
An official website of the United States government
The .gov means it’s official. Federal government websites often end in .gov or .mil. Before sharing sensitive information, make sure you’re on a federal government site.
The site is secure. The https:// ensures that you are connecting to the official website and that any information you provide is encrypted and transmitted securely.
- Publications
- Account settings
Preview improvements coming to the PMC website in October 2024. Learn More or Try it out now .
- Advanced Search
- Journal List
- Front Public Health
- PMC10616829
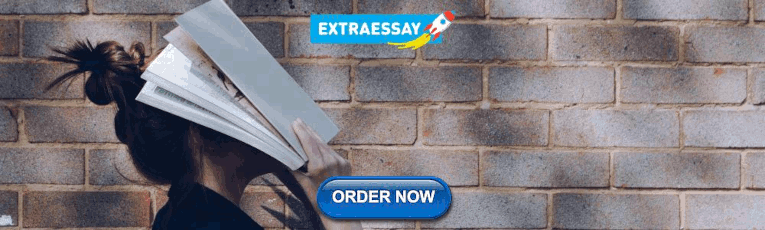
Socioeconomic status and health behavior in children and adolescents: a systematic literature review
Nirmal gautam.
1 School of Business, Faculty of Business, Education, Law and Arts, University of Southern Queensland, Toowoomba, QLD, Australia
2 Centre for Health Research, University of Southern Queensland, Toowoomba, QLD, Australia
Getenet Dessie
3 National Centre for Epidemiology and Population Health, College of Health and Medicine, Australian National University, Canberra, ACT, Australia
4 College of Medicine and Health Sciences, Bahir Dar University, Bahir Dar, Ethiopia
Mohammad Mafizur Rahman
Rasheda khanam, associated data.
The original contributions presented in the study are included in the article/ Supplementary material , further inquiries can be directed to the corresponding author.
Socioeconomic status affects individuals’ health behaviors and contributes to a complex relationship between health and development. Due to this complexity, the relationship between SES and health behaviors is not yet fully understood. This literature review, therefore, aims to assess the association between socioeconomic status and health behaviors in childhood and adolescence. Preferred Reporting for Systematic Review and Meta-Analysis protocol guidelines were used to conduct a systematic literature review. The electronic online databases EBSCO Host, PubMed, Web of Science, and Science Direct were utilized to systematically search published articles. The Joanna Briggs Institute’s critical appeal tool was used to assess the quality of included studies. Eligibility criteria such as study context, study participants, study setting, outcome measures, and key findings were used to identify relevant literature that measured the association between socioeconomic status and health behaviors. Out of 2,391 studies, only 46 met the final eligibility criteria and were assessed in this study. Our review found that children and adolescents with low socioeconomic status face an elevated risk of unhealthy behaviors (e.g., early initiation of smoking, high-energy-dense food, low physical activity, and involvement in drug abuse), in contrast to their counterparts. Conversely, children and adolescents from higher socioeconomic backgrounds exhibit a higher prevalence of health-promoting behaviors, such as increased consumption of fruit and vegetables, dairy products, regular breakfast, adherence to a nutritious diet, and engagement in an active lifestyle. The findings of this study underscore the necessity of implementing specific intervention measures aimed at providing assistance to families from disadvantaged socioeconomic backgrounds to mitigate the substantial disparities in health behavior outcomes in children and adolescents.
1. Introduction
Health behavior constitutes a fundamental facet of individuals’ holistic well-being, exerting a substantial influence on their physical health, mental well-being, and overall longevity ( 1–5 ). Over the past decades, considerable attention has been directed toward comprehending health behaviors and cultivating healthy lifestyles ( 6–9 ). However, socioeconomic status (SES) (e.g., income, education, occupation, social position) pose a significant obstacle to achieving these objectives ( 10 ). Thus, people from low-SES backgrounds are more likely to exhibit a higher rate of smoking tobacco, drinking alcohol, excess weight gain, and a sedentary lifestyle, which has been consistently identified as a pivotal determinant of premature and preventable morbidity and mortality compared to their counterparts ( 11–13 ).
The literature reveals that children and adolescents’ health and health behaviors primarily depend on their parental SES backgrounds ( 14–18 ). It is well documented that children and adolescents from high parental SES backgrounds have more access to education, housing, food, clothing, health services, and social services ( 19–23 ). These advantageous services enhance self-confidence, self-esteem, and self-efficacy, which promotes a healthy lifestyle and reduces the risk of stress in youngsters ( 14 , 15 , 17 ). In contrast, parental support, healthy parental lifestyle, medical services, and social networks are less open to children and adolescents with low parental SES ( 21 , 24–27 ). Hence, poor availability of goods and services increases the likelihood of physical and mental health issues and encourages them to adopt risky behaviors (e.g., smoking, drinking alcohol, illicit drug use, gambling) to deal with their concerns ( 28–33 ). Therefore, parental social, cultural, and economic status are fundamental determinants of health and health behaviors in children and adolescents, which are apparent from the early stages of life and persist into adolescence ( 34 , 35 ).
Globally, millions of children, particularly those with low socioeconomic profiles, do not start their lives in a healthy state ( 36 , 37 ). This could be due to insufficient goods and services, which are the primary causes of impairment in children’s neuro-biological development, resulting in poor social, emotional, psychological, and physiological outcomes ( 38 , 39 ). Thus, focusing on improving support for deprived and underserved populations is a powerful strategy to establish the roots of healthy behaviors in childhood and adolescent development ( 40 ). Therefore, it is recommended that every government adopt a health and health equity policy program to promote positive health behaviors among its population ( 41–44 ).
Numerous studies have been documented in the international literature to determine ( 45 ), analyze ( 46 ), and explored ( 47 , 48 ) the relationship between SES and health behaviors in children and adolescents. For example, in a study conducted by Liu et al. ( 49 ), adolescents hailing from families with lower parental SES exhibited a significantly higher likelihood of [OR = 2.12, 95% CI, 1.49–3.01] cigarette smoking than those from middle and high SES backgrounds. Melotti et al. ( 50 ) explored the association between parental SES and alcohol consumption among adolescents. They discovered an inverse association, revealing that adolescents with low SES had higher odds (OR: 1.26, 95% CI, 1.05–1.52) of consuming alcohol compared to those with high SES backgrounds. Furthermore, a seminal study by Krist et al. ( 51 ) investigated the impact of parental SES on physical activity levels in children and adolescents. The results indicated that those from lower parental SES backgrounds were less likely to engage in regular physical activity (OR = 0.90, 95% CI 0.63–1.29) in comparison to their peers from higher SES backgrounds. Collectively, this extensive body of evidence underscores the pivotal role played by parental SES in shaping the multifaceted landscape of health behaviors among children and adolescents. These disparities in health behaviors often contribute to adverse health outcomes, including a heightened prevalence of chronic diseases such as obesity, diabetes, and cardiovascular diseases ( 52 ).
Given the evidence above, examining the association between parental SES and health behaviors of children and adolescents holds profound scientific significance and societal consequences. Thus, a good understanding of how parental SES influences health behaviors among young individuals enables the identification of vulnerable populations at an early stage, allowing for targeted interventions and preventive measures to combat socioeconomic comorbidities and their consequences during childhood and adolescence. However, to the best of our knowledge, the existing body of literature examining the association between SES and health behaviors (e.g., protective health behaviors and damaging health behaviors) among children and adolescents remains limited ( 45–47 , 53 , 54 ). To address this gap in the literature, this review comprehensively examines the association between SES and health behaviors in children and adolescents aged 3–18 years, utilizing the literature on SES and health behaviors. In this review, health behaviors were examined in two ways: (i) protecting health behaviors and (ii) impairing health behaviors among children and adolescents. Protecting health behaviors is defined as the consumption of fruit vegetables, consumption of dairy products, regular breakfast, and involvement in physical activity during leisure time ( 55 ). Consumption of high-fatty foods (e.g., chips, noodles), high sugary drinks (e.g., fruit juice, Coco Cola, cordial) and engagement in smoking (i.e., tobacco, cannabis), illicit drugs, alcohol consumption, and sedentary activities during their leisure time are defined as unhealthy behaviors exhibited by children and adularescent ( 56 ). In this review study, we were particularly interested to examining two important research questions: (i) Does low socioeconomic status influence risky or impair health behavior patterns among children and adolescents compared to their counterparts? (ii) What is the association between SES and health behaviors in children and adolescents? Through an exploration of these research questions, this study will help to better understand the relationship between SES and health behaviors in children and adolescents. By doing so, this study seeks to provide valuable insights that can inform potential policy interventions aims at enhancing the health behavior outcomes of children and adolescents, particularly those who come from underserved and disadvantaged socioeconomic backgrounds.
This review article followed the Preferred Reporting for Systematic Review and Meta-Analysis Protocol (PRISMA-P) guidelines in order to identify studies that were screened, included and excluded in this review ( 57 ). PRISMA-P helps to provide a guideline for development of protocol for systematic review, and meta-analysis in order to improve the quality and transparency of the studies ( 58 , 59 ). In this review, we used the PRIMA checklist shown in Supplementary Table S3 .
2.1. Data sources and literature search
To identify relevant articles on SES and health behaviors in childhood and adolescence, different electronic databases of various disciplines were searched, including Academic Search, APA PsycArticles, APA PsycInfo, Cumulative Index of Nursing and Allied Health Literature (CINAHL), Health Source, Nursing/Academic Edition, Psychology and Behavioral Sciences Collection, Sociology Source, Sociology Source Ultimate, PubMed, Web of Science, and Science Direct. These electronic databases were searched using the keywords: socioeconomic, “socio-economic,” “health behavior,” “health behavior,” “health behaviors,” “health behaviors,” teen*, adolescent*, and child*” (see Supplementary Table S1 ). The articles were subsequently exported using EndNote citation manager X9 version.
2.2. Criteria of included studies
In this systematic literature review, we included studies that reported an association between SES and health behaviors. Additionally, we considered studies that had at least one specific health behavior, either protecting or impairing health behaviors, such as smoking, drinking alcohol, illicit drugs, physical exercise, consumption of fruit and vegetables, and dietary habits. Consequently, all peer-reviewed prospective, retrospective, quantitative studies from both developed and developing countries, published in the English language were included. Subsequently, national representative surveys (cross-national and longitudinal) consisting of more than 500 samples from the sampled population (i.e., children and adolescents aged 3–18 years) were also included. In this study, we considered children (i.e., 3–12 years) and adolescents (13–18 years of age) as defined by the US Department of Health and Johns Hopkins Medicine, respectively ( 60 , 61 ). In summary, the inclusion criteria for this review study were structured in accordance with the PICOS model, where: “P” = Population (i.e., children and adolescents aged 3 to 18 years old), “I” = intervention (we do not have intervention in this review study), “C” = comparator (i.e., high SES and low SES backgrounds), “O” = Outcome of this study (i.e., health behavior either protecting or impairing health behaviors), and “S” = study design (i.e., cross-sectional, longitudinal only).
2.3. Criteria of excluded studies
Based on predefined eligibility criteria, we evaluated the titles and abstracts of the identified studies. In cases where the studies were found relevant to our review, we thoroughly assessed the full text. However, if the studies were not relevant to our study, such as national income inequalities and health behavior or national per-capita income inequalities and health behaviors in childhood and adolescence, we excluded such studies. Additionally, if the study did not meet the eligibility criteria such as population, comparator, outcome, and study design, we excluded the paper from this study. For example, review articles, pilot studies, reports, dissertations, books, symposia, supplementary, prospective, or intervention studies, and articles published in other languages. Articles published before 2000 were also excluded from this study, primarily because their full-text versions were not readily accessible. Furthermore, articles with a sample size of less than 500 were excluded from the analysis. The decision to exclude studies with a sample size less than 500 was driven by a combination of methodological constraints. Two articles had sample sizes of 246 and 310, respectively; however, these studies found no association between SES and health behaviors in adolescents. Therefore, we decided to exclude them from the final analysis.
2.4. Data extraction
The data extraction was done by two independent reviewers using a data extraction table. The data extraction was based on the Joanna Briggs Institute (JBI) checklist (e.g., country, study setting/context, participant characteristics, outcome measures, and key findings). The final data extraction was based on the phase two screening of studies (i.e., 46 studies) by NG following the PRISMA guidelines ( 57 ), while GD, MMR, and RK approved data extraction.
2.5. Quality assessment of study
The Joanna Briggs Institute (JBI) and System for the Unified Management of the Assessment and Review of Information (SUMARI) is an appraisal tools assist in evaluation of the trustworthiness outcomes of the included studies ( 62–64 ). For the cross-sectional studies, JBI SUMARI apprise as follows: (i) inclusion criteria were clearly defined for the study population; (ii) study subject and study setting were clearly explained; (iii) exposures were scientifically measured; (iv) standard criteria and objectives were used to assess the measurement of the study; (v) confounding factors were identified; (vi) a strategic plan was developed to address the confounding factors; (vii) the results of the studies were reliable and valid; (viii) an appropriate statistical method was used to analyze the study. On the other hand, cohort studies were evaluated as follows: (i) the sample was recruited from the study population; (ii) exposures were measured equally between the exposed and unexposed groups; (iii) exposures were measured in a valid and reliable way; (iv) confounding factors were identified; (v) the statistical plan was used to address the confounding factors; (vi) at the beginning of the study, the sampled population was free from exposures; (vii) outcomes of the study were measured in a valid and reliable way; (viii) follow-up time was sufficiently reported; (ix) follow-up was completed, and if not, reasons were well explained; (x) plans were explored to address the issue of those in the sample lost to follow-up; (xi) the standard statistical method to analyze the study was used. To establish the credibility of our findings, every included study was apprised using the JBI SUMARI and provided the score for each study by two independent reviewers. Studies were included in this review if they had gained a score of 60 percent or above, while studies with a score of less than 60 percent were excluded. However, in the context of this study appraisal, none of the papers met the criteria for a score below 60 percent. As a result, it was not necessary to exclude any studies through the application of the JBI SUMARI appraisal tools (see Supplementary Table S4 ). In alignment with these principles, our review study also employed the JBI SUMARI to contribute to the overall reliability and quality of the review findings ( 65 , 66 ).
Furthermore, the risk of bias in the included studies was evaluated using the 10-item grading scale for prevalence studies developed by Hoy et al. ( 67 ). The study methodology, case definition, prevalence periods, sampling, data collection, reliability, and validity of the investigations were thoroughly scrutinized. Each study was categorized as exhibiting either a low risk of bias (shown by affirmative replies to domain questions) or a high risk of bias (indicated by negative responses to domain questions). In each study, a binary scoring system was used to assign a value of 1 (indicating presence) or 0 (indicating absence) to each domain. The cumulative sum of these domain values was used to derive the overall study quality score. The assessment of bias risk was performed by calculating the total number of high-risk biases in each study. Studies were categorized as having a low risk of bias if they had two or fewer high-risk biases, moderate risk of bias if they had three or four high-risk biases, and high risk of bias if they had five or more high-risk biases. To resolve discrepancies among the reviewers, a consensus-based approach was employed for the final categorization of the risk of bias (see Supplementary Table S5 ).
2.6. Outcome measurement of the study
The outcomes of this study were health behaviors, either through health-protective behaviors (e.g., consumption of fruit and vegetables, consumption of a healthy diet, physical exercise) or impairing health behaviors (e.g., smoking, drinking alcohol, high sedentary lifestyle and illicit drug use), in children and adolescents ( 68 ). Childhood and adolescence are two distinct stages of life that are characterized by diverse features in the respective age groups (e.g., physical and psychological) ( 69 , 70 ). Childhood is a time of rapid and remarkable development, encompassing significant strides in the physical, cognitive, social, and emotional dimensions that outpace the progress seen in other life stages. During this period, children’s innate intellectual curiosity flourishes, driving them to explore the world around them and actively engage in interactive experiences ( 71 , 72 ). On the other hand, adolescence is widely acknowledged as a transformative phase known as the “storm and stress” period, characterized by profound changes in biological, cognitive, psychosocial, and emotional realms ( 73 ). These transformative shifts often give rise to a range of adjustment challenges, including mood swings, propensity for risk-taking behaviors, and conflicts with both parental figures and peers ( 73 ). Thus, considering the relationship between health behaviors in childhood and adolescence is pivotal, therefore, this review study examined the association between parental SES and health behaviors in children and adolescents separately.
2.7. Measure of socioeconomic status (SES)
SES is a multidimensional construct and is measured objectively by income, education, and occupation, or subjectively by prestige, place of residence, ethnic origin, or religious background, covering both objective and subjective measures of SES ( 74–76 ). The stratification of SES into subgroups (e.g., low SES and high SES) was predicted based on goods and materials consumed by the individual in a household, including durable goods such as televisions, bicycles, and vehicles, as well as housing-related attributes such as access to drinking water, food, bathroom facilities, and agricultural and flooring materials ( 77 ). Households that possessed adequate provision of food, water, hand-washing materials, agricultural products, fields for production, and other consumer items for a duration of merely 6 months were categorized as having low-income or low- SES backgrounds. On the other hand, families that possessed an ample supply of food and other products, including consumer goods, to sustain themselves for a period of 12 months or more were classified as affluent or wealthy or high SES backgrounds ( 77 , 78 ). Based on previous literature ( 21 , 22 , 77 , 79 , 80 ), our study defined low SES and high SES backgrounds and examined the association between SES and health behaviors in children and adolescents.
3.1. Description of the study
In this systematic literature review, 2,391 articles were initially retrieved, and 585 duplicates were removed before entering the first phase of screening. A total of 1806 articles were assessed in the first phase of screening based on their titles and abstracts. Thus, in the first screening phase, we retained only 146 articles and rejected 1,660 articles. Consequently, in phase two of the screening, we assessed 146 papers based on their full text. Of these, only 46 articles met the inclusion criteria for further evaluation. Hence, these 46 articles were eligible in our final review, which is shown in Figure 1 .

PRISMA flow diagram.
A total of 46 articles were assessed to examine the association between SES and health behaviors in childhood and adolescence. Most of the studies were from Europe ( n = 36), followed by Asia ( n = 9), North America ( n = 5), and Africa ( n = 2). There has been a noticeable increase in studies on SES and health behavior in childhood and adolescence in the past decade (i.e., 2010 to 2020). From a total of 46 studies, 13 studies ( 79 , 81–92 ) were published between 2000 to 2010, while 33 studies ( 25 , 47–51 , 53 , 93–118 ) were published from 2011 to 2022.
3.2. Parental socioeconomic status and smoking
3.2.1. parental socioeconomic status and smoking among children.
From a total of 46 studies, 18 studies ( 25 , 47 , 49 , 50 , 53 , 79 , 81 , 84 , 86–88 , 93 , 96 , 97 , 104 , 105 , 107 , 109 ) showed an association between SES and smoking behavior in children and adolescents. Out of 18 studies, five reported a positive association between low SES and smoking behavior in children, implying that children with low SES had a greater risk of exposure to early smoking, and experimenting with smoking, compared to those who were from a high SES background ( 25 , 49 , 53 , 104 , 105 ).
3.2.2. Parental socioeconomic status and smoking among adolescents
From a total of 18 studies, 12 studies showed a positive association between low SES and smoking behaviors in adolescents, indicating that adolescents with low SES had a higher risk of smoking behaviors than those with a high SES background ( 50 , 79 , 81 , 84 , 86–88 , 93 , 96 , 97 , 107 , 109 ), while one study found a negative association between low SES and smoking behavior ( 47 ). In summary, a majority of 17 studies (94.44%) supported that children (5 studies, 27.77%) and adolescents (12 studies, 66.66%) from low SES backgrounds were more likely to be exposed to, have tried, or smoked daily, compared to those with high SES (see Table 1 ).
Studies examining the association between parental SES and smoking.
3.3. Parental socioeconomic status and drinking alcohol
3.3.1. parental socioeconomic status and drinking alcohol among children.
Out of 46 studies, 18 studies ( 25 , 47 , 50 , 53 , 79 , 83–85 , 91 , 97 , 100–102 , 104 , 105 , 107 , 108 , 115 ) assessed the association between SES and drinking alcohol. Of them, seven studies reported a positive association between high SES and drinking alcohol in children ( 25 , 53 , 83 , 102 , 104 , 105 , 115 ). This implies that younger children from higher SES backgrounds are more likely to experiment with drinking alcohol compared with their counterparts. One study found a negative association between drinking alcohol and high SES ( 100 ).
3.3.2. Parental socioeconomic status and drinking alcohol among adolescents
With regard to SES and drinking alcohol by adolescents, from 18 studies, six reported a negative association between drinking alcohol and high SES ( 50 , 85 , 91 , 101 , 107 , 108 ). Conversely, three studies reported a positive association between drinking alcohol and high SES ( 47 , 79 , 97 ). This positive association indicates that adolescents from a high SES background were found to have a higher chance of drinking alcohol than their counterparts. Moreover, a study by Richter et al. ( 84 ) reported that adolescents from Western, Northern, Southern (except Spain), Central and Eastern (except Latvia) Europe, and from a low SES background, were found to have a lower chance of drinking alcohol compared to their counterparts; however, adolescents from Scotland, Wales, Norway, Finland, Denmark, Greece, Italy, Malta, Hungary, Russia, and Ukraine were only statistically significant. In summary, out of 18 studies, children (seven studies, or 38.88%) and adolescents (three studies, or 16.66%) from a high SES background had a higher chance of drinking alcohol than those from a low SES background, while six studies (33.33%) found a negative association between a high SES background and drinking alcohol in adolescents ( Table 2 ).
Studies examining the association between parental SES and alcohol consumption.
3.4. Parental socioeconomic status and physical activity (PA)
3.4.1. parental socioeconomic status and physical activity (pa) among children.
We identified 15 studies ( 25 , 48 , 51 , 53 , 81 , 89 , 92 , 94 , 97 , 98 , 104 , 105 , 110 , 113 , 117 ) that examined the association between SES and PA. From a total of 15 studies, seven found a positive association between high SES and PA in children ( 25 , 48 , 51 , 98 , 104 , 110 , 117 ); however, two reported a negative association between high SES and PA ( 53 , 105 ). This negative association implies that those children from a high SES background had a lower chance of being physically active compared to their counterparts.
3.4.2. Parental socioeconomic status and physical activity (PA) among adolescents
Five studies found a positive association between high-SES and physical activity in adolescents ( 89 , 92 , 94 , 97 , 113 ). These findings implied that the adolescents from high parental SES backgrounds were more physically active compared to their counterparts, while one study found a negative association between high SES and PA ( 81 ). In summary, children from high-SES backgrounds in seven studies (46.66%) and adolescents in five studies (33.33%) had a higher chance of being more physically active than those from low- SES backgrounds ( Table 3 ).
Studies examining the association between parental SES and physical activity (PA).
3.5. Parental socioeconomic status and fruits/vegetables
3.5.1. parental socioeconomic status and fruits/vegetables among children.
Fourteen studies ( 48 , 53 , 79 , 81 , 82 , 84 , 89 , 90 , 95 , 99 , 106 , 110 , 111 , 118 ) were identified that examined the association between SES and fruit and vegetable consumption. Ten of these studies found a positive link between high SES and children’s consumption of fruits and vegetables ( 48 , 53 , 82 , 84 , 95 , 99 , 106 , 110 , 111 , 118 ), which implies that children from a high SES background had a higher chance of consuming high amounts of fruits and vegetables compared to those from low SES backgrounds.
3.5.2. Parental socioeconomic status and fruits/vegetables among adolescents
From 14 studies that examined the association between SES and the consumption of fruits and vegetables, four studies found a positive association between high SES and the consumption of fruit and vegetables in adolescents ( 79 , 81 , 89 , 90 ). A study by Vereecken et al. ( 82 ) reported that adolescents from Europe (West, South, North, Central, East), North America, and Asia with low SES were less likely to consume fruits and vegetables than those from high-SES backgrounds. Adolescents from low- SES families in Western Europe, Northern Europe, Southern Europe, North-Eastern Europe (except Estonia, Hungary, Latvia, Russia, and Ukraine), North America, and Asia were less likely to consume vegetables than their counterparts. Overall, high SES was associated with greater rates of fruit and vegetable consumption among children (10 studies or 71.42%) and adolescents (4 studies or 28.57%) compared to low SES (see Table 4 ).
Studies examining the association between SES and fruits and vegetables consumption.
3.6. Parental socioeconomic status and dietary habits
3.6.1. parental socioeconomic status and dietary habits among children.
A total of 46 studies were included in this review study. Of these, 18 studies ( 25 , 48 , 79 , 81 , 82 , 89 , 90 , 97 , 99 , 104–106 , 111 , 112 , 114 , 116–118 ) examined the association between SES and healthy diet habits in childhood and adolescence. Of a total of 18 studies, six studies revealed a positive association between high SES and healthy diet habits (e.g., animal products, nutritious food, balanced diet, breakfast) in children ( 25 , 48 , 104 , 111 , 116 , 117 ). Consequently, another four studies reported a positive association between high SES and unhealthy diet (e.g., Biscuits, pastries, irregular breakfast, sweet foods, and soft drinks) ( 82 , 89 , 106 , 114 ), while three studies found a negative association between high SES and low consumption of unhealthy dietary foods ( 99 , 105 , 118 ).
3.6.2. Parental socioeconomic status and dietary habits among adolescents
Out of 18 studies that examined the association between SES and dietary habits, five studies reported a positive association between high SES and consumption of healthy dietary food in adolescents ( 79 , 81 , 90 , 97 , 112 ), implying that adolescents with high parental SES had a higher probability of consuming a high proportion of dairy products, a regular breakfast, a healthy or nutritious diet, a balanced diet, and a low proportion of high-fat diet than those with low SES (see Table 5 ). In summary, six (33.33%) and five studies (27.77%) were positively associated with high SES and healthy dietary habits in childhood and adolescence, respectively.
Studies examining the association between parental SES and diet habits.
3.7. Parental socioeconomic status and cannabis, marijuana, and illicit drug use by adolescents
In this literature review, we assessed three studies ( 47 , 101 , 103 ) out of 46 that examined the relationship between SES and cannabis use and illicit drugs. Of these, one study reported a negative association between high SES and cannabis use ( 47 ), while another found a negative relationship between high SES and marijuana consumption ( 101 ). Similarly, two other studies found a negative association between high SES and illicit drugs used by adolescents ( 101 , 103 ). This indicates that adolescents with low SES were found to consume cannabis, marijuana, and illicit drugs more frequently than adolescents from high SES backgrounds. In summary, 66.66%, or two studies, reported a negative association between high SES and illicit drug used in adolescents (see Table 6 ).
Studies examining the association between SES and cannabis, and illicit drug use.
4. Discussion
Our study produced evidence, mostly from cross-sectional and longitudinal studies, that consistently demonstrates a strong relationship between SES and health behaviors. SES plays a major and well-documented influence in the onset and progression of chronic diseases in children and adolescents. Lower-income children and adolescents may have less access to frequent check-ups, preventative care, and early disease identification, increasing their risk of acquiring chronic disorders. Furthermore, SES influences the availability and cost of healthy food. Low-income families may struggle to offer adequate diets, which can contribute to poor eating habits, obesity, and linked chronic illnesses such as type 2 diabetes ( 52 , 119 , 120 ). Childhood and adolescence are regarded as pivotal stages in the development of life foundations in the general population. Focusing on younger ages is important because health behaviors can be learned and consolidated during these ages, affecting an individual’s health for the rest of their life. Therefore, this review study aims to examine the association between SES and health behaviors in children and adolescents.
The findings of this review demonstrate that children and adolescents from low SES backgrounds are more likely to engaged in unhealthy behaviors, such as smoking, alcohol consumption, physical inactivity, poor dietary choices, and drug use, when compared to their peers from higher SES backgrounds. These disparities in multiple health behaviors among children and adolescents may be possibly due to low parental SES, which limits access to healthy food, physical activity, and health education. Lower parental SES also correlates with low health literacy, which may lead to a lack of awareness about the importance of healthy behaviors. These findings align with existing literature, highlighting the impact of economic constraints in low-SES households, hindering access to health-promoting resources. Limited financial resources often result in inadequate nutrition, reduced physical activity opportunities, and lower educational attainment, contributing to reduced health awareness ( 120–124 ). This knowledge and resource gap influences healthy behaviors in individuals, children, and adolescents. As a result, it is critical to emphasize the need for tailored interventions for disadvantaged children and adolescents.
In the context of SES and smoking behavior among children and adolescents, the results of this review study reported that children and adolescents with low parental SES backgrounds had heightened vulnerability to early exposure to smoking during childhood and early initiation of smoking during adolescence. This susceptibility may be attributed to a confluence of factors, including pervasive tobacco advertising, normalization of smoking within their social environments, easy access to cigarettes, limited social support for smoking cessation, heightened nicotine dependence, and the burden of stressful life circumstances. These findings substantiate the existing body of literature, which consistently demonstrates an elevated prevalence of smoking within lower SES strata, often attributed to factors such as a paucity of robust social support networks and the presence of stress-inducing lifestyles. Furthermore, children from low SES backgrounds are approximately 6.6 times more likely to be exposed to secondhand smoke within their parental residences compared to their counterparts in high- SES households ( 125–127 ). This discrepancy underscores the urgent need for targeted interventions to mitigate the adverse consequences of smoking in vulnerable populations, particularly during the critical period of life.
On the other hand, alcohol consumption was significantly higher among adolescents with high SES. These findings align with those of previous research, reinforcing the robust association between SES and adolescent alcohol consumption. Specifically, adolescents from high parental SES backgrounds exhibit increased odds of alcohol use (OR = 1.4, 95% CI = 1.19–1.78) ( 128 ). Recent research by Torchyan et al. ( 129 ) further substantiates this phenomenon, indicating a heightened likelihood of weekly alcohol consumption (OR = 1.24, 95% CI = 1.16–1.32) among high-SES adolescents when compared to their counterparts ( 129 ). This persistent pattern has been confirmed in other studies, indicating that high-SES adolescents are more likely to consume alcohol under parental supervision than their counterparts, ( 130 ). This could be explained through social and cultural activities (business meetings, and, party celebrations), the availability of alcohol at home, and the availability of pocket money to purchase alcohol ( 131 ). This review underlines early alcohol initiation among children with high SES, which is often influenced by parental drinking behavior ( 132 ). Thus, parental discretion in alcohol consumption around children is pivotal in preventing negative alcohol-related behavior ( 133 ). Therefore, this study suggests that parents should take care while drinking alcohol in front of their children to prevent them from developing bad alcohol-related behavior.
In addition, our review study found that children and adolescents with high SES were more likely to be engaged in physical exercise. These findings are consistent with previous studies and led to the conclusion that parental SES (OR = 2.73, 95% CI = 2.18, 3.42) were significantly ( p < 0.05) associated with participation in indoor and outdoor physical activities by children and adolescents ( 134 , 135 ). If parents had a good family income, there would be a higher chance of availability of goods and material resources at home ( 136 ). This availability of materials would make it possible to be more engaged in physical activity rather than spending more time watching television or being inactive ( 137 ). However, children and adolescents from lower parental SES backgrounds were found to participate less often in physical activity but were more often involved in sedentary behaviors. Previous studies have found that poor parental SES backgrounds were significantly associated with poor physical activity, high sedentary activity, and high screen time in children and adolescents ( 113 , 138 , 139 ). Moreover, there was ample evidence to suggest that more children and adolescents from deprived SES were more likely to spend more time inside the home due to a lack of a secure neighborhood, lack of green areas for sports and recreational activities, and the cost associated with physical activity ( 140 , 141 ).
In relation to SES and diet (e.g., dairy products, fruit, vegetables, breakfast, soft drinks, and high-fat diet), we found that higher SES was positively associated with the consumption of breakfast, dairy products, fruit, vegetables, and a balanced diet, but negatively associated with consumption of sugar, sugar items, high- fat diet, and soft drinks. The findings of this review study are consistent with those of other studies that show that parental SES has a strong influence on children’s diet ( 142 ). These findings show that children and adolescents from high-SES families consume a healthy proportion of calories ( β = 1.86, SE = 0.76) rather than high-energy foods ( 143 ). Children and adolescents from low-SES families may have few food options and may even face food insecurity and scarcity at home ( 144 ). Therefore, low parental SES may lead to low price food, high-fat diet, high- salt food, energy-dense food, and low intake of regular breakfast, dairy products, fruits, and vegetables during childhood and adolescence ( 144–147 ).
Moreover, in the context of SES and cannabis and illicit drug abuse in adolescents, there was a correlation between the consumption of cannabis and illicit drug abuse and low parental SES, poor schooling, unsafe neighborhoods, and stressful daily life events. Thus, it is important to consider how parental SES particularly affects an adolescent’s health behaviors. This review study revealed that 66.66% of the adolescents had consumed illicit drugs. These conditions increase the risk of physical, psychological, social, and emotional competence in adolescents. These findings could help to provide information about how low parental SES affects children and adolescents, and thus motivate authorities to carry out preventative activities and appropriate rules, regulations, and policies to promote healthy lifestyles in children and adolescents ( 148 ). Overall, risky health behaviors were found to be a major concern, particularly in children and adolescents with low parental SES. These conditions may increase the risk of poor health and development in childhood and adolescence. Therefore, to control these issues, an appropriate strategy helps to protect and prevent risky health behaviors in children and adolescents and gives them equal rights, services, and facilities to fight against inequalities. Hence, this study indicated that parental SES play a significant role in developing social and emotional competence and positive health outcomes in children and adolescents. Therefore, authorities and government bodies should pay more attention to the health and health behaviors of every individual, including children and adolescents, and provide support to children and adolescents, especially those with low parental SES.
