
An official website of the United States government
The .gov means it’s official. Federal government websites often end in .gov or .mil. Before sharing sensitive information, make sure you’re on a federal government site.
The site is secure. The https:// ensures that you are connecting to the official website and that any information you provide is encrypted and transmitted securely.
- Publications
- Account settings
Preview improvements coming to the PMC website in October 2024. Learn More or Try it out now .
- Advanced Search
- Journal List
- Springer Nature - PMC COVID-19 Collection

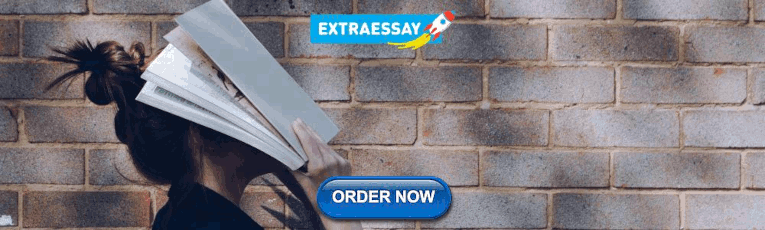
Student satisfaction and interaction in higher education
Wan hoong wong.
1 Singapore Institute of Management, Singapore, Singapore
2 University of Western Australia, Perth, Australia
Elaine Chapman
Associated data.
Data will be made available on request to the corresponding author.
No non-commercial software or custom code used in the study.
Given the pivotal role of student satisfaction in the higher education sector, myriad factors contributing to higher education satisfaction have been examined in the literature. Within this literature, one lesser-researched factor has been that of the quality and types of interpersonal interactions in which students engage. As existing literature has yet to fully explore the contributions made by different forms of interaction to student satisfaction in higher education, this study aimed to provide a more fine-grained analysis of how different forms of interaction between students, their peers and their instructors relate to different aspects of student satisfaction. A total of 280 undergraduate students from one of the largest higher education institutions in Singapore participated in the study. Results provided an in-depth analysis of eight aspects of student satisfaction (i.e. satisfaction with the program, teaching of lecturers, institution, campus facilities, student support provided, own learning, overall university experience and life as a university student in general) and suggested that the different aspects of student satisfaction were associated with three different forms of interaction: student–student formal, student–student informal and student-instructor.
Introduction
In higher education (HE), student satisfaction is vital both for the success of institutions and for that of individual students, particularly in our current global climate. Rapid technological advancements, in particular, have intensified competition in the HE sector in recent years. In Singapore and other countries presently, not only do HE institutions need to compete for students with branch campuses of foreign institutions set up locally, but also with digital platforms that offer massive open online courses (MOOCs) that allow students to learn without being attached to specific institutions. By 2015, there were already about 220 international branch campuses of overseas universities in operation worldwide (Maslen, 2015 ).
In this cut-throat context, maximising student satisfaction has become a primary focus of many universities and colleges, irrespective of their physical location. Such move is no surprise considering that student satisfaction is now often used as a measure of HE institutions’ performance (Jereb et al., 2018 ; McLeay et al., 2017 ). As reflected in recent studies on the impact of COVID-19 (Coronavirus Disease 2019) upon the HE sector, student satisfaction has been incorporated as an index to measure the extent to which attendant disruptions to services have affected the quality of HE services received by students (e.g. Duraku & Hoxha, 2020 ; Shahzad et al., 2020 ). However, this does not imply that HE institutions have no other considerations when it comes to making student satisfaction their top priorities to pursue service excellence. As discussed in the subsequent section, giving what students want most to keep them satisfied can have undesirable effects on students as well as on institutions. Hence, any discussion that frames the debate on student satisfaction should go beyond its role as a metric to measure HE institutions’ performance.
The vital role of student satisfaction in higher education
Students’ satisfaction with the quality of the education services they receive is a crucial index of the performance of HE institutions in today’s world (Butt & Rehman, 2010 ; Santini et al., 2017 ; Weingarten et al., 2018 ). Student satisfaction figures are also used as a means by which to distribute precious resources across HE institutions in many countries. For instance, the Australian government has recently announced the adoption of the performance-based funding (PBF) scheme to be used in future years, in which the provision of funding to Australian universities will be based, in part, on the quality of the overall student experience (Australian Department of Education, Skills and Employment, 2020 ). Student satisfaction is one of the indices that may be used to measure the overall student experience within this scheme (Commonwealth of Australia, 2019 ).
Be it academic programs or the peripheral student support services, the ability to provide high-quality services to students has been regarded by many scholars as crucial for HE institutions to withstand the increasingly competitive HE environments in which they must now operate (Butt & Rehman, 2010 ; Lapina et al., 2016 ; McLeay et al., 2017 ; Paul & Pradhan, 2019 ). Higher service quality, driven by outstanding learning processes and high levels of satisfaction with the services delivered, has been deemed to be what will “set a HE institution apart” from its rivals (McLeay et al., 2017 ).
Numerous more immediate commercial benefits derived from high levels of student satisfaction have also been highlighted in the literature. When satisfied with the quality of services provided, students are more likely to continue with their enrolled institutions and recommend them to prospective students (Mihanović et al., 2016 ). Student loyalty is another reward that HE institutions can gain from having highly satisfied students. As loyal students are more likely to engage in alumni activities, greater alumni engagement can in turn benefit the institutions through the provision of direct financial support, as well as attractive employment opportunities for current graduates (Paul & Pradhan, 2019 ; Senior et al., 2017 ).
Concurrently, with rising government interventions in various countries to regulate their HE sectors, there have been increasing calls for HE institutions to improve their service quality (Hou et al., 2015 ; Dill and Beerkens, 2013 ). As a result, the use of quality assurance regimes by governments to regulate HE has become more prominent worldwide (Jarvis, 2014 ). In a recent report, it was estimated that the tuition fees for bachelor programs in some OECD countries (Organization for Economic Cooperation and Development) have risen as much as 20% between 2007 and 2017 (OECD, 2019 ). With substantial cost outlays involved in the provision of HE, concerns over returns on financial investments are not limited to students and parents, but apply also to entire governments (Lapina et al., 2016 ; Weingarten et al., 2018 ). With the costs of providing HE continue to rise, many HE providers are becoming increasingly concerned about how best to meet the needs and expectations of students and keep them satisfied (Weingarten et al., 2018 ). Such concerns were valid particularly when student satisfaction was linked numerous institutional aspects. As discovered in a study conducted by De Jager and Gbadamosi ( 2010 ) on 391 students from two African universities, student satisfaction was reported to relate significantly to various institutional aspects including academic reputation, accommodation and scholarship, location and logistics, sports reputation and facilities and safety and security.
Student satisfaction is not only crucial to institutions, but also to learners themselves. Students’ satisfaction with their learning experiences is not, however, related simply to the feelings they have about the quality of the education services they receive. Within the HE literature, high levels of student satisfaction have also been linked to the attainment of important learning outcomes in HE. For instance, scholars have recognised that student satisfaction may influence outcomes such as academic achievement, retention and student motivation (Aldridge & Rowley, 1998 ; Duque, 2014 ; Mihanović et al., 2016 ; Nastasić et al., 2019 ). Evidently, such hypothesised links between satisfaction and key learning outcomes have received some empirical support to date. For instance, positive associations between student satisfaction and student performance have been reported in two HE-based studies, with one using student grades as performance indicators (van Rooij et al., 2018 ) and the other using mastery of knowledge and general success of the faculty as performance indicators (Mihanović et al., 2016 ). In contrast, strong negative associations between student satisfaction and attrition were reported by Duque ( 2014 ).
However, in considering student satisfaction with the quality of education services, the literature highlighted some key concerns of treating students as customers. Focusing on satisfying students in the same way companies satisfying their customers may result in HE institutions emphasising less on what students need most as learners, such as achieving learning outcomes or training to be work-ready, and more on what they want most in order to feel satisfied as fee payers (Calma and Dickson-Deane, 2020 ). What students want best for themselves may not necessarily be beneficial for them to attain quality education, for that they may adopt short-term perspective and prefer assessment that they can score well rather than those that they can learn well (Guilbault, 2016 ). Additionally, with a customer mindset, students may also feel they are entitled to be awarded a degree for the fees they have paid, resulting in shifting their responsibility to learn and be engaged to institutions (Budd, 2017 ). If student expectations are to be met by institutions in order to keep “customers” satisfied, this can subsequently lead to grade inflation (Hassel & Lourey, 2005 ). As such, although it is crucial for HE institutions to improve the quality of education services provided to students by meeting their expectations and keeping them satisfied, it should be noted that such action may generate undesirable effects if students were treated squarely as customers.
The construct of student satisfaction and its predictive factors
In general, student satisfaction can be viewed as a short-term attitude, which relates to students’ subjective evaluations of the extent to which their expectations of given educational experiences have been met or exceeded (Elliot & Healy, 2001 ; Elliot & Shin, 2002 ). As students form numerous expectations in relation to their educational experiences, many scholars conceptualise student satisfaction as a multidimensional construct (Hanssen & Solvoll, 2015 ; Jereb et al., 2018 ; Nastasić et al., 2019 ; Weerasinghe et al., 2017 ).
In Sirgy et al.’s ( 2010 ) framework, for instance, overall satisfaction with college life was broken down into three components, which represented satisfaction with academic aspects, social aspects and college facilities and services. Similarly, in investigating university students’ views of their academic studies, Wach et al. ( 2016 ) measured satisfaction using items across three dimensions of satisfaction. These related to the content of learning (i.e. the joy and satisfaction felt by students on their chosen preferred majors), the conditions of learning (i.e. students’ satisfaction with the terms and conditions of the academic programs) and personal coping with learning (i.e. students’ satisfaction with their own ability to cope with academic stress).
The recognition that student satisfaction is a multidimensional construct is also evident in the identification of numerous dimensions that contribute to HE students’ overall satisfaction levels. Academic aspects comprise one such set of key contributors to student satisfaction in HE. These relate to considerations such as the perceived quality of teaching, feedback provided by instructors, teaching styles of instructors, quality of learning experiences and class sizes (Aldemir & Gülcan, 2004 ; Butt & Rehman, 2010 ; Duque, 2014 ; Jereb et al., 2018 ; Nastasić et al., 2019 ; Paul & Pradhan, 2019 ; Weerasinghe et al., 2017 ). More general attributes of the courses in which students are enrolled (e.g. curriculum, course content and teaching materials) have also been cited as significant (Aldemir & Gülcan, 2004 ; Butt & Rehman, 2010 ; Duque, 2014 ; Weerasinghe et al., 2017 ).
Empirical studies have attested to the relevance of the above-named attributes in determining HE students’ satisfaction levels (Aldemir & Gülcan, 2004 ; Bell & Brooks, 2018 ; Butt & Rehman, 2010 ; Nastasić et al., 2019 ; Siming et al., 2015 ). However, this list is by no means exhaustive in describing the factors that students will consider in providing satisfaction ratings. More generic, institution-wide attributes, such as ease of access to student services and the level of infrastructure support provided by an institution (e.g. transportation and boarding services, internet access and administrative services), as well as the facilities it offers (e.g. teaching facilities, leisure and sports facilities, IT facilities and study areas) have also been recognised by scholars to contribute to HE students’ satisfaction levels (Aldemir & Gülcan, 2004 ; Butt & Rehman, 2010 ; Duque, 2014 ; Hanssen & Solvoll, 2015 ; Jereb et al., 2018 ; Weerasinghe et al., 2017 ). Less tangible aspects of students’ experiences such as the reputation and impressions of the institution (Butt & Rehman, 2010 ; Duque, 2014 ; Hanssen & Solvoll, 2015 ; Jereb et al., 2018 ), student centeredness and campus climate (Elliot & Healy, 2001 ) and students’ own life experiences while at college (Mihanović et al., 2016 ; Nastasić et al., 2019 ; Weerasinghe et al., 2017 ) have also been noted.
Interaction and student satisfaction in higher education
Beyond the factors above, the recent student satisfaction literature has highlighted the potential role played by students’ interpersonal interactions in HE as a key predictor of student satisfaction levels. This is to be expected, given the vital role of interpersonal interaction in learning. According to the social constructivist paradigm, learning is an inherently social process, in which interpersonal interactions are critical in the construction of knowledge and understanding (Pritchard & Woollard, 2013 ). Studies have affirmed that HE students recognise the importance of interpersonal interactions with their classmates and university staff in furthering their content learning (Hurst et al., 2013 ). In Burgess et al.’s ( 2018 ) comprehensive study using the data of millions of university students from the UK’s National Student Survey (NSS), the aspect of “social life and meeting people” was recognised as one of the key determinants contributing to university satisfaction despite it has not been included in their study.
In general, two forms of interaction have been examined in relation to student satisfaction in HE: student-faculty and student–student interactions. The proposed importance of student-faculty interactions in determining student satisfaction levels in HE is reflected in both the theoretical and the empirical literature. In one very early review, Pascarella ( 1980 ) reported that student-faculty informal contact was positively associated with college satisfaction, alongside other educational outcomes. Similarly, in Aldemir and Gülcan’s ( 2004 ) conceptual framework, the authors included the variable “communication with instructors both in and outside classroom” as one of the factors that contributed to university students’ satisfaction levels. This variable was then found empirically significant in predicting satisfaction levels in a sample of more than 300 Turkish university students.
Table Table1 1 provides a broad summary of empirical studies that have examined either student-faculty or student–student interpersonal interactions as predictors of student satisfaction in HE. Collectively, these studies have reported significant associations between satisfaction and both types of interactions. Although most have focused on the context of online learning, as Kuo et al. ( 2014 ) argued, high-quality interactions are important in all forms of education, whether technology-based or more traditional.
Empirical studies on interaction and student satisfaction in HE
The crucial role of interaction in HE has been underscored more recently by the concerns over the loss of social contact and socialisation following the suspension of in-person classes due to the COVID-19 outbreak which has impacted the students negatively (UNESCO International Institute for Higher Education in Latin America and the Caribbean, 2020 ). HE students have also raised their own concerns over the quality of education they receive in online, as compared to in-person formats, which differ primarily in terms of the level of interpersonal interaction they afford (Ang, 2020 ). Such concerns make clear the perceived significance of interpersonal interaction in the overall HE learning experience.
It should be noted that there is a need to consider the role of students’ demographic background in examining the relationship between interaction and student satisfaction, with the evidence in the literature indicating that students’ demographic profiles can moderate the types and levels of interpersonal interactions they have with their peers or instructors. In a study conducted by Kim and Sax ( 2009 ) using data on 58,281 US students, differences in the frequency of student-faculty interactions were attributed to gender, alongside other demographic variables (race, social class and first-generation status). Similarly, in a study by Criado-Gomis et al. ( 2012 ) on 1000 graduates from two Spanish universities, significant differences were seen in the quality of interactions between male and female students. At around the same time, Ke and Kwak’s ( 2013 ) study of 392 students from a US university indicated that age was significantly correlated with the perceived quality of peer interactions that occurred in online learning environments.
Rationale and aims of the present study
The literature suggests a wide range of attributes that may contribute to student satisfaction levels in HE. This aligns with the propositions of Jereb et al. ( 2018 ), who underscored the complexity of student satisfaction and the myriad factors that influence it. Existing scholarly work is yet to provide a complete understanding on the different aspects of HE students’ satisfaction and establish concrete links between these aspects and the different forms of interpersonal interactions in which HE students may engage.
From Table Table1, 1 , studies conducted thus far have tended to focus on measuring student satisfaction using generic or unidimensional measures. Similarly, the measurement of interpersonal interaction has typically been restricted to only two dimensions (student–student or student-instructor), though evidence from the literature suggests a need to divide these further into formal and informal forms (Kraemer, 1997 ; Mamiseishvili, 2011 ; Meeuwisse et al., 2010 ). By taking a broader view of student satisfaction and interpersonal interactions, the present study aimed to provide a more fine-grained analysis of how different aspects of HE students’ satisfaction levels may relate to different forms of interpersonal interaction.
It has been noted that what contributes to student satisfaction levels can be highly contextual. In defining overall student satisfaction in HE, Duque ( 2014 ) contended that overall satisfaction with an organisation will be based on all encounters and experiences a consumer has with that particular organisation. It is acknowledged, therefore, that the results presented in this paper may be particular to the context in which the study was conducted (the details of the participating institution and the participants selected for this research were provided in the “ Method ” section below).
Three research questions were formulated to guide the research conducted in this study:
- How satisfied were the students with different aspects of the HE institution studied, and how did satisfaction levels vary across these different aspects?
- How did different forms of interpersonal interaction relate to different aspects of these students’ satisfaction levels?
- Did the types of interpersonal interaction in which students engaged vary with students’ gender and age?
Participants and setting
Students participated in this research were enrolled 14 international undergraduate degree programs from the UK, offered by the participating institution. The institution is one of the largest private HE institutions in Singapore at the time of the study. Established in the 1960s, the institution admits approximately 17,000 local and foreign students. Its physical campus offers a variety of facilities such as library, performing art theatre, cafeterias and sport facilities. The institution also offers a wide range of services such as counselling and career advisory services.
These students were invited to participate in an online survey in the middle of the 2018–2019 academic year, to report their satisfaction levels with different aspects of their learning experiences, and on the forms of interpersonal interactions in which they typically engaged. In all, 280 students provided complete responses to the survey. Of this sample, 105 (37.50%) were males and 175 (62.50%) were females. The respondents were aged between 18 to 40 years old, with an overall mean of 22.79 years ( SD = 2.40). One hundred and ninety-seven (70.36%) were continuing students, while 83 (29.64%) were final year students.
Student satisfaction
Drawing upon the existing literature, the present study focused on eight aspects of student satisfaction, classified into three categories (academic, institution and university life). Each of these eight aspects was represented by a single item in the student satisfaction measure, to which students responded on a 7-point rating scale (see Table Table2 2 below). The rated satisfaction scores obtained for all the eight aspects form the eight dependent variables, each to be predicted by the four interaction variables (see next section).
Item statements measuring student satisfaction levels on eight different aspects
Note: Item 7 and item 8 may appear similar, but the two items are not the same. While item 7 focuses more on the experiences in attaining university education, item 8 relates more broadly to the overall university life lived out by a student
Interaction
Following Meeuwisse et al.’s ( 2010 ) model, four forms of interaction were measured in the study, each representing a different dimension of interpersonal interaction. Each was measured by a number of items in the interpersonal interaction measure, as shown in Table Table3. 3 . In the survey, respondents were asked to select the items that related to them, based on their own experiences of interacting with their peers and instructors/lecturers. Therefore, for each respondent, the total score obtained for each of the four types of interpersonal interaction was simply a summed total based on selected items within each type. The four scores obtained formed the four independent variables (i.e. predictors) used to predict each of the eight satisfaction variables (the dependent variables) explained above.
Item statements measuring four different forms of interaction
Note: Not all item statements are included in the table. For each form of interaction, only one of the items is presented here for reference
The online survey was hosted on the Qualtrics platform, a web-based survey tool that allows respondents to answer online survey questions. Institutional ethics approval was first obtained prior to conducting the survey. Email invitations were sent to students to participate in the online survey. The purpose of the survey, time required to answer the survey, identity confidentiality and data protection assurances were all stated in the email. Participants were asked to consent to participate before entering the survey. Two email reminders following the initial invitations were also sent to increase participation rates. A pilot study, conducted before the launch of the survey, indicated that the instructions and questions within the survey were clear to a pilot sample of 14 students who attended the same university as the intended survey participants.
The analysis was divided into three parts to address the three research questions. Descriptive statistics and repeated measures analysis of variance (ANOVA) were used to analyse and compare levels of student satisfaction across the eight aspects identified, to address research question 1 (How satisfied were the students with different aspects of the HE institution studied, and how did satisfaction levels vary across these different aspects?). This provided a broad overview of student satisfaction levels within the specific HE institution in which the study was conducted.
Stepwise regression and correlation analyses were conducted to address research question 2 (How did different forms of interpersonal interaction relate to different aspects of these students’ satisfaction levels?) via SPSS V26. Using the satisfaction ratings for each identified aspect of student experience (program, institution, student’s own learning, teaching of lecturers, student support provided, life as a university student in general, campus facilities and overall university experience) as the dependent variables and scores for the four types of interpersonal interaction (student–student formal interaction, student–student informal interaction, student-instructor formal interaction and student-instructor formal interaction) as independent variables, eight regression models were formulated. Results obtained were interpreted and analysed to uncover more specific relationships between different dimensions of student satisfaction and interpersonal interaction.
Bivariate correlations between two demographic variables (age and gender) and the four interaction variables were examined to address research question 3 (Did the types of interpersonal interaction in which students engaged vary with students’ gender and age?). The results obtained were analysed to reveal how the age and gender of the students were associated with their engagement in different forms of interpersonal interaction, and thus, suggest how these variables might contribute differentially to student satisfaction levels.
Five multivariate outliers were detected using the Mahalanobis distances, tested at a significance level of 0.001 and subsequently removed from the analysis. This is because the presence of outliers can distort the statistical analysis performed (Tabachnick & Fidell, 2013 ). This resulted in only 275 cases being used in the analyses for the study.
Student satisfaction on different aspects of the institution
The descriptive statistics presented in Table Table4 4 suggest that in general, respondents were favourable about their institution in terms of the different satisfaction elements surveyed. The mean satisfaction score for all eight aspects was higher than 4 (midpoint of the rating scale). For seven of the eight aspects of satisfaction, the median and mode scores were recorded at 5. The proportion of respondents with ratings of 5 and above was in the range of 59.27–76.36% for the same seven aspects.
Descriptive statistics of the eight aspects of student satisfaction ( n = 275)
Comparing different aspects of satisfaction, the respondents were most satisfied with the two academic aspects, particularly with the academic program in which they were enrolled. For both “program” and “teaching of the lecturers”, the mean scores were the highest among all the eight aspects of student satisfaction, and more than 70% of the respondents gave a rating of 5 or above for these two aspects.
They were least satisfied with the items referring to their university lives while studying at the institution. The range of mean scores for the three aspects within this set (4.36–4.78) was generally lower than for other aspects of satisfaction (academic, 4.96–5.12; institution, 4.49–4.85). The item “My life as a university student in general” attracted a particularly low number of positive ratings, with mean, median and mode scores ranked lowest for this item among all the eight aspects of satisfaction surveyed. Less than 50% of respondents gave a rating of 5 or above for this aspect. Among the three aspects surveyed, however, the respondents were most satisfied with “my own learning”.
Among all the three institutional aspects surveyed, “institution” was the aspect with the highest mean score, and the only item in this group in which more than 60% of respondents gave a rating of 5 or above. Satisfaction levels for “campus facilities” and “Student support provided” were notably lower, even when compared with some of the university life aspects.
The results from the repeated measures ANOVA showed a significant difference across the eight satisfaction mean scores, F (7,268) = 14.79, p < 0.001. The effect size (partial η 2 ) was 0.28, indicating that some 28% of variance in satisfaction scores was attributable to the type of satisfaction measure used. The pairwise comparisons also showed that each of the satisfaction mean scores was significantly different from at least two other mean satisfaction mean scores. The mean scores for satisfaction in terms of “program” and “my life as a university student in general”, in particular, were different from the mean satisfaction scores of the other six aspects measured (Table (Table5 5 ).
Pairwise comparisons of different satisfaction mean scores ( n = 275)
* p < 0.05
Interaction and student satisfaction
The bivariate correlations between the eight satisfaction variables and the four interaction variables are provided in Table Table6. 6 . To explore relationships between these eight variables and the interaction variables, separate stepwise regression analyses were performed, in each case, with satisfaction ratings as the dependent variable, and the four interaction variables entered as predictors.
Descriptive statistics and bivariate correlations of variables examined in the stepwise regression analysis ( n = 275)
** p < 0.01
Relationships between interaction and student satisfaction with academic aspects
The two stepwise regressions performed for satisfaction scores associated with academic aspects are shown in Table Table7. 7 . In both cases, the analysis stopped after one step. For satisfaction ratings related to the “program”, student–student informal interactions were identified as the only significant predictor, while for satisfaction with “teaching of the lecturers”, student-instructor formal interaction was identified as the only significant predictor.
Stepwise regression: student satisfaction on interaction ( n = 275)
Relationships between interaction and student satisfaction with institutional aspects
Three stepwise regressions were performed with the satisfaction scores for “institution”, “campus facilities” and “student support provided” as the dependent variables, respectively. Again, the analysis stopped after one step for satisfaction related to the “institution” and also for “student support provided”. Student–student informal interactions were identified as the only significant predictor of both ratings. There were no significant predictors of satisfaction in terms of “campus facilities”.
Relationships between interaction and student satisfaction with university life
Three stepwise regressions were performed for the satisfaction scores related to “my own learning”, “my overall university experience” and “my life as a university student in general” as the dependent variables. Again, the analysis stopped after one step in all three cases. Student–student informal interaction was identified as the only significant predictor of satisfaction on all three aspects of the students’ self-reflective experiences. The association between student–student informal interaction and satisfaction with respect to “my own learning” and “my overall university experience” was slightly stronger than for “my life as a university student in general”.
Comparison between the four forms of interaction in predicting student satisfaction
From the regression analysis, each of the four interaction variables was identified as a significant predictor of at least one aspect of student satisfaction. At the same time, no aspects of satisfaction were predicted by more than one predictor. Furthermore, satisfaction with campus facilities was not predicted by any of the four interaction variables.
Overall, student–student informal interaction was the only variable that significantly predicted more than one satisfaction aspect (one academic aspect, one institutional aspect and all three university life aspects). None of the other three interaction variables significantly predicted more than one satisfaction aspect, with student–student formal interaction significantly predicting only “student support provided”. Student-instructor formal interactions significantly predicted ratings in terms of “teaching of the lecturers”. Student-instructor informal interactions did not significantly predict any of the satisfaction variables.
Associations between demographic and interaction variables
Table Table8 8 presents bivariate correlations between the four interaction variables and the two demographic variables of age and gender. Significant positive correlations were found between the two demographic variables and student-instructor formal instruction. The positive correlation between gender and student-instructor formal interaction indicates that male students were likely to have more formal interactions with their instructors than were female students. In the case of age, the significant positive correlation indicates that older students (this can be taken as those above the mean age of 22.79 years in the participating institution) were more likely to have formal interactions with their lecturers than were younger students (those below the mean age of 22.79 years in the participating institution).
Bivariate correlations between interaction and demographic variables
No significant correlations were found between the two demographic variables and any other interaction variables.
The present study aimed not only to provide a more in-depth analysis of different aspects of HE students’ satisfaction in one HE institution in Singapore, but also to provide a more nuanced analysis of relationships between these aspects and different forms of interpersonal interactions in which HE students engaged. The following sections discuss the findings of the study in greater depth, to address the three formulated research questions.
Student satisfaction on academics, institutions and university life
In addressing research question 1 (How satisfied were the students with different aspects of the HE institution studied, and how did satisfaction levels vary across these different aspects?), while the findings show that students were generally satisfied with the different aspects surveyed, satisfaction levels were not the same across different aspects. This reinforces the notion that student satisfaction in HE ought to be treated as a multidimensional construct. Findings also confirmed that the differences across various satisfaction levels were statistically significant.
More importantly, the findings suggest that general satisfaction as university students requires the fulfilments in aspects beyond what most institutions are currently providing to their students. As reflected in the results, the respondents’ life satisfaction as university students was noticeably lower than satisfaction attained for all other aspects. Not only did “my life as a university student in general” have the lowest satisfaction mean score among all satisfaction aspects measured, it was also noted that its mode and median scores were lower than the midpoint score of 4. As depicted in Rode et al.’s ( 2005 ) and Sirgy et al.’s ( 2010 ) frameworks, HE students’ life satisfaction depends upon on satisfaction attained from a wide range of academic and non-academic aspects.
Analysing the different aspects of satisfaction separately as was done here would provide more nuanced feedback to HE institutions, empowering them further to focus on various aspects of the services they provide to enhance student learning experiences and satisfaction. In this case, the fact that satisfaction with student support was notably lower than the ratings obtained for most of the other satisfaction aspects, the results strongly suggest that enhancing the usual institutional aspects such as campus facilities or administrative services will not be the most effective approach to enhancing student satisfaction. As indicated in Kakada’s ( 2019 ) study, student satisfaction was found positively related to technology, academic, social and service supports provided.
The role of interaction in higher education student satisfaction
Through research question 2 (How did different forms of interpersonal interaction relate to different aspects of these students’ satisfaction levels?), the study aimed to provide a more nuanced analysis of relationships between interpersonal interactions and student satisfaction in HE, by examining how each specific form of interaction related to different aspects of student satisfaction.
Results indicated that student satisfaction in HE was not only explained by who the students interacted with (peers vs. instructors), but also how they interacted with these individuals (either in a formal or an informal format). With three forms of interaction (student–student formal, student–student informal and student-instructor formal) found to be significant predictors of different aspects of satisfaction, this suggests that how the students engage with their peers and lecturers was also vital in explaining their satisfaction with their HE experiences. This further reaffirms the notion that both formal and informal interactions are crucial in HE as posited in Tinto’s, 1975 model of college student attrition (Tinto, 1975 ).
The results also underscored the relative importance of different forms of interpersonal interaction in explaining different aspects of student satisfaction. From the results, student–student informal interaction was significantly associated with satisfaction in all three aspects studied—academic, institution and university life. This suggests that student–student interaction could be the most critical form of interaction in terms of student satisfaction levels. Other forms of interaction were also significantly associated with specific aspects of satisfaction. Student-instructor formal interaction was a significant predictor of HE students’ satisfaction with the teaching of their instructors, while student–student formal interaction significantly predicted satisfaction with the student support provided by the institution. As such, the different forms of interaction appear to play complementary roles in predicting students’ overall satisfaction levels. With consideration on the specificity of different forms of interaction and different aspects of satisfaction, this adds greater depth to the existing literature in discussing the role of student–student and student-instructor interactions as predictors of student satisfaction as most past studies tend to draw limited distinction between different forms of interactions, or between different aspects of satisfaction (see Chang & Smith, 2008 ; Palmer & Koenig-Lewis, 2011 ).
The more granular level of findings on the relationship between interaction and student satisfaction have several possible implications for practice in the HE context. First, the findings suggest a need for HE institutions to recognise the vital role of student–student informal interactions as a predictor of HE students’ satisfaction levels. This finding aligns with the propositions of other scholars in the field. For example, Meeuwisse et al. ( 2010 ) posed that students’ informal relationships with their peers are vital in developing their sense of belonging. In a separate study conducted by Senior and Howard ( 2014 ), it was found that collaborative learning was fostered through friendship groups in which students interact with one another to develop conceptual understanding. From a broader perspective, this finding is consistent with the notion that peers play a significant role in HE student development. In Astin’s 1993 landmark study involving more than 20,000 college students, it was suggested that peer influences had contributed significantly to the growth and development of undergraduate students (Feldman & Astin, 1994 ). Thus, HE institutions who wish to bolster student satisfaction levels could identify ways to establish structures that foster more frequent and higher quality informal student–student interactions. As indicated in Burnett et al.’s ( 2007 ) study, the frequency and intensity of interaction between students and instructors and peers contributed to the students’ satisfaction levels.
Second, with the findings indicating the need to improve student support, the institution concerned should incorporate the element of formal student–student interaction in the provision of student support. One such initiative is the peer-to-peer support programs. Within the literature, such support programs have been reported to have positive impacts on HE learning in different studies (Arco-Tirado et al., 2019 ; Backer et al., 2015 ; Munley et al., 2010 ). As the institution concerned has already put in place a peer tutoring program (called Peer-Assisted Learning Program), it could also consider fostering greater student–student formal interaction in other support areas to further improve student satisfaction, as recommended by Kakada et al. ( 2019 ).
Age and gender were also found to be significantly associated with student-instructor formal interactions, which implies that institutions could consider age and gender differences in designing such structures. For example, given that male students were likely to have a greater number of formal interactions with their lecturers, HE institutions may need to consider more differentiated practices that faculty members can adopt to ensure that new female students engage regularly in formal interactions with them.
