
An official website of the United States government
The .gov means it’s official. Federal government websites often end in .gov or .mil. Before sharing sensitive information, make sure you’re on a federal government site.
The site is secure. The https:// ensures that you are connecting to the official website and that any information you provide is encrypted and transmitted securely.
- Publications
- Account settings
Preview improvements coming to the PMC website in October 2024. Learn More or Try it out now .
- Advanced Search
- Journal List
- Wiley - PMC COVID-19 Collection

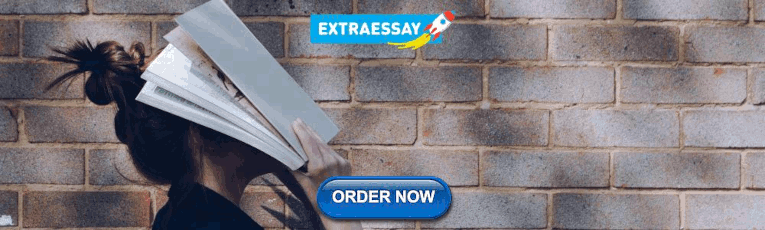
A literature review of the economics of COVID‐19
Abel brodeur.
1 Department of Economics, University of Ottawa, Ottawa Ontario, Canada
Suraiya Bhuiyan
Associated data.
Table B: COVID‐19 ‐ Timeline
Figure A: Cumulative COVID‐19 Cases and Deaths – Global Pandemic (as on 30 November 2020)
Table C: Cumulative Cases: Top 10 Countries (as of 30 November 2020)
The goal of this piece is to survey the developing and rapidly growing literature on the economic consequences of COVID‐19 and the governmental responses, and to synthetize the insights emerging from a very large number of studies. This survey: (i) provides an overview of the data sets and the techniques employed to measure social distancing and COVID‐19 cases and deaths; (ii) reviews the literature on the determinants of compliance with and the effectiveness of social distancing; (iii) mentions the macroeconomic and financial impacts including the modelling of plausible mechanisms; (iv) summarizes the literature on the socioeconomic consequences of COVID‐19, focusing on those aspects related to labor, health, gender, discrimination, and the environment; and (v) summarizes the literature on public policy responses.
1. INTRODUCTION
The World was gripped by a pandemic over the first half of 2020, of which the second wave emerged in the Fall. It was identified as a new coronavirus (severe acute respiratory syndrome coronavirus 2, or SARS‐CoV‐2), and later renamed as Coronavirus Disease‐19 or COVID‐19 (Qiu et al., 2020 ). While COVID‐19 originated in the city of Wuhan in the Hubei province of China, it has spread rapidly across the World, resulting in a human tragedy and in tremendous economic damage. By the end of November 2020, there had been close to 63 million reported cases of COVID‐19 globally and over 1.4 million deaths.
Pandemics are anything but new, and they have had severe, adverse economic impacts in the past; COVID‐19 is not expected to be any different (see the Online Appendix for a brief history of past pandemics and their socioeconomic consequences). Given the rapid spread of COVID‐19, countries across the World have adopted several public health measures intended to prevent its spread, including social distancing (Fong et al., 2020 ). According to Mandavilli ( 2020 ), this strategy saved thousands of lives, both during other pandemics, such as the Spanish flu of 1918, and more recently a flu outbreak that occurred in Mexico City in 2009. As part of social distancing measures, businesses, schools, community centers, and nongovernmental organization (NGOs) were required to close down, mass gatherings have been prohibited, and lockdown measures have been imposed in many countries, allowing travel only for essential needs. 1 The goal of these measures is to facilitate a “flattening the curve,” that is, a reduction in the number of new daily cases of COVID‐19 in order to halt their exponential growth and, hence, reduce pressure on medical services (John Hopkins University, 2020 ).
The spread of COVID‐19 has resulted in a considerable slowdown in economic activities. According to an early forecast of The World Bank ( 2020 ), global GDP in 2020 relative to 2019 is forecasted to fall by 5.2%. Similarly, the OECD ( 2020 ) forecasts a fall in global GDP by 6 to 7.6%, depending on whether or not a second wave of COVID‐19 emerges. In its latest forecast, the International Monetary Fund ( 2020 ) projected a contraction of 4.4% in light of the stronger than expected recoveries in advanced economies which lifted lockdowns during May and June of 2020. This was mainly the result of the unprecedented fiscal, monetary, and regulatory responses in these countries that helped to maintain household disposable income, protect cash flows for firms, and support credit provisions.
The economic implications will be wide ranging and uncertain, with different effects expected on labor markets, production supply chains, financial markets, and GDP levels. The negative effects may vary by the stringency of the social distancing measures (e.g., lockdowns and related restrictions), their length of implementation, and the degree of compliance with them. In addition, the pandemic and the subsequent interventions may well lead to higher levels of mental health distress, increased economic inequality, and particularly harsh effects on certain socio‐demographic groups.
The goal of this piece is to survey the emerging and already vast literature on the economic consequences of COVID‐19, and to synthesize the insights contained in a growing number of studies. Figure 1 illustrates the number of National Bureau of Economic Research (NBER) working articles that have been released related to the pandemic between March and November of 2020. 2 By the end of November 2020, there had been 247 articles related to COVID‐19. Similarly, 204 discussion articles on the pandemic were released by the IZA Institute of Labor Economics (IZA) from March to November of 2020. 3

COVID‐19 publications in 2020 in the NBER working paper series. [Color figure can be viewed at wileyonlinelibrary.com ]
Source : Authors’ compilation drawn from the NBER website
This article will focus on five broad areas: (i) the measurement of the spread of COVID‐19 and social distancing activities, (ii) the effectiveness and compliance with social distancing regulations, (iii) the economic impacts of COVID‐19 and the mechanisms giving rise to them, (iv) the socioeconomic consequences of lockdowns, and (v) the policy measures and regulations that have been implemented in response to the pandemic. One topic that we do not cover explicitly is the interface between COVID‐19 and financial markets. This omission is due partly to space constraints, but also to the fact that the outcomes in financial markets that are related to COVID‐19 are extremely volatile, and therefore, any analysis contained in our survey would be ephemeral.
The rest of the article is structured as follows. Section 2 provides an outline of the measurement of COVID‐19 spread and of social distancing actions by documenting and describing the most popular data sources. Section 3 discusses the socioeconomic determinants and the effectiveness of social distancing activities. Section 4 focuses on the economic and financial impacts including modelling of the plausible behavioral mechanisms. Section 5 reviews the literature on the socioeconomic consequences of social distancing measures, focusing on the labor‐related, health‐related, gender‐related, discriminatory, and environmental aspects. Section 6 consists of a summary of the economic impact of the policy responses. Section 7 provides the conclusion.
2. MEASUREMENT OF COVID‐19 AND SOCIAL DISTANCING ACTIONS
2.1. measurement of covid‐19 spread.
Before reviewing the potential economic impact and socioeconomic consequences, it is important to contextualize the data related to COVID‐19, without which it would not be possible to assess the scope of the pandemic. Timely and reliable data inform us of how and where the disease is spreading, what impact the pandemic has on the lives of people around the World, and to what extent the counter measures that are taken are successful (Roser et al., 2020 ).
Four key indicators are: (i) the total number of tests carried out, (ii) the number of confirmed COVID‐19 cases, (iii) the number of confirmed COVID‐19 deaths, and (iv) the number of people who have recovered from COVID‐19. These numbers are provided by different local, regional, and national health agencies/ministries across countries. However, for research and educational purposes, the data are accumulated by the Center for Systems Science and Engineering at Johns Hopkins University. 4 The database provides the figures as well as visual maps of the distribution of cases across the World. They are reported at the provincial level for China, at the city level for the United States of America, Australia, and Canada, and at the country level for all other countries (Dong et al., 2020 ). The data are corroborated with the WHO, 5 the Center for Disease Control (CDC) in the United States, and the European Center for Disease Control (ECDC).
Based on these figures, the Case Fatality Rate (CFR) is calculated as the number of confirmed deaths divided by the number of confirmed cases, which gives the mortality rate. 6 However, Roser et al. ( 2020 ) caution against taking the CFR numbers at face value to assess mortality risks, 7 because the CFR is based on the number of confirmed cases. Due to limited and sporadic testing capacities, not all COVID‐19 cases can be confirmed. Moreover, the CFR reflects the incidence of the disease in a particular context at a particular point in time. Therefore, CFRs are subject to changes over time and are sensitive to the location and population characteristics.
Recent studies indicate that there are large measurement errors associated with COVID‐19 case numbers. Using data on influenza‐like illnesses (ILI) from the CDC, Silverman et al. ( 2020 ) show that ILIs can be a useful predictor of COVID‐19 cases in the United States. The authors find that there was an escalation in the number of ILI patients during March of 2020. These cases could not be properly identified as COVID‐19 cases due to the lack of testing capabilities during the early stages of the pandemic's progression. The authors suggest that the surge in ILIs may have corresponded to 8.7 million new COVID‐19 cases between March 8 and March 28, most of which were probably not diagnosed. Based on imputation, that figure suggests that almost 80% of all actual cases in the United States during that time period were never diagnosed.
While the dataset mentioned above focuses on counts and tests, the COVID Tracking Project 8 in the United States provides additional data on patients who have been hospitalized, are in intensive care units (ICUs), and are on ventilator support for each of the 50 states. It also grades each state on data quality. Recently, it has included the COVID Racial Data Tracker , 9 which shows the race and the ethnicity of individuals affected by COVID‐19. All of these combined measures and statistics provide a more comprehensive perspective of the spread of the pandemic in the United States.
2.2. Measurement of social distancing
Compared to measuring the spread of the virus, social distancing is not easy to quantify. We determined from the literature that there are three main techniques that are employed: (i) developing and calculating measures of the mobility of the population, (ii) modelling proxies, and (iii) calculating indices. Proxies and indices are based on data related to the observed spread of infection and to the implementation of social distancing policies, respectively. On the other hand, the movements of people are based on their observed travelling patterns. Mobility measures have been used extensively in recent months to discern mobility patterns during the pandemic (Nguyen et al., 2020 ). However, mobility data providers have slight differences in their methodologies. Table 1 provides a summary of how different mobility data providers compile their data.
Social distancing— Mobility measures and how they work
Mobility data are more dynamic and are available at a daily frequency. They can also be used to measure the effect of social distancing on other variables, such as adherence to shelter‐in‐place policies or labor employment patterns (Gupta et al., 2020 ). They also offer key insights into human behavior. For example, “Safegraph” data suggest that social activity in the United States started declining substantially and rapidly well before lockdown measures were imposed (Farboodi et al., 2020 ).
Outside of the United States, a large number of studies have relied on Google LLC Community Mobility Reports. For China, mobility has been mostly measured using data from Baidu Inc. For example, Kraemer et al. ( 2020 ) document how COVID‐19 spread in China using Baidu Inc. data. They investigate travel history from Wuhan to other cities in China, finding that the spatial distribution of cases in other cities was correlated with individual peoples’ travel histories. However, after the implementation of social distancing measures in these cities, the correlation no longer held. Therefore, the authors conclude that local lockdowns rather than travel restrictions helped to mitigate the spread and transmission of COVID‐19 in cities outside Wuhan. See Coelho et al. ( 2020 ) for an examination of the spread of COVID‐19 in Brazil using daily air travel statistics from the Official Airline Guide to measure mobility.
Mobility data do have their own limitations and are not frequently used in the case of epidemics, even though they might be useful (Oliver et al., 2020 ). Mobility data are a proxy for time spent in different locations. They do not allow one to determine the situational context of the contacts that are reported, which are needed to understand the spread of COVID‐19, that is whether they occur in the workplace or in the general community (Martín‐Calvo et al., 2020 ). Those two situations involve different levels of the inherent risk of transmission. In regards to the productive activities of the individuals that are tracked, information on the context is also indeterminate. For those who are working virtually from their homes, for instance, these measures do not capture the value‐added stemming from the time that they allocate to their jobs in the labor market. It is also likely that the quality of these measures can deteriorate when overall unemployment rates and job disruptions are high (Gupta et al., 2020 ). 10 Telecom operator data are deemed to be more representative than locational data, as the former are not limited to people with smartphones, GPS locators, and histories of travel using GPS location (Lomas, 2020 ).
Social media has also been used to measure mobility patterns. Galeazzi et al. (2020) analyze the effect of lockdowns in France, Italy, and United Kingdom on national mobility patterns by exploiting geolocalized data observed from 13 million Facebook users. The authors predictably find that people transition toward localized, short‐ range mobility patterns instead of international, long‐range patterns. However, mobility patterns display heterogeneity across countries. In France and the United Kingdom, mobility is more “concentrated” around huge, central metropolises that are largely disconnected from the provinces, which helps to reduce transmission of the virus. In Italy, on the other hand, the population is more “distributed” across clusters around four major cities that remain interconnected, thus permitting persistent spread.
3. SOCIAL DISTANCING: DETERMINANTS, EFFECTIVENESS, AND COMPLIANCE
A large range of social distancing policies have been implemented, ranging from full‐scale lockdowns to voluntary self‐compliance measures. 11 For example, Sweden imposed relatively light restrictions (Juranek & Zoutman, 2020 ). Large‐scale events were prohibited, and restaurants and bars were restricted to table service only; however, private businesses were generally allowed to operate freely. The population was encouraged to stay at home if they were feeling unwell and to limit social interactions if possible (T.M. Andersen et al., 2020 bib18 ).
People tend to adopt social distancing practices when there is a specific incentive to do so in terms of risk to health and financial cost (Makris, 2020 ). Maloney and Taskin ( 2020 ) attribute voluntary, cooperative actions to either fear of infection or to a sense of social responsibility. Stringent social distancing measures tend to be implemented in countries with a greater proportion of elderly residents, a higher population density, a greater proportion of employees working in vulnerable occupations, higher degrees of democratic freedom, a higher incidence of international travel, and greater distances from the Equator (e.g., Jinjarak et al., 2020 ). Appealing to a game theoretic approach, Cui et al. ( 2020 ) argue that states sharing economic ties will be “tipped” to reach a Nash equilibrium, whereby all other states comply with shelter‐in‐place policies. 12
Social distancing policy determinants have been linked to political party characteristics, political beliefs, and partisan differences (Baccini & Brodeur, 2021 ; Barrios & Hochberg, forthcoming; Murray & Murray, 2020 ). Barrios and Hochberg (forthcoming) correlate the risk perception for contracting COVID‐19 with partisan differences. They find that, in the absence of the imposition of social distancing, counties in the United States which had higher vote shares for Donald Trump are less likely to engage in social distancing. This persists even when mandatory stay‐at‐home measures are implemented across states. Allcott et al. ( 2020 ) find a similar pattern. In addition, the authors show through surveys that Democratic and Republican supporters have different risk perceptions about contracting COVID‐19, and hence hold divergent views regarding the importance of following social distancing measures. These stylized facts make it hard to estimate the causal effect of COVID‐19 on electoral outcomes (Baccini et al., 2021 ).
Researchers are trying to determine the effectiveness of social distancing policies in reducing social interactions and ultimately infections and deaths. Abouk and Heydari ( 2021 ) show that reductions in outside‐the‐home social interactions in the United States are driven by a combination of governmental regulations and voluntary measures, with a strong causal impact for the implementation of statewide stay‐at‐home orders, but more moderate impacts for nonessential business closures and limitations placed on bars/restaurants. Ferguson et al. ( 2020 ) argue that multiple interventions are required in order to have a substantial desired impact on transmission. The optimal mitigation strategy, which is a combination of case isolations, home quarantining, and social distancing of high‐risk groups, would reduce the number of deaths by half and the demand for beds in ICUs by two‐thirds in the United Kingdom and the United States.
Some studies focus on the impact of social distancing on COVID‐19 cases, hospitalizations, etc. For example, Fang et al. ( 2020 ) argue that if lockdown policies had not been imposed in Wuhan, then the infection rates would have been 65% higher in cities outside of Wuhan. Hartl et al. ( 2020 ) show that growth rate of COVID‐19 cases in Germany dropped from 26.7 to 13.8% within 7 days after implementation of lockdowns in the country. Greenstone and Nigam ( 2020 ) project that 3 to 4 months of adherence to social distancing regulations would reduce the number of cases in the United States by 1.7 million by October of 2021, 630,000 of which would translate into averted overcrowding of ICUs in hospitals. Friedson et al. ( 2020 ) argue that early intervention in California helped to reduce significantly the numbers of COVID‐19 cases and deaths during the first 3 weeks following its enactment. Note that this set of interventions falls well short of an economic shutdown.
Similarly, Dave, Friedson, Matsuzawa, Sabia, et al. ( 2020 ) find that counties in Texas that adopted shelter‐in‐place orders earlier than the statewide shelter‐in‐place order experienced a 19 to 26% fall in the rates of COVID‐19 case growth 2 weeks after implementation of such orders. M. Andersen et al. ( 2020 ) find that temporary paid sick leave, a federal mandate enacted in the United States, which allowed private and public employees 2 weeks of paid leave, led to increased compliance with stay‐at‐home orders. On a more global scale, Hsiang et al. ( 2020 ) show that social distancing interventions prevented or delayed around 62 million confirmed cases, corresponding to the aversion of roughly 530 million total infections in China, South Korea, Italy, Iran, France, and the United States within 7 days.
Another important related issue is the determinants of compliance behavior (e.g., Coelho et al., 2020 ; Fan et al., 2020 ). The documented socioeconomic determinants of the degree of compliance with social distancing (lockdowns or safer‐at‐home orders) include, among other factors, income level, trust, and social capital, public discourse, and to some extent, news channel viewership. The degree of ethnic diversity is another documented socioeconomic determinant of social distancing (Egorov et al., 2021 ). Galasso et al. ( 2020 ) rely on survey data from eight OECD countries and provide evidence that women are more likely than men to agree with restrictive public policy measures and to comply with them. Chiou and Tucker ( 2020 ) show that Americans living in higher‐income regions with access to high‐speed internet are more likely to comply with social distancing directives. Coven and Gupta ( 2020 ) find that residents of low‐income neighborhoods in New York City comply less with shelter‐in‐place activities during non‐working hours. According to the authors, this pattern is consistent with the fact that low‐income populations are more likely to be front line, “essential” workers and are also are more likely to make frequent retail shopping visits for essentials, making for two compounded effects. People with lower income levels, less flexible work arrangements (e.g., the inability to work remotely), and a lack of accessible interior space outside of bedrooms are less likely to engage in social distancing (Papageorge et al., 2020 ). Last, Bonaccorsi et al. ( 2020 ) analyze the heterogeneous impacts of lockdowns by socioeconomic conditions of people in Italy. Using mobility data from Facebook, they provide evidence that mobility reduction is higher in municipalities which have stronger fiscal capacity and also those which have lower per‐capita income levels. The authors conclude that the pandemic has disproportionately affected poor individuals within municipalities with strong fiscal capacity in Italy.
Individual beliefs and social preferences should also be taken into consideration, as they affect behavior and compliance. Based on an experimental setup with participants in the United States and the United Kingdom, Akesson et al. ( 2020 ) conclude that individuals overestimated the infectiousness of COVID‐19 relative to expert suggestions. If they were exposed to expert opinion, individuals were prone to correct their beliefs. However, the more infectious COVID‐19 was deemed to be, the less likely they were to undertake social distancing measures. This was perhaps due to the belief that the individual will contract COVID‐19 regardless of his/her social distancing practices. Briscese et al. ( 2020 ) model the impact of “lockdown extension” on compliance using a representative sample of residents from Italy. The authors find that if a given hypothetical extension is shorter than expected (i.e., a positive surprise), the residents are more willing to engage in self‐isolation. Therefore, to ensure compliance, these authors suggest that it is imperative for the government or local authorities to work on communication and to manage peoples’ expectations. Campos‐Mercade et al. ( 2021 ) examine the relationship between social preferences and social distancing compliance. The authors find that people who exhibit prosocial behavior (in this instance individuals who claim that they do not want to expose others to risks) are more likely to follow social distancing measures and other health‐related guidelines.
Bargain and Aminjonov ( 2020 ) demonstrate that residents in European regions with high levels of trust decrease their mobility related to non‐necessary activities compared to regions with lower levels of trust. Brück et al. ( 2020 ) document a negative relationship between being in contact with sick people and trust in people and institutions. Similarly, Brodeur et al. ( 2020 ) find that counties in the United States exhibiting relatively more trust in others decrease their mobility significantly once a lockdown policy is implemented. They also provide evidence that the estimated effect on postlockdown compliance is especially large if people tend to place trust in the media, and relatively smaller if they tend to trust in science, medicine, or government.
Researchers also think about this chain of causality in reverse. Aksoy Eichengreen, and Saka ( 2020 ) find that individuals’ degrees of exposure to epidemics (especially during the ages 18 to 25) has a negative effect on their confidence in political institutions. These individuals are also less likely to have confidence in their health care systems during the times of pandemics. Barrios et al. ( 2021 ) and Durante et al. ( 2021 ) provide evidence that regions with stronger civic culture engaged in more voluntary social distancing. Aksoy, Ganslmeier, and Poutvaara ( 2020 ) find that a high level of public attention (measured through the share of Google shares containing matters related to COVID‐19) has a significant correlation with the timing of implementation of social distancing measures. This relationship is mostly applicable for countries with high quality of institutions. Last, Bartscher et al. ( 2020 ) show that higher levels of social capital (proxied through voter turnout in parliamentary elections) lead to fewer cases per capita accumulated from mid‐March to mid‐May in selected European countries and United Kingdom.
Daniele et al. ( 2020 ) investigate the effect of the COVID‐19 shock on sociopolitical attitudes as opposed to the impact of latter on the spread of the virus. Employing a randomized survey flow design for 8,000 respondents in Germany, Italy, Netherlands and Spain, the authors find that COVID‐19 has led to a deterioration in the levels of interpersonal and institutional trust. It has also lowered support for the European Union in general and for social welfare spending financed by taxes. The authors conclude that these results are driven by the “economic insecurity” rather than the “health” dimensions resulting from the crisis.
Simonov et al. ( 2020 ) analyze the causal effect of cable news viewership on social distancing compliance. The authors examine the average partial effect of Fox News viewership, a news channel that has mostly refuted expert recommendations from leaders of the United States and global public health communities on the severity of COVID‐19 and on compliance, and find that a 1 percentage point increase in Fox News viewership reduced the propensity to stay at home by 8.9 percentage points. Bursztyn et al. ( 2020 ) show that greater exposure to the Hannity show compared to the Tucker Carlson Tonight show in Fox News is associated with larger COVID‐19 case numbers and deaths. This is because the former TV host downplayed the importance of COVID‐19, while the latter provided a serious warning on the same topic during early February. The variation between the messages in the two shows led to changes in behavior in response to COVID‐19.
Table 2 provides a summary of the literature related to the determinants (i.e., factors which influence implementation of social distancing as a policy measure), compliance with social distancing (i.e., whether people are actually following social distancing measures), and their effectiveness (i.e., evidence of success in reducing COVID‐19 cases).
Determinants, compliance and effectiveness of social distancing measures: Summary of studies
4. MACROECONOMIC IMPACTS AND PLAUSIBLE MECHANISMS
4.1. plausible mechanisms for macroeconomic impact.
To understand the potential negative economic impact of COVID‐19, it is important to comprehend the economic transmission channels through which the shocks will adversely affect the economy. According to Carlsson‐Szlezak et al. ( 2020a , b ), there are three main transmission channels. The first is the direct impact, which is related to reduced consumption of goods and services. Prolonged lengths of the pandemic and the concomitant social distancing measures might reduce consumer confidence by keeping consumers at home, wary of discretionary spending, and pessimistic about their long‐term economic prospects. The second one is the indirect impact working through financial market shocks and their effects on the real economy. Household wealth will likely fall, savings will increase, and consumption spending will decrease further. The third consists of supply‐side disruptions; as restrictions halt or hamper production activities, they will negatively impact supply chains, labor demand, and employment, leading to prolonged periods of lay‐offs and rising unemployment. In particular, Baldwin ( 2020 ) discusses the expectation shock by which a “wait‐and‐see” attitude is adopted by economic agents. The author argues that this is common during economic climates characterized by uncertainties, as there is less confidence in markets and in engaging in economic transactions. Ultimately, the intensity of the shock is determined by the underlying epidemiological properties of the virus, consumer behaviour, and firm behavior in the face of adversity and uncertainty, and public policy responses. To understand the implications of the spread of the virus and the consequent social distancing measures on economic activities, a number of researchers have integrated canonical epidemiology models such as the susceptible, infected, resolved model (SIR) with macroeconomic models (see the Online Appendix for a detailed review of these models).
Gourinchas ( 2020 , p. 33) summarizes the effect on the economy by stating: “A modern economy is a complex web of interconnected parties: employees, firms, suppliers, consumers, and financial intermediaries. Everyone is someone else's employee, customer, lender, etc.” Due to the very high degrees of interconnectiveness and specialization of productive activities, a breakdown in the supply chains and the circular flows will have cascading effects. Baldwin ( 2020 ) describes the impact of COVID‐19 and subsequent social distancing measures on the macroeconomy within a circular flow framework.
It is also important to understand the processes that generate recoveries from economic crises. Carlsson‐Szlezak et al. ( 2020a ) explain different types of recovery in the aftermath of negative shocks through the concept of “shock geometry.” There are three broad scenarios of economic recoveries, which we mention in ascending order of their severity. First, there is the most optimistic one labelled “V‐shaped,” whereby aggregate output is displaced and quickly recovers to its pre‐crisis path. Second, there is the “U‐shaped” path, whereby output drops swiftly but does not return swiftly to its precrisis path. The gap between the former trajectory of output and the actual one remains large for quite some time, but recovery eventually occurs. Third, in the case of the very grim “L‐shaped” path, output drops and reaches a trough, but subsequent growth rates remain very low. The gap between the former and the new output paths continues to widen. Another scenario of economic recovery often mentioned is the “K‐shaped” one, which occurs when, following a recession, different parts of the economy recover at different rates, times, or magnitudes.
Carlsson‐Szlezak et al. ( 2020b ) state that after previous pandemics, such as the 1918 Spanish Influenza, the 1958 Asian Influenza, the 1968 Hong Kong influenza, and the 2002 SARS outbreak, economies have tended to experience “V‐shaped” recoveries. However, the pattern for the COVID‐19 economic recovery is not expected to be straightforward. This is because the effects on employment due to social distancing measures and lockdowns are expected to be much larger. According to Gourinchas ( 2020 ), during a short period, as much as 50% of the working population might not be able to find work. Moreover, even if no containment measures are implemented, a recession would occur anyway, fueled by the precautionary and/or risk‐averse behavior of households and firms faced with the uncertainty of dealing with a pandemic as well as with an inadequate public health response (Gourinchas, 2020 ).
Guerrieri et al. ( 2020 ) show that in a multisector model with certain assumptions, such as incomplete markets, low substitutability across sectors, and liquidity‐constrained consumers, COVID‐19 imparts a supply shock which works through lockdowns, layoffs, firm closures, etc. The subsequent impact would be a drop in aggregate demand and a demand‐deficient recession, that is, a “Keynesian supply shock.”
Baqaee and Farhi ( 2020 ) analyze the impact through a disaggregated Keynesian model comprised of multiple sectors, factors of production, and input‐output linkages with different features, such as nominal wage rigidities and credit constraints. They find that negative supply shocks are stagflationary, whereas negative demand shocks are deflationary. The policy implications are somewhat ambiguous. Policies that boost aggregate supply (e.g., providing subsidies to businesses, relaxing lockdowns, etc.) might not be effective in increasing demand in certain demand‐constrained sectors. Similarly, demand‐inducing policies (e.g., lower interest rates, more generous social insurance, etc.) might lead to supply shortages and inflationary pressures in certain sectors.
4.2. Quantitative macroeconomic impacts
As the pandemic unfolds, many researchers have been thinking about the economic impact from a historical perspective. Ludvigson et al. ( 2020 ) try to quantify the macroeconomic impact of costly disasters (natural and manmade) and translate them into estimates of the impact of COVID‐19. They find that in a fairly conservative scenario, pandemics, such as COVID‐19, are tantamount to large, multiple‐period exogenous shocks. Using a “costly disaster” index, the authors find that COVID‐19 is constituted of multi‐period shocks in the United States, which leads to a 12.75% drop in industrial production, a 17% loss in service employment, sustained and drastic reductions in air travel, and macroeconomic uncertainties which linger for up to 5 months. Jordà et al. ( 2020 ) analyze the rate of return on the real natural interest rate (the level of real returns on safe assets resulting from the demand and supply of investment capital in a noninflationary environment) from the 14 th century to 2018. Theoretically, a pandemic is supposed to induce a downward negative shock to the real natural interest rate. This is because investment demand decreases due to excess capital per labor unit (i.e., a scarcity of labor being utilized), while savings flows increase due to either precautionary reasons or to replace lost wealth. The authors find that the natural rate of interest may be about 2 percentage points lower than it would otherwise have been some 20 years after the pandemic, and only return to counterfactual levels after 40 years.
Analysis based on historical data, however, might not be relevant in this case. According to Baker et al. ( 2020 ), COVID‐19 has led to massive spikes in uncertainty, and there are no close historical parallels. Because of the speed of evolution and timely requirements of data, the authors suggest that one should utilize forward‐looking uncertainty measures to ascertain its impact on the economy. They formulate the uncertainty measure from the Standard & Poor's 500 Volatility Index (VIX) and the news‐based economic policy uncertainty (EPU) index developed by Baker et al. ( 2016 ). Using a real business cycle model, the authors find that a COVID‐19 shock leads to a year‐over‐year contraction of GDP by 11% in fourth quarter of 2020. According to the authors, more than half of the contraction is caused by COVID‐19‐induced uncertainty. Based on a similar approach, Altig et al. ( 2020 ) conduct an analysis of different forward‐looking uncertainty measures during the pandemic. Coibion et al. ( 2020a ) use surveys to assess the macroeconomic expectations of households in the United States. They find that it is primarily lockdowns, rather than the infections themselves, that lead to declines in consumption spending and employment, lower inflationary expectations, increased uncertainty, and lower mortgage payments being made.
Eichenbaum et al. ( 2020 ) model the interactions between economic decisions and the spread of the virus. They find that, without any mitigation measures, aggregate consumption falls by 9.3% over a 32‐week period. On the other hand, labor supply or hours worked follow a U‐shaped pattern, with a peak decline of 8.25% in the 32nd week from the start of the pandemic. These reductions decrease peak infection rates and death tolls from 7% and 0.30% to 5% and 0.26% respectively, but worsen the magnitude of the recession. Infected people fail to internalize the impact of their choices on the spread of the virus. Therefore, the optimal containment policy increases the severity of the recession but saves lives. 13
Mulligan ( 2020 ) assesses the opportunity cost of “shutdowns” in order to document the macroeconomic impact of COVID‐19. Within the National Accounting Framework for the United States, the author extrapolates the welfare loss stemming from “nonworking days,” the fall in the labor‐capital ratio resulting from the absence/layoff of workers, and the resulting idle capacity of workplaces. After accounting for dead‐weight losses stemming from fiscal stimulus, the replacement of normal import and export flows with black market activities, and the effect on nonmarket activities (lost productivity, missed schooling for children and young adults), the author finds the welfare loss to be approximately $7 trillion per year of shutdown. Medical innovations, such as vaccine development, contact tracing, and workplace risk mitigation can help to offset the welfare loss by around $2 trillion per year of shutdown.
