- Browse All Articles
- Newsletter Sign-Up
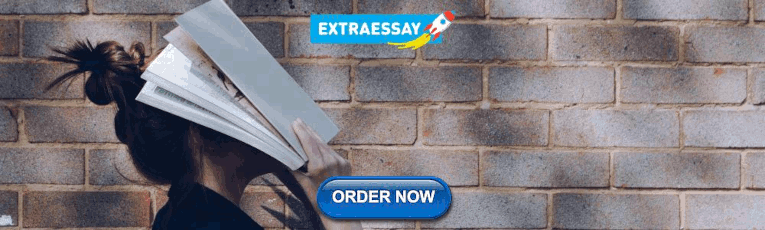
BanksandBanking ā
No results found in working knowledge.
- Were any results found in one of the other content buckets on the left?
- Try removing some search filters.
- Use different search filters.

- SUGGESTED TOPICS
- The Magazine
- Newsletters
- Managing Yourself
- Managing Teams
- Work-life Balance
- The Big Idea
- Data & Visuals
- Reading Lists
- Case Selections
- HBR Learning
- Topic Feeds
- Account Settings
- Email Preferences
Case Study: Will a Bankās New Technology Help or Hurt Morale?
- Leonard A. Schlesinger

A CEO weighs the growth benefits of AI against the downsides of impersonal decision making.
Beth Daniels, the CEO of Michiganās Vanir Bancorp, sat silent as her chief human resources officer and chief financial officer traded jabs. The trio had founded their community bank three years earlier with the mission of serving small-business owners, particularly those on the lower end of the credit spectrum. After getting a start-up off the ground in a mature, heavily regulated industry, they were a tight-knit, battle-tested team. But the current meeting was turning into a civil war.

- Leonard A. Schlesinger is the Baker Foundation Professor at Harvard Business School, where he serves as chair of its practice-based faculty.
Partner Center
- Starting Your Business How To Create a Full-Fledged Ecommerce Website: 7 Steps October 25, 2022
- Starting Your Business Omnichannel E-commerce: What You Need to Succeed October 28, 2022
- Starting Your Business The Guide To Opening An Online Retail Store [With 8 Steps To Follow] October 28, 2022
Digital transformation in banking: A complete guide
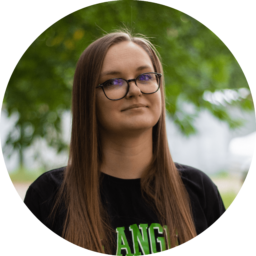
August 02, 2023
Digital transformation is a challenge for the banking industry, but it is necessary to adapt to the modern world where customers expect fast, efficient, and convenient services. Traditional approaches no longer meet the needs of the modern consumer. So, banks that want to remain competitive must abandon conservative methods and fully immerse themselves in the process of digital transformation.
This article discusses what is digital transformation in banking, key factors driving digital transformation , and successful examples of digital transformation in the banking industry.
What is digital transformation in banking?
5 key factors driving digital transformation in banking, technologies that drive digital transformation in banking, successful examples of digital transformation in the banking industry, how can soloway tech help you digitally transform your business.
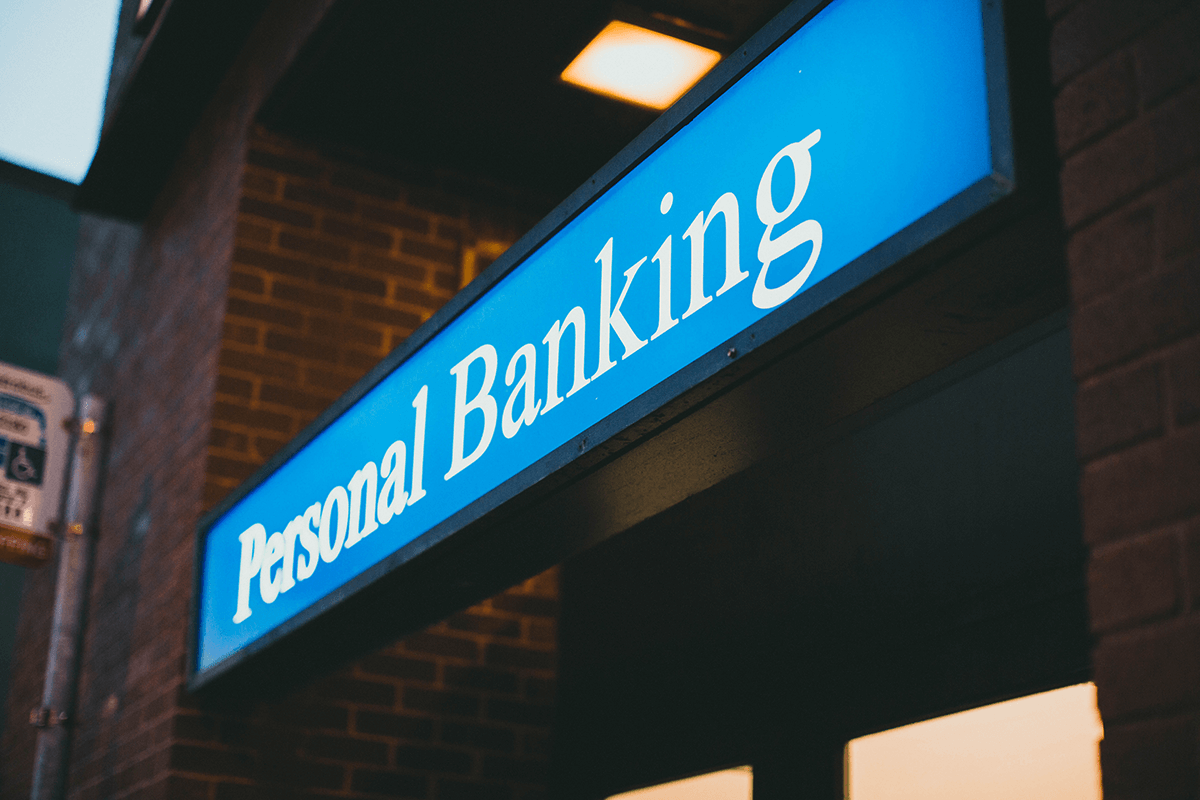
Digital transformation in banking refers to applying new digital technologies and strategies to change and improve banking operations. This includes various changes to increase efficiency, meet customer needs, improve operational effectiveness, and develop new digital products and services.
Mobile applications and personal cabinets on the website are vivid examples of banks’ digital transformation. It is enough to press the buttons on a smartphone or computer to open an account, take out a loan, or order a new plastic card. The services are available not only to individuals but also to legal entities. The accounting departments use client-bank programs to transfer salaries to employees, pay taxes, and receive money from customers.
When you call the hotline of your financial organization, you are answered not by a specialist but by a robot. Virtual assistants have replaced some employees. Moreover, some US banks operate without branches at all. There are employees only in the head office, and customer transactions are conducted exclusively through the Internet.
It is more convenient for people to work with banks remotely, so credit institutions invest a lot of money in digital transformation. It is important that the interface of applications is user-friendly and understandable and transactions are fast. This will attract more customers and, accordingly, increase the profits of the financial company.
Pros and cons of digital transformation in banking
Digital transformation in banking is developing at a rapid pace. It has objective advantages:
- Services of financial organizations are available from anywhere in the world
- The cost of remote operations is cheaper
- There are no queues
- Improved customer service
- Improved operational efficiency
- Big Data and analytics
- Innovation and new opportunities
But there are disadvantages too:
- Dependence on the Internet
- Vulnerability of security systems and regular hacker attacks
- Inaccessibility for some customers
- Threat of job losses
- Dependence on technology
Technology should become a tool that will give banks more flexibility in decision-making and reduce risks.
Fintech companies, which have recently created large-scale services with significantly more interaction points with the client than the classic banking business, are taking the lead. Given that over the last 10 years, the banking industry has experienced a serious tightening of regulatory requirements, fintech is becoming a severe competitor for banks. The solution that banks have found is to change their business model with a focus on digitalization, create their own ecosystems, and develop non-financial services.
Ecosystems are a new global standard for business development and a major stage in the development of the economy. They aggregate data on producers and consumers and help optimize the resources of both. There is no turning back. Creating ecosystems seems to be a common vertical integration strategy for banks when related businesses are pulled up to the core business.
We highlight 5 key factors driving digital transformation in banking:
- Customer experience. Providing convenience and personalization for customers is a crucial factor in digital transformation. Banks should develop and implement innovative digital channels, such as mobile apps, online banking, chatbots, and others, to facilitate access to financial services and improve customer satisfaction.
- Automation and process optimization. The use of automation technologies, such as robo-advisors, machine learning, and artificial intelligence, helps reduce routine operations, lower costs, and improve efficiency. This can include automating lending, foreign exchange, internal audit, and more.
- Evolving regulatory landscape. Regulatory changes and initiatives have pushed banks to adopt digital transformation. Open banking regulations, data protection regulations (such as GDPR), and initiatives promoting competition and innovation have compelled banks to invest in technology to comply with regulations, foster innovation, and enhance transparency.
- Competitive pressure. Fintech startups and tech giants have disrupted the traditional banking landscape. These non-traditional players offer innovative and agile financial services, posing a competitive threat to traditional banks. To remain competitive, banks invest in digital technologies to improve their offerings, provide unique value propositions, and stay ahead of the competition.
- Enhanced customer insights. Digital transformation enables banks to gain deeper insights into customer behavior, preferences, and needs. By analyzing customer data, banks can offer personalized services, targeted marketing campaigns, and customized product recommendations, leading to higher customer satisfaction and loyalty.
These factors interact with each other and require a comprehensive approach for successful digital transformation in the banking industry.
An important point is cybercrime. The emergence of new technologies has left hackers with many loopholes for hacking into networks and devices. At the current growth rate, cyberattack damage will amount to about $10.5 trillion annually by 2025 āa 300% increase from 2015.
However, cyber threats are not slowing down digital transformation. On the contrary, they drive it (this applies to banks and other organizations). The search for vulnerabilities is a never-ending process that contributes to developing security systems.
The main principle of the fight against cybercrime in many banks is that the fight should be at all levels. It means from the protection of external perimeters to specific systems at specific addresses and ports. This includes protection against DoS attacks, firewalls, full control of the bank’s systems, control of viruses to avoid data leakage, etc.
Technologies are evolving at an incredible pace. Artificial Intelligence (AI), Big Data, Blockchain, and other innovations transform how we live, work, and do business.
For example, artificial intelligence allows banks to automate processes and make customer interaction more personalized and efficient. Machine learning can analyze large amounts of data, identify patterns and trends that help make better decisions and predict risks. Machine learning and neural networks also greatly help in document recognition and remote customer verification.
Big Data analysis is becoming a valuable tool in the banking sector, allowing banks to identify patterns, trends, and useful insights hidden in huge amounts of data. It can be used to develop personalized products and services, improve decision-making, detect fraud , and understand and predict customer behavior.