Overall, the more nuanced analyses provided by the present study not only expand previous understandings of student satisfaction and interaction in the context of HE, but also offer practical insights upon which HE institutions can draw to elevate their students’ satisfaction levels. From the findings, it is suggested that HE institutions should evaluate more specifically different aspects of student satisfaction on a regular basis, as well as focusing upon enhancing the quality and quantity of interpersonal interactions in which students regularly engage.
It should be noted that, given the highly contextualised nature of student satisfaction research (Santini et al., 2017 ), the generality of the present study may be limited to universities that are similar to the one that participated in the present study. Future research should, therefore, seek to determine whether the results of the present study generalise to other contexts. The study could also be replicated with students studying at other levels (e.g. the postgraduate level) or those with particular profiles (e.g. international students, students at risk or students from minority ethnic group).
Also, as a construct that relates closely to attitudes and expectations, student satisfaction is likely to change over time. As such, research on student satisfaction in HE should be a continual process as no single study—conducted at a specific timepoint—can entirely capture the changing nature of student satisfaction over time. Thus, HE institutions themselves are likely to be in the best position to evaluate the factors which predict their own students’ satisfaction levels, ideally, as an element of regular, ongoing quality improvement efforts.
Data availability
Code availability, declarations.
This research was approved by the University of Western Australia Human Ethics Committee, Approval Number RA/4/20/4756.
All participants were required to provide consent for participation online, prior to entering the survey.
All participants were required to provide consent for publication online, prior to entering the survey.
The authors declare no competing interests.
Publisher's note
Springer Nature remains neutral with regard to jurisdictional claims in published maps and institutional affiliations.
- Aldemir C, Gülcan Y. Students satisfaction in higher education: A Turkish case. Higher Education Management and Policy. 2004; 16 (2):109–122. doi: 10.1787/hemp-v16-art19-en. [ CrossRef ] [ Google Scholar ]
- Aldridge S, Rowley J. Measuring customer satisfaction in higher education. Quality Assurance in Education. 1998; 6 (4):197–204. doi: 10.1108/09684889810242182. [ CrossRef ] [ Google Scholar ]
- Ang, J. (2020, August 23). Hopes up for S’poreans eager to return to Aussie unis. The Straits Times . Retrieved September 21, 2020, from https://www.straitstimes.com/singapore/education/hopes-up-for-sporeans-eager-to-return-to-aussie-unis
- Arco-Tirado, J., Fernández-Martín, F., & Hervás-Torres, M. (2019). Evidence-based peer-tutoring program to improve students’ performance at the university. Studies in Higher Education (Dorchester-on-Thames) , 1–13. 10.1080/03075079.2019.1597038
- Australia. Department of Education, Skills and Employment. (2020). Performance-based funding for the Commonwealth Grant Scheme . Retrieved January 2, 2021, from https://www.education.gov.au/performance-based-funding-commonwealth-grant-scheme
- Backer LD, Keer HV, Valcke M. Promoting university students metacognitive regulation through peer learning: The potential of reciprocal peer tutoring. Higher Education. 2015; 70 (3):469–486. doi: 10.1007/s10734-014-9849-3. [ CrossRef ] [ Google Scholar ]
- Bell A, Brooks C. What makes students satisfied? A discussion and analysis of the UK’s national student survey. Journal of Further and Higher Education. 2018; 42 (8):1118–1142. doi: 10.1080/0309877X.2017.1349886. [ CrossRef ] [ Google Scholar ]
- Budd R. Undergraduate orientations towards higher education in Germany and England: Problematizing the notion of “student as customer” Higher Education. 2017; 73 (1):23–37. doi: 10.1007/s10734-015-9977-4. [ CrossRef ] [ Google Scholar ]
- Burgess A, Senior C, Moores E. A 10-year case study on the changing determinants of university student satisfaction in the UK. PLoS ONE. 2018; 13 (2):e0192976–e0192976. doi: 10.1371/journal.pone.0192976. [ PMC free article ] [ PubMed ] [ CrossRef ] [ Google Scholar ]
- Burnett K, Bonnici LJ, Miksa SD, Kim J. Frequency, intensity and topicality in online learning: An exploration of the interaction dimensions that contribute to student satisfaction in online learning. Journal of Education for Library and Information Science. 2007; 48 (1):21–35. [ Google Scholar ]
- Butt B, Rehman K. A study examining the students satisfaction in higher education. Procedia - Social and Behavioral Sciences. 2010; 2 (2):5446–5450. doi: 10.1016/j.sbspro.2010.03.888. [ CrossRef ] [ Google Scholar ]
- Calma A, Dickson-Deane C. The student as customer and quality in higher education. International Journal of Educational Management. 2020; 34 (8):1221–1235. doi: 10.1108/IJEM-03-2019-0093. [ CrossRef ] [ Google Scholar ]
- Chang SH, Smith RA. Effectiveness of personal interaction in a learner-centered paradigm distance education class based on student satisfaction. Journal of Research on Technology in Education. 2008; 40 (4):407–426. doi: 10.1080/15391523.2008.10782514. [ CrossRef ] [ Google Scholar ]
- Commonwealth of Australia. (2019). Performance-based funding for the Commonwealth Grant Scheme: Report for the Minister for Education – June 2019 . Retrieved September 8, 2020, from https://docs.education.gov.au/system/files/doc/other/ed19-0134_-_he-_performance-based_funding_review_acc.pdf
- Criado-Gomis, A., Iniesta-Bonillom, M. A., & Sanchez-Fernandez, R. (2012). Quality of student-faculty interaction at university: An empirical approach of gender and ICT usage. Socialinės technologijos, 2 (2), 249–262. Retrieved November 23, 2020, from https://core.ac.uk/download/pdf/197244734.pdf
- De Jager JW, Gbadamosi G. Specific remedy for specific problem: Measuring service quality in South African higher education. Higher Education. 2010; 60 (3):251–267. doi: 10.1007/s10734-009-9298-6. [ CrossRef ] [ Google Scholar ]
- Dill DD, Beerkens M. Designing the framework conditions for assuring academic standards: Lessons learned about professional, market, and government regulation of academic quality. Higher Education. 2013; 65 (3):341–357. doi: 10.1007/s10734-012-9548-x. [ CrossRef ] [ Google Scholar ]
- Duque L. A framework for analysing higher education performance: Students’ satisfaction, perceived learning outcomes, and dropout intentions. Total Quality Management & Business Excellence. 2014; 25 (1–2):1–21. doi: 10.1080/14783363.2013.807677. [ CrossRef ] [ Google Scholar ]
- Duraku, Z. H., & Hoxha, L. (2020). The impact of COVID-19 on higher education: A study of interaction among students' mental health, attitudes toward online learning, study skills, and changes in students' life. Retrieved September 18, 2020, from https://www.researchgate.net/publication/341599684_The_impact_of_COVID-19_on_higher_education_A_study_of_interaction_among_students'_mental_health_attitudes_toward_online_learning_study_skills_and_changes_in_students'_life
- Elliott KM, Healy MA. Key factors influencing student satisfaction related to recruitment and retention. Journal of Marketing for Higher Education. 2001; 10 (4):1–11. doi: 10.1300/J050v10n04_01. [ CrossRef ] [ Google Scholar ]
- Elliott KM, Shin D. Student satisfaction: An alternative approach to assessing this important concept. Journal of Higher Education Policy and Management. 2002; 24 (2):197–209. doi: 10.1080/1360080022000013518. [ CrossRef ] [ Google Scholar ]
- Feldman KA, Astin AW. What matters in college? Four critical years revisited [Review of What Matters in College? Four Critical Years Revisited, by A. W. Astin] The Journal of Higher Education. 1994; 65 (5):615–622. doi: 10.2307/2943781. [ CrossRef ] [ Google Scholar ]
- Guilbault M. Students as customers in higher education: Reframing the debate. Journal of Marketing for Higher Education. 2016; 26 (2):132–142. doi: 10.1080/08841241.2016.1245234. [ CrossRef ] [ Google Scholar ]
- Hanssen T, Solvoll G. The importance of university facilities for student satisfaction at a Norwegian University. Facilities. 2015; 33 (13/14):744–759. doi: 10.1108/F-11-2014-0081. [ CrossRef ] [ Google Scholar ]
- Hassel H, Lourey J. The dea(r)th of student responsibility. College Teaching. 2005; 53 (1):2–13. doi: 10.3200/CTCH.53.1.2-13. [ CrossRef ] [ Google Scholar ]
- Hou AYC, Ince M, Tsai S, Chiang CL. Quality assurance of quality assurance agencies from an Asian perspective: Regulation, autonomy and accountability. Asia Pacific Education Review. 2015; 16 (1):95–106. doi: 10.1007/s12564-015-9358-9. [ CrossRef ] [ Google Scholar ]
- Hurst, B., Wallace, R., & Nixon, S. (2013). The impact of social interaction on student learning. Reading Horizons, 52 (4), 375–398. Retrieved October 11, 2020, from https://bearworks.missouristate.edu/cgi/viewcontent.cgi?article=1022&context=articles-coe
- Jarvis D. Regulating higher education: Quality assurance and neo-liberal managerialism in higher education—A critical introduction. Policy and Society. 2014; 33 (3):155–166. doi: 10.1016/j.polsoc.2014.09.005. [ CrossRef ] [ Google Scholar ]
- Jereb E, Jerebic J, Urh M. Revising the importance of factors pertaining to student satisfaction in higher education. Organizacija. 2018; 51 (4):271–285. doi: 10.2478/orga-2018-0020. [ CrossRef ] [ Google Scholar ]
- Johnson ZS, Cascio R, Massiah CA. Explaining student interaction and satisfaction: An empirical investigation of delivery mode influence. Marketing Education Review. 2014; 24 (3):227–238. doi: 10.2753/MER1052-8008240304. [ CrossRef ] [ Google Scholar ]
- Kakada, P., Deshpande, Y., & ShilpaBisen (2019). Technology support, social support, academic support, service support, and student satisfaction. J ournal of Information Technology Education, 18 , 549–70. Retrieved February 10, 2021, from http://www.jite.org/documents/Vol18/JITEv18ResearchP549-570Kakada5813.pdf
- Ke F, Kwak D. Online learning across ethnicity and age: A study on learning interaction participation, perception, and learning satisfaction. Computers and Education. 2013; 61 :43–51. doi: 10.1016/j.compedu.2012.09.003. [ CrossRef ] [ Google Scholar ]
- Kim Y, Sax L. Student–faculty interaction in research universities: Differences by student gender, race, social class, and first-generation status. Research in Higher Education. 2009; 50 (5):437–459. doi: 10.1007/s11162-009-9127-x. [ CrossRef ] [ Google Scholar ]
- Kraemer BA. The academic and social integration of Hispanic students into college. The Review of Higher Education. 1997; 20 (2):163–179. doi: 10.1353/rhe.1996.0011. [ CrossRef ] [ Google Scholar ]
- Kuo Y-C, Walker AE, Schroder KE, Belland BR. Interaction, Internet self-efficacy, and self-regulated learning as predictors of student satisfaction in online education courses. The Internet and Higher Education. 2014; 20 :35–50. doi: 10.1016/j.iheduc.2013.10.001. [ CrossRef ] [ Google Scholar ]
- Kurucay M, Inan FA. Examining the effects of learner-learner interactions on satisfaction and learning in an online undergraduate course. Computers & Education. 2017; 115 :20–37. doi: 10.1016/j.compedu.2017.06.010. [ CrossRef ] [ Google Scholar ]
- Lapina I, Roga R, Müürsepp P. Quality of higher education: International students’ satisfaction and learning experience. International Journal of Quality and Service Sciences. 2016; 8 (3):263–278. doi: 10.1108/IJQSS-04-2016-0029. [ CrossRef ] [ Google Scholar ]
- Mamiseishvili K. Academic and social integration and persistence of international students at U.S. two-year institutions. Community College Journal of Research and Practice. 2011; 36 (1):15–27. doi: 10.1080/10668926.2012.619093. [ CrossRef ] [ Google Scholar ]
- Maslen, G. (2015, February 20). While branch campuses proliferate, many fail . University World News. Retrieved December 15, 2020, from http://www.universityworldnews.com/article.php?story=20150219113033746
- McLeay F, Robson A, Yusoff M. New applications for importance-performance analysis (IPA) in higher education. Journal of Management Development. 2017; 36 (6):780–800. doi: 10.1108/JMD-10-2016-018. [ CrossRef ] [ Google Scholar ]
- Meeuwisse M, Severiens SE, Born MP. Learning environment, interaction, sense of belonging and study success in ethnically diverse student groups. Research in Higher Education. 2010; 51 (6):528–545. doi: 10.1007/s11162-010-9168-1. [ CrossRef ] [ Google Scholar ]
- Mihanović Z, Batinić A, Pavičić J. The link between students’ satisfaction with faculty, overall students’ satisfaction with student life and student performances. Review of Innovation and Competitiveness. 2016; 2 (1):37–60. doi: 10.32728/ric.2016.21/3. [ CrossRef ] [ Google Scholar ]
- Munley VG, Garvey E, McConnell MJ. The effectiveness of peer tutoring on student achievement at the university level. The American Economic Review. 2010; 100 (2):277–282. doi: 10.1257/aer.100.2.277. [ CrossRef ] [ Google Scholar ]
- Nastasić A, Banjević K, Gardašević D. Student satisfaction as a performance indicator of higher education institution. Mednarodno Inovativno Poslovanje. 2019; 11 (2):67–76. doi: 10.32015/JIBM/2019-11-2-8. [ CrossRef ] [ Google Scholar ]
- OECD OECD at a glance: OECD indicators. OECD Publishing, Paris. 2019 doi: 10.1787/f8d7880d-en. [ CrossRef ] [ Google Scholar ]
- Palmer A, Koenig-Lewis N. The effects of pre-enrolment emotions and peer group interaction on students’ satisfaction. Journal of Marketing Management. 2011; 27 (11–12):1208–1231. doi: 10.1080/0267257X.2011.614955. [ CrossRef ] [ Google Scholar ]
- Pascarella ET. Student-faculty informal contact and college outcomes. Review of Educational Research. 1980; 50 (4):545–595. doi: 10.3102/00346543050004545. [ CrossRef ] [ Google Scholar ]
- Paul R, Pradhan S. Achieving student satisfaction and student loyalty in higher education: A focus on service value dimensions. Services Marketing Quarterly. 2019; 40 (3):245–268. doi: 10.1080/15332969.2019.1630177. [ CrossRef ] [ Google Scholar ]
- Pritchard, A., & Woollard, J. (2013). Psychology for the classroom: Constructivism and social learning . Routledge. Retrieved February 19, 2021, from https://ebookcentral-proquest-com.ezproxy.library.uwa.edu.au/lib/uwa/detail.action?docID=515360
- Rode JC, Arthaud-Day ML, Mooney CH, Near JP, Baldwin TT, Bommer WH, Rubin RS. Life satisfaction and student performance. Academy of Management Learning & Education. 2005; 4 (4):421–433. doi: 10.5465/AMLE.2005.19086784. [ CrossRef ] [ Google Scholar ]
- Santini F, Ladeira W, Sampaio C, da Silva Costa G. Student satisfaction in higher education: A meta-analytic study. Journal of Marketing for Higher Education. 2017; 27 (1):1–18. doi: 10.1080/08841241.2017.1311980. [ CrossRef ] [ Google Scholar ]
- Senior C, Howard C. Learning in friendship groups: Developing students’ conceptual understanding through social interaction. Frontiers in Psychology. 2014; 5 :1–8. doi: 10.3389/fpsyg.2014.01031. [ PMC free article ] [ PubMed ] [ CrossRef ] [ Google Scholar ]
- Senior C, Moores E, Burgess A. “I can’t get no satisfaction”: Measuring student satisfaction in the age of a consumerist higher education. Frontiers in Psychology. 2017; 8 :1–3. doi: 10.3389/fpsyg.2017.00980. [ PMC free article ] [ PubMed ] [ CrossRef ] [ Google Scholar ]
- Shahzad, A., Hassan, R., Aremu, A., Hussain, A., & Lodhi, R. (2020 ). Effects of COVID-19 in E-learning on higher education institution students: The group comparison between male and female. Quality & Quantity, 1– 22. 10.1007/s11135-020-01028-z [ PMC free article ] [ PubMed ]
- Sher, A. (2009). Assessing the relationship of student-instructor and student-student interaction to student learning and satisfaction in web-based online learning environment. Journal of Interactive Online Learning, 8 (2), 102–120. Retrieved October 17, 2020, from https://www.semanticscholar.org/paper/Assessing-the-Relationship-of-Student-Instructor-to-Sher/7810cfba73c549ffc94437375b9e6e8f84336af5
- Siming, L., Niamatullah, Gao, J., Xu, D., & Shafi, K. (2015). Factors leading to students’ Satisfaction in the higher learning institutions. Journal of Education and Practice 6 (31), 114–118. Retrieved March 6, 2021, from https://files.eric.ed.gov/fulltext/EJ1083362.pdf
- Sirgy M, Lee D, Grzeskowiak S, Yu G, Webb D, El-Hasan K, Jesus Garcia Vega J, Ekici A, Johar J, Krishen A, Kangal A, Swoboda B, Claiborne C, Maggino F, Rahtz D, Canton A, Kuruuzum A. Quality of College Life (QCL) of students: Further validation of a measure of well-being. Social Indicators Research. 2010; 99 (3):375–390. doi: 10.1007/s11205-010-9587-6. [ CrossRef ] [ Google Scholar ]
- Tabachnick B, Fidell L. Using multivariate statistics. 6. Pearson Education; 2013. [ Google Scholar ]
- Tinto V. Dropout from higher education: A theoretical synthesis of recent research. Review of Educational Research. 1975; 45 (1):89–125. doi: 10.3102/00346543045001089. [ CrossRef ] [ Google Scholar ]
- UNESCO International Institute for Higher Education in Latin America and the Caribbean (IESALC). (2020). COVID-19 and higher education: Today and tomorrow. Impact analysis, policy responses and recommendations . Retrieved February 24, 2021, from http://www.iesalc.unesco.org/en/wp-content/uploads/2020/04/COVID-19-EN-090420-2.pdf
- van Rooij E, Jansen E, van de Grift W. First-year university students’ academic success: The importance of academic adjustment. European Journal of Psychology of Education. 2018; 33 (4):749–767. doi: 10.1007/s10212-017-0347-8. [ CrossRef ] [ Google Scholar ]
- Wach F, Karbach J, Ruffing S, Brünken R, Spinath F. University students’ satisfaction with their academic studies: Personality and motivation matter. Frontiers in Psychology. 2016; 7 (55):1–12. doi: 10.3389/fpsyg.2016.00055. [ PMC free article ] [ PubMed ] [ CrossRef ] [ Google Scholar ]
- Weerasinghe, I.S., Fernando, S., & Lalitha, R. (2017). Students’ satisfaction in higher education. American Journal of Educational Research, 5 (5), 533 – 539. Retrieved September 2, 2020, from https://papers.ssrn.com/sol3/papers.cfm?abstract_id=2976013
- Weingarten, H., Hicks, M., & Kaufman, A. (2018). Assessing quality in postsecondary education: International perspectives . Kingston, Ontario, Canada: School of Policy Studies, Queen’s University. Retrieved October 19, 2020, from https://www-jstor-org.ezproxy.library.uwa.edu.au/stable/j.ctv8bt1
Click through the PLOS taxonomy to find articles in your field.
For more information about PLOS Subject Areas, click here .
Loading metrics
Open Access
Peer-reviewed
Research Article
Academic student satisfaction and perceived performance in the e-learning environment during the COVID-19 pandemic: Evidence across ten countries
Contributed equally to this work with: Damijana Keržič, Jogymol Kalariparampil Alex, Roxana Pamela Balbontín Alvarado, Denilson da Silva Bezerra, Maria Cheraghi, Beata Dobrowolska, Adeniyi Francis Fagbamigbe, MoezAlIslam Ezzat Faris, Thais França, Belinka González-Fernández, Luz Maria Gonzalez-Robledo, Fany Inasius, Sujita Kumar Kar, Kornélia Lazányi, Florin Lazăr, Juan Daniel Machin-Mastromatteo, João Marôco, Bertil Pires Marques, Oliva Mejía-Rodríguez, Silvia Mariela Méndez Prado, Alpana Mishra, Cristina Mollica, Silvana Guadalupe Navarro Jiménez, Alka Obadić, Daniela Raccanello, Md Mamun Ur Rashid, Dejan Ravšelj, Nina Tomaževič, Chinaza Uleanya, Lan Umek, Giada Vicentini, Özlem Yorulmaz, Ana-Maria Zamfir, Aleksander Aristovnik
Roles Conceptualization, Investigation, Methodology, Project administration, Supervision, Visualization, Writing – original draft, Writing – review & editing
Affiliations Faculty of Public Administration, University of Ljubljana, Ljubljana, Slovenia, Faculty of Social Sciences, University of Ljubljana, Ljubljana, Slovenia

Roles Writing – review & editing
Affiliation Faculty of Educational Sciences, Walter Sisulu University, Mthatha, South Africa
Roles Investigation, Writing – original draft, Writing – review & editing
Affiliation Faculty of Education and Humanities, University of Bío Bío, Concepción, Chile
Affiliation Department of Oceanography and Limnology, Federal University of Maranhão, São Luís, Brazil
Roles Investigation
Affiliation Social Determinant of Health Research Center, Department of Public Health, School of Health, Ahvaz Jundishapur University of Medical Sciences, Ahvaz, Iran
Affiliation Faculty of Health Sciences, Medical University of Lublin, Lublin, Poland
Roles Formal analysis, Investigation, Writing – review & editing
Affiliation Department of Epidemiology and Medical Statistics, Faculty of Public Health, College of Medicine, University of Ibadan, Ibadan, Nigeria
Roles Investigation, Writing – review & editing
Affiliation Department of Clinical Nutrition and Dietetics, College of Health Sciences, University of Sharjah, Sharjah, United Arab Emirates
Affiliation Centre for Research and Studies in Sociology, Cies-Iscte, Portugal
Affiliation Department of Sciences and Engineering, Universidad Iberoamericana Puebla/Red Citeg, Mexico City, Mexico
Affiliation Facultad de Medicina, Universidad Autónoma del Estado de Morelos, Morelos, Mexico
Affiliation Faculty of Economic and Communication, Bina Nusantara University, West Jakarta, Indonesia
Roles Writing – original draft, Writing – review & editing
Affiliation Department of Psychiatry, King George’s Medical University, Lucknow, India
Affiliation John von Neumann Faculty of Informatics, Obuda University, Budapest, Hungary
Affiliation Faculty of Sociology and Social Work, University of Bucharest, Bucharest, Romania
Affiliation Faculty of Philosophy and Letters, Universidad Autónoma de Chihuahua, Chihuahua, Mexico
Roles Formal analysis, Investigation, Methodology, Visualization, Writing – original draft, Writing – review & editing
Affiliation William James Centre for Research, ISPA—Instituto Universitário, Lisbon, Portugal
Affiliation Higher Institute of Engineering of Porto, Polytechnic Institute of Porto, Porto, Portugal
Roles Conceptualization, Investigation, Writing – original draft, Writing – review & editing
Affiliation División de Investigación Clínica, Centro de Investigación Biomédica de Michoacán, Instituto Mexicano del Seguro Social, Mexico, Mexico
Affiliation Faculty of Social Sciences and Humanities, ESPOL Polytechnic University, Guayaquil, Ecuador
Affiliation Faculty of Community Medicine, KIMS, Bhubaneswar, KIIT University, Bhubaneswar, India
Roles Data curation, Formal analysis, Methodology, Writing – original draft, Writing – review & editing
Affiliation Department of Statistical Sciences, Sapienza University of Rome, Rome, Italy
Affiliation DTI-CUCEA & Instituto de Astronomía y Meteorología—CUCEI, Universidad de Guadalajara, Guadalajara, Mexico
Affiliation Faculty of Economics and Business, University of Zagreb, Zagreb, Croatia
Affiliation Department of Human Sciences, University of Verona, Verona, Italy
Affiliation Department of Agricultural Extension and Rural Development, Patuakhali Science and Technology University, Barisal, Bangladesh
Roles Data curation
Affiliation Faculty of Public Administration, University of Ljubljana, Ljubljana, Slovenia
Affiliation Business Management, University of South Africa (UNISA), Pretoria, South Africa
Roles Data curation, Investigation, Methodology, Resources, Validation, Writing – original draft, Writing – review & editing
Affiliation Department of Econometrics, Faculty of Economics, University of Istanbul, Istanbul, Turkey
Affiliation National Scientific Research Institute for Labour and Social Protection, Bucharest, Romania
- [ ... ],
Roles Conceptualization, Funding acquisition, Investigation, Project administration, Supervision, Writing – review & editing
* E-mail: [email protected]
- [ view all ]
- [ view less ]
- Damijana Keržič,
- Jogymol Kalariparampil Alex,
- Roxana Pamela Balbontín Alvarado,
- Denilson da Silva Bezerra,
- Maria Cheraghi,
- Beata Dobrowolska,
- Adeniyi Francis Fagbamigbe,
- MoezAlIslam Ezzat Faris,
- Thais França,
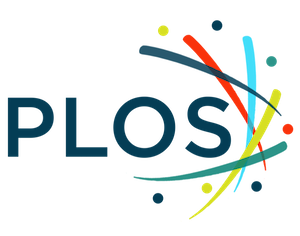
- Published: October 20, 2021
- https://doi.org/10.1371/journal.pone.0258807
- Reader Comments
The outbreak of the COVID-19 pandemic has dramatically shaped higher education and seen the distinct rise of e-learning as a compulsory element of the modern educational landscape. Accordingly, this study highlights the factors which have influenced how students perceive their academic performance during this emergency changeover to e-learning. The empirical analysis is performed on a sample of 10,092 higher education students from 10 countries across 4 continents during the pandemic’s first wave through an online survey. A structural equation model revealed the quality of e-learning was mainly derived from service quality, the teacher’s active role in the process of online education, and the overall system quality, while the students’ digital competencies and online interactions with their colleagues and teachers were considered to be slightly less important factors. The impact of e-learning quality on the students’ performance was strongly mediated by their satisfaction with e-learning. In general, the model gave quite consistent results across countries, gender, study fields, and levels of study. The findings provide a basis for policy recommendations to support decision-makers incorporate e-learning issues in the current and any new similar circumstances.
Citation: Keržič D, Alex JK, Pamela Balbontín Alvarado R, Bezerra DdS, Cheraghi M, Dobrowolska B, et al. (2021) Academic student satisfaction and perceived performance in the e-learning environment during the COVID-19 pandemic: Evidence across ten countries. PLoS ONE 16(10): e0258807. https://doi.org/10.1371/journal.pone.0258807
Editor: Dejan Dragan, Univerza v Mariboru, SLOVENIA
Received: July 21, 2021; Accepted: October 5, 2021; Published: October 20, 2021
Copyright: © 2021 Keržič et al. This is an open access article distributed under the terms of the Creative Commons Attribution License , which permits unrestricted use, distribution, and reproduction in any medium, provided the original author and source are credited.
Data Availability: The data presented in this study are available in Supporting Information (see S1 Dataset ).
Funding: This research and the APC were funded by the Slovenian Research Agency grant number P5-0093. The funders had no role in study design, data collection and analysis, decision to publish, or preparation of the manuscript.
Competing interests: The authors have declared that no competing interests exist.
Introduction
COVID-19, as a global public health crisis, has been brutal on the economy, education and food security of people all around the world, regardless of national boundaries. Affected sectors include tertiary education, featuring one of the worst disruptions during the lockdown periods given that most countries have tried to keep their essential economic activities running. Still, such activities did not extend to higher education institutions (HEIs), which were closed completely after the suspension of face-to-face activities in an effort to avoid the virus spreading among their students and staff and, in turn, the general population.
Nevertheless, HEIs have continued to offer education by using various digital media, e-learning platforms and video conferencing systems. The result is that e-learning has become a compulsory educational process. Many HEIs were even encountering this mode of delivery for the first time, making the transition particularly demanding for them since no time was available to organize and adapt to the new landscape for education. Both teachers and students today find themselves in a new environment, where some seem better at adapting than others. This means the quality of teaching and learning call for special consideration. In this article, the term “e-learning” refers to all forms of delivery for teaching and learning purposes that rely on different information communication technologies (ICTs) during the COVID-19 lockdown.
To understand COVID-19’s impact on the academic sphere, especially on students’ learning effectiveness, we explored the factors influencing how students have perceived their academic performance since HEIs cancelled their onsite classes. Students’ satisfaction in e-learning environments has been studied ever since the new mode of delivery via ICT first appeared (e.g. [ 1 ]), with researchers having tried to reveal factors that shape success with the implementation of e-learning systems (e.g. [ 2 – 4 ]), yet hitherto little attention has been paid to this topic in the current pandemic context. This study thus aims to fill this gap by investigating students’ e-learning experience in this emergency shift. Therefore, the questions we address in the paper are:
- R1: Which factors have contributed to students’ greater satisfaction with the e-learning during the COVID-19 pandemic?
- R2: Are there any differences between factors influencing quality of the e-learning regarding countries, gender, and fields of study?
- R3: How does the students’ satisfaction with the transition to e-learning during the COVID-19 pandemic relate to their academic performance?
According to previous research and considering the new circumstances (e.g. [ 5 – 7 ]), we propose a model for explaining students’ perceived academic performance. In order to identify relevant variables positively affecting students’ performance, we use data from the multi-country research study “Impacts of the COVID-19 Pandemic on Life of Higher Education Students”, coordinated by the Faculty of Public Administration, University of Ljubljana, Slovenia [ 8 ]. Structural equation modelling (SEM) is applied to explore the causal relationships among latent concepts, measured by several observed items. Since the SEM approach has a long history of successful applications in research, especially in the social sciences [ 9 , 10 ] and also in the educational context [ 11 ], it offers a suitable statistical framework that allows us to define a conceptual model containing interrelated variables connected to e-learning’s effect on students’ performance [ 9 , 10 ].
This study significantly contributes to understanding of students’ satisfaction and performance in the online environment. The research findings may be of interest to higher education planners, teachers, support services and students all around the world.
E-learning and the COVID-19 pandemic
According to the International Association of Universities (IAU), over 1.5 billion students and young people around the globe have been affected by the suspension of school and university classes due to the pandemic [ 12 ]. Thus, to maintain continuity in learning while working on containing the pandemic, countries have had to rely hugely on the e-learning modality, which may be defined as learning experiences with the assistance of online technologies. However, most HEIs were unprepared to effectively deal with the abrupt switch from on-site classes to on-line platforms, either due to infrastructure unavailability or the lack of suitable pedagogic projects [ 13 , 14 ]. To understand the mechanism and depth of the effects of COVID-19, many research studies have been carried out across the world.
Before COVID-19, as new technologies were developed, different e-learning modalities like blended learning and massive open online courses were gradually spreading around the world during the last few decades [ 15 , 16 ]. Hence, e-learning was deeply rooted in adequate planning and instructional design based on the available theories and models. It should be noted at the outset that what has been installed at many HEIs during the pandemic cannot even be considered e-learning, but emergency remote teaching, which is not necessarily as efficient and effective as a well-established and strategically organized system [ 17 ]. Still, all over the world online platforms, for example MS Teams, Moodle, Google Classroom, and Blackboard are in use. Although e-learning offers some educational continuity when it comes to academic learning, technical education has suffered doubly since the social distancing requirements have disrupted the implementation of both practical and work-based learning activities, which are critical for educational success [ 18 ].
According to Puljak et al. [ 19 ], while students have mostly been satisfied with how they have adapted to e-learning, they have missed the lectures and personal communication with their teachers. They declared that e-learning could not replace regular learning experiences; only 18.9% of students were interested in e-learning exclusively in the long run. Inadequate readiness among teachers and students to abruptly switch from face-to-face teaching to a digital platform has been reported [ 20 ].
