Behavioral Finance
- Reference work entry
- First Online: 22 November 2023
- Cite this reference work entry
- Pedro Manuel Nogueira Reis 7 &
- António Pedro Soares Pinto 7
38 Accesses
Behavioural finance ; Investor sentiment
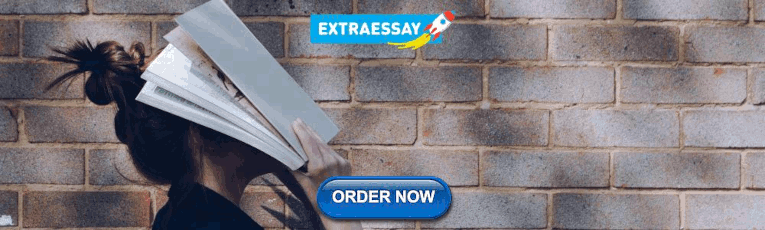
Definition/Description
The holy grail of academic finance is to identify those factors that are best able to explain expected returns. The capital asset pricing model (CAPM) proposed by Sharpe ( 1964 ) and Lintner ( 1965 ) sought to calculate the risk premia inherent to financial assets. Researchers correspondingly study a variety of risk factors to best explain and predict the expected returns, including works by Fama and French ( 2016 , 2018 ), and Barillas and Shanken ( 2018 ) among many others. In addition to company fundamentals and the macroeconomic environment, a new branch of finance has emerged for forecasting expected returns based on investor sentiment as one of the main drivers inducing return co-movements. Optimism or pessimism may drive investor behaviors that condition their interactions with the markets and therefore impacting on stock returns. This investor sentiment can derive from their belief or otherwise in the future...
This is a preview of subscription content, log in via an institution to check access.
Access this chapter
- Available as PDF
- Read on any device
- Instant download
- Own it forever
- Available as EPUB and PDF
- Durable hardcover edition
- Dispatched in 3 to 5 business days
- Free shipping worldwide - see info
Tax calculation will be finalised at checkout
Purchases are for personal use only
Institutional subscriptions
Baker, M., & Wurgler, J. (2006). Investor sentiment and the cross – Section of stock returns. The Journal of Finance, 61 (4), 1645–1680.
Article Google Scholar
Baker, M., & Wurgler, J. (2007). Investor sentiment in the stock market. Journal of Economic Perspectives, 21 (2), 129–152.
Barillas, F., & Shanken, J. (2018). Comparing asset pricing models. Journal of Finance, 73 (2), 715–754. https://doi.org/10.1111/jofi.12607 .
Benhabib, J., Liu, X., & Wang, P. (2016). Sentiments, financial markets, and macroeconomic fluctuations. Journal of Financial Economics, 120 (2), 420–443. https://doi.org/10.1016/j.jfineco.2016.01.008 .
Chen, R., Yu, J., Jin, C., & Bao, W. (2019). Internet finance investor sentiment and return comovement. Pacific-Basin Finance Journal, 56 , 151–161.
Fama, E. F. (1960). Efficient market hypothesis. Doctoral dissertation, Ph.D. thesis, Ph.D. dissertation, University of Chicago Graduate School of Business.
Google Scholar
Fama, E. F., & French, K. R. (2016). Dissecting anomalies with a five-factor model. Review of Financial Studies, 29 (1), 69–103.
Fama, E. F., & French, K. R. (2018). Choosing factors. Journal of Financial Economics, 128 (2), 234–252. https://doi.org/10.1016/j.jfineco.2018.02.012 .
Kahneman, D., & Tversky, A. (1990). 6. Prospect theory: An analysis of decision under risk. In Rationality in action: Contemporary approaches (p. 140). Cambridge: Cambridge University Press.
Lintner, J. (1965). The valuation of risk assets and the selection of risky investments in stock portfolios and capital budgets. Review of Economics and Statistics, 2 , 13–37.
Loughran, T., & Mcdonald, B. (2016). Textual analysis in accounting and finance: A survey. Journal of Accounting Research, 54 (4), 1187–1230. https://doi.org/10.1111/1475-679X.12123 .
Piccione, M., & Spiegler, R. (2014). Manipulating market sentiment. Economics Letters, 122 (2), 370–373. https://doi.org/10.1016/j.econlet.2013.12.021 .
Pompian, M. M. (2011). Behavioral finance and wealth management: How to build investment strategies that account for investor biases (Vol. 667). Hoboken: Wiley.
Sharpe, W. F. (1964). Capital asset prices: A theory of market equilibrium under conditions of risk. Journal of Finance, 19 (3), 425–442.
Zhou, G. (2018). Measuring investor sentiment. Annual Review of Finance Economics, 10 , 239–259. https://doi.org/10.1146/annurev-financial-110217-022725 .
Download references
Author information
Authors and affiliations.
School of Management, Polytechnic Institute of Viseu, Viseu, Portugal
Pedro Manuel Nogueira Reis & António Pedro Soares Pinto
You can also search for this author in PubMed Google Scholar
Corresponding author
Correspondence to António Pedro Soares Pinto .
Editor information
Editors and affiliations.
Guildhall Faculty of Business and Law London Metropolitan University, London Metropolitan University, London, UK
Samuel O. Idowu
BFH - Bern, Bern, Switzerland
René Schmidpeter
College of Business, Loyola University New Orleans, New Orleans, LA, USA
Nicholas Capaldi
International Training Centre of the IL, International Labor Organization, Turin, Italy
Liangrong Zu
Department of Economics, Society and Politics, University of Urbino Carlo Bo, Urbino, Italy
Mara Del Baldo
Instituto Politécnico da Guarda, Guarda, Portugal
Section Editor information
School of Law & Justice, University of Canberra, Canberra, Australia
Benedict Sheehy
Rights and permissions
Reprints and permissions
Copyright information
© 2023 Springer Nature Switzerland AG
About this entry
Cite this entry.
Reis, P.M.N., Pinto, A.P.S. (2023). Behavioral Finance. In: Idowu, S.O., Schmidpeter, R., Capaldi, N., Zu, L., Del Baldo, M., Abreu, R. (eds) Encyclopedia of Sustainable Management. Springer, Cham. https://doi.org/10.1007/978-3-031-25984-5_985
Download citation
DOI : https://doi.org/10.1007/978-3-031-25984-5_985
Published : 22 November 2023
Publisher Name : Springer, Cham
Print ISBN : 978-3-031-25983-8
Online ISBN : 978-3-031-25984-5
eBook Packages : Business and Management Reference Module Humanities and Social Sciences Reference Module Business, Economics and Social Sciences
Share this entry
Anyone you share the following link with will be able to read this content:
Sorry, a shareable link is not currently available for this article.
Provided by the Springer Nature SharedIt content-sharing initiative
- Publish with us
Policies and ethics
- Find a journal
- Track your research
This website uses cookies.
By clicking the "Accept" button or continuing to browse our site, you agree to first-party and session-only cookies being stored on your device to enhance site navigation and analyze site performance and traffic. For more information on our use of cookies, please see our Privacy Policy .
- Journal of Economic Perspectives
- Winter 2003
From Efficient Markets Theory to Behavioral Finance
- Robert J. Shiller
- Article Information
- Comments ( 0 )
JEL Classification
- G14 Information and Market Efficiency; Event Studies; Insider Trading
- Harvard Business School →
- Behavioral Finance & Financial Stability →
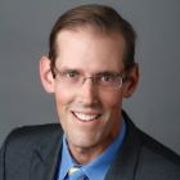
Malcolm P. Baker
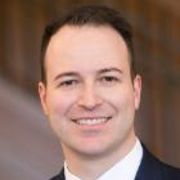
Marco Di Maggio
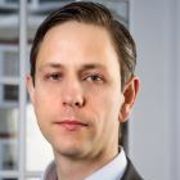
Robin Greenwood
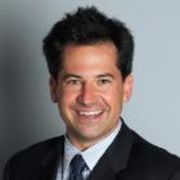
Samuel G. Hanson
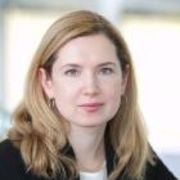
Victoria Ivashina
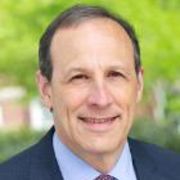
David S. Scharfstein
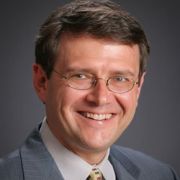
Andrei Shleifer
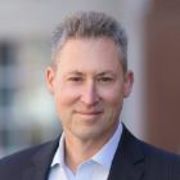
Erik Stafford
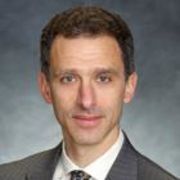
Jeremy C. Stein
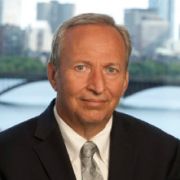
Lawrence Summers
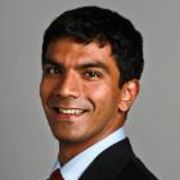
Adi Sunderam
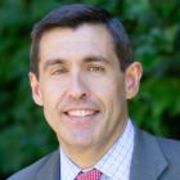
Luis M. Viceira
Post doctoral.
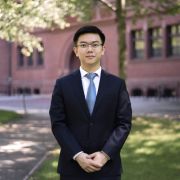
Doctoral Students
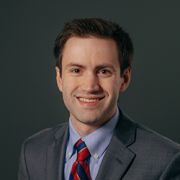
Michael Blank
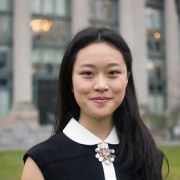
Spencer Kwon
University of Minnesota Twin Cities
University digital conservancy, behavioral finance: fifa world cup expectations and stock market success, view/download file, persistent link to this item, journal title, journal issn, volume title, published date, description, collections, series/report number, funding information, isbn identifier, doi identifier, previously published citation, suggested citation.
