Purdue Online Writing Lab Purdue OWL® College of Liberal Arts
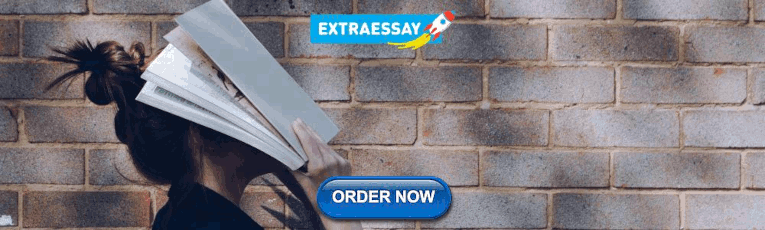
Writing a Literature Review
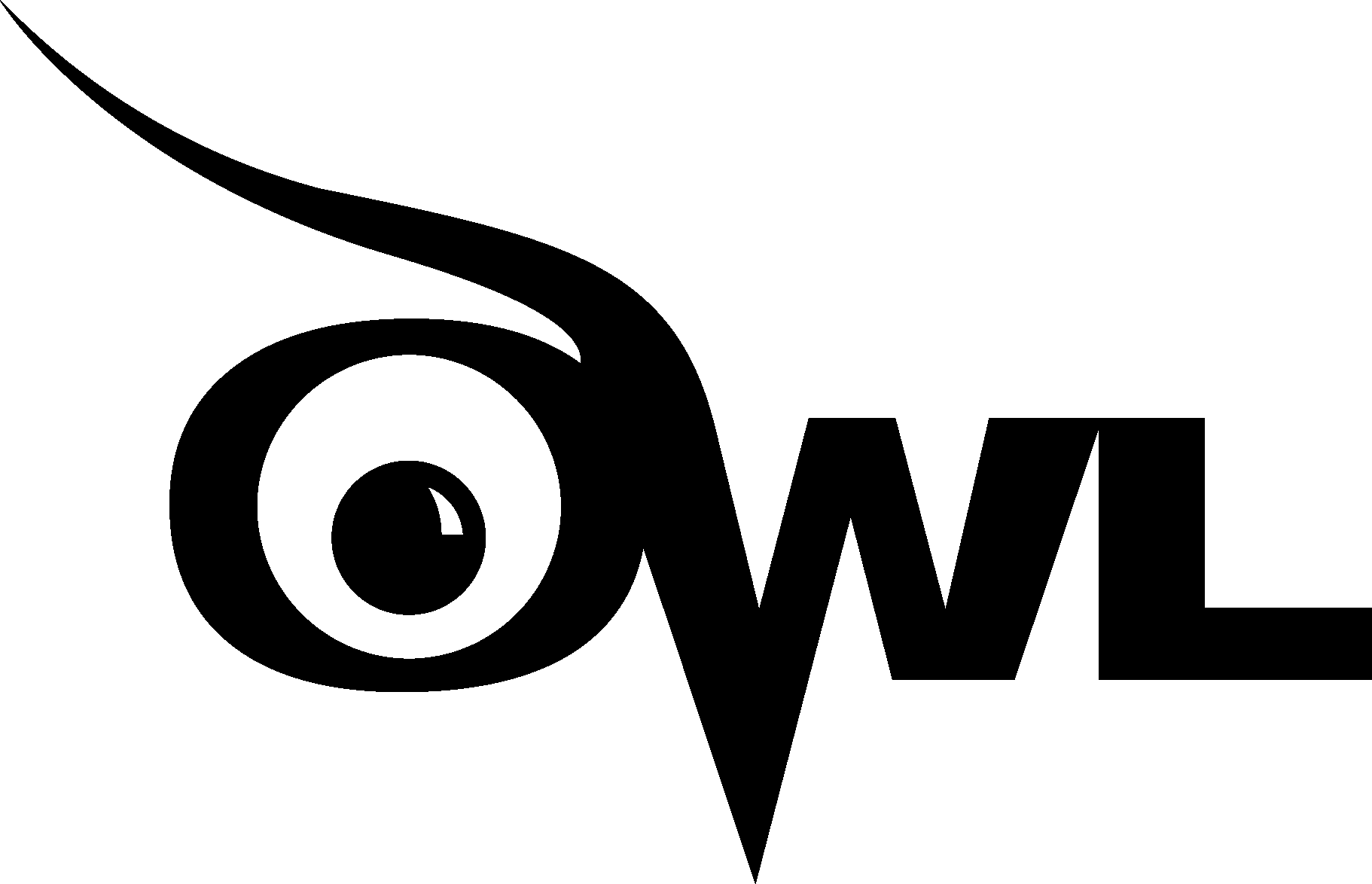
Welcome to the Purdue OWL
This page is brought to you by the OWL at Purdue University. When printing this page, you must include the entire legal notice.
Copyright ©1995-2018 by The Writing Lab & The OWL at Purdue and Purdue University. All rights reserved. This material may not be published, reproduced, broadcast, rewritten, or redistributed without permission. Use of this site constitutes acceptance of our terms and conditions of fair use.
A literature review is a document or section of a document that collects key sources on a topic and discusses those sources in conversation with each other (also called synthesis ). The lit review is an important genre in many disciplines, not just literature (i.e., the study of works of literature such as novels and plays). When we say “literature review” or refer to “the literature,” we are talking about the research ( scholarship ) in a given field. You will often see the terms “the research,” “the scholarship,” and “the literature” used mostly interchangeably.
Where, when, and why would I write a lit review?
There are a number of different situations where you might write a literature review, each with slightly different expectations; different disciplines, too, have field-specific expectations for what a literature review is and does. For instance, in the humanities, authors might include more overt argumentation and interpretation of source material in their literature reviews, whereas in the sciences, authors are more likely to report study designs and results in their literature reviews; these differences reflect these disciplines’ purposes and conventions in scholarship. You should always look at examples from your own discipline and talk to professors or mentors in your field to be sure you understand your discipline’s conventions, for literature reviews as well as for any other genre.
A literature review can be a part of a research paper or scholarly article, usually falling after the introduction and before the research methods sections. In these cases, the lit review just needs to cover scholarship that is important to the issue you are writing about; sometimes it will also cover key sources that informed your research methodology.
Lit reviews can also be standalone pieces, either as assignments in a class or as publications. In a class, a lit review may be assigned to help students familiarize themselves with a topic and with scholarship in their field, get an idea of the other researchers working on the topic they’re interested in, find gaps in existing research in order to propose new projects, and/or develop a theoretical framework and methodology for later research. As a publication, a lit review usually is meant to help make other scholars’ lives easier by collecting and summarizing, synthesizing, and analyzing existing research on a topic. This can be especially helpful for students or scholars getting into a new research area, or for directing an entire community of scholars toward questions that have not yet been answered.
What are the parts of a lit review?
Most lit reviews use a basic introduction-body-conclusion structure; if your lit review is part of a larger paper, the introduction and conclusion pieces may be just a few sentences while you focus most of your attention on the body. If your lit review is a standalone piece, the introduction and conclusion take up more space and give you a place to discuss your goals, research methods, and conclusions separately from where you discuss the literature itself.
Introduction:
- An introductory paragraph that explains what your working topic and thesis is
- A forecast of key topics or texts that will appear in the review
- Potentially, a description of how you found sources and how you analyzed them for inclusion and discussion in the review (more often found in published, standalone literature reviews than in lit review sections in an article or research paper)
- Summarize and synthesize: Give an overview of the main points of each source and combine them into a coherent whole
- Analyze and interpret: Don’t just paraphrase other researchers – add your own interpretations where possible, discussing the significance of findings in relation to the literature as a whole
- Critically Evaluate: Mention the strengths and weaknesses of your sources
- Write in well-structured paragraphs: Use transition words and topic sentence to draw connections, comparisons, and contrasts.
Conclusion:
- Summarize the key findings you have taken from the literature and emphasize their significance
- Connect it back to your primary research question
How should I organize my lit review?
Lit reviews can take many different organizational patterns depending on what you are trying to accomplish with the review. Here are some examples:
- Chronological : The simplest approach is to trace the development of the topic over time, which helps familiarize the audience with the topic (for instance if you are introducing something that is not commonly known in your field). If you choose this strategy, be careful to avoid simply listing and summarizing sources in order. Try to analyze the patterns, turning points, and key debates that have shaped the direction of the field. Give your interpretation of how and why certain developments occurred (as mentioned previously, this may not be appropriate in your discipline — check with a teacher or mentor if you’re unsure).
- Thematic : If you have found some recurring central themes that you will continue working with throughout your piece, you can organize your literature review into subsections that address different aspects of the topic. For example, if you are reviewing literature about women and religion, key themes can include the role of women in churches and the religious attitude towards women.
- Qualitative versus quantitative research
- Empirical versus theoretical scholarship
- Divide the research by sociological, historical, or cultural sources
- Theoretical : In many humanities articles, the literature review is the foundation for the theoretical framework. You can use it to discuss various theories, models, and definitions of key concepts. You can argue for the relevance of a specific theoretical approach or combine various theorical concepts to create a framework for your research.
What are some strategies or tips I can use while writing my lit review?
Any lit review is only as good as the research it discusses; make sure your sources are well-chosen and your research is thorough. Don’t be afraid to do more research if you discover a new thread as you’re writing. More info on the research process is available in our "Conducting Research" resources .
As you’re doing your research, create an annotated bibliography ( see our page on the this type of document ). Much of the information used in an annotated bibliography can be used also in a literature review, so you’ll be not only partially drafting your lit review as you research, but also developing your sense of the larger conversation going on among scholars, professionals, and any other stakeholders in your topic.
Usually you will need to synthesize research rather than just summarizing it. This means drawing connections between sources to create a picture of the scholarly conversation on a topic over time. Many student writers struggle to synthesize because they feel they don’t have anything to add to the scholars they are citing; here are some strategies to help you:
- It often helps to remember that the point of these kinds of syntheses is to show your readers how you understand your research, to help them read the rest of your paper.
- Writing teachers often say synthesis is like hosting a dinner party: imagine all your sources are together in a room, discussing your topic. What are they saying to each other?
- Look at the in-text citations in each paragraph. Are you citing just one source for each paragraph? This usually indicates summary only. When you have multiple sources cited in a paragraph, you are more likely to be synthesizing them (not always, but often
- Read more about synthesis here.
The most interesting literature reviews are often written as arguments (again, as mentioned at the beginning of the page, this is discipline-specific and doesn’t work for all situations). Often, the literature review is where you can establish your research as filling a particular gap or as relevant in a particular way. You have some chance to do this in your introduction in an article, but the literature review section gives a more extended opportunity to establish the conversation in the way you would like your readers to see it. You can choose the intellectual lineage you would like to be part of and whose definitions matter most to your thinking (mostly humanities-specific, but this goes for sciences as well). In addressing these points, you argue for your place in the conversation, which tends to make the lit review more compelling than a simple reporting of other sources.
Get science-backed answers as you write with Paperpal's Research feature
What is a Literature Review? How to Write It (with Examples)
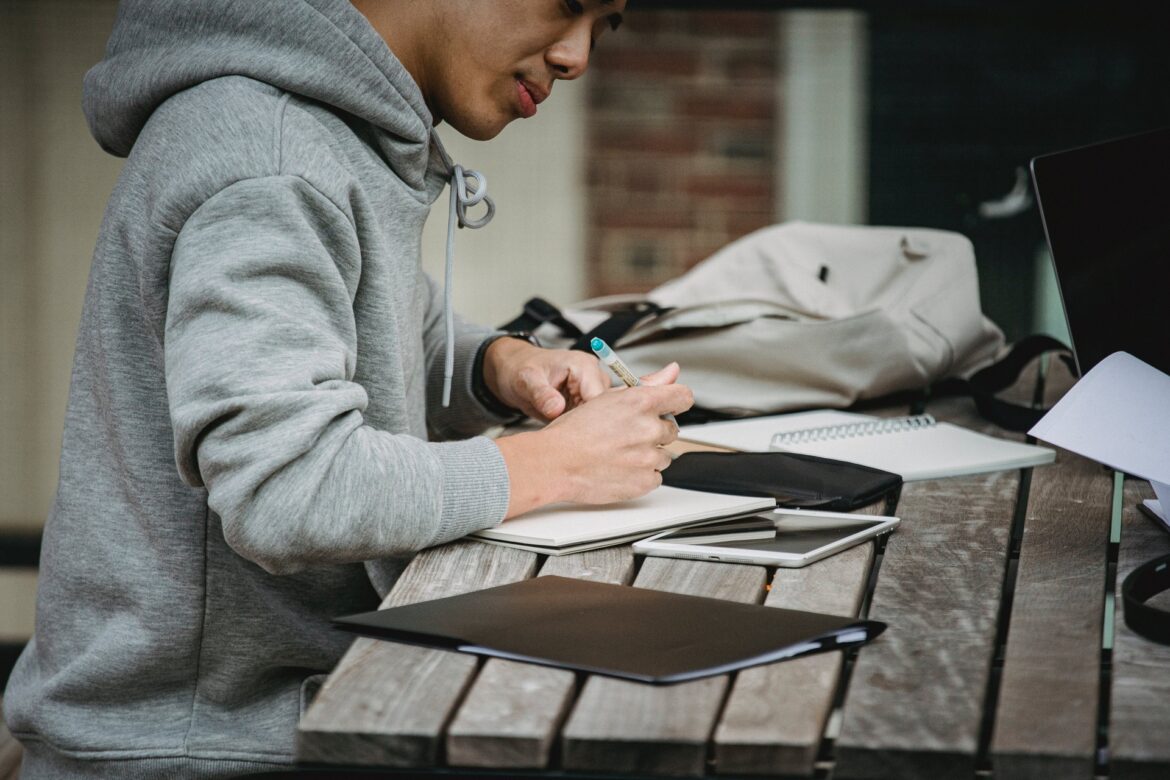
A literature review is a critical analysis and synthesis of existing research on a particular topic. It provides an overview of the current state of knowledge, identifies gaps, and highlights key findings in the literature. 1 The purpose of a literature review is to situate your own research within the context of existing scholarship, demonstrating your understanding of the topic and showing how your work contributes to the ongoing conversation in the field. Learning how to write a literature review is a critical tool for successful research. Your ability to summarize and synthesize prior research pertaining to a certain topic demonstrates your grasp on the topic of study, and assists in the learning process.
Table of Contents
- What is the purpose of literature review?
- a. Habitat Loss and Species Extinction:
- b. Range Shifts and Phenological Changes:
- c. Ocean Acidification and Coral Reefs:
- d. Adaptive Strategies and Conservation Efforts:
- How to write a good literature review
- Choose a Topic and Define the Research Question:
- Decide on the Scope of Your Review:
- Select Databases for Searches:
- Conduct Searches and Keep Track:
- Review the Literature:
- Organize and Write Your Literature Review:
- Frequently asked questions
What is a literature review?
A well-conducted literature review demonstrates the researcher’s familiarity with the existing literature, establishes the context for their own research, and contributes to scholarly conversations on the topic. One of the purposes of a literature review is also to help researchers avoid duplicating previous work and ensure that their research is informed by and builds upon the existing body of knowledge.
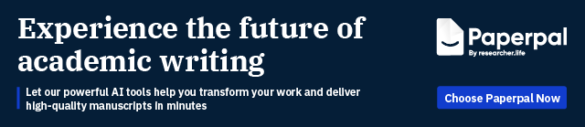
What is the purpose of literature review?
A literature review serves several important purposes within academic and research contexts. Here are some key objectives and functions of a literature review: 2
- Contextualizing the Research Problem: The literature review provides a background and context for the research problem under investigation. It helps to situate the study within the existing body of knowledge.
- Identifying Gaps in Knowledge: By identifying gaps, contradictions, or areas requiring further research, the researcher can shape the research question and justify the significance of the study. This is crucial for ensuring that the new research contributes something novel to the field.
- Understanding Theoretical and Conceptual Frameworks: Literature reviews help researchers gain an understanding of the theoretical and conceptual frameworks used in previous studies. This aids in the development of a theoretical framework for the current research.
- Providing Methodological Insights: Another purpose of literature reviews is that it allows researchers to learn about the methodologies employed in previous studies. This can help in choosing appropriate research methods for the current study and avoiding pitfalls that others may have encountered.
- Establishing Credibility: A well-conducted literature review demonstrates the researcher’s familiarity with existing scholarship, establishing their credibility and expertise in the field. It also helps in building a solid foundation for the new research.
- Informing Hypotheses or Research Questions: The literature review guides the formulation of hypotheses or research questions by highlighting relevant findings and areas of uncertainty in existing literature.
Literature review example
Let’s delve deeper with a literature review example: Let’s say your literature review is about the impact of climate change on biodiversity. You might format your literature review into sections such as the effects of climate change on habitat loss and species extinction, phenological changes, and marine biodiversity. Each section would then summarize and analyze relevant studies in those areas, highlighting key findings and identifying gaps in the research. The review would conclude by emphasizing the need for further research on specific aspects of the relationship between climate change and biodiversity. The following literature review template provides a glimpse into the recommended literature review structure and content, demonstrating how research findings are organized around specific themes within a broader topic.
Literature Review on Climate Change Impacts on Biodiversity:
Climate change is a global phenomenon with far-reaching consequences, including significant impacts on biodiversity. This literature review synthesizes key findings from various studies:
a. Habitat Loss and Species Extinction:
Climate change-induced alterations in temperature and precipitation patterns contribute to habitat loss, affecting numerous species (Thomas et al., 2004). The review discusses how these changes increase the risk of extinction, particularly for species with specific habitat requirements.
b. Range Shifts and Phenological Changes:
Observations of range shifts and changes in the timing of biological events (phenology) are documented in response to changing climatic conditions (Parmesan & Yohe, 2003). These shifts affect ecosystems and may lead to mismatches between species and their resources.
c. Ocean Acidification and Coral Reefs:
The review explores the impact of climate change on marine biodiversity, emphasizing ocean acidification’s threat to coral reefs (Hoegh-Guldberg et al., 2007). Changes in pH levels negatively affect coral calcification, disrupting the delicate balance of marine ecosystems.
d. Adaptive Strategies and Conservation Efforts:
Recognizing the urgency of the situation, the literature review discusses various adaptive strategies adopted by species and conservation efforts aimed at mitigating the impacts of climate change on biodiversity (Hannah et al., 2007). It emphasizes the importance of interdisciplinary approaches for effective conservation planning.
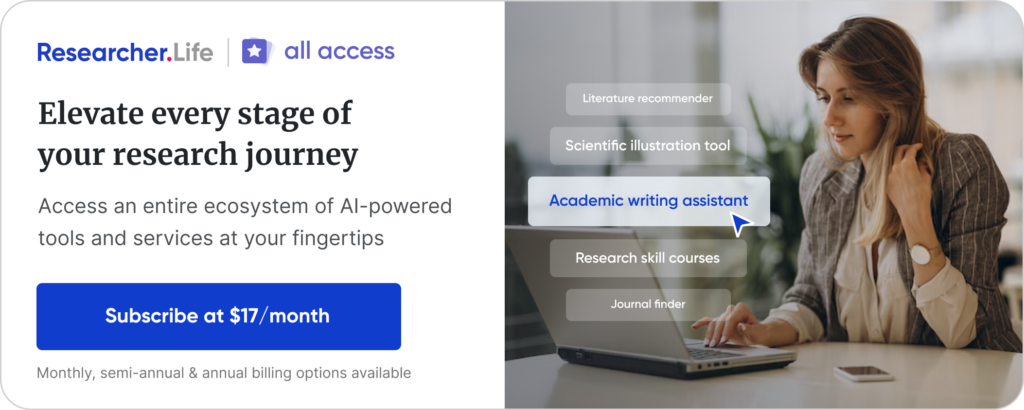
How to write a good literature review
Writing a literature review involves summarizing and synthesizing existing research on a particular topic. A good literature review format should include the following elements.
Introduction: The introduction sets the stage for your literature review, providing context and introducing the main focus of your review.
- Opening Statement: Begin with a general statement about the broader topic and its significance in the field.
- Scope and Purpose: Clearly define the scope of your literature review. Explain the specific research question or objective you aim to address.
- Organizational Framework: Briefly outline the structure of your literature review, indicating how you will categorize and discuss the existing research.
- Significance of the Study: Highlight why your literature review is important and how it contributes to the understanding of the chosen topic.
- Thesis Statement: Conclude the introduction with a concise thesis statement that outlines the main argument or perspective you will develop in the body of the literature review.
Body: The body of the literature review is where you provide a comprehensive analysis of existing literature, grouping studies based on themes, methodologies, or other relevant criteria.
- Organize by Theme or Concept: Group studies that share common themes, concepts, or methodologies. Discuss each theme or concept in detail, summarizing key findings and identifying gaps or areas of disagreement.
- Critical Analysis: Evaluate the strengths and weaknesses of each study. Discuss the methodologies used, the quality of evidence, and the overall contribution of each work to the understanding of the topic.
- Synthesis of Findings: Synthesize the information from different studies to highlight trends, patterns, or areas of consensus in the literature.
- Identification of Gaps: Discuss any gaps or limitations in the existing research and explain how your review contributes to filling these gaps.
- Transition between Sections: Provide smooth transitions between different themes or concepts to maintain the flow of your literature review.
Conclusion: The conclusion of your literature review should summarize the main findings, highlight the contributions of the review, and suggest avenues for future research.
- Summary of Key Findings: Recap the main findings from the literature and restate how they contribute to your research question or objective.
- Contributions to the Field: Discuss the overall contribution of your literature review to the existing knowledge in the field.
- Implications and Applications: Explore the practical implications of the findings and suggest how they might impact future research or practice.
- Recommendations for Future Research: Identify areas that require further investigation and propose potential directions for future research in the field.
- Final Thoughts: Conclude with a final reflection on the importance of your literature review and its relevance to the broader academic community.
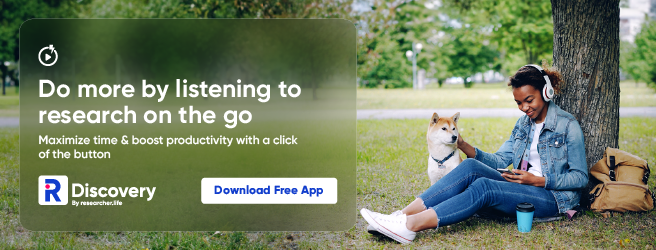
Conducting a literature review
Conducting a literature review is an essential step in research that involves reviewing and analyzing existing literature on a specific topic. It’s important to know how to do a literature review effectively, so here are the steps to follow: 1
Choose a Topic and Define the Research Question:
- Select a topic that is relevant to your field of study.
- Clearly define your research question or objective. Determine what specific aspect of the topic do you want to explore?
Decide on the Scope of Your Review:
- Determine the timeframe for your literature review. Are you focusing on recent developments, or do you want a historical overview?
- Consider the geographical scope. Is your review global, or are you focusing on a specific region?
- Define the inclusion and exclusion criteria. What types of sources will you include? Are there specific types of studies or publications you will exclude?
Select Databases for Searches:
- Identify relevant databases for your field. Examples include PubMed, IEEE Xplore, Scopus, Web of Science, and Google Scholar.
- Consider searching in library catalogs, institutional repositories, and specialized databases related to your topic.
Conduct Searches and Keep Track:
- Develop a systematic search strategy using keywords, Boolean operators (AND, OR, NOT), and other search techniques.
- Record and document your search strategy for transparency and replicability.
- Keep track of the articles, including publication details, abstracts, and links. Use citation management tools like EndNote, Zotero, or Mendeley to organize your references.
Review the Literature:
- Evaluate the relevance and quality of each source. Consider the methodology, sample size, and results of studies.
- Organize the literature by themes or key concepts. Identify patterns, trends, and gaps in the existing research.
- Summarize key findings and arguments from each source. Compare and contrast different perspectives.
- Identify areas where there is a consensus in the literature and where there are conflicting opinions.
- Provide critical analysis and synthesis of the literature. What are the strengths and weaknesses of existing research?
Organize and Write Your Literature Review:
- Literature review outline should be based on themes, chronological order, or methodological approaches.
- Write a clear and coherent narrative that synthesizes the information gathered.
- Use proper citations for each source and ensure consistency in your citation style (APA, MLA, Chicago, etc.).
- Conclude your literature review by summarizing key findings, identifying gaps, and suggesting areas for future research.
The literature review sample and detailed advice on writing and conducting a review will help you produce a well-structured report. But remember that a literature review is an ongoing process, and it may be necessary to revisit and update it as your research progresses.
Frequently asked questions
A literature review is a critical and comprehensive analysis of existing literature (published and unpublished works) on a specific topic or research question and provides a synthesis of the current state of knowledge in a particular field. A well-conducted literature review is crucial for researchers to build upon existing knowledge, avoid duplication of efforts, and contribute to the advancement of their field. It also helps researchers situate their work within a broader context and facilitates the development of a sound theoretical and conceptual framework for their studies.
Literature review is a crucial component of research writing, providing a solid background for a research paper’s investigation. The aim is to keep professionals up to date by providing an understanding of ongoing developments within a specific field, including research methods, and experimental techniques used in that field, and present that knowledge in the form of a written report. Also, the depth and breadth of the literature review emphasizes the credibility of the scholar in his or her field.
Before writing a literature review, it’s essential to undertake several preparatory steps to ensure that your review is well-researched, organized, and focused. This includes choosing a topic of general interest to you and doing exploratory research on that topic, writing an annotated bibliography, and noting major points, especially those that relate to the position you have taken on the topic.
Literature reviews and academic research papers are essential components of scholarly work but serve different purposes within the academic realm. 3 A literature review aims to provide a foundation for understanding the current state of research on a particular topic, identify gaps or controversies, and lay the groundwork for future research. Therefore, it draws heavily from existing academic sources, including books, journal articles, and other scholarly publications. In contrast, an academic research paper aims to present new knowledge, contribute to the academic discourse, and advance the understanding of a specific research question. Therefore, it involves a mix of existing literature (in the introduction and literature review sections) and original data or findings obtained through research methods.
Literature reviews are essential components of academic and research papers, and various strategies can be employed to conduct them effectively. If you want to know how to write a literature review for a research paper, here are four common approaches that are often used by researchers. Chronological Review: This strategy involves organizing the literature based on the chronological order of publication. It helps to trace the development of a topic over time, showing how ideas, theories, and research have evolved. Thematic Review: Thematic reviews focus on identifying and analyzing themes or topics that cut across different studies. Instead of organizing the literature chronologically, it is grouped by key themes or concepts, allowing for a comprehensive exploration of various aspects of the topic. Methodological Review: This strategy involves organizing the literature based on the research methods employed in different studies. It helps to highlight the strengths and weaknesses of various methodologies and allows the reader to evaluate the reliability and validity of the research findings. Theoretical Review: A theoretical review examines the literature based on the theoretical frameworks used in different studies. This approach helps to identify the key theories that have been applied to the topic and assess their contributions to the understanding of the subject. It’s important to note that these strategies are not mutually exclusive, and a literature review may combine elements of more than one approach. The choice of strategy depends on the research question, the nature of the literature available, and the goals of the review. Additionally, other strategies, such as integrative reviews or systematic reviews, may be employed depending on the specific requirements of the research.
The literature review format can vary depending on the specific publication guidelines. However, there are some common elements and structures that are often followed. Here is a general guideline for the format of a literature review: Introduction: Provide an overview of the topic. Define the scope and purpose of the literature review. State the research question or objective. Body: Organize the literature by themes, concepts, or chronology. Critically analyze and evaluate each source. Discuss the strengths and weaknesses of the studies. Highlight any methodological limitations or biases. Identify patterns, connections, or contradictions in the existing research. Conclusion: Summarize the key points discussed in the literature review. Highlight the research gap. Address the research question or objective stated in the introduction. Highlight the contributions of the review and suggest directions for future research.
Both annotated bibliographies and literature reviews involve the examination of scholarly sources. While annotated bibliographies focus on individual sources with brief annotations, literature reviews provide a more in-depth, integrated, and comprehensive analysis of existing literature on a specific topic. The key differences are as follows:
References
- Denney, A. S., & Tewksbury, R. (2013). How to write a literature review. Journal of criminal justice education , 24 (2), 218-234.
- Pan, M. L. (2016). Preparing literature reviews: Qualitative and quantitative approaches . Taylor & Francis.
- Cantero, C. (2019). How to write a literature review. San José State University Writing Center .