The closure of universities and schools due to the COVID-19 pandemic has led to several adverse consequences for students, such as interrupted learning, giving students fewer opportunities to grow and develop [ 21 ]. This shift has resulted in various psychological changes among both students and teachers [ 22 ] and greatly affected their performance. Tutoring system in higher education is an established model of support, advice, and guidance for students in higher education with a purpose to improve motivation and success and prevent drop-out. Pérez-Jorge et al. [ 23 ] studied the effectiveness of the university tutoring system during the Covid-19 pandemic. The relation between tutor and student is based on collaboration and communication, which required to adopting quickly to the new situations using different communication technology. The research focused on four different forms of tutoring: in person, by e-mail, using virtual tutoring (Hangout/Google Meet) and WhatsApp. They pointed out that synchronous models and frequent daily communication are essential for effective and successful tutoring system where application WhatsApp, with synchronous communication by messages and video calls, is the form with which students were most satisfied and gain the most from it.
The goal of shifting teaching and learning over to online platforms is to minimize in-person interactions to reduce the risk of acquiring COVID-19 through physical contact. The form of interaction has also moved from offline mode to online mode. Students interact with each other in online platforms for their close group and also for larger groups [ 24 , 25 ]. Many clinical skills are learned through direct interactions with patients and caregivers, one area that has been badly affected by the switch to e-learning platforms [ 26 – 28 ].
Student satisfaction with e-learning
Student satisfaction has been shown to be a reliable proxy for measuring the success of implementing ICT-based initiatives in e-learning environments. Scholars have documented a strong relationship between how students perceive their academic performance and how satisfied students are with their e-learning environments [ 1 , 29 – 31 ].
The literature reveals important antecedents related to students’ satisfaction with e-learning training, such as online interactions [ 32 , 33 ], computer efficiency [ 34 , 35 ], online skills [ 36 ], teacher support [ 34 , 37 , 38 ], course design [ 29 , 39 ], teacher feedback [ 40 ], quality of information and activity [ 1 ] and technical support [ 34 , 36 , 41 ]. During the COVID-19 pandemic, environmental aspects like temperature, lighting and noise have been identified as significant determinants of students’ e-learning performance [ 42 ].
Sun et al. [ 1 ] consider the effect of overall quality–as a holistic construct–on satisfaction with the e-learning system. Their research identifies several quality factors that facilitate e-learning through factors associated with: learners (mental health, self-efficacy and attitude of the learner), teachers/instructors (attitude and response timelines assigned by the teacher), technology (quality of technology and the Internet), curriculum (quality and flexibility of the curriculum), design (usefulness and complexity of the design) and environment (interactiveness and assessment diversity). This pandemic has challenged HEIs around the world since e-learning requires physical equipment such as computers, servers, learning and communication platforms, but also software applications, operating systems and experts in the use of these technologies. However, teachers must also possess sufficient digital competencies if they are to use ICT effectively in the learning process.
One of the most relevant factors related to success in implementing e-learning relates to how online education is conducted [ 19 ]. This includes receiving timely feedback, teachers’ efforts to be organized, delivering online lectures (and recording them), adapting instructions to this learning model, and helping students follow the courses and look for feedback on their experiences. In some cases, students have not been appropriately guided to follow their courses, overloaded with too many assignments, while there has been a general concern about the lack or loss of practical instruction, which has thus not entirely been covered in their e-learning experiences.
According to Chopra et al. [ 37 ], timely feedback and responses to students’ actions are key to effective online delivery. Another study also found a positive association between e-service and information quality with students’ satisfaction [ 43 ]. Based on interviews with teachers and students from Jordan, Almaiah et al. [ 44 ] found that it is crucial to analyse students and teachers’ use and adoption of systems, while their critical challenges included: (1) change management, students’ and teachers’ resistance, since many prefer traditional learning; (2) ICT literacy; (3) students’ self-efficacy and motivation; and (4) technical issues around systems’ accessibility, availability, usability, reliability, personalization, and service quality, mainly because perceived ease of use might benefit students’ performance and their efficacy while using e-learning systems. Perceived ease of use influences both system adoption and perceived usefulness and was clearly an important aspect since many participants complained that the e-learning system implemented was neither easy to use nor flexible, and this affected their experience regarding technical issues.
An Indian study reports a decline in teacher–student interaction when teaching moved across to online platforms [ 22 ]. Hence, greater autonomy is required from students, along with self-regulation and skills to learn online for effective learning [ 45 ].
Yet, students’ expertise in computer use and different learning platforms deeply influences their participation in e-learning [ 34 ]. Similarly, Wu et al. [ 35 ] emphasize the lack of adequate computer skills as an important impediment to effective online delivery. It is important to note that not only the lack of soft skills but also not having adequate hardware can obstruct e-learning. The Hungarian Rectors’ Conference [ 46 ], on the basis of 42 Hungarian HEIs’ responses, reported that the experiences with e-learning were generally positive. Still, the main issues involved the lack of technical preparation and equipment; in particular, many students did not have adequate equipment or Internet access. The levels of the students’ satisfaction with the e-learning was also reported to be better among students in developed countries than their counterparts in developing ones [ 26 ]. Similarly, resource-scarce settings struggle with the unavailability of digital platforms for education, limited Internet access, poor Internet speed, high cost of Internet and inadequate expertise to work via digital platforms [ 14 ]. The infrastructure resources in developing countries are incomparable to developed ones because there is a lack of technological infrastructure for e-learning like computers, connectivity and electricity on top of deficient skills and the active participation of both students and teachers due to insufficient ICT literacy [ 47 ].
To strengthen e-learning, the following strategies have been suggested as useful:
- To use a wide variety of learning strategies [ 48 ].
- To use tools that allow students to collaboratively build knowledge, discuss, co-construct and interact with the content [ 49 ].
- To incorporate social media in e-learning so as to provide an adequate and more engaging learning space [ 50 ].
- To use flexible and scaffolded online resources so as to acquire new technical skills that may be useful for future working opportunities [ 51 ].
- To provide adequate technological infrastructure and equipment for e-learning [ 26 ].
Students’ satisfaction and performance
Several comprehensive models have also been developed for studying e-learning performance. The technology acceptance model (TAM) provides an easy way to assess the effects of two beliefs–perceived usefulness and perceived ease of use–on users’ intention to utilize a certain technology, hence providing a good prediction of students’ participation and involvement in e-learning, which in turn influences their performance [ 52 ].
Rizun and Strzelecki [ 53 ] employed an extension of the TAM, which suggests that acceptance of e-learning is related to enjoyment and self-efficacy. According to DeLone and McLean [ 54 ], system usage–the degree to which an individual uses the capabilities of a given information system in terms of frequency, nature and duration of use–has a direct connection with users’ satisfaction and their online performance. By applying DeLone and McLean’s Model (D&M model) of Information Systems Success, Aldholay et al. [ 55 ] were able to prove that system, service and information quality related to e-learning have significant positive effects on system usage, that thereby predicts a user’s satisfaction and has a positive impact on their performance.
Recently, Al-Fraihat et al. [ 41 ] used a multidimensional and comprehensive model and found seven types of quality factors that influence the success of e-learning systems, namely: technical system quality, information quality, service quality, education system quality, support system quality, learner quality, and instructor quality as antecedents of perceived satisfaction, perceived usefulness, use and benefits of e-learning. Moreover, Baber [ 56 ] relates students’ perception of their learning outcomes and their satisfaction to factors like students’ motivation, course structure, the instructor’s knowledge and facilitation.
Cidral et al. [ 34 ] proposed 11 different constructs of effective e-learning, among which we can mention individual skills, system requirements, and interaction-focused elements. System use and user satisfaction were shown to exert the greatest positive impact on individuals’ performance through e-learning. In a similar study, Hassanzadeh et al. [ 57 ] identified the following factors as responsible for success with e-learning: use of the system, loyalty to the system, benefits of using the system, intention to use, technical system quality, service quality, user satisfaction, goal achievement, and content and information quality.
Rashid and Yadav [ 58 ] draw attention to several critical issues that may affect the effectiveness of e-learning: students’ possibility to have access to and to afford e-learning technologies; the need for educators to be properly trained in the use of the technologies; teachers’ autonomy and trust; and the quality of the communication among higher education stakeholders. Moreover, Deloitte [ 59 ] highlights the importance of institutional support in the successful delivery of e-learning.
Constructs of the conceptual model and research hypotheses
This study proposes a conceptual model for analysing students’ perceived academic performance during the period of the COVID-19 pandemic, which forced the transition from on-site to on-line teaching and learning. In this research, we combine the theoretical results of previous studies on e-learning with the emergency changeover to various online modes of delivery in response to the pandemic lockdown. The proposed conceptual model builds on the model of students’ satisfaction with e-learning suggested by Sun et al. [ 1 ] as well as the D&M model [ 60 ], which was used to describe different information systems’ success, including the e-learning system [ 41 ]. Cidral et al. [ 34 ] studied similar key apects of quality e-learning systems.
In the conceptual model we propose second-order multidimensional construct E-learning Quality of five components. Based on the literature [ 1 , 34 , 37 ] the construct connects three aspects of quality: learner, teacher and system.
Two factors associated with students’ satisfaction corresponding to the learner dimension are included in our proposed model: Home Infrastructure and Computer Skills . The rapid transition to online study meant students were relocated to a home environment where many did not enjoy suitable conditions to study, both a quiet place and digital equipment with access to (high-performance) Internet, which is indispensable for effective online study. Therefore, the latent variable Home Infrastructure covers the ICT conditions at home, i.e. having one’s own computer or access to one, the required software, a webcam, and a stable (and fast) Internet connection [ 37 ]. The greater the students’ previous knowledge and experience in using digital media, the easier the transition to e-learning has been. Computer Skills describe students’ expertise in using computers and different learning platforms, which is particularly important for active participation in the online delivery mode [ 34 , 35 ].
The teacher dimension refers to the organization of teaching in a new e-learning environment. Studies show the organization and delivery of study material is important for student satisfaction and performance. Three constructs related to teachers are defined in the model. Mode of Delivery corresponds to the different forms used in online lectures, tutorials or practical classes providing learning materials and assignments, such as videoconference, audio recording, forum or e-mail [ 57 ]. Teachers play a valuable active role in the online environment by guiding students through the learning contents and providing them with timely responses and information. Equally important are prepared assignments that encourage and motivate students to independently learn at home. Online Instruction focuses on teachers’ active role and attitude to online teaching. The construct is explained by Information Quality and two other aspects assessed in our questionnaire, namely preparing regular assignments and being open to students’ suggestions [ 34 , 41 , 61 ]. Information Quality measures teachers’ responsiveness to the students, such as timely feedback or answering questions in an e-learning environment [ 34 , 37 ]. We also propose a second-order construct System Quality , composed of learner and teacher dimensions: Home Infrastructure and Mode of Delivery .
Previous studies reveal that IT service support has a positive influence on users’ perceptions of their satisfaction with the system. As the transition to online study happened quickly and without prior training, the support of both the IT and the administrative service is vital for ensuring that students are satisfied with their new learning environment [ 34 , 37 , 41 , 57 , 61 , 62 ]. In our model, Service Quality refers to the aspect of administrative, technical and learning assistance. To compensate for the lack of social contact while studying from home, various forms of online interactions are possible. Teacher–student or student–student interactions were shown to be important factors of satisfaction with the e-learning system [ 34 , 41 , 61 ]. The construct Online Interactions describes how often a student communicates with colleagues from the course, the teachers or the administrative staff.
To summarize, E-learning Quality is multidimensional construct of five components Students’ Computer Skills , System Quality , which reflects the Mode of Delivery and Home Infrastructure ,. Online Instruction assessed through Information Quality , Online Service Quality and Online Interactions with colleagues, teachers and staff. We hypothesize:
- H1: Students’ Computer Skills is correlated with Home Infrastructure .
During the COVID-19 pandemic, teaching and learning were completely implemented in the online environment and thus we include the quality dimension, which measures several important aspects of the e-learning system: system quality, information quality, service quality, learner digital quality and interaction quality. Models measuring the success of the information system (also the e-learning system) are usually based on the D&M model, where user satisfaction and quality dimension play an important role [ 34 , 41 , 57 , 61 ]. The construct Perceived Student Satisfaction is manifested by students’ satisfaction with the organization of e-learning (i.e. lectures, tutorials, seminars, mentorships) and with the support of the teachers and the student counselling service [ 34 , 57 ]. Perceived Student Performance aims to capture students’ benefits of using an e-learning system. It measures students’ opinion of their performance and whether it has worsened with the transition to the online learning mode [ 34 , 41 , 57 ]. The proposed model’s structural part includes three constructs: E-learning Quality , Perceived Student Satisfaction and Perceived Student Performance . We may reasonably assume the quality of the e-learning system has a positive effect on satisfaction with the online education environment system, leading to the system’s greater use and thus to improve the student performance. It is unlikely that one can perform well without use of the system.
This leads to three hypotheses being proposed:
- H2: E-learning Quality has a positive effect on Perceived Student Satisfaction .
- H3: Perceived Student Satisfaction has a positive effect on Perceived Student Performance .
- H4: E-learning Quality has an indirect (mediated by Perceived Student Satisfaction ) positive effect on Perceived Student Performance .
Therefore, we propose the conceptual model presented in Fig 1 . and construct description in Table 1 .
- PPT PowerPoint slide
- PNG larger image
- TIFF original image
https://doi.org/10.1371/journal.pone.0258807.g001
https://doi.org/10.1371/journal.pone.0258807.t001
Materials and methods
Design and procedure.
The data for this study come from a very comprehensive and large-scale global student survey entitled “Impacts of the COVID-19 Pandemic on Life of Higher Education Students”, aimed at examining how students perceive the impacts of the pandemic’s first wave in early 2020 on various aspects of their lives on a global level [ 8 ]. This project was originally promoted by the Faculty of Public Administration, University of Ljubljana (Slovenia), which, thanks to the support of international partners, was able to be disseminated worldwide. The online questionnaire was adapted and extended from the European Students’ Union [ 63 ] survey. It was formed by 39 questions, mainly including closed-ended questions (see S1 Questionnaire ). It focused on socio-demographic, geographic and other aspects pertaining to the life of university students, such as academic online work and life, social life, emotional life, personal circumstances, changes in habits, the roles and measures of institutions, as well as personal reflections on COVID-19 [ 64 ]. Initially, the online questionnaire was designed in English and later translated into six different languages (Italian, North Macedonian, Portuguese, Romanian, Spanish, Turkish). The translation of the questionnaire was carried out by native speakers, being proficient in English. The web-based survey was launched via the open-source web application 1KA (One Click Survey; www.1ka.si ) on 5 May 2020 and remained open until 15 June 2020, that is, in a period when most nations were experiencing the onerous restrictions imposed by the lockdown. Participation in the study reached global proportions by exceeding the milestone of 30,000 responses submitted by students from more than 130 countries on all six continents. The entire dataset was first analysed by Aristovnik et al. [ 8 ].
Participants
The survey was intended for all higher education students at least 18 years of age, representing the target population of this study. The sampling technique used is non-probabilistic, specifically convenience sampling through university communication systems around the world and social media. The students were informed about the details of the study and gave their informed consent before participating. Due to this study’s specific focus on academic online work and life, it only includes student data with respect to selected parts of the questionnaire. However, since the respondents were not obliged to complete the questionnaire in full, the number of respondents varied across questions. Accordingly, a complete-case-analysis approach was applied to mitigate missing data issues [ 65 ]. With the assumption of “missing completely at random”, meaning the complete cases are a random sample of the originally identified set of cases, a complete-case approach is the most common method for handling missing data in many research fields, including educational and epidemiologic research [ 66 , 67 ]. In order to assure a more robust analysis and perform reliable comparisons on the national level, this study focuses on the 10 countries (Chile, Ecuador, India, Italy, Mexico, Poland, Portugal, Romania, Slovenia, Turkey) that provided at least 500 answers with regard to different aspects of students’ academic life.
The final dataset consisted of 10,092 participants or students enrolled in HEIs, of whom 92% were attending a full-time study course. They were at least 18 years old, with a median age of 23 years (IQR [21.0, 24.0]), and about two-thirds of them (67%) being female. Most respondents (82%) were pursuing a bachelor’s degree, 16% a master’s degree, and 2% a doctoral course. Twelve percent were majoring in a study course in the Arts and Humanities, 37% in the Social Sciences, 32% in the Applied Sciences and 19% in the Natural and Life Sciences. Detailed information on the sample, i.e. the number of respondents and participants’ sociodemographic characteristics by country, are given in Table 1 .
This study primarily focuses on how COVID-19 has affected different aspects of students’ academic life. Specifically, students reported their experiences with the organization of teaching and administrative services, along with their satisfaction, expectations and perceived impacts on their university career. This involves a total of 34 survey items, representing a basis for measuring the 9 latent constructs used in our proposed conceptual model. Individual satisfaction and concern levels were measured on a 5-point Likert scale, from 1 (lowest value) to 5 (highest value) [ 68 ]. A more detailed description, including the set of measuring items and their characteristics, is found in Table 2 .
https://doi.org/10.1371/journal.pone.0258807.t002
Ethical considerations
All participants were informed about the details of the study and gave their informed consent before participating. By clicking on a button ‘next page’ participants agreed to participate in the survey. Study participation was anonymous and voluntary, and students could withdraw from the study without any consequences. For data-protection reasons, the online survey was open to people aged 18 or over and enrolled in a higher education institution. The procedures of this study comply with the provisions of the Declaration of Helsinki regarding research on human participants. Ethical Committees of several of the higher education institutions involved approved this study, such as the University of Verona (protocol number: 152951), ISPA–Instituto Universitário (Ethical Clearance Number: I/035/05/2020), University of Arkansas (IRB protocol number: 2005267431), Walter Sisulu University (Ethical Clearance Number: REC/ST01/2020) and Fiji National University (CHREC ID: 252.20).
Data analysis
We implemented the SEM with use of the lavaan package (v.0.6.4, [ 69 ]) in the R statistical environment (v.4.0.2, [ 70 ]). A two-step approach was followed. In the first step, we checked the fit of the measurement model to all the latent variables; in the second step, we checked the fit of the structural model. The Comparative Fit Index (CFI), Tucker-Lewis Index (TLI), Root Mean Square Error of Approximation (RMSEA) and Square Root Mean Residual (SRMR) were used as goodness of fit indices. The fit was deemed appropriate for CFI and TLI above .90, and for RMSEA and SRMR values below .06 and .08, respectively (e.g. [ 71 , 72 ]).
We assessed the reliability of the first-order and second-order factors with McDonald’s omega ( ω ) and ω L2 , respectively, and convergent validity with Average Variance Extracted (AVE) using the semTools package (v.0.5.3, [ 73 ]). Omega and AVE values above .70 and .50 were indicative of good reliability and convergent validity, respectively [ 72 , 74 , 75 ].
Invariance analysis was performed [ 72 ] by comparing the difference in the fit of a series of sequentially constrained models from configural (Conf), intercepts (Intercpy), loadings (Load), means (Means), to regression coefficients (Regr). Invariance was assumed for nonsignificant Δ χ 2 or, preferentially, ΔCFI<-.01 for two sequentially constrained models.
Preliminary analyses
Factor loadings and factor reliabilities for the first- and second-order constructs used in the model are given in Table 3 . All factor loadings for the first-order constructs were statistically significant for p < .001 and larger than the usual .50 cut-off value. Reliability, as measured by McDonald’s ω , ranged from .67 (for Online Instruction ) to .94 (for Mode of Delivery ). The second-order constructs have lower reliability values, which is explained by the reduced number of indicators in some of these constructs. For the first-order constructs, AVE ranged from .55 (for Online Interactions ) to .80 (for Mode of Delivery ). As seen from the reliability measures, the second-order constructs, especially the ones with few indicators, displayed lower AVE. Moreover, in Fig 2 , we show the path coefficients calculated for each hypothesis.
https://doi.org/10.1371/journal.pone.0258807.g002
https://doi.org/10.1371/journal.pone.0258807.t003
Model of student perceived performance
The overall model under the e-learning regime due to the COVID-19 pandemic is depicted in Fig 2 . The estimated model had a good fit to the 10,092 students from the 10 countries that provided more than 500 valid responses ( χ 2 (519) = 5213.6, p < .001, CFI = .990, TLI = .989, RMSEA = .063, SRMR = .049) with all structural paths significant at p < .001. The model explained 55% (R 2 = .55, p < .001) of the students’ perceived performance. Major determinants of E-learning Quality were Service Quality ( β = .96, p < .001) and overall System Quality ( β = .90, p < .001). Online Interactions with colleagues and teachers ( β = .34, p < .001) and the students’ Computer Skills ( β = .46, p < .001) had a lower impact on the e-learning system’s overall quality.
Country invariance
The analysis of invariance revealed configural invariance (CFI = .900, TLI = .900, RMSEA = .070, SRMR = .060) for the 10 countries. However, no weak measurement invariance (equal loadings between countries) was observed (Δ χ 2 Load (243) = 510.93, p < .001; ΔCFI Load = -.03). Thus, the proposed conceptual model was fit to the 10 participating countries individually. Table 4 summarizes the structural standardized coefficients and fit indices obtained for each country.
https://doi.org/10.1371/journal.pone.0258807.t004
Overall, the models displayed an acceptable fit for all countries (CFI and TLI greater or equal to .850) and for most countries RMSEA and SRMR less or equal than .05 and .06, respectively). The model explained from 35% (India) to 59% (Portugal) of the Perceived Student Performance variation within countries. The overall mean explained variance was 42%.
Gender and areas of study invariance
Invariance analysis of the model revealed strong metric invariance for gender according to the ΔCFI criteria (ΔCFI Load = -.001; ΔCFI Intercpt = -.001), but not for the Δ χ 2 criteria (Δ χ 2 Load (26) = 62.253; p < .001; Δ χ 2 Intercpt (26) = 79.824; p < .001). However, for large sample sizes inflation of χ 2 is well known, thus recent research has adopted different criteria, including the ΔCFI as described in the methods section. Using the gender ΔCFI criteria, invariance was also observed for factor means ΔCFI Means <-.001) and structural regression coefficients (ΔCFI Means = -.001).
The model displayed strong metric invariance for the areas of study (Arts and Humanities, Social Sciences, Applied Sciences, Natural Sciences) according to the ΔCFI criteria (ΔCFI Load = -.002; ΔCFI Intercpt = -.003). Using the same criteria, invariance was also observed for factor means ΔCFI Means = -.001) and structural regression coefficients (ΔCFI Means <-.001).
Therefore, we conclude that the model is invariant for gender and areas of study, implying that we can apply it for both genders and all four areas of study.
The goal of this research was to analyse which factors influenced students’ perceived academic performance after switching their academic activities over to the online mode, as imposed by the lockdown in response to COVID-19 in 2020. To this end, a global study including 62 countries was conducted. In this paper, we presented the results of 10 countries that provided more than 500 valid responses.
The study results show that the impact of computer skills is less influential for e-learning quality compared to other factors like system quality, which is the most determinative factor. These results are aligned with previous studies (e.g. [ 34 , 37 ]), which found that system quality is positively related to a user’s perceived satisfaction, but are contrary to Al-Fraihat et al. [ 41 ] who did not detect any significant system quality impact. Our data also show that different modes of delivery positively influenced system quality. On the other hand, even though the quality and diversity of the home infrastructure revealed some impact on the system quality, it is a less determinative factor. These results suggest that students respond better to diversity in learning formats, but it seems that having suitable infrastructure is not so important.
As concerns online instruction, we found that it is one of the three major determinant of e-learning quality and, therefore, for students’ perceived satisfaction and performance. Online instruction can be assessed by the construct information quality, as well as by considering other factors like the teacher’s active role and attitude to online teaching, preparation of regular assignments and openness to the students’ suggestions [ 34 , 41 , 61 ]. Information quality can be explained by teachers’ responsiveness to the students, such as timely feedback or answering questions in an e-learning environment [ 19 , 34 , 37 ].
The active role of teachers and their responsiveness and feedback seem crucial for the students’ satisfaction with the online instruction since the teacher/instructor is a key element of success with the e-learning environment [ 76 ]. Sun et al. [ 1 ] investigated the instructor’s role in the success of e-learning, focusing on two specific indicators: instructor response timelines, and instructor attitude to e-learning. They found a positive and significant relationship between these aspects and the satisfaction of students. Similar findings were outlined by Cidral et al. [ 34 ], who documented a positive relationship between instructor attitude to e-learning and user satisfaction. In addition, Al-Fraihat et al. [ 41 ] and Mtebe and Raphael [ 77 ] established a positive relationship between the instructor’s quality and students’ perceived satisfaction with an e-learning system. Moreover, the quality of information provided by the instructor/teacher has been considered to be a determinant of perceived satisfaction in previous studies that support our findings [ 29 , 37 , 41 , 43 , 78 , 79 ]. According to Al-Fraihat et al. [ 41 ], it is essential to provide students with clear, updated and sufficient information and quality content.
Regarding online service quality, we found that it was a major determinant of the students’ perceived e-learning quality. This allows us to infer that administrative, technical and learning assistance through tutors and the library is very important for students’ greater satisfaction and, in consequence, students’ higher perceived satisfaction and performance. This result is contrary to Cidral et al. [ 34 ], yet consistent with the findings of Al-Fraihat et al. [ 41 ], Hassanzadeh et al. [ 57 ] and Chopra [ 37 ], who state that providing quality services might increase the level of satisfaction, making it crucial to have personnel available to support students with their technical issues and satisfy their needs, generating positive feelings towards the e-learning system.
The construct online interactions describes how often a student communicates with colleagues from the course, the teachers or the administrative staff [ 34 , 41 ]. This factor was considered to be one of the least determinative of overall satisfaction-learning quality and, consequently, least able to explain the conceptual model of perceived student performance. It seems the new emergency remote teaching and learning scenario [ 17 ] has affected the frequency of student interactions with colleagues and teachers [ 19 , 22 ], which may explain why it is less important for perceived e-learning quality. Our results suggest these interactions are still needed for a successful student performance in an e-learning environment, although they are less determinative than other factors.
The first hypothesis (H1) about the influence of the students’ computer skills on e-learning quality and The first hypothesis (H1), referring to the intercorrelation between students’ computer skills with the quality and variety of the IT infrastructure at home, were confirmed. The correlation is only moderate. In other terms, students who possess different digital media and better-quality infrastructure at home had greater digital competencies, which then favoured their perceived e-learning quality and, thus, the students’ perceived satisfaction and performance under the e-learning mode.
Taking all five dimensions of e-learning quality into consideration, the second hypothesis (H2) is also confirmed because this factor (e-learning quality) has a very strong positive effect on perceived student satisfaction. Students who are more satisfied with the quality of their e-learning experience are generally more satisfied with their education, which further more positively influences their perceived academic performance (see H3). Students more satisfied with their online education also perform better at school. The result highlights the role of students’ satisfaction in their academic performance [ 60 ]. At the same time, we may infer that students who use the online learning mode more frequently perceive their educational performance is higher.
The last hypothesis (H4) is also confirmed. E-learning quality has an indirect (mediated by perceived student satisfaction) positive effect on perceived student performance. The overarching research question of our study is thereby confirmed: the better the quality of the e-learning system, the more satisfied students are with their academic performances.
Regarding the country comparisons (see Table 2 ) and considering the overall model’s lack of invariance and irrespective of the country differences, the results show that students’ perceived satisfaction is largely predicted by the quality of the school’s service and the quality of the overall system. However, it is worth discussing some of the outliers shown in Table 2 .
Concerning computer skills, one can observe a significant difference between India and the other countries. This result might have been influenced by the fact that the majority of Indian students participating in the study have a technical background, pursuing Engineering or Medical Sciences. Hence, their proficiency in computing is expected to be high. On the other side, among Romanian students the impact of computer skills on e-learning quality is the lowest, which may be explained by the structure of the Romanian sample, comprising more Social Sciences students who prefer face-to-face interactions over the use of different platforms for online teaching, which has increased the workload compared to the previous situation.
While examining the results for the construct system quality, we see that, although most countries show similar structural standardized coefficients, Portugal has a slightly highest coefficient compared to the rest of the countries. This result might be caused by the fact that Portugal had already been through a process of creating a very strong online higher education infrastructure [ 80 ], meaning the students’ transition to this modality has been quite smooth and they do not seem to perceive any significant change.
With respect to online interactions, India has a significantly higher coefficient than the corresponding values for the other countries. This result may be explained by the fact that the average university class size in India is 150–250 students, making it very difficult for the students to interact with each other or with the teachers in a personal way. In the new e-learning scenario, teachers are more available for flexible consultation time. In addition, many of the teaching strategies that lecturers are relying on encourage collaborative work. Yet, in contrast, Slovenia has the lowest coefficient for this factor, which can be attributed to the fact that, even before the pandemic outbreak, e-learning was widespread in higher education, including blended learning, and thus the students do not consider that online interactions have increased or changed due to the pandemic.
Regarding online instruction, Turkey has the lowest coefficient of the 10 countries. The high number of Turkish students per academic, which exceeds the OECD average [ 81 ] makes it difficult for academics to give individual feedback to all of their students.
Regarding gender and areas of study, the proposed model proved to be invariant for both factors, which confirms its relevance in explaining students’ perceived academic performance through the quality of the e-learning infrastructure as mediated by students’ perceived satisfaction.
Although no significant difference in the results is found by gender, the number of female participants is remarkably higher than for males in all countries. Although the causes of this result lie beyond the scope of this study, it would be worth analysing them in future research.
Conclusions
Our study has provided insights into latent factors explaining students’ perceived academic performance during the first wave of COVID-19 pandemic, which forced the transition to online education. The results confirmed all of the hypotheses and the proposed conceptual model was revealed to be reliable.
According to the study results, the quality of e-learning during the COVID-19 pandemic’s first wave was mainly derived from service quality with administrative, technical and learning assistance through tutors and the library, teachers’ active role in the process of online education with their responsiveness and timely feedback, and overall system quality with the mode of delivery and IT infrastructure. Students’ digital competencies and online interactions with colleagues and teachers were shown to be slightly less important factors, yet still statistically significant. Moreover, our study shows that the impact of e-learning quality on student performance is strongly mediated by student satisfaction with e-learning.
Understanding the factors that influenced students’ performance after the urgent introduction of e-learning may be important for decision-makers and all those involved in implementation in any future new similar circumstances. Thus, the results of our study imply a clear strategy for education, research and policy. Investment in the development of digital competencies, of both students and academic staff, together with initiatives supporting research and interdisciplinary innovative collaboration within the scope of different aspects of higher online education, are recommended and should be encouraged.
Limitations
This study has several limitations that should be considered. First, the convenience sampling methodology, which limits the generalizability of the results. The calculated results are based on a sample, which includes students from 10 countries, although European countries prevail. It is clear that the countries are on different levels of economic development and have differently organized and developed higher education systems. Further, no data come from low-income countries, where students might have a problem with an Internet connection and access to appropriate equipment [ 82 , 83 ]. In addition, to access the online questionnaire students first needed to have electronic devices and an Internet connection, which could cause selection bias.
Another important limitation of this study is the time in which the data were collected. Not all countries were in the same pandemic phase or lockdown period, which might impact the student responses. Therefore, our study does not give a full picture of the students’ perceived satisfaction and performance during e-learning in the time of the first wave of the pandemic.
Future work
Future research could attempt to cluster countries by their economic development level given that e-learning quality and students’ perceived satisfaction and performance with online education depend on IT technology development and IT tools’ access and affordability [ 83 ]. In the future, studies should include representative countries on all levels of development and economic growth to further test the proposed model and look for differences in the area of students’ perceived satisfaction and performance with e-learning. This may help generate evidence for policymakers to invest in developing online education infrastructure in low- and middle-income countries.
Further, although digitalization in HEIs has been confirmed as significant and essential for the higher education system’s functioning during the lockdown [ 84 ] and e-learning has offered some kind of continuity of academic education, it does not meet all of the needs for practical and work-based learning, e.g. in medical and health or technical sciences education, especially when viewed in the long run [ 85 – 87 ]. In future research, more emphasis should be placed on analysing students’ perceived satisfaction and performance with online education in the context of differences between fields of study, particularly in relation to the nature of education (theoretical vs. practical) and the competencies that are supposed to be developed during education.
Future research may also consider differences between local and international students’ perceived satisfaction and performance. According to the EMN/OECD report [ 88 ], the COVID-19 pandemic has imposed more difficult situations on international students than local students in terms of psychological and financial issues. This may well impact their academic outcomes. Such analysis could also compare the adaptation to the online education environment of students whose training is in their mother language and students for which the training is in a second language.
