- Search Menu
- Sign in through your institution
- Supplements
- Cohort Profiles
- Education Corner
- Author Guidelines
- Submission Site
- Open Access
- About the International Journal of Epidemiology
- About the International Epidemiological Association
- Editorial Team
- Editorial Board
- Advertising and Corporate Services
- Journals Career Network
- Self-Archiving Policy
- Dispatch Dates
- Contact the IEA
- Journals on Oxford Academic
- Books on Oxford Academic
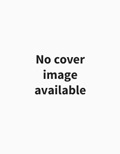
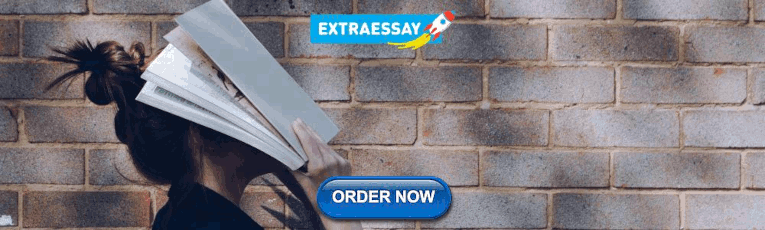
Article Contents
Cardiovascular and associated metabolic disease.
- < Previous
Physical activity and health: current issues and research needs
- Article contents
- Figures & tables
- Supplementary Data
Adrianne E Hardman, Physical activity and health: current issues and research needs, International Journal of Epidemiology , Volume 30, Issue 5, October 2001, Pages 1193–1197, https://doi.org/10.1093/ije/30.5.1193
- Permissions Icon Permissions
A substantial body of evidence now demonstrates the burden of ill-health attributable to sedentary living. This is most compelling for coronary heart disease (CHD) and, combined with the high prevalence of inactivity, 1 provides the rationale for Professor Morris's claim that exercise is 'today's best buy in public health'. 2 Besides a reduced risk of CHD, evidence is secure for many other health gains from physical activity; these include a reduced risk of stroke, 3, 4 type II diabetes, 5, 6 colon cancer, 7, 8 and hip fracture. 9, 10 There is evidence enough to justify the further development of public health policies to promote physical activity. The difficulty is with the specifics of what to promote and prescribe.
This paper is concerned with future contributions by research to an evidence-based rationale for exercise recommendations—both to the public at large and to individuals. It is clear that physically active people have a lower disease risk than sedentary individuals but the components of activity which determine particular health gains are poorly understood. Thus the 'dose-response' relationships for physical activity are the subject of current research interest. Intuitively, these will not be the same for different health outcomes and this is one reason why further study of the associated mechanisms is important. Understanding the underlying mechanisms will clarify the relative importance of intensity, frequency, duration and mode of exercise for specified health gains. It will also help us to distinguish the effects of exercise per se from those of co-existing behaviours and to identify stages of life during which levels of particular types of activity are critical for given health outcomes. This paper presents a personal view of research needs.
How important is intensity?
The rate of energy expenditure (in oxygen uptake units) of common physical activities is expressed in METS. One MET is equivalent to the resting metabolic rate, assumed to be 3.5 ml oxygen per kg of body mass per minute.
Oxygen uptake reserve is obtained by subtracting one MET (3.5 ml . kg .–1 min –1 ) from the maximal oxygen uptake.
Its importance in the epidemiology of physical activity is evidenced by data from British civil servants. 12 Whereas only frequent vigorous exercise (defined as liable to entail peaks of energy expenditure of ≥7.5 kcal.min –1 [31.5 kJ.min –1 ]) was associated with protection against heart attack in men aged 45–54 at entry, there was a dose-response relationship for a lesser degree of such exercise (either <2 sessions per week or not so intense, e.g. 'fairly brisk' walking for >30 min. per day) among older men aged 55–64 at entry. Thus, for example, older men reporting moderately intense activity such as 'much stair climbing' (not judged sufficiently vigorous to be included in the 'vigorous aerobic' cluster of activities) showed a coronary rate which was significantly lower than that in less active men. Protection among younger men was limited to those reporting frequent vigorous aerobic exercise. This finding suggests that the key features of cardio-protective exercise include its intensity relative to individual capacity. V • O 2 max declines, on average, by about 10% per decade in middle-aged and older people, 13 so exercise of a given MET value represents a higher relative intensity for older people. Where the number of individuals surveyed permit, one approach 14 may be to express the MET value of the activity in relation to age-related average values for oxygen uptake reserve.
Frequency of exercise
Recent recommendations 15, 16 are for exercise on '… most, preferably all, days of the week', underlining the importance of frequent exercise. This notion reflects increasing recognition of the acute effects of exercise, i.e. altered physiological or metabolic responses lasting between several hours and a few days after a session of exercise. These include a decrease in blood pressure, 17 improved insulin sensitivity 18 and decreases in plasma triglycerides. 19 The time-courses over which they disappear are poorly understood, however. Some information is available, for example the attenuation of the postprandial rise in plasma triglycerides following a standard high-fat meal has been reported to disappear within 60 hours of an exercise session. 20 Improved insulin sensitivity may persist for a little longer. 21 More information is required, however, as the duration of these effects dictates the frequency with which exercise sessions must be taken if favourable postprandial responses are to be maintained. Similarly, the determinants of the magnitude of acute effects of exercise need to be elucidated. Theoretically, this may be enhanced by training 22 because training permits more frequent and longer exercise sessions to be accomplished without fatigue. To the author's knowledge, this proposition has seldom been tested. 23
Pattern of exercise
Epidemiological studies have found an inverse relationship between the total energy expended in leisure time physical activity and health outcomes. These include a lower risk of all-cause mortality, 24 cardiovascular morbidity and mortality, 24, 25 type II diabetes, 6 hypertension, 26 and site-specific cancers. 27, 28 Some activities contributing to high totals of energy expenditure seem likely to have been performed at least partly on an intermittent basis, for example walking, 29 climbing stairs, 25, 30 gardening, 29 and repair work. 24 Survey evidence therefore suggests that several short sessions of moderate physical activity during the day influence health outcomes in a positive manner, at least when they contribute to a high total energy expenditure.
Scientific evidence for the efficacy of this pattern of exercise as a means of eliciting chronic (training) effects is limited however, both in the number of randomly controlled trials (three to the author's knowledge) and scope (the only common outcome measure was fitness). 31 Evidence is limited to scientific studies with outcome measures primarily of fitness and/or fatness. Only one study reported the effect of exercise pattern on acute health-related responses. This found similar reductions in plasma triglycerides with three, 10-minute bouts of brisk walking at intervals during the day and one, 30-minute bout in sedentary people consuming normal meals. 32
Further research is clearly required before the principle of accumulating exercise in short bouts throughout the day can be endorsed with confidence.
Energy expenditure and energy turnover
The product of intensity, frequency and duration of exercise—sometimes described as the total 'volume' of exercise (a difficult term)—yields the total gross energy expenditure. Some evidence points to this as an important determinant of health gains. In addition to the surveys referred to above, this includes the finding from the US Runners' Health Study that running mileage was six times more important in predicting high density lipoprotein cholesterol concentration than running speed. 33 This was not the case for associations with blood pressure or waist circumference, however, where running speed was the more important determinant. 33 Total energy expenditure may also be the main determinant of some acute effects of exercise. Two examples are relevant. First, the increase in glucose disposal rate was similar following exercise at 50% or 75% V • O 2 max when the total energy expended was held constant. 34 Second, the attenuation of postprandial plasma triglycerides by prior exercise was strikingly similar following a long bout of low intensity exercise and a shorter bout of moderate exercise expending the same energy. 35 This topic, again under-researched, is related to that of the accumulation of exercise (referred to above) because that enshrines the notion that the total energy expenditure is all-important.
Of course, in free-living people, an increased level of physical activity is invariably associated with an increase in energy intake so that energy turnover is increased. Speculatively, a higher energy turnover may constitute a metabolically desirable state because of effects on the pathways concerned with the disposition, storage and degradation of muscle energy substrates. Evidence for the health gains from such a state include the finding that men who were classified as obese by body mass index (BMI) but who had a high level of physical fitness had lower cardiovascular and total mortality rates than lean men who were unfit. 36 Similarly, although both high BMI and a high energy intake were associated with increased risk of colon cancer among inactive people, this was not the case among physically active individuals. 8 This finding suggests that a high energy intake does not confer increased risk of this cancer in the presence of a high expenditure.
The suggestion that a high energy turnover is metabolically advantageous is not new. The term 'metabolic fitness' was introduced by Després and Lamarche, 37 on the basis of a series of studies showing that change to plasma lipoprotein lipids and body fatness were achieved through high-volume, low intensity training in the absence of increases in V • O 2 max. Efforts to test this hypothesis through comparing the effects of 'lifestyle' activity with those of traditional exercise programmes have recently been reported 38– 40 but information is needed for a variety of health outcomes in different populations.
Over the last decade, epidemiological data on physical activity (a behaviour) has been complemented by findings based on physical fitness (a set of attributes related to the ability to perform exercise). These studies show a dose-response relationship so that, although men in the highest fitness groups consistently show the lowest coronary attack and total mortality rates, moderate levels of fitness also confer a statistically significant and clinically important reduction in risk. 41, 42 Physical fitness, because it is probably a more objective measure than physical activity is an attractive outcome measure. Its use could be extended of course if it could be measured satisfactorily outside the laboratory. A low-cost, rapid, non-intimidating method for this would allow large surveys with the statistical power to detect, for example, effects in sub-groups and effects of specific activities. Walking tests such as the UKK Institute's 2 km protocol 43 are attractive for both practical and theoretical reasons. Performance on these tests measures not only functional capacity (V • O 2 max, the most frequently used laboratory measure), but also endurance. This is defined as the capability to sustain aerobic exercise using a high proportion of V • O 2 max. Endurance is more sensitive to changes in physical activity level than V • O 2 max and, because it derives largely from metabolic adaptations in muscle, may be a more important determinant of related health gains.
As mentioned, epidemiological studies show associations between fitness and a variety of health outcomes. The need to elucidate the relationships between the 'dose' and pattern of activity and the health outcome has been mentioned above. Fitness (particularly endurance) is labile and so rather easily changed through short-term interventions. It therefore offers a means of studying these dose-response relations indirectly (but inexpensively), serving a link between the behaviour and health outcomes.
Most epidemiological studies have classified physical activities according to estimated energy expenditure—either totals or threshold rates. Recommendations to the public (whether direct or via health professionals), however, need to promote activities rather than energy expenditures. Walking is an obvious example. It is popular, inexpensive and carries a low risk of injury. It is often the most commonly reported activity, particularly among women 44 and older men. 12 Some landmark studies, including those by Professor Morris's group, 12 have published separate analyses for walking. 25 In British civil servants brisk walking accounted for over half of the exercise which was protective against heart attack in 55–64-year-old men. 12 Protection from attack among fairly brisk walkers was not significantly affected by controlling for participation in sports and cycling or for a lot of other CHD predictive factors. In recent years more data has become available, however. In the US Nurses Health Study, for example, walking was inversely associated with coronary events; women in the highest quintile group for walking (≥3 h per week at a brisk pace) had a multivariate relative risk of 0.65 (95% CI : 0.47–0.91). 45 Similarly, healthy older men in the Honolulu Heart Study who walked >1.5 miles per day had half the coronary risk of those who walked <0.25 miles per day. 46 Walking has also been reported to be associated with a lower risk of type II diabetes 47 (independently of participation in vigorous activity).
These observations are consistent with reports that moderate levels of fitness, associated with a reduction in all-cause mortality, are attainable through brisk or fast walking. 48, 49 Bearing in mind that sedentary people seldom exert themselves at more than 30–35% of V • O 2 max, 50 such walking is sufficiently vigorous to improve fitness in a majority of people whose health is at risk because of their inactivity.
Walking is especially suitable for older people and the functional gains it elicits will likely improve quality of life. It is plainly acceptable for them, and carries a low risk of injury. In 13 weeks of training by walking, only one injury was sustained among 57 healthy men and women their 70s. 51 Among older people, regular walking has been associated with lower rates of hospitalization, 52 lower plasma triglycerides and higher bone mineral density. 53
Because it is accessible to all but the very frail, more information on the specific benefits from walking—according to pace and distance—is sorely needed.
Studies of the associations between physical activity habits and disease outcomes must be complemented by research into the underlying mechanisms. Not only does this increase confidence that such associations may be causal but it helps us to understand the relative importance of the different components of exercise as mediators of specified health gains. For cardiovascular disease much is known of the potential contribution from exercise-induced changes to blood lipids, with recent information about considerable effects on the dynamic postprandial phase. Other mechanisms must be involved, however, because patients with CHD get improved myocardial perfusion (and decreased risk of further episodes) without net regression. 54
Recent findings suggest effects on the acute phases of the disease. (This would be concordant with observations that only continuing, current exercise confers a lower risk; past exercise has no effect. 12, 55 ) These include improved flow-mediated dilatation. 56 There may be links here with lipoprotein metabolism because flow-mediated dilatation is impaired by high plasma triglycerides, in proportion to concentration. 57
Mechanisms need elucidating in other areas, for instance skeletal health. Is the lower risk of hip fracture among physically active older women due to a decreased risk of falling and/or to an effect on bone mineral density? Is physical activity level particularly important during the years when bone formation predominates? The relationship between physical activity and a reduced risk of colon cancer is among the most consistent finding in the epidemiological literature. Is the mechanism systemic (reduced growth-promoting milieu) or local (increased colonic peristalsis)? Women who regularly engage in exercise may have a lower risk of breast cancer. 58 Speculation on potential mechanisms has involved endocrine factors and/or improved weight maintenance. Depending on the answers to such questions, some forms and regimens of exercise may be more effective than others in the achievement of particular objectives.
Physical inactivity is a waste of human potential for health and well-being and its high prevalence is a cause for concern. Its potential contribution to positive health (not merely the absence of disease but associated with a capacity to enjoy life and to withstand challenges 16 ) is considerable. So much is known—yet we need to understand much more. The effective 'dose' of exercise needed to elicit effects likely to be of clinical importance must be defined and this information translated into practical advice readily understood by the population at risk. Ten years after Professor Morris's plea for 'physiology and epidemiology to get together', 12 the need for co-operative efforts from these disciplines is even more urgent.
'Thank you'
I thank Professor Morris for posing thought-provoking questions and for stimulating discussion of these. His contributions—to research, to the National Fitness Survey for England, and to the development of public health policies—are valued by so many. It continues to be an education and a privilege to work with him.
National Fitness Survey: Main Findings. London: Sports Council and Health Education Authority, 1992.
Morris JN. Exercise in the prevention of coronary heart disease: today's best buy in public health. Med Sci Sports Exerc 1994 ; 26 : 807 –14.
Wannamethee G, Shaper AG. Physical activity and stroke in British middle-aged men. Br Med J 1992 ; 304 : 597 –601.
Lee I-M, Paffenbarger RS. Physical activity and stroke incidence. The Harvard Alumni Health Study. Stroke 1998 ; 29 : 2049 –54.
Haapanen N, Miilunpalo S, Vuori I, Oja P, Pasanen M. Association of leisure time physical activity with the risk of coronary heart disease, hypertension and diabetes in middle-aged men and women. Int J Epidemiol 1997 ; 26 : 739 –47.
Helmrich SP, Ragland DR, Leung RW, Paffenbarger RS. Physical activity and reduced occurrence of non-insulin-dependent diabetes mellitus. N Engl J Med 1991 ; 325 : 147 –52.
Giovannucci E, Ascherio A, Rimm EB, Colditz GA, Stampfer MJ, Willett WC. Physical activity, obesity, and risk for colon cancer and adenoma in men. Ann Int Med 1995 ; 122 : 327 –34.
Slattery ML, Potter J, Caan B et al . Energy balance and colon cancer—beyond physical activity. Cancer Res 1997 ; 57 : 75 –80.
Cummings SR, Nevitt MC, Browner WS et al . Risk factors for hip fracture in white women. N Engl J Med 1995 ; 332 : 767 –73.
Dargen-Molina P, Favier F, Grandjean H et al . Fall-related factors and risk of hip fracture: the EPIDOS prospective study. Lancet 1996 ; 348 : 145 –49.
Swain DP, Leutholtz BC. Heart rate reserve is equivalent to %V • O 2 Reserve, not to %V • O 2max . Med Sci Sports Exerc 1997 ; 29 : 410 –14.
Morris JN, Clayton DG, Everitt MG, Semmence AM, Burgess EH. Exercise in leisure time: coronary attack and death rates. Br Heart J 1990 ; 63 : 325 –34.
Hagberg JM. Effect of training on the decline of V • O 2max with aging. Fed Proc 1987 ; 46 : 1830 –33.
Howley ET. Type of activity: resistance, aerobic, anaerobic and leisure-time versus occupational physical activity. Med Sci Sports Exerc 2001 (in press).
More People, More Active, More Often . Report of Physical Activity Task Force. London: Department of Health, 1995.
Physical Activity and Health. A Report of the Surgeon General . US Departments of Health and Human Services. Centers for Disease Control and Prevention. Atlanta, GA, 1996.
Pescatello LS, Fargo AE, Leach CN, Scherzer HH. Short-term effect of dynamic exercsie on arterial blood pressure. Circulation 1991 ; 83 : 1557 –61.
Dela F, Mikines KJ, von Linstow M, Secher NH, Galbo H. Effect of training on insulin-mediated glucose uptake in human muscle. Am J Physiol 1992 ; 263 : E1134 –43.
Tsetsonis NV, Hardman AE, Mastana SS. Acute effects of exercise on postprandial lipemia: a comparative study in trained and untrained middle-aged women. Am J Clin Nutr 1997 ; 65 : 525 –33.
Hardman AE, Lawrence JEM, Herd SL. Postprandial lipemia in endurance-trained people during a short interruption to training. J Appl Physiol 1998 ; 84 : 1895 –901.
King DS, Baldus RJ, Sharp RL, Kesl LD, Feltmeyer TL, Riddle MS. Time course for exercise-induced alterations in insulin action and glucose tolerance in middle-aged people. J Appl Physiol 1995 ; 78 : 17 –22.
Haskell WL. Health consequences of physical activity: understanding and challenges regarding dose-response. Med Sci Sports Exerc 1994 ; 26 : 649 –60.
Crouse SF, O'Brien BC, Grandjean PW, Lowe RC, Rohack JJ, Green JS. Effects of training and a single session of exercise on lipids and apolipoproteins in hypercholesterolemic men. J Appl Physiol 1997 ; 83 : 2019 –28.
Haapanen N, Miilunpalo S, Vuori I, Oja P, Pasanen M. Characteristics of leisure time physical activity associated with decreased risk of premature all-cause and cardiovascular disease mortality in middle-aged men. Am J Epidemiol 1996 ; 143 : 870 –80.
Paffenbarger RS, Hyde RT, Wing AL, Hsieh C-C. Physical activity, all-cause mortality, and longevity of college alumni. N Engl J Med 1986 ; 314 : 605 –13.
Paffenbarger RS, Wing AL, Hyde RT, Jung DL. Physical activity and incidence of hypertension in college alumni. Am J Epidemiol 1983 ; 117 : 247 –57.
Thune I, Lund E. Physical activity and risk of colorectal cancer in men and women. Br J Cancer 1996 ; 73 : 1134 –40.
Thune I, Brenn T, Lund E, Gaard M. Physical activity and the risk of breast cancer. N Engl J Med 1997 ; 336 : 1269 –75.
Magnus K, Matroos A, Strackee J. Walking, cycling, or gardening, with or without seasonal interruption, in relation to coronary events. Am J Epidemiol 1979 ; 110 : 724 –33.
Paffenbarger RS, Hyde RT, Wing AL, Lee I-M, Jung DL, Kampert JB. The association of changes in physical activity level and other lifestyle characteristics with mortality among men. N Engl J Med 1993 ; 328 : 538 –45.
Hardman AE. Issues of fractionalization (intermittent versus continuous exercise). Med Sci Sports Exerc 2001 ; 33 (6Suppl.): S421 –27.
Murphy MH, Nevill AM, Hardman AE. Different patterns of brisk walking are equally effective in decreasing postprandial lipaemia. Int J Obesity 2000 ; 24 : 1303 –09.
Williams PT. Relationships of heart disease risk factors to exercise quantity and intensity. Arch Int Med 1998 ; 158 : 237 –45.
Braun B, Zimmermann MB, Kretchmer N. Effects of exercise intensity on insulin sensitivity in women with non-insulin-dependent diabetes mellitus. J Appl Physiol 1995 ; 78 : 300 –06.
Tsetsonis NV, Hardman AE. Reduction in postprandial lipemia after walking: influence of exercise intensity. Med Sci Sports Exerc 1996 ; 28 : 1235 –42.
Wei M, Kampert JB, Barlow CE et al . Relationship between low cardiorespiratory fitness and mortality in normal-weight, overweight, and obese men. J Am Med Assoc 1999 ; 282 : 1547 –53.
Després J-P, Lamarche B. Low-intensity endurance exercise training, plasma lipoproteins and the risk of coronary heart disease. J Int Med 1994 ; 236 : 7 –22.
Andersen RE. Effects of lifestyle activity vs structured aerobic exercise in obese women. A randomized trial. J Am Med Assoc 1999 ; 281 : 335 –40.
Jakicic JM, Winters C, Lang W, Wing RR. Effects of intermittent exercise and use of home exercise equipment on adherence, weight loss, and fitness in overweight women. A randomized trial. J Am Med Assoc 1999 ; 282 : 1554 –60.
Dunn AL, Marcus BH, Kampert JB, Garcia ME, Kohl HW, Blair SN. Comparison of lifestyle and structured interventions to increase physical activity and cardiorespiratory fitness. J Am Med Assoc 1999 ; 281 : 327 –34.
Lakka TA, Venäläinen JM, Raurama R, Salonen R, Tuomilehto J, Salonen JT. Relation of leisure-time physical activity and cardiorespiratory fitness to the risk of acute myocardial infarction in men. N Engl J Med 1994 ; 330 : 1549 –54.
Blair SN, Kampert JB, Kohl HW et al . Influences of cardiorespiratory fitness and other precursors on cardiovascular disease and all-cause mortality in men and women. J Am Med Assoc 1996 ; 276 : 205 –10.
Oja P, Laukkanen R, Pasanen M, Tyry T, Vuori I. A 2-km walking test for assessing cardiorespiratory fitness in healthy adults. Int J Sports Med 1991 ; 12 : 356 –62.
Lemaitre RN, Heckbert SR, Psaty BM, Siscovick DS. Leisure-time physical activity and the risk of nonfatal myocardial infarction in postmenopausal women. Arch Int Med 1995 ; 155 : 2302 –08.
Manson J, Hu FB, Rich-Edwards JW et al . A prospective study of walking as compared with vigorous exercise in the prevention of coronary heart disease in women. N Engl J Med 1999 ; 341 : 650 –58.
Hakim AA, Curb JD, Petrovitch H et al . Effects of walking on coronary heart disease in elderly men. The Honolulu Heart Program. Circulation 1999 ; 100 : 9 –13.
Hu FB, Sigal RJ, Rich-Edwards JW et al . Walking compared with vigorous physical activity and risk of type 2 diabetes in women. J Am Med Assoc 1999 ; 282 : 1433 –39.
Stensel DJ, Brooke-Wavell K, Hardman AE, Jones PRM, Norgan NG. The influence of a one year programme of brisk walking on endurance fitness and body composition in previously sedentary men aged 42–59 years. Eur J Appl Physiol 1994 ; 68 : 531 –37.
Hardman AE, Jones PRM, Norgan NG, Hudson A. Brisk walking improves endurance fitness without changing body fatness in previously sedentary women. Eur J Appl Physiol 1992 ; 65 : 354 –59.
Franklin BA, Blair SN, Haskell WL, Thompson PD, Van Camp SP. Exercise and cardiac complications. Do the benefits outweigh the risks? Physician Sportsmed 1994 ; 22 : 56 –68.
Pollock ML, Carroll JF, Graves JE et al . Injuries and adherence to walk/jog and resistance training programs in the elderly. Med Sci Sports Exerc 1991 ; 23 : 1194 –200.
LaCroiz AZ, Leveille SG, Hecht JA, Grothaus LC, Wagner EH. Does walking decrease the risk of cardiovascular disease hospitalizations and death in older adults? J Am Geriatr Soc 1996 ; 44 : 113 –20.
Frändin K, Grimby G, Mellström D, Svanborg D. Walking habits and health-related factors in a 70-year-old population. Gerontology 1991 ; 37 : 281 –88.
Schuler G, Hambrecht R, Schlierf G et al . Regular physical exercise and low-fat diet: effects on the progression of coronary artery disease. Circulation 1992 ; 86 : 1 –11.
Paffenbarger RS, Wing AL, Hyde RT. Physical activity as an index of heart attack risk in college alumni. Am J Epidemiol 1978 ; 108 : 161 –75.
Hambrecht R, Wolf A, Gielen S et al . Effect of exercise on coronary endothelial function in patients with coronary artery disease. N Engl J Med 2000 ; 342 : 454 –60.
Vogel RA, Corretti MC, Plotnick GD. Effect of a single high-fat meal on endothelial function in healthy subjects. Am J Cardiol 1997 ; 79 : 350 –54.
Bernstein L, Henderson BE, Hanisch R, Sullivan-Halley J, Ross RK. Physical exercise and reduced risk of breast cancer in young women. J Natl Cancer Inst 1994 ; 86 : 1403 –08.
Email alerts
Citing articles via, looking for your next opportunity.
- About International Journal of Epidemiology
- Recommend to your Library
Affiliations
- Online ISSN 1464-3685
- Copyright © 2024 International Epidemiological Association
- About Oxford Academic
- Publish journals with us
- University press partners
- What we publish
- New features
- Open access
- Institutional account management
- Rights and permissions
- Get help with access
- Accessibility
- Advertising
- Media enquiries
- Oxford University Press
- Oxford Languages
- University of Oxford
Oxford University Press is a department of the University of Oxford. It furthers the University's objective of excellence in research, scholarship, and education by publishing worldwide
- Copyright © 2024 Oxford University Press
- Cookie settings
- Cookie policy
- Privacy policy
- Legal notice
This Feature Is Available To Subscribers Only
Sign In or Create an Account
This PDF is available to Subscribers Only
For full access to this pdf, sign in to an existing account, or purchase an annual subscription.
Advertisement
Healthy minds live in healthy bodies – effect of physical health on mental health: Evidence from Australian longitudinal data
- Open access
- Published: 04 April 2022
- Volume 42 , pages 18702–18713, ( 2023 )
Cite this article
You have full access to this open access article
- Tinh Doan ORCID: orcid.org/0000-0002-2297-8187 1 ,
- Lyndall Strazdins 1 &
- Dan Chateau 1
15k Accesses
8 Citations
14 Altmetric
Explore all metrics
It is well known that physical and mental health are closely related, with growing evidence for biological and behavioural pathways. Mostly the research has focussed on mental health as the key driver of this inter-connection; the extent physical health shapes mental health has received less attention. We aim to derive robust estimates of the unique role physical health may play in shaping mental health outcomes. To do so we use a novel approach, incorporating longitudinal and instrumental variable methods which can address the reciprocal relationship between physical and mental health, and the endogeneity of physical health, before estimating the physical to mental health pathway. A sample of 209,442 observations (or 24,966 unique individuals) aged 15 and over spanning 18 years (2002–2019) was extracted from the Household Income and Labour Dynamics in Australian Survey (HILDA). We find that physical activity and health shocks erode mental health via their impact on physical health with a one point improvement (or worsening) in physical health scores (0–100) resulting in a rise (or decline) of 0.43 points (or 43%) in mental health score.
Similar content being viewed by others
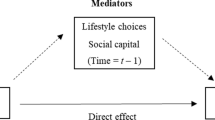
Well-Being and Physical Health: A Mediation Analysis
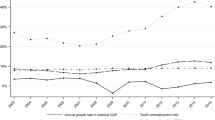
Longitudinal Trends in Self-Rated Health During Times of Economic Uncertainty in Italy
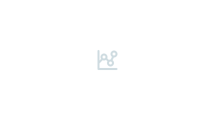
Employment status and perceived health condition: longitudinal data from Italy
Avoid common mistakes on your manuscript.
Introduction
Mental and physical health goes hand in hand, and it is widely agreed that they can be interrelated. However, most studies of their interplay have focused on effects of mental health on physical health, with less attention paid to the reverse (i.e., impact of physical health on mental health). This mental to physical health pathway was highlighted in Prince et al. ( 2007 ) ‘ No health without mental health ’, and a range of studies demonstrated how shocks to mental health from job loss, loss of family or relatives, family violence, abuse and bullying would change health behaviours (sleep, eating, and exercise) and hence underlying physical health (e.g., increased blood pressure, heart rate, decreased mobility, pain, migraines etc.). Such studies trace the pathways and associations, often starting from broader social and economic circumstances, which through their impact on mental health generate physical illness (Fadlon & Nielsen, 2019 ; Hoang et al., 2019 ; Margolis, 2013 ; Saxena et al., 2005 ; Averina et al., 2005 ; Lasser et al., 2000 ; Spiegel & Giese-Davis, 2003 ; De Groot et al., 2001 ).
Mental and physical health pathways
There are, however, multiple linkages and potential pathways between mental and physical health. For example, positive psychological well-being can help reduce the risk of serious physical problems including heart attacks and strokes (Vázquez et al., 2009 ). In contrast, poor mental health can lead to damaging behaviors or inhibit health behaviors (substance abuse, physical inactivity for instance), leading to poor physical health. For instance, people with mental illness are much more likely to suffer from sleeping disorders (Averina et al., 2005 ), and they are more likely to smoke than people without a mental illness (Lasser et al., 2000 ). This in turn increases risks for health concerns such as cancer or cardiovascular diseases (Fadlon & Nielsen, 2019 ; Hoang et al., 2019 ; Margolis, 2013 ; Saxena et al., 2005 ). Depression is also found to be linked to some chronic diseases, such as cancer (Spiegel & Giese-Davis, 2003 ), diabetes (De Groot et al., 2001 ) and asthma (Di Marco et al., 2010 ) and has been considered by some to have a causal role (e.g., Maghout-Juratli et al., 2010 ). It is also argued that people with mental illness may find it difficult to access adequate healthcare (Eisenberg et al., 2007 ), compounding their physical health status. Vaillant ( 1979 ) showed that better mental health in the past was strongly linked to good current physical health. This relationship was still strongly positive even after controlling for alcohol and tobacco use, and obesity. Poor mental health increase risk for communicable and non-communicable diseases, unintentional and intentional injuries (Prince et al., 2007 ).
In many studies the direction of influence is assumed to be from mental to physical health. However, some findings point to a complex and possibly reciprocal relationship. According to a 20-year follow-up study conducted by Paffenbarger et al. ( 1994 ) on 21,569 Harvard alumni, the depression rate was lower among participants with regular physical activity such as sports players and higher among those who are smokers or heavy drinkers, who are classified as poor physical health. Similarly, Camacho et al. ( 1991 ), using three waves of data of the Almeda County during 1965–1983, found a lower risk of depression among those with better physical health and regular physical activity. An investigation in the middle-age population in the Biobank, UK found that people who had more than one physical health condition (multi-morbidity), tended to have worse mental health than their physically healthier counterparts (Ronaldson et al., 2021 ). Further, Ohrnberger et al. ( 2017a ) investigated 11,203 older adults from 2002 to 2013 and found that better physical health in the past has a strong association with current mental health, and the relationship was even stronger than that from education or physical activity.
Another potential pathway is via adverse shocks or events such as accidents or traumatic injuries, which might worsen physical and mental health simultaneously or through the distress caused by the physical injuries or conditions and functional impairments (Kessler et al., 1997 ; Flett et al., 2002 ; Holman et al., 2008 ; Koren et al., 2001 ; Ullman & Siegel, 1996 ). Trauma is also the cause of some chronic and acute physical disorders including cardiovascular illness (Geerse et al., 2006 ) or immune functioning (McEwen, 2005 ) that may in turn affect mental health in the long term. Olafson et al. ( 2021 ) showed that ICU hospitalized patients used more medications for their mental health than before their hospitalization, and also more than the general population or non-ICU hospitalized cohorts.
Very few studies address the possibility of reciprocal causality between mental and physical health, despite this being integral to theories such as allostatic load. Chronic stresses, or physical shocks and trauma can alter body physiology including the endocrine system (McEwen & Wingfield, 2003 ). For example, Miller et al. ( 2007 ) found that cortisol levels for trauma exposed individuals were related to timing after the events, and that trauma events create both too much and too little cortisol, and then health outcomes related to both too much and too little cortisol. Trauma events also result in rising heart rate, and rising blood pressure and even lead to long-term high blood pressure (Pole, 2007 ; Buckley & Kaloupek, 2001 ), affecting the immune system (McEwen, 2005 ), increasing risk of musculoskeletal disorders after health shocks such as accidents (Dorn et al., 2008 ). Acute trauma affects adversely physical health as well as mental health, and this is widely evidenced in the literature (D’Andrea et al., 2011 ).
Methodology to address reciprocal pathways and endogeneity
Both theory and evidence point to complex, reciprocal linkages between mental and physical health. However few psychological or population health studies explicitly model them, and current estimates of the direction and strength of influence are likely biased. Ohrnberger et al. ( 2017b ) looking at the relationship between physical and mental health, would be the most comprehensive study to date. They analysed the direct and indirect effects of past mental health on present physical health, and past physical health on present mental health using lifestyle choices and social capital in a mediation framework. However, their model (simultaneous equation modelling-SEM) could only provide estimates of relationships between two health measures but not the direction of influence because the SEM design does not allow for causal inference (Dilalla, 2000 ). The SEM methodology is not able to address bias caused by potential reverse causality between mental health and physical health variables, a gap this current study seeks to address. We therefore incorporate time-differenced estimator and longitudinal instrumental variable (IV) methods into our modelling of physical and mental health associations, using the Australian nationally representative longitudinal data.
The longitudinal instrumental variable (IV) method can correct for biases caused by endogeneity of physical health, and the reverse relationship between mental health and physical health. These methods are widely used in economic research, and are potentially valuable techniques for psychology and population health research, where reciprocal and reverse relationships are likely important for testing assumptions of causality. Most existing public health studies use Randomised Control Trials (RCTs) or experiments to estimate directions of influence. Unlike RCTs, the IV method is a quasi or natural experimental method using available secondary datasets (see Angrist & Krueger, 2001 ; Wooldridge, 2010 ) and can addresses the bias caused by endogeneity of physical health and reverse causality between mental health and physical health before modelling the effect of physical health on mental health. Unlike RCT, which is often expensive to implement, the quasi experiment approach enables effective use of existing data sets, a less costly design option. Using this method we seek to robustly estimate the unbiased or pure effects of physical health on mental health, adjusting for reverse and reciprocal relationships to test the following hypotheses:
Research hypotheses
H 1. Physical exercise and health shocks e.g., accidents strongly affect physical health
H 2 . Changes in physical health status strongly impact mental health
Data and method
Data and analysis sample.
The data used in current analysis is from the Household, Income and Labour Dynamics in Australia (HILDA) survey. The HILDA survey is a household-based longitudinal study of a nationally representative sample of Australians, surveying more than 7000 households and 17,000 individuals each year since 2001. The survey combines detailed information on household and individual circumstances with measures of health, employment, income, health shock events. Attrition rates are low; with more than 90% of respondents in any wave responding in the next wave. For a detailed discussion of the survey design, see Watson and Wooden ( 2012 ) and for user manuals, technical papers and related publications, see https://melbourneinstitute.unimelb.edu.au/hilda .
Because some of our variables used in the analysis are not available in wave 2001 (e.g., smoking status), we restricted our sample to waves 2–19 (2002–2019). Our analysis was restricted to individuals aged 15 and over. The final data set has 209,442 observations (from 24,966 unique individuals).
