have some college education as well as those who graduated from high school but did not attend college. Adults who have not completed high school are not included in the analysis of noncollege adults. About 6% of young adults have not completed high school. Trends in some labor market outcomes for those who have not finished high school are impacted by changes in the foreign-born share of the U.S. population. The Census data used in this analysis did not collect information on nativity before 1994.
Some college includes those with an associate degree and those who attended college but did not obtain a degree.
The some college or less population refers to adults who have some college education, those with a high school diploma only and those who did not graduate high school.
A full-time, full-year worker works at least 50 weeks per year and usually 35 hours a week or more.
The labor force includes all who are employed and those who are unemployed but looking for work.
The labor force participation rate is the share of a population that is in the labor force.
Young adults living independently refers to those who are not living in the home of either of their parents.
Household income is the sum of incomes received by all members of the household ages 15 and older. Income is the sum of earnings from work, capital income such as interest and dividends, rental income, retirement income, and transfer income (such as government assistance) before payments for such things as personal income taxes, Social Security and Medicare taxes, union dues, etc. Non-cash transfers such as food stamps, health benefits, subsidized housing and energy assistance are not included. As household income is pretax, it does not include stimulus payments or tax credits for earned income and children/dependent care.
Net worth, or wealth, is the difference between the value of what a household owns (assets) and what it owes (debts).
All references to party affiliation include those who lean toward that party. Republicans include those who identify as Republicans and those who say they lean toward the Republican Party. Democrats include those who identify as Democrats and those who say they lean toward the Democratic Party.
At a time when many Americans are questioning the value of a four-year college degree, economic outcomes for young adults without a degree are improving.
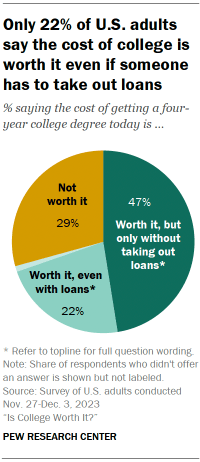
After decades of falling wages, young U.S. workers (ages 25 to 34) without a bachelor’s degree have seen their earnings increase over the past 10 years. Their overall wealth has gone up too, and fewer are living in poverty today.
Things have also improved for young college graduates over this period. As a result, the gap in earnings between young adults with and without a college degree has not narrowed.
The public has mixed views on the importance of having a college degree, and many have doubts about whether the cost is worth it, according to a new Pew Research Center survey.
- Only one-in-four U.S. adults say it’s extremely or very important to have a four-year college degree in order to get a well-paying job in today’s economy. About a third (35%) say a college degree is somewhat important, while 40% say it’s not too or not at all important.
- Roughly half (49%) say it’s less important to have a four-year college degree today in order to get a well-paying job than it was 20 years ago; 32% say it’s more important, and 17% say it’s about as important as it was 20 years ago.
- Only 22% say the cost of getting a four-year college degree today is worth it even if someone has to take out loans. Some 47% say the cost is worth it only if someone doesn’t have to take out loans. And 29% say the cost is not worth it.
These findings come amid rising tuition costs and mounting student debt . Views on the cost of college differ by Americans’ level of education. But even among four-year college graduates, only about a third (32%) say college is worth the cost even if someone has to take out loans – though they are more likely than those without a degree to say this.
Four-year college graduates (58%) are much more likely than those without a college degree (26%) to say their education was extremely or very useful in giving them the skills and knowledge they needed to get a well-paying job. (This finding excludes the 9% of respondents who said this question did not apply to them.)
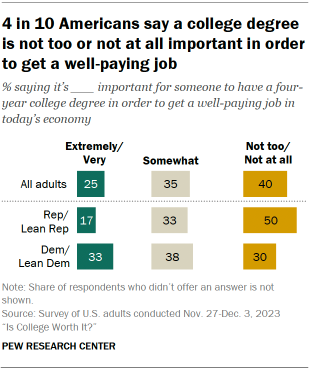
Views on the importance of college differ widely by partisanship. Republicans and Republican-leaning independents are more likely than Democrats and Democratic leaners to say:
- It’s not too or not at all important to have a four-year college degree in order to get a well-paying job (50% of Republicans vs. 30% of Democrats)
- A college degree is less important now than it was 20 years ago (57% vs. 43%)
- It’s extremely or very likely someone without a four-year college degree can get a well-paying job (42% vs. 26%)
At the same time that the public is expressing doubts about the value of college, a new Center analysis of government data finds young adults without a college degree are doing better on some key measures than they have in recent years.
A narrow majority of workers ages 25 to 34 do not have a four-year college degree (54% in 2023). Earnings for these young workers mostly trended downward from the mid-1970s until roughly a decade ago.
Outcomes have been especially poor for young men without a college degree. Other research has shown that this group saw falling labor force participation and sagging earnings starting in the early 1970s , but the last decade has marked a turning point.
This analysis looks at young men and young women separately because of their different experiences in the labor force.
Trends for young men
- Labor force participation: The share of young men without a college degree who were working or looking for work dropped steadily from 1970 until about 2014. Our new analysis suggests things have stabilized somewhat for this group over the past decade. Meanwhile, labor force participation among young men with a four-year degree has remained mostly flat.
- Full-time, full-year employment: The share of employed young men without a college degree who are working full time and year-round has varied somewhat over the years – trending downward during recessions. It’s risen significantly since the Great Recession of 2007-09, with the exception of a sharp dip in 2021 due to the COVID-19 pandemic. For employed young men with a college degree, the share working full time, full year has remained more stable over the years.
