
An official website of the United States government
The .gov means it’s official. Federal government websites often end in .gov or .mil. Before sharing sensitive information, make sure you’re on a federal government site.
The site is secure. The https:// ensures that you are connecting to the official website and that any information you provide is encrypted and transmitted securely.
- Publications
- Account settings
Preview improvements coming to the PMC website in October 2024. Learn More or Try it out now .
- Advanced Search
- Journal List
- J Biomed Phys Eng
- v.12(3); 2022 Jun
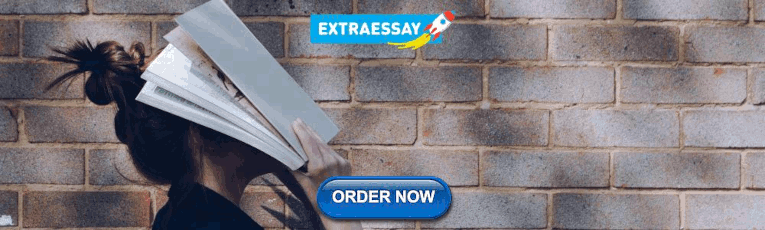
Prediction of Breast Cancer using Machine Learning Approaches
Reza rabiei.
1 PhD, Department of Health Information Technology and Management, School of Allied Medical Sciences, Shahid Beheshti University of Medical Sciences, Tehran, Iran
Seyed Mohammad Ayyoubzadeh
2 PhD, Department of Health Information Technology and Management, School of Allied Medical Sciences, Tehran University of Medical Science, Tehran, Iran
Solmaz Sohrabei
3 MSc, Department Deputy of Development, Management and Resources, Office of Statistic and Information Technology Management, Zanjan University of Medical Sciences, Zanjan, Iran
Marzieh Esmaeili
Alireza atashi.
4 PhD, Department of E-Health, Virtual School, Tehran University of Medical Sciences, Medical Informatics Research Group, Clinical Research Department, Breast Cancer Research Center, Motamed Cancer Institute, ACECR, Tehran, Iran
Background:
Breast cancer is considered one of the most common cancers in women caused by various clinical, lifestyle, social, and economic factors. Machine learning has the potential to predict breast cancer based on features hidden in data.
This study aimed to predict breast cancer using different machine-learning approaches applying demographic, laboratory, and mammographic data.
Material and Methods:
In this analytical study, the database, including 5,178 independent records, 25% of which belonged to breast cancer patients with 24 attributes in each record was obtained from Motamed cancer institute (ACECR), Tehran, Iran. The database contained 5,178 independent records, 25% of which belonged to breast cancer patients containing 24 attributes in each record. The random forest (RF), neural network (MLP), gradient boosting trees (GBT), and genetic algorithms (GA) were used in this study. Models were initially trained with demographic and laboratory features (20 features). The models were then trained with all demographic, laboratory, and mammographic features (24 features) to measure the effectiveness of mammography features in predicting breast cancer.
RF presented higher performance compared to other techniques (accuracy 80%, sensitivity 95%, specificity 80%, and the area under the curve (AUC) 0.56). Gradient boosting (AUC=0.59) showed a stronger performance compared to the neural network.
Conclusion:
Combining multiple risk factors in modeling for breast cancer prediction could help the early diagnosis of the disease with necessary care plans. Collection, storage, and management of different data and intelligent systems based on multiple factors for predicting breast cancer are effective in disease management.
Introduction
Breast cancer is considered a multifactorial disease and the most common cancer in women worldwide [ 1 , 2 ] with approximately 30% of all female cancers [ 3 , 4 ] (i.e. 1.5 million women are diagnosed with breast cancer each year, and 500,000 women die from this disease in the world). Over the past 30 years, this disease has increased, while the death rate has decreased. However, the reduction in mortality due to mammography screening is estimated at 20% and improvement in cancer treatment is estimated at 60% [ 5 , 6 ].
Diagnostic mammography can assess abnormal breast cancer tissue in patients with subtle and inconspicuous malignancy signs. Due to a large number of images, this method cannot effectively be used in assessing cancer suspected areas. According to a report, approximately 50% of breast cancers were not detected in screenings of women with very dense breast tissue [ 7 ]. However, about a quarter of women with breast cancer are diagnosed negatively within two years of screening. Therefore, the early and timely diagnosis of breast cancer is crucial [ 8 ].
Most mammography-based breast cancer screening is performed at regular intervals - usually annually or every two years - for all women. This “A fix screening program for everyone” is not effective in diagnosing cancer at the individual level and may impair the effectiveness of screening programs [ 9 ]. On the other hand, experts suggest that considering other risk factors along with mammography screening can help a more accurate diagnosis of women at risk [ 9 - 11 ]. Moreover, effective risk prediction through modeling can not only help radiologists in setting up a personal screening for patients and encouraging them to participate in the program for early detection but also help identify high-risk patients [ 12 , 13 ].