5. Strength and limitations
This study comprehensively examined the association between SES and health behaviors, including protecting and impairing health behaviors in children and adolescents across the world. Moreover, this study used multiple databases and followed a structural research process that provided transparent, unbiased, and reliable information on SES and health behaviors. In summary, this study’s strengths lie in its comprehensive approach, use of multiple databases, adherence to a structured research process, and commitment to providing unbiased information. However, this study had certain limitations. The heterogeneity of the studies makes Meta-Analysis not possible. Another limitation is no risk of bias and no registration in “PROSPERO,” was remedied by that two researchers have independently assessed each article, and that a Quality Score was provided ( Supplementary Table S4 ) as well as a “PRISMA” approach ( Supplementary Table S3 ).
6. Conclusion
The current study revealed a robust association between low parental SES and a myriad of unhealthy behaviors (high smoking behavior, low physical exercise, illicit drug use, low consumption of fruit and vegetables, and unhealthy diet) in children and adolescents. However, alcohol consumption was more common in adolescents with a high parental SES. Based on these significant findings, this study underscores the urgent need for an appropriate intervention program that caters to the unique needs of children and adolescents from low-SES families. By implementing measures such as free all-day schools with complementary meals, free after-school activities, and supervised homework sessions, we can bridge the gaps in opportunities, capabilities, and productivity, thereby fostering healthier and more promising futures for these individuals.
Data availability statement
Author contributions.
NG: conceptualization, data curation, methodology, software, formal analysis, and original drafting. GD: methodology, reviewing, editing, supervision, and validation. MR: reviewing, editing, supervision, and validation. RK: conceptualization, reviewing, editing, supervision, and validation. All authors contributed to the article and approved the submitted version.
Acknowledgments
We are profoundly grateful for the invaluable support and proofreading expertise provided by the late Barbara Harmes. Our deepest respect goes out to Tricia Kelly, Rowena McGregor, Margaret Bremner, and all the dedicated librarians at USQ for their unwavering assistance in the library. Additionally, we extend our heartfelt appreciation to the School of Business at the University of Southern Queensland, Toowoomba, Australia, for creating an environment conducive to the successful completion of this study.
Abbreviations
Conflict of interest.
The authors declare that the research was conducted in the absence of any commercial or financial relationships that could be construed as a potential conflict of interest.
Publisher’s note
All claims expressed in this article are solely those of the authors and do not necessarily represent those of their affiliated organizations, or those of the publisher, the editors and the reviewers. Any product that may be evaluated in this article, or claim that may be made by its manufacturer, is not guaranteed or endorsed by the publisher.
Supplementary material
The Supplementary material for this article can be found online at: https://www.frontiersin.org/articles/10.3389/fpubh.2023.1228632/full#supplementary-material
Socioeconomic Status (SES)
- Reference work entry
- pp 6210–6212
- Cite this reference work entry
- Cynthia M. H. Villalba 3 , 4
1516 Accesses
2 Citations
Family background ; Home background
Socioeconomic status (SES) can be defined firstly as a construct that represents social and economic background of an individual or group unit (e.g., household). Secondly, the sociological concept addresses relative position in a particular social structure as it is based on the assumption that an unequal status structure exists in all societies (Hollingshead, 1975 ). It normally includes acquired (e.g., wealth, status, and prestige, following Haug, 1977 ) versus biological characteristics of an individual such as age or ethnic origin. At household level, it can include demographic data such as place of residence. It is a construct designed to group context-specific, meaningful, and statistically valid indicators, either as a composite indicator or by using single indicators/variables, or even a single proxy variable.
Description
Socioeconomic status (SES) or social background has become one of the most prominent explanatory factors...
This is a preview of subscription content, log in via an institution to check access.
Access this chapter
- Available as PDF
- Read on any device
- Instant download
- Own it forever
- Available as EPUB and PDF
- Durable hardcover edition
- Dispatched in 3 to 5 business days
- Free shipping worldwide - see info
Tax calculation will be finalised at checkout
Purchases are for personal use only
Institutional subscriptions
Adler, N. E., Boyce, T., Chesney, M. A., Cohen, S., Folkman, S., Kahn, R. L., et al. (1994). Socioeconomic status and health: The challenge of the gradient. American Psychologist, 49 (1), 15.
Google Scholar
Bourdieu, P., & Passeron, J. C. (1977). Reproduction in society, education and culture (Trans. R.). (English edition) Sage Publications Ltd., London.
Bradley, R. H., & Corwyn, R. F. (2002). Socioeconomic status and child development. Annual Review of Psychology, 53 , 371–399.
Breen, R., & Jonsson, J. O. (2005). Inequality of opportunity in comparative perspective: Recent research on educational attainment and social mobility. Annual Review of Sociology , 31 , 223–243.
Coleman, J. S. (1988). Social capital in the creation of human capital. American Journal of Sociology , 94 , 95–120.
Currie, C. E., Elton, R. A., Todd, J., & Platt, S. (1997). Indicators of socioeconomic status for adolescents: The WHO Health Behaviour in School-aged Children Survey. Health Education Research, 12 (3), 385–397.
Entwistle, D.R., & Astone, N.M. (1994). Some practical guidelines for measuring youth race/ethnicity and socioeconomic status. Child Development , 65 , 1521–40.
Erikson, R., & Jonsson, J. O. (1998). Social origin as an interest-bearing asset: Family background and labour-market rewards among employees in Sweden. Acta Sociologica, 41 (1), 19–36.
Haug, M. R. (1977). Measurement in social stratification. Annual Review of Sociology, 3 , 51–77.
Hauser, R. M. (1994). Measuring socioeconomic status in studies of child development. Child Development, 65 , 1541–1545.
Hollingshead, A.B. (1975). Four factor index of social status. Unpublished working paper. Yale University, Department of Sociology , New Haven, CT.
McMillan, J., & Western, J. (2000). Measurement of the socio-economic status of Australian higher education students. Higher Education, 39 (2), 223–247.
Schultz, W. (2005, April 7–11). Measuring the socio-economic background of students and its effects on achievement in PISA 2000 and PISA 2003 . Paper prepared for the Annual Meetings of the American Educational Research Association, San Francisco.
Schulz, W., Ainley, J., Fraillon, J., Kerr, D., & Losito, B. (2010). ICCS 2009 International Report: Civic knowledge, attitudes and engagement among lower secondary school students in thirty-eight countries. International Association for the Evaluation of Educational Achievement (IEA), Amsterdam.
Vyas, S., & Kumaranayake, L. (2006). Constructing socio-economic status indices: How to use principal components analysis. Health Policy and Planning, 21 (6), 459–468.
Warschauer, M. (2013). Language and the digital divide. In The Encyclopedia of Applied Linguistics .
Download references
Author information
Authors and affiliations.
Institute of International Education, Stockholm University (Sweden) IACM/FORTH, Stockholm, Sweden
Cynthia M. H. Villalba
Institute of International Education, Educational Research and Evaluation Group (Greece), Heraklion, Greece
You can also search for this author in PubMed Google Scholar
Corresponding author
Correspondence to Cynthia M. H. Villalba .
Editor information
Editors and affiliations.
University of Northern British Columbia, Prince George, BC, Canada
Alex C. Michalos
(residence), Brandon, MB, Canada
Rights and permissions
Reprints and permissions
Copyright information
© 2014 Springer Science+Business Media Dordrecht
About this entry
Cite this entry.
Villalba, C.M.H. (2014). Socioeconomic Status (SES). In: Michalos, A.C. (eds) Encyclopedia of Quality of Life and Well-Being Research. Springer, Dordrecht. https://doi.org/10.1007/978-94-007-0753-5_2805
Download citation
DOI : https://doi.org/10.1007/978-94-007-0753-5_2805
Publisher Name : Springer, Dordrecht
Print ISBN : 978-94-007-0752-8
Online ISBN : 978-94-007-0753-5
eBook Packages : Humanities, Social Sciences and Law
Share this entry
Anyone you share the following link with will be able to read this content:
Sorry, a shareable link is not currently available for this article.
Provided by the Springer Nature SharedIt content-sharing initiative
- Publish with us
Policies and ethics
- Find a journal
- Track your research
- Open access
- Published: 26 September 2017
Different indicators of socioeconomic status and their relative importance as determinants of health in old age
- Alexander Darin-Mattsson ORCID: orcid.org/0000-0001-5491-1510 1 ,
- Stefan Fors 1 &
- Ingemar Kåreholt 1 , 2
International Journal for Equity in Health volume 16 , Article number: 173 ( 2017 ) Cite this article
187k Accesses
181 Citations
53 Altmetric
Metrics details
Socioeconomic status has been operationalised in a variety of ways, most commonly as education, social class, or income. In this study, we also use occupational complexity and a SES-index as alternative measures of socioeconomic status. Studies show that in analyses of health inequalities in the general population, the choice of indicators influence the magnitude of the observed inequalities. Less is known about the influence of indicator choice in studies of older adults. The aim of this study is twofold: i) to analyse the impact of the choice of socioeconomic status indicator on the observed health inequalities among older adults, ii) to explore whether different indicators of socioeconomic status are independently associated with health in old age.
We combined data from two nationally representative Swedish surveys, providing more than 20 years of follow-up. Average marginal effects were estimated to compare the association between the five indicators of SES, and three late-life health outcomes: mobility limitations, limitations in activities of daily living (ADL), and psychological distress.
All socioeconomic status indicators were associated with late-life health; there were only minor differences in the effect sizes. Income was most strongly associated to all indicators of late-life health, the associations remained statistically significant when adjusting for the other indicators. In the fully adjusted models, education contributed to the model fits with 0–3% (depending on the outcome), social class with 0–1%, occupational complexity with 1–8%, and income with 3–18%.
Conclusions
Our results indicate overlapping properties between socioeconomic status indicators in relation to late-life health. However, income is associated to late-life health independently of all other variables. Moreover, income did not perform substantially worse than the composite SES-index in capturing health variation. Thus, if the primary objective of including an indicator of socioeconomic status is to adjust the model for socioeconomic differences in late-life health rather than to analyse these inequalities per se, income may be the preferable indicator. If, on the other hand, the primary objective of a study is to analyse specific aspects of health inequalities, or the mechanisms that drive health inequalities, then the choice of indicator should be theoretically guided.
Studies of social determinants of health have repeatedly found socioeconomic inequalities in health. People with lower socioeconomic status (SES) have, on average, poorer health and die younger than those with more favourable SES. Socioeconomic inequalities in health persist into old age [ 1 , 2 , 3 , 4 ]. The results from a comparative study showed that the magnitude of inequality in morbidity in eleven European countries varied, however, health inequalities in all age groups were observed in all countries [ 3 ]. As most morbidity and mortality occurs in old age, these inequalities may affect a substantial proportion of the older population and increase the economic burden of public spending as the population ages.
In studies of health inequalities in later life, SES is most commonly operationalised as either education, social class, or income – and often without providing a rationale for the choice of indicator [ 5 , 6 , 7 ].
The overarching aim of this study was to explore how the three most common indicators of SES (education, social class, and income) are associated with health in old age. We also included occupational complexity as an alternative indicator of SES, as recent research suggests that complexity is a key driver of labour market stratification [ 8 , 9 , 10 ]. Education, social class, occupational complexity, and income all have overlapping properties, but they may also be independently associated with health in old age. Therefore, we explored the relation between these variables, a composite measure of the variables, and change in mobility limitations, activities of daily living, and psychological distress from working ages to old age.
Education, social class, occupational complexity, income, and health in old age
The individuals’ highest attained level of education is generally reached in early adulthood, and serves to bridge socioeconomic conditions across generations [ 11 ]. Research suggest that the associations between education and health is driven by increases in human capital, psychosocial resources, living conditions, better health care and lifestyle, and selection (direct and indirect) [ 12 ]. Studies show that people with lower levels of education tend to have a more rapid health decline in old age [ 13 ]. However, educational level often show a weaker association with health in old age than other indices, such as wealth, income, tenure, and deprivation [ 14 , 15 , 16 ].
Most often, social class is identified using occupation as the stratifying principle. Many class schemas primarily distinguish occupations depending on ownership (i.e, between employers and employees). Thereafter, groups of employers and employees are distinguished depending on size and type of organization, skill requirements, power relations, and working conditions [ 17 , 18 ]. Besides being associated with current income, social class is also associated with: i) income security, ii) short term income stability, and iii) long term income development [ 19 , 20 ]. Results on the association between social class and late-life health are ambiguous. Duncan et al. [ 15 ] did not find any association between social class and mortality in older age, while other studies have [ 1 , 21 ].
Some research have suggested that skill requirements, productivity and efficiency may provide a better explanation of social stratification at the labour market than conventional class theories [ 8 , 9 , 10 ]. Using Swedish data, Tåhlin found that the occupational complexity level is more strongly associated to the earned wages than traditional social class schemas [ 9 , 10 ]. Tåhlin further argues that the level of occupational complexity associated with a given occupation can be used as a proxy for efficiency and productivity. Against this background, we have included a measure of occupational complexity as an alternative indicator of SES.
Income is often considered to be a straightforward indicator of material resources, and income is, robustly and positively, associated with longevity [ 22 , 23 ]. Income might affect the health of people by enabling those with high income to lead healthy lifestyles, while those at the lower rungs of the income distribution have fewer of these enabling resources [ 24 ]. Income has an important role as an enabling resource for health care access and use in the Andersen health behavioural model [ 25 , 26 ]. On the other hand, poor health can also result in lower incomes [ 27 ]. Hence, the association between income and health is likely shaped by bi-directional causal mechanisms. In the literature, measures indicating financial situation is commonly most strongly associated to health and mortality in old age, when compared to e.g. educational level and social class [ 14 , 15 , 16 ].
The rationale for including specific indicators of SES in studies of health in old age may vary. It could be to monitor, or to understand, how the social patterning of resources affect health. In this case, it is important to consider the different pathways and mechanism that education, social class, income, and occupational complexity might have on health. Another reason could be to merely adjust a model for as much socioeconomic variance as possible, without any specific interest in the mechanisms driving the health inequalities. Research suggest that a composite measures of individual level indicators could be appropriate for this purpose [ 28 ]. The findings from a previous study, based on the same data as the present study, suggested that a composite measure of individual level variables of SES was more suitable for this purpose than any of the individual variables [ 29 ]. On the other hand, using a composite measure of individual level variables of SES, may obscure the underlying mechanisms and prevent progression in the understanding of how different aspects of SES contributes to health.
Education, social class, occupational complexity, and income are inherently associated, as education is associated with occupational position and complexity, which in turn is associated with income [ 8 , 30 ]. In addition, socioeconomic disadvantage tend to accumulate over the life course, both between and within socioeconomic domains [ 31 ]. Evidence suggest that there are certain, sensitive, periods in life where exposure to socioeconomic disadvantages may have increased and sustained effect on health throughout the life course [ 32 ].
Studies of the working age population have showed that different indicators of SES are differently associated with different health outcomes, which suggest that different underlying mechanisms may generate these associations [ 5 , 33 ]. These findings suggest that the choice of indicator may be of importance for the results and the interpretations, when studying socioeconomic inequalities in health. Less is known of how different SES indicators relate to health in old age.
A thorough exploration of how the association between SES and health in old age varies by indicator of SES may provide important insights into the mechanisms generating socioeconomic inequalities in late-life health. As most commonly used indictors of SES are rooted in educational and occupational stratification, we chose to assess these variables in late working life when people tend to have reached their peak positions, in terms of educational level, social class, and income.
In the present study, late life health is assessed in terms of mobility limitations, limitations in activities of daily living (ADL), and psychological distress. These are all common areas of health problems in later life, and the burden of these afflictions in terms of societal costs is substantial [ 34 ]. Mobility limitations and ADL limitations are rare among people under the age of 40, but after the age of 40 the prevalence increase with age. Mobility and ADL limitations, and psychological distress are all strong predictors of need for social services and institutionalization [ 35 , 36 , 37 ]. Moreover, psychological distress also predict other health outcomes with special relevance for the old age population, such as dementia, mortality, cardiovascular diseases, and cerebrovascular disease [ 38 , 39 , 40 ].
All analyses were conducted on linkages drawn from two data sources: the Swedish Level of Living Surveys (LNU) and the Swedish Longitudinal Study of Living Conditions of the Oldest Old (SWEOLD). Each linkage entail data from one wave of LNU with follow-up in a wave of SWEOLD. LNU and SWEOLD are both longitudinal social surveys, based on random samples of the Swedish population. The first wave of LNU was carried out in 1968 using a nationally representative sample of individuals aged 18 to 75 years. The sample was then followed-up in 1974, 1981, 1991, 2000, and 2010 [ 41 ]. The response rates for the waves used in the present study varies between 78.3% and 90.8% (n ≈ 6000–8000). In LNU, respondents were asked about a wide variety of topics, including their present health status, educational achievements, and occupation. SWEOLD is a continuation of LNU: it includes those who have ‘aged out’ of the LNU sample (i.e. those who are 75 years or older), and who were previously included in the LNU sample. SWEOLD has been conducted in 1992, 2002, 2004, and 2011; and the response rates varied between 84.4% and 95.4% (n ≈ 600–1000). In the 2004 wave, the age-span included was wider, and included those aged 69 years and older [ 42 ].
The independent variables were assessed at baseline and dependent variables in the designated follow-up. Respondents from LNU 1968 were followed-up in SWEOLD 1992 (linkage 1), respondents from LNU 1981 were followed-up in SWEOLD 2002 (linkage 2) and SWEOLD 2004 (linkage 3), and respondents from LNU 1991 were followed-up in SWEOLD 2011 (linkage 4).
The four linkages were analysed separately and as pooled data. The magnitude of the associations between the independent variables and the outcomes varied by linkage, but the differences were small and not systematic. Thus, we present the results from the full sample, with all the linkages pooled.
The study was restricted to people who were 46 to 64 years at baseline, and who were alive and participated in the designated follow-up ( n = 2342). The age restriction was based on the possibility to be included in a follow-up in old age (SWEOLD data). Social class and occupational complexity level is based on occupation, therefore we included only those that were in paid employment (or self-employed) at baseline in the main analyses ( n = 2027; 87% of the total sample). However, we performed separate analyses for those who were not in paid employment (or self-employed) at baseline (Table 3 ).
In the analyses of mobility limitations, the study sample was restricted to people without any mobility limitations at baseline ( n = 1772), and in the analyses of psychological distress, to people without psychological distress at baseline ( n = 1616). Sensitivity analyses, comparing the estimates to estimates based on the full sample showed similar results. Respondents who did not answer the questions about the outcome variables were excluded, which resulted in a different number of observations in the analyses of mobility limitations ( n = 1763) and psychological distress ( n = 1596). Since ADL limitations was not assessed in the LNU (baseline) all respondents were analysed studying ADL limitations ( n = 2027).
Measurements
Mobility limitations were assessed by the question: ‘Can you walk 100 meters at a fairly brisk pace without problems?’ and ‘Can you climb stairs (up and down) without problems?’ The response alternatives were ‘yes’ and ‘no’. Responses were summarised in an index that ranged from 0 (no problems) to 2 (problems with both tasks).
Limitations in activity of daily living (ADL) were assessed by five questions: ‘Can you eat by yourself?’, ‘Can you go to the toilet by yourself?’, ‘Can you dress and undress yourself?’, ‘Can you get in and out of bed by yourself?’, and ‘Can you wash your hair by yourself?’. The response alternatives were ‘yes, manage completely by myself’, ‘yes, with help’, and ‘no, not at all’. The responses were summarised in an index from 0 (managed all five tasks without help) to 10 (not able to perform any of the tasks).
Psychological distress was assessed by a general question: ‘Have you had any of the following diseases or disorders during the last 12 months?’, followed by a multi-item list of symptoms and disorders. We calculated an index based on the responses regarding anxiety and depressive symptoms. The response alternatives were ‘no’, ‘yes, slight’, and ‘yes, severe’. The responses were summarised in an index ranging from 0 (no problems) to 4 (severe psychological distress).
Education was measured as educational attainment. Self-reported educational attainment was divided into three groups: high, medium, or low. Upper secondary school or above was considered a high level of educational attainment. Compulsory school complemented with vocational training was considered a medium level of education. Attending compulsory school only or no schooling was considered as low level of education.
Social class was based on self-reported occupation at baseline, classified in accordance with the Swedish socioeconomic index (SEI), which is very similar to the Erikson, Goldthorpe, and Portocarero’s (EGP) schema [ 43 ]. The social classes were collapsed into three groups: low (unskilled and skilled blue-collar workers, small farmers and entrepreneurs without employees); medium (lower-level white-collar workers, farmers and entrepreneurs with 1 to 19 employees); and high (intermediate and upper-level white-collar workers, farmers and entrepreneurs with 20 or more employees, and academic professionals).
Occupational complexity was assessed by assigning a ‘substantive complexity’ score to each occupational category. The substantive complexity scores indicate the level of intellectual flexibility, engagement, and skills needed to perform working tasks of greater or lesser complexity. The measure of substantive complexity used in this study was developed by Roos and Treiman [ 44 ], and is based on the U.S. Dictionary of Occupational Titles (DOT) and the U.S. Census 1970. The DOT included 46 worker characteristics, assessed by job analysts. Roos and Treiman performed a principal component factor analysis and found a factor, including eight of the characteristics (general educational development, specific vocational preparation, complexity of work with data, intelligence aptitude, verbal aptitude, numerical aptitude, abstract interest in the job, and temperament for repetitive and continuous processes), that they called ‘substantive complexity’. This measure forms the basis for our measure of occupational complexity [ 44 ]. These scores have later been matched to Swedish occupational categories. See Andel et al. [ 45 ] for a description of the matching procedure and Darin-Mattsson et al. [ 29 ] for a more thorough description of occupational complexity. Occupational complexity ranges 0–10, the scale was divided into three categories on the complexity scale: 0–3.3 = low complexity, 3.4–6.4 = medium complexity, and 6.5–10 = high complexity.
Individual income was assessed from Swedish tax registers the year before baseline. Income was standardised to the purchasing power of 1991, log transformed and divided into quintiles. To increase the comparability of the models and to increase model fit, we divided income into three categories. We collapsed the two lowest quintiles into category 1 and quintiles three and four into category 2. Category 3 consisted of the fifth quintile, which included those with the highest incomes. This categorization was data-driven and based on tests of spline lines, which showed that the used categorization gave the best fit of the data.
We also used all of the SES indicators described above to construct a composite measure of SES (the SES-index ) . We used education, social class, occupational complexity, and income as classified above, and summarized them. The index was then divided into tertiles. This was done to investigate whether a composite measure could, statistically, capture as much, or more, of the variance in late-life health as the individual indicators.
Covariates in all analyses were age, sex, and linkage. Age and sex was assessed by self-reports during the interview. Age was measured by birth year and given continuous representation in all analyses. Sex was categorized as either woman or man.
Statistical methods
The results are presented in terms of Average Marginal Effects (AME) multiplied by 100. AMEs are estimated as the average difference in probability of the given outcome across all observations with covariates at their observed values. Thus, the estimates can be interpreted as the differences in the probability of the outcome in percentage points. We use AMEs, rather than odds ratios or β-coefficients, as they are comparable across models, intuitively interpretable, and provide absolute measures of inequality [ 46 ]. The AMEs from ordered logistic regression should be interpreted as the difference in the probability of reporting an outcome one-step higher on the scale than the outcome in the reference group. The proportional odds assumption was tested with the gologit2 command in Stata [ 47 ], and the assumption was accepted.
In addition, we used McKelvey & Zavoina’s pseudo-R2 to compare estimates of explained variance from different models using the same dataset [ 48 ]. To study how each of the main independent variables contributed to model fit, we calculated the change in pseudo-R2 obtained by adding each independent variables (education, social class, income, and occupational complexity) one at a time to a model including only the outcomes and adjustments for sex, age, and linkage. For each indicator of socioeconomic status, we also calculated the change in pseudo-R2 associated to the exclusion of that variable from the full model (model 2).
We also ran all analyses stratified by sex. We found only small, statistically non-significant, differences between women and men except in the association between social class and mobility limitations (see results).
Our study design allow individuals to be included in several linkages. Thus, 282 people were included in both linkage 2 and 3 (<15% of the sample) and the indicators of health in old age could be clustered on individual level. As this could lead to artificially low standard errors, we used cluster-correlated robust estimates of variance in the analyses [ 49 ].
We also used multiple imputation to impute missing data on mobility limitations at baseline (113 imputations were included). Sensitivity analyses showed none or small differences between the imputed and non-imputed data. There were no internal non-responses of psychological distress at baseline in the analytical sample. No imputations were made on the outcome variables.
Spearman’s correlations between the independent variables were moderate. The correlation between education and social class was 0.51; between education and income, 0.38; between education and occupational complexity, 0.29; between class and income, 0.39; between class and occupational complexity, 0.38; and between income and occupational complexity, 0.22. All correlations were statistically significant ( p < 0.001). Correlations between the indicators differed between the linkages, and there was a general trend towards minor, statistically non-significant, increases in the correlations over time. Model 2 was tested for multicollinearity with the VIF command in Stata, and the result indicated no multicollinearity.
Descriptive statistics are presented in Table 1 . There were more women than men among the respondents, and women reported more problems in all three outcomes. Most respondents (around 50%) had low education and social class, but medium occupational complexity. The mean age at follow-up was 79 years. There were no statistically significant differences in psychological distress by age group. However, limitations in mobility and ADL increased with age. In general, the respondents with the highest level of the SES indicators had the least problems while the lowest group had the worst health outcomes, with one exception – people with medium social class had significantly lower ADL limitations than people with high or low social class. The association between ADL limitations and occupational complexity was not significant, and there were no statistically significant differences in psychological distress by education or social class.
The associations between education, social class, occupational complexity, income, the SES-index, and health in later life were all analysed separately in model 1 (Table 2 ), adjusted only for age, sex, and linkage. In model 2 (Table 2 ), all the SES indicators were analysed simultaneously in relation to each health outcome. We also adjusted the associations between each individual SES indicator and each health outcome, by the other indicators of SES one-by-one. For these results, see Additional file 1 : Table S1 and Additional file 2 : Table S2.
At this point, it is worth noting that the effect sizes in the main analyses are dependent on the scales of the outcome variables. As the health outcomes are scaled differently, this means that the estimates for the different outcomes are not comparable.
Education was significantly and negatively associated with both mobility limitations and psychological distress, but not with ADL limitations (Table 2 ; model 1). Including education increased the model fit by 12% in the analyses of mobility limitations, 3% for ADL limitations, and 15% for psychological distress. However, the inclusion of the variable did not significantly improve the model fit for ADL limitations and psychological distress. Only the high-educated group deviated significantly from the other groups, with better health. Adjusted for the other SES indicators (Table 2 ; model 2), education was no longer significantly associated with the health outcomes, and contributed with only 1% to the total model fit for mobility limitations, 0% for ADL limitations, and 3% for psychological distress (Table 2 ; model 2).
- Social class
People with low social class had an increased risk of all outcomes compared to the other two groups (Table 2 ; model 1). Social class contributed to the model fit with approximately the same magnitude as education. Analyses stratified by sex showed no differences in the probability of mobility limitations between men from the middle and upper social classes, however, the difference between women from the middle and upper social classes were statistically significant (AME 4.83, p = 0.002). In the fully adjusted model (Table 2 ; model 2), social class did not contribute to any explained variance at all.
- Occupational complexity
People who held occupations with a low complexity level had an elevated risk for mobility limitations and psychological distress in old age, compared to those who held an occupation characterised by high complexity. On the other hand, there were no statistically significant differences in ADL limitations, by complexity level. However, the inclusion of the variable improved the model fit significantly. Occupational complexity contributed less to the explained variance in the models than education, with the exception of the models for psychological distress. In the fully adjusted model (Table 2 ; model 2), there were no statistically significant associations between occupational complexity and late-life health.
Income was statistically significantly associated with all outcomes. The high-income group consequently had the lowest probabilities of adverse health of all income groups. Income contributed to model fit by 13% for mobility limitations, 7% for ADL limitations, and 27% for psychological distress (Table 2 ). Income was the only indicator that remained statistically significantly associated with the health outcomes in the fully adjusted models (model 2). In addition, income contributed the most to the model fits in model 2: 7% to mobility limitations, 3% to ADL limitations, and 18% to psychological distress.
The high SES-index group had better health than the other groups. The SES-index increased model fit more than any of the other indicators in the models of mobility limitations, about the same as income for ADL limitations, and less than income for psychological distress.
Including all the indicators of SES simultaneously increased model fit for mobility limitations by 29%, compared to a model only adjusted for age, sex, and linkage. The individual contribution of each variable sum up to an increased model fit of 46% (education 12% + social class 12% + occupational complexity 9% + income 13%). The difference between 46 and 29% indicate that the properties of the socioeconomic indicators overlap. The composite measure of the variables (SES-index) explained less variance than simultaneously including all SES indicators. A similar pattern emerged for ADL limitations. The contribution, when including all SES indicators simultaneously was 12%, while the sum of the individual contributions amounted to 19%, and the SES-index only explained 6%. The same pattern holds when psychological distress is the outcome, the summed increase in model fit for all SES indicators was 72%, whereas the combined increase was 37%, and the SES-index increased model fit by 20%. These result suggests that properties of the indicators overlap when analysing psychological distress.
People not in paid employment at baseline
Only people who held a paid occupation at baseline were included in the above analyses (Table 2 ). In Table 3 , we analysed those who did not have a paid occupation in relation to those who held a paid occupation at baseline.
The results show that individuals who did not hold a paid occupation at baseline had higher probabilities of health problems in old age, compared to those who held paid occupations at baseline. The increased probabilities of health problems among those without a paid occupation at baseline was of the magnitude of 7.46 percentage points for mobility limitations, 8.62 percentage points for ADL limitations, and 7.33 percentage points for psychological distress.
We found that education, social class, occupational complexity, income, and a composite measure of SES (the SES-index) all were significantly associated with late life health. Income was most strongly associated with adverse health in late life. Income was also the only variable that remained statistically significantly associated with health when adjusted for the other separate SES variables. The results also indicate that income captured as much variance in the health outcomes as the composite measure, based on a series of SES indicators.
As with all studies, these results should be interpreted with caution. We explored the independent associations between several interrelated indicators of SES and late-life health in a mid-sized sample. Therefore, it is possible that the study lacked sufficient statistical power to efficiently detect all relevant associations.
As in all studies based on older adults, the results may be affected by selective survival [ 50 ]. As we studied inequality in health among those surviving into old age, inclusion in our target population was contingent on survival into old age. Thus, our estimates might be attenuated by the higher mortality rates among those with lower SES than among those with higher SES. Selective survival also implies that the indicators that exhibit the weakest associations with late-life health might be more strongly associated with health and mortality earlier in life, and vice versa. Similarly, there was also a selection into employment in mid-life that may affect our findings. This selection is typically stronger among women; as women are less likely than men to be employed in paid occupation. Hence, women were more likely than men to be excluded from our main analyses and end up in the not employed category. Our results show that those who were not employed at baseline were at an increased risk for poorer late-life health compared to those who were employed at baseline.
The study is based on data from social surveys. The health measures used are based on self-reports, rather than clinical examinations. It is not certain that the findings from this study can be generalized to other health outcomes. For example, the patterns might differ substantially for lifetime prevalence of coronary heart disease, cancer or diabetes.
We only explored individual-level variables of SES in this study. Previous research has suggested that, under some circumstances, household SES may be a more suitable indicator of women’s SES than individual SES [ 51 ]. As a sensitivity measure, we therefore also ran the models using household social class. The results were similar (all association went in the same direction and were approximately of the same magnitude), possibly because our main analyses were restricted to people with a paid occupation at baseline. We also tested household income for those cases where it was available. The associations between household income and individual income in relation to late-life health were similar.
Unfortunately, we were not able to assess wealth. Wealth is an indicator of financial resources accumulated over the life course (including inheritances), and the patterning of wealth in old age might therefore differ substantially from the patterning of incomes.
