
An official website of the United States government
The .gov means it’s official. Federal government websites often end in .gov or .mil. Before sharing sensitive information, make sure you’re on a federal government site.
The site is secure. The https:// ensures that you are connecting to the official website and that any information you provide is encrypted and transmitted securely.
- Publications
- Account settings
Preview improvements coming to the PMC website in October 2024. Learn More or Try it out now .
- Advanced Search
- Journal List
- J Med Internet Res
- v.22(11); 2020 Nov

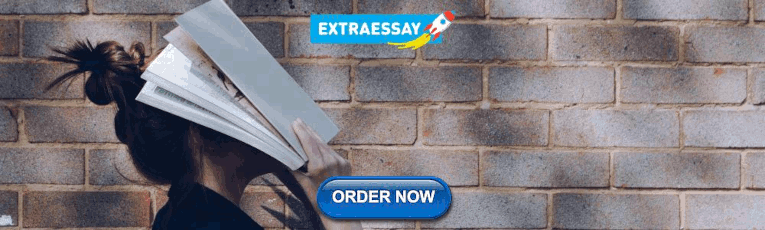
The Internet of Things: Impact and Implications for Health Care Delivery
Jaimon t kelly.
1 Menzies Health Institute Queensland, Griffith University, Gold Coast, Australia
2 Centre of Applied Health Economics, Griffith University, Brisbane, Australia
Katrina L Campbell
3 Metro North Hospital and Health Service, Brisbane, Australia
Enying Gong
4 School of Population and Global Health, The University of Melbourne, Melbourne, Australia
Paul Scuffham
Associated data.
Examples of Internet of Things devices that can support health service delivery.
Examples of how smart homes can improve health care delivery.
Scenarios where Internet of Things can be used to improve health system efficiency.
The Internet of Things (IoT) is a system of wireless, interrelated, and connected digital devices that can collect, send, and store data over a network without requiring human-to-human or human-to-computer interaction. The IoT promises many benefits to streamlining and enhancing health care delivery to proactively predict health issues and diagnose, treat, and monitor patients both in and out of the hospital. Worldwide, government leaders and decision makers are implementing policies to deliver health care services using technology and more so in response to the novel COVID-19 pandemic. It is now becoming increasingly important to understand how established and emerging IoT technologies can support health systems to deliver safe and effective care. The aim of this viewpoint paper is to provide an overview of the current IoT technology in health care, outline how IoT devices are improving health service delivery, and outline how IoT technology can affect and disrupt global health care in the next decade. The potential of IoT-based health care is expanded upon to theorize how IoT can improve the accessibility of preventative public health services and transition our current secondary and tertiary health care to be a more proactive, continuous, and coordinated system. Finally, this paper will deal with the potential issues that IoT-based health care generates, barriers to market adoption from health care professionals and patients alike, confidence and acceptability, privacy and security, interoperability, standardization and remuneration, data storage, and control and ownership. Corresponding enablers of IoT in current health care will rely on policy support, cybersecurity-focused guidelines, careful strategic planning, and transparent policies within health care organizations. IoT-based health care has great potential to improve the efficiency of the health system and improve population health.
Introduction
The challenges presented by an aging population with multiple chronic conditions are ubiquitous worldwide [ 1 ]. The medical, lifestyle, and personal health needs across aging populations will continue to place a burden on health care resources. Meeting these challenges requires a focus on empowering populations to self-manage their health through health innovation to improve well-being and attenuate health resource burden [ 2 ].
Background of Digital Devices and the Internet of Things
Entering the 2020 decade, more devices are connected to the internet than ever before, and this will continue to grow at a rapid trajectory. Worldwide, more than 21 billion devices have been estimated to be connected to the internet in 2020, which is 5 times the number of devices 4 years prior [ 3 ]. The Internet of Things (IoT) can be defined in its simplest scenario as a network that connects uniquely identifiable devices (or things ) to the internet, enabling them to collect, send, store, and receive data [ 4 ]. From a health care perspective, IoT can be considered as any device that can collect health-related data from individuals, including computing devices, mobile phones, smart bands and wearables, digital medications, implantable surgical devices, or other portable devices, which can measure health data and connect to the internet [ 5 ].
The growth of IoT technology has driven interest in a wide range of health practices to improve population health more specifically [ 6 ]. Recent reviews have overviewed the various services and applications of IoT in health care (eg, eHealth, mobile health [mHealth], ambient assisted living, semantic devices, wearable devices and smartphones, and community-based health care) [ 5 , 7 ]. These services have been detailed extensively and can have many applications across single condition and cluster condition management, including, for example, the ability to track and monitor health progress remotely by health care professionals, improve self-management of chronic conditions, assist in the early detection of abnormalities, fast-track symptom identification and clinical diagnoses, deliver early intervention, and improve adherence to prescriptions [ 8 ]. These applications can make better use of health care resources and provide quality and low-cost medical care.
Health Systems Are Changing
With the 2020 public health response to the novel COVID-19 pandemic to effectively shut down traditional modes of health service delivery worldwide, efforts to reduce implementation barriers to technology-supported health delivery highlight the potential to reframe traditional models of care into virtual and distance modalities [ 9 ]. In response, many countries have successfully implemented technology-supported services to maintain health care practices and social distancing [ 10 ]. As global leaders consider policies that potentially provide more access to technology-supported health services in response to (and considerations post) the current COVID-19 crisis, it is becoming increasingly important to understand how established and emerging IoT technologies can support health systems to deliver safe and effective care in either a complementary or an alternative way during times of crisis or health epidemics [ 11 ].
This viewpoint paper will overview current technologies in health care, outline how IoT devices are improving health service delivery, and outline how IoT technologies can affect global health care in the next decade. This viewpoint paper also overviews how the disruption in health care from IoT can lead to improved access and equitable primary, secondary, and tertiary smart health care, which is more proactive, continuous, and coordinated.
IoT-Based Health Care Architecture
The architecture of IoT in health care delivery essentially consists of 3 basic layers [ 12 ]: (1) the perception layer, (2) the network layer, and (3) the application layer. It is not our intention to extensively detail these layers; however, a summary and the related health implications are provided in the following sections.
Perception Layer: Sensing Systems That Collect Data
Perception and identification technologies are the foundation of IoT. Sensors are devices that can perceive changes in an environment and can include, for example, radio frequency identification (RFID), infrared sensors, cameras, GPS, medical sensors, and smart device sensors. These sensors allow for comprehensive perception through object recognition, location recognition, and geographic recognition and can convert this information to digital signals, which is more convenient for network transmission [ 12 , 13 ]. Sensor technologies allow for treatments to be monitored in real time and facilitate the acquisition of a multitude of physiological parameters about a patient so that diagnoses and high-quality treatment can be fast-tracked. There are many examples of potentially lifesaving IoT sensor devices; however, not all devices are clinically tested or have been proved to be safe or effective. A summary of IoT devices that may support and improve health service delivery is provided in Multimedia Appendix 1 [ 14 - 47 ].
Network Layer: Data Communication and Storage
The network level of IoT technologies includes wired and wireless networks, which communicate and store processed (layer 1) information either locally or at a centralized location. Communication between things can occur over low, medium, and high frequencies, the latter being the predominant focus of IoT. These include short-range communication technologies, such as RFID, wireless sensor networks, Bluetooth, Zigbee, low-power Wi-Fi, and global system for mobile communications [ 12 ]. High-frequency fourth-generation (4G) cellular networks have seen even more communication potential, and evolving 5G networks are becoming more readily available and are expected to be a major driver of the growth of IoT applications for health care, with the potential to provide reliable connection up to thousands of devices at the same time [ 48 ].
Communicated data are stored locally (often decentralized) or sent to a centralized cloud server. Cloud-based computing to support the delivery of health services has many benefits, as it is ubiquitous, flexible, and scalable in terms of data acquisition, storage, and transmission between devices connected to the cloud [ 49 ]. The use of the cloud can be foreseen to support data-intensive electronic medical records (EMRs), patient portals, medical IoT devices (which can include smartphone apps), and the big data analytics driving decision support systems and therapeutic strategies [ 5 ]. However, with more cloud apps entering the health market, it is just as important that an evidence base supports its effectiveness and safety and can deal with the security of health data and the reliability and transparency of that data by third parties. Furthermore, it has been suggested that centralized cloud storage will present issues in the future to users, such as excessive data accumulation and latency because of the distance between IoT devices and data centers.
Decentralized data processing and networking approaches may improve the scalability of IoT in health care. Edge cloud is a newer cloud computing concept that allows IoT sensors and network gateways to process and analyze data themselves (ie, at the edge ) in a decentralized fashion, reducing the amount of data required to be communicated and managed at a centralized location [ 12 , 50 ]. Similarly, blockchain storage uses a decentralized approach to data storage, creating independent blocks containing individual sets of information, which forms a dependent link in a collective block, which in turn creates a network regulated by patients rather than a third party [ 51 ]. There are examples of platforms engineering blockchain for medical practice already [ 51 , 52 ]; however, research on edge cloud and blockchains in health care is still limited and is an important area for future research.
Application Layer
The application layer interprets and applies data and is responsible for delivering application-specific services to the user [ 12 ]. Some of the most promising medical applications that IoT provides are through artificial intelligence (AI). The scientific applications of AI have proliferated, including image analysis, text recognition with natural language processing, drug activity design, and prediction of gene mutation expression [ 53 ]. AI has the capability to read available EMR data, including medical history, physical, laboratory, imaging, and medications, and contextualize these data to generate treatment and/or diagnosis decisions and/or possibilities. For example, IBM Watson uses AI to read both structured and unstructured text in the EMR, read images to highlight primary and incidental findings, and compile relevant medical literature in response to clinical queries [ 54 ].
IoT-based health care and use of deep machine learning can assist health professionals in seeing the unseeable and providing new and enhanced diagnostic capability. Although diagnostic confidence may never reach 100%, combining machines and clinician expertise reliably enhances system performance. For example, compared with the diagnostic evaluation by 54 ophthalmologists and senior residents, applying AI to retinal images improved the detection and grading of diabetic retinopathy and macular edema, achieving high specificities (98%) and sensitivities (90%) [ 55 ]. AI and deep learning can also optimize disease management, can provide big data and analysis generated from mHealth apps and IoT devices, and are starting to see adoption in health care [ 56 ]. Some examples of this include predicting risk, future medical outcomes, and care decisions in diabetes and mental health [ 57 ] and predicting the progression of congestive heart failure [ 58 , 59 ], bone disease [ 60 ], Alzheimer disease [ 61 ], benign and malignant tumor classification [ 62 , 63 ], and cardiac arrhythmias [ 64 ].
Expanding the Functions and Scope of IoT to Provide Smart Health Care
IoT is an infrastructure that enables smart health services to operate. When health data are collected by IoT sensors, communicated, and stored, this enables data analytics and smart health care, which can improve risk factor identification, disease diagnoses, treatment, and remote monitoring and empower people to self-manage.
Smart health care services make use of advancements in information technologies, such as IoT, big data analytics, cloud computing, AI, and deep machine learning, to transform traditional health care delivery to be a more efficient, convenient, and a more personalized system [ 65 ]. Current developments in information computer technologies have allowed the development of health care solutions with more intelligent prediction capabilities both in and out of the hospital. We are seeing the use of virtual models to transfer care provided in hospitals to the home through the use of sensors and devices that allow remote review and monitoring of patients in their homes or treated in hospitals and creates a continuum among these through cloud access [ 7 ]. More recently, the 2020 public health efforts around the world to mitigate the spread of COVID-19 have (at least temporarily) led governments and policy makers to remove implementation and remuneration barriers to enable health care professionals to use virtual models of care for people who need it [ 9 ]. IoT also provides the opportunity to improve the quality and efficiency of the entire ecosystem of service delivery, including hospital management, medical asset management, monitoring of the workflow of staff, and optimization of medical resources based on patient flow [ 66 , 67 ].
How IoT Can Improve Health Service Delivery
Primary health care becoming more accessible.
A focus on disease prevention must become a priority this decade, as the burden of disease attributable to modifiable risk factors is greater than ever before [ 1 , 68 ]. IoT in health care has the potential to improve population health and transition our health care model to a true hybrid model of primary, secondary, and tertiary care, where the health system can use its existing workforce in new and more efficient ways. Transforming health delivery in this way is crucial to improving self-management for people with chronic conditions, as even among high health care users, more than 90% of lifestyle self-management is done by patients themselves, outside of hospitals, and in clinical settings [ 69 , 70 ].
There is a clear public demand for easy-to-access health information. For example, in a 2015 US survey, 58% (931/1604) of smartphone users downloaded a health-related app for their lifestyle self-management [ 71 ]. AI has also driven the availability of point-of-care health information, such as chatbots (or AI doctors), which can deliver lifestyle and medical advice. Examples of these established AI bots are Woebot, Your.Md, Babylon, and HealthTap, where a patient can input their symptoms and advice is generated instantly [ 72 ]. However, more than half of the most highly rated apps make medical claims that are not approved [ 73 ], with no formal process of approving apps or informing consumer choice [ 74 ], and much remains to be done to understand the potential of chatbots to improve health. Therefore, a reliable digital health evidence base is essential [ 75 ]. If health professionals have evidence-based digital resources, devices, and mobile apps readily at their disposal, digital prescriptions could become an enabler of wider adoption of IoT in health care and facilitate a wider population focus on disease prevention.
At the individual level, IoT offers the opportunity to link and potentially learn from nonhealth IoT technologies to monitor daily activities, provide support with information, and promote behavior changes ( Multimedia Appendix 2 ). In addition, IoT and data linkage create great potential of transparent, evidence-based decision making, which may be able to drive the shift of disease patterns and increase the well-being of citizens at scale. The integration of urban infrastructures, IoT technologies, and cloud computing allows the collection and analysis of a vast quantity of different human and non–human-related data. These data could provide valuable information about population-level surveillance in diseases and accidents, risk factors, and environmental conditions [ 76 ], which is difficult to collect through the traditional human-reported disease surveillance system and can be of particular benefit in pandemic responses [ 77 ]. For example, in Taiwan, big data analytics applied to electronic data (GPS, closed-circuit television surveillance, and credit card payments) in the community and personal mobile data have been effectively used to contact trace, communicate, and isolate potential contacts during the global COVID-19 pandemic [ 78 ]. Through IoT and data linkage, decision makers are likely to be able to make evidence-based decisions in promoting healthy social and built environments, safe transportation systems, high-quality public services, and smart health care and emergency response systems [ 76 , 79 , 80 ].
Secondary and Tertiary Health Care That Is Proactive, Continuous, and Coordinated
An IoT-based health care system enables the overall health care systems to move past a traditional model of service delivery, which is often reactive, intermittent, and uncoordinated, to a more proactive, continuous, and coordinated approach [ 81 ]. Such an approach is favorable because it offers the opportunity to provide high-quality care that is less invasive and appealing to patients and health care professionals. This change in the health care system landscape is also highly appealing for policy makers because it can greatly enhance the efficiency (and subsequently reduce resource use) of the health system [ 82 ] and also provide the health system flexibility to shift its models of care and delivery of services as required on an individual or population-wide basis. Multimedia Appendix 3 summarizes 7 examples of how IoT can improve the coordination of health services and likely improve our health system efficiency.
Enablers and Barriers to Address for IoT-Based Health Care
Policy support.
Policy support is one of the most important environmental enablers of IoT. Many countries already have policies in place for eHealth (eg, web-based and software programs to deliver health services) [ 83 , 84 ] and either have or are in the process of developing policies for IoT infrastructure, investment, and/or implementation in health care. For example, China, India, Indonesia, Japan, Malaysia, the Philippines, Singapore, Thailand, the European Union, the United States, and Vietnam currently have relevant policies in place for IoT [ 85 ]. Australia is also in the process of establishing a policy for IoT development and investment [ 86 ].
Technology That Is Accessible and Easy to Use
The ubiquitous nature of technology means that consumers and health care professionals have greater access to digital resources than ever before [ 87 ]. However, it is also important for health systems to be aware of the inequities that may eventuate from the widespread implementation of IoT for health care, including individuals who may not be able to afford or access technology hardware or reliable internet services because of geographic location or financial disadvantage. Similarly, if individuals do not perceive the technology as user friendly , experience poor connections, or do not feel the initiative has been designed in consultation with them (both patients and health professionals), then this often results in frustration and reluctance to use such services [ 88 , 89 ].
Cybersecurity-Focused Guidelines for Robust and Resilient Market Adoption
Cyber risk is a major obstacle to the broad adoption of IoT [ 90 ]. The privacy of patients must be ensured to prevent unauthorized identification and tracking. From this perspective, the higher the level of autonomy and intelligence of the things, the more the challenges for the protection of identities and privacy.
Confidence and Acceptability
There is a gap in public awareness and understanding of data safety in cloud-stored health information. This is of concern, as it is the single biggest threat to the adoption of IoT from a societal perspective. The premise of IoT is clear to society; however, what is not clear to people is the actual value that IoT delivers to them personally from a health care perspective [ 91 , 92 ]. The potential threat of breached confidentiality may never go away; however, the perceived value to consumers needs to outweigh these concerns to confidently engage with IoT-supported health infrastructure [ 90 ]. The confidence and acceptability of IoT by health care professionals are similarly important. There is a diverse range of factors that affect clinicians’ acceptability of technology-supported programs, including the characteristics of the technology (eg, accuracy, compatibility with usual systems, and ease of use), individual’s attitudes and knowledge (eg, familiarity and impact on professional security), external factors (eg, patient and health professional interaction), and organization readiness (eg, training and reimbursement) [ 93 ].
Privacy and Security
IoT might allow opportunities for cyberattacks and for personal data to be collected inappropriately. IoT-based applications are vulnerable to cyberattacks for 2 basic reasons: (1) most of the communications are wireless, which makes eavesdropping very easy; and (2) most of the IoT components are characterized by low energy, and therefore, they can hardly implement complex schemes on their own to ensure security. The National Institute of Standards and Technology has recently released a draft security guide and recommendations for IoT devices, which will see an emphasis on data security in IoT devices [ 94 ]; however, whether such a guideline can or will be enforced across IoT health devices is unclear.
Data Storage, Control, and Ownership
To move forward in IoT-based health care, transparency and enforced codes of practice regarding where centralized cloud data are stored and who owns the data, needs to be considered For example, does the data host have viewing rights to someone’s data and are these data completely controlled by individuals or are they never deleted from the cloud, despite a user’s request? Another important consideration is the sharing of data across states or territories and internationally. Privacy, security, and confidentiality of data control and storage should be federally enforced, but international hosts and suppliers may not be required to follow any such code. Therefore, the use of these platforms requires strategic planning and transparent guidelines to develop and implement robust IoT-based health care policies and models of care.
Interoperability and Standardization Protocols
Issues around the interoperability and standardization of IoT and health care systems are a big threat to the wider adoption of IoT for health care systems. Lack of standardization threatens the development of IoT in the health setting context, as the industry and manufacturers are yet to reach a consensus regarding wireless communication protocols and standards for machine-to-machine communication. Without a unified, standardized, and interoperable system, the adoption of IoT into health care will be greatly hindered and is unlikely to have international reach [ 95 ]. Semantic interoperability in IoT is a necessary condition for big data techniques to support decision-making processes [ 96 ]. It is increasingly common for each new technology startup, device, or system manufacturer to define their own specific architecture, protocols, and data formats, which are unable to communicate with the health care environment unless they are appreciably redeveloped or adapted to interoperate with hospital IoT platforms [ 96 ]. This creates Vertical Silos [ 97 ], which demands the development of new features for granting interoperability between different systems. The future and full potential of IoT-enabled health care relies on addressing interoperability, of which some frameworks do exist [ 98 ]. Achieving interoperability across IoT platforms can provide a safer, more accessible, productive, and satisfying experience for clinicians and patients alike.
Remuneration
Finally, remuneration for technology-assisted health care has historically been challenging [ 99 ] and differs appreciably across different countries. This is likely to be even more complex for IoT-delivered health care, where reimbursement considerations have not been established (and this is unlikely until the abovementioned points are addressed). As international health systems establish robust policies and guidelines on cybersecurity and address the issues surrounding interoperability and standardization protocols, reimbursement and regulatory considerations across single-payer and multipayer systems should become a key priority to ensuring successful, effective, and cost-effective IoT health care models can be implemented in practice.
Conclusions
From this viewpoint, the potential of IoT is summarized as a growing area of research in health care. These developments provide a great opportunity for health care systems to proactively predict health issues and diagnose, treat, and monitor patients both in and out of the hospital. As the adoption of technology-supported health services increases to enable health systems to deliver flexible models of care, an increasing number of traditional health service delivery practices will be complemented or replaced through IoT. However, the implementation of IoT in health care will rely on a clear and robust code of practice for the management of data, privacy, confidentiality, and cybersecurity concerning the supply and use of IoT devices in health care. There are still important gaps for future research to address, which relate to the IoT technology itself, the health system, and the users of IoT technology. Specific future research on IoT technology needs to address how IoT devices can be designed with standardized protocols and interoperability with international and cross-state health systems. More research is also needed on the efficiency of blockchain storage compared with centralized cloud-based storage solutions in the context of IoT-supported health care delivery. From a health system perspective, there is a need for clinical guidelines on digital health prescriptions and robust policy regarding remuneration for primary and secondary care services provided through IoT. Finally, more research is needed to determine the acceptability and digital literacy of consumers and clinicians in the context of using IoT to improve the delivery and overall experience of health care. Although this viewpoint is a summary of selected literature only and not based on an exhaustive systematic review of the literature, we believe that addressing these areas for future research will go a long way to enable a wider uptake of IoT, which can ultimately save health care dollars and improve patient-centered care.
Acknowledgments
This research received no specific funding. JK was supported through a Griffith University Postdoctoral Research Fellowship. PS was partially funded through a National Health and Medical Research Council Senior Research Fellowship (#1136923). EG was supported by the Melbourne Graduate Research Scholarship. The authors wish to thank Dr Tilman Dingler for his assistance in the network section of this paper.
Abbreviations
Multimedia appendix 1, multimedia appendix 2, multimedia appendix 3.
Authors' Contributions: Each author contributed to the conception and design of this paper. JK conducted the literature searches and drafted the first draft of the manuscript. EG, KC, and PS revised the manuscript. All authors read and approved the final manuscript.
Conflicts of Interest: None declared.
Advertisement
Internet of things in Healthcare: a conventional literature review
- Review Paper
- Published: 20 July 2023
- Volume 13 , pages 699–719, ( 2023 )
Cite this article
- Brijendra Singh ORCID: orcid.org/0000-0003-2608-3388 1 ,
- Daphne Lopez 1 &
- Rabie Ramadan 2
492 Accesses
4 Citations
Explore all metrics
The power of the Internet has impacted various industries, especially the healthcare industry. With the rapid population growth and advancement of various sensor technologies and smart devices, the demand for quality healthcare services has increased in the past few years. At the same time, Internet of Things (IoT) technology makes intelligent objects communicate with each other by connecting all the objects without human intervention. IoT facilitated services and technologies in healthcare to provide low-cost and efficient service delivery to patients and consumers. It reduces the patient’s hospital stay time and efficient information exchange by providing cost-effective healthcare services. This systematic review analyzes different technologies, core applications, datasets, algorithms, challenges, and limitations of IoT in the healthcare sector.
Database search is performed to review IoT healthcare-enabling technologies, algorithms, applications, and IoT-centric architectures. Search has been conducted over popular databases like Springer, Elsevier, and IEEE Xplore. We have downloaded around 544 research papers, out of which we have selected only 116 based on our interests.
We identified different challenges in healthcare IoT applications as security and privacy, scalability, high cost, energy efficiency, quality of service, network architecture, mobility, and interoperability. However, data security and privacy issues are significant challenges in the healthcare sector as they deal with patients’ confidential information. Also, we proposed a novel architecture that covers most IoT healthcare requirements. IoT healthcare applications are also detailed. In addition, it reviews most of the healthcare datasets and the utilized algorithms.