Content distributed via the University Digital Conservancy may be subject to additional license and use restrictions applied by the depositor. By using these files, users agree to the Terms of Use . Materials in the UDC may contain content that is disturbing and/or harmful. For more information, please see our statement on harmful content in digital repositories .
- Help & FAQ
Essays on behavioral finance
- Center Ph. D. Students
- Research Group: Finance
Research output : Thesis › Doctoral Thesis
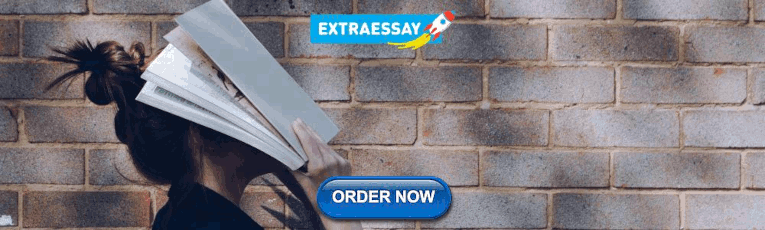
Access to Document
- PhD thesis Ayse Terzi Final published version, 732 KB
Fingerprint
- Behavioral Finance Business & Economics 100%
- Reference Point Business & Economics 91%
- Decision Making under Risk Business & Economics 55%
- Asset Markets Business & Economics 46%
- Demographic Factors Business & Economics 44%
- Discounting Business & Economics 42%
- Personality Traits Business & Economics 42%
- Private Information Business & Economics 39%
T1 - Essays on behavioral finance
AU - Terzi, Ayse
N1 - Series: CentER Dissertation Series Volume: 516
N2 - This thesis deals with a range of topics in experimental and behavioral finance. The first part investigates the role of personal inclination in reference point employment by individuals. The second part extends this questions by focusing on the role of personality traits and demographic factors in driving reference point exhibition in decision making under risk. The third part documents the role of ambiguity in the dissemination of private information in asset markets. Finally, the last part explores differences in time discounting under risk and ambiguity.
AB - This thesis deals with a range of topics in experimental and behavioral finance. The first part investigates the role of personal inclination in reference point employment by individuals. The second part extends this questions by focusing on the role of personality traits and demographic factors in driving reference point exhibition in decision making under risk. The third part documents the role of ambiguity in the dissemination of private information in asset markets. Finally, the last part explores differences in time discounting under risk and ambiguity.
M3 - Doctoral Thesis
SN - 978 90 5668 517 1
T3 - CentER Dissertation Series
PB - CentER, Center for Economic Research
CY - Tilburg
Academia.edu no longer supports Internet Explorer.
To browse Academia.edu and the wider internet faster and more securely, please take a few seconds to upgrade your browser .
Enter the email address you signed up with and we'll email you a reset link.
- We're Hiring!
- Help Center
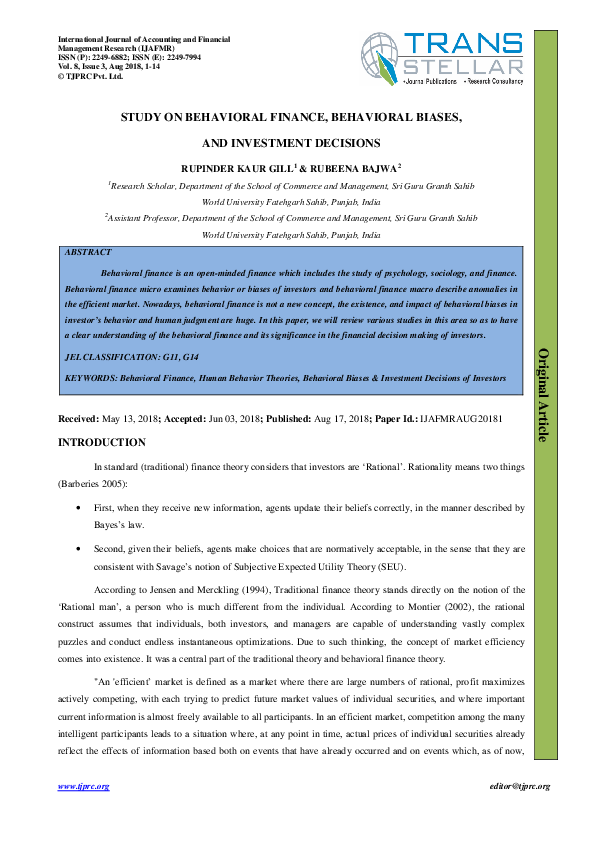
STUDY ON BEHAVIORAL FINANCE, BEHAVIORAL BIASES, AND INVESTMENT DECISIONS

Behavioral finance is an open-minded finance which includes the study of psychology, sociology, and finance. Behavioral finance micro examines behavior or biases of investors and behavioral finance macro describe anomalies in the efficient market. Nowadays, behavioral finance is not a new concept, the existence, and impact of behavioral biases in investor's behavior and human judgment are huge. In this paper, we will review various studies in this area so as to have a clear understanding of the behavioral finance and its significance in the financial decision making of investors. JEL CLASSIFICATION: G11, G14
Related Papers
José G. Vargas-Hernández
This paper focuses on the study of one of the most recent and successful technology-based startups, whose nature is the hospitality industry. Airbnb is part of the model peer to peer of the collaborative economy, which means it is participating in a Community market based on trust the host-guest experience for traveling richer than it would simply stay in a hotel. For the development of this work the research methods will be descriptive and analytical due to the interest of not only explain the origin and strategies that practices the company but also doing an analysis of the tendency of users, both the hosts and guests about their perception of the services offered and whether it will become the preferred way to rent a space. The trends show that the explosive growth since its inception continues to be a trend, as long as the regulations imposed by different governments are met and that trust between users stays strong.
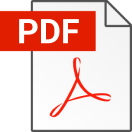
Fitry Andry
Raja Asad Azad
Traditional finance theory is based on the principle of maximization of utility and explains how choices are made by rational people. Although the theory provides numerous insights, observation of actual behavior of people was seen to be different from what the theory predicted. The homo economicus is in reality a homo sapien who has emotions and beliefs that help to filter the content from his or her environment. These beliefs and preferences that arise due to cogni-tive limitations, presence of emotions, and various psychological motives guide or bias his or her decisions. Much literature states that the biases should be corrected as they negatively impact financial behaviour and individual's well‐being. However, evolutionary psychology considers biases as design features of human mind. Thus, biases are not always bad, as at times, these biases can help the individual investor to choose the best course of action from the multiple possibilities and enable committing the less costly mistakes, thereby helping the individual to achieve satisficing behaviour. This paper aims to explore the investor biases and see whether they are related to the financial satisfaction of the individuals. Financial satisfaction is the measure of satisfaction with one's financial situation. The results showed that overconfidence bias, reliance on expert bias, and self‐control bias have a positive and significant association with financial satisfaction levels. Association of a few other biases with financial satisfaction was also observed under certain control conditions. This study provides further insights on investor behavior and paves the way for various possibilities for future research.
IJAR Indexing
Research has proved that investors in the equity market are not consistently rational. Emotions influence their decision making process in the complex environment of equity market, in the form of behavioral biases. This paper reviews five important behavioral biases exhibited by investors in the equity market. The behavioral biases reviewed include, representativeness, anchoring, gambler?s fallacy, availability and optimism. The literature available for each of the biases is reviewed and hence this paper draws attention to a new dimension in finance.
AARF Publications Journals
Asian Journal of Economics, Business and Accounting
This study investigates the existence of heuristics biases in Colombo Stock Exchange and their effect on investment performance from individual investor's point of view. In specific, the effects of anchoring, availability bias, gamblers fallacy, overconfidence and representativeness are investigated. Further, the study inspects whether the heuristics biases differ between younger and older investors. The primary data were collected by survey from 425 individual investors. The data were analyzed using multivariate analysis such as Confirmatory Factor Analysis (CFA) and Structure Equation Modeling (SEM). The results show that there is a statistically significant effect of anchoring, availability bias, overconfidence and representativeness bias on investment performance. However, gamblers fallacy not significantly affects investment performance. Furthermore, statistically significant differences are found between the answers of younger and older investors. This study, hopefully, will help investors to be aware of the impact of their own heuristics bias on their decision making in the stock market, thus increasing the rationality of investment decisions for enhanced market efficiency.
IJREAM EDITOR
Finance is the system that includes the granting of money and credit, making of investments and provision of banking facilities. Behavioral finance is a new academic discipline which seeks to apply the insights of the psychologists to understand the behavior of both investors and financial markets. This study analyse the Investors behavior through 600 respondents using Factor analysis test. The results of the study show that the 16 variables selected for the study had been reduced to 5 factor models using the principle component analysis such as Market Dynamics, Logical Analysis , Herding Bias, Regret Aversion and Heuristic Bias. Thus, Behavioral finance is becoming a primary part of the decision making process, since it influences investors' behavior greatly.
Nada Ibrahim
This study investigates the existence of behavioral biases in Amman Stock Exchange and their effect on investment performance from investor's point of view. In specific, the effects of overconfidence bias, familiarity bias, loss aversion bias, disposition bias, availability bias, representativeness bias, confirmation bias and herding bias are investigated. Moreover, the study inspects whether the behavioral biases differ between males and females. The results show that there is a statistically significant effect of overconfidence bias, familiarity bias, availability bias, representativeness bias and herding bias on investment performance (p≤5%). Moreover, disposition bias, confirmation bias and loss aversion bias significantly affect investment performance but at a critical level of (p≤10%). No statistically significant differences are found between the answers of males and females.
