Click through the PLOS taxonomy to find articles in your field.
For more information about PLOS Subject Areas, click here .
Loading metrics
Open Access
Peer-reviewed
Research Article
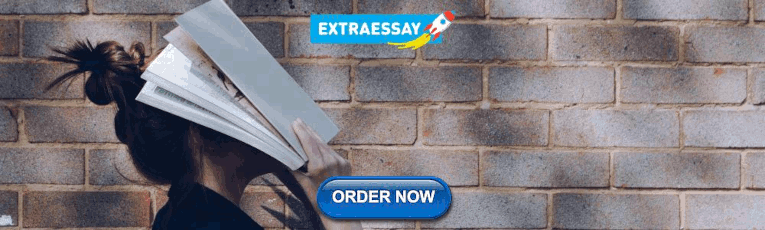
A domain-knowledge modeling of hospital-acquired infection risk in Healthcare personnel from retrospective observational data: A case study for COVID-19
Roles Conceptualization, Data curation, Formal analysis, Investigation, Methodology, Software, Supervision, Validation, Visualization, Writing – original draft, Writing – review & editing
Affiliations Department of Industrial and Management Systems Engineering, University of South Florida, Tampa, FL, United States of America, Department of Industrial and Manufacturing Engineering, North Dakota State University, Fargo, North Dakota, United States of America

Roles Conceptualization, Investigation, Validation, Visualization, Writing – review & editing
Affiliations University of North Dakota, Fargo, North Dakota, United States of America, Sanford Hospital, Fargo, North Dakota, United States of America
Roles Conceptualization, Methodology, Writing – review & editing
Affiliation Department of Biological Sciences, University of Notre Dame, Notre Dame, Indiana, United States of America
Roles Conceptualization, Methodology, Validation, Writing – review & editing
Affiliation Department of Industrial and Systems Engineering, North Carolina A&T State University, Greensboro, North Carolina, United States of America
Roles Conceptualization, Writing – review & editing
Affiliation Department of Industrial and Manufacturing Engineering, North Dakota State University, Fargo, North Dakota, United States of America
Roles Conceptualization, Funding acquisition, Investigation, Methodology, Supervision, Validation, Visualization, Writing – review & editing
* E-mail: [email protected]
- Phat K. Huynh,
- Arveity R. Setty,
- Quan M. Tran,
- Om P. Yadav,
- Nita Yodo,
- Trung Q. Le
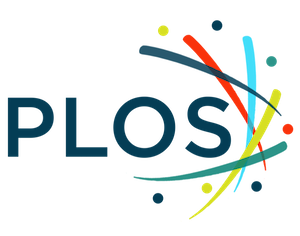
- Published: November 21, 2022
- https://doi.org/10.1371/journal.pone.0272919
- Peer Review
- Reader Comments
Introduction
Hospital-acquired infections of communicable viral diseases (CVDs) have been posing a tremendous challenge to healthcare workers globally. Healthcare personnel (HCP) is facing a consistent risk of viral infections, and subsequently higher rates of morbidity and mortality.
Materials and methods
We proposed a domain-knowledge-driven infection risk model to quantify the individual HCP and the population-level risks. For individual-level risk estimation, a time-variant infection risk model is proposed to capture the transmission dynamics of CVDs. At the population-level, the infection risk is estimated using a Bayesian network model constructed from three feature sets, including individual-level factors, engineering control factors, and administrative control factors. For model validation, we investigated the case study of the Coronavirus disease, in which the individual-level and population-level infection risk models were applied. The data were collected from various sources such as COVID-19 transmission databases, health surveys/questionaries from medical centers, U.S. Department of Labor databases, and cross-sectional studies.
Regarding the individual-level risk model, the variance-based sensitivity analysis indicated that the uncertainty in the estimated risk was attributed to two variables: the number of close contacts and the viral transmission probability. Next, the disease transmission probability was computed using a multivariate logistic regression applied for a cross-sectional HCP data in the UK, with the 10-fold cross-validation accuracy of 78.23%. Combined with the previous result, we further validated the individual infection risk model by considering six occupations in the U.S. Department of Labor O*Net database. The occupation-specific risk evaluation suggested that the registered nurses, medical assistants, and respiratory therapists were the highest-risk occupations. For the population-level risk model validation, the infection risk in Texas and California was estimated, in which the infection risk in Texas was lower than that in California. This can be explained by California’s higher patient load for each HCP per day and lower personal protective equipment (PPE) sufficiency level.
The accurate estimation of infection risk at both individual level and population levels using our domain-knowledge-driven infection risk model will significantly enhance the PPE allocation, safety plans for HCP, and hospital staffing strategies.
Citation: Huynh PK, Setty AR, Tran QM, Yadav OP, Yodo N, Le TQ (2022) A domain-knowledge modeling of hospital-acquired infection risk in Healthcare personnel from retrospective observational data: A case study for COVID-19. PLoS ONE 17(11): e0272919. https://doi.org/10.1371/journal.pone.0272919
Editor: Anete Trajman, Federal University of Rio de Janeiro, BRAZIL
Received: January 11, 2022; Accepted: July 28, 2022; Published: November 21, 2022
Copyright: © 2022 Huynh et al. This is an open access article distributed under the terms of the Creative Commons Attribution License , which permits unrestricted use, distribution, and reproduction in any medium, provided the original author and source are credited.
Data Availability: The data and the description can be accessible via: https://github.com/PhatKHuynh/COVID-19-datasets .
Funding: This work was supported by National Institute of General Medical Sciences of the National Institutes of Health (NIH) under Award Number U54GM128729 and National Science Foundation under Award Number 2119691. The funders had no role in study design, data collection and analysis, decision to publish, or preparation of the manuscript.
Competing interests: The authors have declared that no competing interests exist.
1. Introduction
Nosocomial infections (i.e., hospital-acquired infections) of communicable viral diseases (CVDs) (e.g., influenza virus, hepatitis A virus, and rotavirus infections) have posed huge challenges to public health organizations. Healthcare personnel (HCP) experience the highest risk [ 1 – 3 ] because of the direct or indirect contact with infected patients and virus-contaminated surfaces. Subsequently, these HCP may become widespread the virus to non-infectious patients, coworkers, and their family members. Although there has been an increasing number of hospital outbreaks of CVDs over the last decade, current containment and preventive measures in hospital settings usually overlook asymptomatic individuals and “super spreader” events [ 4 , 5 ]. Hence a quantitative estimation of the infection risk in HCP is critical to mitigate and subsequently prevent nosocomial infections in hospitals. Furthermore, a precise measure of HCP infection risk is also important to address the epidemiological issues in hospital settings and provide information for personal protective equipment (PPE) allocation, safety plans for HCP, and staffing strategies.
Modeling nosocomial HCP infections in hospitals has been based on mathematical models to qualitatively capture the dynamics of CVDs and the effects of different control measures [ 6 , 7 ] at population level. One traditional model of disease spread is the compartmental SEIR (Susceptible-Exposed-Infected-Recovered) epidemiological model [ 8 ]. It divides a population into four different compartments or sub-groups (susceptible, exposed, infected, and recovered individuals) and employs deterministic ordinary differential equations to model the spread of a CVD. In the literature, there are many variants of this model (e.g., SIS, SIRD, MSIR, and MSEIR model). These models consider the population as homogenous without individual interactions (e.g., patients and HCP); therefore, they fail to capture the individual contact process and the effects of individual risk and protective factors [ 9 ]. In other words, only the patient flow dynamics are captured at the population level, but the individual-level information is neglected. To address the “homogenous population” assumption and capture better transmission dynamics, spatial epidemic models [ 10 , 11 ] and metapopulation models [ 12 , 13 ] have been introduced, which can be considered as “heterogeneous models”. The spatial modeling approach incorporates the spatial information of disease occurrences and transmission behaviors to estimate of the probability of infection transmission from an infectious to a susceptible individual at a certain distance. The metapopulation models are the integration of the compartmental models and spatial epidemic models, which allows us to simulate the behaviors of a large population given a well-defined spatial distribution. In this approach, the entire population is separated into two different subpopulations with coupled disease transmission dynamics based on the subpopulation characteristics, and each subpopulation is mixed homogeneously. In addition, network models [ 14 , 15 ] have been proposed to model the contact network elaborately without having the assumption that all individuals interact with each other with a regular random pattern [ 16 ]. The contact network concept was founded by the mathematical graph theory, and a graph as two fundamental components: vertices and edges. In the context of epidemiological modeling, a vertex can represent an individual, a subpopulation, or even an entire country, an edge is the link between vertices, which captures the disease transmissions and individual interactions. Despite the tremendous efforts in advancing the population-based mathematical epidemiological model, the advanced models, such as “heterogeneous models” and network models, are still limited in terms of modeling and characterizing the individuals’ movements and interactions.
To overcome the limitations of the population-based models, individual-based models have been proposed to fully capture the complexity of individual behaviors and interactions with other individuals. First, complex systems approaches using cellular automata (CA) theory have been proposed to model location-specific dynamics of susceptible populations and the probabilistic nature of disease transmission [ 17 , 18 ], which takes into account the individual movements of the populations. However, The major drawback of CA models is their insufficiency in characterizing the spatial-temporal information of individuals’ movements and interactions [ 19 ]. Agent-based modeling (ABM) was proposed to address the limitations of CA models by accounting for the individual-level movement of disease carriers and the contact network of people [ 20 ]. Although the ABM approach can capture the spread of a CVD in a spatial region (e.g., hospital) over time and estimate the risk of viral infection, it requires a large amount of information of individuals’ movement and high computational cost. Moreover, individuals’ movements are highly restricted in hospital settings, especially for patients who have positive test results for infectious diseases.
