Cohort Studies: Design, Analysis, and Reporting
Affiliations.
- 1 Department of Quantitative Health Sciences, Lerner Research Institute, Cleveland Clinic, Cleveland, OH. Electronic address: [email protected].
- 2 Department of Quantitative Health Sciences, Lerner Research Institute, Cleveland Clinic, Cleveland, OH.
- PMID: 32658655
- DOI: 10.1016/j.chest.2020.03.014
Cohort studies are types of observational studies in which a cohort, or a group of individuals sharing some characteristic, are followed up over time, and outcomes are measured at one or more time points. Cohort studies can be classified as prospective or retrospective studies, and they have several advantages and disadvantages. This article reviews the essential characteristics of cohort studies and includes recommendations on the design, statistical analysis, and reporting of cohort studies in respiratory and critical care medicine. Tools are provided for researchers and reviewers.
Keywords: bias; cohort studies; confounding; prospective; retrospective.
Copyright © 2020 American College of Chest Physicians. Published by Elsevier Inc. All rights reserved.
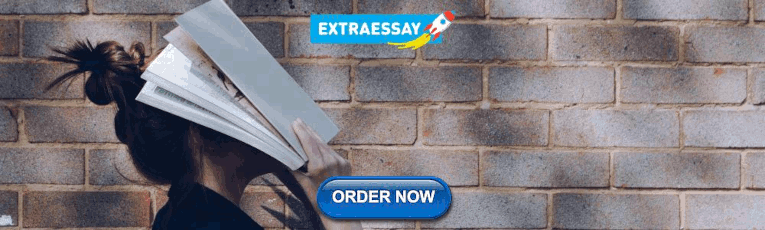
Publication types
- Cohort Studies*
- Data Interpretation, Statistical
- Guidelines as Topic
- Research Design / statistics & numerical data*
- Type 2 Diabetes
- Heart Disease
- Digestive Health
- Multiple Sclerosis
- Diet & Nutrition
- Supplements
- Health Insurance
- Public Health
- Patient Rights
- Caregivers & Loved Ones
- End of Life Concerns
- Health News
- Thyroid Test Analyzer
- Doctor Discussion Guides
- Hemoglobin A1c Test Analyzer
- Lipid Test Analyzer
- Complete Blood Count (CBC) Analyzer
- What to Buy
- Editorial Process
- Meet Our Medical Expert Board
What Is a Cohort Study?
A cohort study often looks at 2 (or more) groups of people that have a different attribute (for example, some smoke and some don't) to try to understand how the specific attribute affects an outcome. The goal is to understand the relationship between one group's shared attribute (in this case, smoking) and its eventual outcome.
pixelfit/Getty Images
Cohort Study Design
There are two categories of evidence-based human medical research:
Experimental research: This involves a controlled process through which each participant in a clinical trial is exposed to some type of intervention or situation—like a drug, vaccine, or environmental exposure. Sometimes there is also a control group that is not exposed for comparison. The results come from tracking the effects of the exposure or intervention over a set period of time.
Observational research: This is when there is no intervention. The researchers simply observe the participants' exposure and outcomes over a set period of time in an attempt to identify potential factors that could affect a variety of health conditions.
Cohort studies are longitudinal, meaning that they take place over a set period of time—frequently, years—with periodic check-ins with the participants to record information like their health status and health behaviors.
They can be either:
- Prospective: Start in the present and continue into the future
- Retrospective: Start in the present, but look to the past for information on medical outcomes and events
Purpose of Cohort Studies
The purpose of cohort studies is to help advance medical knowledge and practice, such as by getting a better understanding of the risk factors that increase a person's chances of getting a particular disease.
Participants in cohort studies are grouped together based on having a shared characteristic—like being from the same geographic location, having the same occupation, or having a diagnosis of the same medical condition.
Each time the researchers check-in with participants in cohort trials, they're able to measure their health behaviors and outcomes over a set period of time. For example, a study could involve two cohorts: one that smokes and the other that doesn't. As the data is collected over time, the researchers would have a better idea of whether there appears to be a link between a behavior—in this case, smoking—and a particular outcome (like lung cancer, for example).
Strengths of Cohort Studies
Much of the medical profession's current knowledge of disease risk factors comes from cohort studies. In addition to showing disease progression, cohort studies also help researchers calculate the incidence rate, cumulative incidence, relative risk, and hazard ratio of health conditions.
- Size : Large cohort studies with many participants usually give researchers more confident conclusions than small studies.
- Timeline : Because they track the progression of diseases over time, cohort studies can also be helpful in establishing a timeline of a health condition and determining whether specific behaviors are potential contributing factors to disease.
- Multiple measures : Often, cohort studies allow researchers to observe and track multiple outcomes from the same exposure. For example, if a cohort study is following a group of people undergoing chemotherapy, researchers can study the incidence of nausea and skin rashes in the patients. In this case, there is one exposure (chemotherapy) and multiple outcomes (nausea and skin rashes).
- Accuracy : Another strength of cohort studies—specifically, prospective cohort studies—is that researchers might be able to measure the exposure variable, other variables, and the participants' health outcomes with relative accuracy.
- Consistency : Outcomes measured in a study can be done uniformly.
Retrospective cohort studies have their own benefits, namely that they can be conducted relatively quickly, easily, and cheaply than other types of research.
Weaknesses of Cohort Studies
While cohort studies are an essential part of medical research, they are not without their limitations.
These can include:
- Time: Researchers aren't simply bringing participants into the lab for one day to answer a few questions. Cohort studies can last for years—even decades—which means that the costs of running the study can really add up.
- Self-reporting: Even though retrospective cohort studies are less costly, they come with their own significant weakness in that they might rely on participants' self-reporting of past conditions, outcomes, and behaviors. Because of this, it can be more difficult to get accurate results.
- Drop-out: Given the lengthy time commitment required to be a part of a cohort study, it's not unusual for participants to drop out of this type of research. Though they have every right to do that, having too many people leave the study could potentially increase the risk of bias.
- Behavior alteration: Another weakness of cohort studies is that participants may alter their behavior in ways they wouldn't otherwise if they were not part of a study, which could alter the results of the research.
- Potential for biases: Even the most well-designed cohort studies won't achieve results as robust as those reached via randomized controlled trials. This is because by design—i.e. people put into groups based on certain shared traits—there is an inherent lack of randomization.
A Word From Verywell
Medicines, devices, and other treatments come to the market after many years of research. There's a long journey between the first tests of early formulations of a drug in a lab, and seeing commercials for it on TV with a list of side effects read impossibly quickly.
Think about the last time you had a physical. Your healthcare provider likely measured several of your vital signs and gave you a blood test, then reported back to you about the various behaviors you may need to change in order to reduce your risk of developing certain diseases. Those risk factors aren't just guesses; many of them are the result of cohort studies.
Song JW, Chung KC. Observational studies: cohort and case-control studies . Plast Reconstr Surg . 2010;126(6):2234-2242. doi:10.1097/PRS.0b013e3181f44abc.
Barrett D, Noble H. What are cohort studies? Evidence-Based Nursing . 2019;22(4):95-96. doi:10.1136/ebnurs-2019-103183
Wang X, Kattan MW. Cohort studies: design, analysis, and reporting . CHEST . 2020;158(1):S72-S78. doi: 10.1016/j.chest.2020.03.014.
Setia MS. Methodology series module 1: cohort studies. Indian J Dermatol . 2016;61(1):21-25. doi:10.4103/0019-5154.174011.
By Elizabeth Yuko, PhD Yuko has a doctorate in bioethics and medical ethics and is a freelance journalist based in New York.
Thank you for visiting nature.com. You are using a browser version with limited support for CSS. To obtain the best experience, we recommend you use a more up to date browser (or turn off compatibility mode in Internet Explorer). In the meantime, to ensure continued support, we are displaying the site without styles and JavaScript.
- View all journals
- Explore content
- About the journal
- Publish with us
- Sign up for alerts
- Published: 13 January 2022
Cohort studies investigating the effects of exposures: key principles that impact the credibility of the results
- Anna Miroshnychenko 1 ,
- Dena Zeraatkar 1 , 2 ,
- Mark R. Phillips ORCID: orcid.org/0000-0003-0923-261X 1 ,
- Sophie J. Bakri 3 ,
- Lehana Thabane ORCID: orcid.org/0000-0003-0355-9734 1 , 4 ,
- Mohit Bhandari ORCID: orcid.org/0000-0001-9608-4808 1 , 5 &
- Varun Chaudhary ORCID: orcid.org/0000-0002-9988-4146 1 , 5
for the Retina Evidence Trials InterNational Alliance (R.E.T.I.N.A.) Study Group
Eye volume 36 , pages 905–906 ( 2022 ) Cite this article
7588 Accesses
2 Citations
1 Altmetric
Metrics details
- Outcomes research
What are cohort studies?
Cohort studies are observational studies that follow groups of patients with different exposures forward in time and determine outcomes of interest in each exposure group or that investigate the effect of one or more participant characteristics on prognostic outcomes [ 1 ]. The focus of this editorial is on cohort studies that investigate the effects of exposures that may be associated with an increased or a decreased occurrence of the outcome of interest. Cohort studies may be prospective or retrospective in design. In prospective cohort studies, investigators enroll participants, assess exposure status, initiate follow up, and measure the outcome of interest in the future. In retrospective cohort studies, data on both the exposures and outcome of interest have been previously collected.
Purpose of cohort studies
While large well-designed randomized controlled trials (RCTs) represent the optimal design for making inferences about the effects of exposures or interventions on health outcomes, they are often not feasible to conduct—due to costs or challenges of recruiting patients with rare conditions and following patients for sufficient durations. Further, patients included in RCTs may not be representative of patients encountered in practice and the effectiveness of therapies in strict clinical trials may be different than when implemented in routine practice. In such circumstances, well-designed observational studies, which include cohort studies, can play an important role in producing evidence to guide clinical care decisions in ophthalmology. Cohort studies can also be conducted to generate hypotheses and establishing questions for future RCTs.
The differentiating characteristics between observational (e.g., cohort study) and experimental (e.g., RCT) study designs are that in the former the investigator does not intervene and rather “observes” and examines the relationship or association between an exposure and outcome. Examples of cohort studies in ophthalmology include evaluation of a possible association between exposure to ambient air pollution and age-related cataract [ 2 ]; or assessment of the impact of eye preserving therapies for patients with advanced retinoblastoma [ 3 ].
Key determinants of credibility (i.e., internal validity) in cohort studies
Readers considering applying evidence from cohort studies should be mindful of the following factors that affect the credibility or internal validity of cohort studies.
Factors that decrease the credibility of cohort studies
Cohort studies are at serious risk of confounding bias and so adjusting or accounting for confounding factors is a priority in these studies. Confounding occurs when the exposure of interest is associated with another factor that also influences the outcome of interest. Investigators can use various design (e.g., matching) and statistical methods (e.g., adjusted analyses based on regression methods) to deal with known, measured confounders. Readers should assess whether the authors accounted for known confounders of the relationship under investigation in either their design or statistical analysis. Readers should be mindful, however, that possibility of residual confounding caused by unknown or unmeasured confounders always remains.
Inappropriate selection of participants into the cohort study can result in selection bias. Selection bias occurs when selection of participants is related to both the intervention and outcome. Bias in measurement of exposure/outcome, or detection bias, can arise when outcome assessors are aware of intervention status, different methods are used to assess outcomes in the different intervention groups, and/or the exposure status is misclassified differentially or non-differentially (i.e., the probability of individuals being misclassified is different or equal between groups in a study, respectively).
Missing data may also affect the credibility of cohort studies. Bias due to missing data in prospective and retrospective studies arises when follow up data are missing for individuals initially included in the study. Participants with missing outcome data may differ importantly from those with complete data (e.g., they may be healthier or may not have experienced adverse events).
Last, credibility of a cohort study may be affected by the reporting of results. Selective reporting arises when investigators selectively report results in studies in such a way so that the study report highlights or emphasizes evidence supporting a particular hypothesis and does not report or understates evidence supporting an alternative hypothesis. Investigators may selectively report results for timepoints or measures that produced results consistent with their preconceived beliefs or results that were newsworthy and disregard results for timepoints or measures that produced results that were inconsistent with their beliefs or considered not newsworthy. Publication bias refers to the propensity for studies with anomalous, interesting, or statistically significant results to be published at higher rates or to be published more rapidly or to be published in journals with higher visibility.
Factors that increase the credibility of cohort studies
Three uncommon situations can sometimes make us more certain of findings of cohort studies—in some circumstances, these situations can make us as confident of evidence from cohort studies as we would be for evidence from a rigorous RCT. First, when the observed effect is large (typically a relative risk (RR) > 2 or RR < 0.5), biases, such as confounding, are less likely to completely explain the observed effect. Second, we may be more certain of results when we observe a dose-response gradient: biases in non-randomized studies (e.g., confounding and errors in the classification of the exposure) are unlikely to produce spurious dose-response associations., when all suspected biases are believed to act against the observed direction of effect, we can be more certain that the observed effect is not due to the suspected biases. It is, however, difficult to anticipate with sufficient certainty the direction in which effects are likely biased in complex epidemiological studies. Because situations that make us more certain of findings of cohort studies occur infrequently, cohort studies usually provide only low to very low certainty evidence [ 4 ].
Applicability (i.e., external validity) in cohort studies
If the populations, exposures, or outcomes investigated in cohort studies differ from the those of interest in routine or typical settings, the evidence may not be applicable or externally valid. Such judgements depend on whether differences between studies and the question of interest would lead to an appreciable change in the direction or magnitude of effect. Generally, observational studies (e.g., cohort studies) have higher external validity than experimental studies (e.g., RCTs) [ 5 ].
Cohort studies follow a population exposed or not exposed to a potential causal agent forward in time and assess outcomes. Cohort studies are beneficial because these studies allow the investigators to observe a possible association between an exposure and outcome of interest in a population that cannot be randomly subjected to an exposure due to ethical, methodological, or feasibility limitations. Cohort studies, however, have several limitations that should be acknowledged and minimized if possible.
Barrett D, Noble H. What are cohort studies? Evid-Based Nurs. 2019;22:95–6.
Article Google Scholar
Shin J, Lee H, Kim H. Association between exposure to ambient air pollution and age-related cataract: a nationwide population-based retrospective cohort study. Int J Environ Res Public Health. 2020;17:9231.
Article CAS Google Scholar
Zhou C, Wen X, Ding Y, Ding J, Jin M, Liu Z, et al. Eye-preserving therapies for advanced retinoblastoma: a multicenter cohort of 1678 patients in China. Ophthalmology.2021;S0161-6420:00683–7.
Google Scholar
Guyatt GH, Oxman AD, Vist G, Kunz R, Brozek J, Alonso-Coello P, et al. GRADE guidelines: 4. Rating the quality of evidence-study limitations (risk of bias). J Clin Epidemiol. 2011;64:407–15.
Rothwell PM. External validity of randomised controlled trials: “to whom do the results of this trial apply?”. Lancet.2005;365:82–93.
Download references
Author information
Authors and affiliations.
Department of Health Research Methods, Evidence, and Impact, McMaster University, Hamilton, ON, Canada
Anna Miroshnychenko, Dena Zeraatkar, Mark R. Phillips, Lehana Thabane, Mohit Bhandari, Varun Chaudhary & Lehana Thabane
Department of Biomedical Informatics, Harvard Medical School, Boston, MA, USA
Dena Zeraatkar
Department of Ophthalmology, Mayo Clinic, Rochester, MN, USA
Sophie J. Bakri
Biostatistics Unit, St. Joseph’s Healthcare-Hamilton, Hamilton, ON, Canada
Lehana Thabane & Lehana Thabane
Department of Surgery, McMaster University, Hamilton, ON, Canada
Mohit Bhandari, Varun Chaudhary, Varun Chaudhary & Mohit Bhandari
Retina Consultants of Texas (Retina Consultants of America), Houston, TX, USA
Charles C. Wykoff
Blanton Eye Institute, Houston Methodist Hospital, Houston, TX, USA
NIHR Moorfields Biomedical Research Centre, Moorfields Eye Hospital, London, UK
Sobha Sivaprasad
Cole Eye Institute, Cleveland Clinic, Cleveland, OH, USA
Peter Kaiser
Retinal Disorders and Ophthalmic Genetics, Stein Eye Institute, University of California, Los Angeles, CA, USA
David Sarraf
The Retina Service at Wills Eye Hospital, Philadelphia, PA, USA
Sunir J. Garg
Center for Ophthalmic Bioinformatics, Cole Eye Institute, Cleveland Clinic, Cleveland, OH, USA
Rishi P. Singh
Cleveland Clinic Lerner College of Medicine, Cleveland, OH, USA
Department of Ophthalmology, University of Bonn, Boon, Germany
Frank G. Holz
Singapore Eye Research Institute, Singapore, Singapore
Tien Y. Wong
Singapore National Eye Centre, Duke-NUD Medical School, Singapore, Singapore
Centre for Eye Research Australia, Royal Victorian Eye and Ear Hospital, East Melbourne, VIC, Australia
Robyn H. Guymer
Department of Surgery (Ophthalmology), The University of Melbourne, Melbourne, VIC, Australia
You can also search for this author in PubMed Google Scholar
- Varun Chaudhary
- , Mohit Bhandari
- , Charles C. Wykoff
- , Sobha Sivaprasad
- , Lehana Thabane
- , Peter Kaiser
- , David Sarraf
- , Sophie J. Bakri
- , Sunir J. Garg
- , Rishi P. Singh
- , Frank G. Holz
- , Tien Y. Wong
- & Robyn H. Guymer
Contributions
AM was responsible for writing, critical review and feedback on manuscript. DZ was responsible for writing, critical review and feedback on manuscript. MRP was responsible for conception of idea, critical review and feedback on manuscript. SJB was responsible for critical review and feedback on manuscript. LT was responsible for critical review and feedback on manuscript. MB was responsible for conception of idea, critical review and feedback on manuscript. VC was responsible for conception of idea, critical review and feedback on manuscript.
Corresponding author
Correspondence to Varun Chaudhary .
Ethics declarations
Competing interests.
SJB: Consultant: Adverum, Allegro, Alimera, Allergan, Apellis, Eyepoint, ilumen, Kala, Genentech, Novartis, Regenexbio, Roche, Zeiss – unrelated to this study. MB: Research funds: Pendopharm, Bioventus, Acumed – unrelated to this study. VC: Advisory Board Member: Alcon, Roche, Bayer, Novartis; Grants: Bayer, Novartis – unrelated to this study. Rest authors have nothing to disclose.
Additional information
Publisher’s note Springer Nature remains neutral with regard to jurisdictional claims in published maps and institutional affiliations.
*A list of authors and their affiliations appears at the end of the paper.
Rights and permissions
Reprints and permissions
About this article
Cite this article.
Miroshnychenko, A., Zeraatkar, D., Phillips, M.R. et al. Cohort studies investigating the effects of exposures: key principles that impact the credibility of the results. Eye 36 , 905–906 (2022). https://doi.org/10.1038/s41433-021-01897-0
Download citation
Received : 26 November 2021
Revised : 30 November 2021
Accepted : 06 December 2021
Published : 13 January 2022
Issue Date : May 2022
DOI : https://doi.org/10.1038/s41433-021-01897-0
Share this article
Anyone you share the following link with will be able to read this content:
Sorry, a shareable link is not currently available for this article.
Provided by the Springer Nature SharedIt content-sharing initiative
Quick links
- Explore articles by subject
- Guide to authors
- Editorial policies

Open Access is an initiative that aims to make scientific research freely available to all. To date our community has made over 100 million downloads. It’s based on principles of collaboration, unobstructed discovery, and, most importantly, scientific progression. As PhD students, we found it difficult to access the research we needed, so we decided to create a new Open Access publisher that levels the playing field for scientists across the world. How? By making research easy to access, and puts the academic needs of the researchers before the business interests of publishers.
We are a community of more than 103,000 authors and editors from 3,291 institutions spanning 160 countries, including Nobel Prize winners and some of the world’s most-cited researchers. Publishing on IntechOpen allows authors to earn citations and find new collaborators, meaning more people see your work not only from your own field of study, but from other related fields too.
Brief introduction to this section that descibes Open Access especially from an IntechOpen perspective
Want to get in touch? Contact our London head office or media team here
Our team is growing all the time, so we’re always on the lookout for smart people who want to help us reshape the world of scientific publishing.
Home > Books > Cohort Studies in Health Sciences
Prospective Cohort Studies in Medical Research
Submitted: 05 December 2017 Reviewed: 15 March 2018 Published: 05 November 2018
DOI: 10.5772/intechopen.76514
Cite this chapter
There are two ways to cite this chapter:
From the Edited Volume
Cohort Studies in Health Sciences
Edited by R. Mauricio Barría
To purchase hard copies of this book, please contact the representative in India: CBS Publishers & Distributors Pvt. Ltd. www.cbspd.com | [email protected]
Chapter metrics overview
3,690 Chapter Downloads
Impact of this chapter
Total Chapter Downloads on intechopen.com

Total Chapter Views on intechopen.com
Overall attention for this chapters
Cohort studies are the analytical design of observational studies that are epidemiologically used to identify and quantify the relationship between exposure and outcome. Due to the longitudinal design, cohort studies have several advantages over other types of observational studies. The purpose of this chapter is to cover the various characteristics of prospective cohort studies. This chapter is divided into three main sections. In the first we introduce the concept and ranking of cohort studies, as well as the advantages and disadvantages. In the second we focus on the design of cohort studies, mainly its prospective aspect, and the distinguishing features from the retrospective type. The section also covers the essential characteristics of a cohort study design and its varied applications in medical research. In the third we go over examples of prospective studies in the medical field. For each, an overview of the study design is given, along with a random selection of study findings/impact, strengths and weaknesses.
- observational study
- cohort study
- prospective cohort study
- longitudinal study
- study design
- epidemiology
- medical research
Author Information
Samer hammoudeh.
- Hamad Medical Corporation, Doha, Qatar
Wessam Gadelhaq
Ibrahim janahi *.
*Address all correspondence to: [email protected]
1. Cohort studies
1.1. introduction.
The term “cohort” originates from Latin “cohors” [ 1 ]. A term that was used in the military back in Roman times, which referred to a unit that is comprised of 300–600 men, of which each 10 cohorts were named a legion [ 2 ]. In the field of epidemiology, Frost was the first to introduce the term “cohort study” back in 1935 [ 3 ]. Cohort refers to a group of individuals that share a common factor or a defining characteristic [ 4 , 5 ], or in other words, cohort is a certain component of a specific population that can be measured and followed throughout time [ 6 ]. Cohort studies are classified under the non-experimental type of studies [ 4 ], which are observational by default [ 7 ].
A cohort study follows people as groups, two or more, from exposure to outcome [ 2 , 8 ]. The two groups would be categorized based on their exposure status to “exposed” and “unexposed” [ 4 , 9 , 10 ]. If there were multiple groups then these would be categorized either by the type or level of exposure [ 4 ]. The main characteristic of a cohort study is that it follows participants in a forward manner, from the presence of the exposure to the presence of the outcome [ 2 , 9 , 10 , 11 ]. Or as De Rango describes it: using a longitudinal pattern, a cohort study, follows a group or groups of individuals over time in order to ascertain the incidence of a predetermined outcome after being exposed to a certain factor, whether being a risk factor, medication, or intervention [ 12 ]. Cohort studies can either be prospective (concurrent) or retrospective (non-concurrent) [ 9 ].
1.2. Ranking of cohort studies
Researchers agree that cohort studies, as related to the hierarchy of evidence, rank below meta-analysis, systematic review and randomized controlled trial, but rank higher than case–control studies, cross sectional studies, case series/reports [ 13 , 14 , 15 , 16 ]. As newer models or classifications of the hierarchy of evidence have emerged, where meta-analysis and systematic reviews have been removed from the hierarchy and repositioned as a magnifying glass or a lens through which evidence from other types of studies can be viewed or scrutinized; cohort studies remain below randomized controlled trials and higher than the other types [ 17 ]. Cohort studies provide information on the relationship between exposure and outcome when a randomized controlled trial is not possible to conduct for whatever reason [ 6 , 15 ].
1.3. Advantages of cohort studies
Cohort studies are the design of choice when randomization is not practical or ethical [ 6 , 18 ]. They are also useful in the study of infections [ 9 ] and for hypothesis generation [ 19 ]. Due to the design of cohort studies, and since temporal sequence is present, both incidence rate and cumulative incidence can be calculated [ 2 , 8 , 20 , 21 , 22 ]. They also allow for the measurement of relative risk (RR) [ 2 , 8 , 23 ], hazard ratio [ 8 ], and attributable risk [ 8 , 23 ]. Furthermore, they allow for the study of multiple outcomes that can be associated with a single type of exposure [ 2 , 20 ] or multiple exposures [ 18 ]. Additionally, they allow for the study of rare exposures [ 2 , 18 , 20 ]. Finally, cohort studies have lower risk of encountering survivor bias [ 2 ], and recall bias [ 9 , 21 ]. Survivor bias occurs when focusing only on those who survived or made it through a certain criteria or point, and ignoring those that didn’t, such as studying rapidly fatal diseases [ 2 ].
1.4. Disadvantages of cohort studies
Among the disadvantages of cohort studies is selection bias, which may occur when the participants are not representative of the population or of the patient grouping that they fall under. This in turn will influence how well or not the results can be generalized to the rest of the population, in what is known as external validity [ 2 , 12 , 18 , 24 , 25 ]. This will be covered later in section three of this chapter under aspects of cohort studies. Another disadvantage is that causation cannot be established from cohort studies [ 18 , 20 ], as it would require an experimental design in order to determine any causal effect [ 20 ]. However, due to the longitudinal design of cohort studies, they may aid in studying a certain causal hypothesis [ 20 ]. A third disadvantage is that they require a large sample size, which might pose an issue when dealing with outcomes that take a long time to develop [ 10 ]. Finally, cohort studies cannot be used to study rare outcomes [ 23 ].
2. Prospective cohort studies
2.1. types of cohort studies.
Cohort studies are either prospective or retrospective [ 1 , 2 , 18 ]. In the former, the researcher would assess exposure at baseline and then follow the person over time in order to determine the outcome such as the development of a disease [ 9 , 18 , 20 , 21 , 26 ]. In the latter, the order is reversed, as a cohort is established after the follow up has been conducted, or the outcome has developed, and exposure is then assessed in a retrospective manner [ 9 , 18 , 20 , 21 , 27 ]. Merrill indicates that the outcome status at the start of the study is what determines the overall study type. If the outcome has not yet developed then it is a prospective study, and if the outcome has already developed then it is a retrospective study [ 23 ]. Cohort studies can also be classified based on whether or not participants are replaced once they are lost. If those that drop out or are lost to follow up are replaced with new participants, then this would be classified as a dynamic or an open cohort. In the case that those lost do not get replaced, then it would be classified as a fixed or closed cohort [ 4 , 20 ].
2.1.1. Prospective cohort studies
Prospective cohort studies, as the name indicates, observes a group of people after being exposed to a certain factor in order to investigate the outcome, following the natural sequence of time, starting with the present and looking forward in time [ 12 , 18 , 20 ], which in turn provides true risk (absolute) estimates for the groups under investigation [ 26 ]. It is considered the gold standard among observational studies [ 8 ]. Under this type of study, the researcher would have control over data collection methodology, as well as the overall cohort study set up, which gives prospective cohort studies an advantage over retrospective cohort studies [ 9 ]. Further advantages and disadvantages of prospective cohort studies are discussed below.
2.1.1.1. Advantages of prospective cohort studies
Euser et al. highlight the major advantage of prospective cohort studies as being accurate in regards to the information collected about exposures, endpoints, and confounders [ 18 ]. Others list the following as advantages of prospective cohort studies; first: the exposure has already been measured before the outcome has occurred, which allows for the assessment of temporal sequence [ 28 ]. This allows for the calculation of incidence and the determination of the disease process [ 2 , 12 , 20 , 23 ]. Second: elimination of recall bias, as there is no need for any recollection of information since the data is being collected in a prospective manner [ 7 ]. However, Kip et al. reported that recall bias can pose an issue in prospective cohort studies if the exposure is self-reported, brief, and requires multiple measurements, such as stress episodes [ 29 ]. Third: It allows for the study of exposures were randomization is not practical or ethical [ 12 ]. Fourth: it allows for the study of rare exposures [ 20 ]. Fifth: it allows for the study of multiple outcomes [ 20 , 26 ].
2.1.1.2. Disadvantages of prospective cohort studies
Among the disadvantages of prospective cohort studies is the loss to follow up, which is common among cohort studies. This can ultimately lead to differential loss to follow up among those exposed and unexposed, which in turn can complicate the interpretation of the results [ 2 , 7 , 12 , 18 , 24 ]. Another disadvantage is that they are time consuming if follow up periods are far apart. This would be resource consuming as well, which would make prospective cohort studies not suitable for the study of outcomes that take long time to develop [ 18 , 20 , 24 , 26 ]. A third disadvantage is that they are expensive to conduct [ 18 , 20 , 30 ]. The third section of this chapter is dedicated to providing examples of prospective cohort studies.
2.1.2. Retrospective cohort studies
As previously described, retrospective cohort studies, also known as historic [ 28 ] or historical [ 24 ] cohorts, use data that has already been collected, such as databases of healthcare records, in order to investigate the association between the exposure and the outcome [ 22 , 24 , 26 , 28 ]. Although the outcome has already occurred, the design of retrospective cohort studies is similar to those of prospective cohort studies [ 22 ]. They also have similar advantages and disadvantages [ 26 , 28 ]. Hess indicates that retrospective studies in general are useful as pilot studies for future prospective studies [ 31 ].
Retrospective cohort studies have advantages and disadvantages. They are time efficient and cheap since the data has been collected previously and is available for scrutiny [ 18 , 20 , 26 ]. Additionally, since the exposure has already been measured before the outcome has occurred, this allows for the assessment of temporal sequence [ 28 ]. However, retrospective cohort studies use information that has been collected in the past for another objective other than the current study [ 18 ], and in some cases, collected for a purpose that is not related to medical research [ 9 ]. Due to this factor, the investigator lacks control over the collection of data [ 24 , 26 , 27 ]. Additionally, the measurement of exposure and outcome might be inconsistent or inaccurate, which can become a source of bias [ 24 , 27 , 28 , 31 , 32 ].
High plasma phosphate as a risk factor for decline in renal function and mortality in pre-dialysis patients [ 18 , 33 ]. In this study, Voormolen et al. followed the clinical course among incident pre-dialysis patients, using medical charts, to study the decline in kidney function and its association with plasma phosphate levels [ 18 , 33 ].
Assessment of female sex as a risk factor in atrial fibrillation in Sweden: nationwide retrospective cohort study [ 28 , 34 ]. In this study, Friberg et al. investigated gender differences in the incidence of stroke among those with atrial fibrillation using the Swedish hospital discharge registry [ 28 , 34 ].
Outcomes of care by hospitalists, general Internists, and family physicians [ 35 ]. In this study, Lindenauer et al. collected data from various hospitals in the USA, and compared the outcome of patients treated by the three types of care provider [ 35 ].
2.1.3. Aspects of cohort studies
2.1.3.1. validity.
Validity is the epidemiological assessment to the lack of systematic error [ 4 , 11 ]. There are two types of validity: internal validity and external validity [ 4 , 11 , 25 ]. Internal validity refers to the inferences made from the study that are related to the same source population [ 4 , 5 , 11 , 25 , 36 ], as to whether or not the study has measured what it had originally planned on measuring [ 25 , 36 ]. For an example, if the exposure caused the observed change in the outcome, then the study would be considered to have high internal validity [ 11 ]. On the other hand, if the observed change in the outcome was caused by a systematic error (bias), then the study would be considered to have low internal validity [ 11 ]. Threats or violations to internal validity will be discussed later in this section under bias.
External validity refers to the degree to which the study results can be generalized to other populations [ 4 , 5 , 11 , 25 , 36 ]. For example, if the study participants were not representative of the general population, then the study results cannot be generalizable to others [ 12 ]. The highest level of external validity occurs when the results can be generalized to three other domains: other populations, other environments, and other times [ 36 ]. External validity can be improved by using random selection [ 37 ].
It is essential to have internal validity in order to establish external validity; that is the study must have internal validity in the first place in order to have external validity [ 4 , 11 ]. For an example, if the exposure caused the observed change in the outcome, then the results can be generalizable to others. If the observed change was caused by any other factor, then the results cannot be generalized to others [ 4 , 11 ]. Based on the validity hierarchy, cohort studies are considered to have low internal validity, while the external validity is high [ 11 , 16 ].
2.1.3.2. Bias
Bias is a study systematic error in the design, conduct, or analysis that can be categorized into three main categories: selection bias, information bias, and confounding [ 4 , 25 , 38 ]. Selection bias occurs when the sample chosen for the study is not obtained randomly, so that the sample chosen is no longer representative of the overall population [ 4 , 25 , 38 , 39 ]. This type of bias includes three types: attrition bias, non-respondent bias, and the healthy entrant effect [ 38 ]. Attrition bias, or loss to follow up bias, occurs due to dropouts or death, which can be encountered in studies with long follow up durations (prospective) [ 23 ]. Non-respondent bias occurs when those that respond are different than those that don’t respond. For example, nonsmokers are more likely to return questionnaires about smoking than smokers are [ 25 ]. The healthy entrant effect or the healthy worker effect occurs when there are differences between those that are exposed and those that are not exposed. For an example, when comparing working individuals to the general population, as workers are more likely to be healthier than the general population. In order to avoid this type of bias, it is recommended to use two similar groups, such as using two groups of working individuals [ 23 ].
Information bias (measurement bias) [ 25 ], occurs when the data obtained is being recorded inaccurately [ 4 , 25 , 38 , 39 , 40 ]. This type of bias can be differential (nonrandom) or nondifferential (random) as related to the outcome [ 4 , 9 , 23 , 25 ]. The former is dependent on other variables and leads to overestimation or underestimation of any possible association, while the latter is independent from other variables and leads to underestimation of any possible association [ 4 , 9 , 23 ], and if the exposure was dichotomous, this type leads to bias towards the null [ 9 ]. Non differential is more commonly encountered in cohort studies [ 9 ]. Information bias can be reduced by using standardized assessment tools that have been validated [ 9 ]. Information bias is also known as classification bias, observation bias [ 25 ], or misclassification bias [ 23 ].