Other researchers have examined the supply side. Bonadio et al. ( 2020 ) use a quantitative framework to simulate a global lockdown as a contraction in labor supply for 64 countries. The authors find that the average decline in real GDP constitutes a major contraction in economic activity, with a large share attributed to disruptions in global supply chains. Elenev et al. ( 2020 ) model the impact of COVID‐19 as a fall in worker productivity and as a decline in labor supply, which both adversely affect firm revenue. The fall in revenue and the subsequent non‐repayment of debt‐servicing obligations spur a wave of corporate defaults, which might also bring down financial intermediaries. Céspedes et al. ( 2020 ) formulate a minimalist economic model in which the virus also leads to losses in productivity. The authors predict a vicious cycle triggered by the loss of productivity causing lower collateral values, in turn limiting the amount of borrowing activity, subsequently leading to decreased employment, followed by a further decline in productivity. The shock is thus magnified through an “unemployment and asset price deflation doom loop” (see Fornaro & Wolf, 2020 ).
Consumption pattern responses and debt responses from pandemic shocks had not been analyzed prior to COVID‐19 (Baker et al., 2020 ). Using transaction‐level household data, these authors find that households sharply increased their spending during the initial period in specific sectors such as retail and food spending. These increases, however, were followed by a decrease in overall spending. Similarly, Chang and Meyerhoefer ( 2020 ) show that consumers in Taiwan have increased food purchases from online platforms. Binder ( 2020 ) conduct an online survey of 500 United States consumers to investigate their concerns and responses related to COVID‐19, which indicated those items of consumption on which they were spending either more or less. They find that 28% of the respondents in that survey postponed future travel plans, and that 40% forewent food purchases. Interestingly, Binder ( 2020 ) finds from the surveys that consumers tend to associate graver concerns about COVID‐19 with higher inflationary expectations, a sentiment which serves as a proxy for “pessimism” or “bad times.”
Clemens and Veuger ( 2020 ) focus on the declines in government sales and income tax collections across US states. According to the authors, COVID‐19 has led to a substantial decline in consumption levels compared to income levels. This pattern is unlike the case in previous recessions, during which income decreased more than consumption. The authors find that the COVID‐19 pandemic will reduce the states’ tax collections by $42 billion in the second quarter of 2020. For fiscal year 2021, the authors anticipate an overall decline in sales and income tax revenues of $106 billion with heterogenous losses across US states.
McKibbin and Fernando ( 2020 ) estimate the aggregate economic costs. Using a hybrid DSGE/CGE global model, the authors model COVID‐19 as a negative shock to labor supply, consumption spending, financial markets, but as a positive shock to government expenditure, particularly stemming from health‐related expenditures. The authors outline seven different scenarios and provide a range of estimates of the increase in mortality and the fall in GDP for a number of countries across the world. In the case of the most contained outbreak, the number of deaths reaches around 15 million, while the reduction in global GDP is around $2.4 trillion in 2020.
Eppinger et al. ( 2020 ) use a quantitative international trade model with input‐output linkages for 43 countries to assess the impact of COVID‐19 supply shock on global value chains. They find that due to the supply shock, China experienced a welfare loss of 30% with moderate (positive or negative) spillover to other countries. Estimating a simulation consisting of a counterfactual scenario described as “without global value chains,” the authors find that welfare losses are reduced for some countries by as much as 40%, while they are magnified for others.
The economic impact of shocks, such as pandemics, is usually measured with aggregate time series data. However, these datasets are available only after a certain lag. In order to analyze the economic impact at a higher frequency, Lewis et al. ( 2020 ) developed a weekly economic index (WEI) using 10 different economic variables to track the economic impact of COVID‐19 in the United States. These authors report that between March 21 and March 28, the WEI declined by 6.19%. This was driven by a decline in consumer confidence, a fall in fuel sales, a rise in unemployment insurance (UI) claims, and changes in other variables. Similarly, Demirguc‐Kunt et al. ( 2020 ) estimate the economic impact of social distancing measures via three high‐frequency proxies (electricity consumption, nitrogen dioxide emissions, and mobility records). The authors find that social distancing measures led to a 10% decline in economic activity (as measured by electricity usage and emissions) across European and Central Asian countries between January and April. Chetty et al. ( 2020 ) develop a real‐time economic tracker using daily statistics on consumption, employment, business revenue, job postings, and other variables. The authors show that the initial slowdown in economic activity was partly driven by reductions in consumption by high‐income individuals. These spending shocks negatively affected business revenues catering to high‐income individuals. Subsequently, low‐income individuals working for these businesses lose much of their incomes and reduce their consumption levels. Kapteyn et al. ( 2020 ) tracked a representative sample of 7,000 respondents in Los Angeles County, California every 2 weeks to assess the impact of COVID‐19 over time.
Brinca et al. ( 2020 ) estimate the labor demand and supply shocks occurring in different sectors in the US economy employing a Bayesian structural vector autoregression model. They find that the decrease in work hours can be attributed to negative labor supply shock, a result that they suggest has important policy design implications. A negative labor supply shock is directly related to the lockdown and might be mitigated once such policies are lifted.
5. SOCIOECONOMIC CONSEQUENCES OF COVID‐19
We now review studies documenting the socioeconomic consequences of COVID‐19 and the ensuing lockdowns. Social distancing and lockdown measures have been shown to adversely affect labor markets, mental health and well‐being, racial inequality, and gender‐related outcomes. The environmental implications, while likely to be positive overall, also deserve careful analysis.
5.1. Labor market outcomes
A large number of studies document the effects on the variables of hours of work and job losses (e.g., Kahn et al., 2020 ). The major increases in unemployment observed in the United States are driven partly by lockdowns and social distancing policies (Rojas et al., 2020 ). Accounting for cross‐state variation in the timing of business closures and stay‐at‐home mandates in the United States, Gupta et al. ( 2020 ) find that the employment rate in the United States falls by about 1.7 percentage points for every extra 10 days that a state experienced a stay‐at‐home mandate during the period of March 12th to April 12th.
Coibion et al. ( 2020b ) find that the level of unemployment and job losses in the United States is more severe than one might judge based on the rise in UI claims, which is to be expected given the low coverage rate of the UI regimes in the United States. They also project a severe fall in the labor participation rate in the long run accompanied by an increase in the number of “discouraged workers” (jobless workers who have stopped actively searching for work, effectively withdrawing from the labor force). This phenomenon might be due to the disproportionate impact of COVID‐19 on the older population. Aum et al. ( 2020a , 2021b ) find that an increase in infections leads to a drop in local employment even in the absence of lockdowns in South Korea, whose government did not mandate them. This estimated impact was higher for countries, such as the United States and the United Kingdom, where mandatory lockdown measures were imposed.
Adams‐Prassl et al. ( 2020a ) analyze the inequality of the distributions of job and income losses based on the type of job held and on individual characteristics for the United States and the United Kingdom. The authors find that workers who can perform none of their employment tasks from home are more likely to lose their job. This study also finds that younger individuals and people without a university education were significantly more likely to experience drops in their income. Yasenov ( 2020 ) finds that workers with lower levels of education, younger adults, and immigrants are concentrated in occupations whose tasks are less likely to be performed from home. Similarly, Alstadsæter et al. ( 2020 ) find that the pandemic shock in Norway has a strong socioeconomic gradient, as it has disproportionately affected the financially vulnerable population, including parents with younger children.
Béland, Brodeur, and Wright ( 2020 ) discuss heterogeneous effects across occupations and workers in the United States, showing that occupations that have a higher share of workers working remotely were less affected by COVID‐19. On the other hand, occupations with relatively more workers working in proximity to others were more affected. They also find that occupations classified as “more exposed to disease” are less affected, which is possibly due to the number of essential workers in these occupations. Based on these results, it can be reasonably expected that workers might change (or students might select different) occupations in the medium term. Bui et al. ( 2020 ) focus on the impact of COVID‐19 on older workers in the United States. Using CPS data, they show that older workers who are over 65 years of age, especially women, are facing higher unemployment in this COVID‐19 recession compared to previous ones.
Kahn et al. ( 2020 ) show that firms in the United States dramatically reduced job vacancies from the second week of March 2020 and thereafter. The authors find that the job vacancy declines occurred simultaneously with increasing UI claims. Notably, the labor market declines (proxied through reductions in job vacancies and increases in UI claims) were uniform across states, with no notable differences across states which experienced the spread of the pandemic, or implemented stay‐at‐home orders, earlier than others. The study also finds that the reductions in job vacancies were uniform across industries and occupations, except for those in front line jobs, such as nursing. Baert et al. ( 2020a ) investigate the impact of COVID‐19 on career prospects through surveys conducted in Belgium. They document concerns that were expressed about job losses and missing out on promotions, especially among migrant workers.
Fairlie ( 2020 ) analyzes the impact of COVID‐19 on the number of small businesses in the United States. Using the April 2020 CPS data, the author finds that the number of active business owners declined by 22% between February and April 2020. While most major industries faced large drop in business, the authors also find that female and immigrant‐owned businesses were disproportionately affected.
With the enforcement of social distancing measures, work from home has become increasingly prevalent. The degree to which economic activity is impaired by such social distancing measures depends largely on the capacity of firms to maintain business processes from the homes of workers (Alipour et al. 2020 ; Papanikolaou & Schmidt, 2020 ). Additionally, working from home or working remotely are much more common and are thought to cause lower productivity losses in industries that are staffed by better educated and better paid workers (Bartik et al., 2020 ). Brynjolfsson et al. ( 2020 ) find that the increase in cases per 100k individuals is associated with a significant rise in the fraction of workers switching to remote work and the fall in the fraction of workers commuting to work in the United States. Interestingly, the authors find that people working from home are more likely to claim UI (if they are laid off) than people who are still commuting to work and are likely working in industries providing essential services.
Dingel and Neiman ( 2020 ) analyze the feasibility of jobs that can be done from home. They find that 37% of jobs can be feasibly performed from home. A different but related context for the feasibility of work from home is the extent to which the job involves face‐to‐face (F2F) interaction. According to Avdiu and Nayyar ( 2020 ), the job‐characteristic variables of home‐based work (HBW) and F2F interaction differ along three main dimensions, namely: (i) temporal (short run vs. medium run); (ii) the primary channel of effects (supply and demand of labor for the occupation/tasks); and (iii) the relevant margins of adjustment (intensive vs. extensive). They argue that the supply of labor in industries with HBW capabilities and low F2F interactions (e.g., professional, scientific, and technical services) might be the least affected. Nevertheless, those industries and occupations with HBW capabilities and high F2F interactions are likely to experience negative productivity shocks. As lockdown restrictions are lifted, industries with low HBW capabilities and low F2F interactions (e.g., manufacturing, transportation, and warehousing) might be able to recover relatively quickly. The risk of infection through physical proximity can be mitigated by wearing personal protective equipment (PPE) and by taking other relevant precautionary measures. However, those industries with low HBW capabilities and high F2F interactions (e.g., accommodation and food services, arts entertainment and recreation) are likely to experience slower recoveries, as consumers might be apprehensive about patronizing them, for example, cinemas and restaurants. Using a web survey in Belgium, Baert et al. ( 2020b ) find that a majority of respondents thought that teleworking and digital conferencing were here to stay and will become more common in the postpandemic period.
From the firm's perspective, there are large short‐term effects of temporary closures, such as the (perhaps permanent) loss of productive workers and declines in job postings, all of which are characterized by strong heterogeneity across industries. Bartik et al. ( 2020 ) survey a small number of firms in the United States and document that several of them have temporarily closed shop and reduced their number of employees compared to January 2020. The surveyed firms were not optimistic about the efficacy of the fiscal stimulus implemented by the federal government of the United States. Campello et al. ( 2020 ) find that job losses have been more severe for industries with highly concentrated labor markets (i.e., where hiring is dominated by a few employers), nontradable sectors (e.g., construction, health services), and credit‐constrained firms. Hassan et al. ( 2020 ) discern a pattern of heterogeneity with respect to firm resilience across industries around the World. Based on earnings call reports, they provide evidence that some firms are expecting increased business opportunities in the midst of the global disruption (e.g., firms which make medical supplies or others whose competitors are facing negative impressions after the outbreak due to their association with regions where case numbers are high). Barrero et al. ( 2020 ) measure the reallocation of labor in response to the pandemic‐induced demand response (e.g., increased hiring by delivery companies, delivery‐oriented restaurant/fast food chains, technology companies).
To conclude this subsection, a large number of studies try to predict labor market outcomes by exploiting high frequency data (e.g., Adams‐Prassl et al. 2020a , Chetty et al., 2020 ). For instance, Bartik et al. ( 2020 ) and Kurmann et al. ( 2020 ) rely on worker‐firm matched daily data drawn from “Homebase,” a scheduling and time clock software provider, to construct real‐time data for small businesses. Other studies have also used high‐frequency electricity market data to estimate the short‐run impacts of COVID‐19 on economic activity (e.g., Fezzi & Fanghella, 2020 ).
5.2. Health outcomes
The impact of the pandemic on physical health and mortality has been documented in many studies (e.g., Goldstein & Lee, 2020 ; Lin & Meissner, 2020 ). Knittel and Ozaltun ( 2020 ) document a positive correlation between the share of elderly population, the incidence of commuting via public transportation, and the number of COVID‐19 deaths in the United States. In contrast, the authors provide evidence that obesity rates, the number of ICU beds per capita, and poverty rates are not related to the death rate. Chatterji and Li ( 2021 ) document the effect of the pandemic on the US health care sector. The authors find that it is associated with a 67% decline in the total number of outpatient visits per provider by the week of April 12th‐18th 2020 relative to the same week in prior years. This might have negative health consequences, especially among individuals with chronic health conditions. Hermosilla et al. ( 2020 ) show that COVID‐19 has crowded out non‐COVID‐19‐related health care demands in China. Others, such as Alé‐Chilet et al. ( 2020 ), explore the drop in emergency cases in hospitals around the world.
Nevertheless, during a crisis, such as the COVID‐19 pandemic, it is common for everyone to experience increased levels of distress and anxiety, particularly the sentiment of social isolation (American Medical Association, 2020 ). A growing number of studies document worsening mental health status and levels of well‐being (Adams‐Prassl et al. 2020b ; Brodeur, Clark, Fleche, & Powdthavee, 2021 ; Davillas & Jones, 2020 ; de Pedraza et al., 2020 , and Tubadji et al., 2020 ). According to Lu et al. ( 2020 ), social distancing or lockdown measures are likely to affect psychological well‐being through a lack of access to essential household supplies, discriminatory treatment, or exclusion by neighbors. They assert that maintaining a positive attitude (in terms of severity perceptions, the credibility of real‐time updates of information, and confidence in social distancing measures) can help reduce depression. Hamermesh ( 2020 ) also provides evidence that, adjusted for numerous demographic and economic variables, happiness levels during the COVID‐19 pandemic are affected by how people spend time and with whom. In the opposite case, using an experimental set‐up, Bogliacino et al. ( 2020 ) find that a negative shock triggered by COVID‐19 lowers cognitive functionality and increases risk aversion and the propensity to punish others, that is negative reciprocity. Public mental health is also affected by the cognitive bias related to the diffusion of public death toll statistics (Tubadji et al., 2020 ). These needs are all the less likely to be addressed given the lower levels of provision of health care and social work services.
Using the Canadian Perspective Survey Series, Béland, Brodeur, Mikola, and Wright ( 2020 ) find that those who missed work not due to COVID‐19, and those who were already unemployed, showed declines in mental health. Using panel data in the United Kingdom, Etheridge and Spantig ( 2020 ) report a large deterioration in the state of mental health, with much larger effects for women.
The implementation of lockdown policy also adversely affected public mental health. Armbruster and Klotzbücher ( 2020 ) demonstrate that there were increases in the demand for psychological assistance (through helpline calls) due to lockdown measures imposed in Germany. The authors find that these calls were mainly driven by mental health issues such as loneliness and depression. Brodeur, Clark, Fleche, & Powdthavee et al. ( 2021 ) show that there has been a substantial increase in the search intensity on Google for “boredom” and “loneliness” during the postlockdown period in nine Western European countries and the United States during the first few weeks of lockdowns. Using experimental surveys, Codagnone Bogliacino, Gómez, and Charris et al. ( 2020 ) find that about 43% of the population in Italy, Spain, and United Kingdom are at high risk of developing mental health problems; not only because of the negative economic shock, but also due to conditions of long‐standing economic weakness and vulnerability in those countries.
Fetzer et al. ( 2020 ) find that there has been broad public support for COVID‐19 containment measures. However, some of the respondents believe that the general public fails to adhere to health measures, and that the governmental response has been insufficient. These respondents have a tendency to exhibit a poorer state of mental health. If governments are seen to take decisive actions, however, then the respondents altered their perceptions about governments and other citizens, which in turn improved their state of mental health.
5.3. Gender and racial inequality
A growing literature points out that COVID‐19 has had an unequal impact between genders and across races in OECD countries; specifically, women and racial minorities, such as African‐Americans and Latinos, have been unduly and adversely affected. While it is thought that prior recessions typically affected men more than women, many studies provide evidence that COVID‐19 has large negative effects on women's labor market outcomes (Adams‐Prassl et al., 2020a ; Forsythe et al., 2020 ; Yasenov, 2020 ). Alon et al. ( 2020 ) argue that women's employment is concentrated in sectors such as health care and education. Moreover, the closure of schools and daycare centers led directly to increased childcare needs, which would have a negative impact on working and/or single mothers. For example, based on household surveys in Spain, Farré et al. ( 2020 ) find that while men increased their participation in household work and childcare duties during lockdowns, the burden of these tasks fell disproportionately on women.
Couch et al. ( 2020a ) examine the variation in unemployment shocks among minority groups in the United States. The authors find that Latino groups were disproportionately affected by the pandemic. They attribute the difference to an unfavorable occupational distribution (e.g., Latino workers tend to work in nonessential services) and to lower skill levels among them. Borjas and Cassidy ( 2020 ) determine that the COVID‐19 shock led to a fall in employment rates of immigrant men compared to native men in United States, which was in contrast to the historical pattern observed during previous recessions. The immigrants’ relatively high rate of job loss was attributed to the fact that immigrants were less likely to hold jobs that could be performed remotely from home. The likelihood of being unemployed during March 2020 was significantly higher for racial and ethnic minorities in the United States (Montenovo et al., 2020 ). In a similar vein, McLaren ( 2020 ) finds that minorities’ population shares in a county strongly correlate with COVID‐19‐related deaths in the United States. After controlling for the factors of education, jobs, and travel patterns, the correlation holds for the African‐American and the First‐Nations populations. The author shows that these racial disparities between African‐Americans, First Nations peoples, and others can be partially attributed to differentials in public transit usage patterns.
Couch et al. ( 2020b ) find that COVID‐19 has unduly affected women compared to men in the United States. Using employment to population ratios and number of hours from the CPS data, the authors find that women with school‐age children faced greater declines in employment and work hours compared to men between April and August 2020. The reductions in work hours and employment can be explained by additional childcare responsibilities, job and skill characteristics, and lower numbers of women involved in “essential” industries.
Schild et al. ( 2020 ) find that COVID‐19 occasioned a rise of Sino‐phobia across the internet, particularly when western countries started showing signs of infection. Bartos et al. ( 2020 ) document the causal effect of economic hardships on hostility against certain ethnic groups in the context of COVID‐19 using an experimental approach. The authors find that the COVID‐19 pandemic magnifies sentiments of hostility and discrimination against foreigners, especially those from Asia.
5.4. Environmental outcomes
The global lockdown and the considerable slowdown of economic activities are expected to have a positive effect on the environment (Almond et al., 2020 ; Cicala et al., 2020 ). He et al. ( 2020 ) show that lockdown measures in China led to a remarkable improvement in air quality. The Air Quality Index and the fine particulate matter (PM 2.5 ) concentrations were brought down by 25% within weeks of the lockdown, with larger effects recorded in colder, richer, and more industrialized cities. Similarly, Almond et al. ( 2020 ) focused on air pollution and the release of greenhouse gases in China during the post‐COVID‐19 period. They determined that, while nitrogen dioxide (NO 2 ) emissions fell precipitously, sulphur dioxide emissions (SO 2 ) did not decrease. For China as a whole, PM 2.5 emissions fell by 22%; however, ozone concentrations increased by 40%. These variations show that there is not necessarily an unambiguous improvement in air pollution due to the economic slowdown. The reduction can be attributed to less travel in personal vehicles causing lower nitrous oxide (NO 2 ) emissions.
Brodeur, Cook, & Wright ( 2021 ) examine the causal effect of “safer‐at‐home” policies on air pollution across US counties. They find that “safer‐at‐home” policies decreased air pollution (measured as PM 2.5 emissions) by almost 25% on average, with larger effects for populous counties. Cicala et al. ( 2020 ) focus on the health and mortality benefits of reduced vehicle travel and electricity consumption in the United States due to stay‐at‐home policies, suggesting that reductions in emissions from less travel and from lower electricity usage reduced deaths by over 360 per month.
On the other hand, Andree ( 2020 ) focuses on the effect of pollution on cases, finding that PM 2.5 levels are a highly significant predictor of COVID‐19 incidence using data from 355 municipalities in the Netherlands. In terms of COVID‐19‐related deaths, Knittel and Ozaltun ( 2020 ) find no evidence that pollution levels are related to death rates in the United States.
Based on the research discussed in section 5 above, Table 3 provides a summary of these strands of the literature dealing with the socioeconomic and environmental outcomes resulting from social distancing actions, stay‐at‐home orders, and/or lockdowns including a listing of the statistical measures and methodologies that were utilized.
Socioeconomic outcomes of COVID‐19 lockdowns: Summary of studies
6. POLICY MEASURES
The economic literature deals with a wide assortment of policy measures. We organize our presentation into six broad topics: (i) the types of policy measures, (ii) the determinants of government policy, (iii) optimal testing methods, (iv) the lockdown measures and their associated factors, (v) the lifting of the lockdown measures, and (vi) the economic stimulus measures.
To mitigate the negative effects of public health controls on the economy and to sustain and promote public welfare, governments all around the World have implemented a variety of policies within a very short time frame. These include fiscal, monetary, and financial policy measures (Gourinchas, 2020 ). The economic measures vary across counties in terms of breadth and scope, and they target households, firms, health systems, and/or banks (Weder di Mauro, 2020 ).
Using a database of economic policies implemented by 166 countries, Elgin et al. ( 2020 ) employ the technique of Principal Component Analysis (PCA) to develop their COVID‐19 Economic Stimulus Index. The authors correlate the standardized index with predictors of governmental response, such as population characteristics (e.g., median age), public health‐related measures (e.g., the number of hospital beds per capita), and economic variables (e.g., GDP per capita). They find that the economic stimulus is larger for countries with higher COVID‐19 infections, older median ages, and higher GPD‐per‐capita levels. In addition, the authors develop a “Stringency Index,” which includes measures such as school closures and travel restrictions. They find that the “Stringency Index” is not a significant predictor of their economic stimulus index, which suggests that public health measures do not drive economic stimulus measures (Weder di Mauro, 2020 ).
On a similar note, Porcher ( 2020 ) has created an index of public health measures using the PCA technique. The index is based on 10 common public health policies implemented across 180 countries to mitigate the spread of COVID‐19. The index is designed to measure the stringency of the public health response across countries. The author finds that, abstracting from the COVID‐19 case numbers and deaths, countries which have better public‐health systems and effective governance tend to have less stringent public health measures.
C. Cheng et al. ( 2020 ) develop the “CoronaNet–COVID‐19 Government Response Database,” which accounts for policy announcements made by countries globally since 31 December 2019. The information that is contained in this data base is categorized according to: (i) type of policy, (ii) national versus subnational enforcement, (iii) people and geographic region targeted by the policy, and (iv) the time frame within which it is implemented. Table 4 provides a description of the government response database for 125 countries. Counts are tabulated according to 15 types of interventions for two variables: cumulative number of policies (of that type) implemented and the number of countries which have implemented it. It also displays that average value for the degree of enforcement.
Summary statistics of COVID‐19 government response dataset
Source: C. Cheng et al. ( 2020 ).
There is substantial variation across policy measures. The policy most governments have implemented is “external border restriction,” that is, restricting access to entry through ports. It has been imposed by 186 countries; the second most common policy measure, implemented by 169 countries, is “school closures.” However, in terms of the frequency of implementation across all countries, the type of “obtaining or securing health resources” has the highest level. This includes the provision of materials (e.g., face masks), personnel (e.g., doctors, nurses), and infrastructure (e.g., hospitals). The second most frequently implemented policy is “restrictions on nonessential businesses.” In terms of stringency of policy enforcement, “emergency declaration” and the formation of a “new task force” or an “administrative reconfiguration to tackle pandemic” are implemented with 100% stringency.
Due to these major differences between policy responses across countries and over time, the authors use a dynamic Bayesian item‐response approach to measure the implied economic, social, and political cost of implementing a particular policy over time. They also develop a supplementary measure labelled the “Policy Activity Index,” which assigns a higher rank for policy measures to countries that are more willing to implement a “costly” policy. Based on that index, the authors determine that school closure is the costliest to implement followed by mandatory business closure and social distancing policies. Moreover, internal border restrictions are viewed as more costly compared to external border restriction.
The topic of optimal testing methods has received a great deal of attention in the media and, to some extent, in academia. A well‐known proposal defended by Paul Romer and many others is a comprehensive “test and isolate” policy, which would effectively reduce the effective reproduction number and allow the economy to operate more openly. 14 Taipale et al. ( 2020 ) formalize this proposal and argue that the epidemic would collapse at a sufficient rate of testing and isolation, and that concurrent testing would outperform random sampling of individuals. Other proposals for optimal testing include regular testing of people in groups that are more likely to be exposed to COVID‐19 (e.g., Cleevely et al., 2020 ; Gollier & Gossner, 2020 ), multi‐stage group testing (e.g., Eberhardt et al., 2020 ), and testing on exit from quarantine instead of upon entry (e.g., Wells et al. 2020 ).
The topic of optimal lockdown policies has been investigated mostly by using epidemiology macroeconomic models, some of which are oriented around the dichotomy between the case in which the choices (and responses) are all made by private agents and the case in which the choices are made by a social planner (Acemoglu et al., forthcoming; Alvarez et al., forthcoming; Berger et al., forthcoming; Bethune & Korinek 2020 ; Eichenbaum et al., 2020 ). Jones et al. ( 2020 ) argue that in contrast to private agents, the social planner will seek to front‐load mitigation strategies: that is to impose strict lockdowns from the beginning to reduce the spread of infection and let the economy to fall into a deep recession. This is because their model's set‐up not only considers the concomitant health care costs and congestion in hospitals, but also rightly considers the fact that workers need time to become productive for a work‐from‐home situation. The outcomes are dependent on the assumed values of the parameters that are inputted into these models. The optimal policy choice reflects the rate of time preference, epidemiological factors, the value of statistical life, the rate at which death rate increases in the infected population, the hazard rate for a vaccine discovery, the learning effects in the health care sector, and the severity of output losses due to a lockdown (Gonzalez‐Eiras & Niepelt, 2020 ). The intensity of the lockdown depends on the gradient of the fatality rate as a function of the number of infected individuals and on the assumed value of a statistical life. The absence of testing increases the economic costs of the lockdown and shortens the duration of the optimal lockdown (Alvarez et al., forthcoming). Chang and Velasco ( 2020 ) argue that the optimality of policies depends on peoples’ expectations. For instance, fiscal transfers must be large enough to induce people to stay at home in order to reduce the degree of contagion; otherwise they might not change their behavior in efforts to reduce the risk of infection. Their analysis also contains a critique of the use of SIR models, as the parameters used in that class of models (which remain fixed in value) would shift as individuals change their behavior in response to policy. Kozlowski et al. ( 2020 ) investigate the scarring effect on perceptions (i.e. the change in belief about the probability of an extreme but negative or tail‐risk event) of COVID‐19, and find that revisions in belief about tail‐risk events among economic agents will lead to a larger and more persistent negative impact on the economy in the long run.
When the daily death rates and case numbers decline, policies regarding reopening the economy are of primary importance. Gregory et al. ( 2020 ) describe the lockdown measure as a “loss of productivity,” whereby relationships between employers and laborers are suspended, terminated, or continued. They further explain that postpandemic, the speed and the type (V‐shaped or L‐shaped) of recovery depend on at least three factors: (i) the fraction of workers who, at the beginning of the lockdown, enter unemployment while maintaining a relationship with their employer, (ii) the rate at which inactive relationships between employers and employees dissolve during the lockdown, and (iii) the rate at which workers who, at the end of the lockdown, are not recalled by their previous employer can find new, stable jobs elsewhere (Gregory et al., 2020 ).
Harris ( 2020 ) points out the importance of utilizing several status indicators (e.g., results of partial voluntary testing, guidelines for eligibility of testing, daily hospitalization rates) in order to decide upon the course of action on reopening the economy. Kopecky and Zha ( 2020 ) state that decreases in deaths are either due to implementation of social distancing measures or to herd immunity; it is hard to identify and disentangle those factors using standard SIR models. They argue that with the “identification problem,” there will be considerable uncertainty about the conditions for restarting the economy. Only comprehensive testing can help resolve this ambiguity by quickly and accurately identifying new cases so that future outbreaks could be contained by isolation and contact‐tracing measures (Kopecky & Zha, 2020 ).
Agarwal et al. ( 2020 ) rely on synthetic control methods to investigate the effect of counterfactual mobility restriction interventions in United States. Using the daily death data from different countries, the authors create different “synthetic mobility United States” variables. These are applied to predict a counterfactual scenario and to understand the trade‐off between different levels of mobility interventions on death levels in United States. They find that a small decrease in mobility reduces the number of deaths; however, after registering a 40% drop in mobility, the benefits derived from mobility restrictions (in terms of the number of deaths) diminish. Using a counterfactual scenario, the authors find that lifting severe mobility restrictions but retaining moderate mobility restrictions (e.g., by imposing limitations in retail and public transport locations) might effectively reduce the number of deaths in United States. Others, such as Rampini ( 2020 ), make the case for the sequential lifting of lockdown measures for the younger population at the initial stages, followed by the older population at later stages. The authors state that the lower effect on the younger population group is a fortunate coincidence, and thus, the economic consequences of interventions can be greatly reduced by adopting a sequential approach. Oswald and Powdthavee ( 2020 ) make a similar case for releasing the younger population from mobility restrictions first on the grounds of higher economic efficiency (as they are more likely to be in the labor force) and their greater resilience against infections.
As some US states reopened, some researchers turned their focus on the immediate consequences. Nguyen et al. ( 2020 ) find that 4 days after reopening, mobility has increased by 6 to 8%, with greater increases across states which were late adopters of lockdown measures. These findings have important implications for the resurgence of cases, hospital capacity utilization, and further deaths. Dave, Friedson, Matsuzawa, and McNichols et al. ( 2020 ) analyze the effect of lifting the shelter‐in‐place order in Wisconsin, after the Wisconsin Supreme Court abolished it, on social distancing and the number of cases and find no statistically significant impact. W. Cheng et al. ( 2020 ) find that employment activity in the United States increased in May due to reopening in some states, mainly as a result of people who resumed working at their previous job. However, they find that the longer employees are separated from their firms, the more their re‐employment probabilities decline.
In regards to the aggregate macroeconomy, Gourinchas ( 2020 ) states that without substantial, timely, and stimulative macroeconomic intervention, the output lost from the economic downturn will be greatly amplified, especially as economic agents react to the negative shock by reducing consumption spending, investment spending, and engaging in lower credit transactions. The author suggests that there should be cross‐regional variation in government responses based on country characteristics. With high amounts of government debt and historically low interest levels existing in most developed countries, Bianchi et al. ( 2020 ) recommend a coordinated monetary and fiscal policy to address the COVID‐19 economic fallout. They recommend that fiscal policy should be used to enact an emergency budget with a ceiling placed on the debt‐to‐GDP ratio. This measure would increase aggregate spending, raise the inflation rate, and reduce real interest rates. The monetary authorities would need to coordinate with fiscal policy authorities by adopting an above‐normal inflation target. In the long run, governments would try to balance the budget, and future monetary policy would aim to bring inflation back to normal levels.
