
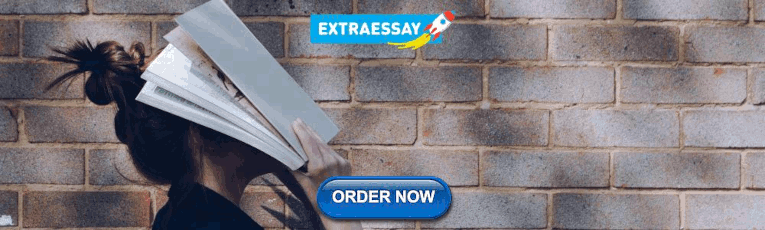
IMRAD Format For Research Papers: The Complete Guide

Thank you for reading DrAiMD’s Substack. This post is public so feel free to share it.
Writing a strong research paper is key to succeeding in academia, but it can be overwhelming to know where to start. That’s where the IMRAD format comes in. IMRAD provides a clear structure to help you organize and present your research logically and coherently. In this comprehensive guide, we’ll explain the IMRAD format, why it’s so important for research writing, and how to use it effectively. Follow along to learn the ins and outs of crafting papers in the gold-standard IMRAD structure. In this article, I’ll walk you through the IMRAD format step-by-step. I’ll explain each section, how to write it, and what to avoid. By the end of this article, you’ll be able to write a research paper that is clear, concise, and well-organized.
What is IMRAD Format?
IMRAD stands for Introduction, Methods, Results, and Discussion . It’s a way of organizing a scientific paper to make the information flow logically and help readers easily find key details. The IMRAD structure originated in medical journals but is now the standard format for many scientific fields.
Thanks for reading DrAiMD’s Substack! Subscribe for free to receive new posts and support my work.
Here’s a quick overview of each section’s purpose:
Introduction : Summary of prior research and objective of your study
Methods : How you carried out the study
Results : Key findings and analysis
Discussion : Interpretation of results and implications
Most papers also include an abstract at the beginning and a conclusion at the end to summarize the entire report.
Why is the IMRAD Format Important?
Using the IMRAD structure has several key advantages:
It’s conventional and familiar. Since I MRAD is so widely used , it helps ensure editors, reviewers, and readers can easily find the details they need. This enhances clarity and comprehension.
It emphasizes scientific rigor. The methods and results sections encourage thorough reporting of how you conducted the research. This supports transparency, credibility, and reproducibility.
It encourages precision. The structure necessitates concise writing focused only on the core aims and findings. This avoids rambling or repetition.
It enables efficient reading. Readers can quickly skim to the sections most relevant to them, like only reading the methods. IMRAD facilitates this selective reading.
In short, the IMRAD format ensures your writing is clear, precise, rigorous, and accessible – crucial qualities in scientific communication.
When Should You Use IMRAD Format?
The IMRAD structure is ideal for:
Primary research papers that report new data and findings
Review papers that comprehensively summarize prior research
Grant proposals requesting funding for research
IMRAD is not typically used for other paper types like:
Editorials and opinion pieces
Popular science articles for general audiences
Essays analyzing a topic rather than presenting new data
So, if you are writing a scholarly scientific paper based on experiments, investigations, or observational studies, the IMRAD format is likely expected. Embrace this conventional structure to help communicate your exciting discoveries.
Now that we’ve covered the key basics let’s dive into how to write each section of an IMRAD paper.
The abstract is a succinct summary of your entire paper, typically around 200 words. Many readers will only read the abstract, so craft it carefully to function as a standalone piece highlighting your most important points.
Elements to include:
Research problem, question, or objectives
Methods and design
Major findings or developments
Conclusions and implications
While written first, refine the abstract last to accurately encapsulate your final paper. A clear precise abstract can help attract readers and set the tone for your work. Take a look at our complete guide to abstract writing here !
INTRODUCTION
The Introduction provides the necessary background context and sets up the rationale for your research. Start by briefly summarizing the core findings from previous studies related to your topic to orient readers to the field. Provide more detail on the specific gaps, inconsistencies, or unanswered questions in the research your study aims to address. Then, clearly state your research questions, objectives, experimental hypotheses, and overall purpose or anticipated contributions. The Introduction establishes why your research is needed and clarifies your specific aims. Strive for a concise yet comprehensive overview that lets readers learn more about your fascinating study. Writing a good introduction is like writing a good mini-literature review on a subject. Take a look at our complete guide to literature review writing here!
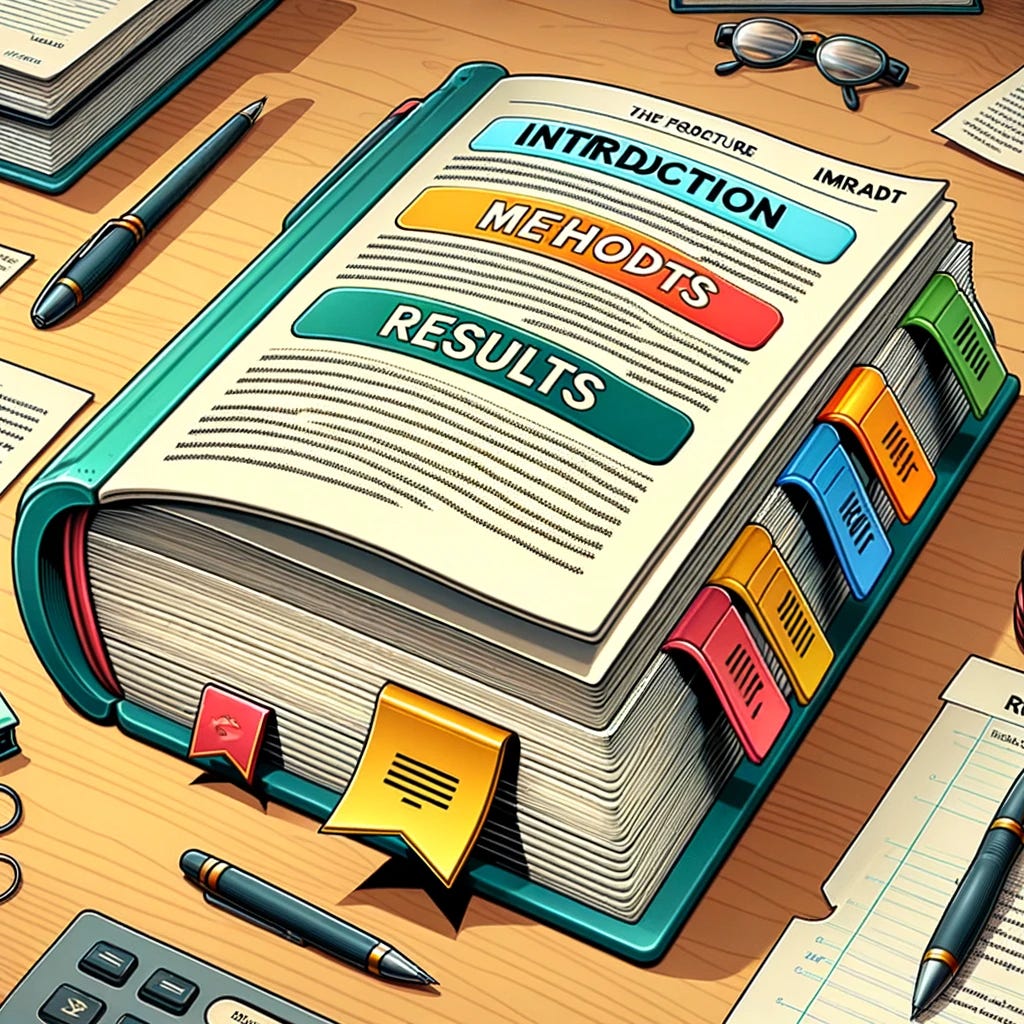
The methods section is the nuts and bolts, where you comprehensively describe how you carried out the research. Sufficient detail is crucial so others can assess your work and reproduce the study. Take a look at our complete guide to writing an informative and tight literature review here!
Research Design
Start by explaining the overall design and approach. Specify:
Research types like experimental, survey, observational, etc.
Study duration
Sample size
Control vs experimental groups
Clarify the variables, treatments, and factors involved.
Participants
Provide relevant characteristics of the study population or sample, such as:
Health status
Geographic location
For human studies, include recruitment strategies and consent procedures.
List any instruments, tests, assays, chemicals, or other materials utilized. Include details like manufacturers and catalog numbers.
Chronologically explain each step of the experimental methods. Be precise and thorough to enable replication. Use past tense and passive voice.
Data Analysis
Describe any statistical tests, data processing, or software used to analyze the data.
The methods section provides the roadmap of your research journey. Strive for clarity and completeness. Now we’re ready for the fun part – the results!
This section shares the key findings and data from your study without interpretation. The results should mirror the methods used.
Report Findings Concisely
Use text, figures, and tables to present the core results:
Focus only on key data directly related to your objectives
Avoid lengthy explanations and extraneous details
Highlight the most groundbreaking findings
Use Visuals to Present Complex Data
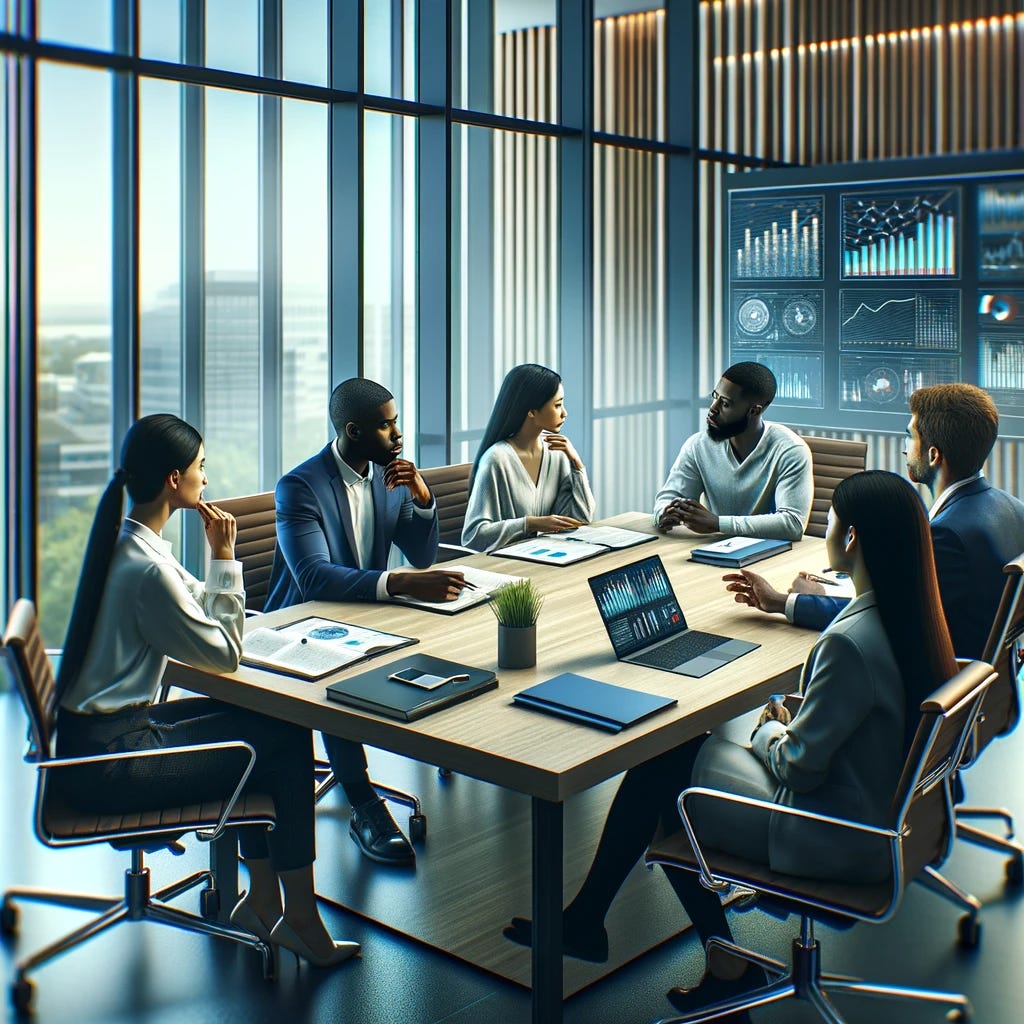
Tables and figures efficiently communicate more complex data:
Tables organize detailed numerical or textual data
Figures vividly depict relationships like graphs, diagrams, photos
Include clear captions explaining what is shown
Refer to each visual in the text
Reporting your results objectively lays the groundwork for the next section – making sense of it all through discussion.
Here, you interpret the data, explain the implications, acknowledge limitations, and make recommendations for future research. The discussion allows you to show the greater meaning of your study.
Interpret the Findings
Analyze the results in the context of your initial hypothesis and prior studies:
How do your findings compare to past research? Are they consistent or contradictory?
What conclusions can you draw from the data?
What theories or mechanisms could explain the outcomes?
Discuss the Implications
Address the impact and applications of the research:
How do the findings advance scientific understanding or technical capability?
Can the results improve processes, design, or policies in related fields?
What innovations or new research directions do they enable?
Identify Limitations and Future Directions
No study is perfect, so discuss potential weaknesses and areas for improvement:
Were there any methodological limitations that could influence the results?
Can the research be expanded by testing new variables or conditions?
How could future studies build on your work? What questions remain unanswered?
A thoughtful discussion emphasizes the meaningful contributions of your research.
The conclusion recaps the significance of your study and key takeaways. Like the abstract, many readers may only read your opening and closing, so ensure the conclusion packs a punch.