Paperpal is an AI writing assistant that help academics write better, faster with real-time suggestions for in-depth language and grammar correction. Trained on millions of research manuscripts enhanced by professional academic editors, Paperpal delivers human precision at machine speed.
Try it for free or upgrade to Paperpal Prime , which unlocks unlimited access to premium features like academic translation, paraphrasing, contextual synonyms, consistency checks and more. It’s like always having a professional academic editor by your side! Go beyond limitations and experience the future of academic writing. Get Paperpal Prime now at just US$19 a month!
Related Reads:
- Empirical Research: A Comprehensive Guide for Academics
- How to Write a Scientific Paper in 10 Steps
- Life Sciences Papers: 9 Tips for Authors Writing in Biological Sciences
- What is an Argumentative Essay? How to Write It (With Examples)
6 Tips for Post-Doc Researchers to Take Their Career to the Next Level
Self-plagiarism in research: what it is and how to avoid it, you may also like, measuring academic success: definition & strategies for excellence, what is academic writing: tips for students, why traditional editorial process needs an upgrade, paperpal’s new ai research finder empowers authors to..., what is hedging in academic writing , how to use ai to enhance your college..., ai + human expertise – a paradigm shift..., how to use paperpal to generate emails &..., ai in education: it’s time to change the..., is it ethical to use ai-generated abstracts without....
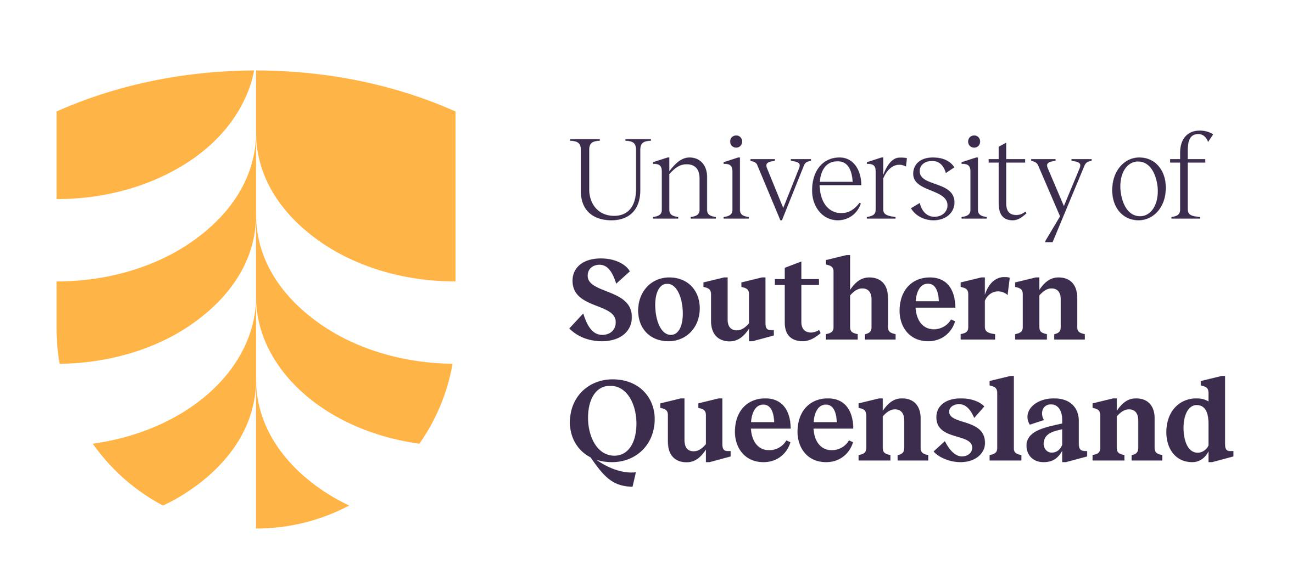
Want to create or adapt books like this? Learn more about how Pressbooks supports open publishing practices.
7 Writing a Literature Review
Hundreds of original investigation research articles on health science topics are published each year. It is becoming harder and harder to keep on top of all new findings in a topic area and – more importantly – to work out how they all fit together to determine our current understanding of a topic. This is where literature reviews come in.
In this chapter, we explain what a literature review is and outline the stages involved in writing one. We also provide practical tips on how to communicate the results of a review of current literature on a topic in the format of a literature review.
7.1 What is a literature review?
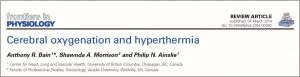
Literature reviews provide a synthesis and evaluation of the existing literature on a particular topic with the aim of gaining a new, deeper understanding of the topic.
Published literature reviews are typically written by scientists who are experts in that particular area of science. Usually, they will be widely published as authors of their own original work, making them highly qualified to author a literature review.
However, literature reviews are still subject to peer review before being published. Literature reviews provide an important bridge between the expert scientific community and many other communities, such as science journalists, teachers, and medical and allied health professionals. When the most up-to-date knowledge reaches such audiences, it is more likely that this information will find its way to the general public. When this happens, – the ultimate good of science can be realised.
A literature review is structured differently from an original research article. It is developed based on themes, rather than stages of the scientific method.
In the article Ten simple rules for writing a literature review , Marco Pautasso explains the importance of literature reviews:
Literature reviews are in great demand in most scientific fields. Their need stems from the ever-increasing output of scientific publications. For example, compared to 1991, in 2008 three, eight, and forty times more papers were indexed in Web of Science on malaria, obesity, and biodiversity, respectively. Given such mountains of papers, scientists cannot be expected to examine in detail every single new paper relevant to their interests. Thus, it is both advantageous and necessary to rely on regular summaries of the recent literature. Although recognition for scientists mainly comes from primary research, timely literature reviews can lead to new synthetic insights and are often widely read. For such summaries to be useful, however, they need to be compiled in a professional way (Pautasso, 2013, para. 1).
An example of a literature review is shown in Figure 7.1.
Video 7.1: What is a literature review? [2 mins, 11 secs]
Watch this video created by Steely Library at Northern Kentucky Library called ‘ What is a literature review? Note: Closed captions are available by clicking on the CC button below.
Examples of published literature reviews
- Strength training alone, exercise therapy alone, and exercise therapy with passive manual mobilisation each reduce pain and disability in people with knee osteoarthritis: a systematic review
- Traveler’s diarrhea: a clinical review
- Cultural concepts of distress and psychiatric disorders: literature review and research recommendations for global mental health epidemiology
7.2 Steps of writing a literature review
Writing a literature review is a very challenging task. Figure 7.2 summarises the steps of writing a literature review. Depending on why you are writing your literature review, you may be given a topic area, or may choose a topic that particularly interests you or is related to a research project that you wish to undertake.
Chapter 6 provides instructions on finding scientific literature that would form the basis for your literature review.
Once you have your topic and have accessed the literature, the next stages (analysis, synthesis and evaluation) are challenging. Next, we look at these important cognitive skills student scientists will need to develop and employ to successfully write a literature review, and provide some guidance for navigating these stages.
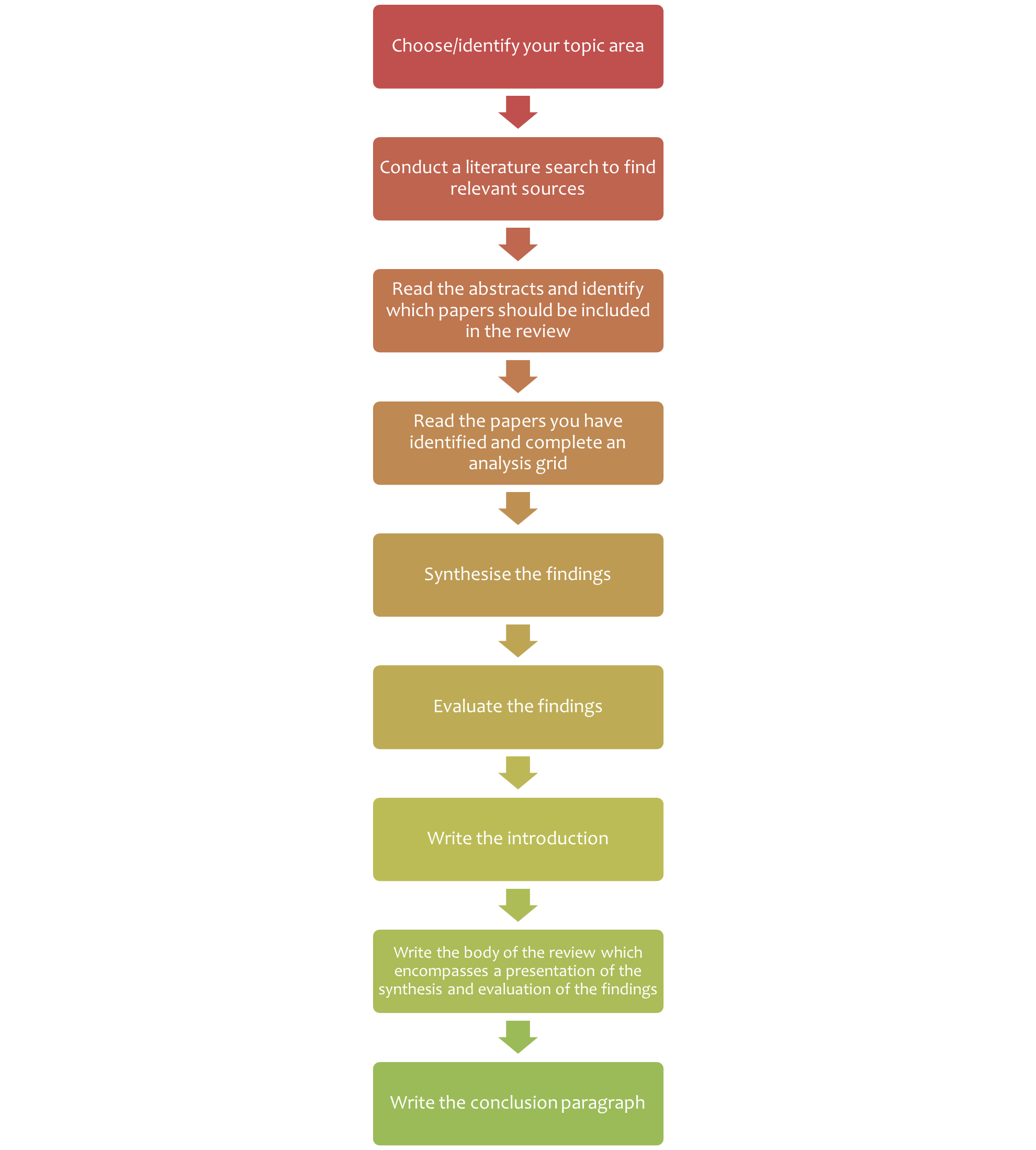
Analysis, synthesis and evaluation
Analysis, synthesis and evaluation are three essential skills required by scientists and you will need to develop these skills if you are to write a good literature review ( Figure 7.3 ). These important cognitive skills are discussed in more detail in Chapter 9.
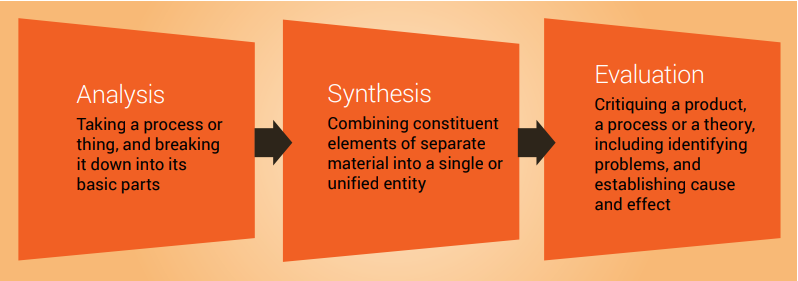
The first step in writing a literature review is to analyse the original investigation research papers that you have gathered related to your topic.
Analysis requires examining the papers methodically and in detail, so you can understand and interpret aspects of the study described in each research article.
An analysis grid is a simple tool you can use to help with the careful examination and breakdown of each paper. This tool will allow you to create a concise summary of each research paper; see Table 7.1 for an example of an analysis grid. When filling in the grid, the aim is to draw out key aspects of each research paper. Use a different row for each paper, and a different column for each aspect of the paper ( Tables 7.2 and 7.3 show how completed analysis grid may look).
Before completing your own grid, look at these examples and note the types of information that have been included, as well as the level of detail. Completing an analysis grid with a sufficient level of detail will help you to complete the synthesis and evaluation stages effectively. This grid will allow you to more easily observe similarities and differences across the findings of the research papers and to identify possible explanations (e.g., differences in methodologies employed) for observed differences between the findings of different research papers.
Table 7.1: Example of an analysis grid
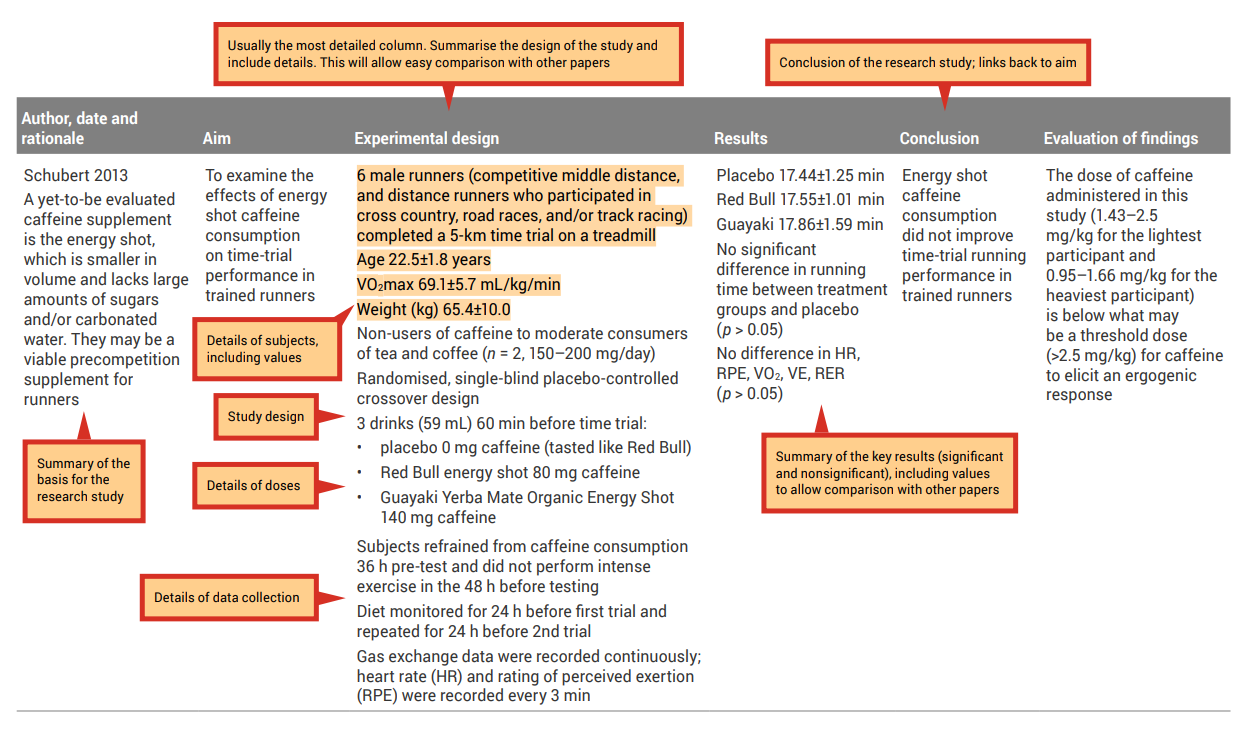
Table 7.3: Sample filled-in analysis grid for research article by Ping and colleagues
Source: Ping, WC, Keong, CC & Bandyopadhyay, A 2010, ‘Effects of acute supplementation of caffeine on cardiorespiratory responses during endurance running in a hot and humid climate’, Indian Journal of Medical Research, vol. 132, pp. 36–41. Used under a CC-BY-NC-SA licence.
Step two of writing a literature review is synthesis.
Synthesis describes combining separate components or elements to form a connected whole.
You will use the results of your analysis to find themes to build your literature review around. Each of the themes identified will become a subheading within the body of your literature review.
A good place to start when identifying themes is with the dependent variables (results/findings) that were investigated in the research studies.
Because all of the research articles you are incorporating into your literature review are related to your topic, it is likely that they have similar study designs and have measured similar dependent variables. Review the ‘Results’ column of your analysis grid. You may like to collate the common themes in a synthesis grid (see, for example Table 7.4 ).
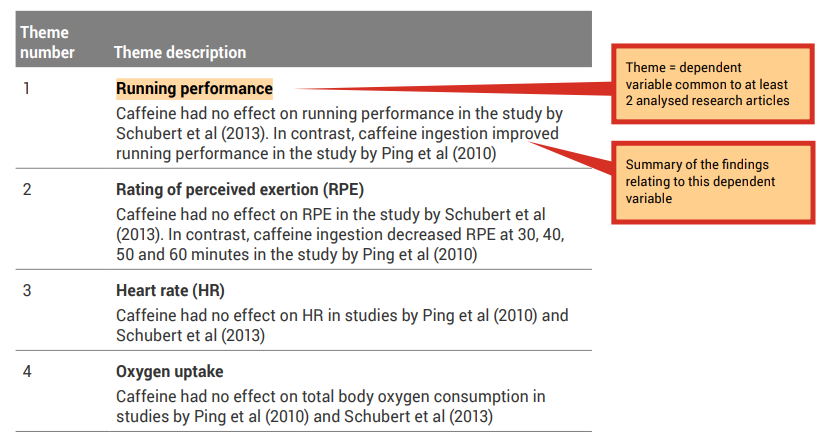
Step three of writing a literature review is evaluation, which can only be done after carefully analysing your research papers and synthesising the common themes (findings).
During the evaluation stage, you are making judgements on the themes presented in the research articles that you have read. This includes providing physiological explanations for the findings. It may be useful to refer to the discussion section of published original investigation research papers, or another literature review, where the authors may mention tested or hypothetical physiological mechanisms that may explain their findings.
When the findings of the investigations related to a particular theme are inconsistent (e.g., one study shows that caffeine effects performance and another study shows that caffeine had no effect on performance) you should attempt to provide explanations of why the results differ, including physiological explanations. A good place to start is by comparing the methodologies to determine if there are any differences that may explain the differences in the findings (see the ‘Experimental design’ column of your analysis grid). An example of evaluation is shown in the examples that follow in this section, under ‘Running performance’ and ‘RPE ratings’.
When the findings of the papers related to a particular theme are consistent (e.g., caffeine had no effect on oxygen uptake in both studies) an evaluation should include an explanation of why the results are similar. Once again, include physiological explanations. It is still a good idea to compare methodologies as a background to the evaluation. An example of evaluation is shown in the following under ‘Oxygen consumption’.
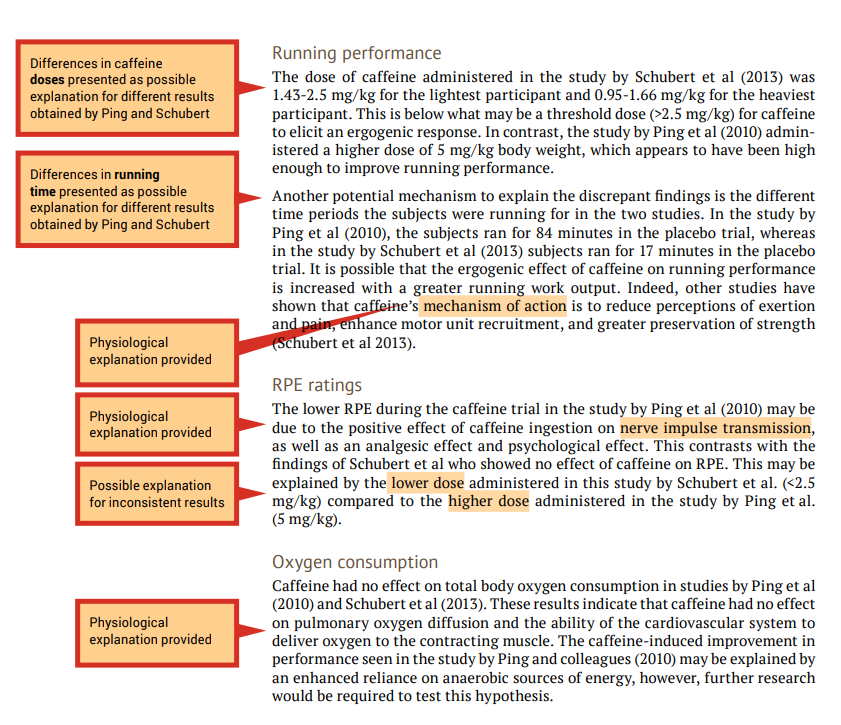
7.3 Writing your literature review
Once you have completed the analysis, and synthesis grids and written your evaluation of the research papers , you can combine synthesis and evaluation information to create a paragraph for a literature review ( Figure 7.4 ).
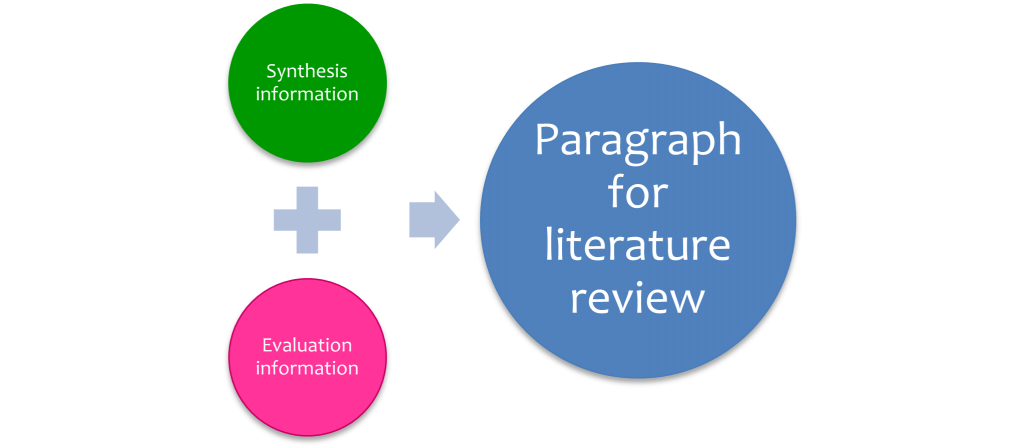
The following paragraphs are an example of combining the outcome of the synthesis and evaluation stages to produce a paragraph for a literature review.
Note that this is an example using only two papers – most literature reviews would be presenting information on many more papers than this ( (e.g., 106 papers in the review article by Bain and colleagues discussed later in this chapter). However, the same principle applies regardless of the number of papers reviewed.
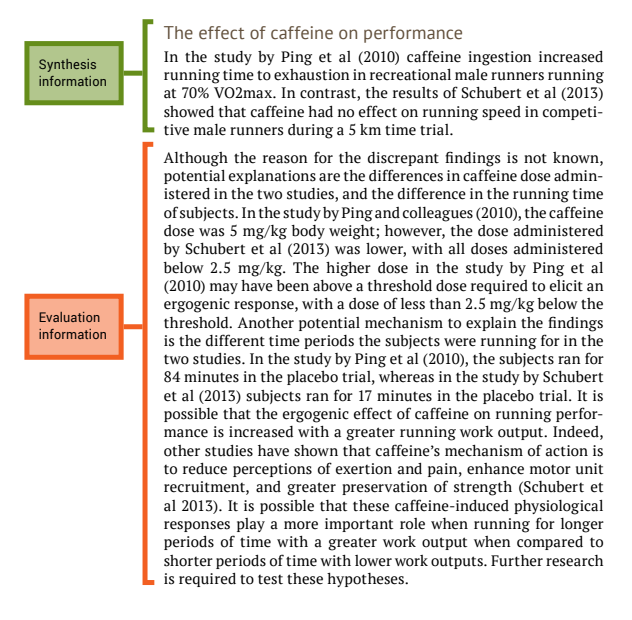
The next part of this chapter looks at the each section of a literature review and explains how to write them by referring to a review article that was published in Frontiers in Physiology and shown in Figure 7.1. Each section from the published article is annotated to highlight important features of the format of the review article, and identifies the synthesis and evaluation information.
In the examination of each review article section we will point out examples of how the authors have presented certain information and where they display application of important cognitive processes; we will use the colour code shown below:

This should be one paragraph that accurately reflects the contents of the review article.
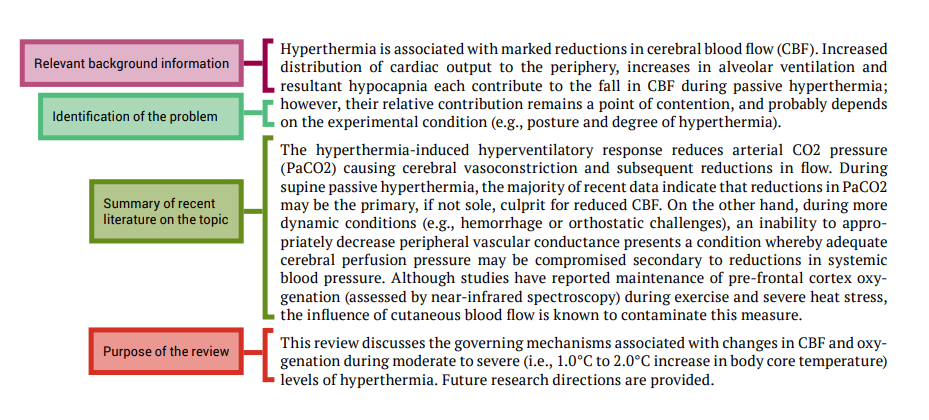
Introduction
The introduction should establish the context and importance of the review
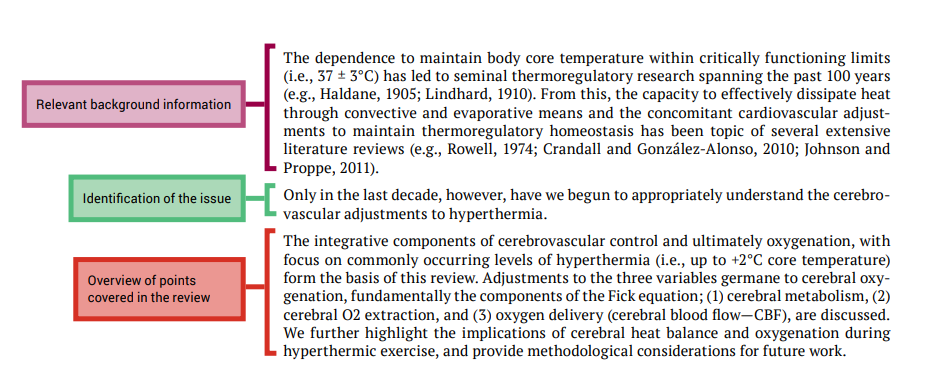
Body of literature review
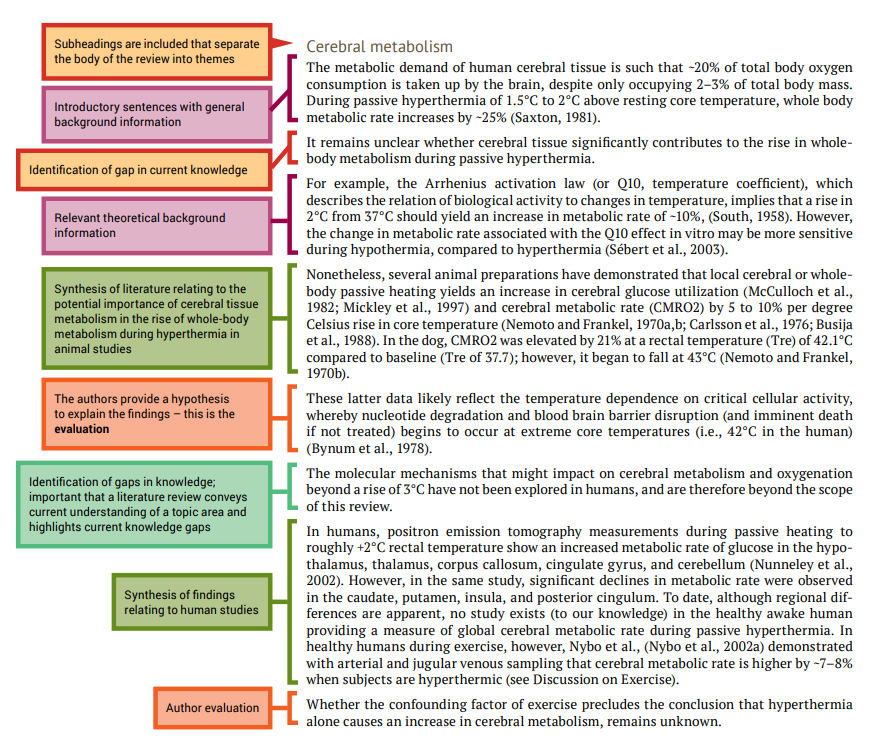
The reference section provides a list of the references that you cited in the body of your review article. The format will depend on the journal of publication as each journal has their own specific referencing format.
