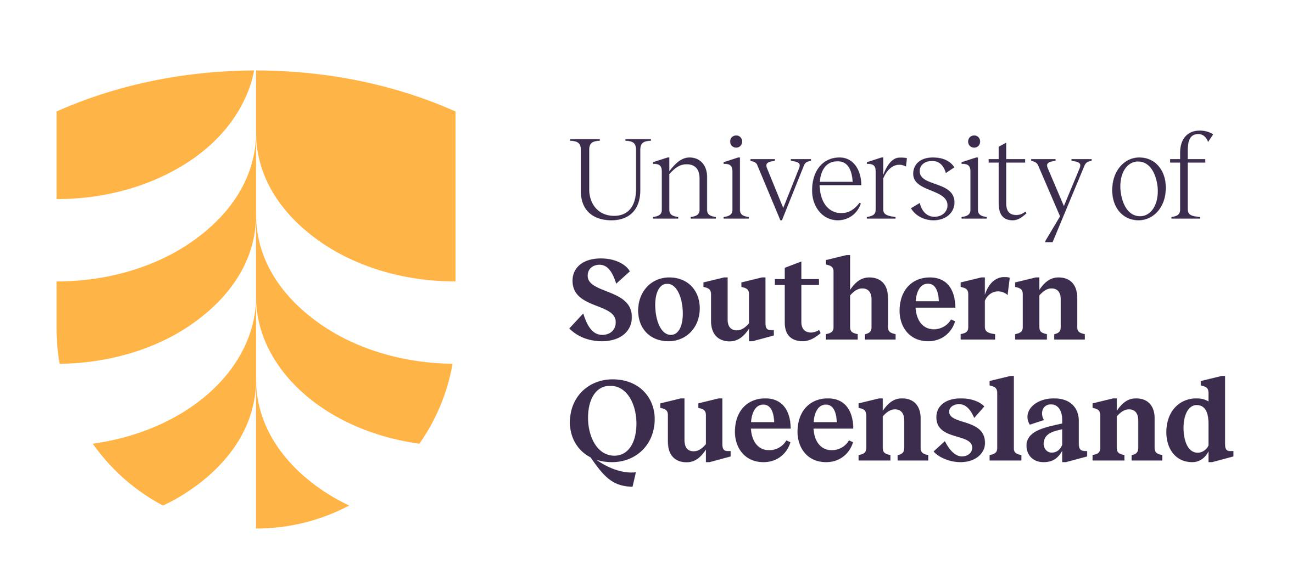
Want to create or adapt books like this? Learn more about how Pressbooks supports open publishing practices.
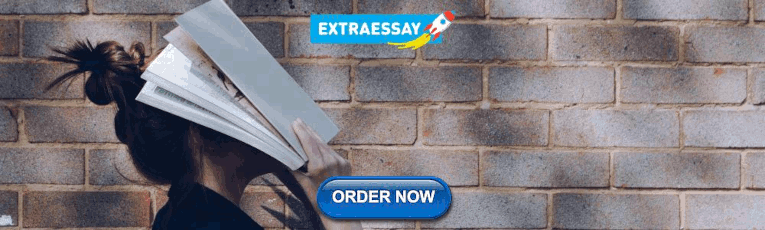
Section 7.1: Mediation and Moderation Models
Learning Objectives
At the end of this section you should be able to answer the following questions:
- Define the concept of a moderator variable.
- Define the concept of a mediator variable.
As we discussed in the lesson on correlations and regressions, understanding associations between psychological constructs can tell researchers a great deal about how certain mental health concerns and behaviours affects us on an emotional level. Correlation analyses focus on the relationship between two variables, and regression is the association of multiple independent variables with a single dependant variable.
Some predictor variables interact in a sequence, rather than impacting the outcome variable singly or as a group (like regression).
Moderation and mediation is a form of regression that allows researchers to analyse how a third variable effects the relationship of the predictor and outcome variable.
PowerPoint: Basic Mediation Model
Consider the Basic Mediation Model in this slide:
- Chapter Seven – Basic Mediation Model
We know that high levels of stress can negatively impact health, we also know that a high level of social support can be beneficial to health. With these two points of knowledge, could it be that social support might provide a protective factor from the effects of stress on health? Thinking about a sequence of effects, perhaps social support can mediate the effect of stress on health.
Mediation is a more complicated extension of multiple regression procedures. Mediation examines the pattern of relationships among three variables (Simple Mediation Model), and can be used on four or more variables.
Examples of Research Questions
Here are some examples of research questions that could use a mediation analysis.
- If an intervention increases secure attachment among young children, do behavioural problems decrease when the children enter school?
- Does physical abuse in early childhood lead to deviant processing of social information that leads to aggressive behaviour?
- Do performance expectations start a self-fulfilling prophecy that affects behaviour?
- Can changes in cognitive attributions reduce depression?
PowerPoint: Three Mediation Figures
Consider the Three Figures Illustrating Mediation from the following slides:
- Chapter Seven – Three Mediation Figures
Looking at this conceptual model, you can see the direct effect of X on Y. You can also see the effect of M on Y. What we are interested in is the effects of X on Y, accounting for the effects of M.
An example mediation model is that of the mediating effect of health-related behaviours on conscientiousness and overall physical health. Conscientiousness, or the personality trait associated with hardworking has relationship with overall physical health, but if an individual is hardworking, but does not perform health-related behaviours like exercise or diet control, then they are likely to be less healthy. From this, we can assume that health-related behaviours mediates the relationship between conscientiousness and physical health.
Statistics for Research Students Copyright © 2022 by University of Southern Queensland is licensed under a Creative Commons Attribution 4.0 International License , except where otherwise noted.
Share This Book
Master ISS literature search: Phrasing the question
Introduction
- Phrasing the question
Search engines
- Search strategy and techniques
Getting hold of the full text
Selecting and evaluating sources
- Referring in APA Style
Summary and feedback
On this page:
Formulating a research question
Inclusion and exclusion criteria
Developing your research question
Other pages:
Search strategy and search techniques
Referring in APA style
You can go from one page to the other by clicking on the tabs in the black top bar (for example, go to the tab Search engines) or use the next/previous buttons at the bottom of the page.
Read the pages from top to bottom.
You can navigate to specific topics or pages by clicking the links in the Index.
Make sure your research question is clear, specific or focused, and answerable.
Clear: your question provides enough information that readers can easily understand its purpose without needing additional explanation. Focused and answerable: your question can be answered thoroughly in the space the writing task allows.
You can use the PICO model (especially suitable for clinical questions) to help you with phrasing the research question:
- P opulation/ P roblem: What are the characteristics of the population or problem (demographics, risk factors, age group, etc)?
- I ntervention: What is the intervention under consideration for this population?
- C omparison: What is the alternative to the intervention
- O utcome: What are the included outcomes (e.g. quality of life, adverse effects)?
Or use the acronym PAC : P opulation, A ssociation, C onstruct. This tool is more suitable for other types of research design: To what extent is [construct 1] associated with [construct 2] in [types of people and setting if specified].
Or the well known W ho, W hat, H ow questions. For example: How does the What affect the Who?
Inclusion and exclusion criteria
Now you need to specify your inclusion and exclusion criteria.
Inclusion criteria describe specific characteristics which a study must meet to be included in your literature review. Exclusion criteria describe anything that would make a study ineligible to be included.
Determining your inclusion/exclusion criteria will partly run parallel with creating your research question. This is because the question already partly defines which characteristics are important, and at the same time you will enable yourself to accentuate your research question by determining your inclusion/exclusion criteria.
Think about:
- Population characteristics (e.g. adolescents, immigrants)
- Problem specifics (e.g. drug use (general) vs cannabis use/XTC (more specific)
- Setting or location (e.g. school(type), rural/urban, country)
- Study design (e.g. qualitative/quantitative, cross-sectional studies)
- Reported outcome (e.g.
And more practical:
- Language (e.g. only English?)
- Publication year (e.g. only the last 10 years)
- Type of publication (e.g. only academic, peer-reviewed articles)
This section of the LibGuide originates from the master Youth Studies (-2023), it may not represent the current curriculum of your master.
For your systematic literature review you will need, besides a clear main question (in which you research the effect of an independent variable on a dependent variable), also subquestions (think of a mediation or moderation effect).
A research question in which mediation is researched could be:
- Is the relation between impopularity and symptoms of depression in adolescents mediated by the use of social network sites?
You research the direct relation between impopularity (independent variable) and symptoms of depression (dependent variable) and the indirect relation between these two variables via social network sites (mediator). Schematically a representation of mediation could look like this (in which 1 is the original relation and 4 the relation checked before the mediator):
Moderation (interaction effect)
A research question in which moderation (interaction effect) is researched could be:
- To what extent is socio-economic status related to cannabis use in adolescents and is this relation moderated by their parents style of upbringing?
You research the relation between socio-economic status (independent variable) and cannabis use (dependent variable) and check if this relation is influenced by education/upbringing (moderator).
Schematically a representation of moderation could look like this:
Translation:
onafhankelijke variabele = independent variable afhankelijke variabele = dependent variable
- << Previous: Introduction
- Next: Search engines >>
- Last Updated: May 15, 2024 12:01 PM
- URL: https://libguides.library.uu.nl/ASW-master-Youth-Studies
Click through the PLOS taxonomy to find articles in your field.
For more information about PLOS Subject Areas, click here .
Loading metrics
Open Access
Peer-reviewed
Research Article
Anxiety, Affect, Self-Esteem, and Stress: Mediation and Moderation Effects on Depression
Affiliations Department of Psychology, University of Gothenburg, Gothenburg, Sweden, Network for Empowerment and Well-Being, University of Gothenburg, Gothenburg, Sweden
Affiliation Network for Empowerment and Well-Being, University of Gothenburg, Gothenburg, Sweden
Affiliations Department of Psychology, University of Gothenburg, Gothenburg, Sweden, Network for Empowerment and Well-Being, University of Gothenburg, Gothenburg, Sweden, Department of Psychology, Education and Sport Science, Linneaus University, Kalmar, Sweden
* E-mail: [email protected]
Affiliations Network for Empowerment and Well-Being, University of Gothenburg, Gothenburg, Sweden, Center for Ethics, Law, and Mental Health (CELAM), University of Gothenburg, Gothenburg, Sweden, Institute of Neuroscience and Physiology, The Sahlgrenska Academy, University of Gothenburg, Gothenburg, Sweden
- Ali Al Nima,
- Patricia Rosenberg,
- Trevor Archer,
- Danilo Garcia
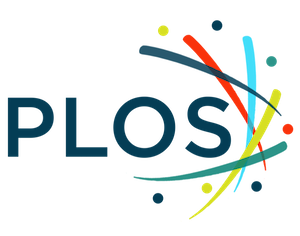
- Published: September 9, 2013
- https://doi.org/10.1371/journal.pone.0073265
- Reader Comments
23 Sep 2013: Nima AA, Rosenberg P, Archer T, Garcia D (2013) Correction: Anxiety, Affect, Self-Esteem, and Stress: Mediation and Moderation Effects on Depression. PLOS ONE 8(9): 10.1371/annotation/49e2c5c8-e8a8-4011-80fc-02c6724b2acc. https://doi.org/10.1371/annotation/49e2c5c8-e8a8-4011-80fc-02c6724b2acc View correction
Mediation analysis investigates whether a variable (i.e., mediator) changes in regard to an independent variable, in turn, affecting a dependent variable. Moderation analysis, on the other hand, investigates whether the statistical interaction between independent variables predict a dependent variable. Although this difference between these two types of analysis is explicit in current literature, there is still confusion with regard to the mediating and moderating effects of different variables on depression. The purpose of this study was to assess the mediating and moderating effects of anxiety, stress, positive affect, and negative affect on depression.
Two hundred and two university students (males = 93, females = 113) completed questionnaires assessing anxiety, stress, self-esteem, positive and negative affect, and depression. Mediation and moderation analyses were conducted using techniques based on standard multiple regression and hierarchical regression analyses.
Main Findings
The results indicated that (i) anxiety partially mediated the effects of both stress and self-esteem upon depression, (ii) that stress partially mediated the effects of anxiety and positive affect upon depression, (iii) that stress completely mediated the effects of self-esteem on depression, and (iv) that there was a significant interaction between stress and negative affect, and between positive affect and negative affect upon depression.
The study highlights different research questions that can be investigated depending on whether researchers decide to use the same variables as mediators and/or moderators.
Citation: Nima AA, Rosenberg P, Archer T, Garcia D (2013) Anxiety, Affect, Self-Esteem, and Stress: Mediation and Moderation Effects on Depression. PLoS ONE 8(9): e73265. https://doi.org/10.1371/journal.pone.0073265
Editor: Ben J. Harrison, The University of Melbourne, Australia
Received: February 21, 2013; Accepted: July 22, 2013; Published: September 9, 2013
Copyright: © 2013 Nima et al. This is an open-access article distributed under the terms of the Creative Commons Attribution License, which permits unrestricted use, distribution, and reproduction in any medium, provided the original author and source are credited.
Funding: The authors have no support or funding to report.
Competing interests: The authors have declared that no competing interests exist.
Introduction
Mediation refers to the covariance relationships among three variables: an independent variable (1), an assumed mediating variable (2), and a dependent variable (3). Mediation analysis investigates whether the mediating variable accounts for a significant amount of the shared variance between the independent and the dependent variables–the mediator changes in regard to the independent variable, in turn, affecting the dependent one [1] , [2] . On the other hand, moderation refers to the examination of the statistical interaction between independent variables in predicting a dependent variable [1] , [3] . In contrast to the mediator, the moderator is not expected to be correlated with both the independent and the dependent variable–Baron and Kenny [1] actually recommend that it is best if the moderator is not correlated with the independent variable and if the moderator is relatively stable, like a demographic variable (e.g., gender, socio-economic status) or a personality trait (e.g., affectivity).
Although both types of analysis lead to different conclusions [3] and the distinction between statistical procedures is part of the current literature [2] , there is still confusion about the use of moderation and mediation analyses using data pertaining to the prediction of depression. There are, for example, contradictions among studies that investigate mediating and moderating effects of anxiety, stress, self-esteem, and affect on depression. Depression, anxiety and stress are suggested to influence individuals' social relations and activities, work, and studies, as well as compromising decision-making and coping strategies [4] , [5] , [6] . Successfully coping with anxiety, depressiveness, and stressful situations may contribute to high levels of self-esteem and self-confidence, in addition increasing well-being, and psychological and physical health [6] . Thus, it is important to disentangle how these variables are related to each other. However, while some researchers perform mediation analysis with some of the variables mentioned here, other researchers conduct moderation analysis with the same variables. Seldom are both moderation and mediation performed on the same dataset. Before disentangling mediation and moderation effects on depression in the current literature, we briefly present the methodology behind the analysis performed in this study.