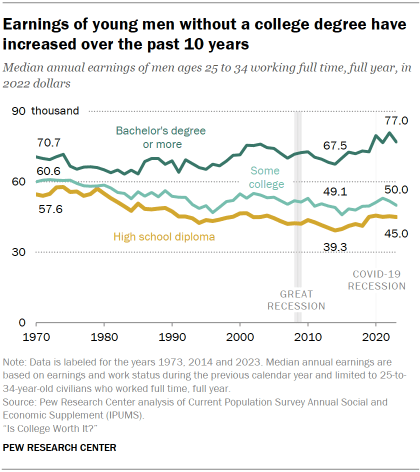
- Median annual earnings: Since 2014, earnings have risen for young men with some college education and for those whose highest attainment is a high school diploma. Even so, earnings for these groups remain below where they were in the early 1970s. Earnings for young men with a bachelor’s degree have also trended up, for the most part, over the past 10 years.
- Poverty: Among young men without a college degree who are living independently from their parents, the share in poverty has fallen significantly over the last decade. For example, 12% of young men with a high school diploma were living in poverty in 2023, down from a peak of 17% in 2011. The share of young men with a four-year college degree who are in poverty has also fallen and remains below that of noncollege young men.
Trends for young women
- Labor force participation: The shares of young women with and without a college degree in the labor force grew steadily from 1970 to about 1990. Among those without a college degree, the share fell after 2000, and the drop-off was especially sharp for young women with a high school diploma. Since 2014, labor force participation for both groups of young women has increased.
- Full-time, full-year employment: The shares of employed young women working full time and year-round, regardless of their educational attainment, have steadily increased over the decades. There was a decline during and after the Great Recession and again (briefly) in 2021 due to the pandemic. Today, the shares of women working full time, full year are the highest they’ve ever been across education levels.
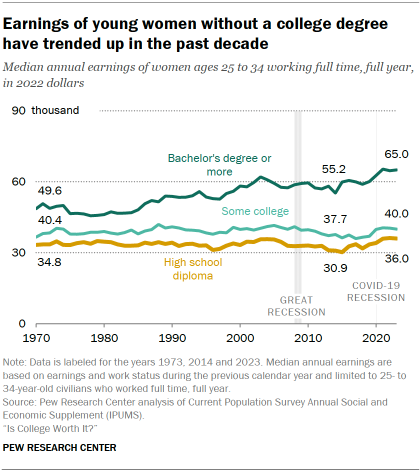
- Median annual earnings: Median earnings for young women without a college degree were relatively flat from 1970 until about a decade ago. These women did not experience the steady decline in earnings that noncollege young men did over this period. By contrast, earnings have grown over the decades for young women with a college degree. In the past 10 years, earnings for women both with and without a college degree have risen.
- Poverty: As is the case for young men without a college degree, the share of noncollege young women living in poverty has fallen substantially over the past decade. In 2014, 31% of women with a high school diploma who lived independently from their parents were in poverty. By 2023, that share had fallen to 21%. Young women with a college degree remain much less likely to be in poverty than their counterparts with less education.
Sign up for our weekly newsletter
Fresh data delivery Saturday mornings
Sign up for The Briefing
Weekly updates on the world of news & information
- Business & Workplace
- Economic Conditions
- Higher Education
- Income & Wages
- Recessions & Recoveries
- Student Loans
Half of Latinas Say Hispanic Women’s Situation Has Improved in the Past Decade and Expect More Gains
From businesses and banks to colleges and churches: americans’ views of u.s. institutions, fewer young men are in college, especially at 4-year schools, key facts about u.s. latinos with graduate degrees, private, selective colleges are most likely to use race, ethnicity as a factor in admissions decisions, most popular, report materials.
1615 L St. NW, Suite 800 Washington, DC 20036 USA (+1) 202-419-4300 | Main (+1) 202-857-8562 | Fax (+1) 202-419-4372 | Media Inquiries
Research Topics
- Email Newsletters
ABOUT PEW RESEARCH CENTER Pew Research Center is a nonpartisan fact tank that informs the public about the issues, attitudes and trends shaping the world. It conducts public opinion polling, demographic research, media content analysis and other empirical social science research. Pew Research Center does not take policy positions. It is a subsidiary of The Pew Charitable Trusts .
© 2024 Pew Research Center

- Condition of Education Digest of Education Statistics Projections of Education Statistics Topical Studies
- National Assessment of Educational Progress (NAEP) Program for the International Assessment of Adult Competencies (PIAAC)
- International Activities Program (IAP)
- Early Childhood Longitudinal Study (ECLS) National Household Education Survey (NHES)
- Common Core of Data (CCD) Secondary Longitudinal Studies Program Education Demographic and Geographic Estimates (EDGE) National Teacher and Principal Survey (NTPS) more...
- Library Statistics Program
- Baccalaureate and Beyond (B&B) Career/Technical Education Statistics (CTES) Integrated Postsecondary Education Data System (IPEDS) National Postsecondary Student Aid Study (NPSAS) more...
- Common Education Data Standards (CEDS) National Forum on Education Statistics Statewide Longitudinal Data Systems Grant Program - (SLDS) more...
- Distance Learning Dataset Training National Postsecondary Education Cooperative (NPEC) Statistical Standards Program more...