It is important to accurately cite references in research papers to acknowledge your sources and ensure credit is appropriately given to authors of work you have referred to. An accurate and comprehensive reference list also shows your readers that you are well-read in your topic area and are aware of the key papers that provide the context to your research.
It is important to keep track of your resources and to reference them consistently in the format required by the publication in which your work will appear. Most scientists will use reference management software to store details of all of the journal articles (and other sources) they use while writing their review article. This software also automates the process of adding in-text references and creating a reference list. In the review article by Bain et al. (2014) used as an example in this chapter, the reference list contains 106 items, so you can imagine how much help referencing software would be. Chapter 5 shows you how to use EndNote, one example of reference management software.
Click the drop down below to review the terms learned from this chapter.
Copyright note:
- The quotation from Pautasso, M 2013, ‘Ten simple rules for writing a literature review’, PLoS Computational Biology is use under a CC-BY licence.
- Content from the annotated article and tables are based on Schubert, MM, Astorino, TA & Azevedo, JJL 2013, ‘The effects of caffeinated ‘energy shots’ on time trial performance’, Nutrients, vol. 5, no. 6, pp. 2062–2075 (used under a CC-BY 3.0 licence ) and P ing, WC, Keong , CC & Bandyopadhyay, A 2010, ‘Effects of acute supplementation of caffeine on cardiorespiratory responses during endurance running in a hot and humid climate’, Indian Journal of Medical Research, vol. 132, pp. 36–41 (used under a CC-BY-NC-SA 4.0 licence ).
Bain, A.R., Morrison, S.A., & Ainslie, P.N. (2014). Cerebral oxygenation and hyperthermia. Frontiers in Physiology, 5 , 92.
Pautasso, M. (2013). Ten simple rules for writing a literature review. PLoS Computational Biology, 9 (7), e1003149.
How To Do Science Copyright © 2022 by University of Southern Queensland is licensed under a Creative Commons Attribution-NonCommercial-ShareAlike 4.0 International License , except where otherwise noted.
Share This Book
Thank you for visiting nature.com. You are using a browser version with limited support for CSS. To obtain the best experience, we recommend you use a more up to date browser (or turn off compatibility mode in Internet Explorer). In the meantime, to ensure continued support, we are displaying the site without styles and JavaScript.
- View all journals
- Explore content
- About the journal
- Publish with us
- Sign up for alerts
- CAREER FEATURE
- 04 December 2020
- Correction 09 December 2020
How to write a superb literature review
Andy Tay is a freelance writer based in Singapore.
You can also search for this author in PubMed Google Scholar
Literature reviews are important resources for scientists. They provide historical context for a field while offering opinions on its future trajectory. Creating them can provide inspiration for one’s own research, as well as some practice in writing. But few scientists are trained in how to write a review — or in what constitutes an excellent one. Even picking the appropriate software to use can be an involved decision (see ‘Tools and techniques’). So Nature asked editors and working scientists with well-cited reviews for their tips.
Access options
Access Nature and 54 other Nature Portfolio journals
Get Nature+, our best-value online-access subscription
24,99 € / 30 days
cancel any time
Subscribe to this journal
Receive 51 print issues and online access
185,98 € per year
only 3,65 € per issue
Rent or buy this article
Prices vary by article type
Prices may be subject to local taxes which are calculated during checkout
doi: https://doi.org/10.1038/d41586-020-03422-x
Interviews have been edited for length and clarity.
Updates & Corrections
Correction 09 December 2020 : An earlier version of the tables in this article included some incorrect details about the programs Zotero, Endnote and Manubot. These have now been corrected.
Hsing, I.-M., Xu, Y. & Zhao, W. Electroanalysis 19 , 755–768 (2007).
Article Google Scholar
Ledesma, H. A. et al. Nature Nanotechnol. 14 , 645–657 (2019).
Article PubMed Google Scholar
Brahlek, M., Koirala, N., Bansal, N. & Oh, S. Solid State Commun. 215–216 , 54–62 (2015).
Choi, Y. & Lee, S. Y. Nature Rev. Chem . https://doi.org/10.1038/s41570-020-00221-w (2020).
Download references
Related Articles
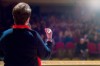
- Research management
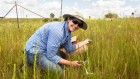
Want to make a difference? Try working at an environmental non-profit organization
Career Feature 26 APR 24
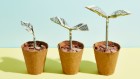
Scientists urged to collect royalties from the ‘magic money tree’
Career Feature 25 APR 24
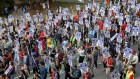
NIH pay rise for postdocs and PhD students could have US ripple effect
News 25 APR 24
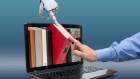
Algorithm ranks peer reviewers by reputation — but critics warn of bias
Nature Index 25 APR 24
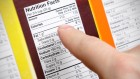
Researchers want a ‘nutrition label’ for academic-paper facts
Nature Index 17 APR 24
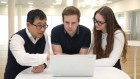
How young people benefit from Swiss apprenticeships
Spotlight 17 APR 24
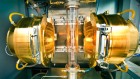
Retractions are part of science, but misconduct isn’t — lessons from a superconductivity lab
Editorial 24 APR 24
ECUST Seeking Global Talents
Join Us and Create a Bright Future Together!
Shanghai, China
East China University of Science and Technology (ECUST)
Position Recruitment of Guangzhou Medical University
Seeking talents around the world.
Guangzhou, Guangdong, China
Guangzhou Medical University
Junior Group Leader
The Imagine Institute is a leading European research centre dedicated to genetic diseases, with the primary objective to better understand and trea...
Paris, Ile-de-France (FR)
Imagine Institute
Director of the Czech Advanced Technology and Research Institute of Palacký University Olomouc
The Rector of Palacký University Olomouc announces a Call for the Position of Director of the Czech Advanced Technology and Research Institute of P...
Czech Republic (CZ)
Palacký University Olomouc
Course lecturer for INFH 5000
The HKUST(GZ) Information Hub is recruiting course lecturer for INFH 5000: Information Science and Technology: Essentials and Trends.
The Hong Kong University of Science and Technology (Guangzhou)
Sign up for the Nature Briefing newsletter — what matters in science, free to your inbox daily.
Quick links
- Explore articles by subject
- Guide to authors
- Editorial policies
- USC Libraries
- Research Guides
Organizing Your Social Sciences Research Paper
- 5. The Literature Review
- Purpose of Guide
- Design Flaws to Avoid
- Independent and Dependent Variables
- Glossary of Research Terms
- Reading Research Effectively
- Narrowing a Topic Idea
- Broadening a Topic Idea
- Extending the Timeliness of a Topic Idea
- Academic Writing Style
- Applying Critical Thinking
- Choosing a Title
- Making an Outline
- Paragraph Development
- Research Process Video Series
- Executive Summary
- The C.A.R.S. Model
- Background Information
- The Research Problem/Question
- Theoretical Framework
- Citation Tracking
- Content Alert Services
- Evaluating Sources
- Primary Sources
- Secondary Sources
- Tiertiary Sources
- Scholarly vs. Popular Publications
- Qualitative Methods
- Quantitative Methods
- Insiderness
- Using Non-Textual Elements
- Limitations of the Study
- Common Grammar Mistakes
- Writing Concisely
- Avoiding Plagiarism
- Footnotes or Endnotes?
- Further Readings
- Generative AI and Writing
- USC Libraries Tutorials and Other Guides
- Bibliography
A literature review surveys prior research published in books, scholarly articles, and any other sources relevant to a particular issue, area of research, or theory, and by so doing, provides a description, summary, and critical evaluation of these works in relation to the research problem being investigated. Literature reviews are designed to provide an overview of sources you have used in researching a particular topic and to demonstrate to your readers how your research fits within existing scholarship about the topic.
Fink, Arlene. Conducting Research Literature Reviews: From the Internet to Paper . Fourth edition. Thousand Oaks, CA: SAGE, 2014.
Importance of a Good Literature Review
A literature review may consist of simply a summary of key sources, but in the social sciences, a literature review usually has an organizational pattern and combines both summary and synthesis, often within specific conceptual categories . A summary is a recap of the important information of the source, but a synthesis is a re-organization, or a reshuffling, of that information in a way that informs how you are planning to investigate a research problem. The analytical features of a literature review might:
- Give a new interpretation of old material or combine new with old interpretations,
- Trace the intellectual progression of the field, including major debates,
- Depending on the situation, evaluate the sources and advise the reader on the most pertinent or relevant research, or
- Usually in the conclusion of a literature review, identify where gaps exist in how a problem has been researched to date.
Given this, the purpose of a literature review is to:
- Place each work in the context of its contribution to understanding the research problem being studied.
- Describe the relationship of each work to the others under consideration.
- Identify new ways to interpret prior research.
- Reveal any gaps that exist in the literature.
- Resolve conflicts amongst seemingly contradictory previous studies.
- Identify areas of prior scholarship to prevent duplication of effort.
- Point the way in fulfilling a need for additional research.
- Locate your own research within the context of existing literature [very important].
Fink, Arlene. Conducting Research Literature Reviews: From the Internet to Paper. 2nd ed. Thousand Oaks, CA: Sage, 2005; Hart, Chris. Doing a Literature Review: Releasing the Social Science Research Imagination . Thousand Oaks, CA: Sage Publications, 1998; Jesson, Jill. Doing Your Literature Review: Traditional and Systematic Techniques . Los Angeles, CA: SAGE, 2011; Knopf, Jeffrey W. "Doing a Literature Review." PS: Political Science and Politics 39 (January 2006): 127-132; Ridley, Diana. The Literature Review: A Step-by-Step Guide for Students . 2nd ed. Los Angeles, CA: SAGE, 2012.
Types of Literature Reviews
It is important to think of knowledge in a given field as consisting of three layers. First, there are the primary studies that researchers conduct and publish. Second are the reviews of those studies that summarize and offer new interpretations built from and often extending beyond the primary studies. Third, there are the perceptions, conclusions, opinion, and interpretations that are shared informally among scholars that become part of the body of epistemological traditions within the field.
In composing a literature review, it is important to note that it is often this third layer of knowledge that is cited as "true" even though it often has only a loose relationship to the primary studies and secondary literature reviews. Given this, while literature reviews are designed to provide an overview and synthesis of pertinent sources you have explored, there are a number of approaches you could adopt depending upon the type of analysis underpinning your study.
Argumentative Review This form examines literature selectively in order to support or refute an argument, deeply embedded assumption, or philosophical problem already established in the literature. The purpose is to develop a body of literature that establishes a contrarian viewpoint. Given the value-laden nature of some social science research [e.g., educational reform; immigration control], argumentative approaches to analyzing the literature can be a legitimate and important form of discourse. However, note that they can also introduce problems of bias when they are used to make summary claims of the sort found in systematic reviews [see below].
Integrative Review Considered a form of research that reviews, critiques, and synthesizes representative literature on a topic in an integrated way such that new frameworks and perspectives on the topic are generated. The body of literature includes all studies that address related or identical hypotheses or research problems. A well-done integrative review meets the same standards as primary research in regard to clarity, rigor, and replication. This is the most common form of review in the social sciences.
Historical Review Few things rest in isolation from historical precedent. Historical literature reviews focus on examining research throughout a period of time, often starting with the first time an issue, concept, theory, phenomena emerged in the literature, then tracing its evolution within the scholarship of a discipline. The purpose is to place research in a historical context to show familiarity with state-of-the-art developments and to identify the likely directions for future research.
Methodological Review A review does not always focus on what someone said [findings], but how they came about saying what they say [method of analysis]. Reviewing methods of analysis provides a framework of understanding at different levels [i.e. those of theory, substantive fields, research approaches, and data collection and analysis techniques], how researchers draw upon a wide variety of knowledge ranging from the conceptual level to practical documents for use in fieldwork in the areas of ontological and epistemological consideration, quantitative and qualitative integration, sampling, interviewing, data collection, and data analysis. This approach helps highlight ethical issues which you should be aware of and consider as you go through your own study.
Systematic Review This form consists of an overview of existing evidence pertinent to a clearly formulated research question, which uses pre-specified and standardized methods to identify and critically appraise relevant research, and to collect, report, and analyze data from the studies that are included in the review. The goal is to deliberately document, critically evaluate, and summarize scientifically all of the research about a clearly defined research problem . Typically it focuses on a very specific empirical question, often posed in a cause-and-effect form, such as "To what extent does A contribute to B?" This type of literature review is primarily applied to examining prior research studies in clinical medicine and allied health fields, but it is increasingly being used in the social sciences.
Theoretical Review The purpose of this form is to examine the corpus of theory that has accumulated in regard to an issue, concept, theory, phenomena. The theoretical literature review helps to establish what theories already exist, the relationships between them, to what degree the existing theories have been investigated, and to develop new hypotheses to be tested. Often this form is used to help establish a lack of appropriate theories or reveal that current theories are inadequate for explaining new or emerging research problems. The unit of analysis can focus on a theoretical concept or a whole theory or framework.
NOTE : Most often the literature review will incorporate some combination of types. For example, a review that examines literature supporting or refuting an argument, assumption, or philosophical problem related to the research problem will also need to include writing supported by sources that establish the history of these arguments in the literature.
Baumeister, Roy F. and Mark R. Leary. "Writing Narrative Literature Reviews." Review of General Psychology 1 (September 1997): 311-320; Mark R. Fink, Arlene. Conducting Research Literature Reviews: From the Internet to Paper . 2nd ed. Thousand Oaks, CA: Sage, 2005; Hart, Chris. Doing a Literature Review: Releasing the Social Science Research Imagination . Thousand Oaks, CA: Sage Publications, 1998; Kennedy, Mary M. "Defining a Literature." Educational Researcher 36 (April 2007): 139-147; Petticrew, Mark and Helen Roberts. Systematic Reviews in the Social Sciences: A Practical Guide . Malden, MA: Blackwell Publishers, 2006; Torracro, Richard. "Writing Integrative Literature Reviews: Guidelines and Examples." Human Resource Development Review 4 (September 2005): 356-367; Rocco, Tonette S. and Maria S. Plakhotnik. "Literature Reviews, Conceptual Frameworks, and Theoretical Frameworks: Terms, Functions, and Distinctions." Human Ressource Development Review 8 (March 2008): 120-130; Sutton, Anthea. Systematic Approaches to a Successful Literature Review . Los Angeles, CA: Sage Publications, 2016.
Structure and Writing Style
I. Thinking About Your Literature Review
The structure of a literature review should include the following in support of understanding the research problem :
- An overview of the subject, issue, or theory under consideration, along with the objectives of the literature review,
- Division of works under review into themes or categories [e.g. works that support a particular position, those against, and those offering alternative approaches entirely],
- An explanation of how each work is similar to and how it varies from the others,
- Conclusions as to which pieces are best considered in their argument, are most convincing of their opinions, and make the greatest contribution to the understanding and development of their area of research.
The critical evaluation of each work should consider :
- Provenance -- what are the author's credentials? Are the author's arguments supported by evidence [e.g. primary historical material, case studies, narratives, statistics, recent scientific findings]?
- Methodology -- were the techniques used to identify, gather, and analyze the data appropriate to addressing the research problem? Was the sample size appropriate? Were the results effectively interpreted and reported?
- Objectivity -- is the author's perspective even-handed or prejudicial? Is contrary data considered or is certain pertinent information ignored to prove the author's point?
- Persuasiveness -- which of the author's theses are most convincing or least convincing?
- Validity -- are the author's arguments and conclusions convincing? Does the work ultimately contribute in any significant way to an understanding of the subject?
II. Development of the Literature Review
Four Basic Stages of Writing 1. Problem formulation -- which topic or field is being examined and what are its component issues? 2. Literature search -- finding materials relevant to the subject being explored. 3. Data evaluation -- determining which literature makes a significant contribution to the understanding of the topic. 4. Analysis and interpretation -- discussing the findings and conclusions of pertinent literature.
Consider the following issues before writing the literature review: Clarify If your assignment is not specific about what form your literature review should take, seek clarification from your professor by asking these questions: 1. Roughly how many sources would be appropriate to include? 2. What types of sources should I review (books, journal articles, websites; scholarly versus popular sources)? 3. Should I summarize, synthesize, or critique sources by discussing a common theme or issue? 4. Should I evaluate the sources in any way beyond evaluating how they relate to understanding the research problem? 5. Should I provide subheadings and other background information, such as definitions and/or a history? Find Models Use the exercise of reviewing the literature to examine how authors in your discipline or area of interest have composed their literature review sections. Read them to get a sense of the types of themes you might want to look for in your own research or to identify ways to organize your final review. The bibliography or reference section of sources you've already read, such as required readings in the course syllabus, are also excellent entry points into your own research. Narrow the Topic The narrower your topic, the easier it will be to limit the number of sources you need to read in order to obtain a good survey of relevant resources. Your professor will probably not expect you to read everything that's available about the topic, but you'll make the act of reviewing easier if you first limit scope of the research problem. A good strategy is to begin by searching the USC Libraries Catalog for recent books about the topic and review the table of contents for chapters that focuses on specific issues. You can also review the indexes of books to find references to specific issues that can serve as the focus of your research. For example, a book surveying the history of the Israeli-Palestinian conflict may include a chapter on the role Egypt has played in mediating the conflict, or look in the index for the pages where Egypt is mentioned in the text. Consider Whether Your Sources are Current Some disciplines require that you use information that is as current as possible. This is particularly true in disciplines in medicine and the sciences where research conducted becomes obsolete very quickly as new discoveries are made. However, when writing a review in the social sciences, a survey of the history of the literature may be required. In other words, a complete understanding the research problem requires you to deliberately examine how knowledge and perspectives have changed over time. Sort through other current bibliographies or literature reviews in the field to get a sense of what your discipline expects. You can also use this method to explore what is considered by scholars to be a "hot topic" and what is not.
III. Ways to Organize Your Literature Review
Chronology of Events If your review follows the chronological method, you could write about the materials according to when they were published. This approach should only be followed if a clear path of research building on previous research can be identified and that these trends follow a clear chronological order of development. For example, a literature review that focuses on continuing research about the emergence of German economic power after the fall of the Soviet Union. By Publication Order your sources by publication chronology, then, only if the order demonstrates a more important trend. For instance, you could order a review of literature on environmental studies of brown fields if the progression revealed, for example, a change in the soil collection practices of the researchers who wrote and/or conducted the studies. Thematic [“conceptual categories”] A thematic literature review is the most common approach to summarizing prior research in the social and behavioral sciences. Thematic reviews are organized around a topic or issue, rather than the progression of time, although the progression of time may still be incorporated into a thematic review. For example, a review of the Internet’s impact on American presidential politics could focus on the development of online political satire. While the study focuses on one topic, the Internet’s impact on American presidential politics, it would still be organized chronologically reflecting technological developments in media. The difference in this example between a "chronological" and a "thematic" approach is what is emphasized the most: themes related to the role of the Internet in presidential politics. Note that more authentic thematic reviews tend to break away from chronological order. A review organized in this manner would shift between time periods within each section according to the point being made. Methodological A methodological approach focuses on the methods utilized by the researcher. For the Internet in American presidential politics project, one methodological approach would be to look at cultural differences between the portrayal of American presidents on American, British, and French websites. Or the review might focus on the fundraising impact of the Internet on a particular political party. A methodological scope will influence either the types of documents in the review or the way in which these documents are discussed.
Other Sections of Your Literature Review Once you've decided on the organizational method for your literature review, the sections you need to include in the paper should be easy to figure out because they arise from your organizational strategy. In other words, a chronological review would have subsections for each vital time period; a thematic review would have subtopics based upon factors that relate to the theme or issue. However, sometimes you may need to add additional sections that are necessary for your study, but do not fit in the organizational strategy of the body. What other sections you include in the body is up to you. However, only include what is necessary for the reader to locate your study within the larger scholarship about the research problem.
Here are examples of other sections, usually in the form of a single paragraph, you may need to include depending on the type of review you write:
- Current Situation : Information necessary to understand the current topic or focus of the literature review.
- Sources Used : Describes the methods and resources [e.g., databases] you used to identify the literature you reviewed.
- History : The chronological progression of the field, the research literature, or an idea that is necessary to understand the literature review, if the body of the literature review is not already a chronology.
- Selection Methods : Criteria you used to select (and perhaps exclude) sources in your literature review. For instance, you might explain that your review includes only peer-reviewed [i.e., scholarly] sources.
- Standards : Description of the way in which you present your information.
- Questions for Further Research : What questions about the field has the review sparked? How will you further your research as a result of the review?
IV. Writing Your Literature Review
Once you've settled on how to organize your literature review, you're ready to write each section. When writing your review, keep in mind these issues.
Use Evidence A literature review section is, in this sense, just like any other academic research paper. Your interpretation of the available sources must be backed up with evidence [citations] that demonstrates that what you are saying is valid. Be Selective Select only the most important points in each source to highlight in the review. The type of information you choose to mention should relate directly to the research problem, whether it is thematic, methodological, or chronological. Related items that provide additional information, but that are not key to understanding the research problem, can be included in a list of further readings . Use Quotes Sparingly Some short quotes are appropriate if you want to emphasize a point, or if what an author stated cannot be easily paraphrased. Sometimes you may need to quote certain terminology that was coined by the author, is not common knowledge, or taken directly from the study. Do not use extensive quotes as a substitute for using your own words in reviewing the literature. Summarize and Synthesize Remember to summarize and synthesize your sources within each thematic paragraph as well as throughout the review. Recapitulate important features of a research study, but then synthesize it by rephrasing the study's significance and relating it to your own work and the work of others. Keep Your Own Voice While the literature review presents others' ideas, your voice [the writer's] should remain front and center. For example, weave references to other sources into what you are writing but maintain your own voice by starting and ending the paragraph with your own ideas and wording. Use Caution When Paraphrasing When paraphrasing a source that is not your own, be sure to represent the author's information or opinions accurately and in your own words. Even when paraphrasing an author’s work, you still must provide a citation to that work.
V. Common Mistakes to Avoid
These are the most common mistakes made in reviewing social science research literature.
- Sources in your literature review do not clearly relate to the research problem;
- You do not take sufficient time to define and identify the most relevant sources to use in the literature review related to the research problem;
- Relies exclusively on secondary analytical sources rather than including relevant primary research studies or data;
- Uncritically accepts another researcher's findings and interpretations as valid, rather than examining critically all aspects of the research design and analysis;
- Does not describe the search procedures that were used in identifying the literature to review;
- Reports isolated statistical results rather than synthesizing them in chi-squared or meta-analytic methods; and,
- Only includes research that validates assumptions and does not consider contrary findings and alternative interpretations found in the literature.
Cook, Kathleen E. and Elise Murowchick. “Do Literature Review Skills Transfer from One Course to Another?” Psychology Learning and Teaching 13 (March 2014): 3-11; Fink, Arlene. Conducting Research Literature Reviews: From the Internet to Paper . 2nd ed. Thousand Oaks, CA: Sage, 2005; Hart, Chris. Doing a Literature Review: Releasing the Social Science Research Imagination . Thousand Oaks, CA: Sage Publications, 1998; Jesson, Jill. Doing Your Literature Review: Traditional and Systematic Techniques . London: SAGE, 2011; Literature Review Handout. Online Writing Center. Liberty University; Literature Reviews. The Writing Center. University of North Carolina; Onwuegbuzie, Anthony J. and Rebecca Frels. Seven Steps to a Comprehensive Literature Review: A Multimodal and Cultural Approach . Los Angeles, CA: SAGE, 2016; Ridley, Diana. The Literature Review: A Step-by-Step Guide for Students . 2nd ed. Los Angeles, CA: SAGE, 2012; Randolph, Justus J. “A Guide to Writing the Dissertation Literature Review." Practical Assessment, Research, and Evaluation. vol. 14, June 2009; Sutton, Anthea. Systematic Approaches to a Successful Literature Review . Los Angeles, CA: Sage Publications, 2016; Taylor, Dena. The Literature Review: A Few Tips On Conducting It. University College Writing Centre. University of Toronto; Writing a Literature Review. Academic Skills Centre. University of Canberra.
Writing Tip
Break Out of Your Disciplinary Box!
Thinking interdisciplinarily about a research problem can be a rewarding exercise in applying new ideas, theories, or concepts to an old problem. For example, what might cultural anthropologists say about the continuing conflict in the Middle East? In what ways might geographers view the need for better distribution of social service agencies in large cities than how social workers might study the issue? You don’t want to substitute a thorough review of core research literature in your discipline for studies conducted in other fields of study. However, particularly in the social sciences, thinking about research problems from multiple vectors is a key strategy for finding new solutions to a problem or gaining a new perspective. Consult with a librarian about identifying research databases in other disciplines; almost every field of study has at least one comprehensive database devoted to indexing its research literature.
Frodeman, Robert. The Oxford Handbook of Interdisciplinarity . New York: Oxford University Press, 2010.
Another Writing Tip
Don't Just Review for Content!
While conducting a review of the literature, maximize the time you devote to writing this part of your paper by thinking broadly about what you should be looking for and evaluating. Review not just what scholars are saying, but how are they saying it. Some questions to ask:
- How are they organizing their ideas?
- What methods have they used to study the problem?
- What theories have been used to explain, predict, or understand their research problem?
- What sources have they cited to support their conclusions?
- How have they used non-textual elements [e.g., charts, graphs, figures, etc.] to illustrate key points?
When you begin to write your literature review section, you'll be glad you dug deeper into how the research was designed and constructed because it establishes a means for developing more substantial analysis and interpretation of the research problem.
Hart, Chris. Doing a Literature Review: Releasing the Social Science Research Imagination . Thousand Oaks, CA: Sage Publications, 1 998.
Yet Another Writing Tip
When Do I Know I Can Stop Looking and Move On?
Here are several strategies you can utilize to assess whether you've thoroughly reviewed the literature:
- Look for repeating patterns in the research findings . If the same thing is being said, just by different people, then this likely demonstrates that the research problem has hit a conceptual dead end. At this point consider: Does your study extend current research? Does it forge a new path? Or, does is merely add more of the same thing being said?
- Look at sources the authors cite to in their work . If you begin to see the same researchers cited again and again, then this is often an indication that no new ideas have been generated to address the research problem.
- Search Google Scholar to identify who has subsequently cited leading scholars already identified in your literature review [see next sub-tab]. This is called citation tracking and there are a number of sources that can help you identify who has cited whom, particularly scholars from outside of your discipline. Here again, if the same authors are being cited again and again, this may indicate no new literature has been written on the topic.
Onwuegbuzie, Anthony J. and Rebecca Frels. Seven Steps to a Comprehensive Literature Review: A Multimodal and Cultural Approach . Los Angeles, CA: Sage, 2016; Sutton, Anthea. Systematic Approaches to a Successful Literature Review . Los Angeles, CA: Sage Publications, 2016.
- << Previous: Theoretical Framework
- Next: Citation Tracking >>
- Last Updated: Apr 24, 2024 10:51 AM
- URL: https://libguides.usc.edu/writingguide
Have a language expert improve your writing
Run a free plagiarism check in 10 minutes, automatically generate references for free.
- Knowledge Base
- Dissertation
- What is a Literature Review? | Guide, Template, & Examples
What is a Literature Review? | Guide, Template, & Examples
Published on 22 February 2022 by Shona McCombes . Revised on 7 June 2022.
What is a literature review? A literature review is a survey of scholarly sources on a specific topic. It provides an overview of current knowledge, allowing you to identify relevant theories, methods, and gaps in the existing research.
There are five key steps to writing a literature review:
- Search for relevant literature
- Evaluate sources
- Identify themes, debates and gaps
- Outline the structure
- Write your literature review
A good literature review doesn’t just summarise sources – it analyses, synthesises, and critically evaluates to give a clear picture of the state of knowledge on the subject.
Instantly correct all language mistakes in your text
Be assured that you'll submit flawless writing. Upload your document to correct all your mistakes.
