- Privacy Policy

Home » Framework Analysis – Method, Types and Examples
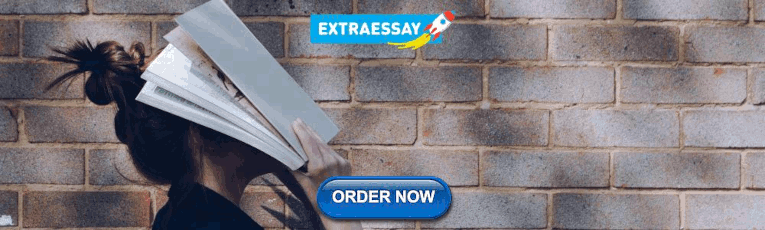
Framework Analysis – Method, Types and Examples
Table of Contents

Framework Analysis
Definition:
Framework Analysis is a qualitative research method that involves organizing and analyzing data using a predefined analytical framework. The analytical framework is a set of predetermined themes or categories that are derived from the research questions or objectives. The framework provides a structured approach to data analysis and can help to identify patterns, themes, and relationships in the data.
Steps in Framework Analysis
Here are the general steps in Framework Analysis:
Familiarization
Get familiar with the data by reading and re-reading it. This step helps you to become immersed in the data and to get a sense of its content, structure, and scope.
Identify a Coding Framework
Identify a coding framework or set of themes that will be used to analyze the data. These themes can be derived from existing literature or theories or developed based on the data itself.
Code the data by applying the coding framework to the data. This involves breaking down the data into smaller units and assigning each unit to a particular theme or category.
Chart or summarize the data by creating tables or matrices that display the distribution and frequency of each theme or category across the data set.
Mapping and interpretation
Analyze the data by examining the relationship between different themes or categories, and by exploring the implications and meanings of the findings in relation to the research question.
Verification
Verify the accuracy and validity of the findings by checking them against the original data, comparing them with other sources of data, and seeking feedback from others.
Report the findings by presenting them in a clear, concise, and organized manner. This involves summarizing the key themes, presenting supporting evidence, and providing interpretations and recommendations based on the findings.
Framework Analysis Conducting Guide
Here is a step-by-step guide to conducting framework analysis:
- Define the research question: The first step in conducting framework analysis is to clearly define the research question or objective that you want to investigate.
- Develop the analytical framework: Develop a coding framework or a set of predetermined themes or categories that are relevant to the research question. These themes or categories can be derived from existing literature or theories, or they can be developed based on the data collected.
- Data collection: Collect the data using a suitable method such as interviews, focus groups, surveys or observation.
- Familiarization: Transcribe and familiarize yourself with the data. Read through the data several times and take notes to identify any patterns, themes or issues that are emerging.
- Coding : Code the data by identifying key themes or categories and assigning each piece of information to a specific theme or category.
- Charting: Create charts or tables that display the frequency and distribution of each theme or category. This helps to summarize the data and identify patterns.
- Mapping and interpretation: Analyze the data to identify patterns, relationships, and themes. Interpret the findings in light of the research objectives and provide explanations for any significant patterns or themes that have emerged.
- Validation : Validate the findings by sharing them with others and seeking feedback. This can help to ensure that the findings are robust and reliable.
- Report writing: Write a report that summarizes the findings, includes quotes or examples from the data to support the findings and provides recommendations for future research.
Applications of Framework Analysis
Framework Analysis has a wide range of applications in research, including:
- Policy analysis: Framework Analysis can be used to analyze policies and policy documents to identify key themes, patterns, and underlying assumptions.
- Social science research: Framework Analysis is commonly used in social science research to analyze qualitative data from interviews, focus groups, and other sources.
- Health research: Framework Analysis can be used to analyze qualitative data from health research studies, such as patient and provider perspectives, to identify themes and patterns.
- Environmental research : Framework Analysis can be used to analyze qualitative data from environmental research studies to identify themes and patterns related to environmental attitudes, behaviors, and practices.
- Education research: Framework Analysis can be used to analyze qualitative data from educational research studies to identify themes and patterns related to teaching practices, student learning, and educational policies.
- Market research: Framework Analysis can be used to analyze qualitative data from market research studies to identify themes and patterns related to consumer attitudes, behaviors, and preferences.
Examples of Framework Analysis
Here are some examples of Framework Analysis in various research contexts:
- Health Research: A study on the experiences of cancer survivors might use Framework Analysis to identify themes related to the psychological, social, and physical aspects of survivorship. Themes might include coping strategies, social support, and health outcomes.
- Education Research: A study on the impact of a new teaching approach might use Framework Analysis to identify themes related to the implementation of the approach, the effectiveness of the approach, and barriers to its implementation. Themes might include teacher attitudes, student engagement, and logistical challenges.
- Environmental Research : A study on the factors that influence pro-environmental behaviors might use Framework Analysis to identify themes related to environmental attitudes, behaviors, and practices. Themes might include social norms, personal values, and perceived barriers to behavior change.
- Policy Analysis: A study on the implementation of a new policy might use Framework Analysis to identify themes related to policy development, implementation, and outcomes. Themes might include stakeholder perspectives, organizational structures, and policy effectiveness.
- Social Science Research: A study on the experiences of immigrant families might use Framework Analysis to identify themes related to the challenges and opportunities faced by immigrant families in their new country. Themes might include language barriers, cultural differences, and social support.
When to use Framework Analysis
Framework Analysis is a useful method for analyzing qualitative data when the research questions require an in-depth exploration of a particular phenomenon, concept, or experience. It is particularly useful when:
- The research involves multiple sources of qualitative data, such as interviews, focus groups, or documents, that need to be analyzed and compared.
- The research questions require a systematic and structured approach to data analysis that enables the identification of patterns, themes, and relationships in the data.
- The research involves a large and complex dataset that requires a method for organizing and synthesizing the data in a meaningful way.
- The research aims to generate new insights and understandings from the data, rather than testing pre-existing hypotheses or theories.
- The research requires a method that is transparent, replicable, and verifiable, as Framework Analysis provides a clear framework for data analysis and reporting.
Purpose of Framework Analysis
The purpose of Framework Analysis is to systematically organize and analyze qualitative data in a structured and transparent manner. The method is designed to identify patterns, themes, and relationships in the data that are relevant to the research question or objective. By using a rigorous and transparent approach to data analysis, Framework Analysis enables researchers to generate new insights and understandings from the data, and to provide a clear and structured presentation of the findings.
The method is particularly useful for analyzing large and complex qualitative datasets that require a method for organizing and synthesizing the data in a meaningful way. It can be used to explore a wide range of research questions and objectives across various fields, including health research, social science research, education research, policy analysis, and environmental research, among others.
Overall, the purpose of Framework Analysis is to provide a systematic and transparent method for analyzing qualitative data that enables researchers to generate new insights and understandings from the data in a rigorous and structured manner.
Characteristics of Framework Analysis
Some Characteristics of Framework Analysis are:
- Systematic and Structured Approach: Framework Analysis provides a systematic and structured approach to data analysis that involves a series of steps that are followed in a predetermined order.
- Transparency and Replicability: Framework Analysis emphasizes transparency and replicability, as it involves a clearly defined process for data analysis that can be applied consistently across different datasets and research questions.
- Flexibility : Framework Analysis is flexible and adaptable to a wide range of research contexts and objectives, as it can be used to analyze qualitative data from various sources and to explore different research questions.
- In-depth Exploration of the Data: Framework Analysis enables an in-depth exploration of the data, as it involves a thorough and detailed analysis of the data to identify patterns, themes, and relationships.
- Applicable to Large and Complex Datasets: Framework Analysis is particularly useful for analyzing large and complex qualitative datasets, as it provides a method for organizing and synthesizing the data in a meaningful way.
- Data-Driven: Framework Analysis is data-driven, as it focuses on the analysis and interpretation of the data rather than on pre-existing hypotheses or theories.
- Emphasis on Contextual Understanding : Framework Analysis emphasizes contextual understanding, as it involves a detailed examination of the data to identify the social, cultural, and environmental factors that may influence the phenomena under investigation.
Advantages of Framework Analysis
Some Advantages of Framework Analysis are as follows:
- Transparency : Framework Analysis provides a clear and structured approach to data analysis, which makes the process transparent and easy to follow. This ensures that the findings can be easily replicated or verified by other researchers.
- Thorough Analysis : Framework Analysis enables a thorough and detailed analysis of the data, which allows for the identification of patterns, themes, and relationships that may not be apparent through other methods.
- Contextual Understanding: Framework Analysis emphasizes the importance of understanding the context in which the data was collected, which enables a more nuanced interpretation of the findings.
- Collaborative Analysis: Framework Analysis can be used as a collaborative method for data analysis, as it allows multiple researchers to work together to analyze the data and develop a shared understanding of the findings.
- Efficient and Time-saving: Framework Analysis can be an efficient and time-saving method for analyzing qualitative data, as it provides a structured and organized approach to data analysis that can help researchers manage and synthesize large datasets.
- Comprehensive Reporting: Framework Analysis can help ensure that the research findings are comprehensive and well-reported, as the method provides a clear framework for presenting the results.
Limitations of Framework Analysis
Some Limitations of Framework Analysis are as follows:
- Subjectivity : Framework Analysis relies on the interpretation of the researchers, which can introduce subjectivity into the analysis process.
- Time-consuming : Framework Analysis can be a time-consuming method for data analysis, particularly when working with large and complex datasets.
- Limited ability to generate new theory : Framework Analysis is a deductive approach that relies on pre-existing theories and concepts to guide the analysis, which may limit the ability to generate new theoretical insights.
- Risk of oversimplification: The structured approach of Framework Analysis can lead to oversimplification of the data, as complex issues may be reduced to predefined categories or themes.
- Limited ability to capture the complexity of the data : The predefined categories or themes used in Framework Analysis may not be able to capture the full complexity of the data, particularly when dealing with nuanced or context-specific phenomena.
- Limited use with non-textual data : Framework Analysis is primarily designed for analyzing qualitative textual data and may not be as effective for analyzing non-textual data such as images, videos, or audio recordings.
About the author
Muhammad Hassan
Researcher, Academic Writer, Web developer
You may also like

Documentary Analysis – Methods, Applications and...

ANOVA (Analysis of variance) – Formulas, Types...

Uniform Histogram – Purpose, Examples and Guide

Symmetric Histogram – Examples and Making Guide

Correlation Analysis – Types, Methods and...

Inferential Statistics – Types, Methods and...
Understanding and solving intractable resource governance problems.
- In the Press
- Conferences and Talks
- Exploring models of electronic wastes governance in the United States and Mexico: Recycling, risk and environmental justice
- The Collaborative Resource Governance Lab (CoReGovLab)
- Water Conflicts in Mexico: A Multi-Method Approach
- Past projects
- Publications and scholarly output
- Research Interests
- Higher education and academia
- Public administration, public policy and public management research
- Research-oriented blog posts
- Stuff about research methods
- Research trajectory
- Publications
- Developing a Writing Practice
- Outlining Papers
- Publishing strategies
- Writing a book manuscript
- Writing a research paper, book chapter or dissertation/thesis chapter
- Everything Notebook
- Literature Reviews
- Note-Taking Techniques
- Organization and Time Management
- Planning Methods and Approaches
- Qualitative Methods, Qualitative Research, Qualitative Analysis
- Reading Notes of Books
- Reading Strategies
- Teaching Public Policy, Public Administration and Public Management
- My Reading Notes of Books on How to Write a Doctoral Dissertation/How to Conduct PhD Research
- Writing a Thesis (Undergraduate or Masters) or a Dissertation (PhD)
- Reading strategies for undergraduates
- Social Media in Academia
- Resources for Job Seekers in the Academic Market
- Writing Groups and Retreats
- Regional Development (Fall 2015)
- State and Local Government (Fall 2015)
- Public Policy Analysis (Fall 2016)
- Regional Development (Fall 2016)
- Public Policy Analysis (Fall 2018)
- Public Policy Analysis (Fall 2019)
- Public Policy Analysis (Spring 2016)
- POLI 351 Environmental Policy and Politics (Summer Session 2011)
- POLI 352 Comparative Politics of Public Policy (Term 2)
- POLI 375A Global Environmental Politics (Term 2)
- POLI 350A Public Policy (Term 2)
- POLI 351 Environmental Policy and Politics (Term 1)
- POLI 332 Latin American Environmental Politics (Term 2, Spring 2012)
- POLI 350A Public Policy (Term 1, Sep-Dec 2011)
- POLI 375A Global Environmental Politics (Term 1, Sep-Dec 2011)
Writing theoretical frameworks, analytical frameworks and conceptual frameworks
Three of the most challenging concepts for me to explain are the interrelated ideas of a theoretical framework, a conceptual framework, and an analytical framework. All three of these tend to be used interchangeably. While I find these concepts somewhat fuzzy and I struggle sometimes to explain the differences between them and clarify their usage for my students (and clearly I am not alone in this challenge), this blog post is an attempt to help discern these analytical categories more clearly.
A lot of people (my own students included) have asked me if the theoretical framework is their literature review. That’s actually not the case. A theoretical framework , the way I define it, is comprised of the different theories and theoretical constructs that help explain a phenomenon. A theoretical framework sets out the various expectations that a theory posits and how they would apply to a specific case under analysis, and how one would use theory to explain a particular phenomenon. I like how theoretical frameworks are defined in this blog post . Dr. Cyrus Samii offers an explanation of what a good theoretical framework does for students .
For example, you can use framing theory to help you explain how different actors perceive the world. Your theoretical framework may be based on theories of framing, but it can also include others. For example, in this paper, Zeitoun and Allan explain their theoretical framework, aptly named hydro-hegemony . In doing so, Zeitoun and Allan explain the role of each theoretical construct (Power, Hydro-Hegemony, Political Economy) and how they apply to transboundary water conflict. Another good example of a theoretical framework is that posited by Dr. Michael J. Bloomfield in his book Dirty Gold, as I mention in this tweet:
In Chapter 2, @mj_bloomfield nicely sets his theoretical framework borrowing from sociology, IR, and business-strategy scholarship pic.twitter.com/jTGF4PPymn — Dr Raul Pacheco-Vega (@raulpacheco) December 24, 2017
An analytical framework is, the way I see it, a model that helps explain how a certain type of analysis will be conducted. For example, in this paper, Franks and Cleaver develop an analytical framework that includes scholarship on poverty measurement to help us understand how water governance and poverty are interrelated . Other authors describe an analytical framework as a “conceptual framework that helps analyse particular phenomena”, as posited here , ungated version can be read here .
I think it’s easy to conflate analytical frameworks with theoretical and conceptual ones because of the way in which concepts, theories and ideas are harnessed to explain a phenomenon. But I believe the most important element of an analytical framework is instrumental : their purpose is to help undertake analyses. You use elements of an analytical framework to deconstruct a specific concept/set of concepts/phenomenon. For example, in this paper , Bodde et al develop an analytical framework to characterise sources of uncertainties in strategic environmental assessments.
A robust conceptual framework describes the different concepts one would need to know to understand a particular phenomenon, without pretending to create causal links across variables and outcomes. In my view, theoretical frameworks set expectations, because theories are constructs that help explain relationships between variables and specific outcomes and responses. Conceptual frameworks, the way I see them, are like lenses through which you can see a particular phenomenon.
A conceptual framework should serve to help illuminate and clarify fuzzy ideas, and fill lacunae. Viewed this way, a conceptual framework offers insight that would not be otherwise be gained without a more profound understanding of the concepts explained in the framework. For example, in this article, Beck offers social movement theory as a conceptual framework that can help understand terrorism . As I explained in my metaphor above, social movement theory is the lens through which you see terrorism, and you get a clearer understanding of how it operates precisely because you used this particular theory.
Dan Kaminsky offered a really interesting explanation connecting these topics to time, read his tweet below.
I think this maps to time. Theoretical frameworks talk about how we got here. Conceptual frameworks discuss what we have. Analytical frameworks discuss where we can go with this. See also legislative/executive/judicial. — Dan Kaminsky (@dakami) September 28, 2018
One of my CIDE students, Andres Ruiz, reminded me of this article on conceptual frameworks in the International Journal of Qualitative Methods. I’ll also be adding resources as I get them via Twitter or email. Hopefully this blog post will help clarify this idea!
You can share this blog post on the following social networks by clicking on their icon.
Posted in academia .
Tagged with analytical framework , conceptual framework , theoretical framework .
By Raul Pacheco-Vega – September 28, 2018
7 Responses
Stay in touch with the conversation, subscribe to the RSS feed for comments on this post .
Thanks, this had some useful clarifications for me!
I GOT CONFUSED AGAIN!
No need to be confused!
Thanks for the Clarification, Dr Raul. My cluttered mind is largely cleared, now.
Thanks,very helpful
I too was/am confused but this helps 🙂
Thank you very much, Dr.
Leave a Reply Cancel Some HTML is OK
Name (required)
Email (required, but never shared)
or, reply to this post via trackback .
About Raul Pacheco-Vega, PhD
Find me online.