Rohit Kumar
Finance has been studied around the globe from ages but the dimensions of behavioral science have been related with finance only a few decades before. This led to evolution of behavioral finance, where effect of human emotions, cognitive errors and psychology on investment decision is studied. The main objective of this study was to explore the individual investors’ investment preference i.e., utilitarian or value-expressive. Moreover, the extent to which their investment decision is dominated by their investment preference has been studied. The relationship between demographic factors and investment preference of an individual has also been examined. The results show that the individual investors at Indian stock exchange, in general, are more value-expressive than utilitarian. Their investment decisions are affected by many behavioral biases as well as with certain demographic factors.
Metamorphosis- A Journal of Management Research
Ansted I Joseph , Anu Antony
Investors exhibit irrational behaviour in their decision-making. The decision-making process itself is considered to be a cognitive process as the investors have to make a decision based on various alternatives available to them. The researchers have found that the investors’ decision-making was adversely affected by the various psychological/behavioural factors. The current study was carried forward to identify the effect of the behavioural factors affecting the investment decision of the investors. Five behavioural factors, namely overconfidence bias, representative bias, regret aversion, mental accounting, and herd behaviour, were considered to study the behavioural biases of the investors. The study sample was taken from investors of Kerala, and the analytical hierarchy process (AHP) method was used to analyse the intensity of behavioural factors affecting the investment decision. Based on the priority vector, it was found that the investors of Kerala were highly influenced with overconfidence bias and regret aversion. Herd behaviour had less effect on their decision-making.
RELATED PAPERS
Indus Foundation International Journals UGC Approved
AARF Publications Journals , Dr. P.S.Ravindra
Muhammad Mubin , Sumra Mughal
Abey Francis
Imran Umer Chhapra
Mustafa Okur
Magdalena Mikołajek-Gocejna
DeBondt, W.F.M. and Thaler, R.H. (1995), “Financial decision making in markets and firms: a behavioral perspective”,Handbooks inOperations Research and Management Science, Vol. 9 No. 13, pp. 385-410.
Asif Ali 8221-FMS/MBA/F19
Advances in Cognitive Systems
Jaap Spronk
Sana Jameel
Jacob Niyoyita Mahina
Salman Shahid
Zulqarnain Asab
Journal of Behavioral Decision Making
John Nofsinger
Ashesh Kaushik
AMARI Mouna
Suzane Strehlau
Sebastian Arumi
rayhan kabir
Victoria Bogdan
Decision Support Systems
Richard Deaves
HANDBOOK OF FINANCE: VOLUME 2: INVESTMENT MANAGEMENT AND FINANCIAL MANAGEMENT, Frank J. Fabozzi, ed., John Wiley & Sons, pp. 85-111, 2008.
Victor Ricciardi
IAEME Publication
Duncan Elly
IOSR Journals
INFORMATION …
SHILI BABAEI
Marwa Zouaoui
- We're Hiring!
- Help Center
- Find new research papers in:
- Health Sciences
- Earth Sciences
- Cognitive Science
- Mathematics
- Computer Science
- Academia ©2024
- Trading Skills
- Trading Psychology
Behavioral Finance: Biases, Emotions and Financial Behavior
- Search Search Please fill out this field.
What Is Behavioral Finance?
Understanding behavioral finance, behavioral finance concepts.
- Biases Studied
- The Stock Market
- Behavioral Finance FAQs
Adam Hayes, Ph.D., CFA, is a financial writer with 15+ years Wall Street experience as a derivatives trader. Besides his extensive derivative trading expertise, Adam is an expert in economics and behavioral finance. Adam received his master's in economics from The New School for Social Research and his Ph.D. from the University of Wisconsin-Madison in sociology. He is a CFA charterholder as well as holding FINRA Series 7, 55 & 63 licenses. He currently researches and teaches economic sociology and the social studies of finance at the Hebrew University in Jerusalem.
:max_bytes(150000):strip_icc():format(webp)/adam_hayes-5bfc262a46e0fb005118b414.jpg)
Yarilet Perez is an experienced multimedia journalist and fact-checker with a Master of Science in Journalism. She has worked in multiple cities covering breaking news, politics, education, and more. Her expertise is in personal finance and investing, and real estate.
:max_bytes(150000):strip_icc():format(webp)/YariletPerez-d2289cb01c3c4f2aabf79ce6057e5078.jpg)
- Behavioral Finance: Biases, Emotions and Financial Behavior CURRENT ARTICLE
- Introduction to Behavioral Finance
- Understanding Investor Behavior
- Market Psychology
- Power of the Masses Drives the Market
- Read the Market's Psychological State
- Herd Instinct
- When Fear and Greed Take Over
- Behavioral Biases and How to Avoid Them
- How to Avoid Emotional Investing
- Psychological Traps Investors Should Avoid
- Psychological Quirks That Affect Your Trading
- Removing the Barriers to Successful Investing
- Break Bad Trading Habits and Follow Your Rules
- Random Reinforcement: Why Most Traders Fail
- How to Develop a Trading Brain
- Let Your Profits Run
- The Art of Cutting Your Losses
- Positive Feedback
- Loss Psychology
- Psychological Coping Strategies for Handling Losses
- Regret Avoidance
- Technical Analysis That Indicates Market Psychology
- Psychology of Support and Resistance Zones
- Investing vs. Gambling
- The Downward Spiral of Trading Addiction
- The Casino Mentality In Trading
Behavioral finance, a subfield of behavioral economics , proposes that psychological influences and biases affect the financial behaviors of investors and financial practitioners. Moreover, influences and biases can be the source for the explanation of all types of market anomalies and specifically market anomalies in the stock market, such as severe rises or falls in stock price. As behavioral finance is such an integral part of investing, the Securities and Exchange Commission has staff specifically focused on behavioral finance.
Key Takeaways
- Behavioral finance is an area of study focused on how psychological influences can affect market outcomes.
- Behavioral finance can be analyzed to understand different outcomes across a variety of sectors and industries.
- One of the key aspects of behavioral finance studies is the influence of psychological biases.
- Some common behavioral financial aspects include loss aversion, consensus bias, and familiarity tendencies.
- The efficient market theory which states all equities are priced fairly based on all available public information is often debunked for not incorporating irrational emotional behavior.
Behavioral finance can be analyzed from a variety of perspectives. Stock market returns are one area of finance where psychological behaviors are often assumed to influence market outcomes and returns but there are also many different angles for observation. The purpose of the classification of behavioral finance is to help understand why people make certain financial choices and how those choices can affect markets.
Within behavioral finance, it is assumed that financial participants are not perfectly rational and self-controlled but rather psychologically influential with somewhat normal and self-controlling tendencies. Financial decision-making often relies on the investor's mental and physical health. As an investor's overall health improves or worsens, their mental state often changes. This impacts their decision-making and rationality towards all real-world problems, including those specific to finance.
One of the key aspects of behavioral finance studies is the influence of biases. Biases can occur for a variety of reasons. Biases can usually be classified into one of five key concepts. Understanding and classifying different types of behavioral finance biases can be very important when narrowing in on the study or analysis of industry or sector outcomes and results.
Read about Investopedia's 10 Rules of Investing by picking up a copy of our special issue print edition.
Behavioral finance typically encompasses five main concepts:
- Mental accounting : Mental accounting refers to the propensity for people to allocate money for specific purposes.
- Herd behavior : Herd behavior states that people tend to mimic the financial behaviors of the majority of the herd. Herding is notorious in the stock market as the cause behind dramatic rallies and sell-offs.
- Emotional gap : The emotional gap refers to decision-making based on extreme emotions or emotional strains such as anxiety, anger, fear, or excitement. Oftentimes, emotions are a key reason why people do not make rational choices.
- Anchoring : Anchoring refers to attaching a spending level to a certain reference. Examples may include spending consistently based on a budget level or rationalizing spending based on different satisfaction utilities.
- Self-attribution : Self-attribution refers to a tendency to make choices based on overconfidence in one's own knowledge or skill. Self-attribution usually stems from an intrinsic knack in a particular area. Within this category, individuals tend to rank their knowledge higher than others, even when it objectively falls short.
Behavioral finance is exploited through credit card rewards, as consumers are more likely to be willing to spend points, rewards, or miles as opposed to paying for transactions with direct cash.
Some Biases Revealed by Behavioral Finance
Breaking down biases further, many individual biases and tendencies have been identified for behavioral finance analysis. Some of these include:
Confirmation Bias
Confirmation bias is when investors have a bias toward accepting information that confirms their already-held belief in an investment. If information surfaces, investors accept it readily to confirm that they're correct about their investment decision—even if the information is flawed.
Experiential Bias
An experiential bias occurs when investors' memory of recent events makes them biased or leads them to believe that the event is far more likely to occur again. For this reason, it is also known as recency bias or availability bias.
For example, the financial crisis in 2008 and 2009 led many investors to exit the stock market. Many had a dismal view of the markets and likely expected more economic hardship in the coming years. The experience of having gone through such a negative event increased their bias or likelihood that the event could reoccur. In reality, the economy recovered, and the market bounced back in the years to follow.
Loss Aversion
Loss aversion occurs when investors place a greater weighting on the concern for losses than the pleasure from market gains. In other words, they're far more likely to try to assign a higher priority to avoiding losses than making investment gains.
As a result, some investors might want a higher payout to compensate for losses. If the high payout isn't likely, they might try to avoid losses altogether even if the investment's risk is acceptable from a rational standpoint.
Applying loss aversion to investing, the so-called disposition effect occurs when investors sell their winners and hang onto their losers. Investors' thinking is that they want to realize gains quickly. However, when an investment is losing money, they'll hold onto it because they want to get back to even or their initial price. Investors tend to admit they are correct about an investment quickly (when there's a gain).
However, investors are reluctant to admit when they made an investment mistake (when there's a loss). The flaw in disposition bias is that the performance of the investment is often tied to the entry price for the investor. In other words, investors gauge the performance of their investment based on their individual entry price disregarding fundamentals or attributes of the investment that may have changed.