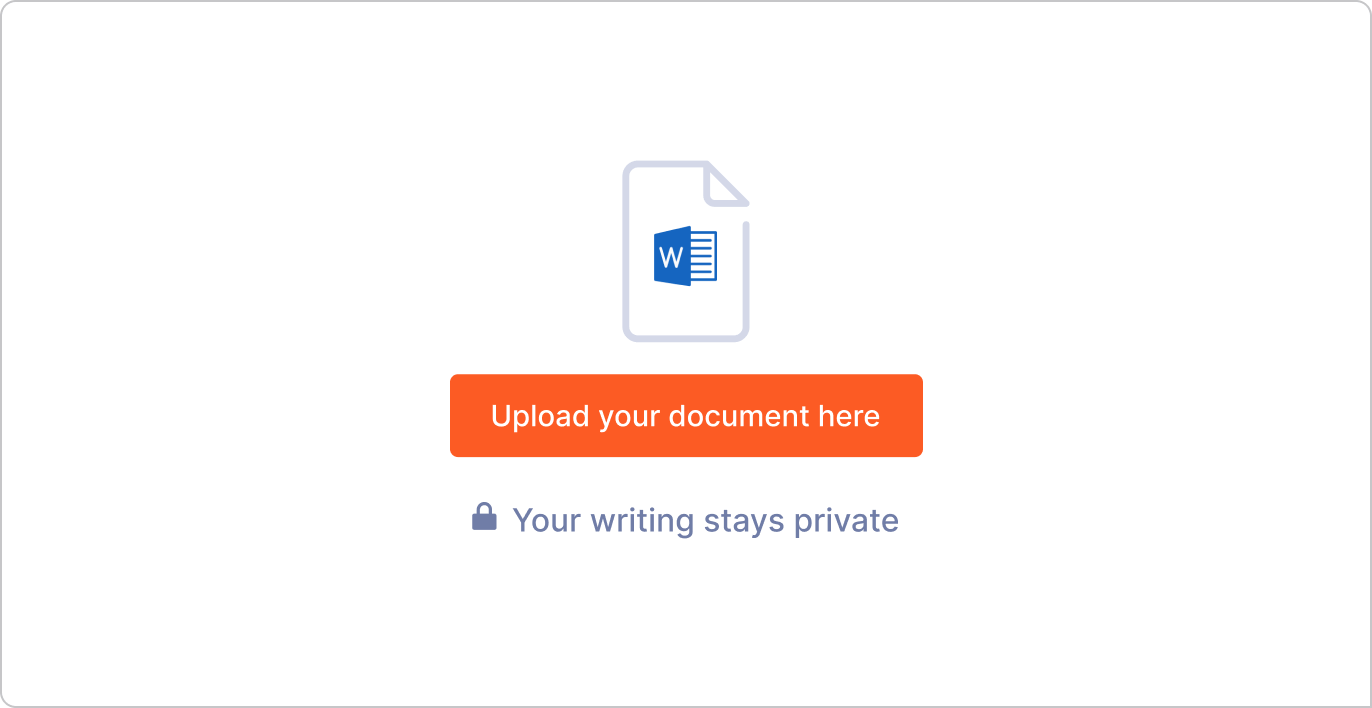
Table of contents
Why write a literature review, examples of literature reviews, step 1: search for relevant literature, step 2: evaluate and select sources, step 3: identify themes, debates and gaps, step 4: outline your literature review’s structure, step 5: write your literature review, frequently asked questions about literature reviews, introduction.
- Quick Run-through
- Step 1 & 2
When you write a dissertation or thesis, you will have to conduct a literature review to situate your research within existing knowledge. The literature review gives you a chance to:
- Demonstrate your familiarity with the topic and scholarly context
- Develop a theoretical framework and methodology for your research
- Position yourself in relation to other researchers and theorists
- Show how your dissertation addresses a gap or contributes to a debate
You might also have to write a literature review as a stand-alone assignment. In this case, the purpose is to evaluate the current state of research and demonstrate your knowledge of scholarly debates around a topic.
The content will look slightly different in each case, but the process of conducting a literature review follows the same steps. We’ve written a step-by-step guide that you can follow below.
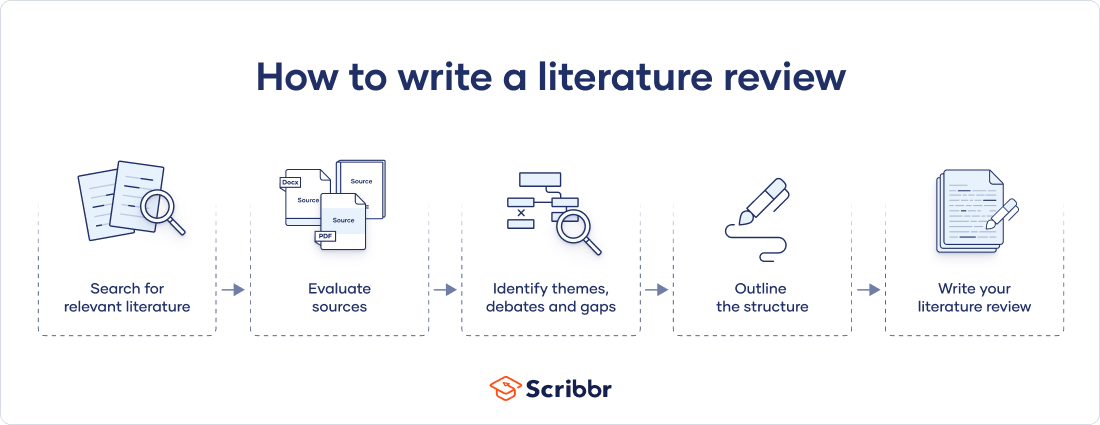
The only proofreading tool specialized in correcting academic writing
The academic proofreading tool has been trained on 1000s of academic texts and by native English editors. Making it the most accurate and reliable proofreading tool for students.
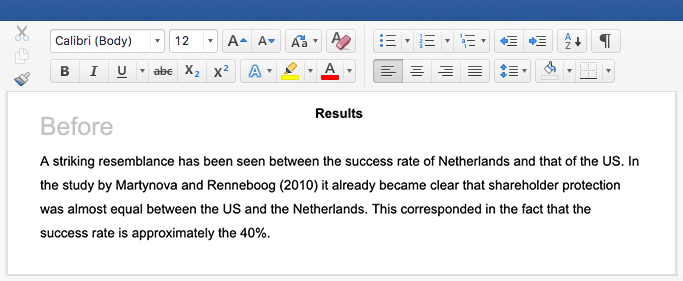
Correct my document today
Writing literature reviews can be quite challenging! A good starting point could be to look at some examples, depending on what kind of literature review you’d like to write.
- Example literature review #1: “Why Do People Migrate? A Review of the Theoretical Literature” ( Theoretical literature review about the development of economic migration theory from the 1950s to today.)
- Example literature review #2: “Literature review as a research methodology: An overview and guidelines” ( Methodological literature review about interdisciplinary knowledge acquisition and production.)
- Example literature review #3: “The Use of Technology in English Language Learning: A Literature Review” ( Thematic literature review about the effects of technology on language acquisition.)
- Example literature review #4: “Learners’ Listening Comprehension Difficulties in English Language Learning: A Literature Review” ( Chronological literature review about how the concept of listening skills has changed over time.)
You can also check out our templates with literature review examples and sample outlines at the links below.
Download Word doc Download Google doc
Before you begin searching for literature, you need a clearly defined topic .
If you are writing the literature review section of a dissertation or research paper, you will search for literature related to your research objectives and questions .
If you are writing a literature review as a stand-alone assignment, you will have to choose a focus and develop a central question to direct your search. Unlike a dissertation research question, this question has to be answerable without collecting original data. You should be able to answer it based only on a review of existing publications.
Make a list of keywords
Start by creating a list of keywords related to your research topic. Include each of the key concepts or variables you’re interested in, and list any synonyms and related terms. You can add to this list if you discover new keywords in the process of your literature search.
- Social media, Facebook, Instagram, Twitter, Snapchat, TikTok
- Body image, self-perception, self-esteem, mental health
- Generation Z, teenagers, adolescents, youth
Search for relevant sources
Use your keywords to begin searching for sources. Some databases to search for journals and articles include:
- Your university’s library catalogue
- Google Scholar
- Project Muse (humanities and social sciences)
- Medline (life sciences and biomedicine)
- EconLit (economics)
- Inspec (physics, engineering and computer science)
You can use boolean operators to help narrow down your search:
Read the abstract to find out whether an article is relevant to your question. When you find a useful book or article, you can check the bibliography to find other relevant sources.
To identify the most important publications on your topic, take note of recurring citations. If the same authors, books or articles keep appearing in your reading, make sure to seek them out.
You probably won’t be able to read absolutely everything that has been written on the topic – you’ll have to evaluate which sources are most relevant to your questions.
For each publication, ask yourself:
- What question or problem is the author addressing?
- What are the key concepts and how are they defined?
- What are the key theories, models and methods? Does the research use established frameworks or take an innovative approach?
- What are the results and conclusions of the study?
- How does the publication relate to other literature in the field? Does it confirm, add to, or challenge established knowledge?
- How does the publication contribute to your understanding of the topic? What are its key insights and arguments?
- What are the strengths and weaknesses of the research?
Make sure the sources you use are credible, and make sure you read any landmark studies and major theories in your field of research.
You can find out how many times an article has been cited on Google Scholar – a high citation count means the article has been influential in the field, and should certainly be included in your literature review.
The scope of your review will depend on your topic and discipline: in the sciences you usually only review recent literature, but in the humanities you might take a long historical perspective (for example, to trace how a concept has changed in meaning over time).
Remember that you can use our template to summarise and evaluate sources you’re thinking about using!
Take notes and cite your sources
As you read, you should also begin the writing process. Take notes that you can later incorporate into the text of your literature review.
It’s important to keep track of your sources with references to avoid plagiarism . It can be helpful to make an annotated bibliography, where you compile full reference information and write a paragraph of summary and analysis for each source. This helps you remember what you read and saves time later in the process.
You can use our free APA Reference Generator for quick, correct, consistent citations.
Prevent plagiarism, run a free check.
To begin organising your literature review’s argument and structure, you need to understand the connections and relationships between the sources you’ve read. Based on your reading and notes, you can look for:
- Trends and patterns (in theory, method or results): do certain approaches become more or less popular over time?
- Themes: what questions or concepts recur across the literature?
- Debates, conflicts and contradictions: where do sources disagree?
- Pivotal publications: are there any influential theories or studies that changed the direction of the field?
- Gaps: what is missing from the literature? Are there weaknesses that need to be addressed?
This step will help you work out the structure of your literature review and (if applicable) show how your own research will contribute to existing knowledge.
- Most research has focused on young women.
- There is an increasing interest in the visual aspects of social media.
- But there is still a lack of robust research on highly-visual platforms like Instagram and Snapchat – this is a gap that you could address in your own research.
There are various approaches to organising the body of a literature review. You should have a rough idea of your strategy before you start writing.
Depending on the length of your literature review, you can combine several of these strategies (for example, your overall structure might be thematic, but each theme is discussed chronologically).
Chronological
The simplest approach is to trace the development of the topic over time. However, if you choose this strategy, be careful to avoid simply listing and summarising sources in order.
Try to analyse patterns, turning points and key debates that have shaped the direction of the field. Give your interpretation of how and why certain developments occurred.
If you have found some recurring central themes, you can organise your literature review into subsections that address different aspects of the topic.
For example, if you are reviewing literature about inequalities in migrant health outcomes, key themes might include healthcare policy, language barriers, cultural attitudes, legal status, and economic access.
Methodological
If you draw your sources from different disciplines or fields that use a variety of research methods , you might want to compare the results and conclusions that emerge from different approaches. For example:
- Look at what results have emerged in qualitative versus quantitative research
- Discuss how the topic has been approached by empirical versus theoretical scholarship
- Divide the literature into sociological, historical, and cultural sources
Theoretical
A literature review is often the foundation for a theoretical framework . You can use it to discuss various theories, models, and definitions of key concepts.
You might argue for the relevance of a specific theoretical approach, or combine various theoretical concepts to create a framework for your research.
Like any other academic text, your literature review should have an introduction , a main body, and a conclusion . What you include in each depends on the objective of your literature review.
The introduction should clearly establish the focus and purpose of the literature review.
If you are writing the literature review as part of your dissertation or thesis, reiterate your central problem or research question and give a brief summary of the scholarly context. You can emphasise the timeliness of the topic (“many recent studies have focused on the problem of x”) or highlight a gap in the literature (“while there has been much research on x, few researchers have taken y into consideration”).
Depending on the length of your literature review, you might want to divide the body into subsections. You can use a subheading for each theme, time period, or methodological approach.
As you write, make sure to follow these tips:
- Summarise and synthesise: give an overview of the main points of each source and combine them into a coherent whole.
- Analyse and interpret: don’t just paraphrase other researchers – add your own interpretations, discussing the significance of findings in relation to the literature as a whole.
- Critically evaluate: mention the strengths and weaknesses of your sources.
- Write in well-structured paragraphs: use transitions and topic sentences to draw connections, comparisons and contrasts.
In the conclusion, you should summarise the key findings you have taken from the literature and emphasise their significance.
If the literature review is part of your dissertation or thesis, reiterate how your research addresses gaps and contributes new knowledge, or discuss how you have drawn on existing theories and methods to build a framework for your research. This can lead directly into your methodology section.
A literature review is a survey of scholarly sources (such as books, journal articles, and theses) related to a specific topic or research question .
It is often written as part of a dissertation , thesis, research paper , or proposal .
There are several reasons to conduct a literature review at the beginning of a research project:
- To familiarise yourself with the current state of knowledge on your topic
- To ensure that you’re not just repeating what others have already done
- To identify gaps in knowledge and unresolved problems that your research can address
- To develop your theoretical framework and methodology
- To provide an overview of the key findings and debates on the topic
Writing the literature review shows your reader how your work relates to existing research and what new insights it will contribute.
The literature review usually comes near the beginning of your dissertation . After the introduction , it grounds your research in a scholarly field and leads directly to your theoretical framework or methodology .
Cite this Scribbr article
If you want to cite this source, you can copy and paste the citation or click the ‘Cite this Scribbr article’ button to automatically add the citation to our free Reference Generator.
McCombes, S. (2022, June 07). What is a Literature Review? | Guide, Template, & Examples. Scribbr. Retrieved 22 April 2024, from https://www.scribbr.co.uk/thesis-dissertation/literature-review/
Is this article helpful?
Shona McCombes
Other students also liked, how to write a dissertation proposal | a step-by-step guide, what is a theoretical framework | a step-by-step guide, what is a research methodology | steps & tips.

How to Write a Good Scientific Literature Review
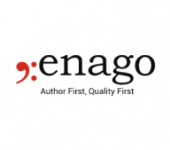
Nowadays, there is a huge demand for scientific literature reviews as they are especially appreciated by scholars or researchers when designing their research proposals. While finding information is less of a problem to them, discerning which paper or publication has enough quality has become one of the biggest issues. Literature reviews narrow the current knowledge on a certain field and examine the latest publications’ strengths and weaknesses. This way, they are priceless tools not only for those who are starting their research, but also for all those interested in recent publications. To be useful, literature reviews must be written in a professional way with a clear structure. The amount of work needed to write a scientific literature review must be considered before starting one since the tasks required can overwhelm many if the working method is not the best.
Designing and Writing a Scientific Literature Review
Writing a scientific review implies both researching for relevant academic content and writing , however, writing without having a clear objective is a common mistake. Sometimes, studying the situation and defining the work’s system is so important and takes equally as much time as that required in writing the final result. Therefore, we suggest that you divide your path into three steps.
Define goals and a structure
Think about your target and narrow down your topic. If you don’t choose a well-defined topic, you can find yourself dealing with a wide subject and plenty of publications about it. Remember that researchers usually deal with really specific fields of study.
It is time to be a critic and locate only pertinent publications. While researching for content consider publications that were written 3 years ago at the most. Write notes and summarize the content of each paper as that will help you in the next step.
Time to write
Check some literature review examples to decide how to start writing a good literature review . When your goals and structure are defined, begin writing without forgetting your target at any moment.
Related: Conducting a literature survey? Wish to learn more about scientific misconduct? Check out this resourceful infographic.
Here you have a to-do list to help you write your review :
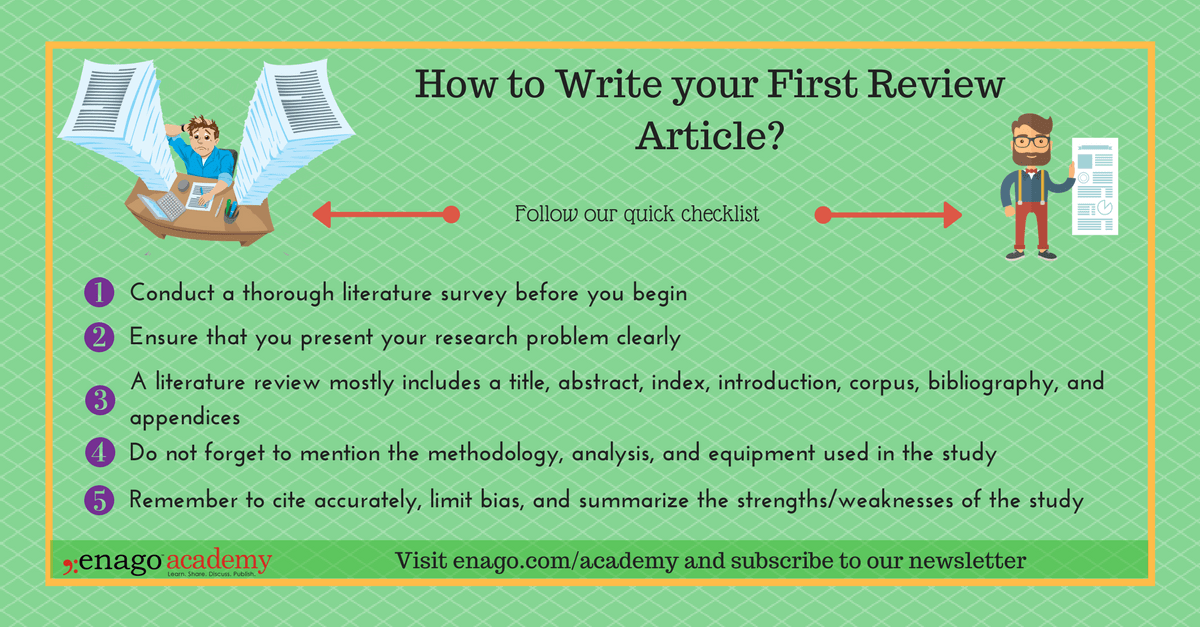
- A scientific literature review usually includes a title, abstract, index, introduction, corpus, bibliography, and appendices (if needed).
- Present the problem clearly.
- Mention the paper’s methodology, research methods, analysis, instruments, etc.
- Present literature review examples that can help you express your ideas.
- Remember to cite accurately.
- Limit your bias
- While summarizing also identify strengths and weaknesses as this is critical.
Scholars and researchers are usually the best candidates to write scientific literature reviews, not only because they are experts in a certain field, but also because they know the exigencies and needs that researchers have while writing research proposals or looking for information among thousands of academic papers. Therefore, considering your experience as a researcher can help you understand how to write a scientific literature review.
Have you faced challenges while drafting your first literature review? How do you think can these tips help you in acing your next literature review? Let us know in the comments section below! You can also visit our Q&A forum for frequently asked questions related to copyrights answered by our team that comprises eminent researchers and publication experts.
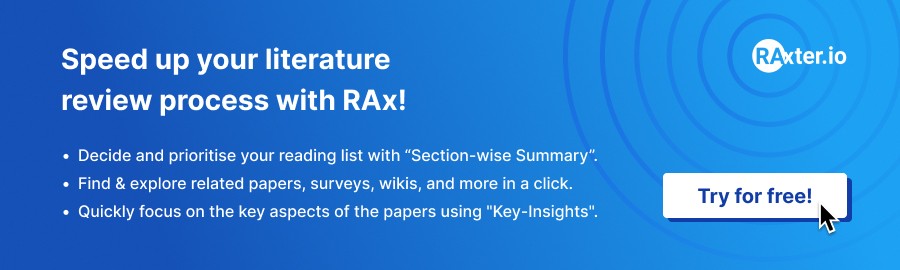
Thank you for your information. It adds knowledge on critical review being a first time to do it, it helps a lot.
yes. i would like to ndertake the course Bio ststistics
Rate this article Cancel Reply
Your email address will not be published.
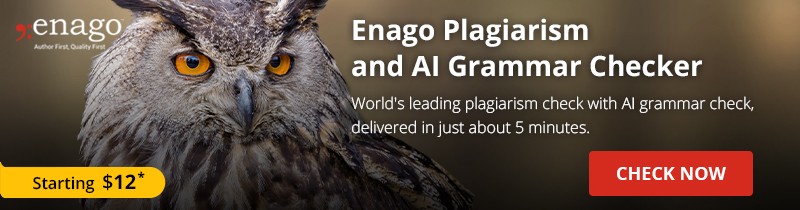
Enago Academy's Most Popular Articles
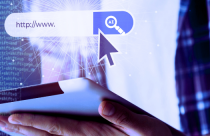
- Reporting Research
AI Assistance in Academia for Searching Credible Scholarly Sources
The journey of academia is a grand quest for knowledge, more specifically an adventure to…
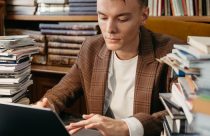
- Manuscripts & Grants
Writing a Research Literature Review? — Here are tips to guide you through!
Literature review is both a process and a product. It involves searching within a defined…
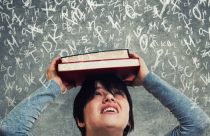
- AI in Academia
How to Scan Through Millions of Articles and Still Cut Down on Your Reading Time — Why not do it with an AI-based article summarizer?
Researcher 1: “It’s flooding articles every time I switch on my laptop!” Researcher 2: “Why…
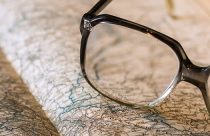
How to Master at Literature Mapping: 5 Most Recommended Tools to Use
This article is also available in: Turkish, Spanish, Russian, and Portuguese
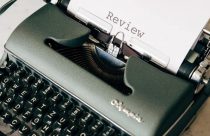
- Old Webinars
- Webinar Mobile App
Improving Your Chances of Publication in International Peer-reviewed Journals
Types of literature reviews Tips for writing review articles Role of meta-analysis Reporting guidelines
How to Scan Through Millions of Articles and Still Cut Down on Your Reading Time —…

Sign-up to read more
Subscribe for free to get unrestricted access to all our resources on research writing and academic publishing including:
- 2000+ blog articles
- 50+ Webinars
- 10+ Expert podcasts
- 50+ Infographics
- 10+ Checklists
- Research Guides
We hate spam too. We promise to protect your privacy and never spam you.
I am looking for Editing/ Proofreading services for my manuscript Tentative date of next journal submission:
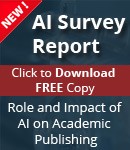
What should universities' stance be on AI tools in research and academic writing?
- Search Menu
- Advance Articles
- Editor's Choice
- CME Reviews
- Best of 2021 collection
- Abbreviated Breast MRI Virtual Collection
- Contrast-enhanced Mammography Collection
- Author Guidelines
- Submission Site
- Open Access
- Self-Archiving Policy
- Accepted Papers Resource Guide
- About Journal of Breast Imaging
- About the Society of Breast Imaging
- Guidelines for Reviewers
- Resources for Reviewers and Authors
- Editorial Board
- Advertising Disclaimer
- Advertising and Corporate Services
- Journals on Oxford Academic
- Books on Oxford Academic

Article Contents
- Introduction
- Selection of a Topic
- Scientific Literature Search and Analysis
- Structure of a Scientific Review Article
- Tips for Success
- Acknowledgments
- Conflict of Interest Statement
- < Previous
A Step-by-Step Guide to Writing a Scientific Review Article
- Article contents
- Figures & tables
- Supplementary Data
Manisha Bahl, A Step-by-Step Guide to Writing a Scientific Review Article, Journal of Breast Imaging , Volume 5, Issue 4, July/August 2023, Pages 480–485, https://doi.org/10.1093/jbi/wbad028
- Permissions Icon Permissions
Scientific review articles are comprehensive, focused reviews of the scientific literature written by subject matter experts. The task of writing a scientific review article can seem overwhelming; however, it can be managed by using an organized approach and devoting sufficient time to the process. The process involves selecting a topic about which the authors are knowledgeable and enthusiastic, conducting a literature search and critical analysis of the literature, and writing the article, which is composed of an abstract, introduction, body, and conclusion, with accompanying tables and figures. This article, which focuses on the narrative or traditional literature review, is intended to serve as a guide with practical steps for new writers. Tips for success are also discussed, including selecting a focused topic, maintaining objectivity and balance while writing, avoiding tedious data presentation in a laundry list format, moving from descriptions of the literature to critical analysis, avoiding simplistic conclusions, and budgeting time for the overall process.
- narrative discourse
Email alerts
Citing articles via.
- Recommend to your Librarian
- Journals Career Network
Affiliations
- Online ISSN 2631-6129
- Print ISSN 2631-6110
- Copyright © 2024 Society of Breast Imaging
- About Oxford Academic
- Publish journals with us
- University press partners
- What we publish
- New features
- Open access
- Institutional account management
- Rights and permissions
- Get help with access
- Accessibility
- Advertising
- Media enquiries
- Oxford University Press
- Oxford Languages
- University of Oxford
Oxford University Press is a department of the University of Oxford. It furthers the University's objective of excellence in research, scholarship, and education by publishing worldwide
- Copyright © 2024 Oxford University Press
- Cookie settings
- Cookie policy
- Privacy policy
- Legal notice
This Feature Is Available To Subscribers Only
Sign In or Create an Account
This PDF is available to Subscribers Only
For full access to this pdf, sign in to an existing account, or purchase an annual subscription.
How to write a good scientific review article
Affiliation.
- 1 The FEBS Journal Editorial Office, Cambridge, UK.
- PMID: 35792782
- DOI: 10.1111/febs.16565
Literature reviews are valuable resources for the scientific community. With research accelerating at an unprecedented speed in recent years and more and more original papers being published, review articles have become increasingly important as a means to keep up to date with developments in a particular area of research. A good review article provides readers with an in-depth understanding of a field and highlights key gaps and challenges to address with future research. Writing a review article also helps to expand the writer's knowledge of their specialist area and to develop their analytical and communication skills, amongst other benefits. Thus, the importance of building review-writing into a scientific career cannot be overstated. In this instalment of The FEBS Journal's Words of Advice series, I provide detailed guidance on planning and writing an informative and engaging literature review.
© 2022 Federation of European Biochemical Societies.
Publication types
- Review Literature as Topic*
Research in the Biological and Life Sciences: A Guide for Cornell Researchers: Literature Reviews
- Books and Dissertations
- Databases and Journals
- Locating Theses
- Resource Not at Cornell?
- Citing Sources
- Staying Current
- Measuring your research impact
- Plagiarism and Copyright
- Data Management
- Literature Reviews
- Evidence Synthesis and Systematic Reviews
- Writing an Honors Thesis
- Poster Making and Printing
- Research Help
What is a Literature Review?
A literature review is a body of text that aims to review the critical points of current knowledge on a particular topic. Most often associated with science-oriented literature, such as a thesis, the literature review usually proceeds a research proposal, methodology and results section. Its ultimate goals is to bring the reader up to date with current literature on a topic and forms that basis for another goal, such as the justification for future research in the area. (retrieved from http://en.wikipedia.org/wiki/Literature_review )
Writing a Literature Review
The literature review is the section of your paper in which you cite and briefly review the related research studies that have been conducted. In this space, you will describe the foundation on which your research will be/is built. You will:
- discuss the work of others
- evaluate their methods and findings
- identify any gaps in their research
- state how your research is different
The literature review should be selective and should group the cited studies in some logical fashion.
If you need some additional assistance writing your literature review, the Knight Institute for Writing in the Disciplines offers a Graduate Writing Service .
Demystifying the Literature Review
For more information, visit our guide devoted to " Demystifying the Literature Review " which includes:
- guide to conducting a literature review,
- a recorded 1.5 hour workshop covering the steps of a literature review, a checklist for drafting your topic and search terms, citation management software for organizing your results, and database searching.