My Research Output
- Google Scholar Profile
- Academia.Edu
- ResearchGate
My Social Networks
- Polycentricity Network
Recent Posts
- “State-Sponsored Activism: Bureaucrats and Social Movements in Brazil” – Jessica Rich – my reading notes
- Reading Like a Writer – Francine Prose – my reading notes
- Using the Pacheco-Vega workflows and frameworks to write and/or revise a scholarly book
- On framing, the value of narrative and storytelling in scholarly research, and the importance of asking the “what is this a story of” question
- The Abstract Decomposition Matrix Technique to find a gap in the literature
Recent Comments
- Hazera on On framing, the value of narrative and storytelling in scholarly research, and the importance of asking the “what is this a story of” question
- Kipi Fidelis on A sequential framework for teaching how to write good research questions
- Razib Paul on On framing, the value of narrative and storytelling in scholarly research, and the importance of asking the “what is this a story of” question
- Jonathan Wilcox on An improved version of the Drafts Review Matrix – responding to reviewers and editors’ comments
- Catherine Franz on What’s the difference between the Everything Notebook and the Commonplace Book?
Follow me on Twitter:
Proudly powered by WordPress and Carrington .
Carrington Theme by Crowd Favorite
- Infographics
- Youtube Summary
- Youtube to Blog
Beginner's Guide to Analytical Frameworks
- Introduction
Analytical frameworks are essential tools for understanding complex problems, making informed decisions, and developing effective strategies. This guide is designed to introduce beginners to the fundamental concepts of analytical frameworks, exploring its various types, and providing insights into how they can be applied in practical scenarios. Whether you're a student, a professional, or someone with a keen interest in analytics, this article will equip you with the knowledge to confidently navigate the world of analytical frameworks.
- Key Highlights
Importance of analytical frameworks in problem-solving
Overview of different types of analytical frameworks
Step-by-step guide on applying analytical frameworks
Real-world examples of analytical framework application
Tips for choosing the right framework for your needs
- Understanding Analytical Frameworks
Before we delve into the intricacies of analytical frameworks, let's establish a foundational understanding. These frameworks are not just tools; they are the compasses that guide us through the complex landscapes of data, decisions, and strategic planning. They empower us to dissect and understand multifaceted problems, ensuring our decisions are informed, strategic, and impactful. This section paves the way for a deeper exploration, illuminating the what, the why, and the how of analytical frameworks.
Definition and Purpose of Analytical Frameworks
At their core, analytical frameworks are structured tools used to systematically analyze and interpret information. They serve multiple purposes across various fields, from business strategy and market analysis to social research and policy development. For instance, a business might use a SWOT (Strengths, Weaknesses, Opportunities, Threats) analysis to evaluate its competitive position in the market or to plan its growth strategy.
These frameworks help by providing a clear methodology for breaking down complex issues into manageable components, making it easier to identify the root causes of problems, understand relationships, and predict future trends. By applying an analytical framework like the PESTLE (Political, Economic, Social, Technological, Legal, Environmental) analysis, organizations can assess external factors that could impact their operations, enabling proactive rather than reactive strategies.
Importance in Decision-Making
The power of analytical frameworks lies in their ability to aid in making informed decisions . By systematically breaking down complex problems, these frameworks ensure that every aspect of a situation is considered. For example, Porter’s Five Forces framework allows businesses to analyze their industry's competitive forces to strategize accordingly.
This methodical approach to decision-making not only enhances the quality of the decisions but also increases the decision-makers' confidence. It transforms guesswork into a structured analysis, providing a clear path from problem identification to solution implementation. In essence, analytical frameworks turn the daunting task of decision-making into a clear, manageable process.
Common Misconceptions
Despite their utility, several misconceptions about analytical frameworks persist. One common belief is that they are only useful for large corporations or complex research projects. However, these tools are equally beneficial for small businesses or even individual decision-making processes. They scale to fit the problem at hand, whether it's choosing a new product line or planning a career move.
Another misconception is that using an analytical framework guarantees success. While these frameworks can significantly improve the decision-making process, they are not a magic bullet. Success also depends on the quality of input data and the decision-maker's judgment. It's crucial to approach these frameworks as guides rather than definitive answers to complex problems. By clearing these misunderstandings, beginners can more effectively leverage analytical frameworks to their advantage.
- Exploring Analytical Frameworks: A Beginner's Guide
In the vast expanse of business strategy and analysis, understanding and applying the right analytical frameworks can be the beacon that guides organizations through the complexities of decision-making. This section delves into some of the most influential frameworks, offering a doorway for beginners to step into the realm of strategic analysis.
Diving Deep into SWOT Analysis
SWOT Analysis stands as a cornerstone in strategic planning, offering a clear framework to evaluate the Strengths, Weaknesses, Opportunities, and Threats associated with a project or in a business scenario. Its application spans across various sectors, from business strategy to personal career planning.
Practical Application: Imagine a startup in the tech industry. By applying SWOT, they can pinpoint their innovative technology (Strength), limited market presence (Weakness), emerging markets (Opportunity), and established competitors (Threat). This comprehensive analysis aids in crafting strategic plans that leverage strengths, address weaknesses, exploit opportunities, and mitigate threats.
Benefits: The simplicity and versatility of SWOT Analysis make it an essential tool for strategic planning. It provides actionable insights that can guide decision-making processes, from market entry strategies to product development.
Unpacking PESTLE Analysis
PESTLE Analysis offers a macro-environmental framework, analyzing Political, Economic, Social, Technological, Legal, and Environmental factors impacting businesses. It's particularly valuable for assessing market growth or decline, business position, and direction for operations.
Example in Action: Consider a multinational corporation assessing a new market entry. Through PESTLE, they evaluate the political stability of the country, economic trends , cultural attitudes towards foreign businesses, technological infrastructure , legal barriers to entry , and environmental regulations . This holistic view enables informed strategic decisions, aligning business objectives with external realities.
Real-World Application Examples: By understanding the broader macro-environmental factors, businesses can anticipate changes and adapt strategies accordingly. For instance, a company might shift its supply chain strategy in anticipation of changes in environmental regulations.
Exploring Porter’s Five Forces
Porter’s Five Forces provides a framework for analyzing the competitive forces in an industry, including competition intensity, potential of new entrants, power of suppliers, power of customers, and threat of substitute products . It’s especially useful for evaluating the strategic position of a business within its industry.
Application Insight: An e-commerce business may use Porter’s Five Forces to assess its competitive landscape. By analyzing the bargaining power of suppliers (can suppliers dictate terms?), bargaining power of customers (do customers have choices?), threat of new entrants (is it easy for new players to enter the market?), threat of substitutes (are there alternative products?), and competitive rivalry (how intense is the competition?), the business can identify strategic opportunities and threats.
Relevance and Application: This framework helps businesses understand the dynamics of their industry and shape strategies that exploit competitive advantages or address vulnerabilities. For example, a business might decide to focus on customer loyalty programs to counter strong competition and the threat of substitutes.
- Applying Analytical Frameworks Effortlessly
Understanding the theoretical aspects of analytical frameworks is a critical first step. However, the real power of these frameworks is unleashed only when they are applied effectively to real-world scenarios. This section is designed to bridge the gap between theory and practice, offering a concise, step-by-step guide that caters to the application of these frameworks across various situations.
A Step-by-Step Guide to Applying Analytical Frameworks
Identify the Objective : Begin by clearly defining what you wish to achieve with the analysis. Setting a clear objective guides the selection of the appropriate framework.
Select the Right Framework : Based on the objective, choose a framework that best fits the problem at hand. For instance, SWOT Analysis is ideal for evaluating project feasibility, while PESTLE Analysis can help understand the macro-environmental factors affecting a project.
Gather Relevant Data : Collect all necessary information that will feed into the framework. This could range from internal performance metrics to external market research.
Apply the Framework : Methodically fill in the sections of the chosen framework with the collected data. This process often reveals insights that were not apparent before.
Analyze the Results : Look for patterns, strengths, weaknesses, opportunities, and threats that emerge from the analysis.
Make Informed Decisions : Use the insights gained to guide strategic decisions, ensuring they are backed by systematic analysis.
Real-World Applications: Case Studies on Analytical Frameworks
Analytical frameworks have been successfully applied across industries to drive strategic decisions. For example, a tech startup might use SWOT Analysis to navigate its market entry strategy, identifying key opportunities and threats in the competitive landscape. Similarly, a multinational corporation might employ PESTLE Analysis to assess the viability of entering a new geographical market, taking into consideration political, economic, social, technological, legal, and environmental factors. These real-world applications underscore the versatility and utility of analytical frameworks in providing structured insights that inform strategic decisions.
Avoiding Common Pitfalls in Applying Analytical Frameworks
While analytical frameworks are powerful tools, their effectiveness can be compromised by common mistakes. Here are a few to avoid:
Overlooking Context : Applying a framework without adjusting for the specific context of the problem can lead to misleading conclusions. Always tailor the framework to fit the unique aspects of the scenario.
Data Overload : While thorough data collection is crucial, overwhelming the framework with too much information can obscure key insights. Focus on data that directly impacts the objective.
Ignoring External Inputs : Solely relying on internal perspectives can limit the scope of analysis. Incorporate external viewpoints and data sources to enrich the analysis.
By being mindful of these pitfalls and adopting a structured approach to applying analytical frameworks, organizations can significantly enhance their decision-making processes.
- Choosing the Right Analytical Framework
Selecting the most suitable analytical framework from the plethora available can seem like a daunting task. This section is aimed at demystifying this process, providing clear, actionable insights that would guide you in identifying the framework that best matches the unique requirements of your project or problem. Let's dive into the factors that should influence your decision and how to compare different frameworks effectively.
Factors to Consider
Understanding Your Objectives: The first step in choosing the right analytical framework is crystal clear clarity on what you aim to achieve. Are you looking to understand consumer behavior, or are you more interested in the competitive landscape of your industry? For instance, a SWOT Analysis might be perfect for strategic planning, while Porter’s Five Forces could provide deeper insights into competitive dynamics.
Complexity of the Data: The nature and complexity of the data at hand also play a crucial role. A framework like PESTLE Analysis is fantastic for macro-environmental insights but might be too broad if your data is highly specific or technical. In such cases, a more niche framework, perhaps a statistical model or a custom-built analytical tool, might be more appropriate.
Resource Availability: Consider the resources you have at your disposal, including time, financial resources, and expertise. Some frameworks require more extensive data collection and analysis than others. For example, conducting a comprehensive Market Analysis might be resource-intensive, but essential for entering new markets.
Comparing Frameworks
Comparing different analytical frameworks involves looking at their strengths and weaknesses in various contexts. For example, while SWOT Analysis offers a broad overview of internal and external factors, it might not delve deeply into the specifics of competitive rivalry, where Porter’s Five Forces shines.
Practical Application: Consider how each framework has been applied in real-world scenarios similar to yours. For instance, PESTLE Analysis has been widely used by businesses looking to expand internationally to understand the macro-environmental factors affecting their operation in new markets.
Customization and Flexibility: Some frameworks offer more flexibility and can be customized to better fit specific projects. Understanding the extent to which a framework can be tailored to meet your needs is crucial. A mix-and-match approach, combining elements from different frameworks, could also provide a comprehensive analysis.
In conclusion, the choice of analytical framework is not one-size-fits-all. It requires a careful consideration of your project's specific needs, the nature of the data, and the resources available to you. By systematically comparing the options, you can select the most suitable framework to gain valuable insights and drive informed decisions.
- Best Practices and Tips for Mastering Analytical Frameworks
As we wrap up our beginner's guide to analytical frameworks, it's crucial to focus on best practices and tips that will empower enthusiasts to not only understand but also effectively implement these frameworks in their projects or analyses. The journey from learning to mastery involves continuous improvement and the effective application of strategies that complement the frameworks' strengths.
Crafting Effective Implementation Strategies
Implementing analytical frameworks effectively requires a blend of strategic planning and practical application. Start by defining clear objectives for your analysis, ensuring that the chosen framework aligns with your goals. For instance, if your project involves evaluating competitive market dynamics, Porter’s Five Forces provides a structured way to assess competitors, potential entrants, and substitute products.
- Engage stakeholders early in the process to gather diverse insights and foster buy-in. A collaborative approach enhances the relevance and acceptance of the analysis.
- Iterate and adapt your analysis as you gather more data or as the situation evolves. Flexibility is key to uncovering nuanced insights.
Remember, the value of an analytical framework lies in its application to real-world scenarios. For example, applying SWOT Analysis to a startup might reveal opportunities for innovation that were not initially apparent. Regularly revisiting and refining your analysis ensures that your strategies remain aligned with changing circumstances.
Embracing Continuous Learning and Improvement
The landscape of analytical frameworks is ever-evolving, with new methodologies emerging and existing ones being refined. Staying at the forefront of this evolution requires a commitment to continuous learning .
- Participate in online forums and communities , such as Reddit’s r/dataisbeautiful or Kaggle , to exchange insights with peers.
- Attend workshops and webinars that focus on the latest trends in analytical frameworks.
- Practice regularly by applying frameworks to diverse scenarios, even outside your immediate project needs. This not only enhances your skill but also your adaptability.
For example, exploring how PESTLE Analysis can be applied to understand the impact of global events on local markets can provide valuable insights into external factors affecting businesses.
Exploring Additional Resources for Deeper Insights
Diving deeper into analytical frameworks necessitates access to quality resources. Here is a curated list to kickstart your journey beyond this guide:
- Books : 'Competitive Strategy' by Michael E. Porter offers in-depth insights into industry analysis.
- Courses : Platforms like Coursera and edX offer courses on strategic business analytics.
- Online Resources : Websites like MindTools provide practical guides on numerous frameworks.
Leveraging these resources can significantly enhance your understanding and application of analytical frameworks. Whether you're a student, a professional, or an enthusiast, the journey towards mastering these tools is ongoing and deeply rewarding.
Analytical frameworks are powerful tools that, when understood and applied correctly, can significantly enhance the quality of your decisions and strategies. This guide has aimed to provide a comprehensive overview for beginners, covering everything from the basics to practical application tips. With this knowledge, you are now better equipped to navigate the complex world of analytical frameworks, ready to tackle challenges with a structured and informed approach.
Q: What is an analytical framework?
A: An analytical framework is a structured approach used to dissect complex problems, analyze data, and make decisions. It involves applying specific models or strategies to systematically understand and address challenges.
Q: Why are analytical frameworks important?
A: Analytical frameworks are important because they provide a systematic method for analyzing problems and making informed decisions. They help break down complex issues into manageable parts, facilitating clearer understanding and more effective solutions.
Q: Can you give examples of analytical frameworks?
A: Yes, some common examples include SWOT Analysis (Strengths, Weaknesses, Opportunities, Threats), PESTLE Analysis (Political, Economic, Social, Technological, Legal, Environmental), and Porter’s Five Forces .
Q: How do I choose the right analytical framework?
A: Choosing the right analytical framework depends on your specific needs, the nature of the problem, and the context in which you are operating. Consider factors such as the framework’s relevance to your industry, its complexity, and your own familiarity with it.
Q: What are common pitfalls in applying analytical frameworks?
A: Common pitfalls include overly complicating the analysis, using the wrong framework for your specific situation, or relying too heavily on the framework without considering unique aspects of your problem. Avoid these by being mindful of your choice and application.
Q: How can I effectively implement an analytical framework?
A: To effectively implement an analytical framework, start by clearly defining your problem. Gather and analyze relevant data, apply the chosen framework systematically, and use your findings to inform your decision-making or strategy development.
Q: Are analytical frameworks only useful for businesses?
A: No, analytical frameworks can be applied in various fields beyond business, including public policy, education, healthcare, and personal decision-making. They are versatile tools for problem-solving and analysis in any complex situation.
Table of Contents
Share this article
Related Articles
Mastering Subtotals in Excel: A Beginner's Guide
Learn how to efficiently create subtotal summaries in Excel 2007 with our comprehensive beginner's guide. Master this essential skill for data analysis.
Mastering Excel Borders: A Beginner's Guide
Learn how to apply and customize borders in Excel with this comprehensive guide for beginners. Enhance your spreadsheets now.
Count Substrings in Excel: A Beginner's Guide
Learn to easily count the occurrences of a substring within a string in Excel, a must-read guide for Excel beginners.
Unlock Advanced AI Analytics
Elevate your analytics with Skills.ai. Our platform offers AI-driven insights, enabling you to customize data and create tailored reports effortlessly. Start your journey towards smarter analytics today!
"Skills.ai is the ultimate analytics partner, providing efficient and intelligent solutions." "An invaluable tool for data scientists, the platform's self-driven analytics reduce time to insights significantly."