Mediation and moderation
Baron and Kenny [1] postulated several criteria for the analysis of a mediating effect: a significant correlation between the independent and the dependent variable, the independent variable must be significantly associated with the mediator, the mediator predicts the dependent variable even when the independent variable is controlled for, and the correlation between the independent and the dependent variable must be eliminated or reduced when the mediator is controlled for. All the criteria is then tested using the Sobel test which shows whether indirect effects are significant or not [1] , [7] . A complete mediating effect occurs when the correlation between the independent and the dependent variable are eliminated when the mediator is controlled for [8] . Analyses of mediation can, for example, help researchers to move beyond answering if high levels of stress lead to high levels of depression. With mediation analysis researchers might instead answer how stress is related to depression.
In contrast to mediation, moderation investigates the unique conditions under which two variables are related [3] . The third variable here, the moderator, is not an intermediate variable in the causal sequence from the independent to the dependent variable. For the analysis of moderation effects, the relation between the independent and dependent variable must be different at different levels of the moderator [3] . Moderators are included in the statistical analysis as an interaction term [1] . When analyzing moderating effects the variables should first be centered (i.e., calculating the mean to become 0 and the standard deviation to become 1) in order to avoid problems with multi-colinearity [8] . Moderating effects can be calculated using multiple hierarchical linear regressions whereby main effects are presented in the first step and interactions in the second step [1] . Analysis of moderation, for example, helps researchers to answer when or under which conditions stress is related to depression.
Mediation and moderation effects on depression
Cognitive vulnerability models suggest that maladaptive self-schema mirroring helplessness and low self-esteem explain the development and maintenance of depression (for a review see [9] ). These cognitive vulnerability factors become activated by negative life events or negative moods [10] and are suggested to interact with environmental stressors to increase risk for depression and other emotional disorders [11] , [10] . In this line of thinking, the experience of stress, low self-esteem, and negative emotions can cause depression, but also be used to explain how (i.e., mediation) and under which conditions (i.e., moderation) specific variables influence depression.
Using mediational analyses to investigate how cognitive therapy intervations reduced depression, researchers have showed that the intervention reduced anxiety, which in turn was responsible for 91% of the reduction in depression [12] . In the same study, reductions in depression, by the intervention, accounted only for 6% of the reduction in anxiety. Thus, anxiety seems to affect depression more than depression affects anxiety and, together with stress, is both a cause of and a powerful mediator influencing depression (See also [13] ). Indeed, there are positive relationships between depression, anxiety and stress in different cultures [14] . Moreover, while some studies show that stress (independent variable) increases anxiety (mediator), which in turn increased depression (dependent variable) [14] , other studies show that stress (moderator) interacts with maladaptive self-schemata (dependent variable) to increase depression (independent variable) [15] , [16] .
The present study
In order to illustrate how mediation and moderation can be used to address different research questions we first focus our attention to anxiety and stress as mediators of different variables that earlier have been shown to be related to depression. Secondly, we use all variables to find which of these variables moderate the effects on depression.
The specific aims of the present study were:
- To investigate if anxiety mediated the effect of stress, self-esteem, and affect on depression.
- To investigate if stress mediated the effects of anxiety, self-esteem, and affect on depression.
- To examine moderation effects between anxiety, stress, self-esteem, and affect on depression.
Ethics statement
This research protocol was approved by the Ethics Committee of the University of Gothenburg and written informed consent was obtained from all the study participants.
Participants
The present study was based upon a sample of 206 participants (males = 93, females = 113). All the participants were first year students in different disciplines at two universities in South Sweden. The mean age for the male students was 25.93 years ( SD = 6.66), and 25.30 years ( SD = 5.83) for the female students.
In total, 206 questionnaires were distributed to the students. Together 202 questionnaires were responded to leaving a total dropout of 1.94%. This dropout concerned three sections that the participants chose not to respond to at all, and one section that was completed incorrectly. None of these four questionnaires was included in the analyses.
Instruments
Hospital anxiety and depression scale [17] ..
The Swedish translation of this instrument [18] was used to measure anxiety and depression. The instrument consists of 14 statements (7 of which measure depression and 7 measure anxiety) to which participants are asked to respond grade of agreement on a Likert scale (0 to 3). The utility, reliability and validity of the instrument has been shown in multiple studies (e.g., [19] ).
Perceived Stress Scale [20] .
The Swedish version [21] of this instrument was used to measures individuals' experience of stress. The instrument consist of 14 statements to which participants rate on a Likert scale (0 = never , 4 = very often ). High values indicate that the individual expresses a high degree of stress.
Rosenberg's Self-Esteem Scale [22] .
The Rosenberg's Self-Esteem Scale (Swedish version by Lindwall [23] ) consists of 10 statements focusing on general feelings toward the self. Participants are asked to report grade of agreement in a four-point Likert scale (1 = agree not at all, 4 = agree completely ). This is the most widely used instrument for estimation of self-esteem with high levels of reliability and validity (e.g., [24] , [25] ).
Positive Affect and Negative Affect Schedule [26] .
This is a widely applied instrument for measuring individuals' self-reported mood and feelings. The Swedish version has been used among participants of different ages and occupations (e.g., [27] , [28] , [29] ). The instrument consists of 20 adjectives, 10 positive affect (e.g., proud, strong) and 10 negative affect (e.g., afraid, irritable). The adjectives are rated on a five-point Likert scale (1 = not at all , 5 = very much ). The instrument is a reliable, valid, and effective self-report instrument for estimating these two important and independent aspects of mood [26] .
Questionnaires were distributed to the participants on several different locations within the university, including the library and lecture halls. Participants were asked to complete the questionnaire after being informed about the purpose and duration (10–15 minutes) of the study. Participants were also ensured complete anonymity and informed that they could end their participation whenever they liked.
Correlational analysis
Depression showed positive, significant relationships with anxiety, stress and negative affect. Table 1 presents the correlation coefficients, mean values and standard deviations ( sd ), as well as Cronbach ' s α for all the variables in the study.
- PPT PowerPoint slide
- PNG larger image
- TIFF original image
https://doi.org/10.1371/journal.pone.0073265.t001
Mediation analysis
Regression analyses were performed in order to investigate if anxiety mediated the effect of stress, self-esteem, and affect on depression (aim 1). The first regression showed that stress ( B = .03, 95% CI [.02,.05], β = .36, t = 4.32, p <.001), self-esteem ( B = −.03, 95% CI [−.05, −.01], β = −.24, t = −3.20, p <.001), and positive affect ( B = −.02, 95% CI [−.05, −.01], β = −.19, t = −2.93, p = .004) had each an unique effect on depression. Surprisingly, negative affect did not predict depression ( p = 0.77) and was therefore removed from the mediation model, thus not included in further analysis.
The second regression tested whether stress, self-esteem and positive affect uniquely predicted the mediator (i.e., anxiety). Stress was found to be positively associated ( B = .21, 95% CI [.15,.27], β = .47, t = 7.35, p <.001), whereas self-esteem was negatively associated ( B = −.29, 95% CI [−.38, −.21], β = −.42, t = −6.48, p <.001) to anxiety. Positive affect, however, was not associated to anxiety ( p = .50) and was therefore removed from further analysis.
A hierarchical regression analysis using depression as the outcome variable was performed using stress and self-esteem as predictors in the first step, and anxiety as predictor in the second step. This analysis allows the examination of whether stress and self-esteem predict depression and if this relation is weaken in the presence of anxiety as the mediator. The result indicated that, in the first step, both stress ( B = .04, 95% CI [.03,.05], β = .45, t = 6.43, p <.001) and self-esteem ( B = .04, 95% CI [.03,.05], β = .45, t = 6.43, p <.001) predicted depression. When anxiety (i.e., the mediator) was controlled for predictability was reduced somewhat but was still significant for stress ( B = .03, 95% CI [.02,.04], β = .33, t = 4.29, p <.001) and for self-esteem ( B = −.03, 95% CI [−.05, −.01], β = −.20, t = −2.62, p = .009). Anxiety, as a mediator, predicted depression even when both stress and self-esteem were controlled for ( B = .05, 95% CI [.02,.08], β = .26, t = 3.17, p = .002). Anxiety improved the prediction of depression over-and-above the independent variables (i.e., stress and self-esteem) (Δ R 2 = .03, F (1, 198) = 10.06, p = .002). See Table 2 for the details.
https://doi.org/10.1371/journal.pone.0073265.t002
A Sobel test was conducted to test the mediating criteria and to assess whether indirect effects were significant or not. The result showed that the complete pathway from stress (independent variable) to anxiety (mediator) to depression (dependent variable) was significant ( z = 2.89, p = .003). The complete pathway from self-esteem (independent variable) to anxiety (mediator) to depression (dependent variable) was also significant ( z = 2.82, p = .004). Thus, indicating that anxiety partially mediates the effects of both stress and self-esteem on depression. This result may indicate also that both stress and self-esteem contribute directly to explain the variation in depression and indirectly via experienced level of anxiety (see Figure 1 ).
Changes in Beta weights when the mediator is present are highlighted in red.
https://doi.org/10.1371/journal.pone.0073265.g001
For the second aim, regression analyses were performed in order to test if stress mediated the effect of anxiety, self-esteem, and affect on depression. The first regression showed that anxiety ( B = .07, 95% CI [.04,.10], β = .37, t = 4.57, p <.001), self-esteem ( B = −.02, 95% CI [−.05, −.01], β = −.18, t = −2.23, p = .03), and positive affect ( B = −.03, 95% CI [−.04, −.02], β = −.27, t = −4.35, p <.001) predicted depression independently of each other. Negative affect did not predict depression ( p = 0.74) and was therefore removed from further analysis.
The second regression investigated if anxiety, self-esteem and positive affect uniquely predicted the mediator (i.e., stress). Stress was positively associated to anxiety ( B = 1.01, 95% CI [.75, 1.30], β = .46, t = 7.35, p <.001), negatively associated to self-esteem ( B = −.30, 95% CI [−.50, −.01], β = −.19, t = −2.90, p = .004), and a negatively associated to positive affect ( B = −.33, 95% CI [−.46, −.20], β = −.27, t = −5.02, p <.001).
A hierarchical regression analysis using depression as the outcome and anxiety, self-esteem, and positive affect as the predictors in the first step, and stress as the predictor in the second step, allowed the examination of whether anxiety, self-esteem and positive affect predicted depression and if this association would weaken when stress (i.e., the mediator) was present. In the first step of the regression anxiety ( B = .07, 95% CI [.05,.10], β = .38, t = 5.31, p = .02), self-esteem ( B = −.03, 95% CI [−.05, −.01], β = −.18, t = −2.41, p = .02), and positive affect ( B = −.03, 95% CI [−.04, −.02], β = −.27, t = −4.36, p <.001) significantly explained depression. When stress (i.e., the mediator) was controlled for, predictability was reduced somewhat but was still significant for anxiety ( B = .05, 95% CI [.02,.08], β = .05, t = 4.29, p <.001) and for positive affect ( B = −.02, 95% CI [−.04, −.01], β = −.20, t = −3.16, p = .002), whereas self-esteem did not reach significance ( p < = .08). In the second step, the mediator (i.e., stress) predicted depression even when anxiety, self-esteem, and positive affect were controlled for ( B = .02, 95% CI [.08,.04], β = .25, t = 3.07, p = .002). Stress improved the prediction of depression over-and-above the independent variables (i.e., anxiety, self-esteem and positive affect) (Δ R 2 = .02, F (1, 197) = 9.40, p = .002). See Table 3 for the details.
https://doi.org/10.1371/journal.pone.0073265.t003
Furthermore, the Sobel test indicated that the complete pathways from the independent variables (anxiety: z = 2.81, p = .004; self-esteem: z = 2.05, p = .04; positive affect: z = 2.58, p <.01) to the mediator (i.e., stress), to the outcome (i.e., depression) were significant. These specific results might be explained on the basis that stress partially mediated the effects of both anxiety and positive affect on depression while stress completely mediated the effects of self-esteem on depression. In other words, anxiety and positive affect contributed directly to explain the variation in depression and indirectly via the experienced level of stress. Self-esteem contributed only indirectly via the experienced level of stress to explain the variation in depression. In other words, stress effects on depression originate from “its own power” and explained more of the variation in depression than self-esteem (see Figure 2 ).
https://doi.org/10.1371/journal.pone.0073265.g002
Moderation analysis
Multiple linear regression analyses were used in order to examine moderation effects between anxiety, stress, self-esteem and affect on depression. The analysis indicated that about 52% of the variation in the dependent variable (i.e., depression) could be explained by the main effects and the interaction effects ( R 2 = .55, adjusted R 2 = .51, F (55, 186) = 14.87, p <.001). When the variables (dependent and independent) were standardized, both the standardized regression coefficients beta (β) and the unstandardized regression coefficients beta (B) became the same value with regard to the main effects. Three of the main effects were significant and contributed uniquely to high levels of depression: anxiety ( B = .26, t = 3.12, p = .002), stress ( B = .25, t = 2.86, p = .005), and self-esteem ( B = −.17, t = −2.17, p = .03). The main effect of positive affect was also significant and contributed to low levels of depression ( B = −.16, t = −2.027, p = .02) (see Figure 3 ). Furthermore, the results indicated that two moderator effects were significant. These were the interaction between stress and negative affect ( B = −.28, β = −.39, t = −2.36, p = .02) (see Figure 4 ) and the interaction between positive affect and negative affect ( B = −.21, β = −.29, t = −2.30, p = .02) ( Figure 5 ).
https://doi.org/10.1371/journal.pone.0073265.g003
Low stress and low negative affect leads to lower levels of depression compared to high stress and high negative affect.
https://doi.org/10.1371/journal.pone.0073265.g004
High positive affect and low negative affect lead to lower levels of depression compared to low positive affect and high negative affect.
https://doi.org/10.1371/journal.pone.0073265.g005
The results in the present study show that (i) anxiety partially mediated the effects of both stress and self-esteem on depression, (ii) that stress partially mediated the effects of anxiety and positive affect on depression, (iii) that stress completely mediated the effects of self-esteem on depression, and (iv) that there was a significant interaction between stress and negative affect, and positive affect and negative affect on depression.
Mediating effects
The study suggests that anxiety contributes directly to explaining the variance in depression while stress and self-esteem might contribute directly to explaining the variance in depression and indirectly by increasing feelings of anxiety. Indeed, individuals who experience stress over a long period of time are susceptible to increased anxiety and depression [30] , [31] and previous research shows that high self-esteem seems to buffer against anxiety and depression [32] , [33] . The study also showed that stress partially mediated the effects of both anxiety and positive affect on depression and that stress completely mediated the effects of self-esteem on depression. Anxiety and positive affect contributed directly to explain the variation in depression and indirectly to the experienced level of stress. Self-esteem contributed only indirectly via the experienced level of stress to explain the variation in depression, i.e. stress affects depression on the basis of ‘its own power’ and explains much more of the variation in depressive experiences than self-esteem. In general, individuals who experience low anxiety and frequently experience positive affect seem to experience low stress, which might reduce their levels of depression. Academic stress, for instance, may increase the risk for experiencing depression among students [34] . Although self-esteem did not emerged as an important variable here, under circumstances in which difficulties in life become chronic, some researchers suggest that low self-esteem facilitates the experience of stress [35] .
