- En español – ExME
- Em português – EME
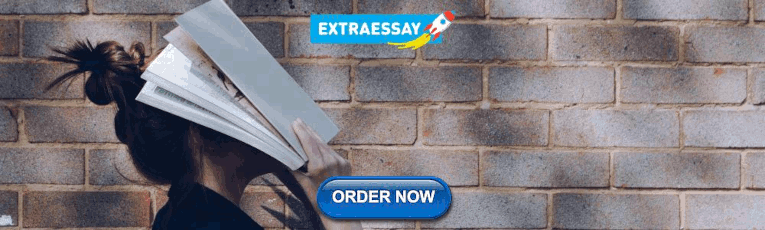
Multivariate analysis: an overview
Posted on 9th September 2021 by Vighnesh D
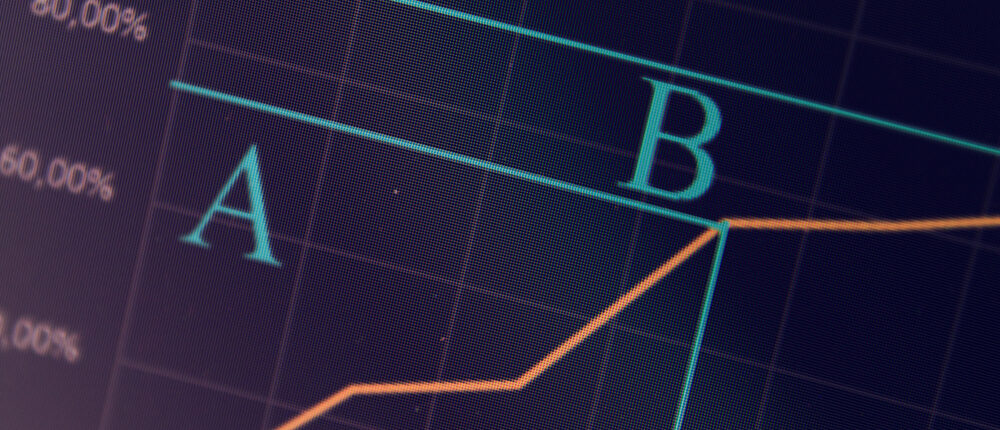
Data analysis is one of the most useful tools when one tries to understand the vast amount of information presented to them and synthesise evidence from it. There are usually multiple factors influencing a phenomenon.
Of these, some can be observed, documented and interpreted thoroughly while others cannot. For example, in order to estimate the burden of a disease in society there may be a lot of factors which can be readily recorded, and a whole lot of others which are unreliable and, therefore, require proper scrutiny. Factors like incidence, age distribution, sex distribution and financial loss owing to the disease can be accounted for more easily when compared to contact tracing, prevalence and institutional support for the same. Therefore, it is of paramount importance that the data which is collected and interpreted must be done thoroughly in order to avoid common pitfalls.
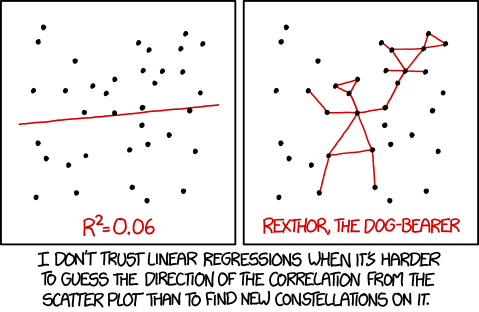
Image from: https://imgs.xkcd.com/comics/useful_geometry_formulas.png under Creative Commons License 2.5 Randall Munroe. xkcd.com.
Why does it sound so important?
Data collection and analysis is emphasised upon in academia because the very same findings determine the policy of a governing body and, therefore, the implications that follow it are the direct product of the information that is fed into the system.
Introduction
In this blog, we will discuss types of data analysis in general and multivariate analysis in particular. It aims to introduce the concept to investigators inclined towards this discipline by attempting to reduce the complexity around the subject.
Analysis of data based on the types of variables in consideration is broadly divided into three categories:
- Univariate analysis: The simplest of all data analysis models, univariate analysis considers only one variable in calculation. Thus, although it is quite simple in application, it has limited use in analysing big data. E.g. incidence of a disease.
- Bivariate analysis: As the name suggests, bivariate analysis takes two variables into consideration. It has a slightly expanded area of application but is nevertheless limited when it comes to large sets of data. E.g. incidence of a disease and the season of the year.
- Multivariate analysis: Multivariate analysis takes a whole host of variables into consideration. This makes it a complicated as well as essential tool. The greatest virtue of such a model is that it considers as many factors into consideration as possible. This results in tremendous reduction of bias and gives a result closest to reality. For example, kindly refer to the factors discussed in the “overview” section of this article.
Multivariate analysis is defined as:
The statistical study of data where multiple measurements are made on each experimental unit and where the relationships among multivariate measurements and their structure are important
Multivariate statistical methods incorporate several techniques depending on the situation and the question in focus. Some of these methods are listed below:
- Regression analysis: Used to determine the relationship between a dependent variable and one or more independent variable.
- Analysis of Variance (ANOVA) : Used to determine the relationship between collections of data by analyzing the difference in the means.
- Interdependent analysis: Used to determine the relationship between a set of variables among themselves.
- Discriminant analysis: Used to classify observations in two or more distinct set of categories.
- Classification and cluster analysis: Used to find similarity in a group of observations.
- Principal component analysis: Used to interpret data in its simplest form by introducing new uncorrelated variables.
- Factor analysis: Similar to principal component analysis, this too is used to crunch big data into small, interpretable forms.
- Canonical correlation analysis: Perhaps one of the most complex models among all of the above, canonical correlation attempts to interpret data by analysing relationships between cross-covariance matrices.
ANOVA remains one of the most widely used statistical models in academia. Of the several types of ANOVA models, there is one subtype that is frequently used because of the factors involved in the studies. Traditionally, it has found its application in behavioural research, i.e. Psychology, Psychiatry and allied disciplines. This model is called the Multivariate Analysis of Variance (MANOVA). It is widely described as the multivariate analogue of ANOVA, used in interpreting univariate data.
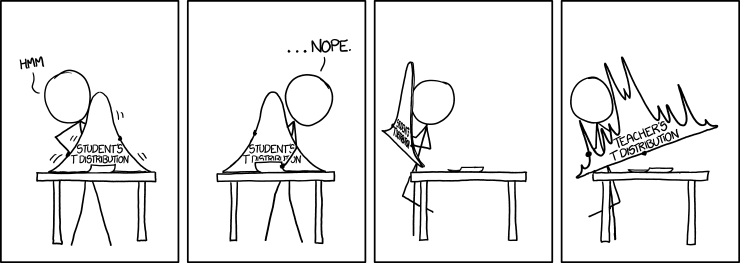
Image from: https://imgs.xkcd.com/comics/t_distribution.png under Creative Commons License 2.5 Randall Munroe. xkcd.com.
Interpretation of results
Interpretation of results is probably the most difficult part in the technique. The relevant results are generally summarized in a table with an associated text. Appropriate information must be highlighted regarding:
- Multivariate test statistics used
- Degrees of freedom
- Appropriate test statistics used
- Calculated p-value (p < x)
Reliability and validity of the test are the most important determining factors in such techniques.
Applications
Multivariate analysis is used in several disciplines. One of its most distinguishing features is that it can be used in parametric as well as non-parametric tests.
Quick question: What are parametric and non-parametric tests?
- Parametric tests: Tests which make certain assumptions regarding the distribution of data, i.e. within a fixed parameter.
- Non-parametric tests: Tests which do not make assumptions with respect to distribution. On the contrary, the distribution of data is assumed to be free of distribution.
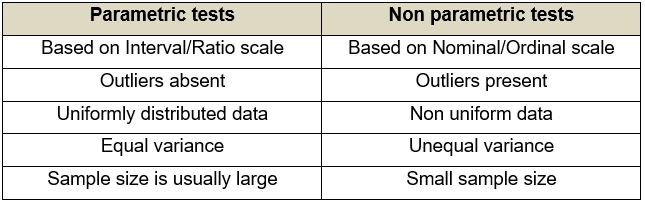
Uses of Multivariate analysis: Multivariate analyses are used principally for four reasons, i.e. to see patterns of data, to make clear comparisons, to discard unwanted information and to study multiple factors at once. Applications of multivariate analysis are found in almost all the disciplines which make up the bulk of policy-making, e.g. economics, healthcare, pharmaceutical industries, applied sciences, sociology, and so on. Multivariate analysis has particularly enjoyed a traditional stronghold in the field of behavioural sciences like psychology, psychiatry and allied fields because of the complex nature of the discipline.
Multivariate analysis is one of the most useful methods to determine relationships and analyse patterns among large sets of data. It is particularly effective in minimizing bias if a structured study design is employed. However, the complexity of the technique makes it a less sought-out model for novice research enthusiasts. Therefore, although the process of designing the study and interpretation of results is a tedious one, the techniques stand out in finding the relationships in complex situations.
References (pdf)
Leave a Reply Cancel reply
Your email address will not be published. Required fields are marked *
Save my name, email, and website in this browser for the next time I comment.
No Comments on Multivariate analysis: an overview
I got good information on multivariate data analysis and using mult variat analysis advantages and patterns.
Great summary. I found this very useful for starters
Thank you so much for the dscussion on multivariate design in research. However, i want to know more about multiple regression analysis. Hope for more learnings to gain from you.
Thank you for letting the author know this was useful, and I will see if there are any students wanting to blog about multiple regression analysis next!
When you want to know what contributed to an outcome what study is done?
Dear Philip, Thank you for bringing this to our notice. Your input regarding the discussion is highly appreciated. However, since this particular blog was meant to be an overview, I consciously avoided the nuances to prevent complicated explanations at an early stage. I am planning to expand on the matter in subsequent blogs and will keep your suggestion in mind while drafting for the same. Many thanks, Vighnesh.
Sorry, I don’t want to be pedantic, but shouldn’t we differentiate between ‘multivariate’ and ‘multivariable’ regression? https://stats.stackexchange.com/questions/447455/multivariable-vs-multivariate-regression https://www.ajgponline.org/article/S1064-7481(18)30579-7/fulltext
Subscribe to our newsletter
You will receive our monthly newsletter and free access to Trip Premium.
Related Articles
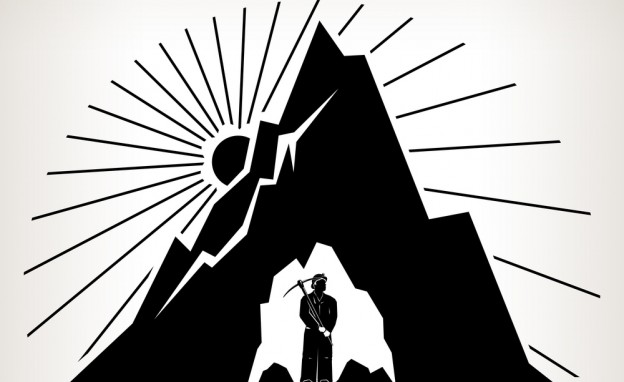
Data mining or data dredging?
Advances in technology now allow huge amounts of data to be handled simultaneously. Katherine takes a look at how this can be used in healthcare and how it can be exploited.
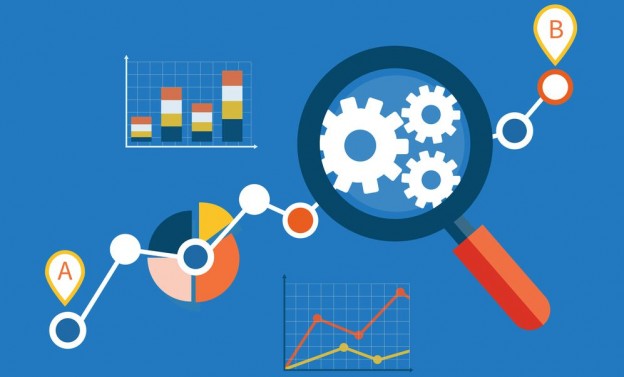
Nominal, ordinal, or numerical variables?
How can you tell if a variable is nominal, ordinal, or numerical? Why does it even matter? Determining the appropriate variable type used in a study is essential to determining the correct statistical method to use when obtaining your results. It is important not to take the variables out of context because more often than not, the same variable that can be ordinal can also be numerical, depending on how the data was recorded and analyzed. This post will give you a specific example that may help you better grasp this concept.
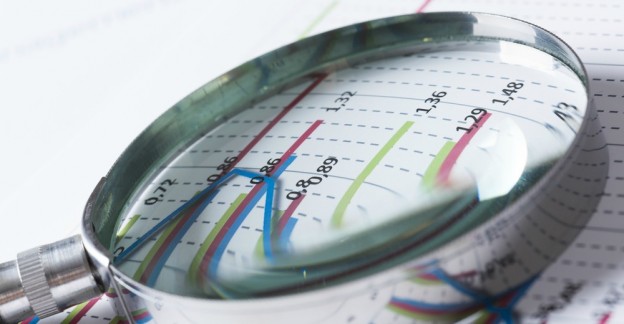
Data analysis methods
A description of the two types of data analysis – “As Treated” and “Intention to Treat” – using a hypothetical trial as an example
- Subject List
- Take a Tour
- For Authors
- Subscriber Services
- Publications
- African American Studies
- African Studies
- American Literature
- Anthropology
- Architecture Planning and Preservation
- Art History
- Atlantic History
- Biblical Studies
- British and Irish Literature
- Childhood Studies
- Chinese Studies
- Cinema and Media Studies
- Communication
- Criminology
- Environmental Science
- Evolutionary Biology
- International Law
- International Relations
- Islamic Studies
- Jewish Studies
- Latin American Studies
- Latino Studies
- Linguistics
- Literary and Critical Theory
- Medieval Studies
- Military History
- Political Science
- Public Health
- Renaissance and Reformation
- Social Work
- Urban Studies
- Victorian Literature
- Browse All Subjects
How to Subscribe
- Free Trials
In This Article Expand or collapse the "in this article" section Multivariate Research Methodology
Introduction, general overviews.
- Multivariate Assumptions
- Multivariate Effect Sizes
- Multivariate Analysis of Variance
- Discriminant Analysis
- Canonical Correlation Analysis
- Exploratory Factor Analysis
- Principal Components Analysis and Common Factor Analysis
- Factor Retention
- Rotation Criteria
- Confirmatory Factor Analysis
- Structural Equation Modeling
- Multidimensional Scaling
- Nonparametric Multivariate Analysis
Related Articles Expand or collapse the "related articles" section about
About related articles close popup.
Lorem Ipsum Sit Dolor Amet
Vestibulum ante ipsum primis in faucibus orci luctus et ultrices posuere cubilia Curae; Aliquam ligula odio, euismod ut aliquam et, vestibulum nec risus. Nulla viverra, arcu et iaculis consequat, justo diam ornare tellus, semper ultrices tellus nunc eu tellus.
- Educational Statistics for Longitudinal Research
- Methodologies for Conducting Education Research
- Quantitative Research Designs in Educational Research
- Social Science and Education Research
- Statistical Assumptions
Other Subject Areas
Forthcoming articles expand or collapse the "forthcoming articles" section.
- Black Women in Academia
- Girls' Education in the Developing World
- History of Education in Europe
- Find more forthcoming articles...
- Export Citations
- Share This Facebook LinkedIn Twitter
Multivariate Research Methodology by Robin K. Henson , Krystal Hinerman LAST REVIEWED: 28 April 2016 LAST MODIFIED: 28 April 2016 DOI: 10.1093/obo/9780199756810-0145
Broadly defined, multivariate research methods involve the inclusion of more than one outcome in a singular analysis. Instead of conducting a series of univariate analysis, one for each outcome, multivariate analyses consider all the outcomes of interest at the same time. When compared to multiple univariate analysis, the multivariate approach allows for the reduction in Type I error, for an increase in power, and for the relationship between the outcome variables to be expressed. Perhaps most importantly from an applied perspective, the multivariate approach allows the researcher to analyze the data in a way that is most reflective of the actual research context and environment. In education, research scenarios involving multiple outcomes could include examples such as how a character education program impacts student self-efficacy, attitudes, and behavior, or how mentoring influences reading, math, and science performance. Beyond more applied uses of multivariate designs, multivariate analysis can extend to include models such as those specific to testing structural validity of instruments designed to measure latent constructs such as intelligence and personality or to predicting group placement. In other words, multivariate research methods are warranted in most situations where multiple dependent variables are being considered in the same research scenario. In order to appropriately analyze data gathered under a multivariate design, researchers must shift away from univariate analysis into the multivariate analysis framework. The sections in this article are intended as an introduction to the applications, considerations, and mechanics of conducting multivariate research. Although the sections are presented as discrete categories, the topics often overlap and inform each other. The sections are General Overviews of multivariate topics, Multivariate Assumptions , Multivariate Effect Sizes , Multivariate Analysis of Variance (MANOVA), Discriminant Analysis (DA), Canonical Correlation Analysis (CCA), Exploratory Factor Analysis (EFA), Principal Components Analysis and Common Factor Analysis , Factor Retention , Rotation Criteria , Confirmatory Factor Analysis (CFA), Structural Equation Modeling (SEM), Multidimensional Scaling (MDS), and Nonparametric Multivariate Analysis .
The text and articles in this section provide overviews and introductions to major concepts for consideration while conducting multivariate analysis. Huberty and Morris 1989 and Huberty 1994 emphasize the reason for conducting a multivariate analysis instead of a series of univariate analyses. Tabachnick and Fidell 2012 and Stevens 2009 provide comprehensive introductions to understanding and conducting multivariate analysis. Grimm and Yarnold 1995 and Grimm and Yarnold 2000 include introductory chapters on a broad range of multivariate topics written by several experts in the field. Written in a conversational style, Harris 2001 introduces multivariate analysis to the novice researcher, while Johnson and Wichern 2007 provides in-depth chapters for those with stronger statistical backgrounds. Meyers, et al. 2013 presents introductions and step-by-step analysis examples using SPSS (Statistical Package for the Social Sciences). Raykov and Marcoulides 2008 provides several applied examples that include syntax for SPSS, SAS, and MPlus. Loehlin 2004 introduces latent variable models, including factor, path, and structural equation analysis.
Grimm, L. G., and P. R. Yarnold, eds. 1995. Reading and understanding multivariate statistics . Washington, DC: American Psychological Association.
Written for those with little to no exposure to multivariate analyses but a basic understanding of univariate statistics. Includes chapter topics such as path analysis, multidimensional scaling, analysis of cross-classified data, logistic regression, DA, MANOVA, and meta-analysis. Each chapter includes suggestions for further reading and a glossary of terms and symbols.
Grimm, L. G., and P. R. Yarnold, eds. 2000. Reading and understanding MORE multivariate statistics . Washington, DC: American Psychological Association.
An extension of the previous edition focusing on conceptual understanding. Includes chapters on generalizability theory, item response theory, assessing validity of measurement, cluster analysis, SEM, CCA, repeated measures analyses, and survival analysis. Each chapter includes suggestions for further reading and a glossary of terms and symbols.
Harris, R. J. 2001. A primer of multivariate statistics . 3d ed. New York: Psychology Press.
Presented in a conversational style, includes discussion of the theoretical reasoning behind multivariate analysis as well has how the analyses are conducted. Emphasizes the importance of testing the interpretations of latent variables produced through multivariate analyses.
Huberty, C. J. 1994. Why multivariate analyses? Educational and Psychological Measurement 54:620–627.
DOI: 10.1177/0013164494054003005
Overviews the definition and purpose of multivariate analyses. Emphasizes the importance of choosing the appropriate response variables. Refutes the idea of an obligatory multivariate analysis followed by a series of univariate analysis as protection against Type I error.
Huberty, C. J., and J. D. Morris. 1989. Multivariate analysis versus multiple univariate analyses. Psychological Bulletin 105:302–308.
DOI: 10.1037/0033-2909.105.2.302
Discusses the methodological argument surrounding multiple ANOVAs versus a MANOVA as it relates to Type I error. Emphasizes that analysis decisions should be based on the established research questions. Multivariate analysis is more appropriate for research questions involving outcome variable selection, variable ordering, and variable system structure.
Johnson, R. A., and D. W. Wichern. 2007. Applied multivariate statistical analysis . 6th ed. Upper Saddle River, NJ: Pearson.
Written from more of a business perspective for those with a stronger statistical background. Presents in-depth chapters on matrix algebra, sampling, and multivariate normal distribution in addition to commonly applied multivariate analyses. Includes examples with SAS syntax and output for two-way MANOVA, PCA, factor analysis, and DA.
Loehlin, J. C. 2004. Latent variable models: An introduction to factor, path, and structural equation analysis . 4th ed. Mahwah, NJ: Lawrence Erlbaum.
Provides a comprehensive overview of latent variable modeling as it pertains to factor analysis, path analysis, and structural equation modeling. Includes both introductory and advanced topics, accessibly presented using path diagrams, applied examples from published research, and illustrative exercises. Both the novice and more advanced user will find the explanations and extensive references useful.
Meyers, L. S., G. Gamst, and A. J. Guarino. 2013. Applied multivariate research: Design and interpretation . Los Angeles: SAGE.
Presented using conversational narrative, provides an introduction of multivariate research to the novice to intermediate user. Includes numerous graphical illustrations including path diagrams and segments of output. Readers will find the step-by-step approach with screenshots of SPSS menus and output helpful to conducting and interpreting analysis.
Raykov, T., and G. A. Marcoulides. 2008. An introduction to applied multivariate analysis . New York: Routledge.
Written as an introductory applied text, covers commonly used multivariate analyses while focusing on necessary methodological considerations such as data screening, meeting assumptions, and missing data. Includes explanations through extensions of univariate concepts. Examples of data analysis in SPSS, SAS, and MPlus included.
Stevens, J. 2009. Applied multivariate statistics for the social sciences . 5th ed. New York: Routledge.
Comprehensive text covering many background topics such as matrix algebra and multiple regression as well as the major multivariate analyses such as MANOVA, DA, factor analysis, CCA, and multivariate categorical data analysis. Each chapter begins with a brief introduction to the topic and includes example data sets with instructions for conducting the analysis and interpreting output in SPSS and SAS.
Tabachnick, B. G., and L. S. Fidell. 2012. Using multivariate statistics . 6th ed. Boston: Pearson Education.
A comprehensive applied review including conceptual background and discussion of analytical decisions, as well as a demonstration of how to conduct multivariate analysis. Demonstrations include DA, logistic regression, survival analysis, CCA, PCA, factor analysis, SEM, and multilevel linear modeling. Includes SPSS and SAS syntax and sample output.
back to top
Users without a subscription are not able to see the full content on this page. Please subscribe or login .
Oxford Bibliographies Online is available by subscription and perpetual access to institutions. For more information or to contact an Oxford Sales Representative click here .
- About Education »
- Meet the Editorial Board »
- Academic Achievement
- Academic Audit for Universities
- Academic Freedom and Tenure in the United States
- Action Research in Education
- Adjuncts in Higher Education in the United States
- Administrator Preparation
- Adolescence
- Advanced Placement and International Baccalaureate Courses
- Advocacy and Activism in Early Childhood
- African American Racial Identity and Learning
- Alaska Native Education
- Alternative Certification Programs for Educators
- Alternative Schools
- American Indian Education
- Animals in Environmental Education
- Art Education
- Artificial Intelligence and Learning
- Assessing School Leader Effectiveness
- Assessment, Behavioral
- Assessment, Educational
- Assessment in Early Childhood Education
- Assistive Technology
- Augmented Reality in Education
- Beginning-Teacher Induction
- Bilingual Education and Bilingualism
- Black Undergraduate Women: Critical Race and Gender Perspe...
- Blended Learning
- Case Study in Education Research
- Changing Professional and Academic Identities
- Character Education
- Children’s and Young Adult Literature
- Children's Beliefs about Intelligence
- Children's Rights in Early Childhood Education
- Citizenship Education
- Civic and Social Engagement of Higher Education
- Classroom Learning Environments: Assessing and Investigati...
- Classroom Management
- Coherent Instructional Systems at the School and School Sy...
- College Admissions in the United States
- College Athletics in the United States
- Community Relations
- Comparative Education
- Computer-Assisted Language Learning
- Computer-Based Testing
- Conceptualizing, Measuring, and Evaluating Improvement Net...
- Continuous Improvement and "High Leverage" Educational Pro...
- Counseling in Schools
- Critical Approaches to Gender in Higher Education
- Critical Perspectives on Educational Innovation and Improv...
- Critical Race Theory
- Crossborder and Transnational Higher Education
- Cross-National Research on Continuous Improvement
- Cross-Sector Research on Continuous Learning and Improveme...
- Cultural Diversity in Early Childhood Education
- Culturally Responsive Leadership
- Culturally Responsive Pedagogies
- Culturally Responsive Teacher Education in the United Stat...
- Curriculum Design
- Data Collection in Educational Research
- Data-driven Decision Making in the United States
- Deaf Education
- Desegregation and Integration
- Design Thinking and the Learning Sciences: Theoretical, Pr...
- Development, Moral
- Dialogic Pedagogy
- Digital Age Teacher, The
- Digital Citizenship
- Digital Divides
- Disabilities
- Distance Learning
- Distributed Leadership
- Doctoral Education and Training
- Early Childhood Education and Care (ECEC) in Denmark
- Early Childhood Education and Development in Mexico
- Early Childhood Education in Aotearoa New Zealand
- Early Childhood Education in Australia
- Early Childhood Education in China
- Early Childhood Education in Europe
- Early Childhood Education in Sub-Saharan Africa
- Early Childhood Education in Sweden
- Early Childhood Education Pedagogy
- Early Childhood Education Policy
- Early Childhood Education, The Arts in
- Early Childhood Mathematics
- Early Childhood Science
- Early Childhood Teacher Education
- Early Childhood Teachers in Aotearoa New Zealand
- Early Years Professionalism and Professionalization Polici...
- Economics of Education
- Education For Children with Autism
- Education for Sustainable Development
- Education Leadership, Empirical Perspectives in
- Education of Native Hawaiian Students
- Education Reform and School Change
- Educator Partnerships with Parents and Families with a Foc...
- Emotional and Affective Issues in Environmental and Sustai...
- Emotional and Behavioral Disorders
- English as an International Language for Academic Publishi...
- Environmental and Science Education: Overlaps and Issues
- Environmental Education
- Environmental Education in Brazil
- Epistemic Beliefs
- Equity and Improvement: Engaging Communities in Educationa...
- Equity, Ethnicity, Diversity, and Excellence in Education
- Ethical Research with Young Children
- Ethics and Education
- Ethics of Teaching
- Ethnic Studies
- Evidence-Based Communication Assessment and Intervention
- Family and Community Partnerships in Education
- Family Day Care
- Federal Government Programs and Issues
- Feminization of Labor in Academia
- Finance, Education
- Financial Aid
- Formative Assessment
- Future-Focused Education
- Gender and Achievement
- Gender and Alternative Education
- Gender, Power and Politics in the Academy
- Gender-Based Violence on University Campuses
- Gifted Education
- Global Mindedness and Global Citizenship Education
- Global University Rankings
- Governance, Education
- Grounded Theory
- Growth of Effective Mental Health Services in Schools in t...
- Higher Education and Globalization
- Higher Education and the Developing World
- Higher Education Faculty Characteristics and Trends in the...
- Higher Education Finance
- Higher Education Governance
- Higher Education Graduate Outcomes and Destinations
- Higher Education in Africa
- Higher Education in China
- Higher Education in Latin America
- Higher Education in the United States, Historical Evolutio...
- Higher Education, International Issues in
- Higher Education Management
- Higher Education Policy
- Higher Education Research
- Higher Education Student Assessment
- High-stakes Testing
- History of Early Childhood Education in the United States
- History of Education in the United States
- History of Technology Integration in Education
- Homeschooling
- Inclusion in Early Childhood: Difference, Disability, and ...
- Inclusive Education
- Indigenous Education in a Global Context
- Indigenous Learning Environments
- Indigenous Students in Higher Education in the United Stat...
- Infant and Toddler Pedagogy
- Inservice Teacher Education
- Integrating Art across the Curriculum
- Intelligence
- Intensive Interventions for Children and Adolescents with ...
- International Perspectives on Academic Freedom
- Intersectionality and Education
- Knowledge Development in Early Childhood
- Leadership Development, Coaching and Feedback for
- Leadership in Early Childhood Education
- Leadership Training with an Emphasis on the United States
- Learning Analytics in Higher Education
- Learning Difficulties
- Learning, Lifelong
- Learning, Multimedia
- Learning Strategies
- Legal Matters and Education Law
- LGBT Youth in Schools
- Linguistic Diversity
- Linguistically Inclusive Pedagogy
- Literacy Development and Language Acquisition
- Literature Reviews
- Mathematics Identity
- Mathematics Instruction and Interventions for Students wit...
- Mathematics Teacher Education
- Measurement for Improvement in Education
- Measurement in Education in the United States
- Meta-Analysis and Research Synthesis in Education
- Methodological Approaches for Impact Evaluation in Educati...
- Mindfulness, Learning, and Education
- Mixed Methods Research
- Motherscholars
- Multiliteracies in Early Childhood Education
- Multiple Documents Literacy: Theory, Research, and Applica...
- Multivariate Research Methodology
- Museums, Education, and Curriculum
- Music Education
- Narrative Research in Education
- Native American Studies
- Nonformal and Informal Environmental Education
- Note-Taking
- Numeracy Education
- One-to-One Technology in the K-12 Classroom
- Online Education
- Open Education
- Organizing for Continuous Improvement in Education
- Organizing Schools for the Inclusion of Students with Disa...
- Outdoor Play and Learning
- Outdoor Play and Learning in Early Childhood Education
- Pedagogical Leadership
- Pedagogy of Teacher Education, A
- Performance Objectives and Measurement
- Performance-based Research Assessment in Higher Education
- Performance-based Research Funding
- Phenomenology in Educational Research
- Philosophy of Education
- Physical Education
- Podcasts in Education
- Policy Context of United States Educational Innovation and...
- Politics of Education
- Portable Technology Use in Special Education Programs and ...
- Post-humanism and Environmental Education
- Pre-Service Teacher Education
- Problem Solving
- Productivity and Higher Education
- Professional Development
- Professional Learning Communities
- Program Evaluation
- Programs and Services for Students with Emotional or Behav...
- Psychology Learning and Teaching
- Psychometric Issues in the Assessment of English Language ...
- Qualitative Data Analysis Techniques
- Qualitative, Quantitative, and Mixed Methods Research Samp...
- Qualitative Research Design
- Queering the English Language Arts (ELA) Writing Classroom
- Race and Affirmative Action in Higher Education
- Reading Education
- Refugee and New Immigrant Learners
- Relational and Developmental Trauma and Schools
- Relational Pedagogies in Early Childhood Education
- Reliability in Educational Assessments
- Religion in Elementary and Secondary Education in the Unit...
- Researcher Development and Skills Training within the Cont...
- Research-Practice Partnerships in Education within the Uni...
- Response to Intervention
- Restorative Practices
- Risky Play in Early Childhood Education
- Scale and Sustainability of Education Innovation and Impro...
- Scaling Up Research-based Educational Practices
- School Accreditation
- School Choice
- School Culture
- School District Budgeting and Financial Management in the ...
- School Improvement through Inclusive Education
- School Reform
- Schools, Private and Independent
- School-Wide Positive Behavior Support
- Science Education
- Secondary to Postsecondary Transition Issues
- Self-Regulated Learning
- Self-Study of Teacher Education Practices
- Service-Learning
- Severe Disabilities
- Single Salary Schedule
- Single-sex Education
- Single-Subject Research Design
- Social Context of Education
- Social Justice
- Social Network Analysis
- Social Pedagogy
- Social Studies Education
- Sociology of Education
- Standards-Based Education
- Student Access, Equity, and Diversity in Higher Education
- Student Assignment Policy
- Student Engagement in Tertiary Education
- Student Learning, Development, Engagement, and Motivation ...
- Student Participation
- Student Voice in Teacher Development
- Sustainability Education in Early Childhood Education
- Sustainability in Early Childhood Education
- Sustainability in Higher Education
- Teacher Beliefs and Epistemologies
- Teacher Collaboration in School Improvement
- Teacher Evaluation and Teacher Effectiveness
- Teacher Preparation
- Teacher Training and Development
- Teacher Unions and Associations
- Teacher-Student Relationships
- Teaching Critical Thinking
- Technologies, Teaching, and Learning in Higher Education
- Technology Education in Early Childhood
- Technology, Educational
- Technology-based Assessment
- The Bologna Process
- The Regulation of Standards in Higher Education
- Theories of Educational Leadership
- Three Conceptions of Literacy: Media, Narrative, and Gamin...
- Tracking and Detracking
- Traditions of Quality Improvement in Education
- Transformative Learning
- Transitions in Early Childhood Education
- Tribally Controlled Colleges and Universities in the Unite...
- Understanding the Psycho-Social Dimensions of Schools and ...
- University Faculty Roles and Responsibilities in the Unite...
- Using Ethnography in Educational Research
- Value of Higher Education for Students and Other Stakehold...
- Virtual Learning Environments
- Vocational and Technical Education
- Wellness and Well-Being in Education
- Women's and Gender Studies
- Young Children and Spirituality
- Young Children's Learning Dispositions
- Young Children's Working Theories
- Privacy Policy
- Cookie Policy
- Legal Notice
- Accessibility
Powered by:
- [66.249.64.20|185.66.14.133]
- 185.66.14.133
An Introduction to Multivariate Analysis
Data analytics is all about looking at various factors to see how they impact certain situations and outcomes. When dealing with data that contains more than two variables, you’ll use multivariate analysis.
Multivariate analysis isn’t just one specific method—rather, it encompasses a whole range of statistical techniques. These techniques allow you to gain a deeper understanding of your data in relation to specific business or real-world scenarios.
So, if you’re an aspiring data analyst or data scientist, multivariate analysis is an important concept to get to grips with.
In this post, we’ll provide a complete introduction to multivariate analysis. We’ll delve deeper into defining what multivariate analysis actually is, and we’ll introduce some key techniques you can use when analyzing your data. We’ll also give some examples of multivariate analysis in action.
Want to skip ahead to a particular section? Just use the clickable menu.
- What is multivariate analysis?
- Multivariate data analysis techniques (with examples)
- What are the advantages of multivariate analysis?
- Key takeaways and further reading
Ready to demystify multivariate analysis? Let’s do it.
1. What is multivariate analysis?
In data analytics, we look at different variables (or factors) and how they might impact certain situations or outcomes.
For example, in marketing, you might look at how the variable “money spent on advertising” impacts the variable “number of sales.” In the healthcare sector, you might want to explore whether there’s a correlation between “weekly hours of exercise” and “cholesterol level.” This helps us to understand why certain outcomes occur, which in turn allows us to make informed predictions and decisions for the future.
There are three categories of analysis to be aware of:
- Univariate analysis , which looks at just one variable
- Bivariate analysis , which analyzes two variables
- Multivariate analysis , which looks at more than two variables
As you can see, multivariate analysis encompasses all statistical techniques that are used to analyze more than two variables at once. The aim is to find patterns and correlations between several variables simultaneously—allowing for a much deeper, more complex understanding of a given scenario than you’ll get with bivariate analysis.