Machine learning, as a modeling approach, represents the process of extracting knowledge from data and discovering hidden relationships [ 14 ], widely used in healthcare in recent years [ 15 ] to predict different diseases [ 16 - 18 ]. Some studies only used demographic risk factors (lifestyle and laboratory data) in predicting breast cancer [ 19 , 20 ], and several studies predicted based on mammographic stereotypes [ 21 ] or used data from patient biopsy [ 22 ]. Others showed the application of genetic data in predicting breast cancer [ 23 ].
A major challenge in predicting breast cancer is the creation of a model for addressing all known risk factors [ 24 - 26 ]. Current prediction models might only focus on the analysis of mammographic images or demographic risk factors without other critical factors. In addition, these models, which are accurate enough for identifying high-risk women, could result in multiple screening and invasive sampling with magnetic resonance imaging (MRI) and ultrasound. The financial and psychological burden could be experienced by patients [ 27 - 29 ].
The effective prediction of breast cancer risk requires different factors, including demographic, laboratory, and mammographic risk factors [ 24 , 25 , 30 , 31 ]. Therefore, multifactorial models with many risk factors in their analysis can be effective in assessing the risk of breast cancer through more accurate analysis [ 32 , 33 ]. The current study aimed to predict breast cancer using different machine learning approaches considering various factors in modeling.
Material and Methods
In this analytical study, the database was obtained from a clinical breast cancer research center (Motamed cancer institute) in Tehran, Iran. The research was conducted in 4 stages: data collection, data pre-processing, modeling, and model evaluation.
Data Collection
In the first stage, 5178 records of people, referred to the research center over the past 10 years (2011-2021), were prepared retrospectively. Each record covered 24 features (11 demographic features, 9 laboratory features, and 4 mammography features) ( Table 1 ), all labeled to indicate the presence or absence of breast cancer, of which 1,295 records (25%) were identified as breast cancer.
The relevant features of breast cancer
DCIS: Ductal carcinoma in situ, IDC: Invasive ductal carcinoma, ILC: Invasive lobular carcinoma
Data preprocessing
The second step was associated with data preprocessing in which five records related to men were removed, and a total of 1290 records remained. Some of the patients’ laboratory features that were outside the considered range were repositioned in the central registry as their laboratory results were available. In addition, for records with missing values, the method of maximum frequency or the same mod was used. Finally, the Synthetic Minority Oversampling Technique (SMOTE) was used to balance the training data due to the difference in the number of study class records.
Modeling for breast cancer prediction
In the third step, the Scikit-Learn 0.18.2 library, NumPy v1.20, TPOT, and Python open-source programming were used for modeling. Three leaners, i.e. Random forest (RF), Gradient Boosting trees (GBT), and Multi-layer Perceptron (MLP) were applied to the dataset. In addition, the K-Fold (K=3) validation was used to gain the optimized hyper-parameter of each model in the genetic algorithm step. In the final evaluation, the train-test split method (75% for training and 25% for testing) was used to more accurately estimate the performance of the model. In this study, a genetic algorithm (GA) with a population of 5, the number of children 50, and the number of 10 generations with the criterion of the highest accuracy in model selection were used to optimize values for variables. Further, these models were then trained with demographic and laboratory features (20 features). Finally, the model was trained with all demographic, laboratory, and mammography features (24 features) to measure the effect of mammography features in predicting breast cancer. In the current study, MLP hidden layers numbers were considered 10, and the alpha value for the training rate was 0.01-0.2. The sigmoid and hyperbolic tangent functions were selected for activation function. The value of the solver optimizer function was set to a gradient-based optimizer method, such as Adam and Stochastic Gradient Descent (SGD) to find the optimal weights. In the GBT model, the learning rate was considered 0.01-0.2, and the maximum depth was regarded as 3, 5, and 8. The buoyancy level learning was 0.1 and the estimator value for the gradient boosting was 10. In the random forest (RF) model, the minimum number of sheets required to split an external node was considered 4 and 12. The estimator value was 151, and the node evaluation parameter to prevent splitting (min_samples_split) was considered 5 and 10. The block diagram for the methods is shown in Figure 1 .

Block diagram of methods
Random Forest (RF)
As a non-parametric approach, the RF uses the classification method. For each set of data, the RF performs categorization at high speed and applies a large number of decision trees [ 34 ]. In each tree, there is a random number of input variables, then all the trees are combined for a better inference from the variables [ 35 ].
Gradient Boosting Trees (GBT)
This algorithm is one of the reinforcement gradient algorithms with a very good performance in classification and performs the best classification for each of the data [ 36 ]. In this method, the trees are trained one after another; each subset tree is taught primarily with data erroneously predicted by the previous tree. This process continuously reduces the model error since each model is sequentially improved against the weaknesses of the previous model [ 37 , 38 ].
