View Answer Keys
View the correct answers for activities in the learning path.
This procedure is for activities that are not provided by an app in the toolbar.
Some MindTap courses contain only activities provided by apps.
- Click an activity in the learning path.
Numbers, Facts and Trends Shaping Your World
Read our research on:
Full Topic List
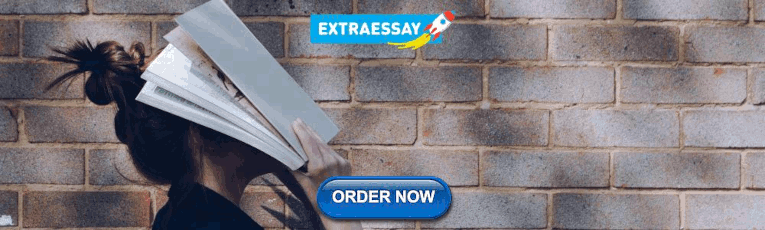
Regions & Countries
- Publications
- Our Methods
- Short Reads
- Tools & Resources
Read Our Research On:
- Facebook Algorithms and Personal Data
About half of Facebook users say they are not comfortable when they see how the platform categorizes them, and 27% maintain the site’s classifications do not accurately represent them
Table of contents.
- Acknowledgments
- Methodology
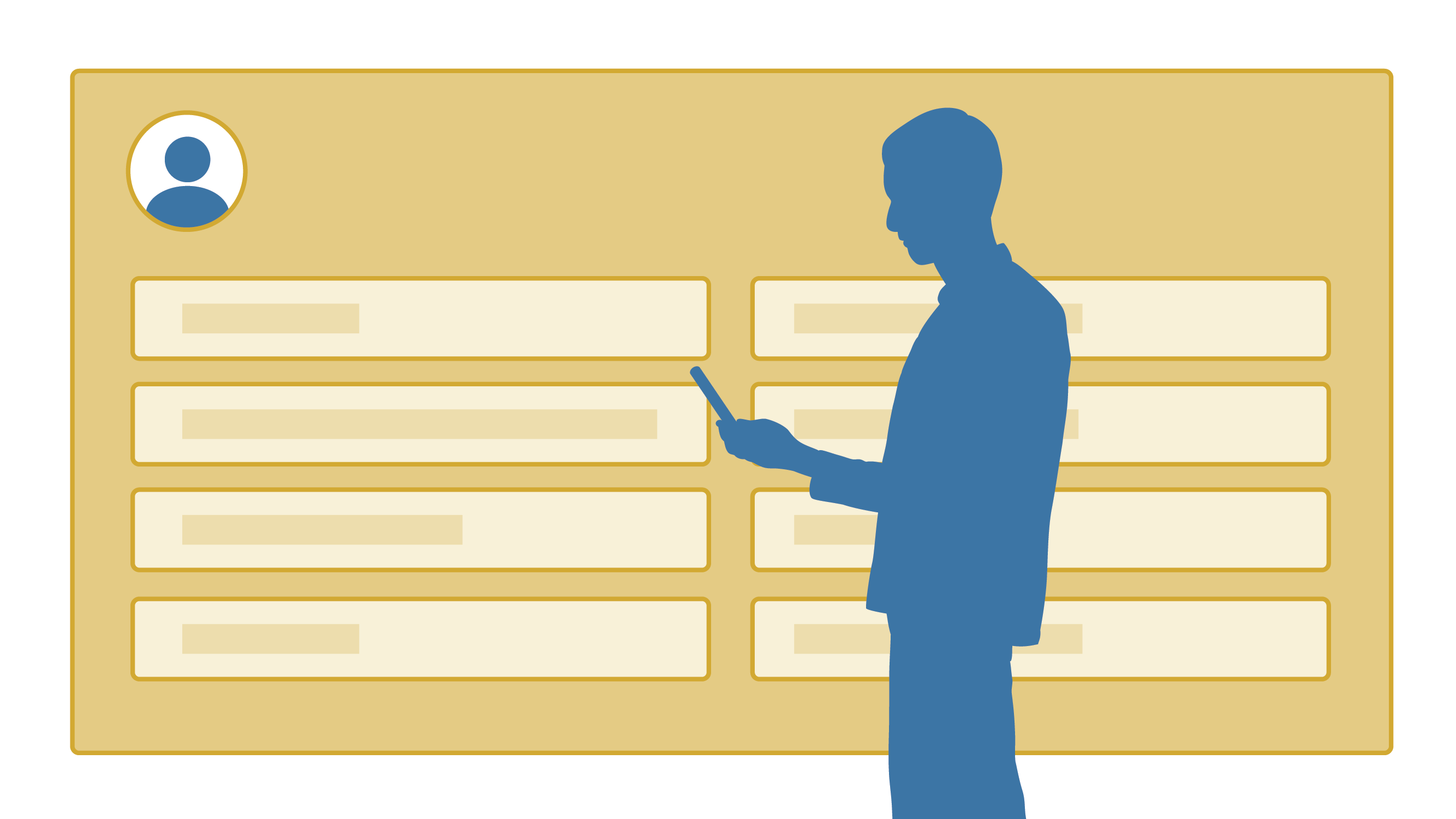
Most commercial sites, from social media platforms to news outlets to online retailers, collect a wide variety of data about their users’ behaviors. Platforms use this data to deliver content and recommendations based on users’ interests and traits, and to allow advertisers to target ads to relatively precise segments of the public. But how well do Americans understand these algorithm-driven classification systems, and how much do they think their lives line up with what gets reported about them? As a window into this hard-to-study phenomenon, a new Pew Research Center survey asked a representative sample of users of the nation’s most popular social media platform, Facebook, to reflect on the data that had been collected about them. (See more about why we study Facebook in the box below .)
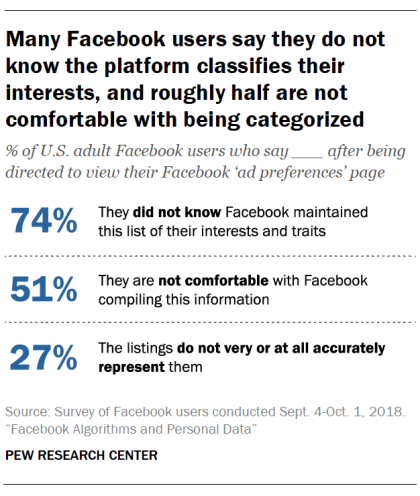
Facebook makes it relatively easy for users to find out how the site’s algorithm has categorized their interests via a “ Your ad preferences ” page. 1 Overall, however, 74% of Facebook users say they did not know that this list of their traits and interests existed until they were directed to their page as part of this study.
When directed to the “ad preferences” page, the large majority of Facebook users (88%) found that the site had generated some material for them. A majority of users (59%) say these categories reflect their real-life interests, while 27% say they are not very or not at all accurate in describing them. And once shown how the platform classifies their interests, roughly half of Facebook users (51%) say they are not comfortable that the company created such a list.
The survey also asked targeted questions about two of the specific listings that are part of Facebook’s classification system: users’ political leanings, and their racial and ethnic “affinities.”
In both cases, more Facebook users say the site’s categorization of them is accurate than say it is inaccurate. At the same time, the findings show that portions of users think Facebook’s listings for them are not on the mark.
When it comes to politics, about half of Facebook users (51%) are assigned a political “affinity” by the site. Among those who are assigned a political category by the site, 73% say the platform’s categorization of their politics is very or somewhat accurate, while 27% say it describes them not very or not at all accurately. Put differently, 37% of Facebook users are both assigned a political affinity and say that affinity describes them well, while 14% are both assigned a category and say it does not represent them accurately.
