
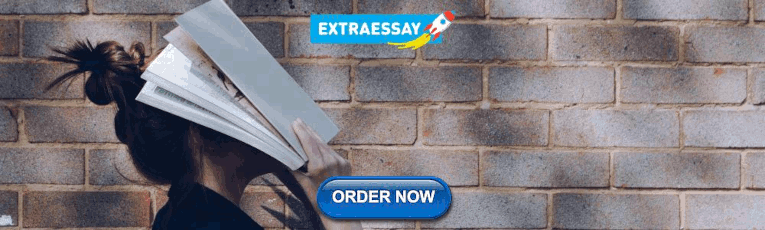
Qualitative Data Analysis Methods 101:
The “big 6” methods + examples.
By: Kerryn Warren (PhD) | Reviewed By: Eunice Rautenbach (D.Tech) | May 2020 (Updated April 2023)
Qualitative data analysis methods. Wow, that’s a mouthful.
If you’re new to the world of research, qualitative data analysis can look rather intimidating. So much bulky terminology and so many abstract, fluffy concepts. It certainly can be a minefield!
Don’t worry – in this post, we’ll unpack the most popular analysis methods , one at a time, so that you can approach your analysis with confidence and competence – whether that’s for a dissertation, thesis or really any kind of research project.
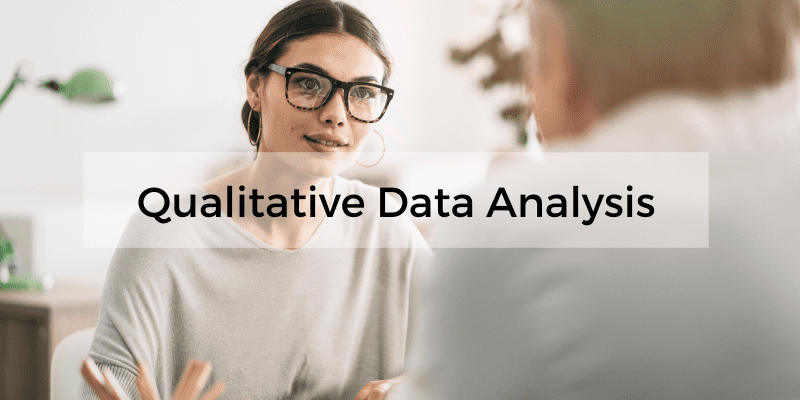
What (exactly) is qualitative data analysis?
To understand qualitative data analysis, we need to first understand qualitative data – so let’s step back and ask the question, “what exactly is qualitative data?”.
Qualitative data refers to pretty much any data that’s “not numbers” . In other words, it’s not the stuff you measure using a fixed scale or complex equipment, nor do you analyse it using complex statistics or mathematics.
So, if it’s not numbers, what is it?
Words, you guessed? Well… sometimes , yes. Qualitative data can, and often does, take the form of interview transcripts, documents and open-ended survey responses – but it can also involve the interpretation of images and videos. In other words, qualitative isn’t just limited to text-based data.
So, how’s that different from quantitative data, you ask?
Simply put, qualitative research focuses on words, descriptions, concepts or ideas – while quantitative research focuses on numbers and statistics . Qualitative research investigates the “softer side” of things to explore and describe , while quantitative research focuses on the “hard numbers”, to measure differences between variables and the relationships between them. If you’re keen to learn more about the differences between qual and quant, we’ve got a detailed post over here .
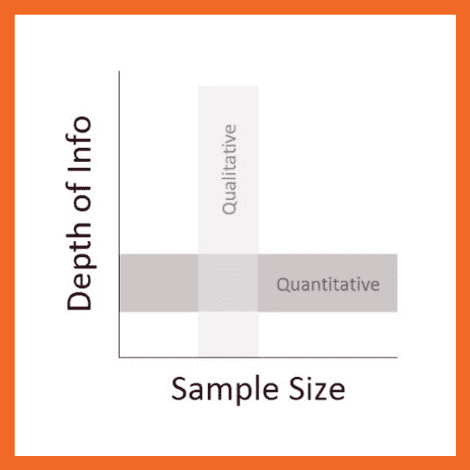
So, qualitative analysis is easier than quantitative, right?
Not quite. In many ways, qualitative data can be challenging and time-consuming to analyse and interpret. At the end of your data collection phase (which itself takes a lot of time), you’ll likely have many pages of text-based data or hours upon hours of audio to work through. You might also have subtle nuances of interactions or discussions that have danced around in your mind, or that you scribbled down in messy field notes. All of this needs to work its way into your analysis.
Making sense of all of this is no small task and you shouldn’t underestimate it. Long story short – qualitative analysis can be a lot of work! Of course, quantitative analysis is no piece of cake either, but it’s important to recognise that qualitative analysis still requires a significant investment in terms of time and effort.
Need a helping hand?
In this post, we’ll explore qualitative data analysis by looking at some of the most common analysis methods we encounter. We’re not going to cover every possible qualitative method and we’re not going to go into heavy detail – we’re just going to give you the big picture. That said, we will of course includes links to loads of extra resources so that you can learn more about whichever analysis method interests you.
Without further delay, let’s get into it.
The “Big 6” Qualitative Analysis Methods
There are many different types of qualitative data analysis, all of which serve different purposes and have unique strengths and weaknesses . We’ll start by outlining the analysis methods and then we’ll dive into the details for each.
The 6 most popular methods (or at least the ones we see at Grad Coach) are:
- Content analysis
- Narrative analysis
- Discourse analysis
- Thematic analysis
- Grounded theory (GT)
- Interpretive phenomenological analysis (IPA)
Let’s take a look at each of them…
QDA Method #1: Qualitative Content Analysis
Content analysis is possibly the most common and straightforward QDA method. At the simplest level, content analysis is used to evaluate patterns within a piece of content (for example, words, phrases or images) or across multiple pieces of content or sources of communication. For example, a collection of newspaper articles or political speeches.
With content analysis, you could, for instance, identify the frequency with which an idea is shared or spoken about – like the number of times a Kardashian is mentioned on Twitter. Or you could identify patterns of deeper underlying interpretations – for instance, by identifying phrases or words in tourist pamphlets that highlight India as an ancient country.
Because content analysis can be used in such a wide variety of ways, it’s important to go into your analysis with a very specific question and goal, or you’ll get lost in the fog. With content analysis, you’ll group large amounts of text into codes , summarise these into categories, and possibly even tabulate the data to calculate the frequency of certain concepts or variables. Because of this, content analysis provides a small splash of quantitative thinking within a qualitative method.
Naturally, while content analysis is widely useful, it’s not without its drawbacks . One of the main issues with content analysis is that it can be very time-consuming , as it requires lots of reading and re-reading of the texts. Also, because of its multidimensional focus on both qualitative and quantitative aspects, it is sometimes accused of losing important nuances in communication.
Content analysis also tends to concentrate on a very specific timeline and doesn’t take into account what happened before or after that timeline. This isn’t necessarily a bad thing though – just something to be aware of. So, keep these factors in mind if you’re considering content analysis. Every analysis method has its limitations , so don’t be put off by these – just be aware of them ! If you’re interested in learning more about content analysis, the video below provides a good starting point.
QDA Method #2: Narrative Analysis
As the name suggests, narrative analysis is all about listening to people telling stories and analysing what that means . Since stories serve a functional purpose of helping us make sense of the world, we can gain insights into the ways that people deal with and make sense of reality by analysing their stories and the ways they’re told.
You could, for example, use narrative analysis to explore whether how something is being said is important. For instance, the narrative of a prisoner trying to justify their crime could provide insight into their view of the world and the justice system. Similarly, analysing the ways entrepreneurs talk about the struggles in their careers or cancer patients telling stories of hope could provide powerful insights into their mindsets and perspectives . Simply put, narrative analysis is about paying attention to the stories that people tell – and more importantly, the way they tell them.
Of course, the narrative approach has its weaknesses , too. Sample sizes are generally quite small due to the time-consuming process of capturing narratives. Because of this, along with the multitude of social and lifestyle factors which can influence a subject, narrative analysis can be quite difficult to reproduce in subsequent research. This means that it’s difficult to test the findings of some of this research.
Similarly, researcher bias can have a strong influence on the results here, so you need to be particularly careful about the potential biases you can bring into your analysis when using this method. Nevertheless, narrative analysis is still a very useful qualitative analysis method – just keep these limitations in mind and be careful not to draw broad conclusions . If you’re keen to learn more about narrative analysis, the video below provides a great introduction to this qualitative analysis method.
QDA Method #3: Discourse Analysis
Discourse is simply a fancy word for written or spoken language or debate . So, discourse analysis is all about analysing language within its social context. In other words, analysing language – such as a conversation, a speech, etc – within the culture and society it takes place. For example, you could analyse how a janitor speaks to a CEO, or how politicians speak about terrorism.
To truly understand these conversations or speeches, the culture and history of those involved in the communication are important factors to consider. For example, a janitor might speak more casually with a CEO in a company that emphasises equality among workers. Similarly, a politician might speak more about terrorism if there was a recent terrorist incident in the country.
So, as you can see, by using discourse analysis, you can identify how culture , history or power dynamics (to name a few) have an effect on the way concepts are spoken about. So, if your research aims and objectives involve understanding culture or power dynamics, discourse analysis can be a powerful method.
Because there are many social influences in terms of how we speak to each other, the potential use of discourse analysis is vast . Of course, this also means it’s important to have a very specific research question (or questions) in mind when analysing your data and looking for patterns and themes, or you might land up going down a winding rabbit hole.
Discourse analysis can also be very time-consuming as you need to sample the data to the point of saturation – in other words, until no new information and insights emerge. But this is, of course, part of what makes discourse analysis such a powerful technique. So, keep these factors in mind when considering this QDA method. Again, if you’re keen to learn more, the video below presents a good starting point.
QDA Method #4: Thematic Analysis
Thematic analysis looks at patterns of meaning in a data set – for example, a set of interviews or focus group transcripts. But what exactly does that… mean? Well, a thematic analysis takes bodies of data (which are often quite large) and groups them according to similarities – in other words, themes . These themes help us make sense of the content and derive meaning from it.
Let’s take a look at an example.
With thematic analysis, you could analyse 100 online reviews of a popular sushi restaurant to find out what patrons think about the place. By reviewing the data, you would then identify the themes that crop up repeatedly within the data – for example, “fresh ingredients” or “friendly wait staff”.
So, as you can see, thematic analysis can be pretty useful for finding out about people’s experiences , views, and opinions . Therefore, if your research aims and objectives involve understanding people’s experience or view of something, thematic analysis can be a great choice.
Since thematic analysis is a bit of an exploratory process, it’s not unusual for your research questions to develop , or even change as you progress through the analysis. While this is somewhat natural in exploratory research, it can also be seen as a disadvantage as it means that data needs to be re-reviewed each time a research question is adjusted. In other words, thematic analysis can be quite time-consuming – but for a good reason. So, keep this in mind if you choose to use thematic analysis for your project and budget extra time for unexpected adjustments.
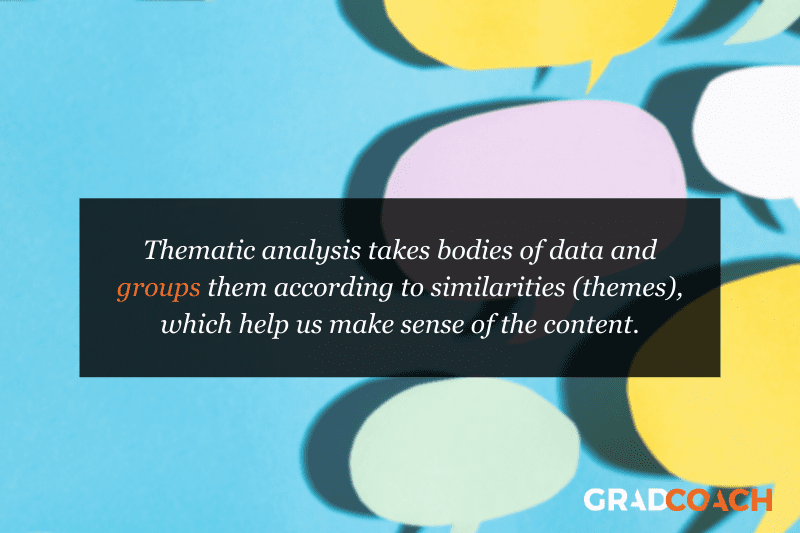
QDA Method #5: Grounded theory (GT)
Grounded theory is a powerful qualitative analysis method where the intention is to create a new theory (or theories) using the data at hand, through a series of “ tests ” and “ revisions ”. Strictly speaking, GT is more a research design type than an analysis method, but we’ve included it here as it’s often referred to as a method.
What’s most important with grounded theory is that you go into the analysis with an open mind and let the data speak for itself – rather than dragging existing hypotheses or theories into your analysis. In other words, your analysis must develop from the ground up (hence the name).
Let’s look at an example of GT in action.
Assume you’re interested in developing a theory about what factors influence students to watch a YouTube video about qualitative analysis. Using Grounded theory , you’d start with this general overarching question about the given population (i.e., graduate students). First, you’d approach a small sample – for example, five graduate students in a department at a university. Ideally, this sample would be reasonably representative of the broader population. You’d interview these students to identify what factors lead them to watch the video.
After analysing the interview data, a general pattern could emerge. For example, you might notice that graduate students are more likely to read a post about qualitative methods if they are just starting on their dissertation journey, or if they have an upcoming test about research methods.
From here, you’ll look for another small sample – for example, five more graduate students in a different department – and see whether this pattern holds true for them. If not, you’ll look for commonalities and adapt your theory accordingly. As this process continues, the theory would develop . As we mentioned earlier, what’s important with grounded theory is that the theory develops from the data – not from some preconceived idea.
So, what are the drawbacks of grounded theory? Well, some argue that there’s a tricky circularity to grounded theory. For it to work, in principle, you should know as little as possible regarding the research question and population, so that you reduce the bias in your interpretation. However, in many circumstances, it’s also thought to be unwise to approach a research question without knowledge of the current literature . In other words, it’s a bit of a “chicken or the egg” situation.
Regardless, grounded theory remains a popular (and powerful) option. Naturally, it’s a very useful method when you’re researching a topic that is completely new or has very little existing research about it, as it allows you to start from scratch and work your way from the ground up .
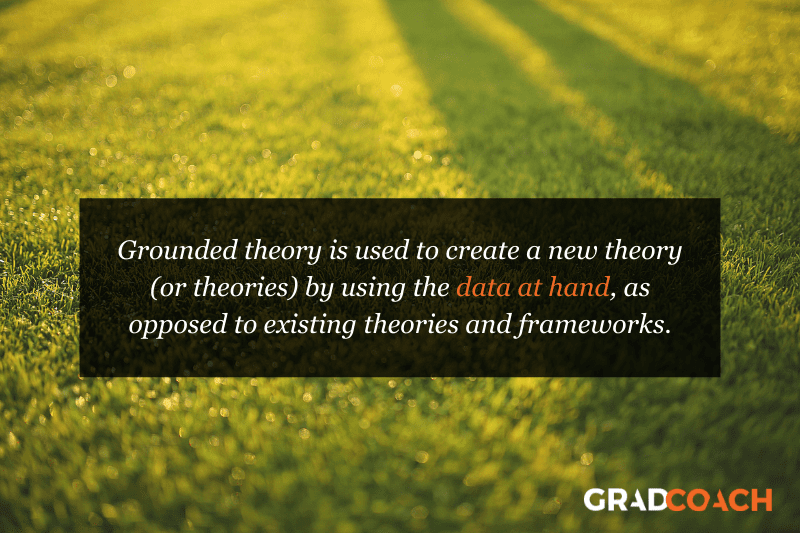
QDA Method #6: Interpretive Phenomenological Analysis (IPA)
Interpretive. Phenomenological. Analysis. IPA . Try saying that three times fast…
Let’s just stick with IPA, okay?
IPA is designed to help you understand the personal experiences of a subject (for example, a person or group of people) concerning a major life event, an experience or a situation . This event or experience is the “phenomenon” that makes up the “P” in IPA. Such phenomena may range from relatively common events – such as motherhood, or being involved in a car accident – to those which are extremely rare – for example, someone’s personal experience in a refugee camp. So, IPA is a great choice if your research involves analysing people’s personal experiences of something that happened to them.
It’s important to remember that IPA is subject – centred . In other words, it’s focused on the experiencer . This means that, while you’ll likely use a coding system to identify commonalities, it’s important not to lose the depth of experience or meaning by trying to reduce everything to codes. Also, keep in mind that since your sample size will generally be very small with IPA, you often won’t be able to draw broad conclusions about the generalisability of your findings. But that’s okay as long as it aligns with your research aims and objectives.
Another thing to be aware of with IPA is personal bias . While researcher bias can creep into all forms of research, self-awareness is critically important with IPA, as it can have a major impact on the results. For example, a researcher who was a victim of a crime himself could insert his own feelings of frustration and anger into the way he interprets the experience of someone who was kidnapped. So, if you’re going to undertake IPA, you need to be very self-aware or you could muddy the analysis.
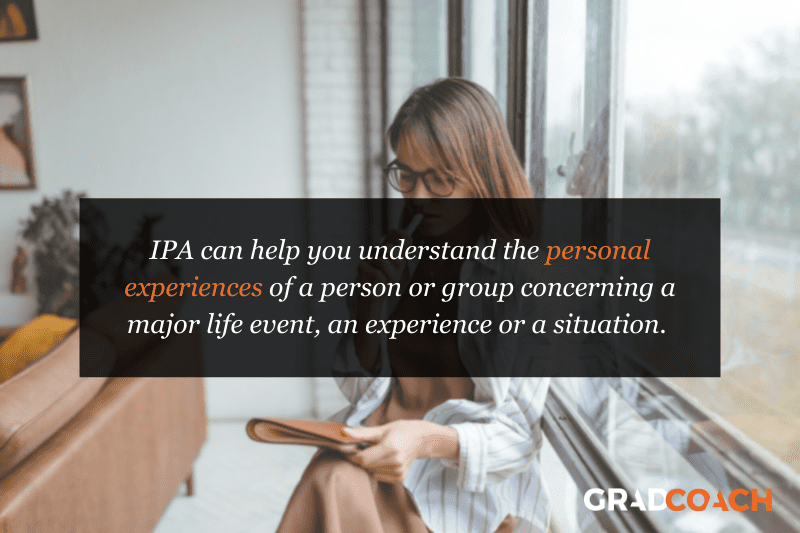
How to choose the right analysis method
In light of all of the qualitative analysis methods we’ve covered so far, you’re probably asking yourself the question, “ How do I choose the right one? ”
Much like all the other methodological decisions you’ll need to make, selecting the right qualitative analysis method largely depends on your research aims, objectives and questions . In other words, the best tool for the job depends on what you’re trying to build. For example:
- Perhaps your research aims to analyse the use of words and what they reveal about the intention of the storyteller and the cultural context of the time.
- Perhaps your research aims to develop an understanding of the unique personal experiences of people that have experienced a certain event, or
- Perhaps your research aims to develop insight regarding the influence of a certain culture on its members.
As you can probably see, each of these research aims are distinctly different , and therefore different analysis methods would be suitable for each one. For example, narrative analysis would likely be a good option for the first aim, while grounded theory wouldn’t be as relevant.
It’s also important to remember that each method has its own set of strengths, weaknesses and general limitations. No single analysis method is perfect . So, depending on the nature of your research, it may make sense to adopt more than one method (this is called triangulation ). Keep in mind though that this will of course be quite time-consuming.
As we’ve seen, all of the qualitative analysis methods we’ve discussed make use of coding and theme-generating techniques, but the intent and approach of each analysis method differ quite substantially. So, it’s very important to come into your research with a clear intention before you decide which analysis method (or methods) to use.
Start by reviewing your research aims , objectives and research questions to assess what exactly you’re trying to find out – then select a qualitative analysis method that fits. Never pick a method just because you like it or have experience using it – your analysis method (or methods) must align with your broader research aims and objectives.
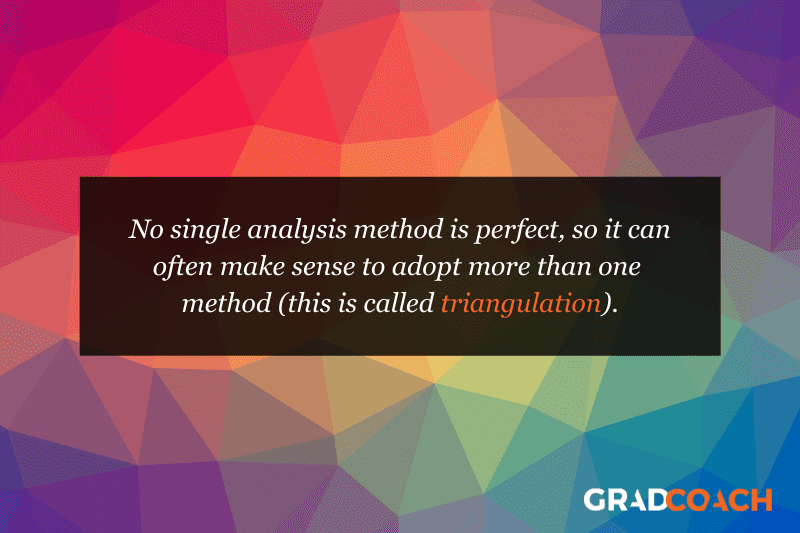
Let’s recap on QDA methods…
In this post, we looked at six popular qualitative data analysis methods:
- First, we looked at content analysis , a straightforward method that blends a little bit of quant into a primarily qualitative analysis.
- Then we looked at narrative analysis , which is about analysing how stories are told.
- Next up was discourse analysis – which is about analysing conversations and interactions.
- Then we moved on to thematic analysis – which is about identifying themes and patterns.
- From there, we went south with grounded theory – which is about starting from scratch with a specific question and using the data alone to build a theory in response to that question.
- And finally, we looked at IPA – which is about understanding people’s unique experiences of a phenomenon.
Of course, these aren’t the only options when it comes to qualitative data analysis, but they’re a great starting point if you’re dipping your toes into qualitative research for the first time.
If you’re still feeling a bit confused, consider our private coaching service , where we hold your hand through the research process to help you develop your best work.
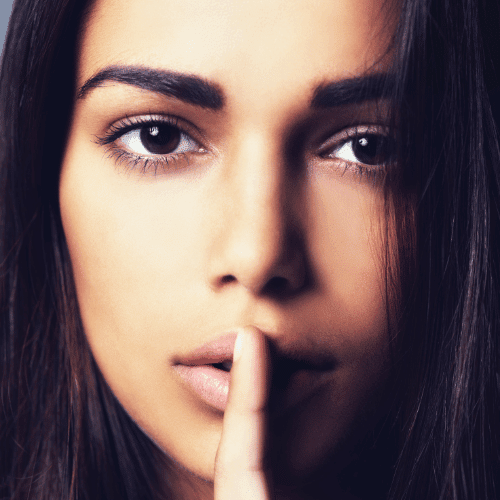
Psst... there’s more!
This post was based on one of our popular Research Bootcamps . If you're working on a research project, you'll definitely want to check this out ...
You Might Also Like:
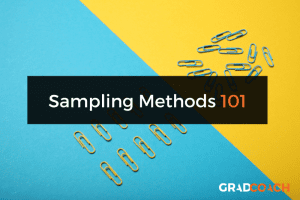
84 Comments
This has been very helpful. Thank you.
Thank you madam,
Thank you so much for this information
I wonder it so clear for understand and good for me. can I ask additional query?
Very insightful and useful
Good work done with clear explanations. Thank you.
Thanks so much for the write-up, it’s really good.
Thanks madam . It is very important .
thank you very good
This has been very well explained in simple language . It is useful even for a new researcher.
Great to hear that. Good luck with your qualitative data analysis, Pramod!
This is very useful information. And it was very a clear language structured presentation. Thanks a lot.
Thank you so much.
very informative sequential presentation
Precise explanation of method.
Hi, may we use 2 data analysis methods in our qualitative research?
Thanks for your comment. Most commonly, one would use one type of analysis method, but it depends on your research aims and objectives.
You explained it in very simple language, everyone can understand it. Thanks so much.
Thank you very much, this is very helpful. It has been explained in a very simple manner that even a layman understands
Thank nicely explained can I ask is Qualitative content analysis the same as thematic analysis?
Thanks for your comment. No, QCA and thematic are two different types of analysis. This article might help clarify – https://onlinelibrary.wiley.com/doi/10.1111/nhs.12048
This is my first time to come across a well explained data analysis. so helpful.
I have thoroughly enjoyed your explanation of the six qualitative analysis methods. This is very helpful. Thank you!
Thank you very much, this is well explained and useful
i need a citation of your book.
Thanks a lot , remarkable indeed, enlighting to the best
Hi Derek, What other theories/methods would you recommend when the data is a whole speech?
Keep writing useful artikel.
It is important concept about QDA and also the way to express is easily understandable, so thanks for all.
Thank you, this is well explained and very useful.
Very helpful .Thanks.
Hi there! Very well explained. Simple but very useful style of writing. Please provide the citation of the text. warm regards
The session was very helpful and insightful. Thank you
This was very helpful and insightful. Easy to read and understand
As a professional academic writer, this has been so informative and educative. Keep up the good work Grad Coach you are unmatched with quality content for sure.
Keep up the good work Grad Coach you are unmatched with quality content for sure.
Its Great and help me the most. A Million Thanks you Dr.
It is a very nice work
Very insightful. Please, which of this approach could be used for a research that one is trying to elicit students’ misconceptions in a particular concept ?
This is Amazing and well explained, thanks
great overview
What do we call a research data analysis method that one use to advise or determining the best accounting tool or techniques that should be adopted in a company.
Informative video, explained in a clear and simple way. Kudos
Waoo! I have chosen method wrong for my data analysis. But I can revise my work according to this guide. Thank you so much for this helpful lecture.
This has been very helpful. It gave me a good view of my research objectives and how to choose the best method. Thematic analysis it is.
Very helpful indeed. Thanku so much for the insight.
This was incredibly helpful.
Very helpful.
very educative
Nicely written especially for novice academic researchers like me! Thank you.
choosing a right method for a paper is always a hard job for a student, this is a useful information, but it would be more useful personally for me, if the author provide me with a little bit more information about the data analysis techniques in type of explanatory research. Can we use qualitative content analysis technique for explanatory research ? or what is the suitable data analysis method for explanatory research in social studies?
that was very helpful for me. because these details are so important to my research. thank you very much
I learnt a lot. Thank you
Relevant and Informative, thanks !
Well-planned and organized, thanks much! 🙂
I have reviewed qualitative data analysis in a simplest way possible. The content will highly be useful for developing my book on qualitative data analysis methods. Cheers!
Clear explanation on qualitative and how about Case study
This was helpful. Thank you
This was really of great assistance, it was just the right information needed. Explanation very clear and follow.
Wow, Thanks for making my life easy
This was helpful thanks .
Very helpful…. clear and written in an easily understandable manner. Thank you.
This was so helpful as it was easy to understand. I’m a new to research thank you so much.
so educative…. but Ijust want to know which method is coding of the qualitative or tallying done?
Thank you for the great content, I have learnt a lot. So helpful
precise and clear presentation with simple language and thank you for that.
very informative content, thank you.
You guys are amazing on YouTube on this platform. Your teachings are great, educative, and informative. kudos!
Brilliant Delivery. You made a complex subject seem so easy. Well done.
Beautifully explained.
Thanks a lot
Is there a video the captures the practical process of coding using automated applications?
Thanks for the comment. We don’t recommend using automated applications for coding, as they are not sufficiently accurate in our experience.
content analysis can be qualitative research?
THANK YOU VERY MUCH.
Thank you very much for such a wonderful content
do you have any material on Data collection
What a powerful explanation of the QDA methods. Thank you.
Great explanation both written and Video. i have been using of it on a day to day working of my thesis project in accounting and finance. Thank you very much for your support.
very helpful, thank you so much
Submit a Comment Cancel reply
Your email address will not be published. Required fields are marked *
Save my name, email, and website in this browser for the next time I comment.
- Print Friendly
- AI & NLP
- Churn & Loyalty
- Customer Experience
- Customer Journeys
- Customer Metrics
- Feedback Analysis
- Product Experience
- Product Updates
- Sentiment Analysis
- Surveys & Feedback Collection
- Try Thematic
Welcome to the community
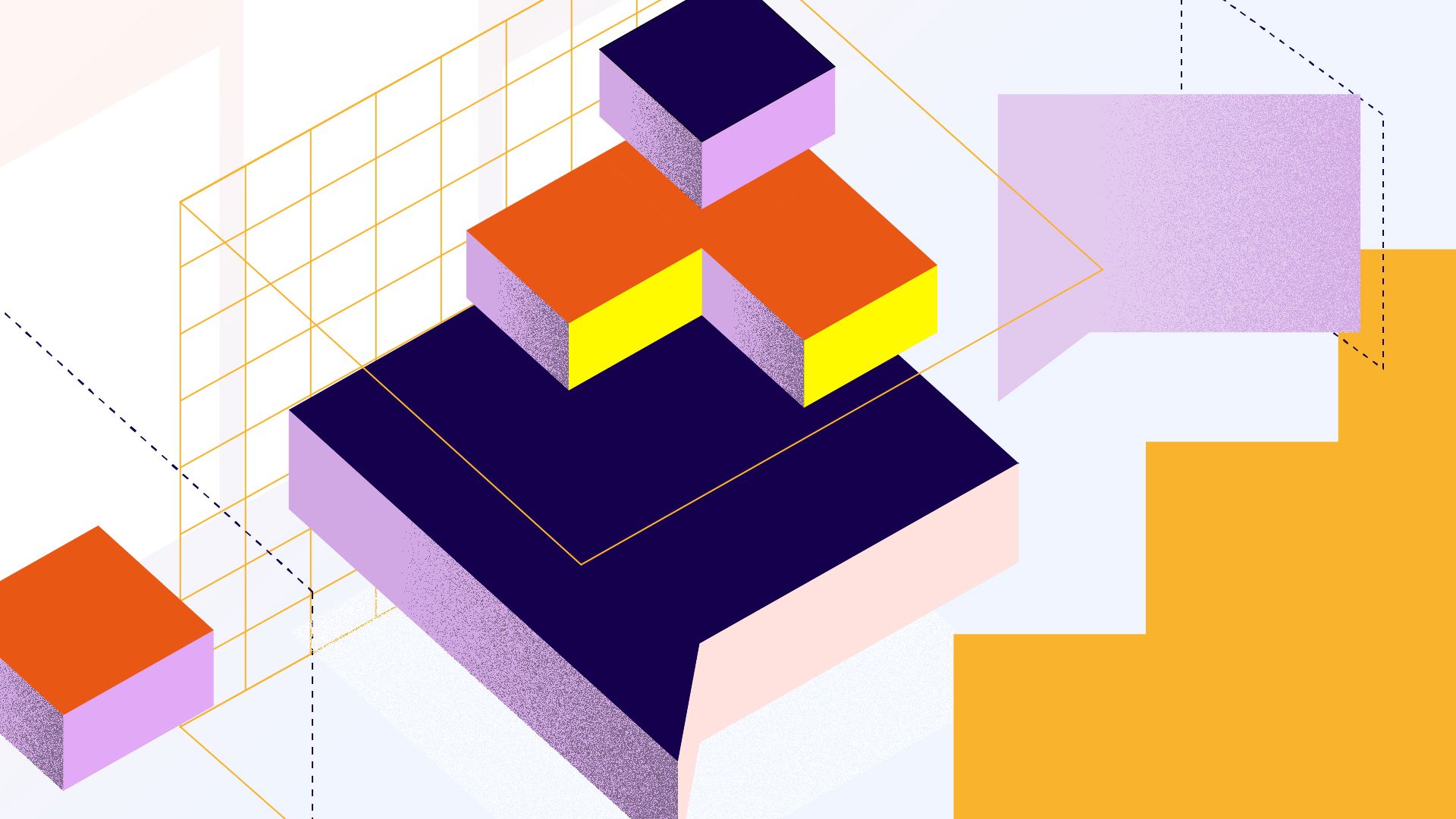
Qualitative Data Analysis: Step-by-Step Guide (Manual vs. Automatic)
When we conduct qualitative methods of research, need to explain changes in metrics or understand people's opinions, we always turn to qualitative data. Qualitative data is typically generated through:
- Interview transcripts
- Surveys with open-ended questions
- Contact center transcripts
- Texts and documents
- Audio and video recordings
- Observational notes
Compared to quantitative data, which captures structured information, qualitative data is unstructured and has more depth. It can answer our questions, can help formulate hypotheses and build understanding.
It's important to understand the differences between quantitative data & qualitative data . But unfortunately, analyzing qualitative data is difficult. While tools like Excel, Tableau and PowerBI crunch and visualize quantitative data with ease, there are a limited number of mainstream tools for analyzing qualitative data . The majority of qualitative data analysis still happens manually.
That said, there are two new trends that are changing this. First, there are advances in natural language processing (NLP) which is focused on understanding human language. Second, there is an explosion of user-friendly software designed for both researchers and businesses. Both help automate the qualitative data analysis process.
In this post we want to teach you how to conduct a successful qualitative data analysis. There are two primary qualitative data analysis methods; manual & automatic. We will teach you how to conduct the analysis manually, and also, automatically using software solutions powered by NLP. We’ll guide you through the steps to conduct a manual analysis, and look at what is involved and the role technology can play in automating this process.
More businesses are switching to fully-automated analysis of qualitative customer data because it is cheaper, faster, and just as accurate. Primarily, businesses purchase subscriptions to feedback analytics platforms so that they can understand customer pain points and sentiment.
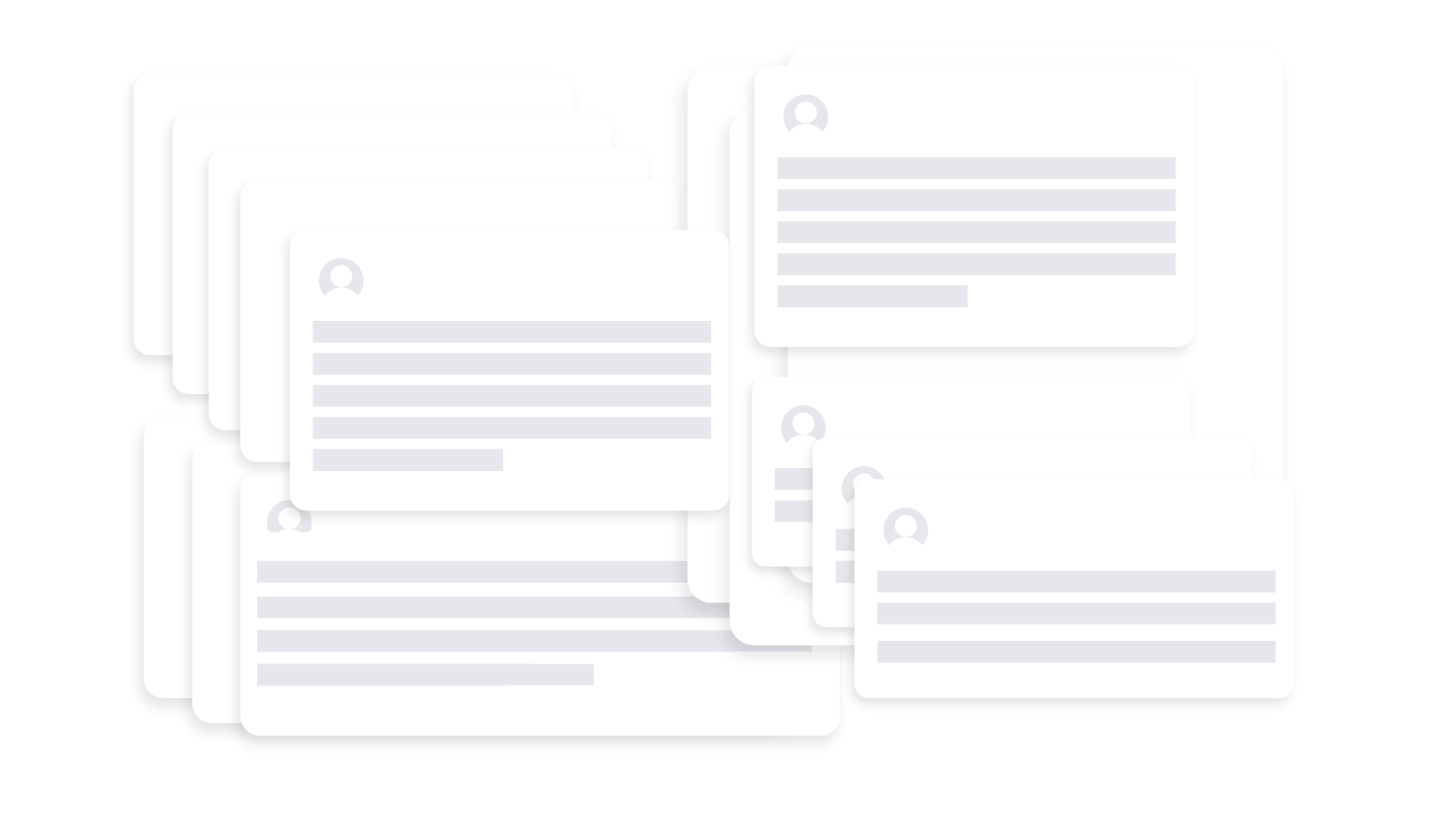
We’ll take you through 5 steps to conduct a successful qualitative data analysis. Within each step we will highlight the key difference between the manual, and automated approach of qualitative researchers. Here's an overview of the steps:
The 5 steps to doing qualitative data analysis
- Gathering and collecting your qualitative data
- Organizing and connecting into your qualitative data
- Coding your qualitative data
- Analyzing the qualitative data for insights
- Reporting on the insights derived from your analysis
What is Qualitative Data Analysis?
Qualitative data analysis is a process of gathering, structuring and interpreting qualitative data to understand what it represents.
Qualitative data is non-numerical and unstructured. Qualitative data generally refers to text, such as open-ended responses to survey questions or user interviews, but also includes audio, photos and video.
Businesses often perform qualitative data analysis on customer feedback. And within this context, qualitative data generally refers to verbatim text data collected from sources such as reviews, complaints, chat messages, support centre interactions, customer interviews, case notes or social media comments.
How is qualitative data analysis different from quantitative data analysis?
Understanding the differences between quantitative & qualitative data is important. When it comes to analyzing data, Qualitative Data Analysis serves a very different role to Quantitative Data Analysis. But what sets them apart?
Qualitative Data Analysis dives into the stories hidden in non-numerical data such as interviews, open-ended survey answers, or notes from observations. It uncovers the ‘whys’ and ‘hows’ giving a deep understanding of people’s experiences and emotions.
Quantitative Data Analysis on the other hand deals with numerical data, using statistics to measure differences, identify preferred options, and pinpoint root causes of issues. It steps back to address questions like "how many" or "what percentage" to offer broad insights we can apply to larger groups.
In short, Qualitative Data Analysis is like a microscope, helping us understand specific detail. Quantitative Data Analysis is like the telescope, giving us a broader perspective. Both are important, working together to decode data for different objectives.
Qualitative Data Analysis methods
Once all the data has been captured, there are a variety of analysis techniques available and the choice is determined by your specific research objectives and the kind of data you’ve gathered. Common qualitative data analysis methods include:
Content Analysis
This is a popular approach to qualitative data analysis. Other qualitative analysis techniques may fit within the broad scope of content analysis. Thematic analysis is a part of the content analysis. Content analysis is used to identify the patterns that emerge from text, by grouping content into words, concepts, and themes. Content analysis is useful to quantify the relationship between all of the grouped content. The Columbia School of Public Health has a detailed breakdown of content analysis .
Narrative Analysis
Narrative analysis focuses on the stories people tell and the language they use to make sense of them. It is particularly useful in qualitative research methods where customer stories are used to get a deep understanding of customers’ perspectives on a specific issue. A narrative analysis might enable us to summarize the outcomes of a focused case study.
Discourse Analysis
Discourse analysis is used to get a thorough understanding of the political, cultural and power dynamics that exist in specific situations. The focus of discourse analysis here is on the way people express themselves in different social contexts. Discourse analysis is commonly used by brand strategists who hope to understand why a group of people feel the way they do about a brand or product.
Thematic Analysis
Thematic analysis is used to deduce the meaning behind the words people use. This is accomplished by discovering repeating themes in text. These meaningful themes reveal key insights into data and can be quantified, particularly when paired with sentiment analysis . Often, the outcome of thematic analysis is a code frame that captures themes in terms of codes, also called categories. So the process of thematic analysis is also referred to as “coding”. A common use-case for thematic analysis in companies is analysis of customer feedback.
Grounded Theory
Grounded theory is a useful approach when little is known about a subject. Grounded theory starts by formulating a theory around a single data case. This means that the theory is “grounded”. Grounded theory analysis is based on actual data, and not entirely speculative. Then additional cases can be examined to see if they are relevant and can add to the original grounded theory.
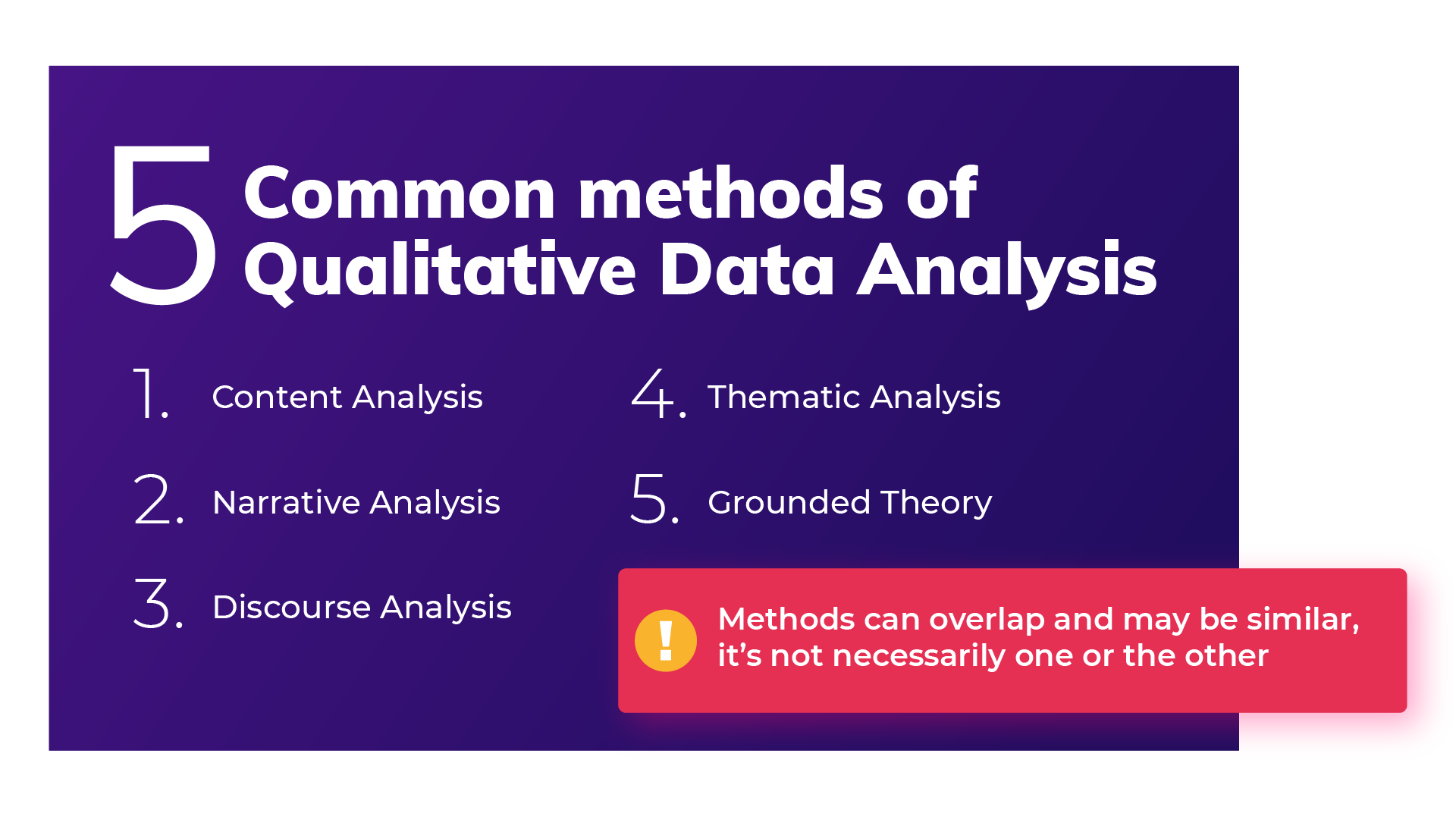
Challenges of Qualitative Data Analysis
While Qualitative Data Analysis offers rich insights, it comes with its challenges. Each unique QDA method has its unique hurdles. Let’s take a look at the challenges researchers and analysts might face, depending on the chosen method.
- Time and Effort (Narrative Analysis): Narrative analysis, which focuses on personal stories, demands patience. Sifting through lengthy narratives to find meaningful insights can be time-consuming, requires dedicated effort.
- Being Objective (Grounded Theory): Grounded theory, building theories from data, faces the challenges of personal biases. Staying objective while interpreting data is crucial, ensuring conclusions are rooted in the data itself.
- Complexity (Thematic Analysis): Thematic analysis involves identifying themes within data, a process that can be intricate. Categorizing and understanding themes can be complex, especially when each piece of data varies in context and structure. Thematic Analysis software can simplify this process.
- Generalizing Findings (Narrative Analysis): Narrative analysis, dealing with individual stories, makes drawing broad challenging. Extending findings from a single narrative to a broader context requires careful consideration.
- Managing Data (Thematic Analysis): Thematic analysis involves organizing and managing vast amounts of unstructured data, like interview transcripts. Managing this can be a hefty task, requiring effective data management strategies.
- Skill Level (Grounded Theory): Grounded theory demands specific skills to build theories from the ground up. Finding or training analysts with these skills poses a challenge, requiring investment in building expertise.
Benefits of qualitative data analysis
Qualitative Data Analysis (QDA) is like a versatile toolkit, offering a tailored approach to understanding your data. The benefits it offers are as diverse as the methods. Let’s explore why choosing the right method matters.
- Tailored Methods for Specific Needs: QDA isn't one-size-fits-all. Depending on your research objectives and the type of data at hand, different methods offer unique benefits. If you want emotive customer stories, narrative analysis paints a strong picture. When you want to explain a score, thematic analysis reveals insightful patterns
- Flexibility with Thematic Analysis: thematic analysis is like a chameleon in the toolkit of QDA. It adapts well to different types of data and research objectives, making it a top choice for any qualitative analysis.
- Deeper Understanding, Better Products: QDA helps you dive into people's thoughts and feelings. This deep understanding helps you build products and services that truly matches what people want, ensuring satisfied customers
- Finding the Unexpected: Qualitative data often reveals surprises that we miss in quantitative data. QDA offers us new ideas and perspectives, for insights we might otherwise miss.
- Building Effective Strategies: Insights from QDA are like strategic guides. They help businesses in crafting plans that match people’s desires.
- Creating Genuine Connections: Understanding people’s experiences lets businesses connect on a real level. This genuine connection helps build trust and loyalty, priceless for any business.
How to do Qualitative Data Analysis: 5 steps
Now we are going to show how you can do your own qualitative data analysis. We will guide you through this process step by step. As mentioned earlier, you will learn how to do qualitative data analysis manually , and also automatically using modern qualitative data and thematic analysis software.
To get best value from the analysis process and research process, it’s important to be super clear about the nature and scope of the question that’s being researched. This will help you select the research collection channels that are most likely to help you answer your question.
Depending on if you are a business looking to understand customer sentiment, or an academic surveying a school, your approach to qualitative data analysis will be unique.
Once you’re clear, there’s a sequence to follow. And, though there are differences in the manual and automatic approaches, the process steps are mostly the same.
The use case for our step-by-step guide is a company looking to collect data (customer feedback data), and analyze the customer feedback - in order to improve customer experience. By analyzing the customer feedback the company derives insights about their business and their customers. You can follow these same steps regardless of the nature of your research. Let’s get started.
Step 1: Gather your qualitative data and conduct research (Conduct qualitative research)
The first step of qualitative research is to do data collection. Put simply, data collection is gathering all of your data for analysis. A common situation is when qualitative data is spread across various sources.
Classic methods of gathering qualitative data
Most companies use traditional methods for gathering qualitative data: conducting interviews with research participants, running surveys, and running focus groups. This data is typically stored in documents, CRMs, databases and knowledge bases. It’s important to examine which data is available and needs to be included in your research project, based on its scope.
Using your existing qualitative feedback
As it becomes easier for customers to engage across a range of different channels, companies are gathering increasingly large amounts of both solicited and unsolicited qualitative feedback.
Most organizations have now invested in Voice of Customer programs , support ticketing systems, chatbot and support conversations, emails and even customer Slack chats.
These new channels provide companies with new ways of getting feedback, and also allow the collection of unstructured feedback data at scale.
The great thing about this data is that it contains a wealth of valubale insights and that it’s already there! When you have a new question about user behavior or your customers, you don’t need to create a new research study or set up a focus group. You can find most answers in the data you already have.
Typically, this data is stored in third-party solutions or a central database, but there are ways to export it or connect to a feedback analysis solution through integrations or an API.
Utilize untapped qualitative data channels
There are many online qualitative data sources you may not have considered. For example, you can find useful qualitative data in social media channels like Twitter or Facebook. Online forums, review sites, and online communities such as Discourse or Reddit also contain valuable data about your customers, or research questions.
If you are considering performing a qualitative benchmark analysis against competitors - the internet is your best friend. Gathering feedback in competitor reviews on sites like Trustpilot, G2, Capterra, Better Business Bureau or on app stores is a great way to perform a competitor benchmark analysis.
Customer feedback analysis software often has integrations into social media and review sites, or you could use a solution like DataMiner to scrape the reviews.
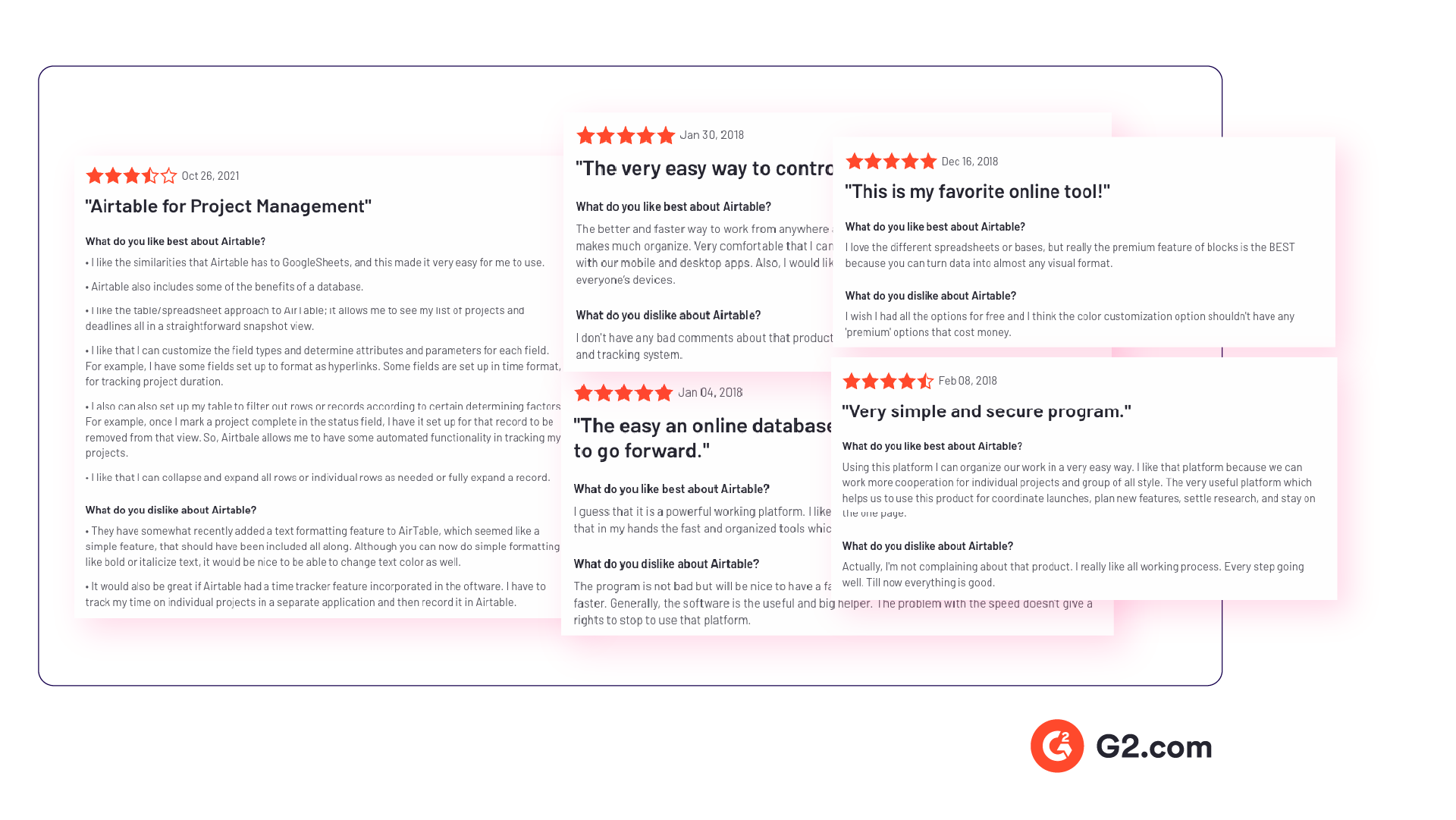
Step 2: Connect & organize all your qualitative data
Now you all have this qualitative data but there’s a problem, the data is unstructured. Before feedback can be analyzed and assigned any value, it needs to be organized in a single place. Why is this important? Consistency!
If all data is easily accessible in one place and analyzed in a consistent manner, you will have an easier time summarizing and making decisions based on this data.
The manual approach to organizing your data
The classic method of structuring qualitative data is to plot all the raw data you’ve gathered into a spreadsheet.
Typically, research and support teams would share large Excel sheets and different business units would make sense of the qualitative feedback data on their own. Each team collects and organizes the data in a way that best suits them, which means the feedback tends to be kept in separate silos.
An alternative and a more robust solution is to store feedback in a central database, like Snowflake or Amazon Redshift .
Keep in mind that when you organize your data in this way, you are often preparing it to be imported into another software. If you go the route of a database, you would need to use an API to push the feedback into a third-party software.
Computer-assisted qualitative data analysis software (CAQDAS)
Traditionally within the manual analysis approach (but not always), qualitative data is imported into CAQDAS software for coding.
In the early 2000s, CAQDAS software was popularised by developers such as ATLAS.ti, NVivo and MAXQDA and eagerly adopted by researchers to assist with the organizing and coding of data.
The benefits of using computer-assisted qualitative data analysis software:
- Assists in the organizing of your data
- Opens you up to exploring different interpretations of your data analysis
- Allows you to share your dataset easier and allows group collaboration (allows for secondary analysis)
However you still need to code the data, uncover the themes and do the analysis yourself. Therefore it is still a manual approach.
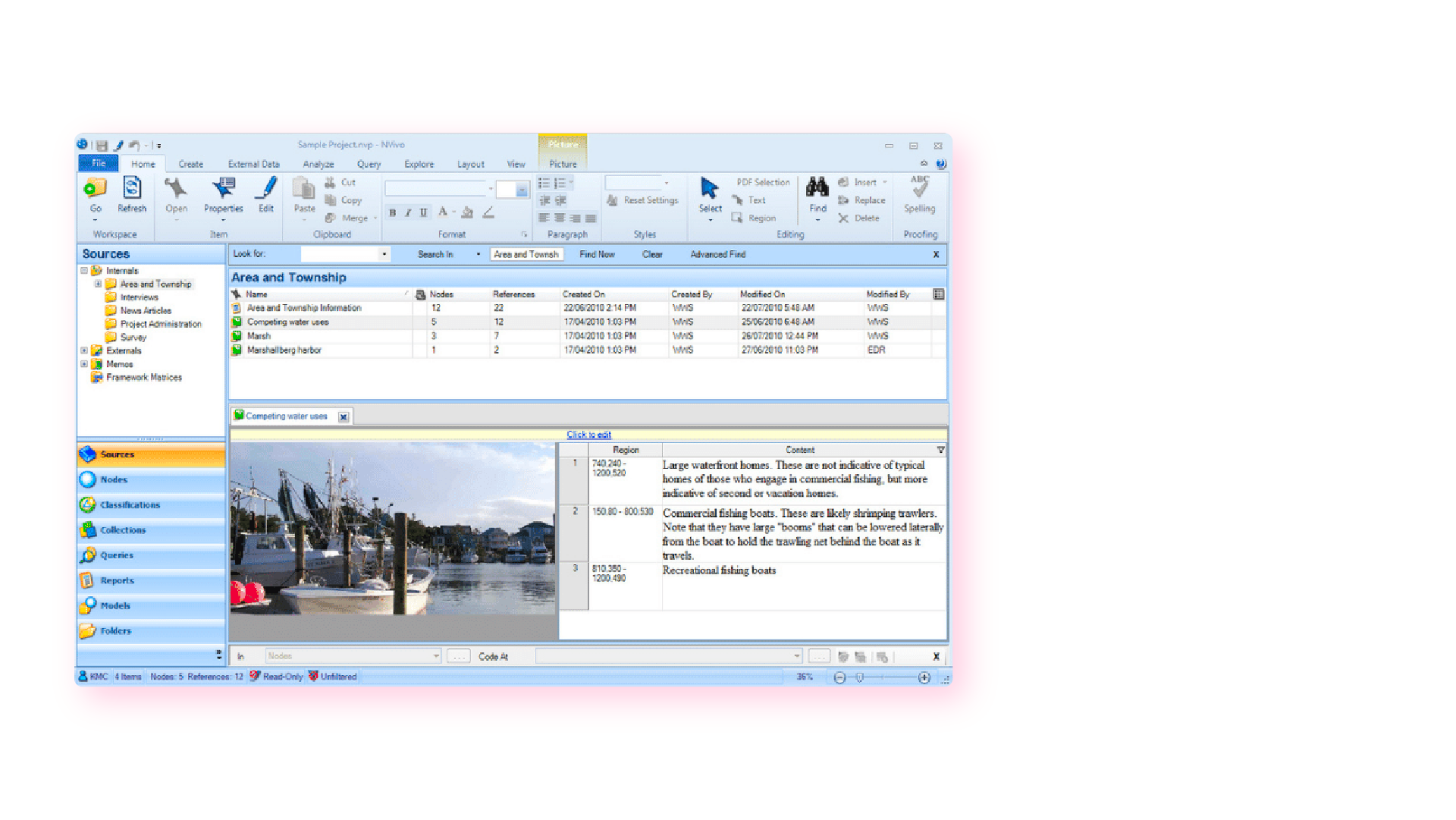
Organizing your qualitative data in a feedback repository
Another solution to organizing your qualitative data is to upload it into a feedback repository where it can be unified with your other data , and easily searchable and taggable. There are a number of software solutions that act as a central repository for your qualitative research data. Here are a couple solutions that you could investigate:
- Dovetail: Dovetail is a research repository with a focus on video and audio transcriptions. You can tag your transcriptions within the platform for theme analysis. You can also upload your other qualitative data such as research reports, survey responses, support conversations, and customer interviews. Dovetail acts as a single, searchable repository. And makes it easier to collaborate with other people around your qualitative research.
- EnjoyHQ: EnjoyHQ is another research repository with similar functionality to Dovetail. It boasts a more sophisticated search engine, but it has a higher starting subscription cost.
Organizing your qualitative data in a feedback analytics platform
If you have a lot of qualitative customer or employee feedback, from the likes of customer surveys or employee surveys, you will benefit from a feedback analytics platform. A feedback analytics platform is a software that automates the process of both sentiment analysis and thematic analysis . Companies use the integrations offered by these platforms to directly tap into their qualitative data sources (review sites, social media, survey responses, etc.). The data collected is then organized and analyzed consistently within the platform.
If you have data prepared in a spreadsheet, it can also be imported into feedback analytics platforms.
Once all this rich data has been organized within the feedback analytics platform, it is ready to be coded and themed, within the same platform. Thematic is a feedback analytics platform that offers one of the largest libraries of integrations with qualitative data sources.
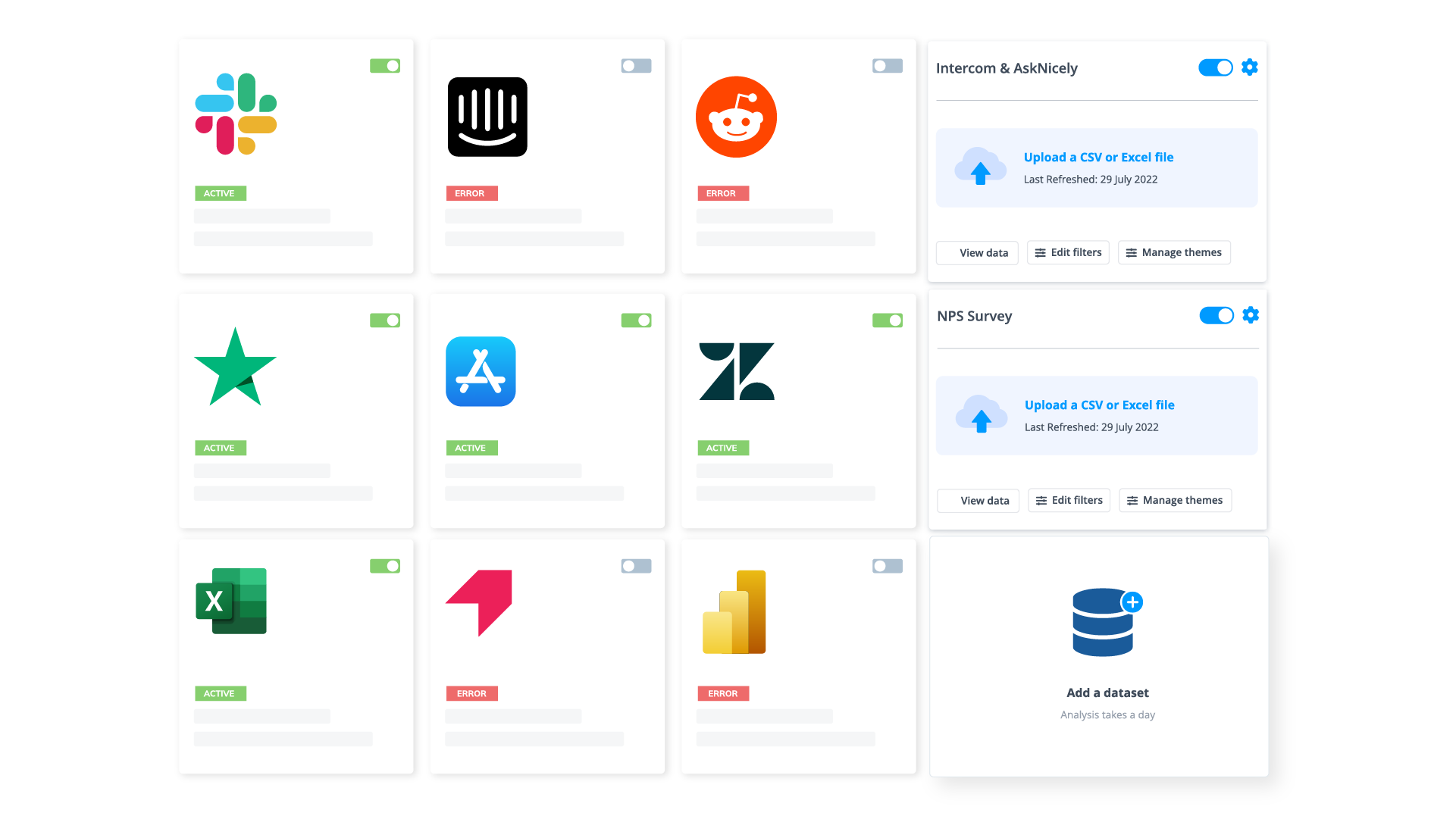
Step 3: Coding your qualitative data
Your feedback data is now organized in one place. Either within your spreadsheet, CAQDAS, feedback repository or within your feedback analytics platform. The next step is to code your feedback data so we can extract meaningful insights in the next step.
Coding is the process of labelling and organizing your data in such a way that you can then identify themes in the data, and the relationships between these themes.
To simplify the coding process, you will take small samples of your customer feedback data, come up with a set of codes, or categories capturing themes, and label each piece of feedback, systematically, for patterns and meaning. Then you will take a larger sample of data, revising and refining the codes for greater accuracy and consistency as you go.
If you choose to use a feedback analytics platform, much of this process will be automated and accomplished for you.
The terms to describe different categories of meaning (‘theme’, ‘code’, ‘tag’, ‘category’ etc) can be confusing as they are often used interchangeably. For clarity, this article will use the term ‘code’.
To code means to identify key words or phrases and assign them to a category of meaning. “I really hate the customer service of this computer software company” would be coded as “poor customer service”.
How to manually code your qualitative data
- Decide whether you will use deductive or inductive coding. Deductive coding is when you create a list of predefined codes, and then assign them to the qualitative data. Inductive coding is the opposite of this, you create codes based on the data itself. Codes arise directly from the data and you label them as you go. You need to weigh up the pros and cons of each coding method and select the most appropriate.
- Read through the feedback data to get a broad sense of what it reveals. Now it’s time to start assigning your first set of codes to statements and sections of text.
- Keep repeating step 2, adding new codes and revising the code description as often as necessary. Once it has all been coded, go through everything again, to be sure there are no inconsistencies and that nothing has been overlooked.
- Create a code frame to group your codes. The coding frame is the organizational structure of all your codes. And there are two commonly used types of coding frames, flat, or hierarchical. A hierarchical code frame will make it easier for you to derive insights from your analysis.
- Based on the number of times a particular code occurs, you can now see the common themes in your feedback data. This is insightful! If ‘bad customer service’ is a common code, it’s time to take action.
We have a detailed guide dedicated to manually coding your qualitative data .
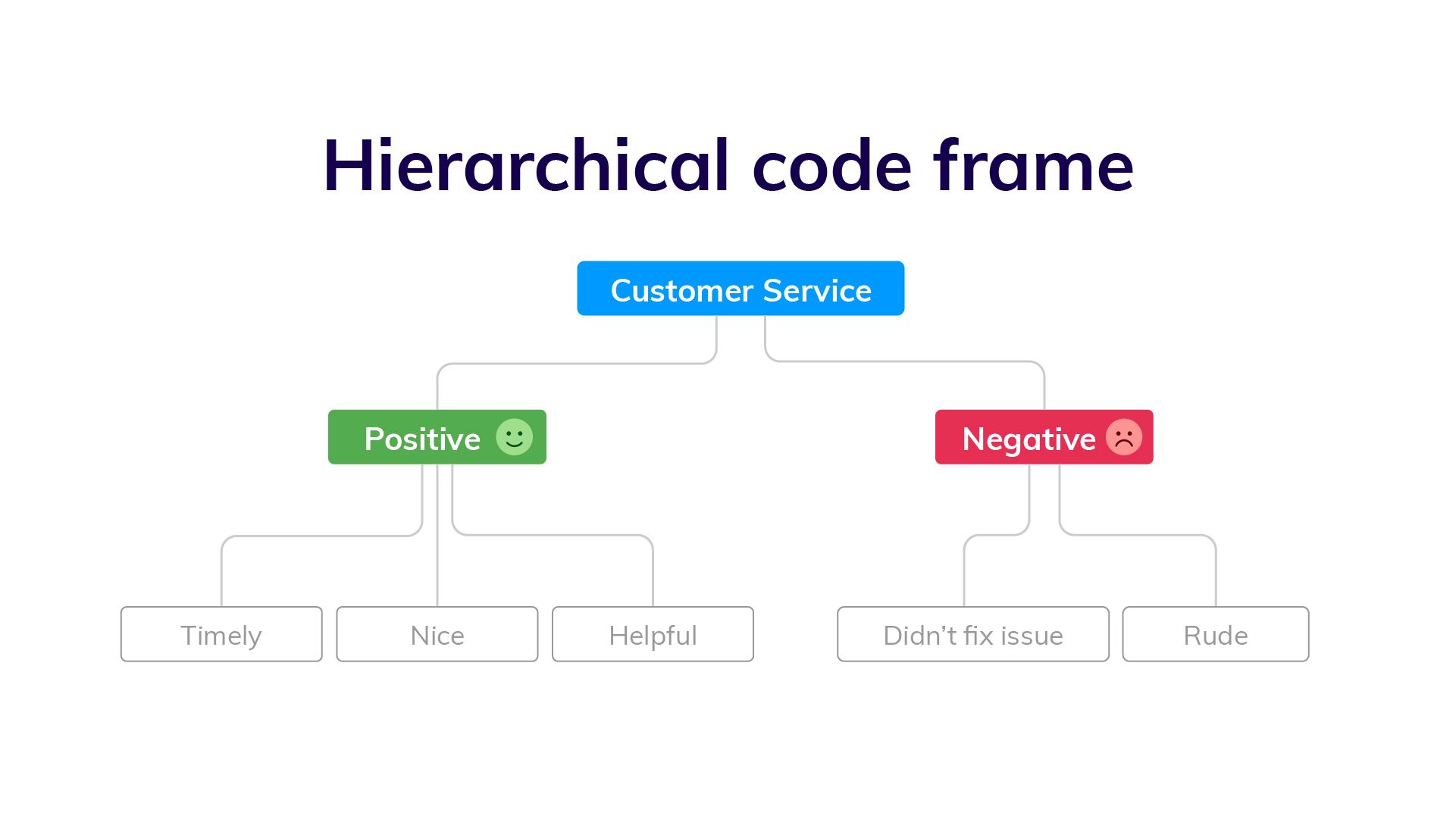
Using software to speed up manual coding of qualitative data
An Excel spreadsheet is still a popular method for coding. But various software solutions can help speed up this process. Here are some examples.
- CAQDAS / NVivo - CAQDAS software has built-in functionality that allows you to code text within their software. You may find the interface the software offers easier for managing codes than a spreadsheet.
- Dovetail/EnjoyHQ - You can tag transcripts and other textual data within these solutions. As they are also repositories you may find it simpler to keep the coding in one platform.
- IBM SPSS - SPSS is a statistical analysis software that may make coding easier than in a spreadsheet.
- Ascribe - Ascribe’s ‘Coder’ is a coding management system. Its user interface will make it easier for you to manage your codes.
Automating the qualitative coding process using thematic analysis software
In solutions which speed up the manual coding process, you still have to come up with valid codes and often apply codes manually to pieces of feedback. But there are also solutions that automate both the discovery and the application of codes.
Advances in machine learning have now made it possible to read, code and structure qualitative data automatically. This type of automated coding is offered by thematic analysis software .
Automation makes it far simpler and faster to code the feedback and group it into themes. By incorporating natural language processing (NLP) into the software, the AI looks across sentences and phrases to identify common themes meaningful statements. Some automated solutions detect repeating patterns and assign codes to them, others make you train the AI by providing examples. You could say that the AI learns the meaning of the feedback on its own.
Thematic automates the coding of qualitative feedback regardless of source. There’s no need to set up themes or categories in advance. Simply upload your data and wait a few minutes. You can also manually edit the codes to further refine their accuracy. Experiments conducted indicate that Thematic’s automated coding is just as accurate as manual coding .
Paired with sentiment analysis and advanced text analytics - these automated solutions become powerful for deriving quality business or research insights.
You could also build your own , if you have the resources!
The key benefits of using an automated coding solution
Automated analysis can often be set up fast and there’s the potential to uncover things that would never have been revealed if you had given the software a prescribed list of themes to look for.
Because the model applies a consistent rule to the data, it captures phrases or statements that a human eye might have missed.
Complete and consistent analysis of customer feedback enables more meaningful findings. Leading us into step 4.
Step 4: Analyze your data: Find meaningful insights
Now we are going to analyze our data to find insights. This is where we start to answer our research questions. Keep in mind that step 4 and step 5 (tell the story) have some overlap . This is because creating visualizations is both part of analysis process and reporting.
The task of uncovering insights is to scour through the codes that emerge from the data and draw meaningful correlations from them. It is also about making sure each insight is distinct and has enough data to support it.
Part of the analysis is to establish how much each code relates to different demographics and customer profiles, and identify whether there’s any relationship between these data points.
Manually create sub-codes to improve the quality of insights
If your code frame only has one level, you may find that your codes are too broad to be able to extract meaningful insights. This is where it is valuable to create sub-codes to your primary codes. This process is sometimes referred to as meta coding.
Note: If you take an inductive coding approach, you can create sub-codes as you are reading through your feedback data and coding it.
While time-consuming, this exercise will improve the quality of your analysis. Here is an example of what sub-codes could look like.
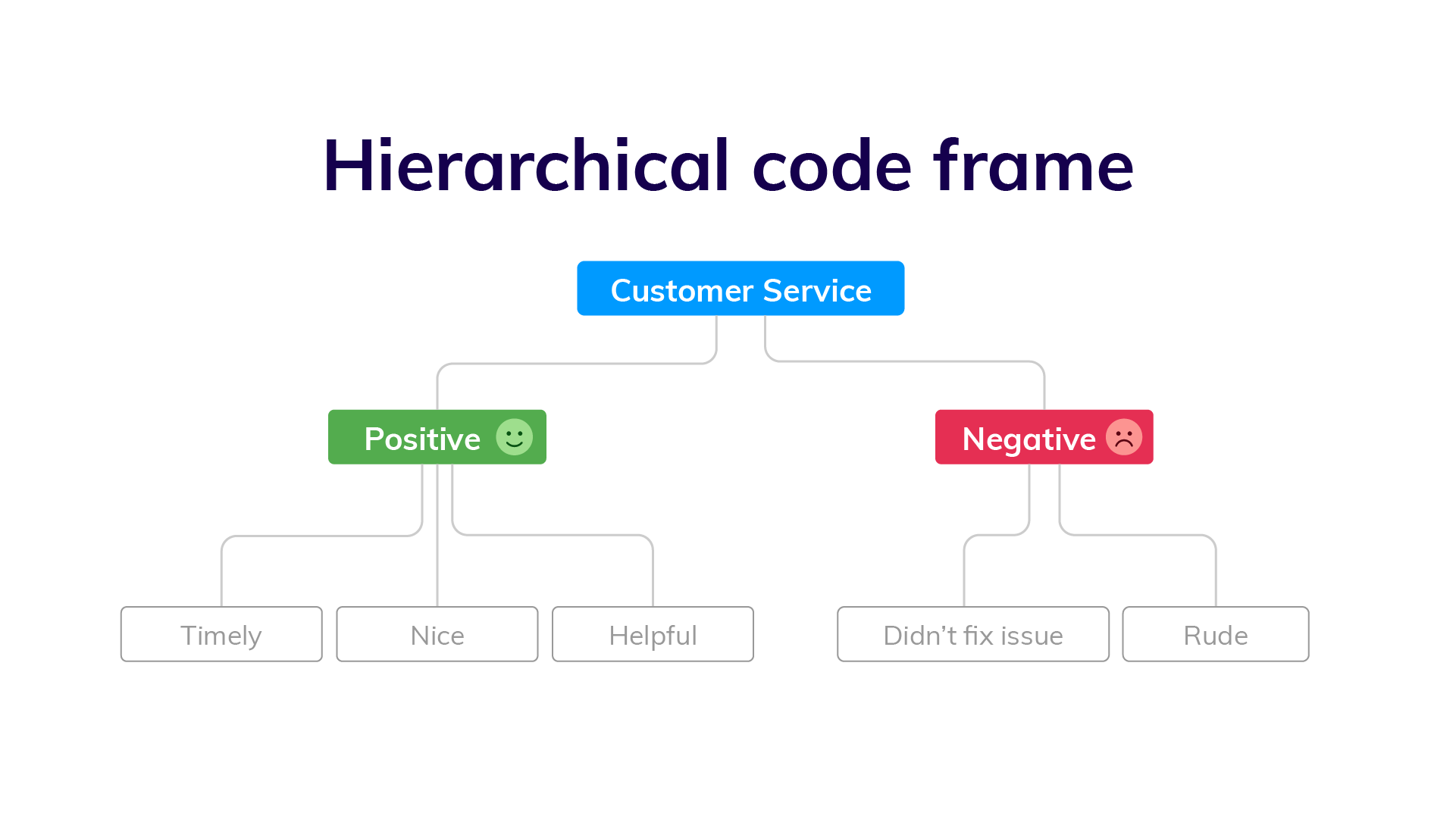
You need to carefully read your qualitative data to create quality sub-codes. But as you can see, the depth of analysis is greatly improved. By calculating the frequency of these sub-codes you can get insight into which customer service problems you can immediately address.
Correlate the frequency of codes to customer segments
Many businesses use customer segmentation . And you may have your own respondent segments that you can apply to your qualitative analysis. Segmentation is the practise of dividing customers or research respondents into subgroups.
Segments can be based on:
- Demographic
- And any other data type that you care to segment by
It is particularly useful to see the occurrence of codes within your segments. If one of your customer segments is considered unimportant to your business, but they are the cause of nearly all customer service complaints, it may be in your best interest to focus attention elsewhere. This is a useful insight!
Manually visualizing coded qualitative data
There are formulas you can use to visualize key insights in your data. The formulas we will suggest are imperative if you are measuring a score alongside your feedback.
If you are collecting a metric alongside your qualitative data this is a key visualization. Impact answers the question: “What’s the impact of a code on my overall score?”. Using Net Promoter Score (NPS) as an example, first you need to:
- Calculate overall NPS
- Calculate NPS in the subset of responses that do not contain that theme
- Subtract B from A
Then you can use this simple formula to calculate code impact on NPS .
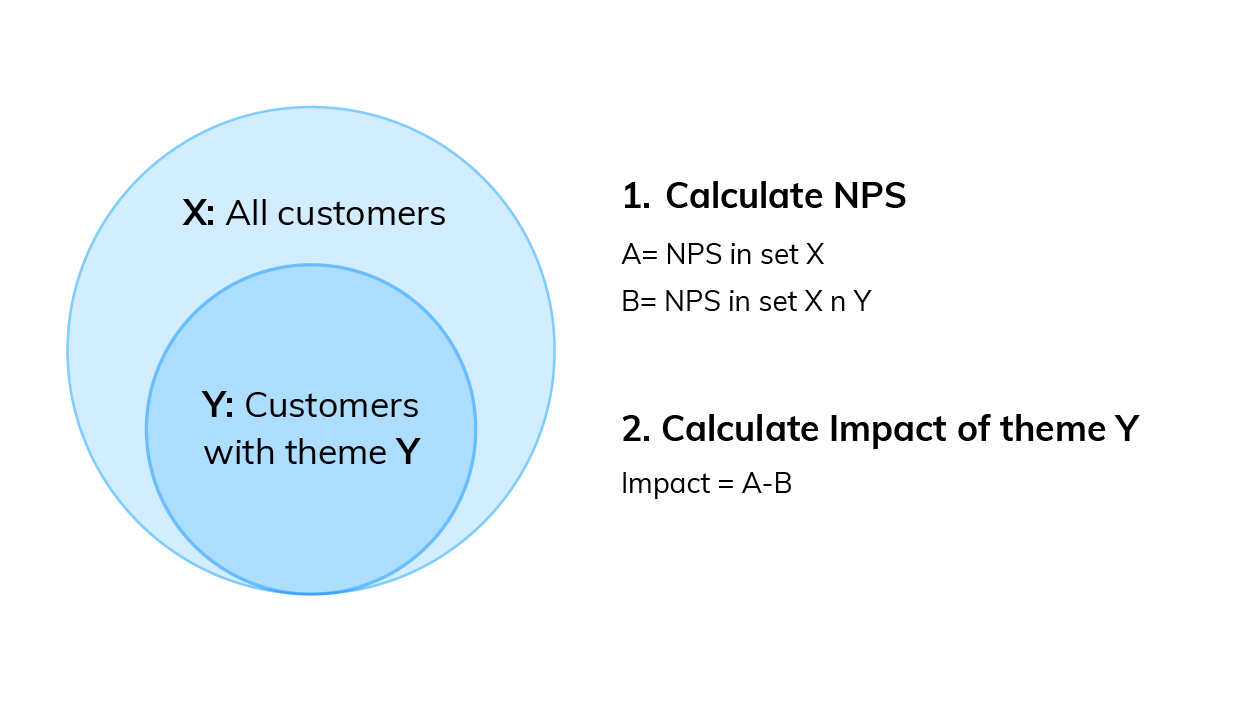
You can then visualize this data using a bar chart.
You can download our CX toolkit - it includes a template to recreate this.
Trends over time
This analysis can help you answer questions like: “Which codes are linked to decreases or increases in my score over time?”
We need to compare two sequences of numbers: NPS over time and code frequency over time . Using Excel, calculate the correlation between the two sequences, which can be either positive (the more codes the higher the NPS, see picture below), or negative (the more codes the lower the NPS).
Now you need to plot code frequency against the absolute value of code correlation with NPS. Here is the formula:
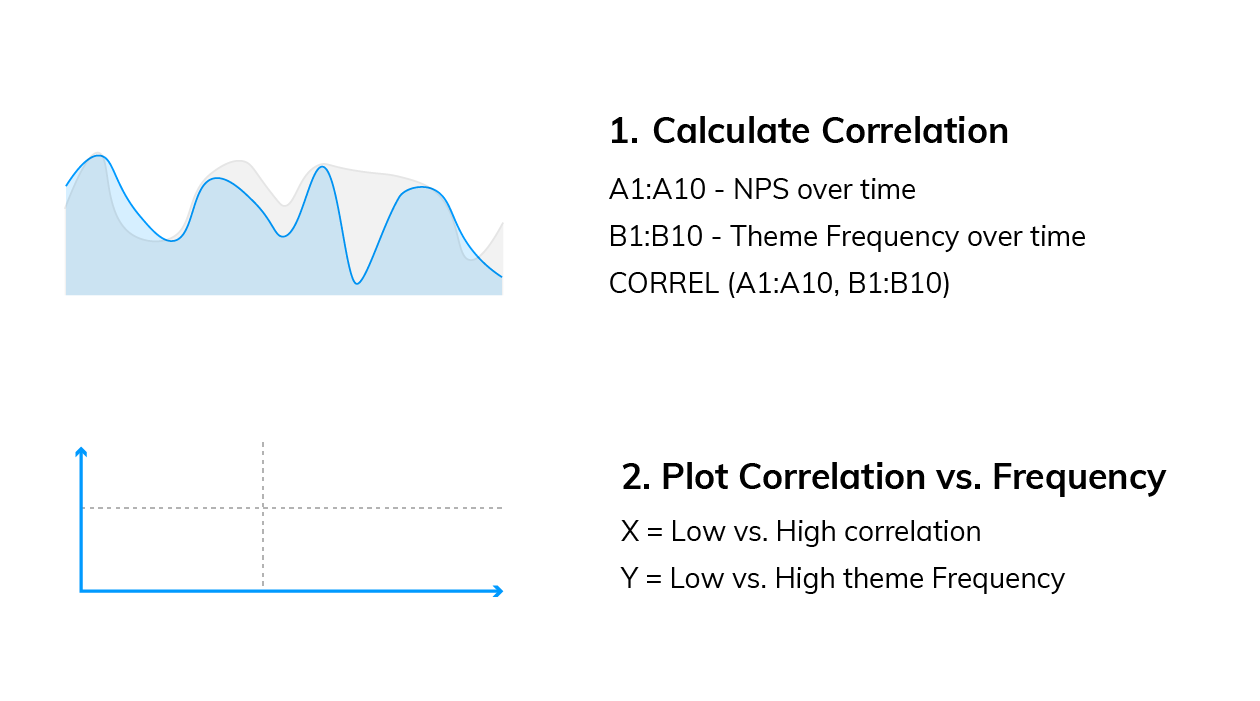
The visualization could look like this:
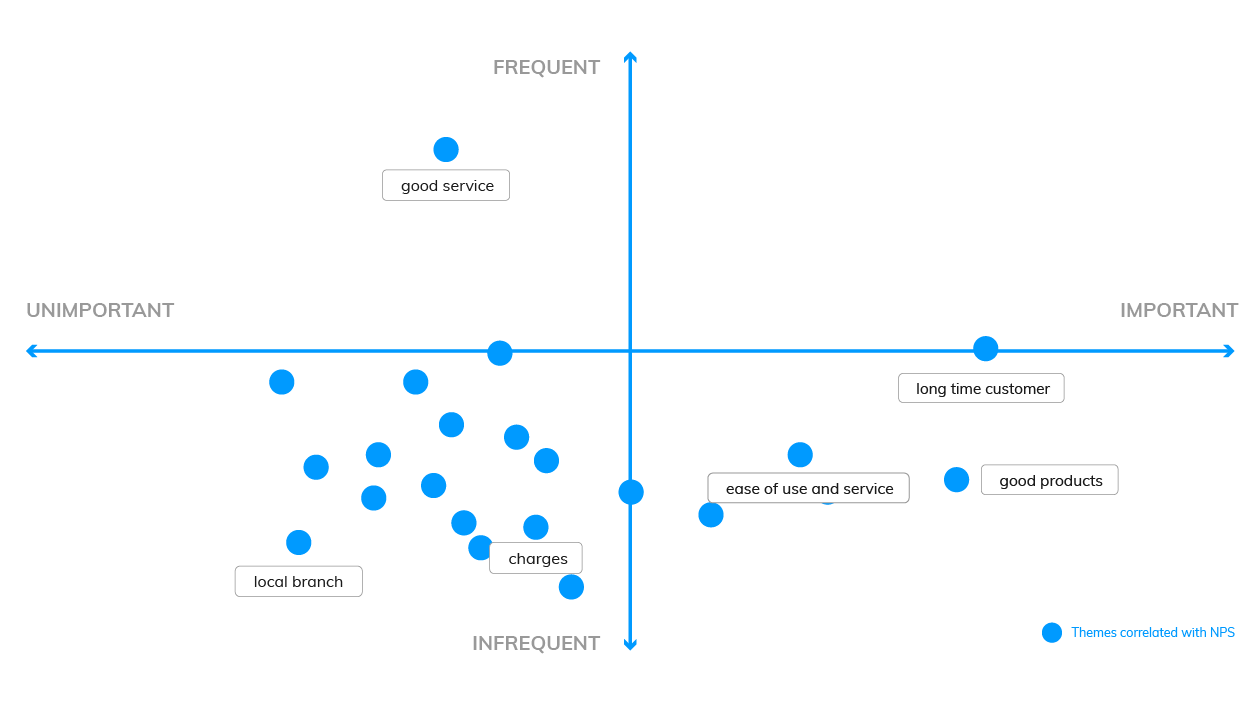
These are two examples, but there are more. For a third manual formula, and to learn why word clouds are not an insightful form of analysis, read our visualizations article .
Using a text analytics solution to automate analysis
Automated text analytics solutions enable codes and sub-codes to be pulled out of the data automatically. This makes it far faster and easier to identify what’s driving negative or positive results. And to pick up emerging trends and find all manner of rich insights in the data.
Another benefit of AI-driven text analytics software is its built-in capability for sentiment analysis, which provides the emotive context behind your feedback and other qualitative textual data therein.
Thematic provides text analytics that goes further by allowing users to apply their expertise on business context to edit or augment the AI-generated outputs.
Since the move away from manual research is generally about reducing the human element, adding human input to the technology might sound counter-intuitive. However, this is mostly to make sure important business nuances in the feedback aren’t missed during coding. The result is a higher accuracy of analysis. This is sometimes referred to as augmented intelligence .
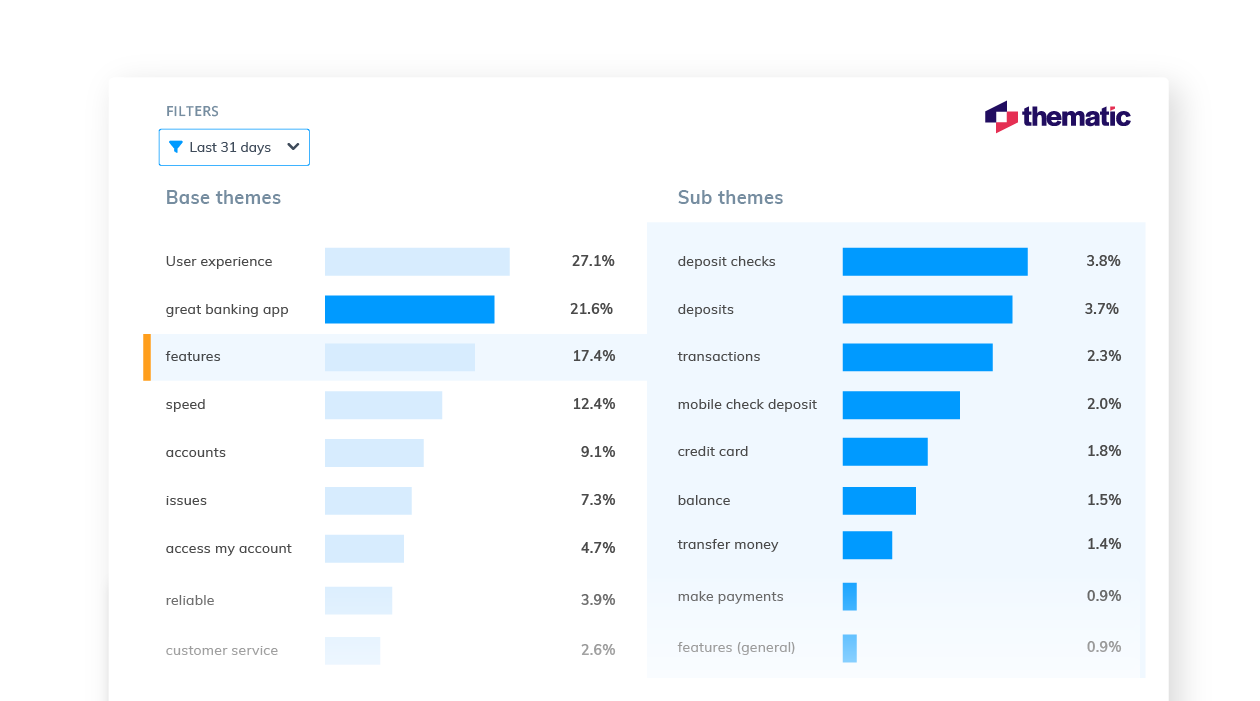
Step 5: Report on your data: Tell the story
The last step of analyzing your qualitative data is to report on it, to tell the story. At this point, the codes are fully developed and the focus is on communicating the narrative to the audience.
A coherent outline of the qualitative research, the findings and the insights is vital for stakeholders to discuss and debate before they can devise a meaningful course of action.
Creating graphs and reporting in Powerpoint
Typically, qualitative researchers take the tried and tested approach of distilling their report into a series of charts, tables and other visuals which are woven into a narrative for presentation in Powerpoint.
Using visualization software for reporting
With data transformation and APIs, the analyzed data can be shared with data visualisation software, such as Power BI or Tableau , Google Studio or Looker. Power BI and Tableau are among the most preferred options.
Visualizing your insights inside a feedback analytics platform
Feedback analytics platforms, like Thematic, incorporate visualisation tools that intuitively turn key data and insights into graphs. This removes the time consuming work of constructing charts to visually identify patterns and creates more time to focus on building a compelling narrative that highlights the insights, in bite-size chunks, for executive teams to review.
Using a feedback analytics platform with visualization tools means you don’t have to use a separate product for visualizations. You can export graphs into Powerpoints straight from the platforms.
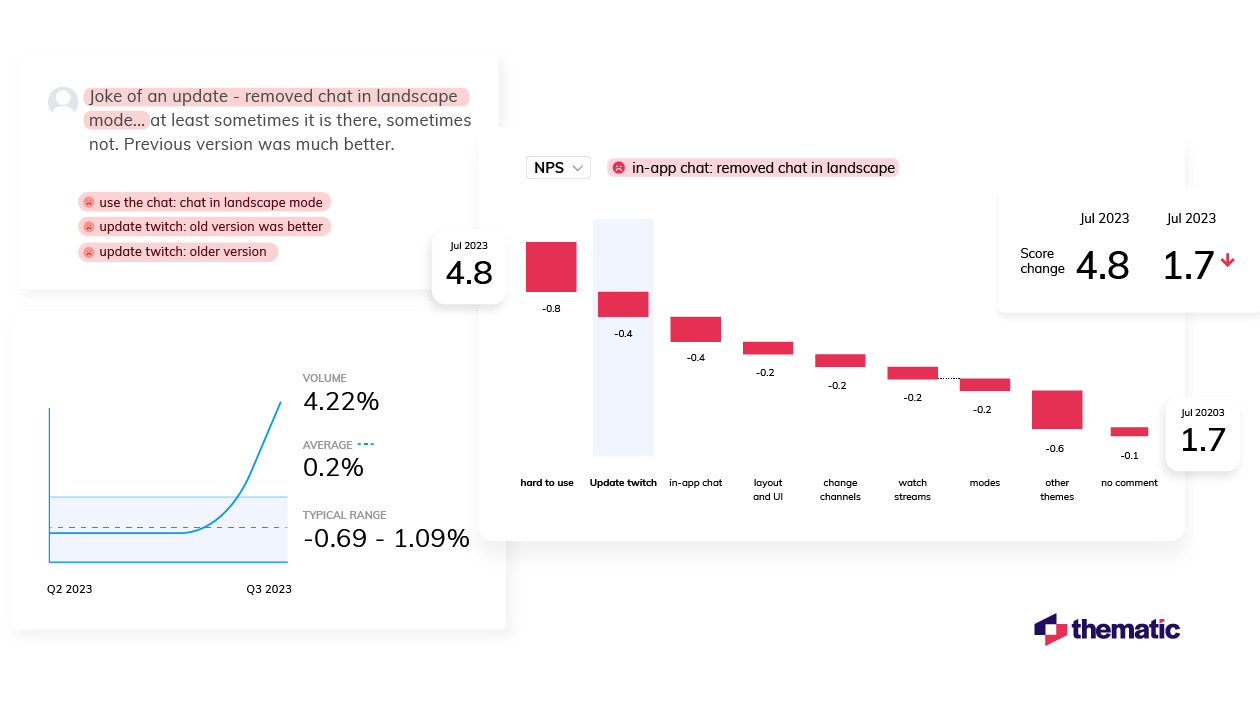
Conclusion - Manual or Automated?
There are those who remain deeply invested in the manual approach - because it’s familiar, because they’re reluctant to spend money and time learning new software, or because they’ve been burned by the overpromises of AI.
For projects that involve small datasets, manual analysis makes sense. For example, if the objective is simply to quantify a simple question like “Do customers prefer X concepts to Y?”. If the findings are being extracted from a small set of focus groups and interviews, sometimes it’s easier to just read them
However, as new generations come into the workplace, it’s technology-driven solutions that feel more comfortable and practical. And the merits are undeniable. Especially if the objective is to go deeper and understand the ‘why’ behind customers’ preference for X or Y. And even more especially if time and money are considerations.
The ability to collect a free flow of qualitative feedback data at the same time as the metric means AI can cost-effectively scan, crunch, score and analyze a ton of feedback from one system in one go. And time-intensive processes like focus groups, or coding, that used to take weeks, can now be completed in a matter of hours or days.
But aside from the ever-present business case to speed things up and keep costs down, there are also powerful research imperatives for automated analysis of qualitative data: namely, accuracy and consistency.
Finding insights hidden in feedback requires consistency, especially in coding. Not to mention catching all the ‘unknown unknowns’ that can skew research findings and steering clear of cognitive bias.
Some say without manual data analysis researchers won’t get an accurate “feel” for the insights. However, the larger data sets are, the harder it is to sort through the feedback and organize feedback that has been pulled from different places. And, the more difficult it is to stay on course, the greater the risk of drawing incorrect, or incomplete, conclusions grows.
Though the process steps for qualitative data analysis have remained pretty much unchanged since psychologist Paul Felix Lazarsfeld paved the path a hundred years ago, the impact digital technology has had on types of qualitative feedback data and the approach to the analysis are profound.
If you want to try an automated feedback analysis solution on your own qualitative data, you can get started with Thematic .
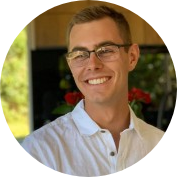
Community & Marketing
Tyler manages our community of CX, insights & analytics professionals. Tyler's goal is to help unite insights professionals around common challenges.
We make it easy to discover the customer and product issues that matter.
Unlock the value of feedback at scale, in one platform. Try it for free now!
- Questions to ask your Feedback Analytics vendor
- How to end customer churn for good
- Scalable analysis of NPS verbatims
- 5 Text analytics approaches
- How to calculate the ROI of CX
Our experts will show you how Thematic works, how to discover pain points and track the ROI of decisions. To access your free trial, book a personal demo today.
Recent posts
When two major storms wreaked havoc on Auckland and Watercare’s infrastructurem the utility went through a CX crisis. With a massive influx of calls to their support center, Thematic helped them get inisghts from this data to forge a new approach to restore services and satisfaction levels.
Become a qualitative theming pro! Creating a perfect code frame is hard, but thematic analysis software makes the process much easier.
Qualtrics is one of the most well-known and powerful Customer Feedback Management platforms. But even so, it has limitations. We recently hosted a live panel where data analysts from two well-known brands shared their experiences with Qualtrics, and how they extended this platform’s capabilities. Below, we’ll share the
Have a language expert improve your writing
Run a free plagiarism check in 10 minutes, automatically generate references for free.
- Knowledge Base
- Methodology
- What Is Qualitative Research? | Methods & Examples
What Is Qualitative Research? | Methods & Examples
Published on 4 April 2022 by Pritha Bhandari . Revised on 30 January 2023.
Qualitative research involves collecting and analysing non-numerical data (e.g., text, video, or audio) to understand concepts, opinions, or experiences. It can be used to gather in-depth insights into a problem or generate new ideas for research.
Qualitative research is the opposite of quantitative research , which involves collecting and analysing numerical data for statistical analysis.
Qualitative research is commonly used in the humanities and social sciences, in subjects such as anthropology, sociology, education, health sciences, and history.
- How does social media shape body image in teenagers?
- How do children and adults interpret healthy eating in the UK?
- What factors influence employee retention in a large organisation?
- How is anxiety experienced around the world?
- How can teachers integrate social issues into science curriculums?
Table of contents
Approaches to qualitative research, qualitative research methods, qualitative data analysis, advantages of qualitative research, disadvantages of qualitative research, frequently asked questions about qualitative research.
Qualitative research is used to understand how people experience the world. While there are many approaches to qualitative research, they tend to be flexible and focus on retaining rich meaning when interpreting data.
Common approaches include grounded theory, ethnography, action research, phenomenological research, and narrative research. They share some similarities, but emphasise different aims and perspectives.
Prevent plagiarism, run a free check.
Each of the research approaches involve using one or more data collection methods . These are some of the most common qualitative methods:
- Observations: recording what you have seen, heard, or encountered in detailed field notes.
- Interviews: personally asking people questions in one-on-one conversations.
- Focus groups: asking questions and generating discussion among a group of people.
- Surveys : distributing questionnaires with open-ended questions.
- Secondary research: collecting existing data in the form of texts, images, audio or video recordings, etc.
- You take field notes with observations and reflect on your own experiences of the company culture.
- You distribute open-ended surveys to employees across all the company’s offices by email to find out if the culture varies across locations.
- You conduct in-depth interviews with employees in your office to learn about their experiences and perspectives in greater detail.
Qualitative researchers often consider themselves ‘instruments’ in research because all observations, interpretations and analyses are filtered through their own personal lens.
For this reason, when writing up your methodology for qualitative research, it’s important to reflect on your approach and to thoroughly explain the choices you made in collecting and analysing the data.
Qualitative data can take the form of texts, photos, videos and audio. For example, you might be working with interview transcripts, survey responses, fieldnotes, or recordings from natural settings.
Most types of qualitative data analysis share the same five steps:
- Prepare and organise your data. This may mean transcribing interviews or typing up fieldnotes.
- Review and explore your data. Examine the data for patterns or repeated ideas that emerge.
- Develop a data coding system. Based on your initial ideas, establish a set of codes that you can apply to categorise your data.
- Assign codes to the data. For example, in qualitative survey analysis, this may mean going through each participant’s responses and tagging them with codes in a spreadsheet. As you go through your data, you can create new codes to add to your system if necessary.
- Identify recurring themes. Link codes together into cohesive, overarching themes.
There are several specific approaches to analysing qualitative data. Although these methods share similar processes, they emphasise different concepts.
Qualitative research often tries to preserve the voice and perspective of participants and can be adjusted as new research questions arise. Qualitative research is good for:
- Flexibility
The data collection and analysis process can be adapted as new ideas or patterns emerge. They are not rigidly decided beforehand.
- Natural settings
Data collection occurs in real-world contexts or in naturalistic ways.
- Meaningful insights
Detailed descriptions of people’s experiences, feelings and perceptions can be used in designing, testing or improving systems or products.
- Generation of new ideas
Open-ended responses mean that researchers can uncover novel problems or opportunities that they wouldn’t have thought of otherwise.
Researchers must consider practical and theoretical limitations in analysing and interpreting their data. Qualitative research suffers from:
- Unreliability
The real-world setting often makes qualitative research unreliable because of uncontrolled factors that affect the data.
- Subjectivity
Due to the researcher’s primary role in analysing and interpreting data, qualitative research cannot be replicated . The researcher decides what is important and what is irrelevant in data analysis, so interpretations of the same data can vary greatly.
- Limited generalisability
Small samples are often used to gather detailed data about specific contexts. Despite rigorous analysis procedures, it is difficult to draw generalisable conclusions because the data may be biased and unrepresentative of the wider population .
- Labour-intensive
Although software can be used to manage and record large amounts of text, data analysis often has to be checked or performed manually.
Quantitative research deals with numbers and statistics, while qualitative research deals with words and meanings.
Quantitative methods allow you to test a hypothesis by systematically collecting and analysing data, while qualitative methods allow you to explore ideas and experiences in depth.
There are five common approaches to qualitative research :
- Grounded theory involves collecting data in order to develop new theories.
- Ethnography involves immersing yourself in a group or organisation to understand its culture.
- Narrative research involves interpreting stories to understand how people make sense of their experiences and perceptions.
- Phenomenological research involves investigating phenomena through people’s lived experiences.
- Action research links theory and practice in several cycles to drive innovative changes.
Data collection is the systematic process by which observations or measurements are gathered in research. It is used in many different contexts by academics, governments, businesses, and other organisations.
There are various approaches to qualitative data analysis , but they all share five steps in common:
- Prepare and organise your data.
- Review and explore your data.
- Develop a data coding system.
- Assign codes to the data.
- Identify recurring themes.
The specifics of each step depend on the focus of the analysis. Some common approaches include textual analysis , thematic analysis , and discourse analysis .
Cite this Scribbr article
If you want to cite this source, you can copy and paste the citation or click the ‘Cite this Scribbr article’ button to automatically add the citation to our free Reference Generator.
Bhandari, P. (2023, January 30). What Is Qualitative Research? | Methods & Examples. Scribbr. Retrieved 14 May 2024, from https://www.scribbr.co.uk/research-methods/introduction-to-qualitative-research/
Is this article helpful?
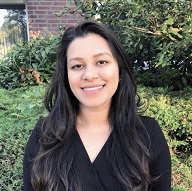
Pritha Bhandari