- Assessments K-12 Students K-12 Teachers and Faculty K-12 Schools College Students College Faculty College Institutions Mapping
- Delta Cost Project IPEDS Data Center How to apply for Restricted Use License Online Codebook
- ACS-ED Tables Data Lab Elementary Secondary Information System International Data Explorer IPEDS Data Center NAEP Data Explorer
- ACS Dashboard College Navigator Private Schools Public School Districts Public Schools Search for Schools and Colleges
- NAEP State Profiles (nationsreportcard.gov) Public School District Finance Peer Search Education Finance Statistics Center IPEDS Data Center
- NAEP Question Tool NAAL Questions Tool
- ACS-ED Dashboard ACS-ED Maps Locale Lookup MapEd SAFEMap School and District Navigator
- Bibliography ED Data Inventory
- Assessments Early Childhood Elementary and Secondary Postsecondary and Beyond Resources Special Topics
- Search for Schools and Colleges College Navigator Other Search Tools Public Schools Public School Districts Private Schools
- NCES Blog What's New at NCES Conferences/Training NewsFlash Funding Opportunities Press Releases StatChat
- Search Publications and Products Annual Reports Restricted-use Data Licenses Recent Publications By Subject Index A-Z By Survey & Program Areas Data Products Last 6 Months
- About NCES Commissioner Contact NCES Staff Help
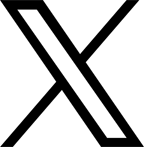
Explore NAEP results! By clicking "continue" you will be leaving the National Assessment of Educational Progress (NAEP) operational website and opening The Nation's Report Card (NRC) website. Explore NAEP results about students' performance, and access a suite of data tools.
The NAEP Long-Term Trend Assessment Results for Reading & Mathematics are here!

Explore by Subject

Explore Results
What is naep.
The Nation’s Report Card is a resource—a common measure of student achievement—because it offers a window into the state of our K-12 education system and what our children are learning. When students, their parents, teachers, and principals participate in the Nation’s Report Card—the largest nationally representative and continuing assessment of what America's students know and can do in various subject areas—they are helping to inform decisions about how to improve the education system in our country.
Your browser does not support iFrame.
- Publications
- IES Centers
- Data Training
- School Search
- IES Policies and Standards
- ED Data Inventory
- IES Diversity Statement
- NCES Statistical Standards
- Peer Review Process
- Privacy and Security Policies
- Public Access Policy
- U.S. Department of Education
- Additional Resources
- Organizational Chart
Tennessee teacher shortage: Analysis gives insight on what's helping, what needs work

Tackling Tennessee's ongoing teacher shortage is the focus of a newly released policy memo from the State Collaborative on Reforming Education, also known as SCORE .
The collaborative was founded in 2009 by former U.S. Sen. Bill Frist as an independent, nonprofit and nonpartisan institution focused on education research and advocacy. The analysis, released Monday, included data from a diverse mix of 15 Tennessee school districts, along with insight into what's driving the teacher shortage and what state and local leaders can do to address it.
"With growing concerns about the educator pipeline and its impact on student learning, Tennessee has an urgent need to better understand how the state is attracting, developing, retaining, and maximizing its educator workforce," the report stated.
The analysis shared insight into the state's education labor markets, the impact of teacher salaries and other factors, what's driving teachers to leave the field, diversity disparities between teachers and students and what can be done to address all those things.
Here are four key takeaways from the analysis.
How Tennessee teacher shortages compare to the rest of the nation
Statewide, the analysis estimated there are around 60,000 teachers in the workforce, with more than 1,000 vacancies. That number, based on 2022 data from the Tennessee Department of Education, reflects unfilled teaching positions that led to the lack of course availability.
"Alarmingly, one-third of these vacancies were in the K-5 grade band," the analysis said.
However, not all school districts were struggling with vacancies. Nearly a third of districts reported no vacancies in the 2022-23 school year. Tennessee's vacancies are also not an outlier in the United States, with the state's shortage on par with the average nationwide, according to the analysis.
Insight on teacher turnover and salaries
Teacher turnover rates are most persistent among newer hires, especially those who lacked resources like coaching, mentoring and peer support, the analysis showed. A growing number of teachers are also undecided about their plans to stay in the profession.
State leaders recently set a goal to make the starting teacher salary $50,000 a year by 2026 and invested an additional $1 billion into public education. Still, nine out of the 15 districts included in the analysis had average staff salaries that fell below the family median income in their respective counties. Taking all the districts into account, the average staff salary sat at $2,068 above that median.
Teacher pay has been central in the push to address shortages in Tennessee and nationwide. In fact, salaries increased far more rapidly from 2021-2023 than in the three years prior, the analysis showed.
More: Tennessee names its top performing schools for 2022-23. See if yours made the list
But when it comes to why teachers are leaving the profession, salary actually ranked fifth on the list. Here are the top five reasons teachers said they decided not to teach the following year:
- 40% said they were leaving on their own for other reasons
- 37% said they were leaving because of leadership
- 27% said they were leaving due to the workload
- 26% said they were leaving due to the culture and climate among teachers and staff
- 23% said they were leaving due to salary
Stark racial, ethnic disparities among teachers, students
The majority of the educators in the 15 districts studied are white, according to the analysis. While that matches up with Tennessee as a whole, a stark disparity is revealed when it compares to student demographics. Here are some of the demographics and numbers the analysis compared among the districts studied:
- 73% of educators are white, versus 37% of students
- 23% of educators are Black, compared to 41% of students
- 2% of educators are Hispanic, compared to 18% of students
- 1% of educators are Asian, compared to 3% of students
A push for state, local action
Things like special permits to issue emergency teaching credentials, salary increases and efforts to strengthen training for teaching programs for new and existing educators have helped the shortage, the analysis said. But there's still more work to be done.