For some users, Facebook also lists a category called “multicultural affinity.” According to third-party online courses about how to target ads on Facebook, this listing is meant to designate a user’s “affinity” with various racial and ethnic groups, rather than assign them to groups reflecting their actual race or ethnic background. Only about a fifth of Facebook users (21%) say they are listed as having a “multicultural affinity.” Overall, 60% of users who are assigned a multicultural affinity category say they do in fact have a very or somewhat strong affinity for the group to which they are assigned, while 37% say their affinity for that group is not particularly strong. Some 57% of those who are assigned to this category say they do in fact consider themselves to be a member of the racial or ethnic group to which Facebook assigned them.
These are among the findings from a survey of a nationally representative sample of 963 U.S. Facebook users ages 18 and older conducted Sept. 4 to Oct. 1, 2018, on GfK’s KnowledgePanel.
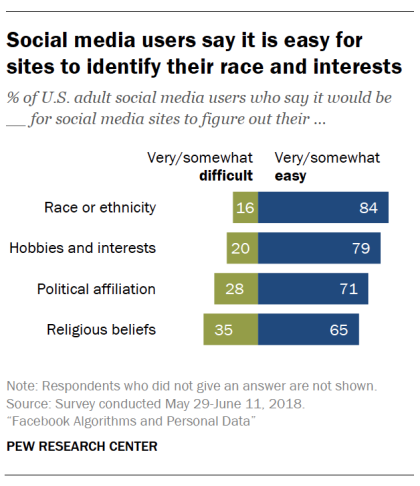
A second survey of a representative sample of all U.S. adults who use social media – including Facebook and other platforms like Twitter and Instagram – using Pew Research Center’s American Trends Panel gives broader context to the insights from the Facebook-specific study.
This second survey, conducted May 29 to June 11, 2018, reveals that social media users generally believe it would be relatively easy for the platforms they use to determine key traits about them based on the data they have amassed about their behaviors. Majorities of social media users say it would be very or somewhat easy for these platforms to determine their race or ethnicity (84%), their hobbies and interests (79%), their political affiliation (71%) or their religious beliefs (65%). Some 28% of social media users believe it would be difficult for these platforms to figure out their political views, nearly matching the share of Facebook users who are assigned a political listing but believe that listing is not very or not at all accurately.
Why we study Facebook
Pew Research Center chose to study Facebook for this research on public attitudes about digital tracking systems and algorithms for a number of reasons. For one, the platform is used by a considerably bigger number of Americans than other popular social media platforms like Twitter and Instagram. Indeed, its global user base is bigger than the population of many countries. Facebook is the third most trafficked website in the world and fourth most in the United States . Along with Google , Facebook dominates the digital advertising market, and the firm itself elaborately documents how advertisers can micro-target audience segments. In addition, the Center’s studies have shown that Facebook holds a special and meaningful place in the social and civic universe of its users.
The company allows users to view at least a partial compilation of how it classifies them on the page called “ Your ad preferences .” It is relatively simple to find this page, which allows researchers to direct Facebook users to their preferences page and ask them about what they see.
Users can find their own preferences page by following the directions in the Methodology section of this report. They can opt out of being categorized this way for ad targeting, but they will still get other kinds of less-targeted ads on Facebook.
Most Facebook users say they are assigned categories on their ad preferences page
A substantial share of websites and apps track how people use digital services, and they use that data to deliver services, content or advertising targeted to those with specific interests or traits. Typically, the precise workings of the proprietary algorithms that perform these analyses are unknowable outside the companies who use them. At the same time, it is clear the process of algorithmically assessing users and their interests involves a lot of informed guesswork about the meaning of a user’s activities and how those activities add up to elements of a user’s identity.
Facebook, the most prominent social network in the world, analyzes scores of different dimensions of its users’ lives that advertisers are then invited to target. The company allows users to view at least a partial compilation of how it classifies them on the page called “ Your ad preferences .” The page, which is different for each user, displays several types of personal information about the individual user, including “your categories” – a list of a user’s purported interests crafted by Facebook’s algorithm. The categorization system takes into account data provided by users to the site and their engagement with content on the site, such as the material they have posted, liked, commented on and shared.
These categories might also include insights Facebook has gathered from a user’s online behavior outside of the Facebook platform. Millions of companies and organizations around the world have activated the Facebook pixel on their websites. The Facebook pixel records the activity of Facebook users on these websites and passes this data back to Facebook. This information then allows the companies and organizations who have activated the pixel to better target advertising to their website users who also use the Facebook platform. Beyond that, Facebook has a tool allowing advertisers to link offline conversions and purchases to users – that is, track the offline activity of users after they saw or clicked on a Facebook ad – and find audiences similar to people who have converted offline. (Users can opt out of having their information used by this targeting feature.)
Overall, the array of information can cover users’ demographics, social networks and relationships, political leanings, life events, food preferences, hobbies, entertainment interests and the digital devices they use. Advertisers can select from these categories to target groups of users for their messages. The existence of this material on the Facebook profile for each user allows researchers to work with Facebook users to explore their own digital portrait as constructed by Facebook.
The Center’s representative sample of American Facebook users finds that 88% say they are assigned categories in this system, while 11% say that after they are directed to their ad preferences page they get a message saying, “You have no behaviors.”
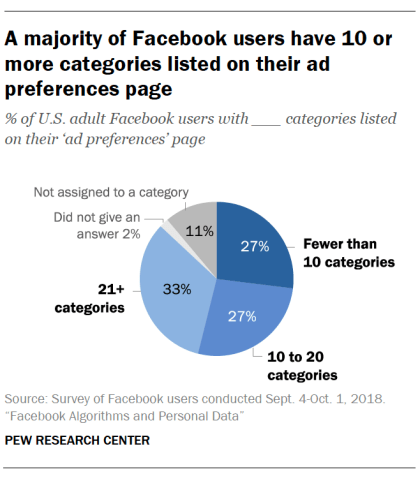
Some six-in-ten Facebook users report their preferences page lists either 10 to 20 (27%) or 21 or more (33%) categories for them, while 27% note their list contains fewer than 10 categories.
Those who are heavier users of Facebook and those who have used the site the longest are more likely to be listed in a larger number of personal interest categories. Some 40% of those who use the platform multiple times a day are listed in 21 or more categories, compared with 16% of those who are less-than-daily users. Similarly, those who have been using Facebook for 10 years or longer are more than twice as likely as those with less than five years of experience to be listed in 21 or more categories (48% vs. 22%).
74% of Facebook users say they did not know about the platform’s list of their interests
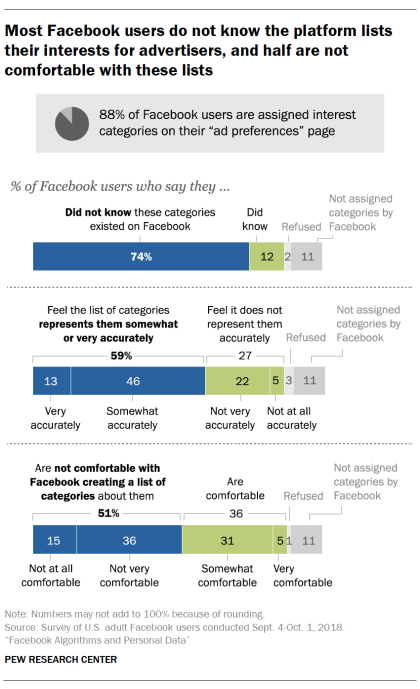
About three-quarters of Facebook users (74%) say they did not know this list of categories existed on Facebook before being directed to the page in the Center’s survey, while 12% say they were aware of it. 2 Put differently, 84% of those who reported that Facebook had categorized their interests did not know about it until they were directed to their ad preferences page.
When asked how accurately they feel the list represents them and their interests, 59% of Facebook users say the list very (13%) or somewhat (46%) accurately reflects their interests. Meanwhile, 27% of Facebook users say the list not very (22%) or not at all accurately (5%) represents them.