Moderator effects/interaction effects
The present study showed that the interaction between stress and negative affect and between positive and negative affect influenced self-reported depression symptoms. Moderation effects between stress and negative affect imply that the students experiencing low levels of stress and low negative affect reported lower levels of depression than those who experience high levels of stress and high negative affect. This result confirms earlier findings that underline the strong positive association between negative affect and both stress and depression [36] , [37] . Nevertheless, negative affect by itself did not predicted depression. In this regard, it is important to point out that the absence of positive emotions is a better predictor of morbidity than the presence of negative emotions [38] , [39] . A modification to this statement, as illustrated by the results discussed next, could be that the presence of negative emotions in conjunction with the absence of positive emotions increases morbidity.
The moderating effects between positive and negative affect on the experience of depression imply that the students experiencing high levels of positive affect and low levels of negative affect reported lower levels of depression than those who experience low levels of positive affect and high levels of negative affect. This result fits previous observations indicating that different combinations of these affect dimensions are related to different measures of physical and mental health and well-being, such as, blood pressure, depression, quality of sleep, anxiety, life satisfaction, psychological well-being, and self-regulation [40] – [51] .
Limitations
The result indicated a relatively low mean value for depression ( M = 3.69), perhaps because the studied population was university students. These might limit the generalization power of the results and might also explain why negative affect, commonly associated to depression, was not related to depression in the present study. Moreover, there is a potential influence of single source/single method variance on the findings, especially given the high correlation between all the variables under examination.
Conclusions
The present study highlights different results that could be arrived depending on whether researchers decide to use variables as mediators or moderators. For example, when using meditational analyses, anxiety and stress seem to be important factors that explain how the different variables used here influence depression–increases in anxiety and stress by any other factor seem to lead to increases in depression. In contrast, when moderation analyses were used, the interaction of stress and affect predicted depression and the interaction of both affectivity dimensions (i.e., positive and negative affect) also predicted depression–stress might increase depression under the condition that the individual is high in negative affectivity, in turn, negative affectivity might increase depression under the condition that the individual experiences low positive affectivity.
Acknowledgments
The authors would like to thank the reviewers for their openness and suggestions, which significantly improved the article.
Author Contributions
Conceived and designed the experiments: AAN TA. Performed the experiments: AAN. Analyzed the data: AAN DG. Contributed reagents/materials/analysis tools: AAN TA DG. Wrote the paper: AAN PR TA DG.
- View Article
- Google Scholar
- 3. MacKinnon DP, Luecken LJ (2008) How and for Whom? Mediation and Moderation in Health Psychology. Health Psychol 27 (2 Suppl.): s99–s102.
- 4. Aaroe R (2006) Vinn över din depression [Defeat depression]. Stockholm: Liber.
- 5. Agerberg M (1998) Ut ur mörkret [Out from the Darkness]. Stockholm: Nordstedt.
- 6. Gilbert P (2005) Hantera din depression [Cope with your Depression]. Stockholm: Bokförlaget Prisma.
- 8. Tabachnick BG, Fidell LS (2007) Using Multivariate Statistics, Fifth Edition. Boston: Pearson Education, Inc.
- 10. Beck AT (1967) Depression: Causes and treatment. Philadelphia: University of Pennsylvania Press.
- 21. Eskin M, Parr D (1996) Introducing a Swedish version of an instrument measuring mental stress. Stockholm: Psykologiska institutionen Stockholms Universitet.
- 22. Rosenberg M (1965) Society and the Adolescent Self-Image. Princeton, NJ: Princeton University Press.
- 23. Lindwall M (2011) Självkänsla – Bortom populärpsykologi & enkla sanningar [Self-Esteem – Beyond Popular Psychology and Simple Truths]. Lund:Studentlitteratur.
- 25. Blascovich J, Tomaka J (1991) Measures of self-esteem. In: Robinson JP, Shaver PR, Wrightsman LS (Red.) Measures of personality and social psychological attitudes San Diego: Academic Press. 161–194.
- 30. Eysenck M (Ed.) (2000) Psychology: an integrated approach. New York: Oxford University Press.
- 31. Lazarus RS, Folkman S (1984) Stress, Appraisal, and Coping. New York: Springer.
- 32. Johnson M (2003) Självkänsla och anpassning [Self-esteem and Adaptation]. Lund: Studentlitteratur.
- 33. Cullberg Weston M (2005) Ditt inre centrum – Om självkänsla, självbild och konturen av ditt själv [Your Inner Centre – About Self-esteem, Self-image and the Contours of Yourself]. Stockholm: Natur och Kultur.
- 34. Lindén M (1997) Studentens livssituation. Frihet, sårbarhet, kris och utveckling [Students' Life Situation. Freedom, Vulnerability, Crisis and Development]. Uppsala: Studenthälsan.
- 35. Williams S (1995) Press utan stress ger maximal prestation [Pressure without Stress gives Maximal Performance]. Malmö: Richters förlag.
- 37. Garcia D, Kerekes N, Andersson-Arntén A–C, Archer T (2012) Temperament, Character, and Adolescents' Depressive Symptoms: Focusing on Affect. Depress Res Treat. DOI:10.1155/2012/925372.
- 40. Garcia D, Ghiabi B, Moradi S, Siddiqui A, Archer T (2013) The Happy Personality: A Tale of Two Philosophies. In Morris EF, Jackson M-A editors. Psychology of Personality. New York: Nova Science Publishers. 41–59.
- 41. Schütz E, Nima AA, Sailer U, Andersson-Arntén A–C, Archer T, Garcia D (2013) The affective profiles in the USA: Happiness, depression, life satisfaction, and happiness-increasing strategies. In press.
- 43. Garcia D, Nima AA, Archer T (2013) Temperament and Character's Relationship to Subjective Well- Being in Salvadorian Adolescents and Young Adults. In press.
- 44. Garcia D (2013) La vie en Rose: High Levels of Well-Being and Events Inside and Outside Autobiographical Memory. J Happiness Stud. DOI: 10.1007/s10902-013-9443-x.
- 48. Adrianson L, Djumaludin A, Neila R, Archer T (2013) Cultural influences upon health, affect, self-esteem and impulsiveness: An Indonesian-Swedish comparison. Int J Res Stud Psychol. DOI: 10.5861/ijrsp.2013.228.

Kluwer Mediation Blog
Research on mediation – why its tricky and why we need to do it.
In the next few weeks, my first cohort of Masters in Conflict Management students will be submitting their research dissertations. Meanwhile next year’s are beginning to think about the research topics. For me, this is terribly exciting. I get to see the results and outcomes of the research completed, and help the new students choose interesting topics. I get to work with enthusiastic, interested and passionate students and help them explore new areas of study.
For the students themselves, I’m not sure exciting is quite how they would describe how they feel, certainly not those in the final stages of editing and proof reading. Research can be a frustrating, isolating and thankless task. Obstacles must be overcome, cul de sacs navigated and hours spent reading books and articles that may end up not being relevant. And then there are the supervisors to deal with….
Research in conflict and mediation, in particular, poses its own unique challenges. Some of these might be off-putting to prospective students so it is worth addressing these in a little more detail to establish how they can be overcome.
First of all, where to begin!!?? How do you choose a narrow enough area in a field as wide as conflict and mediation? As academics we talk about identifying a gap in the research. Mediation is such an under-researched area that it consists more of gaps than research results. This is a fantastic opportunity for students on the one hand, but frustrating on the other. For postgraduate level research, the research question must be narrow enough to address within the confines of a Masters dissertation or PhD thesis. This can mean the difference between researching, say, barriers to family mediation and barriers to family mediation in court annexed programmes in Norway (or wherever). It can be challenging for students, particularly those who have not worked in the field, to identify sufficiently narrow gaps without some guidance from tutors. Having then identified a topic, the overall dearth of research can mean that relevant literature is difficult to source. Reading into the context of the research can prove tricky and sometimes relevant literature will only be available in the context of other jurisdictions or indeed other disciplines. Some of the most relevant literature can be in the form of unpublished research dissertations which can be difficult to access if they are located in other universities – a request to students everywhere – publish your research people!!!
Again, tutors with subject matter expertise can help out a lot here, as can looking outside the traditional media of books and journals to source relevant writing. Conflict is a subject that spans a number of different disciplines – law, business, social studies, psychology, so thinking outside the frame of the “home” department can be useful. When looking for other unpublished research in this area, identify universities that have relevant departments or schools and see can they be visited to do a search through dissertations. This can even be useful at the conceptual stage, to see how other students have framed their research questions.
The next challenge may come after identification of a research question, and is related to the nature of mediation itself. In my experience, what researchers are most interested in is what happens in the mediation room; the experience of the parties, the interventions of the mediators, the dynamic in the room. Research subjects, and data, are difficult to source however due to, first of all, the confidential nature of mediation, meaning that mediators, services and other gatekeepers will rarely allow researchers access to clients, and rightly so. Parties themselves may be reluctant to engage in research for many reasons, including wanting to forget a difficult episode in their lives as quickly as possible, concerns about confidentiality and fear of conflicts or at least negative emotions being stirred up again. Even if one party agrees, the other may not. Where parties do agree, the researcher will need to overcome significant ethical issues and may not gain the ethical approval required from their institution.
This issue prevails not just at postgraduate level but in all research contexts in this area. It is not an easy one to overcome. Students may have more luck with larger, possibly funded services where appropriate safeguards can be put in place, than with private clients. Certain aspects of research relating to mediation parties may prove easier to achieve, such as research on demographics, attendance rates or similar. A careful consideration of the appropriate research methodology might assist. Using an anonymous online survey might be easier for parties to contemplate than engaging in face to face interviews. Thinking outside the box may also be of assistance. Maybe engaging with practitioners to ascertain what they feel might affect parties to mediation or what they feel about certain aspects of the parties’ experience could be just as interesting and relevant, though from a different angle of course, as engaging with parties directly. One of the great joys of research is how many different ways there are to do it.
Finally, and practically, the issue of resources must be mentioned. Conflict studies and mediation are often thrown into the melting pot that is known as the humanities. And when it comes to the humanities, research funding tends to be scarce, at least when compared with other disciplines. Seeking postgraduate funding for research into mediation is, somewhat ironically given how often cost savings are cited as a reason for promoting mediation, a thankless and often fruitless task. Despite the benefits that are clear to us working in the area, it is not generally identified as an area worth funding research into. I definitely don’t have an easy answer for this one but, again, a bit of lateral thinking might help. As I’ve already said, conflict and mediation is a huge field that has tributaries into many other disciplines. Some of these other disciplines do benefit from funding. So in thinking about a research topic, if funding, or rather the lack thereof, is a barrier, it could be worth thinking about these areas and seeing where they and mediation interface and what research might be worth doing in this interface. A colleague of mine put this suggestion a little more bluntly saying “if you put technology in the title you’ll be sorted..” While I’m not suggesting this will bring a funding windfall, it is worth thinking about.
None of these challenges should put potential research students off. Research in conflict and mediation is urgently needed to inform our practices, to drive policy at governmental level and to assist in understanding this complex area. It is also intensely rewarding. Even if a small study on a narrow topic might feel like it is insignificant, it will contribute to knowledge in this area and give other researchers something to build on. It is so important that we not only carry out this research but also that we publish or otherwise share it with our peers and with those in other disciplines. It is time we put a greater effort into filling all those gaps we identify. And to my long suffering students, the finishing line is in sight, stay strong…
________________________
To make sure you do not miss out on regular updates from the Kluwer Mediation Blog, please subscribe here .
Profile Navigator and Relationship Indicator Access 17,000+ data-driven profiles of arbitrators, expert witnesses, and counsels, derived from Kluwer Arbitration's comprehensive collection of international cases and awards and appointment data of leading arbitral institutions, to uncover potential conflicts of interest. Learn how Kluwer Arbitration can support you.
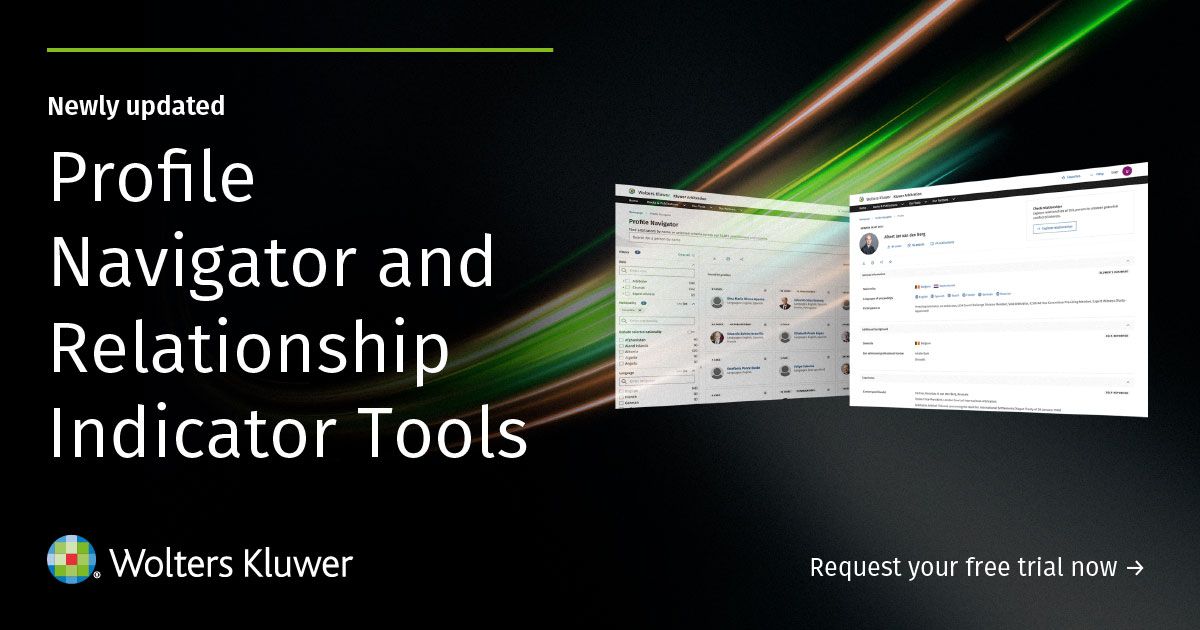
Sabine, your comments are spot on. One potentially rich source for research is the results of the Global Pound Conference Series (www.globalpoundonference.org) which has just finished its final ‘live’ session in London. Here’s the RFP calling for assistance with data analysis and related work: https://imimediation.org/request-for-proposals-global-pound-conference-series
Sabine, Are you aware of any researchers looking for online mediation sessions to review? I served as volunteer mediator in small claims venue for more than 300 cases years ago. After participating with the virtual mediation lab I would like to serve again as a volunteer mediator in the virtual realm. Working with researchers seems to be the most viable means for me to do this. There are issues concerning confidentiality and simulation verses actual mediation. My target for starting to offer virtual mediations is September or November 2017. I hope to allocate time for 4 to 8 matters per month and continue for 18 to 36 months. I am looking for the right team of people for this to come to fruition. The cost of the zoom meeting room and the website are minimal. The site is under construction, needing some revision and refining.
Leave a Reply Cancel reply
Your email address will not be published. Required fields are marked *
Save my name, email, and website in this browser for the next time I comment.

