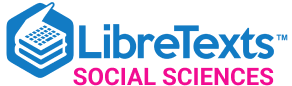
- school Campus Bookshelves
- menu_book Bookshelves
- perm_media Learning Objects
- login Login
- how_to_reg Request Instructor Account
- hub Instructor Commons
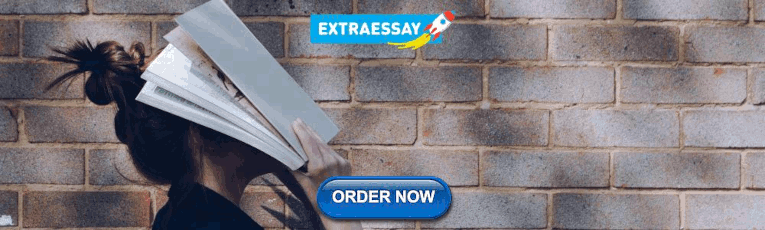
Margin Size
- Download Page (PDF)
- Download Full Book (PDF)
- Periodic Table
- Physics Constants
- Scientific Calculator
- Reference & Cite
- Tools expand_more
- Readability
selected template will load here
This action is not available.
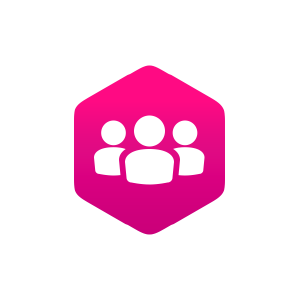
7.3: Types of Reasoning
- Last updated
- Save as PDF
- Page ID 67186
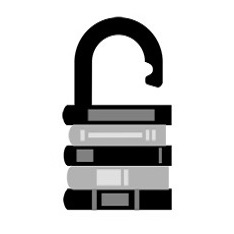
- Jim Marteney
- Los Angeles Valley College via ASCCC Open Educational Resources Initiative (OERI)
\( \newcommand{\vecs}[1]{\overset { \scriptstyle \rightharpoonup} {\mathbf{#1}} } \)
\( \newcommand{\vecd}[1]{\overset{-\!-\!\rightharpoonup}{\vphantom{a}\smash {#1}}} \)
\( \newcommand{\id}{\mathrm{id}}\) \( \newcommand{\Span}{\mathrm{span}}\)
( \newcommand{\kernel}{\mathrm{null}\,}\) \( \newcommand{\range}{\mathrm{range}\,}\)
\( \newcommand{\RealPart}{\mathrm{Re}}\) \( \newcommand{\ImaginaryPart}{\mathrm{Im}}\)
\( \newcommand{\Argument}{\mathrm{Arg}}\) \( \newcommand{\norm}[1]{\| #1 \|}\)
\( \newcommand{\inner}[2]{\langle #1, #2 \rangle}\)
\( \newcommand{\Span}{\mathrm{span}}\)
\( \newcommand{\id}{\mathrm{id}}\)
\( \newcommand{\kernel}{\mathrm{null}\,}\)
\( \newcommand{\range}{\mathrm{range}\,}\)
\( \newcommand{\RealPart}{\mathrm{Re}}\)
\( \newcommand{\ImaginaryPart}{\mathrm{Im}}\)
\( \newcommand{\Argument}{\mathrm{Arg}}\)
\( \newcommand{\norm}[1]{\| #1 \|}\)
\( \newcommand{\Span}{\mathrm{span}}\) \( \newcommand{\AA}{\unicode[.8,0]{x212B}}\)
\( \newcommand{\vectorA}[1]{\vec{#1}} % arrow\)
\( \newcommand{\vectorAt}[1]{\vec{\text{#1}}} % arrow\)
\( \newcommand{\vectorB}[1]{\overset { \scriptstyle \rightharpoonup} {\mathbf{#1}} } \)
\( \newcommand{\vectorC}[1]{\textbf{#1}} \)
\( \newcommand{\vectorD}[1]{\overrightarrow{#1}} \)
\( \newcommand{\vectorDt}[1]{\overrightarrow{\text{#1}}} \)
\( \newcommand{\vectE}[1]{\overset{-\!-\!\rightharpoonup}{\vphantom{a}\smash{\mathbf {#1}}}} \)
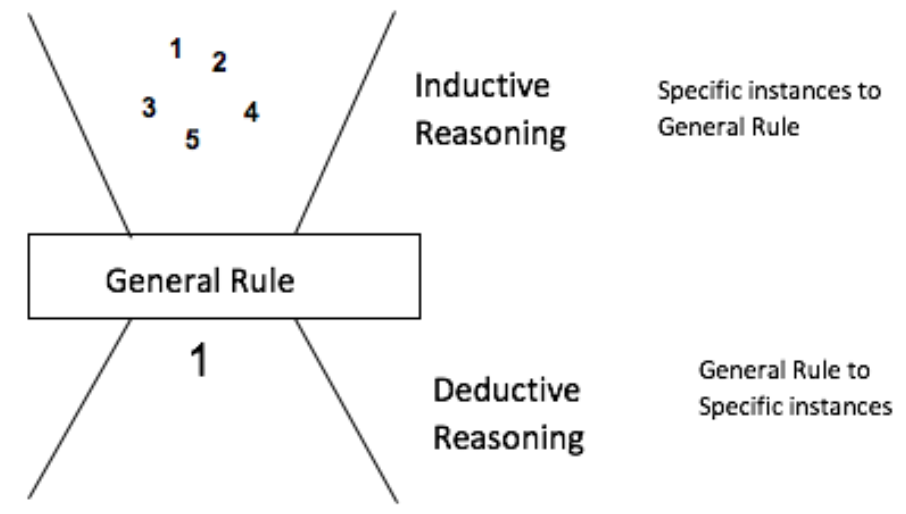
Inductive Reasoning
Inductive reasoning is the process of reasoning from specifics to a general conclusion related to those specifics. You have a series of facts and/or observations. From all of this data you make a conclusion or as the graphic above calls it, a "General Rule." Inductive reasoning allows humans to create generalizations about people, events, and things in their environment. There are five methods of inductive reasoning: example, cause, sign, comparison , and authority .
Example Reasoning
Example reasoning involves using specific instances as a basis for making a valid conclusion. In this approach, specific instances 1, 2, and 3 lead to a generalized conclusion about the whole situation. For example: I have a Sony television, a Sony stereo, a Sony car radio, a Sony video system, and they all work well. It is clear that Sony produces superior electronic products. Or, I have taken four good professors at this college, Mr. Smith, Mrs. Ortiz, Dr. Willard, and Ms. Richard; therefore, I can conclude that the professors at this college are good.
Tests for Reasoning by Example
Some audiences may find one enough, while others may need many more. For instance, the Neilson Ratings that are used to measure the television viewing preferences of 300 million Americans are determined by roughly 3,000 homes scattered throughout the United States. Yet, the television industry, which uses them to set advertising rates, accepts the 3,000 examples as enough to validate the conclusions.
- The examples must be typical of the whole. They must be representative of the topic about which the conclusion is reached, not fringe examples. For example, you come to college and take one English class whose instructor you find disappointing. You conclude that all 300 instructors at this particular college are poor teachers from this one class from this one Department. The sample might not be representative of the whole population of instructors.
- Important counter examples must be accounted for. If the counter examples mitigate against the examples used, the generalization is threatened. What if a good friend of yours also took another English class and was pleased by the experience. He found that his instructor was an excellent teacher. His example becomes a counter one to the specific instance you used to draw your conclusion, which is now very much in doubt.
- The examples must be relevant to the time period of your argument . If you are dealing with something recent, you need recent examples. If you are trying to prove something in the 1850's, examples from that period are appropriate. If you took the English class 30 years ago, it would be difficult to draw a valid conclusion about the nature of teachers at the college today without using recent examples. Likewise, recent examples may not be reflective of the way the college was 30 years ago.
Causal Reasoning
Causal Reasoning is based on the idea that for every action there is a reaction. Stated very simply, a cause is anything that is directly responsible for producing something else, usually termed the effect. There are two forms of causal reasoning:
The goal of causal reasoning is to figure out how or why something happened. For instance, you did well on a test because you studied two days in advance. I could then predict that if you study two days in advance of the next test, you will do well. In causal reasoning, the critical thinker is trying to establish a predictive function between two directly related variables. If we can figure out how and why things occur, we can then try to predict what will happen in the future.
- Cause to effect, a known cause or causes is capable of producing some unknown effect or effects
- Effect to cause, some known effect(s) has/have been produced by some unknown cause or causes.
Tests of Causal Reasoning
- The cause must be capable of producing the effect described, and vice versa. Has causality really been established or is it just coincidence? Is the cause really capable of producing the effect and vice versa? There must be a direct connection between the cause and the effect that can be demonstrated using empirical evidence. For example, many people mistake superstition for causal reasoning. Is the source of good luck the rubbing of a rabbit’s foot? Is the cause of bad luck really the fact that you walked under a ladder or broke the mirror? Did wearing that shirt really cause your team to win five games in a row? The critical thinker must make a clear distinction between a valid causal occurrence and sheer coincidence.
- Cumulative causal reasoning increases the soundness of the conclusion . The more times the causal pattern has happened, the greater the strength given to the causal reasoning, leading to a more valid conclusion. If this is the first time this association has ever been asserted the advocate will have to use more evidence to support the soundness of the causal reasoning advanced.
- Counter causal factors must also be accounted for. The advocate must be aware of the other inherent causal factors that could disrupt the relationship between the cause and effect presented. A claim was made by a father that his son committed suicide, because he was influenced to do so by the songs of a particular rock musician. If we assume that such a causal association exists, we also need to know if there are any other factors that could disrupt the connection: Was the son using drugs; had he tried to commit suicide before; were there family problems; did he listen to other artists and other types of music; did he have peer problems; did he have relationship problems; was he having problems in school, etc.? Each one of these, individually, might be enough to destroy the direct causal relationship that is attempting to be established.
In Massachusetts, Michelle Carter is on trial for manslaughter. As a teenager, she texted her boyfriend, Roy, and encouraged him to commit suicide. And he did. Her defense attorney is arguing that Roy had mental problems, was already suicidal, and that the texts did not cause him to take his life. The prosecution is arguing that the text did cause Roy to kill himself. This is going to be a difficult case to resolve. As stated by Daniel Medwed, a Northeastern University law professor, “ Causation is going to be a vital part of this case, can the prosecution prove that she caused him to kill himself in this way? Would he have done it anyway ?” 1
Sign Reasoning
Sign reasoning involves inferring a connection between two related situations. The theory is that the presence or absence of one indicates the presence or absence of the other. In other words, the presence of an attribute is a signal that something else, the substance, exists. One doesn't cause the other to exist, but instead is a sign that it exists. Football on television is a sign that Fall has arrived. Football on television does not cause Fall to arrive; they just arrive at the same time. A flag is flying at half-staff. is a sign that that there has been a tragedy or a significant person has died. The flag flying at half-staff did not cause the death. It is a sign that the situation occurred.
Sign Reasoning in Poker
Quite a few players' posture betrays the nature of their cards. An unconscious change in their sitting position, such as leaning forward, likely indicates a strong hand. With a weak hand they often show less body tension, for example, having hanging shoulders.
If someone has concealed his mouth with his hand, he often holds a weak hand - he wants to hide his emotions. In a sense, he does not want his expression to betray his hand. The same is true for a player who is reluctant to glance at you: he is worried that his eyes might indicate he is afraid.
Particularly for beginners, a quick glance at his cards is a reliable tell. The tell here is an unconscious one, brief look at the player's own cards. If, for example, the flop brings 3 hearts and the player looks at his cards, it is unlikely he has the flush.
This is because with an off-suit hand, a beginner usually takes no notice of the suits at first glance. Only with a suited hand will they remember the suit. Thus, you can often assume here that they have at most one heart. 2
Tests of Sign Reasoning
- Other substance/attribute relationships must be considered. Is there another substance that might have the same attributes? Could the sending of roses to your wife be a sign of something other than love? Can the same signs indicate the presence of a valid second or third substance?
- Cumulative sign reasoning produces a more probable connection. The more often this substance/attribute relationship occurs, the more likely it is to repeat itself. If this is the first time you have noticed the association, you will need a good deal of evidence to demonstrate that it really is a valid sign argument.
Comparison Reasoning
Comparison reasoning is also known as reasoning by analogy. This type of reasoning involves drawing comparisons between two similar things, and concluding that, because of the similarities involved, what is correct about one is also correct of the other. There was once an ad for alligator meat that presented this comparison; "When you try alligator meat just remember what is considered exotic food today may often become normal fare in the future. This was the case with lobster. About 75 years ago, lobster was thought of as poor man's food; many New Englanders would not even think of eating it. Today, of course, lobster is a delicacy savored by many people." This type of reasoning wants us to conclude that alligator meat is to humans today, as lobster meat was to humans 75 years ago. And since lobster is now a delicacy so will alligator meat. There are two types of comparisons: figurative and literal.
- Literal comparisons attempt to establish a link between similar classifications; cars to cars, states to states, people to people. For instance, you can compare a Ford compact car with a Toyota compact car; the lottery in one state with the lottery in another state; how your parents treat you with how your best friend is treated by her parents. In these comparisons, similar classifications are being used for the purposes of making the analogy. Literal comparisons can provide logical proof for the point being made and thus can increase the validity of the argument.
- Figurative comparisons attempt to link similarities between two cases from different classifications. Jim Baker of the Bush 2000 campaign, argued after the 5-4 Supreme Court decision awarding the state of Florida to Bush, “Saying George W. Bush stole the Presidency from Al Gore is like saying someone tried to steer the Titanic after it had already hit the iceberg.” Figurative comparisons carry no weight in terms of providing logical proof for an argument. They can, however, be very effective for the purpose of illustration and persuading an audience.
The line between a Literal and Figurative analogy is not clear. Instead of a comparison being totally figurative or totally literal, the comparison can be viewed in degrees using the following continuum.
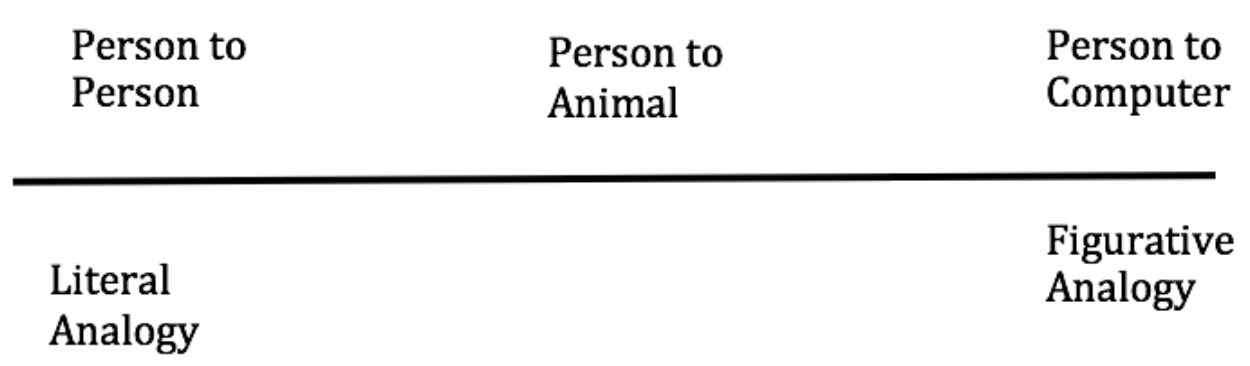
There are few literal comparisons that can be made between a person and a computer. A person to an animal may have some overlapping actual similarities. While comparing one person to another person suggests a Literal Analogy. The more towards the figurative side the comparison is, the less the argument is logically valid. The more towards the literal side the comparison is, the more logically valid the argument is.
Tests for comparison reasoning
- To be considered as proof, the analogy must be a literal one. The further advocates move away from figurative comparisons and toward the literal comparison end of the continuum, the more validity they secure for their argument. Figurative comparisons carry no logical argumentative influence at all.
- The cases need to contain significant points of similarity. The greater the number of important or major similar points between the cases, the easier it is to establish the comparison as a sound one. However, no matter how many points of similarity can be established between the two cases, major points of differences can destroy the analogy.
- Cumulative comparison reasoning will produce a more probable conclusion. The greater the number of cases a person can use for the purpose of comparison, the more valid the comparison. If a student has been to more than one college or has had many instructors, he or she can evaluate the quality of the teachers by comparing them. The validity of his or her conclusion is increased as the number of teachers compared increases.
Children often try to convince a parent to let them do or try something the parent is opposed to by comparing themselves to another child. They point out they are the same age as the other child, they are in the same grade in school, the child lives in the same neighborhood as they do, thus they should be allowed to do what the other child is allowed to do. This seems to be a very effective argument by comparison until the parent says, you are not that child or we are not their parents. To the parents, these points of difference destroy the comparison the child is trying to make.
Poor Figurative Analogy May 23, 2016
(CNN) Veterans Affairs Secretary Bob McDonald downplayed Monday the time it takes for veterans to receive medical treatment by comparing the "experience" of waiting for health care to Disneyland guests waiting for a ride.
"When you go to Disney, do they measure the number of hours you wait in line? Or what's important?" McDonald told reporters at a Christian Science Monitor
breakfast in Washington. "What's important is what's your satisfaction with the experience?"
American Legion National Commander Dale Barnett excoriated McDonald: "The American Legion agrees that the VA secretary's analogy between Disneyland and VA wait times was an unfortunate comparison because people don't die while waiting to go on Space Mountain." 3
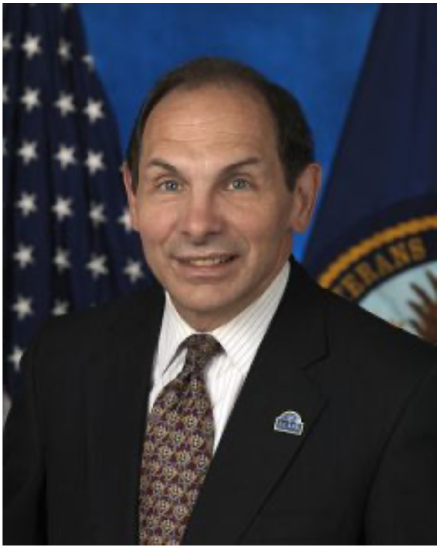
Reasoning from Authority
Reasoning from Authority is used when a person argues that a particular claim is justified, because, it is held or advocated by a credible source. That credible source can be a person or organization. Basically, the authority possesses some credentials that qualify the source as an authority. Thus, you accept the argument because someone you feel is an authority tells you so. You can use this type of argument in two ways. First, you can ask that an argument be accepted simply because someone you consider an authority advocates it. People grant authority status to other people they think have more knowledge than they do: students to teachers, patients to doctors, and clients to lawyers. Children often argue this way when they justify a position by saying “because my mommy or daddy said so.”
Second, you can support your arguments with the credibility of another person. Here you are attempting to transfer the positive ethos from the credible source to the position you are advocating. Advertisers do this when they get popular athletes and entertainers to promote their products. The advertisers are hoping that your positive view of these people will transfer to their product, thus producing higher sales for the products. You may be persuaded to see a particular movie, attend a certain play, or eat at a restaurant because, it was advocated by a well-known critic.
Tests for reasoning from authority
- The authority must be credible . That is, the authority must possess the necessary qualifications for the target audience in order for the source to be used as justification for a point of view. If challenged, the advocate must be prepared to defend the expertise and ethos of his or her authority.
- Views of counter authorities must be taken into account. The advocate must be aware of the other “experts” or highly credible sources who take an opposite position from the one being advocated. If he or she fails to do this, the argument breaks down into a battle over whose expert or authority should be accepted as being the most accurate.
- Cumulative views of authorities increase the validity of the reasoning . Citing more than one expert or authority will increase the likelihood that your position will be viewed as the most valid one being argued.
Important conclusion: Since the process of reasoning by induction usually involves arriving at a conclusion based on a limited sampling, the conclusion to an inductive argument can never be totally certain. Why? Because no matter which type of inductive reasoning is used, nor how carefully critical thinkers adhere to the tests of each reasoning pattern, critical thinkers can never sample the totality of the population used to infer the generalization about that population.
Thus, conclusions drawn from inductive reasoning are always only probable. To use induction effectively, an advocate must demonstrate that the specifics are compelling, and thus justify the conclusion, but never claim that the conclusion is guaranteed in all situations.
Deductive Reasoning
Deductive reasoning is the process of reasoning from general statements, or rules, to a certain, specific, and logical conclusion. Deductive arguments begin with a general statement that has already been arrived at inductively. Unlike inductive reasoning, where the conclusion may be very valid, but is always only probable, the conclusion reached by deductive reasoning is logically certain.
A deductive argument offers two or more premises that lead to a conclusion directly related to those premises. As long as the two premises are sound, there can be no doubt that the final statement is correct. The final statement is a matter of logical certainty.
Deductive arguments are not spoken of as “true” or “false,” but as “sound” or “unsound.” A sound argument is one in which the premises guarantee the conclusion, and an unsound argument is one in which the premises do not guarantee the conclusion.
An advocate who uses deduction to frame an argument must be certain that the general statement is accepted as correct and then must demonstrate the relationship between this general statement and the specific claim, thus proving beyond a doubt the conclusion.
A deductive argument has three parts: a major premise, a minor premise, and a conclusion. This form is called a syllogism.
The major premise is a general statement. For example: All telemarketers are obnoxious . The subject section of the major premise (All telemarketers) is known as the antecedent; the predicate section of the major premise (are obnoxious) is known as the consequent.
The minor premise is a statement of a specific instance related to the major premise:
The person on the phone is a telemarketer.
The conclusion is the statement derived from the minor premises relationship to the major premise: The person on the phone is obnoxious .
An effective deductive argument is one in which your audience accepts the general statement and is then logically compelled by the development of the argument to accept your conclusion.
Thus, we use inductive reasoning to create generalizations or major premises, and we can use deductive reasoning to apply those generalizations to specific situations.
The final step in checking the strength of reasoning is to make sure there are no fallacies. Often, correcting for fallacies is the missing piece to creating and evaluating logical arguments
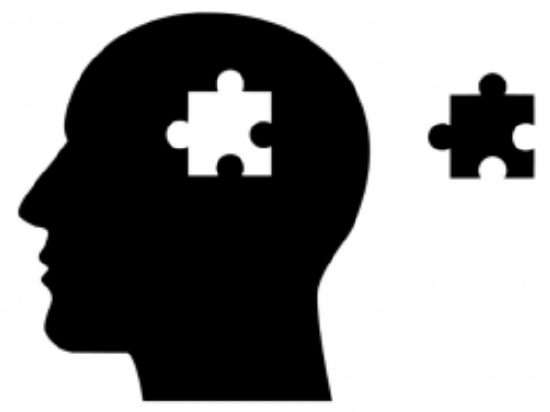
- Associated Press. ''Just do it, babe': Teen's texts to suicidal boyfriend revealed." New York Post , 9 Sept. 2015, https://nypost.com/2015/09/09/teen-c...st-do-it-babe/ . Accessed 6 November 2019.
- "Poker tells - hidden body language. To bluff or not to bluff?" PokerStrategy.com , https://www.pokerstrategy.com/strategy/live-poker/poker-tells-body-language/ . Accessed 6 November 2019.
- Griffin, Drew. "VA Secretary Disneyland-wait time comparison draws ire." CNN , 23 May 2016, https://www.cnn.com/2016/05/23/politics/veterans-affairs-secretary-disneyland-wait-times/index.html . Accessed 6 November 2019.
Thank you for visiting nature.com. You are using a browser version with limited support for CSS. To obtain the best experience, we recommend you use a more up to date browser (or turn off compatibility mode in Internet Explorer). In the meantime, to ensure continued support, we are displaying the site without styles and JavaScript.
- View all journals
- Explore content
- About the journal
- Publish with us
- Sign up for alerts
- Review Article
- Published: 26 April 2024
The development of human causal learning and reasoning
- Mariel K. Goddu ORCID: orcid.org/0000-0003-4969-7948 1 , 2 , 3 &
- Alison Gopnik 4 , 5
Nature Reviews Psychology volume 3 , pages 319–339 ( 2024 ) Cite this article
1592 Accesses
169 Altmetric
Metrics details
- Human behaviour
Causal understanding is a defining characteristic of human cognition. Like many animals, human children learn to control their bodily movements and act effectively in the environment. Like a smaller subset of animals, children intervene: they learn to change the environment in targeted ways. Unlike other animals, children grow into adults with the causal reasoning skills to develop abstract theories, invent sophisticated technologies and imagine alternate pasts, distant futures and fictional worlds. In this Review, we explore the development of human-unique causal learning and reasoning from evolutionary and ontogenetic perspectives. We frame our discussion using an ‘interventionist’ approach. First, we situate causal understanding in relation to cognitive abilities shared with non-human animals. We argue that human causal understanding is distinguished by its depersonalized (objective) and decontextualized (general) representations. Using this framework, we next review empirical findings on early human causal learning and reasoning and consider the naturalistic contexts that support its development. Then we explore connections to related abilities. We conclude with suggestions for ongoing collaboration between developmental, cross-cultural, computational, neural and evolutionary approaches to causal understanding.
This is a preview of subscription content, access via your institution
Access options
Subscribe to this journal
Receive 12 digital issues and online access to articles
55,14 € per year
only 4,60 € per issue
Buy this article
- Purchase on Springer Link
- Instant access to full article PDF
Prices may be subject to local taxes which are calculated during checkout
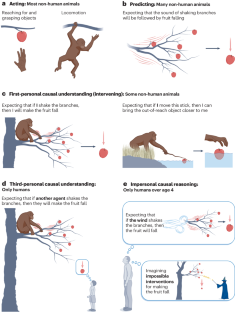
Similar content being viewed by others
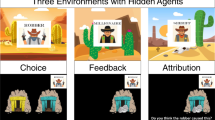
The rational use of causal inference to guide reinforcement learning strengthens with age
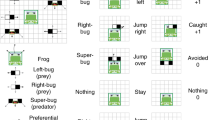
Causal reductionism and causal structures
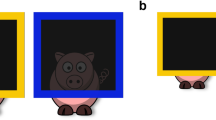
Children transition from simple associations to explicitly reasoned social learning strategies between age four and eight
Prüfer, K. et al. The bonobo genome compared with the chimpanzee and human genomes. Nature 486 , 527–531 (2012).
PubMed PubMed Central Google Scholar
Gopnik, A. & Wellman, H. M. Reconstructing constructivism: causal models, Bayesian learning mechanisms, and the theory theory. Psychol. Bull. 138 , 1085–1108 (2012). This paper provides an introduction to the interventionist approach formalized in terms of causal Bayes nets.
Gopnik, A. & Schulz, L. (eds.) Causal Learning: Psychology, Philosophy and Computation (Oxford Univ. Press, 2007). This edited volume provides an interdisciplinary overview of the interventionist approach.
Pearl, J. Causality (Cambridge Univ. Press, 2009).
Penn, D. C. & Povinelli, D. J. Causal cognition in human and nonhuman animals: a comparative, critical review. Annu. Rev. Psychol. 58 , 97–118 (2007).
PubMed Google Scholar
Schölkopf, B. et al. Toward causal representation learning. Proc. IEEE 109 , 612–634 (2021).
Google Scholar
Spirtes, P., Glymour, C. & Scheines, R. in Causation, Prediction, and Search Lecture Notes in Statistics Vol 81, 103–162 (Springer, 1993).
Gopnik, A. et al. A theory of causal learning in children: causal maps and Bayes nets. Psychol. Rev. 111 , 3–32 (2004). This seminal paper reviews the causal Bayes nets approach to causal reasoning and suggests that children construct mental models that help them understand and predict causal relations.
Pearl, J. & Mackenzie, D. The Book of Why: The New Science of Cause and Effect (Basic Books, 2018). This book provides a high-level overview of approaches to causal inference from theoretical computer science for a general audience.
Lombrozo, T. & Vasil, N. in Oxford Handbook of Causal Reasoning (ed. Waldmann, M.) 415–432 (Oxford Univ. Press, 2017).
Woodward, J. Making Things Happen: A Theory of Causal Explanation (Oxford Univ. Press, 2005). This book outlines the ‘interventionist’ approach to causation and causal explanation in philosophy.
Shanks, D. R. & Dickinson, A. Associative accounts of causality judgment. Psychol. Learn. Motiv. 21 , 229–261 (Elsevier, 1988).
Carey, S. The Origin of Concepts (Oxford Univ. Press, 2009).
Michotte, A. The Perception of Causality (Routledge, 2017).
Leslie, A. M. The perception of causality in infants. Perception 11 , 173–186 (1982). This canonical study shows that 4.5-month-old and 8-month-old infants are sensitive to spatiotemporal event configurations and contingencies that adults also construe as causal.
Leslie, A. M. Spatiotemporal continuity and the perception of causality in infants. Perception 13 , 287–305 (1984).
Danks, D. Unifying the Mind: Cognitive Representations as Graphical Models (MIT Press, 2014).
Godfrey‐Smith, P. in The Oxford Handbook of Causation (eds Beebee, H., Hitchcock, C. & Menzies, P.) 326–338 (Oxford Academic, 2009).
Gweon, H. & Schulz, L. 16-month-olds rationally infer causes of failed actions. Science 332 , 1524–1524 (2011).
Woodward, J. Causation with a Human Face: Normative Theory and Descriptive Psychology (Oxford Univ. Press, 2021). This book integrates philosophical and psychological literatures on causal reasoning to provide both normative and descriptive accounts of causal reasoning.
Rozenblit, L. & Keil, F. The misunderstood limits of folk science: an illusion of explanatory depth. Cogn. Sci . 26 , 521–562 (2002).
Ismael, J. How Physics Makes us Free (Oxford Univ. Press, 2016).
Woodward, J. in Causal Learning: Psychology, Philosophy, and Computation (eds Gopnik, A. & Schulz, L.) Oxford Series in Cognitive Development 19–36 (Oxford Academic, 2007).
Godfrey-Smith, P. Other Minds: The Octopus, the Sea, and the Deep Origins of Consciousness (Farrar, Straus and Giroux, 2016).
Körding, K. P. et al. Causal inference in multisensory perception. PLoS One 2 , e943 (2007).
Wei, K. & Körding, K. P. in Sensory Cue Integration (eds Trommershäuser, J., Kording, K. & Landy, M. S.) Computational Neuroscience Series 30–45 (Oxford Academic, 2011).
Haith, A. M. & Krakauer, J. W. in Progress in Motor Control: Neural, Computational and Dynamic Approaches (eds Richardson, M. J., Riley, M. A. & Shockley, K.) 1–21 (Springer, 2013).
Krakauer, J. W. & Mazzoni, P. Human sensorimotor learning: adaptation, skill, and beyond. Curr. Opin. Neurobiol. 21 , 636–644 (2011).
Adolph, K. E. An ecological approach to learning in (not and) development. Hum. Dev. 63 , 180–201 (2020).
Adolph, K. E., Hoch, J. E. & Ossmy, O. in Perception as Information Detection (eds Wagman, J. B. & Blau, J. J. C.) 222–236 (Routledge, 2019).
Riesen, A. H. The development of visual perception in man and chimpanzee. Science 106 , 107–108 (1947).
Spelke, E. S. What Babies Know: Core Knowledge and Composition Vol. 1 (Oxford Univ. Press, 2022).
Pearce, J. M. & Bouton, M. E. Theories of associative learning in animals. Annu. Rev. Psychol. 52 , 111–139 (2001).
Wasserman, E. A. & Miller, R. R. What’s elementary about associative learning? Annu. Rev. Psychol. 48 , 573–607 (1997).
Sutton, R. S. & Barto, A. G. Reinforcement learning: an introduction. Robotica 17 , 229–235 (1999).
Gershman, S. J., Markman, A. B. & Otto, A. R. Retrospective revaluation in sequential decision making: a tale of two systems. J. Exp. Psychol. Gen. 143 , 182–194 (2014).
Gershman, S. J. Reinforcement learning and causal models. In Oxford Handbook of Causal Reasoning (ed. Waldmann, M.) 295 (Oxford Univ. Press, 2017).
Taylor, A. H. et al. Of babies and birds: complex tool behaviours are not sufficient for the evolution of the ability to create a novel causal intervention. Proc. R. Soc. B 281 , 20140837 (2014). This study shows that complex tool use does not entail the ability to understand and create novel causal interventions: crows do not learn causal interventions from observing the effects of their own accidental behaviours.
Povinelli, D. J. & Penn, D. C. in Tool Use and Causal Cognition (eds McCormack, T., Hoerl, C. & Butterfill, S.) 69–88 (Oxford Univ. Press, 2011).
Povinelli, D. J. & Henley, T. More rope tricks reveal why more task variants will never lead to strong inferences about higher-order causal reasoning in chimpanzees. Anim. Behav. Cogn. 7 , 392–418 (2020).
Tomasello, M. & Call, J. Primate Cognition (Oxford Univ. Press, 1997).
Visalberghi, E. & Tomasello, M. Primate causal understanding in the physical and psychological domains. Behav. Process. 42 , 189–203 (1998).
Völter, C. J., Sentís, I. & Call, J. Great apes and children infer causal relations from patterns of variation and covariation. Cognition 155 , 30–43 (2016).
Tennie, C., Call, J. & Tomasello, M. Untrained chimpanzees ( Pan troglodytes schweinfurthii ) fail to imitate novel actions. Plos One 7 , e41548 (2012).