Conclusions
Based on the reviewed articles in this paper, it is has been concluded that IoT devices are beneficial in the healthcare applications, regardless of their limitations in terms of energy, processing, and storage. It is a promising technology that can save time and effort to the practitioners and doctors. Several architectures and technologies are discussed as well as the limitations, which enables researchers to tackle those limitations. However, the future of IoT healthcare holds immense potential for high-security enabled and pervasive applications. Analyzing vast healthcare data using deep learning techniques can play a vital role in extracting valuable insights. To leverage the power of deep learning, it is crucial to design a well-structured cloud architecture capable of implementing these techniques and predicting future trends. While researchers have proposed various frameworks for IoT healthcare, there remains a need to assess the feasibility and effectiveness of these proposed frameworks. Furthermore, it is essential to address the specific requirements and intricacies when designing detailed architectural patterns for healthcare applications. Overall, the future of IoT healthcare is expansive and offers exciting research opportunities for scholars and experts in the field. By embracing innovative technologies and addressing the specific needs of the healthcare industry, we can unlock the full potential of IoT in revolutionizing healthcare delivery and improving patient outcomes.
This is a preview of subscription content, log in via an institution to check access.
Access this article
Price includes VAT (Russian Federation)
Instant access to the full article PDF.
Rent this article via DeepDyve
Institutional subscriptions
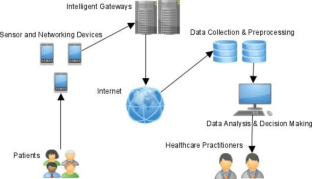
Similar content being viewed by others
The Medical Internet of Things: A Review of Intelligent Machine Learning and Deep Learning Applications for Leveraging Healthcare
Convergence Between IoT and AI for Smart Health and Predictive Medicine
Recent Trends in IoT–Based Smart Healthcare Applying ML and DL
Data availability.
Not applicable.
Code Availability
Mohanty SN, Ramya KC, Rani SS, Gupta D, Shankar K, Lakshmanaprabu SK, Khanna A. An efficient lightweight integrated Blockchain (ELIB) model for IoT security and privacy. Future Generation Computer Systems. 2020;102:1027–37.
Article Google Scholar
Tewari A, Gupta BB. Security, privacy and trust of different layers in internet-of-things (IoTs) framework. Future generation computer systems. 2020;108:909–20.
Shvachko K, Kuang H, Radia S, Chansler R. (2010, May). The hadoop distributed file system. In 2010 IEEE 26th symposium on mass storage systems and technologies (MSST) (pp. 1–10). IEEE.
Nurdin MRF, Hadiyoso S, Rizal A. (2016, September). A low-cost Internet of Things (IoT) system for multi-patient ECG’s monitoring. In 2016 International Conference on Control, Electronics, Renewable Energy and Communications (ICCEREC) (pp. 7–11). IEEE.
Tanwar S, Parekh K, Evans R. Blockchain-based electronic healthcare record system for healthcare 4.0 applications. J Inform Secur Appl. 2020;50:102407.
Google Scholar
Awan, K. M., Ashraf, N., Saleem, M. Q., Sheta, O. E., Qureshi, K. N., Zeb, A., … Sadiq,A. S. (2019). A priority-based congestion-avoidance routing protocol using IoT-based heterogeneous medical sensors for energy efficiency in healthcare wireless body area networks. International Journal of Distributed Sensor Networks , 15 (6), 1550147719853980.
Shameer K, Badgeley MA, Miotto R, Glicksberg BS, Morgan JW, Dudley JT. Translational bioinformatics in the era of real-time biomedical, health care and wellness data streams. Brief Bioinform. 2017;18(1):105–24.
Maksimović M, Vujović V, Periśić B. (2015, June). A custom Internet of Things healthcare system. In 2015 10th iberian conference on information systems and technologies (CISTI) (pp. 1–6). IEEE.
Sarwesh P, Shet NSV, Chandrasekaran K. (2017). Energy efficient Network Design for IoT Healthcare Applications. Internet of things and Big Data Technologies for Next Generation Healthcare (35–61). Springer, Cham.
Chapter Google Scholar
Gómez J, Oviedo B, Zhuma E. Patient monitoring system based on internet of things. Procedia Comput Sci. 2016;83:90–7.
Hossain MS, Muhammad G. Cloud-assisted industrial internet of things (iiot)–enabled framework for health monitoring. Comput Netw. 2016;101:192–202.
Abdulmohsin Hammood D, Rahim HA, Alkhayyat A, Ahmad RB. Body-to-body Cooperation in Internet of Medical Things: toward Energy Efficiency Improvement. future Internet. 2019;11(11):239.
Gia TN, Jiang M, Sarker VK, Rahmani AM, Westerlund T, Liljeberg P, Tenhunen H. (2017, June). Low-cost fog-assisted healthcare IoT system with energy-efficient sensor nodes. In 2017 13th international wireless communications and mobile computing conference (IWCMC) (pp. 1765–1770). IEEE.
Otoom M, Otoum N, Alzubaidi MA, Etoom Y, Banihani R. An IoT-based framework for early identification and monitoring of COVID-19 cases. Biomed Signal Process Control. 2020;62:102149.
Kumari A, Tanwar S, Tyagi S, Kumar N. Fog computing for Healthcare 4.0 environment: Opportunities and challenges. Comput Electr Eng. 2018;72:1–13.
Sood SK, Mahajan I. Wearable IoT sensor based healthcare system for identifying and controlling chikungunya virus. Comput Ind. 2017;91:33–44.
Wan J, Al-awlaqi MA, Li M, O’Grady M, Gu X, Wang J, Cao N. Wearable IoT enabled real-time health monitoring system. EURASIP J Wirel Commun Netw. 2018;2018(1):298.
Anzanpour A, Rahmani AM, Liljeberg P, Tenhunen H. (2015, October). Context-aware early warning system for in-home healthcare using internet-of-things. International Internet of Things Summit (517–22). Springer, Cham.
Abdelgawad A, Yelamarthi K, Khattab A. (2016, November). IoT-based health monitoring system for active and assisted living. In International Conference on Smart Objects and Technologies for Social Good (pp. 11–20). Springer, Cham.
Singh SK, Rathore S, Park JH. Blockiotintelligence: a blockchain-enabled intelligent IoT architecture with artificial intelligence. Future Generation Computer Systems. 2020;110:721–43.
Tripathi G, Ahad MA, Paiva S. (2020, March). S2HS-A blockchain based approach for smart healthcare system. In Healthcare (Vol. 8, 1, 100391). Elsevier.
Pinto S, Cabral J, Gomes T. (2017, March). We-care: An IoT-based health care system for elderly people. In 2017 IEEE International Conference on Industrial Technology (ICIT) (pp. 1378–1383). IEEE.
Domingos D, Respício A, Martinho R. (2020). Reliability of IoT-aware BPMN healthcare processes. Virtual and Mobile Healthcare: breakthroughs in Research and Practice (793–821). IGI Global.
Dhanvijay MM, Patil SC. (2020). Optimized mobility management protocol for the IoT based WBAN with an enhanced security. Wireless Netw, 1–19.
Ullah F, Habib MA, Farhan M, Khalid S, Durrani MY, Jabbar S. Semantic interoperability for big-data in heterogeneous IoT infrastructure for healthcare. Sustainable cities and society. 2017;34:90–6.
Jabbar S, Ullah F, Khalid S, Khan M, Han K. (2017). Semantic interoperability in heterogeneous IoT infrastructure for healthcare. Wireless Communications and Mobile Computing , 2017 .
Oryema B, Kim HS, Li W, Park JT. (2017, January). Design and implementation of an interoperable messaging system for IoT healthcare services. In 2017 14th IEEE Annual Consumer Communications & Networking Conference (CCNC) (pp. 45–52). IEEE.
Mavrogiorgou A, Kiourtis A, Perakis K, Pitsios S, Kyriazis D. (2019). IoT in healthcare: Achieving interoperability of high-quality data acquired by IoT medical devices. Sensors , 19 (9), 1978.
Pace, P., Gravina, R., Aloi, G., Fortino, G., Ibanez-Sanchez, G., Traver, V., … Yacchirema,D. C. (2017, June). IoT platforms interoperability for active and assisted living healthcare services support. In 2017 Global Internet of Things Summit (GIoTS) (pp. 1–6). IEEE.
Pushpa PV, Riyaz R. (2018, November). Internet of Things based Context Aware Remote Health Care Services. In 2018 IEEE Symposium Series on Computational Intelligence (SSCI) (pp. 799–806). IEEE.
Abuseta Y. (2020). A Context Aware Framework for IoT Based Healthcare Monitoring Systems. arXiv preprint arXiv:2008.10341 .
Zemmoudj S, Bermad N, Omar M. (2019, June). CAPM: Context-Aware Privacy Model for IoT-Based Smart Hospitals. In 2019 15th International Wireless Communications & Mobile Computing Conference (IWCMC) (pp. 1139–1144). IEEE.
Zemmoudj S, Bermad N, Omar M. Context-aware pseudonymization and authorization model for IoT-based smart hospitals. J Ambient Intell Humaniz Comput. 2019;10(11):4473–90.
Reddy RV, Murali D, Rajeshwar J. (2019). Context-aware middleware architecture for IoT-based smart healthcare applications. Innovations in Computer Science and Engineering (557–67). Springer, Singapore.
Zemmoudj S, Bermad N, Omar M. (2019, December). CA-ADP: Context-Aware Authorization and Delegation Protocol for IoT-based healthcare smart systems. In 2019 International Conference on Internet of Things, Embedded Systems and Communications (IINTEC) (pp. 203–208). IEEE.
Ghosh AM, Halder D, Hossain SA. (2016, May). Remote health monitoring system through IoT. In 2016 5th International Conference on Informatics, Electronics and Vision (ICIEV) (pp. 921–926). IEEE.
Akkaş MA, Sokullu R, Çetin HE. Healthcare and patient monitoring using IoT. Internet of Things. 2020;11:100173.
Valanarasu MR. Smart and secure IoT and AI integration framework for hospital environment. J ISMAC. 2019;1(03):172–9.
Kordestani H, Barkaoui K, Zahran W. (2020, August). HapiChain: a blockchain-based framework for patient-centric telemedicine. In 2020 IEEE 8th International Conference on Serious Games and Applications for Health (SeGAH) (pp. 1–6). IEEE.
Yoon W, Ha M, Kwon K, Kim D. (2014, October). 6Lo bluetooth low energy for patient-centric healthcare service on the Internet of things. In Proc. of the Int. Conf. on the Internet of Things .
Magsi H, Sodhro AH, Chachar FA, Abro SAK, Sodhro GH, Pirbhulal S. (2018, March). Evolution of 5G in Internet of medical things. In 2018 international conference on computing, mathematics and engineering technologies (iCoMET) (pp. 1–7). IEEE.
Mavrogiorgou A, Kiourtis A, Kyriazis D. (2019, November). Delivering Reliability of Data Sources in IoT Healthcare Ecosystems. In 2019 25th Conference of Open Innovations Association (FRUCT) (pp. 211–219). IEEE.
Antar F, Jain M, Dugyala H, Nelluri D, Morelos-Zaragoza R. (2017, August). Reliability improvements to healthcare patient RFID tracking with multiple tags. In 2017 IEEE SmartWorld, Ubiquitous Intelligence & Computing, Advanced & Trusted Computed, Scalable Computing & Communications, Cloud & Big Data Computing, Internet of People and Smart City Innovation (SmartWorld/SCALCOM/UIC/ATC/CBDCom/IOP/SCI) (pp. 1–5). IEEE.
Vergutz A, Noubir G, Nogueira M. Reliability for Smart Healthcare: A Network Slicing Perspective. IEEE Network. 2020;34(4):91–7.
Mukherjee R, Kundu A, Mukherjee I, Gupta D, Tiwari P, Khanna A, Shorfuzzaman M. (2021). IoT-cloud based healthcare model for COVID-19 detection: an enhanced k-Nearest neighbour classifier based approach. Computing, 1–21.
Khan MA. An IoT framework for heart disease prediction based on MDCNN classifier. IEEE Access. 2020;8:34717–27.
Satpathy S, Mohan P, Das S, Debbarma S. A new healthcare diagnosis system using an IoT-based fuzzy classifier with FPGA. J Supercomputing. 2020;76(8):5849–61.
Memon MH, Li JP, Haq AU, Memon MH, Zhou W. (2019). Breast cancer detection in the IOT health environment using modified recursive feature selection. wireless communications and mobile computing, 2019.
Kumar PM, Lokesh S, Varatharajan R, Babu GC, Parthasarathy P. Cloud and IoT based disease prediction and diagnosis system for healthcare using fuzzy neural classifier. Future Generation Computer Systems. 2018;86:527–34.
Saini R, Kumar P, Kaur B, Roy PP, Dogra DP, Santosh KC. Kinect sensor-based interaction monitoring system using the BLSTM neural network in healthcare. Int J Mach Learn Cybernet. 2019;10(9):2529–40.
Bharadwaj H, Krishna, et al. A review on the role of machine learning in enabling IoT Based Healthcare Applications. " IEEE Access. 2021;9:38859–90.
Yuehong YIN, et al. The internet of things in healthcare: an overview. J Industrial Inform Integr. 2016;1:3–13.
Haghi M, Neubert S, Geissler A, Fleischer H, Stoll N, Stoll R, Thurow K. A flexible and pervasive IoT based Healthcare platform for physiological and environmental parameters monitoring. IEEE Internet of Things Journal. 2020;1–1. https://doi.org/10.1109/jiot.2020.2980432 .
Ganchev I, et al. editors. Enhanced living environments: algorithms, architectures, platforms, and systems. Volume 11369. Springer; 2019.
Zhou G, Wang Y, Cui L. “Biomedical sensor, device and measurement systems.“ Adv Bioeng 177 (2015).
Ryu S. Impact sound-based surface identification using smart audio sensors with deep neural networks. IEEE Sens J. 2020;20(18):10936–44.
Do H, Manh KC, Welch, Sheng W. “SoHAM: A Sound-Based Human Activity Monitoring Framework for Home Service Robots.“ IEEE Trans Autom Sci Eng (2021).
Noor MB, Taj NZ, Zenia, Shamim Kaiser M. Challenges ahead in Healthcare Applications for Vision and Sensors.“ vision, sensing and analytics: integrative approaches. Cham: Springer; 2021. pp. 397–413.
Ahmed I, Jeon G, Piccialli F. “A Deep Learning-based Smart Healthcare System for Patient’s Discomfort Detection at the Edge of Internet of Things.“ IEEE Internet of Things Journal (2021).
Mavinkattimath SG, Khanai R, Dattaprasad A Torse. “A survey on secured wireless body sensor networks.“ 2019 International Conference on Communication and, Processing S. (ICCSP). IEEE, 2019.
Belle A et al. “Big data analytics in healthcare.“ BioMed research international 2015 (2015).
Surya L. “An exploratory study of AI and Big Data, and it’s future in the United States.“ International Journal of Creative Research Thoughts (IJCRT), ISSN (2015): 2320–2882.
Krishnamoorthy S, Dua A, Gupta S. Role of emerging technologies in future IoT-driven Healthcare 4.0 technologies: a survey, current challenges and future directions. J Ambient Intell Humaniz Comput. 2023;14(1):361–407.
Sharma P, Namasudra S, Chilamkurti N, Kim BG, Crespo G, R. Blockchain-based privacy preservation for IoT-enabled healthcare system. ACM Trans Sens Networks. 2023;19(3):1–17.
Kumar P, Kumar R, Gupta GP, Tripathi R, Jolfaei A, Islam AN. A blockchain-orchestrated deep learning approach for secure data transmission in IoT-enabled healthcare system. J Parallel Distrib Comput. 2023;172:69–83.
Al-Saggaf AA, Sheltami T, Alkhzaimi H, Ahmed G. Lightweight two-factor-based user authentication protocol for iot-enabled healthcare ecosystem in quantum computing. Arab J Sci Eng. 2023;48(2):2347–57.
Das S, Namasudra S. A novel hybrid encryption method to secure healthcare data in IoT-enabled healthcare infrastructure. Comput Electr Eng. 2022;101:107991.
Kamruzzaman MM, Yan B, Sarker MNI, Alruwaili O, Wu M, Alrashdi I. (2022). Blockchain and fog computing in IoT-driven healthcare services for smart cities. Journal of Healthcare Engineering, 2022.
Ranjan A, Kale AA, Kumar R, Raj S. (2023). Review of Big Data, Internet of Things (IoT) and Real-time Analytics in Healthcare. Internet of Things (IoT) and Real-time Analytics in Healthcare (April 22, 2023).
Reisman M. EHRs: the challenge of making electronic data usable and interoperable. Pharm Ther. 2017;42(9):572.
Murphy GF, Hanken MA, Waters KA. (1999). Electronic health records: changing the vision. (No Title).
Pirbhulal S, Zhang H, Alahi E, Ghayvat ME, Mukhopadhyay H, Zhang SC, Y. T., Wu W. A novel secure IoT-based smart home automation system using a wireless sensor network. Sensors. 2017;17(1):69.
Lee, G. H., Moon, H., Kim, H., Lee, G. H., Kwon, W., Yoo, S., … Hahn, S. K. (2020).Multifunctional materials for implantable and wearable photonic healthcare devices. Nature Reviews Materials , 5 (2), 149–165.
Yang, G., Xie, L., Mäntysalo, M., Zhou, X., Pang, Z., Da Xu, L., … Zheng, L. R. (2014).A health-IoT platform based on the integration of intelligent packaging, unobtrusive bio-sensor, and intelligent medicine box. IEEE transactions on industrial informatics , 10 (4), 2180–2191.
Alihamidi I, Madi AA, Addaim A. (2019, October). Proposed Architecture of e-health IOT. In 2019 International Conference on Wireless Networks and Mobile Communications (WINCOM) (pp. 1–7). IEEE.
Maktoubian J, Ansari K. An IoT architecture for preventive maintenance of medical devices in healthcare organizations. Health and Technology. 2019;9(3):233–43.
Aski VJ, Sonawane SS, Soni U. (2019). IoT enabled ubiquitous healthcare data acquisition and monitoring system for personal and medical usage powered by cloud application: an architectural overview. Recent developments in machine learning and data analytics (1–15). Springer, Singapore.
Ahmadi H, Arji G, Shahmoradi L, Safdari R, Nilashi M, Alizadeh M. The application of internet of things in healthcare: a systematic literature review and classification. Universal Access in the Information Society; 2018. pp. 1–33.
Azzawi MA, Hassan R, Bakar KAA. A review on internet of things (IoT) in healthcare. Int J Appl Eng Res. 2016;11(20):10216–21.
Joyia GJ, Liaqat RM, Farooq A, Rehman S. Internet of medical things (IOMT): applications, benefits and future challenges in healthcare domain. J Commun. 2017;12(4):240–7.
Baker SB, Xiang W, Atkinson I. Internet of things for smart healthcare: Technologies, challenges, and opportunities. IEEE Access. 2017;5:26521–44.
Woo MW, Lee J, Park K. A reliable IoT system for personal healthcare devices. Future Generation Computer Systems. 2018;78:626–40.
Milovanovic D, Bojkovic Z. Cloud-based IoT healthcare applications: requirements and recommendations. Int J Internet Things Web Serv. 2017;2:60–5.
Maksimović M. (2018). Implementation of fog computing in IoT-based healthcare system. Jita-Journal of Information Technology and Aplications, 14(2).
Farahani B, Firouzi F, Chang V, Badaroglu M, Constant N, Mankodiya K. Towards fog-driven IoT eHealth: promises and challenges of IoT in medicine and healthcare. Future Generation Computer Systems. 2018;78:659–76.
Moosavi SR, Gia TN, Nigussie E, Rahmani AM, Virtanen S, Tenhunen H, Isoaho J. End-to-end security scheme for mobility enabled healthcare internet of things. Future Generation Computer Systems. 2016;64:108–24.
Gohar M, Choi JG, Koh SJ. CoAP-based group mobility management protocol for the internet-of-things in WBAN environment. Future Generation Computer Systems. 2018;88:309–18.
Din S, Paul A, Hong WH, Seo H. Constrained application for mobility management using embedded devices in the internet of things based urban planning in smart cities. Sustainable Cities and Society. 2019;44:144–51.
Jung JH, Choi DK, Kim JI, Koh SJ. (2019, January). Mobility management for healthcare services in coap-based iot networks. In 2019 International Conference on Information Networking (ICOIN) (pp. 7–12). IEEE.
Babu BS, Srikanth K, Ramanjaneyulu T, Narayana IL. IoT for healthcare. Int J Sci Res. 2016;5(2):322–6.
Luo E, Bhuiyan MZA, Wang G, Rahman MA, Wu J, Atiquzzaman M. Privacyprotector: privacy-protected patient data collection in IoT-based healthcare systems. IEEE Commun Mag. 2018;56(2):163–8.
Yeh KH. A secure IoT-based healthcare system with body sensor networks. IEEE Access. 2016;4:10288–99.
Subasi A, Radhwan M, Kurdi R, Khateeb K. (2018, February). IoT based mobile healthcare system for human activity recognition. In 2018 15th learning and technology conference (L&T) (pp. 29–34). IEEE.
Ahmadi H, Arji G, Shahmoradi L, Safdari R, Nilashi M, Alizadeh M. The application of internet of things in healthcare: a systematic literature review and classification. Univ Access Inf Soc. 2019;18(4):837–69.
Marques G, Pitarma R, Garcia M, N., Pombo N. Internet of things architectures, technologies, applications, challenges, and future directions for enhanced living environments and healthcare systems: a review. Electronics. 2019;8(10):1081.
Selvaraj S, Sundaravaradhan S. Challenges and opportunities in IoT healthcare systems: a systematic review. SN Appl Sci. 2020;2(1):1–8.
Gupta V, Singh Gill H, Singh P, Kaur R. An energy efficient fog-cloud based architecture for healthcare. J Stat Manage Syst. 2018;21(4):529–37.
Abdelmoneem RM, Benslimane A, Shaaban E, Abdelhamid S, Ghoneim S. (2019, May). A cloud-fog based architecture for iot applications dedicated to healthcare. In ICC 2019–2019 IEEE International Conference on Communications (ICC) (pp. 1–6). IEEE.
Magsi H, Sodhro AH, Zahid N, Pirbhulal S, Wang L, Al-Rakhami MS. A Novel Adaptive Battery-Aware Algorithm for Data Transmission in IoT-Based Healthcare Applications. Electronics. 2021;10(4):367.
Raghupathi W, Raghupathi V. Big data analytics in healthcare: promise and potential. Health Inform Sci Syst. 2014;2(1):3.
Ahmed E, Yaqoob I, Hashem IAT, Khan I, Ahmed AIA, Imran M, Vasilakos AV. The role of big data analytics in internet of things. Comput Netw. 2017;129:459–71.
Brezulianu A, Geman O, Zbancioc MD, Hagan M, Aghion C, Hemanth DJ, Son LH. IoT based heart activity monitoring using inductive sensors. Sensors. 2019;19(15):3284.
Fog computing and the. Internet of things: extend the cloud to where the things are(2016), p. 6 www.cisco.com.
Lubrin E, Lawrence E, Navarro KF. (2005, July). Wireless remote healthcare monitoring with motes. In International Conference on Mobile Business (ICMB’05) (pp. 235–241). IEEE.
Gordon WJ, Catalini C. Blockchain technology for healthcare: facilitating the transition to patient-driven interoperability. Comput Struct Biotechnol J. 2018;16:224–30.
Raj C, Jain C, Arif W. (2017, March). HEMAN: Health monitoring and nous: An IoT based e-health care system for remote telemedicine. In 2017 International Conference on Wireless Communications, Signal Processing and Networking (WiSPNET) (pp. 2115–2119). IEEE.
Stradolini F, Tamburrano N, Modoux T, Tuoheti A, Demarchi D, Carrara S. (2018, May). IoT for telemedicine practices enabled by an Android™ application with cloud system integration. In 2018 IEEE international symposium on circuits and systems (ISCAS) (pp. 1–5). IEEE.
Lavanya R, Nivetha M, Revasree K, Sandhiya K. (2018, March). Smart chair-a telemedicine based health monitoring system. In 2018 second international conference on electronics, communication and aerospace technology (ICECA) (pp. 459–463). IEEE.