An official website of the United States government
The .gov means it’s official. Federal government websites often end in .gov or .mil. Before sharing sensitive information, make sure you’re on a federal government site.
The site is secure. The https:// ensures that you are connecting to the official website and that any information you provide is encrypted and transmitted securely.
- Publications
- Account settings
Preview improvements coming to the PMC website in October 2024. Learn More or Try it out now .
- Advanced Search
- Journal List
- Perspect Clin Res
- v.14(1); Jan-Mar 2023
- PMC10003579
Introduction to qualitative research methods – Part I
Shagufta bhangu.
Department of Global Health and Social Medicine, King's College London, London, United Kingdom
Fabien Provost
Carlo caduff.
Qualitative research methods are widely used in the social sciences and the humanities, but they can also complement quantitative approaches used in clinical research. In this article, we discuss the key features and contributions of qualitative research methods.
INTRODUCTION
Qualitative research methods refer to techniques of investigation that rely on nonstatistical and nonnumerical methods of data collection, analysis, and evidence production. Qualitative research techniques provide a lens for learning about nonquantifiable phenomena such as people's experiences, languages, histories, and cultures. In this article, we describe the strengths and role of qualitative research methods and how these can be employed in clinical research.
Although frequently employed in the social sciences and humanities, qualitative research methods can complement clinical research. These techniques can contribute to a better understanding of the social, cultural, political, and economic dimensions of health and illness. Social scientists and scholars in the humanities rely on a wide range of methods, including interviews, surveys, participant observation, focus groups, oral history, and archival research to examine both structural conditions and lived experience [ Figure 1 ]. Such research can not only provide robust and reliable data but can also humanize and add richness to our understanding of the ways in which people in different parts of the world perceive and experience illness and how they interact with medical institutions, systems, and therapeutics.

Examples of qualitative research techniques
Qualitative research methods should not be seen as tools that can be applied independently of theory. It is important for these tools to be based on more than just method. In their research, social scientists and scholars in the humanities emphasize social theory. Departing from a reductionist psychological model of individual behavior that often blames people for their illness, social theory focuses on relations – disease happens not simply in people but between people. This type of theoretically informed and empirically grounded research thus examines not just patients but interactions between a wide range of actors (e.g., patients, family members, friends, neighbors, local politicians, medical practitioners at all levels, and from many systems of medicine, researchers, policymakers) to give voice to the lived experiences, motivations, and constraints of all those who are touched by disease.
PHILOSOPHICAL FOUNDATIONS OF QUALITATIVE RESEARCH METHODS
In identifying the factors that contribute to the occurrence and persistence of a phenomenon, it is paramount that we begin by asking the question: what do we know about this reality? How have we come to know this reality? These two processes, which we can refer to as the “what” question and the “how” question, are the two that all scientists (natural and social) grapple with in their research. We refer to these as the ontological and epistemological questions a research study must address. Together, they help us create a suitable methodology for any research study[ 1 ] [ Figure 2 ]. Therefore, as with quantitative methods, there must be a justifiable and logical method for understanding the world even for qualitative methods. By engaging with these two dimensions, the ontological and the epistemological, we open a path for learning that moves away from commonsensical understandings of the world, and the perpetuation of stereotypes and toward robust scientific knowledge production.

Developing a research methodology
Every discipline has a distinct research philosophy and way of viewing the world and conducting research. Philosophers and historians of science have extensively studied how these divisions and specializations have emerged over centuries.[ 1 , 2 , 3 ] The most important distinction between quantitative and qualitative research techniques lies in the nature of the data they study and analyze. While the former focus on statistical, numerical, and quantitative aspects of phenomena and employ the same in data collection and analysis, qualitative techniques focus on humanistic, descriptive, and qualitative aspects of phenomena.[ 4 ]
For the findings of any research study to be reliable, they must employ the appropriate research techniques that are uniquely tailored to the phenomena under investigation. To do so, researchers must choose techniques based on their specific research questions and understand the strengths and limitations of the different tools available to them. Since clinical work lies at the intersection of both natural and social phenomena, it means that it must study both: biological and physiological phenomena (natural, quantitative, and objective phenomena) and behavioral and cultural phenomena (social, qualitative, and subjective phenomena). Therefore, clinical researchers can gain from both sets of techniques in their efforts to produce medical knowledge and bring forth scientifically informed change.
KEY FEATURES AND CONTRIBUTIONS OF QUALITATIVE RESEARCH METHODS
In this section, we discuss the key features and contributions of qualitative research methods [ Figure 3 ]. We describe the specific strengths and limitations of these techniques and discuss how they can be deployed in scientific investigations.

Key features of qualitative research methods
One of the most important contributions of qualitative research methods is that they provide rigorous, theoretically sound, and rational techniques for the analysis of subjective, nebulous, and difficult-to-pin-down phenomena. We are aware, for example, of the role that social factors play in health care but find it hard to qualify and quantify these in our research studies. Often, we find researchers basing their arguments on “common sense,” developing research studies based on assumptions about the people that are studied. Such commonsensical assumptions are perhaps among the greatest impediments to knowledge production. For example, in trying to understand stigma, surveys often make assumptions about its reasons and frequently associate it with vague and general common sense notions of “fear” and “lack of information.” While these may be at work, to make such assumptions based on commonsensical understandings, and without conducting research inhibit us from exploring the multiple social factors that are at work under the guise of stigma.
In unpacking commonsensical understandings and researching experiences, relationships, and other phenomena, qualitative researchers are assisted by their methodological commitment to open-ended research. By open-ended research, we mean that these techniques take on an unbiased and exploratory approach in which learnings from the field and from research participants, are recorded and analyzed to learn about the world.[ 5 ] This orientation is made possible by qualitative research techniques that are particularly effective in learning about specific social, cultural, economic, and political milieus.
Second, qualitative research methods equip us in studying complex phenomena. Qualitative research methods provide scientific tools for exploring and identifying the numerous contributing factors to an occurrence. Rather than establishing one or the other factor as more important, qualitative methods are open-ended, inductive (ground-up), and empirical. They allow us to understand the object of our analysis from multiple vantage points and in its dispersion and caution against predetermined notions of the object of inquiry. They encourage researchers instead to discover a reality that is not yet given, fixed, and predetermined by the methods that are used and the hypotheses that underlie the study.
Once the multiple factors at work in a phenomenon have been identified, we can employ quantitative techniques and embark on processes of measurement, establish patterns and regularities, and analyze the causal and correlated factors at work through statistical techniques. For example, a doctor may observe that there is a high patient drop-out in treatment. Before carrying out a study which relies on quantitative techniques, qualitative research methods such as conversation analysis, interviews, surveys, or even focus group discussions may prove more effective in learning about all the factors that are contributing to patient default. After identifying the multiple, intersecting factors, quantitative techniques can be deployed to measure each of these factors through techniques such as correlational or regression analyses. Here, the use of quantitative techniques without identifying the diverse factors influencing patient decisions would be premature. Qualitative techniques thus have a key role to play in investigations of complex realities and in conducting rich exploratory studies while embracing rigorous and philosophically grounded methodologies.
Third, apart from subjective, nebulous, and complex phenomena, qualitative research techniques are also effective in making sense of irrational, illogical, and emotional phenomena. These play an important role in understanding logics at work among patients, their families, and societies. Qualitative research techniques are aided by their ability to shift focus away from the individual as a unit of analysis to the larger social, cultural, political, economic, and structural forces at work in health. As health-care practitioners and researchers focused on biological, physiological, disease and therapeutic processes, sociocultural, political, and economic conditions are often peripheral or ignored in day-to-day clinical work. However, it is within these latter processes that both health-care practices and patient lives are entrenched. Qualitative researchers are particularly adept at identifying the structural conditions such as the social, cultural, political, local, and economic conditions which contribute to health care and experiences of disease and illness.
For example, the decision to delay treatment by a patient may be understood as an irrational choice impacting his/her chances of survival, but the same may be a result of the patient treating their child's education as a financial priority over his/her own health. While this appears as an “emotional” choice, qualitative researchers try to understand the social and cultural factors that structure, inform, and justify such choices. Rather than assuming that it is an irrational choice, qualitative researchers try to understand the norms and logical grounds on which the patient is making this decision. By foregrounding such logics, stories, fears, and desires, qualitative research expands our analytic precision in learning about complex social worlds, recognizing reasons for medical successes and failures, and interrogating our assumptions about human behavior. These in turn can prove useful in arriving at conclusive, actionable findings which can inform institutional and public health policies and have a very important role to play in any change and transformation we may wish to bring to the societies in which we work.
Financial support and sponsorship
Conflicts of interest.
There are no conflicts of interest.
Mastering Qualitative Data Analysis: The Step-by-Step Process & 5 Essential Methods
12 min read
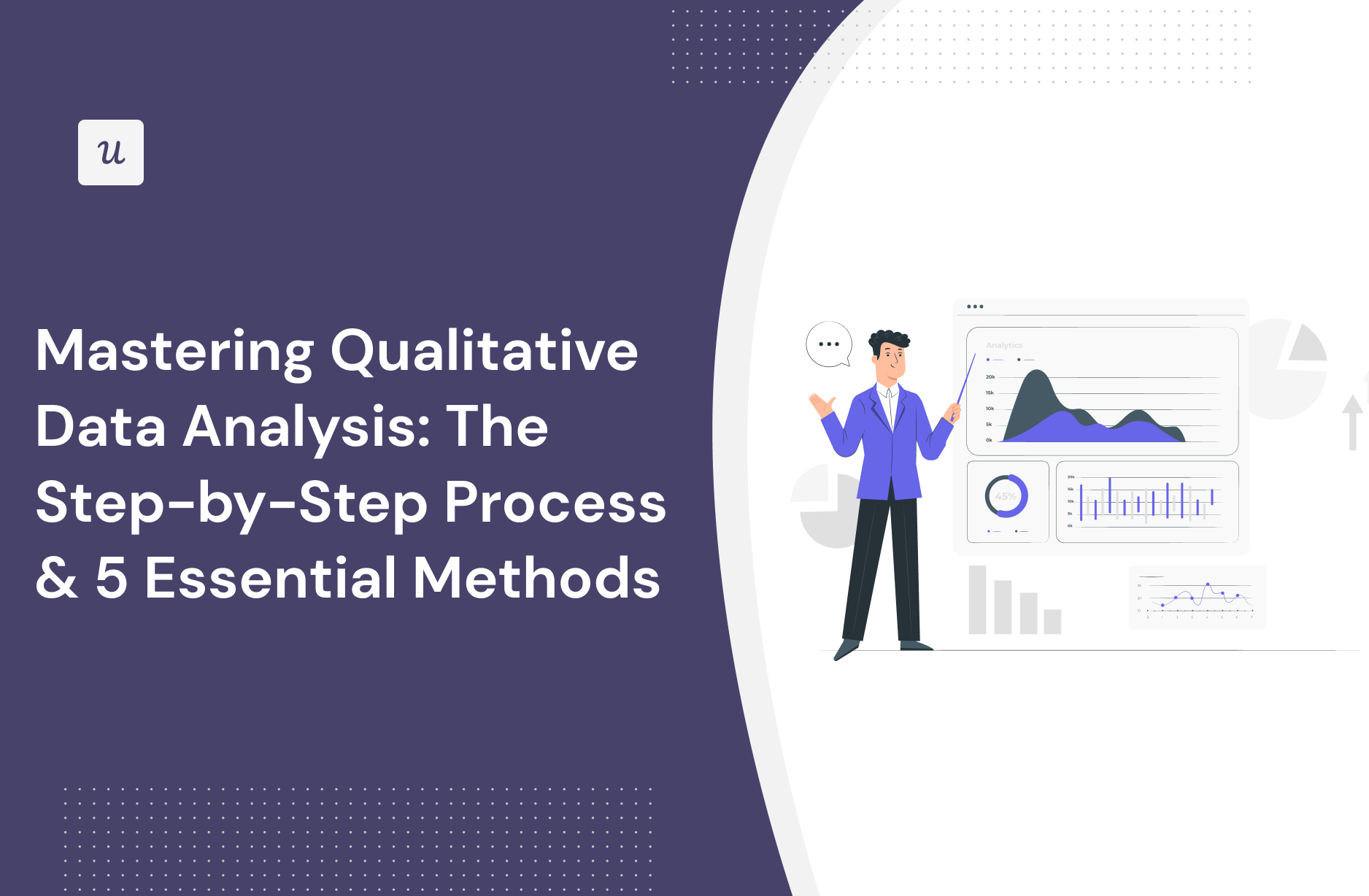
Wondering how to analyze qualitative data and get actionable insights? Search no further!
This article will help you analyze qualitative data and fuel your product growth . We’ll walk you through the following steps:
- 5 Qualitative data analysis methods.
- 5 Steps to analysing qualitative data.
- How to act on research findings.
Let’s get started!
- Qualitative data analysis turns non-numerical data into insights, including customer feedback , surveys, and interviews.
- Qualitative data provides rich insights for refining strategies and uncovering growth opportunities.
- The benefits of qualitative data analysis include deep insight, flexibility, contextual understanding, and amplifying participant voices.
- Challenges include data overload, reliability, and validity concerns, as well as time-intensive nature.
- Qualitative and quantitative data analysis differ in analyzing numerical vs. non-numerical data.
- Qualitative data methods include content analysis, narrative analysis, discourse analysis, thematic analysis, and grounded theory analysis.
- Content analysis involves systematically analyzing text to identify patterns and themes.
- Narrative analysis interprets stories to understand customer feelings and behaviors.
- The thematic analysis identifies patterns and themes in data.
- Grounded theory analysis generates hypotheses from data.
- Choosing a method depends on research questions, data type, context, expertise, and resources.
- The qualitative data analysis process involves defining questions, gathering data, organizing, coding, and making hypotheses.
- Userpilot facilitates qualitative data collection through surveys and offers NPS dashboard analytics.
- Building in-app experiences based on qualitative insights enhances user experience and drives satisfaction.
- The iterative qualitative data analysis process aims to refine understanding of the customer base.
- Userpilot can automate data collection and analysis, saving time and improving customer understanding. Book a demo to learn more!