Familiarity Bias
The familiarity bias is when investors tend to invest in what they know , such as domestic companies or locally owned investments. As a result, investors are not diversified across multiple sectors and types of investments, which can reduce risk. Investors tend to go with investments that they have a history or have familiarity with.
Familiarity bias can occur in so many ways. You may resist investing in a specific company because of what industry it is in, where it operates, what products it sells, who oversees the management of the company, who its clientele base is, how it performs its marketing, and how complex its accounting is.
Behavioral Finance in the Stock Market
The efficient market hypothesis (EMH) says that at any given time in a highly liquid market , stock prices are efficiently valued to reflect all the available information. However, many studies have documented long-term historical phenomena in securities markets that contradict the efficient market hypothesis and cannot be captured plausibly in models based on perfect investor rationality.
The EMH is generally based on the belief that market participants view stock prices rationally based on all current and future intrinsic and external factors. When studying the stock market, behavioral finance takes the view that markets are not fully efficient. This allows for the observation of how psychological and social factors can influence the buying and selling of stocks.
The understanding and usage of behavioral finance biases can be applied to stock and other trading market movements on a daily basis. Broadly, behavioral finance theories have also been used to provide clearer explanations of substantial market anomalies like bubbles and deep recessions. While not a part of EMH, investors and portfolio managers have a vested interest in understanding behavioral finance trends. These trends can be used to help analyze market price levels and fluctuations for speculation as well as decision-making purposes.
What Does Behavioral Finance Tell Us?
Behavioral finance helps us understand how financial decisions around things like investments, payments, risk, and personal debt, are greatly influenced by human emotion, biases, and cognitive limitations of the mind in processing and responding to information.
How Does Behavioral Finance Differ From Mainstream Financial Theory?
Mainstream theory, on the other hand, makes the assumptions in its models that people are rational actors, that they are free from emotion or the effects of culture and social relations, and that people are self-interested utility maximizers. It also assumes, by extension, that markets are efficient and firms are rational profit-maximizing organizations. Behavioral finance counters each of these assumptions.
How Does Knowing About Behavioral Finance Help?
By understanding how and when people deviate from rational expectations, behavioral finance provides a blueprint to help us make better, more rational decisions when it comes to financial matters.
What Is an Example of a Finding in Behavioral Finance?
Investors are found to systematically hold on to losing investments far too long than rational expectations would predict, and they also sell winners too early. This is known as the disposition effect, and is an extension of the concept of loss aversion to the domain of investing. Rather than locking in a paper loss, investors holding lose positions may even double down and take on greater risk in hopes of breaking even.
:max_bytes(150000):strip_icc():format(webp)/GettyImages-1186875474-5bd82696d460477481060d73de210380.jpg)
- Terms of Service
- Editorial Policy
- Privacy Policy
- Your Privacy Choices
- My Shodhganga
- Receive email updates
- Edit Profile
Shodhganga : a reservoir of Indian theses @ INFLIBNET
- Shodhganga@INFLIBNET
- Sri Chandrasekharendra Saraswathi Viswa Mahavidyalaya
- Department of Management Studies
Items in Shodhganga are licensed under Creative Commons Licence Attribution-NonCommercial-ShareAlike 4.0 International (CC BY-NC-SA 4.0).

- Application Requirements
- Student Experience Q&A
- Business Analytics Certificate
- Finance Certificate Programs
- Tuition & Financial Aid
- International and Transfer Students
- Military Friendly
- How to Apply
- Accreditation
- Leadership Development Resources
- MBA Career Outcomes
- MSBA Career Outcomes
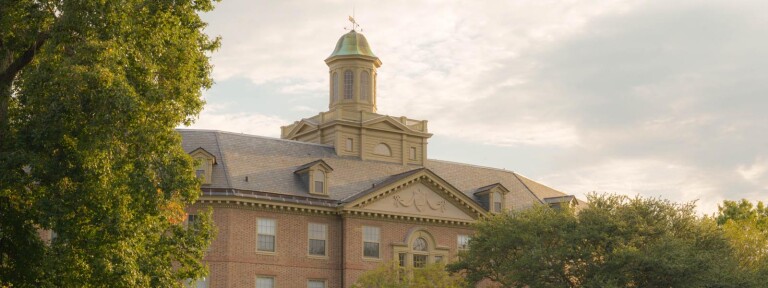
The Role of Behavioral Finance: Investor Psychology, Market Anomalies and Decision-Making
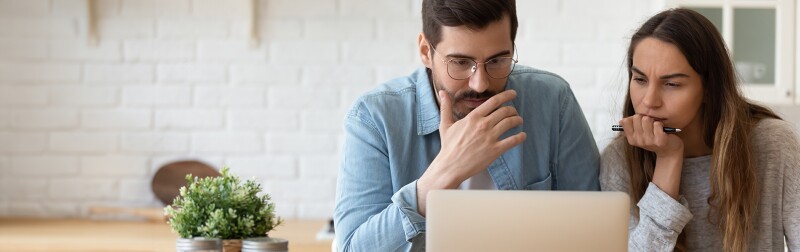
People often think of economics as a rational field, but emotions play a bigger role than you might think. Behavioral finance, the study of how psychology influences financial decisions, can tell us a lot about economic trends and individual choices.
So what is behavioral finance , exactly? And how do psychology and decision-making intersect? This article will break down the key impact of behavioral finance on investor psychology, market anomalies and decision-making in finance.
Understanding the Behavioral Finance Fundamentals
Behavioral finance is a subfield of behavioral economics or the study of irrational behavior in financial decision-making. Studying behavioral economics is one way to explain why economic systems don’t always work the way you might expect them to. In other words, markets are often irrational because they’re driven by people who make irrational decisions.
The key to understanding behavioral finance, or behavioral economics, is understanding how psychological biases can affect the decision-making process around finances. Those biases include: 1
- Confirmation bias: People tend to believe statements that align with their current beliefs
- Repetition bias: Frequently repeated statements often seem more credible
- Risk aversion: People are more likely to make a low-risk decision over a high-risk decision
- Herd effect: The herd effect is the inclination to follow the crowd or do what’s popular
These biases apply to everyday decisions, such as choosing where to have lunch or sharing a social media post, but they also impact financial decision-making.
Behavioral Finance vs. Traditional Finance Theory
Traditional finance theory relies on rational choice theory: The idea that individuals will always make decisions that align with their best interests. 2 Behavioral finance, on the other hand, seeks to understand why people might make choices that don’t ultimately serve them. This offers a more nuanced view of economics and can explain flaws and anomalies that traditional finance theory doesn’t account for.
People don’t always act rationally, and investors are no different. That’s why understanding behavioral finance concepts is one of the most important soft skills in finance .
Investor Psychology: Influences and Effects
You don’t have to be an expert in psychology to make smart investments. However, understanding the relationship between investor psychology and market trends can help you make educated predictions about future performance, address your own biases and invest wisely.
Emotions and cognitive biases play a role in even a well-educated investor’s choices. That includes the biases listed above, as well as external factors such as cultural influences, which can impact an investor’s sense of loss aversion and patience. 3 The herd effect is a particularly important concept in investor psychology—trading an asset simply because it’s viewed as a hot commodity can lead to asset bubbles and have a large-scale impact on the wider market. 4
Exploring Stock Market Anomalies Through a Behavioral Lens
Can behavioral finance explain major stock market anomalies in finance? Yes—and no. Like any field, behavioral finance is not a perfect science, and it can’t always predict a market anomaly before it happens. However, you can look back at financial events through a behavioral lens in order to better understand what happened.
Remember that the herd effect can lead to market bubbles and crashes. For example, consider the dot-com bubble of the late 1990s, in which a frenzy over the internet led to an over-investment in unprofitable companies. 5 Psychological theories can also explain anomalies in asset pricing, such as the GameStop phenomenon of 2020, in which a small group of investors drove up the price of GameStop’s stock during the COVID-19 lockdowns. 6
Behavioral finance and market decisions aren’t always easy to understand. However, an understanding of psychology can help everyday investors make sense of seemingly random events.
Decision-Making Strategies in Behavioral Finance
You may have heard of the five principles of finance , which can improve your financial literacy. When it comes to behavioral finance, however, there are a few more advanced theories that can help improve your decision-making.
Prospect theory is one example. This theory aims to predict how people will assess risk based on expected outcomes rather than absolute outcomes. 7 Prospect theory can be used to support predictive market models and to address one’s own biases as an individual investor. Heuristics—the process of simplifying complex decisions—and biases should also be addressed in investment decision-making.
Some helpful strategies for overcoming your own biases and cognitive limitations in investing include:
- Discussing investment decisions with multiple unrelated advisors
- Avoiding impulsive decisions, especially when influenced by trends
- Assessing your financial position before making a decision
- Investing in a diverse portfolio to offset potentially risky decisions
- Getting an education in behavioral finance and investing
Real-World Applications of Behavioral Finance
Aside from informing individual investment decisions, how does the study of behavioral finance apply in real life?
For one thing, behavioral finance plays a role in large-scale investment management. Finance professionals who manage large investment portfolios will benefit from a thorough, up-to-date education in behavioral finance.
Another example is behavioral bias in retirement savings. Research in behavioral finance has significantly impacted retirement savings, highlighting how inertia and loss aversion influence retirement planning. Studies have shown that automatically enrolling employees in retirement savings plans increases participation rates, as many individuals exhibit status quo bias and would not opt-in on their own. 8 Similarly, adjustments in the default contribution rates can counteract loss aversion, as individuals tend to stick with default settings even if they are suboptimal.
A behavioral finance education can also support a successful career in financial advising—helping individuals mitigate their biases and make smarter, well-informed investments.
An Online Master’s in Behavioral Finance
Behavioral finance is an ever-evolving field that supports a number of financial careers. If you’re interested in learning more about the challenges, theories and strategies of this field, consider an Online Master of Science in Finance (MSF) degree from William & Mary.
