(Stanford users can avoid this Captcha by logging in.)
- Send to text email RefWorks EndNote printer
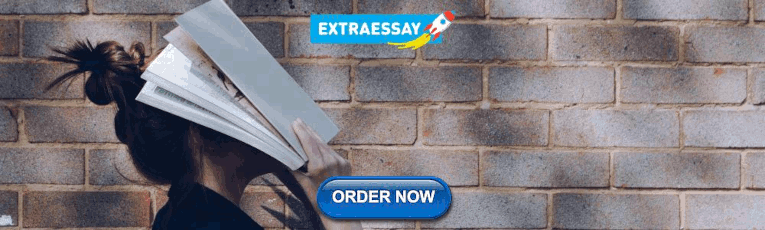
The Oxford handbook of qualitative research in American music education
Available online.
- EBSCO Academic Comprehensive Collection
More options
- Find it at other libraries via WorldCat
- Contributors
Description
Creators/contributors, contents/summary.
- 1 - Introduction. Colleen Conway
- Part One: Defining Qualitative Research in American Music Education
- 2- Qualitative Research in Music Education: Concepts, Goals, and Characteristics. Koji Matsunobu and Liora Bresler
- 3 - History of Qualitative Research American Music Education. Colleen Conway and Chad West
- 4 - Epistemology and Qualitative Research in Music Education. Randall Allsup
- 5 - Theoretical Frameworks in Music Education Qualitative Research. John Scheib
- 6 - Changing the Conversation: Considering Quality in Music Education Qualitative Research. Mitchell Robinson
- Part Two: Variety in Qualitative Approaches in Music Education
- 7 - Case Study Research in Music Education. Janet Barrett
- 8 - Ethnographic Research in Music Education. Patti Krueger
- 9 - Phenomenological Research in Music Education. Ryan Hourigan and Scott Edgar
- 10 - Narrative Inquiry in Music Education. Sandra Stauffer
- 11 - Practitioner Inquiry in Music Education. Janet Robbins
- 12 - Use of Qualitative Research Within Mixed Methods Designs. Kate Fitzpatrick
- Part Three: Qualitative Data Collection and Analysis in Music Education
- 13 - Collecting and Analyzing Observation of Music Teaching and Learning Data. Margaret Schmidt
- 14 - Conducting and Analyzing Individual Interviews. Kathryn Roulston
- 15 - Conducting and Analyzing Focus Group Interview Data. John Eros
- 16 - Collecting, Generating, and Analyzing Multimodal and Multimedia Data: From Journals to Video Recordings. Evan Tobias
- 17 - Uses of Technology in Music Education Qualitative Research. Peter Webster
- 18 - Music-Making as Data: Collection and Analysis. Kristen Pellegrino
- Part Four: Qualitative Research Within Selected Areas of Music Education
- 19 - Early Childhood Music Education. Alison Reynolds
- 20 - General Music. Ann Marie Stanley
- 21- Instrumental Music. Chad West
- 22 - Instrumental Music. Margaret Berg
- 23 - Choral Music. Bridget Sweet
- 24 - Critical Analysis of Qualitative Research on Learning to Teach Music In Preservice Music Teacher Education. Mark Campbell and Linda Thompson
- 25 - Music Teacher Professional Development. Colleen Conway and Scott Edgar
- 26 - Adult and Community Music Education. Nathan Kruse and Erin Hansen
- 27 - Qualitative Research Examining Students with Exceptionalities in Music Education. Ryan Hourigan
- 28 - Qualitative Research to Examine Underserved Populations. Bruce Carter
- 29 - World Music in the Music Education Classroom. Yiannis Miralis
- Part Five: Ethics, Publishing and the Future of Qualitative Research in Music Education
- 30 - Ethics and Qualitative Research in Music Education. Judy Birk and Cynthia Shindledecker
- 31 - The Politics of Publication: Voices, Venues and Ethics. Mitchell Robinson
- 32 - Qualitative Research Experientially and Aesthetically. Liora Bresler
- 33 - Future Possibilities for Qualitative Research in Music Education. Janet Barrett.
- (source: Nielsen Book Data)
Bibliographic information
Browse related items.