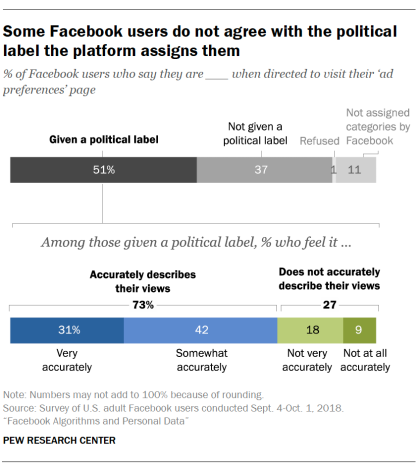
Yet even with a majority of users noting that Facebook at least somewhat accurately assesses their interests, about half of users (51%) say they are not very or not at all comfortable with Facebook creating this list about their interests and traits. This means that 58% of those whom Facebook categorizes are not generally comfortable with that process. Conversely, 5% of Facebook users say they are very comfortable with the company creating this list and another 31% declare they are somewhat comfortable.
There is clear interplay between users’ comfort with the Facebook traits-assignment process and the accuracy they attribute to the process. About three-quarters of those who feel the listings for them are not very or not at all accurate (78%) say they are uncomfortable with lists being created about them, compared with 48% of those who feel their listing is accurate.
Facebook’s political and ‘racial affinity’ labels do not always match users’ views
It is relatively common for Facebook to assign political labels to its users. Roughly half (51%) of those in this survey are given such a label. Those assigned a political label are roughly equally divided between those classified as liberal or very liberal (34%), conservative or very conservative (35%) and moderate (29%).
Among those who are assigned a label on their political views, close to three-quarters (73%) say the listing very accurately or somewhat accurately describes their views. Meanwhile, 27% of those given political classifications by Facebook say that label is not very or not at all accurate.
There is some variance between what users say about their political ideology and what Facebook attributes to them. 3 Specifically, self-described moderate Facebook users are more likely than others to say they are not classified accurately. Among those assigned a political category, some 20% of self-described liberals and 25% of those who describe themselves as conservative say they are not described well by the labels Facebook assigns to them. But that share rises to 36% among self-described moderates.
In addition to categorizing users’ political views, Facebook’s algorithm assigns some users to groups by “multicultural affinity,” which the firm says it assigns to people whose Facebook activity “aligns with” certain cultures. About one-in-five Facebook users (21%) say they are assigned such an affinity.
The use of multicultural affinity as a tool for advertisers to exclude certain groups has created controversies. Following pressure from Congress and investigations by ProPublica , Facebook signed an agreement in July 2018 with the Washington State Attorney General saying it would no longer let advertisers unlawfully exclude users by race, religion, sexual orientation and other protected classes.
In this survey, 43% of those given an affinity designation are said by Facebook’s algorithm to have an interest in African American culture, and the same share (43%) is assigned an affinity with Hispanic culture. One-in-ten are assigned an affinity with Asian American culture. Facebook’s detailed targeting tool for ads does not offer affinity classifications for any other cultures in the U.S., including Caucasian or white culture.
Of those assigned a multicultural affinity, 60% say they have a “very” or “somewhat” strong affinity for the group they were assigned, compared with 37% who say they do not have a strong affinity or interest. 4 And 57% of those assigned a group say they consider themselves to be a member of that group, while 39% say they are not members of that group.
- The linked page can only be viewed by those with Facebook accounts. ↩
- The Facebook users who said they had no listed categories were not asked this question or the other questions in this survey that specifically involve category listings. ↩
- For Facebook and advertisers, the actual label may be less important than how people are grouped. Specifically, a person may not label themselves a “liberal,” but their interests and activities might resemble those of other users who are self-described liberals. The common goal for advertisers is to target users based on their likely interests, regardless of what they might call themselves. ↩
- When survey respondents said they were assigned more than one multicultural affinity, they were asked their interest and membership in a random selection of one of those groups. ↩
Sign up for our weekly newsletter
Fresh data delivery Saturday mornings
Sign up for The Briefing
Weekly updates on the world of news & information
- Business & Workplace
- Civic Activities Online
- Emerging Technology
- Technology Adoption
5 key themes in Americans’ views about AI and human enhancement
Ai and human enhancement: americans’ openness is tempered by a range of concerns, what is machine learning, and how does it work, the longer and more often people use facebook, the more ad preferences the site lists about them, how does a computer ‘see’ gender, most popular, report materials.
- American Trends Panel Wave 35
1615 L St. NW, Suite 800 Washington, DC 20036 USA (+1) 202-419-4300 | Main (+1) 202-857-8562 | Fax (+1) 202-419-4372 | Media Inquiries
Research Topics
- Age & Generations
- Coronavirus (COVID-19)
- Economy & Work
- Family & Relationships
- Gender & LGBTQ
- Immigration & Migration
- International Affairs
- Internet & Technology
- Methodological Research
- News Habits & Media
- Non-U.S. Governments
- Other Topics
- Politics & Policy
- Race & Ethnicity
- Email Newsletters
ABOUT PEW RESEARCH CENTER Pew Research Center is a nonpartisan fact tank that informs the public about the issues, attitudes and trends shaping the world. It conducts public opinion polling, demographic research, media content analysis and other empirical social science research. Pew Research Center does not take policy positions. It is a subsidiary of The Pew Charitable Trusts .
Copyright 2024 Pew Research Center
6.894 : Interactive Data Visualization
Assignment 2: exploratory data analysis.
In this assignment, you will identify a dataset of interest and perform an exploratory analysis to better understand the shape & structure of the data, investigate initial questions, and develop preliminary insights & hypotheses. Your final submission will take the form of a report consisting of captioned visualizations that convey key insights gained during your analysis.
Step 1: Data Selection
First, you will pick a topic area of interest to you and find a dataset that can provide insights into that topic. To streamline the assignment, we've pre-selected a number of datasets for you to choose from.
However, if you would like to investigate a different topic and dataset, you are free to do so. If working with a self-selected dataset, please check with the course staff to ensure it is appropriate for the course. Be advised that data collection and preparation (also known as data wrangling ) can be a very tedious and time-consuming process. Be sure you have sufficient time to conduct exploratory analysis, after preparing the data.
After selecting a topic and dataset – but prior to analysis – you should write down an initial set of at least three questions you'd like to investigate.
Part 2: Exploratory Visual Analysis
Next, you will perform an exploratory analysis of your dataset using a visualization tool such as Tableau. You should consider two different phases of exploration.
In the first phase, you should seek to gain an overview of the shape & stucture of your dataset. What variables does the dataset contain? How are they distributed? Are there any notable data quality issues? Are there any surprising relationships among the variables? Be sure to also perform "sanity checks" for patterns you expect to see!
In the second phase, you should investigate your initial questions, as well as any new questions that arise during your exploration. For each question, start by creating a visualization that might provide a useful answer. Then refine the visualization (by adding additional variables, changing sorting or axis scales, filtering or subsetting data, etc. ) to develop better perspectives, explore unexpected observations, or sanity check your assumptions. You should repeat this process for each of your questions, but feel free to revise your questions or branch off to explore new questions if the data warrants.
- Final Deliverable
Your final submission should take the form of a Google Docs report – similar to a slide show or comic book – that consists of 10 or more captioned visualizations detailing your most important insights. Your "insights" can include important surprises or issues (such as data quality problems affecting your analysis) as well as responses to your analysis questions. To help you gauge the scope of this assignment, see this example report analyzing data about motion pictures . We've annotated and graded this example to help you calibrate for the breadth and depth of exploration we're looking for.
Each visualization image should be a screenshot exported from a visualization tool, accompanied with a title and descriptive caption (1-4 sentences long) describing the insight(s) learned from that view. Provide sufficient detail for each caption such that anyone could read through your report and understand what you've learned. You are free, but not required, to annotate your images to draw attention to specific features of the data. You may perform highlighting within the visualization tool itself, or draw annotations on the exported image. To easily export images from Tableau, use the Worksheet > Export > Image... menu item.
The end of your report should include a brief summary of main lessons learned.