Multi-Layer Perceptron (MLP)
As a deep artificial neural network, the MLP is composed of an input layer for receiving the signal, an output layer used for prediction, and in between those two, some hidden layers are acting as the computation engine. The MLP is trained by a backpropagation algorithm, which is part of the supervised networks. In this network, data are driven from input nodes to output nodes. If there is an error in the output, this error must be somehow returned from the output to the input, and this corrects the weights. The most commonly used method for this is the post-diffusion algorithm [ 39 , 40 ].
Genetic Algorithm (GA)
As a subset of the evolutionary computing algorithm, GA is directly associated with artificial intelligence and used for solving optimization problems through the evolution process [ 41 , 42 ]. To obtain the best answer, the GA applies the best survival rule to a series of problems for patterning the best solution for problems [ 43 , 44 ]. In each generation, the optimal solution is achieved based on a natural biological process and by selecting the best chromosomes for creating the subsequent generation to solve the problem optimally [ 45 ].
Model Evaluation
The test results of the database samples (confusion matrix) are shown in Table 2 . In the final stage, the performance of the created models was measured by different criteria. The classification of samples is one of the common criteria in evaluating and measuring the ability of classifiers, the degree of separation or accuracy, and the separation of classes [ 46 ]. In this study, accuracy, sensitivity, specificity, and the area under the receiver operating characteristic (ROC) curve were used to measure the overall performance of the classifiers.
Confusion matrix of a binominal classifier
TN: True Negative, FN: False Negative, FP: False Positive, TP: True Positive
A total of 1290 records containing 24 demographic, laboratory, and mammographic features related to breast cancer were used in the study; the weight of the features based on their degree of importance is shown in (the weights are between (0.0 - 1) ( Figure 2 ). Family history of breast cancer, personal history of breast cancer, breast density, and age of diagnosis is 5 important factors in the diagnosis of this disease.

The weight of the features in breast cancer prediction
The performance of the models shown based on the ROC area under the curve demonstrated the Gradient Boosting Trees (GBT) as the model with the highest performance. The modeling results using RF, GBT, and MLP are shown in Table 3 , and the comparison of their ROC curve is demonstrated in Figure 3 and Table 4 .
Performance comparison of the breast cancer prediction models
AUC: Area under the ROC curve, ROC: Receiver operating characteristic

Receiver operating characteristic (ROC) curve of models
Area under the Receiver operating characteristic (ROC) curve
GBT: Gradient Boosting Tree, MLP: Multi-Layer-Perceptron, RF: Random Forest
According to the findings of the current study, the mammographic features along with other features could improve the performance of models. The RF model showed the highest sensitivity (95%), but was more efficient due to the sensitivity of breast cancer diagnosis, models, such as gradient boosting with higher specificity (86%).
In a study by Rosner et al. [ 47 , 48 ], the findings showed that family and personal history of breast cancer were two of the key influential factors in breast cancer, which are consistent with the findings of the current study as these two factors demonstrated the highest weight (0.92 and 0.89) compared to other factors. Breast density and age are influential in tumor appearance and increase the proportion of breast cancers [ 49 ] with the weights (0.80, 0.80), respectively. However, the hysterectomy feature was used along with other risk factors that could influence the performance of models. The study by Chow et al. assessed the risk of breast cancer after hysterectomy and showed a statistical significance between hysterectomy and breast cancer [ 50 ].
The use of optimization algorithms with feature weighting and proper adjustment of classification parameters could improve the performance of classification algorithms [ 51 ]. Studies reported that the classifiers that used GA in feature selection demonstrated better performance compared to those that did not use the GA. For the prediction of breast cancer, Bhattacharya et al. [ 52 ] approached three machine learning algorithms and used GA for feature selection; the findings of this study showed that the GA led to an improved performance for models created. In a study by Sakri et al. [ 53 ] to predict breast cancer recurrence in 198 instances with 34 clinical attributes, the GA was used for optimization. The Naive Bayes accuracy, sensitivity, specificity, and area under the ROC curve were reported at 70%, 81%, 79%, and 0.82, respectively in this study. Kumar et al. [ 54 ] used GA on a breast cancer dataset containing 611 records with 10 features to predict breast cancer survival and the reported accuracy, and ROC were 88% and 0.966 for GA, showing a better performance compared to Naive Bayes, DT, and K-nearest neighbor (KNN); in their study conducted to classify the masses observed in mammographic stereotypes, Thawkar and Ingolikar [ 55 ] used a dataset composed of 651 records with 25 mammography features. In the current study, the models were optimized by GA, and the ROC, accuracy, sensitivity, and specificity were 0.974, 95%, 96.14%, and 93.94% for RF, respectively. In the studies noted above, the modeling was performed using one set of influencing factors.