- Stanford Home
- Maps & Directions
- Search Stanford
- Emergency Info
- Terms of Use
- Non-Discrimination
- Accessibility
© Stanford University , Stanford , California 94305 .
- LOGIN & Help
Qualitative Research in Music Education: Concepts, Goals and Characteristics
- Curriculum and Instruction
- School of Music
- Office of the Provost
Research output : Chapter in Book/Report/Conference proceeding › Chapter
- ethnography
- phenomenology
- emergent research design
- senses in fieldwork
- polyphonic texts
Online availability
- 10.1093/oxfordhb/9780199844272.013.002
Library availability
Fingerprint.
- music lessons Social Sciences 100%
- qualitative research Social Sciences 68%
- trustworthiness Social Sciences 19%
- genre Social Sciences 15%
- music Social Sciences 14%
- interpretation Social Sciences 10%
T1 - Qualitative Research in Music Education
T2 - Concepts, Goals and Characteristics
AU - Bresler, Liora
AU - Matsunobu, Koji
PY - 2014/7
Y1 - 2014/7
N2 - Qualitative research is an umbrella term for a wide array of genres. Recognizing the differences in intellectual traditions, purposes, units of analysis, and foci of qualitative research, this chapter examines the shared assumptions within qualitative inquiry, its broad goals, key concepts, general characteristics, and methods. The epistemological, methodological, and ethical underpinnings of qualitative research are discussed through current research examples in music education. Central to the discussion is reflexive, recursive, responsive, and collaborative aspects of qualitative research, as well as its emergent research design and interpretive process. Related issues to be discussed are the criteria of process and product, including trustworthiness and validity of interpretation, the situated nature of the researcher, subjectivity, the role of senses, and empathetic, case-specific understanding. We also discuss how the processes and characteristics of music and sound can inform the ways we conduct and think of qualitative research.
AB - Qualitative research is an umbrella term for a wide array of genres. Recognizing the differences in intellectual traditions, purposes, units of analysis, and foci of qualitative research, this chapter examines the shared assumptions within qualitative inquiry, its broad goals, key concepts, general characteristics, and methods. The epistemological, methodological, and ethical underpinnings of qualitative research are discussed through current research examples in music education. Central to the discussion is reflexive, recursive, responsive, and collaborative aspects of qualitative research, as well as its emergent research design and interpretive process. Related issues to be discussed are the criteria of process and product, including trustworthiness and validity of interpretation, the situated nature of the researcher, subjectivity, the role of senses, and empathetic, case-specific understanding. We also discuss how the processes and characteristics of music and sound can inform the ways we conduct and think of qualitative research.
KW - case study
KW - ethnography
KW - phenomenology
KW - emergent research design
KW - empathy
KW - senses in fieldwork
KW - polyphonic texts
KW - ethics
U2 - 10.1093/oxfordhb/9780199844272.013.002
DO - 10.1093/oxfordhb/9780199844272.013.002
M3 - Chapter
SN - 9780199844272
BT - The Oxford Handbook of Qualitative Research in American Music Education
A2 - Conway, Colleen M
PB - Oxford University Press
- Search Menu
- Browse content in Arts and Humanities
- Browse content in Archaeology
- Anglo-Saxon and Medieval Archaeology
- Archaeological Methodology and Techniques
- Archaeology by Region
- Archaeology of Religion
- Archaeology of Trade and Exchange
- Biblical Archaeology
- Contemporary and Public Archaeology
- Environmental Archaeology
- Historical Archaeology
- History and Theory of Archaeology
- Industrial Archaeology
- Landscape Archaeology
- Mortuary Archaeology
- Prehistoric Archaeology
- Underwater Archaeology
- Urban Archaeology
- Zooarchaeology
- Browse content in Architecture
- Architectural Structure and Design
- History of Architecture
- Residential and Domestic Buildings
- Theory of Architecture
- Browse content in Art
- Art Subjects and Themes
- History of Art
- Industrial and Commercial Art
- Theory of Art
- Biographical Studies
- Byzantine Studies
- Browse content in Classical Studies
- Classical History
- Classical Philosophy
- Classical Mythology
- Classical Literature
- Classical Reception
- Classical Art and Architecture
- Classical Oratory and Rhetoric
- Greek and Roman Epigraphy
- Greek and Roman Law
- Greek and Roman Papyrology
- Greek and Roman Archaeology
- Late Antiquity
- Religion in the Ancient World
- Digital Humanities
- Browse content in History
- Colonialism and Imperialism
- Diplomatic History
- Environmental History
- Genealogy, Heraldry, Names, and Honours
- Genocide and Ethnic Cleansing
- Historical Geography
- History by Period
- History of Emotions
- History of Agriculture
- History of Education
- History of Gender and Sexuality
- Industrial History
- Intellectual History
- International History
- Labour History
- Legal and Constitutional History
- Local and Family History
- Maritime History
- Military History
- National Liberation and Post-Colonialism
- Oral History
- Political History
- Public History
- Regional and National History
- Revolutions and Rebellions
- Slavery and Abolition of Slavery
- Social and Cultural History
- Theory, Methods, and Historiography
- Urban History
- World History
- Browse content in Language Teaching and Learning
- Language Learning (Specific Skills)
- Language Teaching Theory and Methods
- Browse content in Linguistics
- Applied Linguistics
- Cognitive Linguistics
- Computational Linguistics
- Forensic Linguistics
- Grammar, Syntax and Morphology
- Historical and Diachronic Linguistics
- History of English
- Language Acquisition
- Language Evolution
- Language Reference
- Language Variation
- Language Families
- Lexicography
- Linguistic Anthropology
- Linguistic Theories
- Linguistic Typology
- Phonetics and Phonology
- Psycholinguistics
- Sociolinguistics
- Translation and Interpretation
- Writing Systems
- Browse content in Literature
- Bibliography
- Children's Literature Studies
- Literary Studies (Asian)
- Literary Studies (European)
- Literary Studies (Eco-criticism)
- Literary Studies (Romanticism)
- Literary Studies (American)
- Literary Studies (Modernism)
- Literary Studies - World
- Literary Studies (1500 to 1800)
- Literary Studies (19th Century)
- Literary Studies (20th Century onwards)
- Literary Studies (African American Literature)
- Literary Studies (British and Irish)
- Literary Studies (Early and Medieval)
- Literary Studies (Fiction, Novelists, and Prose Writers)
- Literary Studies (Gender Studies)
- Literary Studies (Graphic Novels)
- Literary Studies (History of the Book)
- Literary Studies (Plays and Playwrights)
- Literary Studies (Poetry and Poets)
- Literary Studies (Postcolonial Literature)
- Literary Studies (Queer Studies)
- Literary Studies (Science Fiction)
- Literary Studies (Travel Literature)
- Literary Studies (War Literature)
- Literary Studies (Women's Writing)
- Literary Theory and Cultural Studies
- Mythology and Folklore
- Shakespeare Studies and Criticism
- Browse content in Media Studies
- Browse content in Music
- Applied Music
- Dance and Music
- Ethics in Music
- Ethnomusicology
- Gender and Sexuality in Music
- Medicine and Music
- Music Cultures
- Music and Religion
- Music and Media
- Music and Culture
- Music Education and Pedagogy
- Music Theory and Analysis
- Musical Scores, Lyrics, and Libretti
- Musical Structures, Styles, and Techniques
- Musicology and Music History
- Performance Practice and Studies
- Race and Ethnicity in Music
- Sound Studies
- Browse content in Performing Arts
- Browse content in Philosophy
- Aesthetics and Philosophy of Art
- Epistemology
- Feminist Philosophy
- History of Western Philosophy
- Metaphysics
- Moral Philosophy
- Non-Western Philosophy
- Philosophy of Science
- Philosophy of Language
- Philosophy of Mind
- Philosophy of Perception
- Philosophy of Action
- Philosophy of Law
- Philosophy of Religion
- Philosophy of Mathematics and Logic
- Practical Ethics
- Social and Political Philosophy
- Browse content in Religion
- Biblical Studies
- Christianity
- East Asian Religions
- History of Religion
- Judaism and Jewish Studies
- Qumran Studies
- Religion and Education
- Religion and Health
- Religion and Politics
- Religion and Science
- Religion and Law
- Religion and Art, Literature, and Music
- Religious Studies
- Browse content in Society and Culture
- Cookery, Food, and Drink
- Cultural Studies
- Customs and Traditions
- Ethical Issues and Debates
- Hobbies, Games, Arts and Crafts
- Lifestyle, Home, and Garden
- Natural world, Country Life, and Pets
- Popular Beliefs and Controversial Knowledge
- Sports and Outdoor Recreation
- Technology and Society
- Travel and Holiday
- Visual Culture
- Browse content in Law
- Arbitration
- Browse content in Company and Commercial Law
- Commercial Law
- Company Law
- Browse content in Comparative Law
- Systems of Law
- Competition Law
- Browse content in Constitutional and Administrative Law
- Government Powers
- Judicial Review
- Local Government Law
- Military and Defence Law
- Parliamentary and Legislative Practice
- Construction Law
- Contract Law
- Browse content in Criminal Law
- Criminal Procedure
- Criminal Evidence Law
- Sentencing and Punishment
- Employment and Labour Law
- Environment and Energy Law
- Browse content in Financial Law
- Banking Law
- Insolvency Law
- History of Law
- Human Rights and Immigration
- Intellectual Property Law
- Browse content in International Law
- Private International Law and Conflict of Laws
- Public International Law
- IT and Communications Law
- Jurisprudence and Philosophy of Law
- Law and Politics
- Law and Society
- Browse content in Legal System and Practice
- Courts and Procedure
- Legal Skills and Practice
- Primary Sources of Law
- Regulation of Legal Profession
- Medical and Healthcare Law
- Browse content in Policing
- Criminal Investigation and Detection
- Police and Security Services
- Police Procedure and Law
- Police Regional Planning
- Browse content in Property Law
- Personal Property Law
- Study and Revision
- Terrorism and National Security Law
- Browse content in Trusts Law
- Wills and Probate or Succession
- Browse content in Medicine and Health
- Browse content in Allied Health Professions
- Arts Therapies
- Clinical Science
- Dietetics and Nutrition
- Occupational Therapy
- Operating Department Practice
- Physiotherapy
- Radiography
- Speech and Language Therapy
- Browse content in Anaesthetics
- General Anaesthesia
- Neuroanaesthesia
- Browse content in Clinical Medicine
- Acute Medicine
- Cardiovascular Medicine
- Clinical Genetics
- Clinical Pharmacology and Therapeutics
- Dermatology
- Endocrinology and Diabetes
- Gastroenterology
- Genito-urinary Medicine
- Geriatric Medicine
- Infectious Diseases
- Medical Toxicology
- Medical Oncology
- Pain Medicine
- Palliative Medicine
- Rehabilitation Medicine
- Respiratory Medicine and Pulmonology
- Rheumatology
- Sleep Medicine
- Sports and Exercise Medicine
- Clinical Neuroscience
- Community Medical Services
- Critical Care
- Emergency Medicine
- Forensic Medicine
- Haematology
- History of Medicine
- Browse content in Medical Dentistry
- Oral and Maxillofacial Surgery
- Paediatric Dentistry
- Restorative Dentistry and Orthodontics
- Surgical Dentistry
- Browse content in Medical Skills
- Clinical Skills
- Communication Skills
- Nursing Skills
- Surgical Skills
- Medical Ethics
- Medical Statistics and Methodology
- Browse content in Neurology
- Clinical Neurophysiology
- Neuropathology
- Nursing Studies
- Browse content in Obstetrics and Gynaecology
- Gynaecology
- Occupational Medicine
- Ophthalmology
- Otolaryngology (ENT)
- Browse content in Paediatrics
- Neonatology
- Browse content in Pathology
- Chemical Pathology
- Clinical Cytogenetics and Molecular Genetics
- Histopathology
- Medical Microbiology and Virology
- Patient Education and Information
- Browse content in Pharmacology
- Psychopharmacology
- Browse content in Popular Health
- Caring for Others
- Complementary and Alternative Medicine
- Self-help and Personal Development
- Browse content in Preclinical Medicine
- Cell Biology
- Molecular Biology and Genetics
- Reproduction, Growth and Development
- Primary Care
- Professional Development in Medicine
- Browse content in Psychiatry
- Addiction Medicine
- Child and Adolescent Psychiatry
- Forensic Psychiatry
- Learning Disabilities
- Old Age Psychiatry
- Psychotherapy
- Browse content in Public Health and Epidemiology
- Epidemiology
- Public Health
- Browse content in Radiology
- Clinical Radiology
- Interventional Radiology
- Nuclear Medicine
- Radiation Oncology
- Reproductive Medicine
- Browse content in Surgery
- Cardiothoracic Surgery
- Gastro-intestinal and Colorectal Surgery
- General Surgery
- Neurosurgery
- Paediatric Surgery
- Peri-operative Care
- Plastic and Reconstructive Surgery
- Surgical Oncology
- Transplant Surgery
- Trauma and Orthopaedic Surgery
- Vascular Surgery
- Browse content in Science and Mathematics
- Browse content in Biological Sciences
- Aquatic Biology
- Biochemistry
- Bioinformatics and Computational Biology
- Developmental Biology
- Ecology and Conservation
- Evolutionary Biology
- Genetics and Genomics
- Microbiology
- Molecular and Cell Biology
- Natural History
- Plant Sciences and Forestry
- Research Methods in Life Sciences
- Structural Biology
- Systems Biology
- Zoology and Animal Sciences
- Browse content in Chemistry
- Analytical Chemistry
- Computational Chemistry
- Crystallography
- Environmental Chemistry
- Industrial Chemistry
- Inorganic Chemistry
- Materials Chemistry
- Medicinal Chemistry
- Mineralogy and Gems
- Organic Chemistry
- Physical Chemistry
- Polymer Chemistry
- Study and Communication Skills in Chemistry
- Theoretical Chemistry
- Browse content in Computer Science
- Artificial Intelligence
- Computer Architecture and Logic Design
- Game Studies
- Human-Computer Interaction
- Mathematical Theory of Computation
- Programming Languages
- Software Engineering
- Systems Analysis and Design
- Virtual Reality
- Browse content in Computing
- Business Applications
- Computer Security
- Computer Games
- Computer Networking and Communications
- Digital Lifestyle
- Graphical and Digital Media Applications
- Operating Systems
- Browse content in Earth Sciences and Geography
- Atmospheric Sciences
- Environmental Geography
- Geology and the Lithosphere
- Maps and Map-making
- Meteorology and Climatology
- Oceanography and Hydrology
- Palaeontology
- Physical Geography and Topography
- Regional Geography
- Soil Science
- Urban Geography
- Browse content in Engineering and Technology
- Agriculture and Farming
- Biological Engineering
- Civil Engineering, Surveying, and Building
- Electronics and Communications Engineering
- Energy Technology
- Engineering (General)
- Environmental Science, Engineering, and Technology
- History of Engineering and Technology
- Mechanical Engineering and Materials
- Technology of Industrial Chemistry
- Transport Technology and Trades
- Browse content in Environmental Science
- Applied Ecology (Environmental Science)
- Conservation of the Environment (Environmental Science)
- Environmental Sustainability
- Environmentalist Thought and Ideology (Environmental Science)
- Management of Land and Natural Resources (Environmental Science)
- Natural Disasters (Environmental Science)
- Nuclear Issues (Environmental Science)
- Pollution and Threats to the Environment (Environmental Science)
- Social Impact of Environmental Issues (Environmental Science)
- History of Science and Technology
- Browse content in Materials Science
- Ceramics and Glasses
- Composite Materials
- Metals, Alloying, and Corrosion
- Nanotechnology
- Browse content in Mathematics
- Applied Mathematics
- Biomathematics and Statistics
- History of Mathematics
- Mathematical Education
- Mathematical Finance
- Mathematical Analysis
- Numerical and Computational Mathematics
- Probability and Statistics
- Pure Mathematics
- Browse content in Neuroscience
- Cognition and Behavioural Neuroscience
- Development of the Nervous System
- Disorders of the Nervous System
- History of Neuroscience
- Invertebrate Neurobiology
- Molecular and Cellular Systems
- Neuroendocrinology and Autonomic Nervous System
- Neuroscientific Techniques
- Sensory and Motor Systems
- Browse content in Physics
- Astronomy and Astrophysics
- Atomic, Molecular, and Optical Physics
- Biological and Medical Physics
- Classical Mechanics
- Computational Physics
- Condensed Matter Physics
- Electromagnetism, Optics, and Acoustics
- History of Physics
- Mathematical and Statistical Physics
- Measurement Science
- Nuclear Physics
- Particles and Fields
- Plasma Physics
- Quantum Physics
- Relativity and Gravitation
- Semiconductor and Mesoscopic Physics
- Browse content in Psychology
- Affective Sciences
- Clinical Psychology
- Cognitive Psychology
- Cognitive Neuroscience
- Criminal and Forensic Psychology
- Developmental Psychology
- Educational Psychology
- Evolutionary Psychology
- Health Psychology
- History and Systems in Psychology
- Music Psychology
- Neuropsychology
- Organizational Psychology
- Psychological Assessment and Testing
- Psychology of Human-Technology Interaction
- Psychology Professional Development and Training
- Research Methods in Psychology
- Social Psychology
- Browse content in Social Sciences
- Browse content in Anthropology
- Anthropology of Religion
- Human Evolution
- Medical Anthropology
- Physical Anthropology
- Regional Anthropology
- Social and Cultural Anthropology
- Theory and Practice of Anthropology
- Browse content in Business and Management
- Business Strategy
- Business Ethics
- Business History
- Business and Government
- Business and Technology
- Business and the Environment
- Comparative Management
- Corporate Governance
- Corporate Social Responsibility
- Entrepreneurship
- Health Management
- Human Resource Management
- Industrial and Employment Relations
- Industry Studies
- Information and Communication Technologies
- International Business
- Knowledge Management
- Management and Management Techniques
- Operations Management
- Organizational Theory and Behaviour
- Pensions and Pension Management
- Public and Nonprofit Management
- Strategic Management
- Supply Chain Management
- Browse content in Criminology and Criminal Justice
- Criminal Justice
- Criminology
- Forms of Crime
- International and Comparative Criminology
- Youth Violence and Juvenile Justice
- Development Studies
- Browse content in Economics
- Agricultural, Environmental, and Natural Resource Economics
- Asian Economics
- Behavioural Finance
- Behavioural Economics and Neuroeconomics
- Econometrics and Mathematical Economics
- Economic Systems
- Economic History
- Economic Methodology
- Economic Development and Growth
- Financial Markets
- Financial Institutions and Services
- General Economics and Teaching
- Health, Education, and Welfare
- History of Economic Thought
- International Economics
- Labour and Demographic Economics
- Law and Economics
- Macroeconomics and Monetary Economics
- Microeconomics
- Public Economics
- Urban, Rural, and Regional Economics
- Welfare Economics
- Browse content in Education
- Adult Education and Continuous Learning
- Care and Counselling of Students
- Early Childhood and Elementary Education
- Educational Equipment and Technology
- Educational Strategies and Policy
- Higher and Further Education
- Organization and Management of Education
- Philosophy and Theory of Education
- Schools Studies
- Secondary Education
- Teaching of a Specific Subject
- Teaching of Specific Groups and Special Educational Needs
- Teaching Skills and Techniques
- Browse content in Environment
- Applied Ecology (Social Science)
- Climate Change
- Conservation of the Environment (Social Science)
- Environmentalist Thought and Ideology (Social Science)
- Natural Disasters (Environment)
- Social Impact of Environmental Issues (Social Science)
- Browse content in Human Geography
- Cultural Geography
- Economic Geography
- Political Geography
- Browse content in Interdisciplinary Studies
- Communication Studies
- Museums, Libraries, and Information Sciences
- Browse content in Politics
- African Politics
- Asian Politics
- Chinese Politics
- Comparative Politics
- Conflict Politics
- Elections and Electoral Studies
- Environmental Politics
- European Union
- Foreign Policy
- Gender and Politics
- Human Rights and Politics
- Indian Politics
- International Relations
- International Organization (Politics)
- International Political Economy
- Irish Politics
- Latin American Politics
- Middle Eastern Politics
- Political Methodology
- Political Communication
- Political Philosophy
- Political Sociology
- Political Behaviour
- Political Economy
- Political Institutions
- Political Theory
- Politics and Law
- Public Administration
- Public Policy
- Quantitative Political Methodology
- Regional Political Studies
- Russian Politics
- Security Studies
- State and Local Government
- UK Politics
- US Politics
- Browse content in Regional and Area Studies
- African Studies
- Asian Studies
- East Asian Studies
- Japanese Studies
- Latin American Studies
- Middle Eastern Studies
- Native American Studies
- Scottish Studies
- Browse content in Research and Information
- Research Methods
- Browse content in Social Work
- Addictions and Substance Misuse
- Adoption and Fostering
- Care of the Elderly
- Child and Adolescent Social Work
- Couple and Family Social Work
- Developmental and Physical Disabilities Social Work
- Direct Practice and Clinical Social Work
- Emergency Services
- Human Behaviour and the Social Environment
- International and Global Issues in Social Work
- Mental and Behavioural Health
- Social Justice and Human Rights
- Social Policy and Advocacy
- Social Work and Crime and Justice
- Social Work Macro Practice
- Social Work Practice Settings
- Social Work Research and Evidence-based Practice
- Welfare and Benefit Systems
- Browse content in Sociology
- Childhood Studies
- Community Development
- Comparative and Historical Sociology
- Economic Sociology
- Gender and Sexuality
- Gerontology and Ageing
- Health, Illness, and Medicine
- Marriage and the Family
- Migration Studies
- Occupations, Professions, and Work
- Organizations
- Population and Demography
- Race and Ethnicity
- Social Theory
- Social Movements and Social Change
- Social Research and Statistics
- Social Stratification, Inequality, and Mobility
- Sociology of Religion
- Sociology of Education
- Sport and Leisure
- Urban and Rural Studies
- Browse content in Warfare and Defence
- Defence Strategy, Planning, and Research
- Land Forces and Warfare
- Military Administration
- Military Life and Institutions
- Naval Forces and Warfare
- Other Warfare and Defence Issues
- Peace Studies and Conflict Resolution
- Weapons and Equipment