Recommended Data Sources
To get up and running quickly with this assignment, we recommend exploring one of the following provided datasets:
World Bank Indicators, 1960–2017 . The World Bank has tracked global human developed by indicators such as climate change, economy, education, environment, gender equality, health, and science and technology since 1960. The linked repository contains indicators that have been formatted to facilitate use with Tableau and other data visualization tools. However, you're also welcome to browse and use the original data by indicator or by country . Click on an indicator category or country to download the CSV file.
Chicago Crimes, 2001–present (click Export to download a CSV file). This dataset reflects reported incidents of crime (with the exception of murders where data exists for each victim) that occurred in the City of Chicago from 2001 to present, minus the most recent seven days. Data is extracted from the Chicago Police Department's CLEAR (Citizen Law Enforcement Analysis and Reporting) system.
Daily Weather in the U.S., 2017 . This dataset contains daily U.S. weather measurements in 2017, provided by the NOAA Daily Global Historical Climatology Network . This data has been transformed: some weather stations with only sparse measurements have been filtered out. See the accompanying weather.txt for descriptions of each column .
Social mobility in the U.S. . Raj Chetty's group at Harvard studies the factors that contribute to (or hinder) upward mobility in the United States (i.e., will our children earn more than we will). Their work has been extensively featured in The New York Times. This page lists data from all of their papers, broken down by geographic level or by topic. We recommend downloading data in the CSV/Excel format, and encourage you to consider joining multiple datasets from the same paper (under the same heading on the page) for a sufficiently rich exploratory process.
The Yelp Open Dataset provides information about businesses, user reviews, and more from Yelp's database. The data is split into separate files ( business , checkin , photos , review , tip , and user ), and is available in either JSON or SQL format. You might use this to investigate the distributions of scores on Yelp, look at how many reviews users typically leave, or look for regional trends about restaurants. Note that this is a large, structured dataset and you don't need to look at all of the data to answer interesting questions. In order to download the data you will need to enter your email and agree to Yelp's Dataset License .
Additional Data Sources
If you want to investigate datasets other than those recommended above, here are some possible sources to consider. You are also free to use data from a source different from those included here. If you have any questions on whether your dataset is appropriate, please ask the course staff ASAP!
- data.boston.gov - City of Boston Open Data
- MassData - State of Masachussets Open Data
- data.gov - U.S. Government Open Datasets
- U.S. Census Bureau - Census Datasets
- IPUMS.org - Integrated Census & Survey Data from around the World
- Federal Elections Commission - Campaign Finance & Expenditures
- Federal Aviation Administration - FAA Data & Research
- fivethirtyeight.com - Data and Code behind the Stories and Interactives
- Buzzfeed News
- Socrata Open Data
- 17 places to find datasets for data science projects
Visualization Tools
You are free to use one or more visualization tools in this assignment. However, in the interest of time and for a friendlier learning curve, we strongly encourage you to use Tableau . Tableau provides a graphical interface focused on the task of visual data exploration. You will (with rare exceptions) be able to complete an initial data exploration more quickly and comprehensively than with a programming-based tool.
- Tableau - Desktop visual analysis software . Available for both Windows and MacOS; register for a free student license.
- Data Transforms in Vega-Lite . A tutorial on the various built-in data transformation operators available in Vega-Lite.
- Data Voyager , a research prototype from the UW Interactive Data Lab, combines a Tableau-style interface with visualization recommendations. Use at your own risk!
- R , using the ggplot2 library or with R's built-in plotting functions.
- Jupyter Notebooks (Python) , using libraries such as Altair or Matplotlib .
Data Wrangling Tools
The data you choose may require reformatting, transformation or cleaning prior to visualization. Here are tools you can use for data preparation. We recommend first trying to import and process your data in the same tool you intend to use for visualization. If that fails, pick the most appropriate option among the tools below. Contact the course staff if you are unsure what might be the best option for your data!
Graphical Tools
- Tableau Prep - Tableau provides basic facilities for data import, transformation & blending. Tableau prep is a more sophisticated data preparation tool
- Trifacta Wrangler - Interactive tool for data transformation & visual profiling.
- OpenRefine - A free, open source tool for working with messy data.
Programming Tools
- JavaScript data utilities and/or the Datalib JS library .
- Pandas - Data table and manipulation utilites for Python.
- dplyr - A library for data manipulation in R.
- Or, the programming language and tools of your choice...
The assignment score is out of a maximum of 10 points. Submissions that squarely meet the requirements will receive a score of 8. We will determine scores by judging the breadth and depth of your analysis, whether visualizations meet the expressivenes and effectiveness principles, and how well-written and synthesized your insights are.
We will use the following rubric to grade your assignment. Note, rubric cells may not map exactly to specific point scores.
Submission Details
This is an individual assignment. You may not work in groups.
Your completed exploratory analysis report is due by noon on Wednesday 2/19 . Submit a link to your Google Doc report using this submission form . Please double check your link to ensure it is viewable by others (e.g., try it in an incognito window).
Resubmissions. Resubmissions will be regraded by teaching staff, and you may earn back up to 50% of the points lost in the original submission. To resubmit this assignment, please use this form and follow the same submission process described above. Include a short 1 paragraph description summarizing the changes from the initial submission. Resubmissions without this summary will not be regraded. Resubmissions will be due by 11:59pm on Saturday, 3/14. Slack days may not be applied to extend the resubmission deadline. The teaching staff will only begin to regrade assignments once the Final Project phase begins, so please be patient.
- Due: 12pm, Wed 2/19
- Recommended Datasets
- Example Report
- Visualization & Data Wrangling Tools
- Submission form
CSE 163, Summer 2020: Homework 3: Data Analysis
In this assignment, you will apply what you've learned so far in a more extensive "real-world" dataset using more powerful features of the Pandas library. As in HW2, this dataset is provided in CSV format. We have cleaned up the data some, but you will need to handle more edge cases common to real-world datasets, including null cells to represent unknown information.
Note that there is no graded testing portion of this assignment. We still recommend writing tests to verify the correctness of the methods that you write in Part 0, but it will be difficult to write tests for Part 1 and 2. We've provided tips in those sections to help you gain confidence about the correctness of your solutions without writing formal test functions!
This assignment is supposed to introduce you to various parts of the data science process involving being able to answer questions about your data, how to visualize your data, and how to use your data to make predictions for new data. To help prepare for your final project, this assignment has been designed to be wide in scope so you can get practice with many different aspects of data analysis. While this assignment might look large because there are many parts, each individual part is relatively small.
Learning Objectives
After this homework, students will be able to:
- Work with basic Python data structures.
- Handle edge cases appropriately, including addressing missing values/data.
- Practice user-friendly error-handling.
- Read plotting library documentation and use example plotting code to figure out how to create more complex Seaborn plots.
- Train a machine learning model and use it to make a prediction about the future using the scikit-learn library.
Expectations
Here are some baseline expectations we expect you to meet:
Follow the course collaboration policies
If you are developing on Ed, all the files are there. The files included are:
- hw3-nces-ed-attainment.csv : A CSV file that contains data from the National Center for Education Statistics. This is described in more detail below.
- hw3.py : The file for you to put solutions to Part 0, Part 1, and Part 2. You are required to add a main method that parses the provided dataset and calls all of the functions you are to write for this homework.
- hw3-written.txt : The file for you to put your answers to the questions in Part 3.
- cse163_utils.py : Provides utility functions for this assignment. You probably don't need to use anything inside this file except importing it if you have a Mac (see comment in hw3.py )
If you are developing locally, you should navigate to Ed and in the assignment view open the file explorer (on the left). Once there, you can right-click to select the option to "Download All" to download a zip and open it as the project in Visual Studio Code.
The dataset you will be processing comes from the National Center for Education Statistics. You can find the original dataset here . We have cleaned it a bit to make it easier to process in the context of this assignment. You must use our provided CSV file in this assignment.
The original dataset is titled: Percentage of persons 25 to 29 years old with selected levels of educational attainment, by race/ethnicity and sex: Selected years, 1920 through 2018 . The cleaned version you will be working with has columns for Year, Sex, Educational Attainment, and race/ethnicity categories considered in the dataset. Note that not all columns will have data starting at 1920.