Statistical models have been used as an alternative to mathematical models to quantify the population-averaged effects of protective or risk factors on the time-variant infection risk of HCP. Statistical models capture the disease transmission dynamics within the hospital, HCP-related risk factors of infection, and other patients and HCP as sources of infection [ 21 ]. Two classes of statistical models, namely measure of association and statistical survival analysis, have been proposed to estimate HCP infection risk. The measure of association approaches quantifies the relationship between the exposed and diseased HCP groups by using the adjusted odds ratio (aOR), risk difference (RD), and relative risk (RR) as the risk measures [ 2 , 22 – 25 ]. To capture the changes of HCP’s characteristics and infection risk over time, survival analysis models are used to estimate the HCP infection risk and the expected duration of time until a viral infection occurs [ 26 , 27 ]. Although time-dependent variables have been considered in the survival analysis models, the stochastic nature of epidemiological dynamics and individual interactions have not been investigated.
To overcome the above research gaps, this paper proposes a probabilistic domain-knowledge-driven model of the infection risk of CVDs for HCP, which is a hybrid multi-scale approach that combines mechanistic modeling and statistical modeling approaches. The term “domain-knowledge-driven model” [ 28 ] refers to a class of statistical or machine learning models that leverage the expert knowledges and embed them into the models to enhance the performance, understanding, and validity. The domain knowledge we incorporated in our proposed probabilistic framework includes modes of CVD transmissions, significant risk and protective factors of the infection risk, and disease transmission dynamics through patient compartments. The proposed model was formulated for the infection risk estimation at both individual and population levels with respect to three modes of transmissions: 1) direct contact of susceptible HCP with other infectious individuals including patients and coworkers, 2) airborne viruses, and 3) contaminated equipment and surfaces. The individual-level risk model was built based on the population grouping in the SEIR model with the consideration of the time-varying confounders to capture the dynamical contagious disease transmission mechanism. At the population-level, three subsets of features, which are introduced in Sub-section 2.2, were constructed and represented by a Bayesian network [ 29 ], from which the probability of transmission from patients to HCP was estimated. The main contributions of this paper are 1) a novel probabilistic model characterizes the dynamics of the disease transmission in HCP over time and 2) a domain-knowledge-driven risk analysis model that quantifies both the individual-level and population-level infection risk. The remainder of the manuscript is organized as follows: Section 2 discusses the model formulation and model validation; the results with sensitivity analysis and the case study on the COVID-19 are presented in Section 3, discussion and conclusions are provided in Sections 4 and 5.
2. Materials and methods
The proposed framework consists of two sub-models: (1) an individual-level infection risk model that quantifies the risk of infection of an HCP, and (2) a population-level model that estimates the infection risk under working conditions at a medical facility. The output from the first sub-model serves as an input for the estimation of the population infection risk in the second model. Other inputs, such as engineering control and administrative factors, were also considered in the estimation of population risk.
2.1. Individual-level infection risk model
2.2. Population risk indicator model
- PPT PowerPoint slide
- PNG larger image
- TIFF original image
https://doi.org/10.1371/journal.pone.0272919.g001
3. Results and covid-19 case study
3.1. sensitivity analysis using simulated data.
The variance-based sensitivity analysis was utilized to study the uncertainty of HCP’s potential infection risk output caused by the variance of the input variables.
3.1.1. The measure of sensitivity of to and close contact sequence.
https://doi.org/10.1371/journal.pone.0272919.g002
3.1.2. Response surface of the mean and variance of .
https://doi.org/10.1371/journal.pone.0272919.g003
3.2. Model validation using COVID-19 case study
3.2.1. case study description..
Data sets of HCPs with COVID-19 were used to validate the proposed model. Access to these data sources can be provided per requests or via the cited references. The validation was performed on three main components: the viral transmission probability model, the individual-level infection risk model, and the population-level risk model. The HCP’s occupational infection risk to COVID-19, interim guidance regarding risk assessment and universal PPE policy issued by the CDC [ 41 ], and the risk factors for severe acute respiratory syndrome coronavirus (SARS-CoV-2) transmission in hospital settings from previous studies were also included to develop the model for the case study.
The major factors resulting in high risk for HCPs are 1) exposure to COVID-19 patients without using appropriate PPE, 2) involvement in aerosol-generating procedures and the interventions performed by physicians or nurses, and 3) contact with patients and colleagues during the incubation period. Many studies suggested that there is a significant association between PPE use and infection risk and that masks are the most consistent contributing measure to reduce the risk [ 34 , 35 ]. A similar association was observed for other PPE, such as gowns, gloves, and eye protection. Other exposures and treatment practices (e.g., intubation involvement, patient care, or having contact with secretions) were found to link with increased infection risk for HCPs [ 36 , 37 ]. Finally, given the implementation of a universal PPE policy, the high risk of infection among HCP also arises from contacting asymptomatic patients and colleagues who are in the early phase of viral infections [ 24 ].
Different regression models, including logistic regression, log-binomial, and Poisson, were used with the defined risk measures to estimate the COVID-19 infection risk among HCP groups [ 23 – 25 , 38 – 45 ]. Statistical survival analysis models were also used to estimate the HCP’s risk of contracting SARS COV-2 viruses and the expected duration of time until viral infection occurs. Shah et al. [ 27 ] modeled hospital admission of healthcare workers with COVID-19 using Cox regression and conditional logistic regression. Long Nguyen et al. [ 26 ] assessed the COVID-19 infection risk among healthcare workers in contrast to the general community by examining the effect of PPE on risk. They also used Cox proportional hazards model to calculate multivariate-adjusted hazard ratios (HRs) of a positive test. However, the major limitations of these models are: 1) the individual-specific characteristics, e.g., occupation [ 46 ], type of PPE used, experience level, and exposure duration to COVID-19 patients, are not considered [ 26 , 27 ], and 2) the simple formalism of the models without time-varying stochastic transmissions oversimplifies the complex contagious mechanism of SARS COV-2.
3.2.2. Data description.
Data collected from multiple sources, namely COVID-19 transmission databases, health surveys/questionaries, U.S. Department of Labor databases, and cross-sectional study of UK-based healthcare workers, are illustrated in Table 1 .
https://doi.org/10.1371/journal.pone.0272919.t001
3.2.3. Model variable selection.
Variables from recent findings of SARS-CoV-2 as introduced in Sub-section 3.2.1, were used to select the features. The validation was performed on three main components: the viral transmission probability model, the individual-level infection risk model, and the population-level risk model. Regarding the viral transmission probability model, we included the following covariates in the model: Age , Cancer , Resp , Obes , Smoker , Allied _ prof , Dental _ staff , Doctor , Pub _ trans , C _ contact , AGP , PPE _ train , Lacked _ PPE , Cont _ wo _ PPE , and Imp _ PPE . These are significant factors suggested by the original cross-sectional study [ 54 ]. The description of these variables is summarized in S1 Table . To validate the individual-level infection risk model, the U.S. Department of Labor O*Net database was employed to quantify the risk score for healthcare-related occupations, where virus exposure time and duration and working environment were considered. For the population-level risk model, the PPE sufficiency level, regional infection risk and the hospitalization data of HCP were selected to estimate population-level infection risk in California and Texas medical centers [ 49 , 50 ] and implement a surrogate method for model validation. The description of these variables is summarized in S1 Table .
3.2.4. Model validation of viral transmission probability estimation using multivariate logistic regression.
To validate the logistic regression introduced in Sub-section 2.1., we considered different protective and risk factors for COVID-19 in the data set of UK-based healthcare workers [ 54 ] and modelled the association between these covariates and the COVID-19 infection status using multivariable logistic regression. The data set provides 6263 responses in which a composite outcome was present in 1,806 (29.4%) HCP, of whom 49 (0.8%) HCP were admitted to hospitals, 459 (7.5%) were tested positive for SARS-CoV-2, and 1,776 (28.9%) HCP were self-isolated. The covariates included in the model were reported in Sub-section 3.2.3. The estimated coefficients with their standard errors (SEs) and their statistical significance indicated by p-value are shown in Table 2 .
https://doi.org/10.1371/journal.pone.0272919.t002
According the table, the most significant variables (p-value < 0.001) that influence the disease transmission probability are Age , Obes , Allied _ prof , Dental _ staff , Pub _ trans , C _ contact , AGP , Lacked _ PPE , and cont _ wo _ PPE . The model goodness-of-fit was further assessed by the Akaike information criterion (AIC) and 10-fold cross validation. The AIC value for the above model was 7317.70 and that for the null model was 7449.75. The 10-fold cross validation accuracy was calculated to be 78.23%, which showed that the performance on test data was relatively good.
3.2.5. Model validation of the individual-level infection risk.
Lastly, the total number of contacts | C (∙) | was fixed to be 5 and the value ϕ was set to 20. Next, the risk was estimated using Eq ( 12 ), and the results are summarized in Table 3 .
https://doi.org/10.1371/journal.pone.0272919.t003
3.2.6. Model validation of the population-level infection risk.
The population-level infection risk was validated based on the total of confirmed COVID-19 cases of HCP reported to the CDC. The number of positive COVID-19 cases of HCP in the US up to April 9, 2020, is presented in Fig 4 .
https://doi.org/10.1371/journal.pone.0272919.g004
https://doi.org/10.1371/journal.pone.0272919.t004
4. Discussion
Hospital-acquired infections of communicable viral diseases are posing a challenge to healthcare workers globally. HCP is facing a consistent risk of hospital-acquired infections, and subsequently higher rates of morbidity and mortality. Therefore, mitigating and preventing nosocomial infections in hospitals is an urgent and important task to lower the risk of contracting CVDs for HCP, guarantee adequate availability of PPE and develop well-informed strategies to protect health-care workers from contracting CVDs. In this paper, we have developed a proposed probabilistic model characterizes the dynamics of the disease transmission in HCP over time, in which the domain-knowledge-driven risk analysis framework can quantify both the individual-level and population-level infection risk. We validated the model at both levels using two main approaches, namely the variance-based sensitivity analysis using the simulated data and the COVID-19 case study. The sensitivity analysis indicated that the uncertainty in the HCP infection risk is attributed to 2 variables: the number of close contacts and the viral transmission probability. The COVID-19 case study showed that the occupations with the highest risk are registered nurses, medical assistants, and respiratory therapists. In addition, the results indicated the significant risk and protective factors of the COVID-19 transmission risk of HCP.