"Tennessee has been a pioneer in educator labor market innovation for decades," the analysis read. "With the ongoing and longstanding staffing challenges, it is time for Tennessee leaders to boldly design and implement the holistic staffing solutions that best serve students for years to come."
More: Tennessee lawmakers take on education: Key K-12 bills to watch in 2024
It mapped out key actions that state and local leaders could take to attract new talent, empower and retain current educators and better understand the forces at play in the education industry on a national, state and local level. Those include:
- Gathering better data that is highly localized to each district
- Focusing on supporting newer teachers
- Revisiting policies on compensation and staffing, including differentiated compensation
- Looking into staffing structures in Tennessee and across the nation that can inform strategy on how to foster a reliable, effective and diverse education workforce
Read the full report for yourself
The analysis, along with other reports from SCORE, can be found at tnscore.org/resources/strengthening-tennessees-educator-labor-market .
UN report says that education, social safety nets vital for Asia to grow rich, cope with aging
A report by the United Nations says that as economies in Asia and the Pacific slow and grow older, countries need to do more to ensure that workers get the education, training and social safety nets needed to raise incomes and ensure social equity
TOKYO — As economies in Asia and the Pacific slow and grow older, countries need to do more to ensure that workers get the education, training and social safety nets needed to raise incomes and ensure social equity, a United Nations report said Tuesday.
The report by the International Labor Organization said that growth in productivity has slowed, hurting incomes and undermining the purchasing power of the region’s 2 billion workers. By improving productivity, governments can boost incomes and better prepare for the aging of their work forces, the report said.

- Election 2024
- Entertainment
- Newsletters
- Photography
- Personal Finance
- AP Investigations
- AP Buyline Personal Finance
- AP Buyline Shopping
- Press Releases
- Israel-Hamas War
- Russia-Ukraine War
- Global elections
- Asia Pacific
- Latin America
- Middle East
- Election Results
- Delegate Tracker
- AP & Elections
- Auto Racing
- 2024 Paris Olympic Games
- Movie reviews
- Book reviews
- Personal finance
- Financial Markets
- Business Highlights
- Financial wellness
- Artificial Intelligence
- Social Media
UN report says that education, social safety nets vital for Asia to grow rich, cope with aging
FILE - Commuters walk in a passageway during a rush hour at Shinagawa Station Wednesday, Feb. 14, 2024, in Tokyo. As economies in Asia and the Pacific slow and grow older, countries need to do more to ensure that workers get the education, training and social safety nets needed to raise incomes and ensure social equity, a United Nations report said Tuesday, May 28. (AP Photo/Eugene Hoshiko, File)
FILE - Pedicab drivers move on a street in Manila, Philippines, on May 6, 2022. As economies in Asia and the Pacific slow and grow older, countries need to do more to ensure that workers get the education, training and social safety nets needed to raise incomes and ensure social equity, a United Nations report said Tuesday, May 28, 2024. (AP Photo/Aaron Favila, File)
FILE - Laborers carry wood piles for preparations of the Make In India summit in Mumbai, India, on Feb. 9, 2016 . As economies in Asia and the Pacific slow and grow older, countries need to do more to ensure that workers get the education, training and social safety nets needed to raise incomes and ensure social equity, a United Nations report said Tuesday, May 28, 2024. (AP Photo/Rafiq Maqbool, File)
- Copy Link copied

TOKYO (AP) — As economies in Asia and the Pacific slow and grow older, countries need to do more to ensure that workers get the education, training and social safety nets needed to raise incomes and ensure social equity, a United Nations report said Tuesday.
The report by the International Labor Organization said that growth in productivity has slowed, hurting incomes and undermining the purchasing power of the region’s 2 billion workers. By improving productivity, governments can boost incomes and better prepare for the aging of their work forces, the report said.
Two in three workers in the region were in informal employment in 2023, such as day labor, lacking the kinds of protections that come from formal jobs.
“The lack of job opportunities that meet decent work criteria, including good incomes, not only jeopardizes social justice in the region, but it also presents a risk factor for the labor market outlook,” the report said.
Showing the potential for improvement, labor productivity grew at an average annual rate of 4.3% in 2004-2021, helping to raise incomes per worker in terms of purchasing power parity, which compares standards of living in different countries using a common currency, to $15,700 from $7,700. But it has slowed in the past decade, the report said, hindering progress toward greater affluence.
It highlighted various challenges, especially unemployment among young people not in school, which is more than triple the adult rate, at 13.7%.
Increasing use of artificial intelligence and other automation technology will cause some people to lose their jobs, it said, with women engaged in clerical and information technology work most likely to be affected as companies roll back their reliance on offshore call centers that have provided good quality jobs in countries like the Philippines and India.
Other factors such as trade disputes and political turmoil threaten to disrupt jobs in some industries, but aging poses an even bigger challenge as countries grow old before they become affluent.
The ratio of people in Asia aged older than 65 to those 15–64 years old is projected to double to nearly a third by 2050 from about 15% in 2023, the ILO report says.
In places like Japan, short-handed employers have moved to alleviate work loads by using robots and computerized ordering in restaurants, cutting hours and installing self-checkout machines.
The report noted that a key reason why some countries face labor shortages despite having ample numbers of unemployed or underemployed workers is a mismatch between jobs and skills and education.