Our provided hw3-nces-ed-attainment.csv looks like: (⋮ represents omitted rows):
Column Descriptions
- Year: The year this row represents. Note there may be more than one row for the same year to show the percent breakdowns by sex.
- Sex: The sex of the students this row pertains to, one of "F" for female, "M" for male, or "A" for all students.
- Min degree: The degree this row pertains to. One of "high school", "associate's", "bachelor's", or "master's".
- Total: The total percent of students of the specified gender to reach at least the minimum level of educational attainment in this year.
- White / Black / Hispanic / Asian / Pacific Islander / American Indian or Alaska Native / Two or more races: The percent of students of this race and the specified gender to reach at least the minimum level of educational attainment in this year.
Interactive Development
When using data science libraries like pandas , seaborn , or scikit-learn it's extremely helpful to actually interact with the tools your using so you can have a better idea about the shape of your data. The preferred practice by people in industry is to use a Jupyter Notebook, like we have been in lecture, to play around with the dataset to help figure out how to answer the questions you want to answer. This is incredibly helpful when you're first learning a tool as you can actually experiment and get real-time feedback if the code you wrote does what you want.
We recommend that you try figuring out how to solve these problems in a Jupyter Notebook so you can actually interact with the data. We have made a Playground Jupyter Notebook for you that has the data uploaded. At the top-right of this page in Ed is a "Fork" button (looks like a fork in the road). This will make your own copy of this Notebook so you can run the code and experiment with anything there! When you open the Workspace, you should see a list of notebooks and CSV files. You can always access this launch page by clicking the Jupyter logo.
Part 0: Statistical Functions with Pandas
In this part of the homework, you will write code to perform various analytical operations on data parsed from a file.
Part 0 Expectations
- All functions for this part of the assignment should be written in hw3.py .
- For this part of the assignment, you may import and use the math and pandas modules, but you may not use any other imports to solve these problems.
- For all of the problems below, you should not use ANY loops or list/dictionary comprehensions. The goal of this part of the assignment is to use pandas as a tool to help answer questions about your dataset.
Problem 0: Parse data
In your main method, parse the data from the CSV file using pandas. Note that the file uses '---' as the entry to represent missing data. You do NOT need to anything fancy like set a datetime index.
The function to read a CSV file in pandas takes a parameter called na_values that takes a str to specify which values are NaN values in the file. It will replace all occurrences of those characters with NaN. You should specify this parameter to make sure the data parses correctly.
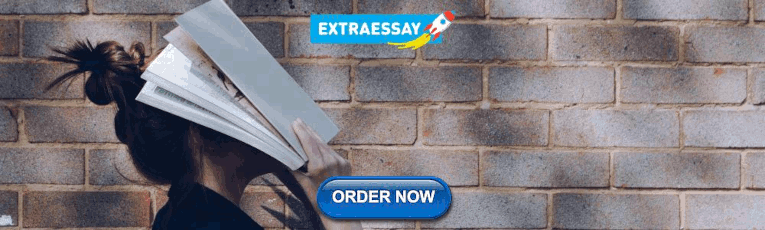
Problem 1: compare_bachelors_1980
What were the percentages for women vs. men having earned a Bachelor's Degree in 1980? Call this method compare_bachelors_1980 and return the result as a DataFrame with a row for men and a row for women with the columns "Sex" and "Total".
The index of the DataFrame is shown as the left-most column above.
Problem 2: top_2_2000s
What were the two most commonly awarded levels of educational attainment awarded between 2000-2010 (inclusive)? Use the mean percent over the years to compare the education levels in order to find the two largest. For this computation, you should use the rows for the 'A' sex. Call this method top_2_2000s and return a Series with the top two values (the index should be the degree names and the values should be the percent).
For example, assuming we have parsed hw3-nces-ed-attainment.csv and stored it in a variable called data , then top_2_2000s(data) will return the following Series (shows the index on the left, then the value on the right)
Hint: The Series class also has a method nlargest that behaves similarly to the one for the DataFrame , but does not take a column parameter (as Series objects don't have columns).
Our assert_equals only checks that floating point numbers are within 0.001 of each other, so your floats do not have to match exactly.
Optional: Why 0.001?
Whenever you work with floating point numbers, it is very likely you will run into imprecision of floating point arithmetic . You have probably run into this with your every day calculator! If you take 1, divide by 3, and then multiply by 3 again you could get something like 0.99999999 instead of 1 like you would expect.
This is due to the fact that there is only a finite number of bits to represent floats so we will at some point lose some precision. Below, we show some example Python expressions that give imprecise results.
Because of this, you can never safely check if one float is == to another. Instead, we only check that the numbers match within some small delta that is permissible by the application. We kind of arbitrarily chose 0.001, and if you need really high accuracy you would want to only allow for smaller deviations, but equality is never guaranteed.
Problem 3: percent_change_bachelors_2000s
What is the difference between total percent of bachelor's degrees received in 2000 as compared to 2010? Take a sex parameter so the client can specify 'M', 'F', or 'A' for evaluating. If a call does not specify the sex to evaluate, you should evaluate the percent change for all students (sex = ‘A’). Call this method percent_change_bachelors_2000s and return the difference (the percent in 2010 minus the percent in 2000) as a float.
For example, assuming we have parsed hw3-nces-ed-attainment.csv and stored it in a variable called data , then the call percent_change_bachelors_2000s(data) will return 2.599999999999998 . Our assert_equals only checks that floating point numbers are within 0.001 of each other, so your floats do not have to match exactly.
Hint: For this problem you will need to use the squeeze() function on a Series to get a single value from a Series of length 1.
Part 1: Plotting with Seaborn
Next, you will write functions to generate data visualizations using the Seaborn library. For each of the functions save the generated graph with the specified name. These methods should only take the pandas DataFrame as a parameter. For each problem, only drop rows that have missing data in the columns that are necessary for plotting that problem ( do not drop any additional rows ).
Part 1 Expectations
- When submitting on Ed, you DO NOT need to specify the absolute path (e.g. /home/FILE_NAME ) for the output file name. If you specify absolute paths for this assignment your code will not pass the tests!
- You will want to pass the parameter value bbox_inches='tight' to the call to savefig to make sure edges of the image look correct!
- For this part of the assignment, you may import the math , pandas , seaborn , and matplotlib modules, but you may not use any other imports to solve these problems.
- For all of the problems below, you should not use ANY loops or list/dictionary comprehensions.
- Do not use any of the other seaborn plotting functions for this assignment besides the ones we showed in the reference box below. For example, even though the documentation for relplot links to another method called scatterplot , you should not call scatterplot . Instead use relplot(..., kind='scatter') like we showed in class. This is not an issue of stylistic preference, but these functions behave slightly differently. If you use these other functions, your output might look different than the expected picture. You don't yet have the tools necessary to use scatterplot correctly! We will see these extra tools later in the quarter.
Part 1 Development Strategy
- Print your filtered DataFrame before creating the graph to ensure you’re selecting the correct data.
- Call the DataFrame describe() method to see some statistical information about the data you've selected. This can sometimes help you determine what to expect in your generated graph.
- Re-read the problem statement to make sure your generated graph is answering the correct question.
- Compare the data on your graph to the values in hw3-nces-ed-attainment.csv. For example, for problem 0 you could check that the generated line goes through the point (2005, 28.8) because of this row in the dataset: 2005,A,bachelor's,28.8,34.5,17.6,11.2,62.1,17.0,16.4,28.0
Seaborn Reference
Of all the libraries we will learn this quarter, Seaborn is by far the best documented. We want to give you experience reading real world documentation to learn how to use a library so we will not be providing a specialized cheat-sheet for this assignment. What we will do to make sure you don't have to look through pages and pages of documentation is link you to some key pages you might find helpful for this assignment; you do not have to use every page we link, so part of the challenge here is figuring out which of these pages you need. As a data scientist, a huge part of solving a problem is learning how to skim lots of documentation for a tool that you might be able to leverage to solve your problem.