Online Resources
- A Guide to Library Research at Cornell University
- Literature Reviews: An Overview for Graduate Students North Carolina State University
- The Literature Review: A Few Tips on Conducting Written by Dena Taylor, Director, Health Sciences Writing Centre, and Margaret Procter, Coordinator, Writing Support, University of Toronto
- How to Write a Literature Review University Library, University of California, Santa Cruz
- Review of Literature The Writing Center, University of Wisconsin-Madison
Print Resources
- << Previous: Writing
- Next: Evidence Synthesis and Systematic Reviews >>
- Last Updated: Oct 25, 2023 11:28 AM
- URL: https://guides.library.cornell.edu/bio

Something went wrong when searching for seed articles. Please try again soon.
No articles were found for that search term.
Author, year The title of the article goes here
LITERATURE REVIEW SOFTWARE FOR BETTER RESEARCH

“This tool really helped me to create good bibtex references for my research papers”
Ali Mohammed-Djafari
Director of Research at LSS-CNRS, France
“Any researcher could use it! The paper recommendations are great for anyone and everyone”
Swansea University, Wales
“As a student just venturing into the world of lit reviews, this is a tool that is outstanding and helping me find deeper results for my work.”
Franklin Jeffers
South Oregon University, USA
“One of the 3 most promising tools that (1) do not solely rely on keywords, (2) does nice visualizations, (3) is easy to use”
Singapore Management University
“Incredibly useful tool to get to know more literature, and to gain insight in existing research”
KU Leuven, Belgium
“Seeing my literature list as a network enhances my thinking process!”
Katholieke Universiteit Leuven, Belgium
“I can’t live without you anymore! I also recommend you to my students.”
Professor at The Chinese University of Hong Kong
“This has helped me so much in researching the literature. Currently, I am beginning to investigate new fields and this has helped me hugely”
Aran Warren
Canterbury University, NZ
“It's nice to get a quick overview of related literature. Really easy to use, and it helps getting on top of the often complicated structures of referencing”
Christoph Ludwig
Technische Universität Dresden, Germany
“Litmaps is extremely helpful with my research. It helps me organize each one of my projects and see how they relate to each other, as well as to keep up to date on publications done in my field”
Daniel Fuller
Clarkson University, USA
“Litmaps is a game changer for finding novel literature... it has been invaluable for my productivity.... I also got my PhD student to use it and they also found it invaluable, finding several gaps they missed”
Varun Venkatesh
Austin Health, Australia
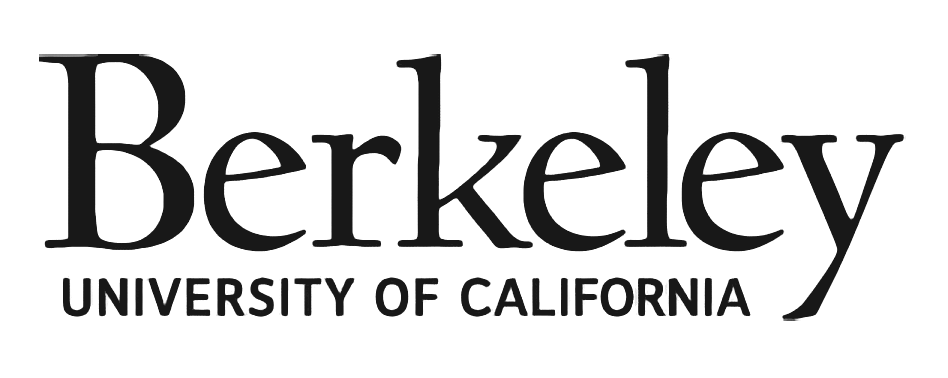
Our Course: Learn and Teach with Litmaps
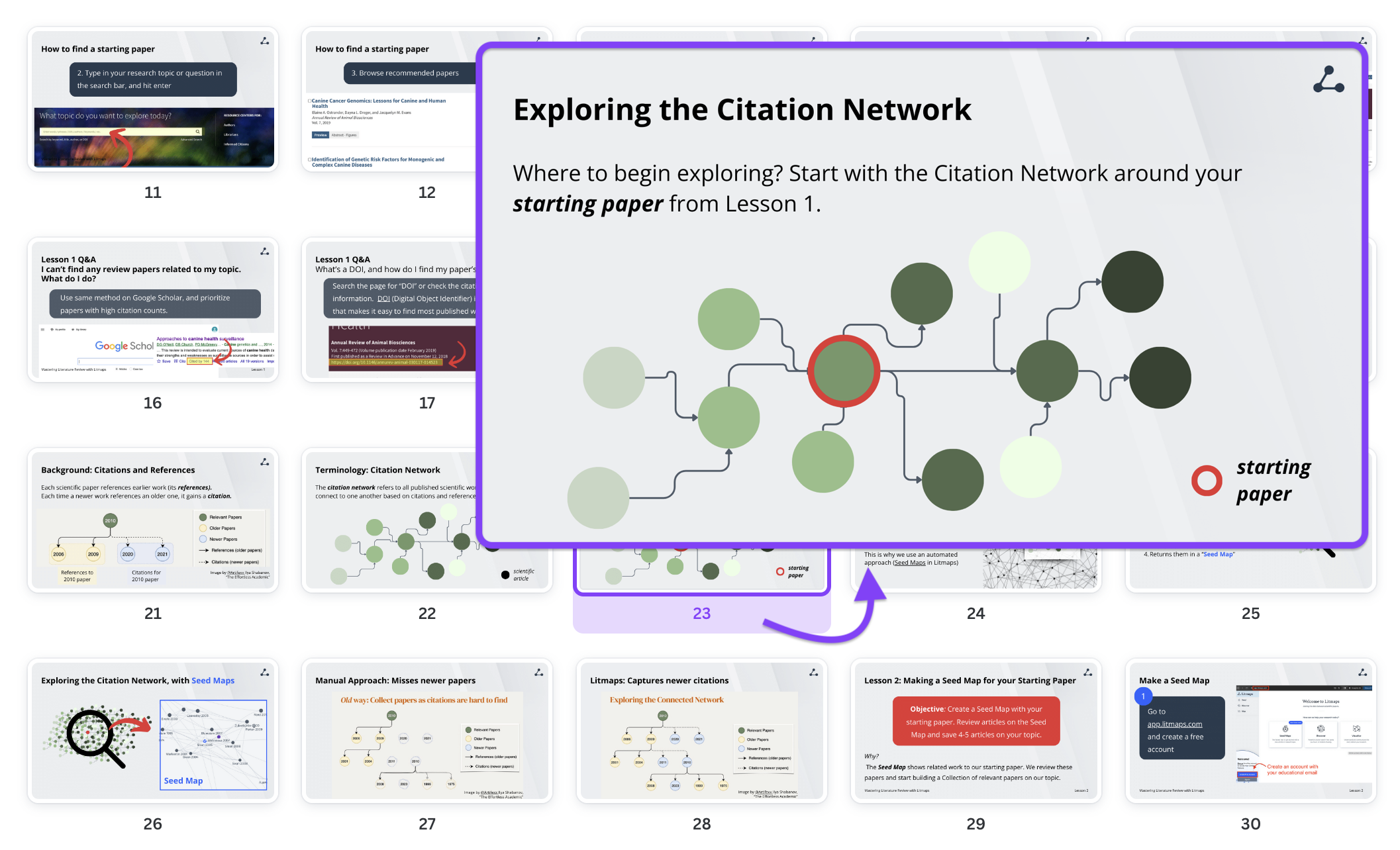
- About WordPress
- Get Involved
- WordPress.org
- Documentation
- Learn WordPress
SRJ Student Resource
Literature review vs research articles: how are they different.
Unlock the secrets of academic writing with our guide to the key differences between a literature review and a research paper! 📚 Dive into the world of scholarly exploration as we break down how a literature review illuminates existing knowledge, identifies gaps, and sets the stage for further research. 🌐 Then, gear up for the adventure of crafting a research paper, where you become the explorer, presenting your unique insights and discoveries through independent research. 🚀 Join us on this academic journey and discover the art of synthesizing existing wisdom and creating your own scholarly masterpiece! 🎓✨
We are always accepting submissions! Submit work within SRJ’s scope anytime while you’re a graduate student.
Leave a Reply Cancel reply
The act of commenting on this site is an opt-in action and San Jose State University may not be held liable for the information provided by participating in the activity.
Your email address will not be published. Required fields are marked *
Save my name, email, and website in this browser for the next time I comment.
Your go-to destination for graduate student research support
- Open access
- Published: 24 April 2024
Breast cancer screening motivation and behaviours of women aged over 75 years: a scoping review
- Virginia Dickson-Swift 1 ,
- Joanne Adams 1 ,
- Evelien Spelten 1 ,
- Irene Blackberry 2 ,
- Carlene Wilson 3 , 4 , 5 &
- Eva Yuen 3 , 6 , 7 , 8
BMC Women's Health volume 24 , Article number: 256 ( 2024 ) Cite this article
100 Accesses
Metrics details
This scoping review aimed to identify and present the evidence describing key motivations for breast cancer screening among women aged ≥ 75 years. Few of the internationally available guidelines recommend continued biennial screening for this age group. Some suggest ongoing screening is unnecessary or should be determined on individual health status and life expectancy. Recent research has shown that despite recommendations regarding screening, older women continue to hold positive attitudes to breast screening and participate when the opportunity is available.
All original research articles that address motivation, intention and/or participation in screening for breast cancer among women aged ≥ 75 years were considered for inclusion. These included articles reporting on women who use public and private breast cancer screening services and those who do not use screening services (i.e., non-screeners).
The Joanna Briggs Institute (JBI) methodology for scoping reviews was used to guide this review. A comprehensive search strategy was developed with the assistance of a specialist librarian to access selected databases including: the Cumulative Index to Nursing and Allied Health Literature (CINAHL), Medline, Web of Science and PsychInfo. The review was restricted to original research studies published since 2009, available in English and focusing on high-income countries (as defined by the World Bank). Title and abstract screening, followed by an assessment of full-text studies against the inclusion criteria was completed by at least two reviewers. Data relating to key motivations, screening intention and behaviour were extracted, and a thematic analysis of study findings undertaken.
A total of fourteen (14) studies were included in the review. Thematic analysis resulted in identification of three themes from included studies highlighting that decisions about screening were influenced by: knowledge of the benefits and harms of screening and their relationship to age; underlying attitudes to the importance of cancer screening in women's lives; and use of decision aids to improve knowledge and guide decision-making.
The results of this review provide a comprehensive overview of current knowledge regarding the motivations and screening behaviour of older women about breast cancer screening which may inform policy development.
Peer Review reports
Introduction
Breast cancer is now the most commonly diagnosed cancer in the world overtaking lung cancer in 2021 [ 1 ]. Across the globe, breast cancer contributed to 25.8% of the total number of new cases of cancer diagnosed in 2020 [ 2 ] and accounts for a high disease burden for women [ 3 ]. Screening for breast cancer is an effective means of detecting early-stage cancer and has been shown to significantly improve survival rates [ 4 ]. A recent systematic review of international screening guidelines found that most countries recommend that women have biennial mammograms between the ages of 40–70 years [ 5 ] with some recommending that there should be no upper age limit [ 6 , 7 , 8 , 9 , 10 , 11 , 12 ] and others suggesting that benefits of continued screening for women over 75 are not clear [ 13 , 14 , 15 ].
Some guidelines suggest that the decision to end screening should be determined based on the individual health status of the woman, their life expectancy and current health issues [ 5 , 16 , 17 ]. This is because the benefits of mammography screening may be limited after 7 years due to existing comorbidities and limited life expectancy [ 18 , 19 , 20 , 21 ], with some jurisdictions recommending breast cancer screening for women ≥ 75 years only when life expectancy is estimated as at least 7–10 years [ 22 ]. Others have argued that decisions about continuing with screening mammography should depend on individual patient risk and health management preferences [ 23 ]. This decision is likely facilitated by a discussion between a health care provider and patient about the harms and benefits of screening outside the recommended ages [ 24 , 25 ]. While mammography may enable early detection of breast cancer, it is clear that false-positive results and overdiagnosis Footnote 1 may occur. Studies have estimated that up to 25% of breast cancer cases in the general population may be over diagnosed [ 26 , 27 , 28 ].
The risk of being diagnosed with breast cancer increases with age and approximately 80% of new cases of breast cancer in high-income countries are in women over the age of 50 [ 29 ]. The average age of first diagnosis of breast cancer in high income countries is comparable to that of Australian women which is now 61 years [ 2 , 4 , 29 ]. Studies show that women aged ≥ 75 years generally have positive attitudes to mammography screening and report high levels of perceived benefits including early detection of breast cancer and a desire to stay healthy as they age [ 21 , 30 , 31 , 32 ]. Some women aged over 74 participate, or plan to participate, in screening despite recommendations from health professionals and government guidelines advising against it [ 33 ]. Results of a recent review found that knowledge of the recommended guidelines and the potential harms of screening are limited and many older women believed that the benefits of continued screening outweighed the risks [ 30 ].
Very few studies have been undertaken to understand the motivations of women to screen or to establish screening participation rates among women aged ≥ 75 and older. This is surprising given that increasing age is recognised as a key risk factor for the development of breast cancer, and that screening is offered in many locations around the world every two years up until 74 years. The importance of this topic is high given the ambiguity around best practice for participation beyond 74 years. A preliminary search of Open Science Framework, PROSPERO, Cochrane Database of Systematic Reviews and JBI Evidence Synthesis in May 2022 did not locate any reviews on this topic.
This scoping review has allowed for the mapping of a broad range of research to explore the breadth and depth of the literature, summarize the evidence and identify knowledge gaps [ 34 , 35 ]. This information has supported the development of a comprehensive overview of current knowledge of motivations of women to screen and screening participation rates among women outside the targeted age of many international screening programs.
Materials and methods
Research question.
The research question for this scoping review was developed by applying the Population—Concept—Context (PCC) framework [ 36 ]. The current review addresses the research question “What research has been undertaken in high-income countries (context) exploring the key motivations to screen for breast cancer and screening participation (concepts) among women ≥ 75 years of age (population)?
Eligibility criteria
Participants.
Women aged ≥ 75 years were the key population. Specifically, motivations to screen and screening intention and behaviour and the variables that discriminate those who screen from those who do not (non-screeners) were utilised as the key predictors and outcomes respectively.
From a conceptual perspective it was considered that motivation led to behaviour, therefore articles that described motivation and corresponding behaviour were considered. These included articles reporting on women who use public (government funded) and private (fee for service) breast cancer screening services and those who do not use screening services (i.e., non-screeners).
The scope included high-income countries using the World Bank definition [ 37 ]. These countries have broadly similar health systems and opportunities for breast cancer screening in both public and private settings.
Types of sources
All studies reporting original research in peer-reviewed journals from January 2009 were eligible for inclusion, regardless of design. This date was selected due to an evaluation undertaken for BreastScreen Australia recommending expansion of the age group to include 70–74-year-old women [ 38 ]. This date was also indicative of international debate regarding breast cancer screening effectiveness at this time [ 39 , 40 ]. Reviews were also included, regardless of type—scoping, systematic, or narrative. Only sources published in English and available through the University’s extensive research holdings were eligible for inclusion. Ineligible materials were conference abstracts, letters to the editor, editorials, opinion pieces, commentaries, newspaper articles, dissertations and theses.
This scoping review was registered with the Open Science Framework database ( https://osf.io/fd3eh ) and followed Joanna Briggs Institute (JBI) methodology for scoping reviews [ 35 , 36 ]. Although ethics approval is not required for scoping reviews the broader study was approved by the University Ethics Committee (approval number HEC 21249).
Search strategy
A pilot search strategy was developed in consultation with an expert health librarian and tested in MEDLINE (OVID) and conducted on 3 June 2022. Articles from this pilot search were compared with seminal articles previously identified by the members of the team and used to refine the search terms. The search terms were then searched as both keywords and subject headings (e.g., MeSH) in the titles and abstracts and Boolean operators employed. A full MEDLINE search was then carried out by the librarian (see Table 1 ). This search strategy was adapted for use in each of the following databases: Cumulative Index to Nursing and Allied Health Literature (CINAHL), Medical Literature Analysis and Retrieval System Online (MEDLINE), Web of Science and PsychInfo databases. The references of included studies have been hand-searched to identify any additional evidence sources.
Study/source of evidence selection
Following the search, all identified citations were collated and uploaded into EndNote v.X20 (Clarivate Analytics, PA, USA) and duplicates removed. The resulting articles were then imported into Covidence – Cochrane’s systematic review management software [ 41 ]. Duplicates were removed once importation was complete, and title and abstract screening was undertaken against the eligibility criteria. A sample of 25 articles were assessed by all reviewers to ensure reliability in the application of the inclusion and exclusion criteria. Team discussion was used to ensure consistent application. The Covidence software supports blind reviewing with two reviewers required at each screening phase. Potentially relevant sources were retrieved in full text and were assessed against the inclusion criteria by two independent reviewers. Conflicts were flagged within the software which allows the team to discuss those that have disagreements until a consensus was reached. Reasons for exclusion of studies at full text were recorded and reported in the scoping review. The Preferred Reporting Items of Systematic Reviews extension for scoping reviews (PRISMA-ScR) checklist was used to guide the reporting of the review [ 42 ] and all stages were documented using the PRISMA-ScR flow chart [ 42 ].
Data extraction
A data extraction form was created in Covidence and used to extract study characteristics and to confirm the study’s relevance. This included specific details such as article author/s, title, year of publication, country, aim, population, setting, data collection methods and key findings relevant to the review question. The draft extraction form was modified as needed during the data extraction process.
Data analysis and presentation
Extracted data were summarised in tabular format (see Table 2 ). Consistent with the guidelines for the effective reporting of scoping reviews [ 43 ] and the JBI framework [ 35 ] the final stage of the review included thematic analysis of the key findings of the included studies. Study findings were imported into QSR NVivo with coding of each line of text. Descriptive codes reflected key aspects of the included studies related to the motivations and behaviours of women > 75 years about breast cancer screening.
In line with the reporting requirements for scoping reviews the search results for this review are presented in Fig. 1 [ 44 ].
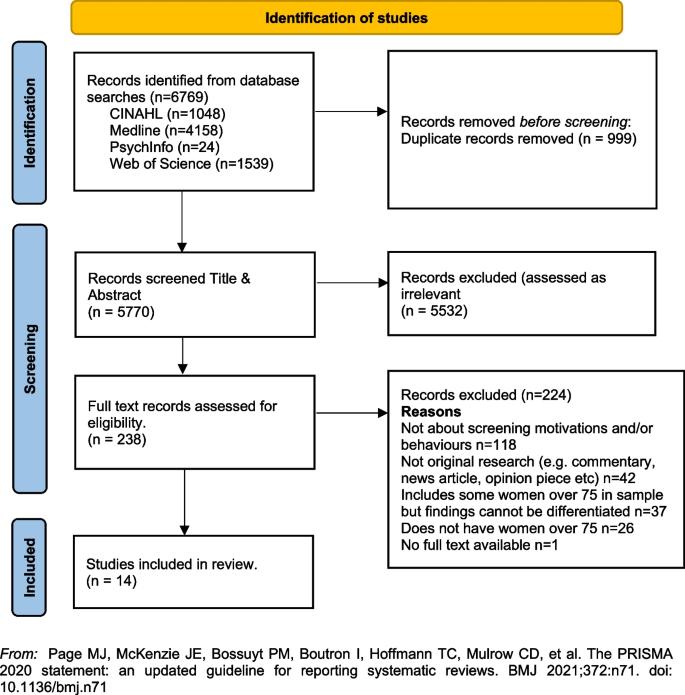
PRISMA Flowchart. From: Page MJ, McKenzie JE, Bossuyt PM, Boutron I, Hoffmann TC, Mulrow CD, et al. The PRISMA 2020 statement: an updated guideline for reporting systematic reviews. BMJ 2021;372:n71. https://doi.org/10.1136/bmj.n71
A total of fourteen [ 14 ] studies were included in the review with studies from the following countries, US n = 12 [ 33 , 45 , 46 , 47 , 48 , 49 , 50 , 51 , 52 , 53 , 54 , 55 ], UK n = 1 [ 23 ] and France n = 1 [ 56 ]. Sample sizes varied, with most containing fewer than 50 women ( n = 8) [ 33 , 45 , 46 , 48 , 51 , 52 , 55 ]. Two had larger samples including a French study with 136 women (a sub-set of a larger sample) [ 56 ], and one mixed method study in the UK with a sample of 26 women undertaking interviews and 479 women completing surveys [ 23 ]. One study did not report exact numbers [ 50 ]. Three studies [ 47 , 53 , 54 ] were undertaken by a group of researchers based in the US utilising the same sample of women, however each of the papers focused on different primary outcomes. The samples in the included studies were recruited from a range of locations including primary medical care clinics, specialist medical clinics, University affiliated medical clinics, community-based health centres and community outreach clinics [ 47 , 53 , 54 ].
Data collection methods varied and included: quantitative ( n = 8), qualitative ( n = 5) and mixed methods ( n = 1). A range of data collection tools and research designs were utilised; pre/post, pilot and cross-sectional surveys, interviews, and secondary analysis of existing data sets. Seven studies focused on the use of a Decision Aids (DAs), either in original or modified form, developed by Schonberg et al. [ 55 ] as a tool to increase knowledge about the harms and benefits of screening for older women [ 45 , 47 , 48 , 49 , 52 , 54 , 55 ]. Three studies focused on intention to screen [ 33 , 53 , 56 ], two on knowledge of, and attitudes to, screening [ 23 , 46 ], one on information needs relating to risks and benefits of screening discontinuation [ 51 ], and one on perceptions about discontinuation of screening and impact of social interactions on screening [ 50 ].
The three themes developed from the analysis of the included studies highlighted that decisions about screening were primarily influenced by: (1) knowledge of the benefits and harms of screening and their relationship to age; (2) underlying attitudes to the importance of cancer screening in women's lives; and (3) exposure to decision aids designed to facilitate informed decision-making. Each of these themes will be presented below drawing on the key findings of the appropriate studies. The full dataset of extracted data can be found in Table 2 .
Knowledge of the benefits and harms of screening ≥ 75 years
The decision to participate in routine mammography is influenced by individual differences in cognition and affect, interpersonal relationships, provider characteristics, and healthcare system variables. Women typically perceive mammograms as a positive, beneficial and routine component of care [ 46 ] and an important aspect of taking care of themselves [ 23 , 46 , 49 ]. One qualitative study undertaken in the US showed that few women had discussed mammography cessation or the potential harms of screening with their health care providers and some women reported they would insist on receiving mammography even without a provider recommendation to continue screening [ 46 ].
Studies suggested that ageing itself, and even poor health, were not seen as reasonable reasons for screening cessation. For many women, guidance from a health care provider was deemed the most important influence on decision-making [ 46 ]. Preferences for communication about risk and benefits were varied with one study reporting women would like to learn more about harms and risks and recommended that this information be communicated via physicians or other healthcare providers, included in brochures/pamphlets, and presented outside of clinical settings (e.g., in community-based seniors groups) [ 51 ]. Others reported that women were sometimes sceptical of expert and government recommendations [ 33 ] although some were happy to participate in discussions with health educators or care providers about breast cancer screening harms and benefits and potential cessation [ 52 ].
Underlying attitudes to the importance of cancer screening at and beyond 75 years
Included studies varied in describing the importance of screening, with some attitudes based on past attendance and some based on future intentions to screen. Three studies reported findings indicating that some women intended to continue screening after 75 years of age [ 23 , 45 , 46 ], with one study in the UK reporting that women supported an extension of the automatic recall indefinitely, regardless of age or health status. In this study, failure to invite older women to screen was interpreted as age discrimination [ 23 ]. The desire to continue screening beyond 75 was also highlighted in a study from France that found that 60% of the women ( n = 136 aged ≥ 75) intended to pursue screening in the future, and 27 women aged ≥ 75, who had never undergone mammography previously (36%), intended to do so in the future [ 56 ]. In this same study, intentions to screen varied significantly [ 56 ]. There were no sociodemographic differences observed between screened and unscreened women with regard to level of education, income, health risk behaviour (smoking, alcohol consumption), knowledge about the importance and the process of screening, or psychological features (fear of the test, fear of the results, fear of the disease, trust in screening impact) [ 56 ]. Further analysis showed that three items were statistically correlated with a higher rate of attendance at screening: (1) screening was initiated by a physician; (2) the women had a consultation with a gynaecologist during the past 12 months; and (3) the women had already undergone at least five screening mammograms. Analysis highlighted that although average income, level of education, psychological features or other types of health risk behaviours did not impact screening intention, having a mammogram previously impacted likelihood of ongoing screening. There was no information provided that explained why women who had not previously undergone screening might do so in the future.
A mixed methods study in the UK reported similar findings [ 23 ]. Utilising interviews ( n = 26) and questionnaires ( n = 479) with women ≥ 70 years (median age 75 years) the overwhelming result (90.1%) was that breast screening should be offered to all women indefinitely regardless of age, health status or fitness [ 23 ], and that many older women were keen to continue screening. Both the interview and survey data confirmed women were uncertain about eligibility for breast screening. The survey data showed that just over half the women (52.9%) were unaware that they could request mammography or knew how to access it. Key reasons for screening discontinuation were not being invited for screening (52.1%) and not knowing about self-referral (35.1%).
Women reported that not being invited to continue screening sent messages that screening was no longer important or required for this age group [ 23 ]. Almost two thirds of the women completing the survey (61.6%) said they would forget to attend screening without an invitation. Other reasons for screening discontinuation included transport difficulties (25%) and not wishing to burden family members (24.7%). By contrast, other studies have reported that women do not endorse discontinuation of screening mammography due to advancing age or poor health, but some may be receptive to reducing screening frequency on recommendation from their health care provider [ 46 , 51 ].
Use of Decision Aids (DAs) to improve knowledge and guide screening decision-making
Many women reported poor knowledge about the harms and benefits of screening with studies identifying an important role for DAs. These aids have been shown to be effective in improving knowledge of the harms and benefits of screening [ 45 , 54 , 55 ] including for women with low educational attainment; as compared to women with high educational attainment [ 47 ]. DAs can increase knowledge about screening [ 47 , 49 ] and may decrease the intention to continue screening after the recommended age [ 45 , 52 , 54 ]. They can be used by primary care providers to support a conversation about breast screening intention and reasons for discontinuing screening. In one pilot study undertaken in the US using a DA, 5 of the 8 women (62.5%) indicated they intended to continue to receive mammography; however, 3 participants planned to get them less often [ 45 ]. When asked whether they thought their physician would want them to get a mammogram, 80% said “yes” on pre-test; this figure decreased to 62.5% after exposure to the DA. This pilot study suggests that the use of a decision-aid may result in fewer women ≥ 75 years old continuing to screen for breast cancer [ 45 ].
Similar findings were evident in two studies drawing on the same data undertaken in the US [ 48 , 53 ]. Using a larger sample ( n = 283), women’s intentions to screen prior to a visit with their primary care provider and then again after exposure to the DA were compared. Results showed that 21.7% of women reduced their intention to be screened, 7.9% increased their intentions to be screened, and 70.4% did not change. Compared to those who had no change or increased their screening intentions, women who had a decrease in screening intention were significantly less likely to receive screening after 18 months. Generally, studies have shown that women aged 75 and older find DAs acceptable and helpful [ 47 , 48 , 49 , 55 ] and using them had the potential to impact on a women’s intention to screen [ 55 ].
Cadet and colleagues [ 49 ] explored the impact of educational attainment on the use of DAs. Results highlight that education moderates the utility of these aids; women with lower educational attainment were less likely to understand all the DA’s content (46.3% vs 67.5%; P < 0.001); had less knowledge of the benefits and harms of mammography (adjusted mean ± standard error knowledge score, 7.1 ± 0.3 vs 8.1 ± 0.3; p < 0.001); and were less likely to have their screening intentions impacted (adjusted percentage, 11.4% vs 19.4%; p = 0.01).
This scoping review summarises current knowledge regarding motivations and screening behaviours of women over 75 years. The findings suggest that awareness of the importance of breast cancer screening among women aged ≥ 75 years is high [ 23 , 46 , 49 ] and that many women wish to continue screening regardless of perceived health status or age. This highlights the importance of focusing on motivation and screening behaviours and the multiple factors that influence ongoing participation in breast screening programs.