In 1939, Paul Lazarsfeld found that indices constructed with different information (that is, indices based on whether a person paid income tax, owned a car, or whether a person had a telephone in his or her home) resulted in approximately the same distribution of economic status throughout the population. He concluded that it is both theoretically and empirically probable that indices of economic status are interchangeable [ 52 ]. Geyer et al. [ 5 ] tested Lazarsfeld’s hypothesis using education, social class, and income as predictors of four different health outcomes in two populations aged 25–64 years. They found that each indicator had an independent effect on the outcomes but that the effect sizes and strengths of the associations varied by indicator. Education was the strongest predictor for diabetes and income strongest predicted all-cause mortality, while results were mixed for myocardial infarction morbidity and mortality. Thus, they argued that indicators of SES are not interchangeable in relation to health. In contrast, our results showed no independent effects by education, social class or occupational complexity on health in old age. Only income was independently associated with health in old age. In addition, Geyer and colleagues found that different indicators were differently associated to different outcomes. In contrast, our results showed that income was most strongly associated to all health outcomes, except compared with the SES-index. Torssander and Erikson [ 33 ] found similar results as Geyer and colleagues in relation to mortality risk in the Swedish population aged 35–59. Each indicator of SES was clearly associated with risk of death for both women and men. They suggested that each indicator’s crude association with mortality was in line with Lazarsfeld’s hypothesis on the interchangeability of indices, only if one assume that these indicators measure a general latent construct. On the other hand, the authors argue that if each indicator has an independent function, using them to map a latent construct would result in a loss of information [ 33 ]. This idea, that using one of these indicators interchangeably to indicate a latent concept of SES may result in a loss of information relevant for social stratification and health and policy implications, have been supported by others [ 6 , 53 ]. Few studies have explored this issue in relation to health in old age, but Avlund et al. [ 14 ] found that different indicators of SES were individually associated to different health outcomes in old age. Our results show that income was the only indicator independently associated to late-life health, and that the indicators are otherwise statistically interchangeable. The indicators had approximately the same association to the outcomes, and their contribution to the model fits were comparable.
In line with previous studies [ 14 , 15 , 16 ], we also found that the most direct measure of economic differences (in this case, income) was most strongly and robustly associated with adverse health in old age.
A novel contribution of our study was the introduction of occupational complexity as an alternative indicator of SES in studies of health inequalities in old age. However, our results did not suggest that occupational complexity was a stronger determinant of late-life health than education, social class, income or a composite measure of SES (the SES-index).
Further research is needed to confirm the robustness of these findings and to explore the causal mechanisms underlying the associations between different aspects of socioeconomic status and late-life health.
This study investigated the most commonly used indicators of SES in health research in relation to three health outcomes in old age. We also included a less traditional measure strongly associated with SES (occupational complexity), and a composite measure based on several indicators of SES (the SES-index). The study contributes to the literature by doing an in depth investigation of how the SES indicators relate to each other, and to late-life health, and by testing the predictive value of two novel measures of SES. In sum, our results suggests that income explain more variance in late-life health than any of the other SES indicators, with a predictive capacity that is equal, or even better, than that of a composite measure including a range of indicators. In sum, our results suggests that if the primary objective of including an indicator of SES, in studies of health in old age, is to merely adjust the model for socioeconomic differences income may be the preferred choice. If, on the other hand, the primary objective of the study is to examine health inequalities or the mechanisms that drive health inequalities in old age per se, then the choice of indicator should be made on the basis of a theoretical model that considers the unique properties of the different indicators.
Fors S, Lennartsson C, Lundberg O. Health inequalities among older adults in Sweden 1991–2002. Eur J Pub Health. 2007;18:138–43.
Article Google Scholar
Fors S, Thorslund M. Enduring inequality: Educational disparities in health among the oldest old in Sweden 1992-2011. Int J Public Health. 2015;60:91.
Article PubMed Google Scholar
Huisman M, Kunst AE, Mackenbach JP. Socioeconomic inequalities in morbidity among the elderly; a European overview. Soc Sci Med. 2003;57:861–73.
Hoffmann R. Socioeconomic differences in old age mortality. Springer Science & Business Media; 2008.
Geyer S, Hemström Ö, Peter R, Vågerö D. Education, income, and occupational class cannot be used interchangeably in social epidemiology. Empirical evidence against a common practice. J. Epidemiol. Community Dent Health. 2006;60:804–10.
Google Scholar
Goldthorpe JH. Analysing social inequality: a critique of two recent contributions from economics and epidemiology. Eur Sociol Rev. 2009;26:731–44.
Ploubidis GB, Benova L, Grundy E, Laydon D, DeStavola B. Lifelong socio economic position and biomarkers of later life health: Testing the contribution of competing hypotheses. Soc Sci Med. 2014;119:258–65.
Le Grand C, Tåhlin M. Class, occupation, wages, and skills: The iron law of labor market inequality. Cl. Stratif. Anal. Emerald Group Publishing Limited. 2013;30:3–46.
Tåhlin M. Vertical differentiation of work tasks: conceptual and measurement issues. Empir Res Vocat Educ Train. 2011;3:55–70.
Tåhlin M. Class clues. Eur Sociol Rev. 2007;23:557–72.
Mirowsky J, Ross CE. Education, social status, and health. New York: Transaction Publishers; 2003.
Hayward MD, Hummer RA, Sasson I. Trends and group differences in the association between educational attainment and US adult mortality: Implications for understanding education’s causal influence. Soc Sci Med. 2015;127:8–18.
Dupre ME. Educational Differences in Age-Related Patterns of Disease: Reconsidering the Cumulative Disadvantage and Age-As-Leveler Hypotheses ∗ . J Health Soc Behav. 2007;48:1–15.
Avlund K, Holstein BE, Osler M, Damsgaard MT, Holm-Pedersen P, Rasmussen NK. Social position and health in old age: the relevance of different indicators of social position. Scand J Soc Med. 2003;31:126–36.
Duncan GJ, Daly MC, McDonough P, Williams DR. Optimal indicators of socioeconomic status for health research. Am J Public Health. 2002;92:1151–7.
Article PubMed PubMed Central Google Scholar
Grundy E, Holt G. The socioeconomic status of older adults: How should we measure it in studies of health inequalities? J Epidemiol Community Health. 2001;55:895–904.
Article CAS PubMed PubMed Central Google Scholar
Goldthorpe JH. On sociology. 2. Illustration and retrospect. Standford: Stanford University Press; 2007.
Rose D, Harrison E. Social class in Europe: An introduction to the European socio-economic classification. NewYork: Routledge; 2010.
Goldthorpe JH. The economic basis of social class. 2004;
Watson D, Whelan CT, Maître B. Class and poverty: cross-sectional and dynamic analysis of income poverty and life-style deprivation. Social Class in Europe: An Introduction to the European Socio-Economic Classification. London: Routledge; 2009.
Enroth L, Raitanen J, Hervonen A, Jylhä M. Do socioeconomic health differences persist in nonagenarians? J. Gerontol. B. Psychol. Sci. Soc. Sci. 2013;68:837–47.
Chetty R, Stepner M, Abraham S, Lin S, Scuderi B, Turner N, et al. The association between income and life expectancy in the United States, 2001-2014. JAMA. 2016;315:1750–66.
Rehnberg J, Fritzell J. The shape of the association between income and mortality in old age: A longitudinal Swedish national register study. SSM-Popul Health. 2016;2:750–6.
Marmot M. The influence of income on health: views of an epidemiologist. Health Aff. (Millwood). 2002;21:31–46.
Aday LA, Andersen R. A framework for the study of access to medical care. Health Serv Res. 1974;9:208.
CAS PubMed PubMed Central Google Scholar
Andersen RM. Revisiting the behavioral model and access to medical care: does it matter? J Health Soc Behav. 1995;36:1–10.
Muennig P. Health selection vs. causation in the income gradient: What can we learn from graphical trends? J. Health Care Poor Underserved. 2008;19:574–9.
Galobardes B, Lynch J, Smith GD. Measuring socioeconomic position in health research. Br Med Bull. 2007;1:21–37.
Darin-Mattsson A, Andel R, Fors S, Kåreholt I. Are Occupational Complexity and Socioeconomic Position Related to Psychological Distress 20 Years Later? J Aging Health. 2015;27:1266–85.
Mirowsky J, Ross CE. Education, cumulative advantage, and health. Ageing Int. 2005;30:27.
Dannefer D. Cumulative advantage/disadvantage and the life course: Cross-fertilizing age and social science theory. J. Gerontol. B. Psychol. Sci. Soc. Sci. 2003;58:S327–37.
Ben-Shlomo Y, Kuh D. A life course approach to chronic disease epidemiology: conceptual models, empirical challenges and interdisciplinary perspectives. 2002;
Torssander J, Erikson R. Stratification and mortality—A comparison of education, class, status, and income. Eur Sociol Rev. 2009;26:465–74.
Ratigan A, Kritz-Silverstein D, Barrett-Connor E. Sex differences in the association of physical function and cognitive function with life satisfaction in older age: The Rancho Bernardo Study. Maturitas. 2016;89:29–35.
Ahacic K, Parker M, Thorslund M. Mobility limitations in the Swedish population from 1968 to 1992: age, gender and social class differences. Aging Milan Italy. 2000;12:190–8.
CAS Google Scholar
Larsson K, Thorslund M, Kåreholt I. Are public care and services for older people targeted according to need? Applying the behavioural model on longitudinal data of a Swedish urban older population. Eur J Ageing. 2006;3:22–33.
Molarius A, Berglund K, Eriksson C, Eriksson HG, Lindén-Boström M, Nordström E, et al. Mental health symptoms in relation to socio-economic conditions and lifestyle factors–a population-based study in Sweden. BMC Public Health. 2009;9:302.
Batty GD, Russ TC, Stamatakis E, Kivimäki M. Psychological distress and risk of peripheral vascular disease, abdominal aortic aneurysm, and heart failure: pooling of sixteen cohort studies. Atherosclerosis. 2014;236:385–8.
Article CAS PubMed Google Scholar
Russ TC, Stamatakis E, Hamer M, Starr JM, Kivimäki M, Batty GD. Association between psychological distress and mortality: individual participant pooled analysis of 10 prospective cohort studies. BMJ. 2012;345:e4933.
Wilson R, Barnes L, Bennett D, Li Y, Bienias J, De Leon CM, et al. Proneness to psychological distress and risk of Alzheimer disease in a biracial community. Neurology. 2005;64:380–2.
Fritzell J, Lundberg O. Health inequalities and welfare resources: continuity and change in Sweden. Bristol: Policy Press; 2007.
Lennartsson C, Agahi N, Hols-Salén L, Kelfve S, Kåreholt I, Lundberg O, et al. Data resource profile: the Swedish Panel Study of living conditions of the oldest old (SWEOLD). Int J Epidemiol. 2014;43:731–8.
Erikson R, Goldthorpe JH. The constant flux: A study of class mobility in industrial societies. USA: Oxford University Press; 1992.
Roos PA, Treiman DJ. 1980. Worker functions and work traits for the 1970 U.S. census classification. In A. Miller (Ed.),Work, jobs and occupations:336–389. Washington, DC: National Academy Press; 1980.
Andel R, Crowe M, Pedersen NL, Mortimer J, Crimmins E, Johansson B, et al. Complexity of work and risk of Alzheimer’s disease: a population-based study of Swedish twins. J Gerontol B Psychol Sci Soc Sci. 2005;60:P251–8.
Mood C. Logistic regression: Why we cannot do what we think we can do, and what we can do about it. Eur Sociol Rev. 2010;26:67–82.
Williams R. Generalized ordered logit/partial proportional odds models for ordinal dependent variables. Stata J. 2006;6:58.
DeMaris A. Explained variance in logistic regression: A Monte Carlo study of proposed measures. Sociol Methods Res. 2002;31:27–74.
Hardin JW, Hilbe JM, Hilbe J. Generalized linear models and extensions. Texas: Stata press; 2007.
Markides KS, Machalek R. Selective survival, aging and society. Arch Gerontol Geriatr. 1984;3:207–22.
Erikson R. Social class of men, women and families. Sociology. 1984;18:500–14.
Lazarsfeld PF. Interchangeability of indices in the measurement of economic influences. J Appl Psychol. 1939;23:33.
Deaton A. Policy implications of the gradient of health and wealth. Health Aff (Millwood). 2002;21:13–30.
Download references
Acknowledgements
Not applicable.
This research was funded by the Marianne and Marcus Wallenberg (MMW) Foundation (Grant MMW 2011.0036, 2016.0081) and the Swedish Research Council for Health, Working Life, and Welfare (Grant 2012–0761, 2012–1704, and 2016–00241).
Availability of data and materials
It is possible to apply for LNU data at the Swedish Institute for Social Research ( http://www.sofi.su.se/english/research/three-research-departments/lnu-level-of-living/apply-for-lnu-data ) and SWEOLD data at Aging Research Center (ARC) ( http://www.sweold.se/dataaccessengelsk.htm ).
Author information
Authors and affiliations.
Aging Research Centre (ARC), Karolinska Institutet & Stockholm University, Gävlegatan 16, 113 30, Stockholm, Sweden
Alexander Darin-Mattsson, Stefan Fors & Ingemar Kåreholt
Institute of Gerontology, School of Health and Welfare, Aging Research Network-Jönköping (ARN-J), Jönköping University, Jönköping, Sweden
Ingemar Kåreholt
You can also search for this author in PubMed Google Scholar
Contributions
The authors contributed as follows: AD-M: conception and design, analysis and interpretation of data, drafting and revising, and approval; SF: conception and design, interpretation, critical revision, and approval; IK: conception and design, analysis and interpretation of data, critical revision, and approval. All authors read and approved the final manuscript.
Corresponding author
Correspondence to Alexander Darin-Mattsson .
Ethics declarations
Ethics approval and consent to participate.
This study was approved by the ethical board in Stockholm (dnr: 2010/403–31/4) and we only analysed non-identifiable data. Written consent to use data for scientific purposes, including publication, was obtained from all survey respondents.
Consent for publication
Competing interests.
The authors declare that they have no competing interests.
Publisher’s Note
Springer Nature remains neutral with regard to jurisdictional claims in published maps and institutional affiliations.
Additional files
Additional file 1: table s1..
Average marginal effects (AMEs) times 100 of reporting more health problems than the reference group and model fit (R2 change). (DOCX 17 kb)
Additional file 2: Table S2.
Rights and permissions.
Open Access This article is distributed under the terms of the Creative Commons Attribution 4.0 International License ( http://creativecommons.org/licenses/by/4.0/ ), which permits unrestricted use, distribution, and reproduction in any medium, provided you give appropriate credit to the original author(s) and the source, provide a link to the Creative Commons license, and indicate if changes were made. The Creative Commons Public Domain Dedication waiver ( http://creativecommons.org/publicdomain/zero/1.0/ ) applies to the data made available in this article, unless otherwise stated.
Reprints and permissions
About this article
Cite this article.
Darin-Mattsson, A., Fors, S. & Kåreholt, I. Different indicators of socioeconomic status and their relative importance as determinants of health in old age. Int J Equity Health 16 , 173 (2017). https://doi.org/10.1186/s12939-017-0670-3
Download citation
Received : 31 January 2017
Accepted : 21 September 2017
Published : 26 September 2017
DOI : https://doi.org/10.1186/s12939-017-0670-3
Share this article
Anyone you share the following link with will be able to read this content:
Sorry, a shareable link is not currently available for this article.
Provided by the Springer Nature SharedIt content-sharing initiative
- Socioeconomic indicators
- Late-life health
International Journal for Equity in Health
ISSN: 1475-9276
- General enquiries: [email protected]
An IERI – International Educational Research Institute Journal
- Open access
- Published: 06 June 2020
The measure of socio-economic status in PISA: a review and some suggested improvements
- Francesco Avvisati ORCID: orcid.org/0000-0001-9354-5234 1
Large-scale Assessments in Education volume 8 , Article number: 8 ( 2020 ) Cite this article
41k Accesses
101 Citations
7 Altmetric
Metrics details
This article reviews the history of the measure of socio-economic status in PISA and identifies theoretical underpinnings of the index of economic, social and cultural status (ESCS). It then highlights multiple changes in the instruments and scaling methods used by PISA over time, and suggests ways of resolving the tensions behind some of these changes and thereby stabilise the measure of ESCS. A stable definition and operational procedure to derive the ESCS index appears essential to compare the ESCS-achievement relationship over time. Some of the suggestions included in this article were already implemented in the 2018 cycle.
Introduction
The index of economic, cultural and social status (ESCS) is probably, just after student achievement scores, the most used variable in reports and in secondary analysis of data from the Programme for International Student Assessment (PISA). Based on student reports to the context questionnaire, it helps address relevant questions about educational opportunity and inequalities in learning outcomes. While well-established and sometimes used as a reference (if not a standard) for the measurement of socio-economic status (SES) in national assessments of school-aged children (INVALSI 2017 , p. 70; Cowan et al. 2012 ), the ESCS index has also been criticised.
Some scholars, in particular, have challenged the validity, reliability or comparability of the current measurement of socio-economic status or of its components in PISA, calling for revisions and extensions of the index (Rutkowski and Rutkowski 2013 ; Pokropek et al. 2017 ; Willms and Tramonte 2015 ).
This article reviews the history and use of ESCS, and formulates recommendations to strengthen its measurement, based on a set of reporting priorities. The significant advances in statistical methodologies since the early 2000s, and more importantly, concerns about the validity of the current measure of socio-economic status in PISA and of the inferences that are made based on it, justify an effort to revisit this variable. The review takes the PISA 2015 database as a starting point: some of the recommendations in this review were shared, in a preliminary version, with the PISA 2018 consortium and were already implemented in the PISA 2018 database.
What is ESCS, and why is it useful?
The PISA index of economic, social and cultural status is closely related to other measures of socio-economic status (SES) that are commonly used in the education literature, in particular within the North American tradition. A report by the “American Psychological Association Task Force on Socioeconomic Status” (APA 2007 ) described three alternative approaches to define and analyse socio-economic differences in education and in other domains. A first approach (the “materialist” view of SES) summarises the relationship between outcomes (e.g. education) and socio-economic status through the relationship with quantifiable characteristics such as income and wealth. Researchers within this tradition often focus on essential resources and define cut-offs (poverty line, “free-school meal status”) on continuous measures. A second approach (“gradient approaches”) emphasises relative status, and conceives socio-economic status as a unidimensional ranking of individuals in society; this ranking can be informed by multiple dimensions, including, sometimes, subjective ones [such as subjective perceptions of one’s status, measured, for example, through the MacArthur scale (Goodman et al. 2001 )]. A third approach focuses on hierarchies of power and privilege and their reproduction (“class models”); researchers following this approach, which is more closely linked to theories of social stratification [e.g. (Weber 1922 )], focus on categorical measures, which can be ordered or unordered [for example, “the intellectual elite” vs “the business elite”; (Piketty 2018 )].
PISA questionnaire frameworks do not provide an explicit theoretical foundation and definition of economic, social and cultural status (ESCS). The index is operationally defined in the technical reports. For example, the first ESCS variable (constructed for PISA 2000) was simply described as a convenient way to facilitate reporting: “To facilitate the analysis, this chapter combines into a single index the different economic, social and cultural aspects of family background that were examined separately in Chapter 6” (OECD 2001 , p. 185).
The ideas and definition that underpin the construction of ESCS must therefore be found in the broader literature, including secondary literature about PISA. According to Doug Willms, who worked on the construction of the first ESCS variable in PISA, ESCS is closely related to the “gradient approach”. Indeed, in reviewing the measure of socio-economic status used in PISA (Willms and Tramonte 2015 ; Willms 2006 ), he referred to the definition of socio-economic status by Mueller and Parcel ( 1981 , p. 14):
Socio - economic status is the relative position of a family or individual in a social system in which individuals are ranked according to their access to or control over wealth, power and status.
This definition highlights the relative nature of socio-economic scores as reflecting the hierarchical ranking that characterises modern human societies, while “wealth, power and status” refer to the three components of social stratification highlighted in Weber ( 1922 ). In reviewing the broader literature about socio-economic status and achievement, Sirin ( 2005 , p. 418) refers to this same definition as one that can be applied to most studies.
The gradient approach alone however cannot explain PISA’s desire to anchor the measure of socio-economic status on a common scale for all countries and for all years, so as to enable comparisons of “status” across individuals belonging to distinct national societies. The need for such a common scale appears driven by a different definition, which conceptualises socio-economic status not only as one’s position, but as a direct measure of the amount of valued resources and capital that individuals can access and control. This view of “socio-economic status” corresponds to the definition provided by the panel of experts convened at the request of the National Assessment Governing Board to provide recommendations concerning socio-economic status (Cowan et al. 2012 ):
SES can be defined broadly as one’s access to financial, social, cultural and human capital resources. […]
In sum, the current measure of ESCS and its use in PISA analysis appear inspired by both the materialist view and by gradient approaches. A possible definition of ESCS in PISA therefore is:
ESCS is a measure of students’ access to family resources (financial capital, social capital, cultural capital and human capital) which determine the social position of the student’s family/household.
While commonly used in education research, inferences that rest on a composite measure of socio-economic status—whether conceived as a relative measure of position, or as a unidimensional proxy for different kinds of resources—have also been criticised by prominent scholars (Deaton 2002 ; O’Connell 2019 ). Angus Deaton, for example, formulated this critique as follows:
“we have a correlation between socioeconomic status and health and evidence that the correlation is causal, at least in part. [What this implies for policy, however,] depends on what we mean by ‘socioeconomic status’, a term that is convenient as a shorthand for a wide range of possibilities, including income, education, rank, or social class, but that is useless for thinking about policy in the absence of an instrument that acts on them all” (Deaton 2002 ).
A similar critique has also been formulated, within the context of analyses based on PISA data, by Keskpaik and Rocher ( 2011 ), who suggest that the individual components of ESCS provide a more useful description of equity in school systems than the unidimensional analysis based on ESCS: a critique that emphasises the multi-dimensional nature of ESCS and is also closely related to “class models”, more typical of the European tradition.
From a measurement perspective, socio-economic status is often conceptualised as a formative latent variable, rather than a reflective latent variable; and the short discussion in this section reflects different views as to whether it should be seen as a causal formative construct (a latent variable that is caused by the observed variables through which it is measured) or simply as a composite formative construct (a convenient, and entirely artificial summary of somewhat unrelated measures) (Bollen and Bauldry 2011 ).
Our view is that a composite measure of socio-economic status has mainly a practical utility in analysis, avoiding problems that would otherwise arise due to the correlated nature of individual components or due to their interactive effects. In particular, an index such as PISA’s ESCS can be used to build synthetic, high-level descriptive indicators of inequality of opportunity and of outcomes in education, or as a “control” variable to account for the possible confounding effect of pre-existing individual differences on the outcome of interest. While the measure of PISA is inspired by both the gradient and the materialist approach, I suggest to consider ESCS mostly as an artificial composite. In doing so, I make both the individual components and the algebraic operations through which the components are combined part of the definition of ESCS, and redirect questions about the validity, reliability and comparability of the index to its individual components, where such questions are more tractable.
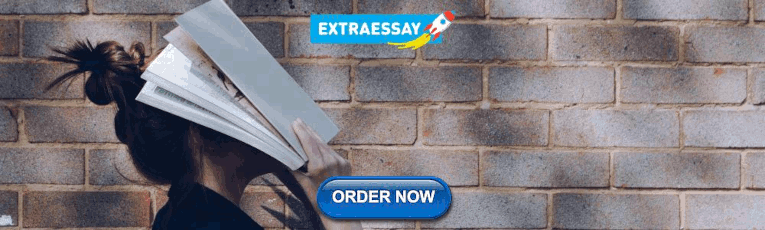
What are the components of socio-economic status?
The definition of ESCS as a composite inspired by the North American tradition of SES measurement suggests constructing ESCS by combining into a single score distinct measures of the financial, social, cultural and human capital resources available to students (and perhaps other resources which are relevant to the family’s position in a particular social hierarchy); this composite score can be thought of as an approximation of individuals’ ranking in a national and global society.
In modern, industrialised societies, it has been common (at least since the first half of the twentieth century) to use formal education credentials, occupation titles converted to a status or prestige scale, and income as the individual components through which socio-economic status is measured. Cowan et al. ( 2012 ) note:
Traditionally, a student’s SES has included, as components, parental educational attainment, parental occupational status, and household or family income, with appropriate adjustment for household or family composition. […]
Education, occupation and income are sometimes referred to as the “big three” (Willms and Tramonte 2019 ); Sirin ( 2005 , p. 418) notes that there is substantial agreement among researchers on the “tripartite nature of SES that incorporates parental income, parental education, and parental occupation as the three main indicators of SES”. Ensminger and Fothergill ( 2003 ) note that “education, income and occupation” are the “three most common measures of SES”, but does not recommend combining them into one scale.
The PISA measure of socio-economic status (ESCS) has traditionally been built as a weighted average of three indices: parental educational attainment (in years), parental occupational status on the “International Socio-Economic Index” (ISEI) scale (Ganzeboom 2010 ; Ganzeboom et al. 1992 ), and a measure of “household possessions”. Two of the three components that inform the composite score of ESCS—parental years of education and parental occupational status—coincide with those used “traditionally”, according to Cowan et al. ( 2012 ). The third component—an index of household possessions, based on the possession or consumption of durable goods—can be thought of as a measure of the household’s income, or more precisely, of its “permanent” component (Friedman 1957 ).
What reporting goals should inform the measure of socio-economic status in PISA?
Having established the artificial nature of ESCS as a “convenient summary”, its construction should be guided by the validity of the inferences and conclusions that are based on it. A review of existing reports leads to identify the following desirable features for the measure of socio-economic status in PISA: Footnote 1
The PISA data set should include a measure of socio-economic status that supports an analysis of the relationship between student socio-economic status and achievement, through a limited number of key indicators;
The PISA data set should include a measure of socio-economic status that enables valid comparisons of the relationship between student socio-economic status and achievement across countries and, within countries, over time;
The PISA data set should include a measure of socio-economic status that enables to classify some students as “vulnerable” or “disadvantaged”, in order to analyse the concentration of such students in particular schools and compare their prevalence, and distribution, over time.
The PISA data set should include one or more measures of socio-economic status to account for individual differences in endowments and prior achievement, in particular when analysing the relationship between achievement and schooling variables (school tracks, school type, teaching practices, learning practices, opportunity-to-learn variables, …).
The PISA data set should include a school-level measure of student advantage/disadvantage, which enables to classify some schools as “advantaged” or “disadvantaged” and which can be used in regression analysis (including for the analysis of individual outcomes);
The development of indicators which capture the essential aspects of the relationship between socio-economic status and achievement, and which enable countries to monitor changes in this relationship over time and to compare themselves to other systems, are valued PISA outputs. Indeed, the relationship between students’ socio-economic profile and their performance is an important indicator of the fairness of education systems, i.e. the extent to which good or adverse circumstances (factors that are outside of students’ own control) influence their opportunities to access quality education and reach good learning outcomes; and distributive values such as equality, adequacy and benefitting the less advantaged are at the heart of many education policy decisions (Brighouse et al. 2015 ).
These needs create a strong rationale for summarising the information about the different components of socio-economic status into a single variable. While it is possible to measure the strength of the relationship between socio-economic status and outcomes in terms of the variation explained by multi-dimensional measures of socio-economic status, this would preclude simple visualisations of this relationship. Multi-dimensional measures also pose challenges for analysing gaps in the average performance of students at different “levels” of socio-economic status (e.g. in different quarters or quintiles), as this would either result in a multiplicity of measures (one per dimension) or in the need to specify distinct discrete profiles or classes to define the levels; in contrast, a single continuous measure allows to simply visualise this relationship and to use simple indicators, such as the gap between the top and bottom quarter of ESCS or the average gap along the continuum (or “slope of the socio-economic gradient”).
Neither of the first two needs mentioned above provides a strong rationale, however, for preferring a particular scale or for assuming a particular distribution for the composite measure of socio-economic status. For example, to avoid choosing a particular scale, a rank- or percentile-measure (which would result in a uniform distribution of socio-economic status) could be used in every country. Non-linear transformations of the composite score, of course, do not result in the same conclusions about the shape of the relationship, and would implicitly re-define the meaning of the “slope”, i.e. of the average gap along the continuum (while a robust, non-parametric definition of the “strength” would be unaffected by such transformations).
Additional reporting needs must be invoked to justify reporting the composite measure of socio-economic status on an interval scale with the same origin in all countries and all cycles. In particular, a common scale presents the advantage of enabling further analyses, such as decomposing the changes in slopes (by distinguishing compositional changes, driven by differences in the underlying distribution of socio-economic status, from shifts in the relationship of socio-economic status and performance). It can also support the development of additional indicators such as the “level” of the socio-economic gradient, i.e. the average outcomes of students at particular points in the distribution of socio-economic status—although due to the artificial nature (and scale) of ESCS, interpretability remains an issue.
The definition of a category of “vulnerable” students, based on some combination of resource indicators, can be a natural by-product of the construction of ESCS or proceed independently. In particular, the definition of levels of vulnerability could explicitly take into account multiple resources and dimensions, without the need to combine them into one measure. At the same time, if the emphasis is on relative disadvantage, a measure that assigns weights to the different dimensions in order to provide a single ranking of students is necessary. In order to enable meaningful comparisons over time or between countries, a composite measure of socio-economic status—or one of its components—should then be reported on an interval scale with the same measurement unit and origin in all countries and over time.
Finally, ESCS is often used as a control variable in regression analyses based on the PISA dataset. The cross-sectional nature of PISA data poses important challenges for the interpretation of analyses that relate resources and processes to outcomes. Measures of family background and socio-economic status can account for possible confounding factors that may create spurious relationships between the outcomes of schooling and the type of school- and out-of-school experiences students have. In such analyses, the direct relationship between outcomes and socio-economic status is of little interest. In some cases, the rationale for including measures of socio-economic status is not to “account” for confounding factors, but simply to increase the precision of estimates; for example, gender can often be expected to be unrelated to family socio-economic status, yet the inclusion of socio-economic status in a regression analysis increases the precision with which gender gaps are estimated.
It is in general preferable to introduce the different components of socio-economic status independently in regression analyses, in order to optimally reduce the variation to be explained and to interpret coefficients on a natural scale, rather than the artificial scale created by the ESCS aggregate. While the different components are related, they are conceptually distinct, and the empirical correlation among different components is unlikely to cause multi-collinearity issues in the large samples that are typical of PISA analysis. However, if interest lies in examining interactive effects (e.g. whether the gender gap is higher among advantaged than among disadvantaged students), focusing on a single measure of socio-economic advantage (which could be a composite measure) greatly facilitates interpretation.
Many policy questions in education are at the school level: how should resources be allocated between schools? When should an additional class be created, or an existing class be closed (and merged with other classes)? Who should decide on the recruitment of new teachers? In analyses related to these questions, it is often interesting to have a school-level measure of advantage and disadvantage. In fact, in many countries, some administrative measures of school advantage exist, because of their use in the funding formula or in staff allocation decisions; for example, “Title I” status in the United States, or the inclusion of the school in a “priority education zone” (ZEP) or “priority education network” (REP) in France. School-level measures that are aggregations of student-level variables, such as the percentage of students eligible for the “National School Lunch Program” (NLSP) in the United States, the percentage of students eligible for “Free School Meals” (FSM) in England, or the percentage of students with low-educated parents, with an immigrant background, or with limited proficiency in the national language, are also often used.
In multi-national studies like PISA, administrative measures are typically not available and not comparable across countries. Instead, one may construct school-level measures of socio-economic (dis)advantage either based on the student sample, or based on specific questions about the entire student body asked to students, teachers, or principals/school administrators. Because of the small within-school samples of students used in PISA, proportions based on the characteristics of sampled students can be affected by significant measurement error, and it may be preferable to use means or medians of continuous measures, rather than proportions of categorical measures. Including more than one measure at the school level can also be problematic, particularly in school-level analyses, due to the small number of schools per country and the high correlation among such measures that can be expected.
In small schools, measures of school advantage that are based on a single grade or cohort of students may give a noisy vision of the school socio-economic profile. The error in the mean estimate is inversely related to the number of students in the sample. In addition, because PISA samples are age-based (as opposed to grade-based), in some cases the PISA sample can give a biased vision of the school profile; such is the case, for example, when the students eligible for PISA are atypical in terms of their grade attainment. Footnote 2
To overcome this problem, school principals could be asked to provide a measure of socio-economic status that refers to the entire student body or to all students in a particular target grade—for example, the proportion of students that lack the basic necessities or advantages of life, such as adequate housing, nutrition or medical care. Indeed, a similar question was introduced in the PISA 2015 School Questionnaire (SC048) and is used in the “Teaching and Learning International Survey” (TALIS).
Another solution for secondary users of PISA data is to restrict the sample for analyses involving school-level aggregates to schools where “average socio-economic status” is better measured, or to conduct sensitivity analyses. The “Effective Teacher Policies” report, for example, restricted its analysis of school advantage/disadvantage to schools that include the modal level of schooling for 15-year-old students (OECD 2018a , p. 87). The “Equity in Education report” addressed the issue of measurement error (and, to some extent, bias) by restricting the analysis of the relationship between student performance and school socio-economic profile (mean and standard error) to schools in which 10 or more students had a valid ESCS index; sensitivity analyses were conducted (OECD 2018b , p. 137).
How can the current measure of ESCS be improved?
The PISA 2015 Technical Report (OECD 2017 , pp. 339–340) describes ESCS as “a composite score derived via principal component analysis (PCA) from the indicators parental education (PARED), highest parental occupation (HISEI), and household possessions (HOMEPOS) including books in the home”:
The next sections will review the instruments and procedures used to derive the composite ESCS index in greater detail. While these instruments have remained similar to when it was first developed, some changes were introduced over time; some of these changes do not appear to reflect methodological advances or new reporting needs. In reviewing each step in the construction of ESCS, the aim is to formulate recommendations for future PISA questionnaires and databases.