We recommend to read the documentation in the following order:
- Start by skimming the examples to see the possible things the function can do. Don't spend too much time trying to figure out what the code is doing yet, but you can quickly look at it to see how much work is involved.
- Then read the top paragraph(s) that give a general overview of what the function does.
- Now that you have a better idea of what the function is doing, go look back at the examples and look at the code much more carefully. When you see an example like the one you want to generate, look carefully at the parameters it passes and go check the parameter list near the top for documentation on those parameters.
- It sometimes (but not always), helps to skim the other parameters in the list just so you have an idea what this function is capable of doing
As a reminder, you will want to refer to the lecture/section material to see the additional matplotlib calls you might need in order to display/save the plots. You'll also need to call the set function on seaborn to get everything set up initially.
Here are the seaborn functions you might need for this assignment:
- Bar/Violin Plot ( catplot )
- Plot a Discrete Distribution ( distplot ) or Continuous Distribution ( kdeplot )
- Scatter/Line Plot ( relplot )
- Linear Regression Plot ( regplot )
- Compare Two Variables ( jointplot )
- Heatmap ( heatmap )
Make sure you read the bullet point at the top of the page warning you to only use these functions!
Problem 0: Line Chart
Plot the total percentages of all people of bachelor's degree as minimal completion with a line chart over years. To select all people, you should filter to rows where sex is 'A'. Label the x-axis "Year", the y-axis "Percentage", and title the plot "Percentage Earning Bachelor's over Time". Name your method line_plot_bachelors and save your generated graph as line_plot_bachelors.png .
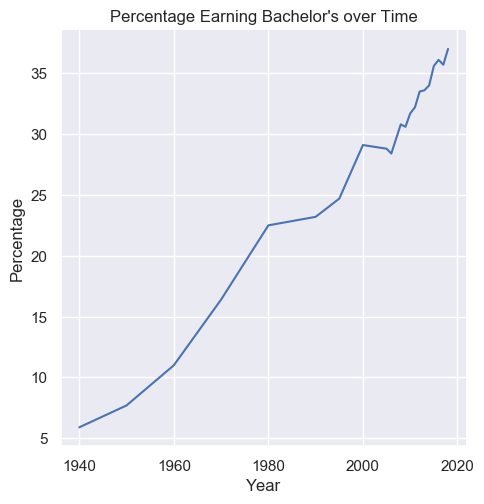
Problem 1: Bar Chart
Plot the total percentages of women, men, and total people with a minimum education of high school degrees in the year 2009. Label the x-axis "Sex", the y-axis "Percentage", and title the plot "Percentage Completed High School by Sex". Name your method bar_chart_high_school and save your generated graph as bar_chart_high_school.png .
Do you think this bar chart is an effective data visualization? Include your reasoning in hw3-written.txt as described in Part 3.
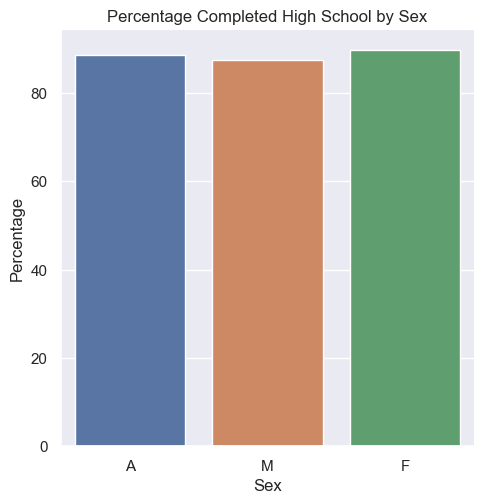
Problem 2: Custom Plot
Plot the results of how the percent of Hispanic individuals with degrees has changed between 1990 and 2010 (inclusive) for high school and bachelor's degrees with a chart of your choice. Make sure you label your axes with descriptive names and give a title to the graph. Name your method plot_hispanic_min_degree and save your visualization as plot_hispanic_min_degree.png .
Include a justification of your choice of data visualization in hw3-written.txt , as described in Part 3.
Part 2: Machine Learning using scikit-learn
Now you will be making a simple machine learning model for the provided education data using scikit-learn . Complete this in a function called fit_and_predict_degrees that takes the data as a parameter and returns the test mean squared error as a float. This may sound like a lot, so we've broken it down into steps for you:
- Filter the DataFrame to only include the columns for year, degree type, sex, and total.
- Do the following pre-processing: Drop rows that have missing data for just the columns we are using; do not drop any additional rows . Convert string values to their one-hot encoding. Split the columns as needed into input features and labels.
- Randomly split the dataset into 80% for training and 20% for testing.
- Train a decision tree regressor model to take in year, degree type, and sex to predict the percent of individuals of the specified sex to achieve that degree type in the specified year.
- Use your model to predict on the test set. Calculate the accuracy of your predictions using the mean squared error of the test dataset.
You do not need to anything fancy like find the optimal settings for parameters to maximize performance. We just want you to start simple and train a model from scratch! The reference below has all the methods you will need for this section!
scikit-learn Reference
You can find our reference sheet for machine learning with scikit-learn ScikitLearnReference . This reference sheet has information about general scikit-learn calls that are helpful, as well as how to train the tree models we talked about in class. At the top-right of this page in Ed is a "Fork" button (looks like a fork in the road). This will make your own copy of this Notebook so you can run the code and experiment with anything there! When you open the Workspace, you should see a list of notebooks and CSV files. You can always access this launch page by clikcing the Jupyter logo.
Part 2 Development Strategy
Like in Part 1, it can be difficult to write tests for this section. Machine Learning is all about uncertainty, and it's often difficult to write tests to know what is right. This requires diligence and making sure you are very careful with the method calls you make. To help you with this, we've provided some alternative ways to gain confidence in your result:
- Print your test y values and your predictions to compare them manually. They won't be exactly the same, but you should notice that they have some correlation. For example, I might be concerned if my test y values were [2, 755, …] and my predicted values were [1022, 5...] because they seem to not correlate at all.
- Calculate your mean squared error on your training data as well as your test data. The error should be lower on your training data than on your testing data.
Optional: ML for Time Series
Since this is technically time series data, we should point out that our method for assessing the model's accuracy is slightly wrong (but we will keep it simple for our HW). When working with time series, it is common to use the last rows for your test set rather than random sampling (assuming your data is sorted chronologically). The reason is when working with time series data in machine learning, it's common that our goal is to make a model to help predict the future. By randomly sampling a test set, we are assessing the model on its ability to predict in the past! This is because it might have trained on rows that came after some rows in the test set chronologically. However, this is not a task we particularly care that the model does well at. Instead, by using the last section of the dataset (the most recent in terms of time), we are now assessing its ability to predict into the future from the perspective of its training set.
Even though it's not the best approach to randomly sample here, we ask you to do it anyways. This is because random sampling is the most common method for all other data types.
Part 3: Written Responses
Review the source of the dataset here . For the following reflection questions consider the accuracy of data collected, and how it's used as a public dataset (e.g. presentation of data, publishing in media, etc.). All of your answers should be complete sentences and show thoughtful responses. "No" or "I don't know" or any response like that are not valid responses for any questions. There is not one particularly right answer to these questions, instead, we are looking to see you use your critical thinking and justify your answers!
- Do you think the bar chart from part 1b is an effective data visualization? Explain in 1-2 sentences why or why not.
- Why did you choose the type of plot that you did in part 1c? Explain in a few sentences why you chose this type of plot.
- Datasets can be biased. Bias in data means it might be skewed away from or portray a wrong picture of reality. The data might contain inaccuracies or the methods used to collect the data may have been flawed. Describe a possible bias present in this dataset and why it might have occurred. Your answer should be about 2 or 3 sentences long.
Context : Later in the quarter we will talk about ethics and data science. This question is supposed to be a warm-up to get you thinking about our responsibilities having this power to process data. We are not trying to train to misuse your powers for evil here! Most misuses of data analysis that result in ethical concerns happen unintentionally. As preparation to understand these unintentional consequences, we thought it would be a good exercise to think about a theoretical world where you would willingly try to misuse data.