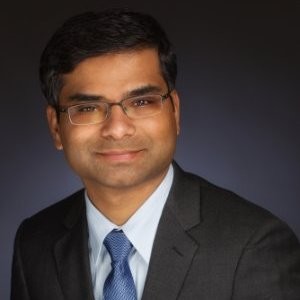
Ram Ravichandran
- Skip to main content
- Skip to primary sidebar
- Skip to footer
- QuestionPro

- Solutions Industries Gaming Automotive Sports and events Education Government Travel & Hospitality Financial Services Healthcare Cannabis Technology Use Case NPS+ Communities Audience Contactless surveys Mobile LivePolls Member Experience GDPR Positive People Science 360 Feedback Surveys
- Resources Blog eBooks Survey Templates Case Studies Training Help center

Home Market Research Research Tools and Apps
Analytical Research: What is it, Importance + Examples
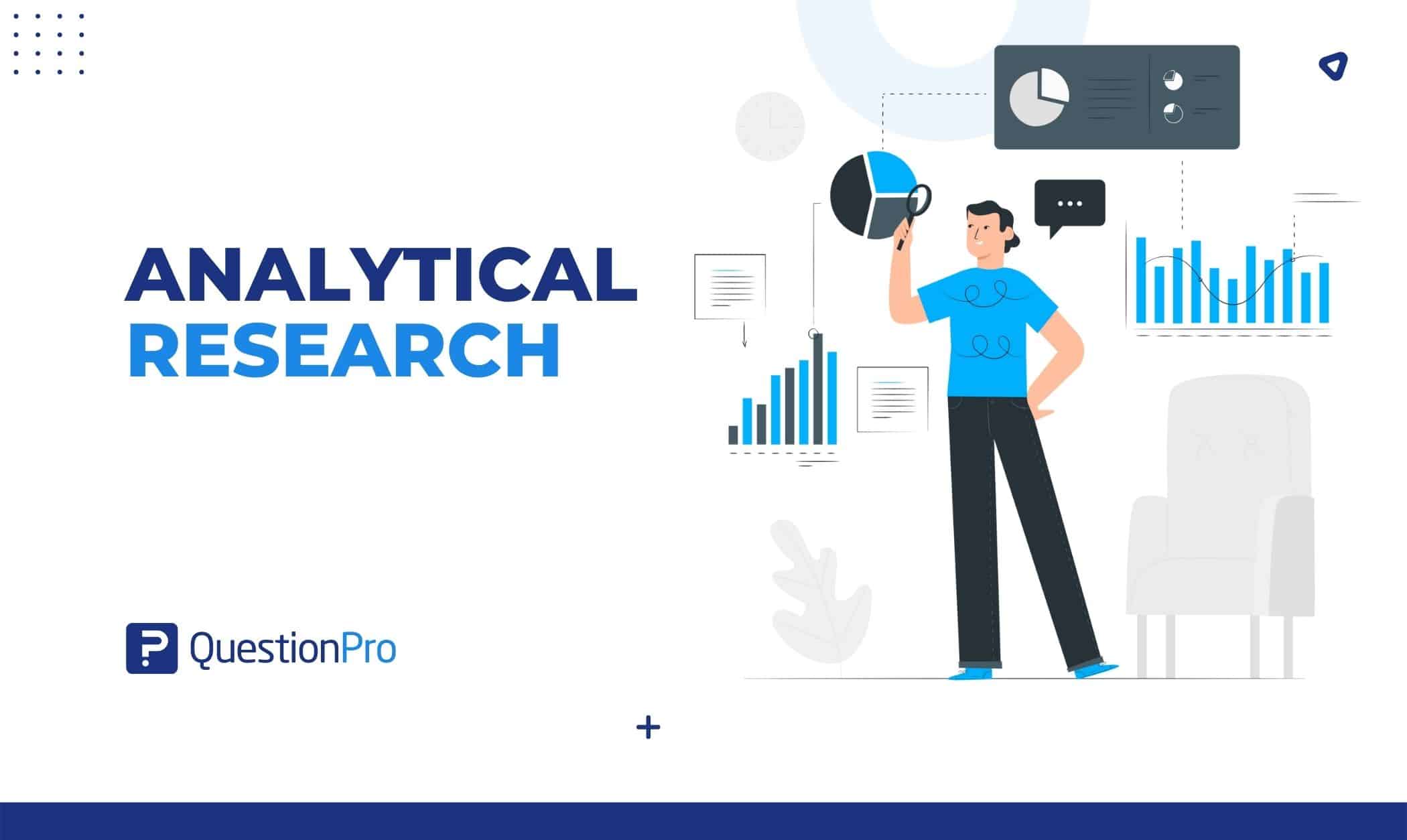
Finding knowledge is a loose translation of the word “research.” It’s a systematic and scientific way of researching a particular subject. As a result, research is a form of scientific investigation that seeks to learn more. Analytical research is one of them.
Any kind of research is a way to learn new things. In this research, data and other pertinent information about a project are assembled; after the information is gathered and assessed, the sources are used to support a notion or prove a hypothesis.
An individual can successfully draw out minor facts to make more significant conclusions about the subject matter by using critical thinking abilities (a technique of thinking that entails identifying a claim or assumption and determining whether it is accurate or untrue).
What is analytical research?
This particular kind of research calls for using critical thinking abilities and assessing data and information pertinent to the project at hand.
Determines the causal connections between two or more variables. The analytical study aims to identify the causes and mechanisms underlying the trade deficit’s movement throughout a given period.
It is used by various professionals, including psychologists, doctors, and students, to identify the most pertinent material during investigations. One learns crucial information from analytical research that helps them contribute fresh concepts to the work they are producing.
Some researchers perform it to uncover information that supports ongoing research to strengthen the validity of their findings. Other scholars engage in analytical research to generate fresh perspectives on the subject.
Various approaches to performing research include literary analysis, Gap analysis , general public surveys, clinical trials, and meta-analysis.
Importance of analytical research
The goal of analytical research is to develop new ideas that are more believable by combining numerous minute details.
The analytical investigation is what explains why a claim should be trusted. Finding out why something occurs is complex. You need to be able to evaluate information critically and think critically.
This kind of information aids in proving the validity of a theory or supporting a hypothesis. It assists in recognizing a claim and determining whether it is true.
Analytical kind of research is valuable to many people, including students, psychologists, marketers, and others. It aids in determining which advertising initiatives within a firm perform best. In the meantime, medical research and research design determine how well a particular treatment does.
Thus, analytical research can help people achieve their goals while saving lives and money.
Methods of Conducting Analytical Research
Analytical research is the process of gathering, analyzing, and interpreting information to make inferences and reach conclusions. Depending on the purpose of the research and the data you have access to, you can conduct analytical research using a variety of methods. Here are a few typical approaches:
Quantitative research
Numerical data are gathered and analyzed using this method. Statistical methods are then used to analyze the information, which is often collected using surveys, experiments, or pre-existing datasets. Results from quantitative research can be measured, compared, and generalized numerically.
Qualitative research
In contrast to quantitative research, qualitative research focuses on collecting non-numerical information. It gathers detailed information using techniques like interviews, focus groups, observations, or content research. Understanding social phenomena, exploring experiences, and revealing underlying meanings and motivations are all goals of qualitative research.
Mixed methods research
This strategy combines quantitative and qualitative methodologies to grasp a research problem thoroughly. Mixed methods research often entails gathering and evaluating both numerical and non-numerical data, integrating the results, and offering a more comprehensive viewpoint on the research issue.
Experimental research
Experimental research is frequently employed in scientific trials and investigations to establish causal links between variables. This approach entails modifying variables in a controlled environment to identify cause-and-effect connections. Researchers randomly divide volunteers into several groups, provide various interventions or treatments, and track the results.
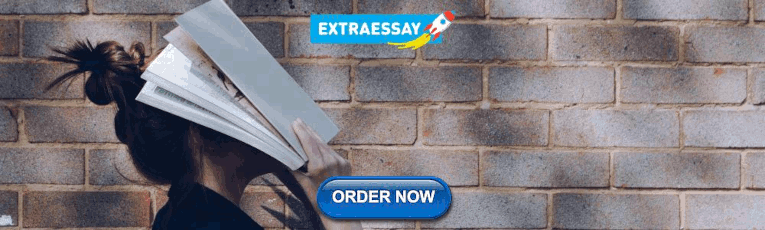
Observational research
With this approach, behaviors or occurrences are observed and methodically recorded without any outside interference or variable data manipulation . Both controlled surroundings and naturalistic settings can be used for observational research . It offers useful insights into behaviors that occur in the actual world and enables researchers to explore events as they naturally occur.
Case study research
This approach entails thorough research of a single case or a small group of related cases. Case-control studies frequently include a variety of information sources, including observations, records, and interviews. They offer rich, in-depth insights and are particularly helpful for researching complex phenomena in practical settings.
Secondary data analysis
Examining secondary information is time and money-efficient, enabling researchers to explore new research issues or confirm prior findings. With this approach, researchers examine previously gathered information for a different reason. Information from earlier cohort studies, accessible databases, or corporate documents may be included in this.
Content analysis
Content research is frequently employed in social sciences, media observational studies, and cross-sectional studies. This approach systematically examines the content of texts, including media, speeches, and written documents. Themes, patterns, or keywords are found and categorized by researchers to make inferences about the content.
Depending on your research objectives, the resources at your disposal, and the type of data you wish to analyze, selecting the most appropriate approach or combination of methodologies is crucial to conducting analytical research.
Examples of analytical research
Analytical research takes a unique measurement. Instead, you would consider the causes and changes to the trade imbalance. Detailed statistics and statistical checks help guarantee that the results are significant.
For example, it can look into why the value of the Japanese Yen has decreased. This is so that an analytical study can consider “how” and “why” questions.
Another example is that someone might conduct analytical research to identify a study’s gap. It presents a fresh perspective on your data. Therefore, it aids in supporting or refuting notions.
Descriptive vs analytical research
Here are the key differences between descriptive research and analytical research:
The study of cause and effect makes extensive use of analytical research. It benefits from numerous academic disciplines, including marketing, health, and psychology, because it offers more conclusive information for addressing research issues.
QuestionPro offers solutions for every issue and industry, making it more than just survey software. For handling data, we also have systems like our InsightsHub research library.
You may make crucial decisions quickly while using QuestionPro to understand your clients and other study subjects better. Make use of the possibilities of the enterprise-grade research suite right away!
LEARN MORE FREE TRIAL
MORE LIKE THIS
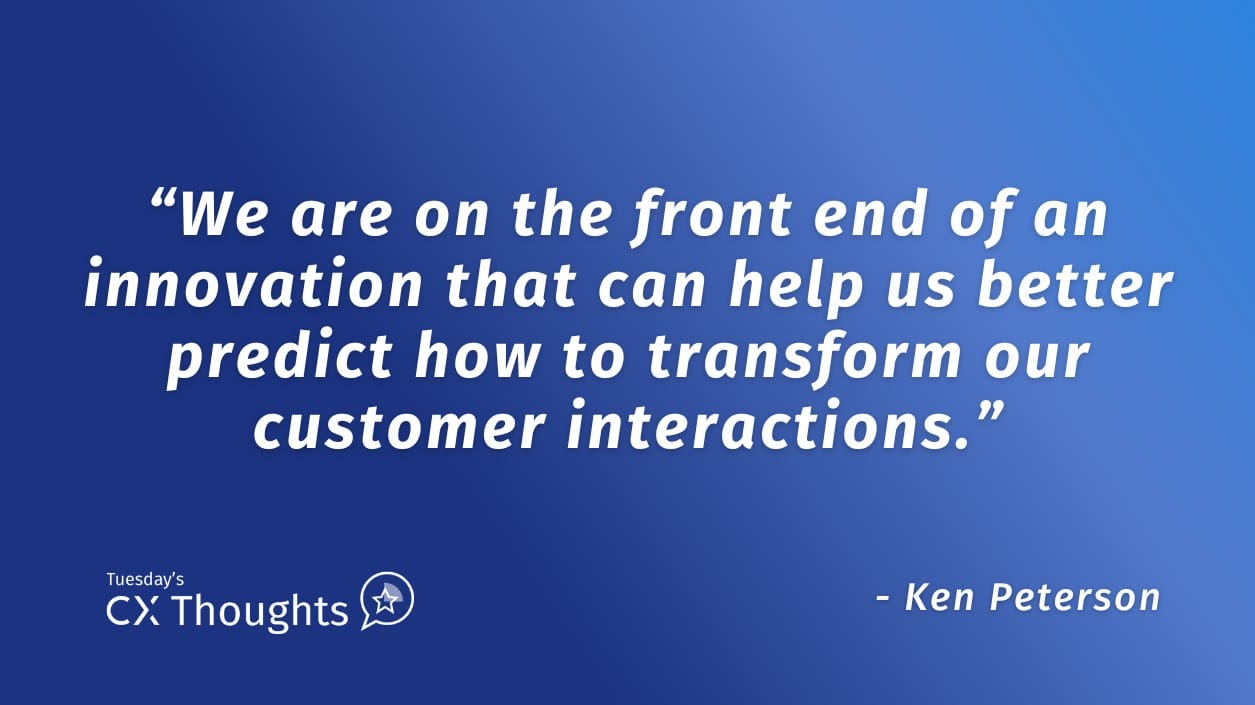
How Can I Help You? — Tuesday CX Thoughts
Jun 5, 2024
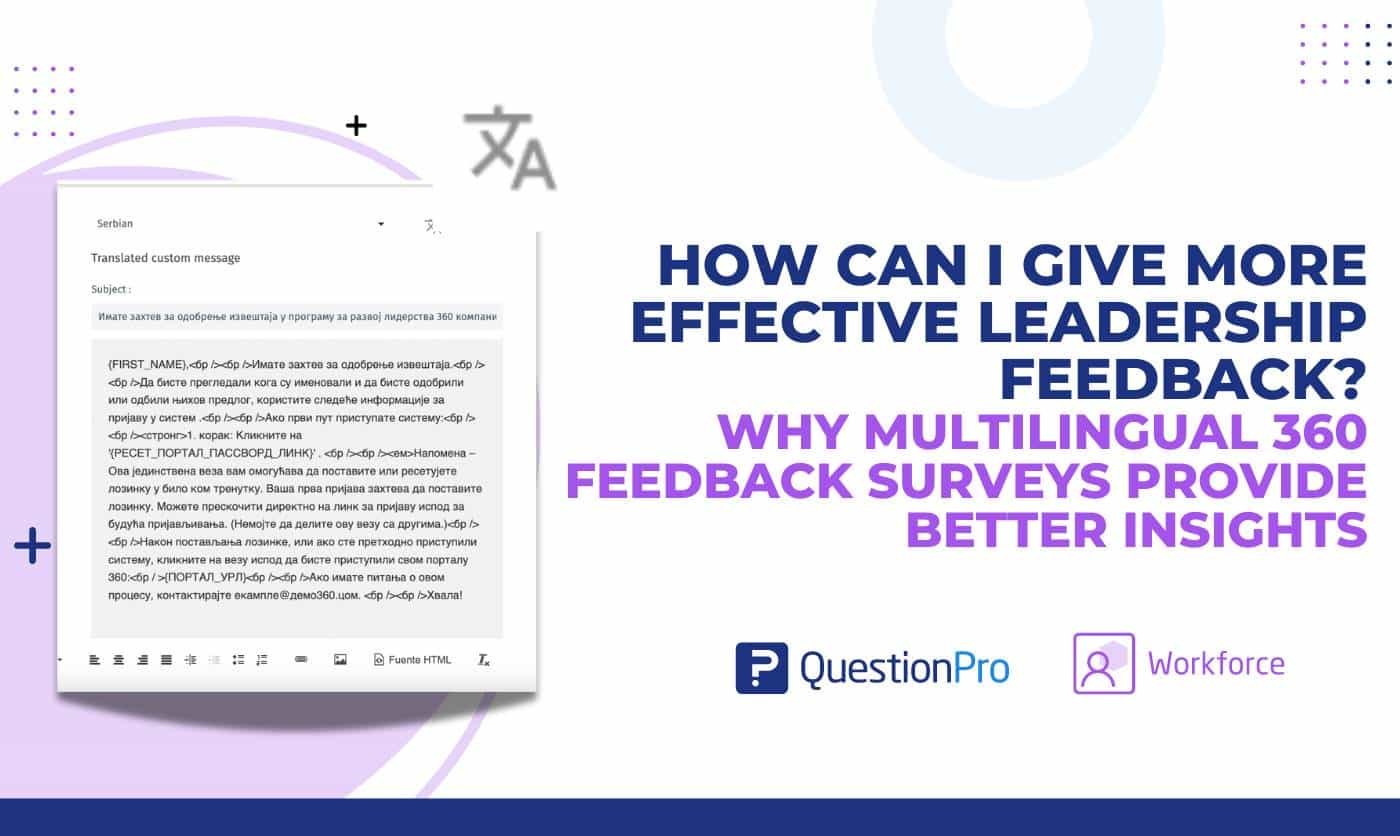
Why Multilingual 360 Feedback Surveys Provide Better Insights
Jun 3, 2024
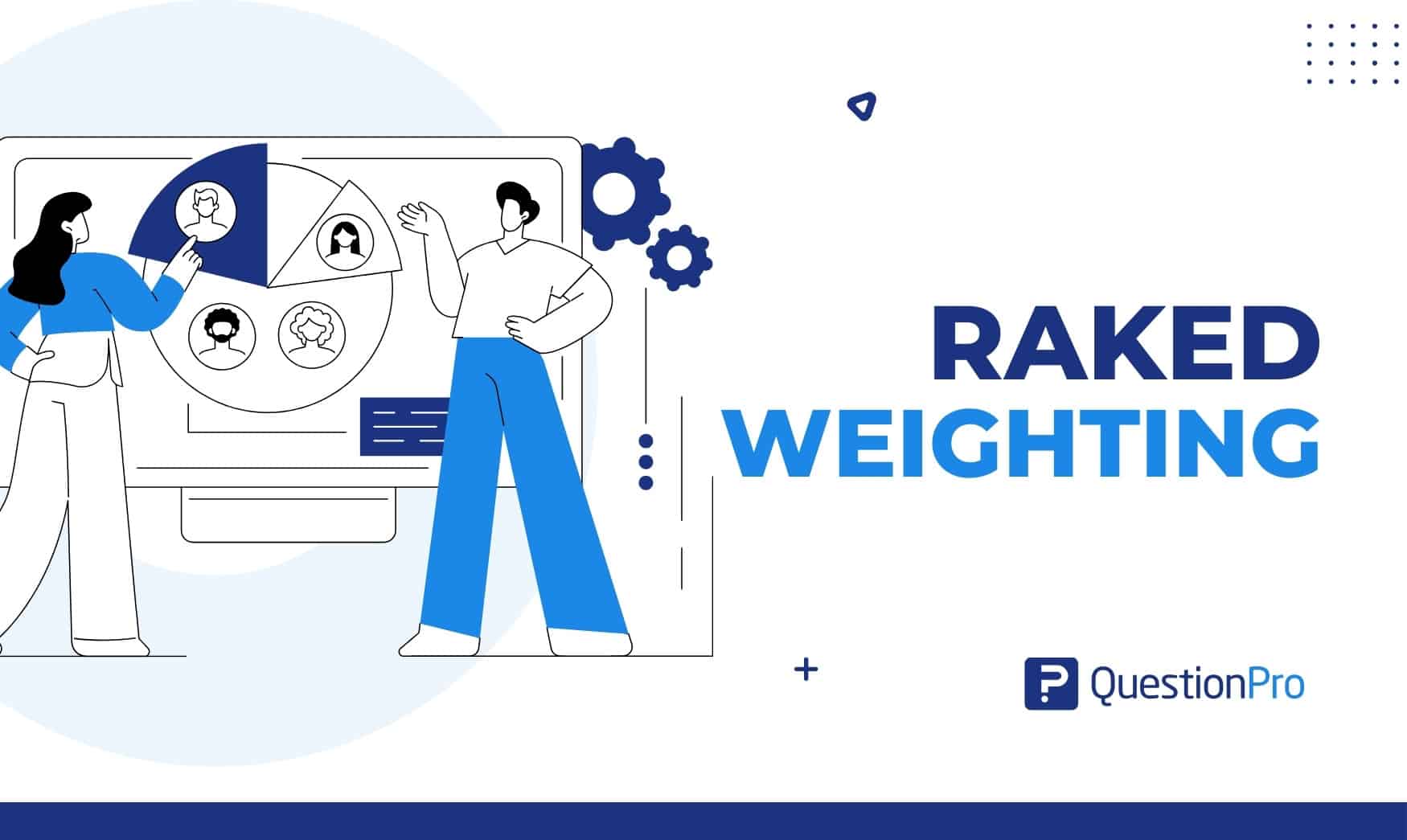
Raked Weighting: A Key Tool for Accurate Survey Results
May 31, 2024
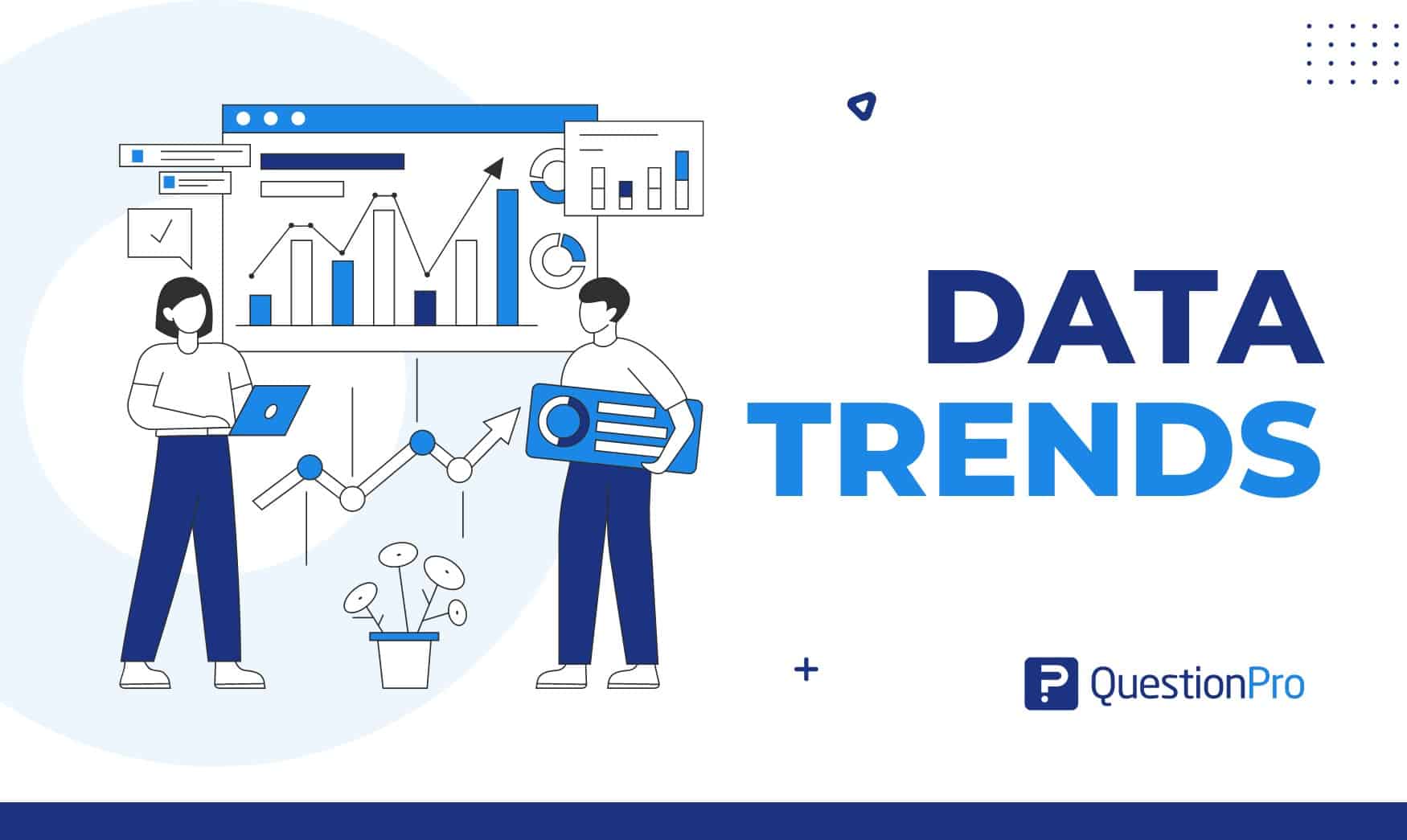
Top 8 Data Trends to Understand the Future of Data
May 30, 2024
Other categories
- Academic Research
- Artificial Intelligence
- Assessments
- Brand Awareness
- Case Studies
- Communities
- Consumer Insights
- Customer effort score
- Customer Engagement
- Customer Experience
- Customer Loyalty
- Customer Research
- Customer Satisfaction
- Employee Benefits
- Employee Engagement
- Employee Retention
- Friday Five
- General Data Protection Regulation
- Insights Hub
- Life@QuestionPro
- Market Research
- Mobile diaries
- Mobile Surveys
- New Features
- Online Communities
- Question Types
- Questionnaire
- QuestionPro Products
- Release Notes
- Research Tools and Apps
- Revenue at Risk
- Survey Templates
- Training Tips
- Uncategorized
- Video Learning Series
- What’s Coming Up
- Workforce Intelligence
- USC Libraries
- Research Guides
Organizing Your Social Sciences Research Paper
- Theoretical Framework
- Purpose of Guide
- Design Flaws to Avoid
- Independent and Dependent Variables
- Glossary of Research Terms
- Reading Research Effectively
- Narrowing a Topic Idea
- Broadening a Topic Idea
- Extending the Timeliness of a Topic Idea
- Academic Writing Style
- Applying Critical Thinking
- Choosing a Title
- Making an Outline
- Paragraph Development
- Research Process Video Series
- Executive Summary
- The C.A.R.S. Model
- Background Information
- The Research Problem/Question
- Citation Tracking
- Content Alert Services
- Evaluating Sources
- Primary Sources
- Secondary Sources
- Tiertiary Sources
- Scholarly vs. Popular Publications
- Qualitative Methods
- Quantitative Methods
- Insiderness
- Using Non-Textual Elements
- Limitations of the Study
- Common Grammar Mistakes
- Writing Concisely
- Avoiding Plagiarism
- Footnotes or Endnotes?
- Further Readings
- Generative AI and Writing
- USC Libraries Tutorials and Other Guides
- Bibliography
Theories are formulated to explain, predict, and understand phenomena and, in many cases, to challenge and extend existing knowledge within the limits of critical bounded assumptions or predictions of behavior. The theoretical framework is the structure that can hold or support a theory of a research study. The theoretical framework encompasses not just the theory, but the narrative explanation about how the researcher engages in using the theory and its underlying assumptions to investigate the research problem. It is the structure of your paper that summarizes concepts, ideas, and theories derived from prior research studies and which was synthesized in order to form a conceptual basis for your analysis and interpretation of meaning found within your research.