Confounding: confounding is a distortion of the effect [ 4 , 25 ] that may lead to overestimation or underestimation of an effect, or even reversing the direction of an effect [ 4 ]. A confounding factor is a risk factor that is associated with the exposure and influences the outcome, however, is not related to the causation sequence [ 4 , 25 , 39 ]. Unlike selection and information bias, confounding can be controlled for prior to study initiation, or after study completion [ 25 ]. Controlling for confounding factors can be accomplished through: restriction, matching, stratification, and using multivariate techniques [ 23 , 25 , 27 ].
Restriction would involve excluding those with the confounding factor [ 23 , 25 ]. If the confounding factor is categorical, then participants that fall within that category would be excluded [ 4 ], such as if smoking was considered to be a confounding factor, then those that smoke would be excluded [ 25 ]. If the confounding factor was continuous, such as age, then a range of that variable would be used to restrict the confounding [ 4 ]. Matching would involve choosing two groups that are similar to each other as much as possible [ 23 , 25 , 41 ], such as matching by gender or age [ 39 ]. Matching can be either individual matching or frequency matching. The former involves matching on an individual participant level, while the latter refers to matching on a group level [ 4 ]. Overmatching may occur when matching is being used, which may reflect on the statistical efficiency, validity, or cost efficiency of the study [ 4 ]. After the completion of the study, and during the analysis stage, stratification can be used to control for confounding by dividing the groups into several subgroups that are based on the confounding factor [ 23 , 25 , 39 , 41 ]. Multivariate techniques are also used during the analysis stage and allow for the control of multiple factors [ 25 , 39 , 41 ].
2.1.3.3. Exposure and risk
Exposure must be determined using a clear and accurate definition [ 2 , 22 ], which in some cases may involve levels of exposure [ 2 ]. This helps in eliminating possible selection bias [ 2 ]. The challenge becomes greater when there are multiple exposure assessments over an extended period of time [ 30 ]. The validity and the cost are two important aspects that must be taken into consideration when selecting an exposure measurement tool [ 30 ].
Both groups, those exposed and those that are not exposed should be at risk of eventually developing the outcome at some stage [ 2 ]. The exclusion criteria should exclude those that are not at risk of developing the outcome [ 24 ]. For an example, a study investigating the role of antipsychotics in the development of diabetes, should exclude those with diabetes to start with, since they are not at risk [ 10 ]. This helps in eliminating possible selection bias [ 2 ].
2.1.3.4. Outcomes
Outcomes should have a clear and specific definition from the beginning of the study [ 2 , 22 ], which must be measurable as well [ 2 , 22 ]. Outcomes should also be measured in a similar manner across all participants [ 2 , 22 ]. This helps in eliminating possible information bias [ 2 ]. It is recommended to use measurement tools that have been previously validated when dealing with secondary data, and to blind those who are assessing the outcome when dealing with primary data [ 10 ].
2.1.3.5. Controls
The comparison group or controls (unexposed group) should be similar to the exposed group in all possible aspects, but differ in regards to the exposure itself [ 2 ]. Three types of controls can be used, with the first being the most preferable: internal comparisons, other external cohorts, and the general population [ 2 ].
2.1.3.6. Follow up
To avoid loss to follow up and its consequent effects on the validity of the study results; measures should be taken in order to minimize the attrition rate [ 2 , 22 , 24 , 27 , 42 ]. Some of these actions include excluding those that are at high risk of not committing to the study, providing incentives for participation, collecting personal information that would allow or facilitate future contact, and maintaining ongoing contact on regular basis during the conduction period of the study [ 2 , 23 , 24 , 27 ]. The maximum acceptable limit for loss to follow up is 20% [ 23 , 24 , 42 ].
2.1.3.7. Precision
Precision is based on the absence of random error or chance [ 4 , 11 ]. This random variation can be due to the sample itself, or how it was selected, or how it was measured [ 4 , 11 ]. Standard deviations and confidence intervals are useful in determining the precision of a study, as a large standard deviation or a wide confidence interval would indicate low precision [ 11 ]. Random error or variation can be reduced by increasing the sample size [ 4 , 27 , 43 ], improving how you sample and how you measure, in addition to using the appropriate statistical methods [ 43 ].
2.1.3.8. Analysis of data
The main statistical term or product of cohort studies is the relative risk or risk ratio [ 6 , 21 ], which represents the risk of developing the outcome among those that are exposed in relation to those that are not exposed [ 20 ]. An RR that is equivalent to 1 indicates an absence of any type of association. An RR that is greater than 1 would indicate that there is a positive correlation between the exposure and risk of developing a disease. An RR that is smaller than 1 would indicate the presence of a protective effect between the exposure and the outcome [ 12 ]. Other outcome measures include: hazard ratios, survival curves, and life-table rates [ 2 ]. Some of the common statistical analysis involving cohort studies include: analysis of variance (ANOVA), multivariate analysis of variance (MANOVA), mixed effect regression model, and generalized estimating equation models [ 7 ].
2.1.3.9. Reporting
The reporting of prospective cohort studies should follow the STROBE guidelines [ 12 ], which also apply to other observational studies [ 41 , 44 ]. This acronym stands for: Strengthening the Reporting of Observational Studies in Epidemiology. These guidelines were designed by a group of international scholars including journal editors, epidemiologists, statisticians and researchers in order to set universal standards when reporting observational studies. It is comprised of a 22 item checklist that precisely dictates what should be reported under each section of an article [ 44 , 45 , 46 , 47 ]. Sessler and Imrey indicate that the most crucial ones are related to the study: objectives, methodology, definitions, source of data, statistical analysis, participants, and results [ 41 ]. Further information can be found at http://www.strobe-statement.org/ .
Bookwala et al. outlined three main factors that aid in evaluating prospective cohort studies in their article titled “the three-minute appraisal of a prospective cohort study”. These are related to (1) comparison groups selection; (2) the impact of confounding variables; (3) type of analytical strategy used [ 48 ]. Finally, the equator network (which is supported by the University of Oxford, UK, and aims to improve the quality and transparency of health research) provides guidelines and instructions for the reporting of various kinds of studies. These can be found at www.equator-network.org . Additional information regarding what to look for in a cohort study, as well as evaluation checklists can be found elsewhere [ 2 , 8 , 11 , 25 , 39 , 48 , 49 ]. The next section of this chapter will cover examples of famous prospective cohort studies from the medical field.
3. Examples of prospective cohort studies
3.1. the framingham heart study, 3.1.1. overview.
The Framingham heart study, initiated in 1948 by The National Heart Institute (currently the National Heart, Lung, and Blood Institute) [ 50 ], is considered to be the longest, ongoing, prospective cohort study in the history of the USA [ 51 ]. Others view it as a live model that illustrates the cohort design [ 52 ]. The study was based on the hypothesis that arteriosclerosis and hypertensive cardiovascular disease are the result of several causation factors combined rather than an individual factor [ 53 ]. Based on this, the aim of the study was to investigate the factors that contribute to the development of cardiovascular disease (CVD) by following a large cohort of individuals over a long period of time [ 50 ]. Back then in 1951, when the first article about the study was published, little was known about arteriosclerosis and hypertensive cardiovascular disease [ 53 ].
The original cohort included 5209 participants, ages 30–62 years, that were recruited at the beginning of the study in the town of Framingham, Massachusetts, USA [ 50 ]. The same cohort has been followed since initiation every two years for physical, laboratory, and lifestyle examinations [ 50 ]. The second generation, the offspring cohort, was recruited in 1971 and included 5124 participants. While 1994 witnessed the enrollment of the first Omni cohort (n = 506), in order to diversify the study population. More recently in 2002, the third generation cohort (n = 4095) was enrolled, while in 2003 the new offspring cohort (n = 103), and the second Omni group (n = 410) was enrolled [ 50 ]. The study continues to follow these cohorts every 2–6 years [ 54 ]. This multi generation, multi ethnicity, enrollment design aided significantly in the study of genetics in relation to a wide range of factors and illnesses [ 51 , 54 ].
Based on the Framingham study data, since initiation and through November 2017, a total of 3561 articles have been published so far [ 55 ]. The accumulation of knowledge that has risen from this study has shed the light on cardiovascular disease risk factors [ 50 , 51 , 56 ], by further expanding on our understanding of chronic illnesses such as diabetes, obesity, metabolic syndrome and nonalcoholic fatty liver disease [ 51 , 57 ]. Such risk factors include high blood pressure, high cholesterol levels, smoking, obesity, diabetes, and physical inactivity [ 50 , 57 ].
The study was the basis of which the Framingham risk score was built on [ 56 ]. Initially published by Wilson et al. in 1998 [ 58 ], it allows for the calculation of a 10 year risk estimate of developing coronary heart disease (CHD) based on the levels of different variables [ 56 , 58 ]. This would allow for the undertaking of preventive measures [ 56 ]. Later on in 2002, the Adult Treatment Panel of the National Cholesterol Education Program used the risk score as a foundation for its risk calculator [ 56 ].
3.1.2. Study findings
The study website ( https://www.framinghamheartstudy.org/about-fhs/research-milestones.php ) covers a long list of findings, among those; cigarette smoking was discovered to increase ones risk of developing heart disease back in 1960. In 1970, high blood pressure was discovered to increase ones risk of stroke. In 1988, the beneficial effects of HDL cholesterol were discovered. In 2002, the study found that obesity is considered a risk factor leading to heart failure. More recently in 2010 sleep apnea was linked to a higher risk of stroke [ 59 ]. More information and a full list of research milestones can be found elsewhere [ 59 ].
3.1.3. Strengths and weaknesses
In addition to what had been previously discussed regarding the benefits of the prospective design of the study, a high retention rate is among the strengths of the Framingham Heart Study as participants continue to return for their follow up visits despite the years [ 54 ]. Among the weaknesses is that the study was conducted in one population residing in one locality [ 7 ], which in turn reflects on the ability to generalize findings to other populations [ 58 ]. Another weakness is that the study cohort was not randomly selected, as investigators had to use volunteers in order to obtain the necessary sample. The final cohort ended up being more healthy when compared to the general population [ 7 , 60 ].
3.2. The Nurses’ Health Study (NHS)
3.2.1. overview.
This National Institutes of Health (NIH) funded study started in 1976 [ 61 ], and as of today includes more than 275,000 participants and counting, as the Nurses’ Health Study 3 is still recruiting subjects [ 62 ]. The study looks into the risk factors that have been implicated in major chronic diseases among women [ 62 ]. Initially, the study focused on heart disease, cancer, smoking, and contraceptive methods [ 61 ]. As the study evolved, it investigated many other lifestyle factors, characteristics, and diseases [ 61 , 63 ].
The original cohort of the study has been followed up on by mail every two years, with a minimum response rate of 90% [ 61 ]. The second cohort, under NHS 2, was enrolled in 1989 and included 116,430 women. These also were followed up on using mail every two years. A food frequency questionnaire was added in 1991 and was mailed out every four years, with a response rate of 85–90%. Later on blood and urine samples were collected from participants [ 61 ]. The third cohort, under NHS 3, was enrolled in 2010 and is still enrolling, with a goal of diversifying the study population to include other ethnic backgrounds [ 61 ].
3.2.2. Study findings
The study website ( http://www.nurseshealthstudy.org/about-nhs/key-contributions-scientific-knowledge ) covers numerous study findings, such as reporting lower risk of colon cancer and polyps with higher levels of vitamin D [ 64 ]. Also among the findings, Giovannucci et al. reported lower risk rates of colon cancer with longer duration of aspirin usage [ 65 ]. Baer et al. reported on mortality related risk factors among the NHS cohort [ 66 ]. Other findings related to breast cancer, CHD, stroke, colon cancer, hip fracture, cognitive function, and eye disease, in relation to cigarette smoking, oral contraceptives, post-menopausal hormone therapy obesity, alcohol, and diet can be found elsewhere [ 64 , 67 , 68 , 69 , 70 , 71 , 72 , 73 , 74 , 75 , 76 , 77 , 78 , 79 ]. More recently Colditz et al. summarized the findings and impact of the three NHS studies in an article published in the American Journal of Public Health [ 80 ].
3.2.3. Strengths and weaknesses
With focus on women, it is considered to be the longest and largest running prospective cohort study that investigates the role of lifestyle on health [ 63 ]. Among the strengths of this study is that it included multiple assessments of the various lifestyle characteristics and exposure factors [ 63 , 80 ], in turn, it also contributed to the methodology of lifestyle assessment in general, which has been used in other studies [ 63 , 80 ]. Additionally, it allowed for the calculation of mortality rates [ 63 ]. As for the weaknesses, white women dominated the original cohort, which reflects on the generalizability of the study results [ 4 , 63 ].
3.3. The Caerphilly Prospective Study (CAPS)
3.3.1. overview.
Also known as the Caerphilly Heart Disease Study, this study was conducted in Caerphilly, South Wales, UK, and focused on ischemic heart disease (IHD) in relation to hormones, hemostatic factors, and lipids [ 81 ]. As the study evolved, other investigations were included which looked into cognitive function, stroke and hearing problems [ 81 ].
The study included four phases. In the first phase, 2512 males, ages 45–59 years, were recruited in 1979. The procedures included blood tests, electrocardiogram (ECG), clinical history, lifestyle and IHD related questionnaires [ 81 ]. The second phase ran from 1984 to 1988 and included 447 males. An audiometry test was added to the list of investigations that were included in the first phase [ 81 ]. Phase 3 took place from 1989 to 1993 and added a cognitive function test and a bleeding time test [ 81 ]. Phase 4 was conducted from 1993 to 1997, which included the audiometry and cognitive function tests originally included in the second and third phases, respectively [ 81 ]. Follow up was conducted at a later stage through mail. The study has accumulated in a total of 150 studies and counting [ 81 ].
3.3.2. Study findings
Among the findings of the Caerphilly Prospective study; Elwood et al. showed that adopting a healthy lifestyle was associated with lower rates of chronic disease, as well as less cognitive impairment and dementia [ 82 ]. In other findings, Mertens et al. reported an inverse association between CVD and adopting a healthy diet [ 83 ], while Bolton et al. reported an inverse association between mid-life lung function and arterial stiffness among men [ 84 ]. Additional findings can be found elsewhere [ 85 , 86 , 87 , 88 , 89 , 90 , 91 ].
3.4. Conclusion
The three sections of this chapter covered the two types of cohort studies. Observational studies in general and cohort studies in specific are a good source of information when an experiment is not feasible. Prospective cohort studies provide valuable information when studying the relationship between exposure and outcome. As with any type of study, prospective cohort studies come with advantages and disadvantages that need to be taken into consideration when interpreting the results of these studies.
Conflict of interest
The author(s) declare no conflict of interest.
Abbreviations
- 1. Morabia A. A History of Epidemiologic Methods and Concepts. Birkhäuser: Switzerland, Basel; 2004
- 2. Grimes DA, Schulz KF. Cohort studies: Marching towards outcomes. The Lancet. 2002; 359 (9303):341-345
- 3. Doll R. Cohort studies: History of the method. I. Prospective cohort studies. Sozial- und Praventivmedizin. 2001; 46 (2):75-86
- 4. Rothman KJ, Greenland S, Lash TL. Modern Epidemiology. 3rd ed. Philadelphia: Wolters Kluwer Health/Lippincott Williams & Wilkins, c2008; 2008
- 5. Bryman A. Social research methods. 4th ed. Oxford; New York: Oxford University Press; 2012
- 6. Mann CJ. Observational research methods. Research design II: Cohort, cross sectional, and case-control studies. Emergency Medicine Journal. 2003; 20 (1):54
- 7. Caruana EJ, Roman M, Hernández-Sánchez J, Solli P. Longitudinal studies. Journal of Thoracic Disease. 2015; 7 (11):E537-EE40
- 8. Thiese MS. Observational and interventional study design types; an overview. Biochemia Medica. 2014; 24 (2):199-210
- 9. Miller AB, Goff DC, BammannDr K, Wild P. Cohort Studies. In: Ahrens W, Pigeot I, editors. Handbook of Epidemiology. New York, NY: Springer; 2014. pp. 259-291
- 10. Gamble J-M. An introduction to the fundamentals of cohort and case–control studies. The Canadian Journal of Hospital Pharmacy. 2014; 67 (5):366-372
- 11. Carlson MDA, Morrison RS. Study design, precision, and validity in observational studies. Journal of Palliative Medicine. 2009; 12 (1):77-82
- 12. De Rango P. Prospective cohort studies. European Journal of Vascular and Endovascular Surgery. 2016; 51 (1):151
- 13. Costantino G, Montano N, Casazza G. When should we change our clinical practice based on the results of a clinical study? The hierarchy of evidence. Internal and Emergency Medicine. 2015; 10 (6):745-747
- 14. Burns PB, Rohrich RJ, Chung KC. The levels of evidence and their role in evidence-based medicine. Plastic and Reconstructive Surgery. 2011; 128 (1):305-310
- 15. Petrisor BA, Bhandari M. The hierarchy of evidence: Levels and grades of recommendation. Indian Journal of Orthopaedics. 2007; 41 (1):11-15
- 16. Perry-Parrish C, Dodge R. Research and statistics: Validity hierarchy for study design and study type. Pediatrics in Review. 2010; 31 (1):27-29
- 17. Murad MH, Asi N, Alsawas M, Alahdab F. New evidence pyramid. Evidence-Based Medicine. 2016
- 18. Euser AM, Zoccali C, Jager KJ, Dekker FW. Cohort studies: Prospective versus retrospective. Nephron Clinical practice. 2009; 113 (3):c214-c217
- 19. Noordzij M, Dekker FW, Zoccali C, Jager KJ. Study designs in clinical research. Nephron Clinical Practice. 2009; 113 (3):c218-cc21
- 20. Levin KA. Study design IV: Cohort studies. Evidence-Based Dentistry. 2006; 7 :51
- 21. Munnangi S, Boktor SW. Epidemiology, Study Design. Treasure Island (FL): StatPearls Publishing LLC; 2017
- 22. Setia MS. Methodology Series Module 1: Cohort Studies. Indian Journal of Dermatology. 2016; 61 (1):21-25
- 23. Merrill RM. Introduction to Epidemiology. 6th ed. Burlington, MA: Jones & Bartlett Learning; 2012
- 24. Song JW, Chung KC. Observational studies: cohort and case-control studies. Plastic and Reconstructive Surgery. 2010; 126 (6):2234-2242
- 25. Grimes DA, Schulz KF. Bias and causal associations in observational research. Lancet. 2002; 359 (9302):248-252
- 26. Jekel JF. Epidemiology, Biostatistics, and preventive medicine. 3rd ed. Philadelphia: Saunders/Elsevier, c2007; 2007
- 27. Hulley SB, Cummings SR, Browner WS, Grady DG, Newman TB. Designing Clinical Research. Wolters Kluwer/Lippincott Williams & Wilkins; 2013
- 28. Sedgwick P. Retrospective cohort studies: advantages and disadvantages. BMJ: British Medical Journal. 2014; 348
- 29. Kip KE, Cohen F, Cole SR, Wilhelmus KR, Patrick DL, Blair RC, et al. Recall bias in a prospective cohort study of acute time-varying exposures: example from the herpetic eye disease study. Journal of Clinical Epidemiology. 2001; 54 (5):482-487
- 30. White E, Hunt JR, Casso D. Exposure measurement in cohort studies: the challenges of prospective data collection. Epidemiologic Reviews. 1998; 20 (1):43-56
- 31. Hess DR. Retrospective studies and chart reviews. Respiratory Care. 2004; 49 (10):1171
- 32. Sedgwick P. Prospective cohort studies: Advantages and disadvantages. BMJ: British Medical Journal. 2013; 347
- 33. Voormolen N, Noordzij M, Grootendorst DC, Beetz I, Sijpkens YW, van Manen JG, et al. High plasma phosphate as a risk factor for decline in renal function and mortality in pre-dialysis patients. Nephrology, dialysis, transplantation : official publication of the European Dialysis and Transplant Association - European Renal Association 2007;22(10):2909-2916
- 34. Friberg L, Benson L, Rosenqvist M, Lip GYH. Assessment of female sex as a risk factor in atrial fibrillation in Sweden: Nationwide retrospective cohort study. BMJ: British Medical Journal. 2012; 344
- 35. Lindenauer PK, Rothberg MB, Pekow PS, Kenwood C, Benjamin EM, Auerbach AD. Outcomes of care by hospitalists, general internists, and family physicians. The New England Journal of Medicine. 2007; 357 (25):2589-2600
- 36. Goodwin KA, Goodwin CJ. Research in Psychology: Methods and Design. 8th ed. Hoboken, NJ: Wiley; 2017
- 37. Trochim W, Donnelly JP. The Research Methods Knowledge Base. 3rd ed. Boston, MA: Cengage Learning; 2006
- 38. Sedgwick P. Bias in observational study designs: Prospective cohort studies. BMJ: British Medical Journal. 2014; 349
- 39. Hammer GP, du Prel J-B, Blettner M. Avoiding bias in observational studies: Part 8 in a series of articles on evaluation of scientific publications. Deutsches Ärzteblatt International. 2009; 106 (41):664-668
- 40. Boyko EJ. Observational research--Opportunities and limitations. Journal of Diabetes and its Complications. 2013; 27 (6):642-648
- 41. Sessler DI, Imrey PB. Clinical research methodology 2: Observational clinical research. Anesthesia & Analgesia. 2015; 121 (4):1043-1051
- 42. Dettori JR. Loss to follow-up. Evidence-Based Spine-Care Journal. 2011; 2 (1):7-10
- 43. Schoenbach V, Rosamond W. Understanding the Fundamentals of Epidemiology: An Evolving Text. University of North Carolina at Chapel Hill; 2000
- 44. von Elm E, Altman DG, Egger M, Pocock SJ, Gøtzsche PC, Vandenbroucke JP. The Strengthening the Reporting of Observational Studies in Epidemiology (STROBE) statement: Guidelines for reporting observational studies. Preventive Medicine. 2007; 45 (4):247-251
- 45. Vandenbroucke JP, von Elm E, Altman DG, Gøtzsche PC, Mulrow CD, Pocock SJ, et al. Strengthening the Reporting of Observational Studies in Epidemiology (STROBE): Explanation and elaboration. PLoS Medicine. 2007; 4 (10):e297
- 46. Vandenbroucke JP, Elm E, Altman DG, et al. Strengthening the reporting of observational studies in epidemiology (strobe): Explanation and elaboration. Annals of Internal Medicine. 2007; 147 (8):W-163-WW-94
- 47. Vandenbroucke JP, von Elm E, Altman DG, Gøtzsche PC, Mulrow CD, Pocock SJ, et al. Strengthening the Reporting of Observational Studies in Epidemiology (STROBE): Explanation and elaboration. Epidemiology 2007; 18 (6):805-835
- 48. Bookwala A, Hussain N, Bhandari M. The three-minute appraisal of a prospective cohort study. Indian Journal of Orthopaedics. 2011; 45 (4):291-293
- 49. Tooth L, Ware R, Bain C, Purdie DM, Dobson A. Quality of reporting of observational longitudinal research. American Journal of Epidemiology. 2005; 161 (3):280-288
- 50. FHS. History of the Framingham Heart Study 2017. Available from: https://www.framinghamheartstudy.org/about-fhs/history.php
- 51. Long MT, Fox CS. The Framingham Heart Study--67 years of discovery in metabolic disease. Nature Reviews Endocrinology. 2016; 12 (3):177-183
- 52. Oppenheimer GM. Framingham Heart Study: the first 20 years. Progress in Cardiovascular Diseases. 2010; 53 (1):55-61
- 53. Dawber TR, Meadors GF, Moore FE. Epidemiological approaches to heart disease: The Framingham Study. American Journal of Public Health and the Nations Health. 1951; 41 (3):279-286
- 54. Tsao CW, Vasan RS. Cohort Profile: The Framingham Heart Study (FHS): overview of milestones in cardiovascular epidemiology. International Journal of Epidemiology. 2015; 44 (6):1800-1813
- 55. FHS. Framingham Heart Study Bibliography 2017. Available from: https://www.framinghamheartstudy.org/fhs-bibliography/index.php
- 56. Mahmood SS, Levy D, Vasan RS, Wang TJ. The Framingham Heart Study and the epidemiology of cardiovascular diseases: A historical perspective. Lancet. 2014; 383 (9921):999-1008
- 57. Hajar R. Framingham contribution to cardiovascular disease. Heart Views : The Official Journal of the Gulf Heart Association. 2016; 17 (2):78-81
- 58. Wilson PW, D'Agostino RB, Levy D, Belanger AM, Silbershatz H, Kannel WB. Prediction of coronary heart disease using risk factor categories. Circulation. 1998; 97 (18):1837-1847
- 59. FHS. Research Milestones 2017. Available from: https://www.framinghamheartstudy.org/about-fhs/research-milestones.php
- 60. FHS. Epidemiological Background and Design: The Framingham Study 2017. Available from: https://www.framinghamheartstudy.org/about-fhs/background.php
- 61. NHS. History 2016. Available from: http://www.nurseshealthstudy.org/about-nhs/history
- 62. NHS. About NHS 2016. Available from: http://www.nurseshealthstudy.org/about-nhs
- 63. Colditz GA, Manson JE, Hankinson SE. The Nurses' Health Study: 20-year contribution to the understanding of health among women. Journal of Women's Health. 1997; 6 (1):49-62
- 64. NHS. Key contributions to scientific knowledge 2016. Available from: http://www.nurseshealthstudy.org/about-nhs/key-contributions-scientific-knowledge
- 65. Giovannucci E, Egan KM, Hunter DJ, Stampfer MJ, Colditz GA, Willett WC, et al. Aspirin and the risk of colorectal cancer in women. The New England Journal of Medicine. 1995; 333 (10):609-614
- 66. Baer HJ, Glynn RJ, Hu FB, Hankinson SE, Willett WC, Colditz GA, et al. Risk factors for mortality in the nurses' health study: A competing risks analysis. American Journal of Epidemiology. 2011; 173 (3):319-329
- 67. Bernstein AM, Pan A, Rexrode KM, Stampfer M, Hu FB, Mozaffarian D, et al. Dietary protein sources and the risk of stroke in men and women. Stroke. 2012; 43 (3):637-644
- 68. Chiuve SE, Fung TT, Rexrode KM, Spiegelman D, Manson JE, Stampfer MJ, et al. Adherence to a low-risk, healthy lifestyle and risk of sudden cardiac death among women. JAMA. 2011; 306 (1):62-69
- 69. Liu S, Willett WC, Stampfer MJ, Hu FB, Franz M, Sampson L, et al. A prospective study of dietary glycemic load, carbohydrate intake, and risk of coronary heart disease in US women. The American Journal of Clinical Nutrition. 2000; 71 (6):1455-1461
- 70. Hu FB, Manson JE, Stampfer MJ, Colditz G, Liu S, Solomon CG, et al. Diet, lifestyle, and the risk of type 2 diabetes mellitus in women. The New England Journal of Medicine. 2001; 345 (11):790-797
- 71. Hu FB, Bronner L, Willett WC, Stampfer MJ, Rexrode KM, Albert CM, et al. Fish and omega-3 fatty acid intake and risk of coronary heart disease in women. JAMA. 2002; 287 (14):1815-1821
- 72. Ayas NT, White DP, Manson JE, Stampfer MJ, Speizer FE, Malhotra A, et al. A prospective study of sleep duration and coronary heart disease in women. Archives of Internal Medicine. 2003; 163 (2):205-209
- 73. Curhan GC, Willett WC, Knight EL, Stampfer MJ. Dietary factors and the risk of incident kidney stones in younger women: Nurses' Health Study II. Archives of Internal Medicine. 2004; 164 (8):885-891
- 74. Holmes MD, Chen WY, Feskanich D, Kroenke CH, Colditz GA. Physical activity and survival after breast cancer diagnosis. JAMA. 2005; 293 (20):2479-2486
- 75. Eliassen AH, Missmer SA, Tworoger SS, Spiegelman D, Barbieri RL, Dowsett M, et al. Endogenous steroid hormone concentrations and risk of breast cancer among premenopausal women. Journal of the National Cancer Institute. 2006; 98 (19):1406-1415
- 76. Hunter DJ, Kraft P, Jacobs KB, Cox DG, Yeager M, Hankinson SE, et al. A genome-wide association study identifies alleles in FGFR2 associated with risk of sporadic postmenopausal breast cancer. Nature Genetics. 2007; 39 (7):870-874
- 77. Song H, Ramus SJ, Tyrer J, Bolton KL, Gentry-Maharaj A, Wozniak E, et al. A genome-wide association study identifies a new ovarian cancer susceptibility locus on 9p22.2. Nature Genetics. 2009; 41 (9):996-1000
- 78. Beral V, Doll R, Hermon C, Peto R, Reeves G. Ovarian cancer and oral contraceptives: collaborative reanalysis of data from 45 epidemiological studies including 23, 257 women with ovarian cancer and 87, 303 controls. Lancet. 2008; 371 (9609):303-314
- 79. Sarwar N, Gao P, Seshasai SR, Gobin R, Kaptoge S, Di Angelantonio E, et al. Diabetes mellitus, fasting blood glucose concentration, and risk of vascular disease: A collaborative meta-analysis of 102 prospective studies. Lancet. 2010; 375 (9733):2215-2222
- 80. Colditz GA, Philpott SE, Hankinson SE. The impact of the Nurses’ Health Study on population health: Prevention, translation, and control. American Journal of Public Health. 2016; 106 (9):1540-1545
- 81. Bristol Uo. Caerphilly Prospective Study: About 2017. Available from: https://www.bristol.ac.uk/population-health-sciences/projects/caerphilly/about/
- 82. Elwood P, Galante J, Pickering J, Palmer S, Bayer A, Ben-Shlomo Y, et al. Healthy lifestyles reduce the incidence of chronic diseases and dementia: Evidence from the Caerphilly Cohort Study. PLoS One. 2013; 8 (12):e81877
- 83. Mertens E, Markey O, Geleijnse JM, Lovegrove JA, Givens DI. Adherence to a healthy diet in relation to cardiovascular incidence and risk markers: evidence from the Caerphilly Prospective Study. European Journal of Nutrition. 2017
- 84. Bolton CE, Cockcroft JR, Sabit R, Munnery M, McEniery CM, Wilkinson IB, et al. Lung function in mid-life compared with later life is a stronger predictor of arterial stiffness in men: the Caerphilly Prospective Study. International Journal of Epidemiology. 2009; 38 (3):867-876
- 85. Patterson CC, Smith AE, Yarnell JW, Rumley A, Ben-Shlomo Y, Lowe GD. The associations of interleukin-6 (IL-6) and downstream inflammatory markers with risk of cardiovascular disease: The Caerphilly Study. Atherosclerosis. 2010; 209 (2):551-557
- 86. Gallacher J, Bayer A, Lowe G, Fish M, Pickering J, Pedro S, et al. Is sticky blood bad for the brain?: Hemostatic and inflammatory systems and dementia in the Caerphilly Prospective Study. Arteriosclerosis, Thrombosis, and Vascular Biology. 2010; 30 (3):599-604
- 87. Sarkar C, Gallacher J, Webster C. Built environment configuration and change in body mass index: The Caerphilly Prospective Study (CaPS). Health & Place. 2013; 19 :33-44
- 88. McEniery CM, Spratt M, Munnery M, Yarnell J, Lowe GD, Rumley A, et al. An analysis of prospective risk factors for aortic stiffness in men: 20-year follow-up from the Caerphilly prospective study. Hypertension (Dallas, Tex: 1979). 2010; 56 (1):36-43
- 89. Livingstone KM, Lovegrove JA, Cockcroft JR, Elwood PC, Pickering JE, Givens DI. Does dairy food intake predict arterial stiffness and blood pressure in men?: Evidence from the Caerphilly Prospective Study. Hypertension (Dallas, Tex: 1979). 2013; 61 (1):42-47
- 90. Eicher JD, Xue L, Ben-Shlomo Y, Beswick AD, Johnson AD. Replication and hematological characterization of human platelet reactivity genetic associations in men from the Caerphilly Prospective Study (CaPS). Journal of Thrombosis and Thrombolysis. 2016; 41 (2):343-350
- 91. Gallacher JE, Yarnell JW, Sweetnam PM, Elwood PC, Stansfeld SA. Anger and incident heart disease in the caerphilly study. Psychosomatic Medicine. 1999; 61 (4):446-453
© 2018 The Author(s). Licensee IntechOpen. This chapter is distributed under the terms of the Creative Commons Attribution 3.0 License , which permits unrestricted use, distribution, and reproduction in any medium, provided the original work is properly cited.
Continue reading from the same book
Published: 19 September 2018
By Muriel Ramirez-Santana
6001 downloads
By Cristhian Saavedra Santiesteban
1120 downloads
By René Mauricio Barría
1849 downloads
Log in using your username and password
- Search More Search for this keyword Advanced search
- Latest content
- Current issue
- Write for Us
- BMJ Journals More You are viewing from: Google Indexer
You are here
- Volume 22, Issue 4
What are cohort studies?
- Article Text
- Article info
- Citation Tools
- Rapid Responses
- Article metrics

- http://orcid.org/0000-0003-4308-4219 David Barrett 1 ,
- Helen Noble 2
- 1 Faculty of Health Sciences , University of Hull , Hull , UK
- 2 School of Nursing and Midwifery , Queen’s University Belfast , Belfast , UK
- Correspondence to Dr David Barrett, Faculty of Health Sciences, University of Hull, Hull HU6 7RX, UK; D.I.Barrett{at}hull.ac.uk
https://doi.org/10.1136/ebnurs-2019-103183
Statistics from Altmetric.com
Request permissions.
If you wish to reuse any or all of this article please use the link below which will take you to the Copyright Clearance Center’s RightsLink service. You will be able to get a quick price and instant permission to reuse the content in many different ways.