Bigio et al. ( 2020 ) focus on the cases for government transfers versus credit subsidy policies. They determine that the optimal mix between them depends on the level of financial development in the economy. According to these authors, economies with a developed financial system should utilize stimulative credit policies. On the other hand, developing economies should engage in more transfer spending. Guerrieri et al. ( 2020 ) show that the optimal economic policy response for the “Keynesian supply shock” induced by COVID‐19 would be to combine expansionary monetary policy and bolster social insurance programs for employees in the affected sectors. Unconventional policies, such as wage subsidies, helicopter drops of liquid assets, equity injections, and loan guarantees, can keep the economy in a full employment, high‐productivity equilibrium (Céspedes et al., 2020 ). These policies can break the cycle of negative feedback loops between corporate defaults and potential insolvency of financial intermediaries, which could culminate in a meltdown in financial markets (Elenev et al., 2020 ). Didier et al. ( 2020 ) discuss the policy menu, priorities, and trade‐offs of providing direct financing to firms.
Chetty et al. ( 2020 ) analyze the causal effect of policies implemented in the United States on households and businesses. They find that stimulus payments delivered through the Coronavirus Aid, Relief, and Economic Security (CARES) Act increased consumption spending, and that this spending was directed toward durable goods, which require low physical interaction at various stages of production. As a result, this spending is not directed toward small‐ and medium‐size businesses whose revenues were very adversely affected. On the other hand, loans to small businesses from the Paycheck Protection Program did little to restore employment among businesses. According to their analysis, the economic recovery depends on restoring consumer confidence and targeting income replacement programs rather than uniform lump‐sum stimulus payments.
Codagnone, Bogliacino, and Gómez, Folkvord et al. ( 2020 ) focus on the expectations of stakeholders with regards to the postlockdown period. Using an experimental survey in Spain, Italy, and United Kingdom, the authors find that exposure to the COVID‐19 shock and the ensuing lockdown led to pessimistic expectations about job opportunities, greater drawdowns of savings than before, and a deterioration in social relations which might be instrumental in finding job opportunities in the long run. The authors conclude that the fiscal policy measures might be insufficient in managing these expectations amidst uncertainties. They call on policy makers to draft contingency plans for exiting the lockdown—not only in terms of public expenditures earmarked for postlockdown operations, but also in terms of public health strategies to tackle a second wave of COVID‐19.
7. CONCLUSIONS
This study delved into the research related to the economics of COVID‐19 that has been released over a short time period. Our primary aim is to synthesize and to bring coherence and structure to the very rapidly growing body of relevant scientific evidence. By providing an annotated list of dozens of articles along with a brief capsule of their content, we hope to facilitate further research in the many strands of the COVID‐related literature. For readers who are interested in this critically important and pressing topic, this piece also provides an informative summary of the state of knowledge at the time of writing.
Before covering the impacts of COVID‐19, we documented the most popular data sources that are exploited to measure the known cases and deaths resulting from COVID‐19, as well as the social distancing activities. We first pointed out that the numbers of reported cases and deaths are subject to measurement error due to many factors, including testing capacity constraints and lags. Mobility measures that are based on GPS coordinates emitted from cell phones have been used extensively to measure social distancing. However, there are certain caveats that apply, particularly in terms of privacy concerns and the representativeness of data. The article also reviewed separate research related to social distancing activity itself, particularly in regards to its determinants, its efficacy in mitigating the spread of COVID‐19, and compliance with these orders. Going forward, social distancing actions and their measurements will continue to figure prominently in academic research and policy development.
We divided our coverage of the impact of the macroeconomy into two subsections, the first of which deals with the propagation mechanisms. The stay‐at‐home orders have very adverse effects on supply chains as well as on employment, which in turn causes drastic declines in consumption spending for many goods and services. The resultant declines in consumer and investor confidence reinforce negative multiplier effects in a downward spiral between labor and output markets, which can be partially attenuated by stimulative fiscal and monetary policies. Since the trajectory for the macroeconomy depends critically on the degree of spread of the virus itself, some researchers have integrated that element into their models. We reviewed the three potential “shapes” for the macroeconomic recovery: the highly optimistic yet implausible “V” path, the somewhat favorable “U” path, and the pessimistic yet more likely “L” path.
The second aspect of the macroeconomic impact of COVID‐19 that we discussed involves the forecasts. It is thought that the lockdown and social distancing measures wreak greater economic harm than the spread of the virus itself. The tremendous uncertainty regarding the path of the virus is compounded with a high degree of economic uncertainty such that these projections are subject to very wide confidence intervals and constant revisions. Some articles have attempted to address the longer‐term negative impacts on macro variables such as capital formation, productivity, and government finances. Other studies have focused on changes in patterns of consumption, employment, savings, and consumer debt by exploiting real‐time data.
In terms of the socioeconomic consequences of COVID‐19, we focus on the impact of the pandemic and the social distancing measures on outcomes in four areas: the labor market, mental health and well‐being, racial and gender inequality, and the environment. In terms of the labor market outcomes, research has shown that there is a high degree of heterogeneity in the pattern of job losses. The pandemic has caused a major shift toward work from home and away from positions involving F2F interactions with either the public or coworkers. Due to technological features and the nature of the services rendered, there are only a certain number of jobs that can be “feasibly” done from home and do not require F2F interactions. This contributes to the disproportionate effect of the pandemic on workers in certain industries and occupations, many of which have a relatively high concentration of lower‐skilled and/or less educated workers.
Social distancing measures have led to serious deteriorations in the levels of mental health, family stress, and domestic violence. Health care services for non‐COVID patients have been crowded out in many instances. There has been a marked rise in observed racial discrimination and sentiments of hostility toward certain ethnic groups. A growing number of studies also document that women have been adversely affected by the loss of child care and educational services for their children. The only seemingly positive consequence of social distancing/lockdown measures is the decrease in air pollution levels and the incidence of accidents involving motor vehicles. However, the impacts on the environment are multi‐faceted, and thus there remains a fair amount of ambiguity.
The goal of our piece was to survey and summarize the findings of the literature on the economics of COVID‐19. This was a very challenging task, as the literature is growing and evolving fast, and the pandemic is far from over at the time of writing. There are a few qualifications that are worth mentioning. First, very few of the research articles surveyed have undergone normal scientific review processes. Second, we mostly did not comment on methodology, which necessitates caution in interpretations. Finally, due to time as well as space constraints, we offer little in the way of critical analysis. Nonetheless, we hope this survey will facilitate further research in the many strands of the COVID‐related literature.
Supporting information
Table A: Major Pandemics: Historical Timeline
Brodeur A, Gray D, Islam A, Bhuiyan S. A literature review of the economics of COVID‐19 . Journal of Economic Surveys . 2021; 35 :1007–1044. 10.1111/joes.12423 [ PMC free article ] [ PubMed ] [ CrossRef ] [ Google Scholar ]
1 Social distancing (or physical distancing) is defined as maintaining physical space between yourself and other people residing outside one's home. To practice social/physical distancing: (i) stay at least 6 feet (about 2 arms’ lengths) from other people, (ii) do not gather in groups, and (iii) avoid crowded places and mass gatherings.
2 The list of NBER working article is available at this URL: https://www.nber.org/wp_covid19.html
3 The list of IZA discussion articles is available at this URL: https://covid‐19.iza.org/publications
4 See the link for the numbers and visual representation. Retrieved from https://coronavirus.jhu.edu
5 See WHO COVID‐19 Dashboard: https://covid19.who.int.
6 Refer to Johns Hopkins University ( 2020b ) for CFR data across countries.
7 See the link for further details: https://ourworldindata.org/mortality‐risk‐covid .
8 See the link for further details: https://covidtracking.com/data .
9 See the link for further details: https://covidtracking.com/race .
10 Mobility measures track work locations based on movements to a workplace from a reference point such as their home. However, if a person works from home or becomes unemployed, there will not be a distinct workplace reference point. Hence, the quality of mobility measures is expected to deteriorate.
11 The WHO Health System Response Monitor provides a cross country analysis and other details: https://analysis.covid19healthsystem.org/ .
12 According to the authors, if all members of a set choose to implement shelter‐in‐place policies, then the best response for agents is to follow. Hence, even in the absence of a federal mandate, the members of this “tipping set” can drive all others to adopt shelter‐in‐place policies.
13 The interaction between economic and epidemiological models is described in more details in the Online Appendix.
14 Further details on the “test and isolate” policy is available at the URL: https://paulromer.net/covid‐sim‐part1.
15 See the link for further details: https://www.google.com/covid19/mobility .
16 See the link for further details: https://www.unacast.com/covid19 .
17 See the link for further details: https://www.safegraph.com/dashboard/covid19‐commerce‐patterns .
18 See the link for further details: http://research.baidu.com/Blog/index‐view?id=13 .
- Abouk, R. , & Heydari, B. (2021). The immediate effect of COVID‐19 policies on social distancing behavior in the United States . Public Health Reports . 10.1177/0033354920976575 [ PMC free article ] [ PubMed ] [ CrossRef ] [ Google Scholar ]
- Acemoglu, D. , Chernozhukov, V. , Werning, I. , & Whinston, M. D. (forthcoming). A multi‐risk sir model with optimally targeted lockdown . American Economic Review: Insights .
- Adams‐Prassl, A. , Boneva, T. , Golin, M. , & Rauh, C. (2020a). Inequality in the impact of the coronavirus shock: Evidence from real time surveys . Journal of Public Economics , 189 , 104245. [ Google Scholar ]
- Adams‐Prassl, A. , Boneva, T. , Golin, M. , & Rauh, C. (2020b). The impact of the coronavirus lockdown on mental health: Evidence from the US. Cambridge Working Papers in Economics. University of Cambridge, Cambridge, MA . Retrieved from http://www.econ.cam.ac.uk/researchfiles/repec/cam/pdf/cwpe2037.pdf
- Agarwal, A. , Alomar, A. , Sarker, A. , Shah, D. , Shen, D. , & Yang, C. (2020). Two burning questions on COVID‐19: Did shutting down the economy help? Can we (Partially) reopen the economy without risking the second wave? arXiv.org, 2005.00072. Retrieved from http://arxiv.org/abs/2005.00072
- Akesson, J. , Ashworth‐Hayes, S. , Hahn, R. , Metcalfe, R. D. , & Rasooly, I. (2020). Fatalism, beliefs, and behaviors during the COVID‐19 pandemic. NBER Working Paper No. 27245. National Bureau of Economic Research, Cambridge, MA. [ PMC free article ] [ PubMed ] [ Google Scholar ]
- Aksoy, C. G. , Eichengreen, B. , & Saka, O. (2020). The political scar of epidemics. IZA Discussion Paper No. 13351. Institute of Labor Economics, Bonn, Germany. [ Google Scholar ]
- Aksoy, C. G. , Ganslmeier, M. , & Poutvaara, P. (2020). Public attention and policy responses to COVID‐19 pandemic. IZA Discussion Paper No. 13427. Institute of Labor Economics, Bonn, Germany. http://ftp.iza.org/dp13427.pdf [ Google Scholar ]
- Alé‐Chilet, J. , Atal, J. P. , & Dominguez‐Rivera, P. (2020). Activity and the incidence of emergencies: Evidence from daily data at the onset of a pandemic. PIER Working Paper 20‐016. University of Pennsylavania, Philadelphia, PA . Retrieved from https://economics.sas.upenn.edu/system/files/working‐papers/20‐016%20PIER%20Paper%20Submission%20_NEW.pdf
- Alipour, J.‐V. , Falck, O. , & Schüller, S. (2020). Germany's capacities to work from home. IZA Discussion Paper No. 13152. Institute of Labor Economics, Bonn, Germany. Retrieved from http://ftp.iza.org/dp13152.pdf [ Google Scholar ]
- Allcott, H. , Boxell, L. , Conway, J. C. , Gentzkow, M. , Thaler, M. , & Yang, D. Y. (2020). Polarization and public health: Partisan differences in social distancing during the coronavirus pandemic . Journal of Public Economics , 191 , 104254. [ PMC free article ] [ PubMed ] [ Google Scholar ]
- Almond, D. , Du, X. , & Zhang, S. (2020). Did COVID‐19 improve air quality near Hubei? NBER Working Paper No. 27086. National Bureau of Economic Research, Cambridge, MA. [ Google Scholar ]
- Alon, T. M. , Doepke, M. , Olmstead‐Rumsey, J. , & Tertilt, M. (2020). The impact of COVID‐19 on gender equality. NBER Working Paper No. 26947. National Bureau of Economic Research, Cambridge, MA. [ Google Scholar ]
- Alstadsæter, A. , Bratsberg, B. , Eielsen, G. , Kopczuk, W. , Markussen, S. , Raaum, O. , & Røed, K. (2020). The first weeks of the Coronavirus crisis: Who got hit, when and why? Evidence from Norway. NBER Working Paper No. 27131. National Bureau of Economic Research, Cambridge, MA. 10.3386/w27131 [ CrossRef ] [ Google Scholar ]
- Altig, D. , Baker, S. R. , Barrero, J. M. , Bloom, N. , Bunn, P. , Chen, S. , Davis, S. J. , Leather, J. , Meyer, B. H. , Mihaylov, E. , Mizen, P. , Parker, N. B. , Renault, T. , Smietanka, P. , & Thwaites, G. (2020). Economic uncertainty before and during the COVID‐19 pandemic . Journal of Public Economics , 191 , 104274. [ PMC free article ] [ PubMed ] [ Google Scholar ]
- Alvarez, F. E. , Argente, D. , & Lippi, F. (forthcoming). A simple planning problem for covid‐19 lockdown, testing and tracing . American Economic Review: Insights . [ Google Scholar ]
- American Medical Association . (2020). Managing mental health during COVID‐19. American Medical Association. Retrieved https://www.ama‐assn.org/delivering‐care/public‐health/managing‐mental‐health‐during‐covid‐19 [ Google Scholar ]
- Andersen, M. , Maclean, J. C. , Pesko, M. F. , & Simon, K. I. (2020). Effect of a federal paid sick leave mandate on working and staying at home: Evidence from cellular device data. NBER Working Paper No. 27138. National Bureau of Economic Research, Cambridge, MA. [ Google Scholar ]
- Andersen, T. M. , Schröder, P. J. H. , & Svarer, M. (2020). Designing Reopening Strategies in the Aftermath of COVID‐19 Lockdowns: Some Principles with an Application to Denmark. IZA Policy Paper No. 158. Institute of Labor Economics, Bonn, Germany. [ Google Scholar ]
- Andree, B. P. J. (2020). Incidence of COVID‐19 and connections with air pollution exposure: Evidence from the Netherlands. Policy Research Working Paper No. 9221. The World Bank, Washington, DC. Retrieved from http://documents.worldbank.org/curated/en/462481587756439003/Incidence-of-COVID-19-and-Connections-with-Air-Pollution-Exposure-Evidence-from-the-Netherlands [ Google Scholar ]
- Armbruster, S. , & Klotzbücher, V. (2020). Lost in lockdown? COVID‐19, social distancing, and mental health in Germany. Discussion Paper No. 2020–04. University of Freiburg, Wilfried Guth Endowed Chair for Constitutional Political Economy and Competition Policy, Germany. [ Google Scholar ]
- Aum, S. , Lee, S. Y. , & Shin, Y. (2020). COVID‐19 doesn't need lockdowns to destroy jobs: The effect of local outbreaks in Korea. NBER Working Paper No. 27264. National Bureau of Economic Research, Cambridge, MA . [ PMC free article ] [ PubMed ]
- Aum, S. , Lee, S. Y. , & Shin, Y. (2021). Inequality of fear and self‐quarantine: Is there a trade‐off between GDP and public health? Journal of Public Economics , 194 , 104354. 10.1016/j.jpubeco.2020.104354 [ CrossRef ] [ Google Scholar ]
- Avdiu, B. , & Nayyar, G. (2020). When face‐to‐face interactions become an occupational hazard: Jobs in the time of COVID‐19. Policy Research Working Paper No. 9240. The World Bank, Washington, DC. Retrieved from http://documents.worldbank.org/curated/en/173701589222966874/When-Face-to-Face-Interactions-Become-an-Occupational-Hazard-Jobs-in-the-Time-of-COVID-19 [ Google Scholar ]
- Baccini, L. , & Brodeur, A. (2021). Explaining governors’ response to the Covid‐19 pandemic in the United States . American Politics Research , 49 ( 2 ), 215–220. [ Google Scholar ]
- Baccini, L. , Brodeur, A. , & Weymouth, S. (2021) The COVID‐19 pandemic and the 2020 U.S. presidential election . Journal of Population Economics , 34 , 739–767. [ PMC free article ] [ PubMed ] [ Google Scholar ]
- Baert, S. , Lippens, L. , Moens, E. , Sterkens, P. , & Weytjens, J. (2020a). How do we think the COVID‐19 crisis will affect our careers (if any remain)? IZA Discussion Paper No. 13164. Institute of Labor Economics, Bonn, Germany. Retrieved from http://ftp.iza.org/dp13164.pdf [ Google Scholar ]
- Baert, S. , Lippens, L. , Moens, E. , Sterkens, P. , & Weytjens, J. (2020b). The COVID‐19 crisis and telework: A research survey on experiences, expectations and hopes. IZA Discussion Paper No. 13229. Institute of Labor Economics, Bonn, Germany. Retrieved from http://ftp.iza.org/dp13229.pdf [ PMC free article ] [ PubMed ] [ Google Scholar ]
- Baker, S. R. , Bloom, N. , & Davis, S. J. (2016). Measuring economic policy uncertainty . Quarterly Journal of Economics , 131 ( 4 ), 1593–1636. [ Google Scholar ]
- Baker, S. R. , Bloom, N. , Davis, S. J. , & Terry, S. J. (2020). COVID‐induced economic uncertainty. NBER Working Paper No. 26983. National Bureau of Economic Research. Cambridge, MA. [ Google Scholar ]
- Baker, S. R. , Farrokhnia, R. A. , Meyer, S. , Pagel, M. , & Yannelis, C. (2020). How does household spending respond to an epidemic? Consumption during the 2020 COVID‐19 pandemic . The Review of Asset Pricing Studies , 10 ( 4 ), 834–862. 10.1093/rapstu/raaa009 [ CrossRef ] [ Google Scholar ]
- Baldwin, R. (2020). Keeping the lights on: Economic medicine for a medical shock . VoxEU.Org . Retrieved from https://voxeu.org/article/how-should-we-think-about-containing-covid-19-economic-crisis
- Baqaee, D. , & Farhi, E. (2020). Supply and demand in disaggregated keynesian economies with an application to the Covid‐19 crisis. NBER Working Paper No. 27152. National Bureau of Economic Research, Cambridge, MA. [ Google Scholar ]
- Bargain, O. , & Aminjonov, U. (2020). Trust and compliance to public health policies in times of COVID‐19 . Journal of Public Economics , 192 , 104316. [ PMC free article ] [ PubMed ] [ Google Scholar ]
- Barrero, J. M. , Bloom, N. , & Davis, S. J. (2020). COVID‐19 is also a reallocation shock , BPEA Conference Drafts, June 25 2020. Brookings Papers on Economic Activity. Retrieved from https://www.brookings.edu/wp-content/uploads/2020/06/Barrero-et-al-conference-draft.pdf
- Barrios, J. M. , & Hochberg, Y. (forthcoming). Risk perception through the lens of politics in the time of the COVID‐19 pandemic . Journal of Financial Economics . [ PMC free article ] [ PubMed ] [ Google Scholar ]
- Barrios, J. M. , Benmelech, E. , Hochberg, Y. V. , Sapienza, P. , & Zingales, L. (2021). Civic capital and social distancing during the COVID‐19 pandemic . Journal of Public Economics , 193 , 104310. 10.1016/j.jpubeco.2020.104310 [ PMC free article ] [ PubMed ] [ CrossRef ] [ Google Scholar ]
- Bartik, A. W. , Bertrand, M. , Cullen, Z. , Glaeser, E. L. , Luca, M. , & Stanton, C. (2020). The impact of COVID‐19 on small business outcomes and expectations . Proceedings of the National Academy of Sciences , 117 ( 30 ), 17656–17666. 10.1073/pnas.2006991117 [ PMC free article ] [ PubMed ] [ CrossRef ] [ Google Scholar ]
- Bartik, A. W. , Cullen, Z. B. , Glaeser, E. L. , Luca, M. , & Stanton, C. T. (2020). What jobs are being done at home during the Covid‐19 crisis? Evidence from firm‐level surveys. NBER Working Paper No. 27422. National Bureau of Economic Research, Cambridge, MA. [ Google Scholar ]
- Bartos, V. , Bauer, M. , Cahlikova, J. , & Chytilová, J. (2020). COVID‐19 crisis fuels hostility against foreigners. IZA Discussion Paper No. 13250. Institute of Labor Economics, Bonn, Germany. [ Google Scholar ]
- Bartscher, A. K. , Seitz, S. , Siegloch, S. , Slotwinski, M. , & Wehrhöfer, N. (2020). Social capital and the spread of Covid‐19: Insights from European countries. IZA Discussion Paper No. 13310. Institute of Labor Economics, Bonn, Germany. Retrieved from http://ftp.iza.org/dp13310.pdf [ PMC free article ] [ PubMed ] [ Google Scholar ]
- Béland, L.‐P. , Brodeur, A. , & Wright, T. (2020). The short‐term economic consequences of Covid‐19: Exposure to disease, remote work and government response. IZA Discussion Paper No. 13159. Institute of Labor Economics, Bonn, Germany. Retrieved from http://ftp.iza.org/dp13159.pdf [ Google Scholar ]
- Béland, L.‐P. , Brodeur, A. , Mikola, D. , & Wright, T. (2020). The short‐term economic consequences of Covid‐19: Occupation tasks and mental health in Canada . IZA Discussion Paper No. 13254. Institute of Labor Economics, Bonn, Germany. Retrieved from http://ftp.iza.org/dp13254.pdf [ Google Scholar ]
- Berger, D. , Herkenhoff, K. , Huang, C. , & Mongey, S. (forthcoming). Testing and Reopening in an SEIR Model . Journal of Economic Dynamics . 10.1016/j.red.2020.11.003 [ CrossRef ] [ Google Scholar ]
- Bethune, Z. A. , & Korinek, A. (2020). Covid‐19 infection externalities: Pursuing herd immunity or containment? Covid Economics, Vetted and Real Time Papers, 11 , 1. Retrieved from https://cepr.org.uk/sites/default/files/CovidEconomics11.pdf#page=6 [ Google Scholar ]
- Bianchi, F. , Faccini, R. , & Melosi, L. (2020). Monetary and fiscal policies in times of large debt: Unity is strength (Revised May 2020) . Federal Reserve Bank of Chicago Research Paper Series . 10.21033/wp-2020-13 [ CrossRef ]
- Bigio, S. , Zhang, M. , & Zilberman, E. (2020). Transfers vs credit policy: Macroeconomic policy trade‐offs during Covid‐19. NBER Working Paper No. 27118. National Bureau of Economic Research, Cambridge, MA. [ Google Scholar ]
- Binder, C. (2020). Coronavirus fears and macroeconomic expectations . Review of Economics and Statistics , 102 : 721–730. [ Google Scholar ]
- Bogliacino, F. , Codagnone, C. , Montealegre, F. , Folkvord, F. , Gómez, C. E. , Charris, R. A. , Liva, G. , Villanueva, F. L. , & Veltri, G. A. (2020). Negative shocks predict change in cognitive function and preferences: Assessing the negative affect and stress hypothesis in the context of the COVID‐19 pandemic and the lockdown mitigation strategy [Preprint]. SocArXiv. 10.31235/osf.io/qhkf9 [ PMC free article ] [ PubMed ] [ CrossRef ]
- Bonaccorsi, G. , Pierri, F. , Cinelli, M. , Flori, A. , Galeazzi, A. , Porcelli, F. , Schmidt, A. L. , Valensise, C. M. , Scala, A. , Quattrociocchi, W. , & Pammolli, F. (2020). Economic and social consequences of human mobility restrictions under COVID‐19 . Proceedings of the National Academy of Sciences , 117 ( 27 ), 15530–15535. [ PMC free article ] [ PubMed ] [ Google Scholar ]
- Bonadio, B. , Huo, Z. , Levchenko, A. A. , & Pandalai‐Nayar, N. (2020). Global supply chains in the pandemic. NBER Working Paper No. 27224. National Bureau of Economic Research, Cambridge, MA. [ PMC free article ] [ PubMed ] [ Google Scholar ]
- Borjas, G. J. , & Cassidy, H. (2020). The adverse effect of the COVID‐19 labor market shock on immigrant employment. NBER Working Paper No. 27243. National Bureau of Economic Research, Cambridge, MA. [ Google Scholar ]
- Brinca, P. , Duarte, J. B. , & Faria e Castro, M. (2020). Measuring labor supply and demand shocks during COVID‐19. Working papers 2020‐011E. Federal Reserve Bank, St. Louis, MO. [ PMC free article ] [ PubMed ] [ Google Scholar ]
- Briscese, G. , Lacetera, N. , Macis, M. , & Tonin, M. (2020). Compliance with COVID‐19 social‐distancing measures in Italy: The role of expectations and duration. IZA Discussion Paper No. 13092. Institute of Labor Economics, Bonn, Germany. Retrieved from http://ftp.iza.org/dp13092.pdf [ Google Scholar ]
- Brodeur, A. , Clark, A. , Fleche, S. , & Powdthavee, N. (2021). COVID‐19, lockdowns and well‐being: Evidence from Google trends . Journal of Public Economics , 193 , 104346. [ PMC free article ] [ PubMed ] [ Google Scholar ]
- Brodeur, A. , Cook, N. , & Wright, T. (2021). On the effects of Covid‐19 safer‐at‐home policies on social distancing, car crashes and pollution . Journal of Environmental Economics and Management , 102427 . 10.1016/j.jeem.2021.102427 [ PMC free article ] [ PubMed ] [ CrossRef ] [ Google Scholar ]
- Brodeur, A. , Grigoryeva, I. , & Kattan, L. (2020). Stay‐at‐home orders, social distancing and trust. IZA Discussion Paper No. 13234. Institute of Labor Economics, Bonn, Germany. Retrieved from http://ftp.iza.org/dp13234.pdf [ PMC free article ] [ PubMed ] [ Google Scholar ]
- Brück, T. , Ferguson, N. , Justino, P. , & Stojetz, W. (2020). Trust in the time of corona. IZA Discussion Paper No. 13386. Institute of Labor Economics, Bonn, Germany. Retrieved from http://ftp.iza.org/dp13386.pdf [ Google Scholar ]
- Brynjolfsson, E. , Horton, J. J. , Ozimek, A. , Rock, D. , Sharma, G. , & TuYe, H.‐Y. (2020). COVID‐19 and remote work: An early look at US data. NBER Working Paper No. 27344. National Bureau of Economic Research, Cambridge, MA. 10.3386/w27344 [ CrossRef ] [ Google Scholar ]
- Bui, T. T. M. , Button, P. , & Picciotti, E. G. (2020). Early evidence on the impact of Coronavirus disease 2019 (COVID‐19) and the recession on older workers . Public Policy & Aging Report , 30 ( 4 ), 154–159. [ PMC free article ] [ PubMed ] [ Google Scholar ]
- Bursztyn, L. , Rao, A. , Roth, C. P. , & Yanagizawa‐Drott, D. H. (2020). Misinformation during a pandemic. NBER Working Paper No. 27417. National Bureau of Economic Research, Cambridge, MA. Retrieved from https://www.nber.org/papers/w27417.pdf [ Google Scholar ]
- Campello, M. , Kankanhalli, G. , & Muthukrishnan, P. (2020). Corporate hiring under COVID‐19: Labor market concentration, downskilling, and income inequality. NBER Working Paper No. 27208. National Bureau of Economic Research, Cambridge, MA. Retrieved from https://www.nber.org/papers/w27208.pdf [ Google Scholar ]
- Campos‐Mercade, P. , Meier, A. N. , Schneider, F. H. , & Wengström, E. (2021). Prosociality predicts health behaviors during the COVID‐19 pandemic . Journal of Public Economics , 195 , 104367. 10.1016/j.jpubeco.2021.104367 [ PMC free article ] [ PubMed ] [ CrossRef ] [ Google Scholar ]
- Carlsson‐Szlezak, P. , Reeves, M. , & Swartz, P. (2020a). Understanding the economic shock of Coronavirus . Harvard Business Review . Retrieved from https://hbr.org/2020/03/understanding-the-economic-shock-of-coronavirus
- Carlsson‐Szlezak, P. , Reeves, M. , & Swartz, P. (2020b). What coronavirus could Mea `1n for the global economy . Harvard Business Review . Retrieved from https://hbr.org/2020/03/what-coronavirus-could-mean-for-the-global-economy
- Céspedes, L. F. , Chang, R. , & Velasco, A. (2020). The macroeconomics of a pandemic: A minimalist model. NBER Working Paper No. 27228. National Bureau of Economic Research, Cambridge, MA. [ Google Scholar ]
- Chang, H. H. , & Meyerhoefer, C. D. (2020). COVID‐19 and the demand for online food shopping services: Empirical evidence from Taiwan . American Journal of Agricultural Economics . 103 ( 2 ), 448–465. 10.1111/ajae.12170 [ CrossRef ] [ Google Scholar ]
- Chang, R. , & Velasco, A. (2020). Economic policy incentives to preserve lives and livelihoods. NBER Working Paper No. 27020. National Bureau of Economic Research, Cambridge, MA. [ Google Scholar ]
- Chatterji, P. , & Li, Y. (2021). Effects of the COVID‐19 Pandemic on outpatient providers in the US . Medical Care . 59 ( 1 ), 58–61. 10.1097/MLR.0000000000001448 [ PubMed ] [ CrossRef ] [ Google Scholar ]
- Cheng, C. , Barceló, J. , Hartnett, A. S. , Kubinec, R. , & Messerschmidt, L. (2020). COVID‐19 government response event dataset (CoronaNet v.1.0) . Nature Human Behaviour , 4 ( 7 ), 756–768. [ PubMed ] [ Google Scholar ]
- Cheng, W. , Carlin, P. , Carroll, J. , Gupta, S. , Rojas, F. L. , Montenovo, L. , Nguyen, T. D. , Schmutte, I. M. , Scrivner, O. , Simon, K. I. , Wing, C. , & Weinberg, B. (2020). Back to business and (re)employing workers? Labor market activity during state COVID‐19 reopenings. NBER Working Paper No. 27419. National Bureau of Economic Research, Cambridge, MA .