Finally, the survey is based on the subjective opinion of students, also with regard to their academic performance. Therefore, to objectify the results further research entailing analysis of the relationship between students’ satisfaction with online education and their learning outcomes expressed in the form of grades may reveal interesting results. Namely, recent analyses suggest that students have been receiving higher grades during the pandemic compared to the on-site education before the pandemic, which may increase their satisfaction with the e-education [ 82 ].
Supporting information
S1 questionnaire..
https://doi.org/10.1371/journal.pone.0258807.s001
S1 Dataset.
https://doi.org/10.1371/journal.pone.0258807.s002
Acknowledgments
We wish to thank all the numerous international partners with data collection: Yusuf Alpayadin; Sorin Gabriel Anton; Roberto Burro; Silvia Cantele; Özkan Cikrikci; Michela Cortini; Manuel Gradim de Oliveira Gericota; Iusein Ervin; Stefania Fantinelli; Paulo Ferrinho; Sandeep Grover; Aleksandar Kešeljević; Poliana Leru; Piotr Major; João Matias; Marek Milosz; Andronic Octavian; Izabela Ostoj; Justyna Podgórska-Bednarz; Vijayalakshmi Reddy; Maya Roche; Ana Sofia Rodrigues; Piotr Rzymski; Oana Săndulescu; Rinku Sanjeev; Ganesh Kamath Sevagur; Parag Suresh Amin; Rajanikanta Swain. We would also like to thank anonymous global survey participants for their valuable insights into the lives of students, which they shared selflessly. Finally, we would like to acknowledge the CovidSocLab project ( http://www.covidsoclab.org/ ) as a working platform for collaboration.
- View Article
- Google Scholar
- PubMed/NCBI
- 11. Khine MS. Application of structural equation modeling in educational research and practice. Rotterdam: SensePublishers; 2013.
- 12. IUA. COVID-19: Higher education challenges and responses. International Association of Universities. 2020. Available from: https://www.iau-aiu.net/COVID-19-Higher-Education-challenges-and-responses
- 18. OECD. Education at a Glance 2020. OECD indicators. OECD Publishing. 2020. https://doi.org/10.1787/69096873-en
- 45. Schleicher A. The impact of COVID-19 on education: Insights from education at a glance 2020. OECD. 2020. Available from: https://www.oecd.org/education/the-impact-of-covid-19-on-education-insights-education-at-a-glance-2020.pdf
- 46. Hungarian Rectors’ Conference. Hungarian response to COVID-19. In Regional/National Perspectives on the Impact of COVID-19 on Higher Education (pp. 25–30). International Association of Universities, 2020. Available from: https://www.iau-aiu.net/IMG/pdf/iau_covid-19_regional_perspectives_on_the_impact_of_covid-19_on_he_july_2020_.pdf
- 47. Aung TN, Khaing SS. Challenges of implementing e-learning in developing countries: A review. In: Zin T.; Lin J.W.; Pan J.S.; Tin P.; Yokota M., editors. Genetic and evolutionary computing: Advances in intelligent systems and computing. Springer. 2016; 388, pp. 405–411. https://doi.org/10.1007/978-3-319-23207-2_41
- 59. Deloitte. Understanding the impact of COVID-19 on higher education institutions. 2020. Available from: https://www2.deloitte.com/ie/en/pages/covid-19/articles/covid-19-on-higher-education.html
- 63. European Students’ Union. ESU’s survey on student life during the Covid-19 pandemic. 2020. Available from: https://eua.eu/partners-news/492-esu%E2%80%99s-survey-on-student-life-during-the-Covid-19-pandemic.html
- 70. R Core Team. R: A language and environment for statistical computing. R Foundation for Statistical Computing. 2020. Available from: https://www.R-project.org/
- 72. Marôco J. Análise de equações estruturais: Fundamentos teóricos, software & aplicações. (2nd ed.). Report Number. 2014.
- 73. Jorgensen TD, Pornprasertmanit S, Schoemann A, Rosseel Y. semTools: Useful tools for structural equation modeling. R package version 0.5–3. 2020. Available from: https://CRAN.R-project.org/package=semTools
- 80. Krueger K. Reinventing learning in Portugal: An ecosystem approach report of the 2013 CoSN Delegation to Portugal. CoSN. 2013. Available from: https://cosn.org/sites/default/files/pdf/ReinventingLearning_Portugal_April14.pdf
- 81. Eurostat. Tertiary education statistics. 2020. Available from: https://ec.europa.eu/eurostat/statistics-explained/index.php?title=Tertiary_education_statistics&oldid=507549
- 88. EMN/OECD. Impact of COVID-19 on international students in EU and OECD member states–EMN-OECD Inform. Brussels: European Migration Network. 2020. Available from: https://ec.europa.eu/home-affairs/sites/homeaffairs/files/00_eu_inform2_students_final_en.pdf
Advertisement
Learner satisfaction, engagement and performances in an online module: Implications for institutional e-learning policy
- Published: 11 November 2020
- Volume 26 , pages 2623–2656, ( 2021 )
Cite this article
- Yousra Banoor Rajabalee ORCID: orcid.org/0000-0003-4885-610X 1 , 2 &
- Mohammad Issack Santally ORCID: orcid.org/0000-0003-3745-2150 1 , 2
76k Accesses
127 Citations
20 Altmetric
Explore all metrics
There has been debates related to online and blended learning from a perspective of learner experiences in terms of student satisfaction, engagement and performances. In this paper, we analyze student feedback and report the findings of a study of the relationships between student satisfaction and their engagement in an online course with their overall performances. The module was offered online to 844 university students in the first year across different disciplines, namely Engineering, Science, Humanities, Management and Agriculture. It was assessed mainly through continuous assessments and was designed using a learning-by-doing pedagogical approach. The focus was on the acquisition of new skills and competencies, and their application in authentic mini projects throughout the module. Student feedback was coded and analyzed for 665 students both from a quantitative and qualitative perspective. The association between satisfaction and engagement was significant and positively correlated. Furthermore, there was a weak but positive significant correlation between satisfaction and engagement with their overall performances. Students were generally satisfied with the learning design philosophy, irrespective of their performance levels. Students, however, reported issues related to lack of tutor support and experiencing technical difficulties across groups. The findings raise implications for institutional e-learning policy making to improve student experiences. The factors that are important relate to the object of such policies, learning design models, student support and counseling, and learning analytics.
Similar content being viewed by others
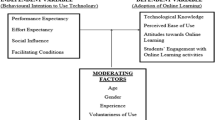
Adoption of online mathematics learning in Ugandan government universities during the COVID-19 pandemic: pre-service teachers’ behavioural intention and challenges
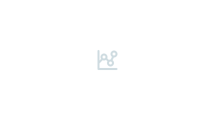
Online learning in higher education: exploring advantages and disadvantages for engagement
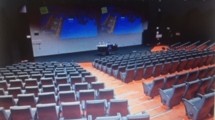
Learning environments’ influence on students’ learning experience in an Australian Faculty of Business and Economics
Avoid common mistakes on your manuscript.
1 Introduction
The world is going through tough times with the Covid-19 pandemic. Inevitably, there has been severe impacts on education systems around the globe. Schools and universities were closed, and millions of kids, adolescents and young adults have been out of schools and universities. Nichols ( 2003 ) pointed out that the Internet could be seen as (i) another delivery medium, (ii) as a medium to add value to the existing educational transaction or, (iii) as a way to transform the teaching and learning process. The research and discourse surrounding quality of online learning provisions, student engagement and satisfaction has been ongoing by both proponents and opponents of online learning (Biner et al. 1994 ; Rienties et al. 2018 ). With the abrupt shift and uptake of online learning, due to the Covid-19 pandemic, such discourse finds its relevance much beyond the classic academic research and debate. It is linked to the future of teaching and learning in technology-enabled learning environments. Arguably, the adoption of technology has disrupted the traditional teaching practices as teachers often find it difficult to adjust and connect their existing pedagogy with technology (Sulisworo 2013 ). Similarly, if informed policy decisions are not taken, this can affect the knowledge transfer processes as well as reduce the efficiency of teaching and learning processes (Ezugwu et al. 2016 ).
One of the challenges of online learning relates to students’ learning experiences and achievement. Sampson et al. ( 2010 ) stated that students’ satisfaction and outcomes are good indicators for assessing the quality and effectiveness of online programs. It is of concern for institutions to know whether its students, in general, are satisfied with their learning experience (Kember and Ginns 2012 ). Another essential element for quality online education is learner engagement. Learner engagement refers to the effort the learner makes to promote his or her psychological commitment to stay engaged in the learning process, to acquire knowledge and build his or her critical thinking (Dixson 2015 ). While there are different conceptualisations of student engagement (Zepke and Leach 2010 ), advocates of learning analytics tend to lay emphasis on the analysis of platform access logs including clicks on learning resources when it comes to student engagement in online learning (Rienties et al. 2018 ). The proposition is that being active online through logins, active sessions and clicks actually reflects actual engagement in an online course and result in better student performances. However, this model mainly works in classic online modules, and there is limited availability of literature measuring students’ engagement in activity-based hybrid learning environments where there is a mix online and offline activities (Rajabalee et al. 2020 ).
In this research, the aim was to investigate the relationships between students’ reported engagement, their satisfaction levels and their overall performances in an online module that was offered to first-year University students of different disciplines (Science, Engineering, Agriculture, Humanities, Management). The learning design followed an activity-based learning approach, where there was a total of nine learning activities to complete over two semesters. The focus was on skills development and competency-based learning via a learning-by-doing approach. There were 844 students enrolled on the module, and they were supported by a group of seven tutors. The end of module feedback, comprising mainly of open-ended questions, aligned with the Online Student Engagement (OSE) model, and the Online Learning Consortium model of student satisfaction were coded and analysed accordingly. Furthermore, the correlation between student satisfaction, engagement and their performances were established.
The findings of this research contribute to the existing knowledge through new insights into determining students’ engagement in online courses that follow an activity-based learning design approach. It is observed in this study, in line with other research that learning dispositions, such as the reported engagement, perceived satisfaction and student feedback elements could be useful dimensions to add to a learning design ecosystem to improve student learning experiences with the objective to move towards a competency and outcomes-based learning model. Based on the results and findings, the implications for institutional e-learning policy are discussed.
2 Literature review
2.1 learner satisfaction and experiences in e-learning environments.
Learner satisfaction and experiences are crucial elements that contribute to the quality and acceptance of e-learning in higher education institutions (Sampson et al. 2010 ). Dziuban et al. ( 2015 ) reported that the Online Learning Consortium (formerly known as Sloan Consortium) considered student satisfaction of online learning in higher education as an essential element to measure the quality of online courses. Different factors influence learner satisfaction such as their digital literacy levels, their social and professional engagements, the learner support system including appropriate academic guidance and the course learning design (Allen et al. 2002 ).
According to Moore ( 2009 ), factors such as the use of learning strategies, learning difficulties, peer-tutor support, ability to apply knowledge and achievement of learning outcomes indicate those elements that impact on the overall satisfaction of students in online learning. A learning strategy is a set of tasks through which learners plan and organize their engagement in a way to facilitate knowledge acquisition and understanding (Ismail 2018 ). Enhancing the learning process with appropriate learning strategies may contribute to better outcomes and performances (Thanh and Viet 2016 ). Aung and Ye ( 2016 ) reported that students’ success and achievement were positively related to student satisfaction.
Students, in online courses, often experience learning difficulties, which encompass a range of factors such as digital literacy, conceptual understanding, technical issues and ease of access (Gillett-Swan 2017 ). These difficulties if not overcome on time, tend to reduce learning effectiveness and motivation, and may also affect their overall satisfaction (Ni 2013 ). Learner support may include instructional or technical support, where tutors and other student peers engage collectively to help students tackle issues that they encounter during the course. Such support, especially when students face technical difficulties is vital to overcome challenges and impacts on overall student satisfaction (Markova et al. 2017 ). The ability of students to apply knowledge, and to achieve the intended learning outcomes, also impacted on student satisfaction and quality of learning experience (Mihanović et al. 2016 ).
Student perception is the way students perceive and look at a situation from a personal perspective and experience. When students have a positive perception, they are more likely to feel satisfied with the course (Lee 2010 ). It is, therefore, crucial to understand how students think about a course, certainly to determine its implications on their academic experiences. A negative feeling is an emotion that students sometimes express concerning their learning experience. It could be in the form of anxiety, uneasiness, demotivation and apprehension or in terms of their readiness to use technology (Yunus et al. 2016 ). At the same time, negative feeling tends to have an impact on student online learning experience and their satisfaction (Abdous 2019 ). Learner autonomy relates to students’ independence in learning. It indicates how students take their responsibility and initiative for self-directed learning and organize their schedules. Cho and Heron ( 2015 ) argued that learner autonomy in online courses influences student experiences and satisfaction.
2.2 Measuring student satisfaction in online courses
Student satisfaction is an essential indicator of students’ overall academic experiences and achievement (Virtanen et al. 2017 ). There are different instruments to measure student satisfaction in an online environment. Using survey questionnaires is generally standard practice for measuring learner satisfaction. Over the years, a variety of tools such as Course Experience Questionnaire (Ramsden 1991 ), National Student Survey (Ashby et al. 2011 ) and Students’ Evaluations of Educational Quality (Marsh 1982 ), were developed and used to measure student satisfaction. The Satisfaction of Online Learning (SOL) is an instrument that was established to measure student’s satisfaction in online mathematics courses (Davis 2017 ). It consisted of eight specific components, comprising of effectiveness and timeliness of the feedback, use of discussion boards in the classroom, dialogue between instructors and students, perception of online experiences, instructor characteristics, the feeling of a learning community and computer-mediated communication. The Research Initiative for Teaching Effectiveness (RITE) developed an instrument that focussed on the dynamics of student satisfaction with online learning (Dziuban et al. 2015 ). RITE assessed two main components namely, learning engagement and interaction value and, encompasses items such as student satisfaction, success, retention, reactive behaviour patterns, demographic profiles and strategies for success. Zerihun et al. ( 2012 ), further argued that most assessments of student satisfaction are based on teacher performance rather than on how student learning occurred. Li et al. ( 2016 ) used the Student Experience on a Module (SEaM) questionnaire, where questions were categorized under three themes, to explore the construct of student satisfaction. The three themes contain inquiries related to the (1) overall module, (2) teaching, learning and assessment and (3) tutor feedback.
2.3 Learner engagement in online courses
One of the critical elements affecting the quality of online education is the need to ensure that learners are effectively and adequately engaged in the educational process (Robinson and Hullinger 2008 ; Sinclair et al. 2017 ). Learner engagement refers to the effort the learner makes to promote his or her psychological commitment to stay engaged in the learning process to acquire knowledge and build his or her critical thinking (Dixson 2015 ). It is also associated with the learner’s feeling of personal motivation in the course, to interact with the course contents, tutors and peers, respectively (Czerkawski and Lyman 2016 ). There are different models to measure learner engagement in learning contexts. Lauría et al. ( 2012 ) supported the fact that the number of submitted assignments, posts in forums, and completion of online quizzes can quantify learner’s regularity in MOOCs. Studies using descriptive statistics reported that consistency and persistence in learning activities are related to learner engagement and successful performance (Greller et al. 2017 ). Learner engagement is also about exploring those activities that require online or platform presence (Anderson et al. 2014 ). Those online activities can be in the form of participation in discussion forums, wikis, blogs, collaborative assignments, online quizzes which require a level of involvement from the learner. Lee et al. ( 2019 ) reported that indicators of student engagement, such as psychological motivation, peer collaboration, cognitive problem solving, interaction with tutors and peers, can help to improve student engagement and ultimately assist tutors in effective curriculum design.
2.4 Measuring learner engagement in online courses
Kuh ( 2003 ) developed the National Survey of Student Engagement (NSSE) benchmarks to evaluate students’ engagement through their skills, emotion, interaction and performance, applicable mainly to the traditional classroom settings. Another model relevant to the classroom environment is the Classroom Survey of Student Engagement (CLASSE) developed by Smallwood ( 2006 ). The Student Course Engagement Questionnaire (SCEQ) proposed by Handelsman et al. ( 2005 ), uses the psychometric procedure to obtain information from the students’ perspective to quantify students’ engagement in an individual course.
Roblyer and Wiencke’s ( 2004 ) proposed the Rubric for Assessing Interactive Qualities of Distance Courses (RAIQDC) which was designed as an instructive tool, to determine the degree of tutor-learner interactivity in a distance learning environment. Dixson ( 2010 ) developed the Online Student Engagement (OSE) scale model using the SCEQ model of Handelsman et al. ( 2005 ) as the base model. It aimed at measuring students’ engagement through their learning experiences, skills, participation, performance, and emotion in an online context. Dixson ( 2015 ) validated the OSE using the concept of behavioural engagement comprising of what was earlier described as observational and application learning behaviours. Dixson ( 2015 ) reported a significant correlation between application learning behaviours and OSE scale and a non-significant association between observation learning behaviours and OSE. Kahu ( 2013 ) critically examined student engagement models from different perspectives, namely behavioral, psychological, socio-cultural and holistic perspective. While the framework proposed is promising for a holistic approach to student engagement in a broader context of schooling, the OSE model as proposed by Dixson ( 2015 ) aligns quite well with the conceptual arguments of Kahu ( 2013 ) in the context of students’ engagement in online courses.
Gelan et al. ( 2018 ) measure online engagement by the number of times students log in the VLE to follow a learning session. They also found that students who tend to show higher regularity level in their online interaction and by attending more learning session were successful, compared to non-successful students. Ma et al. ( 2015 ) used learning analytics to track data related to teaching and learning activities to build an interaction-activity model to demonstrate how the instructor’s role has an impact on students’ engagement activities. An analysis of student emotions through their participation in forums and their performance in online courses can serve as the basis to model student engagement (Kagklis et al. 2015 ). They further observed that the students’ participation in forums was not directly associated with their performances. The reason was that most students preferred to emphasize working on their coursework as they will be given access to their exam, upon completion of a cumulative number of assignments and obtaining their grades. Therefore, although students tend to slow down their participation, they were still considered engaged in the online course.
Activity-based learning is an approach where the learner plays an active role in his or her learning through participation, experimentation and exploration of different learning activities. It involves learning-by-doing, learning-by-questioning and learning-by-solving problems where the learners consolidate their acquired knowledge by applying their skills learnt in a relevant learning situation (Biswas et al. 2018 ). These activities can be in the form of concept mapping, written submission and brainstorming discussions (Fallon et al. 2013 ). The study of Fallon et al. ( 2013 ) used the NSSE (National Survey of Student Engagement) questionnaire to measure and report on students’ engagement in learning materials and activities. They found encouraging results whereby they could establish that students responded positively to the activity-based learning approach, and there has been an enhancement in students’ participation and engagement. In line with this, Kugamoorthy ( 2017 ) postulated that the activity-based learning approach has motivated and increased student participation in learning activities as well as improved self-learning practices and higher cognitive skills. Therefore, student participation in activity-based learning model encourages students to think critically and develop their practical skills when they learn actively and comprehensively by involving cognitive, affective and psychomotor domains.
2.5 Student performances, satisfaction and their engagement in online courses
Research has demonstrated that activities that encouraged online and social presence, enhance and build learner confidence and increase performance are critical factors in engagement (Anderson et al. 2014 ; Dixson 2015 ). Furthermore, Strang ( 2017 ) found that when students are encouraged to complete online activities such as self-assessment quizzes, this promotes their learning and engagement and hence result in higher grades. Tempelaar et al. ( 2017 ) postulated that factors such as cultural differences, learning styles, learning motivations and emotions might impact on learner performances. Smith et al. ( 2012 ) deduced that students’ pace of learning and engagement with learning materials are indicators of their performance and determinants of learning experience and satisfaction. Macfadyen and Dawson ( 2012 ) found that variables such as discussion forum posts and completed assignments, can be used as practical predictors of learner performance, and thus can be used to help in learners’ retention and in improving their learning experiences. Pardo et al. ( 2017 ) utilized self-reported and observed data to investigate they can predict academic performance and understand why some students tend to perform better. They used a blended learning module the collected data related to students’ motivational, affective and cognitive aspects while observed data was related to students’ engagement captured from activities and interactions on learning management system. They deduced that students adopting a positive self-regulated strategy participated more frequently in online events, which could explain why some students perform better than others.
3 The research context
The module that was selected for this study was a first-year online module offered to students of the first year across disciplines. The module used an activity-based learning design consisting of nine learning activities. There were no written exams, and the first eight learning activities counted as continuous assessment, and the ninth activity counting as an end of module assessment. The module focused on the learning-by-doing approach, through authentic assignments such as developing a website, use an authoring tool, engage in critical reflection through blogging and YouTube video posts, general forum discussions as well as drill and practice questions such as online MCQs. The learning design principle that guided the pedagogical approach was the knowledge acquisition, application and construction cycle through sharing & reflective practice (Rajabalee et al. 2020 ). Although the module was fully online, it is necessary to point out, that not all of the learning activities necessitated persistent online presence for completion. For instance, students could download specific instructions from the e-learning platform, carry out the learning activities on their laptops, and then upload the final product for marking. The students further completed an end-of-module feedback activity using an instrument designed by the learning designers. The questions in the feedback activity were mainly open-ended and were in line with the OSE questionnaire and the Sloan instrument to measure student satisfaction in online courses. The approach was not survey based, but mainly taking a more in-depth qualitative approach as proposed by Kahu ( 2013 ). In this study, the research questions are set as follows:
To what extent does performances and engagement of students impact on students’ satisfaction in the online module.
How did students feel concerning the delivery of the module, their learning outcomes and their overall experience?
4 Methodology
The approach was to engage in an exploratory research study. The aim was to retrieve and analyze the data collected and accessible for an online module through the application of descriptive learning analytics. Such data are related to student satisfaction, their reported engagement in the online module and their overall performances. This study was based on the actual population of students who enrolled on the module. Consequently, there was no sampling done. Enrolment was optional as the module was offered as a ‘General Education’ course to first year students. It was open to students in all disciplines. All the students come from the national education system of Mauritius having completed the Higher School Certificate. The age group of the students were between 19 and 21. The student feedback was an integral component of the module and counted as part of a learning activity. Students who followed this module had initially agreed that information related to their participation and contributions in the course be used for research purposes in an anonymous manner. All student records were completely anonymized prior to classification and analysis of data.
4.1 Profile of participants
The students came from different disciplines, as highlighted in Table 1 . All participants had the required digital literacy skills, and they have followed the Information Technology introductory course as well as the national IC3 (Internet and Core Computing Certification) course at Secondary Level. Seven tutors and students facilitated the module with student groups ranging from 100 to 130 per group. The role of the tutors was mainly to act as a facilitator for the learning process and to mark learning activities and to provide feedback to the students. The Table 1 below, contains appropriate information about the participants across disciplines and gender for the module. Table 2 provides the information about the 179 students who did not complete the student feedback activity of the module.
4.2 Methods
This research used a mixed-method approach, given the nature of the research question. The primary method was quantitative data-gathering and analysis through measures of the degree of association between variables. It was also essential to process qualitative data that was available through student feedback. The qualitative research studied student satisfaction and perceptions concerning their online engagement via the end of module feedback questions. The quantitative part mainly focused on applied statistics such as t-tests and correlation testing to find the relationships between variables such as learner engagement, performance and level of participation. The quantitative aspects of the analysis were used in conjunction with the findings from the qualitative research analysis to understand better the underlying issues and theoretical underpinnings related to the learning environment and the learning processes of the students.
4.3 Student performance model
The Student Performance Model in this research has been initially conceptualized in line with the literature as a function of engagement, satisfaction and continuous assessment marks. The continuous assessment consisted of eight learning activities as follows:
The final assessment (activity 9) was a mini project where students were expected to apply the knowledge acquired through the continuous learning activities (1–8). Each student mark is moderated by another tutor in an independent manner as per the regulations of the University.
4.4 Defining and measuring student engagement
The literature reports several ways to measure students’ engagement in classroom settings as well as in online learning environments. The Online Student Engagement (OSE) questionnaire is one such instrument. However, it is a self-report of students’ perceived engagement done in survey style using a Likert scale. For the current module, there were two constraints to apply to the OSE to determine the students’ perceived engagement. The first constraint was that the module was not running in an experimental context. Therefore, at the time of conception and delivery, it was not predetermined that student engagement would be a variable to be measured. The second constraint was that the module followed the activity-based learning design model. The OSE mainly seeks feedback from students where the classic e-learning model is applied where the content is at the heart of the learning process. For the current module, the researchers had to adopt a different approach to extract reported student engagement data, from the end of module feedback activity.
4.5 Measuring student satisfaction through the end of module feedback activity
The course designers, therefore, wanted a different way for constructive feedback to be given by students through the elaboration of a set of open-ended guided questions. The students had to report on their experiences in the course from (i) the learning outcomes achievement perspective, (ii) the learner support processes including tutor and peer support (iii) their learning strategies and ways of tackling the different learning activities, and (iv) learning difficulties encountered and how they engaged in resolving and overcoming such challenges, in line with the Sloan Consortium Quality in Online Education Framework (Moore 2009 ). From such type of feedback, the tutoring team and the course designers would be able to understand better how the students engaged in the course from a qualitative perspective, and what were their satisfaction levels after completing the module.
Such information was therefore obtained mainly in qualitative form as students would mostly narrate about their learning experiences in the course. There are a series of approaches for qualitative data analysis, which process data sets through a systematic review. For this particular research, there were three possibilities in terms of the study of the qualitative data gathered through the feedback questions given to the students, namely grounded theory, phenomenology and content analysis. After careful consideration concerning the research questions and the literature, content analysis was deemed more appropriate for this research as it is a method of analyzing data which are obtained or collected from open-ended questions, surveys, interviews and observations (Creswell 2009 ). It uses a systematic approach when analyzing contents and documents.
For this study, deductive content analysis was used as a research approach to explore the learners’ feedback and experiences and to make meaning to the data. Firstly, concerning the engagement of students, the aim was to extract relevant meaning from data that could form codes related to the Online Student Engagement (OSE) scale as defined in the literature. Secondly, codes were obtained concerning the data related to students’ satisfaction as described in the paragraph above from the responses received. Finally, there was a need to move to quantitative content analysis to be able to conduct descriptive statistical analyses to answer the relevant research question. Table 3 below highlights the related themes to group the codes for both the perceived students’ engagement and satisfaction.
4.6 Classification of student satisfaction and engagement levels
Based on the questions in the reflective activity that guided learners’ reflection for their feedback, the researchers established a classification for the perceived level of engagement and the perceived satisfaction level of the students. The instrument used is provided as an annex. As regards to student engagement, the overall engagement is defined as a combination of (i) the learning strategies employed by the student to complete the learning activities; (ii) the involvement of the student in peer and tutor communication; (iii) the achievement of the learning outcomes reflected in their performances; and (iv) their ability to apply their knowledge acquired to demonstrate skills and competencies. They were used to study perceptions of learners in the online course and to describe their level of satisfaction, as shown in Tables 4 and 5 below. The researchers adopted single coding as the coding approach. However, where uncertainties occurred or in cases of ambiguity, the tutor group validated these elements, including the themes that emerged from the coding process. In this process, therefore, inter-coder reliability could not be calculated. Single-coder reliability has been argued by Potter and Levine-Donnerstein ( 1999 , p. 265) to be more reliable when the complexity of the task is low as compared to high complexity tasks where multiple-coder approach would be more reliable. In this research, the coding process was not complicated, as it mainly related to codes and themes established from student satisfaction and their engagement from two well-defined instruments from the literature such as the OSE and the Sloan Quality Guidelines. The single-coder approach was, therefore justified in this case.
The classification and explanatory rubrics in Tables 4 and 5 below were established through consensus with the tutor team and taking into consideration the relevant literature on student satisfaction and engagement. To classify the level of each student, the extracted codes from each student entry were used as guideline to decide on the classification. Each theme as described in Table 3 above carries a maximum of 2 points and has been further classified as follows:
A score of 0 is set if theme is not relevant (i.e. there is no codes) to the feedback of the student.
A score of 1 if the theme is partly relevant (i.e. not more than half of the codes) to the feedback of the student.
A score of 2 if the theme is fully relevant (i.e. more than half of the codes) to the feedback of the student.
4.7 Limitations of the research
In this research, actual data that were available were retrieved and analyzed for a module that was not designed to be offered in an experimental context. Student feedback was therefore a classic process of questions elaborated by the learning design team to gather information to judge the learning experience of participants. While self-reporting tools like the Online Student Engagement (OSE) would have been helpful to compensate, the fact that the course had already taken place, meant that the OSE questionnaire was not administered beforehand. This deficiency was addressed through the student feedback data-collection which was designed during the courseware development process and was aligned with established models of student satisfaction that gathered information from the students with respect to their own perceived engagement in the course. Through a qualitative analysis obtained by coding the responses of the students, the issue of student engagement has been adequately addressed from that perspective. Another limitation relates to the number of students who completed the feedback activity. As the exercise was not compulsory, not all students completed the student feedback, so the coding and analysis of feedback is limited only to those who effectively responded. Sampling was not a significant concern here, as the research subjects were not selected through a sampling technique, but responses sought from whole cohort. The results of the research with respect to the questions where the student feedback is available cannot be generalized as being representative of the whole cohort and have to be interpreted with this constraint in mind. Finally, the findings of this research relate to a course which was designed to suit a diverse set of student profiles and specific findings cannot be considered to be applicable to other modules in different specific contexts and following different pedagogical designs.
5 Findings & Results
5.1 descriptive statistics.
Out of the 844 students, 179 did not participate in the feedback process, and consequently, there is no related data for them to compute their perceived satisfaction and their reported engagement level. As can be seen from the tables below, the majority of students reported being moderately satisfied (44.7%). On the reported engagement level, 32.2% reported being moderately engaged as compared to 29.4% and 17.2% who reported high and low engagement, respectively. Those that were missing have further been classified as ‘Not Reported’ for the and were excluded from further analysis.
The coding for the perceived satisfaction and reported engagement was done as per the themes in Table 3 . For each theme, the students’ feeling for each code was rated on a scale [0,1,2] and the rubric in Table 5 . A value of 0 relates to a reported low score of the feedback, 1 for an average rating, and 2 for high score feedback. A sum of the components is carried out to get the cumulative score for each set of themes under Engagement and Satisfaction. Given that Engagement had only four themes, the maximum possible score was eight while for satisfaction, the eight themes would cumulate to a maximum possible total of 16. The Skewness test (near to zero) and the Kurtosis value (−1, −0.9) for both variables reveals that the distribution can reasonably be assumed to follow a normal distribution (Tables 6 and 7 ).
The box plots below illustrate the distribution for the reported engagement and perceived satisfaction for this group concerning Gender and Discipline. In both plots, the median line for gender is lower for males.
The box plot below represents the distribution for the reported engagement and the perceived satisfaction of students of this cohort. The reported seems to be lower than the reported engagement levels.
5.2 RQ1: To what extent does performances and engagement of students impact on students’ satisfaction in the online module.
We test three hypotheses for this research question.
5.2.1 Hypothesis #1: There a significant difference between mean satisfaction levels and engagement level of students, within disciplines and from the gender perspective.
A one-way ANOVA was conducted to compare the mean satisfaction levels of students from different disciplines. Normality checks and Levene’s test were carried out, and the assumptions met. There was no significant difference in the perceived satisfaction of students across disciplines [F (4,660) = 0.098, p = 0.983]. Similarly, there were no significant differences between the reported engagement levels of student across disciplines. [F(4,660) = 0.355, p = 0.840]. Furthermore, there were no significant differences concerning gender for both the perceived satisfaction and the reported engagement level of the students in this cohort as per the ANOVA Table 8 below.
5.2.2 Hypothesis #2: There is a correlation between students’ satisfaction level and reported engagement level for the current cohort.
Correlation analysis was used to measure the degree association between the perceived satisfaction level and their reported engagement in the module. Since the reported engagement and the perceived satisfaction were inferred from the same feedback questionnaire, through different codes and themes, it is observed that there was a strong positive correlation between the two variables. The variance inflation factor (VIF) values nearing to 1 suggested that collinearity was not a problem as per the Table 9 below.