Measures of mental health and physical health
Our outcome of interest is mental health (MH), which was constructed from five questions from the Short Form 36 (SF-36) (Ware et al., 2000 ), which is included in the HILDA survey. Three items assess nervousness and depression (‘Have you been a very nervous person?’, ‘Have you felt so down in the dumps that nothing could cheer you up?’ and ‘Have you felt downhearted and blue?’) and the other two happiness and calm (‘Have you felt calm and peaceful?’ and ‘Have you been a happy person?’). The score for mental health was calculated by summing scores of these above 5 questions and then transforming to a 0–100 scale, with a higher score representing better mental health. The primary variable of interest is physical health component score (PCS) from the SF-36, which covers 21 questions divided into several components including physical functioning (constructed from 10 questions about activities such as lifting, carrying, climbing, bending, kneeling, walking, bathing…), physical limitations (or role physical, constructed from 4 questions about physical limitations that affect work or activities: ‘Cut down the amount of time spent on work or other activities’; ‘Accomplished less than would like’; ‘Were limited in the kind of work’; ‘Had difficulty performing work or other activities’), bodily pain (2 questions about bodily pain and how it interferes with normal work), and general health (5 questions about self-assessed health and one’s own health relative to others’ health such as ‘Get sick a little easier than other people’; ‘As healthy as anybody I know’; ‘Expect my health to get worse’; ‘My health is excellent’). PCS is obtained by averaging over the component scores. These component scores were computed using the same method applied for mental health above.
Instrumental variables
One of our models was an instrumental variable (IV) estimator (we will provide detailed discussion in Section 3.3 below) in which we used lag of physical activity level, lag of long term health condition, and length of health shock events (a duration between year the health shock occurred to contemporary year) as instruments to predict physical health ( PCS ) before estimating the effect of PCS on MH . Physical activity was frequency of physical activity per week. Participants responded to a six-category question “In general, how often do you participate in moderate or intensive physical activity for at least 30 min?”: (1) Not at all; (2) Less than once a week; (3) One to two times a week; (4) Three times a week; (5) More than 3 times a week (but not every day); and (6) Every day. We regrouped these responses into 4 categories: sedentary (combining 1 and 2), low (combining 3 and 4), moderate (5), and high activity (6). We used 4 categories to capture physical health-relevant variation in activity, and used lag of physical activity to avoid the reciprocal relationship between current physical activity and PCS . The lag of the response to a question on long-term health condition (yes/no; ‘do you have any long-term health condition, impairment or disability that restricts you in your everyday activities, and has lasted or is likely to last, for 6 months or more?’) to predict physical health was also included. The lag was used to address the reciprocal relationship between the long term health condition and physical health. The last instrument was the length from shock events to physical health. We used the length (years) to explore the lasting effect of the shock events to physical health. For people who had no physical health shocks, the length was set to null (year). These events include conditions affecting the use of feet and legs, arms and fingers, restricting physical activity and work, causing back pain, migraines, chronic or recurring pains, developing long-term effect as result of head injuries, stroke or brain damage. We tested the IV relevance and exclusion assumptions, and the results showed that these IVs are valid and are very strong predictors of physical health (see more in the Result section below).
Table 1 presents basic statistics for key variables for the analysis sample. Because we used different levels of variables’ lags in a series of model specifications, only the contemporary value for these variables is presented.
Estimation methods
The determinants of mental health have been well explored in the existing literature (Allen et al., 2014 ; Maunder & Hunter, 2008 ). They include physical activity (Ohrnberger et al., 2017b ), social attachment (Maunder & Hunter, 2008 ), education (Ohrnberger et al., 2017a ), and income and employment (Stuckler et al., 2009 ). The relationship between physical health and mental health can be explained by two main underlying mechanisms: namely psychological channels and biological channels (Gaudlitz et al., 2013 ). From the psychological point of view, physical health can boost individual self-esteem by the appreciation of other people or the society on the level of fitness or physical abilities, physical self-concept, etc. that helps to protect them from anxiety and negative feelings (Knapen et al., 2005 ). Moreover, good physical functioning is commonly associated with social engagement that is shown to be protective from mental health problems, and high levels of leisure-time activities such as physical exercise can help mitigate the risk of depression or anxiety (Pasco et al., 2011 ). From the biological perspective, good physical health is also believed to increase the availability of the enzyme tryptophan in the brain, which stimulates serotonin synthesis and helps to mitigate anxiety or mental disorders (Gaudlitz et al., 2013 ).
Mental health ( MH ) disorders such as psychological distress, depression or anxiety are also affected by numerous factors apart from physical health status including individual and household demographic and economic profiles such as age, gender, education, race, language barrier, marital status, employment status, income, surrounding environment such as socio-economic disadvantage as well as regional-specific factors such as regional unemployment rate (Andrade et al., 2014 ; Cohen et al., 2009 ; Caldwell et al., 2004 ; Norstrand et al., 2013 ; Nieuwenhuijsen et al., 2010 ; Warr, 1984 ).
Our mental health prediction model is based on individual and household demographic and socio-economic characteristics and physical health as discussed above. Accordingly, the model for MH prediction is as follow:
Where MH it is mental health score of individual i in year t . PCS it is physical health component score ( PCS ), X 1 is a vector of the individual and household characteristics (age, age squared, gender, marital status, migration background, education, smoking, alcohol drinking, labour force status, home ownership, household equivalized disposable income, household socioeconomic disadvantage deciles). X 2 is a vector of extra control variables including regional unemployment rate, urbanity, and state and year dummy variables.
There is a potential mutual or reciprocal relationship between PCS and MH. The endogeneity of PCS suggests a non-zero correlation between PCS and error terms in the Ordinary Least Squares (OLS) estimation of eq. ( 1 ), resulting in bias in the estimated coefficient of the effect of PCS on MH.
To overcome this bias in the OLS estimate, we employed a series of estimation techniques. First , we used the first and second lags of PCS (prior physical health) in place of PCS in eq. ( 1 ). This approach ensures PCS is pre-determined or exogenous to contemporary mental health (MH) status. Second, longitudinal models (e.g. fixed effect (FE)) were used to estimate the effect of PCS variation within individuals on MH variation over time. The longitudinal methods can address the bias resulting from unobserved heterogeneity. However, longitudinal strategies cannot address reverse causality (Lakdawalla & Philipson, 2007 ). Third, a similar approach to the FE model is the time-differenced estimator that estimates the effect of change in PCS on change in MH, but this approach also fails to address reverse causality. We modified this approach by adding the first and second lag of the variation (time-differences) in PCS. This approach would capture the lasting effect of physical health on mental health. Further, longer differenced estimators (in this paper, we used one-year and then two-year differences) Footnote 1 can reduce the bias from measurement error (Greene, 1993 ; Griliches & Hausman, 1986 ). Finally, we applied an instrumental variable (IV) estimator to identify the causal relationship. In this method, physical health (PCS) is instrumented by physical activity level (lag), long-term health conditions (lag), Footnote 2 and length of shocks to physical health and its squared term (to capture PCS worsening and then recovering after the health shocks) in the first stage of estimation. Footnote 3 These IVs are more likely to affect physical health conditions (relevance assumption), but the residuals/error terms in the model of MH are unlikely to be correlated (directly) with these factors (exclusion assumption). These three IVs passed the tests for the relevance and exclusion assumptions so that they are valid instruments (see more details in Section 4). In the second stage, the predicted PCS was included in estimation equation to estimate the causal effect of PCS on MH. Because the standard IV estimator assumes the error terms are homoscedastic and non-autocorrelated, this can lead to inconsistent estimates of standard errors. We thus applied GMM (generalized method of moment) IV regression to obtain consistent estimates of coefficients and asymptotic standard errors. The advantage of GMM IV over standard IV estimator is that if heteroskedasticity is present, the GMM estimator is more efficient than the standard IV estimator, whereas if heteroskedasticity is not present, the GMM estimator is no worse asymptotically than the IV estimator (Baum et al., 2003 ).
We first started with a simple Ordinary Least Squares (OLS) estimator in which we controlled for a full range of observed risk factors associated with mental health. Second, we modified the OLS model to include lags of PCS to address reverse causality between PCS and MH (mutual effect). Third, we ran Fixed Effect (FE) and time-differenced estimators (first and second time-differenced). Fourth, we employed the IV estimator which we believed to provide the most reliable estimates of the causal effects in our data.
Although we used various approaches, we expected estimates to provide consistent findings (although magnitudes of estimates from different models may vary, as each model captures different levels of health variation, as well as having different sample sizes).
OLS estimates
The first column in Table 2 , tells us that MH is associated with PCS, each score increase in PCS is associated with about 0.4 score points increase in MH. In columns 2 and 3, each score rise in the range (0–100) leads to an increase of 0.3173–0.3309 score points. When we added both first and second lag of PCS in the last model (column 4), the total effect of each score rise in PCS on MH is about 0.38 score points (0.2206 + 0.1627). All of the estimates are highly statistically significant at 1% confidence level (or p < 0.0001). The OLS estimates especially in column 1 are likely biased due to endogeneity of PCS, that is, correlation between PCS and the error term of eq. 1 (due to unobserved confounding factors).
Longitudinal approach and time-differenced estimates
Fixed effect and time-differenced estimates using one-year and two-year differences show a very consistent finding that physical health significantly affects mental health. The estimates of the effect of PCS on MH on columns 1, 2 and 4 are very consistent both in terms of magnitude and sign (a small variation in coefficients is due to changes in sample size). However, FE or time-differenced models may still give biased estimates due to a reciprocal relationship between change in MH and change in PCS. We are not able to definitively attribute the change in MH to the change in PCS. To overcome this, in columns 3 and 5, we allowed changes in PCS prior to changes in MH by adding lags of change in PCS. This enables us to more confidently make inferences that a physical health change leads to a change in mental health. The estimates show that changes in PCS result in changes in MH, although the effect is smaller. This is expected because the lags of time-difference in PCS create larger time gaps between when physical health is observed and when mental health is observed. The effect of PCS on MH may therefore fade out (Table 3 ).
Instrumental variable (IV) estimates
We employed the GMM IV estimator in a joint estimation procedure to estimate the effect of physical health (PCS) on mental health (MH) and the results are presented in Table 4 .
In IV model, the population is divided into subgroups ( g ) who share the same values for unobserved attributes. Suppose an intervention leads to a change in mean PCS of group g (∆PCSg ), and let β 1 g is the effect of PCS on MH of group g when there is no intervention. Suppose the intervention (the instruments) affects only treatment group who are identical to those in comparison group, that is, both groups those have the same unobserved attributes would have the same PCS level and MH outcome in the absence of the intervention. The instrument e.g., shocks to health play a role like intervention that is why IV method is named as a quasi or natural experiment.
The validity of the IV estimator relies heavily on an assumption that the instruments Zs (intervention) are uncorrelated with unobserved attributes of individuals that affects MH, that is, cov ( Z i , ε i ) = 0. In the case of experiment of Zi (random assignment of the intervention), the difference in mean MH between treatment/intervention and control/comparison group will not be exacerbated by IV estimator, but this is not completely the case of quasi or natural experiments (Card, 1999 ). This problem is the limitation of IV estimator. The IV estimates in the presence of weak instruments (weakly correlated with PCS, but possibly correlated with the residual of the MH equation), the estimates would be very imprecise and seriously inconsistent (Belzil, 2007 ). Thus, the weak IV test is needed to ensure that IV estimation does not provide imprecise estimates of effect of physical health on mental health.
We conducted a series of the IV tests and the results are presented in the bottom panel of Table 4 . The first test was an endogeneity test of the endogenous regressor ( PCS ), and its null hypothesis is that PCS is exogenous. The test result rejected the null hypothesis (p < 0.0001) suggesting that physical health PCS is endogenous and the OLS estimator for eq. ( 1 ) produces a biased estimate of the effect of PCS on MH. The next test is to test the power of prediction of the three excluded instruments Z in the first stage of estimation. The hypothesis of the instruments jointly equalling zero in the first stage was rejected (with a very high F-value of 7835.9, p < 0.0001), and the test for weak instruments (Anderson-Rubin Wald test) rejected the hypothesis of weak instruments (p < 0.0001). Further, all coefficients of excluded instruments in the first stage estimation (column 1, Table 4 ) are significant at the 1% level. We also conducted a test for IV redundancy to see if any of the excluded IVs are redundant, and the test showed that the IVs were not redundant (p < 0.0001). Another important test was to test if the equation is weakly identified. The Kleibergen-Paap rank Wald F-statistic of 7835.5 was much higher than the Stock-Yogo weak identification test critical values at 5% maximal IV relative bias of 19.28. This suggested that our model equation is not weakly identified. Finally, the Sargan and Basmann test for over-identification shows that our model is over-identified (that is, we have more valid IVs than number of equations, in our case two equations). In sum, all of the tests that were conducted to determine the validity of the IV model indicate that we have identified strong instruments that enable us to be confident in our IV estimates presented in Table 4 .
Our IV estimates show that higher levels of prior physical activity were a strong predictor of PCS. For example, comparing to those having a sedentary physical activity level, people who engaged in moderate or intensive physical activity one to three times a week (low physical activity) were about 4.8 points higher in PCS, which increased to 7.3 points for those who exercised more than 3 times a week (moderate physical activity), and 8.3 points for those who exercised every day (high physical activity). Having prior long-term health conditions was associated with a decline of 15.4 points in their PCS. For people who had physical health shocks their PCS declined by 0.97 points per year since the shock occurred. However, PCS decline flattened and recovered after some time as the squared term of the time since shock is positive and statistically significant. In a model using a dummy variable for lag of physical health shocks, it showed that following one year after the shock, PCS declined by 11.3 points (we did not report the full estimates here but will be available upon request).
After adjusting for the endogeneity of physical health using the IV method, our estimate showed that physical health strongly affects mental health. Each point improvement in PCS led to an increase of 0.43 points in MH. In contrast, worsening physical health by one point resulted in a decline of 0.43 points in mental health. Any shocks that are harmful to people’s physical health appear to cause significant deterioration to their mental health.
Conclusion and discussion
The main body of mental health research focuses on individual, household and socioeconomic risk factors as predictors of mental health, or on the impact of mental health on physical health. Another body of the literature looks at the simple relationship or correlation between physical and mental health. Much less is known about the causal influence of physical health on mental health. In this paper, we used comprehensive estimation methods to address the reciprocal relationship between physical health and mental health, and endogeneity of physical health.
There has been a paradigm shift on how health is conceived: mental and physical health are now viewed as interlinked and mutually influential. However, research evidence and approaches have lagged behind this shift, especially research on the extent physical health shocks and conditions are determinants of mental health conditions and symptoms. A key barrier has been the lack of feasible methods that can robustly test for influence by modelling these complex endogenous and reciprocal pathways. In this paper, we used comprehensive estimation methods (time-differenced and IV estimators) drawing from econometric approaches and applied them to this public health problem. These methods help address the reciprocal relationship between physical health and mental health, and endogeneity of physical health. We found that factors such as physical exercise, long-term health conditions and shocks to physical health strongly affect physical health. Any improvement (or worsening) in physical health then strongly affects mental health afterward. Based on responses to health questions from the SF-36, we found that a one-point improvement (or worsening) in physical health scores (0–100) will result in a rise (or decline) of 0.43 points (or 43%) in mental health score.
Our findings, based on modelling systems of influence, provide estimates of likely causal paths and shed new light on the significance of physical health–related factors as drivers of mental health. For example, Ohrnberger et al. ( 2017b ), Roberts et al. ( 2018 ), and Ronaldson et al. ( 2021 ) found the relationship between poor physical health and mental health illness, depression, anxiety or risk for mental health disorders. Our finding delivers a precise estimate of this causal effect of physical health on mental health, consistent with Olafson et al. ( 2021 ) evidence that that worsening physical health due to trauma events or hospitalization leads to higher mental health medication. Their study was on a cohort of ICU-hospitalized patients, while our analysis was conducted on a nationally representative sample of Australian population aged 15 and over.
The instruments in the IV model such as prior (or lag of) physical exercise and shocks to physical health were found to have strong effects on individual physical health status. These instruments play a role as meditators between physical health and mental health. The effect of these instruments on physical health is consistent with the existing literature. For instance, D’Andrea et al. ( 2011 ) found that shocks to health such as trauma affected adversely physical health. Acute trauma exposure leads to negative physical health consequences such as significant disruption to gastrointestinal functioning, the cardiovascular system, immunological functioning, the reproductive system, the musculoskeletal system, neuroendocrine functioning, brain structure and functioning. Camacho et al. ( 1991 ), Gaudlitz et al. ( 2013 ) and Ohrnberger et al. ( 2017b ) showed that physical activity helped reduce risks for physical health and hence mental health.
Our analysis using IV models (quasi or natural experiment) goes beyond just looking at simple association between physical health and mental health. It offers causal relationship inference for policy on mental health that could be both feasible and (relatively) immediate where there is existing data. Adapting this analytic strategy would benefit other areas where random controlled trials (RCT) and experimental methods may be practically impossible or prohibitively expensive, and may take many years to undertake.
In Australia, a recent study (Young et al., 2017 ) found a significant correlation between physical health and mental health status. The study indicated that the majority of participants reported the awareness of the importance of physical health to their mental health and wellbeing. An earlier study by Creamer et al. ( 2001 ) on 10,641 participants in 1997 found that post-traumatic stress disorders lead to poorer physical state and caused a higher level of depression and anxiety among Australian households. Improving the physical health for general population in particular, for those with mental health issues is important in order to improve their wellbeing in Australia. People diagnosed with mental illness have relatively poor physical health and often die earlier than their counterparts in the general population. People living with mental illness in rural Australia have three times the risk of premature death than the total population (Roberts et al., 2018 ).
Strategies aiming to help improve physical health in the population ultimately will improve mental health. Morgan et al. ( 2021 ) showed that most strategies out of 61 programs and initiatives in Australia, which were reviewed in their study, aiming at improving mental health have primarily addressed stigma and discrimination towards people with mental illness and their families, or for culturally and linguistically diverse communities with limited evidence of effectiveness. Other studies in Australia or elsewhere focused on improving service use accessibility, treatment and social supports (Allen et al., 2014 ; Andrade et al., 2014 ; Cohen et al., 2009 ; Caldwell et al., 2004 ), but missed the role of physical health and physical health-related risk factors/health behaviour. Intervention and preventative strategies therefore should also focus on promoting a healthy life via physical exercise, and safer environments at work and outside work to reduce risks to physical health, as our study showed that health behaviours such as exercise and shocks (i.e., risk) to health strongly affect physical health, and worsening physical health leads to significant declines in mental health.
One limitation may be that the HILDA mental health measure used in the current study is simplistic, and the gold standard is diagnosis-validated data. However, the health measures in the SF-36 were validated with Australian data. For example, Sanson-Fisher and Perkins ( 1998 ) showed that physical functioning, role-physical, general health, and bodily pain scales were found to be strongly correlated with the physical dimension, and mental health measure was strongly correlated with the mental health dimension in psychometric testing, using the International Quality of Life Assessment (IQOLA) methods (Ware & Gandek, 1998b ). In addition, Butterworth and Crosier ( 2004 ) demonstrated and provided evidence for the validity of the SF-36 data in the HILDA Survey, and supported its use as a general outcome measure of physical and mental health status in Australia. To further validate the HILDA mental health measure used in the current analysis, we checked the correlation between Kessler psychological distress (K10) Footnote 4 (also collected in HILDA) and the mental health variable for the years with available K10 psychological distress data, the correlation was found to be very high (0.82, statistically significant, p < 0.0001) suggesting that using either the K10 score or mental health variable captures well individual’s mental health status. Unfortunately, the K10 data in the HILDA were collected in a few years with time gaps (in waves 2007, 2009, 2011, 2013, 2015, 2017, 2019). The K10 psychological distress data with time gaps thus were not suitable for our longitudinal data modelling.
Our study underscores the importance of redesigning health systems to truly address the interplay between physical and mental health. Usually services are designed to offer separate, specialist teams and treatment for physical and mental health. Our study shows that physical health promotion, health problems, risks and shocks are important drivers of physical health changes and ultimately mental health, as is the reverse, and these dual impacts need to be assessed, monitored and addressed as ‘business as usual’. Our study supports calls for embedding dual mental and physical health supports and treatment in all health services as a system and policy reform.
One may want to use longer time differences but it reduces sample size significantly.
We also ran a model with contemporary physical activity and long-term health conditions, producing consistent effects of these factors on PCS. Details are available upon request.
We also ran a model using a dummy variable for prior physical health shocks, with modest changes to the results.
Kessler psychological distress scale (K10) score was constructed from 10 questions about mental health: depressed; everything was an effort; so nervous that nothing could calm you down; so restless that you could not sit still; hopeless; nervous; restless or fidgety; so sad that nothing could cheer you up; tired out for no good reasons; worthless .
Allen, J., Balfour, R., Bell, R., & Marmot, M. (2014). Social determinants of mental health. International Review of Psychiatry, 26 (4), 392–407.
Article PubMed Google Scholar
Andrade, H., Alonso, J., Mneimneh, Z., et al. (2014). Barriers to mental health treatment: results from the WHO world mental health surveys. Psychological Medicine, 44 (2014), 1303–1317.
Angrist, J., & Krueger, A. (2001). Instrumental variables and the search for identification: From supply and demand to natural experiments. Journal of Economic Perspectives, 15 (4), 69–85. https://doi.org/10.1257/jep.15.4.69
Article Google Scholar
Averina, M., Nilssen, O., Brenn, T., Brox, J., Arkhipovsky, L., & Kalinin, G. (2005). Social and lifestyle determinants of depression, anxiety, sleeping disorders and self-evaluated quality of life in Russia. Social Psychiatry and Psychiatric Epidemiology, 40 (7), 511–518. https://doi.org/10.1007/s00127-005-0918-x
Baum, C., Schaffer, M., & Stillman, S. (2003). Instrumental variables and GMM: Estimation and testing. Stata Journal, 3 (1), 1–31. https://doi.org/10.1177/2F1536867X0300300101
Belzil, C. (2007). The return to schooling in structural dynamic models: a survey. European Economic Review, 51 (5), 1059–1105. https://doi.org/10.1016/j.euroecorev.2007.01.008
Buckley, C., & Kaloupek, G. (2001). A meta-analytic examination of basal cardiovascular activity in post-traumatic stress disorder. Psychosomatic Medicine, 63 (4), 585–594.
Butterworth, P., & Crosier, T. (2004). The validity of the SF-36 in an Australian National Household Survey: demonstrating the applicability of the Household Income and Labour Dynamics in Australia (HILDA) Survey to examination of health inequalities. BMC Public Health, 4 (1), 1–11. https://doi.org/10.1186/1471-2458-4-44
Caldwell, M., Jorm, F., & Dear, G. (2004). Suicide and mental health in rural, remote and metropolitan areas in Australia. Medical Journal of Australia, 181 (7), S10–S14. https://doi.org/10.5694/j.1326-5377.2004.tb06348.x
Camacho, C., Roberts, E., Lazarus, B., Kaplan, A., & Cohen, D. (1991). Physical activity and depression: evidence from the Alameda County Study. American journal of epidemiology, 134 (2), 220–231. https://doi.org/10.1093/oxfordjournals.aje.a116074
Card, D. (1999). The causal effect of education on earnings. Handbook of Labor Economics, 3 , 1801–1863.
Cohen, A., Gilman, S., Houck, P., Szanto, K., & Reynolds, F. (2009). Socioeconomic status and anxiety as predictors of antidepressant treatment response and suicidal ideation in older adults. Social Psychiatry and Psychiatric Epidemiology, 44 (2009), 272–277.
Creamer, M., Burgess, P., & McFarlane, C. (2001). Post-traumatic stress disorder: findings from the Australian National Survey of Mental Health and Well-being. Psychological Medicine, 31 (7), 1237–1247. https://doi.org/10.1017/S0033291701004287
D’Andrea, W., Sharma, R., Zelechoski, A., & Spinazzola, J. (2011). Physical health problems after single trauma exposure: when stress takes root in the body. Journal of the American Psychiatric Nurses Association, 17 (6), 378–392. https://doi.org/10.1177/2F1078390311425187
De Groot, M., Anderson, R., Freedland, K. E., Clouse, R. E., & Lustman, P. J. (2001). Association of depression and diabetes complications: a meta-analysis. Psychosomatic Medicine, 63 (4), 619–630.
Di Marco, F., Verga, M., Santus, P., Giovannelli, F., Busatto, P., Neri, M., ... & Centanni, S. (2010). Close correlation between anxiety, depression, and asthma control. Respiratory Medicine, 104 (1), 22–28. https://doi.org/10.1016/j.rmed.2009.08.005
DiLalla, L. F. (2000). Structural equation modeling: Uses and issues. In Handbook of applied multivariate statistics and mathematical modeling (pp. 439–464). Academic Press. https://doi.org/10.1016/B978-012691360-6/50016-1
Book Google Scholar
Dorn, T., Yzermans, C., Spreeuwenberg, M., Schilder, A., & van der Zee, J. (2008). A cohort study of the long-term impact of a fire disaster on the physical and mental health of adolescents. Journal of Traumatic Stress, 21 (2), 239–242. https://doi.org/10.1002/jts.20328
Eisenberg, D., Golberstein, E., & Gollust, S. E. (2007). Help-seeking and access to mental health care in a university student population. Medical Care , 43 (7), 594–601.
Fadlon, I., & Nielsen, H. (2019). Family health behaviors. American Economic Review, 109 (9), 3162–3191. https://doi.org/10.1257/aer.20171993
Flett, A., Kazantzis, N., Long, R., MacDonald, C., & Millar, M. (2002). Traumatic events and physical health in a New Zealand community sample. Journal of Traumatic Stress, 15 (4), 303–312. https://doi.org/10.1023/A:1016251828407
Gaudlitz, K., von Lindenberger, B.-L., Zschucke, E., & Ströhle, A. (2013). Mechanisms underlying the relationship between physical activity and anxiety: Human data. In P. Ekkekakis (Ed.), Routledge handbook of physical activity and mental health (pp. 139–151). Oxfordshire.
Google Scholar
Geerse, G.-J., van Gurp, L. C., Wiegant, V. M., & Stam, R. (2006). Individual reactivity to the open-field predicts the expression of stress-induced behavioural and somatic pain sensitisation. Behavioural Brain Research, 174 (1), 112–118. https://doi.org/10.1016/j.bbr.2006.07.010
Greene, W. (1993). Econometric Analysis (Vol. 1993). Prentice Hall.
Griliches, Z., & Hausman, A. (1986). Errors in variables in panel data. Journal of Econometrics, 31 (1), 93–118. https://doi.org/10.1016/0304-4076(86)90058-8
Hoang, D., Kristoffersen, I., & Li, I. (2019). All in the mind? Estimating the effect of mental health on health behaviours. Social Science & Medicine, 225 (2019), 69–84. https://doi.org/10.1016/j.socscimed.2019.02.017
Holman, E., Silver, R., Poulin, M., Andersen, J., Gil-Rivas, V., & McIntosh, D. N. (2008). Terrorism, acute stress, and cardiovascular health: A 3-year national study following the September 11th attacks. Archives of General Psychiatry, 65 (1), 73–80. https://doi.org/10.1001/archgenpsychiatry.2007.6
Kessler, C., Anthony, C., Blazer, G., Bromet, E., Eaton, W., Kendler, K.,. .. Zhao, S. (1997). The US national comorbidity survey: Overview and future directions. Epidemiologia e Psichiatria Sociale, 6 (1), 4–16.
Knapen, J., Van de Vliet, P., Van Coppenolle, H., et al. (2005). Comparison of changes in physical self-concept, global self-esteem, depression and anxiety following two different psychomotor therapy programs in nonpsychotic psychiatric inpatients. Psychotherapy and psychosomatics, 74 (6), 353–361. https://doi.org/10.1159/000087782
Koren, D., Arnon, I., & Klein, E. (2001). Long term course of chronic posttraumatic stress disorder in traffic accident victims: a three-year prospective follow-up study. Behaviour Research and Therapy, 39 (12), 1449–1458. https://doi.org/10.1016/S0005-7967(01)00025-0
Lakdawalla, D., & Philipson, T. (2007). Labor supply and weight. Journal of Human Resources, 42 (1), 85–116. https://doi.org/10.3368/jhr.XLII.1.85
Lasser, K., Boyd, W., Woolhandler, S., Himmelstein, U., McCormick, D., & Bor, H. (2000). Smoking and mental illness: a population-based prevalence study. JAMA, 284 (20), 2606–2610. https://doi.org/10.1001/jama.284.20.2606
Maghout-Juratli, S., Janisse, J., Schwartz, K., & Arnetz, B. B. (2010). The causal role of fatigue in the stress-perceived health relationship: a MetroNet study. The Journal of the American Board of Family Medicine, 23 (2), 212–219.
Margolis, R. (2013). Health shocks in the family: Gender differences in smoking changes. Journal of Aging and Health, 25 (5), 882–903. https://doi.org/10.1177/2F0898264313494411
Maunder, G., & Hunter, J. (2008). Attachment relationships as determinants of physical health. Journal of the American Academy of Psychoanalysis and Dynamic Psychiatry, 36 (1), 11–32. https://doi.org/10.1521/jaap.2008.36.1.11
McEwen, S. (2005). Stressed or stressed out: What is the difference? Journal of Psychiatry & Neuroscience, 30 (5), 315.
McEwen, S., & Wingfield, C. (2003). The concept of allostasis in biology and biomedicine. Hormones and Behavior, 43 (1), 2–15. https://doi.org/10.1016/S0018-506X(02)00024-7
Miller, E., Chen, E., & Zhou, S. (2007). If it goes up, must it come down? Chronic stress and the hypothalamicpituitary-adrenocortical axis in humans. Psychological Bulletin, 133 (1), 25–45.
Morgan, J., Wright, J., & Reavley, J. (2021). Review of Australian initiatives to reduce stigma towards people with complex mental illness: what exists and what works? International Journal of Mental Health Systems, 15 , 10 (2021).
Article PubMed PubMed Central Google Scholar
Nieuwenhuijsen, K., Bruinvels, D., & Frings-Dresen, M. (2010). Psychosocial work environment and stress-related disorders, a systematic review. Occupational Medicine, 60 (4), 277–286. https://doi.org/10.1093/occmed/kqq081
Norstrand, A., Glicksman, A., Lubben, J., & Kleban, M. (2012). The Role of the Social Environment on Physical and Mental Health of Older Adults. Journal of Housing for the Elderly, 26 (1-3), 209–307.
Ohrnberger, J., Fichera, E., & Sutton, M. (2017a). The dynamics of physical and mental health in the older population. The Journal of the Economics of Ageing, 9 , 52–62. https://doi.org/10.1016/j.jeoa.2016.07.002
Ohrnberger, J., Fichera, E., & Sutton, M. (2017b). The relationship between physical and mental health: A mediation analysis. Social Science & Medicine, 195 , 42–49. https://doi.org/10.1016/j.socscimed.2017.11.008
Olafson, K., Marrie, R., Bolton, J., et al. (2021). The 5-year pre- and post-hospitalization treated prevalence of mental disorders and psychotropic medication use in critically ill patients: a Canadian population-based study. Intensive Care Medicine, 47 (12), 1450–1461.
Paffenbarger, R., Lee, M., & Leung, R. (1994). Physical activity and personal characteristics associated with depression and suicide in American college men. Acta Psychiatrica Scandinavica, 89 (s377), 16–22. https://doi.org/10.1111/j.1600-0447.1994.tb05796.x
Pasco, A., Williams, J., Jacka, N., Henry, J., et al. (2011). Habitual physical activity and the risk for depressive and anxiety disorders among older men and women. International psychogeriatrics, 23 (2), 292–298.
Pole, N. (2007). The psychophysiology of posttraumatic stress disorder: A meta-analysis. Psychological Bulletin, 133 (5), 725–746 https://psycnet.apa.org/doi/10.1037/0033-2909.133.5.725
Prince, M., Patel, V., Saxena, S., Maj, M., Maselko, J., Phillips, M., & Rahman, A. (2007). No health without mental health. The Lancet, 370 (9590), 859–877. https://doi.org/10.1016/S0140-6736(07)61238-0
Roberts, R., Lockett, H., Bagnall, C., Maylea, C., & Hopwood, M. (2018). Improving the physical health of people living with mental illness in Australia and New Zealand. Australian Journal of Rural Health, 26 (5), 354–362. https://doi.org/10.1111/ajr.12457
Ronaldson, A., Arias de la Torre, J., Prina, M., et al. (2021). Associations between physical multimorbidity patterns and common mental health disorders in middle-aged adults: A prospective analysis using data from the UK Biobank. The Lancet Regional Health - Europe, 8 (2021), 100,149. https://doi.org/10.1016/j.lanepe.2021.100149
Sanson-Fisher, R., & Perkins, J. (1998). Adaptation and validation of the SF-36 Health Survey for use in Australia. Journal of clinical epidemiology, 51 (11), 961–967. https://doi.org/10.1016/S0895-4356(98)00087-0
Saxena, S., Van Ommeren, M., Tang, C., & Armstrong, P. (2005). Mental health benefits of physical activity. Journal of Mental Health, 14 (5), 445–451. https://doi.org/10.1080/09638230500270776
Spiegel, D., & Giese-Davis, J. (2003). Depression and cancer: mechanisms and disease progression. Biological psychiatry, 54 (3), 269–282.
Stuckler, D., Basu, S., Suhrcke, M., Coutts, A., & McKee, M. (2009). The public health effect of economic crises and alternative policy responses in Europe: an empirical analysis. The Lancet, 374 (9686), 315–323. https://doi.org/10.1016/S0140-6736(09)61124-7
Ullman, E., & Siegel, M. (1996). Traumatic events and physical health in a community sample. Journal of traumatic Stress, 9 (4), 703–720.
Vaillant, E. (1979). Natural history of male psychologic health: Effects of mental health on physical health. New England Journal of Medicine, 301 (23), 1249–1254. https://doi.org/10.1056/NEJM197912063012302
Vázquez, C., Hervás, G., Rahona, J. J., & Gómez, D. (2009). Psychological well-being and health. Contributions of positive psychology. Annuary of Clinical and Health Psychology, 5 (2009), 15–27.
Ware, J., & Gandek, B. (1998a). Overview of the SF-36 Health Survey and the International Quality of Life Assessment (IQOLA) Project. Journal of Clinical Epidemiology, 51 (11), 903–912. https://doi.org/10.1016/S0895-4356(98)00081-X
Ware, J., & Gandek, B. (1998b). Methods for testing data quality, scaling assumptions, and reliability: The IQOLA Project approach. Journal of Clinical Epidemiology, 51 (11), 945–952. https://doi.org/10.1016/S0895-4356(98)00085-7
Ware, J. E., Kosinski, M., & Gandek, B. (2000). SF-36 health survey: manual and interpretation guide Lincoln. RI: QualityMetric Incorporated .
Warr, P. (1984). Job Loss, Unemployment and Psychological Well-Being. In V. L. Allen & E. van de Vliert (Eds.), Role Transitions: Explorations and Explanations (pp. 263–285).
Chapter Google Scholar
Watson, N., & Wooden, M. P. (2012). The HILDA survey: a case study in the design and development of a successful household panel survey. Longitudinal and Life Course Studies, 3 (3), 369–381.
Wooldridge, J. (2010). Econometric Analysis of Cross Section and Panel Data. Econometric Analysis of Cross Section and Panel Data . MIT Press.
Young, J., Praskova, A., Hayward, N., & Patterson, S. (2017). Attending to physical health in mental health services in Australia: a qualitative study of service users’ experiences and expectations. Health & Social Care in the Community, 25 (2), 602–611. https://doi.org/10.1111/hsc.12349
Download references
Acknowledgments
This paper uses unit record data from the Household, Income and Labour Dynamics in Australia (HILDA) Survey 2002–2019. The HILDA Project was initiated and is funded by the Australian Government Department of Social Services (DSS) and is managed by the Melbourne Institute of Applied Economic and Social Research (Melbourne Institute). The findings and views reported in this paper, however, are those of the authors and should not be attributed to the universities, DSS or the Melbourne Institute. The authors are responsible for all findings and views expressed in this paper.
Open Access funding enabled and organized by CAUL and its Member Institutions
Author information
Authors and affiliations.
Research School of Population Health, Australian National University, Canberra, Australia
Tinh Doan, Lyndall Strazdins & Dan Chateau
RMIT University, Hanoi, Vietnam
You can also search for this author in PubMed Google Scholar
Corresponding author
Correspondence to Tinh Doan .
Ethics declarations
Conflict of interest.
We declare that there is no conflict of interest in this study.
Ethical approval
This paper uses secondary data (HILDA) and there is no requirement for ethical review .
Additional information
Publisher’s note.
Springer Nature remains neutral with regard to jurisdictional claims in published maps and institutional affiliations.