Blockchain is another innovative technology that can tremendously change the banking industry. Most of the current problems in the banking sector are related to the human factor. In particular, they include high commission costs and time spent on money transfers and transactions, internal and external fraud, human error, leakage of personal data, and much more. There are several main areas where blockchain technology can be used in the banking industry:
- Smart contracts
- International payments, settlements for foreign trade transactions, and internal payments
- Transactions with securities
- National digital currency
Other technologies that drive digital transformation in banking include Cloud Computing, Internet of Things (IoT), Robotic Process Automation (RPA), Biometrics, and Open Banking APIs.
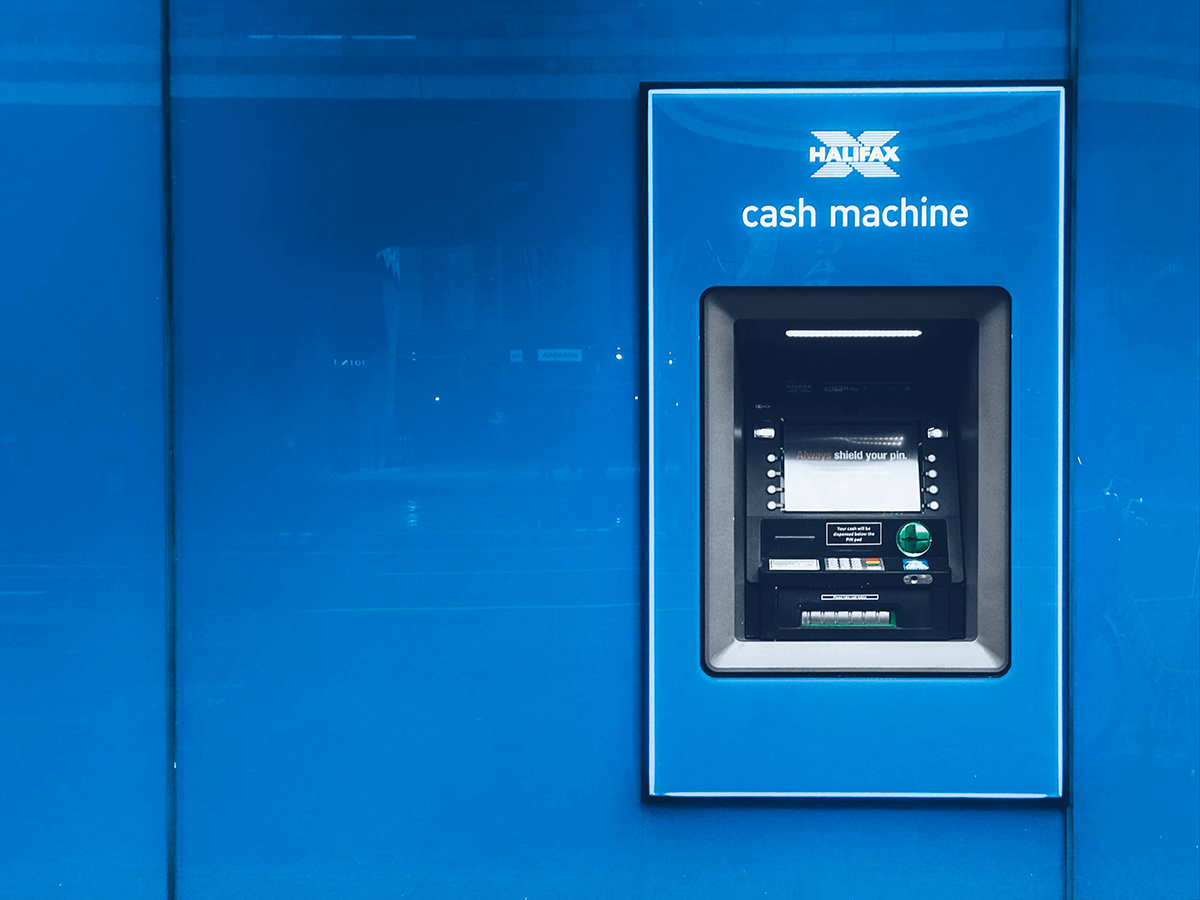
Many success stories of digital transformation in banking demonstrate how digitalization improves customer banking experience and operational efficiency. For example:
- DBS Bank (Singapore). DBS Bank is considered one of the leaders in digital transformation. They have developed a digital platform, DBS Digibank, which provides customers with a wide range of banking services through mobile apps and online banking. They actively use artificial intelligence and analytics to provide personalized recommendations and improve customer experience.
- JPMorgan Chase (USA). JPMorgan Chase has embraced digital transformation to improve operational efficiency and customer service. They have developed their proprietary digital platform, Chase Mobile Banking, which allows customers to perform various banking transactions through mobile devices. They also actively apply machine learning and analytics to better analyze data and deliver services.
- ING Bank (Netherlands). ING Bank has moved from a traditional bank to a digital organization. They provide customers convenient online services and mobile apps and actively use data analytics to provide personalized offers and improve customer experience. They have also implemented digital tools within the bank to streamline processes and improve efficiency.
- BBVA (Spain). BBVA focused on digital transformation and innovation to improve customer experience and banking processes. They developed the BBVA Digital Banking platform, which provides customers with a wide range of services through mobile apps and online banking. They have also implemented blockchain technology to improve the security and efficiency of financial transactions.
- Ally Bank (USA). Ally Bank is an example of a successful digital transformation. They provide a full range of banking services through an online platform, including account opening, lending, investments, and mortgages. Ally Bank actively utilizes digital channels and tools to provide convenience and accessibility to customers.
These examples demonstrate how banks use digital technologies to increase the availability of services, improve customer experience, and optimize their operations.
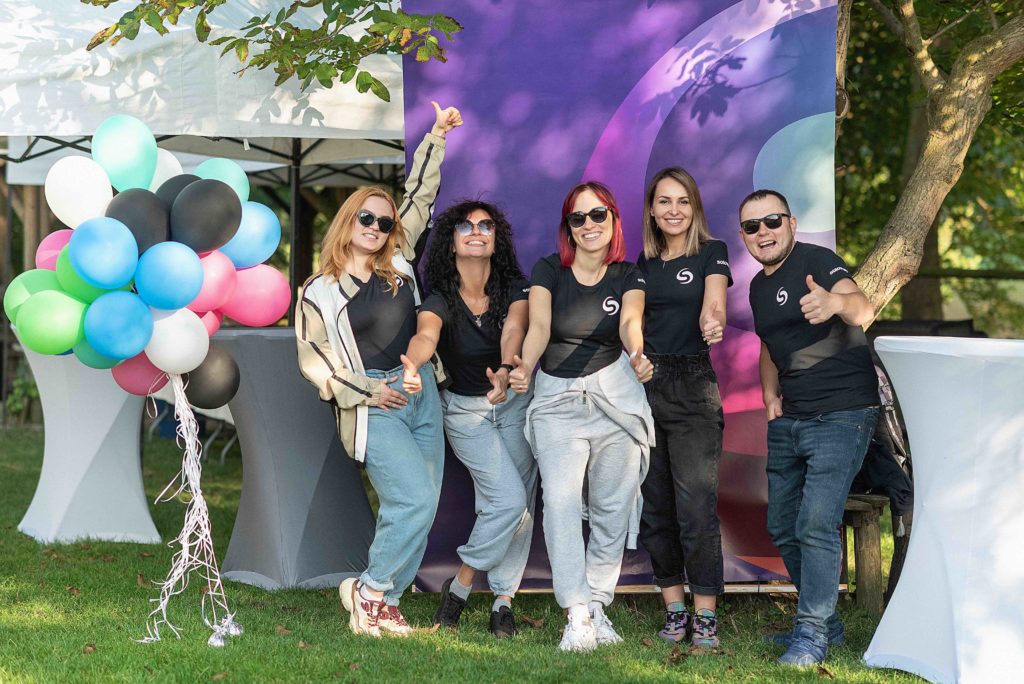
At SoloWay Tech, we specialize in providing comprehensive digital transformation and consulting services to help businesses thrive in the digital age. With our expertise and industry knowledge, we can guide your organization through the complex digital transformation process, enabling you to unlock new opportunities and achieve sustainable growth. We can:
- Consult regarding the digital transformation of your business
- Develop a digital transformation strategy
- Design digital customer experience
- Optimize business processes
- Automate business processes
- Re-engineer legacy apps
- Develop innovative products and services
- Implement end-to-end ML and AI engines
- Engineer IoT
- Build Big Data infrastructure
- Consult regarding the best implementation of IT infrastructure in your business.
At SoloWay Tech, we understand that each business has unique challenges and requirements. Our collaborative approach, deep industry expertise, and proven methodologies empower us to tailor our services to your specific needs, enabling you to achieve sustainable growth and competitive advantage through digital transformation.
Embark on your digital transformation journey with SoloWay Tech and unlock the full potential of your business in the digital era. Contact us today to learn more about our services and how we can help you drive innovation, efficiency, and success.
Digital transformation has become imperative for the banking industry to adapt to the evolving needs and expectations of customers in the modern world. The shift towards digitalization offers numerous advantages, such as enhanced customer experiences, improved operational efficiency, access to Big Data analytics, and new opportunities for innovation. However, there are also challenges to consider, including cybersecurity risks, potential job losses, and dependence on technology.
To embark on a successful digital transformation journey, businesses may seek the expertise of companies like SoloWay Tech that specialize in assisting organizations in their digitalization efforts. With the right guidance and implementation strategies, banks can harness the power of digital technologies to stay competitive, meet customer expectations, and drive innovation in the ever-evolving banking landscape.
Similar articles
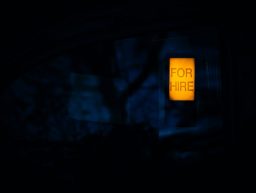
The Ultimate Guide to HR Digital Transformation
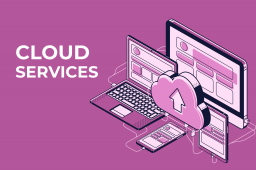
The Role of the Cloud in Digital Transformation
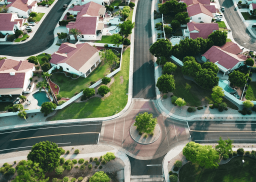
Blockchain for Real Estate: Use Cases and Examples
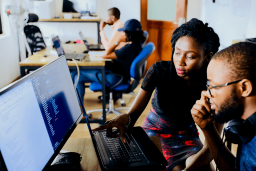
Tech Audit: Who Needs It And How To Do It Properly
We use cookies to provide you with a better on website experience, privacy setting.
This website uses cookies to improve your experience while you navigate through the website.
View the Cookie Policy
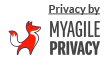
Fill the form below and Do your Solo
Company name, how can we help you.
To read this content please select one of the options below:
Please note you do not have access to teaching notes, impact of big data analytics onĀ banking: a case study.
Journal of Enterprise Information Management
ISSN : 1741-0398
Article publication date: 23 November 2022
Issue publication date: 7 March 2023
The paper aims to help enterprises gain valuable knowledge about big data implementation in practice and improve their information management ability, as they accumulate experience, to reuse or adapt the proposed method to achieve a sustainable competitive advantage.