The scatter plot below illustrates the spread of values for the reported engagement and the perceived satisfaction of students.
From the figure, it can be deduced that the perceived satisfaction of a student in a module will depend on his or her reported engagement level. The more a student feels engaged in the course, he or she will be more satisfied. However, this deduction emanates from self-report instruments used by the student to report on his or her learning experiences.
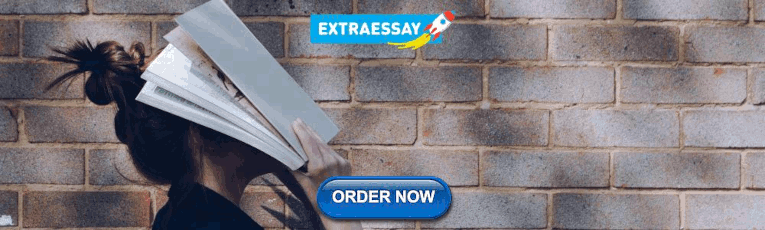
5.2.3 Hypothesis #3: There is a correlation between students’ satisfaction level and their performances.
The scatter plot below illustrates the mark distribution for both the continuous learning activities and the final learning activity with respect to the satisfaction of the students.
Given that final performance marks and the reported satisfaction could be assumed to follow a normal distribution. In contrast, the continuous learning marks followed an asymmetric distribution, and two separate correlation tests were carried out. The Pearson correlation coefficient was calculated for the final performance and reported satisfaction and the Kendal Tau non-parametric test for the continuous assessment and the reported satisfaction. The correlations for both cumulative assessment and final mark with the reported satisfaction is significant ( p < 0.01) and this has been shown in Tables 10 and 11 below.
5.3 RQ 2: How did students feel, concerning the delivery of the module, their learning outcomes and their overall experience?
Only 665 students provided their feedback in a narrative as per the questionnaire provided to them. The rationale of this qualitative part of the study was to examine the relationships between students’ perception of their learning experience towards this module and their performance levels. The overall performance in the final assessment demonstrated that high performers were 22.4% ( n = 149), average performers were 63.8% ( n = 424), and low performers were 13.8% ( n = 92) of the students. In terms of gender, 35.6% ( n = 237) of the students who provided their feedback were male, and 64.4% ( n = 428) were female. Feedback data gathered was then organized and coded. Overall, the total number of 2366 of codes were recorded. While high performers in the final assessment contributed an average of 3.9 total codes, average performers contributed an average of 3.5, and low performers contributed an average of 3.3 total codes. Table 12 contains a descriptive summary of each code.
Table 13 explains how each level of students in the final assessment reported their feedback under the different codes devised. Hence, the coded statements were compared with the students’ performances from each level (High, Average, Low). For example, out of 208 codes categorized as ‘IT skills acquired’, 25% were reported by high performers in the final assessment. In contrast, 62.02% were reported by average performers, and low performers reported 12.98%.
Table 14 explains how each level of students in the cumulative assessment activities reported their feedback under the different codes devised. The coded statements were compared with the students’ performances in Activities 1 to 8 from each level (High, Average, Low). For example, out of a total of 130 codes categorized as ‘Developed learner autonomy’, 76.15% of the codes were reported by high performers in the cumulative assessment. In contrast, 23.08% were reported by average performers, and low performers reported 0.77%.
Table 15 explains how students from each discipline reported their feedback under the different codes devised. The coded statements were compared within disciplines. For example, out of a total of 192 codes categorized as ‘had a negative feeling about the course’, both Engineering and Science disciplines reported 28.13% of the codes. In contrast, 23.44% were reported by Law and Management, Humanities reported 16.67%, and Agriculture disciplines reported 3.65%.
The pie chart below illustrates the code distributions with respect to the % of occurrences in the feedback.
20.4% of reported codes demonstrated that students had built an overall positive perception from the module, and 18.9% were related to having attained positive achievement. Most of the themes, (except ‘negative feelings about the course’, ‘mixed feeling and experiences’ and ‘encountered technical difficulty’) would contribute to give a positive indication of perceived satisfaction in the course
“…The experience, skills and knowledge that I have acquired in this module will no doubt be of great help to me in the future. I am already applying some of the things I have learned here in my studies, for example, concept mapping. I learned from the you-tubing activity that I can actually create simple animations to convey information in a more interesting manner... There is so much more to learn about educational technologies, but so far this module has been a very enriching experience …” (Student B4157, female, Science discipline, High performer category in Cumulative Assessment, Final Assessment = 6.5, Cumulative Assessment = 8.7)
“…This is one of the modules I have mostly appreciated during my 1st year in the university… During the course, I have been able to learn numerous things … However, this has not just been a module, it has been a self-development course as far as I am concerned; Through this coursework, I have gained the experience needed to efficiently and effectively use technology, multimedia tools and employ modern ICT in education. As an end note, I would like to congratulate the members of the department for their excellent support, guidance and having offered us such a pleasant module to work on…” (Student B7772, male, Engineering discipline, Average performer category in Final Assessment, Final Assessment = 6, Cumulative Assessment = 8.125)
“…This module helps in widening our knowledge. It helps in making practical use of new assets that was once unused and unknown. E.g. the cartoon maker, multimedia assignments. Also, it is an interactive module where different people share their views. In this way students widen their knowledge as well as share their knowledge… Personally, I really learn a lot from this module. I got to explore my own hidden talents and discover new applications. I think this module will be a real help in the future…” (Student ID B2842, female, Science discipline, Low performer category in Final Assessment, Final Assessment = 4, Cumulative Assessment = 7.9)
8.8% of the 2366 codes related to the different ICT-related skills that students acquired in the module. While many of these related to the use of social media, forums as well as software and not excluding computer-mediated communication the code was named “IT skills acquired”
“…my idea of this module was plainly that I will get to learn new IT software… There are too many benefits I obtained from this module. I have also been able to use the software, apply IT to education, and it is fun as well as fruitful…” (Student B2480, female, Law & Management discipline, High performer category in Final Assessment, Final Assessment = 8, Cumulative Assessment = 8.225)
“…I think that this module has increased my creativity level, and my technology knowledge is broader than before. Also, through constantly editing my work on Microsoft word, this has improved my writing… To be able to work out the units, I have done some research on Google and gone through the given materials thoroughly…” (Student B6609, female, Engineering discipline, High performer category in Cumulative Assessment, Final Assessment = 7, Cumulative Assessment = 7.8125)
The above comment highlights how ICT skills such as repeated use of word processing software which seems a simple process could result in improved writing skills, and that Google search was also a skill that was valued by students. In contrast, in the comment below, it was evident that for other students, the development of advanced digital skills was valued and welcome.
“…I have also developed the skills to create and manage educational technologies materials including websites and cartoon software. Through this coursework, I have gained the experience needed to efficiently and effectively use technology, multimedia tools and employ modern ICT in education…” (Student B7772, male, Engineering discipline, Average performer category in Final Assessment, Final Assessment = 6, Cumulative Assessment = 8.125)
“…Actually, it helped me in using and managing technological processes… this was an interesting module which helped me to improve my learning skill technologically…” (Student B4126, female, Science discipline, Average performer category in Cumulative Assessment, Final Assessment = 6.5, Cumulative Assessment = 6.3125)
“…this module was a challenge to me, but I ended up enjoying the different activities offered. It helped in improving my IT skills…” (Student A2406, female, Humanities discipline, Low performer category in Cumulative Assessment, Final Assessment = 7, Cumulative Assessment = 4.8625)
Students in their feedback further reported critical thinking, creativity and practical skills as well as learner autonomy. While 8.1% were reported as ‘Developed creative/practical skills’, 2.9% were recorded as ‘Developed critical thinking/reflective ability’ and 5.5% as ‘Developed learner autonomy’ of the total codes. Critical thinking, creativity and acquisition of practical skills were the core competencies to be developed for this module.
“…this module has been an aid to me in developing the skill of being able to criticize a piece of my own work or others; to be analytical about every simple details, to be able to make a constructive opinion. As benefit, I have also much appreciated the fact that all the basic knowledge/information for the different tasks were always already provided…” (Student B9533, female, Science discipline, High performer category in both Final and Cumulative Assessment, Final Assessment = 7.5, Cumulative Assessment = 7.4)
“…With the various activities proposed, I came to learn to analyze things with a more critical eye and as far as I could, provide constructive criticism on several aspects which stood out to me. This not only helped me in this particular module but in my other classes as well with quite a few topics overlapping and which gave me an edge and a number of different viewpoints on these…” (Student C0295, female, Law & Management discipline, Low performer category in Final Assessment, Final Assessment = 5, Cumulative Assessment = 7.5625)
The fact that learners were in an online module, practically on their own with minimum tutor interaction, required them to take charge of their learning process. Students reported how they had to solve problems on their own, including the planning of the time to work on the module to meet deadlines and to make sufficient effort to acquire the minimum required competencies and to ensure successful completion of the module.
“…Educational technology has indeed increased my knowledge as well as improved my learning skills. It indeed motivated me in my learning process as one can learn at his own pace and at any time within the day. It helped me to assume my responsibility as a student and to submit assignments within the given delay time…” (Student B1497, female, Engineering discipline, High performer category in Cumulative Assessment, Final Assessment = 6, Cumulative Assessment = 8.475)
“…I am now definitely a fanboy of the e-learning system. The reasons are flexibility; work at your own pace, at your own time and in your own way!” (Student B0167, male, Science discipline, High performer category in Final Assessment, Final Assessment = 8.5, Cumulative Assessment = 6.9375)
“…One benefit from this module was that I was able to do all the work at my own pace and feel free to do it whenever I had time. There was no constant pressure, there was a deadline to be respected, and I only had to manage my time to submit my work, and it was done without any pressure…” (Student B1779, female, Humanities discipline, Average performer category in Cumulative Assessment, Final Assessment = 7, Cumulative Assessment = 7.0375)
“…Taking on the role of leader for group work get to you to mature a lot and be more responsible, but it takes a lot of hard work… I never thought I would know so much one day…developing self-discipline…” (Student A2640, female, Law & Management discipline, Low performer category in Cumulative Assessment, Final Assessment = 7, Cumulative Assessment = 4.7625)
As it can be seen by the above comments, depending on learner preferences, self-paced independent learning is often welcome by students, and the need to assume responsibilities is an interesting value proposition that can result in more autonomy and commitment of the learner. The other aspect, which was prevailing among the codes obtained, was “Learning Strategies – Personal development” with 6.1% and ‘Social interaction/communication’ which was 14.4% of the codes. Learners reported how they tackled the different learning activities, and how they overcame any barrier and interacted with other learners and tutors through the forum discussion for support (Figs. 1 , 2 , 3 , 4 and 5 ).
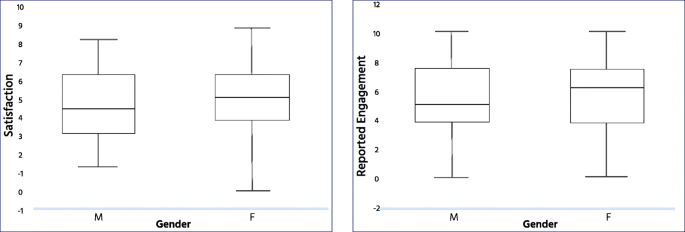
Distribution of perceived satisfaction and reported engagement w.r.t Gender
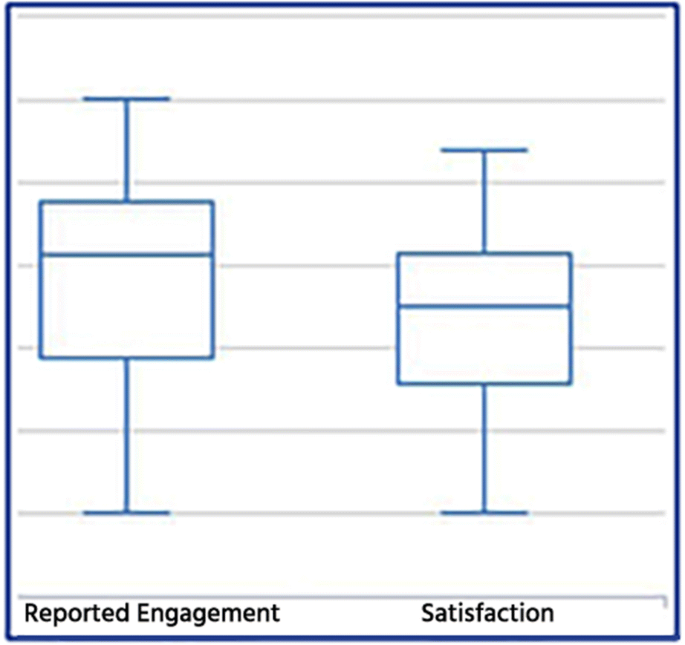
Distribution of perceived satisfaction and reported engagement
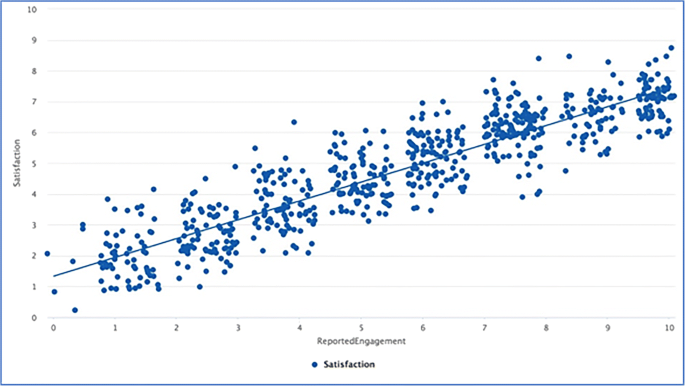
Scatter plot – reported engagement v/s perceived satisfaction
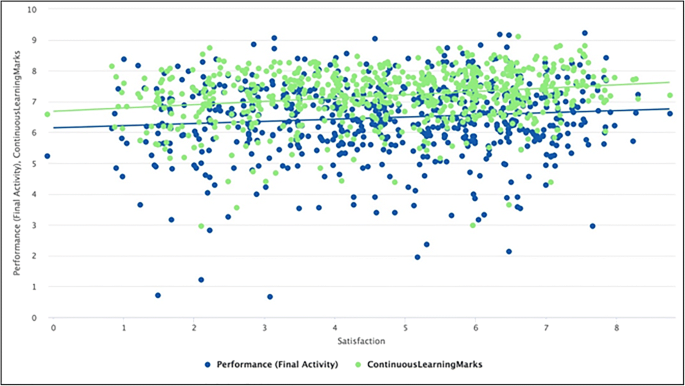
Scatter plot for Cumulative Assessment and Final Assessment w.r.t students’ satisfaction
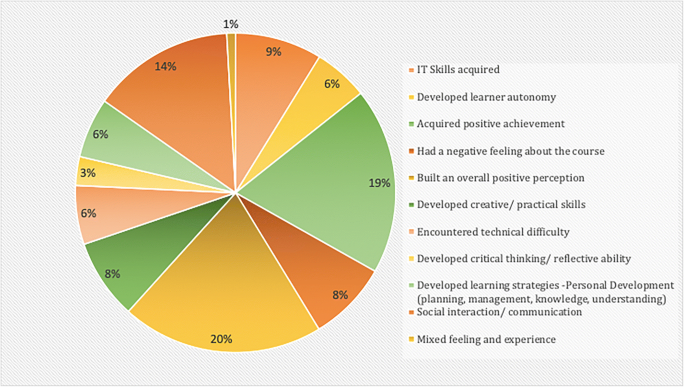
Number of occurrences per code
“…This module has taught me many things, especially in terms of time management and developing a pedagogical approach to my work. This is something I never really paid attention to before working on Educational Technologies assignments. For once I could put myself in my teachers and lecturers’ places and comprehend the different approaches they have to take when explaining a certain topic!” (Student B2456, female, Agriculture discipline, High performer category in Cumulative Assessment, Final Assessment = 7, Cumulative Assessment = 7.375)
“…the module helps us in our personal development as well as introduces us to what is necessary in education if ever, we are interested in the teaching field…” (Student ID A4709, female, Science discipline, High performer category in Final Assessment, Final Assessment = 7.5, Cumulative Assessment = 7.5625)
“…This module has given me great experience…learnt strategies before doing any journals like I have done the outlines first in order to avoid messing the ideas and go out of subject…” (Student ID B8920, female, Humanities discipline, Low performer category in both Final and Cumulative Assessment, Final Assessment = 2.567, Cumulative Assessment = 4.0625)
“…Each weekend, I dedicated 4 hours to do the homework… I planned my work on Saturdays and carried it out on Sundays. I gained better planning and better time management skills…” (Student A4901, female, Law & Management discipline, Average performer category in Cumulative Assessment, Final Assessment = 5, Cumulative Assessment = 6.125)
The students described techniques that helped them to learn and achieve the outcomes. As can be seen, by the above comment, a feeling of fun was apparent given that students had to learn in different ways such as inquiry-based learning which gave them a degree of flexibility and variety of learning processes. E.g. there were consequential learning outcomes, which resulted in a particular competency about dealing with, different image formats. Furthermore, as could be seen in many comments, students understood the concept of “just-in-time” learning where they could acquire specific skills through research on Google. They could even view tutorials on YouTube at the time of execution of a particular task related to an assignment (e.g. conversion to ZIP format before uploading an assignment on the platform).
8.1% of the codes, however, mentioned some form of negative feeling and inadequate learning experience overall. These were mainly related to students not finding the pertinence of the module, lack of digital skills, or who had communication issues with peers and tutors. At the same time, another 6% of the codes highlighted technical difficulty experienced by students due to poor Internet connection or difficulty in solving technical issues including installation and configuration of software or uploading of their assignments.
“…I did encounter several difficulties. I would not understand how to use a program or as for the eXe software, I could not save my works…at times I had to do the activities again and again. It was tiring…” (Student B2180, male, Engineering discipline, High performer category in Cumulative Assessment, Final Assessment = 6, Cumulative Assessment = 7.8)
“…it is difficult for me to complete it alone, I am not used to the different tool on the computer, sections has been more complicated, difficult to go throughout the steps without a basic knowledge of how to use the different functions on the screen of the computer…” (Student B5681, female, Humanities discipline, Average performer category in Cumulative Assessment, Final Assessment = 6.3, Cumulative Assessment = 6.375)
“… less teacher-student interaction, less student-student interaction, in all there is a lack of communication, there were lack of feedback from our tutors about the learning activities being done. No result of how we were working…” (Student B2107, male, Engineering discipline, Average performer category in Final Assessment, Final Assessment = 6, Cumulative Assessment = 6.6875)
“…Trouble with assignment…It was a disaster…I did the activities 1 to 4, 9 and 13 and even the feedback I am not sure what I did wrong because this site holds record of only 2 of my uploads…I think it is lacking in the communication department...I think that the forum is not effective…” (Student B3527, female, Humanities discipline, Low performer category in Cumulative Assessment, Final Assessment = 6, Cumulative Assessment = 4.9875)
The codes representing a negative feeling and the occurrence of technical difficulties can provide interesting insights into either a range of pre-emptive or just-in-time measures that can be taken by course developers, tutors and administrators to provide timely support to the learners during the course itself. This may significantly improve the learning experience and overall perception of learners as if they are detected early, they can prevent dropouts, frustrations and poor performances from occurring. However, the positive side concerning the current module is that the codes representing negative feelings and technical difficulties represent 14.1% only of the total number of codes generated. Many of those who expressed that they had technical difficulties also highlighted what they did to overcome them. It is important to mention however, that in this module, the experience of technical difficulties and developing the necessary skills to deal with, then are part of the core learning outcomes, as many educators precisely abandon technology or show reluctance to embrace technology-enabled teaching precisely because of their lack of confidence in their own digital skills. Finally, 0.9% of total codes were reported as ‘Mixed feeling and experience’ where the students had neither a positive nor a negative experience in the course.
“…even if instructions were given, I used to find some activities really difficult…Overall it was a fun as well as difficult experience…” (Student A1261, female, Humanities discipline, High performer category in Cumulative Assessment, Final Assessment = 6.5, Cumulative Assessment = 7.175)
“I had difficulty to meet the deadlines as I was more stressed by my first-year core modules. I was also not very familiar with a lot of the computer directed tasks… I am quite satisfied with the work…” (Student A2967, female, Humanities discipline, High performer category in Final Assessment, Final Assessment = 7.5, Cumulative Assessment = 6.9375)
“At the beginning of the module, I find quite interesting. Then, it was very tough… The storyboard was very interesting, yet I found quite problems on drawing the storyboard but fortunately, after many difficulties I succeeded in doing it…” (Student B6023, female, Law & Management discipline, Average performer category in Final Assessment, Final Assessment = 7, Cumulative Assessment = 7.225)
“So, the only thing I can finally say is that educational technology’s module is neither so difficult nor easy…” (Student B3016, female, Humanities discipline, Low performer category in Final Assessment, Final Assessment = 5, Cumulative Assessment = 7.95)
In summary, while there are some cases where students still complained about the lack of tutor responses and interactions while other students commended the independence they were given and found tutors’ support to be more than adequate. It further emerged that the majority of the students irrespective of overall performances reported a high level of satisfaction. The level of satisfaction was, therefore, not directly related to the performances as it could be observed that high performers could also express mitigated feelings. In contrast, some low performers reported a positive sense of satisfaction.
6 Discussion
Student engagement is an important issue in higher education and has been the subject of interest from research, practitioner and policy-making perspectives. There are different models of engagement that have been studied and proposed. The reliability of self-reported data of students and the lack of a holistic model incorporating multiple dimensions have been the subject of critical analysis by researchers (Kahu 2013 ). The issue of engagement has also been widely discussed in the context of online learning and different instruments which are mainly survey-based such as the OSE model (Dixson 2015 ) have been developed. The challenge of a reliable model to define student engagement in online courses remains based on the findings of this research as well. In terms of practical course design, there is a need for learning designers to define beforehand, the student engagement model that would be applied prior to the start of a course, and then conceive their learning activities accordingly.
A positive, but weak association was established between reported engagement with respect to the continuous learning marks and the performances in the final activity. If reported engagement in this context can be defined as the extent to which the student felt connected and committed to the module, it does not necessarily imply that their performances (by way of marks achieved) would reflect that. The findings related to the association of the reported engagement of students concerning the different learning domains, however, contradict the findings of Dixson ( 2015 ) who reported a significant correlation between application learning behaviours and OSE scale and a non-significant correlation between observation learning behaviours and OSE. Regarding the reported satisfaction and engagement, it was observed that the higher level of reported engagement resulted in higher levels of satisfaction from the students. However, since the same feedback instrument was used to derive codes related to engagement and satisfaction, this might explain the relatively strong association between the two. This finding is however coherent with the claims of Hartman and Truman-Davis ( 2001 ) and Dziuban et al. ( 2015 ) who established that there is a significant correlation in the amount and quality of learner interaction with learner satisfaction.
It was also observed that tutor support had played an important part in shaping the students’ level of satisfaction as some students expressed negative feelings when the tutor support was not adequate. Proper academic guidance, as reported in the literature, is a contributing factor in learners’ performances, achievement and satisfaction (Earl-Novell 2006 ). While it has been established in the literature that in general student satisfaction is not linked to performances, a significant positive correlation was observed in this study between perceived satisfaction and both continuous learning marks and the final performance marks. However, the degree of association, as measured by the correlation coefficient (.108) was weak. In this respect, further analysis through linear regression revealed that perceived satisfaction was not a significant predictor of performances.
If the intention behind the adoption of e-learning is to improve the teaching and learning experiences of on-campus students as argued by Moore ( 2009 ) and Abdous ( 2019 ), institutional policies will need to focus mainly on digital learning and technology-enabled pedagogies. This is in line with the critical approach taken by Kahu ( 2013 ) arguing that student engagement should be about developing competencies in a holistic manner goes beyond the notion of just ‘getting qualifications’. In this research, the activity-based learning design was at the heart of the offer of such a course. The Internet acted mainly as a means to transform the teaching and learning process (Nichols 2003 ) as skills acquisition, and competency-based outcomes were critical to the learning design. The findings show that irrespective of the overall performances of the students, the majority of them appreciated the learning design, the educational experience, but not necessarily the fact that it was online. Therefore, such approaches mean that institutional leaders should reflect on how to design online courses using competency-based design to better engage students to improve student satisfaction and overall experiences. In that context, there is a need ensure that learning design guidelines is at the heart of the e-learning related policies. The core idea is to engage in a paradigm shift from teacher to learner-centred methods. Learner-centred approaches further imply that the right balance has to be established between mass-customization (one-size-fits-all), and personalized learner support within such environments. Learner support is an essential aspect of quality assurance to be taken into account in technology-enabled learning policies (Sinclair et al. 2017 ).
In this research, descriptive analytics was used to analyze data related to student performances, satisfaction and reported engagement. In line with Macfadyen and Dawson ( 2012 ), we can see a learning analytics approach has helped to give some constructive meaning to the data gathered on the e-Learning platforms to understand better our students’ learning patterns and experiences. Therefore, learning analytics is an essential disposition that institutional e-learning policies have to consider. Sentiment analysis, for instance can add value to the learner support framework, as it allows the tutor(s) to focus his or her efforts on supporting primarily those who are experiencing difficulties while maintaining a minimum level of interaction with those independent learners. This argument has been supported in the literature by different authors (Lehmann et al. 2014 ; Tempelaar et al. 2015 ).
The module under study relied mainly on asynchronous tutor intervention when it comes to learner support. Such a model of tutor support has been predominant in online distance education (Guri-Rosenblit 2009 ). However, with the exponential development in Internet infrastructure and video conferencing technologies, real-time synchronous tutor intervention is more and more being adopted, giving rise to the concept of “Distributed Virtual Learning (DVL)”. DVL allows for tutor-student interaction in real-time, especially where students report problems, or when built-in analytics such as sentiment analysis can flag students who are at risk. The concepts that embody DVL have to be duly taken into consideration by policymakers.
7 Conclusion
From this research, it emerged that students’ satisfaction and their engagement are essential elements defining their learning experiences. Analysis of the feedback revealed that technical difficulties and lack of tutor support create a sense of frustration even if the student ultimately performs well. It is important that such emotions are captured just-in-time during the time the module is offered as timely action can then be taken to address student concerns. At a time, where institutions are moving to e-learning to ensure continuity of educational services, there are important policy implications for the longer-term effectiveness in terms of learning outcomes and student experience.
Abdous, M. (2019). Influence of satisfaction and preparedness on online students’ feelings of anxiety. The Internet and Higher Education, 41 , 34–44. https://doi.org/10.1016/j.iheduc.2019.01.001 .
Article Google Scholar
Allen, M., Bourhis, J., & Burrell, N. (2002). Comparing student satisfaction with distance education to traditional classrooms in higher education: A meta-analysis. American Journal of Distance Education., 16 (2), 83–97. https://doi.org/10.1207/S15389286AJDE1602_3 .
Anderson, A., Huttenlocher, D., Kleinberg, J. & Leskovec, J. (2014). Engaging with massive online courses. In Proceedings of the 23rd international conference on World wide web (pp. 687-698). ACM. https://doi.org/10.1145/2566486.2568042 .
Ashby, A., Richardson, J., & Woodley, A. (2011). National student feedback surveys in distance education: An investigation at the UK Open University. Open Learning: The Journal of Open, Distance and e-Learning., 26 (1), 5–25. https://doi.org/10.1080/02680513.2011.538560 .
Aung, J., & Ye, Y. (2016). The relationship between the levels of students’ satisfaction and their achievement at Kant Kaw education Center in Myanmar. Scholar: Human Sciences, 8 (1), 38 Retrieved from http://repository.au.edu/handle/6623004553/17994 .
Google Scholar
Biner, P., Dean, R., & Mellinger, A. (1994). Factors underlying distance learner satisfaction with televised college-level courses. American Journal of Distance Education., 8 (1), 60–71. https://doi.org/10.1080/08923649409526845 .
Biswas, A., Das, S., & Ganguly, S. (2018). Activity-based learning (ABL) for engaging engineering students. In Industry Interactive Innovations in Science, Engineering and Technology (pp. 601-607) . Singapore: Springer. https://doi.org/10.1007/978-981-10-3953-9_58 .
Book Google Scholar
Cho, M., & Heron, M. (2015). Self-regulated learning: The role of motivation, emotion, and use of learning strategies in students’ learning experiences in a self-paced online mathematics course. Distance Education., 36 (1), 80–99. https://doi.org/10.1080/01587919.2015.1019963 .
Creswell, J. (2009). Research design: Qualitative, quantitative, and mixed methods approaches (3rd ed.) . Thousand Oaks, CA: Sage Publications Retrieved from: http://www.drbrambedkarcollege.ac.in/sites/default/files/research-design-ceil.pdf .
Czerkawski, B. C., & Lyman, E. W. (2016). An instructional design framework for fostering student engagement in online learning environments. TechTrends, 60 (6), 532–539. https://doi.org/10.1007/s11528-016-0110-z .
Davis, A. (2017). Measuring student satisfaction in online mathematics courses--RESEARCH. Kentucky Journal of Excellence in College Teaching and Learning., 4 (2) Retrieved from: https://encompass.eku.edu/kjectl/vol14/iss/2 .
Dixson, M. (2010). Creating effective student engagement in online courses: What do students find engaging? Journal of the Scholarship of Teaching and Learning, pp. , 1–13 Retrieved from https://scholarworks.iu.edu/journals/index.php/josotl/article/view/1744 .
Dixson, M. (2015). Measuring student engagement in the online course: The online student engagement scale (OSE). Online Learning, 19 (4). https://doi.org/10.24059/olj.v19i4.561 .
Dziuban, C., Moskal, P., Thompson, J., Kramer, L., DeCantis, G., & Hermsdorfer, A. (2015). Student satisfaction with online learning: Is it a psychological contract? Online Learning, 19 (2). https://doi.org/10.24059/olj.v19i2.496 .
Earl-Novell, S. (2006). Determining the extent to which program structure features and integration mechanisms facilitate or impede doctoral student persistence in mathematics. International Journal of Doctoral Studies, 1 , 52–55. https://doi.org/10.2894/560 .
Ezugwu, A., Ofem, P., Rathod, P., Agushaka, J., & Haruna, S. (2016). An empirical evaluation of the role of information and communication Technology in Advancement of teaching and learning. Procedia Computer Science, 92 , 568–577. https://doi.org/10.1016/j.procs.2016.07.384 .
Fallon, E., Walsh, S., & Prendergast, T. (2013). An activity-based approach to the learning and teaching of research methods: Measuring student engagement and learning. Irish Journal of Academic Practice, 2 (1), 2. https://doi.org/10.21427/D7Q72W .
Gelan, A., Fastré, G., Verjans, M., Martin, N., Janssenswillen, G., Creemers, M., Lieben, J., Depaire, B., & Thomas, M. (2018). Affordances and limitations of learning analytics for computer-assisted language learning: A case study of the VITAL project. Computer Assisted Language Learning., 31 (3), 294–319. https://doi.org/10.1080/09588221.2017.1418382 .
Gillett-Swan, J. (2017). The challenges of online learning: Supporting and engaging the isolated learner. Journal of Learning Design., 10 (1), 20–30. https://doi.org/10.5204/jld.v9i3.293 .
Greller, W., Santally, M., Boojhawon, R., Rajabalee, Y., & Kevin, R. (2017). Using learning analytics to investigate student performance in blended learning courses. Journal of Higher Education Development–ZFHE, 12 (1) Retrieved from: http://www.zfhe.at/index.php/zfhe/article/view/1004 .
Guri-Rosenblit, S. (2009). Distance education in the digital age. Common Misconceptions and Challenging Tasks. Journal of Distance Education. Retrieved from: https://eric.ed.gov/?id=EJ851907
Handelsman, M., Briggs, W., Sullivan, N., & Towler, A. (2005). A measure of college student course engagement. The Journal of Educational Research., 98 (3), 184–192. https://doi.org/10.3200/JOER.98.3.184-192 .