We validated the individual-level infection risk model, implemented the model using the two-parameter regressive equation, and estimated the individual risk for six occupations. The results highly depend on the pre-defined parameters, which can be estimated in healthcare settings when data are available. It was shown that healthcare workers and nurses are frequently in close contact with COVID-19 patients, which therefore increases the risk for acquiring SARS-CoV-2 virus [ 57 ]. Because HCP can acquire infection through various pathways apart from direct patient care, such as exposure to colleagues, family members, or people in the community, the time-varying risk estimation in the model can provide informed decisions for screening HCP for COVID-19 before workplace entry. The individual risk model can be improved and more specific to better model the transmission dynamics, e.g., a model that incorporates the quantification of indoor airborne infection risks using a probabilistic framework [ 58 ]. In addition, we do not assume that the recovered patients confer immunity to reinfection when being released from isolation This statement can be further clarified that even the patients are fully recovered after getting contracted with COVID-19 (or any communicable viral diseases in general) and being released back to the population, they are still under the risk of reinfection with the same disease strain or other strains. However, reinfection with the same strain is very rare. Hence, if the HCP were recovered from the disease, they might get infected again; however, they still confer some degree of immunity from subsequent infection. Therefore, we can consider adding a new group of patients in our model called “reinfected patients”. Moreover, the same idea can be applied for the vaccinated population, in which people have vaccine-induced immunity.
For model validation at the population level, we considered two case studies to estimate the risk of infection of HCP in Texas and California states. Both states have a high number of lab-confirmed SARS-CoV-2 patients. The average number of hospitalizations in Texas and California were 16843 cases/day and 4219 cases/day, respectively. However, the infection risk in Texas was 0.0084 which was lower than the risk in California (0.0132). This was mainly due to the difference in patient load for each HCP per day and the two states’ PPE sufficiency level. From Table 4 , the average PPE sufficiency level in California was only 0.744 as opposed to 0.9355 in Texas, and the average percentage of ICU beds available per 100,100 people in Texas was significantly higher than that in California, which implies heavier patient loads in California. The model also made some important assumptions: 1) close contacts with COVID-19 patients are independent and there is no viral transmission among HCP, and 2) protective/risk factors are well-defined and sufficient to estimate the risk of infection.
5. Conclusion and future work
The paper proposed a time-variant infection risk analysis model to characterize the dynamic of the disease infection risk in HCP over time and a domain-knowledge-driven infection risk to quantify the complexities of HCP’s risk of CVDs in healthcare settings. The infection risk analysis model for HCP was estimated at both individual and population levels. The individual-level risk model was built based on the population grouping concept of the well-established epidemiological SEIR model with the consideration of the time-varying confounders to capture the dynamical contagious disease transmission mechanism. At the population-level, three subsets of features were constructed and represented by a Bayesian network, from which the probability of viral transmission from patients to HCP was estimated. To validate our methods, we have incorporated the data from multiple data sources from the US, the UK, and Taiwan for the COVID-19 case study, which contains the information about potential factors that affect COVID-19 transmission mechanism; and the domain knowledge of similar contagious diseases such as SARS or MERS from the relevant studies to estimate the risk of COVID-19 infection of HCP. For individual-level risk estimation, the model was founded on the SEIR compartmental model and developed for the occupational-specific and individualized infection risk model. As a result, the model can accurately capture the infection risk varying over time under the control of those individual time-varying confounders, and it is also able to account for the intrinsic stochastic transmission mechanisms. At the population level, the Bayesian network formalism can accommodate the limited data scenario, and it can update the parameters when more data are available. The results from two case studies are interpretable at the population level, which showed infection risk in California is higher than in Texas because of the heavier patient loadings and shortage of PPE. The major limitations of the CDC’s interim guideline for risk assessment, which is inadequate in quantifying the risk of infection in an individualized HCP, have been addressed by our model. The model would significantly endorse the PPE allocation and safety plans for HCP and enhance the crisis-level staffing strategies in facilities with the staffing shortages. Longitudinal experimental designs are required to collect more COVID-19 data among HCP to validate the proposed model properly. Future work would involve: 1) model assumption validation when more data are available and sufficient, 2) model modification and reformulation if the assumptions are violated (e.g., independence assumption and new vaccinated or “reinfected” population), and 3) validating the model with the other related case studies of communicable viral diseases.
Summary table
What was already known on the topic
- Hospital-acquired infections of communicable viral diseases are posing a challenge to healthcare workers globally.
- Healthcare personnel (HCP) is facing a consistent risk of hospital-acquired infections, and subsequently higher rates of morbidity and mortality.
- Mitigating and preventing nosocomial infections in hospitals is an urgent and important task to lower the risk of contracting CVDs for HCP.
- Previous mathematical models and statistical methods to model the nosocomial infection risk in HCP fail to capture the time-dependent disease transmission processes and the effects of individual risk and protective factors.
- What this study added to our knowledge
- Our proposed probabilistic model characterizes the dynamics of the disease transmission in HCP over time
- The knowledge-driven risk analysis framework can quantify both the individual-level and population-level infection risk.
- The sensitivity analyses indicated that the uncertainty in the HCP infection risk is attributed to 2 variables: the number of close contacts and the viral transmission probability.
- The COVID-19 case study showed that the occupations with the highest risk are registered nurses, medical assistants, and respiratory therapists.
- The results indicated that age, smoking status, occupation, PPE use, using public transport, close contacts with patients, and having regular exposure to aerosol-generating procedures are significant factors.
Supporting information
S1 table. characteristics of the selected features and their associated databases..
https://doi.org/10.1371/journal.pone.0272919.s001
S1 File. The expectation and variance of the potential individual risk.
https://doi.org/10.1371/journal.pone.0272919.s002
- View Article
- Google Scholar
- PubMed/NCBI
A full competing risk analysis of hospital-acquired infections can easily be performed by a case-cohort approach
Affiliations.
- 1 Institute for Medical Biometry and Statistics, Center for Medical Biometry and Medical Informatics, Medical Center University of Freiburg, Stefan-Meier-Str. 26, 79104 Freiburg, Germany. Electronic address: [email protected].
- 2 ICU Department University Hospital Arnau de Vilanova, Lleida, Spain and Universitat Autónoma de Barcelona, Spain.
- 3 Service of Intensive Care Medicine, Hospital de Galdakao-Usansolo, Labeaga s/n. 48960. Galdakao, Bizkaia, Spain.
- 4 Service of Intensive Care Medicine, Parc de Salut Mar, Universitat Autonoma de Barcelona IMIM (GREPAC - Grup Recerca Patologia Crítica) Passeig Marítim, 25-29. 08003 Barcelona, Spain.
- 5 Institute for Medical Biometry and Statistics, Center for Medical Biometry and Medical Informatics, Medical Center University of Freiburg, Stefan-Meier-Str. 26, 79104 Freiburg, Germany.
- PMID: 26633600
- DOI: 10.1016/j.jclinepi.2015.11.011
Objectives: We provide a case-cohort approach and show that a full competing risk analysis is feasible even in a reduced data set. Competing events for hospital-acquired infections are death or discharge from the hospital because they preclude the observation of such infections.
Study design and setting: Using surveillance data of 6,568 patient admissions (full cohort) from two Spanish intensive care units, we propose a case-cohort approach which uses only data from a random sample of the full cohort and all infected patients (the cases). We combine established methodology to study following measures: event-specific as well as subdistribution hazard ratios for all three events (infection, death, and discharge), cumulative hazards as well as incidence functions by risk factor, and also for all three events.
Results: Compared with the values from the full cohort, all measures are well approximated with the case-cohort design. For the event of interest (infection), event-specific and subdistribution hazards can be estimated with the full efficiency of the case-cohort design. So, standard errors are only slightly increased, whereas the precision of estimated hazards of the competing events is inflated according to the size of the subcohort.
Conclusion: The case-cohort design provides an appropriate sampling design for studying hospital-acquired infections in a reduced data set. Potential effects of risk factors on the competing events (death and discharge) can be evaluated.
Keywords: Competing risk model; Cox proportional hazards model; Cumulative incidence function; Fine and Gray model; Multistate model; Nosocomial infections; Sampling designs; Subdistribution.
Copyright © 2015 Elsevier Inc. All rights reserved.
Publication types
- Multicenter Study
- Research Support, Non-U.S. Gov't
- Cohort Studies
- Cross Infection / epidemiology*
- Epidemiologic Research Design*
- Hospital Mortality*
- Intensive Care Units / statistics & numerical data
- Models, Statistical
- Patient Discharge / statistics & numerical data*
- Proportional Hazards Models
- Risk Assessment
- Risk Factors
- Spain / epidemiology
Outcome of hospital-acquired bloodstream infection: interplay between bacteria, host, and healthcare system organization
- Published: 27 May 2024
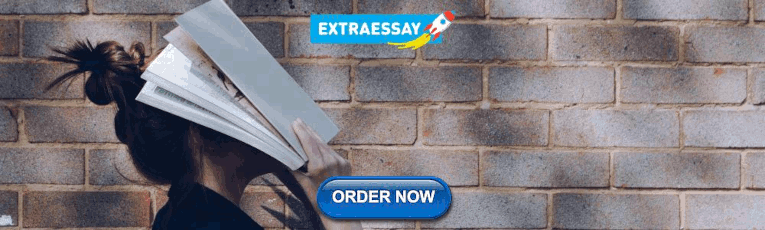
Cite this article
- Nicolas Mongardon ORCID: orcid.org/0000-0003-2756-4052 1 , 2 , 3 &
- Jean-Louis Vincent 4
729 Accesses
13 Altmetric
Explore all metrics
Avoid common mistakes on your manuscript.