Whiten, A., Horner, V., Litchfield, C. A. & Marshall-Pescini, S. How do apes ape? Anim. Learn. Behav. 32 , 36–52 (2004).
Moore, R. Imitation and conventional communication. Biol. Phil. 28 , 481–500 (2013).
Meltzoff, A. N. & Marshall, P. J. Human infant imitation as a social survival circuit. Curr. Opin. Behav. Sci. 24 , 130–136 (2018).
Boesch, C. & Boesch, H. Optimisation of nut-cracking with natural hammers by wild chimpanzees. Behaviour 83 , 265–286 (1983).
Chappell, J. & Kacelnik, A. Tool selectivity in a non-primate, the New Caledonian crow ( Corvus moneduloides ). Anim. Cogn. 5 , 71–78 (2002).
Weir, A. A., Chappell, J. & Kacelnik, A. Shaping of hooks in New Caledonian crows. Science 297 , 981–981 (2002).
Wimpenny, J. H., Weir, A. A., Clayton, L., Rutz, C. & Kacelnik, A. Cognitive processes associated with sequential tool use in New Caledonian crows. PLoS One 4 , e6471 (2009).
Manrique, H. M., Gross, A. N.-M. & Call, J. Great apes select tools on the basis of their rigidity. J. Exp. Psychol. Anim. Behav. Process. 36 , 409–422 (2010).
Mulcahy, N. J., Call, J. & Dunbar, R. I. Gorillas ( Gorilla gorilla ) and orangutans ( Pongo pygmaeus ) encode relevant problem features in a tool-using task. J. Comp. Psychol. 119 , 23–32 (2005).
Sanz, C., Call, J. & Morgan, D. Design complexity in termite-fishing tools of chimpanzees ( Pan troglodytes ). Biol. Lett. 5 , 293–296 (2009).
Visalberghi, E. et al. Selection of effective stone tools by wild bearded capuchin monkeys. Curr. Biol. 19 , 213–217 (2009).
Seed, A., Hanus, D. & Call, J. in Tool Use and Causal Cognition (eds McCormack, T., Hoerl, C. & Butterfill, S.) 89–110 (Oxford Univ. Press, 2011).
Völter, C. J. & Call, J. in APA Handbook of Comparative Psychology: Perception, Learning, and Cognition (eds Call, J. et al.) 643–671 (American Psychological Association, 2017). This chapter provides a comprehensive overview of causal and inferential reasoning in non-human animals, highlighting (1) the difference between prediction versus causal knowledge and (2) the organization of non-human animals’ knowledge (stimulus- and/or context-specific versus general and structured).
Völter, C. J., Lambert, M. L. & Huber, L. Do nonhumans seek explanations? Anim. Behav. Cogn. 7 , 445–451 (2020).
Call, J. Inferences about the location of food in the great apes ( Pan paniscus , Pan troglodytes , Gorilla gorilla , and Pongo pygmaeus ). J. Comp. Psychol. 118 , 232–241 (2004).
Call, J. Apes know that hidden objects can affect the orientation of other objects. Cognition 105 , 1–25 (2007).
Hanus, D. & Call, J. Chimpanzees infer the location of a reward on the basis of the effect of its weight. Curr. Biol. 18 , R370–R372 (2008).
Hanus, D. & Call, J. Chimpanzee problem-solving: contrasting the use of causal and arbitrary cues. Anim. Cogn. 14 , 871–878 (2011).
Petit, O. et al. Inferences about food location in three cercopithecine species: an insight into the socioecological cognition of primates. Anim. Cogn. 18 , 821–830 (2015).
Heimbauer, L. A., Antworth, R. L. & Owren, M. J. Capuchin monkeys ( Cebus apella ) use positive, but not negative, auditory cues to infer food location. Anim. Cogn. 15 , 45–55 (2012).
Schloegl, C., Schmidt, J., Boeckle, M., Weiß, B. M. & Kotrschal, K. Grey parrots use inferential reasoning based on acoustic cues alone. Proc. R. Soc. B 279 , 4135–4142 (2012).
Schloegl, C., Waldmann, M. R. & Fischer, J. Understanding of and reasoning about object–object relationships in long-tailed macaques? Anim. Cogn. 16 , 493–507 (2013).
Schmitt, V., Pankau, B. & Fischer, J. Old world monkeys compare to apes in the primate cognition test battery. PLoS One 7 , e32024 (2012).
Völter, C. J. & Call, J. Great apes ( Pan paniscus , Pan troglodytes , Gorilla gorilla , Pongo abelii ) follow visual trails to locate hidden food. J. Comp. Psychol. 128 , 199–208 (2014).
Blaisdell, A. P., Sawa, K., Leising, K. J. & Waldmann, M. R. Causal reasoning in rats. Science 311 , 1020–1022 (2006).
Leising, K. J., Wong, J., Waldmann, M. R. & Blaisdell, A. P. The special status of actions in causal reasoning in rats. J. Exp. Psychol. Gen. 137 , 514–527 (2008).
Flavell, J. H. The Developmental Psychology Of Jean Piaget (Van Nostrand, 1963).
Piaget, J. The Construction of Reality in the Child (Routledge, 2013).
Henrich, J., Heine, S. J. & Norenzayan, A. Most people are not WEIRD. Nature 466 , 29–29 (2010).
Ayzenberg, V. & Behrmann, M. Development of visual object recognition. Nat. Rev. Psychol. 3 , 73–90 (2023).
Bronson, G. W. Changes in infants’ visual scanning across the 2- to 14-week age period. J. Exp. Child. Psychol. 49 , 101–125 (1990).
Aslin, R. Ν. in Eye Movements . Cognition and Visual Perception (eds Fisher, D. F., Monty, R. A. & Senders, J. W.) 31–51 (Routledge, 2017).
Miranda, S. B. Visual abilities and pattern preferences of premature infants and full-term neonates. J. Exp. Child. Psychol. 10 , 189–205 (1970).
Haith, M. M., Hazan, C. & Goodman, G. S. Expectation and anticipation of dynamic visual events by 3.5-month-old babies. Child Dev. 59 , 467–479 (1988).
Harris, P. & MacFarlane, A. The growth of the effective visual field from birth to seven weeks. J. Exp. Child. Psychol. 18 , 340–348 (1974).
Cohen, L. B. & Amsel, G. Precursors to infants’ perception of the causality of a simple event. Infant. Behav. Dev. 21 , 713–731 (1998).
Leslie, A. M. & Keeble, S. Do six-month-old infants perceive causality? Cognition 25 , 265–288 (1987).
Oakes, L. M. & Cohen, L. B. Infant perception of a causal event. Cogn. Dev. 5 , 193–207 (1990). This canonical study shows that 10-month-olds, but not 6-month-olds, discriminate between causal versus non-causal events.
Kotovsky, L. & Baillargeon, R. Calibration-based reasoning about collision events in 11-month-old infants. Cognition 51 , 107–129 (1994).
Kominsky, J. F. et al. Categories and constraints in causal perception. Psychol. Sci. 28 , 1649–1662 (2017).
Spelke, E. S., Breinlinger, K., Macomber, J. & Jacobson, K. Origins of knowledge. Psychol. Rev. 99 , 605 (1992).
Baillargeon, R. in Language, Brain, and Cognitive Development: Essays in Honor of Jacques Mehler (ed. Dupoux, E.) 341–361 (MIT Press, 2001).
Hespos, S. J. & Baillargeon, R. Infants’ knowledge about occlusion and containment events: a surprising discrepancy. Psychol. Sci. 12 , 141–147 (2001).
Spelke, E. S. Principles of object perception. Cogn. Sci. 14 , 29–56 (1990).
Sobel, D. M. & Kirkham, N. Z. Blickets and babies: the development of causal reasoning in toddlers and infants. Dev. Psychol. 42 , 1103–1115 (2006).
Sobel, D. M. & Kirkham, N. Z. Bayes nets and babies: infants’ developing statistical reasoning abilities and their representation of causal knowledge. Dev. Sci. 10 , 298–306 (2007).
Bell, S. M. & Ainsworth, M. D. S. Infant crying and maternal responsiveness. Child Dev . 43 , 1171–1190 (1972).
Jordan, G. J., Arbeau, K., McFarland, D., Ireland, K. & Richardson, A. Elimination communication contributes to a reduction in unexplained infant crying. Med. Hypotheses 142 , 109811 (2020).
Nakayama, H. Emergence of amae crying in early infancy as a possible social communication tool between infants and mothers. Infant. Behav. Dev. 40 , 122–130 (2015).
Meltzoff, A. N. & Moore, M. K. in The Body and the Self (eds Bermúdez, J. L., Marcel, A. J. & Eilan, N.) 3–69 (MIT Press, 1995).
Rovee, C. K. & Rovee, D. T. Conjugate reinforcement of infant exploratory behavior. J. Exp. Child. Psychol. 8 , 33–39 (1969).
Hillman, D. & Bruner, J. S. Infant sucking in response to variations in schedules of feeding reinforcement. J. Exp. Child. Psychol. 13 , 240–247 (1972).
DeCasper, A. J. & Spence, M. J. Prenatal maternal speech influences newborns’ perception of speech sounds. Infant. Behav. Dev. 9 , 133–150 (1986).
Watson, J. S. & Ramey, C. T. Reactions to response-contingent stimulation in early infancy. Merrill-Palmer Q. Behav. Dev. 18 , 219–227 (1972).
Rovee-Collier, C. in Handbook of Infant Development 2nd edn (ed. Osofsky, J. D.) 98–148 (John Wiley & Sons, 1987).
Twitchell, T. E. The automatic grasping responses of infants. Neuropsychologia 3 , 247–259 (1965).
Wallace, P. S. & Whishaw, I. Q. Independent digit movements and precision grip patterns in 1–5-month-old human infants: hand-babbling, including vacuous then self-directed hand and digit movements, precedes targeted reaching. Neuropsychologia 41 , 1912–1918 (2003).
Von Hofsten, C. Mastering reaching and grasping: the development of manual skills in infancy. Adv. Psychol . 61 , 223–258 (1989).
Witherington, D. C. The development of prospective grasping control between 5 and 7 months: a longitudinal study. Infancy 7 , 143–161 (2005).
Needham, A., Barrett, T. & Peterman, K. A pick-me-up for infants’ exploratory skills: early simulated experiences reaching for objects using ‘sticky mittens’ enhances young infants’ object exploration skills. Infant. Behav. Dev. 25 , 279–295 (2002).
van den Berg, L. & Gredebäck, G. The sticky mittens paradigm: a critical appraisal of current results and explanations. Dev. Sci. 24 , e13036 (2021).
Keen, R. The development of problem solving in young children: a critical cognitive skill. Annu. Rev. Psychol. 62 , 1–21 (2011). This paper provides an overview of the developmental trajectory of ‘problem-solving’skills in young children, integrating findings from perception and motor development studies with cognitive problem-solving studies.
Claxton, L. J., McCarty, M. E. & Keen, R. Self-directed action affects planning in tool-use tasks with toddlers. Infant. Behav. Dev. 32 , 230–233 (2009).
McCarty, M. E., Clifton, R. K. & Collard, R. R. The beginnings of tool use by infants and toddlers. Infancy 2 , 233–256 (2001).
Gopnik, A. & Meltzoff, A. N. Semantic and cognitive development in 15- to 21-month-old children. J. Child. Lang. 11 , 495–513 (1984).
Gopnik, A. & Meltzoff, A. N. in The Development of Word Meaning: Progress in Cognitive Development Research (eds Kuczaj, S. A. & Barrett, M. D.) 199–223 (Springer, 1986).
Gopnik, A. & Meltzoff, A. N. Words, Thoughts, and Theories (Mit Press, 1997).
Tomasello, M. in Early Social Cognition: Understanding Others in the First Months of Life (ed. Rochat, P.) 301–314 (Lawrence Erlbaum Associates, 1999).
Tomasello, M. & Farrar, M. J. Joint attention and early language. Child Dev . 57 , 1454–1463 (1986).
Gopnik, A. Words and plans: early language and the development of intelligent action. J. Child. Lang. 9 , 303–318 (1982). This paper proposes that language acquisition tracks with conceptual developments in infants’ and toddlers’ abilities in goal-directed action and planning.
Meltzoff, A. N. Infant imitation and memory: nine-month-olds in immediate and deferred tests. Child. Dev. 59 , 217–225 (1988).
Meltzoff, A. N. Infant imitation after a 1-week delay: long-term memory for novel acts and multiple stimuli. Dev. Psychol. 24 , 470–476 (1988).
Gergely, G., Bekkering, H. & Király, I. Rational imitation in preverbal infants. Nature 415 , 755–755 (2002).
Meltzoff, A. N., Waismeyer, A. & Gopnik, A. Learning about causes from people: observational causal learning in 24-month-old infants. Dev. Psychol. 48 , 1215–1228 (2012). This study demonstrates that 2-year-old and 3-year-old children learn novel causal relations from observing other agents’ interventions (observational causal learning).
Waismeyer, A., Meltzoff, A. N. & Gopnik, A. Causal learning from probabilistic events in 24‐month‐olds: an action measure. Dev. Sci. 18 , 175–182 (2015).
Stahl, A. E. & Feigenson, L. Observing the unexpected enhances infants’ learning and exploration. Science 348 , 91–94 (2015). This study demonstrates that 11-month-old children pay special visual and exploratory attention to objects that appear to violate the laws of physics as the result of an agent’s intervention.
Perfors, A., Tenenbaum, J. B., Griffiths, T. L. & Xu, F. A tutorial introduction to Bayesian models of cognitive development. Cognition 120 , 302–321 (2011).
Gopnik, A. & Bonawitz, E. Bayesian models of child development. Wiley Interdiscip. Rev. Cognit. Sci. 6 , 75–86 (2015). This paper is a technical introduction and tutorial in the Bayesian framework.
Gopnik, A., Sobel, D. M., Schulz, L. E. & Glymour, C. Causal learning mechanisms in very young children: two-, three-, and four-year-olds infer causal relations from patterns of variation and covariation. Dev. Psychol. 37 , 620–629 (2001).
Schulz, L. E. & Bonawitz, E. B. Serious fun: preschoolers engage in more exploratory play when evidence is confounded. Dev. Psychol. 43 , 1045–1050 (2007).
Gopnik, A. & Sobel, D. M. Detecting blickets: how young children use information about novel causal powers in categorization and induction. Child. Dev. 71 , 1205–1222 (2000).
Schulz, L. E., Gopnik, A. & Glymour, C. Preschool children learn about causal structure from conditional interventions. Dev. Sci. 10 , 322–332 (2007).
Walker, C. M., Gopnik, A. & Ganea, P. A. Learning to learn from stories: children’s developing sensitivity to the causal structure of fictional worlds. Child. Dev. 86 , 310–318 (2015).
Schulz, L. E., Bonawitz, E. B. & Griffiths, T. L. Can being scared cause tummy aches? Naive theories, ambiguous evidence, and preschoolers’ causal inferences. Dev. Psychol. 43 , 1124–1139 (2007).
Kushnir, T. & Gopnik, A. Young children infer causal strength from probabilities and interventions. Psychol. Sci. 16 , 678–683 (2005).
Walker, C. M. & Gopnik, A. Toddlers infer higher-order relational principles in causal learning. Psychol. Sci. 25 , 161–169 (2014). This paper shows that 18–30-month-old infants can learn relational causal rules and generalize them to novel stimuli.
Sobel, D. M., Yoachim, C. M., Gopnik, A., Meltzoff, A. N. & Blumenthal, E. J. The blicket within: preschoolers’ inferences about insides and causes. J. Cogn. Dev. 8 , 159–182 (2007).
Schulz, L. E. & Sommerville, J. God does not play dice: causal determinism and preschoolers’ causal inferences. Child. Dev. 77 , 427–442 (2006).
Schulz, L. E. & Gopnik, A. Causal learning across domains. Dev. Psychol. 40 , 162–176 (2004).
Seiver, E., Gopnik, A. & Goodman, N. D. Did she jump because she was the big sister or because the trampoline was safe? Causal inference and the development of social attribution. Child. Dev. 84 , 443–454 (2013).
Vasilyeva, N., Gopnik, A. & Lombrozo, T. The development of structural thinking about social categories. Dev. Psychol. 54 , 1735–1744 (2018).
Kushnir, T., Xu, F. & Wellman, H. M. Young children use statistical sampling to infer the preferences of other people. Psychol. Sci. 21 , 1134–1140 (2010).
Kushnir, T. & Gopnik, A. Conditional probability versus spatial contiguity in causal learning: preschoolers use new contingency evidence to overcome prior spatial assumptions. Dev. Psychol. 43 , 186–196 (2007).
Kimura, K. & Gopnik, A. Rational higher‐order belief revision in young children. Child. Dev. 90 , 91–97 (2019).
Goddu, M. K. & Gopnik, A. Learning what to change: young children use “difference-making” to identify causally relevant variables. Dev. Psychol. 56 , 275–284 (2020).
Gopnik, A. et al. Changes in cognitive flexibility and hypothesis search across human life history from childhood to adolescence to adulthood. Proc. Natl Acad. Sci. USA 114 , 7892–7899 (2017).
Lucas, C. G., Bridgers, S., Griffiths, T. L. & Gopnik, A. When children are better (or at least more open-minded) learners than adults: developmental differences in learning the forms of causal relationships. Cognition 131 , 284–299 (2014). This paper shows that young children learn and generalize unusual causal relationships more readily than adults do.
Goddu, M. K., Lombrozo, T. & Gopnik, A. Transformations and transfer: preschool children understand abstract relations and reason analogically in a causal task. Child. Dev. 91 , 1898–1915 (2020).
Magid, R. W., Sheskin, M. & Schulz, L. E. Imagination and the generation of new ideas. Cogn. Dev. 34 , 99–110 (2015).
Liquin, E. G. & Gopnik, A. Children are more exploratory and learn more than adults in an approach–avoid task. Cognition 218 , 104940 (2022).
Erickson, J. E., Keil, F. C. & Lockhart, K. L. Sensing the coherence of biology in contrast to psychology: young children’s use of causal relations to distinguish two foundational domains. Child Dev. 81 , 390–409 (2010).
Keil, F. C. Concepts, Kinds, and Cognitive Development (MIT Press, 1992).
Carey, S. Conceptual Change in Childhood (MIT Press, 1987).
Gelman, S. A. The Essential Child: Origins of Essentialism in Everyday Thought (Oxford Univ. Press, 2003).
Ahl, R. E., DeAngelis, E. & Keil, F. C. “I know it’s complicated”: children detect relevant information about object complexity. J. Exp. Child. Psychol. 222 , 105465 (2022).
Chuey, A. et al. No guts, no glory: underestimating the benefits of providing children with mechanistic details. npj Sci. Learn. 6 , 30 (2021).
Keil, F. C. & Lockhart, K. L. Beyond cause: the development of clockwork cognition. Curr. Dir. Psychol. Sci. 30 , 167–173 (2021).
Chuey, A., Lockhart, K., Sheskin, M. & Keil, F. Children and adults selectively generalize mechanistic knowledge. Cognition 199 , 104231 (2020).
Lockhart, K. L., Chuey, A., Kerr, S. & Keil, F. C. The privileged status of knowing mechanistic information: an early epistemic bias. Child Dev. 90 , 1772–1788 (2019).
Kominsky, J. F., Zamm, A. P. & Keil, F. C. Knowing when help is needed: a developing sense of causal complexity. Cogn. Sci. 42 , 491–523 (2018).
Mills, C. M. & Keil, F. C. Knowing the limits of one’s understanding: the development of an awareness of an illusion of explanatory depth. J. Exp. Child Psychol. 87 , 1–32 (2004).
Goldwater, M. B. & Gentner, D. On the acquisition of abstract knowledge: structural alignment and explication in learning causal system categories. Cognition 137 , 137–153 (2015).
Rottman, B. M., Gentner, D. & Goldwater, M. B. Causal systems categories: differences in novice and expert categorization of causal phenomena. Cogn. Sci. 36 , 919–932 (2012).
Bonawitz, E. B. et al. Just do it? Investigating the gap between prediction and action in toddlers’ causal inferences. Cognition 115 , 104–117 (2010). This study demonstrates that the ability to infer causal relations from observations of correlational information without an agent’s involvement or the use of causal language develops at around the age of four years.
Herrmann, E., Call, J., Hernández-Lloreda, M. V., Hare, B. & Tomasello, M. Humans have evolved specialized skills of social cognition: the cultural intelligence hypothesis. Science 317 , 1360–1366 (2007).
Tomasello, M. Becoming Human: A Theory of Ontogeny (Harvard Univ. Press, 2019).
Henrich, J. The Secret of our Success (Princeton Univ. Press, 2015).
Hesslow, G. in Contemporary Science and Natural Explanation: Commonsense Conceptions of Causality (ed. Hilton, D. J.) 11–32 (New York Univ. Press, 1988).
Woodward, J. The problem of variable choice. Synthese 193 , 1047–1072 (2016).
Khalid, S., Khalil, T. & Nasreen, S. A survey of feature selection and feature extraction techniques in machine learning. In 2014 Science and Information Conf . 372–378 (IEEE, 1988).
Bonawitz, E., Denison, S., Griffiths, T. L. & Gopnik, A. Probabilistic models, learning algorithms, and response variability: sampling in cognitive development. Trends Cogn. Sci. 18 , 497–500 (2014).
Bonawitz, E., Denison, S., Gopnik, A. & Griffiths, T. L. Win–stay, lose–sample: a simple sequential algorithm for approximating Bayesian inference. Cogn. Psychol. 74 , 35–65 (2014).
Denison, S., Bonawitz, E., Gopnik, A. & Griffiths, T. L. Rational variability in children’s causal inferences: the sampling hypothesis. Cognition 126 , 285–300 (2013).
Samland, J., Josephs, M., Waldmann, M. R. & Rakoczy, H. The role of prescriptive norms and knowledge in children’s and adults’ causal selection. J. Exp. Psychol. Gen. 145 , 125–130 (2016).
Samland, J. & Waldmann, M. R. How prescriptive norms influence causal inferences. Cognition 156 , 164–176 (2016).
Phillips, J., Morris, A. & Cushman, F. How we know what not to think. Trends Cogn. Sci. 23 , 1026–1040 (2019).
Gureckis, T. M. & Markant, D. B. Self-directed learning: a cognitive and computational perspective. Perspect. Psychol. Sci. 7 , 464–481 (2012).
Saylor, M. & Ganea, P. Active Learning from Infancy to Childhood (Springer, 2018).
Goddu, M. K. & Gopnik, A. in The Cambridge Handbook of Cognitive Development (eds Houdé, O. & Borst, G.) 299–317 (Cambridge Univ. Press, 2022).
Gopnik, A. Scientific thinking in young children: theoretical advances, empirical research, and policy implications. Science 337 , 1623–1627 (2012).
Weisberg, D. S. & Sobel, D. M. Constructing Science: Connecting Causal Reasoning to Scientific Thinking in Young Children (MIT Press, 2022).
Xu, F. Towards a rational constructivist theory of cognitive development. Psychol. Rev. 126 , 841 (2019).
Xu, F. & Kushnir, T. Infants are rational constructivist learners. Curr. Dir. Psychol. Sci. 22 , 28–32 (2013).
Lapidow, E. & Bonawitz, E. What’s in the box? Preschoolers consider ambiguity, expected value, and information for future decisions in explore-exploit tasks. Open. Mind 7 , 855–878 (2023).
Kidd, C., Piantadosi, S. T. & Aslin, R. N. The Goldilocks effect: human infants allocate attention to visual sequences that are neither too simple nor too complex. PLoS One 7 , e36399 (2012).
Ruggeri, A., Swaboda, N., Sim, Z. L. & Gopnik, A. Shake it baby, but only when needed: preschoolers adapt their exploratory strategies to the information structure of the task. Cognition 193 , 104013 (2019).
Sim, Z. L. & Xu, F. Another look at looking time: surprise as rational statistical inference. Top. Cogn. Sci. 11 , 154–163 (2019).
Sim, Z. L. & Xu, F. Infants preferentially approach and explore the unexpected. Br. J. Dev. Psychol. 35 , 596–608 (2017).
Siegel, M. H., Magid, R. W., Pelz, M., Tenenbaum, J. B. & Schulz, L. E. Children’s exploratory play tracks the discriminability of hypotheses. Nat. Commun. 12 , 3598 (2021).
Schulz, E., Wu, C. M., Ruggeri, A. & Meder, B. Searching for rewards like a child means less generalization and more directed exploration. Psychol. Sci. 30 , 1561–1572 (2019).
Schulz, L. Infants explore the unexpected. Science 348 , 42–43 (2015).
Perez, J. & Feigenson, L. Violations of expectation trigger infants to search for explanations. Cognition 218 , 104942 (2022).
Cook, C., Goodman, N. D. & Schulz, L. E. Where science starts: spontaneous experiments in preschoolers’ exploratory play. Cognition 120 , 341–349 (2011). This study demonstrates that preschoolers spontaneously perform causal interventions that are relevant to disambiguating multiple possible causal structures in their free play.
Lapidow, E. & Walker, C. M. Learners’ causal intuitions explain behavior in control of variables tasks. Dev. Psychol. (in the press).
Lapidow, E. & Walker, C. M. Rethinking the “gap”: self‐directed learning in cognitive development and scientific reasoning. Wiley Interdiscip. Rev. Cogn. Sci. 13 , e1580 (2022). This theory paper provides a complementary viewpoint to ‘child-as-scientist’, or Bayesian ‘rational constructivist’, account, arguing that children seek to identify and generate evidence for causal relations that are robust across contexts (and thus will be reliable for causal intervention).
Lapidow, E. & Walker, C. M. Informative experimentation in intuitive science: children select and learn from their own causal interventions. Cognition 201 , 104315 (2020).
Moeller, A., Sodian, B. & Sobel, D. M. Developmental trajectories in diagnostic reasoning: understanding data are confounded develops independently of choosing informative interventions to resolve confounded data. Front. Psychol. 13 , 800226 (2022).
Fernbach, P. M., Macris, D. M. & Sobel, D. M. Which one made it go? The emergence of diagnostic reasoning in preschoolers. Cogn. Dev. 27 , 39–53 (2012).
Buchanan, D. W. & Sobel, D. M. Mechanism‐based causal reasoning in young children. Child. Dev. 82 , 2053–2066 (2011).
Sobel, D. M., Benton, D., Finiasz, Z., Taylor, Y. & Weisberg, D. S. The influence of children’s first action when learning causal structure from exploratory play. Cogn. Dev. 63 , 101194 (2022).
Lapidow, E. & Walker, C. M. The Search for Invariance: Repeated Positive Testing Serves the Goals of Causal Learning (Springer, 2020).
Klayman, J. Varieties of confirmation bias. Psychol. Learn. Motiv. 32 , 385–418 (1995).
Zimmerman, C. The development of scientific thinking skills in elementary and middle school. Dev. Rev. 27 , 172–223 (2007).
Rule, J. S., Tenenbaum, J. B. & Piantadosi, S. T. The child as hacker. Trends Cogn. Sci. 24 , 900–915 (2020).
Burghardt, G. M. The Genesis of Animal Play: Testing the Limits (MIT Press, 2005).
Chu, J. & Schulz, L. E. Play, curiosity, and cognition. Annu. Rev. Dev. Psychol. 2 , 317–343 (2020).
Schulz, L. The origins of inquiry: inductive inference and exploration in early childhood. Trends Cogn. Sci. 16 , 382–389 (2012).
Harris, P. L., Kavanaugh, R. D., Wellman, H. M. & Hickling, A. K. in Monographs of the Society for Research in Child Development https://doi.org/10.2307/1166074 (Society for Research in Child Development, 1993).
Weisberg, D. S. in The Oxford Handbook of the Development of Imagination (ed. Taylor, M.) 75–93 (Oxford Univ. Press, 2013).
Gopnik, A. & Walker, C. M. Considering counterfactuals: the relationship between causal learning and pretend play. Am. J. Play. 6 , 15–28 (2013).
Weisberg, D. S. & Gopnik, A. Pretense, counterfactuals, and Bayesian causal models: why what is not real really matters. Cogn. Sci. 37 , 1368–1381 (2013).
Root-Bernstein, M. M. in The Oxford Handbook of the Development of Imagination (ed. Taylor, M.) 417–437 (Oxford Univ. Press, 2013).
Buchsbaum, D., Bridgers, S., Skolnick Weisberg, D. & Gopnik, A. The power of possibility: causal learning, counterfactual reasoning, and pretend play. Phil. Trans. R. Soc. B 367 , 2202–2212 (2012).
Wente, A., Gopnik, A., Fernández Flecha, M., Garcia, T. & Buchsbaum, D. Causal learning, counterfactual reasoning and pretend play: a cross-cultural comparison of Peruvian, mixed-and low-socioeconomic status US children. Phil. Trans. R. Soc. B 377 , 20210345 (2022).
Buchsbaum, D., Gopnik, A., Griffiths, T. L. & Shafto, P. Children’s imitation of causal action sequences is influenced by statistical and pedagogical evidence. Cognition 120 , 331–340 (2011).
Csibra, G. & Gergely, G. ‘Obsessed with goals’: functions and mechanisms of teleological interpretation of actions in humans. Acta Psychol. 124 , 60–78 (2007).
Kelemen, D. The scope of teleological thinking in preschool children. Cognition 70 , 241–272 (1999).
Casler, K. & Kelemen, D. Young children’s rapid learning about artifacts. Dev. Sci. 8 , 472–480 (2005).
Casler, K. & Kelemen, D. Reasoning about artifacts at 24 months: the developing teleo-functional stance. Cognition 103 , 120–130 (2007).
Ruiz, A. M. & Santos, L. R. 6. in Tool Use in Animals: Cognition and Ecology 119–133 (Cambridge Univ. Press, 2013).
Walker, C. M., Rett, A. & Bonawitz, E. Design drives discovery in causal learning. Psychol. Sci. 31 , 129–138 (2020).
Butler, L. P. & Markman, E. M. Finding the cause: verbal framing helps children extract causal evidence embedded in a complex scene. J. Cogn. Dev. 13 , 38–66 (2012).
Callanan, M. A. et al. Exploration, explanation, and parent–child interaction in museums. Monogr. Soc. Res. Child. Dev. 85 , 7–137 (2020).
McHugh, S. R., Callanan, M., Jaeger, G., Legare, C. H. & Sobel, D. M. Explaining and exploring the dynamics of parent–child interactions and children’s causal reasoning at a children’s museum exhibit. Child Dev . https://doi.org/10.1111/cdev.14035 (2023).
Sobel, D. M., Letourneau, S. M., Legare, C. H. & Callanan, M. Relations between parent–child interaction and children’s engagement and learning at a museum exhibit about electric circuits. Dev. Sci. 24 , e13057 (2021).
Willard, A. K. et al. Explain this, explore that: a study of parent–child interaction in a children’s museum. Child Dev. 90 , e598–e617 (2019).
Daubert, E. N., Yu, Y., Grados, M., Shafto, P. & Bonawitz, E. Pedagogical questions promote causal learning in preschoolers. Sci. Rep. 10 , 20700 (2020).
Yu, Y., Landrum, A. R., Bonawitz, E. & Shafto, P. Questioning supports effective transmission of knowledge and increased exploratory learning in pre‐kindergarten children. Dev. Sci. 21 , e12696 (2018).
Walker, C. M. & Nyhout, A. in The Questioning Child: Insights From Psychology and Education (eds Butler, L. P., Ronfard, S. & Corriveau, K. H.) 252–280 (Cambridge Univ. Press, 2020).
Weisberg, D. S. & Hopkins, E. J. Preschoolers’ extension and export of information from realistic and fantastical stories. Infant. Child. Dev. 29 , e2182 (2020).
Tillman, K. A. & Walker, C. M. You can’t change the past: children’s recognition of the causal asymmetry between past and future events. Child. Dev. 93 , 1270–1283 (2022).
Rottman, B. M., Kominsky, J. F. & Keil, F. C. Children use temporal cues to learn causal directionality. Cogn. Sci. 38 , 489–513 (2014).
Tecwyn, E. C., Mazumder, P. & Buchsbaum, D. One- and two-year-olds grasp that causes must precede their effects. Dev. Psychol. 59 , 1519–1531 (2023).
Liquin, E. G. & Lombrozo, T. Explanation-seeking curiosity in childhood. Curr. Opin. Behav. Sci. 35 , 14–20 (2020).
Mills, C. M., Legare, C. H., Bills, M. & Mejias, C. Preschoolers use questions as a tool to acquire knowledge from different sources. J. Cogn. Dev. 11 , 533–560 (2010).
Ruggeri, A., Sim, Z. L. & Xu, F. “Why is Toma late to school again?” Preschoolers identify the most informative questions. Dev. Psychol. 53 , 1620–1632 (2017).
Ruggeri, A. & Lombrozo, T. Children adapt their questions to achieve efficient search. Cognition 143 , 203–216 (2015).
Legare, C. H. & Lombrozo, T. Selective effects of explanation on learning during early childhood. J. Exp. Child. Psychol. 126 , 198–212 (2014).
Legare, C. H. Exploring explanation: explaining inconsistent evidence informs exploratory, hypothesis‐testing behavior in young children. Child Dev. 83 , 173–185 (2012).
Walker, C. M., Lombrozo, T., Legare, C. H. & Gopnik, A. Explaining prompts children to privilege inductively rich properties. Cognition 133 , 343–357 (2014).
Vasil, N., Ruggeri, A. & Lombrozo, T. When and how children use explanations to guide generalizations. Cogn. Dev. 61 , 101144 (2022).