An example of multivariate analysis
Let’s imagine you’re interested in the relationship between a person’s social media habits and their self-esteem. You could carry out a bivariate analysis, comparing the following two variables:
- How many hours a day a person spends on Instagram
- Their self-esteem score (measured using a self-esteem scale)
You may or may not find a relationship between the two variables; however, you know that, in reality, self-esteem is a complex concept. It’s likely impacted by many different factors—not just how many hours a person spends on Instagram. You might also want to consider factors such as age, employment status, how often a person exercises, and relationship status (for example). In order to deduce the extent to which each of these variables correlates with self-esteem, and with each other, you’d need to run a multivariate analysis.
So we know that multivariate analysis is used when you want to explore more than two variables at once. Now let’s consider some of the different techniques you might use to do this.
2. Multivariate data analysis techniques and examples
There are many different techniques for multivariate analysis, and they can be divided into two categories:
- Dependence techniques
- Interdependence techniques
So what’s the difference? Let’s take a look.
Multivariate analysis techniques: Dependence vs. interdependence
When we use the terms “dependence” and “interdependence,” we’re referring to different types of relationships within the data. To give a brief explanation:
Dependence methods
Dependence methods are used when one or some of the variables are dependent on others. Dependence looks at cause and effect; in other words, can the values of two or more independent variables be used to explain, describe, or predict the value of another, dependent variable? To give a simple example, the dependent variable of “weight” might be predicted by independent variables such as “height” and “age.”
In machine learning, dependence techniques are used to build predictive models. The analyst enters input data into the model, specifying which variables are independent and which ones are dependent—in other words, which variables they want the model to predict, and which variables they want the model to use to make those predictions.
Interdependence methods
Interdependence methods are used to understand the structural makeup and underlying patterns within a dataset. In this case, no variables are dependent on others, so you’re not looking for causal relationships. Rather, interdependence methods seek to give meaning to a set of variables or to group them together in meaningful ways.
So: One is about the effect of certain variables on others, while the other is all about the structure of the dataset.
With that in mind, let’s consider some useful multivariate analysis techniques. We’ll look at:
Multiple linear regression
Multiple logistic regression, multivariate analysis of variance (manova), factor analysis, cluster analysis.
Multiple linear regression is a dependence method which looks at the relationship between one dependent variable and two or more independent variables. A multiple regression model will tell you the extent to which each independent variable has a linear relationship with the dependent variable. This is useful as it helps you to understand which factors are likely to influence a certain outcome, allowing you to estimate future outcomes.
Example of multiple regression:
As a data analyst, you could use multiple regression to predict crop growth. In this example, crop growth is your dependent variable and you want to see how different factors affect it. Your independent variables could be rainfall, temperature, amount of sunlight, and amount of fertilizer added to the soil. A multiple regression model would show you the proportion of variance in crop growth that each independent variable accounts for.
Source: Public domain via Wikimedia Commons
Logistic regression analysis is used to calculate (and predict) the probability of a binary event occurring. A binary outcome is one where there are only two possible outcomes; either the event occurs (1) or it doesn’t (0). So, based on a set of independent variables, logistic regression can predict how likely it is that a certain scenario will arise. It is also used for classification. You can learn about the difference between regression and classification here .
Example of logistic regression:
Let’s imagine you work as an analyst within the insurance sector and you need to predict how likely it is that each potential customer will make a claim. You might enter a range of independent variables into your model, such as age, whether or not they have a serious health condition, their occupation, and so on. Using these variables, a logistic regression analysis will calculate the probability of the event (making a claim) occurring. Another oft-cited example is the filters used to classify email as “spam” or “not spam.” You’ll find a more detailed explanation in this complete guide to logistic regression .
Multivariate analysis of variance (MANOVA) is used to measure the effect of multiple independent variables on two or more dependent variables. With MANOVA, it’s important to note that the independent variables are categorical, while the dependent variables are metric in nature. A categorical variable is a variable that belongs to a distinct category—for example, the variable “employment status” could be categorized into certain units, such as “employed full-time,” “employed part-time,” “unemployed,” and so on. A metric variable is measured quantitatively and takes on a numerical value.
In MANOVA analysis, you’re looking at various combinations of the independent variables to compare how they differ in their effects on the dependent variable.
Example of MANOVA:
Let’s imagine you work for an engineering company that is on a mission to build a super-fast, eco-friendly rocket. You could use MANOVA to measure the effect that various design combinations have on both the speed of the rocket and the amount of carbon dioxide it emits. In this scenario, your categorical independent variables could be:
- Engine type, categorized as E1, E2, or E3
- Material used for the rocket exterior, categorized as M1, M2, or M3
- Type of fuel used to power the rocket, categorized as F1, F2, or F3
Your metric dependent variables are speed in kilometers per hour, and carbon dioxide measured in parts per million. Using MANOVA, you’d test different combinations (e.g. E1, M1, and F1 vs. E1, M2, and F1, vs. E1, M3, and F1, and so on) to calculate the effect of all the independent variables. This should help you to find the optimal design solution for your rocket.
Factor analysis is an interdependence technique which seeks to reduce the number of variables in a dataset. If you have too many variables, it can be difficult to find patterns in your data. At the same time, models created using datasets with too many variables are susceptible to overfitting. Overfitting is a modeling error that occurs when a model fits too closely and specifically to a certain dataset, making it less generalizable to future datasets, and thus potentially less accurate in the predictions it makes.
Factor analysis works by detecting sets of variables which correlate highly with each other. These variables may then be condensed into a single variable. Data analysts will often carry out factor analysis to prepare the data for subsequent analyses.
Factor analysis example:
Let’s imagine you have a dataset containing data pertaining to a person’s income, education level, and occupation. You might find a high degree of correlation among each of these variables, and thus reduce them to the single factor “socioeconomic status.” You might also have data on how happy they were with customer service, how much they like a certain product, and how likely they are to recommend the product to a friend. Each of these variables could be grouped into the single factor “customer satisfaction” (as long as they are found to correlate strongly with one another). Even though you’ve reduced several data points to just one factor, you’re not really losing any information—these factors adequately capture and represent the individual variables concerned. With your “streamlined” dataset, you’re now ready to carry out further analyses.
Another interdependence technique, cluster analysis is used to group similar items within a dataset into clusters.
When grouping data into clusters, the aim is for the variables in one cluster to be more similar to each other than they are to variables in other clusters. This is measured in terms of intracluster and intercluster distance. Intracluster distance looks at the distance between data points within one cluster. This should be small. Intercluster distance looks at the distance between data points in different clusters. This should ideally be large. Cluster analysis helps you to understand how data in your sample is distributed, and to find patterns.
Learn more: What is Cluster Analysis? A Complete Beginner’s Guide
Cluster analysis example:
A prime example of cluster analysis is audience segmentation. If you were working in marketing, you might use cluster analysis to define different customer groups which could benefit from more targeted campaigns. As a healthcare analyst , you might use cluster analysis to explore whether certain lifestyle factors or geographical locations are associated with higher or lower cases of certain illnesses. Because it’s an interdependence technique, cluster analysis is often carried out in the early stages of data analysis.
Source: Chire, CC BY-SA 3.0 via Wikimedia Commons
More multivariate analysis techniques
This is just a handful of multivariate analysis techniques used by data analysts and data scientists to understand complex datasets. If you’re keen to explore further, check out discriminant analysis, conjoint analysis, canonical correlation analysis, structural equation modeling, and multidimensional scaling.
3. What are the advantages of multivariate analysis?
The one major advantage of multivariate analysis is the depth of insight it provides. In exploring multiple variables, you’re painting a much more detailed picture of what’s occurring—and, as a result, the insights you uncover are much more applicable to the real world.
Remember our self-esteem example back in section one? We could carry out a bivariate analysis, looking at the relationship between self-esteem and just one other factor; and, if we found a strong correlation between the two variables, we might be inclined to conclude that this particular variable is a strong determinant of self-esteem. However, in reality, we know that self-esteem can’t be attributed to one single factor. It’s a complex concept; in order to create a model that we could really trust to be accurate, we’d need to take many more factors into account. That’s where multivariate analysis really shines; it allows us to analyze many different factors and get closer to the reality of a given situation.
4. Key takeaways and further reading
In this post, we’ve learned that multivariate analysis is used to analyze data containing more than two variables. To recap, here are some key takeaways:
- The aim of multivariate analysis is to find patterns and correlations between several variables simultaneously
- Multivariate analysis is especially useful for analyzing complex datasets, allowing you to gain a deeper understanding of your data and how it relates to real-world scenarios
- There are two types of multivariate analysis techniques: Dependence techniques, which look at cause-and-effect relationships between variables, and interdependence techniques, which explore the structure of a dataset
- Key multivariate analysis techniques include multiple linear regression, multiple logistic regression, MANOVA, factor analysis, and cluster analysis—to name just a few
So what now? For a hands-on introduction to data analytics, try this free five-day data analytics short course . And, if you’d like to learn more about the different methods used by data analysts, check out the following:
- What is data cleaning and why does it matter?
- SQL cheatsheet: Learn your first 8 commands
- A step-by-step guide to the data analysis process
- Technical Support
- Find My Rep
You are here
Making Sense of Multivariate Data Analysis An Intuitive Approach
- John Spicer
- Description
See what’s new to this edition by selecting the Features tab on this page. Should you need additional information or have questions regarding the HEOA information provided for this title, including what is new to this edition, please email [email protected] . Please include your name, contact information, and the name of the title for which you would like more information. For information on the HEOA, please go to http://ed.gov/policy/highered/leg/hea08/index.html .
For assistance with your order: Please email us at [email protected] or connect with your SAGE representative.
SAGE 2455 Teller Road Thousand Oaks, CA 91320 www.sagepub.com
“This book serves as a resource for readers who want to have an overall view of what encompasses multivariate analyses. The author has discussed some important issues rather philosophically (e.g., theory vs. data analysis). These points are valuable even for readers who have extensive training with multivariate analyses.”
“This book is a helpful guide to reading and understanding multivariate data analysis results in social and psychological research.”
"Spicer's book is a superb overview of multivariate statistics, but without formulas. Even though he is trying to offer a nontechnical overview of multivariate analyses, he doesn't shortchange the reader in any way. As much as you might know about stat, you'll learn some more here."
This book is easy to understand although multivariate analysis is complicated. Students find it very helpful especially when interpreting the analytic results.
The approach of using published articles as illustrations and non-mathematical languages are definitely useful for social science students to understand the complexity of mutlivariate analysis in context.
It is a straightforward presentation of analysis procedures included in this course. I have used a number of QASS series publications in teaching data analysis for almost 20 years. I had them as texts when I was a PhD student. Generally more accepted by students than more in-depth treatments.
- Assumes no prior statistical knowledge
- Relies mainly on verbal exposition rather than symbols and formulae
- Carries the reader from basic to advanced ideas in a relatively short space to demonstrate their continuity
- Highlights the underlying strategies that unite all common multivariate techniques
- Encourages the reader to reflect critically on the limitations of multivariate analysis in the broad research context
- Uses examples from contemporary research on subjective well-being drawn from a variety of areas in the behavioral and social sciences
Sample Materials & Chapters
Chapter 1. What Makes A Difference?
Chapter 3. Accounting for Differences in a Complex World
Chapter 5. Logistic Regression and Discriminant Analysis
For instructors
Select a purchasing option.

This title is also available on SAGE Research Methods , the ultimate digital methods library. If your library doesn’t have access, ask your librarian to start a trial .

Want to create or adapt books like this? Learn more about how Pressbooks supports open publishing practices.
Quantitative Data Analysis With SPSS
14 Quantitative Analysis with SPSS: Multivariate Crosstabs
Mikaila Mariel Lemonik Arthur
Producing a multivariate crosstabulation is exactly the same as producing a bivariate crosstabulation, except that an additional variable is added. Note that, due to the limitations of the crosstabulation approach, you are not actually looking at the relationships between all three variables simultaneously (and this approach is limited to three variables). Rather, you are looking at how controlling for a third variable—your “Layer” or control variable—changes the relationship between the independent and dependent variable in your analysis. What SPSS produces, then, is basically a stack of crosstabulation tables with your independent and dependent variables, one for each category of your control variable, along with statistical significance and association values for each category of your control variable. This chapter will review how to produce and interpret a multivariate crosstabulation. It uses variables with fairly few categories for ease of interpretation. Do note that when using variables with many categories, results can become quite complex and lengthy, and due to small numbers of cases left in each cell of the very lengthy tables, statistical significance is likely to be reduced. Thus, analysts should take care to consider whether the relationship(s) they are interested in are suitable for this type of analysis, and may want to consider recoding variables (see the chapter on data management ) with many categories into somewhat fewer categories to facilitate analysis.
To produce a multivariate crosstabulation, follow the same steps as you
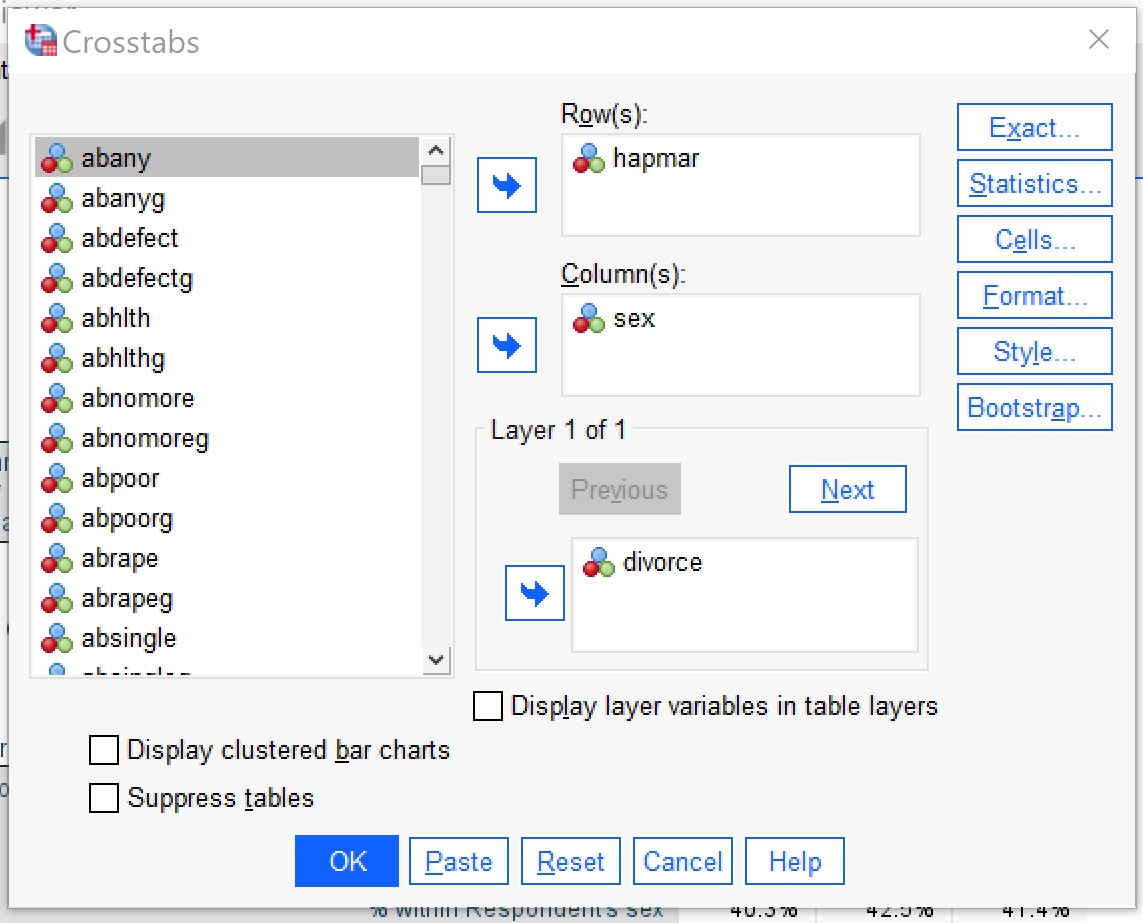
would follow to produce a bivariate crosstabulation —put the independent variable in the columns box, the dependent variable in the rows box, select column percentages under cells, and select chi square and an appropriate measure of association under statistics. Note that the measure of association you choose should be the same one that you would choose for a bivariate analysis with the same independent and dependent variables, as the third variable is a control variable and does not alter the criteria upon which the decision about measures of association is made. The one thing you need to add in order to produce a multivariate crosstabulation is that you add your third variable, the control variable, to the Layer box in the crosstabs dialog. Figure 1 shows what this would look like for a crosstabulation with the independent variable SEX, the dependent variable HAPMAR, and the control variable DIVORCE. In other words, this analysis is exploring whether being male or female influences respondents’ feelings of happiness in their marriages, controlling for whether or not they have ever been divorced.
First, consider the crosstabulation table. As you can see, this table really consists of three tables stacked on top of each other. Each of these three tables considers the relationship between sex and the happiness of the respondent’s marriage, but there is one table for those who have ever been divorced, one table for those who have never been divorced, and one table for everyone. Comparing the percentages across the rows, we can make the following observations:
- Among those who have ever been divorced , males are slightly more likely to be very happy in their marriage, while females are somewhat more likely to be not too happy.
- Among those who have not ever been divorced, males are more likely to be very happy in their marriage, while females are more likely to be pretty happy and are somewhat more likely to be not too happy.
- Among the entire sample, males are more likely to be very happy in their marriages, while females are more likely to be pretty happy and somewhat more likely to be not too happy.
- Overall, then, the results suggest men are happier in their marriages than women.
Next, we turn to statistical significance. At the p<0.05 level, we can observe that this analysis produces significant results for those who have never been divorced and for the entire sample, but not for those who have been divorced. Turning to the association, we find a weak association—the figures for those who have been divorced, those who have not been divorced, and the entire population are quite similar.
Thus, we can conclude that women who have never been divorced are, on average, less happy in their marriages than men who have never been divorced, but that among those who have been divorced, the relationship between sex and marital happiness is not statistically significant.
Select three variables of interest. Answer the following questions:
- Which is the independent variable, which is the dependent variable, and which is the control variable?
- What is the research hypothesis for this analysis? What do you predict will be the relationship between the independent variable and the dependent variable, and how will the control variable impact this relationship?
- What is the null hypothesis for this analysis?
- What confidence level (p value) have you chosen?
- Which measure of association is most appropriate for this relationship?
Next, use SPSS to produce a multivariate crosstabulation according to the instructions in this chapter. Interpret the crosstabulation. First, answer the following questions for each of the stacked crosstabulations of your independent and dependent variable (one for each category of the control variable, plus one for everyone):
- Is the relationship between the independent and dependent variables statistically significant?
- Can the null hypothesis be rejected?
- How strong is the association between the two variables?
- Looking at that pattern of percentages across the rows, what can you determine about the nature of the relationship between the two variables?
Then, compare your results across the different categories of the control variable.
- What does this tell you about how the control variable impacts the relationship between the independent and dependent variables?
- Is there support for your research hypothesis?
Media Attributions
- crosstabs dialog multivariate © IBM SPSS is licensed under a All Rights Reserved license
Social Data Analysis Copyright © 2021 by Mikaila Mariel Lemonik Arthur is licensed under a Creative Commons Attribution-NonCommercial-ShareAlike 4.0 International License , except where otherwise noted.
All Courses
- Interview Questions
- Free Courses
- Career Guide
- PGP in Data Science and Business Analytics
- PG Program in Data Science and Business Analytics Classroom
- PGP in Data Science and Engineering (Data Science Specialization)
- PGP in Data Science and Engineering (Bootcamp)
- PGP in Data Science & Engineering (Data Engineering Specialization)
- Master of Data Science (Global) – Deakin University
- MIT Data Science and Machine Learning Course Online
- Master’s (MS) in Data Science Online Degree Programme
- MTech in Data Science & Machine Learning by PES University
- Data Analytics Essentials by UT Austin
- Data Science & Business Analytics Program by McCombs School of Business
- MTech In Big Data Analytics by SRM
- M.Tech in Data Engineering Specialization by SRM University
- M.Tech in Big Data Analytics by SRM University
- PG in AI & Machine Learning Course
- Weekend Classroom PG Program For AI & ML
- AI for Leaders & Managers (PG Certificate Course)
- Artificial Intelligence Course for School Students
- IIIT Delhi: PG Diploma in Artificial Intelligence
- Machine Learning PG Program
- MIT No-Code AI and Machine Learning Course
- Study Abroad: Masters Programs
- MS in Information Science: Machine Learning From University of Arizon
- SRM M Tech in AI and ML for Working Professionals Program
- UT Austin Artificial Intelligence (AI) for Leaders & Managers
- UT Austin Artificial Intelligence and Machine Learning Program Online
- MS in Machine Learning
- IIT Roorkee Full Stack Developer Course
- IIT Madras Blockchain Course (Online Software Engineering)
- IIIT Hyderabad Software Engg for Data Science Course (Comprehensive)
- IIIT Hyderabad Software Engg for Data Science Course (Accelerated)
- IIT Bombay UX Design Course – Online PG Certificate Program
- Online MCA Degree Course by JAIN (Deemed-to-be University)
- Cybersecurity PG Course
- Online Post Graduate Executive Management Program
- Product Management Course Online in India
- NUS Future Leadership Program for Business Managers and Leaders
- PES Executive MBA Degree Program for Working Professionals
- Online BBA Degree Course by JAIN (Deemed-to-be University)
- MBA in Digital Marketing or Data Science by JAIN (Deemed-to-be University)
- Master of Business Administration- Shiva Nadar University
- Post Graduate Diploma in Management (Online) by Great Lakes
- Online MBA Programs
- Cloud Computing PG Program by Great Lakes
- University Programs
- Stanford Design Thinking Course Online
- Design Thinking : From Insights to Viability
- PGP In Strategic Digital Marketing
- Post Graduate Diploma in Management
- Master of Business Administration Degree Program
- MS in Business Analytics in USA
- MS in Machine Learning in USA
- Study MBA in Germany at FOM University
- M.Sc in Big Data & Business Analytics in Germany
- Study MBA in USA at Walsh College
- MS Data Analytics
- MS Artificial Intelligence and Machine Learning
- MS in Data Analytics
- Master of Business Administration (MBA)
- MS in Information Science: Machine Learning
- MS in Machine Learning Online
- MS in Computer Science
- MS in Computer Science and MS in Data Analytics
- MIT Data Science Program
- AI For Leaders Course
- Data Science and Business Analytics Course
- Cyber Security Course
- PG Program Online Artificial Intelligence Machine Learning
- PG Program Online Cloud Computing Course
- Data Analytics Essentials Online Course
- MIT Programa Ciencia De Dados Machine Learning
- MIT Programa Ciencia De Datos Aprendizaje Automatico
- Program PG Ciencia Datos Analitica Empresarial Curso Online
- Mit Programa Ciencia De Datos Aprendizaje Automatico
- Online Data Science Business Analytics Course
- Online Ai Machine Learning Course
- Online Full Stack Software Development Course
- Online Cloud Computing Course
- Cybersecurity Course Online
- Online Data Analytics Essentials Course
- Ai for Business Leaders Course
- Mit Data Science Program
- No Code Artificial Intelligence Machine Learning Program
- MS Information Science Machine Learning University Arizona
- Wharton Online Advanced Digital Marketing Program
- Multivariate Analysis Overview
- What is Multivariate Analysis?
- The Evolution of Multivariate Analysis
- Multivariate Analysis: An Overview
- Key Applications of Multivariate Analysis
- Advantages and Disadvantages of Multivariate Analysis
- Classification Chart of Multivariate Techniques
- Comprehensive Model Building Process
- Key Multivariate Techniques
- Model Building Process in Multivariate Analysis
- Multivariate Analysis FAQs
Overview of Multivariate Analysis | What is Multivariate Analysis and Model Building Process?
Multivariate Analysis (MVA) is a powerful statistical method that examines multiple variables to understand their impact on a specific outcome. This technique is crucial for analyzing complex data sets and uncovering hidden patterns across diverse fields such as weather forecasting, marketing, and healthcare. By exploring the relationships between several variables at once, MVA provides deeper insights and more accurate predictions, enhancing decision-making in data-driven industries. In this blog, we’ll explore the foundations, applications, and methods of multivariate analysis, highlighting its significance in modern data analysis.
Multivariate Analysis (MVA) is an essential statistical process used to understand the impact of multiple variables on a single outcome. This technique is fundamental in fields where complex interrelationships among data need to be studied, such as in weather forecasting where variables like temperature, humidity, and wind speed all influence weather conditions. Also, take up a Multivariate Time Series Forecasting In R to learn more about the concept.
Multivariate analysis has a rich history, beginning with John Wishart’s 1928 paper on sample covariance matrices. Over the decades, significant developments were made by statisticians like R.A. Fischer and Hotelling, initially applying these methods in psychology, education, and biology. The mid-20th century saw a technological boom with the advent of computers, expanding the application of multivariate analysis into new fields such as geology and meteorology. This period marked a shift from theoretical exploration to practical, computational applications.
Watch this youtube video for better understanding of Multivariate Analysis. It will provide you with an overview of the analysis.
The versatility of multivariate analysis is evident across various sectors:
- Healthcare: During the COVID-19 pandemic, multivariate analysis was crucial in forecasting disease spread and outcomes based on multiple health and environmental variables.
- Real Estate: Analysts use multivariate analysis to predict housing prices based on factors such as location, local infrastructure, and market conditions.
- Marketing: Techniques like conjoint analysis help determine consumer preferences and the trade-offs consumers make between different product attributes.
- The main advantage of multivariate analysis is that since it considers more than one factor of independent variables that influence the variability of dependent variables, the conclusion drawn is more accurate.
- The conclusions are more realistic and nearer to the real-life situation.
Disadvantages
- The main disadvantage of MVA includes that it requires rather complex computations to arrive at a satisfactory conclusion.
- Many observations for a large number of variables need to be collected and tabulated; it is a rather time-consuming process.
Selection of the appropriate multivariate technique depends upon-
a) Are the variables divided into independent and dependent classification?
b) If Yes, how many variables are treated as dependents in a single analysis?
c) How are the variables, both dependent and independent measured?
Multivariate analysis technique can be classified into two broad categories viz., This classification depends upon the question: are the involved variables dependent on each other or not?
If the answer is yes: We have Dependence methods. If the answer is no: We have Interdependence methods.
Dependence technique: Dependence Techniques are types of multivariate analysis techniques that are used when one or more of the variables can be identified as dependent variables and the remaining variables can be identified as independent.
Also Read: What is Big Data Analytics?
Model building in multivariate analysis involves several critical steps:
- Defining the Problem: Clearly defining what you need to solve or understand is the first step in multivariate analysis.
- Data Collection: Gathering adequate data for all variables considered relevant to the analysis.
- Data Preparation: Ensuring data cleanliness and proper format for analysis.
- Choosing the Right Model: Selecting a statistical model that fits the data and problem. This might include regression analysis, conjoint analysis, or discriminant analysis, depending on the complexity and nature of the problem.
- Model Testing and Validation: Rigorous testing to validate the model’s assumptions and its predictive power.
- Implementation and Monitoring: Applying the model in real-world scenarios and continually monitoring its effectiveness.
Below is a tabular overview of the primary multivariate analysis techniques, detailing their purposes and typical applications:
Building a model in multivariate analysis involves several critical steps:
- Defining Objectives: Clearly stating what you want to achieve with the analysis.
- Choosing Techniques: Selecting the appropriate statistical techniques based on the data and objectives.
- Assumption Testing: Checking assumptions like normality, homoscedasticity, linearity, and absence of multicollinearity.
- Model Estimation and Validation: Estimating the model parameters and validating the model through various diagnostic tests.
- Interpretation: Drawing conclusions from the model and applying these insights to make informed decisions.
Advantages Over Univariate and Bivariate Analysis
Unlike univariate or bivariate analysis, which examine one or two variables respectively, multivariate analysis provides a more comprehensive view by considering multiple variables simultaneously. This holistic approach leads to insights that are more detailed and closely aligned with the complexities of real-world scenarios.
Multivariate analysis is a powerful tool that, when applied correctly, can offer deep insights into complex data sets across various industries. It enables analysts to make more informed decisions, predict future trends, and optimize outcomes based on a thorough understanding of the interplay between multiple factors. For professionals looking to enhance their analytical skills, learning multivariate analysis through structured courses and hands-on practice is highly recommended.
If you are a beginner in the field of data science and wish to kick-start your career, taking up free online courses can help you grasp the introductory concepts in a comprehensive manner. Great Learning Academy offers a Data Science Foundations Free Online Course that can help you become job-ready. Some of the skills you will gain by the end of the course are linear programming, hands-on experience, and analytics landscape.
Three categories of multivariate analysis are: Cluster Analysis, Multiple Logistic Regression, and Multivariate Analysis of Variance.
Multivariate analysis is helpful in effectively minimizing the bias.
Multivariate refers to multiple dependent variables that result in one outcome. This means that a majority of our real-world problems are multivariate. For example, based on the season, we cannot predict the weather of any given year. Several factors play an important role in predicting the same. Such as, humidity, precipitation, pollution, etc.
There are several applications of multivariate analysis. It allows us to handle a huge dataset and discover hidden data structures that contribute to a better understanding and easy interpretation of data. There are various multivariate techniques that can be selected depending on the task at hand.
Multivariate analysis talks about two or more variables. It analyses which ones are correlated with a specific outcome. Whereas bivariate analysis talks about only two paired datasets and studies whether there is a relationship between them.

Top Free Courses

What is Time Complexity And Why Is It Essential?

Learn Pattern Program in Python – Examples and Code Explanation

A Complete understanding of LASSO Regression

Top 30 Python Libraries To Know

Python Dictionary Append: How To Add Key/Value Pair?

¿Qué es la Ciencia de Datos? – Una Guía Completa [2024]
Leave a comment cancel reply.
Your email address will not be published. Required fields are marked *
Save my name, email, and website in this browser for the next time I comment.
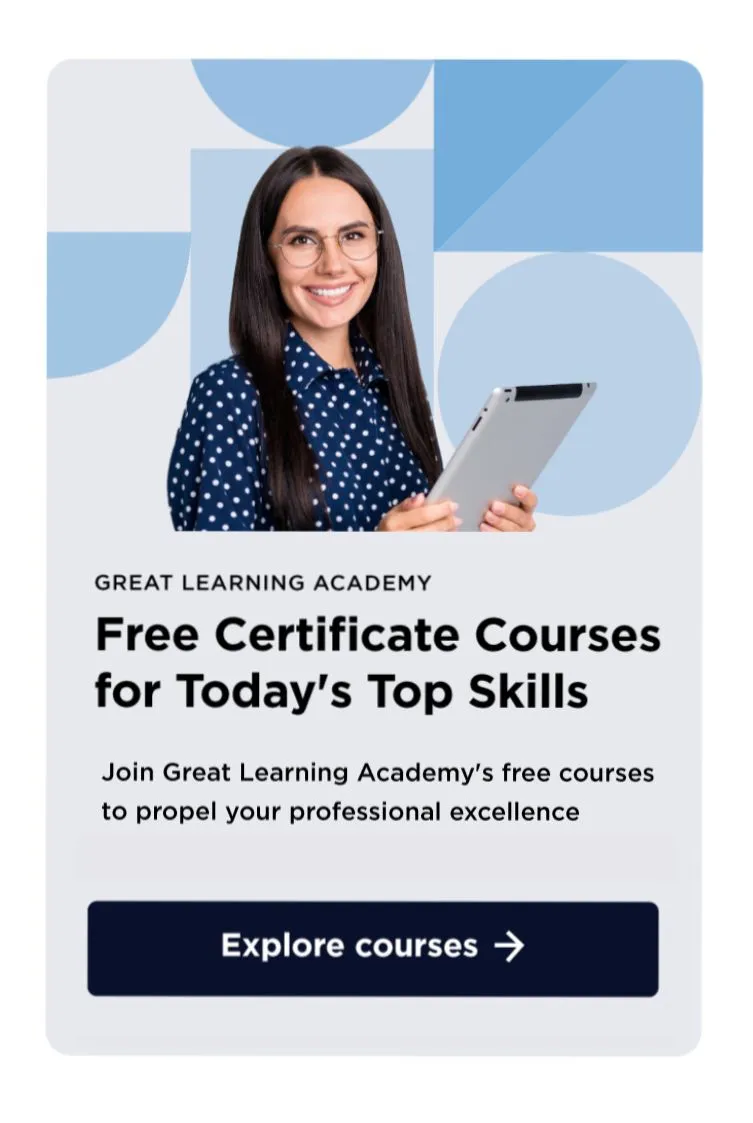
Table of contents
Multivariate analysis — definition, methods, and examples

Multivariate analysis allows you to find patterns between variables, helping you better understand the effects that different factors have on each other and the relationships between them. It represents a critical tool for marketers looking for ways to get deeper insight into the outcome of campaign decisions.
Business owners can also use multivariate analysis to better determine the impact of complex market and global factors on everything from sales to consumer behavior.
This article will demonstrate how multivariate analysis can help you determine how different variables interact with each other in complex scenarios, and the power that comes with identifying patterns and the relationships between those variables. It will also detail multiple methods, examples, and possible use cases to help you understand the strengths of each.
Specifically, it will explain:
- What multivariate analysis is
Multiple linear regression
Multiple logistic regressions, multivariate analysis of variance (manova), factor analysis, cluster analysis, discriminant analysis, conjoint analysis, correlation analysis, what is multivariate analysis.
Multivariate analysis is used to find patterns and correlations between multiple factors by analyzing two or more variables at once. Experts generally group the various approaches of multivariate analysis into two camps — dependent techniques for those instances when the outlined variables depend on one another, and independent techniques for when they do not.
There are many ways to conduct a multivariate analysis. Below we’ll explore some of the most common methods.
Multiple linear regression is an example of a dependent technique that looks at the relationship between one dependent variable and two or more independent variables. For instance, say a couple decides to sell their home. The price they can get for it depends as a variable on many independent factors, including location, time of year, interest rates, and the existence of similar listings in the area.
Let’s think about another example. You want to understand how social media spend across multiple platforms is likely to affect future traffic to your website. In this scenario, the amount and type of spend and platform type are all independent variables, and web traffic is the dependent variable.
Multiple logistic regressions, meanwhile, examine the probability of one of two events occurring. Will one’s partner accept an offer of marriage? Will a child graduate from college? In both scenarios, myriad factors may contribute to the final outcome — has the partner expressed interest in marriage previously? Does the child study hard? Ultimately, both come down to a simple yes or no.
For a business owner trying to determine how likely a certain kind of client is to contract with her demolition company for a job, multiple logistic regression is the way to go.
Multivariate analysis of variance (MANOVA) tests the difference in the effect of multiple independent variables on multiple dependent variables. Say, for example, a marketer wants to study the impact of pairing a price reduction with an increase in campaign budget — both independent variables — on the sales of a certain face cream.
Moving inventory isn’t her only concern, however. She also wants to know what the new price and spend will do to her total revenues on the cream for that period. Both sales and revenues represent dependent variables. In this case, a MANOVA would prove most helpful.