Deciphering sepsis outcomes has been the subject of intense research for decades. Its focus has predominantly been on the characteristics of the host (underlying comorbidities, genetic background) or the pathogen (virulence, antimicrobial resistance patterns) [ 1 ]. The impact of the healthcare system and of hospital or intensive care unit (ICU) organization has hardly been investigated. However, these factors potentially have a major effect on outcomes, both for community-acquired infections (with possible influence of a myriad of country-level factors, e.g., vaccination, incidence of obesity, number of general physicians, …) and for nosocomial infections (with possible influence of, e.g., hand hygiene measures, antibiotic stewardship programs…) [ 2 ].
In a study published in the current issue of Intensive Care Medicine, Buetti et al. evaluated the possible impact of center and country factors on the adequacy of early antimicrobial therapy, as a process indicator, and 28-day mortality in patients with healthcare-associated bloodstream infections (HABSI) [ 3 ]. For this purpose, they reviewed data from the EUROBACT-2 study, a large prospective, multicenter, observational study that included 2600 patients from 333 ICUs in 52 countries [ 4 ]. The current study excluded centers with less than 10 patients or over < 3 months, giving 2209 patients with an HABSI from 201 ICUs in 47 countries (52% of the ICUs were in European countries). HABSI was defined as a positive blood culture (at least two positive blood cultures for common contaminants) sampled more than 48 h after hospital admission. This approach has the advantage of providing a strict, objective criterion for diagnosis, compared to the relatively loose criteria often used in studies on nosocomial infections.
Overall, 51% of patients received adequate antimicrobial therapy (a regimen that included at least one antimicrobial with in vitro activity for the microorganism on the first day) and the 28-day mortality was 38%. A large proportion (80%) of the HABSI were ICU-acquired, mainly from respiratory and intravascular catheter infections. Administration of adequate antimicrobial therapy was associated with availability of early or daily therapeutic drug monitoring (TDM) for aminoglycosides, weekly performance of surveillance cultures for multidrug-resistant organism carriage, and increasing human development index (HDI), a surrogate composite marker of country development that incorporates life expectancy, education level, and national income per capita. The presence of intermediate care beds, early or daily availability of TDM for aminoglycosides, 24/7 availability of a clinical pharmacist, prevalence of vancomycin-resistant enterococci in the ICU, and HDI values were associated with mortality (Table 1 ).
The authors should be commended for providing such a nice up-to-date screenshot of how healthcare organization can impact HABSI prognosis. This study highlights dramatic real-life data, e.g., only 51% of patients received adequate antimicrobial therapy within the first 24 h. Why was this proportion so low and is there room for improvement? Adequacy was defined as the administration at the correct dose and route of at least one drug with in vitro activity against the pathogen, and was reviewed by a panel of experts (although the authors did not provide information about the gold-standards). From the results, we can suggest several possible explanations for this low rate.
A first reason may be that physicians simply failed to select the best antimicrobial agent for that patient. This could be due to lack of knowledge, a misinterpretation of the severity of the problem, or of the specific clinical context of the infection. Information about MDR carriage, for example, can be important to decide whether or not extended-spectrum β-lactamase-producing Enterobacteriaceae (ESBLE) should be covered in empiric treatment, and was associated in this study with greater administration of adequate antibiotic treatment. The role of weekly surveillance cultures for infection prevention purposes in the ICU, also shown here to be associated with antibiotic adequacy, remains a matter of debate. Guidelines for infection control measures to reduce transmission of ESBLE and multidrug-resistant Gram-negative bacteria recommend performing rectal screening at admission and then weekly thereafter in ICU patients only in specific epidemic or endemic settings [ 5 ]. Buetti’s findings bring so an important brick in the wall of infection control strategies by advocating for active surveillance cultures in the ICU. This measure goes far beyond collective purposes, to offer the individual interest of guiding antimicrobial therapy. Indeed, knowing the personal ecology of the patient is pertinent because of the excellent negative predictive value of negative rectal swabs which enables carbapenem sparing in non-severely ill patients [ 6 ]. Conversely, the digestive carriage of ESBLE strongly suggests the need for empiric administration of carbapenem in patients with septic shock.
A second reason may be that the dosing and route of administration were suboptimal. Indeed, this study highlights a number of factors supporting this possibility, Including what can be seen as complementary measures to ensuring optimal antibiotic dosing, i.e., good access to TDM and good availability of clinical pharmacists. Although beta-lactams, vancomycin, and aminoglycoside levels are now routinely monitored in many institutions, TDM of almost all other antibiotics is about to be part of routine care [ 7 ]. It is increasingly recognized that personalized antibiotic dosing in critically ill patients is associated with better outcomes, especially in the early phase of sepsis [ 8 ]. Although the optimal mode of administration of beta-lactam antibiotics, i.e., short versus prolonged or continuous infusion, is not entirely settled [ 9 , 10 ], questions of stability at room temperature, dilutions, dosing simulations, and drug interactions are best addressed with the collaboration of a clinical pharmacist [ 11 ]. Overall, the exchanges between a multidisciplinary team (infection control physician, microbiologist, clinical pharmacists, and infectious diseases physicians) are central to optimize patient care and antimicrobial stewardship [ 12 ]. They will be likely enhanced by the new technologies and artificial intelligence which are exponentially entering into the laboratory to accelerate delivery of identification and antimicrobial susceptibility testing of the pathogen [ 13 ]. Interestingly, this study also highlights the impact of availability of intermediate care unit beds on reducing mortality, possibly because they enable more intensive monitoring and patient management to be provided than is possible on the general ward [ 14 ]. Reducing nosocomial infections may also be conveyed by a reduction to the risk exposure: as catheters were the second source of BSI, it is a paramount importance to recall that one of the aim of the daily round is to consider whether central catheter, or each tube and drain, can be removed.
At a more global level, the degree of development of the country, as assessed using the HDI, was associated with adequacy of antibiotic therapy and with mortality. Although perhaps not surprising, this is an important observation at a period when healthcare investment is increasingly challenged by policy makers [ 15 ]. Finally, the cost-effectiveness of the different strategies to improve healthcare-related outcomes is crucial and may represent a barrier to their implementation, especially in middle- and low-income countries. This issue deserves to develop infection control strategies based on available and affordable resources at a country level, with a target tailored to the baseline risk and level of nosocomial infections.
In summary, this interesting study highlights several aspects of healthcare organization that can have clinical implications not only for the intensivist but also for the wider hospital management and healthcare authorities.
Vincent J-L, Sakr Y, Singer M et al (2020) Prevalence and outcomes of infection among patients in intensive care units in 2017. JAMA 323:1478–1487. https://doi.org/10.1001/jama.2020.2717
Article PubMed PubMed Central Google Scholar
Ginestra JC, Coz Yataco AO, Dugar SP, Dettmer MR (2024) Hospital-onset sepsis warrants expanded investigation and consideration as a unique clinical entity. Chest S0012–3692(24):00039–00044. https://doi.org/10.1016/j.chest.2024.01.028
Article Google Scholar
Buetti N, Tabah A, Setti N et al (2024) The role of centre and country factors on process and outcome indicators in critically ill patients with hospital-acquired bloodstream infections. Intensive Care Med. https://doi.org/10.1007/s00134-024-07348-0
Article PubMed Google Scholar
Tabah A, Buetti N, Staiquly Q et al (2023) Epidemiology and outcomes of hospital-acquired bloodstream infections in intensive care unit patients: the EUROBACT-2 international cohort study. Intensive Care Med 49:178–190. https://doi.org/10.1007/s00134-022-06944-2
Article CAS PubMed PubMed Central Google Scholar
Tacconelli E, Cataldo MA, Dancer SJ et al (2014) ESCMID guidelines for the management of the infection control measures to reduce transmission of multidrug-resistant Gram-negative bacteria in hospitalized patients. Clin Microbiol Infect 20(Suppl 1):1–55. https://doi.org/10.1111/1469-0691.12427
Baba H, Nimmo GR, Allworth AM et al (2011) The role of surveillance cultures in the prediction of susceptibility patterns of Gram-negative bacilli in the intensive care unit. Eur J Clin Microbiol Infect Dis 30:739–744. https://doi.org/10.1007/s10096-010-1146-1
Article CAS PubMed Google Scholar
Liu L, Zhang L, Zheng X et al (2023) LC-MS/MS-based multiplex antibacterial platform for therapeutic drug monitoring in intensive care unit patients. Front Pharmacol 14:1116071. https://doi.org/10.3389/fphar.2023.1116071
Ollivier J, Carrié C, d’Houdain N et al (2019) Are standard dosing regimens of ceftriaxone adapted for critically ill patients with augmented creatinine clearance? Antimicrob Agents Chemother 63:e02134-e2218. https://doi.org/10.1128/AAC.02134-18
Li X, Long Y, Wu G et al (2023) Prolonged vs intermittent intravenous infusion of β-lactam antibiotics for patients with sepsis: a systematic review of randomized clinical trials with meta-analysis and trial sequential analysis. Ann Intensive Care 13:121. https://doi.org/10.1186/s13613-023-01222-w
Dulhunty JM, Roberts JA, Davis JS et al (2015) A multicenter randomized trial of continuous versus intermittent β-lactam infusion in severe sepsis. Am J Respir Crit Care Med 192:1298–1305. https://doi.org/10.1164/rccm.201505-0857OC
Chai MG, Roberts JA, Kelly CF et al (2023) Efficiency of dosing software using Bayesian forecasting in achieving target antibiotic exposures in critically ill patients, a prospective cohort study. Anaesth Crit Care Pain Med 42:101296. https://doi.org/10.1016/j.accpm.2023.101296
Janssen RME, Oerlemans AJM, van der Hoeven JG et al (2023) Decision-making regarding antibiotic therapy duration: an observational study of multidisciplinary meetings in the intensive care unit. J Crit Care 78:154363. https://doi.org/10.1016/j.jcrc.2023.154363
Lakbar I, Singer M, Leone M (2023) 2030: will we still need our microbiologist? Intensive Care Med 49:1232–1234. https://doi.org/10.1007/s00134-023-07186-6
Vincent J-L, Rubenfeld GD (2015) Does intermediate care improve patient outcomes or reduce costs? Crit Care 19:89. https://doi.org/10.1186/s13054-015-0813-0
Wallace DJ, Donohue JM, Angus DC et al (2020) Association between state medicaid expansion and emergency access to acute care hospitals in the United States. JAMA Netw Open 3:e2025815. https://doi.org/10.1001/jamanetworkopen.2020.25815
Download references
Author information
Authors and affiliations.