The generally high regard attributed to screening among women aged ≥ 75 years presents a complex challenge for health professionals who are focused on potential harm (from available national and international guidelines) in ongoing screening for women beyond age 75 [ 18 , 20 , 57 ]. Included studies highlight that many women relied on the advice of health care providers regarding the benefits and harms when making the decision to continue breast screening [ 46 , 51 , 52 ], however there were some that did not [ 33 ]. Having a previous pattern of screening was noted as being more significant to ongoing intention than any other identified socio-demographic feature [ 56 ]. This is perhaps because women will not readily forgo health care practices that they have always considered important and that retain ongoing importance for the broader population.
For those women who had discontinued screening after the age of 74 it was apparent that the rationale for doing so was not often based on choice or receipt of information, but rather on factors that impact decision-making in relation to screening. These included no longer receiving an invitation to attend, transport difficulties and not wanting to be a burden on relatives or friends [ 23 , 46 , 51 ]. Ongoing receipt of invitations to screen was an important aspect of maintaining a capacity to choose [ 23 ]. This was particularly important for those women who had been regular screeners.
Women over 75 require more information to make decisions regarding screening [ 23 , 52 , 54 , 55 ], however health care providers must also be aware that the element of choice is important for older women. Having a capacity to choose avoids any notion of discrimination based on age, health status, gender or sociodemographic difference and acknowledges the importance of women retaining control over their health [ 23 ]. It was apparent that some women would choose to continue screening at a reduced frequency if this option was available and that women should have access to information facilitating self-referral [ 23 , 45 , 46 , 51 , 56 ].
Decision-making regarding ongoing breast cancer screening has been facilitated via the use of Decision Aids (DAs) within clinical settings [ 54 , 55 ]. While some studies suggest that women will make a decision regardless of health status, the use of DAs has impacted women’s decision to screen. While this may have limited benefit for those of lower educational attainment [ 48 ] they have been effective in improving knowledge relating to harms and benefits of screening particularly where they have been used to support a conversation with women about the value of screening [ 54 , 55 , 56 ].
Women have identified challenges in engaging in conversations with health care providers regarding ongoing screening, because providers frequently draw on projections of life expectancy and over-diagnosis [ 17 , 51 ]. As a result, these conversations about screening after age 75 years often do not occur [ 46 ]. It is likely that health providers may need more support and guidance in leading these conversations. This may be through the use of DAs or standardised checklists. It may be possible to incorporate these within existing health preventive measures for this age group. The potential for advice regarding ongoing breast cancer screening to be available outside of clinical settings may provide important pathways for conversations with women regarding health choices. Provision of information and advice in settings such as community based seniors groups [ 51 ] offers a potential platform to broaden conversations and align sources of information, not only with health professionals but amongst women themselves. This may help to address any misconception regarding eligibility and access to services [ 23 ]. It may also be aligned with other health promotion and lifestyle messages provided to this age group.
Limitations of the review
The searches that formed the basis of this review were carried in June 2022. Although the search was comprehensive, we have only captured those studies that were published in the included databases from 2009. There may have been other studies published outside of these periods. We also limited the search to studies published in English with full-text availability.
The emphasis of a scoping review is on comprehensive coverage and synthesis of the key findings, rather than on a particular standard of evidence and, consequently a quality assessment of the included studies was not undertaken. This has resulted in the inclusion of a wide range of study designs and data collection methods. It is important to note that three studies included in the review drew on the same sample of women (283 over > 75)[ 49 , 53 , 54 ]. The results of this review provide valuable insights into motivations and behaviours for breast cancer screening for older women, however they should be interpreted with caution given the specific methodological and geographical limitations.
Conclusion and recommendations
This scoping review highlighted a range of key motivations and behaviours in relation to breast cancer screening for women ≥ 75 years of age. The results provide some insight into how decisions about screening continuation after 74 are made and how informed decision-making can be supported. Specifically, this review supports the following suggestions for further research and policy direction:
Further research regarding breast cancer screening motivations and behaviours for women over 75 would provide valuable insight for health providers delivering services to women in this age group.
Health providers may benefit from the broader use of decision aids or structured checklists to guide conversations with women over 75 regarding ongoing health promotion/preventive measures.
Providing health-based information in non-clinical settings frequented by women in this age group may provide a broader reach of information and facilitate choices. This may help to reduce any perception of discrimination based on age, health status or socio-demographic factors.
Availability of data and materials
All data generated or analysed during this study is included in this published article (see Table 2 above).
Cancer Australia, in their 2014 position statement, define “overdiagnosis” in the following way. ‘’Overdiagnosis’ from breast screening does not refer to error or misdiagnosis, but rather refers to breast cancer diagnosed by screening that would not otherwise have been diagnosed during a woman’s lifetime. “Overdiagnosis” includes all instances where cancers detected through screening (ductal carcinoma in situ or invasive breast cancer) might never have progressed to become symptomatic during a woman’s life, i.e., cancer that would not have been detected in the absence of screening. It is not possible to precisely predict at diagnosis, to which cancers overdiagnosis would apply.” (accessed 22. nd August 2022; https://www.canceraustralia.gov.au/resources/position-statements/overdiagnosis-mammographic-screening ).
World Health Organization. Breast Cancer Geneva: WHO; 2021 [Available from: https://www.who.int/news-room/fact-sheets/detail/breast-cancer#:~:text=Reducing%20global%20breast%20cancer%20mortality,and%20comprehensive%20breast%20cancer%20management .
International Agency for Research on Cancer (IARC). IARC Handbooks on Cancer Screening: Volume 15 Breast Cancer Geneva: IARC; 2016 [Available from: https://publications.iarc.fr/Book-And-Report-Series/Iarc-Handbooks-Of-Cancer-Prevention/Breast-Cancer-Screening-2016 .
Australian Institute of Health and Welfare. Cancer in Australia 2021 [Available from: https://www.canceraustralia.gov.au/cancer-types/breast-cancer/statistics .
Breast Cancer Network Australia. Current breast cancer statistics in Australia 2020 [Available from: https://www.bcna.org.au/media/7111/bcna-2019-current-breast-cancer-statistics-in-australia-11jan2019.pdf .
Ren W, Chen M, Qiao Y, Zhao F. Global guidelines for breast cancer screening: A systematic review. The Breast. 2022;64:85–99.
Article PubMed PubMed Central Google Scholar
Cardoso F, Kyriakides S, Ohno S, Penault-Llorca F, Poortmans P, Rubio IT, et al. Early breast cancer: ESMO Clinical Practice Guidelines for diagnosis, treatment and follow-up. Ann Oncol. 2019;30(8):1194–220.
Article CAS PubMed Google Scholar
Hamashima C, Hattori M, Honjo S, Kasahara Y, Katayama T, Nakai M, et al. The Japanese guidelines for breast cancer screening. Jpn J Clin Oncol. 2016;46(5):482–92.
Article PubMed Google Scholar
Bevers TB, Helvie M, Bonaccio E, Calhoun KE, Daly MB, Farrar WB, et al. Breast cancer screening and diagnosis, version 3.2018, NCCN clinical practice guidelines in oncology. J Natl Compr Canc Net. 2018;16(11):1362–89.
Article Google Scholar
He J, Chen W, Li N, Shen H, Li J, Wang Y, et al. China guideline for the screening and early detection of female breast cancer (2021, Beijing). Zhonghua Zhong liu za zhi [Chinese Journal of Oncology]. 2021;43(4):357–82.
CAS PubMed Google Scholar
Cancer Australia. Early detection of breast cancer 2021 [cited 2022 25 July]. Available from: https://www.canceraustralia.gov.au/resources/position-statements/early-detection-breast-cancer .
Schünemann HJ, Lerda D, Quinn C, Follmann M, Alonso-Coello P, Rossi PG, et al. Breast Cancer Screening and Diagnosis: A Synopsis of the European Breast Guidelines. Ann Intern Med. 2019;172(1):46–56.
World Health Organization. WHO Position Paper on Mammography Screening Geneva WHO. 2016.
Google Scholar
Lansdorp-Vogelaar I, Gulati R, Mariotto AB. Personalizing age of cancer screening cessation based on comorbid conditions: model estimates of harms and benefits. Ann Intern Med. 2014;161:104.
Lee CS, Moy L, Joe BN, Sickles EA, Niell BL. Screening for Breast Cancer in Women Age 75 Years and Older. Am J Roentgenol. 2017;210(2):256–63.
Broeders M, Moss S, Nystrom L. The impact of mammographic screening on breast cancer mortality in Europe: a review of observational studies. J Med Screen. 2012;19(suppl 1):14.
Oeffinger KC, Fontham ETH, Etzioni R, Herzig A, Michaelson JS, Shih YCT, et al. Breast cancer screening for women at average risk: 2015 Guideline update from the American cancer society. JAMA - Journal of the American Medical Association. 2015;314(15):1599–614.
Walter LC, Schonberg MA. Screening mammography in older women: a review. JAMA. 2014;311:1336.
Article CAS PubMed PubMed Central Google Scholar
Braithwaite D, Walter LC, Izano M, Kerlikowske K. Benefits and harms of screening mammography by comorbidity and age: a qualitative synthesis of observational studies and decision analyses. J Gen Intern Med. 2016;31:561.
Braithwaite D, Mandelblatt JS, Kerlikowske K. To screen or not to screen older women for breast cancer: a conundrum. Future Oncol. 2013;9(6):763–6.
Demb J, Abraham L, Miglioretti DL, Sprague BL, O’Meara ES, Advani S, et al. Screening Mammography Outcomes: Risk of Breast Cancer and Mortality by Comorbidity Score and Age. Jnci-Journal of the National Cancer Institute. 2020;112(6):599–606.
Demb J, Akinyemiju T, Allen I, Onega T, Hiatt RA, Braithwaite D. Screening mammography use in older women according to health status: a systematic review and meta-analysis. Clin Interv Aging. 2018;13:1987–97.
Qaseem A, Lin JS, Mustafa RA, Horwitch CA, Wilt TJ. Screening for Breast Cancer in Average-Risk Women: A Guidance Statement From the American College of Physicians. Ann Intern Med. 2019;170(8):547–60.
Collins K, Winslow M, Reed MW, Walters SJ, Robinson T, Madan J, et al. The views of older women towards mammographic screening: a qualitative and quantitative study. Br J Cancer. 2010;102(10):1461–7.
Welch HG, Black WC. Overdiagnosis in cancer. J Natl Cancer Inst. 2010;102(9):605–13.
Hersch J, Jansen J, Barratt A, Irwig L, Houssami N, Howard K, et al. Women’s views on overdiagnosis in breast cancer screening: a qualitative study. BMJ : British Medical Journal. 2013;346:f158.
De Gelder R, Heijnsdijk EAM, Van Ravesteyn NT, Fracheboud J, Draisma G, De Koning HJ. Interpreting overdiagnosis estimates in population-based mammography screening. Epidemiol Rev. 2011;33(1):111–21.
Monticciolo DL, Helvie MA, Edward HR. Current issues in the overdiagnosis and overtreatment of breast cancer. Am J Roentgenol. 2018;210(2):285–91.
Shepardson LB, Dean L. Current controversies in breast cancer screening. Semin Oncol. 2020;47(4):177–81.
National Cancer Control Centre. Cancer incidence in Australia 2022 [Available from: https://ncci.canceraustralia.gov.au/diagnosis/cancer-incidence/cancer-incidence .
Austin JD, Shelton RC, Lee Argov EJ, Tehranifar P. Older Women’s Perspectives Driving Mammography Screening Use and Overuse: a Narrative Review of Mixed-Methods Studies. Current Epidemiology Reports. 2020;7(4):274–89.
Austin JD, Tehranifar P, Rodriguez CB, Brotzman L, Agovino M, Ziazadeh D, et al. A mixed-methods study of multi-level factors influencing mammography overuse among an older ethnically diverse screening population: implications for de-implementation. Implementation Science Communications. 2021;2(1):110.
Demb J, Allen I, Braithwaite D. Utilization of screening mammography in older women according to comorbidity and age: protocol for a systematic review. Syst Rev. 2016;5(1):168.
Housten AJ, Pappadis MR, Krishnan S, Weller SC, Giordano SH, Bevers TB, et al. Resistance to discontinuing breast cancer screening in older women: A qualitative study. Psychooncology. 2018;27(6):1635–41.
Arksey H, O’Malley L. Scoping studies: towards a methodological framework. Int J Soc Res Methodol. 2005;8(1):19–32.
Peters M, Godfrey C, McInerney P, Munn Z, Tricco A, Khalil HAE, et al. Chapter 11: Scoping reviews. JBI Manual for Evidence Synthesis 2020 [Available from: https://jbi-global-wiki.refined.site/space/MANUAL .
Peters MD, Godfrey C, McInerney P, Khalil H, Larsen P, Marnie C, et al. Best practice guidance and reporting items for the development of scoping review protocols. JBI evidence synthesis. 2022;20(4):953–68.
Fantom NJ, Serajuddin U. The World Bank’s classification of countries by income. World Bank Policy Research Working Paper; 2016.
Book Google Scholar
BreastScreen Australia Evaluation Taskforce. BreastScreen Australia Evaluation. Evaluation final report: Screening Monograph No 1/2009. Canberra; Australia Australian Government Department of Health and Ageing; 2009.
Nelson HD, Cantor A, Humphrey L. Screening for breast cancer: a systematic review to update the 2009 U.S. Preventive Services Task Force recommendation2016.
Woolf SH. The 2009 breast cancer screening recommendations of the US Preventive Services Task Force. JAMA. 2010;303(2):162–3.
Covidence systematic review software. [Internet]. Veritas-Health-Innovation 2020. Available from: https://www.covidence.org/ .
Tricco AC, Lillie E, Zarin W, O’Brien KK, Colquhoun H, Levac D, et al. PRISMA Extension for Scoping Reviews (PRISMA-ScR): Checklist and Explanation. Ann Intern Med. 2018;169(7):467–73.
Tricco AC, Lillie E, Zarin W, O’Brien K, Colquhoun H, Kastner M, et al. A scoping review on the conduct and reporting of scoping reviews. BMC Med Res Methodol. 2016;16(1):15.
Page MJ, McKenzie JE, Bossuyt PM, Boutron I, Hoffmann TC, Mulrow CD, et al. The PRISMA 2020 statement: an updated guideline for reporting systematic reviews. BMJ. 2021;372:n71.
Beckmeyer A, Smith RM, Miles L, Schonberg MA, Toland AE, Hirsch H. Pilot Evaluation of Patient-centered Survey Tools for Breast Cancer Screening Decision-making in Women 75 and Older. Health Behavior and Policy Review. 2020;7(1):13–8.
Brotzman LE, Shelton RC, Austin JD, Rodriguez CB, Agovino M, Moise N, et al. “It’s something I’ll do until I die”: A qualitative examination into why older women in the U.S. continue screening mammography. Canc Med. 2022;11(20):3854–62.
Article CAS Google Scholar
Cadet T, Pinheiro A, Karamourtopoulos M, Jacobson AR, Aliberti GM, Kistler CE, et al. Effects by educational attainment of a mammography screening patient decision aid for women aged 75 years and older. Cancer. 2021;127(23):4455–63.
Cadet T, Aliberti G, Karamourtopoulos M, Jacobson A, Gilliam EA, Primeau S, et al. Evaluation of a mammography decision aid for women 75 and older at risk for lower health literacy in a pretest-posttest trial. Patient Educ Couns. 2021;104(9):2344–50.
Cadet T, Aliberti G, Karamourtopoulos M, Jacobson A, Siska M, Schonberg MA. Modifying a mammography decision aid for older adult women with risk factors for low health literacy. Health Lit Res Prac. 2021;5(2):e78–90.
Gray N, Picone G. Evidence of Large-Scale Social Interactions in Mammography in the United States. Atl Econ J. 2018;46(4):441–57.
Hoover DS, Pappadis MR, Housten AJ, Krishnan S, Weller SC, Giordano SH, et al. Preferences for Communicating about Breast Cancer Screening Among Racially/Ethnically Diverse Older Women. Health Commun. 2019;34(7):702–6.
Salzman B, Bistline A, Cunningham A, Silverio A, Sifri R. Breast Cancer Screening Shared Decision-Making in Older African-American Women. J Natl Med Assoc. 2020;112(5):556–60.
PubMed Google Scholar
Schoenborn NL, Pinheiro A, Kistler CE, Schonberg MA. Association between Breast Cancer Screening Intention and Behavior in the Context of Screening Cessation in Older Women. Med Decis Making. 2021;41(2):240–4.
Schonberg MA, Kistler CE, Pinheiro A, Jacobson AR, Aliberti GM, Karamourtopoulos M, et al. Effect of a Mammography Screening Decision Aid for Women 75 Years and Older: A Cluster Randomized Clinical Trial. JAMA Intern Med. 2020;180(6):831–42.
Schonberg MA, Hamel MB, Davis RB. Development and evaluation of a decision aid on mammography screening for women 75 years and older. JAMA Intern Med. 2014;174:417.
Eisinger F, Viguier J, Blay J-Y, Morère J-F, Coscas Y, Roussel C, et al. Uptake of breast cancer screening in women aged over 75years: a controversy to come? Eur J Cancer Prev. 2011;20(Suppl 1):S13-5.
Schonberg MA, Breslau ES, McCarthy EP. Targeting of Mammography Screening According to Life Expectancy in Women Aged 75 and Older. J Am Geriatr Soc. 2013;61(3):388–95.
Download references
Acknowledgements
We would like to acknowledge Ange Hayden-Johns (expert librarian) who assisted with the development of the search criteria and undertook the relevant searches and Tejashree Kangutkar who assisted with some of the Covidence work.
This work was supported by funding from the Australian Government Department of Health and Aged Care (ID: Health/20–21/E21-10463).
Author information
Authors and affiliations.
Violet Vines Centre for Rural Health Research, La Trobe Rural Health School, La Trobe University, P.O. Box 199, Bendigo, VIC, 3552, Australia
Virginia Dickson-Swift, Joanne Adams & Evelien Spelten
Care Economy Research Institute, La Trobe University, Wodonga, Australia
Irene Blackberry
Olivia Newton-John Cancer Wellness and Research Centre, Austin Health, Melbourne, Australia
Carlene Wilson & Eva Yuen
Melbourne School of Population and Global Health, Melbourne University, Melbourne, Australia
Carlene Wilson
School of Psychology and Public Health, La Trobe University, Bundoora, Australia
Institute for Health Transformation, Deakin University, Burwood, Australia
Centre for Quality and Patient Safety, Monash Health Partnership, Monash Health, Clayton, Australia
You can also search for this author in PubMed Google Scholar
Contributions
VDS conceived and designed the scoping review. VDS & JA developed the search strategy with librarian support, and all authors (VDS, JA, ES, IB, CW, EY) participated in the screening and data extraction stages and assisted with writing the review. All authors provided editorial support and read and approved the final manuscript prior to submission.
Corresponding author
Correspondence to Joanne Adams .
Ethics declarations
Competing interests.
The authors declare no competing interests.
Ethics approval and consent to participate
Ethics approval and consent to participate was not required for this study.
Consent for publication
Consent for publication was not required for this study.
Competing interest
The authors declare they have no competing interests.
Additional information
Publisher’s note.
Springer Nature remains neutral with regard to jurisdictional claims in published maps and institutional affiliations.
Rights and permissions
Open Access This article is licensed under a Creative Commons Attribution 4.0 International License, which permits use, sharing, adaptation, distribution and reproduction in any medium or format, as long as you give appropriate credit to the original author(s) and the source, provide a link to the Creative Commons licence, and indicate if changes were made. The images or other third party material in this article are included in the article's Creative Commons licence, unless indicated otherwise in a credit line to the material. If material is not included in the article's Creative Commons licence and your intended use is not permitted by statutory regulation or exceeds the permitted use, you will need to obtain permission directly from the copyright holder. To view a copy of this licence, visit http://creativecommons.org/licenses/by/4.0/ . The Creative Commons Public Domain Dedication waiver ( http://creativecommons.org/publicdomain/zero/1.0/ ) applies to the data made available in this article, unless otherwise stated in a credit line to the data.
Reprints and permissions
About this article
Cite this article.
Dickson-Swift, V., Adams, J., Spelten, E. et al. Breast cancer screening motivation and behaviours of women aged over 75 years: a scoping review. BMC Women's Health 24 , 256 (2024). https://doi.org/10.1186/s12905-024-03094-z
Download citation
Received : 06 September 2023
Accepted : 15 April 2024
Published : 24 April 2024
DOI : https://doi.org/10.1186/s12905-024-03094-z
Share this article
Anyone you share the following link with will be able to read this content:
Sorry, a shareable link is not currently available for this article.
Provided by the Springer Nature SharedIt content-sharing initiative
- Breast cancer
- Mammography
- Older women
- Scoping review
BMC Women's Health
ISSN: 1472-6874
- Submission enquiries: [email protected]
- General enquiries: [email protected]
Help | Advanced Search
Computer Science > Neural and Evolutionary Computing
Title: a survey of decomposition-based evolutionary multi-objective optimization: part ii -- a data science perspective.
Abstract: This paper presents the second part of the two-part survey series on decomposition-based evolutionary multi-objective optimization where we mainly focus on discussing the literature related to multi-objective evolutionary algorithms based on decomposition (MOEA/D). Complementary to the first part, here we employ a series of advanced data mining approaches to provide a comprehensive anatomy of the enormous landscape of MOEA/D research, which is far beyond the capacity of classic manual literature review protocol. In doing so, we construct a heterogeneous knowledge graph that encapsulates more than 5,400 papers, 10,000 authors, 400 venues, and 1,600 institutions for MOEA/D research. We start our analysis with basic descriptive statistics. Then we delve into prominent research/application topics pertaining to MOEA/D with state-of-the-art topic modeling techniques and interrogate their sptial-temporal and bilateral relationships. We also explored the collaboration and citation networks of MOEA/D, uncovering hidden patterns in the growth of literature as well as collaboration between researchers. Our data mining results here, combined with the expert review in Part I, together offer a holistic view of the MOEA/D research, and demonstrate the potential of an exciting new paradigm for conducting scientific surveys from a data science perspective.
Submission history
Access paper:.
- Other Formats
References & Citations
- Google Scholar
- Semantic Scholar
BibTeX formatted citation

Bibliographic and Citation Tools
Code, data and media associated with this article, recommenders and search tools.
- Institution
arXivLabs: experimental projects with community collaborators
arXivLabs is a framework that allows collaborators to develop and share new arXiv features directly on our website.
Both individuals and organizations that work with arXivLabs have embraced and accepted our values of openness, community, excellence, and user data privacy. arXiv is committed to these values and only works with partners that adhere to them.
Have an idea for a project that will add value for arXiv's community? Learn more about arXivLabs .

An official website of the United States government
The .gov means it’s official. Federal government websites often end in .gov or .mil. Before sharing sensitive information, make sure you’re on a federal government site.
The site is secure. The https:// ensures that you are connecting to the official website and that any information you provide is encrypted and transmitted securely.
- Publications
- Account settings
Preview improvements coming to the PMC website in October 2024. Learn More or Try it out now .
- Advanced Search
- Journal List
- Springer Nature - PMC COVID-19 Collection

Scientific paper recommendation systems: a literature review of recent publications
Christin katharina kreutz.
1 Cologne University of Applied Sciences, Cologne, Germany
Ralf Schenkel
2 Trier University, Trier, Germany
Scientific writing builds upon already published papers. Manual identification of publications to read, cite or consider as related papers relies on a researcher’s ability to identify fitting keywords or initial papers from which a literature search can be started. The rapidly increasing amount of papers has called for automatic measures to find the desired relevant publications, so-called paper recommendation systems. As the number of publications increases so does the amount of paper recommendation systems. Former literature reviews focused on discussing the general landscape of approaches throughout the years and highlight the main directions. We refrain from this perspective, instead we only consider a comparatively small time frame but analyse it fully. In this literature review we discuss used methods, datasets, evaluations and open challenges encountered in all works first released between January 2019 and October 2021. The goal of this survey is to provide a comprehensive and complete overview of current paper recommendation systems.
Introduction
The rapidly increasing number of publications leads to a large quantity of possibly relevant papers [ 6 ] for more specific tasks such as finding related papers [ 28 ], finding ones to read [ 109 ] or literature search in general to inspire new directions and understand the state-of-the-art approaches [ 46 ]. Overall researchers typically spend a large amount of time on searching for relevant related work [ 7 ]. Keyword-based search options are insufficient to find relevant papers [ 9 , 52 , 109 ], they require some form of initial knowledge about a field. Oftentimes, users’ information needs are not explicitly specified [ 56 ] which impedes this task further.
To close this gap, a plethora of paper recommendation systems have been proposed recently [ 37 , 39 , 88 , 104 , 117 ]. These systems should fulfil different functions: for junior researchers systems should recommend a broad variety of papers, for senior ones the recommendations should align more with their already established interests [ 9 ] or help them discover relevant interdisciplinary research [ 100 ]. In general paper recommendation approaches positively affect researchers’ professional lives as they enable finding relevant literature more easily and faster [ 50 ].
As there are many different approaches, their objectives and assumptions are also diverse. A simple problem definition of a paper recommendation system could be the following: given one paper recommend a list of papers fitting the source paper [ 68 ]. This definition would not fit all approaches as some specifically do not require any initial paper to be specified but instead observe a user as input [ 37 ]. Some systems recommend sets of publications fitting the queried terms only if these papers are all observed together [ 60 , 61 ], most of the approaches suggest a number of single publications as their result [ 37 , 39 , 88 , 117 ], such that any single one of these papers satisfies the information need of a user fully. Most approaches assume that all required data to run a system is present already [ 37 , 117 ] but some works [ 39 , 88 ] explicitly crawl general publication information or even abstracts and keywords from the web.
In this literature review we observe papers recently published in the area of scientific paper recommendation between and including January 2019 and October 2021 1 . We strive to give comprehensive overviews on their utilised methods as well as their datasets, evaluation measures and open challenges of current approaches. Our contribution is fourfold:
- We propose a current multidimensional characterisation of current paper recommendation approaches.
- We compile a list of recently used datasets in evaluations of paper recommendation approaches.
- We compile a list of recently used evaluation measures for paper recommendation.
- We analyse existing open challenges and identify current novel problems in paper recommendation which could be specifically helpful for future approaches to address.
In the following Sect. 2 we describe the general problem statement for paper recommendation systems before we dive into the literature review in Sect. 3 . Section 4 gives insight into datasets used in current work. In the following Sect. 5 different definitions of relevance, relevance assessment as well as evaluation measures are analysed. Open challenges and objectives are discussed in detail in Sect. 7 . Lastly Sect. 8 concludes this literature review.
Problem statement
Over the years different formulations for a problem statement of a paper recommendation system have emerged. In general they should specify the input for the recommendation system, the type of recommendation results, the point in time when the recommendation will be made and which specific goal an approach tries to achieve. Additionally, the target audience should be specified.
As input we can either specify an initial paper [ 28 ], keywords [ 117 ], a user [ 37 ], a user and a paper [ 5 ] or more complex information such as user-constructed knowledge graphs [ 109 ]. Users can be modelled as a combination of features of papers they interacted with [ 19 , 21 ], e.g. their clicked [ 26 ] or authored publications [ 22 ]. Papers can for example be represented by their textual content [ 88 ].
As types of recommendation we could either specify single (independent) papers [ 37 ] or a set of papers which is to be observed completely to satisfy the information need [ 61 ]. A study by Beierle et al. [ 18 ] found that existing digital libraries recommend between three and ten single papers, in their case the optimal number of suggestions to display to users was five to six.
As for the point in time , most work focuses on immediate recommendation of papers. Only a few approaches also consider delayed suggestion 2 via newsletter for example [ 56 ].
In general, recommended papers should be relevant in one way or another to achieve certain goals . The intended goal of authors of papers could, e.g. either be to recommend papers which should be read [ 109 ] by a user or recommend papers which are simply somehow related to an initial paper [ 28 ], by topic, citations or user interactions.
Different target audiences , for example junior or senior researcher, have different demands from paper recommendation systems [ 9 ]. Usually paper recommendation approaches target single users but there are also works which strive to recommend papers for sets of users [ 110 , 111 ].