With a fully online education, you’ll have the opportunity to get educated and boost your career without taking time away from your work and family. And, when you choose William & Mary’s Online MSF program, you have the unique opportunity to graduate with both your master’s degree and a specialized finance certificate—either a Corporate Finance certificate or an Investment Management certificate—in as few as 16 months. Earn two credentials and get a deep dive into finance more quickly and thoroughly than you would with a typical MBA.
Speak to an admissions outreach advisor today to learn more about the benefits of the Online MSF.
- Retrieved on April 23, 2024 from blackrock.com/lu/individual/education/behavioural-finance
- Retrieved on April 23, 2024 from investopedia.com/terms/r/rational-choice-theory.asp
- Retrieved on April 23, 2024 from toptal.com/finance/financial-analysts/investor-psychology-behavioral-biases
- Retrieved on April 23, 2024 from capital.com/herd-bias
- Retrieved on April 23, 2024 from investopedia.com/terms/d/dotcom-bubble.asp
- Retrieved on April 23, 2024 from cnbc.com/2021/01/30/psychology-of-gamestop-stock-phenomenon.html
- Retrieved on April 23, 2024 from behavioraleconomics.com/resources/mini-encyclopedia-of-be/prospect-theory/
- Retrieved on April 23, 2024, from asppa.org/news/autoenrollment-boon-research-finds
Return to Online Business Blog
William & Mary has engaged Everspring , a leading provider of education and technology services, to support select aspects of program delivery.
- Introduction
- Conclusions
- Article Information
BMI indicates body mass index (calculated as weight in kilograms divided by height in meters squared).
The data points represent means. Error bars represent the SEs.
The data points indicate estimated marginal means for weekly loss of control eating, overeating, and binge eating, and the shaded areas represent the 95% CIs. Scores range from 0 (not at all) to 10 (very much), with higher scores indicating greater intensity of each behavior per week.
Trial Protocol
eTable 1. Descriptive Statistics and Reliabilities of Primary and Secondary Outcomes
eTable 2. Baseline Differences in Demographic Characteristics
eTable 3. Weekly Trends in Eating Disorder–Related Behaviors
eTable 4. Sensitivity Analyses for Primary and Secondary Outcomes
eTable 5. Moderator Analyses of Participant Characteristics on the Primary Outcome
eTable 6. Temporal Trajectories in Ecological Momentary Assessment Data
eTable 7. Negative Effects Attributed to the Web-Based Intervention
eTable 8. Dropout Over Time in the Control and Intervention Group
Data Sharing Statement
- Digital Interventions to Close the Treatment Gap for Binge Eating JAMA Network Open Invited Commentary May 16, 2024 Andrea K. Graham, PhD
See More About
Sign up for emails based on your interests, select your interests.
Customize your JAMA Network experience by selecting one or more topics from the list below.
- Academic Medicine
- Acid Base, Electrolytes, Fluids
- Allergy and Clinical Immunology
- American Indian or Alaska Natives
- Anesthesiology
- Anticoagulation
- Art and Images in Psychiatry
- Artificial Intelligence
- Assisted Reproduction
- Bleeding and Transfusion
- Caring for the Critically Ill Patient
- Challenges in Clinical Electrocardiography
- Climate and Health
- Climate Change
- Clinical Challenge
- Clinical Decision Support
- Clinical Implications of Basic Neuroscience
- Clinical Pharmacy and Pharmacology
- Complementary and Alternative Medicine
- Consensus Statements
- Coronavirus (COVID-19)
- Critical Care Medicine
- Cultural Competency
- Dental Medicine
- Dermatology
- Diabetes and Endocrinology
- Diagnostic Test Interpretation
- Drug Development
- Electronic Health Records
- Emergency Medicine
- End of Life, Hospice, Palliative Care
- Environmental Health
- Equity, Diversity, and Inclusion
- Facial Plastic Surgery
- Gastroenterology and Hepatology
- Genetics and Genomics
- Genomics and Precision Health
- Global Health
- Guide to Statistics and Methods
- Hair Disorders
- Health Care Delivery Models
- Health Care Economics, Insurance, Payment
- Health Care Quality
- Health Care Reform
- Health Care Safety
- Health Care Workforce
- Health Disparities
- Health Inequities
- Health Policy
- Health Systems Science
- History of Medicine
- Hypertension
- Images in Neurology
- Implementation Science
- Infectious Diseases
- Innovations in Health Care Delivery
- JAMA Infographic
- Law and Medicine
- Leading Change
- Less is More
- LGBTQIA Medicine
- Lifestyle Behaviors
- Medical Coding
- Medical Devices and Equipment
- Medical Education
- Medical Education and Training
- Medical Journals and Publishing
- Mobile Health and Telemedicine
- Narrative Medicine
- Neuroscience and Psychiatry
- Notable Notes
- Nutrition, Obesity, Exercise
- Obstetrics and Gynecology
- Occupational Health
- Ophthalmology
- Orthopedics
- Otolaryngology
- Pain Medicine
- Palliative Care
- Pathology and Laboratory Medicine
- Patient Care
- Patient Information
- Performance Improvement
- Performance Measures
- Perioperative Care and Consultation
- Pharmacoeconomics
- Pharmacoepidemiology
- Pharmacogenetics
- Pharmacy and Clinical Pharmacology
- Physical Medicine and Rehabilitation
- Physical Therapy
- Physician Leadership
- Population Health
- Primary Care
- Professional Well-being
- Professionalism
- Psychiatry and Behavioral Health
- Public Health
- Pulmonary Medicine
- Regulatory Agencies
- Reproductive Health
- Research, Methods, Statistics
- Resuscitation
- Rheumatology
- Risk Management
- Scientific Discovery and the Future of Medicine
- Shared Decision Making and Communication
- Sleep Medicine
- Sports Medicine
- Stem Cell Transplantation
- Substance Use and Addiction Medicine
- Surgical Innovation
- Surgical Pearls
- Teachable Moment
- Technology and Finance
- The Art of JAMA
- The Arts and Medicine
- The Rational Clinical Examination
- Tobacco and e-Cigarettes
- Translational Medicine
- Trauma and Injury
- Treatment Adherence
- Ultrasonography
- Users' Guide to the Medical Literature
- Vaccination
- Venous Thromboembolism
- Veterans Health
- Women's Health
- Workflow and Process
- Wound Care, Infection, Healing
Get the latest research based on your areas of interest.
Others also liked.
- Download PDF
- X Facebook More LinkedIn
Pruessner L , Timm C , Barnow S , Rubel JA , Lalk C , Hartmann S. Effectiveness of a Web-Based Cognitive Behavioral Self-Help Intervention for Binge Eating Disorder : A Randomized Clinical Trial . JAMA Netw Open. 2024;7(5):e2411127. doi:10.1001/jamanetworkopen.2024.11127
Manage citations:
© 2024
- Permissions
Effectiveness of a Web-Based Cognitive Behavioral Self-Help Intervention for Binge Eating Disorder : A Randomized Clinical Trial
- 1 Department of Psychology, Heidelberg University, Heidelberg, Germany
- 2 Department of Psychology, University of Osnabrück, Osnabrück, Germany
- Invited Commentary Digital Interventions to Close the Treatment Gap for Binge Eating Andrea K. Graham, PhD JAMA Network Open
Question Does a web-based cognitive behavioral self-help intervention improve outcomes in patients with binge eating disorder (BED)?
Findings In this randomized clinical trial involving 154 patients with BED, access to a web-based cognitive behavioral self-help intervention was superior to a waiting-list condition. The intervention significantly reduced the number of objective binge eating episodes compared with the control group.
Meaning These findings highlight the value of web-based cognitive behavioral self-help interventions as a promising solution to support individuals with BED and address the substantial treatment gap in this population.
Importance Binge eating disorder (BED) is one of the most frequent eating pathologies and imposes substantial emotional and physical distress, yet insufficient health care resources limit access to specialized treatment. Web-based self-help interventions emerge as a promising solution, offering more accessible care.
Objective To examine the effectiveness of a web-based cognitive behavioral self-help intervention for individuals with BED.
Design, Setting, and Participants This 2-arm, parallel-group randomized clinical trial conducted from January 15, 2021, to August 3, 2022, in Germany and other German-speaking countries enrolled patients aged 18 to 65 years who met the diagnostic criteria for BED (according to the Diagnostic and Statistical Manual of Mental Disorders [Fifth Edition]). Data analysis occurred between January 27 and September 4, 2023, following our statistical analysis plan.
Interventions Participants were randomized to a web-based self-help intervention or a waiting-list control condition.
Main Outcomes and Measures The primary outcome was a change in objective binge eating episodes from baseline to after treatment. Secondary outcomes included global eating pathology, clinical impairment, work capacity, well-being, comorbid psychopathology, self-esteem, and emotion regulation.
Results A total of 1602 patients were screened, of whom 154 (mean [SD] age, 35.93 [10.59] years; 148 female [96.10%]) fulfilled the criteria for BED and were randomized (77 each to the intervention and control groups). The web-based intervention led to significant improvements in binge eating episodes (Cohen d , −0.79 [95% CI, −1.17 to −0.42]; P < .001), global eating psychopathology (Cohen d , −0.71 [95% CI, −1.07 to −0.35]; P < .001), weekly binge eating (Cohen d , −0.49 [95% CI, −0.74 to −0.24]; P < .001), clinical impairment (Cohen d , −0.75 [95% CI, −1.13 to −0.37]; P < .001), well-being (Cohen d , 0.38 [95% CI, 0.01 to 0.75]; P = .047), depression (Cohen d , −0.49 [95% CI, −0.86 to −0.12]; P = .01), anxiety (Cohen d , −0.37 [95% CI, −0.67 to −0.07]; P = .02), self-esteem (Cohen d , 0.36 [95% CI, 0.13 to 0.59]; P = .003), and emotion regulation (difficulties: Cohen d , −0.36 [95% CI, −0.65 to −0.07]; P = .01 and repertoire: Cohen d , 0.52 [95% CI, 0.19 to 0.84]; P = .003).