Li C, Hu X, Zhang L. The IoT-based heart disease monitoring system for pervasive healthcare service. Procedia Comput Sci. 2017;112:2328–34.
Xia T, Song X, Zhang H, Song X, Kanasugi H, Shibasaki R. Measuring spatio-temporal accessibility to emergency medical services through big GPS data. Health Place. 2019;56:53–62.
Yao X, Farha F, Li R, Psychoula I, Chen L, Ning H. (2020). Security and privacy issues of physical objects in the IoT: challenges and opportunities. Digit Commun Networks.
Wu T, Wu F, Redoute JM, Yuce MR. An autonomous wireless body area network implementation towards IoT connected healthcare applications. IEEE Access. 2017;5:11413–22.
Rahimi Moosavi S, Nguyen Gia T, Rahmani AM, Nigussie E, Virtanen S, Isoaho J, Tenhunen H. (2015). SEA: a secure and efficient authentication and authorization architecture for IoT-based healthcare using smart gateways. In Procedia Computer Science (Vol. 52, pp. 452–459). Elsevier.
Elhoseny M, Ramírez-González G, Abu-Elnasr OM, Shawkat SA, Arunkumar N, Farouk A. Secure medical data transmission model for IoT-based healthcare systems. Ieee Access. 2018;6:20596–608.
Gope P, Hwang T. BSN-Care: a secure IoT-based modern healthcare system using body sensor network. IEEE Sens J. 2015;16(5):1368–76.
Dhanvijay MM, Patil SC. Internet of things: a survey of enabling technologies in healthcare and its applications. Comput Netw. 2019;153:113–31.
Alaiad A, Zhou L. Patients’ adoption of WSN-based smart home healthcare systems: an integrated model of facilitators and barriers. IEEE Trans Prof Commun. 2017;60(1):4–23.
Geller T, David YB, Khmelnitsky E, Ben-Gal I, Ward A, Miller D, Bambos N. (2019, May). Learning health state transition probabilities via wireless body area networks. In ICC 2019–2019 IEEE International Conference on Communications (ICC) (pp. 1–6). IEEE.
Marques G, Pitarma R. An indoor monitoring system for ambient assisted living based on internet of things architecture. Int J Environ Res Public Health. 2016;13(11):1152.
Tariq M, Majeed H, Beg MO, Khan FA, Derhab A. Accurate detection of sitting posture activities in a secure IoT based assisted living environment. Future Generation Computer Systems. 2019;92:745–57.
Lavanya S, Lavanya G, Divyabharathi J. (2017, March). Remote prescription and I-Home healthcare based on IoT. In 2017 international conference on innovations in green energy and healthcare technologies (IGEHT) (pp. 1–3). IEEE.
Yang L, Ge Y, Li W, Rao W, Shen W. (2014, May). A home mobile healthcare system for wheelchair users. In Proceedings of the 2014 IEEE 18th international conference on computer supported cooperative work in design (CSCWD) (pp. 609–614). IEEE.
Hassan MM, Gumaei A, Aloi G, Fortino G, Zhou M. A smartphone-enabled fall detection framework for elderly people in connected home healthcare. IEEE Network. 2019;33(6):58–63.
Verma P, Sood SK. Fog assisted-IoT enabled patient health monitoring in smart homes. IEEE Internet of Things Journal. 2018;5(3):1789–96.
Mungmunpuntipantip R, Wiwanitkit V. GPS COVID-19 TrackerApplied Informatics Technology for Prevention of Disease spreading. Int J Prev Med. 2020;11(7):11–88.
Aziz K, Tarapiah S, Ismail SH, Atalla S. (2016, March). Smart real-time healthcare monitoring and tracking system using GSM/GPS technologies. In 2016 3rd MEC International Conference on Big Data and Smart City (ICBDSC) (pp. 1–7). IEEE.
Download references
Authors have no conflict of interest.
Author information
Authors and affiliations.
School of Information Technology and Engineering, Vellore Institute of Technology, Vellore, India
Brijendra Singh & Daphne Lopez
Computer Engineering Department, Cairo University, Giza, Egypt
Rabie Ramadan
You can also search for this author in PubMed Google Scholar
Contributions
All the authors have contributed significantly towards research work.
Corresponding author
Correspondence to Brijendra Singh .
Ethics declarations
Conflict of interest, additional information, publisher’s note.
Springer Nature remains neutral with regard to jurisdictional claims in published maps and institutional affiliations.
Rights and permissions
Springer Nature or its licensor (e.g. a society or other partner) holds exclusive rights to this article under a publishing agreement with the author(s) or other rightsholder(s); author self-archiving of the accepted manuscript version of this article is solely governed by the terms of such publishing agreement and applicable law.
Reprints and permissions
About this article
Singh, B., Lopez, D. & Ramadan, R. Internet of things in Healthcare: a conventional literature review. Health Technol. 13 , 699–719 (2023). https://doi.org/10.1007/s12553-023-00771-1
Download citation
Received : 06 April 2023
Accepted : 14 July 2023
Published : 20 July 2023
Issue Date : September 2023
DOI : https://doi.org/10.1007/s12553-023-00771-1
Share this article
Anyone you share the following link with will be able to read this content:
Sorry, a shareable link is not currently available for this article.
Provided by the Springer Nature SharedIt content-sharing initiative
- Wearable sensors
- Body Sensor Network (BSN)
- Find a journal
- Publish with us
- Track your research
This paper is in the following e-collection/theme issue:
Published on 10.11.2020 in Vol 22 , No 11 (2020) : November
The Internet of Things: Impact and Implications for Health Care Delivery
Authors of this article:

- Jaimon T Kelly 1, 2 , BHlthSc, Mast Nutr&Diet, PhD ;
- Katrina L Campbell 1, 2, 3 , PhD ;
- Enying Gong 4 , MSc ;
- Paul Scuffham 1, 2 , PhD
1 Menzies Health Institute Queensland, Griffith University, Gold Coast, Australia
2 Centre of Applied Health Economics, Griffith University, Brisbane, Australia
3 Metro North Hospital and Health Service, Brisbane, Australia
4 School of Population and Global Health, The University of Melbourne, Melbourne, Australia
Corresponding Author:
Jaimon T Kelly, BHlthSc, Mast Nutr&Diet, PhD
Menzies Health Institute Queensland
Griffith University
G40 Griffith Health Centre
Level 8.86 Gold Coast campus
Gold Coast, 4222
Phone: 61 5678001 ext 8001
Email: [email protected]
The Internet of Things (IoT) is a system of wireless, interrelated, and connected digital devices that can collect, send, and store data over a network without requiring human-to-human or human-to-computer interaction. The IoT promises many benefits to streamlining and enhancing health care delivery to proactively predict health issues and diagnose, treat, and monitor patients both in and out of the hospital. Worldwide, government leaders and decision makers are implementing policies to deliver health care services using technology and more so in response to the novel COVID-19 pandemic. It is now becoming increasingly important to understand how established and emerging IoT technologies can support health systems to deliver safe and effective care. The aim of this viewpoint paper is to provide an overview of the current IoT technology in health care, outline how IoT devices are improving health service delivery, and outline how IoT technology can affect and disrupt global health care in the next decade. The potential of IoT-based health care is expanded upon to theorize how IoT can improve the accessibility of preventative public health services and transition our current secondary and tertiary health care to be a more proactive, continuous, and coordinated system. Finally, this paper will deal with the potential issues that IoT-based health care generates, barriers to market adoption from health care professionals and patients alike, confidence and acceptability, privacy and security, interoperability, standardization and remuneration, data storage, and control and ownership. Corresponding enablers of IoT in current health care will rely on policy support, cybersecurity-focused guidelines, careful strategic planning, and transparent policies within health care organizations. IoT-based health care has great potential to improve the efficiency of the health system and improve population health.
Introduction
The challenges presented by an aging population with multiple chronic conditions are ubiquitous worldwide [ 1 ]. The medical, lifestyle, and personal health needs across aging populations will continue to place a burden on health care resources. Meeting these challenges requires a focus on empowering populations to self-manage their health through health innovation to improve well-being and attenuate health resource burden [ 2 ].
Background of Digital Devices and the Internet of Things
Entering the 2020 decade, more devices are connected to the internet than ever before, and this will continue to grow at a rapid trajectory. Worldwide, more than 21 billion devices have been estimated to be connected to the internet in 2020, which is 5 times the number of devices 4 years prior [ 3 ]. The Internet of Things (IoT) can be defined in its simplest scenario as a network that connects uniquely identifiable devices (or things ) to the internet, enabling them to collect, send, store, and receive data [ 4 ]. From a health care perspective, IoT can be considered as any device that can collect health-related data from individuals, including computing devices, mobile phones, smart bands and wearables, digital medications, implantable surgical devices, or other portable devices, which can measure health data and connect to the internet [ 5 ].
The growth of IoT technology has driven interest in a wide range of health practices to improve population health more specifically [ 6 ]. Recent reviews have overviewed the various services and applications of IoT in health care (eg, eHealth, mobile health [mHealth], ambient assisted living, semantic devices, wearable devices and smartphones, and community-based health care) [ 5 , 7 ]. These services have been detailed extensively and can have many applications across single condition and cluster condition management, including, for example, the ability to track and monitor health progress remotely by health care professionals, improve self-management of chronic conditions, assist in the early detection of abnormalities, fast-track symptom identification and clinical diagnoses, deliver early intervention, and improve adherence to prescriptions [ 8 ]. These applications can make better use of health care resources and provide quality and low-cost medical care.
Health Systems Are Changing
With the 2020 public health response to the novel COVID-19 pandemic to effectively shut down traditional modes of health service delivery worldwide, efforts to reduce implementation barriers to technology-supported health delivery highlight the potential to reframe traditional models of care into virtual and distance modalities [ 9 ]. In response, many countries have successfully implemented technology-supported services to maintain health care practices and social distancing [ 10 ]. As global leaders consider policies that potentially provide more access to technology-supported health services in response to (and considerations post) the current COVID-19 crisis, it is becoming increasingly important to understand how established and emerging IoT technologies can support health systems to deliver safe and effective care in either a complementary or an alternative way during times of crisis or health epidemics [ 11 ].
This viewpoint paper will overview current technologies in health care, outline how IoT devices are improving health service delivery, and outline how IoT technologies can affect global health care in the next decade. This viewpoint paper also overviews how the disruption in health care from IoT can lead to improved access and equitable primary, secondary, and tertiary smart health care, which is more proactive, continuous, and coordinated.
IoT-Based Health Care Architecture
The architecture of IoT in health care delivery essentially consists of 3 basic layers [ 12 ]: (1) the perception layer, (2) the network layer, and (3) the application layer. It is not our intention to extensively detail these layers; however, a summary and the related health implications are provided in the following sections.
Perception Layer: Sensing Systems That Collect Data
Perception and identification technologies are the foundation of IoT. Sensors are devices that can perceive changes in an environment and can include, for example, radio frequency identification (RFID), infrared sensors, cameras, GPS, medical sensors, and smart device sensors. These sensors allow for comprehensive perception through object recognition, location recognition, and geographic recognition and can convert this information to digital signals, which is more convenient for network transmission [ 12 , 13 ]. Sensor technologies allow for treatments to be monitored in real time and facilitate the acquisition of a multitude of physiological parameters about a patient so that diagnoses and high-quality treatment can be fast-tracked. There are many examples of potentially lifesaving IoT sensor devices; however, not all devices are clinically tested or have been proved to be safe or effective. A summary of IoT devices that may support and improve health service delivery is provided in Multimedia Appendix 1 [ 14 - 47 ].
Network Layer: Data Communication and Storage
The network level of IoT technologies includes wired and wireless networks, which communicate and store processed (layer 1) information either locally or at a centralized location. Communication between things can occur over low, medium, and high frequencies, the latter being the predominant focus of IoT. These include short-range communication technologies, such as RFID, wireless sensor networks, Bluetooth, Zigbee, low-power Wi-Fi, and global system for mobile communications [ 12 ]. High-frequency fourth-generation (4G) cellular networks have seen even more communication potential, and evolving 5G networks are becoming more readily available and are expected to be a major driver of the growth of IoT applications for health care, with the potential to provide reliable connection up to thousands of devices at the same time [ 48 ].
Communicated data are stored locally (often decentralized) or sent to a centralized cloud server. Cloud-based computing to support the delivery of health services has many benefits, as it is ubiquitous, flexible, and scalable in terms of data acquisition, storage, and transmission between devices connected to the cloud [ 49 ]. The use of the cloud can be foreseen to support data-intensive electronic medical records (EMRs), patient portals, medical IoT devices (which can include smartphone apps), and the big data analytics driving decision support systems and therapeutic strategies [ 5 ]. However, with more cloud apps entering the health market, it is just as important that an evidence base supports its effectiveness and safety and can deal with the security of health data and the reliability and transparency of that data by third parties. Furthermore, it has been suggested that centralized cloud storage will present issues in the future to users, such as excessive data accumulation and latency because of the distance between IoT devices and data centers.
Decentralized data processing and networking approaches may improve the scalability of IoT in health care. Edge cloud is a newer cloud computing concept that allows IoT sensors and network gateways to process and analyze data themselves (ie, at the edge ) in a decentralized fashion, reducing the amount of data required to be communicated and managed at a centralized location [ 12 , 50 ]. Similarly, blockchain storage uses a decentralized approach to data storage, creating independent blocks containing individual sets of information, which forms a dependent link in a collective block, which in turn creates a network regulated by patients rather than a third party [ 51 ]. There are examples of platforms engineering blockchain for medical practice already [ 51 , 52 ]; however, research on edge cloud and blockchains in health care is still limited and is an important area for future research.
Application Layer
The application layer interprets and applies data and is responsible for delivering application-specific services to the user [ 12 ]. Some of the most promising medical applications that IoT provides are through artificial intelligence (AI). The scientific applications of AI have proliferated, including image analysis, text recognition with natural language processing, drug activity design, and prediction of gene mutation expression [ 53 ]. AI has the capability to read available EMR data, including medical history, physical, laboratory, imaging, and medications, and contextualize these data to generate treatment and/or diagnosis decisions and/or possibilities. For example, IBM Watson uses AI to read both structured and unstructured text in the EMR, read images to highlight primary and incidental findings, and compile relevant medical literature in response to clinical queries [ 54 ].
IoT-based health care and use of deep machine learning can assist health professionals in seeing the unseeable and providing new and enhanced diagnostic capability. Although diagnostic confidence may never reach 100%, combining machines and clinician expertise reliably enhances system performance. For example, compared with the diagnostic evaluation by 54 ophthalmologists and senior residents, applying AI to retinal images improved the detection and grading of diabetic retinopathy and macular edema, achieving high specificities (98%) and sensitivities (90%) [ 55 ]. AI and deep learning can also optimize disease management, can provide big data and analysis generated from mHealth apps and IoT devices, and are starting to see adoption in health care [ 56 ]. Some examples of this include predicting risk, future medical outcomes, and care decisions in diabetes and mental health [ 57 ] and predicting the progression of congestive heart failure [ 58 , 59 ], bone disease [ 60 ], Alzheimer disease [ 61 ], benign and malignant tumor classification [ 62 , 63 ], and cardiac arrhythmias [ 64 ].
Expanding the Functions and Scope of IoT to Provide Smart Health Care
IoT is an infrastructure that enables smart health services to operate. When health data are collected by IoT sensors, communicated, and stored, this enables data analytics and smart health care, which can improve risk factor identification, disease diagnoses, treatment, and remote monitoring and empower people to self-manage.
Smart health care services make use of advancements in information technologies, such as IoT, big data analytics, cloud computing, AI, and deep machine learning, to transform traditional health care delivery to be a more efficient, convenient, and a more personalized system [ 65 ]. Current developments in information computer technologies have allowed the development of health care solutions with more intelligent prediction capabilities both in and out of the hospital. We are seeing the use of virtual models to transfer care provided in hospitals to the home through the use of sensors and devices that allow remote review and monitoring of patients in their homes or treated in hospitals and creates a continuum among these through cloud access [ 7 ]. More recently, the 2020 public health efforts around the world to mitigate the spread of COVID-19 have (at least temporarily) led governments and policy makers to remove implementation and remuneration barriers to enable health care professionals to use virtual models of care for people who need it [ 9 ]. IoT also provides the opportunity to improve the quality and efficiency of the entire ecosystem of service delivery, including hospital management, medical asset management, monitoring of the workflow of staff, and optimization of medical resources based on patient flow [ 66 , 67 ].
How IoT Can Improve Health Service Delivery
Primary health care becoming more accessible.
A focus on disease prevention must become a priority this decade, as the burden of disease attributable to modifiable risk factors is greater than ever before [ 1 , 68 ]. IoT in health care has the potential to improve population health and transition our health care model to a true hybrid model of primary, secondary, and tertiary care, where the health system can use its existing workforce in new and more efficient ways. Transforming health delivery in this way is crucial to improving self-management for people with chronic conditions, as even among high health care users, more than 90% of lifestyle self-management is done by patients themselves, outside of hospitals, and in clinical settings [ 69 , 70 ].
There is a clear public demand for easy-to-access health information. For example, in a 2015 US survey, 58% (931/1604) of smartphone users downloaded a health-related app for their lifestyle self-management [ 71 ]. AI has also driven the availability of point-of-care health information, such as chatbots (or AI doctors), which can deliver lifestyle and medical advice. Examples of these established AI bots are Woebot, Your.Md, Babylon, and HealthTap, where a patient can input their symptoms and advice is generated instantly [ 72 ]. However, more than half of the most highly rated apps make medical claims that are not approved [ 73 ], with no formal process of approving apps or informing consumer choice [ 74 ], and much remains to be done to understand the potential of chatbots to improve health. Therefore, a reliable digital health evidence base is essential [ 75 ]. If health professionals have evidence-based digital resources, devices, and mobile apps readily at their disposal, digital prescriptions could become an enabler of wider adoption of IoT in health care and facilitate a wider population focus on disease prevention.
At the individual level, IoT offers the opportunity to link and potentially learn from nonhealth IoT technologies to monitor daily activities, provide support with information, and promote behavior changes ( Multimedia Appendix 2 ). In addition, IoT and data linkage create great potential of transparent, evidence-based decision making, which may be able to drive the shift of disease patterns and increase the well-being of citizens at scale. The integration of urban infrastructures, IoT technologies, and cloud computing allows the collection and analysis of a vast quantity of different human and non–human-related data. These data could provide valuable information about population-level surveillance in diseases and accidents, risk factors, and environmental conditions [ 76 ], which is difficult to collect through the traditional human-reported disease surveillance system and can be of particular benefit in pandemic responses [ 77 ]. For example, in Taiwan, big data analytics applied to electronic data (GPS, closed-circuit television surveillance, and credit card payments) in the community and personal mobile data have been effectively used to contact trace, communicate, and isolate potential contacts during the global COVID-19 pandemic [ 78 ]. Through IoT and data linkage, decision makers are likely to be able to make evidence-based decisions in promoting healthy social and built environments, safe transportation systems, high-quality public services, and smart health care and emergency response systems [ 76 , 79 , 80 ].
Secondary and Tertiary Health Care That Is Proactive, Continuous, and Coordinated
An IoT-based health care system enables the overall health care systems to move past a traditional model of service delivery, which is often reactive, intermittent, and uncoordinated, to a more proactive, continuous, and coordinated approach [ 81 ]. Such an approach is favorable because it offers the opportunity to provide high-quality care that is less invasive and appealing to patients and health care professionals. This change in the health care system landscape is also highly appealing for policy makers because it can greatly enhance the efficiency (and subsequently reduce resource use) of the health system [ 82 ] and also provide the health system flexibility to shift its models of care and delivery of services as required on an individual or population-wide basis. Multimedia Appendix 3 summarizes 7 examples of how IoT can improve the coordination of health services and likely improve our health system efficiency.
Enablers and Barriers to Address for IoT-Based Health Care
Policy support.
Policy support is one of the most important environmental enablers of IoT. Many countries already have policies in place for eHealth (eg, web-based and software programs to deliver health services) [ 83 , 84 ] and either have or are in the process of developing policies for IoT infrastructure, investment, and/or implementation in health care. For example, China, India, Indonesia, Japan, Malaysia, the Philippines, Singapore, Thailand, the European Union, the United States, and Vietnam currently have relevant policies in place for IoT [ 85 ]. Australia is also in the process of establishing a policy for IoT development and investment [ 86 ].
Technology That Is Accessible and Easy to Use
The ubiquitous nature of technology means that consumers and health care professionals have greater access to digital resources than ever before [ 87 ]. However, it is also important for health systems to be aware of the inequities that may eventuate from the widespread implementation of IoT for health care, including individuals who may not be able to afford or access technology hardware or reliable internet services because of geographic location or financial disadvantage. Similarly, if individuals do not perceive the technology as user friendly , experience poor connections, or do not feel the initiative has been designed in consultation with them (both patients and health professionals), then this often results in frustration and reluctance to use such services [ 88 , 89 ].
Cybersecurity-Focused Guidelines for Robust and Resilient Market Adoption
Cyber risk is a major obstacle to the broad adoption of IoT [ 90 ]. The privacy of patients must be ensured to prevent unauthorized identification and tracking. From this perspective, the higher the level of autonomy and intelligence of the things, the more the challenges for the protection of identities and privacy.
Confidence and Acceptability
There is a gap in public awareness and understanding of data safety in cloud-stored health information. This is of concern, as it is the single biggest threat to the adoption of IoT from a societal perspective. The premise of IoT is clear to society; however, what is not clear to people is the actual value that IoT delivers to them personally from a health care perspective [ 91 , 92 ]. The potential threat of breached confidentiality may never go away; however, the perceived value to consumers needs to outweigh these concerns to confidently engage with IoT-supported health infrastructure [ 90 ]. The confidence and acceptability of IoT by health care professionals are similarly important. There is a diverse range of factors that affect clinicians’ acceptability of technology-supported programs, including the characteristics of the technology (eg, accuracy, compatibility with usual systems, and ease of use), individual’s attitudes and knowledge (eg, familiarity and impact on professional security), external factors (eg, patient and health professional interaction), and organization readiness (eg, training and reimbursement) [ 93 ].
Privacy and Security
IoT might allow opportunities for cyberattacks and for personal data to be collected inappropriately. IoT-based applications are vulnerable to cyberattacks for 2 basic reasons: (1) most of the communications are wireless, which makes eavesdropping very easy; and (2) most of the IoT components are characterized by low energy, and therefore, they can hardly implement complex schemes on their own to ensure security. The National Institute of Standards and Technology has recently released a draft security guide and recommendations for IoT devices, which will see an emphasis on data security in IoT devices [ 94 ]; however, whether such a guideline can or will be enforced across IoT health devices is unclear.
Data Storage, Control, and Ownership
To move forward in IoT-based health care, transparency and enforced codes of practice regarding where centralized cloud data are stored and who owns the data, needs to be considered For example, does the data host have viewing rights to someone’s data and are these data completely controlled by individuals or are they never deleted from the cloud, despite a user’s request? Another important consideration is the sharing of data across states or territories and internationally. Privacy, security, and confidentiality of data control and storage should be federally enforced, but international hosts and suppliers may not be required to follow any such code. Therefore, the use of these platforms requires strategic planning and transparent guidelines to develop and implement robust IoT-based health care policies and models of care.
Interoperability and Standardization Protocols
Issues around the interoperability and standardization of IoT and health care systems are a big threat to the wider adoption of IoT for health care systems. Lack of standardization threatens the development of IoT in the health setting context, as the industry and manufacturers are yet to reach a consensus regarding wireless communication protocols and standards for machine-to-machine communication. Without a unified, standardized, and interoperable system, the adoption of IoT into health care will be greatly hindered and is unlikely to have international reach [ 95 ]. Semantic interoperability in IoT is a necessary condition for big data techniques to support decision-making processes [ 96 ]. It is increasingly common for each new technology startup, device, or system manufacturer to define their own specific architecture, protocols, and data formats, which are unable to communicate with the health care environment unless they are appreciably redeveloped or adapted to interoperate with hospital IoT platforms [ 96 ]. This creates Vertical Silos [ 97 ], which demands the development of new features for granting interoperability between different systems. The future and full potential of IoT-enabled health care relies on addressing interoperability, of which some frameworks do exist [ 98 ]. Achieving interoperability across IoT platforms can provide a safer, more accessible, productive, and satisfying experience for clinicians and patients alike.