For each component, changes in the questionnaire items, and changes in the rules used to derive variables from questionnaire responses will be examined. For the composite measure, changes in the imputation procedure and changes in the weighting scheme will be examined.
Improving the measurement of parents’ or care-takers’ education attainment
The measurement of education attainment in 2015.
In 2015, the “parental education” component of ESCS was measured based on questions about father’s and mother’s level of schooling and father’s and mother’s post-secondary educational qualifications. Footnote 3 These questions were used to identify the highest level of education completed by each parent on a 7-point scale based on the International Standard Classification of Education (ISCED) 1997 (None; ISCED Level 1; ISCED Level 2; ISCED Level 3B or 3C; ISCED Level 3A or 4; ISCED Level 5B; ISCED Level 5A or 6). The highest of the two values was then selected and converted to a years-of-education equivalent (PARED), using conversion tables based on national ISCED mappings, provided by national project managers and documented in an appendix to the technical report.
The following issues affecting the validity, reliability and comparability across countries and over time of the current measurement of “parental education” can be identified.
Increase in immigrant populations
The substantial increase, in many countries, in the proportion of students with an immigrant background, defies the assumptions behind using national and time-invariant mappings to put educational qualifications (which immigrants may have earned in their home countries) on an SES scale.
Over-reporting of post-secondary qualifications compared to national statistics
At the country level, the proportion of students who report their mothers (fathers) to have tertiary education credentials correlates highly with the proportion of 35–54 year-old women (men) who have such credentials, at least for countries covered by the OECD Education at a Glance database. The linear correlation coefficient is 0.86 for mothers/women, and 0.78 for fathers/men. In general, the rate of tertiary qualifications reported for fathers is higher than the corresponding rate among 35–44-year-old men (and similarly for mothers), suggesting that students over-report tertiary degrees. The extent to which this occurs may vary across countries: Poland and Greece, for example, have similar rates of tertiary attainment among men (below 30%); but only about 20% of students report tertiary degrees for their fathers in Poland, Footnote 4 compared to over 40% in Greece. On the other hand, in Russia, more than 80% of students report their fathers to have a tertiary qualification, while labour-force surveys indicate that only about 45% of men to have such qualifications (Fig. 1 ).
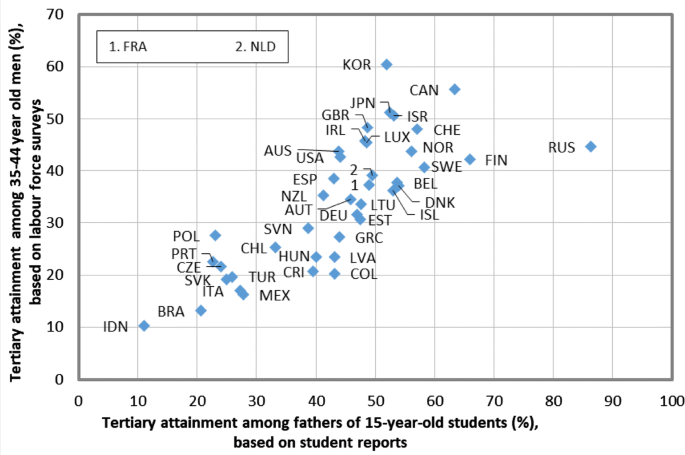
(Source: OECD, PISA 2015 Database; Education at a Glance Database ( https://stats.oecd.org/OECDStat_Metadata/ShowMetadata.ashx?Dataset=EAG_NEAC ))
Tertiary attainment of fathers (PISA) and of 35–44 year-old men (EAG). Pearson’s linear correlation coefficient between the two series is 0.78 (N = 41)
Inconsistency between “level of schooling” and “post-secondary qualifications”
The PISA 2015 measure was based on distinct answer formats for qualifications up to upper-secondary level (ISCED Level 3) and for post-secondary qualifications. By virtue of the hierarchical nature of the ISCED classification, in order to have an ISCED Level 4 degree or higher it is necessary, in theory, to have earned an ISCED Level 3 qualification. Footnote 5 However, a significant number of students report a level lower than ISCED Level 3 as their parents’ highest level of schooling, and at the same time report their parents as having post-secondary qualifications.
Missing answers
The percentage of students with missing reports on both their father’s and mother’s education—and thus for whom no “parental education” component could be computed—is relatively low, but varies considerably across countries and years. The median missing rate across countries for PARED was 1.9% in 2015 (inter-decile range: 0.6–4.5%). The highest missing rate was observed in Germany (17.5%), Footnote 6 the lowest missing rate in Romania (0.04%). The percentage of missing answers fluctuated over time with no clear trend, and no particular change related, for example, to the introduction of computer-based questionnaires in 2015 (Fig. 2 ).
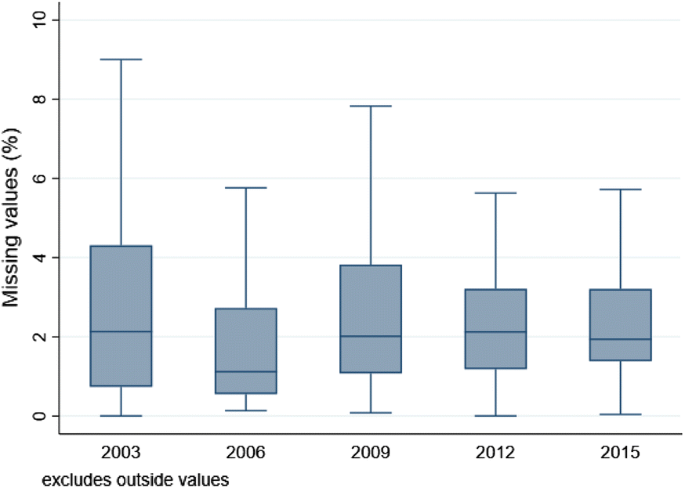
(Source: PISA 2003–2015 database)
Percentage of respondents with missing parental education component. The figure represents the median, interquartile range (shaded area) and adjacent values for country-level missing rates in PARED. Adjacent values are defined as the most extreme missing rates within 1.5 times the interquartile range from the nearest quartile. The following countries have missing rates that exceed the upper adjacent value: 2003: Canada (10.9), Germany (11.0), Luxembourg (13.1), New Zealand (11.9); 2006: Germany (6.2), United Kingdom (8.7), Israel (7.2), Luxembourg (6.8), New Zealand (7.9); 2009: Germany (13.0), United Kingdom (8.0), New Zealand (9.3), Panama (16.7); 2012: Germany (21.5), United Kingdom (7.6), Luxembourg (6.8), New Zealand (8.9); 2015: Brazil (6.6), Germany (17.5), United Kingdom (9.3), New Zealand (8.9)
Misreporting
Researchers have questioned whether students provide valid responses regarding their parents’ education. Studies that were able to compare reports by multiple raters have found that students’ reports of parental education have relatively low correlations with parents’ self reports, lower than, for example, correlations among reports of parents’ occupations; in addition, there appears to be considerable variability across countries in inter-rater agreement (Willms and Tramonte 2019 ; Lien et al. 2001 ; Looker 1989 ; Jerrim and Micklewright 2014 ; Schulz 2005 ).
Changes over time in questionnaire instruments
The international versions of the parental education questions used different wording and response formats in PISA 2000, 2003, 2006 and 2009, and have since not changed until 2018 (Box 1 ).
However, more subtle changes have been introduced all along in national questionnaires, in harmonisation rules to map back national adaptations to international response options, and in rules to derive the year-of-education equivalence:
The mapping of ISCED levels to years of schooling changed a first time in 2006, and was subsequently revised in every cycle in consultation with countries.
Changes in national adaptations of the international questions—e.g. to distinguish a greater number of tertiary qualifications (bachelor, masters, …)—and/or to the mapping of national response options into international variables (“harmonisation”) are poorly documented, but may affect the comparability of data over time. Footnote 7
Alternative measures
Some issues related to the measurement of parental education are difficult to solve: for example, the misreporting of parental education by students. Other issues may be addressed more simply in revised procedures or instruments, such as:
Considering students’ answers about post-secondary qualifications only for those students who reported their parents’ highest level of schooling to be at least (lower) secondary education. This has the potential to reduce mis-reporting and the over-reporting of tertiary qualifications, in particular in developing countries where universal primary education was not yet the rule in the parents’ generation. In future questionnaires, this filter rule could be automatically applied at the time of data collection.
Using a single, international conversion to convert major ISCED levels into their (approximate) years-of-education equivalent. In order to minimise the differences with established patterns, this international conversion could be initially determined by using the modal years of education across countries for each ISCED level. This would eliminate a source of mistakes in the calculation of ESCS and limit arbitrary differences between countries with similar educational structures. The comparability of the parental education component of socio-economic status across countries and over time would therefore rely directly on the comparability of the education levels, before their conversion on an SES scale.
Table 4 ( Appendix ) uses PISA 2015 data to explore the impact of these suggested changes on the measure of parental years of education in PISA, and on the relationship with performance.
Results included in Table 4 ( Appendix ) suggest that the introduction of a filter on secondary education and of a common conversion from ISCED levels to years of education would only minimally affect within-country analyses, and has the potential to improve the cross-country comparability of PARED and its use a component of an international measure of socio-economic status. The alternative that incorporates both changes (PARED2) in particular has marginally higher concurrent and criterion validity, as indicated by correlations with country-level measures based on labour-force surveys, with other ESCS components, and with science performance. The correlation with country-level measures based on labour-force surveys measures increases only minimally, across all countries, but a larger increase is observed for lower-income countries (where over-reporting may be more of an issue). The correlation with the “household possessions” component of ESCS (variable HOMEPOS) was investigated in particular on the share of students with values in the top and bottom international quintile of these measures. Indeed, one use of ESCS as an internationally comparable scale is to generate international categories (typically “deciles” or “quintiles”) of resources; and the analysis typically focuses on the extremes (bottom decile, top decile, etc.). Because parental education (PARED) is the most discrete component of ESCS, the actual values used to convert the top and bottom education category onto the ESCS scale loom large on the percentage of students who are classified in the top and bottom quintiles of ESCS. In particular, in 2015, using the national conversion, countries such as Australia, Israel, France and New Zealand (which have relatively small values, for the PARED conversion of tertiary education) ended up having fewer students than one would expect in the top quintile. An international conversion of education levels to the SES scale would result in international rankings of students on the scale that no longer depend on the values chosen to convert tertiary education into years of education, but directly on the qualifications level distinguished in questionnaires. For the purpose of trend analyses, both suggested changes—using a filter on post-secondary education qualifications and a conversion from ISCED levels to years of education that is common to all countries—could be applied retrospectively to past datasets. Trend comparability of the “education” component of socio-economic status would rely directly on the comparability (over time) of major levels of education distinguished in questionnaires; where this comparability can no longer be guaranteed for all education levels distinguished in questionnaires, because of changes in education structures, it may be necessary to merge questionnaire levels into broader categories that remain comparable.
Parents’ occupational status
The measurement of occupational status in 2015.
The “parental occupation” component of ESCS is measured based on open-ended questions about father’s and mother’s job title/occupation (questions ST014Q01TA and ST014Q02TA in PISA 2015). Countries are then required to use the information provided by students to assign a code based on the International Standard Classification of Occupation (ISCO) to each student. This international classification developed by the International Labour Organisation (ILO) distinguishes over 400 occupations (4-digit codes, e.g. “carpenters”, “stonemasons”, “roofers”, “plasterers”), grouped into 28 groups (defined by the first two digits of their codes, e.g. “Building and related trades workers, excluding electricians”) and 10 major groups (first digit, e.g. “craft and related trades workers”). PISA has expanded the list of ISCO codes with special codes for unemployed and inactive parents (9701 “Doing housework, bringing up children”, 9702 “Learning, studying”, 9703 “Retired, pensioner, on unemployment benefits”). The ISCO codes provided by countries (which form a nominal scale) are then converted into an ordinal or interval scale using prestige rankings or income rankings based on international studies; PISA refers to the “International Socio-Economic Index of occupational status” (ISEI) developed by Ganzeboom ( 2010 ). The highest value for either parent is used in the ESCS composite (HISEI).
Similar to what is proposed above for the education component of socio-economic status, the occupation component already relies on a common mapping, across all countries, of detailed occupational categories on an SES scale. The comparability of the occupation component of socio-economic status across countries and over time therefore relies on the comparability of the occupational codes.
Accuracy of coding
There are no standards about the quality of coding procedures. Some countries do not code occupations to the four-digit level: until 2009, Japan coded occupations only at the 2-digit level; in 2015, the United Kingdom coded occupations only at the 3-digit level.
Validity of the ISEI conversion across time and countries
The prestige, skill level and income of certain occupations can vary significantly across countries and levels of development (to take just one example, “cattle farmer” can correspond to very different income and skill levels, depending on the national context); but Ganzeboom’s work (Ganzeboom et al. 1992 ; Ganzeboom 2010 ) is based on a more restricted set of countries than the set of countries participating in PISA (the most recent conversion of occupational codes to ISEI codes is based on 42 countries or subnational entities that participated in the International Social Survey Programme between 2002 and 2007). Furthermore, the relative prestige and income of certain occupations and the educational requirements to access certain occupations is also subject to change over time (e.g. “teacher”), creating tensions for the conversion of ISCO codes to an ordinal or interval scale.
Missing data
The percentage of students with missing reports on father’s and mother’s occupation is relatively high, and varies significantly across countries. The median missing rate across countries for mother’s occupation (OCOD1) is 12.8% in 2015 (inter-decile range: 6.8–23.8%). The highest missing rate is observed in Algeria (74.8%), the lowest missing rate in Viet Nam (2.0%). The median missing rate across countries for father’s occupation (OCOD2) is 16.6% in 2015 (inter-decile range: 24.5–8.7%). The highest missing rate is observed in Thailand (30.3%), the lowest missing rate in Viet Nam (5.7%). While single-parent households may explain the high levels of missingness to some extent, some scholars attribute high rates of missing data to the open-ended nature of the question (Willms and Tramonte 2015 ). Figure 3 shows that the median rate of missingness has increased over time, and in particular after 2009. The high level of missingness in low-achieving countries suggests that the response burden for this question may be too high for children with low literacy levels.
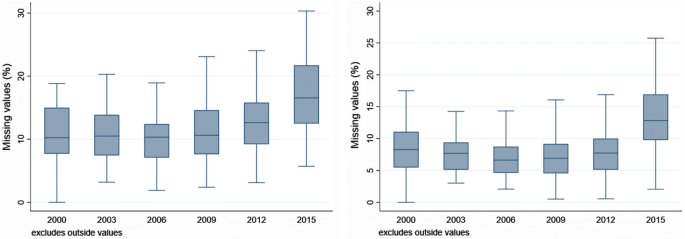
(Source: PISA 2000–2015 database)
Percentage of respondents with missing ISCO codes for parental occupation. Share of missing values for fathers’ (left) and mothers’ (right) occupation codes. The figure represents the median, interquartile range (shaded area) and adjacent values for country-level missing rates in OCOD2 (Father ISCO code) and OCOD1 (Mother ISCO code). Adjacent values are defined as the most extreme missing rates within 1.5 times the interquartile range from the nearest quartile. The following countries have missing rates that exceed the upper adjacent value: Fathers: 2000: Israel (31.1), Japan (65.0), United States (28.3); 2003: Japan (25.4); 2006: Israel (21.7), Japan (22.0), Qatar (44.8); 2009: Georgia (30.3), Panama (25.3), Himachal Pradesh (India) (32.8), Tamil Nadu (India) (26.3); 2012: Albania (31.7), Austria (100), Japan (26.8); 2015: none. Mothers: 2000: Brasil (26.7), Israel (25.5), Japan (66.5), United States (23.1); 2003: Canada (16.4), Germany (16.7), Japan (21.3), Netherlands (15.8), New Zealand (17.8); 2006: Israel (16.5), Japan (16.3), Qatar (31.4); 2009: Canada (16.8), Germany (16.7), Japan (16.2), QTN (24.8); 2012: Albania (27.4), Austria (100), Germany (22.3); 2015: Algeria (74.8), United Kingdom (28.3), Chinese Taipei (37.0), Thailand (29.1), Tunisia (66.2)
In addition, until 2015, no score on the ISEI scale was assigned for the three codes that PISA added to the list of ISCO codes (“Doing housework, bringing up children”, “Learning, studying”, “Retired, pensioner, on unemployment benefits”). As a result, even after combining father’s and mother’s reports in a HISEI value (and thus limiting the impact of single-parent households), missing rates for this component of ESCS remain high. The median missing rate across countries for HISEI is 9.3% in 2015 (inter-decile range: 4.7–17.7%). The highest missing rate is observed in Algeria (24.0%), the lowest missing rate in Viet Nam (4.7%) (Fig. 4 ).
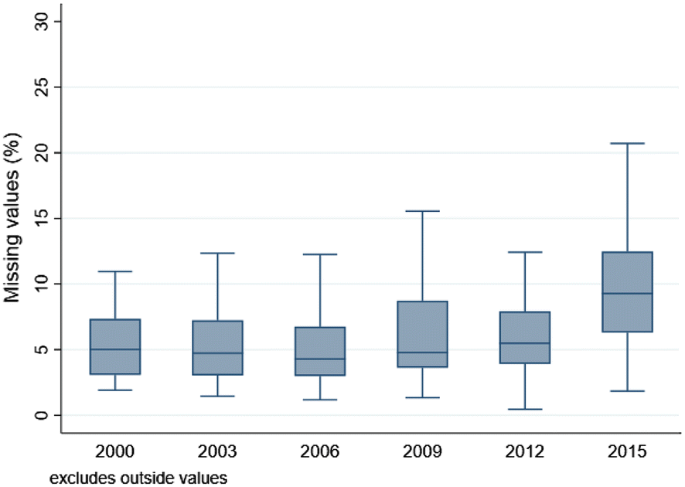
Percentage of respondents with missing parental occupation component of ESCS. The figure represents the median, interquartile range (shaded area) and adjacent values for HISEI. Adjacent values are defined as the most extreme missing rates within 1.5 times the interquartile range from the nearest quartile. The following countries have missing rates that exceed the upper adjacent value: 2000: Israel (26.0), Japan (62.5), United States (14.6); 2003: New Zealand (14.1); 2006: Azerbaidjan (17.0), Israel (16.1), Jordan (20.7), Qatar (41.2); 2009: Georgia (22.4), Himachal Pradesh (India) (32.6), Panama (17.0), Qatar (19.4), Tamil Nadu (India) (23.4); 2012: Germany (19.5), Jordan (21.8), Montenegro (16.2), Qatar (18.3), United Arab Emirates (15.0). 2015: Algeria (23.4), Thailand (22.8)
Coding open-ended questions is costly and requires relatively specialised knowledge that national PISA centres do not necessarily have in house.
Two possible changes to PISA procedures and instruments can address the main issues identified about the parental occupation component of ESCS.
Assign an (approximate) ISEI code to “non-occupations” identified by the pseudo-ISCO codes 9701, 9702 and 9703 (“Doing housework, bringing up children”, “Learning, studying”, “Retired, pensioner, on unemployment benefits”). A possibility is to use the lowest ISEI code for these occupations (11); this would limit the impact of this change for households where only one of the parents has such a code. Following a similar logic, but to avoid extreme values, I suggest using 17 as the ISEI value for these occupations, as this corresponds to the ISEI value used in PISA for occupations generically classified as “elementary occupations” (ISCO08 equal to “9000”). The extension of the ISEI scale to account for the special ISCO codes used in PISA can eliminate one source of cross-country differences in missing rates and thereby improve cross-country comparability.
Reduce the coding scheme to 1-digit or 2-digit codes only or use a closed response format to collect information about occupations. The impact of using only 1-digit or 2-digit coding can be explored by recoding existing data to this level of precision only. In contrast, the impact on the validity and comparability of the data collected from closed response questions about occupations can only be investigated based on field-trial data in which both question formats are administered. While the close response format may significantly reduce missingness and costs, it may also have a higher reading load (since all response categories need to be read) and induce socially desirable answers (e.g. due to order effects) to a greater extent than a text-entry field. Reducing the coding scheme to one or two digits would significantly reduce the cost of ISCO coding. It may also contribute to greater cross-country comparability of occupational codes as a result of higher, and more consistent coder reliability. In the absence of information about the current level of coder reliability, however, these gains remain speculative.
Table 5 (in Appendix ) uses PISA 2015 data to explore the impact of some of the suggested changes on the parental occupation component of socio-economic status, and on its relationship with performance.
Results indicate that the inclusion of pseudo-ISEI values for the additional occupation codes created by PISA (housewife, etc.) not only reduces missingness for this component, but also results in a higher correlation with science achievement ( Appendix , Table 5 ). By reducing the share of students for whom this component is missing, the inclusion of such pseudo-ISEI values can change the country-level average HISEI (and as a consequence, average ESCS) significantly in some cases, as shown by Jordan, whose mean value decreases by about one fourth of a standard deviation.
Results also suggests that analyses based on the parental occupation component of socio-economic status are relatively robust to changes in the coding scheme that would significantly reduce the data-collection costs for countries. In particular, the use of a more limited number of codes—either 34 two-digit codes or 10 one-digit codes—would have only a limited impact on country rankings and on the estimated correlations with achievement ( Appendix , Table 5 ).
- Household possessions
The measurement of household possessions in 2015
In 2015, the “household possessions” component of socio-economic status was based on 25 items in questions ST011 (16 dichotomous items, including 3 chosen by each country), ST012 (8 polytomous items, with a four-point scale) and ST013 (one polytomous item, with a 6-point scale) (see Table 1 ).
A one-dimensional generalised partial credit model was fitted to the data, with some items receiving country-specific item parameters. This was the case for the three national items in ST011, but also for the two indicators about the possession of “classic literature” and “books of poetry”, for which the meaning and the national examples included in the item stem may vary significantly across countries. Items that showed strong evidence of misfit for particular countries were also assigned national items; this was the case for the indicator on “educational software” in Japan.
The following issues affecting the validity, reliability and comparability across countries and over time of the current measurement of household possessions can be identified.
Validity of household possessions in cross-country comparisons
A measure of income or consumption for cross-country comparison should be expected to correlate highly with other measures of national income or household income. The country means of the PISA 2015 measure of household possession have a moderate positive correlation (0.65) with per-capita gross national income measures. The correlation is even higher if small countries with large natural resource revenues or financial sectors are excluded, or if income is expressed in a logarithmic scale (Fig. 5 ).
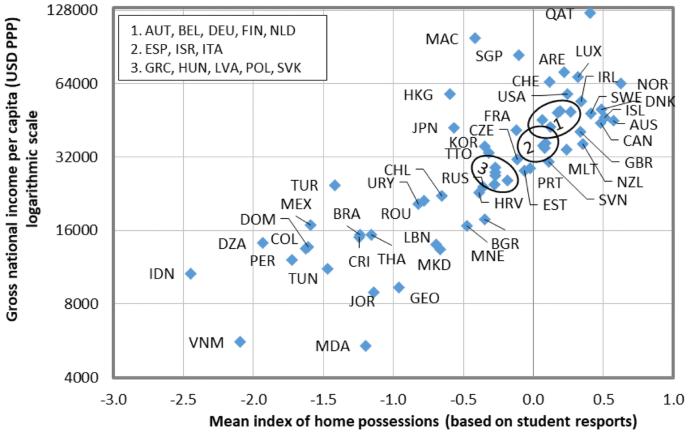
(Source: PISA 2015 Database and https://data.worldbank.org )
Correlation of average HOMEPOS (PISA) and GNI per capita (World Bank). Pearson’s linear correlation coefficient between the two series is equal to 0.65 (0.80 when GNI per capita is measured on a logarithmic scale)
Similarly, the percentage of 15-year-old students with a low level on the international household possession scale (values of HOMEPOS below − 1.77) correlates strongly (r = 0.85) with the percentage of the general population living below the World Bank upper middle-income International Poverty Line, set at USD 5.50 (PPP) (Fig. 6 ).
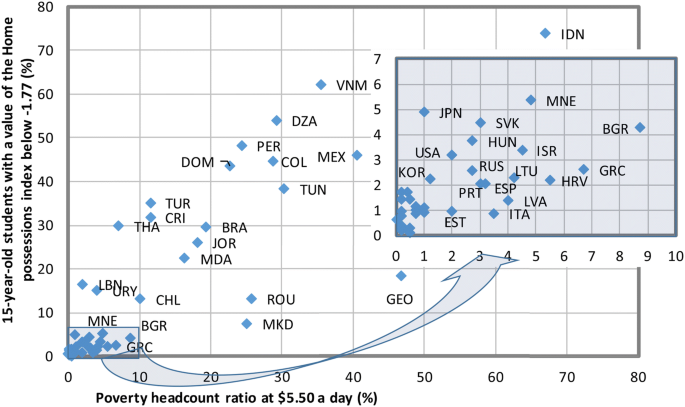
(Source: PISA 2015 database and https://data.worldbank.org )
Students with low household possessions and international poverty rates. The smaller, shaded chart shows an enlargement of the shaded area in the larger chart. Pearson’s linear correlation coefficient between the two series is 0.85. Values for Australia, Austria, Belgium, Canada, Cyprus, the Czech Republic, Germany, Denmark, Finland, France, Ireland, Iceland, Luxembourg, Malta, the Netherlands, Norway, Slovenia, Sweden, Switzerland and the United Kingdom, all countries with a poverty headcount ratio at or below 1%, are also shown but not labelled in the charts
The percentage of students with missing reports about their household possessions is relatively low, compared to other components of ESCS. The use of a proxy of family income instead of direct questions about this therefore seems successful at overcoming problems associated with missing answers. Nevertheless, there has been an increase in the rate of missing answers in 2012 and 2015, compared to previous surveys, and driven in particular by higher missing rates in Germany (Fig. 7 ). Footnote 8
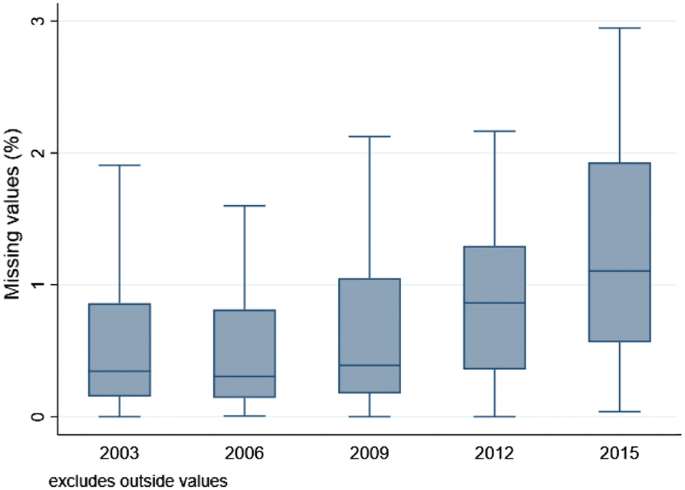
Percentage of respondents with missing household possessions component. The figure represents the median, interquartile range (shaded area) and adjacent values for country-level missing rates in the household possession index (HOMEPOS). Adjacent values are defined as the most extreme missing rates within 1.5 times the interquartile range from the nearest quartile. The following countries have missing rates that exceed the upper adjacent value: 2003: Brasil (2.11), Canada (9.78), Czech Republic (4.14), Germany (5.67), United Kingdom (3.72), Netherlands (3.16); 2006: Bulgaria (2.06), Canada (3.53), Chile (2.05), Germany (3.15), Israel (4.03), Norway (1.98), Qatar (4.47); 2009: Brasil (5.68), Germany (7.04), Panama (6.43); 2012: Albania (6.90), Germany (15.13), Iceland (2.73), Israel (2.86), Qatar (3.24); 2015: Brazil (5.22), Germany (12.64), Russia (4.07), Tunisia (4.17)
Changes in measurement instruments
There have been numerous changes over the years in the instruments used to measure household possessions.
The most visible change is the change in the set of international items in the household possession scale and in the order of items within each question (Table 1 ). The position of the questions within the questionnaire (at the beginning or at the end) has also changed over cycles.
A second type of change is the change in the answer categories for “Books at home”, which were changed in PISA 2003 to better match those used in other educational studies like TIMSS and PIRLS (Table 2 ).
Less visible changes have also happened over the years. In particular:
In 2003, the second set of questions about household possessions [Question 18, items (a) to (e)], asking students to count the “number of… at home”, could not be included in the computation of ESCS since they were deleted from the student data file (OECD 2005a , p. 246). The suppression of student responses was necessary because preliminary analyses revealed that students were confused by the data-entry codes that were printed next to the answer boxes, and which contradicted the answer categories for students provided above the boxes. It must be noted that these small numbers were re-introduced in 2015 for paper-based instruments (in use in a small minority of countries), but no similar action was taken (Fig. 8 ). In 2018, two-digit data-entry codes (“01”, “02”, “03”, “04”) were used in countries that continued to administer paper-based instruments.
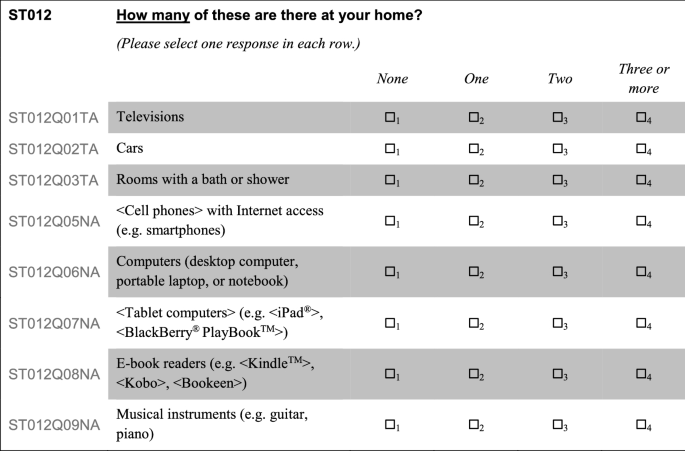
The problem with PISA 2015 paper-based instruments. The data-entry subscripts next to answer boxes are intended to help coders during data entry; they contradict however the labels provided above for respondents, leading to possible confusion. In PISA 2000, 2006, 2009 and 2012, only the last answer box (“three or more”) had a data-entry subscript
In 2006, the item “A room with a bath or shower” was suppressed from the database and the computation of the household possessions scale; the reason for this suppression is not documented in the technical documentation.
Most national items (dichotomous, country-specific items in ST011) have been modified over time.
Change in measurement models
The scaling model and the scaling procedures for the household possession index have changed in almost every cycle of PISA.
For the first PISA database and report (OECD 2001 ), three distinct indices (family wealth, cultural possessions and home educational resources) were derived, and no “overall” household possession index was created to summarise the items in the three indices and the books at home question. The household possession index was first created in PISA 2003, by combining items used in the family wealth, cultural possessions and home educational resources indices with the “books in the home” indicator (OECD 2005b ).
In 2006 and 2009, a country-specific measurement model was assumed for household possessions; scores were put on the same scale through an equating procedure (mean equating on a set of item difficulties in 2006; a linear transformation based on country means in a concurrent scaling in 2009) (OECD 2009 , 2012 ).
In 2009 and 2012, the calibration samples for HOMEPOS were drawn from multiple cycles (in 2012, a higher number of observations from the most recent cycle than from previous cycles was included) (OECD 2009 , 2012 ). In all other cycles, only observations from the most recent cycle contributed to these steps.
In 2012, the scaling of HOMEPOS was performed in two steps: national questionnaire items were not included in the first scaling run, but only in a second run (which was performed separately for each country) where all parameters for the international items were constrained to the values obtained in the first step (OECD 2014 ).
Until 2012, a partial credit model was used in the scaling of HOMEPOS (OECD 2014 ); in 2015, in line with other background questionnaire indices, a generalised partial credit model (including a “slope” or discrimination parameter) was used (OECD 2017 ). The evidence from 2015 shows significant variation in the discrimination parameter, even among items with the same (dichotomous or polytomous) response format: discrimination is only 0.59 for “books to help with your school work”, but 2.45 for “a link to the Internet”.
In 2015, for the first time, all countries’ data were used to scale HOMEPOS; until then, the scaling model for HOMEPOS was calibrated using observations from OECD countries only (OECD 2017 ).
Measurement equivalence across countries and over time
Several scholars have highlighted the weakness of the evidence in favour of a common measure of household possessions in PISA that is valid for all countries (Rutkowski and Rutkowski 2013 ; Pokropek et al. 2017 ).