Sometimes too many variables find their way into a dataset. When that happens, it can be difficult to identify any clear patterns in all the information spread out in front of you. Trim back some of those variables using factor analysis, and you can begin to make sense of your data.
Do this by combining closely correlated variables, such as condensing a target audience’s income and education into “socioeconomic status” — or multiple behaviors, such as leaving a positive review and signing up for your newsletter into “customer loyalty.”
Like factor analysis, cluster analysis is a technique you can use to turn down the noise in your data to zero in on what’s most important. Only instead of grouping similar factors together, cluster analysis includes combining similar observations. A scientist performs cluster analysis when, upon discovering new types of deep-sea fish, she organizes them into a single species based on shared traits.
Applied to marketing, cluster analysis represents a powerful market segmentation tool that — when done regularly using powerful, automated software — can help teams deliver personalized experiences based on similar relevant behaviors.
In contrast to factor and cluster analysis, both of which represent independent techniques, discriminant analysis is a dependent technique. Put another way, cluster and factor analysis are exploratory, allowing you to determine the grouping pattern that maximizes similarities within groups — and, by extension, differences between groups.
Discriminant analysis is also concerned with simplifying datasets through classification. However, unlike the previous two types of analysis, it’s a dependent technique. This means it begins with a specific dependent variable, leaving you to focus on the impact your independent variable or variables have on distinguishing between groups. For example, let’s say you want to look at repeat buyers. The repeat buyers represent your dependent variable, a group that you can then examine more closely using any independent variables you have available to you — be it age, location, gender, or something else.
Conjoint analysis refers to the process of surveying consumers to identify which part of a product or service they value most. Used widely in market research, conjoint analysis provides real-world insight into which factors consumers consider most important when making a purchase. Such data is invaluable for minimizing guesswork when it comes to rolling out new products or services (or when tweaking old ones).
Not all feedback deserves equal weight, however. Only invest in a survey that is sure to reach consumers that match your target audience for the product. Just asking the right people isn’t enough. For a response of any real value, you’ll want to be sure to survey as many individuals as possible so as to root out outlying opinions and establish a statistically significant trend.
Equally important for actionable outputs is your survey’s format. Offer too many options, and the respondent becomes overwhelmed. Too few, however, and the survey may fail to pick up on key nuances in consumer preference. One solution researchers have developed to solve this problem is that of asking respondents to rank paired attributes. Do they value clothing that is “affordable and on trend” or “high quality and durable?” This way, the individual taking the survey only has to weigh two options at any given time.
Rarely does life ever offer up just two choices, however, which is why more and more marketers find themselves opting for choice-based conjoint analysis. In this approach, respondents must select one from a handful of full-profile concepts.
For example, say you want to test out an idea for a new winter jacket. Choice-based conjoint analysis would require you to design between three and five different jackets, each with distinct features for respondents to choose from. In the case of a clear winner, you are ready to move forward with confidence knowing that at least compared to the other possibilities, the jacket performs well with your target audience.
Oftentimes the responses are a bit more muddied, however, in which case iteration based on the results of a second or even third survey can help refine your data.
Just because two variables are correlated does not imply causation. Ice cream sales and pool drownings both increase in the summer, but one does not lead to the other. Correlation analysis is designed to help determine the relationship between two variables, including whether or not they are directly linked.
There are three main types of correlation analysis. Pearson’s correlation coefficient is used for linearly related variables — that is to say, as one variable goes up, the other follows (and vice versa). This relationship is measured using a number between 0 and 1, with 1 representing a perfectly linear relationship.
Spearman’s rank-order correlation is used to organize data defined by a set order, also known as ordinal association. For example, say the same runners face off in a series of matches and you want to determine the correlation between contests. You would use their results and Spearman’s rank-order correlation to calculate your answer.
Finally, Kendall’s tau correlation allows you to determine how dependent two variables are on one another, with 0 indicating complete independence and 1 the complete dependence. Note that this goes beyond occurrence. As in the ice cream and drowning example, just because two variables increase in frequency in tandem does not mean they are dependent on each other for happening. Similarly, just because your web traffic and web sales tend to rise and fall in tandem does not necessarily mean one causes another.
For marketers and business owners, correlation analysis can help take the guesswork out of where a company should invest in order to boost its bottom line. Suppose you notice that those consumers who test-drive the cars at your dealership for a minimum of 15 minutes are twice as likely to purchase a vehicle that same day. You want to know if it’s that extra time in the car that pushes them over the edge, or if customers are simply driving the cars longer because they already know they’re inclined to sign the dotted line.
Correlation analysis can help clarify the relationship between the two and possibly uncover a critical consumer behavior for driving sales.
Conducting your own multivariate analyses
Multivariate analysis helps you understand complex scenarios by uncovering patterns and relationships between multiple variables.
The term itself may sound like a highly technical and specialized skill. But in truth, most of us are engaged in some form of multivariate analysis all day long. New parents try to tease out how nap lengths, feeding intervals, and sleep environment combine to influence the number of times a child wakes up during the night. Homeowners must consider a dozen factors — some personal, some global — when deciding whether or not to refinance.
Likewise, marketers understand intuitively that sales and revenue are the result of many factors, ranging from price and campaign spending all the way up to inflation and global market trends.
Ready to get started on your own multivariate analysis? Cluster analysis is a great way to use your existing customer segmentation data to find correlations that might reveal a group that could benefit from a targeted campaign.
Adobe Target allows you to quickly perform multivariate testing, helping you to deliver more personalized experiences to your customers.
Watch an overview video to find out how Adobe Target can help you conduct your own multivariate analyses.
https://business.adobe.com/blog/basics/define-data-analytics
https://business.adobe.com/blog/basics/cluster-analysis-definition
https://business.adobe.com/blog/basics/market-segmentation-examples

Multivariate analysis: the need for data, and other problems
- PMID: 1125504
- DOI: 10.1192/bjp.126.3.237
Multivariate analyses are an aid to, not a substitute for critical thinking in the area of data analysis. Meaningful results can only be produced by these methods if careful consideration is given to questions of sample size, variable type, variable distribution etc., and accusations of subjectivity in interpretation can only be overcome by replication. The computer revolution has produced many problems for statisticans, not least of which is the ease with which experiments may access packages of programs for multivariate analysis, and so bypass a "difficult" (by which is meant one who will not do simply as he is told) statistician. Of course there are many abusers of univariate statistical methods. Here, however, the abuses are not likely to lead to such seriously misleading results as in the multivariate case. Perhaps a major cause of the continuing misuse of statistical methods is the insistence of many journal editors in psychology and related areas, on articles being laced with multivariate analyses, and on encouraging the pedantic use of signifance levels, i.e. the inevitable p less than minus, as if such inclusions lent an air of respectability to their journal which it might not otherwise have had. Research workers in these fields would be better encouraged to devote more time to an initial screening of their data using simple graphical techniques, to ensure that their data are at least approximately suitable for more complicated multivariate analyses.
- Psychometrics
- Research Design
- Statistics as Topic*

An official website of the United States government
The .gov means it’s official. Federal government websites often end in .gov or .mil. Before sharing sensitive information, make sure you’re on a federal government site.
The site is secure. The https:// ensures that you are connecting to the official website and that any information you provide is encrypted and transmitted securely.
- Publications
- Account settings
Preview improvements coming to the PMC website in October 2024. Learn More or Try it out now .
- Advanced Search
- Journal List
- J Thorac Dis
- v.7(3); 2015 Mar
Multivariate analysis in thoracic research
Noemí mengual-macenlle, pedro j. marcos, rafael golpe, diego gonzález-rivas.
Multivariate analysis is based in observation and analysis of more than one statistical outcome variable at a time. In design and analysis, the technique is used to perform trade studies across multiple dimensions while taking into account the effects of all variables on the responses of interest. The development of multivariate methods emerged to analyze large databases and increasingly complex data. Since the best way to represent the knowledge of reality is the modeling, we should use multivariate statistical methods. Multivariate methods are designed to simultaneously analyze data sets, i.e., the analysis of different variables for each person or object studied. Keep in mind at all times that all variables must be treated accurately reflect the reality of the problem addressed. There are different types of multivariate analysis and each one should be employed according to the type of variables to analyze: dependent, interdependence and structural methods. In conclusion, multivariate methods are ideal for the analysis of large data sets and to find the cause and effect relationships between variables; there is a wide range of analysis types that we can use.
Introduction
With the development of information technology and communication, it is now easier to make processes of collection, storage and transportation of large—both in volume and complexity—databases from observation or experimentation. A multivariate approach will help to illuminate the inter interrelatedness between and within sets of variables.
Multivariate analysis in a broad sense is the set of statistical methods aimed simultaneously analyze datasets. That is, for each individual or object being studied, analyzed several variables. The essence of multivariate thinking is to expose the inherent structure and meaning revealed within these sets if variables through application and interpretation of various statistical methods.
There are two determining factors that have to take into account when doing a multivariate approach ( 1 ): (I) the multidimensional nature of the data matrix and (II) the purpose of trying it, preserving its complex structure. This is based on the belief that the variables are interrelated, so that only the set of the same test may provide a better understanding of the studied object obtaining information univariate and bivariate statistical methods are unable to achieve. The joint treatment of the variables will faithfully reflect the reality of the problem addressed ( 2 ).
Types of multivariate methods
Multivariate methods can be classified based on the types of variables ( 3 ) ( Figure 1 ):

Classification of multivariate methods.
- Regression analysis: It is typically used to predict the behavior of certain variables from others. Generally, it does not describe the relationship between variables because it ignores the possible random variations in the value of the independent variable and they are not derived from the variation of the dependent variables. An example would be to analyze a sample of 500 smokers aged 50 years and establish the relationship between lung cancer (outcome or dependent variable) and some of its basic characteristics and habits: number of cigarettes per day, number of years of smoking, forced expiratory volume at first second (FEV1), etc.
- Survival analysis: It consists of a set of appropriate statistical techniques where each subject is followed for a certain period. Here the independent variable is the time to the event (time to death for survival analysis, but also can be time to recurrence, time to discharge, etc.). This model will allow us to study the relationship between a set of explanatory variables and the incidence of the event of interest and also to predict the survival chances of a given subject from the pattern of values taken in the predictors. The most widely used model in health sciences is the proportional hazards model of Cox. An example would be a study for ex-smokers relapse (outcome variable) in their consumption according to their employment status, the presence of mental illness, if they have other toxic habits and years of smoking (explanatory variables).
- Analysis of variance: a method for comparing various measures in different situations that is used when the full sample is divided into several groups based on one or more non-metric independent variables and the dependent variables analyzed are metric. An example could be to evaluate the efficacy of different drugs to control dual bronchodilator chronic obstructive pulmonary disease (COPD), compared with inhaled corticosteroid and a bronchodilator drug in a population of patients with COPD; these patients were randomly distributed. The first group would be provided a placebo, the second an inhaled corticosteroid and a bronchodilator; the third, it would be administered twice bronchodilator therapy and the results are evaluated.
- Canonical correlation: it is based on to try to analyze simultaneously multiple dependent and independent metric variables by calculating linear combinations of each set of variables, looking for the possible existence of relationship between two sets of variables. Its objectives are to determine whether two sets of variables (measurements on the same objectives) are independent from each other or, conversely, to determine the magnitude of the relationships that may exist between the two sets. A possible example would be to determine if lifestyle and dietary habits have an effect on health by measuring different variables such as weight, blood pressure and dyslipidemia.
- Discriminant analysis: it provides rules for classifying new observations of their group of origin, based on the information provided by the values it takes the independent variables. In other words, it indicates which variables differentiate the groups. The objective of this analysis is to find the linear combination of independent variables that best differentiate the groups. We would establish which patients would benefit and which lung cancers are not of a formal lobectomy—versus atypical resection—in stage I.
- Logistic regression models: Logistic regression measures the relationship between a categorical dependent variable and one or more independent variables, which are usually (but not necessarily) continuous, by using probability scores as the predicted values of the dependent variable ( 5 ). The aim would be to explore a set of possible predictors to define those that are important in explaining a particular dependent variable and build a prognostic index to predict response or outcome (Y) from a set of explanatory variables (X), help control the effects of confounding variables to estimate the specific effect of one or more factors (Xi) on a variable (Y), detect and assess interaction effects between two or more explanatory variables. The regression analysis may be applied for example in a study of lung cancer [dependent variable (Y)] from studying snuff consumption (X1), asbestos exposure (X2) and exposure to radon (X3).
- Conjoint analysis: It is a method that analyzes the effect of non-metric independent variables in metric or non-metric variables. Unlike the analysis of variance, the dependent variables may be non-metric and the analyst sets non-metric values of the variables. The goal is to understand how individuals form their preferences for objects or stimuli, estimating the relative importance attached to each of the attributes or characteristics of it. This model would explain the preferences of patients with lung cancer when choosing a treatment.
- Factor analysis and principal component analysis: this technique allows to analyze interrelationships among a large number of metric variables explaining these relationships in terms of a smaller number of variables called factors if they are unobservable or components if they are observable. It removes existing redundancies between the initial set of observed variables. A possible example would be to study a group of items from a scale and label them under a single dimension or factor assessment.
- Multidimensional scales: It transforms judgments of similarity or preference distances represented in multidimensional space. The objectives are: proximity between objects used to perform a spatial representation of them and identify the underlying dimensions. An example is the spatial representation of the similarities between the various chemotherapy treatments lung cancer in order to know the relative positioning of each of them.
- Cluster analysis: This is a method for ranking entities; whether individuals or variables in a small number of groups so that the observations within a group are very similar and very different from the rest. Unlike discriminant analysis, the number and composition of these groups is unknown. This method could build a sanitary map in Spain according to the incidence of the different types of lung cancer.
- Multidimensional scales.
- Cluster analysis.
- Correspondence analysis: It is applied to multidimensional contingency tables and pursues a similar multidimensional but simultaneously representing the rows and columns of the contingency tables scales goal. Its goals are to reduce the data (non-metric variables); from the relationship between observed variables, identifying dimensions or latent variables; deepen the relations established between two or more categorical variables. A possible example would be to get a perceptual positioning map showing the association between lung cancer and the underlying dimensions.
- Log-linear models: It is based on applying to multidimensional contingency tables and multidimensional relationships modeled dependence of the observed variables that seek to explain the noted frequencies. They allow the researchers to test different models that posit types of relationships between two or more categorical variables.
- Structural methods: These methods assume that the variables are divided into two groups: the dependent variables and the independent. They aim to analyze how the independent variables affect the dependent variables and the relationships of the variables in the two groups together. For example, consider how the resources of the fast lane of lung neoplasms with perceptions that patients have of it are used.
Stages of realization of a multivariate analysis
The steps (I) to perform a multivariate analyze can be summarized in:
- State the objectives of the analysis. Define problem in its conceptual terms, objectives and multivariate techniques that are going to be employed.
- Design analysis. To determine the sample size and estimation techniques those are going to be employed.
- Decide what to do with the missing data.
- Perform the analysis. Identify outliers and influential observations whose influence on the estimates and goodness of fit should be analyzed.
- Interpret the results. These interpretations can lead to redefine the variables or the model which can return back to steps (III) and (IV).
- Validate the results. At this point, we must establish the validity of the results obtained by analyzing other results obtained with the sample is generalized to the population from which it comes.
Multivariate analysis example
Wells et al . ( 6 ) published in New England Journal of Medicine a study were they hypothesized that a computed tomographic (CT) metric of pulmonary vascular disease [pulmonary artery enlargement, as determined by a ratio of the diameter of the pulmonary artery to the diameter of the aorta (PA: A ratio) of >1] would be associated with previous severe COPD exacerbations . A univariate logistic regression was used to determine the associations between patient characteristics (including the PA: A ratio) and the occurrence of a severe exacerbation of COPD in the year before enrollment. Variables showing a univariate association with severe exacerbations (at P<0.10) were included in stepwise backward multivariate logistic models to adjust for confounders. These models included also variables previously reported to be independently associated with acute exacerbations of COPD in the ECLIPSE study as gastro-esophageal reflux disease (GERD), lower values for the forced expiratory volume in 1 second (FEV1), a history of acute exacerbations of COPD within the previous year, increased white-cell count, and decreased quality of life as measured by the St. George’s Respiratory Questionnaire (SGRQ) score (which ranges from 0 to 100, with higher scores indicating worse quality of life and with a minimal clinically important difference of 4 points). Authors found significant univariate associations between severe exacerbations and younger age, black race, use of supplemental oxygen, congestive heart failure, sleep apnea, thromboembolic disease, GERD, asthma, chronic bronchitis, employment in a hazardous job. Thanks to the development of a multivariate model, it will not only let to handle many covariates, it will let to asses potential confounders and also test for interaction or effect modification. Multiple logistic-regression analyses showed continued significant independent associations between severe exacerbations and younger age, lower FEV1 values, higher score on the SGRQ, and a PA: A ratio of more than 1.
Conclusions
This article provides a brief overview of the importance of using multivariate studies in the health sciences and the different types of existing methods and their application depending on the type of variables to deal with. In addition, it described the steps to follow to design a multivariate study.
Acknowledgements
Disclosure : The authors declare no conflict of interest.
Thank you for visiting nature.com. You are using a browser version with limited support for CSS. To obtain the best experience, we recommend you use a more up to date browser (or turn off compatibility mode in Internet Explorer). In the meantime, to ensure continued support, we are displaying the site without styles and JavaScript.
- View all journals
- My Account Login
- Explore content
- About the journal
- Publish with us
- Sign up for alerts
- Systematic Review
- Open access
- Published: 01 June 2024
Multivariate brain-behaviour associations in psychiatric disorders
- S. Vieira ORCID: orcid.org/0000-0002-6770-2934 1 , 2 , 3 ,
- T. A. W. Bolton 1 , 4 ,
- M. Schöttner ORCID: orcid.org/0000-0002-4521-9837 1 ,
- L. Baecker ORCID: orcid.org/0000-0002-6218-0292 2 ,
- A. Marquand ORCID: orcid.org/0000-0001-5903-203X 5 , 6 , 7 ,
- A. Mechelli 2 &
- P. Hagmann 1
Translational Psychiatry volume 14 , Article number: 231 ( 2024 ) Cite this article
885 Accesses
19 Altmetric
Metrics details
- Human behaviour
- Psychiatric disorders
Mapping brain-behaviour associations is paramount to understand and treat psychiatric disorders. Standard approaches involve investigating the association between one brain and one behavioural variable (univariate) or multiple variables against one brain/behaviour feature (‘single’ multivariate). Recently, large multimodal datasets have propelled a new wave of studies that leverage on ‘doubly’ multivariate approaches capable of parsing the multifaceted nature of both brain and behaviour simultaneously. Within this movement, canonical correlation analysis (CCA) and partial least squares (PLS) emerge as the most popular techniques. Both seek to capture shared information between brain and behaviour in the form of latent variables. We provide an overview of these methods, review the literature in psychiatric disorders, and discuss the main challenges from a predictive modelling perspective. We identified 39 studies across four diagnostic groups: attention deficit and hyperactive disorder (ADHD, k = 4, N = 569), autism spectrum disorders (ASD, k = 6, N = 1731), major depressive disorder (MDD, k = 5, N = 938), psychosis spectrum disorders (PSD, k = 13, N = 1150) and one transdiagnostic group (TD, k = 11, N = 5731). Most studies (67%) used CCA and focused on the association between either brain morphology, resting-state functional connectivity or fractional anisotropy against symptoms and/or cognition. There were three main findings. First, most diagnoses shared a link between clinical/cognitive symptoms and two brain measures, namely frontal morphology/brain activity and white matter association fibres (tracts between cortical areas in the same hemisphere). Second, typically less investigated behavioural variables in multivariate models such as physical health (e.g., BMI, drug use) and clinical history (e.g., childhood trauma) were identified as important features. Finally, most studies were at risk of bias due to low sample size/feature ratio and/or in-sample testing only. We highlight the importance of carefully mitigating these sources of bias with an exemplar application of CCA.
Similar content being viewed by others
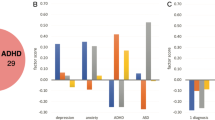
Striatal connectopic maps link to functional domains across psychiatric disorders
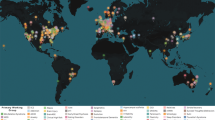
ENIGMA and global neuroscience: A decade of large-scale studies of the brain in health and disease across more than 40 countries
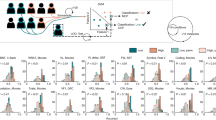
Brain–phenotype models fail for individuals who defy sample stereotypes
Introduction.
Mapping the association between brain and behaviour has been a focus of psychiatric research for many decades. However, the analytical methods to achieve this have changed substantially over time. Historically, mass-univariate analysis, where many single brain features are linked to a single behavioural phenotype, have dominated the literature [ 1 , 2 , 3 , 4 ]. However, there is growing concern about the reproducibility of these findings, especially when carried out in typically small samples [ 5 , 6 ]. This approach also does not take into account the mutual dependencies between different brain regions, nor is it consistent with the current view that behaviour is best explained by distributed neural networks, rather than localised regions [ 7 , 8 , 9 , 10 ]. Therefore, multivariate methods are better suited to capture brain–behaviour relationships. Within this context, two main families of methods are becoming increasingly popular: 1) mapping multiple brain features to one behavioural feature (i.e., ‘many-to-one’ associations) and 2) discovering latent brain-behaviour associations from multiple brain and multiple behaviour features (i.e., ‘many-to-many’ associations or ‘doubly multivariate’ methods). The former has enjoyed considerable interest in the last two decades, with a wealth of studies investigating how neural features can predict a range of univariate psychiatric outcomes such as functioning [ 11 , 12 ], diagnosis [ 13 , 14 , 15 ] and response to treatment [ 16 , 17 ] using popular approaches such as support vector machine (SVM) [ 18 , 19 , 20 ]. Within the second group, there are many approaches that could be used in principle, such as independent component analysis (ICA) and its variants (e.g., parallel ICA, joint ICA or linked ICA) [ 21 ], multilevel clustering [ 22 ], canonical correlation analysis (CCA) [ 23 ] and partial least squares [ 24 ] (PLS). The latter two emerge as the most established and popular techniques in brain-behaviour studies, as evidenced by several recent studies in the general population [ 25 ] and tutorials tailored to brain-behaviour investigations [ 26 , 27 ]. There are many variations of CCA and PLS [ 27 ]. The two most used types of PLS in neuroimaging are Partial Least Squares Correlation (PLSC) and Partial Least Squares Regression (PLSR). PLSC is a correlational technique that estimates associations between two sets of data (e.g., behaviour and brain morphology), while PLSR is a regression technique that predicts one set of data from another (e.g., predicts behaviour from brain activity). Multiset CCA (mCCA) and mCCA+ICA are variations of CCA and have mostly been used to investigate the association between different imaging modalities or incorporate a behavioural constraint (use behavioural data to guide the fusion between imaging modalities) [ 28 , 29 , 30 , 31 ]. Here we focus on standard CCA and PLSC (henceforth PLS) (and their regularised variations) as they are the most commonly used approaches to investigate brain-behaviour associations [ 25 , 32 ]. Both seek to capture shared information, in the form of latent variables, between two sets of multivariate data. Early applications in neuroimaging aimed at combining different imaging modalities, a process also known as multimodal fusion [ 33 ]. More recently, the same approach is being used to map brain and behaviour associations. This is achieved by attributing weights to the brain and behavioural variables such that their linear combination maximises the correlation (CCA) or covariance (PLS) between the resulting latent variables (see Box 1 for an overview; for a more in-depth explanation see Tabachnick et al., [ 34 ]). The main advantage of this approach is that the multivariate nature of both brain and behaviour can be modelled simultaneously. Indeed, although the standard use of ‘many-to-one’ approaches in psychiatric imaging acknowledges the multifaceted nature of brain data, they do not take into account that different aspects of behaviour also interact with each other [ 35 ]. Therefore, approaches such as CCA and PLS are better suited to honour the complex brain-behaviour associations by allowing to uncover joint multivariate relationships.
Although CCA/PLS were introduced many decades ago, they are ´data-hungry’ and can be computationally demanding. The release of large publicly available imaging datasets with comprehensive behavioural assessments, such as the Adolescent Brain Cognitive Development [ 36 ], Human Connectome Project [ 37 ] and UK BioBank [ 38 ], has propelled a renewed interest in the use of these methods to investigate brain-behaviour associations [ 39 , 40 , 41 , 42 , 43 ]. For example, specific brain patterns have been linked to perinatal and early life events, sociocognition, and urbanicity [ 44 , 45 , 46 ], different types of psychopathology [ 47 ], as well as physical features, lifestyle and cognition [ 48 ]. Notably, these methods may be particularly useful in psychiatry. Mental health disorders are best understood as a complex pattern of both neural and behavioural changes. For example, psychosis is characterised by widespread changes in brain morphology, structure and connectivity [ 49 , 50 , 51 ], but also by a pattern of positive, negative, mood and cognitive symptoms, insight and functioning, all relevant for diagnosis and treatment [ 52 ]. However, despite the consensus of the multivariate brain-behaviour interaction in psychiatric disorders, these studies remain rare; multimodal data is expensive and requires expertise in several data modalities (e.g., neuroimaging, cognition, psychopathology) as well as in the analytical methods to integrate them. Parallel to this, there is also a growing interest in investigating the generalisability and stability of brain-behaviour associations in psychiatry [ 27 , 53 , 54 ]. This is partly in response to the replication crisis in psychiatry/psychology [ 25 ] combined with the pursuit of translational clinical tools [ 55 ]. In practice, this means that the typical descriptive analytical framework, where the aim is to find above-chance associations in-sample, is being challenged and more emphasis is being given to predictive approaches, where the goal is to test whether a brain-behaviour association is stable and can be generalised to new data [ 56 , 57 ]. The aim of this systematic review is to summarise the applications of commonly used ‘doubly’ multivariate methods, namely CCA and PLS, to investigate brain-behaviour associations in psychiatric disorders within this context, as well as discuss main challenges and future directions.
Box 1 Overview of canonical correlation analysis and partial least squares
CCA and PLS are data-driven techniques that can extract latent associations between a set of multivariate brain data and a set of multivariate behavioural data. The former can comprise, for example, functional connectivity for selected brain regions, while the latter may include, e.g ., information about symptoms, cognition, medication, duration of illness and psychiatric history. Simply put, CCA and PLS quantify the association between brain and behaviour by finding the weights, for each dataset, that maximise the link between the two.
Formally, consider brain and behaviour data matrices X and Y , of respective sizes S × n x and S × n y , with S the number of subjects with available measurements and n x / n y the number of brain/behavioural measures at hand. CCA and PLS extract associated weight vectors w x and w y , of respective sizes n x × 1 and n y × 1. The products L x = X · w x and L y = Y · w y are, respectively, weighted combinations of the brain and behavioural measures for each subject; they thus have size S × 1 and are known as latent variables . CCA and PLS find the weights that maximize Pearson’s correlation (for the former) or covariance (for the latter) between the brain and behavioural latent variables, thus unravelling low-dimensional brain/behaviour associations. Multiple overlapping associations can be extracted, at most equal to the number of measures available in the smaller dataset; i.e., min( n x , n y ). All the weight vectors extracted on either the brain or the behaviour side jointly form an orthonormal basis, so that each association thus draws on distinct parts of the input data. Their respective strength ( i.e ., effect size) is typically reported with Pearson’s correlation coefficient for CCA, and with the fraction of explained covariance for PLS. The ones with smallest strength may be negligible and should be discarded, as typically done by assessing the statistical significance of each association.
The terminology of the CCA/PLS elements varies. In CCA, each pair of latent variables is called a canonical mode , while a weight vector and a latent variable are referred to as a canonical vector and a canonical variable / variate , respectively. In PLS, they are respectively called salience and score . One can also retrieve the relative contributions of brain and behavioural measures to the association by quantifying the absolute correlation between the original and the latent variables, yielding so-called structure coefficients (in CCA) or loadings (in general). The interpretation of brain-behaviour associations is done by inspecting the magnitude of the weights/loadings for the brain and behavioural variables. The weights are adjusted for the within-dataset correlations, thus indicating the relative contribution of each variable to the latent variable.
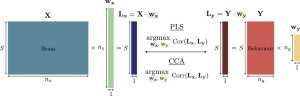
Brain-behaviour associations in psychiatric disorders
Our systematic review identified 39 studies that investigated brain-behaviour associations in mental health disorders using CCA/PLS (see supplementary methods for search strategy and data extraction) across four diagnostic groups: four in attention deficit and hyperactivity disorder (ADHD, N = 569), six in autism spectrum disorders (ASD, N = 1731), five in major depressive disorder (MDD; N = 938) and thirteen in psychosis, including three in individuals at clinical high-risk (CHR-P, N = 278), five in first episode (FEP, N = 455) and five in established schizophrenia (SZ, N = 417). An additional eleven studies were carried out in large samples including several diagnoses (Transdiagnostic; N = 5731) including SZ, bipolar disorder, ADHD and ASD. Twenty-six studies (67%) used CCA (or regularised CCA). The main characteristics of the included studies are summarised in Tables S2 – S6 . Figure 1 shows the brain-behaviour associations that were investigated for each diagnostic group and total sample, while Fig. 2 displays, for the same groups, the frequency of statistically significant associations. Overall, studies focused mostly on the association between either brain morphology, resting-state functional connectivity or fractional anisotropy against symptoms and/or cognition. A wide range of additional behavioural domains were also investigated, namely demographics, clinical information and history as well as physical health (Fig. 1 ).
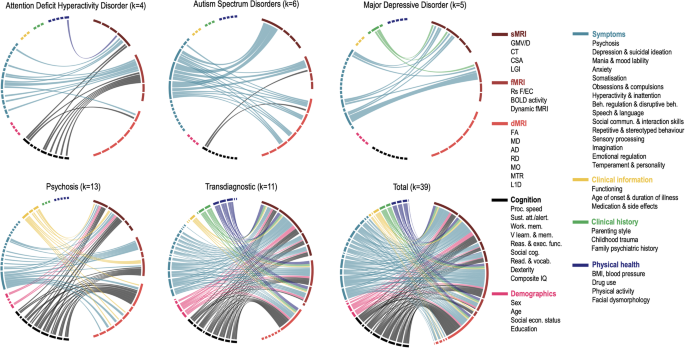
The size of a connection is proportional to the number of reported models for a given association. Labels are listed clockwise. ADHD: attention deficit hyperactivity disorder, ASD autism spectrum disorders, MDD major depressive disorder, PSD psychosis spectrum disorders, TD transdiagnostic group, sMRI structural MRI, GMV/D grey matter volume/density, CT cortical thickness, CSA cortical surface area, LGI local gyrification indices, fMRI functional MRI, rsFC resting-state functional connectivity, BOLD blood-oxygenation-level-dependent signal, dMRI diffusion MRI, FA fractional anisotropy, MD mean diffusivity, AD axial diffusivity; RD radial diffusivity; MO mode of anisotropy, MTR magnetization transfer ratio; L1D L1 diffusivity.
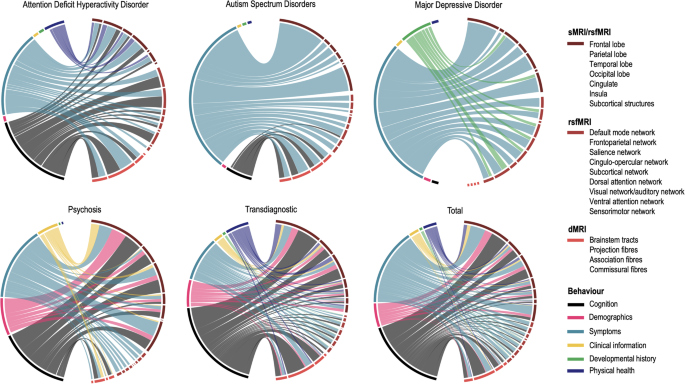
The thickness of the connections is proportional to the number of reported associations within each diagnostic group. Labels are listed clockwise.
Behaviour was associated with widespread regions, networks and white matter tracts across the brain for all diagnostic groups. In ADHD, cognition and symptoms were mostly linked with the default mode and frontoparietal networks and white matter association fibres, whereas in ASD, symptoms were mostly associated with the morphology of frontal and subcortical regions, as well as white matter association fibres. Similarly, depressive symptoms in MDD were also associated mostly with frontal regions but also with limbic regions and the cingulate cortex. In psychosis, all types of behavioural features were more visibly associated with frontal regions, followed by temporal and subcortical regions. Finally, the prevalence of frontal regions was also clear in the transdiagnostic group, especially in the association with cognition; white matter association fibres were also linked with cognition and symptoms. Notably, there was no clear association between specific patterns of functional connectivity and behaviour (Fig. 2 ). In the next sections, the brain-behaviour associations for each diagnostic group are presented in more detail.
Attention deficit and hyperactivity disorder
Four studies investigated the association between ADHD symptoms/ general cognition and either cortical gyrification [ 58 ], resting-state functional connectivity [ 59 , 60 ] or fractional anisotropy [ 61 ]. The former also included measurements of facial dysmorphology typically associated with pre-natal alcohol exposure alongside full scale IQ, behavioural, emotional and cognitive regulation, and inattention and hyperactivity/impulsivity scores. Results showed one significant latent variable, in which lower gyrification in prefrontal, insular, cingulate, temporal, and parietal cortices was associated with greater facial dysmorphia and hyperactivity/impulsivity, as well as poorer IQ and behavioural regulation [ 58 ]. Lin et al. [ 59 ] found one significant canonical mode where higher hyperactivity/impulsivity and lower cognitive function were associated with higher connectivity predominantly between the default mode, frontoparietal, cingulo-opercular ( e.g ., insula and thalamus) and subcortical ( e.g ., pallidum and putamen) networks. Luo et al. [ 60 ] also found several canonical links between several cognitive domains, ADHD and other symptoms ( e.g ., depression, anxiety, social difficulties) with the default mode and frontoparietal networks. Finally, Tsai et al. [ 61 ] reported one significant canonical mode, where higher white matter microstructural integrity in multiple brain systems, mostly in association fibres ( e.g ., cingulum, inferior fronto-occipital, uncinate and longitudinal fasciculi), was negatively associated with attention dysregulation, aggression, anxiety, depressive and ADHD symptoms, and positively associated with cognitive function.