Elements to cover:
Restate the research problem and objectives
Summarize the major findings and main points
Emphasize broader implications and applications
The conclusion provides the perfect opportunity to drive home the importance of your work. End on a high note that resonates with readers.
The IMRAD format organizes research papers into logical sections that improve scientific communication. By following the Introduction-Methods-Results-and-Discussion structure, you can craft clear, credible, and impactful manuscripts. Use IMRAD to empower readers to comprehend and assess your exciting discoveries efficiently. With this gold-standard format under your belt, your next great paper is within reach.

Ready for more?
- Communicating in STEM Disciplines
- Features of Academic STEM Writing
- STEM Writing Tips
- Academic Integrity in STEM
- Strategies for Writing
- Science Writing Videos – YouTube Channel
- Educator Resources
- Lesson Plans, Activities and Assignments
- Strategies for Teaching Writing
- Grading Techniques
IMRAD (Introduction, Methods, Results and Discussion)
Academic research papers in STEM disciplines typically follow a well-defined I-M-R-A-D structure: Introduction, Methods, Results And Discussion (Wu, 2011). Although not included in the IMRAD name, these papers often include a Conclusion.
Introduction
The Introduction typically provides everything your reader needs to know in order to understand the scope and purpose of your research. This section should provide:
- Context for your research (for example, the nature and scope of your topic)
- A summary of how relevant scholars have approached your research topic to date, and a description of how your research makes a contribution to the scholarly conversation
- An argument or hypothesis that relates to the scholarly conversation
- A brief explanation of your methodological approach and a justification for this approach (in other words, a brief discussion of how you gather your data and why this is an appropriate choice for your contribution)
- The main conclusions of your paper (or the “so what”)
- A roadmap, or a brief description of how the rest of your paper proceeds
The Methods section describes exactly what you did to gather the data that you use in your paper. This should expand on the brief methodology discussion in the introduction and provide readers with enough detail to, if necessary, reproduce your experiment, design, or method for obtaining data; it should also help readers to anticipate your results. The more specific, the better! These details might include:
- An overview of the methodology at the beginning of the section
- A chronological description of what you did in the order you did it
- Descriptions of the materials used, the time taken, and the precise step-by-step process you followed
- An explanation of software used for statistical calculations (if necessary)
- Justifications for any choices or decisions made when designing your methods
Because the methods section describes what was done to gather data, there are two things to consider when writing. First, this section is usually written in the past tense (for example, we poured 250ml of distilled water into the 1000ml glass beaker). Second, this section should not be written as a set of instructions or commands but as descriptions of actions taken. This usually involves writing in the active voice (for example, we poured 250ml of distilled water into the 1000ml glass beaker), but some readers prefer the passive voice (for example, 250ml of distilled water was poured into the 1000ml beaker). It’s important to consider the audience when making this choice, so be sure to ask your instructor which they prefer.
The Results section outlines the data gathered through the methods described above and explains what the data show. This usually involves a combination of tables and/or figures and prose. In other words, the results section gives your reader context for interpreting the data. The results section usually includes:
- A presentation of the data obtained through the means described in the methods section in the form of tables and/or figures
- Statements that summarize or explain what the data show
- Highlights of the most important results
Tables should be as succinct as possible, including only vital information (often summarized) and figures should be easy to interpret and be visually engaging. When adding your written explanation to accompany these visual aids, try to refer your readers to these in such a way that they provide an additional descriptive element, rather than simply telling people to look at them. This can be especially helpful for readers who find it hard to see patterns in data.
The Discussion section explains why the results described in the previous section are meaningful in relation to previous scholarly work and the specific research question your paper explores. This section usually includes:
- Engagement with sources that are relevant to your work (you should compare and contrast your results to those of similar researchers)
- An explanation of the results that you found, and why these results are important and/or interesting
Some papers have separate Results and Discussion sections, while others combine them into one section, Results and Discussion. There are benefits to both. By presenting these as separate sections, you’re able to discuss all of your results before moving onto the implications. By presenting these as one section, you’re able to discuss specific results and move onto their significance before introducing another set of results.
The Conclusion section of a paper should include a brief summary of the main ideas or key takeaways of the paper and their implications for future research. This section usually includes:
- A brief overview of the main claims and/or key ideas put forth in the paper
- A brief discussion of potential limitations of the study (if relevant)
- Some suggestions for future research (these should be clearly related to the content of your paper)
Sample Research Article
Resource Download
Wu, Jianguo. “Improving the writing of research papers: IMRAD and beyond.” Landscape Ecology 26, no. 10 (November 2011): 1345–1349. http://dx.doi.org/10.1007/s10980-011-9674-3.
Further reading:
- Organization of a Research Paper: The IMRAD Format by P. K. Ramachandran Nair and Vimala D. Nair
- George Mason University Writing Centre’s guide on Writing a Scientific Research Report (IMRAD)
- University of Wisconsin Writing Centre’s guide on Formatting Science Reports
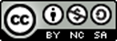
Structure of a Research Paper

Structure of a Research Paper: IMRaD Format
I. The Title Page
- Title: Tells the reader what to expect in the paper.
- Author(s): Most papers are written by one or two primary authors. The remaining authors have reviewed the work and/or aided in study design or data analysis (International Committee of Medical Editors, 1997). Check the Instructions to Authors for the target journal for specifics about authorship.
- Keywords [according to the journal]
- Corresponding Author: Full name and affiliation for the primary contact author for persons who have questions about the research.
- Financial & Equipment Support [if needed]: Specific information about organizations, agencies, or companies that supported the research.
- Conflicts of Interest [if needed]: List and explain any conflicts of interest.
II. Abstract: “Structured abstract” has become the standard for research papers (introduction, objective, methods, results and conclusions), while reviews, case reports and other articles have non-structured abstracts. The abstract should be a summary/synopsis of the paper.
III. Introduction: The “why did you do the study”; setting the scene or laying the foundation or background for the paper.
IV. Methods: The “how did you do the study.” Describe the --
- Context and setting of the study
- Specify the study design
- Population (patients, etc. if applicable)
- Sampling strategy
- Intervention (if applicable)
- Identify the main study variables
- Data collection instruments and procedures
- Outline analysis methods
V. Results: The “what did you find” --
- Report on data collection and/or recruitment
- Participants (demographic, clinical condition, etc.)
- Present key findings with respect to the central research question
- Secondary findings (secondary outcomes, subgroup analyses, etc.)
VI. Discussion: Place for interpreting the results
- Main findings of the study
- Discuss the main results with reference to previous research
- Policy and practice implications of the results
- Strengths and limitations of the study
VII. Conclusions: [occasionally optional or not required]. Do not reiterate the data or discussion. Can state hunches, inferences or speculations. Offer perspectives for future work.
VIII. Acknowledgements: Names people who contributed to the work, but did not contribute sufficiently to earn authorship. You must have permission from any individuals mentioned in the acknowledgements sections.
IX. References: Complete citations for any articles or other materials referenced in the text of the article.
- IMRD Cheatsheet (Carnegie Mellon) pdf.
- Adewasi, D. (2021 June 14). What Is IMRaD? IMRaD Format in Simple Terms! . Scientific-editing.info.
- Nair, P.K.R., Nair, V.D. (2014). Organization of a Research Paper: The IMRAD Format. In: Scientific Writing and Communication in Agriculture and Natural Resources. Springer, Cham. https://doi.org/10.1007/978-3-319-03101-9_2
- Sollaci, L. B., & Pereira, M. G. (2004). The introduction, methods, results, and discussion (IMRAD) structure: a fifty-year survey. Journal of the Medical Library Association : JMLA , 92 (3), 364–367.
- Cuschieri, S., Grech, V., & Savona-Ventura, C. (2019). WASP (Write a Scientific Paper): Structuring a scientific paper. Early human development , 128 , 114–117. https://doi.org/10.1016/j.earlhumdev.2018.09.011

Research Paper Basics: IMRaD
- Finding Databases in GALILEO
- Finding Journals in GALILEO
- Finding Materials in GIL-Find
- ProQuest Research Companion
- How to Search JSTOR
- Scholarly/Peer-Reviewed vs. Popular
- Tutorial: Why Citations Matter
- Literature Review
- Annotated Bibliography
- Podcast Studio
- Reserve a Room
- Share Your Work
- Finding Images
- Using RICOH Boards
- Writing Guides
- The Research Process
What is IMRaD?
IMRaD is an acronym for Introduction , Methods , Results , and Discussion . It describes the format for the sections of a research report. The IMRaD (or IMRD) format is often used in the social sciences, as well as in the STEM fields.
Credit: IMRD: The Parts of a Research Paper by Wordvice Editing Service on YouTube
Outline of Scholarly Writing
With some variation among the different disciplines, most scholarly articles of original research follow the IMRD model, which consists of the following components:
Introduction
- Statement of Problem (i.e. "the Gap")
- Plan to Solve the Problem
Method & Results
- How Research was Done
- What Answers were Found
- Interpretation of Results (What Does It Mean?)
- Implications for the Field
This form is most obvious in scientific studies, where the methods are clearly defined and described, and data is often presented in tables or graphs for analysis.
In other fields, such as history, the method and results may be embedded in a narrative, perhaps describing and interpreting events from archival sources. In this case, the method is the selection of archival sources and how they were interpreted, while the results are the interpretation and resultant story.
In full-length books, you might see this general pattern followed over the entire book, within each chapter, or both.

Credit: Howard-Tilton Memorial Library at Tulane University. This work is licensed under a Creative Commons Attribution-NonCommercial 4.0 International License .
IMRAD Format
- Writing Center | George Mason University
- IMRAD Outlining | Excelsior College
- Florida Atlantic University Libraries
- << Previous: Annotated Bibliography
- Next: Group Project Tools >>
- Last Updated: Apr 18, 2024 9:25 PM
- URL: https://libguides.ccga.edu/researchbasics
Gould Memorial Library College of Coastal Georgia One College Drive Brunswick, GA 31520 (912) 279-5874 Library Hours Camden Center Library College of Coastal Georgia 8001 Lakes Blvd / Wildcat Blvd Kingsland, GA 31548 (912) 510-3332 Library Hours
The Visual Communication Guy
Learn Visually. Communicate Powerfully.

- About The VCG
- Contact Curtis
- Five Paragraph Essay
- IMRaD (Science)
- Indirect Method (Bad News)
- Inverted Pyramid (News)
- Martini Glass
- Narrative Format
Rogerian Method
- Toulmin Method
- Apostrophes
- Exclamation Marks (Points)
- Parentheses
- Periods (Full Stops)
- Question Marks
- Quotation Marks
- Plain Language
- APPEALS: ETHOS, PATHOS, LOGOS
- CLUSTER ANALYSIS
- FANTASY-THEME
- GENERIC CRITICISM
- IDEOLOGICAL CRITICISM
- NEO-ARISTOTELIAN
- O.P.T.I.C. (VISUAL ANALSYIS)
- S.O.A.P.S.T.O.N.E. (WRITTEN ANALYSIS)
- S.P.A.C.E.C.A.T. (RHETORICAL ANALYSIS)
- BRANCHES OF ORATORY
- FIGURES OF SPEECH
- FIVE CANONS
- LOGICAL FALLACIES
- Information Design Rules
- Arrangement
- Organization
- Negative Space
- Iconography
- Photography
- Which Chart Should I Use?
- “P” is for PREPARE
- "O" is for OPEN
- "W" is for WEAVE
- “E” is for ENGAGE
- PRESENTATION EVALUTION RUBRIC
- POWERPOINT DESIGN
- ADVENTURE APPEAL
- BRAND APPEAL
- ENDORSEMENT APPEAL
- HUMOR APPEAL
- LESS-THAN-PERFECT APPEAL
- MASCULINE & FEMININE APPEAL
- MUSIC APPEAL
- PERSONAL/EMOTIONAL APPEAL
- PLAIN APPEAL
- PLAY-ON-WORDS APPEAL
- RATIONAL APPEAL
- ROMANCE APPEAL
- SCARCITY APPEAL
- SNOB APPEAL
- SOCIAL APPEAL
- STATISTICS APPEAL
- YOUTH APPEAL
- The Six Types of Résumés You Should Know About
- Why Designing Your Résumé Matters
- The Anatomy of a Really Good Résumé: A Good Résumé Example
- What a Bad Résumé Says When It Speaks
- How to Write an Amazing Cover Letter: Five Easy Steps to Get You an Interview
- Make Your Boring Documents Look Professional in 5 Easy Steps
- Business Letters
- CONSUMER PROFILES
- ETHNOGRAPHY RESEARCH
- FOCUS GROUPS
- OBSERVATIONS
- SURVEYS & QUESTIONNAIRES
- S.W.O.T. ANALYSES
- USABILITY TESTS
- CITING SOURCES: MLA FORMAT
- MLA FORMAT: WORKS CITED PAGE
- MLA FORMAT: IN-TEXT CITATIONS
- MLA FORMAT: BOOKS & PAMPHLETS
- MLA FORMAT: WEBSITES AND ONLINE SOURCES
- MLA FORMAT: PERIODICALS
- MLA FORMAT: OTHER MEDIA SOURCES
- Course Syllabi
- Checklists and Peer Reviews (Downloads)
- Communication
- Poster Prints
- Poster Downloads
- Handout & Worksheet Downloads
- QuickGuide Downloads
- Downloads License Agreements
How to Organize a Paper: The IMRaD Format

What is the IMRaD Format?
The IMRaD (often pronounced “im-rad”) format is a scientific writing structure that includes four or five major sections: introduction (I); research methods (M); results (R); analysis (a); and discussion (D). The IMRaD format is the most commonly used format in scientific article and journal writing and is used widely across most scientific and research fields.