Walker, C. M., Bonawitz, E. & Lombrozo, T. Effects of explaining on children’s preference for simpler hypotheses. Psychon. Bull. Rev. 24 , 1538–1547 (2017).
Walker, C. M. & Lombrozo, T. Explaining the moral of the story. Cognition 167 , 266–281 (2017).
Walker, C. M., Lombrozo, T., Williams, J. J., Rafferty, A. N. & Gopnik, A. Explaining constrains causal learning in childhood. Child. Dev. 88 , 229–246 (2017).
Gopnik, A. Childhood as a solution to explore–exploit tensions. Phil. Trans. R. Soc. B 375 , 20190502 (2020).
Wente, A. O. et al. Causal learning across culture and socioeconomic status. Child. Dev. 90 , 859–875 (2019).
Carstensen, A. et al. Context shapes early diversity in abstract thought. Proc. Natl Acad. Sci. USA 116 , 13891–13896 (2019). This study provides evidence for developmentally early emerging cross-cultural differences in learning ‘individual’ versus ‘relational’ causal rules in children from individualist versus collectivist societies.
Ross, N., Medin, D., Coley, J. D. & Atran, S. Cultural and experiential differences in the development of folkbiological induction. Cogn. Dev. 18 , 25–47 (2003).
Inagaki, K. The effects of raising animals on children’s biological knowledge. Br. J. Dev. Psychol. 8 , 119–129 (1990).
Cole, M. & Bruner, J. S. Cultural differences and inferences about psychological processes. Am. Psychol. 26 , 867–876 (1971).
Rogoff, B. & Morelli, G. Perspectives on children’s development from cultural psychology. Am. Psychol. 44 , 343–348 (1989).
Rogoff, B. Adults and peers as agents of socialization: a highland Guatemalan profile. Ethos 9 , 18–36 (1981).
Shneidman, L., Gaskins, S. & Woodward, A. Child‐directed teaching and social learning at 18 months of age: evidence from Yucatec Mayan and US infants. Dev. Sci. 19 , 372–381 (2016).
Callanan, M., Solis, G., Castañeda, C. & Jipson, J. in The Questioning Child: Insights From Psychology and Education (eds Butler, L. P., Ronfard, S. & Corriveau, K. H.) 73–88 (Cambridge Univ. Press, 2020).
Gauvain, M., Munroe, R. L. & Beebe, H. Children’s questions in cross-cultural perspective: a four-culture study. J. Cross-Cult. Psychol. 44 , 1148–1165 (2013).
Adolph, K. E. & Hoch, J. E. Motor development: embodied, embedded, enculturated, and enabling. Annu. Rev. Psychol. 70 , 141–164 (2019).
Schleihauf, H., Herrmann, E., Fischer, J. & Engelmann, J. M. How children revise their beliefs in light of reasons. Child. Dev. 93 , 1072–1089 (2022).
Vasil, N. et al. Structural explanations lead young children and adults to rectify resource inequalities. J. Exp. Child Psychol. 242 , 105896 (2024).
Koskuba, K., Gerstenberg, T., Gordon, H., Lagnado, D. & Schlottmann, A. What’s fair? How children assign reward to members of teams with differing causal structures. Cognition 177 , 234–248 (2018).
Bowlby, J. The Bowlby–Ainsworth attachment theory. Behav. Brain Sci. 2 , 637–638 (1979).
Tottenham, N., Shapiro, M., Flannery, J., Caldera, C. & Sullivan, R. M. Parental presence switches avoidance to attraction learning in children. Nat. Hum. Behav. 3 , 1070–1077 (2019).
Frankenhuis, W. E. & Gopnik, A. Early adversity and the development of explore–exploit tradeoffs. Trends Cogn. Sci. 27 , 616–630 (2023).
Van IJzendoorn, M. H. & Kroonenberg, P. M. Cross-cultural patterns of attachment: a meta-analysis of the strange situation. Child Dev. 59 , 147–156 (1988).
Gopnik, A. Explanation as orgasm. Minds Mach. 8 , 101–118 (1998).
Gottlieb, S., Keltner, D. & Lombrozo, T. Awe as a scientific emotion. Cogn. Sci. 42 , 2081–2094 (2018).
Valdesolo, P., Shtulman, A. & Baron, A. S. Science is awe-some: the emotional antecedents of science learning. Emot. Rev. 9 , 215–221 (2017).
Keil, F. C. Wonder: Childhood and the Lifelong Love of Science (MIT Press, 2022).
Perez, J. & Feigenson, L. Stable individual differences in infants’ responses to violations of intuitive physics. Proc. Natl Acad. Sci. USA 118 , e2103805118 (2021).
Goddu, M. K., Sullivan, J. N. & Walker, C. M. Toddlers learn and flexibly apply multiple possibilities. Child. Dev. 92 , 2244–2251 (2021).
Cisek, P. Resynthesizing behavior through phylogenetic refinement. Atten. Percept. Psychophys. 81 , 2265–2287 (2019).
Pezzulo, G. & Cisek, P. Navigating the affordance landscape: feedback control as a process model of behavior and cognition. Trends Cogn. Sci. 20 , 414–424 (2016).
Cisek, P. Cortical mechanisms of action selection: the affordance competition hypothesis. Phil. Trans. R. Soc. B 362 , 1585–1599 (2007).
Tomasello, M. The Evolution of Agency: Behavioral Organization From Lizards to Humans (MIT Press, 2022).
Beck, S. R., Robinson, E. J., Carroll, D. J. & Apperly, I. A. Children’s thinking about counterfactuals and future hypotheticals as possibilities. Child. Dev. 77 , 413–426 (2006).
Robinson, E. J., Rowley, M. G., Beck, S. R., Carroll, D. J. & Apperly, I. A. Children’s sensitivity to their own relative ignorance: handling of possibilities under epistemic and physical uncertainty. Child. Dev. 77 , 1642–1655 (2006).
Leahy, B. P. & Carey, S. E. The acquisition of modal concepts. Trends Cogn. Sci. 24 , 65–78 (2020).
Mody, S. & Carey, S. The emergence of reasoning by the disjunctive syllogism in early childhood. Cognition 154 , 40–48 (2016).
Shtulman, A. & Carey, S. Improbable or impossible? How children reason about the possibility of extraordinary events. Child Dev. 78 , 1015–1032 (2007).
Redshaw, J. & Suddendorf, T. Children’s and apes’ preparatory responses to two mutually exclusive possibilities. Curr. Biol. 26 , 1758–1762 (2016).
Phillips, J. S. & Kratzer, A. Decomposing modal thought. Psychol. Rev. (in the press).
Vetter, B. Abilities and the epistemology of ordinary modality. Mind (in the press).
Kahneman, D. & Tversky, A. Variants of uncertainty. Cognition 11 , 143–157 (1982).
Rafetseder, E., Schwitalla, M. & Perner, J. Counterfactual reasoning: from childhood to adulthood. J. Exp. Child Psychol. 114 , 389–404 (2013).
Beck, S. R. & Riggs, K. J. Developing thoughts about what might have been. Child Dev. Perspect. 8 , 175–179 (2014).
Kominsky, J. F. et al. The trajectory of counterfactual simulation in development. Dev. Psychol. 57 , 253 (2021). This study uses a physical collision paradigm to demonstrate that the content of children’s counterfactual judgements changes over development.
Gerstenberg, T. What would have happened? Counterfactuals, hypotheticals and causal judgements. Phil. Trans. R. Soc. B 377 , 20210339 (2022).
Gerstenberg, T., Goodman, N. D., Lagnado, D. A. & Tenenbaum, J. B. A counterfactual simulation model of causal judgments for physical events. Psychol. Rev. 128 , 936 (2021).
Nyhout, A. & Ganea, P. A. The development of the counterfactual imagination. Child Dev. Perspect. 13 , 254–259 (2019).
Nyhout, A. & Ganea, P. A. Mature counterfactual reasoning in 4- and 5-year-olds. Cognition 183 , 57–66 (2019).
Moll, H., Meltzoff, A. N., Merzsch, K. & Tomasello, M. Taking versus confronting visual perspectives in preschool children. Dev. Psychol. 49 , 646–654 (2013).
Moll, H. & Tomasello, M. Three-year-olds understand appearance and reality — just not about the same object at the same time. Dev. Psychol. 48 , 1124–1132 (2012).
Moll, H. & Meltzoff, A. N. How does it look? Level 2 perspective‐taking at 36 months of age. Child. Dev. 82 , 661–673 (2011).
Gopnik, A., Slaughter, V. & Meltzoff, A. in Children’s Early Understanding of Mind: Origins and Development (eds Lewis, C. & Mitchell, P.) 157–181 (Routledge, 1994).
Gopnik, A. & Astington, J. W. Children’s understanding of representational change and its relation to the understanding of false belief and the appearance–reality distinction. Child Dev. 59 , 26–37 (1988).
Doherty, M. & Perner, J. Metalinguistic awareness and theory of mind: just two words for the same thing? Cogn. Dev. 13 , 279–305 (1998).
Wimmer, H. & Perner, J. Beliefs about beliefs: representation and constraining function of wrong beliefs in young children’s understanding of deception. Cognition 13 , 103–128 (1983).
Flavell, J. H., Speer, J. R., Green, F. L., August, D. L. & Whitehurst, G. J. in Monographs of the Society for Research in Child Development 1–65 (Society for Research in Child Development, 1981).
Wellman, H. M., Cross, D. & Watson, J. Meta‐analysis of theory‐of‐mind development: the truth about false belief. Child. Dev. 72 , 655–684 (2001).
Kelemen, D. & DiYanni, C. Intuitions about origins: purpose and intelligent design in children’s reasoning about nature. J. Cogn. Dev. 6 , 3–31 (2005).
Kelemen, D. Why are rocks pointy? Children’s preference for teleological explanations of th natural world. Dev. Psychol. 35 , 1440 (1999).
Vihvelin, K. Causes, Laws, and Free Will: Why Determinism Doesn’t Matter (Oxford Univ. Press, 2013).
Yiu, E., Kosoy, E. & Gopnik, A. Transmission versus truth, imitation versus innovation: what children can do that large language and language-and-vision models cannot (yet). Persp. Psychol. Sci . https://doi.org/10.1177/17456916231201401 (2023).
Kosoy, E. et al. Towards understanding how machines can learn causal overhypotheses. Preprint at arXiv https://doi.org/10.48550/arXiv.2206.08353 (2022).
Frank, M. C. Baby steps in evaluating the capacities of large language models. Nat. Rev. Psychol. 2 , 451–452 (2023).
Frank, M. C. Bridging the data gap between children and large language models. Trends Cogn. Sci . 27 , https://doi.org/10.1016/j.tics.2023.08.007 (2023).
Schmidhuber, J. A possibility for implementing curiosity and boredom in model-building neural controllers. In Proc. Int. Conf. on Simulation of Adaptive Behavior: From Animals to Animats 222–227 (MIT Press, 1991).
Volpi, N. C. & Polani, D. Goal-directed empowerment: combining intrinsic motivation and task-oriented behaviour. IEEE Trans. Cogn. Dev. Syst. 15 , 361–372 (2020).
Salge, C., Glackin, C. & Polani, D. in Guided Self-Organization: Inception . Emergence, Complexity and Computation Vol. 9 (ed. Prokopenko, M.) 67–114 (Springer, 2014).
Klyubin, A. S., Polani, D. & Nehaniv, C. L. Empowerment: a universal agent-centric measure of control. In 2005 IEEE Congr. on Evolutionary Computation 128–135 (IEEE, 2005).
Gopnik, A. Empowerment as causal learning, causal learning as empowerment: a bridge between Bayesian causal hypothesis testing and reinforcement learning. Preprint at https://philsci-archive.pitt.edu/id/eprint/23268 (2024).
Rovee-Collier, C. K., Sullivan, M. W., Enright, M., Lucas, D. & Fagen, J. W. Reactivation of infant memory. Science 208 , 1159–1161 (1980).
Kominsky, J. F., Li, Y. & Carey, S. Infants’ attributions of insides and animacy in causal interactions. Cogn. Sci. 46 , e13087 (2022).
Lakusta, L. & Carey, S. Twelve-month-old infants’ encoding of goal and source paths in agentive and non-agentive motion events. Lang. Learn. Dev. 11 , 152–175 (2015).
Saxe, R., Tzelnic, T. & Carey, S. Knowing who dunnit: infants identify the causal agent in an unseen causal interaction. Dev. Psychol. 43 , 149–158 (2007).
Saxe, R., Tenenbaum, J. & Carey, S. Secret agents: inferences about hidden causes by 10- and 12-month-old infants. Psychol. Sci. 16 , 995–1001 (2005).
Liu, S., Brooks, N. B. & Spelke, E. S. Origins of the concepts cause, cost, and goal in prereaching infants. Proc. Natl Acad. Sci. USA 116 , 17747–17752 (2019).
Liu, S. & Spelke, E. S. Six-month-old infants expect agents to minimize the cost of their actions. Cognition 160 , 35–42 (2017).
Nyhout, A. & Ganea, P. A. What is and what never should have been: children’s causal and counterfactual judgments about the same events. J. Exp. Child. Psychol. 192 , 104773 (2020).
Legare, C. H. The contributions of explanation and exploration to children’s scientific reasoning. Child. Dev. Perspect. 8 , 101–106 (2014).
Denison, S. & Xu, F. Twelve‐to 14‐month‐old infants can predict single‐event probability with large set sizes. Dev. Sci. 13 , 798–803 (2010).
Denison, S., Reed, C. & Xu, F. The emergence of probabilistic reasoning in very young infants: evidence from 4.5-and 6-month-olds. Dev. Psychol. 49 , 243 (2013).
Alderete, S. & Xu, F. Three-year-old children’s reasoning about possibilities. Cognition 237 , 105472 (2023).
Scholl, B. J. & Tremoulet, P. D. Perceptual causality and animacy. Trends Cogn. Sci. 4 , 299–309 (2000).
Hood, B., Carey, S. & Prasada, S. Predicting the outcomes of physical events: two‐year‐olds fail to reveal knowledge of solidity and support. Child. Dev. 71 , 1540–1554 (2000).
Hood, B. M., Hauser, M. D., Anderson, L. & Santos, L. Gravity biases in a non‐human primate? Dev. Sci. 2 , 35–41 (1999).
Hood, B. M. Gravity does rule for falling events. Dev. Sci. 1 , 59–63 (1998).
Hood, B. M. Gravity rules for 2-to 4-year olds? Cognit. Dev. 10 , 577–598 (1995).
Woodward, A. L. Infants selectively encode the goal object of an actor’s reach. Cognition 69 , 1–34 (1998).
Woodward, A. L. Infants’ grasp of others’ intentions. Curr. Dir. Psychol. Sci. 18 , 53–57 (2009).
Woodward, A. L., Sommerville, J. A., Gerson, S., Henderson, A. M. & Buresh, J. The emergence of intention attribution in infancy. Psychol. Learn. Motiv. 51 , 187–222 (2009).
Meltzoff, A. N. ‘Like me’: a foundation for social cognition. Dev. Sci. 10 , 126–134 (2007).
Gerson, S. A. & Woodward, A. L. The joint role of trained, untrained, and observed actions at the origins of goal recognition. Infant. Behav. Dev. 37 , 94–104 (2014).
Gerson, S. A. & Woodward, A. L. Learning from their own actions: the unique effect of producing actions on infants’ action understanding. Child. Dev. 85 , 264–277 (2014).
Sommerville, J. A., Woodward, A. L. & Needham, A. Action experience alters 3-month-old infants’ perception of others’ actions. Cognition 96 , B1–B11 (2005).
Liu, S. & Almeida, M. Knowing before doing: review and mega-analysis of action understanding in prereaching infants. Psychol. Bull. 149 , 294–310 (2023).
Download references
Acknowledgements
The authors acknowledge their funding sources: the Alexander von Humboldt Foundation, the Templeton World Charity Foundation (0434), the John Templeton Foundation (6145), the Defense Advanced Research Projects Agency (047498-002 Machine Common Sense), the Department of Defense Multidisciplinary University Initiative (Self-Learning perception Through Real World Interaction), and the Canadian Institute for Advanced Research Catalyst Award. For helpful discussions, comments, and other forms of support, the authors thank: S. Boardman, E. Bonawitz, B. Brast-McKie, D. Buchsbaum, M. Deigan, J. Engelmann, T. Friend, T. Gerstenberg, S. Kikkert, A. Kratzer, E. Lapidow, B. Leahy, T. Lombrozo, J. Phillips, H. Rakoczy, L. Schulz, D. Sobel, E. Spelke, H. Steward, B. Vetter, M. Waldmann and E. Yiu.
Author information
Authors and affiliations.
Department of Philosophy, Stanford University, Stanford, CA, USA
Mariel K. Goddu
Institut für Philosophie, Freie Universität Berlin, Berlin, Germany
Centre for Advanced Study in the Humanities: Human Abilities, Berlin, Germany
Department of Psychology, University of California, Berkeley, Berkeley, CA, USA
Alison Gopnik
Department of Philosophy Affiliate, University of California, Berkeley, Berkeley, CA, USA
You can also search for this author in PubMed Google Scholar
Contributions
M.K.G. wrote the article and created the figures. A.G. contributed substantially to discussion of the content and to multiple revisions. A.G. and M.K.G. together reviewed and edited the manuscript before submission.
Corresponding authors
Correspondence to Mariel K. Goddu or Alison Gopnik .
Ethics declarations
Competing interests.
The authors declare no competing interests.
Peer review
Peer review information.
Nature Reviews Psychology thanks Jonathan Kominsky and the other, anonymous, reviewer(s) for their contribution to the peer review of this work.
Additional information
Publisher’s note Springer Nature remains neutral with regard to jurisdictional claims in published maps and institutional affiliations.
Rights and permissions
Springer Nature or its licensor (e.g. a society or other partner) holds exclusive rights to this article under a publishing agreement with the author(s) or other rightsholder(s); author self-archiving of the accepted manuscript version of this article is solely governed by the terms of such publishing agreement and applicable law.
Reprints and permissions
About this article
Cite this article.
Goddu, M.K., Gopnik, A. The development of human causal learning and reasoning. Nat Rev Psychol 3 , 319–339 (2024). https://doi.org/10.1038/s44159-024-00300-5
Download citation
Accepted : 11 March 2024
Published : 26 April 2024
Issue Date : May 2024
DOI : https://doi.org/10.1038/s44159-024-00300-5
Share this article
Anyone you share the following link with will be able to read this content:
Sorry, a shareable link is not currently available for this article.
Provided by the Springer Nature SharedIt content-sharing initiative
Quick links
- Explore articles by subject
- Guide to authors
- Editorial policies
Sign up for the Nature Briefing newsletter — what matters in science, free to your inbox daily.

PHIL102: Introduction to Critical Thinking and Logic
Causal Reasoning
Read this section to investigate the complications of causality, particularly as it relates to correlation. Sometimes, two correlated events share a common cause, and sometimes, correlation is accidental. Complete the exercises to practice determining sufficient evidence for causation and determining accidental correlation. Check your answers against the key.
Causal reasoning
When I strike a match, it will produce a flame. It is natural to take the striking of the match as the cause that produces the effect of a flame. But what if the matchbook is wet? Or what if I happen to be in a vacuum in which there is no oxygen (such as in outer space)? If either of those things is the case, then the striking of the match will not produce a flame. So it isn't simply the striking of the match that produces the flame, but a combination of the striking of the match together with a number of other conditions that must be in place in order for the striking of the match to create a flame. Which of those conditions we call the "cause" depends in part on the context. Suppose that I'm in outer space striking a match (suppose I'm wearing a space suit that supplies me with oxygen but that I'm striking the match in space, where there is no oxygen). I continuously strike it but no flame appears (of course). But then someone (also in a space suit) brings out a can of compressed oxygen that they spray on the match while I strike it. All of a sudden a flame is produced. In this context, it looks like it is the spraying of oxygen that causes flame, not the striking of the match. Just as in the case of the striking of the match, any cause is more complex than just a simple event that produces some other event. Rather, there are always multiple conditions that must be in place for any cause to occur. These conditions are called background conditions . That said, we often take for granted the background conditions in normal contexts and just refer to one particular event as the cause. Thus, we call the striking of the match the cause of the flame. We don't go on to specify all the other conditions that conspired to create the flame (such as the presence of oxygen and the absence of water). But this is more for convenience than correctness. For just about any cause, there are a number of conditions that must be in place in order for the effect to occur. These are called necessary conditions (recall the discussion of necessary and sufficient conditions from chapter 2, section 2.7). For example, a necessary condition of the match lighting is that there is oxygen present. A necessary condition of a car running is that there is gas in the tank. We can use necessary conditions to diagnose what has gone wrong in cases of malfunction. That is, we can consider each condition in turn in order to determine what caused the malfunction. For example, if the match doesn't light, we can check to see whether the matches are wet. If we find that the matches are wet then we can explain the lack of the flame by saying something like, "dropping the matches in the water caused the matches not to light". In contrast, a sufficient condition is one which, if present, will always bring about the effect. For example, a person being fed through an operating wood chipper is sufficient for causing that person's death (as was the fate of Steve Buscemi's character in the movie Fargo).
Because the natural world functions in accordance with natural laws (such as the laws of physics), causes can be generalized. For example, any object near the surface of the earth will fall towards the earth at 9.8 m/s2 unless impeded by some contrary force (such as the propulsion of a rocket). This generalization applies to apples, rocks, people, wood chippers and every other object. Such causal generalizations are often parts of explanations. For example, we can explain why the airplane crashed to the ground by citing the causal generalization that all unsupported objects fall to the ground and by noting that the airplane had lost any method of propelling itself because the engines had died. So we invoke the causal generalization in explaining why the airplane crashed. Causal generalizations have a particular form:
For any x, if x has the feature(s) F , then x has the feature G
For example:
For any human, if that human has been fed through an operating wood chipper , then that human is dead .
For any engine, if that engine has no fuel , then that engine will not operate .
For any object near the surface of the earth, if that object is unsupported and not impeded by some contrary force , then that object will fall towards the earth at 9.8 m/s 2 .
Being able to determine when causal generalizations are true is an important part of becoming a critical thinker. Since in both scientific and every day contexts we rely on causal generalizations in explaining and understanding our world, the ability to assess when a causal generalization is true is an important skill. For example, suppose that we are trying to figure out what causes our dog, Charlie, to have seizures. To simplify, let's suppose that we have a set of potential candidates for what causes his seizures. It could be either:
or some combination of these things. Suppose we keep a log of when these things occur each day and when his seizures (S) occur. In the table below, I will represent the absence of the feature by a negation. So in the table below, "~A" represents that Charlie did not eat human food on that day; "~B" represents that he did not get a bath and shampoo that day; "~S" represents that he did not have a seizure that day. In contrast, "B" represents that he did have a bath and shampoo, whereas "C" represents that he was given a flea treatment that day. Here is how the log looks:
How can we use this information to determine what might be causing Charlie to have seizures? The first thing we'd want to know is what feature is present every time he has a seizure. This would be a necessary (but not sufficient) condition. And that can tell us something important about the cause. The necessary condition test says that any candidate feature (here A, B, C, or D) that is absent when the target feature (S) is present is eliminated as a possible necessary condition of S.3 In the table above, A is absent when S is present, so A can't be a necessary condition (i.e., day 1). D is also absent when S is present (day 4) so D can't be a necessary condition either. In contrast, B is never absent when S is present—that is every time S is present, B is also present. That means B is a necessary condition, based on the data that we have gathered so far. The same applies to C since it is never absent when S is present. Notice that there are times when both B and C are absent, but on those days the target feature (S) is absent as well, so it doesn't matter.
The next thing we'd want to know is which feature is such that every time it is present, Charlie has a seizure. The test that is relevant to determining this is called the sufficient condition test. The sufficient condition test says that any candidate that is present when the target feature (S) is absent is eliminated as a possible sufficient condition of S. In the table above, we can see that no one candidate feature is a sufficient condition for causing the seizures since for each candidate (A, B, C, D) there is a case (i.e. day) where it is present but that no seizure occurred. Although no one feature is sufficient for causing the seizures (according to the data we have gathered so far), it is still possible that certain features are jointly sufficient . Two candidate features are jointly sufficient for a target feature if and only if there is no case in which both candidates are present and yet the target is absent. Applying this test, we can see that B and C are jointly sufficient for the target feature since any time both are present, the target feature is always present. Thus, from the data we have gathered so far, we can say that the likely cause of Charlie's seizures are when we both give him a bath and then follow that bath up with a flea treatment. Every time those two things occur, he has a seizure (sufficient condition); and every time he has a seizure, those two things occur (necessary condition). Thus, the data gathered so far supports the following causal conditional:
Any time Charlie is given a shampoo bath and a flea treatment, he has a seizure.
Although in the above case, the necessary and sufficient conditions were the same, this needn't always be the case. Sometimes sufficient conditions are not necessary conditions. For example, being fed through a wood chipper is a sufficient condition for death, but it certainly isn't necessary! (Lot's of people die without being fed through a wood chipper, so it can't be a necessary condition of dying). In any case, determining necessary and sufficient conditions is a key part of determining a cause.
When analyzing data to find a cause it is important that we rigorously test each candidate. Here is an example to illustrate rigorous testing. Suppose that on every day we collected data about Charlie he ate human food but that on none of the days was he given a bath and shampoo, as the table below indicates.
Given this data, A trivially passes the necessary condition test since it is always present (thus, there can never be a case where A is absent when S is present). However, in order to rigorously test A as a necessary condition, we have to look for cases in which A is not present and then see if our target condition S is present. We have rigorously tested A as a necessary condition only if we have collected data in which A was not present. Otherwise, we don't really know whether A is a necessary condition. Similarly, B trivially passes the sufficient condition test since it is never present (thus, there can never be a case where B is present but S is absent). However, in order to rigorously test B as a sufficient condition, we have to look for cases in which B is present and then see if our target condition S is absent. We have rigorously tested B as a sufficient condition only if we have collected data in which B is present. Otherwise, we don't really know whether B is a sufficient condition or not.
In rigorous testing , we are actively looking for (or trying to create) situations in which a candidate feature fails one of the tests. That is why when rigorously testing a candidate for the necessary condition test, we must seek out cases in which the candidate is not present, whereas when rigorously testing a candidate for the sufficient condition test, we must seek out cases in which the candidate is present. In the example above, A is not rigorously tested as a necessary condition and B is not rigorously tested as a sufficient condition. If we are interested in finding a cause, we should always rigorously test each candidate. This means that we should always have a mix of different situations where the candidates and targets are sometimes present and sometimes absent.
The necessary and sufficient conditions tests can be applied when features of the environment are wholly present or wholly absent. However, in situations where features of the environment are always present in some degree, these tests will not work (since there will never be cases where the features are absent and so rigorous testing cannot be applied). For example, suppose we are trying to figure out whether CO 2 is a contributing cause to higher global temperatures. In this case, we can't very well look for cases in which CO 2 is present but high global temperatures aren't (sufficient condition test), since CO 2 and high temperatures are always present to some degree. Nor can we look for cases in which CO 2 is absent when high global temperatures are present (necessary condition test), since, again, CO 2 and high global temperatures are always present to some degree. Rather, we must use a different method, the method that J.S. Mill called the method of concomitant variation . In concomitant variation we look for how things vary vis-à-vis each other. For example, if we see that as CO 2 levels rise, global temperatures also rise, then this is evidence that CO 2 and higher temperatures are positively correlated. When two things are positively correlated , as one increases, the other also increases at a similar rate (or as one decreases, the other decreases at a similar rate). In contrast, when two things are negatively correlated , as one increases, the other decreases at similar rate (or vice versa). For example, if as a police department increased the number of police officers on the street, the number of crimes reported decreases, then number of police on the street and number of crimes reported would be negatively correlated. In each of these examples, we may think we can directly infer the cause from the correlation - the rising CO2 levels are causing the rising global temperatures and the increasing number of police on the street is causing the crime rate to drop. However, we cannot directly infer causation from correlation. Correlation is not causation. If A and B are positively correlated, then there are four distinct possibilities regarding what the cause is:
- A is the cause of B
- B is the cause of A
- Some third thing, C, is the cause of both A and B increasing
- The correlation is accidental
In order to infer what causes what in a correlation, we must rely on our general background knowledge (i.e., things we know to be true about the world), our scientific knowledge, and possibly further scientific testing. For example, in the global warming case, there is no scientific theory that explains how rising global temperatures could cause rising levels of CO 2 but there is a scientific theory that enables us to understand how rising levels of CO 2 could increase average global temperatures. This knowledge makes it plausible to infer that the rising CO 2 levels are causing the rising average global temperatures. In the police/crime case, drawing on our background knowledge we can easily come up with an inference to the best explanation argument for why increased police presence on the streets would lower the crime rate - the more police on the street, the harder it is for criminals to get away with crimes because there are fewer places where those crimes could take place without the criminal being caught. Since criminals don't want to risk getting caught when they commit a crime, seeing more police around will make them less likely to commit a crime. In contrast, there is no good explanation for why decreased crime would cause there to be more police on the street. In fact, it would seem to be just the opposite: if the crime rate is low, the city should cut back, or at least remain stable, on the number of police officers and put those resources somewhere else. This makes it plausible to infer that it is the increased police officers on the street that is causing the decrease in crime.
Sometimes two things can be correlated without either one causing the other. Rather, some third thing is causing them both. For example, suppose that Bob discovers a correlation between waking up with all his clothes on and waking up with a headache. Bob might try to infer that sleeping with all his clothes on causes headaches, but there is probably a better explanation than that. It is more likely that Bob's drinking too much the night before caused him to pass out in his bed with all his clothes on, as well as his headache. In this scenario, Bob's inebriation is the common cause of both his headache and his clothes being on in bed.
Sometimes correlations are merely accidental, meaning that there is no causal relationship between them at all. For example, Tyler Vigen4 reports that the per capita consumption of cheese in the U.S. correlates with the number of people who die by becoming entangled in their bedsheets:

And the number of Mexican lemons imported to the U.S. correlates with the number of traffic fatalities:

Clearly neither of these correlations are causally related at all - they are accidental correlations . What makes them accidental is that we have no theory that would make sense of how they could be causally related. This just goes to show that it isn't simply the correlation that allows us to infer a cause, but, rather, some additional background theory, scientific theory, or other evidence that establishes one thing as causing another. We can explain the relationship between correlation and causation using the concepts of necessary and sufficient conditions (first introduced in chapter 2): correlation is a necessary condition for causation, but it is not a sufficient condition for causation.
Our discussion of causes has shown that we cannot say that just because A precedes B or is correlated with B, that A caused B. To claim that since A precedes or correlates with B, A must therefore be the cause of B is to commit what is called the false cause fallacy . The false cause fallacy is sometimes called the "post hoc" fallacy. "Post hoc" is short for the Latin phrase, "post hoc ergo propter hoc," which means "after this therefore because of this". As we've seen, false cause fallacies occur any time someone assumes that two events that are correlated must be in a causal relationship, or that since one event precedes another, it must cause the other. To avoid the false cause fallacy, one must look more carefully into the relationship between A and B to determine whether there is a true cause or just a common cause or accidental correlation. Common causes and accidental correlations are more common than one might think.

MIT Root Cause Analysis
You are here, using causal thinking to solve problems.
Causal thinking can be used to examine a host of issue or negatives events, from those with a big impact to smaller recurring problems that hint at systemic problems. But how do we think causally in a less formal context, or in our everyday meetings? This doesn't have to be too structured, but you can take a page from how we approach problem solving in formal root cause analyses:
- Establish the ground rules and agree that you're there to find causes and not point fingers.
- Have someone take charge of facilitating the discussion and someone who's willing to take notes.
- Agree on how to define what the problem that occured is before you begin solving it.
- Openly share what you know - each of you potentially holds a piece of the puzzle.
- This is a creative process so be open to thinking about the problem in a new way.
- Respect each other's ideas and time.
- Don't be afraid of turning over every stone as you brainstorm what might have caused the issue.
- Come up with some reasonable suggestions on how to eliminate the problem, or ways of minimizing it if it recurrs.
RCA Toolbox
- Process Map
- Best Practices
- Case Studies
- Video Resources

An official website of the United States government
The .gov means it’s official. Federal government websites often end in .gov or .mil. Before sharing sensitive information, make sure you’re on a federal government site.
The site is secure. The https:// ensures that you are connecting to the official website and that any information you provide is encrypted and transmitted securely.
- Publications
- Account settings
Preview improvements coming to the PMC website in October 2024. Learn More or Try it out now .
- Advanced Search
- Journal List
- Korean J Med Educ
- v.31(4); 2019 Dec
Reasoning processes in clinical reasoning: from the perspective of cognitive psychology
Hyoung seok shin.
Department of Medical Education, Korea University College of Medicine, Seoul, Korea
Clinical reasoning is considered a crucial concept in reaching medical decisions. This paper reviews the reasoning processes involved in clinical reasoning from the perspective of cognitive psychology. To properly use clinical reasoning, one requires not only domain knowledge but also structural knowledge, such as critical thinking skills. In this paper, two types of reasoning process required for critical thinking are discussed: inductive and deductive. Inductive and deductive reasoning processes have different features and are generally appropriate for different types of tasks. Numerous studies have suggested that experts tend to use inductive reasoning while novices tend to use deductive reasoning. However, even experts sometimes use deductive reasoning when facing challenging and unfamiliar problems. In clinical reasoning, expert physicians generally use inductive reasoning with a holistic viewpoint based on a full understanding of content knowledge in most cases. Such a problem-solving process appears as a type of recognition-primed decision making only in experienced physicians’ clinical reasoning. However, they also use deductive reasoning when distinct patterns of illness are not recognized. Therefore, medical schools should pursue problem-based learning by providing students with various opportunities to develop the critical thinking skills required for problem solving in a holistic manner.