Design/methodology/approach
Guided by the theory of technological frames of reference (TFR) and transaction cost theory (TCT), this paper describes a real-world case study in the banking industry to explain how to help enterprises leverage big data analytics for changes. Through close integration with bank's daily operations and strategic planning, the case study shows how the analytics team frame the challenge and analyze the data with two analytic models ā customer segmentation (unsupervised) and product affinity prediction (supervised), to initiate the adoption of big data analytics in precise marketing.
The study reported relevant findings from a longitudinal data analysis and identified some key success factors. First, non-technical factors, for example intuitive analytics results, appropriate evaluation baseline, multiple-wave implementation and selection of marketing channels critically influence big data implementation progress in organizations. Second, a successful campaign also relies on technical factors. For example, the clustering analytics could promote customers' response rates, and the product affinity prediction model could boost efficient transaction and lower time costs.
Originality/value
For theoretical contribution, this paper verified that the outstanding characteristics of online mutual fund platforms brought up by Nagle, Seamans and Tadelis (2010) could not guarantee organizations' competitive advantages from the aspect of TCT.
- Transaction cost theory
- Big data analytics
- Enterprise information management
- BankingĀ industry
- Precise marketing
He, W. , Hung, J.-L. and Liu, L. (2023), "Impact of big data analytics onĀ banking: a case study", Journal of Enterprise Information Management , Vol. 36 No. 2, pp. 459-479. https://doi.org/10.1108/JEIM-05-2020-0176
Emerald Publishing Limited
Copyright © 2022, Emerald Publishing Limited
Related articles
Weāre listening — tell us what you think, something didnāt workā¦.
Report bugs here
All feedback is valuable
Please share your general feedback
Join us on our journey
Platform update page.
Visit emeraldpublishing.com/platformupdate to discover the latest news and updates
Questions & More Information
Answers to the most commonly asked questions here
- Candlesticks Book
- Stock Compare
- Superstar Portfolio
- Stock Buckets
- Nifty Heatmap
- *Now Available in Hindi

HDFC Bank Case Study 2021 – Industry, SWOT, Financials & Shareholding
by Jitendra Singh | Mar 4, 2021 | Case Study , Stocks | 1 comment

HDFC Bank Case Study and analysis 2021: In this article, we will look into the fundamentals of HDFC Bank, focusing on both qualitative and quantitative aspects. Here, we will perform the SWOT Analysis of HDFC Bank, Michael Porterās 5 Force Analysis, followed by looking into HDFC Bank’s key financials. We hope you will find the HDFC Bank case study helpful.
Disclaimer: This article is only for informational purposes and should not be considered any kind of advisory/advice.Ā Please perform your independent analysis before investing in stocks, or take the help of your investment advisor. The data is collected fromĀ Trade Brains Portal .
Table of Contents
About HDFC Bank and its Business Model
Incorporated in 1994, HDFC Bank is one of the earliest private sector banks to get approval from RBI in this segment. HDFC Bank has a pan India presence with over 5400+ banking outlets in 2800+ cities, having a wide base of more than 56 million customers and all its branches interlinked on an online real-time basis.
HDFC Limited is the promoter of the company, which was established in 1977. HDFC Bank came up with its 50 crore-IPO in March 1996, receiving 55 times subscription. Currently, HDFC Bank is the largest bank in India in terms of market capitalization (Nearly Rs 8.8 Lac Cr.). HDFC Securities and HDB Financial Services are the subsidiary companies of the bank.

HDFC Bank primarily provides the following services:
Note: If you want to learn Candlesticks and Chart Trading from Scratch, here’s the best book available on Amazon ! Get the book now!
- Retail Banking (Loan Products, Deposits, Insurance, Cards, Demat services, etc.)
- Wholesale Banking (Commercial Banking. Investment Banking, etc.)
- Treasury (Forex, Debt Securities, Asset Liability Management)
HDFC Bank Case Study – Industry Analysis
There are 12 PSU banks, 22 Private sector banks, 1485 urban cooperative banks, 56 regional rural banks, 46 foreign banks and 96,000 rural cooperative banks in India. The total number of ATMs in India has constantly seen a rise and there are 209,110 ATMs in India as of August 2020, which are expected to further grow to 407,000 by the end of 2021.
In the last four years, bank credit recorded a growth of 3.57% CAGR, surging to $1698.97 billion as of FY20. At the same time, deposits rose with a CAGR of 13.93% reaching $1.93trillion by FY20. However, the growth in total deposits to GDB has fallen to 7.9% in FY20 owing to pandemic crises, which was above 9% before it.
Due to strong economic activity and growth, rising salaries, and easier access to credit, the credit demand has surged resulting in the Credit to GDP ratio advancing to 56%. However, it is still far less than the developed economies of the world. Even in China, it is revolving around 150 to 200%.
As of FY20, India’s Retail lending to GDP ratio is 18% , whereas in developed economies (US, UK) it varies between 70% – 80%).
Michael Porter’s 5 Force Analysis of HDFC Bank
1. rivalry amongst competitors.
- The banking sector has evolved very rapidly in the past few years with technology coming in, and now it is not only limited to depositing and lending but various categories of loans and advances, digital services, insurance schemes, cards, broking services, etc.; hence, the banks face stiff competition from its rivals.
2. A Threat by Substitutes
- For services like mutual funds, investments, insurances, categorized loans, etc., banks are not the only option these days because a lot of niche players have put their foot in the specialized category, surging the threat by substitutes for the banks.
- Another threat for the traditional banks is NEO Banks. TheĀ Neo Banks Ā are virtualĀ banks that operate online, are completely digital, and have a minimum physical presence.
3. Barriers to Entry
- Banks run in a highly regulated sector. Strict regulatory norms, huge initial capital requirements and winning the trust of people make it very tough for new players to come out as a national level bank in India. However, if a company enters as a niche player, there are relatively fewer entry barriers.
- With RBI approving the functioning of new small finance banks, payment banks and entry of foreign banks, the competition has further intensified in the Indian banking sector.
4. Bargaining Power of Suppliers
- The only supply which banks need is capital and they have four sources for the capital supply viz. deposits from customers, mortgage securities, loans, and loans from financial institutions. Customer deposits enjoy higher bargaining power as it is totally dependent on income and availability of options.
- Financial Institutions need to hedge inflation, and banks are liable to the rules and regulations of the RBI which makes them a safer bet; hence, they have less bargaining power.
5. Bargaining Power of Customers
- In modern days, customers not only expect proper banking but also the quality and faster services. With the advent of digitalization and the entry of new private banks and foreign banks, the bargaining power of customers has increased a lot.
- In terms of lending, creditworthy borrowers enjoy a high level of bargaining power as there is a large availability of banks and NBFCs which are ready to offer attractive loans and services at low switching and other costs.
HDFC Bank Case Study – SWOT Analysis
Now, moving forward in our HDFC Bank case study, we will perform the SWOT analysis.
1. Strengths
- Currently, HDFC Bank is the leader in the retail loan segment (personal, car and home loans) and credit card business, increasing its market share each year
- The HDFC tag has become a sign of trust in the people as HDFC has come out as a pioneer not only in banking, but loans, insurances, mutual funds, AMC and brokerage.
- HDFC Bank has always been an institution of its words as it has, without fail, delivered its guidance and this has created a strong brand loyalty in the market for them.
- HDFC Bank has very well leveraged the technology to help its profitability, only 34% transaction via Internet Banking in 2010 to 95% transaction in 2020.
2. Weaknesses
- HDFC bank doesn’t have a significant rural presence as compared to its peers. Since its inception, it has focused mainly on high-end clients. However, the focus is shifting in the recent period as nearly 50% of its branches are now in semi-urban and rural areas.
3. Opportunities
- The average age of the Indian population is around 28 years and more than 65% of the population is below 35, with increasing disposable income and rising urbanization, the demand for retail loans is expected to increase. HDFC Bank, being a leader in retail lending, can make the best out of this opportunity.
- With modernization in farming and a rise in rural and semi-urban disposable income, consumer spending is expected to rise. HDFC Bank can increase its market share in these segments by grabbing this opportunity. Currently, the bank has only 21% of the branches in rural areas.
- A lot of niche players have set up their strong branches in respective segments, which has shown stiff competition and has shrined the market share and profit margin for the company. Example – Gold Loans, Mutual Funds , Brokerage, etc.
- In-Vehicle Financing (which is HDFC Bank’s major source of lending income), most of the leading vehicle companies are providing the same service, which is a threat to the bank’s business.
Asian Paints Case Study 2021 – Industry, SWOT, Financials & Shareholding
HDFC Bank’s Management
HDFC Bank has set high standards in corporate governance since its inception.
Right from sticking to their words to proper book writing, HDFC has never compromised with the banking standards, and all the credit goes to Mr. Aditya Puri, the man behind HDFC Bank, who took the bank to such great heights that today its market capitalization is more than that of Goldman Sachs and Morgan Stanley of the US.
In 2020, after 26 years of service, he retired from his position in the bank and passed on the baton of Managing Director to Mr. Shasidhar Jagadishan. He joined the bank as a Manager in the finance function in 1996 and with an experience of over 29 years in banking, Jagadishan has led various segments of the sector in the past.
Financial Analysis of HDFC Bank
- 48% of the total revenue for HDFC bank comes from Retail Banking, followed by Wholesale Banking (27%), Treasury (12%), and 13% of the total comes from other sources.
- Industries receive a maximum share of loans issued by HDFC bank, which is 31.7%, followed by Personal Loans and Services both at a 28.7% share of the total. Only 10.9% of the total loans are issued to Agricultural and allied activities.
- HDFC Bank has a 31.3% market share in credit card transactions, showing a growth of 0.23% from the previous fiscal year, which makes it the market leader, followed by SBI.
- HDFC Bank is the market leader in large corporate Banking and Mid-Size Corporate Banking with 75% and 60% share respectively.
- In Mobile Banking Transaction, the market share of HDFC bank is 11.8%, which has seen a degrowth of 0.66% in the current fiscal year.
- With each year, HDFC Bank has shown increasing net profit, which makes the 1-year profit growth (24.57%) greater than both 3-year CAGR (21.75%) and 5-year CAGR (20.78%).
- Capital Adequacy Ratio, which is a very important figure for any bank stands at 18.52% for HDFC Bank.
- As of Sept 2020 HDFC, is at the second position in bank advances with a 10.1% market share, which has shown a rise from 9.25% a year ago. SBI tops this list with a 22.8% market share, Bank of Baroda is at the third spot with a 6.68% share, followed by Kotak Mahindra Bank (6.35%).
- HDFC Bank is again at the second spot in the market share of Bank deposits with 8.6%. SBI leads with a nearly 24.57% market share. PNB holds 7.5% of the market share in this category, coming out as the third followed by Bank of Baroda with 6.89%.
HDFC Bank Financial Ratios
1. profitability ratios.
- As of FY20, the net profit margin for the bank stands at 22.87%, which has seen a continuous rise for the last 4 fiscal years. This a very positive sign for the bank’s profitability.
- The Net Interest Margin (NIM) has been fluctuating from the range of 3.85% to 4.05% in the last 5 fiscal years. Currently, it stands at 3.82% as of FY20.
- Since FY16, there has been a constant fall in RoE, right from the high of 18.26% to 16.4% as of FY20.
- RoA has been more or less constant for the company, currently at 1.89%, which is a very positive sign.