- < Previous chapter
- Next chapter >

11 Considerations of Quality in Qualitative Research
- Published: February 2023
- Cite Icon Cite
- Permissions Icon Permissions
This chapter describes how excellence and quality can be demonstrated in qualitative research and provides criteria for discerning these qualities as a consumer of qualitative reports. Researchers are advised to make carefully considered choices about the terms they adopt, such as “validation,” “verification,” “credibility,” and “trustworthiness,” for describing qualitative inquiry. The chapter discusses design elements and validation strategies that researchers can use to enhance the credibility of their research, as well as ways that research participants and external reviewers can be involved in these processes. Strategic actions that researchers can take include pursuing triangulation or crystallization, presenting negative case analysis, engaging in reflexive practices, and taking steps to recognize and manage their own subjectivity. Participants can be actively engaged in validation through member reflections, by interacting with the researcher in the field over a prolonged period of time, or through more intense collaboration with researchers in various phases of the research. Additional validation strategies call for external reviewers to audit the research process and products and for readers to assess the potential transferability of research findings based on thick description provided by the researcher. We conclude the chapter with guiding questions readers can use to discern quality in the research reports they consume.
Signed in as
Institutional accounts.
- Google Scholar Indexing
- GoogleCrawler [DO NOT DELETE]
Personal account
- Sign in with email/username & password
- Get email alerts
- Save searches
- Purchase content
- Activate your purchase/trial code
- Add your ORCID iD
Institutional access
Sign in with a library card.
- Sign in with username/password
- Recommend to your librarian
- Institutional account management
- Get help with access
Access to content on Oxford Academic is often provided through institutional subscriptions and purchases. If you are a member of an institution with an active account, you may be able to access content in one of the following ways:
IP based access
Typically, access is provided across an institutional network to a range of IP addresses. This authentication occurs automatically, and it is not possible to sign out of an IP authenticated account.
Sign in through your institution
Choose this option to get remote access when outside your institution. Shibboleth/Open Athens technology is used to provide single sign-on between your institution’s website and Oxford Academic.
- Click Sign in through your institution.
- Select your institution from the list provided, which will take you to your institution's website to sign in.
- When on the institution site, please use the credentials provided by your institution. Do not use an Oxford Academic personal account.
- Following successful sign in, you will be returned to Oxford Academic.
If your institution is not listed or you cannot sign in to your institution’s website, please contact your librarian or administrator.
Enter your library card number to sign in. If you cannot sign in, please contact your librarian.
Society Members
Society member access to a journal is achieved in one of the following ways:
Sign in through society site
Many societies offer single sign-on between the society website and Oxford Academic. If you see ‘Sign in through society site’ in the sign in pane within a journal:
- Click Sign in through society site.
- When on the society site, please use the credentials provided by that society. Do not use an Oxford Academic personal account.
If you do not have a society account or have forgotten your username or password, please contact your society.
Sign in using a personal account
Some societies use Oxford Academic personal accounts to provide access to their members. See below.
A personal account can be used to get email alerts, save searches, purchase content, and activate subscriptions.
Some societies use Oxford Academic personal accounts to provide access to their members.
Viewing your signed in accounts
Click the account icon in the top right to:
- View your signed in personal account and access account management features.
- View the institutional accounts that are providing access.
Signed in but can't access content
Oxford Academic is home to a wide variety of products. The institutional subscription may not cover the content that you are trying to access. If you believe you should have access to that content, please contact your librarian.
For librarians and administrators, your personal account also provides access to institutional account management. Here you will find options to view and activate subscriptions, manage institutional settings and access options, access usage statistics, and more.
Our books are available by subscription or purchase to libraries and institutions.
- About Oxford Academic
- Publish journals with us
- University press partners
- What we publish
- New features
- Open access
- Rights and permissions
- Accessibility
- Advertising
- Media enquiries
- Oxford University Press
- Oxford Languages
- University of Oxford
Oxford University Press is a department of the University of Oxford. It furthers the University's objective of excellence in research, scholarship, and education by publishing worldwide
- Copyright © 2024 Oxford University Press
- Cookie settings
- Cookie policy
- Privacy policy
- Legal notice
This Feature Is Available To Subscribers Only
Sign In or Create an Account
This PDF is available to Subscribers Only
For full access to this pdf, sign in to an existing account, or purchase an annual subscription.
Music: Research Methods
- Popular Music
- Databases and indexes
- Call numbers
Research Methods
- Dictionaries and encyclopedias
- Composition and Creative Music Technology
- Music, culture and place
- Music history
- Music in Australia
- Teaching Music
- Drumming Traditions of West Africa
- Digital collections
- Special collections
- Academic writing
- Introduction
- Qualitative and Quantitative Methodology
- Artistic Research
- Autoethnography
- Creative Practice
- Ethnography
- Indigenous Methodologies
- Narrative Inquiry
- Practice as Research
- Oxford Handbooks
- Methodology of Music Research
Many books about research methodology introduce the reader to a range of existing methods. This page selects online resources relevant to music and interdisciplinary studies and some books only have a chapter, or a case study, that applies to music, performance or creative practice. If you are seeking a particular kind of research methodology, you can meet a librarian online , or email [email protected].
SAGE research methods online SAGE Research Methods Online (SRMO) is a research methods tool created to help researchers, faculty and students with their research projects. Researchers can browse by methods to help them design research projects, understand particular methods or identify a new method. Since SRMO focuses on methodology rather than disciplines, it is best to use music as a keyword in order to find applications to music research. You can also use the Methods Map to read definitions of key terminology, or to find narrower definitions for qualitative data analysis or research design.
Sage research methods foundations SAGE Research Methods Foundations provides a concise introduction to methods and research terms for those who are new to research in general or to that particular method. While a general search can provide an overwhelming number of results, SAGE Research Methods Foundations offers a targeted list of entries to guide users through the content. The navigation menu puts the entry in context, so users can easily find more general topics related to a method or continue on to more specific sub-topics. There is an A-Z section on the innovators of methods, with profiles that evaluate their contributions. The content ranges from Participatory and Arts-Based Research, Analysis of Material Culture, Narrative Research, Autobiography to Sampling.
The Sage Encyclopedia of Qualitative Research Methods includes the following entry: Music in Qualitative Research
The SAGE Handbook of Digital Dissertations and Theses includes the following chapter: Practice-as-Research in Music Performance
The Orpheus Institute Series aims to enhance and advance discourse in the field of artistic research in music and to generate future work. A number of titles in the series are found below. The library has access to all the titles through JSTOR .
Oxford Handbooks Online brings together the world's leading scholars to write review essays that evaluate the current thinking on a field or topic. You can search simultaneously across all handbooks by keywords. Methodologies are discussed in the following selectied chapters:
Toward a Methodology for Research into the Revival of Musical Life after War, Natural Disaster, Bans on All Music, or Neglect by Margaret Kartomi from The Oxford handbook of music revival (2014) Music-Making As Data: Collection and Analysis by Kristen Pellegrino from The Oxford Handbook of Qualitative Research in American Music Education (2014) Creating a Framework for Music Making and Leisure: Max Kaplan Leads the Way by Marie McCarthy from the Oxford handbook of music making and leisure (2017) Introduction: Situating Country Music Studies by Travis D. Stimeling from The Oxford handbook of country music studies (2017)
Methodology of Music Research is a series published by Peter Lang in Switzerland.
- Research Catalogue A non-commercial, collaboration and publishing platform for artistic research provided by the Society for Artistic Research which is free to use for artists and researchers. It aims to provide resources for teaching purposes, student assessment, peer review workflows and research funding administration.
- << Previous: Call numbers
- Next: Dictionaries and encyclopedias >>
Empirical Analysis of Gestural Sonic Objects Combining Qualitative and Quantitative Methods
- Conference paper
- Open Access
- First Online: 10 May 2024
- Cite this conference paper
You have full access to this open access conference paper
- Federico Visi 5 , 6 ,
- Rodrigo Schramm 5 ,
- Kerstin Frödin 5 ,
- Åsa Unander-Scharin 5 &
- Stefan Östersjö 5
Part of the book series: Current Research in Systematic Musicology ((CRSM,volume 12))
Included in the following conference series:
- International Seminar on Sonic Design
567 Accesses
In this chapter, we describe a series of studies related to our research on using gestural sonic objects in music analysis. These include developing a method for annotating the qualities of gestural sonic objects on multimodal recordings; ranking which features in a multimodal dataset are good predictors of basic qualities of gestural sonic objects using the Random Forests algorithm; and a supervised learning method for automated spotting designed to assist human annotators. The subject of our analyses is a performance of Fragmente 2 , a choreomusical composition based on the Japanese composer Makoto Shinohara’s solo piece for tenor recorder Fragmente (1968). To obtain the dataset, we carried out a multimodal recording of a full performance of the piece and obtained synchronised audio, video, motion, and electromyogram (EMG) data describing the body movements of the performers. We then added annotations on gestural sonic objects through dedicated qualitative analysis sessions. The task of annotating gestural sonic objects on the recordings of this performance has led to a meticulous examination of related theoretical concepts to establish a method applicable beyond this case study. This process of gestural sonic object annotation—like other qualitative approaches involving manual labelling of data—has proven to be very time-consuming. This motivated the exploration of data-driven, automated approaches to assist expert annotators.
You have full access to this open access chapter, Download conference paper PDF
- Gestural sonic object
- multimodal analysis
- machine learning
- music performance
- choreomusical composition
1 Introduction
The chapter begins with an introduction to central topics: the gestural sonic object, multimodal analysis of music performance, and machine learning in music practice and analysis. Then we describe the analysed piece and the methods adopted for data collection and analysis before reporting on the results of feature ranking for sound and gestural modalities and automated spotting of gestural sonic objects qualities. We discuss the implications of using the notion of gestural sonic objects in artistic practice and present some practical and conceptual considerations arising from our experience with annotating gestural sonic objects. Finally, we propose some interpretation of the feature ranking results and overall implications of these studies.
1.1 Gestural Sonic Objects
The sonic object is generally associated with the electroacoustic composition practice known as musique concrète, particularly with the work of Pierre Schaeffer and his collaborators (Schaeffer, 1966 ). Essentially, sonic objects are defined as fragments of musical sound approximately in the 0.5–5 s duration range that can be perceived holistically as a coherent and meaningful unit (Godøy, 2018). The concept was extended from an embodied perspective informed by motor theory by Rolf Inge Godøy ( 2006 ). From this viewpoint, sonic objects are extended with the gestural affordances of musical sound into gestural sonic objects . We consider the concept of gestural sonic object as a useful tool for research and artistic practice, as it allows for an analysis that uses perception as the starting point for explorations of sound and body movement in music. This resonates with the attitude of Schaeffer and collaborators, as described by Godøy, who notes that subjective perception of sound is the most important tool for research, while correlations between subjective perception and acoustic signals are mapped only at a later stage (Godøy, 2018, p. 762).
Godøy (2018, p. 768) also notes that the “motor theory suggests that production schemas are projected onto what we hear”, indicating that characteristics of the gesture involved in sound production may affect how the resulting sound is experienced. The idea of such resonances between gesture and produced sound is investigated further by Godøy et al. ( 2016 ). This is done in relation to the three basic dynamic envelopes of sonic objects suggested by Schaeffer: sustained (continuous transfer of energy from the body to the instrument, resulting in a more or less continuous sound), impulsive (sudden peak of effort resulting in a sudden attack in the sound followed by a decay), and iterative (rapid back and forth motion, resulting in fast ripple-like features in the sound). These categories are effectively illustrated by Godøy et al. ( 2016 ) using the graphical representation we report in Fig. 1 . Similarities between sound and motion related to these typological categories are central to the analysis we propose in this chapter.
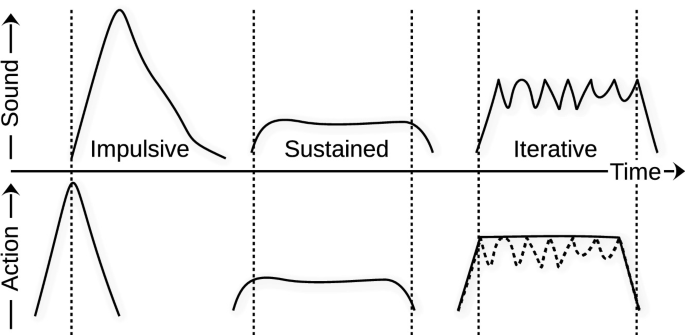
Schematic illustration of the three basic dynamic typological categories of sound (top) and the corresponding motion effort types (bottom) from Godøy et al. ( 2016 ).
In a project titled Music in Movement, Östersjö ( 2016 ) initiated a series of multimedia productions that sought to combine the practices of musical composition and choreography, building on a multimodal understanding of music perception and on an analytical approach to performance built on the concept of gestural sonic objects. This entailed researching how qualitative and quantitative data could be combined in the composition process. The outcome was a series of works comprising choreographies (performed by musicians, with and without their instruments), new music (for Vietnamese and Western instruments), installations, and video art, all drawn from analysis of gesture as seen in “Go To Hell”, a multimedia production based on Östersjö’s performance of the guitar composition Toccata Orpheus by Rolf Riehm ( 1990 ). In a PhD project carried out as a part of Music in Movement, Nguyễn ( 2019 ) further observes how “gesture in musical performance can be reflective of societal constructions of gender, but also holds the potential to create a platform for critique and the proposition of social change” (p. 42). Her artistic PhD project explored how the analysis of gestural sonic objects can provide the material for a compositional practice driven by the aim of producing artworks that also enact a performative critique of embodied practices of composition and performance. Such artistic application of a multimodal analysis of gestural sonic objects also informs the work discussed in the present chapter.