- Chetty, R. , Friedman, J. N. , Hendren, N. , Stepner, M. , & Team, T. O. I. (2020). How did COVID‐19 and stabilization policies affect spending and employment? A new real‐time economic tracker based on private sector data. NBER Working Paper No. 27431. National Bureau of Economic Research, Cambridge, MA. [ Google Scholar ]
- Chiou, L. , & Tucker, C. (2020). Social distancing, internet access and inequality. NBER Working Paper No. 26982. National Bureau of Economic Research, Cambridge, MA. [ Google Scholar ]
- Cicala, S. , Holland, S. P. , Mansur, E. T. , Muller, N. Z. , & Yates, A. J. (2020). Expected health effects of reduced air pollution from COVID‐19 social distancing. NBER Working Paper No. 27135. National Bureau of Economic Research, Cambridge, MA. [ Google Scholar ]
- Cleevely, M. , Susskind, D. , Vines, D. , Vines, L. , & Wills, S. (2020). A workable strategy for COVID‐19 testing: Stratified periodic testing rather than universal random testing . Oxford Review of Economic Policy , 36 ( Supplement_1 ), S14–S37. [ Google Scholar ]
- Clemens, J. , & Veuger, S. (2020). Implications of the Covid‐19 Pandemic for State Government Tax Revenues . National Tax Journal . 73 ( 3 ), 619–644. 10.17310/ntj.2020.3.01 [ CrossRef ] [ Google Scholar ]
- Coelho, F. C. , Lana, R. M. , Cruz, O. G. , Villela, D. A. M. , Bastos, L. S. , Piontti, A. P. y , Davis, J. T. , Vespignani, A. , Codeço, C. T. , & Gomes, M. F. C. (2020). Assessing the spread of COVID‐19 in Brazil: Mobility, morbidity and social vulnerability . Plos One , 15 ( 9 ), e0238214. [ PMC free article ] [ PubMed ] [ Google Scholar ]
- Codagnone, C. , Bogliacino, F. , Gómez, C. , Charris, R. , Montealegre, F. , Liva, G. , Lupiáñez‐Villanueva, F. , Folkvord, F. , & Veltri, G. A. (2020). Assessing concerns for the economic consequence of the COVID‐19 response and mental health problems associated with economic vulnerability and negative economic shock in Italy, Spain, and the United Kingdom . Plos One , 15 ( 10 ), e0240876. [ PMC free article ] [ PubMed ] [ Google Scholar ]
- Codagnone, C. , Bogliacino, F. , Gómez, C. E. , Folkvord, F. , Liva, G. , Charris, R. A. , Montealegre, F. , Lupiáñez‐Villanueva, F. , & Veltri, G. A. (2020). Restarting “normal” life after Covid‐19 and the lockdown: Evidence from Spain, the United Kingdom, and Italy [Preprint]. SocArXiv. 10.31235/osf.io/vd4cq [ PMC free article ] [ PubMed ] [ CrossRef ]
- Coibion, O. , Gorodnichenko, Y. , & Weber, M. (2020). The cost of the Covid‐19 crisis: Lockdowns, macroeconomic expectations, and consumer spending. NBER Working Paper No. 27141. National Bureau of Economic Research, Cambridge, MA. [ Google Scholar ]
- Coibion, O. , Gorodnichenko, Y. , & Weber, M. (2020b). Labor markets during the COVID‐19 crisis: A preliminary view. NBER Working Paper No. 27017. National Bureau of Economic Research, Cambridge, MA. [ Google Scholar ]
- Couch, K. A. , Fairlie, R. W. , & Xu, H. (2020a). Early evidence of the impacts of COVID‐19 on minority unemployment . Journal of Public Economics , 192 , 104287. [ PMC free article ] [ PubMed ] [ Google Scholar ]
- Couch, K. A. , Fairlie, R. W. , & Xu, H. (2020b). Gender and the COVID‐19 labor market downturn. stanford institute for economic policy research. Working Paper No. 20–037. [ Google Scholar ]
- Coven, J. , & Gupta, A. (2020). Disparities in mobility responses to COVID‐19. NYU Stern Working Paper, 2020. Retrieved from https://static1.squarespace.com/static/56086d00e4b0fb7874bc2d42/t/5ebf201183c6f016ca3abd91/1589583893816/DemographicCovid.pdf [ Google Scholar ]
- Cui, Z. , Heal, G. , & Kunreuther, H. (2020). Covid‐19, shelter‐in place strategies and tipping. NBER Working Paper No. 27124. National Bureau of Economic Research, Cambridge, MA. [ Google Scholar ]
- Daniele, G. , Martinangeli, A. F. M. , Passarelli, F. , Sas, W. , & Windsteiger, L. (2020). Wind of change? Experimental survey evidence on the Covid‐19 shock and socio‐political attitudes in Europe. CESifo Working Paper No. 8517. Center for Economic Studies and Ifo Institute (CESifo). Retrieved from https://www.econstor.eu/handle/10419/223589 [ Google Scholar ]
- Dave, D. , Friedson, A. , Matsuzawa, K. , Sabia, J. J. , & Safford, S. (2020a). Were urban cowboys enough to control COVID‐19? Local shelter‐in‐place orders and coronavirus case growth . Journal of Urban Economics , 103294 . 10.1016/j.jue.2020.103294 [ PMC free article ] [ PubMed ] [ CrossRef ] [ Google Scholar ]
- Dave, D. M. , Friedson, A. I. , Matsuzawa, K. , McNichols, D. , & Sabia, J. J. (2020). Did the Wisconsin Supreme court restart a COVID‐19 epidemic? Evidence from a natural experiment. NBER Working Paper No. 27322. National Bureau of Economic Research, Cambridge, MA. [ Google Scholar ]
- Davillas, A. , & Jones, A. M. (2020). The COVID‐19 pandemic and its impact on inequality of opportunity in psychological distress in the UK. ISER Working Paper Series No. 2020–07. Institute for Social and Economic Research. Retrieved from https://www.iser.essex.ac.uk/research/publications/working-papers/iser/2020-07.pdf [ Google Scholar ]
- de Pedraza, P. , Guzi, M. , & Tijdens, K. (2020). Life dissatisfaction and anxiety in COVID‐19 pandemic . EUR 30243 EN, Publications Office of the European Union, Luxembourg, 2020, ISBN 978‐92‐76‐19341‐8. 10.2760/755327 [ CrossRef ]
- Demirguc‐Kunt, A. , Lokshin, M. M. , & Torre, I. (2020). The sooner, the better: The early economic impact of non‐pharmaceutical interventions during the COVID‐19 pandemic. Policy Research Working Paper No. 9257. The World Bank, Washington, DC. Retrieved from http://documents.worldbank.org/curated/en/636851590495700748/The-Sooner-the-Better-The-Early-Economic-Impact-of-Non-Pharmaceutical-Interventions-during-the-COVID-19-Pandemic [ Google Scholar ]
- Didier, T. , Huneeus, F. , Larrain, M. , & Schmukler, S. L. (2020). Financing firms in hibernation during the COVID‐19 pandemic. Policy Research Working Paper No. 9236. The World Bank, Washington, DC. Retrieved from http://documents.worldbank.org/curated/en/818801588952012929/Financing-Firms-in-Hibernation-during-the-COVID-19-Pandemic [ Google Scholar ]
- Dingel, J. I. , & Neiman, B. (2020). How many jobs can be done at home? Journal of Public Economics , 189 , 104235. [ PMC free article ] [ PubMed ] [ Google Scholar ]
- Dong, E. , Du, H. , & Gardner, L. (2020). An interactive web‐based dashboard to track COVID‐19 in real time Lancet . Infectious Diseases , 20 ( 5 ), 533–534. [ PMC free article ] [ PubMed ] [ Google Scholar ]
- Durante, R. , Guiso, L. , & Gulino, G. (2021). Asocial capital: Civic culture and social distancing during COVID‐19 . Journal of Public Economics , 194 , 104342. 10.1016/j.jpubeco.2020.104342 [ PMC free article ] [ PubMed ] [ CrossRef ] [ Google Scholar ]
- Eberhardt, J. N. , Breuckmann, N. P. , & Eberhardt, C. S. (2020). Multi‐stage group testing improves efficiency of large‐scale COVID‐19 screening . Journal of Clinical Virology , 104382 . 10.1016/j.jcv.2020.104382 [ PMC free article ] [ PubMed ] [ CrossRef ] [ Google Scholar ]
- Egorov, G. , Enikolopov, R. , Makarin, A. , & Petrova, M. (2021). Divided we stay home: Social distancing and ethnic diversity . Journal of Public Economics , 194 , 104328. 10.1016/j.jpubeco.2020.104328 [ CrossRef ] [ Google Scholar ]
- Eichenbaum, M. S. , Rebelo, S. , & Trabandt, M. (2020). The macroeconomics of epidemics NBER Working Paper No. 26882. National Bureau of Economic Research, Cambridge, MA. [ Google Scholar ]
- Elenev, V. , Landvoigt, T. , & Van Nieuwerburgh, S. (2020). Can the Covid bailouts save the economy? NBER Working Paper No. 27207. National Bureau of Economic Research, Cambridge, MA. [ Google Scholar ]
- Elgin, C. , Basbug, G. , & Yalaman, A. (2020). Economic policy responses to a pandemic: Developing the Covid‐19 economic stimulus index . COVID Economics, Vetted and Real‐Time Papers , 3 , 40–53. [ Google Scholar ]
- Eppinger, P. S. , Felbermayr, G. , Krebs, O. , & Kukharskyy, B. (2020). Covid‐19 shocking global value chains. Kiel Working Paper No. 2167. Kiel Institute for the World Economy, Germany. Retrieved from https://www.econstor.eu/handle/10419/224061 [ Google Scholar ]
- Etheridge, B. , & Spantig, L. (2020). The gender gap in mental well‐being during the Covid‐19 outbreak: Evidence from the UK. ISER Working Paper Series No. 2020–08. Institute for Social and Economic Research. Retrieved from https://www.iser.essex.ac.uk/research/publications/working-papers/iser/2020-08.pdf [ Google Scholar ]
- Fairlie, R. (2020). The impact of COVID‐19 on small business owners: Evidence from the first three months after widespread social‐distancing restrictions . Journal of Economics & Management Strategy , 29 ( 4 ), 727–740. [ PMC free article ] [ PubMed ] [ Google Scholar ]
- Fan, Y. , Orhun, A. Y. , & Turjeman, D. (2020). Heterogeneous actions, beliefs, constraints and risk tolerance during the COVID‐19 pandemic. NBER Working Paper No. 27211. National Bureau of Economic Research, Cambridge, MA. [ Google Scholar ]
- Fang, H. , Wang, L. , & Yang, Y. (2020). Human mobility restrictions and the spread of the novel coronavirus (2019‐nCov) in China . Journal of Public Economics , 191 , 104272. 10.1016/j.jpubeco.2020.104272 [ PMC free article ] [ PubMed ] [ CrossRef ] [ Google Scholar ]
- Farboodi, M. , Jarosch, G. , & Shimer, R. (2020). Internal and external effects of social distancing in a pandemic. NBER Working Paper No. 27059. National Bureau of Economic Research, Cambridge, MA. [ Google Scholar ]
- Farré, L. , Fawaz, Y. , González, L. , & Graves, J. (2020). How the COVID‐19 lockdown affected gender inequality in paid and unpaid work in Spain. IZA Discussion Paper No. 13434. Institute of Labor Economics, Bonn, Germany. Retrieved from http://ftp.iza.org/dp13434.pdf [ Google Scholar ]
- Ferguson, N. , Laydon, D. , Nedjati Gilani, G. , Imai, N. , Ainslie, K. , Baguelin, M. , Bhatia, S. , Boonyasiri, A. , Cucunuba Perez, Z. , Cuomo‐Dannenburg, G. , Dighe, A. , Dorigatti, I. , Fu, H. , Gaythorpe, K. , Green, W. , Hamlet, A. , Hinsley, W. , Okell, L. , Van Elsland, S. , …, & Ghani, A. (2020). Impact of non‐pharmaceutical interventions (NPIs) to reduce COVID19 mortality and healthcare demand. Imperial College London. [ Google Scholar ]
- Fetzer, T. R. , Witte, M. , Hensel, L. , Jachimowicz, J. , Haushofer, J. , Ivchenko, A. , Caria, S. , Reutskaja, E. , Roth, C. P. , Fiorin, S. , Gómez, M. , Kraft‐Todd, G. , Götz, F. M. , & Yoeli, E. (2020). Global behaviors and perceptions at the onset of the COVID‐19 pandemic. NBER Working Paper No. 27082. National Bureau of Economic Research, Cambridge, MA. [ Google Scholar ]
- Fezzi, C. , & Fanghella, V. (2020). Real‐time estimation of the short‐run impact of COVID‐19 on economic activity using electricity market data . Environmental and Resource Economics , 76 ( 4 ), 885–900. [ PMC free article ] [ PubMed ] [ Google Scholar ]
- Fong, M. W. , Gao, H. , Wong, J. Y. , Xiao, J. , Shiu, E. Y. C. , Ryu, S. , & Cowling, B. J. (2020). Nonpharmaceutical measures for pandemic influenza in nonhealthcare settings—Social distancing measures . Emerging Infectious Diseases , 26 ( 5 ), 976–984. [ PMC free article ] [ PubMed ] [ Google Scholar ]
- Fornaro, L. , & Wolf, M. (2020). Covid‐19 coronavirus and macroeconomic policy. Barcelona GSE Working Paper No. 1168. Barcelona Graduate School of Economics, Barcelona, Spain. Retrieved from https://fondazionecerm.it/wp-content/uploads/2020/04/GSE-Covid-19-Coronavirus-and-Macroeconomic-Policy.pdf [ Google Scholar ]
- Forsythe, E. , Kahn, L. B. , Lange, F. , & Wiczer, D. (2020). Labor demand in the time of COVID‐19: Evidence from vacancy postings and UI claims . Journal of Public Economics , 189 , 104238. [ PMC free article ] [ PubMed ] [ Google Scholar ]
- Friedson, A. I. , McNichols, D. , Sabia, J. J. , & Dave, D. (2020). Did California's shelter‐in‐place order work? Early Coronavirus‐related public health effects. NBER Working Paper No. 26992. National Bureau of Economic Research, Cambridge, MA. [ Google Scholar ]
- Galasso, V. , Pons, V. , Profeta, P. , Becher, M. , Brouard, S. , & Foucault, M. (2020). Gender differences in COVID‐19 attitudes and behavior: Panel evidence from eight countries . Proceedings of the National Academy of Sciences , 117 ( 44 ), 27285. [ PMC free article ] [ PubMed ] [ Google Scholar ]
- Galeazzi, A. , Cinelli, M. , Bonaccorsi, G. , Pierri, F. , Schmidt, A. L. , Scala, A. , Pammolli, F. , & Quattrociocchi, W. (2020). Human mobility in response to COVID‐19 in France, Italy and UK . ArXiv:2005.06341 [Physics] . Retrieved from http://arxiv.org/abs/2005.06341 [ PMC free article ] [ PubMed ]
- Goldstein, J. R. , & Lee, R. D. (2020). Demographic perspectives on the mortality of COVID‐19 and other epidemics . Proceedings of the National Academy of Sciences , 117 ( 36 ), 22035. [ PMC free article ] [ PubMed ] [ Google Scholar ]
- Gollier, C. , & Gossner, O. ((2020). Group testing against Covid‐19 . Retrieved from http://eprints.lse.ac.uk/104228/3/covid_economics.pdf
- Gonzalez‐Eiras, M. , & Niepelt, D. (2020). On the optimal “Lockdown” during an epidemic. Working Paper No. 20.01. Swiss National Bank Study Center Gerzensee. Retrieved from https://szgerzensee.ch/fileadmin/Dateien_Anwender/Dokumente/working_papers/wp-2001.pdf [ Google Scholar ]
- Gourinchas, P.‐O. (2020). Flattening the pandemic and recession curves . Mitigating the COVID Economic Crisis: Act Fast and Do Whatever. Retrieved from http://viet-studies.net/kinhte/COVIDEconomicCrisis.pdf#page=38
- Greenstone, M. , & Nigam, V. (2020). Does social distancing matter? . University of Chicago, Becker Friedman Institute for Economics; Working Paper No. 2020–26. Retrieved from http://iepecdg.com.br/wp-content/uploads/2020/04/SSRN-id3561244.pdf [ Google Scholar ]
- Gregory, V. , Menzio, G. , & Wiczer, D. G. (2020). Pandemic recession: L or V‐shaped? Quarterly Review , 40 ( 1 ). 10.21034/qr.4011 [ CrossRef ] [ Google Scholar ]
- Guerrieri, V. , Lorenzoni, G. , Straub, L. , & Werning, I. (2020). Macroeconomic implications of COVID‐19: Can negative supply shocks cause demand shortages? NBER Working Paper No. 26918. National Bureau of Economic Research, Cambridge, MA. 10.3386/w26918 [ CrossRef ] [ Google Scholar ]
- Gupta, S. , Montenovo, L. , Nguyen, T. D. , Rojas, F. L. , Schmutte, I. M. , Simon, K. I. , Weinberg, B. A. , & Wing, C. (2020). Effects of social distancing policy on labor market outcomes. NBER Working Paper No. 27280. National Bureau of Economic Research, Cambridge, MA. [ Google Scholar ]
- Hamermesh, D. S. (2020). Life satisfaction, loneliness and togetherness, with an application to Covid‐19 lock‐downs . Review of Economics of the Household , 18 : 983–1000. [ PMC free article ] [ PubMed ] [ Google Scholar ]
- Harris, J. E. (2020). Reopening Under COVID‐19: What to Watch For. NBER Working Paper No. 27166. National Bureau of Economic Research, Cambridge, MA. [ Google Scholar ]
- Hartl, T. , Wälde, K. , & Weber, E. (2020). Measuring the impact of the German public shutdown on the spread of Covid‐19 . Center for Economic Policy Research , 1 , 25–42. [ Google Scholar ]
- Hassan, T. A. , Hollander, S. , van Lent, L. , & Tahoun, A. (2020). Firm‐level exposure to epidemic diseases: Covid‐19, SARS, and H1N1. NBER Working Paper No. 26971. National Bureau of Economic Research, Cambridge, MA. [ Google Scholar ]
- He, G. , Pan, Y. , & Tanaka, T. (2020). COVID‐19, city lockdowns, and air pollution: Evidence from China. HKUST IEMS Working Paper No. 2019‐72, HongKong .
- Hermosilla, M. , Ni, J. , Wang, H. , & Zhang, J. (2020). Unmet needs: Healthcare crowd‐out during the COVID‐19 pandemic . Retrieved from https://ssrn.com/abstract=3607594 or 10.2139/ssrn.3607594 [ CrossRef ]
- Hsiang, S. , Allen, D. , Annan‐Phan, S. , Bell, K. , Bolliger, I. , Chong, T. , Druckenmiller, H. , Huang, L. Y. , Hultgren, A. , Krasovich, E. , Lau, P. , Lee, J. , Rolf, E. , Tseng, J. , & Wu, T. (2020). The effect of large‐scale anti‐contagion policies on the COVID‐19 pandemic . Nature , 584 ( 7820 ), 262–267. [ PubMed ] [ Google Scholar ]
- International Monetary Fund . (2020). World economic outlook update, October 2020: A long and difficult ascent . IMF. Retrieved from https://www.imf.org/en/Publications/WEO/Issues/2020/09/30/world-economic-outlook-october-2020
- Jinjarak, Y. , Ahmed, R. , Nair‐Desai, S. , Xin, W. , & Aizenman, J. (2020). Accounting for global COVID‐19 diffusion patterns, January–April 2020 . Economics of Disasters and Climate Change , 4 ( 3 ), 515–559. [ PMC free article ] [ PubMed ] [ Google Scholar ]
- John Hopkins University . (2020). New cases of COVID‐19 in world countries . Johns Hopkins Coronavirus Resource Center. Retrieved from https://coronavirus.jhu.edu/data/new-cases [ Google Scholar ]
- Jones, C. J. , Philippon, T. , & Venkateswaran, V. (2020). Optimal mitigation policies in a pandemic: Social distancing and working from home. NBER Working Paper No. 26984. National Bureau of Economic Research, Cambridge, MA. [ Google Scholar ]
- Jordà, Ò. , Singh, S. R. , & Taylor, A. M. (2020). Longer‐run economic consequences of pandemics. NBER Working Paper No. 26934. National Bureau of Economic Research, Cambridge, MA. [ Google Scholar ]
- Juranek, S. , & Zoutman, F. (2020). The effect of social distancing measures on the demand for intensive care: Evidence on COVID‐19 in Scandinavia. NHH Discussion Paper 02/2020 . Retrieved from https://openaccess.nhh.no/nhh-xmlui/bitstream/handle/11250/2652920/0220.pdf?sequence=1&isAllowed=y
- Kahn, L. B. , Lange, F. , & Wiczer, D. G. (2020). Labor demand in the time of COVID‐19: Evidence from vacancy postings and UI claims. NBER Working Paper No. 27061. National Bureau of Economic Research, Cambridge, MA. [ PMC free article ] [ PubMed ] [ Google Scholar ]
- Kapteyn, A. , Angrisani, M. , Bennett, D. , Bruin, W. B. de, Darling, J. , Gutsche, T. , Liu, Y. , Meijer, E. , Perez‐Arce, F. , Schaner, S. , Thomas, K. , & Weerman, B. (2020). Tracking the effect of the COVID‐19 pandemic on the lives of American households . Survey Research Methods , 14 ( 2 ), 179–186. [ Google Scholar ]
- Knittel, C. R. , & Ozaltun, B. (2020). What does and does not correlate with COVID‐19 death rates. NBER Working Paper No. 27391. National Bureau of Economic Research, Cambridge, MA. Retrieved from http://www.nber.org/papers/w27391 [ Google Scholar ]
- Kopecky, K. A. , & Zha, T. (2020). Impacts of COVID‐19: Mitigation efforts versus herd immunity. Policy Hub No. 03–2020. Federal Reserve Bank of Atlanta. Retrieved from https://www.frbatlanta.org/-/media/documents/research/publications/policy-hub/2020/04/17/impacts-of-covid-19-mitigation-efforts-versus-herd-immunity.pdf [ Google Scholar ]
- Kozlowski, J. , Veldkamp, L. , & Venkateswaran, V. (2020). Scarring body and mind: The long‐term belief‐scarring effects of COVID‐19. NBER Working Paper No. 27439. National Bureau of Economic Research, Cambridge, MA. [ Google Scholar ]
- Kraemer, M. U. G. , Yang, C.‐H. , Gutierrez, B. , Wu, C.‐H. , Klein, B. , Pigott, D. M. , Group, O. C.‐19 D. W. , Plessis, L. d. , Faria, N. R. , Li, R. , Hanage, W. P. , Brownstein, J. S. , Layan, M. , Vespignani, A. , Tian, H. , Dye, C. , Pybus, O. G. , & Scarpino, S. V. (2020). The effect of human mobility and control measures on the COVID‐19 epidemic in China . Science , 368 ( 6490 ), 493–497. [ PMC free article ] [ PubMed ] [ Google Scholar ]
- Kurmann, A. , Lale, E. , & Ta, L. (2020). The impact of COVID‐19 on US employment and hours: Real‐time estimates with homebase data . Retreived from http://www.andrekurmann.com/hb_covid .
- Lewis, D. , Mertens, K. , & Stock, J. H. (2020). U.S. economic activity during the early weeks of the SARS‐Cov‐2 outbreak. NBER Working Paper No. 26954. National Bureau of Economic Research, Cambridge, MA. [ Google Scholar ]
- Lin, P. Z. , & Meissner, C. M. (2020). A note on long‐run persistence of public health outcomes in pandemics. NBER Working Paper No. 27119. National Bureau of Economic Research, Cambridge, MA. [ Google Scholar ]
- Lomas, N . (2020). Google is now publishing coronavirus mobility reports, feeding off users’ location history. TechCrunch. Retrieved from https://social.techcrunch.com/2020/04/03/google-is-now-publishing-coronavirus-mobility-reports-feeding-off-users-location-history/ [ Google Scholar ]
- Lu, H. , Nie, P. , & Qian, L. (2020). Do quarantine experiences and attitudes towards COVID‐19 affect the distribution of mental health in China? A quantile regression analysis . Applied Research in Quality of Life. 10.1007/s11482-020-09851-0 [ PMC free article ] [ PubMed ] [ CrossRef ]
- Ludvigson, S. C. , Ma, S. , & Ng, S. (2020). Covid19 and the macroeconomic effects of costly disasters. NBER Working Paper No. 26987. National Bureau of Economic Research, Cambridge, MA. [ Google Scholar ]
- Makris, M. (2020). Covid and social distancing with a heterogenous population. School of Economics Discussion Paper No. 2002. University of Kent. Retrieved from https://www.kent.ac.uk/economics/repec/2002.pdf [ Google Scholar ]
- Maloney, W. F. , & Taskin, T. (2020). Determinants of social distancing and economic activity during COVID‐19: A global view (No. WPS9242; pp. 1–23). The World Bank. Retrieved from http://documents.worldbank.org/curated/en/325021589288466494/Determinants-of-Social-Distancing-and-Economic-Activity-during-COVID-19-A-Global-View [ Google Scholar ]
- Mandavilli, A. (2020). Wondering about social distancing? The New York Times . Retrieved from https://www.nytimes.com/2020/03/16/smarter-living/coronavirus-social-distancing.html
- Martín‐Calvo, D. , Aleta, A. , Pentland, A. , Moreno, Y. , & Moro, E. (2020). Effectiveness of social distancing strategies for protecting a community from a pandemic with a data‐driven contact network based on census and real‐world mobility data . MIT Connection Science . Retrieved from https://connection.mit.edu/sites/default/files/publication-pdfs/Preliminary_Report_Effectiveness_of_social_distance_strategies_COVID-19%20%281%29.pdf
- McKibbin, W. J. , & Fernando, R. (2020). The global macroeconomic impacts of COVID‐19: seven scenarios. CAMA Working Paper No. 19/2020. The Center for Applied Macroeconomic Analysis, Australian National University. Retrieved from https://cama.crawford.anu.edu.au/sites/default/files/publication/cama_crawford_anu_edu_au/2020-03/19_2020_mckibbin_fernando_0.pdf [ Google Scholar ]
- McLaren, J. (2020). Racial disparity in COVID‐19 deaths: Seeking economic roots with census data. NBER Working Paper No. 27407. National Bureau of Economic Research, Cambridge, MA. Retrieved from http://www.nber.org/papers/w27407 [ Google Scholar ]
- Montenovo, L. , Jiang, X. , Rojas, F. L. , Schmutte, I. M. , Simon, K. I. , Weinberg, B. A. , & Wing, C. (2020). Determinants of disparities in Covid‐19 job losses. NBER working paper No. 27132. National Bureau of Economic Research, Cambridge, MA. [ Google Scholar ]
- Mulligan, C. B. (2020). Economic activity and the value of medical innovation during a pandemic. NBER Working Paper No. 27060. National Bureau of Economic Research, Cambridge, MA. [ Google Scholar ]
- Murray, G. R. , & Murray, S. M. (2020). Following doctors’ advice: explaining the issuance of stay‐at‐home orders related to the coronavirus disease 2019 (COVID‐19) by U.S. governors (OSF Preprints No. 92ay6). Center for Open Science. 10.31219/osf.io/92ay6 [ CrossRef ]
- Nguyen, T. D. , Gupta, S. , Andersen, M. , Bento, A. , Simon, K. I. , & Wing, C. (2020). Impacts of state reopening policy on human mobility. NBER Working Paper No. 27235. National Bureau of Economic Research, Cambridge, MA. [ Google Scholar ]
- OECD . (2020). OECD Economic Outlook, Volume 2020 Issue 1: Preliminary version | OECD iLibrary . Retrieved from https://www.oecd-ilibrary.org/sites/0d1d1e2e-en/index.html?itemId=/content/publication/0d1d1e2e-en
- Oliver, N. , Letouzé, E. , Sterly, H. , Delataille, S. , De Nadai, M. , Lepri, B. , Lambiotte, R. , Benjamins, R. , Cattuto, C. , Colizza, V. , de Cordes, N. , Fraiberger, S. P. , Koebe, T. , Lehmann, S. , Murillo, J. , Pentland, A. , Pham, P. N. , Pivetta, F. , Salah, A. A. , & Vinck, P. (2020). Mobile phone data and COVID‐19: Missing an opportunity? ArXiv:2003.12347 . Retrieved from https://arxiv.org/ftp/arxiv/papers/2003/2003.12347.pdf [ PMC free article ] [ PubMed ]
- Oswald, A. J. , & Powdthavee, N. (2020). The case for releasing the young from lockdown: A briefing paper for policymakers. IZA Discussion Papers No. 13113. Institute of Labor Economics. Retrieved from http://ftp.iza.org/dp13113.pdf [ Google Scholar ]
- Papageorge, N. W. , Zahn, M. V. , Belot, M. , van den Broek‐Altenburg, E. , Choi, S. , Jamison, J. C. , & Tripodi, E. (2020). Socio‐demographic factors associated with self‐protecting behavior during the Covid‐19 pandemic . Journal of Population Economics , 34 , 691–738. 10.1007/s00148-020-00818-x [ PMC free article ] [ PubMed ] [ CrossRef ] [ Google Scholar ]
- Papanikolaou, D. , & Schmidt, L. D. W. (2020). Working remotely and the supply‐side impact of Covid‐19. NBER Working Paper No. 27330. National Bureau of Economic Research, Cambridge, MA. [ Google Scholar ]
- Porcher, S. (2020). “Contagion”: The determinants of governments’ public health responses to COVID‐19 all around the world . HAL Archives. Retrieved from https://halshs.archives-ouvertes.fr/halshs-02567286/document
- Qiu, Y. , Chen, X. , & Shi, W. (2020). Impacts of social and economic factors on the transmission of coronavirus disease 2019 (COVID‐19) in China . Journal of Population Economics , 1–46. 10.1007/s00148-020-00778-2 [ PMC free article ] [ PubMed ] [ CrossRef ] [ Google Scholar ]
- Rampini, A. A. (2020). Sequential lifting of COVID‐19 interventions with population heterogeneity. NBER Working Paper No. 27063. National Bureau of Economic Research, Cambridge, MA. [ Google Scholar ]
- Rojas, F. L. , Jiang, X. , Montenovo, L. , Simon, K. I. , Weinberg, B. A. , & Wing, C. (2020). Is the cure worse than the problem itself? Immediate labor market effects of COVID‐19 case rates and school closures in the U.S. NBER working paper No. 27127. National Bureau of Economic Research, Cambridge, MA. [ Google Scholar ]
- Roser, M. , Ritchie, H. , Ortiz‐Ospina, E. , & Hasell, J. (2020). Coronavirus pandemic (COVID‐19) . Our World in Data . Retrieved from https://ourworldindata.org/coronavirus
- Schild, L. , Ling, C. , Blackburn, J. , Stringhini, G. , Zhang, Y. , & Zannettou, S. (2020). “Go eat a bat, Chang!”: An early look on the emergence of sinophobic behavior on web communities in the face of COVID‐19 . ArXiv:2004.04046 . Retrieved from http://arxiv.org/abs/2004.04046
- Silverman, J. D. , Hupert, N. , & Washburne, A. D. (2020). Using influenza surveillance networks to estimate state‐specific prevalence of SARS‐CoV‐2 in the United States . Science Translational Medicine . 10.1126/scitranslmed.abc1126 [ PMC free article ] [ PubMed ] [ CrossRef ] [ Google Scholar ]
- Simonov, A. , Sacher, S. K. , Dubé, J.‐P. H. , & Biswas, S. (2020). The persuasive effect of Fox news: Non‐compliance with social distancing during the Covid‐19 pandemic. NBER Working Paper No. 27237. National Bureau of Economic Research, Cambridge, MA. [ Google Scholar ]
- Taipale, J. , Romer, P. , & Linnarsson, S. (2020). Population‐scale testing can suppress the spread of COVID‐19 . MedRxiv, 2020.04.27.20078329. 10.1101/2020.04.27.20078329 [ CrossRef ]
- Tubadji, A. , Boy, F. , & Webber, D. (2020). Narrative economics, public policy and mental health . Center for Economic Policy Research , 20 , 109–131. [ Google Scholar ]
- Weder di Mauro, B. (2020). Macroeconomics of the flu. Center for Economic Policy Research. Retrieved from http://repository.graduateinstitute.ch/record/298218 [ Google Scholar ]
- Wells, C. R. , Townsend, J. P. , Pandey, A. , Krieger, G. , Singer, B. , McDonald, R. H. , Moghadas, S. M. , & Galvani, A. P. (2020). Optimal COVID‐19 quarantine and testing strategies . MedRxiv, 2020.10.27.20211631. 10.1101/2020.10.27.20211631 [ PMC free article ] [ PubMed ] [ CrossRef ]
- World Bank . (2020). Global Economic Prospects . Washington, DC: World Bank. Retrieved from https://openknowledge.worldbank.org/handle/10986/33748 [ Google Scholar ]
- Yasenov, V. I. (2020). Who can work from home? IZA Discussion Paper No. 13197. Institute of Labor Economics, Cambridge, MA. Retrieved from http://ftp.iza.org/dp13197.pdf [ Google Scholar ]

The Economic Impact of COVID-19 around the World
This article provides an account of the worldwide economic impact of the COVID-19 shock. In 2020, it severely impacted output growth and employment, particularly in middle-income countries. Governments responded primarily by increasing expenditure, supported by an expansion of the supply of money and debt. These policies did not put upward pressure on prices until 2021. International trade was severely disrupted across all regions in 2020 but subsequently recovered. For 2021, we find that the adverse effects of the COVID-19 shock on output and prices were significant and persistent, especially in emerging and developing countries.