- statistics and research methods
In 1951, Richard Doll and Austin Bradford-Hill commenced a ground-breaking research project by writing to all registered doctors in the UK to ask about their smoking habits. The British Doctors Study recruited and followed-up over 40 000 participants, monitoring mortality rates and causes of death over the subsequent years and decades. Even by the time of the first set of preliminary results in 1954, there was evidence to link smoking with lung cancer and increased mortality. 1 Over the following decades, the study provided further definitive evidence of the health risks from smoking, and was extended to explore other causes of death (eg, heart disease) and other behavioural variables (eg, alcohol intake).
The Doctors Health Survey is one of the largest, most ambitious and best-known cohort studies and demonstrates the value of this approach in supporting our understanding of disease risk. However, as a method, cohort studies can have much wider applications. This article provides an overview of cohort studies, identifying the opportunities and challenges they present to researchers, and the role they play in developing the evidence base for nursing and healthcare more broadly.
Cohort studies are a type of longitudinal study —an approach that follows research participants over a period of time (often many years). Specifically, cohort studies recruit and follow participants who share a common characteristic, such as a particular occupation or demographic similarity. During the period of follow-up, some of the cohort will be exposed to a specific risk factor or characteristic; by measuring outcomes over a period of time, it is then possible to explore the impact of this variable (eg, identifying the link between smoking and lung cancer in the British Doctors Study.) Cohort studies are, therefore, of particular value in epidemiology, helping to build an understanding of what factors increase or decrease the likelihood of developing disease.
Though the most high-profile types of cohort studies are usually related to large epidemiological research studies, they are not the only application of this method. Within nursing research, cohort studies have focused on the progress of nurses through their education and careers. Li et al —as part of the European NEXT study group—recruited almost 6500 female nurses who, at the time of recruitment, had no intention to leave the profession. The study followed the cohort up for a year, identifying that 8% developed the intention to leave nursing, often due to issues such as poor salary or limited promotion prospects. 4
Usually, cohort studies should adopt a purely observational approach. However, some research is labelled as a cohort study while exploring the effectiveness of specific interventions. For example, Lansperger et al explored nurse practitioner (NP)-led critical care in a large university hospital in the USA. They collected data on all patients who were admitted to the intensive care unit over a 3-year period. Patients from this cohort were cared for by teams led by either doctors or NPs, and outcomes (primarily 90-day mortality) were monitored. By comparing the groups, the researchers established that outcomes were similar regardless of whether patient care was led by a doctor or an NP. 5
Strengths and weaknesses of cohort studies
Cohort studies are an effective and robust method of establishing cause and effect. As they are usually large in size, researchers are able to draw confident conclusions regarding the link between risk factors and disease. In many cases, because participants are often free of disease at the commencement of the study, cohort studies are particularly useful at identifying the timelines over which certain behaviours can contribute to disease.
However, the nature of cohort studies can cause challenges. Collecting prospective data on thousands of participants over many years (and sometimes decades) is complex, time-consuming and expensive. Participants may drop out, increasing the risk of bias; equally, it is possible that the behaviour of participants may alter because they are aware that they are part of a study cohort. The analysis of data from these large-scale studies is also complex, with large numbers of confounding variables making it difficult to link cause and effect. Where cohort (or ‘cohort-like’) studies link to a specific intervention (as in the case of the Lansperger et al study into nursing practitioner-led critical care 5 ), the lack of randomisation to different arms of the study makes the approach less robust than randomised controlled trials.
One way of making a cohort study less time-consuming is to carry it out retrospectively. This is a more pragmatic approach, as it can be completed more quickly using historical data. For example, Wray et al used a retrospective cohort study to identify factors that were associated with non-continuation of students on nursing programmes. By exploring characteristics in five previous cohorts of students, they were able to identify that factors such as being older and/or local were linked to higher levels of continuation. 6
However, this retrospective approach increases the risk of bias in the sampling of the cohort, with greater likelihood of missing data. Retrospective cohort studies are also weakened by the fact that the data fields available are not designed with the study in mind—instead, the researcher simply has to make use of whatever data are available, which may hinder the quality of the study.
Reporting and critiquing of cohort studies
When reporting a cohort study, it is recommended that STROBE guidance 7 is followed. STROBE is an international, collaborative enterprise which includes experts with experience in the organisation and of dissemination of observational studies, including cohort studies. The aim is to STrengthen the Reporting of OBservational studies in Epidemiology. The STROBE checklist for cohort studies - available at https://www.strobe-statement.org/fileadmin/Strobe/uploads/checklists/STROBE_checklist_v4_combined.pdf - includes detail related to the introduction/methods/results/discussion of the study.
Critical appraisal of any cohort study is essential to identify the strengths and weaknesses of the study and to determine the usefulness and validity of the study findings. Components of critical appraisal in relation to cohort studies include evaluation of the study design in relation to the research question, assessment of the methodology, suitability of statistical methods used, conflicts of interest and how relevant the research is to practice. 8–10
Cohort studies are the cornerstone of epidemiological research, providing an understanding of risk factors for disease based on findings in thousands of participants over many years. Disease prevention guidelines used by nurses and other healthcare professionals across the globe are based on the evidence from high-profile studies, such as the British Doctors Study, the Framingham Heart Study and the Nurses’ Health Study. However, cohort studies offer opportunities outside epidemiology: in nursing research, the approach is useful in exploring areas such as factors that influence students’ progression through their programme or nurses’ progression through their career.
This approach to research does bring with it some important challenges—often related to their size, complexity and longevity. However, with careful planning and implementation, cohort studies can make valuable contributions to the development of evidence-based healthcare.
- Colditz GA ,
- Philpott SE ,
- Hankinson SE
- Galatsch M ,
- Siegrist J , et al
- Landsperger JS ,
- Semler MW ,
- Wang L , et al
- Aspland J ,
- Barrett D , et al
- von Elm E ,
- Altman DG ,
- Egger M , et al
- Rochon PA ,
- Gurwitz JH ,
- Sykora K , et al
- Critical Appraisal Skills Programme
Competing interests None declared.
Patient consent for publication Not required.
Provenance and peer review Commissioned; internally peer reviewed.
Read the full text or download the PDF:
Cohort Study: Definition, Designs & Examples
Julia Simkus
Editor at Simply Psychology
BA (Hons) Psychology, Princeton University
Julia Simkus is a graduate of Princeton University with a Bachelor of Arts in Psychology. She is currently studying for a Master's Degree in Counseling for Mental Health and Wellness in September 2023. Julia's research has been published in peer reviewed journals.
Learn about our Editorial Process
Saul Mcleod, PhD
Editor-in-Chief for Simply Psychology
BSc (Hons) Psychology, MRes, PhD, University of Manchester
Saul Mcleod, PhD., is a qualified psychology teacher with over 18 years of experience in further and higher education. He has been published in peer-reviewed journals, including the Journal of Clinical Psychology.
Olivia Guy-Evans, MSc
Associate Editor for Simply Psychology
BSc (Hons) Psychology, MSc Psychology of Education
Olivia Guy-Evans is a writer and associate editor for Simply Psychology. She has previously worked in healthcare and educational sectors.
On This Page:
A cohort study is a type of longitudinal study where a group of individuals (cohort), often sharing a common characteristic or experience, is followed over an extended period of time to study and track outcomes, typically related to specific exposures or interventions.
In cohort studies, the participants must share a common factor or characteristic such as age, demographic, or occupation. A “cohort” is a group of subjects who share a defining characteristic.
Cohort studies are observational, so researchers will follow the subjects without manipulating any variables or interfering with their environment.
This type of study is beneficial for medical researchers, specifically in epidemiology, as scientists can use data from cohort studies to understand potential risk factors or causes of a disease.
Before any appearance of the disease is investigated, medical professionals will identify a cohort, observe the target participants over time, and collect data at regular intervals.
Weeks, months, or years later, depending on the duration of the study design, the researchers will examine any factors that differed between the individuals who developed the condition and those who did not.
They can then determine if an association exists between an exposure and an outcome and even identify disease progression and relative risk.
Retrospective
- A retrospective cohort study is a type of observational research that uses existing past data to identify two groups of individuals—those with the risk factor or exposure (cohort) and without—and follows their outcomes backward in time to determine the relationship.
- In a retrospective study , the subjects have already experienced the outcome of interest or developed the disease before starting the study.
- The researchers then look back in time to identify a cohort of subjects before developing the disease and use existing data, such as medical records, to discover any patterns.
Prospective
A prospective cohort study is a type of longitudinal research where a group of individuals sharing a common characteristic (cohort) is followed over time to observe and measure outcomes, often to investigate the effect of suspected risk factors.
In a prospective study , the investigators will design the study, recruit subjects, and collect baseline data on all subjects before they have developed the outcomes of interest.
- The subjects are followed and observed over a period of time to gather information and record the development of outcomes.
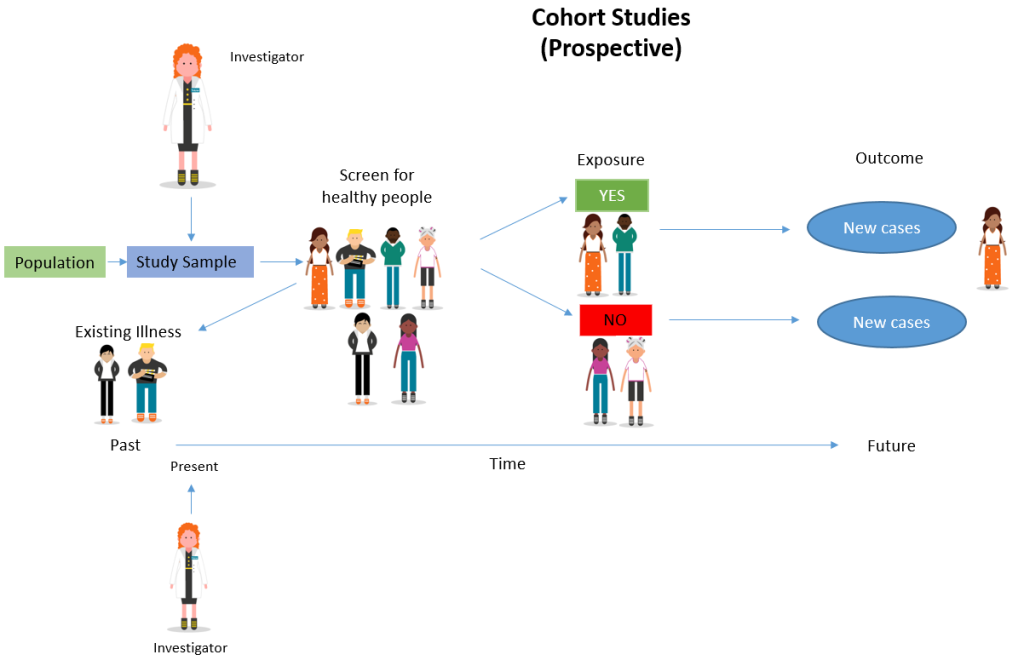
Determine cause-and-effect relationships
Because researchers study groups of people before they develop an illness, they can discover potential cause-and-effect relationships between certain behaviors and the development of a disease.
Provide extensive data
Cohort studies enable researchers to study the causes of disease and identify multiple risk factors associated with a single exposure. These studies can also reveal links between diseases and risk factors.
Enable studies of rare exposures
Cohort studies can be very useful for evaluating the effects and risks of rare diseases or unusual exposures, such as toxic chemicals or adverse effects of drugs.
Can measure a continuously changing relationship between exposure and outcome
Because cohort studies are longitudinal, researchers can study changes in levels of exposure over time and any changes in outcome, providing a deeper understanding of the dynamic relationship between exposure and outcome.
Limitations
Time consuming and expensive.
Cohort studies usually require multiple months or years before researchers are able to identify the causes of a disease or discover significant results. Because of this, they are often more expensive than other types of studies. Retrospective studies, though, tend to be cheaper and quicker than prospective studies as the data already exists.
Require large sample sizes
Cohort studies require large sample sizes in order for any relationships or patterns to be meaningful. Researchers are unable to generate results if there is not enough data.
Prone to bias
Because of the longitudinal nature of these studies, it is common for participants to drop out and not complete the study. The loss of follow-up in cohort studies means researchers are more likely to estimate the effects of an exposure on an outcome incorrectly.
Unable to discover why or how a certain factor is associated with a disease
Cohort studies are used to study cause-and-effect relationships between a disease and an outcome. However, they do not explain why the factors that affect these relationships exist. Experimental studies are required to determine why a certain factor is associated with a particular outcome.
The Framingham Heart Study
Studied the effects of diet, exercise, and medications on the development of hypertensive or arteriosclerotic cardiovascular disease, in a longitudinal population-based cohort.
The Whitehall Study
The initial prospective cohort study examined the association between employment grades and mortality rates of 17139 male civil servants over a period of ten years, beginning in 1967. When the Whitehall Study was conducted, there was no requirement to obtain ethical approval for scientific studies of this kind.
The Nurses’ Health Study
Researched long-term effects of nurses” nutrition, hormones, environment, and work-life on health and disease development.
The British Doctors Study
This was a prospective cohort study that ran from 1951 to 2001, investigating the association between smoking and the incidence of lung cancer.
The Black Women’s Health Study
Gathered information about the causes of health problems that affect Black women.
Millennium Cohort Study
Found evidence to show how various circumstances in the first stages of life can influence later health and development. The study began with an original sample of 18,818 cohort members.
The Danish Cohort Study of Psoriasis and Depression
Studied the association between psoriasis and the onset of depression.
The 1970 British Cohort Study
Followed the lives of around 17,000 people born in England, Scotland, and Wales in a single week of 1970.
Frequently Asked Questions
1. are case-control studies and cohort studies the same.
While both studies are commonly used among medical professionals to study disease, they differ.
Case-control studies are performed on individuals who already have a disease (cases) and compare them with individuals who share similar characteristics but do not have the disease (controls).
In cohort studies, on the other hand, researchers identify a group before any of the subjects have developed the disease. Then after an extended period, they examine any factors that differed between the individuals who developed the condition and those who did not.
2. What is the difference between a cross-sectional study and a cohort study?
Like case-control and cohort studies, cross-sectional studies are also used in epidemiology to identify exposures and outcomes and compare the rates of diseases and symptoms of an exposed group with an unexposed group.
However, cross-sectional studies analyze information about a population at a specific point in time, while cohort studies are carried out over longer periods.
3. What is the difference between cohort and longitudinal studies?
A cohort study is a specific type of longitudinal study. Another type of longitudinal study is called a panel study which involves sampling a cross-section of individuals at specific intervals for an extended period.
Panel studies are a type of prospective study, while cohort studies can be either prospective or retrospective.
Barrett D, Noble H. What are cohort studies? Evidence-Based Nursing 2019; 22:95-96.
Kandola, A.A., Osborn, D.P.J., Stubbs, B. et al. Individual and combined associations between cardiorespiratory fitness and grip strength with common mental disorders: a prospective cohort study in the UK Biobank. BMC Med 18, 303 (2020). https://doi.org/10.1186/s12916-020-01782-9
Marmot, M. G., Rose, G., Shipley, M., & Hamilton, P. J. (1978). Employment grade and coronary heart disease in British civil servants. Journal of Epidemiology & Community Health, 32(4), 244-249.
Rosenberg, L., Adams-Campbell, L., & Palmer, J. R. (1995). The Black Women’s Health Study: a follow-up study for causes and preventions of illness. Journal of the American Medical Women’s Association (1972), 50(2), 56-58.
Samer Hammoudeh, Wessam Gadelhaq and Ibrahim Janahi (November 5th 2018). Prospective Cohort Studies in Medical Research, Cohort Studies in Health Sciences, R. Mauricio Barría, IntechOpen, DOI: 10.5772/intechopen.76514. Available from: https://www.intechopen.com/chapters/60939
Setia M. S. (2016). Methodology Series Module 1: Cohort Studies. Indian journal of dermatology, 61(1), 21–25. https://doi.org/10.4103/0019-5154.174011
Zabor, E. C., Kaizer, A. M., & Hobbs, B. P. (2020). Randomized Controlled Trials. Chest, 158(1). https://doi.org/10.1016/j.chest.2020.03.013
Further Information
- Cohort Effect? Definition and Examples
- Barrett, D., & Noble, H. (2019). What are cohort studies?. Evidence-based nursing, 22(4), 95-96.
- The Whitehall Studies
- Euser, A. M., Zoccali, C., Jager, K. J., & Dekker, F. W. (2009). Cohort studies: prospective versus retrospective. Nephron Clinical Practice, 113(3), c214-c217.

Related Articles
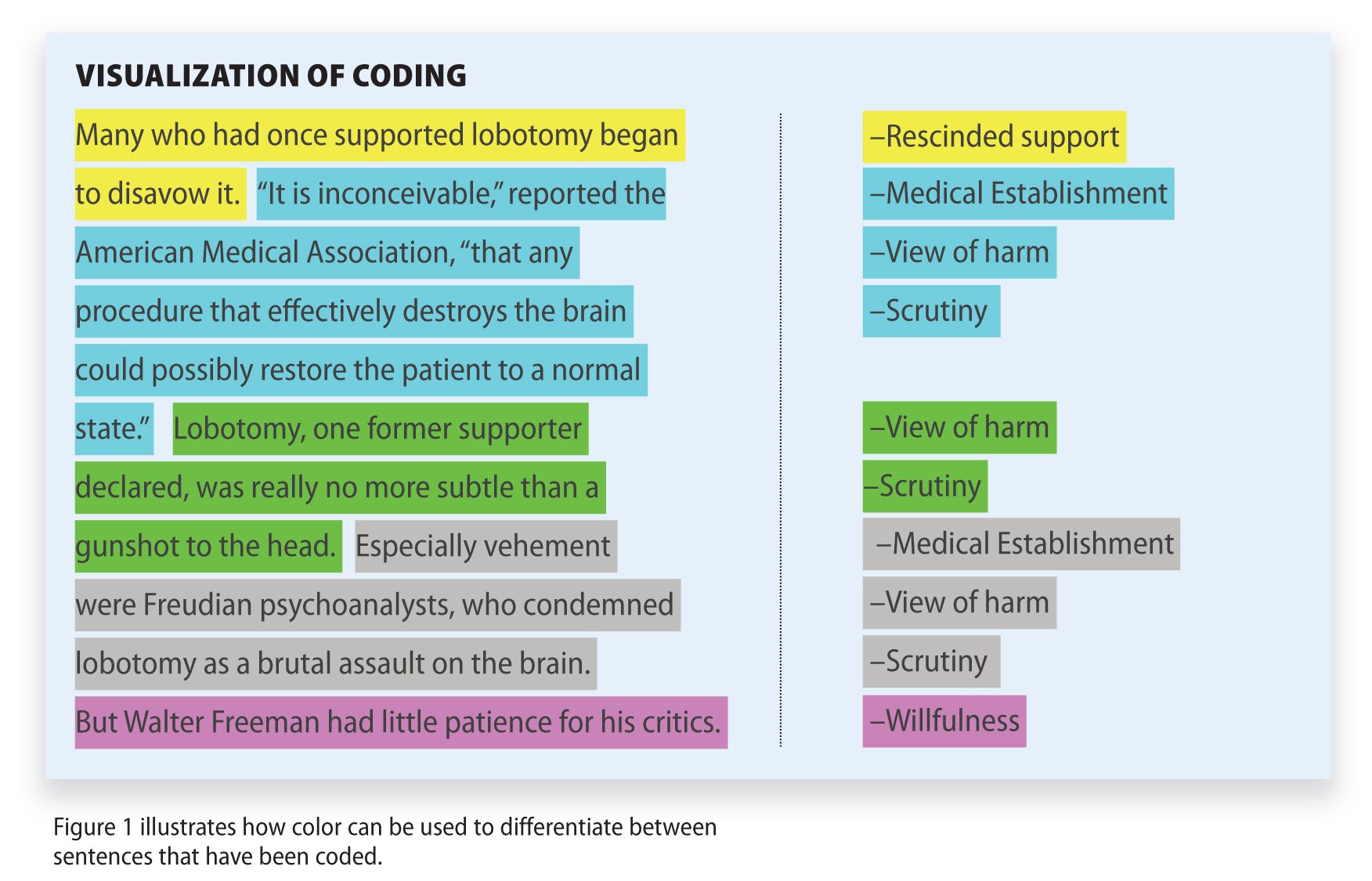
Research Methodology
Qualitative Data Coding
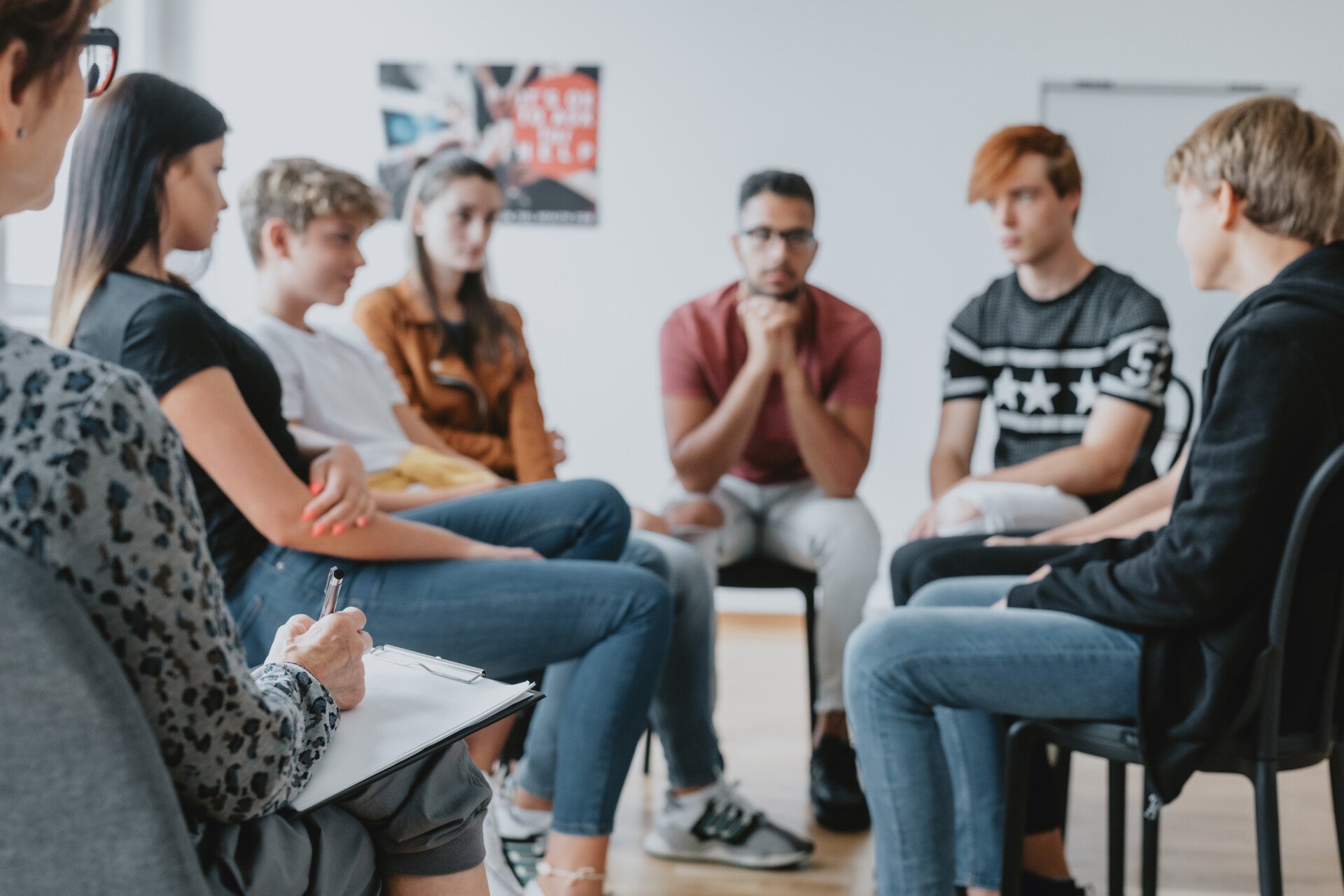
What Is a Focus Group?
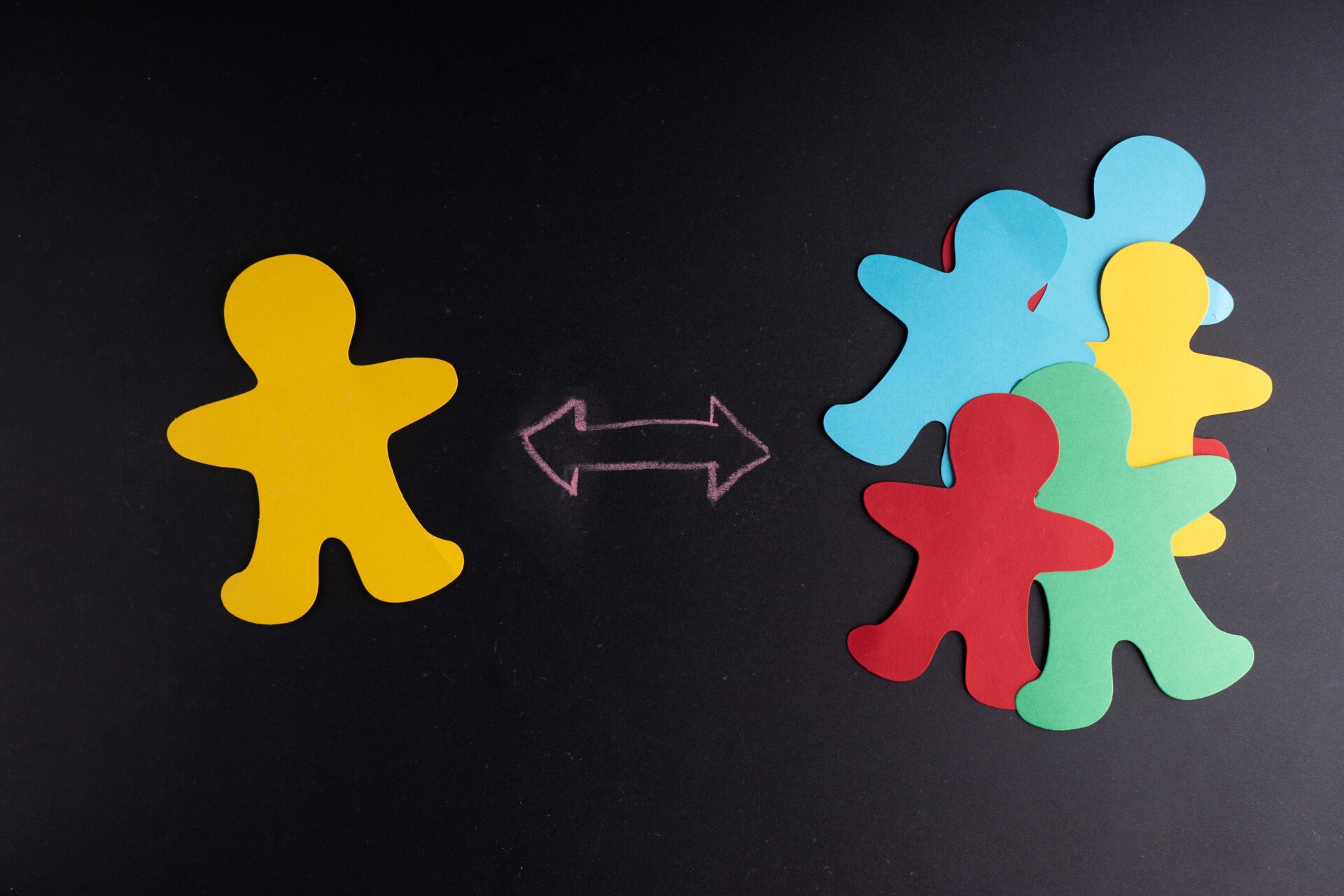
Cross-Cultural Research Methodology In Psychology
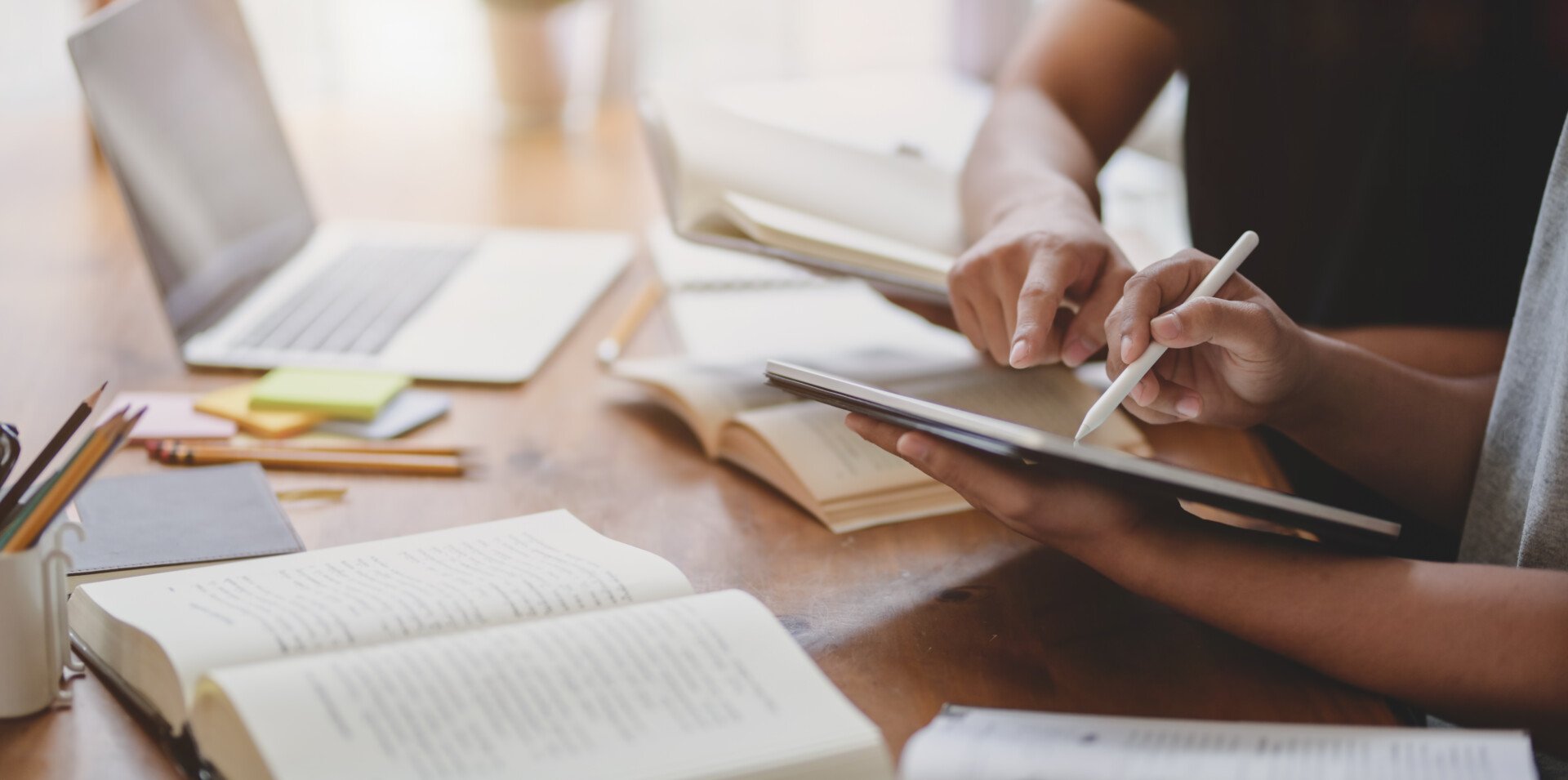
What Is Internal Validity In Research?