2. Operational Ratios
- Gross NPA for the bank has fallen from FY19 (1.36) to 1.26, which a positive sign for the company. A similar improvement is also visible in the Net NPA, currently standing at 0.36.
- The CASA ratio for the bank is 42.23%, which has been seeing a continuous fall since FY17 (48.03%). However, there has been a spike rise in FY17 as in FY16, it was 43.25 and in FY18, again came to the almost same level of 43.5.
- In FY19, Advance Growth witnessed a massive spike from 18.71 level in FY18 rising to 24.47%. However, in FY20, it again fell nearly 4 points, coming down to 21.27%.
HDFC Bank Case Study – Shareholding Pattern
- Promoters hold 26% shares in the bank, which has been almost at the same level for the last many quarters. In the December quarter a years ago, the promoter holding was 26.18%. The marginal fall is only due to Aditya Puri retiring and selling few shares for his post-retirement finance, which he stated.
- FIIs own 39.95% shareholding in the bank, which has been increasing for years in every quarter. HDFC bank has been a darling share in the investor community.
- 21.70% of shares are owned by DIIs as of December Quarter 2020. Although it is less than the SeptQ2020(22.90%), it is still far above the year-ago quarter (21.07).
- Public holding in HDFC bank is 12.95% as of Dec Q2020, which has tanked from the year-ago quarter (14.83%) as FIIs increasing their share, which is evident from the rising share prices.
Closing Thoughts
In this article, we tried to perform a quick HDFC Bank Ā case study. Although there are still many other prospects to look into, however, this guide would have given you a basic idea about HDFC Bank.
What do you think about HDFC Bank fundamentals from the long-term investment point of view? Do let us know in the comment section below. Take care and happy investing!
Start Your Stock Market Journey Today!
Want to learn Stock Market trading and Investing? Make sure to check out exclusive Stock Market courses by FinGrad, the learning initiative by Trade Brains. You can enroll in FREE courses and webinars available on FinGrad today and get ahead in your trading career. Join now!!
Nice can I get full case
Submit a Comment Cancel reply
Your email address will not be published. Required fields are marked *

Search Topic or Keyword
Trending articles.

Recent IPO’s
- Go Digit IPO Review 2024 – GMP, Financials And More
- Aadhar Housing FinanceĀ IPO Review – GMP, Financials And More
- TBO TEKĀ IPO Review – GMP, Financials And More
- IndegeneĀ IPO Review – GMP, Financials, And More
- JNK India IPO Review 2024 – GMP, Financials And More
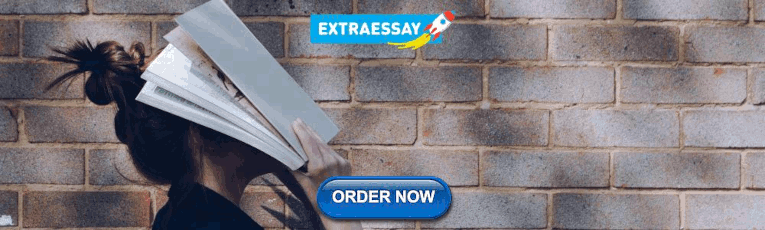
Easiest Stock Screener Tool!
Best stock discovery tool with +130 filters, built for fundamental analysis. Profitability, Growth, Valuation, Liquidity, and many more filters. Search Stocks Industry-wise, Export Data For Offline Analysis, Customizable Filters.
- — Stock Screener
- — Compare Stocks
- — Stock Buckets
- — Portfolio Backtesting
Start your stock analysis journey with Trade Brains Portal today. Launch here !
Keep the Learning On!
Subscribe to Youtube to watch our latest stock market videos. Subscribe here .
- Case Study of the Indian Banking and Financial Services Industry using Strategic Tools
Finance is like Oil to the Engine of the Indian Economy
As finance is the grease and the oil that keeps the engine of any economy running, the BFSI sector assumes importance in this context. While the post independence era witnessed many large private banks that were either family or community run as well as some government owned banks, the nationalization of the banking sector in 1969 and the early 1970s meant that the government was the prime mover as far as banking and finance was concerned.
The situation of government ownership of banks continued well into the 1990s when the first wave of liberalization ensured that banks were now allowed to be privately owned. While multinational banks were always privately owned, most Indian banks were government owned or owned in a quasi governmental manner.
Even after liberalization, the RBI or the Reserve Bank of India proceeded cautiously as far as private ownership of the BFSI sector was concerned. However, this did not deter many firms such as the NBFCs or the Non Banking Financial Companies from operating and indeed, flouting the rules thereby leading to periodic bouts of crises.
Becoming World Class in their Practices and Dealing with Crises
Now, the BFSI sector in India is in a position where it can compete with its peers abroad and elsewhere mainly due to the pioneering efforts of the first wave of post liberalization banks such as HDFC and ICICI. No wonder that the Indian BFSI sector has become a dream job destination for millions of graduates in the technical and managerial institutes.
Having said that, at present, the BFSI sector in India is in crisis due to its profligate lending practices during the boom years of the first decade of the 21st century . Indeed, concomitant with the growth of the Indian Economy and the blistering pace of capacity addition as well as booming industries, the banks and financial institutions threw caution to the winds and engaged in indiscriminate lending without doing their due diligence.
For instance, during the heydays of growth between 2000 and 2008, banks, and financial institutions in India lent to just about anybody and the GFC or the Global Financial Crisis of 2008 resulted in such debts turning bad.
However, it is to the credit of the then ruling dispensation that the 2008 crisis and the global bust did not have major impacts on the Indian BFSI sector due to adequate oversight and regulation by the government in tandem with the RBI.
Having said that, some experts believe that what they did was to merely ākick the can down the roadā without solving the problem and this in turn led to the ballooning of the NPAs or the Non Performing Assets to such an extent that at the moment, absent massive recapitalization, the Indian BFSI sector would be in major trouble soon.
PESTLE Analysis
In India, like in most developing countries, politics is inseparable from business and hence, the Indian BFSI Sector is indeed impacted heavily by the policies and the regulations that are passed by the ruling dispensations at the center . Moreover, given the high incidence of governmental and public sector ownership of the so-called State Run Banks, political interference is natural and a major input into the decision making process at the banks.
While experts have repeatedly called for lesser political interference in the running of the banks, most people agree that it is routine for lenders in the public sector and even the private sector to pay heed to requests from politicians as far as lending and other aspects are concerned. Moreover, the political masters appoint the chairpersons of the banks and financial institutions and this gives them a major say I the day to business practices at these entities.
Economic forces determine the workings of the BFSI sector and it is but natural that the economic environment plays a major role in the fortunes of the sector.
Whether it is liberalization or the GFC or the Demonetization measure, banks and financial institutions are heavily impacted by the macro and the micro economic forces. Indeed, both of them are important unlike in the West where the macro is often the main driver of performance of banks. The reason for this is that the Indian Economy has not yet matured to an extent where micro trends are insignificant.
For instance, small changes in consumer loans and personal finance segments is enough to cause major impacts on the workings and business practices of the firms in the BFSI sector. Having said that, the steady development and integration of the Indian Economy into the broader global economy has resulted in the macro gaining traction as the moving force of the BFSI sector.
The changing socio-cultural profiles and the demographic shifts underway among the Indian consumers does impact the Indian BFSI sector to a great extent. For instance, with the increase in the population of the youth, banks and financial institutions are offering ever more lucrative investment options to this segment.
In addition, Indians are now more risk prone as far as their investment patterns are concerned. This can be seen in the rising numbers of people taking personal, housing, consumer, and other loans to fund their extravagant lifestyles. Moreover, with the rise in consumerism, there has been a concomitant increase in the numbers of Indians holding credit cards and debit cards that are also in tune with the Indian Governmentās Digital India push for payment avenues.
Indeed, taken together, what the changing sociocultural dynamics indicates is that the BFSI sector in India is at a stage where it is mimicking the consumer lending practices that are usually associated with the West.
Technological
It would be an understatement to say that there is a paradox at the heart of the Indian BFSI sector as far as impacts of technological advances are concerned. For instance, while banking is as old as the Indian Republic, it was only recently that the Indian BFSI sector took to technology in a big way. Once having done so, it ensured that its tech offerings were as good as those of the advanced countries with the added advantage of the Indian IT (Information Technology) industry being at the forefront of the adoption of technology in banking and finance.
Having said that, the paradox here is that while a certain percentage (some of would say miniscule) transacts online and the mobile channels with advanced tech, the majority of Indians are simply in the tech wilderness as far as their ability to leverage technology is concerned. Indeed, this is the reason why Demonetization and the concomitant push towards Digital Banking failed to take off as most Indians are not used to using tech enabled banking and payment channels.
Given the fact that the Indian Legal System is cumbersome and long winding, it is natural for banks and financial institutions in India to take the consumers for granted including trying out unconventional and often, legally dubious methods of loan recovery as well as selling of financial products.
Indeed, while there are many cases of fraud that are registered annually, the resolution, or the settlement of such cases is lackluster to say the least.
In addition, with the country having weak laws as far as cybercrime is concerned, the thousands of Indians who fall prey to phishing, cyber fraud, and online scams keeps growing without any meaningful solution to their woes. Thus, it can be said that there is an urgent need to rectify the situation.
Environmental
This aspect does not have much of an impact on the Indian BFSI sector since Green Lending and CSR or Corporate Social Responsibility business practices are yet to take off among the banks and financial institutions. Indeed, it is only recently that the banks and financial institutions started a separate department for these aspects and hence, the sector has a long way to go before it catches up in this regard.
SWOT Analysis
The main strength of the Indian BFSI sector lies in its ability to deliver volumes since India being a large and diverse country, offers the benefits of a humungous customer base. In addition, the Indian BFSI sector also relies on high net worth individuals who are a sizable segment of the population considering the number of Millionaires and Billionaires in the country. Apart from this, the Indian BFSI sector is also heavily driven by corporates who use it for their domestic and international operations.
Having said that, the most notable weakness of the Indian BFSI sector is its informal and unstructured lending and banking processes . Indeed, despite attempts by the RBI and the Finance Ministry, they have been unable to rein in the dubious practices followed by the banks and NBFCs.
For instance, the recent scandals involving well connected businesspersons and the allegations of misconduct that has been leveled indicates that crony capitalism is very much the case as far as the Indian BFSI sector is concerned. This in turn, raises serious questions about its ability to mature into a world class sector which does not bode well for the countryās aspiration to be a global player and a key pillar of the global economy.
Opportunities
On the other hand, there are humungous opportunities for the Indian BFSI Sector since the majority of the population is unbanked and especially in the rural areas where banks and formal financial sector firms do not have a presence.
Indeed, banking for the unbanked offers an unprecedented opportunity for the Indian BFSI sector as can be seen from the success of emerging banks such as Bandhan Bank.
Further, there are many options for the latest generation payments banks and other institutions that are cropping up to take advantage of the mobile and Smartphone penetration to leverage their existing banking channels.