Autism spectrum disorders
The only three studies mostly investigated the association between different ASD symptoms including difficulty in social interaction and communication, stereotyped behaviour and sensory processing against brain morphology [ 62 , 63 ], functional connectivity [ 64 , 65 ] and white matter integrity [ 66 , 67 ]. In a large sample of 325 participants, Mei et al. [ 62 ] found one significant canonical mode, in which stronger negative weights on social communication difficulties and stereotyped behaviour were associated with higher weights on grey matter volume in the lateral occipital and superior parietal lobes, right precentral gyrus, amygdala, hippocampus and parahippocampal gyrus and positively with grey matter volume in the thalamus and putamen. In a separate CCA, the same study also found that higher frequency of sensory symptoms was associated with less volume in the cerebellum, lateral occipital and parietal lobes, precentral and inferior frontal gyri and middle frontal lobe. Using the same sample, Mei et al. [ 66 ] expanded on these findings by combining grey matter volume and white matter microstructure as input features along with core ASD symptoms. The only significant canonical mode was dominated by white matter features in the inferior and superior longitudinal fasciculus, inferior fronto-occipital fasciculus, corticospinal tract and thalamic radiation, which was associated with repetitive and stereotyped behaviour. Similar tracts, including the inferior longitudinal and uncinate fasciculi, corpus callosum, corticospinal and frontal aslant tracts, thalamic radiation and cingulum were also associated with affect, behavioural, and cognitive dysregulation in Ni et al. [ 67 ]. Another study attempted to parse brain-behaviour heterogeneity and found four subtypes in which social difficulties correlated mostly with either fronto-parietal regions, brainstem, temporal-occipital or fronto-subcortical regions [ 63 ]. As for functional connectivity, repetitive and stereotyped behaviour as well as social difficulties were associated mainly with the visual network [ 64 , 65 ].
Major depressive disorder
Overall, four studies covered a wide range of behavioural features, namely depressive and anxiety symptoms, personality traits and childhood trauma, in association with either brain morphology or resting-state functional connectivity. Drysdale et al. [ 68 ] found two clinical profiles – anhedonia & psychomotor retardation and anxiety & insomnia – associated with different functional connectivity networks. The former profile was mostly associated with subcortical structures, as well as the default mode and salience networks, whereas the latter was associated almost exclusively with subcortical regions, especially limbic structures. A similar study with a more comprehensive set of behavioural features (anxiety, depression, personality traits, childhood trauma) found a significant association between functional connectivity in similar networks, especially between subcortical structures, the dorsal attention, sensorimotor, ventral attention networks and childhood trauma [ 69 ]. When investigating the association of the same behavioural features with brain morphology, anxious misery was negatively associated with grey matter volume in several cortical and subcortical structures including the middle cingulate and frontal gyri, frontal pole, bilateral amygdala, right hippocampal and superior frontal gyri [ 70 ]. Conversely, positive personality traits were positively associated with grey matter volume in the central operculum, posterior and middle cingulate gyri, hippocampus, and amygdala, whereas childhood trauma was negatively associated with grey matter volume in the entorhinal cortex and left hippocampal gyrus. Finally, somatisation was associated with functional connectivity in a widespread group of brain regions including the occipital, temporal and frontal lobes as well as the cerebellum.
Thirteen studies investigated the association between either brain morphology, resting-state connectivity or FA against a wide range of cognitive and clinical measures including, amongst others, symptoms, functioning, duration of untreated illness and antipsychotic medication. Three studies did not find a statistically significant brain-behaviour association [ 71 , 72 , 73 ]. Of the remaining studies, frontal brain regions were more prominently involved in all behavioural domains investigated, followed by temporal and subcortical regions. Symptoms were associated with a wide range of connectivity networks, but mostly with the default mode and sensorimotor networks. As for FA, links between association fibres and cognition, symptoms and clinical information were also observed. In CHR-P, prodromal symptoms and poor functioning were associated with white matter microstructure in association fibres (sagittal stratum, uncinate fasciculus and cingulum) and in the anterior corona radiata [ 74 ]. Conversely, cognitive abilities including processing speed, working memory and executive functioning were mostly associated with association fibres (fornix and uncinate fasciculus) and brainstem tracts (superior cerebellar peduncle and medial lemniscus) [ 75 ]. In FEP, the association between several behavioural features ( e.g ., cognition, symptom severity, medication, education) and cortical thickness and hippocampal white matter revealed two sex-specific latent variables. In males, greater verbal memory impairments, fewer years of education, greater antipsychotic medication and positive/negative symptomatology were associated with reduced volumes in limbic structures, reduced thickness in mostly parietal and temporal regions, and higher thickness in several frontal regions and in the precuneus. In females, fewer depressive/negative symptoms and an earlier age of onset were associated with reduced volumes in limbic structures, low thickness in temporal and frontal regions and in the cingulate cortex, as well as higher thickness in parietal and occipital regions. In established SZ, cognitive [ 76 , 77 ] and negative [ 76 ] symptoms were associated with grey matter volume in a widespread network of predominately prefrontal, temporal, parietal and (para)limbic regions. A significant association was also found between psychotic symptoms and more time in states characterised by inactive default mode and executive networks, and with heightened activity in the sensorimotor network [ 78 ].
Transdiagnostic groups
Several studies opted for combining diagnostic groups to identify transdiagnostic biomarkers. Half of the studies focused on psychosis spectrum disorders, namely schizophrenia, schizoaffective disorder and bipolar disorder (with and without psychotic features). The remaining studies investigated mostly a combination of ADHD, ASD as well as mood, anxiety, and borderline personality disorder. The majority of studies investigated cognition, which was mainly associated with the morphology of frontal regions. For example, the superior, inferior, caudal and middle frontal cortices were amongst the features with highest loadings across several brain morphology features, namely grey matter volume, cortical thickness, cortical surface area and local gyrification index in the psychosis spectrum group, ADHD, mood and anxiety disorders [ 79 , 80 ]. As for white matter microstructure, cognition was mostly associated with fractional anisotropy in the superior longitudinal fasciculus, sagittal stratum and fornix [ 81 , 82 ]. Interestingly, in the only two studies with physical measures amongst a wide range of behavioural features [ 41 , 80 ], variables such as BMI, drug use and physical activity had the highest loadings across brain modalities, namely morphology, white matter microstructure and functional activity, as well as across brain regions. Overall, psychopathology ( e.g ., psychosis, anxiety) was associated with a wide range of brain modalities and regions, as expected due to the different diagnostic groups. However, only five studies investigated the association between brain and symptoms, all with different diagnoses and/or brain features, making it difficult to ascertain which associations were more prevalent in the different diagnostic groups/symptoms. Notably, three out of the four functional connectivity studies did not find any statistically significant brain-behaviour association [ 41 , 83 , 84 ]; however, they were also amongst the most robust ones due to their rigorous out-of-sample testing.
Risk of bias
To our knowledge, there are no established tools to systematically assess the risk of bias in ‘doubly’ multivariate brain-behaviour studies. A subjective judgment was made for each study based on three domains that have been consistently highlighted in brain-behaviour studies using these methods: 1) Data dimensionality, 2) Validation strategy, 3) Stability of feature weights. The former was operationalised as the ratio between sample size (N) and total number of brain and behaviour features entered into the CCA/PLS model ( i.e ., effective number of variables, f); an N/f ratio lower than 10 was considered high risk bias [ 34 ]. In terms of validation strategy, in-sample testing only and out-of-sample testing (through resampling or an independent dataset) were considered high and low risk of bias, respectively [ 85 ]. Finally, studies that did not investigate the stability of feature loadings were rated as high bias for the last domain [ 85 ]. If any of the information needed to rate the domains was unclear or missing, the study was rated as ‘unclear’. Most studies (80%) were at high risk of bias for Data dimensionality and 68% were also at high risk due to an in-sample Validation strategy (Fig. 3 , supplementary information).
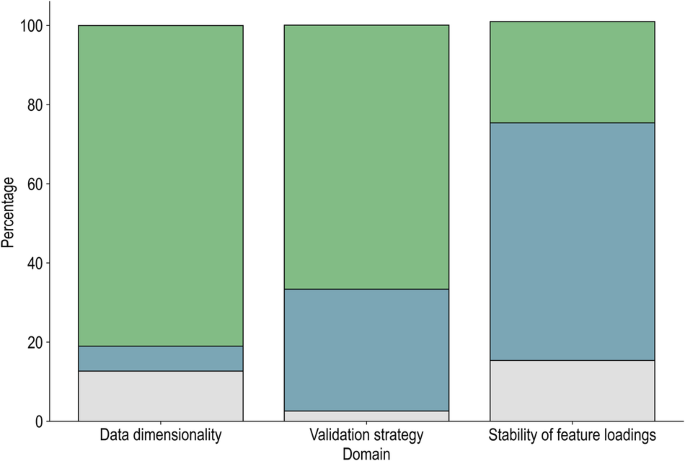
Proportion of reported CCA/PLS models with low (blue), high (green) or unclear (grey) bias for the three domains: Data dimensionality, Validation strategy and Stability of feature loadings.
Generalisability and stability of brain-behaviour associations
The majority of included studies used a descriptive approach to investigate brain-behaviour associations, that is, CCA/PLS models were trained and tested in the same sample. It is not possible to know whether the same effect size would have held (generalisability) and/or whether the weights would have remained similar (stability) when tested in new data. This is further exacerbated by the fact that most studies had small sample sizes relative to the total number of brain-behaviour features. Although many studies used feature selection/dimensionality reduction to address this, it may not have been enough to mitigate the risk of overfitting as most of them still did not meet the recommended guidelines of 10 participants/feature minimum [ 34 ]. More stringent thresholds have been suggested including 42 [ 86 ] and 50 [ 85 ], indicating that the risk of bias may be even greater. Additionally, very few studies used an independent sample to test their findings. This is a more demanding generalisation than resampling, further highlighting the chances of overfitting. The association between small sample sizes and optimistic performance in predictive modelling is well-established [ 6 , 87 ]; it can also be observed here (Fig. 4A ) and in similar studies in healthy controls [ 85 ]. The two limitations, especially when combined, are problematic because CCA and PLS are already prone to overfitting; i.e ., brain-behaviour associations tend to be much stronger in the sample where the model is trained/fitted ( i.e ., in-sample testing) compared to the associations in a new and unseen sample where the same trained model is applied ( i.e ., out-of-sample testing) [ 54 ]. To illustrate how this can lead to biased latent brain-behaviour associations, we present an exemplar standard CCA between cortical thickness (68 original features; 5 after dimensionality reduction) and cognition (11 features) in the Human Connectome Project (HCP) dataset (N = 842, N/f = 52.6) and compare the i) in- and out-of-sample first canonical correlation and ii) weights across models with increasing ( i.e ., more favourable) N/f ratio (Fig. 4B ; see supplementary methods). The former investigates how the first ( i.e ., strongest) canonical correlation of a CCA model trained on a subsample of the HCP generalises to another subsample of the same dataset; the latter assesses the stability of model weights; i.e ., to what extent feature importance varies across multiple models. Ideally, in- and out-of-sample effect sizes should be similar and weights stable across models. However, as expected, for small N/f ratios, there was a clear discrepancy between the means of the in- and out-of-sample effect sizes, and the latter varied substantially, a strong indicator of poor generalizability. As the N/f ratio increased, the effect sizes began to converge, suggesting a more generalizable model. In the total sample, the mean canonical correlation in- and out-of-sample nearly converged at 0.28 (sd=0.02) and 0.21 (sd=0.06), respectively (Fig. 4C ). This is in line with more realistic effect sizes for brain-behaviour associations [ 6 ]. The stability of the weights (average cosine similarity between feature weights of all 100 iterations for a given N/f ratio; see supplementary methods) follows a similar pattern (Fig. 4D ). The weights from models trained in small ratios varied substantially between models ( i.e ., low cosine similarly) suggesting poor stability of feature importance. For the total sample, the cosine similarity was 0.79, indicating a good weight stability. However, the plot suggests that this could be further improved with a larger sample, which is consistent with more recent suggestions that a minimum N/f ratio of 50 is needed for a stable CCA [ 85 ]. All combined, these results raise important questions about the validity and reliability of most of the published studies and highlight the need for careful testing to avoid inflated/unstable results. Although some studies used feature selection, dimensionality reduction and/or regularised CCA/PLS, it may not have been enough to mitigate the risk of overfitting. Future studies should follow guidelines for out-of-sample testing, investigating and reporting weights stability and mitigating the effects of high-dimensionality [ 27 , 85 , 88 ].
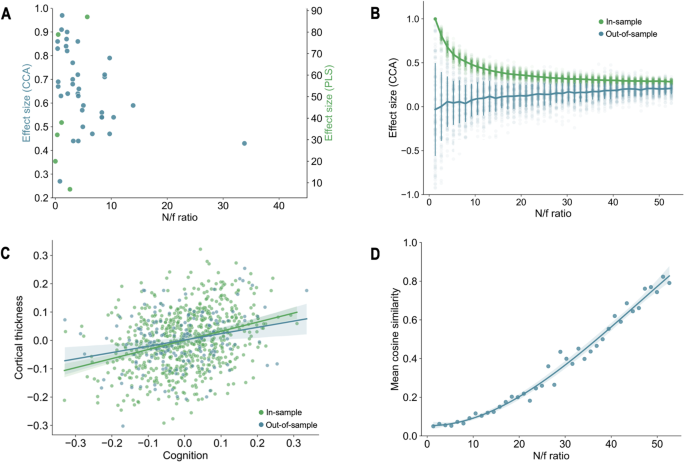
A Association between sample size (N) /effective features (f) ratio and effect size (canonical correlation and explained variance for CCA and PLS, respectively) for the first pair of latent variables in the included studies. B Impact of sample size on in- and out-of-sample effect size in a CCA between cortical thickness of 68 brain regions (reduced to 5 components with principal component analysis) and 11 cognitive tests in the HCP dataset. The CCA was trained and tested iteratively in 100 random subsamples of the same size for increasing sample sizes (x-axis). The first canonical correlation was used to measure effect size (y axis). The green (in-sample) and blue (out-of-sample) lines show the mean first canonical correlation for each sample size and corresponding standard deviation. C First canonical correlation for the total sample ( N = 842) for in- and out- of sample. D Effect of sample size on weight stability. At each sample size, the mean cosine similarity was calculated between the weights of the 100 iterations.
Interpretation of brain-behaviour associations in psychiatry
Interpretability of multivariate models is paramount to understand the underlying mechanisms of psychiatric disorders. A strong and robust association which provides no information about its underlying brain and behavioural features would be of limited clinical utility. The interpretation of CCA/PLS models is typically done by inspecting the model’s weights or loadings; the larger the absolute weight/loading, the more a variable contributed to the overall association (Box 1 ). Importantly, a reliable interpretation of brain-behaviour association requires valid model weights. As shown in Fig. 4D , this may be difficult to secure in small samples. Several studies investigated the stability of weights via bootstrapping and quantified the variability of the weights for each individual variable across iterations. This is a useful strategy as it allows to estimate a confidence interval for each variable weight; however, a more appropriate approach for ’doubly’ multivariate methods would be to report the variability of the entire set of variables’ weights across iterations, using for example cosine similarity. This gives a better estimate of the stability of the entire model’s solution, rather than individual variables. Notably, studies varied in how the most contributing features were identified ( e.g ., top 25% or 5 features, statistically significant features, features with weights above a certain threshold). This is an important limitation of the literature (and by extension, of this review) as it hinders comparisons between studies. In addition, the term ‘brain-behaviour’ should not be confused with any directionality whereby neural features predict behaviour. CCA and PLS-correlation are symmetrical in nature; i.e ., there is no directionality in covariance and correlation. Although sometimes the terms independent/predictor variables and dependent/criterion variables may be used to describe the brain and behaviour datasets, the order of datasets is ultimately arbitrary [ 34 ]. Therefore, the interpretation of associations using these methods should be limited to inferring shared information between brain and behavioural features. Finally, key characteristics of behavioural data and how they can impact the strength and interpretability of associations are less discussed than imaging in brain-behaviour studies [ 89 ]. Notably however, CCA/PLS are sensitive to associations both between and within datasets. In practice, this means that small changes in the behavioural data (either due to the choice and/or quality of variables) may markedly alter the composition of the latent variables and ultimately the strength and interpretation of brain-behaviour associations [ 34 ]. Some of the included studies focused on symptoms or cognition, while others used different combinations of either/both plus demographics, clinical information and history, and/or physical health. However, the choice of behavioural variable matters; for example, cognition has been shown to have more reliable associations with neural features compared to other behavioural variables such as parent-reported behavioural measures [ 90 ]. Similarly, a wider range with less inter-correlated behavioural variables may result in a more diluted brain-behaviour association. Additionally, testing less investigated psychometric properties in psychiatric neuroimaging such as the ability to measure high/low levels and unique/shared variance of the behavioural phenotype (phenotypic resolution and complexity) as well as ability to measure the same construct across different groups (measurement invariance) may help increase the effect size and interpretability of current reliable brain-behaviour associations [ 89 ]. Going forward, studies should report key psychometric properties of behavioural measurements ( e.g ., via item-response theory) in their sample in order to increase transparency of the quality of behavioural data. Very few studies have directly investigated the impact of different imaging modalities on brain-behaviour associations [ 27 , 54 ]. This limited evidence suggests that the behaviour of CCA/PLS is similar between grey matter volume and structural/functional connectivity. However, it is reasonable to expect that modalities/pre-processing pipelines that result in more cohesive ( i.e ., inter-correlated) data across participants will contribute to stronger brain-behaviour associations, much like in behavioural data.
Prediction of clinical outcomes based on brain-behaviour associations
Most studies used either CCA or PLS as the main approach, that is, the aim of the study was to investigate brain-behaviour associations. Although an interesting aim in itself, these methods can also be used to extract low-dimensional brain-behaviour patterns to be inputted into a machine learning model to predict clinical outcomes, such as relapse or response to treatment; this initial step is known as early fusion [ 91 ]. As large brain-behaviour datasets become increasingly accessible, the use CCA/PLS as an early fusion method will likely become more popular. For example, functional connectivity signatures of cognitive performance or symptoms have been used as input for clustering analysis to find subgroups in psychosis [ 92 ], ASD [ 64 ] and depression [ 68 ]. Notably, in a rare effort to replicate findings, the results from the latter study were not reproduced [ 84 ]. In the original study, a subset of brain-behaviour features was selected as input for CCA if they were correlated in the entire sample. The replication study addressed this (and other similar limitations) by including the feature selection step and the CCA in the same cross-validation scheme to ensure that both steps were tested for generalisability. Failure to do this is a common source of bias in machine learning studies that leads to optimistic findings [ 15 , 93 , 94 ]. In line with our exemplar CCA application, these two studies highlight the need and the complexity of implementing rigorous out-of-sample testing in brain-behaviour studies. Early fusion could also be used in conjunction with more popular supervised techniques for classification ( e.g ., SVM) or regression ( e.g ., support vector regression) to predict clinical outcomes of interest such as conversion to illness and severity of symptoms. Another possible application is to model brain-behaviour associations in a reference cohort ( e.g ., healthy controls), map patients against this reference model and quantify the deviation from the expected brain-behaviour interaction [ 95 ]. Given the known heterogeneity in both neural and behavioural presentations in psychiatric disorders, their interaction is also likely to be heterogeneous [ 63 ]. This approach has the advantage of allowing patients to deviate from the expected brain-behaviour interaction in their own unique way, and as such may be a promising approach to model complex and heterogeneous brain-behaviour associations in psychiatry. It would also be interesting to expand on brain-behaviour predictors to other relevant domains such as brain-behaviour-omics associations. This could be achieved via multiset CCA (mCCA), an extension of CCA capable of modelling latent associations between three or more sets of multivariate data [ 96 , 97 , 98 ]. Multi-level clustering [ 99 ] is another promising approach whereby patients are grouped based on multiple domains, e.g ., brain and behaviour, simultaneously. For example, Dwyer et al. found a subset of early psychosis patients with low functioning and reduced brain volume [ 22 ]. Future studies could investigate how membership of different brain-behaviour subtypes is associated with prognosis. Finally, multi-level networks, where brain and behaviour associations are explored by modelling the links between brain and behaviour networks, are also a promising way forward [ 100 ] For example, Hilland et al. developed a joint network of depression-related brain structures and individual depression symptoms [ 101 ].
What to use when
A natural question is which approach should be used: CCA or PLS? Theoretically speaking, the difference lies in the quantity that is being optimized (covariance for PLS versus correlation for CCA). In CCA, cross-modality relationships have been normalized by within-block variance; in other words, dependencies between input variables, on either the brain or the behavior side, have been factored out. For this reason, the optimization tends to emphasize unique contributions that variables from the first set make to the prediction of variables within the second. In contrast, collective contributions across different input variables (that is, redundant relationships) are emphasized with PLS [ 102 ]. In this review most studies (67%) used CCA; this may be because CCA is an older method that derived from the well-established and popular general linear model, whereas PLS is more recent. Experiments investigating the differences between the two have found that CCA and PLS results are most similar when the correlations within each dataset are low; however, the reliability of CCA worsens with high correlations within either dataset [ 102 ]. Notably, PLS has more stable weights in smaller samples [ 90 ], however they are more biased and larger samples are needed to have unbiased results compared to CCA [ 54 ]. Beyond these findings, knowledge is limited regarding whether other characteristics of the data influence the choice of method. Moreover, given that the stability and reliability of both methods varies with type of input data ( e.g ., parent-reported child behaviour versus cognitive data) [ 90 ], each method should be thoroughly investigated for each problem in order to make an informed decision. This should include the strength of within- and between-datasets correlation, testing different numbers of input features ( i.e ., does dimensionality reduction/feature selection improve reliability and stability), in- and out-of-sample associations ( i.e ., quantify the degree of overfitting) and stability of model weights/loadings ( i.e ., using resampling techniques).
Limitations
This review focused on two popular methods – CCA and PLS – to investigate brain-behaviour associations. Other methods can also be used such as multi-level clustering or independent component analysis. However, application of these methods in brain-behaviour studies in psychiatric disorders is still sparse. In our exemplar CCA, we discuss the importance of ‘out-of-sample’ testing using one large sample. Although this is a significant improvement from in-sample testing, the sub-samples used to find the brain-behaviour association and test it for generalizability follow the same recruitment and assessment procedures, which may mask the true strength of the association. Ideally, testing the CCA model in an independent sample would have yielded a result closer to the true association. Finally, this systematic review was not pre-registered; however, the search strategy is shown in the supplementary information.
‘Doubly multivariate’ methods, namely CCA and PLS, are becoming a popular approach to investigate brain-behaviour associations in psychiatry. These methods are attractive because they honour the multivariate nature of both brain and behaviour in health and disease. We found 37 studies that used either CCA or PLS to investigate brain-behaviour associations across several diagnostic groups. Overall, our findings suggest that frontal regions may be a transdiagnostic marker for psychopathology and cognition in psychiatric disorders. Although only a small number of studies investigated physical measures such as BMI, and developmental history such as childhood trauma, evidence suggests that these factors may be associated with specific brain signatures. This highlights the importance of expanding the investigation of brain-behaviour studies to include transdiagnostic factors of psychiatric illness in addition to symptoms and cognition. In addition, the choice and quality of behavioural measures should be scrutinized to similar standards as neuroimaging data; this could be achieved, for example, by reporting key psychometric properties of the behavioural data, such as validity, reliability and measurement invariance. Finally, we speculate that the overall effect sizes of the ‘doubly multivariate’ brain-behaviour associations reported so far may be optimistic. This is mainly due to the lack of rigorous out-of-sample testing and the suboptimal ratio between sample size and number of features, which combined may lead to overfitting. In conclusion, the capacity of ‘doubly multivariate’ models to learn complex associations, makes this a promising approach for investigating how brain and behaviour interact in psychiatric disorders. While there are still important challenges to overcome, the findings reviewed here provide preliminary evidence for the potential role of these methods in the investigation of mental health disorders.
Fusar-Poli P, Borgwardt S, Crescini A, Deste G, Kempton MJ, Lawrie S, et al. Neuroanatomy of vulnerability to psychosis: A voxel-based meta-analysis. Neurosci Biobehav Rev. 2011;35:1175–85.
Article CAS PubMed Google Scholar
Vissink CE, Winter-van Rossum I, Cannon TD, Fusar-Poli P, Kahn RS, Bossong MG. Structural Brain Volumes of Individuals at Clinical High Risk for Psychosis: A Meta-analysis. Biol Psychiatry Glob Open Sci. 2022;2:147–52.
Article PubMed Google Scholar
Luna LP, Radua J, Fortea L, Sugranyes G, Fortea A, Fusar-Poli P, et al. A systematic review and meta-analysis of structural and functional brain alterations in individuals with genetic and clinical high-risk for psychosis and bipolar disorder. Prog Neuro-Psychopharmacology Biol Psychiatry. 2022;117:110540.
Article CAS Google Scholar
Sacher J, Neumann J, Fünfstück T, Soliman A, Villringer A, Schroeter ML. Mapping the depressed brain: A meta-analysis of structural and functional alterations in major depressive disorder. J Affect Disord. 2012;140:142–8.
Kharabian Masouleh S, Eickhoff SB, Hoffstaedter F, Genon S, Initiative ADN. Empirical examination of the replicability of associations between brain structure and psychological variables. Elife. 2019;8:e43464.
Article PubMed PubMed Central Google Scholar
Marek S, Tervo-Clemmens B, Calabro FJ, Montez DF, Kay BP, Hatoum AS, et al. Reproducible brain-wide association studies require thousands of individuals. Nature. 2022;603:654–60.
Article CAS PubMed PubMed Central Google Scholar
Biswal BB, Mennes M, Zuo X-N, Gohel S, Kelly C, Smith SM, et al. Toward discovery science of human brain function. Proc Natl Acad Sci. 2010;107:4734–9.
Mulders PC, van Eijndhoven PF, Schene AH, Beckmann CF, Tendolkar I. Resting-state functional connectivity in major depressive disorder: a review. Neurosci Biobehav Rev. 2015;56:330–44.
Kennedy DP, Courchesne E. The intrinsic functional organization of the brain is altered in autism. Neuroimage. 2008;39:1877–85.
Sheffield JM, Barch DM. Cognition and resting-state functional connectivity in schizophrenia. Neurosci Bio Behav Rev. 2016;61:108–20.
Article Google Scholar
Koutsouleris N, Kambeitz-Ilankovic L, Ruhrmann S, Rosen M, Ruef A, Dwyer DB, et al. Prediction Models of Functional Outcomes for Individuals in the Clinical High-Risk State for Psychosis or With Recent-Onset Depression: A Multimodal, Multisite Machine Learning Analysis. JAMA Psychiatry. 2018;75:1156–72.
Sartori JM, Reckziegel R, Passos IC, Czepielewski LS, Fijtman A, Sodré LA, et al. Volumetric brain magnetic resonance imaging predicts functioning in bipolar disorder: A machine learning approach. J Psychiatr Res. 2018;103:237–43.
Vieira S, Pinaya WHL, Mechelli A. Using deep learning to investigate the neuroimaging correlates of psychiatric and neurological disorders: Methods and applications. Neurosci Bio Behav Rev. 2017;74:58–75.
Quaak M, van de Mortel L, Thomas RM, van Wingen G. Deep learning applications for the classification of psychiatric disorders using neuroimaging data: Systematic review and meta-analysis. NeuroImage Clin. 2021;30:102584.
Wolfers T, Buitelaar JK, Beckmann CF, Franke B, Marquand AF. From estimating activation locality to predicting disorder: A review of pattern recognition for neuroimaging-based psychiatric diagnostics. Neurosci Bio Behav Rev. 2015;57:328–49.
Del Fabro L, Bondi E, Serio F, Maggioni E, D’Agostino A, Brambilla P. Machine learning methods to predict outcomes of pharmacological treatment in psychosis. Transl Psychiatry. 2023;13:75.
Vieira S, Liang X, Guiomar R, Mechelli A. Can we predict who will benefit from cognitive-behavioural therapy? A systematic review and meta-analysis of machine learning studies. Clin Psychol Rev. 2022;97:102193.
Orrù G, Pettersson-Yeo W, Marquand AF, Sartori G, Mechelli A. Using Support Vector Machine to identify imaging biomarkers of neurological and psychiatric disease: A critical review. Neurosci Biobehav Rev. 2012;36:1140–52.
Pereira F, Mitchell T, Botvinick M. Machine learning classifiers and fMRI: A tutorial overview. Neuroimage. 2009;45:S199–S209.
Vapnik V. The Nature of Statistical Learning Theory . Springer, 1995.
Meda SA, Jagannathan K, Gelernter J, Calhoun VD, Liu J, Stevens MC, et al. A pilot multivariate parallel ICA study to investigate differential linkage between neural networks and genetic profiles in schizophrenia. Neuroimage. 2010;53:1007–15.
Dwyer DB, Buciuman M-O, Ruef A, Kambeitz J, Sen Dong M, Stinson C, et al. Clinical, Brain, and Multilevel Clustering in Early Psychosis and Affective Stages. JAMA Psychiatry. 2022;79:677–89.
Hotelling H. Relations between two sets of variates. In: Breakthroughs in statistics: methodology and distribution . Springer, 1992, 162–90.
Wold H. Partial least squares. In Kotz S, Johnson NL (Eds.) Encyclopedia of statistical sciences, Vol. 6 (Wiley, New York, 1985).
Genon S, Eickhoff SB, Kharabian S. Linking interindividual variability in brain structure to behaviour. Nat Rev Neurosci. 2022;23:307–18.
Wang H-T, Smallwood J, Mourao-Miranda J, Xia CH, Satterthwaite TD, Bassett DS, et al. Finding the needle in a high-dimensional haystack: Canonical correlation analysis for neuroscientists. Neuroimage. 2020;216:116745.
Mihalik A, Chapman J, Adams RA, Winter NR, Ferreira FS. Canonical Correlation Analysis and Partial Least Squares for Identifying Brain – Behavior Associations: A Tutorial and a Comparative Study. Biol Psychiatry Cogn Neurosci Neuroimaging. 2022;7:1055–1067.
PubMed Google Scholar
Liang C, Pearlson G, Bustillo J, Kochunov P, Turner JA, Wen X, et al. Psychotic Symptom, Mood, and Cognition-associated Multimodal MRI Reveal Shared Links to the Salience Network Within the Psychosis Spectrum Disorders. Schizophr Bull. 2022;49:172–84.
Article PubMed Central Google Scholar
Sui J, Adali T, Pearlson G, Yang H, Sponheim SR, White T, et al. A CCA+ICA based model for multi-task brain imaging data fusion and its application to schizophrenia. Neuroimage. 2010;51:123–34.
Sui J, He H, Pearlson GD, Adali T, Kiehl KA, Yu Q, et al. Three-way (N-way) fusion of brain imaging data based on mCCA + jICA and its application to discriminating schizophrenia. Neuroimage. 2013;66:119–32.
Sui J, Yu Q, He H, Pearlson GD, Calhoun VD. A Selective Review of Multimodal Fusion Methods in Schizophrenia. Front Hum Neurosci. 2012;6:27.
Krishnan A, Williams LJ, Randal A, Abdi H. Partial Least Squares (PLS) methods for neuroimaging: A tutorial and review. Neuroimage. 2011;56:455–75.
Calhoun VD, Sui J. Multimodal fusion of brain imaging data: A key to finding the missing link(s) in complex mental illness. Biol Psychiatry Cogn Neurosci neuroimaging. 2016;1:230–44.
PubMed PubMed Central Google Scholar
Tabachnick BG, Fidell LS, Ullman JB. (eds) Using multivariate statistics (Boston, MA: pearson, 2013).
Schöttner M, Bolton TAW, Patel J, Nahálka AT, Vieira S, Hagmann P. Exploring the latent structure of behavior using the Human Connectome Project’s data. Sci Rep. 2023;13:713.
Casey BJ, Cannonier T, Conley MI, Cohen AO, Barch DM, Heitzeg MM, et al. The Adolescent Brain Cognitive Development (ABCD) study: Imaging acquisition across 21 sites. Dev Cogn Neurosci. 2018;32:43–54.
Van Essen DC, Smith SM, Barch DM, Behrens TEJ, Yacoub E, Ugurbil K. The WU-Minn Human Connectome Project: An overview. Neuroimage. 2013;80:62–79.
Littlejohns TJ, Holliday J, Gibson LM, Garratt S, Oesingmann N, Alfaro-Almagro F, et al. The UK Biobank imaging enhancement of 100,000 participants: rationale, data collection, management and future directions. Nat Commun. 2020;11:2624.
Modabbernia A, Reichenberg A, Ing A, Moser DA, Doucet GE, Artiges E, et al. Linked patterns of biological and environmental covariation with brain structure in adolescence: a population-based longitudinal study. 2021;26:4905–4918.
Miller KL, Alfaro-almagro F, Bangerter NK, Thomas DL, Yacoub E, Xu J, et al. Multimodal population brain imaging in the UK Biobank prospective epidemiological study. Nat Neurosci. 2016;19:1523–36. https://doi.org/10.1038/nn.4393
Moser DA, Doucet GE, Lee WH, Rasgon A. Multivariate associations among behavioral, clinical, and multimodal imaging phenotypes in patients with psychosis. JAMA. 2018. https://jamanetwork.com/journals/jamapsychiatry/article-abstract/2673930 .
Nicolaisen-Sobesky E, Mihalik A, Kharabian-Masouleh S, Ferreira FS, Hoffstaedter F, Schwender H, et.al. A cross-cohort replicable and heritable latent dimension linking behaviour to multi-featured brain structure. Commun Biol. 2022;26:1297. https://doi.org/10.1038/s42003-022-04244-5 .
Smith SM, Nichols TE, Vidaurre D, Winkler AM, Behrens TEJ, Glasser MF, et al. A positive-negative mode of population covariation links brain connectivity, demographics and behavior. Nat Publ Gr. 2015; 18. https://doi.org/10.1038/nn.4125 .
Alnæs D, Kaufmann T, Marquand AF, Smith SM, Westlye LT. Patterns of sociocognitive stratification and perinatal risk in the child brain. 2020. https://doi.org/10.1073/pnas.2001517117 .
Xu X, Hilal S, Collinson SL, Chong EJY, Ikram MK. Association of magnetic resonance imaging markers of cerebrovascular disease burden and cognition. Stroke 2015. https://doi.org/10.1161/strokeaha.115.010700 .
Xu J, Liu N, Polemiti E, Garcia-Mondragon L, Tang J, Liu X, et al. Effects of urban living environments on mental health in adults. Nat Med. 2023;29:1456–67.
Xia CH, Ma Z, Ciric R, Gu S, Betzel RF, Kaczkurkin AN, et al. Linked dimensions of psychopathology and connectivity in functional brain networks. Nat Commun. 2018;9:3003.
Moser DA, Doucet GE, Ing A, Dima D, Schumann G, Bilder RM, et al. An integrated brain–behavior model for working memory. 2018;23:1974–1980.
Pettersson-Yeo W, Allen P, Benetti S, McGuire P, Mechelli A. Dysconnectivity in schizophrenia: Where are we now? Neurosci Biobehav Rev. 2011;35:1110–24.
Wheeler AL, Voineskos AN. A review of structural neuroimaging in schizophrenia: from connectivity to connectomics. Front Hum Neurosci. 2014;8:653.
Li S, Hu N, Zhang W, Tao B, Dai J, Gong Y, et al. Dysconnectivity of Multiple Brain Networks in Schizophrenia: A Meta-Analysis of Resting-State Functional Connectivity. Front Psychiatry 2019; 10. https://doi.org/10.3389/fpsyt.2019.00482 .
McCutcheon RA, Reis Marques T, Howes OD. Schizophrenia—An Overview. JAMA Psychiatry. 2020;77:201–10.
Mihalik A, Ferreira FS, Moutoussis M, Ziegler G, Adams RA, Rosa MJ, et al. Multiple Holdouts With Stability: Improving the Generalizability of Machine Learning Analyses of Brain – Behavior Relationships. 2020;87:368–376.