In 2015, for the first time the PISA consortium introduced an analysis of measurement invariance for the items included in IRT indices, through the inspection of root-mean-square deviation (RMSD) and mean-deviaton (MD) statistics for each item × group interaction. For the purpose of the index of household possessions (HOMEPOS), analyses on the invariance of item parameters across countries, languages and cycles were conducted and unique parameters were assigned if necessary (OECD, PISA 2015 Technical Report, 2017 , p. 342). These analyses led to the use of more country-specific item parameters in the scaling of HOMEPOS. More recently, Lee and von Davier ( 2020 ) analysed the invariance of item parameters both across countries and over time, using concurrent, multiple-group calibration with partial invariance constraints, and concluded that four items in the scale, all related to technology, functioned differently across the PISA cycles, and (among those used in 2015) four other items (i.e. bathroom, classic literature, poetry books, and TV) functioned differently across the majority of participating countries when used to measure family wealth. Several other items used in 2015 exhibited high levels of misfit for a minority of country/language groups; the most notable are the questions about the number of “cars” (40% of country/language groups requiring unique parameters and the number of “computers” (34%).
Even without turning to fit indices and model-based evaluations of equivalence, theoretical considerations and simple comparisons of the mean levels of different household possessions items indicate possible problems of non-invariance across cycles and time. In particular, the extent to which the possession of certain technological goods at home indicates high social class is likely to change, as their price declines and their novelty fades; while for others, and particularly traditional cultural possessions (including books), new substitutes (e.g. e-books) become available. There are clear indications (Fig. 9 ) for example that the availability of “a link to the Internet” at home has moved from being an item indicating high income to an item indicating the availability of basic resources: the upward trend in the availability of “a link to the Internet” is particularly steep in lower-income countries. The top charts in the same figure also show how the relative order of “Cars”, compared to other durable goods, changes between lower-income and higher-income countries; while the bottom charts show similar variation, across countries, for items related to “classic literature”, “poetry books” and “works of art”. At the same time, Fig. 9 indicates also a certain stability and relatively consistent orderings for most items, particularly those indicating durable goods.

Trends in the possession of distinct household possession items, by national income. Lower income countries include countries with a gross national income below USD 28,500 (PPP). This threshold was chosen in order to have an about equal number of countries in the lower- and higher-income group. Only countries/economies that participated in every PISA cycle are included in the analysis. The lower values (compared to a linear trend line) observed in 2003 for many items may be related to the different response format used in that year, which did not distinguish item-non-response from a “no” answer (see notes under Table 1 )
Misreporting, local dependencies and inconsistencies
The items included in the household possession scale are, to some extent, dependent on each other. For example, it is impossible to have “a computer you can use for school work” (question ST011Q04TA in PISA 2015) in the home if there are “no computers” at all (ST012Q06NA) in the home. Similarly, there are multiple questions about books, which are expected to correlate more highly among them than with any other question.
Alternative instruments and scaling procedures
As indicated previously, there is a long history of changes in the measurement and scaling of the household possession components of ESCS. In the initial years, several changes were made to the instruments (or errors in the instruments corrected). Over time PISA has also oscillated between treating the household possession scale as a national scale and treating it as an international scale.
Of the three components of ESCS, the “household possessions” component has been the subject of most scrutiny by researchers; this criticism has also resulted in constructive advice to improve the measurement of this component.
In particular, Lee and von Davier ( 2020 ) show that the tension between the ideal of strong international comparability of a household-possessions component of socio-economic status and the reality of national specificities in consumption preferences and cost schedules, for example with respect to car ownership, can be successfully navigated by relying on multiple-group concurrent calibration with partial invariance constraints. In other words: the tension between a common measurement model for all country/language groups and the reality of model misfit and differential item functioning can be handled (and at the same time, shown explicitly) in a model in which common item parameters are imposed for the majority (but not the totality) of items and groups. In Table 6 ( Appendix ), I estimate such a partial-invariance model and replicate Lee and von Davier’s ( 2020 ) finding that the resulting scale correlates more strongly, on average, with test performance (an indicator of greater within-country accuracy and of criterion validity), while preserving the overall correlation (at country level) with measures of national income or poverty (an indicator of cross-country comparability and of concurrent validity). Lee and von Davier ( 2020 ) further show that the concurrent calibration approach with partial-invariance constraints can be successfully extended to the time dimension, resulting in a scale that finds the best possible balance between comparability (over time and across countries) and accuracy of scores.
Improvements to the instruments may also be considered in parallel. The periodic phasing out and replacement of certain items could be informed by the results of scaling: those items for which invariance constraints cannot be maintained may be replaced by new ones, thus ensuring that the home-possessions scale remains relevant in the presence of changing consumption patterns. In addition, PISA might consider introducing greater coordination, at regional level, in the selection of “national items” (Rutkowski and Rutkowski 2013 ). One possibility would be to introduce an international set of “optional” items from which countries can choose in order to replace one or more of the national items. These optional items would undergo the same translation and verification procedures as international items, and would be treated in scaling as international items (unless there is evidence of misfit for some or all countries), which are missing by design in countries that chose not to administer them. They would strengthen the comparability of the household possessions scale across countries sharing similar levels of economic development, geography, or cultural background.
Improving the composite score
Handling missing data.
In PISA 2015 and PISA 2018, a stochastic regression-based imputation of the missing component was implemented prior to computing a composite measure of socio-economic status. The imputation is applied for those cases where students have values on two out of three components and assumes a multivariate normal distribution for the three variables as well as that data were missing at random (OECD 2017 , 2020 ). While other imputation procedures can be considered (such as the use of multiple-imputation routines, and the inclusion of auxiliary background information in imputation), these changes are unlikely to be consequential for most analyses in which ESCS is used. It would be useful, however, to include in the dataset the imputed and standardised values that are used in the construction of ESCS, not only to document this step in the construction of ESCS, but also to facilitate the equating of measures from other surveys. This would indeed make the scale transformations required when moving from the distinct components to a composite measure visible and replicable.
Weighting scheme
In all past cycles of PISA, empirical weights based on an international PCA were used to combine the three (or five, in PISA 2000) components of socio-economic status into a single score.
The application of principal component weights implies that different components are weighted equally across countries, but differently over time. It can also be criticised as a somewhat unnecessary complication, which makes the definition of ESCS sample-dependent. For example, the optimal component weights for particular national samples can sometimes be very different from those used in the construction of ESCS, which are based on the international sample.
Alternatively, ESCS could be constructed using arbitrary weights; after all, ESCS is just a convenient summary of a multidimensional construct and the definition of these weights can be included in the operational definition of ESCS. Arbitrary weights would also make the ESCS measure more robust to changes in the sample and its construction easier to replicate based on public use files or on data from other surveys.
But how should the weights be determined? The simplest solution would be to use equal weights to combine the three standardised components. In fact, when looking in detail at the variation of component weights across survey cycles, it is striking that they have remained very similar across all cycles and very close to “equal weights” for all three (standardised) components (Table 3 ). In general, the weight for the HOMEPOS component has always been slightly below the weight for PARED and HISEI, but the difference has never been more than 10%, and has tended to reduce over time (perhaps as a consequence of expanding membership in the OECD and the use of all countries in calibration in 2015).
Given the available evidence, the use of arbitrary equal weights for producing the ESCS composite presents several advantages and would create very limited disruptions for trend analyses. Table 7 (in Appendix ) demonstrates this point by comparing the means and correlations based on the original ESCS composite (using principal-component weights) with those based on an alternative composite, using equal weights: differences are hardly noticeable, not only in the aggregate, but also within countries.
This article provides a rationale and theoretical underpinning for the construction of a composite measure of socio-economic status in PISA and in other large-scale international surveys, and suggests practical ways in which the ESCS variable can be improved to strengthen the arguments supporting its validity and comparability, reduce measurement error and missing values, and facilitate the construction of linked measures based on multiple survey years or based on other datasets.
The review highlights the hybrid nature of ESCS as both a measure of resources and a measure of relative social status; and underlines the utility of such a hybrid, composite measure for the construction of indicators of inequality of opportunity in education. It also identifies situations in which it may be preferable to use the individual components of socio-economic status (rather than the composite measure) in analyses based on PISA (or other similar) data.
While ESCS is conceived of as little more than a “convenient summary” of distinct resources that bear a relationship with an individual’s position in society, in order to allow for meaningful comparisons of the indicators based on ESCS over time and between countries, it is important to ensure that the scaling and measurement quality of ESCS remain comparable across different contexts. Yet, in the past, the operational definition of ESCS has changed in almost every cycle; and the measurement quality of the underlying components has received little attention. This review suggests that the validity and cross-country comparability of the components that are summarised in ESCS is, in fact, relatively high, based on concurrent evidence at the country level, and indicates ways in which it can be improved, while also addressing other aspects of measurement quality (such as missingness and reliability). It also suggests simplifying, and stabilising, the way in which the different components are combined, by abandoning the use of empirical weights (based on principal component analysis) in favour of arbitrary weights.
Availability of data and materials
The datasets and questionnaires analysed in this article are available from http://www.oecd.org/pisa .
A similar review was conducted by Willms and Tramonte ( 2015 ).
In France, Italy and Portugal, for example, PISA-eligible students in lower secondary schools are all behind track, below the expected grade for 15-year olds; they tend to be low-achieving and more often come from immigrant or disadvantaged backgrounds than their (younger) schoolmates, which are not eligible to be sampled in the PISA age-based sample. In other countries, such as Australia or Russia, 15-year-old students in upper secondary schools are all ahead of track. They may be more advantaged than the typical (older) students in the schools they attend.
The corresponding variables in the PISA 2015 dataset are ST005Q01TA, ST006Q01TA, ST007Q01TA and ST008Q01TA.
The low value for Poland may also be related to a mistake in the database; indeed, it seems that ST008, indicating fathers’ post-secondary qualifications in the PISA 2015 dataset for Poland, is identical to ST007, indicating mothers’ education.
In practice, there may be exceptions, and multiple ISCED levels may be combined in a single programme; for example, in the Netherlands the typical upper-secondary vocational track leads to an ISCED Level 4 qualification, with no formal degree to sanction the completion of ISCED Level 3.
The high level of missing answers about parental background in Germany is, in part, due to the presence of a filter in German questionnaires, which implies that these questions are not administered to students from the Land of Berlin whose parents have not given explicit consent to the collection of information about the out-of-school environment.
For example, in PISA 2015, students in Denmark were asked about vocational degrees [“En erhvervsfaglig uddannelse (fx elektriker, butiksassistent, smed, SOSU)”] as part of questions on post-secondary qualifications (ST006/ST008), and Danish questionnaires only included ISCED3A qualifications in ST005/ST007; while in PISA 2018, vocational qualifications were included as an additional option in question ST005/ST007.
This increase coincides with the introduction, in February 2011, of a more stringent data protection law in the Land of Berlin, which requires explicit consent for all data collections, including by public actors.
Abbreviations
Economic, social and cultural status
Gross national income
International Labour Organisation
International Standard Classification of Education
International Standard Classification of Occupations
International Socio-Economic Index
Free School Meal
Index of household possessions (variable in the PISA Database)
Mean deviation
National School Lunch Programme
Organisation for Economic Co-Operation and Development
Parental education (variable in the PISA database)
Principal component analysis
Progress in International Reading Literacy Study
Programme for International Student Assessment
Réseau d’éducation prioritaire (Priority education network)
Root mean square deviation
- Socio-economic status
Teaching and Learning International Survey
Trends in mathematics and science study
Zone d’éducation prioritiaire (Priority education zone)
APA. (2007). Report of the APA task force on socioeconomic status. Retrieved January 18, 2019 from https://www.apa.org/pi/ses/resources/publications/task-force-2006.pdf .
Bollen, K., & Bauldry, S. (2011). Three Cs in measurement models: Causal indicators, composite indicators, and covariates. Psychological Methods, 16 (3), 265–284. https://doi.org/10.1037/a0024448 .
Article Google Scholar
Brighouse, H., Ladd, H., Loeb, S., & Swift, A. (2015). Educational goods and values: A framework for decision makers. Theory and Research in Education, 14 (1), 3–25. https://doi.org/10.1177/1477878515620887 .
Cowan, C. D., Hauser, R. M., Levin, H. M., Beale Spencer, M., & Chapman, C. (2012). Improving the measurement of socioeconomic status for the National Assessment of Educational Progress: A theoretical foundation. Retrieved January 18, 2019 from https://nces.ed.gov/nationsreportcard/pdf/researchcenter/Socioeconomic_Factors.pdf .
Deaton, A. (2002). Policy implications of the gradient of health and wealth. Health Affairs, 21 (2), 13–30. https://doi.org/10.1377/hlthaff.21.2.13 .
Ensminger, M., & Fothergill, K. (2003). A decade of measuring SES: What it tells us and where to go from here. In M. Bornstein & R. Bradley (Eds.), Socioeconomic status, parenting, and child development (pp. 13–27). Mahwah: Lawrence Erlbaum.
Google Scholar
Friedman, M. (1957). The permanent income hypothesis. In A theory of the consumption function (pp. 20–37). Princeton: Princeton University Press. Retrieved January 18, 2019 from https://www.nber.org/chapters/c4405.pdf .
Ganzeboom, H. (2010). How was new ISEI - 08 constructed. Retrieved February 20, 2019 from http://www.harryganzeboom.nl/isco08/qa-isei-08.htm .
Ganzeboom, H., De Graaf, P., & Treiman, D. (1992). A standard international socio-economic index of occupational status. Social Science Research, 21 (1), 1–56. https://doi.org/10.1016/0049-089X(92)90017-B .
Goodman, E., Adler, N., Kawachi, I., Frazier, A., Huang, B., & Colditz, G. (2001). Adolescents’ perceptions of social status: Development and evaluation of a new indicator. Pediatrics, 108 (2), e31–e31. https://doi.org/10.1542/peds.108.2.e31 .
INVALSI. (2017). Rilevazioni nazionali degli apprendimenti 2016–17. Retrieved January 18, 2019 from https://invalsi-areaprove.cineca.it/docs/file/Rapporto_Prove_INVALSI_2017.pdf .
Jerrim, J., & Micklewright, J. (2014). Socio-economic gradients in children’s cognitive skills: Are cross-country comparisons robust to who reports family background? European Sociological Review, 30 (6), 766–781. https://doi.org/10.1093/esr/jcu072 .
Keskpaik, S., & Rocher, T. (2011). La mesure de l’équité dans PISA: pour une décomposition des indices statistiques. Revue Éducation et formations, 80 , 69–78. Retrieved January 29, 2019 from http://cache.media.education.gouv.fr/file/revue_80/30/4/Depp-EetF-2011-80-mesure-equite-pisa-indices-statistiques_203304.pdf .
Lee, S., & von Davier, M. (2020). Improving measurement properties of the PISA home possessions scale through partial invariance modeling. Psychological Test and Assessment Modeling, 62 (1), 55–83. Retrieved from https://www.psychologie-aktuell.com/fileadmin/Redaktion/Journale/ptam-2020-1/04_Lee.pdf
Lien, N., Friestad, C., & Klepp, K.-I. (2001). Adolescents’ proxy reports of parents’ socioeconomic status: How valid are they? Journal of Epidemiology and Community Health, 55 (10), 731–737. https://doi.org/10.1136/jech.55.10.731 .
Looker, E. (1989). Accuracy of proxy reports of parental status characteristics. Sociology of Education, 62 (4), 257. https://doi.org/10.2307/2112830 .
Mueller, C., & Parcel, T. (1981). Measures of socioeconomic status: Alternatives and recommendations. Child Development, 52 (1), 13–30.
O’Connell, M. (2019). Is the impact of SES on educational performance overestimated? Evidence from the PISA survey. Intelligence, 75, 41–47. https://doi.org/10.1016/j.intell.2019.04.005 .
OECD. (2001). Knowledge and skills for life: First results from PISA 2000. In PISA. Paris: OECD Publishing. https://doi.org/10.1787/9789264195905-en .
OECD. (2005a). PISA 2003 data analysis manual: SPSS. In PISA. Paris: OECD Publishing. https://doi.org/10.1787/9789264010666-en .
OECD. (2005b). PISA 2003 technical report. In PISA. Paris: OECD Publishing. https://doi.org/10.1787/9789264010543-en .
OECD. (2009). PISA 2006 technical report. In PISA. Paris: OECD Publishing. https://doi.org/10.1787/9789264048096-en .
OECD. (2012). PISA 2009 technical report. In PISA. Paris: OECD Publishing. https://doi.org/10.1787/9789264167872-en.
OECD. (2014). PISA 2012 technical report. Paris: OECD Publishing. Retrieved December 23, 2019 from https://www.oecd.org/pisa/pisaproducts/PISA-2012-technical-report-final.pdf .
OECD. (2017). PISA 2015 technical report. Retrieved July 31, 2017 from http://www.oecd.org/pisa/data/2015-technical-report/ .
OECD. (2018a). Effective teacher policies: Insights from PISA. In PISA. Paris: OECD Publishing. https://doi.org/10.1787/9789264301603-en .
OECD. (2018b). Equity in education: Breaking down barriers to social mobility. In PISA. Paris: OECD Publishing. https://doi.org/10.1787/9789264073234-en .
OECD. (2020). PISA 2018 technical report . Retrieved from https://www.oecd.org/pisa/data/pisa2018technicalreport/ .
Piketty, T. (2018). Brahmin left vs merchant right: Rising inequality and the changing structure of political conflict. WID. World Working Paper.
Pokropek, A., Borgonovi, F., & McCormick, C. (2017). On the cross-country comparability of indicators of socioeconomic resources in PISA. Applied Measurement in Education, 30 (4), 243–258. https://doi.org/10.1080/08957347.2017.1353985 .
Robitzsch, A., Kiefer, T., & Wu, M. (2019). TAM: test analysis modules. Retrieved from https://CRAN.R-project.org/package=TAM .
Rutkowski, D., & Rutkowski, L. (2013). Measuring socioeconomic background in PISA: One size might not fit all. Research in Comparative and International Education, 8 (3), 259–278. https://doi.org/10.2304/rcie.2013.8.3.259 .
Schulz, W. (2005). Measuring the socio - economic background of students and its effect on achievement in PISA 2000 and PISA 2003. Retrieved May 15, 2019 from https://files.eric.ed.gov/fulltext/ED493510.pdf .
Sirin, S. (2005). Socioeconomic status and academic achievement: A meta-analytic review of research. Review of Educational Research, 75 (3), 417–453. https://doi.org/10.3102/00346543075003417 .
Weber, M. (1922). Wirtschaft und Gesellschaft . Tübingen: Mohr.
Willms, J. (2006). Learning divides: Ten policy questions about the performance and equity of schools and schooling systems. UNESCO Institute for Statistics, Montreal. Retrieved February 20, 2019 from https://unesdoc.unesco.org/ark:/48223/pf0000147066 .
Willms, J., & Tramonte, L. (2015). Towards the development of contextual questionnaires for the PISA for development study. In OECD education working papers (Vol. 2015). Paris: OECD Publishing. https://dx.doi.org/10.1787/5js1kv8crsjf-en .
Willms, J. D., & Tramonte, L. (2019). The measurement and use of socioeconomic status in educational research. In L. E. Suter, B. Denman, & E. Smith (Eds.), The SAGE handbook of comparative studies in education . London: Sage.
Download references
Acknowledgements
Camille Marec provided excellent research assistance for all empirical analyses included in this article. This article benefitted from the suggestions and comments of other members of the PISA Analysis and Reporting team at the OECD, of the PISA 2018 Consortium, and of the PISA Technical Advisory Group—the author wishes to acknowledge, in particular, Jonas Bertling, Janine Buchholz, Alfonso Echazarra, Pauline Givord, Rachel Renbarger, Keith Rust, Leslie Rutkowski and Matthias von Davier. The author is solely responsible for all remaining errors and imprecisions.
The author has not received funding.
Author information
Authors and affiliations.
OECD, Directorate for Education and Skills, 2 Rue André Pascal, 75775, Paris Cedex 16, France
Francesco Avvisati
You can also search for this author in PubMed Google Scholar
Contributions
The author read and approved the final manuscript.
Corresponding author
Correspondence to Francesco Avvisati .
Ethics declarations
Competing interests.
FA was an analyst in the OECD PISA team when he prepared this article. The opinions expressed and arguments employed in this article are those of the author and do not necessarily represent the official view of the OECD or of its member countries.
Additional information
Publisher's note.
Springer Nature remains neutral with regard to jurisdictional claims in published maps and institutional affiliations.
See Tables 4 , 5 , 6 and 7 .
Rights and permissions
Open Access This article is licensed under a Creative Commons Attribution 4.0 International License, which permits use, sharing, adaptation, distribution and reproduction in any medium or format, as long as you give appropriate credit to the original author(s) and the source, provide a link to the Creative Commons licence, and indicate if changes were made. The images or other third party material in this article are included in the article's Creative Commons licence, unless indicated otherwise in a credit line to the material. If material is not included in the article's Creative Commons licence and your intended use is not permitted by statutory regulation or exceeds the permitted use, you will need to obtain permission directly from the copyright holder. To view a copy of this licence, visit http://creativecommons.org/licenses/by/4.0/ .
Reprints and permissions
About this article
Cite this article.
Avvisati, F. The measure of socio-economic status in PISA: a review and some suggested improvements. Large-scale Assess Educ 8 , 8 (2020). https://doi.org/10.1186/s40536-020-00086-x
Download citation
Received : 29 December 2019
Accepted : 27 May 2020
Published : 06 June 2020
DOI : https://doi.org/10.1186/s40536-020-00086-x
Share this article
Anyone you share the following link with will be able to read this content:
Sorry, a shareable link is not currently available for this article.
Provided by the Springer Nature SharedIt content-sharing initiative
- Background questionnaire
ORIGINAL RESEARCH article
Socioeconomic status and psychological well-being: revisiting the role of subjective socioeconomic status.
- 1 Department of Psychology, University of Jaén, Jaén, Spain
- 2 Department of Social Psychology, Faculty of Psychology, Mind, Brain and Behavior Research Center, University of Granada, Granada, Spain
Socioeconomic status (SES) is a complex and multidimensional construct, encompassing both independent objective characteristics (e.g., income or education) and subjective people’s ratings of their placement in the socioeconomic spectrum. Within the growing literature on subjective SES belongingness and psychological well-being, subjective indices of SES have tended to center on the use of pictorial rank-related social ladders where individuals place themselves relative to others by simultaneously considering their income, educational level, and occupation. This approach, albeit consistent with the idea of these social ladders as summative or cognitive SES markers, might potentially constrain individuals’ conceptions of their SES. This research ( N = 368; M age = 39.67, SD = 13.40) is intended to expand prior investigations on SES and psychological well-being by revisiting the role of subjective SES. In particular, it (a) proposes an innovative adaptation of the traditional MacArthur Scale of subjective SES to income, education, and occupation, thus resulting in three separate social ladders; and (b) tests the empirical contribution of such three social ladders to psychological well-being. Overall, our findings showed that the novel education and occupation ladders (excluding the income ladder) are predictive of a significant part of the variance levels of psychological well-being that is not due to canonical objective metrics of SES (i.e., income, education, and occupation), or to the conventional MacArthur Scale of subjective SES. Although preliminary, these results underscore the need to further reconsider (subjective) SES-related conceptualization and measurement strategies to gather a more comprehensive understanding of the SES-psychological well-being link.
Introduction
During the last decade, the psychology of socioeconomic status (SES) or social class, which is broadly characterized as a social stratification system derived from access to various resources (economic, social, etc.; Moya and Fiske, 2017 ), has experienced a remarkable growth (see Manstead, 2018 ). Such increased interest has been fundamentally driven by the onset of the Great Recession, which is connected to the broadening gap between the “haves” and the “have-nots” ( Pfeffer et al., 2013 ). Indeed, in this socioeconomic climate, class disparities and their detrimental wide-ranging consequences across distinct domains are more visible ( Moya and Fiske, 2017 ). Although it could be argued that almost all people’s psychological and social outcomes are largely influenced by their objective or perceived socioeconomic standing, ranging from food preferences ( Baumann et al., 2019 ) and speech patterns ( Kraus et al., 2019 ) to humor-related dispositions ( Navarro-Carrillo et al., 2020 ) and identity ( Easterbrook et al., 2020 ), empirical research has mainly focused on investigating the connections between SES and psychological well-being and health-related aspects (e.g., Howell and Howell, 2008 ; Curhan et al., 2014 ; Präg et al., 2016 ; Huang et al., 2017 ).
Cumulative empirical evidence has highlighted that long-established objective metrics of SES, such as income, educational level, and occupation, only show low to modest correlations with personal well-being indicators ( Diener and Oishi, 2000 ; Howell and Howell, 2008 ). In contrast, a growing number of studies have revealed that subjective assessments of SES exhibit robust associations with well-being and health scores above and beyond objective SES (e.g., Adler et al., 2000 ; Kraus et al., 2013 ; Garza et al., 2017 ; Navarro-Carrillo et al., 2019 ). Within this area, while objective SES is commonly assessed using various indices of material wealth (e.g., income, education), subjective SES is primarily assessed using the MacArthur Scale of Subjective Social Status (MacArthur SSS scale; Adler et al., 2000 ), a pictorial format measure represented by a 10-rung social ladder on which people indicate their socioeconomic standing relative to others in society based on income, educational level, and occupation. Within psychological and health sciences, the development and subsequent consideration of this measure, whose theoretical underpinnings rely upon social comparison processes, have provided a substantial contribution in terms of the clarification of the complex nature of the SES–well-being connection. In particular, researchers have posited that the MacArthur SSS scale, insofar as it allows individuals to capture their own social standing in a personalized manner across the SES components, could represent a cognitive average of classical objective SES indices (i.e., a general marker of a person’s SES), thereby providing a more accurate estimation of SES (see Präg et al., 2016 ).
Given the multidimensional nature of SES mentioned above, the joint assessment of objective and subjective SES indices is invariably recommended ( Kraus and Stephens, 2012 ; Rubin et al., 2014 ), as this approach would facilitate comparisons between the various facets of SES within the framework of their contribution to well-being. Indeed, that constitutes one of the major strands of research in the psychology of SES. Our paper, which is precisely embedded in that research sphere, is aimed at extending prior investigations on the SES–well-being link by presenting and testing an innovative approach to subjective SES evaluation. Although prior empirical findings indicate that conventional objective markers of SES (i.e., income, education, and occupation) are only moderately inter-correlated and thus represent independent (and not interchangeable) components of SES ( Torssander and Erikson, 2010 ), the MacArthur SSS scale considers these objective facets of SES in a simultaneous and undifferentiated manner within the person’s social comparison process. This notion, albeit aligned with the view of the MacArthur SSS scale as a subjective general (and summative) SES marker, does not allow the scientific community to ascertain the specific weight that people attribute to each component of SES when subjectively estimating their socioeconomic position relative to others. Therefore, we aim to address this gap by (a) adapting the MacArthur SSS scale to income, educational level, and occupation and (b) unveiling whether any of these three novel social ladders (one for each SES indicator) are predictive of a significant proportion of the variance of well-being that is not attributable to neither objective measures of SES (i.e., income, education, and occupation) nor the traditional MacArthur SSS scale.
Objective and Subjective Socioeconomic Status
Objective SES has been traditionally defined by access to material and social dimensions ( Oakes and Rossi, 2003 ; Snibbe and Markus, 2005 ). Accordingly, this form of SES is usually operationalized by considering various objective indicators that may ultimately reflect differences in individuals’ access to material and social resources. In particular, among the multiple objective indices of SES, three distinctive aspects emerge quite clearly: income, educational level, and occupation ( Kraus and Stephens, 2012 ; Baker, 2014 ; Manstead, 2018 ).
Income establishes the access path to desired services, material goods, and pleasant experiences, among other things ( Lucas and Schimmack, 2009 ; Kraus and Stephens, 2012 ). In addition, and as a proof of the importance of income, prior research has shown that this indicator is connected with a broad array of psychological variables, such as social trust ( Brandt et al., 2015 ), personality ( Piff, 2014 ), and prosocial tendencies ( Piff and Robinson, 2017 ). Like income, education is widely considered a canonical marker of objective SES. As Snibbe and Markus (2005) synthetized, educational level allows researchers to capture relevant sociocultural and psychosocial-related outcomes (e.g., behavioral patterns, lifestyle). Moreover, higher educational level has been linked to beneficial economic outcomes, such as, for instance, diminished financial hardship ( American Psychological Association, 2007 ). Occupation, for its part, has been argued to be a further proxy for objective SES because of its tight connection to earnings and educational level ( Duncan and Magnuson, 2012 ) and its capacity to differentially shape psychological experiences ( American Psychological Association, 2007 ; Kraus and Stephens, 2012 ). Nevertheless, this indicator of objective SES is used less than income and education in psychological research.
Objective SES is frequently measured in undergraduate samples (by utilizing a global index encompassing family income and parental educational level; Kraus and Keltner, 2009 ; Côté et al., 2017 ) and community-based samples (by including a specific objective indicator or by building a composite index defined by the combination of some of these dimensions, particularly income and educational level; Kraus and Park, 2014 ; Navarro-Carrillo et al., 2018 ). Earlier research revealed that these objective facets of SES are moderately inter-correlated ( Singh-Manoux et al., 2003 ), which suggests that these indices should be distinguishable. In this vein, the Report of the APA Task Force on Socioeconomic Status ( American Psychological Association, 2007 ) stated that “it is generally more informative to assess the different dimensions of SES and understand how each contributes to an outcome under study rather than merge the measures” (p. 11).
SES is not exclusively shaped by material resources. Indeed, current approaches underscore that subjective assessments founded on social comparison processes (e.g., determining one’s own socioeconomic position relative to that of other individuals or groups) play a pivotal role in shaping SES ( Boyce et al., 2010 ; Kraus, 2018 ). Consistent with this emerging perspective, subjective SES is conceptualized as individuals’ perceptions pertaining to their standing in the social hierarchy relative to others ( Adler et al., 2000 ; Kraus et al., 2012 ).
Although different methods of assessing subjective SES exist (see Rubin et al., 2014 ), one of the few such tools explicitly based on a relative social comparison process is the MacArthur SSS scale ( Adler et al., 2000 ). Furthermore, this measure is the dominant means of evaluating subjective SES ( Cundiff and Matthews, 2017 ). Using this graphical 10-rung ladder, which represents ascending positions based on income, educational level, and occupation, individuals estimate their SES by marking the rung where they place themselves relative to others in society in general or in a specific social group or community.
As in the case of objective SES, subjective SES is commonly assessed using the MacArthur SSS scale in undergraduate ( Jury et al., 2019 ; Loeb and Hurd, 2019 ) and community-based ( Bjornsdottir et al., 2017 ; Wang et al., 2019 ) samples. However, studies that use this measure among adolescents are becoming more frequent (e.g., Joffer et al., 2019 ; Moor et al., 2019 ). Prior research has constantly shown that the MacArthur SSS scale exhibits mostly moderated associations with traditional objective SES indicators ( Adler et al., 2000 ; Ostrove et al., 2000 ), thus providing evidence of its conceptual and empirical differentiation from objective SES.
Objective and Subjective Socioeconomic Status and Psychological Well-Being
The analysis of the empirical connection between SES and psychological well-being and health has been the focus of much controversy across the various disciplines interested in addressing this issue. This stems at least in part from the various approaches used to conceptualize and measure SES as a relevant factor for well-being and health. Notwithstanding the above, examining the socioeconomic determinants of psychological well-being—which broadly refers to optimal human functioning and the eagerness to reach meaningful vital objectives ( Ruini and Cesetti, 2019 )—became of particular interest because, throughout different studies, its desirable effects on various personal domains have been substantiated. For instance, higher levels of psychological well-being have been related to positive family experiences and optimal biological functioning ( Ryff, 2014 ), as well as with reduced depression levels ( Ryff and Keyes, 1995 ; Ruini and Cesetti, 2019 ).
Many studies have amassed empirical evidence on the positive relationship between SES (as measured by objective, classical indices of material wealth) and psychological well-being/health-related factors (e.g., Diener and Oishi, 2000 ; Diener and Biswas-Diener, 2002 ; Diener et al., 2003 ; Vera-Villarroel et al., 2015 ). Nevertheless, it is important to note that the strength of such associations is relatively modest. For instance, Howell and Howell (2008) , in a meta-analytic research analyzing the relationship between objective SES and personal well-being in a total of 111 independent samples from 54 countries worldwide, revealed that the average estimated association of these variables was approximately r = 0.13.
Studies that examined the role of subjective SES (as measured by the MacArthur SSS scale or equivalent scales) have provided valuable comprehensive knowledge on the SES–well-being/health connection. Classical empirical works, such as those developed by Adler et al. (2000) , Goodman et al. (2003) , Singh-Manoux et al. (2003) , or Cohen et al. (2008) , established the foundations on which more recent investigations were built. This groundbreaking research has shown that subjective SES is, compared to objective SES, a stronger predictor of psychological functioning indicators (e.g., control over life) and physiological outcomes (e.g., heart rate and sleep latency) among healthy white women ( Adler et al., 2000 ), body mass index among adolescents ( Goodman et al., 2003 ), ill health among civil service employees ( Singh-Manoux et al., 2003 ), and susceptibility to upper respiratory infection among healthy men and women ( Cohen et al., 2008 ). Importantly, these effects of subjective SES on well-being and health-related outcomes were independent of the respondents’ objective SES, thereby by providing solid preliminary support for the independent contribution of subjective socioeconomic standing to well-being/health. Results from subsequent investigations are also in keeping with those mentioned above. Thus, the stronger connection of subjective SES, as compared to conventional objective markers of SES, with various psychological well-being and health-related aspects has recently been proved by valuable research findings. For instance, subjective SES (as measured by a social ladder comparable to the MacArthur SSS scale) was found to be associated with psychological well-being and self-perceived health even after controlling for objective SES across 29 countries ( Präg et al., 2016 ). Along the same lines, Cundiff and Matthews (2017) , after analyzing a total of 31 studies, demonstrated that subjective SES (assessed by the MacArthur SSS scale) had a unique relationship with physical health in adults over and above canonical objective indices of SES. Similarly, Tan et al. (in press) examined the link between objective and subjective SES and well-being in a total of 336 independent samples. Their results not only confirmed that the estimated subjective SES–well-being association was significantly larger (almost twice) than that of the objective SES–well-being association, but also illuminated differences that depended on the type of objective and subjective SES measure. In particular, in terms of objective SES, their data showed that the meta-analytic effect size corresponding to the relationship of income with well-being was higher than that of education. Regarding subjective SES measures, the meta-analytic effect size of the relation between the MacArthur SSS scale and well-being was higher than that of the connection between the perceived SES category and well-being, thus verifying the crucial role of the MacArthur SSS scale.