Fernando Martin is an assistant vice president and economist, Juan M. Sánchez is a vice president and economist, and Olivia Wilkinson is a senior research associate at the Federal Reserve Bank of St. Louis.
INTRODUCTION
For over two years, the world has been battling the health and economic consequences of the COVID-19 pandemic. As of the writing of this article, deaths attributed to COVID-19 have surpassed six-and-a-half million people. Global economic growth was severely impacted: World output by the end of 2021 was more than 4 percentage points below its pre-pandemic trend. International trade was also significantly disrupted at the onset of the pandemic. The pandemic also prompted a strong policy response, resulting in a rise of government deficits and debt as well as widespread increases in the money supply. Finally, after an initial decline, prices have soared, resulting in elevated inflation rates.
This article provides an account of the worldwide economic impact of the COVID-19 shock. This shock was not felt simultaneously around the world, and mitigation policies, both health related and economic, varied substantially across countries. Yet there are some significant similarities in outcomes, especially when considering the pandemic period as a whole. Our analysis focuses on the shock's effects on specific groups of countries, related by their level of development and geographical location.
We find that the COVID-19 shock severely impacted output growth and employment in 2020, particularly in middle-income countries. The government response, mainly consisting of increased expenditure, implied a rise in debt levels. Advanced countries, having easier access to credit markets, experienced the highest increase in indebtedness. All regions also relied on monetary policy to support the fiscal expansion, and hence the money supply increased everywhere. The specific circumstances surrounding the shock implied that the expansionary fiscal and monetary policies did not put upward pressure on prices until 2021. International trade was severely disrupted across all regions in 2020 but subsequently recovered. When extending the analysis to 2021, we find that the adverse effects of the shock on output and prices have been significant and persistent, especially in emerging and developing countries.
Read the full article .
Cite this article

Subscribe to Our Newsletter
Stay current with brief essays, scholarly articles, data news, and other information about the economy from the Research Division of the St. Louis Fed.
SUBSCRIBE TO THE RESEARCH DIVISION NEWSLETTER
Research division.
- Legal and Privacy
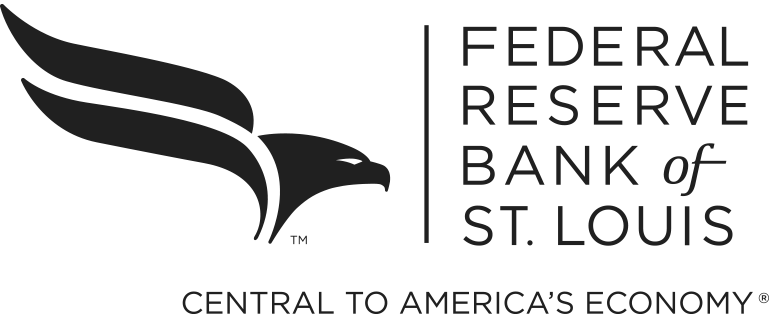
One Federal Reserve Bank Plaza St. Louis, MO 63102
Information for Visitors

You are using an outdated browser. Please upgrade your browser to improve your experience.
- Publications
- IZA Discussion Papers
- A Literature Review of the Economics of COVID-19
IZA DP No. 13411: A Literature Review of the Economics of COVID-19
published in: Journal of Economic Surveys, 2021, 35(4), 1007-1044
The goal of this piece is to survey the emerging and rapidly growing literature on the economic consequences of COVID-19 and government response, and to synthetize the insights emerging from a very large number of studies. This survey (i) provides an overview of the data sets used to measure social distancing and COVID-19 cases and deaths; (ii) reviews the literature on the determinants of compliance and effectiveness of social distancing; (iii) summarizes the literature on the socio-economic consequences of COVID-19 and government interventions, focusing on labor, health, gender, discrimination and environmental aspects; and (iv) discusses policy proposals.
- coronavirus
Cookie settings
These necessary cookies are required to activate the core functionality of the website. An opt-out from these technologies is not available.
In order to further improve our offer and our website, we collect anonymous data for statistics and analyses. With the help of these cookies we can, for example, determine the number of visitors and the effect of certain pages on our website and optimize our content.
- Systematic review update
- Open access
- Published: 16 February 2024
The global economic burden of COVID-19 disease: a comprehensive systematic review and meta-analysis
- Ahmad Faramarzi ORCID: orcid.org/0000-0001-5661-8991 1 ,
- Soheila Norouzi ORCID: orcid.org/0000-0002-3028-7861 1 ,
- Hossein Dehdarirad 2 ,
- Siamak Aghlmand 1 ,
- Hasan Yusefzadeh 1 &
- Javad Javan-Noughabi 3
Systematic Reviews volume 13 , Article number: 68 ( 2024 ) Cite this article
1548 Accesses
1 Citations
7 Altmetric
Metrics details
The COVID-19 pandemic has caused a considerable threat to the economics of patients, health systems, and society.
This meta-analysis aims to quantitatively assess the global economic burden of COVID-19.
A comprehensive search was performed in the PubMed, Scopus, and Web of Science databases to identify studies examining the economic impact of COVID-19. The selected studies were classified into two categories based on the cost-of-illness (COI) study approach: top-down and bottom-up studies. The results of top-down COI studies were presented by calculating the average costs as a percentage of gross domestic product (GDP) and health expenditures. Conversely, the findings of bottom-up studies were analyzed through meta-analysis using the standardized mean difference.
The implemented search strategy yielded 3271 records, of which 27 studies met the inclusion criteria, consisting of 7 top-down and 20 bottom-up studies. The included studies were conducted in various countries, including the USA (5), China (5), Spain (2), Brazil (2), South Korea (2), India (2), and one study each in Italy, South Africa, the Philippines, Greece, Iran, Kenya, Nigeria, and the Kingdom of Saudi Arabia. The results of the top-down studies indicated that indirect costs represent 10.53% of GDP, while the total estimated cost accounts for 85.91% of healthcare expenditures and 9.13% of GDP. In contrast, the bottom-up studies revealed that the average direct medical costs ranged from US $1264 to US $79,315. The meta-analysis demonstrated that the medical costs for COVID-19 patients in the intensive care unit (ICU) were approximately twice as high as those for patients in general wards, with a range from 0.05 to 3.48 times higher.
Conclusions
Our study indicates that the COVID-19 pandemic has imposed a significant economic burden worldwide, with varying degrees of impact across countries. The findings of our study, along with those of other research, underscore the vital role of economic consequences in the post-COVID-19 era for communities and families. Therefore, policymakers and health administrators should prioritize economic programs and accord them heightened attention.
Peer Review reports
Coronavirus disease 2019 (COVID-19) is a respiratory infection instigated by the severe acute respiratory syndrome coronavirus 2 (SARS-COV-2), first identified in Wuhan, China, in December 2019. The disease has since proliferated globally at an alarming rate, prompting the World Health Organization (WHO) to declare a pandemic on March 11, 2020 [ 1 ]. As of February 21, 2023, the global total of confirmed COVID-19 cases stands at 757,264,511, with a death toll of 6,850,594 [ 2 ].
Patients afflicted with COVID-19 exhibit a range of symptoms, including flu-like manifestations, acute respiratory failure, thromboembolic diseases, and organ dysfunction or failure [ 3 ]. Moreover, these patients have had to adapt to significant changes in their environment, such as relocating for quarantine purposes, remote work or job loss, and air-conditioning [ 4 , 5 ].
The COVID-19 pandemic has imposed substantial direct and indirect costs on patients, families, healthcare systems, and communities. These costs fluctuate significantly based on socioeconomic factors, age, disease severity, and comorbidities [ 6 , 7 ]. For instance, a study conducted in the United States of America (USA) estimated the median direct medical cost of a single symptomatic COVID-19 case to be US $3045 during the infection period alone [ 8 ]. Additionally, indirect costs arising from the pandemic, such as lost productivity due to morbidity and mortality, reduced consumer spending, and supply chain disruptions, could be substantial in certain countries [ 9 ]. Studies by Maltezou et al. and Faramarzi et al. revealed that absenteeism costs accounted for a large proportion (80.4%) of total costs [ 10 ] and estimated an average cost of US $671.4 per patient [ 11 ], respectively. Furthermore, the macroeconomic impact of the COVID-19 pandemic is considerably more significant. Data from Europe indicates that the gross domestic product (GDP) fell by an average of 7.4% in 2020 [ 12 ]. Globally, the economic burden of COVID-19 was estimated to be between US $77 billion and US $2.7 trillion in 2019 [ 13 ]. Another study calculated the quarantine costs of COVID-19 to exceed 9% of the global GDP [ 14 ].
Evaluating the cost of COVID-19, encompassing both direct (medical and non-medical) and indirect costs, provides valuable insights for policymakers and healthcare managers to devise effective strategies for resource allocation and cost control, particularly in the post-COVID-19 era. Despite the abundance of literature on COVID-19, only a handful of studies have concentrated on its economic burden. Furthermore, the currency estimates provided in these articles is inconsistent. To address this gap, our study aimed to conduct a systematic review and meta-analysis of the global economic burden of COVID-19. The objectives of this study are twofold: firstly, to estimate the direct and indirect costs of COVID-19 as a percentage of GDP and health expenditure (HE) at the global level, and secondly, to estimate the direct medical costs based on the inpatient ward, which includes both the general ward and the intensive care unit (ICU).
This study was designed according to the Preferred Reporting Items for Systematic Reviews and Meta Analyses (PRISMA) guidelines [ 15 ].
Search strategy and data sources
We performed a comprehensive search in PubMed, Scopus, and Web of Science databases to retrieve studies on the economic burden of COVID-19 disease. To this objective, we conducted a comprehensive search by combining the search terms relating to COVID-19 (coronavirus, 2019-nCoV), as a class, with the terms relating to the economic burden and terms related to it (direct cost, indirect cost, productivity cost, morbidity cost, mortality cost, cost analysis, cost of illness, economic cost, noneconomic cost, financial cost, expenditure, spending). The search was limited to English language publications and human studies that were published before September 19, 2021. The search strategy was validated by a medical information specialist. All search strategies are available in the Additional file 1 .
Screening and selection
Two reviewers independently screened all distinct articles, focusing on the title and abstract and utilizing EndNote software. The reviewers were blinded to each other’s findings during the screening phase. Potential duplicates were identified and scrutinized to exclude identical entries. Any discrepancies between the reviewers were reconciled through consensus or by consulting a third reviewer. The final decision regarding inclusion was determined subsequent to a comprehensive review of the full-text article. The whole process of the study selection was outlined in a flow chart (Fig. 1 ).
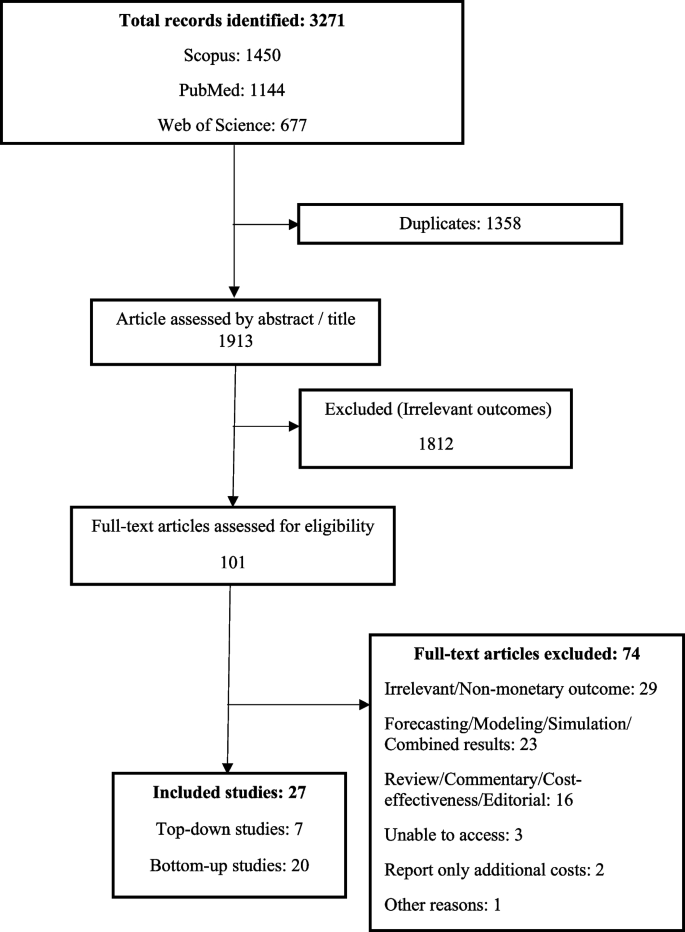
Flowchart depicting the selection of research studies
This systematic review included all original studies that addressed the economic burden of COVID-19, provided they (1) estimated all costs associated with COVID-19, including both direct (medical and non-medical) and indirect (morbidity and mortality) costs and (2) were designed as observational studies or controlled clinical trials. Studies were excluded based on the following criteria: (1) they were review articles, commentaries, editorials, protocols, case studies, case series, animal studies, book chapters, or theses, (2) they estimated costs for a specific disease or action during the COVID-19 pandemic, and (3) they were studies assessing budget impact or economic evaluations.
Data extraction
A specific data extraction template was developed to extract relevant information from every study that satisfied our eligibility criteria. The data extracted covered the general study characteristics (authors, study publication, geographical location of data collection), cost-related information (direct medical cost, direct nonmedical cost, indirect cost, total cost, years of costing, and currency), and participants-related data (sample size and population studied for estimation).
Outcome and quality assessment
The primary outcomes were documented as the standardized mean difference (SMD) accompanied by 95% confidence intervals, representing the direct medical costs borne in general wards as compared to ICU for patients diagnosed with COVID-19. Additionally, another outcome was the estimation of these costs as a proportion of the GDP and health expenditure (HE).
A quality assessment was conducted on all the included studies, utilizing the checklist formulated by Larg and Moss [ 16 ]. This checklist comprises three domains: analytic framework, methodology and data, and analysis and reporting. The quality assessment was independently corroborated by two reviewers. In case of any discrepancies in the quality assessment, resolution was ensured through consensus or consultation with a third reviewer.
Statistical analysis
To analyze the data, we utilized the cost-of-illness (COI) study approach, which involved categorizing the studies into two groups: top-down studies and bottom-up studies. Top-down studies were defined as population-based methods that estimated costs for a specific country or group of countries, while bottom-up studies were defined as person methods that estimated costs per person [ 16 ].
In our methodological approach to the top-down studies, we initially categorized the costs into direct and indirect types. The direct costs comprised both medical and nonmedical expenses, while the indirect costs were related to potential productivity losses stemming from mortality and morbidity. Subsequently, we undertook the adjustment of all costs to the 2020 US dollar value. This was achieved based on the principle of purchasing power parity (PPP), and we utilized the currency conversion factor as recommended by the World Bank for this purpose. We employed the method proposed by Konnopka and König to present the COVID-19 cost to top-down studies. This method, which expresses the costs as a proportion of the gross domestic product (GDP) and health expenditure (HE), eliminates the need for adjustments for inflation or differences in purchasing power [ 17 ]. Moreover, we computed the costs using both an unweighted mean and a population-weighted mean.
In the bottom-up studies, a random-effects model was employed for the meta-analysis, with the SMD serving as the measure of effect size. To mitigate the influence of heterogeneity, all costs were converted to 2020 US dollars based on PPP, utilizing the currency conversion factor suggested by the World Bank. The focus of our analysis was a comparison of the direct medical costs of patients admitted to the general ward versus those in ICU. The SMD was calculated as the measure of effect size, with the sample size acting as the weighting factor. Heterogeneity was assessed through Cochran’s Q test and the I 2 statistic. The Q -test, a classical measure with a chi-square distribution, is calculated as the weighted sum of squared differences between individual study effects and the pooled effects across studies. The I 2 statistic represents the percentage of variation across studies, with threshold values of 25%, 50%, and 75% indicating low, moderate, and high levels of heterogeneity, respectively. To assess possible publication or disclosure bias, we used funnel plots, the Begg-adjusted rank correlation test, and Egger’s test. All statistical analyses were performed using STATA version 14 (Stata Corp, College Station, TX, USA), and P -values less than 0.05 were considered as statistically significant.
The study selection process is illustrated in Figure 1 . The search strategy produced 3271 records (Scopus, 1450; PubMed, 1144; Web of Science, 677), from which 1358 duplicates were eliminated. Out of the remaining 1913 articles, a mere 101 satisfied the inclusion criteria and underwent a full-text review. During this full-text screening, 74 articles were excluded for various reasons, resulting in a final selection of 27 studies included in the systematic review. Among these, 20 were bottom-up studies [ 7 , 10 , 18 , 19 , 20 , 21 , 22 , 23 , 24 , 25 , 26 , 27 , 28 , 29 , 30 , 31 , 32 , 33 , 34 , 35 ], and 7 were top-down studies [ 36 , 37 , 38 , 39 , 40 , 41 , 42 ].
Characteristics of included studies
Table 1 presents the general characteristics of the included studies. Out of the 27 studies, 5 were conducted in the USA; 5 in China; 2 each in Spain, Brazil, South Korea, and India; and 1 each in Italy, South Africa, the Philippines, Greece, Iran, Kenya, Nigeria, and the Kingdom of Saudi Arabia. Based on the methodology employed, 20 studies were categorized as bottom-up studies and seven as top-down studies.
Among the seven top-down studies, only three calculated direct medical costs [ 37 , 38 , 41 ], two studies examined the direct nonmedical costs [ 38 , 41 ], and all but Santos et al. [ 37 ], who did not report these costs, calculated indirect costs. Of the 20 bottom-up studies, all but 1 study [ 31 ] assessed the direct medical costs. Only four studies calculated the direct nonmedical costs [ 10 , 19 , 29 , 34 ], and seven studies reported the indirect costs [ 7 , 10 , 19 , 26 , 29 , 31 , 34 ].
Table 2 presents the specific characteristics of the top-down studies. These studies indicate that the direct costs of COVID-19 span from US $860 million to US $8,657 million, while indirect costs range from US $610 million to US $5,500,000 million. On average, top-down studies estimate the direct costs associated with COVID-19 to constitute 2.73% and 0.39% of healthcare expenditures, based on unweighted and weighted means, respectively. The results also reveal that, on average, indirect costs account for 10.53% of GDP, with a range of 0.02 to 30.90%. Furthermore, the total cost estimated by top-down studies comprises 85.91% of healthcare expenditure and 9.13% of GDP.
Table 3 outlines the specific characteristics of the bottom-up studies. Excluding two studies [ 23 , 27 ], all reported their sample sizes, which varied from 9 to 1,470,721. The mean estimate of direct medical costs ranged from US $1264 to US $79,315. Two studies reported values for direct nonmedical costs [ 19 , 29 ], with means of US $25 and US $71. The mean estimate of indirect costs ranged from US $187 to US $689,556.
Meta-analysis results
The results of the meta-analysis for the direct medical costs are shown in Figure 2 . The results indicate a significant association between the mean cost of direct medical services and the inpatient ward. Specifically, the analysis yielded a standardized mean difference (SMD) of 1.62 ( CI : 0.9–2.35) with a substantial degree of heterogeneity ( Q = 26170, p < 0.0001; I 2 = 100%).
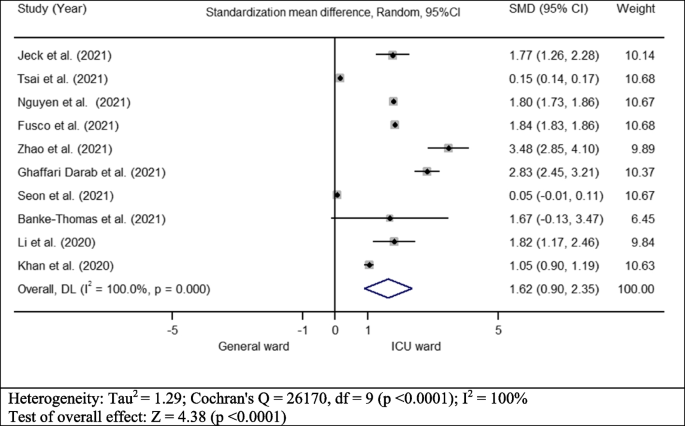
Mean direct medical cost for patient with COVID-19 based on disease severity
Assessment of publication bias
Figure 3 presents the information related to publication bias. The funnel plot, constructed from the studies included, does not suggest the presence of potential publication bias. Moreover, the application of Begg’s and Egger’s tests in the statistical analysis resulted in P-values of 0.788 and 0.789, respectively, indicating an absence of significant bias.
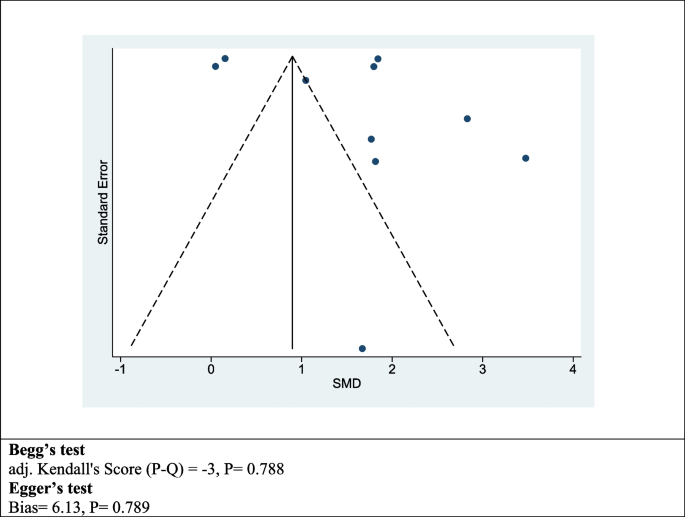
The funnel plots, Begg’s test, and Egger’s test to assessment of publication bias for included studies that assessed the direct medical costs of patients hospitalized in the general ward versus those in the intensive care unit (ICU)
This investigation represents the initial systematic review and meta-analysis conducted on the topic of the global economic impact of COVID-19. Furthermore, it is the first study to evaluate economic burden research related to COVID-19 using both top-down and bottom-up approaches, and it has conducted a meta-analysis of medical direct expenses based on hospitalization wards. In general, studies examining the economic impact of COVID-19 are scarce, with a greater proportion of studies employing a bottom-up approach. More than 30% of these studies were conducted in the USA and China. Patients admitted to the ICU ward exhibited higher costs than those admitted to the general ward.
Admission to the ICU significantly escalated the medical expenditure associated with COVID-19 treatment. This study discovered that the medical costs for COVID-19 patients in the ICU were approximately twice as high as those for patients in general wards, with a range from 0.05 to 3.48 times higher. This finding aligns with existing literature, which suggests that ICU patients with COVID-19 are more likely to require expensive treatments such as mechanical ventilation and extracorporeal membrane oxygenation, compared to those in general wards [ 44 , 45 ]. Consistent with this, other studies have reported an increase in medical expenditures with the hospitalization of COVID-19 patients in the ICU. For instance, a study conducted in the USA found a fivefold increase in costs for patients in the ICU who required invasive mechanical ventilation (IMV), compared to those not in the ICU or without IMV [ 22 ]. Similarly, a study in China reported a 2.5-fold increase in costs for severe COVID-19 patients compared to mild cases [ 30 ]. Given the elevated medical costs associated with treating COVID-19 patients in the ICU or those with severe symptoms, health policymakers must concentrate on implementing programs that promote early diagnosis. Consequently, healthcare providers could initiate treatment at an earlier stage, potentially reducing the severity of the disease and associated costs.
Our research indicates that significant variations in estimated costs would be observed if these costs were reported in PPP, particularly in relation to direct medical expenses. The lowest value was calculated in India, amounting to US $1264, while the highest value was observed in the USA, reaching US $54,165. Furthermore, the calculated medical costs varied across countries. For example, in the USA, direct medical expenditures ranged from US $1701 to US $54,156 [ 21 , 35 ]. In contrast, in China, the reported costs fluctuated between US $5264 and US $79,315 [ 7 , 25 ]. Several factors contribute to this variation in the estimation of direct medical costs. Primarily, direct medical costs cover a spectrum of services, including diagnosis, medication, consumables, inpatient care, and consultation services. Consequently, each study may have estimated the direct medical costs for a subset or the entirety of these services, leading to differences in the estimated costs. For instance, Nguyen et al. demonstrated a nearly threefold increase in direct costs for COVID-19 patients managed with extracorporeal membrane oxygenation (ECMO) compared to patients not receiving ECMO [ 35 ]. This highlights the impact of specific treatments on the overall cost. Secondly, the sample size may vary between studies, resulting in different cost estimates. Larger sample sizes typically provide more accurate and reliable estimates, but they also require more resources to collect and analyze. Lastly, the studies may have estimated costs for patients with varying conditions, such as those in acute status, patients hospitalized in general wards, or those admitted to ICU wards.
In addition to direct medical expenditures, the indirect costs arising from productivity losses due to COVID-19 have substantial societal implications. This study discovered that direct medical expenses attributable to COVID-19 varied from US $860 million (representing 0.11% of China’s healthcare expenditure) as reported by Zhao et al. [ 38 ] in China to US $8657 million (equivalent to 7.4% of Spanish healthcare expenditure) as reported by Gonzalez Lopez et al. [ 41 ] in Spain. On a global scale, direct medical costs due to COVID-19 constituted 2.73% of healthcare expenditure and 0.25% of GDP. The results also unveiled that the indirect costs of the COVID-19 pandemic impacted different countries to varying extents. The minimum value of indirect costs was estimated in Italy [ 40 ] and India [ 39 ] at US $610 million and US $658 million, respectively. Interestingly, when reported as a percentage of GDP, India had a lower cost (0.02% of GDP) compared to China (0.03% of GDP). The maximum value of indirect costs was calculated in the USA at US $5,500,000 million, which accounted for approximately 26.32% of the USA’s GDP [ 36 ]. Despite the numerical value of indirect costs being lower in Spain than in the USA and China, it represented a higher percentage of GDP (30.90%). The resulting pooled estimate indicated that the indirect costs due to COVID-19 were responsible for 10.53% of global GDP. The review underscores the significant economic repercussions of COVID-19. The total costs in the USA accounted for about 157% of healthcare expenditure and 26% of GDP, in China for 80% of healthcare expenditure and 4.28% of GDP, and in Spain for approximately 345% of healthcare expenditure and 32% of GDP. Globally, the total costs of COVID-19 accounted for about 86% of healthcare expenditure and 9.13% of GDP. This highlights the profound economic impact of the pandemic on both healthcare systems and economies worldwide.
Strengths and limitation
Our study possesses several significant strengths. It is the inaugural meta-analysis of the worldwide costs associated with COVID-19, supplementing a systematic review conducted by Richards et al. on the economic burden studies of COVID-19 [ 12 ]. A considerable number of studies was conducted in the USA and China, but our analysis also incorporated studies from other high- and low-income countries, potentially enhancing the generalizability of our findings. Recognizing that economic burden studies often display significant heterogeneity, we endeavored to minimize this by distinguishing between bottom-up and top-down studies and standardizing currencies to US dollars in terms of PPP.
However, our study is not without limitations. As is typical with all meta-analyses of economic burden studies, the most substantial limitation is heterogeneity. This heterogeneity can originate from various factors, including differences in study design, the range of services included in individual studies, the year of estimation, the currencies used for estimation, the study population, among other factors. Our systematic review only incorporated studies that estimated costs for an actual population, thereby excluding a wide array of studies on the economic burden of COVID-19 that employed modeling techniques. Future research could potentially conduct systematic reviews and meta-analyses on cost estimation modeling studies for COVID-19. Lastly, while no publication bias was detected through statistical analysis, our study was limited to papers written in English. As a result, numerous papers published in other languages were inevitably excluded.
Our research indicates that the COVID-19 pandemic has imposed a substantial economic strain worldwide, with the degree of impact varying across nations. The quantity of studies examining the economic repercussions of COVID-19 is limited, with a majority employing a bottom-up methodology. The indirect costs ascribed to COVID-19 constituted 10.53% of the global GDP. In total, the costs linked to COVID-19 represented 9.13% of GDP and 86% of healthcare spending. Moreover, our meta-analysis disclosed that the direct medical expenses for COVID-19 patients in the ICU were almost twice those of patients in general wards. The results of our research, along with those of others, underscore the pivotal role of economic outcomes in the post-COVID-19 era for societies and families. Consequently, it is imperative for policymakers and health administrators to prioritize and pay greater attention to economic programs.
Availability of data and materials
Data sharing is not applicable as no new data were generated during the study. The data analysis file during this study is available from the corresponding author on reasonable request.
Lu H, Stratton CW, Tang YW. Outbreak of pneumonia of unknown etiology in Wuhan, China: the mystery and the miracle. J Med Virol. 2020;92(4):401–2.
Article CAS PubMed PubMed Central Google Scholar
Available from: https://www.who.int/emergencies/diseases/novel-coronavirus-2019 .
Gautier JF, Ravussin Y. A new symptom of COVID-19: loss of taste and smell. Obesity. 2020;28(5):848.
Article CAS PubMed Google Scholar
Delikhoon M, Guzman MI, Nabizadeh R, Norouzian BA. Modes of transmission of severe acute respiratory syndrome-coronavirus-2 (SARS-CoV-2) and factors influencing on the airborne transmission: a review. Int J Environ Res Public Health. 2021;18(2):395.
Alimohammadi M, Abolli S, Ghordouei ME. Perceiving effect of environmental factors on prevalence of SARS-Cov-2 virus and using health strategies: a review. J Adv Environ Health Res. 2022;10(3):187–96.
Article Google Scholar
Li XZ, Jin F, Zhang JG, Deng YF, Shu W, Qin JM, et al. Treatment of coronavirus disease 2019 in Shandong, China: a cost and affordability analysis. Infect Dis Poverty. 2020;9(1):78.
Jin H, Wang H, Li X, Zheng W, Ye S, Zhang S, et al. Economic burden of COVID-19, China, January–March, 2020: a cost-of-illness study. Bull World Health Organ. 2021;99(2):112.
Article PubMed Google Scholar
Bartsch SM, Ferguson MC, McKinnell JA, O'Shea KJ, Wedlock PT, Siegmund SS, et al. The potential health care costs and resource use associated with COVID-19 in the United States. Health Aff (Millwood). 2020;39(6):927–35.
Juranek S, Paetzold J, Winner H, Zoutman F. Labor market effects of COVID-19 in Sweden and its neighbors: evidence from novel administrative data. NHH Dept of Business and Management Science Discussion Paper. (2020/8); 2020.