When Do I Use the IMRaD Format?
If you are writing a paper where you are conducting objective research in order answer a specific question, the IMRaD format will most likely serve your purposes best. The IMRaD format is especially useful if you are conducting primary research (such as experimentation, questionnaires, focus groups, observations, interviews, and so forth), but it can be applied even if you only conduct secondary research (which is research you gather from reading sources like books, magazines, journal articles, and so forth.)
The goal of using the IMRaD format is to present facts objectively, demonstrating a genuine interest and care in developing new understanding about a topic; when using this format, you don’t explicitly state an argument or opinion, but rather, you rely on collected data and previously researched information in order to make a claim.
While there are nuances and adjustments that would be made to the following document types, the IMRaD format is the foundational structure many research-driven documents:
- Recommendation reports
- Plans (such as an integrated marketing plan or project management plan)
How Does the IMRaD Format Work?
As mentioned above, the IMRaD format includes four or five major sections. The little “a” has had multiple interpretations over the years; some would suggest it means nothing other than “and,” as in “Introduction, Methods, Results, and Discussion,” but others have argued that the “a” should be viewed as “Analysis” in papers where the “Results” section may not be immediately clear and a section that analyzes the results is important for reader comprehension. Either way, the “a” often remains in lower-case to indicate that, while it’s often important, it isn’t always necessary. Below, we’ll review the five major sections, with “a” given equal weight to the other sections.
Note that these five sections should always go in the order listed below:
- Statement of the topic you are about to address
- Current state of the field of understanding (often, we call this a literature review and it may even merit having its own section)
- Problem or gap in knowledge (what don’t we know yet or need to know? what does the field still need to understand? what’s been left out of previous research? is this a new issue that needs some direction?)
- Forecast statement that explains, very briefly, what the rest of the paper will entail, including a possible quick explanation of the type of research that needs to be conducted
- Separate each type of research you conducted (interviews, focus groups, experiments, etc.) into sub-sections and only discuss one research method in each sub-section (for clarity and organization, it’s important to not talk about multiple methods at once)
- Be very detailed about your process. If you interviewed people, for example, we need to know how many people you interviewed, what you asked them, what you hoped to learn by interviewing them, why chose to interview over other methods, why you interviewed those people specifically (including providing they demographic information if it’s relevant), and so forth. For other types of data collection, we need to know what your methods were–how long you observed; how frequently you tested; how you coded qualitative data; and so forth.
- Don’t discuss what the research means. You’ll use the next two sections–Analysis and Discussion–to talk about what the research means. To stay organized, simply discuss your research methods. This is the single biggest mistake when writing research papers, so don’t fall into that trap.
- Results: The results section is critical for your audience to understand what the research showed. Use this section to show tables, charts, graphs, quotes, etc. from your research. At this point, you are building your reader towards drawn conclusions, but you are not yet providing a full analysis. You’re simply showing what the data says. Follow the same order as the Methods section–if you put interviews first, then focus groups second, do the same in this section. Be sure, when you include graphics and images, that you label and title every table or graphic (“ Table 3: Interview Results “) and that you introduce them in the body of your text (“As you can see in Figure 1 , seventy-nine percent of respondents…”)
- Analysis: The analysis section details what you and others may learn from the data. While some researchers like to combine this section with the Discussion section, many writers and researchers find it useful to analyze the data separately. In the analysis section, spend time connecting the dots for the reader. What do the interviews say about the way employers think about their employees? What do the observations say about how employees respond to workplace criticism? Can any connections be made between the two research types? It’s important in the Analysis section that you don’t draw conclusions that the research findings don’t suggest. Always stick to what the research says.
- Discussion: Finally, you conclude this paper by suggesting what new knowledge this provides to the field. You’ll often want to note the limitations of your study and what further research still needs to be done. If something alarming or important was discovered, this is where you highlight that information. If you use the IMRaD format to write other types of papers (like a recommendation report or a plan), this is where you put the recommendations or the detailed plan.
Back to the Organization Memo
Other Formats
Indirect Method
Proposal Format
Shop for your perfect poster print or digital download at our online store!
English Editing Research Services

Tips for Writing a Research Paper – IMRaD Structure
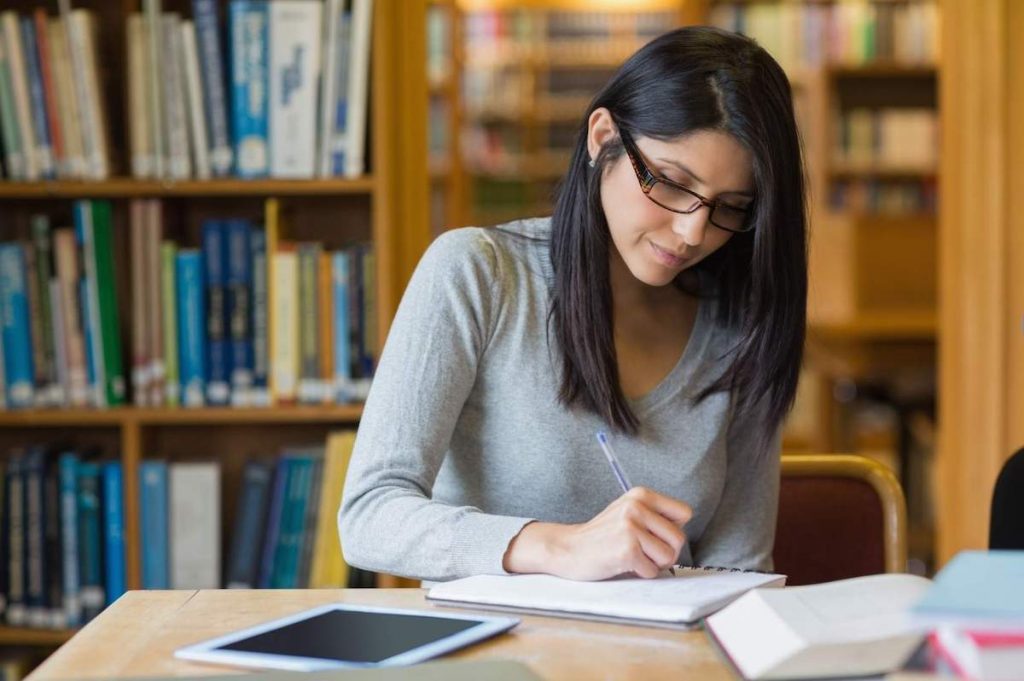
Peer-reviewed academic journals publish a variety of article types, such as research articles that report original research, reviews of the literature, and case reports of a small number of interesting cases. Each article type has its own specific format, and it is important that you use the appropriate one.
1. Know IMRaD
Original research papers usually use the IMRaD formula. This acronym includes the four main sections of a research paper, which answer four basic questions, as follows:
- I ntroduction: Why did you do the study?
- M ethods: What did you do?
- R esults: What did you find? a nd…
- D iscussion: What do your findings mean? How do you advance your field?
According to the International Committee of Medical Journal Editors , the “[ IMRaD ] structure is not an arbitrary publication format but a reflection of the process of scientific discovery.” The full structure is actually TA-IMRaD-RAS , because research papers begin with a Title and Abstract , end with the References, and often also have an Acknowledgment and various Statements. Some features of these additional sections are as follows:
- Title: usually part of the submitted Title Page, which also contains authors’ details and often the word count and number of illustrations (tables and figures)
- Abstract: a summary of the study with or without subheadings such as Introduction, Methods, Results, Conclusion; usually ends with key words
- References: two commonly used styles are numbering in order of appearance (Vancouver) and alphabetical by surname and in date order (Harvard); the style and the position of the reference list depend on the journal
- Acknowledgments : here, you thank people who do not qualify for authorship but who helped you with the research or its analysis, reporting, and presentation
- Statements: declarations of, for example, work contributed by each author, funding source/s, conflicts of interest (reasons for any perceived bias), ethics approval , whether the data can be accessed by others, any supplementary methods/results files online, and whether any of the work has been previously presented; these declarations are sometimes made on the submitted Title Page and may appear at the beginning or end of the published article
Get the full details on using IMRaD in this handy infographic you can download from the Edanz Learning Lab eBooks and infographics .
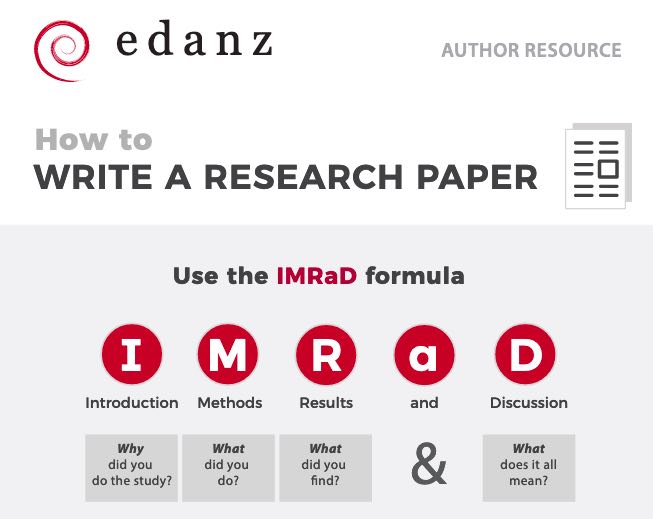
2. Find a target journal early
Refer to the author guidelines of your target journal early on in the writing process. These guidelines explain the journal’s requirements for manuscript preparation, for example:• Word count of the main text• Word count and format of the abstract• Variations of IMRaD structure:o Methods may be at the end or combined with Resultso Results may be combined with Discussion
o Methods, Results, and Discussion may all be combined as one or more sections, with different headings for different parts of the study
- IMRaD section names (for example, Introduction, Background, or no heading for the first section of IMRaD)
- Extra sections: some journals require a Literature Review or Related Work section between the Introduction and Methods; some require Conclusion and Future Work sections after the Discussion
- Number and style of references
- Number and formatting of illustrations and associated text, and placement of illustrations within the main text, at the end, or in separate files
- What statements to include and if there are special online forms to complete
- General formatting (such as double line spacing)
- UK or US spelling
Using the free Edanz Journal Selector will help you find a suitable journal and its online author guidelines.
3. Use the “write” order
To increase your writing efficiency, use TA-MRDI order instead of TA-IMRaD . Otherwise, you may waste time at the start by writing an Introduction that is too long or unrelated to the rest of the paper.
The “write” order of TA-MRDI, with the Introduction written at the end, will allow you to build a focused academic argument and help convince the reader of the need for and importance of your study. The recommended order for writing your research paper is actually based on your illustrations and can be summarized in these 10 steps:
1. Preparation
- Draft your illustrations, put them in a logical order
- Summarize each illustration’s key point
- Use the key points and notes from your initial reading to make a brief IMRaD outline to answer the questions: Why did you do the study? What did you do? What did you find? What does it all mean?
2. Title
Announce the most important feature of your research.
3. Abstract
Summarize the key messages of your IMRaD outline in the abstract ; input text into the Edanz Journal Selector to find a target journal.
4. Methods
Describe the materials/samples, procedures, and analytical methods in the order of your illustrations to allow others to repeat your study.
5. Results
Finalize your illustrations and highlight their main features in the main text.
6. Discussion
Evaluate your results in the context of the published literature, identify strengths and weaknesses, draw conclusions, and include implications and future directions.
7. Introduction
Present enough information for readers to understand your study’s aim, design, conclusions, implications, and importance; the amount of background depends on the target journal readership (for example, generalists vs. specialists).
8. References, etc.
Prepare the References and any Acknowledgment/s and Statements .
Finalize the Title .
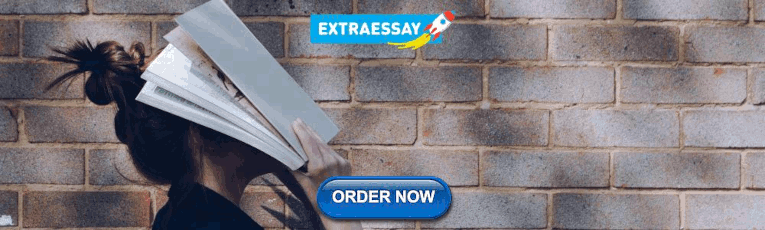
10. Finally…the abstract again
Finalize the Abstract .The “write” order of TA-MRDI will allow you to save time and start writing even while you are still performing the research. As soon as you have analyzed your results, prepare the illustrations and make sure they have corresponding descriptions in the Methods section of the main text. The Methods are factual, recent, and familiar to you, so they should be relatively easy to describe.
In the main text of the Results , you highlight the main features of your data and illustrations, making sure to describe relationships between the data instead of just repeating what is already shown in the illustrations.
In the Discussion , you compare your findings with those already published, and you identify strengths and weaknesses of your research. In this way, you evaluate your results in the context of what is already known in your field, and you can draw conclusions and propose practical and conceptual implications and future research directions.
While you read the relevant literature , you can decide which published articles will help frame your research in the Introduction , especially if your study design and data are of a higher quality than those in the literature.
After writing the Discussion, you will also have a clear idea of the key findings, variables, concepts, theories, and topics that need to be explained to the reader in the Introduction. By writing the Introduction last, you will provide readers with a logical and convincing rationale for your study and help them to understand the relevance and usefulness of your findings.
Complying with the author guidelines of your target journal and being familiar with IMRaD and the “write” order of TA-MRDI will help you prepare your manuscript efficiently and completely.