Service d’Anesthésie-Réanimation et Médecine Péri-Opératoire, DMU CARE, Assistance Publique-Hôpitaux de Paris (AP-HP), Hôpitaux Universitaires Henri Mondor, 94010, Créteil, France
Nicolas Mongardon
Univ Paris Est Créteil, Faculté de Santé, 94010, Créteil, France
U955-IMRB, Equipe 03 “Pharmacologie et Technologies pour les Maladies Cardiovasculaires (PROTECT)”, Inserm, Univ Paris Est Créteil (UPEC), Ecole Nationale Vétérinaire d’Alfort (EnVA), 94700, Maisons-Alfort, France
Department of Intensive Care, Erasme Hospital, Université Libre de Bruxelles, Brussels, Belgium
Jean-Louis Vincent
You can also search for this author in PubMed Google Scholar
Contributions
NM wrote the first draft, and JLV edited it for critical content. Both authors read and approved the final manuscript.
Corresponding author
Correspondence to Nicolas Mongardon .
Ethics declarations
Conflicts of interest.
NM received consultant fees from AOP Health and Baxter. He has no other conflicts of interest related to this manuscript. JLV has no conflicts of interest related to this manuscript.
Additional information
Publisher's note.
Springer Nature remains neutral with regard to jurisdictional claims in published maps and institutional affiliations.
Rights and permissions
Reprints and permissions
About this article
Mongardon, N., Vincent, JL. Outcome of hospital-acquired bloodstream infection: interplay between bacteria, host, and healthcare system organization. Intensive Care Med (2024). https://doi.org/10.1007/s00134-024-07490-9
Download citation
Received : 09 March 2024
Accepted : 10 May 2024
Published : 27 May 2024
DOI : https://doi.org/10.1007/s00134-024-07490-9
Share this article
Anyone you share the following link with will be able to read this content:
Sorry, a shareable link is not currently available for this article.
Provided by the Springer Nature SharedIt content-sharing initiative
- Find a journal
- Publish with us
- Track your research

An official website of the United States government
The .gov means it’s official. Federal government websites often end in .gov or .mil. Before sharing sensitive information, make sure you’re on a federal government site.
The site is secure. The https:// ensures that you are connecting to the official website and that any information you provide is encrypted and transmitted securely.
- Publications
- Account settings
Preview improvements coming to the PMC website in October 2024. Learn More or Try it out now .
- Advanced Search
- Journal List
- Antimicrob Resist Infect Control

An observational case study of hospital associated infections in a critical care unit in Astana, Kazakhstan
Dmitriy viderman.
1 Nazarbayev University School of Medicine (NUSOM), 5/1 Kerey and Zhanibek Khans Street, Astana, Kazakhstan 010000
3 National Research Neurosurgery Center, Astana, Kazakhstan
Yekaterina Khamzina
Zhannur kaligozhin.
2 National Research Center for Oncology and Transplantation, Astana, Kazakhstan
Makhira Khudaibergenova
Agzam zhumadilov, byron crape, azliyati azizan, associated data.
Please contact author for data requests.
Hospital Associated infections (HAI) are very common in Intensive Care Units (ICU) and are usually associated with use of invasive devices in the patients. This study was conducted to determine the prevalence and etiological agents of HAI in a Surgical ICU in Kazakhstan, and to assess the impact of these infections on ICU stay and mortality.
To assess the rate of device-associated infections and causative HAI etiological agents in an ICU at the National Research Center for Oncology and Transplantation (NRCOT) in Astana, Kazakhstan.
This retrospective, observational study was conducted in a 12-bed ICU at the NRCOT, Astana, Kazakhstan. We enrolled all patients who were admitted to the ICU from January, 2014 through November 2015, aged 18 to 90 years of age who developed an HAI.
The most common type of HAI was surgical site infection (SSI), followed by ventilator-associated pneumonia (VAP), catheter-related blood stream infection (BSI) and catheter-associated urinary tract infection (UTI). The most common HAI was SSI with Pseudomonas aeruginosa as the most common etiological agent. The second most common HAI was VAP also with P. aeruginosa followed by BSI which was also associated with P. aeruginosa (in 2014) and Enterococcus faecalis , and Klebsiella pneumoniae (in 2015) as the most common etiological agents causing these infections.
We found that HAI among our study population were predominantly caused by gram-negative pathogens, including P. aeruginosa , K. pneumoniae , and E. coli. To our knowledge, this is the only study that describes ICU-related HAI situation from a country within the Central Asian region. Many developing countries such as Kazakhstan lack surveillance systems which could effectively decrease incidence of HAIs and healthcare costs for their treatment. The epidemiological data on HAI in Kazakhstan currently is underrepresented and poorly reported in the literature. Based on this and previous studies, we propose that the most important interventions to prevent HAI at the NRCOT and similar Healthcare Institutions in Kazakhstan are active surveillance, regular infection control audits, rational and effective antibacterial therapy, and general hygiene measures.
HAI influence the quality of health care and are a major source of adverse outcomes during health care delivery [ 1 , 2 ]. HAI greatly increase morbidity and mortality of patients and healthcare costs [ 3 ]. The burden of HAI in developing countries is significant, whereby the incidence can be up to 15% of total hospitalized patients, and up to 50% among ICU patients [ 4 ]. HAI are challenging to treat because the etiological agents frequently develop multidrug, extensively drug and pandrug-resistance [ 5 ]. HAI have a big economic impact on healthcare by extending ICU stay, hospital stay, and increasing the need for invasive procedures. The most common HAI are primary bloodstream infections (BSI), ventilator-associated pneumonia (VAP), urinary tract infections (UTI) and surgical site infections (SSI), with SSI being the most prevalent in some studies [ 2 , 6 ]. Prevention programs for HAI which could result in positive cost-benefit ratios typically originate with laboratory data from the clinical microbiology laboratory; this provides information regarding the causative pathogenic organisms causing the HAI [ 6 ].
The incidence of HAI in ICUs is about 2 to 5 times higher than those in general inpatient departments due to many associated risk factors [ 1 , 7 ]. Furthermore, antimicrobial resistance rates in ICU are much greater than in general departments [ 7 ]. In order to reduce incidence of HAI, surveillance analysis is an essential step to identify problems and implement interventions [ 8 ]. National surveillance data form the basis for prevention and control of HAI in developed countries such as the USA and Australia, but this is rarely available in many developing countries, including Kazakhstan [ 9 ]. A recent systematic meta-analysis of the burden of HAI in Southeast Asia found that the most common HAI pathogens were mostly gram-negative bacilli, and were predominantly Pseudomonas aeruginosa , Klebsiella species and Acinetobacter baumannii ; these findings are similar to those reported for many other developing countries [ 9 ]. According to the National Healthcare Safety Network (NHSN) report in 2013, ICU-related central-line associated BSI continued to decrease, whereas urinary catheter-associated tract infection rates increased in the majority of ICU types [ 10 ]. Several studies conducted in developing countries similar to ours, reported their findings in the literature; one study from Kuwait reported that their VAP rate was 4.0 per 1000 mechanical ventilator days, the central line–associated BSI rate was 3.5 per 1000 central line days, and the catheter-associated urinary tract infection (CAUTI) rate was 3.3 per 1000 urinary catheter days [ 11 ]. Another study from Ecuador showed that device associated HAIs rates in their ICUs were higher than the United States CDC/NSHN rates and similar to International Nosocomial Infection Control Consortium (INICC) international rates [ 12 ].
In an effort to evaluate the local HAI situation in Kazakhstan, we conducted an observational case study to assess incidence of the different types of HAI over a period of two years in 2014 and 2015. Our goal was to investigate if the patterns regarding type of HAI cases and the etiological agents were consistent from year to year, therefore we performed an analysis and comparison of data from at least two years. The data from the study was retrospectively analyzed in our effort to identify the causative HAI bacterial pathogens. We aimed to assemble and analyze epidemiological data associated with four different types of device associated HAI in an ICU at the National Research Center for Oncology and Transplantation (NRCOT) in Astana, Kazakhstan. The information summarized here will form the basis for a much larger surveillance study that could guide decisions regarding appropriate and potentially more efficacious use of prophylactic control within the ICU. This study could also guide the establishment of a national surveillance program to control and prevent HAI in hospitals in Kazakhstan and worldwide.