Helmer M, Warrington S, Mohammadi-Nejad A-R, Ji JL, Howell A, Rosand B, et al. On the stability of canonical correlation analysis and partial least squares with application to brain-behavior associations. Commun Biol. 2024;7:217.
Mechelli A, Vieira S. From models to tools: clinical translation of machine learning studies in psychosis. npj Schizophr. 2020;6:4.
Shmueli G. To Explain or to Predict? Stat Sci. 2010;25:289–310.
Yarkoni T, Westfall J. Choosing Prediction Over Explanation in Psychology: Lessons From Machine Learning. Perspect Psychol Sci. 2017;12:1100–22.
Kilpatrick LA, Joshi SH, O’Neill J, Kalender G, Dillon A, Best KM, et al. Cortical gyrification in children with attention deficit-hyperactivity disorder and prenatal alcohol exposure. Drug Alcohol Depend. 2021;225:108817.
Lin HY, Cocchi L, Zalesky A, Lv J, Perry A, Tseng WYI, et al. Brain-behavior patterns define a dimensional biotype in medication-naïve adults with attention-deficit hyperactivity disorder. Psychol Med. 2018;48:2399–408.
Luo L, Chen L, Wang Y, Li Q, He N, Yuanyuan Li, et al. Patterns of brain dynamic functional connectivity are linked with attention-deficit/hyperactivity disorder-related behavioral and cognitive dimensions. Psychol Med. 2023;53:6666–77.
Tsai CJ, Lin HY, Tseng IWY, Gau SSF. White matter microstructural integrity correlates of emotion dysregulation in children with ADHD: A diffusion imaging tractography study. Prog Neuro-Psychopharmacology Biol Psychiatry. 2021;110:110325.
Mei T, Llera A, Floris DL, Forde NJ, Tillmann J, Durston S, et al. Gray matter covariations and core symptoms of autism: the EU-AIMS Longitudinal European Autism Project. Mol Autism. 2020;11:1–13.
Zhang J, Fang S, Yao Y, Li F, Luo Q. Parsing the heterogeneity of brain-symptom associations in autism spectrum disorder via random forest with homogeneous canonical correlation. J Affect Disord. 2023;335:36–43.
Buch AM, Vértes PE, Seidlitz J, Kim SH, Grosenick L, Liston C. Molecular and network-level mechanisms explaining individual differences in autism spectrum disorder. 2023. https://doi.org/10.1038/s41593-023-01259-x .
Ilioska I, Oldehinkel M, Llera A, Chopra S, Looden T, Chauvin R, et al. Robust Patterns of Atypical Functional Connectivity in Autism. Biol Psychiatry 2023;94:29–39.
Mei T, Forde NJ, Floris DL, Dell’Acqua F, Stones R, Ilioska I, et al. Autism Is Associated With Interindividual Variations of Gray and White Matter Morphology. Biol Psychiatry Cogn Neurosci Neuroimaging 2022. https://doi.org/10.1016/j.bpsc.2022.08.011 .
Ni HC, Lin HY, Tseng WYI, Gau SSF. Association of self-regulation with white matter correlates in boys with and without autism spectrum disorder. Sci Rep. 2020;10:1–12.
Drysdale AT, Grosenick L, Downar J, Dunlop K, Mansouri F, Meng Y, et al. Resting-state connectivity biomarkers define neurophysiological subtypes of depression. Nat Med. 2017;23:28–38.
Yu M, Linn KA, Shinohara RT, Oathes DJ, Cook PA, Duprat R. Childhood trauma history is linked to abnormal brain connectivity in major depression. 2019. https://doi.org/10.1073/pnas.1900801116 .
Yu M, Cullen N, Linn KA, Oathes DJ, Seok D, Cook PA, et al. Structural brain measures linked to clinical phenotypes in major depression replicate across clinical centres. Mol Psychiatry 2021;26:2764–75.
Dean AM, Goodby E, Ooi C, Nathan PJ, Lennox BR, Scoriels L, et al. Speed of facial affect intensity recognition as an endophenotype of first-episode psychosis and associated limbic-cortical grey matter systems. 2013;43:591–602.
Raghava JM, Mandl RCW, Nielsen MØ, Multimodal assessment of white matter microstructure in antipsychotic-naïve schizophrenia patients and confounding effects of recreational drug use. 2021;15:36–48.
Thomas MB, Raghava JM, Pantelis C, Rostrup E, Nielsen MØ, Jensen MH, et al. ScienceDirect Associations between cognition and white matter microstructure in first-episode antipsychotic-naı patients with schizophrenia and healthy controls: A multivariate pattern analysis. 2021; 9. https://doi.org/10.1016/j.cortex.2021.03.003 .
Krakauer K, Ebdrup BH, Glenthøj BY, Raghava JM, Nordholm D, Randers L. Patterns of white matter microstructure in individuals at ultra-high-risk for psychosis: associations to level of functioning and clinical symptoms. 2017;47:2689–707.
Kristensen TD, Mandl RCW, Raghava JM, Jessen K, Jepsen JRM, Fagerlund B, et al. Widespread higher fractional anisotropy associates to better cognitive functions in individuals at ultra-high risk for psychosis. Hum Brain Mapp. 2019;40:5185–201.
Kirschner M, Shafiei G, Markello RD, Makowski C, Talpalaru A, Hodzic-santor B, et al. Latent Clinical-Anatomical Dimensions of Schizophrenia. Schizophr Bull. 2020;46:1426–38.
Syeda WT, Wannan CMJ, Merritt AH, Raghava JM, Jayaram M, Velakoulis D, et al. Cortico-cognition coupling in treatment resistant schizophrenia. Neuroimage Clin. 2022;35:103064 https://doi.org/10.1016/j.nicl.2022.103064 .
Kottaram A, Zalesky A, Johnston LA, Cocchi L, Ganella EP. Brain network dynamics in schizophrenia: Reduced dynamism of the default mode network. 2019;40:2212–28.
Rodrigue AL, Mcdowell JE, Tandon N, Keshavan MS, Tamminga CA, Pearlson GD, et al. Archival Report Multivariate Relationships Between Cognition and Brain Anatomy Across the Psychosis Spectrum. Biol Psychiatry Cogn Neurosci Neuroimaging. 2018;3:992–1002.
Voldsbekk I, Kjelkenes R, Wolfers T, Dahl A, Lund MJ, Kaufmann T, et al. Developmental Cognitive Neuroscience Shared pattern of impaired social communication and cognitive ability in the youth brain across diagnostic boundaries. Dev Cogn Neurosci. 2023;60:101219.
Tung Y, Lin H, Chen C, Sc M, Shang C, Ph D, et al. Whole Brain White Matter Tract Deviation and Idiosyncrasy From Normative Development in Autism and ADHD and Unaffected Siblings Link With Dimensions Psychopathol Cogn. 2021. https://doi.org/10.1176/appi.ajp.2020.20070999 .
Chien Y, Lin H, Tung Y, Hwang T, Chen C, Wu C Neurodevelopmental model of schizophrenia revisited: similarity in individual deviation and idiosyncrasy from the normative model of whole-brain white matter tracts and shared brain-cognition covariation with ADHD and ASD. 2022. https://doi.org/10.1038/s41380-022-01636-1 .
Ji JL, Helmer M, Fonteneau C, Burt JB, Tamayo Z, Adkinson BD, et al. Mapping brain-behavior space relationships along the psychosis spectrum. 2021;10:e66968.
Dinga R, Schmaal L, Penninx BWJH, Jose M, Tol V, Veltman DJ, et al. Evaluating the evidence for biotypes of depression: Methodological replication and extension of. NeuroImage Clin. 2019;22:101796.
Helmer M, Warrington S, Mohammadi-Nejad AR, Ji JL, Howell A, Rosand B, et al. On the stability of canonical correlation analysis and partial least squares with application to brain-behavior associations. Commun Biol 2024;7:217.
Pituch KA, Stevens JP. Applied multivariate statistics for the social sciences: Analyses with SAS and IBM’s SPSS . Routledge, 2015.
Varoquaux G. Cross-validation failure: Small sample sizes lead to large error bars. Neuroimage. 2017;180:68–77.
Monteiro JM, Rao A, Shawe-taylor J. A multiple hold-out framework for Sparse Partial Least Squares. J Neurosci Methods. 2016;271:182–94.
Tiego J, Martin EA, Deyoung CG, Hagan K, Cooper SE, Pasion R, et al. Precision behavioral phenotyping as a strategy for uncovering the biological correlates of psychopathology. Nat Ment Health. 2023;1:304–15. https://doi.org/10.1038/s44220-023-00057-5
Nakua H, Yu J, Abdi H, Hawco C, Voineskos A, Hill S. Comparing the stability and reproducibility of brain-behaviour relationships found using Canonical Correlation Analysis and Partial Least Squares within the ABCD Sample. 2023.
Vieira S, Pinaya WHL, Garcia-Dias R, Mechelli A. Multimodal integration. In: Machine Learning . Academic Press, 2020, 283–305.
Viviano JD, Buchanan RW, Calarco N, Gold JM, Foussias G, Bhagwat N, et al. Resting-State Connectivity Biomarkers of Cognitive Performance and Social Function in Individuals With Schizophrenia Spectrum Disorder and Healthy Control Subjects. 2018;84:665–74.
Arbabshirani MR, Plis S, Sui J, Calhoun VD. Single subject prediction of brain disorders in neuroimaging: Promises and pitfalls. Neuroimage. 2017;145:137–65.
Scheinost D, Noble S, Horien C, Greene AS, Lake EM, Salehi M, et al. Ten simple rules for predictive modeling of individual differences in neuroimaging. Neuroimage. 2019;193:35–45.
Marquand AF, Rezek I, Buitelaar J, Beckmann CF. Understanding Heterogeneity in Clinical Cohorts Using Normative Models: Beyond Case-Control Studies. Biol Psychiatry. 2016;80:552–61.
Parra LC Multiset Canonical Correlation Analysis simply explained. https://arxiv.org/pdf/1802.03759.pdf (accessed 25 May2020).
Lerman-Sinkoff DB, Kandala S, Calhoun VD, Barch DM, Mamah DT. Transdiagnostic Multimodal Neuroimaging in Psychosis: Structural, Resting-State, and Task Magnetic Resonance Imaging Correlates of Cognitive Control. Biol Psychiatry Cogn Neurosci Neuroimaging. 2019;4:870–80.
Liu S, Wang H, Song M, Lv L, Cui Y, Liu Y, et al. Linked 4-Way Multimodal Brain Differences in Schizophrenia in a Large Chinese Han Population. Schizophr Bull. 2018;45:436–49.
Deshpande G, Jia H. Multi-Level Clustering of Dynamic Directional Brain Network Patterns and Their Behavioral Relevance. Front Neurosci. 2020; 13. https://doi.org/10.3389/fnins.2019.01448 .
Blanken TF, Bathelt J, Deserno MK, Voge L, Borsboom D, Douw L. Connecting brain and behavior in clinical neuroscience: A network approach. Neurosci Biobehav Rev. 2021;130:81–90.
Hilland E, Landrø NI, Kraft B, Tamnes CK, Fried EI, Maglanoc LA, et al. Exploring the links between specific depression symptoms and brain structure: A network study. Psychiatry Clin Neurosci. 2020;74:220–1.
McIntosh AR. Comparison of Canonical Correlation and Partial Least Squares analyses of simulated and empirical data. arXiv preprint arXiv:2107.06867. 2021 Jul 14.
Download references
Acknowledgements
This work was supported by a Sir Henry Wellcome postdoctoral fellowship from the Wellcome Trust to SV (grant number 221638/Z/20/Z). TB, MS, and PH were supported by Swiss National Science Foundation grant #320030_197787. Data were provided by the Human Connectome Project, WU-Minn Consortium (Principal Investigators: David Van Essen and Kamil Ugurbil; 1U54MH091657) funded by the 16 NIH Institutes and Centers that support the NIH Blueprint for Neuroscience Research; and by the McDonnell Center for Systems Neuroscience at Washington University.
Author information
Authors and affiliations.
Department of Radiology, Lausanne University Hospital and University of Lausanne, Lausanne, Switzerland
S. Vieira, T. A. W. Bolton, M. Schöttner & P. Hagmann
Department of Psychosis Studies, Institute of Psychiatry, Psychology and Neuroscience, King’s College London, London, UK
S. Vieira, L. Baecker & A. Mechelli
Center for Research in Neuropsychology and Cognitive Behavioral Intervention, Faculty of Psychology and Educational Sciences, University of Coimbra, Coimbra, Portugal
Neurosurgery Service and Gamma Knife Center, Lausanne University Hospital, Lausanne, Switzerland
T. A. W. Bolton
Donders Institute for Brain, Cognition and Behavior, Radboud University Nijmegen, Nijmegen, The Netherlands
A. Marquand
Department for Cognitive Neuroscience, Radboud University Medical Center Nijmegen, Nijmegen, The Netherlands
Department of Neuroimaging, Institute of Psychiatry, Psychology, & Neuroscience, King’s College London, London, UK
You can also search for this author in PubMed Google Scholar
Contributions
SV: Conceptualization, Data Curation, Analysis, Writing – Original Draft, Writing – Review and Editing, Funding Acquisition. TB: Analysis, Writing – Review and Editing. MS: Data Curation, Writing – Review and Editing. LB: Data Curation, Writing – Review and Editing. AMa, AMe, and PH: Writing – Review and Editing.
Corresponding author
Correspondence to S. Vieira .
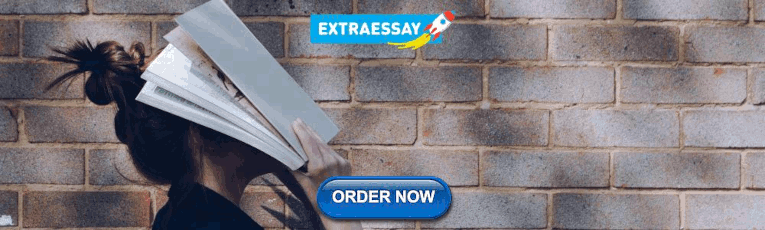
Ethics declarations
Competing interests.
The authors declare no competing interests.
Additional information
Publisher’s note Springer Nature remains neutral with regard to jurisdictional claims in published maps and institutional affiliations.
Supplementary information
Prisma_checklist, supplementary materials, rights and permissions.
Open Access This article is licensed under a Creative Commons Attribution 4.0 International License, which permits use, sharing, adaptation, distribution and reproduction in any medium or format, as long as you give appropriate credit to the original author(s) and the source, provide a link to the Creative Commons licence, and indicate if changes were made. The images or other third party material in this article are included in the article’s Creative Commons licence, unless indicated otherwise in a credit line to the material. If material is not included in the article’s Creative Commons licence and your intended use is not permitted by statutory regulation or exceeds the permitted use, you will need to obtain permission directly from the copyright holder. To view a copy of this licence, visit http://creativecommons.org/licenses/by/4.0/ .
Reprints and permissions
About this article
Cite this article.
Vieira, S., Bolton, T.A.W., Schöttner, M. et al. Multivariate brain-behaviour associations in psychiatric disorders. Transl Psychiatry 14 , 231 (2024). https://doi.org/10.1038/s41398-024-02954-4
Download citation
Received : 26 July 2023
Revised : 15 May 2024
Accepted : 21 May 2024
Published : 01 June 2024
DOI : https://doi.org/10.1038/s41398-024-02954-4
Share this article
Anyone you share the following link with will be able to read this content:
Sorry, a shareable link is not currently available for this article.
Provided by the Springer Nature SharedIt content-sharing initiative
Quick links
- Explore articles by subject
- Guide to authors
- Editorial policies

- Open access
- Published: 29 May 2024
Exploring the use of body worn cameras in acute mental health wards: a mixed-method evaluation of a pilot intervention
- Una Foye 1 , 2 ,
- Keiran Wilson 1 , 2 ,
- Jessica Jepps 1 , 2 ,
- James Blease 1 ,
- Ellen Thomas 3 ,
- Leroy McAnuff 3 ,
- Sharon McKenzie 3 ,
- Katherine Barrett 3 ,
- Lilli Underwood 3 ,
- Geoff Brennan 1 , 2 &
- Alan Simpson 1 , 2
BMC Health Services Research volume 24 , Article number: 681 ( 2024 ) Cite this article
255 Accesses
12 Altmetric
Metrics details
Body worn cameras (BWC) are mobile audio and video capture devices that can be secured to clothing allowing the wearer to record some of what they see and hear. This technology is being introduced in a range of healthcare settings as part of larger violence reduction strategies aimed at reducing incidents of aggression and violence on inpatient wards, however limited evidence exists to understand if this technology achieves such goals.
This study aimed to evaluate the implementation of BWCs on two inpatient mental health wards, including the impact on incidents, the acceptability to staff and patients, the sustainability of the resource use and ability to manage the use of BWCs on these wards.
The study used a mixed-methods design comparing quantitative measures including ward activity and routinely collected incident data at three time-points before during and after the pilot implementation of BWCs on one acute ward and one psychiatric intensive care unit, alongside pre and post pilot qualitative interviews with patients and staff, analysed using a framework based on the Consolidated Framework for Implementation Research.
Results showed no clear relationship between the use of BWCs and rates or severity of incidents on either ward, with limited impact of using BWCs on levels of incidents. Qualitative findings noted mixed perceptions about the use of BWCs and highlighted the complexity of implementing such technology as a violence reduction method within a busy healthcare setting Furthermore, the qualitative data collected during this pilot period highlighted the potential systemic and contextual factors such as low staffing that may impact on the incident data presented.
This study sheds light on the complexities of using such BWCs as a tool for ‘maximising safety’ on mental health settings. The findings suggest that BWCs have a limited impact on levels of incidents on wards, something that is likely to be largely influenced by the process of implementation as well as a range of contextual factors. As a result, it is likely that while BWCs may see successes in one hospital site this is not guaranteed for another site as such factors will have a considerable impact on efficacy, acceptability, and feasibility.
Peer Review reports
Body worn cameras (BWC) are mobile audio and video capture devices that can be secured to clothing allowing the wearer to record some of what they see and hear. In England, these have been introduced in the National Health Service (NHS) as part of a violence reduction strategy [ 1 ] which emphasises the reduction of aggression and violence against staff. The NHS Staff Survey 2022 found that 14.7% of NHS staff had experienced at least one incident of physical violence from patients, relatives or other members of the public in the previous 12 months. Violent attacks on staff were found to contribute to almost half of staff illness [ 2 ]. Levels of violence against staff working in mental health trusts remain much higher than other types of healthcare providers [ 3 ]. Numerous reports internationally highlight the increased risks faced by staff working in psychiatric care [ 4 ], though studies have reported that both ward staff and mental health patients experience violence and feeling unsafe on inpatient wards [ 5 , 6 ].
Body worn cameras have been in use for over a decade within law enforcement, where they hoped to provide transparency and accountability within use-of-force incidents and in the event of citizen complaints against police [ 7 ]. It was believed that video surveillance would help identify integral problems within the organisation, improve documentation of evidence, reduce use-of-force incidents, improve police-community relations, and provide training opportunities for officers [ 8 ]. However, a recent extensive international systematic review by Lum et al. [ 9 ], found that despite the successes noted in early evaluations, the way BWCs are currently used by police may not substantially affect most officer or citizen behaviours. Irrespective of these findings, other public services such as train operators have been implementing BWCs for security purposes, with reductions reported in the number of assaults on railway staff [ 10 ].
A recent systematic review of BWC use in public sector services established that there is a poor evidence base supporting the use of BWCs in the reduction of violence and aggression [ 11 ]. Yet, we are seeing a swift increase in the use of BWCs in mental health settings with that aim, with few studies conducted on the use of BWC technology in inpatient mental health wards, and even fewer studies exploring staff or patients’ views. Two evaluations conducted in England reported mixed results with both increases and decreases in violence and aggression found, and variation between types of wards. There is some suggestion of a reduction in more serious incidents and the use of restraint, but quality of evidence is low [ 12 , 13 ].
The use of BWCs in mental healthcare settings for safety and security remains a contentious topic due to the lack of evidence regarding the influence that such technology has on preventing violence and aggression and the complex philosophical and ethical issues raised, particularly where many patients may lack capacity and/or are detained under mental health legislation [ 14 ]. Additionally, there are concerns that BWCs may be used as a ‘quick fix’ for staff shortages rather than addressing the wider systemic and resourcing issues facing services [ 15 ]. With little independent evaluation of body-worn cameras in mental health settings, many of these concerns remain unanswered. There is also limited understanding of this technology from an implementation perspective. Therefore, in this study we aimed to conduct an independent evaluation of the introduction of BWCs as a violence reduction intervention on two inpatient mental health wards during a six-month pilot period to explore the impact of using the technology, alongside an exploration of the facilitators and barriers to implementation.
Research aim(s)
To evaluate the implementation of BWCs on two inpatient mental health wards, including the impact on incidents, the acceptability to staff and patients, the sustainability of the resource use and ability to manage the use of BWCs on these wards.
Patient and public involvement
The research team included a researcher and independent consultant, each with lived experience of mental health inpatient care. In addition, we recruited and facilitated a six member Lived Experience Advisory Panel (LEAP). This group was made up of patients and carers, some of whom had experienced the use of BWCs. Members were of diverse ethnic backgrounds and included four women and two men. The LEAP provided guidance and support for the research team in developing an understanding of the various potential impacts of the use of BWCs on inpatient mental health wards. Members contributed to the design of the study, development of the interview schedule, practice interviews prior to data collection on the wards, and supported the analysis and interpretation of the data, taking part in coding sessions to identify themes in the interview transcripts. The LEAP met once a month for two hours and was chaired by the Lived Experience Research Assistant and Lived Experience Consultant. Participants in the LEAP were provided with training and paid for their time.
The pilot introduction of the body worn cameras was conducted within a London mental health Trust consisting of four hospital sites with 17 acute wards. The research team were made aware of extensive preparatory work and planning that was conducted at a directorate and senior management level prior to camera implementation, including lived experience involvement and consultation, and the development of relevant policies and protocols inclusive of a human rights assessment and legal consultation.
The pilot period ran from 25th April to 25th October 2022. Reveal (a company who supply BWCs nationally across the UK) provided the Trust with 12 Calla BWCs for a flat fee that covered use of the cameras, cloud-based storage of footage, management software, and any support/maintenance required during the pilot period. Cameras were introduced to two wards based on two hospital sites, with six cameras provided to each of the wards on the same date. Training on using the BWCs was provided by the BWC company to staff working on both wards prior to starting the pilot period. Ward one was a 20-bed male acute inpatient ward, representing the most common ward setting where cameras have been introduced. Ward two was a ten-bed male Psychiatric Intensive Care Unit (PICU), representing smaller and more secure wards in which patients are likely to present as more unwell and where there are higher staff to patient ratios.
To answer our research questions, we used a mixed-methods design [ 16 ]. Using this design allowed us to investigate the impact of implementing BWCs in mental health settings on a range of quantitative and qualitative outcomes. This mixed methods design allows the study to statistically evaluate the effectiveness of using BWCs in these settings on key dependent variables (i.e., rates of violence and aggression, and incidents of conflict and containment) alongside qualitatively exploring the impact that the implementation of such technology has on patients and staff.
To ensure that the study was able to capture the impact and effect of implementation of the cameras, a repeated measures design was utilised to capture data at three phases on these wards:
Pre-pilot data: data prior of the implementation of the BWCs (quantitative and qualitative data).
Pilot period data: data collected during the six-month pilot period when BWCs were implemented on the wards (quantitative and qualitative data).
Post-pilot: data collected after the pilot period ended and cameras had been removed from the wards (quantitative data only).
Quantitative methods
Quantitative data was collected at all three data collection periods:
Pre-period: Data spanning six months prior to the implementation of BWCs (Nov 21 to May 22).
Pilot period: Data spanning the six months of the Trusts pilot period of using BWCs on the wards (June 22 to Nov 22).
Post-pilot: Data spanning the six months following the pilot period, when BWCs had been removed (Dec 22 to May 23).
Quantitative measures
To analyse the impact of BWC implementation, we collected two types of incident data related to violence and aggression and use of containment measures, including BWCs. Combined, these data provide a view of a wide range of incidents and events happening across the wards prior to, during, and after the implementation and removal of the BWCs.
The patient-staff conflict checklist
The Patient-staff Conflict Checklist (PCC-SR) [ 17 ] is an end of shift report that is completed by nurses to collate the frequency of conflict and containment events. This measure has been used successfully in several studies on inpatient wards [ 18 , 19 , 20 ].The checklist consists of 21 conflict behaviour items, including physical and verbal aggression, general rule breaking (e.g., smoking, refusing to attend to personal hygiene), eight containment measures (e.g., special observation, seclusion, physical restraint, time out), and staffing levels. In tests based on use with case note material, the PCC-SR has demonstrated an interrater reliability of 0.69 [ 21 ] and has shown a significant association with rates of officially reported incidents [ 22 ].
The checklist was revised for this study to include questions related to the use of BWCs ( e.g., how many uses of BWCs happened during the shift when a warning was given and the BWC was not used; when a warning was given and the BWC was used; when the BWC was switched on with no warning given ) in order to provide insight into how the cameras were being used on each ward (see appendix 1). Ward staff were asked to complete the checklist online at the end of each shift.
Routinely collected incident data (via datix system)
To supplement the PCC-SR-R, we also used routinely collected incident data from both wards for all three data collection phases. This data is gathered as part of routine practice by ward staff members via the Datix system Datix [ 23 ] is a risk management system used widely across mental health wards and Trusts in the UK to gather information on processes and errors. Previous studies have utilised routinely collect data via this system [ 24 , 25 ]. Incidents recorded in various Datix categories were included in this study (see Table 1 ). Incidents were anonymised before being provided to the research team to ensure confidentiality.
Routinely collected data included:
Recorded incidents of violence and aggression.
Recorded use of restrictive practices including seclusion, restraint, and intra-muscular medication/rapid tranquilisations.
Patient numbers.
Staffing levels.
Numbers of staff attending BWC training.
Quantitative data analysis
Incident reports.
Incident reports retrieved from Datix were binary coded into aggregate variables to examine violence and aggression, self-harm, and other conflict as outlined in Table 1 . Multivariate analyses of variance (MANOVA) were used to identify differences in type of incident (violence against person, violence against object, verbal aggression, self-harm, conflict) for each ward. MANOVA was also used to examine differences in incident outcomes (severity, use of restrictive practice, police involvement) across pre-trial, trial, and post-trial periods for each ward. Incident severity was scored by ward staff on a four-point scale (1 = No adverse outcome, 2 = Low severity, 3 = Moderate severity, 4 = Severe). Use of restrictive practice and police involvement were binary coded for presence or absence. Analyses were conducted using SPSS [ 26 ].
Patient-staff conflict checklist shift-report – revised (PCC-SR-R; )
Data were condensed into weeks for analysis rather than shifts to account for variability in PCC-SR-R submission by shift. Linear regressions assessed the relationship between BWC use and incident outcome (severity, use of restrictive practice, police involvement).
Qualitative methods
We used semi-structured qualitative interviews to explore participants’ experiences of BWCs on the ward to understand the impact of their use as well as to identify any salient issues for patients, staff and visitors that align with the measures utilised within the quantitative aspect of this study. These interviews were conducted at two time points: pre-pilot and at the end of the six-month pilot period.
Sample selection, eligibility, and recruitment
Convenience sampling was used to recruit staff and patients on wards. Researchers approached ward managers to distribute information sheets to staff, who shared that information with patients. Staff self-selected to participate in the study by liaising directly with the research team. Patients that were identified as close to discharge and having capacity to consent were approached by a clinical member of the team who was briefed on the study inclusion criteria (see Table 2 ). The staff member spoke with the patient about the study and provided them with a copy of the information sheet to consider. If patients consented, a member of the research team approached the participant to provide more information on the study and answer questions. After initial contact with the research team, participants were given a 24-hour period to consider whether they wanted to participate before being invited for an interview.
Participants were invited to take part in an interview within a private space on the ward. Interviews were scheduled for one hour with an additional 15 min before and after to obtain informed consent and answer any questions. Participation was voluntary and participants were free to withdraw at any time. To thank patients for their time, we offered a £10 voucher following the interview. Interviews were audio-recorded and saved to an encrypted server. Interview recordings were transcribed by an external company, and the research team checked the transcripts for accuracy and pseudonymised all participants. All transcripts were allocated a unique ID number and imported to MicroSoft Excel [ 27 ] for analysis.
Qualitative data analysis
Qualitative data were analysed using a framework analysis [ 28 ] informed by implementation science frameworks. Our coding framework used the Consolidated Framework for Implementation Research (CFIR) [ 29 ], which is comprised of five major domains including: Intervention Characteristics, Implementation Processes, Outer Setting, Inner Setting, and Characteristics of the Individual. Each domain consists of several constructs that reflect the evidence base of the types of factors that are most likely to influence implementation of interventions. The CFIR is frequently used to design and conduct implementation evaluations and is commonly used for complex health care delivery interventions to understand barriers and facilitators to implementation. Based on its description, the CFIR is an effective model to address our research question, particularly given the complexity of the implementation of surveillance technology such as BWCs in this acute care setting.
The initial analytic stage was undertaken by eight members of the study team with each researcher charting data summaries onto the framework for each of the interviews they had conducted on MicroSoft Excel [ 27 ]. Sub-themes within each broad deductive theme from our initial framework were then derived inductively through further coding and collaborative discussion within the research team, inclusive of Lived Experience Researcher colleagues. Pseudonyms were assigned to each participant during the anonymisation of transcripts along with key identifiers to provide context for illustrative quotes (e.g., P = patient, S = staff, A = acute ward, I = Intensive Care, Pre = pre-BWC implementation interview, Post = Post BWC implementation interview).
All participants gave their informed consent for inclusion before they participated in the study. The study was conducted in accordance with the Declaration of Helsinki, and the protocol was approved by the Health Research Authority: London - Camden & Kings Cross Research Ethics Committee (IRAS Project ID 322,268, REC Reference 23/LO/0337).
Quantitative results
Exploring how body worn cameras were used during the pilot period.
Analysis of the PCC-SR-R provides information about how the BWCs were used on a day-to-day basis during the pilot period. Out of 543 total shift reports completed, BWC use was reported 50 times, indicating that BWCs were used on less than 10% of shifts overall; 78% of those deployments were on the Acute ward (see Figure 1 ). Overall, the majority of deployments happened as activations without a warning being given ( n = 30, 60% of activations), 19 times the BWC was deployed with a warning but the camera was not activated (38%), and only one was the camera activated without a warning being given (2%).
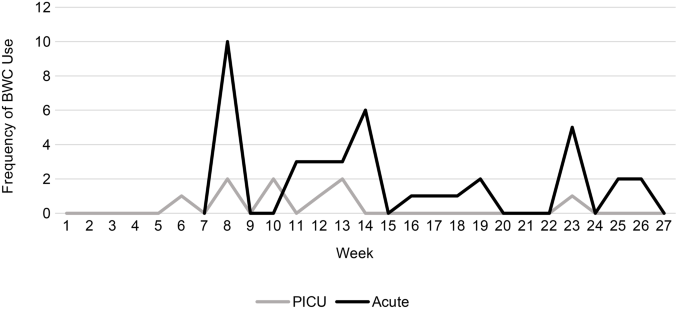
BWC use by ward per week of pilot (no data available before week 6 on Ward 1)
According to the PCC-SR-R, a total of 227 incidents of aggression occurred during the pilot period across both wards (see Table 3 ). Overall, there were small statistically significant correlations between BWC usage and certain types of conflict, aggression, and restrictive practice. Results found that BWC use was positively correlated with verbal aggression and use of physical restraint. BWC use was moderately positively correlated with verbal aggression ( r = .37, p < .001). This indicates that BWCs were more likely to be used in incidents involving verbal aggression, which do not tend to be documented in Datix. Similarly, BWC use was moderately positively correlated with physical restraint ( r = .31, p < .001) indicating that they were also more likely to be used alongside physical restraint.
Exploring the impact of BWCs utilising routinely collected ward data
Acute ward results.
Routine data collected via Datix records were used to examine differences in frequency of conflict and aggression, incident severity, and use of containment measures before, during, and after introduction of BWCs on each trial ward (see Table 4 ).
There was no effect of trial period on incident type ( F (10, 592) = 1.703, p = .077, Wilk’s Λ = 0.945), meaning there was no discernible difference in the type of incidents that occurred (E.g., verbal aggression, physical aggression) before, during, and after the pilot phase.
Incident outcomes
There was an effect of trial period on incident outcomes ( F (6, 596) = 10.900, p < .001, Wilk’s Λ = 0.812). Incident severity was statistically significantly higher in the trial and post-trial periods compared to the pre-trial period. Use of restrictive practice was significantly lower in the post-trial period compared to the pre-trial and trial period. Police involvement was also lower in the post-trial period compared to the pre-trial and trial periods (see Table 5 ).
Results for the psychiatric intensive care unit
There was an effect of trial period on incident type ( F (10, 490) = 4.252, p < .001, Wilk’s Λ = 0.847). Verbal aggression was statistically significantly higher in the post-trial period compared to the pre and trial periods. Self-harm was statistically significantly higher in the trial period compared to the pre-trial and post-trial periods. There were no differences in violence against a person ( p = .162), violence against an object or conflict behaviour (see Table 4 ).
There was a statistically significant difference in incident outcome across the trial periods ( F (6, 494) = 12.907, p < .001, Wilk’s Λ = 0.747). There was no difference in incident severity or police involvement. However, use of restrictive practice was statistically significantly higher in the pre-trial period, reducing in the test period, and reducing further in the post-trial period (see Table 5 ).
Qualitative findings
A total of 22 participants took part in interviews: five patients and 16 staff members. During the pre-pilot interviews a total of nine staff took part (five in the acute ward, four in the PICU ward) and two patients (both from the acute ward). After the pilot period, a total of eight staff took part (four from each ward) and three patients (all from the acute ward). Table 6 includes a full description of participants.
Below we have presented the key themes aligning to the five core CFIR categories of Intervention Characteristics, Characteristics of Individuals, The Process of Implementation, the Inner Setting, and The Outer Setting (see Table 7 ).
Intervention characteristics
Design and usability of wearing a bwc on the ward.
When discussing the use of the BWCs, staff noted a range of design issues related to the cameras that they said impacted on their use and acceptance of the cameras. This included the nature of the camera pulling on clothing necklines (a particular issue for female staff working on male wards), and overheating causing discomfort and irritation to skin, challenges with infection control, as well as the issue of cameras in a mental health setting where they can be easily grabbed, thrown and broken during an incident. Staff often cited these design issues as related to the lack of proactive use of the cameras on the wards.
There were issues around the devices getting overheated or about it going on your clothing, it pulls down the top… we had one person who was leading on it, whenever he was around, of course, the camera was being used, but if he wasn’t there, people weren’t as proactive in using the camera. Petra (f), Staff, A, Post.
There were also issues with staff forgetting to wear the cameras, forgetting to switch them on during incidents, and forgetting to charge them at the end of the shift, reducing the potential use of the cameras by other staff. These were perceived as key logistical issues prior to the pilot and were reported as issues at the end of the pilot by several staff on the wards.