Finally, after drafting your manuscript, remember to revise, edit, and proofread your manuscript. You’re well on your way to outperforming your competition and raising your publication rate .
Structured abstract generator (SAG) model: analysis of IMRAD structure of articles and its effect on extractive summarization
- Open access
- Published: 07 May 2024
Cite this article
You have full access to this open access article
- Ayşe Esra Özkan Çelik ORCID: orcid.org/0000-0002-2553-0361 1 &
- Umut Al 2
120 Accesses
Explore all metrics
An abstract is the most crucial element that may convince readers to read the complete text of a scientific publication. However, studies show that in terms of organization, readability, and style, abstracts are also among the most troublesome parts of the pertinent manuscript. The ultimate goal of this article is to produce better understandable abstracts with automatic methods that will contribute to scientific communication in Turkish. We propose a summarization system based on extractive techniques combining general features that have been shown to be beneficial for Turkish. To construct the data set for this aim, a sample of 421 peer-reviewed Turkish articles in the field of librarianship and information science was developed. First, the structure of the full-texts, and their readability in comparison with author abstracts, were examined for text quality evaluation. A content-based evaluation of the system outputs was then carried out. System outputs, in cases of using and ignoring structural features of full-texts, were compared. Structured outputs outperformed classical outputs in terms of content and text quality. Each output group has better readability levels than their original abstracts. Additionally, it was discovered that higher-quality outputs are correlated with more structured full-texts, highlighting the importance of structural writing. Finally, it was determined that our system can facilitate the scholarly communication process as an auxiliary tool for authors and editors. Findings also indicate the significance of structural writing for better scholarly communication.
Similar content being viewed by others
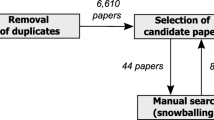
Artificial intelligence to automate the systematic review of scientific literature
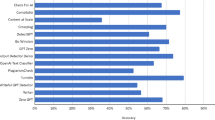
Testing of detection tools for AI-generated text
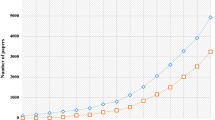
A tale of two databases: the use of Web of Science and Scopus in academic papers
Avoid common mistakes on your manuscript.
1 Introduction
Abstracts are the most important textual tools in enabling potential readers to read the relevant full-texts from the huge stack of electronic information retrieved through the Internet. It is reported that there is a correlation between a scientific article’s readability and impact determined by its subsequent citations or the possibility of being published in a top 5 journal in a relevant subject [ 1 , 2 ]. However, compared to the relevant full-texts, abstracts are even much more subject to readability issues and structural flaws in their contents [ 3 , 4 , 5 , 6 ].
The electronic versions of scientific publications have become more preferred than the printed ones in a short time, with their advanced functionality that accelerates the access and publishing process [ 7 ]. However, electronic formats of scientific publications are almost identical to the printed formats. Thus, the electronic forms of publications have not increased the user experience in terms of readability [ 8 ]. In contrast, online communication brings new challenges to the scientific community for analyzing retrieved documents. These challenges include the distraction caused by being online, the obligation to choose from a stack of related articles, and the difficulty of maintaining focus while navigating through linked web pages [ 9 , 10 , 11 ]. Research has shown that reading and comprehending a lengthy electronic text, which requires scrolling and navigating back and forth, demands more mental effort than reading a printed text [ 12 , 13 ]. Screen reading has been found to be inherently distracting, mainly because of the above mentioned multitasking nature of online reading [ 14 ].
While reading lengthy electronic texts can be challenging, scientific publications are constructed and archived following certain rules, making them highly structured text data [ 15 ]. The components of a scientific article, including title, abstract, keywords, article body, acknowledgments, bibliography, and appendices, each have very specific functions and are located in particular places within a manuscript. The article bodies also follow a well-defined structure over time, largely due to the introduction of the IMRAD (Introduction, Methods, Results, and Discussion) format by Pasteur in 1876 [ 3 ]. The IMRAD format is now widely adopted by the scientific community as it ensures that articles are well-organized and easy to read, regardless of whether they are published in electronic or print format. Each section has a specific role in communicating the research findings as follows:
Introduction: What was studied and why?
Methods: How was the study conducted?
Results: What were the findings?
Discussion: What do the findings mean?
Before reading the body text, readers first encounter titles and sometimes keywords that contain very limited information about the article. Abstracts, on the other hand, are the first and last stop for the reader to learn the content before proceeding to review the full-text. Therefore, for most readers, an article is as interesting as its abstract. Studies have shown that nearly half of the readers of scientific articles who read the abstracts also read the full-texts [ 16 ]. In a study, users’ transaction records of more than 1000 scientists, and 17,000 sessions on ScienceDirect were examined [ 6 , 17 ]. It was found that at least 20% of the users only read abstracts and that they trust the abstracts to select the relevant articles and to provide the necessary preliminary information for their research.
The language used in the abstract should be clear enough so that everyone can understand it, even if they don’t know much about the topic or English isn’t their first language. However, it’s often the case that abstracts are more difficult to read than the main body of an article [ 3 , 4 , 5 , 18 , 19 ]. Moreover, the abstract section should also cover the major information given in the full-text. Studies have found that skipping necessary information in abstracts is a frequently observed problem [ 6 , 20 , 21 , 22 ].
How can abstracts be written to persuade readers to read the full text, especially if the reader has difficulty understanding the abstract? Structured abstract writing may be a solution, as it can improve readability and comprehension by dividing the text into subheadings [ 23 ]. In this way the informativeness of the abstract increases. When compared to unstructured abstracts, structured abstracts have significantly higher information quality [ 24 ]. Further, the indexing performance of the publication increases. It provides ease of access to the user and increased relevance in search results. This facilitates access to the article for all users with varying degrees of familiarity with the subject of the publication. The structural headings can help readers to find and understand the information they need more easily. It is easier for the author to write an abstract using a structured format than a classical one. The author cannot forget to mention all parts of the publication in the abstract. In that manner, abstract full-text consistency increases. It is preferred more by the readers and authors than the classical versions [ 23 ].
Given the critical role of abstracts in scholarly communication, this study is conducted to enhance the informativeness of abstracts by utilizing the high readability of full-text sentences and the structured ordering inherited from the full-text articles.
2 Literature review
The main research topics related to abstracts in the literature deal with organizational issues, readability issues and presentation issues in general. Many researchers have found that abstracts do not follow the structural order followed in the full-text, if the journal does not have a specific policy on this issue.
In the process of deciding whether to read the full text of an academic article, readers are most interested in descriptive information about the research problem, method, or results. Skipping information about these parts in abstracts is a frequently observed problem [ 6 , 20 , 21 , 22 ]. The abstract of a scientific paper often contains long, inverted sentences with conjunctions and intensive use of specific technical terms or jargon related to the field. The conscious preference for such sophisticated language features has resulted in abstracts becoming progressively more difficult to read over time. The readability of an abstract is usually found more difficult than the other parts of the article [ 3 , 4 , 5 , 18 , 19 ]. Although the subject of the presentation is an element that should be considered separately from the readability context [ 25 ], it is difficult to read an abstract written in a single block without paragraphs and subtitles, in fonts smaller than the full-text, and sometimes in italics [ 27 , 27 ]. The abstract formats required by journals vary. The two most dominant formats are classical (or traditional) abstracts and structured abstracts. Classical abstracts which are preferred by most journals, are not produced in a format that will attract the attention of the reader within the scope of the presentation. Abstracts that are written in a single block in an unstructured format, without paragraphs and subheadings, are generally called classical. Structured abstracts must be produced by filling in all the structural titles specified by the journal.
Luhn [ 28 ] carried out his pioneering work in the field of automatic text summarization in order to save the reader time and effort in finding useful information in an article or report when the widespread use of the Internet and information technologies were not yet on the agenda. Since then, the summarization of scientific textual data has become a necessary and crucial task in Natural Language Processing (NLP) [ 29 , 30 ]. However, there are certain difficulties such as the abstract generation, having labeled training and test corpora, and the scaling of collections of large documents.
Research in automatic text summarization has witnessed a proliferation of techniques since the beginning. The process generally involves several stages, including pre-processing the source document, extracting relevant features, and applying a summary generation method or algorithms. In the pre-processing stage, text documents are prepared for the next stages using linguistic techniques such as sentence segmentation, punctuation removal, stop word filtering, stemming, etc. Then, words are converted to numbers for computers to decode language patterns. Common methods include bag-of-words, n-grams, tf-idf, and word embeddings. For feature extraction, some of the commonly used features [ 31 ] that are used at both the word and sentence level to identify and extract salient sentences from documents are listed below:
Word level features
Keywords (content words): Nouns, verbs, adjectives, and adverbs with high TF-IDF scores suggesting sentence importance.
Title words: Sentences containing words from the title are likely to be relevant to the topic of the document.
Cue Phrases: Phrases such as “conclusion”, “because”, “this information”, etc. that indicate structure or importance.
Biased words: Domain-specific words that reflect the topic of the document are considered important.
Capitalized words: Names or acronyms such as “UNICEF” that indicate important entities.
Sentence level features
Sentence Location: Sentences in the document are prioritized due to information hierarchy. For instance, beginning and ending sentences are likely to hold more weight.
Length: Optimal length of sentences plays an important role in identifying excessive detail or lack of information.
Paragraph Location: Similar to sentence location, beginning and ending paragraphs of the document carry higher weight.
Sentence-Sentence Similarity: Sentences with higher similarity to other sentences of the document indicate their importance.
Text summarization methods are typically confined to extractive and abstractive summarization. In extractive text summarization, supervised and unsupervised learning methods are applied. Supervised learning needs a labeled dataset containing both summarized and non-summarized text, while unsupervised learning uses advanced algorithms such as fuzzy-based, graph-based, concept-based, and latent semantics to process input automatically [ 32 ].
Summarization of scientific papers is one of the applications of automatic summarization. Abstract generation-based applications and citation-based applications are two main branches of scientific article summarization. Other applications focus on specific problems such as the summarization of tables, figures, or specific sections of the related article [ 29 ]. Turkish text summarization studies primarily used extractive techniques due to a deficiency of trained corpora, a requirement that is still unmet in languages with limited resources like Turkish [ 33 ].
In addition, in scientific article summarization, single-article summarization with extractive techniques has predominantly been used with the high dominance of combinations of statistical and machine learning approaches, and intrinsic evaluation methods which are largely based on ROUGE (Recall-Oriented Understudy for Gisting Evaluation) metrics [ 29 ]. The ROUGE evaluation of an automated scientific article summarization system that focused on the dataset containing academic articles shows that the extractive algorithms are better than the abstractive algorithms [ 34 ].
Our summarization model is based on a study [ 35 ] that evaluated the performance of 15 different extractive-based sentence selection methods, both individually and combined, on 20 Turkish news documents. The study aimed to select the most important sentences in a document. They analyzed the outputs of the methods based on the summaries of sentences hand-selected by 30 evaluators. The best results were obtained when the sentence position, number of common adjacencies, and inclusion of nouns were combined. While these features were combined in a linear function, their weights were kept equal.
3 Research objectives and questions
We propose a summarization model based on extractive techniques combining general sentence selection features that have been shown by human judgments to be beneficial for Turkish [ 35 ]. Our study aims to assess the suitability of the Turkish librarianship and information science (LIS) corpus for automatic summarization methods by evaluating it from a broad perspective, rather than developing our own method. We focus on the full-text structural order to improve the extractive sentence selection process. Additionally, we compare the readability levels of full texts and abstracts to emphasize the significance of readability in scholarly communication. Raising awareness of this issue is also important, especially among LIS professionals.
The field of LIS is a broad and interdisciplinary field that encompasses a wide range of research topics. That is characterized by integrating research paradigms and methodologies from various disciplines [ 36 ]. This interdisciplinary nature makes LIS an ideal domain to examine the structural layouts of various approaches employed in scientific articles which can be extended to other fields. Due to this characteristic, LIS was selected as the domain in this study.
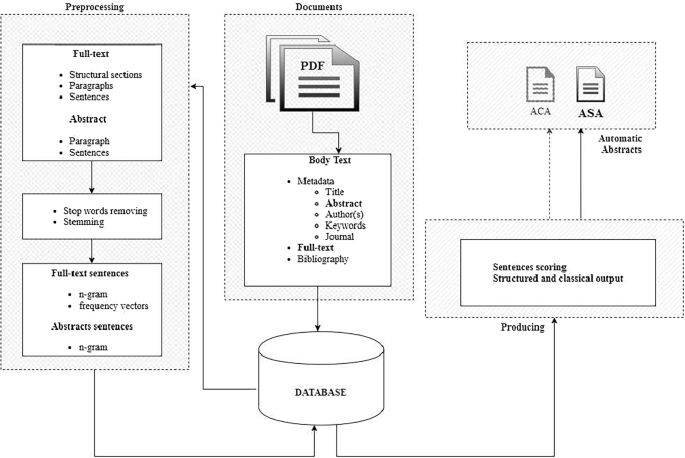
SAG architecture
The main goal of this study is to understand the benefits of generating structured abstracts using extractive methods. We aim to identify the most feasible way to generate abstracts for scholarly communication in Turkish. It is clear that choosing the most important sentences from each structural section of a scientific article and presenting them under the structural headings will facilitate the abstract generation process. Moreover, such structural sectioning increases the semantic integrity and readability of an abstract. Our main hypothesis is “Considering the structural features of full-texts in extracting abstract sentences with automatic methods will increase the quality of the outputs”. The study attempts to answer the following research questions: (1) Are the full-texts of Turkish LIS articles organized taking into consideration the basic structural features that are expected to exist in a scientific publication? (2) What is the readability of the full-texts and the abstracts of Turkish LIS articles, based on the readability scale? (3) Does using full-text structural features in extracting abstracts with automated methods improve output quality?