Introduction
It is hard to describe clinical reasoning in a sentence, because it has been studied by a number of researchers from various perspectives, such as medical education, cognitive psychology, clinical psychology, and so forth, and they have failed to reach an agreement on its basic characteristics [ 1 ]. Accordingly, clinical reasoning has been defined in various ways. Some researchers defined clinical reasoning as a crucial skill or ability that all physicians should have for their clinical decision making, regardless of their area of expertise [ 2 , 3 ]. Others focused more on the processes of clinical reasoning; thus, they defined it as a complex process of identifying the clinical issues to propose a treatment plan [ 4 - 6 ]. However, these definitions are not so different. Taking this into account, it can be concluded that clinical reasoning is used to analyze patients’ status and arrive at a medical decision so that doctors can provide the proper medical treatment.
In reality, properly working clinical reasoning requires three domains of knowledge: diagnostic knowledge, etiological knowledge, and treatment knowledge [ 6 ]. From the perspective of cognitive psychology, structural knowledge is needed to integrate domain knowledge and find solutions based on the learner’s prior knowledge and experience [ 7 ], and structural knowledge can be constructed as a form of mental model by understanding the relations between the interconnected factors involved in clinical issues [ 8 , 9 ]. In this cognitive process, critical thinking skills such as causal reasoning and systems thinking can play a pivotal role in developing deeper understanding of given problem situations. Causal reasoning is the ability to identify causal relationships between sets of causes and effects [ 10 ]. Causality often involves a series or chain of events that can be used to infer or predict the effects and consequences of a particular cause [ 10 - 13 ]. Systems thinking is a thinking paradigm or conceptual framework where understanding is defined in terms of how well one is able to break a complex system down into its component parts [ 14 , 15 ]. It is based on the premise that a system involves causality between factors that are parts of the system as a whole [ 14 ]. Systems thinking is a process for achieving a deeper understanding of complex phenomena that are composed of components that are causally interrelated [ 14 - 16 ]. As a result, causal reasoning and systems thinking are skills that can help people to better understand complex phenomena in order to arrive at effective and targeted solutions that address the root causes of complex problems [ 10 , 12 , 15 ].
If cognitive skills work properly, one can make correct decisions all of the time. However, human reasoning is not always logical, and people often make mistakes in their reasoning. The more difficult the problems with which they are presented, the more likely they are to choose wrong answers that are produced by errors or flaws in the reasoning process [ 17 , 18 ]. Individual differences in reasoning skills—such as systems thinking, causal reasoning, and thinking processes—may influence and explain observed differences in their understanding. Therefore, to better assist learners in solving problems, instructors should focus more on facilitating the reasoning skills required to solve given problems successfully.
In this review paper, the author focuses on the reasoning processes involved in clinical reasoning, given that clinical reasoning is considered as a sort of problem-solving process. Therefore, this paper introduces concepts related to the reasoning processes involved in clinical reasoning and their influences on novices and experts in the field of medical education from the perspective of cognitive psychology. Then, based on the contents discussed, the author will be able to propose specific instructional strategies associated with reasoning processes to improve medical students’ reasoning skills to enhance their clinical reasoning.
Concepts and nature of reasoning processes
Generally, reasoning processes can be categorized into two types: inductive/forward and deductive/backward [ 19 ]. In an inductive reasoning process, one observes several individual facts first, then makes a conclusion about a premise or principle based on these facts. Yet there may be the possibility that a conclusion is not true even though a premise or principle in support of that conclusion is true, because the conclusion is generalized from the facts observed by the learner, but the learner does not observe all relevant examples [ 20 ].
In general, in a deductive reasoning process, according to Johnson-Laird [ 20 ], one establishes a mental model or a set of models to solve given problems considering general knowledge and principles based on a solid foundation. Then, one makes a conclusion or finds a solution based on the mental model or set of models. To verify a mental model, one needs to check the validity of the conclusions or solutions by searching for counterexamples. If one cannot find any counterexamples, the conclusions can be accepted as true and the solutions as valid. Consequently, the initial mental model or set of models can be used for deductive reasoning.
Anderson [ 17 ] proposed three different ways of solving complex problems: means-ends analysis, working backward, and planning by simplification. A means-ends analysis is a process that gets rid of differences between the current state and the ideal state in order to determine sub-goals in solving problems, and the process can be repeated until the major goal is achieved [ 21 - 23 ]. It can be considered an inductive reasoning process, because the distinct feature of means-ends analysis where it achieves sub-goals in consecutive order is similar to inductive reasoning. Working backward is addressed as an opposite concept to means-ends analysis [ 17 ], because it needs to set up a desired result to find causes by measuring the gap between the current state and the ideal state; then, this process is repeated until the root causes of a problem are identified. According to Anderson [ 17 ], means-ends analysis (inductive reasoning) is more useful in finding a solution quickly when a limited number of options are given or many sub-goals should be achieved for the major goal; whereas working backward (deductive reasoning) spends more time removing wrong answers or inferences to find the root causes of a problem. In conclusion, inductive and deductive reasoning processes have different features and can play different roles in solving complex problems.
The use of reasoning processes
A number of researchers across different fields have used inductive and deductive approaches as reasoning processes to solve complex problems or complete tasks. For example, Scavarda et al. [ 24 ] used both approaches in their study to collect qualitative data through interviews with experts, and they found that experts with a deductive approach used a top-down approach and those with an inductive approach used a bottom-up approach to solve a given problem. In a study of Overmars et al. [ 25 ], the results showed that a deductive approach explicitly illustrated causal relations and processes in 39 geographic contexts and it was appropriate for evaluating various possible scenarios; whereas an inductive approach presented associations that did not guarantee causality and was more useful for identifying relatively detailed changes.
Sharma et al. [ 26 ] found that inductive or deductive approaches can both be useful depending on the characteristics of the tasks and resources available to solve problems. An inductive approach is considered a data-driven approach, which is a way to find possible outcomes based on rules detected from undoubted facts [ 26 ]. Therefore, if there is a lot of available data and an output hypothesis, then it is effective to use an inductive approach to discover solutions or unexpected and interesting findings [ 26 , 27 ]. An inductive approach makes it possible to directly reach conclusions via thorough reasoning that involves the following procedures: (1) recognize, (2) select, and (3) act [ 28 ]. These procedures are recurrent, but one cannot know how long they should be continued to complete a task, because a goal is not specified [ 26 ]. Consequently, an inductive approach is useful when analyzing an unstructured data set or system [ 29 ].
On the other hand, a deductive approach sets up a desired goal first, then finds a supporting basis—such as information and rules—for the goals [ 26 ]. For this, a backward approach, which is considered deductive reasoning, gradually gets rid of things proved unnecessary for achieving the goal while reasoning; therefore, it is regarded as a goal-driven approach [ 28 ]. If the output hypothesis is limited and it is necessary to find supporting facts from data, then a deductive approach would be effective [ 26 , 28 ]. This implies that a deductive approach is more appropriate when a system or phenomenon is well-structured and relationships between the components are clearly present [ 29 ]. Table 1 shows a summary of the features and differences of the inductive and deductive reasoning processes.
Features of Inductive and Deductive Reasoning Processes
The classification according to the reasoning processes in the table is dichotomous, but they do not always follow this classification absolutely. This means that each reasoning process shows such tendencies.
Considering the attributes of the two reasoning processes, an inductive approach is effective for exploratory tasks that do not have distinct goals—for example, planning, design, process monitoring, and so on, while a deductive approach is more useful for diagnostic and classification tasks [ 26 ]. In addition, an inductive approach is more useful for discovering solutions from an unstructured system. On the other hand, a deductive approach can be better used to identify root causes in a well-structured context. While both reasoning approaches are useful in particular contexts, it can be suggested that inductive reasoning is more appropriate than deductive reasoning in clinical situations, which focus on diagnosis and treatment of diseases rather than on finding their causes.
Reasoning processes by novices and experts
As mentioned above, which reasoning process is more effective for reaching conclusions can be generally determined depending on the context and purpose of the problem solving. In reality, however, learners’ choices are not always consistent with this suggestion, because they are affected not only by the problem itself, but also by the learner. Assuming that learners or individuals can be categorized into two types, novices and experts, based on their level of prior knowledge and structural knowledge, much research has shown that novices and experts use a different reasoning process for problem solving. For example, in a study of Eseryel et al. [ 30 ], novice instructional designers who possessed theoretical knowledge but little experience showed different patterns of ill-structured problem solving compared to experts with real-life experience. Given that each learner has a different level of prior knowledge relating to particular topics and critical thinking skills, selecting the proper reasoning process for each problem is quite complex. This section focuses on which reasoning process an individual uses depending on their content and structural knowledge.
Numerous studies have examined which reasoning processes are used by experts, who have sufficient content and structural knowledge, and novices, who have little content and structural knowledge, for problem solving. The result of a study of Hong et al. [ 31 ] showed that children generally performed better when using cause-effect inferences (inductive approach) than effect-cause inferences (considered a deductive approach). According to Anderson [ 17 ], people are faced with some difficulties when they solve problems using induction. The first difficulty is in formulating proper hypotheses and the second is that people do not know how to interpret negative evidence when it is given and reach a conclusion based on that evidence [ 17 ]. Nevertheless, most students use a type of inductive reasoning to solve problems that they have not previously faced [ 32 ]. Taken together, the studies suggest that novices generally prefer an inductive approach to a deductive approach for solving problems because they may feel comfortable and natural using an inductive approach but tend to experience difficulties during problem-solving processes. From these findings, it can be concluded that novices are more likely to use inductive reasoning, but it is not always productive.
Nevertheless, there is still a controversy about which reasoning processes are used by experts or novices [ 33 ]. For example, experts in specific domains use an inductive approach to solving problems, but novices, who have a lower level of prior knowledge in specific domains, tend to use a deductive approach [ 23 ]. In contrast, according to Smith [ 34 ], studies in which more familiar problems were used concluded that experts preferred an inductive approach, whereas in studies that employed relatively unfamiliar problems that required more time and effort to solve, experts tended to prefer a deductive approach. In line with this finding, in solving physics problems, experts mostly used inductive reasoning that was faster and had fewer errors for problem solving only when they encountered easy or familiar problems where they could gain a full understanding of the situation quickly, but novices took more time to deductively reason by planning and solving each step in the process of problem solving [ 35 ].
Assuming that an individual’s prior knowledge consists of content knowledge such as knowledge of specific domains as well as structural knowledge such as the critical thinking skills required for problem solving in the relevant field, it seems experts use an inductive approach when faced with relatively easy or familiar problems; while a deductive approach is used for relatively challenging, unfamiliar, or complex problems. In the case of novices, it may be better to use deductive reasoning for problem solving considering that they have a lower level of prior knowledge and that even experts use deductive reasoning to solve complex problems.
Inductive and deductive reasoning in clinical reasoning
In medicine, concepts of inductive and deductive reasoning apply to gathering appropriate information and making a clinical diagnosis considering that the medical treatment process is a form of problem solving. Inductive reasoning is used to make a diagnosis by starting with an analysis of observed clinical data [ 36 , 37 ]. Inductive reasoning is considered as scheme-inductive problem solving in medicine [ 36 ], because in inductive reasoning, one first constructs his/her scheme (also considered a mental model) based on one’s experiences and knowledge. It is generally used for a clinical presentation-based model, which has been most recently applied to medical education [ 38 ].
In contrast, deductive reasoning entails making a clinical diagnosis by testing hypotheses based on systematically collected data [ 39 ]. Deductive reasoning is considered an information-gathering method, because one constructs a hypothesis first then finds supporting or refuting facts from data [ 36 , 40 ]. It has been mostly used for discipline-based, system-based, and case-based models in medical education [ 38 ].
Inductive and deductive reasoning by novice and expert physicians
A growing body of research explores which reasoning processes are mainly used by novices and experts in clinical reasoning. Novice physicians generally use deductive reasoning, because limited knowledge restricts them from using deductive reasoning [ 1 , 38 ]. Also, it is hard to consider deductive reasoning as an approach generally used by experts, since they do not repeatedly test a hypothesis based on limited knowledge in order to move on to the next stage in the process of problem solving [ 38 ]. Therefore, it seems that deductive reasoning is generally used by novices, while inductive reasoning is used by expert physicians in general. However, this may be too conclusive and needs to be further examined in the context of clinical reasoning.
In clinical reasoning, inductive reasoning is more intuitive and requires a holistic view based on a full understanding of content knowledge, including declarative and procedural knowledge, but also structural knowledge; thus, it occurs only when physicians’ knowledge structures of given problems are highly organized [ 38 ]. Expert physicians recognize particular patterns of symptoms through repeated application of deductive reasoning, and the pattern recognition process makes it possible for them to apply inductive reasoning when diagnosing patients [ 10 ]. As experts automate a number of cognitive sequences required for problem solving in their own fields [ 35 ], expert physicians automatically make appropriate diagnoses following a process of clinical reasoning when they encounter patients who have familiar or typical diseases. Such a process of problem solving is called recognition-primed decision making (RPDM) [ 41 , 42 ]. It is a process of finding appropriate solutions to ill-structured problems in a limited timeframe [ 10 ]. In RPDM, expert physicians are aware of what actions should be taken when faced with particular situations based on hundreds of prior experiences [ 10 ]. These prior experiences are called illness scripts in diagnostic medicine [ 10 ], and this is a concept similar to a mental model or schema in problem solving.
However, expert physicians do not always use inductive reasoning in their clinical reasoning. Jonassen [ 10 ] categorized RPDM into three forms of variations in problem solving by experts, and the first form of variation is the simplest and easiest one based on inductive reasoning, as mentioned above. The second type of variation occurs when an encountered problem is somewhat atypical [ 10 ]. Even expert physicians are not always faced with familiar or typical diseases when treating patients. Expert physicians’ RPDM does not work automatically when faced with atypical symptoms, because they do not have sufficient experiences relevant to the atypical symptoms. In this case, it can be said that they have weak illness scripts or mental models of the given symptoms. In the second variation, experts need more information and will attempt to connect it to their prior knowledge and experiences [ 10 ]. Deductive reasoning is involved in this process so that problem solvers can test their hypotheses in order to find new patterns and construct new mental models based on the newly collected data and previous experiences. The third variation of RPDM is when expert physicians have no previous experience or prior knowledge of given problem situations; in other words, no illness script or mental model [ 10 ]. Jonassen [ 10 ] argued that a mental simulation is conducted to predict the consequences of various actions by experts in the third variation. This process inevitably involves repetitive deductive reasoning to test a larger number of hypotheses when making a diagnosis.
Similarly, from the perspective of dual process theory as a decision-making process, decision making is classified into two approaches based on the reasoning style: type 1 and type 2 (or system 1 and system 2) [ 43 , 44 ]. According to Croskerry [ 44 ], the type 1 decision-making process is intuitive and based on experiential-inductive reasoning, while type 2 is an analytical and hypothetico-deductive decision-making process [ 44 , 45 ]. A feature that distinguishes the two processes is whether a physician who encounters a patient’s symptoms succeeds in pattern recognition. If a physician recognizes prominent features of the visual presentation of illness, type 1 processes (or system 1) are operated automatically, whereas type 2 (or system 2) processes work if any distinct feature of illness presentation is not recognized [ 44 ].
Only experienced expert physicians can use RPDM [ 10 , 46 ] or type 1 and 2 processes [ 43 ], because it can occur solely based on various experiences and a wide range of prior knowledge that can be gained as a result of a huge amount of deductive reasoning since they were novices. Consequently, it can be concluded that expert physicians generally use more inductive reasoning when they automatically recognize key patterns of given problems or symptoms, while sometimes they also use deductive reasoning when they additionally need processes of hypothesis testing to recognize new patterns of symptoms.
From the perspective of cognitive processes, clinical reasoning is considered as one of the decision-making processes that finds the best solutions to patients’ illnesses. As a form of decision making for problem solving, two reasoning processes have been considered: inductive and deductive reasoning. Deductive reasoning can be used to make a diagnosis if physicians have insufficient knowledge, sufficient time, and the ability to analyze the current status of their patients. However, in reality, it is inefficient to conduct thorough deductive reasoning at each stage of clinical reasoning because only a limited amount of time is allowed for both physicians and patients to reach a conclusion in most cases. A few researchers have suggested that using deductive reasoning is more likely to result in diagnostic errors than inductive reasoning, because evidence-based research, such as deductive reasoning, focuses mainly on available and observable evidence and rules out the possibility of any other possible factors influencing the patient’s symptoms [ 37 , 38 ]. However, when a physician encounters unfamiliar symptom and the degree of uncertainty is high, deductive reasoning is required to reach the correct diagnosis through analytical and slow diagnostic processes by collecting data from resources [ 44 ]. Taken together, in order to make the most of a limited timeframe and reduce diagnostic errors, physicians should be encouraged to use inductive reasoning in their clinical reasoning as far as possible given that patterns of illness presentation are recognized.
Unfortunately, it is not always easy for novice physicians to apply inductive or deductive reasoning in all cases. Expert physicians have sufficient capabilities to use both inductive and deductive reasoning and can also automate their clinical reasoning based on inductive reasoning, because they have already gathered the wide range of experiences and knowledge required to diagnose various symptoms. Novice physicians should make a greater effort to use inductive reasoning when making diagnoses; however, it takes experiencing countless deductive reasoning processes to structure various illness scripts or strong mental models until they reach a professional level. As a result, teaching not only clinical reasoning as a whole process but also the critical thinking skills required for clinical reasoning is important in medical schools [ 47 ]. For this, medical schools should pursue problem-based learning by providing students with various opportunities to gain content knowledge as well as develop the critical thinking skills —such as data analysis skills, metacognitive skills, causal reasoning, systems thinking, and so forth—required for problem solving in a holistic manner so that they can improve their reasoning skills and freely use both inductive and deductive approaches in any context. Further studies will be reviewed to provide detailed guidelines or teaching tips on how to develop medical students’ critical thinking skills.
Acknowledgments
Conflicts of interest
No potential conflict of interest relevant to this article was reported.
Author contributions
All work was done by HS.

Want to create or adapt books like this? Learn more about how Pressbooks supports open publishing practices.
Chapter 6. Causal Reasoning
Forthcoming – draft chapter outline.
- Necessary & Sufficient Conditions
- Aristotle’s Categories of Causes
- Mill’s Methods of Discovery
- Realism & Antirealism
- Raven’s Paradox
- Underdetermination
How to Think For Yourself Copyright © 2023 by Rebeka Ferreira, Anthony Ferrucci is licensed under a Creative Commons Attribution-NonCommercial-NoDerivatives 4.0 International License , except where otherwise noted.
Share This Book
- Search Menu
- Browse content in Arts and Humanities
- Browse content in Archaeology
- Anglo-Saxon and Medieval Archaeology
- Archaeological Methodology and Techniques
- Archaeology by Region
- Archaeology of Religion
- Archaeology of Trade and Exchange
- Biblical Archaeology
- Contemporary and Public Archaeology
- Environmental Archaeology
- Historical Archaeology
- History and Theory of Archaeology
- Industrial Archaeology
- Landscape Archaeology
- Mortuary Archaeology
- Prehistoric Archaeology
- Underwater Archaeology
- Urban Archaeology
- Zooarchaeology
- Browse content in Architecture
- Architectural Structure and Design
- History of Architecture
- Residential and Domestic Buildings
- Theory of Architecture
- Browse content in Art
- Art Subjects and Themes
- History of Art
- Industrial and Commercial Art
- Theory of Art
- Biographical Studies
- Byzantine Studies
- Browse content in Classical Studies
- Classical History
- Classical Philosophy
- Classical Mythology
- Classical Literature
- Classical Reception
- Classical Art and Architecture
- Classical Oratory and Rhetoric
- Greek and Roman Papyrology
- Greek and Roman Epigraphy
- Greek and Roman Law
- Greek and Roman Archaeology
- Late Antiquity
- Religion in the Ancient World
- Digital Humanities
- Browse content in History
- Colonialism and Imperialism
- Diplomatic History
- Environmental History
- Genealogy, Heraldry, Names, and Honours
- Genocide and Ethnic Cleansing
- Historical Geography
- History by Period
- History of Emotions
- History of Agriculture
- History of Education
- History of Gender and Sexuality
- Industrial History
- Intellectual History
- International History
- Labour History
- Legal and Constitutional History
- Local and Family History
- Maritime History
- Military History
- National Liberation and Post-Colonialism
- Oral History
- Political History
- Public History
- Regional and National History
- Revolutions and Rebellions
- Slavery and Abolition of Slavery
- Social and Cultural History
- Theory, Methods, and Historiography
- Urban History
- World History
- Browse content in Language Teaching and Learning
- Language Learning (Specific Skills)
- Language Teaching Theory and Methods
- Browse content in Linguistics
- Applied Linguistics
- Cognitive Linguistics
- Computational Linguistics
- Forensic Linguistics
- Grammar, Syntax and Morphology
- Historical and Diachronic Linguistics
- History of English
- Language Evolution
- Language Reference
- Language Acquisition
- Language Variation
- Language Families
- Lexicography
- Linguistic Anthropology
- Linguistic Theories
- Linguistic Typology
- Phonetics and Phonology
- Psycholinguistics
- Sociolinguistics
- Translation and Interpretation
- Writing Systems
- Browse content in Literature
- Bibliography
- Children's Literature Studies
- Literary Studies (Romanticism)
- Literary Studies (American)
- Literary Studies (Asian)
- Literary Studies (European)
- Literary Studies (Eco-criticism)
- Literary Studies (Modernism)
- Literary Studies - World
- Literary Studies (1500 to 1800)
- Literary Studies (19th Century)
- Literary Studies (20th Century onwards)
- Literary Studies (African American Literature)
- Literary Studies (British and Irish)
- Literary Studies (Early and Medieval)
- Literary Studies (Fiction, Novelists, and Prose Writers)
- Literary Studies (Gender Studies)
- Literary Studies (Graphic Novels)
- Literary Studies (History of the Book)
- Literary Studies (Plays and Playwrights)
- Literary Studies (Poetry and Poets)
- Literary Studies (Postcolonial Literature)
- Literary Studies (Queer Studies)
- Literary Studies (Science Fiction)
- Literary Studies (Travel Literature)
- Literary Studies (War Literature)
- Literary Studies (Women's Writing)
- Literary Theory and Cultural Studies
- Mythology and Folklore
- Shakespeare Studies and Criticism
- Browse content in Media Studies
- Browse content in Music
- Applied Music
- Dance and Music
- Ethics in Music
- Ethnomusicology
- Gender and Sexuality in Music
- Medicine and Music
- Music Cultures
- Music and Media
- Music and Religion
- Music and Culture
- Music Education and Pedagogy
- Music Theory and Analysis
- Musical Scores, Lyrics, and Libretti
- Musical Structures, Styles, and Techniques
- Musicology and Music History
- Performance Practice and Studies
- Race and Ethnicity in Music
- Sound Studies
- Browse content in Performing Arts
- Browse content in Philosophy
- Aesthetics and Philosophy of Art
- Epistemology
- Feminist Philosophy
- History of Western Philosophy
- Metaphysics
- Moral Philosophy
- Non-Western Philosophy
- Philosophy of Language
- Philosophy of Mind
- Philosophy of Perception
- Philosophy of Science
- Philosophy of Action
- Philosophy of Law
- Philosophy of Religion
- Philosophy of Mathematics and Logic
- Practical Ethics
- Social and Political Philosophy
- Browse content in Religion
- Biblical Studies
- Christianity
- East Asian Religions
- History of Religion
- Judaism and Jewish Studies
- Qumran Studies
- Religion and Education
- Religion and Health
- Religion and Politics
- Religion and Science
- Religion and Law
- Religion and Art, Literature, and Music
- Religious Studies
- Browse content in Society and Culture
- Cookery, Food, and Drink
- Cultural Studies
- Customs and Traditions
- Ethical Issues and Debates
- Hobbies, Games, Arts and Crafts
- Lifestyle, Home, and Garden
- Natural world, Country Life, and Pets
- Popular Beliefs and Controversial Knowledge
- Sports and Outdoor Recreation
- Technology and Society
- Travel and Holiday
- Visual Culture
- Browse content in Law
- Arbitration
- Browse content in Company and Commercial Law
- Commercial Law
- Company Law
- Browse content in Comparative Law
- Systems of Law
- Competition Law
- Browse content in Constitutional and Administrative Law
- Government Powers
- Judicial Review
- Local Government Law
- Military and Defence Law
- Parliamentary and Legislative Practice
- Construction Law
- Contract Law
- Browse content in Criminal Law
- Criminal Procedure
- Criminal Evidence Law
- Sentencing and Punishment
- Employment and Labour Law
- Environment and Energy Law
- Browse content in Financial Law
- Banking Law
- Insolvency Law
- History of Law
- Human Rights and Immigration
- Intellectual Property Law
- Browse content in International Law
- Private International Law and Conflict of Laws
- Public International Law
- IT and Communications Law
- Jurisprudence and Philosophy of Law
- Law and Politics
- Law and Society
- Browse content in Legal System and Practice
- Courts and Procedure
- Legal Skills and Practice
- Primary Sources of Law
- Regulation of Legal Profession
- Medical and Healthcare Law
- Browse content in Policing
- Criminal Investigation and Detection
- Police and Security Services
- Police Procedure and Law
- Police Regional Planning
- Browse content in Property Law
- Personal Property Law
- Study and Revision
- Terrorism and National Security Law
- Browse content in Trusts Law
- Wills and Probate or Succession
- Browse content in Medicine and Health
- Browse content in Allied Health Professions
- Arts Therapies
- Clinical Science
- Dietetics and Nutrition
- Occupational Therapy
- Operating Department Practice
- Physiotherapy
- Radiography
- Speech and Language Therapy
- Browse content in Anaesthetics
- General Anaesthesia
- Neuroanaesthesia
- Clinical Neuroscience
- Browse content in Clinical Medicine
- Acute Medicine
- Cardiovascular Medicine
- Clinical Genetics
- Clinical Pharmacology and Therapeutics
- Dermatology
- Endocrinology and Diabetes
- Gastroenterology
- Genito-urinary Medicine
- Geriatric Medicine
- Infectious Diseases
- Medical Toxicology
- Medical Oncology
- Pain Medicine
- Palliative Medicine
- Rehabilitation Medicine
- Respiratory Medicine and Pulmonology
- Rheumatology
- Sleep Medicine
- Sports and Exercise Medicine
- Community Medical Services
- Critical Care
- Emergency Medicine
- Forensic Medicine
- Haematology
- History of Medicine
- Browse content in Medical Skills
- Clinical Skills
- Communication Skills
- Nursing Skills
- Surgical Skills
- Browse content in Medical Dentistry
- Oral and Maxillofacial Surgery
- Paediatric Dentistry
- Restorative Dentistry and Orthodontics
- Surgical Dentistry
- Medical Ethics
- Medical Statistics and Methodology
- Browse content in Neurology
- Clinical Neurophysiology
- Neuropathology
- Nursing Studies
- Browse content in Obstetrics and Gynaecology
- Gynaecology
- Occupational Medicine
- Ophthalmology
- Otolaryngology (ENT)
- Browse content in Paediatrics
- Neonatology
- Browse content in Pathology
- Chemical Pathology
- Clinical Cytogenetics and Molecular Genetics
- Histopathology
- Medical Microbiology and Virology
- Patient Education and Information
- Browse content in Pharmacology
- Psychopharmacology
- Browse content in Popular Health
- Caring for Others
- Complementary and Alternative Medicine
- Self-help and Personal Development
- Browse content in Preclinical Medicine
- Cell Biology
- Molecular Biology and Genetics
- Reproduction, Growth and Development
- Primary Care
- Professional Development in Medicine
- Browse content in Psychiatry
- Addiction Medicine
- Child and Adolescent Psychiatry
- Forensic Psychiatry
- Learning Disabilities
- Old Age Psychiatry
- Psychotherapy
- Browse content in Public Health and Epidemiology
- Epidemiology
- Public Health
- Browse content in Radiology
- Clinical Radiology
- Interventional Radiology
- Nuclear Medicine
- Radiation Oncology
- Reproductive Medicine
- Browse content in Surgery
- Cardiothoracic Surgery
- Gastro-intestinal and Colorectal Surgery
- General Surgery
- Neurosurgery
- Paediatric Surgery
- Peri-operative Care
- Plastic and Reconstructive Surgery
- Surgical Oncology
- Transplant Surgery
- Trauma and Orthopaedic Surgery
- Vascular Surgery
- Browse content in Science and Mathematics
- Browse content in Biological Sciences
- Aquatic Biology
- Biochemistry
- Bioinformatics and Computational Biology
- Developmental Biology
- Ecology and Conservation
- Evolutionary Biology
- Genetics and Genomics
- Microbiology
- Molecular and Cell Biology
- Natural History
- Plant Sciences and Forestry
- Research Methods in Life Sciences
- Structural Biology
- Systems Biology
- Zoology and Animal Sciences
- Browse content in Chemistry
- Analytical Chemistry
- Computational Chemistry
- Crystallography
- Environmental Chemistry
- Industrial Chemistry
- Inorganic Chemistry
- Materials Chemistry
- Medicinal Chemistry
- Mineralogy and Gems
- Organic Chemistry
- Physical Chemistry
- Polymer Chemistry
- Study and Communication Skills in Chemistry
- Theoretical Chemistry
- Browse content in Computer Science
- Artificial Intelligence
- Computer Architecture and Logic Design
- Game Studies
- Human-Computer Interaction
- Mathematical Theory of Computation
- Programming Languages
- Software Engineering
- Systems Analysis and Design
- Virtual Reality
- Browse content in Computing
- Business Applications
- Computer Security
- Computer Games
- Computer Networking and Communications
- Digital Lifestyle
- Graphical and Digital Media Applications
- Operating Systems
- Browse content in Earth Sciences and Geography
- Atmospheric Sciences
- Environmental Geography
- Geology and the Lithosphere
- Maps and Map-making
- Meteorology and Climatology
- Oceanography and Hydrology
- Palaeontology
- Physical Geography and Topography
- Regional Geography
- Soil Science
- Urban Geography
- Browse content in Engineering and Technology
- Agriculture and Farming
- Biological Engineering
- Civil Engineering, Surveying, and Building
- Electronics and Communications Engineering
- Energy Technology
- Engineering (General)
- Environmental Science, Engineering, and Technology
- History of Engineering and Technology
- Mechanical Engineering and Materials
- Technology of Industrial Chemistry
- Transport Technology and Trades
- Browse content in Environmental Science
- Applied Ecology (Environmental Science)
- Conservation of the Environment (Environmental Science)
- Environmental Sustainability
- Environmentalist Thought and Ideology (Environmental Science)
- Management of Land and Natural Resources (Environmental Science)
- Natural Disasters (Environmental Science)
- Nuclear Issues (Environmental Science)
- Pollution and Threats to the Environment (Environmental Science)
- Social Impact of Environmental Issues (Environmental Science)
- History of Science and Technology
- Browse content in Materials Science
- Ceramics and Glasses
- Composite Materials
- Metals, Alloying, and Corrosion
- Nanotechnology
- Browse content in Mathematics
- Applied Mathematics
- Biomathematics and Statistics
- History of Mathematics
- Mathematical Education
- Mathematical Finance
- Mathematical Analysis
- Numerical and Computational Mathematics
- Probability and Statistics
- Pure Mathematics
- Browse content in Neuroscience
- Cognition and Behavioural Neuroscience
- Development of the Nervous System
- Disorders of the Nervous System
- History of Neuroscience
- Invertebrate Neurobiology
- Molecular and Cellular Systems
- Neuroendocrinology and Autonomic Nervous System
- Neuroscientific Techniques
- Sensory and Motor Systems
- Browse content in Physics
- Astronomy and Astrophysics
- Atomic, Molecular, and Optical Physics
- Biological and Medical Physics
- Classical Mechanics
- Computational Physics
- Condensed Matter Physics
- Electromagnetism, Optics, and Acoustics
- History of Physics
- Mathematical and Statistical Physics
- Measurement Science
- Nuclear Physics
- Particles and Fields
- Plasma Physics
- Quantum Physics
- Relativity and Gravitation
- Semiconductor and Mesoscopic Physics
- Browse content in Psychology
- Affective Sciences
- Clinical Psychology
- Cognitive Psychology
- Cognitive Neuroscience
- Criminal and Forensic Psychology
- Developmental Psychology
- Educational Psychology
- Evolutionary Psychology
- Health Psychology
- History and Systems in Psychology
- Music Psychology
- Neuropsychology
- Organizational Psychology
- Psychological Assessment and Testing
- Psychology of Human-Technology Interaction
- Psychology Professional Development and Training
- Research Methods in Psychology
- Social Psychology
- Browse content in Social Sciences
- Browse content in Anthropology
- Anthropology of Religion
- Human Evolution
- Medical Anthropology
- Physical Anthropology
- Regional Anthropology
- Social and Cultural Anthropology
- Theory and Practice of Anthropology
- Browse content in Business and Management
- Business Ethics
- Business Strategy
- Business History
- Business and Technology
- Business and Government
- Business and the Environment
- Comparative Management
- Corporate Governance
- Corporate Social Responsibility
- Entrepreneurship
- Health Management
- Human Resource Management
- Industrial and Employment Relations
- Industry Studies
- Information and Communication Technologies
- International Business
- Knowledge Management
- Management and Management Techniques
- Operations Management
- Organizational Theory and Behaviour
- Pensions and Pension Management
- Public and Nonprofit Management
- Strategic Management
- Supply Chain Management
- Browse content in Criminology and Criminal Justice
- Criminal Justice
- Criminology
- Forms of Crime
- International and Comparative Criminology
- Youth Violence and Juvenile Justice
- Development Studies
- Browse content in Economics
- Agricultural, Environmental, and Natural Resource Economics
- Asian Economics
- Behavioural Finance
- Behavioural Economics and Neuroeconomics
- Econometrics and Mathematical Economics
- Economic History
- Economic Systems
- Economic Methodology
- Economic Development and Growth
- Financial Markets
- Financial Institutions and Services
- General Economics and Teaching
- Health, Education, and Welfare
- History of Economic Thought
- International Economics
- Labour and Demographic Economics
- Law and Economics
- Macroeconomics and Monetary Economics
- Microeconomics
- Public Economics
- Urban, Rural, and Regional Economics
- Welfare Economics
- Browse content in Education
- Adult Education and Continuous Learning
- Care and Counselling of Students
- Early Childhood and Elementary Education
- Educational Equipment and Technology
- Educational Strategies and Policy
- Higher and Further Education
- Organization and Management of Education
- Philosophy and Theory of Education
- Schools Studies
- Secondary Education
- Teaching of a Specific Subject
- Teaching of Specific Groups and Special Educational Needs
- Teaching Skills and Techniques
- Browse content in Environment
- Applied Ecology (Social Science)
- Climate Change
- Conservation of the Environment (Social Science)
- Environmentalist Thought and Ideology (Social Science)
- Natural Disasters (Environment)
- Social Impact of Environmental Issues (Social Science)
- Browse content in Human Geography
- Cultural Geography
- Economic Geography
- Political Geography
- Browse content in Interdisciplinary Studies
- Communication Studies
- Museums, Libraries, and Information Sciences
- Browse content in Politics
- African Politics
- Asian Politics
- Chinese Politics
- Comparative Politics
- Conflict Politics
- Elections and Electoral Studies
- Environmental Politics
- European Union
- Foreign Policy
- Gender and Politics
- Human Rights and Politics
- Indian Politics
- International Relations
- International Organization (Politics)
- International Political Economy
- Irish Politics
- Latin American Politics
- Middle Eastern Politics
- Political Behaviour
- Political Economy
- Political Institutions
- Political Methodology
- Political Communication
- Political Philosophy
- Political Sociology
- Political Theory
- Politics and Law
- Public Policy
- Public Administration
- Quantitative Political Methodology
- Regional Political Studies
- Russian Politics
- Security Studies
- State and Local Government
- UK Politics
- US Politics
- Browse content in Regional and Area Studies
- African Studies
- Asian Studies
- East Asian Studies
- Japanese Studies
- Latin American Studies
- Middle Eastern Studies
- Native American Studies
- Scottish Studies
- Browse content in Research and Information
- Research Methods
- Browse content in Social Work
- Addictions and Substance Misuse
- Adoption and Fostering
- Care of the Elderly
- Child and Adolescent Social Work
- Couple and Family Social Work
- Developmental and Physical Disabilities Social Work
- Direct Practice and Clinical Social Work
- Emergency Services
- Human Behaviour and the Social Environment
- International and Global Issues in Social Work
- Mental and Behavioural Health
- Social Justice and Human Rights
- Social Policy and Advocacy
- Social Work and Crime and Justice
- Social Work Macro Practice
- Social Work Practice Settings
- Social Work Research and Evidence-based Practice
- Welfare and Benefit Systems
- Browse content in Sociology
- Childhood Studies
- Community Development
- Comparative and Historical Sociology
- Economic Sociology
- Gender and Sexuality
- Gerontology and Ageing
- Health, Illness, and Medicine
- Marriage and the Family
- Migration Studies
- Occupations, Professions, and Work
- Organizations
- Population and Demography
- Race and Ethnicity
- Social Theory
- Social Movements and Social Change
- Social Research and Statistics
- Social Stratification, Inequality, and Mobility
- Sociology of Religion
- Sociology of Education
- Sport and Leisure
- Urban and Rural Studies
- Browse content in Warfare and Defence
- Defence Strategy, Planning, and Research
- Land Forces and Warfare
- Military Administration
- Military Life and Institutions
- Naval Forces and Warfare
- Other Warfare and Defence Issues
- Peace Studies and Conflict Resolution
- Weapons and Equipment

- < Previous chapter
- Next chapter >
7 7 Causal thinking
- Published: March 2011
- Cite Icon Cite
- Permissions Icon Permissions
How do people acquire and use causal knowledge? This chapter argues that causal learning and reasoning are intertwined, and recruit similar representations and inferential procedures. In contrast to covariation‐based approaches to learning, this chapter maintains that people use multiple sources of evidence to discover causal relations, and that the causal representation itself is separate from these informational sources. The key roles of prior knowledge and interventions in learning are also discussed. Finally, this chapter speculates about the role of mental simulation in causal inference. Drawing on parallels with work in the psychology of mechanical reasoning, the notion of a causal mental model is proposed as a viable alternative to reasoning systems based in logic or probability theory alone. The central idea is that when people reason about causal systems they utilize mental models that represent objects, events or states of affairs, and reasoning and inference is carried out by mental simulation of these models.