Some machine-learning studies [ 56 - 62 ] reported higher accuracy (100%) and sensitivity (100%) for breast cancer prediction compared to the present study, which is likely due to using different databases, such as “Wisconsin” and “SEER”. Similar to the database used in the current study, some studies used databases from specific medical or research centers. Behravan and Hartikainen [ 33 ] predicted breast cancer using a database containing 695 records, including demographic risk factors and genetic data; their findings suggested that the XGBoost model with different factors showed improved performance (AUC= 0.788) compared to a model with just one set of factors (AUC= 0.678). In a study by Feld et al. [ 10 ] to predict breast cancer, the modeling was performed on 738 records, including demographic, genetic, and abnormal mammographic data, and the reported AUC was 0.75. Other studies suggest that considering different factors in modeling would improve modeling performance. For example, by Ayvaci MU et al. [ 63 ], the analysis of demographic, mammography, and biopsy data using logistic regression resulted in an AUC of 0.84. Rajendran k et al. [ 64 ] analyzed 2.4 million records of mammography screening and demographic risk factors associated with breast cancer to predict breast cancer using the Naïve Bayes, RF, and C4.5 techniques; the findings indicated the highest AUC (0.993) for Naïve Bayes.
The findings of a study by Atashi et al. [ 65 ] conducted on a database with 4004 records, including demographic risk factors showed the higher performance of the neural network (sensitivity= %80.9, specificity= %99.8, accuracy= %62.8) compared to other approaches, such as C5.0. Mosayebi et al. study [ 66 ] was conducted on a database with 5471 records, including demographic and laboratory features reported for C.50 (accuracy 82%, sensitivity 86%. and specificity 77%). In a study by Jalali et al. [ 67 ] performed on 644 records (with 10 clinical features), the support vector machine (SVM) was reported with the highest sensitivity (94.33%), accuracy (93.72%), and specificity (92.26%). Afshar et al. [ 68 ] studied the survival of breast cancer patients using a dataset with 856 records and 15 clinical features using machine learning models. In this study, C5.0 showed the highest sensitivity (92.21%) and accuracy (84%). In addition, in a similar study by Nourelahi et al. [ 69 ] to predict patient survival on a database consisting of 5673 cases and 41 clinical features, logistic regression presented a sensitivity of 71.85%, specificity of 72.83%, and accuracy of 72.49%. In addition, Tapak et al. [ 70 ] performed a study on a database with 550 records to predict the survival and metastasis of breast cancer and also reported the sensitivity and specificity of 99% for AdaBoost, the findings of the current study suggest that modeling with a variety of related risk factors from different sources could improve the performance of models in breast cancer prediction.
In the current study, limitations are considered as follows: modeling based on records of only one database, and the lack of access to genetic data that could influence the findings of the study. However, different machine learning approaches were used considering demographic, laboratory, and mammography features, resulting in comparing the performance of different approaches in predicting breast cancer.
The proposed machine-learning approaches could predict breast cancer as the early detection of this disease could help slow down the progress of the disease and reduce the mortality rate through appropriate therapeutic interventions at the right time. Applying different machine learning approaches, accessibility to bigger datasets from different institutions (multi-center study), and considering key features from a variety of relevant data sources could improve the performance of modeling.
Authors’ Contribution
R. Rabiei proposed conceptualization and design, supervision of modeling, manuscript drafting, editing, and critical review. Data modeling, interpretation, and manuscript drafting was done by SM. Ayyoubzadeh. S. Sohrabei provided conceptualization and design, data modeling and interpretation, manuscript drafting, and editing. M. Esmaeili presented data interpretation and manuscript drafting. A. Atashi collected data and manuscript drafting. All the authors read, modified, and approved the final version of the manuscript.
Ethical Approval
This study was approved by Clinical Research Department, Breast Cancer Research Center, Motamed Cancer Institute (ACECR), Tehran, Iran, with Approval ID IR, ACECR, IBCRC, REC.1394.68.
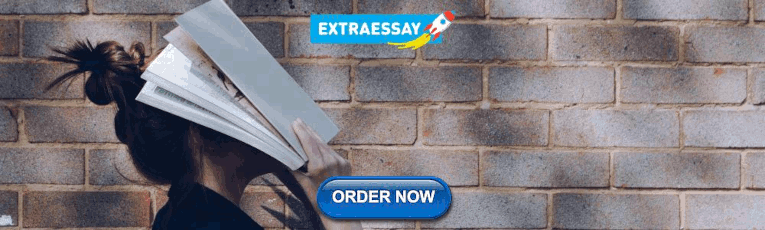
Informed consent
We used anonymous data for modeling and no consent was required for conducting this study.
There was no funding for conducting this study.