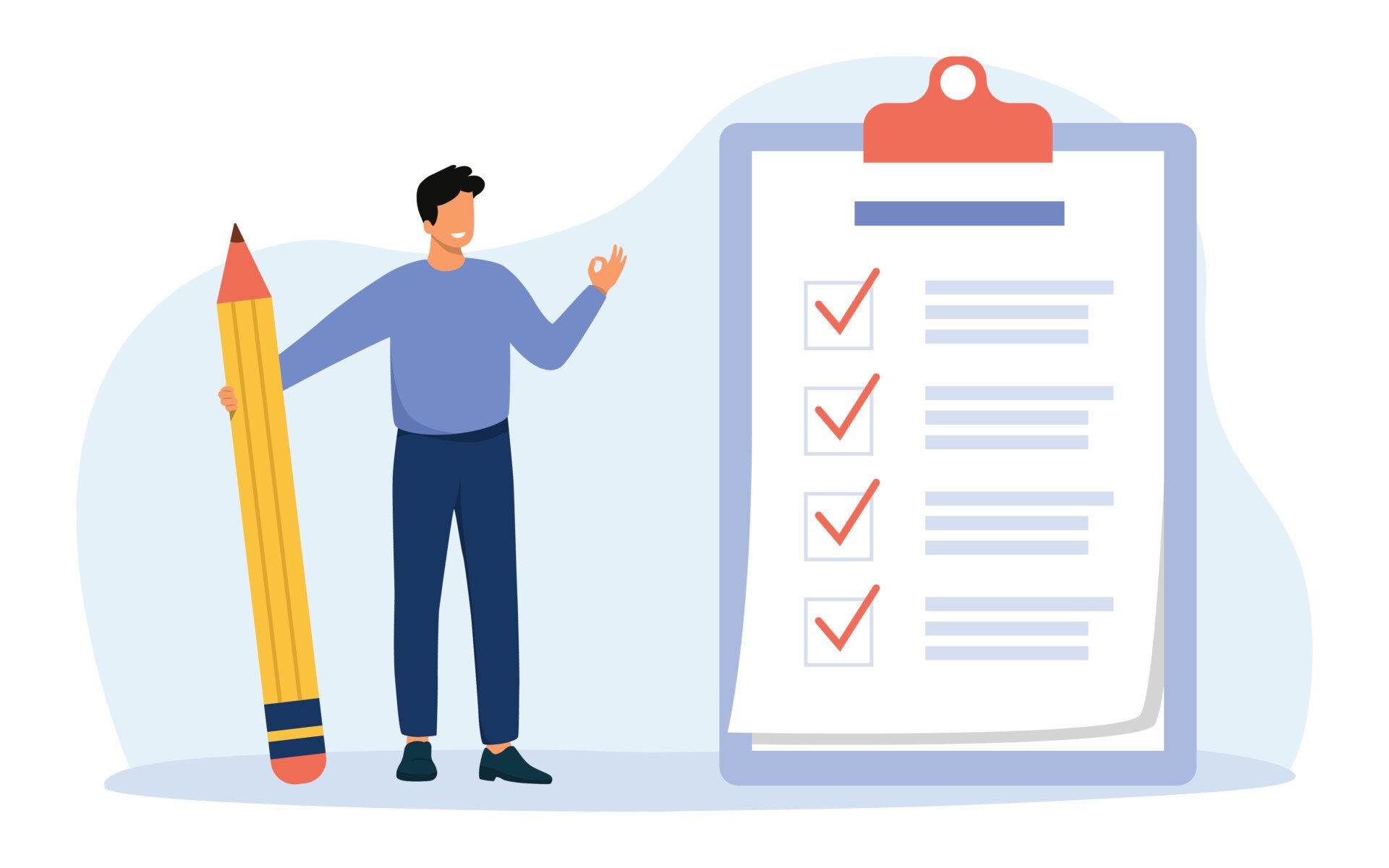
Research Methodology , Statistics
What Is Face Validity In Research? Importance & How To Measure
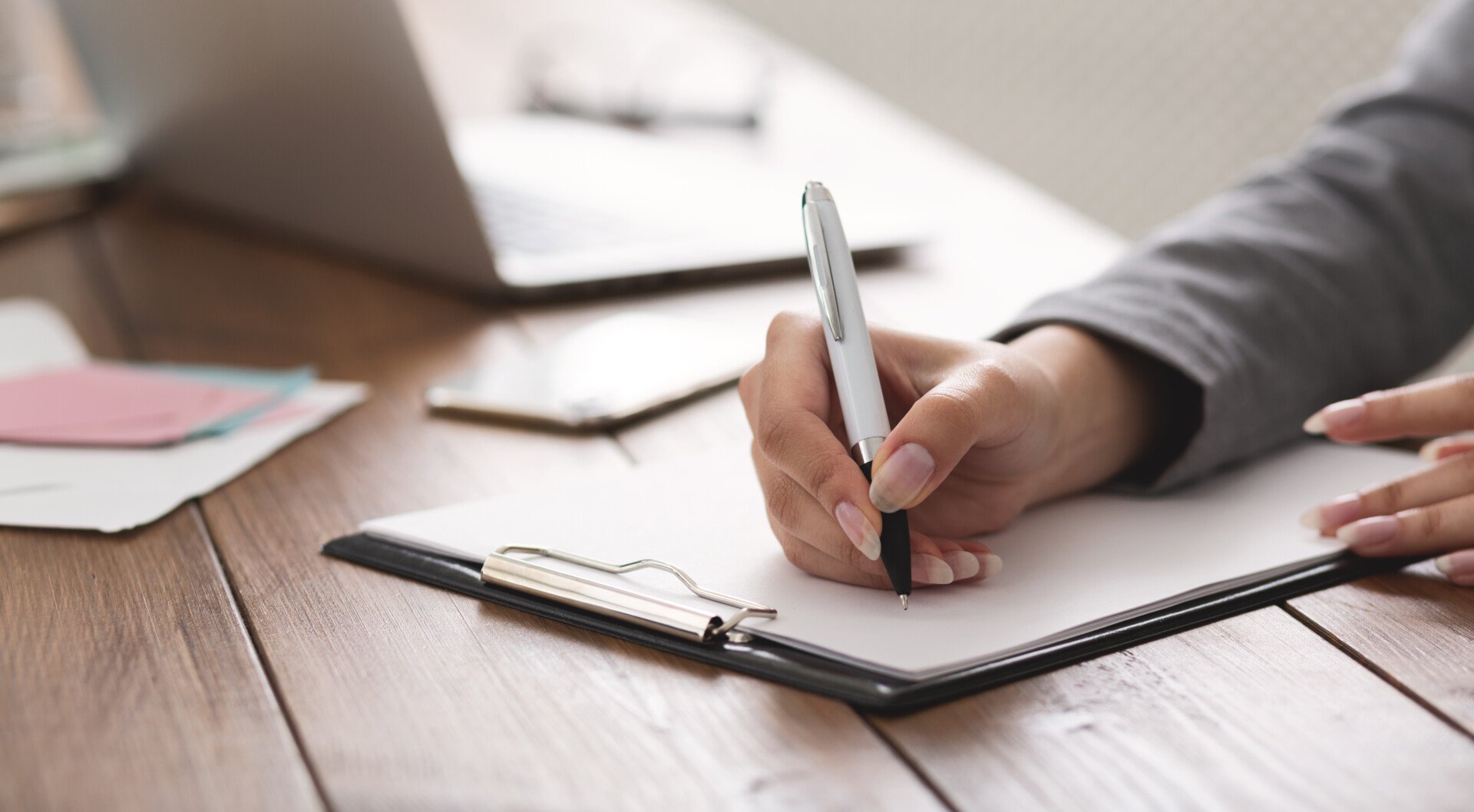
Criterion Validity: Definition & Examples
- Open access
- Published: 19 May 2024
The incidence and factors associated with anemia in elective surgical patients admitted to a surgical intensive care unit: a retrospective cohort study
- Habtie Bantider Wubet 1 ,
- Lidya Haddis Mengistu 2 ,
- Negesse Zurbachew Gobezie 1 ,
- Begizew Yimenu Mekuriaw 3 ,
- Alemie Fentie Mebratie 4 &
- Wosenyeleh Admasu Sahile 2
European Journal of Medical Research volume 29 , Article number: 290 ( 2024 ) Cite this article
Metrics details
Anemia is a frequently reported and commonly documented issue in intensive care units. In surgical intensive care units, more than 90% of patients are found to be anemic. It is a hematologic factor that contributes to extended mechanical ventilation, sepsis, organ failure, longer hospitalizations in critical care units, and higher mortality. Thus, this study aimed to determine the incidence and identify factors associated with anemia in elective surgical patients admitted to the surgical intensive care unit.
A retrospective follow-up study involving 422 hospitalized patients was carried out between December 2019 and December 2022 in the surgical intensive care unit after elective surgery at Tikur-Anbessa Specialized Hospital, Addis Ababa, Ethiopia. Data were gathered from the patients’ charts, and study participants were chosen using methods of systematic random sampling. SPSS 26 (the statistical software for social science, version 26) was used to analyze the data. Bivariable and multivariable binary logistic regression were used to examine associations between variables.
The incidence of anemia in elective surgical patients admitted to the intensive care unit was 69.9% (95% CI 65.4–74.5%). American Society of Anesthesiologists’ class III (ASA III) [AOR: 8.53, 95% CI 1.92–13.8], renal failure [AOR:2.53, 95% CI (1.91–5.81)], malignancy [AOR: 2.59, 95% CI (1.31–5.09)], thoracic surgery [AOR: 4.07, 95% CI (2.11–7.87)], urologic surgery [AOR: 6.22, 95% CI (2.80–13.80)], and neurosurgery [AOR: 4.51, 95% CI (2.53–8.03)] were significantly associated with anemia in surgical patients admitted to the intensive care unit.
More than two-thirds of the intensive care unit-admitted surgical patients experienced anemia. An American Society of Anesthesiologists’ (ASA III score), renal failure, malignancy, thoracic surgery, urologic surgery, and neurosurgery were significantly associated with this condition. Early identification helps to institute preventive and therapeutic measures.
Introduction
The World Health Organization (WHO) defines anemia as having a hemoglobin level of less than 13 g/dl (hematocrit 39) in males and less than 12 g/dl (hematocrit 36) in non-pregnant women. A reduced number of red blood cells results in a decreased ability of the blood to transfer oxygen to the tissues because hemoglobin is essential for carrying oxygen [ 1 ].
Anemia is highly prevalent and frequently observed in intensive care unit (ICU) -admitted patients after surgery. Approximately 67% (2/3) of patients admitted to the ICU on the day of admission have hemoglobin (Hb) less than 12 g/dl and 97% of patients in the ICU have protracted noticeable anemia [ 2 ]. In developing countries, the frequency of anemia is significant due to low socioeconomic status and inadequate healthcare services [ 3 ].
ICU anemia is strongly linked to poor surgical patient outcomes, including a higher risk of oxygen depletion (reduced blood oxygen-carrying capacity), blood transfusion, extended ICU stays, surgical site infections, resource consumption, prolonged need for mechanical ventilation, more complicated surgery, and advanced treatment [ 4 , 5 ]. Furthermore, studies show increased requirements for re-intubation and weaning failure in those patients who are anemic [ 6 ].
Anemia in ICU-admitted patients poses challenges in both patient management and patient outcomes. This hematologic risk factor raises patient mortality and morbidity. Its adverse outcomes include congestive heart failure, respiratory failure, hypoxia, cardiac arrest, multi-organ failure, chronic kidney disease, failure of weaning from a mechanical ventilator, prolonged hospitalization, infection, and a greater chance of dying [ 7 , 8 , 9 ]. Anemia is also a great burden for anesthetists and anesthesiologists, as hemoglobin is one of the clinical parameters that determine anesthesia choice and service delivery to the patient [ 10 ].
Different techniques were tried to cope with patients with anemia as part of the management plan. Most of the time, anemia in ICU-admitted patients is managed by transfusing packed red blood cells, which improves oxygen delivery to the tissue and decreases tissue hypoxia [ 11 ].
However, the transfusion of blood to critically ill patients is potentially dangerous and carries a risk. As blood-transfused patients are immune-compromised most of the time, they are more likely to develop blood transfusion-related complications. Some of the reported complications following transfusion include ALI (acute lung injury), organ dysfunction, systemic infections, and death [ 12 , 13 ]. Transfusion also increases hospital stay and resource consumption [ 14 ].
If anemia exists without obvious factors and if the patient is on certain medications, treatment can be done by withholding the drugs and putting the patient on corticosteroids [ 5 ].
However, anemia is an illness that can be treated and prevented and is frequently managed with fewer adverse effects without the need for blood transfusions if it is identified early to decrease blood transfusion and transfusion-related complications [ 15 ].
Even though anemia is highly prevalent in ICU-admitted patients, few studies have been conducted to investigate factors associated with it. Therefore, the purpose of this study was to ascertain the incidence of anemia and the factors associated with it in elective adult surgical patients admitted to ICU. It is advantageous for doctors and anesthetists to illustrate various anemia-related factors to lower the risk of transfusions, enhance clinical management, and enhance the quality of anesthesia and surgery.
Study design, period, and setting
A retrospective follow-up study involving 422 hospitalized patients was carried out between December 2019 and December 2022 in the surgical intensive care unit after elective surgery at Tikur-Anbessa Specialized Hospital (TASH), Addis Ababa, Ethiopia.
Inclusion and exclusion criteria
Inclusion criteria.
All patients 18 years of age and above who underwent elective surgery and were admitted to the surgical ICU have been included.
Exclusion criteria
No records of baseline hemoglobin or hematocrit
Hemolytic anemia as a direct cause of ICU admission
Congenital causes of anemia such as sickle cell
Patients on treatments for anemia were excluded from the study.
Operational definitions
Hematocrit: the proportion of red blood cells in the blood. Healthy adults typically have hematocrits between 36 and 48% for women and 39–52% for men [ 16 ].
Hemoglobin: is a protein that contains iron in RBCs and is responsible for providing oxygen to the cells. It ranges from 12–16 for females and 13–17 for males [ 16 ].
Prolonged ICU stay: patients who are admitted to the ICU and stay for more than 8 days [ 17 ].
Post-surgery: the period starting from the immediate termination of surgery and till discharge [ 18 ].
Postsurgical anemia: is defined as anemia after surgery measured in terms of reduction of hemoglobin less than 13 g/dl for males and less than 12 g/dl for females according to the WHO [ 19 ].
Post-surgical anemia in the ICU: anemia developed during any period in the intensive care unit stay [ 20 ].
Sample size determination
In the research area, the incidence of anemia among patients hospitalized in the surgical intensive care unit (ICU) and the associated risk factors were unknown. Using the single population formula, the sample size was determined with a 5% margin of error at a 95% confidence interval and a 50% incidence of anemia in surgical patients admitted to the intensive care unit:
In this case, n = (1.96) 2 0.5 (1–0.5)/ (0.05) 2 = 384, where z , 1.96; p , 0.5; CI , 95%; w , margin of error = 0.05.
To account for the non-response rate, we added 10% of the n (i.e., 384 + 38 = 422); as a result, 422 surgical patients who were hospitalized in the intensive care unit were included in the study.
Sampling technique
The study participants were chosen using a systematic random sampling technique. The number of surgical patients admitted to the surgical ICU monthly was 40–45 patients. So, on average, 480 to 540 patients are admitted to the surgical ICU annually. Three years of data were taken retrospectively to establish the incidence of anemia and to search out the risk factors. Thus, in 3 years, 1530 patients were admitted to the surgical intensive care unit.
The sampling interval was based on systematic random sampling, and it was 1530/422 = 4. Therefore; every fourth patient was picked up to be included in the study (Fig. 1 ).
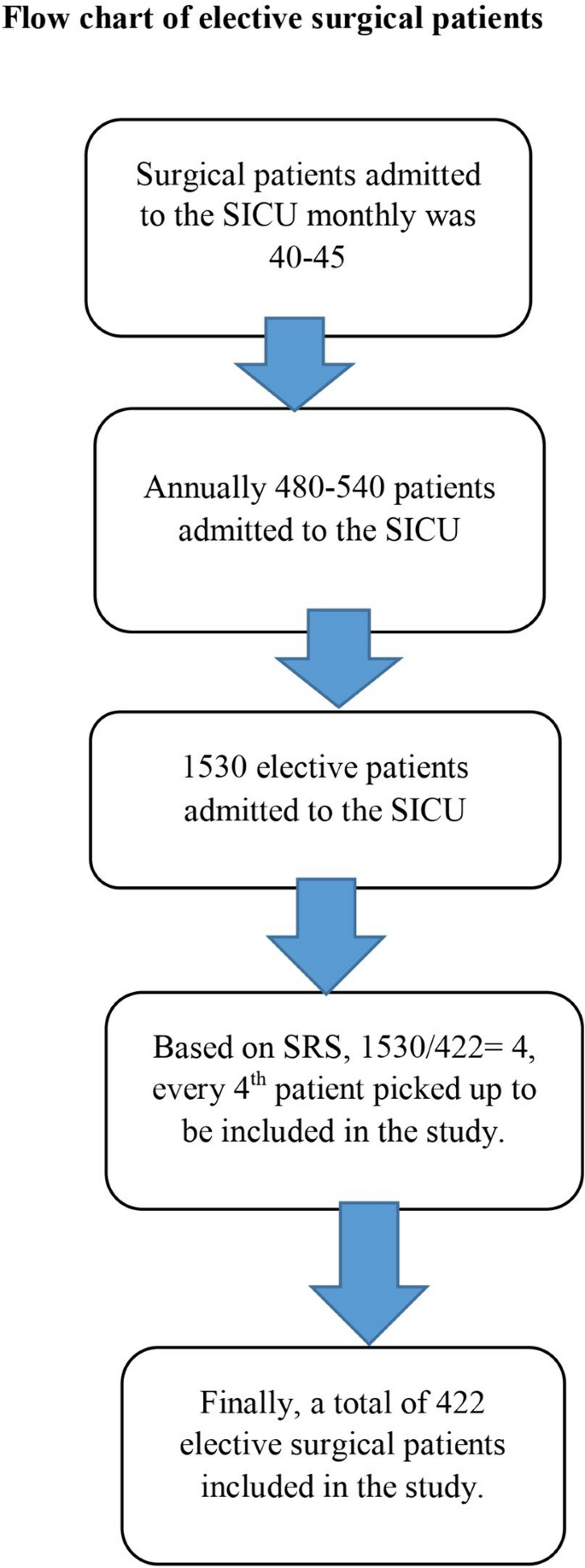
The flow chart of patients admitted to the surgical ICU after elective surgery
Data collection procedure
Data has been collected from the patient’s chart by using a structured checklist. The questionnaire was designed to collect data on socio-demographic variables (age, sex, and residency), hematologic measurements (hemoglobin and hematocrit), previous surgery, medical status (hypertension, diabetes mellitus, renal failure, cancer, RVI, and heart failure), and drug history (Beta-blocker, calcium channel blocker, ACEIs, HAARTs, and chemotherapies). Blood loss, duration of surgery, duration on a mechanical ventilator, duration of ICU stays, and type of anesthesia employed were also investigated in patients’ medical charts.
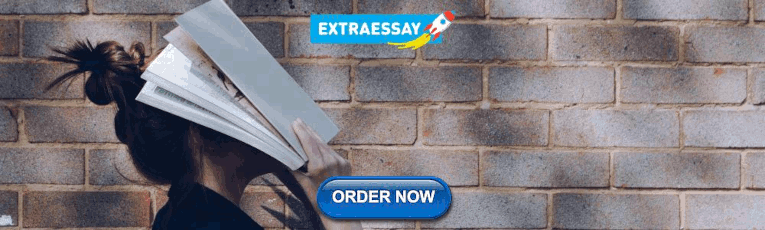
Data processing and analysis
Before being imported into Epidata version 4.6 and exported to SPSS version 26 for analysis, the data was first checked, coded, and entered. The collinearity diagnosis test (variance inflation factors, or VIF) and tolerance were used to screen for multicollinearity. The study employed binary logistic regression analysis to investigate potential relationships between the independent factors and the outcome variable. Variables in bivariable regression with a p-value less than 0.2 are added to multivariable logistic regression to assess the strength of the association. Lastly, variables are considered statistically significant if their p-value is less than 0.05. The model's fitness was evaluated using the Hosmer–Lemeshow goodness of fit test. The continuous variables were expressed using the mean and standard deviation. The categorical data have been expressed using percentages and continuous data using mean.
Socio-demographic and clinical characteristics of study participants
This study was conducted on a total of 422 patients who were admitted to the ICU after elective surgery. Of the total study participants, 174 (41.2%) were men, 229 (54.3%) were from rural areas with a mean age of 48 ( ± 17.7) years. The duration of surgery with mean ± standard deviation was 5.13 ± 1.25 h. The mean time of surgical patients waiting on mechanical ventilators and in the ICU was 8.0 ± 6 and 8.9 ± 6.6 days, respectively. The average volume of blood loss during the surgical procedure was 1380 (± 855) milliliters. Of those surgical patients, the majority of patients were ASA II (58.5%) (Table 1 ).
Coexisting conditions and medical histories of patients admitted to the intensive care unit following elective surgery
Of the total study participants, 148 (35.1%), 90 (21.3%), and 84 (19.9%) study participants had histories of hypertension, cancer, and two or more diseases, respectively. Regarding treatment factors, 107 (25.4%), 102 (24.2%), 96 (22.7%), and 71 (16.8%) had histories of two or more medications, ACEIs, calcium channel blockers, and chemotherapies, respectively (Table 2 ).
Incidence of anemia in surgical intensive care unit
This study found that among patients hospitalized in the surgical intensive care unit following elective surgery, the overall incidence of anemia was 69.9% (95% CI 65.4% to 74.5%). The lowest and highest hemoglobin was noted on the seventh day of ICU admission (9.2 g/dl) and at the time of discharge (10.3 g/dl) respectively (Fig. 2 ).
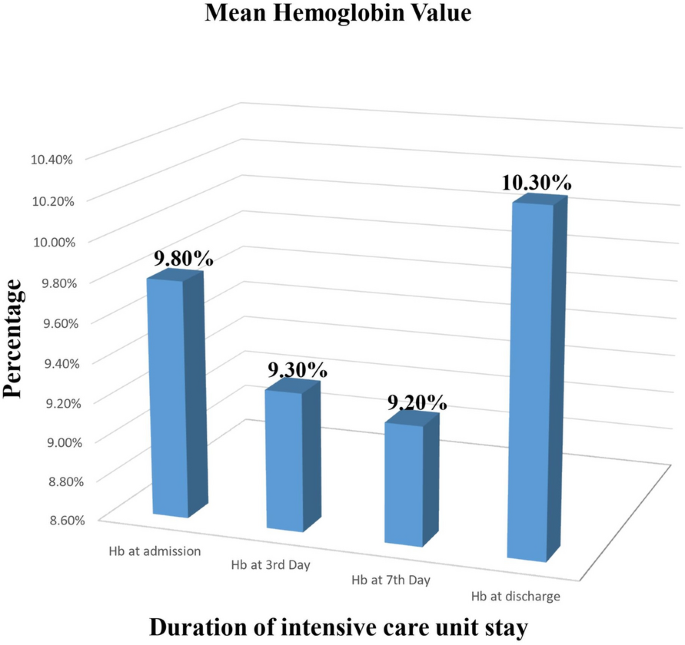
The mean hemoglobin value for patients admitted to the surgical ICU after elective surgery to the length of stay in the ICU
Factors associated with anemia in surgical ICU admitted patients
In a bi-variable logistic regression analysis, ASA class, renal failure, malignancy, heart failure, calcium channel blockers, ACE inhibitors, chemotherapy, thoracic surgery, urologic surgery, and neurologic surgery were discovered to be substantially associated with anemia ( p -value < 0.2) and were candidates for multivariable logistic regression analysis. In multivariate logistic regression, ASA class III, renal failure, malignancy, thoracic surgery, urologic surgery, and neurologic surgery were found to have a significant association with the outcome variable (Table 3 ).
According to this study, the incidence of anemia in elective surgical ICU-admitted patients was 69.9% (95% CI 65.4% to 74.5%). The lowest hemoglobin level was noted on the seventh day of ICU admission (9.2 g/dl).
This study’s findings were consistent with those of other researches in Germany showing that 66.9% of ICU-admitted surgical patients had anemia (i.e. mean hemoglobin level less than 10 g/dl) during their ICU admission, and there was further decrement in mean hemoglobin level as the ICU stay increased [ 21 ].
Similarly, a Polish study found that nearly all patients became anemic during the first three days after ICU admission, and as many as 66% of patients were anemic on the day of admission [ 13 ]. Our study also showed that the first three days of ICU admission had a significant impact on hemoglobin concentration, with the biggest drops in hemoglobin occurring during this time and continuing to decline thereafter.
In contrast, the incidence of anemia at admission in our study was higher than the findings of the studies conducted in Greece (43.1%) [ 22 ], in Spain (63.31%) [ 4 ], in Western Europe (63%) [ 23 ], in the United States (57.8%) [ 24 ], and in Canada (61.3%) [ 25 ]. These differences might happen as a result of the fact differences in sample size and clinical characteristics of the study participants.
The incidence of anemia in our study was lower than in studies in India, reaching 97% within a week of ICU admission with hemoglobin or hematocrit levels below 12 g/dl (36%) [ 2 ]. This difference might have occurred because of differences in sample size and inclusion–exclusion criteria.
Further reports in Germany show the frequency of anemia in ICU-admitted patients was 98%, which is too high [ 26 ]. This variety has occurred because the study participants they included in this investigation were all on mechanical ventilators.
In Niger, the incidence of anemia in patients admitted to surgical ICU was 86.9% [ 27 ]. This was also high as compared with our result. This difference might be due to the inclusion of both emergency and elective surgical patients in their study.
According to this study, ASA physical status III, renal failure, cancer, thoracic, urologic, and neurologic surgery were found to be significantly associated with the incidence of anemia.
Compared to those patients with ASA I and II, patients with ASA status III had an 8.5 times increased risk of developing anemia. Other studies confirmed the ASA physical status class with preexisting co-morbidities increases adverse postoperative surgical outcomes [ 28 ]. As the physical status class of ASA increases, postoperative adverse events like morbidity and mortality increase too [ 28 , 29 ]. As ASA class increases, coexisting medical conditions increase, the stage of disease may advance, and immunity declines resulting in decreased activity of reticuloendothelial cells which contributes to the development of anemia.
According to this study, the risk of developing anemia in surgical patients admitted to ICU was 2.5 times higher in patients with renal failure as compared with those patients without renal failure. This conclusion is consistent with research gained by other authors. Although anemia can occur at various stages of chronic kidney disease (CKD), the incidence of anemia and the severity of CKD are strongly correlated [ 30 ].
Renal hormones such as erythropoietin, which regulates the bone marrow's production of red blood cells, are thought to play a role in renal failure. As kidney disease progresses, other factors that lower red cell survival and inhibit marrow erythropoiesis may also contribute to anemia [ 30 ].
In our study, anemia was 2.6 times more likely to develop in patients having a history of malignancy than in patients who hadn’t a history of malignancy. Similar studies demonstrated that individuals admitted to the ICU with cancer had a higher frequency of anemia [ 31 ].
Compared to patients who were not having thoracic surgery, we found that patients who were having thoracic surgery were four times more likely to experience ICU anemia. Studies support the conclusions we have made. After undergoing pulmonary surgery, a retrospective examination of 465 patients in China revealed that 75.3% of patients in the intensive care unit had a postoperative anemia diagnosis [ 32 ]. This is also true in patients undergoing cardiac surgery in which the prevalence of anemia is high [ 33 ].
Patients who had undergone urologic surgery were 6.2 times more likely to develop anemia in the ICU than those who had not undergone urologic surgery. According to different studies, anemia is significantly associated with urologic surgery, and the magnitude is even higher in renal transplant surgeries [ 34 , 35 ].
ICU anemia was frequently encountered in patients undergoing neurologic surgery, 4.5 times more frequently than in those who hadn’t undergone neurologic surgery. As evidenced by different published reports, anemia is a common scenario in patients undergoing neurologic surgery after ICU admission. This is supported by a study [ 36 , 37 ].
The possible postulated mechanisms of anemia after surgery are described below. Anemia during surgery is a typical clinical scenario. Anemia that develops postoperatively resembles chronic illness anemia and is likely due to the impact that inflammatory mediators generated both before and after surgery have on the growth and survival of RBCs [ 38 , 39 ].
Strengths and limitations of the study
Most of the studies on anemia were pre-operative and cross-sectional studies, so this study identifies the incidence and factors associated with anemia postoperatively after patients have been admitted to the surgical ICU using cohort studies.
This study provides insights and clues about the incidence and associated factors of anemia in ICU-admitted elective surgical patients for future research; as such studies are limited in the area.
Limitations
In this study, we used secondary data, and as a result, some important information regarding factors associated with anemia (e.g., nutritional status and living conditions) was not found during the chart review.
Among surgical patients admitted to the ICU, the incidence of anemia is high. The majority of surgical intensive care unit admitted patients exhibit anemia during their course of stay. ASA physical status class III, renal failure, malignancy, thoracic surgery, urologic surgery, and neurologic surgery significantly increase the likelihood of anemia in surgical patients who are hospitalized in the intensive care unit (ICU). So, early screening and the institution of therapeutic measures are necessary to reduce the adverse effects of anemia. Preoperative screening of ASA physical status and medical conditions, early identifications and treatment of patients with comorbidities before surgery, and minimizing modifiable factors (i.e. duration of surgery and intraoperative blood loss) help to reduce the incidence of anemia in surgical patients admitted to ICU.
Data availability
If a legitimate request is made, the corresponding author will provide the data sets utilized and analyzed during the study.
Abbreviations
Acute lung injury
American Society of Anesthesiologists
Complete blood count
Demographic Health Survey
- Intensive care unit
Institutional review board
Patient blood management
Red blood cell
Surgical intensive care unit
Statistical Package for Social Sciences
Systematic random sampling
Tikur Anbessa Specialized Hospital
World Health Organization
Buttarello M. Laboratory diagnosis of anemia: are the old and new red cell parameters useful in classification and treatment, how? Int J Lab Hematol. 2016;38:123–32.
Article PubMed Google Scholar
Rawal G, Kumar R, Yadav S, Singh A. Anemia in intensive care: a review of current concepts. J Crit Care Med. 2016;2(3):109–14.
Article Google Scholar
Prasanth R. Prevalence of anemia in both developing and developed countries around the world. World J Anemia. 2017;1(2):40–3.
Juárez-Vela R, Andrés-Esteban EM, Gea-Caballero V, Sánchez-González JL, Marcos-Neira P, Serrano-Lázaro A, et al. Related factors of anemia in critically ill patients: a prospective multicenter study. J Clin Med. 2022;11(4):1031.
Article PubMed PubMed Central Google Scholar
Renard D, Rosselet A. Drug-induced hemolytic anemia: pharmacological aspects. Transfus Clin Biol. 2017;24(3):110–4.
Article CAS PubMed Google Scholar
Kim J, Curran BP, Du AL, Gabriel RA, Du A. The association of primary anesthesia type with postoperative transfusion in anemic patients undergoing primary total joint arthroplasty. Cureus. 2022. https://doi.org/10.7759/cureus.24496 .
Kim J, Shin S, Han K, Lee K, Kim J, Choi Y, et al. Relationship between socioeconomic status and anemia prevalence in adolescent girls based on the fourth and fifth Korea national health and nutrition examination surveys. Eur J Clin Nutr. 2014;68(2):253–8.
Tesfaye TS, Tessema F, Jarso H. Prevalence of anemia and associated factors among “apparently healthy” urban and rural residents in Ethiopia: a comparative cross-sectional study. J Blood Med. 2020;11:89–96.
Song X, Liu X-Y, Wang H-R, Guo X-Y, Kashani KB, Ma P-L. Association between anemia and ICU outcomes. Chin Med J. 2021;134(14):1744–6.
Cherry-Bukowiec JR, Engoren M, Wiktor A, Raghavendran K, Napolitano LM. Hepcidin and anemia in surgical critical care: a prospective cohort study. Crit Care Med. 2018;46(6):e567–74.
Vincent J-L, Jaschinski U, Wittebole X, Lefrant J-Y, Jakob SM, Almekhlafi GA, et al. Worldwide audit of blood transfusion practice in critically ill patients. Crit Care. 2018;22:1–9.
Fritsch SJ, Dreher M, Simon T-P, Marx G, Bickenbach J. Haemoglobin value and red blood cell transfusions in prolonged weaning from mechanical ventilation: a retrospective observational study. BMJ Open Respir Res. 2022;9(1): e001228.
Jati SAW, Aditianingsih D, George Y, Risadayanti R. Effect of hemoglobin levels on the success of weaning and extubation processes in critical patients with mechanical ventilation: a systematic review, Bali. J Anesthesiol. 2023;7(4):197–201.
Google Scholar
Prin M, Rui S, Pan S, Kadyaudzu C, Mehta PS, Li G, et al. Anemia at intensive care unit admission and hospital mortality among patients at a referral hospital in Malawi. Am Surg. 2021;87(8):1334–40.
Shander A, Lobel GP, Javidroozi M. Anesthesia for patients with anemia. Anesthesiol Clin. 2016;34(4):711–30.
Zeleke MB, Shaka MF, Anbesse AT, Tesfaye SH. Anemia and its determinants among male and female adolescents in Southern Ethiopia: a comparative cross-sectional study. Anemia. 2020;2020:3906129.
Hermans G, Van Aerde N, Meersseman P, Van Mechelen H, Debaveye Y, Wilmer A, et al. Five-year mortality and morbidity impact of prolonged versus brief ICU stay: a propensity score matched cohort study. Thorax. 2019;74(11):1037–45.
Bowyer A, Royse C. Postoperative recovery and outcomes—what are we measuring and for whom? Anaesthesia. 2016;71:72–7.
Muñoz M, Acheson A, Bisbe E, Butcher A, Gómez-Ramírez S, Khalafallah A, et al. An international consensus statement on the management of postoperative anaemia after major surgical procedures. Anaesthesia. 2018;73(11):1418–31.
Kalra SK, Thilagar B, Khambaty M, Manjarrez E. Post-operative anemia after major surgery: a brief review. Curr Emerg Hosp Med Rep. 2021;9(3):89–95.
Zuther M, Rübsam M-L, Zimmermann M, Zarbock A, Hönemann C. Improved diagnosis of iron deficiency anemia in the critically ill via fluorescence flowcytometric hemoglobin biomarkers. Cells. 2022;12(1):140.
Aydogan M, Ucar M, Yücel A, Karakas B, Gok A, Togal T. Effects of iron deficiency on transfusion requirements in critically ill patients: a preliminary observational study. Crit Care. 2015;19:1–201.
Anand S, Burkenroad A, Glaspy J. Workup of anemia in cancer. Clin Adv Hematol Oncol. 2020;18:640–6.
PubMed Google Scholar
Warner MA, Hanson AC, Frank RD, Schulte PJ, Go RS, Storlie CB, et al. Prevalence of and recovery from anemia following hospitalization for critical illness among adults. JAMA Netw Open. 2020;3(9):e2017843.
James TE, Barty R, Liu Y, Rochwerg B, Heddle N, Siegal DM. Blood loss due to laboratory testing in critical care patients: a retrospective cohort study. Blood. 2018;132:4885.
Ghiani A, Sainis A, Sainis G, Neurohr C. Anemia and red blood cell transfusion practice in prolonged mechanically ventilated patients admitted to a specialized weaning center: an observational study. BMC Pulm Med. 2019;19(1):1–9.
Kron AT, Collins A, Cserti-Gazdewich C, Pendergrast J, Webert K, Lieberman L, et al. A prospective multi-faceted interventional study of blood bank technologist screening of red blood cell transfusion orders: the START study. Transfusion. 2021;61(2):410–22.
Li G, Walco JP, Mueller DA, Wanderer JP, Freundlich RE. Reliability of the ASA physical status classification system in predicting surgical morbidity: a retrospective analysis. J Med Syst. 2021;45:1–8.
Rosa F, Tortorelli A, Quero G, Galiandro F, Fiorillo C, Sollazzi L, et al. The impact of preoperative ASA-physical status on postoperative complications and long-term survival outcomes in gastric cancer patients. Eur Rev Med Pharmacol Sci. 2019;23(17):7383–90.
CAS PubMed Google Scholar
Habib A, Ahmad R, Rehman S. Hematological changes in chronic renal failure patients and the effect of hemodialysis on these parameters. Int J Res Med Sci. 2017;5(11):4998–5003.
Assi HI, Halim NA, Alameh I, Khoury J, Nahra V, Sukhon F, et al. Outcomes of patients with malignancy admitted to the intensive care units: a prospective study. Crit Care Res Pract. 2021;2021:1.
Article CAS Google Scholar
Lang Z, Wu Y, Bao M. Coagulation status and surgical approach as predictors of postoperative anemia in patients undergoing thoracic surgery: a retrospective study. Front Surg. 2021;8: 744810.