FIND A MEDIATOR QUICK LISTS
- Mediators Listed by State/City
- Mediators Listed by Practice Area
- Mediator Services
- Certification
- Mediate University
- Statewide Banner
- Online Meeting Room
- About Mediation
- Public Policy
The Mediator’s Toolkit: Formulating and Asking Questions for Successful Outcomes

Originally published in the ADR Institute of Canada (ADRIC) Canadian Arbitration and Mediation Journal – ADRIC.ca Vol.27. No. 2 Canadian Arbitration & Mediation Journal
THE MEDIATOR’S TOOLKIT: FORMULATING AND ASKING QUESTIONS FOR SUCCESSFUL OUTCOMES
Mediators need to formulate and ask incisive questions that challenge entrenched thinking and shift perspectives. The Mediator’s Toolkit offers a way to do that using questions such as those that Gerry O’Sullivan calls “Journey of Infer- ence Questions.” She suggests that such questions are designed to specifically create new insight for the parties. Here is a brief extract from the book.
The concept of the “ladder of inference” was first developed by Chris Argyris and subsequently presented by Peter Senge in his book The Fifth Discipline. In this book I am calling it the “Journey of Inference” because I consider it to be a continuous circular journey in the mind rather than a journey to the top of a ladder and back down again. See Figure below.
Journey of Inference questions take a party through the information they se- lected during a precipitating event; the interpretations they made about that information; the assumptions they made; and the conclusions they then reached which, in turn, informed any decisions or actions they took. These questions also explore the beliefs of a party and how these beliefs may have influenced his or her Journey of Inference.
This workplace scenario, with the accompanying guidelines and steps, will serve to demonstrate how Journey of Inference questions work.
Ann and Mary have both worked in a hospital laboratory for five years. The laboratory had nine members of staff in total. The working relation- ship between Ann and Mary was good and they even socialized together on occasions. Lately, how- ever, Ann had noticed a slight difference in her relationship with Mary. There was nothing that she could specifically name — it was just a niggly feeling that Ann had had for a few weeks, with nothing to back it up.
Last week when Ann arrived at work, Mary was walking towards her in the hospital corridor. When Ann was about to say hello, she noticed that Mary kept her head down and did not say hello to her. Ann was taken aback by this and continued walking towards the laboratory. Ann’s first thought was that this confirmed her previous suspicions: she interpreted the incident to mean that Mary wanted to avoid her, and she then assumed that Mary did not like her anymore. Ann concluded that Mary probably wished to end their friendship but had no idea why Mary would want to do this, especially without telling her why. As she continued to reflect, Ann became convinced that Mary had been talking about her behind her back to others in the laboratory.
Ann then realized that this was just one more example of the way people behave: they never have the courage to say something to your face, but spend their time thinking negative thoughts about you, while continuing to smile and pretend that everything is OK with the friendship. Then they talk to others about you and try to turn them against you too. Ann immediately decided that she would stop talking to Mary and to all the other staff as well. Ann had experienced this situation many times before and she believed that she knew exactly how to deal with it!
Over the next few days, both Mary and the other staff began to wonder what was wrong with Ann. But they did not approach her, because they noticed she was bubbling over with anger and they knew she could be aggressive at times. They did not want to create a scene, but they all engaged on their own individual Journeys of Inference and took actions in line with their personal past experiences and beliefs.
Over the following days, Ann noticed more and more things that confirmed her suspicion that no one wished to be her friend any more. She even started to proactively look for examples to prove that her beliefs were correct. The situation became steadily worse until one day Ann completely lost her temper with Mary in the hospital cafeteria while dozens of staff looked on. Mary went to the human resources department to make a complaint about Ann, and mediation was proposed.
“All the people she knows probably don’t want to have anything to do with me anymore, but I don’t care.”
“This must mean she is trying to avoid me.”
Selecting data and making inferences is largely an unconscious process, but it can be made conscious through mediation questions. Journey of Inference questions support parties to look for new and clarifying information that may even prove their interpretations and assumptions to have been incorrect. The resulting re-interpretations they make may then be more accurate and balanced.
GUIDELINES FOR ASKING JOURNEY OF INFERENCE QUESTIONS
THE JOURNEY OF INFERENCE QUESTIONING PROCESS CAN STOP AT ANY TIME, IF NECESSARY, FOR EXAMPLE:
— If understanding is reached early in the questioning process — for instance, at interpretations stage.
— If one party is finding the process too intense and difficult.
SAMPLE JOURNEY OF INFERENCE QUESTIONS
There are three steps involved in developing a series of Journey of Inference questions:
Step 1: Hearing the narrative of a party
Step 2: Challenging the narrative
Step 3: Building a possible new narrative
Here are some sample questions that could be asked in the workplace scenario involving Mary and Ann. [This list of questions has been condensed for this article.]
HEARING THE NARRATIVE
— Ann, would you like to tell me what happened, please, when you and Mary passed each other in the corridor? Then what happened?
Selected Data
— What did you observe, Ann? What information or facts did you take from this event?
Interpretations
— When that happened [Mary walking past you with her head down], what did you think it meant? What brought you to this interpretation?
Assumptions
— And what did you think that meant and what assumptions did you make about what might happen? What brought you to that assumption?
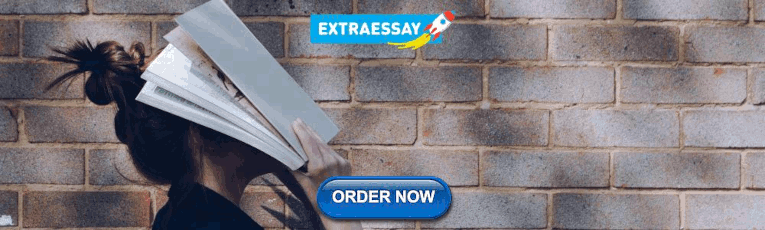
Conclusions and Judgments
— After you made that assumption, what conclusions or judgments did you come to? What brought you to this judgment or conclusion?
— What are your beliefs about the world and how people usually behave in a situation like this?
— How did these beliefs influence the decisions you made or the actions you took afterwards? What did you decide to do?
— And then what happened? What else happened?
After going through Step 1, mediators need to summarize, identify and name to parties the link between the initial interpretations made by a party and the resulting decisions or actions they took.
CHALLENGING THE NARRATIVE
— What had you been thinking/feeling about Mary before/when this happened?
— On what did you base that thinking? What was the tangible evidence for this?
— What had been your expectations of Mary? What influenced those expectations?
— If you had not been concentrating on what you were expecting, what else might you have seen?
— What would others have observed, if they had been there when Mary walked past you with her head down? — On a scale of 0 to 10, with 10 indicating complete certainty, how certain can you be about…?
— What is this uncertainty about? (If the response is less than 10)
— Ann, what did you think might have been Mary’s intention?
— What influenced or contributed to you interpreting what you observed in this way?— How might your stated niggly feeling about your friendship with Mary have influenced what you actually saw and your interpretations? If your friendship had still been good when Mary passed you in the corridor with her head down, what might your interpretations have been?
— If you were to look at yourself and this incident from a balcony, what might you have seen and what interpretations might you have made?
— Is there a time or a circumstance that might result in you interpreting this differently?
Questions can also be asked about the perspective of the other party:
— If asked, what might Mary say about the time you saw her passing you in the hospital corridor?
— What do you think would surprise Mary the most about what you interpreted from this situation, and about what you mentioned about her intent?
— What interpretation might Mary have liked you to make?
— What assumptions did you make after you initially interpreted Mary’s actions in that way?
— What did you think was going to happen?
— What influenced you to make this specific assumption?
— What other assumptions could you have made?
— If you had made a different assumption, what might have been the outcome?
The mediator may continue with more questions about the assumptions made, based on Ann’s responses if relevant.
Conclusions
— After you made that initial assumption, what judgment or conclusion did you come to, Ann?
— What brought you to make this judgment or conclusion? What did this decision mean for you?
— What other conclusions could you have come to?
The mediator may continue with more questions about the conclusions made, and ask the party to rank their alternative conclusions, if relevant.
— What is it you think or believe about life or people that brought you to that conclusion? How has this belief served you in the past? Are there situations where these beliefs may be valid or invalid? What are the distinctions you make between these situations?
— What other beliefs do you have that could have resulted in your reaching a different conclusion?
— You mentioned earlier that after this event you made decisions about how you were going to respond to it and that the conflict escalated and you felt more entrenched. Having reflected on this now, what other decisions or actions could you have taken?
— How might this have impacted on the conflict situation and its progression?
— What might have been the outcomes?
At times, only one party needs to be asked Journey of Inference questions. But in this case, Mary had also made a Journey of Inference, so similar questions needed to be asked of her.
When thepasthasbeen deconstructed and it appears, or is stated, that new learning and insight have been gained by both parties, then it is time to start reflecting on any further misinterpretations that parties may have made.
— Mary and Ann, as the conflict progressed, what do you think each of you may have intended that may have been misinterpreted by the other?
— What do each of you think your misinterpretation may have meant for the other party?
— In what way might this interpretation have led to either of you employing a particular behaviour as a response?
Creating understanding between the parties is further helped by facilitating them to talk about the impact that the conflict is having on them. This may only be done if a mediator knows that each party will listen to the other respectfully.
— How has this conflict impacted on both of you?
— What has been the worst thing for each of you in all this?
— How did the impact of all this influ- ence the thinking of both of you and the actions you took?
— With what kinds of things do you think the other party struggled?
— What do each of you need the other person to know or understand now?
— What might each of you have needed for this to happen differently?
— What could each of you now offer the other?
Facilitating regret and using the past to inform the future
If there has been a paradigm shift in one or both parties, then the following questions may allow for some regret to be shown and may open possibilities for solutions.
— If you were to go back in time with the information that you have now, what might each of you have said/ done differently?
Statement of the new narrative
— If you were to tell this story now to another person, based on the understanding you have both gained, how would you describe this story to them?
Note: Further issues may arise here and may need to be managed.
Agreements regarding the future
— If something like this were to happen again, how would you manage it? What would each of you need from the other? What could each of you offer the other?
— What can be taken from your learning to inform agreements between you for the future?
HAZARD WARNING

The Mediator’s Toolkit: Formulating and Asking Questions for Successful Outcomes Gerry O’Sullivan September, 2018 Trade Paperback $27.99 CAD
GET YOUR MESSAGE TO ADR SPECIALISTS AND USERS ACROSS CANADA!
ADRIC has creative advertising opportunities to promote your services.
Gerry O'Sullivan
Gerry O’Sullivan is a mediator, trainer, and facilitator with over 30 years of experience in conflict resolution and training. She delivers Mediators' Institute of Ireland accredited Certified Professional Mediation Training in Ireland. Gerry is the director of O’Sullivan Solutions and she lives in Castlegregory on the southwest coast of Ireland. MORE >
Featured Members
Read these next, don saposnek: mediation not a separate profession – video.
Don Saposnek discusses his view of mediation as a practice people do in addition to another professional practice such as the pracitce of law, instead of mediation as a cohesive,...
Different Approaches, Same Service – Mediation
The first mediation course is a special moment that each of us holds in our hearts and it represents the foundation of every mediator. The transformational process that we all...
Resources to Help us Evaluate Research
Neuroscience and Conflict Resolution Blog by Stephanie West AllenAs neuro-foolishness and psych-silliness continue to seep into both mainstream media and mediation presentations, resources to help us be discerning users of...

An official website of the United States government
The .gov means it’s official. Federal government websites often end in .gov or .mil. Before sharing sensitive information, make sure you’re on a federal government site.
The site is secure. The https:// ensures that you are connecting to the official website and that any information you provide is encrypted and transmitted securely.
- Publications
- Account settings
Preview improvements coming to the PMC website in October 2024. Learn More or Try it out now .
- Advanced Search
- Journal List
- HHS Author Manuscripts

A General Model for Testing Mediation and Moderation Effects
Amanda j. fairchild.
Department of Psychology, University of South Carolina, Barnwell College, 1512 Pendleton St., Columbia, SC 29208, USA
David P. MacKinnon
Research in Prevention Lab, Department of Psychology, Arizona State University, P.O. Box 871104, Tempe, AZ 85287-1104, USA
This paper describes methods for testing mediation and moderation effects in a dataset, both together and separately. Investigations of this kind are especially valuable in prevention research to obtain information on the process by which a program achieves its effects and whether the program is effective for subgroups of individuals. A general model that simultaneously estimates mediation and moderation effects is presented, and the utility of combining the effects into a single model is described. Possible effects of interest in the model are explained, as are statistical methods to assess these effects. The methods are further illustrated in a hypothetical prevention program example.
Relations between variables are often more complex than simple bivariate relations between a predictor and a criterion. Rather these relations may be modified by, or informed by, the addition of a third variable in the research design. Examples of third variables include suppressors, confounders, covariates, mediators, and moderators ( MacKinnon et al. 2000 ). Many of these third variable effects have been investigated in the research literature, and more recent research has examined the influences of more than one third variable effect in an analysis. The importance of investigating mediation and moderation effects together has been recognized for some time in prevention science, but statistical methods to conduct these analyses are only now being developed. Investigations of this kind are especially valuable in prevention research where data may present several mediation and moderation relations.
Previous research has described the differences between mediation and moderation and has provided methods to analyze them separately (e.g., Dearing and Hamilton 2006 ; Frazier et al. 2004 ; Gogineni et al. 1995 ; Rose et al. 2004 ). More recent research has presented models to simultaneously estimate mediation and moderation to investigate how the effects work together (e.g., Edwards and Lambert 2007 ; MacKinnon 2008 ; Muller et al. 2005 ; Preacher et al. 2007 ). A review of the substantive literature illustrates that few applied research examples have used these models, however. Although analyzing mediation and moderation separately for the same data may be useful, as described later in this paper, simultaneous examination of the effects is often relevant and allows for the investigation of more varied, complex research hypotheses.
What Type of Research Questions Can Be Addressed with the Simultaneous Analysis of Mediation and Moderation Effects?
Is the process by which a program has an effect the same across different types of participants.
In prevention and intervention research, the mediation model has been used to understand the mechanism(s) by which program effects occur. To determine the generalizability of these mechanisms or to explain an unexpectedly small mediated effect it may be of interest to investigate whether the mediation relation, or the indirect effect, holds across different subgroups (e.g., men vs. women or low-risk vs. high-risk). To investigate these hypotheses, a researcher asks whether the indirect effect is moderated, or whether the mediated effect depends on levels of another variable. For example, suppose that a business implements a worksite-wellness program (the independent variable, X) to reduce obesity-related health risks in its employees. Program developers hypothesize that by increasing employee knowledge about the benefits of eating fruits and vegetables (the mediator variable, M), employee consumption of fruits and vegetables will increase (the dependent variable, Y), thus reducing health risk. An estimate of the indirect effect of the program on employee fruit and vegetable consumption through employee knowledge of the benefits of eating fruits and vegetables is unexpectedly low. Through talks with employees, it becomes apparent that participants were more or less motivated to gain and use knowledge from the program to improve their diet based on whether they had a family history of obesity-related illness such as diabetes or cardiovascular disease. Program developers hypothesize that participants’ family history of obesity-related illness may moderate the mediation relation in the data, affecting the influence of the program on employee knowledge of fruits and vegetables and its subsequent impact on fruit and vegetable consumption (See Fig. 1 ).