Google Scholar
Maltezou H, Giannouchos T, Pavli A, Tsonou P, Dedoukou X, Tseroni M, et al. Costs associated with COVID-19 in healthcare personnel in Greece: a cost-of-illness analysis. J Hosp Infect. 2021;114:126–33.
Faramarzi A, Javan-Noughabi J, Tabatabaee SS, Najafpoor AA, Rezapour A. The lost productivity cost of absenteeism due to COVID-19 in health care workers in Iran: a case study in the hospitals of Mashhad University of Medical Sciences. BMC Health Serv Res. 2021;21(1):1169.
Article PubMed PubMed Central Google Scholar
Richards F, Kodjamanova P, Chen X, Li N, Atanasov P, Bennetts L, et al. Economic burden of COVID-19: a systematic review. Clinicoecon Outcomes Res. 2022;14:293–307.
Forsythe S, Cohen J, Neumann P, Bertozzi SM, Kinghorn A. The economic and public health imperatives around making potential coronavirus disease–2019 treatments available and affordable. Value Health. 2020;23(11):1427–31.
Rodela TT, Tasnim S, Mazumder H, Faizah F, Sultana A, Hossain MM. Economic impacts of coronavirus disease (COVID-19) in developing countries; 2020.
Book Google Scholar
Moher D, Liberati A, Tetzlaff J, Altman DG. PRISMA Group* t. Preferred Reporting Items for Systematic Reviews and Meta-Analyses: the PRISMA statement. Ann Intern Med. 2009;151(4):264–9.
Larg A, Moss JR. Cost-of-illness studies: a guide to critical evaluation. Pharmacoeconomics. 2011;29:653–71.
Konnopka A, König H. Economic burden of anxiety disorders: a systematic review and meta-analysis. Pharmacoeconomics. 2020;38:25–37.
Jeck J, Jakobs F, Kron A, Franz J, Cornely OA, Kron F. A cost of illness study of COVID-19 patients and retrospective modelling of potential cost savings when administering remdesivir during the pandemic “first wave” in a German tertiary care hospital. Infection. 2022;50(1):191–201.
Kotwani P, Patwardhan V, Pandya A, Saha S, Patel GM, Jaiswal S, et al. Valuing out-of-pocket expenditure and health related quality of life of COVID-19 patients from Gujarat, India. J Commun Dis (E-ISSN: 2581-351X & P-ISSN: 0019-5138). 2021;53(1):104–9.
Tsai Y, Vogt TM, Zhou F. Patient characteristics and costs associated with COVID-19–related medical care among Medicare fee-for-service beneficiaries. Ann Intern Med. 2021;174(8):1101–9.
Weiner JP, Bandeian S, Hatef E, Lans D, Liu A, Lemke KW. In-person and telehealth ambulatory contacts and costs in a large US insured cohort before and during the COVID-19 pandemic. JAMA Netw Open. 2021;4(3):e212618-e.
Di Fusco M, Shea KM, Lin J, Nguyen JL, Angulo FJ, Benigno M, et al. Health outcomes and economic burden of hospitalized COVID-19 patients in the United States. J Med Econ. 2021;24(1):308–17.
Edoka I, Fraser H, Jamieson L, Meyer-Rath G, Mdewa W. Inpatient care costs of COVID-19 in South Africa’s public healthcare system. Int J Health Policy Manag. 2022;11(8):1354.
PubMed Google Scholar
Tabuñar SM, Dominado TM. Hospitalization expenditure of COVID-19 patients at the university of the Philippines-Philippine general hospital (UP-PGH) with PhilHealth coverage. Acta Med Philipp. 2021;55(2)
Zhao J, Yao Y, Lai S, Zhou X. Clinical immunity and medical cost of COVID-19 patients under grey relational mathematical model. Results Phys. 2021;22:103829.
Ghaffari Darab M, Keshavarz K, Sadeghi E, Shahmohamadi J, Kavosi Z. The economic burden of coronavirus disease 2019 (COVID-19): evidence from Iran. BMC Health Serv Res. 2021;21(1):1–7.
Barasa E, Kairu A, Maritim M, Were V, Akech S, Mwangangi M. Examining unit costs for COVID-19 case management in Kenya. BMJ Glob Health. 2021;6(4):e004159.
Seon J-Y, Jeon W-H, Bae S-C, Eun B-L, Choung J-T, Oh I-H. Characteristics in pediatric patients with coronavirus disease 2019 in Korea. J Korean Med Sci. 2021;36(20):e148.
Banke-Thomas A, Makwe CC, Balogun M, Afolabi BB, Alex-Nwangwu TA, Ameh CA. Utilization cost of maternity services for childbirth among pregnant women with coronavirus disease 2019 in Nigeria’s epicenter. Int J Gynaecol Obstet. 2021;152(2):242–8.
Li X-Z, Jin F, Zhang J-G, Deng Y-F, Shu W, Qin J-M, et al. Treatment of coronavirus disease 2019 in Shandong, China: a cost and affordability analysis. Infect Dis Poverty. 2020;9(03):31–8.
CAS Google Scholar
Kirigia JM, Muthuri RNDK. The fiscal value of human lives lost from coronavirus disease (COVID-19) in China. BMC Res Notes. 2020;13:1–5.
Lee JK, Kwak BO, Choi JH, Choi EH, Kim J-H, Kim DH. Financial burden of hospitalization of children with coronavirus disease 2019 under the national health insurance service in Korea. J Korean Med Sci. 2020;35(24):e224.
Khan AA, AlRuthia Y, Balkhi B, Alghadeer SM, Temsah M-H, Althunayyan SM, et al. Survival and estimation of direct medical costs of hospitalized COVID-19 patients in the Kingdom of Saudi Arabia. Int J Environ Res Public Health. 2020;17(20):7458.
Mas Romero M, Avendaño Céspedes A, Tabernero Sahuquillo MT, Cortés Zamora EB, Gómez Ballesteros C, Sánchez-Flor Alfaro V, et al. COVID-19 outbreak in long-term care facilities from Spain. Many lessons to learn. PLoS One. 2020;15(10):e0241030.
Nguyen NT, Sullivan B, Sagebin F, Hohmann SF, Amin A, Nahmias J. Analysis of COVID-19 patients with acute respiratory distress syndrome managed with extracorporeal membrane oxygenation at US academic centers. Ann Surg. 2021;274(1):40.
Viscusi WK. Economic lessons for COVID-19 pandemic policies. South Econ J. 2021;87(4):1064–89.
Santos HLPC, Maciel FBM, Santos Junior GM, Martins PC, Prado NMBL. Public expenditure on hospitalizations for COVID-19 treatment in 2020, in Brazil. Rev Saude Publica. 2021;55:52.
Zhao J, Jin H, Li X, Jia J, Zhang C, Zhao H, et al. Disease burden attributable to the first wave of COVID-19 in China and the effect of timing on the cost-effectiveness of movement restriction policies. Value Health. 2021;24(5):615–24.
John D, Narassima M, Menon J, Rajesh JG, Banerjee A. Estimation of the economic burden of COVID-19 using disability-adjusted life years (DALYs) and productivity losses in Kerala, India: a model-based analysis. BMJ Open. 2021;11(8):e049619.
Nurchis MC, Pascucci D, Sapienza M, Villani L, D’Ambrosio F, Castrini F, et al. Impact of the burden of COVID-19 in Italy: results of disability-adjusted life years (DALYs) and productivity loss. Int J Environ Res Public Health. 2020;17(12):4233.
González López-Valcárcel B, Vallejo-Torres L. The costs of COVID-19 and the cost-effectiveness of testing. Appl Econ Anal. 2021;29(85):77–89.
Debone D, Da Costa MV, Miraglia SG. 90 days of COVID-19 social distancing and its impacts on air quality and health in Sao Paulo, Brazil. Sustainability. 2020;12(18):7440.
Article CAS Google Scholar
Ghaffari Darab M, Keshavarz K, Sadeghi E, Shahmohamadi J, Kavosi Z. The economic burden of coronavirus disease 2019 (COVID-19): evidence from Iran. BMC Health Serv Res. 2021;21(1):132.
Oliveira TF, Rocha CAO, Santos AGG, Silva Junior LCF, Aquino SHS, Cunha EJO, et al. Extracorporeal membrane oxygenation in COVID-19 treatment: a systematic literature review. Braz J Cardiovasc Surg. 2021;36:388–96.
Petrilli CM, Jones SA, Yang J, Rajagopalan H, O’Donnell L, Chernyak Y, et al. Factors associated with hospital admission and critical illness among 5279 people with coronavirus disease 2019 in New York City: prospective cohort study. BMJ. 2020;369:m1966.
Download references
There was no funding utilized in this study.
Author information
Authors and affiliations.
Department of Health Economics and Management, School of Public Health, Urmia University of Medical Sciences, Urmia, Iran
Ahmad Faramarzi, Soheila Norouzi, Siamak Aghlmand & Hasan Yusefzadeh
Department of Medical Library and Information Science, School of Allied Medical Sciences, Tehran University of Medical Sciences, Tehran, Iran
Hossein Dehdarirad
Social Determinants of Health Research Center, Mashhad University of Medical Sciences, Mashhad, Iran
Javad Javan-Noughabi
You can also search for this author in PubMed Google Scholar
Contributions
AF was principal investigators of designing the study, conducted analysis, and was major contributor in writing the manuscript. SN, HD, and SA conducted the literature search, conducted the screening and data extraction. HY and JJ contributed in designing the study and writing the manuscript. All authors contributed, reviewed, and approved this paper.
Corresponding author
Correspondence to Ahmad Faramarzi .
Ethics declarations
Ethics approval and consent to participate.
This study was approved by the Ethics Committee of the Urmia University of Medical Sciences (IR.UMSU.REC.1400.121).
Consent for publication
Not applicable.
Competing interests
The authors declare that they have no competing interests.
Additional information
Publisher’s note.
Springer Nature remains neutral with regard to jurisdictional claims in published maps and institutional affiliations.
Supplementary information
Additional file 1..
Search strategies
Rights and permissions
Open Access This article is licensed under a Creative Commons Attribution 4.0 International License, which permits use, sharing, adaptation, distribution and reproduction in any medium or format, as long as you give appropriate credit to the original author(s) and the source, provide a link to the Creative Commons licence, and indicate if changes were made. The images or other third party material in this article are included in the article's Creative Commons licence, unless indicated otherwise in a credit line to the material. If material is not included in the article's Creative Commons licence and your intended use is not permitted by statutory regulation or exceeds the permitted use, you will need to obtain permission directly from the copyright holder. To view a copy of this licence, visit http://creativecommons.org/licenses/by/4.0/ . The Creative Commons Public Domain Dedication waiver ( http://creativecommons.org/publicdomain/zero/1.0/ ) applies to the data made available in this article, unless otherwise stated in a credit line to the data.
Reprints and permissions
About this article
Cite this article.
Faramarzi, A., Norouzi, S., Dehdarirad, H. et al. The global economic burden of COVID-19 disease: a comprehensive systematic review and meta-analysis. Syst Rev 13 , 68 (2024). https://doi.org/10.1186/s13643-024-02476-6
Download citation
Received : 13 August 2023
Accepted : 31 January 2024
Published : 16 February 2024
DOI : https://doi.org/10.1186/s13643-024-02476-6
Share this article
Anyone you share the following link with will be able to read this content:
Sorry, a shareable link is not currently available for this article.
Provided by the Springer Nature SharedIt content-sharing initiative
- Cost of illness
- Systematic review
Systematic Reviews
ISSN: 2046-4053
- Submission enquiries: Access here and click Contact Us
- General enquiries: [email protected]
Thank you for visiting nature.com. You are using a browser version with limited support for CSS. To obtain the best experience, we recommend you use a more up to date browser (or turn off compatibility mode in Internet Explorer). In the meantime, to ensure continued support, we are displaying the site without styles and JavaScript.
- View all journals
- My Account Login
- Explore content
- About the journal
- Publish with us
- Sign up for alerts
- Open access
- Published: 04 April 2022
Economic losses from COVID-19 cases in the Philippines: a dynamic model of health and economic policy trade-offs
- Elvira P. de Lara-Tuprio 1 ,
- Maria Regina Justina E. Estuar 2 ,
- Joselito T. Sescon 3 ,
- Cymon Kayle Lubangco ORCID: orcid.org/0000-0002-1292-4687 3 ,
- Rolly Czar Joseph T. Castillo 3 ,
- Timothy Robin Y. Teng 1 ,
- Lenard Paulo V. Tamayo 2 ,
- Jay Michael R. Macalalag 4 &
- Gerome M. Vedeja 3
Humanities and Social Sciences Communications volume 9 , Article number: 111 ( 2022 ) Cite this article
41k Accesses
8 Citations
19 Altmetric
Metrics details
- Science, technology and society
The COVID-19 pandemic forced governments globally to impose lockdown measures and mobility restrictions to curb the transmission of the virus. As economies slowly reopen, governments face a trade-off between implementing economic recovery and health policy measures to control the spread of the virus and to ensure it will not overwhelm the health system. We developed a mathematical model that measures the economic losses due to the spread of the disease and due to different lockdown policies. This is done by extending the subnational SEIR model to include two differential equations that capture economic losses due to COVID-19 infection and due to the lockdown measures imposed by the Philippine government. We then proceed to assess the trade-off policy space between health and economic measures faced by the Philippine government. The study simulates the cumulative economic losses for 3 months in 8 scenarios across 5 regions in the country, including the National Capital Region (NCR), to capture the trade-off mechanism. These scenarios present the various combinations of either retaining or easing lockdown policies in these regions. Per region, the trade-off policy space was assessed through minimising the 3-month cumulative economic losses subject to the constraint that the average health-care utilisation rate (HCUR) consistently falls below 70%, which is the threshold set by the government before declaring that the health system capacity is at high risk. The study finds that in NCR, a policy trade-off exists where the minimum cumulative economic losses comprise 10.66% of its Gross Regional Domestic Product. Meanwhile, for regions that are non-adjacent to NCR, a policy that hinges on trade-off analysis does not apply. Nevertheless, for all simulated regions, it is recommended to improve and expand the capacity of the health system to broaden the policy space for the government in easing lockdown measures.
Similar content being viewed by others
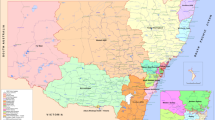
Modelling COVID-19 pandemic control strategies in metropolitan and rural health districts in New South Wales, Australia
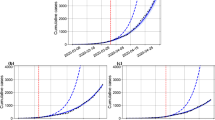
Mathematical modeling of COVID-19 in 14.8 million individuals in Bahia, Brazil
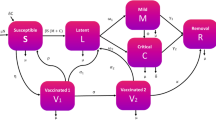
Cost-effectiveness analysis of COVID-19 intervention policies using a mathematical model: an optimal control approach
Introduction.
The Philippine population of 110 million comprises a relatively young population. On May 22, 2021, the number of confirmed COVID-19 cases reported in the country is 1,171,403 with 55,531 active cases, 1,096,109 who recovered, and 19,763 who died. As a consequence of the pandemic, the real gross domestic product (GDP) contracted by 9.6% year-on-year in 2020—the sharpest decline since the Philippine Statistical Agency (PSA) started collecting data on annual growth rates in 1946 (Bangko Sentral ng Pilipinas, 2021 ). The strictest lockdown imposed from March to April 2020 had the most severe repercussions to the economy, but restrictions soon after have generally eased on economic activities all over the country. However, schools at all levels remain closed and minimum restrictions are still imposed in business operations particularly in customer accommodation capacity in service establishments.
The government is poised for a calibrated reopening of business, mass transportation, and the relaxation of age group restrictions. The government expects a strong recovery before the end of 2021, when enough vaccines have been rolled out against COVID-19. However, the economic recovery plan and growth targets at the end of the year are put in doubt with the first quarter of 2021 growth rate of GDP at -4.2%. This is exacerbated by the surge of cases in March 2021 that took the National Capital Region (NCR) and contiguous provinces by surprise, straining the hospital bed capacity of the region beyond its limits. The government had to reinforce stricter lockdown measures and curfew hours to stem the rapid spread of the virus. The country’s economic development authority proposes to ensure hospitals have enough capacity to allow the resumption of social and economic activities (National Economic and Development Authority, 2020 ). This is justified by pointing out that the majority of COVID-19 cases are mild and asymptomatic.
Efforts in monitoring and mitigating the spread of COVID-19 requires understanding the behaviour of the disease through the development of localised disease models operationalized as an ICT tool accessible to policymakers. FASSSTER is a scenario-based disease surveillance and modelling platform designed to accommodate multiple sources of data as input allowing for a variety of disease models and analytics to generate meaningful information to its stakeholders (FASSSTER, 2020 ). FASSSTER’s module on COVID-19 currently provides information and forecasts from national down to city/municipality level that are used for decision-making by individual local government units (LGUs) and also by key government agencies in charge of the pandemic response.
In this paper, we develop a mathematical model that measures the economic losses due to the spread of the disease and due to different lockdown policies to contain the disease. This is done by extending the FASSSTER subnational Susceptible-Exposed-Infectious-Recovered (SEIR) model to include two differential equations that capture economic losses due to COVID-19 infection and due to the lockdown measures imposed by the Philippine government. We then proceed to assess the trade-off policy space faced by the Philippine government given the policy that health-care utilisation rate must not be more than 70%, which is the threshold set by the government before declaring that the health system capacity is at high risk.
We simulate the cumulative economic losses for 3 months in 8 scenarios across 5 regions in the country, including the National Capital Region (NCR) to capture the trade-off mechanism. These 8 scenarios present the various combinations of either retaining or easing lockdown policies in these regions. Per region, the trade-off policy space was assessed through minimising the 3-month cumulative economic losses subject to the constraint that the average health-care utilisation rate (HCUR) consistently falls below 70%. The study finds that in NCR, a policy trade-off exists where the minimum economic losses below the 70% average HCUR comprise 10.66% of its Gross Regional Domestic Product. Meanwhile, for regions that are non-adjacent to NCR, a policy that hinges on trade-off analysis does not apply. Nevertheless, for all simulated regions, it is recommended to improve and expand the capacity of the health system to broaden the policy space for the government in easing lockdown measures.
The sections of the paper proceed as follows: the first section reviews the literature, the second section explains the FASSSTER SEIR model, the third section discusses the economic dynamic model, the fourth section specifically explains the parameters used in the economic model, the fifth section briefly lays out the policy trade-off model, the sixth discusses the methods used in implementing the model, the seventh section presents the results of the simulations, the eighth section discusses and interprets the results, and the final section presents the conclusion.
Review of related literature
Overview of the economic shocks of pandemics.
The onslaught of the Coronavirus Disease 2019 (COVID-19) pandemic since 2020 has disrupted lifestyles and livelihoods as governments restrict mobility and economic activity in their respective countries. Unfortunately, this caused a –3.36% decline in the 2020 global economy (World Bank, 2022 ), which will have pushed 71 million people into extreme poverty (World Bank, 2020 ; 2021 ).
As an economic phenomenon, pandemics may be classified under the typologies of disaster economics. Particularly, a pandemic’s impacts may be classified according to the following (Benson and Clay, 2004 ; Noy et al., 2020 ; Keogh-Brown et al., 2010 ; 2020 ; McKibbin and Fernando, 2020 ; Verikios et al., 2012 ): (a) direct impacts, where pandemics cause direct labour supply shocks due to mortality and infection; (b) indirect impacts on productivity, firm revenue, household income, and other welfare effects, and; (c) macroeconomic impacts of a pandemic.
For most pandemic scenarios, social distancing and various forms of lockdowns imposed by countries around the world had led to substantial disruptions in the supply-side of the economy with mandatory business closures (Maital and Barzani, 2020 ; Keogh-Brown et al., 2010 ). Social distancing will have contracted labour supply as well, thus contributing to contractions in the macroeconomy (Geard et al., 2020 ; Keogh-Brown et al., 2010 ). Thus, in general, the literature points to a pandemic’s impacts on the supply- and demand-side, as well as the displacement of labour supply; thus, resulting in lower incomes (Genoni et al., 2020 ; Hupkau et al., 2020 ; United Nations Development Programme, 2021 ). Often, these shocks result from the lockdown measures; thus, a case of a trade-off condition between economic losses and the number of COVID-19 casualties.
Static simulations for the economic impacts of a pandemic
The typologies above are evident in the analyses and simulations on welfare and macroeconomic losses related to a pandemic. For instance, computable general equilibrium (CGE) and microsimulation analyses for the 2009 H1N1 pandemic and the COVID-19 pandemic showed increases in inequities, welfare losses, and macroeconomic losses due to lockdown and public prevention strategies (Cereda et al., 2020 ; Keogh-Brown et al., 2020 ; Keogh-Brown et al., 2010 ). Public prevention-related labour losses also comprised at most 25% of the losses in GDP in contrast with health-related losses, which comprised only at most 17% of the losses in GDP.
Amidst the COVID-19 pandemic in Ghana, Amewu et al. ( 2020 ) find in a social accounting matrix-based analysis that the industry and services sectors will have declined by 26.8% and 33.1%, respectively. Other studies investigate the effects of the pandemic on other severely hit sectors such as the tourism sector. Pham et al. ( 2021 ) note that a reduction in tourism demand in Australia will have caused a reduction in income of tourism labourers. Meanwhile, in a static CGE-microsimulation model by Laborde, Martin, and Vos ( 2021 ), they show that as the global GDP will have contracted by 5% following the reduction in labour supply, this will have increased global poverty by 20%, global rural poverty by 15%, poverty in sub-Saharan Africa by 23%, and in South Asia by 15%.
However, due to the static nature of these analyses, the clear trade-off between economic and health costs under various lockdown scenarios is a policy message that remains unexplored, as the simulations above only explicitly tackle a pandemic’s macroeconomic effects. This gap is mostly due to these studies’ usage of static SAM- and CGE-based analyses.
Dynamic simulations for the economic impacts of a pandemic
An obvious advantage of dynamic models over static approaches in estimating the economic losses from the pandemic is the capacity to provide forward-looking insights that have practical use in policymaking. Epidemiological models based on systems of differential equations explicitly model disease spread and recovery as movements of population across different compartments. These compartmental models are useful in forecasting the number of infected individuals, critically ill patients, death toll, among others, and thus are valuable in determining the appropriate intervention to control epidemics.
To date, the Susceptible-Infectious-Recovered (SIR) and SEIR models are among the most popular compartmental models used to study the spread of diseases. In recent years, COVID-19 has become an important subject of more recent mathematical modelling studies. Many of these studies deal with both application and refinement of both SIR and SEIR to allow scenario-building, conduct evaluation of containment measures, and improve forecasts. These include the integration of geographical heterogeneities, the differentiation between isolated and non-isolated cases, and the integration of interventions such as reducing contact rate and isolation of active cases (Anand et al., 2020 ; Chen et al., 2020 ; Hou et al., 2020 ; Peng et al., 2020 ; Reno et al., 2020 ).
Typical epidemiological models may provide insight on the optimal lockdown measure to reduce the transmissibility of a virus. However, there is a need to derive calculations on economic impacts from the COVID-19 case projections to arrive at a conclusion on the optimal frontier from the trade-off between health and economic losses. In Goldsztejn, Schwartzman and Nehorai ( 2020 ), an economic model that measures lost economic productivity due to the pandemic, disease containment measures and economic policies is integrated into an SEIR model. The hybrid model generates important insight on the trade-offs between short-term economic gains in terms of productivity, and the continuous spread of the disease, which in turn informs policymakers on the appropriate containment policies to be implemented.
This approach was further improved by solving an optimal control of multiple group SIR model to find the best way to implement a lockdown (Acemoglu et al., 2020 ). Noting the trade-offs between economic outcomes and spread of disease implied in lockdown policies, Acemoglu et al. ( 2020 ) find that targeted lockdown yields the best result in terms of economic losses and saving lives. However, Acemoglu et al. ( 2020 ) only determine the optimal lockdown policy and their trade-off analysis through COVID-associated fatalities. Kashyap et al. ( 2020 ) note that hospitalisations may be better indicators for lockdown and, as a corollary, reopening policies.
Gaps in the literature
With the recency of the pandemic, there is an increasing but limited scholarship in terms of jointly analysing the losses brought about by the pandemic on health and the economy. On top of this, the literature clearly has gaps in terms of having a trade-off model that captures the context of low- and middle-income countries. Devising a trade-off model for said countries is an imperative given the structural and capability differences of these countries from developed ones in terms of responding to the pandemic. Furthermore, the literature has not explicitly looked into the trade-off between economic losses and health-care system capacities, both at a national and a subnational level.
With this, the paper aims to fill these gaps with the following. Firstly, we extend FASSSTER’s subnational SEIR model to capture the associated economic losses given various lockdown scenarios at a regional level. Then, we construct an optimal policy decision trade-off between the health system and the economy in the Philippines’ case at a regional level. From there, we analyse the policy implications across the different regions given the results of the simulations.
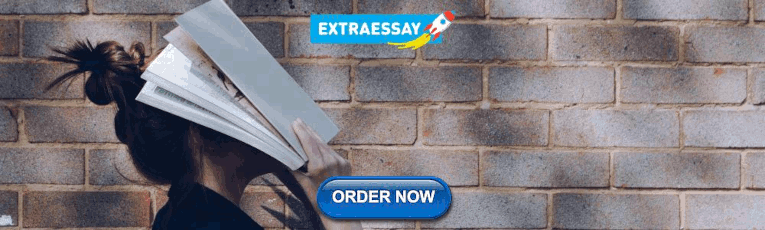
The FASSSTER SEIR model
The FASSSTER model for COVID-19 uses a compartmental model to describe the dynamics of disease transmission in a community, and it is expressed as a system of ordinary differential equations (Estadilla et al., 2021 ):
where β = β 0 (1– λ ), \(\alpha _a = \frac{c}{\tau }\) , \(\alpha _s = \frac{{1 - c}}{\tau }\) , and N ( t ) = S ( t ) = E ( t ) + I a ( t ) + I s ( t ) + C ( t ) + R ( t ).
The six compartments used to divide the entire population, namely, susceptible ( S ), exposed ( E ), infectious but asymptomatic ( I a ), infectious and symptomatic ( I s ), confirmed ( C ), and recovered ( R ), indicate the status of the individuals in relation to the disease. Compartment S consists of individuals who have not been infected with COVID-19 but may acquire the disease once exposed to infectious individuals. Compartment E consists of individuals who have been infected, but not yet capable of transmitting the disease to others. The infectious members of the population are split into two compartments, I a and I s , based on the presence of disease symptoms. These individuals may eventually transition to compartment C once they have been detected, in which case they will be quarantined and receive treatment. The individuals in the C compartment are commonly referred to as active cases. Finally, recovered individuals who have tested negative or have undergone the required number of days in isolation will move out to the R compartment. Given that there had only been rare instances of reinfection (Gousseff et al., 2020 ), the FASSSTER model assumes that recovered individuals have developed immunity from the disease. A description of the model parameters can be found in Supplementary Table S1 .
The model has several nonnegative parameters that govern the movement of individuals along the different compartments. The parameter β represents the effective transmission rate, and it is expressed as a product of the disease transmission rate β 0 and reduction factor 1 − λ . The rate β 0 is derived from an assumed reproduction number R 0 , which varies depending on the region. The parameter λ reflects the effect of mobility restrictions such as lockdowns and compliance of the members of the population to minimum health standards (such as social distancing, wearing of face masks etc.). In addition, the parameter ψ captures the relative infectiousness of asymptomatic individuals in relation to those who exhibit symptoms.
The incubation period τ and fraction of asymptomatic cases c are used to derive the transfer rates α α and α s from the exposed compartment to I a and I s compartments, respectively. Among those who are infectious and asymptomatic, a portion of them is considered pre-symptomatic, and hence will eventually develop symptoms of the disease; this is reflected in the parameter ω. The respective detection rates δ a and δ s of asymptomatic and symptomatic infectious individuals indicate the movement from the undetected infectious compartment to the confirmed compartment. These parameters capture the entire health system capacity to prevent-detect-isolate-treat-reintegrate (PDITR) COVID-19 cases; hence, they will henceforth be referred to as HSC parameters. The recoveries of infectious asymptomatic individuals and among the active cases occur at the corresponding rates θ and r . Death rates due to the disease, on the other hand, are given by ∈ I and ∈ T for the infectious symptomatic and confirmed cases, respectively.
Aside from the aforementioned parameters, the model also utilises parameters not associated with the COVID-19 disease, such as the recruitment rate A into the susceptible population. This parameter represents the birth rate of the population and is assumed to be constant. In addition, a natural death rate per unit of time is applied to all compartments in the model, incorporating the effect of non-COVID-19 related deaths in the entire population.
Economic dynamic model
The trade-off model aims to account for the incurred economic losses following the rise and fall of the number of COVID-19 cases in the country and the implementation of various lockdown measures. The model variables are estimated per day based on the SEIR model estimate of daily cases and are defined as follows. Let Y E ( t ) be the economic loss due to COVID-19 infections (hospitalisation, isolation, and death of infected individuals) and Y E ( t ) be the economic loss due to the implemented lockdown at time t . The dynamics of each economic variable through time is described by an ordinary differential equation. Since each equation depends only on the values of the state variables of the epidemiological model, then it is possible to obtain a closed form solution.
Economic loss due to COVID-19 infections (hospitalisation, isolation, and health)
The economic loss due to hospitalisation, isolation, and death Y E is described by the following differential equation:
where z = annual gross value added of each worker (assumed constant for all future years and for all ages), w = daily gross value added, ι i = % population with ages 0–14 ( i = 1), and labour force with ages 15–34 ( i = 2), 35–49 ( i = 3) and 50–64 ( i = 4), s r = social discount rate, κ = employed to population ratio, T i = average remaining productive years for people in age bracket i , i = 1, 2, 3, 4, and T 5 = average age of deaths from 0–14 years old age group. Note that the above formulation assumes that the young population 0–14 years old will start working at age 15, and that they will work for T 1 −15 years.
Solving Eq. ( 7 ), we obtain for t ≥ 0,
In this equation, the terms on the right-hand side are labelled as (A), (B), and (C). Term (A) is the present value of all future gross value added of 0–14 years old who died due to COVID-19 at time t . Similarly, term (B) is the present value of all future gross value added of people in the labour force who died due to COVID-19 at time t . Term (C) represents the total gross value added lost at time t due to sickness and isolation.
The discounting factors and the population age group shares in (A) and (B) can be simplified further into K 1 and K 2 , where \(K_1 = \iota _1\left( {\frac{{\left( {s_r + 1} \right)^{T_1 + T_5 - 13} - \left( {s_r + 1} \right)}}{{s_r\left( {s_r + 1} \right)^{T_1 + 1}}}} \right)\) and \(K_2 = \mathop {\sum}\nolimits_{i = 2}^4 {\iota _i\left( {\frac{{\left( {s_r + 1} \right)^{T_i + 2} - \left( {s_r + 1} \right)}}{{s_r\left( {s_r + 1} \right)^{T_i + 1}}}} \right)}\) . By letting L 1 = z( K 1 + K 2 ) ∈ I + κw (1 – ∈ I ) and L 2 = z( K 1 + K 2 ) ∈ T + κw (1 – ∈ T ), we have:
Economic losses due to lockdown policies
Equation ( 7 ) measures the losses due mainly to sickness and death from COVID-19. The values depend on the number of detected and undetected infected individuals, C and I s . The other losses sustained by the other part of the population are due to their inability to earn because of lockdown policies. This is what the next variable Y L represents, whose dynamics is given by the differential equation
where φ = the displacement rate, and κ and w are as defined previously.