Rights and permissions
Open Access This article is licensed under a Creative Commons Attribution 4.0 International License, which permits use, sharing, adaptation, distribution and reproduction in any medium or format, as long as you give appropriate credit to the original author(s) and the source, provide a link to the Creative Commons licence, and indicate if changes were made. The images or other third party material in this article are included in the article's Creative Commons licence, unless indicated otherwise in a credit line to the material. If material is not included in the article's Creative Commons licence and your intended use is not permitted by statutory regulation or exceeds the permitted use, you will need to obtain permission directly from the copyright holder. To view a copy of this licence, visit http://creativecommons.org/licenses/by/4.0/ .
Reprints and permissions
About this article
Doan, T., Ha, V., Strazdins, L. et al. Healthy minds live in healthy bodies – effect of physical health on mental health: Evidence from Australian longitudinal data. Curr Psychol 42 , 18702–18713 (2023). https://doi.org/10.1007/s12144-022-03053-7
Download citation
Accepted : 25 March 2022
Published : 04 April 2022
Issue Date : August 2023
DOI : https://doi.org/10.1007/s12144-022-03053-7
Share this article
Anyone you share the following link with will be able to read this content:
Sorry, a shareable link is not currently available for this article.
Provided by the Springer Nature SharedIt content-sharing initiative
- physical health
- mental health
- instrumental variable
- Find a journal
- Publish with us
- Track your research
ORIGINAL RESEARCH article
Thirty years of research on physical activity, mental health, and wellbeing: a scientometric analysis of hotspots and trends.
Parts of this article's content have been modified or rectified in:
Erratum: Thirty years of research on physical activity, mental health, and wellbeing: A scientometric analysis of hotspots and trends
- Read erratum
- 1 Division of Adult Psychiatry, Department of Psychiatry, University Hospitals of Geneva, Thonex, Switzerland
- 2 College of Computing and Informatics, Drexel University, Philadelphia, PA, United States
- 3 Scientific Research Department, GGz Centraal, Amersfoort, Netherlands
- 4 School for Mental Health and Neuroscience, Maastricht University, Maastricht, Netherlands
- 5 Katholieke Universiteit Leuven Department of Rehabilitation Sciences, Leuven, Belgium
- 6 University Psychiatric Center Katholieke Universiteit Leuven, Leuven, Belgium
- 7 Division of Psychology and Mental Health, Manchester Academic Health Science Centre, University of Manchester, Manchester, United Kingdom
- 8 Greater Manchester Mental Health National Health Service Foundation Trust, Manchester Academic Health Science Centre, Manchester, United Kingdom
- 9 Centre for Health, Performance and Wellbeing, Anglia Ruskin University, Cambridge, United Kingdom
- 10 Physiotherapy Department, South London and Maudsley National Health Service Foundation Trust, London, United Kingdom
- 11 Department of Psychological Medicine, Institute of Psychiatry, Psychology and Neuroscience, King's College London, London, United Kingdom
- 12 Discipline of Psychiatry and Mental Health, Medicine and Health, University of New South Wales, Kensington, NSW, Australia
- 13 School of Health Sciences, Medicine and Health, University of New South Wales, Kensington, NSW, Australia
- 14 Department of Sports Methods and Techniques, Federal University of Santa Maria, Santa Maria, Brazil
- 15 Department of Psychiatry, University of Ottawa, Ottawa, ON, Canada
- 16 Department of Mental Health, The Ottawa Hospital, Ottawa, ON, Canada
- 17 Ottawa Hospital Research Institute, Clinical Epidemiology Program, University of Ottawa, Ottawa, ON, Canada
- 18 Department of Child and Adolescent Psychiatry, Charité Universitätsmedizin, Berlin, Germany
The sheer volume of research publications on physical activity, mental health, and wellbeing is overwhelming. The aim of this study was to perform a broad-ranging scientometric analysis to evaluate key themes and trends over the past decades, informing future lines of research. We searched the Web of Science Core Collection from inception until December 7, 2021, using the appropriate search terms such as “physical activity” or “mental health,” with no limitation of language or time. Eligible studies were articles, reviews, editorial material, and proceeding papers. We retrieved 55,353 documents published between 1905 and 2021. The annual scientific production is exponential with a mean annual growth rate of 6.8% since 1989. The 1988–2021 co-cited reference network identified 50 distinct clusters that presented significant modularity and silhouette scores indicating highly credible clusters ( Q = 0.848, S = 0.939). This network identified 6 major research trends on physical activity, namely cardiovascular diseases, somatic disorders, cognitive decline/dementia, mental illness, athletes' performance, related health issues, and eating disorders, and the COVID-19 pandemic. A focus on the latest research trends found that greenness/urbanicity (2014), concussion/chronic traumatic encephalopathy (2015), and COVID-19 (2019) were the most active clusters of research. The USA research network was the most central, and the Chinese research network, although important in size, was relatively isolated. Our results strengthen and expand the central role of physical activity in public health, calling for the systematic involvement of physical activity professionals as stakeholders in public health decision-making process.
Introduction
Physical activity can be considered as medicine and has been used in both the treatment and prevention of a variety of chronic conditions ( 1 ). Longitudinal cohort studies demonstrate that a low cardiorespiratory fitness constitutes the largest attributable fraction for all-cause mortality ( 2 ). There is also overwhelming evidence that low physical activity (i.e., not meeting physical activity recommendations) is considered as an important risk factor for chronic conditions including some cancers, cardiovascular disease, diabetes, dementia, and in particular for a patient with mental illness (schizophrenia, bipolar disorder, or major depressive disorder) ( 3 – 5 ). Patients with mental illness have poor physical health compared with the general population, with reduced life expectancy and a higher risk of premature death beyond suicide, from natural causes ( 6 ). At least partially, among other factors, their poor physical health is due to higher sedentary behavior and lower physical activity compared with the general population ( 7 , 8 ). Physical activity, and its structured form of exercise, seem to affect the brain and mind, beyond physical health, both as a factor associated with poor mental health and quality of life and as a treatment for mental disorders ( 9 ). Indeed, exercise has shown to be efficacious in a number of mental disorders, according to a previous umbrella review pooling 27 systematic reviews ( 10 , 11 ). Exercise is also now seen as a potential preventive or disease-modifying treatment of dementia and brain aging ( 12 ) or as a possible treatment for negative symptoms in schizophrenia ( 13 ).
Importantly, systematic reviews, meta-analysis, and umbrella reviews have offered a deep synthesis of specific research questions addressed within the exponential volume of physical activity literature related to mental health and wellbeing. However, such systematic methods may not be appropriate to encompass hundreds or thousands of new publications per year. In fact, systematic reviews have to be narrow in their inclusion criteria and offer a comprehensive view on a specific and restricted research or clinical question. For instance, a meta-analysis can inform if an intervention is efficacious for a given population on an outcome of interest ( 14 , 15 ) or an umbrella review can assess the credibility of an association between a risk factor and an incident condition ( 16 – 19 ). Nevertheless, none of the two offers an insight on the temporal trend of research, the complex network of topics, authors, publications, networks, institutions, and their bibliometric performance. Gaining such overarching views of how an entire field of research on a particular topic is important and useful, in order to gauge how the academic literature is developing and inform the next steps for the science to pursue.
The integration of developments in data visualization, text mining, and network analysis has permitted the emergence of a new framework and a new generation of research synthesis of both evidence and influence, named research weaving ( 20 ). This framework combines visual analytics and scientometrics to visualize and delineate the development of a field, its underlying intellectual structure and the dynamics of scholarly communication over time ( 21 ). A comprehensive delineation of how scientometrics and bibliometrics overlap and distinct can be found in Hood and Wilson 2001 paper ( 22 ).
To the best of our knowledge, no broad-ranging scientometric study of research trends and influence networks of physical activity, mental health and wellbeing has yet been conducted. Thus, in this article, we present one to bridge the gap.
Materials and methods
Search strategy and data collection.
We searched the Web of Science Core Collection (WOSCC) on December 7, 2021, using a combination of keywords and Medical Subject Headings such as “physical activity,” “mental health,” and “mental illness * .” WOSCC provides full references and complete citations of articles published in major journals since 1900 and is one of the largest comprehensive sources for bibliometric studies ( 23 ). The full protocol with the search key is available on osf.io. This current study protocol is based on a first large-scale scientometric analysis ( 24 ). The database source was limited to the Web of Science Citation Index Expanded. The document types are limited to “article,” “review,” “editorial material,” and “proceeding papers,” without restrictions on language or time. The dataset was extracted from the WOSCC in tag-delimited plain text files.
In order to assess the quality of the reference filtering process and the homogeneity of the dataset, we independently inspected each of the most cited references (604 articles in total), and a randomly selected sample of 10% of included articles to allow a margin of error (i.e., inclusion of non-relevant papers) of 5% with a 95% confidence interval ( Supplementary Table 1 ; Figure 1 ).
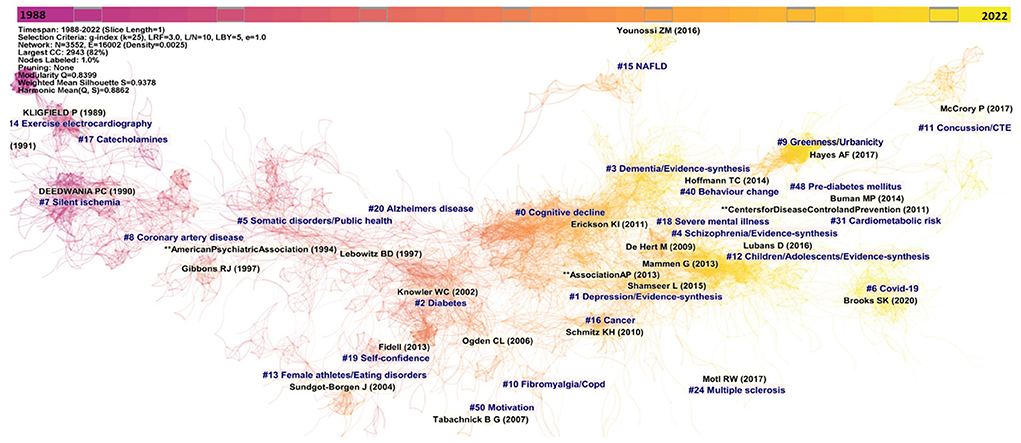
Figure 1 . Co-citation reference network with cluster visualization (1988–2021). The unit of measure are articles and constitutes nodes. Nodes are organized according to year of publication. The size of a node (article) is proportional to the number of times the node has been co-cited. Colored shades indicate the passage of the time, from past (purplish) to the present time (yellowish).
The primary outcome was to visualize research trends on physical activity related to mental health and wellbeing and to characterize the evolution of research trends using networks of co-cited references and networks of co-occurring keywords assigned to relevant publications.
The secondary outcome was to provide clinicians, researchers, and policymakers with a specific unit of measure of the research network (countries, institutions, authors, and journals) and to identify emerging trends and limitations.
Data analysis
Two different software tools for constructing bibliometric networks were used: Bibliometrix R package (3.1.4) ( 25 ) and CiteSpace (version 5.8.R4) ( 21 ). Bibliometric outcomes included citation counts, co-citations, and co-occurrences. A co-citation count is defined as the frequency with which two published articles are cited together by subsequently published articles ( 26 ). Co-occurrence networks are based on how frequently two entities, such as keywords, appear in the same articles.
The Bibliometrix R package was used for the analysis of publication outputs and the trend of growth. CiteSpace was used for the study of several types of networks, namely, networks of co-cited references, networks of co-cited authors, and co-occurrence networks of authors, keywords, institutions, and countries. For instance, the co-cited (authors') institutions network accounts for the cooperation between two or more institutions, which reflects the cooperation between authors and the influence networks.
CiteSpace produces a variety of metrics of significance, with temporal metrics such as citation burstness, structural metrics such as betweenness centrality, modularity, and silhouette score as well as a combination of both, namely, the sigma metric. The betweenness centrality of a node measures the fraction of shortest paths in an underlying network passing through the node ( 27 ). The burstness of the frequency of an entity over time indicates a specific duration of a surge of the frequency ( 28 ). The sigma indicator combines structural and temporal properties of a node, namely, its betweenness centrality and citation burst ( 29 ). Modularity (the Q score) measures the quality of dividing a network into clusters, and the silhouette score (the S score) of a cluster measures the quality of a clustering configuration ( 30 ). The Q score ranges from 0 to +1. The cluster structure is considered significant with a Q score >0.3, and higher values indicate a well-structured network. The S score ranges from −1 to +1. If the S score is >0.3, 0.5, or 0.7, the network is considered homogenous, reasonable, or highly credible, respectively. In addition, we conducted a structural variation analysis that focuses on novel boundary-spanning connections to detect transformative papers ranked on their divergence modularity ( 31 ). These transformative papers can potentially change to the existing structure of knowledge.
We extracted cluster labels from keywords associated with articles that are responsible for the formation of a cluster selected by the likelihood ratio test ( p < 0.001). Each cluster was closely inspected, and eventually cluster labels were improved based on the authors' judgment.
The second level of the data filtering process was applied during the generation of networks within each dataset (e.g., most cited reference) in order to detect duplicates, references without authors, or any non-relevant unit of measure that was excluded (e.g., DSM reference; CIM-10) or merged (e.g., author Motl RW and Motl W Robert).
The g-index was used for all calculations. This index permits to give credit to lowly cited or non-cited papers while giving credit for highly cited papers, thus partially alleviating bias from highly cited papers as seen with the h-index ( 32 ). CiteSpace general parameters are reported in Supplementary Information 1 .
Analysis of publication outputs, major journals, and growth trend prediction
We report a flowchart with detail of the 56,442 retrieved documents from the WOS Science citation index expanded and the different steps of our scientometric study: identification and screening of studies, software analyses, and expert review's interpretation (Supplementary Figure 1 ).
Among the retrieved documents, 1,089 documents were excluded, and 55,353 documents encompassing 1,306,828 references were retained (47,105 articles; 6,671 reviews; 564 editorial material; 1,013 proceeding papers). The data filtering process consisted of the inspection of each 604 highly cited papers, editorial material, and proceeding papers and the inspection of 10% randomly selected titles of the retrieved documents. Only 4% ( n = 224 articles) were not relevant ( Supplementary Figure 1 ).
The retained 55,353 articles were published between 1905 and May 2022 in 24 different languages (95.1% of articles in English). The annual scientific production is still in 2022 exponential with a mean annual growth rate of 6.8% since 1989 ( n = 17) and 2022 ( n = 5,604) ( Supplementary Figures 2, 3 ).
The first article identified was a Franz SI and Hamilton GV article on “the effects of exercise upon the retardation in conditions of depression” published in the American Journal of Insanity ( 33 ).
Analysis of co-citation reference: Clusters of research and most cited papers
Clusters of research.
We constructed a synthesized network of co-cited references based on articles published during the 1988–2021 time period as suggested by CiteSpace after the removal of empty time intervals to optimize time slicing ( Figure 1 ). In this network, each node represents a highly co-cited article. We further explored the latest research trends with the extraction of co-citation networks for the 2016-(May) 2022 time period, and the monthly time sliced networks for the year of 2021 ( Supplementary Figure 4 ). All three networks presented significant modularity and silhouette scores indicating highly credible clusters ( Q = 0.8481, S = 0.9394; Q = 0.7712, S = 0.9445; and Q = 0.4854, S = 0.8376, respectively).
The 1988–2021 network identified 50 different clusters, with a single constellation of 26 clusters that reveals six distinct major trends of research on physical activity, namely cardiovascular disease, somatic disorders, cognitive decline/dementia, mental illness, athletes' performance, related health issues and eating disorders and COVID-19 pandemic.
The earliest research trend identified concerns physical activity and cardiovascular diseases consisting of four distinct clusters during the years 1991 to 1997 as follows, with clusters number (clusters' size decreased from cluster number #0), label, silhouette score, size, pooled mean year of publication, the most representative reference; #14, “exercise electrocardiography” ( S = 0.987; 65; 1987) ( 34 ), #7 “silent ischemia” ( S = 0.964; 145; 1989) ( 35 ), #17 “catecholamine” ( S = 0.987; 46; 1989) ( 36 ), and #8 “coronary artery disease” ( S = 0.962; 141; 1994) ( 37 ). This research trend then vanished until it recently reappeared in the 2016–2021 network with cluster #14 “cardio-metabolic health markers” ( S = 0.991; 6; 2014) ( 38 ), #31 “cardiometabolic risk” ( S = 0.999; 5; 2014) ( 39 ), and continues to evolve, as shown in the 2021 network with cluster #9 “cardiovascular disease” ( S = 0.998; 4; 2016) ( 40 ).
The second major trend of research emerged in 1995 on “somatic disorders/public health,” cluster #5 ( S = 0.953; 238; 1995) ( 41 ) that directly evolved into cluster #2 “diabetes” ( S = 0.918; 289; 2001) ( 42 ) and further develop into a relatively isolated cluster #10 “fibromyalgia/copd” ( S = 0.981; 97; 2005) ( 43 ), compared to a succession of other clusters on somatic disorders #16 “cancer” ( S = 0.993; 52; 2009) ( 44 ), #15 “NAFLD” ( S = 0.998; 55; 2011) ( 45 ), #48 “pre-diabetes” ( S = 0.994; 4; 2012) ( 46 ) and #24 “multiple sclerosis” ( S = 0.999; 9; 2014) ( 47 ).
The third major trend concerned cognitive decline and dementia and started in 1997 with a small cluster #20 “Alzheimer's disease” ( S = 0.993; 16; 1997) ( 48 ), then evolved in a much larger cluster #3 “dementia” ( S = 0.916; 269; 2014) ( 49 ), and the largest cluster of the network, cluster #0 “cognitive decline” ( S = 0.923; 324; 2006) ( 50 ). This cluster continues as the most prominent cluster of the 2016–2021 network #0 “evidence-synthesis/cognitive decline” ( S = 0.938; 221; 2015) ( 51 ) and also extended to a cluster on frailty, #9 “frailty” ( S = 0.991; 15; 2014) ( 52 ).
The fourth major trend on research concerned mental illness. This trend started in 2007 with a small cluster #18 “severe mental illness” ( S = 0.985; 46; 2007) ( 53 ), and rapidly evolved in two major clusters, #1 “depression” ( S = 0.823; 292; 2009) ( 42 ), and #4 “schizophrenia” ( S = 0.912; 267; 2015) ( 54 ). The 2016-2021 network confirmed the importance of this major trend with #2 “evidence-synthesis/depression” ( S = 0.819; 142; 2016) ( 55 ). This trend now mainly focus on evidence-synthesis and became #12 “children/adolescents/evidence-synthesis” ( S = 0.963; 75; 2016) ( 56 ).
The fifth trend concerns physical activity, athlete's performance, related health issues, and eating disorders with a succession of small and isolated clusters: #19 “self-confidence” ( S = 0.995; 38; 1998) ( 57 ), #13 “female athletes/eating disorders” ( S = 0.967; 74; 2000) ( 58 ), #50 “motivation” ( S = 0.997; 4; 2005) ( 59 ), and #11 “concussion/chronic traumatic encephalopathy” ( S 0 = 0.996; 11; 2014) ( 60 ). A focus on the 2021 network reveals the latest cluster of the trend, #7 “elite athletes” ( S = 0.986; 75; 2017) ( 61 ).
The sixth and last trend concerned COVID-19 pandemic and starts with cluster #6 “COVID-19' ( S = 0.968; 26; 2019) ( 62 ), that continues to evolve in the 2016–2021 network with #1 “COVID-19” ( S = 0.987; 172; 2019) ( 63 ), #20 “post-COVID-19/long COVID” ( S = 1; 4; 2019) ( 64 ) and became in 2021 the most important cluster with #0 “COVID” ( S = 0.818; 147; 2019) ( 63 ), and #4 “COVID/children” ( S = 0.837; 59; 2019) ( 65 ).
Finally, two recent isolated clusters that we cannot relate to a specific trend have also emerged: cluster #9 “greenness/urbanicity” ( S = 0.998; 2015) ( 66 ), and #40 “behavior change” ( S = 0.996; 7; 2013) ( 67 ).
The link walkthrough over time between clusters based on burstness dynamics for the 1988–2021 network is available as a video on osf.io.
Most cited papers
We report the top 10 most co-cited references for the 1988–2021 time period in Table 1 . The top three most co-cited articles in our network were the Schuch et al.'s meta-analysis on exercise as a treatment of depression ( 55 ), followed by the Erickson et al.'s randomized-controlled trial (RCT) on exercise increasing the size of the anterior hippocampus in older adults ( 50 ), and the Ngandu et al.'s RCT on the multidomain intervention of diet, exercise, cognitive training, and vascular risk monitoring vs. control to prevent cognitive decline in at-risk elderly people ( 54 ).
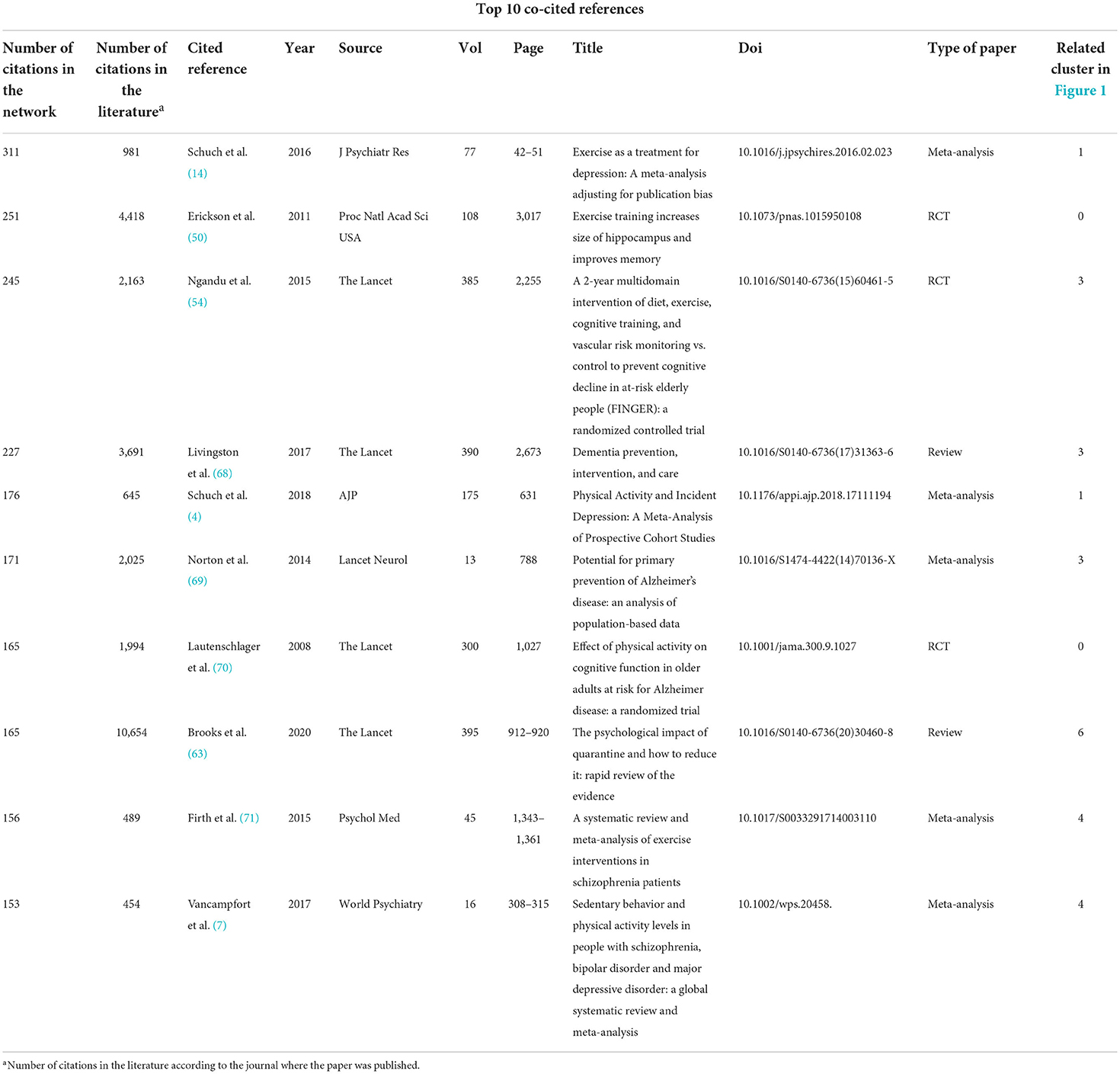
Table 1 . The top 10 most cited journals and reference.
Moreover, we produced the analysis of burstness for the top references of the 1988–2021, 2016–2021, and 2021 time periods ( Supplementary Tables 2P–R ). The analysis of burstness revealed that the top three references with the latest and strongest beginning of citation burst were the Warburton and Bredin paper on health benefits of physical activity ( 72 ), the Brooks et al. paper on the psychological impact of quarantine ( 63 ), and the Stubbs et al. EPA guidance on physical activity as a treatment for severe mental illness ( 11 ).
Another important aspect of scientometric studies is the detection of potentially transformative papers, by conducting a structural variation analysis for the 2016–2021 and the 2021–2021 time period ( Supplementary Table 3 ). For the 2016–2021 time period, the top three identified articles based on the strongest centrality divergence were the Stubbs et al. study on factors influencing physical activity among 204,186 people across 46 low-and middle-income countries ( 73 ), Vancampfort et al.'s meta-analysis on sedentary behavior and physical activity levels in people with severe mental illness ( 7 ), and Vancampfort et al.'s review on physical activity and metabolic disease among people with severe mental illness ( 74 ). For the time period January 2021 to May 2021, the top three studies were the Aguilar et al.'s study on the association between leisure-time exercise and depressive symptoms ( 75 ), the Schuch et al.'s study on the ELSA-Brasil cohort concerning the association between leisure-time, transport, depression and anxiety symptoms ( 76 ), and the van Sluijs et al.'s review on physical activity behaviors during adolescence ( 77 ).
Analysis of co-occurrence of keywords
The use of author keywords can help identify the latest trends of research and choose search keywords for future reviews. The co-occurrence author keywords network for 1988–2021 is shown in Supplementary Figure 5 , and the 2016–2021 time period is shown in Figure 2 . In this network, each node is a highly co-occurring keyword. Both networks presented significant modularity and silhouette scores indicating credible clusters ( Q = 0.3327, S = 0.6823 and Q = 0.3971, S = 0.6614 respectively).
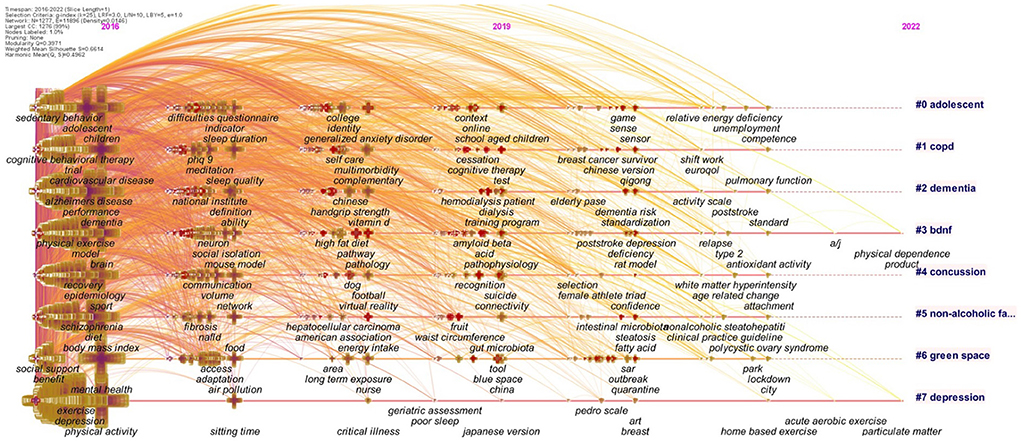
Figure 2 . Co-occurrence authors' keyword network (2016–2021). In this co-occurrence author's keywords analysis, the size of the cross is proportional to the frequency of keyword occurrence.
The 1988–2021 network presented six different clusters: #0 “mental health”; #1 “hippocampus”; #2 “quality of life”; #3 “coronary artery disease”; #4 “obesity,” and #5 “dementia,” and the 2016–2021 network presented seven different clusters: #0 “adolescent”; #1 “copd”; #2 “dementia”; #3 “bdnf”; #4 “concussion”; #5 “non-alcoholic fatty liver disease”; #6 “green space” and #7 “depression”.
The burstness analysis extracted the top 30 co-cited keywords; the latest and strongest beginning of citation bursts for the 1988–2021 network were “quality of life,” “major depression,” “controlled trial,” “meta-analysis,” and “sedentary behavior,” and for the 2016–2021 network were “psychological impact,” “acute respiratory syndrome,” “rat model,” “epidemic,” and “deficiency” ( Supplementary Tables 3S–V ).
Analysis of influence and co-operation network
Co-cited countries and co-cited institutions network.
We produced the co-cited countries and co-cited institutions network ( Figures 3A,B ). Units of measures were authors' countries and authors' institutions. A significant modularity and silhouette score were found ( Q = 0.5321; S = 0.785).
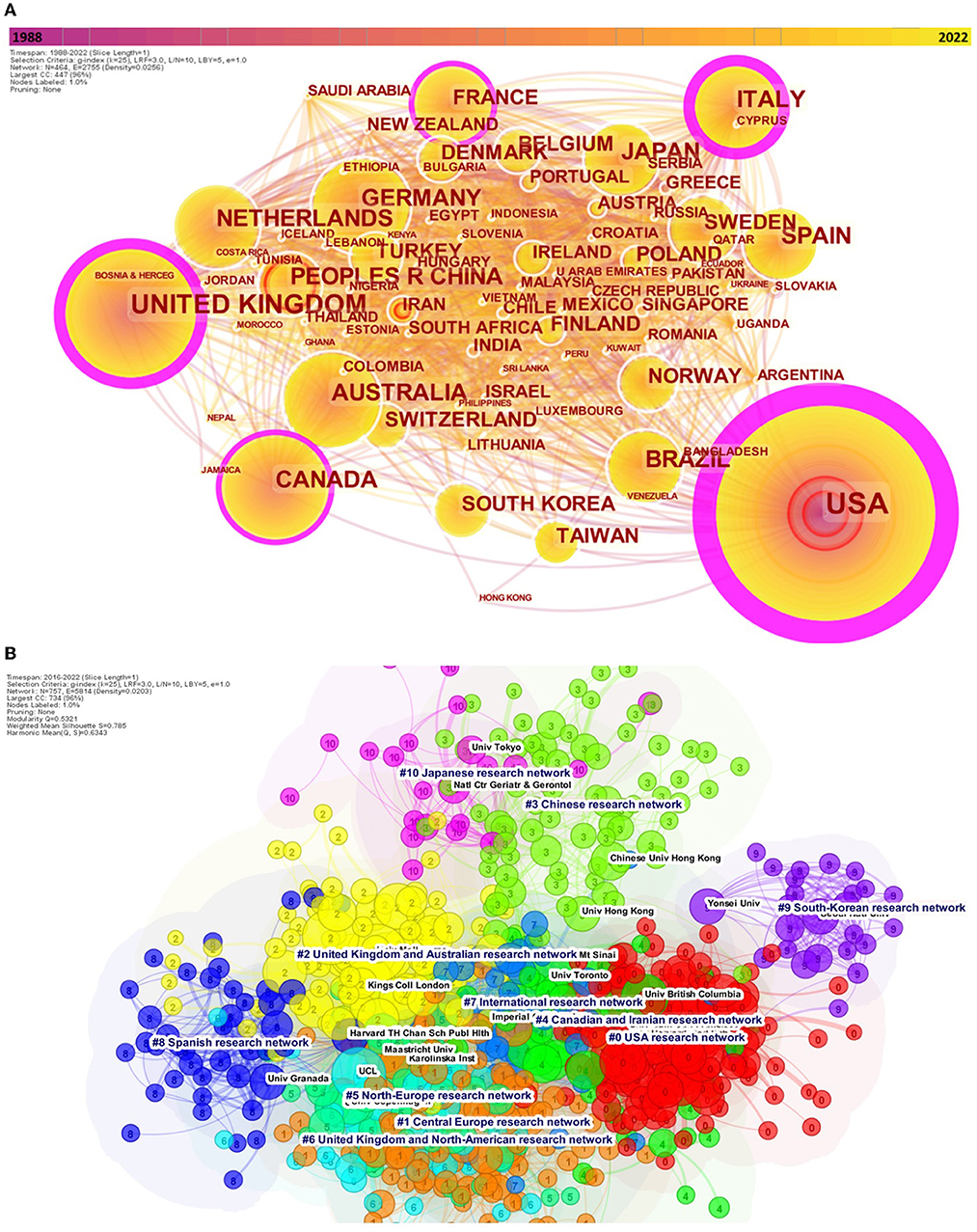
Figure 3 . Co-cited author's countries (A) (1988–2021) and co-cited author's institutions network with corresponding clusters (B) (2016–2021). Both the co-cited author's countries and co-cited author's institutions permits to reveal the collaborative country network. Betweenness centrality organize the network, with the countries presenting the most important centrality being at the center of the network. Nodes are according to each network, countries or institutions. The outermost purple ring denotes the centrality level, and highly central nodes are considered pivotal points in the research field. We limited the nodes to the 80 first countries.
Overall, 176 different countries were identified. In the 1988–2021 network, the country with the most important number of author's citation were the United States of America (USA) ( n = 17,988), followed by the United Kingdom ( n = 5,720) Australia ( n = 4,431), Canada ( n = 3,773), and People's Republic of China ( n = 3,160). Similarly, in the 2016–2021 network, the most cited top countries were identical; however, China was now in fourth place ( Supplementary Figure 6 ; Supplementary Table 4 ). The analysis of burstness reveals confirmed that China was from far the country with the most important strength of burst these last 2 years (231.72), whereas the USA latest important burst date to the 1998–2003 period (83.54) ( Supplementary Tables 2A,B ). The co-cited author's institutions network reveals what institutions are the most cited. We produced the last five-year network (2016–2021) and identified 757 different organizations ( Figure 3B , Supplementary Figure 7 ).
The most central network was the USA research network #0, with the greatest betweenness centrality to other clusters, such as the Central Europe research network #1, or the United Kingdom and Australian research network #2. The Chinese research network #3, although important in size, was relatively isolated, sharing few links with the Japanese research network #10, whereas the Spanish #8 and the South Korean #9 network were more isolated ( Supplementary Table 4 ). The burstness analysis revealed that the five institutions with the latest and strongest strength of citation burst were as follows: Central South University (China), University of Extremadura (Spain), Federal University of Santa Maria (Brazil), University of Paris (France), and University of Lisbon (Portugal) ( Supplementary Tables 2C,D ). The sigma score revealed that the institutions with the greatest scores were Charité (#1; 2016), Medical University of Vienna (#1; 2017), and Peking University (#3; 2016).
Co-authorship, co-cited and co-cited journals network
Our dataset includes 1,306,827 citations with an average of 31.85 citations per document. About 175,508 different authors were found, with an average of 3.17 authors and 5.76 co-authors per document in 4,193 different sources (e.g., books and journals) ( Supplementary Figure 1 ).
We produced the co-authorship networks, which are the social networks encompassing researchers that reflect collaboration among them, each node representing a different highly cited co-author ( Supplementary Figure 8 , Supplementary Table 4 ). The network revealed that French researchers are closely collaborating within France and on physical exercise and aging/depression (#9; 2018). The burstness analysis revealed that the co-authors that were the most participating in articles these last years were Stubbs B, Smith L, De Hert M, Vancampfort D and Probst M ( Supplementary Tables 2G,H ). We further produce the co-cited author network that permits to visualize “who cites who” for the last 5 years (2016–2021 network) was also conducted ( Supplementary Figure 9 ). The burstness analysis revealed that the most co-cited first authors according to our datasets were Brooks SK, Wang CY, Ogden CL, Holmes EA, and Kandola SA. Furthermore, the latest top cited authors (as first authors) with the most important strength of burst were Brooks SK, Schuch FB, Wang CY, Firth J, and Stubbs B ( Supplementary Tables 3I,J ).
The top five journals with the most documents were as follows: the International Journal of Environmental Research and Public Health ( n = 1,164) in first place with a massive raise of documents these last 3 years; PLOS ONE ( n = 1,017); BMC Public Health ( n = 625); BMJ OPEN ( n = 513) and the Journal of Affective Disorders ( n = 453) ( Supplementary Figure 10 ). We conducted the co-cited journal network that retained 2,879 journals and showed the highly cited journals with high betweenness centrality ( Supplementary Figure 11 ).