The practicalities of will they actually turn it on in those sorts of incidents, I don’t know. Just little stuff as well, like if they don’t put it back on the docking station, so you think you’re charging it for next shift but then it’s not charged and the battery is dead, that’s one less camera to use, so little stuff. Jamal (m), Staff, A, Pre.
In relation to usability, staff noted that the cameras were small and easy to use given their simple single switch interface. It was felt that not having to upload and manage the data themselves made cameras more user friendly and usable by staff members. Protocols put into place such as signing the cameras in and out, and allocation for use during shifts were likened to procedures in place for other security measures therefore the implementation of this for the BWCs was viewed as easy for many staff.
It’s just like the ASCOM alarms that we wear. There’s a system to sign in and sign out, and that’s it. Alice (f), Staff, A, Pre.
While staff were generally positive about the usability of the cameras, some were cautious of with concerns for those less confident with technology.
… you have to be conscious that there’s some people – it’s quite easy to use, but I can say that because I’m alright using devices and all that but there’s some that are older age or not that familiar with using devices that may struggle with using it… they’re feeling a bit anxious and a bit scared, if they’re not familiar with it then they won’t use it. Jamal (m), Staff, A, Pre.
Evidence strength and quality: do BWCs change anything?
There were conflicting reports regarding the potential benefits of using BWCs on the wards, with both staff and patients reporting mixed perceptions as to whether the cameras might reduce violence and aggression. In the pre-pilot interviews, some staff reported feeling that the BWCs may have a positive impact on reducing physical violence.
I think it’s going to reduce violence and aggression on the ward…I don’t think they’ll want to punch you…they might be verbally abusive but in terms of physical that might reduce. Sarah (f), Staff, I, Pre.
Patients however noted that the cameras might hold staff to account of their own behaviours and therefore may improve care, however they felt that this impact would wear off after the first few months after which people might forget about the cameras being there.
Now they’ve got the body cams, it’s going to be a lot of changes. They’ll think, ‘Ooh well he’s on tape’. So, it might do something to their conscience, they actually start to listen to patients… until the novelty wears off and it might go back to square one again. Ian (m), Patient, A, Pre.
One staff member suggested that incident rates had reduced following introduction of the BWCs, but they remained unsure as to whether this was due to the cameras, reflecting that violence and aggression on wards can be related to many factors.
I know our violence and aggression has reduced significantly since the start of the cameras pilot… I don’t know, because obviously wearing the camera’s one thing, but if they weren’t in use, I don’t know maybe just the presence of the camera made a difference. But yeah, it’s hard to tell. Petra (f), Staff, A, Post.
In contrast, several staff reported that they had seen limited evidence for such changes.
I used it yesterday. He was aggressive and I used it, but he even when I was using [it] he doesn’t care about the camera… it didn’t make any difference… It doesn’t stop them to do anything, this camera does not stop them to do anything. Abraham (m), Staff, I, Post.
Some staff suggested that in some circumstances the cameras increased patient agitation and created incidents, so there was a need to consider whether the BWCs were going to instigate aggression in some circumstances.
There has been with a few patients because they will threaten you. They will tell you, ‘if you turn it on, I’m gonna smash your head in’. So incidents like that, I will not turn it on… Yeah, or some of them will just tell you, ‘if you come close by, I’m going to pull that off your chest’. So things like that, I just stay back. Ada (f), Staff, A, Post.
One rationale for a potential lack of effectiveness was noted by both staff and patients and was related to the levels of acute illness being experienced by patients which meant that for many they were too unwell to have insight into their own actions or those of staff switching on the cameras.
We’ve had instances where patients are so unwell that they just don’t care. You switch on the camera, whether you switch it on or not, it doesn’t really change the behaviour. ‘All right, okay, whatever switch it on’. They’re so unwell, they’re not really understanding. Petra (f), Staff, A, Post. It might make [staff] feel safer as a placebo effect, but I don’t think it would necessarily make them safer… I think the people that are likely to attack a member of staff are crazy enough that they’re not gonna even consider the camera as a factor. Harry (m), Patient, A, Pre.
This lack of evidence that the cameras were necessarily effective in reducing incident rates or severity of incidents may have had an impact on staff buy-in and the use of the cameras as a result. One staff member reflected that having feedback from senior management about the impact and evidence would have been useful during the pilot period to inform ward staff whether the cameras were influencing things or not.
Staff want feedback. I don’t think we’ve had any since we’ve had the cameras… it would be nice to get feedback from, I don’t know, whoever is watching it, and stuff like that. Ada (f), Staff, A, Post.
Relative advantage: are BWCs effective and efficient for the ward?
Due to a combination of personal beliefs related to BWCs, the lack of evidence of their impact on violence and aggression, and other elements of care and culture on the wards, a number of staff and patients explored alternative interventions and approaches that may be more beneficial than BWCs. Both staff and patients suggested that Closed Circuit Television (CCTV) as an intervention that provided the transparency of using cameras and video footage but with an independent perspective. This was felt by many to remove the bias that could be introduced in BWC use as the video capture didn’t require staff control of the filming.
I feel like [BWCs] puts all the power and trust into the hands of the staff and I feel that it would be better to have CCTV on the ward because CCTV is neutral. Harry (m), Patient, A, Pre. I have control over that [BWC recording] … It kind of gives that split as well between staff and patients. You can tell me or I can tell you when to switch it on. Whereas I feel like a CCTV camera is there all the time. Nobody’s asking to switch it on. It’s there. If you wanted to review the footage you can request it, anyone can request to view the footage for a legitimate reason. Whereas the camera can come across as if you’re threatening. Petra (f), Staff, A, Post.
In addition, some participants reflected that the nature and design of BWCs meant that unless staff were present for an incident it wouldn’t be captured, whereas CCTV has the advantage of being always present.
If there’s CCTV, then it’s the same thing, you get me. Like, if its body worn cameras that people can always do things away from staff. They can always go down to that corridor to have their fight or go to the side where staff ain’t gonna see them to have their fight, but with CCTV you can’t do that. Elijah (m), Patient, A, Post.
In addition to exploring technological and video-based interventions, many staff noted that the key tool to violence reduction had to be the use of de-escalation skills, noting that the use of communication and positive relationships had to be the primary tool before other interventions such as BWCs or CCTV.
We do a lot of verbal de-escalation. So we got our destress room now still open. That has a punch bag, and it’s got sensory tiles, and the aim and hope is that when people do get frustrated, because we’re all human. We all get annoyed at anything or many little things in life. There is the aim that they go into that room and start punching the bag instead of property and damaging furniture. But we also are working really hard on verbal de-escalation and actually trying to listen to patients and talk to them before anything else. And that’s helped a lot. And between this kind of shared, or role modelling, where while we’re showing staff, actually even spending an extra 20 min is okay. If it means you’re not going to end up having to restrain a patient. Petra (f), Staff, A, Post.
By using communication skills and de-escalation techniques skilfully, some staff felt there was no need to utilise the BWCs. One concern with the introduction of the BWCs for staff was that the use of this technology may negatively impact on trust and relationships and the use of de-escalation.
Some situations I feel like it can make a situation worse sometimes… I think a lot of situations can be avoided if you just talk with people…. Trying to find out why they’re angry, trying to just kind of see it from their point of view, understand them… I think maybe additional training for verbal de-escalation is needed first. Patrick (m), Staff, A, Post.
Characteristics of individuals
Staff and patients’ knowledge and beliefs about the intervention.
Overall, there were mixed views among both staff and patients as to whether cameras would reduce incidents, prior to and after the pilot period. When considering the possible impact on violence and aggressive incidents there was a view among staff that there was the need for a nuanced and person-centred view.
All the patients that come in, they’re different you know. They have different perceptions; they like different things… everyone is different. So, it just depends. We might go live, and then we have good feedback because the patients they are open and the understand why we have it, and then as they get discharged and new patients come in it might not go as well. It just depends. Serene (f), Staff, A, Pre.
As a result of the desire to be person-centred in the use of such interventions, one staff member noted that they weighed-up such consequences for the patient before using the BWC and would make decisions not to use the camera where they thought it may have a negative impact.
Actually, with this body worn camera, as I did mention, if a patient is unwell, that doesn’t, the patient will not have the capacity to I mean, say yes, you cannot just put it on like that. Yeah, I know it’s for evidence, but when something happens, you first have to attend to the patient. You first have to attend to the patient before this camera is, for me. Ruby (f), Staff, I, Post.
Some staff questioned the existing evidence and theories as to why BWCs work to reduce incidents, and instead noted that for some people it will instigate an incident, while others may be triggered by a camera.
I’m on the fence of how that is going to work because I know the evidence is that by telling a patient ‘look if you keep escalating I’m gonna have to turn this on’, but I know several of our patients would kind of take that as a dare and escalate just to spite so that you would turn it on. Diana (f), Staff, A, Pre.
In contrast, some staff felt the cameras helped them feel safer on wards due to transparency of footage as evidence for both staff and patients.
They [staff] need to use it for protection, for recording evidence, that type of thing… They can record instances for later evidence. Yeah, for them as well. Safer for them and for patients because you can also have the right to get them to record, because a patient might be in the wrong but sometimes it may be the staff is in the wrong position. And that’s achieving safety for patients as well. Yeah, I think it works both ways. Dylan (m), Patient, A, Post.
Positive buy-in was also related to the potential use of the intervention as a training, learning or reflective tool for staff to improve practice and care and promote positive staff behaviour.
If you know that your actions might be filmed one way or the other, that would make me to step up your behaviour to patients… if you know that your actions can be viewed, if the authority wants to, then you behave properly with patients so I think that will improve the quality of the care to patient. Davide (m), Staff, I, Pre.
While there were some positive attitudes towards the cameras, there remained considerable concerns among participants regarding the transparency of camera use to collate evidence in relation to incidents as it was widely noted that the cameras remain in staff control therefore there is an issue in relation to bias and power.
I do think my gut would say that it wouldn’t necessarily be well received. Because also I think people feel like prisoners in here, that’s how some of the patients have described their experience, so in terms of the power dynamic and also just – I think that can make one feel a bit, even worse, basically, you know? Leslie (m), Staff, A, Pre.
These issues lead to staff reporting they didn’t want to wear the camera.
I’d feel quite uncomfortable wearing one to be honest. Leslie (m), Staff, A, Pre.
The staff control of the cameras had a particular impact on patient acceptability of the intervention as it led to some patients viewing BWCs as being an intervention for staff advantage and staff safety, thus increasing a ‘them and us’ culture and leading to patient resistance to the cameras. This was particularly salient for those with prior negative experiences of police use of cameras or mistrust in staff.
I feel like the fact that the body worn cameras is gonna be similar to how the police use them, if a staff member has negative intent toward a patient, they would be able to instigate an incident and then turn the camera on and use the consequences of what they’ve instigated to expect restraint or injection or whatever else might happen. So, I feel like it would be putting all the power and trust into the hands of the staff and I feel that it would be better to have CCTV on the ward because CCTV is neutral. Whereas, the body worn camera, especially with some of the personality conflicts/bad attitudes, impressions I’ve had from certain members of staff since I’ve been here, I feel like body worn cameras might be abused in that way possible. Harry (m), Patient, A, Pre.
Perceived unintended consequences and impact on care
Prior to the implementation there were concerns from staff that the introduction of BWCs could have consequences beyond the intended use of reducing violence and aggression, unintentionally affecting a range of factors that may impact on the overall delivery of care. There was a key concern regarding the potential negative impact that cameras may have for patients who have paranoia or psychosis as well as for those who may have prior traumatic experiences of being filmed.
It might have negative impacts on these patients because I’m thinking about kind of patients with schizophrenia and things like that who already have paranoid delusions, thinking that people are after them, thinking that people are spying on them, people are watching them, and then seeing kind of cameras around. It might have negative impacts on them. Tayla (f), Staff, I, Pre. When I was admitted I was going through psychosis… I don’t want to be filmed and things like that. So you just see a camera, a guy with a camera on, you are like, are you filming me? Elijah (m), Patient, A, Post.
There was also a considerable concern among both staff and patients that the use of cameras would have a negative impact on the therapeutic relationship between staff and patients. This was felt to be related to the implication that the cameras enhanced a ‘them and us’ dynamic due to the power differential that staff controlling the cameras can create, likened to policing and criminalisation of patients. With the potential of a negative impact on relationships between staff and patients, staff suggested they may be disinclined to use BWCs if it would stop patients speaking to them or approaching them if they needed support.
Yeah, I think it would probably damage [the therapeutic relationship] because I think what’s probably quite helpful is things that maybe create less of a power difference. I think to some extent, [the BWC] might hinder that ability. Like for example imagine going to a therapist and them just like ‘I’ve got this camera in the corner of the room and it’s gonna be filming our session and just in case – or like, just in case I feel that you might get aggressive with me’. Um, I don’t think that’s going to help the therapeutic relationship! Jamal (m), Staff, A, Pre. When you get body worn cameras on there, the relationship as well between staff and patients, is just gonna instantly change because you’re looking like police! Elijah (m), Patient, A, Post.
In contrast, a minority of staff felt that the presence of cameras may improve relationships as they provide transparency of staff behaviour and would encourage staff to behave well and provide high quality care for patients.
It will also help how, improve the way we look at the patients… because if you know that your actions might be filmed one way or the other, that would make me to step up your behaviour you know… you behave properly with patients so I think that will improve the quality of the care to patient. More efficiently, more caring to patient. Davide (m), Staff, I, Pre.
The process of implementation
Planning: top-down implementation.
Staff perceived that BWC implementation directives had been given by senior management or policy stakeholders whom they felt viewed the process from a position of limited understanding due to a lack of ‘frontline’ mental health service experience. This led to a lack of faith amongst staff, and a perception that funds were being misspent.
They sit up there, they just roll it out and see how it works, how it goes. They waste a whole lot of money, millions or whatever, thousands of pounds in it, and then they see that ‘Oh, it’s not gonna work’. They take it back and all of that. Before coming out with it, you need to come speak to us… they just sit up there drinking tea and coffee, and then they’re just like, Oh, yeah, well, let’s do it this way…come stay with these people, work with them, for just I give you a 12 h shift, stay with them. Richard (m), Staff, I, Post.
This was exacerbated when staff felt there was a lack of consultation or explanation.
we don’t always get the ins and outs of certain things…We know that the cameras are coming in and stuff like that, but you know, and obviously it’s gone through every avenue to make sure that it’s fine. But then sometimes we don’t always know the ins and outs to then explain to people why we have the cameras. Patrick (m), Staff, A, Post.
It was also highlighted that due to multiple initiatives being implemented and directives handed down in parallel, staff felt negative towards interventions more widely, with the BWCs being ‘ just another thing to do’ , adding to workload for staff and reducing enthusiasm to use the cameras.
it’s not just to do with the camera, I just think there’s lots of changes happening at once, and there’s loads of new things being constantly introduced that people are just thinking oh it’s another thing. I think that’s what it is more than the camera itself. Alice (f), Staff, A, Pre.
Execution: training, Use and Ward Visibility
Overall, there was a lack of consistency amongst staff in their understanding of the purpose and processes of using the BWCs on the wards.
What do you do, do you record every single thing or, I don’t know. Do you record like, if a patient said, I want to talk to you, confidential, you go sit in a room, do you record things like those or is it just violence and aggression? Ada (f), Staff, A, Post.
The lack of clarity regarding the purpose of the intervention and the appropriate use of the cameras was felt to impact staffs’ attitudes and acceptance of using them and contributed to a lack of transparency or perhaps trust regarding the use of any subsequent video footage.
I think if the importance of the recording was explained a bit more…and how it would improve things, I think people would use it more… that’s why I don’t think it’s always used sometimes… if you’re not sure why some of it’s important, then you’re not going to see the value…I think if you’re gonna keep with them, it’s about updating the training, teaching staff when to use it, then where does that information go? How does that look in terms of improving? Just a bit of transparency, I think. But when you don’t know certain things it’s a bit hard to get behind something or back it, you know? Patrick (m), Staff, A, Post.
The lack of information about the purpose and processes related to the intervention was also seen among patients, with most patients noting that they hadn’t received information about the cameras during their admissions.
No information at all. I don’t think any of the patients know about it. Toby (m), Patient, A, Post.
While training was provided it was widely felt that it was insufficient to provide understanding about the purpose of the cameras or the more in-depth processes beyond operational aspects such as charging and docking. Several staff interviewed were unaware of the training, while others noted that they had an informal run-through by colleagues rather than anything formal.
What training are you talking about?… I wasn’t here, so I was taught by my colleague. I mean, from what I was taught, to operate the camera, and to give a warning to the patient that you’re going to use the camera. Nevis (f), Staff, A, Post.
Longer training with further details beyond operational use was felt to be needed by staff.
I think the training should have to be longer, even if it’s like an hour or something… Like what situations deem the camera to be… more information on the cameras, when to use it, why it’s used, and I think if the importance of the recording was explained a bit more and what it was doing and how that recording would go and how it would improve things. Patrick (m), Staff, A, Post.
Furthermore, there was a need for training to be on a rolling basis given the use of bank staff who were not trained to use the cameras or to understand the proper processes or purpose of using the BWCs, which could leave them vulnerable to misuse or abuse.
We have bank staff [who aren’t trained] so they say ‘I don’t know how to use that camera you are giving me’. Nevis (f), Staff, A, Post.
The inner setting
Ward context: acceptance of violence and aggression is part of the job.
It was widely believed by staff that the nature of working on a mental health ward included accepting that violence and aggression was part of the job. This was not seen as an acceptance of violence but more that the job was providing care for individuals who are mentally unwell, and confusion, fear, frustration and aggression can be part of that. As a result, there was an ambivalence among some staff that the introduction of cameras would change this.
I think like in this line of work, there’s always that potential for like risky behaviours to happen. I’m not sure if putting the camera on will make much difference. Patrick (m), Staff, A, Post.
Staff noted that because of the nature of the job, staff are used to managing these situations and they understood that it was part of the job; therefore, it was unlikely that they would record everything that on paper might be considered an incident.
There’s also enough things that happen here, so I don’t think they would record [the incidents] because it’s just another day here. You know what I’m saying… [staff] can just say, ‘Stop, go back to your room and leave it at that and that kind of be the end of it’. Dylan (m), Patient, A, Post. We are trained for it. Eveline (f), Staff, I, Pre.
This acceptance that incidents are a hazard of mental healthcare was linked to staff’s acknowledgment that many factors make up the complexity of violence and aggression including the nature of individual patients, acuity levels, ward atmosphere, staffing levels, access to activities, leave and outside space. The interplay of multiple factors creates a context in which frustrations and incidents are likely, thus become part of the everyday and ‘normal’ life on the ward for staff and patients alike.
I feel like, you know, how in GP services you say, zero tolerance to abusive language, or any kind of harassment. I don’t think there is that on a psychiatric ward you are kind of expected to take all the abuse and just get on with it. Petra (f), Staff, A, Post.
With staff reported having a higher threshold for these behaviours it was perceived that this was likely to impact on the efficiency of the intervention as staff would be less likely to consider a situation as violent but more ‘ part of the job’ .
Reactive nature of the ward and incidents
Most participants noted that the ward context is always changing with people being admitted and discharged, with daily staff changes and wider turnover of staff, so things are never static and can change at any point. This reflects the dynamic nature of the ward which creates a complex moving picture that staff need to consider and react to.
[the atmosphere] it’s very good at the moment. If you had asked me this two weeks ago, I would say, ‘Oh, my gosh’. But it changes… The type of patient can make your whole ward change… it depends on the client group we have at the time. Nevis (f), Staff, A, Post.
Staff noted that a key limitation of using the cameras to reduce incidents was the reactive nature of the environment and care being provided. This was felt to impact on the feasibility and use of the cameras as staff noted that they often react to what is happening rather than thinking to ‘ put the camera on first ’. It was felt by staff with experience of reacting to incidents that the failure to use BWCs during these processes were linked to staff’s instincts and training to focus on patients as a priority.
Say for instance, you’re in the office, and two patients start fighting, or a patient attacks someone and, all you’re thinking about is to go there to stop the person. You’re not thinking about putting on any camera. You understand? So sometimes it’s halfway through it, somebody might say, ‘Has anybody switched the camera on’? And that’s the time you start recording… If something happens immediately, you’re not thinking about the camera at that time, you’re just thinking to just go, so yeah. Nevis (f), Staff, A, Post.
Incidents happen quickly and often surprise staff, therefore staff react instantly so are not thinking about new processes such as recording on the cameras as this would slow things down or is not in the reactive nature needed by staff during such incidents.
When you’re in the middle of an incident and your adrenaline’s high, you’re focusing on the incident itself. It’s very difficult for you to now remember, remind yourself to switch on the camera because you’re thinking, patient safety, staff safety, who’s coming to relieve you? What’s going on? Who’s at the door? Petra (f), Staff, A, Post.
In addition, the need for an immediate response meant that it was felt that by the time staff remember to, or have the chance to, switch the camera on it was often too late.
Sometimes in the heat of moments and stuff like that, or if the situation’s happening, sometimes you don’t always think to, you know, put your camera on. Patrick (m), Staff, A, Post.
Outer setting
Resources: staffing.
Issues related to staffing were highlighted by several participants as a key problem facing mental health wards thus leading to staff having higher workloads, and higher rates of bank and agency staff being used on shift and feeling burnt-out.
Out of all the wards I’ve been on I’d say this is the worst. It’s primarily because the staff are overworked…it seems like they spend more time doing paperwork than they do interacting with the patients. Harry (m), Patient, A, Pre. We’re in a bit of a crisis at the minute, we’re really, really understaffed. We’re struggling to cover shifts, so the staff are generally quite burnt out. We’ve had a number of people that have just left all at once, so that had an impact… Staff do get frustrated if they’re burnt out from lack of staff and what have you. Alice (f), Staff, A, Pre.
It was noted by one participant that the link of a new intervention with extra workload was likely to have a negative impact on its acceptability due to these increasing demands.
People automatically link the camera to then the additional paperwork that goes alongside it. It’s like, ‘Oh god, if we do this, we’ve got to do that’, and that could play a part. Petra (f), Staff, A, Post.
One staff member noted that the staffing issue meant there were more likely to be bank staff on wards so the care of patients may be affected as temporary staff may be less able to build meaningful therapeutic relationships.
So obviously there is the basic impact on safety of not having adequate staffing, but then there’s the impact of having a lot of bank staff. So obviously when you have permanent staff they get to know the patients more, we’re able to give them the more individualised care that we ideally should be giving them, but we can’t do that with bank staff. Diana (f), Staff, A, Pre.
It was also suggested that staffing levels and mix often made it more difficult to provide activities or facilitate escorted leave which can lead to patients feeling frustrated and becoming more aggressive.
So you know there is enough staff to facilitate the actual shift, so you know when there’s less staff like you say you’ve got people knocking at the door, but then you don’t have staff to take people out on leave straight away, that all has a rippling effect! Serene (f), Staff, A, Pre.
Wider systemic issues
Overall, there was a concern that the introduction of BWCs would not impact on wider, underlying factors that may contribute to frustration, aggression and incidents on wards. Providing a more enhanced level of care and better addressing the needs of patients was felt to be central to helping people but also reducing the frustration that patients feel when on the ward.
… for violence and aggression, [focus on] the mental health side of things like therapy and psychology should be compulsory. It shouldn’t be something you apply for and have to wait three or four weeks for. I think every person should, more than three or four weeks even, months even… we need psychology and therapists. That’s what will stop most violence, because psychologists and a therapist can edit the way that they speak to people because they’ve been given that skill depending on the way the person behaves. So that’s what we need regularly… not like all this dancing therapy, yoga therapy. That’s a person, that you come and you actually sit down and talk through your shit with them. That will help! Elijah (m), Patient, A, Post. There’s a lack of routine and I think there’s a lack of positive interaction between the patient and the staff as well. The only time you interact with a member of staff is if you’re hassling them for something, you have to hassle for every little thing, and it becomes a sort of, frustration inducing and like I’m a very calm person, but I found myself getting very fucking angry, to be honest, on this ward just because out of pure frustration… there’s bigger problems than body worn cameras going on. Harry (m), Patient, A, Pre.
Staff agreed that there was a need to invest in staff and training rather than new technologies or innovations as it is staff and their skills behind the camera.
It’s not the camera that will do all of that. It’s not making the difference. It’s a very good, very beautiful device, probably doing its job in its own way. But it’s more about investing in the staff, giving them that training and making them reflect on every day-to-day shift. Richard (m), Staff, I, Post.
There was felt to be a need to support staff more in delivering care within wards that can be challenging and where patients are unwell to ensure that staff feel safe. While in some circumstances the cameras made some staff feel safer, greater support from management would be more beneficial in making staff feel valued.
In this study exploring the implementation and use of body-worn cameras on mental health wards, we employed two methods for collecting and comparing data on incidents and use of containment measures, including BWCs, on one acute ward and one psychiatric intensive care unit. We found no clear relationship between the use of BWCs and rates or severity of incidents on either ward. While BWCs may be used when there are incidents of both physical and verbal aggression, results indicate that they may also provoke verbal aggression, as was suggested during some interviews within this study. This should be a concern, as strong evidence that being repeatedly subject to verbal aggression and abuse can lead to burnout and withdrawal of care by staff [ 30 ]. These mixed findings reflect results that were reported in two earlier studies of BWCs on mental health wards [ 12 , 13 ]. However, the very low use of the cameras, on just 10 per cent of the shifts where data was obtained, makes it even more difficult to draw any conclusions.
While the data shows limited impact of using BWCs on levels of incidents, we did find that during the pilot period BWC use tended to occur alongside physical restraint, but the direction of relationship is unclear as staff were asked to use BWCs when planning an intervention such as restraint. This relationship with restraint reflected the findings on several wards in a previous study [ 13 ], while contrasting with those reported in a second study that found reductions in incidents involving restraint during the evaluation period [ 12 ]. Such a mix of findings highlights the complexity of using BWCs as a violence reduction method within a busy healthcare setting in which several interacting components and contextual factors, and behaviours by staff and patients can affect outcomes [ 31 ]. The qualitative data collected during this pilot period highlighted the potential systemic and contextual factors such as low staffing that may have a confounding impact on the incident data presented in this simple form.
The findings presented within this evaluation provide some insights into the process of implementing BWCs as a safety intervention in mental health services and highlight some of the challenges and barriers faced. The use of implementation science to evaluate the piloting of BWCs on wards helps to demonstrate how multiple elements including a variety of contextual and systemic factors can have a considerable impact and thus change how a technology may vary not only between hospitals, but even across wards in the same hospital. By understanding the elements that may and do occur during the process of implementing such interventions, we can better understand if and how BWCs might be used in the future.
Within this pilot, extensive preparatory work conducted at a directorate and senior management level did not translate during the process of implementation at a ward level, which appeared to impact on the use of BWCs by individuals on the wards. This highlights that there is a need to utilise implementation science approaches in planning the implementation of new technologies or interventions and to investigate elements related to behavioural change and context rather than just the desired and actual effects of the intervention itself.
While ward staff and patients identified the potential for BWCs to enhance safety on the wards, participants distrusted their deployment and expressed concerns about ethical issues and possible harmful consequences of their use on therapeutic relationships, care provided and patient wellbeing. These themes reflect previous findings from a national interview study of patient and staff perspectives and experiences of BWCs in inpatient mental health wards [ 14 ]. Given these issues, alternatives such as increasing de-escalation skills were identified by staff as possible routes that may be more beneficial in these settings. Furthermore, other approaches such as safety huddles have also been highlighted within the literature as potential means to improve patient safety by looking ahead at what can be attended to or averted [ 32 ].
Furthermore, it is important to consider that the presence of power imbalances and the pre-existing culture on the ward have considerable implications for safety approaches and must be considered, as exemplified by the preferences by both staff and patients in this evaluation for more perceived ‘impartial’ interventions such as CCTV. As identified within previous studies [ 14 ], BWCs can have different implications for psychological safety, particularly for vulnerable patients who already feel criminalised in an environment with asymmetrical power imbalances between staff and patients. This is particularly salient when considering aspects of identity such as race, ethnicity, and gender both in terms of the identities of the patient group but also in terms of the staff/patient relationship.
While preferences in this study note CCTV as more ‘impartial’, work by Desai [ 33 ] draws on the literature about the use of surveillance cameras in other settings (such as public streets) as well as on psychiatric wards and concludes that CCTV monitoring is fraught with difficulties and challenges, and that ‘watching’ patients and staff through the lens of a camera can distort the reality of what is happening within a ward environment. In her recently published book, Desai [ 34 ] develops this theme to explore the impacts of being watched on both patients and staff through her ethnographic research in psychiatric intensive care units. She highlights concerns over the criminalisation of patient behaviour, safeguarding concerns in relation to the way women’s bodies and behaviours are viewed and judged, and the undermining by CCTV of ethical mental health practice by staff who attempt to engage in thoughtful, constructive, therapeutic interactions with patients in face-to-face encounters. Appenzeller et al.’s [ 35 ] review found that whilst the presence of CCTV appeared to increase subjective feelings of safety amongst patients and visitors, there was no objective evidence that video surveillance increases security, and that staff may develop an over-reliance on the technology.
In addition, our findings add to the existing literature which notes that alternative interventions and approaches that address underlying contextual and systemic issues related to improving care on inpatient wards require attention to address the underlying factors related to incidents, e.g., flashpoints [ 36 ]. Evidence suggests that factors leading to incidents can be predicted; therefore, there is a need to enable staff to work in a proactive way to anticipate and prevent incidents rather than view incidents as purely reactive [ 37 , 38 , 39 ]. Such skills-based and relational approaches are likely to impact more on improving safety and reducing incidents by addressing the complex and multi-faceted issue of incidents on inpatient mental health wards [ 40 ].
These findings highlight that interventions such as BWCs are not used within a vacuum, and that hospitals are complex contexts in which there are a range of unique populations, processes, and microsystems that are multi-faceted [ 41 ]. As a result, interventions will encounter both universal, specific, and local barriers that will impact on its functioning in the real world. This is salient because research suggests that camera use inside mental health wards is based on a perception of the violent nature of the mental health patient, a perception that not only influences practice but also impacts how patients experience the ward [ 33 ]. As a result, there needs to be careful consideration of the use of any new and innovative intervention aimed at improving safety within mental health settings that have limited research supporting their efficacy.
Limitations
While the study provides important insights into the efficacy and acceptability of introducing BWCs onto inpatient mental health wards, there were several limitations. Firstly, the analysis of incident data is limited in its nature as it only presents surface level information about incidents without wider contextual information. Results using such data should be cautiously interpreted as they do not account for confounding factors, such as staffing, acuity, ward culture or ward atmosphere, that are likely to contribute to incidents of violence and aggression. For example, while there was a statistically significant decrease in restrictive practice on the PICU across the study period, we know that BWCs were not widely used on that ward, so this is likely due to a confounding variable that was not accounted for in the study design.
Secondly, the study faced limitations in relation to recruitment, particularly with patients. Researchers’ access to wards was challenging due to high staff turnover and high rates of acuity, meaning many patients were not deemed well enough to be able to consent to take part in the study. In addition, the low use of the cameras on wards meant that many patients, and some staff, had not seen the BWCs in use. Similarly, patients had been provided limited information about the pilot, so their ability to engage in the research and describe their own experiences with BWCs was restricted.
Thirdly, analysis captures the active use of the BWC, however it does not fully capture the impact of staff wearing the cameras even where they do not actively use them. While our qualitative analysis provides insight into the limitation of such passive use, it is likely that the presence of the cameras being worn by staff, even when turned off, may have an impact on both staff and patient behaviours. This may explain trends in the data that did not reach significance but warrant further investigation in relation to the presence of BWCs, nonetheless.
Finally, researchers had planned to collect quantitative surveys from staff and patients in relation to their experiences of the ward atmosphere and climate, views related to therapeutic relationships on the ward, levels of burnout among staff, views on care, and attitudes to containment measures. Due to issues related to staff time, patient acuity, and poor engagement from staff leading to challenges accessing the wards, the collection of such survey data was unfeasible, and this element of the study was discontinued. As a result, we have not reported this aspect in our paper. This limitation reflects the busy nature of inpatient mental health wards with pressures on staff and high levels of ill health among patients. As such, traditional methodologies for evaluation are unlikely to elicit data that is comprehensive and meaningful. Alternative approaches may need to be considered.
Future directions
With BWCs being increasingly used across inpatient mental health services [ 14 ], it is important that further research and evaluation is conducted. To date, there is limited data regarding the effectiveness of this technology in relation to violence reduction; however, there may be other beneficial uses in relation to safeguarding and training [ 13 ]. Future research should consider alternative methods that ensure contextual factors are accounted for and that patient voices can be maximised. For example, focus groups with patients currently admitted to a mental health ward or interviews with those who have recently been on a ward that has used the cameras, would bypass problems encountered with capacity to consent in the present study. Furthermore, ethnographic approaches may provide a deeper understanding of the implementation, deployment and impact that BWCs have on wards.
Overall, this research sheds light on the complexities of using BWCs as a tool for ‘maximising safety’ in mental health settings. The findings suggest that BWCs have a limited impact on levels of incidents on wards, something that is likely to be largely influenced by the process of implementation as well as a range of contextual factors, including the staff and patient populations on the wards. As a result, it is likely that while BWCs may see successes in one hospital site this is not guaranteed for another site as such factors will have a considerable impact on efficacy, acceptability, and feasibility. Furthermore, the findings point towards the need for more consideration to be placed on processes of implementation and the complex ethical discussions regarding BWC use from both a patient and a staff perspective.
In conclusion, while there have been advances in digital applications and immersive technologies showing promise of therapeutic benefits for patients and staff more widely, whether BWCs and other surveillance approaches are to be part of that picture remains to be seen and needs to be informed by high-quality, co-produced research that focuses on wider therapeutic aspects of mental healthcare.
Data availability
The data that support the findings of this study are available on request from the corresponding author. The data are not publicly available due to privacy or ethical restrictions.
Department of Health and Social Care. The NHS Long Term Plan. 2019. https://www.longtermplan.nhs.uk/about/ . [Accessed on 01/11/2021].
NHS England. Violence prevention and safety. 2023. https://www.england.nhs.uk/supporting-our-nhs-people/health-and-wellbeing-programmes/violence-prevention-and-safety/#:~:text=The%20impact%20on%20staff%20is,thinking%20about%20leaving%20the%20organisation . [Accessed on 06/07/23].
Royal College of Nursing. Violence and aggression in the NHS: estimating the size and the impact of the problem. London: Royal College of Nursing; 2018.
Google Scholar
Iozzino L, Ferrari C, Large M, Nielssen O, de Girolamo G. Prevalence and risk factors of violence by psychiatric acute inpatients: a systematic review and meta-analysis. PLoS ONE. 2015;10(6):e0128536. https://doi.org/10.1371/journal.pone.0128536 .
Article CAS PubMed PubMed Central Google Scholar
Cranage K, Foster K. Mental health nurses’ experience of challenging workplace situations: a qualitative descriptive study. Int J Ment Health Nurs. 2020;31(3):665–76. https://doi.org/10.1111/inm.12986 .