In our study, we examined articles published in the field of LIS with classical abstracts. The corpus was analyzed to determine whether the full-texts of the articles are more readable and better structured than the classical author abstracts. We generate a simple automatic abstract generator model that chooses the most important sentences from each structural section of each article.
4 Methodology
We utilized an extractive automatic summarization system named, Structured Abstract Generator (SAG), which depends on the extraction of the most important sentences from all structural parts of the full-texts of articles. Figure 1 demonstrates the architecture of the SAG. This section describes the methodology used in the study.
4.1 Data collection and representation
To construct a corpus for the study, Türk Kütüphaneciliği -Turkish Librarianship (TL) and Bilgi Dünyası -Information World (IW), which are major journals in the field of librarianship and information science in Turkey, have been used. Both journals asked the authors to develop classical abstracts. In addition, both journals do not set either an IMRAD or similar clear template for full-texts. However, IW draws a framework in line with the IMRAD regarding the arrangement of the content. All refereed articles written in Turkish were included in the study. Since each journal is open access, there was no problem in accessing these articles. This study is the first in Turkish to conduct a detailed full-text analysis of a large corpus of LIS literature.
In the initial stage, all articles were saved in PDF format with a unique identifier that encoded the journal name, year, volume, and issue information. For example, the identifier BD200011 indicates an article published in the year 2000, which is the 1st volume of the year and the 1st article of the volume in the IW (BD in Turkish) journal.
Once the articles were identified, they were converted into.txt format using UTF-8 character encoding to ensure the correct representation of Turkish characters. Then, article metadata was automatically extracted. This included author names, titles, abstracts, body text, and keywords, which are clear indicators of the content and are located in specific places in the document.
After processing 421 documents from two journals (172 IW, 249 TL), a relational database was created using MySQL. This database enabled the efficient processing of article full-text sentences as vectors, where each component is assigned to the corresponding structural section of the document, as well as the document’s metadata. The IMRAD format, which is the most prominent organizational structure for full-text in scientific writing, was used in this study.
To facilitate further stages, web-based interfaces were developed to enable the monitoring and management of rules governing the structural layout decisions for each article. The development of a web-based system offered inherent advantages in terms of providing flexible work arrangements and enabling quick control over individuals in operator roles. The solution was designed to be compatible with both mobile and desktop devices, enabling the team to operate flexibly and remotely.
The team of operators consisted of six professionals, two undergraduate students, and four PhD students from the Department of Information Management. These individuals had prior expertise regarding the structural components of scientific articles. Two roles were identified for the expert team: operator (4 experts) and administrator (2 experts).
Operators copied and pasted the body text from these interfaces according to IMRAD headings, retaining complete control over the process. After the completion of the IMRAD marking procedure for an article, operators were unable to make any additional modifications using the interface. However, administrators retained the authorization to execute final supervision and operational functions subsequent to this stage. This control was important to ensure that the IMRAD structure of the articles, which was inherited by paragraphs, was determined correctly. To ensure inter-annotator agreement of scholarship decisions, each article was tagged by at least two operators and one expert doctoral student during the manual step.
By implementing this work plan, the expert team successfully achieved the systematic and efficient classification of the boundaries and structural sections (according to the IMRAD format) of each paragraph of the body text. Consequently, the work of carefully adhering to the sequential arrangement of sentences in all articles was successfully completed within a brief timeframe. This hierarchical structure of body text was further applied to the sentence level through the utilization of a relational database. At the end of the two main steps mentioned above, 101,019 sentences were extracted from 421 articles. Next, word frequency vectors and n-gram sequences were obtained using Zemberek [ 37 ] and then stored in the database.
Table 1 shows an example of the data representation for a sentence of an article. The ID BD200011 indicates that the sentence is from the first article of the first volume of the year 2000 of the IW (BD in Turkish) journal. The remaining information refers to the 27th sentence of the 5th paragraph of the 1st IMRAD section of the relevant article. In this study, we used the following section numbers: 1 for Introduction, 2 for Method, 3 for Results, and 4 for Discussion. The title information indicates the title of the paragraph to which this sentence belongs.
4.2 Stemming
Since Turkish has an agglutinative morphology, inflectional or plural suffixes may produce multiple words from one root. Turkish words that appear in different ways in the text but have the same meaning in terms of their roots can be shown in a single way. Due to the high reduction rate provided in the size of the document-term matrix, it is strongly recommended to apply to stemming in Turkish texts [ 38 ]. For root finding, we utilize Zemberek [ 37 ], a natural language processing toolkit for Turkish for root finding. Although sentences of articles had been parsed under the supervision of the operators, we employed data-cleaning methods on the raw data.
After the stemming and data-cleaning processes, word frequency vectors are produced. Table 2 depicts the example of a vector representation of a sentence whose raw data is seen in Table 1 .
4.3 Extractive summarization and evaluation process
Extractive automatic summarization methods include the process of scoring, sorting and selecting sentences in the document. Automatic text summarization approaches and methods are employed to identify key representative sentences from the full-text. Sentences are scored based on their predetermined features, and the significance of each sentence in the document is determined by these scores. Sentence selection functions that bring together each feature by weighting are another stage of the extractive automatic summarization systems. Features used in sentence scoring are as follows.
4.3.1 Sentence position
This feature assumes that the most important information in a text is usually presented at the beginning. It assigns a higher ranking score to sentences that are closer to the beginning of the text, using the following formula
here i is the sequence number of the sentence in the document and n is the number of sentences in the document.
Formula 1 gives, each sentence ranking points from 1 to 0 depending on the order of appearance in the article.
4.3.2 Sentence centrality
Centrality is the most widely used feature in automatic text summarization for a variety of text types and corpora. It is based on finding the degrees of representing the basic information given in the full-text, in terms of the scoring of the sentences. It is calculated by considering how many other sentences in the document are connected to it. There are many different ways to calculate centrality. Within the scope of the study, the centrality of each sentence for a document with n sentences was obtained as in Formula ( 2 ) [ 39 ].
here \(\mathrm {\ i\ne j} \text { and } \textrm{cos}\left( \textrm{s}_\textrm{i}\textrm{,} \textrm{s}_\textrm{j}\right) \mathrm {\ge 0.16}\) .
Sentence centrality based on three factors: the similarity between a sentence \(\textrm{s}_\textrm{i}\) and other sentences \(\textrm{s}_\textrm{j}\) in the document, the number of shared words (n-friends) between \(\textrm{s}_\textrm{i}\) and \(\textrm{s}_\textrm{j}\) , and the presence of common n-grams between them. The resulting sum is then normalized by dividing it by n-1, where n is the number of sentences in the document. An experimentally determined threshold value of \(\textrm{cos}\left( \textrm{s}_\textrm{i}\textrm{,} \textrm{s}_\textrm{j}\right) \mathrm {\ge 0.16}\) was found to be appropriate. Accordingly,
where \(\mathrm {\ i\ne j}\) . Here, the number of shared affinities are calculated as in Formula 3 over sets of sentences similar to both \(\textrm{s}_\textrm{i}\) and \(\textrm{s}_\textrm{j}\) . 2-grams were used for shared n-grams in Formula 4. \(\mathrm {\left| X \right| }\) gives the number of elements of the set X.
The sim value of each sentence is calculated using the cosine similarity measure [ 40 ]. Cosine similarity is one of the most preferred methods to compare two texts and to make decisions over the similarity between them.
Let X and Y be vector representations of the two sentences to be compared. Given the Euclidean norm of X, \(\mathrm {||}\textrm{X}\mathrm {||=} \sqrt{\textrm{x}_\textrm{1}^\textrm{2}+ \textrm{x}_\textrm{2}^\textrm{2} +\cdots + \textrm{x}_\textrm{p}^\textrm{2}\mathrm {\ } }\) and the vector product of X and Y, to be defined by \(\textrm{XY}=\textrm{x}_\textrm{1}\textrm{y}_\textrm{1}+\textrm{x}_\textrm{2}\textrm{y}_\textrm{2}+ \cdots +\textrm{x}_\textrm{p}\textrm{y}_\textrm{p}\) , the cosine value of the angle \(\theta \) between the two vectors gives the similarity value of the two sentences represented by these two vectors as in Formula ( 5 ) [ 41 ].
4.3.3 Noun score
Another feature discussed in this study is whether the sentences contain nouns. The nouns in the texts transmit the information about the content of the text. Therefore, the text summarization system gives points to the sentences containing nouns according to the number of nouns they contain. Zemberek [ 37 ] was used to calculate the score. That score (NS) of each sentence was added to the formula after normalizing by a count of all words of the related sentence.
4.3.4 Ranking score
By combining the linear Formula ( 6 ), which accepts the weights of all three mentioned features as equal, the ranking scores \(\text {RS(}\textrm{s}_\textrm{i}\text {)}\) are calculated as follows.
here i is the sequence number of the sentence \(\textrm{s}_\textrm{i}\) in the document. The word frequency vectors and n-gram sequences stored in the database were used in sentence score calculation.
4.3.5 Generating automatic abstracts
The intended outputs of our system are automatic structured abstracts (ASA). In addition to these outputs, we evaluated the impact of considering structural features on the performance of an extractive-based text summarization system with automatic classical abstracts (ACA) without using structural features, with the same ranking function. The structural section marking of the corpus full-texts is compatible with the widely accepted and well-known IMRAD headings, so the layout of the ASA output of our system is also compatible with IMRAD.
The word limit for our system’s output was determined by reviewing the TL and IW journal guides. The journal TL does not have a word limit for abstracts, while the journal IW has a 250-word limit, which we considered reasonable. Usually, journal guides indicate a word limit for abstracts, with the range being from 150 to 300 words (APA, 2010). As such, we set a 250-word limit for the output of our automated structural abstract system.
For ASAs, the 250-word limit is divided equally among the structural sections of the article. The highest-scoring sentences are selected from each section until the word limit for that section is reached. In this step, sentences are first sorted according to their structural section and then according to their score. For ACAs, the highest-scoring sentences are selected from the entire article until the 250-word limit is reached. In this step, we only sort sentences according to their score.
4.3.6 Evaluation process
In this study, the effect of selecting sentences by considering the structural features of the full-text while generating abstracts was measured using automatic methods. The evaluation is conducted in three stages. Firstly, the distribution of selected sentences for ASA and ACAs within the full text is compared to ensure that the automatic summaries are representative. Next, the full text, original abstract, ASA, and ACA are evaluated for readability to determine whether the automatic summaries are easier to understand than the author summaries. Finally, structural (ASA) and non-structural (ACA) automatic summaries are compared using n-gram co-occurrence between the original abstracts to measure quality and effectiveness. ROUGE scores [ 42 ] are used to compare n-grams in the reference summaries and the extracted summaries as a standard of automatic evaluation of document summarization.
ROUGE evaluation
The ROUGE evaluation approach is based on n-gram co-occurrence, longest common subsequence, and weighted longest common subsequence between the ideal summary and the extracted summary [ 42 ]. The n-grams are ordered terms of length n derived from a given sequence of text used to find the association statistic between reference summary and candidate summary. Formula ( 7 ) calculates the nominal value for each ROUGE-N between the candidate abstract and the reference abstract(s).
where n is the length of n-grams and \(\textrm{Count}_\textrm{match}\) is the maximum number of n-gram overlaps seen in the reference and candidate abstracts [ 42 ]. When X and Y represent two different pieces of text, the overlap between them is calculated as in Formula ( 8 ) [ 43 ]. \(\mathrm {||X||}\) represents the size of the relevant text.
It is a common approach to use abstracts written by the author as reference abstracts in the evaluation process when performing automatic summarization studies for academic articles. Within the scope of this study, author abstracts were used as reference abstracts to calculate the n-gram overlaps of the system outputs with the recall, precision, and F-score scores obtained based on the ROUGE measurements. The ROUGE 2.0 [ 44 ] package was employed in this stage. This comparison is obtained using the mean recall, mean precision, and mean F-score values relative to the ROUGE-1, ROUGE-2, ROUGE-L, and ROUGE-SU4 measurements. The precision value is obtained by dividing the total number of instances included in the ideal and system-generated summaries by the number of instances in the system summary. The recall value is calculated by dividing the number of instances in the ideal and system summaries together by the total number of instances in the ideal summary. The F-score value is obtained by combining the precision and recall values. The simplest way to obtain F-score is to calculate the harmonic mean of these two values [ 45 ]. For more reliable results on a sentence basis, two ROUGE values are used during the evaluation phase. These are ROUGE-L and ROUGE-SU4. ROUGE-L gives the longest common subsequence (LCS) measurement and is calculated by Formula ( 9 ) [ 43 ].
here \(\mathrm {LCS(X, Y)}\) is the length of the longest common subsequence of X and Y. Length values are the length value of the relevant texts whereas \(\textrm{edit}_\textrm{di}\left( \textrm{X, Y}\right) \) is the minimum number of deletion and addition operations which are required to transform X into Y [ 46 ]. The LCS is sensitive to how information is ordered in the text. The disadvantage of ROUGE-L is that it may catch the main word sequence in the text and skip the side subjects that create shorter sequences [ 42 ]. ROUGE-SU4, which evaluates any word pair by allowing arbitrary spaces in the sentence order, measures the 2-gram association created by skipping four 1-grams at most [ 42 ].
Readability of texts
Reading is a complex process that requires readers to make sense of the given message, comprehend it, and finally interpret it [ 47 ]. The suitability of the text for the target audience can be determined through readability calculations.