The top five highly cited journals were Archives of General Psychiatry (JAMA), The Lancet, PLOS ONE, Medicine and Science in Sports and Exercise , and the New England Journal of Medicine ( Table 2 ). The burstness analysis further reveals that five journals with the latest beginning of burst were Frontiers in Psychology, The Lancet Psychiatry, International Journal of Environmental Research and Public Health, Nutrients , and Frontiers in Psychiatry ( Supplementary Tables 2E,F ).
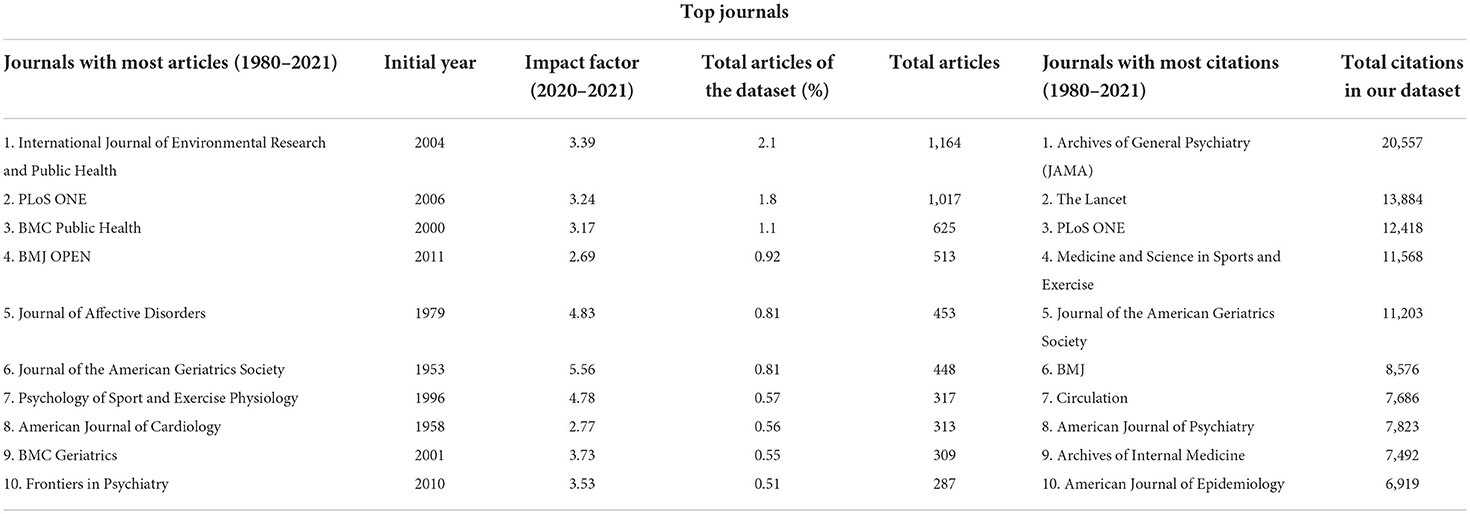
Table 2 . Journals with most articles and citations.
Summary of the main findings
To the best of our knowledge, this is the first broad scientometric that proposes a comprehensive overview of the development of research on physical activity, mental health, and wellbeing.
We retained 55,353 documents revealing an exponential growth of scientific production since the 90s. The USA holds for decades the leading position in research; however, China is very active since 2020 with an important burst of citations, mainly due to publication on COVID-19. The King's College London and Harvard University were the most influential institutions in terms of citation count. In supplement to actual reviews, this scientometric study reveals the influence and collaboration network, which could help researchers to identify major scholarly communities and establish potential research collaboration.
Identification of research trends
The six distinct major trends of research identified expose the history and the latest development of research on physical activity, mental health, and wellbeing. The first major trend of research concerns physical activity and cardiovascular disease, reminding the past and present intertwine. First research focused on cardiovascular disease ( 35 ). The large body of research on evidence synthesis of the last decades that mainly focused on the prevention to treatment role of physical activity for cardiovascular disease started with guidelines for exercise testing ( 37 , 78 ), and that continues to date with consideration of cardiometabolic risk factors ( 39 ).
The extension of prevention and treatment of physical activity to other somatic disorders constituted the second major trend, making levels of physical activity a public health priority ( 41 ), that continues to date ( 79 ). Another trend, which emerged after 2000, is the potential of physical activity for the prevention and treatment of dementia with increased importance of evidence-synthesis studies ( 51 , 80 , 81 ).
Physical activity has also been explored as a potential intervention for the prevention and treatment of dementia. As regards to prevention, it has been demonstrated that physical activity is a protective factor against Alzheimer's disease and other types of dementia ( 82 , 83 ). As a treatment, recently an umbrella review has pooled evidence from as many as 27 systematic reviews, including 18 with meta-analyses, overall reporting on 28,205 participants with mild cognitive impairment or dementia ( 84 ). The authors showed that mind-body intervention and mixed physical activity interventions had a small effect on global cognition, whereas resistance training had a large effect on global cognition in those with mild cognitive impairment. In people affected by dementia, a small effect of physical activity/exercise emerged in improving global cognition in Alzheimer's disease and all types of dementia. Importantly, physical activity/exercise also improved other outcomes not strictly related to cognition, including the risk of falls, and neuropsychiatric symptoms.
Adjacently, a massive body of evidence has organized an important trend of research on the benefits of physical activity for both prevention and treatment of severe mental disorders, in particular depression ( 4 , 85 , 86 ) and schizophrenia ( 71 , 87 ). More recently, the evidence has focused on evidence-synthesis ( 10 , 74 ) and mental health/wellbeing ( 9 ).
Other lesser, although highly relevant trends were also uncovered, such as the importance of physical activity for athlete's performance ( 88 , 89 ). While most of the research efforts in that area have focused on how to optimize performance in the context of professional athletics ( 90 ), perfectionism, and excessive physical activity can also be a symptom of mental disorders, and eating disorders in particular ( 58 ). This research trends now focus on concussion and its consequence (chronic traumatic encephalopathy) ( 60 ).
Finally, a large body of research has focused on physical activity and COVID-19. Physical activity is a protective factor for COVID-19 complications ( 91 ). During COVID-19 research has also focused on restrictions and physical activity ( 63 ). Finally, physical activity's relevance has also been shown to extend beyond the clinical sciences and start to dialogue with greenness and urban planning ( 66 , 92 , 93 ).
Although various trends of research have developed these last decades, we can identify two important gaps, the one of the roles of physical activity in the prevention or treatment of substance-use disorders, and the one regarding the socioeconomic inequalities in access to physical exercise ( 94 ). Meta-review covering this subject ( 10 ) concluded that exercise can improve multiple mental health outcomes in those with alcohol-use disorders and substance-use disorders; however, further research is needed in these conditions, notably with the use of mind-body practices ( 95 , 96 ).
Strengths and limitations
This work has strengths and weaknesses. Strengths are its novel evidence-synthesis approach, complete systematic reviews, and meta-analysis, by providing information on the evolution of research trends over time, the visualization of networks of authors, countries, and institutions, and that go beyond common measures of academic bibliometric performance (i.e., impact factor, H-Index, number of papers or citations). This novel research framework permits repeatable, reproducible, and comparable analysis with less bias than conventional time-consuming reviews that are vulnerable to biased coverage/selection.
Limitations are that, despite the quality check procedures outlined in the methods, this is not a systematic review. Furthermore, gathered data were only obtained from WOSCC, which can limit retrieved publication ( 94 , 97 ). Also, the centrality and number of citations are not necessarily indicative of the quality of a work, as faulty publications can be highly cited because they are frequently criticized as well ( 98 ). Finally, no reporting guidance is available for scientometric studies yet, given their recent introduction in the literature.
In conclusion, researchers have consistently focused on the role of physical activity on cardiovascular disease, other somatic disorders, dementia, mental disorders, athlete's performance, and eating disorders and more recently on COVID-19 pandemic, which clearly shows the role of physical activity as medicine across physical and mental disorders. More recently, the literature has focused on green space, urban planning, and behavior change, further expanding the multidisciplinary reach of physical activity. Taken together our results strengthen and expand the specific and central role of physical activity in public health, calling for the systematic involvement of physical activity professionals as stakeholders in the public health decision-making process.
Data availability statement
The raw data supporting the conclusions of this article will be made available by the authors, without undue reservation.
Author contributions
MSa and MSo: conceptualization and writing—original draft preparation. MSa, CC, and MSo: methodology, formal analysis, and investigation. MSa, CC, OS, JD, DV, JF, LS, BS, SR, FS, and MSo: writing—review and editing. CC and MSo: supervision. All authors contributed to the article and approved the submitted version.
Open access funding was provided by the University of Geneva.
Conflict of interest
Author OS has received advisory board honoraria from Otsuka, Lilly, Lundbeck, Sandoz, and Janssen in an institutional account for research and teaching. Author JF has received consultancy fees from Parachute BH for a separate project. Author BS is on the Editorial Board of Ageing Research Reviews, Mental Health and Physical Activity, the Journal of Evidence Based Medicine and the Brazilian Journal of Psychiatry. Author BS has received honorarium from a co-edited a book on exercise and mental illness, advisory work from ASICS & ParachuteBH for unrelated work. Author MSo has received honoraria/has been a consultant for Angelini, Lundbeck and Otsuka.
The remaining authors declare that the research was conducted in the absence of any commercial or financial relationships that could be construed as a potential conflict of interest.
Publisher's note
All claims expressed in this article are solely those of the authors and do not necessarily represent those of their affiliated organizations, or those of the publisher, the editors and the reviewers. Any product that may be evaluated in this article, or claim that may be made by its manufacturer, is not guaranteed or endorsed by the publisher.
Supplementary Material
The Supplementary Material for this article can be found online at: https://www.frontiersin.org/articles/10.3389/fpubh.2022.943435/full#supplementary-material
1. Thompson WR, Sallis R, Joy E, Jaworski CA, Stuhr RM, Trilk JL. Exercise is medicine. Am J Lifestyle Med. (2020) 14:511–23. doi: 10.1177/1559827620912192
PubMed Abstract | CrossRef Full Text | Google Scholar
2. Lee D-C, Artero EG, Sui X, Blair SN. Mortality trends in the general population: the importance of cardiorespiratory fitness. J Psychopharmacol. (2010) 24(4 Suppl.):27–35. doi: 10.1177/1359786810382057
3. Rezende LFM, Sá TH, Markozannes G, Rey-López JP, Lee IM, Tsilidis KK, et al. Physical activity and cancer: an umbrella review of the literature including 22 major anatomical sites and 770 000 cancer cases. Br J Sports Med. (2018) 52:826–33. doi: 10.1136/bjsports-2017-098391
4. Schuch FB, Vancampfort D, Firth J, Rosenbaum S, Ward PB, Silva ES, et al. Physical activity and incident depression: a meta-analysis of prospective cohort studies. Am J Psychiatry. (2018) 175:631–48. doi: 10.1176/appi.ajp.2018.17111194
5. Svensson M, Brundin L, Erhardt S, Hållmarker U, James S, Deierborg T. Physical activity is associated with lower long-term incidence of anxiety in a population-based, large-scale study. Front Psychiatry. (2021) 12:714014. doi: 10.3389/fpsyt.2021.714014
6. Correll CU, Solmi M, Veronese N, Bortolato B, Rosson S, Santonastaso P, et al. Prevalence, incidence and mortality from cardiovascular disease in patients with pooled and specific severe mental illness: a large-scale meta-analysis of 3,211,768 patients and 113,383,368 controls. World Psychiatry. (2017) 16:163–80. doi: 10.1002/wps.20420
7. Vancampfort D, Firth J, Schuch FB, Rosenbaum S, Mugisha J, Hallgren M, et al. Sedentary behavior and physical activity levels in people with schizophrenia, bipolar disorder and major depressive disorder: a global systematic review and meta-analysis. World Psychiatry. (2017) 16:308–15. doi: 10.1002/wps.20458
8. Hallgren M, Herring MP, Owen N, Dunstan D, Ekblom Ö, Helgadottir B, et al. Exercise, physical activity, and sedentary behavior in the treatment of depression: broadening the scientific perspectives and clinical opportunities. Front Psychiatry. (2016) 7:36. doi: 10.3389/fpsyt.2016.00036
9. Firth J, Solmi M, Wootton RE, Vancampfort D, Schuch FB, Hoare E, et al. A meta-review of “lifestyle psychiatry” the role of exercise, smoking, diet and sleep in the prevention and treatment of mental disorders. World Psychiatry. (2020) 19:360–80. doi: 10.1002/wps.20773
10. Ashdown-Franks G, Firth J, Carney R, Carvalho AF, Hallgren M, Koyanagi A, et al. Exercise as medicine for mental and substance use disorders: a meta-review of the benefits for neuropsychiatric and cognitive outcomes. Sports Med. (2020) 50:151–70. doi: 10.1007/s40279-019-01187-6
11. Stubbs B, Vancampfort D, Hallgren M, Firth J, Veronese N, Solmi M, et al. Epa guidance on physical activity as a treatment for severe mental illness: a meta-review of the evidence and position statement from the European Psychiatric Association (Epa), Supported by the International Organization of Physical Therapists in Mental Health (Ioptmh). Eur Psychiatry. (2018) 54:124–44. doi: 10.1016/j.eurpsy.2018.07.004
12. van der Kolk NM, de Vries NM, Kessels RPC, Joosten H, Zwinderman AH, Post B, et al. Effectiveness of home-based and remotely supervised aerobic exercise in Parkinson's disease: a double-blind, randomised controlled trial. Lancet Neurol. (2019) 18:998–1008. doi: 10.1016/S1474-4422(19)30285-6
13. Sabe M, Kaiser S, Sentissi O. Physical exercise for negative symptoms of schizophrenia: systematic review of randomized controlled trials and meta-analysis. Gen Hosp Psychiatry. (2020) 62:13–20. doi: 10.1016/j.genhosppsych.2019.11.002
14. Schuch FB, Vancampfort D, Richards J, Rosenbaum S, Ward PB, Stubbs B. Exercise as a treatment for depression: a meta-analysis adjusting for publication bias. J Psychiatr Res. (2016) 77:42–51. doi: 10.1016/j.jpsychires.2016.02.023
15. Stubbs B, Vancampfort D, Rosenbaum S, Ward PB, Richards J, Soundy A, et al. Dropout from exercise randomized controlled trials among people with depression: a meta-analysis and meta regression. J Affect Disord. (2016) 190:457–66. doi: 10.1016/j.jad.2015.10.019
16. Solmi M, Köhler CA, Stubbs B, Koyanagi A, Bortolato B, Monaco F, et al. Environmental risk factors and nonpharmacological and nonsurgical interventions for obesity: an umbrella review of meta-analyses of cohort studies and randomized controlled trials. Eur J Clin Invest. (2018) 48:e12982. doi: 10.1111/eci.12982
17. Arango C, Dragioti E, Solmi M, Cortese S, Domschke K, Murray RM, et al. Risk and protective factors for mental disorders beyond genetics: an evidence-based atlas. World Psychiatry. (2021) 20:417–36. doi: 10.1002/wps.20894
18. Gastaldon C, Solmi M, Correll CU, Barbui C, Schoretsanitis G. Risk factors of postpartum depression and depressive symptoms: umbrella review of current evidence from systematic reviews and meta-analyses of observational studies. Br J Psychiatry . (2022). doi: 10.1192/bjp.2021.222. [Epub ahead of print].
19. Solmi M, Radua J, Stubbs B, Ricca V, Moretti D, Busatta D, et al. Risk factors for eating disorders: an umbrella review of published meta-analyses. Rev Bras Psiquiatria. (2021) 43:314–23. doi: 10.1590/1516-4446-2020-1099
20. Nakagawa S, Samarasinghe G, Haddaway NR, Westgate MJ, O'Dea RE, Noble DWA, et al. Research weaving: visualizing the future of research synthesis. Trends Ecol Evol. (2019) 34:224–38. doi: 10.1016/j.tree.2018.11.007
21. Chen C. Citespace II: detecting and visualizing emerging trends and transient patterns in scientific literature. J Am Soc Inf Sci Technol. (2006) 57:359–77. doi: 10.1002/asi.20317
CrossRef Full Text | Google Scholar
22. Hood W, Wilson C. The literature of bibliometrics, scientometrics, and informetrics. Scientometrics. (2001) 52:291–314. doi: 10.1023/A:1017919924342
23. Mongeon P, Paul-Hus A. The journal coverage of Web of Science and Scopus: a comparative analysis. Scientometrics . (2016) 106:213–28. doi: 10.1007/s11192-015-1765-5
24. Sabe M, Pillinger T, Kaiser S, Chen C, Taipale H, Tanskanen A, et al. Half a century of research on antipsychotics and schizophrenia: a scientometric study of hotspots, nodes, bursts, and trends. Neurosci Biobehav Rev. (2022) 136:104608. doi: 10.1016/j.neubiorev.2022.104608
25. Aria M, Cuccurullo C. Bibliometrix: an R-tool for comprehensive science mapping analysis. J Informetr. (2017) 11:959–75. doi: 10.1016/j.joi.2017.08.007
26. Small H. Co-citation in the scientific literature: a new measure of the relationship between two documents. J Am Soc Inf Sci. (1973) 24:265–9. doi: 10.1002/asi.4630240406
27. Freeman LC. A set of measures of centrality based on betweenness. Sociometry. (1977) 40:35–41. doi: 10.2307/3033543
28. Kleinberg J. Bursty and hierarchical structure in streams. Data Min Knowl Discov. (2003) 7:373–97. doi: 10.1023/A:1024940629314
29. Chen C, Ibekwe-SanJuan F, Hou J. The structure and dynamics of cocitation clusters: a multiple-perspective cocitation analysis. J Am Soc Inf Sci Technol. (2010) 61:1386–409. doi: 10.1002/asi.21309
30. Shibata N, Kajikawa Y, Takeda Y, Matsushima K. Detecting emerging research fronts based on topological measures in citation networks of scientific publications. Technovation. (2008) 28:758–75. doi: 10.1016/j.technovation.2008.03.009
31. Chen C. Predictive effects of structural variation on citation counts. J Am Soc Inf Sci Technol. (2012) 63:431–49. doi: 10.1002/asi.21694
32. Egghe L. Theory and practise of the G-index. Scientometrics. (2006) 69:131–52. doi: 10.1007/s11192-006-0144-7
33. Franz SI, Hamilton GV. The effects of exercise upon the retardation in conditions of depression. Am J Psychiatry. (1905) 62:239–56. doi: 10.1176/ajp.62.2.239
34. Lachterman B, Lehmann KG, Abrahamson D, Froelicher VF. “Recovery only” St-segment depression and the predictive accuracy of the exercise test. Ann Intern Med. (1990) 112:11–6. doi: 10.7326/0003-4819-112-1-11
35. Deedwania PC, Carbajal EV. Silent ischemia during daily life is an independent predictor of mortality in stable angina. Circulation. (1990) 81:748–56. doi: 10.1161/01.CIR.81.3.748
36. Moses J, Steptoe A, Mathews A, Edwards S. The effects of exercise training on mental well-being in the normal population: a controlled trial. J Psychosom Res. (1989) 33:47–61. doi: 10.1016/0022-3999(89)90105-0
37. Gibbons RJ, Balady GJ, Beasley JW, Bricker JT, Duvernoy WF, Froelicher VF, et al. Acc/Aha guidelines for exercise testing. A Report of the American College of Cardiology/American Heart Association Task Force on Practice Guidelines (Committee on Exercise Testing). J Am Coll Cardiol. (1997) 30:260–311. doi: 10.1161/01.CIR.96.1.345
38. Buman MP, Winkler EA, Kurka JM, Hekler EB, Baldwin CM, Owen N, et al. Reallocating time to sleep, sedentary behaviors, or active behaviors: associations with cardiovascular disease risk biomarkers, Nhanes 2005-2006. Am J Epidemiol. (2014) 179:323–34. doi: 10.1093/aje/kwt292
39. Chastin SF, Palarea-Albaladejo J, Dontje ML, Skelton DA. Combined effects of time spent in physical activity, sedentary behaviors and sleep on obesity and cardio-metabolic health markers: a novel compositional data analysis approach. PLoS ONE. (2015) 10:e0139984. doi: 10.1371/journal.pone.0139984
40. Carney RM, Freedland KE. Depression and coronary heart disease. Nat Rev Cardiol. (2017) 14:145–55. doi: 10.1038/nrcardio.2016.181
41. Pate RR, Pratt M, Blair SN, Haskell WL, Macera CA, Bouchard C, et al. Physical activity and public health. A Recommendation from the Centers for Disease Control and Prevention and the American College of Sports Medicine. JAMA. (1995) 273:402–7. doi: 10.1001/jama.1995.03520290054029
42. Knowler WC, Barrett-Connor E, Fowler SE, Hamman RF, Lachin JM, Walker EA, et al. Reduction in the incidence of type 2 diabetes with lifestyle intervention or metformin. N Engl J Med. (2002) 346:393–403. doi: 10.1056/NEJMoa012512
43. Wolfe F, Häuser W, Hassett AL, Katz RS, Walitt BT. The development of fibromyalgia–i: examination of rates and predictors in patients with rheumatoid arthritis (Ra). Pain. (2011) 152:291–9. doi: 10.1016/j.pain.2010.09.027
44. Speck RM, Courneya KS, Mâsse LC, Duval S, Schmitz KH. An update of controlled physical activity trials in cancer survivors: a systematic review and meta-analysis. J Cancer Surviv. (2010) 4:87–100. doi: 10.1007/s11764-009-0110-5
45. Chalasani N, Younossi Z, Lavine JE, Diehl AM, Brunt EM, Cusi K, et al. The diagnosis and management of non-alcoholic fatty liver disease: practice guideline by the American Association for the Study of Liver Diseases, American College of Gastroenterology, and the American Gastroenterological Association. Hepatology. (2012) 55:2005–23. doi: 10.1002/hep.25762
46. American Diabetes Association. Standards of medical care in diabetes−2013. Diabetes Care. (2013) 36(Suppl. 1):S11–66. doi: 10.2337/dc13-S011
47. Motl RW. Benefits, safety, and prescription of exercise in persons with multiple sclerosis. Expert Rev Neurother. (2014) 14:1429–36. doi: 10.1586/14737175.2014.983904
48. Henderson VW, Paganini-Hill A, Miller BL, Elble RJ, Reyes PF, Shoupe D, et al. Estrogen for Alzheimer's disease in women: randomized, double-blind, placebo-controlled trial. Neurology. (2000) 54:295–301. doi: 10.1212/WNL.54.2.295
49. Rosenbaum S, Tiedemann A, Sherrington C, Curtis J, Ward PB. Physical activity interventions for people with mental illness: a systematic review and meta-analysis. J Clin Psychiatry. (2014) 75:964–74. doi: 10.4088/JCP.13r08765
50. Erickson KI, Voss MW, Prakash RS, Basak C, Szabo A, Chaddock L, et al. Exercise training increases size of hippocampus and improves memory. Proc Nat Acad Sci USA. (2011) 108:3017. doi: 10.1073/pnas.1015950108
51. Groot C, Hooghiemstra AM, Raijmakers PG, van Berckel BN, Scheltens P, Scherder EJ, et al. The effect of physical activity on cognitive function in patients with dementia: a meta-analysis of randomized control trials. Ageing Res Rev. (2016) 25:13–23. doi: 10.1016/j.arr.2015.11.005
52. Clegg A, Young J, Iliffe S, Rikkert MO, Rockwood K. Frailty in elderly people. Lancet. (2013) 381:752–62. doi: 10.1016/S0140-6736(12)62167-9
53. DeHert M, Schreurs V, Vancampfort D, Van Winkel R. Metabolic syndrome in people with schizophrenia: a review. World Psychiatry. (2009) 8:15–22. doi: 10.1002/j.2051-5545.2009.tb00199.x
54. Ngandu T, Lehtisalo J, Solomon A, Levälahti E, Ahtiluoto S, Antikainen R, et al. A 2 year multidomain intervention of diet, exercise, cognitive training, and vascular risk monitoring versus control to prevent cognitive decline in at-risk elderly people (finger): a randomised controlled trial. Lancet. (2015) 385:2255–63. doi: 10.1016/S0140-6736(15)60461-5
55. Schuch FB, Vancampfort D, Rosenbaum S, Richards J, Ward PB, Veronese N, et al. Exercise for depression in older adults: a meta-analysis of randomized controlled trials adjusting for publication bias. Rev Bras Psiquiatria. (2016) 38:247–54. doi: 10.1590/1516-4446-2016-1915
56. Lubans D, Richards J, Hillman C, Faulkner G, Beauchamp M, Nilsson M, et al. Physical activity for cognitive and mental health in youth: A systematic review of mechanisms. Pediatrics . (2016) 138:e20161642. doi: 10.1542/peds.2016-1642
57. Jones G, Hanton S, Swain A. Intensity and interpretation of anxiety symptoms in elite and non-elite sports performers. Pers Individ Dif. (1994) 17:657–63. doi: 10.1016/0191-8869(94)90138-4
58. Sundgot-Borgen J, Torstveit M. Prevalence of eating disorders in elite athletes is higher than in the general population. Clin J Sport Med. (2004) 14:25–32. doi: 10.1097/00042752-200401000-00005
59. Stoeber J, Rambow A. Perfectionism in adolescent school students: relations with motivation, achievement, and well-being. Pers Individ Dif. (2007) 42:1379–89. doi: 10.1016/j.paid.2006.10.015
60. McKee AC, Daneshvar DH, Alvarez VE, Stein TD. The neuropathology of sport. Acta Neuropathol. (2014) 127:29–51. doi: 10.1007/s00401-013-1230-6
61. Reardon CL, Hainline B, Aron CM, Baron D, Baum AL, Bindra A, et al. Mental health in elite athletes: International Olympic Committee Consensus Statement (2019). Br J Sports Med. (2019) 53:667. doi: 10.1136/bjsports-2019-100715
62. Wang B, Li R, Lu Z, Huang Y. Does comorbidity increase the risk of patients with Covid-19: evidence from meta-analysis. Aging. (2020) 12:6049–57. doi: 10.18632/aging.103000
63. Brooks SK, Webster RK, Smith LE, Woodland L, Wessely S, Greenberg N, et al. The psychological impact of quarantine and how to reduce it: rapid review of the evidence. Lancet. (2020) 395:912–20. doi: 10.1016/S0140-6736(20)30460-8
64. Mazza MG, De Lorenzo R, Conte C, Poletti S, Vai B, Bollettini I, et al. Anxiety and Depression in Covid-19 Survivors: Role of Inflammatory and Clinical Predictors. Brain Behav Immun. (2020) 89:594–600. doi: 10.1016/j.bbi.2020.07.037
65. Biddle SJH, Ciaccioni S, Thomas G, Vergeer I. Physical activity and mental health in children and adolescents: an updated review of reviews and an analysis of causality. Psychol Sport Exerc. (2019) 42:146–55. doi: 10.1016/j.psychsport.2018.08.011
66. Hartig T, Mitchell R, de Vries S, Frumkin H. Nature and health. Annu Rev Public Health. (2014) 35:207–28. doi: 10.1146/annurev-publhealth-032013-182443
67. Hoffmann K, Sobol NA, Frederiksen KS, Beyer N, Vogel A, Vestergaard K, et al. Moderate-to-high intensity physical exercise in patients with Alzheimer's disease: a randomized controlled trial. J Alzheimers Dis. (2016) 50:443–53. doi: 10.3233/JAD-150817
68. Livingston G, Huntley J, Sommerlad A, Ames D, Ballard C, Banerjee S, et al. Dementia prevention, intervention, and care: 2020 report of the Lancet Commission. Lancet. (2020) 396:413–46. doi: 10.1016/S0140-6736(20)30367-6
69. Norton S, Matthews FE, Barnes DE, Yaffe K, Brayne C. Potential for primary prevention of Alzheimer's disease: an analysis of population-based data. Lancet Neurol. (2014) 13:788–94. doi: 10.1016/S1474-4422(14)70136-X
70. Lautenschlager NT, Cox KL, Flicker L, Foster JK, van Bockxmeer FM, Xiao J, et al. Effect of physical activity on cognitive function in older adults at risk for Alzheimer disease: a randomized trial. JAMA. (2008) 300:1027–37. doi: 10.1001/jama.300.9.1027
71. Firth J, Cotter J, Elliott R, French P, Yung AR. A systematic review and meta-analysis of exercise interventions in schizophrenia patients. Psychol Med. (2015) 45:1343–61. doi: 10.1017/S0033291714003110
72. Warburton DER, Bredin SSD. Health benefits of physical activity: a systematic review of current systematic reviews. Curr Opin Cardiol. (2017) 32:541–56. doi: 10.1097/HCO.0000000000000437
73. Stubbs B, Koyanagi A, Schuch FB, Firth J, Rosenbaum S, Gaughran F, et al. Physical activity levels and psychosis: a mediation analysis of factors influencing physical activity target achievement among 204 186 people across 46 low- and middle-income countries. Schizophr Bull. (2017) 43:536–45. doi: 10.1093/schbul/sbw111
74. Vancampfort D, Stubbs B. Physical activity and metabolic disease among people with affective disorders: prevention, management and implementation. J Affect Disord. (2017) 224:87–94. doi: 10.1016/j.jad.2016.07.042
75. Aguilar BAS, Tebar WR, Silva SCB, Gomes LQ, Damato TMM, Mota J, et al. Leisure-time exercise is associated with lower depressive symptoms in community dwelling adults. Eur J Sport Sci. (2022) 22:916–25. doi: 10.1080/17461391.2021.1895892
76. Schuch FB, Werneck AO, Vancampfort D, Stubbs B, Teychene M, Lotufo PA, et al. Cross-sectional associations of leisure and transport related physical activity with depression and anxiety. J Psychiatr Res. (2021) 140:228–34. doi: 10.1016/j.jpsychires.2021.05.053
77. van Sluijs EMF, Ekelund U, Crochemore-Silva I, Guthold R, Ha A, Lubans D, et al. Physical activity behaviours in adolescence: current evidence and opportunities for intervention. Lancet. (2021) 398:429–42. doi: 10.1016/S0140-6736(21)01259-9
78. Thompson PD, Buchner D, Pina IL, Balady GJ, Williams MA, Marcus BH, et al. Exercise and physical activity in the prevention and treatment of atherosclerotic cardiovascular disease: A Statement from the Council on Clinical Cardiology (Subcommittee on Exercise, Rehabilitation, and Prevention) and the Council on Nutrition, Physical Activity, and Metabolism (Subcommittee on Physical Activity). Circulation. (2003) 107:3109–16. doi: 10.1161/01.CIR.0000075572.40158.77
79. WHO. Who Guidelines on Physical Activity and Sedentary Behaviour . Geneva: World Health Organization (2020).
Google Scholar
80. Sofi F, Valecchi D, Bacci D, Abbate R, Gensini GF, Casini A, et al. Physical activity and risk of cognitive decline: a meta-analysis of prospective studies. J Intern Med. (2011) 269:107–17. doi: 10.1111/j.1365-2796.2010.02281.x
81. Colcombe S, Kramer AF. Fitness effects on the cognitive function of older adults: a meta-analytic study. Psychol Sci. (2003) 14:125–30. doi: 10.1111/1467-9280.t01-1-01430
82. Ahlskog JE. Aerobic exercise: evidence for a direct brain effect to slow Parkinson disease progression. Mayo Clinic Proc. (2018) 93:360–72. doi: 10.1016/j.mayocp.2017.12.015
83. Mentis A-FA, Dardiotis E, Efthymiou V, Chrousos GP. Non-genetic risk and protective factors and biomarkers for neurological disorders: a meta-umbrella systematic review of umbrella reviews. BMC Med. (2021) 19:6. doi: 10.1186/s12916-020-01873-7
84. Demurtas J, Schoene D, Torbahn G, Marengoni A, Grande G, Zou L, et al. Physical activity and exercise in mild cognitive impairment and dementia: an umbrella review of intervention and observational studies. J Am Med Direct Assoc. (2020) 21:1415–22.e6. doi: 10.1016/j.jamda.2020.08.031
85. Strawbridge WJ, Deleger S, Roberts RE, Kaplan GA. Physical activity reduces the risk of subsequent depression for older adults. Am J Epidemiol. (2002) 156:328–34. doi: 10.1093/aje/kwf047
86. Rebar AL, Stanton R, Geard D, Short C, Duncan MJ, Vandelanotte C, et al. Meta-meta-analysis of the effect of physical activity on depression and anxiety in non-clinical adult populations. Health Psychol Rev. (2015) 9:366–78. doi: 10.1080/17437199.2015.1022901
87. Vancampfort D, Knapen J, Probst M, Scheewe T, Remans S, De Hert M, et al. Systematic review of correlates of physical activity in patients with schizophrenia. Acta Psychiatr Scand. (2012) 125:352–62. doi: 10.1111/j.1600-0447.2011.01814.x
88. Chen Y, Buggy C, Kelly S. Winning at all costs: a review of risk-taking behaviour and sporting injury from an occupational safety and health perspective. Sports Med. (2019) 5:15. doi: 10.1186/s40798-019-0189-9
89. Wyss T, Boesch M, Roos L, Tschopp C, Frei KM, Annen H, et al. Aerobic fitness level affects cardiovascular and salivary alpha amylase responses to acute psychosocial stress. Sports Med. (2016) 2:33. doi: 10.1186/s40798-016-0057-9
90. Burton D. Advances in sport and exrecise psychology measurement. In: Duda IJL, editor. Measuring Competitive State Anxiety . Morgantown: Fitness Information Technology, Inc. (1998). p. 129–48.
91. Sallis R, Young DR, Tartof SY, Sallis JF, Sall J, Li Q, et al. Physical inactivity is associated with a higher risk for severe covid-19 outcomes: a study in 48 440 adult patients. Br J Sports Med. (2021) 55:1099. doi: 10.1136/bjsports-2021-104080
92. Akpinar A. How is quality of urban green spaces associated with physical activity and health? Urban Forest Urban Green. (2016) 16:76–83. doi: 10.1016/j.ufug.2016.01.011
93. Bedimo-Rung AL, Mowen AJ, Cohen DA. The significance of parks to physical activity and public health: a conceptual model. Am J Prev Med. (2005) 28(2 Suppl. 2):159–68. doi: 10.1016/j.amepre.2004.10.024
94. Stalsberg R, Pedersen AV. Are differences in physical activity across socioeconomic groups associated with choice of physical activity variables to report? Int J Environ Res Public Health. (2018) 15:922. doi: 10.3390/ijerph15050922
95. Wang D, Wang Y, Wang Y, Li R, Zhou C. Impact of physical exercise on substance use disorders: a meta-analysis. PLoS ONE . (2014) 9:e110728. doi: 10.1371/journal.pone.0110728
96. Vancampfort D, Stubbs B, Van Damme T, Smith L, Hallgren M, Schuch F, et al. The efficacy of meditation-based mind-body interventions for mental disorders: a meta-review of 17 meta-analyses of randomized controlled trials. J Psychiatr Res. (2021) 134:181–91. doi: 10.1016/j.jpsychires.2020.12.048
97. Visser M, van Eck NJ, Waltman L. Large-scale comparison of bibliographic data sources: scopus, web of science, dimensions, crossref, and microsoft academic. Quant Sci Stud. (2021) 2:20–41. doi: 10.1162/qss_a_00112
98. Greenberg SA. How citation distortions create unfounded authority: analysis of a citation network. BMJ. (2009) 339:b2680. doi: 10.1136/bmj.b2680
Keywords: physical exercise, mental illness, evidence synthesis, scientometrics, CiteSpace
Citation: Sabe M, Chen C, Sentissi O, Deenik J, Vancampfort D, Firth J, Smith L, Stubbs B, Rosenbaum S, Schuch FB and Solmi M (2022) Thirty years of research on physical activity, mental health, and wellbeing: A scientometric analysis of hotspots and trends. Front. Public Health 10:943435. doi: 10.3389/fpubh.2022.943435
Received: 17 May 2022; Accepted: 15 July 2022; Published: 09 August 2022.
Reviewed by:
Copyright © 2022 Sabe, Chen, Sentissi, Deenik, Vancampfort, Firth, Smith, Stubbs, Rosenbaum, Schuch and Solmi. This is an open-access article distributed under the terms of the Creative Commons Attribution License (CC BY) . The use, distribution or reproduction in other forums is permitted, provided the original author(s) and the copyright owner(s) are credited and that the original publication in this journal is cited, in accordance with accepted academic practice. No use, distribution or reproduction is permitted which does not comply with these terms.