Dhir A, Tempe DK. Anemia and patient blood management in cardiac surgery—literature review and current evidence. J Cardiothorac Vasc Anesth. 2018;32(6):2726–42.
Jun KW, Cho J, Kim MH, Hwang JK, Park SC, Moon IS, et al. Changes in hemostatic factors after kidney transplantation: a retrospective cohort study. Medicine. 2021;100(36): e27179.
Article CAS PubMed PubMed Central Google Scholar
Bamgbola OF. Spectrum of anemia after kidney transplantation: pathophysiology and therapeutic implications. Clin Transplant. 2016;30(10):1185–94.
Vanhala H, Junttila E, Kataja A, Huhtala H, Luostarinen T, Luoto T. Incidence and associated factors of anemia in patients with acute moderate and severe traumatic brain injury. Neurocrit Care. 2022;37(3):629–37.
Kisilevsky A, Gelb A, Bustillo M, Flexman A. Anaemia and red blood cell transfusion in intracranial neurosurgery: a comprehensive review. Br J Anesthesia. 2018;120(5):988–98.
Shander A, Kaufman M, Goodnough LT. How i treat anemia in the perisurgical setting. Blood. 2020;136(7):814–22.
Tanner L, Neef V, Raimann FJ, Störmann P, Marzi I, Lefering R, et al. Influence of anemia in severely injured patients on mortality, transfusion and length of stay: an analysis of the TraumaRegister DGU®. Eur J Trauma Emerg Surg. 2022;48(4):2741–9.
Download references
Acknowledgements
We express our gratitude to Addis Ababa University (AAU) for providing us with the necessary ethical clearance and approval to carry out this study. We would also like to thank all of the data collectors for their incredibly hard work during the data collection procedures.
The governmental, private, or nonprofit sectors did not provide any grants or other financial assistance for this work.
Author information
Authors and affiliations.
Department of Anesthesia, College of Medicine and Health Sciences, Debre Tabor University, Debre Tabor, Ethiopia
Habtie Bantider Wubet & Negesse Zurbachew Gobezie
Department of Anesthesia, College of Medicine and Health Sciences, Addis Ababa University, Addis Ababa, Ethiopia
Lidya Haddis Mengistu & Wosenyeleh Admasu Sahile
Department of Midwifery, College of Health Science, Debre Tabor University, Debre Tabor, Ethiopia
Begizew Yimenu Mekuriaw
Department of Medical Laboratory Science, College of Medicine and Health Sciences, Debre Tabor University, Debre Tabor, Ethiopia
Alemie Fentie Mebratie
You can also search for this author in PubMed Google Scholar
Contributions
H.B. contributed to the idea and planning of the research, data collection, analysis, and interpretation. Scientific advice on the study design, data analysis, and paper writing provided by W.A. and L.H. N.Z. contributed to the manuscript’s development and revision of the research. Data collection, critical manuscript revision for significant intellectual content, and manuscript approval for publication were all completed by B.Y. and A.F. The authors all agreed to take responsibility for all parts of the work and read and approved the final article.
Corresponding author
Correspondence to Habtie Bantider Wubet .
Ethics declarations
Competing interests.
The authors declare no competing interests.
Ethics approval and consent to participate
The study received approval from Addis Ababa University's ethical institutional review board. The study was carried out following the 1994 Helsinki Declaration and its subsequent revisions.
Consent for publication
Not applicable.
Conmpeting interests
There are no conflicting interests that the authors declare.
Additional information
Publisher's note.
Springer Nature remains neutral with regard to jurisdictional claims in published maps and institutional affiliations.
Rights and permissions
Open Access This article is licensed under a Creative Commons Attribution 4.0 International License, which permits use, sharing, adaptation, distribution and reproduction in any medium or format, as long as you give appropriate credit to the original author(s) and the source, provide a link to the Creative Commons licence, and indicate if changes were made. The images or other third party material in this article are included in the article's Creative Commons licence, unless indicated otherwise in a credit line to the material. If material is not included in the article's Creative Commons licence and your intended use is not permitted by statutory regulation or exceeds the permitted use, you will need to obtain permission directly from the copyright holder. To view a copy of this licence, visit http://creativecommons.org/licenses/by/4.0/ . The Creative Commons Public Domain Dedication waiver ( http://creativecommons.org/publicdomain/zero/1.0/ ) applies to the data made available in this article, unless otherwise stated in a credit line to the data.
Reprints and permissions
About this article
Cite this article.
Wubet, H.B., Mengistu, L.H., Gobezie, N.Z. et al. The incidence and factors associated with anemia in elective surgical patients admitted to a surgical intensive care unit: a retrospective cohort study. Eur J Med Res 29 , 290 (2024). https://doi.org/10.1186/s40001-024-01887-4
Download citation
Received : 28 December 2023
Accepted : 15 May 2024
Published : 19 May 2024
DOI : https://doi.org/10.1186/s40001-024-01887-4
Share this article
Anyone you share the following link with will be able to read this content:
Sorry, a shareable link is not currently available for this article.
Provided by the Springer Nature SharedIt content-sharing initiative
European Journal of Medical Research
ISSN: 2047-783X
- Submission enquiries: [email protected]
- General enquiries: [email protected]

An official website of the United States government
The .gov means it’s official. Federal government websites often end in .gov or .mil. Before sharing sensitive information, make sure you’re on a federal government site.
The site is secure. The https:// ensures that you are connecting to the official website and that any information you provide is encrypted and transmitted securely.
- Publications
- Account settings
Preview improvements coming to the PMC website in October 2024. Learn More or Try it out now .
- Advanced Search
- Journal List
- Pediatr Investig
- v.3(4); 2019 Dec

Clinical research study designs: The essentials
Ambika g. chidambaram.
1 Children's Hospital of Philadelphia, Philadelphia Pennsylvania, USA
Maureen Josephson
In clinical research, our aim is to design a study which would be able to derive a valid and meaningful scientific conclusion using appropriate statistical methods. The conclusions derived from a research study can either improve health care or result in inadvertent harm to patients. Hence, this requires a well‐designed clinical research study that rests on a strong foundation of a detailed methodology and governed by ethical clinical principles. The purpose of this review is to provide the readers an overview of the basic study designs and its applicability in clinical research.
Introduction
In clinical research, our aim is to design a study, which would be able to derive a valid and meaningful scientific conclusion using appropriate statistical methods that can be translated to the “real world” setting. 1 Before choosing a study design, one must establish aims and objectives of the study, and choose an appropriate target population that is most representative of the population being studied. The conclusions derived from a research study can either improve health care or result in inadvertent harm to patients. Hence, this requires a well‐designed clinical research study that rests on a strong foundation of a detailed methodology and is governed by ethical principles. 2
From an epidemiological standpoint, there are two major types of clinical study designs, observational and experimental. 3 Observational studies are hypothesis‐generating studies, and they can be further divided into descriptive and analytic. Descriptive observational studies provide a description of the exposure and/or the outcome, and analytic observational studies provide a measurement of the association between the exposure and the outcome. Experimental studies, on the other hand, are hypothesis testing studies. It involves an intervention that tests the association between the exposure and outcome. Each study design is different, and so it would be important to choose a design that would most appropriately answer the question in mind and provide the most valuable information. We will be reviewing each study design in detail (Figure 1 ).

Overview of clinical research study designs
Observational study designs
Observational studies ask the following questions: what, who, where and when. There are many study designs that fall under the umbrella of descriptive study designs, and they include, case reports, case series, ecologic study, cross‐sectional study, cohort study and case‐control study (Figure 2 ).

Classification of observational study designs
Case reports and case series
Every now and then during clinical practice, we come across a case that is atypical or ‘out of the norm’ type of clinical presentation. This atypical presentation is usually described as case reports which provides a detailed and comprehensive description of the case. 4 It is one of the earliest forms of research and provides an opportunity for the investigator to describe the observations that make a case unique. There are no inferences obtained and therefore cannot be generalized to the population which is a limitation. Most often than not, a series of case reports make a case series which is an atypical presentation found in a group of patients. This in turn poses the question for a new disease entity and further queries the investigator to look into mechanistic investigative opportunities to further explore. However, in a case series, the cases are not compared to subjects without the manifestations and therefore it cannot determine which factors in the description are unique to the new disease entity.
Ecologic study
Ecological studies are observational studies that provide a description of population group characteristics. That is, it describes characteristics to all individuals within a group. For example, Prentice et al 5 measured incidence of breast cancer and per capita intake of dietary fat, and found a correlation that higher per capita intake of dietary fat was associated with an increased incidence of breast cancer. But the study does not conclude specifically which subjects with breast cancer had a higher dietary intake of fat. Thus, one of the limitations with ecologic study designs is that the characteristics are attributed to the whole group and so the individual characteristics are unknown.
Cross‐sectional study
Cross‐sectional studies are study designs used to evaluate an association between an exposure and outcome at the same time. It can be classified under either descriptive or analytic, and therefore depends on the question being answered by the investigator. Since, cross‐sectional studies are designed to collect information at the same point of time, this provides an opportunity to measure prevalence of the exposure or the outcome. For example, a cross‐sectional study design was adopted to estimate the global need for palliative care for children based on representative sample of countries from all regions of the world and all World Bank income groups. 6 The limitation of cross‐sectional study design is that temporal association cannot be established as the information is collected at the same point of time. If a study involves a questionnaire, then the investigator can ask questions to onset of symptoms or risk factors in relation to onset of disease. This would help in obtaining a temporal sequence between the exposure and outcome. 7
Case‐control study
Case‐control studies are study designs that compare two groups, such as the subjects with disease (cases) to the subjects without disease (controls), and to look for differences in risk factors. 8 This study is used to study risk factors or etiologies for a disease, especially if the disease is rare. Thus, case‐control studies can also be hypothesis testing studies and therefore can suggest a causal relationship but cannot prove. It is less expensive and less time‐consuming than cohort studies (described in section “Cohort study”). An example of a case‐control study was performed in Pakistan evaluating the risk factors for neonatal tetanus. They retrospectively reviewed a defined cohort for cases with and without neonatal tetanus. 9 They found a strong association of the application of ghee (clarified butter) as a risk factor for neonatal tetanus. Although this suggests a causal relationship, cause cannot be proven by this methodology (Figure 3 ).

Case‐control study design
One of the limitations of case‐control studies is that they cannot estimate prevalence of a disease accurately as a proportion of cases and controls are studied at a time. Case‐control studies are also prone to biases such as recall bias, as the subjects are providing information based on their memory. Hence, the subjects with disease are likely to remember the presence of risk factors compared to the subjects without disease.
One of the aspects that is often overlooked is the selection of cases and controls. It is important to select the cases and controls appropriately to obtain a meaningful and scientifically sound conclusion and this can be achieved by implementing matching. Matching is defined by Gordis et al as ‘the process of selecting the controls so that they are similar to the cases in certain characteristics such as age, race, sex, socioeconomic status and occupation’ 7 This would help identify risk factors or probable etiologies that are not due to differences between the cases and controls.
Cohort study
Cohort studies are study designs that compare two groups, such as the subjects with exposure/risk factor to the subjects without exposure/risk factor, for differences in incidence of outcome/disease. Most often, cohort study designs are used to study outcome(s) from a single exposure/risk factor. Thus, cohort studies can also be hypothesis testing studies and can infer and interpret a causal relationship between an exposure and a proposed outcome, but cannot establish it (Figure 4 ).

Cohort study design
Cohort studies can be classified as prospective and retrospective. 7 Prospective cohort studies follow subjects from presence of risk factors/exposure to development of disease/outcome. This could take up to years before development of disease/outcome, and therefore is time consuming and expensive. On the other hand, retrospective cohort studies identify a population with and without the risk factor/exposure based on past records and then assess if they had developed the disease/outcome at the time of study. Thus, the study design for prospective and retrospective cohort studies are similar as we are comparing populations with and without exposure/risk factor to development of outcome/disease.
Cohort studies are typically chosen as a study design when the suspected exposure is known and rare, and the incidence of disease/outcome in the exposure group is suspected to be high. The choice between prospective and retrospective cohort study design would depend on the accuracy and reliability of the past records regarding the exposure/risk factor.
Some of the biases observed with cohort studies include selection bias and information bias. Some individuals who have the exposure may refuse to participate in the study or would be lost to follow‐up, and in those instances, it becomes difficult to interpret the association between an exposure and outcome. Also, if the information is inaccurate when past records are used to evaluate for exposure status, then again, the association between the exposure and outcome becomes difficult to interpret.
Case‐control studies based within a defined cohort
Case‐control studies based within a defined cohort is a form of study design that combines some of the features of a cohort study design and a case‐control study design. When a defined cohort is embedded in a case‐control study design, all the baseline information collected before the onset of disease like interviews, surveys, blood or urine specimens, then the cohort is followed onset of disease. One of the advantages of following the above design is that it eliminates recall bias as the information regarding risk factors is collected before onset of disease. Case‐control studies based within a defined cohort can be further classified into two types: Nested case‐control study and Case‐cohort study.
Nested case‐control study
A nested case‐control study consists of defining a cohort with suspected risk factors and assigning a control within a cohort to the subject who develops the disease. 10 Over a period, cases and controls are identified and followed as per the investigator's protocol. Hence, the case and control are matched on calendar time and length of follow‐up. When this study design is implemented, it is possible for the control that was selected early in the study to develop the disease and become a case in the latter part of the study.
Case‐cohort Study
A case‐cohort study is similar to a nested case‐control study except that there is a defined sub‐cohort which forms the groups of individuals without the disease (control), and the cases are not matched on calendar time or length of follow‐up with the control. 11 With these modifications, it is possible to compare different disease groups with the same sub‐cohort group of controls and eliminates matching between the case and control. However, these differences will need to be accounted during analysis of results.
Experimental study design
The basic concept of experimental study design is to study the effect of an intervention. In this study design, the risk factor/exposure of interest/treatment is controlled by the investigator. Therefore, these are hypothesis testing studies and can provide the most convincing demonstration of evidence for causality. As a result, the design of the study requires meticulous planning and resources to provide an accurate result.
The experimental study design can be classified into 2 groups, that is, controlled (with comparison) and uncontrolled (without comparison). 1 In the group without controls, the outcome is directly attributed to the treatment received in one group. This fails to prove if the outcome was truly due to the intervention implemented or due to chance. This can be avoided if a controlled study design is chosen which includes a group that does not receive the intervention (control group) and a group that receives the intervention (intervention/experiment group), and therefore provide a more accurate and valid conclusion.
Experimental study designs can be divided into 3 broad categories: clinical trial, community trial, field trial. The specifics of each study design are explained below (Figure 5 ).

Experimental study designs
Clinical trial
Clinical trials are also known as therapeutic trials, which involve subjects with disease and are placed in different treatment groups. It is considered a gold standard approach for epidemiological research. One of the earliest clinical trial studies was performed by James Lind et al in 1747 on sailors with scurvy. 12 Lind divided twelve scorbutic sailors into six groups of two. Each group received the same diet, in addition to a quart of cider (group 1), twenty‐five drops of elixir of vitriol which is sulfuric acid (group 2), two spoonfuls of vinegar (group 3), half a pint of seawater (group 4), two oranges and one lemon (group 5), and a spicy paste plus a drink of barley water (group 6). The group who ate two oranges and one lemon had shown the most sudden and visible clinical effects and were taken back at the end of 6 days as being fit for duty. During Lind's time, this was not accepted but was shown to have similar results when repeated 47 years later in an entire fleet of ships. Based on the above results, in 1795 lemon juice was made a required part of the diet of sailors. Thus, clinical trials can be used to evaluate new therapies, such as new drug or new indication, new drug combination, new surgical procedure or device, new dosing schedule or mode of administration, or a new prevention therapy.
While designing a clinical trial, it is important to select the population that is best representative of the general population. Therefore, the results obtained from the study can be generalized to the population from which the sample population was selected. It is also as important to select appropriate endpoints while designing a trial. Endpoints need to be well‐defined, reproducible, clinically relevant and achievable. The types of endpoints include continuous, ordinal, rates and time‐to‐event, and it is typically classified as primary, secondary or tertiary. 2 An ideal endpoint is a purely clinical outcome, for example, cure/survival, and thus, the clinical trials will become very long and expensive trials. Therefore, surrogate endpoints are used that are biologically related to the ideal endpoint. Surrogate endpoints need to be reproducible, easily measured, related to the clinical outcome, affected by treatment and occurring earlier than clinical outcome. 2
Clinical trials are further divided into randomized clinical trial, non‐randomized clinical trial, cross‐over clinical trial and factorial clinical trial.
Randomized clinical trial
A randomized clinical trial is also known as parallel group randomized trials or randomized controlled trials. Randomized clinical trials involve randomizing subjects with similar characteristics to two groups (or multiple groups): the group that receives the intervention/experimental therapy and the other group that received the placebo (or standard of care). 13 This is typically performed by using a computer software, manually or by other methods. Hence, we can measure the outcomes and efficacy of the intervention/experimental therapy being studied without bias as subjects have been randomized to their respective groups with similar baseline characteristics. This type of study design is considered gold standard for epidemiological research. However, this study design is generally not applicable to rare and serious disease process as it would unethical to treat that group with a placebo. Please see section “Randomization” for detailed explanation regarding randomization and placebo.
Non‐randomized clinical trial
A non‐randomized clinical trial involves an approach to selecting controls without randomization. With this type of study design a pattern is usually adopted, such as, selection of subjects and controls on certain days of the week. Depending on the approach adopted, the selection of subjects becomes predictable and therefore, there is bias with regards to selection of subjects and controls that would question the validity of the results obtained.
Historically controlled studies can be considered as a subtype of non‐randomized clinical trial. In this study design subtype, the source of controls is usually adopted from the past, such as from medical records and published literature. 1 The advantages of this study design include being cost‐effective, time saving and easily accessible. However, since this design depends on already collected data from different sources, the information obtained may not be accurate, reliable, lack uniformity and/or completeness as well. Though historically controlled studies maybe easier to conduct, the disadvantages will need to be taken into account while designing a study.
Cross‐over clinical trial
In cross‐over clinical trial study design, there are two groups who undergoes the same intervention/experiment at different time periods of the study. That is, each group serves as a control while the other group is undergoing the intervention/experiment. 14 Depending on the intervention/experiment, a ‘washout’ period is recommended. This would help eliminate residuals effects of the intervention/experiment when the experiment group transitions to be the control group. Hence, the outcomes of the intervention/experiment will need to be reversible as this type of study design would not be possible if the subject is undergoing a surgical procedure.
Factorial trial
A factorial trial study design is adopted when the researcher wishes to test two different drugs with independent effects on the same population. Typically, the population is divided into 4 groups, the first with drug A, the second with drug B, the third with drug A and B, and the fourth with neither drug A nor drug B. The outcomes for drug A are compared to those on drug A, drug A and B and to those who were on drug B and neither drug A nor drug B. 15 The advantages of this study design that it saves time and helps to study two different drugs on the same study population at the same time. However, this study design would not be applicable if either of the drugs or interventions overlaps with each other on modes of action or effects, as the results obtained would not attribute to a particular drug or intervention.
Community trial
Community trials are also known as cluster‐randomized trials, involve groups of individuals with and without disease who are assigned to different intervention/experiment groups. Hence, groups of individuals from a certain area, such as a town or city, or a certain group such as school or college, will undergo the same intervention/experiment. 16 Hence, the results will be obtained at a larger scale; however, will not be able to account for inter‐individual and intra‐individual variability.
Field trial
Field trials are also known as preventive or prophylactic trials, and the subjects without the disease are placed in different preventive intervention groups. 16 One of the hypothetical examples for a field trial would be to randomly assign to groups of a healthy population and to provide an intervention to a group such as a vitamin and following through to measure certain outcomes. Hence, the subjects are monitored over a period of time for occurrence of a particular disease process.
Overview of methodologies used within a study design
Randomization.
Randomization is a well‐established methodology adopted in research to prevent bias due to subject selection, which may impact the result of the intervention/experiment being studied. It is one of the fundamental principles of an experimental study designs and ensures scientific validity. It provides a way to avoid predicting which subjects are assigned to a certain group and therefore, prevent bias on the final results due to subject selection. This also ensures comparability between groups as most baseline characteristics are similar prior to randomization and therefore helps to interpret the results regarding the intervention/experiment group without bias.
There are various ways to randomize and it can be as simple as a ‘flip of a coin’ to use computer software and statistical methods. To better describe randomization, there are three types of randomization: simple randomization, block randomization and stratified randomization.
Simple randomization
In simple randomization, the subjects are randomly allocated to experiment/intervention groups based on a constant probability. That is, if there are two groups A and B, the subject has a 0.5 probability of being allocated to either group. This can be performed in multiple ways, and one of which being as simple as a ‘flip of a coin’ to using random tables or numbers. 17 The advantage of using this methodology is that it eliminates selection bias. However, the disadvantage with this methodology is that an imbalance in the number allocated to each group as well as the prognostic factors between groups. Hence, it is more challenging in studies with a small sample size.
Block randomization
In block randomization, the subjects of similar characteristics are classified into blocks. The aim of block randomization is to balance the number of subjects allocated to each experiment/intervention group. For example, let's assume that there are four subjects in each block, and two of the four subjects in each block will be randomly allotted to each group. Therefore, there will be two subjects in one group and two subjects in the other group. 17 The disadvantage with this methodology is that there is still a component of predictability in the selection of subjects and the randomization of prognostic factors is not performed. However, it helps to control the balance between the experiment/intervention groups.
Stratified randomization
In stratified randomization, the subjects are defined based on certain strata, which are covariates. 18 For example, prognostic factors like age can be considered as a covariate, and then the specified population can be randomized within each age group related to an experiment/intervention group. The advantage with this methodology is that it enables comparability between experiment/intervention groups and thus makes result analysis more efficient. But, with this methodology the covariates will need to be measured and determined before the randomization process. The sample size will help determine the number of strata that would need to be chosen for a study.
Blinding is a methodology adopted in a study design to intentionally not provide information related to the allocation of the groups to the subject participants, investigators and/or data analysts. 19 The purpose of blinding is to decrease influence associated with the knowledge of being in a particular group on the study result. There are 3 forms of blinding: single‐blinded, double‐blinded and triple‐blinded. 1 In single‐blinded studies, otherwise called as open‐label studies, the subject participants are not revealed which group that they have been allocated to. However, the investigator and data analyst will be aware of the allocation of the groups. In double‐blinded studies, both the study participants and the investigator will be unaware of the group to which they were allocated to. Double‐blinded studies are typically used in clinical trials to test the safety and efficacy of the drugs. In triple‐blinded studies, the subject participants, investigators and data analysts will not be aware of the group allocation. Thus, triple‐blinded studies are more difficult and expensive to design but the results obtained will exclude confounding effects from knowledge of group allocation.
Blinding is especially important in studies where subjective response are considered as outcomes. This is because certain responses can be modified based on the knowledge of the experiment group that they are in. For example, a group allocated in the non‐intervention group may not feel better as they are not getting the treatment, or an investigator may pay more attention to the group receiving treatment, and thereby potentially affecting the final results. However, certain treatments cannot be blinded such as surgeries or if the treatment group requires an assessment of the effect of intervention such as quitting smoking.
Placebo is defined in the Merriam‐Webster dictionary as ‘an inert or innocuous substance used especially in controlled experiments testing the efficacy of another substance (such as drug)’. 20 A placebo is typically used in a clinical research study to evaluate the safety and efficacy of a drug/intervention. This is especially useful if the outcome measured is subjective. In clinical drug trials, a placebo is typically a drug that resembles the drug to be tested in certain characteristics such as color, size, shape and taste, but without the active substance. This helps to measure effects of just taking the drug, such as pain relief, compared to the drug with the active substance. If the effect is positive, for example, improvement in mood/pain, then it is called placebo effect. If the effect is negative, for example, worsening of mood/pain, then it is called nocebo effect. 21
The ethics of placebo‐controlled studies is complex and remains a debate in the medical research community. According to the Declaration of Helsinki on the use of placebo released in October 2013, “The benefits, risks, burdens and effectiveness of a new intervention must be tested against those of the best proven intervention(s), except in the following circumstances:
Where no proven intervention exists, the use of placebo, or no intervention, is acceptable; or
Where for compelling and scientifically sound methodological reasons the use of any intervention less effective than the best proven one, the use of placebo, or no intervention is necessary to determine the efficacy or safety of an intervention and the patients who receive any intervention less effective than the best proven one, placebo, or no intervention will not be subject to additional risks of serious or irreversible harm as a result of not receiving the best proven intervention.
Extreme care must be taken to avoid abuse of this option”. 22
Hence, while designing a research study, both the scientific validity and ethical aspects of the study will need to be thoroughly evaluated.
Bias has been defined as “any systematic error in the design, conduct or analysis of a study that results in a mistaken estimate of an exposure's effect on the risk of disease”. 23 There are multiple types of biases and so, in this review we will focus on the following types: selection bias, information bias and observer bias. Selection bias is when a systematic error is committed while selecting subjects for the study. Selection bias will affect the external validity of the study if the study subjects are not representative of the population being studied and therefore, the results of the study will not be generalizable. Selection bias will affect the internal validity of the study if the selection of study subjects in each group is influenced by certain factors, such as, based on the treatment of the group assigned. One of the ways to decrease selection bias is to select the study population that would representative of the population being studied, or to randomize (discussed in section “Randomization”).
Information bias is when a systematic error is committed while obtaining data from the study subjects. This can be in the form of recall bias when subject is required to remember certain events from the past. Typically, subjects with the disease tend to remember certain events compared to subjects without the disease. Observer bias is a systematic error when the study investigator is influenced by the certain characteristics of the group, that is, an investigator may pay closer attention to the group receiving the treatment versus the group not receiving the treatment. This may influence the results of the study. One of the ways to decrease observer bias is to use blinding (discussed in section “Blinding”).
Thus, while designing a study it is important to take measure to limit bias as much as possible so that the scientific validity of the study results is preserved to its maximum.
Overview of drug development in the United States of America
Now that we have reviewed the various clinical designs, clinical trials form a major part in development of a drug. In the United States, the Food and Drug Administration (FDA) plays an important role in getting a drug approved for clinical use. It includes a robust process that involves four different phases before a drug can be made available to the public. Phase I is conducted to determine a safe dose. The study subjects consist of normal volunteers and/or subjects with disease of interest, and the sample size is typically small and not more than 30 subjects. The primary endpoint consists of toxicity and adverse events. Phase II is conducted to evaluate of safety of dose selected in Phase I, to collect preliminary information on efficacy and to determine factors to plan a randomized controlled trial. The study subjects consist of subjects with disease of interest and the sample size is also small but more that Phase I (40–100 subjects). The primary endpoint is the measure of response. Phase III is conducted as a definitive trial to prove efficacy and establish safety of a drug. Phase III studies are randomized controlled trials and depending on the drug being studied, it can be placebo‐controlled, equivalence, superiority or non‐inferiority trials. The study subjects consist of subjects with disease of interest, and the sample size is typically large but no larger than 300 to 3000. Phase IV is performed after a drug is approved by the FDA and it is also called the post‐marketing clinical trial. This phase is conducted to evaluate new indications, to determine safety and efficacy in long‐term follow‐up and new dosing regimens. This phase helps to detect rare adverse events that would not be picked up during phase III studies and decrease in the delay in the release of the drug in the market. Hence, this phase depends heavily on voluntary reporting of side effects and/or adverse events by physicians, non‐physicians or drug companies. 2
We have discussed various clinical research study designs in this comprehensive review. Though there are various designs available, one must consider various ethical aspects of the study. Hence, each study will require thorough review of the protocol by the institutional review board before approval and implementation.
CONFLICT OF INTEREST
Chidambaram AG, Josephson M. Clinical research study designs: The essentials . Pediatr Invest . 2019; 3 :245‐252. 10.1002/ped4.12166 [ PMC free article ] [ PubMed ] [ CrossRef ] [ Google Scholar ]
- Introduction
- Conclusions
- Article Information
REP indicates Rochester Epidemiology Project.
The prevalence of IPMNs using computed tomography is presented A, in each 4-year period of the study and B, in age group by decade.
Incidence curves for males and females of pancreatic cancer between the Fukuoka-negative (F-N) intraductal papillary mucinous neoplasm (IPMN) population and non-IPMN population. Patients entered the at-risk set according to their age at computed tomography.
CA indicates cancer antigen; REP, Rochester Epidemiology Project.
Age, sex, and stage–adjusted survival in PC arising from IPMNs (IPMN-PC) and non-IPMN PC is presented. HR indicates hazard ratio.
eAppendix 1. Computed Tomography Cohort Exclusion Criteria
eAppendix 2. Clinical and Demographic Data of Computed Tomography Cohort
eAppendix 3. Categories of Suspected and Presumed Intraductal Papillary Mucinous Neoplasm Based on A Priori Definitions
eAppendix 4. Clinical and Demographic Data of Pancreatic Cancer Cohort
eAppendix 5. Pancreatic Cancer Imaging Classification Using A Priori Definitions to Establish Malignant Transformation of Intraductal Papillary Mucinous Neoplasm
eTable 1. Current Procedural Terminology Codes of Abdominal Computed Tomography (CT) Imaging Used to Develop CT Cohort
eTable 2. Index Codes Used to Collect Pancreatic Cancer Cohort
eTable 3. Demographics and Clinical Characteristics of Computed Tomography Cohort
eTable 4. Demographics and Clinical Characteristics of Pancreatic Cancer Cohort
Data Sharing Statement
- IPMNs of the Pancreas: More Epidemiologically Than Clinically Relevant JAMA Network Open Invited Commentary October 17, 2023 Stefano Crippa, MD, PhD; Gabriele Capurso, MD, PhD; Massimo Falconi, MD
See More About
Sign up for emails based on your interests, select your interests.
Customize your JAMA Network experience by selecting one or more topics from the list below.
- Academic Medicine
- Acid Base, Electrolytes, Fluids
- Allergy and Clinical Immunology
- American Indian or Alaska Natives
- Anesthesiology
- Anticoagulation
- Art and Images in Psychiatry
- Artificial Intelligence
- Assisted Reproduction
- Bleeding and Transfusion
- Caring for the Critically Ill Patient
- Challenges in Clinical Electrocardiography
- Climate and Health
- Climate Change
- Clinical Challenge
- Clinical Decision Support
- Clinical Implications of Basic Neuroscience
- Clinical Pharmacy and Pharmacology
- Complementary and Alternative Medicine
- Consensus Statements
- Coronavirus (COVID-19)
- Critical Care Medicine
- Cultural Competency
- Dental Medicine
- Dermatology
- Diabetes and Endocrinology
- Diagnostic Test Interpretation
- Drug Development
- Electronic Health Records
- Emergency Medicine
- End of Life, Hospice, Palliative Care
- Environmental Health
- Equity, Diversity, and Inclusion
- Facial Plastic Surgery
- Gastroenterology and Hepatology
- Genetics and Genomics
- Genomics and Precision Health
- Global Health
- Guide to Statistics and Methods
- Hair Disorders
- Health Care Delivery Models
- Health Care Economics, Insurance, Payment
- Health Care Quality
- Health Care Reform
- Health Care Safety
- Health Care Workforce
- Health Disparities
- Health Inequities
- Health Policy
- Health Systems Science
- History of Medicine
- Hypertension
- Images in Neurology
- Implementation Science
- Infectious Diseases
- Innovations in Health Care Delivery
- JAMA Infographic
- Law and Medicine
- Leading Change
- Less is More
- LGBTQIA Medicine
- Lifestyle Behaviors
- Medical Coding
- Medical Devices and Equipment
- Medical Education
- Medical Education and Training
- Medical Journals and Publishing
- Mobile Health and Telemedicine
- Narrative Medicine
- Neuroscience and Psychiatry
- Notable Notes
- Nutrition, Obesity, Exercise
- Obstetrics and Gynecology
- Occupational Health
- Ophthalmology
- Orthopedics
- Otolaryngology
- Pain Medicine
- Palliative Care
- Pathology and Laboratory Medicine
- Patient Care
- Patient Information
- Performance Improvement
- Performance Measures
- Perioperative Care and Consultation
- Pharmacoeconomics
- Pharmacoepidemiology
- Pharmacogenetics
- Pharmacy and Clinical Pharmacology
- Physical Medicine and Rehabilitation
- Physical Therapy
- Physician Leadership
- Population Health
- Primary Care
- Professional Well-being
- Professionalism
- Psychiatry and Behavioral Health
- Public Health
- Pulmonary Medicine
- Regulatory Agencies
- Reproductive Health
- Research, Methods, Statistics
- Resuscitation
- Rheumatology
- Risk Management
- Scientific Discovery and the Future of Medicine
- Shared Decision Making and Communication
- Sleep Medicine
- Sports Medicine
- Stem Cell Transplantation
- Substance Use and Addiction Medicine
- Surgical Innovation
- Surgical Pearls
- Teachable Moment
- Technology and Finance
- The Art of JAMA
- The Arts and Medicine
- The Rational Clinical Examination
- Tobacco and e-Cigarettes
- Translational Medicine
- Trauma and Injury
- Treatment Adherence
- Ultrasonography
- Users' Guide to the Medical Literature
- Vaccination
- Venous Thromboembolism
- Veterans Health
- Women's Health
- Workflow and Process
- Wound Care, Infection, Healing
Get the latest research based on your areas of interest.
Others also liked.