Conceptual diagram for the moderation of an indirect effect example
Can a Mediation Relation Explain an Interaction Effect in My Data?
Suppose a similar worksite-wellness program was implemented in a larger sister company and program effects had been dependent on whether the participant was a full or part-time employee at the company. To investigate the underlying reasons for this unexpected interaction, or moderation relation, program analysts could investigate a mediation hypothesis where the interaction effect predicts a mediator variable which predicts the outcome, defined here as the mediation of a moderator effect . For example, perhaps in addition to increasing employee knowledge of fruit and vegetable benefits with the wellness curriculum, the program (X) also introduced a work culture, or a social norm (M), of healthy eating which contributed to employee fruit and vegetable consumption (Y; See Fig. 2 ). Program developers hypothesize that the more hours an employee worked in a week determined how much they were subjected to the social norm which ultimately influenced their fruit and vegetable consumption.

Conceptual diagram for the mediation of a moderator effect example
Current Research
The purpose of this article is to provide a straightforward, methodological resource on models to simultaneously test mediation and moderation effects for the substantive researcher. To that end, we organize methods for simultaneously testing mediation and moderation into a single framework that allows for point estimation and construction of confidence intervals. Interpretation and effect computation are provided, and the model is applied to a substantive dataset to illustrate the methods. To ensure common ground for this discussion, basic mediation and moderation effects from which the model is formed are first reviewed.
Review of the Mediation Model
The mediation model offers an explanation for how, or why, two variables are related, where an intervening or mediating variable, M, is hypothesized to be intermediate in the relation between an independent variable, X, and an outcome, Y (See Fig. 3 ). Early presentations of mediation in prevention research (e.g., Baron and Kenny 1986 ; Judd and Kenny 1981a ; 1981b ) illustrated causal step methods to test for mediation, but more recent research has supported tests for statistical mediation based on coefficients from two or more of the following regression equations ( MacKinnon and Dwyer 1993 ):

Path diagram for the single-mediator model. Note. X= the independent variable, Y= the dependent variable, and M= the mediating variable. The mediation model decomposes the total effect of X on Y ( c ), into two parts: the indirect effect of X on Y, quantified by ab (the product of a and b ), and the direct effect of X on Y with the effect of the mediator removed, quantified by c ′. c = ab + c ′
Where c is the overall effect of the independent variable on Y; c ′ is the effect of the independent variable on Y controlling for M; b is the effect of the mediating variable on Y; a is the effect of the independent variable on the mediator; i 1 , i 2 , and i 3 are the intercepts for each equation; and e 1 , e 2 , and e 3 are the corresponding residuals in each equation (see Fig. 3 ).
Although there are alternative ways to estimate mediation, the product of coefficients is most easily applied to complex models and is used in this paper. The product of coefficients test computes the mediated effect as the product of the â and b ̂ coefficients from Eq. 2 and 3 . Sobel (1982 , 1986) derived the variance of â b ̂ product based on the multivariate delta method. This formula has been widely used to estimate the normal theory standard error of â b ̂ :
Where s a ^ 2 is the variance of the â coefficient and s b ^ 2 is the variance of the b ̂ coefficient.
MacKinnon et al. (1998) and MacKinnon and Lockwood (2001) showed that tests for the mediated effect based on normal theory can yield inaccurate confidence limits and significance tests, however, as the product of two normally distributed variables is not itself normally distributed. Alternative tests based on the asymmetric distribution of the product of two normally distributed variables are available and have been shown to outperform traditional methods ( MacKinnon et al. 2002 ; MacKinnon et al. 2004 ). A new program called “PRODCLIN” ( MacKinnon et al. 2007 ) has automated computation of the distribution of the product test for mediation so that it is widely accessible. The researcher need only specify values of â , b ̂ , the standard error of â , the standard error of b ̂ , and the statistical significance level desired.
Assumptions of the mediation model include the usual OLS estimation assumptions (e.g., correct specification of the model’s functional form, no omitted variables, no measurement error; Cohen et al. 2003 ). Mediation analysis also assumes correct causal ordering of the variables, no reverse causality effects, and no XM interaction.
Review of the Moderation Model
The moderation model tests whether the prediction of a dependent variable, Y, from an independent variable, X, differs across levels of a third variable, Z (See Fig. 4 ). Moderator variables affect the strength and/or direction of the relation between a predictor and an outcome: enhancing, reducing, or changing the influence of the predictor. Moderation effects are typically discussed as an interaction between factors or variables, where the effects of one variable depend on levels of the other variable in analysis. Detailed descriptions of moderator effects and a framework for their estimation and interpretation were presented in Aiken and West (1991) .

Alternate path diagram representations of the moderation model. Note. X= the independent variable, Y= the dependent variable, Z= the moderator variable, XZ= the product of X and the moderator variable, β 1 = the effect of X on Y, β 2 = the effect of Z on Y, and β 3 = the effect of XZ on Y
Moderation effects are tested with multiple regression analysis, where all predictor variables and their interaction term are centered prior to model estimation to improve interpretation of regression coefficients. A single regression equation forms the basic moderation model:
Where β 1 is the coefficient relating the independent variable, X, to the outcome, Y, when Z = 0, β 2 is the coefficient relating the moderator variable, Z, to the outcome when X = 0, i 5 the intercept in the equation, and e 5 is the residual in the equation.
The regression coefficient for the interaction term, β 3 , provides an estimate of the moderation effect. If β 3 is statistically different from zero, there is significant moderation of the X-Y relation in the data. Plotting interaction effects aids in the interpretation of moderation to show how the slope of Y on X is dependent on the value of the moderator variable. Regression slopes that correspond to the prediction of Y from X at a single value of Z are termed simple slopes.
Assumptions of the moderation model include OLS regression assumptions, as described earlier, and homogeneity of error variance. The latter assumption requires that the residual variance in the outcome that remains after predicting Y from X is equivalent across values of the moderator variable.
Combining Mediation and Moderation Analyses
Analyzing the models separately.
Much of the work combining mediation and moderation analyses has been presented in the context of prevention program design and development, where examining mediation and moderation effects together aims to improve program implementation by combining theory-driven ideas and empirical evidence. For example, Donaldson (2001) indicates that multivariate relations between variables in a treatment program tend to be one of three types: (a) direct effects, (b) mediated effects, and (c) moderated effects. By combining the examination of these effects in a single analysis, the researcher may not only identify mediating processes through which the program achieves its effects but may also identify effective program components and/or particular characteristics of the participants or the environment that moderate the effectiveness of the program. If the theoretical underpinnings of a treatment or prevention program serve as a starting point for its curriculum, separate analyses of mediation and moderation may be used to iteratively refine program theory. These analyses may be used to collect empirical feedback and to conduct pilot work of the program before large-scale implementation of the curriculum (See Fig. 5 ). Specifically, by examining mediation one is able to investigate how effective a program curriculum was in changing target behaviors, and whether the program aimed to alter appropriate mediators of desired outcomes. Analyzing moderation effects in this context allows the researcher to identify variables that may improve or reduce the program’s ability to alter mediating variables, as well as to examine the external validity, or generalizability, of the model across different groups or settings ( Hoyle and Robinson 2003 ). Hypothesized moderator variables may be more or less amenable to program tailoring, however. Although program subgroups may be formed on moderators such as age or gender with little difficulty, forming program subgroups based on other moderator variables such as ethnicity or family risk may be impractical and/or unethical. Nonetheless, the identification of subgroups for which a program is most effective is useful, and the examination of moderation and mediation effects in this context increases the scientific understanding of behaviors and improves program efficacy. West and Aiken (1997) have argued that these analyses are especially useful after the successful implementation and evaluation of a treatment program. This allows for the continual development and improvement of a program, but after an effective first evaluation.

Refining program theory: an empirical-theoretical exchange. Note. Figure is based on Donaldson (2001) diagram that occurs on p. 472 of that text
Analyzing the Models Simultaneously
By simultaneously investigating mediation and moderation, the effects may not only be disentangled and analyzed separately but can also be evaluated together. There have been two primary effects analyzed in the literature: (a) the mediation of a moderator effect, and (b) the moderation of an indirect effect. The mediation of a moderator effect involves exploring mediating mechanisms to explain an overall interaction of XZ in predicting Y, whereas the moderation of an indirect effect involves investigating whether a mediated relation holds across levels of a fourth, moderating variable. These effects have previously been referred to as mediated-moderation and moderated-mediation in the literature, respectively. These alternative descriptions may enhance the distinction between the two.
Previous models to simultaneously test mediation and moderation effects have been presented with varying notation (e.g., Edwards and Lambert 2007 ; James and Brett 1984 ; Muller et al. 2005 ; Preacher et al. 2007 ) or without testable equations (e.g., Baron and Kenny 1986 ; Wegener and Fabrigar 2000 ), making it difficult to understand similarities and differences among the methods. Moreover the criteria for testing the effects have varied across sources, making it hard to extrapolate recommendations for use. It is possible to create a general model to test these effects, however, that subsumes several previous frameworks by including all possible interactions between variables in the mediation and moderation models ( MacKinnon 2008 ). Such a model unifies the methods into a single presentation where different models are represented as special cases of the larger framework. Three regression equations form the model:
where all predictors in the model are centered at zero to improve interpretation of the lower order coefficients. In Eq. 6 , c 1 is the effect of the independent variable on the outcome when Z = 0 (also the average effect of X on Y because the mean of Z = 0), c 2 is the effect of the moderator variable on the outcome when X = 0 (also the average effect of Z on Y because the mean of X = 0), c 3 is the effect of the interaction between the independent variable and the moderator on the outcome, and i 6 and e 6 are the intercept and the residual in the equation, respectively. In Eq. 7 , a 1 is the effect of the independent variable on the mediator when Z = 0 (also the average effect of X on M because the mean of Z = 0), a 2 is the effect of the moderator variable on the mediator (also the average effect of Z on M because the mean of X = 0), a 3 is the effect of the interaction between the independent and moderator variables on the mediator, and i 7 and e 7 are the intercept and the residual in the equation, respectively. In Eq. 8 , c ′ 1 is the effect of the independent variable on the outcome when M = 0 and Z = 0 (the average effect of X on Y), c ′ 2 is the effect of the moderator on the outcome when X = 0 and M = 0 (the average effect of Z on Y), c ′ 3 is the effect of the interaction between the independent and moderator variables on the outcome when M = 0 (the average effect of XZ on Y), b 1 is the effect of the mediator on the outcome when X = 0 and Z = 0 (the average effect of M on Y), b 2 is the effect of the interaction between the moderator and mediator variables on the outcome when X = 0 (the average effect of MZ on Y), h is the effect of the interaction between the independent and mediator variables on the outcome when Z = 0 (the average effect of XM on Y), and j is the effect of the three-way interaction of the mediating, moderating, and independent variables on the outcome. The intercept and residual in Eq. 8 are coded i 8 and e 8 , respectively. A path diagram for the model is presented in Fig. 6 .

MacKinnon (2008) General Joint Analysis Mode. Note. X= the independent variable, Y= the dependent variable, Z= the moderator variable, M= the mediating variable, XZ= the interaction of X and Z, MZ=the interaction of M and Z, XM= the interaction of X and M, and XMZ= the three-way interaction between X, M, and Z
Assumptions of the general model include assumptions of the mediation and moderation models as described earlier. Issues of causal inference in non-additive models may also require additional stipulations for estimation. Note that the presence of any significant two-way interactions in the model implies that the main effects of X and M do not provide a complete interpretation of effects. The presence of a significant three-way interaction in the model also implies that lower order two-way interactions do not provide a complete interpretation of effects. If there are significant interactions, point estimates can be probed with plots and tests of simple effects to probe the interaction effects. Edwards and Lambert (2007) , Preacher et al. (2007) , and Tein et al. (2004) provide methods to perform these analyses.
Testing effects: Criteria for the moderation of an indirect effect
To examine whether an indirect effect is moderated, it is of interest to investigate whether the mediated effect ( ab ) differs across levels of a fourth, moderating variable. Previous sources have argued that this effect can be defined by either a moderated a path, a moderated b path, or both moderated a and b paths in the mediation model ( James and Brett 1984 ; Muller et al. 2005 ; Preacher et al. 2007 ; Wegener and Fabrigar 2000 ), such that if there is moderation in either path of the indirect effect then the mediated relation depends on the level of a moderator variable. There are circumstances, however, in which a heterogeneous a or b path does not imply a heterogeneous ab product term.
Although significant heterogeneity in either the a or b path may imply significant heterogeneity in the ab product term in some cases, examining moderation of the product term or moderation of both paths versus examining moderation of single paths in the mediation model are not conceptually identical. Consider the following example where a moderated a path in the mediation model means something different from both moderated a and b paths in the model. Presume that X is calcium intake, M is bone density, Y is the number of broken bones, and Z is gender. Calcium intake is known to have an effect on the bone density of women, and the relation between calcium intake and bone density is stronger in women than it is in men (i.e., heterogeneity in the a path in the model). Specifically, men have greater bone density in general and thus yield fewer gains from supplemental calcium intake. However, bone density affects the fragility of bones in a constant way across males and females, such that low bone density leads to more broken bones (i.e., no heterogeneity of the b path in the model). Previous models would deem this scenario as moderation of the indirect effect, arguing that moderation of the a path suffices as a test for the effect. There are two problems with this argument. First, testing the heterogeneity of only the a or b path in the mediation model is not a test of mediation because only a single link in the mediated effect is tested in each case. Second, a heterogeneous a path in this model suggests something different from both heterogeneous a and b paths or a heterogeneous ab product. Heterogeneity in both paths of the mediated effect would suggest that gender not only moderates the effect of calcium intake on bone density, but that gender also moderates the effect of bone density on broken bones. Heterogeneity of the product estimate of the mediated effect would suggest that gender moderates the mechanism by which calcium intake affects bone loss; this may or may not be true based on the research literature. Although the moderation of a single path may imply moderation of the product term in some cases, it is critical to differentiate the scenarios as they correspond to different research hypotheses.
There are also numerical examples that show instances when heterogeneity in individual paths of the mediation model does not imply heterogeneity of the product term. Consider the following mediated effect scenarios in two moderator-based subgroups:
In both scenarios the a and b paths are heterogeneous across groups thus satisfying criteria for the moderation of a mediated effect as defined by Edward and Lambert (2007) , James and Brett (1984) , Morgan-Lopez and MacKinnon (2006) , Preacher et al. (2007) , and Wegener and Fabrigar (2000) . However the ab product is identical across groups, indicating that there is no moderation of the indirect effect. Although tests for the moderation of a mediated effect based on the heterogeneity of individual path coefficients in the mediation model will be more powerful than a test based on the heterogeneity of the product term (given the usual low power to detect interactions), these tests may also have elevated Type 1 error rates.