Conflict of Interest
Advertisement
Breast Cancer Prediction: A Comparative Study Using Machine Learning Techniques
- Original Research
- Published: 01 September 2020
- Volume 1 , article number 290 , ( 2020 )
Cite this article
- Md. Milon Islam ORCID: orcid.org/0000-0002-4535-5978 1 ,
- Md. Rezwanul Haque 1 ,
- Hasib Iqbal 1 ,
- Md. Munirul Hasan 2 ,
- Mahmudul Hasan ORCID: orcid.org/0000-0002-4386-0356 3 &
- Muhammad Nomani Kabir 2
11k Accesses
164 Citations
Explore all metrics
Early detection of disease has become a crucial problem due to rapid population growth in medical research in recent times. With the rapid population growth, the risk of death incurred by breast cancer is rising exponentially. Breast cancer is the second most severe cancer among all of the cancers already unveiled. An automatic disease detection system aids medical staffs in disease diagnosis and offers reliable, effective, and rapid response as well as decreases the risk of death. In this paper, we compare five supervised machine learning techniques named support vector machine (SVM), K-nearest neighbors, random forests, artificial neural networks (ANNs) and logistic regression. The Wisconsin Breast Cancer dataset is obtained from a prominent machine learning database named UCI machine learning database. The performance of the study is measured with respect to accuracy, sensitivity, specificity, precision, negative predictive value, false-negative rate, false-positive rate, F1 score, and Matthews Correlation Coefficient. Additionally, these techniques were appraised on precision–recall area under curve and receiver operating characteristic curve. The results reveal that the ANNs obtained the highest accuracy, precision, and F1 score of 98.57%, 97.82%, and 0.9890, respectively, whereas 97.14%, 95.65%, and 0.9777 accuracy, precision, and F1 score are obtained by SVM, respectively.
This is a preview of subscription content, log in via an institution to check access.
Access this article
Price includes VAT (Russian Federation)
Instant access to the full article PDF.
Rent this article via DeepDyve
Institutional subscriptions
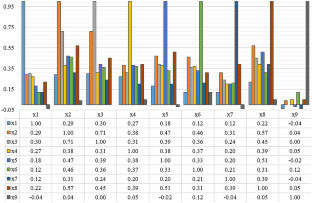
Similar content being viewed by others
Machine Learning Algorithms for Breast Cancer Detection and Prediction
Selecting Best Machine Learning Techniques for Breast Cancer Prediction and Diagnosis
Machine Learning Classifiers Performance Comparison for Breast Cancer Detection
Park SH, Han K. Methodologic guide for evaluating clinical performance and effect of artificial intelligence technology for medical diagnosis and prediction. Radiol Soc N Am. 2018;286(3):800–9.
Google Scholar
Breast Cancer: Statistics, Approved by the Cancer.Net Editorial Board, 04/2017. [Online]. Available: http://www.cancer.net/cancer-types/breast-cancer/statistics . Accessed 26 Aug 2018.
Mori M, Akashi-Tanaka S, Suzuki S, Daniels MI, Watanabe C, Hirose M, Nakamura S. Diagnostic accuracy of contrast-enhanced spectral mammography in comparison to conventional full-field digital mammography in a population of women with dense breasts. Springer. 2016;24(1):104–10.
Kurihara H, Shimizu C, Miyakita Y, Yoshida M, Hamada A, Kanayama Y, Tamura K. Molecular imaging using PET for breast cancer. Springer. 2015;23(1):24–32.
Azar AT, El-Said SA. Probabilistic neural network for breast cancer classification. Neural Comput Appl. 2013;23(6):1737–51.
Article Google Scholar
Nagashima T, Suzuki M, Yagata H, Hashimoto H, Shishikura T, Imanaka N, Miyazaki M. Dynamic-enhanced MRI predicts metastatic potential of invasive ductal breast cancer. Springer. 2002;9(3):226–30.
Park CS, Kim SH, Jung NY, Choi JJ, Kang BJ, Jung HS. Interobserver variability of ultrasound elastography and the ultrasound BI-RADS lexicon of breast lesions. Springer. 2013;22(2):153–60.
Ayon SI, Islam MM, Hossain MR. Coronary artery heart disease prediction: a comparative study of computational intelligence techniques. IETE J Res. 2020;. https://doi.org/10.1080/03772063.2020.1713916 .
Muhammad LJ, Islam MM, Usman SS, Ayon SI. Predictive data mining models for novel coronavirus (COVID-19) infected patients’ recovery. SN Comput Sci. 2020;1(4):206.
Islam MM, Iqbal H, Haque MR, Hasan MK. Prediction of breast cancer using support vector machine and K-Nearest neighbors. In: Proc. IEEE Region 10 Humanitarian Technology Conference (R10-HTC), Dhaka, 2017, pp. 226–229.
Haque MR, Islam MM, Iqbal H, Reza MS, Hasan MK. Performance evaluation of random forests and artificial neural networks for the classification of liver disorder. In: Proc. International Conference on Computer, Communication, Chemical, Material and Electronic Engineering (IC4ME2), Rajshahi, 2018, pp. 1–5.
Ayon SI, Islam MM. Diabetes prediction: a deep learning approach. Int J Inf Eng Electron Bus (IJIEEB). 2019;11(2):21–7.