Try Userpilot and Take Your Qualitative Research to the Next Level
- 14 Day Trial
- No Credit Card Required
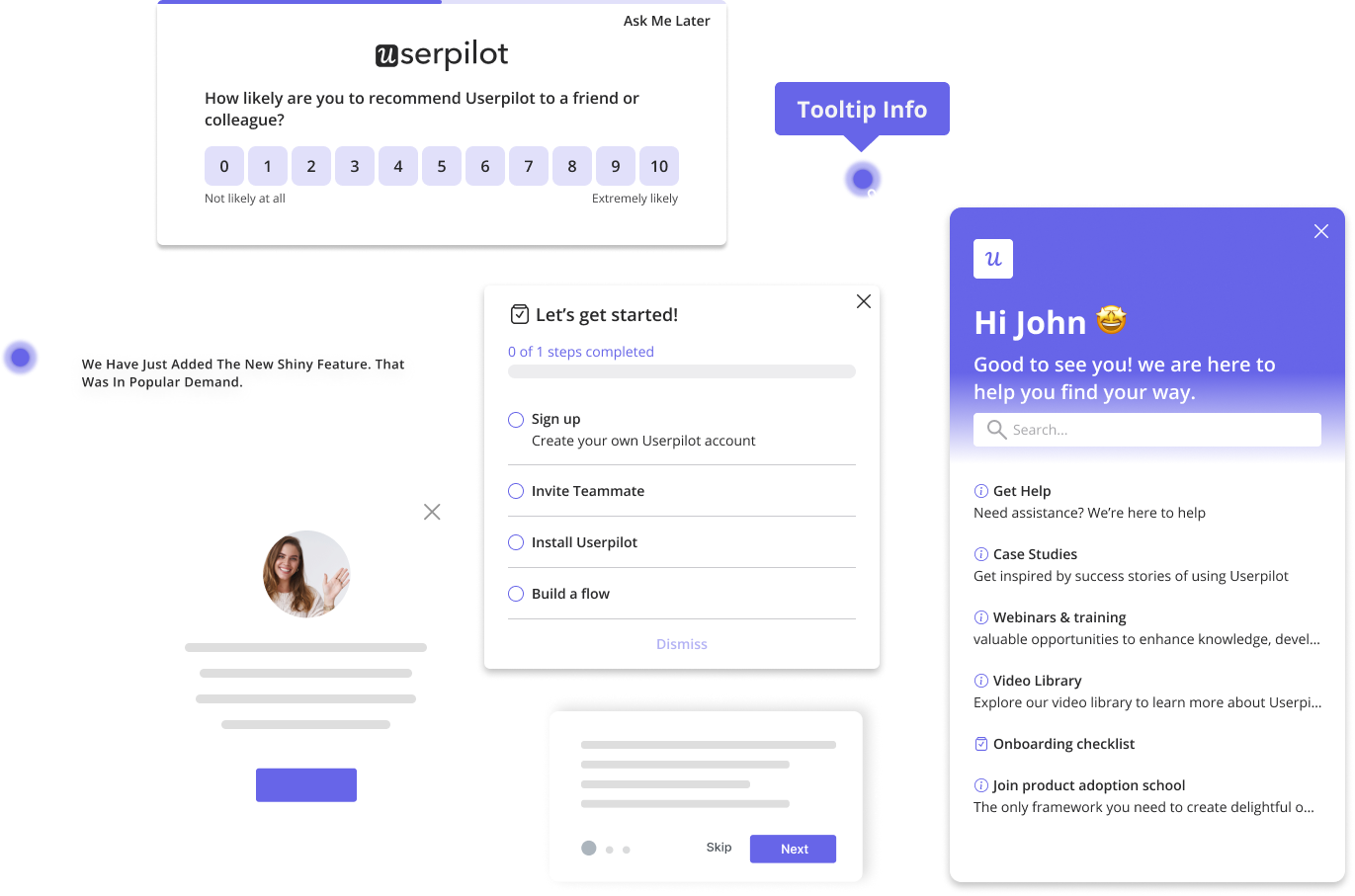
What is a qualitative data analysis?
Qualitative data analysis is the process of turning qualitative data — information that can’t be measured numerically — into insights.
This could be anything from customer feedback, surveys , website recordings, customer reviews, or in-depth interviews.
Qualitative data is often seen as more “rich” and “human” than quantitative data, which is why product teams use it to refine customer acquisition and retention strategies and uncover product growth opportunities.
Benefits of qualitative data analysis
Here are the key advantages of qualitative data analysis that underscore its significance in research endeavors:
- Deep Insight: Qualitative data analysis allows for a deep understanding of complex patterns and trends by uncovering underlying meanings, motivations, and perspectives.
- Flexibility: It offers flexibility in data interpretation, allowing researchers to explore emergent themes and adapt their analysis to new insights.
- Contextual Understanding: Qualitative analysis enables the exploration of contextual factors, providing rich context to quantitative findings and uncovering hidden dynamics.
- Participant Voice: It amplifies the voices of participants, allowing their perspectives and experiences to shape the analysis and resulting interpretations.
Challenges of qualitative data analysis
While qualitative data analysis offers rich insights, it comes with its challenges:
- Data Overload and Management: Qualitative data often comprises large volumes of text or multimedia, posing challenges in organizing, managing, and analyzing the data effectively.
- Reliability and Validity: Ensuring the reliability and validity of qualitative findings can be complex, as there are fewer standardized measures compared to quantitative analysis, requiring meticulous attention to methodological rigor.
- Time-Intensive Nature: Qualitative data analysis can be time-consuming, involving iterative processes of coding, categorizing, and synthesizing data, which may prolong the research timeline and increase resource requirements.
Quantitative data analysis vs. Qualitative data analysis
Here let’s understand the difference between qualitative and quantitative data analysis.
Quantitative data analysis is analyzing numerical data to locate patterns and trends. Quantitative research uses numbers and statistics to systematically measure variables and test hypotheses.
Qualitative data analysis , on the other hand, is the process of analyzing non-numerical, textual data to derive actionable insights from it. This data type is often more “open-ended” and can be harder to conclude from.
However, qualitative data can provide insights that quantitative data cannot. For example, qualitative data can help you understand how customers feel about your product, their unmet needs , and what motivates them.
Other differences include:
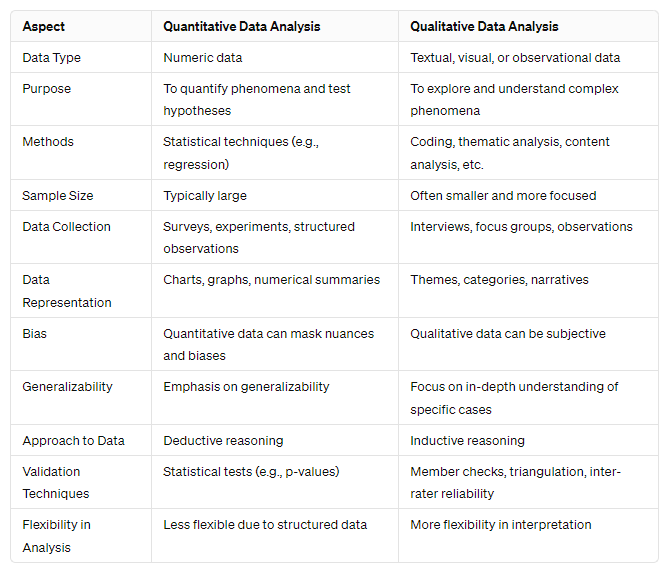
What are the 5 qualitative data analysis methods?
There are 5 main methods of qualitative data analysis. Which one you choose will depend on the type of data you collect, your preferences, and your research goals.
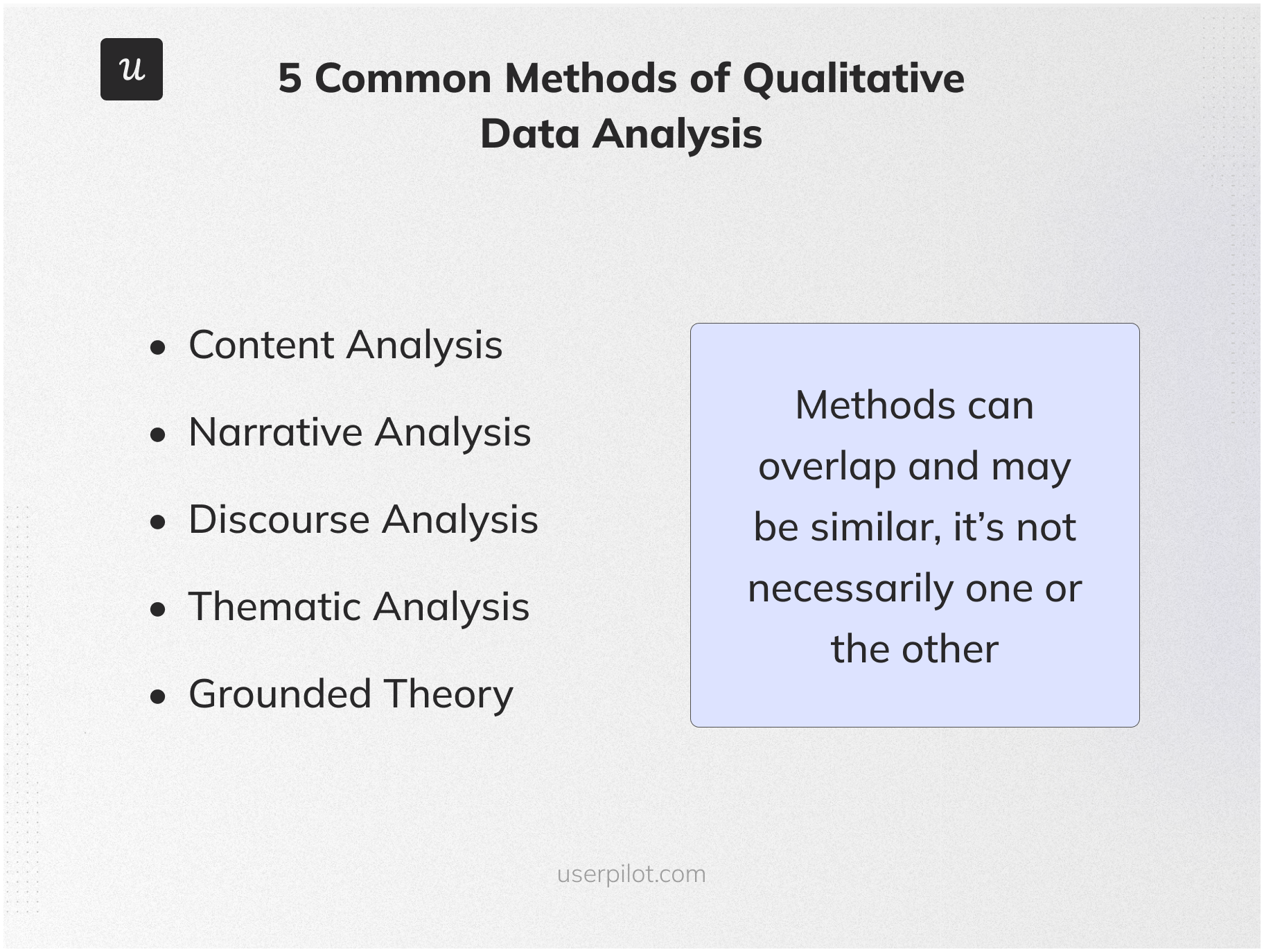
Content analysis
Content analysis is a qualitative data analysis method that systematically analyses a text to identify specific features or patterns. This could be anything from a customer interview transcript to survey responses, social media posts, or customer success calls.
The data is first coded, which means assigning it labels or categories.
For example, if you were looking at customer feedback , you might code all mentions of “price” as “P,” all mentions of “quality” as “Q,” and so on. Once manual coding is done, start looking for patterns and trends in the codes.
Content analysis is a prevalent qualitative data analysis method, as it is relatively quick and easy to do and can be done by anyone with a good understanding of the data.
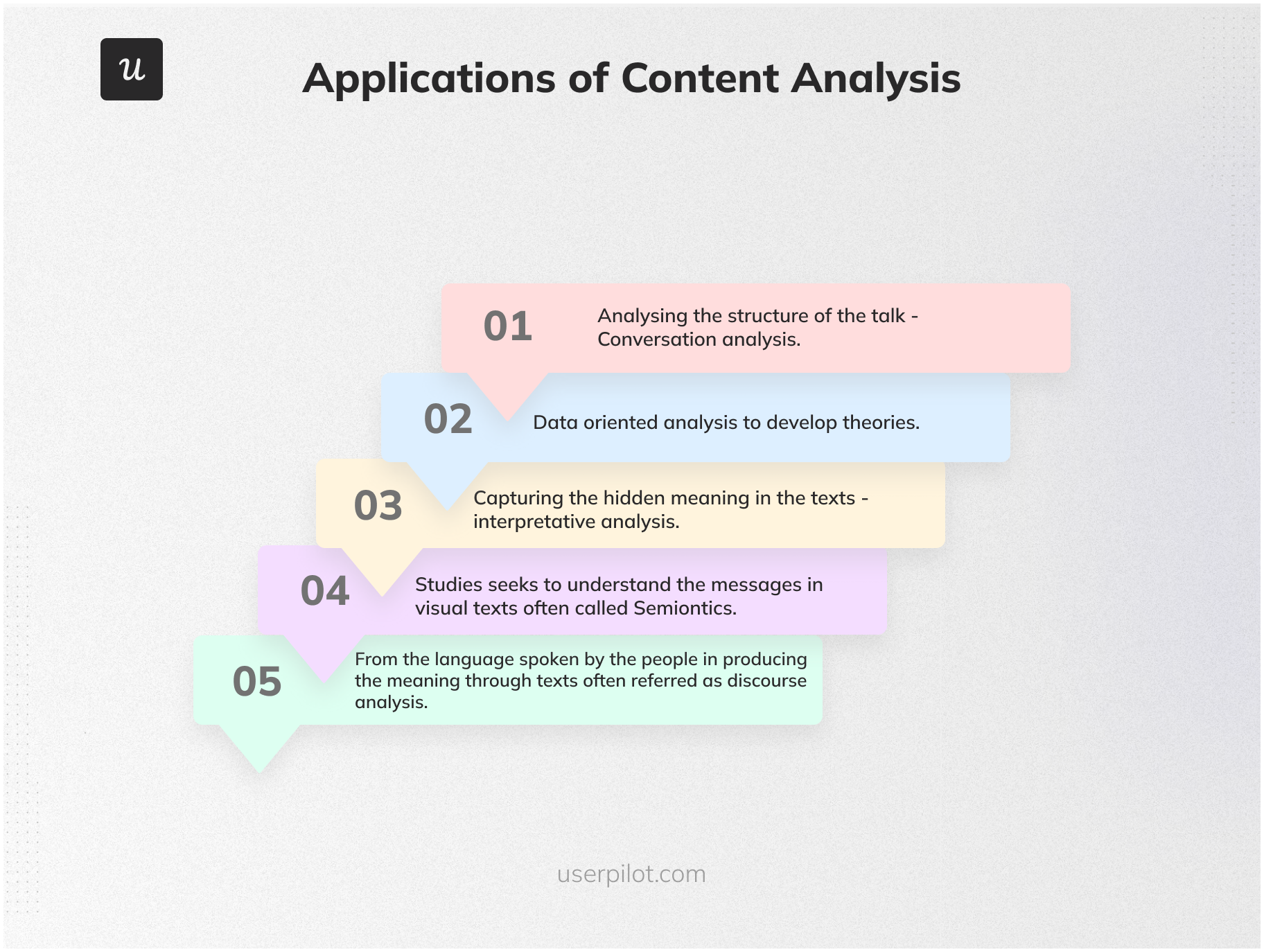
The advantages of content analysis process
- Rich insights: Content analysis can provide rich, in-depth insights into how customers feel about your product, what their unmet needs are, and their motives.
- Easily replicable: Once you have developed a coding system, content analysis is relatively quick and easy because it’s a systematic process.
- Affordable: Content analysis requires very little investment since all you need is a good understanding of the data, and it doesn’t require any special software.
The disadvantages of content analysis process
- Time-consuming: Coding the data is time-consuming, particularly if you have a large amount of data to analyze.
- Ignores context: Content analysis can ignore the context in which the data was collected which may lead to misinterpretations.
- Reductive approach: Some people argue that content analysis is a reductive approach to qualitative data because it involves breaking the data down into smaller pieces.
Narrative analysis
Analysing qualitative data with narrative analysis involves identifying, analyzing, and interpreting customer or research participants’ stories. The input can be in the form of customer interviews, testimonials, or other text data.
Narrative analysis helps product managers to understand customers’ feelings toward the product identify trends in customer behavior and personalize their in-app experiences .
The advantages of narrative analysis
- Provide a rich form of data: The stories people tell give a deep understanding of customers’ needs and pain points.
- Collects unique, in-depth data based on customer interviews or testimonials.
The disadvantages of narrative analysis
- Hard to implement in studies of large numbers.
- Time-consuming: Transcribing customer interviews or testimonials is labor-intensive.
- Hard to reproduce since it relies on unique customer stories.
Discourse analysis
Discourse analysis is about understanding how people communicate with each other. It can be used to analyse written or spoken language. For instance, product teams can use discourse analysis to understand how customers talk about their products on the web.
The advantages of discourse analysis
- Uncovers motivation behind customers’ words.
- Gives insights into customer data.
The disadvantages of disclosure analysis
- Takes a large amount of time and effort as the process is highly specialized and requires training and practice. There’s no “right” way to do it.
- Focuses solely on language.
Thematic analysis
Thematic analysis is a popular qualitative data analysis method that identifies patterns and themes in data. The process of thematic analysis involves coding the data, which means assigning it labels or categories.
It can be paired with sentiment analysis to determine whether a piece of writing is positive, negative, or neutral. This can be done using a lexicon (i.e., a list of words and their associated sentiment scores).
A common use case for thematic analysis in SaaS companies is customer feedback analysis with NPS surveys and NPS tagging to identify patterns among your customer base.
The advantages of thematic analysis
- Doesn’t require training: Anyone with little training on how to label the data can perform thematic analysis.
- It’s easy to draw important information from raw data: Surveys or customer interviews can be easily converted into insights and quantitative data with the help of labeling.
- An effective way to process large amounts of data if done automatically: you will need AI tools for this.
The disadvantages of thematic analysis
- Doesn’t capture complex narratives: If the data isn’t coded correctly, it can be difficult to identify themes since it’s a phrase-based method.
- Difficult to implement from scratch because a perfect approach must be able to merge and organize themes in a meaningful way, producing a set of themes that are not too generic and not too large.
Grounded theory analysis
Grounded theory analysis is a method that involves the constant comparative method, meaning qualitative researchers analyze and code the data on the fly.
The grounded theory approach is useful for product managers who want to understand how customers interact with their products . It can also be used to generate hypotheses about how customers will behave in the future.
Suppose product teams want to understand the reasons behind the high churn rate , they can use customer surveys and grounded theory to analyze responses and develop hypotheses about why users churn and how to reengage inactive ones .
You can filter the disengaged/inactive user segment to make analysis easier.
The advantages of ground theory analysis
- Based on actual data, qualitative analysis is more accurate than other methods that rely on assumptions.
- Analyse poorly researched topics by generating hypotheses.
- Reduces the bias in interpreting qualitative data as it’s analyzed and coded as it’s collected.
The disadvantages of ground theory analysis
- Overly theoretical
- Requires a lot of objectivity, creativity, and critical thinking
Which qualitative data analysis method should you choose?
We have covered different qualitative data analysis techniques with their pros and cons but choosing the appropriate qualitative data analysis method depends on various factors, including:
- Research Question : Different qualitative methods are suitable for different research questions.
- Nature of Data : Consider the type of data you have collected—interview transcripts, reviews, or survey responses—and choose a method that aligns with the data’s characteristics. For instance, thematic analysis is versatile and can be applied to various types of qualitative data, while narrative analysis focuses specifically on stories and narratives.
- Research Context : Take into account the broader context of your research. Some qualitative methods may be more prevalent or accepted in certain fields or contexts.
- Researcher Expertise : Consider your own skills and expertise in qualitative analysis techniques. Some methods may require specialized training or familiarity with specific software tools. Choose a method that you feel comfortable with and confident in applying effectively.
- Research Goals and Resources : Evaluate your research goals, timeline, and resources available for analysis. Some methods may be more time-consuming or resource-intensive than others. Consider the balance between the depth of analysis and practical constraints.
How to perform qualitative data analysis process in steps
With all that theory above, we’ve decided to elicit the essential steps of qualitative research methods and designed a super simple guide for gathering qualitative data.
Let’s dive in!
Step 1: Define your qualitative research questions
The qualitative analysis research process starts with defining your research questions . It’s important to be as specific as possible, as this will guide the way you choose to collect qualitative research data and the rest of your analysis.
Examples are:
- What are the primary reasons customers are dissatisfied with our product?
- How does X group of users feel about our new feature?
- What are our customers’ needs, and how do they vary by segment?
- How do our products fit into our customers’ lives?
- What factors influence the low feature usage rate of the new feature ?
Step 2: Gather your qualitative customer data
Now, you decide what type of data collection to use based on previously defined goals. Here are 5 methods to collect qualitative data for product companies:
- User feedback
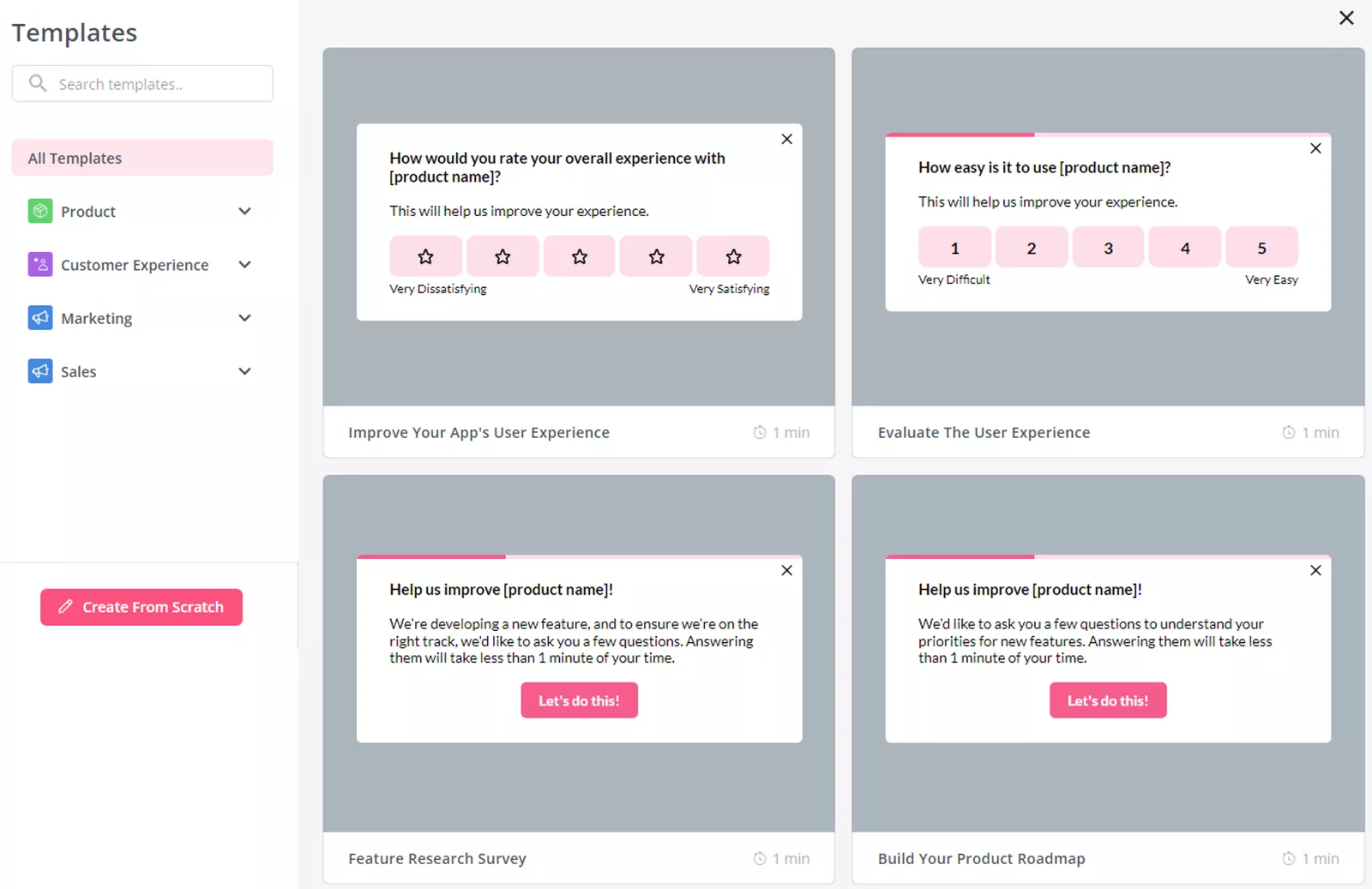
- NPS follow-up questions
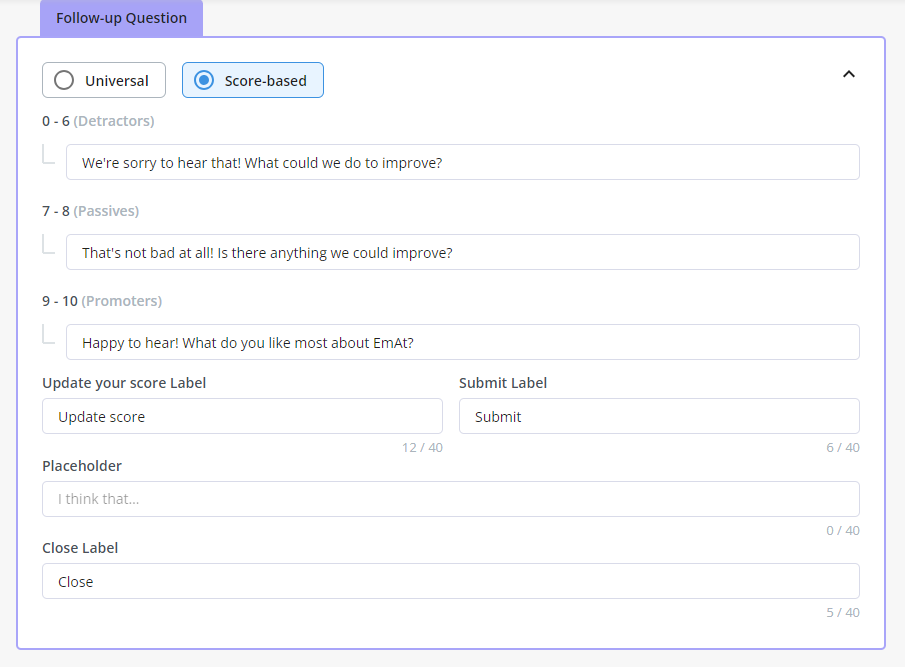
- Review sites
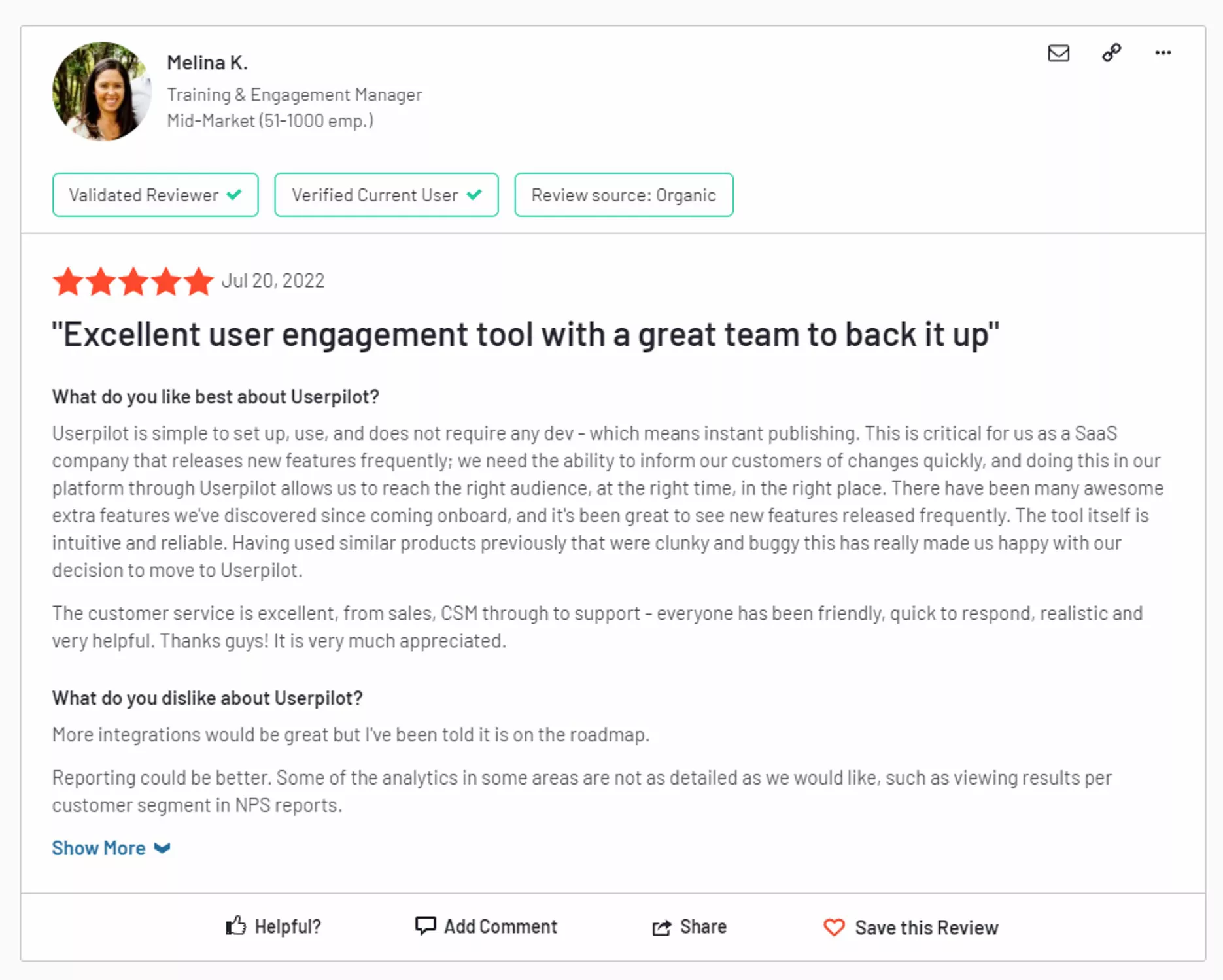
- User interviews
- Focus groups
We recommend using a mix of in-app surveys and in-person interviews. The former helps to collect rich data automatically and on an ongoing basis. You can collect user feedback through in-product surveys, NPS platforms, or use Zoom for live interviews.
The latter enables you to understand the customer experience in the business context as you can ask clarifying questions during the interviews.
Try Userpilot and Easily Collect Qualitative Customer Data
Step 3: organize and categorize collected data.
Before analyzing customer feedback and assigning any value, unstructured feedback data needs to be organized in a single place. This will help you detect patterns and similar themes more easily.
One way to do this is to create a spreadsheet with all the data organized by research questions. Then, arrange the data by theme or category within each research question.
You can also organize NPS responses with Userpilot . This will allow you to quickly calculate scores and see how many promoters, passives, and detractors there are for each research question.
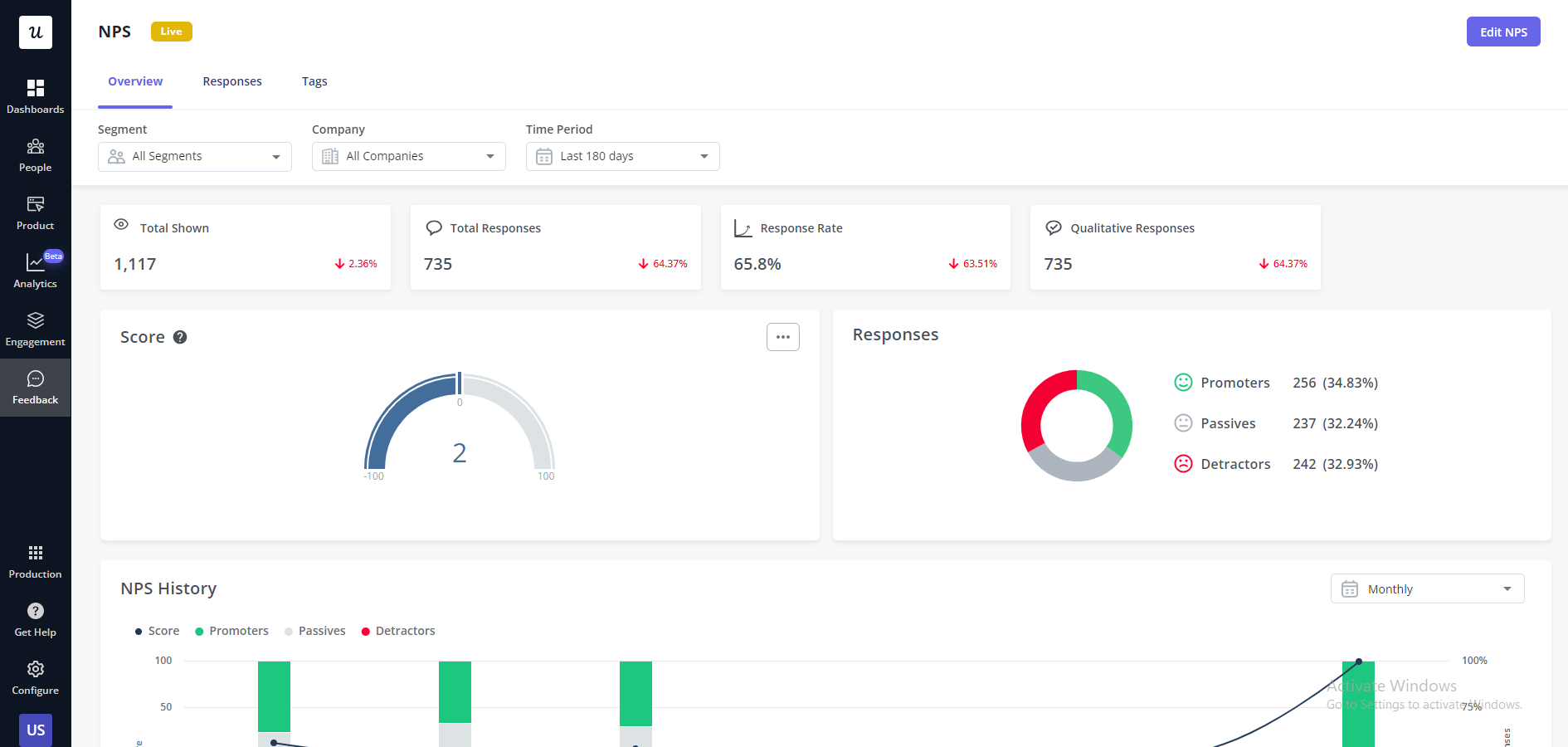
Step 4: Use qualitative data coding to identify themes and patterns
Themes are the building blocks of analysis and help you understand how your data fits together.
For product teams, an NPS survey might reveal the following themes: product defect, pricing, and customer service. Thus, the main themes in SaaS will be around identifying friction points, usability issues, UI issues, UX issues, missing features, etc.
You need to define specific themes and then identify how often they occur. In turn, the pattern is a relationship between 2 or multiple elements (e.g. users who have specific JTBD complain of a specific missing feature).
You can detect those patterns from survey analytics.
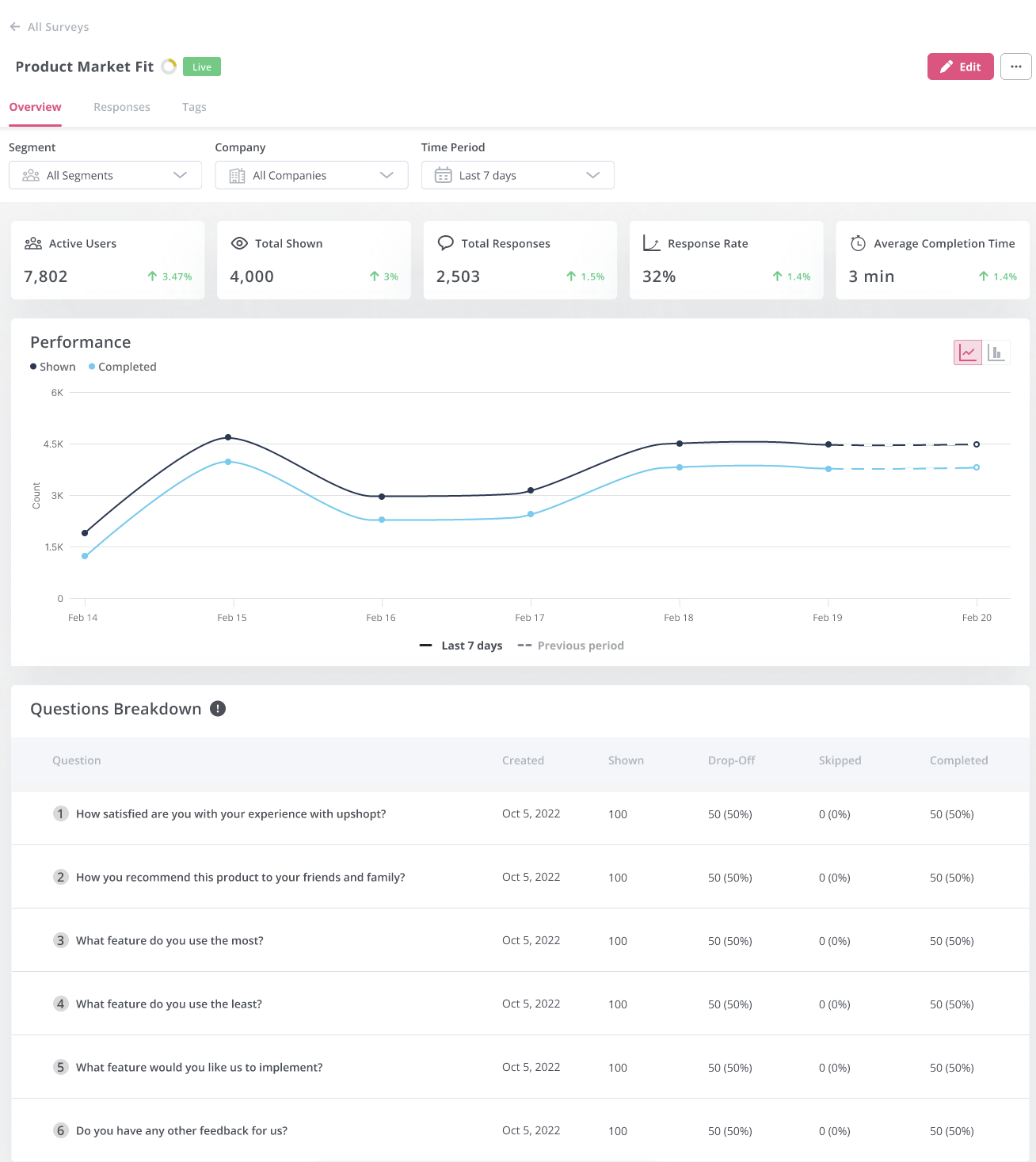
Pair themes with in-app customer behavior and product usage data to understand whether different user segments fall under specific feedback themes.
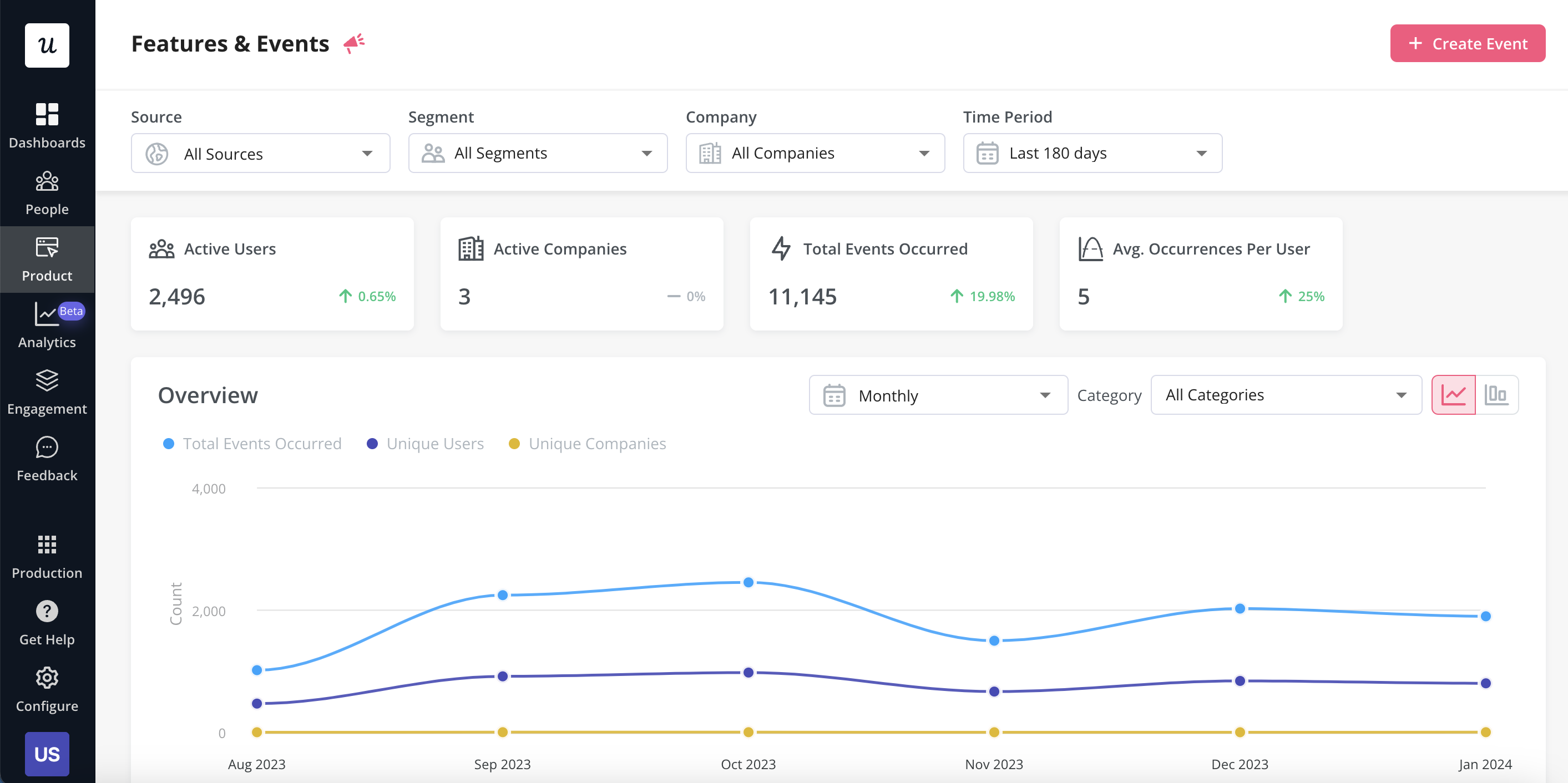
Following this step, you will get enough data to improve customer loyalty .
Step 5: Make hypotheses and test them
The last step in qualitative research is to analyze the data collected to find insights. Segment your users based on in-app behavior, user type, company size, or job to be done to draw meaningful decisions.
For instance, you may notice that negative feedback stems from the customer segment that recently engaged with XYZ features. Just like that, you can pinpoint friction points and the strongest sides of your product to capitalize on.
How to perform qualitative data analysis with Userpilot
Userpilot is a product growth platform that helps product managers collect and analyze qualitative data. It offers a suite of features to make it easy to understand how users interact with your product, their needs, and how you can improve user experience.
When it comes to performing qualitative research, Userpilot is not a qualitative data analysis software but it has some very useful features you could use.
Collect qualitative feedback from users with in-app surveys
Userpilot facilitates the collection of qualitative feedback from users through in-app surveys.
These surveys can be strategically placed within your application to gather insights directly from users while they interact with your product.
By leveraging Userpilot’s in-app survey feature, you can gather valuable feedback on user experiences, preferences, pain points , and suggestions for improvement.
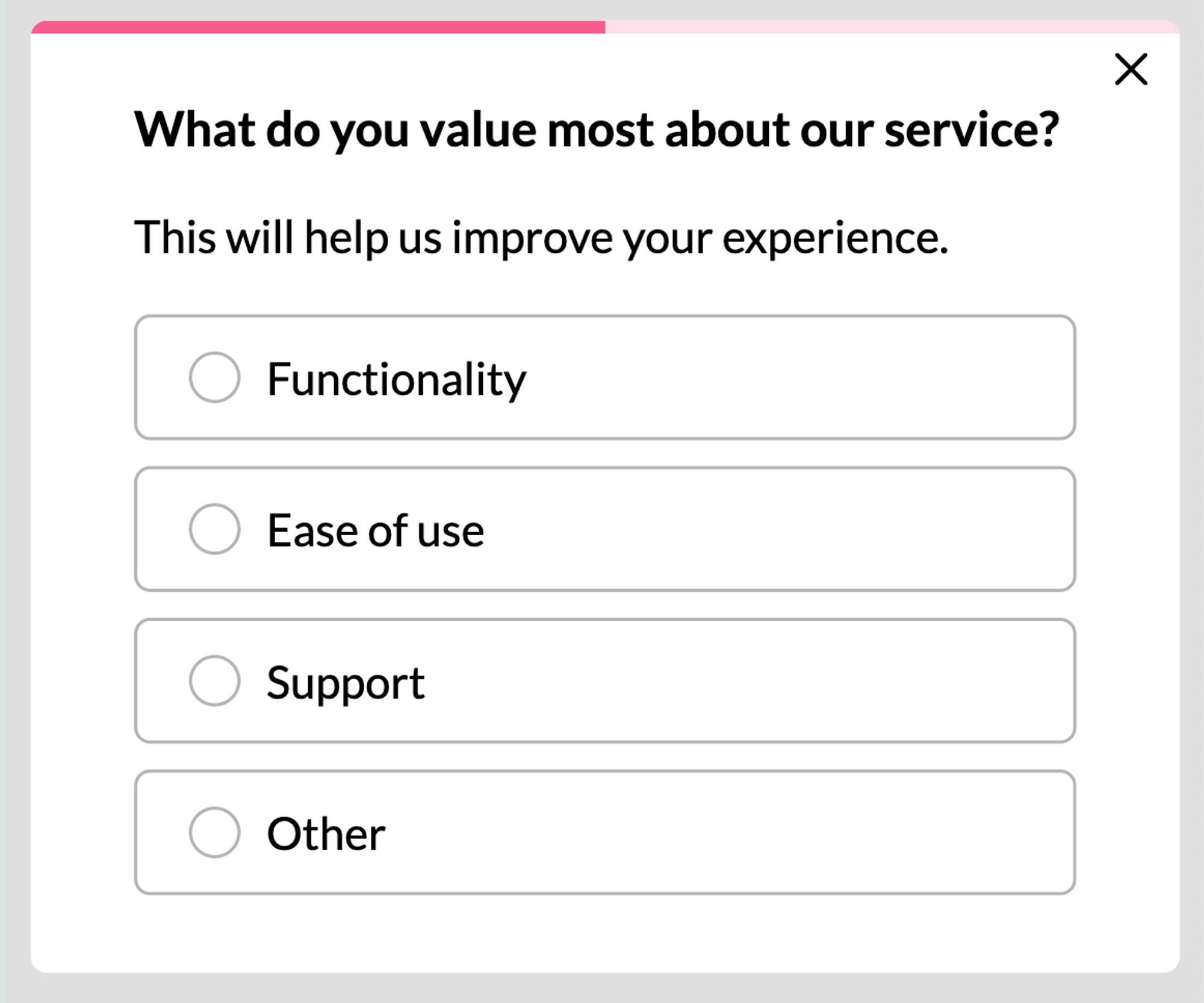
Benefit from NPS dashboard and survey analytics
With Userpilot, you can harness the power of the NPS (Net Promoter Score) dashboard and survey analytics to gain valuable insights into user sentiment and satisfaction levels.
The NPS dashboard provides a comprehensive overview of your NPS scores over time, allowing you to track changes and trends in user loyalty and advocacy.
Additionally, Userpilot’s survey analytics offer detailed insights into survey responses, enabling you to identify common themes, uncover actionable feedback, and prioritize areas for improvement.
Build different in-app experiences based on the insights from qualitative data analysis
By analyzing qualitative feedback collected through in-app surveys, you can segment users based on these insights and create targeted in-app experiences designed to address specific user concerns or enhance key workflows.
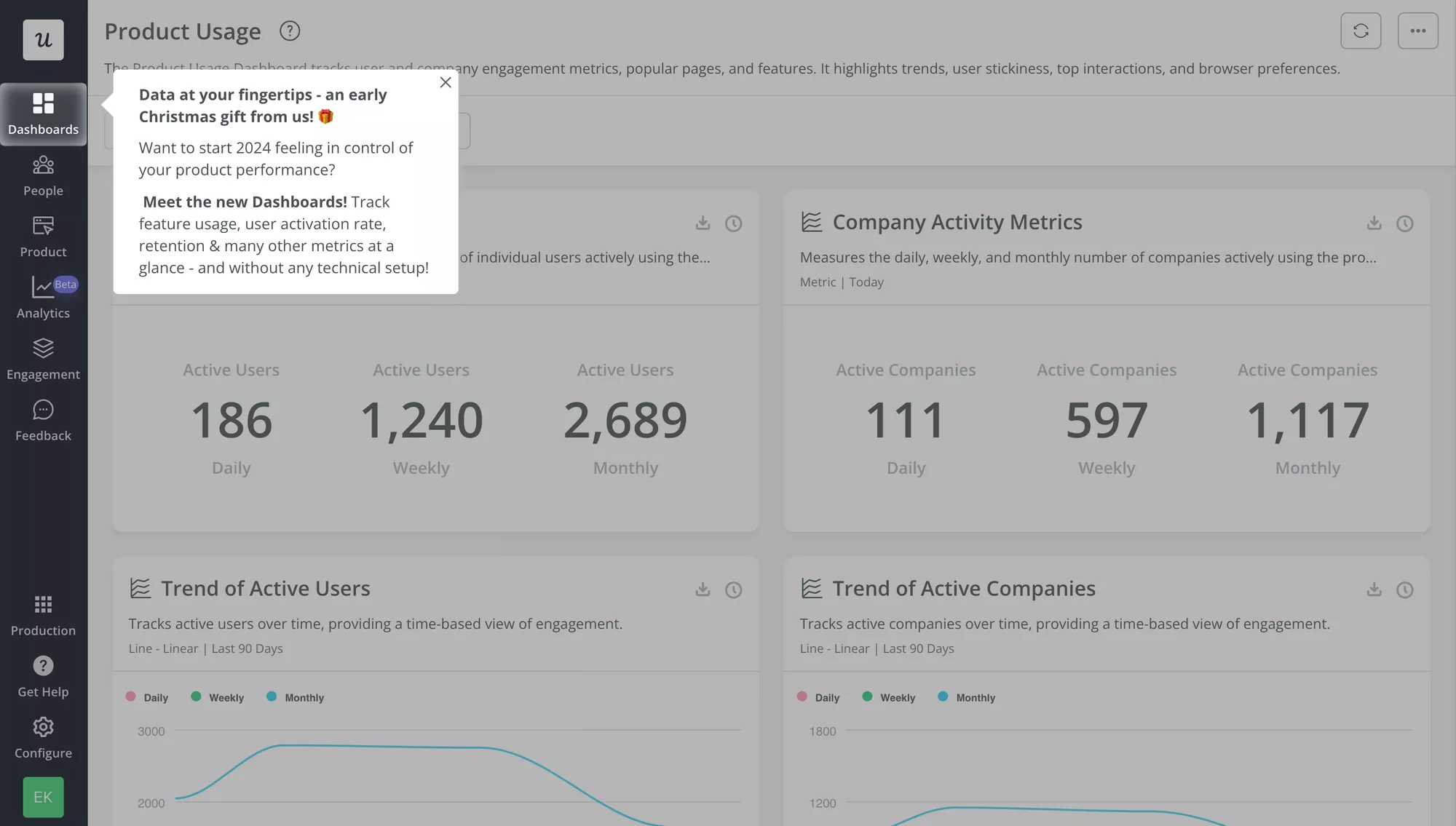
Whether it’s guiding users through new features, addressing common user challenges, or personalizing the user journey based on individual preferences, Userpilot empowers you to deliver a more engaging and personalized user experience that drives user satisfaction and product adoption.
The qualitative data analysis process is iterative and should be revisited as new data is collected. The goal is to constantly refine your understanding of your customer base and how they interact with your product.
Want to get started with qualitative analysis? Get a Userpilot Demo and automate the data collection process. Save time on mundane work and understand your customers better!
Try Userpilot and Take Your Qualitative Data Analysis to the Next Level
Leave a comment cancel reply.
Save my name, email, and website in this browser for the next time I comment.
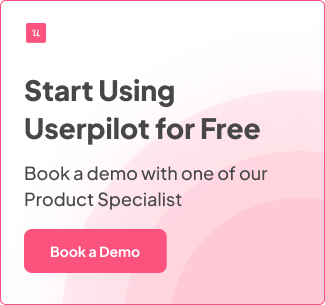
Get The Insights!
The fastest way to learn about Product Growth,Management & Trends.
The coolest way to learn about Product Growth, Management & Trends. Delivered fresh to your inbox, weekly.