Despite potential problems for making inferences on moderation of the ab product from information on the moderation of individual paths, initial simulation work suggests that extending a test of joint significance (where the test for mediation is based on the significance of component paths in the model such that if both â and b ̂ are significant then the mediated effect â b ̂ is deemed significant) to models for mediation and moderation may be acceptable. Specifically, if conclusions about the moderation of â b ̂ are based on whether both â and b ̂ are significantly affected by the moderator variable, Z, Type 1 error rates never exceed .0550 ( Fairchild 2008 ). Effects of the moderator variable on component paths of the mediation model are examined using Eq. 7 and 8 , where â 3 quantifies the effect of Z on the a path and b ̂ 2 quantifies the effect of Z on the b path, respectively (See Fig. 6 ). If both coefficients are significant, it may be claimed that there is significant moderation of the indirect effect. To obtain either a point estimate or confidence limits for the effect, a product of coefficients test can be used.
To estimate a product of coefficients test for moderation of the indirect effect in the case of a dichotomous moderator variable, separate mediation models can be estimated for each group and equivalence of the â b ̂ point estimates can be compared across moderator-based subgroups. An example of a dichotomous moderator variable might be gender or clinical diagnosis. The null hypothesis associated with the test is that the difference between the two mediated effect point estimates in each group is zero:
If the point estimates in each group are statistically different from one another, there is significant moderation of the indirect effect (i.e., heterogeneity in the ab product), such that the mediated effect is moderated by group membership. To test the estimate in Eq. 9 for statistical significance, the difference is divided by a standard error for the estimate to form a z statistic. If the groups are independent, the standard error of the difference between the two coefficients is:
Where s a ^ b ^ group 1 2 is the variance of the mediated effect in group 1 and s a ^ b ^ group 2 2 is the variance of the mediated effect in group 2.To test heterogeneity of the indirect effect in the case of a continuous moderator variable, variance in the estimates of the ab product across levels of the moderator variable is examined. An example of a continuous moderator variable might be individual motivation to improve. Because a z test as shown above can only accommodate moderator variables with two levels (or a small number of levels with contrasts between two variables) and because levels of continuous moderators often do not represent distinct groups, tests with continuous moderators are more complicated. The question becomes how to assess differences in the ab product across a large number of levels of the moderator variable, and the answer to that question is incomplete at this time. Random coefficient models assess variance in regression coefficients across multiple levels such as multiple levels of a moderator. If the moderator is thought of as a higher order variable across which lower order effects (such as ab ) may vary, the random coefficient modeling framework may be suitable to assess variance in the indirect effect across levels of a continuous moderator. Kenny, Korchmaros, and Bolger (2003) describe an estimate of the variance of ab when a and b are correlated for the case of random effects based on Aroian (1947) :
Bauer, Preacher, and Gil (2006) make an important distinction between the variance of ab and the variance of the average value of ab in the multilevel model. It is the variance of ab that is relevant to the question of whether an indirect effect is moderated as we would like to know if there is substantial variability in ab across levels of the moderator variable.
It is also possible to test whether individual paths in the mediation model differ across levels of a moderator variable. These tests can investigate moderation of the direct effect of X on Y ( c ′) or evaluate the generalizability of action and conceptual theory for a program. The null hypothesis to test homogeneity of a program’s direct effect is:
This hypothesis is tested by examining the significance of c ^ 3 ′ in Eq. 8 ; if the regression coefficient is significant, there is significant moderation of the program’s direct effect.
Recall that conceptual theory for a program corresponds to the b path of the mediation model, which defines the theory that links variables or psychological constructs (e.g., M) to behavioral outcomes. The relationships examined in this piece of the model are driven by previous models or theories presented in the literature that explain motivations for behavior. The null hypothesis to test homogeneity of a program’s conceptual theory across levels of the moderator variable is:
This hypothesis is tested by examining the significance of b ̂ 2 in Eq. 8 ; if the regression coefficient is significant there is significant moderation of the program conceptual theory.
Action theory for a program corresponds to the a path of the mediation model, which defines what components of the program are designed to manipulate the mechanisms of change. This piece of the model illustrates how the program intervenes to modify hypothesized mediators. The null hypothesis to test homogeneity of a program’s action theory across levels of the moderator variable is:
This hypothesis is tested by examining the significance of â 3 in Eq. 7 ; if the regression coefficient is significant there is significant moderation of the program action theory. See Chen (1990) for more details on program action and conceptual theory.
Testing effects: Criteria for the mediation of a moderator effect
A test for the mediation of a moderator effect examines whether the magnitude of an overall interaction effect of the independent variable (X) and the moderator variable (Z) on the dependent variable (Y) is reduced once the mediator is accounted for in the model ( Muller et al. 2005 ). Thus, the examination of the mediation of a moderator effect considers the mediation model as a means to explain why a treatment effect of X on Y is moderated by a third variable, Z. In this way, the mediation of a moderator effect hypothesis probes mediation as a possible process that accounts for the interaction of the treatment and the outcome. There is no need to differentiate methods for categorical and continuous moderator variables here.
One way to test whether an XZ interaction in the data is accounted for, at least in part, by a mediating relation is to examine whether the magnitude of the regression coefficient corresponding to the overall interaction effect, c 3 , is reduced once the mediator is added to the model. Using coefficients from Equations 6 and 8 , where ĉ ′ 3 represents the direct effect of the interaction effect on Y once the mediator is included in the model, a point estimate and standard error of this difference can be computed:
Dividing an estimate of the difference in Eq. 15 by its standard error in Eq. 16 provides a test of significance for the estimate. If there is a significant difference in the coefficients then the overall moderation effect of XZ on Y is significantly explained, at least in part, by a mediated relation. The null hypothesis corresponding to this effect is that there is no reduction in the overall interaction once accounting for the mediator:
A test of the mediation of a moderator effect with a product of coefficients estimator circumvents the need for testing the overall interaction of XZ on Y as shown above. Morgan-Lopez and MacKinnon (2006) presented a point estimator and standard error for the product of coefficients method:
The product of coefficients estimator in Eq. 18 illustrates that the effect of the interaction of the independent and moderator variables on the outcome (represented by â 3 ) is transmitted through a mediating variable (represented by b ̂ 1 ). Dividing the estimate of the product by an estimate of its standard error in Eq. 19 provides a test of significance for the estimate based on normal theory. However, as described for the product of coefficients estimator in the single mediator model, asymmetric confidence intervals for the distribution of the product are more accurate than tests based on the normal distribution and should be implemented (note also that a test of joint significance of the coefficients could be conducted). If the product is significantly different from zero, then the moderator effect is explained, at least in part, by a mediating mechanism. The null hypothesis corresponding to this test is that the product of the coefficients is equal to zero:
Numerical Examples
Returning now to the research scenarios presented at the beginning of this paper, numerical examples can be provided to show how these analyses may appear in practice. Two simulated datasets were created to explore the questions. Scenario #1 asked “ Is the process by which a program has an effect the same across different types of participants? ” The researcher investigates whether the indirect effect is moderated to answer this question. Note that although it is necessary to estimate the XM interaction in Equation 8 to avoid bias in the XZ term ( Yzerbyt, Muller, and Judd 2004 ), the XMZ interaction need not be estimated if there is no supporting hypothesis for its existence. Likewise, Equation 6 need not be estimated if overall effects are not part of the research question. Recall that for this example the independent variable, X, was a worksite-wellness program to reduce obesity-related health risk. The mediating variable, M, was knowledge of the benefits of eating fruits and vegetables, and the outcome, Y, was employee consumption of fruits and vegetables. The moderator variable in analysis, Z, was family history of obesity-related illness with 107 employees having no history of disease (i.e., n group1 = 107 ) and 93 employees having a history of disease (i.e., n group2 = 93 ). The null hypothesis for the research question in Scenario #1 is that there is no difference in the indirect effect of the program on employee fruit and vegetable consumption through knowledge of fruit and vegetable benefits across employees who have a family history of disease versus those who have no family history of disease. To test this hypothesis, an estimate of the mediated effect can be computed in each group and compared for differences. Estimating Equations 2 and 3 , the mediated effect, â b ̂ ( s â b ̂ ), for employees with no family history of obesity-related disease was computed as .0004(.0186), with an asymmetric confidence interval of −.0400, .0413, where â ( s â ) = .0041(.2018)and b ̂ ( s b ̂ ) = .0923(.0969) were used as inputs into the PRODCLIN program ( MacKinnon et al. 2007 ). The mediated effect for employees with a family history of obesity-related disease was .2158(.0821), with an asymmetric confidence interval of .0693, .3909, where â ( s â ) = .5479(.1854) and b ̂ ( s b ̂ ) = .3938(.0687) were used as inputs into the PRODCLIN program ( MacKinnon et al. 2007 ). Initial inspection of the indirect effects shows that the mediation relation in the data is significant for individuals with a family history of obesity-related illness (the simple mediated effect for group 1) and nonsignificant for individuals with no family history of disease (the simple mediated effect for group 2). Statistical inspection of the difference between the two indirect effects shows a difference of .2154. Using Eq. 11 , the pooled standard error for this difference was computed as .0421, yielding a z -statistic of 5.1143. Because the obtained z -value is greater than the critical value (1.96) associated with α = .05, there is significant moderation in the indirect effect such that ab is heterogeneous across moderator-based subgroups. Alternatively estimating Equations 7 and 8 and examining a test of joint significance of the â 3 ( s â 3 ) and b ̂ 2 ( s b ̂ 2 ) coefficients to examine whether the indirect effect is moderated yields the same conclusion. Both parameter estimates are significant: â 3 ( s â 3 ) = .2760(.1386) and b ̂ 2 ( s b ̂ 2 ) = .1919(.0697), respectively. Because the moderator variable in this example only had two levels, there is no need to follow up the finding with simple effects; the difference in the mediated effects occurs between the simple mediated effect in group 1 and the simple mediated effect in group 2. Had there been three or more levels of the moderator variable in the example, the researcher could conduct follow up analyses to investigate simple mediated effects at different levels of the moderator variable to see where specific differences occur. Had there also been a significant XM interaction in the data, the researcher would need to estimate simple mediated effects across levels of X as well.
Scenario #2 asked “ Can a mediation relation explain an interaction effect in my data? ” To answer this question, the researcher investigates whether the interaction effect can predict a mediating variable which in turn predicts the outcome. Recall that X was the worksite-wellness program described above, M was a social norm for eating fruits and vegetables, Z was part-time or full-time work status (191 part-time employees, 209 full-time employees), and Y was fruit and vegetable consumption. The null hypothesis for the research question is that a mediating variable cannot explain any part of the interaction. To test this hypothesis, the â 3 b ̂ 1 point estimate is computed for the data and tested for significance. As indicated in the numerical example for Research Scenario #1, although it is necessary to estimate the XM interaction to avoid bias in the XZ term ( Yzerbyt et al. 2004 ), there is no need to model the XMZ interaction if there is no hypothesis to support its estimation. Estimating Eq. 7 and 8 , â 3 b ̂ 1 was computed as .1781 (.0814), with an asymmetric confidence interval of .0192, .3383, where â 3 ( s â 3 ) = .1550(.0706) and b ̂ 1 ( s b ̂ 1 ) = 1.1495(.0405) were used as inputs into the PRODCLIN program ( MacKinnon et al. 2007 ). Failure to include zero in the confidence interval indicates significance of the point estimate, such that the interaction of the program effect and part-time versus full-time work status is explained at least in part by the mediating mechanism of social norms for eating fruits and vegetables. Example SAS datasets, program code and output, and complete worked examples for the general model to simultaneously test mediation and moderation effects are available from the first author.
Issues of Power in Models to Test Mediation and Moderation Effects
Power to detect interaction effects is often low because of the small effect sizes observed in social science ( Aiken and West 1991 ). Models that simultaneously examine mediation and moderation effects are at an even greater disadvantage as they involve several interaction terms as well as estimation of indirect effects. Fairchild (2008) investigated power for the general model using previous arguments made in the literature that interaction effects in real data typically range from explaining 1% to 3% of the variance in the dependent variable ( Champoux and Peters 1987 ; Evans 1985 ; McClelland and Judd 1993 ). Results showed that when the effect size of the â 3 and b ̂ 2 parameters both explained 1% of the variance, a test of joint significance for moderation of the indirect effect required N ≥ 1000 for .8 power. If the effect size of the parameters both explained 3% of the variance, the sample size requirement for .8 power reduced to N ≥ 500. Morgan-Lopez and MacKinnon (2006) showed similar results for tests to examine the mediation of an interaction effect.
The large sample size requirement of these tests makes investigation of mediation and moderation effects in small samples challenging. Effect size analysis usually offers a guide to the practical utility of effects that is independent of significance and sample size, but effect size measures for models that simultaneously analyze mediation and moderation effects are not yet fully researched. Although increasing sample size has historically been most utilized to increase the power of a test, there are several alternatives that may increase power when accessing additional data is not possible ( Beck 1994 ).
Statistical significance of a test is based on four parameters: sample size, effect size, power, and the alpha-level set for the experiment. Thus to achieve .8 power when sample size is fixed either the effect size of the treatment and/or the alpha level of the experiment may be manipulated to increase sensitivity of the research design. For example, rather than implementing the nominal .05 criterion that allows for errors in null-hypothesis-significance-testing 5 times out of every 100, the researcher may choose to evaluate results against a more liberal .10 criterion that allows for errors 10% of the time. Increasing the Type 1 error rate for the study decreases the possibility of making a Type 2 error, or the failure to find a significant effect when one truly exists in the data. This option may be appropriate when an effect of interest is especially important and avoiding Type 2 errors is critical. A second option for increasing power when sample size is limited in a study is to increase the effect size of the manipulation. This option involves increasing the between-group variance on the independent variable in the design and decreasing the within-group variance, or error variance, of the variables ( Beck 1994 ). To increase the between-group variance on the independent variable the researcher may impose an extreme-groups design (see Borich and Godbout 1974 ) or deliver a stronger manipulation so that differences between the treatment and control groups are exaggerated. When the strength of the intervention is not easily increased or formation of extreme groups is not feasible, researchers may opt to focus on decreasing within-group variance, or error variance, in the design. Statistical methods to reduce error variance include improving reliability of the measurement tools used in analysis and implementing analysis of covariance models that partition covariates out of treatment effects. Research design tools that are able to reduce error variance in a model include blocking and matching designs that attempt to equate treatment and control groups on non-intervention dimensions in which they may differ ( Cook and Campbell 1979 ).
Recommendations
To best implement the simultaneous analysis of mediation and moderator effects, the researcher should first identify which third variable model characterizes his or her primary research question. This a priori model identification acts as the starting point of interpretation and can be guided by theory or previous research. If the primary research question is determined to be mediation, the researcher may examine all possible interaction effects in the model or a subset of theoretically-relevant interactions and discuss which moderated effects exist. Methods for examining the moderation of the indirect effect have been discussed in this manuscript, as well as methods for examining moderation in individual links of the mediation model such as the direct effect. Exploring the moderation of either the total or direct effect in the mediation model is simply a means of examining the significance of the individual regression coefficients corresponding to those effects (i.e., c 3 and c ′ 3 , respectively), and any significant interaction effect in the model will be interpreted with reference to mediation. Interactions may be further probed with simple slopes analyses to examine variable relations across levels of the moderator variable ( Edwards and Lambert 2007 ; Preacher et al. 2007 ; Tein et al. 2004 ).