Islam MZ, Islam MM, Asraf A. A combined deep CNN-LSTM network for the detection of novel coronavirus (COVID-19) using X-ray images, 2020. pp. 1–20.
Hasan MK, Islam MM, Hashem MMA. Mathematical model development to detect breast cancer using multigene genetic programming. In: 2016 5th International Conference on Informatics, Electronics and Vision (ICIEV), pp. 574–579, 2016.
Sakri SB, Rashid NBA, Zain ZM. Particle swarm optimization feature selection for breast cancer recurrence prediction. IEEE Access. 2018;6:29637–47.
Juneja K, Rana C. An improved weighted decision tree approach for breast cancer prediction. In: International Journal of Information Technology, 2018.
Yue W, et al. Machine learning with applications in breast cancer diagnosis and prognosis. Designs. 2018;2(2):13.
Banu AB, Subramanian PT. Comparison of Bayes classifiers for breast cancer classification. Asian Pac J Cancer Prev (APJCP). 2018;19(10):2917–20.
Chaurasia V, Pal S, Tiwari B. Prediction of benign and malignant breast cancer using data mining techniques. J Algorithms Comput Technol. 2018;12(2):119–26.
Azar AT, El-Metwally SM. Decision tree classifiers for automated medical diagnosis. Neural Comput Appl. 2012;23(7–8):2387–403.
Senapati MR, Mohanty AK, Dash S, Dash PK. Local linear wavelet neural network for breast cancer recognition. Neural Comput Appl. 2013;22(1):125–31.
Senapati MR, Panda G, Dash PK. Hybrid approach using KPSO and RLS for RBFNN design for breast cancer detection. Neural Comput Appl. 2014;24(3–4):745–53.
Hasan MK, Islam MM, Hashem MMA (2016) Mathematical model development to detect breast cancer using multigene genetic programming. In: Proc. 5th International Conference on Informatics, Electronics and Vision (ICIEV), Dhaka, 2016, pp. 574–579.
Azar AT, El-Said SA. Performance analysis of support vector machines classifiers in breast cancer mammography recognition. Neural Comput Appl. 2013;24(5):1163–77.
Ferreira P, Dutra I, Salvini R, Burnside E. Interpretable models to predict Breast Cancer. In: Proc. IEEE International Conference on Bioinformatics and Biomedicine (BIBM), Shenzhen, 2016, pp. 1507–1511.
Jhajharia S, Verma S, Kumar R. A cross-platform evaluation of various decision tree algorithms for prognostic analysis of breast cancer data. In: Proc. International Conference on Inventive Computation Technologies (ICICT), Coimbatore, 2016, pp. 1–7.
Islam MM, Rahaman A, Islam MR. Development of smart healthcare monitoring system in IoT environment. SN Comput Sci. 2020;1(3):185.
Rahaman A, Islam M, Islam M, Sadi M, Nooruddin S. Developing IoT based smart health monitoring systems: a review. Rev d’Intell Artif. 2019;33(6):435–40.
Breast Cancer Wisconsin (Original) Data Set, [Online]. https://archive.ics.uci.edu/ml/machine-learning-databases/breast-cancer-wisconsin/breast-cancer-wisconsin.data . Accessed 25 Aug 2018.
James G, Witten D, Hastie T, Tibshirani R. An introduction to statistical learning. 1st ed. New York: Springer; 2013.
Book MATH Google Scholar
Guido S, Mller AC. Introduction to machine learning with python. Sebastopol: O’Reilly Media Inc.; 2016.
Dwivedi AK. Performance evaluation of different machine learning techniques for prediction of heart disease. Neural Comput Appl. 2016;29(10):685–93.
Ratner B. Statistical and machine-learning data mining: techniques for better predictive modeling and analysis of big data. Oxford: Chapman and Hall/CRC; 2017.
MATH Google Scholar
Dong L, Wesseloo J, Potvin Y, Li X. Discrimination of mine seismic events and blasts using the fisher classifier, naive bayesian classifier and logistic regression. Rock Mech Rock Eng. 2015;49(1):183–211.
Hosmer DW Jr, Lemeshow S. Applied logistic regression. New York: Wiley; 2004.
Schumacher M, Roner R, Vach W. Neural networks and logistic regression: part I. Comput Stat Data Anal. 1996;21(6):661–82.
Article MATH Google Scholar
Vach W, Roner R, Schumacher M. Neural networks and logistic regression: part II. Comput Stat Data Anal. 1996;21(6):683–701.
Hajmeer M, Basheer I. Comparison of logistic regression and neural network-based classifiers for bacterial growth. Food Microbiol. 2003;20(1):43–55.
Xu Y, Zhu Q, Wang J. Breast cancer diagnosis based on a kernel orthogonal transform. Neural Comput Appl. 2012;21(8):1865–70.
Latchoumi TP, Parthiban L. Abnormality detection using weighed particle swarm optimization and smooth support vector machine. Biomed Res. 2017;28:4749–51.