However, there are dark clouds on the horizon for the Indian BFSI sector especially in terms of the rising bad loans and the NPAs (Non Performing Assets) which can bring down the banking sector if they are not managed in a structured manner. Indeed, it can be said that the Indian BFSI sector is facing an existential crisis as far as the problem of NPAs are concerned . added to this, someday or the other, the sector has to grow beyond its dubious and wink and nudge informal and personal collusion crony capitalist practices if it has to well and truly emerge as a global player.
The Reserve Bank of India and Demonetization
No discussion on the Indian BFSI sector is complete without examining the role of the RBI, the countryās mandated regulator. Starting in its pre independence and post independences periods of regulating the Indian BFSI sector to the privatization wave where it was tasked with maintaining monetary policy and its preeminent role in safeguarding the Indian Economy from external shocks such as the GFC of 2008, the RBI has indeed done a stellar job of stewarding the Indian BFSI sector.
Having said that, its neutrality and independence have been questioned in recent years especially with the Demonetization measure, and this has worrying trends for the future of the Indian BFSI sector.
Indeed, Demonetization could be counted as the most radical measure as far as the Indian Economy in the post independence era is concerned .
Though there were other notable moves such as nationalization and liberalization as well as devaluation of the Indian Rupee, Demonetization beats the other bold moves hollow with its singular thrust of being disruptive in nature.
It would not be an understatement to say that with this measure, the BFSI sector received such a jolt and a shock that the after effects would continue to be felt for years to come.
Authorship/Referencing - About the Author(s)
The article is Written and Reviewed by Management Study Guide Content Team . MSG Content Team comprises experienced Faculty Member, Professionals and Subject Matter Experts. We are a ISO 2001:2015 Certified Education Provider . To Know more, click on About Us . The use of this material is free for learning and education purpose. Please reference authorship of content used, including link(s) to ManagementStudyGuide.com and the content page url.
- History of Modern Banking
- Central Banking in the United States
- Functions of a Central Bank
- Regulatory Role Performed By the Central Bank
- How Reserve Requirements Work ?
- Interbank Lending Markets and Repurchase Agreements
- Types of Products in Commercial Banking
- How Credit Rating Works ?
- Technology and Banking Delivery Channels
- Intermediaries to a Credit Card Transaction
- Fee Based Banking Services
- What Are Smart Cards and How are They Better than Credit Cards ?
- Risks Faced By Banks
- The Kingfisher Airlines Outrage
- What is Islamic Banking ?
- Retail Banking : Demand Deposit Products
- Retail Banking: Time Deposit Products
- Multiplier Effect: How Fractional Reserve Banking Creates Money ?
- Capital Adequacy Ratio
- The Three Basel Accords
- Shadow Banking - Meaning, Functions, Advantages & Disadvantages
- Internet Only Banks
- The ABC of Peer To Peer Credit
- Should āToo Big To Failā Banks be Broken to Pieces ?
- Rigging the LIBOR
- Living in a Cashless Society
- Cashless Economy: Pros and Cons
- The Sinister Motive Behind Cashless Society
- The War on Cash
- Asset Reconstruction Companies
- Lockbox Service Provided by Banks
- Treasury Operations of Banks
- Hire Purchase Agreements
- United States and the Curse of Predatory Lending
- Chinaās Predatory Lending
- Fin Tech: The Future of Banking
- Peer To Peer Lending
- How Technology has been a Game Changer for the Banking and Financial Services Sectors
- The Mega Scam in the Indian Banking System
- Privatization of Indiaās Public Sector Banks
- How dire is India's bad debts problem and what you need to know about it
- Collusion between Private Banks and Central Banks
- Interest Rates and Their Effect on Small Businesses
- Deutsche Bank: The Fall of a Giant
- The Problem with Farm Loan Waivers
- Whats Wrong with European Banks?
- Bank Recapitalization in India
- Will The Fiat Money System Collapse?
- The Rise of Dynamic Discounting
- Demystifying the Mysterious, Glamorous, and Demanding World of Investment Banking
- Indian Banking Sector: Inter-Creditor Pacts
- Why Reserve Bank of India Spooked Investors?
- Why Should Central Banks Be Independent?
- The Downfall of Chanda Kocchar - Indiaās Rockstar Woman CEO
- BB&T and Sun Trust Bank Merger
- The Deutsche Bank Commerzbank Merger
- The Inherent Conflict of Interest in Interest Rates Determination
- The Problem with Postal Banking
- The Wells Fargo Auto Insurance Scandal
- What is FinTech and How it Enables Banking and Financial Firms to Leverage Technology
- The Silicon Valley Bank Collapse: Why Is It Important?
- Impact of the Fall of Silicon Valley Bank
- Rising Interest Rates: The Perfect Storm for The Silicon Valley Bank
- Poor Risk Management at the Silicon Valley Bank
- How Does The Failure of Silicon Valley Bank Affect Stakeholders
- The Role of Bridge Banking in The Silicon Valley Bank Crisis
- Role of Social Media in The Silicon Valley Bank Run
- Can the Silicon Valley Bank Fiasco Cause a Recession?
- Silicon Valley Bank and Easy Money Policy
- Was The Silicon Valley Bank Bailed Out by the Government?
- Bank Term Funding Program (BTFP)
- Problems with Bank Term Funding Program (BTFP)
For enquiries call:
+1-469-442-0620

- Data Science
Top Data Science Use Cases in Banking
Home Blog Data Science Top Data Science Use Cases in Banking

The use of data science in banking and finance is rapidly growing as financial institutions seek to harness the power of big data. Banking organizations are relying on data science in banking and finance more than ever to gain a competitive edge. As a result, integrating data science in banking and finance operations is becoming imperative. Adopting big data technologies offers numerous advantages to banks, including enhanced decision-making, optimized resource allocation, and boosted performance. To grasp the intricate dynamics and effective application of data science in the banking and finance sector, one is advised to enroll in a comprehensive full-time Data Science course . Additionally, as data science in banking and finance continues to evolve, it also promises innovations in fraud detection and personalized customer experiences.
The Growing Role of Data Science in Banking
As far as customer service delivery and operations models are concerned, the banking industry has evolved over the years. Nevertheless, most banks still haven't integrated analytics into their core culture, decisions, and business operations. According to a recent McKinsey report, more than 20 banks in the Middle East, Europe, and Africa are at different levels of analytics maturity. Data analytics efforts were matched with business goals by 30 % of banks surveyed.
How is Data Science Used in Banking and Finance ?
Data Science in banking and finance is revolutionizing the banking industry through its implementation. The banking industry is constantly seeking better ways to identify customers so that they can increase customer loyalty by improving operational efficiency.
For efficient customer interactions, banks are analyzing a large amount of available transaction data to identify patterns.
Data Science in the banking sector āÆuses customer data including previous history, transactions, trends, and communication.
Due to the fact that this data is mostly unstructured, extracting insights from it is a difficult task.
The analysis of data can be done in many ways, such as Machine Learning, data fusion, signal processing, data integration, natural language processing, etc. As part of their customer segmentation processes and fraud detection, data science in banking is used to perform various important tasks.
Data Science Applications in Banking
Petabytes and terabytes of customer information are being stored by some of the world's largest banks every day. It would mean abandoning one of the key development vectors if these data were not used and their analysis was not made available. The motive of data science jobs in banks to employ data science in finance is clear, r, i.e., to increase efficiency as much as possible. However, to understand the data science use cases in banking āÆone needs to go through a part-time Data Science Bootcamp .
Top 9 Use Cases of Data Science in the Banking Domain
Listed below are the top 9 use cases of data science in banking domain :
1. Fraud Detection
Fraud detection applications may damage the bank's reputation, which is why the financial sector spends billions of dollars every year on data science in banking and financial services . Detecting fraudulent activities requires the use of data science to collect, summarize, and predict the customer database. Before data science/big data, it was impossible for data science for banking professionals to analyze customer records to generate accurate information. AI Machine Learning and can assist banks in preventing fraud.āÆ
By using a variety of parameters, such as purchase amount, location, merchant, time and others, data models can be developed to analyze credit card frauds.
2. Managing Customer Data
Data is collected, analyzed, and stored in massive quantities by banks. The goal should not only be to ensure compliance with the law, but rather to learn about their clients in order to generate revenue opportunities using machine learning and data science tools.
A growing number of people are using digital banking nowadays. Consequently, data scientists must isolate the most relevant customer data. Data specialists can then use machine learning models to isolate and process only the most relevant client information to improve business decision-making using the information about customers' preferences, interactions, and behaviors, allowing banks to unlock revenue opportunities by processing only the most relevant information.
3. Risk Modeling
Investment banking data science projects āÆprioritize risk modeling because it regulates banking activities and play a critical role in pricing financial instruments. Corporate finance, mergers and acquisitions, corporate restructuring and reorganizations, and investment banking involve evaluating the net worth of companies to build capital for corporate financing.
This is why the concept of risk modeling has become important to banks, and the best way to assess it is by utilizing the tools and information available through data science. Through the use of Big Data, innovators are now leveraging the power of new technology to make better risk-based decisions through the use of data-driven decision-making processes.
4. Customized Marketing
Personalized and customized marketing is one of the ways in which data science in banking āÆallows for effective customer interaction. To present the most appropriate offers to a particular consumer, self-learning algorithms analyze the accumulated information. The company, therefore, benefits from expanding its product and service offerings, and from increased sales.
5. Customer Lifetime Value Prediction
A business's customer lifetime value estimates the total value that will be derived from their relationship with a customer over the course of their relationship. To generate higher profitability and growth, this measure plays a vital role in creating and sustaining relationships with customers.
It is becoming increasingly difficult for banks to acquire and retain profitable customers. Due to increased competition, banks need to focus their resources efficiently by taking a 360-degree view of each customer. Data science plays a key role here. It is first necessary to consider a wide range of data, such as client attrition and acquisition, the use of various banking services and products, the profitability and volume of those products and services, as well as other client characteristics like geography, demographics, and market.
It is often necessary to clean and manipulate this data to make it usable and meaningful. A bank's clients vary greatly in terms of their profiles, products, and services, and their expectations and behaviors. Generalized linear models, regression trees, classification, and stepwise regression are some of the tools and approaches that data scientists can use to develop a CLV model. To maintain good customer relations and improve profitability and growth, one of the most important processes is to build a predictive model that determines future marketing strategies based on CLV.
6. Customer Segmentation
For the purpose of segmenting their customers, banks perform Customer Segmentation. Customers can be segmented based on their behavior, known as behavioral segmentation, or through their demographic characteristics, known as demographic segmentation.
Religion, age, gender, income, and other factors might be included in the demographic segmentation.
Banking companies benefit from customer segmentation by investing their time and resources in the right places. A variety of application of data science in banking āÆtechniques can be used by banks, such as logistic regression, decision trees, clustering, etc. The CLV can be predicted accordingly for different segments of customers based on these. To identify low-value and high-value segments of customers.
By segmenting their customers, organizations can maximize their sales by utilizing their resources effectively. Furthermore, it is used for improving customer loyalty and providing better customer service.
7. Customer Support
It's imperative to maintain a productive long-term relationship with your customers by providing outstanding customer support. Supporting customers is an important but broad aspect of customer service in the banking industry. As service-based businesses, banks are primarily involved in providing services to their customers. Interacting with customers and responding to their queries and complaints in a timely and thorough manner are part of this task.