Remuneration
Finally, remuneration for technology-assisted health care has historically been challenging [ 99 ] and differs appreciably across different countries. This is likely to be even more complex for IoT-delivered health care, where reimbursement considerations have not been established (and this is unlikely until the abovementioned points are addressed). As international health systems establish robust policies and guidelines on cybersecurity and address the issues surrounding interoperability and standardization protocols, reimbursement and regulatory considerations across single-payer and multipayer systems should become a key priority to ensuring successful, effective, and cost-effective IoT health care models can be implemented in practice.
Conclusions
From this viewpoint, the potential of IoT is summarized as a growing area of research in health care. These developments provide a great opportunity for health care systems to proactively predict health issues and diagnose, treat, and monitor patients both in and out of the hospital. As the adoption of technology-supported health services increases to enable health systems to deliver flexible models of care, an increasing number of traditional health service delivery practices will be complemented or replaced through IoT. However, the implementation of IoT in health care will rely on a clear and robust code of practice for the management of data, privacy, confidentiality, and cybersecurity concerning the supply and use of IoT devices in health care. There are still important gaps for future research to address, which relate to the IoT technology itself, the health system, and the users of IoT technology. Specific future research on IoT technology needs to address how IoT devices can be designed with standardized protocols and interoperability with international and cross-state health systems. More research is also needed on the efficiency of blockchain storage compared with centralized cloud-based storage solutions in the context of IoT-supported health care delivery. From a health system perspective, there is a need for clinical guidelines on digital health prescriptions and robust policy regarding remuneration for primary and secondary care services provided through IoT. Finally, more research is needed to determine the acceptability and digital literacy of consumers and clinicians in the context of using IoT to improve the delivery and overall experience of health care. Although this viewpoint is a summary of selected literature only and not based on an exhaustive systematic review of the literature, we believe that addressing these areas for future research will go a long way to enable a wider uptake of IoT, which can ultimately save health care dollars and improve patient-centered care.
Acknowledgments
This research received no specific funding. JK was supported through a Griffith University Postdoctoral Research Fellowship. PS was partially funded through a National Health and Medical Research Council Senior Research Fellowship (#1136923). EG was supported by the Melbourne Graduate Research Scholarship. The authors wish to thank Dr Tilman Dingler for his assistance in the network section of this paper.
Authors' Contributions
Each author contributed to the conception and design of this paper. JK conducted the literature searches and drafted the first draft of the manuscript. EG, KC, and PS revised the manuscript. All authors read and approved the final manuscript.
Conflicts of Interest
None declared.
Examples of Internet of Things devices that can support health service delivery.
Examples of how smart homes can improve health care delivery.
Scenarios where Internet of Things can be used to improve health system efficiency.
- GBD 2017 DiseaseInjury IncidencePrevalence Collaborators. Global, regional, and national incidence, prevalence, and years lived with disability for 354 diseases and injuries for 195 countries and territories, 1990-2017: a systematic analysis for the Global Burden of Disease Study 2017. Lancet 2018 Nov 10;392(10159):1789-1858 [ FREE Full text ] [ CrossRef ] [ Medline ]
- Mitchell M, Kan L. Digital technology and the future of health systems. Health Syst Reform 2019;5(2):113-120. [ CrossRef ] [ Medline ]
- Internet of Things (IoT) in Healthcare. Research Markets. 2019. URL: https://www.medicaldevice-network.com/comment/bringing-internet-things-healthcare/ [accessed 2020-10-02]
- Mitchell-Box K, Braun KL. Fathers' thoughts on breastfeeding and implications for a theory-based intervention. J Obstet Gynecol Neonatal Nurs 2012;41(6):E41-E50. [ CrossRef ] [ Medline ]
- Dang LM, Piran MJ, Han D, Min K, Moon H. A survey on internet of things and cloud computing for healthcare. Electronics 2019 Jul 9;8(7):768. [ CrossRef ]
- Saarikko T, Westergren UH, Blomquist T. The internet of things: are you ready for what’s coming? Bus Horiz 2017 Sep;60(5):667-676. [ CrossRef ]
- Nazir S, Ali Y, Ullah N, García-Magariño I. Internet of things for healthcare using effects of mobile computing: a systematic literature review. Wireless Commun Mobile Comput 2019 Nov 14;2019:1-20. [ CrossRef ]
- Yin Y, Zeng Y, Chen X, Fan Y. The internet of things in healthcare: an overview. J Ind Inf Integration 2016 Mar;1:3-13. [ CrossRef ]
- Torous J, Jän Myrick K, Rauseo-Ricupero N, Firth J. Digital mental health and covid-19: using technology today to accelerate the curve on access and quality tomorrow. JMIR Ment Health 2020 Mar 26;7(3):e18848 [ FREE Full text ] [ CrossRef ] [ Medline ]
- Fisk M, Livingstone A, Pit SW. Telehealth in the context of covid-19: changing perspectives in Australia, the United Kingdom, and the United States. J Med Internet Res 2020 Jun 9;22(6):e19264 [ FREE Full text ] [ CrossRef ] [ Medline ]
- Ye Q, Zhou J, Wu H. Using information technology to manage the covid-19 pandemic: development of a technical framework based on practical experience in China. JMIR Med Inform 2020 Jun 8;8(6):e19515 [ FREE Full text ] [ CrossRef ] [ Medline ]
- Sethi P, Sarangi S. Internet of things: architectures, protocols, and applications. J Electric Comput Eng 2017:-.
- Wu M, Lu T, Ling F, Sun J. Research on the Architecture of Internet of Things. In: 3rd International Conference on Advanced Computer Theory and Engineering. 2010 Presented at: ICACTE'10; August 20-22, 2010; Chengdu, China. [ CrossRef ]
- Merchant RK, Inamdar R, Quade RC. Effectiveness of population health management using the propeller health asthma platform: a randomized clinical trial. J Allergy Clin Immunol Pract 2016;4(3):455-463. [ CrossRef ] [ Medline ]
- Merchant R, Szefler SJ, Bender BG, Tuffli M, Barrett MA, Gondalia R, et al. Impact of a digital health intervention on asthma resource utilization. World Allergy Organ J 2018;11(1):28 [ FREE Full text ] [ CrossRef ] [ Medline ]
- Levine DM, Ouchi K, Blanchfield B, Diamond K, Licurse A, Pu CT, et al. Hospital-level care at home for acutely ill adults: a pilot randomized controlled trial. J Gen Intern Med 2018 May;33(5):729-736 [ FREE Full text ] [ CrossRef ] [ Medline ]
- Plowman R, Peters-Strickland T, Savage G. Digital medicines clinical review on the safety of tablets with sensors. 2018;17(9):849-852 [ FREE Full text ]
- Naik R, Macey N, West RJ, Godbehere P, Thurston SC, Fox R, et al. First use of an ingestible sensor to manage uncontrolled blood pressure in primary practice: the UK hypertension registry. J Community Med Health Educ 2017;07(01):-. [ CrossRef ]
- Birckhead B, Khalil C, Liu X, Conovitz S, Rizzo A, Danovitch I, et al. Recommendations for methodology of virtual reality clinical trials in health care by an international working group: iterative study. JMIR Ment Health 2019 Jan 31;6(1):e11973 [ FREE Full text ] [ CrossRef ] [ Medline ]
- Eckert M, Volmerg JS, Friedrich CM. Augmented reality in medicine: systematic and bibliographic review. JMIR Mhealth Uhealth 2019 Apr 26;7(4):e10967 [ FREE Full text ] [ CrossRef ] [ Medline ]
- Gerup J, Soerensen CB, Dieckmann P. Augmented reality and mixed reality for healthcare education beyond surgery: an integrative review. Int J Med Educ 2020 Jan 18;11:1-18 [ FREE Full text ] [ CrossRef ] [ Medline ]
- Tashjian VC, Mosadeghi S, Howard AR, Lopez M, Dupuy T, Reid M, et al. Virtual reality for management of pain in hospitalized patients: results of a controlled trial. JMIR Ment Health 2017 Mar 29;4(1):e9 [ FREE Full text ] [ CrossRef ] [ Medline ]
- Chirico A, Lucidi F, De Laurentiis M, Milanese C, Napoli A, Giordano A. Virtual reality in health system: beyond entertainment. A mini-review on the efficacy of VR during cancer treatment. J Cell Physiol 2016 Feb;231(2):275-287. [ CrossRef ] [ Medline ]
- de Carvalho M, Dias T, Duchesne M, Nardi A, Appolinario J. Virtual reality in health system: beyond entertainment. A mini-review on the efficacy of VR during cancer treatment. Behav Sci (Basel) 2017 Jul 9;7(3):- [ FREE Full text ] [ CrossRef ] [ Medline ]
- Lohse KR, Hilderman CG, Cheung KL, Tatla S, van der Loos HF. Virtual reality therapy for adults post-stroke: a systematic review and meta-analysis exploring virtual environments and commercial games in therapy. PLoS One 2014;9(3):e93318 [ FREE Full text ] [ CrossRef ] [ Medline ]
- Valmaggia LR, Latif L, Kempton MJ, Rus-Calafell M. Virtual reality in the psychological treatment for mental health problems: an systematic review of recent evidence. Psychiatry Res 2016 Feb 28;236:189-195. [ CrossRef ] [ Medline ]
- Persky S. Application of virtual reality methods to obesity prevention and management research. J Diabetes Sci Technol 2011 Mar 1;5(2):333-339 [ FREE Full text ] [ CrossRef ] [ Medline ]
- Sangave NA, Aungst TD, Patel DK. Smart connected insulin pens, caps, and attachments: a review of the future of diabetes technology. Diabetes Spectr 2019 Nov;32(4):378-384. [ CrossRef ] [ Medline ]
- Doryab A, Villalba DK, Chikersal P, Dutcher JM, Tumminia M, Liu X, et al. Identifying behavioral phenotypes of loneliness and social isolation with passive sensing: statistical analysis, data mining and machine learning of smartphone and FitBit data. JMIR Mhealth Uhealth 2019 Jul 24;7(7):e13209 [ FREE Full text ] [ CrossRef ] [ Medline ]
- Sposaro F, Tyson G. iFall: an Android application for fall monitoring and response. (1557-170X (Print)) 2020:a. [ CrossRef ]
- Majumder S, Chen L, Marinov O, Chen C, Mondal T, Deen MJ. Noncontact wearable wireless ECG systems for long-term monitoring. IEEE Rev Biomed Eng 2018;11:306-321. [ CrossRef ]
- Kakria P, Tripathi NK, Kitipawang P. A real-time health monitoring system for remote cardiac patients using smartphone and wearable sensors. Int J Telemed Appl 2015;2015:373474 [ FREE Full text ] [ CrossRef ] [ Medline ]
- Dojchinovski D, Ilievski A, Gusev M. editors. Interactive home healthcare system with integrated voice assistant 2019;2019:-. [ CrossRef ]
- Laranjo L, Dunn A, Tong H, Kocaballi A, Chen J, Bashir R, et al. Conversational agents in healthcare: a systematic review. J Am Med Inform Assoc 2018 Sep 1;25(9):1248-1258 [ FREE Full text ] [ CrossRef ] [ Medline ]
- Ilievski A, Dojchinovski D, Gusev M. Interactive Voice Assisted Home Healthcare Systems. In: Proceedings of the 9th Balkan Conference on Informatics. 2019 Presented at: BCI'19; September 26-28, 2019; University of Sofia, Bulgaria. [ CrossRef ]
- Jadczyk T, Kiwic O, Khandwalla RM, Grabowski K, Rudawski S, Magaczewski P, et al. Feasibility of a voice-enabled automated platform for medical data collection: CardioCube. Int J Med Inform 2019 Sep;129:388-393. [ CrossRef ] [ Medline ]
- Chen S, Jones C, Moyle W. Social robots for depression in older adults: a systematic review. J Nurs Scholarsh 2018 Nov;50(6):612-622. [ CrossRef ] [ Medline ]
- van der Putte D, Boumans R, Neerincx M, Rikkert M, de MM. A Social Robot for Autonomous Health Data Acquisition Among Hospitalized Patients: An Exploratory Field Study. In: 14th ACM/IEEE International Conference on Human-Robot Interaction (HRI). 2019 Presented at: HRI'19; March 11-14, 2019; Daegu, Korea (South), Korea (South). [ CrossRef ]
- Wan S, Gu Z, Ni Q. Cognitive computing and wireless communications on the edge for healthcare service robots. Comput Comm 2020 Jan;149:99-106. [ CrossRef ]
- Baur K, Schättin A, de Bruin ED, Riener R, Duarte JE, Wolf P. Trends in robot-assisted and virtual reality-assisted neuromuscular therapy: a systematic review of health-related multiplayer games. J Neuroeng Rehabil 2018 Nov 19;15(1):107 [ FREE Full text ] [ CrossRef ] [ Medline ]
- Tao V, Moy K, Amirfar VA. A little robot with big promise may be future of personalized health care. Pharmacy Today 2016 Sep;22(9):38. [ CrossRef ]
- Ritschel H, Seiderer A, Janowski K, Aslan I, André E. Drink-O-Mender: An Adaptive Robotic Drink Adviser. In: Proceedings of the 3rd International Workshop on Multisensory Approaches to Human-Food Interaction. 2018 Presented at: MHFI'18; October 16, 2018; Boulder, Colorado. [ CrossRef ]
- Moyle W, Jones C, Cooke M, O'Dwyer S, Sung B, Drummond S. Social Robots Helping People With Dementia: Assessing Efficacy of Social Robots in the Nursing Home Environment. In: 6th International Conference on Human System Interactions (HSI). 2013 Presented at: HSI'13; June 6-8, 2013; Sopot, Poland. [ CrossRef ]
- Bhelonde A, Didolkar N, Jangale S, Kulkarni N. Flexible Wound Assessment System for Diabetic Patient Using Android Smartphone. In: International Conference on Green Computing and Internet of Things. 2015 Presented at: ICGCIoT'15; October 8-10, 2015; Noida, India. [ CrossRef ]
- Ashique K, Kaliyadan F, Aurangabadkar SJ. Clinical photography in dermatology using smartphones: an overview. Indian Dermatol Online J 2015;6(3):158-163 [ FREE Full text ] [ CrossRef ] [ Medline ]
- Połap D, Winnicka A, Serwata K, Kęsik K, Woźniak M. An intelligent system for monitoring skin diseases. Sensors (Basel) 2018 Aug 4;18(8):- [ FREE Full text ] [ CrossRef ] [ Medline ]
- Shimizu E, Ogawa Y, Yazu H, Aketa N, Yang F, Yamane M, et al. 'Smart Eye Camera': an innovative technique to evaluate tear film breakup time in a murine dry eye disease model. PLoS One 2019;14(5):e0215130 [ FREE Full text ] [ CrossRef ] [ Medline ]
- Li S, Xu LD, Zhao S. 5G internet of things: a survey. J Ind Inf Integration 2018 Jun;10:1-9. [ CrossRef ]
- Darwish A, Hassanien AE, Elhoseny M, Sangaiah AK, Muhammad K. The impact of the hybrid platform of internet of things and cloud computing on healthcare systems: opportunities, challenges, and open problems. J Ambient Intell Human Comput 2017 Dec 29;10(10):4151-4166. [ CrossRef ]
- Pan J, McElhannon J. Future Edge Cloud and Edge Computing for Internet of Things Applications. IEEE Internet Things J 2018 Feb;5(1):439-449. [ CrossRef ]
- Chen HS, Jarrell JT, Carpenter KA, Cohen DS, Huang X. Blockchain in healthcare: a patient-centered model. Biomed J Sci Tech Res 2019 Aug 8;20(3):15017-15022. [ CrossRef ]
- Park YR, Lee E, Na W, Park S, Lee Y, Lee J. Is blockchain technology suitable for managing personal health records? Mixed-methods study to test feasibility. J Med Internet Res 2019 Feb 8;21(2):e12533 [ FREE Full text ] [ CrossRef ] [ Medline ]
- Miller DD, Brown EW. Artificial intelligence in medical practice: the question to the answer? Am J Med 2018 Feb;131(2):129-133. [ CrossRef ] [ Medline ]
- Artificial Intelligence in Medicine. IBM. 2018. URL: https://www.ibm.com/watson-health/learn/artificial-intelligence-medicine [accessed 2020-02-02]
- Gulshan V, Peng L, Coram M, Stumpe MC, Wu D, Narayanaswamy A, et al. Development and validation of a deep learning algorithm for detection of diabetic retinopathy in retinal fundus photographs. J Am Med Assoc 2016 Dec 13;316(22):2402-2410. [ CrossRef ] [ Medline ]
- Tobore I, Li J, Yuhang L, Al-Handarish Y, Kandwal A, Nie Z, et al. Deep learning intervention for health care challenges: some biomedical domain considerations. JMIR Mhealth Uhealth 2019 Aug 2;7(8):e11966 [ FREE Full text ] [ CrossRef ] [ Medline ]
- Pham T, Tran T, Phung D, Venkatesh S. Predicting healthcare trajectories from medical records: a deep learning approach. J Biomed Inform 2017 May;69:218-229 [ FREE Full text ] [ CrossRef ] [ Medline ]
- Cheng Y, Wang F, Zhang P, Hu J. Risk Prediction With Electronic Health Records: A Deep Learning Approach. In: Proceedings of the 2016 SIAM International Conference on Data Mining. 2016 Presented at: SIAM'16; May 5-7, 2016; Florida, USA. [ CrossRef ]
- Yadav S, Ekbal A, Saha S, Bhattacharyyaditors. Deep Learning Architecture for Patient Data De-identification in Clinical Records. In: Proceedings of the Clinical Natural Language Processing Workshop. 2016 Presented at: ClinicalNLP'16; December 11, 2016; Osaka, Japan. [ CrossRef ]
- Li H, Li X, Ramanathan M, Zhang A. Identifying informative risk factors and predicting bone disease progression via deep belief networks. Methods 2014 Oct 1;69(3):257-265. [ CrossRef ] [ Medline ]
- Suk H, Lee S, Shen D, Alzheimer's Disease Neuroimaging Initiative. Hierarchical feature representation and multimodal fusion with deep learning for AD/MCI diagnosis. Neuroimage 2014 Nov 1;101:569-582 [ FREE Full text ] [ CrossRef ] [ Medline ]
- Lu N, Li T, Ren X, Miao H. A deep learning scheme for motor imagery classification based on restricted boltzmann machines. IEEE Trans Neural Syst Rehabil Eng 2017 Jun;25(6):566-576. [ CrossRef ] [ Medline ]
- Lee C, Chen G, Zhang Z, Chou Y, Hsu C. Is intensity inhomogeneity correction useful for classification of breast cancer in sonograms using deep neural network? J Healthc Eng 2018;2018:8413403 [ FREE Full text ] [ CrossRef ] [ Medline ]
- Mathews SM, Kambhamettu C, Barner KE. A novel application of deep learning for single-lead ECG classification. Comput Biol Med 2018 Aug 1;99:53-62. [ CrossRef ] [ Medline ]
- Tian S, Yang W, Grange JM, Wang P, Huang W, Ye Z. Smart healthcare: making medical care more intelligent. Global Health J 2019 Sep;3(3):62-65. [ CrossRef ]
- Yu L, Lu Y, Zhu X. Smart Hospital based on internet of things. J Netw 2012 Oct 1;7(10):1654-1661. [ CrossRef ]
- Thangaraj M, Ponmalar P, Anuradha S. Internet of Things (IoT) Enabled Smart Autonomous Hospital Management System - a Real World Health Care Use Case With the Technology Drivers. In: IEEE International Conference on Computational Intelligence and Computing Research. 2015 Presented at: IEEE'15; December 10-12, 2015; Madurai, India. [ CrossRef ]
- Australian Burden of Disease Study: Impact and Causes of Illness and Death in Australia 2015. Australian Institute of Health and Welfare. 2015. URL: https://www.aihw.gov.au/reports-data/health-conditions-disability-deaths/burden-of-disease/overview [accessed 2020-10-02]
- de Silva D. Evidence: Helping People Help Themselves. The Heart Foundation. 2011. URL: https://www.health.org.uk/publications/evidence-helping-people-help-themselves [accessed 2020-02-02]
- Vickery DM, Kalmer H, Lowry D, Constantine M, Wright E, Loren W. Effect of a self-care education program on medical visits. J Am Med Assoc 1983 Dec 2;250(21):2952-2956. [ Medline ]
- Pai A. Survey: Percent of Smartphone Users Have Downloaded a Fitness or Health App. Mobile Health News. 2015. URL: https://www.mobihealthnews.com/48273/survey-58-percent-of-smartphone-users-have-downloaded-a-fitness-or-health-app [accessed 2020-04-04]
- Nadarzynski T, Miles O, Cowie A, Ridge D. Acceptability of artificial intelligence (AI)-led chatbot services in healthcare: a mixed-methods study. Digit Health 2019;5:2055207619871808. [ CrossRef ] [ Medline ]
- Wisniewski H, Liu G, Henson P, Vaidyam A, Hajratalli NK, Onnela J, et al. Understanding the quality, effectiveness and attributes of top-rated smartphone health apps. Evid Based Ment Health 2019 Feb;22(1):4-9 [ FREE Full text ] [ CrossRef ] [ Medline ]
- Bates DW, Landman A, Levine DM. Health apps and health policy: what is needed? J Am Med Assoc 2018 Nov 20;320(19):1975-1976. [ CrossRef ] [ Medline ]
- Borycki E. Quality and safety in ehealth: the need to build the evidence base. J Med Internet Res 2019 Dec 19;21(12):e16689 [ FREE Full text ] [ CrossRef ] [ Medline ]
- Pacheco R, Dias, Santinha, Rodrigues, Queirós, Rodrigues. Smart cities and healthcare: a systematic review. Technologies 2019 Aug 16;7(3):58. [ CrossRef ]
- Lai Y, Yeung W, Celi LA. Urban intelligence for pandemic response: viewpoint. JMIR Public Health Surveill 2020 Apr 14;6(2):e18873 [ FREE Full text ] [ CrossRef ] [ Medline ]
- Chen C, Jyan H, Chien S, Jen H, Hsu C, Lee P, et al. Containing covid-19 among 627,386 persons in contact with the diamond princess cruise ship passengers who disembarked in Taiwan: big data analytics. J Med Internet Res 2020 May 5;22(5):e19540 [ FREE Full text ] [ CrossRef ] [ Medline ]
- Wray A, Olstad DL, Minaker LM. Smart prevention: a new approach to primary and secondary cancer prevention in smart and connected communities. Cities 2018 Sep;79:53-69. [ CrossRef ]
- Palmieri F, Ficco M, Pardi S, Castiglione A. A cloud-based architecture for emergency management and first responders localization in smart city environments. Comput Electric Eng 2016 Nov;56:810-830. [ CrossRef ]
- Korzun D. Internet of things meets mobile health systems in smart spaces. In: Internet of Things and Big Data Technologies for Next Generation Healthcare. Switzerland: Springer; 2017.
- Dauwed M, Meri A. In: Ismail Y, editor. IOT Service Utilisation in Healthcare. New York, USA: IntechOpen; 2019.
- World Health Organization. Global Diffusion of Ehealth: Making Universal Health Coverage Achievable: Report of the Third Global Survey on Ehealth. Slitzerland: World Health Organization; 2017.