Although a language-specific formula has not been produced to measure the readability of Turkish texts, an adaptation of the well-known formula called “Flesch Reading Ease” (FRE) [ 48 ] has been widely used since 1997. This adaptation is known as Atesman’s Readability Formula [ 49 ], which calculates the readability of a text based on the average syllable length of the words in the text and the average number of words per sentence.
The Atesman’s Readability Values (ARVs) are calculated with the formulas given in (10) and (11) below:
The readability scale for Turkish texts using ARVs is given in Table 3 .
Academic texts are typically challenging since they contain a lot of jargon specific to the study domain and lengthy sentences with conjunctions. In our study, we have a domain-specific corpus of articles with similar linguistic characteristics. Thus, it is believed that assessing the text’s readability based on the length of sentences and words will be distinctive. While examining the characteristics of the corpus, we calculated the readability values of the body text and traditional abstracts of each article using ARVs. Finally, we compared these calculations with the ARVs of system outputs.
It’s crucial to ensure the corpus texts are structured in a way that supports our analysis. All IMRAD patterns used in the articles are represented in Table 4 . The number of articles in which each pattern is used and the percentages of these articles in the corpus can also be seen in Table 4 .
Figure 2 depicts how the structural order of articles influences the weight distribution of sentences. In Fig. 2 , we see that at least half of the sentences (43.5%) come from articles that use a proper IMRAD format (I, M, R, D). With the addition of sentences coming from articles with an introduction and discussion (I, D) (46.3%), we can say that 89.8% of the sentences come from articles with an acceptable IMRAD structure, as there is a consensus that these types of articles are also suitable for non-experimental social science topics.
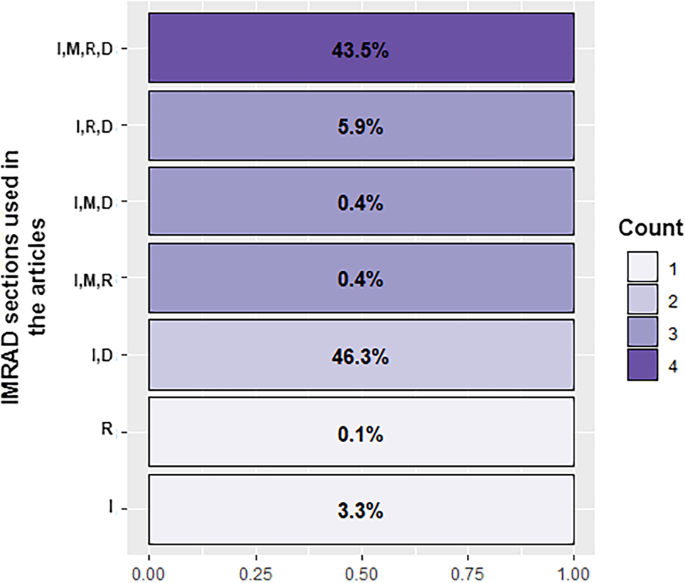
Percentages of IMRAD patterns of the corpus. The color code darkens as the count of sections that are compatible with IMRAD increases
However, it is important to note that every scientific article must contain research question(s) and a method adopted to investigate the question(s). Therefore, the findings about the research question(s) should also be included in the articles. Articles with methods without results (I,M,D), results without methods (I,R,D), or methods and results without discussion (I,M,R) are incompatible with academic writing, as they do not provide a complete account of the research. However, these sentences are the minority of our corpus, constituting only 6.7% (5.9% + 0.4% + 0.4%) of the total. Also, articles consisting of a single IMRAD section including introduction (I), or result (R) remain a minority (3.3% + 0.1% = 3.4%). If such incompatible structural patterns were prevalent, using the SAG system on Turkish LIS articles would be considered inappropriate.
The implications of incompatible structural orders in Turkish LIS articles, particularly those without a method section (I,R,D) (5.9%) or with only an introduction section (I) (3.3%), are worth examining to determine whether they are a domain-specific format or a sign of incomplete content. Having only two IMRAD sections is also worth examining. We defer discussion of these implications to future work, as they are beyond the scope of the present study.
As a result, our corpus reflects the implications of this on the feasibility of extracting automatic structural abstracts.
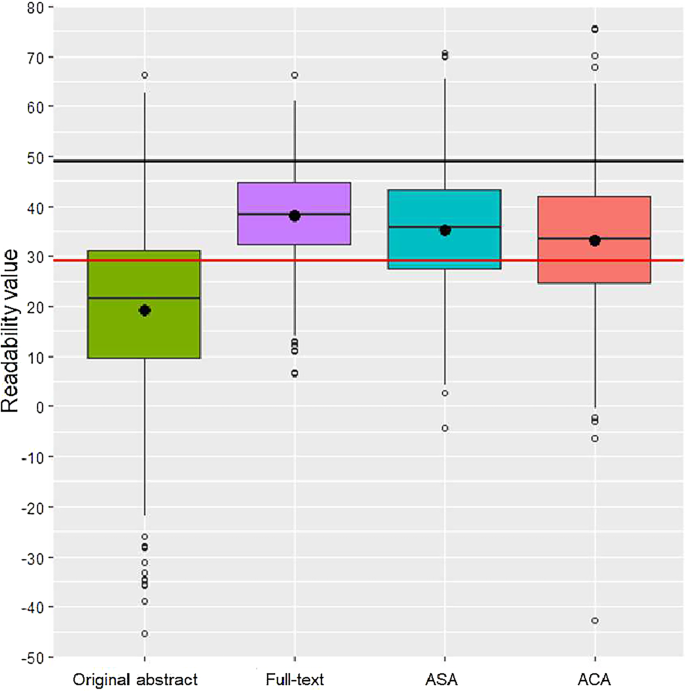
Readability boxplots of abstract, full-text, ASA, ACA
Figure 3 presents boxplots comparing the readability scores of different groups (original abstracts, full-text articles, ASAs, and ACAs) within the corpus. The area between the red horizontal line (y = 29) and the black horizontal line (y = 49) limits the “difficult” area in the graphic depending on the readability scale. The area below the red line indicates “very difficult” readability and the area above the black line indicates “medium difficulty” readability levels. The collection of original abstracts produced by the author is located at the bottom of Fig. 3 which is almost entirely classified as “very difficult”. The full-texts are clearly limited within the “difficult” readability range. The majority of ACA, ASA, and average of the readability values of these texts appear in the “difficult” readability area.
As can be seen in Fig. 3 , an important finding is a divergence between abstracts and full-texts depending on their readability levels. Author abstracts have a “very difficult” readability level on a corpus basis, while the respective full-texts have a level of “difficult” readability. The readability level of the automatic abstracts produced by the SAG is found between the original abstract and the full-text, and they have almost the same readability level as the full-texts. In addition, there is no statistically significant correlation between the ARV values of abstracts and those of the full text (r = 0.18) (Fig. 4 ). Therefore, it can be stated that the authors did not show a similar approach in terms of factors that will affect the readability of the full-text and abstracts of their articles. This finding supports the view that the authors deliberately choose difficult-to-read language features when writing abstracts.
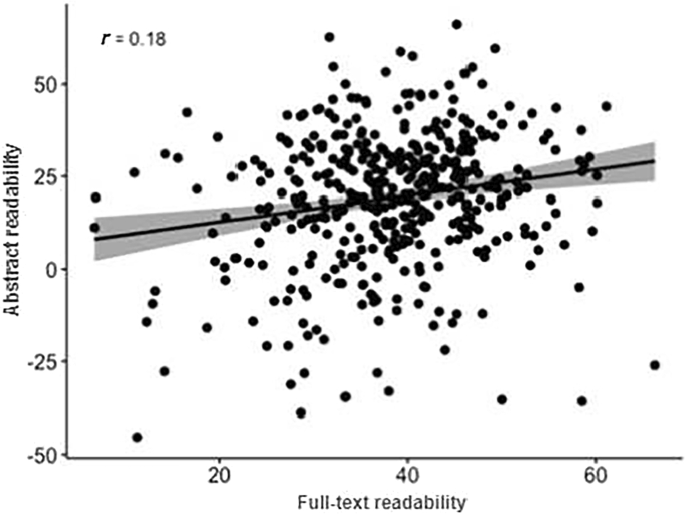
Correlation between the ARV values of abstracts and those of the full-text
It has been seen that the full-text and ASAs distributions based on all IMRAD schemes are proportionally quite similar to each other in Figs. 2 and 5 b. Since ASAs take into account the structural sections of the full-text when selecting sentences, it is not surprising that the system’s structural outputs also reflect the well-structured order of the full-text. However, this graph reveals that, in terms of the amount of structural content in the corpus, the sentence weights of articles with all four IMRAD sections should be represented equally in abstracts. It also suggests that the corpus, which consists of articles selected from the field of LIS and produced with classical abstracts, is actually suitable for structural abstracting.
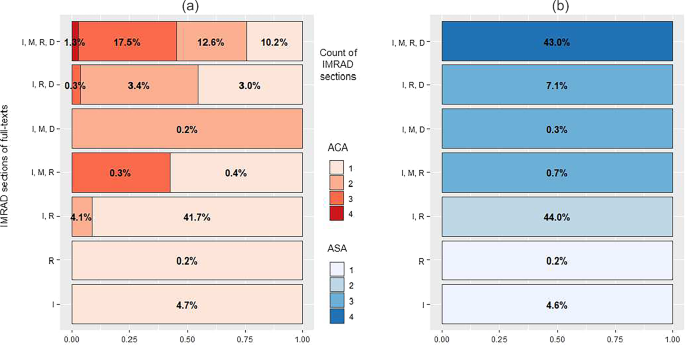
Distribution of ACA ( a ), ASA ( b ) sentences according to the structural formats determined in the corpus. The color code darkens as the count of sections that are compatible with IMRAD increases
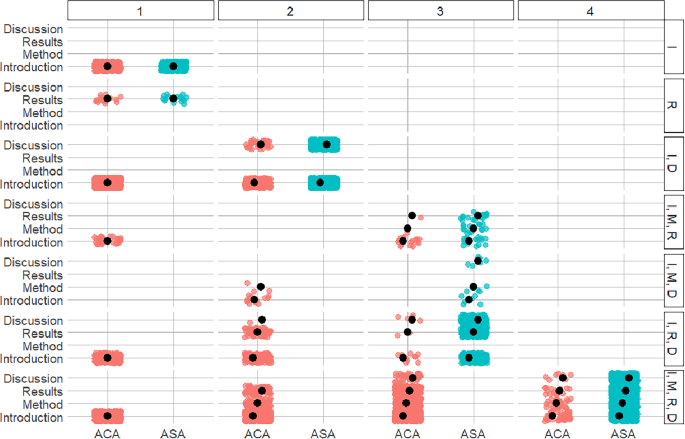
ACA, ASA distribution of IMRAD patterns by their respective full-text IMRAD patterns which are presented on the right-side vertical edge. The numbers above indicate count of IMRAD sections of each output group
On the other hand, Fig. 5 a, which gives the distribution of ACA sentences based on the structural format, differs clearly both from Figs. 3 and 5 b. The weight of the output sentences taken from the articles that have the pattern of four IMRAD sections for ACA is found to be 41.6% (= 1.3% + 17.5% + 12.6% + 10.2%). Only 1.3% of all ACA sentences in the articles with four IMRAD sections consist of four IMRAD sections themselves. Articles with four IMRAD sections account for 17.5% of the ACA sentences in this group, 12.6% for two IMRAD sections, and 10.2% for a single IMRAD section.
When the IMRAD section numbers of ACA’s and the IMRAD patterns of full-texts are examined together within Figs. 5 b, 3 , it is seen that they can have the same IMRAD section numbers as the full-texts with only “I” or “R” IMRAD patterns. For these two relatively small groups, it is not possible to choose sentences from another structural section. Thus, it has been demonstrated that ACAs are far from being fully compatible with the structural order of full-texts.
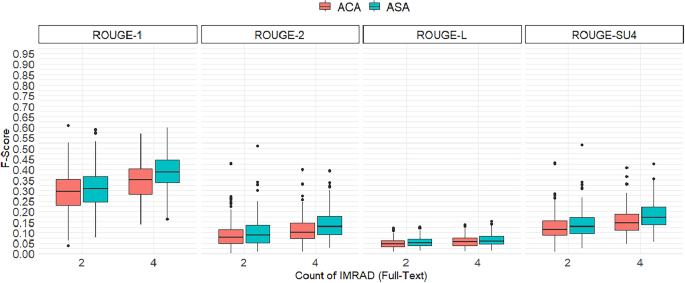
Boxplots of F-scores of the developed system outputs, according to the ROUGE-1, ROUGE-2, ROUGE-L, ROUGE-SU4
Figure 6 shows a graph that displays the distribution of sentences based on their output type and IMRAD patterns. The x-axis represents the abstract type, while the y-axis represents the IMRAD label. The grids at the top show the relationships between different groups of outputs based on the count of IMRAD sections, while the right outer edges of the figure show the relationships between different groups formed based on the IMRAD pattern of the related articles. The labels on the right outer edge represent the abbreviation of IMRAD pattern in the source articles, and the numbers at the top indicate the count of IMRAD in each output group. Each point in the graph shows the distribution of automatic abstract sentences based on the IMRAD count of each output group and IMRAD patterns of the articles from which they are produced.
The grids on the top and right side of the Fig. 6 show how the outputs are grouped based on the number of IMRAD sections and IMRAD pattern, respectively, helping to examine the full-text representativeness between these groups. The projection of each point on the x-axis determines the type of automatic summary in which the relevant sentence is from. Figure 6 displays the distribution of sentences to each output type and IMRAD section.