*Correspondence: Michel Sabe, michel.sabe@hcuge.ch
† These authors have contributed equally to this work and share first authorship
Disclaimer: All claims expressed in this article are solely those of the authors and do not necessarily represent those of their affiliated organizations, or those of the publisher, the editors and the reviewers. Any product that may be evaluated in this article or claim that may be made by its manufacturer is not guaranteed or endorsed by the publisher.
Thank you for visiting nature.com. You are using a browser version with limited support for CSS. To obtain the best experience, we recommend you use a more up to date browser (or turn off compatibility mode in Internet Explorer). In the meantime, to ensure continued support, we are displaying the site without styles and JavaScript.
- View all journals
- My Account Login
- Explore content
- About the journal
- Publish with us
- Sign up for alerts
- Review Article
- Open access
- Published: 18 October 2021
A systematic review of smartphone-based human activity recognition methods for health research
- Marcin Straczkiewicz ORCID: orcid.org/0000-0002-8703-4451 1 ,
- Peter James 2 , 3 &
- Jukka-Pekka Onnela 1
npj Digital Medicine volume 4 , Article number: 148 ( 2021 ) Cite this article
19k Accesses
79 Citations
23 Altmetric
Metrics details
- Predictive markers
- Public health
- Quality of life
Smartphones are now nearly ubiquitous; their numerous built-in sensors enable continuous measurement of activities of daily living, making them especially well-suited for health research. Researchers have proposed various human activity recognition (HAR) systems aimed at translating measurements from smartphones into various types of physical activity. In this review, we summarized the existing approaches to smartphone-based HAR. For this purpose, we systematically searched Scopus, PubMed, and Web of Science for peer-reviewed articles published up to December 2020 on the use of smartphones for HAR. We extracted information on smartphone body location, sensors, and physical activity types studied and the data transformation techniques and classification schemes used for activity recognition. Consequently, we identified 108 articles and described the various approaches used for data acquisition, data preprocessing, feature extraction, and activity classification, identifying the most common practices, and their alternatives. We conclude that smartphones are well-suited for HAR research in the health sciences. For population-level impact, future studies should focus on improving the quality of collected data, address missing data, incorporate more diverse participants and activities, relax requirements about phone placement, provide more complete documentation on study participants, and share the source code of the implemented methods and algorithms.
Similar content being viewed by others
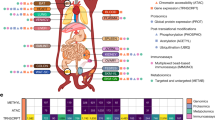
Temporal dynamics of the multi-omic response to endurance exercise training
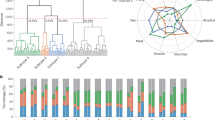
Associations of dietary patterns with brain health from behavioral, neuroimaging, biochemical and genetic analyses
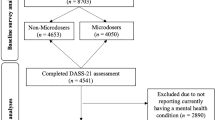
Adults who microdose psychedelics report health related motivations and lower levels of anxiety and depression compared to non-microdosers
Introduction.
Progress in science has always been driven by data. More than 5 billion mobile devices were in use in 2020 1 , with multiple sensors (e.g., accelerometer and GPS) that can capture detailed, continuous, and objective measurements on various aspects of our lives, including physical activity. Such proliferation in worldwide smartphone adoption presents unprecedented opportunities for the collection of data to study human behavior and health. Along with sufficient storage, powerful processors, and wireless transmission, smartphones can collect a tremendous amount of data on large cohorts of individuals over extended time periods without additional hardware or instrumentation.
Smartphones are promising data collection instruments for objective and reproducible quantification of traditional and emerging risk factors for human populations. Behavioral risk factors, including but not limited to sedentary behavior, sleep, and physical activity, can all be monitored by smartphones in free-living environments, leveraging the personal or lived experiences of individuals. Importantly, unlike some wearable activity trackers 2 , smartphones are not a niche product but instead have become globally available, increasingly adopted by users of all ages both in advanced and emerging economies 3 , 4 . Their adoption in health research is further supported by encouraging findings made with other portable devices, primarily wearable accelerometers, which have demonstrated robust associations between physical activity and health outcomes, including obesity, diabetes, various cardiovascular diseases, mental health, and mortality 5 , 6 , 7 , 8 , 9 . However, there are some important limitations to using wearables for studying population health: (1) their ownership is much lower than that of smartphones 10 ; (2) most people stop using their wearables after 6 months of use 11 ; and (3) raw data are usually not available from wearable devices. The last point often forces investigators to rely on proprietary device metrics, which lowers the already low rate of reproducibility of biomedical research in general 12 and makes uncertainty quantification in the measurements nearly impossible.
Human activity recognition (HAR) is a process aimed at the classification of human actions in a given period of time based on discrete measurements (acceleration, rotation speed, geographical coordinates, etc.) made by personal digital devices. In recent years, this topic has been proliferating within the machine learning research community; at the time of writing, over 400 articles had been published on HAR methods using smartphones. This is a substantial increase from just a handful of articles published a few years earlier (Fig. 1 ). As data collection using smartphones becomes easier, analysis of the collected data is increasingly identified as the main bottleneck in health research 13 , 14 , 15 . To tackle the analytical challenges of HAR, researchers have proposed various algorithms that differ substantially in terms of the type of data they use, how they manipulate the collected data, and the statistical approaches used for inference and/or classification. Published studies use existing methods and propose new methods for the collection, processing, and classification of activities of daily living. Authors commonly discuss data filtering and feature selection techniques and compare the accuracy of various machine learning classifiers either on previously existing datasets or on datasets they have collected de novo for the purposes of the specific study. The results are typically summarized using classification accuracy within different groups of activities, such as ambulation, locomotion, and exercise.
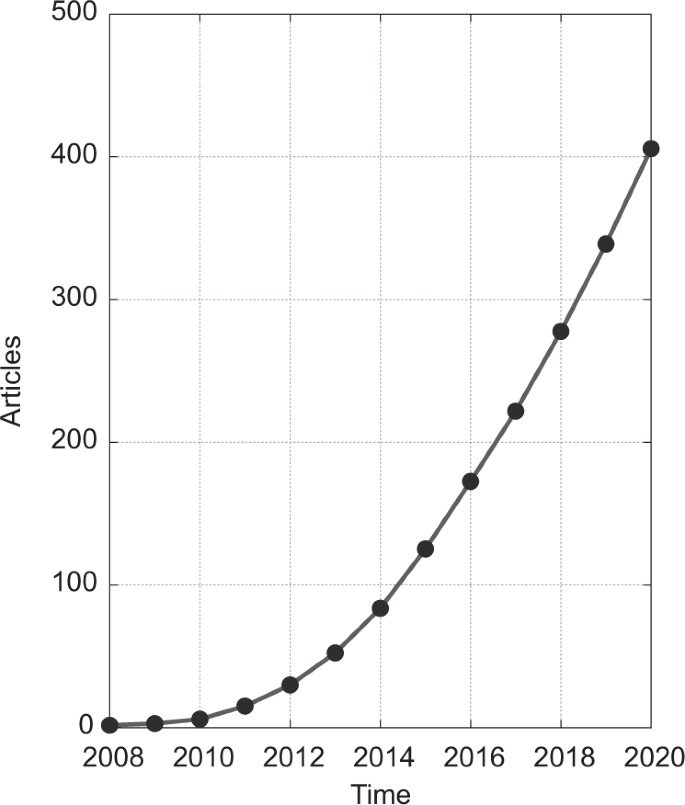
Articles were published between January 2008 and December 2020, based on a search of PubMed, Scopus, and Web of Science databases (for details, see “Methods”).
To successfully incorporate developments in HAR into research in public health and medicine, there is a need to understand the approaches that have been developed and identify their potential limitations. Methods need to accommodate physiological (e.g., weight, height, age) and habitual (e.g., posture, gait, walking speed) differences of smartphone users, as well as differences in the built environment (e.g., buildings and green spaces) that provide the physical and social setting for human activities. Moreover, the data collection and statistical approaches typically used in HAR may be affected by location (where the user wears the phone on their body) and orientation of the device 16 , which complicates the transformation of collected data into meaningful and interpretable outputs.
In this paper, we systematically review the emerging literature on the use of smartphones for HAR for health research in free-living settings. Given that the main challenge in this field is shifting from data collection to data analysis, we focus our analysis on the approaches used for data acquisition, data preprocessing, feature extraction, and activity classification. We provide insight into the complexity and multidimensionality of HAR utilizing smartphones, the types of data collected, and the methods used to translate digital measurements into human activities. We discuss the generalizability and reproducibility of approaches, i.e., the features that are essential and applicable to large and diverse cohorts of study participants. Lastly, we identify challenges that need to be tackled to accelerate the wider utilization of smartphone-based HAR in public health studies.
Our systematic review was conducted by searching for articles published up to December 31, 2020, on PubMed, Scopus, and Web of Science databases. The databases were screened for titles, abstracts, and keywords containing phrases “activity” AND (“recognition” OR “estimation” OR “classification”) AND (“smartphone” OR “cell phone” OR “mobile phone”). The search was limited to full-length journal articles written in English. After removing duplicates, we read the titles and abstracts of the remaining publications. Studies that did not investigate HAR approaches were excluded from further screening. We then filtered out studies that employed auxiliary equipment, like wearable or ambient devices, and studies that required carrying multiple smartphones. Only studies that made use of commercially available consumer-grade smartphones (either personal or loaner) were read in full. We excluded studies that used the smartphone microphone or video camera for activity classification as they might record information about an individual’s surroundings, including information about unconsented individuals, and thus hinder the large-scale application of the approach due to privacy concerns. To focus on studies that mimicked free-living settings, we excluded studies that utilized devices strapped or glued to the body in a fixed position.
Our search resulted in 1901 hits for the specified search criteria (Fig. 2 ). After removal of articles that did not discuss HAR algorithms ( n = 793), employed additional hardware ( n = 150), or utilized microphones, cameras, or body-affixed smartphones ( n = 149), there were 108 references included in this review.
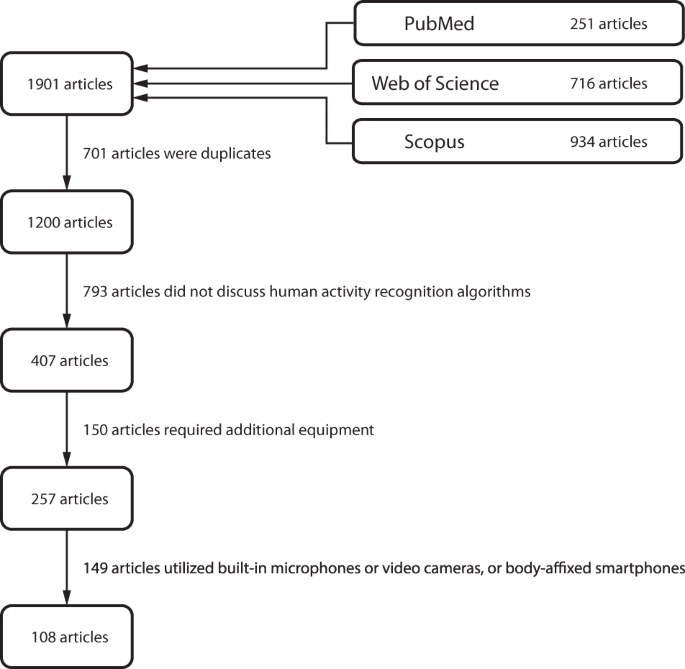
The search was conducted in PubMed, Scopus, and Web of Science databases and included full-length peer-reviewed articles written in English. The search was carried out on January 2, 2021.
Most HAR approaches consist of four stages: data acquisition, data preprocessing, feature extraction, and activity classification (Fig. 3 ). Here, we provide an overview of these steps and briefly point to significant methodological differences among the reviewed studies for each step. Figure 4 summarizes specific aspects of each study. Of note, we decomposed data acquisition processes into sensor type, experimental environment, investigated activities, and smartphone location; we indicated which studies preprocessed collected measurements using signal correction methods, noise filtering techniques, and sensor orientation-invariant transformations; we marked investigations based on the types of signal features they extracted, as well as the feature selection approaches used; we indicated the adopted activity classification principles, utilized classifiers, and practices for accuracy reporting; and finally, we highlighted efforts supporting reproducibility and generalizability of the research. Before diving into these technical considerations, we first provide a brief description of study populations.
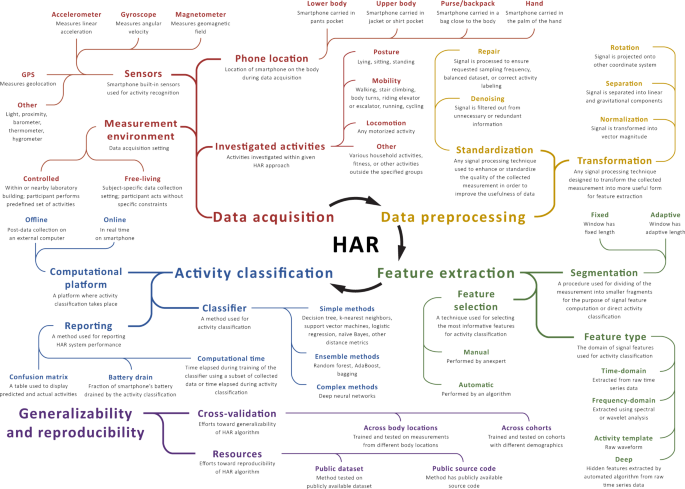
The map displays common aspects of HAR systems together with their operational definitions. The methodological differences between the reviewed studies are highlighted in Figure 4 .
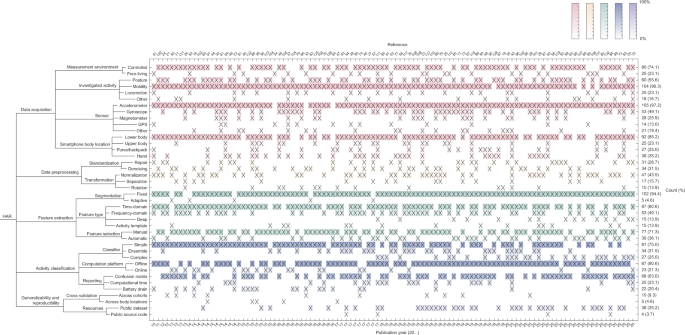
The columns correspond to the 108 reviewed studies and the rows correspond to different technical aspects of each study. Cells marked with a cross (x) indicate that the given study used the given method, algorithm, or approach. Rows have been grouped to correspond to different stages of HAR, such as data processing, and color shading of rows indicates how frequently a particular aspect is present among the studies (darker shade corresponds to higher frequency).
Study populations
We use the term study population to refer to the group of individuals investigated in any given study. In the reviewed studies, data were usually collected from fewer than 30 individuals, although one larger study analyzed data from 440 healthy individuals 17 . Studies often included healthy adults in their 20s and 30s, with only a handful of studies involving older individuals. Most studies did not report the full distribution of ages, only the mean age or the age range of participants (Fig. 5 ). To get a sense of the distribution of participant ages, we attempted to reconstruct an overall approximate age distribution by assuming that the participants in each study are evenly distributed in age between the minimum and maximum ages, which may not be the case. A comparison of the reconstructed age distribution of study participants with nationwide age distributions clearly demonstrates that future HAR research in health settings needs to broaden the age spectrum of the participants. Less effort was devoted in the studies to investigating populations with different demographic and disease characteristics, such as elders 18 , 19 , 20 and individuals with Parkinson’s disease 21 .
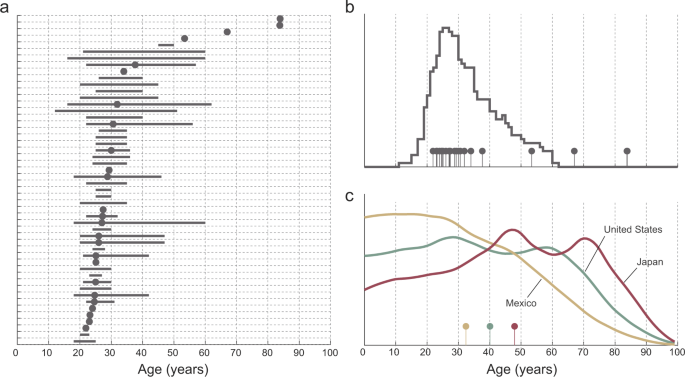
Panel a displays age of the population corresponding to individual studies, typically described by its range (lines) or mean (dots). Panel b displays the reconstructed age distribution in the reviewed studies (see the text). Nationwide age distributions displayed in panel c of three countries offer a stark contrast with the reconstructed distribution of study participant ages.
Data acquisition
We use the term data acquisition to refer to a process of collecting and storing raw sub-second-level smartphone measurements for the purpose of HAR. The data are typically collected from individuals by an application that runs on the device and samples data from built-in smartphone sensors according to a predefined schedule. We carefully examined the selected literature for details on the investigated population, measurement environment, performed activities, and smartphone settings.
In the reviewed studies, data acquisition typically took place in a research facility and/or nearby outdoor surroundings. In such environments, study participants were asked to perform a series of activities along predefined routes and to interact with predefined objects. The duration and order of performed activities were usually determined by the study protocol and the participant was supervised by a research team member. A less common approach involved observation conducted in free-living environments, where individuals performed activities without specific instructions. Such studies were likely to provide more insight into diverse activity patterns due to individual habits and unpredictable real-life conditions. Compared to a single laboratory visit, studies conducted in free-living environments also allowed investigators to monitor behavioral patterns over many weeks 22 or months 23 .
Activity selection is one of the key aspects of HAR. The studies in our review tended to focus on a small set of activities, including sitting, standing, walking, running, and stair climbing. Less common activities involved various types of mobility, locomotion, fitness, and household routines, e.g., slow, normal, and brisk walking 24 , multiple transportation modes, such as by car, bus, tram, train, metro, and ferry 25 , sharp body-turns 26 , and household activities, like sweeping a floor or walking with a shopping bag 27 . More recent studies concentrated solely on walking recognition 28 , 29 . As shown in Fig. 4 , the various measured activities in the reviewed studies can be grouped into classes: “posture” refers to lying, sitting, standing, or any pair of these activities; “mobility” refers to walking, stair climbing, body-turns, riding an elevator or escalator, running, cycling, or any pair of these activities; “locomotion” refers to motorized activities; and “other” refers to various household and fitness activities or singular actions beyond the described groups.
The spectrum of investigated activities determines the choice of sensors used for data acquisition. At the time of writing, a standard smartphone is equipped with a number of built-in hardware sensors and protocols that can be used for activity monitoring, including an accelerometer, gyroscope, magnetometer, GPS, proximity sensor, and light sensor, as well as to collect information on ambient pressure, humidity, and temperature (Fig. 6 ). Accurate estimation of commonly available sensors over time is challenging given a large number of smartphone manufacturers and models, as well as the variation in their adoption in different countries. Based on global statistics on smartphone market shares 30 and specifications of flagship models 31 , it appears that accelerometer, gyroscope, magnetometer, GPS, and proximity and light sensors were fairly commonly available by 2010. Other smartphone sensors were introduced a couple of years later; for example, the barometer was included in Samsung Galaxy S III released in 2012, and thermometer and hygrometer were included in Samsung Galaxy S4 released in 2013.
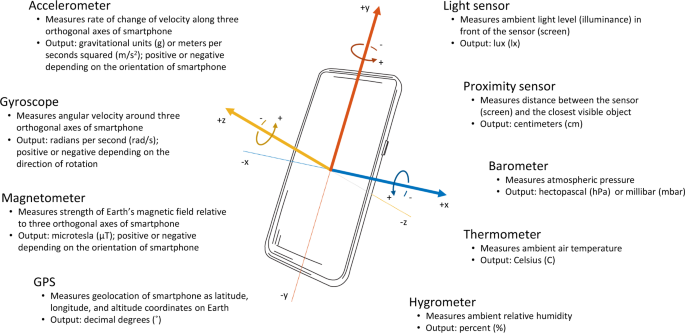
Inertial sensors (accelerometer, gyroscope, and magnetometer) provide measurements with respect to the three orthogonal axes ( x , y , z ) of the body of the phone; the remaining sensors are orientation-invariant.
Our literature review revealed that the most commonly used sensors for HAR are the accelerometer, gyroscope, and magnetometer, which capture data about acceleration, angular velocity, and phone orientation, respectively, and provide temporally dense, high-resolution measurements for distinguishing among activity classes (Fig. 7 ). Inertial sensors were often used synchronously to provide more insight into the dynamic state of the device. Some studies showed that the use of a single sensor can yield similar accuracy of activity recognition as using multiple sensors in combination 32 . To alleviate the impact of sensor position, some researchers collected data using the built-in barometer and GPS sensors to monitor changes in altitude and geographic location 33 , 34 , 35 . Certain studies benefited from using the broader set of capabilities of smartphones; for example, some researchers additionally exploited the proximity sensor and light sensor to allow recognition of a measurement’s context, e.g., the distance between a smartphone and the individual’s body, and changes between in-pocket and out-of-pocket locations based on changes in illumination 36 , 37 . The selection of sensors was also affected by secondary research goals, such as simplicity of classification and minimization of battery drain. In these studies, data acquisition was carried out using a single sensor (e.g., accelerometer 22 ), a small group of sensors (e.g., accelerometer and GPS 38 ), or a purposely modified sampling frequency or sampling scheme (e.g., alternating between data collection and non-collection cycles) to reduce the volume of data collected and processed 39 . Supplementing GPS data with other sensor data was motivated by the limited indoor reception of GPS; satellite signals may be absorbed or attenuated by walls and ceilings 17 up to 60% of the time inside buildings and up to 70% of the time in underground trains 23 .
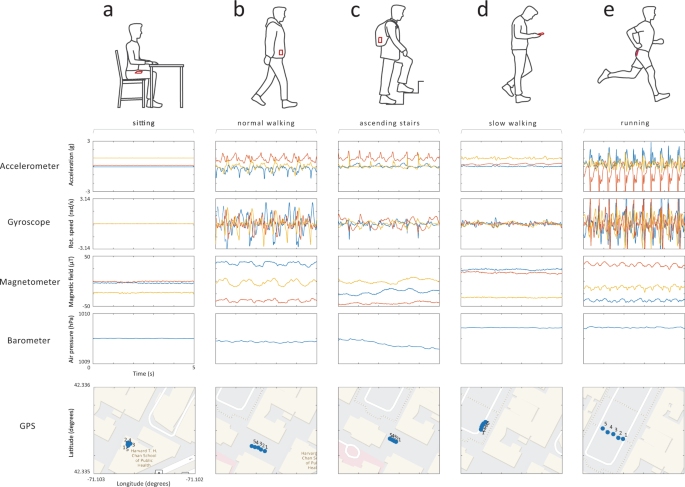
a A person is sitting by the desk with the smartphone placed in the front pants pocket; b a person is walking normally (~1.9 steps per second) with the smartphone placed in a jacket pocket; c a person is ascending stairs with the smartphone placed in the backpack; d a person is walking slowly (~1.4 steps per second) holding the smartphone in hand; e a person is jogging (~2.8 steps per second) with the smartphone placed in back short’s pocket.
Sampling frequency specifies how many observations are collected by a sensor within a 1-s time interval. The selection of sampling frequency is usually performed as a trade-off between measurement accuracy and battery drain. Sampling frequency in the reviewed studies typically ranged between 20 and 30 Hz for inertial sensors and 1 and 10 Hz for the barometer and GPS. The most significant variations were seen in studies where limited energy consumption was a priority (e.g., accelerometer sampled at 1 Hz 40 ) or if investigators used advanced signal processing methods, such as time-frequency decomposition methods, or activity templates that required higher sampling frequency (e.g., accelerometer sampled at 100 Hz 41 ). Some studies stated that inertial sensors sampled at 20 Hz provided enough information to distinguish between various types of transportation 42 , while 10 Hz sampling rate was sufficient to distinguish between various types of mobility 43 . One study demonstrated that reducing the sampling rate from 100 Hz to 12.5 Hz increased the duration of data collection by a factor of three on a single battery charge 44 .
A crucial parameter in the data acquisition process is the smartphone’s location on the body. This is important mainly because of the nonstationary nature of real-life conditions and the strong effect it has on the smartphone’s inertial sensors. The main challenge in HAR in free-living conditions is that data recorded by the accelerometer, gyroscope, and magnetometer sensors differ between the upper and lower body as the device is not affixed to any specific location or orientation 45 . Therefore, it is essential that studies collect data from as many body locations as possible to ensure the generalizability of results. In the reviewed literature, study participants were often instructed to carry the device in a pants pocket (either front or back), although a number of studies also considered other placements, such as jacket pocket 46 , bag or backpack 47 , 48 , and holding the smartphone in the hand 49 or in a cupholder 50 .
To establish the ground truth for physical activity in HAR studies, data were usually annotated manually by trained research personnel or by the study participants themselves 51 , 52 . However, we also noted several approaches that automated this process both in controlled and free-living conditions, e.g., through a designated smartphone application 22 or built-in step counter combined paired with GPS data 53 ., used a built-in step counter and GPS data to produce “weak” labels. The annotation was also done using the built-in microphone 54 , video camera 18 , 20 , or an additional body-worn sensor 29 .
Finally, the data acquisition process in the reviewed studies was carried out on purposely designed applications that captured data. In studies with online activity classification, the collected data did not leave the device, but instead, the entire HAR pipeline was implemented on the smartphone; in contrast, studies using offline classification transmitted data to an external (remote) server for processing using a cellular, Wi-Fi, Bluetooth, or wired connection.
Data preprocessing
We use the term data preprocessing to refer to a collection of procedures aimed at repairing, cleaning, and transforming measurements recorded for HAR. The need for such step is threefold: (1) measurement systems embedded in smartphones are often less stable than research-grade data acquisition units, and the data might therefore be sampled unevenly or there might be missingness or sudden spikes that are unrelated to an individual’s actual behavior; (2) the spatial orientation (how the phone is situated in a person’s pocket, say) of the device influences tri-axial measurements of inertial sensors, thus potentially degrading the performance of the HAR system; and (3) despite careful planning and execution of the data acquisition stage, data quality may be compromised due to other unpredictable factors, e.g., lack of compliance by the study participants, unequal duration of activities in the measurement (i.e., dataset imbalance), or technological issues.
In our literature review, the first group of obstacles was typically addressed using signal processing techniques (in Fig. 4 , see “standardization”). For instance, to alleviate the mismatch between requested and effective sampling frequency, researchers proposed the use of linear interpolation 55 or spline interpolation 56 (Fig. 8 ). Such procedures were imposed on a range of affected sensors, typically the accelerometer, gyroscope, magnetometer, and barometer. Further time-domain preprocessing considered data trimming, carried out to remove unwanted data components. For this purpose, the beginning and end of each activity bout, a short period of activity of a specified kind, were clipped as nonrepresentative for the given activity 46 . During this stage, the researchers also dealt with dataset imbalance, which occurs when there are different numbers of observations for different activity classes in the training dataset. Such a situation makes the classifier susceptible to overfitting in favor of the larger class; in the reviewed studies, this issue was resolved using up-sampling or down-sampling of data 17 , 57 , 58 , 59 . In addition, the measurements were processed for high-frequency noise cancellation (i.e., “denoising”). The literature review identified several methods suitable for this task, including the use of low-pass finite impulse response filters (with a cutoff frequency typically equal to 10 Hz for inertial sensors and 0.1 Hz for barometers) 60 , 61 , which remove the portion of the signal that is unlikely to result from the activities of interest; weighted moving average 55 ; moving median 45 , 62 ; and singular-value decomposition 63 . GPS data were sometimes de-noised based on the maximum allowed positional accuracy 64 .
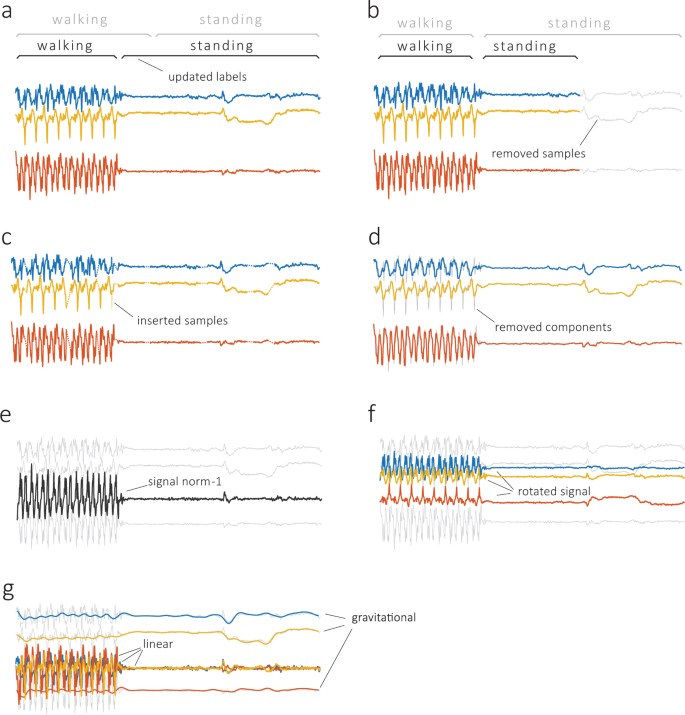
Standardization includes relabeling ( a ), when labels are reassigned to better match transitions between activities; trimming ( b ), when part of the signal is removed to balance the dataset for system training; interpolation ( c ), when missing data are filled in based on adjacent observations; and denoising ( d ), when the signal is filtered from redundant components. The transformation includes normalization ( e ), when the signal is normalized to unidimensional vector magnitude; rotation ( f ), when the signal is rotated to a different coordinate system; and separation ( g ), when the signal is separated into linear and gravitational components. Raw accelerometer data are shown in gray, and preprocessed data are shown using different colors.
Another element of data preprocessing considers device orientation (in Fig. 4 , see “transformation”). Smartphone measurements are sensitive to device orientation, which may be due to clothing, body shape, and movement during dynamic activities 57 . One of the popular solutions reported in the literature was to transform the three-dimensional signal into a univariate vector magnitude that is invariant to rotations and more robust to translations. This procedure was often applied to accelerometer, gyroscope, and magnetometer data. Accelerometer data were also subjected to digital filtering by separating the signal into linear (related to body motions) and gravitational (related to device spatial orientation) acceleration 65 . This separation was typically performed using a high-pass Butterworth filter of low order (e.g., order 3) with a cutoff frequency below 1 Hz. Other approaches transformed tri-axial into bi-axial measurement with horizontal and vertical axes 49 , or projected the data from the device coordinate system into a fixed coordinate system (e.g., the coordinate system of a smartphone that lies flat on the ground) using a rotation matrix (Euler angle-based 66 or quaternion 47 , 67 ).
Feature extraction
We use the term feature extraction to refer to a process of selecting and computing meaningful summaries of smartphone data for the goal of activity classification. A typical extraction scheme includes data visualization, data segmentation, feature selection, and feature calculation. A careful feature extraction step allows investigators not only to understand the physical nature of activities and their manifestation in digital measurements, but also, and more importantly, to help uncover hidden structures and patterns in the data. The identified differences are later quantified through various statistical measures to distinguish between activities. In an alternative approach, the process of feature extraction is automated using deep learning, which handles feature selection using simple signal processing units, called neurons, that have been arranged in a network structure that is multiple layers deep 59 , 68 , 69 , 70 . As with many applications of deep learning, the results may not be easily interpretable.
The conventional approach to feature extraction begins with data exploration. For this purpose, researchers in our reviewed studies employed various graphical data exploration techniques like scatter plots, lag plots, autocorrelation plots, histograms, and power spectra 71 . The choice of tools was often dictated by the study objectives and methods. For example, research on inertial sensors typically presented raw three-dimensional data from accelerometers, gyroscopes, and magnetometers plotted for the corresponding activities of standing, walking, and stair climbing 50 , 72 , 73 . Acceleration data were often inspected in the frequency domain, particularly to observe periodic motions of walking, running, and cycling 45 , and the impact of the external environment, like natural vibration frequencies of a bus or a subway 74 . Locomotion and mobility were investigated using estimates of speed derived from GPS. In such settings, investigators calculated the average speed of the device and associated it with either the group of motorized (car, bus, train, etc.) or non-motorized (walking, cycling, etc.) modes of transportation.
In the next step, measurements are divided into smaller fragments (also, segments or epochs) and signal features are calculated for each fragment (Fig. 9 ). In the reviewed studies, this segmentation was typically conducted using a windowing technique that allows consecutive windows to overlap. The window size usually had a fixed length that varied from 1 to 5 s, while the overlap of consecutive windows was often set to 50%. Several studies that investigated the optimal window size supported this common finding: short windows (1–2 s) were sufficient for recognizing posture and mobility, whereas somewhat longer windows (4–5 s) had better classification performance 75 , 76 , 77 . Even longer windows (10 s or more) were recommended for recognizing locomotion modes or for HAR systems employing frequency-domain features calculated with the Fourier transform (resolution of the resulting frequency spectrum is inversely proportional to window length) 42 . In principle, this calibration aims to closely match the window size with the duration of a single instance of the activity (e.g., one step). Similar motivation led researchers to seek more adaptive segmentation methods. One idea was to segment data based on specific time-domain events, like zero-cross points (when the signal changes value from positive to negative or vice versa), peak points (local maxima), or valley points (local minima), which represent the start and endpoints of a particular activity bout 55 , 57 . This allowed for segments to have different lengths corresponding to a single fundamental period of the activity in question. Such an approach was typically used to recognize quasiperiodic activities like walking, running, and stair climbing 63 .
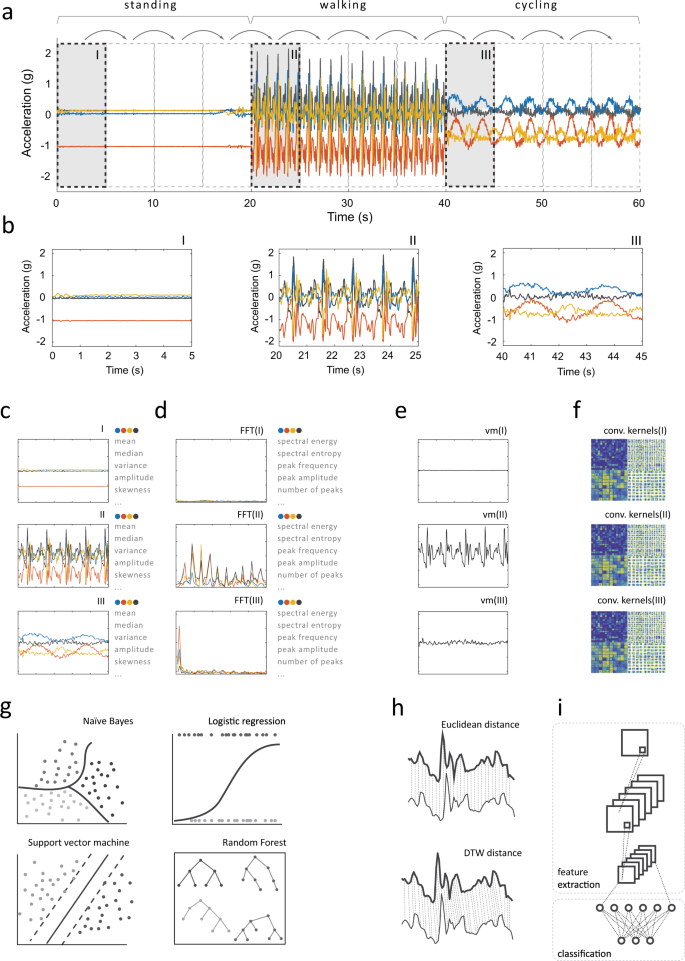
An analyzed measurement ( a ) is segmented into smaller fragments using a sliding window ( b ). Depending on the approach, each segment may then be used to compute time-domain ( c ) or frequency-domain features ( d ), but also it may serve as the activity template ( e ), or as input for deep learning networks that compute hidden (“deep”) features ( f ). The selected feature extraction approach determines the activity classifier: time- and frequency-domain features are paired with machine learning classifiers ( g ) and activity templates are investigated using distance metrics ( h ), while deep features are computed within embedded layers of convolutional neural networks ( i ).