If the statistical model appropriate to answer the primary research question is determined to be moderation, mediation may be investigated as a means to explain an overall moderated treatment effect. To that end, the interpretation of interaction effects in the model focus on whether a mediating mechanism is responsible, at least in part, for overall moderation in the data. As presented in the manuscript, this assessment may be made with either a difference in coefficients ( c ^ 3 − c ^ 3 ′ ) or product of coefficients ( â 3 b ̂ 1 ) estimator. Both tests examine whether there is a decrease in the overall XZ interaction once a mediating variable is modeled. However, given the availability of more accurate significance testing for the product of coefficients estimator, it is recommended that researchers analyze â 3 b ̂ 1 . Preferably, the exploration of possible interactions in either model are well-thought-out and delineated by theory to avoid confusion and unwieldy models, as well as to facilitate interpretation of effects in the model. Regardless of which third variable model is identified with the primary research question, it is necessary to model the XM interaction to avoid bias in the XZ product term ( Yzerbyt, Muller, and Judd 2004 ).
Limitations and Future Directions
The cost of the generalizability of the general model to test mediation and moderation effects is possible inflation of Type I error, lack of power, and difficulty with interpretation of model parameters if several effects are present. The model may be simplified, however, to represent more specialized cases of mediation and moderation joint effects such as baseline by treatment interactions by constraining paths in the model to be zero.
An additional limitation of models with moderation and mediation is the extensive assumptions required for accurate assessment of relations among variables ( Holland 1988 ). The sensitivity of conclusions to violations of assumptions is not yet known and correct conclusions will likely require repeated applications in any substantive research area. In particular, often the X variable is the only variable that represents random assignment, making interpretation of causal relations between other variables in the model susceptible to omitted variable bias. In many applications, the model results may represent descriptive information about how variables are related rather than elucidating true causal relations among variables. Information on true causal relations will require programs of research to replicate and extend results as well as information from other sources such as qualitative information and replication studies in different substantive research areas.
Estimation of the methods and their performance can be investigated for categorical and longitudinal data. Previous work has explored the needs of categorical data in the single mediator model ( MacKinnon and Dwyer 1993 ) and this research may serve as a resource for extending the general model to test mediation and moderation effects into that domain. With regard to longitudinal data, the current model can be applied to two-wave data by using difference scores or residualized change scores, but data with three or more waves cannot yet be accommodated. The addition of cross-lagged effects in longitudinal data frameworks such as autoregressive models or latent growth models increases the possible number of interactions among variables and whether the investigation of all possible cross-lagged effects is valuable or overly cumbersome will need to be determined.
In summary, many questions in prevention science involve how and for whom a program achieves its effects. Mediation and moderation models are ideal for examining these questions. By investigating both mediation and moderation effects in data from prevention programs information about the mechanisms underlying program effects as well as the generalizability of program effects and curricula can be evaluated. Models that simultaneously estimate mediation and moderation effects not only allow for the examination of these questions, but also permit the evaluation of more complex research hypotheses such as whether a moderator effect in the data can be explained by a mediating mechanism, or whether a mediating mechanism depends on the level of another variable. A broad integration of these models into the substantive research literature will enhance the information drawn from prevention work and will inform our knowledge on prevention models.
Contributor Information
Amanda J. Fairchild, Department of Psychology, University of South Carolina, Barnwell College, 1512 Pendleton St., Columbia, SC 29208, USA.
David P. MacKinnon, Research in Prevention Lab, Department of Psychology, Arizona State University, P.O. Box 871104, Tempe, AZ 85287-1104, USA.
- Aiken LS, West SG. Multiple regression: Testing and interpreting interactions. Newbury Park, CA: Sage; 1991. [ Google Scholar ]
- Aroian LA. The probability function of the product of two normally distributed variables. Annals of Mathematical Statistics. 1947; 18 :265–271. doi: 10.1214/aoms/1177730442. [ CrossRef ] [ Google Scholar ]
- Baron RM, Kenny DA. The moderator-mediator variable distinction in social psychological research: Conceptual, strategic, and statistical considerations. Journal of Personality and Social Psychology. 1986; 51 :1173–1182. doi: 10.1037/0022-3514.51.6.1173. [ PubMed ] [ CrossRef ] [ Google Scholar ]
- Bauer DJ, Preacher KJ, Gil KM. Conceptualizing and testing random indirect effects and moderated mediation in multilevel models: New procedures and recommendations. Psychological Methods. 2006; 11 :142–163. [ PubMed ] [ Google Scholar ]
- Beck CT. Achieving statistical power through research design sensitivity. Journal of Advanced Nursing. 1994; 20 :912–916. [ PubMed ] [ Google Scholar ]
- Borich GD, Godbout RC. Extreme groups designs and the evaluation of statistical power. Educational and Psychological Measurement. 1974; 34 :663–675. [ Google Scholar ]
- Champoux JE, Peters WS. Form, effect size, and power in moderated regression analysis. Journal of Occupational Psychology. 1987; 60 :243–255. [ Google Scholar ]
- Chen HT. Theory driven evaluations. Newbury Park, CA: Sage; 1990. [ Google Scholar ]
- Cohen J, Cohen P, West SG, Aiken LA. Applied multiple regression/correlation analysis for the behavioral sciences. 3. Hillsdale, NJ: Lawrence Erlbaum Associates; 2003. [ Google Scholar ]
- Cook TD, Campbell DT. Quasi-experimentation: Design and analysis issues for field settings. Chicago: Rand McNally; 1979. [ Google Scholar ]
- Dearing E, Hamilton LC. Contemporary advances and classic advice for analyzing mediating and moderating variables. Monographs of the Society for Research in Child Development. 2006 December; 71 :88–104. [ Google Scholar ]
- Donaldson SI. Mediator and moderator analysis in program development. In: Sussman S, editor. Handbook of program development for health and behavior research and practice. Thousand Oaks, CA: Sage; 2001. pp. 470–496. [ Google Scholar ]
- Edwards JR, Lambert LS. Methods for integrating moderation and mediation: A general analytical framework using moderated path analysis. Psychological Methods. 2007; 12 :1–22. doi: 10.1037/1082-989X.12.1.1. [ PubMed ] [ CrossRef ] [ Google Scholar ]
- Evans MG. A Monte Carlo study of the effects of correlated method variance in moderated multiple regression analysis. Organizational Behavior and Human Decisions Processes. 1985; 36 :305–323. [ Google Scholar ]
- Fairchild AJ. A comparison of frameworks for the joint analysis of mediation and moderation effects. Arizona State University; Tempe: 2008. Unpublished doctoral dissertation. [ Google Scholar ]
- Frazier PA, Tix AP, Barron KE. Testing moderator and mediator effects in counseling psychology research. Journal of Counseling Psychology. 2004; 51 :115–134. doi: 10.1037/0022-0167.51.1.115. [ CrossRef ] [ Google Scholar ]
- Gogineni A, Alsup R, Gillespie D. Mediation and moderation in social work research. Social Work Research. 1995; 19 :57–63. [ PubMed ] [ Google Scholar ]
- Holland PW. Causal inference, path analysis, and recursive structural equations models. Sociological Methodology. 1988; 18 :449–484. doi: 10.2307/271055. [ CrossRef ] [ Google Scholar ]
- Hoyle RH, Robinson JC. Mediated and moderated effects in social psychological research: Measurement, design, and analysis issues. In: Sansone C, Morf C, Panter AT, editors. Handbook of methods in social psychology. Thousand Oaks, CA: Sage; 2003. pp. 213–233. [ Google Scholar ]
- James LR, Brett JM. Mediators, moderators, and tests for mediation. The Journal of Applied Psychology. 1984; 69 :307–321. doi: 10.1037/0021-9010.69.2.307. [ CrossRef ] [ Google Scholar ]
- Judd CM, Kenny DA. Process analysis: Estimating mediation in treatment evaluations. Evaluation Review. 1981a; 5 :602–619. doi: 10.1177/0193841X8100500502. [ CrossRef ] [ Google Scholar ]
- Judd CM, Kenny DA. Estimating the effects of social intervention. Cambridge, England: Cambridge University Press; 1981b. [ Google Scholar ]
- Kenny DA, Korchmaros JD, Bolger N. Lower level mediation in multilevel models. Psychological Methods. 2003; 8 :115–128. [ PubMed ] [ Google Scholar ]
- MacKinnon DP. Introduction to statistical mediation analysis. Mahwah, NJ: Earlbaum; 2008. [ Google Scholar ]
- MacKinnon DP, Dwyer JH. Estimating mediated effects in prevention studies. Evaluation Review. 1993; 17 :144–158. doi: 10.1177/0193841X9301700202. [ CrossRef ] [ Google Scholar ]
- MacKinnon DP, Fritz MS, Williams J, Lockwood CM. Distribution of the product confidence limits for the indirect effect: Program PRODCLIN. Behavior Research Methods. 2007; 39 :384–389. [ PMC free article ] [ PubMed ] [ Google Scholar ]
- MacKinnon DP, Krull JL, Lockwood CM. Equivalence of the mediation, confounding, and suppression effect. Prevention Science. 2000; 1 :173–181. doi: 10.1023/A:1026595011371. [ PMC free article ] [ PubMed ] [ CrossRef ] [ Google Scholar ]
- MacKinnon DP, Lockwood CM. Distribution of product tests for the mediated effect: Power and Type I error rates. 2001 Unpublished manuscript. [ Google Scholar ]
- MacKinnon DP, Lockwood CM, Hoffman JM. A new method to test for mediation. Paper presented at the annual meeting of the Society for Prevention Research; Park City, UT. 1998. Jun, [ Google Scholar ]
- MacKinnon DP, Lockwood CM, Hoffman JM, West SG, Sheets V. A comparison of methods to test the significance of mediation and other intervening variable effects. Psychological Methods. 2002; 7 :83–104. doi: 10.1037/1082-989X.7.1.83. [ PMC free article ] [ PubMed ] [ CrossRef ] [ Google Scholar ]
- MacKinnon DP, Lockwood CM, Williams J. Confidence limits for the indirect effect: Distribution of the product and resampling methods. Multivariate Behavioral Research. 2004; 39 :99–128. doi: 10.1207/s15327906mbr3901_4. [ PMC free article ] [ PubMed ] [ CrossRef ] [ Google Scholar ]
- McClelland GH, Judd CM. Statistical difficulties of detecting interactions and moderator effects. Psychological Bulletin. 1993; 114 :376–390. [ PubMed ] [ Google Scholar ]
- Morgan-Lopez AA, MacKinnon DP. Demonstration and evaluation of a method for assessing mediated moderation. Behavior Research Methods. 2006; 38 :77–87. [ PubMed ] [ Google Scholar ]
- Muller D, Judd CM, Yzerbyt VY. When moderation is mediated and mediation is moderated. Journal of Personality and Social Psychology. 2005; 89 :852–863. doi: 10.1037/0022-3514.89.6.852. [ PubMed ] [ CrossRef ] [ Google Scholar ]
- Preacher KJ, Rucker DD, Hayes RF. Addressing moderated mediation hypotheses: Theory, methods, and prescriptions. Multivariate Behavioral Research. 2007; 42 :185–227. [ PubMed ] [ Google Scholar ]
- Rose BM, Holmbeck GN, Coakley RM, Franks EA. Mediator and moderator effects in developmental and behavioral pediatric research. Journal of Developmental and Behavioral Pediatrics. 2004; 25 :58–67. doi: 10.1097/00004703-200402000-00013. [ PubMed ] [ CrossRef ] [ Google Scholar ]
- Sobel ME. Asymptotic confidence intervals for indirect effects in structural equation models. Sociological Methodology. 1982; 13 :290–312. doi: 10.2307/270723. [ CrossRef ] [ Google Scholar ]
- Sobel ME. Some new results on indirect effects and their standard errors in covariance structure models. Sociological Methodology. 1986; 16 :159–186. doi: 10.2307/270922. [ CrossRef ] [ Google Scholar ]
- Tein J, Sandler IN, MacKinnon DP, Wolchik SA. How did it work? Who did it work for? Mediation in the context of a moderated prevention effect for children of divorce. Journal of Consulting and Clinical Psychology. 2004; 72 :617–624. doi: 10.1037/0022-006X.72.4.617. [ PMC free article ] [ PubMed ] [ CrossRef ] [ Google Scholar ]
- Wegener DT, Fabrigar LR. Analysis and design for nonexperimental data addressing causal and noncausal hypotheses. In: Reis HT, Judd CM, editors. Handbook of research methods in social and personality psychology. New York: Cambridge University Press; 2000. pp. 412–450. [ Google Scholar ]
- West SG, Aiken LS. Toward understanding individual effects in multiple component prevention programs: Design and analysis strategies. In: Bryant K, Windle M, West S, editors. The science of prevention: Methodological advances from alcohol and substance abuse research. Washington, DC: American Psychological Association; 1997. pp. 167–209. [ Google Scholar ]
- Yzerbyt VY, Muller D, Judd CM. Adjusting researchers’ approach to adjustment: On the use of covariates when testing interactions. Journal of Experimental Social Psychology. 2004; 40 :424–431. doi: 10.1016/j.jesp.2003.10.001. [ CrossRef ] [ Google Scholar ]
Clinical Student Voices
Walking a fine line between facilitation and adjudication in the Harvard Mediation Program
By Manoranjitha Reddy Ani LL.M. ’24
The earliest memories of my childhood are tinged with the idea and practice of ‘law.’ Having witnessed the journey of two litigators (my grandfather and father) throughout my life, I had a perception that litigation is perhaps the best form of dispute resolution, and I did not to explore the alternate ways to resolve a dispute.
While pursuing my LL.M., I attended the ADR Night hosted by the Harvard Negotiation and Mediation Clinical Program , where I learned about the Harvard Mediation Program (HMP) and what it entails. I was intrigued and thought I’d try my hand at mediation. As an international student, I was always conscious of whether I’d ‘fit in’, but HMP is extremely diverse, the recruiting process is transparent, and it recruits people committed to serving the community. It is a student-led organization where the students manage the whole process from recruitment to training, which focuses on building a community. I was elated when I was selected for the training.
I underwent basic training in the Fall of 2023. The training was quite well structured, intense and fun-filled, every session was interactive and informative. We were provided feedback at the end of every session which helped us ameliorate our skills for the next role play. HMP mainly teaches you ways in which one can effectively facilitate conversations between two parties to a dispute by using core skills like active listening, empathy, and neutrality. They focus on building skills required for mediation and tell you how mediation differs from formal adjudication; how one doesn’t need to play the role of a judge to help parties to a dispute reach an amicable settlement. I was intrigued by this method and wanted to explore it further, so pursuant to the training, I started observing in small claims and experienced the practical aspect of it. Subsequently, I also served on the Board of HMP and got to refine the skills I learned through co-teaching modules, coaching role plays and providing feedback during the Spring 2024 Training.