In summary, there is increasingly solid evidence that, beyond the objective material substance of SES, individuals’ subjective perceptions of their position in the socioeconomic hierarchy capture specific differences in well-being/health. However, to the best of our knowledge, no empirical investigation has yet addressed whether the subjective placement within three distinctive graphical social ladders based on income, education, and occupation, rather than within a unique social ladder that considers these three (empirically distinguishing) dimensions of SES together, would uniquely account for psychological well-being scores. Adding to the growing literature on the determination of the ability of various SES indicators to predict well-being, we surmise that these new exploratory approaches could refine subjective SES measurement by elucidating the particular role of such differentiated SES components. Thus, such an approach would facilitate the gathering of comprehensible information pertaining to the need (or absence thereof) for further research to evaluate subjective SES by considering income, education, and occupation using separate social ladders.
Materials and Methods
Participants.
The sample was composed of 368 participants ( M age = 39.67, SD = 13.40, range from 18 to 90), of whom 64.4% were women, 34.5% were men, and 1.1% did not identify themselves as women or men. A total of 19.8% of the participants were single, 17.9% were dating, 11.4% were cohabiting, 42.9% were married, 5.4% were divorced, and 2.4% were widowers. A sensitive power analysis was conducted using linear multiple regressions: fixed model, R 2 deviation from zero in G ∗ Power ( Faul et al., 2009 ) to determine our ability to detect the contribution of each SES indicator on psychological well-being. Taking our sample ( N = 368, α = 0.05) into account, the sensitivity analysis suggests that effect sizes of f 2 ≥ 0.04 are necessary to produce power at the 0.80 level.
A snowball sampling procedure via online administration was used to recruit the participants. Specifically, before the questionnaire was distributed, undergraduate students at a university in southeastern Spain were trained in sampling methods. Collaborators were asked to distribute the questionnaire only to adults of legal age (≥ 18 years). Afterward, they contacted potential respondents (e.g., acquaintances) and provided them with a brief description of the study. Once the participants agreed to participate in the study, they were given access to the online survey. At the beginning of the survey, the participants received information that emphasized the principles of confidentiality and anonymity in this research, their voluntary participation, and the estimated duration. In addition, they were given the e-mail address of one of the researchers in the case they needed to resolve any issues arising from their participation. After signing an informed consent form, the participants completed the questionnaire. Finally, the undergraduate students in charge of distributing the online survey among potential respondents received partial academic credit in exchange for their participation. The study was approved by the ethical committee of the southeastern Spanish university and carried out in compliance with the ethical standards of the Declaration of Helsinki.
Psychological Well-Being
The Spanish adaptation of Ryff’s Psychological Well-Being Scales (PWBS; Díaz et al., 2006 ) was used. It consists of 29 items rated on a 6-point Likert scale ranging from 1 ( strongly disagree ) to 6 ( strongly agree ) that covered six subscales: self-acceptance (e.g., “When I review the story of my life I am happy with how things have turned out”; α = 0.80), positive relationships with others (e.g., “I feel that my friends bring me many things”; α = 0.68), autonomy (e.g., “I have confidence in my opinions even if they are contrary to the general consensus”; α = 0.62), environmental mastery (e.g., “In general, I feel that I am responsible for the situation in which I live”; α = 0.63), purpose in life (e.g., “I have clear the direction and purpose of my life”; α = 0.81), and personal growth (e.g., “I have the feeling that over time I have grown as a person”; α = 0.77). High scores indicated high levels of psychological well-being. The PWBS has six subscales grouped into a second-order factor called global psychological well-being ( Ryff and Keyes, 1995 ). Because the proposed six-dimensional structure with a second-order general factor has been confirmed with Spanish samples ( Díaz et al., 2006 ; Van Dierendonck et al., 2008 ), we also computed the items’ average as a global indicator of psychological well-being (α = 0.88).
Subjective SES
The traditional 10-rung social ladder MacArthur SSS scale ( Adler et al., 2000 ) was administered. Participants were asked to select the rung that represented their position in the social hierarchy relative to others in society in terms of income, educational level, and occupation. High numbers were indicative of higher placement on this social ladder.
MacArthur SSS scale adaptations to income, education, and occupation
Based on the traditional MacArthur SSS scale, we created three pictorial social-related ladders to independently tap people’s subjective perceptions of their (a) income, (b) educational level, and (c) occupational status. Thus, respondents were presented three adapted 10-rung social ladders, one for each SES indicator: (a) Income ladder . This ladder assessed the individuals’ subjective perceptions of their position in the social hierarchy relative to others in society in terms of income-money. Participants were asked to indicate the rung of this ladder on which they believed they stood, considering that individuals at the top of the ladder would have the most income-money, whereas those at the bottom would have the least income-money; (b) Education ladder. This ladder evaluated the individuals’ subjective perceptions of their position in the social hierarchy relative to others in society terms of educational level. Participants were asked to select the rung of this ladder on which they perceived they stood, taking into account that people at the top of the ladder would have the most education, whereas those at the bottom would have the least education; (c) Occupation ladder . This ladder assessed the individuals’ subjective perceptions of their position in the social hierarchy relative to others in society in terms of occupational status. Participants had to select the rung of this ladder on which they perceived they stood, considering that individuals at the top of the ladder would have the best jobs, whereas those at the bottom would have the worst jobs or no job.
Objective SES
Respondents indicated their family’s approximate net monthly income, considering all income sources (e.g., salaries, pensions, scholarships, rental income, etc.). Income was coded into ten categories, from 1 (<650€) to 10 (>5.800€).
Educational level
Participants indicated the highest level of education they had completed. Educational level was coded into eight categories, from 1 (primary school) to 8 (doctoral degree).
Participants indicated which professional occupation best described the type of work they do ( European Social Survey, 2018 ). In this research, occupational status was coded into ten categories, from 1 (unemployed) to 10 (technical professional occupations).
The participants’ distribution in terms of these indices of objective SES is given in the results section.
Statistical Analyses
First, frequency distribution analyses and reliabilities were obtained. Second, Pearson product-moment correlations were performed to test the relationships among the objective and subjective SES indicators and the various psychological well-being dimensions. Before we conducted the hierarchical regression analyses, age, and objective and subjective SES measures were standardized. Then, as an initial check, we confirmed that the collinearity statistics did not exceed the recommended values ( Akinwande et al., 2015 ). Afterward, we performed the hierarchical regression analyses, in which we entered common sociodemographic factors (i.e., gender, age, and marital status) in Step 1 (method: enter). Then, we added objective SES indicators as predictors in Step 2 (method: enter). We included the traditional MacArthur SSS scale in Step 3 (method: enter). Lastly, we entered the new proposed ladders for income, educational level, and occupation in Step 4 (method: enter) to estimate their added value in explaining variance in the criterion variables and to determine their potential unique contribution to psychological well-being above and beyond demographics, objective SES, and the MacArthur SSS scale. We separately introduced self-acceptance, positive relationships with others, autonomy, environmental mastery, purpose in life, personal growth, and global psychological well-being as criteria throughout each regression analysis. We computed the abovementioned analyses using SPSS Version 21.
Description of Objective and Subjective SES Indicators
Regarding the objective SES indicators, the respondents’ income distribution were as follows: <650€ (5.7%), 651€–1300€ (22.8%), 1301€–1950€ (21.7%), 1951€–2600€ (20.9%), 2601€–3250€ (9.5%), 3251€–3900€ (8.4%), 3901€–4550€ (6%), 4551€–5200€ (2.2%), 5201€–5800€ (1.4%), and >5.800 € (1.4%). For educational level, we found the following distribution: primary school (9%), secondary education (5.7%), vocational training (14.4%), bachelor’s degree (9.5%), incomplete university degree (19.6%), university degree (27.4%), master’s degree (10.3%), and doctoral degree (4.1%). Finally, concerning participants’ occupation, we found the following: unemployed (17.4%), agricultural work (e.g., agricultural or livestock worker, day laborer, tractor driver, fisherman, etc.; 3.5%), unskilled worker (e.g., pawn, loading or unloading waiter, unskilled factory worker, etc.; 2.2%), semi-skilled worker (e.g., bricklayer, bus driver, cannery operator, carpenter, metallurgy worker, baker, etc.; 3.5%), skilled worker (e.g., foreman, mechanic, copyist, turner or milling machine, electrician, etc.; 4.6%), occupations related to the service sector (e.g., restaurant owner, police officer, waiter, caretaker, hairdresser, armed forces, etc.; 15.2%), commercials (e.g., sales manager, store owner, store clerk, insurance agent, etc.; 8.2%), office work (e.g., secretary, administrative, accounting, etc.; 6%), senior management administrative occupations (e.g., banking executive, executive of a large company or organization, senior public administration officer, delegate, union, etc.; 3.8%), and technical professional occupations (e.g., doctor, teacher, engineer, artist, financial director, etc.; 33.7%).
The frequency distribution of the subjective SES indicators is represented graphically in Figure 1 . As this figure illustrates, some differences in the distribution rate of the traditional MacArthur SSS scale compared to each novel ladder for income, education, and occupation, as well as between these new ladders, can be observed. For instance, the mean of the traditional MacArthur SSS scale was 6.04 ( SD = 1.47); the means of the income, education, and occupation ladders were 5.25 ( SD = 1.63), 7.15 ( SD = 1.62), and 5.61 ( SD = 2.11), respectively. Responses ranged from 1 to 10 for all ladders.
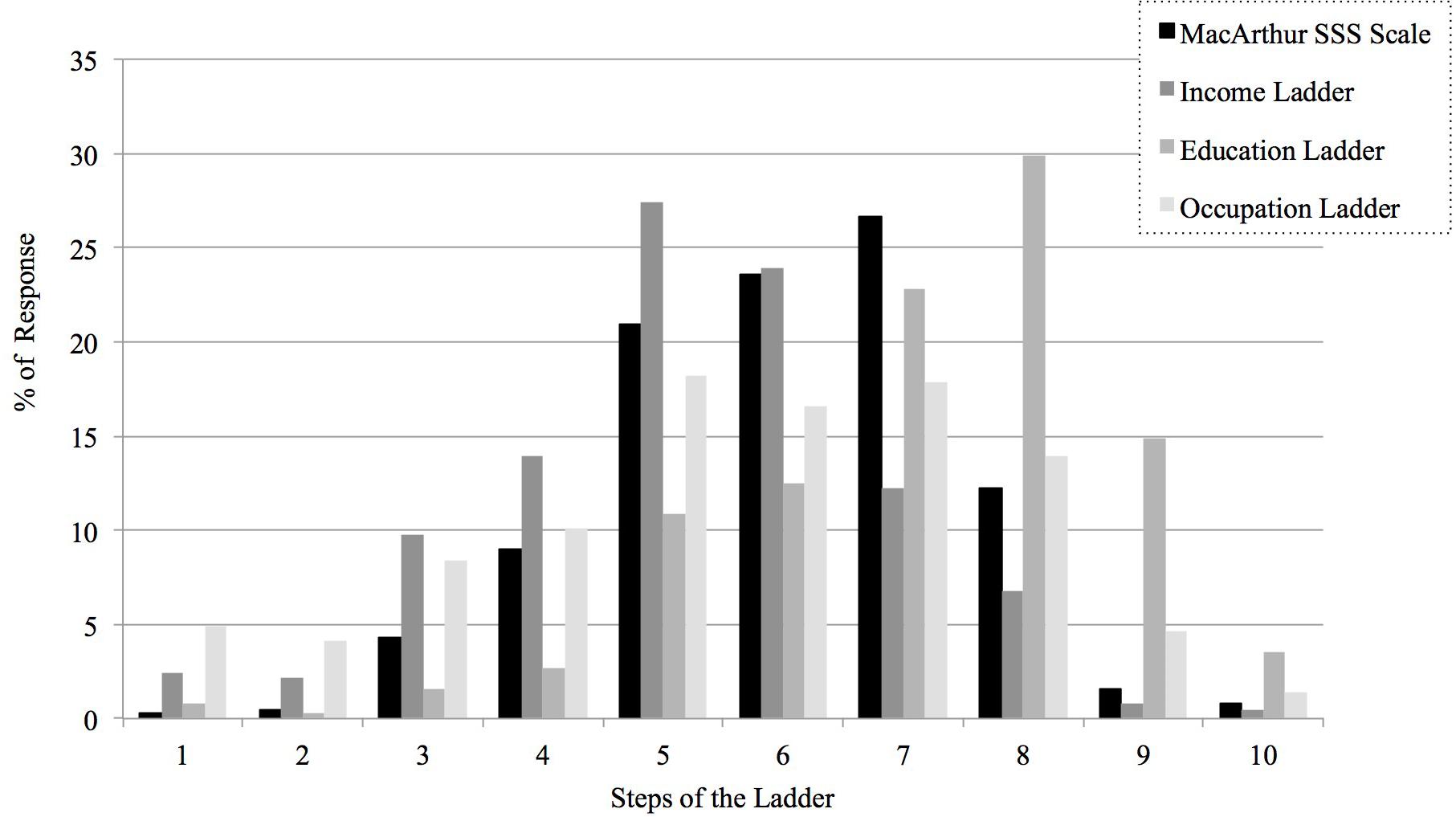
Figure 1. Frequency distribution of each subjective SES indicator.
Associations Between Objective and Subjective SES Indicators
Pearson correlations among objective and subjective SES indicators are given in Table 1 . Objective SES indices (i.e., income, education, and occupation) were positively inter-correlated with each other ( rs ≥ 0.25, p < 0.001). Furthermore, the traditional MacArthur SSS scale was found to be significantly and positively correlated with all the objective SES indicators: r income = 0.30, p < 0.001; r education = 0.12, p = 0.022; and r occupation = 0.24, p < 0.001. In addition, the traditional MacArthur SSS scale was also positively related to the novel income, education, and occupation ladders. Specifically, in this case, the correlations of the traditional MacArthur SSS scale with the income, education, and occupation ladders were higher: r income ladder = 0.62, p < 0.001; r education ladder = 0.36, p < 0.001; and r occupation ladder = 0.55, p < 0.001. Nonetheless, the coefficients were lower than 0.70, ruling out multicollinearity concerns. This pattern of correlations seems to indicate that although the traditional MacArthur SSS scale and the new proposed ladders for income, educational level, and occupation undoubtedly share components, they also differ. This supports the existence of differences between such subjective SES measures. Finally, the income, education, and occupation ladders exhibited significant and positive weak-to-moderate relationships with objective SES factors (i.e., income, education, and occupation). Among these, the only exception was the connection between educational level and the income ladder ( ps > 0.05).
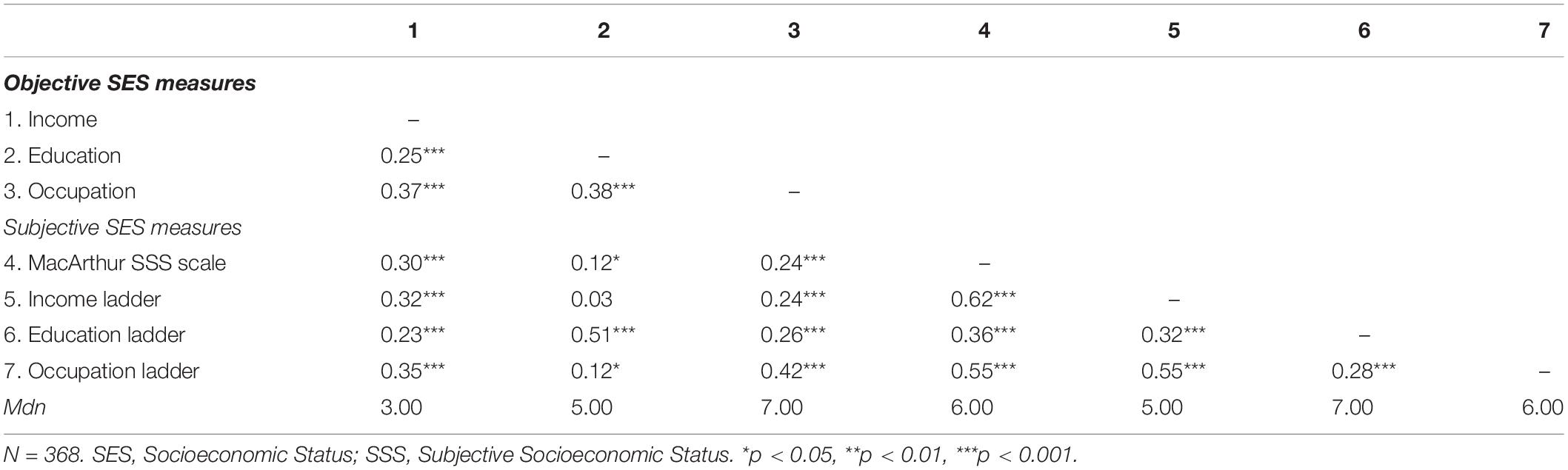
Table 1. Pearson correlations between objective and subjective SES indicators.
Associations Between Objective and Subjective SES Indicators and Psychological Well-Being
Pearson correlations among objective and subjective SES indicators and psychological well-being scales are given in Table 2 . The traditional MacArthur SSS scale did not correlate with autonomy ( r = 0.02, p = 0.712). However, this measure of subjective SES was positively related to self-acceptance, positive relationships with others, environmental mastery, purpose in life, personal growth, and global psychological well-being. Specially, weak correlations coefficients were found for the link between the traditional MacArthur SSS scale and positive relationships with others ( r = 0.15, p = 0.003) and personal growth ( r = 0.16, p = 0.003). More intense associations emerged between the traditional MacArthur SSS scale and self-acceptance, environmental mastery, purpose in life, and global psychological well-being ( rs ≥ 0.25, p < 0.001).
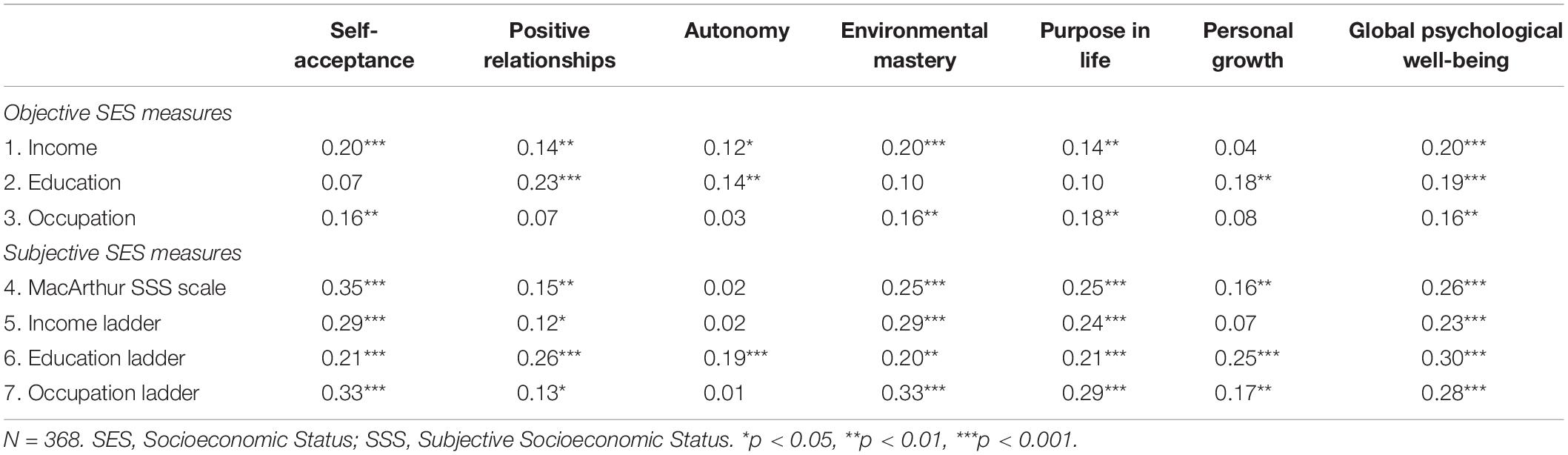
Table 2. Bivariate correlations of objective and subjective SES indicators with psychological well-being.
Focusing on the novel income, education, and occupation ladders, our results showed that the income ladder did not correlate with autonomy ( r = 0.02, p = 0.726) or personal growth ( r = 0.07, p = 0.175). However, it was positively and significantly associated with self-acceptance, positive relationships with others, environmental mastery, purpose in life, and global psychological well-being ( rs ≥ 0.12). Note that the same pattern of correlations was found for income ( rs ≤ 0.20) as an objective feature of SES. Nevertheless, these associations were stronger for the subjective income ladder. Likewise, the education ladder was positively and significantly related with each component of well-being ( rs ≥ 0.19), as well as with the global psychological well-being factor ( rs = 0.30, p < 0.001). By contrast, objective educational level did not correlate with self-acceptance ( r = 0.07, p = 0.172), environmental mastery ( r = 0.10, p = 0.065), and purpose in life ( r = 0.10, p = 0.055). This indicator of objective SES was found to correlate with the rest of measures of psychological well-being ( rs ≤ 0.23); however, these associations were stronger for the education ladder. Finally, the occupation ladder did not correlate with autonomy ( r = 0.01, p = 0.822). Nevertheless, it was positively and significantly associated with self-acceptance, positive relationships with others, environmental mastery, purpose in life, personal growth, and global psychological well-being ( rs ≥ 0.13). A different pattern of associations was found for occupation as an objective indicator of SES. Occupation did not correlate with autonomy, positive relationships with others, and personal growth ( rs ≤ 0.08); however, it showed a positive association with self-acceptance, environmental mastery, purpose in life, and general psychological well-being ( rs ≤ 0.18).
Hierarchical Regression Analyses Predicting Psychological Well-Being
Table 3 through 9 give the findings from the set of multiple hierarchical regression analyses predicting each component of psychological well-being (as well as its general indicator) from demographic factors (i.e., gender, age, and marital status), and the objective and subjective SES measures as predictors.
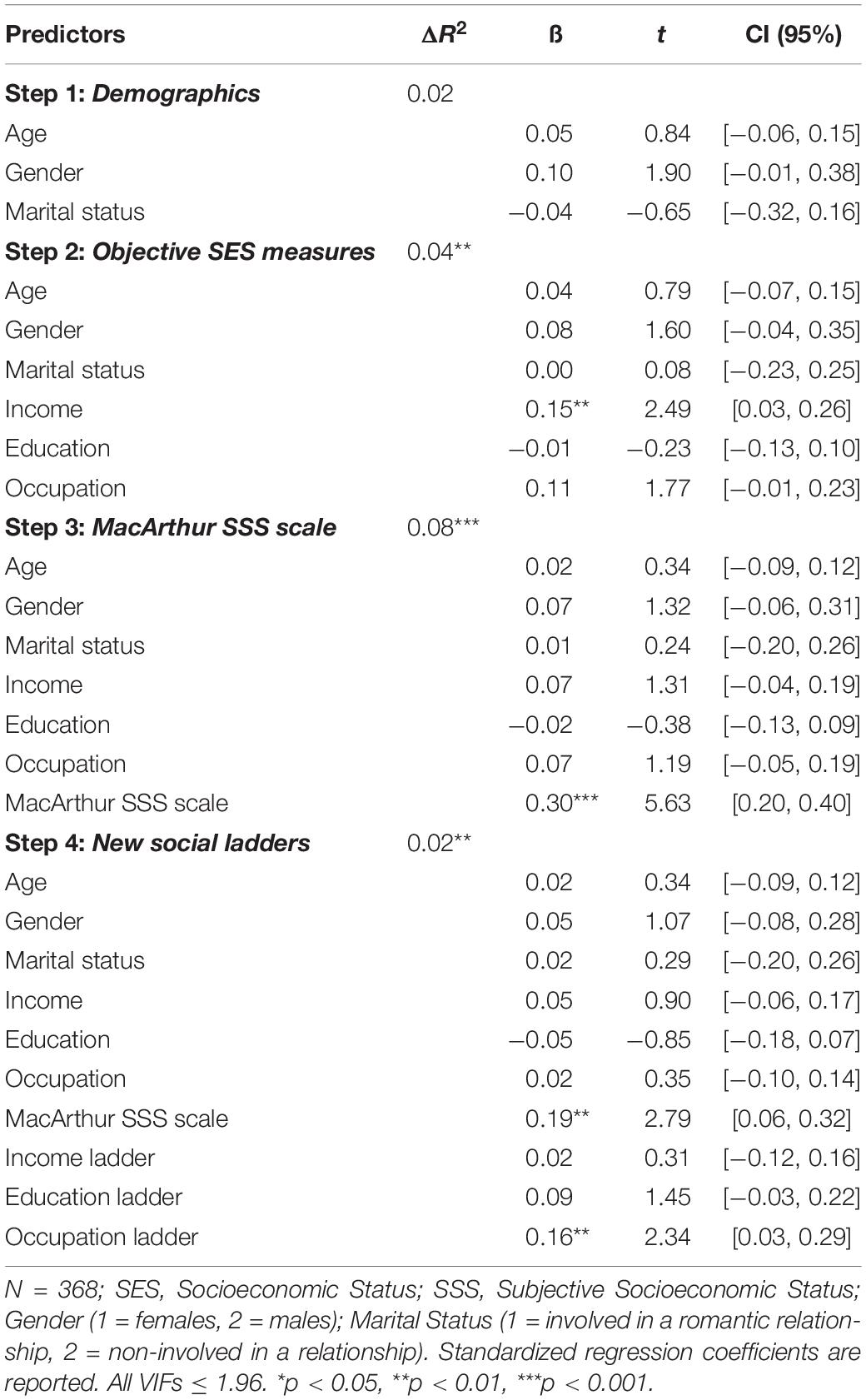
Table 3. Hierarchical regression analysis predicting self-acceptance.
Self-Acceptance
When demographic characteristics were controlled in Step 1 (see Table 3 ), our results revealed that, among the indicators of objective SES entered in Step 2, only income exerted predictive utility (β = 0.15, p = 0.013; 95% CI [0.03, 0.26]) regarding participants’ self-acceptance. Thus, the higher the income, the greater the levels of self-acceptance.
Regarding the traditional MacArthur SSS scale, which was included in Step 3, a respondent’s higher placement on this ladder (β = 0.30, p < 0.001; 95% CI [0.20, 0.40]) was indicative of a greater level of self-acceptance.
When we focused on the income, education, and occupation ladders (see Table 3 ), our results showed that, after controlling for demographics, objective metrics of SES (i.e., income, education, and occupation), and the MacArthur SSS scale, the occupation ladder emerged as a significant predictor of self-acceptance (β = 0.16, p = 0.020; 95% CI [0.03, 0.29]). Therefore, the higher the respondent’s placement in the occupation ladder, the greater their score on self-acceptance. The addition of these new social ladders in Step 4 accounted for incremental criterion variance (2%), F (3,341) = 3.16, p = 0.025.
Positive Relationships
As with self-acceptance (see Table 4 ), income was found to predict positive relationships (β = 0.12, p = 0.044; 95% CI [0.00, 0.23]). However, in this case, educational level also showed predictive utility regarding positive relationships (β = 0.20, p = 0.001; 95% CI [0.08, 0.31]).
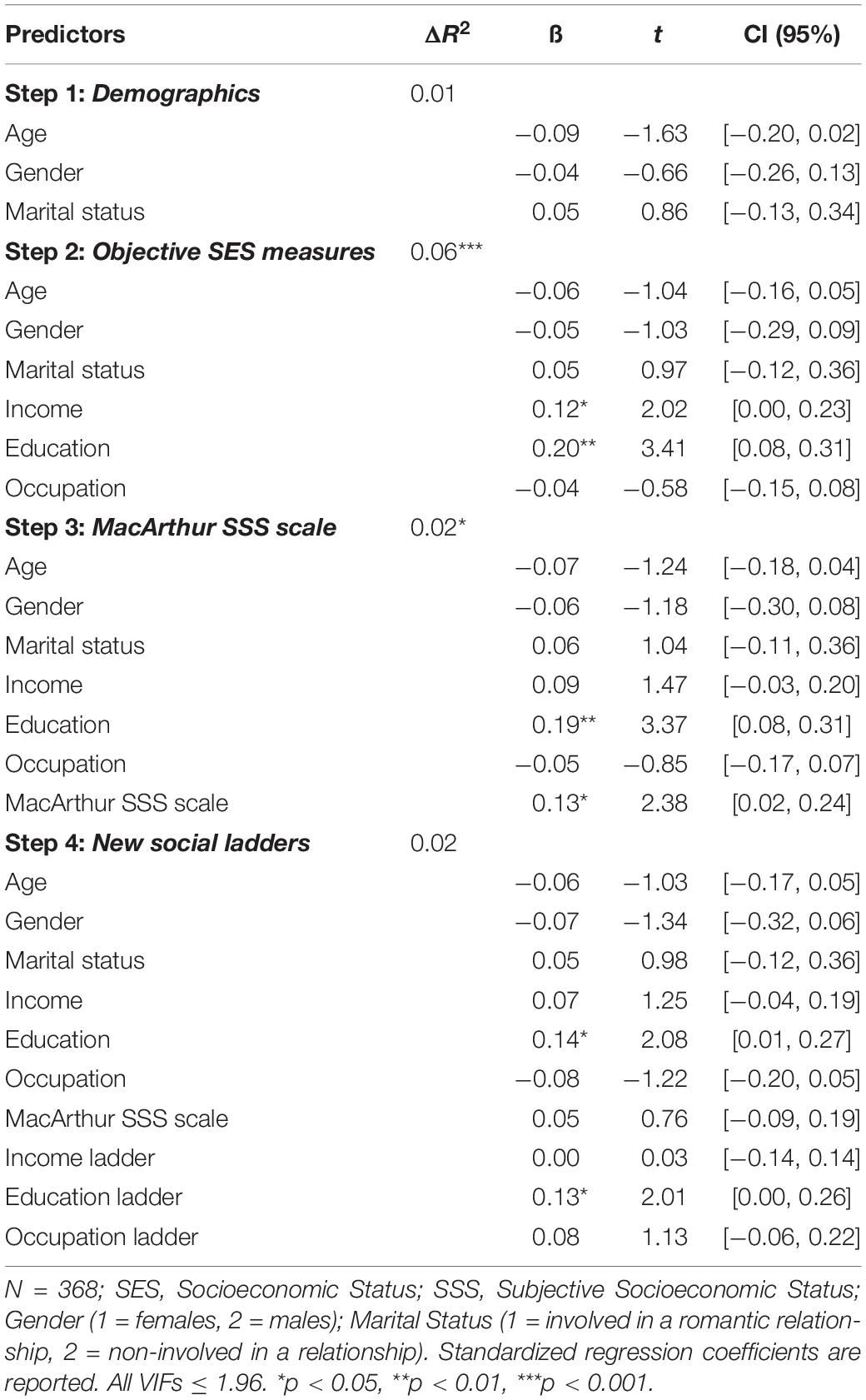
Table 4. Hierarchical regression analysis predicting positive relationships.
The MacArthur SSS scale contributed to the prediction of positive relationships (β = 0.13, p = 0.018; 95% CI [0.02, 0.24]). Thus, participants who placed themselves higher on the MacArthur SSS scale reported increased positive relationships.
As for the novel indicators of subjective SES entered in the last step (see Table 4 ), only the education ladder yielded a significant contribution to the prediction of positive relationships (β = 0.13, p = 0.045; 95% CI [0.00, 0.26]) above and beyond demographics, objective SES indicators, and the MacArthur SSS scale. Albeit not significant, F (3,341) = 2.02, p = 0.112, the inclusion of the income, education, and occupation ladders in the regression equation accounted for a 2% variance in the criterion measure. Furthermore, as shown in Table 4 , the traditional MacArthur SSS scale no longer showed predictive utility regarding positive relationships in this last step (β = 0.05, p = 0.447; 95% CI [−0.09, 0.19]).
As can be seen in Table 5 , and as in all other such previous cases, a higher income was indicative of greater levels of autonomy (β = 0.12, p = 0.040; 95% CI [0.01, 0.23]), even after accounting for respondents’ demographic characteristics.