Article Google Scholar
Jenkin G, Quigg S, Paap H, Cooney E, Peterson D, Every-Palmer S. Places of safety? Fear and violence in acute mental health facilities: a large qualitative study of staff and service user perspectives. PLoS ONE. 2022;17(5):e0266935. https://doi.org/10.1371/journal.pone.0266935 .
Gaub JE, Choate DE, Todak N, Katz CM, White MD. Officer perceptions of body-worn cameras before and after deployment: a study of three departments. Police Q. 2016;19(3):275–302.
Cubitt TI, Lesic R, Myers GL, Corry R. Body-worn video: a systematic review of literature. Australian New Z J Criminol. 2017;50(3):379–96. https://doi.org/10.1177/0004865816638909 .
Lum C, Koper CS, Wilson DB, Stoltz M, Goodier M, Eggins E et al. Body-worn cameras’ effects on police officers and citizen behavior: A systemitic review. Campbell Syst Reviews. 2020;16(3), e1112.
Ariel B, Newton M, McEwan L, Ashbridge GA, Weinborn C, Brants HS. Reducing assaults against Staff using body-worn cameras (BWCs) in Railway stations. Criminal Justice Rev. 2019;44(1):76–93.
Wilson K, Eaton J, Foye U, Ellis M, Thomas E, Simpson A. What evidence supports the use of body worn Cameras in mental health inpatient wards? A systematic review and narrative synthesis of the effects of body worn Cameras in public sector services. Int J Ment Health Nurs. 2021. https://doi.org/10.1111/inm.12954 .
Article PubMed PubMed Central Google Scholar
Ellis T, Shurmer DL, Badham-May S, Ellis-Nee C. The use of body worn video cameras on mental health wards: results and implications from a pilot study. Mental Health Family Med. 2019;15:859–68.
Hardy S, Bennett L, Rosen P, Carroll S, White P, Palmer-Hill S. The feasibility of using body-worn cameras in an inpatient mental health setting. Mental Health Family Med. 2017;13:393–400.
Wilson K, Foye U, Thomas E, Chadwick M, Dodhia S, Allen J, Lynn J, Brennan G, Simpson A. Exploring the use of body-worn cameras in acute mental health wards: a qualitative interview study with mental health patients and staff. Int J Nurs Stud. 2023;140:104456. https://doi.org/10.1016/j.ijnurstu.2023.104456 .
Article PubMed Google Scholar
Baker J, Pryjmachuk S. Will safe staffing in Mental Health nursing become a reality? J Psychiatry Mental Health Nurs. 2016;23(2):75–6. https://doi.org/10.1111/jpm.12282 .
Article CAS Google Scholar
Piano VL, Creswell JW. Understanding Research: A Consumer Guide. 2nd edition. 2015. Boston: Pearson Education, INC.
Bowers L, Simpson A, Alexander J. Patient-staff conflict: results of a survey on acute psychiatric wards. Soc Psychiatry Psychiatr Epidemiol. 2013;38(7):402–8.
Bowers L. The City-128 study of observation and outcomes. BMC Psychiatry. 2007;7(Suppl 1). https://doi.org/10.1186/1471-244X-7-S1-S122 . S122.
Bowers L, Flood C, Brennan G, Allan T. A replication study of the City nurse intervention: reducing conflict and containment on three acute psychiatric wards. J Psychiatr Ment Health Nurs. 2008;15:737–42. https://doi.org/10.1111/j.1365-2850.2008.01294.x .
Article CAS PubMed Google Scholar
Bowers L, James K, Quirk A, Simpson A, Stewart D, Hodsoll J, SUGAR. Reducing conflict and containment rates on acute psychiatric wards: the safewards cluster randomised controlled trial. Int J Nurs Stud. 2015;52(9):1412–22. https://doi.org/10.1016/j.ijnurstu.2015.05.001 . Erratum in: Int J Nurs Stud. 2016;58:102. PMID: 26166187; PMCID: PMC4518134.
Bowers L, Douzenis A, Galeazzi G et al. Disruptive and dangerous behaviour by patients on acute psychiatric wards in three European centres. Social Psychiatry and Psychiatric Epidemiology. 2005;40, 822–828. https://link.springer.com/article/10.1007/s00127-005-0967-1 .
Bowers L, Flood C, Brennan G, LiPang M, Oladapo P. Preliminary outcomes of a trial to reduce conflict and containment on acute psychiatric wards: City nurses. J Psychiatr Ment Health Nurs. 2006;13:165–72. https://doi.org/10.1111/j.1365-2850.2006.00931.x .
Datix. (2019). Products. Retrieved from https://www.rldatix.com/en-uk/products .
Fazel S, Toynbee M, Ryland H, et al. Modifiable risk factors for inpatient violence in psychiatric hospital: prospective study and prediction model. Psychol Med. 2023;53(2):590–6. https://doi.org/10.1017/S0033291721002063 .
Mushcab H, Bunting D, Yami S, Abandi A, Hunt C. An evaluation of Datix implementation for incident reporting at Johns Hopkins Aramco Healthcare. J Patient Saf Risk Manage. 2020;25(2):67–74. https://doi.org/10.1177/2516043520905481 .
SPSS. IBM SPSS statistics for Windows. Armonk, NY: IBM Corp; 2023.
Microsoft Corporation. (2018). Microsoft Excel . Retrieved from https://office.microsoft.com/excel .
Ritchie J, Lewis J. Qualitative. Research Practice. London: Sage; 2003.
Damschroder LJ, Aron DC, Keith RE, et al. Fostering implementation of health services research findings into practice: a consolidated framework for advancing implementation science. Implement Sci. 2009;4:50. https://doi.org/10.1186/1748-5908-4-50 .
Porath CL, Pearson CM. Emotional and behavioral responses to workplace incivility and the impact of hierarchical status. J Appl Soc Psychol. 2009;42(Suppl 1):E326–57. https://doi.org/10.1111/j.1559-1816.2012.01020.x .
Craig P, Dieppe P, Macintyre S, et al. Developing and evaluating complex interventions: the new medical Research Council guidance. BMJ. 2008;337. https://doi.org/10.1136/bmj.a1655 .
Taylor-Watt J, Cruickshank A, Innes J, Brome B, Shah A. Reducing physical violence and developing a safety culture across wards in East London. Br J Mental Health Nurs. 2017. https://doi.org/10.12968/bjmh.2017.6.1.35 .
Desai S. The new stars of CCTV: what is the purpose of monitoring patients in communal areas of psychiatric hospital wards, bedrooms and seclusion rooms? Divers Equality Health Care. 2009;6(1):12. [Google Scholar].
Desai S. Surveillance Practices and Mental Health: the impact of CCTV inside mental health wards. Routledge; 2022.
Appenzeller YE, Appelbaum PS, Trachsel M. Ethical and practical issues in Video Surveillance of Psychiatric Units. Psychiatric Serv. 2020;71(5):480–6. https://doi.org/10.1176/appi.ps.201900397 .
Bowers L. Safewards: a new model of conflict and containment on psychiatric wards. J Psychiatr Ment Health Nurs. 2014;21(6):499–508. https://doi.org/10.1111/jpm.12129 . PMID: 24548312; PMCID: PMC4237187.
Goodman H, Papastavrou Brooks C, Price O, Barley EA. Barriers and facilitators to the effective de-escalation of conflict behaviours in forensic high-secure settings: a qualitative study. Int J Mental Health Syst. 2020;14:59. https://doi.org/10.1186/s13033-020-00392-5 .
Johnson ME, Delaney KR. Keeping the unit safe: the anatomy of escalation. J Am Psychiatr Nurses Assoc. 2007;13:42–52.
Johnson ME, Hauser PM. The practice of expert nurses: accompanying the patient to a calmer space. Issues Ment Health Nurs. 2001;22:651–68.
Hamrin V, Iennaco J, Olsen D. A review of ecological factors affecting inpatient psychiatric unit violence: implications for relational and unit cultural improvements. Issues Ment Health Nurs. 2009;30(4):214 – 26. doi: 10.1080/01612840802701083. PMID: 19363726.
Squires JE, Estabrooks CA, Scott SD, Cummings GG, Hayduk L. The influence of organizational context on the use of research by nurses in Canadian pediatric hospitals. BMC Health Serv Res. 2013;13. https://doi.org/10.1186/1472-6963-13-351 .
Download references
Acknowledgements
We would like to thank The Burdett Trust for Nursing for funding this work. We would also like to acknowledge our wider Lived Experience Advisory Panel and Project Advisory Panel for their contributions and support and would like to thank the staff and service users on the wards we attended for their warmth and participation.
Funding was provided by The Burdett Trust of Nursing. Funders were independent of the research and did not impact findings.
Author information
Authors and affiliations.
Mental Health Nursing, Health Service and Population Research Department, Institute of Psychiatry, Psychology & Neuroscience, King’s College London, Denmark Hill, London, SE5 8AF, UK
Una Foye, Keiran Wilson, Jessica Jepps, James Blease, Geoff Brennan & Alan Simpson
Florence Nightingale Faculty of Nursing, Midwifery & Palliative Care, Mental Health Nursing, King’s College London, London, UK
Una Foye, Keiran Wilson, Jessica Jepps, Geoff Brennan & Alan Simpson
Lived Experience Advisor, London, UK
Ellen Thomas, Leroy McAnuff, Sharon McKenzie, Katherine Barrett & Lilli Underwood
You can also search for this author in PubMed Google Scholar
Contributions
All authors have read and approved the manuscript. Authors AS, UF, KW, GB created the protocol for the study. KW, JJ, UF conducted the recruitment for the study, and conducted the interviews. UF, JJ, JB, LMA, LU, SMK, KB, ET coded data, and contributed to the analysis. All authors supported drafting and development of the manuscript.
Corresponding author
Correspondence to Una Foye .
Ethics declarations
Ethics approval and consent to participate.
All procedures performed in studies involving human participants were in accordance with the ethical standards of the institutional and/or national research committee and with the 1964 Helsinki declaration and its later amendments or comparable ethical. Ethical approval was granted by the Health Research Authority: London - Camden & Kings Cross Research Ethics Committee (IRAS PROJECT ID 322268, REC Reference 23/LO/0337). All participants provided informed consent prior to enrolment in the study, including consent for publication of anonymised quotes.
Consent for publication
Not applicable.
Competing interests
The authors declare no competing interests.
Additional information
Publisher’s note.
Springer Nature remains neutral with regard to jurisdictional claims in published maps and institutional affiliations.
Electronic supplementary material
Below is the link to the electronic supplementary material.
Supplementary Material 1
Supplementary material 2, supplementary material 3, supplementary material 4, supplementary material 5, supplementary material 6, rights and permissions.
Open Access This article is licensed under a Creative Commons Attribution 4.0 International License, which permits use, sharing, adaptation, distribution and reproduction in any medium or format, as long as you give appropriate credit to the original author(s) and the source, provide a link to the Creative Commons licence, and indicate if changes were made. The images or other third party material in this article are included in the article’s Creative Commons licence, unless indicated otherwise in a credit line to the material. If material is not included in the article’s Creative Commons licence and your intended use is not permitted by statutory regulation or exceeds the permitted use, you will need to obtain permission directly from the copyright holder. To view a copy of this licence, visit http://creativecommons.org/licenses/by/4.0/ . The Creative Commons Public Domain Dedication waiver ( http://creativecommons.org/publicdomain/zero/1.0/ ) applies to the data made available in this article, unless otherwise stated in a credit line to the data.
Reprints and permissions
About this article
Cite this article.
Foye, U., Wilson, K., Jepps, J. et al. Exploring the use of body worn cameras in acute mental health wards: a mixed-method evaluation of a pilot intervention. BMC Health Serv Res 24 , 681 (2024). https://doi.org/10.1186/s12913-024-11085-x
Download citation
Received : 03 January 2024
Accepted : 07 May 2024
Published : 29 May 2024
DOI : https://doi.org/10.1186/s12913-024-11085-x
Share this article
Anyone you share the following link with will be able to read this content:
Sorry, a shareable link is not currently available for this article.
Provided by the Springer Nature SharedIt content-sharing initiative
- Implementation
- Body worn cameras
- Qualitative
- Mental health
BMC Health Services Research
ISSN: 1472-6963
- General enquiries: [email protected]
Multilayer quantile graph for multivariate time series analysis and dimensionality reduction
- Regular Paper
- Open access
- Published: 27 May 2024
Cite this article
You have full access to this open access article
- Vanessa Freitas Silva 1 ,
- Maria Eduarda Silva 2 na1 ,
- Pedro Ribeiro 1 na1 &
- Fernando Silva 1 na1
163 Accesses
Explore all metrics
In recent years, there has been a surge in the prevalence of high- and multidimensional temporal data across various scientific disciplines. These datasets are characterized by their vast size and challenging potential for analysis. Such data typically exhibit serial and cross-dependency and possess high dimensionality, thereby introducing additional complexities to conventional time series analysis methods. To address these challenges, a recent and complementary approach has emerged, known as network-based analysis methods for multivariate time series. In univariate settings, quantile graphs have been employed to capture temporal transition properties and reduce data dimensionality by mapping observations to a smaller set of sample quantiles. To confront the increasingly prominent issue of high dimensionality, we propose an extension of quantile graphs into a multivariate variant, which we term “Multilayer Quantile Graphs”. In this innovative mapping, each time series is transformed into a quantile graph, and inter-layer connections are established to link contemporaneous quantiles of pairwise series. This enables the analysis of dynamic transitions across multiple dimensions. In this study, we demonstrate the effectiveness of this new mapping using synthetic and benchmark multivariate time series datasets. We delve into the resulting network’s topological structures, extract network features, and employ these features for original dataset analysis. Furthermore, we compare our results with a recent method from the literature. The resulting multilayer network offers a significant reduction in the dimensionality of the original data while capturing serial and cross-dimensional transitions. This approach facilitates the characterization and analysis of large multivariate time series datasets through network analysis techniques.
Similar content being viewed by others
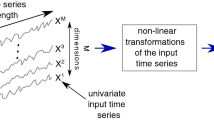
Deep learning for time series classification: a review
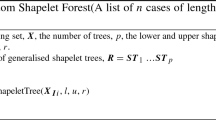
The great multivariate time series classification bake off: a review and experimental evaluation of recent algorithmic advances
Machine Learning Strategies for Time Series Forecasting
Avoid common mistakes on your manuscript.
1 Introduction
In recent years, the prevalence of multidimensional data has surged across various research fields thanks to technological advancements that have enabled the generation of vast datasets using advanced sensing technologies. While time series analysis, a well-established field [ 11 , 17 ], has traditionally focused on the analysis of time-indexed univariate data, the contemporary data landscape now encompasses high-dimensional, multivariate, and panel time series data collected concurrently from numerous sensors. Existing methods for analyzing such data are often constrained, designed for specific domains, and rely on various assumptions, leaving many unresolved challenges and hindering broader applications (see [ 22 ] for more details). For instance, the high dimensionality of the data imposes limitations on computational and memory capacities, rendering the application of conventional methods difficult and often impractical.
Efforts to confront the challenges posed by high-dimensional temporal data have emerged within the realms of data mining, machine learning, and network science. Specifically, network science offers a vast array of both elementary and complex topological features for characterizing various properties of network structures [ 2 , 9 ]. Recent developments have introduced advanced graph structures, known as multilayer networks, which facilitate the modeling of multidimensional data without sacrificing essential properties, including both intra-dimensional and inter-dimensional connections. Multilayer networks are intricate structures capable of establishing internal connections within the same layer/graph and external connections between different layers/graphs. Despite being a relatively recent addition to the field of network science, well-established methods, and methodologies can be readily extended and adapted to this innovative concept [ 14 ].
In this study, our primary focus is on a recent multilayer network-based approach for analyzing and representing multivariate time series data. This approach is relatively novel, especially in the context of constructing multilayer networks. Traditional approaches often involve simplifying multivariate time series into single-layer networks, which can lead to the loss of valuable data information crucial for comprehensive analysis. Furthermore, existing methods that map multivariate time series to multilayer networks have raised questions, as indicated in prior research [ 18 , 20 ]. For instance, the multiplex visibility graphs [ 12 , 15 ] only establish external connections between the same node across different adjacent layers, potentially overlooking direct external connections between different nodes. Another recent example is the multilayer horizontal visibility graphs [ 20 ], which can be computationally intensive and impractical for handling large datasets.
In the realm of univariate time series analysis, quantile graphs [ 8 ] have been proven to be effective in mapping transition properties, offering the advantage of reducing data dimensionality. Given these properties and considering the aforementioned challenges, our work introduces a novel method for mapping multivariate time series data. This method extends the concept of quantile graphs to a multivariate context, resulting in what we term the “Multilayer Quantile Graph” (MQG). The process entails establishing cross-dimensional connections between contemporaneous data quantile samples (that is, between data quantiles at the same timestamp) among the univariate components of the time series dataset.
Our primary objective in this study is twofold: first, to introduce a new multivariate mapping method within the taxonomy of time series mappings [ 20 ], and second, to advance the representation of multivariate time series data using multilayer time series networks. To accomplish this, we evaluate the proposed mapping approach using a synthetic multivariate time series generated from a selected set of multivariate time series models. We analyze high-level topological properties proposed in [ 20 ] through features extracted from the resulting multilayer quantile graphs and use these properties to assess and analyze the mapping method. Additionally, we compare our results with a similar method, the multilayer horizontal visibility graph, which we introduced in our prior work [ 20 ]. We also apply the topological features extracted from MQG on several benchmark multivariate time series datasets. We perform a classification mining task to evaluate their efficacy. Figure 1 illustrates the methodology employed in this study.
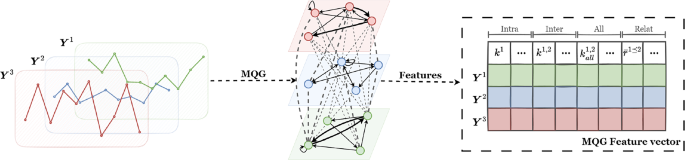
Schematic diagram of the multilayer network-based approach to multivariate time series reducing and mining
In summary, our contributions in this work can be summarized as follows:
A novel concept of a multivariate time series mapping into a multilayer network, capable of capturing characteristics of the underlying time series into the topology of the mapped network;
An implementation of the proposed method that is more efficient than a similar method that we previously proposed [ 20 ];
Empirical experimentation on a diverse set of synthetic and benchmark multivariate time series, showcasing the efficacy and usefulness of our approach.
The remainder of the paper is organized as follows. Section 2 introduces the necessary background and notation to facilitate the understanding of the subsequent sections. Section 3 , provides a concise overview of the main existing multivariate time series mapping approaches. Section 4 proposes a novel multivariate time series mapping method. Section 5 , details the evaluation methodology and describes the experiments conducted. Lastly, in Sect. 6 , we offer our conclusions, provide insights, and outline future research.
2 Preliminaries
To facilitate the comprehension of the paper, we introduce the necessary background and notation on multivariate time series and multilayer networks.
2.1 Multivariate time series
We can think of time series data as collections of observations indexed by time. Formally, we can define a Univariate Time Series (UTS) as a sequence of (scalar) observations time-indexed usually denoted by \(\{{Y}_t\}_{t=1}^{T},\) and a Multivariate Time Series (MTS) as a vector of m observations obtained at each time t , i.e., \(\varvec{Y}_t = [Y_{1,t}, Y_{2,t}, \ldots , Y_{m,t}]^{\prime },\) where \(\prime \) represents the transpose. We denote an MTS by \(\varvec{Y}=\{\varvec{Y}_t\}_{t=1}^{T}\) and the UTS components of the MTS \(\varvec{Y}\) by \(\varvec{Y}^{\alpha }=[Y_{\alpha ,1}, Y_{\alpha ,2}, \ldots , Y_{\alpha , T}]\) with \(\alpha =1, \ldots ,m,\) thus, we can denote an MTS data by its components, \(\varvec{Y}=\{Y^{\alpha }\}_{\alpha =1}^{m}.\)
UTS is ordered in time and usually presents serial correlation as opposed to a random sample. MTS presents not only serial correlation within each UTS component, \(\varvec{Y}^{\alpha },\) but also ( contemporaneous and lagged ) correlation between the different UTS components, \(\varvec{Y}^{\alpha }\) and \(\varvec{Y}^{\beta }, \alpha \ne \beta .\) Thus, analyzing MTS depends on key dependence measures such as the autocorrelation function (ACF), which measures the linear predictability of a UTS, and the cross-correlation function (CCF), which measures the correlation between any two different UTS components of the MTS. The theory of UTS analysis is mature and solid, and although the methods and statistical models extend naturally to the multivariate case new issues and new concepts inevitably arise [ 22 ]. An adequate MTS analysis requires advanced tools, methods, and models for mining information from multiple components that present temporal and cross-sectional correlations and impose high-dimensionality issues.
2.2 Multilayer networks
An alternative time series analysis approach is to map univariate and multivariate time series data to a network representation and use network science methodologies to analyze the original time series. Simply, a network (or graph ) is a mathematical structure, \(G=(V, E),\) that represents a set of elements by nodes, V , and the connections between elements by a set of edges, E .
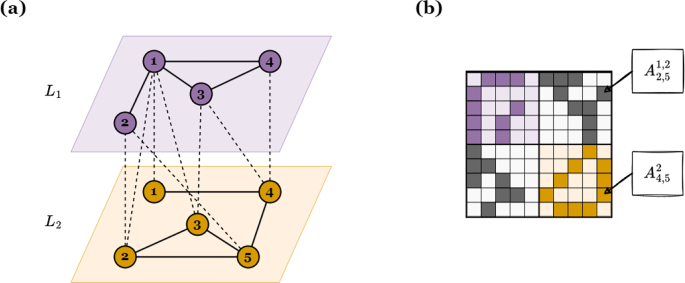
An illustrative example of a a toy multilayer network with five entities \(V= \{1,2,3,4,5\}\) and two elementary layers \(L_1\) and \(L_2\) , and b the corresponding supra-adjacency matrix. The solid lines (colored blocks) represent intra-layer edges and the dashed lines (gray blocks) represent inter-layer edges. Source: Modified from [ 18 ]
A Multilayer Network (MNet) is a more general and complete definition of a network that can model several types of connections between elements of the same and different systems. Formally, a MNet is defined by \(M = (V_M, E_M, V, \varvec{L})\) [ 14 ], where V and \(\varvec{L}\) represent the sets of entities and layers, respectively, and \(V_M\) represents the set of node-layer combinations, \(V_M \subseteq V \times L_1 \times \ldots \times L_m,\) in which a node is present in the corresponding elementary layer \(L_{\alpha } \in \varvec{L}.\) And \(E_M \subseteq V_M \times V_M\) represents the set of edges (pairs of possible combinations of nodes and elementary layers), we call intra-layer edges to the connections between nodes of the same layer, \((v_i^{\alpha }, v_j^{\alpha }),\) and inter-layer edges to the connections between nodes of different layers, \((v_i^{\alpha }, v_j^{\beta })\) with \(\alpha \ne \beta .\) A MNet can be represented by an adjacency tensor of order 4, \(\pmb {\mathcal {A}},\) with tensor element \(\mathcal {A}_{i,j,\alpha ,\beta } = 1\) if \((v_i^{\alpha }, v_j^{\beta }) \in E_M\) and is 0 otherwise [ 14 ]. Another representation is flattening \(\pmb {\mathcal {A}}\) into a supra-adjacency matrix , \(\varvec{A}\) , where intra-layer edges are associated with diagonal element blocks and inter-layer edges with off-diagonal element blocks [ 20 ]. So, we can infer the following types of subgraphs:
Intra-layer graphs , \(G^{\alpha }\) , formed by the diagonal element blocks, \(\left[ \begin{array}{cc} \varvec{A}^{\alpha } &{} \varvec{0}\\ \varvec{0} &{} \varvec{0} \\ \end{array}\right] \) , i.e., intra-layer edges, \(A_{i,j}^{\alpha }\) ,
Inter-layer graphs , \(G^{\alpha ,\beta }\) , formed by off-diagonal element blocks, \(\left[ \begin{array}{cc} \varvec{0} &{} \varvec{A}^{\alpha ,\beta } \\ \varvec{A}^{\beta ,\alpha } &{} \varvec{0} \end{array} \right] , \alpha \ne \beta \) , i.e., inter-layer edges, \(A_{i,j}^{\alpha ,\beta }\) and \(A_{j,i}^{\beta ,\alpha }\) , and no intra-layer edges, \(A_{i,j}^{\alpha } = 0\) and \(A_{i,j}^{\beta } = 0\) , and
All-layer graphs , \(G^{\alpha , \beta }_{all}\) , formed by both on and off-diagonal element blocks, \(\left[ \begin{array}{cc} \varvec{A}^{\alpha } &{} \varvec{A}^{\alpha ,\beta } \\ \varvec{A}^{\beta ,\alpha } &{} \varvec{A}^{\beta } \end{array} \right] , \alpha \ne \beta \) , i.e., intra-layer edges, \(A_{i,j}^{\alpha }\) and \(A_{i,j}^{\beta }\) , and inter-layer edges, \(A_{i,j}^{\alpha ,\beta }\) and \(A_{j,i}^{\beta ,\alpha }\) .
Figure 2 illustrates a simple representation of a multilayer network and the corresponding supra-adjacency matrix.
Network science encompasses a rich array of methodologies, along with a multitude of topological, statistical, spectral, and combinatorial properties, which are instrumental in the analysis and extraction of information from single-layer networks (see [ 2 , 16 ]). These methodologies and properties can be seamlessly extended to the structure of MNet and their respective subgraphs. Additionally, there are emerging methods specifically tailored for the study of MNet structures [ 14 ].
3 Time series mappings
The literature presents a wide range of time series mapping methods for converting both UTS and MTS data into network representations. This innovative framework for time series analysis revolves around a mapping function that can draw inspiration from various concepts, including visibility, transition probability, proximity, time series models, and statistical principles [ 23 ]. These mappings can result either in single-layer Footnote 1 or multilayer networks [ 18 ]. Until now, the predominant focus has been on strategies for mapping UTS into single-layer networks, while the development of mapping MTS has not been as extensive [ 18 ]. In particular, the most commonly employed approach involves techniques that condense MTS data into single-layer networks. In this approach, the node set represents the components of the MTS, denoted as \(Y_{i,t}\) , and the edge set is determined by a statistical model or a correlation measure applied to these UTS components. While this method effectively reduces the dimensionality of MTS data into a more compact structure, namely a single-layer network, it comes at the cost of significant data reduction, preserving only the information captured by the models or measures employed within the mapping function [ 18 ].
Mapping MTS to a MNet represents a cutting-edge and promising approach aimed at retaining more comprehensive data information. Initial efforts concentrated on leveraging multiplex networks (see more in [ 12 , 15 , 18 ]). Specifically, a multiplex network is a particular type of multilayer network defined by a sequence of m graphs, denoted as \(\{G^{\alpha }\}_{\alpha =1}^m = \{(V^{\alpha }, E^{\alpha })\}_{\alpha =1}^m\) , that share the same set of nodes across layers (i.e., \(V^{\alpha } = V^{\beta }\) for all \(\alpha , \beta \) ) and the inter-layer edges can only connect the same nodes in adjacent layers (i.e., \((v_i^{\alpha }, v_j^{\beta })\) with \(i = j\) and \(\alpha \ne \beta \) ) [ 4 ].
Mapping MTS to multiplex networks involves mapping each UTS component onto an individual layer, representing every timestamp (or its corresponding representation) as a unique node, and establishing intra-layer connections based on the fundamental principles of UTS mapping. Additionally, connections between distinct UTS are defined through inter-layer edges that link only contemporaneous nodes across consecutive (adjacent) layers.
In our previous work [ 20 ], we introduced a novel mapping approach based on the concept of visibility and the general definition of multilayer networks. It is called Multilayer Horizontal Visibility Graph (MHVG). This method maps MTS data to an MNet structure, incorporating intra-layer edges derived from UTS visibility mapping and inter-layer edges connecting (directly) lagged nodes between pairs of layers using a novel concept known as cross-visibility. The results of this proposed mapping approach were promising, demonstrating the MNet’s capacity to capture complementary information captured by the created connections between different nodes in different layers. However, one drawback of the MHVG mapping method is its computational intensity, especially when applied to large datasets featuring numerous time series components. MHVG has computational complexity \(\mathcal {O}(m^2T^2)\) which is determined by the procedure that tests the cross-horizontal visibility connections between all pairs of time series in an MTS data.
In this work, we introduce a novel mapping approach aimed at mitigating the computational challenge mentioned above. Our primary objective remains to preserve the MNet structure, which encompasses both intra and inter-layer connections. However, we seek to simultaneously reduce data dimensionality and computational complexity. To achieve this goal, we turn to quantile graphs [ 8 ]. Building upon the concepts introduced in [ 20 ], we extend the QG methodology to fit within an MNet structure, a topic we delve into in the subsequent section.
4 MQG : a novel multivariate time series mapping
A quantile graph (QG) is the result of a UTS mapping technique rooted in the concept of transition probability, which has consistently demonstrated remarkable efficacy in capturing the essential characteristics of UTS data [ 5 , 10 , 19 ]. This method operates by mapping the serial transition probabilities governing the dynamics between UTS data timestamps using a limited set of symbols, typically sample quantiles.
In this section, we introduce an innovative QG algorithm designed to map MTS data into a multilayer quantile graph (MQG). This algorithm entails the establishment of fresh connections among UTS components. These connections are created by extending the concept of transition probability and incorporating sample quantiles from the UTS components. We start by introducing the QG algorithm tailored for UTS data, followed by the unveiling of the MQG algorithm, customized for MTS data.
4.1 Quantile graph
The QG algorithm [ 18 ] (see Algorithm 1) starts to assign the UTS observations to bins that are defined by \(\eta \) sample quantiles, \(q_{1}, q_{2},..., q_{\eta }\) . Each sample quantile, \(q_{i}\) , is mapped to a node \(v_{i}\) of the corresponding graph (a single-layer network) and edges between two nodes \(v_{i}\) and \(v_{j}\) are directed and weighted, \((v_{i}, v_{j}, w_{i,j})\) , with \(w_{i,j}\) corresponding to the transition probability between quantile ranges. The adjacency matrix is a Markov transition matrix: \(\sum _{j=1}^\eta w_{i,j} = 1\) , for each \(i = 1, \ldots , \eta ,\) and the single-layer network is weighted, directed and contains self-loops Footnote 2 . Figure 3 illustrates this mapping method.
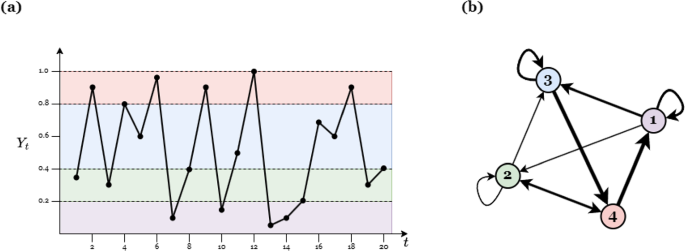
Illustrative example of the quantile graph algorithm for \(\eta = 4\) . a illustrates a toy univariate time series with colored regions representing the different \(\eta \) sample quantiles, and b the corresponding network generated by the quantile graph algorithm. The thicker directed lines represent the edges with greater weights accounting for repeated transitions between quantiles. Source: Reproduced from [ 18 ]
Typically, the number of quantiles ( \(\eta \) ) is significantly smaller than the length of the time series ( \(\eta \ll T\) ). If \(\eta \) is excessively large, the resultant graph may not be connected, resulting in isolated nodes Footnote 3 . Conversely, if \(\eta \) is too small, the QG may exhibit a substantial loss of information, characterized by the assignment of high weights to self-loops.
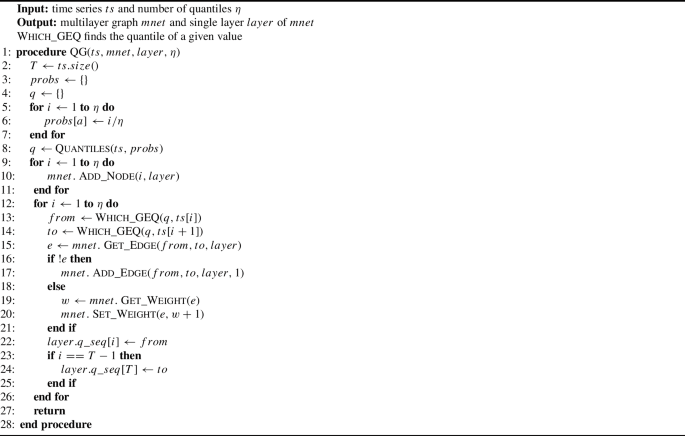
Quantile Graph
4.2 Multilayer quantile graph
The MQG algorithm (see Algorithm 2) builds upon the foundational principles introduced in the preceding QG framework. It starts by mapping all UTS components within MTS data, \(\{\varvec{Y}^{\alpha }\}_{\alpha =1}^m\) , to the corresponding QGs. In this step, for each UTS \(\varvec{Y}^{\alpha }\) , the temporal quantile sequence associated with the resulting QG is stored, and it is denoted as \( \varvec{Q}_{\alpha } = \{q_i^{\alpha ,1}, q_i^{\alpha ,2}, \ldots , q_i^{\alpha ,T}\}\) with \( i = 1, \ldots , \eta \) . Subsequently, for each pair of UTS, denoted as \(\varvec{Y}^{\alpha }\) and \(\varvec{Y}^{\beta }\) , the contemporaneous quantiles ( \(q_i^{\alpha ,t}\) and \(q_j^{\beta ,t}\) at the same time t ) are linked by inter-layer edges representing the cross-dimensions contemporary transitions. For reasons of simplicity, from this point on we will omit the superscript temporal index in the quantile sequence, that is, we will simply denote \( \varvec{Q}_{\alpha } = \{q_i^{\alpha }, q_i^{\alpha }, \ldots , q_i^{\alpha }\}\) .