Abend, Gabriel. "The Meaning of Theory." Sociological Theory 26 (June 2008): 173–199; Kivunja, Charles. "Distinguishing between Theory, Theoretical Framework, and Conceptual Framework: A Systematic Review of Lessons from the Field." International Journal of Higher Education 7 (December 2018): 44-53; Swanson, Richard A. Theory Building in Applied Disciplines . San Francisco, CA: Berrett-Koehler Publishers 2013; Varpio, Lara, Elise Paradis, Sebastian Uijtdehaage, and Meredith Young. "The Distinctions between Theory, Theoretical Framework, and Conceptual Framework." Academic Medicine 95 (July 2020): 989-994.
Importance of Theory and a Theoretical Framework
Theories can be unfamiliar to the beginning researcher because they are rarely applied in high school social studies curriculum and, as a result, can come across as unfamiliar and imprecise when first introduced as part of a writing assignment. However, in their most simplified form, a theory is simply a set of assumptions or predictions about something you think will happen based on existing evidence and that can be tested to see if those outcomes turn out to be true. Of course, it is slightly more deliberate than that, therefore, summarized from Kivunja (2018, p. 46), here are the essential characteristics of a theory.
- It is logical and coherent
- It has clear definitions of terms or variables, and has boundary conditions [i.e., it is not an open-ended statement]
- It has a domain where it applies
- It has clearly described relationships among variables
- It describes, explains, and makes specific predictions
- It comprises of concepts, themes, principles, and constructs
- It must have been based on empirical data [i.e., it is not a guess]
- It must have made claims that are subject to testing, been tested and verified
- It must be clear and concise
- Its assertions or predictions must be different and better than those in existing theories
- Its predictions must be general enough to be applicable to and understood within multiple contexts
- Its assertions or predictions are relevant, and if applied as predicted, will result in the predicted outcome
- The assertions and predictions are not immutable, but subject to revision and improvement as researchers use the theory to make sense of phenomena
- Its concepts and principles explain what is going on and why
- Its concepts and principles are substantive enough to enable us to predict a future
Given these characteristics, a theory can best be understood as the foundation from which you investigate assumptions or predictions derived from previous studies about the research problem, but in a way that leads to new knowledge and understanding as well as, in some cases, discovering how to improve the relevance of the theory itself or to argue that the theory is outdated and a new theory needs to be formulated based on new evidence.
A theoretical framework consists of concepts and, together with their definitions and reference to relevant scholarly literature, existing theory that is used for your particular study. The theoretical framework must demonstrate an understanding of theories and concepts that are relevant to the topic of your research paper and that relate to the broader areas of knowledge being considered.
The theoretical framework is most often not something readily found within the literature . You must review course readings and pertinent research studies for theories and analytic models that are relevant to the research problem you are investigating. The selection of a theory should depend on its appropriateness, ease of application, and explanatory power.
The theoretical framework strengthens the study in the following ways :
- An explicit statement of theoretical assumptions permits the reader to evaluate them critically.
- The theoretical framework connects the researcher to existing knowledge. Guided by a relevant theory, you are given a basis for your hypotheses and choice of research methods.
- Articulating the theoretical assumptions of a research study forces you to address questions of why and how. It permits you to intellectually transition from simply describing a phenomenon you have observed to generalizing about various aspects of that phenomenon.
- Having a theory helps you identify the limits to those generalizations. A theoretical framework specifies which key variables influence a phenomenon of interest and highlights the need to examine how those key variables might differ and under what circumstances.
- The theoretical framework adds context around the theory itself based on how scholars had previously tested the theory in relation their overall research design [i.e., purpose of the study, methods of collecting data or information, methods of analysis, the time frame in which information is collected, study setting, and the methodological strategy used to conduct the research].
By virtue of its applicative nature, good theory in the social sciences is of value precisely because it fulfills one primary purpose: to explain the meaning, nature, and challenges associated with a phenomenon, often experienced but unexplained in the world in which we live, so that we may use that knowledge and understanding to act in more informed and effective ways.
The Conceptual Framework. College of Education. Alabama State University; Corvellec, Hervé, ed. What is Theory?: Answers from the Social and Cultural Sciences . Stockholm: Copenhagen Business School Press, 2013; Asher, Herbert B. Theory-Building and Data Analysis in the Social Sciences . Knoxville, TN: University of Tennessee Press, 1984; Drafting an Argument. Writing@CSU. Colorado State University; Kivunja, Charles. "Distinguishing between Theory, Theoretical Framework, and Conceptual Framework: A Systematic Review of Lessons from the Field." International Journal of Higher Education 7 (2018): 44-53; Omodan, Bunmi Isaiah. "A Model for Selecting Theoretical Framework through Epistemology of Research Paradigms." African Journal of Inter/Multidisciplinary Studies 4 (2022): 275-285; Ravitch, Sharon M. and Matthew Riggan. Reason and Rigor: How Conceptual Frameworks Guide Research . Second edition. Los Angeles, CA: SAGE, 2017; Trochim, William M.K. Philosophy of Research. Research Methods Knowledge Base. 2006; Jarvis, Peter. The Practitioner-Researcher. Developing Theory from Practice . San Francisco, CA: Jossey-Bass, 1999.
Strategies for Developing the Theoretical Framework
I. Developing the Framework
Here are some strategies to develop of an effective theoretical framework:
- Examine your thesis title and research problem . The research problem anchors your entire study and forms the basis from which you construct your theoretical framework.
- Brainstorm about what you consider to be the key variables in your research . Answer the question, "What factors contribute to the presumed effect?"
- Review related literature to find how scholars have addressed your research problem. Identify the assumptions from which the author(s) addressed the problem.
- List the constructs and variables that might be relevant to your study. Group these variables into independent and dependent categories.
- Review key social science theories that are introduced to you in your course readings and choose the theory that can best explain the relationships between the key variables in your study [note the Writing Tip on this page].
- Discuss the assumptions or propositions of this theory and point out their relevance to your research.
A theoretical framework is used to limit the scope of the relevant data by focusing on specific variables and defining the specific viewpoint [framework] that the researcher will take in analyzing and interpreting the data to be gathered. It also facilitates the understanding of concepts and variables according to given definitions and builds new knowledge by validating or challenging theoretical assumptions.
II. Purpose
Think of theories as the conceptual basis for understanding, analyzing, and designing ways to investigate relationships within social systems. To that end, the following roles served by a theory can help guide the development of your framework.
- Means by which new research data can be interpreted and coded for future use,
- Response to new problems that have no previously identified solutions strategy,
- Means for identifying and defining research problems,
- Means for prescribing or evaluating solutions to research problems,
- Ways of discerning certain facts among the accumulated knowledge that are important and which facts are not,
- Means of giving old data new interpretations and new meaning,
- Means by which to identify important new issues and prescribe the most critical research questions that need to be answered to maximize understanding of the issue,
- Means of providing members of a professional discipline with a common language and a frame of reference for defining the boundaries of their profession, and
- Means to guide and inform research so that it can, in turn, guide research efforts and improve professional practice.
Adapted from: Torraco, R. J. “Theory-Building Research Methods.” In Swanson R. A. and E. F. Holton III , editors. Human Resource Development Handbook: Linking Research and Practice . (San Francisco, CA: Berrett-Koehler, 1997): pp. 114-137; Jacard, James and Jacob Jacoby. Theory Construction and Model-Building Skills: A Practical Guide for Social Scientists . New York: Guilford, 2010; Ravitch, Sharon M. and Matthew Riggan. Reason and Rigor: How Conceptual Frameworks Guide Research . Second edition. Los Angeles, CA: SAGE, 2017; Sutton, Robert I. and Barry M. Staw. “What Theory is Not.” Administrative Science Quarterly 40 (September 1995): 371-384.
Structure and Writing Style
The theoretical framework may be rooted in a specific theory , in which case, your work is expected to test the validity of that existing theory in relation to specific events, issues, or phenomena. Many social science research papers fit into this rubric. For example, Peripheral Realism Theory, which categorizes perceived differences among nation-states as those that give orders, those that obey, and those that rebel, could be used as a means for understanding conflicted relationships among countries in Africa. A test of this theory could be the following: Does Peripheral Realism Theory help explain intra-state actions, such as, the disputed split between southern and northern Sudan that led to the creation of two nations?