Conclusion and Relevance In this randomized clinical trial of a web-based self-help intervention for patients with BED, the findings confirmed its effectiveness in reducing binge eating episodes and improving various mental health outcomes, highlighting a scalable solution to bridge the treatment gap for this condition.
Trial Registration ClinicalTrials.gov Identifier: NCT04876183
Binge eating disorder (BED) is one of the most prevalent eating disorders, impacting 1.0% to 2.8% of the population over their lifetimes. 1 - 3 Defined by recurrent episodes of uncontrolled overeating, BED contributes to obesity, hypertension, and type 2 diabetes 4 - 7 and undermines quality of life, occupational performance, and social relationships. 4 , 8 , 9 Without intervention, BED progresses to a chronic condition 10 and may lead to premature death. 8 , 9 , 11
While cognitive behavioral therapy (CBT) has demonstrated its effectiveness as an evidence-based BED intervention, 12 , 13 treatment rates for this disorder are reduced compared with other psychiatric conditions, including anorexia nervosa and bulimia nervosa. 14 - 16 Various barriers prevent individuals from seeking face-to-face psychotherapy, including limited access, clinician unawareness, sociocultural stigma, and treatment costs. 17 As a result, there is a substantial treatment gap for BED.
The rapid evolution of technology provides new avenues for delivering interventions that can address this gap and make evidence-based BED treatments more accessible. 18 - 21 Web-based cognitive behavioral interventions have gained prominence due to their advantages in terms of availability, cost-effectiveness, ease of implementation, and reduced social stigma, thereby circumventing the barriers of conventional BED treatments. 18 , 22 - 24
Although preliminary studies indicate that digital interventions hold promise for addressing BED, there remains a critical need for rigorous scientific investigations under naturalistic conditions. 19 , 25 - 28 This randomized clinical trial aims to fill this gap by examining the effectiveness of a web-based cognitive behavioral self-help intervention for BED compared with a waiting-list control group in an ecologically valid setting. To amplify translational potential and broaden generalizability, we analyzed alterations in key eating disorder symptoms, clinical impairment, well-being, comorbid psychopathology, self-esteem, and emotion regulation.
Given the limited understanding of the outcomes of web-based BED treatments compared with interventions targeting subclinical eating psychopathology 24 , 29 or other mental disorders, 30 - 32 this study evaluated patients with full-threshold BED to enhance clinical applicability. Furthermore, few studies have investigated how these interventions impact participants’ everyday lives. 33 Therefore, our study used ecological momentary assessment (EMA) and weekly symptom monitoring to capture real-time changes in binge eating and its underlying mechanisms.
This randomized clinical trial was conducted from January 15, 2021, to August 3, 2022, to examine the effectiveness of a web-based cognitive behavioral self-help intervention for BED. 34 Participants were randomly assigned to an intervention group with direct access to the web-based treatment or a waiting-list control group. Assessments were completed at baseline (study entrance) and at 6 weeks (midtreatment) and 12 weeks (posttreatment) following baseline. This study followed the Consolidated Standards of Reporting Trials ( CONSORT ) reporting guideline. All participants provided written informed consent, and the study was approved by the institutional review board at Heidelberg University. Upon completing all assessments, participants received a reimbursement of €30 (US $32.40). Data were stored anonymously following European Union regulations.
Nationwide recruitment of participants took place in Germany with additional provisions for the participation of German-speaking individuals from other European countries. Recruitment channels encompassed eating disorder treatment centers, a waiting list of individuals expressing interest in the web-based program, social media platforms, mailing lists, and self-help forums.
Participants completed a digital registration process to undergo eligibility screening, provide informed consent, and schedule a clinical interview, including the Eating Disorder Examination Interview 35 and the Diagnostic Interview for Psychological Disorders. 36 All diagnostic interviews were conducted by trained researchers; supervision was overseen by licensed clinical psychologists (L.P. and C.T.). Interrater reliability was determined during the initial interviews conducted by each interviewer to ensure the accuracy of the diagnostic decisions and consistent implementation of the interviews from the outset of the data collection. This assessment demonstrated significant agreement (with Cohen κ = 0.90 for 19 interviews).
Inclusion criteria encompassed (1) being aged 18 to 65 years, (2) owning a smartphone with internet access, (3) C1-level (advanced) proficiency in German, and (4) a diagnosis of BED based on the Diagnostic and Statistical Manual of Mental Disorders (Fifth Edition). 37 Exclusion criteria entailed (1) a body mass index below 18.5 (calculated as weight in kilograms divided by height in meters squared), (2) current psychotherapy or pharmacotherapy for eating disorders, (3) anorexia nervosa or bulimia nervosa, (4) bipolar disorder or psychotic disorders, (5) acute substance dependence, (6) current severe depressive episodes, and (7) acute suicidality. These exclusion criteria were chosen due to their potential to act as contraindications for web-based self-help interventions. 38 Other comorbidities were not excluded to mirror standard clinical practice.
After the baseline assessment, a 1:1 randomization ratio was executed by an independent researcher unaffiliated with the project using an automated and anonymized process. 39 To uphold allocation concealment, the participants’ identities and group assignments were unknown by the study personnel, including the individual who initiated the randomization. 40 An automated notification informed participants of their randomization results. Moreover, measures used to maintain blinding of the data analysis and clinical interviews, ensuring an unbiased approach throughout the study, are documented in the study protocol ( Supplement 1 ). 34
Participants in the intervention group received access to the 12-week web-based intervention (Selfapy). 34 , 41 - 43 This program is rooted in CBT and uses evidence-based exercises to elucidate risk factors and mechanisms of BED based on a diathesis-stress model. 34 , 41 Central to the program is a core curriculum of 6 mandatory modules focused on self-monitoring of binge eating, psychoeducation, and emotion regulation, complemented by 6 elective specialization areas tailored to participants’ preferences. Each module incorporated a blend of texts, videos, audio, and interactive exercises. 34 , 41 To ensure a personalized and engaging learning experience while maintaining consistent participant progression, the intervention used a sequential module-access strategy, with modules becoming accessible after completing the preceding ones. Email reminders were sent to participants who delayed starting the program to foster initial and sustained engagement, and an integrated messaging feature was provided to facilitate inquiries about the intervention content.
Participants assigned to the control group did not receive access to the web-based intervention during the study. They were notified that they would gain access after a 12-week waiting period. To mimic naturalistic conditions in which individuals seeking help for BED might explore various treatment options, all participants, regardless of group assignment, could seek other types of pharmaceutical and psychological treatments. This design choice also facilitated the ethical consideration of not withholding effective treatment. Importantly, we closely monitored the initiation of other health care services in both groups throughout the study 44 to assess their impact on the intervention’s effectiveness.
The primary outcome was a reduction in objective binge eating episodes from baseline to after treatment (using the Eating Disorder Examination Questionnaire). 45 Secondary outcome measures were global eating psychopathology (using the Eating Disorder Examination Questionnaire); weekly loss of control eating, overeating, and binge eating (using the Weekly Binges Questionnaire) 46 ; clinical impairment (using the Clinical Impairment Assessment Questionnaire scale) 47 ; well-being (using the World Health Organization Well-Being Index-5 questionnaire) 48 ; and work capacity (using the Institute for Medical Technology Assessment Productivity Cost Questionnaire). 49 Exploratory outcomes were depressive symptoms (using the Patient Health Questionnaire-9), 50 anxiety symptoms (using the Generalized Anxiety Disorder Scale-7), 51 self-esteem (using the Rosenberg Self-Esteem Scale), 52 and emotion regulation (using the Difficulties in Emotion Regulation Scale 53 and the Heidelberg Form for Emotion Regulation Strategies 54 ). Detailed descriptions of all scales and psychometric properties are provided in the study protocol ( Supplement 1 ) and eTable 1 in Supplement 2 .
Additional measures included an EMA protocol 55 on participants’ mobile devices for 5 days at baseline and after treatment. Furthermore, the study assessed measures of online intervention attitudes (using the Attitudes Toward Psychological Online Interventions scale), 56 treatment expectations (using the Patients’ Questionnaire on Therapy Expectation and Evaluation scale), 57 adverse effects (using the Negative Effects Questionnaire), 58 and health care service utilization (using the Client Sociodemographic and Service Receipt Inventory). 44
Data analysis occurred between January 27 and September 4, 2023, following our statistical analysis plan. Multilevel models with random intercepts, the fixed effects of time and treatment, and the interaction effect of time and treatment were conducted to examine whether there was a more substantial change in the intervention group compared with the control group in primary and secondary outcomes. These models were selected due to their capacity to address the hierarchical data structure, with observations nested within participants, and their flexibility in accommodating missing data. 59 We assessed the size of the main and interaction effects by estimating Cohen d based on Feingold’s recommendations. 60 To evaluate the clinical significance of our findings, a minimal clinically important difference (MCID) score was computed (MCID = SD b [√(1 − r )]), in which b indicates baseline and r represents the retest reliability of the primary outcome. This method aligns with other trials in the eating disorders field, 61 , 62 ensuring that the MCID reflects a change larger than the measurement error alone. Power analyses 63 based on an intraclass correlation of 0.40, a power of 0.80, and an α level of .05 indicated a required sample of at least 152 participants or more. Statistical analyses were performed using R, version 4.3.1 (R Project for Statistical Computing). A 2-sided P < .05 was considered statistically significant.