In more detail, the MQG involves the following three steps (see Fig. 4 ):
each UTS component, \(\varvec{Y}^{\alpha }, \alpha = 1, \ldots , m,\) is mapped to a QG, \(L_{\alpha },\) by applying the Algorithm 1 (top of Fig. 4 ).
each pair of QGs, \(L_{\alpha }\) and \(L_{\beta }, \alpha ,\beta = 1, \ldots , m\) and \( \alpha \ne \beta ,\) is connected by the corresponding contemporary quantiles, \(q_i^{\alpha }\) and \(q_j^{\beta }, i,j = 1, \ldots , \eta ,\) at the same time \(t = 1, \ldots , T\) (panel (b) of Fig. 4 and Algorithm 3). The quantiles \(q_i^{\alpha }\) and \(q_j^{\beta }\) belong to the temporal sequences \(\varvec{Q}_{\alpha }\) and \(\varvec{Q}_{\beta },\) respectively. The weight \(w_{i,j}^{\alpha ,\beta }\) represents the probability that \(Y_{\alpha ,t}\) and \(Y_{\beta ,t}\) belong to the quantiles \(q_{i}^{\alpha }\) and \(q_{j}^{\beta },\) respectively, at the same time.
an MTS, \(\varvec{Y},\) is mapped into an MQG, M , (panel (c) of Fig. 4 ). Layer set refers to each QG, \(L_{\alpha } \in \varvec{L}, \alpha =1, \ldots , m,\) and the node-layer set refers to the sample quantiles, \(V_M = \{q_i^{\alpha }\}_{i=1}^{\eta }.\) The directed weighted intra-layer edges, \((q_i^{\alpha }, q_j^{\alpha }, w_{i,j}^{\alpha }) \in E_M,\) of individual QG are established in the corresponding layer, \(L_{\alpha },\) and the bidirectional weighted inter-layer edges, \((q_i^{\alpha }, q_j^{\beta }, w_{i,j}^{\alpha ,\beta }) \in E_M, \alpha \ne \beta ,\) of pairwise QGs are established between dimensions pairwise layers, \(L_{\alpha }\) and \(L_{\beta }.\)
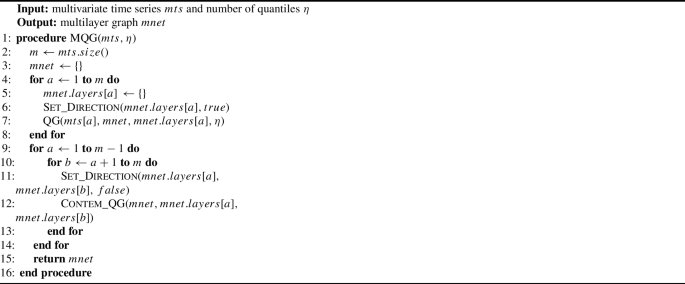
Multilayer Quantile Graph
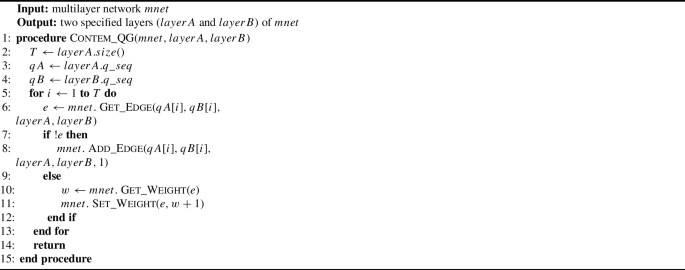
Contemporaneous Quantile Graph
MQG is a directed and weighted MNet. Note that the inter-layer edges are bidirectional, that is, whenever there is a transition from \(q_i^{\alpha }\) to \(q_j^{\beta }\) there is an equivalent transition from \(q_j^{\beta }\) to \(q_i^{\alpha }.\) This symmetry implies that inter-layer edges can also be equivalently represented as undirected edges. To clarify the relationship with the MNet subgraphs described in Sect. 2.2 , we can distinguish two key components within the MQG: a) intra-layer graphs, which correspond to individual QGs, and; b) inter-layer graphs, which correspond to bipartite graphs representing contemporaneous transitions. We will refer to these bipartite graphs as contemporaneous quantile graphs.
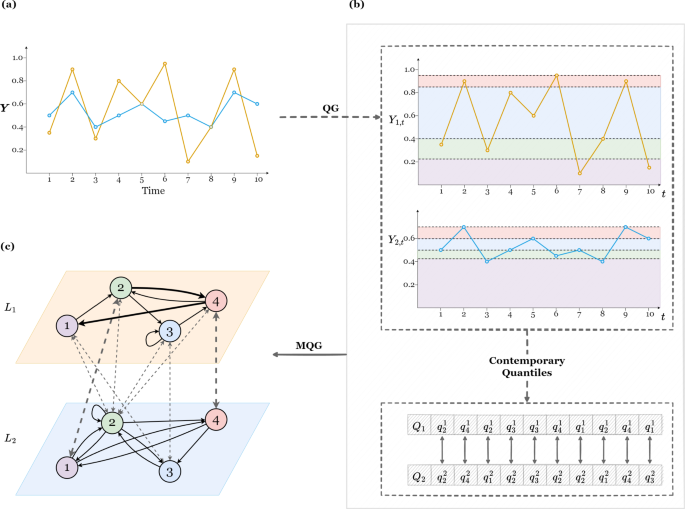
Schematic diagram of the multilayer quantile graph algorithm for \(\eta =4\) : a original time series, b illustration of the intra-layer quantile graphs (colored regions representing the different sample quantiles) and inter-layer contemporaneous edges mapping, c Multilayer quantile graph: black lines represent the intra-layer edges (the QGs), dashed lines the inter-layer edges between nodes contemporaneous quantile nodes, and the thickness of the lines represent the weighted intensities of the edges
4.3 MQG: computational complexity
The computational complexity of the MQG algorithm depends on two variables: T , the time series length, and m , the number of variables. The two for-loops in lines 4 to 8 and lines 9 to 14 of Algorithm 2, determine the algorithm complexity. The first for-loop iterates m times the QG Algorithm 1 (for each time series component), thus it has complexity \(\mathcal {O}(m (\eta T))\) . The second for-loop consists of two nested loops iterating the contemporaneous QG Algorithm 3 through pairs of time series components; thus, it has complexity \(\mathcal {O}(m^2T)\) . This results in a temporal complexity of \(\mathcal {O}(m^2T)\) for the MQG Algorithm 2 which is determined by proceeding the contemporaneous transitions between all pairs of time series in an MTS.
As typically the variable T is much larger than the variable m , the MQG is more efficient in terms of time complexity than the MHVG algorithm.
Regarding spatial complexity, MQG produces a multilayer network with \(m \times \eta \) nodes, while MHVG produces \(m \times T\) nodes. Given that the number of quantiles \(\eta \) is typically much smaller than the length of the time series T , this means that the underlying network representation will also be much smaller, as the number of possible edges in MQG is \(\mathcal {O}((m \times \eta )^2)\) while in MHVG is \(\mathcal {O}((m \times T)^2)\) .
5 Analyzing multivariate times series data via MQG
Analyzing time series data by leveraging features extracted directly and indirectly from the data has emerged as a recent and promising approach in time series data mining [ 13 , 19 , 21 ]. In this section, we will use the topological features introduced in [ 20 ]. These features are based on both intra-layer and inter-layer edges, and our goal is to apply them to analyze the proposed MQG method. The aim is to ascertain whether the information conveyed by inter-layer edges complements the insights gained from intra-layer edges, which is the conventional approach in the literature.
We empirically analyze the proposed MQG algorithm by applying it to a rich MTS dataset and employ data mining techniques to analyze this dataset. We first use a set of synthetic data with different MTS correlation properties, both serial and cross-correlation [ 20 ]. Then, we use different sets of benchmark datasets with a diverse range of data characteristics and with varying dimensions, including differences in time series length and the number of time series components. Before our evaluation, we begin with some considerations about the adopted methodology’s implementation.
5.1 Implementation details
We start by mapping each MTS data \(\varvec{Y}\) into the corresponding MQG using the Algorithm 2 presented in Sect. 4 . We use the formula \(\eta \approx 2T^{1/3}\) defined in [ 6 ] to choose the number of quantiles (an input parameter of the MQG algorithm). We also highlight the subgraphs corresponding to intra-, inter-, and all-layer graphs using the corresponding adjacency submatrices of the resulting MQG, as defined in Sect. 2.2 . Then, we map each MTS data from a dataset of MTSs to the corresponding MQGs (one MQG for each MTS instance) and extract for each MQG the corresponding topological features. For this, we use the high-dimensional topological features presented in [ 20 ] and the methodologies and algorithms described in this work. In short, we compute the following features for each of the resulting MQGs and their subgraphs:
Average degree ( \(\bar{k}\) ): computing the arithmetic mean of the degrees \(k_i\) of all node \(v_i\) in the respective subgraph;
Average path length ( \(\bar{d}\) ): using an algorithm that computes the average shortest path length between all pairs of nodes (of respective subgraphs) using a breadth-first search algorithm;
Modularity ( Q ): computing how good a specific division of the corresponding subgraph into communities is, based on the number of triangles and number of triples;
Number of communities ( S ): using a function that makes use of the known “Louvain” algorithm that finds community structures by multi-level optimization of modularity ( Q ) feature (see [ 3 ] for more details),
Average ratio degree ( \(\bar{r}\) ): computing the arithmetic mean of the ratio degrees \(r_i\) of all node \(v_i\) in the respective subgraph (this is a new topological measure proposed in [ 20 ], where in general the ratio degree is defined as the ratio between inter-layer degree and intra-layer degree of a given node in the network);
Jensen–Shannon divergence ( JSD ): computing the similarity between two degree distributions using the known Jensen–Shannon divergence measure.
We calculate the first four measures ( \(\bar{k}, \bar{d}, Q\) and S ) in the three different possible subgraphs, i.e., intra-, inter-, and all-layer graphs, and the resulting set of features are called intra-features , inter-features and all-features . The last two measures ( \(\bar{r}\) and JSD ) measure the similarity between different connections in the network and are called relational features [ 20 ]. The combination of these feature sets results in a unique vector of features.
We used C++ and its needed set of libraries (such as igraph and standard libraries) to implement the data structure to store an MNet and compute the functions to extract the topological features.
5.2 Synthetic data set: multivariate time series models
We use the six linear and nonlinear bivariate time series models ( \(m=2\) ) summarized in Table 1 and described in detail in [ 20 ]. For each of the six different MTS models, we generate 100 instances of length \(T = 10000\) , and the parameters are chosen so that the data exhibits a range of serial and cross-correlation properties as described in Table 1 . From here on, we refer to this data set as multivariate data generating processes (MDGP) . Footnote 4
In short, MDGP is a diverse set of MTS models with a specific set of properties related to serial and cross-correlation characteristics, namely: white noise (WN) processes representing the noise effects, vector autoregression (VAR) processes representing smooth linear data, and vector generalized autoregressive conditional heteroskedasticity (VGARCH) processes representing nonlinear data with high or low volatility. Furthermore, these processes are designed to represent different levels of correlation, that is, data with and without serial and cross-correlation and data with weak and strong correlation properties both serial and cross, as well as lagged and contemporaneously. The parameters were chosen in order to control these properties. A detailed description of the MDGP and their properties, as well as computational details, can be seen in [ 20 ].
5.2.1 MQG feature space
Particularly, for the MDGP we define \(\eta = 50\) to the number of quantiles parameter, as in [ 7 , 19 ], which is also a value close to the result of the formula \(\eta \approx 2T^{1/3}\) mentioned above. From the resulting diversified vector of 21 high-dimensional topological features (intra-, inter-, all-layer, and relational features) extracted from the resulting multilayer time series networks, MQGs, we perform a principal component analysis (PCA). The obtained PCA feature space is illustrated in Fig. 5 and shows which MNet topological features capture the different properties of the MDGP.
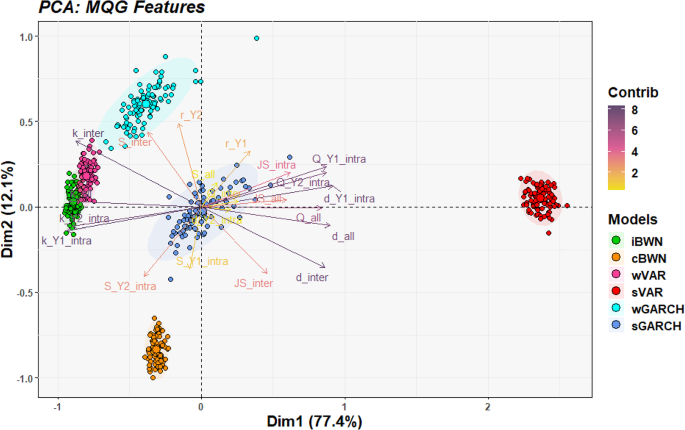
Bi-plot of the first two PCs of MQG topological feature set for the synthetic bivariate dataset. Different colors represent the different multivariate data generating processes and the arrows represent the contribution of the corresponding feature to the PCs: the larger the size, the sharper the color, and the closer to the red the greater the contribution of the feature. Features grouped are positively correlated, while those placed on opposite quadrants are negatively correlated
The feature space is shown in a bi-plot obtained using a total of 21 features with the two PCs explaining 89.5% of the data variance. We can see a good distribution of the characteristics inherent to each sample model. All topological features contribute to the arrangement of the samples which capture different properties of the MTS models. In particular, we can see that the average degree and the number of communities of intra-layer graphs of MQG try to place the WN models in the third quadrant, while the same features for inter-layer graphs try to place the VGARCH and VAR models weakly correlated in the second quadrant. The communities-based features and the average ratio degree seem to contribute to distinguishing the strong and the weak correlation of heteroskedastic models.
5.2.2 MDGP clustering using MQG features
Additionally, we evaluate the MQG feature set (vector of 21 MNet features) in a case study regarding time series clustering. For this task, we rescale the intended topological feature vector into the [0, 1] interval using Min-Max normalization, the PCs are computed (no need of z-score normalization within PCA), and a clustering algorithm, k -means, is applied to the PC’s corresponding to 100% of variance. The clustering results are assessed using appropriate evaluation metrics: Average Silhouette (AS); Adjusted Rand Index (ARI) and Normalized Mutual Information (NMI). Note that AS does not need ground truth, while ARI and NMI do. The range of values for NMI is [0, 1] and for ARI and AS is [-1, 1].
We start by analyzing the usefulness of the MQG feature set by performing a clustering exercise considering different subsets of the MQG feature set. The results are summarized in Table 2 (columns 2, 4, and 6) and indicate that inter-layer edges contain additional information about the MTS data, leading to better clustering results (together with the intra-layer edges). We can also analyze that relational features achieve good clustering results when considered alone. Sub-graphs with both intra-layer edges and inter-layer edges add information that leads to improvements in the clustering results (compare the last three rows with the first two of columns 2, 4, and 6 of Table 2 ). The results show that the contemporaneous quantile graphs (the inter-layer edges from MQG) capture different properties from MTS. Note that the results from the set of intra-layer features are good because the MDGP under analysis involves the same statistical process for the two components of time series whose properties inherent to each process are also captured by the QG mapping methods (as we see in [ 19 ]).
We also compare the results obtained using the MQG feature set with the results obtained in [ 20 ], that is, using the same feature set but for the MHVG mapping method. The columns 3, 5 and 7 of Table 2 summarizes the clustering results obtained in [ 20 ]. The two experiments are made in the same computational environment and using the same methods. We can conclude that features from MQGs are more accurate, almost perfect when we look at the evaluation features ARI and NMI, with a mean value of 0.96, and AS with 0.53, when compared to cross-visibility based mapping that obtains 0.63 to ARI, 0.71 to NMI, and 0.45 to AS feature. So, for the MDGP used in this work, the MQG can be sufficient to cluster the different MTS model samples.
5.3 Real data set: classification using MQG features
To conclude our analysis and demonstrate the applicability and practicality of the proposed method, in this section, we present an application in a multivariate time series classification task (supervised learning). We performed this mining task on real-world and benchmark datasets. We selected 19 datasets from the UEA multivariate time series classification archive [ 1 ], and these datasets have the same length and have no missing values. Table 3 summarizes the general description of each MTS dataset, including dataset size, time series length, number of dimensions/components UTS, number of classes, and the dataset type. The datasets are diverse and exhibit a variety in terms of dimensionality. The dataset size (including both train and test sets) varies between 27 and 10992, time series length \(T \in [8, 2500]\) , the number of UTS components by instance MTS \(m \in [2, 28]\) , and the number of classes ranging from 2 to 26.
Following the implementation details described in Sect. 5.1 , for each dataset and each instance of the dataset, we create the corresponding MQG and extract the associated topological features. The resulting feature vectors were used to perform the classification task for each different dataset. The datasets have associated a training set and a testing set (see columns 2 and 3 of Table 3 ). We used the training set with the corresponding MQG feature vectors and the associated ground-truth classes to train the prediction model (i.e., supervised learning), and we used the testing set with the corresponding MQG feature vectors to make predictions of the classes of this testing set. For consistency, we also performed this classification analysis in MDGP, where we split the dataset into the training set using 80% of the dataset size and the testing set using 20% (see last row of Table 3 ).
We use the random forest ensemble learning method to conduct the training and prediction processes for each dataset. The experiments were performed in R software using the caret package. Results were evaluated using the widely accepted accuracy metric, which computes the ratio of correct predictions to the total number of predictions.
Table 4 presents the accuracy results obtained from the classification analysis carried out for each of the benchmark datasets. The results reflect the different experiments using the proposed feature vectors extracted from MQGs. In general, the most favorable results were achieved when using the entire set of topological features of MQGs, combining all feature subsets. The results indicate that different sets of features capture different data properties, with certain feature sets being more favorable for certain datasets. An interesting task for future work is to add feature selection methods to capture the most representative features of each dataset.
For some datasets the results were good. The characteristics of the dimensions (dataset size, time series length, number of dimensions, or number of classes) of the datasets do not seem to influence these results, except for Handwriting which has a testing set larger than the training set and the number of different classes is also large. However, in general, we consider these results to be very good and promising given the global nature of the features used and, also that we did not use more advanced techniques such as the selection of more representative features and other pre-processing mining techniques, which could improve the results.
6 Conclusion
Methods for mapping multivariate time series into multilayer networks have attracted the attention of researchers in recent times due to their potential. Special interest lies in mappings that generate inter-layer edges thus allowing to capture not only the time dependencies, but also the dependencies between different variables.
In this work, we introduce a multilayer quantile graph as a new multivariate time series mapping method. MQG is based on a transition probability concept extending the traditional concept of quantile graph [ 8 ] for the univariate time series data. The procedure consists of two steps. First, create a reduced multilayer network structure that represents a high-dimensional MTS following the QG mapping method available in the literature. The resulting set of layers/graphs characterizes the serial dynamic transitions of each of the time series components. Second, for each pair of time series components in the MTS, introduce weighted inter-layer edges between corresponding layers that capture the contemporaneous (and lagged) dynamic transitions between the different time series dimensions. MQG was designed to reduce the dimensionality of time series data with high dimensionality and be more computationally efficient and feasible than recently proposed mapping methods. The resulting multilayer networks have smaller dimensions than those obtained by other mapping methods such as the MHVG, and allow the effective reduction in the dimensionality of the MTS. To analyze the proposed multivariate time series mapping, MQG, we consider the specific set of multidimensional topological features proposed by [ 20 ] for MQGs. These features are based on conventional concepts of node centrality, graph distances, clustering, communities, and similarity measures, and are extracted from all the subgraphs of the resulting multilayer network, that is, intra-layer graphs, inter-layer graphs, and all-layer graphs.
To assess the proposed methodology we use the set of MTS models presented in [ 20 ]. The data set consists of 600 synthetic bivariate time series grouped into six different multivariate statistical models. We map the MTS into the MQG and compute the corresponding topological features. The analysis of the set of topological features on the feature space provided by the two principal components shows that different topological features (based on different concepts and different subgraphs of the multilayer network) capture different dynamic properties of the time series models. Furthermore, comparing the feature spaces obtained from MHVG (see [ 20 ]) and from MQG, we can say that the latter enhances the capture of cross-correlation properties.
Finally, we performed a clustering analysis of the synthetic time series based on topological features obtained from MQG and MHVG. The results show that despite the dimensionality reduction, the MQG mapping is sufficient to distinguish the characteristics inherent to each statistical model analyzed in this work and that the inter-layer edges add valuable information to intra-layer features, improving the accuracy of results.
To obtain additional results and demonstrate the application of the proposed mapping method, we perform an experimental analysis of a classification problem in several real and benchmark datasets. For each dataset, we mapped the MTS data instances into the corresponding MQG and we extracted the topological feature vectors. The resulting topological features are used to train a prediction model to perform the prediction of classes of the MTS data. Although the results are not optimal for all data sets, we consider the results to be good given the purpose of this paper. For future work, we intend to improve the classification results obtained, adopting advanced feature selection and data pre-processing techniques. Furthermore, we intend to explore the combination of MQG features with MHVG features.
To conclude, the proposed MQG reduces the dimensionality of the original time series data, reducing the amount of data observations to a smaller number of sample quantiles, preserving the dynamic characteristics of the time series (serially) and between time series (crossly), using probability transitions, during the mapping process. The objectives inherent to the design of this mapping method are quite relevant at a multidisciplinary level where the capabilities of the resulting networks are promising. The MQG algorithm presented in this work represents interconnections (cross-transitions) contemporaneously. However, following the idea presented in [ 7 ], the MQG algorithm can be extended to represent transitions between quantiles corresponding to lagged timestamps from different layers, expanding its capabilities beyond consecutive quantiles. This version may enrich the obtained results, just as in the univariate case [ 7 ]. In our future work, we intend to explore this MQG algorithm version and a more detailed analysis in real-world scenarios.
Data availability
The raw data are available at https://github.com/vanessa-silva/MHVG2MTS .
Recalling the definition of MNet in Sect. 2.2 , a single-layer network, G , is an MNet with \(m=1.\)
A self-loop is an edge that connects a node to itself.
An isolated node is a node that is not connected by an edge to any other node.
MDGP is available at https://github.com/vanessa-silva/MHVG2MTS
Bagnall, A.J., Dau, H.A., Lines, J., Flynn, M., Large, J., Bostrom, A., Southam, P., Keogh, E.J.: The UEA multivariate time series classification archive, (2018). CoRR , arXiv:1811.00075
Barabási, A.-L.: Network Science. Cambridge University Press, Cambridge, United Kingdom (2016)
Google Scholar
Blondel, V.D., Guillaume, J.-L., Lambiotte, R., Lefebvre, E.: Fast unfolding of communities in large networks. J. Stat. Mech: Theory Exp. 2008 (10), P10008 (2008)
Article Google Scholar
Boccaletti, S., Bianconi, G., Criado, R., Del Genio, C.I., Gómez-Gardenes, J., Romance, M., Sendina-Nadal, I., Wang, Z., Zanin, M.: The structure and dynamics of multilayer networks. Phys. Rep. 544 (1), 1–122 (2014)
Article MathSciNet Google Scholar
Campanharo, A., Ramos, F.: Distinguishing different dynamics in electroencephalographic time series through a complex network approach. In: Proceeding Series of the Brazilian Society of Computational and Applied Mathematics , 5 (1), (2017)
Campanharo, A.S., Doescher, E., Ramos, F.M.: Application of quantile graphs to the automated analysis of EEG signals. Neural Process. Lett. 52 , 5–20 (2018)
Campanharo, A.S., Ramos, F.M.: Hurst exponent estimation of self-affine time series using quantile graphs. Phys. A 444 , 43–48 (2016)
Campanharo, A.S., Sirer, M.I., Malmgren, R.D., Ramos, F.M., Amaral, L.A.N.: Duality between time series and networks. PLoS One 6 (8), e23378 (2011)
Costa, L.D.F., Rodrigues, F.A., Travieso, G., Villas Boas, P.R.: Characterization of complex networks: A survey of measurements. Adv. Phys. 56 (1), 167–242 (2007)
de Oliveira Campanharo, A.S.L., Ramos, F.M.: Quantile graphs for the characterization of chaotic dynamics in time series. In: Complex Systems (WCCS), 2015 Third World Conference on, pages 1–4. IEEE (2015)
Douc, R., Moulines, E., Stoffer, D.: Nonlinear Time Series: Theory, 1st edn. Methods and Applications with R Examples. Chapman and Hall/CRC, (2014)
Eroglu, D., Marwan, N., Stebich, M., Kurths, J.: Multiplex recurrence networks. Phys. Rev. E 97 (1), 012312 (2018)
Henderson, T., Fulcher, B.D.: An empirical evaluation of time-series feature sets (2021)
Kivelä, M., Arenas, A., Barthelemy, M., Gleeson, J.P., Moreno, Y., Porter, M.A.: Multilayer networks. J. Complex Netw. 2 (3), 203–271 (2014)
Lacasa, L., Nicosia, V., Latora, V.: Network structure of multivariate time series. Sci. Rep. 5 (1), 15508 (2015)
Peach, R.L., Arnaudon, A., Schmidt, J.A., Palasciano, H.A., Bernier, N.R., Jelfs, K.E., Yaliraki, S.N., Barahona, M.: HCGA: Highly comparative graph analysis for network phenotyping. Patterns 2 (4), 100227 (2021)
Shumway, R.H., Stoffer, D.S.: Time Series Analysis and its Applications: with R examples . 1431-875X. Springer, New York, United States, 4 edition (2017)
Silva, V.F., Silva, M.E., Ribeiro, P., Silva, F.: Time series analysis via network science: Concepts and algorithms. WIREs Data Min. Knowl. Discovery 11 (3), e1404 (2021)
Silva, V.F., Silva, M.E., Ribeiro, P., Silva, F.: Novel features for time series analysis: a complex networks approach. Data Min. Knowl. Disc. 36 , 1062–1101 (2022)
Silva, V.F., Silva, M.E., Ribeiro, P., Silva, F.: MHVG2MTS: Multilayer horizontal visibility graphs for multivariate time series analysis. (2023) arXiv preprint arXiv:2301.02333
Wang, X., Smith, K., Hyndman, R.J.: Characteristic-based clustering for time series data. Data Min. Knowl. Disc. 13 (3), 335–364 (2006)
Wei, W.W.: Multivariate Time Series Analysis and Applications. John Wiley & Sons, Hoboken, New Jersey (2019)
Book Google Scholar
Zou, Y., Donner, R.V., Marwan, N., Donges, J.F., Kurths, J.: Complex network approaches to nonlinear time series analysis. Phys. Rep. 787 , 1–97 (2019)
Download references
Acknowledgements
This work is financed by National Funds through the Portuguese funding agency, FCT—Fundação para a Ciência e a Tecnologia, within project LA/P/0063/2020.
Open access funding provided by FCT|FCCN (b-on). Not applicable.
Author information
Maria Eduarda Silva, Pedro Ribeiro and Fernando Silva have equally contributed to this work.
Authors and Affiliations
CRACS-INESC TEC, Faculdade de Ciências, Universidade do Porto, Porto, Portugal
Vanessa Freitas Silva, Pedro Ribeiro & Fernando Silva
LIAAD-INESC TEC, Faculdade de Economia, Universidade do Porto, Porto, Portugal
Maria Eduarda Silva
You can also search for this author in PubMed Google Scholar
Contributions
The authors contributed equally to this work.
Corresponding author
Correspondence to Vanessa Freitas Silva .
Ethics declarations
Conflict of interest.
The authors declare that they have no conflict of interest.
Additional information
Publisher's note.
Springer Nature remains neutral with regard to jurisdictional claims in published maps and institutional affiliations.
Rights and permissions
Open Access This article is licensed under a Creative Commons Attribution 4.0 International License, which permits use, sharing, adaptation, distribution and reproduction in any medium or format, as long as you give appropriate credit to the original author(s) and the source, provide a link to the Creative Commons licence, and indicate if changes were made. The images or other third party material in this article are included in the article’s Creative Commons licence, unless indicated otherwise in a credit line to the material. If material is not included in the article’s Creative Commons licence and your intended use is not permitted by statutory regulation or exceeds the permitted use, you will need to obtain permission directly from the copyright holder. To view a copy of this licence, visit http://creativecommons.org/licenses/by/4.0/ .
Reprints and permissions
About this article
Silva, V.F., Silva, M.E., Ribeiro, P. et al. Multilayer quantile graph for multivariate time series analysis and dimensionality reduction. Int J Data Sci Anal (2024). https://doi.org/10.1007/s41060-024-00561-6
Download citation
Received : 02 October 2023
Accepted : 06 May 2024
Published : 27 May 2024
DOI : https://doi.org/10.1007/s41060-024-00561-6
Share this article
Anyone you share the following link with will be able to read this content:
Sorry, a shareable link is not currently available for this article.
Provided by the Springer Nature SharedIt content-sharing initiative
- Multivariate time series
- Quantile graphs
- Multilayer networks
- Dimensionality reduction
- Find a journal
- Publish with us
- Track your research
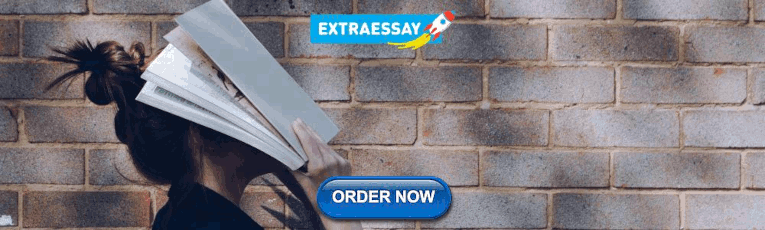
IMAGES
VIDEO
COMMENTS
Conclusion. Multivariate analysis is one of the most useful methods to determine relationships and analyse patterns among large sets of data. It is particularly effective in minimizing bias if a structured study design is employed. However, the complexity of the technique makes it a less sought-out model for novice research enthusiasts.
Abstract. Multivariate analysis is appropriate whenever more than one variable is measured on each sample individual, and overall conclusions about the whole system are sought. Many different multivariate techniques now exist for addressing a variety of objectives. This brief review outlines, in broad terms, some of the more common objectives ...
Broadly defined, multivariate research methods involve the inclusion of more than one outcome in a singular analysis. Instead of conducting a series of univariate analysis, one for each outcome, multivariate analyses consider all the outcomes of interest at the same time. When compared to multiple univariate analysis, the multivariate approach ...
works within the substantive research disciplines of Philosophy, Sociology, Educa-tion, and Economics. An Increasing Impact Over Time. As the total citation count for Multivariate Data Analysis demonstrates, it has had a profound impact in the social sciences as a guide for quantitative analysis. When we
Multivariate analysis, which looks at more than two variables As you can see, multivariate analysis encompasses all statistical techniques that are used to analyze more than two variables at once. The aim is to find patterns and correlations between several variables simultaneously—allowing for a much deeper, more complex understanding of a ...
Described as "causal inference," this involves elements both of theory and of research design. In quantitative studies, it will usually also involve multivariate statistical analysis. ... Multivariate analysis is required to determine whether a variable relationship is indeed causal, or very likely to be, and to inspire confidence in an ...
Abstract. Quantitative data analysis serves as part of an essential process of evidence-making in health and social sciences. It is adopted for any types of research question and design whether it is descriptive, explanatory, or causal. However, compared with qualitative counterpart, quantitative data analysis has less flexibility.
An Intuitive Approach. Making Sense of Multivariate Data Analysis is a short introduction to multivariate data analysis (MDA) for students and practitioners in the behavioral and social sciences. It provides a conceptual overview of the foundations of MDA and of a range of specific techniques including multiple regression, logistic regression ...
These questions can in principle be answered by multiple linear regression analysis. In the multiple linear regression model, Y has normal distribution with mean. The model parameters β 0 + β 1 + +β ρ and σ must be estimated from data. β 0 = intercept. β 1 β ρ = regression coefficients.
2.2. Multivariate Statistical Analysis. Multivariate analysis involves the investigation of multiple variables simultaneously and encompasses a number of techniques that can be used to model data arising from complex systems. Such techniques take on a variety of forms and are used for a number of different tasks.
The instructions for these techniques can be found in the chapter on Quantitative Analysis with SPSS: Correlation. A general rule of thumb is that if a Pearson correlation is above 0.8, this suggests a likely problem with collinearity, though some suggest scrutinizing those pairs of variables with a correlation above 0.7. Figure 1.
Below are the tables SPSS produces for this analysis. After the tables, the text will continue, with an explanation of how one would go about interpreting these results. a. 0 cells (0.0%) have expected count less than 5. The minimum expected count is 36.31. b. 0 cells (0.0%) have expected count less than 5. The minimum expected count is 8.74.
Quantitative data: Multivariate data analysis for answering research questions and hypothesis testing; Phyllis Tharenou, University of South Australia, Ross Donohue, Monash University, Victoria, Brian Cooper, Monash University, Victoria; Book: Management Research Methods; Online publication: 05 June 2012
The Journal of Multivariate Analysis (JMVA) is the central venue for the publication of new, relevant methodology and particularly theoretical developments of multivariate statistics combined with innovative applications pertaining to the analysis and interpretation of multidimensional data. Papers making substantial contributions to regression or time series analysis for multidimensional ...
Definition. In its wider sense, the expression "multivariate statistical analysis" refers to the set of all of the statistical methodologies, techniques, and tools used to analyze jointly two or more statistical variables on a given population. The expression is used as opposite to "univariate statistical analysis," which refers to ...
multivariate analysis. Multivariate methods have had a slightly curious genesis and development. The earliest work, dating from the end of the nineteenth century, was rooted in practical problems arising from social and ed-ucational research (See Educational Statistics, Educational Psychology: Measuring Change Over Time),
North-Holland Publishing Company MULTIVARIATE METHODS FOR QUANTITATIVE AND QUALITATIVE DATA* Wouter J. KELLER and Tom WANSBEEK Netherlands Central Bureau of Statistics, 2270 AZ Voorburg, The Netherlands In this paper several multivariate analysis methods are analyzed by means of the errors-invariables model. ... the research effort in the field ...
Multivariate Analysis (MVA) is an essential statistical process used to understand the impact of multiple variables on a single outcome. This technique is fundamental in fields where complex interrelationships among data need to be studied, such as in weather forecasting where variables like temperature, humidity, and wind speed all influence ...
Multivariate statistical methods in behavioral research 1971 New York McGraw-Hill. Google Scholar. Bock RD and Haggard EA. Whitla DK. The use of multivariate analysis of variance in behavioral research. Handbook of measurement and assessment in behavioral sciences 1968 Reading, Mass Addison-Wesley. Google Scholar. Box GEP and Hunter JS.
Multivariate analysis of variance (MANOVA) tests the difference in the effect of multiple independent variables on multiple dependent variables. Say, for example, a marketer wants to study the impact of pairing a price reduction with an increase in campaign budget — both independent variables — on the sales of a certain face cream.
Multivariate analyses are an aid to, not a substitute for critical thinking in the area of data analysis. Meaningful results can only be produced by these methods if careful consideration is given to questions of sample size, variable type, variable distribution etc., and accusations of subjectivity in interpretation can only be overcome by replication.
Multivariate analysis is based in observation and analysis of more than one statistical outcome variable at a time. In design and analysis, the technique is used to perform trade studies across multiple dimensions while taking into account the effects of all variables on the responses of interest. The development of multivariate methods emerged ...
There is a general understanding that quantitative methods are more trustworthy than methods based uniquely on words and discourse. In this paper, we depart from this thinking to explore how numbers can be used in qualitative research so as to take advantage of its expressive power. We present a technique that enables the application of multivariate data analysis—particularly of ...
PLSC is a correlational technique that estimates associations between two sets of data (e.g., behaviour and brain morphology), while PLSR is a regression technique that predicts one set of data ...
Quantitative data analysis Incident reports. Incident reports retrieved from Datix were binary coded into aggregate variables to examine violence and aggression, self-harm, and other conflict as outlined in Table 1. Multivariate analyses of variance (MANOVA) were used to identify differences in type of incident (violence against person ...
In recent years, there has been a surge in the prevalence of high- and multidimensional temporal data across various scientific disciplines. These datasets are characterized by their vast size and challenging potential for analysis. Such data typically exhibit serial and cross-dependency and possess high dimensionality, thereby introducing additional complexities to conventional time series ...