By using data science, a better automation process can be achieved, making it more precise, personal, direct, and productive, as well as cost-effective in terms of employee time.
8. Real-Time and Predictive Analysis
Banking analytics are becoming increasingly important. Since every banking use case is closely related to analytics and data science in banking āÆtechniques can help improve banks' analytics strategies. Analytics become more sophisticated and accurate as information variety and availability increase.
Data processing has become more affordable and smaller, thus increasing the amount of data available, indicating signals, rather than just noise. To solve problems efficiently and make strategic decisions, it is important to separate true relevant data from noise. Predictive analytics assist in choosing the right technique for solving the problem identified by real-time analytics. Analyzing the bank workflow in advance can greatly improve results and prevent potential problems.
9. Recommendation Engines
As e-commerce and retail industries have grown, recommendation engines have improved. To identify behavioral patterns and recommend products or services, recommender systems are used in the banking sector.āÆ
Letās look at one of the data science case studies in banking . Spanish banking group BBVA, for instance, uses a recommendation engine to offer its users customized suggestions or advice based on their behavior and needs. For managing personal finances and controlling expenses, the bank offers Baby Planner, Commerce360, and Bconomy.
Data science in banking is pivotal for the modern banking sector. It should be at the forefront of banks' decision-making processes, enabling them to derive actionable insights from their client's data. This integration of data science in banking offers a distinctive competitive advantage. Banks should embrace Big Data analytics progressively, beginning with small, manageable steps, which will undoubtedly place them ahead of their competitors. In India, a career as a bank data scientist is particularly lucrative, showcasing significant growth and unparalleled career opportunities.
Data science is a rapidly developing field with many possibilities for using it, and the ability to use machine learning models on real data means that the list of applications is growing every day. So, in order to have a deep knowledge of data science, you can pursue KnowledgeHut's full-time Data Science course .
Frequently Asked Questions (FAQs)
Banks can benefit from data science technologies like AI, natural language processing, and machine learning algorithms in a number of areas, such as fraud detection, risk management, sentiment analysis, and personalized marketing. There has never been a time when data science has disrupted the banking industry like this.
Data scientists have plenty of opportunities in financial services. In contrast to similar roles at pure technology organizations, the career experience can be quite different. The best way to determine whether this industry is right for you is to first consider your interests, work preferences, and long-term goals.
To detect fraudulent activities, data science collects, summarizes, and predicts customer data. Before the advent of data science/big data, it was impossible to analyze customer records to generate accurate information. Banks can prevent fraud with the help of artificial intelligence and machine learning.
Personalized marketing and risk management services of the banking system can be provided by using data science.

Ashish Gulati
Ashish is a techology consultant with 13+ years of experience and specializes in Data Science, the Python ecosystem and Django, DevOps and automation. He specializes in the design and delivery of key, impactful programs.
Avail your free 1:1 mentorship session.
Something went wrong
Upcoming Data Science Batches & Dates

AI-bank of the future: Can banks meet the AI challenge?
In 2016, AlphaGo, a machine, defeated 18-time world champion Lee Sedol at the game of Go, a complex board game requiring intuition, imagination, and strategic thinkingāabilities long considered distinctly human. Since then, artificial intelligence (AI) technologies have advanced even further, 1 AI can be defined as the ability of a machine to perform cognitive functions associated with human minds (e.g., perceiving, reasoning, learning, and problem solving). It includes various capabilities, such as machine learning, facial recognition, computer vision, smart robotics, virtual agents, and autonomous vehicles. See ā Global AI Survey: AI proves its worth, but few scale impact ,ā November 2019, McKinsey.com. and their transformative impact is increasingly evident across industries. AI-powered machines are tailoring recommendations of digital content to individual tastes and preferences, designing clothing lines for fashion retailers, and even beginning to surpass experienced doctors in detecting signs of cancer. For global banking, McKinsey estimates that AI technologies could potentially deliver up to $1 trillion of additional value each year. 2 ā The executiveās AI playbook ,ā McKinsey.com.
Many banks, however, have struggled to move from experimentation around select use cases to scaling AI technologies across the organization. Reasons include the lack of a clear strategy for AI, an inflexible and investment-starved technology core, fragmented data assets, and outmoded operating models that hamper collaboration between business and technology teams. What is more, several trends in digital engagement have accelerated during the COVID-19 pandemic, and big-tech companies are looking to enter financial services as the next adjacency. To compete successfully and thrive, incumbent banks must become āAI-firstā institutions, adopting AI technologies as the foundation for new value propositions and distinctive customer experiences.
In this article, we propose answers to four questions that can help leaders articulate a clear vision and develop a road map for becoming an AI-first bank:
- Why must banks become AI-first?
- What might the AI-bank of the future look like?
- What obstacles prevent banks from deploying AI capabilities at scale?
- How can banks transform to become AI-first?
1. Why must banks become AI-first?
Over several decades, banks have continually adapted the latest technology innovations to redefine how customers interact with them. Banks introduced ATMs in the 1960s and electronic, card-based payments in the ā70s. The 2000s saw broad adoption of 24/7 online banking, followed by the spread of mobile-based ābanking on the goā in the 2010s.
Few would disagree that weāre now in the AI-powered digital age, facilitated by falling costs for data storage and processing, increasing access and connectivity for all, and rapid advances in AI technologies. These technologies can lead to higher automation and, when deployed after controlling for risks, can often improve upon human decision making in terms of both speed and accuracy. The potential for value creation is one of the largest across industries, as AI can potentially unlock $1 trillion of incremental value for banks, annually (Exhibit 1).
Across more than 25 use cases, 3 For an interactive view, visit: www.mckinsey.com/business-functions/mckinsey-analytics/our-insights/the-executives-ai-playbook?page=industries/banking/ AI technologies can help boost revenues through increased personalization of services to customers (and employees); lower costs through efficiencies generated by higher automation, reduced errors rates, and better resource utilization; and uncover new and previously unrealized opportunities based on an improved ability to process and generate insights from vast troves of data.
More broadly, disruptive AI technologies can dramatically improve banksā ability to achieve four key outcomes: higher profits, at-scale personalization, distinctive omnichannel experiences, and rapid innovation cycles. Banks that fail to make AI central to their core strategy and operationsāwhat we refer to as becoming āAI-firstāāwill risk being overtaken by competition and deserted by their customers. This risk is further accentuated by four current trends:
- Rising customer expectations as adoption of digital banking increases. In the first few months of the COVID-19 pandemic, use of online and mobile banking channels across countries has increased by an estimated 20 to 50 percent and is expected to continue at this higher level once the pandemic subsides. Across diverse global markets, between 15 and 45 percent of consumers expect to cut back on branch visits following the end of the crisis. 4 John Euart, Nuno Ferreira, Jonathan Gordon, Ajay Gupta, Atakan Hilal, Olivia White, ā A global view of financial life during COVID-19āan update ,ā July 2020, McKinsey.com. As consumers increase their use of digital banking services, they grow to expect more, particularly when compared to the standards they are accustomed to from leading consumer-internet companies. Meanwhile, these digital experience leaders continuously raise the bar on personalization, to the point where they sometimes anticipate customer needs before the customer is aware of them, and offer highly-tailored services at the right time, through the right channel.
- Leading financial institutionsā use of advanced AI technologies is steadily increasing. Nearly 60 percent of financial-services sector respondents in McKinseyās Global AI Survey report 5 Arif Cam, Michael Chui, Bryce Hall, ā Global AI Survey: AI proves its worth, but few scale impact ,ā November 2019, McKinsey.com. that their companies have embedded at least one AI capability. The most commonly used AI technologies are: robotic process automation (36 percent) for structured operational tasks; virtual assistants or conversational interfaces (32 percent ) for customer service divisions; and machine learning techniques (25 percent) to detect fraud and support underwriting and risk management. While for many financial services firms, the use of AI is episodic and focused on specific use cases, an increasing number of banking leaders are taking a comprehensive approach to deploying advanced AI, and embedding it across the full lifecycle, from the front- to the back-office (Exhibit 2).
- Digital ecosystems are disintermediating traditional financial services. By enabling access to a diverse set of services through a common access point, digital ecosystems have transformed the way consumers discover, evaluate, and purchase goods and services. For example, WeChat users in China can use the same app not only to exchange messages, but also to book a cab, order food, schedule a massage, play games, send money to a contact, and access a personal line of credit. Similarly, across countries, nonbanking businesses and āsuper appsā are embedding financial services and products in their journeys, delivering compelling experiences for customers, and disrupting traditional methods for discovering banking products and services. As a result, banks will need to rethink how they participate in digital ecosystems, and use AI to harness the full power of data available from these new sources.
- Technology giants are entering financial services as the next adjacency to their core business models. Globally, leading technology giants have built extraordinary market advantages: a large and engaged customer network; troves of data, enabling a robust and increasingly precise understanding of individual customers; natural strengths in developing and scaling innovative technologies (including AI); and access to low-cost capital. In the past, tech giants have aggressively entered into adjacent businesses in search of new revenue streams and to keep customers engaged with a fresh stream of offerings. Big-tech players have already gained a foothold in financial services in select domains (especially in payments and, in some cases, lending and insurance), and they may soon look to press their advantages to deepen their presence and build greater scale.
2. What might the AI-bank of the future look like?
To meet customersā rising expectations and beat competitive threats in the AI-powered digital era, the AI-first bank will offer propositions and experiences that are intelligent (that is, recommending actions, anticipating and automating key decisions or tasks), personalized (that is, relevant and timely, and based on a detailed understanding of customersā past behavior and context), and truly omnichannel (seamlessly spanning the physical and online contexts across multiple devices, and delivering a consistent experience) and that blend banking capabilities with relevant products and services beyond banking. Exhibit 3 illustrates how such a bank could engage a retail customer throughout the day. Exhibit 4 shows an example of the banking experience of a small-business owner or the treasurer of a medium-size enterprise.
Internally, the AI-first institution will be optimized for operational efficiency through extreme automation of manual tasks (a āzero-opsā mindset) and the replacement or augmentation of human decisions by advanced diagnostic engines in diverse areas of bank operations. These gains in operational performance will flow from broad application of traditional and leading-edge AI technologies, such as machine learning and facial recognition, to analyze large and complex reserves of customer data in (near) real time.
The AI-first bank of the future will also enjoy the speed and agility that today characterize digital-native companies. It will innovate rapidly, launching new features in days or weeks instead of months. It will collaborate extensively with partners to deliver new value propositions integrated seamlessly across journeys, technology platforms, and data sets.
Would you like to learn more about our Financial Services Practice ?
3. what obstacles prevent banks from deploying ai capabilities at scale.
Incumbent banks face two sets of objectives, which on first glance appear to be at odds. On the one hand, banks need to achieve the speed, agility, and flexibility innate to a fintech. On the other, they must continue managing the scale, security standards, and regulatory requirements of a traditional financial-services enterprise.
Despite billions of dollars spent on change-the-bank technology initiatives each year, few banks have succeeded in diffusing and scaling AI technologies throughout the organization. Among the obstacles hampering banksā efforts, the most common is the lack of a clear strategy for AI. 6 Michael Chui, Sankalp Malhotra, ā AI adoption advances, but foundational barriers remain ,ā November 2018, McKinsey.com. Two additional challenges for many banks are, first, a weak core technology and data backbone and, second, an outmoded operating model and talent strategy.