The literature described a large variety of signal features used for HAR, which can be divided into several categories based on the initial signal processing procedure. This enables one to distinguish between activity templates (i.e., raw signal), deep features (i.e., hidden features calculated within layers of deep neural networks), time-domain features (i.e., statistical measures of time-series data), and frequency-domain features (i.e., statistical measures of frequency representation of time-series data). The most popular features in the reviewed papers were calculated from time-domain signals as descriptive statistics, such as local mean, variance, minimum and maximum, interquartile range, signal energy (defined as the area under the squared magnitude of the considered continuous signal), and higher-order statistics. Other time-domain features included mean absolute deviation, mean (or zero) crossing rate, regression coefficients, and autocorrelation. Some studies described novel and customized time-domain features, like histograms of gradients 78 , and the number of local maxima and minima, their amplitude, and the temporal distance between them 39 . Time-domain features were typically calculated over each axis of the three-dimensional measurement or orientation-invariant vector magnitude. Studies that used GPS also calculated average speed 64 , 79 , 80 , while studies that used the barometer analyzed the pressure derivative 81 .
Signals transformed to the frequency domain were less exploited in the literature. A commonly performed signal decomposition used the fast Fourier transform (FFT) 82 , 83 , an algorithm that converts a temporal sequence of samples to a sequence of frequencies present in that sample. The essential advantage of frequency-domain features over time-domain features is their ability to identify and isolate certain periodic components of performed activities. This enabled researchers to estimate (kinetic) energy within particular frequency bands associated with human activities, like gait and running 51 , as well as with different modes of locomotion 74 . Other frequency-domain features included spectral entropy and parameters of the dominant peak, e.g., its frequency and amplitude.
Activity templates function essentially as blueprints for different types of physical activity. In the HAR systems, we reviewed, these templates were compared to patterns of observed raw measurements using various distance metrics 38 , 84 , such as the Euclidean or Manhattan distance. Given the heterogeneous nature of human activities, activity templates were often enhanced using techniques similar to dynamic time warping 29 , 57 , which measures the similarity of two temporal sequences that may vary in speed. As an alternative to raw measurements, some studies used signal symbolic approximation, which translates a segmented time-series signal into sequences of symbols based on a predefined mapping rule (e.g., amplitude between −1 and −0.5 g represents symbol “a”, amplitude between −0.5 and 0 g represents symbol “b”, and so on) 85 , 86 , 87 .
More recent studies utilized deep features. In these approaches, smartphone data were either fed to deep neural networks as raw univariate or multivariate time series 35 , 48 , 60 or preprocessed into handcrafted time- and frequency-domain feature vectors 82 , 83 . Within the network layers, the input data were then transformed (e.g., using convolution) to produce two-dimensional activation maps that revealed hidden spatial relations between axes and sensors specific to a given activity. To improve the resolution of input data, one study proposed to split the integer and decimal values of accelerometer measurements 41 .
In the reviewed articles, the number of extracted features typically varied from a few to a dozen. However, some studies purposely calculated too many features (sometimes hundreds) and let the analytical method perform variable selection, i.e., identify those features that were most informative for HAR 88 . Support vector machines 81 , 89 , gain ratio 43 , recursive feature elimination 38 , correlation-based feature selection 51 , and principal component analysis 90 were among the popular feature selection/dimension reduction methods used.
Activity classification
We use the term activity classification to refer to a process of associating extracted features with particular activity classes based on the adopted classification principle. The classification is typically performed by a supervised learning algorithm that has been trained to recognize patterns between features and labeled physical activities in the training dataset. The fitted model is then validated on separate observations, using a validation dataset, usually data obtained from the same group of study participants. The comparison between predictions made by the model and the known true labels allows one to assess the accuracy of the approach. This section summarizes the methods used in classification and validation, and also provides some insights into reporting on HAR performance.
The choice of classifier aims to identify a method that has the highest classification accuracy for the collected datasets and for the given data processing environment (e.g., online vs. offline). The reviewed literature included a broad range of classifiers, from simple decision trees 18 , k-nearest neighbors 65 , support vector machines 91 , 92 , 93 , logistic regression 21 , naïve Bayes 94 , and fuzzy logic 64 to ensemble classifiers such as random forest 76 , XGBoost 95 , AdaBoost 45 , 96 , bagging 24 , and deep neural networks 48 , 60 , 82 , 97 , 98 , 99 . Simple classifiers were frequently compared to find the best solution in the given measurement scenario 43 , 53 , 100 , 101 , 102 . A similar type of analysis was implemented for ensemble classifiers 79 . Incremental learning techniques were proposed to adapt the classification model to new data streams and unseen activities 103 , 104 , 105 . Other semi-supervised approaches were proposed to utilize unlabeled data to improve the personalization of HAR systems 106 and data annotation 53 , 70 . To increase the effectiveness of HAR, some studies used a hierarchical approach, where the classification was performed in separate stages and each stage could use a different classifier. The multi-stage technique was used for gradual decomposition of activities (coarse-grained first, then fine-grained) 22 , 37 , 52 , 60 and to handle the predicament of changing sensor location (body location first, then activity) 91 . Multi-instance multi-label approaches were adapted for the classification of complex activities (i.e., activities that consist of several basic activities) 62 , 107 as well as for recognition of basic activities paired with different sensor locations 108 .
Classification accuracy could also be improved by using post-processing, which relies on modifying the initially assigned label using the rules of logic and probability. The correction was typically performed based on activity duration 74 , activity sequence 25 , and activity transition probability and classification confidence 80 , 109 .
The selected method is typically cross-validated, which splits the collected dataset into two or more parts—training and testing—and only uses the part of the data for testing that was not used for training. The literature mentions a few cross-validation procedures, with k -fold and leave-one-out cross-validation being the most common 110 . Popular train-test proportions were 90–10, 70–30, and 60–40. A validation is especially valuable if it is performed using studies with different demographics and smartphone use habits. Such an approach allows one to understand the generalizability of the HAR system to real-life conditions and populations. We found a few studies that followed this validation approach 18 , 21 , 71 .
Activity classification is the last stage of HAR. In our review, we found that analysis results were typically reported in terms of classification accuracy using various standard metrics like precision, recall, and F-score. Overall, the investigated studies reported very high classification accuracies, typically above 95%. Several comparisons revealed that ensemble classifiers tended to outperform individual or single classifiers 27 , 77 , and deep-learning classifiers tended to outperform both individual and ensemble classifiers 48 . More nuanced summaries used the confusion matrix, which allows one to examine which activities are more likely to be classified incorrectly. This approach was particularly useful for visualizing classification differences between similar activities, such as normal and fast walking or bus and train riding. Additional statistics were usually provided in the context of HAR systems designed to operate on the device. In this case, activity classification needed to be balanced among acceptable classifier performance, processing time, and battery drain 44 . The desired performance optimum was obtained by making use of dataset remodeling (e.g., by replacing the oldest observations with the newest ones), low-cost classification algorithms, limited preprocessing, and conscientious feature selection 45 , 86 . Computation time was sometimes reported for complex methods, such as deep neural networks 20 , 82 , 111 and extreme learning machine 112 , as well as for symbolic representation 85 , 86 and in comparative analyses 46 . A comprehensive comparison of results was difficult or impossible, as discussed below.
Over the past decade, many studies have investigated HAR using smartphones. The reviewed literature provides detailed descriptions of essential aspects of data acquisition, data preprocessing, feature extraction, and activity classification. Studies were conducted with one or more objectives, e.g., to limit technological imperfections (e.g., no GPS signal reception indoors), to minimize computational requirements (e.g., for online processing of data directly on the device), and to maximize classification accuracy (all studies). Our review summarizes the most frequently used methods and offers available alternatives.
As expected, no single activity recognition procedure was found to work in all settings, which underlines the importance of designing methods and algorithms that address specific research questions in health while keeping the specifics of the study cohort in mind (e.g., age distribution, the extent of device use, and nature of disability). While datasets were usually collected in laboratory settings, there was little evidence that algorithms trained using data collected in these controlled settings could be generalized to free-living conditions 113 , 114 . In free-living settings, duration, frequency, and specific ways of performing any activity are subject to context and individual ability, and these degrees of freedom need to be considered in the development of HAR systems. Validation of these data in free-living settings is essential, as the true value of HAR systems for public health will come through transportable and scalable applications in large, long-term observational studies or real-world interventions.
Some studies were conducted with a small number of able-bodied volunteers. This makes the process of data handling and classification easier but also limits the generalizability of the approach to more diverse populations. The latter point was well demonstrated in two of the investigated studies. In the first study, the authors observed that the performance of a classifier trained on a young cohort significantly decreases if validated on an older cohort 18 . Similar conclusions can be drawn from the second study, where the observations on healthy individuals did not replicate in individuals with Parkinson’s disease 21 . These facts highlight the role of algorithmic fairness (or fairness of machine learning), the notion that the performance of an algorithm should not depend on variables considered sensitive, such as race, ethnicity, sexual orientation, age, and disability. A highly visible example of this was the decision of some large companies, including IBM, to stop providing facial recognition technology to police departments for mass surveillance 115 , and the European Commission has considered a ban on the use of facial recognition in public spaces 116 . These decisions followed findings demonstrating the poor performance of facial recognition algorithms when applied to individuals with dark-skin tones.
The majority of the studies we reviewed utilized stationary smartphones at a single-body position (i.e., a specific pants pocket), sometimes even with a fixed orientation. However, such scenarios are rarely observed in real-life settings, and these types of studies should be considered more as proofs of concept. Indeed, as demonstrated in several studies, inertial sensor data might not share similar features across body locations 49 , 117 , and smartphone orientation introduces additional artifacts to each axis of measurement which make any distribution-based features (e.g., mean, range, skewness) difficult to use without appropriate data preprocessing. Many studies provided only incomplete descriptions of the experimental setup and study protocol and provided few details on demographics, environmental context, and the details of the performed activities. Such information should be reported as fully and accurately as possible.
Only a few studies considered classification in a context that involves activities outside the set of activities the system was trained on; for example, if the system was trained to recognize walking and running, these were the only two activities that the system was later tested on. However, real-life activities are not limited to a prescribed set of behaviors, i.e., we do not just sit still, stand still, walk, and climb stairs. These classifiers, when applied to free-living conditions, will naturally miss the activities they were not trained on but will also likely overestimate those activities they were trained on. An improved scheme could assume that the observed activities are a sample from a broader spectrum of possible behaviors, including periods when the smartphone is not on a person, or assess the uncertainty associated with the classification of each type of activity 84 . This could also provide for an adaptive approach that would enable observation/interventions suited to a broad range of activities relevant for health, including decreasing sedentary behavior, increasing active transport (i.e., walking, bicycling, or public transit), and improving circadian patterns/sleep.
The use of personal digital devices, in particular smartphones, makes it possible to follow large numbers of individuals over long periods of time, but invariably investigators need to consider approaches to missing sensor data, which is a common problem. The importance of this problem is illustrated in a recent paper that introduced a resampling approach to imputing missing smartphone GPS data; the authors found that relative to linear interpolation—the naïve approach to missing spatial data—imputation resulted in a tenfold reduction in the error averaged across all daily mobility features 118 . On the flip side of missing data is the need to propagate uncertainty, in a statistically principled way, from the gaps in the raw data to the inferences that investigators wish to draw from the data. It is a common observation that different people use their phones differently, and some may barely use their phones at all; the net result is not that the data collected from these individuals are not useful, but instead the data are less informative about the behavior of this individual than they ideally might be. Dealing with missing data and accounting for the resulting uncertainty is important because it means that one does not have to exclude participants from a study because their data fail meet some arbitrary threshold of completeness; instead, everyone counts, and every bit of data from each individual counts.
The collection of behavioral data using smartphones understandably raises concerns about privacy; however, investigators in health research are well-positioned to understand and address these concerns given that health data are generally considered personal and private in nature. Consequently, there are established practices and common regulations on human subjects’ research, where informed consent of the individual to participate is one of the key foundations of any ethically conducted study. Federated learning is a machine learning technique that can be used to train an algorithm across decentralized devices, here smartphones, using only local data (data from the individual) and without the need to exchange data with other devices. This approach appears at first to provide a powerful solution to the privacy problem: the personal data never leave the person’s phone and only the outputs of the learning process, generally parameter estimates, are shared with others. This is where the tension between privacy and the need for reproducible research arises, however. The reason for data collection is to produce generalizable knowledge, but according to an often-cited study, 65% of medical studies were inconsistent when retested and only 6% were completely reproducible 12 . In the studies reviewed here, only 4 out of 108 made the source code or the methods used in the study publicly available. For a given scientific question, studies that are not replicable require the collection of more private and personal data; this highlights the importance of reproducibility of studies, especially in health, where there are both financial and ethical considerations when conducting research. If federated learning provides no possibility to confirm data analyses, to re-analyze data using different methods, or to pool data across studies, it by itself cannot be the solution to the privacy problem. Nevertheless, the technique may act as inspiration for developing privacy-preserving methods that also enable future replication of studies. One possibility is to use publicly available datasets (Table 1 ). If sharing of source code were more common, HAR methods could be tested on these publicly available datasets, perhaps in a similar way as datasets of handwritten digits are used to test classification methods in machine learning research. Although some efforts have been made in this area 42 , 119 , 120 , 121 , the recommended course of action assumes collecting and analyzing data from a large spectrum of sensors on diverse and understudied populations and validating classifiers against widely accepted gold standards.
When accurate, reproducible, and transportable methods coalesce to recognize a range of relevant activity patterns, smartphone-based HAR approaches will provide a fundamental tool for public health researchers and practitioners alike. We hope that this paper has provided to the reader some insights into how smartphones may be used to quantify human behavior in health research and the complexities that are involved in the collection and analysis of such data in this challenging but important field.
Data availability
Aggregated data analyzed in this study are available from the corresponding author upon request.
Code availability
Scripts used to process the aggregated data are available from the corresponding author upon request.
Association, G. The mobile economy 2020. https://www.gsma.com/mobileeconomy/wp-content/uploads/2020/03/GSMA_MobileEconomy2020_Global.pdf (2020).
Mercer, K. et al. Acceptance of commercially available wearable activity trackers among adults aged over 50 and with chronic illness: a mixed-methods evaluation. JMIR mHealth uHealth 4 , e7 (2016).
Article PubMed PubMed Central Google Scholar
Anderson, M. & Perrin, A. Tech adoption climbs among older adults. http://www.pewinternet.org/wp-content/uploads/sites/9/2017/05/PI_2017.05.17_Older-Americans-Tech_FINAL.pdf (2017).
Taylor, K. & Silver, L. Smartphone ownership is growing rapidly around the world, but not always equally. http://www.pewresearch.org/global/wp-content/uploads/sites/2/2019/02/Pew-Research-Center_Global-Technology-Use-2018_2019-02-05.pdf (2019).
Cooper, A. R., Page, A., Fox, K. R. & Misson, J. Physical activity patterns in normal, overweight and obese individuals using minute-by-minute accelerometry. Eur. J. Clin. Nutr. 54 , 887–894 (2000).
Article CAS PubMed Google Scholar
Ekelund, U., Brage, S., Griffin, S. J. & Wareham, N. J. Objectively measured moderate- and vigorous-intensity physical activity but not sedentary time predicts insulin resistance in high-risk individuals. Diabetes Care 32 , 1081–1086 (2009).
Legge, A., Blanchard, C. & Hanly, J. G. Physical activity, sedentary behaviour and their associations with cardiovascular risk in systemic lupus erythematosus. Rheumatology https://doi.org/10.1093/rheumatology/kez429 (2019).
Loprinzi, P. D., Franz, C. & Hager, K. K. Accelerometer-assessed physical activity and depression among U.S. adults with diabetes. Ment. Health Phys. Act. 6 , 79–82 (2013).
Article Google Scholar
Smirnova, E. et al. The predictive performance of objective measures of physical activity derived from accelerometry data for 5-year all-cause mortality in older adults: National Health and Nutritional Examination Survey 2003–2006. J. Gerontol. Ser. A https://doi.org/10.1093/gerona/glz193 (2019).
Wigginton, C. Global Mobile Consumer Trends, 2nd edition. Deloitte, https://www2.deloitte.com/content/dam/Deloitte/us/Documents/technology-media-telecommunications/us-global-mobile-consumer-survey-second-edition.pdf (2017).
Coorevits, L. & Coenen, T. The rise and fall of wearable fitness trackers. Acad. Manag . 2016 , https://doi.org/10.5465/ambpp.2016.17305abstract (2016).
Prinz, F., Schlange, T. & Asadullah, K. Believe it or not: how much can we rely on published data on potential drug targets? Nat. Rev. Drug Discov. 10 , 712 (2011).
Kubota, K. J., Chen, J. A. & Little, M. A. Machine learning for large-scale wearable sensor data in Parkinson’s disease: concepts, promises, pitfalls, and futures. Mov. Disord. 31 , 1314–1326 (2016).
Article PubMed Google Scholar
Iniesta, R., Stahl, D. & McGuffin, P. Machine learning, statistical learning and the future of biological research in psychiatry. Psychol. Med. 46 , 2455–2465 (2016).
Article CAS PubMed PubMed Central Google Scholar
Kuehn, B. M. FDA’s foray into big data still maturing. J. Am. Med. Assoc. 315 , 1934–1936 (2016).
Straczkiewicz, M., Glynn, N. W. & Harezlak, J. On placement, location and orientation of wrist-worn tri-axial accelerometers during free-living measurements. Sensors 19 , 2095 (2019).
Esmaeili Kelishomi, A., Garmabaki, A. H. S., Bahaghighat, M. & Dong, J. Mobile user indoor-outdoor detection through physical daily activities. Sensors 19 , 511 (2019).
Article PubMed Central Google Scholar
Del Rosario, M. B. et al. A comparison of activity classification in younger and older cohorts using a smartphone. Physiol. Meas. 35 , 2269–2286 (2014).
Del Rosario, M. B., Lovell, N. H. & Redmond, S. J. Learning the orientation of a loosely-fixed wearable IMU relative to the body improves the recognition rate of human postures and activities. Sensors 19 , 2845 (2019).
Nan, Y. et al. Deep learning for activity recognition in older people using a pocket-worn smartphone. Sensors 20 , 7195 (2020).
Albert, M. V., Toledo, S., Shapiro, M. & Kording, K. Using mobile phones for activity recognition in Parkinson’s patients. Front. Neurol. 3 , 158 (2012).
Liang, Y., Zhou, X., Yu, Z. & Guo, B. Energy-efficient motion related activity recognition on mobile devices for pervasive healthcare. Mob. Netw. Appl. 19 , 303–317 (2014).
Gjoreski, H. et al. The university of Sussex-Huawei locomotion and transportation dataset for multimodal analytics with mobile devices. IEEE Access 6 , 42592–42604 (2018).
Wu, W., Dasgupta, S., Ramirez, E. E., Peterson, C. & Norman, G. J. Classification accuracies of physical activities using smartphone motion sensors. J. Med. Internet Res. 14 , e130 (2012).
Guvensan, M. A., Dusun, B., Can, B. & Turkmen, H. I. A novel segment-based approach for improving classification performance of transport mode detection. Sensors 18 , 87 (2018).
Pei, L. et al. Human behavior cognition using smartphone sensors. Sensors 13 , 1402–1424 (2013).
Della Mea, V., Quattrin, O. & Parpinel, M. A feasibility study on smartphone accelerometer-based recognition of household activities and influence of smartphone position. Inform. Heal. Soc. Care 42 , 321–334 (2017).
Klein, I. Smartphone location recognition: a deep learning-based approach. Sensors 20 , 214 (2020).
Casado, F. E. et al. Walking recognition in mobile devices. Sensors 20 , 1189 (2020).
O’Dea, S. Global smartphone market share worldwide by vendor 2009–2020. https://www.statista.com/statistics/271496/global-market-share-held-by-smartphone-vendors-since-4th-quarter-2009/ (2021).
GSMArena. https://www.gsmarena.com/ (2021). Accessed 24 March 2021.
Shoaib, M., Bosch, S., Durmaz Incel, O., Scholten, H. & Havinga, P. J. M. Fusion of smartphone motion sensors for physical activity recognition. Sensors 14 , 10146–10176 (2014).
Vanini, S., Faraci, F., Ferrari, A. & Giordano, S. Using barometric pressure data to recognize vertical displacement activities on smartphones. Comput. Commun. 87 , 37–48 (2016).
Wan, N. & Lin, G. Classifying human activity patterns from smartphone collected GPS data: a fuzzy classification and aggregation approach. Trans. GIS 20 , 869–886 (2016).
Gu, Y., Li, D., Kamiya, Y. & Kamijo, S. Integration of positioning and activity context information for lifelog in urban city area. Navigation 67 , 163–179 (2020).
Miao, F., He, Y., Liu, J., Li, Y. & Ayoola, I. Identifying typical physical activity on smartphone with varying positions and orientations. Biomed. Eng. Online 14 , 32 (2015).
Lee, Y.-S. & Cho, S.-B. Layered hidden Markov models to recognize activity with built-in sensors on Android smartphone. Pattern Anal. Appl. 19 , 1181–1193 (2016).
Martin, B. D., Addona, V., Wolfson, J., Adomavicius, G. & Fan, Y. Methods for real-time prediction of the mode of travel using smartphone-based GPS and accelerometer data. Sensors 17 , 2058 (2017).
Oshin, T. O., Poslad, S. & Zhang, Z. Energy-efficient real-time human mobility state classification using smartphones. IEEE Trans. Comput. 64 , 1680–1693 (2015).
Google Scholar
Shin, D. et al. Urban sensing: Using smartphones for transportation mode classification. Comput. Environ. Urban Syst. 53 , 76–86 (2015).
Hur, T. et al. Iss2Image: a novel signal-encoding technique for CNN-based human activity recognition. Sensors 18 , 3910 (2018).
Gjoreski, M. et al. Classical and deep learning methods for recognizing human activities and modes of transportation with smartphone sensors. Inf. Fusion 62 , 47–62 (2020).
Wannenburg, J. & Malekian, R. Physical activity recognition from smartphone accelerometer data for user context awareness sensing. IEEE Trans. Syst. Man, Cybern. Syst. 47 , 3143–3149 (2017).
Yurur, O., Labrador, M. & Moreno, W. Adaptive and energy efficient context representation framework in mobile sensing. IEEE Trans. Mob. Comput. 13 , 1681–1693 (2014).
Li, P., Wang, Y., Tian, Y., Zhou, T.-S. & Li, J.-S. An automatic user-adapted physical activity classification method using smartphones. IEEE Trans. Biomed. Eng. 64 , 706–714 (2017).
PubMed Google Scholar
Awan, M. A., Guangbin, Z., Kim, C.-G. & Kim, S.-D. Human activity recognition in WSN: a comparative study. Int. J. Networked Distrib. Comput. 2 , 221–230 (2014).
Chen, Z., Zhu, Q., Soh, Y. C. & Zhang, L. Robust human activity recognition using smartphone sensors via CT-PCA and online SVM. IEEE Trans. Ind. Inform. 13 , 3070–3080 (2017).
Zhu, R. et al. Efficient human activity recognition solving the confusing activities via deep ensemble learning. IEEE Access 7 , 75490–75499 (2019).
Yang, R. & Wang, B. PACP: a position-independent activity recognition method using smartphone sensors. Inf 7 , 72 (2016).
Gani, M. O. et al. A light weight smartphone based human activity recognition system with high accuracy. J. Netw. Comput. Appl. 141 , 59–72 (2019).
Reddy, S. et al. Using mobile phones to determine transportation modes. ACM Trans. Sens. Networks 6 , 1–27 (2010).
Guidoux, R. et al. A smartphone-driven methodology for estimating physical activities and energy expenditure in free living conditions. J. Biomed. Inform. 52 , 271–278 (2014).
Cruciani, F. et al. Automatic annotation for human activity recognition in free living using a smartphone. Sensors 18 , 2203 (2018).
Micucci, D., Mobilio, M. & Napoletano, P. UniMiB SHAR: A dataset for human activity recognition using acceleration data from smartphones. Appl. Sci. 7 , 1101 (2017).
Derawi, M. & Bours, P. Gait and activity recognition using commercial phones. Comput. Secur. 39 , 137–144 (2013).
Gu, F., Khoshelham, K., Valaee, S., Shang, J. & Zhang, R. Locomotion activity recognition using stacked denoising autoencoders. IEEE Internet Things J. 5 , 2085–2093 (2018).
Chen, Y. & Shen, C. Performance analysis of smartphone-sensor behavior for human activity recognition. IEEE Access 5 , 3095–3110 (2017).
Javed, A. R. et al. Analyzing the effectiveness and contribution of each axis of tri-axial accelerometer sensor for accurate activity recognition. Sensors 20 , 2216 (2020).
Mukherjee, D., Mondal, R., Singh, P. K., Sarkar, R. & Bhattacharjee, D. EnsemConvNet: a deep learning approach for human activity recognition using smartphone sensors for healthcare applications. Multimed. Tools Appl. 79 , 31663–31690 (2020).
Avilés-Cruz, C., Ferreyra-Ramírez, A., Zúñiga-López, A. & Villegas-Cortéz, J. Coarse-fine convolutional deep-learning strategy for human activity recognition. Sensors 19 , 1556 (2019).
Guiry, J. J., van de Ven, P. & Nelson, J. Multi-sensor fusion for enhanced contextual awareness of everyday activities with ubiquitous devices. Sensors 14 , 5687–5701 (2014).
Saha, J., Chowdhury, C., Ghosh, D. & Bandyopadhyay, S. A detailed human activity transition recognition framework for grossly labeled data from smartphone accelerometer. Multimed. Tools Appl . https://doi.org/10.1007/s11042-020-10046-w (2020).
Ignatov, A. D. & Strijov, V. V. Human activity recognition using quasiperiodic time series collected from a single tri-axial accelerometer. Multimed. Tools Appl. 75 , 7257–7270 (2016).
Das, R. D. & Winter, S. Detecting urban transport modes using a hybrid knowledge driven framework from GPS trajectory. ISPRS Int. J. Geo-Information 5 , 207 (2016).
Arif, M., Bilal, M., Kattan, A. & Ahamed, S. I. Better physical activity classification using smartphone acceleration sensor. J. Med. Syst. 38 , 95 (2014).
Heng, X., Wang, Z. & Wang, J. Human activity recognition based on transformed accelerometer data from a mobile phone. Int. J. Commun. Syst. 29 , 1981–1991 (2016).
Gao, Z., Liu, D., Huang, K. & Huang, Y. Context-aware human activity and smartphone position-mining with motion sensors. Remote Sensing 11 , 2531 (2019).
Kang, J., Kim, J., Lee, S. & Sohn, M. Transition activity recognition using fuzzy logic and overlapped sliding window-based convolutional neural networks. J. Supercomput. 76 , 8003–8020 (2020).
Shojaedini, S. V. & Beirami, M. J. Mobile sensor based human activity recognition: distinguishing of challenging activities by applying long short-term memory deep learning modified by residual network concept. Biomed. Eng. Lett. 10 , 419–430 (2020).
Mairittha, N., Mairittha, T. & Inoue, S. On-device deep personalization for robust activity data collection. Sensors 21 , 41 (2021).
Khan, A. M., Siddiqi, M. H. & Lee, S.-W. Exploratory data analysis of acceleration signals to select light-weight and accurate features for real-time activity recognition on smartphones. Sensors 13 , 13099–13122 (2013).
Ebner, M., Fetzer, T., Bullmann, M., Deinzer, F. & Grzegorzek, M. Recognition of typical locomotion activities based on the sensor data of a smartphone in pocket or hand. Sensors 20 , 6559 (2020).
Voicu, R.-A., Dobre, C., Bajenaru, L. & Ciobanu, R.-I. Human physical activity recognition using smartphone sensors. Sensors 19 , 458 (2019).
Hur, T., Bang, J., Kim, D., Banos, O. & Lee, S. Smartphone location-independent physical activity recognition based on transportation natural vibration analysis. Sensors 17 , 931 (2017).
Bashir, S. A., Doolan, D. C. & Petrovski, A. The effect of window length on accuracy of smartphone-based activity recognition. IAENG Int. J. Comput. Sci. 43 , 126–136 (2016).
Lu, D.-N., Nguyen, D.-N., Nguyen, T.-H. & Nguyen, H.-N. Vehicle mode and driving activity detection based on analyzing sensor data of smartphones. Sensors 18 , 1036 (2018).
Wang, G. et al. Impact of sliding window length in indoor human motion modes and pose pattern recognition based on smartphone sensors. Sensors 18 , 1965 (2018).
Jain, A. & Kanhangad, V. Human activity classification in smartphones using accelerometer and gyroscope sensors. IEEE Sens. J. 18 , 1169–1177 (2018).
Bedogni, L., Di Felice, M. & Bononi, L. Context-aware Android applications through transportation mode detection techniques. Wirel. Commun. Mob. Comput. 16 , 2523–2541 (2016).
Ferreira, P., Zavgorodnii, C. & Veiga, L. edgeTrans—edge transport mode detection. Pervasive Mob. Comput. 69 , 101268 (2020).
Gu, F., Kealy, A., Khoshelham, K. & Shang, J. User-independent motion state recognition using smartphone sensors. Sensors 15 , 30636–30652 (2015).
Li, X., Wang, Y., Zhang, B. & Ma, J. PSDRNN: an efficient and effective HAR scheme based on feature extraction and deep learning. IEEE Trans. Ind. Inform. 16 , 6703–6713 (2020).
Zhao, B., Li, S., Gao, Y., Li, C. & Li, W. A framework of combining short-term spatial/frequency feature extraction and long-term IndRNN for activity recognition. Sensors 20 , 6984 (2020).
Huang, E. J. & Onnela, J.-P. Augmented movelet method for activity classification using smartphone gyroscope and accelerometer data. Sensors 20 , 3706 (2020).
Montero Quispe, K. G., Sousa Lima, W., Macêdo Batista, D. & Souto, E. MBOSS: a symbolic representation of human activity recognition using mobile sensors. Sensors 18 , 4354 (2018).
Sousa Lima, W., de Souza Bragança, H. L., Montero Quispe, K. G. & Pereira Souto, E. J. Human activity recognition based on symbolic representation algorithms for inertial sensors. Sensors 18 , 4045 (2018).
Bragança, H., Colonna, J. G., Lima, W. S. & Souto, E. A smartphone lightweight method for human activity recognition based on information theory. Sensors 20 , 1856 (2020).
Saeedi, S. & El-Sheimy, N. Activity recognition using fusion of low-cost sensors on a smartphone for mobile navigation application. Micromachines 6 , 1100–1134 (2015).
Bilal, M., Shaikh, F. K., Arif, M. & Wyne, M. F. A revised framework of machine learning application for optimal activity recognition. Clust. Comput. 22 , 7257–7273 (2019).
Shi, D., Wang, R., Wu, Y., Mo, X. & Wei, J. A novel orientation- and location-independent activity recognition method. Pers. Ubiquitous Comput. 21 , 427–441 (2017).
Antos, S. A., Albert, M. V. & Kording, K. P. Hand, belt, pocket or bag: practical activity tracking with mobile phones. J. Neurosci. Methods 231 , 22–30 (2014).
Shi, J., Zuo, D. & Zhang, Z. Transition activity recognition system based on standard deviation trend analysis. Sensors 20 , 3117 (2020).
Garcia-Gonzalez, D., Rivero, D., Fernandez-Blanco, E. & Luaces, M. R. A public domain dataset for real-life human activity recognition using smartphone sensors. Sensors 20 , 2200 (2020).
Saeedi, S., Moussa, A. & El-Sheimy, N. Context-aware personal navigation using embedded sensor fusion in smartphones. Sensors 14 , 5742–5767 (2014).
Zhang, W., Zhao, X. & Li, Z. A comprehensive study of smartphone-based indoor activity recognition via Xgboost. IEEE Access 7 , 80027–80042 (2019).
Ferrari, A., Micucci, D., Mobilio, M. & Napoletano, P. On the personalization of classification models for human activity recognition. IEEE Access 8 , 32066–32079 (2020).
Zhou, B., Yang, J. & Li, Q. Smartphone-based activity recognition for indoor localization using a convolutional neural network. Sensors 19 , 621 (2019).
Pires, I. M. et al. Pattern recognition techniques for the identification of activities of daily living using a mobile device accelerometer. Electronics 9 , 509 https://www.mdpi.com/2079-9292/9/3/509#cite (2020).
Alo, U. R., Nweke, H. F., Teh, Y. W. & Murtaza, G. Smartphone motion sensor-based complex human activity identification using deep stacked autoencoder algorithm for enhanced smart healthcare system. Sensors 20 , 6300 https://www.mdpi.com/2079-9292/9/3/509#cite (2020).
Otebolaku, A. M. & Andrade, M. T. User context recognition using smartphone sensors and classification models. J. Netw. Comput. Appl. 66 , 33–51 (2016).
Zhuo, S. et al. Real-time smartphone activity classification using inertial sensors—recognition of scrolling, typing, and watching videos while sitting or walking. Sensors 20 , 655 (2020).
Asim, Y., Azam, M. A., Ehatisham-ul-Haq, M., Naeem, U. & Khalid, A. Context-aware human activity recognition (CAHAR) in-the-wild using smartphone accelerometer. IEEE Sens. J. 20 , 4361–4371 (2020).
Zhao, Z., Chen, Z., Chen, Y., Wang, S. & Wang, H. A class incremental extreme learning machine for activity recognition. Cogn. Comput. 6 , 423–431 (2014).
Abdallah, Z. S., Gaber, M. M., Srinivasan, B. & Krishnaswamy, S. Adaptive mobile activity recognition system with evolving data streams. Neurocomputing 150 , 304–317 (2015).
Guo, H., Chen, L., Chen, G. & Lv, M. Smartphone-based activity recognition independent of device orientation and placement. Int. J. Commun. Syst. 29 , 2403–2415 (2016).
Cruciani, F. et al. Personalizing activity recognition with a clustering based semi-population approach. IEEE ACCESS 8 , 207794–207804 (2020).
Saha, J., Ghosh, D., Chowdhury, C. & Bandyopadhyay, S. Smart handheld based human activity recognition using multiple instance multiple label learning. Wirel. Pers. Commun . https://doi.org/10.1007/s11277-020-07903-0 (2020).
Mohamed, R., Zainudin, M. N. S., Sulaiman, M. N., Perumal, T. & Mustapha, N. Multi-label classification for physical activity recognition from various accelerometer sensor positions. J. Inf. Commun. Technol. 17 , 209–231 (2018).
Wang, C., Xu, Y., Liang, H., Huang, W. & Zhang, L. WOODY: a post-process method for smartphone-based activity recognition. IEEE Access 6 , 49611–49625 (2018).
Garcia-Ceja, E. & Brena, R. F. An improved three-stage classifier for activity recognition. Int. J. Pattern Recognit. Artif. Intell . 32 , 1860003 (2018).
Ravi, D., Wong, C., Lo, B. & Yang, G.-Z. A deep learning approach to on-node sensor data analytics for mobile or wearable devices. IEEE J. Biomed. Heal. Inform. 21 , 56–64 (2017).
Chen, Z., Jiang, C. & Xie, L. A novel ensemble ELM for human activity recognition using smartphone sensors. IEEE Trans. Ind. Inform. 15 , 2691–2699 (2019).
van Hees, V. T., Golubic, R., Ekelund, U. & Brage, S. Impact of study design on development and evaluation of an activity-type classifier. J. Appl. Physiol. 114 , 1042–1051 (2013).
Sasaki, J. et al. Performance of activity classification algorithms in free-living older adults. Med. Sci. Sports Exerc. 48 , 941–950 (2016).
Allyn, B. IBM abandons facial recognition products, condemns racially biased surveillance. https://www.npr.org/2020/06/09/873298837/ibm-abandons-facial-recognition-products-condemns-racially-biased-surveillance (2020).
Chee, F. Y. EU mulls five-year ban on facial recognition tech in public areas. https://www.reuters.com/article/uk-eu-ai/eu-mulls-five-year-ban-on-facial-recognition-tech-in-public-areas-idINKBN1ZF2QN (2020).
Saha, J., Chowdhury, C., Chowdhury, I. R., Biswas, S. & Aslam, N. An ensemble of condition based classifiers for device independent detailed human activity recognition using smartphones. Information 9 , 94 (2018).
Barnett, I. & Onnela, J.-P. Inferring mobility measures from GPS traces with missing data. Biostatistics 21 , e98–e112 (2020).
Wang, L. et al. Enabling reproducible research in sensor-based transportation mode recognition with the Sussex-Huawei dataset. IEEE ACCESS 7 , 10870–10891 (2019).
Lee, M. H., Kim, J., Jee, S. H. & Yoo, S. K. Integrated solution for physical activity monitoring based on mobile phone and PC. Healthc. Inform. Res. 17 , 76–86 (2011).