1.2 Multimodal Analysis of Music Performance
Embodied perspectives of human cognition have shifted scholarly understandings of the experience of music (Clayton & Leante, 2013 ; Leman, 2012 ) and have established the notion of music as a multimodal phenomenon, i.e., engaging multiple perceptual channels. Several other studies have employed multimodal data to study music performance with the premise that music is a multimodal phenomenon. To mention a few instances, the quantity of motion has been related to expressiveness (Thompson, 2012 ) and has been used to study the dynamic effects of the bass drum on a dancing audience (Van Dyck et al. 2013 ), while contraction/expansion of the body has been used to estimate expressivity and emotional states (Camurri et al. 2003 ).
In a previous study (Visi et al. 2020 ), we started developing a method for analysing music performance by combining qualitative and quantitative data. We used the stimulated recall technique, affording phenomenological variation through repeated listening. This allowed the listener to approach the listening situation, for instance, from a first- or third-person perspective (Ihde, 2012 ; Stefánsdóttir & Östersjö, 2022 ). The study argued that it is necessary to develop methods for combining qualitative and quantitative to fully understand expressive musical performance. The work presented in this chapter develops the observations by Visi et al. ( 2020 ) by proposing a method for qualitative annotations based on gestural sonic objects and techniques for quantitative data analysis aimed at supporting their empirical analysis of music performance. For the study presented in this chapter, we have recorded multimodal data from a full performance of Fragmente 2 , focusing on the data obtained from the flute player. Gestural sonic objects were annotated in direct collaboration with Frödin and Unander-Scharin, who were also able to provide insight into their experience as composers and performers of the piece.
1.3 Machine Learning in Music Practice and Analysis
Machine learning has been extensively used in the context of music information retrieval, music performance analysis and generative music (Miranda, 2021). Recent machine-learning approaches require large amounts of data to train robust models. This requirement, while commonly addressed in some music-related tasks such as automated music segmentation (McCallum, 2019 ), deep learning-based generative music (Engel et al. 2020 ), and automatic chord recognition (Bortolozzo et al. 2021 ), is often a challenge with multimodal analysis tasks that rely on small datasets that are only partly labelled. To circumvent the limitations caused by the need for large datasets, some interactive machine-learning techniques allow the user to interact with the machine-learning model and the feature selection algorithm to guide the system towards the expected output (McCallum, 2019 ). Alternatively, or in combination with interactive machine learning, automated feature learning can drastically reduce the need for manual feature engineering (Yosinski et al. 2014 ). In this study, we have investigated several methods for automated feature selection (or ranking) and compared prediction results to better understand the relationships between features and gestural sonic object qualities.
Currently, there are several machine-learning approaches to building models that use multimodal data as input for classification tasks (Bishop, 2006 ). However, they usually suffer from overfitting when high data dimensionality is present and only a very low number of samples is available for training. When overfitted, a model can predict samples that are identical or very similar to the ones present in the training dataset, but it fails to generalise the unseen data distribution. In other words, the model memorises the training data instead of learning to classify new data.
There are well-known strategies for avoiding overfitting by means of regularisation and pruning (Duda et al. 2001 ), and the use of an external dataset is a common approach to evaluate the overfitting of a model. When overfitting, the model accuracy over the training/test dataset will usually still increase, while accuracy decreases on the evaluation dataset (unseen data). In this study, we do not have an external dataset for validation, which imposes extra difficulty when selecting the machine learning models and respective feature sets. To mitigate overfitting issues caused by small training datasets, we have considered alternative solutions already applied in the machine learning field, such as domain adaptation (Redko et al. 2019 ), zero/few-shot learning (Fu et al. 2020 ), weak supervision (Paul et al. 2018 ), and robust feature selection (Xie et al. 2019 ).
Unfortunately, domain adaptation and techniques designed to handle weakly labelled datasets still require a considerable amount of training samples to achieve robust models. One could argue that feature engineering and machine learning models could be trained on generic gesture recognition datasets (Estévez-García et al. 2015 ; Ruffieux et al. 2014 ; Tits et al. 2018 ) and then be transferred to the gestural sonic object context. However, the nature of the Fragmente 2 multimodal data recording, which contains a particular configuration of sensors, combining synchronised audio, video, motion, and electromyogram, imposes restrictions and incompatibilities to a direct application of the aforementioned machine learning approaches.
In real-world applications, automated feature extraction methods usually generate redundant and noisy features. Moreover, further analysis of high-dimensional features is problematic as we cannot easily retain the physical meanings of these features. Dimensionality reduction and feature selection-based techniques have the power to discard redundant and noisy features, as well as highlight understandable data properties that can be easily connected to the studied phenomenon.
Given the reasons mentioned above, and to combine dimensionality reduction and feature selection, we have employed a wrapper method (Li et al. 2018 ) as our feature engineering strategy. The wrapper method uses a predefined learning algorithm (Random Forest in our case) to evaluate the quality of selected features based on the predictive performance. The strategy iterates over two steps: a) searching for a subset of features and b) evaluating the selected features. These two steps iterate until a stop criterion is satisfied. This approach worked well in this case of study, however, it is worth mentioning that wrapper methods can have an impractical search space (for d features, it is 2 d ! ) when the number of features is very large. The rationale for a methodology that combines predictive machine learning models and feature selection is that the optimisation of these models is intrinsically connected to a good feature selection.
2 Gestural Sonic Object Multimodal Analysis
2.1 the piece: fragmente 2.
Fragmente 2 is a composition by Kerstin Frödin and Åsa Unander-Scharin for a solo musician and a dancer, based on the Japanese composer Makoto Shinohara’s solo for tenor recorder Fragmente (1968). An initial artistic aim for the two artists was to explore how the musical and choreographic components could be combined in a compositional process in which neither is given less prominence than the other.
Makoto Shinohara (b. 1931) belongs to the first generation of Japanese composers who engaged with the European avant-garde movement, with a particular interest in electronic music and musique concrète. His studio work is also clearly reflected in his compositions for acoustic instruments, and this may explain how analysing musical objects in the score became a central vehicle for creating the new composition. Shinohara’s score to Fragmente is an open-form composition consisting of 14 short fragments, in which extended techniques on the recorder are a central component.
In addition to Shinohara’s 14 fragments, Fragmente 2 contains three additional movement-based fragments, carried out in (relative) silence. The title, Fragmente 2 (2021), suggests that the new composition widens the perspective from the sonic objects in the original score to choreomusical and gestural sonic perspectives. The notion of gestural sonic object was central in the artistic process, which also included analyses of gestural objects in the choreography of the two performers. In Fragmente 2 , the joint compositional work was largely carried out on an object level, counterpointing gestural and sounding materials, while seeking independence for each part. The musical score and the dancer’s choreography have a similar density of activity. Obviously, the musician’s choreography does not hold the same level of refinement as the dancer’s, it is instead worked out from other principles: firstly, what movements were possible to execute while playing, and secondly, how the compositional content could be further enhanced by adding choreographed movement to the musical performance. The creative process made the two artists more aware of the sounds that were produced by their moving bodies, and these were eventually integrated into the compositional structure.
An example of how the compositional methods were directly related to the analysis of different types of objects can be seen in Fig. 2 , which provides a display of how the artists developed what they called “object maps,” which indicate how composed objects are gesturally and temporally related in a particular fragment.
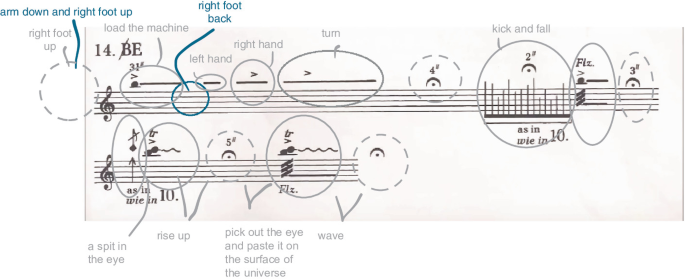
© 1974 Schott Music, London. With kind permission of Schott Music, Mainz, Germany.
Object map of Fragment 14. The musician’s gestural sonic objects are marked with grey circles (dashed circles represent silence), the gestural objects carried out by the musician are further marked in blue, whereas the gestural objects of the dancer are indicated only with grey text. The score is
As seen in object map F14 in Fig. 2 , the fragment begins with gestural objects in both parts, preceding the first sound. These first gestural objects are carried out as synchronised movement; both performers lift their right foot and put it on the left lower leg. As can be seen at the beginning of the recorder player’s part, when the first gestural sonic object is played, a gestural object follows, wherein the musician’s right foot returns to the floor. This leads straight into the next three gestural sonic objects (a repetition of the first note), each synchronised with gestural objects in the dancer’s part. In the second line of Fragment 14, the interaction is different and starts out as cause-and-effect-like relations, leading to a more contrapuntal structure in the final objects. In this particular fragment, the form is derived from an interpretation of the original score, and the choreography both reflects and enhances these structures. While the second line activates a contrapuntal relation, the choreography still follows the original phrasing of the music. It should be noted that the relation between the original score and the new composition is different across fragments and, therefore, not always as closely related to the original piece, but sometimes seeking novel possibilities in how the different objects can be related and combined.
After establishing a working method based on object analysis, the two artists observed that the compositional process could be understood as a set of phenomenological variations of first and third-person perspectives (Ihde, 2012 ) when exploring and performing the relationships between movements and sounds. Hence, methods similar to those applied in the qualitative analysis of the process also form part of the artistic methodology.
Regarding the use of object analysis, the possibility of activating objects in different spatial configurations indicated the structural impact of a particular space in the compositional process. Bodily action in a particular space often decided how sonic and gestural objects were connected, and constitutes one example of phenomenological variation in the artistic process.
2.2 Quantitative Data Collection
We recorded multimodal data throughout a full performance of Fragmente 2 . This included multichannel audio (three channels: separate clip-on condenser microphone for the flute and a stereo recording of the hall ambience) and video (two cameras placed on the left and on the right of the performance space). Full-body motion capture, EMG (finger flexors, oblique muscles, trapezius, and deltoids), and two insole pressure sensors were captured in a configuration similar to the one adopted in a previous study by some of the authors (Visi et al. 2020 ).
We focused the first data collection session on the flute player, obtaining measurements of kinematics, kinetics, and muscle activity using a mobile movement analysis system comprising wireless inertial sensors and EMG electrodes (Noraxon, United States, see Fig. 3 ). Full body kinematics were measured with a wireless MyoMotion (Noraxon, United States) system comprising 16 inertial sensors. Sensors were mounted on the head, upper arms, forearms, hands, upper thoracic (spinal process below C7), lower thoracic (spinal process above L1), sacrum, upper leg, and lower leg and feet. The sampling rate was set to 100 Hz. The ground reaction force from the feet was measured bilaterally with wireless pressure sensor insoles (Medilogic, Germany), with a sampling rate of 100 Hz. Muscle activity was measured with EMG using a Noraxon MiniDTS (Noraxon, United States) wireless eight-sensor system. Skin preparation was done according to the Surface ElectroMyoGraphy for the Non-Invasive Assessment of Muscles (SENIAM) protocol, including shaving and rubbing with chlorhexidine disinfection. Bipolar, self-adhesive Ag/AgCl dual surface electrodes with an inter-electrode distance of 20 mm (Noraxon, United States) were placed on flexor digitorum (Blackwell et al. 1999 ) anterior deltoids, oblique muscles, and upper trapezius bilaterally. The EMG sampling rate was 1,500 Hz. EMG data of the finger flexors allowed us to capture finger movements, which would be difficult to capture by means of optical or inertial sensing. This way, we obtained movement-related data describing key interactions between the musician and the instrument. All the data was synchronised and imported into ELAN (Version 6.4, 2022).
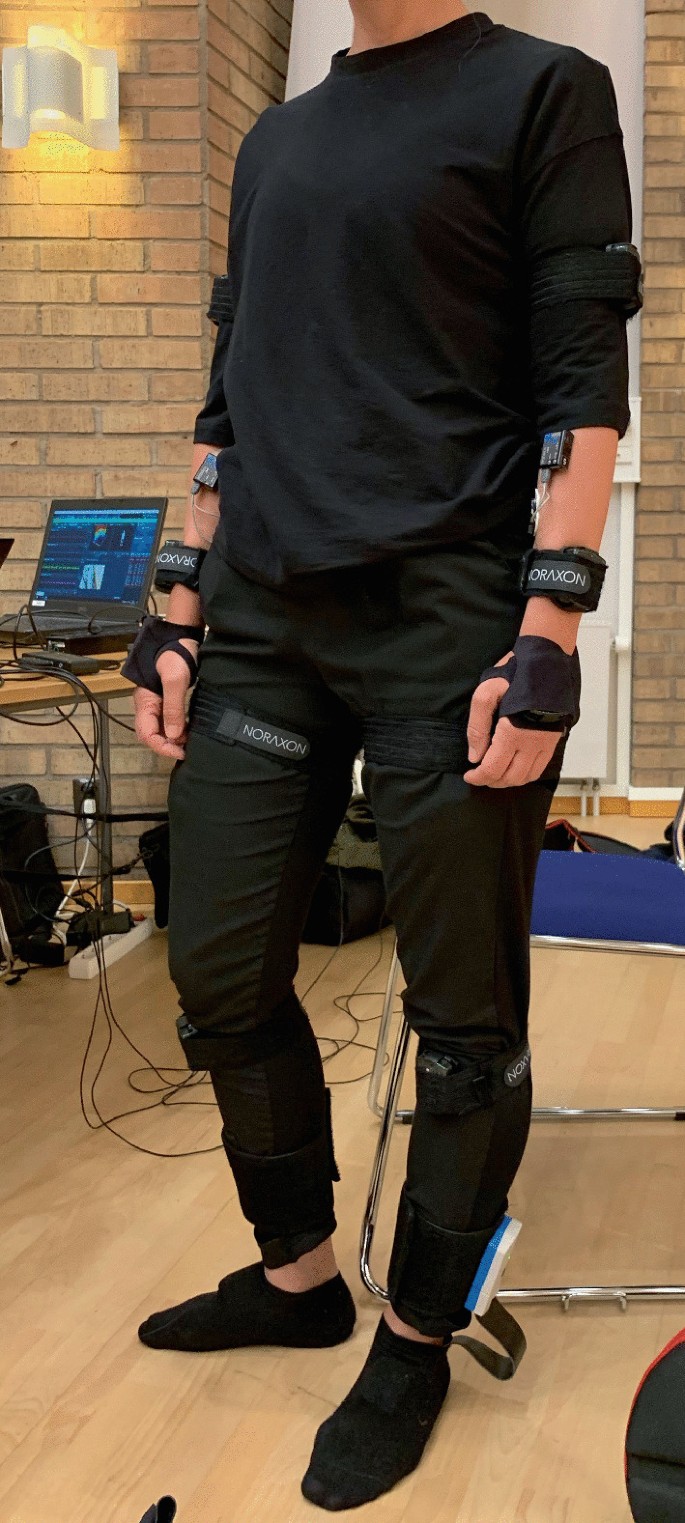
Wireless EMG and motion sensor setup.
2.3 Gestural Sonic Object Qualitative Annotation Method
Qualitative annotations related to gestural sonic object timing and basic typological categories (see Sect. 1.2 ) were added to the ELAN timeline alongside quantitative data during collaborative annotation sessions. We devised a method to annotate gestural sonic objects in an audiovisual recording of a music performance. Firstly, the performance is segmented by identifying salient events occurring in the meso timescale (approx. 0.5 – 5 s), as it is in this range that sequences of tones and movements can form a coherent object with a shape (Godøy, 2018). In this first step, segments in the meso timescale are selected and played back to determine where a gestural sonic object begins and ends. This is not a trivial task, as oftentimes, the boundaries of a gestural sonic object are not obvious. In approaching this empirical analysis task, we often referred to the fundamental characteristics of a gestural sonic object, asking ourselves:
Is the segment long enough to perceive salient basic features such as pitch and timbre as well as elements of rhythm, texture, melody, and harmony?