Kumar UK, Nikhil MBS, Sumangali K. Prediction of breast cancer using voting classifier technique. In: Proc. IEEE International Conference on Smart Technologies and Management for Computing, Communication, Controls, Energy and Materials (ICSTM), Chennai, 2017, pp. 108–114.
Download references
Acknowledgements
This research was partially supported by Universiti Malaysia Pahang (UMP) through UMP Flagship Grant (RDU192206).
Author information
Authors and affiliations.
Department of Computer Science and Engineering, Khulna University of Engineering & Technology, Khulna, 9203, Bangladesh
Md. Milon Islam, Md. Rezwanul Haque & Hasib Iqbal
Faculty of Computing, Universiti Malaysia Pahang, 26300, Gambang, Kuantan, Malaysia
Md. Munirul Hasan & Muhammad Nomani Kabir
Department of Computer Science, Stony Brook University, Stony Brook, NY, 11794-2424, USA
Mahmudul Hasan
You can also search for this author in PubMed Google Scholar
Corresponding author
Correspondence to Md. Milon Islam .
Ethics declarations
Conflict of interest.
On behalf of all authors, the corresponding author states that there is no conflict of interest.
Additional information
Publisher's note.
Springer Nature remains neutral with regard to jurisdictional claims in published maps and institutional affiliations.
This article is part of the topical collection “Advances in Computational Approaches for Artificial Intelligence, Image Processing, IoT and Cloud Applications” guest edited by Bhanu Prakash K N and M. Shivakumar.
Rights and permissions
Reprints and permissions
About this article
Islam, M.M., Haque, M.R., Iqbal, H. et al. Breast Cancer Prediction: A Comparative Study Using Machine Learning Techniques. SN COMPUT. SCI. 1 , 290 (2020). https://doi.org/10.1007/s42979-020-00305-w
Download citation
Received : 11 August 2020
Accepted : 18 August 2020
Published : 01 September 2020
DOI : https://doi.org/10.1007/s42979-020-00305-w
Share this article
Anyone you share the following link with will be able to read this content:
Sorry, a shareable link is not currently available for this article.
Provided by the Springer Nature SharedIt content-sharing initiative
- Breast cancer prediction
- Cancer dataset
- Machine learning
- Support vector machine
- Random forests
- Artificial neural networks
- K-nearest neighbors
- Logistic regression
- Find a journal
- Publish with us
- Track your research
Breast Cancer Detection and Prediction using Machine Learning
Ieee account.
- Change Username/Password
- Update Address
Purchase Details
- Payment Options
- Order History
- View Purchased Documents
Profile Information
- Communications Preferences
- Profession and Education
- Technical Interests
- US & Canada: +1 800 678 4333
- Worldwide: +1 732 981 0060
- Contact & Support
- About IEEE Xplore
- Accessibility
- Terms of Use
- Nondiscrimination Policy
- Privacy & Opting Out of Cookies
A not-for-profit organization, IEEE is the world's largest technical professional organization dedicated to advancing technology for the benefit of humanity. © Copyright 2024 IEEE - All rights reserved. Use of this web site signifies your agreement to the terms and conditions.
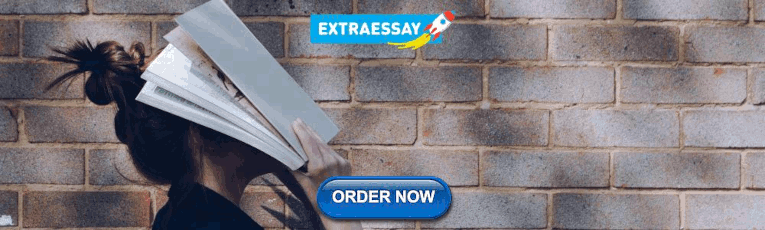
COMMENTS
Afshar et al. [ 68 ] studied the survival of breast cancer patients using a dataset with 856 records and 15 clinical features using machine learning models. In this study, C5.0 showed the highest sensitivity (92.21%) and accuracy (84%). In addition, in a similar study by Nourelahi et al. [ 69 ] to predict patient survival on a database ...
effective treatment to be used and reducing the risks o f. death from breast cancer. Since early detection of cancer. is key to effective treatment of breast cancer we use. various machine ...
Related Works A large number of machine learning algorithms are available for prediction and diagnosis of breast cancer. Some of the machine learning algorithm are Support Vector Machine (SVM), Random Forest, Logistic Regression, Decision tree (C4.5) and K-Nearest Neighbors (KNN Network) etc. A lot of researcher have realized research in breast ...
detect breast cancer. In this research work, we systematically reviewed previous work done on detection and treatment of breast cancer using genetic sequencing or histopathological imaging with the help of deep learning and machine learning. We also provide recommendations to researchers who will work in this field.