HMP has also opened various doors for me as I was introduced to the Harvard Negotiators Project where I got to work and research about by-standers intervention in hate crimes and to the Dispute System Design Clinic (DSD Clinic), which is part of the Harvard Negotiation and Mediation Clinical Program (HNMCP).
In the DSD clinic, I worked on the Brazilian National Council of Justice project during the Spring of 2024. This project involved viewing mediation from a different lens as it was a different form of mediation where the basic skills required were similar to facilitative mediation. The skills acquired through the basic training at HMP alongside additional research about best practices in mediation helped me co-design a Training Manual for judges of Brazil to mediate multi-party land disputes. At DSD Clinic, I learned additional skills like mapping the stakeholders, drafting an interview protocol, conducting interviews and designing work products using the different designing systems. The best part about HMP and HNMCP is that you are guided by your clinical instructors and staff at every step of the project and training.
To reflect on the 9 months I spent in law school, I am forever indebted to the staff of HMP and to my clinical instructors who have constantly supported me throughout the year. The exposure I got at HMP and HNMCP is unparalleled, and I interacted with the best set of peers from around the world. I will certainly miss the fifth floor of Pound Hall (HMP and HNMCP clinical space) where I spent most of my Spring semester. To those upcoming LL.M students who are interested in ADR and particularly mediation, I’d recommend you apply to HMP during the Fall semester as it gives you more time with HMP, and you will be able to mediate a greater number of cases. I intend to use the skills and theoretical knowledge acquired at HMP and HNMCP to mediate more cases in the future alongside litigating in the courts of India.
Filed in: Clinical Student Voices
Tags: Class of 2024 , Harvard Dispute Systems Design Clinic , Harvard Mediation Program , Harvard Negotiation & Mediation Clinical Program
Contact Office of Clinical and Pro Bono Programs
Website: hls.harvard.edu/clinics
Email: [email protected]
Modal Gallery
Gallery block modal gallery.
- Skip to main content
- Keyboard shortcuts for audio player

Your Health
- Treatments & Tests
- Health Inc.
- Public Health
Why writing by hand beats typing for thinking and learning
Jonathan Lambert

If you're like many digitally savvy Americans, it has likely been a while since you've spent much time writing by hand.
The laborious process of tracing out our thoughts, letter by letter, on the page is becoming a relic of the past in our screen-dominated world, where text messages and thumb-typed grocery lists have replaced handwritten letters and sticky notes. Electronic keyboards offer obvious efficiency benefits that have undoubtedly boosted our productivity — imagine having to write all your emails longhand.
To keep up, many schools are introducing computers as early as preschool, meaning some kids may learn the basics of typing before writing by hand.
But giving up this slower, more tactile way of expressing ourselves may come at a significant cost, according to a growing body of research that's uncovering the surprising cognitive benefits of taking pen to paper, or even stylus to iPad — for both children and adults.
Is this some kind of joke? A school facing shortages starts teaching standup comedy
In kids, studies show that tracing out ABCs, as opposed to typing them, leads to better and longer-lasting recognition and understanding of letters. Writing by hand also improves memory and recall of words, laying down the foundations of literacy and learning. In adults, taking notes by hand during a lecture, instead of typing, can lead to better conceptual understanding of material.
"There's actually some very important things going on during the embodied experience of writing by hand," says Ramesh Balasubramaniam , a neuroscientist at the University of California, Merced. "It has important cognitive benefits."
While those benefits have long been recognized by some (for instance, many authors, including Jennifer Egan and Neil Gaiman , draft their stories by hand to stoke creativity), scientists have only recently started investigating why writing by hand has these effects.
A slew of recent brain imaging research suggests handwriting's power stems from the relative complexity of the process and how it forces different brain systems to work together to reproduce the shapes of letters in our heads onto the page.
Your brain on handwriting
Both handwriting and typing involve moving our hands and fingers to create words on a page. But handwriting, it turns out, requires a lot more fine-tuned coordination between the motor and visual systems. This seems to more deeply engage the brain in ways that support learning.
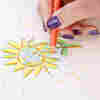
Shots - Health News
Feeling artsy here's how making art helps your brain.
"Handwriting is probably among the most complex motor skills that the brain is capable of," says Marieke Longcamp , a cognitive neuroscientist at Aix-Marseille Université.
Gripping a pen nimbly enough to write is a complicated task, as it requires your brain to continuously monitor the pressure that each finger exerts on the pen. Then, your motor system has to delicately modify that pressure to re-create each letter of the words in your head on the page.
"Your fingers have to each do something different to produce a recognizable letter," says Sophia Vinci-Booher , an educational neuroscientist at Vanderbilt University. Adding to the complexity, your visual system must continuously process that letter as it's formed. With each stroke, your brain compares the unfolding script with mental models of the letters and words, making adjustments to fingers in real time to create the letters' shapes, says Vinci-Booher.
That's not true for typing.
To type "tap" your fingers don't have to trace out the form of the letters — they just make three relatively simple and uniform movements. In comparison, it takes a lot more brainpower, as well as cross-talk between brain areas, to write than type.
Recent brain imaging studies bolster this idea. A study published in January found that when students write by hand, brain areas involved in motor and visual information processing " sync up " with areas crucial to memory formation, firing at frequencies associated with learning.
"We don't see that [synchronized activity] in typewriting at all," says Audrey van der Meer , a psychologist and study co-author at the Norwegian University of Science and Technology. She suggests that writing by hand is a neurobiologically richer process and that this richness may confer some cognitive benefits.
Other experts agree. "There seems to be something fundamental about engaging your body to produce these shapes," says Robert Wiley , a cognitive psychologist at the University of North Carolina, Greensboro. "It lets you make associations between your body and what you're seeing and hearing," he says, which might give the mind more footholds for accessing a given concept or idea.
Those extra footholds are especially important for learning in kids, but they may give adults a leg up too. Wiley and others worry that ditching handwriting for typing could have serious consequences for how we all learn and think.
What might be lost as handwriting wanes
The clearest consequence of screens and keyboards replacing pen and paper might be on kids' ability to learn the building blocks of literacy — letters.
"Letter recognition in early childhood is actually one of the best predictors of later reading and math attainment," says Vinci-Booher. Her work suggests the process of learning to write letters by hand is crucial for learning to read them.
"When kids write letters, they're just messy," she says. As kids practice writing "A," each iteration is different, and that variability helps solidify their conceptual understanding of the letter.
Research suggests kids learn to recognize letters better when seeing variable handwritten examples, compared with uniform typed examples.
This helps develop areas of the brain used during reading in older children and adults, Vinci-Booher found.
"This could be one of the ways that early experiences actually translate to long-term life outcomes," she says. "These visually demanding, fine motor actions bake in neural communication patterns that are really important for learning later on."
Ditching handwriting instruction could mean that those skills don't get developed as well, which could impair kids' ability to learn down the road.
"If young children are not receiving any handwriting training, which is very good brain stimulation, then their brains simply won't reach their full potential," says van der Meer. "It's scary to think of the potential consequences."
Many states are trying to avoid these risks by mandating cursive instruction. This year, California started requiring elementary school students to learn cursive , and similar bills are moving through state legislatures in several states, including Indiana, Kentucky, South Carolina and Wisconsin. (So far, evidence suggests that it's the writing by hand that matters, not whether it's print or cursive.)
Slowing down and processing information
For adults, one of the main benefits of writing by hand is that it simply forces us to slow down.
During a meeting or lecture, it's possible to type what you're hearing verbatim. But often, "you're not actually processing that information — you're just typing in the blind," says van der Meer. "If you take notes by hand, you can't write everything down," she says.
The relative slowness of the medium forces you to process the information, writing key words or phrases and using drawing or arrows to work through ideas, she says. "You make the information your own," she says, which helps it stick in the brain.
Such connections and integration are still possible when typing, but they need to be made more intentionally. And sometimes, efficiency wins out. "When you're writing a long essay, it's obviously much more practical to use a keyboard," says van der Meer.
Still, given our long history of using our hands to mark meaning in the world, some scientists worry about the more diffuse consequences of offloading our thinking to computers.
"We're foisting a lot of our knowledge, extending our cognition, to other devices, so it's only natural that we've started using these other agents to do our writing for us," says Balasubramaniam.
It's possible that this might free up our minds to do other kinds of hard thinking, he says. Or we might be sacrificing a fundamental process that's crucial for the kinds of immersive cognitive experiences that enable us to learn and think at our full potential.
Balasubramaniam stresses, however, that we don't have to ditch digital tools to harness the power of handwriting. So far, research suggests that scribbling with a stylus on a screen activates the same brain pathways as etching ink on paper. It's the movement that counts, he says, not its final form.
Jonathan Lambert is a Washington, D.C.-based freelance journalist who covers science, health and policy.
- handwriting
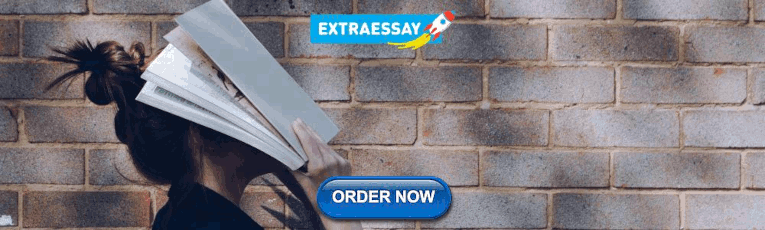
IMAGES
VIDEO
COMMENTS
Section 7.1: Mediation and Moderation Models. At the end of this section you should be able to answer the following questions: Define the concept of a moderator variable. Define the concept of a mediator variable. As we discussed in the lesson on correlations and regressions, understanding associations between psychological constructs can tell ...
Our research questions are delving into the realm of process, ... writing up and reporting mediation analyses, moderation analyses, and conditional process models. Applied researchers will enjoy this work as ... or mediation analysis, whereas questions of are most often answered when through moderation analysis. The goal of mediation analysis ...
Mediation analysis has emerged as a compelling method to disentangle the complex nature of these pathways. ... Likewise, an analysis using the NIE would answer the question, "how much would the Y (e.g., suicide rate) would change on average if the E was controlled at the level it would be with the E present (e.g., with everyone participating ...
Depending on the research question and the study objectives, investigators will aim to test or estimate 1 or more of the following possible effects: exposure-mediator effect, mediator-outcome effect, controlled direct effect, natural direct and indirect effects, 16 interventional direct and indirect effects, 17 or path-specific effects. 1 For ...
Formulating a research question. Make sure your research question is clear, specific or focused, and answerable. Clear: your question provides enough information that readers can easily understand its purpose without needing additional explanation. Focused and answerable: your question can be answered thoroughly in the space the writing task ...
The second question is about moderating variables—variables for which the intervention has a different effect at different values of the moderating variable. More information can be extracted from research studies if measures of mediating and moderating variables are included in the study design and data-collection plan. ... Most programs of ...
The first question asks for a ready-made solution, and is not focused or researchable. The second question is a clearer comparative question, but note that it may not be practically feasible. For a smaller research project or thesis, it could be narrowed down further to focus on the effectiveness of drunk driving laws in just one or two countries.
A good research question is essential to guide your research paper, dissertation, or thesis. All research questions should be: Focused on a single problem or issue. Researchable using primary and/or secondary sources. Feasible to answer within the timeframe and practical constraints. Specific enough to answer thoroughly.
University of Virginia. Moderated mediation analysis is a valuable technique for assessing whether an indirect effect is conditional on values of a moderating variable. We review the basis of moderation and mediation and their integration into a combined model of moderated mediation within a regression framework.
Just as a phenomenon can be caused or influ-enced by more than one IV, a single IV can also influence more than one phenomenon, as illustrated in Figure 4.3. A number of studies have found, for example, that cigarette smoking (IV) can lead to both lung cancer (DV1) and coronary disorders (DV2). Complex hypotheses are common in studies that try ...
varies, while mediation examines why the X-Y relation occurs. • You should use theory to guide the examination of moderation and mediation because the same variables can play the role of mediator or moderator. • Moderation and mediation can be examined simultaneously in mediated moderation and moderated mediation.
1. Testing Mediation with Regression Analysis. Mediation is a hypothesized causal chain in which one variable affects a second variable that, in turn, affects a third variable. The intervening variable, M, is the mediator. It "mediates" the relationship. between a predictor, X, and an outcome. Graphically, mediation can be depicted in the ...
Background Mediation analysis investigates whether a variable (i.e., mediator) changes in regard to an independent variable, in turn, affecting a dependent variable. Moderation analysis, on the other hand, investigates whether the statistical interaction between independent variables predict a dependent variable. Although this difference between these two types of analysis is explicit in ...
Holmbeck, 1997). Methods to assess mediation became particularly popular in psychology after publications by Judd and Kenny (1981) and Baron and Kenny (1986). Today, examples of this simple type of mediation effect are so numer-ous that one can open an issue of virtually any major social science journal and find at least one test of mediation.
As academics we talk about identifying a gap in the research. Mediation is such an under-researched area that it consists more of gaps than research results. This is a fantastic opportunity for students on the one hand, but frustrating on the other. For postgraduate level research, the research question must be narrow enough to address within ...
A mediating variable (or mediator) explains the process through which two variables are related, while a moderating variable (or moderator) affects the strength and direction of that relationship. Including mediators and moderators in your research helps you go beyond studying a simple relationship between two variables for a fuller picture of ...
Many theories in business research postulate a mediator ( M) that transmits the effect of a predictor variable ( X) to an outcome variable ( Y) in a causal sequence such that X causes M and M causes Y. In more general terms, a mediating variable explains the process by which one variable causes another. Theories across many disciplines focus on ...
How do I run a mediation analysis in SPSS? For additional help with statistics. Academic Skills Center General Statistics page ; Academic Skills Center Tutoring website; Still have statistics questions? Ask OASIS; If you are a capstone student needing help with statistics, please visit the Office of Research and Doctoral Services.
There are three steps involved in developing a series of Journey of Inference questions: Step 1: Hearing the narrative of a party. Step 2: Challenging the narrative. Step 3: Building a possible new narrative. Here are some sample questions that could be asked in the workplace scenario involving Mary and Ann.
To access the PROCESS dialog box in (see below) select. The variables in your data file will be listed in the box labelled Data File Variables. Select the outcome variable (in this case Support) and drag it to the box labelled Outcome Variable (Y), or click on .
1. Start with a broad topic. A broad topic provides writers with plenty of avenues to explore in their search for a viable research question. Techniques to help you develop a topic into subtopics and potential research questions include brainstorming and concept mapping.
A general model that simultaneously estimates mediation and moderation effects is presented, and the utility of combining the effects into a single model is described. Possible effects of interest in the model are explained, as are statistical methods to assess these effects. The methods are further illustrated in a hypothetical prevention ...
This project involved viewing mediation from a different lens as it was a different form of mediation where the basic skills required were similar to facilitative mediation. The skills acquired through the basic training at HMP alongside additional research about best practices in mediation helped me co-design a Training Manual for judges of ...
Writing by hand also improves memory and recall of words, laying down the foundations of literacy and learning. In adults, taking notes by hand during a lecture, instead of typing, can lead to ...