As sensitivity analyses, we performed the last observation carried forward approach and the multiple imputations by chained equations technique to deal with missing data. 64 False discovery rate-adjusted P values were calculated using the Bonferroni-Holm correction. 65 Additionally, we explored the impact of potential moderators, such as treatment expectations, 57 online intervention attitudes, adherence, and health care service utilization. 44 Last, we assessed the percentage of individuals in the intervention group who encountered adverse effects. 58
Participant enrollment and study flow are depicted in the CONSORT flow diagram ( Figure 1 ). Of 1602 patients initially screened, 154 participants (mean [SD] age, 35.93 [10.59] years; 148 female [96.10%], 5 male [3.25%], and 1 nonbinary [0.65%]) fulfilled the criteria for BED and were ultimately randomized. Among the total participants, 77 were randomized to the web-based self-help intervention group and 77 to the waiting-list control condition. Table 1 depicts the baseline characteristics, which were comparable across groups (eTable 2 in Supplement 2 ).
Participants in the intervention group revealed a greater reduction in binge eating episodes compared with the control group. The estimated interaction effect between treatment and time, assessing differential changes from baseline to after treatment across the two groups, was −7.91 (95% CI, −11.68 to −4.15), with a Cohen d of −0.79 (95% CI, −1.17 to −0.42; P < .001). Figure 2 illustrates the trajectory of the primary outcome in both groups. In the intervention group, the mean (SD) of binge eating episodes decreased from 14.79 (9.60) at baseline to 6.07 (6.71), marking a significant reduction (coefficient, −9.02 [95% CI, −11.31 to −6.72] and Cohen d , −1.00 [95% CI, −1.30 to −0.70]; P < .001) that surpassed the clinically meaningful threshold (MCID ≥ 3.97 episodes; reliability = 0.84). 62 As expected, the mean (SD) number of binge eating episodes in the control group decreased from 15.01 (10.30) to 14.33 (17.58), indicating no significant change (coefficient, −1.21 [95% CI, −4.34 to 1.93] and Cohen d , −0.09 [95% CI, −0.33 to 0.15]; P = .44).
Table 2 details the treatment effects across all outcomes. The intervention group exhibited significantly reduced global eating psychopathology compared with the control group (Cohen d , −0.71 [95% CI, −1.07 to −0.35]; P < .001). Figure 3 further illustrates that the intervention group experienced significantly greater decreases in weekly loss of control eating (Cohen d , −0.56 [95% CI, −0.81 to −0.31]; P < .001), weekly overeating (Cohen d , −0.44 [95% CI, −0.69 to −0.18]; P < .001), and weekly binge eating (Cohen d , −0.49 [95% CI, −0.74 to −0.24]; P < .001) than the control group (eTable 3 in Supplement 2 ).
Clinical impairment also revealed a notable decrease in the intervention group (Cohen d , −0.75 [95% CI, −1.13 to −0.37]; P < .001) . Additionally, the intervention group experienced a significantly greater improvement in well-being compared with the control group (Cohen d , 0.38 [95% CI, 0.01-0.75]; P = .047). However, there were no meaningful between-group differences regarding changes in work capacity (Cohen d , 0.05 [95% CI, −0.33 to 0.43]; P = .80).
The intervention group revealed significantly more substantial decreases in depression (Cohen d , −0.49 [95% CI, −0.86 to −0.12]; P = .01) and anxiety (Cohen d , −0.37 [95% CI, −0.67 to −0.07]; P = .02) compared with the control group. Furthermore, improvements in self-esteem (Cohen d , 0.36 [95% CI, 0.13 to 0.59]; P = .003) and emotion regulation abilities (difficulties: Cohen d , −0.36 [95% CI, −0.65 to −0.07]; P = .01 and repertoire: Cohen d , 0.52 [95% CI, 0.19 to 0.84]; P = .003) were found in the intervention group compared with the control group.
Sensitivity analyses for all primary and secondary confirmatory outcomes are summarized in eTable 4 in Supplement 2 , reaffirming the results obtained in our primary statistical models. These analyses consistently revealed significant reductions in binge eating episodes, improvements in eating psychopathology, and diminished clinical impairment. However, the impact on well-being did not consistently appear across different sensitivity analyses, indicating less robust effects on this metric. Modeling changes using a continuous time variable replicated the main findings for all outcomes.
Moderators, including baseline symptom severity, treatment expectations, online intervention attitudes, health care service utilization, and demographics, did not impact treatment effects. At baseline, 23 participants (14.94%) received in-person psychotherapy, aligning with typical treatment rates for BED. 66 Participants across both groups showed low current psychotropic medication use (10 participants [6.49%]). Throughout the study, an additional 13 participants (8.44%) in both groups sought in-person psychotherapy, and 2 (1.30%) initiated new psychotropic medication. Treatment-seeking intentions indicated that 26 participants (16.88%) were currently on a waiting list for in-person psychotherapy and aimed to use the web-based intervention to bridge this waiting time. None of these health care service–related variables differed between groups or acted as moderators (eTable 5 in Supplement 2 ).
Additional analyses of EMA data are reported in eTable 6 in Supplement 2 . These indicated that the intervention effects could also be demonstrated momentarily in participants’ everyday lives, revealed by reductions in binge eating, overeating, shape concerns, and weight concerns in the intervention group compared with the control group.
Findings among participants in the intervention group who provided complete data on the web-based program's adverse effects are detailed in eTable 7 in Supplement 2 . While these were overall minimal, the resurgence of distressing memories was recorded as a more frequent adverse effect in 20 of 64 patients (31.25%) at midtreatment and in 8 of 59 patients (13.56%) at posttreatment. These analyses provide a comparative perspective, indicating that negative effects were lower than those reported for face-to-face psychotherapy. 67
The dropout rate was 17.53%, with no significant difference between the intervention and control groups ( χ 2 = 1.62; P = .20) (eTable 8 in Supplement 2 ), indicating comparable retention across study arms. The number of modules completed in the intervention was used as an indicator of treatment dose. Most participants in the intervention group (71 [92.22%]) began the intervention. The participants completed a mean (SD) of 7.65 (4.07) modules, including the 6 core modules and 2 optional secialization areas. Notably, a greater treatment dose, evidenced by completing more modules, was associated with more substantial reductions in eating psychopathology (β, −0.38 [95% CI, −0.63 to −0.12]; P < .001). Additionally, a significant proportion of the control group (61 [79.22%]) enrolled in the program after the study had concluded, highlighting the continued need for the intervention.
Binge eating disorder is a frequent and debilitating disorder with considerable societal and personal burdens. 1 , 7 , 9 However, traditional treatments have faced constraints, such as limited access, stigma, and high cost, 17 , 18 underscoring the need to explore alternative intervention delivery methods. The findings of this randomized clinical trial present new possibilities for addressing this substantial public health challenge by building on the widespread adoption of digital technologies. 18 , 24 , 29
Notably, we found supportive evidence for the effectiveness of a 12-week, web-based cognitive behavioral self-help program for BED. The intervention group outperformed the control group in reducing binge eating episodes and several key outcomes, including global eating psychopathology and clinical impairment. The observed effect sizes mirrored or exceeded those reported in trials investigating other digital interventions for eating disorders 19 , 25 - 28 and in-person guided and unguided self-help interventions for BED. 68 - 70 Furthermore, the effect sizes were consistent with or even surpassed established findings from studies exploring the impact of conventional, in-person CBT interventions, 12 , 13 underlining the clinical relevance of the results. Moreover, by not limiting our inclusion criteria to individuals with comorbid obesity, 69 - 71 our research offers evidence for the broad applicability of web-based interventions in enhancing eating disorder–related outcomes across the BED spectrum.
In addition to the significant reductions in eating psychopathology, the intervention extended its benefits to diverse aspects of participants’ quality of life. Receiving access to the program was linked to improvements in well-being, depressive symptoms, anxiety, self-esteem, and emotion regulation. This expansion beyond eating psychopathology was further substantiated by weekly symptom monitoring and real-time data that were collected in everyday contexts, which revealed that participants in the intervention group experienced tangible improvements in day-to-day symptoms and quality of life.
Together, these findings showcase the potential of digital interventions to enhance participants’ everyday experiences by addressing core eating-related symptomatology and the emotional and psychological mechanisms frequently linked with BED. 72 , 73 To reflect naturalistic conditions, we permitted participants from both study groups to seek additional professional assistance, which may account for the minor improvements in global eating psychopathology and depressive symptoms observed within the control group. Notably, our analysis confirmed that the engagement in psychotherapy or pharmacotherapy following randomization was equally low across both groups and did not moderate the web-based intervention’s outcomes. The positive effects of the intervention further persisted when considering participants’ attitudes toward online interventions, treatment expectations, and demographic characteristics as moderators. Additionally, the effectiveness of self-guided web-based interventions depends on participant motivation, highlighting the importance of monitoring dropout and retention rates. 22 , 71 Our results indicate low dropout levels, underlining our findings’ robustness
Regarding the study’s limitations, it is crucial to acknowledge that our sample’s socioeconomic and demographic composition may reflect disparities in help-seeking behavior and digital literacy among socioeconomic groups. Our study highlights the necessity for targeted strategies to engage male and older populations, who are often underrepresented, in studies of web-based interventions for BED. 19 , 28 Moreover, while self-report measures are indispensable for assessing subjective experiences and complex behaviors, these methods are vulnerable to social desirability. 55 To increase the accuracy of self-reported information, our study complemented traditional questionnaires with real-time EMA and weekly reports. Future research may benefit from additional methodologies to minimize these biases, including double-blind designs and integrating objective measures where feasible. Additionally, the temporal scope of our study, focusing on postintervention changes, calls for future research to extend the assessment period, ideally incorporating a 12-month follow-up to capture the program’s long-term effects. Finally, while our findings posit the effectiveness of the web-based intervention compared with a waiting-list control condition, it is imperative that subsequent investigations include comparisons with alternative control conditions, including face-to-face CBT, 74 , 75 and evaluate the practical implementation of these interventions in health care settings.