Can we perceive the segment as a whole, or is it too long?
Does it feel like a single object or a sequence of objects?
Can we describe a clear shape in the movement of the performer?
Can we describe the performed movement as a single action?
The gestural sonic objects identified through this procedure were then analysed for the purpose of spotting basic typological categories of the dynamic envelopes (impulsive, sustained, iterative) for two modalities. This resulted in seven tiers containing time-based annotations: one indicating the gestural sonic objects and six containing the timings of the dynamic envelopes for each category and modality, as shown in Fig. 4 .
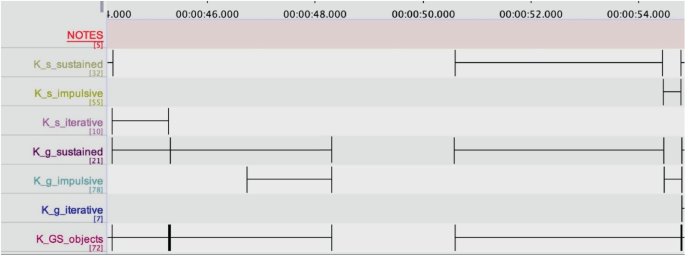
Detail of the ELAN timeline showing tiers identifying gestural sonic objects (bottom) and the basic categories of dynamic envelopes for sound and gesture modalities. The tier labelled “K_GS_objects” contains the beginning and end of gestural sonic objects. The tiers with names starting with “K_g_” contain the start and end points of the respective dynamic envelopes in the gestural domain, while the tiers with names starting with “K_s_” contain the start and end points of the respective dynamic envelope for the sound domain.
For each modality, iterative, sustained, and impulsive components are annotated, thus describing how each gestural sonic object is structured. For the flautist, the analysis focused on movements related to instrumental sound production. In case of doubt or disagreement among the annotators, we referred to the questions above to reach a consensus.
This method for manual annotation of gestural sonic objects developed and tested in the present project was built on earlier experience of stimulated recall analysis (Östersjö, 2020 ; Visi et al. 2020 ). There are important similarities between this method and phenomenological approaches to music research, such as Christensen’s ( 2012 ) method of “experimental listening,” designed as “repeated listenings, guided by deliberately varied music-focusing strategies and hermeneutical strategies, and clarified by intersubjective inquiry” (p. 46). We see our annotation method as an intersubjective inquiry through what could be conceived of as a series of phenomenological variations, making deliberate use of the specific intentionality of the audio and video technologies used in the playback situations (Ihde, 2009 ; Verbeek, 2008 ).
2.4 Feature Ranking Using the Random Forest Algorithm
With the data on the gestural sonic object categories obtained using the qualitative labelling method described above, we explored the quantitative data for the purpose of understanding relationships between the typological categories of gestural sonic objects and data describing sound and body movement. We extracted features from the quantitative data and used the Random Forest algorithm to rank the best predictors for each gestural sonic object category. Random Forest is a popular ensemble learning algorithm that combines multiple decision trees to improve the accuracy and robustness of the model by reducing overfitting and increasing generalisation. It randomly selects feature subsets and data samples to train each decision tree independently before aggregating their predictions to make a final prediction.
From the motion capture data, we extracted low-level descriptors based on kinematic features, including position and its derivatives (velocity, acceleration and jerk) and contraction index. From the pressure sensors, we measured the performer's balance between the feet. From the EMG data, we calculated the root-mean-square (RMS) in order to measure the intensity of muscular activation related to the performer’s finger movement while playing the instrument. From the audio recorded using the microphone mounted on the flute, we used RMS as a measurement of sound energy. We additionally extracted pitch, which is applied to capture the melodic envelope of gesture sound objects. These features contribute to a total of 134 continuous signals (audio and motion) sampled (or resampled to) 1000 times per second. The final dataset contains a single multimodal recording with a duration of 560 s, with 305 gestural sonic object annotations, including their respective gestural and sonic qualities. With the aim to capture different time resolutions of gestural sonic object events in the time sequence, we built the dataset by scanning the signal with sliding windows of multiple durations (10 ms, 100 ms, 500 ms, and 1000 ms), and fixed hop size (20 ms) for all windows.
We extracted statistical descriptors from each analysis window, independently of the signal source. The statistical descriptors reduced the dimensionality of raw data at the cost of losing time localisation. The statistical descriptors are: mean, variance, minimum and maximum values, skewness and kurtosis. The system uses a total of \(K \times N \times M=3216\) features, where \(K=6\) is the number of statistics, \(N\) is the 4 window sizes, and \(M=134\) is the number of input signals. Still, a high dimensional feature set and manual feature selection would not be a reasonable procedure. For this reason, we applied the wrapper method (Li et al. 2018 ), in which we randomly evaluated subset feature combinations by measuring their prediction capacity on a machine-learning model. We first reduced the original feature set dimensionality from 3216 to 50, which is computationally more manageable. To do so, we did not use Principal Component Analysis (PCA) in order to avoid losing the direct interpretation of the original data. Instead, we ranked features through the Random Forest method.
Our initial feature ranking is based on the correlation coefficient among all the variables and their respective individual variance. Thus, features that have a high correlation with several other variables are removed. The resulting feature set is further pruned such that features with very low variance are excluded from the dataset. These methods select the feature subset without any transformation that could distort the original feature interpretation. The reduced feature set is then screened by a wrapper method based on Random Forest, allowing the model to embed nonlinear relationships into a lower dimensional space, giving us a direct view of the most important features. The Random Forest prediction model is implemented with 500 trees, trained to detect gestural sonic objects and their respective qualities. To minimise overfitting, we applied cross-validation (40% for training, 40% for testing, and 20% for validation) and pruning procedure (maximum depth = 8 and maximum number of features at each split = 3 ). The random forest model is configured with Gini impurity for the splitting node procedure. The final feature ranking is based on the average feature score over 1000 random experiments.
2.5 Multimodal Spotting
Based on the feature selection procedure described in the previous section, we also analysed the spotting capabilities of the produced feature selection. Spotting is a technique used to identify specific patterns or events within a larger data set by applying algorithms or filters to the data. In the context of time series data, spotting techniques are often used to identify onsets and offsets of specific events or behaviours, which can then be used to segment the data and extract meaningful insights. In this work, we define the spotting procedure as detecting each starting (onset) and ending (offset) time point of a gestural sonic object.
Since the dataset is based on a single recording and, therefore, is quite small for generalisation, we do not expect to have high accuracy on the onset and offset detections. With this in mind, we trained a Random Forest-based classifier designed to maximise the onset/offset detection accuracy, that strongly penalises false positives. The result, even with a low detection rate of gestural sonic objects, can be used to semi-automatically aid the annotation process of new multimodal recordings. In this case, onset and offset detections can be used as cue points, and these first estimates can be manually confirmed or refined by experts.
We have performed experiments to evaluate the capabilities of minimal feature sets for gestural sonic object classification. The goal was to find a considerably small set of representative features while keeping as high as possible the gestural sonic object classification accuracy. There were two reasons for a small feature set: a) fewer features can help avoid overfitting; b) low data dimensionally is more feasible to interpret.
As mentioned in Sect. 2.4 , we guided the feature selection through an iterative process that ranks the best features while creating a Random Forest-based machine learning model. This process is known as the wrapper method (Li et al. 2018 ). Given our initial high dimensional feature set, the classifier is trained to recognise the gestural sonic object qualities as annotated in the dataset by experts. These qualities consist of the three basic dynamic envelopes for the two gestural modalities, resulting in a total of six classes. We will refer to the gestural sonic object qualities with codes shown in Table 1 .
Annotators might have overlapped some gestural sonic object quality labels during the annotation process. In order to accommodate these cases, the coding scheme also includes possible permutations generated from the initial six gestural sonic object qualities (e.g., [‘s_sus’ AND ‘g_sus’] and [ ‘s_sus’ AND ‘g_imp’]). Figure 5 shows the sample distribution regarding each class in our annotated dataset. We have a total of 17 classes, plus the null class (NC). The NC is related to all data samples that were not labelled by the annotators. This means that part of these samples might not have been correctly assigned to a specific gestural sonic object quality and were unequivocally put in the NC fold. Since the NC is predominant in the dataset, and to avoid the excessive influence of unreliable samples and unbalanced class partitions, we randomly selected and kept only 10% of the original NC data in the final dataset.
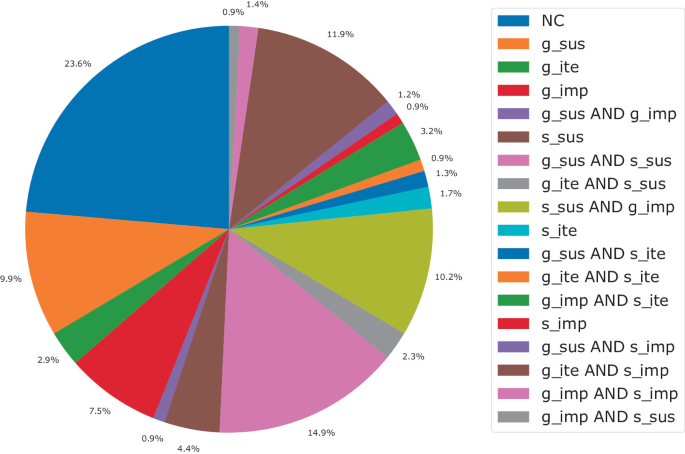
An overview of the gestural sonic object quality class distribution.
3.1 Feature Ranking Per Modality
Throughout the course of the training process, the Random Forest algorithm ranks the features based on their capability to better separate the data distributions. To minimise the inevitable influence of overfitting, we applied pruning to the classification trees. A grid search experiment was used to find the minimal tree depth while keeping accuracy above 90%. Figure 6 shows the classification accuracy versus the tree depth. We found a max depth of 8 as a good compromise, reaching approximately 90% of accuracy.
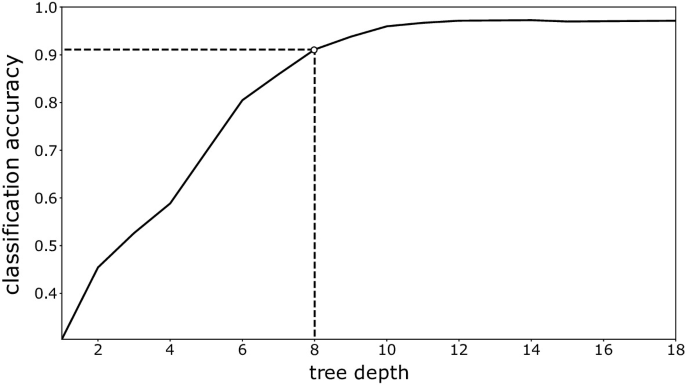
Random Forest pruning: Classifier accuracy is kept over 90% to avoid overfitting.
Once we defined the maximum tree depth, we performed feature selection experiments on the following targets: a) only gesture qualities, b) only sound qualities, and c) gesture and sound qualities. Given the extremely high data dimensionality, no brute force approach would be feasible to find the best small subset of input features. Instead of an exhaustive search, the Random Forest algorithm randomly selects features from the dataset. It is worth mentioning that we cannot guarantee that we will have the optimal final feature subset. In order to increase the chances of a good feature selection, the Random Forest is configured with 500 trees, each doing random feature selection with the configuration described in Sect. 2.4 . We ran each experiment 1000 times with distinct random seeds. This procedure helped to increase the feature variability and gave us a better cover of the feature search space. Tables 2 and 3 summarise the set of features that were mostly chosen across the 1000 experiments and were scored among the top 10 features while predicting qualities in the gesture, sound, and gesture-sound domains, respectively. In other words, we selected the top 10 features based on the top score occurrence frequency of the 3216 features from the initial feature set over all experiments.
In the second experiment, we used the top 50 features from the first experiment. A new Random Forest model was trained on this new subset, and we ranked the resultant top 10 features again. Tables 4 and 5 show the selected top 10 features based on their highest score for gesture, sound, and gesture-sound domains, respectively.
Selecting features using decision trees can be challenging due to the potential for high variance and overfitting, which can lead to suboptimal performance and reduced generalisation ability of the model. A small change in the data can have a big influence on the feature selection. However, based on the k-fold cross-validation and multiple random experiments, we found consistent features that were selected repeatedly most of the time. An additional relevant observation was the importance of the multi-scale/resolution of each feature window analysis. The feature selection process picked not only a specific characteristic of the input signal but also its distinct time resolutions.
3.2 Online Learning Investigation of Gestural Sonic Objects
A challenge has been that, due to the limited amount of data available, we faced a general sensitivity to overfitting. In order to minimise this, incremental and iterative supervision of the annotation process can be integrated with online learning models. A direct application of this kind of strategy could be extracting cue marks that indicate where gesture sonic objects are present in the timeline. Annotators could then validate and correct these cue points to improve the transcription of the recording session. Thus, in addition to the gestural sonic object quality classification task, we also investigated the spotting capabilities of our proposed multimodal feature selection method. The prediction of onsets and offsets for individual gestural sonic object qualities can potentially be used as cue points to assist an iterative and semi-supervised annotation process.
We evaluated the model’s capability to increase its accuracy in the case of using our proposed multimodal feature subset and a Random Forest classifier. Figure 7 shows the accuracy while we increase the ratio of training data versus testing data. This procedure emulates an iterative annotation approach, where annotators iteratively add more valid labels to the dataset. In our experiments, the proposed model has over 70% of classification accuracy with only 3% of the training data. When using 20% of training data, the model improvement increases accuracy to over 85%. It is worth noting that because of tree pruning, the accuracy of our model has asymptotic behaviour at approximately 90%. The asymptotic behaviour of the model's accuracy at approximately 90% suggests that even as more training data is added, the model's performance is unlikely to improve beyond this level. This could be due to the limitations of the features used to train the model or the inherent complexity of the underlying patterns in the data.
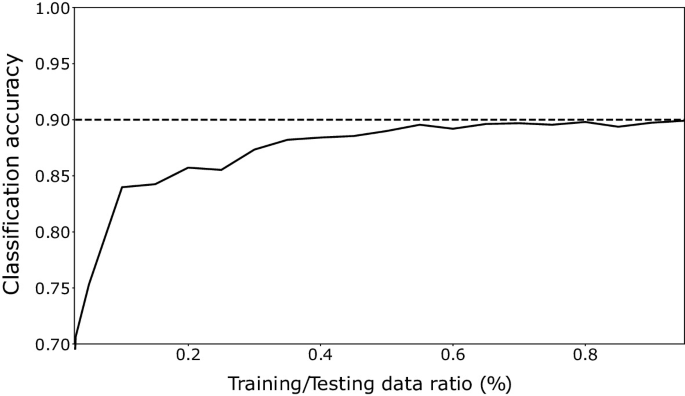
A measurement of accuracy while varying the ratio of training/testing data.
Figures 8 and 9 show the classification result of an online learning approach on an excerpt of the Fragmente 2 piece. This excerpt is 90 s long, and there are 17 gestural sonic objects in the performance section. Gestural sonic object qualities are annotated on the top tiers, and automatically spotted (predicted) onsets/offsets are indicated on the bottom track of each plot. In Fig. 8 , the classifier was trained with a dataset split of 20% for training and 80% for testing, while in Fig. 9 , the data split was 90% for training and 10% for testing. The amount of NC was randomly reduced to 10% of its original distribution. A clear improvement in classification when adding more training samples can be observed. This result paves the way for future work since it supports the assumption that adding new recordings with respective annotations would improve the performance of the gestural sonic object spotting and quality classification.