Congrats! You just got an internship at Evil Corp! Your first task is to come up with an application or analysis that uses this dataset to do something unethical or nefarious. Describe a way that this dataset could be misused in some application or an analysis (potentially using the bias you identified for the last question). Regardless of what nefarious act you choose, evil still has rules: You need to justify why using the data in this is a misuse and why a regular person who is not evil (like you in the real world outside of this problem) would think using the data in this way would be wrong. There are no right answers here of what defines something as unethical, this is why you need to justify your answer! Your response should be 2 to 4 sentences long.
Turn your answers to these question in by writing them in hw3-written.txt and submitting them on Ed
Your submission will be evaluated on the following dimensions:
- Your solution correctly implements the described behaviors. You will have access to some tests when you turn in your assignment, but we will withhold other tests to test your solution when grading. All behavior we test is completely described by the problem specification or shown in an example.
- No method should modify its input parameters.
- Your main method in hw3.py must call every one of the methods you implemented in this assignment. There are no requirements on the format of the output, besides that it should save the files for Part 1 with the proper names specified in Part 1.
- We can run your hw3.py without it crashing or causing any errors or warnings.
- When we run your code, it should produce no errors or warnings.
- All files submitted pass flake8
- All program files should be written with good programming style. This means your code should satisfy the requirements within the CSE 163 Code Quality Guide .
- Any expectations on this page or the sub-pages for the assignment are met as well as all requirements for each of the problems are met.
Make sure you carefully read the bullets above as they may or may not change from assignment to assignment!
A note on allowed material
A lot of students have been asking questions like "Can I use this method or can I use this language feature in this class?". The general answer to this question is it depends on what you want to use, what the problem is asking you to do and if there are any restrictions that problem places on your solution.
There is no automatic deduction for using some advanced feature or using material that we have not covered in class yet, but if it violates the restrictions of the assignment, it is possible you will lose points. It's not possible for us to list out every possible thing you can't use on the assignment, but we can say for sure that you are safe to use anything we have covered in class so far as long as it meets what the specification asks and you are appropriately using it as we showed in class.
For example, some things that are probably okay to use even though we didn't cover them:
- Using the update method on the set class even though I didn't show it in lecture. It was clear we talked about sets and that you are allowed to use them on future assignments and if you found a method on them that does what you need, it's probably fine as long as it isn't violating some explicit restriction on that assignment.
- Using something like a ternary operator in Python. This doesn't make a problem any easier, it's just syntax.
For example, some things that are probably not okay to use:
- Importing some random library that can solve the problem we ask you to solve in one line.
- If the problem says "don't use a loop" to solve it, it would not be appropriate to use some advanced programming concept like recursion to "get around" that restriction.
These are not allowed because they might make the problem trivially easy or violate what the learning objective of the problem is.
You should think about what the spec is asking you to do and as long as you are meeting those requirements, we will award credit. If you are concerned that an advanced feature you want to use falls in that second category above and might cost you points, then you should just not use it! These problems are designed to be solvable with the material we have learned so far so it's entirely not necessary to go look up a bunch of advanced material to solve them.
tl;dr; We will not be answering every question of "Can I use X" or "Will I lose points if I use Y" because the general answer is "You are not forbidden from using anything as long as it meets the spec requirements. If you're unsure if it violates a spec restriction, don't use it and just stick to what we learned before the assignment was released."
This assignment is due by Thursday, July 23 at 23:59 (PDT) .
You should submit your finished hw3.py , and hw3-written.txt on Ed .
You may submit your assignment as many times as you want before the late cutoff (remember submitting after the due date will cost late days). Recall on Ed, you submit by pressing the "Mark" button. You are welcome to develop the assignment on Ed or develop locally and then upload to Ed before marking.
Social Media Syllabus
Teaching students to analyze social data with microsoft social engagement: social media analytics assignment (post 3 of 4).
This post may contain affiliate links. Please read my disclosure for details.

- For CLIENT’S NAME what is the total number of a) shares, b) replies, and c) posts on Twitter during TIME PERIOD?
- (repeat this for however many keywords you have – up to 3)
- In what STATE/COUNTRY were the top posts posted that mention CLIENT?
- Note: if we only have data from Twitter, then just use Twitter.
- What is the sentiment percentages (positive, negative, neutral) for CLIENT?
- Who are the top fans and critics for CLIENT on each platform?
Of course, the above 8 questions are just a sampling of what you could do with the software. In Summary The software can be a bit challenging to use. And I found that students struggled at times to navigate it. It is important to make yourself available in class to help students. Also, because the students had to answer these questions for their client and then for their competitors, it was rather time consuming. Teams that tackled this project in a smart manner, divided up the work and then put their answers together and reviewed them. Some students may feel that this part is somewhat redundant to what they do in Microsoft Excel pivot tables. Questions 1 and 2 from the pivot exercise are similar to questions 1 and 4 from Microsoft Social Engagement, respectively. But, in my point of view, it is different enough and, importantly, it is a different way of analyzing things. Still, because this project overall requires a good deal of work when you consider the pivot tables and the social network mapping ,which we’ll discuss in the next post, you may find it useful to remove some of the above questions. Projects like these can be intimidating and challenging for students. But I truly believe the benefits outweigh the drawbacks. The opportunity for students to learn industry software in the classroom is highly valuable. And it is better for students to dive in while in school than have their first exposure be overwhelming on the job. In the next post, we will discuss part 4 of this assignment which gets students using Netlytic.org to do some basic network mapping of their client’s online network. I will be publishing that post in 2 weeks. Update: You can now read Post #4 on Netlytic . If you haven’t yet, be sure to check out post 1 and post 2 in this series.
Share this:
- Click to share on Twitter (Opens in new window)
- Click to share on Facebook (Opens in new window)
- Click to share on LinkedIn (Opens in new window)
- Click to share on Reddit (Opens in new window)
- Click to share on WhatsApp (Opens in new window)
- Click to share on Pocket (Opens in new window)
- Click to email a link to a friend (Opens in new window)
Leave a Reply Cancel reply
Your email address will not be published. Required fields are marked *
Notify me of follow-up comments by email.
Notify me of new posts by email.
This site uses Akismet to reduce spam. Learn how your comment data is processed .
A Social Media Education Blog by Matthew J. Kushin, Ph.D.
Privacy & cookie policy, privacy overview.
Navigation Menu
Search code, repositories, users, issues, pull requests..., provide feedback.
We read every piece of feedback, and take your input very seriously.
Saved searches
Use saved searches to filter your results more quickly.
To see all available qualifiers, see our documentation .
- Notifications
The following files are work done from Excel Basics for Data Analysis by IBM courtesy of Coursera. Included are two final assignment files that are unedited and edited.
iamAdrianC/Excel_Basics_for_Data_Analysis
Folders and files, repository files navigation, excel_basics_for_data_analysis.
Predictive Analytics: Assignment #2
Martha rose oordt.
For this assignment, I forcasted Monthly Sales for Total Retail from the US Census Bureau. I trained 4 predictive models on data from 2018 to 2021 and tested the models on monthly 2022 data. The predictive models include an ARIMA model, ETS model, an ARIMA dynamic regression model, and an ensemble model averaging the first three models. For my dynamic regression model I used data on US inflation as an external regressor along with a fourier transformation.
Creating Tsibble
Training set (2018-2021) & test set (2022) with cpi lag, time-series plot, seasonal plots.
There is an observed seasonal trend in the data, with retail sales increasing over the course of the year and spiking in December around the holidays. You can see the effects of the pandemic in 2020, as sales dropped significantly in March and April when many businesses were closed and there was a lot of economic uncertainty. Sales quickly pick up again though and actually trend significantly higher in 2021. It would be interesting to compare to data of the service industry to see how retail sales may have been favored over in person services during this time.