- Download PDF
- X Facebook More LinkedIn
de la Fuente J , Chatterjee A , Lui J, et al. Long-Term Outcomes and Risk of Pancreatic Cancer in Intraductal Papillary Mucinous Neoplasms. JAMA Netw Open. 2023;6(10):e2337799. doi:10.1001/jamanetworkopen.2023.37799
Manage citations:
© 2024
- Permissions
Long-Term Outcomes and Risk of Pancreatic Cancer in Intraductal Papillary Mucinous Neoplasms
- 1 Division of Gastroenterology and Hepatology, Mayo Clinic, Rochester, Minnesota
- 2 Department of Internal Medicine, Cleveland Clinic, Cleveland, Ohio
- 3 Department of Internal Medicine, Columbia University Irving Medical Center and the Vagelos College of Physicians and Surgeons, New York, New York
- 4 Department of Radiology, Mayo Clinic, Rochester, Minnesota
- 5 Department of Internal Medicine, Mayo Clinic, Rochester, Minnesota
- 6 Department of Quantitative Health Sciences, Mayo Clinic, Rochester, Minnesota
- 7 Department of Laboratory Medicine and Pathology, Mayo Clinic, Rochester, Minnesota
- 8 Department of Gastroenterology and Hepatology, University of Texas MD Anderson, Houston
- Invited Commentary IPMNs of the Pancreas: More Epidemiologically Than Clinically Relevant Stefano Crippa, MD, PhD; Gabriele Capurso, MD, PhD; Massimo Falconi, MD JAMA Network Open
Question What is the estimated population prevalence of intraductal papillary mucinous neoplasms (IPMNs), associated pancreatic cancer (PC) risk, and proportion of malignant transformation of IPMNs to PC?
Findings In this cohort study of 2114 patients aged 50 years or older, the prevalence of IPMNs on contrast-enhanced computed tomography imaging was 10.9%, more than 80% of which were branch-duct IPMNs with no worrisome or high-risk features, and the risk of PC and overall survival in patients with IPMNs without worrisome or high-risk features was similar to that of patients without IPMNs. Approximately 10% of PCs arose from IPMNs, and patients with these cancers had better outcomes.
Meaning This study found that PC risk was low in patients with Fukuoka-negative IPMNs and similar to that of patients without IPMNs and that while IPMN-associated PC accounted for a small proportion of all PC cases, these patients had better survival.
Importance Intraductal papillary mucinous neoplasms (IPMNs) are pancreatic cysts that can give rise to pancreatic cancer (PC). Limited population data exist on their prevalence, natural history, or risk of malignant transformation (IPMN-PC).
Objective To fill knowledge gaps in epidemiology of IPMNs and associated PC risk by estimating population prevalence of IPMNs, associated PC risk, and proportion of IPMN-PC.
Design, Setting, and Participants : This retrospective cohort study was conducted in Olmsted County, Minnesota. Using the Rochester Epidemiology Project (REP), patients aged 50 years and older with abdominal computed tomography (CT) scans between 2000 and 2015 were randomly selected (CT cohort). All patients from the REP with PC between 2000 and 2019 were also selected (PC cohort). Data were analyzed from November 2021 through August 2023.
Main outcomes and Measures CIs for PC incidence estimates were calculated using exact methods with the Poisson distribution. Cox models were used to estimate age, sex, and stage–adjusted hazard ratios for time-to-event end points.
Results The CT cohort included 2114 patients (1140 females [53.9%]; mean [SD] age, 68.6 [12.1] years). IPMNs were identified in 231 patients (10.9%; 95% CI, 9.7%-12.3%), most of which were branch duct (210 branch-duct [90.9%], 16 main-duct [6.9%], and 5 mixed [2.2%] IPMNs). There were 5 Fukuoka high-risk (F-HR) IPMNs (2.2%), 39 worrisome (F-W) IPMNs (16.9%), and 187 negative (F-N) IPMNs (81.0%). After a median (IQR) follow-up of 12.0 (8.1-15.3) years, 4 patients developed PC (2 patients in F-HR and 2 patients in F-N groups). The PC incidence rate per 100 person years for F-HR IPMNs was 34.06 incidents (95% CI, 4.12-123.02 incidents) and not significantly different for patients with F-N IPMNs compared with patients without IPMNs (0.16 patients; 95% CI, 0.02-0.57 patients vs 0.11 patients; 95% CI, 0.06-0.17 patients; P = .62). The PC cohort included 320 patients (155 females [48.4%]; mean [SD] age, 72.0 [12.3] years), and 9.8% (95% CI, 7.0%-13.7%) had IPMN-PC. Compared with 284 patients with non-IPMN PC, 31 patients with IPMN-PC were older (mean [SD] age, 76.9 [9.2] vs 71.3 [12.5] years; P = .02) and more likely to undergo surgical resection (14 patients [45.2%] vs 60 patients [21.1%]; P = .003) and more-frequently had nonmetastatic PC at diagnosis (20 patients [64.5%] vs 130 patients [46.8%]; P = .047). Patients with IPMN-PC had better survival (adjusted hazard ratio, 0.62; 95% CI, 0.40-0.94; P = .03) than patients with non-IPMN PC.
Conclusions and Relevance In this study, CTs identified IPMNs in approximately 10% of patients aged 50 years or older. PC risk in patients with F-N IPMNs was low and not different compared with patients without IPMNs; approximately 10% of patients with PC had IPMN-PC, and they had better survival compared with patients with non-IPMN PC.
Incidental detection of pancreatic cystic lesions (PCLs) has increased in recent years primarily due to improved resolution and widespread use of cross-sectional imaging. 1 , 2 Intraductal papillary mucinous neoplasms (IPMNs) are the most frequently identified PCLs. 3 - 5 Current international consensus guidelines 4 for management of IPMNs recommend image-based surveillance with the aim to detect clinical and imaging features of advanced neoplasia (high-grade dysplasia or pancreatic cancer). Although a minority of IPMNs warrant surgical resection, our existing knowledge about the prevalence, natural history, and risk of malignancy in IPMNs is largely derived from surgical series 6 , 7 or from patients treated at tertiary centers, 8 - 10 populations which are not representative of the population burden of the disease. 2 , 10 , 11 There is a critical need to define the prevalence and natural history of IPMNs in a population-based cohort to inform surveillance guidelines.
A 2019 systematic review and meta-analysis 12 estimated the pooled population prevalence of PCLs at approximately 8%. The reported prevalence varies across studies depending on the imaging modality used, geographical location, and age of the cohort studied, and most studies include all PCLs not specifically IPMNs. Furthermore, to our knowledge, there are no population-based estimates of the burden of pancreatic cancer (PC) in individuals with IPMNs or the proportion of PCs that develop from or adjacent to an IPMN. This study uses a population-based cohort to assess the prevalence and associated long-term outcomes and PC risk in IPMN. In addition, we investigated the proportion of PCs that arose from IPMNs (IPMN-PCs) and compared their outcomes with those of non-IPMN PCs.
This cohort study was approved by the Mayo Clinic Foundation Institutional Review Board and Olmsted Medical Center Institutional Review Board (IRB). The IRB approved a waiver of the requirement to obtain informed consent in accordance with 45 CFR §46.116 as justified by the investigator and a waiver of Health Insurance Portability and Accountability Act of 1996 (HIPAA) authorization in accordance with applicable HIPAA regulations. The study is reported following the Strengthening the Reporting of Observational Studies in Epidemiology ( STROBE ) reporting guideline.
The Rochester Epidemiology Project (REP) is a medical records linkage system that provides longitudinal, population-based medical data for residents of Olmsted County, Minnesota. The REP database captures more than 95% of the medical care delivered to county residents across all community health care institutions and has served as a valuable resource facilitating population-based research in a variety of diseases. 13 , 14 From the REP database, we created 2 population cohorts to address our aims: the computed tomography (CT) cohort to estimate the population prevalence and natural history of IPMNs and the PC cohort to investigate the proportion of PCs that arose from malignant transformation of IPMNs and compare clinical outcomes with those of non-IPMN PCs.
Using the REP database and procedure codes for abdominal imaging, we identified all unique Olmsted County residents between January 1, 2000, and December 31, 2015, aged 50 years or older who underwent a contrast-enhanced abdomen CT scan (eTable 1 in Supplement 1 ). We divided the 16-year study into 4-year periods and used a computer-generated, stratified random sampling of a total 2500 unique patients: 625 patients from each 4-year period. See exclusion criteria in eAppendix 1 in Supplement 1 .
Clinical and demographic data were entered in the study database (eAppendix 2 in Supplement 1 ). Race was identified from the electronic health record by study team members (A.C. and J.L.). Race options in the databases were Black, Asian, White, other, and unknown. Information regarding race was collected given that this was a population study and the demographics of the population studied may not be generalizable to other populations. CT images were manually reviewed (J.D.L.F. and A.N) for each study individual. If new pancreatic findings or discrepancies from what was described in the original CT scan report were identified, images were reviewed by a second reviewer (H.N. or N.T.) to ensure accuracy. If a PCL was present, it was categorized as an IPMN (branch duct, mixed, or main duct) based on our a priori definitions (eAppendix 3 in Supplement 1 ). 9 , 15 PCLs that met IPMN criteria were further classified as Fukuoka high-risk (F-HR), worrisome (F-W), or negative (F-N) based on current international consensus guidelines. 4 If patients with IPMNs had subsequent cross-sectional imaging of the abdomen (contrast CT or magnetic resonance imaging [MRI]) performed more than 6 months from the date of index imaging, the most recent cross-sectional imaging and clinical data were also recorded.
Using the REP database, we identified all patients aged 18 years or older with confirmed PC in Olmsted County between January 1, 2000, and December 31. 2019. These patients were initially identified using diagnostic codes (eTable 2 in Supplement 1 ). Then, each patient electronic medical record was manually reviewed to ensure accurate diagnosis and determine patients who had pathology-confirmed pancreatic ductal adenocarcinoma or cancer that was clinically treated as PC based on imaging and laboratory testing. Patients who were not Olmsted County residents for 1 year or more were excluded. For each unique patient, we collected relevant demographic, clinical, and staging 16 data (eAppendix 4 in Supplement 1 ).
The cross-sectional imaging at the time of PC diagnosis was reviewed by the study radiologist (N.T.). Each patient was classified using established a priori imaging criteria as definitive IPMN-PC, probable IPMN-PC, possible IPMN-PC, or non-IPMN PC (definitions of classifications used are in eAppendix 5 in Supplement 1 ). For patients in the PC cohort, surgical pathology was reviewed by a gastrointestinal pathologist (R.P.G.) to confirm histology and determine if the PC arose from an IPMN.
All data for our study were stored and managed with a research electronic data capture tool. 17 Continuous data are summarized as mean (SD) unless otherwise noted. Categorical data are presented as frequency (percentage). CIs for binomial proportions were calculated using the Agresti-Coull method. CIs for incidence estimates were calculated using exact methods with the Poisson distribution. We used the 2-sample t test and Pearson χ 2 test to compare independent groups for continuous and discrete variables, respectively. The Stuart-Maxwell test for marginal homogeneity was used to test for differences in discrete variables between baseline and follow-up images. Standardized incidence ratios (SIRs) were calculated to estimate the difference in future risk of PC between patients with and without IPMN cysts in the CT cohort. SIR was calculated by computing incidence rates within age (per year)–, sex-, and IPMN-specific cross-tabulations, then using a generalized linear model (log link) with age (3–degrees of freedom spline), sex, age-sex interaction, and IPMN as explanatory variables, with the log of person-years as an offset. Kaplan-Meier curves for PC risk in the CT cohort were produced using age as the time scale to account for the different age distribution between patients with and without IPMNs. Curves were compared using the score test from a Cox model, with age as the time scale and sex as a stratification variable. Cox models were used to estimate age, sex, and stage–adjusted hazard ratios (HRs) for time-to-event end points (eg, mortality or pancreatic cancer). An adjusted survival curve for IPMN-PC was calculated using estimated survival from the Cox model applied to an IPMN-PC cohort with the covariate distribution of the non-IPMN PC group. All hypothesis tests were 2-tailed with a .05 significance level. Analyses were conducted using R statistical software version 4.1 or higher (R Project for Statistical Computing). Data were analyzed from November 2021 through August 2023.
A total of 2114 patients in the CT cohort (55 Asian [2.6%], 42 Black [2.0%], 1943 White [91.9%], and 74 other race or race not available [3.5%]; 1140 females [53.9%] and 974 males [46.1%]; mean [SD] age, 68.6 [12.1] years) met study eligibility criteria ( Figure 1 ). Of the CT cohort, 231 patients (10.9%; 95% CI, 9.7%-12.3%) were found to have an IPMN while 1883 patients (89.1%; 95% CI, 87.7%-90.3%) did not have an IPMN. Of 231 IPMNs, 80 IPMNs (34.6%) were described in the original radiology report while 151 IPMNs (65.4%) were found after independent review. This prevalence was consistent over the four 4-year periods ( Figure 2 A). The likelihood of discovering an IPMN increased with age, from 4.9% (95% CI, 3.4%-6.8%) in patients aged 50 to 59 years to 8.3% (95% CI, 6.3%-10.9%) in those aged 60 to 69 years, 11.6% (95% CI, 9.0%-14.8%) in those aged 70 to 79 years, 21.2% (95% CI, 17.3%-25.6%) in those aged 80 to 89 years, and 26.0% (95% CI, 17.4%-36.8%) in those aged 90 years or older ( Figure 2 B). Patients with an IPMN compared with patients without an IPMN were older (mean [SD] age, 75.4 [12.1] years vs 67.7 [11.8] years; P < .001) and more often female (147 females [63.6%] vs 993 females [52.7%]; P = .002) and had a lower median (IQR) body mass index (BMI; calculated as weight in kilograms divided by height in meters squared; 26.0 [23.1-29.3] vs 27.7 [24.2-32.1]; P = .002). Race, smoking history, jaundice, history of acute pancreatitis, family history of pancreatic cancer, diabetes history, serum cancer antigen 19-9 (CA 19-9) level, fasting blood glucose level, and hemoglobin A 1c level were similar in the 2 groups (eTable 3 in Supplement 1 ).
Most IPMNs (210 IPMNs [90.9%]) were branch duct, while 16 IPMNs (6.9%) were main duct and 5 IPMNs (2.2%) were mixed. By Fukuoka criteria, 4 187 IPMNs (81.0%) were F-N, 39 IPMNs (16.9%) were F-W, and 5 IPMNs (2.2%) were F-HR. Overall in the CT cohort, 5 patients (0.23%) had an F-HR IPMN. Of 187 F-N cysts, all were branch duct, whereas of 44 F-HR and F-W IPMNs, 16 cysts (36.4%) were main duct and 5 cysts (11.4%) were mixed. The most frequent high-risk and worrisome features detected were obstructive jaundice (4 patients) and a main pancreatic duct (MPD) diameter of 5 to 9 mm (22 patients), respectively. Most IPMNs were solitary (140 IPMNs [60.6%]), and of 217 IPMNs with location identified, they were more frequently located in the head (84 IPMNs [38.7%]) and tail (73 IPMNs [33.6%]) compared with the body (45 IPMNs [20.7%]) and neck (15 IPMNs [6.9%]) ( P < .001 for equal proportions). The mean (SD) size of the largest cyst was 8.8 (5.5) mm. The population prevalence of a cyst greater than 3 cm in diameter was 3 patients (0.1%). Of 217 IPMNs with size measured, 148 cysts (68.2%) were less than 10 mm in diameter. Of 21 IPMNs with a dilated MPD, the mean (SD) MPD diameter was 6.2 (1.3) mm; the population prevalence of an MPD diameter greater than 9 mm was 4 patients (0.2%).
Among patients with IPMNs, 107 individuals (46.3%) received follow-up with at least 1 abdominal cross-sectional imaging (CT or MRI) more than 6 months after the initial contrast CT scan, with a median (IQR) interval of 5.3 (2.2-6.2) years between index CT scan and latest abdominal imaging. A mean (SD) increase in maximal cyst diameter of 1.6 (4.7) mm was noted in 44 patients (41.1%), and 15 patients (14.0%) had additional new cysts identified on follow-up imaging. There were 6 patients (5.6%) who progressed within Fukuoka criteria. Of 86 patients who were F-N at baseline, 6 patients (6.9%) progressed to F-W (4 patients) or F-HR (2 patients). Patients who progressed from F-N to F-HR did so within 5 years of the index CT scan. No F-W IPMNs progressed to F-HR.
In 231 patients with IPMNs, there were 4 PC diagnoses during a median (IQR) follow-up of 12.0 (8.1-15.3) years and 1507 total person-years (2 of 5 patients in F-HR and 2 of 187 patients in F-N). In 1883 patients without IPMNs, there were 17 PC diagnoses during a median (IQR) follow-up of 11.7 (8.4-16.4) years and 15 656 total person-years. Patients with F-N IMPNs who developed PC during surveillance progressed to F-HR, and they both developed PC within 5 years of the index CT scan. The PC incidence rates per 100 person-years for F-HR, F-W, F-N, and non-IPMN groups were 34.06 incidents (95% CI, 4.12-123.02 incidents), less than 0.01 incidents (95% CI, <0.00-1.56 incidents), 0.16 incidents (95% CI, 0.02-0.57 incidents), and 0.11 incidents (95% CI, 0.06-0.17 incidents), respectively ( P < .001). Incidence rates per 100 person-years were not significantly different for patients with F-N IPMNs compared with patients without IPMNs (0.16 incidents; 95% CI, 0.02-0.57 incidents vs 0.11 incidents; 95% CI, 0.06-0.17 incidents; P = .62). Overall, the risk of developing PC during follow-up was not significantly different in patients with F-N IPMNs (SIR = 1.26; 95% CI, 0.28-5.56; P = .76) compared with individuals without IPMNs ( Figure 3 ). The overall survival in these groups was similar (adjusted HR [aHR], 1.05; 95% CI, 0.86-1.28; P = .64) after adjusting for age and sex. Even comparing all patients with non–F-HR IPMNs with patients without IPMNs, the risk of PC (SIR = 1.03; 95% CI, 0.23-4.55; P = .97) and the overall survival (aHR, 1.06; 95% CI, 0.88-1.27; P = .57) were similar after adjusting for age and sex. This cohort of 212 patients with non–F-HR IPMNs consisted primarily of patients with IPMNs less than 10 mm in diameter (145 patients [68.4%]), and IPMNs between 10 and 20 mm (57 patients [26.9%]) and 20 mm or greater (10 patients [4.7%]) were in the minority.
The PC cohort included a total of 320 patients (6 Asian [1.9%], 4 Black [1.2%], 297 White [92.8%], and 13 other race or race not available [4.1%]; 155 females [48.4%] and 165 males [51.6%]; mean [SD] age at time of PC diagnosis, 72.0 [12.3] years) ( Figure 4 ). At PC diagnosis, this population had a median (IQR) serum CA 19-9 level of 351 (44-2515) IU/L and mean (SD) BMI of 26.3 (5.8) and 18 patients (5.7%) had at least 1 first-degree relative with PC. At the time of diagnosis, clinical stage distribution was as follows: 71 patients with stage I (22.2%), 30 patients with stage II (9.4%), 49 patients with stage III (15.3%), 168 patients with stage IV (52.5%), and 2 patients with unknown stage (0.6%) cancer. The median overall survival was 7.0 months (95% CI, 5.5-8.0 months), with significantly longer survival among patients with earlier stage at diagnosis (stage I: 24.4 months; 95% CI, 20.1-40.9 months; stage II: 10.3 months; 95% CI, 7.5-41.2 months; stage III: 7.6 months; 95% CI, 5.6-10.1 months; stage IV: 3.3 months; 95% CI, 2.5-4.8 months; P < .001).
Of 74 patients with PC who underwent surgical resection, 14 individuals (18.9%) were confirmed to have IPMN-PC after pathology expert review. Of patients with radiologic criteria for IPMN-PC, 11 individuals (3.5%) met criteria for definitive IPMN-PC, 15 individuals (4.8%) for probable IPMN-PC, and 21 individuals (6.7%) for possible IPMN-PC, while 266 individuals (85.0%) had non-IPMN PC. Combining patients with pathology-confirmed IPMN-PC and those without pathology confirmation but either definitive or probable IPMN-PC based on imaging, the prevalence of IPMN-PC was 31 patients (9.8%; 95% CI, 7.0%-13.7%). This prevalence of IPMN-PC decreased to 21 patients (6.7%; 95% CI, 4.4%-10.0%) if only definitive IPMN-PC was included from the imaging group. Including only individuals with cross-sectional imaging, surgical pathology, or both, 31 patients with IPMN-PC were older (mean [SD] age, 76.9 [9.2] vs 71.3 [12.5] years; P = .02), more likely to undergo surgical resection (14 patients [45.2%] vs 60 patients [21.1%]; P = .003), more-frequently had nonmetastatic cancer at diagnosis (20 patients [64.5%] vs 130 patients [45.8%]; P = .047), and had significantly improved age, sex, and stage–adjusted survival (aHR, 0.62; 95% CI, 0.40-0.94; P = .03) compared with 284 patients with non-IPMN PC ( Figure 5 ). Demographic and clinical data for patients with IPMN-PC and non-IPMN PC are summarized in eTable 4 in Supplement 1 .
This cohort study found that the estimated population prevalence of IPMNs on CT in individuals aged 50 years or older was 10.9% and this prevalence increased with age. Most of these were branch-duct IPMNs, and fewer than 20% of IPMNs had worrisome or high-risk features. During extended follow-up, development of IPMN-PC was infrequent and the PC risk in patients with IPMNs without worrisome or high-risk features was similar to that of individuals without IPMNs. We further identified that approximately 10% of PCs developed in the background of an IPMN. Compared with patients with non-IPMN PC, individuals with IPMN-PC were less likely to have metastatic disease at diagnosis and had improved overall survival.
This study focused on individuals aged 50 years or older given that PCLs are less likely to be incidentally detected in younger individuals and the risk of PC increases with age. 5 , 10 , 18 - 20 We also specifically assessed IPMNs given that IPMNs are the most prevalent PCLs in clinical practice. 3 - 5 We used contrast-enhanced CT scans given that this is the most widely used cross-sectional abdominal imaging modality in the US 1 and is less impacted by selection bias compared with more pancreas-specific imaging modalities, such as MRI or endoscopic ultrasound. Previous studies 5 , 10 , 19 , 20 using CT imaging reported a PCL prevalence of 1.2% to 5.4%. Our study identified a higher prevalence, likely associated with image review by experts and limiting of the study population to patients aged 50 years and older. Laffan et al, 10 in a CT-based study in the US, described a PCL prevalence in individuals aged 50 to 89 years ranging from 1.5% from 8.7%, while our study found a prevalence of presumed or suspected IPMNs ranging from 4.9% to 21.2% for the same age group. This reported difference may be explained to a certain extent by our study selecting a random cohort from population-based data compared with the single tertiary referral institution in Laffan et al, 10 which specifically excluded individuals with known pancreatic disease in an attempt reduce referral bias.
Multiple studies 8 , 9 , 15 in tertiary centers have found that IPMNs were associated with increased PC risk and that risk was higher in the presence of F-W or F-HR features. Attempts to quantify the risk of malignant transformation of PCLs to PC using large administrative databases have found that overall risk was relatively low. 21 , 22 Specifically, IPMN-PC was more frequently earlier stage at diagnosis and had overall improved survival compared with non-IPMN PC. 23 , 24 Our population-based study’s findings agree with these prior observations. The improved prognosis of IPMN-PC has been attributed to an earlier stage of diagnosis and a greater proportion of nonmetastatic disease, as observed in our study. Moreover, the survival benefit in IPMN-PC has been primarily observed in node-negative disease, further highlighting outcomes associated with stage shift. Although several studies 8 , 9 describe the prevalence of PC in patients with IPMN, the true prevalence in the general population has not been previously reported, to our knowledge. This study provides estimates of the prevalence of IPMN-PC in a population-based PC cohort and addresses a critical gap in our understanding of IPMN-PC epidemiology. Using our CT cohort, we also found that the risk of developing PC was relatively low. This is highlighted further in our study given that patients with F-N IPMNs did not have different rates of PC compared with patients without IPMNs. This finding highlights previous work published by our group finding that F-HR IPMNs were the primary contributors to the risk of developing PC while F-W and F-N IPMNs had a low 5-year PC risk. 15 Although the risk of IPMN-PC is has been extensively described, 8 , 9 our population-based study further demonstrates that most IPMNs did not progress in Fukuoka stage and did not transform into PC, a similar message expressed by the current American Gastroenterological Association pancreatic cyst guidelines, published in 2015, 25 and studies published in 2022 26 and 2016. 27
We acknowledge several limitations in our study. The REP primarily represents a homogenous White population, and inferences from this data may not apply to a population that is more diverse. Most individuals in the CT cohort did not have pathology-confirmed IPMN. We used a priori imaging-based definitions to reduce risk of PCL misclassification. 9 , 15 This is reflective of clinical management given that most IPMNs in clinical practice are presumed or suspected based on imaging and are not biopsy proven. The CT cohort was constructed randomly from a population-based sample of individuals who had undergone cross-sectional imaging. While this potentially introduced selection bias, it provided population-level data and improved on our current epidemiologic knowledge of IPMNs, which is primarily derived from hospital-based referral cohorts. While most CT scans in this population sample were performed for indications unrelated to the pancreas, we did not use indication for imaging as an inclusion or exclusion criterion; therefore, it is possible that some patients in this random sample underwent imaging for a known pancreatic cyst or suspected pancreas cancer. The alternative of an unbiased population-based imaging study to estimate incidental IPMN prevalence would be cost and effort prohibitive and expose study participants to the risk of contrast-enhanced imaging. Another possible limitation of our study was the use of CT instead of MRI. We selected CT imaging given that it is the most widely used imaging modality while recognizing that MRI is better at identifying small IPMNs. However, CT is generally able to detect IPMNs that are clinically relevant, and using MRI as the imaging modality could potentially limit the sample size. A similar population-based study using MRIs may be considered in the future and will be particularly relevant if assessing the incidence of IPMNs. A small subset of the IPMN population in this study underwent long-term imaging follow-up. While this limited the study sample size for assessing long-term outcomes associated with IPMNs, our study provides data on population-level outcomes in contrast to the more widely available referral center–based outcomes in this disease. The small number of PC events in the CT cohort resulted in wide CIs, and inferences based on asymptotic theory may be unreliable. Additionally, to detect IPMN-PCs, we combined imaging and pathologic criteria. Imaging criteria of IPMN-PCs have not been definitively established and may impact the accuracy of our prevalence estimates. However, these imaging criteria for IPMN-PCs were defined a priori in consultation with an expert pancreatic radiologist (N.T.) to avoid the inherent bias of limiting the study to surgically confirmed PC. To further address this limitation, we have included estimates in the subgroup of patients with surgically confirmed PCs.
This cohort study provides population estimates exploring the association between IPMNs and PCs. In the general population, IPMNs on CT were present in approximately 1 of 10 patients aged 50 years or older, of which more than 80% were branch-duct IPMNs without high-risk or worrisome features. Among IPMNs that were F-N at baseline, fewer than 10% developed worrisome or high-risk features on follow-up. PC development in IPMN was a rare event overall. Moreover, in F-N IPMNs, the incidence of PC was not significantly different from that among patients without IPMNs, a key finding that if validated in a larger population could challenge the relevance of extended surveillance in this population of patients with F-N IPMNs who make up most of the IPMN population. We further found that malignant IPMNs comprised approximately 10% of PC diagnoses and that IPMN-PC was earlier stage at diagnosis and associated with improved survival regardless of stage at presentation compared with non-IPMN PC. Results of this study provide insights regarding IPMN prevalence and the associated PC risk and may inform future population surveillance strategies and guidelines for patients with IPMNs.
Accepted for Publication: August 28, 2023.
Published: October 17, 2023. doi:10.1001/jamanetworkopen.2023.37799
Open Access: This is an open access article distributed under the terms of the CC-BY License . © 2023 de la Fuente J et al. JAMA Network Open .
Corresponding Author: Shounak Majumder, MD, Division of Gastroenterology and Hepatology, Mayo Clinic, Rochester, MN 55905 ( [email protected] ).
Author Contributions: Drs de la Fuente and Majumder had full access to all of the data in the study and take responsibility for the integrity of the data and the accuracy of the data analysis.
Concept and design: de la Fuente, Chatterjee, Nehra, Chari, Takahashi, Majumder.
Acquisition, analysis, or interpretation of data: de la Fuente, Chatterjee, Lui, Nehra, Bell, Lennon, Kassmeyer, Graham, Nagayama, Schulte, Doering, Delgado, Vege, Takahashi, Majumder.
Drafting of the manuscript: de la Fuente, Nehra.
Critical review of the manuscript for important intellectual content: All authors.
Statistical analysis: de la Fuente, Lui, Nehra, Bell, Lennon, Kassmeyer, Schulte.
Obtained funding: Majumder.
Administrative, technical, or material support: de la Fuente, Chatterjee, Graham, Doering, Delgado.
Supervision: de la Fuente, Chari, Majumder.
Conflict of Interest Disclosures: Dr Doering reported receiving a minority portion of compensation for licensed intellectual property from Exact Sciences paid to the Mayo Clinic outside the submitted work. Dr Majumder reported receiving grants from the Centene Charitable Foundation and National Cancer Institute during the conduct of the study and research support from Exact Sciences outside the submitted work and having patents pending for Exact Sciences. Mayo Clinic and Exact Sciences have an intellectual property development agreement, and Dr Majumder is listed as an inventor under this agreement and could share future royalties as an employee of Mayo Clinic. No other disclosures were reported.
Funding/Support: The use of research electronic data capture for data collection was made possible by the Mayo Clinic Center for Translational Science Activities through grant UL1TR002377 from the National Center for Advancing Translational Sciences, a component of the National Institutes of Health. This study used resources of the Rochester Epidemiology Project (REP) medical records-linkage system, which is supported by grant AG 058738 from the National Institute on Aging, the Mayo Clinic Research Committee, and fees paid annually by REP users. Dr Majumder was supported by a gift to the Mayo Clinic for the Pancreatic Cancer Early Detection Research Program from the Centene Charitable Foundation and by grant U01 CA210138 from the National Cancer Institute.
Role of the Funder/Sponsor: The funders had no role in the design and conduct of the study; collection, management, analysis, and interpretation of the data; preparation, review, or approval of the manuscript; and decision to submit the manuscript for publication.
Disclaimer: The content of this manuscript is solely the responsibility of the authors and does not necessarily represent the official views of the National Cancer Institute or the National Institutes of Health.
Data Sharing Statement: See Supplement 2 .
Additional Contributions: We would like to acknowledge our programmer analyst, Richard S. Pendegraft, MS (Department of Quantitative Health Sciences, Mayo Clinic), who assisted in selecting the random sample of 2500 patients for manual review. This individual’s services related to this project were supported by Dr Majumder's research grants.
- Register for email alerts with links to free full-text articles
- Access PDFs of free articles
- Manage your interests
- Save searches and receive search alerts
Effect of a fall within three months of admission on delirium in critically Ill elderly patients: a population-based cohort study
- Original Article
- Open access
- Published: 14 May 2024
- Volume 36 , article number 111 , ( 2024 )
Cite this article
You have full access to this open access article
- Fan Bu 1 na1 ,
- Hong-tao Cheng 1 na1 ,
- Zi-lin Wang 1 na1 ,
- Yu-shan Hou 2 ,
- Zhuang Zhuang 1 ,
- Can-yang Li 1 ,
- Ya-qi Wang 1 ,
- Yue Zhang 1 ,
- Jun Lyu 3 , 4 &
- Qi-yuan Lyu 1
189 Accesses
Explore all metrics
Delirium is common among elderly patients in the intensive care unit (ICU) and is associated with prolonged hospitalization, increased healthcare costs, and increased risk of death. Understanding the potential risk factors and early prevention of delirium is critical to facilitate timely intervention that may reverse or mitigate the harmful consequences of delirium.
To clarify the effects of pre-admission falls on ICU outcomes, primarily delirium, and secondarily pressure injuries and urinary tract infections.
The study relied on data sourced from the Medical Information Mart for Intensive Care IV (MIMIC-IV) database. Statistical tests (Wilcoxon rank-sum or chi-squared) compared cohort characteristics. Logistic regression was employed to investigate the association between a history of falls and delirium, as well as secondary outcomes, while Kaplan–Meier survival curves were used to assess short-term survival in delirium and non-delirium patients.
Study encompassed 22,547 participants. Delirium incidence was 40%, significantly higher in patients with a history of falls (54.4% vs. 34.5%, p < 0.001). Logistic regression, controlling for confounders, not only confirmed that a history of falls elevates the odds of delirium (OR: 2.11; 95% CI: 1.97–2.26; p < 0.001) but also showed it increases the incidence of urinary tract infections (OR:1.50; 95% CI:1.40–1.62; p < 0.001) and pressure injuries (OR:1.36; 95% CI:1.26–1.47; p < 0.001). Elderly delirium patients exhibited lower 30-, 180-, and 360-day survival rates than non-delirium counterparts (all p < 0.001).
Conclusions
The study reveals that history of falls significantly heighten the risk of delirium and other adverse outcomes in elderly ICU patients, leading to decreased short-term survival rates. This emphasizes the critical need for early interventions and could inform future strategies to manage and prevent these conditions in ICU settings.
Similar content being viewed by others
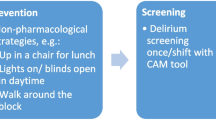
Effectiveness of a Multi-component Delirium Prevention Program Implemented on General Medicine Hospital Units: an Interrupted Time Series Analysis
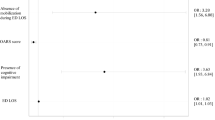
Predictors of delirium in older patients at the emergency department: a prospective multicentre derivation study
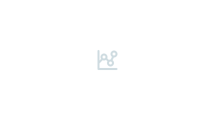
Factors associated with post-operative delirium in hip fracture patients: what should we care
Avoid common mistakes on your manuscript.
Falls have become the second leading cause of death worldwide [ 1 ]. In the United States, approximately 30% of people over the age of 65 experience a fall each year [ 2 ]. Falls not only cause a variety of serious consequences, such as disability, loss of autonomy, and serious neurological damage, but they also increase the risk of death [ 3 , 4 ]. Across all ethnic groups, falls are responsible for a staggering 75% of unintentional deaths in people over the age of 70 [ 5 ]. The financial burden of falls in the U.S. reached approximately $5 billion in 2015, imposing significant financial costs on the healthcare infrastructure [ 6 ]. Therefore, early detection and prevention of falls are essential to promote healthy aging.