- Riazul Islam SM, Daehan K, Humaun Kabir M, Hossain M, Kyung-Sup Kwak. The internet of things for health care: a comprehensive survey. IEEE Access 2015;3:678-708. [ CrossRef ]
- Global Internet of Things Policies 2018-2019. Research and Markets. 2019. URL: https://www.globenewswire.com/news-release/2019/05/30/1859482/0/en/Global-Internet-of-Things-Policies-2018-2019.html [accessed 2020-06-04]
- Horizon Scanning Series: An overview. Australian Council of Learned Academies. 2018. URL: https://acola.org/programs/horizon-scanning-series/ [accessed 2020-04-02]
- Veazie S, Winchell K, Gilbert J, Paynter R, Ivlev I, Eden KB, et al. Rapid evidence review of mobile applications for self-management of diabetes. J Gen Intern Med 2018 Jul;33(7):1167-1176 [ FREE Full text ] [ CrossRef ] [ Medline ]
- Jacob C, Sanchez-Vazquez A, Ivory C. Social, organizational, and technological factors impacting clinicians' adoption of mobile health tools: systematic literature review. JMIR Mhealth Uhealth 2020 Feb 20;8(2):e15935 [ FREE Full text ] [ CrossRef ] [ Medline ]
- Schofield P, Shaw T, Pascoe M. Toward comprehensive patient-centric care by integrating digital health technology with direct clinical contact in Australia. J Med Internet Res 2019 Jun 4;21(6):e12382 [ FREE Full text ] [ CrossRef ] [ Medline ]
- Jalali MS, Kaiser JP, Siegel M, Madnick S. The internet of things promises new benefits and risks: a systematic analysis of adoption dynamics of IoT products. IEEE Secur Privacy 2019 Mar;17(2):39-48. [ CrossRef ]
- Lee H, Park YR, Kim H, Kang NY, Oh G, Jang I, et al. Discrepancies in demand of internet of things services among older people and people with disabilities, their caregivers, and health care providers: face-to-face survey study. J Med Internet Res 2020 Apr 15;22(4):e16614 [ FREE Full text ] [ CrossRef ] [ Medline ]
- Verloo H, Kampel T, Vidal N, Pereira F. Perceptions about technologies that help community-dwelling older adults remain at home: qualitative study. J Med Internet Res 2020 Jun 4;22(6):e17930 [ FREE Full text ] [ CrossRef ] [ Medline ]
- Gagnon M, Ngangue P, Payne-Gagnon J, Desmartis M. m-Health adoption by healthcare professionals: a systematic review. J Am Med Inform Assoc 2016 Jan;23(1):212-220. [ CrossRef ] [ Medline ]
- NIST Releases Draft Security Feature Recommendations for IoT Devices. National Institute of Standards and Technology. 2019. URL: https://www.nist.gov/news-events/news/2019/08/nist-releases-draft-security-feature-recommendations-iot-devices [accessed 2020-02-02]
- Rubí JN, Gondim PR. Iomt platform for pervasive healthcare data aggregation, processing, and sharing based on onem2m and openehr. Sensors (Basel) 2019 Oct 3;19(19):- [ FREE Full text ] [ CrossRef ] [ Medline ]
- Rubí JN, Gondim PR. Interoperable internet of medical things platform for e-health applications. Int J Distrib Sens N 2020 Jan 7;16(1):155014771988959. [ CrossRef ]
- Desai P, Sheth A. Semantic Gateway as a Service Architecture for IoT Interoperability. In: IEEE International Conference on Mobile Services. 2015 Presented at: CMS'15; June 27-July 2, 2015; New York, USA. [ CrossRef ]
- Milosevic Z, Bond A. Digital Health Interoperability Frameworks: Use of RM-ODP Standards. In: 20th International Enterprise Distributed Object Computing Workshop. 2016 Presented at: EDOCW'16; September 5-9, 2016; Vienna, Austria. [ CrossRef ]
- Tuckson RV, Edmunds M, Hodgkins ML. Telehealth. N Engl J Med 2017 Oct 19;377(16):1585-1592. [ CrossRef ] [ Medline ]
Abbreviations
Edited by G Eysenbach; submitted 11.05.20; peer-reviewed by Z Ye, Y Zhang, T Alessa, D Goyal; comments to author 29.06.20; revised version received 16.08.20; accepted 15.09.20; published 10.11.20
©Jaimon T Kelly, Katrina L Campbell, Enying Gong, Paul Scuffham. Originally published in the Journal of Medical Internet Research (http://www.jmir.org), 10.11.2020.
This is an open-access article distributed under the terms of the Creative Commons Attribution License (https://creativecommons.org/licenses/by/4.0/), which permits unrestricted use, distribution, and reproduction in any medium, provided the original work, first published in the Journal of Medical Internet Research, is properly cited. The complete bibliographic information, a link to the original publication on http://www.jmir.org/, as well as this copyright and license information must be included.
- Research article
- Open access
- Published: 08 October 2020
Exploring emerging IoT technologies in smart health research: a knowledge graph analysis
- Xuejie Yang 1 na1 ,
- Xiaoyu Wang ORCID: orcid.org/0000-0003-2085-2924 2 na1 ,
- Xingguo Li 1 na1 ,
- Dongxiao Gu 1 , 3 na1 ,
- Changyong Liang 1 ,
- Kang Li 1 ,
- Gongrang Zhang 1 &
- Jinhong Zhong 1
BMC Medical Informatics and Decision Making volume 20 , Article number: 260 ( 2020 ) Cite this article
12k Accesses
32 Citations
Metrics details
At present, Internet of Things technology has been widely used in various fields, and smart health is also one of its important application areas.
We use the core collection of Web of Science as a data source, using tools such as CiteSpace and bibliometric methods to visually analyze 9561 articles published in the field of smart health research based on the Internet of things (IoT) in 2003–2019, including time distribution, spatial distribution, and literature co-citation analysis and keyword analysis.
The field of smart health research based on IoT has developed rapidly since 2014, but has not yet formed a stable network of authors and institutions. In addition, the knowledge base in this field has been initially formed, and most of the published literatures are multi-theme research.
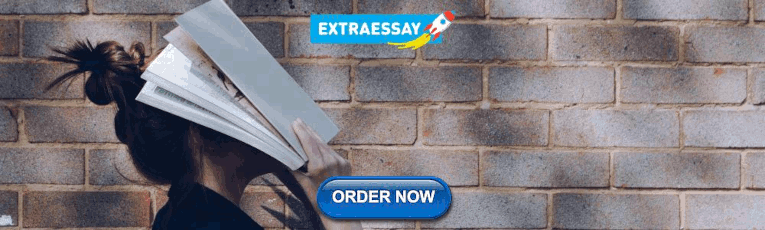
Conclusions
This study discusses the research status, research hotspots and future development trends in this field, and provides important knowledge support for subsequent research.
Peer Review reports
The Internet of Things (IoT) is an important representative of the new generation of information technology. It is the result of rapid development in the field of wireless communications in recent years, and it is a network that extends on the Internet [ 1 ]. It can connect various information sensing devices (such as Radio Frequency Identification, infrared sensors, laser scanners, etc.) to the Internet to realize the “Internet of Everything” [ 2 ]. At present, IoT has been widely used in various fields, such as smart city, smart home, intelligent logistics, intelligent transportation, etc. Among them, smart health is also one of its important application areas. There are countless people who lose their lives every year due to various diseases or health problems. In terms of chronic diseases, the number of people dying from chronic diseases accounts for 60% of the total number of deaths worldwide. People are paying more and more attention to health issues [ 3 ]. Therefore, the use of IoT technology to solve health problems has become one of the research hotspots in the field of smart health.
IoT is connecting physical world with virtual world of Internet. Physical world includes household appliances (such as air purifiers, thermostats, etc.), automobiles, industrial machinery, construction, medical equipment, and human body [ 4 ]. Applying IoT technologies to healthcare will help improve the quality of people life, the level of chronic disease management, danger warning and life-saving interventions. There are lots potential applications of healthcare IoT: (1) Health monitoring . Today’s wearable devices can detect basic activities of human body, analyze human behavior, and measure health status. Smart wearable devices (such as smart watch) can reduce patient anxiety and reduce waste of resources [ 5 ]. This is very different from other sensitive devices for health monitoring in conventional hospitals. (2) Health information support for patients . You can remind patients to take medicine on time through some IoT devices, in clinical. Networking devices such as electrocardiogram, blood oxygen, and blood pressure can improve the continuous measurement, monitoring, and support structure of patients and caregivers, thereby improving clinical outcomes [ 6 , 7 ]. (3) Service improvement . IoT can help connect cars to network systems. If a car has an accident, the system can identify the severity of the accident and help traffic administration department and healthcare emergency center via sending the accident location and direction. This will help the injured people obtain timely assistance [ 8 ]. (4) The collection of information resource for big data analytics . The health IoT can generate massive amounts of health big data. The analysis, mining, and use of health big data can further promote and enhance the development of health IoT [ 9 ].
Since 2003, scholars from all over the world have gradually invested in research in the field of smart health research based on the IoT. In response, some scholars have designed smart wearable systems to solve health problems. Li et al. [ 10 ] established a model of the acceptance of smart wearable system by the elderly, and pointed out the factors influencing their use of smart wearable technology, including self-reported health status. Akbulut et al. [ 11 ] designed a smart wearable system that monitors cardiovascular disease, which provides continuous medical monitoring. Fraise et al. [ 12 ] proposed a multi-agent system (MAS) that uses smart wearable and mobile technology to care for patients in elderly care facilities. In recent years, the development of technology has made smart watches and smart bracelets popular. Previously, Lu et al. [ 13 ] reviewed the application of smart watches in the field of medical health. Through comparative experiments, Hataji et al. [ 14 ] showed that combined treatment could improve the daily physical performance of patients with chronic obstructive pulmonary disease (COPD) under the encouragement of smart watches. Wile et al. [ 15 ] used smart watch devices to distinguish between orthostatic recurrent tremor and primary tremor of Parkinson’s disease. Grym et al. [ 16 ] pointed out that smart wristbands are a viable continuous monitoring tool during pregnancy. Smart home is also an important application of IoT technology in the field of smart health. Dawadi et al. [ 17 ] demonstrated the feasibility of using smart home sensor data and learning-based data analysis to predict clinical scores. Pham et al. [ 18 ] proposed a cloud-based smart home environment (CoSHE) for home healthcare. Ghasemi et al. [ 19 ] proposed a smart home medical system that can diagnose environmental events and health risks quickly and in a timely manner. Alberdi et al. [ 20 ] ‘s experiments show that all mobile, cognitive, and depressive symptoms can be predicted by activity-aware smart home data. In addition, research on disease and health issues through IoT technology is the focus of research in this field. Zhang et al. [ 21 ] proposed a medical data fusion algorithm based on IoT for the particularity of medical IoT data. Hossain et al. [ 22 ] proposed an industrial Internet of Things (IIoT) health monitoring framework that supports cloud computing. Farahani et al. [ 23 ] introduced the overall architecture of the fog-driven IoT e-health ecosystem and discussed the applicability and challenges of the IoT in the field of healthcare.
At present, there are many researches on the application of smart wearables, smart watches, smart bracelets, smart homes and IoT technologies to the field of smart health. However, there is no research to objectively review and visualize all the literature in this field. In order to analyze the development status and future trends of the intelligent health research field based on the IoT systematically, comprehensively, and objectively, this study uses bibliometric methods to visualize the analysis from time distribution, spatial distribution, literature co-citation and keywords based on 9561 literature data in this field from 2003 to 2019. This research provides panoramic knowledge support for researchers in related fields to understand the research status, future trends and hotspots in the field of smart health research based on IoT.
Data sources
The data source for this study was Web of Science (WoS), which selected four core databases of its core collections, including Science Citation Index Expanded, Conference Proceedings Citation Index-Science, and so on. WoS is an important database for obtaining global academic information. It contains more than 13,000 authoritative, high-impact academic journals from around the world, covering the fields of natural sciences, engineering technology, biomedicine, social sciences, arts and humanities. WoS includes references cited in the paper, with a unique citation index, users can use an article, a patent number, a conference document, a journal or a book as a search term to retrieve their citations and easily trace the origin and history of a research document, or track its latest progress. Although the WoS database cannot include all the literature published in this field, it has some representativeness. We invited 5 experts in the field of health IoT to finalize the database and search strategy through Delphi method. The search strategy we used is as follows: TS = (“#1” AND “#2”), Where “#1” is TS = (“internet of things” OR “smart watch*” OR “smart wristband*” OR “smart home*” OR “wearable device*” OR “wearable technolog*” OR “wearable sensor*”), indicating the search term related to the IoT; “#2” indicates TS = (“diseas*” OR “health*“OR “hospital*”), which indicates a search term related to health. In order to ensure that the retrieved documents are related to the retrieval subject, we organized a panel of evaluation consisting of eight Ph.D. candidates in our research area. After excluding irrelevant articles, we finally got 9561 records. (The search time was August 2020).
Research methods and tools
This paper mainly adopts the method of bibliometrics. Bibliometrics refers to the quantitative analysis and management of literature information by mathematical and statistical methods, and then discusses its structure, characteristics and laws [ 24 ]. This study mainly uses HistCite, CiteSpace and MS Excel to visually analyze the relevant literature in the field of smart health research based on IoT. Because HistCite’s statistical function is relatively powerful, it is mainly used to collect relevant data in this paper, and then use Excel software to draw the chart [ 25 ]. CiteSpace is a visualization tool for bibliometrics that focuses on finding key points in the development of a field [ 26 ]. Therefore, this article mainly uses CiteSpace to visualize the authors, institutions, literature co-citation and keywords of the IoT-based smart health research field.
Time distribution map
In order to understand the output of research results in the field of smart health research based on IoT, HistCite was used to statistically analyze the number of scientific literatures in the years from 2003 to 2019, and the trend of annual papers was obtained, as shown in Fig. 1 . As can be seen from the figure, from 2003 to 2019, the annual capacity curve presents an overall growth trend, among which the curve of annual capacity from 2003 to 2014 is relatively flat, and the annual capacity basically conforms to the exponential growth trend, or even below the exponential trend line. In 2014–2019, the annual capacity curve has grown very rapidly, almost showing a linear upward trend, much higher than the index trend line, with a growth rate of 97.88% in 2014–2015. This figure suggests that research in this field will continue to increase in the future and that it will remain a hotspot for future research.
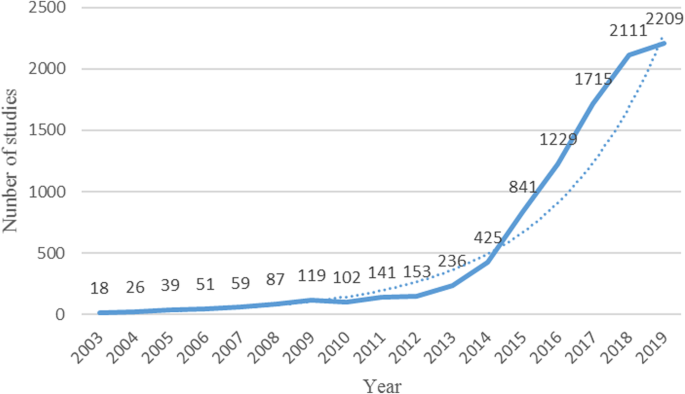
Annual number of published articles
Then, we explored the input of researchers in the field of smart health research based on IoT. The same use of HistCite to statistically analyze the number of scientific research participants in the years from 2003 to 2019, and get the trend of the annual author input, as shown in Fig. 2 . By comparing Figs. 1 and 2 , we can clearly find that their variation trend is roughly the same, in years with a high growth rate of annual capacity, the growth rate of author input is also high. It is also easy to understand that, generally speaking, the annual number of articles and the annual amount of authors input is directly proportional to the relationship.
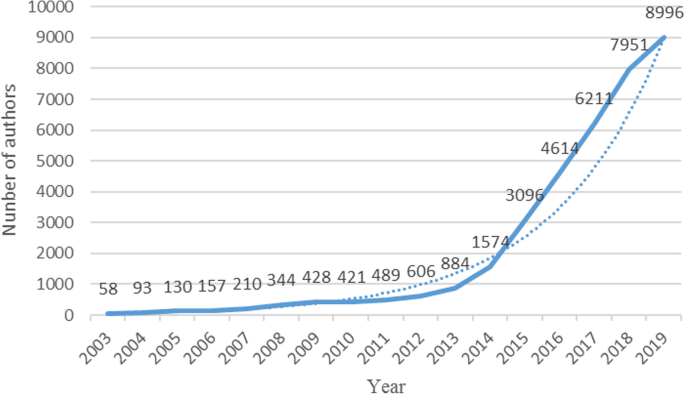
Annual number of authors input
Finally, the input-output ratio of scientific researchers in the field of smart health research based on IoT was understood. We calculated the number of participants in a single paper from 2003 to 2019, and obtained the change trend of the ratio of participants in a single paper, as shown in Fig. 3 . The straight line parallel to the abscissa in the figure is the average number of participants in a single document over the years, which is 3.79. Overall, the annual input-output ratio fluctuated significantly, especially during 2003–2011. This was because the number of documents in previous years was small and the researchers were not fixed. Then the ratio is roughly stable. In the 17 years, only the authors in 2010 and 2019 had an input-output ratio of more than 4. The small number of participants in a single document indicates that in this research field, the contribution rate of individual authors is high, the cooperation between authors is less, the number of authors is not saturated, and there is still much room for improvement.
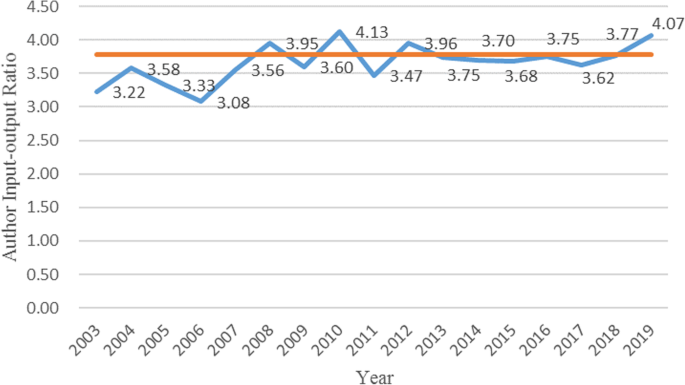
Annual author input-output ratio
Space distribution map
Author distribution.
In order to analyze the author’s cooperative network, we import the preprocessed data into CiteSpace to generate the author cooperation network diagram, as shown in Fig. 4 . The figure shows the author’s name and the relationship between the authors with 10 or more articles. The most published is Bonato and Rahmani, which has published 35 articles. In the figure, the size of the node is proportional to the number of articles issued by the author, the thickness of the connection between the nodes is proportional to the number of cooperation between the authors, and different colors indicate the year of cooperation between different authors. As can be seen in the upper left corner of Fig. 4 , the number of network nodes is 811, the number of connections between nodes is 745, and the density of the network is only 0.0023.
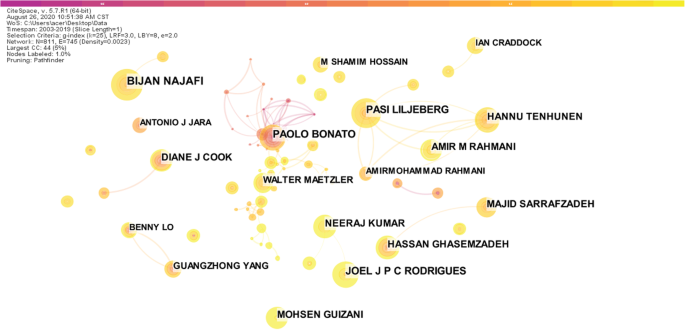
Author collaboration network
Table 1 specifies the authors of the top 10 articles and their related information. In the HistCite software system, the citation frequency is divided into LCS and LGS, where LCS (local citation score) refers to the citation frequency of the document in the database of the field, and GCS (global citation score) refers to the frequency of citation in the Web of Science database [ 27 ]. It can be seen from Table 1 that Bonato and Rahmani have the largest number of publications, and the total quotation has reached 2024 and 908 respectively. The average single paper has been cited more than 15 times, indicating that his papers are not only issued more, but also have higher recognition. The sparseness of network density indicates that the cooperation between authors is not close enough, the field of smart health research based on IoT has not yet formed a stable core author group. And if the authors strengthen cooperation, they can collide with new sparks, promote research and innovation, and make the field flourish.
Institutional distribution
Then we import the pre-processed data into CiteSpace to analyze the organization that publishes the scientific literature, and generate the organization cooperation network diagram, as shown in Fig. 5 . Table 2 shows the top 10 organizations and related information. The organization with the largest number of publications is Chinese Academy of Sciences, which has published 134 scientific papers, 52 more than the second-ranked King Saud University. In addition, there are nearly 200 organizations that have published at least 10 articles, indicating that the research on the field of smart health research based on IoT has received extensive attention from various authoritative academic institutions in the world, showing a hundred schools of contention and a hundred flowers.
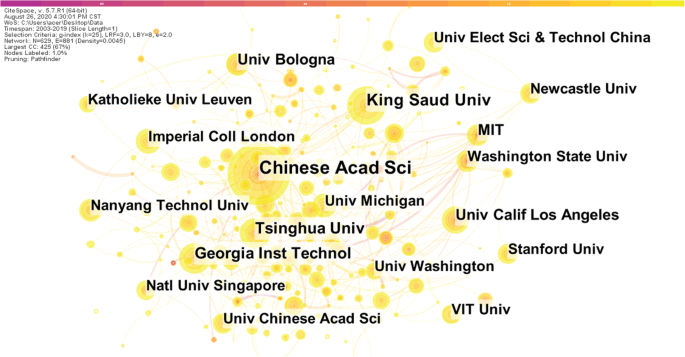
Institution collaboration network
According to Table 2 , Chinese Academy of Sciences not only has the largest number of publications, but its LCS and GCS are also much higher than the second-ranked King Saud University. Although Georgia Institute of Technology is ranked third in the number of publications, its LCS is not high. The average number of citations in the field is only 1.09, indicating that the published articles are not highly recognized by peers. On the contrary, although the numbers of published literatures are not large by Massachusetts Institute of Technology and Washington State University, their average total number of citations have reached 38 and 33 respectively. At the same time, Chinese Academy of Sciences’ LCS and GCS data ranks first among all institutions. These explain that the quality of the literature published by these institutions is very high and is widely supported and highly recognized by researchers and peers.
Research cooperation between institutions is an important way to enhance the research strength of the organization as a whole, to achieve complementary scientific research resources and to share knowledge, and to reflect one of the important indicators of research status in a certain field [ 28 ]. In Fig. 5 , the size of the node is proportional to the number of documents sent by the organization, the thickness of the connection between the nodes is proportional to the number of cooperative researches between the organizations, and different colors indicate the year of collaborative research between the institutions. The number of network nodes is 629, the number of connections between nodes is 881, and the density of the network is 0.0045. It can be seen that in the field of smart health research based on IoT, there is not enough cooperation between institutions, and the relationship between them is not close enough, and a stable and mature institutional cooperation relationship has not yet been formed. Institutions should strengthen cooperation and exchanges, give full play to their respective advantages, and make full use of academic resources to promote innovation in research results and promote the vigorous development of the entire field.
Journal distribution
Finally, we analyzed the journals in the field of smart health research based on IoT. We use HistCite to collect statistics on journals in the field, and Table 3 lists the top 10 journals and related information. Table 3 shows that the journal with the largest number of documents is Sensors , and its LCS and GCS data are also among the best. Interestingly, four of the top 10 journals are from the IEEE Publishing Group: IEEE Access , IEEE Internet of Things Journal , IEEE Sensors Journal and IEEE Journal of Biomedical and Health Informatics. The average single article of IEEE Journal of Biomedical and Health Informatics has been cited as high as 31.7, which is enough to see the journal’s influence in the field of smart health research based on IoT. Conversely, although there are many related articles in the journal of IEEE Access , its average times cited is only 14.9, indicating that the quality and influence of these articles are generally not too high.
Overall, IEEE is the biggest winner in the field of smart health research based on IoT. In addition, there are many journals that focus on the IoT, sensors and health, but there are few journals focusing on the intersection of the IoT and health. This shows that the research on the field of smart health research based on IoT has not yet had a great influence, and the major journals have not paid much attention to it.