The fastest way to learn about Product Growth, Management & Trends.
You might also be interested in ...
How to increase survey response rates: 18 actionable tips.
Adina Timar
50+ Best User Onboarding Tools for SaaS [Updated for 2024 & Categorized]
Userpilot Content Team
SaaS Product Adoption: The Ultimate Guide
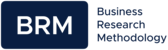
Qualitative Data Analysis
Qualitative data refers to non-numeric information such as interview transcripts, notes, video and audio recordings, images and text documents. Qualitative data analysis can be divided into the following five categories:
1. Content analysis . This refers to the process of categorizing verbal or behavioural data to classify, summarize and tabulate the data.
2. Narrative analysis . This method involves the reformulation of stories presented by respondents taking into account context of each case and different experiences of each respondent. In other words, narrative analysis is the revision of primary qualitative data by researcher.
3. Discourse analysis . A method of analysis of naturally occurring talk and all types of written text.
4. Framework analysis . This is more advanced method that consists of several stages such as familiarization, identifying a thematic framework, coding, charting, mapping and interpretation.
5. Grounded theory . This method of qualitative data analysis starts with an analysis of a single case to formulate a theory. Then, additional cases are examined to see if they contribute to the theory.
Qualitative data analysis can be conducted through the following three steps:
Step 1: Developing and Applying Codes . Coding can be explained as categorization of data. A ‘code’ can be a word or a short phrase that represents a theme or an idea. All codes need to be assigned meaningful titles. A wide range of non-quantifiable elements such as events, behaviours, activities, meanings etc. can be coded.
There are three types of coding:
- Open coding . The initial organization of raw data to try to make sense of it.
- Axial coding . Interconnecting and linking the categories of codes.
- Selective coding . Formulating the story through connecting the categories.
Coding can be done manually or using qualitative data analysis software such as
NVivo, Atlas ti 6.0, HyperRESEARCH 2.8, Max QDA and others.
When using manual coding you can use folders, filing cabinets, wallets etc. to gather together materials that are examples of similar themes or analytic ideas. Manual method of coding in qualitative data analysis is rightly considered as labour-intensive, time-consuming and outdated.
In computer-based coding, on the other hand, physical files and cabinets are replaced with computer based directories and files. When choosing software for qualitative data analysis you need to consider a wide range of factors such as the type and amount of data you need to analyse, time required to master the software and cost considerations.
Moreover, it is important to get confirmation from your dissertation supervisor prior to application of any specific qualitative data analysis software.
The following table contains examples of research titles, elements to be coded and identification of relevant codes:
Qualitative data coding
Step 2: Identifying themes, patterns and relationships . Unlike quantitative methods , in qualitative data analysis there are no universally applicable techniques that can be applied to generate findings. Analytical and critical thinking skills of researcher plays significant role in data analysis in qualitative studies. Therefore, no qualitative study can be repeated to generate the same results.
Nevertheless, there is a set of techniques that you can use to identify common themes, patterns and relationships within responses of sample group members in relation to codes that have been specified in the previous stage.
Specifically, the most popular and effective methods of qualitative data interpretation include the following:
- Word and phrase repetitions – scanning primary data for words and phrases most commonly used by respondents, as well as, words and phrases used with unusual emotions;
- Primary and secondary data comparisons – comparing the findings of interview/focus group/observation/any other qualitative data collection method with the findings of literature review and discussing differences between them;
- Search for missing information – discussions about which aspects of the issue was not mentioned by respondents, although you expected them to be mentioned;
- Metaphors and analogues – comparing primary research findings to phenomena from a different area and discussing similarities and differences.
Step 3: Summarizing the data . At this last stage you need to link research findings to hypotheses or research aim and objectives. When writing data analysis chapter, you can use noteworthy quotations from the transcript in order to highlight major themes within findings and possible contradictions.
It is important to note that the process of qualitative data analysis described above is general and different types of qualitative studies may require slightly different methods of data analysis.
My e-book, The Ultimate Guide to Writing a Dissertation in Business Studies: a step by step approach contains a detailed, yet simple explanation of qualitative data analysis methods . The e-book explains all stages of the research process starting from the selection of the research area to writing personal reflection. Important elements of dissertations such as research philosophy, research approach, research design, methods of data collection and data analysis are explained in simple words. John Dudovskiy
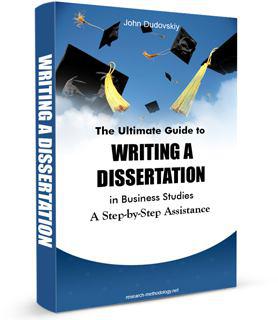
Learn / Guides / Qualitative data analysis guide
Back to guides
5 qualitative data analysis methods
Qualitative data uncovers valuable insights that help you improve the user and customer experience. But how exactly do you measure and analyze data that isn't quantifiable?
There are different qualitative data analysis methods to help you make sense of qualitative feedback and customer insights, depending on your business goals and the type of data you've collected.
Before you choose a qualitative data analysis method for your team, you need to consider the available techniques and explore their use cases to understand how each process might help you better understand your users.
This guide covers five qualitative analysis methods to choose from, and will help you pick the right one(s) based on your goals.
Content analysis
Thematic analysis
Narrative analysis
Grounded theory analysis
Discourse analysis
5 qualitative data analysis methods explained
Qualitative data analysis ( QDA ) is the process of organizing, analyzing, and interpreting qualitative research data—non-numeric, conceptual information, and user feedback—to capture themes and patterns, answer research questions, and identify actions to improve your product or website.
Step 1 in the research process (after planning ) is qualitative data collection. You can use behavior analytics software—like Hotjar —to capture qualitative data with context, and learn the real motivation behind user behavior, by collecting written customer feedback with Surveys or scheduling an in-depth user interview with Engage .
Use Hotjar’s tools to collect feedback, uncover behavior trends, and understand the ‘why’ behind user actions.
1. Content analysis
Content analysis is a qualitative research method that examines and quantifies the presence of certain words, subjects, and concepts in text, image, video, or audio messages. The method transforms qualitative input into quantitative data to help you make reliable conclusions about what customers think of your brand, and how you can improve their experience and opinion.
Conduct content analysis manually (which can be time-consuming) or use analysis tools like Lexalytics to reveal communication patterns, uncover differences in individual or group communication trends, and make broader connections between concepts.
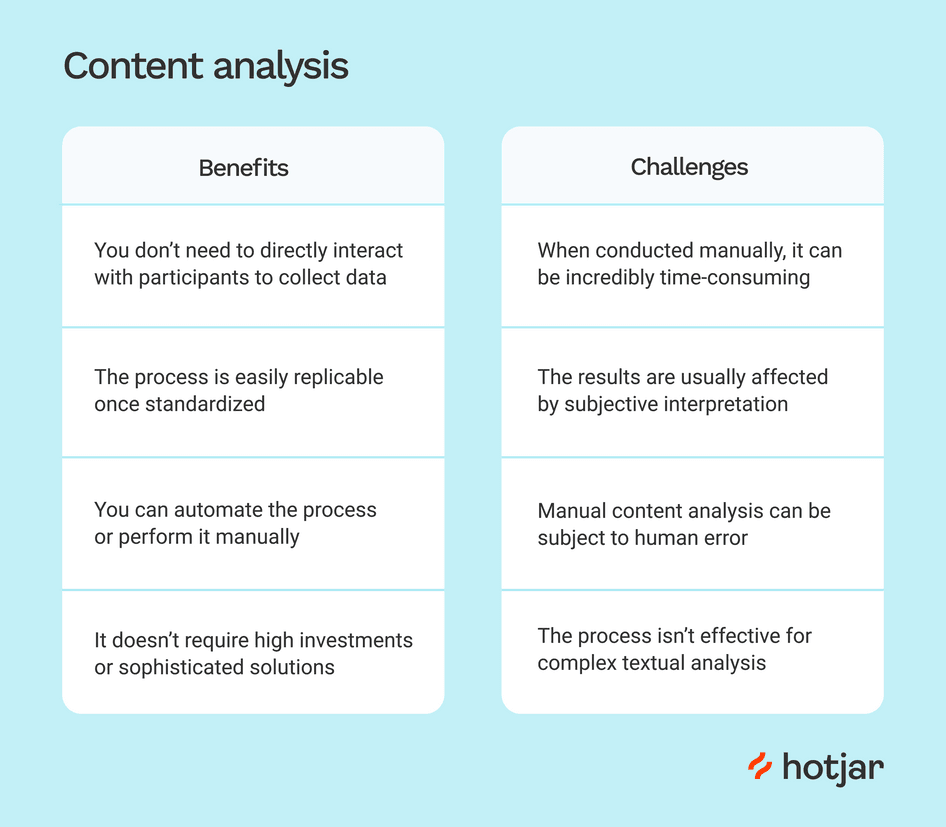
How content analysis can help your team
Content analysis is often used by marketers and customer service specialists, helping them understand customer behavior and measure brand reputation.
For example, you may run a customer survey with open-ended questions to discover users’ concerns—in their own words—about their experience with your product. Instead of having to process hundreds of answers manually, a content analysis tool helps you analyze and group results based on the emotion expressed in texts.
Some other examples of content analysis include:
Analyzing brand mentions on social media to understand your brand's reputation
Reviewing customer feedback to evaluate (and then improve) the customer and user experience (UX)
Researching competitors’ website pages to identify their competitive advantages and value propositions
Interpreting customer interviews and survey results to determine user preferences, and setting the direction for new product or feature developments
Content analysis was a major part of our growth during my time at Hypercontext.
[It gave us] a better understanding of the [blog] topics that performed best for signing new users up. We were also able to go deeper within those blog posts to better understand the formats [that worked].
2. Thematic analysis
Thematic analysis helps you identify, categorize, analyze, and interpret patterns in qualitative study data , and can be done with tools like Dovetail and Thematic .
While content analysis and thematic analysis seem similar, they're different in concept:
Content analysis can be applied to both qualitative and quantitative data , and focuses on identifying frequencies and recurring words and subjects
Thematic analysis can only be applied to qualitative data, and focuses on identifying patterns and themes
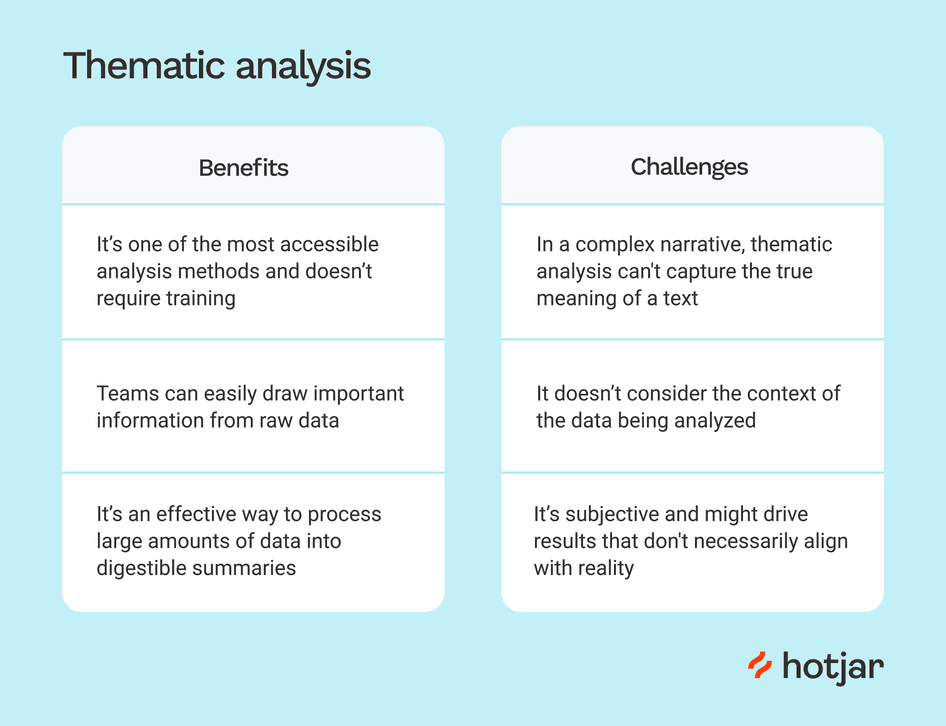
How thematic analysis can help your team
Thematic analysis can be used by pretty much anyone: from product marketers, to customer relationship managers, to UX researchers.
For example, product teams use thematic analysis to better understand user behaviors and needs and improve UX . Analyzing customer feedback lets you identify themes (e.g. poor navigation or a buggy mobile interface) highlighted by users and get actionable insight into what they really expect from the product.
💡 Pro tip: looking for a way to expedite the data analysis process for large amounts of data you collected with a survey? Try Hotjar’s AI for Surveys : along with generating a survey based on your goal in seconds, our AI will analyze the raw data and prepare an automated summary report that presents key thematic findings, respondent quotes, and actionable steps to take, making the analysis of qualitative data a breeze.
3. Narrative analysis
Narrative analysis is a method used to interpret research participants’ stories —things like testimonials , case studies, focus groups, interviews, and other text or visual data—with tools like Delve and AI-powered ATLAS.ti .
Some formats don’t work well with narrative analysis, including heavily structured interviews and written surveys, which don’t give participants as much opportunity to tell their stories in their own words.
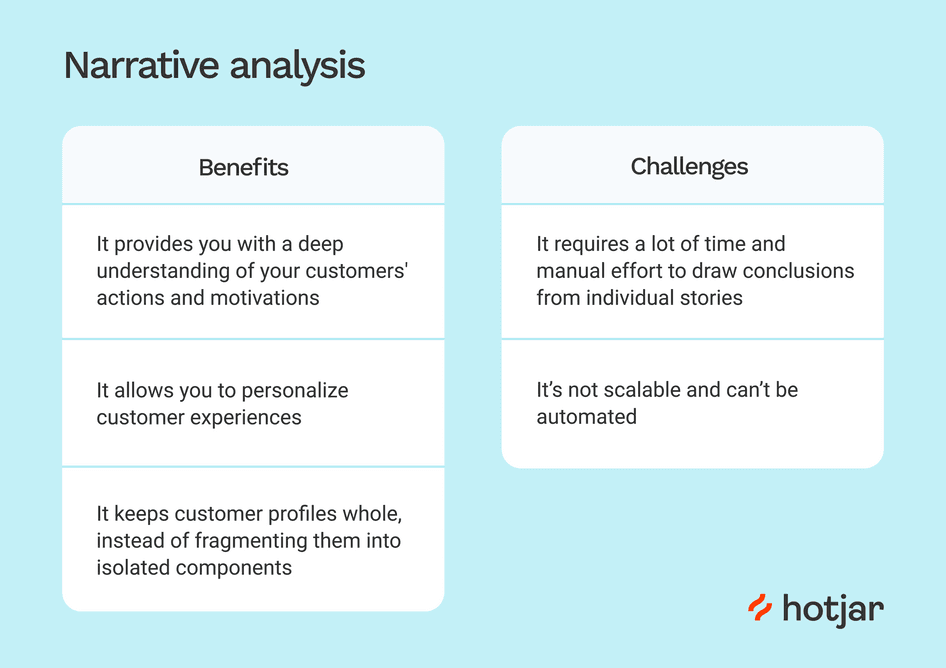
How narrative analysis can help your team
Narrative analysis provides product teams with valuable insight into the complexity of customers’ lives, feelings, and behaviors.
In a marketing research context, narrative analysis involves capturing and reviewing customer stories—on social media, for example—to get in-depth insight into their lives, priorities, and challenges.
This might look like analyzing daily content shared by your audiences’ favorite influencers on Instagram, or analyzing customer reviews on sites like G2 or Capterra to gain a deep understanding of individual customer experiences. The results of this analysis also contribute to developing corresponding customer personas .
💡 Pro tip: conducting user interviews is an excellent way to collect data for narrative analysis. Though interviews can be time-intensive, there are tools out there that streamline the workload.
Hotjar Engage automates the entire process, from recruiting to scheduling to generating the all-important interview transcripts you’ll need for the analysis phase of your research project.
4. Grounded theory analysis
Grounded theory analysis is a method of conducting qualitative research to develop theories by examining real-world data. This technique involves the creation of hypotheses and theories through qualitative data collection and evaluation, and can be performed with qualitative data analysis software tools like MAXQDA and NVivo .
Unlike other qualitative data analysis techniques, this method is inductive rather than deductive: it develops theories from data, not the other way around.
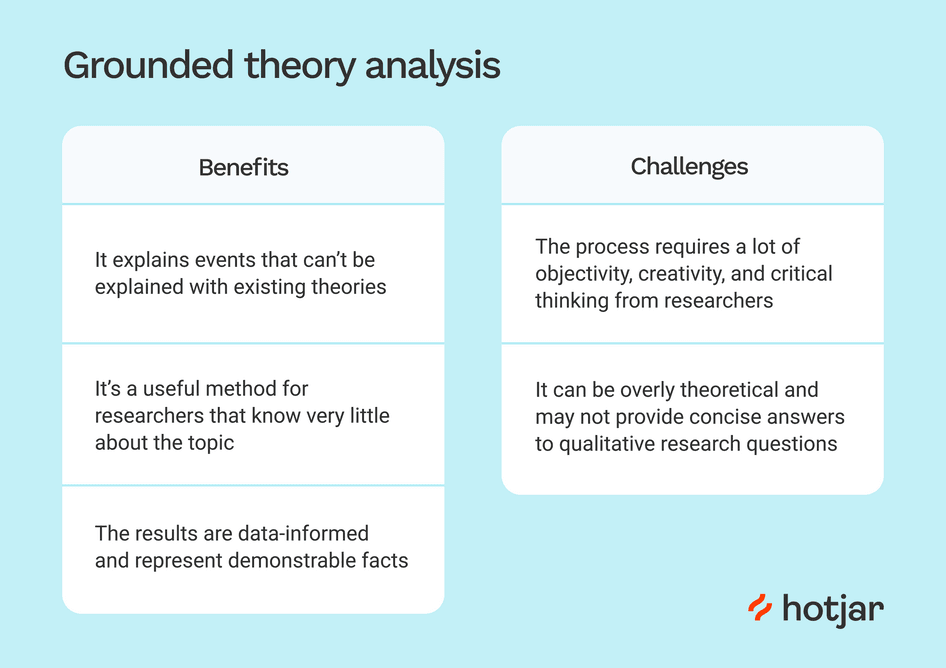
How grounded theory analysis can help your team
Grounded theory analysis is used by software engineers, product marketers, managers, and other specialists who deal with data sets to make informed business decisions.
For example, product marketing teams may turn to customer surveys to understand the reasons behind high churn rates , then use grounded theory to analyze responses and develop hypotheses about why users churn, and how you can get them to stay.
Grounded theory can also be helpful in the talent management process. For example, HR representatives may use it to develop theories about low employee engagement, and come up with solutions based on their research findings.
5. Discourse analysis
Discourse analysis is the act of researching the underlying meaning of qualitative data. It involves the observation of texts, audio, and videos to study the relationships between information and its social context.
In contrast to content analysis, this method focuses on the contextual meaning of language: discourse analysis sheds light on what audiences think of a topic, and why they feel the way they do about it.
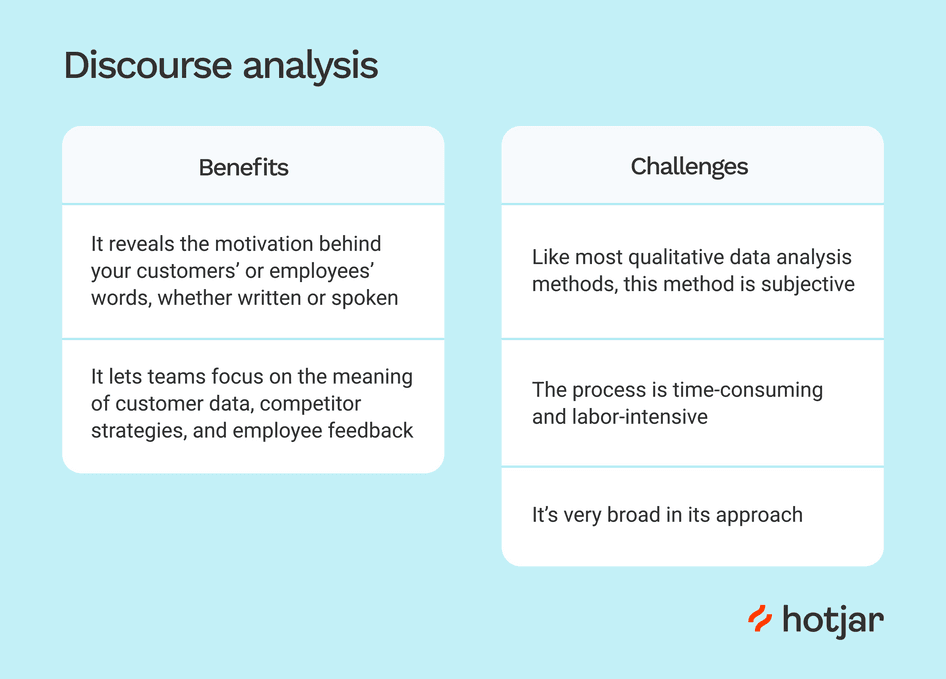
How discourse analysis can help your team
In a business context, this method is primarily used by marketing teams. Discourse analysis helps marketers understand the norms and ideas in their market , and reveals why they play such a significant role for their customers.
Once the origins of trends are uncovered, it’s easier to develop a company mission, create a unique tone of voice, and craft effective marketing messages.
Which qualitative data analysis method should you choose?
While the five qualitative data analysis methods we list above are all aimed at processing data and answering research questions, these techniques differ in their intent and the approaches applied.
Choosing the right analysis method for your team isn't a matter of preference—selecting a method that fits is only possible once you define your research goals and have a clear intention. When you know what you need (and why you need it), you can identify an analysis method that aligns with your research objectives.
Gather qualitative data with Hotjar
Use Hotjar’s product experience insights in your qualitative research. Collect feedback, uncover behavior trends, and understand the ‘why’ behind user actions.
FAQs about qualitative data analysis methods
What is the qualitative data analysis approach.
The qualitative data analysis approach refers to the process of systematizing descriptive data collected through interviews, focus groups, surveys, and observations and then interpreting it. The methodology aims to identify patterns and themes behind textual data, and other unquantifiable data, as opposed to numerical data.
What are qualitative data analysis methods?
Five popular qualitative data analysis methods are:
What is the process of qualitative data analysis?
The process of qualitative data analysis includes six steps:
Define your research question
Prepare the data
Choose the method of qualitative analysis
Code the data
Identify themes, patterns, and relationships
Make hypotheses and act
Qualitative data analysis guide
Previous chapter
QDA challenges
Next chapter
News alert: UC Berkeley has announced its next university librarian
Secondary menu
- Log in to your Library account
- Hours and Maps
- Connect from Off Campus
- UC Berkeley Home
Search form
Research methods--quantitative, qualitative, and more: overview.
- Quantitative Research
- Qualitative Research
- Data Science Methods (Machine Learning, AI, Big Data)
- Text Mining and Computational Text Analysis
- Evidence Synthesis/Systematic Reviews
- Get Data, Get Help!
About Research Methods
This guide provides an overview of research methods, how to choose and use them, and supports and resources at UC Berkeley.
As Patten and Newhart note in the book Understanding Research Methods , "Research methods are the building blocks of the scientific enterprise. They are the "how" for building systematic knowledge. The accumulation of knowledge through research is by its nature a collective endeavor. Each well-designed study provides evidence that may support, amend, refute, or deepen the understanding of existing knowledge...Decisions are important throughout the practice of research and are designed to help researchers collect evidence that includes the full spectrum of the phenomenon under study, to maintain logical rules, and to mitigate or account for possible sources of bias. In many ways, learning research methods is learning how to see and make these decisions."
The choice of methods varies by discipline, by the kind of phenomenon being studied and the data being used to study it, by the technology available, and more. This guide is an introduction, but if you don't see what you need here, always contact your subject librarian, and/or take a look to see if there's a library research guide that will answer your question.
Suggestions for changes and additions to this guide are welcome!
START HERE: SAGE Research Methods
Without question, the most comprehensive resource available from the library is SAGE Research Methods. HERE IS THE ONLINE GUIDE to this one-stop shopping collection, and some helpful links are below:
- SAGE Research Methods
- Little Green Books (Quantitative Methods)
- Little Blue Books (Qualitative Methods)
- Dictionaries and Encyclopedias
- Case studies of real research projects
- Sample datasets for hands-on practice
- Streaming video--see methods come to life
- Methodspace- -a community for researchers
- SAGE Research Methods Course Mapping
Library Data Services at UC Berkeley
Library Data Services Program and Digital Scholarship Services
The LDSP offers a variety of services and tools ! From this link, check out pages for each of the following topics: discovering data, managing data, collecting data, GIS data, text data mining, publishing data, digital scholarship, open science, and the Research Data Management Program.
Be sure also to check out the visual guide to where to seek assistance on campus with any research question you may have!
Library GIS Services
Other Data Services at Berkeley
D-Lab Supports Berkeley faculty, staff, and graduate students with research in data intensive social science, including a wide range of training and workshop offerings Dryad Dryad is a simple self-service tool for researchers to use in publishing their datasets. It provides tools for the effective publication of and access to research data. Geospatial Innovation Facility (GIF) Provides leadership and training across a broad array of integrated mapping technologies on campu Research Data Management A UC Berkeley guide and consulting service for research data management issues
General Research Methods Resources
Here are some general resources for assistance:
- Assistance from ICPSR (must create an account to access): Getting Help with Data , and Resources for Students
- Wiley Stats Ref for background information on statistics topics
- Survey Documentation and Analysis (SDA) . Program for easy web-based analysis of survey data.
Consultants
- D-Lab/Data Science Discovery Consultants Request help with your research project from peer consultants.
- Research data (RDM) consulting Meet with RDM consultants before designing the data security, storage, and sharing aspects of your qualitative project.
- Statistics Department Consulting Services A service in which advanced graduate students, under faculty supervision, are available to consult during specified hours in the Fall and Spring semesters.
Related Resourcex
- IRB / CPHS Qualitative research projects with human subjects often require that you go through an ethics review.
- OURS (Office of Undergraduate Research and Scholarships) OURS supports undergraduates who want to embark on research projects and assistantships. In particular, check out their "Getting Started in Research" workshops
- Sponsored Projects Sponsored projects works with researchers applying for major external grants.
- Next: Quantitative Research >>
- Last Updated: Apr 25, 2024 11:09 AM
- URL: https://guides.lib.berkeley.edu/researchmethods
Your Modern Business Guide To Data Analysis Methods And Techniques

Table of Contents
1) What Is Data Analysis?
2) Why Is Data Analysis Important?
3) What Is The Data Analysis Process?
4) Types Of Data Analysis Methods
5) Top Data Analysis Techniques To Apply
6) Quality Criteria For Data Analysis
7) Data Analysis Limitations & Barriers
8) Data Analysis Skills
9) Data Analysis In The Big Data Environment
In our data-rich age, understanding how to analyze and extract true meaning from our business’s digital insights is one of the primary drivers of success.
Despite the colossal volume of data we create every day, a mere 0.5% is actually analyzed and used for data discovery , improvement, and intelligence. While that may not seem like much, considering the amount of digital information we have at our fingertips, half a percent still accounts for a vast amount of data.
With so much data and so little time, knowing how to collect, curate, organize, and make sense of all of this potentially business-boosting information can be a minefield – but online data analysis is the solution.
In science, data analysis uses a more complex approach with advanced techniques to explore and experiment with data. On the other hand, in a business context, data is used to make data-driven decisions that will enable the company to improve its overall performance. In this post, we will cover the analysis of data from an organizational point of view while still going through the scientific and statistical foundations that are fundamental to understanding the basics of data analysis.
To put all of that into perspective, we will answer a host of important analytical questions, explore analytical methods and techniques, while demonstrating how to perform analysis in the real world with a 17-step blueprint for success.
What Is Data Analysis?
Data analysis is the process of collecting, modeling, and analyzing data using various statistical and logical methods and techniques. Businesses rely on analytics processes and tools to extract insights that support strategic and operational decision-making.
All these various methods are largely based on two core areas: quantitative and qualitative research.
To explain the key differences between qualitative and quantitative research, here’s a video for your viewing pleasure:
Gaining a better understanding of different techniques and methods in quantitative research as well as qualitative insights will give your analyzing efforts a more clearly defined direction, so it’s worth taking the time to allow this particular knowledge to sink in. Additionally, you will be able to create a comprehensive analytical report that will skyrocket your analysis.
Apart from qualitative and quantitative categories, there are also other types of data that you should be aware of before dividing into complex data analysis processes. These categories include:
- Big data: Refers to massive data sets that need to be analyzed using advanced software to reveal patterns and trends. It is considered to be one of the best analytical assets as it provides larger volumes of data at a faster rate.
- Metadata: Putting it simply, metadata is data that provides insights about other data. It summarizes key information about specific data that makes it easier to find and reuse for later purposes.
- Real time data: As its name suggests, real time data is presented as soon as it is acquired. From an organizational perspective, this is the most valuable data as it can help you make important decisions based on the latest developments. Our guide on real time analytics will tell you more about the topic.
- Machine data: This is more complex data that is generated solely by a machine such as phones, computers, or even websites and embedded systems, without previous human interaction.
Why Is Data Analysis Important?
Before we go into detail about the categories of analysis along with its methods and techniques, you must understand the potential that analyzing data can bring to your organization.
- Informed decision-making : From a management perspective, you can benefit from analyzing your data as it helps you make decisions based on facts and not simple intuition. For instance, you can understand where to invest your capital, detect growth opportunities, predict your income, or tackle uncommon situations before they become problems. Through this, you can extract relevant insights from all areas in your organization, and with the help of dashboard software , present the data in a professional and interactive way to different stakeholders.
- Reduce costs : Another great benefit is to reduce costs. With the help of advanced technologies such as predictive analytics, businesses can spot improvement opportunities, trends, and patterns in their data and plan their strategies accordingly. In time, this will help you save money and resources on implementing the wrong strategies. And not just that, by predicting different scenarios such as sales and demand you can also anticipate production and supply.
- Target customers better : Customers are arguably the most crucial element in any business. By using analytics to get a 360° vision of all aspects related to your customers, you can understand which channels they use to communicate with you, their demographics, interests, habits, purchasing behaviors, and more. In the long run, it will drive success to your marketing strategies, allow you to identify new potential customers, and avoid wasting resources on targeting the wrong people or sending the wrong message. You can also track customer satisfaction by analyzing your client’s reviews or your customer service department’s performance.
What Is The Data Analysis Process?

When we talk about analyzing data there is an order to follow in order to extract the needed conclusions. The analysis process consists of 5 key stages. We will cover each of them more in detail later in the post, but to start providing the needed context to understand what is coming next, here is a rundown of the 5 essential steps of data analysis.
- Identify: Before you get your hands dirty with data, you first need to identify why you need it in the first place. The identification is the stage in which you establish the questions you will need to answer. For example, what is the customer's perception of our brand? Or what type of packaging is more engaging to our potential customers? Once the questions are outlined you are ready for the next step.
- Collect: As its name suggests, this is the stage where you start collecting the needed data. Here, you define which sources of data you will use and how you will use them. The collection of data can come in different forms such as internal or external sources, surveys, interviews, questionnaires, and focus groups, among others. An important note here is that the way you collect the data will be different in a quantitative and qualitative scenario.
- Clean: Once you have the necessary data it is time to clean it and leave it ready for analysis. Not all the data you collect will be useful, when collecting big amounts of data in different formats it is very likely that you will find yourself with duplicate or badly formatted data. To avoid this, before you start working with your data you need to make sure to erase any white spaces, duplicate records, or formatting errors. This way you avoid hurting your analysis with bad-quality data.
- Analyze : With the help of various techniques such as statistical analysis, regressions, neural networks, text analysis, and more, you can start analyzing and manipulating your data to extract relevant conclusions. At this stage, you find trends, correlations, variations, and patterns that can help you answer the questions you first thought of in the identify stage. Various technologies in the market assist researchers and average users with the management of their data. Some of them include business intelligence and visualization software, predictive analytics, and data mining, among others.
- Interpret: Last but not least you have one of the most important steps: it is time to interpret your results. This stage is where the researcher comes up with courses of action based on the findings. For example, here you would understand if your clients prefer packaging that is red or green, plastic or paper, etc. Additionally, at this stage, you can also find some limitations and work on them.
Now that you have a basic understanding of the key data analysis steps, let’s look at the top 17 essential methods.
17 Essential Types Of Data Analysis Methods
Before diving into the 17 essential types of methods, it is important that we go over really fast through the main analysis categories. Starting with the category of descriptive up to prescriptive analysis, the complexity and effort of data evaluation increases, but also the added value for the company.
a) Descriptive analysis - What happened.
The descriptive analysis method is the starting point for any analytic reflection, and it aims to answer the question of what happened? It does this by ordering, manipulating, and interpreting raw data from various sources to turn it into valuable insights for your organization.
Performing descriptive analysis is essential, as it enables us to present our insights in a meaningful way. Although it is relevant to mention that this analysis on its own will not allow you to predict future outcomes or tell you the answer to questions like why something happened, it will leave your data organized and ready to conduct further investigations.
b) Exploratory analysis - How to explore data relationships.
As its name suggests, the main aim of the exploratory analysis is to explore. Prior to it, there is still no notion of the relationship between the data and the variables. Once the data is investigated, exploratory analysis helps you to find connections and generate hypotheses and solutions for specific problems. A typical area of application for it is data mining.
c) Diagnostic analysis - Why it happened.
Diagnostic data analytics empowers analysts and executives by helping them gain a firm contextual understanding of why something happened. If you know why something happened as well as how it happened, you will be able to pinpoint the exact ways of tackling the issue or challenge.
Designed to provide direct and actionable answers to specific questions, this is one of the world’s most important methods in research, among its other key organizational functions such as retail analytics , e.g.
c) Predictive analysis - What will happen.
The predictive method allows you to look into the future to answer the question: what will happen? In order to do this, it uses the results of the previously mentioned descriptive, exploratory, and diagnostic analysis, in addition to machine learning (ML) and artificial intelligence (AI). Through this, you can uncover future trends, potential problems or inefficiencies, connections, and casualties in your data.
With predictive analysis, you can unfold and develop initiatives that will not only enhance your various operational processes but also help you gain an all-important edge over the competition. If you understand why a trend, pattern, or event happened through data, you will be able to develop an informed projection of how things may unfold in particular areas of the business.
e) Prescriptive analysis - How will it happen.
Another of the most effective types of analysis methods in research. Prescriptive data techniques cross over from predictive analysis in the way that it revolves around using patterns or trends to develop responsive, practical business strategies.
By drilling down into prescriptive analysis, you will play an active role in the data consumption process by taking well-arranged sets of visual data and using it as a powerful fix to emerging issues in a number of key areas, including marketing, sales, customer experience, HR, fulfillment, finance, logistics analytics , and others.

As mentioned at the beginning of the post, data analysis methods can be divided into two big categories: quantitative and qualitative. Each of these categories holds a powerful analytical value that changes depending on the scenario and type of data you are working with. Below, we will discuss 17 methods that are divided into qualitative and quantitative approaches.
Without further ado, here are the 17 essential types of data analysis methods with some use cases in the business world:
A. Quantitative Methods
To put it simply, quantitative analysis refers to all methods that use numerical data or data that can be turned into numbers (e.g. category variables like gender, age, etc.) to extract valuable insights. It is used to extract valuable conclusions about relationships, differences, and test hypotheses. Below we discuss some of the key quantitative methods.
1. Cluster analysis
The action of grouping a set of data elements in a way that said elements are more similar (in a particular sense) to each other than to those in other groups – hence the term ‘cluster.’ Since there is no target variable when clustering, the method is often used to find hidden patterns in the data. The approach is also used to provide additional context to a trend or dataset.
Let's look at it from an organizational perspective. In a perfect world, marketers would be able to analyze each customer separately and give them the best-personalized service, but let's face it, with a large customer base, it is timely impossible to do that. That's where clustering comes in. By grouping customers into clusters based on demographics, purchasing behaviors, monetary value, or any other factor that might be relevant for your company, you will be able to immediately optimize your efforts and give your customers the best experience based on their needs.
2. Cohort analysis
This type of data analysis approach uses historical data to examine and compare a determined segment of users' behavior, which can then be grouped with others with similar characteristics. By using this methodology, it's possible to gain a wealth of insight into consumer needs or a firm understanding of a broader target group.
Cohort analysis can be really useful for performing analysis in marketing as it will allow you to understand the impact of your campaigns on specific groups of customers. To exemplify, imagine you send an email campaign encouraging customers to sign up for your site. For this, you create two versions of the campaign with different designs, CTAs, and ad content. Later on, you can use cohort analysis to track the performance of the campaign for a longer period of time and understand which type of content is driving your customers to sign up, repurchase, or engage in other ways.
A useful tool to start performing cohort analysis method is Google Analytics. You can learn more about the benefits and limitations of using cohorts in GA in this useful guide . In the bottom image, you see an example of how you visualize a cohort in this tool. The segments (devices traffic) are divided into date cohorts (usage of devices) and then analyzed week by week to extract insights into performance.
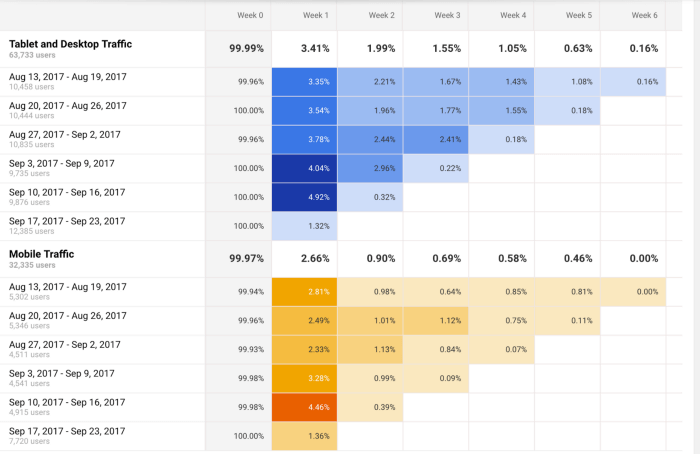
3. Regression analysis
Regression uses historical data to understand how a dependent variable's value is affected when one (linear regression) or more independent variables (multiple regression) change or stay the same. By understanding each variable's relationship and how it developed in the past, you can anticipate possible outcomes and make better decisions in the future.
Let's bring it down with an example. Imagine you did a regression analysis of your sales in 2019 and discovered that variables like product quality, store design, customer service, marketing campaigns, and sales channels affected the overall result. Now you want to use regression to analyze which of these variables changed or if any new ones appeared during 2020. For example, you couldn’t sell as much in your physical store due to COVID lockdowns. Therefore, your sales could’ve either dropped in general or increased in your online channels. Through this, you can understand which independent variables affected the overall performance of your dependent variable, annual sales.
If you want to go deeper into this type of analysis, check out this article and learn more about how you can benefit from regression.
4. Neural networks
The neural network forms the basis for the intelligent algorithms of machine learning. It is a form of analytics that attempts, with minimal intervention, to understand how the human brain would generate insights and predict values. Neural networks learn from each and every data transaction, meaning that they evolve and advance over time.
A typical area of application for neural networks is predictive analytics. There are BI reporting tools that have this feature implemented within them, such as the Predictive Analytics Tool from datapine. This tool enables users to quickly and easily generate all kinds of predictions. All you have to do is select the data to be processed based on your KPIs, and the software automatically calculates forecasts based on historical and current data. Thanks to its user-friendly interface, anyone in your organization can manage it; there’s no need to be an advanced scientist.
Here is an example of how you can use the predictive analysis tool from datapine:

**click to enlarge**
5. Factor analysis
The factor analysis also called “dimension reduction” is a type of data analysis used to describe variability among observed, correlated variables in terms of a potentially lower number of unobserved variables called factors. The aim here is to uncover independent latent variables, an ideal method for streamlining specific segments.
A good way to understand this data analysis method is a customer evaluation of a product. The initial assessment is based on different variables like color, shape, wearability, current trends, materials, comfort, the place where they bought the product, and frequency of usage. Like this, the list can be endless, depending on what you want to track. In this case, factor analysis comes into the picture by summarizing all of these variables into homogenous groups, for example, by grouping the variables color, materials, quality, and trends into a brother latent variable of design.
If you want to start analyzing data using factor analysis we recommend you take a look at this practical guide from UCLA.
6. Data mining
A method of data analysis that is the umbrella term for engineering metrics and insights for additional value, direction, and context. By using exploratory statistical evaluation, data mining aims to identify dependencies, relations, patterns, and trends to generate advanced knowledge. When considering how to analyze data, adopting a data mining mindset is essential to success - as such, it’s an area that is worth exploring in greater detail.
An excellent use case of data mining is datapine intelligent data alerts . With the help of artificial intelligence and machine learning, they provide automated signals based on particular commands or occurrences within a dataset. For example, if you’re monitoring supply chain KPIs , you could set an intelligent alarm to trigger when invalid or low-quality data appears. By doing so, you will be able to drill down deep into the issue and fix it swiftly and effectively.
In the following picture, you can see how the intelligent alarms from datapine work. By setting up ranges on daily orders, sessions, and revenues, the alarms will notify you if the goal was not completed or if it exceeded expectations.
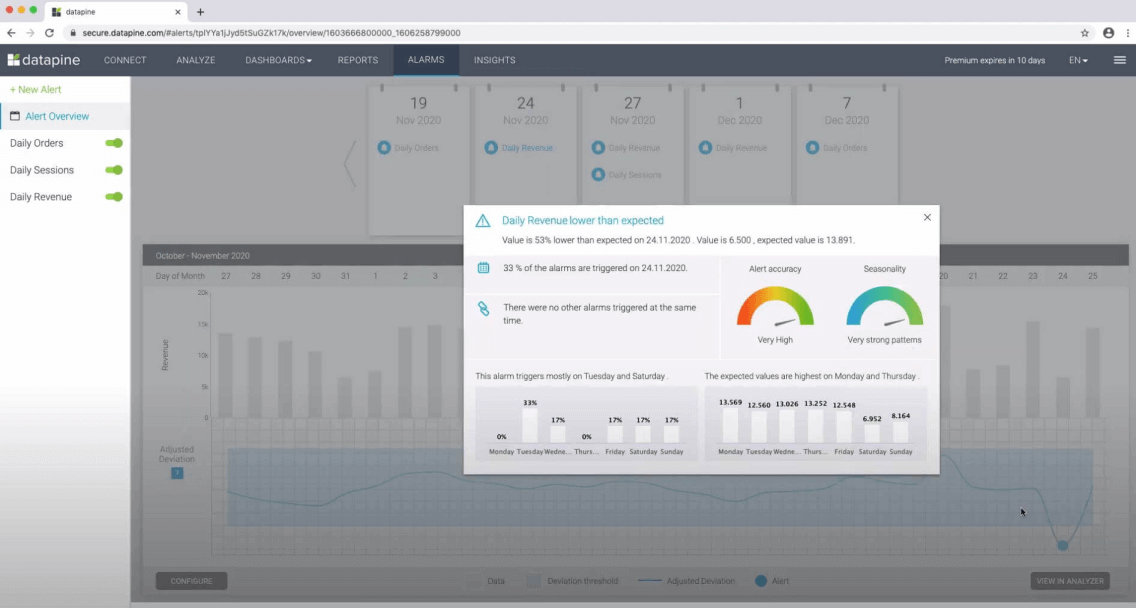
7. Time series analysis
As its name suggests, time series analysis is used to analyze a set of data points collected over a specified period of time. Although analysts use this method to monitor the data points in a specific interval of time rather than just monitoring them intermittently, the time series analysis is not uniquely used for the purpose of collecting data over time. Instead, it allows researchers to understand if variables changed during the duration of the study, how the different variables are dependent, and how did it reach the end result.
In a business context, this method is used to understand the causes of different trends and patterns to extract valuable insights. Another way of using this method is with the help of time series forecasting. Powered by predictive technologies, businesses can analyze various data sets over a period of time and forecast different future events.
A great use case to put time series analysis into perspective is seasonality effects on sales. By using time series forecasting to analyze sales data of a specific product over time, you can understand if sales rise over a specific period of time (e.g. swimwear during summertime, or candy during Halloween). These insights allow you to predict demand and prepare production accordingly.
8. Decision Trees
The decision tree analysis aims to act as a support tool to make smart and strategic decisions. By visually displaying potential outcomes, consequences, and costs in a tree-like model, researchers and company users can easily evaluate all factors involved and choose the best course of action. Decision trees are helpful to analyze quantitative data and they allow for an improved decision-making process by helping you spot improvement opportunities, reduce costs, and enhance operational efficiency and production.
But how does a decision tree actually works? This method works like a flowchart that starts with the main decision that you need to make and branches out based on the different outcomes and consequences of each decision. Each outcome will outline its own consequences, costs, and gains and, at the end of the analysis, you can compare each of them and make the smartest decision.
Businesses can use them to understand which project is more cost-effective and will bring more earnings in the long run. For example, imagine you need to decide if you want to update your software app or build a new app entirely. Here you would compare the total costs, the time needed to be invested, potential revenue, and any other factor that might affect your decision. In the end, you would be able to see which of these two options is more realistic and attainable for your company or research.
9. Conjoint analysis
Last but not least, we have the conjoint analysis. This approach is usually used in surveys to understand how individuals value different attributes of a product or service and it is one of the most effective methods to extract consumer preferences. When it comes to purchasing, some clients might be more price-focused, others more features-focused, and others might have a sustainable focus. Whatever your customer's preferences are, you can find them with conjoint analysis. Through this, companies can define pricing strategies, packaging options, subscription packages, and more.
A great example of conjoint analysis is in marketing and sales. For instance, a cupcake brand might use conjoint analysis and find that its clients prefer gluten-free options and cupcakes with healthier toppings over super sugary ones. Thus, the cupcake brand can turn these insights into advertisements and promotions to increase sales of this particular type of product. And not just that, conjoint analysis can also help businesses segment their customers based on their interests. This allows them to send different messaging that will bring value to each of the segments.
10. Correspondence Analysis
Also known as reciprocal averaging, correspondence analysis is a method used to analyze the relationship between categorical variables presented within a contingency table. A contingency table is a table that displays two (simple correspondence analysis) or more (multiple correspondence analysis) categorical variables across rows and columns that show the distribution of the data, which is usually answers to a survey or questionnaire on a specific topic.
This method starts by calculating an “expected value” which is done by multiplying row and column averages and dividing it by the overall original value of the specific table cell. The “expected value” is then subtracted from the original value resulting in a “residual number” which is what allows you to extract conclusions about relationships and distribution. The results of this analysis are later displayed using a map that represents the relationship between the different values. The closest two values are in the map, the bigger the relationship. Let’s put it into perspective with an example.
Imagine you are carrying out a market research analysis about outdoor clothing brands and how they are perceived by the public. For this analysis, you ask a group of people to match each brand with a certain attribute which can be durability, innovation, quality materials, etc. When calculating the residual numbers, you can see that brand A has a positive residual for innovation but a negative one for durability. This means that brand A is not positioned as a durable brand in the market, something that competitors could take advantage of.
11. Multidimensional Scaling (MDS)
MDS is a method used to observe the similarities or disparities between objects which can be colors, brands, people, geographical coordinates, and more. The objects are plotted using an “MDS map” that positions similar objects together and disparate ones far apart. The (dis) similarities between objects are represented using one or more dimensions that can be observed using a numerical scale. For example, if you want to know how people feel about the COVID-19 vaccine, you can use 1 for “don’t believe in the vaccine at all” and 10 for “firmly believe in the vaccine” and a scale of 2 to 9 for in between responses. When analyzing an MDS map the only thing that matters is the distance between the objects, the orientation of the dimensions is arbitrary and has no meaning at all.
Multidimensional scaling is a valuable technique for market research, especially when it comes to evaluating product or brand positioning. For instance, if a cupcake brand wants to know how they are positioned compared to competitors, it can define 2-3 dimensions such as taste, ingredients, shopping experience, or more, and do a multidimensional scaling analysis to find improvement opportunities as well as areas in which competitors are currently leading.
Another business example is in procurement when deciding on different suppliers. Decision makers can generate an MDS map to see how the different prices, delivery times, technical services, and more of the different suppliers differ and pick the one that suits their needs the best.
A final example proposed by a research paper on "An Improved Study of Multilevel Semantic Network Visualization for Analyzing Sentiment Word of Movie Review Data". Researchers picked a two-dimensional MDS map to display the distances and relationships between different sentiments in movie reviews. They used 36 sentiment words and distributed them based on their emotional distance as we can see in the image below where the words "outraged" and "sweet" are on opposite sides of the map, marking the distance between the two emotions very clearly.

Aside from being a valuable technique to analyze dissimilarities, MDS also serves as a dimension-reduction technique for large dimensional data.
B. Qualitative Methods
Qualitative data analysis methods are defined as the observation of non-numerical data that is gathered and produced using methods of observation such as interviews, focus groups, questionnaires, and more. As opposed to quantitative methods, qualitative data is more subjective and highly valuable in analyzing customer retention and product development.
12. Text analysis
Text analysis, also known in the industry as text mining, works by taking large sets of textual data and arranging them in a way that makes it easier to manage. By working through this cleansing process in stringent detail, you will be able to extract the data that is truly relevant to your organization and use it to develop actionable insights that will propel you forward.
Modern software accelerate the application of text analytics. Thanks to the combination of machine learning and intelligent algorithms, you can perform advanced analytical processes such as sentiment analysis. This technique allows you to understand the intentions and emotions of a text, for example, if it's positive, negative, or neutral, and then give it a score depending on certain factors and categories that are relevant to your brand. Sentiment analysis is often used to monitor brand and product reputation and to understand how successful your customer experience is. To learn more about the topic check out this insightful article .
By analyzing data from various word-based sources, including product reviews, articles, social media communications, and survey responses, you will gain invaluable insights into your audience, as well as their needs, preferences, and pain points. This will allow you to create campaigns, services, and communications that meet your prospects’ needs on a personal level, growing your audience while boosting customer retention. There are various other “sub-methods” that are an extension of text analysis. Each of them serves a more specific purpose and we will look at them in detail next.
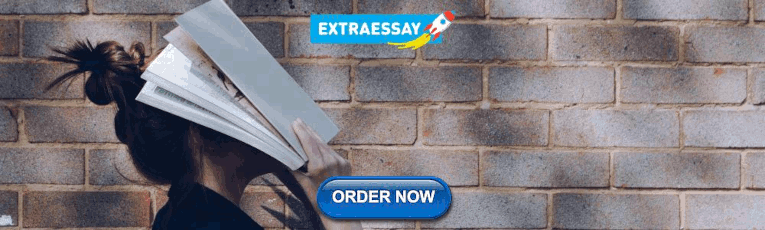
13. Content Analysis
This is a straightforward and very popular method that examines the presence and frequency of certain words, concepts, and subjects in different content formats such as text, image, audio, or video. For example, the number of times the name of a celebrity is mentioned on social media or online tabloids. It does this by coding text data that is later categorized and tabulated in a way that can provide valuable insights, making it the perfect mix of quantitative and qualitative analysis.
There are two types of content analysis. The first one is the conceptual analysis which focuses on explicit data, for instance, the number of times a concept or word is mentioned in a piece of content. The second one is relational analysis, which focuses on the relationship between different concepts or words and how they are connected within a specific context.
Content analysis is often used by marketers to measure brand reputation and customer behavior. For example, by analyzing customer reviews. It can also be used to analyze customer interviews and find directions for new product development. It is also important to note, that in order to extract the maximum potential out of this analysis method, it is necessary to have a clearly defined research question.
14. Thematic Analysis
Very similar to content analysis, thematic analysis also helps in identifying and interpreting patterns in qualitative data with the main difference being that the first one can also be applied to quantitative analysis. The thematic method analyzes large pieces of text data such as focus group transcripts or interviews and groups them into themes or categories that come up frequently within the text. It is a great method when trying to figure out peoples view’s and opinions about a certain topic. For example, if you are a brand that cares about sustainability, you can do a survey of your customers to analyze their views and opinions about sustainability and how they apply it to their lives. You can also analyze customer service calls transcripts to find common issues and improve your service.
Thematic analysis is a very subjective technique that relies on the researcher’s judgment. Therefore, to avoid biases, it has 6 steps that include familiarization, coding, generating themes, reviewing themes, defining and naming themes, and writing up. It is also important to note that, because it is a flexible approach, the data can be interpreted in multiple ways and it can be hard to select what data is more important to emphasize.
15. Narrative Analysis
A bit more complex in nature than the two previous ones, narrative analysis is used to explore the meaning behind the stories that people tell and most importantly, how they tell them. By looking into the words that people use to describe a situation you can extract valuable conclusions about their perspective on a specific topic. Common sources for narrative data include autobiographies, family stories, opinion pieces, and testimonials, among others.
From a business perspective, narrative analysis can be useful to analyze customer behaviors and feelings towards a specific product, service, feature, or others. It provides unique and deep insights that can be extremely valuable. However, it has some drawbacks.
The biggest weakness of this method is that the sample sizes are usually very small due to the complexity and time-consuming nature of the collection of narrative data. Plus, the way a subject tells a story will be significantly influenced by his or her specific experiences, making it very hard to replicate in a subsequent study.
16. Discourse Analysis
Discourse analysis is used to understand the meaning behind any type of written, verbal, or symbolic discourse based on its political, social, or cultural context. It mixes the analysis of languages and situations together. This means that the way the content is constructed and the meaning behind it is significantly influenced by the culture and society it takes place in. For example, if you are analyzing political speeches you need to consider different context elements such as the politician's background, the current political context of the country, the audience to which the speech is directed, and so on.
From a business point of view, discourse analysis is a great market research tool. It allows marketers to understand how the norms and ideas of the specific market work and how their customers relate to those ideas. It can be very useful to build a brand mission or develop a unique tone of voice.
17. Grounded Theory Analysis
Traditionally, researchers decide on a method and hypothesis and start to collect the data to prove that hypothesis. The grounded theory is the only method that doesn’t require an initial research question or hypothesis as its value lies in the generation of new theories. With the grounded theory method, you can go into the analysis process with an open mind and explore the data to generate new theories through tests and revisions. In fact, it is not necessary to collect the data and then start to analyze it. Researchers usually start to find valuable insights as they are gathering the data.
All of these elements make grounded theory a very valuable method as theories are fully backed by data instead of initial assumptions. It is a great technique to analyze poorly researched topics or find the causes behind specific company outcomes. For example, product managers and marketers might use the grounded theory to find the causes of high levels of customer churn and look into customer surveys and reviews to develop new theories about the causes.
How To Analyze Data? Top 17 Data Analysis Techniques To Apply

Now that we’ve answered the questions “what is data analysis’”, why is it important, and covered the different data analysis types, it’s time to dig deeper into how to perform your analysis by working through these 17 essential techniques.
1. Collaborate your needs
Before you begin analyzing or drilling down into any techniques, it’s crucial to sit down collaboratively with all key stakeholders within your organization, decide on your primary campaign or strategic goals, and gain a fundamental understanding of the types of insights that will best benefit your progress or provide you with the level of vision you need to evolve your organization.
2. Establish your questions
Once you’ve outlined your core objectives, you should consider which questions will need answering to help you achieve your mission. This is one of the most important techniques as it will shape the very foundations of your success.
To help you ask the right things and ensure your data works for you, you have to ask the right data analysis questions .
3. Data democratization
After giving your data analytics methodology some real direction, and knowing which questions need answering to extract optimum value from the information available to your organization, you should continue with democratization.
Data democratization is an action that aims to connect data from various sources efficiently and quickly so that anyone in your organization can access it at any given moment. You can extract data in text, images, videos, numbers, or any other format. And then perform cross-database analysis to achieve more advanced insights to share with the rest of the company interactively.
Once you have decided on your most valuable sources, you need to take all of this into a structured format to start collecting your insights. For this purpose, datapine offers an easy all-in-one data connectors feature to integrate all your internal and external sources and manage them at your will. Additionally, datapine’s end-to-end solution automatically updates your data, allowing you to save time and focus on performing the right analysis to grow your company.
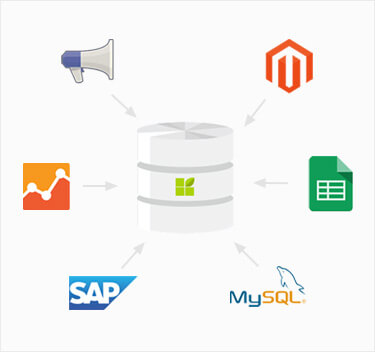
4. Think of governance
When collecting data in a business or research context you always need to think about security and privacy. With data breaches becoming a topic of concern for businesses, the need to protect your client's or subject’s sensitive information becomes critical.
To ensure that all this is taken care of, you need to think of a data governance strategy. According to Gartner , this concept refers to “ the specification of decision rights and an accountability framework to ensure the appropriate behavior in the valuation, creation, consumption, and control of data and analytics .” In simpler words, data governance is a collection of processes, roles, and policies, that ensure the efficient use of data while still achieving the main company goals. It ensures that clear roles are in place for who can access the information and how they can access it. In time, this not only ensures that sensitive information is protected but also allows for an efficient analysis as a whole.
5. Clean your data
After harvesting from so many sources you will be left with a vast amount of information that can be overwhelming to deal with. At the same time, you can be faced with incorrect data that can be misleading to your analysis. The smartest thing you can do to avoid dealing with this in the future is to clean the data. This is fundamental before visualizing it, as it will ensure that the insights you extract from it are correct.
There are many things that you need to look for in the cleaning process. The most important one is to eliminate any duplicate observations; this usually appears when using multiple internal and external sources of information. You can also add any missing codes, fix empty fields, and eliminate incorrectly formatted data.
Another usual form of cleaning is done with text data. As we mentioned earlier, most companies today analyze customer reviews, social media comments, questionnaires, and several other text inputs. In order for algorithms to detect patterns, text data needs to be revised to avoid invalid characters or any syntax or spelling errors.
Most importantly, the aim of cleaning is to prevent you from arriving at false conclusions that can damage your company in the long run. By using clean data, you will also help BI solutions to interact better with your information and create better reports for your organization.
6. Set your KPIs
Once you’ve set your sources, cleaned your data, and established clear-cut questions you want your insights to answer, you need to set a host of key performance indicators (KPIs) that will help you track, measure, and shape your progress in a number of key areas.
KPIs are critical to both qualitative and quantitative analysis research. This is one of the primary methods of data analysis you certainly shouldn’t overlook.
To help you set the best possible KPIs for your initiatives and activities, here is an example of a relevant logistics KPI : transportation-related costs. If you want to see more go explore our collection of key performance indicator examples .