Delirium, characterized by acute neuropsychiatric symptoms such as inattention and sudden cognitive decline, is notably prevalent among elderly inpatients, with incidences reaching 70–87% [ 7 ]. This condition, often overlooked in intensive care unit (ICU) settings due to preexisting conditions in the elderly, can lead to severe consequences including functional impairment, extended hospital stays, cognitive decline, and heightened mortality rates—2–4 times higher than non-ICU settings [ 8 , 9 , 10 ]. At the same time, the management of delirium is associated with high healthcare costs and caregiver burden, the cumulative cost over 30 days for ICU patients with delirium was $17,838 [ 11 ]. This figure is expected to increase as the population continues to age. However, early detection and appropriate care can prevent or mitigate delirium in geriatric patients [ 12 ], potentially reducing healthcare costs, length of hospital stays, and mortality.
While previous literature has recognized the association between falls and delirium [ 13 ], previous studies often included a history of falls as part of a cluster of risk factors without isolating it as a standalone variable. prior investigations have not specifically focused on the unique setting of the ICU.
While prior studies have explored the link between fall history and delirium, they typically treat fall history as part of a broader set of risk factors without specific focus on the ICU context or precise timing of falls. This overlooks the unique dynamics of falls within the ICU setting and their temporal proximity to ICU admission. Moreover, existing research primarily delves into fall risk factors and general outcomes post-fall, rarely connecting falls directly to pressure injury or UTI, especially within the ICU context. ICU patients are more prone to severe outcomes like delirium due to their critical health and complex treatments such as mechanical ventilation [ 14 ]. This study advances the understanding of falls' impact on ICU patients by isolating fall history as a unique variable and exploring its link to specific outcomes like delirium, pressure injuries and urinary tract infections (UTIs). Furthermore, this study integrates survival analysis to explore how pre-admission falls impact mortality and severe outcomes, like delirium, in elderly ICU patients. By focusing on this unique environment, the research aims to provide vital insights into early intervention strategies, significantly enriching the current body of knowledge regarding patient care in critical care settings.
Study design and population
The study relied on data sourced from the Medical Information Mart for Intensive Care IV (MIMIC-IV) database, version 2.0, which is an extensive, freely accessible, and publicly available resource [ 15 ]. The MIMIC-IV database received funding support from the National Institute of Biomedical Imaging and Bioengineering (NIBIB) of the National Institutes of Health (NIH) under grant numbers R01-EB001659 (2003–2013) and R01-EB017205 (2014–2018). Approval for the use of the database was obtained from the institutional review boards of Beth Israel Deaconess Medical Center (Boston, MA) and the Massachusetts Institute of Technology (Cambridge, MA). This study was an analysis of the third-party anonymized publicly available database with pre-existing institutional review board (IRB) approval. The MIMIC-IV database comprehensively captures general patient information, disease-related data, relevant treatments administered, and patients' key blood biochemical indices [ 16 , 17 ].
The subject selection criteria for this study were clearly defined: patients age 65 or older in whom a history of falls in the last 3 months was addressed were included. However, individuals with an ICU stay of less than one day or those without documentation of delirium and severe mental illness were intentionally excluded from this study. Ultimately, a cohort of 22,547 patients was captured in this investigation (Fig. 1 ).
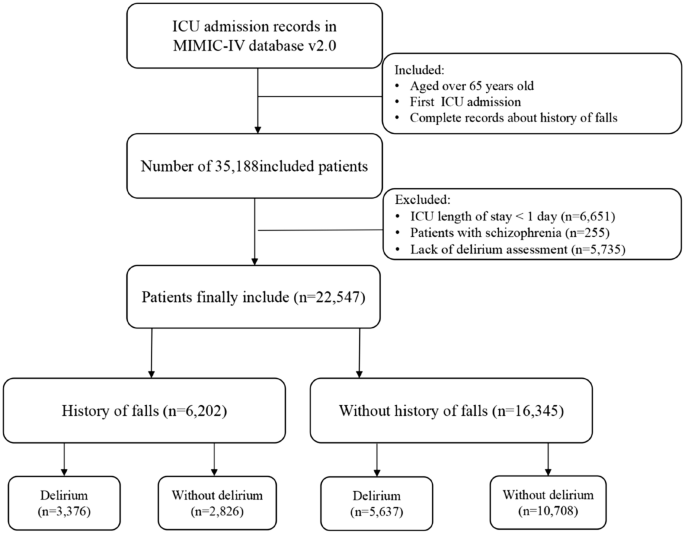
Flowchart of Delirium in Study Participants
Exposure and outcomes
Falls represent unanticipated occurrences beyond an individual's control, characterized by abrupt, involuntary, and unintended shifts in a patient's position resulting in descent to the ground or a lower level compared to their initial position [ 4 ]. The exposure factors in this study were defined as the incidence of unintentional falls among elderly, critically ill patients in the three months prior to ICU admission. A patient's fall history was meticulously assessed upon admission by trained ICU staff through a direct question: "Have you had a fall in the past three months?" An affirmative response to this question identified the patient as having a recent fall history.
Delirium, also referred to as acute encephalopathy, is characterized by changes in consciousness, attention impairment, and cognitive dysfunction [ 18 ]. We, employing the Confusion Assessment Method in the ICU (CAM-ICU) [ 19 ] and a review of physician and nursing notes [ 20 ], conducted the delirium assessment. The assessment of patients' mental status changes during their hospital stay was conducted through a comprehensive review of medical records, including initial and daily observations recorded by nursing staff, evaluations documented in progress notes by nurses or physicians specialized in delirium management, insights from specialized consultations, and summaries provided at discharge. This crucial information is meticulously documented within the "chartevents" form penned by the healthcare provider. Delirium could be defined as a patient who either had 1) a positive CAM-ICU or 2) diagnosed with delirium by a physician based on chart documentation.
Study outcomes included delirium, urinary tract infections and pressure injury, with delirium as the primary outcome and urinary tract infections and pressure injury as secondary outcomes. UTIs were diagnosed according to the International Classification of Diseases, 9th and 10th versions. Pressure injuries were carefully assessed and documented by dedicated intensive care nurses.
Variable collection
Navicat Premium software, version 16, using Structured Query Language, was used for data extraction. The maximum percentage of missing values for any of the extracted variables did not exceed 20%, and multiple imputation was used to deal with these missing data [ 21 ]. The selection of covariates in our study was based on a comprehensive approach that took into account prior literature, clinical expertise, and the availability of data within the database. The following variables were included in this study:
General characteristics: age, sex, marital status, race, language, weight, hospital and ICU length of stay.
Scores: Glasgow Coma Scale (GCS), Sequential Organ Failure Assessment (SOFA), Braden scores and Charlson Comorbidity Index (CCI).
Comorbidities: myocardial infarct, congestive heart failure, dementia, rheumatic disease, mild liver disease, diabetes, renal disease, malignant cancer, severe liver disease, cerebrovascular disease, hypertension, sepsis, depression, pain, paraplegia, peripheral vascular disease and metastatic solid tumor.
Vital signs: heart rate mean, mean blood pressure, respiratory rate mean and temperature mean.
Laboratory parameters: SpO 2 mean, albumin, Blood Urea Nitrogen (BUN) and creatinine.
Treatments and drugs: enteral nutrition, mechanical thrombectomy, dialysis present, vasoactive agents, sedatives and invasive mechanical ventilation.
Outcomes: delirium, pressure injury, urinary tract infection and 30-day, 180-day, and 360-day mortality.
In this study, independent variables with potential impact on outcomes were considered as covariates and adjusted for within regression models based on clinical expertise and literature review. For delirium, adjustments were made for age, sex, race, marital status, dementia, depression, cerebrovascular disease, sedative use, CCI, SOFA, GCS, and Braden scores. For aspiration pneumonia and pressure injuries, adjustments were made for age, sex, race, GCS, CCI, SOFA, and Braden scores.
Statistical methods
This observational study was conducted in adherence to the STROBE (Strengthening the Reporting of Observational Studies in Epidemiology) guidelines to ensure comprehensive and transparent reporting of the research findings [ 22 ]. The study initially performed a descriptive statistical analysis on the collected and organized data. Patients were stratified based on falls presence or absence in the three months before admission. Continuous variables were expressed as medians with interquartile ranges, and differences were compared using the Wilcoxon rank-sum test. Categorical variables were presented as counts and percentages, and group differences were assessed using the chi-squared test. Logistic regression models determined the association between fall history and outcomes, yielding odds ratios (OR) and 95% confidence intervals (CI).
To show the survival rates at 30, 180, and 360 days among the elderly ICU patients who developed delirium, we estimated Kaplan–Meier survival curves and assessed differences in survival distributions between groups using the log-rank test. To examine joint associations, we divided the entire cohort into four groups based on the variables: a history of falls and the presence of delirium. Baseline data for these four groups were tabulated, and between-group differences were assessed using the Kruskal–Wallis H test for continuous variables and the chi-squared test for categorical variables. Hazard ratios (HRs) and 95% confidence intervals for mortality at 30, 180, and 360 days were calculated for the different groups compared with older patients, no history of falls, and no delirium.
Subsequently, this study examined the association between a history of falls and delirium in different subgroups of elderly ICU patients. We examined the association within the subgroups of sex, age, race, marital status, dementia, cerebrovascular disease, and depression. To assess the combined association, we divided the total population into four categories based on history of falls and presence of delirium. The short-term mortality risk in the different groups was calculated in contrast to ischemic stroke patients without fall history and delirium.
Finally, two sensitivity analyses were conducted to assess our findings' robustness. Covariate adjustments were made using propensity score matching (PSM). Propensity scores, derived from logistic regression models incorporating sex, age, race, marital status, body weight, ventilation status, GCS, CCI, and SOFA scores, were estimated. Employing a 1:1 matching algorithm with the nearest neighbor method, the matched population underwent comparison using the Wilcoxon rank-sum test or chi-squared test. To address potential unmeasured confounding factors, the E-value was calculated—an indicator in observational studies gauging the impact of such factors on study outcomes. Higher E-values signify greater result robustness and decreased susceptibility to confounding influences.
Data were processed using R software (version 4.3.0, https://www.r-project.org/ ). A p-value of less than 0.05 was considered indicative of statistical significance.
Baseline characteristics of the study cohort
After careful screening, a total of 22,547 elderly patients were included in the study. Of these, 6,206 patients had experienced a fall within the three months prior to ICU admission. Table 1 A,1B,1C,1D describes the baseline characteristics of the study cohort, categorized by exposure factor (Table 1B,1C,1D in Supplementary Materials). Of the total patient population, 12,078 (53.6%) were male, with a median age of 76.71 years (interquartile range: 70.65–83.82 years) and a median ICU length of stay of 2.44 days (interquartile range: 1.60–4.30 days). Compared to patients without a fall history, those with a fall history had an older median age (79.57 vs. 75.78 years), lower weight (73.00 kg vs. 77.80 kg), and a higher proportion of unmarried individuals (including single, divorced, widowed, etc.). These differences were statistically significant (P < 0.05).
Associations between history of falls and the risks of delirium, urinary tract infection, and pressure injury
Table 2 shows an association between a history of falls and the risk of adverse outcomes in ICU patients. According to the results of the multivariate logistic regression analysis performed, compared to patients without a history of falls, those with such a history had a significantly increased prevalence of delirium during their critical illness, with an adjusted odds ratio (OR) of 2.11 (95% CI: 1.97–2.26). In addition, patients with a history of falls were also at risk for urinary tract infection and pressure injury, with adjusted odds ratios (ORs) of 1.50 (95% CI: 1.40–1.62) and 1.36 (95% CI: 1.26–1.47), respectively. These findings suggested that a history of falls could be an independent risk factor for delirium, UTIs, and pressure injury in the ICU.
In addition, this study compared the survival rates of geriatric critically ill patients, both with and without delirium, at 30, 180, and 360-day intervals (Fig. 2 A,2B,2C). The Kaplan–Meier survival curve demonstrated that elderly patients who developed delirium had significantly lower survival rates at 30, 180, and 360 days compared to those who without delirium (all p < 0.001).
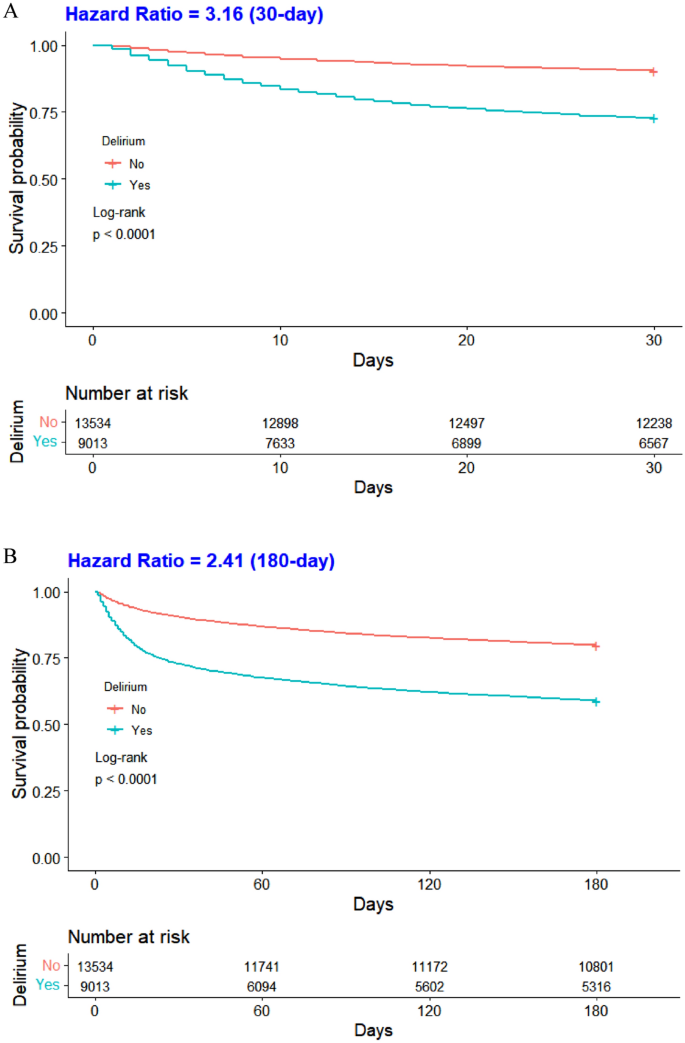
Comparison of Survival Rates of ICU Patients with and without Delirium at 30 (A), 180 (B), and 360 (C)-day Intervals
Subgroup analysis
In this study, a subgroup analysis elucidated the relationship between exposure and the primary outcome (delirium) to further our understanding of the association between a history of falls and delirium within subpopulations. The results show substantial statistical variation across all subgroups, suggesting a persistent association between a history of falls and the incidence of delirium within these boundaries (Fig. 3 ). Apparently, a history of falls may serve as a critical risk factor for delirium in ICU patients, with these findings applicable to individuals across different sex and age groups.
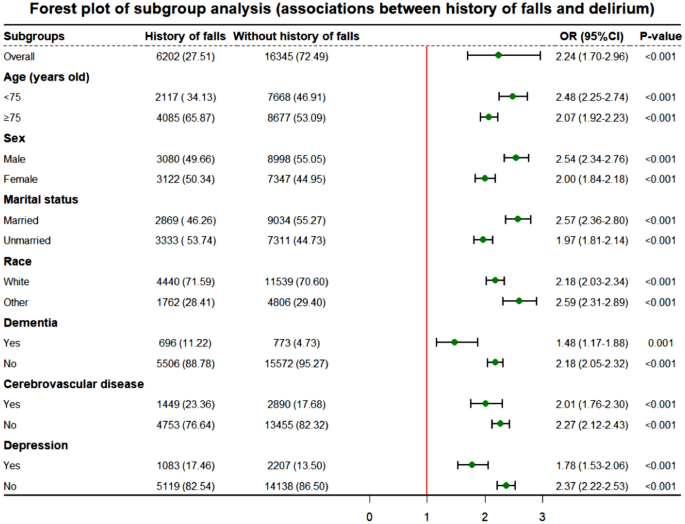
Subgroup analysis of fall history and delirium
Joint association
This study investigated the combined impact of a history of falls and delirium on mortality rates at 30, 180, and 360 days, presented in eTable 3 [Supplementary materials]. Baseline characteristics of the four patient cohorts were detailed in eTable 2 [Supplementary materials], highlighting differences between groups. The multivariate Cox proportional hazards model demonstrated that, compared to older patients without falls and delirium, those with both conditions had the highest mortality risk at 30, 180, and 360 days, with adjusted HRs of 4.07 (3.72, 4.45), 3.07 (2.87, 3.28), and 2.79 (2.62, 2.97), respectively.
Sensitivity analysis
After PSM, most baseline parameters showed no significant differences between the two cohorts. Univariate and multivariate logistic regression analyses on the matched cohort yielded results consistent with the original population (eTable 2 in Supplementary Materials). This underscores the association between a history of falls and delirium, urinary tract infection, and pressure injury in elderly patients during critical illness (all p < 0.05). These outcomes emphasize the robustness and stability of our findings.
This study utilized publicly available MIMIC-IV data from the United States to investigate the association between falls within the previous 3 months and adverse outcomes in ICU patients, focusing on short-term mortality in elderly patients with delirium. Findings revealed that a history of falls within three months before hospital admission heightened the risk of delirium. These results align with prior research [ 23 , 24 ]. A possible explanation may be that falls in older adults typically signal a decline in physical and cognitive function, as evidenced by impaired attention, executive function, and slowed gait [ 25 ]. A one-point decline in the Mini-Mental State Examination score corresponded to a subsequent 20% increase in the risk of falling [ 3 ]. Falls could also induce brain injury and physical trauma, leading to pain, extended hospital stays, and intensified treatment, all factors accelerating delirium onset [ 13 , 26 ]. Identifying and addressing fall-related risk factors is crucial in preventing delirium in ICU patients. Healthcare professionals can utilize a patient's fall history before admission as an indicator for potential delirium, informing targeted interventions alongside other high-risk factors.
In the ICU setting, the interplay between a history of falls and the onset of UTIs and pressure injuries is multifaceted. Findings indicated an increased likelihood of pressure injuries in patients with falls, consistent with prior research [ 27 ]. The explanation lies in fall-related injuries restricting mobility, reducing turning frequency, and predisposing patients to prolonged pressure, thereby compromising skin integrity and fostering pressure ulcers [ 28 ]. Falls, causing brain damage and functional decline, often result in compromised bowel control, contributing to pressure injuries around the buttocks due to combined effects of urine, fecal stimulation, and pressure [ 29 ]. This research also discovered a correlation between a history of falls and urinary tract infections, aligning with the conclusions of prior studies [ 30 ]. Falls in older individuals elevate UTI risks through injuries necessitating catheterization, bacterial colonization, urethral mucosa damage, and a weakened immune response. Reduced mobility, often a consequence of falls, necessitates extended periods of immobilization and potentially the use of urinary catheters, both of which significantly elevate the risk of UTIs. Concurrently, immobilization increases the likelihood of developing pressure injuries due to sustained pressure on certain body areas. In ICUs, complex care protocols such as sedation and the use of physical restraints may inadvertently reduce patient mobility, thereby increasing the likelihood of UTIs and pressure injuries. It is crucial for ICU healthcare professionals to prioritize the prevention of these conditions through careful monitoring and the implementation of specific interventions. Strategies such as frequent repositioning and meticulous urinary catheter management are essential to minimize these risks and improve patient outcomes.
Delirium emerged as an independent risk factor for heightened mortality risk in this study. Patients developing delirium exhibited significantly reduced survival rates at 30, 180, and 360 days compared to their non-delirium counterparts, aligning with prior research [ 31 ]. Delirium represents an episode of acute brain failure and its damage to the brain may not be fully reversible and is closely associated with long-term cognitive decline [ 32 ], which can severely impact a patient's quality of life. Delirium onset is linked with inflammation and neuronal apoptosis, potentially leading to brain atrophy and diminished survival [ 33 ]. Concurrently, delirium-associated complications like aspiration pneumonia and increased restraint use extended hospital stays, elevating ICU infection risks and acting as an independent mortality risk factor [ 34 ]. Each additional hour of delay in hospitalization raised the risk of death by approximately 3% [ 35 ]. The joint effects unveiled a substantial mortality increase at 30, 180, and 360 days in patients with both a history of falls and delirium compared to those without. This survival analysis is pivotal for filling the research void by providing concrete data on mortality risks at critical junctures post-admission, thereby offering a clearer guide for clinical strategies aimed at reducing fall-related fatalities among ICU patients.
Our research demonstrates that a history of falls within three months prior to ICU admission significantly increases the likelihood of delirium, decreases survival rates, and elevates the incidence of secondary outcomes such as pressure ulcers and urinary tract infections in ICU patients. This highlights the critical need for early screening and intervention strategies focusing on fall history to mitigate these risks. By prioritizing fall history in patient evaluations, healthcare professionals can identify high-risk individuals for targeted preventive measures, thereby improving patient care and outcomes in the ICU.
This study boasts several strengths. Firstly, the substantial sample size enhances statistical precision, reducing variance and uncertainty in research findings. Secondly, the innovative assessment method, utilizing the CAM-ICU scale and nursing notes, ensures result stability and reliability. Thirdly, sensitivity analyses confirm the robustness of conclusions. Lastly, explicit definition of fall timing in the Morse Fall Scale score and identification of falls within three months quantify fall history, pinpointing associated high-risk factors [ 36 ]. These strengths significantly bolster the study's credibility.
There are also several limitations to the study. Firstly, its retrospective nature may lead to selection and information biases. Secondly, relying on nursing notes for assessing falls and delirium could result in underreporting. Lastly, the findings from a single tertiary academic medical center in Boston might not be broadly applicable to other settings. To overcome these limitations, future research should adopt a prospective study design could minimize biases associated with retrospective analyses. In addition, expanding the study to include multiple centers with diverse patient populations would improve the generalizability of the findings. Finally, integrating quantitative tools alongside nursing notes could offer a more comprehensive evaluation of falls and delirium, enriching the data quality.
This study demonstrates that a history of falls significantly influences the onset of delirium in elderly ICU patients, also increasing urinary tract infections and pressure injuries. Moreover, patients with delirium exhibited significantly lower short-term survival rates than their healthy counterparts, posing a substantial threat to patient health. These findings highlight the need for healthcare professionals to adopt predictive measures and targeted interventions to enhance patient care and reduce costs, potentially guiding future research to refine management and preventive strategies for delirium and related conditions in the ICU.
Gu Y, Zhang Y, Huang M et al (2018) Your WiFi knows you fall: a channel data-driven device-free fall sensing system. In: 2018 5th IEEE international conference on cloud computing and intelligence systems (CCIS). IEEE, pp 943–947
Joo H, Wang G, Yee SL et al (2017) Economic burden of informal caregiving associated with history of stroke and falls among older adults in the US[J]. Am J Prev Med 53:S197–S204
Article PubMed PubMed Central Google Scholar
Ambrose AF, Paul G, Hausdorff JM (2013) Risk factors for falls among older adults: a review of the literature[J]. Maturitas 75:51–61
Article PubMed Google Scholar
Cheng H, Wang Z, Gu WJ et al (2022) Impact of falls within 3 months on the short-term prognoses of elderly patients in intensive care units: a retrospective cohort study using stabilized inverse probability treatment weighting[J]. Clin Interv Aging 17:1779–1792
Fuller GF (2000) Falls in the elderly[J]. Am Fam Phys 61:2159–2168
CAS Google Scholar
Florence CS, Bergen G, Atherly A et al (2018) The medical costs of fatal falls and fall injuries among older adults[J]. J Am Geriatr Soc 66:693–698
Fong TG, Tulebaev SR, Inouye SK (2009) Delirium in elderly adults: diagnosis, prevention and treatment[J]. Nat Rev Neurol 5:210–220
Ouimet S, Kavanagh BP, Gottfried SB et al (2007) Incidence, risk factors and consequences of ICU delirium[J]. Intensive Care Med 33:66–73
van den Boogaard M, Schoonhoven L, van der Hoeven JG et al (2012) Incidence and short-term consequences of delirium in critically ill patients: a prospective observational cohort study[J]. Int J Nurs Stud 49:775–783
Inouye SK, Westendorp RGJ, Saczynski JS (2014) Delirium in elderly people[J]. Lancet 383:911–922
Vasilevskis EE, Chandrasekhar R, Holtze CH et al (2018) The cost of ICU delirium and coma in the intensive care unit patient[J]. Med Care 56:890–897
Marcantonio ER (2017) Delirium in hospitalized older adults[J]. N Engl J Med 377:1456–1466
Sillner AY, Holle CL, Rudolph JL (2019) The overlap between falls and delirium in hospitalized older adults: a systematic Review[J]. Clin Geriatr Med 35:221–236
Bloomer MJ, Woszczeiko C, Hutchinson AM (2022) Older age, delirium, dementia, frailty, and critical care: care priorities[J]. Intensive Crit Care Nurs 73:103297
Johnson AEW, Bulgarelli L, Shen L et al (2023) MIMIC-IV, a freely accessible electronic health record dataset[J]. Scientific Data 10:1
Article CAS PubMed PubMed Central Google Scholar
Wu WT, Li YJ, Feng AZ et al (2021) Data mining in clinical big data: the frequently used databases, steps, and methodological models[J]. Mil Med Res 8:44
PubMed PubMed Central Google Scholar
Yang J, Li Y, Liu Q et al (2020) Brief introduction of medical database and data mining technology in big data era[J]. J Evid Based Med 13:57–69
Wei F, Cheng H, He R et al (2024) Geriatric nutritional risk index independently predicts delirium in older patients in intensive care units: a multicenter cohort study[J]. Arch Gerontol Geriatr 118:105288
Article CAS PubMed Google Scholar
Barr J, Fraser GL, Puntillo K et al (2013) Clinical practice guidelines for the management of pain, agitation, and delirium in adult patients in the intensive care unit[J]. Crit Care Med 41:263–306
Saczynski JS, Kosar CM, Xu G et al (2014) A tale of two methods: chart and interview methods for identifying delirium[J]. J Am Geriatr Soc 62:518–524
Austin PC, White IR, Lee DS et al (2021) Missing data in clinical research: a tutorial on multiple imputation[J]. Can J Cardiol 37:1322–1331
von Elm E, Altman DG, Egger M et al (2014) The Strengthening the Reporting of Observational Studies in Epidemiology (STROBE) statement: guidelines for reporting observational studies[J]. International J Sur (London, England) 12:1495–1499
Article Google Scholar
Boorsma M, Joling KJ, Frijters DHM et al (2012) The prevalence, incidence and risk factors for delirium in Dutch nursing homes and residential care homes[J]. Int J Geriatr Psychiatry 27:709–715
Zrour C, Haddad R, Zoghbi M et al (2020) Prospective, multi-centric benchmark study assessing delirium: prevalence, incidence and its correlates in hospitalized elderly Lebanese patients[J]. Aging Clin Exp Res 32:689–697
Montero-Odasso M, Speechley M (2018) Falls in cognitively impaired older adults: implications for risk assessment and prevention[J]. J Am Geriatr Soc 66:367–375
MacLullich AM, Ferguson KJ, Miller T et al (2008) Unravelling the pathophysiology of delirium: a focus on the role of aberrant stress responses[J]. J Psychosom Res 65:229–238
Kim J, Lee JY, Lee E (2022) Risk factors for newly acquired pressure ulcer and the impact of nurse staffing on pressure ulcer incidence[J]. J Nurs Manag 30:O1–O9
Doran D, Lefebre N, O’Brien-Pallas L et al (2014) The relationship among evidence-based practice and client dyspnea, pain, falls, and pressure ulcer outcomes in the community setting[J]. Worldviews Evidence-Based Nurs 11:274–283
García-Mayor S, Morilla-Herrera JC, Lupiáñez-Pérez I et al (2018) Peripheral perfusion and oxygenation in areas of risk of skin integrity impairment exposed to pressure patterns. a phase I trial (POTER study)[J]. J Adv Nurs 74:465–471
Amarsy R, Guéret D, Benmansour H et al (2019) Determination of Escherichia coli phylogroups in elderly patients with urinary tract infection or asymptomatic bacteriuria[J]. Clin Microbiol Infect: Off Publ Eur Soc Clin Microbiol Infect Dis 25:839–844
Article CAS Google Scholar
Maldonado JR (2017) Acute brain failure: pathophysiology, diagnosis, management, and sequelae of delirium[J]. Crit Care Clin 33:461–519
Goldberg TE, Chen C, Wang Y et al (2020) Association of delirium with long-term cognitive decline: a meta-analysis[J]. JAMA Neurol 77:1373–1381
van Gool WA, van de Beek D, Eikelenboom P (2010) Systemic infection and delirium: when cytokines and acetylcholine collide[J]. Lancet (London, England) 375:773–775
Aung Thein MZ, Pereira JV, Nitchingham A et al (2020) A call to action for delirium research: Meta-analysis and regression of delirium associated mortality[J]. BMC Geriatr 20:325
Churpek MM, Wendlandt B, Zadravecz FJ et al (2016) Association between ICU transfer delay and hospital mortality: a multicenter investigation[J]. J Hosp Med 11:757–762
Morse JM, Black C, Oberle K et al (1982) A prospective study to identify the fall-prone patient[J]. Soc Sci Med 28:81–86
Download references
This work was supported by the National Natural Science Foundation of China (No.72274078); the Guangdong Provincial Key Laboratory of Traditional Chinese Medicine Informatization (No.2021B1212040007).
Author information
Fan Bu, Hong-tao Cheng and Zi-lin Wang are authors contributed equally.
Authors and Affiliations
School of Nursing, Jinan University, Room 1015, Guangzhou, China
Fan Bu, Hong-tao Cheng, Zi-lin Wang, Zhuang Zhuang, Can-yang Li, Ya-qi Wang, Yue Zhang & Qi-yuan Lyu
Department of Geriatric Psychology, Shandong Daizhuang Hospital, Jining, China
Yu-shan Hou
Department of Clinical Research, The First Affiliated Hospital of Jinan University, Guangzhou, China
Guangdong Provincial Key Laboratory of Traditional Chinese Medicine Informatization, Guangzhou, China
You can also search for this author in PubMed Google Scholar
Contributions
FB, HC and ZW created the study protocol, performed the statistical analyses and wrote the first manuscript draft. QL and JL conceived the study and critically revised the manuscript. YH, ZZ and CL assisted with the study design and performed data collection. FB and HC confirmed the data and assisted with the statistical analyses. YW and YZ contributed to data interpretation and manuscript revision. QL and JL, as senior authors, reviewed and edited all versions of this manuscript. All authors read and approved the final manuscript.
Corresponding authors
Correspondence to Jun Lyu or Qi-yuan Lyu .
Ethics declarations
Conflict of interest.
The authors declare no competing interests.
Human and animal rights and informed consent
Data extracted from the MIMIC-IV database do not require individual informed consent because the research data is publicly available, and all patient data are de-identified.
Data Availability Statement
Data are openly available in a public repository that can be accessed upon completion of the Protecting Human Research Participants web-based training from the National Institutes of Health. The datasets generated and analyzed during the current study are available from the corresponding authors, Jun Lyu and Qi-yuan Lyu, upon reasonable request.
Ethical approval
This is a retrospective study based on MIMIC-IV database. The patient's information has been hidden before the study. There is no need for the patient's informed consent and no ethical conflict
Additional information
Publisher's note.
Springer Nature remains neutral with regard to jurisdictional claims in published maps and institutional affiliations.
Supplementary Information
Below is the link to the electronic supplementary material.
Supplementary file1 (DOCX 47 KB)
Rights and permissions.
Open Access This article is licensed under a Creative Commons Attribution 4.0 International License, which permits use, sharing, adaptation, distribution and reproduction in any medium or format, as long as you give appropriate credit to the original author(s) and the source, provide a link to the Creative Commons licence, and indicate if changes were made. The images or other third party material in this article are included in the article's Creative Commons licence, unless indicated otherwise in a credit line to the material. If material is not included in the article's Creative Commons licence and your intended use is not permitted by statutory regulation or exceeds the permitted use, you will need to obtain permission directly from the copyright holder. To view a copy of this licence, visit http://creativecommons.org/licenses/by/4.0/ .
Reprints and permissions
About this article
Bu, F., Cheng, Ht., Wang, Zl. et al. Effect of a fall within three months of admission on delirium in critically Ill elderly patients: a population-based cohort study. Aging Clin Exp Res 36 , 111 (2024). https://doi.org/10.1007/s40520-024-02740-8
Download citation
Received : 27 December 2023
Accepted : 18 March 2024
Published : 14 May 2024
DOI : https://doi.org/10.1007/s40520-024-02740-8
Share this article
Anyone you share the following link with will be able to read this content:
Sorry, a shareable link is not currently available for this article.
Provided by the Springer Nature SharedIt content-sharing initiative
- Older adults
- History of falls
- Intensive care unit
- Pressure injury
Advertisement
- Find a journal
- Publish with us
- Track your research
- Open access
- Published: 10 May 2024
Clinical features of patients with carcinoma soft tissue metastases as surgical indications: a retrospective cohort study
- Sei Morinaga 1 ,
- Norio Yamamoto 1 ,
- Katsuhiro Hayashi 1 ,
- Akihiko Takeuchi 1 ,
- Shinji Miwa 1 ,
- Kentaro Igarashi 1 ,
- Yuta Taniguchi 1 ,
- Yohei Asano 1 ,
- Takayuki Nojima 2 &
- Hiroyuki Tsuchiya 1
BMC Cancer volume 24 , Article number: 577 ( 2024 ) Cite this article
203 Accesses
8 Altmetric
Metrics details
Soft-tissue metastasis of carcinoma is rare. In the present study, we investigated the surgical indications and clinical features of patients with soft tissue metastases of carcinoma.
In this retrospective cohort study, we enrolled 26 patients with soft tissue carcinoma metastasis referred to our department for treatment. Sex, age, location, size, depth, pain due to the tumor, primary origin, serum C-reactive protein (CRP) level, MRI examinations, diagnosis by a previous physician, carcinoma markers from blood, history of carcinoma, other metastases, performance status (PS), and surgical procedures were documented. Associations between variables and surgery were statistically analyzed.
The primary cancer origin was found to be the lung ( n = 10), kidney ( n = 7), esophagus ( n = 2), stomach ( n = 1), breast ( n = 1), liver ( n = 1), ureter ( n = 1), anus ( n = 1), and unknown ( n = 2). The mean CRP level of all patients was 2.3 mg/dL. Seven tumors (26.9%) were originally suspected to be soft tissue metastases of carcinoma, while 19 tumors (73.1%) were considered soft tissue sarcomas or inflammatory lesions by the previous treating physician. Twenty patients (76.9%) had other metastases. The PS of the 12 patients (46.2%) was zero. Eleven patients (42.3%) underwent surgery for soft tissue metastases. Diagnosis of soft tissue metastasis by a previous physician and good PS ( p < 0.05) were significantly associated with surgery.