“The region still has huge potential for upskilling, productivity improvements and efficiency gains, which can alleviate demographic pressures on the labor market,” it said.
The report noted that more than a third of workers in the region have educational levels too low for their occupations, compared with 18% of workers in high-income countries.
Among other findings:
People in Asia and the Pacific still work more hours than workers in other regions, at 44 hours per week on average, though that is down from more than 47 hours in 2005.
In 2023, nearly 73 million workers in the region lived in extreme poverty, with daily incomes of less than $2.15 in purchasing power parity per person.
Despite raising retirement ages, total labor force participation in the Asia-Pacific region fell from 67% in 1991 to about 61% in 2023. It’s projected to fall to 55% by 2050.
The need for workers to provide long-term care in the region is forecast to more than double to 90 million by 2050 from 46 million in 2023. That would raise the proportion of people working in the field to 4.3% of the total from 2.3% now.
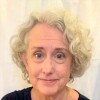
Lebanon: Poverty more than triples over the last decade reaching 44% under a protracted crisis
One of out of every three Lebanese have fallen into poverty in 2022
BEIRUT, May 23, 2024 — Poverty in Lebanon has more than tripled over the past decade reaching 44% of the total population, according to a new World Bank report released today. Based on a recent household survey covering the five governorates of Akkar, Beirut, Bekaa, North Lebanon and most of Mount Lebanon, the report finds that 1 out of every 3 Lebanese in these areas was poverty stricken in 2022, highlighting the critical need to strengthen social safety nets and create jobs to help alleviate poverty and address widening inequality.
The “ Lebanon Poverty and Equity Assessment 2024: Weathering a Protracted Crisis ” examines the current state of poverty and inequality in the country. It documents the impact of the economic and financial crisis on households as well as the effect on labor market dynamics. The report builds on a household survey conducted in collaboration with WFP and UNHCR between December 2022 and May 2023, covering Lebanese, Syrians and other nationals (except for Palestinians in camps and gatherings) in five governorates across Lebanon. Data collected covered demographics, education, employment, health, expenditures, assets, income and coping strategies.
Now in its fifth year, the protracted economic and financial crisis has compelled households to adopt a variety of coping strategies, including cutting back on food consumption and non-food expenses, as well as reducing health expenditures, with likely severe long-term consequences. To better reflect these changes in household behavior, the report adopts a new unofficial poverty line developed for 2022. The existing national poverty line from 2012 no longer captures the current consumption patterns or conditions faced by households in Lebanon today.
The report reveals a significant increase in monetary poverty from 12% in 2012 to 44% in 2022 across surveyed areas. It also highlights poverty being unevenly distributed across the country. Notably, in the North of Lebanon, the poverty rate reached as high as 70% in Akkar, where most residents are employed in the agriculture and construction sectors. Moreover, not only has the share of poor Lebanese nationals tripled to 33 percent from a decade ago, but they have also fallen deeper into poverty with the poverty gap rising from 3% in 2012 to 9.4% in 2022. Concurrently, income inequality appears to have worsened among the Lebanese.
With the rapid expansion of a dollarized cash-based economy, Lebanese households earning in dollars find their purchasing power preserved, while those without access to dollars are increasingly exposed to escalating inflation. Remittances have become a pivotal economic buffer, increasing from an average of 13% of GDP between 2012 and 2019, to about 30% in 2022 (partly due to a denominator effect) and surging by 20% in nominal terms between 2021 and 2022. These financial inflows are playing an increasingly critical role in preventing a segment of the population from falling into poverty.
“Lebanon’s ongoing crisis raises the urgency to better track the evolving nature of households’ well-being in order to develop and adopt the appropriate policies” said Jean-Christophe Carret, World Bank Middle East Country Director . "The Poverty and Equity Assessment highlights the critical need to improve targeting the poor and expand the coverage and depth of social assistance programs to ensure needy households’ access to essential resources including food, healthcare, and education.”
The report also finds that Syrian households have been hard hit by the crisis. Almost nine out of every 10 Syrians are under the poverty line in 2022, while 45% of poor Syrian families have less than acceptable food consumption scores. The majority of working-age Syrians with jobs are engaged in low paying and more precarious informal employment which contributes to impoverishment and food insecurity. While segmented labor markets had mostly mitigated the impact of the demographic surge caused by the influx of Syrian refugees on labor market outcomes for the Lebanese, the 2019 economic crisis has led to Lebanese workers increasingly taking on low-skilled jobs. This shift is partly due to a shrinking pool of better-paying skilled jobs.
The report recommends a series of interventions to help build the resilience of households and their ability to weather the protracted crisis. Looking ahead, social safety nets will continue to play a critical role in helping households meet their basic needs. Comprehensive macro-fiscal reforms will support price stability and provide the fiscal space for social spending. Investing in human capital is also essential in building the resiliency of households, by ensuring and expanding access to quality education and affordable healthcare. Making public transportation more accessible and affordable will facilitate access to schools, healthcare and jobs. Initiatives that link job seekers with formal jobs that better match their skills and productive employment programs that foster entrepreneurship and small business development can also help improve the earning prospects of households and reduce the likelihood of falling into poverty or help climb out of it.
This site uses cookies to optimize functionality and give you the best possible experience. If you continue to navigate this website beyond this page, cookies will be placed on your browser. To learn more about cookies, click here .