However, you may not always be asked by your professor to test a specific theory in your paper, but to develop your own framework from which your analysis of the research problem is derived . Based upon the above example, it is perhaps easiest to understand the nature and function of a theoretical framework if it is viewed as an answer to two basic questions:
- What is the research problem/question? [e.g., "How should the individual and the state relate during periods of conflict?"]
- Why is your approach a feasible solution? [i.e., justify the application of your choice of a particular theory and explain why alternative constructs were rejected. I could choose instead to test Instrumentalist or Circumstantialists models developed among ethnic conflict theorists that rely upon socio-economic-political factors to explain individual-state relations and to apply this theoretical model to periods of war between nations].
The answers to these questions come from a thorough review of the literature and your course readings [summarized and analyzed in the next section of your paper] and the gaps in the research that emerge from the review process. With this in mind, a complete theoretical framework will likely not emerge until after you have completed a thorough review of the literature .
Just as a research problem in your paper requires contextualization and background information, a theory requires a framework for understanding its application to the topic being investigated. When writing and revising this part of your research paper, keep in mind the following:
- Clearly describe the framework, concepts, models, or specific theories that underpin your study . This includes noting who the key theorists are in the field who have conducted research on the problem you are investigating and, when necessary, the historical context that supports the formulation of that theory. This latter element is particularly important if the theory is relatively unknown or it is borrowed from another discipline.
- Position your theoretical framework within a broader context of related frameworks, concepts, models, or theories . As noted in the example above, there will likely be several concepts, theories, or models that can be used to help develop a framework for understanding the research problem. Therefore, note why the theory you've chosen is the appropriate one.
- The present tense is used when writing about theory. Although the past tense can be used to describe the history of a theory or the role of key theorists, the construction of your theoretical framework is happening now.
- You should make your theoretical assumptions as explicit as possible . Later, your discussion of methodology should be linked back to this theoretical framework.
- Don’t just take what the theory says as a given! Reality is never accurately represented in such a simplistic way; if you imply that it can be, you fundamentally distort a reader's ability to understand the findings that emerge. Given this, always note the limitations of the theoretical framework you've chosen [i.e., what parts of the research problem require further investigation because the theory inadequately explains a certain phenomena].
The Conceptual Framework. College of Education. Alabama State University; Conceptual Framework: What Do You Think is Going On? College of Engineering. University of Michigan; Drafting an Argument. Writing@CSU. Colorado State University; Lynham, Susan A. “The General Method of Theory-Building Research in Applied Disciplines.” Advances in Developing Human Resources 4 (August 2002): 221-241; Tavallaei, Mehdi and Mansor Abu Talib. "A General Perspective on the Role of Theory in Qualitative Research." Journal of International Social Research 3 (Spring 2010); Ravitch, Sharon M. and Matthew Riggan. Reason and Rigor: How Conceptual Frameworks Guide Research . Second edition. Los Angeles, CA: SAGE, 2017; Reyes, Victoria. Demystifying the Journal Article. Inside Higher Education; Trochim, William M.K. Philosophy of Research. Research Methods Knowledge Base. 2006; Weick, Karl E. “The Work of Theorizing.” In Theorizing in Social Science: The Context of Discovery . Richard Swedberg, editor. (Stanford, CA: Stanford University Press, 2014), pp. 177-194.
Writing Tip
Borrowing Theoretical Constructs from Other Disciplines
An increasingly important trend in the social and behavioral sciences is to think about and attempt to understand research problems from an interdisciplinary perspective. One way to do this is to not rely exclusively on the theories developed within your particular discipline, but to think about how an issue might be informed by theories developed in other disciplines. For example, if you are a political science student studying the rhetorical strategies used by female incumbents in state legislature campaigns, theories about the use of language could be derived, not only from political science, but linguistics, communication studies, philosophy, psychology, and, in this particular case, feminist studies. Building theoretical frameworks based on the postulates and hypotheses developed in other disciplinary contexts can be both enlightening and an effective way to be more engaged in the research topic.
CohenMiller, A. S. and P. Elizabeth Pate. "A Model for Developing Interdisciplinary Research Theoretical Frameworks." The Qualitative Researcher 24 (2019): 1211-1226; Frodeman, Robert. The Oxford Handbook of Interdisciplinarity . New York: Oxford University Press, 2010.
Another Writing Tip
Don't Undertheorize!
Do not leave the theory hanging out there in the introduction never to be mentioned again. Undertheorizing weakens your paper. The theoretical framework you describe should guide your study throughout the paper. Be sure to always connect theory to the review of pertinent literature and to explain in the discussion part of your paper how the theoretical framework you chose supports analysis of the research problem or, if appropriate, how the theoretical framework was found to be inadequate in explaining the phenomenon you were investigating. In that case, don't be afraid to propose your own theory based on your findings.
Yet Another Writing Tip
What's a Theory? What's a Hypothesis?
The terms theory and hypothesis are often used interchangeably in newspapers and popular magazines and in non-academic settings. However, the difference between theory and hypothesis in scholarly research is important, particularly when using an experimental design. A theory is a well-established principle that has been developed to explain some aspect of the natural world. Theories arise from repeated observation and testing and incorporates facts, laws, predictions, and tested assumptions that are widely accepted [e.g., rational choice theory; grounded theory; critical race theory].
A hypothesis is a specific, testable prediction about what you expect to happen in your study. For example, an experiment designed to look at the relationship between study habits and test anxiety might have a hypothesis that states, "We predict that students with better study habits will suffer less test anxiety." Unless your study is exploratory in nature, your hypothesis should always explain what you expect to happen during the course of your research.
The key distinctions are:
- A theory predicts events in a broad, general context; a hypothesis makes a specific prediction about a specified set of circumstances.
- A theory has been extensively tested and is generally accepted among a set of scholars; a hypothesis is a speculative guess that has yet to be tested.
Cherry, Kendra. Introduction to Research Methods: Theory and Hypothesis. About.com Psychology; Gezae, Michael et al. Welcome Presentation on Hypothesis. Slideshare presentation.
Still Yet Another Writing Tip
Be Prepared to Challenge the Validity of an Existing Theory
Theories are meant to be tested and their underlying assumptions challenged; they are not rigid or intransigent, but are meant to set forth general principles for explaining phenomena or predicting outcomes. Given this, testing theoretical assumptions is an important way that knowledge in any discipline develops and grows. If you're asked to apply an existing theory to a research problem, the analysis will likely include the expectation by your professor that you should offer modifications to the theory based on your research findings.
Indications that theoretical assumptions may need to be modified can include the following:
- Your findings suggest that the theory does not explain or account for current conditions or circumstances or the passage of time,
- The study reveals a finding that is incompatible with what the theory attempts to explain or predict, or
- Your analysis reveals that the theory overly generalizes behaviors or actions without taking into consideration specific factors revealed from your analysis [e.g., factors related to culture, nationality, history, gender, ethnicity, age, geographic location, legal norms or customs , religion, social class, socioeconomic status, etc.].
Philipsen, Kristian. "Theory Building: Using Abductive Search Strategies." In Collaborative Research Design: Working with Business for Meaningful Findings . Per Vagn Freytag and Louise Young, editors. (Singapore: Springer Nature, 2018), pp. 45-71; Shepherd, Dean A. and Roy Suddaby. "Theory Building: A Review and Integration." Journal of Management 43 (2017): 59-86.
- << Previous: The Research Problem/Question
- Next: 5. The Literature Review >>
- Last Updated: May 30, 2024 9:38 AM
- URL: https://libguides.usc.edu/writingguide
- Correspondence
- Open access
- Published: 18 September 2013
Using the framework method for the analysis of qualitative data in multi-disciplinary health research
- Nicola K Gale 1 ,
- Gemma Heath 2 ,
- Elaine Cameron 3 ,
- Sabina Rashid 4 &
- Sabi Redwood 2
BMC Medical Research Methodology volume 13 , Article number: 117 ( 2013 ) Cite this article
524k Accesses
5370 Citations
104 Altmetric
Metrics details
The Framework Method is becoming an increasingly popular approach to the management and analysis of qualitative data in health research. However, there is confusion about its potential application and limitations.
The article discusses when it is appropriate to adopt the Framework Method and explains the procedure for using it in multi-disciplinary health research teams, or those that involve clinicians, patients and lay people. The stages of the method are illustrated using examples from a published study.
Used effectively, with the leadership of an experienced qualitative researcher, the Framework Method is a systematic and flexible approach to analysing qualitative data and is appropriate for use in research teams even where not all members have previous experience of conducting qualitative research.
The Framework Method for the management and analysis of qualitative data has been used since the 1980s [ 1 ]. The method originated in large-scale social policy research but is becoming an increasingly popular approach in medical and health research; however, there is some confusion about its potential application and limitations. In this article we discuss when it is appropriate to use the Framework Method and how it compares to other qualitative analysis methods. In particular, we explore how it can be used in multi-disciplinary health research teams. Multi-disciplinary and mixed methods studies are becoming increasingly commonplace in applied health research. As well as disciplines familiar with qualitative research, such as nursing, psychology and sociology, teams often include epidemiologists, health economists, management scientists and others. Furthermore, applied health research often has clinical representation and, increasingly, patient and public involvement [ 2 ]. We argue that while leadership is undoubtedly required from an experienced qualitative methodologist, non-specialists from the wider team can and should be involved in the analysis process. We then present a step-by-step guide to the application of the Framework Method, illustrated using a worked example (See Additional File 1 ) from a published study [ 3 ] to illustrate the main stages of the process. Technical terms are included in the glossary (below). Finally, we discuss the strengths and limitations of the approach.
Glossary of key terms used in the Framework Method
Analytical framework: A set of codes organised into categories that have been jointly developed by researchers involved in analysis that can be used to manage and organise the data. The framework creates a new structure for the data (rather than the full original accounts given by participants) that is helpful to summarize/reduce the data in a way that can support answering the research questions.
Analytic memo: A written investigation of a particular concept, theme or problem, reflecting on emerging issues in the data that captures the analytic process (see Additional file 1 , Section 7).
Categories: During the analysis process, codes are grouped into clusters around similar and interrelated ideas or concepts. Categories and codes are usually arranged in a tree diagram structure in the analytical framework. While categories are closely and explicitly linked to the raw data, developing categories is a way to start the process of abstraction of the data (i.e. towards the general rather than the specific or anecdotal).
Charting: Entering summarized data into the Framework Method matrix (see Additional File 1 , Section 6).
Code: A descriptive or conceptual label that is assigned to excerpts of raw data in a process called ‘coding’ (see Additional File 1 , Section 3).
Data: Qualitative data usually needs to be in textual form before analysis. These texts can either be elicited texts (written specifically for the research, such as food diaries), or extant texts (pre-existing texts, such as meeting minutes, policy documents or weblogs), or can be produced by transcribing interview or focus group data, or creating ‘field’ notes while conducting participant-observation or observing objects or social situations.
Indexing: The systematic application of codes from the agreed analytical framework to the whole dataset (see Additional File 1 , Section 5).
Matrix: A spreadsheet contains numerous cells into which summarized data are entered by codes (columns) and cases (rows) (see Additional File 1 , Section 6).
Themes: Interpretive concepts or propositions that describe or explain aspects of the data, which are the final output of the analysis of the whole dataset. Themes are articulated and developed by interrogating data categories through comparison between and within cases. Usually a number of categories would fall under each theme or sub-theme [ 3 ].
Transcript: A written verbatim (word-for-word) account of a verbal interaction, such as an interview or conversation.
The Framework Method sits within a broad family of analysis methods often termed thematic analysis or qualitative content analysis. These approaches identify commonalities and differences in qualitative data, before focusing on relationships between different parts of the data, thereby seeking to draw descriptive and/or explanatory conclusions clustered around themes. The Framework Method was developed by researchers, Jane Ritchie and Liz Spencer, from the Qualitative Research Unit at the National Centre for Social Research in the United Kingdom in the late 1980s for use in large-scale policy research [ 1 ]. It is now used widely in other areas, including health research [ 3 – 12 ]. Its defining feature is the matrix output: rows (cases), columns (codes) and ‘cells’ of summarised data, providing a structure into which the researcher can systematically reduce the data, in order to analyse it by case and by code [ 1 ]. Most often a ‘case’ is an individual interviewee, but this can be adapted to other units of analysis, such as predefined groups or organisations. While in-depth analyses of key themes can take place across the whole data set, the views of each research participant remain connected to other aspects of their account within the matrix so that the context of the individual’s views is not lost. Comparing and contrasting data is vital to qualitative analysis and the ability to compare with ease data across cases as well as within individual cases is built into the structure and process of the Framework Method.
The Framework Method provides clear steps to follow and produces highly structured outputs of summarised data. It is therefore useful where multiple researchers are working on a project, particularly in multi-disciplinary research teams were not all members have experience of qualitative data analysis, and for managing large data sets where obtaining a holistic, descriptive overview of the entire data set is desirable. However, caution is recommended before selecting the method as it is not a suitable tool for analysing all types of qualitative data or for answering all qualitative research questions, nor is it an ‘easy’ version of qualitative research for quantitative researchers. Importantly, the Framework Method cannot accommodate highly heterogeneous data, i.e. data must cover similar topics or key issues so that it is possible to categorize it. Individual interviewees may, of course, have very different views or experiences in relation to each topic, which can then be compared and contrasted. The Framework Method is most commonly used for the thematic analysis of semi-structured interview transcripts, which is what we focus on in this article, although it could, in principle, be adapted for other types of textual data [ 13 ], including documents, such as meeting minutes or diaries [ 12 ], or field notes from observations [ 10 ].
For quantitative researchers working with qualitative colleagues or when exploring qualitative research for the first time, the nature of the Framework Method is seductive because its methodical processes and ‘spreadsheet’ approach seem more closely aligned to the quantitative paradigm [ 14 ]. Although the Framework Method is a highly systematic method of categorizing and organizing what may seem like unwieldy qualitative data, it is not a panacea for problematic issues commonly associated with qualitative data analysis such as how to make analytic choices and make interpretive strategies visible and auditable. Qualitative research skills are required to appropriately interpret the matrix, and facilitate the generation of descriptions, categories, explanations and typologies. Moreover, reflexivity, rigour and quality are issues that are requisite in the Framework Method just as they are in other qualitative methods. It is therefore essential that studies using the Framework Method for analysis are overseen by an experienced qualitative researcher, though this does not preclude those new to qualitative research from contributing to the analysis as part of a wider research team.
There are a number of approaches to qualitative data analysis, including those that pay close attention to language and how it is being used in social interaction such as discourse analysis [ 15 ] and ethnomethodology [ 16 ]; those that are concerned with experience, meaning and language such as phenomenology [ 17 , 18 ] and narrative methods [ 19 ]; and those that seek to develop theory derived from data through a set of procedures and interconnected stages such as Grounded Theory [ 20 , 21 ]. Many of these approaches are associated with specific disciplines and are underpinned by philosophical ideas which shape the process of analysis [ 22 ]. The Framework Method, however, is not aligned with a particular epistemological, philosophical, or theoretical approach. Rather it is a flexible tool that can be adapted for use with many qualitative approaches that aim to generate themes.
The development of themes is a common feature of qualitative data analysis, involving the systematic search for patterns to generate full descriptions capable of shedding light on the phenomenon under investigation. In particular, many qualitative approaches use the ‘constant comparative method’ , developed as part of Grounded Theory, which involves making systematic comparisons across cases to refine each theme [ 21 , 23 ]. Unlike Grounded Theory, the Framework Method is not necessarily concerned with generating social theory, but can greatly facilitate constant comparative techniques through the review of data across the matrix.
Perhaps because the Framework Method is so obviously systematic, it has often, as other commentators have noted, been conflated with a deductive approach to qualitative analysis [ 13 , 14 ]. However, the tool itself has no allegiance to either inductive or deductive thematic analysis; where the research sits along this inductive-deductive continuum depends on the research question. A question such as, ‘Can patients give an accurate biomedical account of the onset of their cardiovascular disease?’ is essentially a yes/no question (although it may be nuanced by the extent of their account or by appropriate use of terminology) and so requires a deductive approach to both data collection and analysis (e.g. structured or semi-structured interviews and directed qualitative content analysis [ 24 ]). Similarly, a deductive approach may be taken if basing analysis on a pre-existing theory, such as behaviour change theories, for example in the case of a research question such as ‘How does the Theory of Planned Behaviour help explain GP prescribing?’ [ 11 ]. However, a research question such as, ‘How do people construct accounts of the onset of their cardiovascular disease?’ would require a more inductive approach that allows for the unexpected, and permits more socially-located responses [ 25 ] from interviewees that may include matters of cultural beliefs, habits of food preparation, concepts of ‘fate’, or links to other important events in their lives, such as grief, which cannot be predicted by the researcher in advance (e.g. an interviewee-led open ended interview and grounded theory [ 20 ]). In all these cases, it may be appropriate to use the Framework Method to manage the data. The difference would become apparent in how themes are selected: in the deductive approach, themes and codes are pre-selected based on previous literature, previous theories or the specifics of the research question; whereas in the inductive approach, themes are generated from the data though open (unrestricted) coding, followed by refinement of themes. In many cases, a combined approach is appropriate when the project has some specific issues to explore, but also aims to leave space to discover other unexpected aspects of the participants’ experience or the way they assign meaning to phenomena. In sum, the Framework Method can be adapted for use with deductive, inductive, or combined types of qualitative analysis. However, there are some research questions where analysing data by case and theme is not appropriate and so the Framework Method should be avoided. For instance, depending on the research question, life history data might be better analysed using narrative analysis [ 19 ]; recorded consultations between patients and their healthcare practitioners using conversation analysis [ 26 ]; and documentary data, such as resources for pregnant women, using discourse analysis [ 27 ].