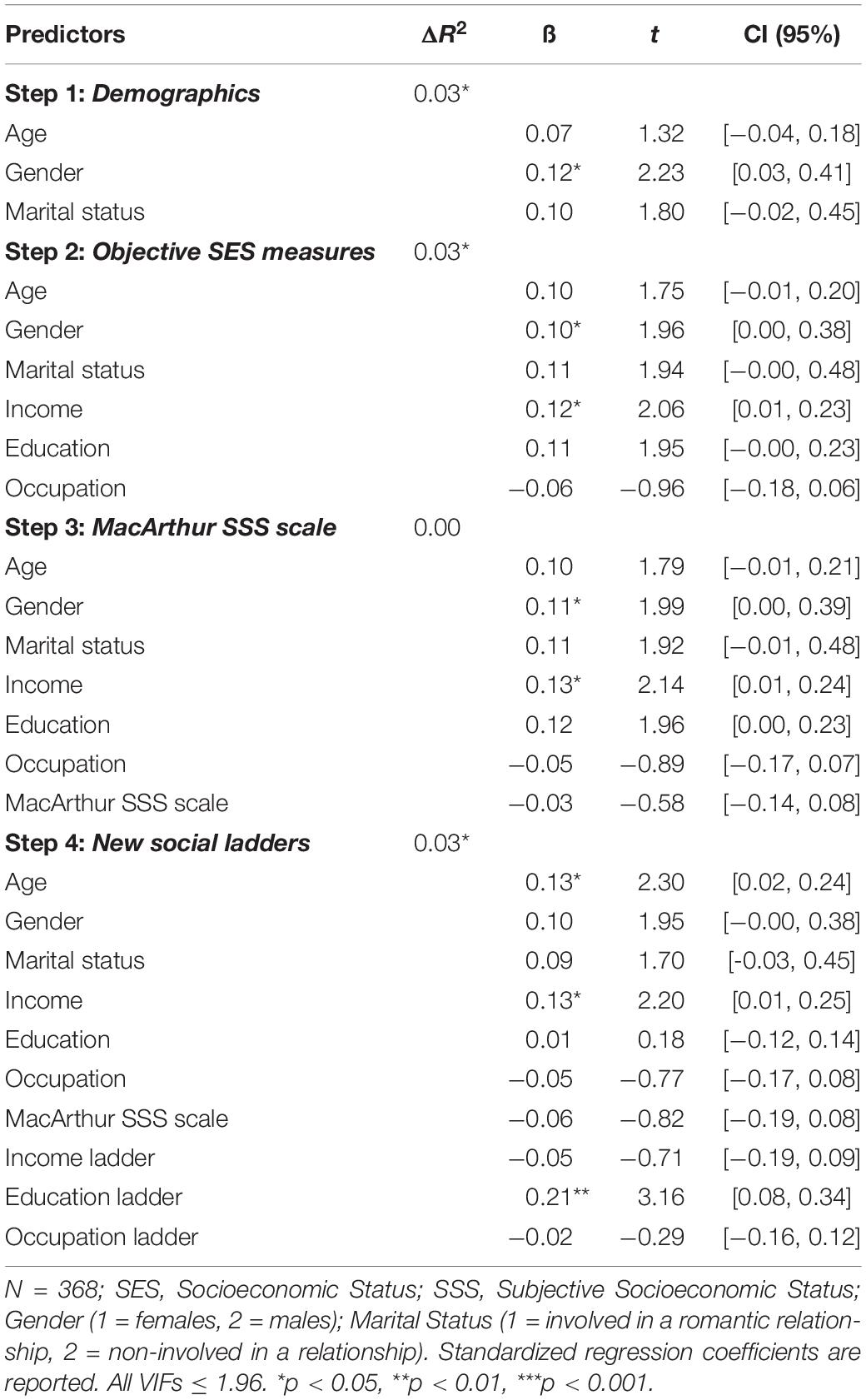
Table 5. Hierarchical regression analysis predicting autonomy.
Turning now to the MacArthur SSS scale (see Table 5 ), our results did not yield a significant contribution of this indicator of subjective SES to the prediction of autonomy (β = −0.03, p = 0.561; 95% CI [−0.14, 0.08]). Moreover, the inclusion of the traditional MacArthur SSS scale in this third step did not significantly account for incremental variance (0%), F (1,344) = 0.34, p = 0.561.
As with positive relationships, the education ladder emerged as a predictor of the participants’ levels of autonomy even after accounting for demographics, objective SES measures, and the MacArthur SSS scale (see Table 5 ). In particular, respondents who placed themselves higher on this education ladder were more inclined to report greater scores on autonomy (β = 0.21, p = 0.002; 95% CI [0.08, 0.34]). Importantly, the addition of the new social ladders (i.e., the income, education, and occupation) in Step 4 accounted for incremental criterion variance (3%), F (3,341) = 3.36, p = 0.019.
Environmental Mastery
As in the preceding cases, income was found to predict environmental mastery beyond demographics (β = 0.15, p = 0.012; 95% CI [0.03, 0.26]). Therefore, higher income was indicative of greater scores on environmental mastery.
As can be seen in Table 6 , the MacArthur SSS scale significantly contributed to the prediction of environmental mastery (β = 0.17, p = 0.003; 95% CI [0.06, 0.27]), even after accounting for demographics and objective SES.
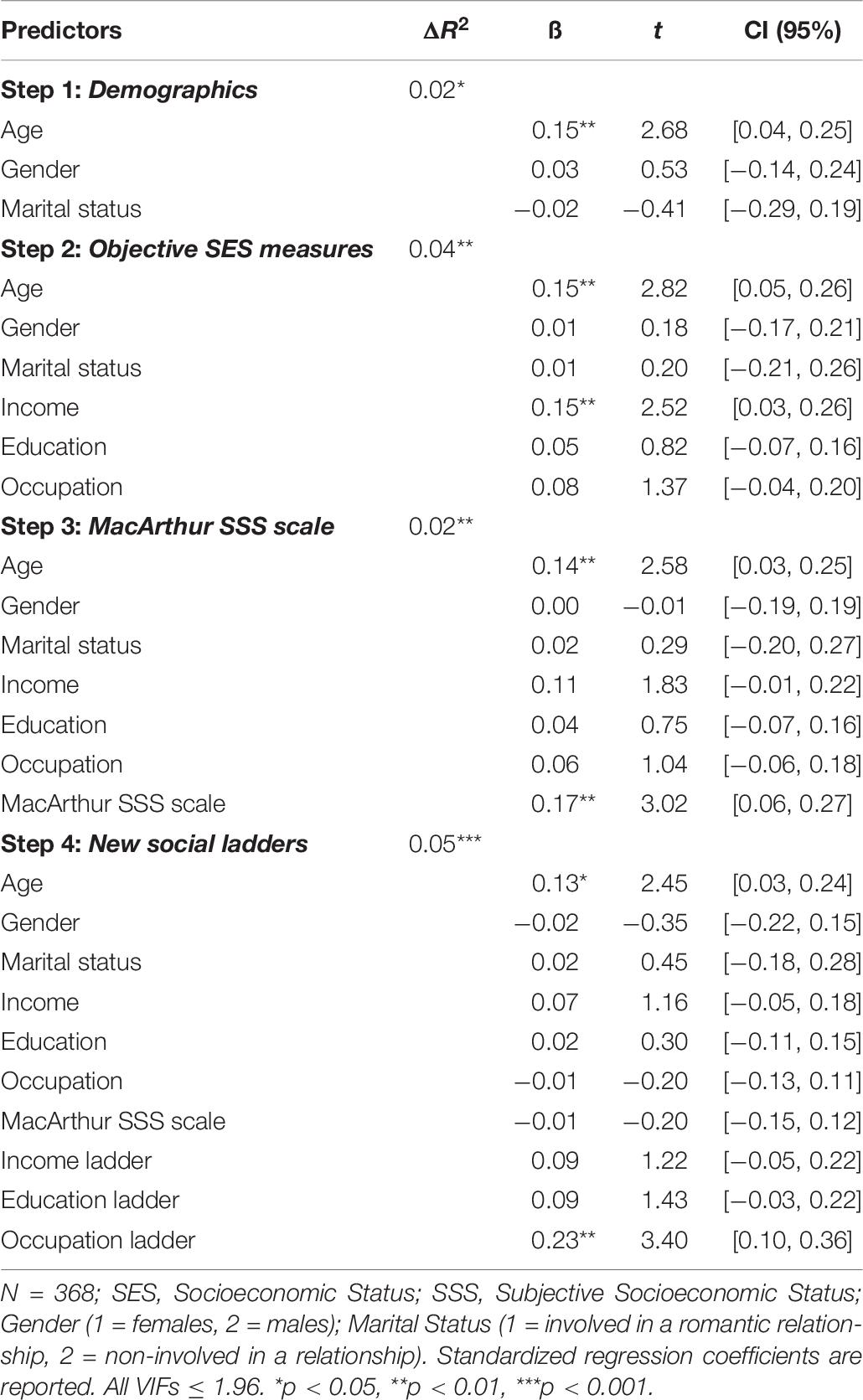
Table 6. Hierarchical regression analysis predicting environmental mastery.
As with self-acceptance, only the occupation ladder yielded a significant contribution to the prediction of environmental mastery (β = 0.23, p = 0.001; 95% CI [0.10, 0.36]) beyond demographics, objective SES indicators, and the traditional MacArthur SSS scale (see Table 6 ). In addition, the inclusion of the income, education, and occupation ladders in this last step accounted for 5% of the variance in the criterion measure, F (3,341) = 6.85, p < 0.001. The regression coefficient of the MacArthur SSS scale was no longer significant in this last step (β = −0.01, p = 0.842; 95% CI [−0.15, 0.12]).
Purpose in Life
Unlike the cases described above, we found none of the objective indices of SES (i.e., income, education, and occupation) predicted purpose in life beyond the respondents’ demographics (see Table 7 ).
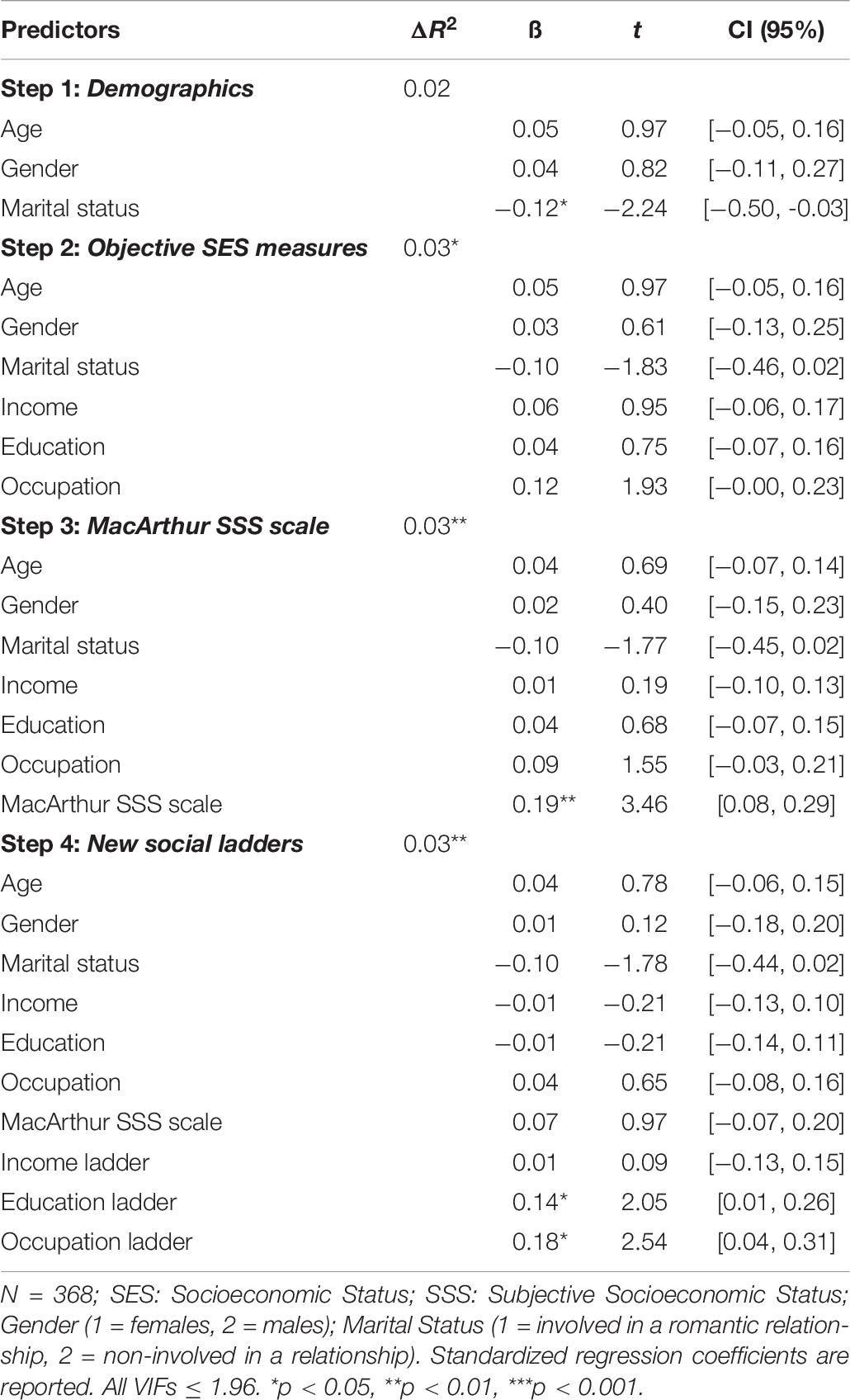
Table 7. Hierarchical regression analysis predicting purpose in life.
The standardized beta coefficient of the MacArthur SSS scale (β = 0.19, p = 0.001; 95% CI [0.08, 0.29]) indicated that this traditional measure of subjective SES was a positive predictor of the participants’ scores on purpose in life beyond demographics and objective SES.
Similar to the previous dimensions, our results corroborated the predictive utility of the occupation and education social ladders regarding purpose in life above and beyond demographics, indicators of objective SES, and the traditional MacArthur SSS scale. As Table 7 illustrates, participants who placed themselves higher on the occupation (β = 0.18, p = 0.012; 95% CI [0.04, 0.31]) and education (β = 0.14, p = 0.041; 95% CI [0.01, 0.26]) ladders were more prone to show greater levels of purpose in life. Moreover, the amount of explained variance of purpose in life increased by 3% in this last step of the regression analysis. The observed increase was statistically significant, F (3,341) = 4.17, p = 0.006. Also, it is worth noting that, in keeping with prior cases, the addition of the new social ladders as predictors caused the MacArthur SSS scale to no longer significantly predict purpose in life (β = 0.07, p = 0.333; 95% CI [−0.07, 0.20]).
Personal Growth
Among the various measures of objective SES (see Table 8 ), our results in this case yielded a significant contribution of education to the prediction of personal growth even after accounting for the respondents’ demographics (β = 0.13, p = 0.026; 95% CI [0.02, 0.25]).
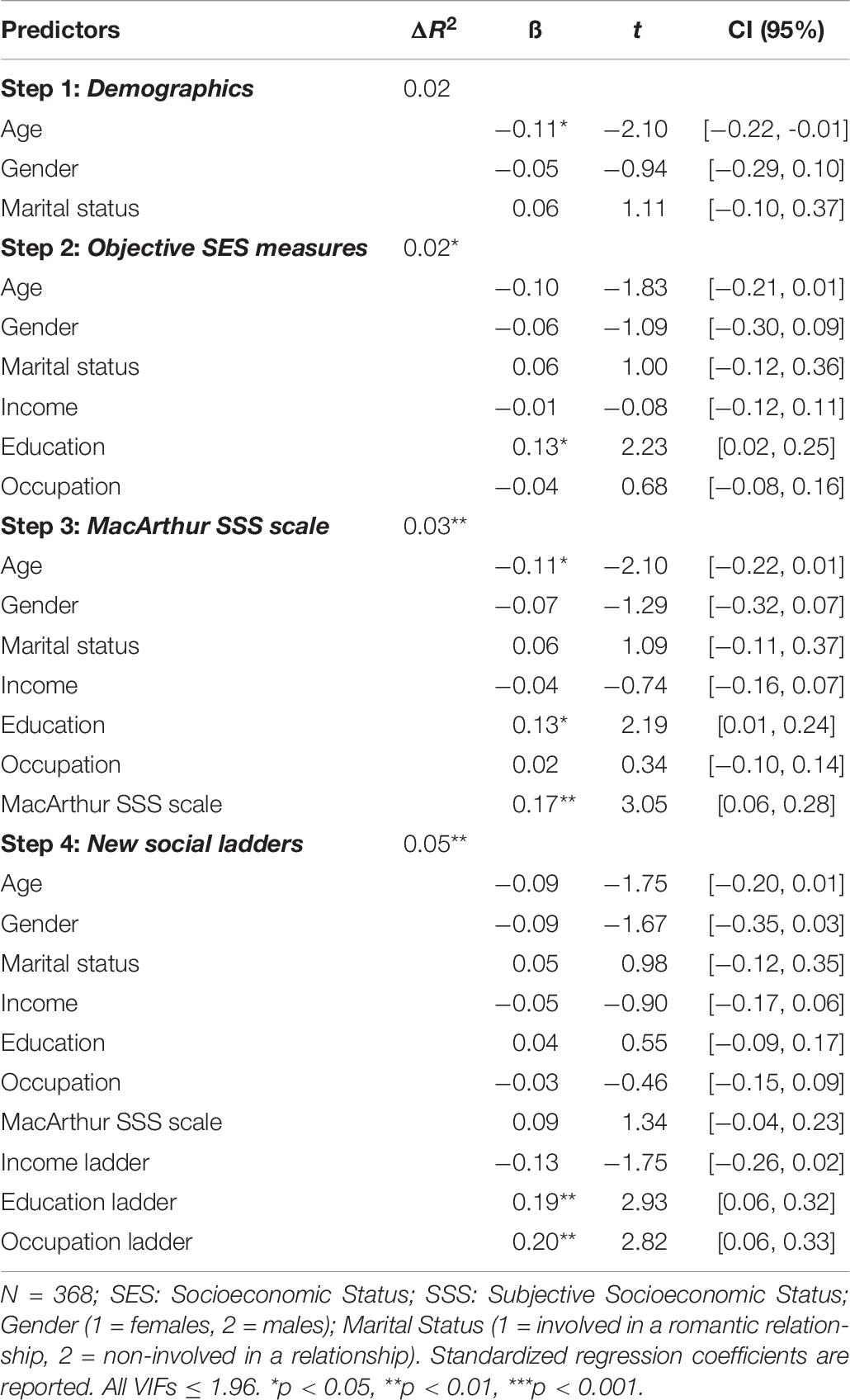
Table 8. Hierarchical regression analysis predicting personal growth.
As can be seen in Table 8 , our results showed that the MacArthur SSS scale emerged as a significant predictor of personal growth beyond demographics and objective SES (β = 0.17, p = 0.002; 95% CI [0.06, 0.28]).
In line with abovementioned results concerning the new proposed ladders for income, educational level, and occupation, our results yielded a significant contribution of the occupation and education social ladders to the prediction of personal growth. As Table 8 illustrates, participants who placed themselves higher on the occupation (β = 0.20, p = 0.005; 95% CI [0.06, 0.33]) and education (β = 0.19, p = 0.004; 95% CI [0.06, 0.32]) ladders reported higher levels of personal growth even after accounting for demographics, objective SES, and the traditional MacArthur SSS scale. This last step of the regression equation explained an additional 5% of the variance in personal growth, F (3,341) = 5.72, p = 0.001. Moreover, we also observed that the standardized beta coefficient of the MacArthur SSS scale did not remain significant (β = 0.09, p = 0.181; 95% CI [−0.04, 0.23]) after the inclusion of the new social ladders.
Global Psychological Well-Being
As Table 9 illustrates, hierarchical regression analyses yielded a significant contribution of income (β = 0.14, p = 0.017; 95% CI [0.02, 0.25]) and education (β = 0.12, p = 0.036; 95% CI [0.01, 0.24]) to the prediction of the global index of psychological well-being beyond demographics.
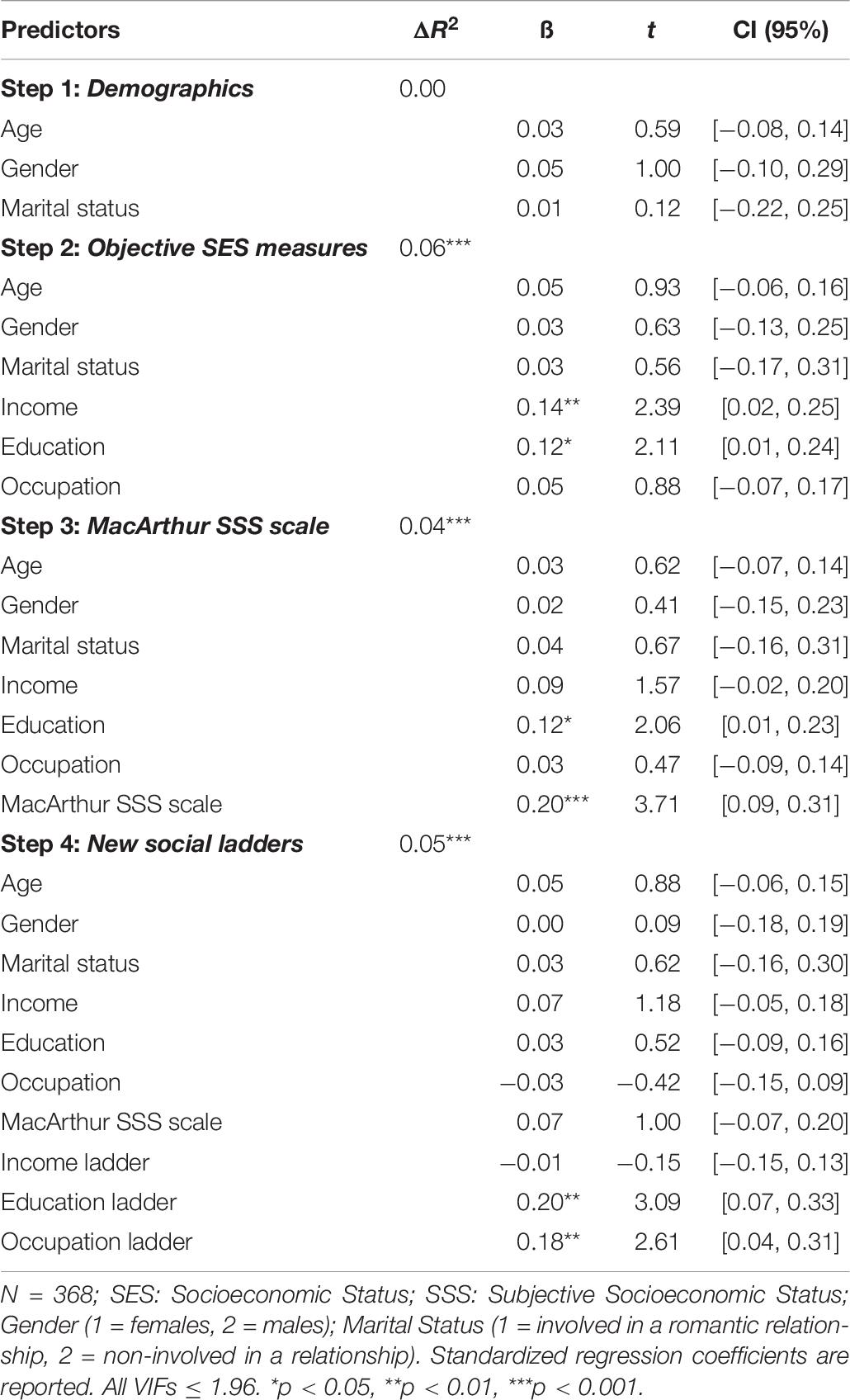
Table 9. Hierarchical regression analysis predicting global psychological well-being.
We also found that the MacArthur SSS scale predicted global psychological well-being. In particular, it was a positive predictor of this global index (β = 0.20, p < 0.001; 95% CI [0.09, 0.31]) beyond demographics and objective SES.
As occurred in earlier cases, standardized beta coefficients indicated that the education (β = 0.20, p = 0.002; 95% CI [0.07, 0.33]) and occupation (β = 0.18, p = 0.010; 95% CI [0.04, 0.31]) ladders were positive predictors of global psychological well-being even after accounting for demographics, objective SES, and the MacArthur SSS scale (see Table 9 ). The amount of explained variance of global psychological well-being increased by 5% in this last step of the regression analysis. The observed increase was statistically significant, F (3,341) = 6.11, p < 0.001. In addition, it is worth noticing that the addition of the new social ladders as predictors caused the MacArthur SSS scale to no longer significantly predict global psychological well-being (β = 0.07, p = 0.319; 95% CI [−0.07, 0.20]).
Lastly, we graphically illustrate the standardized beta coefficients of the different measures of objective and subjective SES in Figure 2 .
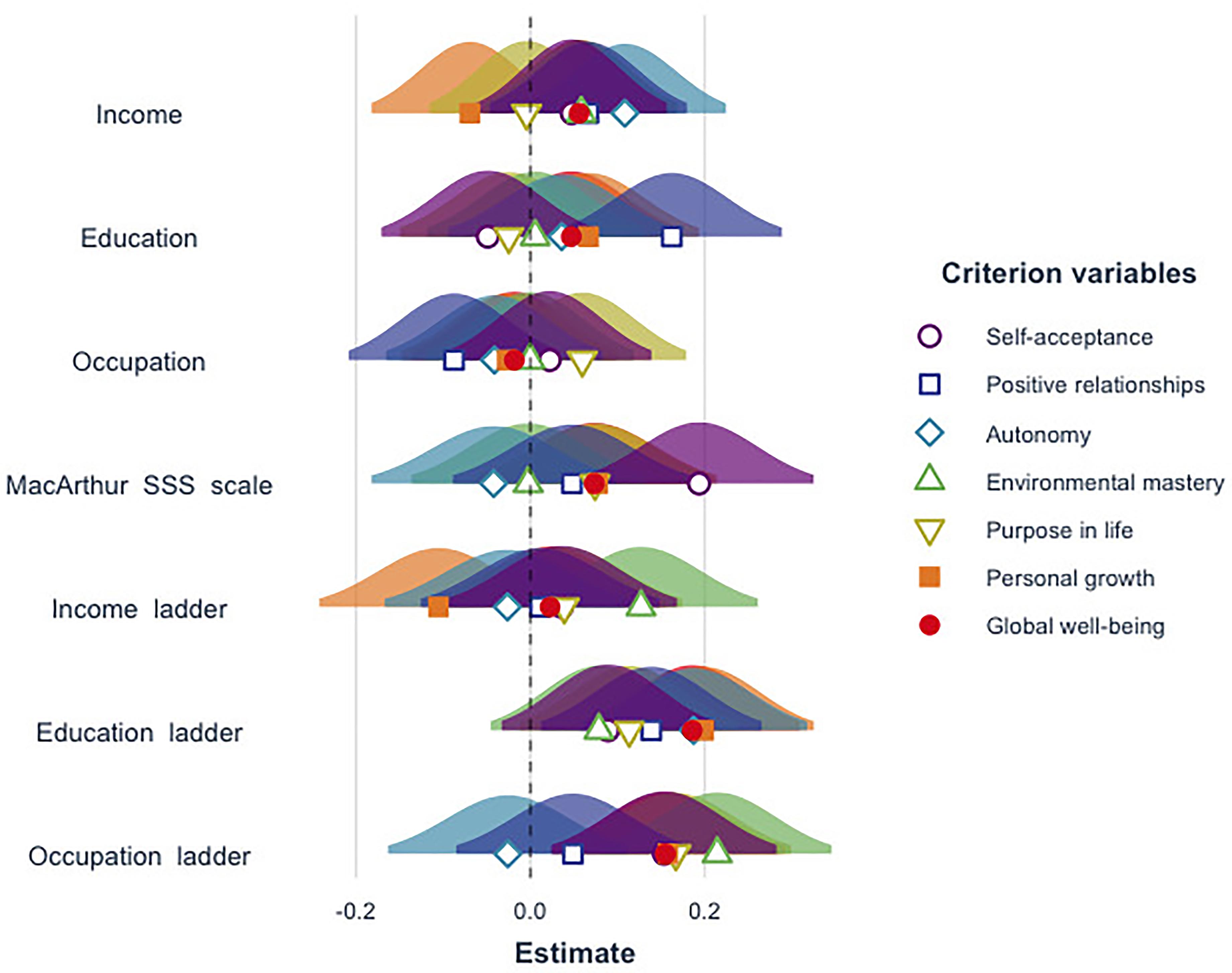
Figure 2. Visual comparison of standardized beta coefficients of the various objective and subjective SES measures.
Prior research has widely converged on the notion that SES is a rather complex and multifaceted construct determined by relatively independent objective indicators (e.g., income, educational level) and individuals’ subjective perceptions of their placement in the socioeconomic hierarchy (see Kraus et al., 2012 ). Nonetheless, within the psychological literature on subjective SES and well-being, subjective assessments of SES have largely focused on the administration of social ladders that simultaneously consider income, educational level, and occupation, potentially constraining individuals’ views of their SES. In an attempt to address this gap, the present research revisited subjective SES measurement by (a) proposing a novel method of assessing subjective SES, namely an adaption of the MacArthur SSS scale, resulting in three independent ladders based on income, educational level, and occupation, and (b) empirically testing the role of these three subjective SES measures in psychological well-being while examining in conjunction objective SES and the traditional MacArthur SSS scale. Hence, this investigation provides the first preliminary data on the empirical contribution of distinctive social ladders focused on income, education, and occupation, as an innovative and broader way of evaluating the effects of subjective SES on various components of psychological well-being.
In aligning with notions recognized in earlier studies, our results clearly confirmed that subjective assessments of SES are better predictors of well-being-related aspects than are objective SES metrics (e.g., Boyce et al., 2010 ; Cundiff and Matthews, 2017 ; Navarro-Carrillo et al., 2019 ). Indeed, among the various components of psychological well-being (i.e., self-acceptance, positive relationships, autonomy, environmental mastery, purpose in life, and personal growth), only the participants’ differences in positive relationships and autonomy with others were significantly explained by an objective SES index while all SES indicators were simultaneously considered. In particular, higher educational level predicted greater scores on positive relationships, and higher income predicted increased autonomy. However, it is important to mention that the education ladder, compared to income, exhibited a higher predictive utility regarding autonomy.
In considering the role of subjective SES indicators, we found that participants’ higher placements on the traditional MacArthur SSS scale only significantly predicted greater scores on self-acceptance when we simultaneously considered the predictive utility of the new proposed ladders for income, educational level, and occupation. Moreover, the traditional MacArthur SSS scale was not a significant predictor of global psychological well-being. In particular, our findings indicated that the novel indicators of subjective SES were stronger predictors of all measures of psychological well-being except self-acceptance than the conventional MacArthur SSS scale. In addition, their inclusion in the hierarchical regression analyses significantly accounted for incremental criterion variance (except in positive relationships) beyond demographics, objective SES, and the MacArthur SSS scale. Interestingly, of these new social ladders, the one linked to income levels, did not emerge as a significant predictor of any of the criterion indicators. Taking into account that income, when compared to other objective facets of SES (e.g., education), has been found to exhibit stronger associations with well-being (e.g., Tan et al., in press ), one might argue that the income ladder should show similar effects. However, according to our data, the education and occupation ladders were identified as consistent predictors of psychological well-being. The education ladder was significantly related to all indicators of psychological well-being except self-acceptance and environmental mastery, which were explained better by the occupation ladder. These results concerning the education ladder could help to elucidate the role of this facet of SES in psychological well-being. Although the contribution of education to well-being has been shown to be relatively limited when it is objectively assessed ( Tan et al., in press ), our findings highlight its relative relevance to psychological well-being when individuals estimate their position within the social hierarchy by comparing their educational level to that of others in society. Previous works have posited that education may precede higher income levels or more prestigious occupations ( Snibbe and Markus, 2005 ). Furthermore, the role of the occupation ladder was almost comparable to that of the education ladder. In particular, we found the occupation ladder predicted all measures of psychological well-being except positive relationships and autonomy, which were explained better by the education ladder. In this case, a similar interpretation to that proposed for the education ladder might be extrapolated for the occupation last. However, the characteristics of the socioeconomic context should not be overlooked. Specifically, this research was conducted in southeastern Spain. This region’s overall socioeconomic reality, even some time after the economic crisis period, remains unfavorable. Thus, within this context of economic difficulties and high unemployment, the perceived value of having a more prestigious occupation might be crucial for individuals’ psychological well-being. Together, our findings revealed that the novel education and occupation ladders are unique predictors of psychological well-being beyond objective SES, and the traditional MacArthur SSS scale.