Knowledge base analysis
Co-citation Networks refers to a knowledge network formed by two scientific documents simultaneously cited by the third article [ 29 ]. Literature co-citation analysis expresses the relationship between documents by the frequency cited by other literatures. That is to say, a certain two documents are cited together by several other documents. The higher the frequency of citations, the closer the relationship between the two documents is, which means that the more the subject backgrounds of the two documents are similar [ 30 ]. Fundamentally speaking, when certain documents, journals, academic groups or individuals are repeatedly quoted by their peers, the knowledge carriers that are cited are essentially recognized by the scientific community in which they are located, thus forming a scientific paradigm. This paradigm relationship can be visualized by analysis of the co-citation network of the literature [ 31 ]. Therefore, through the literature co-citation network analysis, the knowledge base of the research on the field of smart health research based on IoT can be concretely demonstrated.
We import the preprocessed data into CiteSpace, analyze the co-citation relationship between scientific literatures, and generate a co-citation network diagram of the literature, as shown in Fig. 6 . In the figure, each node represents a document that is commonly cited. The size of the node is proportional to the number of times it is cited, the connection between the nodes indicates a co-citation relationship. The thickness of the connection indicates the strength of the co-cited, and the different colors indicate the year in which the document was cited. The number of network nodes is 1239, the number of connections between nodes is 2331, and the density of the network is 0.003.
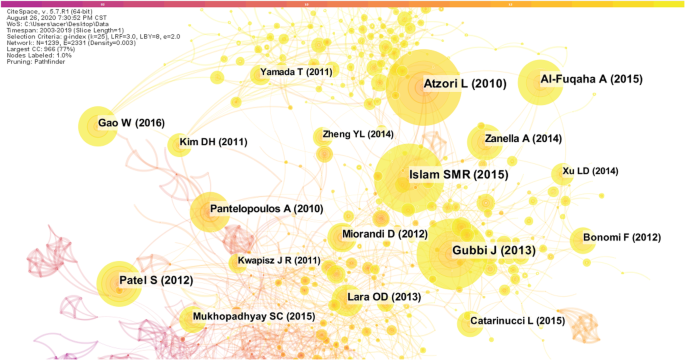
Articles in the co-citation network
Table 4 lists the top 10 co-citation literature and related information. In the literature citation network, Atzori’s article published in Computer Networks in 2010 titled “The internet of things: A survey” was cited as the highest frequency, reaching 290 times [ 1 ]. So far, this article has been cited as a total of 13,691 times in Google Scholar. Gubbi [ 32 ], Islam [ 33 ], Patel [ 34 ], Al-Fuqaha [ 35 ], etc., which are ranked behind, are connected to Atzori [ 1 ]. It shows that the correlation between the top citations in the field is very strong, and the topics of the scientific literature are similar. These documents are all about IoT technology and applications. It can be seen that the current research on IoT technology has been initially matured and standardized. Interestingly, five of the top 10 cited articles were published by the IEEE Publishing Group.
Combined with the above-mentioned journal analysis, it can be said that the IEEE Publishing Group has made tremendous contributions to the development of this field. In addition, in terms of centrality, Atzori’s performance is also very good, the greater the centrality of a node in the network, indicating that it is more important in the network [ 36 ]. Therefore, the comprehensiveness of all aspects can reflect the importance of the document Atzori [ 1 ]. It can be said that this article lays the foundation for the research of smart health research based on IoT. In general, the literature of Fig. 6 has a relatively tightly distributed network, indicating that the knowledge base in this field has been initially formed, which will provide important knowledge support for subsequent research.
Research focus analysis
Research hotspots refer to the focus of research in a certain discipline in a certain period of time. Generally speaking, there is a large number of scientific literatures, academic thoughts, and research groups emerging on a subject [ 37 ]. Kuhn [ 38 ] emphasized that the development of science is an alternating appearance of the conventional science and the scientific revolution, which indicates that the scientific revolution is changing, and that the old and new paradigms are incommensurable. It is precisely because of the existence of incommensurability that the vocabulary system between the old and new paradigms will change accordingly. So, whether the scientific revolution occurs can be judged from whether the vocabulary of the period has changed. The number of co-occurrences of different keywords in the scientific literature can be counted. The level of the co-occurrence frequency can reflect the correlation between the keywords and the hot issues in the specific field during this period [ 39 ]. Therefore, the co-occurrence analysis of keywords can reveal the research structure and research hotspots in specific fields. The research results of Callon et al. [ 40 ] are the earliest applications of co-word analysis. Subsequently, the co-word analysis method has been widely used in the field of information science. Of course, keyword analysis is also based on certain assumptions [ 41 ], including: (1) The selection of keywords is cautious; (2) Multiple keywords in the same document are related to each other and are recognized by the author; (3) If enough authors recognize the relationship between the same keyword, then this relationship can be considered to have a certain meaning in the field; (4) The keyword can reflect the content of the document to a certain extent. When the author chooses the keyword, it is usually affected by other research results. The basic principle of co-word analysis is to count the number of times a group of keywords appears in the same group of documents, then the degree of co-occurrence is measured by the number of co-occurrences, the more co-occurrences, the more closely they are related [ 42 ].
The key word is a high degree of conciseness and generalization of an article, which is the core and essence of the article, frequently high keywords are often used to identify hot topics in a research field. By analyzing keywords, we can intuitively grasp the main research content of a paper, and even the overall research situation in a field [ 43 ]. This study extracts the key words from 9561 documents and conducts frequency statistics and frequency co-occurrence analysis to understand the current structural foundations and research hotspots in the field of smart health research based on IoT, and predict the future development direction of the field. Table 5 lists the top 20 keywords of the co-occurrence frequency. It can be seen that the keyword with the highest frequency is the internet of things, this is very consistent with the topic of this article. The subject of this paper is the IoT and health. The IoT is also the keyword with the highest centrality, indicating that research in this field is basically carried out around the IoT. These keywords with high frequency of occurrence can be divided into four main categories: (1) Keywords related to the IoT technology, such as internet of thing, sensor, wireless sensor network, big data, cloud computing, etc.; (2) Keywords related to health, such as healthcare, health, parkinsons disease, etc.; (3) Keywords related to smart health, such as smart home; (4) Problems arising from research in the field of smart health research based on IoT, such as security.
The co-word network refers to an objective knowledge network that expresses the structure of the scientific knowledge domain, which is composed of co-occurrence between keywords. It can be used to describe the knowledge structure of a subject domain and can reveal the evolution of a disciplinary structure in combination with time series [ 44 ]. We import the pre-processed data into CiteSpace to analyze the keywords of the scientific literature and generate a keyword co-occurrence network diagram, as shown in Fig. 7 . Each node represents a different keyword, and the size of the node is proportional to the frequency of its co-occurrence. The connection between nodes indicates the co-occurrence relationship between two keywords in the same document, and the different colors indicate the years in which different keywords co-occur. The number of network nodes is 889, the number of connections between nodes is 3090, and the density of the network is 0.0078. It can be seen from the figure that the network as a whole is relatively dense, and the co-occurrence relationship between multiple groups of keywords is relatively close, indicating that the research results in the field of intelligent health research based on the Internet of Things are mostly multi-theme research.
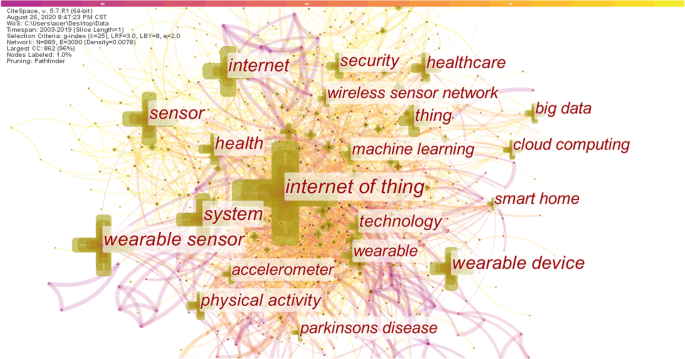
Keyword co-occurrence network
In the future research, the IoT is still the center of research in this field. Focusing on the integration of the IoT and the integration of smart health, it is necessary to solve the security and privacy issues in this field and eliminate concerns for users. IoT technologies often appear at the same time as cloud computing, big data, etc. These emerging technologies are closely related, and artificial intelligence technology cannot lack them. Therefore, in future research, the IoT, big data and cloud computing will frequently appear in the research in this field. Smart homes and smart cities have received a lot of attention in recent years. Smart home is a platform for housing, using IoT technology to connect various devices in the home to achieve convenience, comfort and intelligence. At present, there are few studies on the use of IoT technology to treat specific diseases (such as parkinsons diseases appearing in the top 20 keywords, etc.), so this may be one of the future research trends.
Discussions
Summary of findings.
Using HistCite, CiteSpace, Excel and other analysis tools, the time distribution, spatial distribution, literature citation and research hotspots of knowledge in this field are deeply analyzed and visualized.
(1) In terms of time distribution: the annual load capacity curve and the annual author input curve change trend are roughly the same, and the overall growth trend. In particular, the growth rate since 2014 has been very rapid, almost showing a linear upward trend, much higher than the index trend line. Explain that research in this field will continue to increase in the future and that it will remain a hotspot for future research.
(2) In terms of spatial distribution: a) Author distribution: The author’s cooperation network is sparse, the cooperation between the authors is not close enough, and the stable core author group has not yet formed in this field; b) Institutional distribution: Similarly, the cooperation and cooperation between institutions is not close enough, and a stable and mature institutional cooperation relationship has not yet been formed; c) Journal distribution: There are few journals focusing on the intersection of IoT technology and health. This shows that the research in this field has not yet had a great influence, and the major journals have not paid much attention to it.
(3) In terms of knowledge base analysis: the literature has a relatively tightly indexed network, and the knowledge base in this field has been initially formed, which can provide important knowledge support for subsequent research.
(4) In terms of research hotspot analysis: high-frequency keywords can be divided into four parts. The Internet of Things is a keyword with the highest co-occurrence frequency and the highest centrality. In addition, most of the research results in this field are multi-theme research. Among them, smart home and the use of IoT technology to assist in the treatment of specific diseases are the future research trends.
Future trends
Through the research in this paper, we can find that smart home and smart city are the research hotspots in recent years, and will likely also be the focus of future research.
Smart homes can be a “family health consultant”. For example, the smart home system can realize the “alarm” of the elderly and children, notify the family and locate; The system will automatically start and shut down the air purifier according to the real-time air condition, without manual operation; In addition, the air purifier can be controlled based on the humidity level in the house and the PSI level of asthma and allergic rhinitis patients [ 45 ]. The smart screen in the kitchen can see the children in the living room through the security system, and is equipped with detection equipment for harmful gases such as gas to achieve the function of safety protection; The smart clothing care machine in the cloakroom has the functions of steam sterilization, shaping, drying and other functions to protect the health of users.
The construction of smart health protection system is an important part of the construction of smart cities. The construction of “digital health” system is the focus of promotion. The smart city will build a medical health big data platform, realize the data sharing and sharing of medical and health service organizations, and rely on the smart city cloud platform to form a citizen medical health information big data center, and provide support for promoting three-medicine linkage and achieving graded diagnosis and treatment. In addition, electronic health records of residents in the city will be established to realize the networking of health services in hospitals and clinics throughout the city. And promote remote registration, electronic toll collection, online telemedicine services, graphic and physical examination diagnostic systems, etc., to comprehensively improve the city’s medical and health services.
Future works
The following work will be done in the future studies: (1) The first one it to examine how the Internet of Things actually affects medical accessibility; (2) The second one is to evaluate the objectiveness or reproducibility of reported results as well as relationship between prominent authors and industry; (3) The third one is to use more data resources rather than only WoS data and further validate our search result; (4) The fourth but not the last one is to eliminate irrelevant search results with technical tools rather than the manual methods used on this study.
In order to explore the knowledge base, research hotspots, development status and future research directions of the research on the field of smart health research based on IoT. We conducted a bibliometric analysis of 9561 articles in the Web of Science core database for the 17 years from 2003 to 2019. The results show that the field of smart health research based on IoT has developed rapidly since 2014, but has not yet formed a stable network of authors and institutions. Our research provides panoramic knowledge support for researchers in related fields to understand the research status, future trends and hotspots in the field of smart health research based on IoT.
Availability of data and materials
Data materials are available from the lead author upon request.
Abbreviations
- Internet of things
Multi-agent system
Chronic obstructive pulmonary disease
Cloud-based smart home environment
Industrial Internet of Things
Web of Science
Local citation score
Global citation score
Atzori L, Iera A, Morabito G. The internet of things: a survey. Comput Netw. 2010;54(15):2787–805.
Article Google Scholar
Stankovic JA. Research directions for the internet of things. IEEE Internet Things J. 2014;1(1):3–9.
Zhang G, Li K, Gu D, Wang X, Yang X, Zhu K, Liang G. Visualizing knowledge evolution and hotspots of rural environment and health: a systematic review and research direction. IEEE Access. 2019;7:72538–50.
Greengard S. The internet of things: MIT press; 2015.
Book Google Scholar
Abawajy JH, Hassan MM. Federated internet of things and cloud computing pervasive patient health monitoring system. IEEE Commun Mag. 2017;55(1):48–53.
Fernandes CO, De Lucena CJP. A software framework for remote patient monitoring by using multi-agent systems support. JMIR Med Inform. 2017;5(1):e9.
Article PubMed PubMed Central Google Scholar
Liang C, Gu D, Tao F, et al. Influence of mechanism of patient-accessible hospital information system implementation on doctor–patient relationships: a service fairness perspective. Inf Manag. 2017;54(1):57–72.
Thomas MO, Rad BB. Reliability evaluation metrics for internet of things, car tracking system: a review. Int J Inf Technol Comput Sci (IJITCS). 2017;9(2):1–10.
CAS Google Scholar
Mahdavinejad MS, Rezvan M, Barekatain M, Adibi P, Barnaghi P, Sheth AP. Machine learning for internet of things data analysis: a survey. Digit Commun Netw. 2018;4(3):161–75.
Li J, Ma Q, Chan AH, Man S. Health monitoring through wearable technologies for older adults: smart wearables acceptance model. Appl Ergon. 2019;75:162–9.
Article PubMed Google Scholar
Akbulut FP, Akan A. A smart wearable system for short-term cardiovascular risk assessment with emotional dynamics. Measurement. 2018;128:237–46.
Fraile JA, Zato C, Gil O, de la Prieta F. Multiagent Systems and Wearable Devices: Helping People Live Healthier. In: Augusto JC, Corchado JM, Novais P, Analide C, editors. Ambient Intelligence and Future Trends-International Symposium on Ambient Intelligence (ISAmI 2010). Advances in Soft Computing, vol 72. Berlin, Heidelberg: Springer; 2010.. https://doi.org/10.1007/978-3-642-13268-1_2 .
Lu T-C, Fu C-M, Ma MH-M, Fang C-C, Turner AM. Healthcare applications of smart watches. Appl Clin Inform. 2016;7(03):850–69.
Hataji O, Nishii Y, Ito K, Sakaguchi T, Saiki H, Suzuki Y, D'Alessandro-Gabazza C, Fujimoto H, Kobayashi T, Gabazza EC. Smart watch-based coaching with tiotropium and olodaterol ameliorates physical activity in patients with chronic obstructive pulmonary disease. Exp Therapeutic Med. 2017;14(5):4061–4.
Wile DJ, Ranawaya R, Kiss ZH. Smart watch accelerometry for analysis and diagnosis of tremor. J Neurosci Methods. 2014;230:1–4.
Grym K, Niela-Vilén H, Ekholm E, Hamari L, Azimi I, Rahmani A, Liljeberg P, Löyttyniemi E, Axelin A. Feasibility of smart wristbands for continuous monitoring during pregnancy and one month after birth. BMC Pregnancy Childbirth. 2019;19(1):34.
Dawadi PN, Cook DJ, Schmitter-Edgecombe M. Automated cognitive health assessment from smart home-based behavior data. IEEE J Biomed Health Inform. 2015;20(4):1188–94.
Pham M, Mengistu Y, Do H, Sheng W. Delivering home healthcare through a cloud-based smart home environment (CoSHE). Futur Gener Comput Syst. 2018;81:129–40.
Ghasemi F, Rezaee A, Rahmani AM. Structural and behavioral reference model for IoT-based elderly health-care systems in smart home. Int J Commun Syst. 2019;32(12):e4002.
Alberdi A, Weakley A, Schmitter-Edgecombe M, Cook DJ, Aztiria A, Basarab A, Barrenechea M. Smart home-based prediction of multidomain symptoms related to Alzheimer's disease. IEEE J Biomed Health Inform. 2018;22(6):1720–31.
Zhang W, Yang J, Su H, Kumar M, Mao Y. Medical data fusion algorithm based on internet of things. Pers Ubiquit Comput. 2018;22(5–6):895–902.
Hossain MS, Muhammad G. Cloud-assisted industrial internet of things (iiot)–enabled framework for health monitoring. Comput Netw. 2016;101:192–202.
Farahani B, Firouzi F, Chang V, Badaroglu M, Constant N, Mankodiya K. Towards fog-driven IoT eHealth: promises and challenges of IoT in medicine and healthcare. Futur Gener Comput Syst. 2018;78:659–76.
Qiu J-P, Dong K, Yu H-Q. Comparative study on structure and correlation among author co-occurrence networks in bibliometrics. Scientometrics. 2014;101(2):1345–60.
Garfield E. From the science of science to Scientometrics visualizing the history of science with HistCite software. J Inf Secur. 2009;3(3):173–9.
Google Scholar
Chen C. Searching for intellectual turning points: progressive knowledge domain visualization. Proc Natl Acad Sci. 2004;101(suppl 1):5303–10.
Article CAS PubMed Google Scholar
Emami Z, Hariri N, Khamseh ME, Nooshinfard F. Mapping diabetes research in middle eastern countries during 2007-2013: a scientometric analysis. Med J Islam Repub Iran. 2018;32:84.
PubMed PubMed Central Google Scholar
Gu D, Li J, Li X, Liang C. Visualizing the knowledge structure and evolution of big data research in healthcare informatics. Int J Med Inform. 2017;98:22–32.
Leung XY, Sun J, Bai B. Bibliometrics of social media research: a co-citation and co-word analysis. Int J Hosp Manag. 2017;66:35–45.
Holman D, Lynch R, Reeves A. How do health behaviour interventions take account of social context? A literature trend and co-citation analysis. Health. 2018;22(4):389–410.
Chen C. Science mapping: a systematic review of the literature. J Data Inf Sci. 2017;2(2):1–40.
Gubbi J, Buyya R, Marusic S, Palaniswami M. Internet of things (IoT): a vision, architectural elements, and future directions. Futur Gener Comput Syst. 2013;29(7):1645–60.
Islam SMR, Kwak D, Kabir MH, Hossain M, Kwak K. The internet of things for health care: a comprehensive survey. IEEE Access. 2015;3:678–708.
Patel S, Park H, Bonato P, Chan L, Rodgers M. A review of wearable sensors and systems with application in rehabilitation. J NeuroEng Rehabil. 2012;9(1):21.
Al-Fuqaha A, Guizani M, Mohammadi M, Aledhari M, Ayyash M. Internet of things: a survey on enabling technologies, protocols, and applications. IEEE Commun Surv Tutorials. 2015;17(4):2347–76.
Gu D, Li K, Wang X, Liang C. Visualizing Knowledge Evolution of Emerging Information Technologies in Chronic Diseases Research. In: Chen H, Fang Q, Zeng D, Wu J, editors. Smart Health. ICSH 2018, Wuhan, China. Lecture Notes in Computer Science, vol 10983. Cham: Springer; 2018. https://doi.org/10.1007/978-3-030-03649-2_26 .
Gu D, Li T, Wang X, Yang X, Yu Z. Visualizing the intellectual structure and evolution of electronic health and telemedicine research. Int J Med Inform. 2019;130:103947.
Kuhn Thomas. The Structure of Scientific Revolutions[J]. Philos Books. 1962;4(3):14–16.
Gu D, Li K, Wang X, Li X, Liu F, Jiang L, Zhang F. Discovering and visualizing knowledge evolution of chronic disease research driven by emerging technologies. IEEE Access. 2019;7:72994–3003.
Callon M, Courtial J-P, Turner WA, Bauin S. From translations to problematic networks: an introduction to co-word analysis. Information (International Social Science Council). 1983;22(2):191–235.
Whittaker J. Creativity and conformity in science: titles, keywords and co-word analysis. Soc Stud Sci. 1989;19(3):473–96.
Yan B-N, Lee T-S, Lee T-P. Mapping the intellectual structure of the internet of things (IoT) field (2000–2014): a co-word analysis. Scientometrics. 2015;105(2):1285–300.
Apriliyanti ID, Alon I. Bibliometric analysis of absorptive capacity. Int Bus Rev. 2017;26(5):896–907.
Zhao W, Mao J, Lu K. Ranking themes on co-word networks: exploring the relationships among different metrics. Inf Process Manag. 2018;54(2):203–18.
Gu D, Deng S, Zheng Q, et al. Impacts of case-based health knowledge system in hospital management: the mediating role of group effectiveness. Inf Manag. 2019;56(8):103162.
Download references
Acknowledgements
We would like to thank Fenghong Liu and Sofiya Shaanova for their insightful comments on an earlier version of this manuscript.
The dataset collection and analysis of this research were partially supported by the National Natural Science Foundation of China (NSFC) under grant Nos. 71771077, 71771075 and 72071063, and Fundamental Research Funds for the central universities under grant No. PA2020GDKC0020.
Author information
Xuejie Yang, Xiaoyu Wang, Xingguo Li and Dongxiao Gu contributed equally to this work.
Authors and Affiliations
The School of Management, Hefei University of Technology, 193 Tunxi Road, Hefei, 230009, Anhui, China
Xuejie Yang, Xingguo Li, Dongxiao Gu, Changyong Liang, Kang Li, Gongrang Zhang & Jinhong Zhong
The 1st Affiliated Hospital, Anhui University of Traditional Chinese Medicine, 117 Meishan Road, Hefei, 230031, Anhui, China
Xiaoyu Wang
Key Laboratory of Process Optimization and Intelligent Decision-making of Ministry of Education, Hefei, 230009, Anhui, China
Dongxiao Gu
You can also search for this author in PubMed Google Scholar
Contributions
XJY, XYW and DXG contributed to writing the manuscript. KL and XJY collected, analyzed and interpreted the data. CYL, XGL, GRZ and JHZ revised and improved the manuscript. All authors read and approved the final manuscript.
Corresponding author
Correspondence to Xiaoyu Wang .
Ethics declarations
Ethics approval and consent to participate.
Not applicable.
Consent for publication
Competing interests.
The authors declare that they have no competing interests.
Additional information
Publisher’s note.
Springer Nature remains neutral with regard to jurisdictional claims in published maps and institutional affiliations.
Rights and permissions
Open Access This article is licensed under a Creative Commons Attribution 4.0 International License, which permits use, sharing, adaptation, distribution and reproduction in any medium or format, as long as you give appropriate credit to the original author(s) and the source, provide a link to the Creative Commons licence, and indicate if changes were made. The images or other third party material in this article are included in the article's Creative Commons licence, unless indicated otherwise in a credit line to the material. If material is not included in the article's Creative Commons licence and your intended use is not permitted by statutory regulation or exceeds the permitted use, you will need to obtain permission directly from the copyright holder. To view a copy of this licence, visit http://creativecommons.org/licenses/by/4.0/ . The Creative Commons Public Domain Dedication waiver ( http://creativecommons.org/publicdomain/zero/1.0/ ) applies to the data made available in this article, unless otherwise stated in a credit line to the data.
Reprints and permissions
About this article
Cite this article.
Yang, X., Wang, X., Li, X. et al. Exploring emerging IoT technologies in smart health research: a knowledge graph analysis. BMC Med Inform Decis Mak 20 , 260 (2020). https://doi.org/10.1186/s12911-020-01278-9
Download citation
Received : 24 September 2018
Accepted : 30 September 2020
Published : 08 October 2020
DOI : https://doi.org/10.1186/s12911-020-01278-9
Share this article
Anyone you share the following link with will be able to read this content:
Sorry, a shareable link is not currently available for this article.