7. Omit useless data
Having bestowed your data analysis tools and techniques with true purpose and defined your mission, you should explore the raw data you’ve collected from all sources and use your KPIs as a reference for chopping out any information you deem to be useless.
Trimming the informational fat is one of the most crucial methods of analysis as it will allow you to focus your analytical efforts and squeeze every drop of value from the remaining ‘lean’ information.
Any stats, facts, figures, or metrics that don’t align with your business goals or fit with your KPI management strategies should be eliminated from the equation.
8. Build a data management roadmap
While, at this point, this particular step is optional (you will have already gained a wealth of insight and formed a fairly sound strategy by now), creating a data governance roadmap will help your data analysis methods and techniques become successful on a more sustainable basis. These roadmaps, if developed properly, are also built so they can be tweaked and scaled over time.
Invest ample time in developing a roadmap that will help you store, manage, and handle your data internally, and you will make your analysis techniques all the more fluid and functional – one of the most powerful types of data analysis methods available today.
9. Integrate technology
There are many ways to analyze data, but one of the most vital aspects of analytical success in a business context is integrating the right decision support software and technology.
Robust analysis platforms will not only allow you to pull critical data from your most valuable sources while working with dynamic KPIs that will offer you actionable insights; it will also present them in a digestible, visual, interactive format from one central, live dashboard . A data methodology you can count on.
By integrating the right technology within your data analysis methodology, you’ll avoid fragmenting your insights, saving you time and effort while allowing you to enjoy the maximum value from your business’s most valuable insights.
For a look at the power of software for the purpose of analysis and to enhance your methods of analyzing, glance over our selection of dashboard examples .
10. Answer your questions
By considering each of the above efforts, working with the right technology, and fostering a cohesive internal culture where everyone buys into the different ways to analyze data as well as the power of digital intelligence, you will swiftly start to answer your most burning business questions. Arguably, the best way to make your data concepts accessible across the organization is through data visualization.
11. Visualize your data
Online data visualization is a powerful tool as it lets you tell a story with your metrics, allowing users across the organization to extract meaningful insights that aid business evolution – and it covers all the different ways to analyze data.
The purpose of analyzing is to make your entire organization more informed and intelligent, and with the right platform or dashboard, this is simpler than you think, as demonstrated by our marketing dashboard .

This visual, dynamic, and interactive online dashboard is a data analysis example designed to give Chief Marketing Officers (CMO) an overview of relevant metrics to help them understand if they achieved their monthly goals.
In detail, this example generated with a modern dashboard creator displays interactive charts for monthly revenues, costs, net income, and net income per customer; all of them are compared with the previous month so that you can understand how the data fluctuated. In addition, it shows a detailed summary of the number of users, customers, SQLs, and MQLs per month to visualize the whole picture and extract relevant insights or trends for your marketing reports .
The CMO dashboard is perfect for c-level management as it can help them monitor the strategic outcome of their marketing efforts and make data-driven decisions that can benefit the company exponentially.
12. Be careful with the interpretation
We already dedicated an entire post to data interpretation as it is a fundamental part of the process of data analysis. It gives meaning to the analytical information and aims to drive a concise conclusion from the analysis results. Since most of the time companies are dealing with data from many different sources, the interpretation stage needs to be done carefully and properly in order to avoid misinterpretations.
To help you through the process, here we list three common practices that you need to avoid at all costs when looking at your data:
- Correlation vs. causation: The human brain is formatted to find patterns. This behavior leads to one of the most common mistakes when performing interpretation: confusing correlation with causation. Although these two aspects can exist simultaneously, it is not correct to assume that because two things happened together, one provoked the other. A piece of advice to avoid falling into this mistake is never to trust just intuition, trust the data. If there is no objective evidence of causation, then always stick to correlation.
- Confirmation bias: This phenomenon describes the tendency to select and interpret only the data necessary to prove one hypothesis, often ignoring the elements that might disprove it. Even if it's not done on purpose, confirmation bias can represent a real problem, as excluding relevant information can lead to false conclusions and, therefore, bad business decisions. To avoid it, always try to disprove your hypothesis instead of proving it, share your analysis with other team members, and avoid drawing any conclusions before the entire analytical project is finalized.
- Statistical significance: To put it in short words, statistical significance helps analysts understand if a result is actually accurate or if it happened because of a sampling error or pure chance. The level of statistical significance needed might depend on the sample size and the industry being analyzed. In any case, ignoring the significance of a result when it might influence decision-making can be a huge mistake.
13. Build a narrative
Now, we’re going to look at how you can bring all of these elements together in a way that will benefit your business - starting with a little something called data storytelling.
The human brain responds incredibly well to strong stories or narratives. Once you’ve cleansed, shaped, and visualized your most invaluable data using various BI dashboard tools , you should strive to tell a story - one with a clear-cut beginning, middle, and end.
By doing so, you will make your analytical efforts more accessible, digestible, and universal, empowering more people within your organization to use your discoveries to their actionable advantage.
14. Consider autonomous technology
Autonomous technologies, such as artificial intelligence (AI) and machine learning (ML), play a significant role in the advancement of understanding how to analyze data more effectively.
Gartner predicts that by the end of this year, 80% of emerging technologies will be developed with AI foundations. This is a testament to the ever-growing power and value of autonomous technologies.
At the moment, these technologies are revolutionizing the analysis industry. Some examples that we mentioned earlier are neural networks, intelligent alarms, and sentiment analysis.
15. Share the load
If you work with the right tools and dashboards, you will be able to present your metrics in a digestible, value-driven format, allowing almost everyone in the organization to connect with and use relevant data to their advantage.
Modern dashboards consolidate data from various sources, providing access to a wealth of insights in one centralized location, no matter if you need to monitor recruitment metrics or generate reports that need to be sent across numerous departments. Moreover, these cutting-edge tools offer access to dashboards from a multitude of devices, meaning that everyone within the business can connect with practical insights remotely - and share the load.
Once everyone is able to work with a data-driven mindset, you will catalyze the success of your business in ways you never thought possible. And when it comes to knowing how to analyze data, this kind of collaborative approach is essential.
16. Data analysis tools
In order to perform high-quality analysis of data, it is fundamental to use tools and software that will ensure the best results. Here we leave you a small summary of four fundamental categories of data analysis tools for your organization.
- Business Intelligence: BI tools allow you to process significant amounts of data from several sources in any format. Through this, you can not only analyze and monitor your data to extract relevant insights but also create interactive reports and dashboards to visualize your KPIs and use them for your company's good. datapine is an amazing online BI software that is focused on delivering powerful online analysis features that are accessible to beginner and advanced users. Like this, it offers a full-service solution that includes cutting-edge analysis of data, KPIs visualization, live dashboards, reporting, and artificial intelligence technologies to predict trends and minimize risk.
- Statistical analysis: These tools are usually designed for scientists, statisticians, market researchers, and mathematicians, as they allow them to perform complex statistical analyses with methods like regression analysis, predictive analysis, and statistical modeling. A good tool to perform this type of analysis is R-Studio as it offers a powerful data modeling and hypothesis testing feature that can cover both academic and general data analysis. This tool is one of the favorite ones in the industry, due to its capability for data cleaning, data reduction, and performing advanced analysis with several statistical methods. Another relevant tool to mention is SPSS from IBM. The software offers advanced statistical analysis for users of all skill levels. Thanks to a vast library of machine learning algorithms, text analysis, and a hypothesis testing approach it can help your company find relevant insights to drive better decisions. SPSS also works as a cloud service that enables you to run it anywhere.
- SQL Consoles: SQL is a programming language often used to handle structured data in relational databases. Tools like these are popular among data scientists as they are extremely effective in unlocking these databases' value. Undoubtedly, one of the most used SQL software in the market is MySQL Workbench . This tool offers several features such as a visual tool for database modeling and monitoring, complete SQL optimization, administration tools, and visual performance dashboards to keep track of KPIs.
- Data Visualization: These tools are used to represent your data through charts, graphs, and maps that allow you to find patterns and trends in the data. datapine's already mentioned BI platform also offers a wealth of powerful online data visualization tools with several benefits. Some of them include: delivering compelling data-driven presentations to share with your entire company, the ability to see your data online with any device wherever you are, an interactive dashboard design feature that enables you to showcase your results in an interactive and understandable way, and to perform online self-service reports that can be used simultaneously with several other people to enhance team productivity.
17. Refine your process constantly
Last is a step that might seem obvious to some people, but it can be easily ignored if you think you are done. Once you have extracted the needed results, you should always take a retrospective look at your project and think about what you can improve. As you saw throughout this long list of techniques, data analysis is a complex process that requires constant refinement. For this reason, you should always go one step further and keep improving.
Quality Criteria For Data Analysis
So far we’ve covered a list of methods and techniques that should help you perform efficient data analysis. But how do you measure the quality and validity of your results? This is done with the help of some science quality criteria. Here we will go into a more theoretical area that is critical to understanding the fundamentals of statistical analysis in science. However, you should also be aware of these steps in a business context, as they will allow you to assess the quality of your results in the correct way. Let’s dig in.
- Internal validity: The results of a survey are internally valid if they measure what they are supposed to measure and thus provide credible results. In other words , internal validity measures the trustworthiness of the results and how they can be affected by factors such as the research design, operational definitions, how the variables are measured, and more. For instance, imagine you are doing an interview to ask people if they brush their teeth two times a day. While most of them will answer yes, you can still notice that their answers correspond to what is socially acceptable, which is to brush your teeth at least twice a day. In this case, you can’t be 100% sure if respondents actually brush their teeth twice a day or if they just say that they do, therefore, the internal validity of this interview is very low.
- External validity: Essentially, external validity refers to the extent to which the results of your research can be applied to a broader context. It basically aims to prove that the findings of a study can be applied in the real world. If the research can be applied to other settings, individuals, and times, then the external validity is high.
- Reliability : If your research is reliable, it means that it can be reproduced. If your measurement were repeated under the same conditions, it would produce similar results. This means that your measuring instrument consistently produces reliable results. For example, imagine a doctor building a symptoms questionnaire to detect a specific disease in a patient. Then, various other doctors use this questionnaire but end up diagnosing the same patient with a different condition. This means the questionnaire is not reliable in detecting the initial disease. Another important note here is that in order for your research to be reliable, it also needs to be objective. If the results of a study are the same, independent of who assesses them or interprets them, the study can be considered reliable. Let’s see the objectivity criteria in more detail now.
- Objectivity: In data science, objectivity means that the researcher needs to stay fully objective when it comes to its analysis. The results of a study need to be affected by objective criteria and not by the beliefs, personality, or values of the researcher. Objectivity needs to be ensured when you are gathering the data, for example, when interviewing individuals, the questions need to be asked in a way that doesn't influence the results. Paired with this, objectivity also needs to be thought of when interpreting the data. If different researchers reach the same conclusions, then the study is objective. For this last point, you can set predefined criteria to interpret the results to ensure all researchers follow the same steps.
The discussed quality criteria cover mostly potential influences in a quantitative context. Analysis in qualitative research has by default additional subjective influences that must be controlled in a different way. Therefore, there are other quality criteria for this kind of research such as credibility, transferability, dependability, and confirmability. You can see each of them more in detail on this resource .
Data Analysis Limitations & Barriers
Analyzing data is not an easy task. As you’ve seen throughout this post, there are many steps and techniques that you need to apply in order to extract useful information from your research. While a well-performed analysis can bring various benefits to your organization it doesn't come without limitations. In this section, we will discuss some of the main barriers you might encounter when conducting an analysis. Let’s see them more in detail.
- Lack of clear goals: No matter how good your data or analysis might be if you don’t have clear goals or a hypothesis the process might be worthless. While we mentioned some methods that don’t require a predefined hypothesis, it is always better to enter the analytical process with some clear guidelines of what you are expecting to get out of it, especially in a business context in which data is utilized to support important strategic decisions.
- Objectivity: Arguably one of the biggest barriers when it comes to data analysis in research is to stay objective. When trying to prove a hypothesis, researchers might find themselves, intentionally or unintentionally, directing the results toward an outcome that they want. To avoid this, always question your assumptions and avoid confusing facts with opinions. You can also show your findings to a research partner or external person to confirm that your results are objective.
- Data representation: A fundamental part of the analytical procedure is the way you represent your data. You can use various graphs and charts to represent your findings, but not all of them will work for all purposes. Choosing the wrong visual can not only damage your analysis but can mislead your audience, therefore, it is important to understand when to use each type of data depending on your analytical goals. Our complete guide on the types of graphs and charts lists 20 different visuals with examples of when to use them.
- Flawed correlation : Misleading statistics can significantly damage your research. We’ve already pointed out a few interpretation issues previously in the post, but it is an important barrier that we can't avoid addressing here as well. Flawed correlations occur when two variables appear related to each other but they are not. Confusing correlations with causation can lead to a wrong interpretation of results which can lead to building wrong strategies and loss of resources, therefore, it is very important to identify the different interpretation mistakes and avoid them.
- Sample size: A very common barrier to a reliable and efficient analysis process is the sample size. In order for the results to be trustworthy, the sample size should be representative of what you are analyzing. For example, imagine you have a company of 1000 employees and you ask the question “do you like working here?” to 50 employees of which 49 say yes, which means 95%. Now, imagine you ask the same question to the 1000 employees and 950 say yes, which also means 95%. Saying that 95% of employees like working in the company when the sample size was only 50 is not a representative or trustworthy conclusion. The significance of the results is way more accurate when surveying a bigger sample size.
- Privacy concerns: In some cases, data collection can be subjected to privacy regulations. Businesses gather all kinds of information from their customers from purchasing behaviors to addresses and phone numbers. If this falls into the wrong hands due to a breach, it can affect the security and confidentiality of your clients. To avoid this issue, you need to collect only the data that is needed for your research and, if you are using sensitive facts, make it anonymous so customers are protected. The misuse of customer data can severely damage a business's reputation, so it is important to keep an eye on privacy.
- Lack of communication between teams : When it comes to performing data analysis on a business level, it is very likely that each department and team will have different goals and strategies. However, they are all working for the same common goal of helping the business run smoothly and keep growing. When teams are not connected and communicating with each other, it can directly affect the way general strategies are built. To avoid these issues, tools such as data dashboards enable teams to stay connected through data in a visually appealing way.
- Innumeracy : Businesses are working with data more and more every day. While there are many BI tools available to perform effective analysis, data literacy is still a constant barrier. Not all employees know how to apply analysis techniques or extract insights from them. To prevent this from happening, you can implement different training opportunities that will prepare every relevant user to deal with data.
Key Data Analysis Skills
As you've learned throughout this lengthy guide, analyzing data is a complex task that requires a lot of knowledge and skills. That said, thanks to the rise of self-service tools the process is way more accessible and agile than it once was. Regardless, there are still some key skills that are valuable to have when working with data, we list the most important ones below.
- Critical and statistical thinking: To successfully analyze data you need to be creative and think out of the box. Yes, that might sound like a weird statement considering that data is often tight to facts. However, a great level of critical thinking is required to uncover connections, come up with a valuable hypothesis, and extract conclusions that go a step further from the surface. This, of course, needs to be complemented by statistical thinking and an understanding of numbers.
- Data cleaning: Anyone who has ever worked with data before will tell you that the cleaning and preparation process accounts for 80% of a data analyst's work, therefore, the skill is fundamental. But not just that, not cleaning the data adequately can also significantly damage the analysis which can lead to poor decision-making in a business scenario. While there are multiple tools that automate the cleaning process and eliminate the possibility of human error, it is still a valuable skill to dominate.
- Data visualization: Visuals make the information easier to understand and analyze, not only for professional users but especially for non-technical ones. Having the necessary skills to not only choose the right chart type but know when to apply it correctly is key. This also means being able to design visually compelling charts that make the data exploration process more efficient.
- SQL: The Structured Query Language or SQL is a programming language used to communicate with databases. It is fundamental knowledge as it enables you to update, manipulate, and organize data from relational databases which are the most common databases used by companies. It is fairly easy to learn and one of the most valuable skills when it comes to data analysis.
- Communication skills: This is a skill that is especially valuable in a business environment. Being able to clearly communicate analytical outcomes to colleagues is incredibly important, especially when the information you are trying to convey is complex for non-technical people. This applies to in-person communication as well as written format, for example, when generating a dashboard or report. While this might be considered a “soft” skill compared to the other ones we mentioned, it should not be ignored as you most likely will need to share analytical findings with others no matter the context.
Data Analysis In The Big Data Environment
Big data is invaluable to today’s businesses, and by using different methods for data analysis, it’s possible to view your data in a way that can help you turn insight into positive action.
To inspire your efforts and put the importance of big data into context, here are some insights that you should know:
- By 2026 the industry of big data is expected to be worth approximately $273.4 billion.
- 94% of enterprises say that analyzing data is important for their growth and digital transformation.
- Companies that exploit the full potential of their data can increase their operating margins by 60% .
- We already told you the benefits of Artificial Intelligence through this article. This industry's financial impact is expected to grow up to $40 billion by 2025.
Data analysis concepts may come in many forms, but fundamentally, any solid methodology will help to make your business more streamlined, cohesive, insightful, and successful than ever before.
Key Takeaways From Data Analysis
As we reach the end of our data analysis journey, we leave a small summary of the main methods and techniques to perform excellent analysis and grow your business.
17 Essential Types of Data Analysis Methods:
- Cluster analysis
- Cohort analysis
- Regression analysis
- Factor analysis
- Neural Networks
- Data Mining
- Text analysis
- Time series analysis
- Decision trees
- Conjoint analysis
- Correspondence Analysis
- Multidimensional Scaling
- Content analysis
- Thematic analysis
- Narrative analysis
- Grounded theory analysis
- Discourse analysis
Top 17 Data Analysis Techniques:
- Collaborate your needs
- Establish your questions
- Data democratization
- Think of data governance
- Clean your data
- Set your KPIs
- Omit useless data
- Build a data management roadmap
- Integrate technology
- Answer your questions
- Visualize your data
- Interpretation of data
- Consider autonomous technology
- Build a narrative
- Share the load
- Data Analysis tools
- Refine your process constantly
We’ve pondered the data analysis definition and drilled down into the practical applications of data-centric analytics, and one thing is clear: by taking measures to arrange your data and making your metrics work for you, it’s possible to transform raw information into action - the kind of that will push your business to the next level.
Yes, good data analytics techniques result in enhanced business intelligence (BI). To help you understand this notion in more detail, read our exploration of business intelligence reporting .
And, if you’re ready to perform your own analysis, drill down into your facts and figures while interacting with your data on astonishing visuals, you can try our software for a free, 14-day trial .
- Skip to main content
- Skip to primary sidebar
- Skip to footer
- QuestionPro

- Solutions Industries Gaming Automotive Sports and events Education Government Travel & Hospitality Financial Services Healthcare Cannabis Technology Use Case NPS+ Communities Audience Contactless surveys Mobile LivePolls Member Experience GDPR Positive People Science 360 Feedback Surveys
- Resources Blog eBooks Survey Templates Case Studies Training Help center

Home Market Research
Data Analysis in Research: Types & Methods
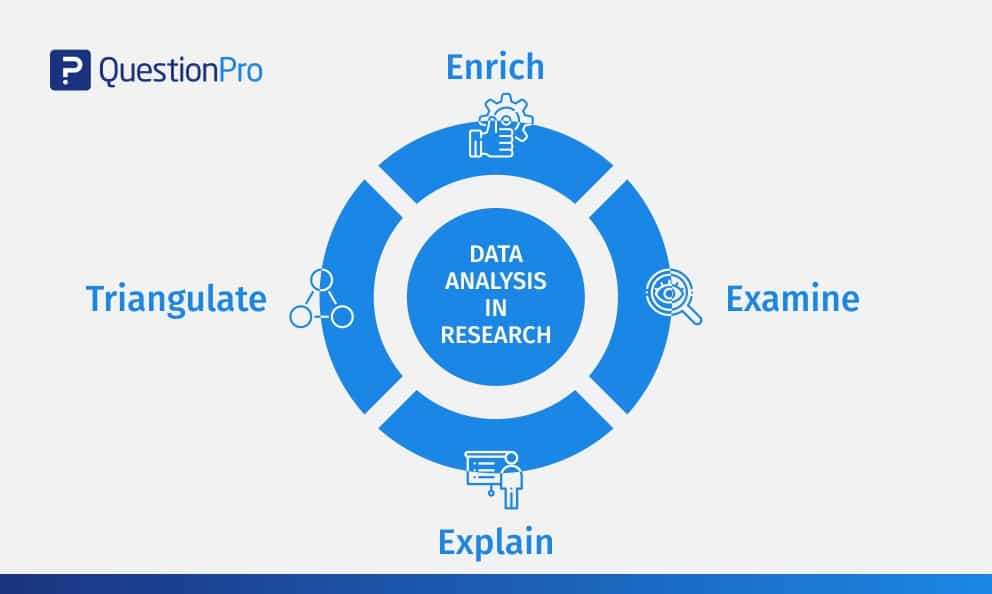
Content Index
Why analyze data in research?
Types of data in research, finding patterns in the qualitative data, methods used for data analysis in qualitative research, preparing data for analysis, methods used for data analysis in quantitative research, considerations in research data analysis, what is data analysis in research.
Definition of research in data analysis: According to LeCompte and Schensul, research data analysis is a process used by researchers to reduce data to a story and interpret it to derive insights. The data analysis process helps reduce a large chunk of data into smaller fragments, which makes sense.
Three essential things occur during the data analysis process — the first is data organization . Summarization and categorization together contribute to becoming the second known method used for data reduction. It helps find patterns and themes in the data for easy identification and linking. The third and last way is data analysis – researchers do it in both top-down and bottom-up fashion.
LEARN ABOUT: Research Process Steps
On the other hand, Marshall and Rossman describe data analysis as a messy, ambiguous, and time-consuming but creative and fascinating process through which a mass of collected data is brought to order, structure and meaning.
We can say that “the data analysis and data interpretation is a process representing the application of deductive and inductive logic to the research and data analysis.”
Researchers rely heavily on data as they have a story to tell or research problems to solve. It starts with a question, and data is nothing but an answer to that question. But, what if there is no question to ask? Well! It is possible to explore data even without a problem – we call it ‘Data Mining’, which often reveals some interesting patterns within the data that are worth exploring.
Irrelevant to the type of data researchers explore, their mission and audiences’ vision guide them to find the patterns to shape the story they want to tell. One of the essential things expected from researchers while analyzing data is to stay open and remain unbiased toward unexpected patterns, expressions, and results. Remember, sometimes, data analysis tells the most unforeseen yet exciting stories that were not expected when initiating data analysis. Therefore, rely on the data you have at hand and enjoy the journey of exploratory research.
Create a Free Account
Every kind of data has a rare quality of describing things after assigning a specific value to it. For analysis, you need to organize these values, processed and presented in a given context, to make it useful. Data can be in different forms; here are the primary data types.
- Qualitative data: When the data presented has words and descriptions, then we call it qualitative data . Although you can observe this data, it is subjective and harder to analyze data in research, especially for comparison. Example: Quality data represents everything describing taste, experience, texture, or an opinion that is considered quality data. This type of data is usually collected through focus groups, personal qualitative interviews , qualitative observation or using open-ended questions in surveys.
- Quantitative data: Any data expressed in numbers of numerical figures are called quantitative data . This type of data can be distinguished into categories, grouped, measured, calculated, or ranked. Example: questions such as age, rank, cost, length, weight, scores, etc. everything comes under this type of data. You can present such data in graphical format, charts, or apply statistical analysis methods to this data. The (Outcomes Measurement Systems) OMS questionnaires in surveys are a significant source of collecting numeric data.
- Categorical data: It is data presented in groups. However, an item included in the categorical data cannot belong to more than one group. Example: A person responding to a survey by telling his living style, marital status, smoking habit, or drinking habit comes under the categorical data. A chi-square test is a standard method used to analyze this data.
Learn More : Examples of Qualitative Data in Education
Data analysis in qualitative research
Data analysis and qualitative data research work a little differently from the numerical data as the quality data is made up of words, descriptions, images, objects, and sometimes symbols. Getting insight from such complicated information is a complicated process. Hence it is typically used for exploratory research and data analysis .
Although there are several ways to find patterns in the textual information, a word-based method is the most relied and widely used global technique for research and data analysis. Notably, the data analysis process in qualitative research is manual. Here the researchers usually read the available data and find repetitive or commonly used words.
For example, while studying data collected from African countries to understand the most pressing issues people face, researchers might find “food” and “hunger” are the most commonly used words and will highlight them for further analysis.
LEARN ABOUT: Level of Analysis
The keyword context is another widely used word-based technique. In this method, the researcher tries to understand the concept by analyzing the context in which the participants use a particular keyword.
For example , researchers conducting research and data analysis for studying the concept of ‘diabetes’ amongst respondents might analyze the context of when and how the respondent has used or referred to the word ‘diabetes.’
The scrutiny-based technique is also one of the highly recommended text analysis methods used to identify a quality data pattern. Compare and contrast is the widely used method under this technique to differentiate how a specific text is similar or different from each other.
For example: To find out the “importance of resident doctor in a company,” the collected data is divided into people who think it is necessary to hire a resident doctor and those who think it is unnecessary. Compare and contrast is the best method that can be used to analyze the polls having single-answer questions types .
Metaphors can be used to reduce the data pile and find patterns in it so that it becomes easier to connect data with theory.
Variable Partitioning is another technique used to split variables so that researchers can find more coherent descriptions and explanations from the enormous data.
LEARN ABOUT: Qualitative Research Questions and Questionnaires
There are several techniques to analyze the data in qualitative research, but here are some commonly used methods,
- Content Analysis: It is widely accepted and the most frequently employed technique for data analysis in research methodology. It can be used to analyze the documented information from text, images, and sometimes from the physical items. It depends on the research questions to predict when and where to use this method.
- Narrative Analysis: This method is used to analyze content gathered from various sources such as personal interviews, field observation, and surveys . The majority of times, stories, or opinions shared by people are focused on finding answers to the research questions.
- Discourse Analysis: Similar to narrative analysis, discourse analysis is used to analyze the interactions with people. Nevertheless, this particular method considers the social context under which or within which the communication between the researcher and respondent takes place. In addition to that, discourse analysis also focuses on the lifestyle and day-to-day environment while deriving any conclusion.
- Grounded Theory: When you want to explain why a particular phenomenon happened, then using grounded theory for analyzing quality data is the best resort. Grounded theory is applied to study data about the host of similar cases occurring in different settings. When researchers are using this method, they might alter explanations or produce new ones until they arrive at some conclusion.
LEARN ABOUT: 12 Best Tools for Researchers
Data analysis in quantitative research
The first stage in research and data analysis is to make it for the analysis so that the nominal data can be converted into something meaningful. Data preparation consists of the below phases.
Phase I: Data Validation
Data validation is done to understand if the collected data sample is per the pre-set standards, or it is a biased data sample again divided into four different stages
- Fraud: To ensure an actual human being records each response to the survey or the questionnaire
- Screening: To make sure each participant or respondent is selected or chosen in compliance with the research criteria
- Procedure: To ensure ethical standards were maintained while collecting the data sample
- Completeness: To ensure that the respondent has answered all the questions in an online survey. Else, the interviewer had asked all the questions devised in the questionnaire.
Phase II: Data Editing
More often, an extensive research data sample comes loaded with errors. Respondents sometimes fill in some fields incorrectly or sometimes skip them accidentally. Data editing is a process wherein the researchers have to confirm that the provided data is free of such errors. They need to conduct necessary checks and outlier checks to edit the raw edit and make it ready for analysis.
Phase III: Data Coding
Out of all three, this is the most critical phase of data preparation associated with grouping and assigning values to the survey responses . If a survey is completed with a 1000 sample size, the researcher will create an age bracket to distinguish the respondents based on their age. Thus, it becomes easier to analyze small data buckets rather than deal with the massive data pile.
LEARN ABOUT: Steps in Qualitative Research
After the data is prepared for analysis, researchers are open to using different research and data analysis methods to derive meaningful insights. For sure, statistical analysis plans are the most favored to analyze numerical data. In statistical analysis, distinguishing between categorical data and numerical data is essential, as categorical data involves distinct categories or labels, while numerical data consists of measurable quantities. The method is again classified into two groups. First, ‘Descriptive Statistics’ used to describe data. Second, ‘Inferential statistics’ that helps in comparing the data .
Descriptive statistics
This method is used to describe the basic features of versatile types of data in research. It presents the data in such a meaningful way that pattern in the data starts making sense. Nevertheless, the descriptive analysis does not go beyond making conclusions. The conclusions are again based on the hypothesis researchers have formulated so far. Here are a few major types of descriptive analysis methods.
Measures of Frequency
- Count, Percent, Frequency
- It is used to denote home often a particular event occurs.
- Researchers use it when they want to showcase how often a response is given.
Measures of Central Tendency
- Mean, Median, Mode
- The method is widely used to demonstrate distribution by various points.
- Researchers use this method when they want to showcase the most commonly or averagely indicated response.
Measures of Dispersion or Variation
- Range, Variance, Standard deviation
- Here the field equals high/low points.
- Variance standard deviation = difference between the observed score and mean
- It is used to identify the spread of scores by stating intervals.
- Researchers use this method to showcase data spread out. It helps them identify the depth until which the data is spread out that it directly affects the mean.
Measures of Position
- Percentile ranks, Quartile ranks
- It relies on standardized scores helping researchers to identify the relationship between different scores.
- It is often used when researchers want to compare scores with the average count.
For quantitative research use of descriptive analysis often give absolute numbers, but the in-depth analysis is never sufficient to demonstrate the rationale behind those numbers. Nevertheless, it is necessary to think of the best method for research and data analysis suiting your survey questionnaire and what story researchers want to tell. For example, the mean is the best way to demonstrate the students’ average scores in schools. It is better to rely on the descriptive statistics when the researchers intend to keep the research or outcome limited to the provided sample without generalizing it. For example, when you want to compare average voting done in two different cities, differential statistics are enough.
Descriptive analysis is also called a ‘univariate analysis’ since it is commonly used to analyze a single variable.
Inferential statistics
Inferential statistics are used to make predictions about a larger population after research and data analysis of the representing population’s collected sample. For example, you can ask some odd 100 audiences at a movie theater if they like the movie they are watching. Researchers then use inferential statistics on the collected sample to reason that about 80-90% of people like the movie.
Here are two significant areas of inferential statistics.
- Estimating parameters: It takes statistics from the sample research data and demonstrates something about the population parameter.
- Hypothesis test: I t’s about sampling research data to answer the survey research questions. For example, researchers might be interested to understand if the new shade of lipstick recently launched is good or not, or if the multivitamin capsules help children to perform better at games.
These are sophisticated analysis methods used to showcase the relationship between different variables instead of describing a single variable. It is often used when researchers want something beyond absolute numbers to understand the relationship between variables.
Here are some of the commonly used methods for data analysis in research.
- Correlation: When researchers are not conducting experimental research or quasi-experimental research wherein the researchers are interested to understand the relationship between two or more variables, they opt for correlational research methods.
- Cross-tabulation: Also called contingency tables, cross-tabulation is used to analyze the relationship between multiple variables. Suppose provided data has age and gender categories presented in rows and columns. A two-dimensional cross-tabulation helps for seamless data analysis and research by showing the number of males and females in each age category.
- Regression analysis: For understanding the strong relationship between two variables, researchers do not look beyond the primary and commonly used regression analysis method, which is also a type of predictive analysis used. In this method, you have an essential factor called the dependent variable. You also have multiple independent variables in regression analysis. You undertake efforts to find out the impact of independent variables on the dependent variable. The values of both independent and dependent variables are assumed as being ascertained in an error-free random manner.
- Frequency tables: The statistical procedure is used for testing the degree to which two or more vary or differ in an experiment. A considerable degree of variation means research findings were significant. In many contexts, ANOVA testing and variance analysis are similar.
- Analysis of variance: The statistical procedure is used for testing the degree to which two or more vary or differ in an experiment. A considerable degree of variation means research findings were significant. In many contexts, ANOVA testing and variance analysis are similar.
- Researchers must have the necessary research skills to analyze and manipulation the data , Getting trained to demonstrate a high standard of research practice. Ideally, researchers must possess more than a basic understanding of the rationale of selecting one statistical method over the other to obtain better data insights.
- Usually, research and data analytics projects differ by scientific discipline; therefore, getting statistical advice at the beginning of analysis helps design a survey questionnaire, select data collection methods , and choose samples.
LEARN ABOUT: Best Data Collection Tools
- The primary aim of data research and analysis is to derive ultimate insights that are unbiased. Any mistake in or keeping a biased mind to collect data, selecting an analysis method, or choosing audience sample il to draw a biased inference.
- Irrelevant to the sophistication used in research data and analysis is enough to rectify the poorly defined objective outcome measurements. It does not matter if the design is at fault or intentions are not clear, but lack of clarity might mislead readers, so avoid the practice.
- The motive behind data analysis in research is to present accurate and reliable data. As far as possible, avoid statistical errors, and find a way to deal with everyday challenges like outliers, missing data, data altering, data mining , or developing graphical representation.
LEARN MORE: Descriptive Research vs Correlational Research The sheer amount of data generated daily is frightening. Especially when data analysis has taken center stage. in 2018. In last year, the total data supply amounted to 2.8 trillion gigabytes. Hence, it is clear that the enterprises willing to survive in the hypercompetitive world must possess an excellent capability to analyze complex research data, derive actionable insights, and adapt to the new market needs.
LEARN ABOUT: Average Order Value
QuestionPro is an online survey platform that empowers organizations in data analysis and research and provides them a medium to collect data by creating appealing surveys.
MORE LIKE THIS
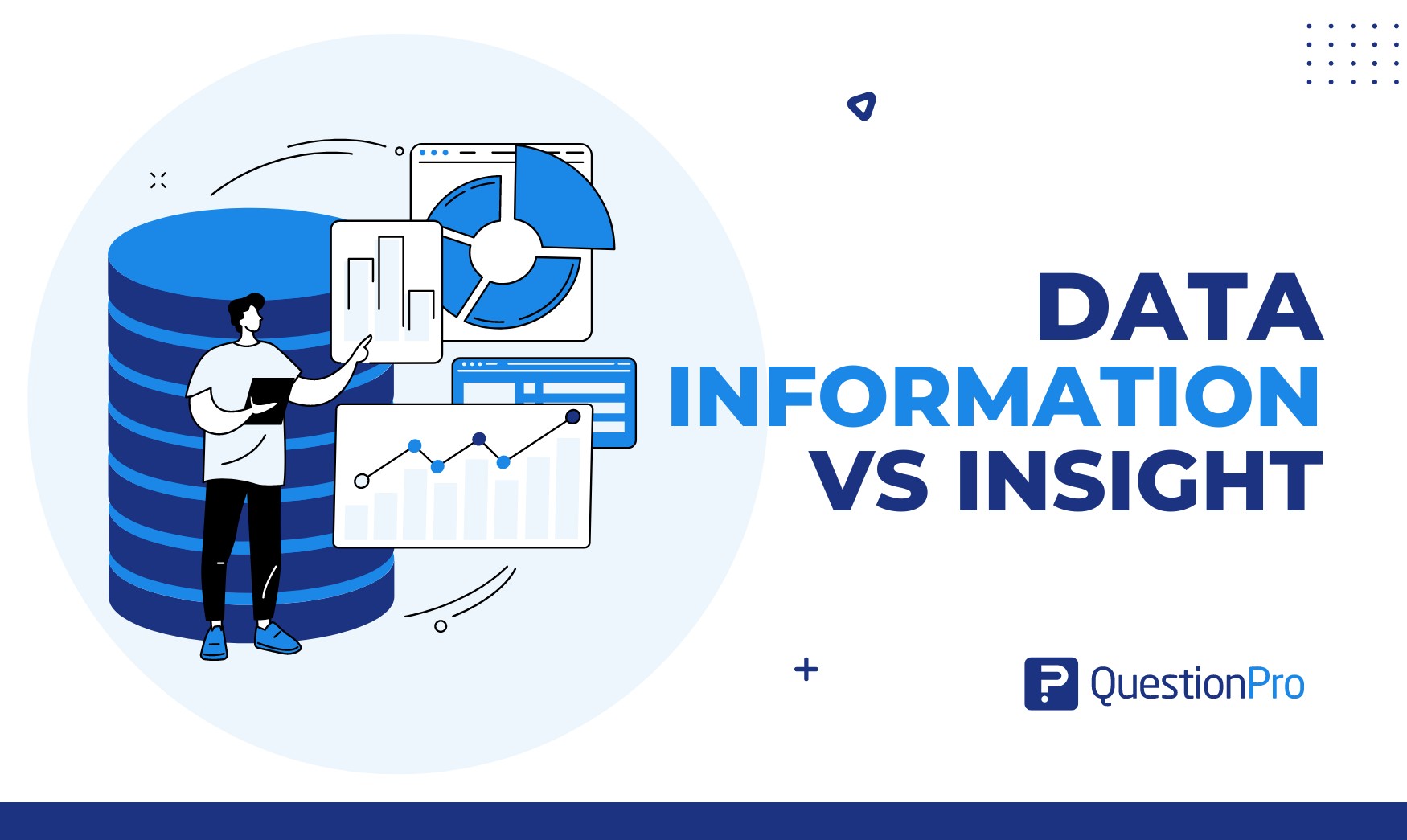
Data Information vs Insight: Essential differences
May 14, 2024
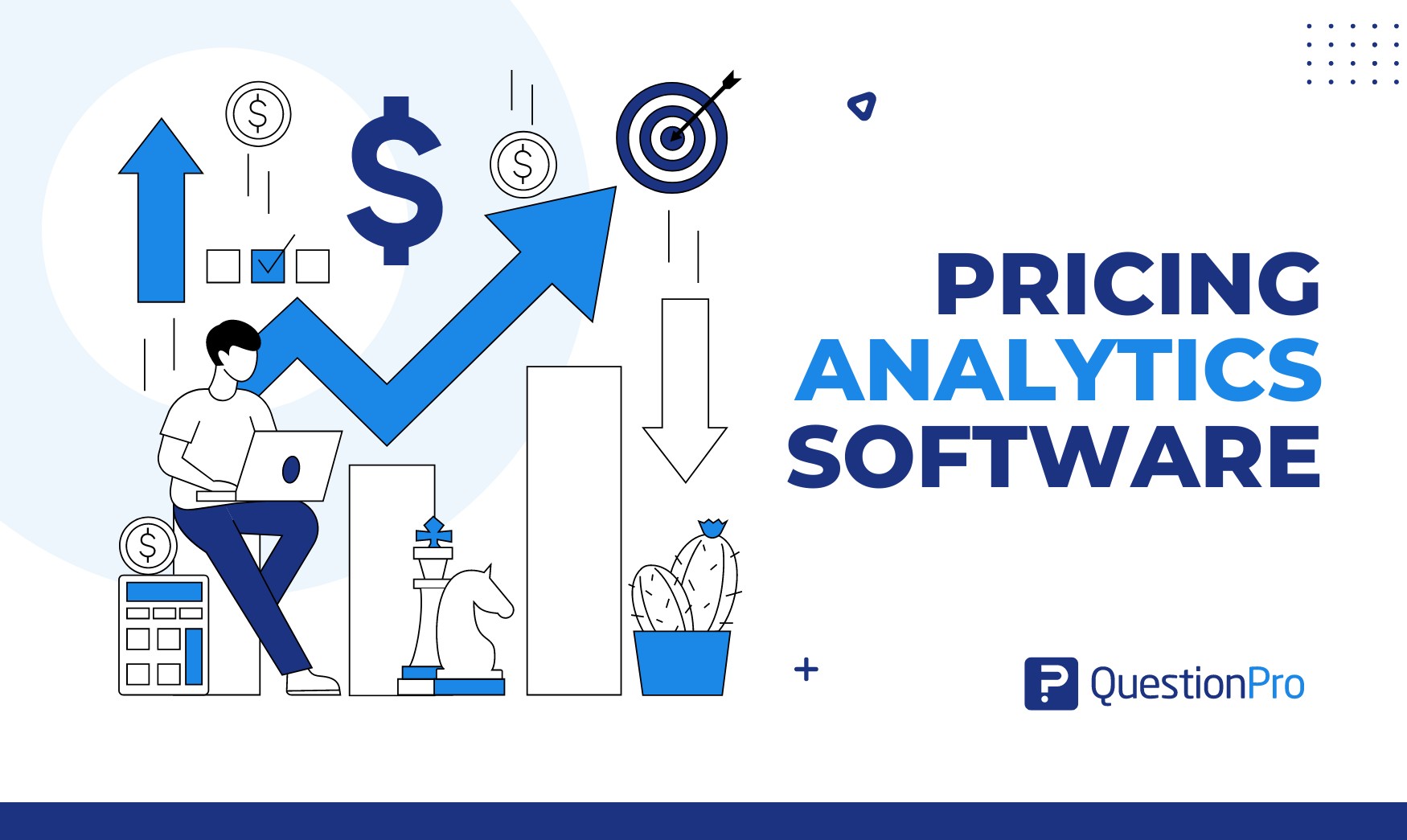
Pricing Analytics Software: Optimize Your Pricing Strategy
May 13, 2024
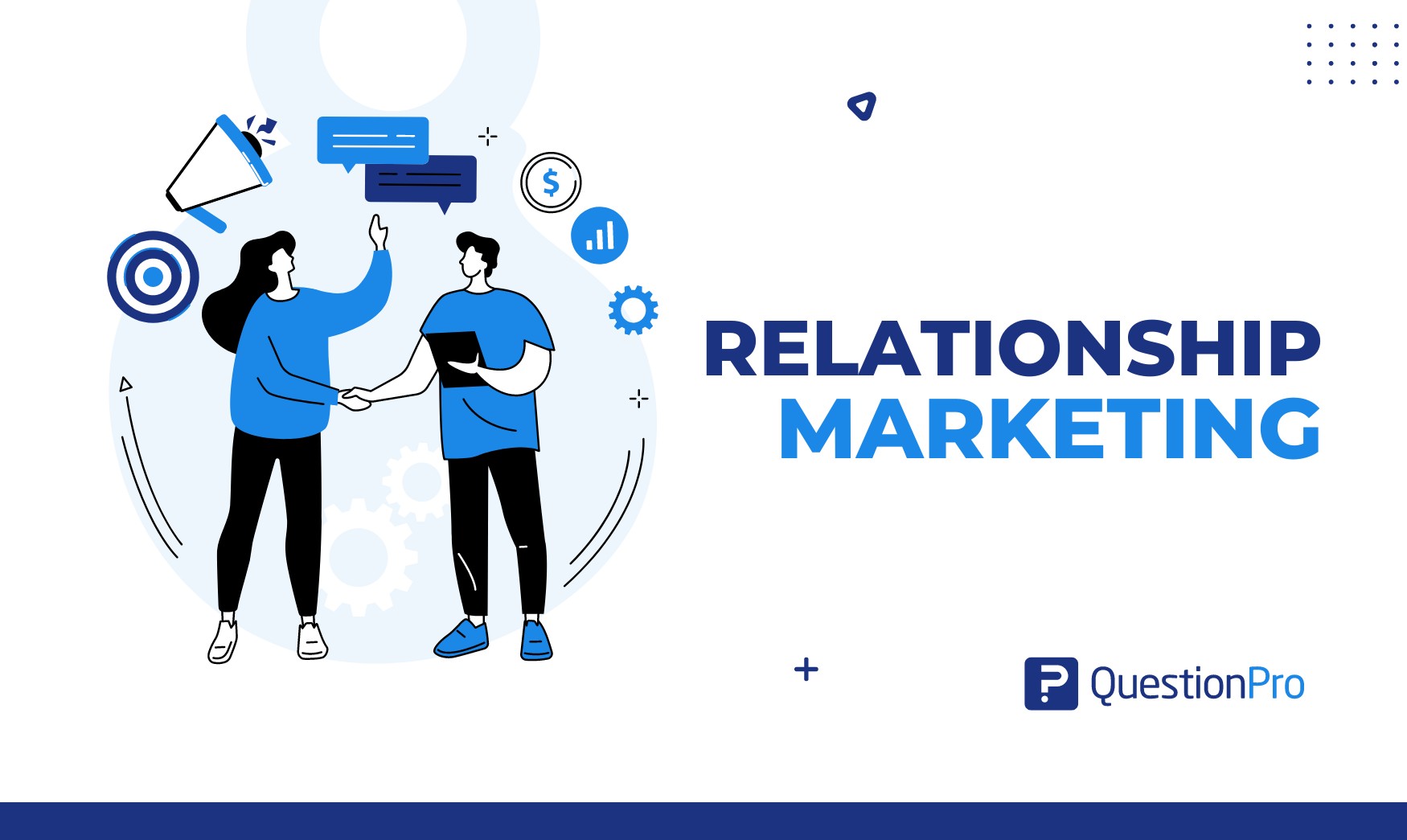
Relationship Marketing: What It Is, Examples & Top 7 Benefits
May 8, 2024
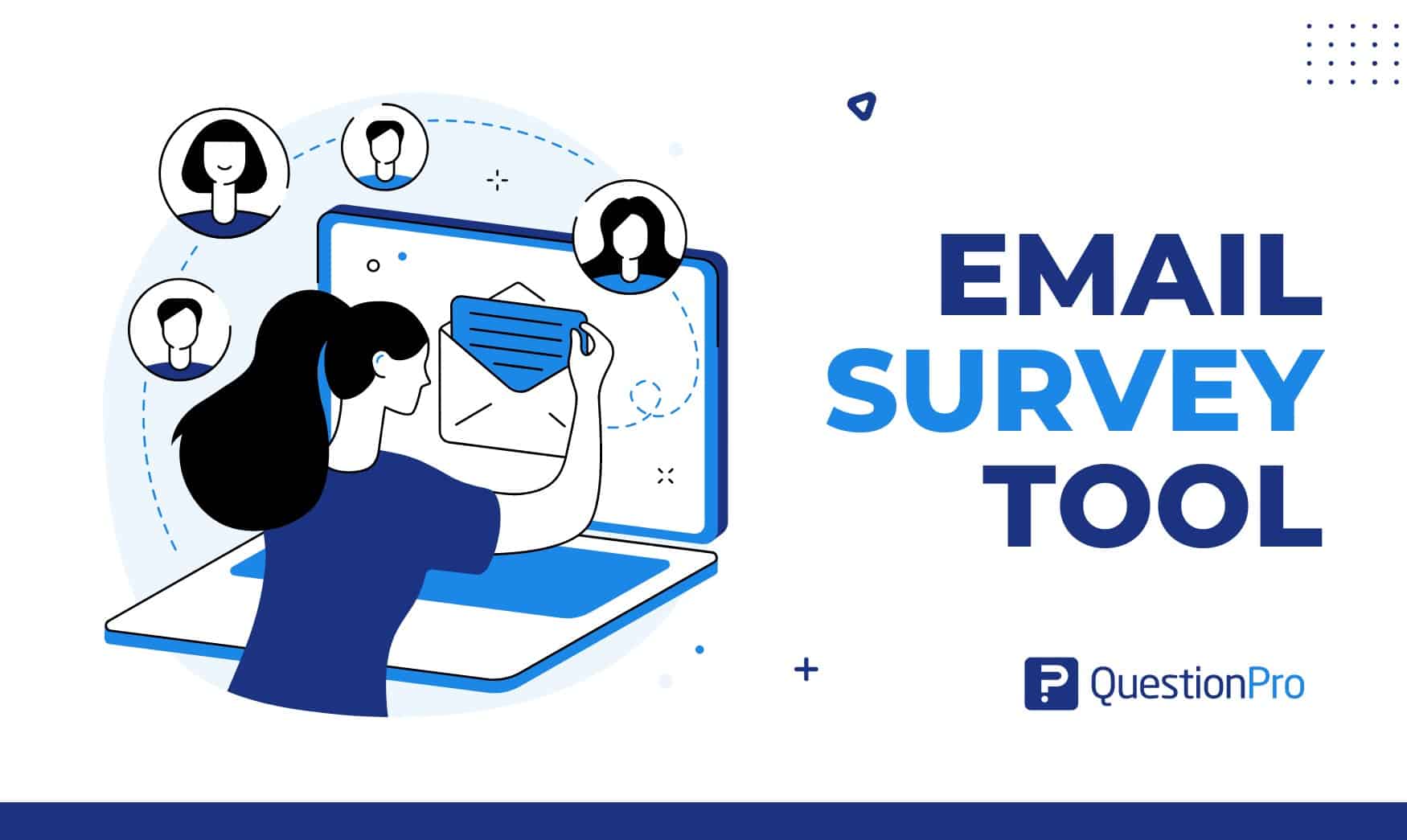
The Best Email Survey Tool to Boost Your Feedback Game
May 7, 2024
Other categories
- Academic Research
- Artificial Intelligence
- Assessments
- Brand Awareness
- Case Studies
- Communities
- Consumer Insights
- Customer effort score
- Customer Engagement
- Customer Experience
- Customer Loyalty
- Customer Research
- Customer Satisfaction
- Employee Benefits
- Employee Engagement
- Employee Retention
- Friday Five
- General Data Protection Regulation
- Insights Hub
- Life@QuestionPro
- Market Research
- Mobile diaries
- Mobile Surveys
- New Features
- Online Communities
- Question Types
- Questionnaire
- QuestionPro Products
- Release Notes
- Research Tools and Apps
- Revenue at Risk
- Survey Templates
- Training Tips
- Uncategorized
- Video Learning Series
- What’s Coming Up
- Workforce Intelligence

CRO Platform
Test your insights. Run experiments. Win. Or learn. And then win.

eCommerce Customer Analytics Platform

Acquisition matters. But retention matters more. Understand, monitor & nurture the best customers.
- Case Studies
- Ebooks, Tools, Templates
- Digital Marketing Glossary
- eCommerce Growth Stories
- eCommerce Growth Show
- Help & Technical Documentation
CRO Guide > Chapter 3.1
Qualitative Research: Definition, Methodology, Limitation, Examples
Qualitative research is a method focused on understanding human behavior and experiences through non-numerical data. Examples of qualitative research include:
- One-on-one interviews,
- Focus groups, Ethnographic research,
- Case studies,
- Record keeping,
- Qualitative observations
In this article, we’ll provide tips and tricks on how to use qualitative research to better understand your audience through real world examples and improve your ROI. We’ll also learn the difference between qualitative and quantitative data.

Table of Contents
Marketers often seek to understand their customers deeply. Qualitative research methods such as face-to-face interviews, focus groups, and qualitative observations can provide valuable insights into your products, your market, and your customers’ opinions and motivations. Understanding these nuances can significantly enhance marketing strategies and overall customer satisfaction.
What is Qualitative Research
Qualitative research is a market research method that focuses on obtaining data through open-ended and conversational communication. This method focuses on the “why” rather than the “what” people think about you. Thus, qualitative research seeks to uncover the underlying motivations, attitudes, and beliefs that drive people’s actions.
Let’s say you have an online shop catering to a general audience. You do a demographic analysis and you find out that most of your customers are male. Naturally, you will want to find out why women are not buying from you. And that’s what qualitative research will help you find out.
In the case of your online shop, qualitative research would involve reaching out to female non-customers through methods such as in-depth interviews or focus groups. These interactions provide a platform for women to express their thoughts, feelings, and concerns regarding your products or brand. Through qualitative analysis, you can uncover valuable insights into factors such as product preferences, user experience, brand perception, and barriers to purchase.
Types of Qualitative Research Methods
1. one-on-one interviews.
- A company might conduct interviews to understand why a product failed to meet sales expectations.
- A researcher might use interviews to gather personal stories about experiences with healthcare.
2. Focus groups
- A focus group could be used to test reactions to a new product concept.
- Marketers might use focus groups to see how different demographic groups react to an advertising campaign.
3. Ethnographic research
- A study of workplace culture within a tech startup.
- Observational research in a remote village to understand local traditions.
4. Case study research
- Analyzing a single school’s innovative teaching method.
- A detailed study of a patient’s medical treatment over several years.
H3: 5. Record keeping
- Historical research using old newspapers and letters.
- A study on policy changes over the years by examining government records.
6. Qualitative observation
- Sight : Observing the way customers visually interact with product displays in a store to understand their browsing behaviors and preferences.
- Smell : Noting reactions of consumers to different scents in a fragrance shop to study the impact of olfactory elements on product preference.
- Touch : Watching how individuals interact with different materials in a clothing store to assess the importance of texture in fabric selection.
- Taste : Evaluating reactions of participants in a taste test to identify flavor profiles that appeal to different demographic groups.
- Hearing : Documenting responses to changes in background music within a retail environment to determine its effect on shopping behavior and mood.
Qualitative Research Real World Examples
1. online grocery shop with a predominantly male audience, 2. software company launching a new product, 3. alan pushkin’s “god’s choice: the total world of a fundamentalist christian school”, 4. understanding buyers’ trends, 5. determining products/services missing from the market, real-time customer lifetime value (clv) benchmark report.
See where your business stands compared to 1,000+ e-stores in different industries.
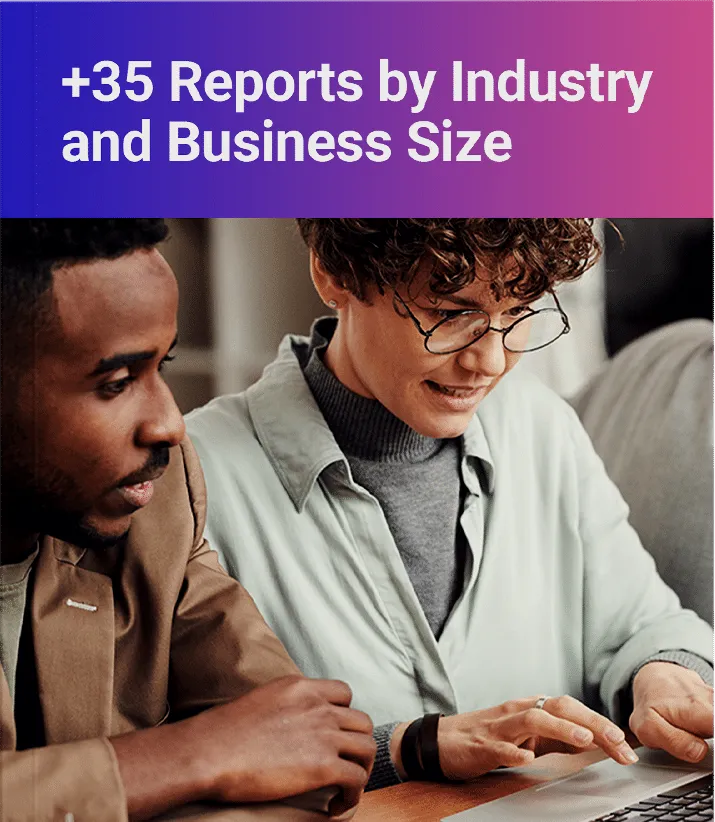
Qualitative Research Approaches
- Narrative : This method focuses on individual life stories to understand personal experiences and journeys. It examines how people structure their stories and the themes within them to explore human existence. For example, a narrative study might look at cancer survivors to understand their resilience and coping strategies.
- Phenomenology : attempts to understand or explain life experiences or phenomena; It aims to reveal the depth of human consciousness and perception, such as by studying the daily lives of those with chronic illnesses.
- Grounded theory : investigates the process, action, or interaction with the goal of developing a theory “grounded” in observations and empirical data.
- Ethnography : describes and interprets an ethnic, cultural, or social group;
- Case study : examines episodic events in a definable framework, develops in-depth analyses of single or multiple cases, and generally explains “how”. An example might be studying a community health program to evaluate its success and impact.
How to Analyze Qualitative Data
1. data collection, 2. data preparation, 3. familiarization.
- Descriptive Coding : Summarize the primary topic of the data.
- In Vivo Coding : Use language and terms used by the participants themselves.
- Process Coding : Use gerunds (“-ing” words) to label the processes at play.
- Emotion Coding : Identify and record the emotions conveyed or experienced.
5. Thematic Development
6. interpreting the data, 7. validation, 8. reporting, limitations of qualitative research, 1. it’s a time-consuming process, 2. you can’t verify the results of qualitative research, 3. it’s a labor-intensive approach, 4. it’s difficult to investigate causality, 5. qualitative research is not statistically representative, quantitative vs. qualitative research.