It is not within the scope of this paper to consider study design or data collection in any depth, but before moving on to describe the Framework Method analysis process, it is worth taking a step back to consider briefly what needs to happen before analysis begins. The selection of analysis method should have been considered at the proposal stage of the research and should fit with the research questions and overall aims of the study. Many qualitative studies, particularly ones using inductive analysis, are emergent in nature; this can be a challenge and the researchers can only provide an “imaginative rehearsal” of what is to come [ 28 ]. In mixed methods studies, the role of the qualitative component within the wider goals of the project must also be considered. In the data collection stage, resources must be allocated for properly trained researchers to conduct the qualitative interviewing because it is a highly skilled activity. In some cases, a research team may decide that they would like to use lay people, patients or peers to do the interviews [ 29 – 32 ] and in this case they must be properly trained and mentored which requires time and resources. At this early stage it is also useful to consider whether the team will use Computer Assisted Qualitative Data Analysis Software (CAQDAS), which can assist with data management and analysis.
As any form of qualitative or quantitative analysis is not a purely technical process, but influenced by the characteristics of the researchers and their disciplinary paradigms, critical reflection throughout the research process is paramount, including in the design of the study, the construction or collection of data, and the analysis. All members of the team should keep a research diary, where they record reflexive notes, impressions of the data and thoughts about analysis throughout the process. Experienced qualitative researchers become more skilled at sifting through data and analysing it in a rigorous and reflexive way. They cannot be too attached to certainty, but must remain flexible and adaptive throughout the research in order to generate rich and nuanced findings that embrace and explain the complexity of real social life and can be applied to complex social issues. It is important to remember when using the Framework Method that, unlike quantitative research where data collection and data analysis are strictly sequential and mutually exclusive stages of the research process, in qualitative analysis there is, to a greater or lesser extent depending on the project, ongoing interplay between data collection, analysis, and theory development. For example, new ideas or insights from participants may suggest potentially fruitful lines of enquiry, or close analysis might reveal subtle inconsistencies in an account which require further exploration.
Procedure for analysis
Stage 1: transcription.
A good quality audio recording and, ideally, a verbatim (word for word) transcription of the interview is needed. For Framework Method analysis, it is not necessarily important to include the conventions of dialogue transcriptions which can be difficult to read (e.g. pauses or two people talking simultaneously), because the content is what is of primary interest. Transcripts should have large margins and adequate line spacing for later coding and making notes. The process of transcription is a good opportunity to become immersed in the data and is to be strongly encouraged for new researchers. However, in some projects, the decision may be made that it is a better use of resources to outsource this task to a professional transcriber.
Stage 2: Familiarisation with the interview
Becoming familiar with the whole interview using the audio recording and/or transcript and any contextual or reflective notes that were recorded by the interviewer is a vital stage in interpretation. It can also be helpful to re-listen to all or parts of the audio recording. In multi-disciplinary or large research projects, those involved in analysing the data may be different from those who conducted or transcribed the interviews, which makes this stage particularly important. One margin can be used to record any analytical notes, thoughts or impressions.
Stage 3: Coding
After familiarization, the researcher carefully reads the transcript line by line, applying a paraphrase or label (a ‘code’) that describes what they have interpreted in the passage as important. In more inductive studies, at this stage ‘open coding’ takes place, i.e. coding anything that might be relevant from as many different perspectives as possible. Codes could refer to substantive things (e.g. particular behaviours, incidents or structures), values (e.g. those that inform or underpin certain statements, such as a belief in evidence-based medicine or in patient choice), emotions (e.g. sorrow, frustration, love) and more impressionistic/methodological elements (e.g. interviewee found something difficult to explain, interviewee became emotional, interviewer felt uncomfortable) [ 33 ]. In purely deductive studies, the codes may have been pre-defined (e.g. by an existing theory, or specific areas of interest to the project) so this stage may not be strictly necessary and you could just move straight onto indexing, although it is generally helpful even if you are taking a broadly deductive approach to do some open coding on at least a few of the transcripts to ensure important aspects of the data are not missed. Coding aims to classify all of the data so that it can be compared systematically with other parts of the data set. At least two researchers (or at least one from each discipline or speciality in a multi-disciplinary research team) should independently code the first few transcripts, if feasible. Patients, public involvement representatives or clinicians can also be productively involved at this stage, because they can offer alternative viewpoints thus ensuring that one particular perspective does not dominate. It is vital in inductive coding to look out for the unexpected and not to just code in a literal, descriptive way so the involvement of people from different perspectives can aid greatly in this. As well as getting a holistic impression of what was said, coding line-by-line can often alert the researcher to consider that which may ordinarily remain invisible because it is not clearly expressed or does not ‘fit’ with the rest of the account. In this way the developing analysis is challenged; to reconcile and explain anomalies in the data can make the analysis stronger. Coding can also be done digitally using CAQDAS, which is a useful way to keep track automatically of new codes. However, some researchers prefer to do the early stages of coding with a paper and pen, and only start to use CAQDAS once they reach Stage 5 (see below).
Stage 4: Developing a working analytical framework
After coding the first few transcripts, all researchers involved should meet to compare the labels they have applied and agree on a set of codes to apply to all subsequent transcripts. Codes can be grouped together into categories (using a tree diagram if helpful), which are then clearly defined. This forms a working analytical framework. It is likely that several iterations of the analytical framework will be required before no additional codes emerge. It is always worth having an ‘other’ code under each category to avoid ignoring data that does not fit; the analytical framework is never ‘final’ until the last transcript has been coded.
Stage 5: Applying the analytical framework
The working analytical framework is then applied by indexing subsequent transcripts using the existing categories and codes. Each code is usually assigned a number or abbreviation for easy identification (and so the full names of the codes do not have to be written out each time) and written directly onto the transcripts. Computer Assisted Qualitative Data Analysis Software (CAQDAS) is particularly useful at this stage because it can speed up the process and ensures that, at later stages, data is easily retrievable. It is worth noting that unlike software for statistical analyses, which actually carries out the calculations with the correct instruction, putting the data into a qualitative analysis software package does not analyse the data; it is simply an effective way of storing and organising the data so that they are accessible for the analysis process.
Stage 6: Charting data into the framework matrix
Qualitative data are voluminous (an hour of interview can generate 15–30 pages of text) and being able to manage and summarize (reduce) data is a vital aspect of the analysis process. A spreadsheet is used to generate a matrix and the data are ‘charted’ into the matrix. Charting involves summarizing the data by category from each transcript. Good charting requires an ability to strike a balance between reducing the data on the one hand and retaining the original meanings and ‘feel’ of the interviewees’ words on the other. The chart should include references to interesting or illustrative quotations. These can be tagged automatically if you are using CAQDAS to manage your data (N-Vivo version 9 onwards has the capability to generate framework matrices), or otherwise a capital ‘Q’, an (anonymized) transcript number, page and line reference will suffice. It is helpful in multi-disciplinary teams to compare and contrast styles of summarizing in the early stages of the analysis process to ensure consistency within the team. Any abbreviations used should be agreed by the team. Once members of the team are familiar with the analytical framework and well practised at coding and charting, on average, it will take about half a day per hour-long transcript to reach this stage. In the early stages, it takes much longer.
Stage 7: Interpreting the data
It is useful throughout the research to have a separate note book or computer file to note down impressions, ideas and early interpretations of the data. It may be worth breaking off at any stage to explore an interesting idea, concept or potential theme by writing an analytic memo [ 20 , 21 ] to then discuss with other members of the research team, including lay and clinical members. Gradually, characteristics of and differences between the data are identified, perhaps generating typologies, interrogating theoretical concepts (either prior concepts or ones emerging from the data) or mapping connections between categories to explore relationships and/or causality. If the data are rich enough, the findings generated through this process can go beyond description of particular cases to explanation of, for example, reasons for the emergence of a phenomena, predicting how an organisation or other social actor is likely to instigate or respond to a situation, or identifying areas that are not functioning well within an organisation or system. It is worth noting that this stage often takes longer than anticipated and that any project plan should ensure that sufficient time is allocated to meetings and individual researcher time to conduct interpretation and writing up of findings (see Additional file 1 , Section 7).
The Framework Method has been developed and used successfully in research for over 25 years, and has recently become a popular analysis method in qualitative health research. The issue of how to assess quality in qualitative research has been highly debated [ 20 , 34 – 40 ], but ensuring rigour and transparency in analysis is a vital component. There are, of course, many ways to do this but in the Framework Method the following are helpful:
Summarizing the data during charting, as well as being a practical way to reduce the data, means that all members of a multi-disciplinary team, including lay, clinical and (quantitative) academic members can engage with the data and offer their perspectives during the analysis process without necessarily needing to read all the transcripts or be involved in the more technical parts of analysis.
Charting also ensures that researchers pay close attention to describing the data using each participant’s own subjective frames and expressions in the first instance, before moving onto interpretation.
The summarized data is kept within the wider context of each case, thereby encouraging thick description that pays attention to complex layers of meaning and understanding [ 38 ].
The matrix structure is visually straightforward and can facilitate recognition of patterns in the data by any member of the research team, including through drawing attention to contradictory data, deviant cases or empty cells.
The systematic procedure (described in this article) makes it easy to follow, even for multi-disciplinary teams and/or with large data sets.
It is flexible enough that non-interview data (such as field notes taken during the interview or reflexive considerations) can be included in the matrix.
It is not aligned with a particular epistemological viewpoint or theoretical approach and therefore can be adapted for use in inductive or deductive analysis or a combination of the two (e.g. using pre-existing theoretical constructs deductively, then revising the theory with inductive aspects; or using an inductive approach to identify themes in the data, before returning to the literature and using theories deductively to help further explain certain themes).
It is easy to identify relevant data extracts to illustrate themes and to check whether there is sufficient evidence for a proposed theme.
Finally, there is a clear audit trail from original raw data to final themes, including the illustrative quotes.
There are also a number of potential pitfalls to this approach:
The systematic approach and matrix format, as we noted in the background, is intuitively appealing to those trained quantitatively but the ‘spreadsheet’ look perhaps further increases the temptation for those without an in-depth understanding of qualitative research to attempt to quantify qualitative data (e.g. “13 out of 20 participants said X). This kind of statement is clearly meaningless because the sampling in qualitative research is not designed to be representative of a wider population, but purposive to capture diversity around a phenomenon [ 41 ].
Like all qualitative analysis methods, the Framework Method is time consuming and resource-intensive. When involving multiple stakeholders and disciplines in the analysis and interpretation of the data, as is good practice in applied health research, the time needed is extended. This time needs to be factored into the project proposal at the pre-funding stage.
There is a high training component to successfully using the method in a new multi-disciplinary team. Depending on their role in the analysis, members of the research team may have to learn how to code, index, and chart data, to think reflexively about how their identities and experience affect the analysis process, and/or they may have to learn about the methods of generalisation (i.e. analytic generalisation and transferability, rather than statistical generalisation [ 41 ]) to help to interpret legitimately the meaning and significance of the data.
While the Framework Method is amenable to the participation of non-experts in data analysis, it is critical to the successful use of the method that an experienced qualitative researcher leads the project (even if the overall lead for a large mixed methods study is a different person). The qualitative lead would ideally be joined by other researchers with at least some prior training in or experience of qualitative analysis. The responsibilities of the lead qualitative researcher are: to contribute to study design, project timelines and resource planning; to mentor junior qualitative researchers; to train clinical, lay and other (non-qualitative) academics to contribute as appropriate to the analysis process; to facilitate analysis meetings in a way that encourages critical and reflexive engagement with the data and other team members; and finally to lead the write-up of the study.
We have argued that Framework Method studies can be conducted by multi-disciplinary research teams that include, for example, healthcare professionals, psychologists, sociologists, economists, and lay people/service users. The inclusion of so many different perspectives means that decision-making in the analysis process can be very time consuming and resource-intensive. It may require extensive, reflexive and critical dialogue about how the ideas expressed by interviewees and identified in the transcript are related to pre-existing concepts and theories from each discipline, and to the real ‘problems’ in the health system that the project is addressing. This kind of team effort is, however, an excellent forum for driving forward interdisciplinary collaboration, as well as clinical and lay involvement in research, to ensure that ‘the whole is greater than the sum of the parts’, by enhancing the credibility and relevance of the findings.
The Framework Method is appropriate for thematic analysis of textual data, particularly interview transcripts, where it is important to be able to compare and contrast data by themes across many cases, while also situating each perspective in context by retaining the connection to other aspects of each individual’s account. Experienced qualitative researchers should lead and facilitate all aspects of the analysis, although the Framework Method’s systematic approach makes it suitable for involving all members of a multi-disciplinary team. An open, critical and reflexive approach from all team members is essential for rigorous qualitative analysis.
Acceptance of the complexity of real life health systems and the existence of multiple perspectives on health issues is necessary to produce high quality qualitative research. If done well, qualitative studies can shed explanatory and predictive light on important phenomena, relate constructively to quantitative parts of a larger study, and contribute to the improvement of health services and development of health policy. The Framework Method, when selected and implemented appropriately, can be a suitable tool for achieving these aims through producing credible and relevant findings.
The Framework Method is an excellent tool for supporting thematic (qualitative content) analysis because it provides a systematic model for managing and mapping the data.
The Framework Method is most suitable for analysis of interview data, where it is desirable to generate themes by making comparisons within and between cases.
The management of large data sets is facilitated by the Framework Method as its matrix form provides an intuitively structured overview of summarised data.
The clear, step-by-step process of the Framework Method makes it is suitable for interdisciplinary and collaborative projects.
The use of the method should be led and facilitated by an experienced qualitative researcher.
Ritchie J, Lewis J: Qualitative research practice: a guide for social science students and researchers. 2003, London: Sage
Google Scholar
Ives J, Damery S, Redwod S: PPI, paradoxes and Plato: who's sailing the ship?. J Med Ethics. 2013, 39 (3): 181-185. 10.1136/medethics-2011-100150.
Article Google Scholar
Heath G, Cameron E, Cummins C, Greenfield S, Pattison H, Kelly D, Redwood S: Paediatric ‘care closer to home’: stake-holder views and barriers to implementation. Health Place. 2012, 18 (5): 1068-1073. 10.1016/j.healthplace.2012.05.003.
Elkington H, White P, Addington-Hall J, Higgs R, Petternari C: The last year of life of COPD: a qualitative study of symptoms and services. Respir Med. 2004, 98 (5): 439-445. 10.1016/j.rmed.2003.11.006.
Murtagh J, Dixey R, Rudolf M: A qualitative investigation into the levers and barriers to weight loss in children: opinions of obese children. Archives Dis Child. 2006, 91 (11): 920-923. 10.1136/adc.2005.085712.
Barnard M, Webster S, O’Connor W, Jones A, Donmall M: The drug treatment outcomes research study (DTORS): qualitative study. 2009, London: Home Office
Ayatollahi H, Bath PA, Goodacre S: Factors influencing the use of IT in the emergency department: a qualitative study. Health Inform J. 2010, 16 (3): 189-200. 10.1177/1460458210377480.
Sheard L, Prout H, Dowding D, Noble S, Watt I, Maraveyas A, Johnson M: Barriers to the diagnosis and treatment of venous thromboembolism in advanced cancer patients: a qualitative study. Palliative Med. 2012, 27 (2): 339-348.
Ellis J, Wagland R, Tishelman C, Williams ML, Bailey CD, Haines J, Caress A, Lorigan P, Smith JA, Booton R, et al: Considerations in developing and delivering a nonpharmacological intervention for symptom management in lung cancer: the views of patients and informal caregivers. J Pain Symptom Manag (0). 2012, 44 (6): 831-842. 10.1016/j.jpainsymman.2011.12.274.
Gale N, Sultan H: Telehealth as ‘peace of mind’: embodiment, emotions and the home as the primary health space for people with chronic obstructive pulmonary disorder. Health place. 2013, 21: 140-147.
Rashidian A, Eccles MP, Russell I: Falling on stony ground? A qualitative study of implementation of clinical guidelines’ prescribing recommendations in primary care. Health policy. 2008, 85 (2): 148-161. 10.1016/j.healthpol.2007.07.011.
Jones RK: The unsolicited diary as a qualitative research tool for advanced research capacity in the field of health and illness. Qualitative Health Res. 2000, 10 (4): 555-567. 10.1177/104973200129118543.
Pope C, Ziebland S, Mays N: Analysing qualitative data. British Med J. 2000, 320: 114-116. 10.1136/bmj.320.7227.114.
Pope C, Mays N: Critical reflections on the rise of qualitative research. British Med J. 2009, 339: 737-739.
Fairclough N: Critical discourse analysis: the critical study of language. 2010, London: Longman
Garfinkel H: Ethnomethodology’s program. Soc Psychol Quarter. 1996, 59 (1): 5-21. 10.2307/2787116.