Provided by the Springer Nature SharedIt content-sharing initiative
- Bibliometrics
- Visual analysis
BMC Medical Informatics and Decision Making
ISSN: 1472-6947
- General enquiries: [email protected]
Internet of Things and its Applications in Healthcare-A Survey
Ieee account.
- Change Username/Password
- Update Address
Purchase Details
- Payment Options
- Order History
- View Purchased Documents
Profile Information
- Communications Preferences
- Profession and Education
- Technical Interests
- US & Canada: +1 800 678 4333
- Worldwide: +1 732 981 0060
- Contact & Support
- About IEEE Xplore
- Accessibility
- Terms of Use
- Nondiscrimination Policy
- Privacy & Opting Out of Cookies
A not-for-profit organization, IEEE is the world's largest technical professional organization dedicated to advancing technology for the benefit of humanity. © Copyright 2024 IEEE - All rights reserved. Use of this web site signifies your agreement to the terms and conditions.

Cost of living help and a future made in Australia
Strengthening medicare and the care economy.
Building a better health system than improves outcomes
Print or save page
On this page
High‑quality health services through Medicare
Boosting access to essential health services
Building a better healthcare system
The Government is investing $2.8 billion to continue its commitment to strengthen Medicare. This includes the $1.2 billion package to address pressures facing the health system, which provides:
- $882.2 million to support older Australians avoid hospital admission, be discharged from hospital earlier and improve their transition out of hospital to other appropriate care.
- $227 million to deliver a further 29 Medicare Urgent Care Clinics and boost support for regional and remote clinics. This will increase the total number of clinics across Australia to 87. Since commencing last year, existing clinics have already provided almost 400,000 bulk‑billed visits.
- $90 million to address health workforce shortages by making it simpler and quicker for international health practitioners to work in Australia.
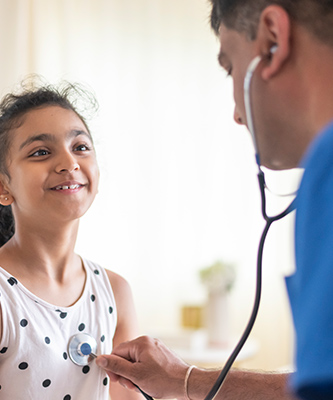
Rohan’s daughter Zoya has been off school with a runny nose and a cough. By 6pm, she is lethargic and has a fever.
Rohan is concerned because his regular GP is now closed. Instead of waiting for hours at the emergency department, he takes Zoya to a Medicare Urgent Care Clinic, without having to make an appointment.
During the bulk billed visit, Zoya is diagnosed with an infection by the doctor and prescribed appropriate medication. Rohan and Zoya leave within an hour of arrival. Zoya makes a full recovery.
Improving health outcomes
Almost half of Australians live with a chronic condition. This Budget will provide $141.1 million for research and services for people living with chronic conditions, including bowel and skin cancer, diabetes and dementia.
To improve health outcomes, the Government is providing:
- Support for Australians to enjoy healthier, more active lives by investing $132.7 million in sport participation and performance programs.
- $825.7 million to ensure Australians can continue to access testing for and vaccinations against COVID‑19. The Government is also ensuring continued access to oral antiviral medicines on the Pharmaceutical Benefits Scheme.
- $41.6 million over two years to continue funding for alcohol and other drug treatment and support services, including the Good Sports alcohol management program for community sporting clubs.
The Government is allocating an additional $411.6 million (for a total $1.6 billion over 13 years) through the Medical Research Future Fund to continue existing research and introduce two new research missions for low‑survival cancers and reducing health inequities.
Improving access to medicines
The Government is investing $3.4 billion for new and amended listings to the Pharmaceutical Benefits Scheme, which means eligible patients can save on treatment costs.
By expanding the Closing the Gap Pharmaceutical Benefits Scheme Co‑payment Program, eligible First Nations patients will have free or cheaper access to all Pharmaceutical Benefits Scheme medicines.
Australians will benefit from $141.1 million to support and expand the National Immunisation Program.
Mental health support
The Government’s $888.1 million mental health package over eight years will help people get the care they need, while relieving pressure on the Better Access initiative and making it easier to access services.
A free, low‑intensity digital service will be established to address the gap for people with mild mental health concerns. From 1 January 2026, Australians will be able to access the service without a referral and receive timely, high‑quality mental health support. Once fully established, 150,000 people are expected to make use of this service each year.
The Government is improving access to free mental health services through a network of walk‑in Medicare Mental Health Centres, built on the established Head to Health network. The upgraded national network of 61 Medicare Mental Health Centres will open by 30 June 2026. They will provide clinical services for adults with moderate‑to‑severe mental health needs.
For Australians with complex mental health needs, funding will be provided for Primary Health Networks to partner with GPs to deliver multidisciplinary, wraparound support services and care coordination.
Improving the aged care system
Providing quality care
The Budget provides $2.2 billion to deliver aged care reforms and continue implementing recommendations from the Royal Commission into Aged Care Quality and Safety.
The new Aged Care Act will put the rights and needs of older people at the centre of the aged care system. The new Act will provide the framework for fundamental change within the aged care sector.
More Home Care Packages
The Government is investing $531.4 million to release an additional 24,100 Home Care Packages in 2024–25. This will help reduce average wait times and enable people to age at home if they prefer to do so.
Improving aged care regulation
Funding of $110.9 million over four years will support an increase in the Aged Care Quality and Safety Commission’s regulatory capabilities.
The Government is investing $1.2 billion in critical digital systems to support the introduction of the new Aged Care Act and contemporary IT systems.
The My Aged Care Contact Centre will receive $37 million to reduce call‑waiting times for people seeking information and access to aged care.
Higher wages for aged care workers
The Government has committed to fund the Fair Work Commission decision to increase the award wage for direct and indirect aged care workers once the final determination is made. This will build on the $11.3 billion already allocated to support the interim 15 per cent wage increase for aged care workers.
The Government is providing $87.2 million for workforce initiatives to attract nurses and other workers into aged care.
Reforming the disability sector
Better and more sustainable services
Getting the National Disability Insurance Scheme (NDIS) back on track
A further $468.7 million is being provided to support people with disability and get the NDIS back on track. This includes:
- $214 million over two years to fight fraud and to co‑design NDIS reforms with people with disability, announced earlier this year
- $160.7 million to upgrade the NDIS Quality and Safeguards Commission’s information technology
- $45.5 million to establish a NDIS Evidence Advisory Committee
- $20 million to start consultation and design on reforms to help NDIS participants and people with disability navigate services.
This builds on $732.9 million provided in the 2023–24 Budget.
In December 2023, National Cabinet agreed to work together to improve the experience of participants and restore the original intent of the Scheme to support people with permanent and significant disability, within a broader ecosystem of supports. This builds on an earlier decision by National Cabinet to ensure Scheme sustainability and achieve an 8 per cent growth target by 1 July 2026, with further moderation as the NDIS matures.
Improving employment for people with disability
A $227.6 million investment will support a new specialised disability employment program to replace the existing Disability Employment Services program by 1 July 2025. This includes a modern digital platform for providers and participants. These reforms will support more people with disability into sustainable work, through a program with greater flexibility, increased individual supports, and better service quality. Eligibility will be expanded to include volunteers outside the income support system and those with less than eight hours per week work capacity.
Delivering essential services
Investing in reliability and security
Strengthening resourcing for Services Australia
The Government is delivering safer and more efficient government services for all Australians.
A $1.8 billion provision will support delivery of customer and payment services. This includes funding for frontline and service delivery staff to manage claims, respond to natural disasters and improve the cyber security environment. The Government is providing $314.1 million over two years to strengthen safety and security at Services Australia centres.
The Government is investing $580.3 million over four years and $139.6 million per year ongoing to sustain the myGov platform and identify potential enhancements. A further $50 million will improve usability, safety and security of the myGov platform and ensure Services Australia can support people to protect their information and privacy.
Strengthening the Australian Taxation Office (ATO) against fraud
There will be $187.4 million to better protect taxpayer data and Commonwealth revenue against fraudulent attacks on the tax and superannuation systems. Funding will upgrade the ATO’s information and communications technologies and increase fraud prevention capabilities to manage increasing risk, prevent revenue loss, and support victims of fraud and cyber crime.
Looking after our veterans
Veterans’ claims processing is prioritised with an additional $186 million for staffing resources and $8.4 million to improve case management and protect against cyber risk. The Government will provide $222 million to harmonise veterans’ compensation and rehabilitation legislation.
A further $48.4 million will be available for Veterans’ Home Care and Community Nursing programs and $10.2 million to provide access to funded medical treatment for ill and injured veterans while their claims for liability are processed.
Back to top

A .gov website belongs to an official government organization in the United States.
A lock ( ) or https:// means you've safely connected to the .gov website. Share sensitive information only on official, secure websites.
- Handwashing
- Hand Hygiene as a Family Activity
- Hand Hygiene FAQs
- Handwashing Facts
- Publications, Data, & Statistics
- Health Promotion Materials
- Global Handwashing Day
- Life is Better with Clean Hands Campaign
- Clinical Safety
- Healthcare Training
- Clean Hands Count Materials
About Handwashing
- Many diseases and conditions are spread by not washing hands with soap and clean, running water.
- Handwashing with soap is one of the best ways to stay healthy.
- If soap and water are not readily available, use a hand sanitizer with at least 60% alcohol to clean your hands.
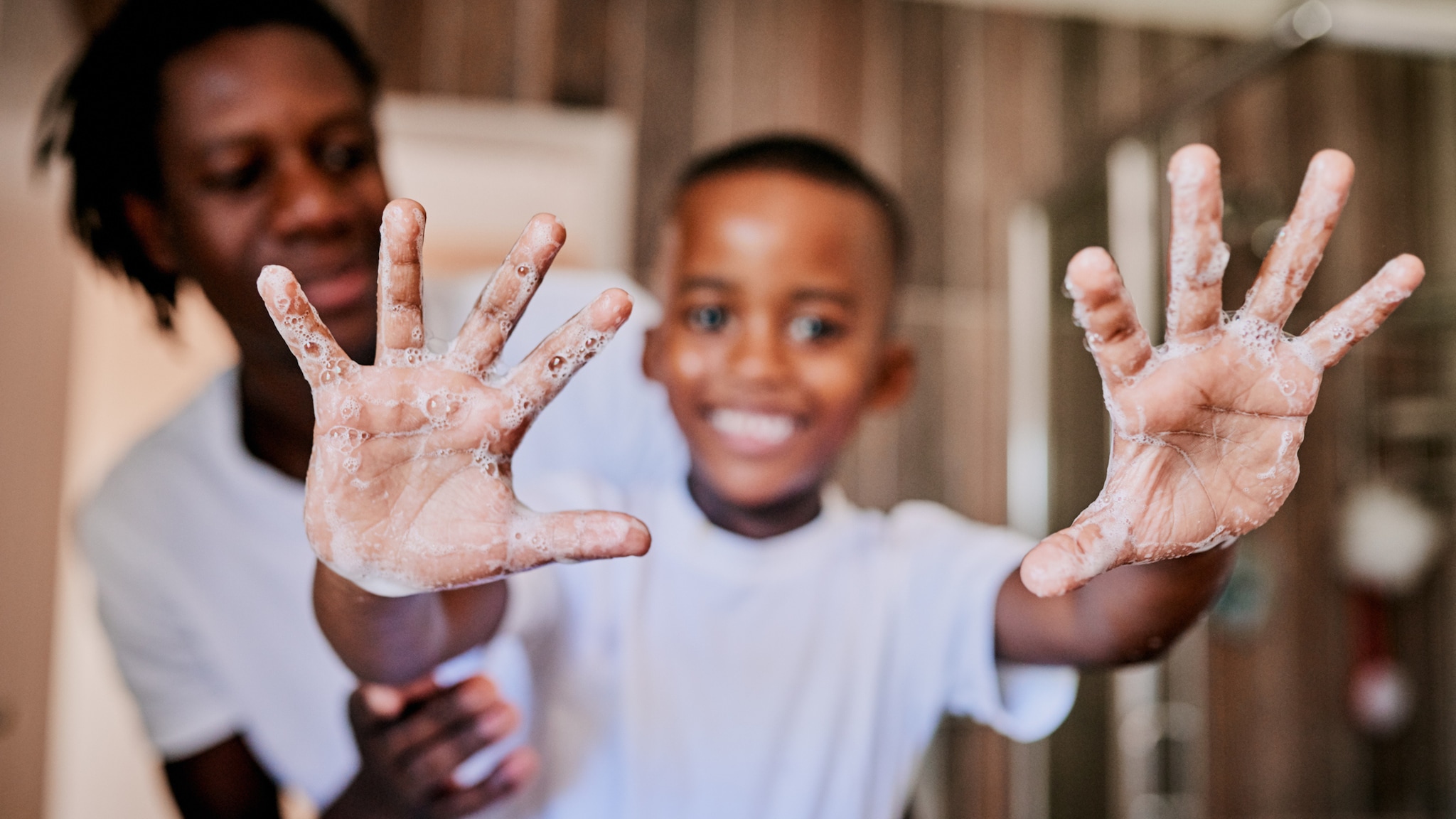
Why it's important
Washing hands can keep you healthy and prevent the spread of respiratory and diarrheal infections. Germs can spread from person to person or from surfaces to people when you:
- Touch your eyes, nose, and mouth with unwashed hands
- Prepare or eat food and drinks with unwashed hands
- Touch surfaces or objects that have germs on them
- Blow your nose, cough, or sneeze into hands and then touch other people's hands or common objects
Key times to wash hands
You can help yourself and your loved ones stay healthy by washing your hands often, especially during these key times when you are likely to get and spread germs:
- Before, during, and after preparing food
- Before and after eating food
- Before and after caring for someone at home who is sick with vomiting or diarrhea
- Before and after treating a cut or wound
- After using the toilet
- After changing diapers or cleaning up a child who has used the toilet
- After blowing your nose, coughing, or sneezing
- After touching an animal, animal feed, or animal waste
- After handling pet food or pet treats
- After touching garbage
How it works
Washing your hands is easy, and it’s one of the most effective ways to prevent the spread of germs. Follow these five steps every time.
- Wet your hands with clean, running water (warm or cold), turn off the tap, and apply soap.
- Lather your hands by rubbing them together with the soap. Lather the backs of your hands, between your fingers, and under your nails.
- Scrub your hands for at least 20 seconds . Need a timer? Hum the “Happy Birthday” song from beginning to end twice.
- Rinse your hands well under clean, running water.
- Dry your hands using a clean towel or an air dryer.
Use hand sanitizer when you can't use soap and water
Washing hands with soap and water is the best way to get rid of germs in most situations. If soap and water are not readily available, you can use an alcohol-based hand sanitizer that contains at least 60% alcohol. You can tell if the sanitizer contains at least 60% alcohol by looking at the product label.
What you can do
CDC has health promotion materials to encourage kids and adults to make handwashing part of their everyday lives.
- Share social media graphics and messages.
- Print stickers and place clings on bathroom mirrors.
- Promote handwashing on or around Global Handwashing Day , celebrated each year on October 15.
- Distribute fact sheets to share information about hand hygiene for specific audiences.
- Frequent Questions About Hand Hygiene
- Hand Hygiene in Healthcare Settings
- The Life is Better with Clean Hands Campaign
Clean Hands
Having clean hands is one of the best ways to avoid getting sick and prevent the spread of germs to others.
For Everyone
Health care providers.
- Introduction
- Article Information
Results are from logistic regression models controlling for age, Hispanic or Latina/x ethnicity, marital status, parity, tobacco use, prenatal visit utilization, stillbirth, and placental abruption. Other race includes Alaska Native, American Indian, Chinese, Filipino, Guam/Chamorro Hawaiian, Indian, Japanese, Korean, Other Asian/Pacific Islander, Samoan, and Vietnamese. In the sample, 4100 patients had a history of substance use, and 33 760 had no history of substance use; 4636 had a urine toxicology test, and 2199 had any positive test result at labor and delivery. Error bars indicate 95% CIs.
Data Sharing Statement
See More About
Select your interests.
- Academic Medicine
- Acid Base, Electrolytes, Fluids
- Allergy and Clinical Immunology
- American Indian or Alaska Natives
- Anesthesiology
- Anticoagulation
- Art and Images in Psychiatry
- Artificial Intelligence
- Assisted Reproduction
- Bleeding and Transfusion
- Caring for the Critically Ill Patient
- Challenges in Clinical Electrocardiography
- Climate and Health
- Climate Change
- Clinical Challenge
- Clinical Decision Support
- Clinical Implications of Basic Neuroscience
- Clinical Pharmacy and Pharmacology
- Complementary and Alternative Medicine
- Consensus Statements
- Coronavirus (COVID-19)
- Critical Care Medicine
- Cultural Competency
- Dental Medicine
- Dermatology
- Diabetes and Endocrinology
- Diagnostic Test Interpretation
- Drug Development
- Electronic Health Records
- Emergency Medicine
- End of Life, Hospice, Palliative Care
- Environmental Health
- Equity, Diversity, and Inclusion
- Facial Plastic Surgery
- Gastroenterology and Hepatology
- Genetics and Genomics
- Genomics and Precision Health
- Global Health
- Guide to Statistics and Methods
- Hair Disorders
- Health Care Delivery Models
- Health Care Economics, Insurance, Payment
- Health Care Quality
- Health Care Reform
- Health Care Safety
- Health Care Workforce
- Health Disparities
- Health Inequities
- Health Policy
- Health Systems Science
- History of Medicine
- Hypertension
- Images in Neurology
- Implementation Science
- Infectious Diseases
- Innovations in Health Care Delivery
- JAMA Infographic
- Law and Medicine
- Leading Change
- Less is More
- LGBTQIA Medicine
- Lifestyle Behaviors
- Medical Coding
- Medical Devices and Equipment
- Medical Education
- Medical Education and Training
- Medical Journals and Publishing
- Mobile Health and Telemedicine
- Narrative Medicine
- Neuroscience and Psychiatry
- Notable Notes
- Nutrition, Obesity, Exercise
- Obstetrics and Gynecology
- Occupational Health
- Ophthalmology
- Orthopedics
- Otolaryngology
- Pain Medicine
- Palliative Care
- Pathology and Laboratory Medicine
- Patient Care
- Patient Information
- Performance Improvement
- Performance Measures
- Perioperative Care and Consultation
- Pharmacoeconomics
- Pharmacoepidemiology
- Pharmacogenetics
- Pharmacy and Clinical Pharmacology
- Physical Medicine and Rehabilitation
- Physical Therapy
- Physician Leadership
- Population Health
- Primary Care
- Professional Well-being
- Professionalism
- Psychiatry and Behavioral Health
- Public Health
- Pulmonary Medicine
- Regulatory Agencies
- Reproductive Health
- Research, Methods, Statistics
- Resuscitation
- Rheumatology
- Risk Management
- Scientific Discovery and the Future of Medicine
- Shared Decision Making and Communication
- Sleep Medicine
- Sports Medicine
- Stem Cell Transplantation
- Substance Use and Addiction Medicine
- Surgical Innovation
- Surgical Pearls
- Teachable Moment
- Technology and Finance
- The Art of JAMA
- The Arts and Medicine
- The Rational Clinical Examination
- Tobacco and e-Cigarettes
- Translational Medicine
- Trauma and Injury
- Treatment Adherence
- Ultrasonography
- Users' Guide to the Medical Literature
- Vaccination
- Venous Thromboembolism
- Veterans Health
- Women's Health
- Workflow and Process
- Wound Care, Infection, Healing
Others Also Liked
- Download PDF
- X Facebook More LinkedIn
Jarlenski M , Shroff J , Terplan M , Roberts SCM , Brown-Podgorski B , Krans EE. Association of Race With Urine Toxicology Testing Among Pregnant Patients During Labor and Delivery. JAMA Health Forum. 2023;4(4):e230441. doi:10.1001/jamahealthforum.2023.0441
Manage citations:
© 2024
- Permissions
Association of Race With Urine Toxicology Testing Among Pregnant Patients During Labor and Delivery
- 1 Department of Health Policy and Management, University of Pittsburgh School of Public Health, Pittsburgh, Pennsylvania
- 2 Friends Research Institute, Baltimore, Maryland
- 3 Department of Obstetrics, Gynecology, and Reproductive Sciences, University of California, San Francisco
- 4 Department of Obstetrics, Gynecology & Reproductive Sciences, University of Pittsburgh School of Medicine, Pittsburgh, Pennsylvania
- 5 Magee-Womens Research Institute, Pittsburgh, Pennsylvania
An estimated 16% of pregnant persons in the US use alcohol (10%) or an illicit substance (6%, including cannabis). 1 Urine toxicology testing (UTT) is often performed at the time of labor and delivery for pregnant patients to evaluate substance use. 2 , 3 We sought to elucidate associations between race and receipt of UTT and a positive test result among pregnant patients admitted to the hospital for delivery.
This cohort study followed the STROBE reporting guideline. Data were extracted from electronic medical records (EMRs) of patients with a live or stillbirth delivery between March 2018 and June 2021 in a large health care system in Pennsylvania. The study was approved by the University of Pittsburgh institutional review board. Informed consent was waived because the research constituted minimal risk. All patients presenting for delivery were verbally screened for substance use using questions adapted from the National Institute on Drug Abuse Quick Screen. 4 Policy specified UTT would be performed for those with a positive screen result, history of substance use in the year prior to delivery, few prenatal visits, or abruption or stillbirth without a clear medical explanation.
We studied 2 binary outcomes: the receipt of UTT (point of care presumptive testing) and a positive test result at delivery. The primary variable of interest, patient race, was conceptualized as a social construct that could manifest in biased or discriminatory delivery of health care. Self-reported race was categorized as Black, White, and other (Alaska Native, American Indian, Chinese, Filipino, Guam/Chamorro Hawaiian, Indian, Japanese, Korean, Other Asian/Pacific Islander, Samoan, and Vietnamese). Substance use history was defined as having a diagnosis of an alcohol, cannabis, opioid, or stimulant use or disorder during pregnancy in the EMR within 1 year prior through delivery. A positive UTT result was defined as at least 1 positive result of a test component, including amphetamines, barbiturates, benzodiazepines, buprenorphine, cocaine, cannabis, methadone, opiates, or phencyclidine. We used multivariable logistic regression models including race and substance use history, adjusting for age, Hispanic or Latina/x ethnicity, marital status, parity, tobacco use, prenatal visit utilization, stillbirth, and placental abruption. We derived mean predicted probabilities of outcomes by race and substance use history. 5 Analyses were conducted using Stata, version 17.
Among 37 860 patients (100% female; mean [SD] age, 29.8 [5.5] years), 16% Black, 76% were White, and 8% were other race ( Table ). Overall, 11% had a history of substance use; opioid use was more common among White patients (40% of all substance use), whereas cannabis use was most common among Black patients (86% of all substance use). The mean predicted probability of having a UTT at delivery was highest among Black patients compared with White patients and other racial groups regardless of history of substance use ( Figure ). For Black patients without a history of substance use, the mean predicted probability of receiving a UTT at delivery was 6.9% (95% CI, 6.4%-7.4%) vs 4.7% (95% CI, 4.4%-4.9%) among White patients. Among Black patients with a history of substance use, the mean predicted probability of receiving a UTT at delivery was 76.4% (95% CI, 74.8%-78.0%) vs 68.7% (95% CI, 67.3%-70.1%) among White patients. In contrast, among those with a history of substance use, the mean predicted probability of having a positive test result was 66.7% (95% CI, 64.8%-68.7%) among White patients and 58.3% (95% CI, 55.5%-61.1%) among Black patients.
In this cohort study, Black patients, regardless of history of substance use, had a greater probability of receiving a UTT at delivery compared with White patients and other racial groups. However, Black patients did not have a higher probability of a positive test result than other racial groups. Limitations of the study include a lack of a sufficient sample size to investigate other racial and ethnic minoritized groups, such as Alaska Native and American Indian patients, and that data were from a single geographical area and may not generalize nationally. To address racial biases, health care systems should examine drug testing practices and adhere to evidence-based practices.
Accepted for Publication: February 4, 2023.