Trending News
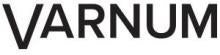
Related Practices & Jurisdictions
- Environmental, Energy & Resources
- Administrative & Regulatory
- All Federal
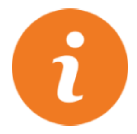
The U.S. Environmental Protection Agency (EPA) has finalized regulations adding seven per- and polyfluoroalkyl substances (PFAS) to the Toxics Release Inventory (TRI) program under the Emergency under Section 313 of the Emergency Planning and Community Right-to-Know Act (EPCRA).
The seven PFAS chemicals include:
- Perfluorohexanoic acid (PFHxA)
- Perfluoropropanoic acid (PFPrA)
- Sodium perfluorohexanoate
- Ammonium perfluorohexanoate, 1,1,1-Trifluoro-N-[(trifluoromethyl)sulfonyl] methanesulfonamide (TFSI)
- Lithium bis[(trifluoromethyl)sulfonyl] azanide, and
- Betaines, dimethyl(.gamma.-.omega.-perfluoro-.gamma.-hydro-C8-18-alkyl).
Facilities that manufacture, process or otherwise use any of these PFAS chemicals above the 100-pound annual threshold must report releases for the 2024 reporting year (along with other chemicals subject to TRI reporting requirements). While TRI reports for the 2024 reporting year are not due until July 1, 2025, regulated facilities should be keeping track of PFAS chemicals now for future reporting.
It should also be noted that pursuant to EPA regulations entitled “Changes to Reporting Requirements for Per- and Polyfluoroalkyl Substances” the PFAS chemicals added to the TRI inventory are designated as “chemicals of special concern.” Chemical of special concern are specifically excluded from utilizing the de minimis exemption, which allows facilities to forego reporting for negligible amounts of chemicals present in mixtures when present at concentrations below 1% (or 0.1% for carcinogens). As a result, regulated facilities utilizing PFAS will be required to track and report very small quantities of PFAS that might be present in products or materials that they manufacture, process or otherwise use.
Current Legal Analysis
More from varnum llp, upcoming legal education events.
Sign Up for e-NewsBulletins

Want to see more like this?
Get our local education coverage delivered directly to your inbox.

- Copy article link
School board hears report on structural analysis of Dennis Lab School's west end campuses
Valerie wells.
- May 28, 2024
Decatur school board discusses the structural analysis of Dennis Lab School's two west end campuses
DECATUR — The saga of building issues with Dennis Lab School continues, with the report Tuesday from structural engineering firm Klingner & Associates on the Mosaic and Kaleidoscope campuses.
Kyle Hannel, project manager and structural engineer for the company, said he personally examined both buildings in December. The company had also been called in for a second opinion on the buildings after the issues were originally discovered in May of last year, but in December, he said, he was able to examine the buildings more thoroughly since the program, students and most furniture has since been removed.
The most serious issues, as reported last year, are the main staircase at the Mosaic campus, and the upper walls and parapets at Kaleidoscope. Those walls are bowing outward as much as six inches out of plumb, he said.
People are also reading…
- Dog sex video probe continues
- Decatur man dies in motorcycle crash
- Coach Olivia Lett leaving Millikin women's basketball program
- Long recovery expected for Mt. Zion teenager after motorcycle crash
- Authorities identify man who died in Monday motorcycle crash in Decatur
- UPDATE: Lanes reopened after fatal I-57 crash
- Decatur water park makes a splash during opening day
- Man with baseball bat damages windows, points gun, cops report
- KPS to become sole owner of Primient after buying Tate & Lyle stake
- Grandmother sentenced in hot food attack on child
- The legend of the Des Peres pickle jar continues to fascinate and delight
- Cold Stone Creamery opens in WoodMound Shopping Center
- Firefighters rescue canoeist from Sangamon River
- On Biz: Cold Stone Creamery, SugaFix Designer Dessert Studio
- Ethel, Paul, and Bill Sly
“We recommend it stays unoccupied until it's repaired,” Hannel said. “There's a safety risk of collapsing. It's up in the air, so if a wall goes down, it could be a total collapse of everything else.”
The staircase at Mosaic, which runs through all three floors in the center of the building, lean heavily and slope more than two degrees. Doors have had to be cut off at the bottom so they swing and don't stick against the floor. In classrooms, floors are spongy, sagging and bounce even when he was the only person in the building, making them in danger of giving way if there were multiple people in the room at once. The staircase should be able to withstand 100 pounds per square foot for the building to be safe for occupancy, especially in case of an emergency when multiple people would have to use them at once to evacuate the building.
The two buildings, Mosaic at 1499 W. Main St. and Kaleidoscope at 520 W. Wood St., were closed on May 31, 2023 and during that summer, the board took measures to move the program to the former Garfield Learning Academy building at 300 Meadow Terrace, renting modular units to increase the space so that all K-8 students could be together on one campus.
A decision on whether it is financially feasible to repair the issues at the two West End buildings is still in the future, as the health/life/safety reports and potential repair costs have yet to be received by the school board.
“We want to look at health/life/safety, too,” Superintendent Rochelle Clark said. “We want to make sure we're giving the total picture to the board when we're looking at costs, and that plays into that alongside the structural analysis. We're going to be working from the back end, putting money to what (information) we have so far when we get the report, but we want to make sure we're looking at health/life/safety, too, for some of the schools we have listed, and making sure we add that cost factor in there as well.”
The administration knows that Dennis is a priority for the district, she said, and she wants to make sure those quotes are uppermost to give them an overall picture of the situation.