Built for stability, banksā core technology systems have performed well, particularly in supporting traditional payments and lending operations. However, banks must resolve several weaknesses inherent to legacy systems before they can deploy AI technologies at scale (Exhibit 5). First and foremost, these systems often lack the capacity and flexibility required to support the variable computing requirements, data-processing needs, and real-time analysis that closed-loop AI applications require. 7 āClosed loopā refers to the fact that the modelsā intelligence is applied to incoming data in near real time, which in turn refines the content presented to the user in near real time. Core systems are also difficult to change, and their maintenance requires significant resources. What is more, many banksā data reserves are fragmented across multiple silos (separate business and technology teams), and analytics efforts are focused narrowly on stand-alone use cases. Without a centralized data backbone, it is practically impossible to analyze the relevant data and generate an intelligent recommendation or offer at the right moment. If data constitute the bankās fundamental raw material, the data must be governed and made available securely in a manner that enables analysis of data from internal and external sources at scale for millions of customers, in (near) real time, at the āpoint of decisionā across the organization. Lastly, for various analytics and advanced-AI models to scale, organizations need a robust set of tools and standardized processes to build, test, deploy, and monitor models, in a repeatable and āindustrialā way.
Banksā traditional operating models further impede their efforts to meet the need for continuous innovation. Most traditional banks are organized around distinct business lines, with centralized technology and analytics teams structured as cost centers. Business owners define goals unilaterally, and alignment with the enterpriseās technology and analytics strategy (where it exists) is often weak or inadequate. Siloed working teams and āwaterfallā implementation processes invariably lead to delays, cost overruns, and suboptimal performance. Additionally, organizations lack a test-and-learn mindset and robust feedback loops that promote rapid experimentation and iterative improvement. Often unsatisfied with the performance of past projects and experiments, business executives tend to rely on third-party technology providers for critical functionalities, starving capabilities and talent that should ideally be developed in-house to ensure competitive differentiation.
4. How can banks transform to become AI-first?
To overcome the challenges that limit organization-wide deployment of AI technologies, banks must take a holistic approach. To become AI-first, banks must invest in transforming capabilities across all four layers of the integrated capability stack (Exhibit 6): the engagement layer, the AI-powered decisioning layer, the core technology and data layer, and the operating model.
As we will explain, when these interdependent layers work in unison, they enable a bank to provide customers with distinctive omnichannel experiences, support at-scale personalization, and drive the rapid innovation cycles critical to remaining competitive in todayās world. Each layer has a unique role to playāunder-investment in a single layer creates a weak link that can cripple the entire enterprise.
The following paragraphs explore some of the changes banks will need to undertake in each layer of this capability stack.
Layer 1: Reimagining the customer engagement layer
Increasingly, customers expect their bank to be present in their end-use journeys, know their context and needs no matter where they interact with the bank, and to enable a frictionless experience. Numerous banking activities (e.g., payments, certain types of lending) are becoming invisible, as journeys often begin and end on interfaces beyond the bankās proprietary platforms. For the bank to be ubiquitous in customersā lives, solving latent and emerging needs while delivering intuitive omnichannel experiences, banks will need to reimagine how they engage with customers and undertake several key shifts.
First, banks will need to move beyond highly standardized products to create integrated propositions that target ājobs to be done.ā 8 Clayton M. Christensen, Taddy Hall, Karen Dillon and David S. Duncan, āKnow your customers ājobs to be done,ā Harvard Business Review , September 2016, hbr.org. This requires embedding personalization decisions (what to offer, when to offer, which channel to offer) in the core customer journeys and designing value propositions that go beyond the core banking product and include intelligence that automates decisions and activities on behalf of the customer. Further, banks should strive to integrate relevant non-banking products and services that, together with the core banking product, comprehensively address the customer end need. An illustration of the ājobs-to-be-doneā approach can be seen in the way fintech Tally helps customers grapple with the challenge of managing multiple credit cards. The fintechās customers can solve several pain pointsāincluding decisions about which card to pay first (tailored to the forecast of their monthly income and expenses), when to pay, and how much to pay (minimum balance versus retiring principal)āa complex set of tasks that are often not done well by customers themselves.
The second necessary shift is to embed customer journeys seamlessly in partner ecosystems and platforms, so that banks engage customers at the point of end use and in the process take advantage of partnersā data and channel platform to increase higher engagement and usage. ICICI Bank in India embedded basic banking services on WhatsApp (a popular messaging platform in India) and scaled up to one million users within three months of launch. 9 āICICI Bank crosses 1 million users on WhatsApp platform,ā Live Mint , July 7, 2020, livemint.com. In a world where consumers and businesses rely increasingly on digital ecosystems, banks should decide on the posture they would like to adopt across multiple ecosystemsāthat is, to build, orchestrate, or partnerāand adapt the capabilities of their engagement layer accordingly.
Third, banks will need to redesign overall customer experiences and specific journeys for omnichannel interaction. This involves allowing customers to move across multiple modes (e.g., web, mobile app, branch, call center, smart devices) seamlessly within a single journey and retaining and continuously updating the latest context of interaction. Leading consumer internet companies with offline-to-online business models have reshaped customer expectations on this dimension. Some banks are pushing ahead in the design of omnichannel journeys, but most will need to catch up.
Reimagining the engagement layer of the AI bank will require a clear strategy on how to engage customers through channels owned by non-bank partners. Banks will need to adopt a design-thinking lens as they build experiences within and beyond the bankās platform, engineering engagement interfaces for flexibility to enable tailoring and personalization for customers, reengineering back-end processes, and ensuring that data-capture funnels (e.g., clickstream) are granularly embedded in the bankās engagement layer. All of this aims to provide a granular understanding of journeys and enable continuous improvement. 10 Jennifer Kilian, Hugo Sarrazin, and Hyo Yeon, ā Building a design-driven culture ,ā September 2015, McKinsey.com.
Layer 2: Building the AI-powered decision-making layer
Delivering personalized messages and decisions to millions of users and thousands of employees, in (near) real time across the full spectrum of engagement channels, will require the bank to develop an at-scale AI-powered decision-making layer. Across domains within the bank, AI techniques can either fully replace or augment human judgment to produce significantly better outcomes (e.g., higher accuracy and speed), enhanced experience for customers (e.g., more personalized interaction and offerings), actionable insights for employees (e.g., which customer to contact first with next-best-action recommendations), and stronger risk management (e.g., earlier detection of likelihood of default and fraudulent activities).
To establish a robust AI-powered decision layer, banks will need to shift from attempting to develop specific use cases and point solutions to an enterprise-wide road map for deploying advanced-analytics (AA)/machine-learning (ML) models across entire business domains. As an illustration, in the domain of unsecured consumer lending alone, more than 20 decisions across the life cycle can be automated. 11 Renny Thomas, Vinayak HV, Raphael Bick, and Shwaitang Singh, ā Ten lessons for building a winning retail and small-business digital lending franchise ,ā November 2019, McKinsey.com. To enable at-scale development of decision models, banks need to make the development process repeatable and thus capable of delivering solutions effectively and on-time. In addition to strong collaboration between business teams and analytics talent, this requires robust tools for model development, efficient processes (e.g., for re-using code across projects), and diffusion of knowledge (e.g., repositories) across teams. Beyond the at-scale development of decision models across domains, the road map should also include plans to embed AI in business-as-usual process. Often underestimated, this effort requires rewiring the business processes in which these AA/AI models will be embedded; making AI decisioning āexplainableā to end-users; and a change-management plan that addresses employee mindset shifts and skills gaps. To foster continuous improvement beyond the first deployment, banks also need to establish infrastructure (e.g., data measurement) and processes (e.g., periodic reviews of performance, risk management of AI models) for feedback loops to flourish.

The executive’s AI playbook
Additionally, banks will need to augment homegrown AI models, with fast-evolving capabilities (e.g., natural-language processing, computer-vision techniques, AI agents and bots, augmented or virtual reality) in their core business processes. Many of these leading-edge capabilities have the potential to bring a paradigm shift in customer experience and/or operational efficiency. While many banks may lack both the talent and the requisite investment appetite to develop these technologies themselves, they need at minimum to be able to procure and integrate these emerging capabilities from specialist providers at rapid speed through an architecture enabled by an application programming interface (API), promote continuous experimentation with these technologies in sandbox environments to test and refine applications and evaluate potential risks, and subsequently decide which technologies to deploy at scale.
To deliver these decisions and capabilities and to engage customers across the full life cycle, from acquisition to upsell and cross-sell to retention and win-back, banks will need to establish enterprise-wide digital marketing machinery. This machinery is critical for translating decisions and insights generated in the decision-making layer into a set of coordinated interventions delivered through the bankās engagement layer. This machinery has several critical elements, which include:
- Data-ingestion pipelines that capture a range of data from multiple sources both within the bank (e.g., clickstream data from apps) and beyond (e.g., third-party partnerships with telco providers)
- Data platforms that aggregate, develop, and maintain a 360-degree view of customers and enable AA/ML models to run and execute in near real time
- Campaign platforms that track past actions and coordinate forward-looking interventions across the range of channels in the engagement layer
Layer 3: Strengthening the core technology and data infrastructure
Deploying AI capabilities across the organization requires a scalable, resilient, and adaptable set of core-technology components. A weak core-technology backbone, starved of the investments needed for modernization, can dramatically reduce the effectiveness of the decision-making and engagement layers. The core-technology-and-data layer has six key elements (Exhibit 7):
- Tech-forward strategy. Banks should have a unified technology strategy that is tightly aligned to business strategy and outlines strategic choices on which elements, skill sets, and talent the bank will keep in-house and those it will source through partnerships or vendor relationships. In addition, the tech strategy needs to articulate how each component of the target architecture will both support the bankās vision to be an AI-first institution and interact with each layer of the capability stack.
- Data management for the AI-enabled world. The bankās data management must ensure data liquidityāthat is, the ability to access, ingest, and manipulate the data that serve as the foundation for all insights and decisions generated in the decision-making layer. Data liquidity increases with the removal of functional silos and allows multiple divisions to operate off the same data, with increased coordination. The data value chain begins with seamless sourcing of data from all relevant internal systems and external platforms. This includes ingesting data into a lake, cleaning and labeling the data required for diverse use cases (e.g., regulatory reporting, business intelligence at scale, AA/ML diagnostics), segregating incoming data (from both existing and prospective customers) to be made available for immediate analysis from data to be cleaned and labeled for future analysis. Furthermore, as banks design and build their centralized data-management infrastructure, they should develop additional controls and monitoring tools to ensure data security, privacy, and regulatory complianceāfor example, timely and role-appropriate access across the organization for various use cases.
- Modern API architecture. APIs are the connective tissue enabling controlled access to services, products, and data, both within the bank and beyond. Within the bank, APIs reduce the need for silos, increase reusability of technology assets, and promote flexibility in the technology architecture. Beyond the bank, APIs accelerate the ability to partner externally, unlock new business opportunities, and enhance customer experiences. While APIs can unlock significant value, it is critical to start by defining where they are to be used and establish centralized governance to support their development and curation. 12 Renny Thomas, Vinayak HV, Raphael Bick, and Shwaitang Singh, ā Ten lessons for building a winning retail and small-business digital lending franchise ,ā November 2019, McKinsey.com.