Overall, the present results show that surgical indications for soft tissue metastasis of carcinoma include diagnosis by the referring physician or good PS of the patients.
Peer Review reports
Soft-tissue metastasis of carcinoma is rare; a previous study described the prevalence of soft tissue metastasis in patients with solid malignancy to be only 0.2–1.2% [ 1 , 2 ]; however, a separate autopsy series study identified a 16–17.5% prevalence of soft tissue metastasis [ 3 , 4 ]. In 2022, 1,918,030 new cancer cases were projected to be diagnosed in the United States [ 5 ]. As of January 1, 2022, more than 18 million Americans were living with a history of cancer [ 6 ]. As advances in medicine continue to progress, the number of cancer survivors is expected to increase; hence, the prevalence of soft tissue metastasis of carcinoma is expected to increase accordingly.
Patients with soft tissue metastasis have a poor prognosis, with a one-year survival of 25–55%, and a two-year survival of only 0–33% [ 7 , 8 ]. Additionally, reports have described that some patients with soft tissue metastasis are commonly initially misdiagnosed as having soft tissue sarcoma or an inflammatory lesion [ 7 , 8 , 9 ]. Hence, accurate diagnosis and proper treatment are of utmost importance in patients with soft tissue metastases. Once the diagnosis of soft tissue metastasis of carcinoma is made, a treatment plan should be promptly designed in cooperation with the department in charge of the primary organ.
Currently, there are limited studies investigating the surgical indications for soft tissue metastases of carcinoma. Therefore, in the present study, we investigated the surgical indications and clinical features of patients with soft tissue metastasis of carcinomas.
Materials and methods
This non-controlled, retrospective analysis was conducted using the data obtained from the medical records of a single institution. This study was approved by the Ethics Committee of our hospital, and informed consent was obtained from all patients. Patients referred to our department and diagnosed with soft tissue carcinoma metastasis between January 2007 and December 2021 were identified and enrolled. Patients with lymph node metastasis or hematologic cancer, such as malignant lymphoma, were excluded. After application of these criteria, 26 patients were included in the study.
We collected the demographic and clinical features of all participants, including sex, age, location, size, depth, pain due to the tumor, primary origin, serum C-reactive protein (CRP) level (Normal range: 0-0.3 mg/dL), magnetic resonance imaging (MRI) examinations (T1-weighted sequence, T2-weighted sequence, and contrast-enhanced MRI), diagnosis of the previous physician, carcinoma markers from blood, history of carcinoma, other metastases, performance status (PS), and surgery for soft tissue metastasis. Tumor depth was defined as superficial or deep in relation to the investing muscular fascia.
All statistical analyses were performed using EZR (Saitama Medical Center, Jichi Medical University, Saitama, Japan). Associations between the variables and surgery for soft tissue metastasis were statistically analyzed using Fisher’s exact test. Statistical significance was set at p -values < 0.05 for all other analyses.
Twenty-six patients, including 22 men and four women, were enrolled in the present study. The mean patient age was 68.6 years (range: 46–93 years). Four metastases were located in the upper extremities, nine in the lower extremities, and 13 in the trunk. The mean metastasis size was 5.2 cm (2.1–13.9 cm). Twenty-four metastases (92.3%) were located beneath the muscle fascia. Thirteen patients (50%) experienced pain due to the metastasis. The primary origin of cancer was the lung in 10 patients, kidney in seven, esophagus in two, stomach in one, breast in one, liver in one, ureter in one, anus in one, and unknown in two. The mean CRP level was 2.3 (0.1–13.6) mg/dL. Seven metastases (26.9%) were suspected as soft tissue metastases of carcinoma, and the remaining 19 (73.1%) were believed to be soft tissue sarcoma or inflammatory lesions by the previous physician. Carcinoma markers from blood were positive in 13 patients (CYFRA in four, CEA in three, SCC in three, PSA in three, CA15-3 in two, ProGRP in one, CA19-9 in one, CA125 in one), negative in six, and unknown in seven. Sixteen patients (61.5%) had a history of carcinoma, 20 patients (76.9%) had other metastases, and the PS of the 12 patients (46.2%) was zero. Overall, 11 patients (42.3%) underwent surgery for soft tissue metastases (Table 1 ).
Of the 26 patients enrolled, 22 underwent MRI, of whom 11 underwent contrast-enhanced MRI. The T1 signals of the 21 metastases (95.4%) presented as homogeneous iso-intensities, while on T2 imaging, 17 metastases (77.3%) were heterogeneous high-intensities. Ring-enhanced lesions were confirmed in five patients (Fig. 1 ), heterogeneous enhanced lesions in five, and homogeneous enhanced lesions in one (Table 2 ).
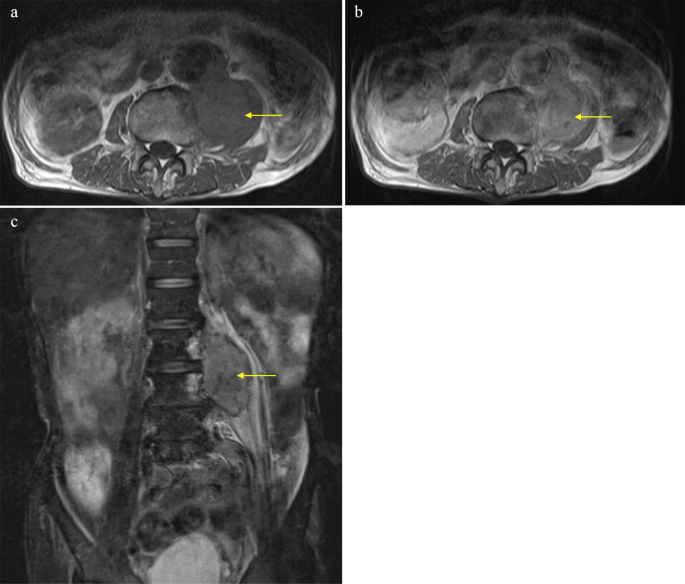
MRI results. ( a ) axial T1-weighted, homogeneous iso-intensities; ( b ) T2-fat suppression, heterogeneous high-intensities; ( c ) axial enhanced, heterogeneous-enhanced lesion
Fisher’s exact test confirmed that a previous diagnosis of soft tissue metastasis and good PS ( p < 0.05) was significantly associated with surgery for soft tissue metastasis (Table 3 ).
In an example case, an 81-year-old man presented with a 3-month history of lower back pain but no swelling documented at a previous hospital. The patient had a medical history of abdominal aortic aneurysm and surgery for ureter cancer five years prior. Magnetic resonance imaging (MRI) revealed a mass in the left iliopsoas muscle (Fig. 1 ).
The T1 signal of the lesion was homogenous iso-intensity, and T2-fat suppression was heterogeneous high-intensity. Contrast-enhanced MRI revealed that the lesion was heterogeneously enhanced. The patient was diagnosed with a left iliopsoas abscess by a previous doctor, and was treated with intravenous antibiotics. However, the patient showed no notable improvement and was subsequently referred to our department. The histological results of the CT-guided biopsy revealed soft tissue metastasis from the ureteral cancer (Fig. 2 ). Surgery for soft tissue metastases was not performed.
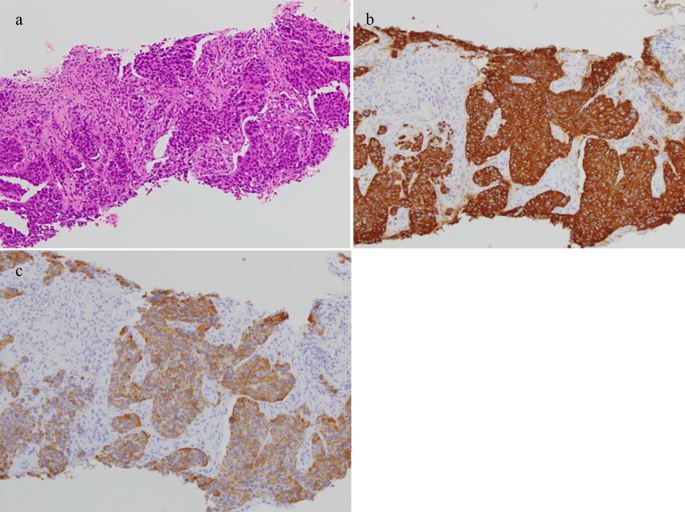
Histological results of the needle biopsy indicated metastasis of urothelial carcinoma ( a ) Hematoxylin eosin staining. The biopsy sample shows positive staining with ( b ) cytokeratin (CK) 7; and ( c ) CK 20
Soft tissue metastases of carcinomas are rare. Prior studies have reported that blood flow is unstable in the skeletal muscles, and tumor cells are destroyed by physical muscle stimulation as lactic acid invades tumor cells [ 10 , 11 ]. Hence, tumor growth rarely occurs in such environments. Unfortunately, there have been relatively few studies with a large number of patients investigating this topic [ 1 , 2 , 8 , 10 , 12 , 13 , 14 ], and as such, there are no guidelines for surgical indications. Therefore, in the present study, we investigated the surgical indications and clinical features of patients with soft tissue metastasis of carcinomas.
Soft tissue metastases from carcinoma were frequently observed in the trunk area in previous large case series [ 2 , 13 ], while the existing literature describes the mean tumor size as 5–8.5 cm [ 1 , 9 , 14 , 15 ]. Moreover, lung cancer is the most common primary carcinoma, followed by kidney and colon cancers [ 8 , 13 ]. In the present study, soft tissue metastases were most common in the trunk, the mean tumor size was 5.2 cm, and the most frequent primary origin was the lungs. Previous reports have described pain as a frequent symptom [ 1 , 7 , 10 , 14 , 16 , 17 ], and research has further suggested that soft tissue metastasis of carcinoma may induce pain owing to inflammation [ 14 ].
The CRP level reflects the inflammatory cytokines produced by cancer cells and the host immune response to cancer cells [ 18 ]. Thirteen patients (50%) experienced pain due to the tumor, and the mean CRP level was 2.3 mg/dL in this analysis. In addition, Ishibashi et al. [ 15 ] reported that an elevated pretreatment CRP value increased to 0.4 mg/dL or above was an independent predictor of poor prognosis in patients with soft tissue metastasis. Therefore, CRP estimation can help surgeons decide whether to pursue radical surgery. In the present analysis, the number of patients with CRP levels > 0.4 mg/dL was 15, and surgical excisions were performed for six of these patients.
MRI for soft tissue metastasis of carcinoma predominantly reveals lesions with poorly defined margins, low T1-signal intensity, high T2-signal intensity, and enhancement with gadolinium [ 19 , 20 ]. Tuoheti et al. [ 1 ] reported that the infiltrative borders of the tumor should be excised to the greatest extent possible after the excision area has been predetermined according to the peritumoral area on the MR image. In our series, wide excisions were performed in all surgical cases. In addition, strong enhancement around the tumor with gadolinium was considered to indicate central necrosis, which has been shown to be a characteristic feature of soft tissue metastasis of carcinoma [ 1 , 12 ]. Moreover, MRI with gadolinium for soft tissue abscesses showed ring-enhanced lesions [ 21 ] and heterogeneous enhancement on MRI with gadolinium has been linked to soft tissue sarcoma [ 22 ]. In the present analysis, 73.1% of the patients with soft metastasis of carcinoma were suspected of having a soft tissue sarcoma or an inflammatory lesion by a previous doctor. Soft tissue metastasis of carcinoma can be mistaken for soft tissue abscess and sarcoma, similar to the case described in this study. Accurate diagnosis of soft tissue metastasis of carcinoma is paramount, as its treatment and prognosis significantly differ from those of other diseases. Determining the organ of origin of metastatic carcinoma should be promptly performed through assessment of clinical information, such as the past history of carcinoma, status of the past carcinoma, radiological findings, and histological results.
An increase in the number of cancer survivors due to advances in medical care has contributed to an increasing number of soft tissue metastases from carcinoma. Tuoheti et al. [ 1 ] previously described the cases of four patients with soft tissue metastasis during a seven-year period (1994–2001), whereas eight patients with soft tissue metastasis were found during the next two years (2001–2003). This sudden increase in patients with soft tissue metastasis within two years may be related to the increased incidence of carcinomas due to aging and other factors. In our analysis, seven patients with soft tissue metastasis of carcinoma were detected during a seven-year period (2007–2013), and 19 patients were detected during an eight-year period (2014–2021).
Although guidelines for bone metastasis of carcinoma exist, there are no standardized treatment guidelines for soft tissue metastasis of carcinoma owing to its rarity. Most soft tissue metastases of carcinoma are detected incidentally during the staging examination [ 2 ]. After detection, treatment of soft tissue metastases depends on the clinical setting in relation to the primary disease (concurrent lesions or recurrence after treatment of primary disease), the symptoms and general condition of the patient, and the histological type and extent of the metastasis (local or disseminated disease) [ 23 ]. In the present analysis, surgeries for soft tissue metastases of carcinoma were performed significantly more often for tumors diagnosed as soft tissue metastasis by the previous physician and patients who had good PS. Studies on cancer have indicated that poor preoperative PS was associated with postoperative complications and morbidities [ 24 , 25 , 26 , 27 ], which implies that patients with poor PS have worse outcomes when undergoing surgery. The results of our study indicate that surgery is more commonly indicated if there is a request for resection from the attending physician of the primary cancer department or if patients have good PS.
Our study has several limitations. First, this was a retrospective study, which would have introduced inevitable bias. Second, this study had a small sample size and multivariate analysis was not applied. Lastly, an analysis of the prognosis of soft tissue metastasis of carcinoma was not conducted. However, the life expectancy of patients with carcinoma depends on the type, and bias was considered.
In summary, this study analyzed the clinical features of soft tissue metastases from carcinoma. Surgical indications include a request for resection from the attending physician of the primary cancer department or good PS of the patients.
Data availability
The datasets supporting the conclusion of this article are included within the article. The underlying datasets are available from the corresponding author on reasonable request.
Tuoheti Y, Okada K, Osanai T, Nishida J, Ehara S, Hashimoto M, Itoi E. Skeletal muscle metastases of Carcinoma: a clinicopathological study of 12 cases. Jpn J Clin Oncol. 2004;34(4):210–4.
Article PubMed Google Scholar
Surov A, Hainz M, Holzhausen HJ, Arnold D, Katzer M, Schmidt J, Spielmann RP, Behrmann C. Skeletal muscle metastases: primary tumours, prevalence, and Radiological features. Eur Radiol. 2010;20(3):649–58.
Pearson C. Incidence and type of pathologic alterations observed in muscle in a routine survey. Neurology. 1969;9:757–66.
Article Google Scholar
Acinas García O, Fernández FA, Satué EG, Buelta L, Val-Bernal JF. Metastasis of malignant neoplasms to skeletal muscle. Rev Esp Oncol. 1984;31(1):57–67.
PubMed Google Scholar
Siegel RL, Miller KD, Fuchs HE, Jemal A, Cancer Statistics. 2022. CA A Cancer J. Clinicians. 2022;72(1):7–33.
Miller KD, Nogueira L, Devasia T, Mariotto AB, Yabroff KR, Jemal A, Kramer J, Siegel RL. Cancer Treatment and Survivorship statistics, 2022. C.A. Cancer J Clin. 2022;72(5):409–36.
Damron TA, Heiner J. Distant soft tissue metastases: a Series of 30 new patients and 91 cases from the literature. Ann Surg Oncol. 2000;7(7):526–34.
Article CAS PubMed Google Scholar
Torigoe T, Terakado A, Suehara Y, Okubo T, Takagi T, Kaneko K, Yazawa Y. Metastatic Soft Tissue Tumors J Cancer Ther. 2011;02(5):746–51.
Google Scholar
Leinung S, Möbius C, Udelnow A, Hauss J, Würl P. Histopathological outcome of 597 isolated soft tissue tumors suspected of soft tissue sarcoma: a single-center 12-Year experience. Eur J Surg Oncol. 2007;33(4):508–11.
Herring CL Jr., Harrelson JM, Scully SP. Metastatic carcinoma to skeletal muscle: a report of 15 patients. Clin Orthop Relat Res. 1998;355:272–81.
Nicolson GL, Poste G. Tumor Implantation and Invasion at Metastatic sites. Int Rev Exp Pathol. 1983;25:77–181.
CAS PubMed Google Scholar
Yoshimura, Y.; Isobe, K.; Koike, T.; Arai, H.; Aoki, K.; Kato, H. Metastatic Carcinoma to Subcutaneous Tissue and Skeletal Muscle: Clinicopathological Features in 11 Cases. Jpn. J. Clin. Oncol. 2011;41(3):358–364.
Plaza, J. A.; Perez-Montiel, D.; Mayerson, J.; Morrison, C.; Suster, S. Metastases to Soft Tissue: A Review of 118 Cases over a 30-Year Period. Cancer. 2008;112(1):193–203.
Tsuchie H, Emori M, Miyakoshi N, Okada K, Nagasawa H, Murahashi Y, Mizushima E, Shimizu J, Yamashita T, Shimada Y. Prognostic factors in patients with Distant Soft Tissue Metastasis of Carcinoma: a clinicopathological study of 16 cases. Med Princ Pract. 2020;29(6):538–43.
Article PubMed PubMed Central Google Scholar
Ishibashi Y, Kobayashi H, Sawada R, Okuma T, Okajima K, Zhang L, Hirai T, Ohki T, Ikegami M, Shinoda Y, Akiyama T, Goto T, Tanaka S. Pretreatment serum C-Reactive protein is a significant prognostic factor in patients with soft tissue metastases. J Orthop Sci. 2021;26(3):478–82.
Haygood TM, Wong J, Lin JC, Li S, Matamoros A, Costelloe CM, Yeung H, Sandler CM, Nunez RF, Kumar R, Madewell JE. Skeletal muscle metastases: a three-part study of a Not-So-Rare entity. Skeletal Radiol. 2012;41(8):899–909.
Herbowski L. Skeletal muscle metastases from Papillary and follicular thyroid carcinomas: an extensive review of the literature. Oncol Lett. 2018;15(5):7083–9.
PubMed PubMed Central Google Scholar
Candido J, Hagemann T, Cancer-Related Inflammation. J Clin Immunol. 2013;33(Suppl 1):S79–84.
Sudo A, Ogihara Y, Shiokawa Y, Fujinami S, Sekiguchi S. Intramuscular Metastasis of Carcinoma. Clin Orthop Relat Res. 1993;296:213–7.
Mignani G, McDonald D, Boriani S, Avella M, Gaiani L, Campanacci M. Soft tissue metastasis from Carcinoma. Case Rep Tumori. 1989;75(6):630–3.
Article CAS Google Scholar
Alaia EF, Chhabra A, Simpfendorfer CS, Cohen M, Mintz DN, Vossen JA, Zoga AC, Fritz J, Spritzer CE, Armstrong DG, Morrison W. B. MRI nomenclature for Musculoskeletal infection. Skeletal Radiol. 2021;50(12):2319–47.
Gruber L, Loizides A, Luger AK, Glodny B, Moser P, Henninger B, Gruber H. Soft-tissue tumor contrast enhancement patterns: diagnostic value and comparison between Ultrasound and MRI. J R Am J Roentgenol. 2017;208(2):393–401.
Atmatzidis S, Chatzimavroudis G, Raptis D, Hatzibougias D, Voloudakis N, Kotoreni G, Pavlidis T, Penlidis M, Koutelidakis IM, Papaziogas B. Solitary soft-tissue metastasis of a pancreatic adenocarcinoma 2 years after curative resection: report of a rare case and a literature review. Am J Case Rep. 2022;23:e934951.
Deslauriers J, Ginsberg RJ, Piantadosi S, Fournier B. Prospective assessment of 30-day operative morbidity for surgical resections in lung cancer. Chest. 1994;106(6 Suppl):S329–30.
Pierce RJ, Copland JM, Sharpe K, Barter CE. Preoperative risk evaluation for lung cancer resection: predicted postoperative product as a predictor of surgical mortality. Am J Respir Crit Care Med. 1994;150(4):947–55.
Au J, el-Oakley R, Cameron EW. Pneumonectomy for bronchogenic carcinoma in the elderly. Eur J Cardiothorac Surg. 1994;8(5):247–50.
Audisio RA, Gennari R, Sunouchi K, Nair HR, Sestini A, Pope D, West C. Preoperative assessment of cancer in elderly patients: a pilot study. Support Cancer Ther. 2003;1(1):55–60.
Download references
Acknowledgements
We would like to thank Editage ( www.editage.com ) for English language editing.
This research received no external funding.
Author information
Authors and affiliations.
Department of Orthopedic Surgery, Graduate School of Medical Sciences, Kanazawa University, 13-1 Takara-machi, Kanazawa, Ishikawa, 920-8640, Japan
Sei Morinaga, Norio Yamamoto, Katsuhiro Hayashi, Akihiko Takeuchi, Shinji Miwa, Kentaro Igarashi, Yuta Taniguchi, Yohei Asano & Hiroyuki Tsuchiya
Department of Diagnostic Pathology, Kanazawa University Hospital, 13-1 Takara-machi, Kanazawa, Ishikawa, 920-8640, Japan
Takayuki Nojima
You can also search for this author in PubMed Google Scholar
Contributions
SM, NY, KH, AT, SM, KI, YT, YA, TN and HT designed the study. SM collected the data. SM and NY analyzed the data and developed the methodology. SM was a major contributor in writing the manuscript. SM analyzed and interpreted the patient data. HT oversaw the study. All authors have read and agreed to the published version of the manuscript.
Corresponding author
Correspondence to Norio Yamamoto .
Ethics declarations
Ethics approval and consent to participate.
This study was conducted in accordance with the Declaration of Helsinki, and was approved by the Ethics Committee of Kanazawa University (Kanazawa, Japan, 113855). Informed consent was obtained from all patients involved in this study.
Consent for publication
Written informed consent was obtained from the study participants to publish this paper, and a copy has been made available to the journal.
Competing interests
The authors declare no competing interests.
Additional information
Publisher’s note.
Springer Nature remains neutral with regard to jurisdictional claims in published maps and institutional affiliations.
Rights and permissions
Open Access This article is licensed under a Creative Commons Attribution 4.0 International License, which permits use, sharing, adaptation, distribution and reproduction in any medium or format, as long as you give appropriate credit to the original author(s) and the source, provide a link to the Creative Commons licence, and indicate if changes were made. The images or other third party material in this article are included in the article’s Creative Commons licence, unless indicated otherwise in a credit line to the material. If material is not included in the article’s Creative Commons licence and your intended use is not permitted by statutory regulation or exceeds the permitted use, you will need to obtain permission directly from the copyright holder. To view a copy of this licence, visit http://creativecommons.org/licenses/by/4.0/ . The Creative Commons Public Domain Dedication waiver ( http://creativecommons.org/publicdomain/zero/1.0/ ) applies to the data made available in this article, unless otherwise stated in a credit line to the data.
Reprints and permissions
About this article
Cite this article.
Morinaga, S., Yamamoto, N., Hayashi, K. et al. Clinical features of patients with carcinoma soft tissue metastases as surgical indications: a retrospective cohort study. BMC Cancer 24 , 577 (2024). https://doi.org/10.1186/s12885-024-12350-2
Download citation
Received : 24 May 2023
Accepted : 07 May 2024
Published : 10 May 2024
DOI : https://doi.org/10.1186/s12885-024-12350-2
Share this article
Anyone you share the following link with will be able to read this content:
Sorry, a shareable link is not currently available for this article.
Provided by the Springer Nature SharedIt content-sharing initiative
- Soft tissue metastasis
- Surgical indication
ISSN: 1471-2407
- Submission enquiries: [email protected]
- General enquiries: [email protected]
Sydney researchers secure over $13 million in NHMRC Clinical Trials and Cohort Studies funding
The National Health and Medical Research Council (NHMRC) Clinical Trials and Cohort Studies scheme supports high-quality clinical trials and cohort studies that address important gaps in knowledge, leading to relevant and implementable findings for the benefit of human health.
Professor Emma Johnston , Deputy Vice-Chancellor (Research) at the University of Sydney, congratulated the grant recipients from the Faculty of Medicine and Health, Professor Jacob George , Professor Emmanuel Stamatakis , Associate Professor Peter Grimison and Dr Ameneh Khatami .
“Jacob, Emmanuel, Peter and Ameneh’s studies aim to address gaps in knowledge that will help the Australian community gain access to better treatments,” said Professor Johnston.
“Their success in this scheme reflects the University's commitment to translating outstanding research into real solutions that enhance health and wellbeing services, practices and policies, and we are proud to partner with the NHMRC in supporting this important work.”
Faculty of Medicine and Health grant recipients
Professor Jacob George has been awarded over $5.9 million to use cellular and spatial genomics to understand liver cancer at the molecular level and build a national cohort for in-depth study, hoping to improve treatment and patient outcomes.
Professor Emmanuel Stamatakis has received over $1.9 million to use wearable device data derived from the Prospective Physical Activity and Sleep consortium to examine the combined associations of physical activity, sitting and sleep with cancer risk.
Associate Professor Peter Grimison has secured over $1.3 million to determine whether giving the same dose of a chemotherapy combination called BEP, Bleomycin, Etoposide Cisplatin, on a two-weekly schedule will be more effective than a three-weekly schedule.
Dr Ameneh Khatami has been awarded over $4.3 million to investigate the use of a locally produced two-phage combination that targets the golden staph bacteria to see if it provides an advantage in addition to antibiotics in treating patients with severe golden staph bloodstream infections.
Related articles
Sydney researchers awarded over $42 million in nhmrc investigator grants, nhmrc partnership grants for sydney health researchers, medicine and health researchers honoured with nhmrc research excellence awards.
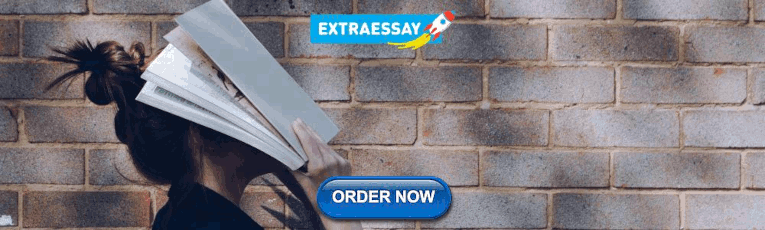
IMAGES
VIDEO
COMMENTS
Cohort studies are one of the most robust forms of medical research. They are well-suited to identifying causes of disease because they look at groups of people before they develop an illness.
At study entry, individual exposures for smoking are determined (smoking pack-years), medical history and cardiovascular health are evaluated. Participants identified at baseline to have heart disease or a history of stroke are excluded from the study. ... Observational research methods—Cohort studies, cross sectional studies, and case ...
A cohort study is a type of observational study that follows a group of participants over a period of time, examining how certain factors (like exposure to a given risk factor) affect their health outcomes. The individuals in the cohort have a characteristic or lived experience in common, such as birth year or geographic area.
Abstract. Cohort studies are types of observational studies in which a cohort, or a group of individuals sharing some characteristic, are followed up over time, and outcomes are measured at one or more time points. Cohort studies can be classified as prospective or retrospective studies, and they have several advantages and disadvantages.
Purpose. Strengths. Weaknesses. A cohort study often looks at 2 (or more) groups of people that have a different attribute (for example, some smoke and some don't) to try to understand how the specific attribute affects an outcome. The goal is to understand the relationship between one group's shared attribute (in this case, smoking) and its ...
A cohort study is a particular form of longitudinal study that samples a cohort (a group of people who share a defining characteristic, ... Shorter term studies are commonly used in medical research as a form of clinical trial, or means to test a particular hypothesis of clinical importance. Such studies typically follow two groups of patients ...
Retrospective Cohort Studies. As an example of a retrospective cohort study, a cohort can be defined to comprise all children born in a health care system between 1980 and 1990. The health care system can be a single hospital, a group of hospitals, an insurance database, or any other database that maintains records of medical information.
The term "cohort" refers to a group of people who have been included in a study by an event that is based on the definition decided by the researcher. For example, a cohort of people born in Mumbai in the year 1980. This will be called a "birth cohort.". Another example of the cohort will be people who smoke.
In clinical research, cohort studies are appropriate when there is evidence to suggest an association between an exposure and an outcome, and the time interval between exposure and the development of outcome is reasonable. Cohort studies are the design of choice for determining the incidence and natural history of a condition.
Cohort studies are observational studies that follow groups of patients with different exposures forward in time and determine outcomes of interest in each exposure group or that investigate the ...
Design, Analysis, and Reporting. Cohort studies are types of observational studies in which a cohort, or a group of individuals sharing some characteristic, are followed up over time, and outcomes are measured at one or more time points. Cohort studies can be classified as prospective or retrospective studies, and they have several advantages ...
The section also covers the essential characteristics of a cohort study design and its varied applications in medical research. In the third we go over examples of prospective studies in the medical field. For each, an overview of the study design is given, along with a random selection of study findings/impact, strengths and weaknesses.
Cohort studies are costly and time consuming. They require not only laboratory equipment and assays but also collaboration from participants and health agencies. Due to cost constraints, they are often confined to a specific population. Nevertheless, they play a crucial role in providing fundamental insights for medical advancements, shedding light on the origins of diseases, and acting in ...
A prospective cohort study is a type of observational study focused on following a group of people (called a cohort) over a period of time, collecting data on their exposure to a factor of interest. Their outcomes are then tracked, in order to investigate the association between the exposure and the outcome. Prospective cohort studies look ...
Despite the ease of conducting cohort studies and the research value of such studies, few centers with long-term research goals conduct such studies. This article provides easy-to- ... design of a clinical study: Part 1. Indian J Psychol Med 2021; 43(2): 177-179. 3. Andrade C. A student's guide to the
Cohort studies are a type of longitudinal study —an approach that follows research participants over a period of time (often many years). Specifically, cohort studies recruit and follow participants who share a common characteristic, such as a particular occupation or demographic similarity. During the period of follow-up, some of the cohort ...
Note Many students confuse retrospective cohort studies with case-control studies. While they are both types of retrospective observational studies, they are not the same. Retrospective cohort studies use secondary research data, such as existing medical records or databases, to identify a group of people with an exposure or risk factor in ...
A cohort study is a type of longitudinal study where a group of individuals (cohort), often sharing a common characteristic or experience, is followed over an extended period of time to study and track outcomes, typically related to specific exposures or interventions. ... (November 5th 2018). Prospective Cohort Studies in Medical Research ...
This cohort study examines data for men and women 40 years and older to determine the association between high-volume leisure-time aerobic physical ... Council on Cardiovascular and Stroke Nursing; Council on Clinical Cardiology; and Stroke Council. ... which is consistent with previous research. 15 For example, in a study of 152 masters ...
Join a Clinical Trial. Whether you are eligible for a research study depends on many things. There are specific requirements to be in research studies. These requirements are different for each clinical trial. Patient Guide to Clinical Trials
Longitudinal cohort study definition. Longitudinal cohort studies follow a designated group of individuals, often from a fixed geographic region, over a period of time to track them for disease outcomes. 1 Participants are selected who are free of the outcome at the beginning of the study, but are at risk for developing the disease. When testing a hypothesis, a group of participants without a ...
Key Points. Question In patients with early multinodular hepatocellular carcinoma (HCC), does liver resection provide a significant survival benefit over percutaneous radiofrequency ablation (PRFA) or transarterial chemoembolization (TACE)?. Findings In a cohort study including 720 patients, liver resection demonstrated significantly higher 1-, 3-, and 5-year survival rates than PRFA and TACE.
Background Anemia is a frequently reported and commonly documented issue in intensive care units. In surgical intensive care units, more than 90% of patients are found to be anemic. It is a hematologic factor that contributes to extended mechanical ventilation, sepsis, organ failure, longer hospitalizations in critical care units, and higher mortality. Thus, this study aimed to determine the ...
Hence, this requires a well‐designed clinical research study that rests on a strong foundation of a detailed methodology and is governed by ethical principles. 2. ... Cohort studies are typically chosen as a study design when the suspected exposure is known and rare, and the incidence of disease/outcome in the exposure group is suspected to ...
Key Points. Question What is the estimated population prevalence of intraductal papillary mucinous neoplasms (IPMNs), associated pancreatic cancer (PC) risk, and proportion of malignant transformation of IPMNs to PC?. Findings In this cohort study of 2114 patients aged 50 years or older, the prevalence of IPMNs on contrast-enhanced computed tomography imaging was 10.9%, more than 80% of which ...
In clinical research, cohort studies are appropriate when there is evidence to suggest an association between an exposure and an outcome, and the time interval between exposure and the development of outcome is reasonable. Cohort studies are the design of choice for determining the incidence and natural history of a condition.
Study design and population. The study relied on data sourced from the Medical Information Mart for Intensive Care IV (MIMIC-IV) database, version 2.0, which is an extensive, freely accessible, and publicly available resource [].The MIMIC-IV database received funding support from the National Institute of Biomedical Imaging and Bioengineering (NIBIB) of the National Institutes of Health (NIH ...
Division of Clinical Epidemiology, Research Center for Medical Sciences, The Jikei University School of Medicine, Tokyo, Japan. ... This was a retrospective cohort study conducted at a family physician teaching clinic located in an urban area within the greater Tokyo region (Kawasaki City), Japan. The population of Kawasaki City was ...
Soft-tissue metastasis of carcinoma is rare. In the present study, we investigated the surgical indications and clinical features of patients with soft tissue metastases of carcinoma. In this retrospective cohort study, we enrolled 26 patients with soft tissue carcinoma metastasis referred to our department for treatment. Sex, age, location, size, depth, pain due to the tumor, primary origin ...
Faculty of Medicine and Health grant recipients. Professor Jacob George has been awarded over $5.9 million to use cellular and spatial genomics to understand liver cancer at the molecular level and build a national cohort for in-depth study, hoping to improve treatment and patient outcomes.. Professor Emmanuel Stamatakis has received over $1.9 million to use wearable device data derived from ...