Image source
Nature of Data:
- Quantitative research : Involves numerical data that can be measured and analyzed statistically.
- Qualitative research : Focuses on non-numerical data, such as words, images, and observations, to capture subjective experiences and meanings.
Research Questions:
- Quantitative research : Typically addresses questions related to “how many,” “how much,” or “to what extent,” aiming to quantify relationships and patterns.
- Qualitative research: Explores questions related to “why” and “how,” aiming to understand the underlying motivations, beliefs, and perceptions of individuals.
Data Collection Methods:
- Quantitative research : Relies on structured surveys, experiments, or observations with predefined variables and measures.
- Qualitative research : Utilizes open-ended interviews, focus groups, participant observations, and textual analysis to gather rich, contextually nuanced data.
Analysis Techniques:
- Quantitative research: Involves statistical analysis to identify correlations, associations, or differences between variables.
- Qualitative research: Employs thematic analysis, coding, and interpretation to uncover patterns, themes, and insights within qualitative data.

Do Conversion Rate Optimization the Right way.
Explore helps you make the most out of your CRO efforts through advanced A/B testing, surveys, advanced segmentation and optimised customer journeys.

Like what you’re reading?
Join the informed ecommerce crowd.
We will never bug you with irrelevant info.
By clicking the Button, you confirm that you agree with our Terms and Conditions .
Continue your Conversion Rate Optimization Journey
- Last modified: January 3, 2023
- Conversion Rate Optimization , User Research
Valentin Radu

We’re a team of people that want to empower marketers around the world to create marketing campaigns that matter to consumers in a smart way. Meet us at the intersection of creativity, integrity, and development, and let us show you how to optimize your marketing.
Our Software
- > Book a Demo
- > Partner Program
- > Affiliate Program
- Blog Sitemap
- Terms and Conditions
- Privacy & Security
- Cookies Policy
- REVEAL Terms and Conditions
- Python For Data Analysis
- Data Science
- Data Analysis with R
- Data Analysis with Python
- Data Visualization with Python
- Data Analysis Examples
- Math for Data Analysis
- Data Analysis Interview questions
- Artificial Intelligence
- Data Analysis Projects
- Machine Learning
- Deep Learning
- Computer Vision
- How to Use Bard for Data Analysis and Insights
- Financial Analysis: Objectives, Methods, and Process
- Time Series Analysis & Visualization in Python
- Difference Between Data Visualization and Data Analytics
- What are the 5 methods of statistical analysis?
- What Is Spatial Analysis, and How Does It Work
- Qualitative and Quantitative Data
- What is Geospatial Data Analysis?
- Data-Driven Design Decisions and Analytics Tools
- Data analysis and Visualization with Python
- Exploratory Data Analysis (EDA) - Types and Tools
- Data Analytics and its type
- Why Data Visualization Matters in Data Analytics?
- What is Data Analysis?
- Data analytics and career opportunities
- Methods of Economic Analysis
- Data Science Methodology and Approach
- Data | Analysis Quiz | Question 1
- What is Exploratory Data Analysis ?
Qualitative Data Analysis Methodologies and Methods
Qualitative data analysis involves interpreting non-numerical data to identify patterns, themes, and insights. There are several methodologies and methods used in qualitative data analysis.
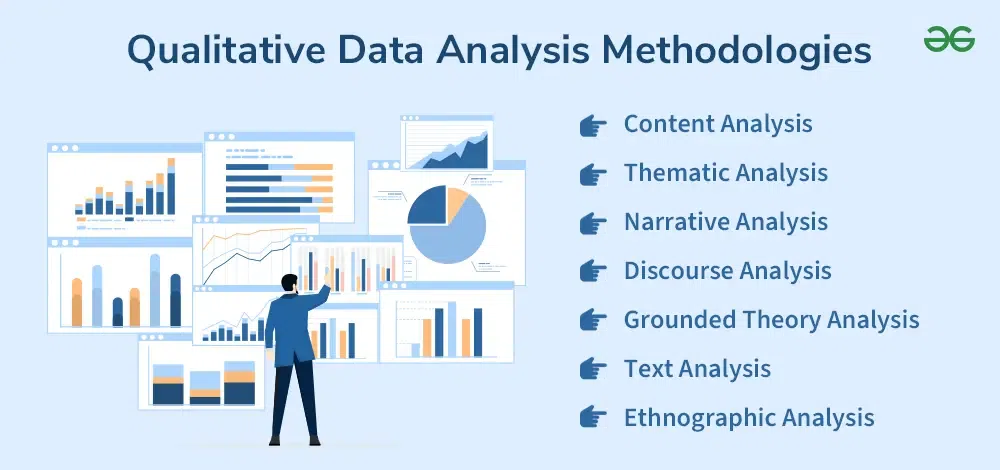
In this article, we will explore qualitative data analysis techniques in great detail, with each method providing a different perspective on how to interpret qualitative data.
Table of Content
Types of Qualitative Data Analysis Methodologies
1. content analysis, 2. thematic analysis, 3. narrative analysis, 4. discourse analysis, 5. grounded theory analysis, 6. text analysis, 7. ethnographic analysis, advantages and disadvantages of different qualitative data analysis methodologies, best practices for qualitative data analysis, qualitative data analysis methods- faq’s.
Lets weigh the benefits and disadvantages of each:
Content analysis involves systematically reading textual content or other types of communication to perceive patterns, themes, and meanings within the content. It provides a dependent technique to inspecting huge volumes of records to discover insights or trends. Researchers categorize and code the content material based on predetermined criteria or emergent themes, taking into consideration quantitative and qualitative interpretation of the facts. Content analysis is regularly an iterative procedure, with researchers revisiting and refining the coding scheme, collecting additional facts, or accomplishing in addition analysis as needed to deepen know-how or cope with new studies questions.
There are 3 fundamental techniques to content analysis:
- Conventional Content Analysis : In conventional content analysis, researchers technique the records with out preconceived categories or theoretical frameworks. Instead, they allow classes and themes to emerge evidently from the statistics through an iterative system of coding and analysis. This technique is exploratory and bendy, allowing for the discovery of latest insights and styles inside the content material.
- Directed Content Analysis : Directed content material analysis entails studying the statistics based totally on existing theories or principles. Researchers start with predefined categories or subject matters derived from theoretical frameworks or previous research findings. The analysis is focused on confirming, refining, or extending present theories in place of coming across new ones. Directed content analysis is specifically beneficial whilst researchers intention to test hypotheses or explore particular concepts in the statistics.
- Summative Content Analysis : Summative content material analysis focuses on quantifying the presence or frequency of precise content within the information. Researchers expand predetermined classes or coding schemes primarily based on predefined criteria, after which systematically code the statistics in line with those classes. The emphasis is on counting occurrences of predefined attributes or topics to provide a numerical summary of the content. Summative content material analysis is frequently used to track modifications over time, examine unique assets of content material, or verify the superiority of specific subject matters inside a dataset.
When to Use Content Analysis?
- Exploratory Research : Content analysis is appropriate for exploratory research in which the goal is to uncover new insights, discover emerging developments, or recognize the breadth of communique on a particular subject matter.
- Comparative Analysis: It is useful for comparative analysis, permitting researchers to compare conversation throughout extraordinary sources, time periods, or cultural contexts.
- Historical Analysis : Content analysis can be carried out to historical research, allowing researchers to analyze ancient files, media content, or archival substances to apprehend conversation styles over the years.
- Policy Analysis: It is valuable for policy analysis, supporting researchers look at the portrayal of problems in media or public discourse and informing coverage-making methods.
- Market Research: Content analysis is usually utilized in market research to investigate advertising and marketing substances, social media content, and customer critiques, presenting insights into patron perceptions and possibilities.
Thematic analysis is a method for identifying, analyzing, and reporting styles or topics within qualitative records. It entails systematically coding and categorizing information to become aware of not unusual issues, styles, or ideas that emerge from the dataset. Researchers interact in a method of inductive reasoning to generate topics that capture the essence of the facts, making an allowance for interpretation and exploration of underlying meanings.
Thematic analysis is appropriate when researchers are seeking for to become aware of, analyze, and document patterns or issues inside qualitative records. It is especially beneficial for exploratory studies where the intention is to find new insights or recognize the breadth of studies and views associated with a specific phenomenon.
Thematic analysis offers a bendy and systematic approach for identifying and reading styles or topics within qualitative statistics, making it a treasured method for exploring complex phenomena and producing insights that inform concept, exercise, and policy.
When to use Thematic analysis?
- Psychology : Thematic analysis is used to explore mental phenomena, which include coping mechanisms in reaction to strain, attitudes towards mental fitness, or stories of trauma.
- Education : Researchers practice thematic analysis to apprehend student perceptions of getting to know environments, teaching methods, or academic interventions.
- Healthcare : Thematic analysis enables take a look at affected person reports with healthcare offerings, attitudes towards treatment alternatives, or obstacles to gaining access to healthcare.
- Market Research: Thematic analysis is applied to research purchaser remarks, perceive product options, or recognize emblem perceptions in marketplace research research.
Narrative analysis entails analyzing and interpreting the memories or narratives that people use to make feel of their stories. It focuses on the shape, content, and which means of narratives to apprehend how people construct and speak their identities, values, and ideals via storytelling. It is especially beneficial for exploring how people assemble and communicate their identities, values, and beliefs through storytelling.
When to use Narrative Analysis?
It’s extensively used throughout numerous disciplines, which includes sociology, psychology, anthropology, literary research, and verbal exchange studies. Some applications of narrative analysis in qualitative statistics analysis methodologies are:
- Understanding Identity Construction : Narrative analysis can be used to explore how people construct their identities through the tales they tell approximately themselves. Researchers can examine the issues, plot systems, and language utilized in narratives to uncover how individuals perceive themselves and their place inside the world.
- Exploring Life Experiences : Researchers frequently use narrative analysis to research the lived reports of people or groups. By inspecting the narratives shared by using members, researchers can advantage insights into the demanding situations, triumphs, and extensive events that shape people’s lives.
- Examining Cultural Meanings and Practices: Narrative analysis can provide treasured insights into cultural meanings and practices. By studying the stories shared within a selected cultural context, researchers can find shared values, ideals, and norms that influence behavior and social interactions.
- Exploring Trauma and Healing : Narrative analysis is usually utilized in studies on trauma and restoration tactics. By studying narratives of trauma survivors, researchers can explore how individuals make experience of their studies, deal with adversity, and embark on trips of restoration and resilience.
- Analyzing Media and Popular Culture : Narrative analysis also can be applied to analyze media texts, inclusive of films, tv suggests, and literature. Researchers can have a look at the narratives constructed within these texts to understand how they reflect and shape cultural beliefs, ideologies, and norms.
Narrative analysis offers a powerful technique for exploring the structure, content, and that means of narratives or stories instructed by people, providing insights into their lived reports, identities, and perspectives. However, researchers need to navigate the interpretive subjectivity, time-extensive nature, and moral concerns related to reading narratives in qualitative studies.
Discourse analysis examines the approaches wherein language is used to construct that means, form social interactions, and reproduce electricity members of the family inside society. It makes a speciality of studying spoken or written texts, in addition to the wider social and cultural contexts in which communique happens. Researchers explore how language displays and shapes social norms, ideologies, and power dynamics.
Discourse analysis is employed when researchers are seeking to investigate social interactions, power dynamics, and identity creation through language. It is applied to take a look at how language shapes social relations, constructs identities, and reflects cultural norms and values.
When to use Discourse Analysis?
- Linguistics and Language Studies : Discourse analysis is foundational to linguistics and language research, where it’s miles used to study language use, communique patterns, and discourse structures. Linguists behavior discourse analysis to investigate how language shapes social interactions, constructs identities, and reflects cultural norms. Discourse analysis facilitates uncover the underlying meanings, ideologies, and energy dynamics embedded in language.
- Media and Communication : Discourse analysis is applied in media and conversation research to have a look at media representations, discursive practices, and ideological frameworks. Researchers conduct discourse analysis to analyze media texts, information coverage, and political speeches, exploring how language constructs and disseminates social meanings and values. Discourse analysis informs media literacy efforts, media grievance, and media coverage debates.
- Political Science : Discourse analysis is applied in political science to look at political rhetoric, public discourse, and policymaking tactics. Researchers behavior discourse analysis to research political speeches, party manifestos, and coverage files, analyzing how language constructs political identities, legitimizes authority, and shapes public opinion. Discourse analysis informs political verbal exchange techniques, political campaigning, and policy advocacy.
Grounded theory analysis is an inductive studies approach used to broaden theories or causes based on empirical data. It includes systematically studying qualitative information to perceive ideas, categories, and relationships that emerge from the statistics itself, rather than testing preconceived hypotheses. Researchers have interaction in a procedure of constant assessment and theoretical sampling to refine and increase theoretical insights.
Grounded theory analysis is hired whilst researchers are seeking for to find styles, relationships, and tactics that emerge from the records itself, with out implementing preconceived hypotheses or theoretical assumptions.
When to use Grounded Theory Analysis?
Grounded concept analysis is applied throughout various disciplines and studies contexts, such as:
- Social Sciences Research : Grounded Theory Analysis is significantly used in sociology, anthropology, psychology, and related disciplines to discover diverse social phenomena together with organization dynamics, social interactions, cultural practices, and societal structures.
- Healthcare Research : In healthcare, Grounded Theory can be implemented to apprehend affected person reviews, healthcare provider-patient interactions, healthcare delivery procedures, and the impact of healthcare guidelines on individuals and communities.
- Organizational Studies : Researchers use Grounded Theory to examine organizational conduct, leadership, place of work subculture, and worker dynamics. It enables in knowledge how groups function and the way they may be advanced.
- Educational Research : In training, Grounded Theory Analysis can be used to discover teaching and getting to know processes, scholar studies, educational regulations, and the effectiveness of educational interventions.
Text analysis involves examining written or verbal communique to extract meaningful insights or styles. It encompasses numerous techniques which includes sentiment analysis, subject matter modeling, and keyword extraction. For instance, in a have a look at on patron opinions of a eating place, textual content analysis is probably used to become aware of established topics along with food first-class, service enjoy, and atmosphere. Key additives and strategies worried in text analysis:
- Sentiment Analysis : This approach includes determining the sentiment expressed in a piece of textual content, whether or not it is high quality, bad, or impartial. Sentiment analysis algorithms use natural language processing (NLP) to analyze the words, phrases, and context within the text to deduce the overall sentiment. For instance, in customer reviews of a eating place, sentiment analysis could be used to gauge purchaser delight levels based totally on the emotions expressed within the critiques.
- Topic Modeling : Topic modeling is a statistical technique used to become aware of the underlying topics or issues present within a group of documents or text statistics. It entails uncovering the latent patterns of co-occurring phrases or terms that constitute awesome topics. Techniques like Latent Dirichlet Allocation (LDA) and Latent Semantic Analysis (LSA) are normally used for topic modeling. In the context of eating place opinions, subject matter modeling should assist identify not unusual subject matters inclusive of meals excellent, provider revel in, cleanliness, etc., across a large corpus of opinions.
- Keyword Extraction : Keyword extraction includes figuring out and extracting the most applicable phrases or phrases from a bit of text that seize its essence or major topics. This technique enables to summarize the important thing content material or subjects mentioned within the textual content. For instance, in eating place analysiss, key-word extraction ought to identify often referred to terms like “scrumptious meals,” “friendly group of workers,” “lengthy wait times,” etc., presenting a quick analysis of customer sentiments and concerns.
When to use Text Analysis?
Text analysis has numerous programs throughout diverse domain names, including:
- Business and Marketing: Analyzing purchaser remarks, sentiment analysis of social media posts, brand monitoring, and market fashion analysis.
- Healthcare: Extracting scientific statistics from scientific notes, analyzing patient comments, and detecting unfavorable drug reactions from textual content information.
- Social Sciences: Studying public discourse, political communique, opinion mining, and discourse analysis in social media.
- Academic Research: Conducting literature analysiss, analyzing studies articles, and identifying rising studies topics and trends.
- Customer Experience : Understanding purchaser sentiments, identifying product or service problems, and improving client satisfaction via text-based totally comments analysis.
Ethnographic analysis involves immersing in a selected cultural or social setting to understand the views, behaviors, and interactions of the human beings within that context. Researchers conduct observations, interviews, and participant observations to gain insights into the culture, practices, and social dynamics of the community under study. It is is suitable when researchers aim to gain an in-depth understanding of a particular cultural or social setting, including the perspectives, behaviors, and interactions of the people within that context. Particularly beneficial for reading complex social phenomena of their natural environment, wherein observations and interactions arise organically.
When to use Ethnographic Analysis?
- Cultural Understanding : Ethnographic analysis is right whilst researchers goal to gain deep insights into the lifestyle, ideals, and social practices of a selected institution or community.
- Behavioral Observation : It is beneficial while researchers want to observe and apprehend the behaviors, interactions, and each day activities of individuals within their natural surroundings.
- Contextual Exploration : Ethnographic analysis is valuable for exploring the context and lived stories of individuals, presenting wealthy, exact descriptions of their social and cultural worlds.
- Complex Social Dynamics: It is suitable whilst analyzing complex social phenomena or phenomena which might be deeply embedded within social contexts, including rituals, traditions, or network dynamics.
- Qualitative Inquiry: Ethnographic analysis is desired while researchers are seeking for to conduct qualitative inquiry targeted on know-how the subjective meanings and perspectives of individuals inside their cultural context.
Ethnographic analysis gives a effective method for analyzing complex social phenomena of their herbal context, offering rich and nuanced insights into the cultural practices, social dynamics, and lived experiences of individuals inside a particular community. However, researchers need to cautiously bear in mind the time commitment, ethical considerations, and potential biases associated with ethnographic studies.
- Clearly Defined Research Question : Ground analysis in a clear and targeted research question. This will manual for information series and preserve you on the right track at some point of analysis.
- Systematic Coding : Develop a coding scheme to categorize facts into significant topics or concepts. Use software gear to assist in organizing and dealing with codes.
- Constant Comparison : Continuously examine new facts with current codes and subject matters to refine interpretations and make sure consistency.
- Triangulation : Validate findings by the use of a couple of records sources, strategies, or researchers to corroborate consequences and beautify credibility.
Refine subject matters and interpretations through engaging in repeated cycles of gathering, coding, and analysis.
Qualitative data analysis techniques are effective means of revealing deep insights and comprehending intricate phenomena in both practice and study. Through the use of rigorous analytical approaches, researchers may convert qualitative data into significant ideas, interpretations, and narratives that further knowledge and support evidence-based decision-making.
Is it possible to mix quantitative and qualitative methodologies for data analysis?
A: In order to triangulate results and get a thorough grasp of study concerns, researchers do, in fact, often use mixed methods techniques.
How can I choose the best approach for analyzing qualitative data for my study?
A: To choose the best approach, take the research topic, the properties of the data, and the theoretical framework into consideration.
What are some tactics I might do to improve the reliability and validity of my qualitative data analysis?
Aim for peer debriefing and member verification to improve validity, and maintain transparency, reflexivity, and methodological coherence throughout the analytic process.
Please Login to comment...
Similar reads.
- Data Analysis
Improve your Coding Skills with Practice
What kind of Experience do you want to share?
Qualitative Data Coding
Saul Mcleod, PhD
Editor-in-Chief for Simply Psychology
BSc (Hons) Psychology, MRes, PhD, University of Manchester
Saul Mcleod, PhD., is a qualified psychology teacher with over 18 years of experience in further and higher education. He has been published in peer-reviewed journals, including the Journal of Clinical Psychology.
Learn about our Editorial Process
Olivia Guy-Evans, MSc
Associate Editor for Simply Psychology
BSc (Hons) Psychology, MSc Psychology of Education
Olivia Guy-Evans is a writer and associate editor for Simply Psychology. She has previously worked in healthcare and educational sectors.
Coding is the process of analyzing qualitative data (usually text) by assigning labels (codes) to chunks of data that capture their essence or meaning. It allows you to condense, organize and interpret your data.
A code is a word or brief phrase that captures the essence of why you think a particular bit of data may be useful. A good analogy is that a code describes data like a hashtag describes a tweet.
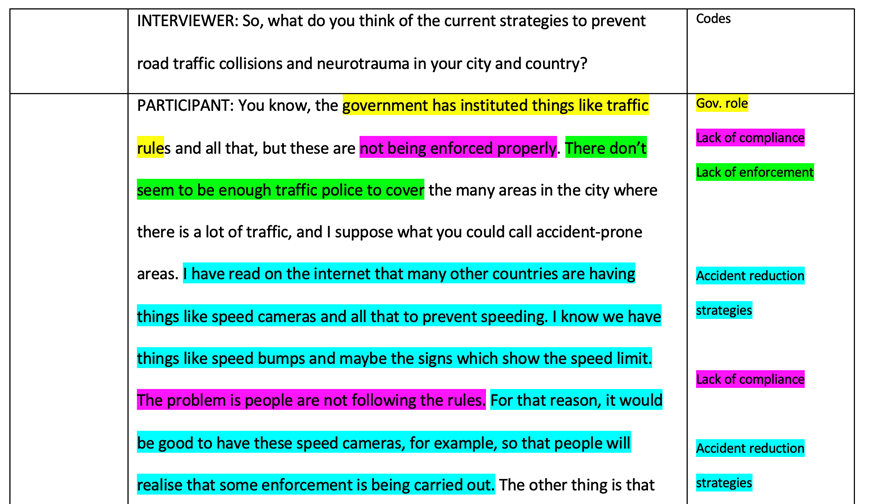
Coding is an iterative process, with researchers refining and revising their codes as their understanding of the data evolves.
The ultimate goal is to develop a coherent and meaningful coding scheme that captures the richness and complexity of the participants’ experiences and helps answer the research questions.
Step 1: Familiarize yourself with the data
- Read through your data (interview transcripts, field notes, documents, etc.) several times. This process is called immersion.
- Think and reflect on what may be important in the data before making any firm decisions about ideas, or potential patterns.
Step 2: Decide on your coding approach
- Will you use predefined deductive codes (based on theory or prior research), or let codes emerge from the data (inductive coding)?
- Will a piece of data have one code or multiple?
- Will you code everything or selectively? Broader research questions may warrant coding more comprehensively.
If you decide not to code everything, it’s crucial to:
- Have clear criteria for what you will and won’t code
- Be transparent about your selection process in research reports
- Remain open to revisiting uncoded data later in analysis
Step 3: Do a first round of coding
- Go through the data and assign initial codes to chunks that stand out
- Create a code name (a word or short phrase) that captures the essence of each chunk
- Keep a codebook – a list of your codes with descriptions or definitions
- Be open to adding, revising or combining codes as you go
Descriptive codes
- In vivo coding / Semantic coding : This method uses words or short phrases directly from the participant’s own language as codes. It deals with the surface-level content, labeling what participants directly say or describe. It identifies keywords, phrases, or sentences that capture the literal content. Participant : “I was just so overwhelmed with everything.” Code : “overwhelmed”
- Process coding : Uses gerunds (“-ing” words) to connote observable or conceptual action in the data. Participant : “I started by brainstorming ideas, then I narrowed them down.” Codes : “brainstorming ideas,” “narrowing down”
- Open coding : A form of initial coding where the researcher remains open to any possible theoretical directions indicated by the data. Participant : “I found the class really challenging, but I learned a lot.” Codes : “challenging class,” “learning experience”
- Descriptive coding : Summarizes the primary topic of a passage in a word or short phrase. Participant : “I usually study in the library because it’s quiet.” Code : “study environment”
Step 4: Review and refine codes
- Look over your initial codes and see if any can be combined, split up, or revised
- Ensure your code names clearly convey the meaning of the data
- Check if your codes are applied consistently across the dataset
- Get a second opinion from a peer or advisor if possible
Interpretive codes
Interpretive codes go beyond simple description and reflect the researcher’s understanding of the underlying meanings, experiences, or processes captured in the data.
These codes require the researcher to interpret the participants’ words and actions in light of the research questions and theoretical framework.
For example, latent coding is a type of interpretive coding which goes beyond surface meaning in data. It digs for underlying emotions, motivations, or unspoken ideas the participant might not explicitly state
Latent coding looks for subtext, interprets the “why” behind what’s said, and considers the context (e.g. cultural influences, or unconscious biases).
- Example: A participant might say, “Whenever I see a spider, I feel like I’m going to pass out. It takes me back to a bad experience as a kid.” A latent code here could be “Feelings of Panic Triggered by Spiders” because it goes beyond the surface fear and explores the emotional response and potential cause.
It’s useful to ask yourself the following questions:
- What are the assumptions made by the participants?
- What emotions or feelings are expressed or implied in the data?
- How do participants relate to or interact with others in the data?
- How do the participants’ experiences or perspectives change over time?
- What is surprising, unexpected, or contradictory in the data?
- What is not being said or shown in the data? What are the silences or absences?
Theoretical codes
Theoretical codes are the most abstract and conceptual type of codes. They are used to link the data to existing theories or to develop new theoretical insights.
Theoretical codes often emerge later in the analysis process, as researchers begin to identify patterns and connections across the descriptive and interpretive codes.
- Structural coding : Applies a content-based phrase to a segment of data that relates to a specific research question. Research question : What motivates students to succeed? Participant : “I want to make my parents proud and be the first in my family to graduate college.” Interpretive Code : “family motivation” Theoretical code : “Social identity theory”
- Value coding : This method codes data according to the participants’ values, attitudes, and beliefs, representing their perspectives or worldviews. Participant : “I believe everyone deserves access to quality healthcare.” Interpretive Code : “healthcare access” (value) Theoretical code : “Distributive justice”
Pattern codes
Pattern coding is often used in the later stages of data analysis, after the researcher has thoroughly familiarized themselves with the data and identified initial descriptive and interpretive codes.
By identifying patterns and relationships across the data, pattern codes help to develop a more coherent and meaningful understanding of the phenomenon and can contribute to theory development or refinement.
For Example
Let’s say a researcher is studying the experiences of new mothers returning to work after maternity leave. They conduct interviews with several participants and initially use descriptive and interpretive codes to analyze the data. Some of these codes might include:
- “Guilt about leaving baby”
- “Struggle to balance work and family”
- “Support from colleagues”
- “Flexible work arrangements”
- “Breastfeeding challenges”
As the researcher reviews the coded data, they may notice that several of these codes relate to the broader theme of “work-family conflict.”
They might create a pattern code called “Navigating work-family conflict” that pulls together the various experiences and challenges described by the participants.
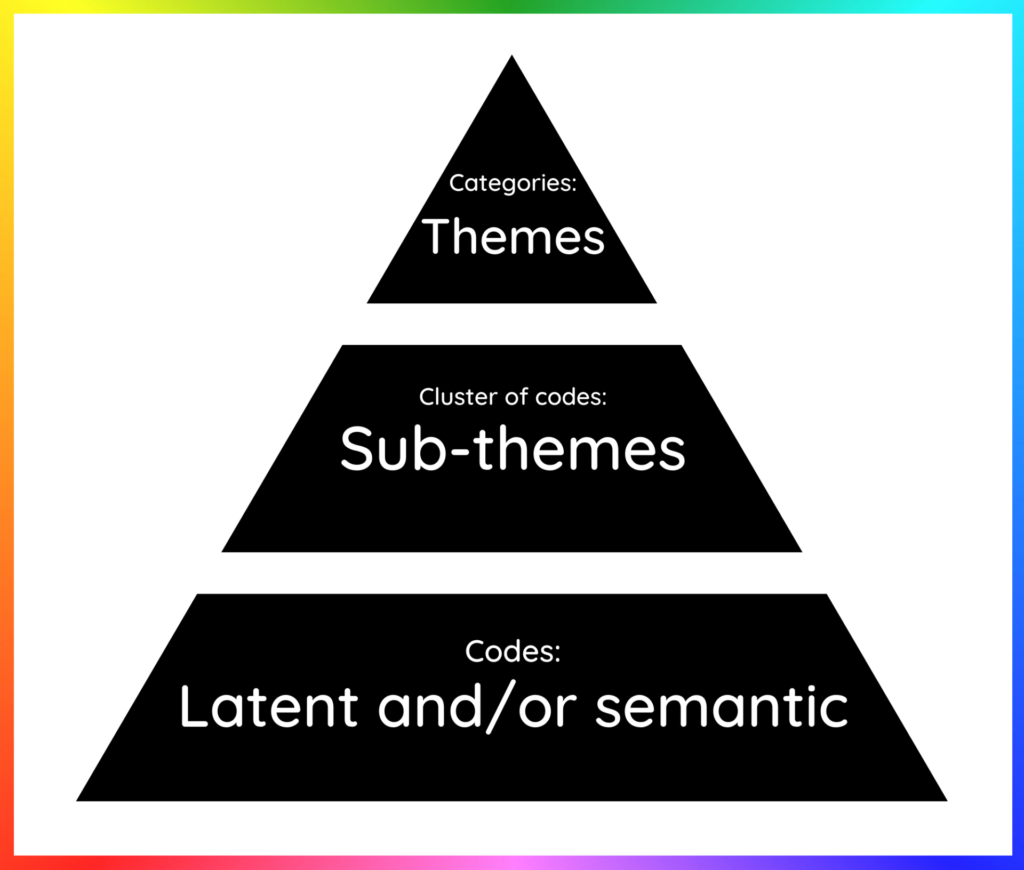
Related Articles
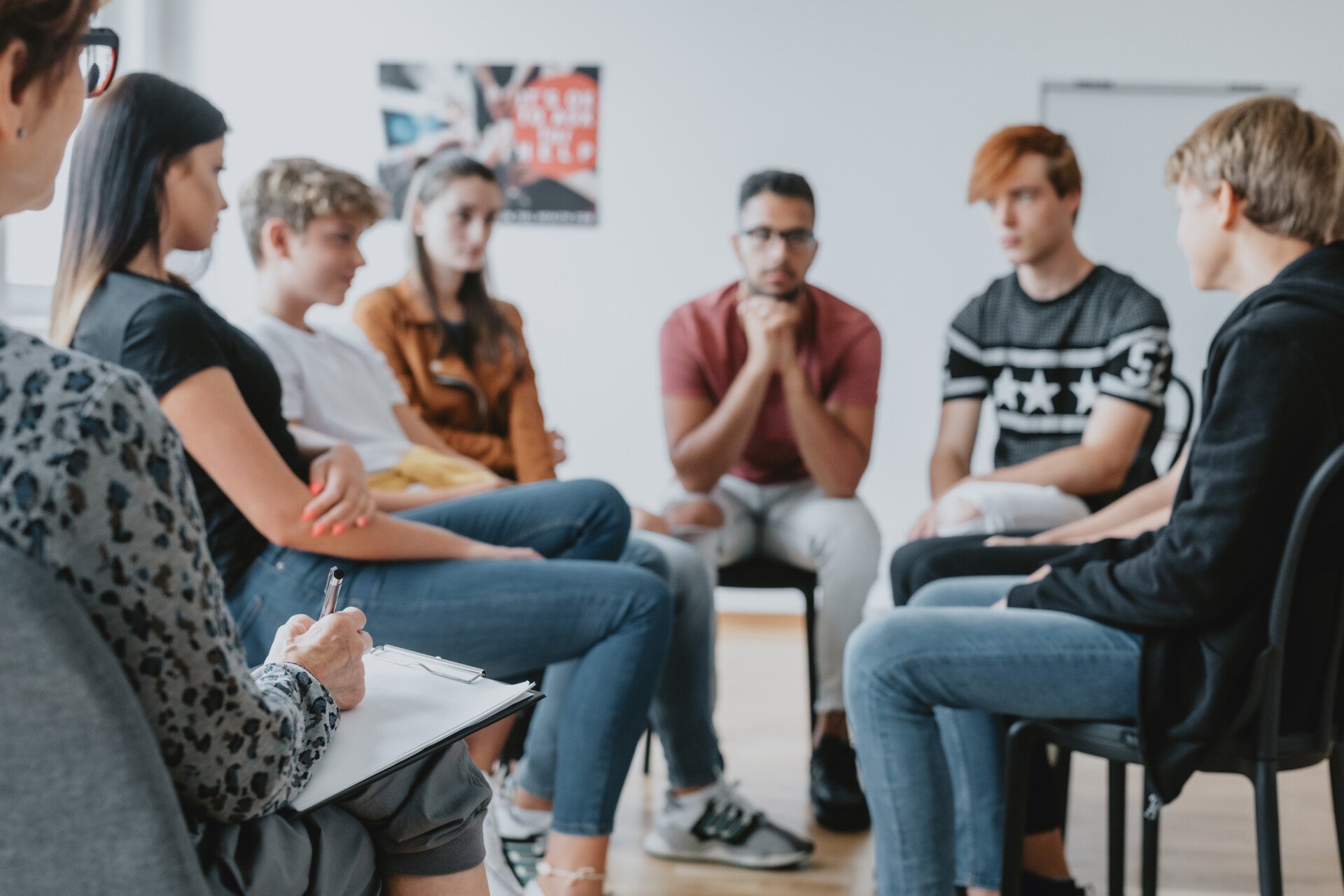
Research Methodology
What Is a Focus Group?
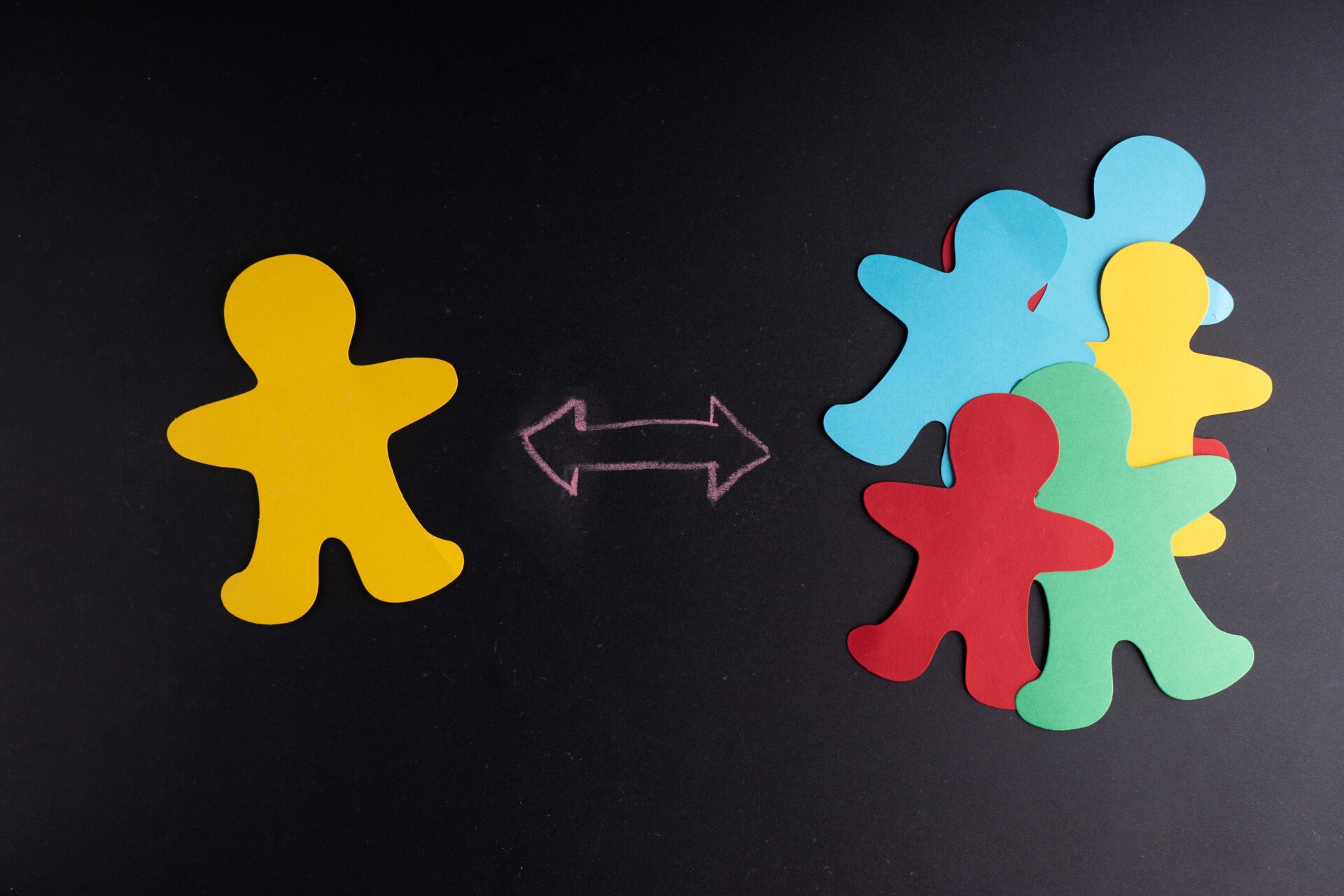
Cross-Cultural Research Methodology In Psychology
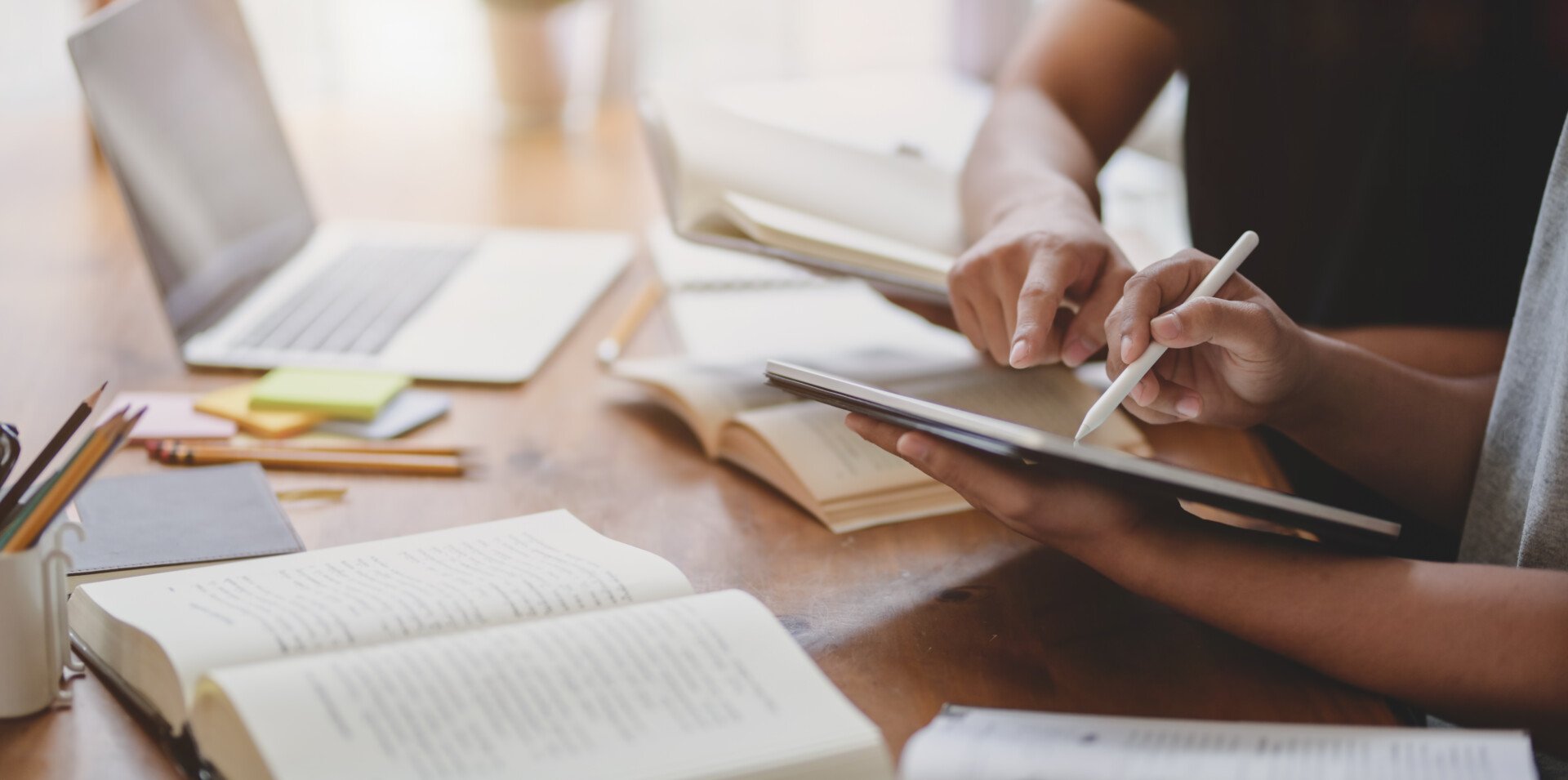
What Is Internal Validity In Research?
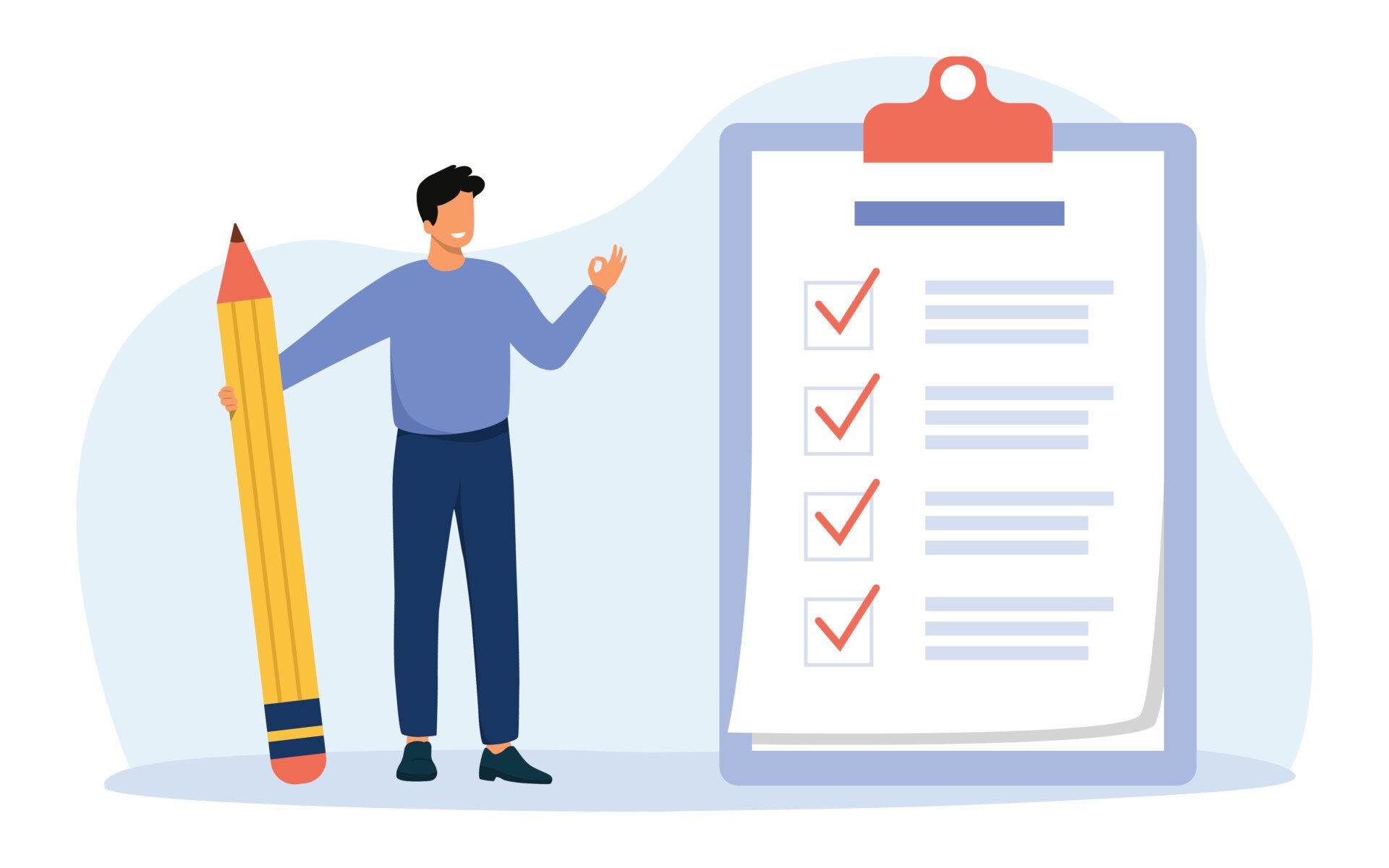
Research Methodology , Statistics
What Is Face Validity In Research? Importance & How To Measure
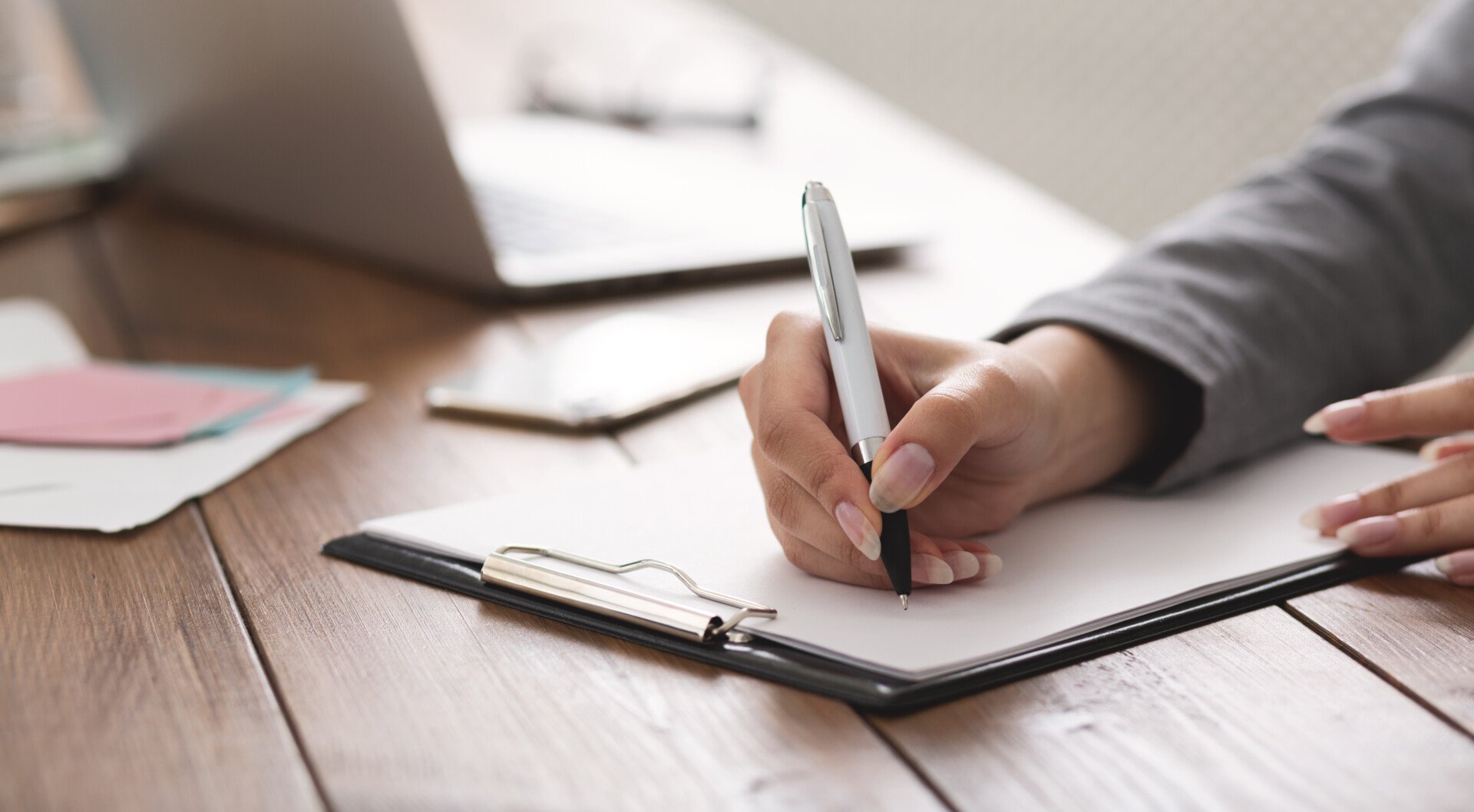
Criterion Validity: Definition & Examples
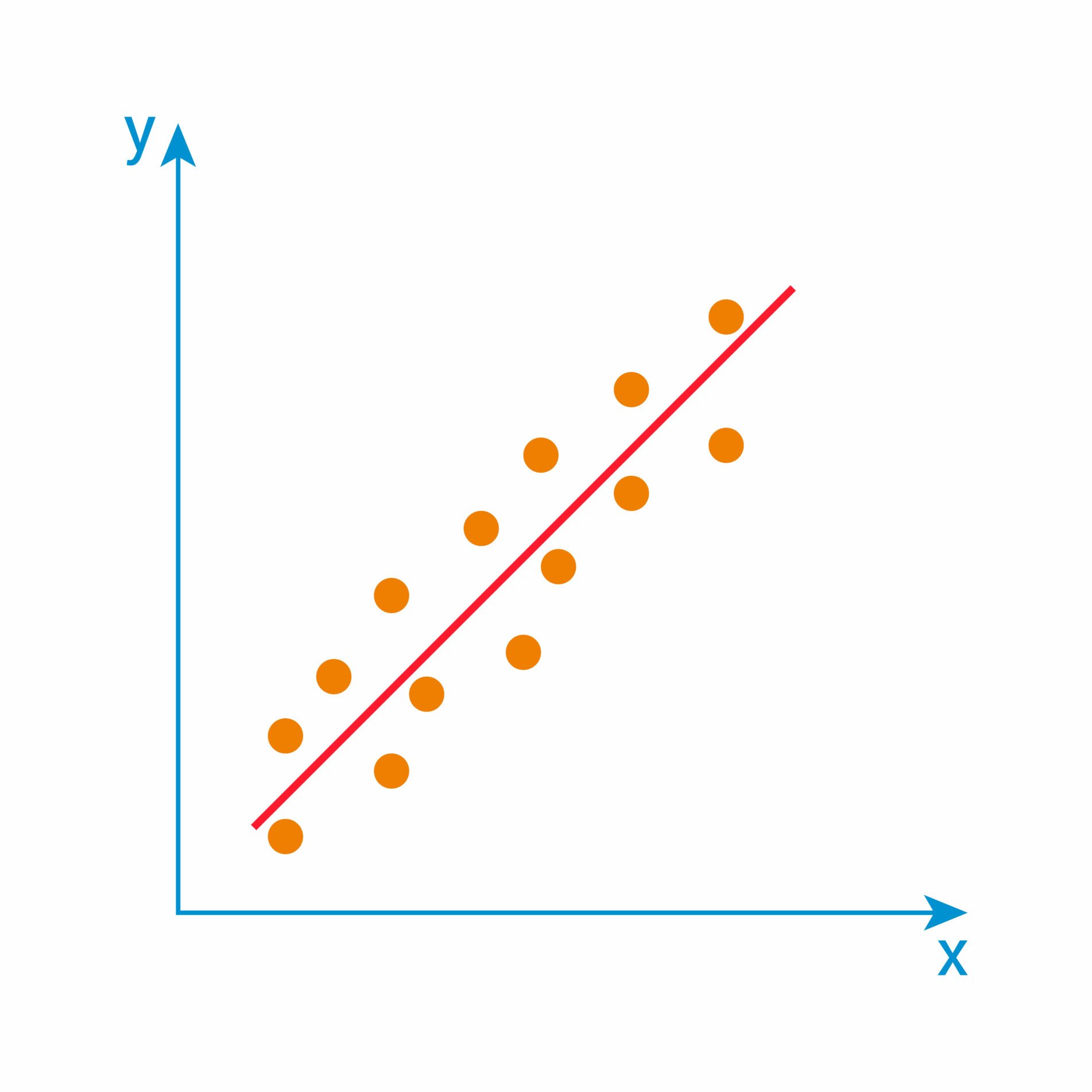
Convergent Validity: Definition and Examples
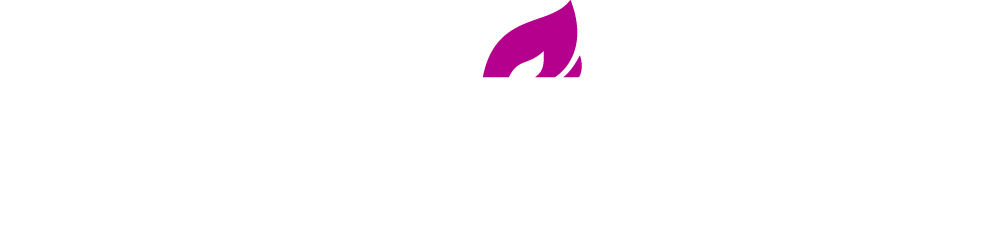
No products in the cart.
What is Qualitative Data Analysis Software (QDA Software)?
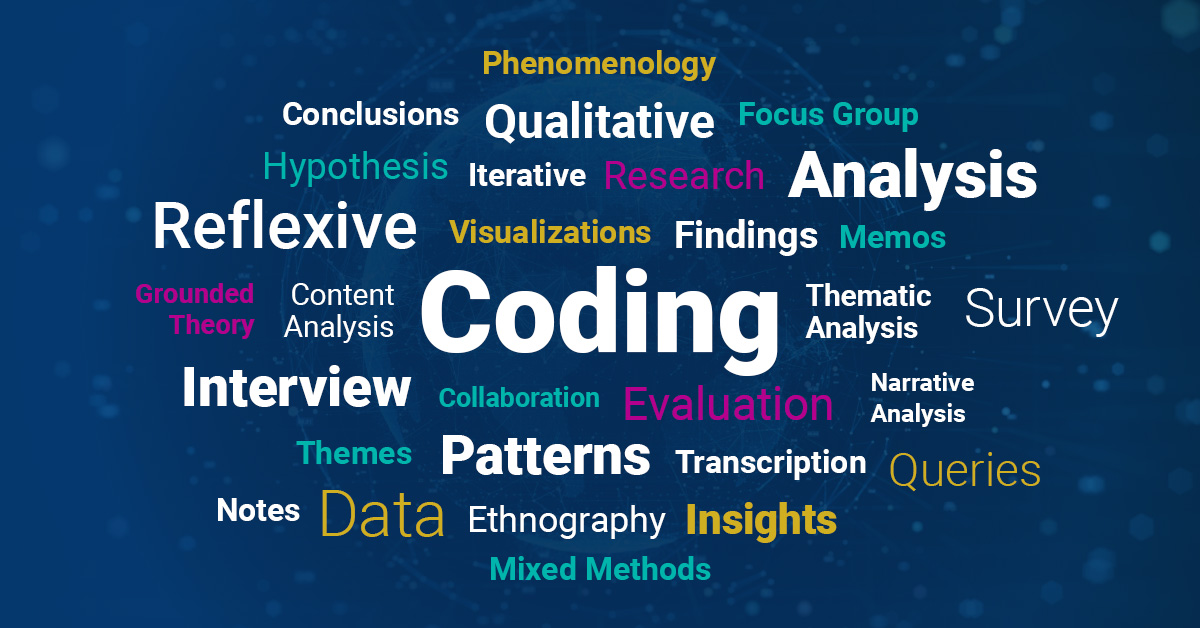
Qualitative Data Analysis Software (QDA software) allows researchers to organize, analyze and visualize their data, finding the patterns in qualitative data or unstructured data: interviews, surveys, field notes, videos, audio files, images, journal articles interviews, web content etc.
Quantitative vs. Qualitative Data Analysis
What is the difference between quantitative and qualitative data analysis. As the name implies, quantitative data analysis has to do with numbers. For example, any time you are doing statistical analysis, you are doing quantitative data analysis. Some examples of quantitative data analysis software are SPSS, STATA, SAS, and Lumivero’s own powerful statistics software, XLSTAT .
In contrast, qualitative analysis "helps you understand people’s perceptions and experiences by systematically coding and analyzing the data", as described in Qualitative vs Quantitative Research 101 . It tends to deal more with words than numbers. It can be useful when working with a lot of rich and deep data and when you aren’t trying to test something very specific. Some examples of qualitative data analysis software are MAXQDA, ATLAS.ti, Quirkos, and Lumivero’s NVivo, the leading tool for qualitative data analysis .
When would you use each one? Well, qualitative data analysis is often used for exploratory research or developing a theory, whereas quantitative is better if you want to test a hypothesis, find averages, and determine relationships between variables. With quantitative research you often want a large sample size to get relevant statistics. In contrast, qualitative research, because so much data in the form of text is involved, can have much smaller sample sizes and still yield valuable insights.
Of course, it’s not always so cut and dry, and many researchers end up taking a «mixed methods» approach, meaning that they combine both types of research. In this case they might use a combination of both types of software programs.
Learn how some qualitative researchers use QDA software for text analysis in the on-demand webinar Twenty-Five Qualitative Researchers Share How-To's for Data Analysis .