The distribution of ACAs and ASAs in full-text sentences, as shown in Fig. 6 , indicates that they are completely different. ACAs are generated without considering the IMRAD structure of the full-text, while ASAs are generated from each IMRAD section. This results in the count of IMRAD sections in ACAs being independent of the count of IMRAD sections in the full-text. For example, ACAs from full-texts with two (I,M), three (I,M,R), and four (I,M,R,D) IMRAD sections may consist of a single (I) IMRAD section.
On the other hand, ASAs are compatible with the full-text and output patterns since they are generated by selecting relevant sentences from the full-text for a specific IMRAD section.
The content-based performance of the SAG is evaluated with n-gram co-occurrences between the system outputs and ideal summaries by ROUGE 2.0 package. At this stage, we used the original summaries as the ideal summaries. It should be noted that the abstracts are relatively short texts that may limit the overlap between the author’s abstracts and the system outputs. On the other hand, the difference between the author’s abstracts and the system outputs may be due to meaning and content, or synonymous words and concepts. Evaluating synonyms in automatic summarization is a difficult task as different synonyms can have different meanings and a word’s meaning can change based on the context in which it is used. Since our study focuses on structural layouts that influence the performance of automatic summarization systems, we have limited our scope to exclude the evaluation of synonyms. As a result, synonyms were not evaluated in the study.
Table 5 shows the mean F-score values for each ROUGE measure, grouped by the count of IMRAD sections in the articles in the corpus. The line labeled “All” refers to the values without grouping the corpus based on IMRAD count. The mean F-score is consistently highest for the count of four IMRAD section groups compared to all other output groups. Additionally, ASAs performed better than ACAs for all F-scores at both four and two IMRAD sections, which are the dominant IMRAD patterns in the corpus.
The highest values of n-gram overlapping with the authors’ abstracts are the ROUGE-1 in all cases. It is also suggested for very short outputs, such as abstracts of scientific articles, that ROUGE-1 alone may be sufficient for evaluating text quality [ 44 ]. The lower values of n-gram overlapping with the abstracts are those in the ROUGE-L. The ROUGE-L deals with the sentence-level structure similarity and identifies the longest string of n-gram associations that occur among the texts it compares. Therefore, it can be argued that short outputs and authors’ abstracts may affect the size of the n-gram association sequences between the sentences. The overall decrease in ROUGE-L scores can also be explained in this way.
In Fig. 7 the results of content-based evaluation are presented. Since the majority of articles in the corpus had two or four IMRAD sections, the performance of the dominant group was compared to better illustrate the effect of IMRAD count on output. The boxplots in each section show the F-scores of the developed system outputs, based on the ROUGE-1, ROUGE-2, ROUGE-L, and ROUGE-SU4 scores. The distributions of the ACA and ASA output groups show similar characteristics in all four score types. It is understood from the graphs that as the count of IMRAD sections in the full-texts increases, the ROUGE scores of both output groups of SAG also increase, and the ASAs have better performances in contrast to the ACAs in all cases.
6 Discussion and conclusion
In this paper, we introduced a Structured Abstract Generator which depends on a simple model for generating high-quality structured abstracts of scientific articles. The purpose of employing such automated methods in extracting abstract sentences from relevant full texts while considering the article structure was to improve the quality of the abstracts. Our system generates structured abstracts (ASAs). We evaluated the impact of considering structural features on the performance of an extractive-based automatic text summarization system with automatically generated classical abstracts (ACAs) without using structural features.
We also present a database that enables the efficient processing of the corpus of 421 Turkish LIS articles in full-text sentences where each component is assigned to the corresponding structural section of the document, as well as the document’s metadata.
First, we explored any factors that could prevent the creation of structured abstracts and showed that our corpus is formatted in a way that enables the automatic generation of structured abstracts. 89.8% of the sentences in our corpus come from articles with an acceptable IMRAD pattern of all four (43.5%) IMRAD sections, or at least two (46.3%) IMRAD sections (Introduction and Discussion). Further research is needed to determine whether having only two IMRAD sections is a domain-specific format or a sign of incomplete content. The other problematic articles were completely incompatible with academic writing are remained in the minority. Our study only examined article structural arrangements with a focus on the sentence selection processes. We leave in-depth studies of articles with missing sections in their structural order according to IMRAD for future work.
Second, the readability levels of the full-texts of articles published in the field of Turkish LIS were calculated, and the corpus was largely classified as “difficult” according to the readability scale. However, the readability value of the abstracts produced by the same authors was significantly at the “very difficult” level. We observed that authors deliberately choose difficult-to-read language features in their abstracts, regardless of the language features they use in full-texts. Both ACA and ASA abstracts were calculated at the same readability level as full-text articles showing that selecting important sentences from full-text articles to generate automatic abstracts improves readability. Despite the reasons that lead authors to write difficult-to-read abstracts, widespread use of tools to select important sentences from the structural sections of full-texts may help to break this habit, which hinders scientific communication, over time.
After assessing the quality of SAG outputs, we found that having a well-organized full text improves the quality of both two output groups of SAG. It was observed that ASAs performed significantly better than ACAs. However, interestingly, ACAs also performed better as the number of structured sections increased, despite being produced without taking into account the structure of the full-text. This could be due to an increase in the structured content of original abstracts, resulting in greater similarity between structured and non-structured automatic abstracts and author abstracts. Alternatively, in the context of information retrieval, it means that authors can produce abstracts that convey information more accurately and have higher recall and precision scores when full-texts structural layout improves. We conclude that it is possible to argue that focusing on structural writing in full-texts alone can contribute to improving the content of the original abstracts produced by the author.
In the near future, we can expect to see various systems such as LLMs (Large Language Models), knowledge graphs, NER (Named Entity Recognition systems) systems, QA (Question Answering) systems, MT (Machine Translation) systems, and text summarization systems being used together to produce high-quality structured abstracts. We may also see the emergence of new tools that are specifically designed to assist researchers in communicating their findings more effectively.
Future research should explore more efficient and effective features for automatic summarization methods to generate summaries of scientific records in different languages and domains. Additionally, future research should investigate how the structure of the full-text can be further optimized to improve the quality of automatic summarization methods. Training domain-specific dictionaries would help to improve the accuracy, readability, and effectiveness of generated abstracts. We plan to train a model to classify structural sections of Turkish articles by employing our data for future research. Thus, we can fully automate the process of producing structured abstracts by learning systems. Different summarization approaches and algorithms should be applied to obtain more readable, high-quality structured abstracts. We also plan studies to train our data to predict the structural order of abstracts. A detailed analysis of user opinions on the readability issue can also be conducted. User studies can also reveal the best sentence weights depending on the structural sections of articles.
Finally, we verified that using structural sentence selection, abstract-generating systems can support scholarly communication as a supplementary tool for authors and editors.
Data availability
Data are available at: https://github.com/esraozzz/SAG/ .
Dowling, M., Hammami, H., Tawil, D., Zreik, O.: Writing energy economics research for impact. Energy J. (2021). https://doi.org/10.5547/01956574.42.3.mdow
Article Google Scholar
Fages, D.M.: Write better, publish better. Scientometrics 122 (3), 1671–1681 (2020). https://doi.org/10.1007/s11192-019-03332-4
Day, R.A.: Bilimsel Makale Nasıl Yazılır Ve Yayımlanır? [How to Write and Publish a Scientific Paper?]. TÜBİTAK, Ankara (1996)
Gazni, A.: Are the abstracts of high impact articles more readable? Investigating the evidence from top research institutions in the world. J. Inf. Sci. 37 (3), 273–281 (2011). https://doi.org/10.1177/0165551511401658
Hartley, J., Pennebaker, J.W., Fox, C.: Abstracts, introductions and discussions: How far do they differ in style? Scientometrics 57 , 389–398 (2003)
Jamar, N., Šauperl, A., Bawden, D.: The components of abstracts: The logical structure of abstracts in the areas of materials science and technology and of library and information science. New Libr. World 115 (1/2), 15–33 (2014). https://doi.org/10.1108/nlw-09-2013-0069
Dewan, P.: Are books becoming extinct in academic libraries? New Libr. World 113 (1/2), 27–37 (2012). https://doi.org/10.1108/03074801211199022
Meadows, A.J.: The scientific paper as an archaeological artefact. J. Inf. Sci. 11 (1), 27–30 (1985). https://doi.org/10.1177/016555158501100104
Carr, N.: Is Google Making Us Stupid? Yale University Press, New Haven (2009). https://doi.org/10.12987/9780300156508-009
Book Google Scholar
Issa, T., Isaias, P.: Internet factors influencing generations Y and Z in Australia and Portugal: a practical study. Inf. Process. Manag. 52 (4), 592–617 (2016). https://doi.org/10.1016/j.ipm.2015.12.006
Merzenich, M.: Going Googly - “On the Brain” with Dr. Michael Merzenich. http://onthebrain.com/2008/08/going-googly/ . Accessed 14 Jun 2023
Singer, L.M., Alexander, P.A.: Reading on paper and digitally: What the past decades of empirical research reveal. Rev. Educ. Res. 87 (6), 1007–1041 (2017). https://doi.org/10.3102/0034654317722961
Wästlund, E.: Experimental Studies of Human-computer Interaction: Working Memory and Mental Workload in Complex Cognition. Department of Psychology, Göthenburg (2007)
Liu, Z.: Reading in the age of digital distraction. J. Doc. 78 (6), 1201–1212 (2021). https://doi.org/10.1108/jd-07-2021-0130
Article MathSciNet Google Scholar
Atanassova, I., Bertin, M., Mayr, P.: Mining scientific papers for bibliometrics: A (very) brief survey of methods and tools. arXiv preprint arXiv:1505.01393 (2015)
Mabe, M.A., Amin, M.: Dr Jekyll and Dr Hyde: author-reader asymmetries in scholarly publishing. ASLIB Proc. 54 (3), 149–157 (2002). https://doi.org/10.1108/00012530210441692
Nicholas, D., Huntington, P., Jamali, H.R.: The use, users, and role of abstracts in the digital scholarly environment. J. Acad. Librariansh. 33 (4), 446–453 (2007). https://doi.org/10.1016/j.acalib.2007.03.004
Plavén-Sigray, P., Matheson, G.J., Schiffler, B.C., Thompson, W.H.: The readability of scientific texts is decreasing over time. eLife (2017). https://doi.org/10.7554/elife.27725
Wang, S., Liu, X., Zhou, J.: Readability is decreasing in language and linguistics. Scientometrics 127 (8), 4697–4729 (2022). https://doi.org/10.1007/s11192-022-04427-1
Atanassova, I., Bertin, M., Larivière, V.: On the composition of scientific abstracts. J. Doc. 72 (4), 636–647 (2016). https://doi.org/10.1108/jdoc-09-2015-0111
Bitri, E., Keseroğlu, H.S.: Türk kütüphaneciliği ve bilgi dünyası dergilerinin özlerine eleştirel bir bakış [A critical view to abstracts of Turkish Librarianship and Information World Journals]. Türk Kütüphaneciliği [Turkish Librarianship] 29 (2), 241–257 (2015)
Šauperl, A., Klasinc, J., Lužar, S.: Components of abstracts: Logical structure of scholarly abstracts in pharmacology, sociology, and linguistics and literature. J. Am. Soc. Inform. Sci. Technol. 59 (9), 1420–1432 (2008). https://doi.org/10.1002/asi.20858
Hartley, J., Betts, L.: The effects of spacing and titles on judgments of the effectiveness of structured abstracts. J. Am. Soc. Inform. Sci. Technol. 58 (14), 2335–2340 (2007). https://doi.org/10.1002/asi.20718
Sharma, S., Harrison, J.E.: Structured abstracts: Do they improve the quality of information in abstracts? Am. J. Orthod. Dentofac. Orthop. 130 (4), 523–530 (2006). https://doi.org/10.1016/j.ajodo.2005.10.023
DuBay, W.H.: The Principles of Readability. ERIC Clearinghouse, Costa Mesa, CA. (2004). https://books.google.com.tr/books?id=Aj0VvwEACAAJ
Ufnalska, S., Hartley, J.: How can we evaluate the quality of abstracts. Eur. Sci. Ed. 35 (3), 69–72 (2009)
Google Scholar
Meadows, A.J.: Communicating Research. Academic Press, New York (1998)
Luhn, H.P.: The automatic creation of literature abstracts. IBM J. Res. Dev. 2 (2), 159–165 (1958). https://doi.org/10.1147/rd.22.0159
Altmami, N.I., Menai, M.E.B.: Automatic summarization of scientific articles: a survey. J. King Saud Univ. Comput. Inf. Sci. 34 (4), 1011–1028 (2022). https://doi.org/10.1016/j.jksuci.2020.04.020
Vilca, G.C.V., Cabezudo, M.A.S.: A study of abstractive summarization using semantic representations and discourse level information. In: Ekštein, K., Matoušek, V. (eds.) Text, Speech, and Dialogue, pp. 482–490. Springer, Cham (2017). https://doi.org/10.1007/978-3-319-64206-2_54
Chapter Google Scholar
Moratanch, N., Chitrakala, S.: A survey on extractive text summarization. In: 2017 International Conference on Computer, Communication and Signal Processing (ICCCSP), pp. 1–6. IEEE, Chennai, India (2017). https://doi.org/10.1109/icccsp.2017.7944061