Merleau-Ponty M: The phenomenology of perception. 1962, London: Routledge and Kegan Paul
Svenaeus F: The phenomenology of health and illness. Handbook of phenomenology and medicine. 2001, Netherlands: Springer, 87-108.
Reissmann CK: Narrative methods for the human sciences. 2008, London: Sage
Charmaz K: Constructing grounded theory: a practical guide through qualitative analysis. 2006, London: Sage
Glaser A, Strauss AL: The discovery of grounded theory. 1967, Chicago: Aldine
Crotty M: The foundations of social research: meaning and perspective in the research process. 1998, London: Sage
Boeije H: A purposeful approach to the constant comparative method in the analysis of qualitative interviews. Qual Quant. 2002, 36 (4): 391-409. 10.1023/A:1020909529486.
Hsieh H-F, Shannon SE: Three approaches to qualitative content analysis. Qual Health Res. 2005, 15 (9): 1277-1288. 10.1177/1049732305276687.
Redwood S, Gale NK, Greenfield S: ‘You give us rangoli, we give you talk’: using an art-based activity to elicit data from a seldom heard group. BMC Med Res Methodol. 2012, 12 (1): 7-10.1186/1471-2288-12-7.
Mishler EG: The struggle between the voice of medicine and the voice of the lifeworld. The sociology of health and illness: critical perspectives. Edited by: Conrad P, Kern R. 1990, New York: St Martins Press, Third
Hodges BD, Kuper A, Reeves S: Discourse analysis. British Med J. 2008, 337: 570-572. 10.1136/bmj.39370.701782.DE.
Sandelowski M, Barroso J: Writing the proposal for a qualitative research methodology project. Qual Health Res. 2003, 13 (6): 781-820. 10.1177/1049732303013006003.
Ellins J: It’s better together: involving older people in research. HSMC Newsletter Focus Serv Users Publ. 2010, 16 (1): 4-
Phillimore J, Goodson L, Hennessy D, Ergun E: Empowering Birmingham’s migrant and refugee community organisations: making a difference. 2009, York: Joseph Rowntree Foundation
Leamy M, Clough R: How older people became researchers. 2006, York: Joseph Rowntree Foundation
Glasby J, Miller R, Ellins J, Durose J, Davidson D, McIver S, Littlechild R, Tanner D, Snelling I, Spence K: Final report NIHR service delivery and organisation programme. Understanding and improving transitions of older people: a user and carer centred approach. 2012, London: The Stationery Office
Saldaña J: The coding manual for qualitative researchers. 2009, London: Sage
Lincoln YS: Emerging criteria for quality in qualitative and interpretive research. Qual Inquiry. 1995, 1 (3): 275-289. 10.1177/107780049500100301.
Mays N, Pope C: Qualitative research in health care: assessing quality in qualitative research. BMJ British Med J. 2000, 320 (7226): 50-10.1136/bmj.320.7226.50.
Seale C: Quality in qualitative research. Qual Inquiry. 1999, 5 (4): 465-478. 10.1177/107780049900500402.
Dingwall R, Murphy E, Watson P, Greatbatch D, Parker S: Catching goldfish: quality in qualitative research. J Health serv Res Policy. 1998, 3 (3): 167-172.
Popay J, Rogers A, Williams G: Rationale and standards for the systematic review of qualitative literature in health services research. Qual Health Res. 1998, 8 (3): 341-351. 10.1177/104973239800800305.
Morse JM, Barrett M, Mayan M, Olson K, Spiers J: Verification strategies for establishing reliability and validity in qualitative research. Int J Qual Methods. 2008, 1 (2): 13-22.
Smith JA: Reflecting on the development of interpretative phenomenological analysis and its contribution to qualitative research in psychology. Qual Res Psychol. 2004, 1 (1): 39-54.
Polit DF, Beck CT: Generalization in quantitative and qualitative research: Myths and strategies. Int J Nurs Studies. 2010, 47 (11): 1451-1458. 10.1016/j.ijnurstu.2010.06.004.
Pre-publication history
The pre-publication history for this paper can be accessed here: http://www.biomedcentral.com/1471-2288/13/117/prepub
Download references
Acknowledgments
All authors were funded by the National Institute for Health Research (NIHR) through the Collaborations for Leadership in Applied Health Research and Care for Birmingham and Black Country (CLAHRC-BBC) programme. The views in this publication expressed are those of the authors and not necessarily those of the NHS, the NIHR or the Department of Health.
Author information
Authors and affiliations.
Health Services Management Centre, University of Birmingham, Park House, 40 Edgbaston Park Road, Birmingham, B15 2RT, UK
Nicola K Gale
School of Health and Population Sciences, University of Birmingham, Edgbaston, Birmingham, B15 2TT, UK
Gemma Heath & Sabi Redwood
School of Life and Health Sciences, Aston University, Aston Triangle, Birmingham, B4 7ET, UK
Elaine Cameron
East and North Hertfordshire NHS Trust, Lister hospital, Coreys Mill Lane, Stevenage, SG1 4AB, UK
Sabina Rashid
You can also search for this author in PubMed Google Scholar
Corresponding author
Correspondence to Nicola K Gale .
Additional information
Competing interests.
The authors declare that they have no competing interests.
Authors’ contributions
All authors were involved in the development of the concept of the article and drafting the article. NG wrote the first draft of the article, GH and EC prepared the text and figures related to the illustrative example, SRa did the literature search to identify if there were any similar articles currently available and contributed to drafting of the article, and SRe contributed to drafting of the article and the illustrative example. All authors read and approved the final manuscript.
Electronic supplementary material
Additional file 1: illustrative example of the use of the framework method.(docx 167 kb), authors’ original submitted files for images.
Below are the links to the authors’ original submitted files for images.
Authors’ original file for figure 1
Authors’ original file for figure 2, authors’ original file for figure 3, authors’ original file for figure 4, authors’ original file for figure 5, authors’ original file for figure 6, rights and permissions.
This article is published under license to BioMed Central Ltd. This is an Open Access article distributed under the terms of the Creative Commons Attribution License ( http://creativecommons.org/licenses/by/2.0 ), which permits unrestricted use, distribution, and reproduction in any medium, provided the original work is properly cited.
Reprints and permissions
About this article
Cite this article.
Gale, N.K., Heath, G., Cameron, E. et al. Using the framework method for the analysis of qualitative data in multi-disciplinary health research. BMC Med Res Methodol 13 , 117 (2013). https://doi.org/10.1186/1471-2288-13-117
Download citation
Received : 17 December 2012
Accepted : 06 September 2013
Published : 18 September 2013
DOI : https://doi.org/10.1186/1471-2288-13-117
Share this article
Anyone you share the following link with will be able to read this content:
Sorry, a shareable link is not currently available for this article.
Provided by the Springer Nature SharedIt content-sharing initiative
- Qualitative research
- Qualitative content analysis
- Multi-disciplinary research
BMC Medical Research Methodology
ISSN: 1471-2288
- General enquiries: [email protected]

An official website of the United States government
The .gov means it’s official. Federal government websites often end in .gov or .mil. Before sharing sensitive information, make sure you’re on a federal government site.
The site is secure. The https:// ensures that you are connecting to the official website and that any information you provide is encrypted and transmitted securely.
- Publications
- Account settings
Preview improvements coming to the PMC website in October 2024. Learn More or Try it out now .
- Advanced Search
- Journal List
- BMJ Open Access

Analytical studies: a framework for quality improvement design and analysis
Conducting studies for learning is fundamental to improvement. Deming emphasised that the reason for conducting a study is to provide a basis for action on the system of interest. He classified studies into two types depending on the intended target for action. An enumerative study is one in which action will be taken on the universe that was studied. An analytical study is one in which action will be taken on a cause system to improve the future performance of the system of interest. The aim of an enumerative study is estimation, while an analytical study focuses on prediction. Because of the temporal nature of improvement, the theory and methods for analytical studies are a critical component of the science of improvement.
Introduction: enumerative and analytical studies
Designing studies that make it possible to learn from experience and take action to improve future performance is an essential element of quality improvement. These studies use the now traditional theory established through the work of Fisher, 1 Cox, 2 Campbell and Stanley, 3 and others that is widely used in biomedicine research. These designs are used to discover new phenomena that lead to hypothesis generation, and to explore causal mechanisms, 4 as well as to evaluate efficacy and effectiveness. They include observational, retrospective, prospective, pre-experimental, quasiexperimental, blocking, factorial and time-series designs.
In addition to these classifications of studies, Deming 5 defined a distinction between analytical and enumerative studies which has proven to be fundamental to the science of improvement. Deming based his insight on the distinction between these two approaches that Walter Shewhart had made in 1939 as he helped develop measurement strategies for the then-emerging science of ‘quality control.’ 6 The difference between the two concepts lies in the extrapolation of the results that is intended, and in the target for action based on the inferences that are drawn.
A useful way to appreciate that difference is to contrast the inferences that can be made about the water sampled from two different natural sources ( figure 1 ). The enumerative approach is like the study of water from a pond. Because conditions in the bounded universe of the pond are essentially static over time, analyses of random samples taken from the pond at a given time can be used to estimate the makeup of the entire pond. Statistical methods, such as hypothesis testing and CIs, can be used to make decisions and define the precision of the estimates.

Environment in enumerative and analytical study. Internal validity diagram from Fletcher et al . 7
The analytical approach, in contrast, is like the study of water from a river. The river is constantly moving, and its physical properties are changing (eg, due to snow melt, changes in rainfall, dumping of pollutants). The properties of water in a sample from the river at any given time may not describe the river after the samples are taken and analysed. In fact, without repeated sampling over time, it is difficult to make predictions about water quality, since the river will not be the same river in the future as it was at the time of the sampling.
Deming first discussed these concepts in a 1942 paper, 8 as well as in his 1950 textbook, 9 and in a 1975 paper used the enumerative/analytical terminology to characterise specific study designs. 5 While most books on experimental design describe methods for the design and analysis of enumerative studies, Moen et al 10 describe methods for designing and learning from analytical studies. These methods are graphical and focus on prediction of future performance. The concept of analytical studies became a key element in Deming's ‘system of profound knowledge’ that serves as the intellectual foundation for improvement science. 11 The knowledge framework for the science of improvement, which combines elements of psychology, the Shewhart view of variation, the concept of systems, and the theory of knowledge, informs a number of key principles for the design and analysis of improvement studies:
- Knowledge about improvement begins and ends in experimental data but does not end in the data in which it begins.
- Observations, by themselves, do not constitute knowledge.
- Prediction requires theory regarding mechanisms of change and understanding of context.
- Random sampling from a population or universe (assumed by most statistical methods) is not possible when the population of interest is in the future.
- The conditions during studies for improvement will be different from the conditions under which the results will be used. The major source of uncertainty concerning their use is the difficulty of extrapolating study results to different contexts and under different conditions in the future.
- The wider the range of conditions included in an improvement study, the greater the degree of belief in the validity and generalisation of the conclusions.
The classification of studies into enumerative and analytical categories depends on the intended target for action as the result of the study:
- Enumerative studies assume that when actions are taken as the result of a study, they will be taken on the material in the study population or ‘frame’ that was sampled.
More specifically, the study universe in an enumerative study is the bounded group of items (eg, patients, clinics, providers, etc) possessing certain properties of interest. The universe is defined by a frame, a list of identifiable, tangible units that may be sampled and studied. Random selection methods are assumed in the statistical methods used for estimation, decision-making and drawing inferences in enumerative studies. Their aim is estimation about some aspect of the frame (such as a description, comparison or the existence of a cause–effect relationship) and the resulting actions taken on this particular frame. One feature of an enumerative study is that a 100% sample of the frame provides the complete answer to the questions posed by the study (given the methods of investigation and measurement). Statistical methods such as hypothesis tests, CIs and probability statements are appropriate to analyse and report data from enumerative studies. Estimating the infection rate in an intensive care unit for the last month is an example of a simple enumerative study.
- Analytical studies assume that the actions taken as a result of the study will be on the process or causal system that produced the frame studied, rather than the initial frame itself. The aim is to improve future performance.
In contrast to enumerative studies, an analytical study accepts as a given that when actions are taken on a system based on the results of a study, the conditions in that system will inevitably have changed. The aim of an analytical study is to enable prediction about how a change in a system will affect that system's future performance, or prediction as to which plans or strategies for future action on the system will be superior. For example, the task may be to choose among several different treatments for future patients, methods of collecting information or procedures for cleaning an operating room. Because the population of interest is open and continually shifts over time, random samples from that population cannot be obtained in analytical studies, and traditional statistical methods are therefore not useful. Rather, graphical methods of analysis and summary of the repeated samples reveal the trajectory of system behaviour over time, making it possible to predict future behaviour. Use of a Shewhart control chart to monitor and create learning to reduce infection rates in an intensive care unit is an example of a simple analytical study.
The following scenarios give examples to clarify the nature of these two types of studies.
Scenario 1: enumerative study—observation
To estimate how many days it takes new patients to see all primary care physicians contracted with a health plan, a researcher selected a random sample of 150 such physicians from the current active list and called each of their offices to schedule an appointment. The time to the next available appointment ranged from 0 to 180 days, with a mean of 38 days (95% CI 35.6 to 39.6).
This is an enumerative study, since results are intended to be used to estimate the waiting time for appointments with the plan's current population of primary care physicians.
Scenario 2: enumerative study—hypothesis generation
The researcher in scenario 1 noted that on occasion, she was offered an earlier visit with a nurse practitioner (NP) who worked with the physician being called. Additional information revealed that 20 of the 150 physicians in the study worked with one or more NPs. The next available appointment for the 130 physicians without an NP averaged 41 days (95% CI 39 to 43 days) and was 18 days (95% CI 18 to 26 days) for the 20 practices with NPs, a difference of 23 days (a 56% shorter mean waiting time).
This subgroup analysis suggested that the involvement of NPs helps to shorten waiting times, although it does not establish a cause–effect relationship, that is, it was a ‘hypothesis-generating’ study. In any event, this was clearly an enumerative study, since its results were to understand the impact of NPs on waiting times in the particular population of practices. Its results suggested that NPs might influence waiting times, but only for practices in this health plan during the time of the study. The study treated the conditions in the health plan as static, like those in a pond.
Scenario 3: enumerative study—comparison
To find out if administrative changes in a health plan had increased member satisfaction in access to care, the customer service manager replicated a phone survey he had conducted a year previously, using a random sample of 300 members. The percentage of patients who were satisfied with access had increased from 48.7% to 60.7% (Fisher exact test, p<0.004).
This enumerative comparison study was used to estimate the impact of the improvement work during the last year on the members in the plan. Attributing the increase in satisfaction to the improvement work assumes that other conditions in the study frame were static.
Scenario 4: analytical study—learning with a Shewhart chart
Each primary care clinic in a health plan reported its ‘time until the third available appointment’ twice a month, which allowed the quality manager to plot the mean waiting time for all of the clinics on Shewhart charts. Waiting times had been stable for a 12-month period through August, but the manager then noted a special cause (increase in waiting time) in September. On stratifying the data by region, she found that the special cause resulted from increases in waiting time in the Northeast region. Discussion with the regional manager revealed a shortage of primary care physicians in this region, which was predicted to become worse in the next quarter. Making some temporary assignments and increasing physician recruiting efforts resulted in stabilisation of this measure.
Documenting common and special cause variation in measures of interest through the use of Shewhart charts and run charts based on judgement samples is probably the simplest and commonest type of analytical study in healthcare. Such charts, when stable, provide a rational basis for predicting future performance.
Scenario 5: analytical study—establishing a cause–effect relationship
The researcher mentioned in scenarios 1 and 2 planned a study to test the existence of a cause–effect relationship between the inclusion of NPs in primary care offices and waiting time for new patient appointments. The variation in patient characteristics in this health plan appeared to be great enough to make the study results useful to other organisations. For the study, she recruited about 100 of the plan's practices that currently did not use NPs, and obtained funding to facilitate hiring NPs in up to 50 of those practices.
The researcher first explored the theories on mechanisms by which the incorporation of NPs into primary care clinics could reduce waiting times. Using important contextual variables relevant to these mechanisms (practice size, complexity, use of information technology and urban vs rural location), she then developed a randomised block, time-series study design. The study had the power to detect an effect of a mean waiting time of 5 days or more overall, and 10 days for the major subgroups defined by levels of the contextual variables. Since the baseline waiting time for appointments varied substantially across practices, she used the baseline as a covariate.
After completing the study, she analysed data from baseline and postintervention periods using stratified run charts and Shewhart charts, including the raw measures and measures adjusted for important covariates and effects of contextual variables. Overall waiting times decreased 12 days more in practices that included NPs than they did in control practices. Importantly, the subgroup analyses according to contextual variables revealed conditions under which the use of NPs would not be predicted to lead to reductions in waiting times. For example, practices with short baseline waiting times showed little or no improvement by employing NPs. She published the results in a leading health research journal.
This was an analytical study because the intent was to apply the learning from the study to future staffing plans in the health plan. She also published the study, so its results would be useful to primary care practices outside the health plan.