The master facilities plan, said board President Bill Clevenger, will be part of a road map that future boards can follow, and will include a rotating schedule of regular maintenance and upgrades that will prevent a repeat of this situation with Dennis' two campuses. Board personnel can potentially change every two years with elections, and without a master plan, things are not addressed in a timely manner, then budgets aren't created that account for those costs as a matter of course.
This story will be updated.
Photos: Open house for Dennis Lab School
Families tour the modular classrooms for fourth through eighth graders during an open house for the temporary location of Dennis Lab School in Decatur on Wednesday.
Special education teacher Ashley Tyler unpacks materials in her new classroom during an open house for Dennis Lab School’s modular classrooms in Decatur on Wednesday.
A classroom is set up in one of the modular classrooms during an open house for the temporary location of Dennis Lab School in Decatur on Wednesday.
A welcome mat decorates the doorway in one of the modular classrooms during an open house for the temporary location of Dennis Lab School in Decatur on Wednesday.
New kindergartener Elaina May, 5, plays on the new playground during an open house for the temporary location of Dennis Lab School in Decatur on Wednesday.
Dublynn Hoerr, Dallas York, Deacon Hoerr, and Patty Gross meet Deacon’s new first-grade teacher, Emily Kelley, second from right, during an open house for the temporary location of Dennis Lab School in Decatur on Wednesday.
Assistant principal Keith Creighton talks to a student in the parking lot between the modular classrooms and the school building during an open house for the new, temporary location of Dennis Lab School in Decatur on Wednesday.
First grader Channing Smith, right, and other students play on the new playground during an open house for the temporary location of Dennis Lab School in Decatur on Wednesday.
Deacon Hoerr and fifth grader Dublynn Hoerr meet Deacon’s new first grade teacher, Emily Kelley, right, during an open house for the temporary location of Dennis Lab School in Decatur on Wednesday.
Families attend an open house for the new, temporary location of Dennis Lab School in Decatur on Wednesday.
Contact Valerie Wells at (217) 421-7982. Follow her on Twitter: @modgirlreporter
Dennis Lab School
- Decatur Public Schools
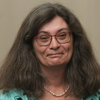
Education Reporter
- Author facebook
- Author twitter
- Author email
Get email notifications on {{subject}} daily!
{{description}}
Email notifications are only sent once a day, and only if there are new matching items.
Followed notifications
Please log in to use this feature, related to this story, dennis lab school at center of whirlwind for decatur schools in 2023.
Superintendent Rochelle Clark deflects on 2023 and looks ahead to the new year.
Dennis Lab School modulars lease extended to three years
Dennis Lab School's modular units will be leased for a minimum of three years after the board voted to extend the lease on Tuesday.
Watch Now: Related Video
Trump criminal trial moves to the jury, june 1 begins hurricane season, here's what to expect | hurricane hunt with joe martucci and sean sublette, us is 'actively engaging' with israel after deadly strike on rafah.
- Notifications
Get up-to-the-minute news sent straight to your device.
News Alerts
Breaking news.
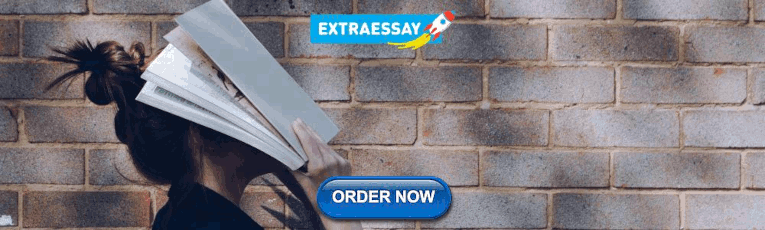
COMMENTS
The Report on the Condition of Education 2021 encompasses key findings from the Condition of Education Indicator System. The Indicator System for 2021 presents 86 indicators, including 22 indicators on crime and safety topics, and can be accessed online through the website or by downloading PDFs for the individual indicators.
The Condition of Education is an annual report to Congress summarizing important developments and trends in the U.S. education system. The report presents 50 indicators on topics ranging from prekindergarten through postsecondary education, as well as labor force outcomes and international comparisons. Discover how you can use the Condition of ...
Last update:20 April 2023. Fewer than 10% of countries have laws that help ensure full inclusion in education, according to UNESCO's 2020 Global Education Monitoring Report: Inclusion and education - All means all. The report provides an in-depth analysis of key factors for exclusion of learners in education systems worldwide including ...
On behalf of the National Center for Education Statistics (NCES), I am pleased to present the 2022 edition of the Condition of Education. The Condition is an annual report mandated by the U.S. Congress that summarizes the latest data on education in the United States.
McKinsey's latest thinking on the issues that matter most in education, from COVID-19 recovery and remote learning, to transforming higher education institutions and building more resilient early childhood, primary, and secondary school systems. Report.
This present volume is the third in a series of education sector analysis (ESA) guidelines following two volumes published in 2014. The series provides methodologies and applied examples for diagnosing education systems and informing national education policies and plans. This volume proposes guidelines to strengthen national capacities in ...
OECD Indicators. Education at a Glance is the authoritative source for information on the state of education around the world. It provides data on the structure, finances and performance of education systems across OECD countries and a number of accession and partner countries. More than 100 charts and tables in this publication - as well as ...
Educational Evaluation and Policy Analysis (EEPA) publishes rigorous, policy-relevant research of interest to those engaged in educational policy analysis, evaluation, and decision making. EEPA is a multidisciplinary journal, and editors consider original research from multiple disciplines, theoretical orientations, and methodologies.