Solving the differential equation, then
Note that [ S ( t ) + E ( t ) + I a ( t ) + R ( t )] is the rest of the population at time t , i.e., other than the active and infectious symptomatic cases. Multiplying this by κ and the displacement rate φ yields the number of employed people in this population who are displaced due to the lockdown policy. Thus, κwφ [ S ( t ) + E ( t ) + I a ( t ) + R ( t )] is the total foregone income due to the lockdown policy.
Economic model parameters
The values of the parameters were derived from a variety of sources. The parameters for employment and gross value added were computed based on the data from the Philippine Statistics Authority ( 2021 , 2020 , 2019a , 2019b ), the Department of Health’s Epidemiology Bureau (DOH-EB) ( 2020 ), the Department of Trade and Industry (DTI) ( 2020a , 2020b ) and the National Economic Development Authority (NEDA) ( 2016 ) (See Supplementary Tables S2 and S3 for the summary of economic parameters).
Parameters determined from related literature
We used the number of deaths from the data of the DOH-EB ( 2020 ) to disaggregate the long-term economic costs of the COVID-related deaths into age groups. Specifically, the COVID-related deaths were divided according to the following age groups: (a) below 15 years old, (b) 15 to 34 years old, (c) 35 to 49 years old, and (d) 50 to 64 years old. The average remaining years for these groups were computed directly from the average age of death of the respective cluster. Finally, we used the social discount rate as determined by NEDA ( 2016 ) to get the present value of the stream of foregone incomes of those who died from the disease.
Parameters estimated from local data
The foregone value added due to labour displacement was estimated as the amount due to workers in a geographic area who were unable to work as a result of strict lockdown measures. It was expected to contribute to the total value added in a given year if the area they reside or work in has not been locked down.
The employed to population ratio κ i for each region i was computed as
where e i was total employment in region i , and Pi was the total population in the region. Both e i and Pi were obtained from the quarterly labour force survey and the census, respectively (Philippine Statistics Authority, 2020 , 2019a , 2019b ).
The annual gross value added per worker z i for region i was computed as
where g ji was the share of sector j in total gross value added of region i , GVA ji was the gross value added of sector j in region i (Philippine Statistics Authority, 2021 ), and e ji was the number of employed persons in sector j of region i . If individuals worked for an average of 22.5 days for each month for 12 months in a year, then the daily gross value added per worker in region i was given by
Apart from this, labour displacement rates were calculated at regional level. The rates are differentiated by economic reopening scenarios from March 2020 to September 2020, from October 2020 to February 2021, and from March 2021 onwards (Department of Trade and Industry, 2020a , 2020b , 2021 ). These were used to simulate the graduate reopening of the economy. From the country’s labour force survey, each representative observation j in a region i is designated with a numerical value in accordance with the percentage operating capacity of the sector where j works in. Given the probability weights p ji , the displacement rate φ i for region i was calculated by
where x ji served as the variable representing the maximum operating capacity designated for j ’s sector of work.
Policy trade-off model
The trade-off between economic losses and health measures gives the optimal policy subject to a socially determined constraint. From the literature, it was pointed out that the optimal policy option would be what minimises total economic losses subject to the number of deaths at a given time (Acemoglu et al., 2020 ). However, for the Philippines’ case, lockdown restrictions are decided based on the intensive care unit and health-care utilisation rate (HCUR). The health system is said to reach its critical levels if the HCUR breaches 70% of the total available bed capacity in intensive care units. Once breached, policymakers would opt to implement stricter quarantine measures.
Given these, a policy mix of various quarantine restrictions may be chosen for as long as it provides the lowest amount of economic losses subject to the constraint that the HCUR threshold is not breached. Since economic losses are adequately captured by the sum of infection-related and lockdown-related losses, Y E ( t ) + Y L ( t ), then policy option must satisfy the constrained minimisation below:
where the objective function is evaluated from the initial time value t 0 to T .
The COVID-19 case information data including the date, location transformed into the Philippine Standard Geographic Code (PSGC), case count, and date reported were used as input to the model. Imputation using predictive mean matching uses the mice package in the R programming language. It was performed to address data gaps including the date of onset, date of specimen collection, date of admission, date of result, and date of recovery. Population data was obtained from the country’s Census of Population and Housing of 2015. The scripts to implement the FASSSTER SEIR model were developed using core packages in R including optimParallel for parameter estimation and deSolve for solving the ordinary differential equations. The output of the model is fitted to historical data by finding the best value of the parameter lambda using the L-BFGS-B method under the optim function and the MSE as measure of fitness (Byrd et al., 1995 ). The best value of lambda is obtained by performing parameter fitting with several bootstraps for each region, having at least 50 iterations until a correlation threshold of at least 90% is achieved. The output generated from the code execution contains values of the different compartments at each point in time. From these, the economic variables Y E ( t ) and Y L ( t ) were evaluated using the formulas in Eq. ( 7 ) and ( 8 ) in their simplified forms, and the parameter and displacement rate values corresponding to the implemented lockdown scenario (Fig. 1 ).
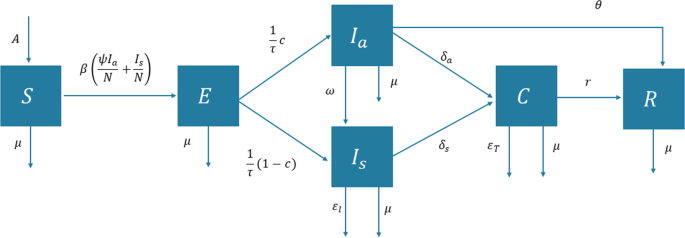
The different population states are represented by the compartments labelled as susceptible (S), exposed (E), infectious but asymptomatic ( I a ), infectious and symptomatic ( I s ), confirmed (C), and recovered (R).
We simulate the economic losses and health-care utilisation capacity (HCUR) for the National Capital Region (NCR), Ilocos Region, Western Visayas, Soccsksargen, and for the Davao Region by implementing various combinations of lockdown restrictions for three months to capture one quarter of economic losses for these regions. The National Capital Region accounts for about half of the Philippines’ gross domestic product, while the inclusion of other regions aim to represent the various areas of the country. The policy easing simulations use the four lockdown policies that the Philippines uses, as seen in Table 1 .
Simulations for the National Capital Region
Table 2 shows the sequence of lockdown measures implemented for the NCR. Each lockdown measure is assumed to be implemented for one month. Two sets of simulations are implemented for the region. The first set assumes a health systems capacity (HSC) for the region at 17.99% (A), while the second is at 21.93% (B). A higher HSC means an improvement in testing and isolation strategies for the regions of concern.
From the sequence of lockdown measures in Table 2 , Fig. 2 shows the plot of the average HCUR as well as the corresponding total economic losses for the two sets of simulations for one quarter. For the scenario at 17.99% HSC (A), the highest loss is recorded at 16.58% of the annual gross regional domestic product (GRDP) while the lowest loss is at 12.19% of its GRDP. Lower average HCUR corresponds to more stringent scenarios starting with Scenario 1. Furthermore, under the scenarios with 21.93% HSC (B), losses and average HCUR are generally lower. Scenarios 1 to 4 from this set lie below the 70% threshold of the HCUR, with the lowest economic loss simulated to be at 9.11% of the GRDP.
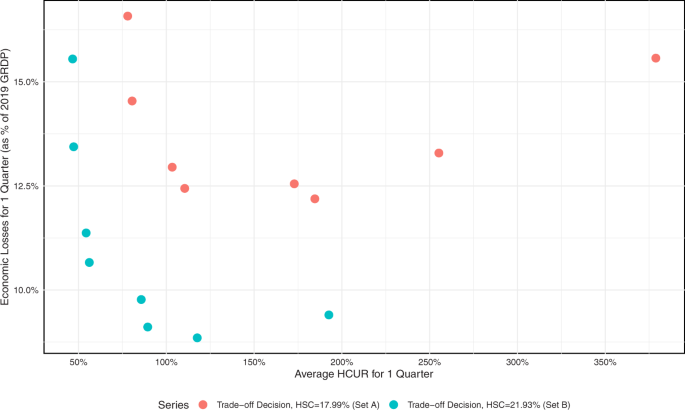
These include the set of trade-off decisions under a health system capacity equal to 17.99%, and another set equal to 21.93% (Source of basic data: Authors’ calculations).
Overall, the trend below shows a parabolic shape. The trend begins with an initial decrease in economic losses as restrictions loosen, but this comes at the expense of increasing HCUR. This is then followed by an increasing trend in losses as restrictions are further loosened. Notably, the subsequent marginal increases in losses in the simulation with 21.93% HSC are smaller relative to the marginal increases under the 17.99% HSC.
Simulations for the Regions Outside of NCR
Table 2 also shows the lockdown sequence for the Ilocos, Western Visayas, Soccsksargen, and Davao regions. The sequence begins with Level III only. Meanwhile, the lowest lockdown measure simulated for the regions is Level I. Two sets of simulations with differing health system capacities for each scenario are done as well.
With this lockdown sequence, Fig. 3 shows the panel of scatter plot between the average HCUR and total economic losses as percentage of the respective GRDP, with both parameters covering one quarter. Similar to the case of the NCR, the average HCUR for the simulations with higher health system capacity (B) is lower than the simulations with lower health system capacity (A). However, unlike in NCR, the regions’ simulations do not exhibit a parabolic shape.
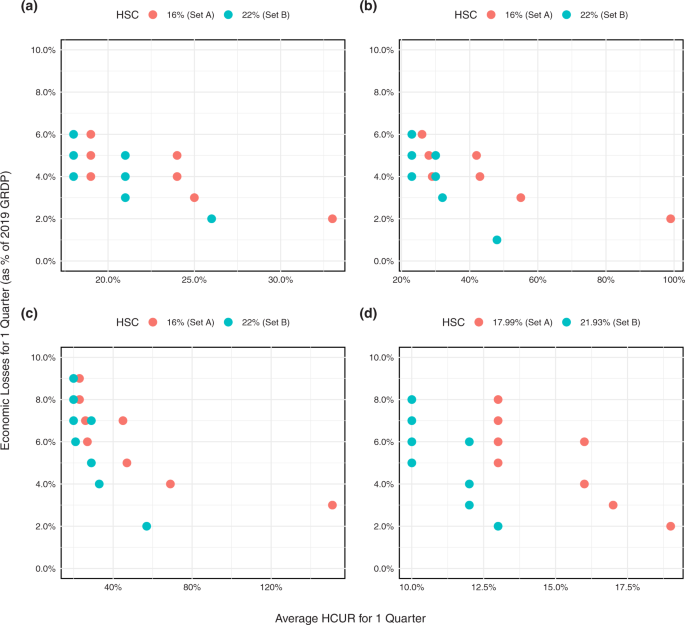
These include trade-offs for a Ilocos Region, b Western Visayas Region, c Soccsksargen Region, and d Davao Region (Source of basic data: Authors’ calculations).
Discussion and interpretation
The hypothetical simulations above clearly capture the losses associated with the pandemic and the corresponding lockdown interventions by the Philippine government. The trend of the simulations clearly shows the differences in the policy considerations for the National Capital Region (NCR) and the four other regions outside of NCR. Specifically, the parabolic trend of the former suggests an optimal strategy that can be attained through a trade-off policy even with the absence of any constraint in finding the said optimal strategy. This trend is borne from the countervailing effects between the economic losses due to COVID-19 infection ( Y E ) and the losses from the lockdown measures ( Y L ) implemented for the region. Specifically, Fig. 4(a), (b) show the composition of economic losses across all scenarios for the NCR simulation under a lower and higher health system capacity (HSC), respectively.
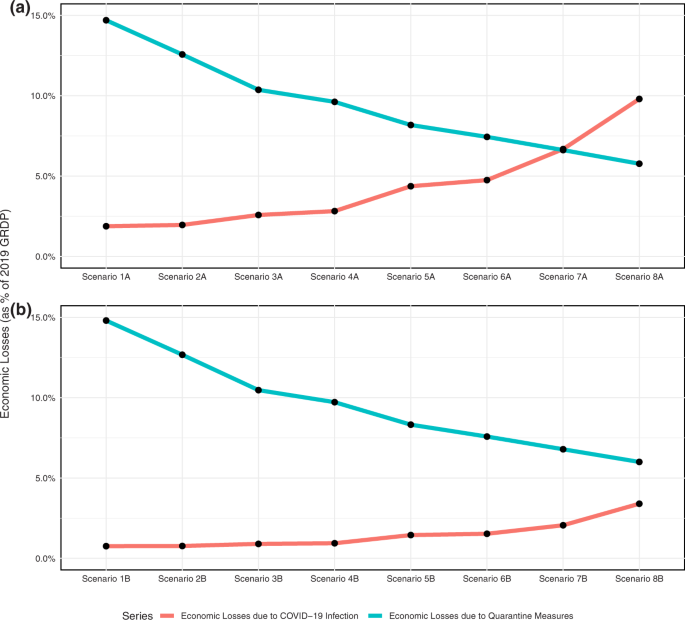
These include losses under a HSC = 17.99% and b HSC = 21.93% in the National Capital Region (Source of basic data: Authors’ calculations).
In both panels of Fig. 4 , as quarantine measures loosen, economic losses from infections ( Y E ) tend to increase while the converse holds for economic losses due to quarantine restrictions ( Y L ). The results are intuitive as loosening restrictions may lead to increased mobility, and therefore increased exposure and infections from the virus. In fact, economic losses from infections ( Y E ) take up about half of the economic losses for the region in Scenario 7A, Fig. 4(a) .
While the same trends can be observed for the scenarios with higher HSC at 21.93%, the economic losses from infections ( Y E ) do not overtake the losses simulated from lockdown restrictions ( Y L ) as seen in Fig. 4(b) . This may explain the slower upward trend of economic losses in Fig. 2 at HSC = 21.93%.
The output of the simulation for the Davao region shows that the economic losses from COVID-19 infections ( Y E ) remain low even as the lockdown restrictions ease down. At the same time, economic losses from lockdown restrictions ( Y L ) show a steady decline with less stringent lockdown measures. Overall, the region experiences a decreasing trend in total economic losses even as the least stringent lockdown measure is implemented for a longer period. This pattern is similar with the regions of Ilocos, Western Visayas, and Soccsksarkgen.
The results of the simulations from Figs. 2 and 3 also demonstrate differing levels of economic losses and health-care utilisation between the two sets of scenarios for NCR and the four other regions. Clearly, lower economic losses and health-care utilisation rates were recorded for the scenarios with higher HSC. Specifically, lower total economic losses can be attributed to a slower marginal increase in losses from infections ( Y E ) as seen in Fig. 4(b) . Thus, even while easing restrictions, economic losses may be tempered with an improvement in the health system.
With the above analysis, the policy trade-off as a constrained minimisation problem of economic losses subject to HCUR above appears to apply in NCR but not in regions outside of NCR. The latter is better off in enhancing prevention, detection, isolation, treatment, and reintegration (PDITR) strategy combined with targeted small area lockdowns, if necessary, without risking any increases in economic losses. But, in all scenarios and anywhere, the enhancement of the HSC through improved PDITR strategies remains vital to avoid having to deal with local infection surges and outbreaks. This also avoids forcing local authorities in a policy bind between health and economic measures to implement. Enhancing PDITR in congested urban centres (i.e., NCR) is difficult especially with the surge in new daily cases. People are forced to defy social distance rules and other minimum health standards in public transportation and in their workplaces that help spread the virus.
We extended the FASSSTER subnational SEIR model to include two differential equations that capture economic losses due to COVID-19 infection and due to the lockdown measures, respectively. The extended model aims to account for the incurred economic losses following the rise and fall of the number of active COVID-19 cases in the country and the implementation of various lockdown measures. In simulating eight different scenarios in each of the five selected regions in the country, we found a tight policy choice in the case of the National Capital Region (NCR) but not in the cases of four other regions far from NCR. This clearly demonstrates the difficult policy decision in the case of NCR in minimising economic losses given the constraint of its intensive care unit (ICU) bed capacity.
On the other hand, the regions far from the NCR have wider policy space towards economic reopening and recovery. However, in all scenarios, the primary significance of improving the health system capacity (HSC) to detect and control the spread of the disease remains in order to widen the trade-off policy space between public health and economic measures.
The policy trade-off simulation results imply different policy approaches in each region. This is also to consider the archipelagic nature of the country and the simultaneous concentration of economic output and COVID-19 cases in NCR and contiguous provinces compared to the rest of the country. Each local region in the country merits exploration of different policy combinations in economic and health measures depending on the number of active COVID-19 cases, strategic importance of economic activities and output specific in the area, the geographic spread of the local population and their places of work, and considering local health system capacities. However, we would like to caution that the actual number of cases could diverge from the results of our simulations. This is because the parameters of the model must be updated regularly driven generally by the behaviour of the population and the likely presence of variants of COVID-19. Given the constant variability of COVID-19 data, we recommend a shorter period of model projections from one to two months at the most.
In summary, this paper showed how mathematical modelling can be used to inform policymakers on the economic impact of lockdown policies and make decisions among the available policy options, taking into consideration the economic and health trade-offs of these policies. The proposed methodology provides a tool for enhanced policy decisions in other countries during the COVID-19 pandemic or similar circumstances in the future.
Data availability
The raw datasets used in this study are publicly available at the Department of Health COVID-19 Tracker Website: https://doh.gov.ph/covid19tracker . Datasets will be made available upon request after completing request form and signing non-disclosure agreement. Code and scripts will be made available upon request after completing request form and signing non-disclosure agreement.
Acemoglu D, Chernozhukov V, Werning I, Whinston M (2020) Optimal targeted lockdowns of a multi-group SIR model. In: National Bureau of Economic Research Working Papers. National Bureau of Economic Research (NBER). https://www.nber.org/system/files/working_papers/w27102/w27102.pdf . Accessed 16 Jun 2021
Amewu S, Asante S, Pauw K, Thurlow J (2020) The economic costs of COVID-19 in Sub-Saharan Africa: insights from a simulation exercise for Ghana. Eur J Dev Res 32(5):1353–1378. https://doi.org/10.1057/s41287-020-00332-6
Article PubMed PubMed Central Google Scholar
Anand N, Sabarinath A, Geetha S, Somanath S (2020) Predicting the spread of COVID-19 using SIR model augmented to incorporate quarantine and testing. Trans Indian Natl Acad Eng 5:141–148. https://doi.org/10.1007/s41403-020-00151-5
Article Google Scholar
Bangko Sentral ng Pilipinas (2021) 2021 Inflation Report First Quarter. https://www.bsp.gov.ph/Lists/Inflation%20Report/Attachments/22/IR1qtr_2021.pdf . Accessed 16 Jun 2021
Benson C, Clay E (2004) Understanding the economic and financial impacts of natural disasters. In: World Bank Disaster Risk Management Paper. World Bank. https://elibrary.worldbank.org/doi/abs/10.1596/0-8213-5685-2 . Accessed Jan 2022
Byrd R, Lu P, Nocedal J, Zhu C (1995) A limited memory algorithm for bound constrained optimization. SIAM J Sci Comput 16:1190–1208. https://doi.org/10.1137/0916069
Article MathSciNet MATH Google Scholar
Cereda F, Rubião R, Sousa L (2020) COVID-19, Labor market shocks, and poverty in brazil: a microsimulation analysis. In: poverty and equity global practice. World Bank. https://openknowledge.worldbank.org/bitstream/handle/10986/34372/COVID-19-Labor-Market-Shocks-and-Poverty-in-Brazil-A-Microsimulation-Analysis.pdf?sequence=1&isAllowed=y . Accessed 21 Feb 2021
Chen D, Lee S, Sang J (2020) The role of state-wide stay-at-home policies on confirmed COVID-19 cases in the United States: a deterministic SIR model. Health Informatics Int J 9(2/3):1–20. https://doi.org/10.5121/hiij.2020.9301
Article CAS Google Scholar
Department of Health-Epidemiology Bureau (2020) COVID-19 tracker Philippines. https://doh.gov.ph/covid19tracker . Accessed 12 Feb 2021
Department of Trade and Industry (2020a) Revised category I-IV business establishments or activities pursuant to the revised omnibus guidelines on community quarantine dated 22 May 2020 Amending for the purpose of memorandum circular 20-22s. https://dtiwebfiles.s3-ap-southeast-1.amazonaws.com/COVID19Resources/COVID-19+Advisories/090620_MC2033.pdf . Accessed 09 Feb 2021
Department of Trade and Industry (2020b) Increasing the allowable operational capacity of certain business establishments of activities under categories II and III under general community quarantine. https://dtiwebfiles.s3-ap-southeast-1.amazonaws.com/COVID19Resources/COVID-19+Advisories/031020_MC2052.pdf . Accessed 09 Feb 2021
Department of Trade and Industry (2021) Prescribing the recategorization of certain business activities from category IV to category III. https://www.dti.gov.ph/sdm_downloads/memorandum-circular-no-21-08-s-2021/ . Accessed 15 Mar 2021
Estadilla C, Uyheng J, de Lara-Tuprio E, Teng T, Macalalag J, Estuar M (2021) Impact of vaccine supplies and delays on optimal control of the COVID-19 pandemic: mapping interventions for the Philippines. Infect Dis Poverty 10(107). https://doi.org/10.1186/s40249-021-00886-5
FASSSTER (2020) COVID-19 Philippines LGU Monitoring Platform. https://fassster.ehealth.ph/covid19/ . Accessed Dec 2020
Geard N, Giesecke J, Madden J, McBryde E, Moss R, Tran N (2020) Modelling the economic impacts of epidemics in developing countries under alternative intervention strategies. In: Madden J, Shibusawa H, Higano Y (eds) Environmental economics and computable general equilibrium analysis. Springer Nature Singapore Pte Ltd., Singapore, pp. 193–214
Chapter Google Scholar
Genoni M, Khan A, Krishnan N, Palaniswamy N, Raza W (2020) Losing livelihoods: the labor market impacts of COVID-19 in Bangladesh. In: Poverty and equity global practice. World Bank. https://openknowledge.worldbank.org/bitstream/handle/10986/34449/Losing-Livelihoods-The-Labor-Market-Impacts-of-COVID-19-in-Bangladesh.pdf?sequence=1&isAllowed=y . Accessed 21 Feb 2021
Goldsztejn U, Schwartzman D, Nehorai A (2020) Public policy and economic dynamics of COVID-19 spread: a mathematical modeling study. PLoS ONE 15(12):e0244174. https://doi.org/10.1371/journal.pone.0244174
Article CAS PubMed PubMed Central Google Scholar
Gousseff M, Penot P, Gallay L, Batisse D, Benech N, Bouiller K, Collarino R, Conrad A, Slama D, Joseph C, Lemaignen A, Lescure F, Levy B, Mahevas M, Pozzetto B, Vignier N, Wyplosz B, Salmon D, Goehringer F, Botelho-Nevers E (2020) Clinical recurrences of COVID-19 symptoms after recovery: viral relapse, reinfection or inflammatory rebound? J Infect 81(5):816–846. https://doi.org/10.1016/j.jinf.2020.06.073
Hou C, Chen J, Zhou Y, Hua L, Yuan J, He S, Guo Y, Zhang S, Jia Q, Zhang J, Xu G, Jia E (2020) The effectiveness of quarantine in Wuhan city against the Corona Virus Disease 2019 (COVID-19): A well-mixed SEIR model analysis. J Med Virol 92(7):841–848. https://doi.org/10.1002/jmv.25827
Article CAS PubMed Google Scholar
Hupkau C, Isphording I, Machin S, Ruiz-Valenzuela J (2020) Labour market shocks during the Covid-19 pandemic: inequalities and child outcomes. In: Covid-19 analysis series. Center for Economic Performance. https://cep.lse.ac.uk/pubs/download/cepcovid-19-015.pdf . Accessed 21 Feb 2021
Inter-Agency Task Force for the Management of Emerging Infectious Diseases (2020) Omnibus Guidelines on the Implementation of Community Quarantine in the Philippines with Amendments as of June 3, 2020. https://www.officialgazette.gov.ph/downloads/2020/06jun/20200603-omnibus-guidelines-on-the-implementation-of-community-quarantine-in-the-philippines.pdf . Accessed 09 Feb 2021
Kashyap S, Gombar S, Yadlowsky S, Callahan A, Fries J, Pinsky B, Shah N (2020) Measure what matters: counts of hospitalized patients are a better metric for health system capacity planning for a reopening. J Am Med Inform Assoc 27(7):1026–1131. https://doi.org/10.1093/jamia/ocaa076
Keogh-Brown M, Jensen H, Edmunds J, Smith R (2020) The impact of Covid-19, associated behaviours and policies on the UK economy: a computable general equilibrium model. SSM Popul Health 12:100651. https://doi.org/10.1016/j.ssmph.2020.100651
Keogh-Brown M, Smith R, Edmunds J, Beutels P (2010) The macroeconomic impact of pandemic influenza: estimates from models of the United Kingdom, France, Belgium and The Netherlands. Eur J Health Econ 11:543–554. https://doi.org/10.1007/S10198-009-0210-1
Article PubMed Google Scholar
Laborde D, Martin W, Vos R (2021) Impacts of COVID-19 on global poverty, food security, and diets: insights from global model scenario analysis. Agri Econ (United Kingdom) 52(3):375–390. https://doi.org/10.1111/agec.12624
Maital S, Barzani E (2020) The global economic impact of COVID-19: a summary of research. https://www.neaman.org.il/en/Files/Global%20Economic%20Impact%20of%20COVID19.pdf . Accessed Jan 2022
McKibbin W, Fernando R (2020) The global macroeconomic impacts of COVID-19: seven scenarios. https://www.brookings.edu/research/the-global-macroeconomic-impacts-of-covid-19-seven-scenarios/ . Accessed Jan 2022
National Economic and Development Authority (2020) Impact of COVID-19 on the economy and the people, and the need to manage risk. https://www.sec.gov.ph/wp-content/uploads/2020/12/2020CG-Forum_Keynote_NEDA-Sec.-Chua_Impact-of-COVID19-on-the-Economy.pdf . Accessed Feb 2021
National Economic Development Authority-Investment Coordination Committee (2016) Revisions on ICC Guidelines and Procedures (Updated Social Discount Rate for the Philippines). http://www.neda.gov.ph/wp-content/uploads/2017/01/Revisions-on-ICC-Guidelines-and-Procedures-Updated-Social-Discount-Rate-for-the-Philippines.pdf . Accessed 12 Feb 2021
Noy I, Doan N, Ferrarini B, Park D (2020) The economic risk of COVID-19 in developing countries: where is it highest? In: Djankov S, Panizza U (eds) COVID-19 in developing economies. Center for Economic Policy Research Press, London, pp. 38–52
Google Scholar
Peng T, Liu X, Ni H, Cui Z, Du L (2020) City lockdown and nationwide intensive community screening are effective in controlling the COVID-19 epidemic: analysis based on a modified SIR model. PLoS ONE 15(8):e0238411. https://doi.org/10.1371/journal.pone.0238411
Pham T, Dwyer L, Su J, Ngo T (2021) COVID-19 impacts of inbound tourism on australian economy. Ann. Tourism Res. 88:103179. https://doi.org/10.1016/j.annals.2021.103179
Philippine Statistics Authority (2019a) 2018 Labor Force Survey (Microdata). https://psa.gov.ph/content/2018-annual-estimates-tables . Accessed 12 Feb 2021
Philippine Statistics Authority (2019b) Updated population projections based on the results of 2015 POPCEN. https://psa.gov.ph/content/updated-population-projections-based-results-2015-popcen . Accessed 12 Feb 2021
Philippine Statistics Authority (2020) Census of population and housing. https://psa.gov.ph/population-and-housing . Accessed May 2020
Philippine Statistics Authority (2021) National Accounts Data Series. https://psa.gov.ph/national-accounts/base-2018/data-series . Accessed 05 Apr 2021
Reno C, Lenzi J, Navarra A, Barelli E, Gori D, Lanza A, Valentini R, Tang B, Fantini MP (2020) Forecasting COVID-19-associated hospitalizations under different levels of social distancing in Lombardy and Emilia-Romagna, Northern Italy: results from an extended SEIR compartmental model. J Clin Med 9(5):1492. https://doi.org/10.3390/jcm9051492
Article CAS PubMed Central Google Scholar
United Nations Development Programme (2021) The Socioeconomic Impact Assessment of COVID-19 in the Bangsamoro Autonomous Region in Muslim Mindanao. UNDP in the Philippines. https://www.ph.undp.org/content/philippines/en/home/library/the-socioeconomic-impact-assessment-of-covid-19-on-the-bangsamor.html . Accessed Nov 2021.
Verikios G, McCaw J, McVernon J, Harris A (2012) H1N1 influenza and the Australian macroeconomy. J Asia Pac Econ 17(1):22–51. https://doi.org/10.1080/13547860.2012.639999
World Bank (2020) Projected Poverty Impacts of COVID-19 (Coronavirus). https://www.worldbank.org/en/topic/poverty/brief/projected-poverty-impacts-of-COVID-19 . Accessed Jan 2022
World Bank (2021) Global economic perspectives. World Bank, Washington DC
World Bank (2022) GDP growth (annual %). https://data.worldbank.org/indicator/NY.GDP.MKTP.KD.ZG . Accessed Jan 2022
Download references
Acknowledgements
We thank Dr. Geoffrey M. Ducanes, Associate Professor, Ateneo de Manila University Department of Economics, for giving us valuable comments in the course of developing the economic model, and Mr. Jerome Patrick D. Cruz, current Ph.D. student, Massachusetts Institute of Technology, for initiating and leading the economic team in FASSSTER in the beginning of the project for their pitches in improving the model. We also thank Mr. John Carlo Pangyarihan for typesetting the manuscript. The project is supported by the Philippine Council for Health Research and Development, United Nations Development Programme and the Epidemiology Bureau of the Department of Health.
Author information
Authors and affiliations.
Department of Mathematics, Ateneo de Manila University, Quezon City, Philippines
Elvira P. de Lara-Tuprio & Timothy Robin Y. Teng
Department of Information Systems and Computer Science, Ateneo de Manila University, Quezon City, Philippines
Maria Regina Justina E. Estuar & Lenard Paulo V. Tamayo
Department of Economics, Ateneo de Manila University, Quezon City, Philippines
Joselito T. Sescon, Cymon Kayle Lubangco, Rolly Czar Joseph T. Castillo & Gerome M. Vedeja
Department of Mathematics, Caraga State University, Butuan City, Philippines
Jay Michael R. Macalalag
You can also search for this author in PubMed Google Scholar
Contributions
All authors contributed to the study conception and design. Model conceptualization, data collection and analysis were performed by EPdL-T, MRJEE, JTS, CKL, CJTC, TRYT, LPT, JMRM, and GMV. All authors commented on previous versions of the manuscript, and read and approved the final manuscript.
Corresponding author
Correspondence to Cymon Kayle Lubangco .
Ethics declarations
Competing interests.
Proponents of the project are corresponding authors in the study. Research and corresponding publications are integral to the development of the models and systems. The authors declare no conflict nor competing interest.
Ethical approval
Not applicable
Informed consent
Additional information.
Publisher’s note Springer Nature remains neutral with regard to jurisdictional claims in published maps and institutional affiliations.
Supplementary information
Supplementary information, rights and permissions.
Open Access This article is licensed under a Creative Commons Attribution 4.0 International License, which permits use, sharing, adaptation, distribution and reproduction in any medium or format, as long as you give appropriate credit to the original author(s) and the source, provide a link to the Creative Commons license, and indicate if changes were made. The images or other third party material in this article are included in the article’s Creative Commons license, unless indicated otherwise in a credit line to the material. If material is not included in the article’s Creative Commons license and your intended use is not permitted by statutory regulation or exceeds the permitted use, you will need to obtain permission directly from the copyright holder. To view a copy of this license, visit http://creativecommons.org/licenses/by/4.0/ .