This randomized clinical trial demonstrated that offering access to a web-based intervention significantly enhanced the daily lives of participants with BED. The provision of accessible and effective treatment options holds promise for improving the everyday experiences of patients with BED, as well as for diminishing its adverse health effects. Providing these programs to those in need of treatment can contribute to alleviating the burden that BED places on patients, their families, and society.
Accepted for Publication: March 7, 2024.
Published: May 16, 2024. doi:10.1001/jamanetworkopen.2024.11127
Open Access: This is an open access article distributed under the terms of the CC-BY-NC-ND License . © 2024 Pruessner L et al. JAMA Network Open .
Corresponding Author: Luise Pruessner, MS, Department of Psychology, Heidelberg University, Hauptstr 47-51, 69117 Heidelberg, Germany ( [email protected] ).
Author Contributions: Ms Pruessner and Mr Hartmann had full access to all of the data in the study and take responsibility for the integrity of the data and the accuracy of the data analysis.
Concept and design: All authors.
Acquisition, analysis, or interpretation of data: Pruessner, Timm, Hartmann.
Drafting of the manuscript: Pruessner.
Critical review of the manuscript for important intellectual content: All authors.
Statistical analysis: Pruessner, Hartmann.
Obtained funding: Pruessner, Timm, Barnow.
Administrative, technical, or material support: Pruessner, Timm.
Supervision: Pruessner, Barnow, Rubel.
Conflict of Interest Disclosures: None reported.
Funding/Support: This study was supported by the European Regional Development Fund awarded to Selfapy. An independent evaluation of the web-based intervention for eating disorders was commissioned by Selfapy to the Department of Psychology at Heidelberg University (to the team of Dr Timm, Prof Barnow, Ms Pruessner, and Mr Hartmann) to ensure adherence to the highest scientific standards. The publication costs were funded by the German Research Foundation (Deutsche Forschungsgemeinschaft) and Heidelberg University.
Role of the Funder/Sponsor: The funder had no role in the design and conduct of the study; collection, management, analysis, and interpretation of the data; preparation, review, or approval of the manuscript; and decision to submit the manuscript for publication.
Data Sharing Statement: See Supplement 3 .
Additional Contributions: We express our appreciation to the following individuals for their significant contributions to participant recruitment and data collection, integral to their thesis work: Maria Brinkhof, BS; Katrin Fischer, MS; Leonie Hans, BS; Tanja Hauser, MS; Nina Helwig, MS; Luisa Jung, BS; Theresa Kloss, BS; Lena Komorowski, MS; Laura Kristalis, MS; Jana Reich, BS; Elena Rettweiler, BS; Marlene Sayer, MS; and Leoni Weintz, MS (all affiliated with Heidelberg University at the time of the study). No additional compensation was provided for their contributions beyond their customary remuneration.
- Register for email alerts with links to free full-text articles
- Access PDFs of free articles
- Manage your interests
- Save searches and receive search alerts
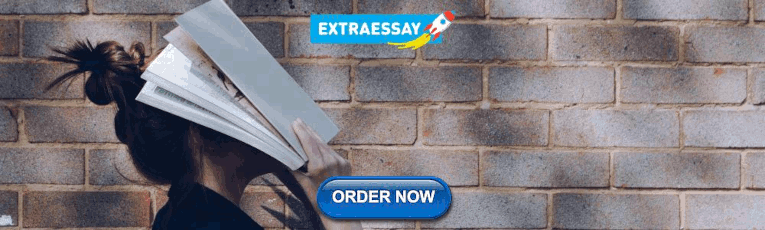
COMMENTS
The field of behavioral finance has attempted to explain a litany of biases, heuristics, and. inefficiencies present in financial markets since its creation in the 1980's. This paper is. structured as a comprehensive literature review of behavioral finance, and includes both the. seminal works as well as more recent papers.
1. Introduction. The traditional finance theory assumes that investors always make rational decisions based on complete information, but behavioral finance argues that investors are influenced by their emotions, biases, and cognitive limitations (Almansour & Arabyat, Citation 2017).The debate between modern finance theory and behavioral finance theory on the influence of non-financial factors ...
The Journal of Behavioral Finance (Q2) was prominent in productivity, having published 36 articles (60% of the sample). The total number of authors in the textual corpus is 152, who worked in 23 countries, predominantly in the United States. As to authorship composition, 91.67% of the papers had two or more authors, and regarding productivity ...
randomness, and herding. This thesis will conclude with applications drawn from the field of behavioral finance that can be applied to both the individual investor and the ... Behavioral finance identifies the potential causes of the recent stock booms and crashes and how they have their roots in human mistakes (Shiller, 2003). Statman (2014)
Behavioral finance is the application of psychology to finance, dedicated to explaining anomalies in the financial market based on research and analysis of human behavior. This paper aims for studying from a conceptual side the main behavioral biases that impact traders operating in the financial market under uncertain circumstances.
Title: Behavioral Finance - Investors' Rationality. Authors: Hannes Bernéus, Carl Sandberg, David Wahlbeck Tutor: Urban Österlund Date: 2008-12-02 Subject terms: Behavioral Finance, Behavioral Economics, Finance, Economic Psychology. Abstract Purpose: The purpose of this thesis is to examine if professional investors are
several renowned researchers, showing how behavioral finance can effect a 'rational' investor's decision making process. By exploring the following categories of internal investor characteristics, biased tendencies, and environmental factors, this thesis aims to show that market participants do not always behave entirely rationally.!!
Behavioral finance applies the field of psychology to finance and became the focus of many studies in the wake of the tech-stock bubble in 2000 (Pompian 2011).Human emotions (investor psychology or cognitive psychology provoking excessive euphoria or fear) and cognitive errors in financial decision-making create psychological biases when assessing the future performance of stock markets ...
Journal of Behavioral Finance, Volume 25, Issue 2 (2024) See all volumes and issues. Volume 25, 2024 Vol 24, 2023 Vol 23, 2022 Vol 22, 2021 Vol 21, 2020 Vol 20, 2019 Vol 19, 2018 Vol 18, 2017 Vol 17, 2016 Vol 16, 2015 Vol 15, 2014 Vol 14, 2013 Vol 13, 2012 Vol 12, 2011 Vol 11, 2010 Vol 10, 2009 Vol 9, 2008 Vol 8, 2007 Vol 7, 2006 Vol 6, 2005 ...
Abstract and Figures. This chapter explores the evolution of modern behavioral finance theories from the traditional framework. It focuses on three main issues. First, it analyzes the importance ...
EliScholar - A Digital Platform for Scholarly Publishing at Yale
Rahmatullah Pashtoon I Declaration of Authorship ) hereby declare that the thesis titled Behavioral Finance and its Impact on Portfolio Investment Decisions - Evidence: India is a bona fide record of the dissertation work carried out by me and submitted to Savithribai Phule Pune University in partial fulfillment of the requirement for Degree ...
Abstract. This Ph.D. dissertation consists of three independent chapters in behavioral finance and corporate finance. The first chapter examines whether and how ethnicity similarity between analysts and executives affect their interactions in conference calls. The second chapter investigates firms' demand for inventor executives, executives ...
Finance literature in this decade and after suggests a more nuanced view of the value of the efficient markets theory, and, starting in the 1990s, a blossoming of research on behavioral finance. Some important developments since 1990 include feedback theories, models of the interaction of smart money with ordinary investors, and evidence on ...
Malcolm Baker is the Robert G. Kirby Professor of Business Administration at the Harvard Business School. He was the Unit Head for finance from 2014 to 2018, and the program director for corporate finance at the National Bureau of Economic Research from 2011 to 2018. His research is in the areas of behavioral finance, corporate finance, and ...
Thesis or Dissertation. Abstract. Behavioral finance, defined as the combination of behavioral and cognitive psychology theory with economics and finance to explain financial decisions, has grown in popularity over the last several years. Behavioral finance has also expanded into sports as researchers seek to find and explain anomalies that ...
Abstract. This thesis deals with a range of topics in experimental and behavioral finance. The first part investigates the role of personal inclination in reference point employment by individuals. The second part extends this questions by focusing on the role of personality traits and demographic factors in driving reference point exhibition ...
Behavioral finance micro examines behavior or biases of investors and behavioral finance macro describe anomalies in the efficient market. Behavioral finance is an open-minded finance which includes the study of psychology, sociology, and finance. ... Master's Thesis in Finance, School of Economics and Management, Lund University. 28. Julious ...
Behavioral finance is a newly developed sub-discipline of Behavioral Economics. The main aim. of behavioral finance is to understand how people make their investment decision and how they. behave ...
Behavioral finance is a field of finance that proposes psychology-based theories to explain stock market anomalies such as severe rises or falls in stock price. Within behavioral finance , it is ...
Shodhganga: a reservoir of Indian theses @ INFLIBNET The Shodhganga@INFLIBNET Centre provides a platform for research students to deposit their Ph.D. theses and make it available to the entire scholarly community in open access.
The Shodhganga@INFLIBNET Centre provides a platform for research students to deposit their Ph.D. theses and make it available to the entire scholarly community in open access. Shodhganga@INFLIBNET. Sri Chandrasekharendra Saraswathi Viswa Mahavidyalaya. Department of Management Studies.
The Role of Behavioral Finance: Investor Psychology, Market Anomalies and Decision-Making. People often think of economics as a rational field, but emotions play a bigger role than you might think. Behavioral finance, the study of how psychology influences financial decisions, can tell us a lot about economic trends and individual choices.
Key Points. Question Does a web-based cognitive behavioral self-help intervention improve outcomes in patients with binge eating disorder (BED)?. Findings In this randomized clinical trial involving 154 patients with BED, access to a web-based cognitive behavioral self-help intervention was superior to a waiting-list condition. The intervention significantly reduced the number of objective ...