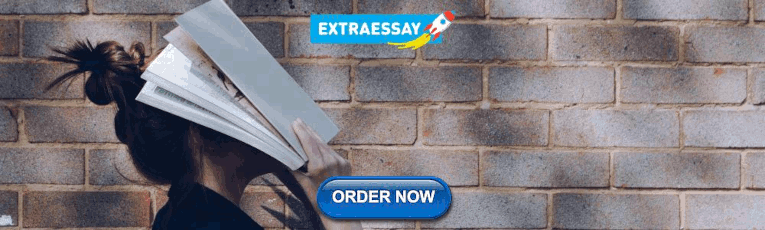
Literature review
In this chapter we first clearly define the scope of our literature review (see Sect. 3.1 ) before we conduct a meta-analysis on the observed papers (see Sect. 3.2 ). Afterwards our categorisation or lack thereof is discussed in depth (see Sect. 3.3 ), before we give short overviews of all paper recommendation systems we found (see Sect. 3.5 ) and some other relevant related work (see Sect. 3.6 ).
To the best of our knowledge the literature reviews by Bai et al. [ 9 ], Li and Zou [ 58 ] and Shahid et al. [ 92 ] are the most recent ones targeting the domain of scientific paper recommendation systems. They were accepted for publication or published in 2019 so they only consider paper recommendation systems up until 2019 at most. We want to bridge the gap between papers published after their surveys were finalised and current work so we only focus on the discussion of publications which appeared between January 2019 and October 2021 when this literature search was conducted.
We conducted our literature search on the following digital libraries: ACM 3 , dblp 4 , GoogleScholar 5 and Springer 6 . Titles of considered publications had to contain either paper , article or publication as well as some form of recommend . Papers had to be written in English to be observed. We judged relevance of retrieved publications by observing titles and abstracts if the title alone did not suffice to assess their topical relevance. In addition to these papers found by systematically searching digital libraries, we also considered their referenced publications if they were from the specified time period and of topical fit. For all papers their date of first publication determines their publication year which decides if they lie in our time observed time frame or not. For example, for journal articles we consider the point in time when they were first published online instead of the date on which they were published in an issue, for conference articles we consider the date of the conference instead a later date when they were published online. Figure 1 depicts the PRISMA [ 79 ] workflow for this study.

PRISMA workflow of our literature review process
We refrain from including works in our study which do not identify as scientific paper recommendation systems such as Wikipedia article recommendation [ 70 , 78 , 85 ] or general news article recommendation [ 33 , 43 , 103 ]. Citation recommendation systems [ 72 , 90 , 124 ] are also out of scope of this literature review. Even though citation and paper recommendation can be regarded as analogous [ 45 ], we argue the differing functions of citations [ 34 ] and tasks of these recommendation systems [ 67 ] should not be mixed with the problem of paper recommendation. Färber and Jatowt [ 32 ] also support this view by stating that both are disjunctive, with paper recommendation pursuing the goal of providing papers to read and investigate while incorporating user interaction data and citation recommendation supporting users with finding citations for given text passages. 7 We also consciously refrain from discussing the plethora of more area-independent recommender systems which could be adopted to the domain of scientific paper recommendation.
Our literature research resulted in 82 relevant papers. Of these, three were review articles. We found 14 manuscripts which do not present paper recommendation systems but are relevant works for the area nonetheless, they are discussed in Sect. 3.6 . This left 65 publications describing paper recommendation systems for us to analyse in the following.
Meta analysis
For papers within our scope, we consider their publication year as stated in the citation information for this meta-analysis. This could affect the publication year of papers compared to the former definition of which papers are included in this survey. For example, for journal articles we do not set the publication year as the point in time when they were first published online, instead for consistency (this data is present in the citation information of papers) for this analysis we use the year the issue was published in which the article is contained. Of the 65 relevant system papers, 21 were published in 2019, 23 were published in 2020 and 21 were published in 2021. On average each paper has 4.0462 authors (std. dev. = 1.6955) and 12.4154 pages (std. dev. = 9.2402). 35 (53.85%) of the papers appeared as conference papers, 27 (41.54%) papers were published in journals and there were two preprints (3.08%) which have not yet been published otherwise. There has been one master’s thesis (1.54%) within scope. The most common venues for publications were the ones depicted in Table 1 . Some papers [ 74 – 76 , 93 , 94 ] described the same approach without modification or extension of the actual paper recommendation methodology, e.g. by providing evaluations 8 . This left us with 62 different paper recommendation systems to discuss.
Top most common venues where relevant papers were published together with their type and number of papers (#p). Other venues had only one associated paper
Categorisation
Former categorisation.
The already mentioned three most recent [ 9 , 58 , 92 ] and one older but highly influential [ 16 ] literature reviews in scientific paper recommendation utilise different categorisations to group approaches. Beel et al. [ 16 ] categorise observed papers by their underlying recommendation principle into stereotyping, content-based filtering, collaborative filtering, co-occurrence, graph-based, global relevance and hybrid models. Bai et al. [ 9 ] only utilise the classes content-based filtering, collaborative filtering, graph-based methods, hybrid methods and other models. Li and Zou [ 58 ] use the categories content-based recommendation, hybrid recommendation, graph-based recommendation and recommendation based on deep learning. Shahid et al. [ 92 ] label approaches by the criterion they identify relevant papers with: content, metadata, collaborative filtering and citations.
The four predominant categories thus are content-based filtering, collaborative filtering, graph-based and hybrid systems. Most of these categories are defined precisely but graph-based approaches are not always characterised concisely: Content-based filtering (CBF) methods are said to be ones where user interest is inferred by observing their historic interactions with papers [ 9 , 16 , 58 ]. Recommendations are composed by observing features of papers and users [ 5 ]. In collaborative filtering (CF) systems the preferences of users similar to a current one are observed to identify likely relevant publications [ 9 , 16 , 58 ]. Current users’ past interactions need to be similar to similar users’ past interactions [ 9 , 16 ]. Hybrid approaches are ones which combine multiple types of recommendations [ 9 , 16 , 58 ].
Graph-based methods can be characterised in multiple ways. A very narrow definition only encompasses ones which observe the recommendation task as a link prediction problem or utilise random walk [ 5 ]. Another less strict definition identifies these systems as ones which construct networks of papers and authors and then apply some graph algorithm to estimate relevance [ 9 ]. Another definition specifies this class as one using graph metrics such as random walk with restart, bibliographic coupling or co-citation inverse document frequency [ 106 ]. Li and Zhou [ 58 ] abstain from clearly characterising this type of systems directly but give examples which hint that in their understanding of graph-based methods somewhere in the recommendation process, some type of graph information, e.g. bibliographic coupling or co-citation strength, should be used. Beel et al. [ 16 ] as well as Bai et al. [ 9 ] follow a similar line, they characterise graph-based methods broadly as ones which build upon the existing connections in a scientific context to construct a graph network.
When trying to classify approaches by their recommendation type, we encountered some problems:
Indications as what type of paper recommendation system works describe themselves with indication if the description is a common used label (c)
- When considering the broadest definition of graph-based methods many recent paper recommendation systems tend to belong to the class of hybrid methods. Most of the approaches [ 5 , 46 , 48 , 49 , 57 , 88 , 105 , 117 ] utilise some type of graph structure information as part of the approach which would classify them as graph-based but as they also utilise historic user-interaction data or descriptions of paper features (see, e.g. Li et al. [ 57 ] who describe their approach as network-based while using a graph structure, textual components and user profiles) which would render them as either CF or CBF also.
Thus we argue the former categories do not suffice to classify the particularities of current approaches in a meaningful way. So instead, we introduce more dimensions by which systems could be grouped.
Current categorisation
Recent paper recommendation systems can be categorised in 20 different dimensions by general information on the approach (G), already existing data directly taken from the papers used (D) and methods which might create or (re-)structure data, which are part of the approach (M):
- (G) Personalisation (person.): The approach produces personalised recommendations. The recommended items depend on the person using the approach, if personalisation is not considered, the recommendation solely depends on the input keywords or paper. This dimension is related to the existence of user profiles.
- (G) Input: The approach requires some form of input, either a paper (p), keywords (k), user (u) or something else, e.g. an advanced type of input (o). Hybrid forms are also possible. In some cases the input is not clearly specified throughout the paper so it is unknown (?).
- (D) Title: The approach utilises titles of papers.
- (D) Abstract (abs.): The approach utilises abstracts of papers.
- (D) Keyword (key.): The approach utilises keywords of papers. These keywords are usually explicitly defined by the authors of papers, contrasting key phrases.
- (D) Text: The approach utilises some type of text of papers which is not clearly specified as titles, abstracts or keywords. In the evaluation this approach might utilise specified text fragments of publications.
- (D) Citation (cit.): The approach utilises citation information, e.g. numbers of citations or co-references.
- (D) Historic interaction (inter.): The approach uses some sort of historic user-interaction data, e.g. previously authored, cited or liked publications. An approach can only include historic user-interaction data if it also somehow contains user profiles.
- (M) User profile (user): The approach constructs some sort of user profile or utilises profile information. Most approaches using personalisation also construct user profiles but some do not explicitly construct profiles but rather encode user information in the used structures.
- (M) Popularity (popul.): The approach utilises some sort of popularity indication, e.g. CORE rank, numbers of citations 9 or number of likes.
- (M) Key phrase (KP): The approach utilises key phrases. Key phrases are not explicitly provided by authors of papers but are usually computed from the titles and abstracts of papers to provide a descriptive summary, contrasting keywords of papers.
- (M) Embedding (emb.): The approach utilises some sort of text or graph embedding technique, e.g. BERT or Doc2Vec.
- (M) Topic model (TM): The approach utilises some sort of topic model, e.g. LDA.
- (M) Knowledge graph (KG): The approach utilises or builds some sort of knowledge graph. This dimension surpasses the mere incorporation of a graph which describes a network of nodes and edges of different types. A knowledge graph is a sub-category of a graph.
- (M) Graph: The approach actively builds or directly uses a graph structure, e.g. a knowledge graph or scientific heterogeneous network. Utilisation of a neural network is not considered in this dimension.
- (M) Meta-path (path): The approach utilises meta-paths. They usually are composed from paths in a network.
- (M) Random Walk (with Restart) (RW): The approach utilises Random Walk or Random Walk with Restart.
- (M) Advanced machine learning (AML): The approach utilises some sort of advanced machine learning component in its core such as a neural network. Utilisation of established embedding methods which themselves use neural networks (e.g. BERT) are not considered in this dimension. We do not consider traditional and simple ML techniques such as k means in this dimension but rather mention methods explicitly defining a loss function, using multi-layer perceptrons or GCNs.
- (M) Crawling (crawl.): The approach conducts some sort of web crawling step.
- (M) Cosine similarity (cosine): The approach utilises cosine similarity at some point.
Of the observed paper recommendation systems, six were general systems or methods which were only applied on the domain of paper recommendation [ 3 , 4 , 24 , 60 , 118 , 121 ]. Two were targeting explicit set-based recommendation of publications where only all papers in the set together satisfy users’ information needs [ 60 , 61 ], two recommend multiple papers [ 42 , 71 ] (e.g. on a path [ 42 ]), all the other approaches focused on recommendation of k single papers. Only two approaches focus on recommendation of papers to user groups instead of single users [ 110 , 111 ]. Only one paper [ 56 ] supports subscription-based recommendation of papers, all other approaches solely regarded a scenario in which papers were suggested straight away.
Table 3 classifies the observed approaches according to the afore discussed dimensions.
Indications whether works utilise the specific data or methods. Papers describing the same approach without extension of the methodology (e.g. only describing more details or an evaluation) are regarded in combination with each other
Comparison of paper recommendation systems in different categories
In this Section, we describe the scientific directions associated with the categories we presented in the previous section as the 65 relevant publications. We focus only on the methodological categories and describe how they are incorporated in the respective approaches.
User profile
32 approaches construct explicit user profiles. They utilise different components to describe users. We differentiate between profiles derived from user interactions and ones derived from papers.
Most user profiles are constructed from users’ actual interactions : unspecified historical interaction [ 30 , 37 , 56 , 57 , 64 , 118 ], the mean of the representation of interacted with papers [ 19 ], time decayed interaction behaviour [ 62 ], liked papers [ 69 , 123 ], bookmarked papers [ 84 , 119 ], read papers [ 111 , 113 ], rated papers [ 3 , 4 , 110 ], clicked on papers [ 24 , 26 , 49 ], categories of clicked papers [ 1 ], features of clicked papers [ 104 ], tweets [ 74 – 76 ], social interactions [ 65 ] and explicitly defined topics of interest tags [ 119 ].
Some approaches derived user profiles from users’ written papers : authored papers [ 5 , 21 , 22 , 55 , 63 , 74 – 76 , 116 ], a partitioning of authored papers [ 27 ], research fields of authored papers [ 41 ] and referenced papers [ 116 ].
We found 13 papers using some type of popularity measure. Those can be defined on authors, venues or papers.
For author-based popularity measures we found unspecified ones [ 65 ] such as authority [ 116 ] as well as ones regarding the citations an author received: citation count of papers [ 22 , 96 , 108 , 119 ], change in citation count [ 25 , 26 ], annual citation count [ 26 ], number of citations related to papers [ 59 ], h-index [ 26 ]. We found two definitions of author’s popularity using the graph structure of scholarly networks, namely the number of co-authors [ 41 ] and a person’s centrality [ 108 ].
For venue-based popularity measures, we found an unspecific reputation notion [ 116 ] as well as incorporation of the impact factor [ 26 , 117 ].
For paper-based popularity measures we encountered some citation-based definitions such as vitality [ 117 ], citation count of papers [ 22 ] and theirs centrality [ 96 ] in the citation network. Additionally, some approaches incorporated less formal interactions: number of downloads [ 56 ], social media mentions [ 119 ] and normalised number of bookmarks [ 84 ].
Only four papers use key phrases in some shape or form: Ahmad and Afzal [ 2 ] construct key terms from preprocessed titles and abstracts using tf-idf to represent papers. Collins and Beel [ 28 ] use the Distiller Framework [ 12 ] to extract uni-, bi- and tri-gram key phrase candidates from tokenised, part-of-speech tagged and stemmed titles and abstracts. Key phrase candidates were weighted and the top 20 represent candidate papers. Kang et al. [ 46 ] extract key phrases from CiteSeer to describe the diversity of recommended papers. Renuka et al. [ 86 ] apply rapid automatic keyword extraction.
In summary, different length key phrases usually get constructed from titles and abstracts with automatic methods such as tf-idf or the Distiller Framework to represent the most important content of publications.
We found a lot of approaches utilising some form of embedding based on existing document representation methods. We distinguish by embedding of papers, users and papers and sophisticated embedding from the proposed approaches.
Among the most common methods was their application on papers : in an unspecified representation [ 30 , 119 ], Word2Vec [ 19 , 37 , 44 , 45 , 55 , 104 , 113 ], Word2Vec of LDA top words [ 24 , 107 ], Doc2vec [ 21 , 28 , 48 , 62 , 63 , 107 ], Doc2Vec of word pairs [ 109 ], BERT [ 123 ] and SBERT [ 5 , 19 ]. Most times these approaches do not mention which part of the paper to use as input but some specifically mention the following parts: titles [ 37 ], titles and abstracts [ 28 , 45 ], titles, abstracts and bodies [ 48 ], keywords and paper [ 119 ].
Few approaches observed user profiles and papers , here Word2Vec [ 21 ] and NPLM [ 29 ] embeddings were used.
Several approaches embed the information in their own model embedding: a heterogeneous information network [ 5 ], a two-layer NN [ 37 ], a scientific social reference network [ 41 ], the TransE model [ 56 ], node embeddings [ 63 ], paper, author and venue embedding [ 116 ], user and item embedding [ 118 ], a GRU and association rule mining model [ 71 ], a GCN embedding of users [ 104 ] and an LSTM model [ 113 ].
Topic model
Eight approaches use some topic modelling component. Most of them use LDA to represent papers’ content [ 3 , 5 , 24 , 27 , 107 , 117 ]. Only two of them do not follow this method: Subathra and Kumar [ 98 ] use LDA on papers to find their top n words, then they use LDA again on these words’ Wikipedia articles. Xie et al. [ 115 ] use a hierarchical LDA adoption on papers, which introduces a discipline classification.
Knowledge graph
Only six of the observed papers incorporate knowledge graphs. Only one uses a predefined one, the Watson for Genomics knowledge graph [ 95 ]. Most of the approaches build their own knowledge graphs, only one asks users to construct the graphs: Wang et al. [ 109 ] build two knowledge graphs, one in-domain and one cross-domain graph. The graphs are user-constructed and include representative papers for the different concepts.
All other approaches do not rely on users building the knowledge graph: Afsar et al. [ 1 ] utilise an expert-built knowledge base as a source for their categorisation of papers, which are then recommended to users. Li et al. [ 56 ] employ a knowledge graph-based embedding of authors, keywords and venues. Tang et al. [ 104 ] link words with high tf-idf weights from papers to LOD and then merge this knowledge graph with the user-paper graph. Wang et al. [ 113 ] construct a knowledge graph consisting of users and papers.
In terms of graphs, we found 33 approaches explicitly mentioning the graph structure they were utilising. We can describe which graph structure is used and which algorithms or methods are applied on the graphs.
Of the observed approaches, most specify some form of (heterogeneous) graph structure . Only a few of them are unspecific and mention an undefined heterogeneous graph [ 63 – 65 ] or a multi-layer [ 48 ] graph. Most works clearly define the type of graph they are using: author-paper-venue-label-topic graph [ 5 ], author-paper-venue-keyword graph [ 56 , 57 ], paper-author graph [ 19 , 29 , 55 , 104 ], paper-topic graph [ 29 ], author-paper-venue graph [ 42 , 121 , 122 ], author graph [ 41 ], paper-paper graph [ 42 , 49 ], citation graph [ 2 , 44 – 46 , 88 , 89 , 106 , 108 , 117 ] or undirected citation graph [ 60 , 61 ]. Some approaches specifically mention usage of co-citations [ 2 , 45 ], bibliographic coupling or both [ 88 , 89 , 96 , 108 ].
As for algorithms or methods used on these graphs , we encountered usage of centrality measures in different graph types [ 41 , 96 , 108 ], some use knowledge graphs (see Sect. 3.4.6 ), some using meta-paths (see Sect. 3.4.8 ), some using random walks e.g. in form of PageRank or hubs and authorities (see Sect. 3.4.9 ), construction of Steiner trees [ 61 ], usage of the graph as input for a GCN [ 104 ], BFS [ 113 ], clustering [ 117 ] or calculation of a closeness degree [ 117 ].
We found only four approaches incorporating meta-paths. Hua et al. [ 42 ] construct author-paper-author and author-paper-venue-paper-author paths by applying beam search. Papers on the most similar paths are recommended to users. Li et al. [ 57 ] construct meta-paths of a max length between users and papers and use random walk on these paths. Ma et al. [ 63 , 64 ] use meta-paths to measure the proximity between nodes in a graph.
Random walk (with restart)
We found twelve approaches using some form of random walk in their methodology. We differentiate between ones using random walk, random walk with restart and algorithms using a random walk component.
Some methods use random walk on heterogeneous graphs [ 29 , 65 ] and weighted multi-layer graphs [ 48 ]. A few approaches use random walk to identify [ 42 , 57 ] or determine the proximity between [ 64 ] meta-paths.
Three approaches explicitly utilise random walk with restart . They determine similarity between papers [ 106 ], identify papers to recommend [ 44 ] or find most relevant papers in clusters [ 117 ].
Some approaches use algorithms which incorporate a random walk component : PageRank [ 107 ] and the identifications of hubs and authorities [ 122 ] with PageRank [ 121 ].
Advanced machine learning
29 approaches utilised some form of advanced machine learning. We encountered different methods being used and some papers specifically presenting novel machine learning models. All of these papers surpass mere usage of a topic model or typical pre-trained embedding method.
We found a multitude of machine learning methods being used, from multi armed bandits [ 1 ], LSTM [ 24 , 37 , 113 ], multi-layer perceptrons [ 62 , 96 , 104 ], (bi-)GRU [ 37 , 69 , 71 , 123 ], matrix factorisation [ 4 , 62 , 69 , 110 , 111 ], gradient ascent or descent [ 41 , 57 , 63 , 116 ], some form of simple neural network [ 30 , 37 , 56 ], some form of graph neural network [ 19 , 49 , 104 ], autoencoder [ 4 ], neural collaborative filtering [ 62 ], learning methods [ 30 , 123 ] to DTW [ 48 ]. Three approaches ranked the papers to recommend [ 56 , 57 , 118 ] with, e.g. Bayesian Personalized Ranking. Two of the observed papers proposed topic modelling approaches [ 3 , 115 ].
Several papers proposed models : a bipartite network embedding [ 5 ], heterogeneous graph embeddings [ 29 , 42 , 48 , 63 ], a scientific social reference network [ 41 ], a paper-author-venue embedding [ 116 ] and a relation prediction model [ 64 ].
We found nine papers incorporating a crawling step as part of their approach. PDFs are oftentimes collected from CiteSeer [ 38 , 46 ] or CiteSeerX [ 2 , 93 , 94 ], in some cases [ 39 , 88 , 110 ] the sources are not explicitly mentioned. Fewer used data sources are Wikipedia for articles explaining the top words from papers [ 98 ] or papers from ACM, IEEE and EI [ 109 ]. Some approaches explicitly mention the extraction of citation information [ 2 , 38 , 39 , 46 , 88 , 93 , 94 ] e.g. to identify co-citations.
Cosine similarity
Some form of cosine similarity was encountered in most (31) paper recommendation approaches. It is often applied between papers, between users, between users and papers and in other forms.
For application between papers we encountered the possibility of using unspecified embeddings: unspecified word or vector representations of papers [ 30 , 48 , 107 , 110 ], papers’ key terms or top words [ 2 , 98 ] and key phrases [ 46 ]. We found some approaches using vector space model variants: unspecified [ 59 ], tf vectors [ 39 , 88 ], tf-idf vectors [ 42 , 95 , 111 ], dimensionality reduced tf-idf vectors [ 86 ] and lastly, tf-idf and entity embeddings [ 56 ]. Some approaches incorporated more advanced embedding techniques: SBERT embeddings [ 5 ], Doc2Vec embeddings [ 28 ], Doc2Vec embeddings with incorporation of their emotional score [ 109 ] and NPLM representations [ 29 ].
Cosine similarity was used between preferences or profiles of users and papers in the following ways: unspecified representations [ 63 , 84 , 113 , 115 ], Boolean representation of users and keywords [ 60 ], tf-idf vectors [ 21 , 74 – 76 ], cf-idf vectors [ 74 – 76 ] and hcf-idf vectors [ 74 – 76 ].
For between users application of cosine similarity, we found unspecified representations [ 41 ] and time-decayed Word2Vec embeddings of users’ papers’ keyword [ 55 ].
Other applications include the usage between input keywords and paper clusters [ 117 ] and between nodes in a graph represented by their neighbouring nodes [ 121 , 122 ].
Paper recommendation systems
The 65 relevant works identified in our literature search are described in this section. We deliberately refrain from trying to structure the section by classifying papers by an arbitrary dimension and instead point to Table 3 to identify those dimensions in which a reader is interested to navigate the following short descriptions. The works are ordered by the surname of the first author and ascending publication year. An exception to this rule are papers presenting extensions of previous approaches with different first authors. These papers are ordered to their preceding approaches.
Afsar et al. [ 1 ] propose KERS, a multi-armed bandit approach for patients to help with medical treatment decision making. It consists of two phases: first an exploration phase identifies categories users are implicitly interested in. This is supported by an expert-built knowledge base. Afterwards an exploitation phase takes place where articles from these categories are recommended until a user’s focus changes and another exploitation phase is initiated. The authors strive to minimise the exploration efforts while maximising users’ satisfaction.
Ahmedi et al. [ 3 ] propose a personalised approach which can also be applied to more general recommendation scenarios which include user profiles. They utilise Collaborative Topic Regression to mine association rules from historic user interaction data.
Alfarhood and Cheng [ 4 ] introduce Collaborative Attentive Autoencoder, a deep learning-based model for general recommendation targeting the data sparsity problem. They apply probabilistic matrix factorisation while also utilising textual information to train a model which identifies latent factors in users and papers.
Ali et al. [ 5 ] construct PR-HNE, a personalised probabilistic paper recommendation model based on a joint representation of authors and publications. They utilise graph information such as citations as well as co-authorships, venue information and topical relevance to suggest papers. They apply SBERT and LDA to represent author embeddings and topic embeddings respectively.
Bereczki [ 19 ] models users and papers in a bipartite graph. Papers are represented by their contents’ Word2Vec or BERT embeddings, users’ vectors consist of representations of papers they interacted with. These vectors are then aggregated with simple graph convolution.
Bulut et al. [ 22 ] focus on current user interest in their approach which utilises k-Means and KNN. Users’ profiles are constructed from their authored papers. Recommended papers are the highest cited ones from the cluster most similar to a user. In a subsequent work they extended their research group to again work in the same domain. Bulut et al. [ 21 ] again focus on users’ features. They represent users as the sum of features of their papers. These representations are then compared with all papers’ vector representations to find the most similar ones. Papers can be represented by TF-IDF, Word2Vec or Doc2Vec vectors.
Chaudhuri et al. [ 25 ] use indirect features derived from direct features of papers in addition to direct ones in their paper recommendation approach: keyword diversification, text complexity and citation analysis. In an extended group Chaudhuri et al. [ 26 ] later propose usage of more indirect features such as quality in paper recommendation. Users’ profiles are composed of their clicked papers. Subsequently they again worked on an approach in the same area but in a slightly smaller group. Chaudhuri et al. [ 24 ] propose the general Hybrid Topic Model and apply it on paper recommendation. It learns users’ preferences and intentions by combining LDA and Word2Vec. They compute user’s interest from probability distributions of words of clicked papers and dominant topics in publications.
Chen and Ban [ 27 ] introduce CPM, a recommendation model based on topically clustered user interests mined from their published papers. They derive user need models from these clusters by using LDA and pattern equivalence class mining. Candidate papers are then ranked against the user need models to identify the best-fitting suggestions.
Collins and Beel [ 28 ] propose the usage of their paper recommendation system Mr. DLib as a recommender as-a-service. They compare representing papers via Doc2Vec with a key phrase-based recommender and TF-IDF vectors.
Du et al. [ 29 ] introduce HNPR, a heterogeneous network method using two different graphs. The approach incorporates citation information, co-author relations and research areas of publications. They apply random walk on the networks to generate vector representations of papers.
Du et al. [ 30 ] propose Polar++, a personalised active one-shot learning-based paper recommendation system where new users are presented articles to vote on before they obtain recommendations. The model trains a neural network by incorporating a matching score between a query article and the recommended articles as well as a personalisation score dependant on the user.
Guo et al. [ 37 ] recommend publications based on papers initially liked by a user. They learn semantics between titles and abstracts of papers on word- and sentence-level, e.g. with Word2Vec and LSTMs to represent user preferences.
Habib and Afzal [ 38 ] crawl full texts of papers from CiteSeer. They then apply bibliographic coupling between input papers and a clusters of candidate papers to identify the most relevant recommendations. In a subsequent work Afzal again used a similar technique. Ahmad and Afzal [ 2 ] crawled papers from CiteSeerX. Cosine similarity of TF-IDF representations of key terms from titles and abstracts is combined with co-citation strength of paper pairs. This combined score then ranks the most relevant papers the highest.
Haruna et al. [ 39 ] incorporate paper-citation relations combined with contents of titles and abstracts of papers to recommend the most fitting publications for an input query corresponding to a paper.
Hu et al. [ 41 ] present ADRCR, a paper recommendation approach incorporating author-author and author-paper citation relationships as well as authors’ and papers’ authoritativeness. A network is built which uses citation information as weights. Matrix decomposition helps learning the model.
Hua et al. [ 42 ] propose PAPR which recommends relevant paper sets as an ordered path. They strive to overcome recommendation merely based on similarity by observing topics in papers changing over time. They combine similarities of TF-IDF paper representations with random-walk on different scientific networks.
Jing and Yu [ 44 ] build a three-layer graph model which they traverse with random-walk with restart in an algorithm named PAFRWR. The graph model consists of one layer with citations between papers’ textual content represented via Word2Vec vectors, another layer modelling co-authorships between authors and the third layer encodes relationships between papers and topics contained in them.
Kanakia et al. [ 45 ] build their approach upon the MAG dataset and strive to overcome the common problems of scalability and cold-start. They combine TF-IDF and Word2Vec representations of the content with co-citations of papers to compute recommendations. Speedup is achieved by comparing papers to clusters of papers instead of all other single papers.
Kang et al. [ 46 ] crawl full texts of papers from CiteSeer and construct citation graphs to determine candidate papers. Then they compute a combination of section-based citation and key phrase similarity to rank recommendations.