Reprints and permissions
About this article
Cite this article.
de Lara-Tuprio, E.P., Estuar, M.R.J.E., Sescon, J.T. et al. Economic losses from COVID-19 cases in the Philippines: a dynamic model of health and economic policy trade-offs. Humanit Soc Sci Commun 9 , 111 (2022). https://doi.org/10.1057/s41599-022-01125-4
Download citation
Received : 06 August 2021
Accepted : 09 March 2022
Published : 04 April 2022
DOI : https://doi.org/10.1057/s41599-022-01125-4
Share this article
Anyone you share the following link with will be able to read this content:
Sorry, a shareable link is not currently available for this article.
Provided by the Springer Nature SharedIt content-sharing initiative
Quick links
- Explore articles by subject
- Guide to authors
- Editorial policies

How serious was it? The impact of preschool closure on mothers’ psychological distress: evidence from the first COVID-19 outbreak
- Published: 15 May 2024
Cite this article
- Izumi Yokoyama 1 &
- Reo Takaku 1
54 Accesses
5 Altmetric
Explore all metrics
During the early days of the initial outbreak of the novel coronavirus disease 2019 (COVID-19) in Japan, preschools were exempt from nationwide school closures, which came into effect on March 2, 2020. In addition, Japan is unique in that it experienced preschool closures without any heavy restrictions on daily life activities, making it possible to separate the effects of preschool closures from those of other anti-COVID-19 policies such as lockdown measures. This study conducted a placebo test that revealed that the decision to close or keep preschools open was left to each facility and the municipality where they were located and was characterized by relatively high randomness. Based on these advantages, we explored the effects of children’s preschool absence during March 2020 on mothers’ psychological distress. Our results showed that preschool closures caused immediate deterioration in mothers’ psychological states, with moderate psychological distress persisting for at least 5 months.
This is a preview of subscription content, log in via an institution to check access.
Access this article
Price includes VAT (Russian Federation)
Instant access to the full article PDF.
Rent this article via DeepDyve
Institutional subscriptions
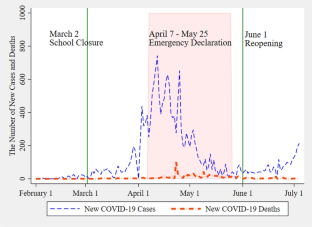
Sources: Ministry of Health, Labor, and Welfare “New coronavirus infection information,” URL: https://covid19.mhlw.go.jp/
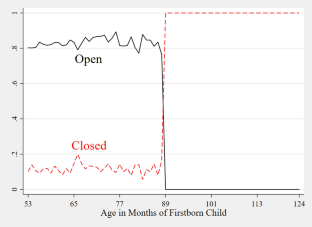
Similar content being viewed by others
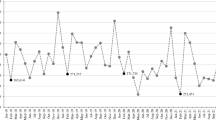
The Impact of Daycare Closures Owing to COVID-19 on Parental Stress: The Case of Japan
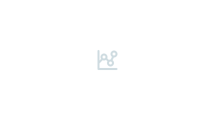
Vulnerability and resilience in children during the COVID-19 pandemic
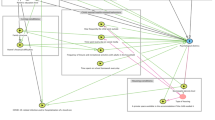
Social determinants associated with psychological distress in children and adolescents during and after the first COVID-19-related lockdown in France: results from the CONFEADO study
Data availability.
We are willing to share our dataset and questionnaire on request.
Some studies found negative effects on mental health soon after the implementation of lockdowns (Armbruster and Klotzbücher, 2020 ; Sibley et al., 2020 ). Sibley et al. ( 2020 ) compared measurements on the Kessler (K6) Psychological Distress Scale (Kessler et al., 2002 ) before and after the lockdown in New Zealand and found a statistically significant increase in psychological distress.
According to (MEXT, 2020 ), 99.9% of elementary schools were closed as of March 10.
For more details on school closures in Japan, see Sects. 2.1 and 2.2 . School closures in other countries are summarized in the Appendix.
Following the removal of the state of emergency declaration on May 25, 2020, which lifted the obligation to close educational facilities, preschools reopened nationwide after June 2020.
For international readers, Ando et al. ( 2020 ) provides a comprehensive overview of the Japanese government’s response to the COVID-19 crisis in terms of the fiscal measures taken between January and June 2020.
We did not impose any restriction on siblings of the firstborn child in the sampling process, because restricting the sample to mothers with only one child would likely bias our results toward families with special features such as low socioeconomic status or strongly career-oriented double-income couples.
In Takaku and Yokoyama ( 2021 ), using the same survey data, we tested whether these sample drops were more likely to occur for mothers who experienced school closures in March 2020 and confirmed that there was no such trend.
Note that there were cases where preschools were closed temporarily in March, possibly due to direct or indirect exposure to COVID-19, but then reopened in April. These cases were omitted from the analysis, because even if we controlled for the potential risk, that is, the number of infected persons by prefecture, the exclusion restrictions would not have been satisfied. Moreover, given that the preschools reopened in April, the infection rate in that prefecture could not have been too high, which was another reason we could not satisfy the exclusion restrictions by controlling for only the number of infected persons by prefecture. Further, since the infection occurred in an area with a low infection rate, infections that occurred around these preschools in March could even be considered close to random. Therefore, discarding these 23 samples from our data would not hurt external validation. There were other cases in which preschools were closed in March but did not open in April. This implies that there was still a high risk of infection in April, meaning that the preschools were located in high-risk areas. Thus, in these cases, the stress caused by this situation could be controlled for by the number of infected persons per prefecture.
The K6 is a truncated form of the 10-item Kessler Psychological Distress Scale (K10). Many economic studies use the K6 and K10 scales in addition to other standard measures of psychological distress and mental health (Cygan-Rehm et al., 2017 ; Gong et al., 2020 ).
As stated in Sect. 3.2 , the difference in K6 scores between the two groups in the previous year reflected the disparity that existed before preschool closure, and this gap was controlled for by the current control variables in the regressions.
This result may have been obtained, because traveling within the same prefecture is relatively easy, given Japan’s developed transportation network, which means that everyone in the same prefecture potentially faces the same infection risk. Thus, obtaining an insignificant coefficient from the placebo test meant that controlling for the potential risk of infection at the prefecture level would be sufficient and that there was no need to also control for a large city dummy variable.
While the true reason behind Prime Minister Abe’s declaration of school closures is not completely clear, hosting the Tokyo Olympics in August 2020 as planned would be a strong motivation to implement school closures. The official explanation in the statement from the Cabinet Office appeared to be based on the belief that infectious diseases spread rapidly among young children, as with influenza, and the government should protect children from this new disease.
American Psychiatric Association. (1994). Diagnostic and statistical manual of mental disorders. DSM IV-4th edition 1994
Ando, M., Furukawa, C., Nakata, D., Sumiya, K., et al. (2020). Fiscal responses to the COVID-19 crisis in Japan: The first six months. National Tax Journal, 73 (3), 901–926.
Article Google Scholar
Armbruster, S., & Klotzbücher, V. (2020). Lost in lockdown? COVID-19, social distancing, and mental health in Germany (No. 2020-04). Diskussionsbeiträge. http://hdl.handle.net/10419/218885 .
Asakawa, S., & Ohtake, F. (2022). Impact of COVID-19 school closures on the cognitive and non-cognitive skills of elementary school students. Research Institute of Economy, Trade and Industry (RIETI): Tech. rep.
Asakawa, S., Ohtake, F., & Sano, S. (2023). The impact of the COVID-19 pandemic on the academic achievement of elementary and junior high school students: Analysis using administrative data from Amagasaki city. Tech. rep.
Baron, E. J., Goldstein, E. G., & Wallace, C. T. (2020). Suffering in silence: How COVID-19 school closures inhibit the reporting of child maltreatment. Journal of Public Economics, 190 (104), 258. https://doi.org/10.1016/j.jpubeco.2020.104258 , http://www.sciencedirect.com/science/article/pii/S0047272720301225
Brodeur, A., Clark, A. E., Fleche, S., & Powdthavee, N. (2021). COVID-19, lockdowns and well-being: Evidence from Google Trends. Journal of Public Economics, 193 (104), 346. https://doi.org/10.1016/j.jpubeco.2020.104346 , http://www.sciencedirect.com/science/article/pii/S0047272720302103
Cabinet Office. (2020). Prime Minister Abe’s Press Conference On February 29. https://www.kantei.go.jp/jp/98_abe/statement/2020/0229kaiken.html , accessed: 2020-09-14
Campedelli, G. M., Aziani, A., & Favarin, S. (2020). Exploring the immediate effects of COVID-19 containment policies on crime: an empirical analysis of the short-term aftermath in Los Angeles. American Journal of Criminal Justic . https://doi.org/10.1007/s12103-020-09578-6
Cygan-Rehm, K., Kuehnle, D., Oberfichtner, M. (2017). Bounding the causal effect of unemployment on mental health: Nonparametric evidence from four countries. Health Economics 26(12):1844–1861, https://doi.org/10.1002/hec.3510 , URL: https://onlinelibrary.wiley.com/doi/abs/10.1002/hec.3510, https://onlinelibrary.wiley.com/doi/pdf/10.1002/hec.3510
Furukawa, T. A., Kawakami, N., Saitoh, M., Ono, Y., Nakane, Y., Nakamura, Y., Tachimori, H., Iwata, N., Uda, H., Nakane, H., et al. (2008). The performance of the Japanese version of the K6 and K10 in the world mental health survey Japan. International journal of methods in psychiatric research, 17 (3), 152–158.
Gong, J., Lu, Y., & Xie, H. (2020). The average and distributional effects of teenage adversity on long-term health. Journal of Health Economics, 71 (102), 288. https://doi.org/10.1016/j.jhealeco.2020.102288 , http://www.sciencedirect.com/science/article/pii/S0167629619304989
Hipp, L., Bünning, M., Munnes, S., & Sauermann, A. (2020). Problems and pitfalls of retrospective survey questions in COVID-19 studies. Survey Research Methods, 14 (2), 109–114. https://doi.org/10.18148/srm/2020.v14i2.7741
Kessler, R. C., Andrews, G., Colpe, L. J., Hiripi, E., Mroczek, D. K., Normand, S. L. T., Walters, E. E., & Zaslavsky, A. M. (2002). Short screening scales to monitor population prevalences and trends in non-specific psychological distress. Psychological Medicine, 32 , 959–976.
Leslie, E., & Wilson, R. (2020). Sheltering in place and domestic violence: Evidence from calls for service during COVID-19. Journal of Public Economics, 189 (104), 241. https://doi.org/10.1016/j.jpubeco.2020.104241 , http://www.sciencedirect.com/science/article/pii/S0047272720301055
MEXT. (2020). What is the mext and Japanese government doing in response to COVID-19? https://www.mext.go.jp/a_menu/coronavirus/index.html . Accessed 14 Sep 2020.
Mohler, G., Bertozzi, A. L., Carter, J., Short, M. B., Sledge, D., Tita, G. E., Uchida, C. D., & Brantingham, P. J. (2020). Impact of social distancing during COVID-19 pandemic on crime in Los Angeles and Indianapolis. Journal of Criminal Justice, 68 (101), 692. https://doi.org/10.1016/j.jcrimjus.2020.101692 . http://www.sciencedirect.com/science/article/pii/S0047235220301860 .
New York Times. (2020). Japan shocks parents by moving to close all schools over Coronavirus. https://www.nytimes.com/2020/02/27/world/asia/japan-schools-coronavirus.html . Accessed 14 Sep 2020.
Nikkei Inc. (2020). More daycare centers are now closed [in Japanese, hoikuen hirogaru kyuen/riyoujishuku]. https://www.nikkei.com/article/DGXMZO57894650Z00C20A4CC1000/ . Accessed 23 Sep 2020.
North, A. (2023). The unexpected pitfalls of work-from-home parenthood. https://www.vox.com/even-better/2023/7/31/23807375/remote-hybrid-wfh-work-parents-moms-parenting , accessed: 2024-03-28
Parker, J.J., Garfield, C.F., Simon, C.D., Bendelow, A., Heffernan, M.E., Davis, M.M., Kan, K. (2023). Teleworking, parenting stress, and the health of mothers and fathers. JAMA Network Open, 6 (11), e2341,844–e2341,844. https://doi.org/10.1001/jamanetworkopen.2023.41844 . https://jamanetwork.com/journals/jamanetworkopen/articlepdf/2811325/parker_2023_ld_230205_1698176578.7112.pdf .
Payne, J.L., & Morgan, A. (2020). COVID-19 and violent crime: A comparison of recorded offence rates and dynamic forecasts (ARIMA) for March 2020 in Queensland, Australia. SocArXiv g4kh7, Center for Open Science, https://doi.org/10.31219/osf.io/g4kh7 , https://ideas.repec.org/p/osf/socarx/g4kh7.html
Pietrobelli, A., Pecoraro, L., Ferruzzi, A., Heo, M., Faith, M., Zoller, T., Antoniazzi, F., Piacentini, G., Fearnbach, S,N., & Heymsfield, S.B. (2020). Effects of COVID-19 lockdown on lifestyle behaviors in children with obesity living in Verona, Italy: A longitudinal study. Obesity, 28 (8):1382–1385. https://doi.org/10.1002/oby.22861 . https://onlinelibrary.wiley.com/doi/abs/10.1002/oby.22861 , https://onlinelibrary.wiley.com/doi/pdf/10.1002/oby.22861 .
Piquero, A. R., Jordan, Riddell SANC., Bishopp, Rand, Reid, J. A., & Piquero, N. L. (2020). Staying home, staying safe? a short-term analysis of COVID-19 on dallas domestic violence. American Journal of Criminal Justice, 45 (4), 601–635.
Rieth, M., & Hagemann, V. (2021). The impact of telework and closure of educational and childcare facilities on working people during COVID-19. Zeitschrift für Arbeits- und Organisationspsychologie, 65 (4), 202–214. https://doi.org/10.1026/0932-4089/a000370
Sakurai, K., Nishi, A., Kondo, K., Yanagida, K., & Kawakami, N. (2011). Screening performance of K6/K10 and other screening instruments for mood and anxiety disorders in Japan. Psychiatry and Clinical Neurosciences, 65 (5), 434–441. https://doi.org/10.1111/j.1440-1819.2011.02236.x . https://onlinelibrary.wiley.com/doi/abs/10.1111/j.1440-1819.2011.02236.x
Sanga, S., McCrary, J. (2020). The impact of the coronavirus lockdown on domestic violence. Working paper
Sibley, C. G., Greaves, L. M., Satherley, N., Wilson, M. S., Overall, N. C., Lee, C. H. J., Milojev, P., Bulbulia, J., Osborne, D., Milfont, T. L., Houkamau, C. A., Duck, I. M., Vickers-Jones, R., & Barlow, F. K. (2020). Effects of the COVID-19 pandemic and nationwide lockdown on trust, attitudes toward government, and well-being. American Psychologist , 75 (5), 618–630. https://doi.org/10.1037/amp0000662 .
Takaku, R., Yokoyama, I. (2021). What the COVID-19 school closure left in its wake: Evidence from a regression discontinuity analysis in Japan. Journal of Public Economics , 195 , 104364.
UNESCO (2020) COVID-19 response. https://en.unesco.org/covid19 . Accessed 14 Sep 2020
Download references
Author information
Authors and affiliations.
Graduate School of Economics, Hitotsubashi University, 2-1, Naka, Kunitachi, Tokyo, 186-8601, Japan
Izumi Yokoyama & Reo Takaku
You can also search for this author in PubMed Google Scholar
Corresponding author
Correspondence to Izumi Yokoyama .
Additional information
Publisher's note.
Springer Nature remains neutral with regard to jurisdictional claims in published maps and institutional affiliations.
This research was supported by the Hitotsubashi Institute for Advanced Study and Grants-in-Aid for Scientific Research (18K12793, PI: Izumi Yokoyama). All errors are our own.
School closures in other countries
This section summarizes school closures in multiple countries. In Japan, all elementary schools closed on March 2, but this requirement was waived for preschools. In contrast, as shown in Table 5 , many developed countries closed schools only gradually, unlike Japan.
For example, in the UK, school closures started on February 20 in some regions, and nationwide closures were implemented approximately one month later. However, nationwide closures were abruptly implemented in Japan despite the fact that only three COVID-19 deaths had occurred by the day of the announcement. Footnote 12
Rights and permissions
Springer Nature or its licensor (e.g. a society or other partner) holds exclusive rights to this article under a publishing agreement with the author(s) or other rightsholder(s); author self-archiving of the accepted manuscript version of this article is solely governed by the terms of such publishing agreement and applicable law.
Reprints and permissions
About this article
Yokoyama, I., Takaku, R. How serious was it? The impact of preschool closure on mothers’ psychological distress: evidence from the first COVID-19 outbreak. JER (2024). https://doi.org/10.1007/s42973-024-00155-8
Download citation
Received : 29 November 2023
Revised : 28 March 2024
Accepted : 02 April 2024
Published : 15 May 2024
DOI : https://doi.org/10.1007/s42973-024-00155-8
Share this article
Anyone you share the following link with will be able to read this content:
Sorry, a shareable link is not currently available for this article.
Provided by the Springer Nature SharedIt content-sharing initiative
- Instrument variable estimation
- Mental health
- Preschool closures
JEL Classifications
- Find a journal
- Publish with us
- Track your research

Cost of living help and a future made in Australia
Strengthening medicare and the care economy.
Building a better health system than improves outcomes
Print or save page
On this page
High‑quality health services through Medicare
Boosting access to essential health services
Building a better healthcare system
The Government is investing $2.8 billion to continue its commitment to strengthen Medicare. This includes the $1.2 billion package to address pressures facing the health system, which provides:
- $882.2 million to support older Australians avoid hospital admission, be discharged from hospital earlier and improve their transition out of hospital to other appropriate care.
- $227 million to deliver a further 29 Medicare Urgent Care Clinics and boost support for regional and remote clinics. This will increase the total number of clinics across Australia to 87. Since commencing last year, existing clinics have already provided almost 400,000 bulk‑billed visits.
- $90 million to address health workforce shortages by making it simpler and quicker for international health practitioners to work in Australia.
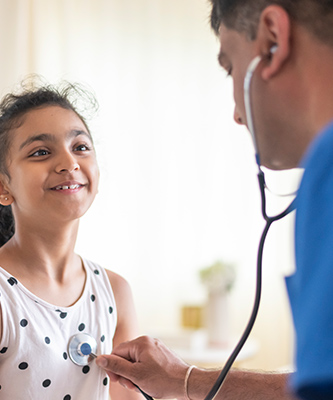
Rohan’s daughter Zoya has been off school with a runny nose and a cough. By 6pm, she is lethargic and has a fever.
Rohan is concerned because his regular GP is now closed. Instead of waiting for hours at the emergency department, he takes Zoya to a Medicare Urgent Care Clinic, without having to make an appointment.
During the bulk billed visit, Zoya is diagnosed with an infection by the doctor and prescribed appropriate medication. Rohan and Zoya leave within an hour of arrival. Zoya makes a full recovery.
Improving health outcomes
Almost half of Australians live with a chronic condition. This Budget will provide $141.1 million for research and services for people living with chronic conditions, including bowel and skin cancer, diabetes and dementia.
To improve health outcomes, the Government is providing:
- Support for Australians to enjoy healthier, more active lives by investing $132.7 million in sport participation and performance programs.
- $825.7 million to ensure Australians can continue to access testing for and vaccinations against COVID‑19. The Government is also ensuring continued access to oral antiviral medicines on the Pharmaceutical Benefits Scheme.
- $41.6 million over two years to continue funding for alcohol and other drug treatment and support services, including the Good Sports alcohol management program for community sporting clubs.
The Government is allocating an additional $411.6 million (for a total $1.6 billion over 13 years) through the Medical Research Future Fund to continue existing research and introduce two new research missions for low‑survival cancers and reducing health inequities.
Improving access to medicines
The Government is investing $3.4 billion for new and amended listings to the Pharmaceutical Benefits Scheme, which means eligible patients can save on treatment costs.
By expanding the Closing the Gap Pharmaceutical Benefits Scheme Co‑payment Program, eligible First Nations patients will have free or cheaper access to all Pharmaceutical Benefits Scheme medicines.
Australians will benefit from $141.1 million to support and expand the National Immunisation Program.
Mental health support
The Government’s $888.1 million mental health package over eight years will help people get the care they need, while relieving pressure on the Better Access initiative and making it easier to access services.
A free, low‑intensity digital service will be established to address the gap for people with mild mental health concerns. From 1 January 2026, Australians will be able to access the service without a referral and receive timely, high‑quality mental health support. Once fully established, 150,000 people are expected to make use of this service each year.
The Government is improving access to free mental health services through a network of walk‑in Medicare Mental Health Centres, built on the established Head to Health network. The upgraded national network of 61 Medicare Mental Health Centres will open by 30 June 2026. They will provide clinical services for adults with moderate‑to‑severe mental health needs.
For Australians with complex mental health needs, funding will be provided for Primary Health Networks to partner with GPs to deliver multidisciplinary, wraparound support services and care coordination.
Improving the aged care system
Providing quality care
The Budget provides $2.2 billion to deliver aged care reforms and continue implementing recommendations from the Royal Commission into Aged Care Quality and Safety.
The new Aged Care Act will put the rights and needs of older people at the centre of the aged care system. The new Act will provide the framework for fundamental change within the aged care sector.
More Home Care Packages
The Government is investing $531.4 million to release an additional 24,100 Home Care Packages in 2024–25. This will help reduce average wait times and enable people to age at home if they prefer to do so.
Improving aged care regulation
Funding of $110.9 million over four years will support an increase in the Aged Care Quality and Safety Commission’s regulatory capabilities.
The Government is investing $1.2 billion in critical digital systems to support the introduction of the new Aged Care Act and contemporary IT systems.
The My Aged Care Contact Centre will receive $37 million to reduce call‑waiting times for people seeking information and access to aged care.
Higher wages for aged care workers
The Government has committed to fund the Fair Work Commission decision to increase the award wage for direct and indirect aged care workers once the final determination is made. This will build on the $11.3 billion already allocated to support the interim 15 per cent wage increase for aged care workers.
The Government is providing $87.2 million for workforce initiatives to attract nurses and other workers into aged care.
Reforming the disability sector
Better and more sustainable services
Getting the National Disability Insurance Scheme (NDIS) back on track
A further $468.7 million is being provided to support people with disability and get the NDIS back on track. This includes:
- $214 million over two years to fight fraud and to co‑design NDIS reforms with people with disability, announced earlier this year
- $160.7 million to upgrade the NDIS Quality and Safeguards Commission’s information technology
- $45.5 million to establish a NDIS Evidence Advisory Committee
- $20 million to start consultation and design on reforms to help NDIS participants and people with disability navigate services.
This builds on $732.9 million provided in the 2023–24 Budget.
In December 2023, National Cabinet agreed to work together to improve the experience of participants and restore the original intent of the Scheme to support people with permanent and significant disability, within a broader ecosystem of supports. This builds on an earlier decision by National Cabinet to ensure Scheme sustainability and achieve an 8 per cent growth target by 1 July 2026, with further moderation as the NDIS matures.
Improving employment for people with disability
A $227.6 million investment will support a new specialised disability employment program to replace the existing Disability Employment Services program by 1 July 2025. This includes a modern digital platform for providers and participants. These reforms will support more people with disability into sustainable work, through a program with greater flexibility, increased individual supports, and better service quality. Eligibility will be expanded to include volunteers outside the income support system and those with less than eight hours per week work capacity.
Delivering essential services
Investing in reliability and security
Strengthening resourcing for Services Australia
The Government is delivering safer and more efficient government services for all Australians.
A $1.8 billion provision will support delivery of customer and payment services. This includes funding for frontline and service delivery staff to manage claims, respond to natural disasters and improve the cyber security environment. The Government is providing $314.1 million over two years to strengthen safety and security at Services Australia centres.
The Government is investing $580.3 million over four years and $139.6 million per year ongoing to sustain the myGov platform and identify potential enhancements. A further $50 million will improve usability, safety and security of the myGov platform and ensure Services Australia can support people to protect their information and privacy.
Strengthening the Australian Taxation Office (ATO) against fraud
There will be $187.4 million to better protect taxpayer data and Commonwealth revenue against fraudulent attacks on the tax and superannuation systems. Funding will upgrade the ATO’s information and communications technologies and increase fraud prevention capabilities to manage increasing risk, prevent revenue loss, and support victims of fraud and cyber crime.
Looking after our veterans
Veterans’ claims processing is prioritised with an additional $186 million for staffing resources and $8.4 million to improve case management and protect against cyber risk. The Government will provide $222 million to harmonise veterans’ compensation and rehabilitation legislation.
A further $48.4 million will be available for Veterans’ Home Care and Community Nursing programs and $10.2 million to provide access to funded medical treatment for ill and injured veterans while their claims for liability are processed.
Back to top
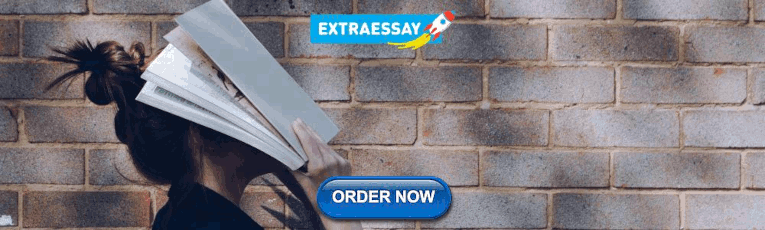
IMAGES
COMMENTS
In order to analyze the economic impact at a higher frequency, Lewis et al. ( 2020) developed a weekly economic index (WEI) using 10 different economic variables to track the economic impact of COVID‐19 in the United States. These authors report that between March 21 and March 28, the WEI declined by 6.19%.
The Journal of Economic Surveys is an international economics journal publishing new ideas in economics, econometrics, economic history and business economics. Abstract The goal of this piece is to survey the developing and rapidly growing literature on the economic consequences of COVID-19 and the governmental responses, and to synthetize the ...
This survey: (i) provides an overview. of the data sets and the techniques employed to mea-. sure social distancing and COVID- cases and deaths; (ii) reviews the literature on the determinants of ...
A literature review exemplifies a conundrum because an effective one cannot be conducted unless a problem statement is established (Ibn-Mohammed ... and McKibbin and Fernando (2020) reported thatthe socio-economic impact of COVID-19 will be felt for many months to come. Download : Download high-res image (298KB) Download : Download full-size ...
For over two years, the world has been battling the health and economic consequences of the COVID-19 pandemic. As of the writing of this article, deaths attributed to COVID-19 have surpassed six-and-a-half million people. Global economic growth was severely impacted: World output by the end of 2021 was more than 4 percentage points below its ...
IZA DP No. 13411: A Literature Review of the Economics of COVID-19. Abel Brodeur, David M. Gray, Anik Islam, Suraiya Jabeen Bhuiyan. published in: Journal of Economic Surveys, 2021, 35 (4), 1007-1044. The goal of this piece is to survey the emerging and rapidly growing literature on the economic consequences of COVID-19 and government response ...
The impact of the COVID-19 pandemic is far-reaching. We selectively review the rapidly growing literature, focusing on (1) the impact of COVID-19 on the labor market, both in terms of overall employment and work-from-home arrangements; (2) how COVID-19 may impact the reorganization of the global supply chain; (3) how COVID-19 accelerated the digitization of the economy, and how this impacts ...
The COVID-19 pandemic has caused a considerable threat to the economics of patients, health systems, and society. This meta-analysis aims to quantitatively assess the global economic burden of COVID-19. A comprehensive search was performed in the PubMed, Scopus, and Web of Science databases to identify studies examining the economic impact of COVID-19.
The outbreak of COVID-19 has showcased detrimental effects on global economy. Human lives were disturbed due to this outbreak. The COVID-19 pandemic has brought fluctuations in the cost of living across the globe and hampered economic activity. The global spread of COVID-19 had significantly restrained economic activity worldwide.
The goal of this piece is to survey the emerging and rapidly growing literature on the economic consequences of COVID-19 and government response, and to synthetize the insights emerging from a very large number of studies. This survey (i) provides an overview of the data sets used to measure social distancing and COVID-19 cases and deaths; (ii ...
Literature Review The Impact of the Epidemic on Total Demand. ... The impact of COVID-19 on the supply side is higher than it was with SARS, which is mainly reflected in two aspects: the delay of reemployment and the confidence of enterprises. ... Older Adults and the Economic Impact of the COVID-19 Pandemic. Journal of Aging & Social Policy ...
A LITERATURE REVIEW AND META-ANALYSIS ... COVID-19 MORTALITY RTALITY SAE./No.200/January 2022 /October 2021 Jonas Herby, Lars Jonung, and Steve H. Hanke. 1 A Literature Review and Meta-Analysis of the ... and as chief economic adviser to Prime Minister Carl Bildt in 1992-94. He holds a PhD in Economics from the University of California, Los ...
The trade-off model aims to account for the incurred economic losses following the rise and fall of the number of COVID-19 cases in the country and the implementation of various lockdown measures.
This paper reviews literatures of the impact of Covid-19 on economy. It is divided the influence into three sections which are macro-economy, stock market and firm-level. All article indicates that Covid-19 pandemic led negative impact on global economy. However, government response action, no matter monetary, fiscal and social policy, can mitigate the negative effects. In addition, companies ...
A major impact of COVID-19 is expected on the Indian economy for fiscal 2020-2021. At the point of writing this article, that is, on 14 April 2020, the lockdown has been extended to 3 May 2020 and in all probabilities is likely to go up to 30 June 2020. So the first quarter will be completely lost.
The COVID-19 pandemic has kept economists busy analyzing many aspects of economic side of the coronavirus impact. This note is meant to present an overview of what economists have analyzed regarding the implications of two of the main components of the CARES Act that affect individuals: the increased UI benefits and the stimulus checks.
The COVID-19 pandemic has provided us with an opportunity to pave the way for introducing digital learning. This article aims to provide a comprehensive report on the impact of the COVID-19 pandemic on online teaching and learning of various papers and indicate the way forward.
The COVID-19 pandemic has immensely impacted the economic, social, and environmental pillars of sustainability in human lives. Due to the scholars' increasing interest in responding to the urgent call for action against the pandemic, the literature of sustainability research considering COVID-19 consequences is very fragmented. Therefore, a comprehensive review of the COVID-19 implications for ...
During the early days of the initial outbreak of the novel coronavirus disease 2019 (COVID-19) in Japan, preschools were exempt from nationwide school closures, which came into effect on March 2, 2020. In addition, Japan is unique in that it experienced preschool closures without any heavy restrictions on daily life activities, making it possible to separate the effects of preschool closures ...
The rest of this paper is organized as follows. Section 2 is dedicated to the literature review. Section 3 presents the fuzzy big data analytics approach proposed in this study. Section 4 details the application of the fuzzy big data analytics approach to forecast the revenue of the catering industry in Taiwan during the COVID-19 pandemic.
The Government is investing $2.8 billion to continue its commitment to strengthen Medicare. This includes the $1.2 billion package to address pressures facing the health system, which provides: $882.2 million to support older Australians avoid hospital admission, be discharged from hospital earlier and improve their transition out of hospital ...
Since the emergence of the Coronavirus Disease 2019 (COVID-19) pandemic, over 494 million confirmed cases and around 6.1 million deaths have been reported globally ().At the beginning of the outbreak, the pandemic presents unprecedented challenges to the health systems and society in general (Legido-Quigley et al., 2020), with health facilities disproportionately affected by inadequate ...