Hartman, J., & Truman-Davis, B. (2001). Factors relating to the satisfaction of faculty teaching online courses at the University of Central Florida. Online education, 2 , 109–128.
Ismail, A. (2018). Empowering your students satisfaction with blended learning: A lesson from the Arabian Gulf University distance teaching and training program. International Journal of Information and Education Technology, 8 (2), 81–94. https://doi.org/10.18178/ijiet.2018.8.2.1019 .
Kagklis, V., Karatrantou, A., Tantoula, M., Panagiotakopoulos, C., & Verykios, V. (2015). A learning analytics methodology for detecting sentiment in student fora: A case study in distance education. European Journal of Open, Distance and E-learning., 18 (2), 74–94. https://doi.org/10.1515/eurodl-2015-0014 .
Kahu, E. (2013). Framing student engagement in higher education, studies in higher education, 38:5, 758-773, https://doi.org/10.1080/03075079.2011.598505 .
Kember, D., & Ginns, P. (2012). Evaluating teaching and learning: A practical handbook for colleges, universities and the scholarship of teaching. Routledge., 66 , 375–377. https://doi.org/10.1007/s10734-012-9557-9 .
Kugamoorthy, S. (2017). Activity based learning: An effective approach for self-regulated learning practices. Department of secondary and tertiary education, faculty of education. The Open University of Sri Lanka
Kuh, G. (2003). What we're learning about student engagement from NSSE: Benchmarks for effective educational practices. Change: The Magazine of Higher Learning., 35 (2), 24–32. https://doi.org/10.1080/00091380309604090 .
Lauría, E., Baron, J., Devireddy, M., Sundararaju, V. and Jayaprakash, S. (2012). Mining academic data to improve college student retention: An open source perspective. In Proceedings of the 2nd International Conference on Learning Analytics and Knowledge (pp. 139-142). ACM. https://doi.org/10.1145/2330601.2330637 .
Lee, J. (2010). Online support service quality, online learning acceptance, and student satisfaction. The Internet and Higher Education, 13 (4), 277–283. https://doi.org/10.1016/j.iheduc.2010.08.002 .
Lee, J., Song, H., & Hong, A. (2019). Exploring factors, and indicators for measuring students’ sustainable engagement in e-learning. Sustainability, 11 (4), 985. https://doi.org/10.3390/su11040985 .
Lehmann, T., Hähnlein, I., & Ifenthaler, D. (2014). Cognitive, metacognitive and motivational perspectives on preflection in self-regulated online learning. Computers in Human Behaviour, 32 , 313–323. https://doi.org/10.1016/j.chb.2013.07.051 .
Li, N., Marsh, V., & Rienties, B. (2016). Modelling and managing learner satisfaction: Use of learner feedback to enhance blended and online learning experience. Decision Sciences Journal of Innovative Education., 14 (2), 216–242. https://doi.org/10.1111/dsji.12096 .
Ma, J., Han, X., Yang, J., & Cheng, J. (2015). Examining the necessary condition for engagement in an online learning environment based on learning analytics approach: The role of the instructor. The Internet and Higher Education, 24 , 26–34. https://doi.org/10.1016/j.iheduc.2014.09.005 .
Macfadyen, L., & Dawson, S. (2012). Numbers are not enough. Why e-learning analytics failed to inform an institutional strategic plan. Educational Technology & Society, 15 (3), 149–163 Retrieved from https://www.jstor.org/stable/pdf/jeductechsoci.15.3.149.pdf .
Markova, T., Glazkova, I., & Zaborova, E. (2017). Quality issues of online distance learning. Procedia-Social and Behavioral . Sciences., 237 , 685–691. https://doi.org/10.1016/j.sbspro.2017.02.043 .
Marsh, H. (1982). SEEQ: A reliable, valid, and useful instrument for collecting Students’ evaluations of university teaching. British journal of educational psychology., 52 (1), 77–95. https://doi.org/10.1111/j.2044-8279.1982.tb02505.x .
Mihanović, Z., Batinić, A. B., & Pavičić, J. (2016). The link between Students' satisfaction with faculty, overall Students’ satisfaction with student life and student performances. Review of Innovation and Competitiveness: A Journal of Economic and Social Research, 2 (1), 37–60. https://doi.org/10.32728/ric.2016.21/3 .
Moore, J. (2009). A synthesis of Sloan-C effective practices. Journal of Asynchronous Learning Networks, 13 (4), 73–97. https://doi.org/10.24059/olj.v13i4.1649 .
Ni, A. (2013). Comparing the effectiveness of classroom and online learning: Teaching research methods. Journal of Public Affairs Education., 19 (2), 199–215. https://doi.org/10.1080/15236803.2013.12001730 .
Nichols, M. (2003). A theory for eLearning. Educational Technology & Society, 6 (2), 1–10 Retrieved from: https://www.jstor.org/stable/jeductechsoci.6.2.1 .
Pardo, A., Han, F., & Ellis, R. A. (2017). Combining university student self-regulated learning indicators and engagement with online learning events to predict academic performance. IEEE Transactions on Learning Technologies, 10 (1), 82–92. https://doi.org/10.1109/TLT.2016.2639508 .
Potter, W. J., & Levine-Donnerstein, D. (1999). Rethinking validity and reliability in content analysis. Journal of Applied Communication Research, 27 (3), 258–284. https://doi.org/10.1080/00909889909365539 .
Rajabalee, Y, B., Santally, M, I., Rennie, F., (2020). Modeling students’ performances in activity-based E-learning from a learning analytics Perspective: Implications and Relevance for Learning Design. International Journal of Distance Education Technologies . https://doi.org/10.4018/IJDET.2020100105
Ramsden, P. (1991). A performance indicator of teaching quality in higher education: The course experience questionnaire. Studies in higher education., 16 (2), 129–150. https://doi.org/10.1080/03075079112331382944 .
Rienties, B., Lewis, T., McFarlane, R., Nguyen, Q., & Toetenel, L. (2018). Analytics in online and offline language learning environments: The role of learning design to understand student online engagement. Computer Assisted Language Learning, 31 (3), 273–293. https://doi.org/10.1080/09588221.2017.1401548 .
Robinson, C., & Hullinger, H. (2008). New benchmarks in higher education: Student engagement in online learning. Journal of Education for Business, 84 (2), 101–108. https://doi.org/10.3200/JOEB.84.2.101-109 .
Roblyer, M., & Wiencke, W. (2004). Exploring the interaction equation: Validating a rubric to assess and encourage interaction in distance courses. Journal of Asynchronous Learning Networks, 8 (4), 24–37.
Sampson, P., Leonard, J., Ballenger, J., & Coleman, J. (2010). Student satisfaction of online courses for educational leadership. Online Journal of Distance Learning Administration, 13 (3) Retrieved from: https://eric.ed.gov/?id=EJ914153 .
Sinclair, P., Levett-Jones, T., Morris, A., Carter, B., Bennett, P., & Kable, A. (2017). High engagement, high quality: A guiding framework for developing empirically informed asynchronous e-learning programs for health professional educators. Nursing & Health Sciences., 19 (1), 126–137. https://doi.org/10.1111/nhs.12322 .
Smallwood, B. (2006). Classroom survey of student engagement. Retrieved from: http://www.unf.edu/acadaffairs/assessment/classe/overview.html .
Smith, V. C., Lange, A., & Huston, D. R. (2012). Predictive modeling to forecast student outcomes and drive effective interventions in online community college courses. Journal of asynchronous learning networks, 16 (3), 51–61. https://doi.org/10.24059/olj.v16i3.275 .
Strang, K. (2017). Beyond engagement analytics: Which online mixed-data factors predict student learning outcomes? Education and Information Technologies., 22 (3), 917–937. https://doi.org/10.1007/s10639-016-9464-2 .
Sulisworo, D. (2013). The paradox on IT literacy and science’s learning achievement in secondary school. International journal of evaluation and research in education (IJERE), 2 (4), 149–152. https://doi.org/10.11591/ijere.v2i4.2732 .
Tempelaar, D. T., Rienties, B., & Giesbers, B. (2015). In search for the most informative data for feedback generation: Learning analytics in a data-rich context. Computers in Human Behavior, 47 , 157–167. https://doi.org/10.1016/j.chb.2014.05.038 .
Tempelaar, D. T., Rienties, B., & Nguyen, Q. (2017). Towards actionable learning analytics using dispositions. IEEE Transactions on Learning Technologies, 10 (1), 6–16. https://doi.org/10.1109/TLT.2017.2662679 .
Thanh, N., & Viet, N. (2016). How to increase student’s satisfaction at higher education institutes (HEIs) today? Journal of Studies in Social Sciences and Humanities, 2 (4), 143–151.
Virtanen, M. A., Kääriäinen, M., Liikanen, E., & Haavisto, E. (2017). The comparison of students’ satisfaction between ubiquitous and web-basedlearning environments. Education and Information Technologies, 22 (5), 2565–2581. https://doi.org/10.1007/s10639-016-9561-2 .
Yunus, K., Wahid, W., Omar, S., & Ab Rashid, R. (2016). Computer phobia among adult university students. International Journal of Applied Linguistics and English Literature, 5 (6), 209–213. https://doi.org/10.7575/aiac.ijalel.v.5n.6p.209 .
Zepke, N., & Leach, L. (2010). Improving student engagement: Ten proposals for action. Active Learning in Higher Education, 11 (3), 167–177.
Zerihun, Z., Beishuizen, J., & Van Os, W. (2012). Student learning experience as indicator of teaching quality. Educational Assessment, Evaluation and Accountability., 24 (2), 99–111. https://doi.org/10.1007/s11092-011-9140-4 .
Download references
Author information
Authors and affiliations.
Mauritius Institute of Education, Moka, Mauritius
Yousra Banoor Rajabalee & Mohammad Issack Santally
University of Mauritius Reduit, Moka, Mauritius
You can also search for this author in PubMed Google Scholar
Corresponding author
Correspondence to Yousra Banoor Rajabalee .
Additional information
Publisher’s note.
Springer Nature remains neutral with regard to jurisdictional claims in published maps and institutional affiliations.
Annex 1 Student Feedback questionnaire
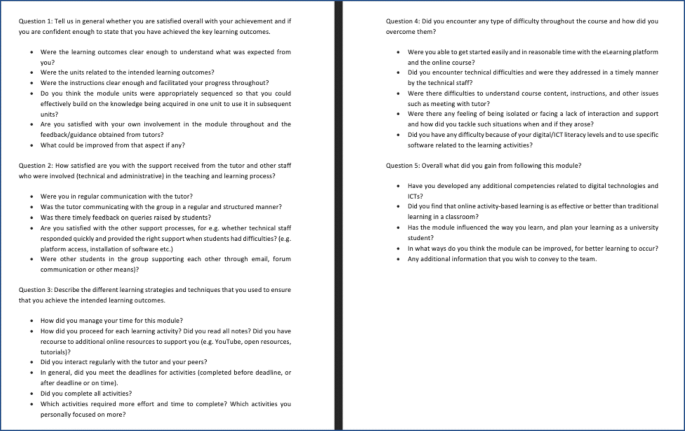
Rights and permissions
Reprints and permissions
About this article
Rajabalee, Y.B., Santally, M.I. Learner satisfaction, engagement and performances in an online module: Implications for institutional e-learning policy. Educ Inf Technol 26 , 2623–2656 (2021). https://doi.org/10.1007/s10639-020-10375-1
Download citation
Received : 07 August 2020
Accepted : 26 October 2020
Published : 11 November 2020
Issue Date : May 2021
DOI : https://doi.org/10.1007/s10639-020-10375-1
Share this article
Anyone you share the following link with will be able to read this content:
Sorry, a shareable link is not currently available for this article.
Provided by the Springer Nature SharedIt content-sharing initiative
- Student satisfaction in online courses
- Online student engagement
- Activity-based learning designs
- E-learning, education technology
- Find a journal
- Publish with us
- Track your research
A Systems View Across Time and Space
- Open access
- Published: 04 March 2022
Student satisfaction survey: a key for quality improvement in the higher education institution
- Anita Kanwar ORCID: orcid.org/0000-0003-4148-3080 1 &
- Meghana Sanjeeva 1
Journal of Innovation and Entrepreneurship volume 11 , Article number: 27 ( 2022 ) Cite this article
63k Accesses
24 Citations
Metrics details
India has witnessed a rapid expansion in the higher education institutions and with this fast pace of growth day by day, competition has set in among the institutes. Before taking admission in an institution student assess the facilities and standard of the institution by referring to the website, other admission portals and by taking peer opinion and public perception. Educational institutions around the world are now requesting students’ feedback on all elements of academic life in the form of a satisfaction feedback questionnaire. The goal of this research is to describe the development and implementation of a survey to assess undergraduate and postgraduate student satisfaction. The Student Satisfaction Survey is a useful and effective instrument that tries to focus resources on areas, where there is low satisfaction but high importance. This paper gives detailed information about the methodology, calculation and outcome of the exercise utilizing Likert scale analysis. With the recent emerging trends, this innovative method offers flexibility to integrate more parameters, group certain parameters to get feedback on a particular issue and transmute the type of questions with changing environment and structure. The analysis is done using a statistical method. The survey result directly highlights the importance and flexibility of method to evaluate overall satisfaction, satisfaction cognate to a single parameter and satisfaction for questions grouped together underlining some paramount aspects of higher education.
Introduction
The Indian higher education system is the third largest in the world. The higher education institutions are governed by the norms set by the affiliating University based on the guidelines provided by the University Grant Commission (UGC). National Assessment and Accreditation Council (NAAC) has been established to strengthen the education system, to ensure complete transparency, to stimulate the academic environment for promotion of quality of teaching–learning and research in higher education institutions (HEI).
Students are the most important stakeholders of any educational institution. Along with students’ progression and placements one of the main indicators of a college's progress is the students’ level of satisfaction. In India HEIs are not only imparting the required skills and improving the abilities of their graduates but are also concentrating to gratify students’ feelings about their scholastic experiences in the institution. There is emphasis on primary activities such as teaching learning, evaluation, research, extension activities, innovation along with emphasis on infrastructure facilities, quality of services, welfare measures for students and staff and overall satisfaction. of overall educational experience.
The vigorous, efficacious and value predicated scholastic system is the backbone of any nation. To progress in the right direction complete knowledge of student diversity, socio economic status, expectations and academic preferences are very useful parameters.
The gratified individual will have greater efficiency and will contribute to further progress of the institution and nation at large. Students who are studying in a higher educational institution seek more quality education and perfection of the system, in terms of approachability of the place, good infrastructure, quality education system, services offered by the institution, additional inputs in the form of value addition and employability enhancement courses etc. As stated by Usman ( 2010 ) the infrastructure facilities are becoming important, because these facilities satisfy student’s perception, esteem and develop them with all the essentials and capabilities to be an efficacious learner.
HEI’s all across the world are increasingly vying for students on a national and international level. They strive to improve student satisfaction to admit and retain students. This can only be accomplished if all of the services that contribute to “academic life” are of sufficient quality. Students satisfaction can be defined as an attitude resulting from an assessment of students’ educational experience, services and facilities provided by the institution. Because students are the important internal judges of performance of the institute, student satisfaction surveys are important and help the HEI to improve and adjust accordingly in the landscape of higher education. It also provides satisfaction to the institute of offering quality education.
Literature review
Mukhtar et. al. ( 2015 ) defined Higher education as education received at a college or university level and is regarded as one of the most essential instruments for a nation's individual, social and economic development. Fortino ( 2012 ) emphasized that creation of prepared minds of students was the main purpose of higher education. Hence, higher education institutions are increasingly recognizing and are placing greater emphasis on meeting the expectations and needs of their customers, that is, the students as rightly pointed out by DeShields et. al. ( 2005 ).
Higher education institutions are facing greater competition to adopt market-oriented methods to separate themselves from their competitors and attract as many students as possible while still meeting the requirements and expectations of present students. As a result, several research studies have been carried out to determine the elements that influence student happiness in higher education.
Elliot and Healy ( 2001 ) defined Students’ satisfaction as a short term attitude, resulting from an evaluation of a students’ educational experiences. It is a multidimensional process that is influenced by a variety of factors. GPA is the most influential factor on student satisfaction, according to Walker-Marshall and Hudson ( 1999 ).
There are personal and institutional factors associated with the concept of student satisfaction. Personal and institutional factors were identified by Appleton-Knapp and Krentler ( 2006 ) as two groups of influences on student satisfaction in higher education. Personal factors include age, gender, employment, preferred learning style, and student GPA, while institutional factors include instruction quality, promptness of instructor feedback, clarity of expectation, and teaching style.
Furthermore, teaching ability, curriculum flexibility, university status and prestige, independence, faculty care, student growth and development, student centeredness, campus climate, institutional effectiveness, and social conditions have been identified as major determinants of student satisfaction in higher education by Douglas et. al. ( 2006 ) and Palacio et. al. ( 2002 ).
Several models and frameworks have been applied by researchers to uplift students’ satisfactions in higher education literature. SERVQUAL is a most popular widely used service quality model which has been applied to measure students’ satisfaction around the world. SERVQUAL is a questionnaire designed, developed, and tested in the business environment by Parasuraman ( 1985 ) to measure service quality and customer satisfaction of a business based on five dimensions: tangibility, reliability, empathy, responsiveness, and assurance. Though widely used in business. It received some criticism from various researchers in higher education literature by scholars.
Elliot and Shin ( 2002 ) developed a more comprehensive student satisfaction inventory covering 11 dimensions and 116 indicators to measure the satisfactions of students in the higher education industry.
Weerasinghe et. al. ( 2017 ) traces the history of several models for student satisfaction derived from the business and higher education arena.
With the development of higher education in the world, the importance of students’ satisfaction has emerged in the literature of higher education. At the beginning, industry based satisfaction models were applied to explain student satisfaction and later developed higher education based models to explain it.
Douglas et. al. ( 2006 ) developed the “Service Product Bundle” method to investigate influences on student’s satisfaction in higher education, considering 12 dimensions.
Though several models are available, it is difficult to directly use any of them due to the heterogeneous nature of our educational system. In India with maximum diversity of religion, culture, demography, language and education system itself, it is all the more difficult to have a single parameter to finalize student satisfaction.
Therefore, there is a need to design a survey suitable to the specific need of a higher education institute. This study is innovative in its approach as it has designed a survey considering the local needs but to meet the global standards. NAAC has given broad guidelines which are suitable for the broad Indian higher education system.
This study endeavors to analyze student satisfaction, using a survey which is designed to obtain feedback on the administrative practices, college infrastructure, teacher quality and additional facilities on the campus. This exercise additionally aims at resoluteness of paramountcy of a variety of practices which were introduced at college to mentor students predicated on their requisites.
NAAC guidelines are taken as the base for designing the questionnaire for obtaining the feedback which is utilized determinately to analyze Student satisfaction. This is also because NAAC is responsible for accreditation of the institution and grading them on a 4-point scale. The questions framed here have direct linkage to the NAAC seven criteria and the corresponding guidelines prescribed from time to time. This paper also highlights the approach, attitude and expectations of students of aided and self-financing courses.
The feedback obtained using google form assesses student satisfaction and experience in the HEI which may lead to better experience leading to overall personality development of the students and will prepare them for the world of work. Another very important observation in this regard is that the students from all socio economic backgrounds prefer better facilities, quality and above all availability of good infrastructures on their campus. Hence most of the questions in the feedback form are predicated on the assessment and quality of the services provided by more minute units/departments of the institution.
To find out the satisfactory level of the students with regard to important parameters.
Methodology
Procedure of the development of the tool.
Four important dimensions viz. Curriculum & Teaching, Infrastructure Facilities, Student Support and Administrative matters were finalized. As the first step towards this exercise, a sample questionnaire is prepared. The sample questions are extensively based on the NAAC parameters, general outlook, type of services and activities offered by the institution and current scenario of higher education institutions in and around India. Some initial basic demographic questions are framed to know the respondents in terms of stream (Arts, Science, commerce, etc.) opted by the student, course in which student has enrolled (degree, diploma, graduate or post graduate etc.) and gender of the student. All other questions are framed to get responses on the 5-point scale designed using Likert scale as described by Norman ( 2010 ), where 1 indicates poor and 5 indicates excellent satisfaction Adnan et al. ( 2016 ) and Hayan and Mokhles ( 2013 )
A number of parameters under each heading were developed but were randomly spread across to get correct responses.
A pilot study was conducted and some parameters were removed.
Later the tool was sent to some experts and necessary changes were made and accordingly the parameters were finalized.
41 questions were framed for which students were asked to give responses on the 5-point scale using Google form.
A simple random sample was selected. The Population of the students for the institute was around 3500. A sample group from one college having both undergraduate and postgraduate students in all the three streams (Arts, Science and Commerce) and having a minimum of 2 years of experience with the institution was identified and more than 500 students were selected for this study.
Data analysis and findings
Survey was rolled out and was kept open for a month. After initial responses were received, a gentle reminder was sent to the students who had not responded. Awareness of this survey was created among student groups to get a better response.
The students were administered the survey online and could participate without disclosing their individual identity to avoid any sort of biased responses from the students or any pressure on the student to give biased response.
The demographic questions were not subjected to any statistical analysis. The percentage wise distribution for each question was directly obtained using Google form analysis data. Table 1 shows the set of responses obtained for one academic year for all the sample questions.
As we have listed, this innovative method offers flexibility to combine together some certain parameters to get feedback on a particular issue. As an example we will endeavour to analyse the academic environment based on inputs from teachers on the basis of their efficacy in transaction of curriculum, approachability, ability to provide additional skill set and knowledge through association activities, career guidance and fairness in examination. For this purpose, we will analyze question number 3, 27, 28, 29, 30, 32 and 34. Table 2 gives responses to obtained effectiveness of the teachers using part of the questionnaire that is using only above listed questions (Fig. 1 ).
Similarly, to find the quality of library services and its effectiveness, question numbers 11 to 16 are combined together and analyzed. Table 3 gives responses obtained to analyze the quality and effectiveness of library services.
Individual parameter effectiveness can be obtained by analyzing individual questions related to the concerned parameter. As an example question number 40 gives overall perception and satisfaction level of students in terms of support which is provided for cultural activities. Here we have matched excellent response with complete satisfaction, very good response with mainly satisfied, good response with just satisfied, average with partially satisfied and poor response with not satisfied. Responses obtained for this aspect show that 11% students are completely satisfied, 25% are mainly satisfied, 40% are just satisfied, 18.4% are partially satisfied and 5.6% are not satisfied at all. The only restriction is sample size should be large enough to get the true picture of satisfaction level as highlighted by Solinas et. al. ( 2012 ) and Silva and Fernandes ( 2012 ). The sample size taken here is more than 500 outgoing students (Figs. 2 and 3 ).
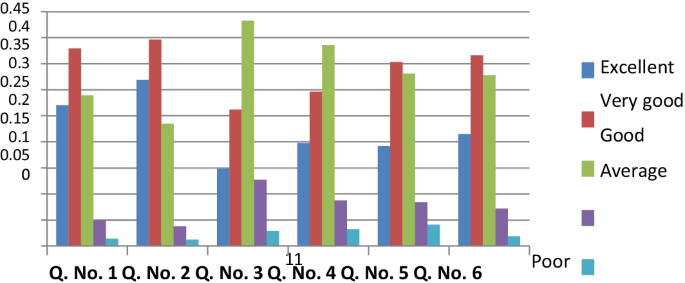
Graphical representation of the responses obtained for the teacher’s effectiveness
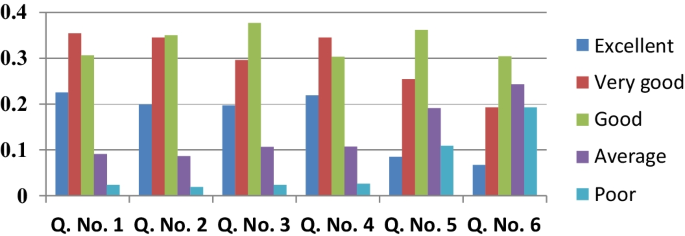
Graphical representation of effectiveness of library services
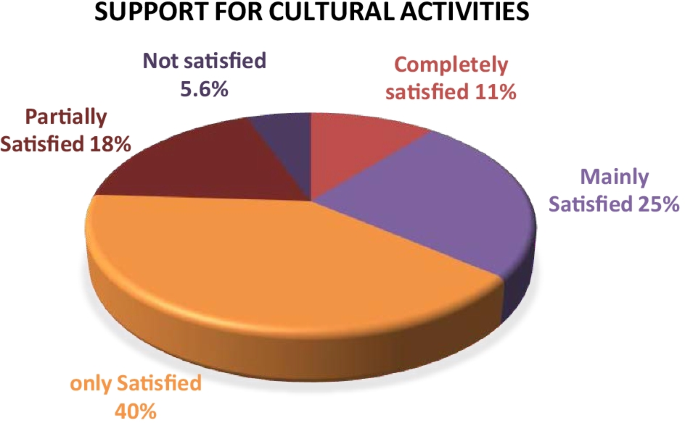
Graphical representation of responses received for support provided for cultural activities
Results and observations
The statistical analysis of above data gives the following result (Table 4 ).
The odd Likert scale has a tendency to give a result in the center scale. Table 4 shows a low standard deviation means that most of the numbers are close to the average. Coefficient of variation tells us about the variability of data. The lower the value of the coefficient of variation, the more precise is the estimate. Although here in all the cases results are pretty good and precise.
The above Table 4 of statistical analysis also indicates that the overall feedback obtained for the institution is Good. The teacher’s effectiveness in delivering the task assigned to them is more towards a very good category. The Quality of library services are also good.
Another important outcome of this activity is if any institution would want to improve the satisfaction of students in the future, they can analyze the individual parameter responses and some reforms and corrective measures can be introduced. It is the responsibility of the institution to analyze, understand and act on that understanding to improve. Since most of the students had rated quality of office services at a lower level, an Enterprise Resource Planning (ERP) packages can be purchased by the institution to increase the efficiency. Similarly, hostel facilities can be extended to students who are in dire need.
In another attempt, students were made aware of the objectives and intended learning outcomes of various parameters and then feedback was taken. This exercise also resulted in improvement of the satisfaction level of the students.
The students’ satisfaction and the whole exercise is an innovative method to obtain students’ feedback on their academic experience, perceptions and expectations from the higher education institution and finally to assess their satisfaction level. It contributes in understanding student’s perception, likes and dislikes and more importantly which educational experience they think of as the most important and which facilities require improvement. The method devised to obtain feedback of students of HEI is very innovative, generic, flexible and easy to adopt by any higher education institution. The questions can be changed and altered based on the requirements of the institution. Various interpretations can be obtained using this technique.
One survey analysis is capable of highlighting many parameters and aspects of higher education institutions. This analysis helps us in determination of parameters which require higher levels of improvement and changes to offer students greater levels of satisfaction. It also helps us in assessing the parameters, where institutions are strong and which can become their strengths. It provides information about actions that can be taken to maintain high levels of satisfaction and improve student learning experiences in the institution. Higher satisfaction level will definitely contribute to better outcomes.
Each question in the questionnaire highlights different aspects of an underlying perception. If few questions are combined together and even Likert scale is used, a reasonably accurate measure of the satisfaction can be obtained and effectiveness of that parameter can be analyzed easily. For instance, Teacher quality in imparting curriculum and giving extra inputs and effectiveness of library services is analyzed in the observation. If this method is used on a regular basis it may provide many insights into satisfaction level of students, changes in student priority, Quality of teachers, factors that really contribute to students’ satisfaction. The study also emphasizes that there is a need to make students aware of objectives and intended learning outcomes. It can help administrators to understand the relative importance and accordingly plan improvement in facilities and resources.
The method developed is a useful tool for selecting the most efficient parameters which help in improvement of experience, which leads to satisfaction. The facilities and services of organizations can then be improved to maximize efficiency. This study presents an easy, reliable and complete quality assessment method to obtain student feedback with no additional cost for any software purchase or training.
Availability of data and materials
All the supporting files are uploaded on the Journal Portal.
Abbreviations
University Grant Commission
National Assessment and Accreditation Council
Higher education institutions
Grade point average
Enterprise Resource Planning
Adnan, A., Mohamed, A., Tarek, A., Mun, S., & Hosny, H. (2016). Measuring student satisfaction with performance enhancement activities: Evidence from business education. International Journal of Information and Education Technology, 6 (10), 741–753.
Article Google Scholar
Appleton-Knapp, S., & Krentler, K. (2006). Measuring student expectations and their effects on satisfaction: The importance of managing student expectations. Journal of Marketing Education, 28 (3), 254–264.
DeShields, O., Kara, A., & Kaynak, E. (2005). Determinants of business student satisfaction and retention in higher education: Applying Herzberg’s two-factor theory. International Journal of Educational Management, 19 (2), 128–139.
Google Scholar
Douglas, J., Douglas, A., & Barnes, B. (2006). Measuring student satisfaction at a UK university. Quality Assurance in Education, 14 (3), 251–267.
Elliott, K., & Healy, M. (2001). Key factors influencing student satisfaction related to recruitment and retention. Journal of Marketing for Higher Education, 10 (4), 1–11.
Elliott, K., & Shin, D. (2002). Student satisfaction: An alternative approach to assessing this important concept. Journal of Higher Education Policy and Management, 24 (2), 197–209.
Fortino, A. (2012). The purpose of higher education: To create prepared minds. Retrieved from https://evolllution.com/opinions/the-purpose-of-higher-educationto-create-prepared-minds
Hayan, D., & Mokhles, A. (2013). The impact of service quality on student satisfaction and behavioral consequences in higher education services. International Journal of Economy, Management and Social Sciences , 2 (6), 285–290.
Mukhtar, U., Anwar, S., Ahmed, U., & Baloch, M. A. (2015). Factors effecting the service quality of public and private sector universities comparatively: An empirical investigation. Researchers World, 6 (3), 132.
Norman, G. (2010). Likert scales, levels of measurement and the “laws” of statistics. Advances in Health Sciences Education, 15 (5), 625–632.
Palacio, A. B., Meneses, G. D., & Pérez, P. J. P. (2002). The configuration of the university image and its relationship with the satisfaction of students. Journal of Educational Administration, 40 (5), 486–505.
Parasuraman, A., Zeithaml, V., & Berry, L. (1985). A conceptual model of service quality and its implications for future research. Journal of Marketing, 49 (4), 41.
Silva, F. H., & Fernandes, P. O. (2012). Empirical study on the student satisfaction in higher education: Importance-satisfaction analysis. WASET Journal, 6 , 1075–1080.
Solinas, G., Masia, M., Maida, G., & Muresu, E. (2012). What really affects student satisfaction? An assessment of quality through a university-wide student survey. Creative Education, 03 (01), 37–40.
Usman, A. (2010). The impact of service quality on students’ satisfaction in higher education institutes of Punjab. Journal of Management Research, 2 (2), 1–11.
Walker-Marshall, A., & Hudson, C. M. (1999). Student satisfaction and student success in the University System of Georgia. AIR 1999 Annual Forum Paper
Weerasinghe, I. S., Lalitha, R., & Fernando, S. (2017). Students’ satisfaction in higher education literature review. American Journal of Educational Research, 5 (5), 533–539.
Download references
Acknowledgements
We are thankful to National Assessment and Accreditation Council, Bangalore for initiating the idea of Student Satisfaction for colleges.
No funding received.
Author information
Authors and affiliations.
Department of Physics, Vivekanand Education Society’s College of Arts, Science and Commerce, Sindhi Society, Chembur, Mumbai, Maharashtra, 400071, India
Anita Kanwar & Meghana Sanjeeva
You can also search for this author in PubMed Google Scholar
Contributions
The first author has been instrumental in the conceptualization, design and development of the tool and the analysis of the research results. The second author has contributed in the manuscript of the paper. Both authors read and approved the final manuscript.
Corresponding author
Correspondence to Anita Kanwar .
Ethics declarations
Competing interests.
The authors declare that they have no competing interests.
Additional information
Publisher's note.
Springer Nature remains neutral with regard to jurisdictional claims in published maps and institutional affiliations.