An illustration of the classification results with a training fold size of 20%. The “predicted” tier (bottom) shows the onsets and offsets automatically predicted for gestural sonic object qualities (impulsive, sustained, iterative) from top tiers K_s (sound) and K_g (gesture).

An illustration of the classification results with a training fold size of 90%. The “predicted” tier (bottom) shows the onsets and offsets automatically predicted for gestural sonic object qualities (impulsive, sustained, iterative) from top tracks K_s (sound) and K_g (gesture).
4 Discussion
The work we presented so far looked at the notion of a gestural sonic object within three different contexts: its use as a conceptual tool for choreomusical composition; the exploration of the concept, and the boundaries of its definition for the development of a method for empirical analysis of embodied music performance; and the use of the data obtained through such analyses for training classifiers capable of predicting the quality of recorded gestural sonic objects through multimodal quantitative data. In this section, we propose some considerations that arose through this interdisciplinary research trajectory that, we believe, might inform further theoretical work.
4.1 Using Gestural Sonic Objects in Artistic Practice
The fact that Shinohara’s score is composed in an object-oriented manner facilitated the artistic process. Therefore, “thinking” in objects became central to the development of the piece: first, in the interpretation of the score; second, in the continued creation of the choreography; and third, in the analysis of gestural sonic objects and gestural objects as they emerged. As noted earlier, it has been possible to enhance the musical structure but also to intentionally explore possible new relations between different object types through an interpretation of the original score built on multimodal object analysis. This object-oriented method, which entailed a close examination of each object, increased the awareness of the choreomusical relation between dancer and musician in performance. It was also instrumental in the rehearsal process since a deepened understanding of the other performer’s part emerged from the analysis. This, in turn, enabled a certain plasticity in the rendering of the individual parts in relation to the whole, as in a closely rehearsed chamber music performance. We find the relation between analysis and creation in the compositional process to be a factor that allowed a deepened interaction between the performers, the original score, and its rework. This relies on an embodied understanding of the original score enabled by a multimodal perspective facilitated by the concept of the gestural sonic object. Such an approach significantly helped to enhance the rhythmical relation between all the parts in performance.
4.2 Annotation Method and Theory: Practical and Conceptual Considerations
Implementing the gestural sonic object annotation method on the recordings of Fragmente 2 has led to some reflections regarding the method itself and the concepts it is based on. Firstly, it provided an occasion for examining the definition of gestural sonic object empirically on multimodal music performance recordings. The definition of a gestural sonic object is relatively broad. Godøy (2018, p. 761) posits that “[a] sonic object may encompass a single tone or chord, a short phrase of several tones and/or chords in succession, a single sound event […], or a more composite but still holistically perceived sound event”. In other words, a sonic object can be many different things; what is crucial is that it is perceived as a coherent entity. The broad definition and the focus on perception entail that, in practice, determining what a sonic object is and what it is not involves a fair amount of subjectivity. The open coding sessions involving multiple observers we ran to annotate Fragmente 2 made this aspect even more evident. Discussing where sonic objects begin and end in the recordings often led to going back to the literature in order to attempt to adhere to the definition as consistently as possible. We paid particular attention to the 0.5–5 s meso timescale when looking at the duration of the annotated objects, and appreciated that segments shorter than this time range effectively lose discernible timbral qualities that would allow us to identify the source of the sound or the overall musical style of the recording. Segments longer than 5 s are experienced as composite sound segments, thus losing the holisticity that characterises sound objects. We were initially doubtful about how to handle long, sustained drone sounds that were several seconds long. Can they be individual objects on their own despite their duration? We do not have a definitive answer, particularly given that we focused on a single composition. However, in the case of the long sustained notes played by the flute in Fragmente 2 , we regularly found small pitch and timbral articulations that, in a way, worked as a “seam”: the point where two long, sustained sound objects fuse. Such aspects were also sometimes found in the corresponding movements of the performer, possibly confirming segmentation and a point of coarticulation at the point where the object fuses. This leads to reflections regarding the “gestural” in gestural sonic objects. The way Godøy extends the notion of the sonic object to comprise gestural and kinematic qualities is underpinned by assumptions of the body being central in the experience of music and the existence of gestural affordances in musical sound (Godøy, 2006 , 2010 ). As exemplified above with the segmentation of long sustained sounds, observing the performer’s movements had a crucial role in forming our understanding of gestural sonic objects in Fragmente 2 . This should come as no surprise, given that gestural sonic objects are multimodal by definition. Yet it was challenging that the sound expressed certain qualities that we could not find in the movement and vice-versa. That led us to label the typological categories of the dynamic envelopes separately, which gave us sufficient flexibility to maintain the labels coherent and consistent even when the envelopes of movement and sound appeared different. The two modalities having different envelopes do not contradict the definition of the gestural sonic object and resonate with other empirical studies (Godøy et al. 2016 ). Another aspect that emerged while developing the method and annotating Fragmente 2 is that, quite frequently, one can find more than one dynamic envelope within the same object without affecting the fact that the object is perceived as a whole. This points to the possibility of gestural sonic objects having an internal dynamic structure that may affect higher-level phenomena such as phase transition, chunking, and phrasing.
In practical terms, the annotation procedure was, as expected, very time-consuming. The annotations of the recordings required more than 10 h of work involving two to four people. We expect the amount of labour required to label a similar recording to decrease significantly as the labelling method is consolidated, given that many of the open coding sessions we carried out were actually focused on developing the method itself. Yet, it is not realistic to think that many researchers and practitioners would be able to invest a similar amount of time to obtain high-quality quantitative data. This calls for tools to support the work of human annotators in ways that help accomplish repetitive tasks whilst not removing human subjectivity from the picture. The way we approached the use of quantitative multimodal data and machine learning algorithms is an attempt to work towards the development of such tools.
4.3 Interpretation of the Feature Ranking Results
The search for multimodal features that can best describe input signals is challenging. Deep learning models have proven to be robust in finding good feature representations as well as producing accurate machine learning models. However, this robustness is tied to the assumption of having access to very large datasets. Unfortunately, this is not the case in the present study. In this work, we have used a series of methods to perform feature extraction as well as feature selection. Initially, we obtained 3216 features from 134 input signals. Such dimensionality is huge compared to the small amount of data samples. Nevertheless, it could also be easily extended to hundreds of thousands of features by applying transformations and additional feature extraction on the input signals. Yet, what is the smallest interpretable feature set that could support a machine learning task to target the classification of gestural sonic objects?
Our approach utilising Random Forest appears to be effective based on the evaluation results. Although we can not ensure the optimal subset feature selection, the proposed iterative process of randomly ranking features by their scores and selection frequency has presented coherent results. The top 10 features, selected among several thousands of experiments, were able to achieve approximately 90% accuracy in the classification of 17 distinct gestural sonic object quality classes. We also kept a very shallow Random Forest model by pruning the trees to a maximum of 8 levels. This helped to diminish overfitting while keeping high accuracy.
The feature rankings in Tables 2 , 3 , 4 and 5 suggest some interpretations. In the first experiment, audio pitch and audio RMS were top-ranked in the sound domain. This supports the expectation that basic sound features such as pitch and loudness have a predominant effect on how annotators label gestural sonic object qualities in the sound domain. There are many other audio features in the time and frequency domains that could also be used (Lerch, 2012 ) and that could be explored in future studies.
In the gesture domain, in the first experiment, features of the EMG of the right finger flexor ranked at the top. In the second experiment, other EMG features, as well as insole sensors and motion features, ranked at the top. This indicates that data related to body motion are better predictors than audio features for the classification in the gesture domain. Among the top-ranked features, RT_finger_flex, M1_insoles_sum, m1_RT_ext_oblique_rms, CI_movmean and audio_pitch were the most frequent. CI_movmean (Contraction Index) also appeared many times on the top-50 rank for both sound and gesture domains, being more frequent in the gesture domain. Notably, in the gesture domain, the classifier also ranked the audio_pitch feature within the top 10. This suggests a cross-modal correlation between audio pitch and gestures that contributes to shaping gestural qualities.
Using multiple time resolutions was an important factor in the feature selection process, as we can see in Tables 4 and 5 . Most selected features were extracted through a sliding window with a hop size of 20 ms and a time duration that covers 1000 ms of the respective input signal. Larger windows were the majority in the gesture domain, while in the sound domain, distinct resolutions were selected for the audio pitch feature. Obviously, large windows can capture longer gesture and sound envelopes, while shorter windows better capture quick performance articulations and details.
5 Conclusions and Implications
We find that the empirical gesture analysis has implications in several contexts. Firstly, this study was a way for us to engage with the gestural sonic object concept in both artistic practice and music analysis, thereby showing its usefulness and, possibly, its limitations.
More broadly, we seek to explore how the combination of qualitative and quantitative analysis and phenomenological variation may enable more dynamic working methods for cross-disciplinary collaboration. We believe that developing multimodal methods for artistic research may be particularly useful in choremusical practices. We are especially interested in the potential of methods that also engage in how the intentionality of audio and video technologies can be addressed through phenomenological variation. This entails an engagement with different modalities of listening and a design that allows for embodied, multimodal and performative approaches to the experience of sound. More empirical analysis beyond the scope of this study would help refine the methods we have proposed and inform further theoretical developments in the study of gestural sonic objects.