Scenario 6: analytical study—implementing improvement
The quality-improvement manager in another health plan wanted to expand the use of NPs in the plan's primary care practices, because published research had shown a reduction in waiting times for practices with NPs. Two practices in his plan already employed NPs. In one of these practices, Shewhart charts of waiting time by month showed a stable process averaging 10 days during the last 2 years. Waiting time averaged less than 7 days in the second practice, but a period when one of the physicians left the practice was associated with special causes.
The quality manager created a collaborative among the plan's primary care practices to learn how to optimise the use of NPs. Physicians in the two sites that employed NPs served as subject matter experts for the collaborative. In addition to making NPs part of their care teams, participating practices monitored appointment supply and demand, and tested other changes designed to optimise response to patient needs. Thirty sites in the plan voluntarily joined the collaborative and hired NPs. After 6 months, Shewhart charts indicated that waiting times in 25 of the 30 sites had been reduced to less than 7 days. Because waiting times in these practices had been stable over a considerable period of time, the manager predicted that future patients would continue to experience reduced times for appointments. The quality manger began to focus on a follow-up collaborative among the backlog of 70 practices that wanted to join.
This project was clearly an analytical study, since its aim was specifically to improve future waiting-time performance for participating sites and other primary care offices in the plan. Moreover, it focused on learning about the mechanisms through which contextual factors affect the impact of NPs on primary care office functions, under practice conditions that (like those in a river) will inevitably change over time.
Statistical theory in enumerative studies is used to describe the precision of estimates and the validity of hypotheses for the population studied. But since these statistical methods provide no support for extrapolation of the results outside the population that was studied, the subject experts must rely on their understanding of the mechanisms in place to extend results outside the population.
In analytical studies, the standard error of a statistic does not address the most important source of uncertainty, namely, the change in study conditions in the future. Although analytical studies need to take into account the uncertainty due to sampling, as in enumerative studies, the attributes of the study design and analysis of the data primarily deal with the uncertainty resulting from extrapolation to the future (generalisation to the conditions in future time periods). The methods used in analytical studies encourage the exploration of mechanisms through multifactor designs, contextual variables introduced through blocking and replication over time.
Prior stability of a system (as observed in graphic displays of repeated sampling over time, according to Shewhart's methods) increases belief in the results of an analytical study, but stable processes in the past do not guarantee constant system behaviour in the future. The next data point from the future is the most important on a graph of performance. Extrapolation of system behaviour to future times therefore still depends on input from subject experts who are familiar with mechanisms of the system of interest, as well as the important contextual issues. Generalisation is inherently difficult in all studies because ‘whereas the problems of internal validity are solvable within the limits of the logic of probability statistics, the problems of external validity are not logically solvable in any neat, conclusive way’ 3 (p. 17).
The diverse activities commonly referred to as healthcare improvement 12 are all designed to change the behaviour of systems over time, as reflected in the principle that ‘not all change is improvement, but all improvement is change.’ The conditions in the unbounded systems into which improvement interventions are introduced will therefore be different in the future from those in effect at the time the intervention is studied. Since the results of improvement studies are used to predict future system behaviour, such studies clearly belong to the Deming category of analytical studies. Quality improvement studies therefore need to incorporate repeated measurements over time, as well as testing under a wide range of conditions (2, 3 and 10). The ‘gold standard’ of analytical studies is satisfactory prediction over time.
Conclusions and recommendations
In light of these considerations, some important principles for drawing inferences from improvement studies include 10 :
- The analysis of data, interpretation of that analysis and actions taken as a result of the study should be closely tied to the current knowledge of experts about mechanisms of change in the relevant area. They can often use the study to discover, understand and evaluate the underlying mechanisms.
- The conditions of the study will be different from the future conditions under which the results will be used. Assessment by experts of the magnitude of this difference and its potential impact on future events should be an integral part of the interpretation of the results of the intervention.
- Show all the data before aggregation or summary.
- Plot the outcome data in the order in which the tests of change were conducted and annotate with information on the interventions.
- Use graphical displays to assess how much of the variation in the data can be explained by factors that were deliberately changed.
- Rearrange and subgroup the data to study other sources of variation (background and contextual variables).
- Summarise the results of the study with appropriate graphical displays.
Because these principles reflect the fundamental nature of improvement—taking action to change performance, over time, and under changing conditions—their application helps to bring clarity and rigour to improvement science.
Acknowledgments
The author is grateful to F Davidoff and P Batalden for their input to earlier versions of this paper.
Competing interests: None.
Provenance and peer review: Not commissioned; externally peer reviewed.
Analytical Framework and Methodology
- First Online: 30 September 2017
Cite this chapter
- Jan-Peter Ferdinand 2
Part of the book series: Contributions to Management Science ((MANAGEMENT SC.))
1010 Accesses
1 Citations
This chapter merges the conceptual and theoretical insights that inform this book in order to operationalize the analytical framework, which is applied to explain how the institutional idiosyncrasies of innovation communities affect entrepreneurship in the field of desktop 3D printing. Additionally, I outline my methodological approach as well as the data sources my analysis draws on. In the context of my empirical investigation, I apply a triangulation that allows me the describe the evolution and change of the 3D printing field, delineate shared understandings on how this issue should be approached appropriately, and finally synthesize the actors’ practical responses to the dilemma of entrepreneurship.
This is a preview of subscription content, log in via an institution to check access.
Access this chapter
- Available as PDF
- Read on any device
- Instant download
- Own it forever
- Available as EPUB and PDF
- Compact, lightweight edition
- Dispatched in 3 to 5 business days
- Free shipping worldwide - see info
- Durable hardcover edition
Tax calculation will be finalised at checkout
Purchases are for personal use only
Institutional subscriptions
Recent receptions of Swidler’s work exaggerate even these tendencies. Especially the framework of “cultural entrepreneurship” (Lounsbury and Glynn 2001), which describes the purposive efforts of actors to produce rationalizing accounts or stories in order “to shape the attention and perceptions of various audiences, justifying the group’s legitimacy and helping to coordinate its expansion to shape the attention” (Wry et al. 2011, p. 450), draws heavily on Swidler’s culture-as-a-toolkit-argument but widely misses her foundations in (institutional) theory.
While the current research on field-configuring events draws on temporally and spatially bounded moments that enable direct interaction among field members (Hardy and Maguire 2010 ; Schüssler et al. 2014 ; Schüßler et al. 2015 ), I consider also incidents like obtained milestones, external shocks, or legal/administrative happenings as potential triggers for discontinuities and change within the field. Of course, these events may also affect the established practices and understandings in connection with the issue of the field (see Hoffman 1999 , p. 353).
Analytically applied, the semiotic chain analysis therefore always entails a normative bias that reproduces the cultural preferences that are prevalent in the particular context of research. Consequently, what empirically turns out as desirable representations of a certain cultural code in my own research embraces the innovation community’s points of view. However, this moral bias is of course leveled in the context of the conceptual and theoretical discussion of my findings.
Barley SR (1983) Semiotics and the study of occupational and organizational cultures. Adm Sci Q 28(3):393–413. doi: 10.2307/2392249
Article Google Scholar
DiMaggio PJ (1997) Culture and cognition. Annu Rev Sociol 23(1):263–287. doi: 10.1146/annurev.soc.23.1.263
Fligstein N (2001a) The architecture of markets: an economic sociology of twenty-first-century capitalist societies, 2nd printing. Princeton University Press, Princeton
Google Scholar
Fligstein N, McAdam D (2012) A theory of fields. Oxford University Press, New York
Book Google Scholar
Friedland R, Alford R (1991) Bringing society back in: symbols, practices and institutional contradictions. In: Powell W, Dimaggio P (eds) The new institutionalism in organizational analysis. University Of Chicago Press, Chicago, pp 232–263
Gartner (2013) Gartner’s 2013 hype cycle for emerging technologies maps out evolving relationship between humans and machines. http://www.gartner.com/newsroom/id/2575515 . Accessed 5 May 2016
Garud R, Jain S, Kumaraswamy A (2002) Institutional entrepreneurship in the sponsorship of common technological standards: the case of Sun Microsystems and Java. Acad Manag J 45(1):196–214. doi: 10.2307/3069292
Geertz C (1973) The interpretation of cultures: selected essays. Basic Books, New York
Giddens A (1984) The constitution of society. University of California Press, Berkley
Hardy C, Maguire S (2010) Discourse, field-configuring events, and change in organizations and institutional fields: narratives of DDT and the Stockholm convention. Acad Manag J 53(6):1365–1392. doi: 10.5465/AMJ.2010.57318384
Hoffman AJ (1999) Institutional evolution and change: environmentalism and the U.S. chemical industry. Acad Manag J 42(4):351–371. doi: 10.2307/257008
Hoffman AJ (2001) Linking organizational and field-level analyses the diffusion of corporate environmental practice. Organ Environ 14(2):133–156. doi: 10.1177/1086026601142001
Lounsbury M, Glynn MA (2001) Cultural entrepreneurship: stories, legitimacy, and the acquisition of resources. Strateg Manag J 22(6–7):545–564. https://doi.org/10.1002/smj.188
Reay T, Jones C (2015) Qualitatively Capturing Institutional Logics. Strateg Organ, June, 1476127015589981. doi: 10.1177/1476127015589981
Schüßler E, Grabher G, Müller-Seitz G (2015) Field-configuring events: arenas for innovation and learning? Ind Innov 22(3):165–172. doi: 10.1080/13662716.2015.1038098
Schüssler E, Rüling C-C, Wittneben BBF (2014) On melting summits: the limitations of field-configuring events as catalysts of change in transnational climate policy. Acad Manag J 57(1):140–171. doi: 10.5465/amj.2011.0812
Scott WR (2001) Institutions and organizations. SAGE, Los Angeles, CA
Swidler A (1986) Culture in action: symbols and strategies. Am Sociol Rev 51(2):273–286. doi: 10.2307/2095521
Weber K (2005a) A toolkit for analyzing corporate cultural toolkits. Poetics 33(3):227–252
Weber K, Heinze KL, DeSoucey M (2008) Forage for thought: mobilizing codes in the movement for grass-fed meat and dairy products. Adm Sci Q 53(3):529–567. doi: 10.2189/asqu.53.3.529
Wry T, Lounsbury M, Glynn MA (2011) Legitimating nascent collective identities: coordinating cultural entrepreneurship. Organ Sci 22(2):449–463. https://doi.org/10.1287/orsc.1100.0613
Download references
Author information
Authors and affiliations.
Technische Universität, Berlin, Germany
Jan-Peter Ferdinand
You can also search for this author in PubMed Google Scholar
Rights and permissions
Reprints and permissions
Copyright information
© 2018 Springer International Publishing AG
About this chapter
Ferdinand, JP. (2018). Analytical Framework and Methodology. In: Entrepreneurship in Innovation Communities. Contributions to Management Science. Springer, Cham. https://doi.org/10.1007/978-3-319-66842-0_4
Download citation
DOI : https://doi.org/10.1007/978-3-319-66842-0_4
Published : 30 September 2017
Publisher Name : Springer, Cham
Print ISBN : 978-3-319-66841-3
Online ISBN : 978-3-319-66842-0
eBook Packages : Business and Management Business and Management (R0)
Share this chapter
Anyone you share the following link with will be able to read this content:
Sorry, a shareable link is not currently available for this article.
Provided by the Springer Nature SharedIt content-sharing initiative
- Publish with us
Policies and ethics
- Find a journal
- Track your research
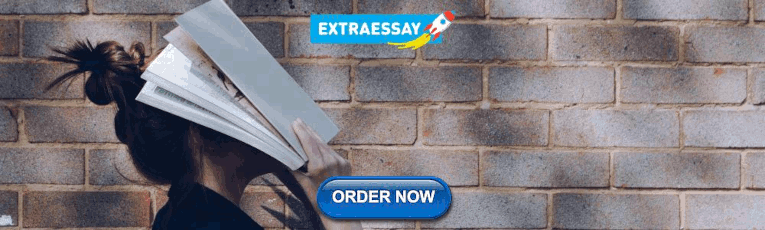
IMAGES
VIDEO
COMMENTS
Framework Analysis. Definition: Framework Analysis is a qualitative research method that involves organizing and analyzing data using a predefined analytical framework. The analytical framework is a set of predetermined themes or categories that are derived from the research questions or objectives. The framework provides a structured approach ...
An analytical framework is, the way I see it, a model that helps explain how a certain type of analysis will be conducted. For example, in this paper, Franks and Cleaver develop an analytical framework that includes scholarship on poverty measurement to help us understand how water governance and poverty are interrelated .
Real-world examples of analytical framework application. Tips for choosing the right framework for your needs . ... This could range from internal performance metrics to external market research. Apply the Framework: Methodically fill in the sections of the chosen framework with the collected data. This process often reveals insights that were ...
A theoretical framework guides the research process like a roadmap for the study, so you need to get this right. Theoretical framework 1,2 is the structure that supports and describes a theory. A theory is a set of interrelated concepts and definitions that present a systematic view of phenomena by describing the relationship among the variables for explaining these phenomena.
For example, it can look into why the value of the Japanese Yen has decreased. This is so that an analytical study can consider "how" and "why" questions. Another example is that someone might conduct analytical research to identify a study's gap. It presents a fresh perspective on your data.
The Framework Method has been developed and used successfully in research for over 25 years, and has recently become a popular analysis method in qualitative health research. The issue of how to assess quality in qualitative research has been highly debated [ 20 , 34 - 40 ], but ensuring rigour and transparency in analysis is a vital component.
Theoretical Framework Example for a Thesis or Dissertation. Published on October 14, 2015 by Sarah Vinz . Revised on July 18, 2023 by Tegan George. Your theoretical framework defines the key concepts in your research, suggests relationships between them, and discusses relevant theories based on your literature review.
A theoretical framework is a foundational review of existing theories that serves as a roadmap for developing the arguments you will use in your own work. Theories are developed by researchers to explain phenomena, draw connections, and make predictions. In a theoretical framework, you explain the existing theories that support your research ...
The theoretical framework adds context around the theory itself based on how scholars had previously tested the theory in relation their overall research design [i.e., purpose of the study, methods of collecting data or information, methods of analysis, the time frame in which information is collected, study setting, and the methodological ...
So you have read tons of papers for your lit review. Now what? Watch this video to learn how to structure this information effectively. It looks at natural a...
ANALYTICAL FRAMEWORK AND RESEARCH METHODOLOGY 3.1 Introduction and objectives In the previous chapter, the research problem addressed in this study was embedded in a larger theoretical and conceptual framework. The issues reviewed helped to identify the research already ... giving examples to support the statement, then asking candidates
Framework Analysis is more prescriptive than other research methodologies as it provides a more step-by-step approach and is primarily used for applied research. The feature that differentiates framework analysis from many other qualitative analysis techniques is its use of a matrix output that enables researchers to systematically analyze data ...
Example from our own empirical research. ... To exemplify the use of the analytical framework suggested, we focus on respondents' perception and anticipation of task share in childcare before first-time parenthood, and any anticipation of changes over time as the child grows up. For illustrative purposes, we selected two couples with similar ...
Developing a conceptual framework in research. Step 1: Choose your research question. Step 2: Select your independent and dependent variables. Step 3: Visualize your cause-and-effect relationship. Step 4: Identify other influencing variables. Frequently asked questions about conceptual models.
Thematic analysis is a research method used to identify and interpret patterns or themes in a data set; it often leads to new insights and understanding (Boyatzis, 1998; Elliott, 2018; Thomas, 2006).However, it is critical that researchers avoid letting their own preconceptions interfere with the identification of key themes (Morse & Mitcham, 2002; Patton, 2015).
research emerged in the past century as a useful framework for social science research, but its history has not been the story of steady, sustained progress along one path. Denzin and Lincoln (1994, 2005) divide the history of 20th-century qualitative social science research, broadly defined, into eight moments.
The Framework Method is becoming an increasingly popular approach to the management and analysis of qualitative data in health research. However, there is confusion about its potential application and limitations. The article discusses when it is appropriate to adopt the Framework Method and explains the procedure for using it in multi-disciplinary health research teams, or those that involve ...
An analytical study is one in which action will be taken on a cause system to improve the future performance of the system of interest. The aim of an enumerative study is estimation, while an analytical study focuses on prediction. Because of the temporal nature of improvement, the theory and methods for analytical studies are a critical ...
The framework method, outlined by Gale et al. (2013), is a highly structured approach to analyzing qualitative data that can be used either inductively or deductively and is not aligned with any ...
When to use thematic analysis. Thematic analysis is a good approach to research where you're trying to find out something about people's views, opinions, knowledge, experiences or values from a set of qualitative data - for example, interview transcripts, social media profiles, or survey responses. Some types of research questions you might use thematic analysis to answer:
Chapter 2. -. Analytical Framework, Study Design, and Methodology. As most of the chapters in this book are based on research that has adopted a clear analytical framework and scientific approach for assessing watershed impacts, it is necessary to discuss the aims of the research and the adopted framework and approach before we go into these ...
Abstract. This chapter merges the conceptual and theoretical insights that inform this book in order to operationalize the analytical framework, which is applied to explain how the institutional idiosyncrasies of innovation communities affect entrepreneurship in the field of desktop 3D printing. Additionally, I outline my methodological ...
This study investigates the influence of operant resources on individual task performance within the framework of knowledge management. Building on existing research, a quantitative model was developed and tested using data from 296 Vietnamese managers and senior employees. Data analysis employed SPSS 21 and AMOS 24 software.