How is Qualitative Data Analysis Software Used for Research?
Qualitative Data Analysis Software works with any qualitative research methodology used by a researcher For example, software for qualitative data analysis can be used by a social scientist wanting to develop new concepts or theories may take a ‘grounded theory’ approach. Or a researcher looking for ways to improve health policy or program design might use ‘evaluation methods’. QDA software analysis tools don't favor a particular methodology — they're designed to facilitate common qualitative techniques no matter what method you use.
NVivo can help you to manage, explore and find patterns in your data and conduct thematic and sentiment analysis, but it cannot replace your analytical expertise.
Qualitative Research as an Iterative Process
Handling qualitative and mixed methods data is not usually a step-by-step process. Instead, it tends to be an iterative process where you explore, code, reflect, memo, code some more, query and so on. For example, this picture shows a path you might take to investigate an interesting theme using QDA software, like NVivo, to analyze data:
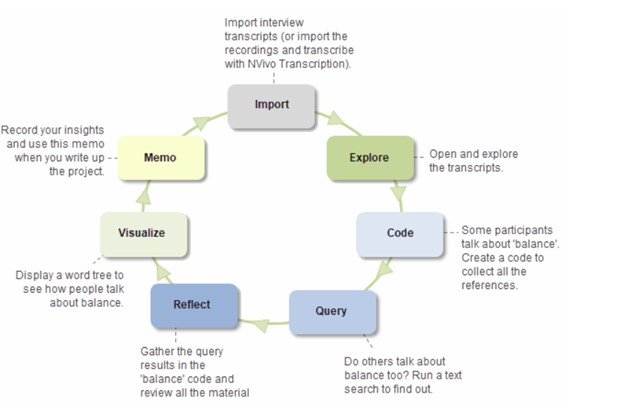
How Do I Choose the Best Approach for My Research Project with QDA Software?
Every research project is unique — the way you organize and analyze the material depends on your methodology, data and research design.
Here are some example scenarios for handling different types of research projects in QDA software — these are just suggestions to get you up and running.
A study with interviews exploring stakeholder perception of a community arts program
Your files consist of unstructured interview documents. You would set up a case for each interview participant, then code to codes and cases. You could then explore your data with simple queries or charts and use memos to record your discoveries.
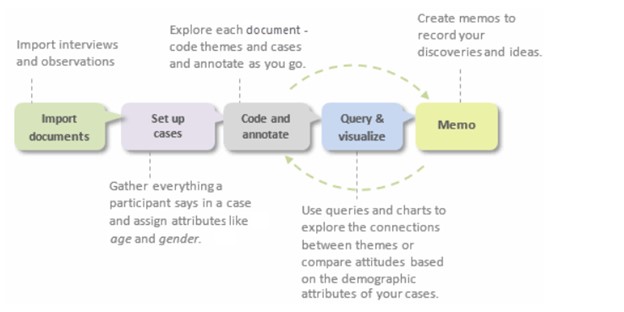
A study exploring community perceptions about climate change using autocoding with AI
Your files consist of structured, consistently formatted interviews (where each participant is asked the same set of questions). With AI, you could autocode the interviews and set up cases for each participant. Then code themes to query and visualize your data.
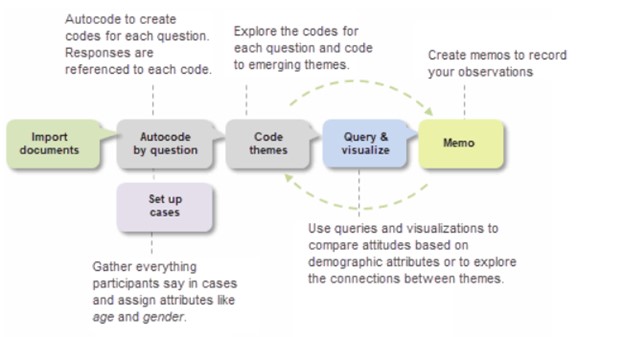
A literature review on adolescent depression
Your files consist of journal articles, books and web pages. You would classify your files before coding and querying them; and then you could critique each file in a memo. With Citavi integration in NVivo, you can import your Citavi references into NVivo.
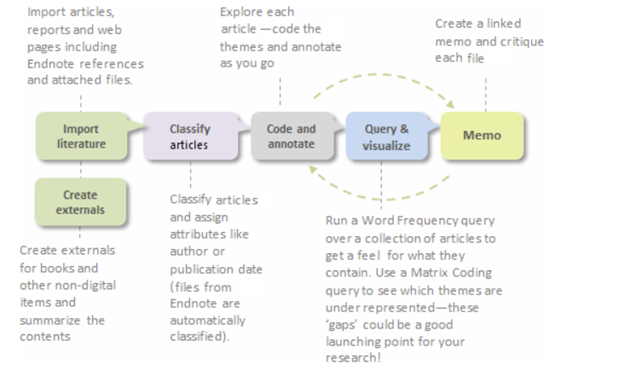
A social media study of the language used by members of an online community
Your files consist of Facebook data captured with NCapture. You would import it as a dataset ready to code and query. Use memos to record your insights.
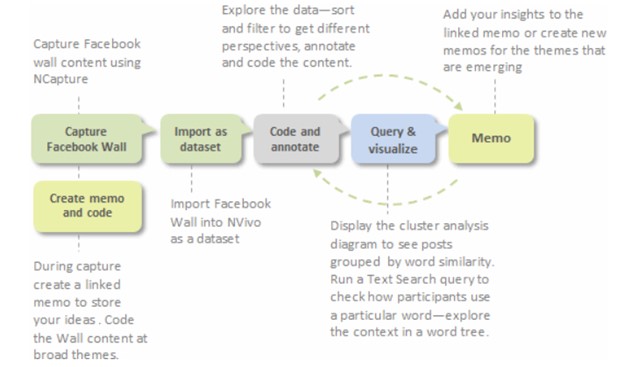
A quick analysis of a local government budget survey
Your file is a large dataset of survey responses. You would import it using the Survey Import Wizard, which prepares your data for analysis. As part of the import, choose to run automated insights with AI to identify and code to themes and sentiment so that you can quickly review results and report broad findings.
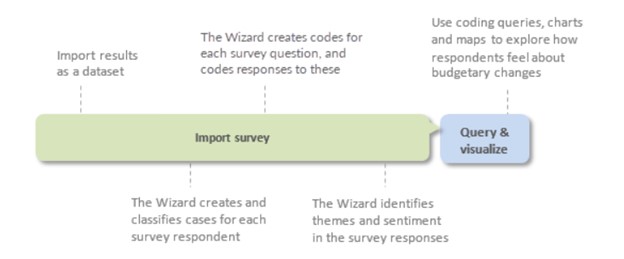
Ways to Get Started with Your Project with Qualitative Analysis Software
Since projects (and researchers) are unique there is no one 'best practice' approach to organizing and analyzing your data but there are some useful strategies to help you get up and running:
- Start now - don't wait until you have collected all the data. Import your research design, grant application or thesis proposal.
- Make a project journa l and state your research questions and record your goals. Why are you doing the project? What is it about? What do you expect to find and why?
- Make a mind map for your preliminary ideas. Show the relationships or patterns you expect to find in your data based on prior experience or preliminary reading.
- Import your interviews, field notes, focus groups —organize these files into folders for easy access.
- Set up an initial code structure based on your early reading and ideas—you could run a Word Frequency query over your data to tease out the common themes for creating your code structure.
- Set up cases for the people, places or other cases in your project.
- Explore your material and code themes as they emerge in your data mining —creating memos and describing your discoveries and interpretations.
- To protect your work, get in the habit of making regular back-ups.
QDA Analysis Tools Help You Work Toward Outcomes that are Robust and Transparent
Using QDA software to organize and analyze your data also increases the 'transparency' of your research outcomes—for example, you can:
- Demonstrate the evolution of your ideas in memos and maps.
- Document your early preconceptions and biases (in a memo or map) and demonstrate how these have been acknowledged and tested.
- Easily find illustrative quotes.
- Always return to the original context of your coded material.
- Save and revisit the queries and visualizations that helped you to arrive at your conclusions.
QDA software, like NVivo, can demonstrate the credibility of your findings in the following ways:
- If you used NVivo for your literature review, run a query or create a chart to demonstrate how your findings compare with the views of other authors.
- Was an issue or theme reported by more than one participant? Run a Matrix Coding query to see how many participants talked about a theme.
- Were multiple methods used to collect the data (interviews, observations, surveys)—and are the findings supported across these text data and video data files? Run a Matrix Coding query to see how often a theme is reported across all your files.

- If multiple researchers analyzed the material — were their findings consistent? Use coding stripes (or filter the contents in a code) to see how various team members have coded the material and run a Coding Comparison query to assess the level of agreement.
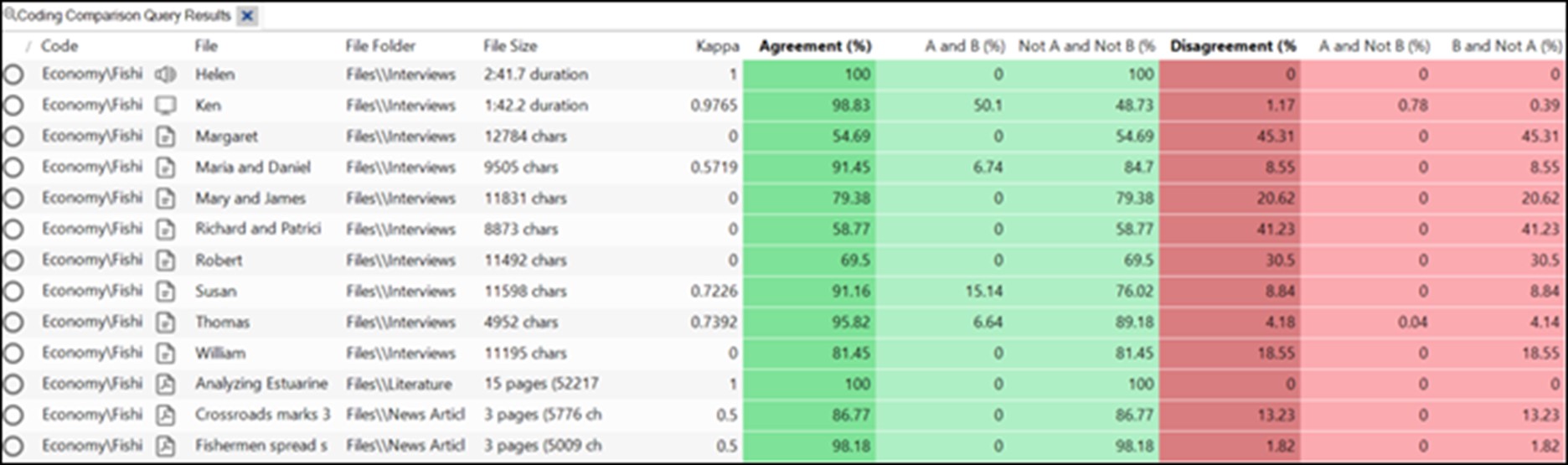
QDA Software Integrations
Many qualitative analysis software options have integration with other software to enhance your research process. NVivo integrates or can be used with the following software:
- NVivo Transcription to save you time and jump start your qualitative data analysis. Learn how in the on-demand webinar Transcription – Go Beyond the Words .
- Reference management software, like Lumivero’s Citavi, for reference management and writing. By combining Citavi and NVivo, you can create complicated searches for certain keywords, terms, and categories and make advanced search syntax, like wildcards, boolean operators, and regular expressions. This integration allows you to take your analyses beyond reference management by developing a central location to collect references and thoughts, analyze literature, and connect empirical data.
- Statistical software, like Lumivero’s XLSTAT , SPSS, or STATA to export your queries from NVivo to run statistical analysis
- Qualtrics, SurveyMonkey to import your survey results into NVivo to start analyzing.
Make Choosing QDA Software Easy — Try NVivo Today!
It's tough choosing QDA software! Test out NVivo, the most cited qualitative data analysis tool, by requesting a free 14-day trial of NVivo to start improving your qualitative and mixed methods research today.
Recent Articles
- Open access
- Published: 13 May 2024
What are the strengths and limitations to utilising creative methods in public and patient involvement in health and social care research? A qualitative systematic review
- Olivia R. Phillips 1 , 2 na1 ,
- Cerian Harries 2 , 3 na1 ,
- Jo Leonardi-Bee 1 , 2 , 4 na1 ,
- Holly Knight 1 , 2 ,
- Lauren B. Sherar 2 , 3 ,
- Veronica Varela-Mato 2 , 3 &
- Joanne R. Morling 1 , 2 , 5
Research Involvement and Engagement volume 10 , Article number: 48 ( 2024 ) Cite this article
226 Accesses
2 Altmetric
Metrics details
There is increasing interest in using patient and public involvement (PPI) in research to improve the quality of healthcare. Ordinarily, traditional methods have been used such as interviews or focus groups. However, these methods tend to engage a similar demographic of people. Thus, creative methods are being developed to involve patients for whom traditional methods are inaccessible or non-engaging.
To determine the strengths and limitations to using creative PPI methods in health and social care research.
Electronic searches were conducted over five databases on 14th April 2023 (Web of Science, PubMed, ASSIA, CINAHL, Cochrane Library). Studies that involved traditional, non-creative PPI methods were excluded. Creative PPI methods were used to engage with people as research advisors, rather than study participants. Only primary data published in English from 2009 were accepted. Title, abstract and full text screening was undertaken by two independent reviewers before inductive thematic analysis was used to generate themes.
Twelve papers met the inclusion criteria. The creative methods used included songs, poems, drawings, photograph elicitation, drama performance, visualisations, social media, photography, prototype development, cultural animation, card sorting and persona development. Analysis identified four limitations and five strengths to the creative approaches. Limitations included the time and resource intensive nature of creative PPI, the lack of generalisation to wider populations and ethical issues. External factors, such as the lack of infrastructure to support creative PPI, also affected their implementation. Strengths included the disruption of power hierarchies and the creation of a safe space for people to express mundane or “taboo” topics. Creative methods are also engaging, inclusive of people who struggle to participate in traditional PPI and can also be cost and time efficient.
‘Creative PPI’ is an umbrella term encapsulating many different methods of engagement and there are strengths and limitations to each. The choice of which should be determined by the aims and requirements of the research, as well as the characteristics of the PPI group and practical limitations. Creative PPI can be advantageous over more traditional methods, however a hybrid approach could be considered to reap the benefits of both. Creative PPI methods are not widely used; however, this could change over time as PPI becomes embedded even more into research.
Plain English Summary
It is important that patients and public are included in the research process from initial brainstorming, through design to delivery. This is known as public and patient involvement (PPI). Their input means that research closely aligns with their wants and needs. Traditionally to get this input, interviews and group discussions are held, but this can exclude people who find these activities non-engaging or inaccessible, for example those with language challenges, learning disabilities or memory issues. Creative methods of PPI can overcome this. This is a broad term describing different (non-traditional) ways of engaging patients and public in research, such as through the use or art, animation or performance. This review investigated the reasons why creative approaches to PPI could be difficult (limitations) or helpful (strengths) in health and social care research. After searching 5 online databases, 12 studies were included in the review. PPI groups included adults, children and people with language and memory impairments. Creative methods included songs, poems, drawings, the use of photos and drama, visualisations, Facebook, creating prototypes, personas and card sorting. Limitations included the time, cost and effort associated with creative methods, the lack of application to other populations, ethical issues and buy-in from the wider research community. Strengths included the feeling of equality between academics and the public, creation of a safe space for people to express themselves, inclusivity, and that creative PPI can be cost and time efficient. Overall, this review suggests that creative PPI is worthwhile, however each method has its own strengths and limitations and the choice of which will depend on the research project, PPI group characteristics and other practical limitations, such as time and financial constraints.
Peer Review reports
Introduction
Patient and public involvement (PPI) is the term used to describe the partnership between patients (including caregivers, potential patients, healthcare users etc.) or the public (a community member with no known interest in the topic) with researchers. It describes research that is done “‘with’ or ‘by’ the public, rather than ‘to,’ ‘about’ or ‘for’ them” [ 1 ]. In 2009, it became a legislative requirement for certain health and social care organisations to include patients, families, carers and communities in not only the planning of health and social care services, but the commissioning, delivery and evaluation of them too [ 2 ]. For example, funding applications for the National Institute of Health and Care Research (NIHR), a UK funding body, mandates a demonstration of how researchers plan to include patients/service users, the public and carers at each stage of the project [ 3 ]. However, this should not simply be a tokenistic, tick-box exercise. PPI should help formulate initial ideas and should be an instrumental, continuous part of the research process. Input from PPI can provide unique insights not yet considered and can ensure that research and health services are closely aligned to the needs and requirements of service users PPI also generally makes research more relevant with clearer outcomes and impacts [ 4 ]. Although this review refers to both patients and the public using the umbrella term ‘PPI’, it is important to acknowledge that these are two different groups with different motivations, needs and interests when it comes to health research and service delivery [ 5 ].
Despite continuing recognition of the need of PPI to improve quality of healthcare, researchers have also recognised that there is no ‘one size fits all’ method for involving patients [ 4 ]. Traditionally, PPI methods invite people to take part in interviews or focus groups to facilitate discussion, or surveys and questionnaires. However, these can sometimes be inaccessible or non-engaging for certain populations. For example, someone with communication difficulties may find it difficult to engage in focus groups or interviews. If individuals lack the appropriate skills to interact in these types of scenarios, they cannot take advantage of the participation opportunities it can provide [ 6 ]. Creative methods, however, aim to resolve these issues. These are a relatively new concept whereby researchers use creative methods (e.g., artwork, animations, Lego), to make PPI more accessible and engaging for those whose voices would otherwise go unheard. They ensure that all populations can engage in research, regardless of their background or skills. Seminal work has previously been conducted in this area, which brought to light the use of creative methodologies in research. Leavy (2008) [ 7 ] discussed how traditional interviews had limits on what could be expressed due to their sterile, jargon-filled and formulaic structure, read by only a few specialised academics. It was this that called for more creative approaches, which included narrative enquiry, fiction-based research, poetry, music, dance, art, theatre, film and visual art. These practices, which can be used in any stage of the research cycle, supported greater empathy, self-reflection and longer-lasting learning experiences compared to interviews [ 7 ]. They also pushed traditional academic boundaries, which made the research accessible not only to researchers, but the public too. Leavy explains that there are similarities between arts-based approaches and scientific approaches: both attempts to investigate what it means to be human through exploration, and used together, these complimentary approaches can progress our understanding of the human experience [ 7 ]. Further, it is important to acknowledge the parallels and nuances between creative and inclusive methods of PPI. Although creative methods aim to be inclusive (this should underlie any PPI activity, whether creative or not), they do not incorporate all types of accessible, inclusive methodologies e.g., using sign language for people with hearing impairments or audio recordings for people who cannot read. Given that there was not enough scope to include an evaluation of all possible inclusive methodologies, this review will focus on creative methods of PPI only.
We aimed to conduct a qualitative systematic review to highlight the strengths of creative PPI in health and social care research, as well as the limitations, which might act as a barrier to their implementation. A qualitative systematic review “brings together research on a topic, systematically searching for research evidence from primary qualitative studies and drawing the findings together” [ 8 ]. This review can then advise researchers of the best practices when designing PPI.
Public involvement
The PHIRST-LIGHT Public Advisory Group (PAG) consists of a team of experienced public contributors with a diverse range of characteristics from across the UK. The PAG was involved in the initial question setting and study design for this review.
Search strategy
For the purpose of this review, the JBI approach for conducting qualitative systematic reviews was followed [ 9 ]. The search terms were (“creativ*” OR “innovat*” OR “authentic” OR “original” OR “inclu*”) AND (“public and patient involvement” OR “patient and public involvement” OR “public and patient involvement and engagement” OR “patient and public involvement and engagement” OR “PPI” OR “PPIE” OR “co-produc*” OR “co-creat*” OR “co-design*” OR “cooperat*” OR “co-operat*”). This search string was modified according to the requirements of each database. Papers were filtered by title, abstract and keywords (see Additional file 1 for search strings). The databases searched included Web of Science (WoS), PubMed, ASSIA and CINAHL. The Cochrane Library was also searched to identify relevant reviews which could lead to the identification of primary research. The search was conducted on 14/04/23. As our aim was to report on the use of creative PPI in research, rather than more generic public engagement, we used electronic databases of scholarly peer-reviewed literature, which represent a wide range of recognised databases. These identified studies published in general international journals (WoS, PubMed), those in social sciences journals (ASSIA), those in nursing and allied health journals (CINAHL), and trials of interventions (Cochrane Library).
Inclusion criteria
Only full-text, English language, primary research papers from 2009 to 2023 were included. This was the chosen timeframe as in 2009 the Health and Social Reform Act made it mandatory for certain Health and Social Care organisations to involve the public and patients in planning, delivering, and evaluating services [ 2 ]. Only creative methods of PPI were accepted, rather than traditional methods, such as interviews or focus groups. For the purposes of this paper, creative PPI included creative art or arts-based approaches (e.g., e.g. stories, songs, drama, drawing, painting, poetry, photography) to enhance engagement. Titles were related to health and social care and the creative PPI was used to engage with people as research advisors, not as study participants. Meta-analyses, conference abstracts, book chapters, commentaries and reviews were excluded. There were no limits concerning study location or the demographic characteristics of the PPI groups. Only qualitative data were accepted.
Quality appraisal
Quality appraisal using the Critical Appraisal Skills Programme (CASP) checklist [ 10 ] was conducted by the primary authors (ORP and CH). This was done independently, and discrepancies were discussed and resolved. If a consensus could not be reached, a third independent reviewer was consulted (JRM). The full list of quality appraisal questions can be found in Additional file 2 .
Data extraction
ORP extracted the study characteristics and a subset of these were checked by CH. Discrepancies were discussed and amendments made. Extracted data included author, title, location, year of publication, year study was carried out, research question/aim, creative methods used, number of participants, mean age, gender, ethnicity of participants, setting, limitations and strengths of creative PPI and main findings.
Data analysis
The included studies were analysed using inductive thematic analysis [ 11 ], where themes were determined by the data. The familiarisation stage took place during full-text reading of the included articles. Anything identified as a strength or limitation to creative PPI methods was extracted verbatim as an initial code and inputted into the data extraction Excel sheet. Similar codes were sorted into broader themes, either under ‘strengths’ or ‘limitations’ and reviewed. Themes were then assigned a name according to the codes.
The search yielded 9978 titles across the 5 databases: Web of Science (1480 results), PubMed (94 results), ASSIA (2454 results), CINAHL (5948 results) and Cochrane Library (2 results), resulting in 8553 different studies after deduplication. ORP and CH independently screened their titles and abstracts, excluding those that did not meet the criteria. After assessment, 12 studies were included (see Fig. 1 ).
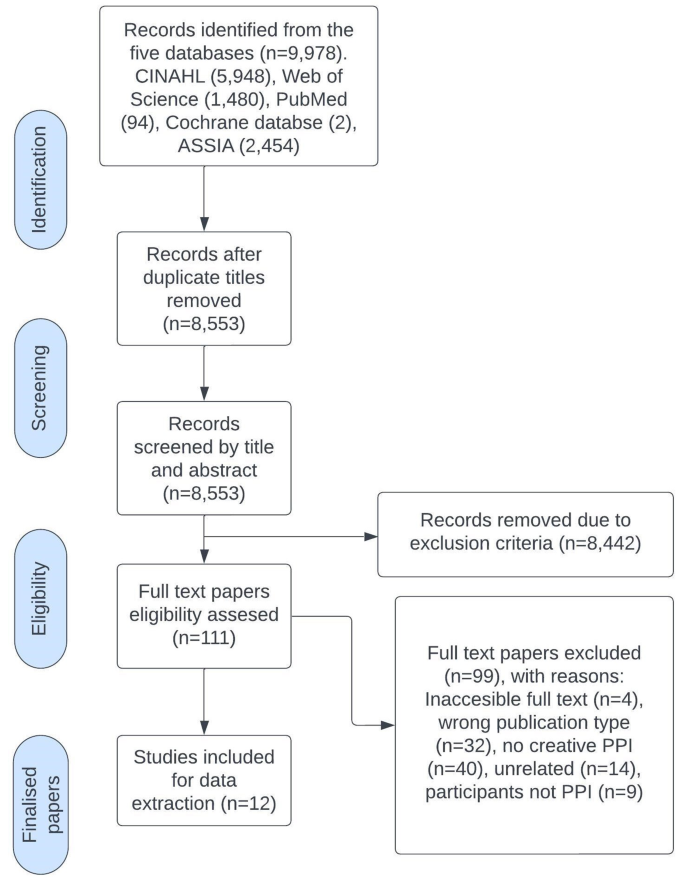
PRISMA flowchart of the study selection process
Study characteristics
The included studies were published between 2018 and 2022. Seven were conducted in the UK [ 12 , 14 , 15 , 17 , 18 , 19 , 23 ], two in Canada [ 21 , 22 ], one in Australia [ 13 ], one in Norway [ 16 ] and one in Ireland [ 20 ]. The PPI activities occurred across various settings, including a school [ 12 ], social club [ 12 ], hospital [ 17 ], university [ 22 ], theatre [ 19 ], hotel [ 20 ], or online [ 15 , 21 ], however this information was omitted in 5 studies [ 13 , 14 , 16 , 18 , 23 ]. The number of people attending the PPI sessions varied, ranging from 6 to 289, however the majority (ten studies) had less than 70 participants [ 13 , 14 , 16 , 17 , 18 , 19 , 20 , 21 , 22 , 23 ]. Seven studies did not provide information on the age or gender of the PPI groups. Of those that did, ages ranged from 8 to 76 and were mostly female. The ethnicities of the PPI group members were also rarely recorded (see Additional file 3 for data extraction table).
Types of creative methods
The type of creative methods used to engage the PPI groups were varied. These included songs, poems, drawings, photograph elicitation, drama performance, visualisations, Facebook, photography, prototype development, cultural animation, card sorting and creating personas (see Table 1 ). These were sometimes accompanied by traditional methods of PPI such as interviews and focus group discussions.
The 12 included studies were all deemed to be of good methodological quality, with scores ranging from 6/10 to 10/10 with the CASP critical appraisal tool [ 10 ] (Table 2 ).
Thematic analysis
Analysis identified four limitations and five strengths to creative PPI (see Fig. 2 ). Limitations included the time and resource intensity of creative PPI methods, its lack of generalisation, ethical issues and external factors. Strengths included the disruption of power hierarchies, the engaging and inclusive nature of the methods and their long-term cost and time efficiency. Creative PPI methods also allowed mundane and “taboo” topics to be discussed within a safe space.
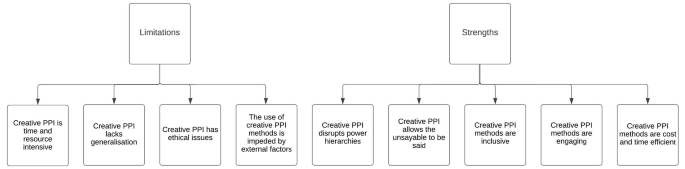
Theme map of strengths and limitations
Limitations of creative PPI
Creative ppi methods are time and resource intensive.
The time and resource intensive nature of creative PPI methods is a limitation, most notably for the persona-scenario methodology. Valaitis et al. [ 22 ] used 14 persona-scenario workshops with 70 participants to co-design a healthcare intervention, which aimed to promote optimal aging in Canada. Using the persona method, pairs composed of patients, healthcare providers, community service providers and volunteers developed a fictional character which they believed represented an ‘end-user’ of the healthcare intervention. Due to the depth and richness of the data produced the authors reported that it was time consuming to analyse. Further, they commented that the amount of information was difficult to disseminate to scientific leads and present at team meetings. Additionally, to ensure the production of high-quality data, to probe for details and lead group discussion there was a need for highly skilled facilitators. The resource intensive nature of the creative co-production was also noted in a study using the persona scenario and creative worksheets to develop a prototype decision support tool for individuals with malignant pleural effusion [ 17 ]. With approximately 50 people, this was also likely to yield a high volume of data to consider.
To prepare materials for populations who cannot engage in traditional methods of PPI was also timely. Kearns et al. [ 18 ] developed a feedback questionnaire for people with aphasia to evaluate ICT-delivered rehabilitation. To ensure people could participate effectively, the resources used during the workshops, such as PowerPoints, online images and photographs, had to be aphasia-accessible, which was labour and time intensive. The author warned that this time commitment should not be underestimated.
There are further practical limitations to implementing creative PPI, such as the costs of materials for activities as well as hiring a space for workshops. For example, the included studies in this review utilised pens, paper, worksheets, laptops, arts and craft supplies and magazines and took place in venues such as universities, a social club, and a hotel. Further, although not limited to creative PPI methods exclusively but rather most studies involving the public, a financial incentive was often offered for participation, as well as food, parking, transport and accommodation [ 21 , 22 ].
Creative PPI lacks generalisation
Another barrier to the use of creative PPI methods in health and social care research was the individual nature of its output. Those who participate, usually small in number, produce unique creative outputs specific to their own experiences, opinions and location. Craven et al. [ 13 ], used arts-based visualisations to develop a toolbox for adults with mental health difficulties. They commented, “such an approach might still not be worthwhile”, as the visualisations were individualised and highly personal. This indicates that the output may fail to meet the needs of its end-users. Further, these creative PPI groups were based in certain geographical regions such as Stoke-on-Trent [ 19 ] Sheffield [ 23 ], South Wales [ 12 ] or Ireland [ 20 ], which limits the extent the findings can be applied to wider populations, even within the same area due to individual nuances. Further, the study by Galler et al. [ 16 ], is specific to the Norwegian context and even then, maybe only a sub-group of the Norwegian population as the sample used was of higher socioeconomic status.
However, Grindell et al. [ 17 ], who used persona scenarios, creative worksheets and prototype development, pointed out that the purpose of this type of research is to improve a certain place, rather than apply findings across other populations and locations. Individualised output may, therefore, only be a limitation to research wanting to conduct PPI on a large scale.
If, however, greater generalisation within PPI is deemed necessary, then social media may offer a resolution. Fedorowicz et al. [ 15 ], used Facebook to gain feedback from the public on the use of video-recording methodology for an upcoming project. This had the benefit of including a more diverse range of people (289 people joined the closed group), who were spread geographically around the UK, as well as seven people from overseas.
Creative PPI has ethical issues
As with other research, ethical issues must be taken into consideration. Due to the nature of creative approaches, as well as the personal effort put into them, people often want to be recognised for their work. However, this compromises principles so heavily instilled in research such as anonymity and confidentiality. With the aim of exploring issues related to health and well-being in a town in South Wales, Byrne et al. [ 12 ], asked year 4/5 and year 10 pupils to create poems, songs, drawings and photographs. Community members also created a performance, mainly of monologues, to explore how poverty and inequalities are dealt with. Byrne noted the risks of these arts-based approaches, that being the possibility of over-disclosure and consequent emotional distress, as well as people’s desire to be named for their work. On one hand, the anonymity reduces the sense of ownership of the output as it does not portray a particular individual’s lived experience anymore. On the other hand, however, it could promote a more honest account of lived experience. Supporting this, Webber et al. [ 23 ], who used the persona method to co-design a back pain educational resource prototype, claimed that the anonymity provided by this creative technique allowed individuals to externalise and anonymise their own personal experience, thus creating a more authentic and genuine resource for future users. This implies that anonymity can be both a limitation and strength here.
The use of creative PPI methods is impeded by external factors
Despite the above limitations influencing the implementation of creative PPI techniques, perhaps the most influential is that creative methodologies are simply not mainstream [ 19 ]. This could be linked to the issues above, like time and resource intensity, generalisation and ethical issues but it is also likely to involve more systemic factors within the research community. Micsinszki et al. [ 21 ], who co-designed a hub for the health and well-being of vulnerable populations, commented that there is insufficient infrastructure to conduct meaningful co-design as well as a dominant medical model. Through a more holistic lens, there are “sociopolitical environments that privilege individualism over collectivism, self-sufficiency over collaboration, and scientific expertise over other ways of knowing based on lived experience” [ 21 ]. This, it could be suggested, renders creative co-design methodologies, which are based on the foundations of collectivism, collaboration and imagination an invalid technique in the research field, which is heavily dominated by more scientific methods offering reproducibility, objectivity and reliability.
Although we acknowledge that creative PPI techniques are not always appropriate, it may be that their main limitation is the lack of awareness of these methods or lack of willingness to use them. Further, there is always the risk that PPI, despite being a mandatory part of research, is used in a tokenistic or tick-box fashion [ 20 ], without considering the contribution that meaningful PPI could make to enhancing the research. It may be that PPI, let alone creative PPI, is not at the forefront of researchers’ minds when planning research.
Strengths of creative PPI
Creative ppi disrupts power hierarchies.
One of the main strengths of creative PPI techniques, cited most frequently in the included literature, was that they disrupt traditional power hierarchies [ 12 , 13 , 17 , 19 , 23 ]. For example, the use of theatre performance blurred the lines between professional and lay roles between the community and policy makers [ 12 ]. Individuals created a monologue to portray how poverty and inequality impact daily life and presented this to representatives of the National Assembly of Wales, Welsh Government, the Local Authority, Arts Council and Westminster. Byrne et al. [ 12 ], states how this medium allowed the community to engage with the people who make decisions about their lives in an environment of respect and understanding, where the hierarchies are not as visible as in other settings, e.g., political surgeries. Creative PPI methods have also removed traditional power hierarchies between researchers and adolescents. Cook et al. [ 13 ], used arts-based approaches to explore adolescents’ ideas about the “perfect” condom. They utilised the “Life Happens” resource, where adolescents drew and then decorated a person with their thoughts about sexual relationships, not too dissimilar from the persona-scenario method. This was then combined with hypothetical scenarios about sexuality. A condom-mapping exercise was then implemented, where groups shared the characteristics that make a condom “perfect” on large pieces of paper. Cook et al. [ 13 ], noted that usually power imbalances make it difficult to elicit information from adolescents, however these power imbalances were reduced due to the use of creative co-design techniques.
The same reduction in power hierarchies was noted by Grindell et al. [ 17 ], who used the person-scenario method and creative worksheets with individuals with malignant pleural effusion. This was with the aim of developing a prototype of a decision support tool for patients to help with treatment options. Although this process involved a variety of stakeholders, such as patients, carers and healthcare professionals, creative co-design was cited as a mechanism that worked to reduce power imbalances – a limitation of more traditional methods of research. Creative co-design blurred boundaries between end-users and clinical staff and enabled the sharing of ideas from multiple, valuable perspectives, meaning the prototype was able to suit user needs whilst addressing clinical problems.
Similarly, a specific creative method named cultural animation was also cited to dissolve hierarchies and encourage equal contributions from participants. Within this arts-based approach, Keleman et al. [ 19 ], explored the concept of “good health” with individuals from Stoke-on Trent. Members of the group created art installations using ribbons, buttons, cardboard and straws to depict their idea of a “healthy community”, which was accompanied by a poem. They also created a 3D Facebook page and produced another poem or song addressing the government to communicate their version of a “picture of health”. Public participants said that they found the process empowering, honest, democratic, valuable and practical.
This dissolving of hierarchies and levelling of power is beneficial as it increases the sense of ownership experienced by the creators/producers of the output [ 12 , 17 , 23 ]. This is advantageous as it has been suggested to improve its quality [ 23 ].
Creative PPI allows the unsayable to be said
Creative PPI fosters a safe space for mundane or taboo topics to be shared, which may be difficult to communicate using traditional methods of PPI. For example, the hypothetical nature of condom mapping and persona-scenarios meant that adolescents could discuss a personal topic without fear of discrimination, judgement or personal disclosure [ 13 ]. The safe space allowed a greater volume of ideas to be generated amongst peers where they might not have otherwise. Similarly, Webber et al. [ 23 ], , who used the persona method to co-design the prototype back pain educational resource, also noted how this method creates anonymity whilst allowing people the opportunity to externalise personal experiences, thoughts and feelings. Other creative methods were also used, such as drawing, collaging, role play and creating mood boards. A cardboard cube (labelled a “magic box”) was used to symbolise a physical representation of their final prototype. These creative methods levelled the playing field and made personal experiences accessible in a safe, open environment that fostered trust, as well as understanding from the researchers.
It is not only sensitive subjects that were made easier to articulate through creative PPI. The communication of mundane everyday experiences were also facilitated, which were deemed typically ‘unsayable’. This was specifically given in the context of describing intangible aspects of everyday health and wellbeing [ 11 ]. Graphic designers can also be used to visually represent the outputs of creative PPI. These captured the movement and fluidity of people and well as the relationships between them - things that cannot be spoken but can be depicted [ 21 ].
Creative PPI methods are inclusive
Another strength of creative PPI was that it is inclusive and accessible [ 17 , 19 , 21 ]. The safe space it fosters, as well as the dismantling of hierarchies, welcomed people from a diverse range of backgrounds and provided equal opportunities [ 21 ], especially for those with communication and memory difficulties who might be otherwise excluded from PPI. Kelemen et al. [ 19 ], who used creative methods to explore health and well-being in Stoke-on-Trent, discussed how people from different backgrounds came together and connected, discussed and reached a consensus over a topic which evoked strong emotions, that they all have in common. Individuals said that the techniques used “sets people to open up as they are not overwhelmed by words”. Similarly, creative activities, such as the persona method, have been stated to allow people to express themselves in an inclusive environment using a common language. Kearns et al. [ 18 ], who used aphasia-accessible material to develop a questionnaire with aphasic individuals, described how they felt comfortable in contributing to workshops (although this material was time-consuming to make, see ‘Limitations of creative PPI’ ).
Despite the general inclusivity of creative PPI, it can also be exclusive, particularly if online mediums are used. Fedorowicz et al. [ 15 ], used Facebook to create a PPI group, and although this may rectify previous drawbacks about lack of generalisation of creative methods (as Facebook can reach a greater number of people, globally), it excluded those who are not digitally active or have limited internet access or knowledge of technology. Online methods have other issues too. Maintaining the online group was cited as challenging and the volume of responses required researchers to interact outside of their working hours. Despite this, online methods like Facebook are very accessible for people who are physically disabled.
Creative PPI methods are engaging
The process of creative PPI is typically more engaging and produces more colourful data than traditional methods [ 13 ]. Individuals are permitted and encouraged to explore a creative self [ 19 ], which can lead to the exploration of new ideas and an overall increased enjoyment of the process. This increased engagement is particularly beneficial for younger PPI groups. For example, to involve children in the development of health food products, Galler et al. [ 16 ] asked 9-12-year-olds to take photos of their food and present it to other children in a “show and tell” fashion. They then created a newspaper article describing a new healthy snack. In this creative focus group, children were given lab coats to further their identity as inventors. Galler et al. [ 16 ], notes that the methods were highly engaging and facilitated teamwork and group learning. This collaborative nature of problem-solving was also observed in adults who used personas and creative worksheets to develop the resource for lower back pain [ 23 ]. Dementia patients too have been reported to enjoy the creative and informal approach to idea generation [ 20 ].
The use of cultural animation allowed people to connect with each other in a way that traditional methods do not [ 19 , 21 ]. These connections were held in place by boundary objects, such as ribbons, buttons, fabric and picture frames, which symbolised a shared meaning between people and an exchange of knowledge and emotion. Asking groups to create an art installation using these objects further fostered teamwork and collaboration, both at an individual and collective level. The exploration of a creative self increased energy levels and encouraged productive discussions and problem-solving [ 19 ]. Objects also encouraged a solution-focused approach and permitted people to think beyond their usual everyday scope [ 17 ]. They also allowed facilitators to probe deeper about the greater meanings carried by the object, which acted as a metaphor [ 21 ].
From the researcher’s point of view, co-creative methods gave rise to ideas they might not have initially considered. Valaitis et al. [ 22 ], found that over 40% of the creative outputs were novel ideas brought to light by patients, healthcare providers/community care providers, community service providers and volunteers. One researcher commented, “It [the creative methods] took me on a journey, in a way that when we do other pieces of research it can feel disconnected” [ 23 ]. Another researcher also stated they could not return to the way they used to do research, as they have learnt so much about their own health and community and how they are perceived [ 19 ]. This demonstrates that creative processes not only benefit the project outcomes and the PPI group, but also facilitators and researchers. However, although engaging, creative methods have been criticised for not demonstrating academic rigour [ 17 ]. Moreover, creative PPI may also be exclusive to people who do not like or enjoy creative activities.
Creative PPI methods are cost and time efficient
Creative PPI workshops can often produce output that is visible and tangible. This can save time and money in the long run as the output is either ready to be implemented in a healthcare setting or a first iteration has already been developed. This may also offset the time and costs it takes to implement creative PPI. For example, the prototype of the decision support tool for people with malignant pleural effusion was developed using personas and creative worksheets. The end result was two tangible prototypes to drive the initial idea forward as something to be used in practice [ 17 ]. The use of creative co-design in this case saved clinician time as well as the time it would take to develop this product without the help of its end-users. In the development of this particular prototype, analysis was iterative and informed the next stage of development, which again saved time. The same applies for the feedback questionnaire for the assessment of ICT delivered aphasia rehabilitation. The co-created questionnaire, designed with people with aphasia, was ready to be used in practice [ 18 ]. This suggests that to overcome time and resource barriers to creative PPI, researchers should aim for it to be engaging whilst also producing output.
That useable products are generated during creative workshops signals to participating patients and public members that they have been listened to and their thoughts and opinions acted upon [ 23 ]. For example, the development of the back pain resource based on patient experiences implies that their suggestions were valid and valuable. Further, those who participated in the cultural animation workshop reported that the process visualises change, and that it already feels as though the process of change has started [ 19 ].
The most cost and time efficient method of creative PPI in this review is most likely the use of Facebook to gather feedback on project methodology [ 15 ]. Although there were drawbacks to this, researchers could involve more people from a range of geographical areas at little to no cost. Feedback was instantaneous and no training was required. From the perspective of the PPI group, they could interact however much or little they wish with no time commitment.
This systematic review identified four limitations and five strengths to the use of creative PPI in health and social care research. Creative PPI is time and resource intensive, can raise ethical issues and lacks generalisability. It is also not accepted by the mainstream. These factors may act as barriers to the implementation of creative PPI. However, creative PPI disrupts traditional power hierarchies and creates a safe space for taboo or mundane topics. It is also engaging, inclusive and can be time and cost efficient in the long term.
Something that became apparent during data analysis was that these are not blanket strengths and limitations of creative PPI as a whole. The umbrella term ‘creative PPI’ is broad and encapsulates a wide range of activities, ranging from music and poems to prototype development and persona-scenarios, to more simplistic things like the use of sticky notes and ordering cards. Many different activities can be deemed ‘creative’ and the strengths and limitations of one does not necessarily apply to another. For example, cultural animation takes greater effort to prepare than the use of sticky notes and sorting cards, and the use of Facebook is cheaper and wider reaching than persona development. Researchers should use their discretion and weigh up the benefits and drawbacks of each method to decide on a technique which suits the project. What might be a limitation to creative PPI in one project may not be in another. In some cases, creative PPI may not be suitable at all.
Furthermore, the choice of creative PPI method also depends on the needs and characteristics of the PPI group. Children, adults and people living with dementia or language difficulties all have different engagement needs and capabilities. This indicates that creative PPI is not one size fits all and that the most appropriate method will change depending on the composition of the group. The choice of method will also be determined by the constraints of the research project, namely time, money and the research aim. For example, if there are time constraints, then a method which yields a lot of data and requires a lot of preparation may not be appropriate. If generalisation is important, then an online method is more suitable. Together this indicates that the choice of creative PPI method is highly individualised and dependent on multiple factors.
Although the limitations discussed in this review apply to creative PPI, they are not exclusive to creative PPI. Ethical issues are a consideration within general PPI research, especially when working with more vulnerable populations, such as children or adults living with a disability. It can also be the case that traditional PPI methods lack generalisability, as people who volunteer to be part of such a group are more likely be older, middle class and retired [ 24 ]. Most research is vulnerable to this type of bias, however, it is worth noting that generalisation is not always a goal and research remains valid and meaningful in its absence. Although online methods may somewhat combat issues related to generalisability, these methods still exclude people who do not have access to the internet/technology or who choose not to use it, implying that online PPI methods may not be wholly representative of the general population. Saying this, however, the accessibility of creative PPI techniques differs from person to person, and for some, online mediums may be more accessible (for example for those with a physical disability), and for others, this might be face-to-face. To combat this, a range of methods should be implemented. Planning multiple focus group and interviews for traditional PPI is also time and resource intensive, however the extra resources required to make this creative may be even greater. Although, the rich data provided may be worth the preparation and analysis time, which is also likely to depend on the number of participants and workshop sessions required. PPI, not just creative PPI, often requires the provision of a financial incentive, refreshments, parking and accommodation, which increase costs. These, however, are imperative and non-negotiable, as they increase the accessibility of research, especially to minority and lower-income groups less likely to participate. Adequate funding is also important for co-design studies where repeated engagement is required. One barrier to implementation, which appears to be exclusive to creative methods, however, is that creative methods are not mainstream. This cannot be said for traditional PPI as this is often a mandatory part of research applications.
Regarding the strengths of creative PPI, it could be argued that most appear to be exclusive to creative methodologies. These are inclusive by nature as multiple approaches can be taken to evoke ideas from different populations - approaches that do not necessarily rely on verbal or written communication like interviews and focus groups do. Given the anonymity provided by some creative methods, such as personas, people may be more likely to discuss their personal experiences under the guise of a general end-user, which might be more difficult to maintain when an interviewer is asking an individual questions directly. Additionally, creative methods are by nature more engaging and interactive than traditional methods, although this is a blanket statement and there may be people who find the question-and-answer/group discussion format more engaging. Creative methods have also been cited to eliminate power imbalances which exist in traditional research [ 12 , 13 , 17 , 19 , 23 ]. These imbalances exist between researchers and policy makers and adolescents, adults and the community. Lastly, although this may occur to a greater extent in creative methods like prototype development, it could be suggested that PPI in general – regardless of whether it is creative - is more time and cost efficient in the long-term than not using any PPI to guide or refine the research process. It must be noted that these are observations based on the literature. To be certain these differences exist between creative and traditional methods of PPI, direct empirical evaluation of both should be conducted.
To the best of our knowledge, this is the first review to identify the strengths and limitations to creative PPI, however, similar literature has identified barriers and facilitators to PPI in general. In the context of clinical trials, recruitment difficulties were cited as a barrier, as well as finding public contributors who were free during work/school hours. Trial managers reported finding group dynamics difficult to manage and the academic environment also made some public contributors feel nervous and lacking confidence to speak. Facilitators, however, included the shared ownership of the research – something that has been identified in the current review too. In addition, planning and the provision of knowledge, information and communication were also identified as facilitators [ 25 ]. Other research on the barriers to meaningful PPI in trial oversight committees included trialist confusion or scepticism over the PPI role and the difficulties in finding PPI members who had a basic understanding of research [ 26 ]. However, it could be argued that this is not representative of the average patient or public member. The formality of oversight meetings and the technical language used also acted as a barrier, which may imply that the informal nature of creative methods and its lack of dependency on literacy skills could overcome this. Further, a review of 42 reviews on PPI in health and social care identified financial compensation, resources, training and general support as necessary to conduct PPI, much like in the current review where the resource intensiveness of creative PPI was identified as a limitation. However, others were identified too, such as recruitment and representativeness of public contributors [ 27 ]. Like in the current review, power imbalances were also noted, however this was included as both a barrier and facilitator. Collaboration seemed to diminish hierarchies but not always, as sometimes these imbalances remained between public contributors and healthcare staff, described as a ‘them and us’ culture [ 27 ]. Although these studies compliment the findings of the current review, a direct comparison cannot be made as they do not concern creative methods. However, it does suggest that some strengths and weaknesses are shared between creative and traditional methods of PPI.
Strengths and limitations of this review
Although a general definition of creative PPI exists, it was up to our discretion to decide exactly which activities were deemed as such for this review. For example, we included sorting cards, the use of interactive whiteboards and sticky notes. Other researchers may have a more or less stringent criteria. However, two reviewers were involved in this decision which aids the reliability of the included articles. Further, it may be that some of the strengths and limitations cannot fully be attributed to the creative nature of the PPI process, but rather their co-created nature, however this is hard to disentangle as the included papers involved both these aspects.
During screening, it was difficult to decide whether the article was utilising creative qualitative methodology or creative PPI , as it was often not explicitly labelled as such. Regardless, both approaches involved the public/patients refining a healthcare product/service. This implies that if this review were to be replicated, others may do it differently. This may call for greater standardisation in the reporting of the public’s involvement in research. For example, the NIHR outlines different approaches to PPI, namely “consultation”, “collaboration”, “co-production” and “user-controlled”, which each signify an increased level of public power and influence [ 28 ]. Papers with elements of PPI could use these labels to clarify the extent of public involvement, or even explicitly state that there was no PPI. Further, given our decision to include only scholarly peer-reviewed literature, it is possible that data were missed within the grey literature. Similarly, the literature search will not have identified all papers relating to different types of accessible inclusion. However, the intent of the review was to focus solely on those within the definition of creative.
This review fills a gap in the literature and helps circulate and promote the concept of creative PPI. Each stage of this review, namely screening and quality appraisal, was conducted by two independent reviewers. However, four full texts could not be accessed during the full text reading stage, meaning there are missing data that could have altered or contributed to the findings of this review.
Research recommendations
Given that creative PPI can require effort to prepare, perform and analyse, sufficient time and funding should be allocated in the research protocol to enable meaningful and continuous PPI. This is worthwhile as PPI can significantly change the research output so that it aligns closely with the needs of the group it is to benefit. Researchers should also consider prototype development as a creative PPI activity as this might reduce future time/resource constraints. Shifting from a top-down approach within research to a bottom-up can be advantageous to all stakeholders and can help move creative PPI towards the mainstream. This, however, is the collective responsibility of funding bodies, universities and researchers, as well as committees who approve research bids.
A few of the included studies used creative techniques alongside traditional methods, such as interviews, which could also be used as a hybrid method of PPI, perhaps by researchers who are unfamiliar with creative techniques or to those who wish to reap the benefits of both. Often the characteristics of the PPI group were not included, including age, gender and ethnicity. It would be useful to include such information to assess how representative the PPI group is of the population of interest.
Creative PPI is a relatively novel approach of engaging the public and patients in research and it has both advantages and disadvantages compared to more traditional methods. There are many approaches to implementing creative PPI and the choice of technique will be unique to each piece of research and is reliant on several factors. These include the age and ability of the PPI group as well as the resource limitations of the project. Each method has benefits and drawbacks, which should be considered at the protocol-writing stage. However, given adequate funding, time and planning, creative PPI is a worthwhile and engaging method of generating ideas with end-users of research – ideas which may not be otherwise generated using traditional methods.
Data availability
No datasets were generated or analysed during the current study.
Abbreviations
Critical Appraisal Skills Programme
The Joanna Briggs Institute
National Institute of Health and Care Research
Public Advisory Group
Public and Patient Involvement
Web of Science
National Institute for Health and Care Research. What Is Patient and Public Involvement and Public Engagement? https://www.spcr.nihr.ac.uk/PPI/what-is-patient-and-public-involvement-and-engagement Accessed 01 Sept 2023.
Department of Health. Personal and Public Involvement (PPI) https://www.health-ni.gov.uk/topics/safety-and-quality-standards/personal-and-public-involvement-ppi#:~:text=The Health and Social Care Reform Act (NI) 2009 placed,delivery and evaluation of services . Accessed 01 Sept 2023.
National Institute for Health and Care Research. Policy Research Programme – Guidance for Stage 1 Applications https://www.nihr.ac.uk/documents/policy-research-programme-guidance-for-stage-1-applications-updated/26398 Accessed 01 Sept 2023.
Greenhalgh T, Hinton L, Finlay T, Macfarlane A, Fahy N, Clyde B, Chant A. Frameworks for supporting patient and public involvement in research: systematic review and co-design pilot. Health Expect. 2019. https://doi.org/10.1111/hex.12888
Article PubMed PubMed Central Google Scholar
Street JM, Stafinski T, Lopes E, Menon D. Defining the role of the public in health technology assessment (HTA) and HTA-informed decision-making processes. Int J Technol Assess Health Care. 2020. https://doi.org/10.1017/S0266462320000094
Article PubMed Google Scholar
Morrison C, Dearden A. Beyond tokenistic participation: using representational artefacts to enable meaningful public participation in health service design. Health Policy. 2013. https://doi.org/10.1016/j.healthpol.2013.05.008
Leavy P. Method meets art: arts-Based Research Practice. New York: Guilford; 2020.
Google Scholar
Seers K. Qualitative systematic reviews: their importance for our understanding of research relevant to pain. Br J Pain. 2015. https://doi.org/10.1177/2049463714549777
Lockwood C, Porritt K, Munn Z, Rittenmeyer L, Salmond S, Bjerrum M, Loveday H, Carrier J, Stannard D. Chapter 2: Systematic reviews of qualitative evidence. Aromataris E, Munn Z, editors. JBI Manual for Evidence Synthesis JBI. 2020. https://synthesismanual.jbi.global . https://doi.org/10.46658/JBIMES-20-03
CASP. CASP Checklists https://casp-uk.net/images/checklist/documents/CASP-Qualitative-Studies-Checklist/CASP-Qualitative-Checklist-2018_fillable_form.pdf (2022).
Braun V, Clarke V. Using thematic analysis in psychology. Qualitative Res Psychol. 2006. https://doi.org/10.1191/1478088706qp063oa
Article Google Scholar
Byrne E, Elliott E, Saltus R, Angharad J. The creative turn in evidence for public health: community and arts-based methodologies. J Public Health. 2018. https://doi.org/10.1093/pubmed/fdx151
Cook S, Grozdanovski L, Renda G, Santoso D, Gorkin R, Senior K. Can you design the perfect condom? Engaging young people to inform safe sexual health practice and innovation. Sex Educ. 2022. https://doi.org/10.1080/14681811.2021.1891040
Craven MP, Goodwin R, Rawsthorne M, Butler D, Waddingham P, Brown S, Jamieson M. Try to see it my way: exploring the co-design of visual presentations of wellbeing through a workshop process. Perspect Public Health. 2019. https://doi.org/10.1177/1757913919835231
Fedorowicz S, Riley V, Cowap L, Ellis NJ, Chambers R, Grogan S, Crone D, Cottrell E, Clark-Carter D, Roberts L, Gidlow CJ. Using social media for patient and public involvement and engagement in health research: the process and impact of a closed Facebook group. Health Expect. 2022. https://doi.org/10.1111/hex.13515
Galler M, Myhrer K, Ares G, Varela P. Listening to children voices in early stages of new product development through co-creation – creative focus group and online platform. Food Res Int. 2022. https://doi.org/10.1016/j.foodres.2022.111000
Grindell C, Tod A, Bec R, Wolstenholme D, Bhatnagar R, Sivakumar P, Morley A, Holme J, Lyons J, Ahmed M, Jackson S, Wallace D, Noorzad F, Kamalanathan M, Ahmed L, Evison M. Using creative co-design to develop a decision support tool for people with malignant pleural effusion. BMC Med Inf Decis Mak. 2020. https://doi.org/10.1186/s12911-020-01200-3
Kearns Á, Kelly H, Pitt I. Rating experience of ICT-delivered aphasia rehabilitation: co-design of a feedback questionnaire. Aphasiology. 2020. https://doi.org/10.1080/02687038.2019.1649913
Kelemen M, Surman E, Dikomitis L. Cultural animation in health research: an innovative methodology for patient and public involvement and engagement. Health Expect. 2018. https://doi.org/10.1111/hex.12677
Keogh F, Carney P, O’Shea E. Innovative methods for involving people with dementia and carers in the policymaking process. Health Expect. 2021. https://doi.org/10.1111/hex.13213
Micsinszki SK, Buettgen A, Mulvale G, Moll S, Wyndham-West M, Bruce E, Rogerson K, Murray-Leung L, Fleisig R, Park S, Phoenix M. Creative processes in co-designing a co-design hub: towards system change in health and social services in collaboration with structurally vulnerable populations. Evid Policy. 2022. https://doi.org/10.1332/174426421X16366319768599
Valaitis R, Longaphy J, Ploeg J, Agarwal G, Oliver D, Nair K, Kastner M, Avilla E, Dolovich L. Health TAPESTRY: co-designing interprofessional primary care programs for older adults using the persona-scenario method. BMC Fam Pract. 2019. https://doi.org/10.1186/s12875-019-1013-9
Webber R, Partridge R, Grindell C. The creative co-design of low back pain education resources. Evid Policy. 2022. https://doi.org/10.1332/174426421X16437342906266
National Institute for Health and Care Research. A Researcher’s Guide to Patient and Public Involvement. https://oxfordbrc.nihr.ac.uk/wp-content/uploads/2017/03/A-Researchers-Guide-to-PPI.pdf Accessed 01 Nov 2023.
Selman L, Clement C, Douglas M, Douglas K, Taylor J, Metcalfe C, Lane J, Horwood J. Patient and public involvement in randomised clinical trials: a mixed-methods study of a clinical trials unit to identify good practice, barriers and facilitators. Trials. 2021 https://doi.org/10.1186/s13063-021-05701-y
Coulman K, Nicholson A, Shaw A, Daykin A, Selman L, Macefield R, Shorter G, Cramer H, Sydes M, Gamble C, Pick M, Taylor G, Lane J. Understanding and optimising patient and public involvement in trial oversight: an ethnographic study of eight clinical trials. Trials. 2020. https://doi.org/10.1186/s13063-020-04495-9
Ocloo J, Garfield S, Franklin B, Dawson S. Exploring the theory, barriers and enablers for patient and public involvement across health, social care and patient safety: a systematic review of reviews. Health Res Policy Sys. 2021. https://doi.org/10.1186/s12961-020-00644-3
National Institute for Health and Care Research. Briefing notes for researchers - public involvement in NHS, health and social care research. https://www.nihr.ac.uk/documents/briefing-notes-for-researchers-public-involvement-in-nhs-health-and-social-care-research/27371 Accessed 01 Nov 2023.
Download references
Acknowledgements
With thanks to the PHIRST-LIGHT public advisory group and consortium for their thoughts and contributions to the design of this work.
The research team is supported by a National Institute for Health and Care Research grant (PHIRST-LIGHT Reference NIHR 135190).
Author information
Olivia R. Phillips and Cerian Harries share joint first authorship.
Authors and Affiliations
Nottingham Centre for Public Health and Epidemiology, Lifespan and Population Health, School of Medicine, University of Nottingham, Clinical Sciences Building, City Hospital Campus, Hucknall Road, Nottingham, NG5 1PB, UK
Olivia R. Phillips, Jo Leonardi-Bee, Holly Knight & Joanne R. Morling
National Institute for Health and Care Research (NIHR) PHIRST-LIGHT, Nottingham, UK
Olivia R. Phillips, Cerian Harries, Jo Leonardi-Bee, Holly Knight, Lauren B. Sherar, Veronica Varela-Mato & Joanne R. Morling
School of Sport, Exercise and Health Sciences, Loughborough University, Epinal Way, Loughborough, Leicestershire, LE11 3TU, UK
Cerian Harries, Lauren B. Sherar & Veronica Varela-Mato
Nottingham Centre for Evidence Based Healthcare, School of Medicine, University of Nottingham, Nottingham, UK
Jo Leonardi-Bee
NIHR Nottingham Biomedical Research Centre (BRC), Nottingham University Hospitals NHS Trust, University of Nottingham, Nottingham, NG7 2UH, UK
Joanne R. Morling
You can also search for this author in PubMed Google Scholar
Contributions
Author contributions: study design: ORP, CH, JRM, JLB, HK, LBS, VVM, literature searching and screening: ORP, CH, JRM, data curation: ORP, CH, analysis: ORP, CH, JRM, manuscript draft: ORP, CH, JRM, Plain English Summary: ORP, manuscript critical review and editing: ORP, CH, JRM, JLB, HK, LBS, VVM.
Corresponding author
Correspondence to Olivia R. Phillips .
Ethics declarations
Ethics approval and consent to participate.
The Ethics Committee of the Faculty of Medicine and Health Sciences, University of Nottingham advised that approval from the ethics committee and consent to participate was not required for systematic review studies.
Consent for publication
Not applicable.
Competing interests
The authors declare no competing interests.
Additional information
Publisher’s note.
Springer Nature remains neutral with regard to jurisdictional claims in published maps and institutional affiliations.
Electronic supplementary material
Below is the link to the electronic supplementary material.
40900_2024_580_MOESM1_ESM.docx
Additional file 1: Search strings: Description of data: the search strings and filters used in each of the 5 databases in this review
Additional file 2: Quality appraisal questions: Description of data: CASP quality appraisal questions
40900_2024_580_moesm3_esm.docx.
Additional file 3: Table 1: Description of data: elements of the data extraction table that are not in the main manuscript
Rights and permissions
Open Access This article is licensed under a Creative Commons Attribution 4.0 International License, which permits use, sharing, adaptation, distribution and reproduction in any medium or format, as long as you give appropriate credit to the original author(s) and the source, provide a link to the Creative Commons licence, and indicate if changes were made. The images or other third party material in this article are included in the article’s Creative Commons licence, unless indicated otherwise in a credit line to the material. If material is not included in the article’s Creative Commons licence and your intended use is not permitted by statutory regulation or exceeds the permitted use, you will need to obtain permission directly from the copyright holder. To view a copy of this licence, visit http://creativecommons.org/licenses/by/4.0/ . The Creative Commons Public Domain Dedication waiver ( http://creativecommons.org/publicdomain/zero/1.0/ ) applies to the data made available in this article, unless otherwise stated in a credit line to the data.
Reprints and permissions
About this article
Cite this article.
Phillips, O.R., Harries, C., Leonardi-Bee, J. et al. What are the strengths and limitations to utilising creative methods in public and patient involvement in health and social care research? A qualitative systematic review. Res Involv Engagem 10 , 48 (2024). https://doi.org/10.1186/s40900-024-00580-4
Download citation
Received : 28 November 2023
Accepted : 25 April 2024
Published : 13 May 2024
DOI : https://doi.org/10.1186/s40900-024-00580-4
Share this article
Anyone you share the following link with will be able to read this content:
Sorry, a shareable link is not currently available for this article.
Provided by the Springer Nature SharedIt content-sharing initiative
- Public and patient involvement
- Creative PPI
- Qualitative systematic review
Research Involvement and Engagement
ISSN: 2056-7529
- General enquiries: [email protected]
Click through the PLOS taxonomy to find articles in your field.
For more information about PLOS Subject Areas, click here .
Loading metrics
Open Access
Peer-reviewed
Research Article
Advanced paramedics’ restraint decision-making when managing acute behavioural disturbance (ABD) in the UK pre-hospital ambulance setting: A qualitative investigation
Roles Conceptualization, Formal analysis, Investigation, Methodology, Project administration, Writing – original draft, Writing – review & editing
* E-mail: [email protected]
Affiliations Department for Health, University of Bath, Bath, Somerset, England, London Ambulance Service NHS Trust, London, England