Mridha, M.F., Lima, A.A., Nur, K., Das, S.C., Hasan, M., Kabir, M.M.: A survey of automatic text summarization: Progress, process and challenges. IEEE Access 9 , 156043–156070 (2021). https://doi.org/10.1109/access.2021.3129786
Baykara, B., Güngör, T.: Abstractive text summarization and new large-scale datasets for agglutinative languages Turkish and Hungarian. Lang. Resour. Eval. 56 (3), 973–1007 (2022). https://doi.org/10.1007/s10579-021-09568-y
Tsonkov, T., Lazarova, G.A., Zmiycharov, V., Koychev, I.: A comparative study of extractive and abstractive approaches for automatic text summarization on scientific texts. In: ERIS, pp. 29–34 (2021)
Güran, A., Arslan, S.N., Kılıç, E., Diri, B.: Sentence selection methods for text summarization. In: 2014 22nd Signal Processing and Communications Applications Conference (SIU). IEEE, Trabzon, Turkey (2014). https://doi.org/10.1109/siu.2014.6830198
Song, N., Chen, K., Zhao, Y.: Understanding writing styles of scientific papers in the IS-LS domain: evidence from abstracts over the past three decades. J. Inform. (2023). https://doi.org/10.1016/j.joi.2023.101377
Akın, A.: Zemberek-NLP, Natural Language Processing Tools for Turkish. (2018). https://github.com/ahmetaa/zemberek-nlp
Tunali, V., Bilgin, T.T.: Türkçe metinlerin kümelenmesinde farklı kök bulma yöntemlerinin etkisinin araştırılması [Examining the impact of different stemming methods on clustering Turkish texts]. In: ELECO’2012 Electric-Electronic and Computer Engineering Symposium, pp. 598–602 (2012)
Binwahlan, M.S., Salim, N., Suanmali, L.: Fuzzy swarm diversity hybrid model for text summarization. Inf. Process. Manag. 46 (5), 571–588 (2010). https://doi.org/10.1016/j.ipm.2010.03.004
Erkan, G., Radev, D.R.: LexRank: graph-based lexical centrality as salience in text summarization. J. Artif. Intell. Res. 22 , 457–479 (2004). https://doi.org/10.1613/jair.1523
Salton, G., Buckley, C.: Term-weighting approaches in automatic text retrieval. Inf. Process. Manag. 24 (5), 513–523 (1988). https://doi.org/10.1016/0306-4573(88)90021-0
Lin, C.-Y.: ROUGE: a package for automatic evaluation of summaries. In: Text Summarization Branches Out, pp. 74–81. Association for Computational Linguistics, Barcelona, Spain (2004). https://aclanthology.org/W04-1013
Saggion, H., Radev, D.R., Teufel, S., Lam, W., Strassel, S.M.: Developing infrastructure for the evaluation of single and multi-document summarization systems in a cross-lingual environment. In: LREC, pp. 747–754 (2002)
Ganesan, K.: Rouge 2.0: updated and improved measures for evaluation of summarization tasks. arXiv preprint arXiv:1803.01937 (2018)
Mani, I., House, D., Klein, G., Hirschman, L., Firmin, T., Sundheim, B.: The TIPSTER SUMMAC text summarization evaluation. In: Proceedings of the Ninth Conference on European Chapter of the Association for Computational Linguistics. Association for Computational Linguistics, USA (1999). https://doi.org/10.3115/977035.977047
Crochemore, M., Rytter, W.: Text Algorithms. Oxford University Press, Oxford, UK (1994)
Özdemir, E.: Eleştirel Okuma [Critical Reading]. Bilgi Publishing, Ankara (2000)
Flesch, R.F.: A new readability yardstick. J. Appl. Psychol. 32 (3), 221–233 (1948). https://doi.org/10.1037/H0057532
Ateşman, E.: Türkçede okunabilirliğin ölçülmesi [Measuring readability in Turkish]. Dil Dergisi [J. Lang.] 58, 71–74 (1997)
Çielik, A.E.: Türkçe akademik yayınlar için yapısal öz çıkarım sistemi [Structured abstract extraction system for Turkish academic publications]. PhD Thesis, Hacettepe University (2021)
Al, U., Sezen, U.: Türkçe atıflar için içerik tabanlıatıf analizi modeli tasarımı [Designing a model for content-based citation analysis for Turkish citations]. TÜBİTAK Sosyal Bilimler Araştırma Grubu-Proje No: SOBAG 115K440). Hacettepe Üniversitesi Bilgi ve Belge Yönetimi Bölümü[Hacettepe University Department of Information Management] (2018)
Download references
Acknowledgements
This article is based on Özkan Çelik’s [ 50 ] Ph.D. dissertation and was supported in part by a research grant from The Scientific and Technological Research Council of Türkiye (Project No: SOBAG 115K440) [ 51 ].
Open access funding provided by the Scientific and Technological Research Council of Türkiye (TÜBİTAK).
Author information
Authors and affiliations.
Library, Hacettepe University, Beytepe, 06800, Ankara, Turkey
Ayşe Esra Özkan Çelik
Department of Information Management, Hacettepe University, Beytepe, 06800, Ankara, Turkey
You can also search for this author in PubMed Google Scholar
Corresponding author
Correspondence to Ayşe Esra Özkan Çelik .
Ethics declarations
Conflict of interest.
The authors declare that they have no conflict of interest.
Additional information
Publisher's note.
Springer Nature remains neutral with regard to jurisdictional claims in published maps and institutional affiliations.
Rights and permissions
Open Access This article is licensed under a Creative Commons Attribution 4.0 International License, which permits use, sharing, adaptation, distribution and reproduction in any medium or format, as long as you give appropriate credit to the original author(s) and the source, provide a link to the Creative Commons licence, and indicate if changes were made. The images or other third party material in this article are included in the article’s Creative Commons licence, unless indicated otherwise in a credit line to the material. If material is not included in the article’s Creative Commons licence and your intended use is not permitted by statutory regulation or exceeds the permitted use, you will need to obtain permission directly from the copyright holder. To view a copy of this licence, visit http://creativecommons.org/licenses/by/4.0/ .
Reprints and permissions
About this article
Özkan Çelik, A.E., Al, U. Structured abstract generator (SAG) model: analysis of IMRAD structure of articles and its effect on extractive summarization. Int J Digit Libr (2024). https://doi.org/10.1007/s00799-024-00402-8
Download citation
Received : 11 July 2023
Revised : 25 March 2024
Accepted : 01 April 2024
Published : 07 May 2024
DOI : https://doi.org/10.1007/s00799-024-00402-8
Share this article
Anyone you share the following link with will be able to read this content:
Sorry, a shareable link is not currently available for this article.
Provided by the Springer Nature SharedIt content-sharing initiative
- Readability
- Scholarly communication
- Automatic text summarization
- Find a journal
- Publish with us
- Track your research
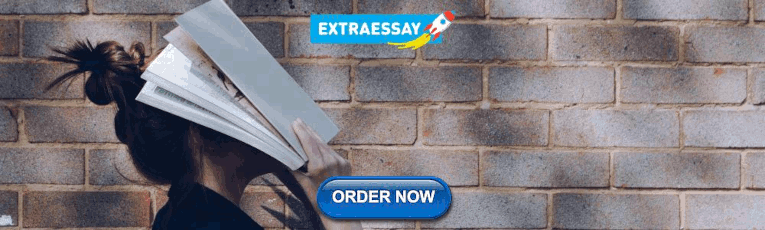
COMMENTS
That's where the IMRAD format comes in. IMRAD provides a clear structure to help you organize and present your research logically and coherently. In this comprehensive guide, we'll explain the IMRAD format, why it's so important for research writing, and how to use it effectively. Follow along to learn the ins and outs of crafting papers ...
Academic research papers in STEM disciplines typically follow a well-defined I-M-R-A-D structure: Introduction, Methods, Results And Discussion (Wu, 2011). Although not included in the IMRAD name, these papers often include a Conclusion. Introduction. Introduction. The Introduction typically provides everything your reader needs to know in ...
Reports of research studies usually follow the IMRAD format. IMRAD (Introduction, Methods, Results, [and] Discussion) is a mnemonic for the major components of a scientific paper. ... Organization of a Research Paper: The IMRAD Format. In: Scientific Writing and Communication in Agriculture and Natural Resources. Springer, Cham. https://doi.org ...
What is an IMRaD report? "IMRaD" format refers to a paper that is structured by four main sections: Introduction, Methods, Results, and Discussion. This format is often used for lab reports as well as for reporting any planned, systematic research in the social sciences, natural sciences, or engineering and computer sciences.
the parts of the IMRAD format as an institutional guideline for research ... Sections of the research paper, ... UB Research and Development Center (2019). IMRAD format guidelines. Baguio City: UBRDC UB Research and Development Center (2015). Thesis/dissertation
It describes the format for the sections of a research report. The IMRaD (or IMRD) format is often used in the social sciences, as well as in the STEM fields. Credit: IMRD: The Parts of a Research Paper by Wordvice Editing Service on YouTube. Outline of Scholarly Writing. With some variation among the different disciplines, most scholarly ...
The IMRaD (often pronounced "im-rad") format is a scientific writing structure that includes four or five major sections: introduction (I); research methods (M); results (R); analysis (a); and discussion (D). The IMRaD format is the most commonly used format in scientific article and journal writing and is used widely across most scientific ...
Results: The IMRAD structure, in those journals, began to be used in the 1940s. In the 1970s, it reached 80% and, in the 1980s, was the only pattern adopted in original papers. Conclusions: Although recommended since the beginning of the twentieth century, the IMRAD structure was adopted as a majority only in the 1970s. The influence of other ...
IMRAD refers to the format in which most biomedical journals publish an original research paper. This framework for a scientific paper spells out how a manuscript should be presented. The letter I stands for Introduction, the M for Methods, the R for Results, the A for And and the D for Discussion. The origin of this format is somewhat hazy ...
IMRAD Outlining. In many of your courses in the sciences and social sciences, such as sociology, psychology, and biology, you may be required to write a research paper using the IMRAD format. IMRAD stands for Introduction, Methods, Results, and Discussion. In this format, you present your research and discuss your methods for gathering research.
Abstract. Most scientific papers are prepared according to a format called IMRAD. The term represents the first letters of the words Introduction, Materials and Methods, Results, And, Discussion. It indicates a pattern or format rather than a complete list of headings or components of research papers; the missing parts of a paper are: Title ...
Introduction Sections in Scientific Research Reports (IMRaD) The goal of the introduction in an IMRaD* report is to give the reader an overview of the literature in the field, show the motivation for your study, and share what unique perspective your research adds. To introduce readers to your material and convince them of the research value ...
Original research papers usually use the IMRaD formula. This acronym includes the four main sections of a research paper, which answer four basic questions, as follows: ... o Methods, Results, and Discussion may all be combined as one or more sections, with different headings for different parts of the study. IMRaD section names (for example ...
IMRAD. In scientific writing, IMRAD or IMRaD ( / ˈɪmræd /) ( Introduction, Methods, Results, and Discussion) [1] is a common organizational structure (a document format). IMRaD is the most prominent norm for the structure of a scientific journal article of the original research type. [2]
The IMRAD layout is a fundamental system that is the basis of all scientific. papers, i.e. the relevant sections representing the acronym are their unavoid-. able parts, although there are some ...
Abstracts can vary in length from one paragraph to several pages, but they follow the IMRaD format and typically spend: • 25% of their space on importance of research (Introduction) • 25% of their space on what you did (Methods) • 35% of their space on what you found: this is the most important part of the abstract (Results)
IMRAD as an adaptable structure for research papers. IMRAD is primarily for original research articles, and has little relevance to other types of papers commonly seen in scientific journals, such as reviews, perspectives, and editorials. Even for research papers, IMRAD is silent about several other components of a modern research paper: title ...
Other parts of your research paper independent of IMRAD include: Tables and figures are the foundation for your story. They are the story. Editors, reviewers, and readers usually look at titles, abstracts, and tables and figures first. Figures and tables should stand alone and tell a complete story.
Abstracts in Scientific Research Papers (IMRaD) Download this guide as a PDF. Return to all guides Abstracts in Scientific Research Papers (IMRaD) An effective abstract in an IMRaD* report provides the reader with a concise, informative summary of the entire paper. An IMRaD abstract should stand on its own; it is not a part of the ...
The scientific research paper follows a specific order and structure, and the core parts that compose this structure are the Introduction, Materials & Method...
IMRaD Results Discussion. Results and Discussion Sections in Scientific Research Reports (IMRaD) After introducing the study and describing its methodology, an IMRaD* report presents and discusses the main findings of the study. In the results section, writers systematically report their findings, and in discussion, they interpret these findings.
Abstract. Most scientific papers are prepared according to a format called IMRAD. The term represents the first letters of the words Introduction, Materials and Methods, Results, And, Discussion ...
IMRAD as an adaptable structure for research papers IMRAD is primarily for original research articles, and has little relevance to other types of papers commonly seen in scientific journals, such as reviews, perspec- ... have become common parts of the IMRAD structure. Even the sequential order of the sections is altered in some journals (e.g ...
slidesmania.com Final Paper Contents - Abstract - Introduction (1-2 pages) - Methods (3-4 pages) - Results and Discussion (2-3 pages) - References (1-3 pages) - Acknowledgements (1 page) - About the Researchers (1-2 pages) - Appendix. slidesmania.com Writing the Introduction - The introduction must be polished, and all changes made must be ...
Articles with four IMRAD sections account for 17.5% of the ACA sentences in this group, 12.6% for two IMRAD sections, and 10.2% for a single IMRAD section. When the IMRAD section numbers of ACA's and the IMRAD patterns of full-texts are examined together within Figs. 5 b, 3 , it is seen that they can have the same IMRAD section numbers as the ...