Finally, we believe the findings on feature ranking can inform future work on feature selection and gestural sonic object analysis by supporting decisions on the type and placement of sensors for multimodal data acquisition. Using supervised learning to automate the annotation of gestural sonic objects could lead to a system to assist annotators and save them hours of labour when annotating gestural sonic objects manually. While we are aware of the implications that the use of machine-learning approaches may have—particularly with regard to the introduction of bias and other costs that data-driven practices may involve (Crawford, 2021 )—we advocate for approaches that assist rather than replace human expert annotators, thereby keeping humans in the loop while enabling new agencies and approaches.
Bishop, C.M.: Pattern Recognition and Machine Learning. Springer (2006)
Google Scholar
Blackwell, J.R., Kornatz, K.W., Heath, E.M.: Effect of grip span on maximal grip force and fatigue of flexor digitorum superficialis. Appl. Ergon. 30 (5), 401–405 (1999). https://doi.org/10.1016/S0003-6870(98)00055-6
Article Google Scholar
Bortolozzo, M., Schramm, R., Jung, C.R.: Improving the classification of rare chords with unlabeled data. In: ICASSP 2021 - 2021 IEEE International Conference on Acoustics, Speech and Signal Processing (ICASSP), pp. 3390–3394 (2021). https://doi.org/10.1109/ICASSP39728.2021.9413701
Camurri, A., Lagerlöf, I., Volpe, G.: Recognizing emotion from dance movement: comparison of spectator recognition and automated techniques. Int. J. Hum. Comput. Stud. 59 (1–2), 213–225 (2003). https://doi.org/10.1016/S1071-5819(03)00050-8
Christensen, E.: Music Listening, Music Therapy, Phenomenology and Neuroscience [PhD]. Aalborg University (2012)
Clayton, M., Leante, L.: Embodiment in music performance. In Clayton, M., Dueck, B., Leante, L. (eds.) Experience and Meaning in Music Performance, pp. 188–207. Oxford University Press (2013). https://doi.org/10.1093/acprof:oso/9780199811328.003.0009
Crawford, K.: Atlas of AI: Power, Politics, and the Planetary Costs of Artificial Intelligence. Yale University Press, New Haven (2021)
Book Google Scholar
Duda, R.O., Hart, P.E., Stork, D.G.: Pattern Classification, 2nd edn. Wiley, Hoboken (2001)
ELAN (Version 6.4). Max Planck Institute for Psycholinguistics; The Language Archive (2022). https://archive.mpi.nl/tla/elan
Engel, J., Hantrakul, L., Gu, C., Roberts, A.: DDSP: differentiable digital signal processing (2020). https://doi.org/10.48550/ARXIV.2001.04643
Estévez-García, R., et al.: Open data motion capture: MOCAP-ULL database. Procedia Comput. Sci. 75 , 316–326 (2015). https://doi.org/10.1016/j.procs.2015.12.253
Fu, Y., et al.: Vocabulary-informed zero-shot and open-set learning. IEEE Trans. Pattern Anal. Mach. Intell. 42 (12), 3136–3152 (2020). https://doi.org/10.1109/TPAMI.2019.2922175
Godøy, R.I.: Gestural-sonorous objects: embodied extensions of Schaeffer’s conceptual apparatus. Organised Sound 11 (2), 149–157 (2006). https://doi.org/10.1017/S1355771806001439
Godøy, R.I.: Gestural affordances of musical sound. In Godøy, R.I., Leman, M. (eds.) Musical Gestures: Sound, Movement, and Meaning. Routledge (2010)
Godøy, R.I.: Sonic object cognition. In: Bader, R. (ed.) Springer Handbook of Systematic Musicology. SH, pp. 761–777. Springer, Heidelberg (2018). https://doi.org/10.1007/978-3-662-55004-5_35
Chapter Google Scholar
Godøy, R.I., Song, M., Nymoen, K., Haugen, M.R., Jensenius, A.R.: Exploring sound-motion similarity in musical experience. J. New Music Res. 45 , 1–13 (2016) https://doi.org/10.1080/09298215.2016.1184689
Ihde, D.: Postphenomenology and Technoscience: The Peking University Lectures. SUNY Press, Albany (2009)
Ihde, D.: Experimental Phenomenology: Multistabilities, 2nd edn. State University of New York Press, Albany (2012)
Leman, M.: Musical gestures and embodied cognition. In: Dutoit, T., Todoroff, T., D’Alessandro, N. (eds.) Actes des Journées d’Informatique Musicale (JIM 2012) (Issue Jim, pp. 5–7). UMONS/numediart (2012). http://www.jim2012.be
Lerch, A.: Audio Content Analysis: An Introduction. Wiley, Hoboken (2012)
Li, J., et al.: Feature selection: a data perspective. ACM Comput. Surv. 50 (6), 1–45 (2018). https://doi.org/10.1145/3136625s
Article MathSciNet Google Scholar
McCallum, M.C.: Unsupervised learning of deep features for music segmentation. In: ICASSP 2019 - 2019 IEEE International Conference on Acoustics, Speech and Signal Processing (ICASSP), pp. 346–350 (2019). https://doi.org/10.1109/ICASSP.2019.8683407
Miranda, E.R. (ed.): Handbook of Artificial Intelligence for Music. Springer, Cham (2021). https://doi.org/10.1007/978-3-030-72116-9
Nguyễn, T.T.: The Choreography of Gender in Traditional Vietnamese Music [PhD]. Lund University (2019)
Östersjö, S.: Go to hell: towards a gesture-based compositional practice. Contemp. Music. Rev. 35 (4–5), 475–499 (2016). https://doi.org/10.1080/07494467.2016.1257625
Östersjö, S.: Listening To The Other. Leuven University Press, Leuven (2020)
Paul, S., Roy, S., Roy-Chowdhury, A.K.: W-TALC: weakly-supervised temporal activity localization and classification. In: Ferrari, V., Hebert, M., Sminchisescu, C., Weiss, Y. (eds.) ECCV 2018. LNCS, vol. 11208, pp. 588–607. Springer, Cham (2018). https://doi.org/10.1007/978-3-030-01225-0_35
Redko, I., Morvant, E., Habrard, A., Sebban, M., Bennani, Y.: Advances in Domain Adaptation Theory. ISTE Press Ltd, Elsevier Ltd (2019)
Riehm, R.: Toccata Orpheus. Ricordi (1990)
Ruffieux, S., Lalanne, D., Mugellini, E., Abou Khaled, O.: A survey of datasets for human gesture recognition. In: Kurosu, M. (ed.) HCI 2014. LNCS, vol. 8511, pp. 337–348. Springer, Cham (2014). https://doi.org/10.1007/978-3-319-07230-2_33
Schaeffer, P.: Traité des objets musicaux: Essai interdisciplines. Edition du Seuil (1966). http://www.seuil.com/livre-9782020026086.htm
Stefánsdóttir, H.S., Östersjö, S.: Listening and mediation: of agency and performantive responsivity in ecological sound art practices. Phenomenol. Pract. 17 (1), 115–136 (2022). https://doi.org/10.29173/pandpr29464
Thompson, M.: The Application of Motion Capture to Embodied Music Cognition Research Marc Thompson [PhD Thesis, University of Jyväskylä] (2012). http://urn.fi/URN:ISBN:978-951-39-4690-6
Tits, M., Laraba, S., Caulier, E., Tilmanne, J., Dutoit, T.: UMONS-TAICHI: a multimodal motion capture dataset of expertise in Taijiquan gestures. Data Brief 19 , 1214–1221 (2018). https://doi.org/10.1016/j.dib.2018.05.088
Van Dyck, E., Moelants, D., Demey, M., Deweppe, A., Coussement, P., Leman, M.: The impact of the bass drum on human dance movement. Music. Percept. 30 (4), 349–359 (2013). https://doi.org/10.1525/mp.2013.30.4.34
Verbeek, P.-P.: Cyborg intentionality: rethinking the phenomenology of human–technology relations. Phenomenol. Cogn. Sci. 7 (3), 387–395 (2008). https://doi.org/10.1007/s11097-008-9099-x
Visi, F.G., Östersjö, S., Ek, R., Röijezon, U.: Method development for multimodal data corpus analysis of expressive instrumental music performance. Front. Psychol. 11 , 576751 (2020). https://doi.org/10.3389/fpsyg.2020.576751
Xie, Q., Luong, M.-T., Hovy, E., Le, Q.V.: Self-training with Noisy Student improves ImageNet classification (2019). https://doi.org/10.48550/ARXIV.1911.04252
Yosinski, J., Clune, J., Bengio, Y., Lipson, H.: How transferable are features in deep neural networks? In: Z. Ghahramani, M. Welling, C. Cortes, N. Lawrence, K. Q. Weinberger (eds.), Advances in Neural Information Processing Systems (vol. 27). Curran Associates, Inc (2014). https://proceedings.neurips.cc/paper/2014/file/375c71349b295fbe2dcdca9206f20a06-Paper.pdf
Download references
Acknowledgements
We would like to thank Ulrik Röijezon for making the multimodal recordings of the Fragmente 2 performances possible.
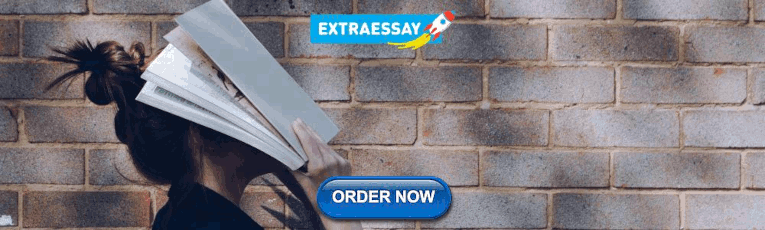
Author information
Authors and affiliations.
Luleå University of Technology, School of Music in Piteå, GEMM (Gesture Embodiment and Machines in Music), Piteå, Sweden
Federico Visi, Rodrigo Schramm, Kerstin Frödin, Åsa Unander-Scharin & Stefan Östersjö
Universität der Künste Berlin, Berlin Open Lab, Berlin, Germany
Federico Visi
You can also search for this author in PubMed Google Scholar
Corresponding author
Correspondence to Federico Visi .
Editor information
Editors and affiliations.
Department of Musicology, University of Oslo, RITMO Centre for Interdisciplinary Studies in Rhythm, Time, and Motion, Oslo, Norway
Alexander Refsum Jensenius
Rights and permissions
Open Access This chapter is licensed under the terms of the Creative Commons Attribution 4.0 International License ( http://creativecommons.org/licenses/by/4.0/ ), which permits use, sharing, adaptation, distribution and reproduction in any medium or format, as long as you give appropriate credit to the original author(s) and the source, provide a link to the Creative Commons license and indicate if changes were made.
The images or other third party material in this chapter are included in the chapter's Creative Commons license, unless indicated otherwise in a credit line to the material. If material is not included in the chapter's Creative Commons license and your intended use is not permitted by statutory regulation or exceeds the permitted use, you will need to obtain permission directly from the copyright holder.
Reprints and permissions
Copyright information
© 2024 The Author(s)
About this paper
Cite this paper.
Visi, F., Schramm, R., Frödin, K., Unander-Scharin, Å., Östersjö, S. (2024). Empirical Analysis of Gestural Sonic Objects Combining Qualitative and Quantitative Methods. In: Jensenius, A.R. (eds) Sonic Design. SSD 2022. Current Research in Systematic Musicology, vol 12. Springer, Cham. https://doi.org/10.1007/978-3-031-57892-2_7
Download citation
DOI : https://doi.org/10.1007/978-3-031-57892-2_7
Published : 10 May 2024
Publisher Name : Springer, Cham
Print ISBN : 978-3-031-57891-5
Online ISBN : 978-3-031-57892-2
eBook Packages : Engineering Engineering (R0)
Share this paper
Anyone you share the following link with will be able to read this content:
Sorry, a shareable link is not currently available for this article.
Provided by the Springer Nature SharedIt content-sharing initiative
- Publish with us
Policies and ethics
- Find a journal
- Track your research
share this!
May 15, 2024
This article has been reviewed according to Science X's editorial process and policies . Editors have highlighted the following attributes while ensuring the content's credibility:
fact-checked
peer-reviewed publication
trusted source
Singing researchers find cross-cultural patterns in music and language
by Max Planck Society
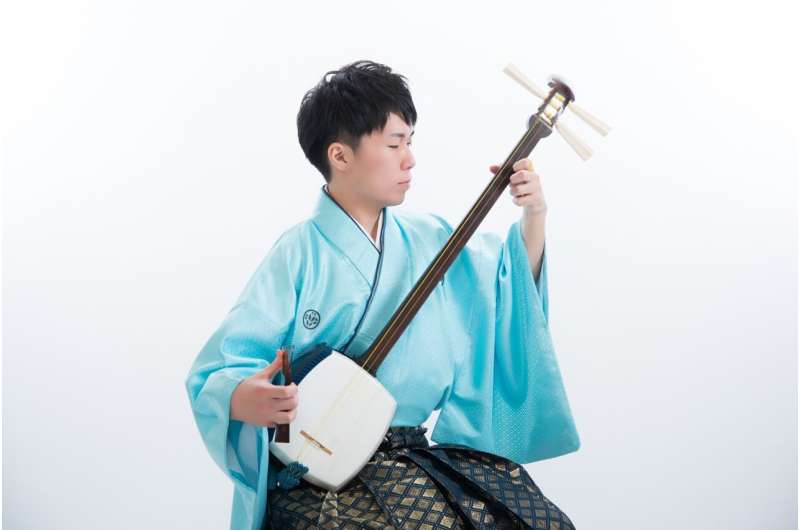
Are acoustic features of music and spoken language shared across cultures? Researchers from the Max Planck Institute for Psycholinguistics in Nijmegen have contributed to a global study of music and speech, published in Science Advances .
An international team of researchers recorded themselves performing traditional music and speaking in their native language . In all 50+ languages, the rhythms of songs and instrumental melodies were slower than those of speech , while the pitches were higher and more stable.
Language and music may share evolutionary functions. Both speech and song have features such as rhythm and pitch. But are similarities and differences between speech and song shared across cultures?
To investigate this question, 75 researchers—speaking 55 languages—were recruited across Asia, Africa, the Americas, Europe and the Pacific. Among them were experts in ethnomusicology, music psychology, linguistics, and evolutionary biology. The researchers were asked to sing, perform instrumentals, recite lyrics and verbally describe songs. The resulting audio samples were analyzed for features such as pitch, timbre and rhythm.
The study provides "strong evidence for cross-cultural regularities," according to senior author Patrick Savage of Waipapa Taumata Rau, University of Auckland, a psychologist and musicologist who sang "Scarborough Fair."
MPI's Limor Raviv, co-author on the study, recorded the Hebrew song "Yerushalayim Shel Zahav." Fellow author Andrea Ravignani from the MPI recorded the Italian song "Bella Ciao," playing the saxophone. The collection also featured the Dutch songs "Hoor de wind waait" and "Dikkertje Dap."
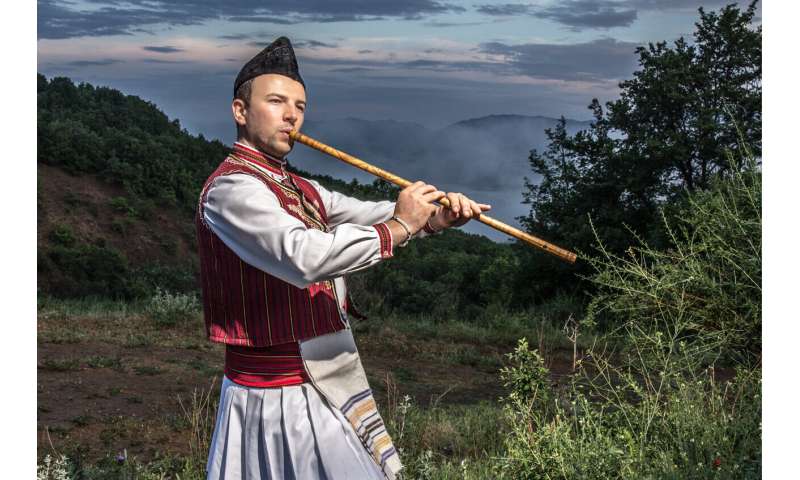
Speculating on underlying reasons for the cross-cultural similarities, Savage suggests songs are more predictably regular than speech because they are used to facilitate synchronization and social bonding.
"Slow, regular, predictable melodies make it easier for us to sing together in large groups," he says. "We're trying to shed light on the cultural and biological evolution of two systems that make us human: music and language."
Journal information: Science Advances
Provided by Max Planck Society
Explore further
Feedback to editors