This retrospective, observational study was conducted from January 2014 through January 2016 in a 12 bed ICU at the National Research Center for Oncology and Transplantation (NRCOT), which is a 280-bed hospital in Astana, Kazakhstan. We undertook this pilot study comparing HAI cases and causative agents over a two year period to assess the current local infection control practices. We hope to understand from this study if intervention in the future would be appropriate to improve upon the current HAI scenario. The main change between 2014 and 2015 was access to better diagnostic capabilities within the hospital laboratory, though there are future plans to implement an improved HAI prevention program. This was considered by us to be a “retrospective” study because data analysis on the most part was done after these were collected (even though the staff collected the data during the patients’ ICU visit). A standard screening protocol for HAI was used [ 13 ]. We tested all patients who spent more than 48 h in the ICU (whereby, all patients with less than 48 h stay in the ICU were excluded from this study). Samples for microbiological culture and analysis were obtained from patients who presented with HAI symptoms. Blood count, blood biochemistry, and blood coagulation tests were performed on all suspected patients who were at high risk of developing HAI following guidelines provided by the Healthcare Infection Control Practices Advisory Committee [ 14 ]. Clinical Pharmacologist and Hospital Infection Specialists visited the ICU every day. Chest radiography, deep tracheal aspirate, bronchoalveolar lavage (BAL) or mini-broncho-alveolar lavage (mini-BAL) were taken for pathogen identification if VAP was suspected. Blood samples, removed intravascular catheters, urine, urinary and wound catheters were also cultured for microbiological analysis if BSI, UTI or SSI were suspected. Samples were cultured using standard microbiological methods; isolated bacteria were identified by standard microbiological methods and tested for antibiotic susceptibility using Kirby-Bauer disk-diffusion technique according to Clinical and Laboratory Standards Institute (CLCI) specifications [ 15 , 16 ].
Study ethics
Ethical clearance for this study was obtained from the Ethics committee of the Institutional Review Board of Nazarbayev University. The study was exempt from being classified as human subject research as no personal information related to any of the patients was made available to the Investigators at any time before, during or after the study.
Study population and microbiological culture and analysis
The patients admitted to the NRCOT ICU were from different surgical departments, which included the department of general, oncology, transplant, vascular, orthopedic, and gynecology surgeries. The patients with a diagnosis of HAI received treatment according to the hospital’s standard protocol of management of HAI. Samples were taken from all patients admitted to the ICU aged 18 to 90 years of age who developed HAI based on clinical, laboratory and instrumental findings throughout the study duration. For microbiological culture and sensitivity testing, lower respiratory tract secretion, blood and urine samples were taken for diagnosis of VAP, catheter-related BSI, and catheter-associated UTI. Blood culture diagnostics was done for all samples from patients with suspected catheter-related blood stream infections. Data relevant to the diagnosis of HAI were taken by the hospital staff, but only microbiological data regarding etiological agents related to device associated HAI were provided for analysis in this study.
Statistical analysis
The rates of development of each type of HAI (BSI, VAP, UTI and SSI) as well as the total rate per 1000 cases were calculated. Relative risk for these groups was assessed using StataMP 13.0 software, and statistically significant cutoff was determined at a p -value of < 0.05, and highly statistically significant cutoff at a p-value of < 0.001. Prevalence of certain isolated strains of bacteria that caused hospital-acquired infections for each year was determined in percent and compared between the years 2014 and 2015 using Chi-square analysis in StataMP 13.0 software. Statistically significant level was determined at a p -value < 0.05; and highly statistically significant level at a p -value of < 0.001.
General overview of ICU patients and the proportions with HAI
A total number of 1257 patients were admitted to the ICU from January 2014 to January 2016 of which, 56.6% (711 patients) were admitted in 2014 compared to the remaining 43.4% (546 patients) in 2015. The mean number of ICU stay was 18 days (with a range of 1–330 days) in 2014, while in 2015 the mean ICU stay was 36 days (with a range of 12–62 days); these variations are also a reflection of some changes in perioperative care that took place in between these two time periods. The change we mention here that led to increased mean number of ICU stay in 2015 compared to 2014 was due to the change in patient population. In 2014, the patient population consisted of patients who were transferred to the ICU after emergency surgeries (which were more than 85% of all transfers to ICU) such as patients who underwent appendectomy, cholecystectomy, hernia repair surgeries, etc. In 2015, the ICU patient population consisted of patients who were transferred to the ICU after oncological surgery (50%) and transplant surgery (30%). The period of ICU stay of patients after emergency surgery was generally shorter than the patients who were moved to the ICU after oncological or transplant surgery. Overall, the patients’ general health conditions were better for the patients in 2014.
During the study period, 249 out of 1257 patients admitted to the ICU developed HAI; the p -value for the development of HAI between 2014 and 2015 was 0.000127 which is highly statistically significant. A total of 114 microbiological cultures were obtained and identified from patients suspected to have had HAI in 2014 compared to 135 cultures isolated in 2015 (with a p -value of 0.6582 which is not significant).
Device associated infections
The HAIs were classified into four categories: BSI, VAP, UTI and SSI. As evident from the Table Table1, 1 , in 2014, SSI constituted the highest percentages of all HAIs followed by VAP, BSI and UTI. When compared to 2014, similar profile of device associated HAIs were observed for 2015, whereby SSI was again the most prominent category that caused HAI, followed by VAP. However in 2015, the percent of observed BSI was equal to the percent of UTI as the least frequent types of HAI.
Types of Hospital Associated infections in percent for each year
BSI : Catheter-associated Blood Stream Infections, VAP : Ventilator-associated Pneumonia, UTI : Catheter-associated Urinary Tract Infections, SSI : Surgical Site Infections
Variation in types of infections
Analysis of HAI categories (BSI, VAP, UTI, and SSI) in 2014 compared to 2015 was conducted and revealed significant variation between certain types of HAI (Fig. 1 ). Specifically, rates of VAP and SSI were found to be statistically different ( p -value < 0.05) between the two years of the study period. Rates of VAP infections were higher in 2015 than in 2014. Similar results were obtained for SSI, whereby the rate of infection was again higher in 2015 ( p -value < 0.05). On the other hand, no difference in rates of BSI and UTI was observed. The total rate of all types of infections was found to be significantly higher in 2015 when compared to the total infection rate in 2014 ( p -value < 0.05). Data was available for the VAP incidence which was determined to be 8.4 per 1000 ventilator days while the incidence of catheter-related blood stream infections was 18 per 1000 line days.

Samples for microbiological culture and analysis were obtained from patients who presented with HAI symptoms. Blood samples, removed intravascular catheters, urine, urinary and wound catheters were also cultured for microbiological analysis if BSI, UTI or SSI were suspected. Comparison of rates of case-specific infections between 2014 and 2015 were performed. Statistically significant level was determined at a p -value < 0.05; and highly statistically significant level at a p -value of < 0.001. Statistically significant difference is noted for VAP, SSI and combined total between years 2014 and 2015 (statistical significance: * p -value< 0.05; ** p -value< 0.001)
Microbiological etiology of HAI
A total number of 249 microbial pathogens were isolated and identified in 2014 and 2015, and the percent distribution of these etiological agents is represented in Fig. 2a and andb. b . The highest percent in 2014 corresponded to Pseudomonas aeruginosa at 23.68%, which was slightly reduced in 2015 to 13.33%. Klebsiella pneumoniae , Escherichia coli , Staphylococcus aureus , Staphylococcus epidermidis, and Acinetobacter baumannii were isolated and identified from many HAI cases for both 2014 and 2015. Unique and relatively widespread isolate for 2014 was Enterobacter aerogenes , representing 17.54% of the cases. Bacterial strains that had an incidence of less than 5% were combined into the subgroup ‘Others’. In 2014 Streptococcus viridans, Citrobacter spp., Enterococcus faecium, Achromobacter spp., Staphylococcus saprophyticus, Candida albicans and Serratia spp. were included in this subgroup. In 2015 however, in addition to the above-mentioned Citrobacter spp. , S. saprophyticus and C. albicans, unique pathogens such as Staphylococcus haemolyticus , Enterococcus cloacae , Candida tropicalis , Burkholderia cepacia , Streptococcus mitis and Stenotrophomonas maltophilia were isolated. When comparison analysis was conducted, highly significant difference between two years ( p -value < 0.001) was identified. Even though many strains were similar for both years, case-wise analysis revealed important differences that should be taken into account. The types of pathogen described originated from cultures isolated from the different HAI categories as shown in Fig. 3a (VAP), Fig. Fig.3b 3b (BSI), Fig. Fig.3c 3c (UTI), and Fig. Fig.3d 3d (SSI), and represented as percent (%) of total number of organisms isolated. We found that HAI were predominantly caused by gram-negative bacterial pathogens, particularly P. aeruginosa and K. pneumoniae . These strains were most frequently associated with all the HAI types particularly SSI and BSI (as the first two most common types of HAI pathogens isolated) though there are some variations between the two years with these two pathogens as well as other types of bacterial pathogens that predominated, such as Staphylococcus epidermidis, E. faecalis and Acinetobacter baumannii . From our study, P. aeruginosa was recognized as a causal agent of the most serious HAI in the ICU. This organism was also causing the majority of VAP and BSIs in two consecutive years. SSI analogously was mainly caused by gram-negative bacteria, of which P. aeruginosa prevailed in 2014, while K. pneumoniae predominated in 2015.

Samples for bacterial culture and analysis were obtained from patients who presented with HAI symptoms. Samples were cultured using standard microbiological methods; isolated bacteria were identified by standard microbiological methods according to Clinical and Laboratory Standards Institute (CLCI) specifications [ 12 , 13 ]. For comparison, Chi-square test was conducted with a p -value < 0.001**. The subgroup ‘Others’ includes rare strains of bacteria (with incidence of < 5%), which were different for each year (comparing 2014 and 2015).