Signed in as
Institutional accounts.
- GoogleCrawler [DO NOT DELETE]
- Google Scholar Indexing
Personal account
- Sign in with email/username & password
- Get email alerts
- Save searches
- Purchase content
- Activate your purchase/trial code
- Add your ORCID iD
Institutional access
Sign in with a library card.
- Sign in with username/password
- Recommend to your librarian
- Institutional account management
- Get help with access
Access to content on Oxford Academic is often provided through institutional subscriptions and purchases. If you are a member of an institution with an active account, you may be able to access content in one of the following ways:
IP based access
Typically, access is provided across an institutional network to a range of IP addresses. This authentication occurs automatically, and it is not possible to sign out of an IP authenticated account.
Sign in through your institution
Choose this option to get remote access when outside your institution. Shibboleth/Open Athens technology is used to provide single sign-on between your institution’s website and Oxford Academic.
- Click Sign in through your institution.
- Select your institution from the list provided, which will take you to your institution's website to sign in.
- When on the institution site, please use the credentials provided by your institution. Do not use an Oxford Academic personal account.
- Following successful sign in, you will be returned to Oxford Academic.
If your institution is not listed or you cannot sign in to your institution’s website, please contact your librarian or administrator.
Enter your library card number to sign in. If you cannot sign in, please contact your librarian.
Society Members
Society member access to a journal is achieved in one of the following ways:
Sign in through society site
Many societies offer single sign-on between the society website and Oxford Academic. If you see ‘Sign in through society site’ in the sign in pane within a journal:
- Click Sign in through society site.
- When on the society site, please use the credentials provided by that society. Do not use an Oxford Academic personal account.
If you do not have a society account or have forgotten your username or password, please contact your society.
Sign in using a personal account
Some societies use Oxford Academic personal accounts to provide access to their members. See below.
A personal account can be used to get email alerts, save searches, purchase content, and activate subscriptions.
Some societies use Oxford Academic personal accounts to provide access to their members.
Viewing your signed in accounts
Click the account icon in the top right to:
- View your signed in personal account and access account management features.
- View the institutional accounts that are providing access.
Signed in but can't access content
Oxford Academic is home to a wide variety of products. The institutional subscription may not cover the content that you are trying to access. If you believe you should have access to that content, please contact your librarian.
For librarians and administrators, your personal account also provides access to institutional account management. Here you will find options to view and activate subscriptions, manage institutional settings and access options, access usage statistics, and more.
Our books are available by subscription or purchase to libraries and institutions.
- About Oxford Academic
- Publish journals with us
- University press partners
- What we publish
- New features
- Open access
- Rights and permissions
- Accessibility
- Advertising
- Media enquiries
- Oxford University Press
- Oxford Languages
- University of Oxford
Oxford University Press is a department of the University of Oxford. It furthers the University's objective of excellence in research, scholarship, and education by publishing worldwide
- Copyright © 2024 Oxford University Press
- Cookie settings
- Cookie policy
- Privacy policy
- Legal notice
This Feature Is Available To Subscribers Only
Sign In or Create an Account
This PDF is available to Subscribers Only
For full access to this pdf, sign in to an existing account, or purchase an annual subscription.
- Original article
- Open access
- Published: 12 January 2022
Causal theory error in college students’ understanding of science studies
- Colleen M. Seifert ORCID: orcid.org/0000-0001-5889-5167 1 ,
- Michael Harrington 1 ,
- Audrey L. Michal 1 &
- Priti Shah 1
Cognitive Research: Principles and Implications volume 7 , Article number: 4 ( 2022 ) Cite this article
6230 Accesses
4 Citations
3 Altmetric
Metrics details
When reasoning about science studies, people often make causal theory errors by inferring or accepting a causal claim based on correlational evidence. While humans naturally think in terms of causal relationships, reasoning about science findings requires understanding how evidence supports—or fails to support—a causal claim. This study investigated college students’ thinking about causal claims presented in brief media reports describing behavioral science findings. How do science students reason about causal claims from correlational evidence? And can their reasoning be improved through instruction clarifying the nature of causal theory error? We examined these questions through a series of written reasoning exercises given to advanced college students over three weeks within a psychology methods course. In a pretest session, students critiqued study quality and support for a causal claim from a brief media report suggesting an association between two variables. Then, they created diagrams depicting possible alternative causal theories. At the beginning of the second session, an instructional intervention introduced students to an extended example of a causal theory error through guided questions about possible alternative causes. Then, they completed the same two tasks with new science reports immediately and again 1 week later. The results show students’ reasoning included fewer causal theory errors after the intervention, and this improvement was maintained a week later. Our findings suggest that interventions aimed at addressing reasoning about causal claims in correlational studies are needed even for advanced science students, and that training on considering alternative causal theories may be successful in reducing casual theory error.
Significance statement
Causal theory error— defined as making a causal claim based on correlational evidence—is ubiquitous in science publications, classrooms, and media reports of scientific findings. Previous studies have documented causal theory error as occurring on a par with correct causal conclusions. However, no previous studies have identified effective interventions to improve causal reasoning about correlational findings. This study examines an example-based intervention to guide students in reasoning about plausible alternative causal theories consistent with the correlational evidence. Following the intervention, advanced college students in a research methods course offered more critiques of causal claims and generated more alternative theories, and then maintained these gains a week later. Our results suggest that causal theory error is common even in college science courses, but interventions focusing on considering alternative theories to a presented causal claim may be helpful. Because behavioral science communications in the media are increasingly available, helping people improve their ability to assess whether evidence from science studies support making changes in behavior, thinking, and policies is an important contribution to science education.
Introduction
Causal claims from research studies shared on social media often exceed the strength of scientific evidence (Haber et al., 2018 ). This occurs in journal articles as well; for example, a recent study in Proceedings of the National Academy of Sciences reported a statistical association between higher levels of optimism and longer life spans, concluding, “…optimism serves as a psychological resource that promotes health and longevity” (Lee et al., 2019 , p. 18,360). Given evidence of association, people (including scientists) readily make a mental leap to infer a causal relationship. This error in reasoning— cum hoc ergo propter hoc (“with this, therefore because of this”)—occurs when two coinciding events are assumed to be related through cause and effect. A press release for the article above offered, “If you’re happy and you know it… you may live longer” (Topor, 2019 ). But will you? What critical thinking is needed to assess this claim?
Defining causal theory error
The tendency to infer causation from correlation—referred to here as causal theory error —is arguably the most ubiquitous and wide-ranging error found in science literature (Bleske-Rechek et al., 2018 ; Kida, 2006 ; Reinhart et al., 2013 ; Schellenberg, 2020 ; Stanovich, 2009 ), classrooms (Kuhn, 2012 ; Mueller & Coon, 2013 ; Sloman & Lagando, 2003 ), and media reports (Adams et al., 2019 ; Bleske-Rechek et al., 2018 ; Sumner et al., 2014 ). While science pedagogy informs us that, “correlation does not imply causation” (Stanovich, 2010 ), the human cognitive system is “built to see causation as governing how events unfold” (Sloman & Lagnado, 2015 , p. 32); consequently, people interpret almost all events through causal relationships (Corrigan & Denton, 1996 ; Hastie, 2015 ; Tversky & Kahneman, 1977 ; Sloman, 2005 ), and act upon them with unwarranted certainty (Kuhn, 2012 ). Claims about causal relationships from correlational findings are used to guide decisions about health, behavior, and public policy (Bott et al., 2019 ; Huggins-Manley et al., 2021 ; Kolstø et al., 2006 ; Lewandowsky et al., 2012 ). Increasingly, science media reports promote causal claims while omitting much of the information needed to evaluate the scientific evidence (Zimmerman et al., 2001 ), and editors may modify media report headlines to make such reports more eye-catching (Jensen, 2008 ). As a result, causal theory error is propagated in a third of press releases and over 80% of their associated media stories (Sumner et al., 2014 ).
The ability to reason about causal relationships is fundamental to science education (Blalock, 1987 ; Jenkins, 1994 ; Kuhn, 1993 , 2005 ; Miller, 1996 ; Ryder, 2001 ), and U.S. standards aim to teach students to critically reason about covariation starting in middle school (Lehrer & Schauble, 2006 ; National Science Education Standards, 1996; Next Generation Science Standards, 2013 ). To infer causation, a controlled experiment involving direct manipulation and random assignment to treatment and control conditions is the “gold standard” (Hatfield et al., 2006 ; Koch & Wüstemann, 2014 ; Reis & Judd, 2000 ; Sullivan, 2011 ). However, for many research questions, collecting experimental evidence from human subjects is expensive, impractical, or unethical (Bensley et al., 2010 ; Stanovich, 2010 ), so causal conclusions are sometimes drawn without experimental evidence (Yavchitz et al., 2012 ). A prominent example is the causal link between cigarette smoking and causes of death: “For reasons discussed, we are of the opinion that the associations found between regular cigarette smoking and death … reflect cause and effect relationships” (Hammond & Horn, 1954 , p. 1328). Nonexperimental evidence (e.g., longitudinal data)—along with, importantly, the lack of plausible alternative explanations—sometimes leads scientists to accept a causal claim supported only by correlational evidence (Bleske-Rechek et al., 2018 ; Marinescu et al., 2018 ; Pearl & Mackenzie, 2018 ; Reinhart et al., 2013 ; Schellenberg, 2020 ). With no hard-and-fast rules regarding the evidence necessary to conclude causation, the qualities of the evidence offered are evaluated to determine the appropriateness of causal claims from science studies (Kuhn, 2012 ; Morling, 2014 ; Picardi & Masick, 2013 ; Steffens et al., 2014 ; Sloman, 2005 ).
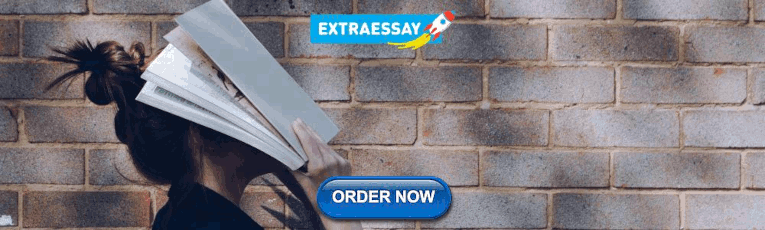
Theory-evidence coordination in causal reasoning
To reason about claims from scientific evidence, Kuhn and colleagues propose a broad-level process of theory-evidence coordination (Kuhn, 1993 ; Kuhn et al., 1988 ), where people use varied strategies to interpret the implications of evidence and align it with their theories (Kuhn & Dean, 2004 ; Kuhn et al., 1995 ). In one study, people read a scenario about a study examining school features affecting students' achievement (Kuhn et al, 1995 , p. 29). Adults examined presented data points and detected simple covariation to (correctly) conclude that, “having a teacher assistant causes higher student achievement” (Kuhn et al, 1995 ). Kuhn ( 2012 ) suggests theory-evidence coordination includes generating some conception as to why an association “makes sense” by drawing on background knowledge to make inferences, posit mechanisms, and consider the plausibility of a causal conclusion (Kuhn et al., 2008 ). Assessments of causal relationship are biased in the direction of prior expectations and beliefs (e.g., Billman et al., 1992 ; Fugelsang & Thompson, 2000 , 2003 ; Wright & Murphy, 1984 ). In general, people focus on how, “…the evidence demonstrates (or at least illustrates) the theory’s correctness, rather than that the theory remains likely to be correct in spite of the evidence” (Kuhn & Dean, 2004 , p. 273).
However, in causal theory error, the process of theory-evidence coordination may require less attention to evidence. Typically, media reports of science studies present a summary of associational evidence that corroborates a presented theoretical causal claim (Adams et al., 2019 ; Bleske-Rechek et al., 2018 ; Mueller, 2020 ; Sumner et al., 2014 ). Because the evidence is often summarized as a probabilistic association (for example, a statistical correlation between two variables), gathering more evidence from instances cannot confirm or disconfirm the causal claim (unlike reasoning about instances, as in Kuhn et al., 1995 ). Instead, the reasoner must evaluate a presented causal theory by considering alternative theories also corresponding with the evidence, disproving internal validity (that the presented causal claim is the only possibility). In the student achievement example (Kuhn et al., 1995 ), a causal theory error occurs by failing to consider that the theory—”having a teacher assistant causes higher student achievement”—is not the only causal theory consistent with the evidence; for example, they may co-occur in schools due to a third variable, such as school funding. In the case of causal theory error, the presented causal claim is consistent with the evidence; however, the non-experimental evidence cannot support only the presented causal claim. Rather than an error in coordinating theory and evidence (Kuhn, 1993 ; Kuhn et al., 1995 ), we propose that causal theory error arises from failure to examine the uniqueness of a given causal theory .
Theory-evidence coordination is often motivated by contextually rich, elaborate, and familiar content along with existing beliefs, but these may also interfere with proficient scientific thinking (Billman et al., 1992 ; Fugelsang & Thompson, 2000 , 2003 ; Halpern, 1998 ; Koehler, 1993 ; Kuhn et al., 1995 ; Wright & Murphy, 1984 ). Everyday contexts more readily give rise to personal beliefs, prior experiences, and emotional responses (Shah et al., 2017 ); when congruent or desirable with a presented claim, these may increase plausibility and judgments of quality (Michal et al., 2021 ; Shah et al., 2017 ). Analytic, critical thinking may occur more readily when information conflicts with existing beliefs (Evans & Curtis-Holmes, 2005 ; Evans, 2003a , b ; Klaczynski, 2000 ; Kunda, 1990 ; Nickerson, 1998 ; Sá et al., 1999 ; Sinatra et al., 2014 ). Consequently, learning to recognize causal theory error may require guiding students in “reasoning through” their own related beliefs to identify how they may align—and not align—with the evidence given. Our approach in this study takes advantage of students’ ability to access familiar contexts and world knowledge to create their own alternative causal theories.
Prevalence of causal theory error
Prior research on causal reasoning has typically examined the formation of causal inferences drawn from observing feature co-occurrence in examples (e.g., Kuhn et al., 1995 ; Cheng, 1997 ; Fugelsang & Thompson, 2000 , 2003 ; Griffiths & Tenenbaum, 2005 ; see also Sloman & Lagnado, 2015 ). Fewer studies have asked people to evaluate causal claims based on summary evidence (such as stating there is a correlation between two variables). A study by Steffens and colleagues ( 2014 ) asked college students to judge the appropriateness of claims from evidence in health science reports. Students were more likely to reject causal claims (e.g., ‘School lunches cause childhood obesity”) from correlational than from experimental studies. However, each report in the study explicitly stated, “Random assignment is a gold standard for experiments, because it rules out alternative explanations. This procedure [does, does not] allow us to rule out alternative explanations” (Steffens et al., 2014 ; p. 127). Given that each reported study was labelled as allowing (or not allowing) a causal claim (Steffens et al., 2014 ), these findings may overestimate people’s ability to avoid causal theory errors.
A field study recruiting people in restaurants to evaluate research reports provides evidence that causal theory errors are quite common. In this study (Bleske-Rechek et al., 2015 ), people read a research scenario linking two variables (e.g., playing video games and aggressive playground behavior) set in either experimental (random assignment to groups) or non-experimental (survey) designs. Then, they selected appropriate inferences among statements including causal links (e.g., “Video games cause more aggression”), reversed causal links (“Aggression causes more video game playing”), and associations (e.g., “Boys who spend more time playing video games tend to be more aggressive”). Across three scenarios, 63% of people drew causal inferences from non-experimental data, just as often as from experimental findings. Further, people were more likely to infer directions of causality that coincided with common-sense notions (e.g., playing games leads to more aggression rather than the reverse) (Bleske-Rechek et al., 2015 ).
Another study by Xiong et al. ( 2020 ) found similarly high rates of endorsing causal claims from correlational evidence. With a crowdsourced sample, descriptions of evidence were presented in text format, such as, "When students eat breakfast very often (more than 4 times a week), their GPA is around 3.5; while when students eat breakfast not very often (less than four times a week), their GPA is around 3.0,” or with added bar graphs, line graphs, or scatterplots (Xiong, et al., 2020 , p. 853). Causal claims (e.g., “If students were to eat breakfast more often, they would have higher GPAs”) were endorsed by 67–76% of adults compared to 85% (on average) for correlational claims (“Students who more often eat breakfast tend to have higher GPA”) (Xiong, et al., 2020 , p. 858). Finally, Adams et al. ( 2017 ) suggested people do not consistently distinguish among descriptions of “moderate” causal relationships, such as, “might cause,” and “associated with,” even though the wording establishes a logical distinction between causation and association. These studies demonstrate that causal theory error in interpreting science findings is pervasive, with most people taking away an inappropriate causal conclusion from associational evidence (Zweig & Devoto, 2015 ).
Causal theory error in science students
However, compared to the general public, those with college backgrounds (who on average have more science education) have been shown to have better science evaluation skills (Amsel et al., 2008 ; Huber & Kuncel, 2015 ; Kosonen & Winne, 1995 ; Norcross et al., 1993 ). College students make better causal judgments when reasoning about science, including taking in given information, reasoning through to a conclusion, and tracking what they know and don’t know from it (Koehler, 1993 ; Kuhn, 2012 ). Might science literacy (Miller, 1996 ) help college students be more successful in avoiding causal theory error? Norris et al. ( 2003 ) found that only about a third of college students can correctly distinguish between causal and correlational statements in general science texts. And teaching scientific content alone may not improve scientific reasoning, as in cross-cultural studies showing Chinese students outperform U.S. students on measures of science content but perform equally on measures of scientific reasoning (Bao et al., 2009 ; Crowell & Schunn, 2016 ). College-level science classes (as many as eight) fail to predict performance on everyday reasoning tasks compared to high school students (Norris & Phillips, 1994 ; Norris et al., 2003 ). Despite more science education, college students still struggle to accurately judge whether causal inferences are warranted (Norris et al., 2003 ; Rodriguez et al., 2016a , b ).
Because media reports typically describe behavioral studies (e.g., Bleske-Rechek et al., 2018 ; Haber et al., 2018 ), psychology students may fare better (Hall & Seery, 2006 ). Green and Hood ( 2013 ) suggest psychology students’ epistemological beliefs benefit from an emphasis on critical thinking, research methods, and integrating knowledge from multiple theories. Hofer ( 2000 ) found that first year psychology students believe knowledge is less certain and more changing in psychology than in science more generally. And Renken and colleagues ( 2015 ) found that psychology-specific epistemological beliefs, such as the subjective nature of knowledge, influence students' academic outcomes. Psychology exposes students to both correlational and experimental studies, fostering distinctions about the strength of empirical evidence (Morling, 2014 ; Reinhart et al., 2013 ; Stanovich, 2009 ). Mueller and Coon ( 2013 ) found students in an introductory psychology class interpreted correlational findings with just 28% error on average, improving to just 7% error at the end of the term. To consider causal theory error among psychology students, we recruited a convenience sample of advanced undergraduate majors in a research methods course. These students may be more prepared to evaluate causal claims in behavioral studies reported in the media.
Correcting causal theory error
To attempt to remedy causal theory error, the present study investigates whether students’ reasoning about causal claims in science studies can be improved through an educational intervention. In a previous classroom study, Mueller and Coon ( 2013 ) introduced a special curriculum over a term in an introductory psychology course. By emphasizing how to interpret correlational findings, the rate of causal theory error decreased by 21%. Our study used a similar pre/post design to assess base rates of causal theory error and determine the impact of a single, short instructional intervention. Our intervention was based on guidelines from science learning studies identifying example-based instruction (Shafto et al., 2014 ; Van Gog & Rummel, 2010 ) and self-explanation (Chi et al., 1989 ). Renkl and colleagues found examples improve learning by promoting self-explanations of concepts through spontaneous, prompted, and trained strategies (Renkl et al., 1998 ; Stark et al., 2002 , 2011 ). Even incorrect examples can be beneficial in learning to avoid errors (Durkin & Rittle-Johnson, 2012 ; Siegler & Chen, 2008 ). Based on evidence that explicit description of the error within an example facilitates learning (Große & Renkl, 2007 ), our intervention presented a causal theory error made in an example and explained why the specific causal inference was not warranted given the evidence (Stark et al., 2011 ).
Following Berthold and Renkl’s paradigm ( 2009 , 2010 ), our intervention incorporated working through an extended example using world knowledge (Kuhn, 2012 ). To facilitate drawing on their own past experiences, the example study selected was relevant for recent high school graduates likely to have pre-existing beliefs and attitudes (both pro and con) toward the presented causal claim (Bleske-Rechek et al., 2015 ; Michal et al., 2021 ). Easily accessible world knowledge related to the study findings may assist students in identifying how independent thinking outside of the presented information can inform their assessment of causal claims. We encouraged students to think on their own about possible causal theories by going “beyond the given information” (Bruner, 1957 ). Open-ended prompts asked students to think through what would happen in the absence of the stated cause, whether a causal mechanism is evident, and whether the study groups differed in some way. Then, students generated their own alternative causal theories, including simple cause-chain and reverse cause-chain models, and potential third variables causing both (common causes) (Pearl, 1995 , 2000 ; Shah et al., 2017 ).
To support students in identifying alternative causal theories, our intervention included visualizing causal models through the creation of diagrams. Creating diagrams after reading scientific material has been linked with better understanding of causal and dynamic relationships (Ainsworth & Loizou, 2003 ; Bobek & Tversky, 2016 ; Gobert & Clement, 1999 ). Students viewing diagrams generate more self-explanations (Ainsworth & Loizou, 2003 ), and training students to construct their own diagrams (“drawing to learn”) may promote additional frames for understanding difficult concepts (Ainsworth & Scheiter, 2021 ). Diagramming causal relationships may help students identify causal theories and assist them in considering alternatives. For simplicity, students diagrammed headlines from actual media reports making a causal claim; for example, ‘‘Sincere smiling promotes longevity” (Mueller, 2020 ). Assessing causal theory error with headlines minimizes extraneous study descriptions that may alter interpretations (Adams et al., 2017 ; Mueller & Coon, 2013 ).
We expected our short intervention to support students in learning to avoid causal theory error; specifically, we predicted that students would be more likely to notice when causal claims from associational evidence were unwarranted through increased consideration of alternative causal theories following the intervention.
Participants
Students enrolled in a psychology research methods course at a large midwestern U.S. university were invited to participate in the study over three consecutive weeks during lecture sessions. The students enrolled during their third or fourth year of college study after completing introductory and advanced psychology courses and a prerequisite in statistics. The three study sessions ( pretest, intervention, and post-test ) occurred in weeks 3–5 of the 14-week course, prior to any instruction or readings on correlational or experimental methodology. Of 240 students enrolled, 97 (40%) completed the voluntary questionnaires for the study in all three sessions and were included in the analyses.
Intervention
The text-based intervention explained how to perform theory-evidence coordination when presented with a causal claim through an extended example (see Appendix 1 ). The example described an Educational Testing Service (ETS) study reporting that 84% of top-tier workers (receiving the highest pay) had taken Algebra 2 classes in high school. Based on this evidence, legislatures in 20 states raised high school graduation requirements to include Algebra 2 (Carnevale et al., 2009 ). The study’s lead author, Anthony Carnevale, acknowledged this as a causal theory error, noting, “The causal relationship is very, very weak. Most people don’t use Algebra 2 in college, let alone in real life. The state governments need to be careful with this” (Whoriskey, 2011 ).
The intervention presented a short summary of the study followed by a series of ten questions in a worksheet format. The first two questions addressed the evidence for the causal claim and endorsement of the causal theory error, followed by five questions to prompt thinking about alternative explanations for the observed association, including reasoning by considering counterfactuals, possible causal mechanisms, equality of groups, self-selection bias, and potential third variables. For the final three questions, students were shown causal diagrams to assess their endorsement of the causal claim, the direction of causation, and potential third variables. The intervention also explicitly described this as an error in reasoning and ended with advice about the need to consider alternative causes when causal claims are made from associational evidence.
Dependent measures
To investigate both students’ understanding of theory-evidence coordination in evaluating a study and their ability to generate alternative theories to the causal claim, we included two tasks—Study Critique and Diagram Generation—in each session (pretest, intervention, and post-test) as repeated measures (see Fig. 1 ). Each task included brief study descriptions paraphrased from actual science media reports (Mueller, 2020 ). To counter item-specific effects, three separate problem sets were generated pairing Critique and Diagram problems at random (see Appendix 2 ). The three problem sets were counterbalanced across students by assigning them at random to alternate forms so that each set of problems occurred equally frequently at each session.

Graphical depiction of questionnaire content for each session in the longitudinal study
In the Study Critique task, a short media story (between 100 and 150 words) was presented for evaluation; for example:
“…Researchers have recently become interested in whether listening to music actually helps students pay attention and learn new information. A recent study was conducted by researchers at a large midwestern university. Students ( n = 450) were surveyed prior to final exams in several large, lecture-based courses, and asked whether they had listened to music while studying for the final. The students who listened to music had, on average, higher test scores than students who did not listen to music while studying. The research team concluded that students who want to do well on their exams should listen to music while studying.”
First, two rating items assessed the perceived quality of the study and its support for the claim with 5-point Likert scales, with “1” indicating low-quality and an unsupported claim, and “5” indicating high-quality and a supported claim. For both scales, higher scores indicate causal theory error (i.e., high quality study does support the causal claim). Next, an open-ended question asked for “a critical evaluation of the study’s method and claims, and what was good and bad about it” (see Appendix 3 ). Responses were qualitatively coded using four emergent themes: (1) pointing to causal theory error (correlation is not causation), (2) the study methodology (not experimental), (3) the identification of alternative theories such as third variables, and (4) additional studies are needed to support a causal claim. Each theme addresses a weakness in reasoning from the study’s correlational evidence to make a causal claim. Other issues mentioned infrequently and not scored as critiques included sample size, specialized populations, lack of doctor’s recommendation, and statistical analyses. Two authors worked independently to code a subset of the responses (10%), and the Cohen's Kappa value computed using SPSS was \(\kappa\) = 0.916, indicating substantial agreement beyond chance on the Landis and Koch ( 1977 ) benchmarks. One author then scored the remaining responses. Higher scores on this Critique Reasons measure indicate greater ability to critique causal claims from associational data.
The Diagram Generation task included instructions with sample causal diagrams (Elwert, 2013 ) to illustrate how to depict relationships between variables (see Appendix 4 ); all students completed these correctly, indicating they understood the task. Then, a short “headline” causal claim was presented, such as, “Smiling increases longevity,” (Abel & Kruger, 2010 ) and students were asked to diagram possible relationships between the two variables. The Alternative Theories measure captured the number of distinct alternative causal relationships generated in diagrams, scored as a count of deductive categories including: (a) direct causal chain, (b) reverse direction chain, (c) common cause (a third variable causes both), and (d) multiple-step chains, with possible scores ranging from 0 to 4. Two authors worked independently to code a subset of the responses (10%), and the Cohen's Kappa value computed using SPSS was \(\kappa\) = 0.973, indicating substantial agreement beyond chance on the Landis and Koch ( 1977 ) benchmarking scale. One author then scored the remainder of the responses. A higher Alternative Theories score reflects greater ability to generate different alternative causal explanations for an observed association.
The study took place during lecture sessions for an advanced psychology course over three weeks in the first third of the term. At the beginning of the lecture each week, students were asked to complete a study questionnaire. In the pretest session, students were randomly assigned to one of three alternate forms, and they received corresponding forms in the intervention and post-test sessions so that the specific science reports and headlines included in each student’s questionnaires were novel to them across sessions. Students were given 10 min to complete the intervention worksheet and 15 min to complete each questionnaire.
Understanding the intervention
To determine how students understood the intervention and whether it was successful, we performed a qualitative analysis of open-ended responses. The example study presented summary data (percentages) of former students in high- and low-earning careers who took high school algebra and concluded that taking advanced algebra improves career earnings. Therefore, correct responses on the intervention reject this causal claim to avoid causal theory error. However, 66% of the students reported in their open-ended responses that they felt the study was convincing, and 63% endorsed the causal theory error as a “good decision” by legislators. To examine differences in students' reasoning, we divided the sample based on responses to these two initial questions.