Roles Conceptualization, Formal analysis, Supervision, Writing – review & editing
Affiliation London Ambulance Service NHS Trust, London, England
Roles Conceptualization, Formal analysis, Methodology, Supervision, Writing – review & editing
Affiliation Department of Psychology, University of Bath, Bath, Somerset, England
- Jaqualine Lindridge,
- Timothy Edwards,
- Leda Blackwood
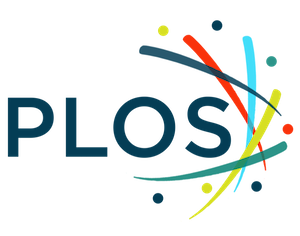
- Published: May 16, 2024
- https://doi.org/10.1371/journal.pone.0302524
- Peer Review
- Reader Comments
Acute behavioural disturbance (ABD), sometimes called ‘excited delirium’, is a medical emergency. In the UK, some patients presenting with ABD are managed by advanced paramedics (APs), however little is known about how APs make restraint decisions. The aim of this research is to explore the decisions made by APs when managing restraint in the context of ABD, in the UK pre-hospital ambulance setting. Seven semi-structured interviews were undertaken with APs. All participants were experienced APs with post-registration, post-graduate advanced practice education and qualifications. The resulting data were analysed using reflexive thematic analysis, informed by critical realism. We identified four interconnected themes from the interview data. Firstly, managing complexity and ambiguity in relation to identifying ABD patients and determining appropriate treatment plans. Secondly, feeling vulnerable to professional consequences from patients deteriorating whilst in the care of APs. Thirdly, negotiating with other professionals who have different roles and priorities. Finally, establishing primacy of care in relation to incidents which involve police officers and other professionals. A key influence was the need to characterise incidents as medical, as an enabler to establishing clinical leadership and decision-making control. APs focused on de-escalation techniques and sought to reduce physical restraint, intervening with pharmacological interventions if necessary to achieve this. The social relationships and interactions with patients and other professionals at the scene were key to success. Decisions are a source of anxiety, with fears of professional detriment accompanying poor patient outcomes. Our results indicate that APs would benefit from education and development specifically in relation to making ABD decisions, acknowledging the context of inter-professional relationships and the potential for competing and conflicting priorities. A focus on joint, high-fidelity training with the police may be a helpful intervention.
Citation: Lindridge J, Edwards T, Blackwood L (2024) Advanced paramedics’ restraint decision-making when managing acute behavioural disturbance (ABD) in the UK pre-hospital ambulance setting: A qualitative investigation. PLoS ONE 19(5): e0302524. https://doi.org/10.1371/journal.pone.0302524
Editor: Peivand Bastani, Flinders University, AUSTRALIA
Received: June 1, 2023; Accepted: April 7, 2024; Published: May 16, 2024
Copyright: © 2024 Lindridge et al. This is an open access article distributed under the terms of the Creative Commons Attribution License , which permits unrestricted use, distribution, and reproduction in any medium, provided the original author and source are credited.
Data Availability: Data are available via the University of Bath Research Data Archive and are available to researchers on application via: Lindridge, J., Edwards, T., Blackwood, L., 2024. Dataset for "Advanced paramedics' restraint decision-making when managing acute behavioural disturbance (ABD): A UK based qualitative investigation.". Bath: University of Bath Research Data Archive. https://doi.org/10.15125/BATH-01326 .
Funding: The author(s) received no specific funding for this work.
Competing interests: The authors have declared that no competing interests exist.
Introduction and background
Acute behavioural disturbance (ABD) is a term used to describe a state of significant agitation or disordered behaviour, with or without physiological compromise [ 1 ] and is the term most widely used by professionals in the UK [ 1 – 3 ]. Restraint and the management of ABD is a controversial area of policy and practice internationally [ 4 , 5 ]. Whilst physical restraint tends to be the preserve of the police, paramedics in some areas administer pharmacological restraint in the form of ketamine [ 6 ], anti-psychotics and/ or benzodiazepines to patients presenting with agitation [ 7 ]. Sadly, some deaths in the context of ABD have been linked to both physical restraint delivered by law enforcement professionals [ 8 , 9 ] and pharmacological restraint delivered by paramedics in the US [ 10 ]. In the UK, the role of some paramedics in restraint decision-making is evolving, with the recent introduction of pharmacological restraint in some Emergency Medical Services (EMS) [ 11 ], following the development of advanced paramedics (APs) and growing concerns in this area of practice. ABD patients typically present initially to police services in public spaces or in police custody [ 12 ]. APs usually attend later, often at the request of the police themselves. APs may arrive at a chaotic scene [ 13 ] and make restraint decisions, although they will not normally be responsible for carrying out physical restraint. Little is known about how APs make restraint decisions.
A narrative literature review was undertaken to identify studies which could shed light on what might influence APs when determining the need for restraint in the context of ABD. Studies relevant to decision-making in two key contexts were sought. Firstly, the restraint of patients experiencing ABD, or a similar problem, in any clinical practice setting or by any healthcare professional group, and secondly, paramedic and AP decision-making more generally. To clarify the difference between paramedics and APs, in the UK, paramedics are registered healthcare professionals who work autonomously within a defined scope of practice. APs are experienced paramedics, with post-registration, post-graduate advanced practice qualifications who within some UK EMS providers are selectively tasked to suspected ABD incidents to provide senior clinical leadership and, where indicated, a pharmacological intervention which is outside of the scope of practice of general paramedics. Whilst APs have an advanced scope of practice and educational background, they remain paramedics by profession and research relating to the paramedic profession was also sought for this reason. Throughout this paper, clinicians are defined as skilled and qualified healthcare workers. The term ‘ambulance clinician’ is a generic label which typically refers to a paramedic or qualified emergency medical technician (EMT) working in the UK NHS ambulance setting.
Research has identified that paramedics have felt unprepared by their formal training to manage behavioural and mental health emergencies and tend to rely on tacit knowledge and their general professional experience [ 14 , 15 ], it is not known if this extends to paramedics working at an advanced practice level. Tacit knowledge has been described as “… knowledge-in-practice developed from direct experience and action; highly pragmatic and situation specific; subconsciously understood and applied; difficult to articulate; usually shared through interactive conversation and shared experience . ” [ 16 ]. Furthermore, restraint-related research in the in-patient psychiatric setting has highlighted that other healthcare professionals rely on tacit knowledge when making restraint decisions [ 17 ], an issue which may be particularly relevant to APs whose scope of practice has been recently developed to include pharmacological restraint [ 11 ].
Research on paramedic decision-making more broadly finds professional vulnerability and a fear of repercussions is a dominant theme with paramedics fearing disciplinary investigation and litigation in relation to managing patients who self-harm [ 14 ]; making non-conveyance decisions [ 18 – 21 ]; and cardiac arrest management [ 22 , 23 ]. More generally, there is anxiety around practice in what has been described as a ‘hostile environment’ of discipline and blame within UK ambulance services [ 24 ]. Paramedics also fear a lack of support from their employers in a range of circumstances [ 20 , 25 ]. Although some of these studies included paramedics working at a specialist or enhanced level [ 20 – 22 , 25 ], it is unclear whether and how transition to AP status affects professional fears and vulnerability. Some studies suggest that decisions in the ambulance practice arena may be more complicated than treatment guidelines account for [ 20 , 26 ], with guidelines failing to respond to the degree of ambiguity and variation which ambulance clinicians encounter in practice. In a similar vein, access to limited information has been identified as a barrier to decision-making in cardiac arrest management [ 27 ] and end of life care [ 28 ]. This suggests that the decision-making landscape in which APs operate is challenging.
Current thinking is that paramedic decision-making is based on atomised individual-level ‘rational’ processes, with studies focusing on cognitive processing [ 29 – 31 ]. However, decisions made in relation to pre-hospital restraint take place in a complex social environment which includes bystanders, other professionals, such as the police, and other ambulance clinicians. The context in which these decisions are made is important. Brandling et al . observed the influence of police officers on clinical decisions in the context of cardiac arrest [ 23 ], with ambulance clinicians (including paramedics) finding it difficult to overturn police decisions in relation to whether to start resuscitation. Other studies have also acknowledged the pressure felt from bystanders or others at the scene. Burrell et al . found that ambulance clinicians encountered pressure from bystanders when responding to patients experiencing seizures in the public place, particularly in respect of providing pharmacological interventions to control seizure activity [ 18 ]. Murphy-Jones and Timmons reported that ambulance clinicians experienced pressure from family, care home staff and other paramedics to convey patients at the end of their life to hospital [ 28 ], even where that appeared contrary to the wishes of the patient. Issues such as these are likely to be relevant to the experience of APs arriving at scenes with access to additional medicines outside of the scope of practice of general paramedics.
Several studies report that nurses consider restraint to be a necessary, but undesirable activity that is contrary to their caring role [ 17 , 32 , 33 ], however little is known on the views of APs in making restraint decisions, and no studies specifically relating to APs were identified.
How APs make restraint decisions in the context of ABD is an important topic with real-world implications for patients and professionals [ 34 ]. Current evidence suggests decisions may be complex [ 20 , 22 , 26 ], and a deeper understanding may be beneficial in developing care in this area of practice. Whilst there has been research undertaken on restraint in other professions [ 32 ], there is a lack of research relating to APs as a specific professional group working in the clinical setting of interest, the pre-hospital ambulance setting.
The aim of this study is to explore the decisions made by APs when managing a restraint incident in the context of ABD, and to identify opportunities to improve performance in this area of practice. Recognising the variation in terminology relating to pharmacological interventions in ABD, the term ‘chemical sedation’ will be used throughout this report to describe the administration of sedative or anti-psychotic agents as it is the term most frequently used by those undertaking the intervention.
Study design and methods
Research philosophy.
This is an exploratory, qualitative study of APs practicing in a large, metropolitan UK emergency medical service (EMS). This study was conducted from a critical realist standpoint, following Campbell [ 35 ] and Maxwell’s [ 36 ] approach which combines ontological realism and epistemological constructivism. Ontological realists believe that reality, and the components of reality, exist independently of consciousness [ 37 ], and epistemological constructivists believe that our understanding of reality is a construction based on human perception [ 36 ]. An alternative to positivism developed by Roy Bhaskar, critical realism was summed up by Sturgiss and Clark as stating that the ‘ …evidence we observe can come close to reality but is always a fallible , social and subjective account of reality . ” [ 38 ] Here, we take the position that the phenomenon of decision-making in the context of ABD is real, and that APs make sense of this phenomenon in a subjective and socially constructed way.
Research context
London Ambulance Service National Health Service (NHS) Trust (LAS), provides free at-the-point-of-access emergency medical and ambulance services across London, a large metropolitan city in the UK. Accessed via a single emergency telephone number, contacts are triaged using the Medical Priority Dispatch System (MPDS ™ ). The typical response to a high-acuity emergency is the attendance of an emergency ambulance, staffed by a paramedic and emergency medical technician, with or without the additional attendance of a solo paramedic attending by car or motorcycle. LAS has a well-established critical care advanced practice service, and additionally deploys APs to suspected cases of ABD, with a recent study identifying that 237 patients were coded as presenting with ABD by APs in a 12-month period [ 13 ]. APs are permitted to administer chemical sedation to patients using Patient Group Directions (PGDs), a legal framework which enables registered health care professionals to administer parenteral medicines without a prescription in the UK.
Sampling and recruitment
The study was advertised using internal bulletins, social media, and word of mouth. Seven APs were recruited using convenience sampling. Overall, approximately 18% of the relevant study population participated. Participants were recruited between May and November 2021.
There is no consensus on the sample size required for qualitative studies [ 39 ]. Braun and Clarke suggest that researchers “… make an in-situ decision about the final sample size , shaped by adequacy (richness , complexity) of the data for addressing the research question…” [ 40 ]. Our research focussed on a specific area of clinical practice and the “… experiences , knowledge , or properties …” of our participants were highly specific in relation to it as key informants [ 37 , 40 , 41 ]. All participants were able to discuss several cases each from their personal experience in depth, producing good quality interview dialogue.
Data collection and processing
Seven semi-structured, in-depth interviews were undertaken, lasting between 52 and 66 minutes. The interviews examined participants’ experiences by enabling them to tell stories about ABD incidents they had attended. Interviews were conducted with the least structure and interviewer input as practicable to allow flexibility for interviewees to lead the discussion and talk about what was meaningful for them and for the interviewer to be flexible in eliciting new information [ 42 ]. Participants were asked to tell the interviewer about a time they had to decide whether to restrain a person with ABD or not, and probes were used to explore the interactions between those at scene, how participants felt about managing ABD, and why they chose to manage the cases in the way that they did. APs are often natural story tellers who frequently engage in reflective practice and often describe the incidents they attend in peer-to-peer conversations, this was evident in interviews with all participants speaking freely and in depth about their experiences. As a paramedic themselves, the interviewer was an insider researcher and therefore shared a degree of professional experience, identity and language with participants [ 43 ]. Insider research benefits from early acceptance of the interviewer and can facilitate a level of openness and access to data which can be difficult to obtain from outsider research [ 44 , 45 ]. The conversations were acquaintance interviews which enabled a good rapport to be established quickly [ 44 ]. It was also recognised that there is risk in insider research for interviewees to assume similarity with researchers and limit their explanations, and for researchers to confuse their own perceptions with those of participants [ 44 ]. To avoid this, informal member checking took place to confirm understanding of participants’ points. This was achieved by the interviewer summarising and restating points and inviting the participant to confirm accuracy.
Interviews were conducted and digitally recorded using Microsoft Teams, before being transcribed intelligent verbatim. The seven interviews generated 234 pages of data, consisting of 65,246 words. Each interview produced rich and complex data with a high level of depth. Based on the specificity of the participants and the quality of interview dialogue, these interviews were deemed to have generated sufficient relevant data to enable the development of meaningful themes [ 40 ]. All data were stored on managed and secure computer servers. Authors JL and LB had access to information which could identify participants during data collection, up to the point of anonymisation. Author TE had access to anonymised data only, as access to participant personal information was not required for both supervisors.
Data analysis
Braun and Clarke’s [ 46 ] method of reflexive thematic analysis (RTA) was used throughout the study. RTA is a method of theoretically flexible analysis which uses systematic coding of data to identify and interpret patterns and develop themes and acknowledges the part played by researchers in knowledge production. RTA values “ …a subjective , situated , aware and questioning researcher…” [ 46 ]. The three authors involved in the analysis were the Chief Investigator who is a woman consultant paramedic with an interest in medical ethics, professional duty and patient safety; a man consultant paramedic with extensive experience in developing and providing advanced paramedic critical care practice; and a senior woman social psychologist who conducts research on contextually situated social interactions and decision-making. The different perspectives and subjectivities of the co-authors were examined through dialogue throughout data analysis, as part of researcher reflectivity. Throughout the analysis, the Chief Investigator made memos noting their reflections and interpretations of the data, situated within their own context and view of the topic.
We used RTA inductively, with an emphasis on broad thematic patterning. Data analysis began following the first interview, and followed an exploratory and iterative approach [ 47 ]. The CI first familiarised themself with the data through listening to recordings during transcription and repeated reading of transcripts. This was followed by coding and theming stages; these stages involved all authors in sharing and discussing interpretations and returning to the data. The development of themes and an analytic narrative was a collaborative process [ 48 ].
Ethical approval
Ethical approval for this study was provided by the Heath Research Authority (IRAS: 265010).
Participants
Of the seven participants, one was female and six were male, noting that most APs in the study population were male at the time of the research. To protect anonymity, gender-neutral pseudonym names have been used. All participants were experienced APs with clinical education at the post graduate level and were adults of working age. Within the study site, it is part of the scope of practice for APs specialising in critical care to administer ketamine (an NMDA receptor antagonist used for sedation), haloperidol (an antipsychotic) and/ or midazolam (a benzodiazepine) to patients experiencing ABD, autonomously and in accordance with relevant PGDs.
We identified four themes from the dataset. The first, managing complexity and ambiguity, encompassed difficulties in differentiating ABD from other differential diagnoses and determining the right clinical management plan, including use of chemical sedation. The second theme, feeling vulnerable to professional consequences, related to participants’ concerns regarding the high-risk nature of the syndrome of ABD, and the potential for professional repercussions if cases led to poor patient outcomes. The third theme, recognising and establishing primacy of care, encompassed making leadership and accountability connections between professionals and the patient. The fourth, and final theme, needing to negotiate with other professionals, related to the interactions between APs and other clinicians or police officers at the incident scene and APs’ perceptions of achieving agreement on how to manage ABD patients. These four themes are reported in the following section, with participants’ data extracts presented alongside the associated analytic narrative.
Managing complexity and ambiguity.
The theme of managing complexity and ambiguity encompassed challenges with differentiating ABD from other forms of behavioural agitation and weighing up what to do, including identifying the right pharmacological intervention for patients who required chemical sedation.
For example, ‘Eddie’ described trying to differentiate which patients presenting with agitation were potentially experiencing ABD, to determine how they should be managed clinically.
“So I try to engage with the male , […] and it became clear that although the male was having some paranoid thoughts , […] he would have little moments where he would engage with me , and look at me and actually would say something sensible . ” ‘Eddie’
Here, ‘Eddie’ sheds light on an important differentiating sign of ABD, the ability to ‘engage’ with the patient and establish if they have a loss of insight. Other participants also referred to the ability to ‘engage’ with ABD patients as a differentiating sign, such as ‘Jordan’ who described assessing how a patient connected with his appearance during one interaction: “…he knows what he’s saying , he reckons I’ve got a big […] your brain is working…” . There is ambiguity in differentiating ABD from other forms of agitation which APs must navigate to make treatment decisions. Participants appeared to find the ability to engage with patients helpful in reducing this uncertainty.
As APs with chemical sedation within their scope of practice, many of the participants were particularly concerned with the decisions they made in relation to this intervention. ‘Pat’ described a case where they used chemical sedation to manage an ABD patient, and this acted as an enabler to good care.
“We had control , literally control of the scene and were able to […] assess him properly . Let’s do a top to toe , check for any injuries , have we missed anything , all the things you’d like to do with these patients , but you can’t ’cause you can’t get near them . ” ‘Pat’
The use of chemical sedation here allows ‘Pat’ to ‘literally [take] control’ and creates an opportunity to examine the patient. Prior to the chemical sedation taking effect, the police were in effect controlling the scene, and the combination of agitation and restraint prevented clinical assessment, prolonging the lack of information in relation to the patient’s physiological condition, increasing the complexity of the scenario and heightening the clinical risk. The use of chemical sedation has allowed access to the patient, and thus access to information which in turns begins to reduce the complexity and ambiguity of the incident and assists in determining subsequent ongoing management.
In making restraint decisions, ‘Charlie’ spoke about their concerns in relation to how much information they had about patients’ underlying physiology and how this affected their choices, particularly in relation to chemical sedation.
“What you are doing is […] taking an action , be that physical restraint or chemical sedation to a patient who you’ve got no idea what their baseline physiology is , and you’ve therefore got no idea how they will respond to those agents . ” ‘Charlie’
‘Charlie’ is particularly concerned about the unpredictability of the patient’s clinical course which results from a lack of information, and the increased risks which result. ‘Jordan’ was also concerned about this issue, adding that “… you just don’t know how people respond , sometimes you give somebody 5 mg of midazolam , and they’re GCS 3 [unresponsive to verbal and physical stimulus] and need airway management , but sometimes people take 15 mg of that stuff , and they’re still really agitated , really aggressive and fighting the police . ” There is an inherent uncertainty here about the outcome of ‘ taking an action ’ which is an important context for decision-making. APs are making decisions which have serious consequences and are doing so in the knowledge that they have a limited influence of the outcomes.
The above accounts speak to ambiguity of diagnosis. We end with ‘Charlie’ who describes a case in which they were dealing with legal ambiguities and the need to make a defensible decision.
“There is a level of immediacy , there is no least restrictive option and […] the Mental Capacity Act gives us a basis of action […] and we are acting in his best interests . ” ‘Charlie’
In this example, ‘Charlie’ has used a systematic and structured approach to work through relevant issues to their decision. This appears to have simplified the decision and reassured ‘Charlie’ that there is a legal basis for restraint, reducing ambiguity.
Feeling vulnerable to professional consequences.
The feeling vulnerable to professional consequences theme incorporates the concerns which participants spoke of in relation to the high-risk nature of these incidents, and what that might mean for professionals should the patient have a poor outcome, in particular concerns relating to medicolegal processes.
In the first example in this theme, ‘Eddie’ recalls a case from early in their advanced practice career where they used chemical sedation, and the patient stopped breathing for a time immediately after the drugs were administered.
“And to this day I can still remember exactly what happened in that case . Because for those few seconds where it looked like she’d stopped breathing , that was absolutely terrifying , because my immediate thought was—I’ve done this . By sedating the patient , and have I given the right drugs , have I made a drug error , have I given too much ? And actually , I double checked , and triple checked my drug dosages prior to administering the drugs . And still I thought I may have made an error , but I hadn’t . ” ’Eddie’
‘Eddie’ is fearful of the patient coming to harm and their first thought was that they had “ killed the patient ”. There is an emphasis here on ‘Eddie’s’ actions potentially causing harm to the patient, and a fear so impactful they can recall the incident in detail many years later. ‘Eddie’ appears to focus initially on the potential for personal error, rather than other potential sources of the patient’s apnoea. They express fear and doubt, even though they know that they have followed the correct procedures.
’Eddie’ also reflected on how similar procedures are managed in different healthcare services and added that unlike other clinical settings APs work in relative isolation when chemical sedation is concerned. Whilst a team of ambulance professionals work together to care for the patient, the AP is typically the only clinician there with specialist knowledge and experience of the procedure, concentrating the responsibility on their shoulders—as ‘Eddie’ said, “ this is on me .” This perhaps contributes to a sense of professional vulnerability due to a lack of immediate shared decision-making with other clinicians.
’Pat’ was also concerned about managing ABD cases in terms of providing chemical sedation, particularly around the risk of deterioration and how APs would experience associated investigations.
“ABD terrifies me . […] . We know at some point we are going to sedate somebody who will die . And that may be as a result of the sedation or […] they were going to die anyway . But we will never know that . And I fear being involved in that I really do . […] There’s internal investigations to go through . Then there’s an external process . There’s probably a referral to HCPC . […] Thinking that someone at some point is going to uncover something you should or shouldn’t have done . […] It sounds terrible , but this , this could be my last ever job . You know , this could be the job that breaks me . ” ’Pat’
There are two key issues here, a fear of a patient dying and a fear around the investigatory processes which would follow that. Firstly, like ‘Eddie’, ‘Pat’ is ‘ terrified ’ and expresses ‘ fear ’ about a patient dying. In saying ‘ we will never know’ we see that there is an intrinsic ambiguity in causes of deterioration, and this was an important burden for participants. Secondly, the idea of a death creates great apprehension for ‘Pat’, who refers to multiple investigations following such an eventuality. ‘Pat’ also expects a summary or arbitrary fitness to practice referral to the Health and Care Professions Council (HCPC), the regulatory body for paramedics in the United Kingdom and anticipates considerable personal critique and judgement.
Here we see mistrust of the investigatory process itself. ‘Pat’ speculates that video evidence might slightly contradict remembered evidence, suggesting that a trivial inaccuracy might bring their credibility into question in court: “‘… you didn’t do that at ‘52 [minutes past the hour] , you did it at ‘53 [minutes past the hour] , so how much of the rest of your statement is inaccurate ? ” . ‘Pat’ appears to be concerned about being vilified and unfairly criticised in an adversarial investigation process.
There is a fear that their career would not survive such a scenario. ‘Pat’ anticipates being “ suspended ” from duty suggesting that “It’s a very ambulance thing , isn’t it , a very paramedic thing that we associate discipline and error in the same bracket . ” This impacts how ‘Pat’ feels about error making, with an emphasis on punishment rather than learning, creating additional fear and anxiety.
In the next example, ‘Charlie’ talks about what they anticipate happening from an investigatory point of view, should a patient with suspected ABD suffer a cardiac arrest in the context of an AP administering sedation.
“When you look at the blood gases of these patients and when you look at their physiology you just realise they are so , so sick some of them . But , we are going to go through that in detail , and we’ll wheel out ‘experts’ who’ve never seen these patients in the community before […] and we will be critiqued to that . ” ’Charlie’
‘Charlie’ sheds additional insight into anxiety about the investigatory process. Specifically, what is articulated is mistrust in a process where people who lack APs experience of clinical practice in context, may be wheeled out as ‘ experts ’. There are two issues here. One is that the authority to critique is placed in the hands of these ‘ experts ’ and that their lack of relevant experience may be detrimental to the outcome. The second is an implicit failure to recognise and validate APs’ professional identities and their specialist knowledge and expertise.
Not all participants shared ‘Pat’ and ‘Charlie’s’ fear of the investigatory process. ‘Glenn’ below spoke positively about the support they would receive from their employer in such an event.
“I have real confidence that providing I do the right thing at the right time , that I will have a consultant doctor in the form of [a medical director] turn up after me in the court , and say ‘I think that’s about right and that’s what we wanted people to do and this is why’ . I wouldn’t do this if I didn’t feel that there was support behind it . ” ’Glenn’
‘Glenn’ brings insight about the importance of employer assistance and support. Acknowledging that a serious event may occur where bold after-event employer support would be needed. ‘Glenn’ is confident that their employer would back them up ‘ in court’ . This confidence is predicated on ‘Glenn’ doing ‘ the right thing at the right time’ , which is an important caveat given the ambiguity hampering diagnosis and treatment planning highlighted earlier. Our analysis cannot speak to why ‘Glenn’ experiences confidence where ‘Pat’ and ‘Charlie’ do not. It does, however, suggest the importance of employers creating a culture of high psychological safety in which the risks which APs and their patients face are understood, and support is made explicit.
Needing to negotiate with other professionals.
The needing to negotiate with other professionals theme comprised challenges experienced by APs when dealing with police and other ambulance clinicians at the scene of ABD incidents, and focussed on how participants experienced these interactions.
‘Charlie’, for example, attended a suspected ABD case in which the police are already at the scene and found their interactions to be straightforward in this case.
“I said I’m really worried this is ABD , and I’m really concerned about what might be […] the cause of this , and the police officer went , who was the kind of lead officer until the Sergeant arrived subsequently , said to me ‘no I think this is ABD , this is like exactly like the video . ’” ’Charlie’
In this incident, ‘Charlie’ connects with the police officer on their clinical concerns, developing a sense of urgency. The police officer validates ‘Charlie’s’ clinical impression. Here, it appears that the sharing of information between professionals has facilitated cooperation, and enabled a helpful relationship based on a shared recognition of the situation, insofar as the patient is an ABD patient for the purposes of management.
In the next example, ‘Eddie’ recounts an incident they attended, in which a patient suspected of having ABD had been subdued by several police officers and was restrained physically, as well as mechanically with handcuffs and leg restraints. The participant suggested to the police that they reduce the level of physical restraint, after which the patient’s agitation reduced.
“That was met with a little bit of resistance from the police . They had had to fight with him quite a lot to get him under control , and there was me saying , ‘right , thanks for all your hard work officers , but now you can let go . ’ And there was some concern that obviously he may then become very agitated , and they would have to start from square one in terms of restraint . ” ’Eddie’
‘Eddie’ acknowledges the events which have occurred prior to their arrival and understands the consequences for the police if reduction in restraint is unsuccessful–they will have to ‘ start from square one’ . The police hold an important gate-keeping role here and recognising that their responsibilities and concerns differ from those of APs appears to have been an important factor in fostering cooperation in this situation. ‘Eddie’ goes on to emphasise the importance of providing meaningful explanations to the police: “ This is why , and if it goes wrong , this is what we will do . So really explaining to police why I want them to do it , not just ‘do it’ . ” , echoing the approaches described by other participants.
In the following scenario, ‘Jordan’ has arrived at an incident where a man who has taken a large amount of cocaine and is agitated and is subject to significant physical restraint. During the struggle to bring the man under control, a police officer has been injured and the police are explicit in their expectation that ‘Jordan’ will provide chemical sedation, however’ Jordan’ does not agree that the patient requires this intervention.
“He said ‘we’re not going to stop physically restraining them until you sedate him’ . I was thinking I was being coached into chemical sedation and he says , ‘we’ve had you guys out before , we’ve never had this problem before , just give him […] sedation . I said listen , this is me , I’m deciding whether I’m going to sedate this patient . You’re not telling me what to do and at the moment the only thing I think is causing this agitation is you physically restraining him . ” ’Jordan’
As in the previous example, there is a dynamic which involves one professional group influencing the actions of another. In this case, it is the police attempting to influence the actions of APs, rather than the other way around as described earlier.
‘Jordan’ withholds chemical sedation because it is not clinically indicated. The police are seeking an alternative to physical restraint in a difficult case, perhaps highlighting the different lenses through which police officers and APs view these events, reinforcing the need for professionals to manage both agreement and disagreement on what course of action to take.
It seems that mutual sharing of information contributes to successful negotiations between professional groups. The establishment of professional connections between professional groups, which respond to different professional concerns and recognises the different ways police and APs view these events fosters cooperation.
Recognising and establishing primacy of care.
The recognising and establishing primacy of care theme encompasses the participants’ experiences of conceptualising the person with ABD as a patient and engaging with their professional duty of care for them. In this first example, ‘Eddie’ talks about the way police and APs as distinct professional groups view ABD patients.
“I think the police can sometimes see them as a bad person , whereas from a clinical perspective , I see these patients as very sick , and this is a medical emergency and actually this person doesn’t really understand what they’re doing…” ‘Eddie’
Here, ‘Eddie’ sheds light on the importance of how the person is categorised, specifically as a ‘patient’ with ABD rather than as a person who is characterised as ‘ bad ’. ‘Eddie’ emphasises the importance of keeping a focus on patients being ‘ critically unwell ’ to avoid getting “ caught up in this escalation where the patient is getting more and more violent , ’cause then you , you can get angry at the patient…” . ‘Eddie’ is specifically concerned here about how conceptualising ABD patients as ‘bad’ rather than sick might affect how professionals engage with them. They highlight the potential for a vicious cycle of agitation, resistance, and restraint, and are worried that the patient’s agitation will trigger stress and anger within themselves which might influence how they respond to the patient. The professional identity of police and APs again appears to have an important impact on how they interpret ABD patient presentations, and how they respond to them, and it appears that both professional groups have a need to maintain self-control when dealing with difficult cases involving ABD.
In the following example, ‘Aubrey’ describes an incident where police are also in attendance and have commenced restraining a person.
“I’m not a huge fan of spit hoods , I’d rather use an oxygen mask . You know and reminding the police that they’re sick and not bad , and that they are patient not a criminal and you know , just creating that culture on scene . ” ’Aubrey’
‘Aubrey’ volunteers a dislike of spit hoods (also known as spit guards), which are available to some UK police officers to protect them from detainees spitting on or at them. ‘Aubrey’ associates spit hoods with law enforcement. In this scenario, the symbolism of the oxygen mask suggests a clinical encounter, whereas the symbolism of a spit hood characterises a policing encounter.
‘Aubrey’ acknowledges that the police have developed their knowledge and approach to ABD patients and suggests that ‘ reminding ’ police that agitation in the context of ABD is clinically rather than behaviourally driven is helpful. This again characterises the incident as clinical rather than a law enforcement issue and suggests that ‘Aubrey’ perceives that the behaviours of the police may veer towards traditional policing behaviours at times in these scenarios. This appears to necessitate action to bring others back to the shared understanding which will facilitate cooperation between police and APs at the scene. ‘Aubrey’ speaks of creating a clinical culture at the scene, which they indicate might be different than a policing culture, but which facilitates the cooperation of the police and APs around a shared understanding of the person as patient first, and detainee second.
In the next example, ‘Glenn’ describes attending ABD incidents with the police and how they interact at the scene.
“It’s a clinical problem , not a law enforcement problem so typically what happens is , the police in the more extreme cases will send a supervisor . So you find yourself that supervisor , you make sure that their body camera is turned on . You find a couple of ambulance staff . And you get a little huddle together and one of the very early things you say is this is now my problem , and I just need your help to sort the problem out […] I find that once you once you use some very clear explicit language around that to say this is my problem , we can [manage] them under the capacity act , I’m responsible . ” ’Glenn’
Here, ‘Glenn’ is positioning themselves as a leader and decision-maker. They establish ownership of the situation and ownership of the consequences. ‘Glenn’ is relieving the police of some responsibility, perhaps serving to alleviate some of their professional concerns regarding potential consequences should the patient have a poor outcome. ‘Glenn’ is presenting themselves as an enabler, in a context where police are concerned about death after police contact and the consequences that brings both in human terms for the patient, their families and the professionals involved, and in relation to the burdens of prolonged investigation. The adoption of a leadership position, taking responsibility for the scenario and clearly articulating what the ‘ask’ is of the police appears helpful in developing cooperation.
Like ‘Charlie’s’ example above, ‘Glenn’ also grounds their decision-making in relevant legislation. This may serve to connect the clinical perspective of the APs with the legal lens of the police and addresses how the responders might justify restricting the patient’s liberty in legal terms. ‘Glenn’ is connecting with the police on common ground, the use of the Mental Capacity Act (2005).
‘Harper’ touched on the issue of more junior paramedics and their interactions with the police.
“If you’ve got an inexperienced member of staff , they’re more likely to be led by the police . […] They may not even know that we should have primacy of care and we can actually say to the police , look you’re restraining him wrong , you need to do it this way or however you want to phrase it . I suppose it goes wider to that societal thing where you always obey police . They were swayed by the police informing them that he was a violent patient , so to be careful , but actually at the time there was quite a lot of police officers restraining him . […] They then just took that for granted even though they didn’t do their own assessment . ” ‘Harper’
In discussing their observations of less experienced paramedics, ‘Harper’ makes several attributions in suggesting that they may be easily influenced by police officers. ‘Harper’ attributes a lack of appreciation of who should have primacy of care to inexperience and suggests that wider social norms about obeying the police may ‘ sway ’ inexperienced clinicians against questioning them. At the point of cardiac arrest, the patient in this case became unambiguously a patient experiencing a clinical emergency which should be clinically led, with policing issues secondary. For ‘Harper’ however, the case was unambiguously a clinical emergency prior to this stage.
‘Harper’ sheds light on the importance of professional curiosity. In this specific case, ‘Harper’ postulates that the paramedics were influenced by the warnings of the police and as a result have not explored the severity of the patient’s illness for themselves, and in doing so overlooked the need to intervene and establish clinical leadership of the incident. Noting this is an attribution, an alternative view might be that the paramedics were respecting the expertise of police in recognising and managing violence.
The purpose of this study was to explore the factors which influence APs when making restraint decisions for patients experiencing ABD, how they understand and manage these influences in practice and to explore the nature of any concerns for practitioners. This research was motivated by increasing professional interest in ABD, as well as new and emerging practice in this area relating to pharmacological interventions and a gap in the literature in relation to what influences decision-making in restraint situations.
Our findings echo the international literature highlighting the sense of vulnerability experienced by paramedics working in the ambulance setting in relation to decision-making [ 14 , 18 – 23 , 25 , 26 , 28 ]. ABD as a concept refers to agitation which may result from multiple causes and several differential diagnoses [ 3 ], this contributes to a complex and ambiguous decision-making context, which is complicated by a lack of information on which APs are required to make important risk-benefit decisions. This ambiguity appears closely linked with concerns about fair and balanced investigation in the event of a patient deteriorating whilst in AP care, and the degree to which clinicians expect their employers to support them after an adverse event. There was a sense that participants did not always feel trusted or respected as professionals, echoing research that finds paramedics perceive a culture of discipline and blame within UK ambulance services [ 24 ]. Whilst our research attested to anxieties APs feel in relation to investigatory processes, not all participants felt this way with some expressing trust and confidence in employer support. Further research may assist in understanding what might contribute to these different perspectives on trust and psychological safety in the workplace.
We found that key influences on decision-making in ABD arose from the social and professional relationships between responders at ABD incident scenes. Thus, our understanding of decision-making needs to shift away from the notion of an atomised individual-level process. The sharing of information and validation of each other’s professional expertise was an important factor in successful joint working and reduced the complexity and ambiguity of incidents. Recognising the different professional goals and concerns of police and APs, and the expression of mutual respect was relevant in APs being able to decide to reduce physical restraint. The relationships between APs and the police identifying and categorising people presenting with ABD as patients rather than perpetrators was also relevant. Participants took active steps to situate incidents as healthcare encounters. This context was key to locating ABD incidents in the span of control of APs within power gradients at scene where it was essential for APs to establish ownership of management decisions which were clinical in nature, including and especially those relating to restraint. One potential contributing factor is how clinicians navigate the traditional social authority and expertise in managing violence and aggression which the police hold, with their duty of care as healthcare professionals. This may be a particular issue where the police have arrived first and defined the context of the scene prior to the arrival of APs. This may be of particular importance in the context of ABD where the maintenance of law and order and providing medical care are, to an extent, entwined. This sets the scene for negotiations with police, predominantly in relation to reduction or cessation of physical restraint, or the initiation of chemical sedation, all with the goal of increasing safety for both the patient and the responders and to facilitate safe onward transfer and clinical care.
APs are experienced paramedics with extended education and training which includes preparation for attending ABD patients. APs are specifically prepared to be ‘the clinical lead’ in the cases they attend and wear different coloured epaulettes to assist other responders in identifying them at the scene of incidents. It might be reasonable to expect a newly qualified paramedic to be less confident in challenging another professional group with relative social authority and expertise in managing agitation and restraint, and perhaps be less likely to effectively establish primacy of care where leadership or the ‘ownership’ of an incident is contested or unclear. APs negotiated clinical leadership and primacy of care at ABD incidents by fostering a shared understanding of the nature of the problem and its associated risks with other professionals at the scene. As was the case in studies relating to restraint in the in-patient setting [ 32 ], as experienced practitioners APs were comfortable trying less ‘hands on’ techniques. Chemical sedation appeared to be favoured by police over physical restraint, and this led at times to APs feeling ‘coached’ into providing a pharmacological intervention and tensions arose where APs determined it was not a clinically appropriate course of action. This perhaps highlights the different lenses through which police and APs view chemical sedation as an intervention.
Implications for practice
This study adds to the evidence base which highlights a sense of professional vulnerability experienced by paramedics working in the ambulance setting in relation to high-risk decision-making. This may have negative effects on the health and wellbeing of staff, and is a patient safety issue [ 49 ]. More cultural change is needed in ambulance services to improve psychological safety to address this.
Our analysis calls attention to the importance of recognising the role of context and social relations in shaping how decisions are made in the pre-hospital ambulance setting. Failure to consider how decisions are made in the context of these social relationships may negatively influence patient safety. In preparing for clinical practice, issues around inter-professional negotiation, assertiveness and primacy of care represent important opportunities for development in pre and post qualification education and training. This is of particular importance in preparing APs for the complexities and risk associated with chemical sedation. A focus on inter-professional training with the police, alongside high-fidelity training and observation may be helpful interventions in achieving this.
Limitations and opportunities for future research
This study took place in a single setting, which is described here to enhance transferability and is not intended to be generalisable to every ambulance service. The LAS employs a small group of around 40 APs who staff four response cars on a 24/7 basis with targeted deployment to suspected ABD incidents, seeing 237 in a year according to one study [ 13 ]. The exposure of ambulance clinicians to suspected ABD cases may vary between different ambulance services.
This was a small study in nomothetic, statistical terms, but not in idiographic terms [ 50 ] where the focus was understanding experiences and sense-making within a small community of professionals. More critical for evidentiary adequacy in this study is adequate discrepant case analysis and adequate variety in kinds of evidence [ 51 ]. In relation to the first, participants were self-selected volunteers, and this may reflect a personal interest in ABD which may affect the data collected. In relation to the second, a single data collection technique was used. Although the interviews were in-depth and produced a rich data corpus, it is recognised that the inclusion of observational techniques would have enhanced the robustness of the study’s findings, by providing an additional source of evidence and reducing reliance on participants recalling their experiences. Ethnographic studies of the full decision-making process from emergency call to handover of care may shed further light on AP decision-making.
This study only included APs, therefore the perspectives of other professionals involved in ABD restraint decisions were not available. Studies exploring the perspectives of general paramedics and EMTs who are also involved in managing ABD patients at incidents scenes, as well as bystanders and emergency department clinicians who receive ABD patients would bring important perspectives. It is also recognised that the perspectives and priorities of police officers are important factors and form part of the context in which APs make restraint decisions, this is also an important opportunity for future research.
This study provides new and in-depth understanding of the decisions made by APs in relation to ABD patients. This is particularly important given recent concerns about death in the context of restraint [ 52 , 53 ]. Our results highlight the uncertainty which surround ABD decisions, increasing their risk, and indicates that the ability to navigate this uncertainty will increase the safety of these decisions in practice.
We found that the decisions APs make in relation to ABD patients are complex and are influenced by several different factors. A key influence was the need to characterise incidents as medical, which participants used as an enabler to establish leadership and decision-making control. APs focused on de-escalation techniques and sought to reduce physical restraint where possible, intervening with pharmacological interventions if necessary to achieve this. The social relationships and interactions with patients and other professionals at the scene were key to successful outcomes and formed an important context for decision-making. We determined that restraint decisions in ABD incidents are a cause of concern for some APs. A lack of psychological safety was also important, with fears of professional detriment accompanying a poor patient outcome.
Acknowledgments
We would like to thank all our study participants for their time and contributions.
- 1. Humphries C, Aw-Yong M, Cowburn P, Deakin C, Grundlingh J, Rix K, et al. Acute Behavioural Disturbance in Emergency Departments. Best Practice Guidelines. London: Royal College of Emergency Medicine; 2022.
- 2. College of Paramedics. Position statement: Acute behavioural disturbance2018 11/11/2018. https://www.collegeofparamedics.co.uk/news/position-statement-management-of-acute-behavioural-disturbance-abd .
- 3. Gorton A, Kalk N, Payne-James J, Rix K, Shelley S, Stark M, et al. Acute behavioural disturbance: guidelines on management in police custody. London: Faculty of Forensic and Legal Medicine; 2022.
- View Article
- PubMed/NCBI
- Google Scholar
- 8. Courts and Tribunals Judiciary. Regulation 28 report to prevent future deaths report: Darren Cumberbatch. UK: Courts and Tribunal Judiciary; 2019.
- 9. Courts and Tribunals Judiciary. Regulation 28 report to prevent future deaths: Neal Saunders. UK: Courts and Tribnunal Judiciary; 2022.
- 10. Broncucia-Jordan M. Chief Coroner Amended Report—Elijah McClain. Adam and Broomfield Counties: Office of the Coroner; 2021.
- 24. McCann L. The Paramedic at Work: A sociology of a new profession. UK: Oxford University Press; 2022.
- 34. Fisher JD, Freeman K, Clarke A, Spurgeon P, Smyth M, Perkins GD, et al. Health Services and Delivery Research. Patient safety in ambulance services: a scoping review. Southampton (UK): NIHR Journals Library; 2015.
- 35. Campbell DT. Evolutionary Epistemology. In: Overman ES, editor. Methodology and epistemology for social science: selected papers. Chicago: University of Chicago Press; 1988.
- 36. Maxwell JA. A realist approach for qualitative research. London: SAGE; 2012. xiii, 222 p. p.
- 37. Patton MQ. Qualitative research & evaluation methods: integrating theory and practice. 4th ed. Thousand Oaks, Calif.; London: SAGE; 2015. xxi, 806 p. p.
- 39. Rudestam KE, Newton RR. Surviving your dissertation: a comprehensive guide to content and process. 3rd ed. Thousand Oaks, Calif.; London: SAGE; 2007. viii, 317 p. p.
- 42. Bowling A. Research methods in health: investigating health and health services. Fourth edition. ed. Maidenhead: Open University Press; 2014. xvii, 512 pages p.
- 46. Braun V, Clarke V. Thematic Analysis: A practical guide. London: Sage; 2022.
- 51. Erickson F. Qualitative methods in research on teaching. In: Wittrock MC, editor. Handbook of research on teaching. 3rd ed. New York: Macmillan; 1986. p. 119–61.
- 52. Angiolini E. Report of the Independent Review of Deaths and Serious Incidents in Police Custody. UK: Home Office, 2017.
- 53. Prasad R. I can’t breath: Race, death and British Policing. UK: INQUEST, 2023.
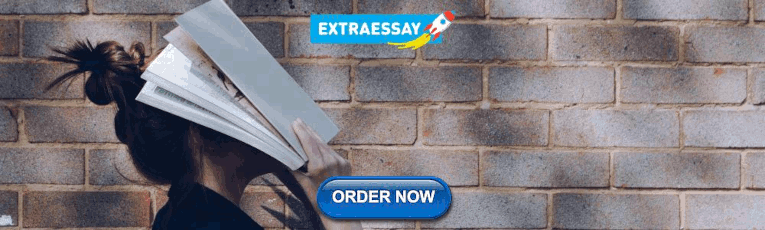
IMAGES
VIDEO
COMMENTS
Qualitative data analysis methods. Wow, that's a mouthful. ... As you can probably see, each of these research aims are distinctly different, and therefore different analysis methods would be suitable for each one. For example, narrative analysis would likely be a good option for the first aim, while grounded theory wouldn't be as relevant. ...
Qualitative data analysis is a systematic process of examining non-numerical data to extract meaning, patterns, and insights. In contrast to quantitative analysis, which focuses on numbers and statistical metrics, the qualitative study focuses on the qualitative aspects of data, such as text, images, audio, and videos.
Qualitative research methods. Each of the research approaches involve using one or more data collection methods.These are some of the most common qualitative methods: Observations: recording what you have seen, heard, or encountered in detailed field notes. Interviews: personally asking people questions in one-on-one conversations. Focus groups: asking questions and generating discussion among ...
Qualitative Research. Qualitative research is a type of research methodology that focuses on exploring and understanding people's beliefs, attitudes, behaviors, and experiences through the collection and analysis of non-numerical data. It seeks to answer research questions through the examination of subjective data, such as interviews, focus ...
Qualitative Data Analysis methods. Once all the data has been captured, there are a variety of analysis techniques available and the choice is determined by your specific research objectives and the kind of data you've gathered. Common qualitative data analysis methods include: Content Analysis. This is a popular approach to qualitative data ...
For many researchers unfamiliar with qualitative research, determining how to conduct qualitative analyses is often quite challenging. Part of this challenge is due to the seemingly limitless approaches that a qualitative researcher might leverage, as well as simply learning to think like a qualitative researcher when analyzing data. From framework analysis (Ritchie & Spencer, 1994) to content ...
How to conduct qualitative research? Given that qualitative research is characterised by flexibility, openness and responsivity to context, the steps of data collection and analysis are not as separate and consecutive as they tend to be in quantitative research [13, 14].As Fossey puts it: "sampling, data collection, analysis and interpretation are related to each other in a cyclical ...
Aims of Qualitative Data Analysis The analysis of qualitative data can have several aims. The first aim may be to describe a phenomenon in some or greater detail. The phenomenon can be the subjective experi-ences of a specific individual or group (e.g. the way people continue to live after a fatal diagnosis). This can focus on the case (indi-
I will discuss some of the different types of qualitative data analysis before focusing on computer pro- ... The distinctive features of qualitative data collection methods that you studied in Chapter 9 are also reflected . ... of a text provided by the subjects of research; other researchers, with different backgrounds, could come to ...
Qualitative research methods. Each of the research approaches involve using one or more data collection methods.These are some of the most common qualitative methods: Observations: recording what you have seen, heard, or encountered in detailed field notes. Interviews: personally asking people questions in one-on-one conversations. Focus groups: asking questions and generating discussion among ...
INTRODUCTION. Qualitative research methods refer to techniques of investigation that rely on nonstatistical and nonnumerical methods of data collection, analysis, and evidence production. Qualitative research techniques provide a lens for learning about nonquantifiable phenomena such as people's experiences, languages, histories, and cultures.
Limited statistical analysis: Qualitative data is often not suitable for statistical analysis, which limits the ability to draw quantitative conclusions from the data. Limited comparability: Qualitative data collection methods are often non-standardized, which makes it difficult to compare findings across different studies or contexts.
There are 5 main methods of qualitative data analysis. Which one you choose will depend on the type of data you collect, your preferences, and your research goals. Content analysis. Content analysis is a qualitative data analysis method that systematically analyses a text to identify specific features or patterns.
5. Grounded theory. This method of qualitative data analysis starts with an analysis of a single case to formulate a theory. Then, additional cases are examined to see if they contribute to the theory. Qualitative data analysis can be conducted through the following three steps: Step 1: Developing and Applying Codes.
We then address the different data collection techniques that can be used within the approach and the suitable types of data analysis. We also demonstrate how, when conducting qualitative research, qualitative researchers are continually making decisions and those decision-making processes are informed by the preceding steps in the research ...
5 qualitative data analysis methods explained. Qualitative data analysis is the process of organizing, analyzing, and interpreting qualitative research data—non-numeric, conceptual information, and user feedback—to capture themes and patterns, answer research questions, and identify actions to improve your product or website.Step 1 in the research process (after planning) is qualitative ...
About Research Methods. This guide provides an overview of research methods, how to choose and use them, and supports and resources at UC Berkeley. As Patten and Newhart note in the book Understanding Research Methods, "Research methods are the building blocks of the scientific enterprise. They are the "how" for building systematic knowledge.
Thematic analysis is a method of data analysis in qualitative research that most researchers use, and it is flexible because it can be applied and utilized broadly across various epistemologies ...
There are a wide variety of qualitative data analysis methods and techniques and the most popular and best known of them are: 1. Grounded Theory Analysis. The grounded analysis is a method and approach that involves generating a theory through the collection and analysis of data. That theory explains how an event or aspect of the social world ...
Gaining a better understanding of different techniques and methods in quantitative research as well as qualitative insights will give your analyzing efforts a more clearly defined direction, so it's worth taking the time to allow this particular knowledge to sink in. Additionally, you will be able to create a comprehensive analytical report that will skyrocket your analysis.
When collecting and analyzing data, quantitative research deals with numbers and statistics, while qualitative research deals with words and meanings. Both are important for gaining different kinds of knowledge. Quantitative research. Quantitative research is expressed in numbers and graphs. It is used to test or confirm theories and assumptions.
Methods used for data analysis in qualitative research. There are several techniques to analyze the data in qualitative research, but here are some commonly used methods, Content Analysis: It is widely accepted and the most frequently employed technique for data analysis in research methodology. It can be used to analyze the documented ...
Types of Qualitative Research Methods Qualitative research methods are designed in a manner that helps reveal the behavior and perception of a target audience regarding a particular topic. The most frequently used qualitative analysis methods are one-on-one interviews, focus groups, ethnographic research, case study research, record keeping ...
Research methods are specific procedures for collecting and analyzing data. Developing your research methods is an integral part of your research design. When planning your methods, there are two key decisions you will make. First, decide how you will collect data. Your methods depend on what type of data you need to answer your research question:
Qualitative data analysis involves interpreting non-numerical data to identify patterns, themes, and insights. There are several methodologies and methods used in qualitative data analysis. In this article, we will explore qualitative data analysis techniques in great detail, with each method providing a different perspective on how to ...
Qualitative Data Coding. Coding is the process of analyzing qualitative data (usually text) by assigning labels (codes) to chunks of data that capture their essence or meaning. It allows you to condense, organize and interpret your data. A code is a word or brief phrase that captures the essence of why you think a particular bit of data may be ...
The type of analysis you plan to conduct also dictates your choice of method. Qualitative data analysis is interpretive and seeks to understand the underlying reasons and motivations. In contrast ...
Published: Oct. 23, 2023. Qualitative Data Analysis Software (QDA software) allows researchers to organize, analyze and visualize their data, finding the patterns in qualitative data or unstructured data: interviews, surveys, field notes, videos, audio files, images, journal articles interviews, web content etc.
Background There is increasing interest in using patient and public involvement (PPI) in research to improve the quality of healthcare. Ordinarily, traditional methods have been used such as interviews or focus groups. However, these methods tend to engage a similar demographic of people. Thus, creative methods are being developed to involve patients for whom traditional methods are ...
The different perspectives and subjectivities of the co-authors were examined through dialogue throughout data analysis, as part of researcher reflectivity. ... Data analysis began following the first interview, and followed an exploratory and iterative ... Qualitative research & evaluation methods: integrating theory and practice. 4th ed ...