Various Breast Cancer Imaging modalities including Mammography, Histopathology, Ultrasound, MRI, PET/CT, and Thermography has been discussed briefly with advantages and disadvantages of each image modality. Various Machine Learning, Deep Learning and Deep Reinforcement Learning algorithms including both supervised and unsupervised approaches ...
Breast cancer is a common cause of female mortality in developing countries. Early detection and treatment are crucial for successful outcomes. Breast cancer develops from breast cells and is considered a leading cause of death in women. This disease is classified into two subtypes: invasive ductal carcinoma (IDC) and ductal carcinoma in situ (DCIS). The advancements in artificial intelligence ...
Many cancer detection systems have been developed recently employing ml algorithms and biosensors. Additionally becoming more prevalent over time are breast screening tests and sophisticated data analysis techniques. Pre-processing is required for the major-ity of databases, depending on the ML model that was employed.
organs is frequent and could be through the bloodstream. Different techniques are used to capture breast cancer such as Ultrasound. Sonography, Computerized T hermography, Biopsy (Histological ...
Artificial intelligence (AI) and particularly the introduction of deep learning convolutional neural networks brings new potential in computer-aided detection systems. In the context of breast cancer, several AI-based systems have been developed and assessed over the last decade, attempting to improve benefits in breast cancer screening accuracy and efficiency. This study focuses on mature ...
13. BREAST CANCER PREDICTION USING. MACHINE LEARNING. Ramik Rawal. School of Computer Science and Engineering (SCOPE), Vellore Institute of. Technology, Gorbachev Road, Vellore, Tamil Nadu 632014 ...
Breast cancer is a serious medical condition that requires early detection for successful treatment. Mammography is a commonly used imaging technique for breast cancer screening, but its analysis can be time-consuming and subjective. This study explores the use of deep learning-based methods for mammogram analysis, with a focus on improving the performance of the analysis process. The study is ...
Early detection of disease has become a crucial problem due to rapid population growth in medical research in recent times. With the rapid population growth, the risk of death incurred by breast cancer is rising exponentially. Breast cancer is the second most severe cancer among all of the cancers already unveiled. An automatic disease detection system aids medical staffs in disease diagnosis ...
Breast cancer is diagnosed in over 2 million people worldwide each year., according to cancer research. accounting for the majority of all cancer diagnoses and deaths., rendering it a major public health issue. However, in its early stages, it is still a curable cancer. Early detection of breast cancer, combined with prompt and effective treatment, improves patients' prognosis and recovery ...
Machine learning has become a prevalent tool in medical applications, particularly in identifying cancer cell types. Breast cancer, a prominent cause of female mortality, can be mitigated through early detection of cancerous cells. Diagnostic tests such as MRI, mammogram, ultrasound, and biopsy are commonly employed for this purpose.
Globally, Breast cancer is one of the most common types of cancer in women. Additional research is required to address the challenges and limitations of traditional detection process, as well as to create standardized processes for data collection and analysis of breast cancer by utilizing mammograms and other medical imaging data. Several research has been undertaken in recent years to create ...
This thesis revisits the problem of ve year survivability predictions for breast cancer using machine learning tools. This work is distinguishable from the past experiments based on the size of the training data, the unbalanced distribution of data in minority and majority classes, and modi ed data cleaning procedures.
Cancer is one of the most prominent cause of fatalities around the world, accounting over 1 crore deaths in past year out of which 22.6% deaths were due to Breast cancer (BC).
This paper presents a Machine Learning model to perform automated diagnosis for breast cancer. This method employed CNN as a classifier model and Recursive Feature Elimination (RFE) for feature selection. Also, five algorithms SVM, Random Forest, KNN, Logistic Regression, Naïve Bayes classifier have been compared in the paper.
Cancer death is one of humanity's major problems in the developing world. Even though there are numerous ways to prevent it from occurring in the first place, some cancer types remain unrepeatable. Due to the absence of adequate forecasting, clinicians are unable to devise a treatment plan that will improve patient mortality rate. Hence, the requisite of time is to develop the technique which ...
The thesis titled Early Detection of Breast Cancer Using Machine Learning Submitted by: Wasi Mohammad Fuad Student ID: 14201029 of Academic Year 2018 has been found as satisfactory and accepted as partial fulfillment of the requirement for the Degree of Computer Science and Engineering 1. Dr. Md. Ashraful Alam Assistant Professor BRAC University 2.
Abstract Machine learning techniques have been used at great lengths in learning a hypothesis from the confirmed samples to help the clinical specialists in making a computer aided diagnosis. Recognition of images as cancer or non-cancer is involved in cancer detection and for this purpose preprocessing of images, feature extraction and classification as well as analysis regarding performance ...
Intrusion Detection Using Big Data and Deep Learning Techniques. ... A comparative study of breast cancer tumor classification by classical machine learning methods and deep learning method ... In contemporary times, machine learning is being used in almost every field due to its better performance. Here, we consider different machine learning ...