ARIMA with regressors
Here I used CPI as an external regressor, ensuring the model used a lag of 12 months. Additionally, I used fourier terms to capture seasonal patterns. Including the fourier terms significantly reduces the bias of the model.
Accuracy Metrics and Discussion
The ensemble model performs best with regard to variability, with the smallest RMSE of 12,021. Its bias measurements (ME and MAE) are also better than both the ARIMA and ETS models, however overall, the ARIMA with CPI as a regressor has the significantly bias, with a mean error of -32. The dynamic regression model does not perform as well regarding variability however with a RMSE of 29,578. Using CPI as a regressor and a fourier transformation smooths out the forecast more than the other models, however it does not account for seasonal fluctuations as well as the other models. Due to the different strengths of each model, the ensemble model averages out errors in predictions across the three models.
- Applied Analytics - Assignment 2
- Last updated almost 2 years ago
- Hide Comments (–) Share Hide Toolbars
Twitter Facebook Google+
Or copy & paste this link into an email or IM:
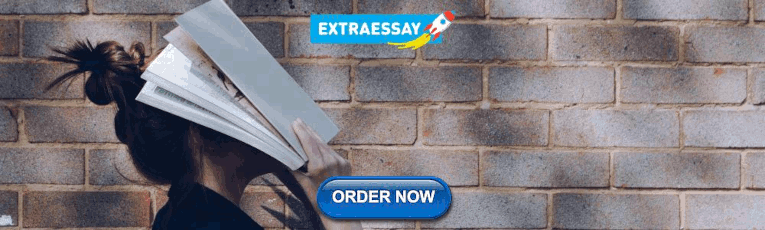
IMAGES
VIDEO
COMMENTS
View the correct answers for activities in the learning path. This procedure is for activities that are not provided by an app in the toolbar. Some MindTap courses contain only activities provided by apps. Click an activity in the learning path. Turn on Show Correct Answers. View Aplia Answer Keys. View the correct answers for Aplia™ activities.
AP Biology facebook analytics assignment: part congratulations! you just landed job as junior social media manager at buhi bags! buhi supply co., an and retail
Most Facebook users say they are assigned categories on their ad preferences page. A substantial share of websites and apps track how people use digital services, and they use that data to deliver services, content or advertising targeted to those with specific interests or traits. Typically, the precise workings of the proprietary algorithms that perform these analyses are unknowable outside ...
Assignment 2: Exploratory Data Analysis. In this assignment, you will identify a dataset of interest and perform an exploratory analysis to better understand the shape & structure of the data, investigate initial questions, and develop preliminary insights & hypotheses. Your final submission will take the form of a report consisting of ...
Analytics' to help you complete the following tasks: Create two dashboards as follows: One dashboard using the tabbed template that has 4 small rectangles at the top and a large rectangle. below - rename this dashboard tab to . Sales. One dashboard using the 2 x 2 rectangle areas tabbed template - rename this dashboard tab to Service.
Visualizing Data Using Spreadsheets, Creating Visualizations and Dashboards with Spreadsheets, Creating Visualizations and Dashboards with Cognos Analytics - minmmwin/Data-Visualization-and-Dashboards-with-Excel-and-Cognos
Step 2: The Assignment Bright Ink is interested in the Facebook post performance from January to March 2021. Since Bright Ink cannot provide you admin access to their Facebook page to access Facebook Insights data, they have provided a data export from Facebook Insights for your convenience. Bright Ink has asked you to answer the following questions using data from the Facebook Insights data ...
In the first part of the final assignment, you will use provided sample data to create some visualizations using Excel for the web. In the second part of the final assignment, you will create some visualizations and add them to a dashboard using Cognos Analytics or Google Looker Studio. ... Creating Visualizations Using Cognos Analytics • 75 ...
Objectives: The goal of this assignment is to apply techniques from social media analytics, text mining and social network analysis to analyze online discourse and network data from social media about a particular event, company, product or service in order to. Objective 1: identify main topical themes (e.g., what customers are saying about a ...
hw3.py: The file for you to put solutions to Part 0, Part 1, and Part 2. You are required to add a main method that parses the provided dataset and calls all of the functions you are to write for this homework. hw3-written.txt: The file for you to put your answers to the questions in Part 3.
Datasets for this assignment will be collected by students using Netlytic. 2) Students will select two companies/organizations/groups to conduct a comparative analysis of social networks by examining social media activity. 3) Identify 2 relevant social media platforms used by both entities and use Netlytic to collect publicly available social ...
This is post #3 in a four part series about a new assignment that I'm using this semester in my Communication research class (all posts on that class). That assignment is a 3-part social media analytics project. Each part is related but unique, allowing students to pick up a new skill set. In this post we'll discuss part 2 of the assignment.
The following files are work done from Excel Basics for Data Analysis by IBM courtesy of Coursera. Included are two final assignment files that are unedited and edited. - iamAdrianC/Excel_Basics_for_Data_Analysis
Facebook Analytics Assignment: Part 1. Congratulations! You just landed a job as a junior social media manager at Buhi Bags! Buhi Supply Co., an e-commerce and retail bag supplier. It specializes in backpacks, duffel bags, messenger bags, pouches, totes, and travel bags. Your first assignment is to analyze the company's most recent Facebook ...
Here the sample data used in this final assignment can be found, in a data module called . Auto group data module. Right-click on Auto group data module and select Create Dashboard . Guidelines for the Submission. Use the course videos and hands-on lab from Module 2 Lesson 2 'Creating Dashboards Using IBM Cognos Analytics' to help you ...
View Facebook Analytics Assignment.docx from MARKETING 653 at Ahmedabad University. CHAPTER 10 Facebook Analytics Assignment By Nathan David Overview LEARNING OBJECTIVES Practice navigating ... Facebook Analytics Assignment.docx - CHAPTER 10 Facebook... Doc Preview. Pages 3. Identified Q&As 11. Total views 54. Ahmedabad University. MARKETING ...
SCHOOL OF COMPUTING DEPARTMENT OF COMPUTER SCIENCE AND ENGINEERING 1152CS194/ TEXT AND SOCIAL MEDIA ANALYTICS Assignment-2 Questions Faculty Name: Dr.J.Visumathi Slot: S4 CO3 Illustrate how to extract key phrases, named entities, and graph structures to reason about data in text and build a dialog framework to enable chatbots and language- driven interaction K1 CO4 Interpret the basic concepts ...
For this assignment, I forcasted Monthly Sales for Total Retail from the US Census Bureau. I trained 4 predictive models on data from 2018 to 2021 and tested the models on monthly 2022 data. The predictive models include an ARIMA model, ETS model, an ARIMA dynamic regression model, and an ensemble model averaging the first three models. For my ...
StatAnalytica - Statistics & Analytics Assignment Help. 17,288 likes · 2 talking about this. Stat Analytica is the best statistics assignment & homework help provider in the USA, Canada, UK,...
Applied Analytics - Assignment 2; by Belle A; Last updated almost 2 years ago; Hide Comments (-) Share Hide Toolbars × Post on: Twitter Facebook Google+ Or copy & paste this link into an email or IM: ...
5/17/23, 12:54 PM about:blank about:blank 1/7 Peer-Graded Assignment: Final Assignment - Part 2 Estimated time needed: 45 minutes You have now completed the first part of this final assignment. In this second part of the final assignment, you will create some visualizations and add them to a dashboard using Cognos Analytics. Software Used in this Assignment The hands-on lab in this ...
This document contains an assignment submitted by Gabriel Theodorus Silalahi analyzing traffic data from January to September 2021 and 2020 for a website. It includes: 1) An analysis of traffic sources which found direct as the highest revenue source, social as the highest conversion rate, and display as the highest bounce rate. Recommendations are made to boost social traffic and engagement ...
PowerBuddy for Learning. PowerBuddy for Learning is the personal assistant for teaching and learning. PowerBuddy makes educators' lives easier by helping them easily create high-quality assignments and instructional content. Students benefit from an always-available personalized assistant to support them in the way they choose to learn.