Published: April 14, 2023. doi:10.1001/jamahealthforum.2023.0441
Open Access: This is an open access article distributed under the terms of the CC-BY License . © 2023 Jarlenski M et al. JAMA Health Forum .
Corresponding Author: Marian Jarlenski, PhD, MPH, University of Pittsburgh School of Public Health, 130 DeSoto St, A619, Pittsburgh, PA 15261 ( [email protected] ).
Author Contributions: Dr Jarlenski and Mr Shroff had full access to all of the data in the study and take responsibility for the integrity of the data and the accuracy of the data analysis.
Concept and design: Jarlenski, Terplan, Krans.
Acquisition, analysis, or interpretation of data: All authors.
Drafting of the manuscript: Jarlenski, Krans.
Critical revision of the manuscript for important intellectual content: All authors.
Statistical analysis: Shroff, Terplan, Brown-Podgorski, Krans.
Obtained funding: Jarlenski, Krans.
Administrative, technical, or material support: Krans.
Supervision: Jarlenski, Krans.
Conflict of Interest Disclosures: Dr Roberts reported receiving grants from the Foundation for Opioid Response Efforts and the University of California, San Francisco CSF Bixby Center for Global Reproductive Health and National Center of Excellence in Women's Health outside the submitted work. Dr Krans reported receiving grants from the National Institutes of Health, Merck, and Gilead outside the submitted work. No other disclosures were reported.
Funding/Support: This work was supported by grant R01DA049759 from the National Institute on Drug Abuse (Dr Jarlenski and Krans).
Role of the Funder/Sponsor: The National Institute on Drug Abuse had no role in the design and conduct of the study; collection, management, analysis, and interpretation of the data; preparation, review, or approval of the manuscript; and decision to submit the manuscript for publication.
Data Sharing Statement: See Supplement .
- IEEE CS Standards
- Career Center
- Subscribe to Newsletter
- IEEE Standards
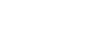
- For Industry Professionals
- For Students
- Launch a New Career
- Membership FAQ
- Membership FAQs
- Membership Grades
- Special Circumstances
- Discounts & Payments
- Distinguished Contributor Recognition
- Grant Programs
- Find a Local Chapter
- Find a Distinguished Visitor
- Find a Speaker on Early Career Topics
- Technical Communities
- Collabratec (Discussion Forum)
- Start a Chapter
- My Subscriptions
- My Referrals
- Computer Magazine
- ComputingEdge Magazine
- Let us help make your event a success. EXPLORE PLANNING SERVICES
- Events Calendar
- Calls for Papers
- Conference Proceedings
- Conference Highlights
- Top 2024 Conferences
- Conference Sponsorship Options
- Conference Planning Services
- Conference Organizer Resources
- Virtual Conference Guide
- Get a Quote
- CPS Dashboard
- CPS Author FAQ
- CPS Organizer FAQ
- Find the latest in advanced computing research. VISIT THE DIGITAL LIBRARY
- Open Access
- Tech News Blog
- Author Guidelines
- Reviewer Information
- Guest Editor Information
- Editor Information
- Editor-in-Chief Information
- Volunteer Opportunities
- Video Library
- Member Benefits
- Institutional Library Subscriptions
- Advertising and Sponsorship
- Code of Ethics
- Educational Webinars
- Online Education
- Certifications
- Industry Webinars & Whitepapers
- Research Reports
- Bodies of Knowledge
- CS for Industry Professionals
- Resource Library
- Newsletters
- Women in Computing
- Digital Library Access
- Organize a Conference
- Run a Publication
- Become a Distinguished Speaker
- Participate in Standards Activities
- Peer Review Content
- Author Resources
- Publish Open Access
- Society Leadership
- Boards & Committees
- Local Chapters
- Governance Resources
- Conference Publishing Services
- Chapter Resources
- About the Board of Governors
- Board of Governors Members
- Diversity & Inclusion
- Open Volunteer Opportunities
- Award Recipients
- Student Scholarships & Awards
- Nominate an Election Candidate
- Nominate a Colleague
- Corporate Partnerships
- Conference Sponsorships & Exhibits
- Advertising
- Recruitment
- Publications
- Education & Career
CVPR Technical Program Features Presentations on the Latest AI and Computer Vision Research
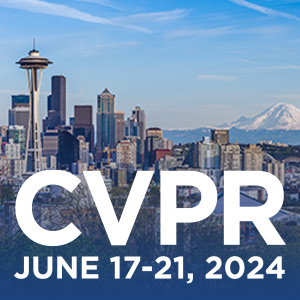
LOS ALAMITOS, Calif., 16 May 2024 – Co-sponsored by the IEEE Computer Society (CS) and the Computer Vision Foundation (CVF), the 2024 Computer Vision and Pattern Recognition (CVPR) Conference is the preeminent event for research and development (R&D) in the hot topic areas of computer vision, artificial intelligence (AI), machine learning (ML), augmented, virtual and mixed reality (AR/VR/MR), deep learning, and related fields. Over the past decade, these areas have seen significant growth, and the emphasis on this sector by the science and engineering community has fueled an increasingly competitive technical program.
This year, the CVPR Program Committee received 11,532 paper submissions—a 26% increase over 2023—but only 2,719 were accepted, resulting in an acceptance rate of just 23.6%. Of those accepted papers, only 3.3% were slotted for oral presentations based on nominations from the area chairs and senior area chairs overseeing the program.
“CVPR is not only the premiere conference in computer vision, but it’s also among the highest-impact publication venues in all of science,” said David Crandall, Professor of Computer Science at Indiana University, Bloomington, Ind., U.S.A., and CVPR 2024 Program Co-Chair. “Having one’s paper accepted to CVPR is already a major achievement, and then having it selected as an oral presentation is a very rare honor that reflects its high quality and potential impact.”
Taking place 17-21 June at the Seattle Convention Center in Seattle, Wash., U.S.A., CVPR offers oral presentations that speak to both fundamental and applied research in areas as diverse as healthcare applications, robotics, consumer electronics, autonomous vehicles, and more. Examples include:
- Pathology: Transcriptomics-guided Slide Representation Learning in Computational Pathology *– Training computer systems for pathology requires a multi-modal approach for efficiency and accuracy. New work from a multi-disciplinary team at Harvard University (Cambridge, Mass., U.S.A.), the Massachusetts Institute of Technology (MIT; Cambridge, Mass., U.S.A.), Emory University (Atlanta, Ga., U.S.A.) and others employs modality-specific encoders, and when applied on liver, breast, and lung samples from two different species, they demonstrated significantly better performance when compared to current baselines.
- Robotics: SceneFun3D: Fine-Grained Functionality and Affordance Understanding in 3D Scenes – Creating realistic interactions in 3D scenes has been troublesome from a technology perspective because it has been difficult to manipulate objects in the scene context. Research from ETH Zürich (Zürich, Switzerland), Google (Mountainview, Calif., U.S.A.), Technical University of Munich (TUM; Munich, Germany), and Microsoft (Redmond, Wash., U.S.A.) has begun bridging that divide by creating a large-scale dataset with more than 14.8k highly accurate interaction annotations for 710 high-resolution real-world 3D indoor scenes. This work, as the paper concludes, has the potential to “stimulate advancements in embodied AI, robotics, and realistic human-scene interaction modeling.”
- Virtual Reality: URHand: Universal Relightable Hands – Teams from Codec Avatars Lab at Meta (Menlo Park, Calif., U.S.A.) and Nanyang Technological University (Singapore) unveil a hand model that generalizes to novel viewpoints, poses, identities, and illuminations, which enables quick personalization from a phone scan. The resulting images make for a more realistic experience of reaching, grabbing, and interacting in a virtual environment.
- Human Avatars: Semantic Human Mesh Reconstruction with Textures – Working to create realistic human models, teams at Nanjing University (Nanjing, China) and Texas A&M University (College Station, Texas, U.S.A.) designed a method of 3-D human mesh reconstruction that is capable of producing high-fidelity and robust semantic renderings that outperform state-of-the-art methods. The paper concludes, “This approach bridges existing monocular reconstruction work and downstream industrial applications, and we believe it can promote the development of human avatars.”
- Text-to-Image Systems: Ranni: Taming Text-to-Image Diffusion for Accurate Instruction – Existing text-to-image models can misinterpret more difficult prompts, but now, new research from Alibaba Group (Hangzhou, Zhejiang, China) and Ant Group (Hangzhou, Zhejiang, China) has made strides in addressing that issue via a middleware layer. This approach, which they have dubbed Ranni, supports the text-to-image generator in better following instructions. As the paper sums up, “Ranni shows potential as a flexible chat-based image creation system, where any existing diffusion model can be incorporated as the generator for interactive generation.”
- Autonomous Driving: Producing and Leveraging Online Map Uncertainty in Trajectory Prediction – To enable autonomous driving, vehicles must be pre-trained on the geographic region and potential pitfalls. High-definition (HD) maps have become a standard part of a vehicle’s technology stack, but current approaches to those maps are siloed in their programming. Now, work from a research team from the University of Toronto (Toronto, Ontario, Canada), Vector Institute (Toronto, Ontario, Canada), NVIDIA Research (Santa Clara, Calif., U.S.A.), and Stanford University (Palo Alto, Calif., U.S.A.) enhances current methodologies by incorporating uncertainty, resulting in up to 50% faster training convergence and up to 15% better prediction performance.
“As the field’s leading event, CVPR introduces the latest research in all areas of computer vision,” said Crandall. “In addition to the oral paper presentations, there will be thousands of posters, dozens of workshops and tutorials, several keynotes and panels, and countless opportunities for learning and networking. You really have to attend the conference to get the full scope of what’s next for computer vision and AI technology.”
Digital copies of all final technical papers* will be available on the conference website by the week of 10 June to allow attendees to prepare their schedules. To register for CVPR 2024 as a member of the press and/or request more on a specific paper, visit https://cvpr.thecvf.com/Conferences/2024/MediaPass or email [email protected]. For more information on the conference, visit https://cvpr.thecvf.com/ .
*Papers linked in this press release refer to pre-print publications. Final, citable papers will be available just prior to the conference.
About the CVPR 2024 The Computer Vision and Pattern Recognition Conference (CVPR) is the preeminent computer vision event for new research in support of artificial intelligence (AI), machine learning (ML), augmented, virtual and mixed reality (AR/VR/MR), deep learning, and much more. Sponsored by the IEEE Computer Society (CS) and the Computer Vision Foundation (CVF), CVPR delivers the important advances in all areas of computer vision and pattern recognition and the various fields and industries they impact. With a first-in-class technical program, including tutorials and workshops, a leading-edge expo, and robust networking opportunities, CVPR, which is annually attended by more than 10,000 scientists and engineers, creates a one-of-a-kind opportunity for networking, recruiting, inspiration, and motivation.
CVPR 2024 takes place 17-21 June at the Seattle Convention Center in Seattle, Wash., U.S.A., and participants may also access sessions virtually. For more information about CVPR 2024, visit cvpr.thecvf.com .
About the Computer Vision Foundation The Computer Vision Foundation (CVF) is a non-profit organization whose purpose is to foster and support research on all aspects of computer vision. Together with the IEEE Computer Society, it co-sponsors the two largest computer vision conferences, CVPR and the International Conference on Computer Vision (ICCV). Visit thecvf.com for more information.
About the IEEE Computer Society Engaging computer engineers, scientists, academia, and industry professionals from all areas and levels of computing, the IEEE Computer Society (CS) serves as the world’s largest and most established professional organization of its type. IEEE CS sets the standard for the education and engagement that fuels continued global technological advancement. Through conferences, publications, and programs that inspire dialogue, debate, and collaboration, IEEE CS empowers, shapes, and guides the future of not only its 375,000+ community members, but the greater industry, enabling new opportunities to better serve our world. Visit computer.org for more information.
Recommended by IEEE Computer Society
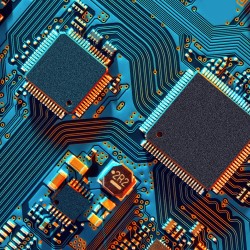
The IEEE International Roadmap for Devices and Systems (IRDS) Emerges as a Global Leader for Chips Acts Visions and Programs
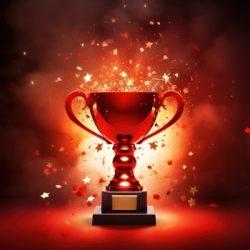
IEEE Computer Society Announces 2024 Class of Fellow
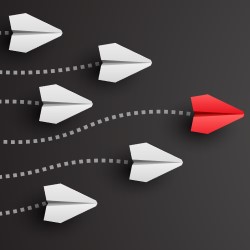
IEEE CS Releases 20 in their 20s List, Identifying Emerging Leaders in Computer Science and Engineering
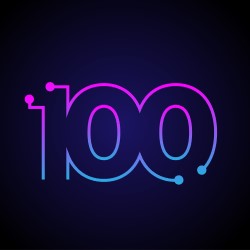
IEEE CS Authors, Speakers, and Leaders Named to Inaugural TIME100 Most Influential People in Artificial Intelligence List
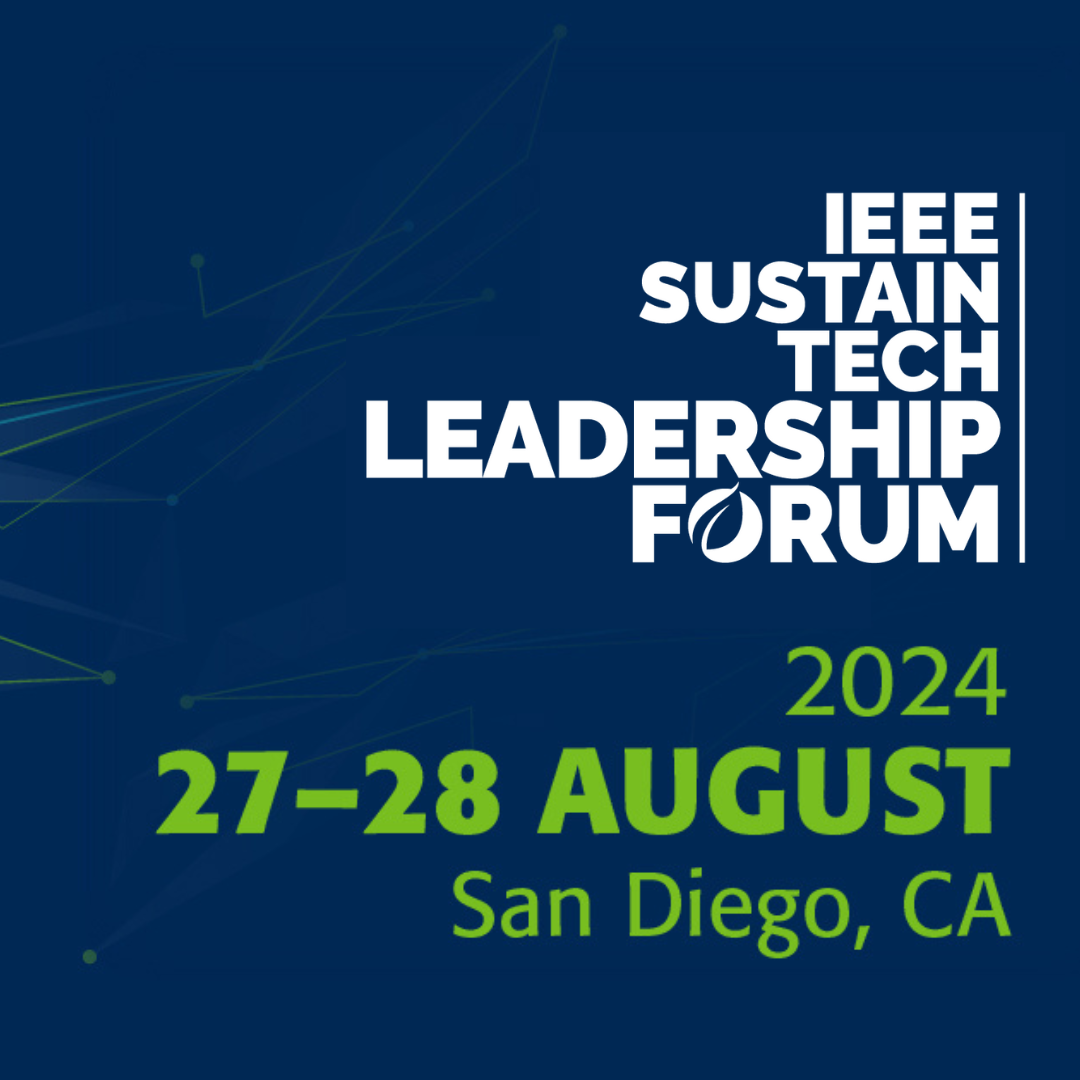
IEEE SustainTech Leadership Forum 2024: Unlocking the Future of Sustainable Technology for Buildings and Factories in the Built Environment
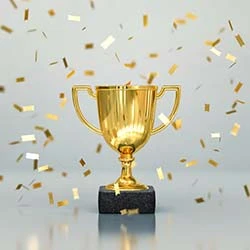
J. Gregory Pauloski and Rohan Basu Roy Named Recipients of 2023 ACM/IEEE CS George Michael Memorial HPC Fellowships
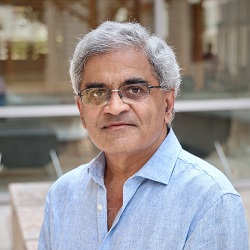
Keshav Pingali Selected to Receive ACM-IEEE CS Ken Kennedy Award
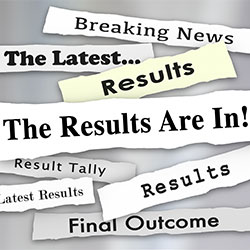
Hironori Washizaki Elected IEEE Computer Society 2025 President
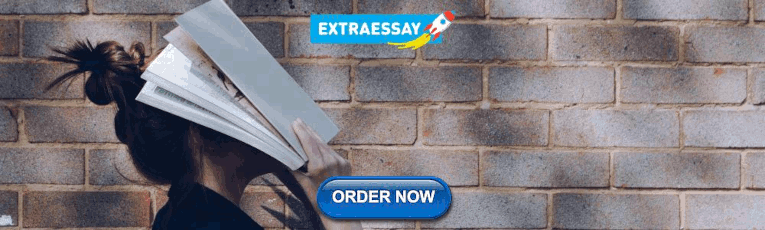
IMAGES
VIDEO
COMMENTS
The Internet of Things (IoT) is a system of wireless, interrelated, and connected digital devices that can collect, send, and store data over a network without requiring human-to-human or human-to-computer interaction. The IoT promises many benefits to streamlining and enhancing health care delivery to proactively predict health issues and ...
Standardization is a key issue limiting progress in this area, and thus this paper proposes a standard model for application in future IoT healthcare systems. This survey paper then presents the state-of-the-art research relating to each area of the model, evaluating their strengths, weaknesses, and overall suitability for a wearable IoT ...
Several academic papers have previously discussed the potential of IoT in healthcare. Traditional literature reviews and systematic literature reviews were used by researchers to summarize IoT research in healthcare, as shown in Table 1.Several aspects of IoT adoption in healthcare have been examined, ranging from exploring the privacy and security issues in IoT-enabling healthcare systems [1 ...
The integration of IoT technology in healthcare has garnered substantial attention, driving extensive research and development efforts. In this comprehensive overview, we summarize key insights, emerging applications, trends, the evolving landscape of IoT in healthcare technologies, security issues, and challenges, drawing from a range of authoritative sources in the field.
Integrating Internet of Things (IoT) technologies in the healthcare industry represents a transformative shift with tangible benefits. This paper provides a detailed examination of IoT adoption in healthcare, focusing on specific sensor types and communication methods. It underscores successful real-world applications, including remote patient ...
The rest of this paper is organized as Fig. 1: Section 2 describes the background, and Section 3 explains the related reviews and surveys in HIoT. Section 4 provides the research methodology, and Section 5 presents a taxonomy of the selected approaches and compares their features. In Section 6, the analysis of the results is discussed.In addition, Section 7 discusses open issues and future trends.
After thoroughly reviewing IoT healthcare-related research articles, the paper's findings shed light on the significance of IoT-enabling technologies in this domain. It emphasizes the importance of wearable sensors, body sensor networks, big data analytics, fog, and cloud computing, pervasive/ubiquitous computing, blockchain, communication ...
5. Identifying useful datasets for IoT healthcare. 6. Reviewing popular algorithms for IoT healthcare data analysis. 7.Identifying the issues and challenges of IoT in healthcare. The paper follows the following roadmap: Sect. 2 discusses materials and methods, Sect.3 presents related work, and Sect. 4 focuses on IoT healthcare enabling ...
The Internet of Things (IoT) is a system of wireless, interrelated, and connected digital devices that can collect, send, and store data over a network without requiring human-to-human or human-to-computer interaction. The IoT promises many benefits to streamlining and enhancing health care delivery to proactively predict health issues and diagnose, treat, and monitor patients both in and out ...
The Internet of Things (IoT) has had a profound impact on the healthcare sector by paving the way for the creation of smart healthcare systems that improve patient monitoring, diagnosis, and individualized care. The purpose of this study is to provide a review of the state of the art in IoT-based healthcare system architectures. In this study, we take a look at the current state of the art of ...
Applications, Selected Cases. and Challenges. Rehab A. Rayan, Christos Tsagkaris, and Romash B. Iryna. 1 Introduction. The Internet of Things (IoT) is a term that has numerous uses, technologies ...
Time distribution map. In order to understand the output of research results in the field of smart health research based on IoT, HistCite was used to statistically analyze the number of scientific literatures in the years from 2003 to 2019, and the trend of annual papers was obtained, as shown in Fig. 1.As can be seen from the figure, from 2003 to 2019, the annual capacity curve presents an ...
Introduction. The Internet of Medical Things (IoMT) is the blend of medical devices with the Internet of Things (IoT). IoMTs are the future of current healthcare systems where every medical device will be connected and monitored over the Internet via healthcare professionals. This offers a faster and lower cost of health care as it evolves.
Success Factors and Challenges of Internet of Things in Healthcare. Our paper review produced six success factors and challenges of IoT in Healthcare. We describe them below: Cost of IoT in Healthcare. While the IoT in healthcare brings many benefits already discussed in this paper, many health care organizations have been slow to adopt it.
This survey paper then presents the state-of-the-art research relating to each area of the model, evaluating their strengths, weaknesses, and overall suitability for a wearable IoT healthcare system.
An Implementation of IoT for Healthcare. Ravi Kishore Kodali, Govinda Swamy and Boppana Lakshmi. Department of Electronic s and Communication En gineering. National Institute of Technology, W ...
Abstract: In this paper, the Internet of Things(IoT) and its applications in the healthcare industry have been discussed. The amalgamation of IoT with healthcare has instigated an increase in the improvement of devices for personalized healthcare management like smart watches, wearable sensors, etc. which have eliminated the need of expensive medical tools, painful procedures and reduced the ...
The Government is allocating an additional $411.6 million (for a total $1.6 billion over 13 years) through the Medical Research Future Fund to continue existing research and introduce two new research missions for low‑survival cancers and reducing health inequities. Improving access to medicines
Most IoT-enabling technologies are general purposed and need to be adapted to comply with the specific requirements of each sector [4].Hence, any technological solution in healthcare should observe requirements such as maximum availability of information resources (to support healthcare anytime), critical security of health information, persistence of large data volume, variability of data and ...
Share information about the importance of handwashing. Why it's important. Washing hands can keep you healthy and prevent the spread of respiratory and diarrheal infections.
Data were extracted from electronic medical records (EMRs) of patients with a live or stillbirth delivery between March 2018 and June 2021 in a large health care system in Pennsylvania. The study was approved by the University of Pittsburgh institutional review board. Informed consent was waived because the research constituted minimal risk.
This year, the CVPR Program Committee received 11,532 paper submissions—a 26% increase over 2023—but only 2,719 were accepted, resulting in an acceptance rate of just 23.6%. Of those accepted papers, only 3.3% were slotted for oral presentations based on nominations from the area chairs and senior area chairs overseeing the program.