The majority ( n = 67) either endorsed the causal claim as convincing or agreed with the decision based on it (or both), and these students were more likely to cite “strong correlation” (25%; χ 2 = 9.88, p = 0.0012, Φ = 0.171) and “foundational math is beneficial” (35%); χ 2 = 8.278, p = 0.004, Φ = 0.164) as reasons. Students who rejected the causal claim (did not find the study convincing nor the decision supported; n = 33) showed signs of difficulty in articulating reasons for this judgment; however, 27% clearly identified causal theory error more often in their reasons ( χ 2 = 10.624, p = 0.001, Φ = 0.183). As Table 1 shows, the two groups' responses differ more initially (consistent with their judgments about the causal claim), but become more aligned on later questions.
On the final two questions, larger differences occur when considering possible alternative “third variables” responsible for both higher earnings and taking more algebra (“smarter,” “richer,” “better schools,” and “headed to college anyway”). More of these potential “third variables” were endorsed as plausible more often by students initially rejecting compared to accepting the causal claim. On a final question, in which three alternative causal diagrams (direct cause, reverse cause, third variable) were presented to illustrate possible causal relationships between Algebra 2 and top tier jobs, over 75% of both groups endorsed a third variable model. Based on these open-ended response measures, it appears most students understood the intervention, and most were able to reason about and accept alternatives to the stated causal claim.
Analysis of causal theory error
We planned to conduct repeated measures ANOVAs with linear contrasts based on expected improvements on all three dependent variables ( Study Ratings, Critique Reasons , and Alternative Theories ) across Sessions (pre-test, immediately post-intervention, and delayed post-test). In this repeated-measures design, each student completed three questionnaires including the same six test problems appearing in differing orders. Approximately equal numbers of students completed each of three forms ( n = 31, 34, 32). A repeated measures analysis with Ordering (green, white, or yellow) as a between-groups factor found no main effects, with no significant differences for Ratings ( F (2, 94) = 0.996, p = 0.373), Reasons ( F (2, 93) = 2.64, p = 0.08), or Theories ( F (2, 93) = 1.84, p = 0.167). No further comparisons were made comparing order groups.
Study critiques: Ratings
The Ratings measures included two assessments: (1) the perceived quality of the study and (2) its support for the causal claim, with higher scores indicating causal theory error (i.e., a “high” quality study and “good” support for a causal claim). At pretest, ratings averaged above the midpoint of the 5-point rating scale for both quality and support, indicating that on average, students considered the study to be of good quality with moderate support for the causal claim. Immediately following the intervention, students ratings showed similar endorsments of study quality and support for a causal claim (see Table 2 ).
However, on the post-test one week later, ratings for both quality and support showed significant decreases. Planned linear contrasts showed a small improvement in rejecting a causal claim over the three sessions in both quality and support ratings, indicating less causal theory error at post-test. Using Tukey’s LSD test, the quality and support ratings in the post-test session were both significantly different from the pretest session ( p = 0.015; 0.043) and intervention session ( p = 0.014; 0.027), while the pretest and intervention means did not differ ( p = 0.822; 0.739), respectively.
Both study quality and causal support ratings remained at pre-test levels after the intervention, but decreased in the final post-test session. About half (48%) of these advanced psychology students rated studies with correlational evidence as “supporting” a causal claim (above scale midpoint) on the pretest, with about the same number at the intervention session; however, at the post-test session, above mid-point support declined to 37%. This suggests students may view the ratings tasks as assessing consistency between theory and evidence (the A → B causal claim matches the study findings). For example, one student gave the study the highest quality rating and a midscale rating for support, but wrote in their open-ended critique that the study was, “bad—makes causation claims.” Another gave ratings of “4” on both scales but wrote that, “The study didn’t talk about other factors that could explain causal links, and as a correlation study, it can’t determine cause.” These responses suggest students may recognize a correspondenc e between the stated theory and the evidence in a study, yet reject the stated causal claim as unique . On the post-test, average ratings decreased, perhaps because students became more critical about what “support for a causal claim” entails. To avoid causal theory error, people must reason that the causal theory offered in the claim is not a unique explanation for the association, and this may not be reflected in ratings of study quality and support for causal claims.
Study critiques: Reasons
A second measure of causal reasoning was the number of Critique Reasons in the open-ended responses, scored as a count of coded themes related to causality (ranging from 0 to 4). These included stating that the study (a) was only correlational, (b) was not an experiment, (c) included other variables affecting the outcomes, and (d) required additional studies to support a causal claim. A planned linear contrast indicated a small improvement in Critiques scores after the pretest session, F (1, 94) = 9.318. p < 0.003, η p 2 = 0.090, with significant improvement from pretest ( M = 1.25; SD = 0.854) to intervention ( M = 1.56, SD = 0.841) and post-test ( M = 1.58, SD = 0.875). The intervention and post-test means were not different by Tukey’s LSD test ( p = 0.769), but both differed from the pretest (intervention p = 0.009, post-test p = 0.003). The percentage of students articulating more than one correct reason to reject a causal claim increased from 31% on the pre-test to 47% immediately after the intervention, and this improvement was maintained on the post-test one week later, as shown in Fig. 2 .
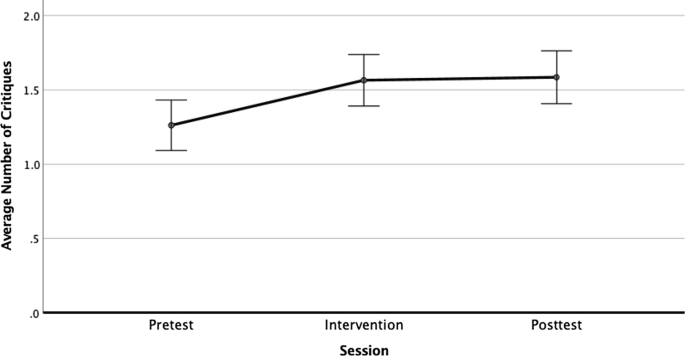
Average number of critique reasons in students’ open-ended responses across sessions. Error bars represent within-subjects standard error of the mean (Cousineau, 2005 )
When considering “what’s good, and what’s bad” about the study, students were more successful in identifying causal theory error following the intervention, and they maintained this gain at the post-test. For example, in response to the claim that “controlling mothers cause kids to overeat,” one student wrote, “There may be a correlation between the 2 variables, but that does not necessarily mean that one causes the other. There could be another answer to why kids have more body fat.” Another wrote, “This is just a correlation, not a causal study. There could be another variable that might be playing a role. Maybe more controlling mothers make their kids study all day and don’t let them play, which could lead to fat buildup. So, the controlling mother causes lack of exercise, which leads to fat kids.” This suggests students followed the guidance in the intervention by questioning whether the study supports the causal claim as unique, and generated their own alternative causal theories.
Alternative theories
In the Diagram task, students created their own representations of possible alternative causal relationships for a causal claim. These causal theories were counted by category, including: (a) direct causal chain, (b) reverse direction chain, (c) common cause (a third variable causes both), and (d) multiple-step chains (intervening causal steps), with scores ranging from 0 to 4 (the same diagram with different variables did not increase the score). A planned linear contrast showed a significant increase in the number of alternative theories included in students’ diagrams ( F (1,93) = 30.935, p < 0.001, η p 2 = 0.25) from pre-test ( M = 1.68, SD = 0.985) to intervention ( M = 2.44, SD = 1.429), and the gain was maintained at post-test ( M = 2.40, SD = 1.469) (see Fig. 3 ). The pretest mean was significantly different (by Tukey’s LSD ) from both the intervention and the post-test mean (both p’s < 0.001), but the intervention and post-test means were not significantly different, p = 0.861. Students provided more (and different) theories about potential causes immediately after the intervention, and this improvement was maintained on the post-test a week later.
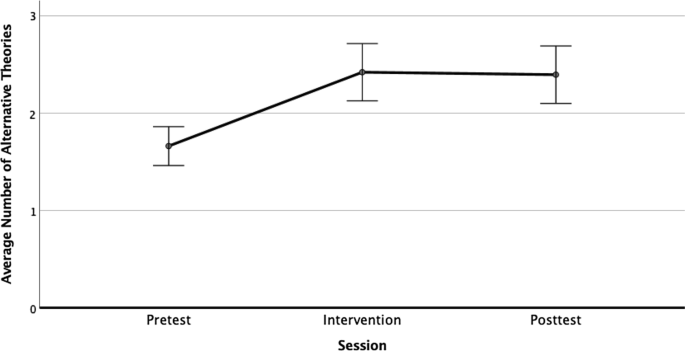
Average number of alternative causal theories generated by students across sessions. Error bars represent within-subjects standard error of the mean (Cousineau, 2005 )
On the Diagram task, students increased the number of different alternative theories generated after the intervention, including direct cause, reversed cause-chain, third variables causing both (common cause), and multiple causal steps. This may reflect an increased ability to consider alternative forms of causal theories to the presented causal theory. Students also maintained this gain of 43% in generating alternatives on the post-test measure one week later. Most tellingly, while 34% offered just one alternative theory on the pre-test, no students gave just one alternative following the intervention or at post-test. While many described reverse-direction or third-variable links as alternatives (see Fig. 4 , left), some offered more novel, complex causal patterns (Fig. 4 , right). This improved ability to generate alternative theories to a presented causal claim suggests students may have acquired a foundational approach for avoiding causal theory errors in the future.
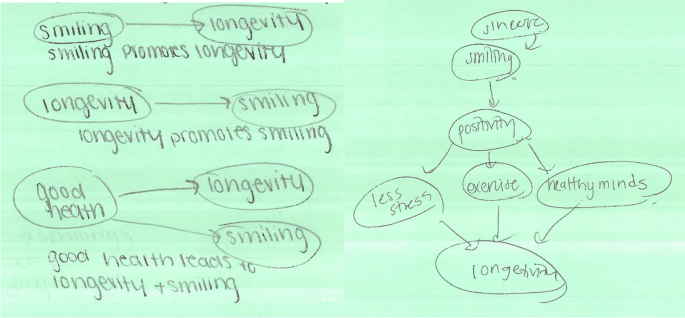
Example diagrams depicting alternative causal theories from a student in the post-test session. On the left, diagrams showing direct cause, reverse cause, and third-variable theories; on right, a more complex theory with multiple steps and paths
The present study documents students’ improvement in avoiding causal theory error when reasoning about scientific findings following a simple, short intervention. The intervention guided students through an extended example of a causal theory error, and included questions designed to scaffold students' thinking about whether a causal claim uniquely accounts for an observed association. Immediately following the intervention and in a delayed test, students increased their reports of problems with causal claims, and generated more alternative causal theories. While causal theory errors have been documented in multiple-choice measures (Bleske-Rechek et al., 2018 ; Mueller & Coon, 2013 ; Xiong et al., 2020 ), this study examined evidence of causal theory errors in students’ open-ended responses to claims in science media reports. This is the first documented intervention associated with significant improvement in avoiding causal theory error from science reports.
Qualities of successful interventions
The intervention was designed following recommendations from example-based instruction (Shafto et al., 2014 ; Siegler & Chen, 2008 ; Van Gog & Rummel, 2010 ). Worked examples may be especially important in learning to reason about new problems (Renkl et al., 1998 ), along with explicitly stating the causal theory error and explanation within the example (Große & Renkl, 2007 ; Stark et al., 2011 ). Our intervention also emphasized recognizing causal theory errors in others ’ reasoning, which may be easier to learn than attempting to correct one’s own errors. Finally, introducing diagramming as a means for visually representing causal theories (Ainsworth & Loizou, 2003 ; Bobek & Tversky, 2016 ; Gobert & Clement, 1999 ) may have been helpful in generating alternative theories by using real-world knowledge to “go beyond the information given” (Bruner, 1957 ; Waldmann et al., 2006 ). To facilitate this thinking, the extended example was selected for relevance to students based in their own experiences (e.g., interest in math driving their course selections), found to be important in other studies (Bleske-Rechek et al., 2015 ; Michal et al., 2021 ). The success of the intervention may be facilitated by an example of causal theory error that “hit close to home” for students through relevance, personal experience, and prior beliefs and attitudes.
In particular, our intervention emphasizing alternative causal theories may assist students in learning to reason about causal claims from correlational studies. Considering multiple causal theories requires original thinking because students must posit additional variables (e.g., third variables not stated in the problem) and unstated causal relationships (e.g., reversed causes, multiple causes and effects). When successful, their alternative theories may help to raise doubt about the internal validity of the study because the presented causal theory is not unique. The findings show that after the intervention, students provided more of their own competing theories, confirming the existence of alternative causes consistent with the correlational evidence. In reasoning about causes and associations, the process of theory-evidence coordination appears to require less attention to evidence and more attention to alternative theories. In the context of evaluating summaries of science reports (such as those frequently found in the media), considering theory-evidence correspondence cannot disconfirm causal claims; instead, reasoning about other causal theories consistent with the evidence may help to identify when a causal theory is not unique, avoiding causal theory error.
The intervention was immediately followed by decrease in causal theory error appearing in students’ study critiques and alternative theories. However, almost half of the students still rated studies with correlational evidence as “high quality” and “supporting a causal claim” immediately after the intervention even while raising issues with the claim in their written reasons for their ratings. This suggests students may interpret “study quality” and “support for claim” ratings questions by assessing the consistency of the correlational evidence and causal theory, while also recognizing that the study cannot uniquely account for the finding without experimental methodology. This suggests open-ended questions may provide information about causal reasoning processes not evident from ratings of support or endorsement of the causal claim alone, as in multiple choice measures (Shou & Smithson, 2015 ).
In prior work on causal reasoning, people viewed a series of presented data points to arrive at a causal theory through a process of theory-evidence coordination (Kuhn, 1993 ; Kuhn et al., 1995 ), and use varied strategies to determine whether evidence aligns with theories (Kuhn & Dean, 2004 ; Kuhn et al., 1995 ). But when summary findings from a study are presented (as in the present study; see also Adams et al., 2017 ; Bleske-Rechek et al., 2015 ; Xiong et al., 2020 ), it is not possible to examine inconsistencies in presented evidence to test alternative theories; instead, the theory-evidence coordination process may focus on addressing whether a presented theory uniquely accounts for the observed association. Further studies are needed to better understand how to support students in considering correlational findings, and how people reason about theory and evidence from study summaries in science communications.
Effective science learning interventions
These results are consistent with prior studies showing that the use of visualizations can improve science learning (Mayer, 2005 , 2020 ). As noted by Gobert and Clement ( 1999 ), creating diagrams from scientific material has been linked with better understanding of causation and dynamics, and drawing has been shown to assist learners in some circumstances (Fiorella & Zhang, 2018 ). In the diagram generation task in our study, students were asked to create their own drawings of possible causal relationships as alternatives to a presented causal claim. Considering their own models of alternative causal theories in the intervention appeared to have a positive effect on students’ later reasoning. Self-generated explanations supported by visual representations have been shown to improve learners’ understanding of scientific data (Ainsworth & Loizou, 2003 ; Bobek & Tversky, 2016 ; Gobert & Clement, 1999 ; Rhodes et al., 2014 ; Tal & Wansink, 2016 ). Previous studies on causal theory error have employed close-ended tasks (Adams et al., 2017 ; Bleske-Rocher et al., 2018 ; Xiong et al., 2020 ), but our findings suggest structuring the learning tasks to allow students to bring their own examples to bear may be especially impactful (Chi et al., 1994 ; Pressley et al., 1992 ).
Studies of example-based instruction (Shafto et al., 2014 ; Van Gog & Rummel, 2010 ) show improvement in learning through spontaneous, prompted, and trained self-explanations of examples (Ainsworth & Loizou, 2003 ; Chi, 2000 ; Chi et al., 1989 ). In particular, the present study supports the importance of examples to illustrate errors in scientific reasoning. Learning from examples of error has been successful in other studies (Durkin & Rittle-Johnson, 2012 ; Ohlsson, 1996 ; Seifert & Hutchins, 1992 ; Siegler & Chen, 2008 ), and instructing students on recognizing common errors in reasoning about science may hold promise (Stadtler et al., 2013 ). Because students may struggle to recognize errors in their own thinking, it may be helpful to provide experience through exposure to others’ errors to build recognition skills. There is some suggestion that science students are better able to process “hedges” in study claims, where less direct wording (such as “associated” and “predicts”) resulted in lower causality ratings compared to direct (“makes”) claims (Adams et al., 2017 ; Durik et al., 2008 ). Learning about hedges and qualifiers used in science writing may help students understand the probabilistic nature of correlational evidence (Butler, 1990 ; Durik et al., 2008 ; Horn, 2001 ; Hyland, 1998 ; Jensen, 2008 ; Skelton, 1988 ).
These results are also consistent with theories of cognitive processes in science education showing reasoning is often motivated by familiar content and existing beliefs that may influence scientific thinking (Billman et al., 1992 ; Fugelsang & Thompson, 2000 , 2003 ; Koehler, 1993 ; Kuhn et al., 1995 ; Wright & Murphy, 1984 ). In this study, when asked to explain their reasons for why the study is convincing, students also invoked heuristic thinking in responses, such as, “…because that’s what I’ve heard before,” or “…because that makes sense to me.” As a quick alternative to careful, deliberate reasoning (Kahneman, 2011 ), students may gloss over the need to consider unstated alternative relationships among the variables, leading to errors in reasoning (Shah et al., 2017 ). Furthermore, analytic thinking approaches like causal reasoning require substantial effort due to limited cognitive resources (Shah et al., 2017 ); so, students may take a heuristic approach when considering evidence and favor it when it fits with previous beliefs (Michal et al., 2021 ; Shah et al., 2017 ). Some studies suggest analytic thinking may be fostered by considering information conflicting with beliefs (Evans & Curtis-Holmes, 2005 ; Evans, 2003a , b ; Klaczynski, 2000 ; Koehler, 1993 ; Kunda, 1990 ; Nickerson, 1998 ; Sá et al., 1999 ; Sinatra et al., 2014 ).
Limitations and future research
Our study examined understanding causal claims from scientific studies within a classroom setting, providing a foundation for students’ later spontaneous causal reasoning in external settings. However, application of these skills outside of the classroom may be less successful. For students, connecting classroom learning about causal theory errors to causal claims arising unexpectedly in other sources is likely more challenging (Hatfield et al., 2006 ). There is evidence that students can make use of statistical reasoning skills gained in class when approached through an unexpected encounter in another context (Fong et al., 1986 ), but more evidence is needed to determine whether the benefits of this intervention extend to social media reports.
As in Mueller and Coon’s ( 2013 ) pre/post classroom design, our study provided all students with the intervention by pedagogical design. While the study took place early in the term, it is possible that students showed improvement over the sessions due to other course activities (though correlational versus experimental design was not yet addressed). Additional experimental studies are needed to rule out possible external influences due to the course instruction; for example, students may, over the two-week span of the study, have become more skeptical about claims from studies given their progress in learning about research methods. As a consequence, students may have become critical of all studies (including those with experimental evidence) rather than identifying concerns specific to claims from correlational studies. In addition, the advanced psychology students in this study may have prior knowledge about science experiments and more readily understand the intervention materials, but other learners may find them less convincing or more challenging. Psychology students may also experience training distinguishing them from other science students, such as an emphasis on critical thinking (Halpern, 1998 ), research methods (Picardi & Masick, 2013 ), and integrating knowledge from multiple theories (Green & Hood, 2013 ; Morling, 2014 ). They may also hold different epistemological beliefs, such as that knowledge is less certain and more changing (Hofer, 2000 ) or more subjective in nature (Renken et al., 2015 ). Future research with more diverse samples at varying levels of science education may suggest how novice learners may benefit from instruction on causal theory error and how it may impact academic outcomes.
The longitudinal design of this classroom study extended across 2 weeks and resulted in high attrition; with attendance optional, only 40% of the enrolled students attended all three sessions and were included in the study. However, this subsample scored similarly ( M = 146.25, SD = 13.4) to nonparticipants ( M = 143.2, SD = 13.7) on final course scores, t (238) = 1.16, p = .123. Non-graded participation may also have limited students’ motivation during the study; for example, the raw number of diagrams generated dropped after the first session (though quality increased in later sessions). Consequently, the findings may underestimate the impact of the intervention when administered as part of a curriculum. Further studies are also needed to determine the impact of specific intervention features, consider alternative types of evidence (such as experimental studies), and examine the qualities of examples that motivate students to create alternative theories.
Finally, the present study examines only science reports of survey studies with just two variables and a presumed causal link, as commonly observed in media reports focusing on just 1 predictor and 1 outcome variable (Mueller, 2020 ). For both science students and the public, understanding more complex causal models arising from varied evidence will likely require even more support for assessment of causal claims (Grotzer & Shane Tutwiler, 2014 ). While the qualities of evidence required to support true causal claims is clear (Stanovich, 2009 , 2010 ), causal claims in non-experimental studies are increasingly widespread in the science literature (Baron & Kenny, 1986 ; Brown et al., 2013 ; Kida, 2006 ; Morling, 2014 ; Reinhart et al., 2013 ; Schellenberg, 2020 ). Bleske-Rechek et al. ( 2018 ) found that half of psychology journal articles offered unwarranted direct causal claims from non-experimental evidence, with similar findings in health studies (Lazarus et al., 2015 ). That causal claims from associational evidence appear in peer-reviewed journals (Bleske-Rechek et al., 2018 ; Brown et al., 2013 ; Lazarus et al., 2015 ; Marinescu et al., 2018 ) suggests a new characterization is needed that acknowledges how varied forms of evidence are used in scientific arguments for causal claims. As Hammond and Horn ( 1954 ) noted about cigarette smoking and death, accumulated associational evidence in the absence of alternative causal theories may justify the assumption of a causal relationship even in the absence of a true experiment.
Implications
Learning to assess the quality of scientific studies is a challenging agenda for science education (Bromme & Goldman, 2014 ). Science reports in the media appear quite different than those in journals, and often include limited information to help the reader recognize the study design as correlational or experimental, or to detect features such as random assignment to groups (Adams et al., 2017 ; Morling, 2014 ). Studies described in media reports often highlight a single causal claim and ignore alternatives even when identified explicitly in the associated journal article (Adams et al., 2019 ; Mueller & Coon, 2013 ; Sumner et al., 2014 ). Reasoning “in a vacuum” is often required given partial information in science summaries, and reasoning about the potential meaning behind observed associations is a key skill to gain from science education. In fact, extensive training in scientific methods (e.g., random assignment, experiment, randomized controlled trials) may not be as critical as acknowledging the human tendency to see a cause behind associated events (Ahn et al., 1995 ; Johnson & Seifert, 1994 ; Sloman, 2005 ). Consequently, causal theory errors are ubiquitous in many diverse settings where underlying causes are unknown, and learning to use more caution in drawing causal conclusions in general is warranted.
However, leaving the task of correcting causal theory error to the learner falls short; instead, both science and media communications need to provide more accurate information (Adams et al., 2017 , 2019 ; Baram-Tsabari & Osborne, 2015 ; Bott et al., 2019 ; Yavchitz et al., 2012 ). Our findings suggest that science media reports should include both alternative causal theories and explicit warnings about causal theory error. More cautious claims and explicit caveats about associations provide greater clarity to media reports without harming interest or uptake of information (Adams, et al., 2019 ; Bott et al., 2019 ), while exaggerated causal claims in journal articles has been linked to exaggeration in media reports (Sumner et al., 2014 ). For example, press releases about health science studies aligning claims (in the headline and text) with the type of evidence presented (correlational vs. randomized controlled trial) resulted in later media articles with more cautious headlines and claims (Adams et al., 2019 ). Hedges and qualifiers are frequently used in academic writing to accurately capture the probabilistic nature of conclusions and are evaluated positively in these contexts (Horn, 2001 ; Hyland, 1998 ; Jensen, 2008 ; Skelton, 1988 ). For example, Durik and colleagues ( 2008 ) found that hedges qualifying interpretative statements did not lead to more negative perceptions of research. Prior work has demonstrated that headlines alone can introduce misinformation (Dor, 2003 ; Ecker et al., 2014a , b ; Lewandowsky et al., 2012 ), so qualifiers must appear with causal claims.
The implications of our study for teaching about causal theory error are particularly important for psychology and other social, behavioral, and health sciences where associational evidence is frequently encountered (Adams et al., 2019 ; Morling, 2014 ). Science findings are used to advance recommendations for behavior and public policies in a wide variety of areas (Adams et al., 2019 ). While many people believe they understand science experiments, the point of true experiments—to identify a cause for a given effect—may not be sufficiently prominent in science education (Durant, 1993 ). Addressing science literacy may require changing the focus toward recognizing causal theory error rather than creating expectations that all causal claims can be documented through experimental science. Because people see associations all around them (Sloman, 2005 ; Sloman & Lagnado, 2015 ), it is critical that science reports acknowledge that their presented findings stop far short of a "gold standard" experiment (Adams et al., 2019 ; Sumner et al., 2014 ; Hatfield et al., 2006 ; Koch & Wüstemann, 2014 ; Reis & Judd, 2000 ; Sullivan, 2011 ). Understanding the low base rate of true experiments in science and the challenges in establishing causal relationships is key to appreciating the value of the scientific enterprise. Science education must aim to create science consumers able to engage in argument from evidence (NTSA Framework, 2012 , p. 73), recognizing that interpretation is required even with true experiments through evaluating internal and external validity. By encouraging people to consider the meaning of scientific evidence in the world, they may be more likely to recognize causal theory errors in everyday life. The present study provides some evidence for a small step in this direction.
Conclusions
The tendency to infer causation from correlation—referred to here as causal theory error —is arguably the most ubiquitous and wide-ranging error found in science literature, classrooms, and media reports. Evaluating a probabilistic association from a science study requires a different form of theory-evidence coordination than in other causal reasoning tasks; in particular, evaluating a presented causal claim from a correlational study requires assessing the plausibility of alternative causal theories also consistent with the evidence. This study provides evidence that college students commit frequent causal theory error in interpreting science reports as captured in open-ended reasoning about the validity of a presented causal claim. This is the first identified intervention associated with significant, substantial changes in students’ ability to avoid causal theory error from claims in science reports. Because science communications are increasingly available in media reports, helping people improve their ability to assess whether studies support potential changes in behavior, thinking, and policies is an important direction for cognitive research and science education.
Availability of data and materials
All data and materials are available upon reasonable request.
Abel, E. L., & Kruger, M. L. (2010). Smile intensity in photographs predicts longevity. Psychological Science, 21 (4), 542–544. https://doi.org/10.1177/0956797610363775
Article PubMed Google Scholar
Adams, R. C., Challenger, A., Bratton, L., Boivin, J., Bott, L., Powell, G., Williams, A., Chambers, C. D., & Sumner, P. (2019). Claims of causality in health news: A randomised trial. BMC Medicine, 17 (1), 1–11.
Article Google Scholar
Adams, R. C., Sumner, P., Vivian-Griffiths, S., Barrington, A., Williams, A., Boivin, J., Chambers, C. D., & Bott, L. (2017). How readers understand causal and correlational expressions used in news headlines. Journal of Experimental Psychology: Applied, 23 (1), 1–14.
PubMed Google Scholar
Ahn, W. K., Kalish, C. W., Medin, D. L., & Gelman, S. A. (1995). The role of covariation versus mechanism information in causal attribution. Cognition, 54 (3), 299–352.
Ainsworth, S., & Loizou, A. (2003). The effects of self-explaining when learning with text or diagrams. Cognitive Science, 27 (4), 669–681. https://doi.org/10.1207/s15516709cog2706_6
Ainsworth, S. E., & Scheiter, K. (2021). Learning by drawing visual representations: Potential, purposes, and practical implications. Current Directions in Psychological Science, 30 (1), 61–67. https://doi.org/10.1177/0963721420979582
Amsel, E., Klaczynski, P. A., Johnston, A., Bench, S., Close, J., Sadler, E., & Walker, R. (2008). A dual-process account of the development of scientific reasoning: The nature and development of metacognitive intercession skills. Cognitive Development, 23 (4), 452–471. https://doi.org/10.1016/j.cogdev.2008.09.002
Bao, L., Cai, T., Koenig, K., Fang, K., Han, J., Wang, J., et al. (2009). Learning and scientific reasoning. Science, 323 (5914), 586–587.
Baram-Tsabari, A., & Osborne, J. (2015). Bridging science education and science communication research. Journal of Research in Science Teaching, 52 (2), 135–144. https://doi.org/10.1002/tea.21202
Baron, R. M., & Kenny, D. A. (1986). The moderator-mediator variable distinction in social psychological research: Conceptual, strategic, and statistical considerations. Journal of Personality and Social Psychology, 51 , 1173–1182.
Bensley, D. A., Crowe, D. S., Bernhardt, P., Buckner, C., & Allman, A. L. (2010). Teaching and assessing critical thinking skills for argument analysis in psychology. Teaching of Psychology, 37 (2), 91–96. https://doi.org/10.1080/00986281003626656
Berthold, K., & Renkl, A. (2009). Instructional aids to support a conceptual understanding of multiple representations. Journal of Educational Psychology, 101 (1), 70–87. https://doi.org/10.1037/a0013247
Berthold, K., & Renkl, A. (2010). How to foster active processing of explanations in instructional communication. Educational Psychology Review, 22 (1), 25–40. https://doi.org/10.1007/s10648-010-9124-9
Billman, D., Bornstein, B., & Richards, J. (1992). Effects of expectancy on assessing covariation in data: “Prior belief” versus “meaning.” Organizational Behavior and Human Decision Processes, 53 (1), 74–88.
Blalock, H. M., Jr. (1987). Some general goals in teaching statistics. Teaching Sociology, 15 (2), 164–172.
Bleske-Rechek, A., Gunseor, M. M., & Maly, J. R. (2018). Does the language fit the evidence? Unwarranted causal language in psychological scientists’ scholarly work. The Behavior Therapist, 41 (8), 341–352.
Google Scholar
Bleske-Rechek, A., Morrison, K. M., & Heidtke, L. D. (2015). Causal inference from descriptions of experimental and non-experimental research: Public understanding of correlation-versus-causation. Journal of General Psychology, 142 (1), 48–70.
Bobek, E., & Tversky, B. (2016). Creating visual explanations improves learning. Cognitive Research: Principles and Implications, 1 , 27. https://doi.org/10.1186/s41235-016-0031-6
Bott, L., Bratton, L., Diaconu, B., Adams, R. C., Challenger, A., Boivin, J., Williams, A., & Sumner, P. (2019). Caveats in science-based news stories communicate caution without lowering interest. Journal of Experimental Psychology: Applied, 25 (4), 517–542.
Bromme, R., & Goldman, S. R. (2014). The public’s bounded understanding of science. Educational Psychologist, 49 (2), 59–69. https://doi.org/10.1080/00461520.2014.921572
Brown, A. W., Brown, M. M. B., & Allison, D. B. (2013). Belief beyond the evidence: Using the proposed effect of breakfast on obesity to show 2 practices that distort scientific evidence. American Journal of Clinical Nutrition, 98 , 1298–1308. https://doi.org/10.3945/ajcn.113.064410
Bruner, J. S. (1957). Going beyond the information given. In J. S. Bruner, E. Brunswik, L. Festinger, F. Heider, K. F. Muenzinger, C. E. Osgood, & D. Rapaport (Eds.), Contemporary approaches to cognition (pp. 41–69). Cambridge, MA: Harvard University Press. [Reprinted in Bruner, J. S. (1973), Beyond the information given (pp. 218–238). New York: Norton].
Butler, C. S. (1990). Qualifications in science: Modal meanings in scientific texts. In W. Nash (Ed.), The writing scholar: Studies in academic discourse (pp. 137–170). Sage Publications.
Carnevale, A., Strohl, J., & Smith, N. (2009). Help wanted: Postsecondary education and training required. New Directions for Community Colleges, 146 , 21–31. https://doi.org/10.1002/cc.363
Cheng, P. (1997). From covariation to causation: A causal power theory. Psychological Review, 104 , 367–405.
Chi, M. T. H. (2000). Self-explaining expository texts: The dual process of generating inferences and repairing mental models. In R. Glaser (Ed.), Advances in instructional psychology: Educational design and cognitive science (pp. 161–238). Erlbaum.
Chi, M. T. H., Bassok, M., Lewis, M. W., Reimann, P., & Glaser, R. (1989). Self-explanations: How students study and use examples in learning to solve problems. Cognitive Science, 13 (2), 145–182. https://doi.org/10.1016/0364-0213(89)90002-5
Chi, M. T., De Leeuw, N., Chiu, M. H., & LaVancher, C. (1994). Eliciting self-explanations improves understanding. Cognitive Science, 18 (3), 439–477. https://doi.org/10.1207/s15516709cog1803_3
Corrigan, R., & Denton, P. (1996). Causal understanding as a developmental primitive. Developmental Review, 16 , 162–202.
Cousineau, D. (2005). Confidence intervals in within-subject designs: A simpler solution to Loftus and Masson’s method. Tutorials in Quantitative Methods for Psychology, 1 , 42–45. https://doi.org/10.20982/tqmp.01.1.p042
Crowell, A., & Schunn, C. (2016). Unpacking the relationship between science education and applied scientific literacy. Research in Science Education, 46 (1), 129–140. https://doi.org/10.1007/s11165-015-9462-1
Dor, D. (2003). On newspaper headlines as relevance optimizers. Journal of Pragmatics, 35 (5), 695–721.