Blue Origin flies thrill seekers to space after two year hiatus

Composition of gut microbiota could influence decision-making
May 18, 2024

Researchers realize multiphoton electron emission with non-classical light

Saturday Citations: Mediterranean diet racks up more points; persistent quantum coherence; vegan dogs

Physicists propose path to faster, more flexible robots

Scientists develop new geochemical 'fingerprint' to trace contaminants in fertilizer
May 17, 2024

Study reveals how a sugar-sensing protein acts as a 'machine' to switch plant growth—and oil production—on and off

Researchers develop world's smallest quantum light detector on a silicon chip

How heat waves are affecting Arctic phytoplankton

Horse remains show Pagan-Christian trade networks supplied horses from overseas for the last horse sacrifices in Europe
Relevant physicsforums posts, cover songs versus the original track, which ones are better, today's fusion music: t square, cassiopeia, rei & kanade sato, new direction....
10 hours ago
Bach, Bach, and more Bach please
14 hours ago
What are your favorite Disco "Classics"?
Who is your favorite jazz musician and what is your favorite song.
More from Art, Music, History, and Linguistics
Related Stories

Song lyrics have become simpler and more repetitive since 1980, study finds
Mar 28, 2024

New research shows how cultural transmission shapes the evolution of music
Mar 22, 2023

Analysis of Japanese and English folk songs finds cross-cultural regularities in music evolution
Feb 4, 2022

Baby talk seems consistent across cultures, from Tanzania to Beijing
Jul 19, 2022

Speaking a tonal language could boost your melodic ability, but at the cost of rhythm
Apr 26, 2023

Music plagiarism: Trial by algorithm?
Oct 10, 2023
Recommended for you

Military rank affects medical care, offering societal insights: Study
May 16, 2024

Study finds saying 'please' may not be so polite in everyday requests

Mechanistic model shows how much gossip is needed to foster social cooperation

The power of ambiguity: Using computer models to understand the debate about climate change
May 13, 2024

Study finds avoiding social media before an election has little to no effect on people's political views

Researchers develop algorithms to understand how humans form body part vocabularies
Let us know if there is a problem with our content.
Use this form if you have come across a typo, inaccuracy or would like to send an edit request for the content on this page. For general inquiries, please use our contact form . For general feedback, use the public comments section below (please adhere to guidelines ).
Please select the most appropriate category to facilitate processing of your request
Thank you for taking time to provide your feedback to the editors.
Your feedback is important to us. However, we do not guarantee individual replies due to the high volume of messages.
E-mail the story
Your email address is used only to let the recipient know who sent the email. Neither your address nor the recipient's address will be used for any other purpose. The information you enter will appear in your e-mail message and is not retained by Phys.org in any form.
Newsletter sign up
Get weekly and/or daily updates delivered to your inbox. You can unsubscribe at any time and we'll never share your details to third parties.
More information Privacy policy
Donate and enjoy an ad-free experience
We keep our content available to everyone. Consider supporting Science X's mission by getting a premium account.
E-mail newsletter
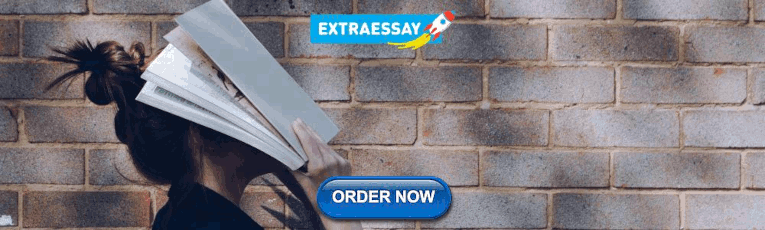
IMAGES
VIDEO
COMMENTS
The past twenty years have been a coming of age for qualitative research in music education. From a marginal, pariah methodology, qualitative research has become a legitimate, central methodology, with its own conferences, research journals, and venues. Mainstream qualitative genres such as case study and ethnography have proliferated ( Lane 2011).
Abstract. Qualitative research can contribute significantly to both applied, and to basic research, opening intellectual avenues that have not been explored in the past. In this paper, I present a unified view of five prominent qualitative methdologies ('genres'): educational criticism; formative research; and action research in the applied ...
The purpose of this article is to offer a brief overview of fundamental concepts and. terminology associated with qualitative research in music education, including philosophical. underpinnings, common methodologies, data collection and analysis methods, and important. considerations for ensuring rigor and accuracy of findings.
The Handbook of Qualitative Research in American Music Education is a resource for music education researchers, music education graduate students, and P-16 music teachers. Qualitative research has become an increasingly popular research approach in music education in the last 20 years and until now there has been no source that clarifies terms ...
This chapter reviews the basic theory and method of qualitative research in music education. Qualitative approaches come with various names and descriptions: case study, field study, ethnographic research, naturalistic, phenomenological, interpretive, symbolic interactionist, or just plain descriptive. The roots of qualitative research methods ...
In this article, I review qualitative research methodology and its origins and methods, discuss topics that have been studied by music education researchers using qualitative research methods, and show possible ways that qualitative research methods might be used to investigate topics in music education. ... My intent is to provide some points ...
While the past 20 years have seen a marked increase in the use and acceptance of qualitative inquiry within the field of music education research, there has not been a singular volume addressing an...
2. Become familiar with various methods of qualitative approaches to inquiry, including narrative, phenomenological, grounded theory, ethnographic, and case study research. 3. Design and carry out a music education research study utilizing an appropriate method of qualitative inquiry. Required Textbooks American Psychological Association. (2010).
Qualitative research is avail-. able as an important means of inquiry for identifying and finding solutions. to many problems in music education, and intelligent discussion of its apparent strengths and weaknesses and reports of its applications can. strengthen the entire research community.
The handbook outlines the history of qualitative research in music education and explores the contemporary use of qualitative approaches in examining issues related to music teaching and learning. It includes 32 chapters and is divided into five parts. Part I defines qualitative research and examines historical, philosophical and ethical issues ...
Qualitative research is an umbrella term for a wide array of genres. Recognizing the differences in intellectual traditions, purposes, units of analysis, and foci of qualitative research, this chapter examines the shared assumptions within qualitative inquiry, its broad goals, key concepts, general characteristics, and methods.
Journal of Research in Music Education, 64(1), 45-70. doi: 10.1177/0022429415627989 Begin thinking about a topic for your final paper 1/13 FMEA - no class 1/20 Qualitative research design: Examination of the five approaches • Read: Creswell Ch. 3-5; Turner, D. W. (2010). Qualitative interview design: A practical guide for novice investigators.
Abstract. This chapter discusses the widespread prevalence of case studies and the role they play in music education research. It has five goals: to address divergent definitions of case study; to represent key dimensions of case studies drawn from these definitions in a diagram; to address common criticisms and misconceptions related to tensions between theoretical and context-dependent ...
This article explores the use of music as a potential research tool, drawing on research carried out by the author. Interest in arts-based research methods has grown in recent years, as one consequence of an extended epistemology that recognizes different forms of knowledge (Reason, 1988; Reason 1994).This explicitly draws on the evocative power of the arts in enhancing representation ...
Increasing numbers of music education researchers have begun to use qualitative methods to examine research topics using interviews, observations, documents, and archival data. In this article, I review qualitative research methodology and its origins and methods, discuss topics that have been studied by music education researchers using qualitative research methods, and show possible ways ...
2. Become familiar with various methods of qualitative approaches to inquiry, including narrative, phenomenological, grounded theory, ethnographic, and case study research. 3. Design and carry out a music education research study utilizing an appropriate method of qualitative inquiry. Required Textbooks American Psychological Association. (2019).
Qualitative Research in Music Education 4(1) 63. Introduction. Leonard Bernstein was known as the academy award-nominated film composer, the concert pianist, the maestro who clashed with orchestra members, and "the ideal explainer of music, both classical and modern" (Horowitz, 1993, para. 1).
Writing on eudaemonic well-being, Ryff (2017) points to the need to understand better how the arts promote well-being across society. Indeed, there is an established body of research that links engagement with music to enhanced mental well-being (Fancourt & Finn, 2019).A recent systematic review, specifically focused on music and well-being, concluded that there is reliable evidence for ...
ing a qualitative a pproach to analyze the effects that music can have on the course of people's lives. W e begin with a discussion of evidence from music psychology research about
Bresler and Stake (1992) list seven characteristics of qualitative research: 1) holistic, 2) empirical, 3) descriptive, 4) interpretative, and 5) empathic; in addition, 6) some researchers ...
Miksza, Peter, and others, 'Considerations of Quality in Qualitative Research', Music Education Research: An Introduction (New York, 2023; online edn, ... As qualitative research became more prominent in the social sciences, early attempts at answering such questions were often couched in language consistent with quantitative and positivist ...
The Sage Encyclopedia of Qualitative Research Methods includes the following entry: Music in Qualitative Research. The SAGE Handbook of Digital Dissertations and Theses includes the following chapter: Practice-as-Research in Music Performance. Music Research: A Handbook by Laurie J. Sampsel. Call Number: 780.72 S192M 2019.
This article reports the results of a qualitative study into the breadth, content, and rationale of musical preferences. In-depth interviews were conducted with adults (age range 18-73 years) at home with their music collections, which acted as an aide memoire to their preferred styles.
In a project titled Music in Movement, Östersjö initiated a series of multimedia productions that sought to combine the practices of musical composition and choreography, building on a multimodal understanding of music perception and on an analytical approach to performance built on the concept of gestural sonic objects.This entailed researching how qualitative and quantitative data could be ...
A wide range of psychological approaches have been used to explore musical preferences, yet few studies have focused on people's own preferred music. This article reports the results of a qualitative study into the breadth, content, and rationale of musical preferences. In-depth interviews were conducted with adults (age range 18-73 years) at home with their music collections, which acted ...
New research shows how cultural transmission shapes the evolution of music Mar 22, 2023 Analysis of Japanese and English folk songs finds cross-cultural regularities in music evolution