The types of HAI pathogens shown in Fig. Fig.2 2 were subdivided into the types of HAI samples they were isolated from; a VAP, b BSI, c UTI, and d SSI and represented as percent (%) of total number of bacteria isolated. Samples were cultured using standard microbiological methods; isolated bacteria were identified by standard microbiological methods according to Clinical and Laboratory Standards Institute (CLCI) specifications [ 12 , 13 ]
In this study, we have shown that healthcare-associated infections constitute a major healthcare burden in Kazakhstan, which is a rapidly developing nation. Kazakhstan is one of the five Central Asian countries (which also include Kyrgyzstan, Tajikistan, Turkmenistan and Uzbekistan) that achieved independence in 1991 after the collapse of the Soviet Union [ 17 , 18 ] . At independence, these Central Asian Post-Soviet (CAPS) countries were faced with many problems; progress to build stronger economies varies between countries. Collapse of the Soviet Union led Kazakhstan as well as the other CAPS countries to economic recession. During the Soviet Union era, there were no wide informational, medical and pharmaceutical exchanges with other countries. On the other hand, the dissolution of the Soviet Union mobilized these countries to democracy which initiated important exchanges of products and knowledge with the other countries. Greater successes have been noted for Kazakhstan, Turkmenistan and Uzbekistan, which are the CAPS countries with the richest natural resources [ 17 ]. The healthcare systems of these CAPS countries have also gone through many decades of profound revolutions, whereby the rate and quality of progress of the healthcare sectors vary between these countries [ 19 ]. Since independence, the CAPS countries have steadily been receiving more attention at the international arena, especially due to the economic and geographical significance of the region. Over the last two decades, Kazakhstan has made significant progress in economy as well as healthcare sector, although there is still much room for improvement.
To our knowledge, this is the only study to be reported in an international journal describing ICU-related HAI situation from the Central Asian region. In our study, HAI represents a significant healthcare problem with ICU related HAI incidence being about 2 to 5 times higher than the incidence in other in-hospital departments [ 7 ]. In our study, patients frequently had the following universal host-related risk factors predisposing to HAI which included advanced age, severity of disease, hyperglycemia, neutropenia, immunosuppressive therapy, cancer diagnosis, and malnutrition. Majority of the patients had at least three of these risk factors. The most common type of HAI in our study was SSI whereby the rate increased significantly ( p -value < 0.05) when comparing the year 2014 to 2015 (Table 1 and Fig. Fig.1). 1 ). Between both years, no intervention was introduced in terms of recommendations to improve upon infection control practices which may have been one of the reason there were no observed decrease in device-associated HAI rates. This high incidence of HAI associated with SSI was due to the fact that the Astana NRCOT is a multidisciplinary hospital focusing on multiple types of surgeries. In most cases, patients also had poor nutritional and altered immune status due to devastating diseases, which were either cancer, kidney and liver failure, diabetes, and coexisting infections at the remote body site. Multiple studies stated that the above mentioned medical conditions predispose patients to the development of SSI [ 20 , 21 ]. Medical procedures in the hospital were typically prolonged, and patients were prone to intraoperative hypothermia, increasing the risk for SSI [ 22 ]. In our setting, colorectal surgery was the most frequently performed surgery, which is recognized as a risk factor for SSI worldwide [ 23 ].
From our study, VAP was identified as the second most common type of HAI. For patients on mechanical ventilation however, VAP was the leading type of infection, which is consistent with reports from multiple other studies [ 24 , 25 ]. Majority of the patients who developed VAP had COPD, advanced age, H 2 -antagonists and antibiotics use, and Multiple Organ System Failure [ 26 ]. Similar to the SSI associated HAI described above, the rates of VAP also increased significantly when comparing 2014 to 2015 (Table 1 and Fig. Fig.1); 1 ); this may have also been due to absence of intervention that was implemented to reduce the number of VAP cases in the studied ICU. The third most common type of HAI identified in our study was BSI. Almost all patients who developed BSI had the following risk factors; central vein catheterization, malnutrition and surgery, and infection with highly antibiotic resistant pathogens. These findings are supported by evidence from other studies, which report BSI to occur in 5% of all ICU patients [ 26 , 27 ].
We found that HAI were predominantly caused by gram-negative pathogens, including P. aeruginosa , K. pneumoniae , and E. coli. These strains were most frequently associated with VAP, SSI, BSI and UTI. From our study, P. aeruginosa was recognized as a causal agent of the most serious HAI in the ICU [ 28 ]. This organism was also causing the majority of VAP and BSI in two consecutive years [ 9 ]. SSI analogously was mainly caused by gram-negative bacteria, of which P. aeruginosa prevailed in 2014, while K. pneumoniae predominated in 2015. Another interesting finding was with regards to the differences between the length of ICU patient stay, the patient population type, rate of HAI as well as the types of pathogens isolated in 2014 when compared to 2015. Since the period of ICU stay of patients after emergency surgeries was much shorter than after oncological or transplant surgeries, the overall period of ICU stay was longer in 2015. Furthermore, ICU patients’ general condition was better among patients in 2014. Our results showed that the rate of HAIs was increased and the distribution of pathogen also changed in 2015 compared to 2014. We propose that the difference between patient populations in the ICU partially explained the reason for increased number and rates of HAI in 2015 (Table 1 and Fig. Fig.1), 1 ), as well as the differences in the types of HAI pathogens that were isolated when these pathogen types were compared between the two years of study. With regards to increased HAI rate, both the oncological and transplant patient populations were immunocompromised due to the surgical procedures, and these patients also received immunosuppressant drugs; this led to further decrease in their immune status. Plus, these patients stayed longer in the ICU thereby had higher risk of acquiring ICU-related HAIs. Interestingly, more HAI pathogens that are more commonly associated with multidrug resistance belonging to the “ESKAPE” pathogen group were identified in 2015. This included P. aeruginosa, E. faecalis, K. pneumoniae and A. baumannii (Fig. 2 ); this is not a surprising finding given that these pathogens are associated with many HAI infections within the last few decades [ 29 ].
There are limitations to this study, which include the fact that data collected did not include patients’ age, sex, and other demographic variables, which might have contributed towards generation of more precise and valuable conclusions. For future studies, the design could include collection of these and other relevant information that would enhance the quality of data and conclusions that can be derived from the study. In this study, some organisms identified were usually not considered common causative agents (such as Candida , Enterococcus faecalis, Staphylococcus epidermidis and Streptococcus mitis for VAP as in Fig. Fig.3a, 3a , and S. epidermidis and S. viridans for UTI as shown in Fig. Fig.3c). 3c ). Such unexpected findings further support our conclusion that there is an urgent and definitive need to improve upon HAI prevention practices which include incorporation of surveillance protocols that meet international standards that can more accurately detect and identify the correct etiological agent (which includes incorporation of quality control measures and established Standard operating procedure) in Kazakhstan and other developing countries within the region. These findings indicate that the surveillance protocols mentioned in the method section were not fully implemented during the study period, which is a major limitation of this study. Therefore the findings from this study demonstrates the need to improve training of infection control personnel regarding the use of and implementation of more stringent practices that meet the international HAI infection control and surveillance guidelines.
HAI is a critical problem for health-care providers worldwide, which should receive appropriate attention for further management. The integrated HAI control program was introduced more than 3 decades ago for the first time, being able to reduce both incidence of infections and related healthcare costs [ 30 ]. Unfortunately, many developing countries such as Kazakhstan lack surveillance systems which could effectively decrease incidence of HAIs and healthcare costs for their treatment. Currently, the international guidelines on prevention, diagnosis and treatment of HAI are not actively practiced, and access to newer antibacterial agents is not readily available in Kazakhstan. Within Kazakhstan, there are National and Regional Medical Centers (hospitals). There are no published epidemiological data on HAI from the Regional Medical Centers, therefore, the epidemiological characteristics of HAI from these Centers are unknown. If we compare the National Medical Centers with Regional hospitals, there is an unequal supply of medical equipment and uneven qualification of medical doctors and staff in the latter. For example, several National Medical Centers have internationally educated doctors and staff as well as equipment resembling those that can be found in the best World Class Medical Centers. These medical Centers can be effective in monitoring, diagnosing, treating and preventing the HAI, whereas many Regional (and rural) hospitals do not even follow any guidelines on diagnosis, treatment and preventions of HAIs. The National Medical Centers frequently receive severely ill patients with multiple undiagnosed multi- and pandrug resistant HAI from the Regional Medical Centers.
We propose that the most important recommended measures to prevent HAI at the NRCOT and similar Healthcare Institutions are rational antibacterial therapy, regular infection control audits, and general hygiene measures. Numerous studies in developing countries have shown that the International Nosocomial Infection Control Consortium (INICC) multidimensional infection control strategy with practice bundles can decrease the rate of HAI. Rosenthal et al. found that implementation of a multidimensional infection control strategy can significantly reduce the central line-associated BSI rates in the PICUs of developing countries [ 31 ]. In another study, Tao et al. demonstrated that a multidimensional infection control intervention for VAP contributed to a significant cumulative reduction in the VAP rate in their ICUs [ 32 ].
This is a first surveillance study on HAI at the NRCOT since its foundation eight years ago. An active surveillance system monitoring incidence and prevalence of HAI as preventative measures is necessary, and this study represents a first step towards this goal.