Education sector analysis. An education sector analysis (ESA) is an in-depth, holistic diagnosis of an education system. It assists with understanding how an education system (and its subsectors) works, why it works that way, and how to improve it. An ESA provides the evidence base for decision-making and is the first step in preparing an ...
Analytics is a critical enabler to help colleges and universities solve tough problems—but leaders in higher-education institutions must devote just as much energy to acting on the insights from the data as they do on enabling analysis of the data. Implementation requires significant changes in culture, policy, and processes.
Descriptive analysis in education: A guide for researchers. Whether the goal is to identify and describe trends and variation in populations, create new measures of key phenomena, or describe samples in studies aimed at identifying causal effects, description plays a critical role in the scientific process in general and education research in ...
Analysis has already revealed deep losses, with international reading scores declining from 2016 to 2021 by more than a year of schooling. These losses may translate to a 0.68 percentage point in global GDP growth. The staggering effects of school closures reach beyond learning. This generation of children could lose a combined total of US$21 ...
This report was prepared for the Institute of Education Sciences (IES) by Decision Information Resources, Inc. under Contract ED-IES-12-C-0057, Analytic Technical Assistance and Development. The content of the publication does not necessarily reflect the views or policies of ... Descriptive analysis in education: A guide for researchers ...
The Future of Education and Skills 2030 reports on curriculum redesign address six common policy issues: 1. What students learn matters: towards a 21 st century curriculum. 2. Curriculum overload: A way forward. 3. Equity through curriculum innovations. 4. Curriculum flexibility and autonomy.
About the Strategic Data Project. SDP partners with state and local K-12 education agencies to build capacity for managing, analyzing, and communicating with data. SDP cultivates analytic talent through a two-year fellowship program, in-person and online trainings, and widely-accessible tools and resources.
Learning analytics in education design: a guide 5. Figure 1: Learning Analytics Cycle (Source: SURF, 2013) Visualisation and analytics. Subsequently, all the data is analy sed and interpreted ...
Cost analysis is often a building block for further economic evaluation, including cost-feasibility analysis (CFA), cost-effectiveness analysis (CEA) and cost-benefit analysis (CBA). These other approaches can— • Clarify whether an education agency has the resources available to implement a program.
Teaching Analytics (TA) is a new theoretical approach, which combines teaching expertise, visual analytics and design-based research to support teacher's diagnostic pedagogical ability to use data and evidence to improve the quality of teaching. TA is now gaining prominence because it offers enormous opportunities to the teachers. It also identifies optimal ways in which teaching performance ...
What is an Evaluation Report? An evaluation report is a document that presents the findings, conclusions, and recommendations of an evaluation, which is a systematic and objective assessment of the performance, impact, and effectiveness of a program, project, policy, or intervention. The report typically includes a description of the evaluation's purpose, scope, methodology, and data sources ...
Education research is instrumental in improving the quality of education systems. It is the most useful tool for assessing the spectrum of learning challenges, outcomes and impact of policy and practice from early childhood to adult education. In sub-Saharan Africa (SSA), where around 70% of populations are under.
The analysis in this report is based on three data sources. The labor force, earnings, hours, household income and poverty characteristics come from the U.S. Census Bureau's Annual Social and Economic Supplement of the Current Population Survey. The findings on net worth are based on the Federal Reserve's Survey of Consumer Finances.
The Nation's Report Card is a resource—a common measure of student achievement—because it offers a window into the state of our K-12 education system and what our children are learning. When students, their parents, teachers, and principals participate in the Nation's Report Card—the largest nationally representative and continuing ...
Statewide, the analysis estimated there are around 60,000 teachers in the workforce, with more than 1,000 vacancies. That number, based on 2022 data from the Tennessee Department of Education ...
A report by the United Nations says that as economies in Asia and the Pacific slow and grow older, countries need to do more to ensure that workers get the education, training and social safety ...
FILE - Commuters walk in a passageway during a rush hour at Shinagawa Station Wednesday, Feb. 14, 2024, in Tokyo. As economies in Asia and the Pacific slow and grow older, countries need to do more to ensure that workers get the education, training and social safety nets needed to raise incomes and ensure social equity, a United Nations report said Tuesday, May 28.
One of out of every three Lebanese have fallen into poverty in 2022 . BEIRUT, May 23, 2024 — Poverty in Lebanon has more than tripled over the past decade reaching 44% of the total population, according to a new World Bank report released today. Based on a recent household survey covering the five governorates of Akkar, Beirut, Bekaa, North Lebanon and most of Mount Lebanon, the report finds ...
U.N. report says education, social safety nets vital for Asia to grow rich, cope with aging Commuters walk in a passageway during a rush hour at Shinagawa Station on Wednesday, Feb. 14, 2024, in ...
The U.S. Environmental Protection Agency (EPA) has finalized regulations adding seven per- and polyfluoroalkyl substances (PFAS) to the Toxics Release Inventory (TRI) program under the Emergency ...
In October each year, the Mid-Year Trends report is released to provide updated figures and analysis for the initial six months of the current year (from 1 January to 30 June). These figures are preliminary, and the final data is included in the subsequent Global Trends report released in June of the following year.
School board hears report on structural analysis of Dennis Lab School's west end campuses. Valerie Wells. 21 mins ago. Contact Valerie Wells at (217) 421-7982. Follow her on Twitter: @modgirlreporter.