Rights and permissions
Open Access This article is licensed under a Creative Commons Attribution 4.0 International License, which permits use, sharing, adaptation, distribution and reproduction in any medium or format, as long as you give appropriate credit to the original author(s) and the source, provide a link to the Creative Commons licence, and indicate if changes were made. The images or other third party material in this article are included in the article's Creative Commons licence, unless indicated otherwise in a credit line to the material. If material is not included in the article's Creative Commons licence and your intended use is not permitted by statutory regulation or exceeds the permitted use, you will need to obtain permission directly from the copyright holder. To view a copy of this licence, visit http://creativecommons.org/licenses/by/4.0/ .
Reprints and permissions
About this article
Cite this article.
Kanwar, A., Sanjeeva, M. Student satisfaction survey: a key for quality improvement in the higher education institution. J Innov Entrep 11 , 27 (2022). https://doi.org/10.1186/s13731-022-00196-6
Download citation
Received : 19 April 2021
Accepted : 12 January 2022
Published : 04 March 2022
DOI : https://doi.org/10.1186/s13731-022-00196-6
Share this article
Anyone you share the following link with will be able to read this content:
Sorry, a shareable link is not currently available for this article.
Provided by the Springer Nature SharedIt content-sharing initiative
- Higher education institution
- Student satisfaction
- Undergraduate
- Postgraduate

- Special Issues
- Conferences
- Turkish Journal of Analysis and Number Theory Home
- Current Issue
- Browse Articles
- Editorial Board
- Abstracting and Indexing
- Aims and Scope
- American Journal of Educational Research
- International Journal of Celiac Disease
- American Journal of Medical Case Reports
- American Journal of Public Health Research
- World Journal of Agricultural Research
- Turkish Journal of Analysis and Number Theory
- Social Science
- Medicine & Healthcare
- Earth & Environmental
- Agriculture & Food Sciences
- Business, Management & Economics
- Biomedical & Life Science
- Mathematics & Physics
- Engineering & Technology
- Materials Science & Metallurgy
- Quick Submission
- Apply for Editorial Position
- Propose a special issue
- Launch a new journal
- Authors & Referees
- Advertisers
- Open Access
- Full-Text PDF
- Full-Text HTML
- Full-Text Epub
- Full-Text XML
- IM Salinda Weerasinghe, R. Lalitha, S. Fernando. Students’ Satisfaction in Higher Education Literature Review. American Journal of Educational Research . Vol. 5, No. 5, 2017, pp 533-539. https://pubs.sciepub.com/education/5/5/9 ">Normal Style
- Weerasinghe, IM Salinda, R. Lalitha, and S. Fernando. 'Students’ Satisfaction in Higher Education Literature Review.' American Journal of Educational Research 5.5 (2017): 533-539. ">MLA Style
- Weerasinghe, I. S. , Lalitha, R. , & Fernando, S. (2017). Students’ Satisfaction in Higher Education Literature Review. American Journal of Educational Research , 5 (5), 533-539. ">APA Style
- Weerasinghe, IM Salinda, R. Lalitha, and S. Fernando. 'Students’ Satisfaction in Higher Education Literature Review.' American Journal of Educational Research 5, no. 5 (2017): 533-539. ">Chicago Style
Students’ Satisfaction in Higher Education Literature Review
Students’ satisfaction can be defined as a short-term attitude resulting from an evaluation of students’ educational experience, services and facilities. Earlier it was measured by common satisfaction frameworks but later higher education specify satisfaction models were developed. The objective of this review is to render all available constructive literature about students’ satisfaction with a sound theoretical and empirical background. Data were collected from refereed journals and conference papers, and are constructively analyzed from different point of views to filter a sound background for future studies. The first section of the paper discuss students’ satisfaction, satisfaction models and frameworks used by previous researchers around the world and second section explain the empirical findings of previous studies in real world context.
1. Higher Education
Higher education is the education at a college or university level is perceived as one of most important instruments for individual social and economic development of a nation 39 . The primary purpose of higher education are creation of knowledge and dissemination for the development of world through innovation and creativity 21 . As well, Fortino, 23 claimed creation of prepared minds of students as purpose of higher education. Hence, higher education institutions are increasingly recognizing and are placing greater emphasis on meeting the expectations and needs of their customers, that is, the students 16 . So, successful completion and enhancement of students’ education are the major reasons for the existence of higher educational institutions. This positive development in higher education shows the importance of educational institutions understanding student satisfaction in a competitive environment 65 . Now the higher education industry is strongly affected by globalization. This has increased the competition among higher education institutions to adopt market-oriented strategies to be differentiate themselves from their competitors to attract as many students as possible satisfying current students’ needs and expectation. Therefore, numerous studies have been conducted to identify the factors influencing student satisfaction in higher education.
2. Satisfaction
Satisfaction is a feeling of happiness that obtain when a person fulfilled his or her needs and desires 55 . It is a state felt by a person who has experienced performance or an outcome that fulfilled his or her expectations 27 . Accordingly, satisfaction can be defined as an experience of fulfillments of an expected outcomes Hon, 26 . Person will satisfy when he /she achieves the expectations, hence it is a willful accomplishment which result in one’s contentment 51 . Satisfaction refers to the feeling of pleasure or disappointment resulting from comparing perceived performance in relation to the expectation Kotler & Keller, 32 . Customers will satisfy when services fit with their expectation 48 . Hence, it is a function of relative level of expectation connecting with people’s perception 39 . When a person perceives that service encountered as good, he would satisfy on the other hand person will dissatisfy when his or her perception crash with the service expectation. Therefore, satisfaction is a perception of pleasurable fulfilment of a service 42 .
3. Student Satisfaction
Students’ satisfaction as a short term attitude, resulting from an evaluation of a students’ educational experiences 19 . It is a positive antecedent of student loyalty 41 and is the result and outcome of an educational system (Zeithaml, 1988). Again Elliot & Shin 20 define student satisfaction as students’ disposition by subjective evaluation of educational outcomes and experience. Therefore, student satisfaction can be defined as a function of relative level of experiences and perceived performance about educational service 39 during the study period, Carey, et al 10 . By considering all, students’ satisfaction can be defined as a short-term attitude resulting from an evaluation of students’ educational experience, services and facilities.
Students’ satisfaction is a multidimensional process which is influenced by different factors. According to Walker-Marshall & Hudson (1999) Grate Point Average (GPA) is the most influential factor on student satisfaction. Marzo-Navarro, et al. 36 , Appleton-Knapp & Krentler 9 identified two groups of influences on student satisfaction in higher education as personal and institutional factors. Personal factors cover age, gender, employment, preferred learning style, student’s GPA and institutional factors cover quality of instructions, promptness of the instructor’s feedback, clarity of expectation, teaching style. Wilkins & Balakrishnan 64 identified quality of lecturers, quality of physical facilities and effective use of technology as key determinant factors of student satisfaction. As well as, student satisfaction in universities is greatly influenced by quality of class room, quality of feedback, lecturer-student relationship, interaction with fellow students, course content, available learning equipment, library facilities and learning materials Journal of Higher Education, 57(1), pp. 1-21." class="coltj"> 24 , Review of Higher Education, 24(3), pp. 309-332." class="coltj"> 33 , Higher Education, 63 (5), pp. 565-81." class="coltj"> 60 . In addition to that, teaching ability, flexible curriculum, university status and prestige, independence, caring of faculty, student growth and development, student centeredness, campus climate, institutional effectiveness and social conditions have been identified as major determinants of student satisfaction in higher education Quality Assurance in Education, pp. 251-267." class="coltj"> 17 , Journal of Educational Administration, 40(5), pp. 486-505." class="coltj"> 45 .
This section presents few models and frameworks applied by researchers to uplift students’ satisfactions in higher education literature. The models and frameworks have been arranged on chronological order of years to identify how focus has changed from past to now.
SERVQUAL is a most popular widely used service quality model which has been applying to measure students’ satisfaction around the world. SERVQUAL is a questionnaire that has been designed, developed and tested in business environment, by Parasuman in 1985 to measure service quality and customer satisfaction of a business taking five dimensions into consideration as tangibility, reliability, empathy, responsiveness and assurance 63 . That questionnaire was administrated by twice, one to measure customer expectation and next to gain customer perception 63 . Though it is widely applied in industry, is much criticized in higher education literature by scholars like; Teas (1992), Buttle (1996), Asubonteng, et al (1996), Pariseau & McDaniel (1997), Aldridge & Rowley (1998), Waugh, 63 . Being a government university in a non-profit service industry, it is difficult to apply business focused service quality model to measure student’s satisfaction as it is. For an example, the model more focuses on service providers’ quality than tangibility. In a university environment, student satisfaction is determined by multiple factors in which quality of service providers is a small part.
The investment theory of students’ satisfaction of Hatcher, Prus, Kryter and Fitzgerald illustrated the behavior of students’ satisfaction with academic performance from investment point of view. According to the theory, student perceives their time, energy and effort as investment and seek a return form that. Accordingly, students will satisfy if they are rewarded in relation to the investment they made 12 . The SERVQUAL measures students’ satisfaction from organizational point of views but the satisfaction of student is influenced by students’ side also such as their dedication, perception, results, attitudes…etc. The gap was filled by Noel-Levitz in 1994 developing “Noel-Levitz Student Satisfaction Index” for higher education which covers faculty services, academic experience, student support facilities, campus life and social integration. Later, Keaveney and Young (1997) introduced Keaveney and Young’s satisfaction model for higher education. It measures the impact of college experience on students’ satisfaction along faculty services, advising staff and class type considering experience as a mediating variable. But the model is too narrowed into few variables and largely ignored university facilities, lectures, non-academic staffs and services in assessing satisfaction. Going beyond mediating models, Dollard, Cotton and de Jongein introduced “Happy - Productive Theory” in 2002 with a moderating variable. According to the model students’ satisfaction is moderated by students’ distress. Consequently, student satisfaction goes up when distress is low and satisfaction goes down when distress is high. The models were too narrowed into small part of satisfaction.
Elliot & Shin developed more comprehensive student satisfaction inventory in 2002 covering 11 dimensions and 116 indicators to measure the satisfactions of students in higher education industry. The dimensions were academic advising effectiveness, campus climate, campus life, campus support services, concern for individual, instructional effectiveness, recruitment and effectiveness of financial aids, registration effectiveness, campus safety and security, service excellence and student centeredness. This index covers all services provided by academic and non-academic staff to students as well has touched physical facilities and other related services being affected to students in a university environment. Similarly, Douglas, et al developed “Service Product Bundle” method in 2006 to investigate influences on student’s satisfaction in higher education, taking 12 dimensions in to consideration which were professional and comfortable environment, student assessments and learning experiences, classroom environment, lecture and tutorial facilitating goods, textbooks and tuition fees, student support facilities, business procedures, relationship with teaching staff, knowledgeable and responsiveness of faculty, staff helpfulness, feedback and class sizes. The dimensions were arranged under four variables; physical goods, facilitating goods, implicit services and explicit service. Unlike the SERVQUAL, Service Product Bundle method provides a more comprehensive range of variables that influence student satisfaction in higher education.
Jurkowitsch, et al. 28 developed a framework to assess students’ satisfaction and its impact, in higher education. In this framework service performance, university performance, relationships with student, university standing works as antecedents of satisfaction and promotion works the successor. Later, Alves and Raposo developed a conceptual model to assess students’ satisfaction in 2010. According to the model student’s satisfaction in higher education is determined by institute’s image, student expectations, perceived technical quality, functional quality and perceived value. These influences can be identified directly or indirectly through other variables. The model further illustrated student loyalty and word of mouth as the main successors of satisfaction. When student satisfaction upsurge, he will psychologically bound with university and its activities. That represent level of loyalty he or she has. Consequences will be spread among friends, relatives, prospect students and interested parties then and there as word of mouth. The main criticism for the model is that it has largely ignored main functions of a university; teaching and learning in measuring satisfaction of students but it has been developed adding two successors of satisfaction as loyalty and world of mouth.
Moving from conventional satisfaction models, student’s satisfaction are now measured by hybrid models. Shuxin, et al. 58 developed a conseptual model integrating two mainstream analysis: factor analysis and path analysis. Direct path of the model explains the impact of perceived quality on student loyalty and indirect path describes the impact of perceived quality and student expectation on loyalty through student satisfaction. Recently, Hanssen & Solvoll 25 develop a conceptual model combining satisfaction model and facility model. The satisfaction model was developed to explain how different factors influence on students’ overall satisfaction and facility model was developed to explain influence of university facilities on student overall satisfaction. According to the model, student satisfaction work as dependent variable of overall model and host city, job prospects, costs of studying, reputation, physical facility are working as independent variables of the satisfaction model. Facility model of the framework, is used to identify the facilities at institute that are most influential in formation of student overall satisfaction, therefore dependent variable (university facility) of facility model is used as one of explanatory variables in satisfaction model. The model has more focus on university facilities and little attention was paid into teaching, learning and administrative process of institutes but it revealed a new path for scholars precisely combing two separate models for satisfaction literature.
Different scholars have used different models to assess students’ satisfaction in higher education and every model is more or less criticized by scholars. As a result, old models have been gradually developed with new insight. Following table summarized the satisfaction models developed by various scholars to measure student satisfaction in higher education.
Table 1. Students’ Satisfaction Models

- Download as PowerPoint Slide Tables index View option Full Size Next Table
According to Table 1 , it seems that various scholars have been taking tremendous efforts to satisfy students in higher education touching different areas of satisfaction using various frameworks and models throughout last few decades. At the beginning, researchers have applied industry satisfaction models and later developed higher education based models to measure the satisfaction. The models have been developed using different dimensions into consideration and been applied in different geographical areas at different times. As a result, same dimensions have shown contradictory relationships with students’ satisfaction at different situations and different dimension have shown similar behaviors with students’ satisfaction around the world. These contrasts have been empirically tested by following scholars through their studies.
Table 2 . Summery of Satisfaction Models

- Download as PowerPoint Slide Tables index View option Full Size Previous Table
4. Empirical Research Findings
A study conducted by Garcl a-Aracil 24 in eleven European Countries, found that student satisfaction across different European Countries was relatively stable despite the differences in education systems. The study further realized that contacts with fellow students, course content, learning equipment, stocking of libraries, teaching quality and teaching/learning materials have significant influence on the students’ satisfaction. Wilkins & Balakrishnan 64 founnd that quality of lecturers, quality and availability of resources and effective use of technology have significant influence on students’ satisfaction in transnational higher education in United Arab Emirates. The study further revealed that there are significant differences of satisfactions at undergraduate and postgraduate levels. Karna & Julin 30 conducted a study on staff and students’ satisfaction about university facilities in Finland. The study found that core university activities, such as research and teaching facilities, have greater impacts on overall students’ and staff satisfaction than supportive facilities. Further, study found that both academic and students perceive physical facilities are more important than general infrastructures in which library facilities are the best explanatory factor of overall satisfaction. In addition, study indicated that students satisfied with factors related to comfortable learning environment, public spaces, campus accessibility and staff satisfied with laboratory and teaching facilities. Finally, overall results indicated that the factors related to the research and teaching activities have the greatest impacts on the overall satisfaction of both groups in Finland.
Douglas 17 measured students’ satisfaction at Faculty of Business and Law, Liverpool John Moores University Malaysia. The study found that physical facilities of university are not significantly important with regards to students’ satisfaction but it works as key determinant of students’ choice in selecting universities. Yusoff et al, 65 identified12 underlying variables that significantly influence students’ satisfaction in Malaysian higher education setting. Accordingly, professional comfortable environment, student assessment and learning experiences, classroom environment, lecture and tutorial facilitating goods, textbooks and tuition fees, student support facilities, business procedures, relationship with the teaching staff, knowledgeable and responsive faculty, staff helpfulness, feedback, and class sizes make significant impact on students’ satisfaction. The study further identified that year of study, program of study and semester grade have significant impact on student support facilities and class sizes. Martirosyan 35 examined the impact of selected variables on students’ satisfaction in Armenia. Light of the study identified reasonable curriculum and faculty services as key determinants of student satisfaction. As well, study found negative relationships of faculty teaching styles and graduate teaching assistants with students’ satisfaction. The study also examined the effects of demographic variables on students’ satisfaction. Out of the several variables associated with student satisfaction, type of institution effect on students’ satisfaction significantly in which students from private institutions reported a significantly higher satisfaction level than their peers at public institutions. Andrea and Benjamin 8 , examined students' satisfaction with university location based on Dunedin city, New Zealand. The study indicated that students at the University of Otago perceive accommodation, socializing, sense of community, safety and cultural scene as most important attributes of university location. The study further identified shopping and dining, appeal and vibrancy, socializing and sense of community and public transport as key drivers of overall satisfaction with the university location. DeShields Jr. in 2005 to investigate the factors contributing to student satisfaction and retention based on Herzberg’s two-factor theory. It found that student who have positive college experience are more satisfy with the university than that of students who haven’t experiences.
Kanan & Baker 29 attempted to examine the efficacy of academic educational programs based on Palestinian developing universities. The study found that academic programs make significantly impact on students’ satisfaction. Navarro 41 examined the impact of degree program on students’ satisfaction in Spanish University System. The result indicated that teaching staff, teaching methods and course administration have significant effect on students’ satisfaction in Spanish University System. Palacio, et al., 44 investigated the impact of university image on students’ satisfaction. The study found that university image of Spanish University System make a significant impact on students’ satisfaction. Malik, et al. 34 explored the impact of service quality on students’ satisfaction in higher education and it was found that cooperation, kindness of administrative staff, responsiveness of the educational system play a vital role in determining students’ satisfaction. Pathmini, et al 49 identified reliability, curriculum and empathy as major determinant factor of student satisfaction in regional state universities. The findings further accentuated that administrators of regional universities should focus their attention more on these three factors other than tangibility, competence and delivery. Farahmandian, et al. 22 investigated the levels of students’ satisfaction and service quality of International Business School, University Teknologi Malaysia. According to the findings, academic advising, curriculum, teaching quality, financial assistance, tuition fee and university facilities have significant impact on students’ satisfaction. Khan 31 discussed the impact of service quality on levels of students’ satisfaction at Heailey College of Commerce, Pakistan. The findings indicated that except tangibility, other dimension of service quality have a significant impact on students’ satisfaction. It means that students don’t rate institute on the basis of building and physical appearance but on quality of education. Study further explored that students willing to put extra efforts on education when the level of satisfaction is high.
Alvis and Rapaso 6 , investigated the influence of university image on student satisfaction and loyalty in Portugal. The findings of the study indicated that university image has both direct and indirect effect on student satisfaction and loyalty. Nasser et al 40 investigated university student knowledge about services and program in relation to their satisfaction at Lebanese Catholic College. The study found that student those who have high knowledge on university procedure, rules and regulation, may hold greater educational value and thus have greater satisfaction levels. Hanssen & Solvoll, 25 identified that reputation of the institution, attractiveness of host university city and quality of facilities have strong influencing powers on students’ satisfaction however job prospects failed to influence significantly on the satisfaction in Norwegian university system. Study further identified that social areas, auditoriums and libraries are the physical factors that most strongly influence on students satisfaction. Ali, et al., 4 found academic aspect, non-academic aspect, and access, reputation, and program issues as greater influencing factors of students’ satisfaction.
With the development of higher education in the world, the importance of students’ satisfaction was emerged in the literature of higher education. At the beginning, industry based satisfaction models were applied to explain student satisfaction and later developed higher education based models to explain it. The paper was discussed the theoretical and empirical literature of higher education with the intension of enhancing existing stock of knowledge. The theoretical review proved that satisfaction is a psychological process and is affected by many factors in different settings.

Cite this article:
Normal style, chicago style.
- Google-plus

- View in article Full Size
- Open access
- Published: 10 May 2024
Adherence to sleep recommendations is associated with higher satisfaction with life among Norwegian adolescents
- Erik Grasaas 1 ,
- Sergej Ostojic 1 &
- Henriette Jahre 2
BMC Public Health volume 24 , Article number: 1288 ( 2024 ) Cite this article
191 Accesses
Metrics details
Sleep plays a crucial role in the health and well-being of adolescents; however, inadequate sleep is frequently reported in numerous countries. This current paper aimed to describe sleep duration, factors impacting sleep, consequences of insufficient sleep and satisfaction with life in Norwegian adolescents, stratified by sex and by adherence to the 8-hour sleep recommendation, and to examine potential associations between adherence to the 8-hours sleep recommendation and satisfaction with life.
This is a cross-sectional study using data from the Norwegian Ungdata Survey, collected in 2021. Adolescents from five Norwegian counties were included, comprising a total of 32,161 upper secondary school students. Study variables were collected through an electronic questionnaire administered during school hours and all data are anonymous. Descriptive data of sleep patterns are presented, and linear regressions were conducted adjusting for SES, perceived stress, physical activity level, over-the-counter analgesics use, grade level and screen time.
73% of adolescents did not adhere to the 8-hours of sleep recommendation per night, with similar results for girls and boys. 64% reported tiredness at school (minimum 1–2 days weekly) and 62% reported that screen time negatively affected their ability to sleep. 23% reported that gaming affected their sleep, with a higher prevalence in boys than girls. Satisfaction with life score was 7.0 ± 1.9 points (out of 10) for the total sample, with higher scores for boys (7.3 ± 1.8 points) than girls (6.9 ± 1.9 points). Regressions revealed a positive association with satisfaction with life (B = 0.31, 95% [0.15 to 0.48]) in adolescents adhering to sleep recommendation of 8h compared to the ones not adhering to the sleep recommendation.
Conclusions
Most Norwegian adolescents fail to adhere to the 8-hours of sleep recommendation and the majority feel tired at school or during activities. More than half of adolescents reported that screen time negatively affected their ability to sleep. Adhering to the sleep recommendation was associated with higher life satisfaction. Our findings highlight the importance of sufficient sleep in adolescents, while future research is needed to examine other sleep related measures on adolescents´ satisfaction with life.
Peer Review reports
Sleep is recognized as a crucial factor for children’s and adolescents’ health and wellbeing [ 1 ]. Sleep recommendation vary with age and according to the US National Sleep Foundation teenagers are recommended 8–10 h of sleep [ 2 ]. However, when Gariepy and colleagues examined sleep patterns in 24 European and North American Countries, including 165,793 adolescents, findings revealed that insufficient sleep is prevalent in many countries [ 3 ]. Insufficient sleep impacts the daytime functioning in adolescents, leading to various negative consequences in their lives [ 4 ]. Extensive research evidence has reported that insufficient sleep among adolescents increases the risk of physical, psychosocial, and behavioral problems, and is associated with worse health outcomes [ 4 , 5 , 6 , 7 , 8 , 9 ].
When examining sleep duration in adolescents, research evidence refers to both the time in bed (TIB) and the sleep onset time (SOT) until wakening as estimates of sleep duration. It is suggested that TIB might overestimate the sleep duration in adolescence [ 10 ], as adolescents don’t immediately fall asleep when they go to bed. The latency time from going to bed to SOT was reported to be on average around 17 min for older adolescents in 2002 [ 11 ]. However, considering the commonality of screen time use before bedtime nowadays, it is presumed that this average time has increased [ 12 , 13 ]. A recent Norwegian sleep study reported the average time between going to bed and SOT was over one hour, revealing that eight in ten adolescents in upper secondary school actually failed to obtain the minimum recommended amount of sleep (8 h) on school days [ 10 ].
Research evidence points to several causes of insufficient sleep in adolescence, which are commonly categorized into internal- and external factors. External factors may include reduced parental involvement, excessive homework or activities, perceived stress, and screen time usage, whereas internal factors refer to puberty and biological processes such as a shift in the circadian rhythm [ 4 , 14 , 15 , 16 , 17 , 18 ]. Regardless of its causes, insufficient sleep is reported to impact all aspects of adolescents’ daily life and wellbeing [ 4 , 5 , 6 , 7 , 9 , 14 , 16 , 17 , 19 , 20 , 21 ]. A well-known indicator of subjective well-being is Life Satisfaction measure, which serves as a useful complement for comparing data across ages and countries, and is assessed to evaluate their life as a whole rather than their current feelings [ 22 ]. Satisfaction with life is therefore a well-known measure to indicate happiness across countries and time [ 22 ]. According to Diener, the measure reflects the cognitive judgment of one´s satisfaction with life [ 23 ]. It has been reported that girls tend to report lower satisfaction with life compared to boys during adolescence, along with a general decrease in satisfaction with life throughout this period [ 24 ].
Since most Norwegian adolescents do not meet the recommended 8 h of sleep [ 10 ], it is crucial to investigate potential consequences for this age group. Since life satisfactions is a good indicator of adolescent’s well-being, and a proxy for happiness it would be interesting to investigate the relationship between sleep duration and satisfaction with life using large dataset with high response rate. Such research can provide substantial insights for both practice and policy development, potentially emphasizing the significance of adhering to the sleep recommendations in Norway. The main aims of the present study were (1) to describe sleep duration, factors impacting sleep, consequences of insufficient sleep and satisfaction with life in Norwegian adolescents, stratified by sex and by adherence to the 8-hour sleep recommendation, and (2) to examine potential association between adherence to the 8-hours of sleep recommendation and satisfaction with life in Norwegian adolescents.
We hypothesized that adolescents adhering to the 8-hour sleep recommendation would have a more positive association to satisfaction with life compared to adolescents sleeping seven hours or less.
This study is reported according to the Strengthening the Reporting of Observational Studies in Epidemiology (STROBE) guidelines [ 25 ].
Study design
This is a cross-sectional study using data from the Norwegian Ungdata Survey, collected in 2021. Ungdata is conducted by Norwegian Social Research (NOVA) at Oslo Metropolitan University in collaboration with regional center for drug rehabilitation (KoRus) and the municipal sector’s organization (KS). It is a quality-assured system for carrying out repeated national surveys among pupils in lower and upper secondary schools related to all aspects of health and wellbeing [ 26 ].
The Ungdata survey includes adolescents from lower and upper secondary schools from almost all municipalities in Norway. The survey consists of a comprehensive electronic questionnaire, with a mandatory basic module for all the municipalities, and a set of optional, predefined questions, which municipalities and counties can choose from. In addition, self-composed questions may also be added by the municipalities, counties or collaborating universities. The Ungdata project is financed from the national budget through grants from the Norwegian Directorate of Health [ 26 ].
Ungdata is a free survey offered to all Norwegian counties and their respective municipalities. The yearly sampling is administered by including specific counties. Within the next two following years, the rest of the counties are recruited. According to Ungdata, within a three-year period, close to all Norwegian municipalities have participated in the survey [ 27 ]. Therefore, the national presented findings from Ungdata usually comprises data from the last three years, which results in a representative study sample for the whole target population. However, according to Ungdata, the survey from 2021 should be assessed more separately, due to the pandemic and due to the record high participation of municipalities this year [ 27 ]. In the supplementary information material provided by Ungdata, there are coding for different counties, municipalities, and schools. Indicating that schools were the primary sampling unit. However, the Ungdata dataset does not include a variable including the separate schools.
Study setting
The surveys take place during one school hour (45–55 min) and are carried out electronically by the respective teacher. Pupils who are not interested in taking the survey are provided other schoolwork. The research evidence extracted from the Ungdata Survey is well suited for planning and initiating work towards adolescents and public health [ 26 ].
Participants
Norwegian adolescents from upper secondary school (16–19 years of age) are included in this study. The response rate was 67% from the whole country [ 27 ]. Adolescents from five counties ( n = 32,161) are included in this specific study because they were the only counties that included sleep in their questionnaire (optional question). Number of participants were lower in questions regarding screentime/gaming affecting their sleep, as these questions were included in only two and three counties, respectively.
Exposure: sleep
Sleep duration was measured using the question “ How many hours of sleep did you get last night”. Seven response alternatives were provided, ranging from, 6 h or less or hourly up to 12 h or more. These response alternatives were recoded into a dichotomous variable to determine whether participants met (8 h or more) or did not meet (7 h or less) the international recommendations for sleep in adolescents [ 2 ]. Problems falling asleep and being tired in school or in activities was measured using four response alternatives, “no days”, “1–2 days”, “3–4 days” and “5 days or more”. If screentime or gaming affected their sleep was measured with two response alternatives, “yes” or “no”. These questions were formulated as: Has screentime affected you to not getting enough sleep and has gaming affected you to not getting enough sleep?
Outcome: satisfaction with life
Satisfaction with life was assessed using the question: “On a scale from 0 to10, how happy are you with your life these days?” Higher scores indicated greater satisfaction with life. This question on satisfaction with life was originally employed in a large Norwegian study called “Young in Oslo in 2018” [ 28 ], including 25,348 adolescents. Using a single-item measure for satisfaction with life has across samples demonstrated a substantial degree of validity and performed similar to the multiple-item satisfaction with life scale [ 29 ]. Especially in adolescence, its reported that a single-item life satisfaction measures perform as well the satisfaction with life scale [ 30 ].
Demographic variables and covariates
The Ungdata study includes demographic measures such as gender, grade level, respective county and municipality, and measures of socioeconomic status (SES). SES is measured by several questions related to parental educational level, books in their home and their level of prosperity. A total sum is calculated based on these three categories and recoded into values from 0 to 3, off which 0 represent lowest SES and 3 the highest SES [ 31 ]. This measure is reported as a validated construct of SES [ 26 ]. As the Ungdata Survey is anonymous, data on age is not available. For overview of study variables and response rate, see Table 1 .
Perceived stress level, physical activity level and use of over-the-counter analgesics (OTCA) are included as categorical covariates in the regression analysis [ 26 ].
Perceived stress level was measured by using the question “ Have you experience so much pressure the last week that you had problems managing it?” . Four response alternatives were provided, “not at all”, “to a small degree”, “to a large degree” and “to a very large degree” [ 26 ]. Perceived stress was found as a relevant psychological covariate in Norwegian adolescents due to the link to exposure and outcome [ 32 , 33 ].
Physical activity level was measured using the question “ How often are you so physical active that you become short of breath or sweaty? ” Six response alternatives were provided from “rare”, to different times a week, up to “at least 5 times a week” [ 26 ].
The use over OTCA was measured by using the question “How often have you used non-prescription drugs (Paracet, Ibux and similar) during the last month?” . Five response alternatives were provided ranging from “no times”, different times a week to “daily” [ 26 ].
Ethical consideration
Participation in the Ungdata survey is voluntary and informed written consent were provided by the adolescents. All questions from Ungdata included in this current study is approved by the Norwegian Agency for Shared Services in Education and Research (ref. 821,474), known as SIKT [ 34 ]. As the survey is conducted in May-June, adolescents in upper secondary school were 16 years or older and did not need parental consent. The study is conducted in accordance with the Helsinki Declaration.
Statistical analyses
All statistical analyses were conducted using IBM SPSS Statistics for Windows, Version 25.0 (IBM Corp., Armonk, NY, USA). For the descriptive measures, continuous variables are described using means and standard deviations (SDs), and categorical variables are presented with counts and percentages. Sleep variables are presented for the total study sample and stratified into girls and boys, and into those who achieved the recommended sleep duration or not. Linear regressions analyses were conducted to examine the association between achieving the recommended sleep duration (8 h or more) or not and satisfaction with life. Stratified regressions analyses for girls and boys were conducted to investigate potential sex differences in the associations. One sample proportion test revealed high precision (CI) in estimates across the descriptive study variables. Both crude and multiple regression analysis adjusted for SES, perceived stress, physical activity level, OTCA use, grade level and screen time are presented. The results are presented with beta coefficients with 95% confidence intervals and R-squared (R 2 ). P -values < 0.05 were considered statistically significant, and all tests were two‐sided. Sensitivity analysis using 7-hours as a cut-off were used to check the robustness of the results. Due to the large sample size and relatively small number of missing, no imputation or bootstrapping was considered necessary.
In total, 32,161 adolescents from five Counties in Norway were included in the analyses. Response rate remained high in selected study variables ranging from 92.5 to 99.9% (Table 1 ). More boys than girls participated (53% versus 47%), 42% of the participants were from 1st grade, 35% from 2nd grade, and 23% from 3rd grade (Table 2 ).