Fahim, M., Fatima, I., Lee, S. & Park, Y.-T. EFM: evolutionary fuzzy model for dynamic activities recognition using a smartphone accelerometer. Appl. Intell. 39 , 475–488 (2013).
Yurur, O., Liu, C. H. & Moreno, W. Light-weight online unsupervised posture detection by smartphone accelerometer. IEEE Internet Things J. 2 , 329–339 (2015).
Awan, M. A., Guangbin, Z., Kim, H.-C. & Kim, S.-D. Subject-independent human activity recognition using Smartphone accelerometer with cloud support. Int. J. Ad Hoc Ubiquitous Comput. 20 , 172–185 (2015).
Chen, Z., Wu, J., Castiglione, A. & Wu, W. Human continuous activity recognition based on energy-efficient schemes considering cloud security technology. Secur. Commun. Netw. 9 , 3585–3601 (2016).
Guo, J. et al. Smartphone-based patients’ activity recognition by using a self-learning scheme for medical monitoring. J. Med. Syst. 40 , 140 (2016).
Walse, K. H., Dharaskar, R. V. & Thakare, V. M. A study of human activity recognition using AdaBoost classifiers on WISDM dataset. IIOAB J. 7 , 68–76 (2016).
Lee, K. & Kwan, M.-P. Physical activity classification in free-living conditions using smartphone accelerometer data and exploration of predicted results. Comput. Environ. Urban Syst. 67 , 124–131 (2018).
Ahmad, N. et al. SARM: salah activities recognition model based on smartphone. Electronics 8 , 881 (2019).
Usman Sarwar, M. et al. Recognizing physical activities having complex interclass variations using semantic data of smartphone. Softw. Pract. Exp . 51 , 532–549 (2020).
Kwapisz, J. R., Weiss, G. M. & Moore, S. A. Activity recognition using cell phone accelerometers. in Proceedings of the Fourth International Workshop on Knowledge Discovery from Sensor Data 10–18 https://doi.org/10.1145/1964897.1964918 (Association for Computing Machinery, 2010).
Sharma, A., Singh, S. K., Udmale, S. S., Singh, A. K. & Singh, R. Early transportation mode detection using smartphone sensing data. IEEE Sens. J . 1 , https://doi.org/10.1109/JSEN.2020.3009312 (2020).
Chen, Z. et al. Smartphone sensor-based human activity recognition using feature fusion and maximum full a posteriori. IEEE Trans. Instrum. Meas. 69 , 3992–4001 (2020).
Vavoulas, G. Chatzaki, C. Malliotakis, T. Pediaditis, M. & Tsiknakis, M. The MobiAct Dataset: recognition of activities of daily living using smartphones. In Proceedings of the International Conference on Information and Communication Technologies for Ageing Well and e-Health (eds. Röcker, C., Ziefle, M., O’Donoghue, J, Maciaszek, L. & Molloy W.) Vol. 1: ICT4AWE, (ICT4AGEINGWELL 2016) 143–151, https://www.scitepress.org/ProceedingsDetails.aspx?ID=VhZYzluZTNE=&t=1 (SciTePress, 2016).
Shoaib, M., Bosch, S., Incel, O. D., Scholten, H. & Havinga, P. J. M. Complex human activity recognition using smartphone and wrist-worn motion sensors. Sensors 16 , 426 (2016).
Lockhart, J. W. et al. Design considerations for the WISDM smart phone-based sensor mining architecture. in Proceedings of the Fifth International Workshop on Knowledge Discovery from Sensor Data. 25–33 https://doi.org/10.1145/2003653.2003656 (Association for Computing Machinery, 2011).
Vaizman, Y., Ellis, K. & Lanckriet, G. Recognizing detailed human context in the wild from smartphones and smartwatches. IEEE Pervasive Comput. 16 , 62–74 (2017).
Sztyler, T. & Stuckenschmidt, H. On-body localization of wearable devices: an investigation of position-aware activity recognition. in 2016 IEEE International Conference on Pervasive Computing and Communications (PerCom) 1–9 https://ieeexplore.ieee.org/document/7456521 (IEEE, 2016).
Malekzadeh, M., Clegg, R. G., Cavallaro, A. & Haddadi, H. Mobile sensor data anonymization. in Proceedings of the International Conference on Internet of Things Design and Implementation. 49–58 https://doi.org/10.1145/3302505.3310068 (ACM, 2019).
Carpineti, C., Lomonaco, V., Bedogni, L., Felice, M. D. & Bononi, L. Custom dual transportation mode detection by smartphone devices exploiting sensor diversity. in 2018 IEEE International Conference on Pervasive Computing and Communications Workshops (PerCom Workshops) 367–372 https://ieeexplore.ieee.org/document/8480119 (IEEE, 2018).
Ichino, H., Kaji, K., Sakurada, K., Hiroi, K. & Kawaguchi, N. HASC-PAC2016: large scale human pedestrian activity corpus and its baseline recognition. in Proceedings of the 2016 ACM International Joint Conference on Pervasive and Ubiquitous Computing: Adjunct. 705–714 https://doi.org/10.1145/2968219.2968277 (Association for Computing Machinery, 2016).
Download references
Acknowledgements
Drs. Straczkiewicz and Onnela are supported by NHLBI award U01HL145386 and NIMH award R37MH119194. Dr. Onnela is also supported by the NIMH award U01MH116928. Dr. James is supported by NCI award R00CA201542 and NHLBI award R01HL150119.
Author information
Authors and affiliations.
Department of Biostatistics, Harvard T.H. Chan School of Public Health, Boston, MA, 02115, USA
Marcin Straczkiewicz & Jukka-Pekka Onnela
Department of Population Medicine, Harvard Medical School and Harvard Pilgrim Health Care Institute, Boston, MA, 02215, USA
Peter James
Department of Environmental Health, Harvard T.H. Chan School of Public Health, Boston, MA, 02115, USA
You can also search for this author in PubMed Google Scholar
Contributions
M.S. conducted the review, prepared figures, and wrote the initial draft. P.J. and J.P.O. revised the manuscript. J.P.O. supervised the project. All authors reviewed the manuscript.
Corresponding author
Correspondence to Marcin Straczkiewicz .
Ethics declarations
Competing interests.
The authors declare no competing interests.
Additional information
Publisher’s note Springer Nature remains neutral with regard to jurisdictional claims in published maps and institutional affiliations.
Rights and permissions
Open Access This article is licensed under a Creative Commons Attribution 4.0 International License, which permits use, sharing, adaptation, distribution and reproduction in any medium or format, as long as you give appropriate credit to the original author(s) and the source, provide a link to the Creative Commons license, and indicate if changes were made. The images or other third party material in this article are included in the article’s Creative Commons license, unless indicated otherwise in a credit line to the material. If material is not included in the article’s Creative Commons license and your intended use is not permitted by statutory regulation or exceeds the permitted use, you will need to obtain permission directly from the copyright holder. To view a copy of this license, visit http://creativecommons.org/licenses/by/4.0/ .
Reprints and permissions
About this article
Cite this article.
Straczkiewicz, M., James, P. & Onnela, JP. A systematic review of smartphone-based human activity recognition methods for health research. npj Digit. Med. 4 , 148 (2021). https://doi.org/10.1038/s41746-021-00514-4
Download citation
Received : 24 March 2021
Accepted : 13 September 2021
Published : 18 October 2021
DOI : https://doi.org/10.1038/s41746-021-00514-4
Share this article
Anyone you share the following link with will be able to read this content:
Sorry, a shareable link is not currently available for this article.
Provided by the Springer Nature SharedIt content-sharing initiative
This article is cited by
Unlocking the potential of smartphone and ambient sensors for adl detection.
- Marija Stojchevska
- Mathias De Brouwer
- Femke Ongenae
Scientific Reports (2024)
Self-supervised learning for human activity recognition using 700,000 person-days of wearable data
- Aiden Doherty
npj Digital Medicine (2024)
Activity recognition based on smartphone sensor data using shallow and deep learning techniques: A Comparative Study
- Asif Iqbal Middya
- Sarvajit Kumar
- Sarbani Roy
Multimedia Tools and Applications (2024)
A “one-size-fits-most” walking recognition method for smartphones, smartwatches, and wearable accelerometers
- Marcin Straczkiewicz
- Emily J. Huang
- Jukka-Pekka Onnela
npj Digital Medicine (2023)
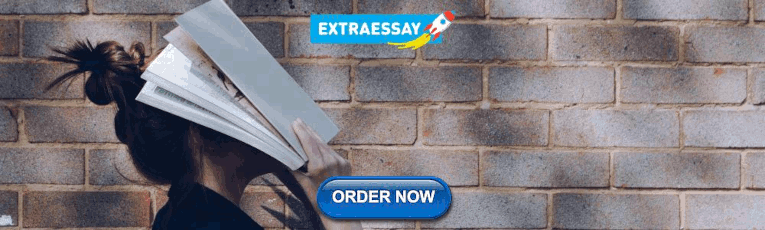
Data filtering and deep learning for enhanced human activity recognition from UWB radars
- Julien Maitre
- Kévin Bouchard
- Sébastien Gaboury
Journal of Ambient Intelligence and Humanized Computing (2023)
Quick links
- Explore articles by subject
- Guide to authors
- Editorial policies
Sign up for the Nature Briefing newsletter — what matters in science, free to your inbox daily.

- Search by keyword
- Search by citation
Page 1 of 502
Exploring food poverty experiences in the German Twitter-Sphere
This study investigates the subjective perceptions of food poverty in Germany by analysing Twitter discourse using the German-language hashtag #IchBinArmutsbetroffen (#IamPovertyAffected) and examines the exte...
- View Full Text
Incomplete tuberculosis reporting and registration to the surveillance system in southwestern China of Yunnan Province: an inventory survey
The real-world tuberculosis (TB) surveillance data was generally incomplete due to underreporting and underdiagnosis. The inventory study aimed to assess and quantify the incompletion of surveillance systems i...
Epidemiological characteristics of neuroendocrine neoplasms in Beijing: a population-based retrospective study
The incidence of neuroendocrine neoplasms (NENs) is rising rapidly worldwide. However, there are few reports on these heterogeneous diseases in China. Our study aimed to explore the epidemiological characteris...
Epidemiologic profile of inflammatory bowel disease in Eastern Mediterranean Region (EMRO) countries: a systematic review and meta-analysis
Inflammatory bowel disease (IBD) consists of two main types: Crohn’s disease (CD) and ulcerative colitis (UC). The epidemiology of IBD patients has not been comprehensively studied in EMRO countries; therefore...
The association between gender equality and climate adaptation across the globe
Climate change has a disproportionate impact on women in comparison to men, and women have a key role to play in climate adaptation. However, evidence is lacking on how gender inequalities may be associated wi...
Public perspectives on COVID-19 public health and social measures in Japan and the United Kingdom: a qualitative study
The COVID-19 pandemic, caused by SARS-CoV-2, was one of the greatest modern public health crises that the world has faced. Countries undertook sweeping public health and social measures (PHSM); including envir...
Exercise, grit, and life satisfaction among Korean adolescents: a latent growth modeling analysis
Life satisfaction among Korean students is declining substantially, and multifaceted improvement efforts are required.
Multi-level analysis of intimate partner violence and its determinants among reproductive age group women in Ethiopia: evidence from Ethiopian Demographic Health Survey, 2016
Intimate partner violence (IPV) is recognized as a main public health challenge, with serious consequences for women’s physical, mental, sexual, and reproductive health. Despite its public health importance, m...
Determinants of premenstrual dysphoric disorder and associated factors among regular undergraduate students at Hawassa University Southern, Ethiopia, 2023: institution-based cross-sectional study
Premenstrual dysphoric disorder (PMDD) is a condition causing severe emotional, physical, and behavioral symptoms before menstruation. It greatly hinders daily activities, affecting academic and interpersonal ...
Exploring the working life of people with multiple sclerosis during the COVID-19 pandemic in Sweden
The COVID-19 pandemic led to vast changes in working life and conditions in which we work. These changes may affect people with multiple sclerosis (PwMS) differently. We aimed to describe the working situation...
The effect of replacing sedentary behavior with different intensities of physical activity on depression and anxiety in Chinese university students: an isotemporal substitution model
Previous research has suggested that engaging in regular physical activity (PA) can help to reduce symptoms of depression and anxiety in university students. However, there is a lack of evidence regarding the ...
Bone fracture is associated with incident myocardial infarction in long-term follow-up
The association between bone fracture and cardiovascular diseases is examined in this study. While basic research has established a connection between fractures and heart attacks through the linkage between bo...
Socio-demographic predictors of not having private dental insurance coverage: machine-learning algorithms may help identify the disadvantaged
For accessing dental care in Canada, approximately 62% of the population has employment-based insurance, 6% have some publicly funded coverage, and 32% have to pay out-of pocket. Those with no insurance or pub...
Machine learning algorithms using national registry data to predict loss to follow-up during tuberculosis treatment
Identifying patients at increased risk of loss to follow-up (LTFU) is key to developing strategies to optimize the clinical management of tuberculosis (TB). The use of national registry data in prediction mode...
#Bartender: portrayals of popular alcohol influencer’s videos on TikTok ©
Despite widespread use of the short-video social media platform TikTok © , limited research investigates how alcohol is portrayed on the platform. Previous research suggests that a driver of alcohol content on TikT...
Energy, nutrient and overall healthiness of processed packaged foods in Fiji, a comparison between 2018 and 2020
In Fiji, packaged foods are becoming increasingly available. However, it is unknown if nutrition composition of these foods has changed. This study aims to assess changes in energy, nutrient content and health...
A cohort study of self-perception of ageing and all-cause mortality among older adults in China: a multiple mediators analysis
Positive self-perception of aging (SPA) is a well-known predictor of longevity, while how and to what extent SPA is linked with all-cause mortality among older adults is still unclear. This study aims to eluci...
Family cohesion and quality of life significantly affecting personality changes in adult epilepsy patients: a case-control study
The goal of epilepsy treatment is not only to control convulsive seizures but also to improve the quality of life of patients. This study aimed to investigate personality changes and the risk factors for their...
Assessment of handwashing impact on detection of SARS-CoV-2, Staphylococcus aureus , Escherichia coli on hands in rural and urban settings of Côte d’Ivoire during COVID-19 pandemic
Handwashing is the first line of hygiene measures and one of the oldest methods of preventing the spread of infectious diseases. Despite its efficacy in the health system, handwashing is often inadequately pra...
Feasibility and acceptability of a cohort study baseline data collection of device-measured physical behaviors and cardiometabolic health in Saudi Arabia: expanding the Prospective Physical Activity, Sitting and Sleep consortium (ProPASS) in the Middle East
Physical behaviors such physical activity, sedentary behavior, and sleep are associated with mortality, but there is a lack of epidemiological data and knowledge using device-measured physical behaviors.
Risk factors and their association network for young adults’ suicidality: a cross-sectional study
Understanding the intricate influences of risk factors contributing to suicide among young individuals remains a challenge. The current study employed interpretable machine learning and network analysis to unr...
Cold waves and fine particulate matter in high-altitude Chinese cities: assessing their interactive impact on outpatient visits for respiratory disease
Extreme weather events like heatwaves and fine particulate matter (PM 2.5 ) have a synergistic effect on mortality, but research on the synergistic effect of cold waves and PM 2.5 on outpatient visits for respirator...
Process evaluation of implementation of the early stages of a whole systems approach to obesity in a small Island
The small Atlantic island of St Helena is a United Kingdom Overseas Territory (UKOT) with a high prevalence of childhood obesity (over a quarter of 4–5 and 10–11 year olds) and, anecdotally, adulthood obesity ...
Risk of not being in employment, education or training (NEET) in late adolescence is signalled by school readiness measures at 4–5 years
Not being in employment, education, or training (NEET) is associated with poor health (physical and mental) and social exclusion. We investigated whether England’s statutory school readiness measure conducted ...
The state of health in the European Union (EU-27) in 2019: a systematic analysis for the Global Burden of Disease study 2019
The European Union (EU) faces many health-related challenges. Burden of diseases information and the resulting trends over time are essential for health planning. This paper reports estimates of disease burden...
The association between non-alcoholic fatty liver disease and urinary incontinence among adult females in the United States
Non-alcoholic fatty liver disease (NAFLD) and urinary incontinence (UI) are both highly prevalent and age-related diseases. Nevertheless, the link between NAFLD and UI is unclear. Hence, the study was designed...
Factors influencing risk perception during Public Health Emergencies of International Concern (PHEIC): a scoping review
The unknownness and dread potential of a risk event shapes its perceived risk. A public health emergency of international concern (PHEIC) declaration by the World Health Organisation (WHO) is a signal for such...
Examining determinants of stunting in Urban and Rural Indonesian: a multilevel analysis using the population-based Indonesian family life survey (IFLS)
In Indonesia, chronic malnutrition leading to stunted growth in children represents a significant issue within the public health domain. The prevalence of stunting varies between urban and rural areas, reflect...
Associated lifestyle factors of elevated plasma aldosterone concentration in community population, gender-stratified analysis of a cross-sectional survey
Aldosterone plays important parts in development of cardio-metabolic diseases as end product of renin-angiotensin-aldosterone system. However, factors elevating circulating aldosterone are not clear, and lifes...
Human resource shortage in India’s health sector: a scoping review of the current landscape
For healthcare delivery to be optimally effective, health systems must possess adequate levels and we must ensure a fair distribution of human resources aimed at healthcare facilities. We conducted a scoping r...
Effects of electronic screen exposure time on hypertensive disorders in pregnancy: a retrospective cohort study
We previously conducted a case-control study and found that exposure to electronic screen before nocturnal sleep was associated with hypertensive disorders in pregnancy (HDP). Hence, we carried out this cohort...
The relationship between oxidative balance scores and chronic diarrhea and constipation: a population-based study
Oxidative stress is closely related to gut health. Exposures to oxidative stress in one’s diet and lifestyle can be evaluated by the oxidative balance score (OBS). However, the relationship between OBS and int...
The effect of physical fitness on psychological health: evidence from Chinese university students
Despite frequent discussions on the link between physical and mental health, the specific impact of physical fitness on mental well-being is yet to be fully established.
New sagittal abdominal diameter and transverse abdominal diameter based equations to estimate visceral fat area in type 2 diabetes patients
Computed Tomography (CT) or Magnetic Resonance Imaging (MRI) are considered gold standards for measuring visceral fat area (VFA). However, their relatively high prices and potential radiation exposure limit th...
Lag effect of ambient temperature on respiratory emergency department visits in Beijing: a time series and pooled analysis
Although the association between ambient temperature and mortality of respiratory diseases was numerously documented, the association between various ambient temperature levels and respiratory emergency depart...
The effect of soy isoflavones supplementation on metabolic status in patients with non-alcoholic fatty liver disease: a randomized placebo controlled clinical trial
Non-alcoholic fatty liver disease (NAFLD) accounts as a crucial health concern with a huge burden on health and economic systems. The aim of this study is to evaluate the effect of soy isoflavones supplementat...
Smokeless tobacco consumption among women of reproductive age: a systematic review and meta-analysis
Smokeless tobacco (SLT) poses many negative health impacts. Despite its longstanding presence in societies across the world, the health implications of SLT have only been rigorously studied in recent decades. ...
Prevalence of HIV-related stigma manifestations and their contributing factors among people living with HIV in Sweden – a nationwide study
With access to antiretroviral therapy (ART) HIV infection is a chronic manageable condition and non-sexually transmissible. Yet, many people living with HIV still testify about experiencing HIV-related stigma...
Disease burden of mental disorders among children and adolescents considering both co-morbidities and suicide in Northeastern China
Few studies have assessed the burden of mental disorders among children and adolescents considering the impact of co-morbidities and suicide on disability adjusted life years (DALYs).
Factors associated with antiretroviral treatment adherence among people living with HIV in Guangdong Province, China: a cross sectional analysis
Understanding factors associated with antiretroviral treatment (ART) adherence is crucial for ART success among people living with HIV (PLHIV) in the “test and treat” era. Multiple psychosocial factors tend to...
Postpartum depression symptoms: prevalence, risk factors, and childbirth experiences in Palestine
Worldwide mothers are suffering from postpartum depression (PPD) which impairs mothers’ well-being, children, and families, and leads to adverse outcomes for mothers and their growing newborns. Low and middle-...
COVID-19 vaccine intentions and attitudes in Black American emerging adults with asthma
Emerging adults (aged 18–29) are less likely to receive the COVID-19 vaccine than any other adult age group. Black Americans are less likely than non-Hispanic white Americans to be fully vaccinated against COV...
Physical activity and functional social support in community-dwelling older adults: a scoping review
Globally, the population of older people is increasing and is estimated to reach nearly 2.1 billion by 2050. Physical activity (PA) is one of the key components for successful ageing. However, PA decreases wit...
Structural relations of illness perception, fatigue, locus of control, self-efficacy, and coping strategies in patients with multiple sclerosis: a cross-sectional study
Patients with multiple sclerosis (MS) experience extensive problems due to fatigue and illness perception. Reducing these problems may improve these patients’ quality of life (QoL). Accordingly, the current st...
Community medical service construction: identifying factors that influence medical choice for patients with non-communicable chronic diseases in the Southwest China
Community medical institutions play a vital role in China’s healthcare system. While the number of these institutions has increased in recent years, their construction contents remain insufficient. The potenti...
Impact of COVID-19 on women living with HIV who are survivors of intimate partner violence
Women living with HIV (WLWH) experience higher rates of intimate partner violence (IPV) compared to women without HIV, but there has been minimal research to date on the impact of the COVID-19 pandemic on the ...
Prediction of adolescent weight status by machine learning: a population-based study
Adolescent weight problems have become a growing public health concern, making early prediction of non-normal weight status crucial for effective prevention. However, few temporal prediction tools for adolesce...
Air pollution, life’s essential 8, and risk of severe non-alcoholic fatty liver disease among individuals with type 2 diabetes
The impacts of long-term exposure to air pollution on the risk of subsequent non-alcoholic fatty liver disease (NAFLD) among participants with type 2 diabetes (T2D) is ambiguous. The modifying role of Life’s E...
Assessment of secular trends of three major gynecologic cancers burden and attributable risk factors from 1990 to 2019: an age period cohort analysis
This study aims to assess the long-term trends in the burden of three major gynecologic cancers(GCs) stratified by social-demographic status across the world from 1990 to 2019. To assess the trends of risk fac...
Cognition as mediator of pulmonary function and risk of sarcopenia among older adults
The relationship between lung function and sarcopenia remains ambiguous. The primary aim of this study was to investigate the potential association between lung function and sarcopenia in the older adults, as ...
Important information
Editorial board
For authors
For editorial board members
For reviewers
- Manuscript editing services
Annual Journal Metrics
2022 Citation Impact 4.5 - 2-year Impact Factor 4.7 - 5-year Impact Factor 1.661 - SNIP (Source Normalized Impact per Paper) 1.307 - SJR (SCImago Journal Rank)
2023 Speed 32 days submission to first editorial decision for all manuscripts (Median) 173 days submission to accept (Median)
2023 Usage 24,332,405 downloads 24,308 Altmetric mentions
- More about our metrics
Peer-review Terminology
The following summary describes the peer review process for this journal:
Identity transparency: Single anonymized
Reviewer interacts with: Editor
Review information published: Review reports. Reviewer Identities reviewer opt in. Author/reviewer communication
More information is available here
- Follow us on Twitter
BMC Public Health
ISSN: 1471-2458
- General enquiries: [email protected]
Help | Advanced Search
Computer Science > Machine Learning
Title: a systematic review and meta-analysis on sleep stage classification and sleep disorder detection using artificial intelligence.
Abstract: Sleep is vital for people's physical and mental health, and sound sleep can help them focus on daily activities. Therefore, a sleep study that includes sleep patterns and disorders is crucial to enhancing our knowledge about individuals' health status. The findings on sleep stages and sleep disorders relied on polysomnography and self-report measures, and then the study went through clinical assessments by expert physicians. However, the evaluation process of sleep stage classification and sleep disorder has become more convenient with artificial intelligence applications and numerous investigations focusing on various datasets with advanced algorithms and techniques that offer improved computational ease and accuracy. This study aims to provide a comprehensive, systematic review and meta-analysis of the recent literature to analyze the different approaches and their outcomes in sleep studies, which includes works on sleep stages classification and sleep disorder detection using AI. In this review, 183 articles were initially selected from different journals, among which 80 records were enlisted for explicit review, ranging from 2016 to 2023. Brain waves were the most commonly employed body parameters for sleep staging and disorder studies. The convolutional neural network, the most widely used of the 34 distinct artificial intelligence models, comprised 27%. The other models included the long short-term memory, support vector machine, random forest, and recurrent neural network, which consisted of 11%, 6%, 6%, and 5% sequentially. For performance metrics, accuracy was widely used for a maximum of 83.75% of the cases, the F1 score of 45%, Kappa of 36.25%, Sensitivity of 31.25%, and Specificity of 30% of cases, along with the other metrics. This article would help physicians and researchers get the gist of AI's contribution to sleep studies and the feasibility of their intended work.
Submission history
Access paper:.
- Other Formats
References & Citations
- Google Scholar
- Semantic Scholar
BibTeX formatted citation

Bibliographic and Citation Tools
Code, data and media associated with this article, recommenders and search tools.
- Institution
arXivLabs: experimental projects with community collaborators
arXivLabs is a framework that allows collaborators to develop and share new arXiv features directly on our website.
Both individuals and organizations that work with arXivLabs have embraced and accepted our values of openness, community, excellence, and user data privacy. arXiv is committed to these values and only works with partners that adhere to them.
Have an idea for a project that will add value for arXiv's community? Learn more about arXivLabs .
Log in using your username and password
- Search More Search for this keyword Advanced search
- Latest content
- Current issue
- For authors
- New editors
- BMJ Journals More You are viewing from: Google Indexer
You are here
- Online First
- Active workplace design: current gaps and future pathways
- Article Text
- Article info
- Citation Tools
- Rapid Responses
- Article metrics

- http://orcid.org/0000-0001-9384-5456 Mohammad Javad Koohsari 1 , 2 , 3 ,
- Andrew T Kaczynski 4 ,
- Akitomo Yasunaga 5 ,
- Tomoya Hanibuchi 6 ,
- Tomoki Nakaya 7 ,
- Gavin R McCormack 8 ,
- Koichiro Oka 2
- 1 School of Advanced Science and Technology , Japan Advanced Institute of Science and Technology , Nomi , Japan
- 2 Faculty of Sport Sciences , Waseda University , Tokorozawa , Japan
- 3 School of Exercise and Nutrition Sciences , Deakin University , Geelong , Victoria , Australia
- 4 Arnold School of Public Health , University of South Carolina , Columbia , South Carolina , USA
- 5 Faculty of Health Sciences , Aomori University of Health and Welfare , Aomori , Japan
- 6 Graduate School of Letters , Kyoto University , Kyoto , Japan
- 7 Graduate School of Environmental Studies , Tohoku University , Sendai , Japan
- 8 Department of Community Health Sciences , University of Calgary , Calgary , Alberta , Canada
- Correspondence to Dr Mohammad Javad Koohsari, Japan Advanced Institute of Science and Technology, Nomi, Japan; koohsari{at}jaist.ac.jp
https://doi.org/10.1136/bjsports-2024-108146
Statistics from Altmetric.com
Request permissions.
If you wish to reuse any or all of this article please use the link below which will take you to the Copyright Clearance Center’s RightsLink service. You will be able to get a quick price and instant permission to reuse the content in many different ways.
- Public health
- Sedentary Behavior
- Health promotion
Introduction
Insufficient physical activity and excessive sitting time among office-based workers have been linked to various health risks and economic consequences. While health promotion interventions are important, the role of workplace design in encouraging active behaviours is increasingly recognised. However, significant gaps exist in knowledge about how workplace design influences these behaviours. This paper identifies the need to investigate the interactive effects of workplace norms and culture and the role of building layouts on workers’ behaviours, as well as the need for more accurate behavioural measures. Bridging these gaps is crucial for designing workplace interventions and promoting active, healthy and productive work environments.
Workplace design: encouraging movement in workplace settings
Existing gaps and future directions, interactive effects of workplace social environments.
Workplace social environments such as norms and culture can significantly influence sedentary behaviours among office-based workers 4 and can affect how workplace design influences workers’ behaviour. Most previous studies have tested the effects of workplace design on employees’ active and sedentary behaviours within Western contexts, 5 leaving a gap in how these relationships vary in other geographical settings with unique workplace norms and cultures. For instance, in a workplace where extended sitting is a cultural norm, employees may still predominantly engage in sedentary behaviour, regardless of having activity-promoting features in their workplace. Conversely, an activity-promoting environment might help mitigate norms towards sitting or even produce multiplicative positive effects in contexts where activity in the workplace is already customary. Conducting studies across varied geographical settings is necessary to identify similarities and differences in the impact of workplace norms and design on workers’ active and sedentary behaviours. Cross-cultural studies can shed light on the generalisability of findings and help develop customised interventions that address specific norms and cultural challenges. Future research can also employ mixed methods to gain a more thorough understanding of the complex interplay between workplace design, norms and culture, and employees’ behaviour. Additionally, the rise of home and hybrid working arrangements indicates that office social norms could extend to home work environments. For example, a culture of regular stretch breaks in the office might encourage similar practices at home, influencing physical activity behaviours remotely. Understanding the detailed relationship between workplace design, norms and employee behaviour is critical for developing targeted contextually relevant interventions that promote active workplace environments.
Precision in tracking workplace behaviours
Accurately measuring employees’ active and sitting behaviours and identifying the ‘locations’ where these behaviours occur is essential to understand their relationships with workplace design attributes. Global positioning systems (GPS) have been commonly used in combination with accelerometer devices to measure and spatially track people’s active and sedentary behaviour in outdoor environments, such as neighbourhoods and cities. 6 Nevertheless, GPS signals have limited accuracy or can be disrupted within indoor environments, resulting in less precise location data.
An indoor positioning system (IPS) can address the limitations of GPS in indoor environments. 7 IPS is a wayfinding technology that uses existing low-cost WiFi and Bluetooth to provide precise locations of individuals inside buildings. The IPS can be integrated with activity-tracking wearable devices, such as accelerometers, pedometers and heart rate monitors, as well as traditional methods like behavioural mapping. This integration allows for the collection of employees’ location data, movement patterns, activity intensities and other biometric data within workplaces. Additionally, the synergy between IPS and wearable devices effectively differentiates between occupational and leisure physical activities in workplaces. This distinction is key to better understanding the health paradox of the different health effects of these two types of physical activities. 8 Furthermore, with the growth of artificial intelligence (AI), there has been a unique opportunity to employ geospatial AI (GeoAI) in workplace environments and health research. GeoAI techniques aim to integrate innovations in spatial sciences with AI, particularly deep learning. 9 The joint application of IPS and GeoAI would enable precise location data of individuals within the workplace while using the power of spatial analysis. GeoAI can analyse workers’ movement patterns derived from IPS in combination with geospatial layers such as spatial layouts, access to common places, and light conditions. For instance, a GeoAI trained by tracking data on people’s movements in various indoor environments would predict people’s movements and derive estimates of the amount of sedentary behaviour of employed people only from planned indoor layout. This analysis allows for identifying hotspots or areas within the workplace where active and sedentary behaviour is prevalent.
Beyond individual design elements: exploring the influence of building layout on workplace behaviour
Most previous studies have primarily examined individual design elements but fail to consider how the overall spatial layout influences movement and behaviour. Building layout encompasses the spatial arrangement of building elements such as walls, doors, windows, and access ways, and plays a fundamental role in defining the functionality of interior spaces. Once a building layout has been established, making substantial alterations to it becomes challenging or, in some cases, impossible. Therefore, designing (and, if feasible, retrofitting) building interiors to promote health is imperative, but it is still unclear which workplace layouts are most supportive of workers’ active behaviours.
The urban design theory of space syntax has the potential to partially address this gap in knowledge. Space syntax uses a set of graph-based estimators to quantify spatial layouts. 10 It offers a framework to investigate the impact of building layout factors, such as workstation arrangement, common area location, and space accessibility, on workers’ movement patterns and behaviours. It goes beyond isolated design elements and considers the spatial configuration as a whole ( figure 1 ). Additionally, more research on ‘how’ people use and perceive their workspaces could complement the space syntax evaluations of building design.
- Download figure
- Open in new tab
- Download powerpoint
Space syntax examines building layouts as a whole, using the graph theory: (A)a schematic workplace layout, (B)space syntax axial lines (i.e., longest and fewest lines traversing all spaces) of the layout, and (C)the connectivity of all spaces based on the graph theory.
Conclusions
Future research should investigate the interactive effects of workplace norms and culture on behaviour and conduct cross-cultural studies to identify similarities and differences. Innovative measurement methods can also be employed to accurately measure behaviours and locations where those behaviours occur within workplaces. Additionally, exploring the influence of spatial layout, and using the urban design theory of space syntax, can offer valuable insights into the design of work environments that facilitate workers’ engagement in active behaviours.
Ethics statements
Patient consent for publication.
Not applicable.
Ethics approval
- Sugiyama T ,
- Eakin EE , et al
- Yoshikawa A ,
- Qiu L , et al
- Koohsari MJ ,
- Liao Y , et al
- Waters CN ,
- Chu AHY , et al
- Hadgraft N ,
- Clark BK , et al
- Katapally TR ,
- Pollard B ,
- Engelen L ,
- Held F , et al
- Holtermann A ,
- Hansen JV ,
- Burr H , et al
- Laden F , et al
- Hillier B ,
Contributors MJK conceived the idea and wrote the initial draft of the manuscript. All authors contributed to the writing and assisted with the analysis and interpretation. All authors have read and approved the final manuscript and agree with the order of the presentation of authors.
Funding MJK is supported by the JSPS KAKENHI (grant 23K09701). KO is supported by the JSPS Grants-in-Aid for Scientific Research program (grant 20H04113).
Competing interests None declared. In particular, none of the authors has a financial interest in the Space Syntax Limited company.
Provenance and peer review Not commissioned; externally peer reviewed.
Read the full text or download the PDF:
Dog Ownership’s Benefits for Cardiovascular Health Research Paper
Introduction, benefits of pet ownership.
A person’s health and life expectancy depend on the heart’s condition, a vital organ in our body. Heart disease can be prevented by staying active, eating a nutritious diet, walking outside, getting adequate sleep, and giving up unhealthy habits. However, having a pet significantly impacts heart health and is another important aspect of cardiac care. So keeping a pet is an excellent method of maintaining good heart health. Owning a dog has been linked to lower cardiovascular risk, and is frequently related to decreased blood pressure, an improved lipid profile, and reduced sympathetic responses to stress. There are conflicting findings in the evidence connecting dog ownership and mortality. Although the relationship between dog ownership and mortality has been studied, an improvement in survival has been shown when a dog is a part of the household.
Since the American Heart Association recommends that people exercise for at least 30 minutes every day, five days a week, the increase in exercise or physical activity is the most likely explanation for the low prevalence of high blood pressure among pet owners. With pets around, much physical work was involved, such as bathing, feeding, walking the animals, and cleaning their cages and aquariums. Regular exercise helps a pet owner’s heart function better and pump blood to the body. A healthy heart pumps blood more efficiently, lessening the arteries’ strain and lowering blood pressure. It is believed that having pets indirectly motivates pet owners to work out more, improving their cardiovascular health.
According to estimations by Maugeri et al., 42% of the participants had a pet of some kind, with 24.3% having a dog and 17.9% having another animal (2019). Pet owners, specifically dog owners, were more likely to report optimum levels of physical activity, diet, blood glucose, and smoking than non-pet owners (median, 10; interquartile range, 3 vs. median, 9; interquartile range, 3; P=0.006). This led to higher CVH scores. Dog owners were more likely to report engaging in recommended levels of physical activity and diet than owners of other pets. Similar findings were seen when comparing dog owners vs. non-dog owners. After adjusting for confounders, dog owners had higher CVH scores than non-pet owners, other pet owners, and non-dog owners (=0.342; SE=0.122; P=0.005, =0.309; SE=0.151; P=0.041).
It has been demonstrated that owning a dog lowers blood pressure. According to the American Heart Association (2019), dog owners are 54% more likely to meet the recommended levels of physical activity than the average person without a pet. As a result, pets make their owners more physically active, which lowers the likelihood that they may develop hypertension. Maugeri et al. (2019) reported that blood pressure in hypertensive individuals with dogs recovered to normal in many cases without using antihypertensive medications. To promote physical activity and lower blood pressure, dog owners are encouraged to move around more outside.