Durant, J. R. (1993). What is scientific literacy? In J. R. Durant & J. Gregory (Eds.), Science and culture in Europe (pp. 129–137). Science Museum.
Durik, A. M., Britt, M. A., Reynolds, R., & Storey, J. (2008). The effects of hedges in persuasive arguments: A nuanced analysis of language. Journal of Language and Social Psychology, 27 (3), 217–234.
Durkin, K., & Rittle-Johnson, B. (2012). The effectiveness of using incorrect examples to support learning about decimal magnitude. Learning and Instruction, 22 (3), 206–214. https://doi.org/10.1016/j.learninstruc.2011.11.001
Ecker, U. K., Lewandowsky, S., Chang, E. P., & Pillai, R. (2014a). The effects of subtle misinformation in news headlines. Journal of Experimental Psychology: Applied, 20 , 323–335. https://doi.org/10.1037/xap0000028
Ecker, U. K., Swire, B., & Lewandowsky, S. (2014b). Correcting misinformation—A challenge for education and cognitive science. In D. N. Rapp & J. L. G. Braasch (Eds.), Processing inaccurate information: Theoretical and applied perspectives from cognitive science and the educational sciences (pp. 13–38). Cambridge, MA: MIT Press.
Elwert, F. (2013). Graphical causal models. In S. L. Morgan (Ed.), Handbook of causal analysis for social research , Handbooks of Sociology and Social Research. Springer. https://doi.org/10.1007/978-94-007-6094-3_13
Evans, D. (2003a). Hierarchy of evidence: A framework for ranking evidence evaluating healthcare interventions. Journal of Clinical Nursing, 12 (1), 77–84. https://doi.org/10.1016/j.learninstruc.2011.11.001
Evans, J. (2003b). In two minds: Dual-process accounts of reasoning. Trends in Cognitive Science, 7 (10), 454–469. https://doi.org/10.1016/j.tics.2003.08.012
Evans, J., & Curtis-Holmes, J. (2005). Rapid responding increases belief bias: Evidence for the dual-process theory of reasoning. Thinking & Reasoning, 11 (4), 382–389. https://doi.org/10.1080/13546780542000005
Fiorella, L., & Zhang, Q. (2018). Drawing boundary conditions for learning by drawing. Educational Psychology Review, 30 (3), 1115–1137. https://doi.org/10.1007/s10648-018-9444-8
Fong, G., Krantz, D., & Nisbett, R. (1986). The effects of statistical training on thinking about everyday problems. Cognitive Psychology, 18 (3), 253–292. https://doi.org/10.1016/0010-0285(86)90001-0
Fugelsang, J. A., & Thompson, V. A. (2000). Strategy selection in causal reasoning: When beliefs and covariation collide. Canadian Journal of Experimental Psychology, 54 , 13–32.
Fugelsang, J. A., & Thompson, V. A. (2003). A dual-process model of belief and evidence interactions in causal reasoning. Memory & Cognition, 31 , 800–815.
Gobert, J., & Clement, J. (1999). Effects of student-generated diagrams versus student-generated summaries on conceptual understanding of causal and dynamic knowledge in plate tectonics. Journal of Research in Science Teaching, 36 (1), 39–53.
Green, H. J., & Hood, M. (2013). Significance of epistemological beliefs for teaching and learning psychology: A review. Psychology Learning & Teaching, 12 (2), 168–178.
Griffiths, T. L., & Tenenbaum, J. B. (2005). Structure and strength in causal induction. Cognitive Psychology, 51 (4), 334–384.
Große, C. S., & Renkl, A. (2007). Finding and fixing errors in worked examples: Can this foster learning outcomes? Learning and Instruction, 17 (6), 612–634. https://doi.org/10.1016/j.learninstruc.2007.09.008
Grotzer, T. A., & Shane Tutwiler, M. (2014). Simplifying causal complexity: How interactions between modes of causal induction and information availability lead to heuristic-driven reasoning. Mind, Brain, and Education, 8 (3), 97–114.
Haber, N., Smith, E. R., Moscoe, E., Andrews, K., Audy, R., Bell, W., et al. (2018). Causal language and strength of inference in academic and media articles shared in social media (CLAIMS): A systematic review. PLoS ONE, 13 (5), e0196346. https://doi.org/10.1371/journal.pone.0196346
Article PubMed PubMed Central Google Scholar
Hall, S. S., & Seery, B. L. (2006). Behind the facts: Helping students evaluate media reports of psychological research. Teaching of Psychology, 33 (2), 101–104. https://doi.org/10.1207/s15328023top3302_4
Halpern, D. F. (1998). Teaching critical thinking for transfer across domains: Disposition, skills, structure training, and metacognitive monitoring. American Psychologist, 53 (4), 449–455. https://doi.org/10.1037/0003-066X.53.4.449
Hammond, E. C., & Horn, D. (1954). The relationship between human smoking habits and death rates: A follow-up study of 187,766 men. Journal of the American Medical Association, 155 (15), 1316–1328. https://doi.org/10.1001/jama.1954.03690330020006
Hastie, R. (2015). Causal thinking in judgments. In G. Keren and G. Wu (Eds.), The Wiley Blackwell handbook of judgment and decision making , First Edition (pp. 590–628). Wiley. https://doi.org/10.1002/9781118468333.ch21
Hatfield, J., Faunce, G. J., & Soames Job, R. F. (2006). Avoiding confusion surrounding the phrase “correlation does not imply causation.” Teaching of Psychology, 33 (1), 49–51.
Hofer, B. K. (2000). Dimensionality and disciplinary differences in personal epistemology. Contemporary Educational Psychology, 25 , 378–405. https://doi.org/10.1006/ceps.1999.1026
Horn, K. (2001). The consequences of citing hedged statements in scientific research articles. BioScience, 51 (12), 1086–1093.
Huber, C. R., & Kuncel, N. R. (2015). Does college teach critical thinking? A meta-analysis. Review of Educational Research, 20 (10), 1–38. https://doi.org/10.3102/0034654315605917
Huggins-Manley, A. C., Wright, E. A., Depue, K., & Oberheim, S. T. (2021). Unsupported causal inferences in the professional counseling literature base. Journal of Counseling and Development, 99 (3), 243–251. https://doi.org/10.1002/jcad.12371
Hyland, K. (1998). Boosting, hedging and the negotiation of academic knowledge. Text & Talk, 18 (3), 349–382.
Jenkins, E. W. (1994). Scientific literacy. In T. Husen & T. N. Postlethwaite (Eds.), The international encyclopedia of education (2nd ed., Vol. 9, pp. 5345–5350). Pergamon Press.
Jensen, J. D. (2008). Scientific uncertainty in news coverage of cancer research: Effects of hedging on scientists’ and journalists’ credibility. Human Communication Research, 34 , 347–369.
Johnson, H. M., & Seifert, C. M. (1994). Sources of the continued influence effect: When discredited information in memory affects later inferences. Journal of Experimental Psychology: Learning, Memory, and Cognition, 20 (6), 1420–1436.
Kahneman, D. (2011). Thinking, fast and slow . Farrar, Straus & Giroux.
Kida, T. E. (2006). Don’t believe everything you think: The 6 basic mistakes we make in thinking . Prometheus Books.
Klaczynski, P. A. (2000). Motivated scientific reasoning biases, epistemological beliefs, and theory polarization: A two-process approach to adolescent cognition. Child Development, 71 (5), 1347–1366. https://doi.org/10.1111/1467-8624.00232
Koch, C., & Wüstemann, J. (2014). Experimental analysis. In The Oxford handbook of public accountability (pp. 127–142).
Koehler, J. J. (1993). The influence of prior beliefs on scientific judgments of evidence quality. Organizational Behavior and Human Decision Processes, 56 , 28–55.
Kolstø, S. D., Bungum, B., Arnesen, E., Isnes, A., Kristensen, T., Mathiassen, K., & Ulvik, M. (2006). Science students’ critical examination of scientific information related to socio-scientific issues. Science Education, 90 (4), 632–655. https://doi.org/10.1002/sce.20133
Kosonen, P., & Winne, P. H. (1995). Effects of teaching statistical laws on reasoning about everyday problems. Journal of Educational Psychology, 87 (1), 33. https://doi.org/10.1037/0022-0663.87.1.33
Kuhn, D. (1993). Connecting scientific and informal reasoning. Merrill-Palmer Quarterly, 39 (1), 74–103.
Kuhn, D. (2005). Education for thinking . Harvard University Press.
Kuhn, D. (2012). The development of causal reasoning. Wires Cognitive Science, 3 , 327–335. https://doi.org/10.1002/wcs.1160
Kuhn, D., Amsel, E., O’Loughlin, M., Schauble, L., Leadbeater, B., & Yotive, W. (1988). Developmental psychology series. The development of scientific thinking skills . Academic Press.
Kuhn, D., & Dean, D., Jr. (2004). Connecting scientific reasoning and causal inference. Journal of Cognition and Development, 5 (2), 261–288.
Kuhn, D., Garcia-Mila, M., Zohar, A., & Andersen, C. (1995). Strategies of knowledge acquisition. Monographs of the Society for Research in Child Development, 60 , i 157.
Kuhn, D., Iordanou, K., Pease, M., & Wirkala, C. (2008). Beyond control of variables: What needs to develop to achieve skilled scientific thinking? Cognitive Development, 23 , 435–451.
Kunda, Z. (1990). The case for motivated reasoning. Psychological Bulletin, 108 (3), 480–498. https://doi.org/10.1037/0033-2909.108.3.480
Landis, J. R., & Koch, G. G. (1977). The measurement of observer agreement for categorical data. Biometrics, 33 , 159–174.
Lazarus, C., Haneef, R., Ravaud, P., & Boutron, I. (2015). Classification and prevalence of spin in abstracts of non-randomized studies evaluating an intervention. BMC Medical Research Methodology, 15 , 85. https://doi.org/10.1186/s12874-015-0079-x
Lee, L. O., James, P., Zevon, E. S., Kim, E. S., Trudel-Fitzgerald, C., Spiro, A., III., Grodstein, F., & Kubzansky, L. D. (2019). Optimism is associated with exceptional longevity in 2 epidemiologic cohorts of men and women. Proceedings of the National Academy of Sciences, 116 (37), 18357–18362. https://doi.org/10.1073/pnas.1900712116
Lehrer, R., & Schauble, L. (2006). Scientific thinking and science literacy. In W. Damon, & R. Lerner (Series Eds.) & K. A. Renninger, & I. E. Sigel (Vol. Eds.), Handbook of child psychology Vol. 4: Child psychology in practice (6th ed.). New York: Wiley. https://doi.org/10.1002/9780470147658.chpsy0405 .
Lewandowsky, S., Ecker, U. K., Seifert, C. M., Schwarz, N., & Cook, J. (2012). Misinformation and its correction: Continued influence and successful debiasing. Psychological Science in the Public Interest, 13 (3), 106–131. https://doi.org/10.1177/1529100612451018
Marinescu, I. E., Lawlor, P. N., & Kording, K. P. (2018). Quasi-experimental causality in neuroscience and behavioural research. Nature Human Behaviour, 2 (12), 891–898.
Mayer, R. E. (2005). Cognitive theory of multimedia learning. The Cambridge handbook of multimedia learning, 41 , 31–48.
Mayer, R. E. (2020). Multimedia learning . Cambridge University Press.
Book Google Scholar
Michal, A. L., Zhong, Y., & Shah, P. (2021). When and why do people act on flawed science? Effects of anecdotes and prior beliefs on evidence-based decision-making. Cognitive Research: Principles and Implications, 6 , 28. https://doi.org/10.1186/s41235-021-00293-2
Miller, J. D. (1996). Scientific literacy for effective citizenship: Science/technology/society as reform in science education . SUNY Press.
Morling, B. (2014). Research methods in psychology: Evaluating a world of information . W.W. Norton and Company
Mueller, J. (2020). Correlation or causation? Retrieved June 1, 2021, from http://jfmueller.faculty.noctrl.edu/100/correlation_or_causation.htm
Mueller, J. F., & Coon, H. M. (2013). Undergraduates’ ability to recognize correlational and causal language before and after explicit instruction. Teaching of Psychology, 40 (4), 288–293. https://doi.org/10.1177/0098628313501038
Next Generation Science Standards Lead States. (2013). Next generation science standards: For states, by states . The National Academies Press.
Nickerson, R. S. (1998). Confirmation bias: A ubiquitous phenomenon in many guises. Review of General Psychology, 2 (2), 175–220. https://doi.org/10.1037/1089-2680.2.2.175
Norcross, J. C., Gerrity, D. M., & Hogan, E. M. (1993). Some outcomes and lessons from a cross-sectional evaluation of psychology undergraduates. Teaching of Psychology, 20 (2), 93–96. https://doi.org/10.1207/s15328023top2002_6
Norris, S. P., & Phillips, L. M. (1994). Interpreting pragmatic meaning when reading popular reports of science. Journal of Research in Science Teaching, 31 (9), 947–967. https://doi.org/10.1002/tea.3660310909
Norris, S. P., Phillips, L. M., & Korpan, C. A. (2003). University students’ interpretation of media reports of science and its relationship to background knowledge, interest, and reading difficulty. Public Understanding of Science, 12 (2), 123–145. https://doi.org/10.1177/09636625030122001
NTSA Framework (2012). Retrieved June 1, 2021 from https://ngss.nsta.org/practices.aspx?id=7
Ohlsson, S. (1996). Learning from error and the design of task environments. International Journal of Educational Research, 25 (5), 419–448.
Pearl, J. (1995). Causal diagrams for empirical research. Biometrika, 82 (4), 669–688.
Pearl, J. (2000). Causality: Models, reasoning, and inference . Cambridge University Press.
Pearl, J., & Mackenzie, D. (2018). The book of why: The new science of cause and effect . Basic Books.
Picardi, C. A., & Masick, K. D. (2013). Research methods: Designing and conducting research with a real-world focus . SAGE Publications.
Pressley, M., Wood, E., Woloshyn, V. E., Martin, V., King, A., & Menke, D. (1992). Encouraging mindful use of prior knowledge: Attempting to construct explanatory answers facilitates learning. Educational Psychologist, 27 (1), 91–109.
Reinhart, A. L., Haring, S. H., Levin, J. R., Patall, E. A., & Robinson, D. H. (2013). Models of not-so-good behavior: Yet another way to squeeze causality and recommendations for practice out of correlational data. Journal of Educational Psychology, 105 , 241–247.
Reis, H. T., & Judd, C. M. (2000). Handbook of research methods in social and personality psychology . Cambridge University Press.
Renken, M. D., McMahan, E. A., & Nitkova, M. (2015). Initial validation of an instrument measuring psychology-specific epistemological beliefs. Teaching of Psychology, 42 (2), 126–136.
Renkl, A., Stark, R., Gruber, H., & Mandl, H. (1998). Learning from worked-out examples: The effects of example variability and elicited self-explanations. Contemporary Educational Psychology, 23 (1), 90–108. https://doi.org/10.1006/ceps.1997.0959
Rhodes, R. E., Rodriguez, F., & Shah, P. (2014). Explaining the alluring influence of neuroscience information on scientific reasoning. Journal of Experimental Psychology: Learning, Memory, and Cognition, 40 (5), 1432–1440. https://doi.org/10.1037/a0036844
Rodriguez, F., Ng, A., & Shah, P. (2016a). Do college students notice errors in evidence when critically evaluating research findings? Journal on Excellence in College Teaching, 27 (3), 63–78.
Rodriguez, F., Rhodes, R. E., Miller, K., & Shah, P. (2016b). Examining the influence of anecdotal stories and the interplay of individual differences on reasoning. Thinking & Reasoning, 22 (3), 274–296. https://doi.org/10.1080/13546783.2016.1139506
Ryder, J. (2001). Identifying science understanding for functional scientific literacy. Studies in Science Education, 36 (1), 1–44. https://doi.org/10.1080/03057260108560166
Sá, W. C., West, R. F., & Stanovich, K. E. (1999). The domain specificity and generality of belief bias: Searching for a generalizable critical thinking skill. Journal of Educational Psychology, 91 (3), 497–510. https://doi.org/10.1037/0022-0663.91.3.497
Schellenberg, E. G. (2020). Correlation = causation? Music training, psychology, and neuroscience. Psychology of Aesthetics, Creativity, and the Arts, 14 (4), 475–480.
Seifert, C. M., & Hutchins, E. L. (1992). Error as opportunity: Learning in a cooperative task. Human-Computer Interaction, 7 (4), 409–435.
Shafto, P., Goodman, N. D., & Griffiths, T. L. (2014). A rational account of pedagogical reasoning: Teaching by, and learning from, examples. Cognitive Psychology, 71 (1), 55–89. https://doi.org/10.1016/j.cogpsych.2013.12.004
Shah, P., Michal, A., Ibrahim, A., Rhodes, R., & Rodriguez, F. (2017). What makes everyday scientific reasoning so challenging? The Psychology of Learning and Motivation, 66 , 251–299. https://doi.org/10.1016/bs.plm.2016.11.006
Shou, Y., & Smithson, M. (2015). Effects of question formats on causal judgments and model evaluation. Frontiers in Psychology , 6 , Article 467. https://doi.org/10.3389/fpsyg.2015.00467 .
Siegler, R. S., & Chen, Z. (2008). Differentiation and integration: Guiding principles for analyzing cognitive change. Developmental Science, 11 (4), 433–448. https://doi.org/10.1111/j.1467-7687.2008.00689.x
Sinatra, G. M., Kienhues, D., & Hofer, B. (2014). Addressing challenges to public understanding of science: Epistemic cognition, motivated reasoning, and conceptual change. Educational Psychologist, 49 (2), 123–138. https://doi.org/10.1080/00461520.2014.916216
Skelton, J. (1988). The care and maintenance of hedges. ELT Journal, 42 (1), 37–43.
Sloman, S. A. (2005). Causal models . Oxford University Press.
Sloman, S. A., & Lagnado, D. A. (2003). Causal invariance in reasoning and learning. The Psychology of Learning and Motivation, 44 , 287–325.
Sloman, S., & Lagnado, D. A. (2015). Causality in thought. Annual Review of Psychology, 66 , 223–247.
Stadtler, M., Scharrer, L., Brummernhenrich, B., & Bromme, R. (2013). Dealing with uncertainty: Readers’ memory for and use of conflicting information from science texts as function of presentation format and source expertise. Cognition and Instruction, 31 (2), 130–150. https://doi.org/10.1080/07370008.2013.769996
Stanovich, K. E. (2009). What intelligence tests miss: The psychology of rational thought . Yale University.
Stanovich, K. E. (2010). How to think straight about psychology (9th ed.). Allyn & Bacon.
Stark, R., Kopp, V., & Fischer, M. R. (2011). Case-based learning with worked examples in complex domains: Two experimental studies in undergraduate medical education. Learning and Instruction, 21 (1), 22–33. https://doi.org/10.1016/j.learninstruc.2009.10.001
Stark, R., Mandl, H., Gruber, H., & Renkl, A. (2002). Conditions and effects of example elaboration. Learning and Instruction, 12 (1), 39–60. https://doi.org/10.1016/s0959-4752(01)00015-9
Steffens, B., Britt, M. A., Braasch, J. L., Strømsø, H., & Bråten, I. (2014). Memory for scientific arguments and their sources: Claim–evidence consistency matters. Discourse Processes, 51 , 117–142.
Sullivan, G. M. (2011). Getting off the “gold standard”: Randomized controlled trials and education research. Journal of Graduate Medical Education, 3 (3), 285–289. https://doi.org/10.4300/JGME-D-11-00147.1
Sumner, P., Vivian-Griffiths, S., Boivin, J., Williams, A., Venetis, C. A., Davies, A., Ogden, J., Whelan, L., Hughes, B., Dalton, B., Boy, F., & Chambers, C. D. (2014). The association between exaggeration in health related science news and academic press releases: Retrospective observational study. British Medical Journal, 2014 (349), g7015. https://doi.org/10.1136/bmj.g7015
Tal, A., & Wansink, B. (2016). Blinded with science: Trivial graphs and formulas increase ad persuasiveness and belief in product efficacy. Public Understanding of Science, 25 (1), 117–125. https://doi.org/10.1177/0963662514549688
Topor, D. D. (2019). If you’re happy and you know it… you may live longer. Harvard Health Blog, Harvard Medical School (10.16.2019). Retrieved June 1, 2021, from https://www.health.harvard.edu/blog/if-you-are-happy-and-you-know-it-you-may-live-longer-2019101618020
Trefil, J. (2008). Science education for everyone: Why and what? Liberal Education , 94 (2), 6–11. Retrieved 6/15/2021 from: https://www.aacu.org/publications-research/periodicals/science-education-everyone-why-and-what
Tversky, A., & Kahneman, D. (1977). Causal thinking in judgment under uncertainty. In J. Hintikka & R. E. Butts (Eds.), Basic problems in methodology and linguistics (pp. 167–190). Springer.
Chapter Google Scholar
Van Gog, T., & Rummel, N. (2010). Example-based learning: Integrating cognitive and social-cognitive research perspectives. Educational Psychology Review, 22 (2), 155–174. https://doi.org/10.1007/s10648-010-9134-7
Waldmann, M. R., Hagmayer, Y., & Blaisdell, A. P. (2006). Beyond the information given: Causal models in learning and reasoning. Current Directions in Psychological Science, 15 (6), 307–311. https://doi.org/10.1111/j.1467-8721.2006.00458.x
Whoriskey, P. (2011). Requiring algebra 2 in high school gains momentum. The Washington Post. Retrieved 6/15/2021 from https://www.washingtonpost.com/business/economy/requiring_algebra_ii_in_high_school_gains_momentum_nationwide/2011/04/01/AF7FBWXC_story.html?noredirect=on&utm_term=.a153d444a4bd
Wright, J. C., & Murphy, G. L. (1984). The utility of theories in intuitive statistics: The robustness of theory-based judgments. Journal of Experimental Psychology: General, 113 , 301–322.
Xiong, C., Shapiro, J., Hullman, J., & Franconeri, S. (2020). Illusion of causality in visualized data. IEEE Transactions on Visualization and Computer Graphics, 26 (1), 853–862. https://doi.org/10.1109/TVCG.2019.2934399
Yavchitz, A., Boutron, I., Bafeta, A., Marroun, I., Charles, P., Mantz, J., & Ravaud, P. (2012). Misrepresentation of randomized controlled trials in press releases and news coverage: A cohort study. PLoS Medicine, 9 , e1001308.
Zimmerman, C., Bisanz, G. L., Bisanz, J., Klein, J. S., & Klein, P. (2001). Science at the supermarket: A comparison of what appears in the popular press, experts’ advice to readers, and what students want to know. Public Understanding of Science, 10 (1), 37–58.
Zweig, M., & Devoto, E. (2015). Observational studies—Does the language fit the evidence? Association versus causation. Health News Review . Retrieved 6/15/2021 from https://www.healthnewsreview.org/toolkit/tips-for-understanding-studies/does-the-language-fit-the-evidence-association-versus-causation/
Download references
Acknowledgements
Meghan Harrington and Devon Shalom assisted with data analysis for the project. We are grateful to Eytan Adar and Hariharan Subramonyam for their conceptual contributions to the project, and to the editor and reviewers for their comments on revisions.
This research was supported by IES R305A170489 from the Institute for Educational Sciences at the US Department of Education.
Author information
Authors and affiliations.
Department of Psychology, University of Michigan, 530 Church St, Ann Arbor, MI, 48109, USA
Colleen M. Seifert, Michael Harrington, Audrey L. Michal & Priti Shah
You can also search for this author in PubMed Google Scholar
Contributions
PS and CS made substantial contributions to the conception of the work; PS, CS, and AM designed the materials; CS and MH contributed the acquisition, analysis, and interpretation of data; MH, CS, and PS drafted the work and AM added substantive revisions. All authors read and approved the final manuscript.
Corresponding author
Correspondence to Colleen M. Seifert .
Ethics declarations
Ethics approval and consent to participate.
This project was approved the Institutional Review Board at the University of Michigan and deemed exempt from IRB oversight (HUM00090207).
Consent for publication
Not applicable.
Competing interests
All authors declare that they have no competing interests.
Additional information
Publisher's note.
Springer Nature remains neutral with regard to jurisdictional claims in published maps and institutional affiliations.
Intervention in session 2
(Labels shown in italics did not appear in participants’ materials.)
Please read the following packet and answer the questions to the best of your ability as you go along.
Algebra 2 and career success
In 2006, researchers at Educational Testing Service (ETS) conducted an experiment in which they followed students for 12 years starting in 8th grade. They found that 84% of the top-tier workers (receiving the highest pay) had taken Algebra 2 classes in high school. In contrast, only 50% of those in the lowest pay tier had taken Algebra 2. This result suggests that requiring students to take Algebra 2 would benefit all students and can prepare them for better jobs after high school. Twenty states, including Michigan, have passed laws making Algebra 2 a graduation requirement for all of their high school students.
1. Evidence quality Does the ETS study convince you that taking Algebra 2 would be beneficial for students? Why or why not?
2. Causal theory error Do you think that requiring Algebra 2 based on the ETS study was a good decision for the state? Why or why not?
The Algebra 2 requirement has now fallen out of favor in some states, and they are now reversing or watering down their Algebra 2 requirement. In Michigan, students can now take a “Career Technical Education” course instead of Algebra 2.
3. Counterfactual How do you think that no required Algebra 2 course will affect Michigan students’ career opportunities?
Some people (and legislators) view the ETS study as proving that taking Algebra 2 causes students to have better chances of getting top-tier jobs. That certainly seems plausible because having math skills should help you get a good job!
4. Causal mechanism Why might taking Algebra 2 lead to students getting a better job?
However, it is not necessarily true that taking Algebra 2 would lead directly to getting better jobs. It is possible that the connection between Algebra 2 and higher job status is that the students who took Algebra 2 differed in other ways from people who did not.
5. Equality of groups In what ways might students in Algebra 2 differ from students who don’t take it? Who chooses to take Algebra 2? Try to think of other differences that might lead some students toward top-tier jobs. List at least 2 different things.
6. Self-selection Think back to the ETS study, when students had to choose whether to take Algebra 2. Why would a high school student decide to take it, and why would a student decide not to take it? Try to think of at least one new reason a student would take it, and one reason not to take it.
7. Third variables Think about the reasons listed below, and judge whether each reason might also explain why algebra students end up in better jobs. Mark each sentence with a “T” for true and “F” for false based on whether you think it is a good reason.
A: Students who chose Algebra 2 were also smarter, so they did well in school and got better jobs. B: Students who chose Algebra 2 were also going to college, so they ended up in better jobs. C: Students who chose Algebra 2 were from richer families who help them end up in better jobs. D: Students who chose Algebra 2 went to better high schools (with more math classes), and therefore they ended up in better jobs.
From the previous questions, notice that there are other reasons that students might both have taken Algebra 2 and gotten a top-tier job. It might look like what the students learned in Algebra 2 helped them get good jobs, but it could have been one of these other reasons that was the real cause.
Description of causal theory error The Michigan legislators seem to make a mistake in their decision making (as all people sometimes do) called the “correlation-to-causation” error. Just because two things are related (like Algebra 2 and better jobs), you can’t decide that one causes the other. Taking Algebra 2 and getting a good job may both be caused by something else; for example, being good in school, being in a good school, or having parents that are doctors. This is called a “third variable” explanation, where two things “go together” because of some other (third) cause.
Take a moment to pause and think: Most of us already know that “correlation does not imply causation;” but many times, especially when the cause makes sense, we forget to question our assumptions and make a causal theory error .
If any two variables A and B are related, it can mean one of several things:
It could mean that A causes B.
It could mean that B causes A.
It could mean that C causes both A and B. Or, A and B both can cause C.
It could be really complicated, in which A causes B which causes more A, and so on. Or, that A causes B, but C also impacts B. Whenever you evaluate evidence, it is important to think through these various possibilities.
In this next exercise, you will have a chance to evaluate evidence like the ETS study about Algebra 2. You will be asked to draw simple pictures to show possible reasons why two variables like Algebra 2 and job quality are related.

8. Correcting causal theory error Does this model make sense? Y or N
You already wrote down why Algebra 2 might help people get good jobs; for example, good jobs may require math skills.
Now consider:

9. Direction of causation Does this model make sense? Y or N
Probably not, because taking Algebra 2 usually happens before graduating and getting a job. So that is not a likely cause.
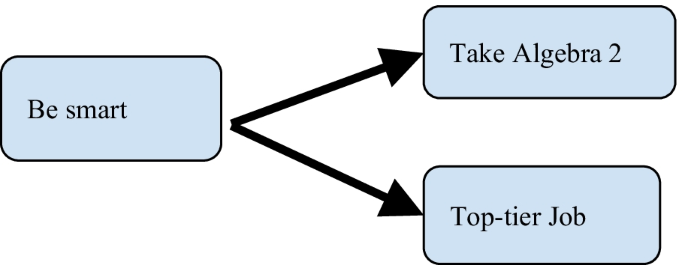
10. Identify third variable Does this model make sense? Y or N
It’s a good one… But you might have an even more complicated model in mind, like this next one:
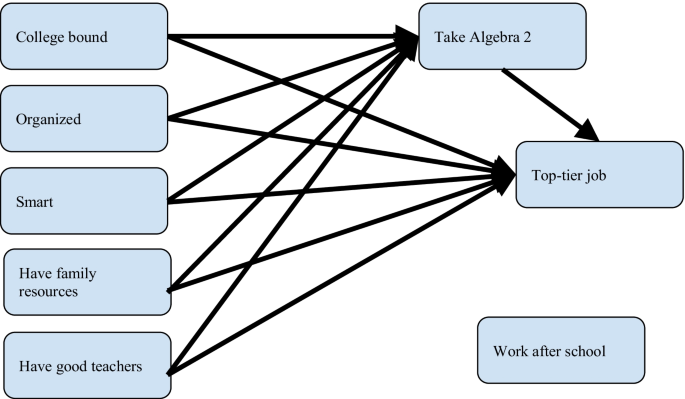
Clearly, there are many other causes that may help you end up in a top-tier job. So how do you decide which ones to choose?
Complex causal models How do scientists decide which is the right model? They tend to use other information, like variables they know are potentially relevant, clear causal explanations, other known relationships, and so forth. In other words, a simple correlation is not enough information to know for sure, but when combined with more information, you may be able to piece together a convincing explanation. But this takes some work on your part, so be ready to slow down and think when you consider evidence.
Advice When you read a media article about a scientific study, you may not have all the information you need to evaluate a causal model. But when you’re reading about a causal model, be extra careful if the cause seems to “make sense:” That is a good time to question yourself about other possible causes for the observed evidence. Ready to try this right now?
Task materials
Silence or music.
Although some people prefer to work in silence, many people opt to listen to music while working or studying. In fact, a brief glimpse into a library or coffee shop will reveal dozens of individuals poring over their laptops and books with earphones wedged into their ears. Researchers have recently become interested in whether listening to music actually helps students pay attention and learn new information. A recent study was conducted by researchers at a large midwestern university. Students ( n = 450) were surveyed prior to final exams in several large, lecture-based courses, and asked whether or not they had listened to music while studying for the final. The students who listened to music had, on average, higher test scores than students who did not listen to music while studying. The research team concluded that students who want to do well on their exams should listen to music while studying.
Computers-glasses
A recent study funded by the National Institute of Health links extended computer use to an increase in glasses and contacts use. Researchers recruited 600 employees from the NYC area and administered a survey on computer use. Of the respondents who used the computer for 30+ h a week, 2/3 wore corrective lenses or contacts and had severe myopia (nearsightedness) or hyperopia (farsightedness). In contrast, people who did not use computers extensively at work were less likely to report wearing corrective lenses: only 10% of people who used computers less than 30 h a week at their jobs required corrective lenses. To avoid harming your eyes, the researchers recommend avoiding too many hours using a computer each day. If it is impossible to avoid screen time on the job, they recommend speaking with an ophthalmologist about what you might do to counteract the negative consequences of screen time.
Parent-self control
An important aspect of parenting is to help children develop their self-control skills. Developmental scientists have long been interested in how parenting practices impact children’s ability to make their own choices. As part of a recent study, researchers measured children's body fat and surveyed mothers about the amount of control they exert over their children's eating. The results of this study, conducted with 400 children aged 3 to 5, found that those with the most body fat had the most “controlling” mothers when it came to the amount of food eaten. This shows that, “when mothers exert more control over their children's eating, the children display less self-control,” researchers said. Researchers recommend that parents should avoid being too controlling, and let their children learn to develop their own skills.
Church-health
Now, please diagram possible relationships between variables suggested by this news headline: “Church attendance boosts immunity.” Be sure to label the circles and links in your diagrams.
Then, write a caption below each diagram to describe the relationships.
Smiling-longevity
Now, please diagram possible relationships between variables suggested by this news headline: “Sincere smiling promotes longevity.” Be sure to label the circles and links in your diagrams.
Breast-feeding and Intelligence
Now, please diagram possible relationships between variables suggested by this news headline: “Breast-fed children found smarter.” Be sure to label the circles and links in your diagrams.
Study critique task
Please rate the quality of the study described above.
To what extent do you think that the study supports the conclusion that one should listen to music while studying?
Please write your critical evaluation of the study and its conclusions: What is good, and bad, about this news report?
Example response showing causal theory error:
“Good: Studies different groups, reaches a reasonable conclusion based off of the analysed data. Bad: Makes no reference to the type of food the mothers were controlling the children to eat, etc.”