Descriptive data of sleep in Norwegian adolescents
Descriptive data of sleep variables are presented in Table 3 . 73% of adolescents did not adhere to the 8-hours of sleep recommendation, with similar results for girls and boys. 62% of respondents reported experiencing difficulties falling asleep on at least one day or more. This issue was more prevalent among girls (68%) than boys (56%). Feeling tired at school at least once a week was reported by 64%, by 71% of the girls and by 56% of the boys. 62% of participants stated that screen time negatively affected their ability to get enough sleep, 66% of girls and 57% of boys reported this. 23% of the adolescents reported that gaming affected their ability to get enough sleep, 11% of the girls and 38% of the boys. Satisfaction with life score was 7.0 ± 1.9 points for the total sample (Table 3 ), with higher scores for boys (7.3 ± 1.8 points) than in girls (6.9 ± 1.9 points) (Table 3 ).
Descriptive measures stratified by adhereing to the 8-hours of sleep recommendations or not showed that 54% of adolescents receiving more than 8 h of sleep had no problems with falling asleep, while 32% of adolescents that did not achieve sleep recommendation had these struggles.
50% of adolescents adhering to the recommended sleep duration reported that they never felt tired at school or in other activities, whereas this was reported by 30% of those who did not adhere to the recommendations. Screen time was descriptively reported to affect sufficient sleep in 45% of those who met the recommendations, and in 67% of those who did not. Gaming was descriptively reported to affect sleep for 15% of those who slept 8 h or more, and 26% in those who slept less (Table 4 ).
Associations between adhering to sleep recommendation or not on satisfaction with life
Adjusted multiple regression analysis stratified by sex showed that adhering to the recommended 8 h of sleep was positively associated with satisfaction with life in girls (B = 0.33; 95% CI [0.11–0.56]) and in boys (B = 0.27; 95% CI [0.02–0.52]) compared to those who did not adhere to the sleep recommendation (Table 5 ).
Crude regression analyses revealed a positive association between adhering to the 8-hours of sleep recommendation and satisfaction with life (B = 0.64; 95% CI [0.59–0.68]). Adjusted multiple regression analyses remained significant after adjusting for SES, perceived stress, physical activity level, OTCA use, grade level, screen time and sex (Table 6 ).
Sensitivity analyses
Adjusted sensitivity analysis using 7 h of sleep as a cut-off showed a stronger association with lower life satisfaction than 8 h of sleep for the total sample (B = 0.51 versus B = 0.31). Similar findings of stronger associations using 7 h cut-off were revealed in stratified analyses by gender, in boys (B = 0.39 versus 0.27) and girls (B = 0.60 versus B = 0.33).
In this study, we aimed to describe sleep duration, factors impacting sleep, consequences of insufficient sleep and satisfaction with life in Norwegian adolescents and examine possible associations between adherence to the 8-hours of sleep recommendation and satisfaction with life. Findings revealed that 73% of adolescents did not meet the recommended sleep duration of at least 8 h per night, with similar results for girls and boys. 64% reported that they felt tired at school or in activities, however more prevalent in girls than boys. Screen time had a negative impact for getting enough sleep in 62% and was more prevalent among girls than boys. Gaming disturbed sleep in 23% and was more prevalent among boys. Satisfaction with life score was 7 out of 10 for the total study sample, with somewhat higher scores for boys than girls. Adhering to the 8-hours sleep recommendation was positively associated with satisfaction with life, and there were similar findings in girls and boys. All findings remained statistically significant after adjusting for SES, perceived stress, physical activity level and OTCA use.
Our findings, revealing that 73% of the adolescents did not adhere to the 8-hours of sleep recommendation, are higher compared to international data, which shows that across countries, 32–86% of adolescents meet sleep recommendations [ 3 ]. However, not adhering to the sleep recommendation appears to be common in Norway. In a Norwegian study by Saxvig and colleagues, it was revealed that 84.8% of adolescents aged 16–17 did not adhere to the recommendation of 8-hour sleep [ 10 ]. These findings show a slightly higher prevalence compared to this current study, which may be due to several methodological differences in self-reporting. Saxvig and colleagues presents findings of sleep duration during schooldays, whereas the question provided by Ungdata refers to “how many hours did you sleep last night?”. Assuming that some Ungdata surveys were conducted on Mondays, the findings may be less comparable to data from schooldays, as adolescents commonly report a relatively large discrepancy between sleep duration on schooldays and weekends [ 35 ]. A recent Norwegian study from 2023 reported that younger Norwegian adolescents tend to sleep one and a half hours longer on weekends compared to schooldays [ 36 ]. Despite this, our findings point to the commonality of failing to obtain the recommendation of 8 h of sleep in the everyday life of Norwegian adolescents.
Estimating sleep duration by self-report in adolescence is challenging due to observed discrepancies between self-reported sleep and objectively measured sleep. However, research evidence suggests that adolescents aged 13–17 years may more precisely estimate their own sleep duration compared to when their parents report on their behalf, as parents tend to report an idealized version [ 37 ]. Objective measures, including actigraphy and the currently considered gold standard, polysomnography, offer potential clinical advantages compared to self-reporting [ 38 ]. However, these advantages are primarily related to pathological conditions, such as accurate diagnosis of sleep disorders and treatment monitoring. Lucas-Thompson and colleagues investigated the between- and within-person associations between self-reported and actigraph-measured nighttime sleep duration in adolescence [ 39 ]. The findings indicated that adolescents reporting longer average nighttime sleep also exhibited longer average actigraph measured sleep duration [ 39 ], suggesting that self-reporting in large samples of adolescence is likely to have high validity. Still, there are potential biases that should be discussed, which could be threating the validity of the study, such as self-report bias, including recall bias or social desirability bias. Despite the study is anonymous, there is no guarantee that adolescents´ didn’t under or overestimate their scores based on poor recollection or because of being afraid of observant classmates. Other relevant bias to mention is selection bias. Although the study includes the majority of Norwegian adolescents, findings may not accurately reflect the total target population.
Interestingly, our descriptive findings revealed similar sleep duration in girls and boys, which is in accordance with international data and other Norwegian sleep studies [ 10 , 36 , 40 ]. However, our descriptive findings revealed some differences in terms of feeling tired (sleepiness). Only 29% of girls reported they never felt sleepy during school or in activities, whereof 44% of the boys reported the same. There might be underlying mechanisms related to sleep quality or productivity differences between girls and boys that might interfere, or it could be related to other aspects of adolescents’ life, such as difference in physical activity levels and gender preferences for activities provided at schools. Nevertheless, Forest and colleagues also reported gender differences in daytime sleepiness during school and social activities in adolescents, with girls perceiving more interference from poor sleep on daytime functioning compared to boys [ 41 ]. Findings indicate other measures than sleep duration is needed for understanding daytime functioning in girls and boys. A meta-analytic review from a school setting, showed that sleepiness revealed the strongest association to school performance, followed by adolescents sleep quality and sleep duration [ 42 ].
Another gender difference was that more girls than boys reported that screen time negatively impacted their ability to sleep. It is reported that time spent in front of a screen usually comes at the expense of sleep [ 43 ]. The inability to sleep and screen time use at night are physiologically linked to the brightness and type of light, and such activity inhibit melatonin production, disrupt the circadian rhythm, and consequently affect adolescents´ feeling of sleepiness before bedtime [ 44 ]. Therefore, the systematic review by Hale et al., explicitly advises to limit or reduce screen time exposure, especially before or during bedtime hours, to minimize any harmful effects of screen time on sleep and well-being [ 13 ]. Moreover, Hale and colleagues reported that adolescents spend about 7 h per day in front of a screen [ 13 ]. Gaming might also contribute to the total screen time in adolescence. In our study, more boys than girls reported that gaming affected their ability to sleep. Time spent on video gaming in adolescence is also reported to be negatively associated with sleep duration [ 45 ].
It is interesting to link the differences in daytime sleepiness between girls and boys to the differences in satisfaction with life, as we suspect that there could be coinciding factors at play. Given that girls tend to experience more tiredness and sleepiness, it would presumably influence their subjective well-being and satisfaction with life, as sleepiness is strongly associated with adolescents’ overall quality of life [ 46 ]. Extensive research evidence has reported gender differences in health-related quality of life (HRQOL) and satisfaction with life, wherein girls tend to report lower scores than boys [ 24 , 47 , 48 , 49 , 50 ]. Moreover, our findings of satisfaction with life align with the “Better Life index” score from the OECD, which reports 7.3 as an average score for Norwegians [ 22 ]. Interestingly, in our study, both girls and those not adhering to the sleep recommendations had coinciding satisfaction with life scores below 7.0.
As hypothesized, the findings showed that adolescents adhering to the 8-hour sleep recommendation had higher life satisfaction compared to adolescents sleeping 7 h or less. Quite similar results were found in both girls and boys, despite a slightly lower p -value was revealed among girls compared to boys, both associations remained significant after adjusting for relevant covariates. Indicating respective associations relevant for the total study sample. Interestingly, a Norwegian study by Ness and Saksvik-Lehouillier investigated the relationship between sleep and satisfaction with life in Norwegian university students. Their results indicated that all sleep parameters, such as sleep quality, less variability in rise time, less variability in sleep duration, longer mean sleep duration were associated with better satisfaction with life. However, less variability of sleep duration was identified as a significant predictor for life satisfaction and not mean sleep duration, indicating that less variability of sleep duration might be more relevant to well-being than sleep duration itself [ 51 ]. Research evidence also reports higher risks of negative health outcomes with higher variability in sleep duration from weekdays to weekends in adolescents [ 35 , 52 ]. Further, a recent Norwegian study reported that sleep duration on weekdays was positively associated with all aspects of adolescents´ HRQOL, whereas sleep duration on weekends revealed mostly nonsignificant findings regarding aspects of HRQOL [ 36 ]. These findings highlight the vulnerability of using only one general sleep duration measure to understand the complexity between sleep and satisfaction with life. Nevertheless, our findings reinforce the importance of the 8-hours sleep recommendation for Norwegian adolescents. Sleep is a multifaceted concept, including different measures such as sleep variability, sleep quality and sleepiness, all of which can have distinct impacts on adolescents’ satisfaction with life. Therefore, it is worth exploring the possibility of sleep recommendations that encompass not only sleep duration, but also explicitly address sleep variability and daytime sleepiness in adolescence in the future.
Strengths and limitations
The primary strength of this study lies in its large sample size, comprising adolescents from both urban and rural regions of Norway, collected within a school-based setting. Additionally, the high response rate (99%) regarding variables related to sleep and life satisfaction enhances the study’s reliability. These factors suggest that the findings could be generalizable for a broader population of Norwegian adolescents attending school. The question regarding sleep duration is based on SOT until awakening time, which is considered an accurate estimation of sleep duration [ 10 ]. Further, Ungdata dataset is cleaned and they have several procedures for identifying unserious answers [ 26 ]. Moreover, reporting according to STROBE guidelines [ 25 ] should be considered a strength, as it provides transparency and accurate reporting of study method and results.
This study also has some limitations. The cross-sectional nature of the study hinders us from determining any causal inference between sleep duration and life satisfaction. Further, another limitation is the use of non-validated instruments regarding sleep as the respective questions in Ungdata derives from an unknown origin [ 27 ]. Moreover, the sleep questions did not distinguish between weekdays and weekends, which might have affected the results. Another limitation is that the scope of this paper was focused on adhering to sleep recommendations or not, and as a result, the sleep duration variable was dichotomized. This dichotomization reduced variability in data and excluded other potential sleep-related variables that could have impacted adolescents’ satisfaction with life. Another limitation is due to study variables are measured over different time frames, as exposure is measured within last day and outcome over a few days. The predicting sleep variable would be more robust if data was provided over a longer period, which would convey a better understanding of sleep variability and average sleep duration. Moreover, we do not have any information on the non-responders, which increases the risk of selection bias. Finally, despite significant statistical associations, caution should be exercised when interpeting the findings for clinical relevance. Still, sensitivity analysis using 7 h of sleep as a cut-off shows a stronger association with lower life satisfaction than 8 h of sleep. This might indicate that less sleep is more strongly related to lower life satisfaction. This should be further explored in future studies investigating sleep as a continuous variable. However, we chose to dichotomize the variable according to sleep recommendations to make it clear and easy to interpret for adolescents, practitioners, and policymakers.
Perspectives
This study showed that the majority of adolescents did not adhere to the 8-hours of sleep recommendation, and many of them reported feeling tired at school or in activities. Screen time and gaming were identified as descriptive factors affecting adolescent’s ability to get enough sleep. Our study added new findings to the research literature by uncovering that sleep recommendations were positively associated with higher life satisfaction by controlling for several relevant covariates in a large sample of Norwegian adolescents, underpinning essential information for people working with adolescents and caregivers. Finally, practice and policy aiming at increasing health and satisfaction with life in adolescents should include and highlight sleep recommendations.
This cross-sectional study demonstrated that almost three out of four Norwegian adolescents did not meet the sleep recommendations, and close to two thirds reported that they feel tired at school or in activities. Screen time negatively affected their ability to get enough sleep. Findings revealed a positive association between adhering to the 8-hours of sleep recommendation and satisfaction with life. These findings reinforce the importance of adhering the sleep recommendation for Norwegian adolescents. Adolescence is a critical time wherein insufficient sleep can have significant consequences. Further research is needed to examine other sleep related measures to adolescents’ satisfaction with life.
Data availability
The dataset that support the findings of this study is available upon reasonable request from the Norwegian Agency for Shared Services in Education and Research (SIKT) [ 34 ]. Dataset citation required from SIKT: https://doi.org/10.18712/NSD-NSD3007-V3 .
Abbreviations
time in bed
sleep onset time
confidence interval
standard deviation
socioeconomic status
Norwegian Social Research
regional center for drug rehabilitation
the municipal sector´s organization
Strengthening The Reporting Of Observational Studies
Norwegian Agency for Shared Services in Education and Research
better policies for better lives.
Matricciani L, et al. Children’s sleep and health: a meta-review. Sleep Med Rev. 2019;46:136–50.
Article PubMed Google Scholar
Hirshkowitz M, et al. National Sleep Foundation’s updated sleep duration recommendations: final report. Sleep Health. 2015;1(4):233–43.
Gariepy G, et al. How are adolescents sleeping? Adolescent sleep patterns and Sociodemographic Differences in 24 European and North American Countries. J Adolesc Health. 2020;66(6s):S81–8.
Owens JA, Weiss MR. Insufficient sleep in adolescents: causes and consequences. Minerva Pediatr. 2017;69(4):326–36.
Lee YJ, et al. Insufficient sleep and suicidality in adolescents. Sleep. 2012;35(4):455–60.
Article CAS PubMed PubMed Central Google Scholar
Palmer CA, et al. Associations among adolescent sleep problems, emotion regulation, and affective disorders: findings from a nationally representative sample. J Psychiatr Res. 2018;96:1–8.
Shochat T, Cohen-Zion M, Tzischinsky O. Functional consequences of inadequate sleep in adolescents: a systematic review. Sleep Med Rev. 2014;18(1):75–87.
Owens J. Insufficient sleep in adolescents and young adults: an update on causes and consequences. Pediatrics. 2014;134(3):e921–32.
Konjarski M, et al. Reciprocal relationships between daily sleep and mood: a systematic review of naturalistic prospective studies. Sleep Med Rev. 2018;42:47–58.
Saxvig IW, et al. Sleep in older adolescents. Results from a large cross-sectional, population-based study. J Sleep Res. 2021;30(4):e13263.
Thorleifsdottir B, et al. Sleep and sleep habits from childhood to young adulthood over a 10-year period. J Psychosom Res. 2002;53(1):529–37.
Article CAS PubMed Google Scholar
Baiden P, Tadeo SK, Peters KE. The association between excessive screen-time behaviors and insufficient sleep among adolescents: findings from the 2017 youth risk behavior surveillance system. Psychiatry Res. 2019;281:112586.
Hale L, Guan S. Screen time and sleep among school-aged children and adolescents: a systematic literature review. Sleep Med Rev. 2015;21:50–8.
Roeser K, et al. Relationship of sleep quality and health-related quality of life in adolescents according to self- and proxy ratings: a questionnaire survey. Front Psychiatry. 2012;3:76.
Article PubMed PubMed Central Google Scholar
Schmidt RE, Van der M, Linden. The relations between Sleep, personality, behavioral problems, and School Performance in adolescents. Sleep Med Clin. 2015;10(2):117–23.
Yeo SC, et al. Associations of sleep duration on school nights with self-rated health, overweight, and depression symptoms in adolescents: problems and possible solutions. Sleep Med. 2019;60:96–108.
Gradisar M, Gardner G, Dohnt H. Recent worldwide sleep patterns and problems during adolescence: a review and meta-analysis of age, region, and sleep. Sleep Med. 2011;12(2):110–8.
Jakobsson M, Josefsson K, Högberg K. Reasons for sleeping difficulties as perceived by adolescents: a content analysis. Scand J Caring Sci. 2020;34(2):464–73.
Chaput JP, et al. Systematic review of the relationships between sleep duration and health indicators in school-aged children and youth. Appl Physiol Nutr Metab. 2016;41(6 Suppl 3):S266–82.
Gustafsson ML, et al. Association between amount of sleep, daytime sleepiness and health-related quality of life in schoolchildren. J Adv Nurs. 2016;72(6):1263–72.
Paiva T, Gaspar T, Matos MG. Sleep deprivation in adolescents: correlations with health complaints and health-related quality of life. Sleep Med. 2015;16(4):521–7.
Satisfaction with life. Accessed 03.10.23 . https://www.oecdbetterlifeindex.org/topics/life-satisfaction/
Diener E. Subjective well-being. Psychol Bull. 1984;95(3):542–75.
Chen X et al. Gender differences in life satisfaction among children and adolescents: a Meta-analysis. J Happiness Stud, 2020. 21.
von Elm E, et al. The strengthening the reporting of Observational studies in Epidemiology (STROBE) Statement: guidelines for reporting observational studies. Ann Intern Med. 2007;147(8):573–7.
Article Google Scholar
Frøyland LR. Ungdata – Lokale ungdomsundersøkelser. Dokumentasjon av variablene i spørreskjemaet. NOVA. 2017 .
Bakken A. Ungdata 2021. Nasjonale resultater 2021.
05.10. 2023, A. Young in Oslo in 2018 . https://www.oslomet.no/forskning/forskningsprosjekter/ung-i-oslo-2018
Cheung F, Lucas RE. Assessing the validity of single-item life satisfaction measures: results from three large samples. Qual Life Res. 2014;23(10):2809–18.
Jovanović V. The validity of the satisfaction with Life Scale in adolescents and a comparison with single-item life satisfaction measures: a preliminary study. Qual Life Res. 2016;25(12):3173–80.
Bakken A, Frøyland LR, Sletten MA. Sosiale forskjeller i unges liv. Hva sier Ungdata-undersøkelsene? NOVA Rapport 3/2016:, 2016.
Grasaas E, et al. The relationship between stress and health-related quality of life and the mediating role of self-efficacy in Norwegian adolescents: a cross-sectional study. Health Qual Life Outcomes. 2022;20(1):162.
Thorsén F, et al. Sleep in relation to psychiatric symptoms and perceived stress in Swedish adolescents aged 15 to 19 years. Scand J Child Adolesc Psychiatr Psychol. 2020;8:10–7.
PubMed PubMed Central Google Scholar
Norwegian Agency for Shared Services in Education and Research (SIKT) . Accessed 05.10.2023; https://sikt.no/en/home
Kim J et al. The impact of Weekday-to-Weekend Sleep Differences on Health Outcomes among adolescent students. Child (Basel), 2022. 9(1).
Grasaas E, et al. Sleep duration in schooldays is associated with health-related quality of life in Norwegian adolescents: a cross-sectional study. BMC Pediatr. 2023;23(1):473.
Short MA, et al. Estimating adolescent sleep patterns: parent reports versus adolescent self-report surveys, sleep diaries, and actigraphy. Nat Sci Sleep. 2013;5:23–6.
Matthews KA, et al. Similarities and differences in estimates of sleep duration by polysomnography, actigraphy, diary, and self-reported habitual sleep in a community sample. Sleep Health. 2018;4(1):96–103.
Lucas-Thompson RG, Crain TL, Brossoit RM. Measuring sleep duration in adolescence: comparing subjective and objective daily methods. Sleep Health. 2021;7(1):79–82.
Saelee R, et al. Racial/Ethnic and Sex/Gender Differences in Sleep Duration Trajectories from Adolescence to Adulthood in a US National Sample. Am J Epidemiol. 2023;192(1):51–61.
Forest G, et al. Gender differences in the interference of sleep difficulties and daytime sleepiness on school and social activities in adolescents. Sleep Med. 2022;100:79–84.
Dewald JF, et al. The influence of sleep quality, sleep duration and sleepiness on school performance in children and adolescents: a meta-analytic review. Sleep Med Rev. 2010;14(3):179–89.
Cheung CHM, et al. Daily touchscreen use in infants and toddlers is associated with reduced sleep and delayed sleep onset. Sci Rep. 2017;7(1):46104.
Wood B, et al. Light level and duration of exposure determine the impact of self-luminous tablets on melatonin suppression. Appl Ergon. 2013;44(2):237–40.
Pérez-Chada D et al. Screen use, sleep duration, daytime somnolence, and academic failure in school-aged adolescents. PLoS ONE, 2023. 18(2 February).
Ahmadi Z, Omidvar S. The quality of sleep and daytime sleepiness and their association with quality of school life and school achievement among students. J Educ Health Promot. 2022;11:159.
Meade T, Dowswell E. Health-related quality of life in a sample of Australian adolescents: gender and age comparison. Qual Life Res. 2015;24(12):2933–8.
Rabbitts JA, et al. Association between widespread Pain scores and functional impairment and health-related quality of life in clinical samples of children. J Pain. 2016;17(6):678–84.
Bisegger C, et al. Health-related quality of life: gender differences in childhood and adolescence. Soz Praventivmed. 2005;50(5):281–91.
Michel G, et al. Age and gender differences in health-related quality of life of children and adolescents in Europe: a multilevel analysis. Qual Life Res. 2009;18(9):1147–57.
Ness TEB, Saksvik-Lehouillier I. The relationships between life satisfaction and Sleep Quality, Sleep Duration and Variability of Sleep in University students. Journal of European Psychology Students; 2018.
Kim SJ, et al. Relationship between weekend catch-up sleep and poor performance on attention tasks in Korean adolescents. Arch Pediatr Adolesc Med. 2011;165(9):806–12.
Download references
Acknowledgements
We wish to thank all the adolescents participating in Ungdata, NOVA and KoRus for giving us access to the data and the Norwegian Directorate of Health for funding the survey.
The Ungdata project is financed from the Norwegian national budget through grants from the Norwegian Directorate of Health [ 26 ].
Open access funding provided by University of Agder
Author information
Authors and affiliations.
Department of Nutrition and Public Health, Faculty of Health and Sport Sciences, University in Agder, Kristiansand, Postbox 422, 4604, Norway
Erik Grasaas & Sergej Ostojic
Department of Rehabilitation Science and Health Technology, Center for Intelligent Musculoskeletal health, Oslo Metropolitan University, Oslo, Norway
Henriette Jahre
You can also search for this author in PubMed Google Scholar
Contributions
All authors contributed to manuscript preparation. EG provided the first original draft of the manuscript and conducted the statistical analysis. SO and HJ contributed to the conceptualization, design and interpretation of findings. All read and approved the final manuscript.
Corresponding author
Correspondence to Erik Grasaas .
Ethics declarations
Ethics approval and consent to participate.
All study procedures were performed in accordance with the 1964 Helsinki declaration for ethical standards in research. Informed consent to participate was obtained from all of the participants. Permission to access and use data were given by Norwegian Agency for Shared Sevices in Education and Research (SIKT) on the 29.09.2023.
Consent for publication
Not applicable.
Competing interests
The authors declare no competing interests.
Additional information
Publisher’s note.
Springer Nature remains neutral with regard to jurisdictional claims in published maps and institutional affiliations.
Electronic supplementary material
Below is the link to the electronic supplementary material.
Supplementary Material 1
Supplementary material 2, rights and permissions.
Open Access This article is licensed under a Creative Commons Attribution 4.0 International License, which permits use, sharing, adaptation, distribution and reproduction in any medium or format, as long as you give appropriate credit to the original author(s) and the source, provide a link to the Creative Commons licence, and indicate if changes were made. The images or other third party material in this article are included in the article’s Creative Commons licence, unless indicated otherwise in a credit line to the material. If material is not included in the article’s Creative Commons licence and your intended use is not permitted by statutory regulation or exceeds the permitted use, you will need to obtain permission directly from the copyright holder. To view a copy of this licence, visit http://creativecommons.org/licenses/by/4.0/ . The Creative Commons Public Domain Dedication waiver ( http://creativecommons.org/publicdomain/zero/1.0/ ) applies to the data made available in this article, unless otherwise stated in a credit line to the data.
Reprints and permissions
About this article
Cite this article.
Grasaas, E., Ostojic, S. & Jahre, H. Adherence to sleep recommendations is associated with higher satisfaction with life among Norwegian adolescents. BMC Public Health 24 , 1288 (2024). https://doi.org/10.1186/s12889-024-18725-1
Download citation
Received : 02 November 2023
Accepted : 28 April 2024
Published : 10 May 2024
DOI : https://doi.org/10.1186/s12889-024-18725-1
Share this article
Anyone you share the following link with will be able to read this content:
Sorry, a shareable link is not currently available for this article.
Provided by the Springer Nature SharedIt content-sharing initiative
- Adolescents
- Satisfaction with life
- Quality of life
BMC Public Health
ISSN: 1471-2458
- Submission enquiries: [email protected]
- General enquiries: [email protected]
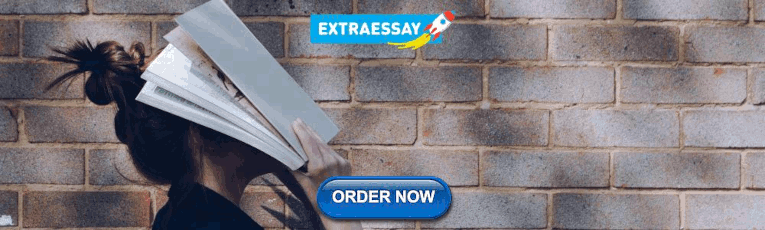
COMMENTS
Given the pivotal role of student satisfaction in the higher education sector, myriad factors contributing to higher education satisfaction have been examined in the literature. Within this literature, one lesser-researched factor has been that of the quality and types of interpersonal interactions in which students engage. As existing literature has yet to fully explore the contributions made ...
The research study encompasses payment of fees, choice factors, and student-consumer expectation and satisfaction in the context of higher education in the U.K. The data was collected through a national survey questionnaire made available online to respondents in their first year of university. 4.2.
Abstract and Figures. The purpose of this research is to review student satisfaction by examining a variety of relevant papers. This article provides a systematic literature review on student ...
Student satisfaction is a simple but dynamic phenomenon. Measuring student satisfaction can be difficult due to the complexities of higher education and the uncertainty revolving around "students as customers". 55 It is challenging to satisfy students, but student satisfaction can give a competitive edge to institutions. Campuses that ...
Students' satisfaction can be defined as a short-term attitude resulting from an evaluation of students' educational experience, services and facilities. Earlier it was measured by common ...
The vital role of student satisfaction in higher education. Students' satisfaction with the quality of the education services they receive is a crucial index of the performance of HE institutions in today's world (Butt & Rehman, 2010; Santini et al., 2017; Weingarten et al., 2018).Student satisfaction figures are also used as a means by which to distribute precious resources across HE ...
Teacher behaviors are known to influence student learning including cognitive and affective learning, participation, involvement, motivation, academic performance, and satisfaction (Frisby & Marin, 2010; Granitz et al., 2009; M. Hill & Epps, 2010).The behavior exhibited by a teacher can influence the student's learning environment which in turn influences the student's satisfaction and ...
This research aimed to contribute knowledge that could be used to improve undergraduate student satisfaction in Higher Education (HE). There are a number of reasons for researching student satisfaction at the present time. Firstly, student satisfaction is a current focus of debate and interest, both within universities, and in the HE literature.
Introduction. Student satisfaction has become both an enduring focus of scholarly research (Turner Citation 2023) and 'a primary focus of many universities and colleges' (Wong and Chapman Citation 2023, 958), with its assessment now 'both a component of external accountability and an internal driver of university policy' (Muijs and Bokhove Citation 2017, 907).
4. There is a strong level of student satisfaction with student services: 3.49: 0.781: 45.3: 5. Student services provides learning opportunities different from the classroom but nonetheless important to my personal growth and development: 3.37: 0.908: 40.7: 6. Personally, I understand how to use student services to my advantage: 3.29: 0.824: 41 ...
Abstract. This study examines the students' satisfaction in higher education in Pakistan. The study focuses on the factors like teachers' expertise, courses offered, learning environment and classroom facilities. Students' response measured through an adapted questionnaire on a 5-point likert scale. The sample size of the study consisted ...
ISSN 2222-1735 (Paper) ISSN 2222-288X (Online) Vol.6, No.31, 2015 ... and campus services and facilities have a relationship with student satisfaction. This research study focused on the sample size of 200 people from the population of university students in Pakistan n=200. From non-probability sampling designs convenience sampling was used for ...
The outbreak of the COVID-19 pandemic has dramatically shaped higher education and seen the distinct rise of e-learning as a compulsory element of the modern educational landscape. Accordingly, this study highlights the factors which have influenced how students perceive their academic performance during this emergency changeover to e-learning. The empirical analysis is performed on a sample ...
Abstract. This study examines the role of satisfaction on students' academic performance and investigates the relationship between satisfaction of students and academic performance and explores other factors that contribute academic performance. A correlation research was used. The study population was the third and the last year students of ...
There has been debates related to online and blended learning from a perspective of learner experiences in terms of student satisfaction, engagement and performances. In this paper, we analyze student feedback and report the findings of a study of the relationships between student satisfaction and their engagement in an online course with their overall performances. The module was offered ...
The goal of this research is to describe the development and implementation of a survey to assess undergraduate and postgraduate student satisfaction. The Student Satisfaction Survey is a useful and effective instrument that tries to focus resources on areas, where there is low satisfaction but high importance. This paper gives detailed ...
In this sense, a more engaged student experience is an improved student experience. 6. Discussion. This study shows that more engaged students are more satisfied, and that more satisfied students are more engaged. There is a strong positive relationship between engagement and satisfaction, whichever way causation runs.
These findings differ from the existing research (Kahu, 2013; Marzo-. Navarro et al., 2005) which argues that student satisfaction leads to quality education. This. paper reveals that the ...
Students' satisfaction with online classes also depends on the socio-economic background of the students. ... Wrote the paper. Funding statement. This research did not receive any specific grant from funding agencies in the public, commercial, or not-for-profit sectors. Data availability statement. The data that has been used is confidential.
The Student Outcomes Survey is an annual national survey of vocational education and training (VET) students. Since 1995, participants have been asked to rate their satisfaction with different aspects of their training, grouped under three main themes: teaching, assessment, and generic skills and learning experiences.
4. Empirical Research Findings. A study conducted by Garcl a-Aracil 24 in eleven European Countries, found that student satisfaction across different European Countries was relatively stable despite the differences in education systems. The study further realized that contacts with fellow students, course content, learning equipment, stocking of libraries, teaching quality and teaching ...
The first section of the paper discuss students' satisfaction, satisfaction models and frameworks used by previous researchers around the world and second section explain the empirical findings of previous studies in real world context. ... (May 28, 2017). American Journal of Educational Research, Vol. 5, No. 5, p. 533-539, 2017, Available at ...
Abstract. This study examines the students' satisfaction in higher education in Pakistan. The study focuses on the factors like teachers' expertise, courses offered, learning environment and ...
Sleep plays a crucial role in the health and well-being of adolescents; however, inadequate sleep is frequently reported in numerous countries. This current paper aimed to describe sleep duration, factors impacting sleep, consequences of insufficient sleep and satisfaction with life in Norwegian adolescents, stratified by sex and by adherence to the 8-hour sleep recommendation, and to examine ...
Students Motivational essays about Khan Sir could revolve around his impactful teaching methodologies, dedication to education, and his ability to inspire मतलब countless students. Khan Sir,...