Limitations and Future Research Directions
Although the current findings allow open up a new strand of research in the psychological literature on SES and well-being, some limitations should be acknowledged while suggesting further research directions. First, it is worth mentioning that this study used non-probabilistic sampling (i.e., snowball sampling procedure), which constrains our results’ potential generalization. It should also be noted here that, although the present sample consisted of well-educated individuals of relatively higher occupational status, respondents’ characteristics in terms of income generally were equivalent to the reference population ( INE, 2019 ). Overall, future research should use probabilistic sampling procedures to collect samples that are as representative as possible. In addition, we followed a non-experimental methodology in this research. Hence, causal inferences regarding our findings must not be made. Thus, further research should use experimental or longitudinal designs to determine the potential causal effects of the proposed subjective SES measures on psychological well-being. Second, although we included different well-established dimensions of psychological well-being ( Ryff and Keyes, 1995 ; Díaz et al., 2006 ) as relevant criteria, it would be advisable to evaluate further well-being or health-related factors (e.g., self-perceived health status or physical health). By doing so, future researchers could rule out the possibility that our results are due in some way to the type of criteria included, thereby extending and complementing this study. Third, our research was carried out in a specific sociocultural context (Spain). Although suggestive, and consistent with the notion that subjective assessments of SES are better predictors of well-being than objective SES indicators, our findings do not allow us to articulate a comprehensive explanation of why subjective SES outperform objective SES in explaining people’s well-being differences. Nonetheless, these results could be useful for subsequent empirical studies aimed at unraveling this issue. Lastly, the present findings should be expanded with new investigations to test these connections in other countries, as well as potential moderators from inequality levels ( Cheung and Lucas, 2016 ) to cultural dimensions ( Curhan et al., 2014 ).
This research offers valuable preliminary insight into the fields of SES and well-being and health by presenting a new approach for subjective SES estimation and testing the empirical contribution of this innovative measurement strategy to psychological well-being. In particular, our findings confirmed that the novel education and occupation ladders are predictive of a significant proportion of the variance levels of psychological well-being that are not due to objective SES metrics (i.e., income, education, and occupation) or the conventional MacArthur SSS scale, underlining the need to validate and expand the present results across samples for the sake of a better understanding of the SES–well-being/health connection.
Data Availability Statement
The datasets generated for this study are available on request to the corresponding author.
Ethics Statement
This research study was approved by the ethical committee of the southeast Spanish university and carried out in compliance with the Ethical Standards of the 1964 Declaration of Helsinki.
Author Contributions
GN-C, MA-F, and IV-S collected the data of the study. GN-C, MA-F, MM, and IV-S analyzed and interpreted the data and reviewed and edited the manuscript. GN-C and MA-F wrote the original draft.
This research was made possible thanks to the financing provided by the Spanish Ministry of Economy, Industry, and Competitiveness for the R&D project “Macrosocial realities (economic crisis and social class) and psychosocial processes: Trust, welfare, altruism, and politics” [PSI-2017-83966-R (MINECO/AEI/FEDER/UE)].
Conflict of Interest
The authors declare that the research was conducted in the absence of any commercial or financial relationships that could be construed as a potential conflict of interest.
Adler, N. E., Epel, E. S., Castellazzo, G., and Ickovics, J. R. (2000). Relationship of subjective and objective social status with psychological and physiological functioning: preliminary data in healthy, White women. Health Psychol. 19, 586–592. doi: 10.1037/0278-6133.19.6.586
PubMed Abstract | CrossRef Full Text | Google Scholar
Akinwande, M. O., Dikko, H. G., and Samson, A. (2015). Variance inflation factor: as a condition for the inclusion of suppressor variable(s) in regression analysis. Open J. Stat. 5, 754–767. doi: 10.4236/ojs.2015.57075
CrossRef Full Text | Google Scholar
American Psychological Association (2007). Report of the APA Task Force On Socioeconomic Status. Washington, DC: American Psychological Association.
Google Scholar
Baker, E. H. (2014). “Socioeconomic status, definition,” in The Wiley Blackwell Encyclopedia of Health, Illness, Behavior, And Society , eds W. C. Cockerham, R. Dingwall, and S. R. Quah (Hoboken, NJ: Wiley-Blackwell), 2210–2214. doi: 10.1002/9781118410868.wbehibs395
Baumann, S., Szabo, M., and Johnston, J. (2019). Understanding the food preferences of people of low socioeconomic status. J. Consum. Cult. 19, 316–339. doi: 10.1177/1469540517717780
Bjornsdottir, R. T., Alaei, R., and Rule, N. O. (2017). The perceptive proletarian: subjective social class predicts interpersonal accuracy. J. Nonverb. Behav. 41, 185–201. doi: 10.1007/s10919-016-0248-6
Boyce, C. J., Brown, G. D. A., and Moore, S. C. (2010). Money and happiness: rank of income, not income, affects life satisfaction. Psychol. Sci. 21, 471–475. doi: 10.1177/0956797610362671
Brandt, M. J., Wetherell, G., and Henry, P. J. (2015). Changes in income predict change in social trust: a longitudinal analysis. Polit. Psychol. 36, 761–768. doi: 10.1111/pops.12228
Cheung, F., and Lucas, R. E. (2016). Income inequality is associated with stronger social comparison effects: the effect of relative income on life satisfaction. J. Pers. Soc. Psychol. 110, 332–341. doi: 10.1037/pspp0000059
Cohen, S., Alper, C. M., Doyle, W. J., Adler, N., Treanor, J. J., and Turner, R. B. (2008). Objective and subjective socioeconomic status and susceptibility to the common cold. Health Psychol. 27, 268–274. doi: 10.1037/0278-6133.27.2.268
Côté, S., Kraus, M. W., Carpenter, N. C., Piff, P. K., Beermann, U., and Keltner, D. (2017). Social affiliation in same-class and cross-class interactions. J. Exp. Psychol. Gen. 146, 269–285. doi: 10.1037/xge0000258
Cundiff, J. M., and Matthews, K. A. (2017). Is subjective social status a unique correlate of physical health? A meta-analysis. Health Psychol. 36, 1109–1125. doi: 10.1037/hea0000534
Curhan, K. B., Levine, C. S., Markus, H. R., Kitayama, S., Park, J., Karasawa, M., et al. (2014). Subjective and objective hierarchies and their relations to psychological well-being: a US/Japan comparison. Soc. Psychol. Pers. Sci. 5, 855–864. doi: 10.1177/1948550614538461
Díaz, D., Rodríguez-Carvajal, R., Blanco, A., Moreno-Jiménez, B., Gallardo, I., Valle, C., et al. (2006). Adaptación española de la escala de bienestar psicológico de Ryff [Spanish adaptation of Ryff’s psychological well-being scales]. Psicothema 18, 572–577.
Diener, E., and Biswas-Diener, R. (2002). Will money increase subjective well-being? Soc. Indic. Res. 57, 119–169. doi: 10.1023/A:1014411319119
Diener, E., and Oishi, S. (2000). “Money and happiness: income and subjective well-being across nations,” in Culture and Subjective Well-Being , eds E. Diener and E. M. Suh (Cambridge, MA: MIT Press), 185–218.
Diener, E., Oishi, S., and Lucas, R. E. (2003). Personality, culture, and subjective well-being: emotional and cognitive evaluations of life. Annu. Rev. Psychol. 54, 403–425. doi: 10.1146/annurev.psych.54.101601.145056
Duncan, G. J., and Magnuson, K. (2012). Socioeconomic status and cognitive functioning: moving from correlation to causation. Wiley Interdiscip. Rev. Cogn. Sci. 3, 377–386. doi: 10.1002/wcs.1176
Easterbrook, M., Kuppens, T., and Manstead, A. S. R. (2020). Socioeconomic status and the structure of the self-concept. Br. J. Soc. Psychol. 59, 66–86. doi: 10.1111/bjso.12334
European Social Survey (2018). ESS Round 9 Source Questionnaire. London: University of London.
Faul, F., Erdfelder, E., Buchner, A., and Lang, A. G. (2009). Statistical power analyses using G ∗ Power 3.1: tests for correlation and regression analyses. Behav. Res. Methods 41, 1149–1160. doi: 10.3758/brm.41.4.1149
Garza, J. R., Glenn, B. A., Mistry, R. S., Ponce, N. A., and Zimmerman, F. J. (2017). Subjective social status and self-reported health among US-born and immigrant Latinos. J. Immigr. Minor Health 19, 108–119. doi: 10.1007/s10903-016-0346-x
Goodman, E., Adler, N. E., Daniels, S. R., Morrison, J. A., Slap, G. B., and Dolan, L. M. (2003). Impact of objective and subjective social status on obesity in a biracial cohort of adolescents. Obes. Res. 11, 1018–1026. doi: 10.1038/oby.2003.140
Howell, R. T., and Howell, C. J. (2008). The relation of economic status to subjective wellbeing in developing countries: a meta-analysis. Psychol. Bull. 134, 536–560. doi: 10.1037/0033-2909.134.4.536
Huang, S., Hou, J., Sun, L., Dou, D., Liu, S., and Zhang, H. (2017). The effects of objective and subjective socioeconomic status on subjective well-being among rural-to-urban migrants in china: the moderating role of subjective social mobility. Front. Psychol. 8:819. doi: 10.3389/fpsyg.2017.00819
INE (2019). Inedatabase . Available online at: https://www.ine.es/jaxiT3/Datos.htm?t=24992#Wtabs-tabla (accessed April 27, 2019).
Joffer, J., Flacking, R., Bergström, E., Randell, E., and Jerdén, L. (2019). Self-rated health, subjective social status in school and socioeconomic status in adolescents: a cross-sectional study. BMC Public Health 19:785. doi: 10.1186/s12889-019-7140-3
Jury, M., Aelenei, C., Chen, C., Darnon, C., and Elliot, A. J. (2019). Examining the role of perceived prestige in the link between students’ subjective socioeconomic status and sense of belonging. Group Process. Intergr. Relat. 22, 356–370. doi: 10.1177/1368430219827361
Kraus, M. W. (2018). “Beggars do not envy millionaires: social comparison, socioeconomic status, and subjective well-being,” in Handbook of Well-Being Noba Scholar Handbook Series: Subjective Well-Being , eds E. Diener, S. Oishi, and L. Tay (Salt Lake City, UT: DEF publishers).
Kraus, M. W., and Keltner, D. (2009). Signs of socioeconomic status: a thin-slicing approach. Psychol. Sci. 20, 99–106. doi: 10.1111/j.1467-9280.2008.02251.x
Kraus, M. W., and Park, J. W. (2014). The undervalued self: social class and self-evaluation. Front. Psychol. 5:1404. doi: 10.3389/fpsyg.2014.01404
Kraus, M. W., Piff, P. K., Mendoza-Denton, R., Rheinschmidt, M. L., and Keltner, D. (2012). Social class, solipsism, and contextualism: how the rich are different from the poor. Psychol. Rev. 119, 546–572. doi: 10.1037/a0028756
Kraus, M. W., and Stephens, N. M. (2012). A road map for an emerging psychology of social class. Soc. Pers. Psychol. Compass. 6, 642–656. doi: 10.1111/j.1751-9004.2012.00453.x
Kraus, M. W., Tan, J. J. X., and Tannenbaum, M. B. (2013). The social ladder: a rank-based perspective on social class. Psychol. Inq. 24, 81–96. doi: 10.1080/1047840X.2013.778803
Kraus, M. W., Torrez, B., Park, J. W., and Ghayebi, F. (2019). Evidence for the reproduction of social class in brief speech. Proc. Natl. Acad. Sci. U.S.A. 116, 22998–23003. doi: 10.1073/pnas.1900500116
Loeb, E., and Hurd, N. M. (2019). Subjective social status, perceived academic competence, and academic achievement among underrepresented students. J. Coll. Stud. Ret. 21, 150–165. doi: 10.1177/1521025117696821
Lucas, R. E., and Schimmack, U. (2009). Income and well-being: how big is the gap between the rich and the poor? J. Res. Pers. 43, 75–78. doi: 10.1016/j.jrp.2008.09.004
Manstead, A. S. (2018). The psychology of social class: how socioeconomic status impacts thought, feelings, and behaviour. Br. J. Soc. Psychol. 57, 267–291. doi: 10.1111/bjso.12251
Moor, I., Kuipers, M. A., Lorant, V., Pförtner, T. K., Kinnunen, J. M., Rathmann, K., et al. (2019). Inequalities in adolescent self-rated health and smoking in Europe: comparing different indicators of socioeconomic status. J. Epidemiol. Commun. Health 73, 963–970. doi: 10.1136/jech-2018-211794
Moya, M., and Fiske, S. T. (2017). The social psychology of the great recession and social class divides. J. Soc. Issues 73, 8–22. doi: 10.1111/josi.12201
Navarro-Carrillo, G., Torres-Marín, J., and Carretero-Dios, H. (2020). Class-based differences in the use of (aggressive) humor: the mediating role of empathic concern. Pers. Individ. Differ. 159:109868. doi: 10.1016/j.paid.2020.109868
Navarro-Carrillo, G., Valor-Segura, I., and Moya, M. (2018). Do you trust strangers, close acquaintances, and members of your ingroup? Differences in trust based on social class in Spain. Soc. Indic. Res. 135, 585–597. doi: 10.1007/s11205-016-1527-7
Navarro-Carrillo, G., Valor-Segura, I., and Moya, M. (2019). The consequences of the perceived impact of the Spanish economic crisis on subjective well-being: the explanatory role of personal uncertainty. Curr. Psychol. doi: 10.1007/s12144-019-00506-4
Oakes, J. M., and Rossi, P. H. (2003). The measurement of SES in health research: current practice and steps toward a new approach. Soc. Sci. Med. 56, 769–784. doi: 10.1016/S0277-9536(02)00073-4
Ostrove, J. M., Adler, N. E., Kuppermann, M., and Washington, A. E. (2000). Objective and subjective assessments of socioeconomic status and their relationship to self-rated health in an ethnically diverse sample of pregnant women. Health Psychol. 19, 613–618. doi: 10.1037/0278-6133.19.6.613
Pfeffer, F. T., Danziger, S., and Schoeni, R. F. (2013). Wealth disparities before and after the great recession. Ann. Am. Acad. Political Soc. Sci. 650, 98–123. doi: 10.1177/0002716213497452
Piff, P. K. (2014). Wealth and the inflated self. Pers. Soc. Psychol. Bull. 40, 34–43. doi: 10.1177/0146167213501699
Piff, P. K., and Robinson, A. R. (2017). Social class and prosocial behavior: current evidence, caveats, and questions. Curr. Opin Psychol. 18, 6–10. doi: 10.1016/j.copsyc.2017.06.003
Präg, P., Mills, M. C., and Wittek, R. (2016). Subjective socioeconomic status and health in cross-national comparison. Soc. Sci. Med. 149, 84–92. doi: 10.1016/j.socscimed.2015.11.044
Rubin, M., Denson, N., Kilpatrick, S., Matthews, K. E., Stehlik, T., and Zyngier, D. (2014). “I am working-class” subjective self-definition as a missing measure of social class and socioeconomic status in higher education research. Educ. Res. 43, 196–200. doi: 10.3102/0013189X14528373
Ruini, C., and Cesetti, G. (2019). Spotlight on eudaimonia and depression. A systematic review of the literature over the past 5 years. Psychol. Res. Behav. Manag. 12, 767–792. doi: 10.2147/PRBM.S178255
Ryff, C. D. (2014). Psychological well-being revisited: advances in the science and practice of eudaimonia. Psychother. Psychosom. 83, 10–28. doi: 10.1159/000353263
Ryff, C. D., and Keyes, C. L. M. (1995). The structure of psychological well-being revisited. J. Pers. Soc. Psychol. 69, 719–727. doi: 10.1037/0022-3514.69.4.719
Singh-Manoux, A., Adler, N. E., and Marmot, M. G. (2003). Subjective social status: its determinants and its association with measures of ill-health in the Whitehall II study. Soc. Sci. Med. 56, 1321–1333. doi: 10.1016/S0277-9536(02)00131-4
Snibbe, A. C., and Markus, H. R. (2005). You can’t always get what you want: educational attainment, agency, and choice. J. Pers. Soc. Psychol. 88, 703–720. doi: 10.1037/0022-3514.88.4.703
Tan, J. J. X., Kraus, M., Carpenter, N., and Adler, N. E. (in press). The association between objective and subjective socioeconomic standing and subjective well-being: a meta-analytic review. Psychol. Bull.
Torssander, J., and Erikson, R. (2010). Stratification and mortality—A comparison of education, class, status, and income. Eur. Sociol. Rev. 26, 465–474. doi: 10.1093/esr/jcp034
Van Dierendonck, D., Díaz, D., Rodríguez-Carvajal, R., Blanco, A., and Moreno-Jiménez, B. (2008). Ryff’s six-factor model of psychological well-being, a Spanish exploration. Soc. Indic. Res. 87, 473–479. doi: 10.1007/s11205-007-9174-7
Vera-Villarroel, P., Celis-Atenas, K., Lillo, S., Contreras, D., Díaz-Pardo, N., Torres, J., et al. (2015). Towards a model of psychological well-being. The role of socioeconomic status and satisfaction with income in Chile. Univer. Psychol. 14, 1055–1066. doi: 10.11144/Javeriana.upsy14-3.tmpw
Wang, Z., Jetten, J., and Steffens, N. K. (2019). The more you have, the more you want? Higher social class predicts a greater desire for wealth and status. Eur. J. Soc. Psychol. doi: 10.1002/ejsp.2620
Keywords : subjective socioeconomic status, objective socioeconomic status, socioeconomic status, psychological well-being, social class
Citation: Navarro-Carrillo G, Alonso-Ferres M, Moya M and Valor-Segura I (2020) Socioeconomic Status and Psychological Well-Being: Revisiting the Role of Subjective Socioeconomic Status. Front. Psychol. 11:1303. doi: 10.3389/fpsyg.2020.01303
Received: 16 March 2020; Accepted: 18 May 2020; Published: 10 June 2020.
Reviewed by:
Copyright © 2020 Navarro-Carrillo, Alonso-Ferres, Moya and Valor-Segura. This is an open-access article distributed under the terms of the Creative Commons Attribution License (CC BY) . The use, distribution or reproduction in other forums is permitted, provided the original author(s) and the copyright owner(s) are credited and that the original publication in this journal is cited, in accordance with accepted academic practice. No use, distribution or reproduction is permitted which does not comply with these terms.
*Correspondence: Ginés Navarro-Carrillo, [email protected] ; María Alonso-Ferres, [email protected]
Disclaimer: All claims expressed in this article are solely those of the authors and do not necessarily represent those of their affiliated organizations, or those of the publisher, the editors and the reviewers. Any product that may be evaluated in this article or claim that may be made by its manufacturer is not guaranteed or endorsed by the publisher.
Academia.edu no longer supports Internet Explorer.
To browse Academia.edu and the wider internet faster and more securely, please take a few seconds to upgrade your browser .
Enter the email address you signed up with and we'll email you a reset link.
- We're Hiring!
- Help Center
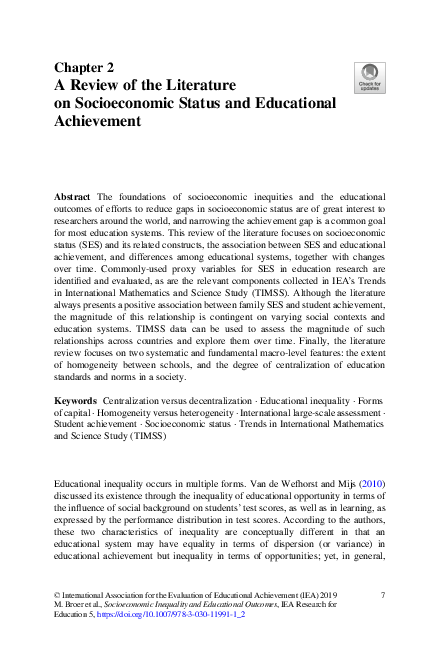
A Review of the Literature on Socioeconomic Status and Educational Achievement

2019, IEA Research for Education
Related Papers
International Association for the Evaluation of Educational Achievement (IEA)
Markus Broer , Frank T Fonseca
This open-access book focuses on trends in educational inequality using twenty years of grade 8 student data collected from 13 education systems by the IEA’s Trends in Mathematics and Science Study (TIMSS) between 1995 and 2015. While the overall positive association between family socioeconomic status (SES) and student achievement is well documented in the literature, the magnitude of this relationship is contingent on social contexts and is expected to vary by education system. Research on how such associations differ across societies and how the strength of these relationships has changed over time is limited. This study, therefore, addresses an important research and policy question by examining changes in the inequality of educational outcomes due to SES over this 20-year period, and also examines the extent to which the performance of students from disadvantaged backgrounds has improved over time in each education system. Education systems generally aim to narrow the achievement gap between low- and high-SES students and to improve the performance of disadvantaged students. However, the lack of quantifiable and comprehensible measures makes it difficult to assess and monitor the effect of such efforts. In this study, a novel measure of SES that is consistent across all TIMSS cycles allows students to be categorized into different socioeconomic groups. This measure of SES may also contribute to future research using TIMSS trend data. Readers will gain new insight into how educational inequality has changed in the education systems studied and how such change may relate to the more complex picture of macroeconomic changes in those societies.
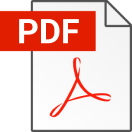
Frontiers in Education
Ola Helenius
We reassess the relation between students’ socioeconomic status (SES) and their achievement by treating SES as multidimensional instead of unidimensional. We use data from almost 600,000 students in 77 countries participating in the 2018 PISA assessment of student achievement in math, science, and reading. The composite measure of SES that PISA uses can be broken down into six component variables that we here use as simultaneous predictors of achievement. This analysis yields several new insights. First, in the typical society, two predictors (books at home and parents’ highest occupational status) clearly outperform the rest. Second, a new composite measure based only on these two components often reveals substantially larger achievement gaps than those reported by PISA. Third, the analysis revealed remarkable differences between societies in the relation between achievement and wealth possessions. In most societies, the independent effect of wealth possessions on student achieveme...
Socioeconomic Inequality and Educational Outcomes
Psychological Bulletin
Nathuram Chaudhary
IRA International Journal of Education and Multidisciplinary Studies (ISSN 2455–2526)
Kazım Çelik
gerald hammond
Abstract Education in our country is one of the major topics for discussion. The conversation around education focuses on the failures of our teachers and students in our schools as compared to foreign countries institutions of higher learning. In a nation of divisive politics, education is an area that both major parties can agree to criticize. Critics claims that our schools are failing to prepare our nations youth for the 21st century world. While many of our nations schools, when examined, are indeed falling well short on standards established by local, State, and Federal officials. What is the truth? Are the schools failing our children? In order to understand the reason why too many students still fail to pass academic measurements, it is necessary to understand and define the major causes. Poverty and its effects on our student’s academic performance have long been identified as one of the leading causes of our academic ineptitude. But is it that simple? What is driving this factor? Surely no one is choosing to be poor, there must be a larger factor-taking place in our culture. This study seeks to show the link between poverty and low academic achievement, but it also strives to show that poverty is not a result of ignorance or inferiority that affects one group over another. Poverty is a part of learned culture, for those raised in this culture of despair and hopelessness there is little chance of escape
IEA Research for Education
Markus Broer
pradip suryawanshi
The following pages will present the influence that socioeconomic status has on school performance. Depending on culture, region and country, the socioeconomic status has an significant impact on school performance and it is seen as a good indicator of it. Method: Participants: a group of 100 young students age between 18 and 24 years old (M.=20.19; S.D.=1.54), all of them aged over 18, being in their fourth year of high school; Instruments: in order to validate the hypothesis we used a socioeconomic questionnaire of our own, since the concept covers several financial factors such as family, parents' academic level, lifestyle, family influence, the number of people in the house. For the academic performance measurement we used the average grades of the students. This group was given a questionnaire measuring the socioeconomic status. School performance was assessed by consulting the students' class books School performance correlates directly proportional to the duration of hours spent learning per day (r =0.221, p <0.05). Another statistically significant correlation is the one between school performance and extracurricular activities (r =-0.30, p <0.01) After the results were analyzed, we were able to determine that school performance is, indeed, influenced by the hours spent learning, free time, the presence of siblings in the family and the family home place, (in the rural or urban area), all of which are metrics for the socioeconomic status.
Dr Yashpal D Netragaonkar
" The present market based global village puts up a barrier in front of those who " cannot read or write or count, and cannot follow written instructions " (Sen, 1998). Education is the basic requirement and the 'Fundamental Right' of the citizens of a nation. While Higher Education is important; the Elementary Education system serves as the base over which the Superstructure of the whole education system is built up. Student's education is closely linked to their life chances, income, and well being. Therefore, it is important to have a clear understanding of what benefits or hinders his/her educational attainment. There are several relevant areas that are most commonly linked to academic performance while the most influencing factor is SocioEconomic Status of the family. Socioeconomic status (SES) is often measured as a combination of education, income, and occupation. Low SES and its correlates, such as lower education, poverty, and poor health, ultimately affect our society as a whole. The main aim of the present paper is to produce a comprehensive literature review of reliable research evidence on the relationship between students' educational attainment and parents' socio economic status.
RELATED PAPERS
Annals of Noninvasive Electrocardiology
Kirsi Lauerma
Physical Review E
Maria Nuevo
Frontiers in Endocrinology
Mauricio Mondaca
Sustainable Development and Planning VII
Denise Bijoux
Tawazun: Jurnal Pendidikan Islam
Ujang Wahyudin
Journal of Surgical Case Reports
suresh kumar Sah
Microscopy and Microanalysis
Paola Cacchio
Revista Clínica Española
Antonia García-Martín
Chris Clarke
American Heart Journal
jose sevilla
Abhishek Das
UKentucky毕业证书 肯塔基大学学位证
Environment, Development and Sustainability
Johan Nordensvard
西安大略大学毕业证文凭学历认证办理 加拿大UWO成绩单学历证书
O Público e o Privado. Revista do Programa de Pós-Graduação em Sociologia da Universidade Estadual do Ceará
Regina Martínez Casas
VNU Journal of Science: Computer Science and Communication Engineering
Dinh Luan Tran
Experimental and Toxicologic Pathology
Björn Jacobsen
Journal of American History
Current Opinion in Oncology
芝加哥大学学位证 UChicago毕业证成绩单
RELATED TOPICS
- We're Hiring!
- Help Center
- Find new research papers in:
- Health Sciences
- Earth Sciences
- Cognitive Science
- Mathematics
- Computer Science
- Academia ©2024
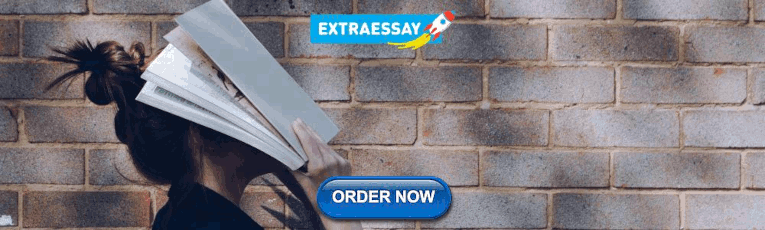
COMMENTS
outcomes of efforts to reduce gaps in socioeconomic status are of great interest to. researchers around the world, and narrowing the achievement gap is a common goal. for most education systems ...
Socioeconomic status (SES; or social class) is considered an important determinant of psychological and life outcomes. ... SUBMIT PAPER. Close Add email alerts. You are adding the following journal to your email alerts. ... Chideya S., Marchi K. S., Metzler M., Posner S. (2005). Socioeconomic status in health research: One size does not fit all ...
Abstract. The foundations of socioeconomic inequities and the educational outcomes of efforts to reduce gaps in socioeconomic status are of great interest to researchers around the world, and narrowing the achievement gap is a common goal for most education systems. This review of the literature focuses on socioeconomic status (SES) and its ...
Introduction. During the last decade, the psychology of socioeconomic status (SES) or social class, which is broadly characterized as a social stratification system derived from access to various resources (economic, social, etc.; Moya and Fiske, 2017), has experienced a remarkable growth (see Manstead, 2018).Such increased interest has been fundamentally driven by the onset of the Great ...
1. Introduction. Extensive research has shed light on the relationship between one's socioeconomic status and their level of health and overall quality of life [].In addition, research conducted over the course of the past two decades has uncovered a correlation between social capital and quality of life [2,3].In recent years, efforts to identify and address the social determinants of people ...
2.7. Measure of socioeconomic status (SES) SES is a multidimensional construct and is measured objectively by income, education, and occupation, or subjectively by prestige, place of residence, ethnic origin, or religious background, covering both objective and subjective measures of SES .
Socioeconomic status (SES) or social background has become one of the most prominent explanatory factors in disciplines such as health, child development, and educational research due to its associations with health outcomes and cognitive and socio-emotional domains (Bradley & Corwyn, 2002; Schultz, 2005).This may in part be due to an increasing need for research on social inequality and its ...
This paper reviews the evidence on the well-known positive association between socioeconomic status and health. We focus on four dimensions of socioeconomic status -- education, financial resources, rank, and race and ethnicity -- paying particular attention to how the mechanisms linking health to each of these dimensions diverge and coincide.
This paper reviews the evidence on the well-known positive association between socioeconomic status and health. We focus on four dimensions of socioeconomic status -- education, financial resources, ... research. II. Socioeconomic Status and Health: Some Facts In this section, we motivate the discussion by describing the relationship between SES
We searched PubMed for research on low socioeconomic status and morbidity, without language or date restrictions, up to Feb 10, 2019, and identified thousands of studies using the search terms "socioeconomic" in combination with "cancer", "infection", "cardiovascular", "coronary heart disease", "stroke", and ...
Conceptualizing and measuring socio-economic status (SES) The understanding of students' socio-economic conditions became a major concern for educational researchers when low academic performance at school was observed in students whose parents had low income, low levels of education, and were employed at low-status jobs (Cowan et al., 2012).
This study suggests focused treatments for low-income students. Policymakers and educators may reduce the achievement gap and promote fair education by understanding how Socioeconomic status ...
Socioeconomic status has been operationalised in a variety of ways, most commonly as education, social class, or income. In this study, we also use occupational complexity and a SES-index as alternative measures of socioeconomic status. Studies show that in analyses of health inequalities in the general population, the choice of indicators influence the magnitude of the observed inequalities.
The correlations between a low family socioeconomic status (SES) and adolescents' poor academic outcomes have been widely documented. ... SUBMIT PAPER. The Journal of Early Adolescence. Impact Factor: 2.1 / 5-Year Impact Factor: 2.7 . JOURNAL HOMEPAGE. SUBMIT PAPER. Close ... Journal of Educational Research, 104, 241-252. Crossref. ISI ...
Family socioeconomic status (SES), a combined measure of parental education, parental occupation, and family income (Stevens & Featherman, 1981), is considered an important factor in predicting children's optimal development (Loft & Waldfogel, 2021), academic achievement (Ogg & Anthony, 2020) and children's learning behaviors (Luo & Gao ...
THE EFFECT OF SOCIO-ECONOMIC STATUS ON ACADEMIC ACHIEVEMENT A Thesis by Jennifer Barry Bachelor of Arts, Wichita State University, 2005 ... Research has found that socioeconomic status, parental involvement, and family size are particularly important family factors (Majorbanks 1996). Peer influences can also affect student performance.
This article reviews the history of the measure of socio-economic status in PISA and identifies theoretical underpinnings of the index of economic, social and cultural status (ESCS). It then highlights multiple changes in the instruments and scaling methods used by PISA over time, and suggests ways of resolving the tensions behind some of these changes and thereby stabilise the measure of ESCS.
The Effects of SES, Parent-Child Relationship, and Learning Motivation on Reading Ability. According to the test method of the moderated mediation model (Baron and Kenny, 1986; Wen et al., 2006; Preacher et al., 2007; Wen and Ye, 2014), we first tested whether the direct path between SES and reading ability was moderated by learning motivation.The model (Model 1) was a saturated model.
Not a complete research paper, just wanted to share this as a little help to those in a little need. Download ... Rodriguez-Hernandez Carlos Felipe, Cascallar Eduardo, Kyndt Eva.- Socio-economic status and academic performance in higher education : a systematic review Educational research review - ISSN 1747-938X - Oxford, Elsevier sci ltd, 29 ...
1 Department of Psychology, University of Jaén, Jaén, Spain; 2 Department of Social Psychology, Faculty of Psychology, Mind, Brain and Behavior Research Center, University of Granada, Granada, Spain; Socioeconomic status (SES) is a complex and multidimensional construct, encompassing both independent objective characteristics (e.g., income or education) and subjective people's ratings of ...
The main areas that have been taken into account in this research paper include, socio-economic status of rural women, labour force participation of women, factors imposing detrimental effects ...
Academia.edu is a platform for academics to share research papers. A Review of the Literature on Socioeconomic Status and Educational Achievement ... Yang, Y. (2003). Dimensions of socio-economic status and their relationship to mathematics and science achievement at individual and collective levels. Scandinavian Journal of Educational Research ...
Abstract. Socio-economic status (SES) is conceptualized as the social and economic standing or class of an. individual or family. Socio-economic status of farmers is an important variable in ...
impact, and socio-economic implications, especially for small-scale farmers in developing nations. This paper examines the multifaceted issues surrounding GMO foods, exploring the ethical concerns ...