- Intelligent infrastructure. As companies in diverse industries increase the share of workload handled on public and private cloud infrastructure, there is ample evidence that cloud-based platforms allow for the higher scalability and resilience crucial to an AI-first strategy. 13 Arul Elumalai and Roger Roberts, ā Unlocking business acceleration in a hybrid cloud world ,ā August 2019, McKinsey.com. Additionally, cloud-based infrastructure reduces costs for IT maintenance and enables self-serve models for development teams, which enable rapid innovation cycles by providing managed services (e.g., setting up new environments in minutes instead of days).
Layer 4: Transitioning to the platform operating model
The AI-first bank of the future will need a new operating model for the organization, so it can achieve the requisite agility and speed and unleash value across the other layers. While most banks are transitioning their technology platforms and assets to become more modular and flexible, working teams within the bank continue to operate in functional silos under suboptimal collaboration models and often lack alignment of goals and priorities.
The platform operating model envisions cross-functional business-and-technology teams organized as a series of platforms within the bank. Each platform team controls their own assets (e.g., technology solutions, data, infrastructure), budgets, key performance indicators, and talent. In return, the team delivers a family of products or services either to end customers of the bank or to other platforms within the bank. In the target state, the bank could end up with three archetypes of platform teams. Business platforms are customer- or partner-facing teams dedicated to achieving business outcomes in areas such as consumer lending, corporate lending, and transaction banking. Enterprise platforms deliver specialized capabilities and/or shared services to establish standardization throughout the organization in areas such as collections, payment utilities, human resources, and finance. And enabling platforms enable the enterprise and business platforms to deliver cross-cutting technical functionalities such as cybersecurity and cloud architecture.
By integrating business and technology in jointly owned platforms run by cross-functional teams, banks can break up organizational silos, increasing agility and speed and improving the alignment of goals and priorities across the enterprise.
The journey to becoming an AI-first bank entails transforming capabilities across all four layers of the capability stack. Ignoring challenges or underinvesting in any layer will ripple through all, resulting in a sub-optimal stack that is incapable of delivering enterprise goals.
A practical way to get started is to evaluate how the bankās strategic goals (e.g., growth, profitability, customer engagement, innovation) can be materially enabled by the range of AI technologiesāand dovetailing AI goals with the strategic goals of the bank. Once this alignment is in place, bank leaders should conduct a comprehensive diagnostic of the bankās starting position across the four layers, to identify areas that need key shifts, additional investments and new talent. They can then translate these insights into a transformation roadmap that spans business, technology, and analytics teams.
Equally important is the design of an execution approach that is tailored to the organization. To ensure sustainability of change, we recommend a two-track approach that balances short-term projects that deliver business value every quarter with an iterative build of long-term institutional capabilities. Furthermore, depending on their market position, size, and aspirations, banks need not build all capabilities themselves. They might elect to keep differentiating core capabilities in-house and acquire non-differentiating capabilities from technology vendors and partners, including AI specialists.
For many banks, ensuring adoption of AI technologies across the enterprise is no longer a choice, but a strategic imperative. Envisioning and building the bankās capabilities holistically across the four layers will be critical to success.
Suparna Biswas is a partner, Shwaitang Singh is an associate partner, and Renny Thomas is a senior partner, all in McKinsey’s Mumbai office. Brant Carson is a partner in the Sydney office, and Violet Chung is a partner in the Hong Kong office. The authors would like to thank Milan Mitra, Anushi Shah, Arihant Kothari, and Yihong Wu for their contributions to this article.
Explore a career with us
Related articles.
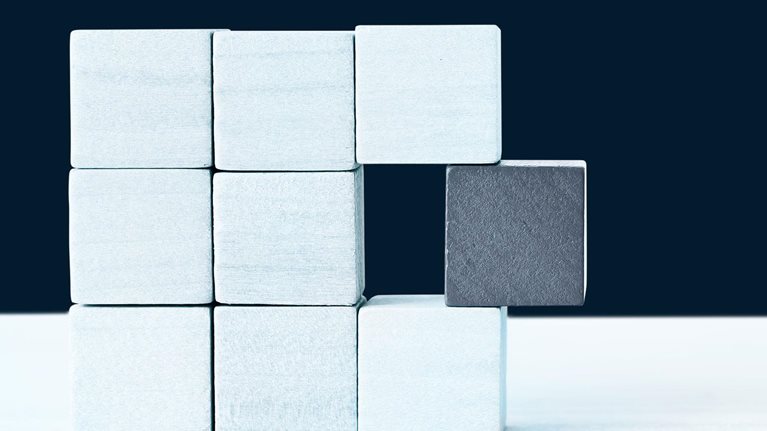
Next-gen technology transformation in financial services

Global AI Survey: AI proves its worth, but few scale impact
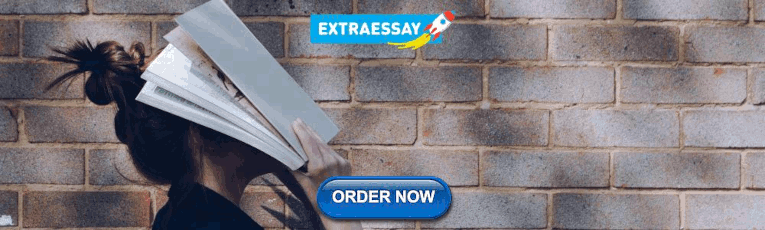
IMAGES
VIDEO
COMMENTS
Between 2008 and 2014, the Top 4 banks sharply decreased their lending to small business. This paper examines the lasting economic consequences of this contraction, finding that a credit supply shock from a subset of lenders can have surprisingly long-lived effects on real activity. 26 Jun 2017. Working Paper Summaries.
Case Study: Will a Bank's New Technology Help or Hurt Morale? ... , IT management, Burnout and Financial service sector. Leonard A. Schlesinger is the Baker ...
3. ITTI Digital Back-Office: Complete Digital Transformation šš» 1.2K applause. Case study by UXDA | Financial UX design architects. The main challenge of ITTI Digital when they started working with UXDA was to disrupt the banking industry by creating a never-before-seen core-banking solution 100% focused on the employees: a solution that would take into account all bank employees' pain ...
Partly as a result of the rise of FinTechs, banking is a sector that is facing significant disruption. In this case study, we identify some of the innovations that are being made both by young start-ups and long-established banks. We explore emerging opportunities in...
Big Data analysis is becoming a valuable tool in the banking sector, allowing banks to identify patterns, trends, and useful insights hidden in huge amounts of data. It can be used to develop personalized products and services, improve decision-making, detect fraud , and understand and predict customer behavior.
A clear strategy centered on high-priority applications. Three elements are essential to the strategy. First, banks need an analytics-ready mind-set. Analytics transforms everyday work in surprising ways, so leaders must open their minds to the possibilities. Our core beliefs about advanced analytics can help. 2.
With revenues of $2.3 trillion, corporate and commercial banking accounts for a significant share of total global banking revenues (Exhibit 1). In the United States, for instance, corporate and commercial banking revenues have advanced at double the rate of GDP growth. 1 Federal Reserve Bank of St. Louis; IHS Global Insight; McKinsey PanoramaāGlobal Banking Pools.
Design/methodology/approach. Guided by the theory of technological frames of reference (TFR) and transaction cost theory (TCT), this paper describes a real-world case study in the banking industry to explain how to help enterprises leverage big data analytics for changes. Through close integration with bank's daily operations and strategic ...
The study revealed five areas where banks can improve returns: Align analytics priorities to strategic vision. ... sharpening analytics efforts could lead to an increase in earnings of as much as $1 trillion annually for the global banking industry. The benefits would be widespread, but about one-third of the gains would come in reduced fraud ...
In the HDFC Bank case study, we have performed the SWOT Analysis, Porter's 5 Force Analysis and looked into key financials. Being the largest private bank.. ... HDFC Bank Case Study - Industry Analysis. There are 12 PSU banks, 22 Private sector banks, 1485 urban cooperative banks, 56 regional rural banks, 46 foreign banks and 96,000 rural ...
Further, the study concludes that ownership is matters in case of Indian commercial banking industry. Private sector banks are idle banks for growth of public and foreign sector banks. View full-text
Investment banking case studies are a part of the hiring process of the investment banking sector. This is the round of interview where organizations try to assess the ability of an individual to work efficiently on a particular real-life scenario.
A Case Study in the Banking Sector: An Ontology for the Selection of Agile and Lean Software Development Methodologies. Conference paper; First Online: 01 April 2021; pp 536-551; Cite this conference paper; ... Onto-logy is applied in two organizations in the banking sector, allowing recommendations to be inferred for the use of Agile ...
This study applied a bibliographic approach to date to evaluate research and available literature on big data in the banking sector. Future studies must further expand this bibliometric analysis scope by using, for example, more documentation, impact factors of the journal, and advanced citation to reveal more insights on this area of study ...
The Indian Banking and Financial Services Industry or the BFSI Sector has come a long way from the time of independence to the Nationalization phase in the 1970s and the liberalization of the economy in the 1990s to the present when it plays a prominent role in the economy of the country. This case analyses the evolution of the Indian BFSI sector along with a Strategic Analysis of the same ...
direction in which the digital banking bandwagon is heading. As per a study by e - Marketer, a US based. market r esearch firm, the number of smart phone users in India was 291.6 million by the ...
The banking sector also focuses on innovative AI technologies to improve customer services as well as overall growth by generating more revenue. ... T.D.; Pudaruth, S.; Ramdin, P. Factors influencing the adoption of internet banking: A case study of commercial banks in Mauritius. World J. Sci. Technol. Sustain. Dev. 2012, 9, 204-234. [Google ...
Listed below are the top 9 use cases of data science in banking domain: 1. Fraud Detection. Fraud detection applications may damage the bank's reputation, which is why the financial sector spends billions of dollars every year on data science in banking and financial services. Detecting fraudulent activities requires the use of data science to ...
For global banking, McKinsey estimates that AI technologies could potentially deliver up to $1 trillion of additional value each year. 2. Many banks, however, have struggled to move from experimentation around select use cases to scaling AI technologies across the organization. Reasons include the lack of a clear strategy for AI, an inflexible ...
IRJHIS2111015 | International Research Journal of Humanities and Interdisciplinary Studies (IRJHIS) | 90 enabled, but a business driver. The growth of the internet, cell phones and communication ... Recent Developments in Banking Sector: 1. Internet: The Internet is a network of computers. Data can be sent and received in any part of the world.
among the youth need to be accomplished through favorable curriculums. Further studies need to be done on this topic of savings encompassing more savings options available other than the ones mentioned in the study. More studies also need to be done to investigate youth savings on a larger group of youths beyond the banking sector.
International Journal of Computer Sciences and Engin eering Open Access. Research Paper Vol-6, Special Issue-8, Oct 2018 E-ISSN: 2347 -2693. A CASE STUDY ON DATA MINING APPLICATIONS ON BANKING ...