Conclusions
We conclude that HAI is one of the major problems in healthcare provision in Kazakhstan. SSI, VAP and BSI are the predominant types of HAI. Gram-negative bacteria such as P. aeruginosa , K. pneumoniae and E. coli are the most common causative agents of HAI. The most important risk factors are advanced age, severity of disease, hyperglycemia, neutropenia, immunosuppressive therapy, cancer diagnosis, and malnutrition. For more precise determination of its causality, an ongoing HAI surveillance program is needed to decrease HAI incidence and prevalence at the NRCOT and other healthcare institutions throughout the country. Kazakhstan has made significant effort and progress since independence to establish a healthcare system that is already an improvement over the centralized Semashko health system model inherited from the Soviet Union at independence [ 19 ]. One of the ways to continue on the positive trajectory of change for an improved healthcare system is implementation of an efficient active surveillance system for HAI that should be implemented nationwide. The system should include an organized and controlled data collection performed by trained personnel. The data collected should include not only infection-related data, but also patient-related data such as risk factors and history of present illness (such as history of HAI, diagnostic tests, surgeries and invasive methods, as well as the antimicrobial drugs used). A system of monthly HAI analysis and report should also be implemented. Such proactive surveillance system coupled with efficient infection control programs would be highly beneficial to control and reduce the rates of HAI within all Regional and National Medical Centers throughout Kazakhstan.
Acknowledgements
The Authors would like to acknowledge the staff for their contributions including sample retrieval, blinding and randomizing, as well as providing information on sample types available prior to randomizing. The Authors also would like to thank Reviewers for their valuable comments.
Nazarbayev University School of Medicine.
Availability of data and materials
Author’s contribution.
DV, AA and YK participated in the study design. ZK and DV participated in the data collection. YK and BC performed the advanced statistical analyses and prepared the associated Figures and Table. YK, DV and AA wrote the manuscript. YK provided technical assistance related to manuscript development. DV and AA conceived of the study, reviewed the data, provided feedback for Figures and Table, and edited the manuscript. AZ and MK provided the technical support to data collection. All authors reviewed and approved the final version of manuscript.
Ethics approval and consent to participate
Competing interests.
The authors declare that they have no competing interests.
Publisher’s Note
Springer Nature remains neutral with regard to jurisdictional claims in published maps and institutional affiliations.
Contributor Information
Dmitriy Viderman, Email: [email protected] .
Yekaterina Khamzina, Email: zk.ude.un@anizmahky .
Zhannur Kaligozhin, Email: [email protected] .
Makhira Khudaibergenova, Email: ur.liam@86ariham .
Agzam Zhumadilov, Email: ur.liam@hzmazga .
Byron Crape, Email: [email protected] .
Azliyati Azizan, Phone: +7-7172-69-4633, Email: [email protected] .
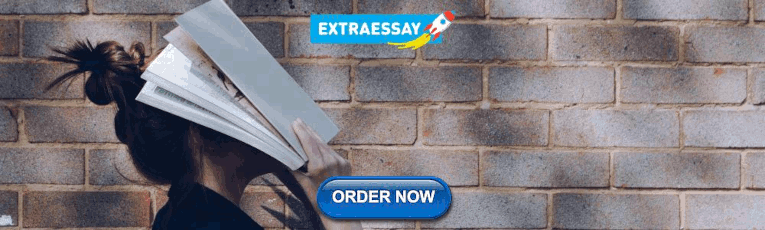
COMMENTS
* Hospital-acquired infections (HAIs) pose a significant threat to health care and healthcare organizations. * HAIs cause patient safety, ethical, regulatory, financial, and legal risk. * The HAI workgroup at one organization is leading improvement efforts that require every individual in the organization to be engaged in the improvement efforts.
Strategies to Prevent Healthcare-Associated Infections. HCAIs (their prevention and control) are a significant global public health burden about which concerns have been raised from all healthcare stakeholders, including health professionals, patients, and the public. 45 - 48 Their impact has dramatically increased because of the advent of multidrug-resistant pathogenic microorganisms. 49 ...
* Hospital-acquired infections (HAIs) pose a significant threat to health care and healthcare organizations. * HAIs cause patient safety, ethical, regulatory, financial, and legal risk. * The HAI workgroup at one organization is leading improvement efforts that require every individual in the organization to be engaged in the improvement efforts.
The HCP's occupational infection risk to COVID-19, interim guidance regarding risk assessment and universal PPE policy issued by the CDC , and the risk factors for severe acute respiratory syndrome coronavirus (SARS-CoV-2) transmission in hospital settings from previous studies were also included to develop the model for the case study.
A domain-knowledge modeling of hospital-acquired infection risk in Healthcare personnel from retrospective observational data: A case study for COVID-19 PLoS One. 2022 Nov 21;17(11 ... factors, engineering control factors, and administrative control factors. For model validation, we investigated the case study of the Coronavirus disease, in ...
Resolving the challenges presented by healthcare-associated infections requires a 'whole healthcare economy' perspective encompassing the interactions between biological, therapeutic, and structural factors. The importance and influence of organizational characteristics is receiving increasing attention. This article reviews some keys ...
Introduction Hospital-acquired infections of communicable viral diseases (CVDs) have been posing a tremendous challenge to healthcare workers globally. Healthcare personnel (HCP) is facing a consistent risk of viral infections, and subsequently higher rates of morbidity and mortality. Materials and methods We proposed a domain-knowledge-driven infection risk model to quantify the individual ...
A survey carried out by the World health organization in 2010 showed that only 16% ... Risk factors for hospital-acquired infections in teaching hospitals of Amhara regional state, Ethiopia: A matched-case control study. PloS one. 2017; 12 (7):e0181145. Epub 2017/07/19. doi: 10.1371/journal.pone.0181145 ; PubMed Central PMCID: PMC5515417. ...
Introduction. Hospital-acquired infections (HAIs) are those infections, which are not present at the time of admission and acquired during the course of hospital settings manifested 48-72 h after admission, more prevalent in critical care settings.[1 2] World Health Organisation (WHO) reported overall 5.1-11.6% prevalence rate of HAIs in high-income countries (HICs).[]
Purpose In the critically ill, hospital-acquired bloodstream infections (HA-BSI) are associated with significant mortality. Granular data are required for optimizing management, and developing guidelines and clinical trials. Methods We carried out a prospective international cohort study of adult patients (≥ 18 years of age) with HA-BSI treated in intensive care units (ICUs) between June ...
This National Healthcare Safety Network (NHSN) surveillance case study is part of a case-study series in the American Journal of Infection Control (AJIC). These cases reflect some of the complex patient scenarios Infection preventionists (IPs) have encountered in their daily surveillance of health care-associated infections (HAI) using NHSN definitions. Objectives have been previously published.1
Hospital-acquired infections, also known as healthcare-associated infections (HAI), are nosocomially acquired infections that are typically not present or might be incubating at the time of admission. These infections are usually acquired after hospitalization and manifest 48 hours after admission to the hospital.
According to the World Health Organization, "health care-associated infections (HAIs) represent the most common adverse event among hospitalized patients."1 According to the latest research, over 2.5 million HAI episodes occur every year in Europe, with more than 90,000 deaths attributed to the 6 most common types: healthcare-associated pneumonia, health care-associated urinary tract ...
Competing events for hospital-acquired infections are death or discharge from the hospital because they preclude the observation of such infections. Study design and setting: Using surveillance data of 6,568 patient admissions (full cohort) from two Spanish intensive care units, we propose a case-cohort approach which uses only data from a ...
Patients affected by SARS-CoV-2 infection can demonstrate COVID-19, which is associated with a high rate of hospitalization, admission to the ICU, 1. and death. 2. For several weeks, starting February 20, 2020, Italy was the epicenter of the first COVID-19 outbreak in the Western world. 3.
Most of the studies on risk factors for hospital-acquired infection employed by cross-sectional methods, that has a limitation of statistical power by comparing the groups internally. The introduction of bias in matched case control study is lower than in the cross-sectional design . Matched case control study design is assumed to generate ...
A study on hospital acquired infection and prevention in CCU at College of Medicine &JNM Hospital March 2019 IOSR Journal of Dental and Medical Sciences 18(3):51-72
An infection acquired in hospital by a patient who was admitted for a reason other than that infection (1). An in-fection occurring in a patient in a hospital or other health care facility in whom the infection was not present or incu-bating at the time of admission. This includes infections acquired in the hospital but appearing after ...
The burden of health care-associated infection worldwide. 29 April 2010. Health care-associated infection (HAI), also referred to as "nosocomial" or "hospital" infection, is an infection occurring in a patient during the process of care in a hospital or other health care facility which was not present or incubating at the time of admission.
In a study published in the current issue of Intensive Care Medicine, Buetti et al. evaluated the possible impact of center and country factors on the adequacy of early antimicrobial therapy, as a process indicator, and 28-day mortality in patients with healthcare-associated bloodstream infections (HABSI) [].For this purpose, they reviewed data from the EUROBACT-2 study, a large prospective ...
Hospital-acquired infections, also known as healthcare-associated infections (HAI), are nosocomially acquired infections that are typically not present or might be incubating at the time of admission. These infections are usually acquired after hospitalization and manifest 48 hours after admission to the hospital. The infections are monitored closely by agencies such as the National Healthcare ...
Hospital-acquired infections pose a significant threat to health care and healthcare organizations. HAIs cause patient safety, ethical, regulatory, financial, and legal risk. The rate of HAIs in 2016 did not align with the mission, vision, or strategic plan of the organization and action had to be taken. The HAI workgroup is leading improvement ...
Background. HAI influence the quality of health care and are a major source of adverse outcomes during health care delivery [1, 2].HAI greatly increase morbidity and mortality of patients and healthcare costs [].The burden of HAI in developing countries is significant, whereby the incidence can be up to 15% of total hospitalized patients, and up to 50% among ICU patients [].
Surveillance of healthcare-associated infections (HAIs) driven by risk-assessment is the cornerstone of effective infection prevention and control (IPC) programs.1. Surveillance is a standardized process of continuous data collection, management, analysis, and interpretation, followed by disseminating findings and recommendations to relevant stakeholders.2 The later include health care ...