Example response avoiding causal theory error:
“The study was conducted well for a correlation finding but not for causation. Therefore, the conclusions the news report and study made cannot be supported.”
Causal diagram task
Instructions.
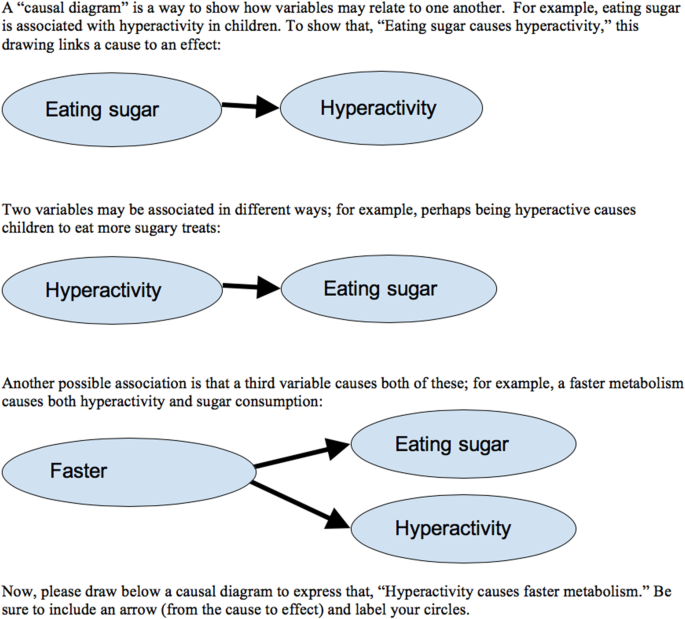
Diagramming task and example student responses:
Example responses.
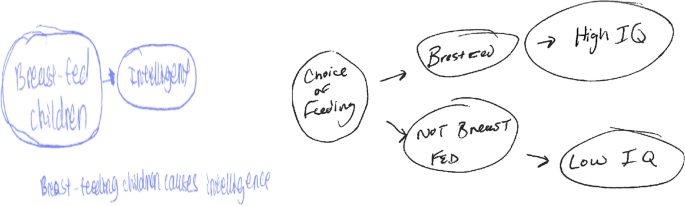
Rights and permissions
Open Access This article is licensed under a Creative Commons Attribution 4.0 International License, which permits use, sharing, adaptation, distribution and reproduction in any medium or format, as long as you give appropriate credit to the original author(s) and the source, provide a link to the Creative Commons licence, and indicate if changes were made. The images or other third party material in this article are included in the article's Creative Commons licence, unless indicated otherwise in a credit line to the material. If material is not included in the article's Creative Commons licence and your intended use is not permitted by statutory regulation or exceeds the permitted use, you will need to obtain permission directly from the copyright holder. To view a copy of this licence, visit http://creativecommons.org/licenses/by/4.0/ .
Reprints and permissions
About this article
Cite this article.
Seifert, C.M., Harrington, M., Michal, A.L. et al. Causal theory error in college students’ understanding of science studies. Cogn. Research 7 , 4 (2022). https://doi.org/10.1186/s41235-021-00347-5
Download citation
Received : 15 June 2021
Accepted : 27 November 2021
Published : 12 January 2022
DOI : https://doi.org/10.1186/s41235-021-00347-5
Share this article
Anyone you share the following link with will be able to read this content:
Sorry, a shareable link is not currently available for this article.
Provided by the Springer Nature SharedIt content-sharing initiative
- Theory-evidence coordination
- Correlation and causation
- Causal inference
- Science education
- Science communication
The Foundations of Strategic Thinking: Effectual, Strategic, and Causal Reasoning
Cite this chapter.
- John Pisapia 4 ,
- Lara Jelenc 5 &
- Annie Mick 4
Part of the book series: Contributions to Management Science ((MANAGEMENT SC.))
1862 Accesses
3 Citations
In this chapter, we dissect the differences between strategic planning and strategic thinking and suggest that traditional methods of planning no longer yield the benefits as in the past. Our analysis lays this failure on the use of a causal reasoning logic that alone no longer benefits organizations. Then we also examine foundational beliefs underpinning strategic thinking by examining the connections among the logic of entrepreneurial, causal, and strategic reasoning. In this analysis we distinguish two binary forms of thinking—causal and effectual—to frame our discussion, and then in the Hegelian tradition we press on to form a higher category of transcendent reconciliation through dialectic synthesis to introduce strategic reasoning. We end by picturing how strategic thinking concepts can form a new organizational change model that supersedes traditional planning. We call this model the strategic thinking protocol, which incorporates the logics of yesterday, today, and tomorrow.
“The world as we have created it is a process of our thinking. It cannot be changed without changing our thinking.” —Albert Einstein
This is a preview of subscription content, log in via an institution to check access.
Access this chapter
- Available as EPUB and PDF
- Read on any device
- Instant download
- Own it forever
- Compact, lightweight edition
- Dispatched in 3 to 5 business days
- Free shipping worldwide - see info
- Durable hardcover edition
Tax calculation will be finalised at checkout
Purchases are for personal use only
Institutional subscriptions
Similar content being viewed by others
Logical Incrementalism
Theory Building in Strategic Management
Axelrod, R., Axelrod, E., Jacobs, J., & Beedon, J. (2006). Beat the odds and succeed in organizational change. Consulting to Management, 17 (2), 6–9.
Google Scholar
Basadur, M. (2004). Leading others to think innovatively together: Creative leadership. The Leadership Quarterly, 15 , 103–121.
Article Google Scholar
Bonn, I. (2001). Developing strategic thinking as a core competence. Management Decision, 39 (1), 63–70.
Bonn, I. (2005). Improving strategic thinking: A multilevel approach. Leadership and Organization Development Journal, 26 (5), 336–354.
Covey, S. (2004). The 8th habit: From effectiveness to greatness . New York: Free Press.
Graetz, F. (2002). Strategic thinking versus strategic planning: Towards understanding the complementarities. Management Decision, 40 (5/6), 456–462.
Heracleous, L. (2003). Strategy and organization . Cambridge: University Press.
Holland, J. (1995). Hidden order: How adaptation builds complexity . New York: Helix Books.
Jelenc, L. (2008). The impact of strategic management and strategic thinking on the performance of Croatian entrepreneurial practice . Doctoral dissertation, University of Ljubljana, Slovenia.
Kim, W. C., & Mauborgne, R. (2005). Blue ocean strategy: How to create uncontested market space and make the competition irrelevant . Boston, MA: Harvard Business School Press.
Liedtka, J. M. (1998). Linking strategic thinking with strategic planning. Strategy and Leadership, 26 (4), 30–35.
Mintzberg, H. (1994). The rise and fall of strategic planning: Reconceiving the roles for planning, plans, planners . New York: Free Press.
O’Shannassy, T. (2003). Modern strategic management: Balancing strategic thinking and strategic planning for internal and external stakeholders. Singapore Management Review, 25 (1), 53–67.
Pisapia, J. (2009). The strategic leader: New tactics in a globalizing world . Charlotte, NC: Information Age Publishers.
Sarasvathy, S. (2001). Causation and effectuation: Toward a theoretical shift from economic inevitability to entrepreneurial contingency. Academy of Management Review, 26 (2), 243–263.
Sarasvathy, S. (2008). Effectuation: Elements of entrepreneurial expertise . Northampton, MA: Edward Elgar.
Book Google Scholar
Sarasvathy, S., & Saras, D. (2004). Making it happen, beyond theories of the firm to theories of firm design. Entrepreneurship Theory and Practice, 28 (6), 519–531.
Sirkin, H., Keenan, P., Jackson, A., Kotter, J., Beer, M., Nohria, N., et al. (2005). Lead change—successfully (3rd ed.). Cambridge: HBR Article Collection.
Sloan, J. (2014). Learning to think strategically (2nd ed.). London: Routledge.
Download references
Acknowledgment
Lara Jelenc’s work has been fully supported by the University of Rijeka, Croatia, under the project number 13.02.1.3.07 and 13.02.2012.
Author information
Authors and affiliations.
Department of Educational Leadership and Research Methodology, Florida Atlantic University, Boca Raton, FL, USA
John Pisapia & Annie Mick
Faculty of Economics, University of Rijeka, Ivana Filipovića 4, 51000, Rijeka, Croatia
Lara Jelenc
You can also search for this author in PubMed Google Scholar
Corresponding author
Correspondence to Lara Jelenc .
Editor information
Editors and affiliations.
Economics and Business Economics, University of Dubrovnik, Dubrovnik, Croatia
Ivona Vrdoljak Raguž
Faculty of Economics and Business, University of Zagreb, Zagreb, Croatia
Najla Podrug
Faculty of Economics, University of Rijeka, Rijeka, Croatia
Rights and permissions
Reprints and permissions
Copyright information
© 2016 Springer International Publishing Switzerland
About this chapter
Pisapia, J., Jelenc, L., Mick, A. (2016). The Foundations of Strategic Thinking: Effectual, Strategic, and Causal Reasoning. In: Vrdoljak Raguž, I., Podrug, N., Jelenc, L. (eds) Neostrategic Management. Contributions to Management Science. Springer, Cham. https://doi.org/10.1007/978-3-319-18185-1_4
Download citation
DOI : https://doi.org/10.1007/978-3-319-18185-1_4
Publisher Name : Springer, Cham
Print ISBN : 978-3-319-18184-4
Online ISBN : 978-3-319-18185-1
eBook Packages : Business and Management Business and Management (R0)
Share this chapter
Anyone you share the following link with will be able to read this content:
Sorry, a shareable link is not currently available for this article.
Provided by the Springer Nature SharedIt content-sharing initiative
- Publish with us
Policies and ethics
- Find a journal
- Track your research
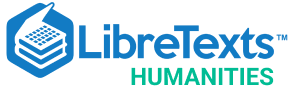
- school Campus Bookshelves
- menu_book Bookshelves
- perm_media Learning Objects
- login Login
- how_to_reg Request Instructor Account
- hub Instructor Commons
Margin Size
- Download Page (PDF)
- Download Full Book (PDF)
- Periodic Table
- Physics Constants
- Scientific Calculator
- Reference & Cite
- Tools expand_more
- Readability
selected template will load here
This action is not available.
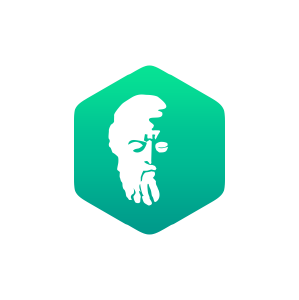
9.1: Hypothetical Reasoning
- Last updated
- Save as PDF
- Page ID 223910
\( \newcommand{\vecs}[1]{\overset { \scriptstyle \rightharpoonup} {\mathbf{#1}} } \)
\( \newcommand{\vecd}[1]{\overset{-\!-\!\rightharpoonup}{\vphantom{a}\smash {#1}}} \)
\( \newcommand{\id}{\mathrm{id}}\) \( \newcommand{\Span}{\mathrm{span}}\)
( \newcommand{\kernel}{\mathrm{null}\,}\) \( \newcommand{\range}{\mathrm{range}\,}\)
\( \newcommand{\RealPart}{\mathrm{Re}}\) \( \newcommand{\ImaginaryPart}{\mathrm{Im}}\)
\( \newcommand{\Argument}{\mathrm{Arg}}\) \( \newcommand{\norm}[1]{\| #1 \|}\)
\( \newcommand{\inner}[2]{\langle #1, #2 \rangle}\)
\( \newcommand{\Span}{\mathrm{span}}\)
\( \newcommand{\id}{\mathrm{id}}\)
\( \newcommand{\kernel}{\mathrm{null}\,}\)
\( \newcommand{\range}{\mathrm{range}\,}\)
\( \newcommand{\RealPart}{\mathrm{Re}}\)
\( \newcommand{\ImaginaryPart}{\mathrm{Im}}\)
\( \newcommand{\Argument}{\mathrm{Arg}}\)
\( \newcommand{\norm}[1]{\| #1 \|}\)
\( \newcommand{\Span}{\mathrm{span}}\) \( \newcommand{\AA}{\unicode[.8,0]{x212B}}\)
\( \newcommand{\vectorA}[1]{\vec{#1}} % arrow\)
\( \newcommand{\vectorAt}[1]{\vec{\text{#1}}} % arrow\)
\( \newcommand{\vectorB}[1]{\overset { \scriptstyle \rightharpoonup} {\mathbf{#1}} } \)
\( \newcommand{\vectorC}[1]{\textbf{#1}} \)
\( \newcommand{\vectorD}[1]{\overrightarrow{#1}} \)
\( \newcommand{\vectorDt}[1]{\overrightarrow{\text{#1}}} \)
\( \newcommand{\vectE}[1]{\overset{-\!-\!\rightharpoonup}{\vphantom{a}\smash{\mathbf {#1}}}} \)
Suppose I’m going on a picnic and I’m only selecting items that fit a certain rule. You want to find out what rule I’m using, so you offer up some guesses at items I might want to bring:
An Egg Salad Sandwich
A grape soda
Suppose now that I tell you that I’m okay with the first two, but I won’t bring the third. Your next step is interesting: you look at the first two, figure out what they have in common, and then you take a guess at the rule I’m using. In other words, you posit a hypothesis. You say something like
Do you only want to bring things that are yellow or tan?
Notice how at this point your hypothesis goes way beyond the evidence. Bananas and egg salad sandwiches have so much more in common than being yellow/tan objects. This is how hypothetical reasoning works: you look at the evidence, add a hypothesis that makes sense of that evidence (one among many hypotheses available), and then check to be sure that your hypothesis continues to make sense of new evidence as it is collected.
Suppose I now tell you that you haven’t guessed the right rule. So, you might throw out some more objects:
A key lime pie
A jug of orange juice
I then tell you that the first two are okay, but again the last item is not going with me on this picnic.
It’s solid items! Solid items are okay, but liquid items are not.
Again, not quite. Try another set of items. You are still convinced that it has to do with the soda and the juice being liquid, so you try out an interesting tactic:
An ice cube
Some liquid water
Some water Vapor
The first and last items are okay, but not the middle one. Now you think you’ve got me. You guess that the rule is “anything but liquids,” but I refuse to tell you whether you got it right. You’re pretty confident at this point, but perhaps you’re not certain . In principle, there could always be more evidence that upsets your hypothesis. I might say that the ocean is okay but a fresh water lake isn’t, and that would be very confusing for you. You’ll never be quite certain that you’ve guessed my rule correctly because it’s always in principle possible that I have a super complex rule that is more complex than your hypothesis.
So in hypothetical reasoning what we’re doing is making a leap from the evidence we have available to the rule or principle or theory which explains that evidence. The hypothesis is the link between the two. We have some finite evidence available to us, and we hypothesize an explanation. The explanation we posit either is or is not the true explanation, and so we’re using the hypothesis as a bridge to get onto the true explanation of what is happening in the world.
The hypothetical method has four stages. Let’s illustrate each with an example. You are investigating a murder and have collected a lot of evidence but do not yet have a guess as to who the killer might be.
1. The occurrence of a problem
Example \(\PageIndex{1}\)
Someone has been murdered and we need to find out who the killer is so that we might bring them to justice.
2. Formulating a hypothesis
Example \(\PageIndex{2}\)
After collecting some evidence, you weigh the reasons in favor of thinking that each suspect is indeed the murderer, and you decide that the spouse is responsible.
3. Drawing implications from the hypothesis
Example \(\PageIndex{3}\)
If the spouse was the murderer, then a number of things follow. The spouse must have a weak alibi or their alibi must rest on some falsehood. There is likely to be some evidence on their property or among their belongings that links the spouse to the murder. The spouse likely had motive. etc., etc., etc.
We can go on for ages, but the basic point is that once we’ve got an idea of what the explanation for the murder is (in this case, the hypothesis is that the spouse murdered the victim), we can ask ourselves what the world would have to be like for that to have been true. Then we move onto the final step:
4. Test those implications.
Example \(\PageIndex{4}\)
We can search the murder scene, try to find a murder weapon, run DNA analysis on the organic matter left at the scene, question the spouse about their alibi and possible motives, check their bank accounts, talk to friends and neighbors, etc. Once we have a hypothesis, in other words, that hypothesis drives the search for new evidence—it tells us what might be relevant and what irrelevant and therefore what is worth our time and what is not.
The Logic of Hypothetical Reasoning
If the spouse did it, then they must have a weak alibi. Their alibi is only verifiable by one person: the victim. So they do have a weak alibi. Therefore...they did it? Not quite.
Just because they have a weak alibi doesn’t mean they did it. If that were true, anyone with a weak alibi would be guilty for everything bad that happened when they weren’t busy with a verifiable activity.
Similarly, if your car’s battery is dead, then it won’t start. This doesn’t mean that whenever your car doesn’t start, the battery is dead. That would be a wild and bananas claim to make (and obviously false), but the original conditional (the first sentence in this paragraph) isn’t wild and bananas. In fact, it’s a pretty normal claim to make and it seems obviously true.
Let’s talk briefly about the logic of hypothetical reasoning so we can discover an important truth.
If the spouse did it, then their alibi will be weak
Their alibi is weak
So, the spouse did it
This is bad reasoning. How do we know? Well, here’s the logical form:
If A, then B
Therefore, A
This argument structure—called “affirming the consequent”—is invalid because there are countless instances of this general structure that have true premises and a false conclusion. Consider the following examples:
Example \(\PageIndex{5}\)
If I cook, I eat well
I ate well tonight, so I cooked.
Example \(\PageIndex{6}\)
If Eric runs for student president, he’ll become more popular.
Eric did become more popular, so he must’ve run for student president.
Maybe I ate well because I’m at the finest restaurant in town. Maybe I ate well because my brother cooked for me. Any of these things is possible, which is the root problem with this argument structure. It infers that one of the many possible antecedents to the conditional is the true antecedent without giving any reason for choosing or preferring this antecedent.
More concretely, affirming the consequent is the structure of an argument that states that a) one thing will explain an event, and b) that the event in question in fact occurred, and then concludes that c) the one thing that would’ve explained the event is the correct explanation of the event.
More concretely still, here’s yet another example of affirming the consequent:
Example \(\PageIndex{7}\)
My being rich would explain my being popular
I am in fact popular,
Therefore I am in fact rich
I might be popular without having a penny to my name. People sometimes root for underdogs, or respond to the right kind of personality regardless of their socioeconomic standing, or respect a good sense of humor or athletic prowess.
If I were rich, though, that would be one potential explanation for my being popular. Rich people have nice clothes, cool cars, nice houses, and get to have the kinds of experiences that make someone a potentially popular person because everyone wants to hear the cool stories or be associated with the exciting life they lead. Perhaps, people often seem to think, they’ll get to participate in the next adventure if they cozy up to the rich people. Rich kids in high school can also throw the best parties (if we’re honest, and that’s a great source of popularity).
But If I’m not rich, that doesn’t mean I’m not popular. It only means that I’m not popular because I’m rich .
Okay, so we’ve established that hypothetical reasoning has the logical structure of affirming the consequent. We’ve further established that affirming the consequent is an invalid deductive argumentative structure. Where does this leave us? Is the hypothetical method bad reasoning ?!?!?!? Nope! Luckily not all reasoning is deductive reasoning.
Remember that we’re discussing inductive reasoning in this chapter. Inductive reasoning doesn’t obey the rules of deductive logic. So it’s no crime for a method of inductive reasoning to be deductively invalid. The crime against logic would be to claim that we have certain knowledge when we only use inductive reasoning to justify that knowledge. The upshot? Science doesn’t produce certain knowledge—it produces justified knowledge, knowledge to a more or less high degree of certitude, knowledge that we can rely on and build bridges on, knowledge that almost certainly won’t let us down (but it doesn’t produce certain knowledge).
We can, though, with deductive certainty, falsify a hypothesis. Consider the murder case: if the spouse did it, then they’d have a weak alibi. That is, if the spouse did it, then they wouldn’t have an airtight alibi because they’d have to be lying about where they were when the murder took place. If it turns out that the spouse does have an airtight alibi, then your hypothesis was wrong.
Let’s take a look at the logic of falsification:
If the spouse did it, then they won’t have an airtight alibi
They have an airtight alibi
So the spouse didn’t do it
Now it’s possible that the conditional premise (the first premise) isn’t true, but we’ll assume it’s true for the sake of the illustration. The hypothesis was that the spouse did it and so the spouse’s alibi must have some weakness.
It’s also possible that our detective work hasn’t been thorough enough and so the second premise is false. These are important possibilities to keep in mind. Either way, here’s the logical form (a bit cleaned up and simplified):
Therefore not A
This is what argument pattern? That’s right! You’re so smart! It’s modus tollens or “the method of denying”. It’s a type of argument where you deny the implications of something and thereby deny that very thing. It’s a deductively valid argument form (remember from our unit on natural deduction?), so we can falsify hypotheses with deductive certainty: if your hypothesis implies something with necessity, and that something doesn’t come to pass, then your hypothesis is wrong.
Your hypothesis is wrong. That is, your hypothesis as it stands was wrong. You might be like one of those rogue and dogged detectives in the television shows that never gives up on a hunch and ultimately discovers the truth through sheer stubbornness and determination. You might think that the spouse did it, even though they’ve got an airtight alibi. In that case, you’ll have to alter your hypothesis a bit.
The process of altering a hypothesis to react to potentially falsifying evidence typically involves adding extra hypotheses onto your original hypothesis such that the original hypothesis no longer has the troubling implications which turned out not to be true. These extra hypotheses are called ad hoc hypotheses.
As an example, Newton’s theory of gravity had one problem: it made a sort of wacky prediction. So the idea was that gravity was an instantaneous attractive force exerted by all massive bodies on all other bodies. That is, all bodies attract all other bodies regardless of distance or time. The result of this should be that all massive bodies should smack into each other over time (after all, they still have to travel towards one another). But we don’t witness this. We should see things crashing towards the center of gravity of the universe at incredible speeds, but that’s not what’s happening. So, by the logic of falsification, Newton’s theory is simply false.
But Newton had a trick up his sleeve: he claimed that God arranged things such that the heavenly bodies are so far apart from one another that they are prevented from crashing into one another. Problem solved! God put things in the right spatial orientation such that the theory of gravity is saved: they won’t crash into each other because they’re so far apart! Newton employed an ad hoc hypothesis to save his theory from falsification.
Abductive Reasoning
There’s one more thing to discuss while we’re still on the topic of hypothetical reasoning or reasoning using hypotheses. ‘Abduction’ is a fancy word for a process or method sometimes called “inference to the best explanation. The basic idea is that we have a bunch of evidence, we try to explain it, and we find that we could explain it in multiple ways. Then we find the “best” explanation or hypothesis and infer that this is the true explanation.
For example, say we’re playing a game that’s sort of like the picnic game from before. I give you a series of numbers, and then you give me more series of numbers so that I can confirm or deny that each meets the rule I have in mind. So I say:
And then you offer the following series (serieses?):
60, 90, 120
Each of these series tests a particular hypothesis. The first tests whether the important thing is that the numbers start with 2, 3, and 4. The second tests whether the rule is to add 10 each successive number in the series. The third tests a more complicated hypothesis: add half of the first number to itself to get the second number, then add one third of the second number to itself to get the third number.
Now let’s say I tell you that only the third series is acceptable. What now?
Well, our hypothesis was pretty complex, but it seems pretty good. I can infer that this is the correct rule. Alternatively, I might look at other hypotheses which fit the evidence equally well: 1x, 1.5x, 2x? or maybe it’s 2x, 3x, 4x? What about x, 1.5x, x\(^2\)? These all make sense of the data, but are they equal apart from that?
Let’s suppose we can’t easily get more data with which to test our various hypotheses. We’ve got 4 to choose from and nothing in the evidence suggests that one of the hypotheses is better than the others—they all fit the evidence perfectly. What do we do?
One thing we could do is choose which hypothesis is best for reasons other than fit with the evidence. Maybe we want a simpler hypothesis, or maybe we want a more elegant hypothesis, or one which suggests more routes for investigation. These are what we might call “theoretical virtues”—they’re the things we want to see in a theory. The process of abduction is the process of selecting the hypothesis that has the most to offer in terms of theoretical virtues: the simplest, most elegant, most fruitful, most general, and so on.
In science in particular, we value a few theoretical virtues over others: support by the empirical evidence available, replicability of the results in a controlled setting by other scientists, ideally mathematical precision or at least a lack of vagueness, and parsimony or simplicity in terms of the sorts of things the hypothesis requires us to believe in.
Confirmation Bias
This is a great opportunity to discuss confirmation bias, or the natural tendency we have to seek out evidence which supports our beliefs and to ignore evidence which gets in the way of our beliefs. We’ll discuss cognitive biases more in Chapter 10, but since we’re dealing with the relationship between evidence and belief, this seems like a good spot to pause and reflect on how our minds work.
The way our minds work naturally, it seems, is to settle on a belief and then work hard to maintain that belief whatever happens. We come to believe that global warming is anthropogenic—is caused by human activities—and then we’re happy to accept a wide variety of evidence for the claim. If the evidence supports our belief, in other words, we don’t take the time or energy to really investigate exactly how convincing that evidence is. If we already believe the conclusion of an inference, in other words, we are much less likely to test or analyze the inference.
Alternatively, when we see pieces of evidence or arguments that appear to point to the contrary, we are either more skeptical of that evidence or more critical of that argument. For instance, if someone notes that the Earth goes through normal cycles of warming and ice ages and warming again, we immediately will look for ways to explain how this warming period is different than others in the past. Or we might look at the period of the cycles to find out if this is happening at the “right” time in our geological history for it not to be caused by humankind. In other words, we’re more skeptical of arguments or evidence that would defeat or undermine our beliefs, but we’re less skeptical and critical of arguments and evidence that supports our beliefs.
Here are some questions to reflect on as you try to decide how guilty you are of confirmation bias in your own reasoning:
Questions for Reflection:
1. Which news sources do you trust? Why?
2. What’s your process for exploring a topic—say a political or scientific or news topic?
3. How do you decide what to believe about a new subject?
4. When other people express an opinion about someone you don’t know, do you withhold judgment? How well do you do so?
5. Are you harder on arguments and evidence that would shake up your beliefs?
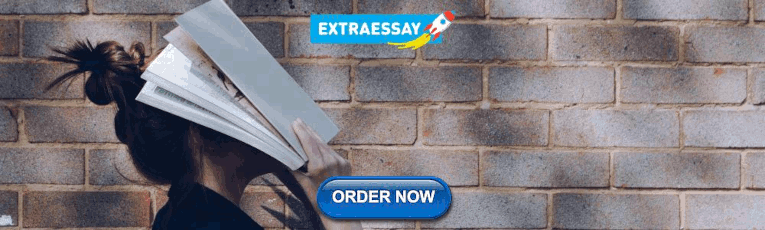
IMAGES
VIDEO
COMMENTS
Causal reasoning is the process of identifying causality: the relationship between a cause and its effect.The study of causality extends from ancient philosophy to contemporary neuropsychology; assumptions about the nature of causality may be shown to be functions of a previous event preceding a later one.The first known protoscientific study of cause and effect occurred in Aristotle's Physics.
9.2: Causal Reasoning. Page ID. We make causal judgments all the time. We think that the world is full of things causing other things to happen. He made me do it. The woman lost the race because she was anemic. The frog jumped off the leaf, causing it to shake and shower dew drops onto the ground below.
The critical thinker must make a clear distinction between a valid causal occurrence and sheer coincidence. Cumulative causal reasoning increases the soundness of the conclusion. The more times the causal pattern has happened, the greater the strength given to the causal reasoning, leading to a more valid conclusion.
Humans have a unique capacity for objective and general causal understanding. In this Review, Goddu and Gopnik describe the development of causal learning and reasoning abilities during evolution ...
Causal Reasoning. Read this section to investigate the complications of causality, particularly as it relates to correlation. Sometimes, two correlated events share a common cause, and sometimes, correlation is accidental. Complete the exercises to practice determining sufficient evidence for causation and determining accidental correlation.
Abstract. Causal reasoning is one of our most central cognitive competencies, enabling us to adapt to our world. Causal knowledge allows us to predict future events, or diagnose the causes of observed facts. We plan actions and solve problems using knowledge about cause-effect relations. Without our ability to discover and empirically test ...
A causal argument is an important argument type, as people are often looking for reasons as to why things have happened but may not be sure or have all of the necessary information. In your causal argument, you get the chance to make these things clear. An argumentative essay focused on why the U.S. has a high number of children who are "food ...
There are four well-established categories of informal reasoning: generalization, analogy, causal reasoning, and abduction. ... Approaches to Improving Reasoning through Critical Thinking. Recall that the goal of critical thinking is not just to study what makes reasons and statements good, but to help us improve our ability to reason, that is ...
A Miniguide to Critical Thinking (Lau) 1: Chapters 1.9: Causation ... Some common mistakes in causal reasoning. Genetic fallacy- Thinking that if some item X comes from a source with a certain property,then X must have the same property as well. But the conclusion does not follow, e.g.Eugenics was practised by the Nazis so it is obviously ...
Section 7 Causal and Counterfactual Reasoning; 29 Causal Thinking; 30 Causation; 31 Propensities and Counterfactuals: The Loser That Almost Won; Section 8 Argumentation; PART II INTERACTIONS OF REASONING IN HUMAN ... Causal thinking and the representation of narrative events. Journal of Memory and Language, 24, 612-630.CrossRef Google Scholar ...
Causal knowledge plays a crucial role in human thought, but the nature of causal representation and inference remains a puzzle. Can human causal inference be captured by relations of probabilistic dependency, or does it draw on richer forms of representation? This article explores this question by reviewing research in reasoning, decision making, various forms of judgment, and attribution.
This is a creative process so be open to thinking about the problem in a new way. Respect each other's ideas and time. Don't be afraid of turning over every stone as you brainstorm what might have caused the issue. Come up with some reasonable suggestions on how to eliminate the problem, or ways of minimizing it if it recurrs. Have fun.
The Importance of Causal Claims (and hence of Causal Reasoning) • Causal claims allow me to make predictions and decisions! • Please note that 'A causes B' is used to express that B is more likely to happen with A than without A - E.g. 'smoking causes cancer' is true if smoking makes you more likely to get cancer than not smoking.
First, we'll cover Hypothetical Reasoning, which is the kind of reasoning that people call the "scientific method.". After that, we'll cover the basics of Causal Reasoning, Statistical Generalization, and Arguing from Analogy. We'll also explore the logic of each a bit and look at some pitfalls of each kind of reasoning. This page ...
In this cognitive process, critical thinking skills such as causal reasoning and systems thinking can play a pivotal role in developing deeper understanding of given problem situations. Causal reasoning is the ability to identify causal relationships between sets of causes and effects [ 10 ].
1.4 The Relationship between Critical Thinking and Philosophical Inquiry §2 The Process of Critical Thinking. 2.1 Recognizing Assumptions; 2.2 Analyzing Arguments; 2.3 Evaluating Evidence; ... Causal Reasoning FORTHCOMING - DRAFT CHAPTER OUTLINE. Necessary & Sufficient Conditions; Types of Causation Aristotle's Categories of Causes;
Drawing on parallels with work in the psychology of mechanical reasoning, the notion of a causal mental model is proposed as a viable alternative to reasoning systems based in logic or probability theory alone. The central idea is that when people reason about causal systems they utilize mental models that represent objects, events or states of ...
A causal argumentis an inductive argument whose conclusion contains a causal claim. There are several inductive patterns of reasoning used to assess causal connections. These include the Method of Agreement, the Method of Difference, the Method of Agreement and Difference, and the Method of Concomitant Variation.
When reasoning about science studies, people often make causal theory errors by inferring or accepting a causal claim based on correlational evidence. While humans naturally think in terms of causal relationships, reasoning about science findings requires understanding how evidence supports—or fails to support—a causal claim. This study investigated college students' thinking about ...
CAUSAL REASONING 3 Improving Causal Reasoning in a College Science Course Accessibility of scientific information has never been better; as digital media has expanded, people have become regular consumers of scientific claims from research journals, television, magazines, blogs, or even word of mouth (Bromme & Goldman, 2014; Baram-Tsabari &
A critical thinker must be a master in fallacy detection. Statistical and Causal Reasoning. Two very specific kinds of reasoning, statistical and causal reasoning, are high-lighted, since they are fairly ubiquitous and of great importance, but at the same time are very much subject to pitfalls and abuse.
beliefs underpinning strategic thinking by examining the connections among the logic of entrepreneurial, causal, and strategic reasoning. In this analysis we distin-guish two binary forms of thinking—causal and effectual—to frame our discussion, and then in the Hegelian tradition we press on to form a higher category of transcen-
Understanding syllogisms, a form of reasoning where a conclusion is drawn from two given or assumed propositions (premises), can significantly sharpen your critical thinking skills. Each premise ...
9.1: Hypothetical Reasoning. Suppose I'm going on a picnic and I'm only selecting items that fit a certain rule. You want to find out what rule I'm using, so you offer up some guesses at items I might want to bring: Suppose now that I tell you that I'm okay with the first two, but I won't bring the third.
Critical thinking is the ability to think clearly and rationally, understanding the logical connection between ideas. It's a way of deciding whether a claim is always true, sometimes true ...
Inductive reasoning is a method of logical thinking that involves creating generalizations based on specific observations or experiences. In the realm of Business Intelligence (BI), you often ...
1 Inductive Basics. Inductive reasoning is a method of thinking that involves creating generalizations based on specific instances. In the context of BI, you use this type of reasoning to predict ...