Being a dog owner is an excellent method to enhance mental health, as poor mental health is a significant risk factor for heart attacks. The hormones endorphins, oxytocin, dopamine, and serotonin, known as natural antidepressants, rise when people communicate with animals, and the stress hormone cortisol is also reduced. Additionally, having a pet requires constant attention, which diverts attention from issues and lonely thoughts and prevents depression-inducing emotions like hopelessness and indifference. As a result, having pets makes people’s life happier, more satisfying, and prosperous, where stress is reduced. Additionally, having a pet gives a person with a mental illness a strong sense of ontological stability, dramatically speeding up the progression of diseases, including depression, schizophrenia, bipolar disorder, and PTSD. Having a dog is frequently linked to better mental health, which is vital for cardiac treatment.
Fewer health issues over time are associated with increased physical activity. Owning a dog can significantly improve a person’s physical health because dogs need to be walked daily. Health professionals claim that moderate exercise for at least 20 minutes daily is required to prevent various chronic ailments, including heart disease and high blood pressure (Mein & Grant, 2018). People who own dogs are less likely to get cardiovascular disease than non-dog owners because most dogs spend at least ten minutes a day walking and playing with their pets. A dog can give people the inspiration they need to go for a walk if they are too lazy to go to the gym or find it challenging to keep to a demanding fitness schedule. The best part is that dog owners do not consider walking a workout because they know that their pets require daily walks for mental and physical stimulation.
Even those who did not own dogs, including those who did not acknowledge other pets, displayed better cardiovascular health (CVH), an association mediated by increased physical activity and a healthier diet. Although the beneficial relationship between owning a dog and engaging in physical activity has been highlighted, more research needs to be done on the relationship between pet ownership and eating habits. It is yet to be determined how having a pet may help with eating healthily.
Dog ownership, in particular, is linked to higher levels of physical activity, social support, and better recovery from catastrophic cardiovascular events. Pet ownership is connected to a lower risk of cardiovascular disease in the general US population, according to a theory put out by Krittanawong et al. (2020). The prevalence of cardiovascular risk factors, such as dyslipidemia, hypertension, and diabetes, is inversely correlated with dog ownership in those without CVD. Ownership of a dog is linked to a higher survival rate in people with coronary artery disease.
Lastly, having a pet can lower cholesterol and triglyceride levels, which is also good for the heart. Being in charge of feeding your pet encourages you to develop a regular eating schedule for yourself, which can help prevent insulin resistance and excessive triglyceride levels. According to Maugeri et al. (2019), altering one’s diet and upping physical activity can result in weight loss, which is one of the best strategies to keep cholesterol levels under control. Dog owners then alter their diets in favor of healthy options, whether consciously or unconsciously, which benefits them on both levels. Therefore, having pets improves nutrition and lowers cholesterol and triglyceride levels, improving heart health.
In conclusion, pet owners claim that pets eventually transform from being amusing creatures into actual family members who, like other family members, impact people’s physical and mental well-being. Getting a dog is a terrific way to improve your heart health and lower your blood pressure, stress, cholesterol, and triglycerides. Numerous pieces of research back up the notion that getting a pet may be an excellent way to boost cardiovascular health. The advantages of owning a pet exceed the potential added responsibilities by a wide margin. Having a pet may lower one’s risk of dying from any cause, but heart disease is hazardous. Additionally, some research findings suggest that owning a pet may reduce the chance of getting hypertension and enhance blood pressure control in people who already have the condition. Additionally, evidence indicates that owning a pet may improve a patient’s prognosis following a myocardial infarction or stroke. Ownership of pets is one of the most significant cardioprotective methods because it reduces sympathetic nervous system activity.
American Heart Association. (2019). Can your pet help you be healthier? American Heart Association. Web.
Krittanawong, C., Kumar, A., Wang, Z., Jneid, H., Virani, S. S., & Levine, G. N. (2020). Pet Ownership and Cardiovascular Health in the US General Population . The American Journal of Cardiology , 125 (8), 1158–1161. Web.
Maugeri, A., Medina-Inojosa, J. R., Kunzova, S., Barchitta, M., Agodi, A., Vinciguerra, M., & Lopez-Jimenez, F. (2019). Dog Ownership and Cardiovascular Health: Results From the Kardiovize 2030 Project . Mayo Clinic Proceedings: Innovations, Quality & Outcomes , 3 (3), 268–275. Web.
Mein, G., & Grant, R. (2018). A cross-sectional exploratory analysis between pet ownership, sleep, exercise, health and neighborhood perceptions: the Whitehall II cohort study . BMC Geriatrics , 18 (1). Web.
- Debates on Whether Dog is the Best Pet or not
- Pets Keeping: Benefits for Children
- Cats vs. Dogs: Are You a Cat or a Dog Person?
- Domestic Cats’ Reactions to Their Owners
- Good and Bad Aspects of a Pet (Puppy)
- Cats and Their Role in Mental Health Support
- Advantages of Adopting Rescue Dogs
- Dog Training Techniques Step by Step
- Chicago (A-D)
- Chicago (N-B)
IvyPanda. (2024, May 24). Dog Ownership's Benefits for Cardiovascular Health. https://ivypanda.com/essays/dog-ownerships-benefits-for-cardiovascular-health/
"Dog Ownership's Benefits for Cardiovascular Health." IvyPanda , 24 May 2024, ivypanda.com/essays/dog-ownerships-benefits-for-cardiovascular-health/.
IvyPanda . (2024) 'Dog Ownership's Benefits for Cardiovascular Health'. 24 May.
IvyPanda . 2024. "Dog Ownership's Benefits for Cardiovascular Health." May 24, 2024. https://ivypanda.com/essays/dog-ownerships-benefits-for-cardiovascular-health/.
1. IvyPanda . "Dog Ownership's Benefits for Cardiovascular Health." May 24, 2024. https://ivypanda.com/essays/dog-ownerships-benefits-for-cardiovascular-health/.
Bibliography
IvyPanda . "Dog Ownership's Benefits for Cardiovascular Health." May 24, 2024. https://ivypanda.com/essays/dog-ownerships-benefits-for-cardiovascular-health/.

An official website of the United States government
The .gov means it’s official. Federal government websites often end in .gov or .mil. Before sharing sensitive information, make sure you’re on a federal government site.
The site is secure. The https:// ensures that you are connecting to the official website and that any information you provide is encrypted and transmitted securely.
- Publications
- Account settings
Preview improvements coming to the PMC website in October 2024. Learn More or Try it out now .
- Advanced Search
- Journal List
- Am J Lifestyle Med
- v.10(3); May-Jun 2016
Positive Psychology and Physical Health
Positive psychology is the scientific study of a healthy and flourishing life. The goal of positive psychology is to complement and extend the traditional problem-focused psychology that has proliferated in recent decades. Positive psychology is concerned with positive psychological states (eg, happiness), positive psychological traits (eg, talents, interests, strengths of character), positive relationships, and positive institutions. We describe evidences of how topics of positive psychology apply to physical health. Research has shown that psychological health assets (eg, positive emotions, life satisfaction, optimism, life purpose, social support) are prospectively associated with good health measured in a variety of ways. Not yet known is whether positive psychology interventions improve physical health. Future directions for the application of positive psychology to health are discussed. We conclude that the application of positive psychology to health is promising, although much work remains to be done.
‘. . . a happy, engaged, and fulfilling psychological and social life is not just a consequence of good health, it is what leads people to live a healthy and long life.’
Health is a state of complete physical, mental, and social well-being and not merely the absence of disease or infirmity. —World Health Organization 1
Most would agree with this statement, but over the years, it has been largely a slogan. More recently, theory, research, and applications from the perspective of positive psychology have helped articulate the meaning of health and well-being. Positive psychology is an umbrella term for the scientific study of the various contributors to a healthy and thriving life for the self and others (eg, positive emotions, life meaning, engaging work, and close relationships). 2 It is the study of strengths, assets, and positive attributes. The topics of concern to positive psychology are broad indicators of psychological, social, and societal well-being.
Research has shown that not only are physical, mental, and social well-beings important components for complete health, but they are also interconnected. Evidence is accumulating that a happy, engaged, and fulfilling psychological and social life is not just a consequence of good health, it is what leads people to live a healthy and long life.
This article provides a brief overview of what positive psychology is and addresses how theories, findings, and especially applications from positive psychology might pertain to physical health.
What Is Positive Psychology?
Positive psychology is a perspective within psychology that studies optimal experience, people being and doing their best. 2 - 4 It challenges the assumptions of the disease model. Positive psychology assumes that life entails more than avoiding or undoing problems and that explanations of the good life must do more than reverse accounts of problems. Someone without symptoms or disorders may or may not be living well. Positive psychology urges attention to what is taking place on the other side of the zero point of being problem-free. It calls for as much focus on strength as on weakness, as much interest in building the best things in life as in repairing the worst, and as much attention to fulfilling the lives of healthy people as to healing the wounds of the distressed. Research findings from positive psychology are intended to contribute to a more complete and balanced scientific understanding of human experiences and ways to foster thriving in individuals, communities, and societies.
One of the triggers for the introduction of positive psychology was the realization that since World War II, psychology as a field had devoted much of its effort to identifying, treating, and—occasionally—preventing problems such as anxiety and depression. 3 The yield of these problem-focused efforts has been impressive, but a myopic view of the human condition has resulted. It is as if psychology has viewed people as only fragile and flawed.
The goal of positive psychology is to complement and extend the problem-focused psychology, and an important idea from positive psychology is that one way to solve problems is by identifying and leveraging individual and societal strengths and assets. 5
The topics of concern to positive psychology can be divided into 4 related topics 2 , 3 :
- Positive subjective experiences (happiness, gratification, fulfillment, flow)
- Positive individual traits (strengths of character, talents, interests, values)
- Positive interpersonal relationships (friendship, marriage, colleagueship)
- Positive institutions (families, schools, businesses, communities)
The value of positive psychology is to use the scientific method to sort through various claims and hypotheses about what it means to live well or poorly and to identify the relevant circumstances in each case.
Positive Psychology and Health
Can physical health be clarified by a positive perspective in the same way that psychological well-being has been clarified? To return to the definition of health by the World Health Organization with which this article began, 1 a positive perspective urges us to look beyond the mere absence of disease and infirmity to define what it means to be healthy in positive terms. 6 - 8 Positive health can be characterized not only as a long and disease-free life but additionally in terms of
- Less frequent and briefer ailments
- Greater recuperative ability
- Rapid wound healing
- More physiological reserves
- Chronic but nondebilitating diseases
Familiar within the field of epidemiology are the population-level concepts of DALYs (disability adjusted life years), HALYs (health-adjusted life years), and QALYs (quality-adjusted life years), which combine measures of morbidity and mortality into the same index. 9 Along these lines, HLEs (happiness-adjusted life expectancies) measure the quality of life in a nation by forming the product of the average life expectancy and the average happiness (aka subjective well-being, life satisfaction) in that nation. 10 Positive health concerns itself with the individual-level analogues of these constructs.
The field of positive health as we envision it overlaps with allied approaches concerned with disease prevention, health promotion , and wellness. The value of positive health as an approach in its own right is that it makes explicit the need to consider good health as opposed to the absence of poor health. Studies of “wellness” often end up being studies of illness, much as studies of mental “health” are often studies of mental illness.
Concern with positive health leads to an examination of health assets , individual-level factors that produce positive health in one or more of the ways that it might be defined, over-and-above the frequently studied risk factors for poor health, like high cholesterol, obesity, smoking, excessive alcohol use, and a sedentary lifestyle. 8 Among the psychosocial risk factors frequently examined with respect to poor health are anger, anxiety, depression, and social isolation. By the logic of a positive perspective, the mere absence of these negative states and traits is not all that matters for physical health. Important as well are positive states and traits, and the contribution of these in their own right needs to be studied, controlling for negative states and traits as well as other usual-suspect risk factors.
There have been extensive studies on negative psychological factors such as stress, depression, hostility, and their effects on increased risk of various health problems. 11 However, less known is whether certain positive psychological factors play a protective role against health risks. Research has shown that positive and negative emotions are not opposite and are only modestly correlated. 12 , 13 Experiencing each of those emotions is also involved in the activation of different brain regions. 14
For the past several years, researchers have examined the contributions of health assets, especially psychological ones, to good health, while they have controlled for established risk factors. Researchers need to examine both risk factors and health assets to understand relationships between both positive and negative psychological factors as they together contribute to health outcomes.
Carefully conducted research shows that positive health assets indeed predict good health assessed in a variety of ways. 15 Among the positive psychology health assets foreshadowing good health are
- Positive emotions 16 , 17
- Life satisfaction 17 , 18
- Optimism 19 - 22
- Forgiveness 23
- Self-regulation 24
- Vitality and zest 25
- Life meaning and purpose 26 - 29
- Helping others and volunteering 30 - 32
- Good social relationships 33 - 35
- Spirituality and religiosity 36 , 37
There has been growing evidence that positive psychological characteristics affect health and longevity using various research methods including longitudinal prospective and experimental designs.
Perhaps, among the most well-known long-term studies that showed the possible link between positive psychological assets and health outcomes is The Nun Study. 38 A group of American nuns who were members of the School Sisters of Notre Dame wrote autobiographical essays in their early 20s when they joined the Sisterhood. Six decades later, researchers who had accessed the convent archive scored the emotional content of 180 essays in terms of positivity, and investigated whether they were related to the mortality of nuns. Indeed, positive emotional content was significantly related to longevity. The nuns who expressed more positive emotions (those in the upper 25%) in their essays, strikingly, lived on average 10 years longer than those expressing fewer positive emotions (those in the bottom 25%). In other words, happier nuns lived longer than less happy (but not depressed) nuns. Putting this in context, unhealthy behavior like smoking costs on average 7 years of one’s life. 2
In another experimental study, Cohen and his colleagues examined the relationships between positive emotions and the vulnerability of catching the common cold. 39 With 334 healthy adult volunteers in the community, they first measured both positive emotional experience, such as happy, pleased, lively, and relaxed, and negative emotional experiences, such as depressed, anxious, and hostile, over a few weeks using self-reports. Afterward, participants were invited to the study lab and exposed to rhinoviruses through nasal drops and monitored in quarantine for the development of the common cold. The researchers found that higher positive emotional experiences were related to lower risk of developing a cold and fewer reports of symptoms, while negative emotional experiences were not significantly related to catching a cold, but associated with reports of more symptoms. In short, this study showed that experiencing positive emotions was linked to greater resistance to developing the common cold.
The health benefits of positive psychological assets have been documented in different cultural settings as well. For instance, in Japanese culture, the most commonly used indicator of subjective well-being is the sense of “life worth living” ( ikigai ). 29 In a population-based prospective cohort study with 43, 391 adults in Ohsaki, Japan, lack of the sense of “life worth living” ( ikigai ) was significantly associated with higher risk of all-cause mortality over time. Those who reported having an ikigai in their life in a survey were more likely to be alive at a 7-year follow-up compared to their counterparts who did not find a sense of ikigai . Interestingly, the increase in mortality risk was due to an increase in mortality from cardiovascular disease and external causes such as suicide, but not to morality from cancer. Having a sense of “life worth living” ( ikigai ) often means having a purpose in life and realizing the value of being alive which could serve as a motivation for living.
In our own study with US adults, having life purpose played a protective role for heart health. 27 At a 2-year follow-up, the higher level of life purpose was prospectively related to lower risks of incidence of myocardial infarction among people with coronary heart disease at the baseline.
One of the ways to achieve a sense of life meaning and purpose is through helping others and doing regular volunteer work in communities. Research has shown that among elders, people who volunteer regularly are healthier and live longer. 30 , 31 In a longitudinal study with a nationally representative sample of community-dwelling older US adults, a study found that volunteerism predicted a lower risk of hypertension 4 years later. 31 That is, those who had volunteered at least 200 hours in the previous 12 months were less likely to develop hypertension risk compared to those who did not volunteer. However, lower levels of volunteering did not decrease the health risk of hypertension. It seems that dosage and intentions of volunteering matters for its health benefits. In another study, people who regularly volunteered for self-oriented motives did not exhibit lower risk for mortality 4 years later, while those who regularly and frequently volunteered for other-oriented motives showed lower risk of subsequent mortality. 32 In conclusion, it is good to be good!
One of the well-studied health-related positive psychology topics is optimism. Optimism is sometimes seen as pollyannaism, a naively rosy view of the world coupled with a “don’t worry, be happy” attitude. However, optimism the way researchers study it is a disposition to an expectation that the future will entail more positive events than negative ones. 40 Optimists are neither in denial nor naive about challenges and difficulties in life. They simply attend to and acknowledge the positive.
Empirical research shows that optimism—usually assessed with self-report surveys—relates to good health and a long life. According to research, among asymptomatic men with HIV, optimism slowed the onset of AIDS over an 18-month follow-up. 41 , 42 Over an 8-year follow-up, optimism predicted better pulmonary function among older men, even when smoking was controlled. 43 In a longitudinal study of older men and women, optimism predicted not only better health but also lower levels of pain. 44 Our own research group recently reported a study of a large nationally representative sample of older adults (aged >50 years) in the United States showing that over a 2-year period, optimism predicted a lower likelihood of stroke, even after controlling for chronic illnesses, self-rated health, and relevant sociodemographic, biological, and psychological factors. 45
In addition, the importance of social support and positive relationships on good health and well-being has long been documented. Supportive social relationships were associated with longevity, less cognitive decline with aging, greater resistance to infectious disease, and better management of chronic illnesses. 33 - 35
Enough well-designed studies exist in support of the premise that health assets predict good health to warrant further investigation. Research so far provides compelling evidence that positive psychological health assets predict or are associated with various health outcomes and longevity among healthy populations. However, what is relatively unknown are the effects of positive health assets for recovery and long-term health outcomes among those with serious health problems such as cancer.
Furthermore, before we consider interventions that deliberately encourage these assets in order to reduce morbidity and mortality and to increase physical well-being, there are issues that need to be addressed. 46
First, assuming that health assets do play a causal role, what are the mechanisms? Research to date has often been stark, usually demonstrating an association over time but not clarifying how it happens. We assume the pathways are multiple, from biological to emotional to cognitive to behavioral to social. For example, in the case of optimism, biologically, it has been linked to better immune system functioning, and behaviorally, people who are optimistic engage in healthier behaviors. They eat healthy, exercise, do not smoke or drink, and seek medical care when they need. Socially, optimists have better and more frequent social contacts. All of these are associated with health benefits.
It is unknown which pathway bears the most traffic or whether the mechanisms vary as a function of the specific health outcome (eg, cardiovascular disease vs the common cold) or as a function of the individual’s age, gender, or lifestyle. Again, the best a researcher can do is to identify plausible mechanisms in a given study and explicitly investigate their role as mediators.
Second, are health assets a cause of good health or merely a correlated marker of its real causes? Indeed, the array of positive health assets is challenging for researchers, who cannot study or control all possible assets in the same investigation. No single study relying on correlational data can be definitive, so it is the overall body of research investigating health assets that must be examined to draw causal conclusions. 17
Third, do the apparent benefits of health assets generalize to all kinds of health outcomes? Much of the relevant research has ascertained general health and all-cause mortality, and some of this work has relied only on self-reported information about health status. When researchers look at specific health outcomes assessed in more objective ways, psychological health assets seem to be more predictive of cardiovascular health than they are of freedom from cancer. So the benefits of health assets may be disease specific. A wider variety of diseases needs to be investigated from the positive health perspective. Moreover, research is not clear about the relative contribution of health assets to disease onset, to disease progression, and/or to recovery.
Positive Psychology Interventions for Physical Health
Researchers and practitioners have begun to develop intervention strategies based on positive psychology to increase positive psychological assets such as positive emotions or life satisfaction to bolster physical health. Whether increasing positive psychological assets will turn to better health outcomes is inconclusive. These intervention efforts targeting health assets in order to lead to better health not only have practical significance but also theoretical importance because appropriately done intervention studies would strengthen the claim that health assets actually cause good health.
We refer to interventions informed by positive psychology as positive psychology interventions. Sometimes positive psychology interventions entail a specific technique, like counting one’s blessings at the end of the day or using one’s signature strengths of character in novels ways. 47 At other times, the intervention uses a more-elaborated therapy package that combines different techniques, such as “Well-Being Therapy,” 48 and Quality of Life Therapy , 49 among others.
Intervention studies allow us to conclude that interventions informed by positive psychology can indeed change positive psychological states and traits, sometimes in lasting ways. 47 , 48 An important qualification is that long-term benefits do not result from one-shot interventions unless these lead to a change in how someone habitually lives. 18 Perhaps, what is required is a sustained lifestyle change.
On the face of it, intervening to increase a health asset should also have benefits for physical health, given the association between health assets and health outcomes. However, this argument does not embody a syllogism. Health assets may not be direct causes of good health, and even if they are, changing them may not result in better health. Said another way, we do not yet know if the health benefits of deliberately cultivated happiness or optimism or life meaning have the same benefits as their naturally occurring counterparts. 50
Needed is intervention research that incudes physical health as an explicitly measured outcome. While it is interesting and important to show that a positive psychology intervention increases the psychological well-being of medial patients, the more exciting issue is whether the intervention also affects their physical health. If so, how quickly would health benefits be evident? And what is the mechanism by which the intervention has an effect?
In studying the mechanisms by which a positive psychology intervention influences physical health, the role played by mundane behavior should not be neglected. 46 There are well-documented “healthy” ways of behaving, 51 including sleeping 8 hours a night, eating balanced meals, not smoking, not drinking to excess, and exercising regularly.
We suspect that positive psychology interventions, when successful, lead people not only to think and feel in more positive ways but also to behave in more healthy ways. 40 , 46 For example, optimistic people are more actively engaged with the world and are better problem solvers than their pessimistic counterparts. They have more frequent and higher quality social contacts as well as more social support. All of these factors may lead to healthier behaviors and habits and eventually to better health.
Positive psychology intervention studies for better health outcomes are in their infancy. So far, nearly all positive psychology interventions primarily targeted changing health-related behaviors such as physical activity, not the health outcome directly. Researchers have shown that positive psychology interventions influence some of the biological and behavioral processes implicated in good health. For example, inducing positive emotions speeds cardiovascular recovery following a stressful event. 52 Training in mindfulness meditation can boost immune function. 53 Psychosocial resilience training targeting positive emotions, cognitive flexibility, social support, life meaning, and active coping reduces total cholesterol among middle-aged adults. 54 Researchers have begun to investigate how positive affect and affirmation influence physical activity and medication adherence among patients with coronary artery disease, 55 asthma, 56 and hypertension. 57 The next step in each case is to show that such interventions also increase good health as opposed to its possible precursors.
Using a randomized controlled clinical trial, a group of researchers recently developed an intervention strategy that enhances positive affect and self-affirmation (PA/SA) and applied it to 3 different high-risk clinical populations (eg, hypertension, asthma, coronary artery disease) to change their health-related behaviors. 55 - 57
In each clinical trial, patients were randomly assigned to either the patient education (PE) control group or the positive-affect/self-affirmation (PA/SA) intervention group. For the control group, each patient received an educational workbook, a pedometer, and a behavior contract for a physical activity goal. For the intervention group, each received PE control components and additionally, a PA/SA workbook chapter, bimonthly induction of PA/SA by telephone, and small mailed gifts. Patients in the PA intervention group were taught how to self-induce positive affect and self-affirmation using a workbook chapter, received bimonthly inducement of PA/SA by telephone, and unexpected small gifts (PA) mailed bimonthly several weeks before follow-up calls. During PA/SA induction phone calls, patients were told to “think about things that make you feel good” and take a moment each day to enjoy positive thoughts (PA), and to think about “proud moments” in their personal lives if they have a difficult time exercising (SA). For both groups, data were collected through a standardized bimonthly telephone follow-up for 12 months.
Using this research design, researchers conducted 3 parallel studies. In study 1, 55 patients were recruited right after percutaneous coronary intervention to increase physical activity among people with coronary artery disease. Compared to the control group, patients in the intervention group engaged in significantly more physical activities. In study 2, 56 physical activity among asthma patients who participated in the study was improved without differences between control and intervention groups. There was no significant effectiveness of intervention. In study 3, 57 the intervention effect on enhancing medication adherence among hypertensive African Americans was examined. Patients in both control and intervention groups received a culturally appropriate hypertension self-management workbook, a behavioral contract, and bimonthly telephone calls to help them better handle barriers to medication adherence. In addition, patients in the PA/SA intervention group received small gifts and bimonthly telephone calls to help them utilize positive thoughts into their daily routine and foster self-affirmation. At the 12-month follow-up, the intervention group showed a significantly higher level of medication adherence compared to the control group (42% vs 36%). The reduction of blood pressure was found among participants without significant differences across groups.
Mixed results from these intervention studies leave questions that need to be clarified with more studies to better understand the effectiveness of a positive psychology intervention on the different health outcomes before they are implemented in health practices.
Another line of positive psychology intervention research that attracted significant attention in recent years is applications of mindfulness meditation. It is assumed that meditations induce positive affect and lead to good health. A study led by a neuroscientist, Richard Davidson, demonstrated that mindfulness meditation produces changes in brain and immune function in a positive way. 53 In this study, 25 healthy employees at a work site received an 8-week intensive clinical training in mindfulness meditation. A weekly training class met for about 3 hours, and a silent 7-hour retreat was held during week 6 of the training. In addition, participants were instructed to perform home meditation practices for 1 hour each day, 6 days a week with the guided audiotapes. Brain electrical activity was measured at the baseline, the end of training, and 4 months after training. Also at the end of training, participants were vaccinated with influenza vaccine. Results from the meditation group were compared to those of the wait-list control group. Among the meditation group, brain activity in the left-sided anterior, associated with positive affect, was significantly increased. They also found significant increases in antibody concentrations to influenza vaccine in the meditation group. Interestingly, the size of increase in left-sided activation predicted the size of antibody concentration rise to the vaccine.
In sum, the effects of positive psychology interventions on health outcomes are inconclusive. As stated before, positive psychology interventions seem to be more effective on reducing health risks among healthy individuals in the short term. However, its long-term health effects, especially, on a population with different health problems, is not clear.
Conclusions
Positive psychology is a perspective that urges scientific attention to strengths and assets that contribute to health and a flourishing life. We have described what positive psychologists have learned about the relationships between positive psychological assets and physical health in the past decade.
To date, the application of positive psychology to health is promising, although much work remains to be done. On the positive side, research shows that what we call positive psychological health assets (eg, positive emotions, life satisfaction, optimism, positive relationships, life purpose) are prospectively associated with good health measured in a variety of ways. Also on the positive side, interventions have been developed that increase these assets; lasting effects require a lifestyle change.
Not yet known is whether positive psychology interventions improve physical health, reducing morbidity and mortality, speeding recovery from illness, and so on. Investigators are beginning to study the health effects of such interventions. Studies to date suggest that positive psychology interventions reduce some of the biological and behavioral processes that affect health, but the next step is to study good health per se.
We urge an open mind about the eventual success of such interventions. It is important not to get too far ahead of the data. Perhaps these interventions will work as intended, perhaps not. For example, the lesson from studies of psychological interventions targeting negative states and traits such as anger and depression in the hope of reducing cardiovascular disease, with which they are associated, is instructive. The success of these interventions is checkered at best. 46 Whether the deliberate cultivation of positive health assets such as positive emotions or life purpose will be more successful in promoting good health than the reduction of psychological risk factors is not known, but is a question worth addressing.
In conclusion, growing evidence suggests that positive psychological assets are linked to health and longevity. However, more studies are necessary to learn more about when, why, how, and for whom positive psychological assets plays a role in good health and whether interventions that enhance these assets will yield health benefits. We urge a skeptical yet fair-minded attitude on the part of researchers and practitioners and that they pay particular attention to underlying mechanisms. Meanwhile, freedom from disease and longevity are not the only goals of life. Quality of life matters in addition to quantity of life. It is clear from research that experiencing frequent positive emotions, having sense of life purpose, paying attention to what is positive in life, and living a more socially integrated life is linked to one’s quality of life across the lifespan. Thus, helping people cultivate positive psychological and social assets in life has potential for leading to happier, more meaningful, and healthier lives.
Acknowledgments
Christopher Peterson unexpectedly died on October 9, 2012, during the revision of this article. We are deeply indebted to his scholarly contributions and mentorship not only to this work but also to the foundation of positive psychology and its applications to various fields. His intellectual and personal contributions will continue to inspire and guide the positive psychology and positive health community.
Support for the preparation of this article was provided by the Robert Wood Johnson Foundation’s Pioneer Portfolio, which supports innovative ideas that may lead to breakthroughs in the future of health and health care. The Pioneer Portfolio funding was administered through a Positive Health grant to the Positive Psychology Center at the University of Pennsylvania, Martin Seligman, Director.
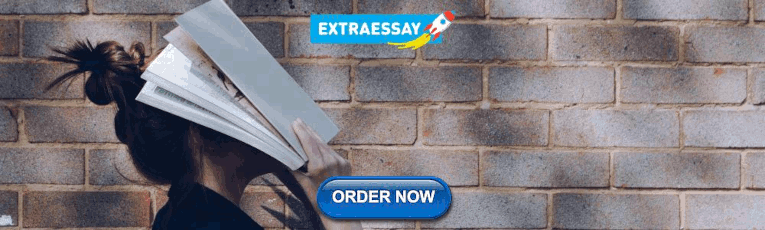
COMMENTS
Background Sedentary lifestyle is a major risk factor for noncommunicable diseases such as cardiovascular diseases, cancer and diabetes. It has been estimated that approximately 3.2 million deaths each year are attributable to insufficient levels of physical activity. We evaluated the available evidence from Cochrane systematic reviews (CSRs) on the effectiveness of exercise/physical activity ...
In this paper, we intend to describe sport's physiological and psychosocial health benefits, stemming both from physical activity and from sport participation per se. This narrative review summarizes research and presents health-related data from Swedish authorities.
Health is a state of complete physical, mental and social well-being and not merely absence of disease [ 1 ]. Fitness is an ability to execute daily functional activities with optimal performance, endurance, and strength to manage minimalist of disease, fatigue, stress and reduced sedentary behavior [ 2 ]. In the modern era with advancement in ...
INTRODUCTION. The World Health Organization (WHO) definition of health encompasses both the absence of disease and the presence of mental and social well-being [].Separate theoretical traditions distinguish between evaluative well-being (e.g., life satisfaction and quality of life [QoL]) and experienced well-being (e.g., happiness and positive affect) [].
Since ancient times, the health benefits of regular physical activity/exercise have been recognized and the classic studies of Morris and Paffenbarger provided the epidemiological evidence in support of such an association. Cardiorespiratory fitness, often measured by maximal oxygen uptake, and habitual physical activity levels are inversely related to mortality. Thus, studies exploring the ...
JPAH is a monthly journal that publishes commentaries, original research, and review papers examining the relationship between physical activity and health, studying physical activity as an exposure as well as an outcome. The journal also invites papers that examine the behavioral, community, and environmental interventions that may affect ...
changes take place or have already taken place, such as menopause and andropause, which involve. diverse psychological impacts and, frequently, physiological changes. A loss of bone mass, for ...
This paper is concerned with future contributions by research to an evidence-based rationale for exercise recommendations—both to the public at large and to individuals. It is clear that physically active people have a lower disease risk than sedentary individuals but the components of activity which determine particular health gains are ...
The aim of this paper is to fill this gap by proposing a mediation framework and estimating the mediating effects of lifestyle choices and social capital in the relationships between physical and mental health in the older population. The analysis is nested within the health economic framework of health production and consumption.
It is well known that physical and mental health are closely related, with growing evidence for biological and behavioural pathways. Mostly the research has focussed on mental health as the key driver of this inter-connection; the extent physical health shapes mental health has received less attention. We aim to derive robust estimates of the unique role physical health may play in shaping ...
Moreover, we produced the analysis of burstness for the top references of the 1988-2021, 2016-2021, and 2021 time periods (Supplementary Tables 2P-R).The analysis of burstness revealed that the top three references with the latest and strongest beginning of citation burst were the Warburton and Bredin paper on health benefits of physical activity (), the Brooks et al. paper on the ...
Smartphones are now nearly ubiquitous; their numerous built-in sensors enable continuous measurement of activities of daily living, making them especially well-suited for health research.
In The Lancet Psychiatry, Chekroud and colleagues1 presented a large cross-sectional examination of physical activity and mental health. Despite imprecision about the terms mental health and exercise in the study—and the cross-sectional design—the findings overall match the existing body of longitudinal research showing that regular physical activity is associated with better mental health.2
physical activity, physical exercise, menta l health, psychological health. After se lecting papers related to the criteria, 20 studies were selected to meet the needs of this systematic re view.
A key relationship between mental and physical health has been hypothesized and observed since ancient times with the Latin motto "mens sana in corpore sano" (healthy mind in a healthy body). ... Furthermore, surveys and qualitative research papers (including reviews of the literature, guidelines, and consensus statements) were considered ...
The association between physical activity and mental health among adults and old adults. The details of the association between PA and mental health among adults and old adults are summarised in Table 1, 27 studies were included [24,25,26,27,28,29,30,31,32,33,34,35,36,37,38,39,40,41,42,43,44,45,46,47,48,49, 51]. Participants characteristics and date of filling the questionnaires
Aim of the paper: To review the positive effects of physical activity on psychological well-being and its possible neurobiological underpinnings, as well as its impact on several neuropsychiatric ...
INTRODUCTION. Sleep is vital for health and well-being in children, adolescents, and adults. 1-3 Healthy sleep is important for cognitive functioning, mood, mental health, and cardiovascular, cerebrovascular, and metabolic health. 4 Adequate quantity and quality of sleep also play a role in reducing the risk of accidents and injuries caused by sleepiness and fatigue, including workplace ...
Despite frequent discussions on the link between physical and mental health, the specific impact of physical fitness on mental well-being is yet to be fully established. Shuzhen Ma, Yanqi Xu, Simao Xu and Zhicheng Guo. BMC Public Health 2024 24 :1365. Research Published on: 21 May 2024.
Sleep is vital for people's physical and mental health, and sound sleep can help them focus on daily activities. Therefore, a sleep study that includes sleep patterns and disorders is crucial to enhancing our knowledge about individuals' health status. The findings on sleep stages and sleep disorders relied on polysomnography and self-report measures, and then the study went through clinical ...
The categories and codes were transferred to the excel spreadsheet and compared. In case of divergences, a consensus was found through joint discussion. With regard to the conceptualisation of health, papers were classified as presenting a narrow conceptualisation of health if they exclusively proposed physical aspects of health as content for PE.
Abstract. In addition to the apparent physical health benefits, physical activity also affects mental health positively. Physically inactive individuals have been reported to have higher rates of morbidity and healthcare expenditures. Commonly, exercise therapy is recommended to combat these challenges and preserve mental wellness.
The global issue of substance abuse demands ongoing initiatives aligned with the United Nations Sustainable Development Goals. With drug use remaining prevalent worldwide, interventions are critical to addressing the associated health challenges and societal implications. Exercise and physical activities have emerged as integral components of substance use disorder (SUD) treatment, offering ...
Individualized information and methods to promote good physical health are required for this group in jeopardy from physical morbidity and mortality. Discover the world's research 25+ million members
Insufficient physical activity and excessive sitting time among office-based workers have been linked to various health risks and economic consequences. While health promotion interventions are important, the role of workplace design in encouraging active behaviours is increasingly recognised. However, significant gaps exist in knowledge about how workplace design influences these behaviours.
Based on the type, timing and severity of the applied stimulus, stress can exert various actions on the body ranging from alterations in homeostasis to life-threatening effects and death. In many cases, the pathophysiological complications of disease arise from stress and the subjects exposed to stress, e.g. those that work or live in stressful ...
Sexual and gender minorities (SGMs) experience a higher mental health burden compared to their cisgender, heterosexual counterparts. Role models and mentors are important for wellbeing and development; however, little evidence exists exploring their impact on SGM people. This systematic scoping review identifies their association with mental and physical wellbeing. Eight databases (Medline ...
Dog ownership, in particular, is linked to higher levels of physical activity, social support, and better recovery from catastrophic cardiovascular events. Pet ownership is connected to a lower risk of cardiovascular disease in the general US population, according to a theory put out by Krittanawong et al. (2020).
Positive psychology is an umbrella term for the scientific study of the various contributors to a healthy and thriving life for the self and others (eg, positive emotions, life meaning, engaging work, and close relationships). 2 It is the study of strengths, assets, and positive attributes. The topics of concern to positive psychology are broad ...