Kong et al. [ 48 ] present VOPRec, a model combining textual components in form of Doc2vec and Paper2Vec paper representations with citation network information in form of Struc2vec. Those networks of papers connect the most similar publications based on text and structure. Random walk on these graphs contributes to the goal of learning vector representations.
L et al. [ 49 ] base their recommendation on lately accessed papers of users as they assume future accessed papers are similar to recently seen ones. They utilise a sliding window to generate sequences of papers, on those they construct a GNN to aggregate neighbouring papers to identify users’ interests.
Li et al. [ 56 ] introduce a subscription-based approach which learns a mapping between users’ browsing history and their clicks in the recommendation mails. They learn a re-ranking of paper recommendations by using its metadata, recency, word representations and entity representations by knowledge graphs as input for a neural network. Their defined target audience are new users.
Li et al. [ 55 ] present HNTA a paper recommendation method utilising heterogeneous networks and changing user interests. Paper similarities are calculated with Word2Vec representations of words recommended for each paper. Changing user interest is modelled with help of an exponential time decay function on word vectors.
Li et al. [ 57 ] utilise user profiles with a history of preferences to construct heterogeneous networks where they apply random walks on meta-paths to learn personalised weights. They strive to discover user preference patterns and model preferences of users as their recently cited papers.
Lin et al. [ 59 ] utilise authors’ citations and years they have been publishing papers in their recommendation approach. All candidate publications are matched against user-entered keywords, the two factors of authors of these candidate publications are combined to identify the overall top recommendations.
Liu et al. [ 60 ] explicitly do not require all recommended publications to fit the query of a user perfectly. Instead they state the set of recommended papers fulfils the information need only in the complete form. Here they treat paper recommendation as a link prediction problem incorporating publishing time, keywords and author influence. In a subsequent work, part of the previous research group again observes the same problem. In this work Liu et al. [ 61 ] propose an approach utilising numbers of citations (author popularity) and relationships between publications in an undirected citation graph. They compute Steiner trees to identify the sets of papers to recommend.
Lu et al. [ 62 ] propose TGMF-FMLP, a paper recommendation approach focusing on the changing preferences of users and novelty of papers. They combine category attributes (such as paper type, publisher or journal), a time-decay function, Doc2Vec representations of the papers’ content and a specialised matrix factorisation to compute recommendations.
Ma et al. [ 64 ] introduce HIPRec, a paper recommendation approach on heterogeneous networks of authors, papers, venues and topics specialised on new publications. They use the most interesting meta-paths to construct significant meta-paths. With these paths and features from these paths they train a model to identify new papers fitting users. Together with another researcher Ma further pursued this research direction. Ma and Wang [ 63 ] propose HGRec, a heterogeneous graph representation learning-based model working on the same network. They use meta-path-based features and Doc2Vec paper embeddings to learn the node embeddings in the network.
Manju et al. [ 65 ] attempt to solve the cold-start problem with their paper recommendation approach coding social interactions as well as topical relevance into a heterogeneous graph. They incorporate believe propagation into the network and compute recommendations by applying random walk.
Mohamed Hassan et al. [ 69 ] adopt an existing tag prediction model which relies on a hierarchical attention network to capture semantics of papers. Matrix factorisation then identifies the publications to recommend.
Nair et al. [ 71 ] propose C-SAR, a paper recommendation approach using a neural network. They input GloVe embeddings of paper titles into their Gated Recurrent Union model to compute probabilities of similarities of papers. The resulting adjacency matrix is input to an association rule mining a priori algorithm which generates the set of recommendations.
Nishioka et al. [ 74 , 75 ] state serendipity of recommendations as their main objective. They incorporate users’ tweets to construct profiles in hopes to model recent interests and developments which did not yet manifest in users’ papers. They strive to diversity the list of recommended papers. In more recent work Nishioka et al. [ 76 ] explained their evaluation more in depth.
Rahdari and Brusilovsky [ 84 ] observe paper recommendation for participants of scientific conferences. Users’ profiles are composed of their past publications. Users control the impact of features such as publication similarity, popularity of papers and its authors to influence the ordering of their suggestions.
Renuka et al. [ 86 ] propose a paper recommendation approach utilising TF-IDF representations of automatically extracted keywords and key phrases. They then either use cosine similarity between vectors or a clustering method to identify the most similar papers for an input paper.
Sakib et al. [ 89 ] present a paper recommendation approach utilising second-level citation information and citation context. They strive to not rely on user profiles in the paper recommendation process. Instead they measure similarity of candidate papers to an input paper based on co-occurred or co-occurring papers. In a follow-up work with a bigger research group Sakib et al. [ 88 ] combine contents of titles, keywords and abstracts with their previously mentioned collaborative filtering approach. They again utilise second-level citation relationships between papers to find correlated publications.
Shahid et al. [ 94 ] utilise in-text citation frequencies and assume a reference is more important to a referencing paper the more often it occurs in the text. They crawl papers from CiteSeerX to retrieve the top 500 citing papers. In a follow-up work with a partially different research group Shahid et al. [ 93 ] evaluate the previously presented approach with a user study.
Sharma et al. [ 95 ] propose IBM PARSe, a paper recommendation system for the medical domain to reduce the number of papers to review for keeping an existing knowledge graph up-to-date. Classifiers identify new papers from target domains, named entity recognition finds relevant medical concepts before papers’ TF-IDF vectors are compared to ones in the knowledge graph. New publications most similar to already relevant ones with matching entities are recommended to be included in the knowledge base.
Subathra and Kumar [ 98 ] constructed an paper recommendation system which applies LDA on Wikipedia articles twice. Top related words are computed using pointwise mutual information before papers are recommended for these top words.
Tang et al. [ 104 ] introduce CGPrec, a content-based and knowledge graph-based paper recommendation system. They focus on users’ sparse interaction history with papers and strive to predict papers on which users are likely to click. They utilise Word2Vec and a Double Convolutional Neural Network to emulate users’ preferences directly from paper content as well as indirectly by using knowledge graphs.
Tanner et al. [ 106 ] consider relevance and strength of citation relations to weigh the citation network. They fetch citation information from the parsed full texts of papers. On the weighted citation networks they run either weighted co-citation inverse document frequency, weighted bibliographic coupling or random walk with restart to identify the highest scoring papers.
Tao et al. [ 107 ] use embeddings and topic modelling to compute paper recommendations. They combine LDA and Word2Vec to obtain topic embeddings. Then they calculate most similar topics for all papers using Doc2Vec vector representations and afterwards identify the most similar papers. With PageRank on the citation network they re-rank these candidate papers.
Waheed et al. [ 108 ] propose CNRN, a recommendation approach using a multilevel citation and authorship network to identify recommendation candidates. From these candidate papers ones to recommend are chosen by combining centrality measures and authors’ popularity. Highly correlated but unrelated Shi et al. [ 96 ] present AMHG, an approach utilising a multilayer perceptron. They also construct a multilevel citation network as described before with added author relations. Here they additionally utilise vector representations of publications and recency.
Wang et al. [ 113 ] introduce a knowledge-aware path recurrent network model. An LSTM mines path information from the knowledge graphs incorporating papers and users. Users are represented by their downloaded, collected and browsed papers, papers are represented by TF-IDF representations of their keywords.
Wang et al. [ 109 ] require users to construct knowledge graphs to specify the domain(s) and enter keywords for which recommended papers are suggested. From the keywords they compute initially selected papers. They apply Doc2Vec and emotion-weighted similarity between papers to identify recommendations.
Wang et al. [ 110 ] regard paper recommendation targeting a group of people instead of single users and introduce GPRAH_ER. They employ a two-step process which first individually predicts papers for users in the group before recommended papers are aggregated. Here users in the group are not considered equal, different importance and reliability weights are assigned such that important persons’ preferences are more decisive of the recommended papers. Together with a different research group two authors again pursued this definition of the paper recommendation problem. Wang et al. [ 111 ] recommend papers for groups of users in an approach called GPMF_ER. As with the previous approach they compute TF-IDF vectors of keywords of papers to calculate most similar publications for each user. Probabilistic matrix factorisation is used to integrate these similarities in a model such that predictive ratings of all users and papers can be obtained. In the aggregation phase the number of papers read by a user is determined to replace the importance component.
Xie et al. [ 116 ] propose JTIE, an approach incorporating contents, authors and venues of papers to learn paper embeddings. Further, directed citation relations are included into the model. Based on users’ authored and referenced papers personalised recommendations are computed. They consider explainability of recommendations. In a subsequent work part of the researchers again work on this topic. Xie et al. [ 115 ] specify on recommendation of papers from different areas for user-provided keywords or papers. They use hierarchical LDA to model evolving concepts of papers and citations as evidence of correlation in their approach.
Yang et al. [ 117 ] incorporate the age of papers and impact factors of venues as weights in their citation network-based approach named PubTeller. Papers are clustered by topic, the most popular ones from the clusters most similar to the query terms are recommendation candidates. In this approach, LDA and TF-IDF are used to represent publications.
Yu et al. [ 118 ] propose ICMN, a general collaborative memory network approach. User and item embeddings are composed by incorporating papers’ neighbourhoods and users’ implicit preferences.
Zavrel et al. [ 119 ] present the scientific literature recommendation platform Zeta Alpha, which bases their recommended papers on examples tagged in user-defined categories. The approach includes these user-defined tags as well as paper content embeddings, social media mentions and citation information in their ensemble learning approach to recommend publications.
Zhang et al. [ 121 ] propose W-Rank, a general approach weighting edges in a heterogeneous author, paper and venue graph by incorporating citation relevance and author contribution. They apply their method on paper recommendation. Network- (via citations) and semantic-based (via AWD) similarity between papers is combined for weighting edges between papers, harmonic counting defines weights of edges between authors and papers. A HITS-inspired algorithm computes the final authority scores. In a subsequent work in a slightly smaller group they focus on a specialised approach for paper recommendation. Here Zhang et al. [ 122 ] strive to emulate a human expert recommending papers. They construct a heterogeneous network with authors, papers, venues and citations. Citation weights are determined by semantic- and network-level similarity of papers. Lastly, recommendation candidates are re-ranked while combining the weighted heterogeneous network and recency of papers.
Zhao et al. [ 123 ] present a personalised approach focusing on diversity of results which consists of three parts. First LFM extracts latent factor vectors of papers and users from the users’ interactions history with papers. Then BERT vectors are constructed for each word of the papers, with those vectors as input and the latent factor vectors as label a BiGRU model is trained. Lastly, diversity and a user’s rating weights determine the ranking of recommended publications for the specific user.
Other relevant work
We now briefly discuss some papers which did not present novel paper recommendation approaches but are relevant in the scope of this literature review nonetheless.
Surrounding paper recommendation
Here we present two works which could be classified as ones to use on top of or in combination with existing paper recommendation systems: Lee et al. [ 51 ] introduce LIMEADE, a general approach for opaque recommendation systems which can for example be applied on any paper recommendation system. They produce explanations for recommendations as a list of weighted interpretable features such as influential paper terms.
Beierle et al. [ 18 ] use the recommendation-as-a-service provider Mr. DLib to analyse choice overload in user evaluations. They report several click-based measures and discuss effects of different study parameters on engagement of users.
(R)Evaluations
The following four works can be grouped as ones which provide (r)evaluations of already existing approaches. Their results could be useful for the construction of novel systems: Ostendorff [ 77 ] suggests considering the context of paper similarity in background, methodology and findings sections instead of undifferentiated textual similarity for scientific paper recommendation.
Mohamed Hassan et al. [ 68 ] compare different text embedding methods such as BERT, ELMo, USE and InferSent to express semantics of papers. They perform paper recommendation and re-ranking of recommendation candidates based on cosine similarity of titles.
Le et al. [ 50 ] evaluate the already existing paper recommendation system Mendeley Suggest, which provides recommendations with different collaborative or content-based approaches. They observe different usage behaviours and state utilisation of paper recommendation systems does positively effect users’ professional lives.
Barolli et al. [ 11 ] compare similarities of paper pairs utilising n-grams, tf-idf and a transformer based on BERT. They model cosine similarities of these pairs into a paper connection graph and argue for the combination of content-based and graph-based methods in the context of COVID-19 paper recommendation systems.
Living labs
Living labs help researchers conduct meaningful evaluations by providing an environment, in which recommendations produced by experimental systems are shown to real users in realistic scenarios [ 14 ]. We found three relevant works for the area of scientific paper recommendation: Beel et al. [ 14 ] proposed a living lab for scholarly recommendation built on top of Mr. DLib, their recommender-as-a-service system. They log users’ actions such as clicks, downloads and purchases for related recommended papers. Additionally, they plan to extend their living lab to also incorporate research grant or research collaborator recommendation.
Gingstad et al. [ 36 ] propose ArXivDigest, an online living lab for explainable and personalised paper recommendations from arXiv. Users can either be suggested papers while browsing their website or via email as a subscription-type service. Different approaches can be hooked into ArXivDigest, the recommendations generated by them can then be evaluated by users. A simple text-based baseline compares user-input topics with articles. Target values of evaluations are users’ clicked and saved papers.
Schaer et al. [ 91 ] held the Living Labs for Academic Search (LiLAS) where they hosted two shared tasks: dataset recommendation for scientific papers and ad-hoc multi-lingual retrieval of most relevant publications regarding specific queries. To overcome the gap between real-world and lab-based evaluations they allowed integrating participants’ systems into real-world academic search systems, namely LIVIO and GESIS Search.
Multilingual/cross-lingual recommendation
The previous survey by Li and Zhou [ 58 ] identifies cross-language paper recommendation as a future research direction. The following two works could be useful for this aspect: Keller and Munz [ 47 ] present their results of participating on the CLEF LiLAS challenge where they tackled recommendation of multilingual papers based on queries. They utilised a pre-computed ranking approach, Solr and pseudo-relevance feedback to extend queries and identify fitting papers.
Safaryan et al. [ 87 ] compare different already existing techniques for cross-language recommendation of publications. They compare word by word translation, linear projection from a Russian to an English vector representation, VecMap alignment and MUSE word embeddings.
Related recommendation systems
Some recommendation approaches are slightly out of scope of pure paper recommendation systems but could still provide inspiration or relevant results: Ng [ 73 ] proposes CBRec, a children’s book recommendation system utilising matrix factorisation. His goal is to encourage good reading habits of children. The approach combines readability levels of users and books with TF-IDF representations of books to find ones which are similar to ones which a child may have already liked.
Patra et al. [ 80 ] recommend publications relevant for datasets to increase reusability. Those papers could describe the dataset, use it or be related literature. The authors represent datasets and articles as vectors and use cosine similarity to identify the best fitting papers. Re-ranking them with usage of Word2Vec embeddings results in the final recommendation.
As the discussed paper recommendation systems utilise different inputs or components of scientific publications and pursue slightly different objectives, datasets to experiment on are also of diverse nature. We do not consider datasets of approaches which do not contain an evaluation [ 60 , 119 ] or do not evaluate the actual paper recommendation [ 2 , 25 , 38 , 84 , 86 ] such as the cosine similarity between a recommended and an initial paper [ 2 , 86 ], the clustering quality on the constructed features [ 25 ] or the Jensen Shannon Divergence between probability distributions of words between an initial and recommended papers [ 38 ]. We also do not discuss datasets where only the data sources are mentioned but no remarks are made regarding the size or composition of the dataset [ 21 , 104 ] or ones where we were not able to identify actual numbers [ 65 ]. Table 4 gives an overview of datasets used in the evaluation of the considered discussed methods. Many of the datasets are unavailable only few years after publication of the approach. Most approaches utilise their own modified version of a public dataset which makes exact replication of experiments hard. In the following the main underlying data sources and publicly available datasets are discussed. Non-publicly available datasets are briefly described in Table 5 .
Overview of datasets utilised in most recent related work with (unofficial) names, public availability of the possibly modified dataset which was used (A?), and a list of papers it was used in. Datasets are grouped by their underlying data source if possible
Description of private datasets utilised in most recent related work with (unofficial) names. Datasets are grouped by their underlying data source if possible
We used the following abbreviations: user(s) u , paper(s) p , interaction(s) i , author(s) a , venue(s) v , reference(s) r , citation(s) c , term(s) t
dblp-based datasets
The dblp computer science bibliography (dblp) is a digital library offering metadata on authors, papers and venues from the area of computer science and adjacent fields [ 54 ]. They provide publicly available short-time stored daily and longer-time stored monthly data dumps 10 .
The dblp + Citations v1 dataset [ 105 ] builds upon a dblp version from 2010 mapped on AMiner. It contains 1,632,442 publications with 2,327,450 citations.
The dblp + Citations v11 dataset 11 builds upon dblp. It contains 4,107,340 papers, 245,204 authors, 16,209 venues and 36,624,464 citations
These datasets do not contain supervised labels provided by human annotators even though the citation information could be used as interaction data.
SPRD-based datasets
The Scholarly Paper Recommendation Dataset (abbreviation: SPRD) 12 was constructed by collecting publications written by 50 researchers of different seniority from the area of computer science which are contained in dblp from 2000 to 2006 [ 58 , 101 , 102 ]. The dataset contains 100,351 candidate papers extracted from the ACM Digital Library as well as citations and references for papers. Relevance assessments of papers relevant to their current interests of the 50 researchers are also included.
A subset of SPRD, SPRD_Senior , which contains only the data of senior researchers can also be constructed [ 99 ].
These datasets specifically contain supervised labels provided by human annotators in the form of sets of papers, which researchers found relevant for themselves.
CiteULike-based datasets
CiteULike [ 20 ] was a social bookmarking site for scientific papers. It contained papers and their metadata. Users were able to include priorities, tags or comments for papers on their reading list. There were daily data dumps available from which datasets could be constructed.
Citeulike-a [ 112 ] 13 contains 5,551 users, 16,980 papers with titles and abstracts from 2004 to 2006 and their 204,986 interactions between users and papers. Papers are represented by their title and abstract.
Citeulike-t [ 112 ] 14 contains 7,947 users, 25,975 papers and 134,860 user-paper interactions. Papers are represented by their pre-processed title and abstract.
These datasets contain labelled data as they build upon CiteULike, which provides bookmarked papers of users.
ACM-based datasets
The ACM Digital Library (ACM) is a semi-open digital library offering information on scientific authors, papers, citations and venues from the area of computer science 15 . They offer an API to query for information. Datasets building upon this source do not contain supervised labels provided by annotators even though the citation information could be used as interaction data.
Scopus-based datasets
Scopus is a semi-open digital library containing metadata on authors, papers and affiliations in different scientific areas 16 . They offer an API to query for data. Datasets building upon this source usually do not contain labels provided by annotators.
AMiner-based datasets
ArnetMiner (AMiner) [ 105 ] is an open academic search system modelling the academic network consisting of authors, papers and venues from all areas 17 . They provide an API to query for information. Datasets building upon this source usually do not contain labelled user interaction data.
AAN-based datasets
The ACL Anthology Network (AAN) [ 81 – 83 ] is a networked database containing papers, authors and citations from the area of computational linguistics 18 . It consists of three networks representing paper-citation relations, author-collaboration relations and the author-citation relations. The original dataset contains 24,766 papers and 124,857 citations [ 71 ]. Datasets building upon this source usually do not contain labelled user interaction data even though the paper-citation, author-collaboration or author-citation relationships could be utilised to replace this data.
Sowiport-based datasets
Sowiport was an open digital library containing information on publications from the social sciences and adjacent fields [ 15 , 40 ]. The dataset linked papers by their attributes such as authors, publishers, keywords, journals, subjects and citation information. Via author names, keywords and venue titles the network could be traversed by triggering them to start a new search [ 40 ]. Sowiport co-operated with the recommendation-as-a-service system Mr. DLib [ 28 ]. Datasets building upon this source usually contain labelled user interaction data, the clicked papers of users.
CiteSeerX-based datasets
CiteSeerX [ 35 , 114 ] is a digital library focused on metadata and full-texts of open access literature 19 . It is the overhauled form of the former digital library CiteSeer. Datasets building upon this source usually do not inherently contain labelled user interaction data.
Patents-based datasets
The Patents dataset provides information on patents and trademarks granted by the United States Patent and Trademark Office 20 . Datasets building upon this source usually do not contain labelled user interaction data.
Hep-TH-based datasets
The original unaltered Hep-TH [ 53 ] dataset 21 stems from the area of high energy physics theory. It contains papers in a graph which were published between 1993 and 2003. It was released as part of KDD Cup 2003. Datasets building upon this source usually do not contain labelled user interaction data.
MAG-based datasets
The Microsoft Academic Graph (MAG) [ 97 ] was an open scientific network containing metadata on academic communication activities 22 . Their heterogeneous graph consists of nodes representing fields of study, authors, affiliations, papers and venues. Datasets building upon this source usually do not contain labelled user interaction data besides citation information.
The following datasets have no common underlying data source: The BBC 23 dataset contains 2,225 BBC news articles which stem from 5 topics. This dataset does not contain labelled user interaction data.
PRSDataset 24 contains 2,453 users, 21,940 items and 35,969 pairs of users and items. This dataset contains user-item interactions.
The performance of a paper recommendation system can be quantified by measuring how well a target value has been approximated by the recommended publications. Relevancy estimations of papers can come from different sources, such as human ratings or datasets. Different interactions derived from clicked or liked papers determine the target values which a recommendation system should approximate. The quality of the recommendation can be described by evaluation measures such as precision or MRR. For example, a dataset could provide information on clicked papers, that are then deemed relevant. The target value which should be approximated with the recommender system are those clicked papers, and the percentage of the recommendations which are contained in the clicked papers could then be reported as the system’s precision.
Due to the vast differences in approaches and datasets used to apply the methods, there is also a spectrum of used evaluation measures and objectives. In this section, first we observe different notions of relevance of recommended papers and individual assessment strategies for relevance. Afterwards we analyse commonly used evaluation measures and list ones which are only rarely encountered in evaluation of paper recommendation systems. Lastly we shed light on the different types of evaluation which authors conducted.
In this discussion we again only consider paper recommendation systems which also evaluate their actual approach. We disregard approaches which do evaluate other properties [ 2 , 25 , 38 , 84 , 86 , 122 ] or contain no evaluation [ 60 , 119 ]. Thus we observe 54 different approaches in this analysis.
Relevance and assessment
Relevance of recommended publications can be evaluated against multiple target values: clicked papers [ 24 , 56 , 104 ], references [ 44 , 115 ], references of recently authored papers [ 57 ], papers an author interacted with in the past [ 49 ], degree-of-relevancy which is determined by citation strength [ 94 ], a ranking based on future citation numbers [ 121 ] as well as papers accepted [ 26 ] or deemed relevant by authors [ 39 , 88 ].
Assessing the relevance of recommendations can also be conducted in different ways: the top n papers recommended by a system can be judged by either a referee team [ 109 ] or single persons [ 26 , 74 , 75 ]. Other options for relevance assessment are the usage of a dataset with user ratings [ 39 , 88 ] or emulation of users and their interests [ 1 , 57 ].
Table 6 holds information on utilised relevance indicators and target values which indicate relevance for the 54 discussed approaches. Relevancy describes the method that defines which of the recommended papers are relevant:
- Human rating: The approach is evaluated using assessments of real users of results specific to the approach.
- Dataset: The approach is evaluated using some type of assessment of a target value which is not specific to the approach but from a dataset. The assessment was either conducted for another approach and re-used or it was collected independent of an approach.
- Papers: The approach is evaluated by some type of assessment of a target value which is directly generated from the papers contained in the dataset such as citations or their keywords.
The target values in Table 6 describe the entities which the approach tried to approximate:
- Clicked: The approximated target value is derived from users’ clicks on papers.
- Read: The approximated target value is derived from users’ read papers.
- Cited: The approximated target value is derived from cited papers.
- Liked: The approximated target value is derived from users’ liked papers.
- Relevancy: The approximated target value is derived from users’ relevance assessment of papers.
- Other user: The approximated target value is derived from other entities associated with a user input, e.g. acceptance of users, users’ interest and relevancy of the recommended papers’ topics.
- Other automatic: The approximated target value is automatically derived from other entities, e.g. user profiles, papers with identical references, degree-of-relevancy, keywords extracted from papers, papers containing the query keywords in the optimal Steiner tree, neighbouring (cited and referencing) papers, included keywords, the classification tag, future citation numbers and an unknown measure derived from a dataset. We refrain from trying to introduce sub-categories for this broad field.
Only three approaches evaluate against multiple target values [ 21 , 30 , 104 ]. Six approaches (11.11%) utilise clicks of users, only one approach (1.85%) uses read papers as target value. Even though cited papers are not the main objective of paper recommendation systems but rather citation recommendation systems, this target was approximated by 13 (24.07%) of the observed systems. Ten approaches (18.52%) evaluated against liked papers, 15 (27.78%) against relevant papers and 13 (24.07%) against some other target value, either user input (three, 5.55%) or automatically derived (ten, 18.52%).
Indications whether approaches utilise the specified relevancy definitions, target values of evaluations and evaluation measures
Evaluation measures
We differentiate between commonly used and rarely used evaluation measures for the task of scientific paper recommendation. They are described in the following sections. Table 6 holds indications of utilised evaluation measures for the 54 discussed approaches. Measures are the methods used to evaluate the approach’s ability to approximate the target value which can be of type precision, recall, f1 measure, nDCG, MRR, MAP or another one.
Out of the observed systems, twelve 25 approaches [ 1 , 28 , 30 , 49 , 59 , 64 , 69 , 71 , 74 – 76 , 107 , 115 , 116 ] (22.22%) only report one single measure, all others report at least two different ones.
Commonly used evaluation measures
Bai et al. [ 9 ] identify precision (P), recall (R), F1 , nDCG , MRR and MAP as evaluation features which have been used regularly in the area of paper recommendation systems. Table 7 gives usage percentages of each of these measures in observed related work.
Common evaluation measures and percentage of observed evaluations of paper recommendation systems in which they were applied
Percentages are rounded to two decimal places
Alfarhood and Cheng [ 4 ] argue against the use of precision when utilising implicit feedback. If a user gives no feedback for a paper it could either mean disinterest or that a user does not know of the existence of the specific publication.
Rarely used evaluation measures
We found a plethora of rarer used evaluation measures which have either been utilised only by the work they were introduced in or to evaluate few approaches. Our analysis in this aspect might be highly influenced by the narrow time frame we observe. Novel measures might require more time to be adopted by a broader audience. Thus we differentiate between novel rarely used evaluation measures and ones where authors do not explicitly claim they are novel. A list of rare but already defined evaluation measures can be found in Table 8 . In total 25 approaches (46.3%) did use an evaluation measure not considered common.
Overview of rare existing measures used in evaluations of observed approaches
Novel rarely used Evaluation Measures. In our considered approaches we only encountered three novel evaluation measures: Recommendation quality as defined by Chaudhuri et al. [ 26 ] is the acceptance of recommendations by users rated on a Likert scale from 1 to 10.
TotNP_EU is a measure defined by Manju et al. [ 65 ] specifically introduced for measuring performance of approaches regarding the cold start problem. It indicates the number of new publications suggested to users with a prediction value above a certain threshold.
TotNP_AVG is another measure defined by Manju et al. [ 65 ] for measuring performance of approaches regarding the cold start problem. It indicates the average number of new publications suggested to users with a prediction value above a certain threshold.
Evaluation types
Evaluations can be classified into different categories. We follow the notion of Beel and Langer [ 17 ] who differentiate between user studies, online evaluations and offline evaluations. They define user studies as ones where users’ satisfaction with recommendation results is measured by collecting explicit ratings. Online evaluations are ones where users do not explicitly rate the recommendation results; relevancy is derived from e.g. clicks. In offline evaluations a ground truth is used to evaluate the approach.
From the 54 observed approaches we found four using multiple evaluation types [ 29 , 46 , 92 , 94 , 109 ]. Twelve (22.22%) were conducting user studies which describe the size and composition of the participant group. 26 Only two approaches [ 28 , 65 ] (3.7%) in the observed papers were evaluated with an online evaluation. We found 44 approaches (81.48%) providing an offline evaluation. Offline evaluations being the most common form of evaluation is unsurprising as this tendency has also been observed in an evaluation of general scientific recommender systems [ 23 ]. Offline evaluations are fast and do not require users [ 23 ]. Nevertheless the margin by which this form of evaluation is conducted could be rather surprising.
A distinction in lab-based vs. real world user studies can be conducted [ 16 , 17 ]. User studies where participants rate recommendations according to some criteria and are aware of the study are lab-based, all others are considered real-world studies. Living labs [ 14 , 36 , 91 ] for example enable real-world user studies. On average the lab-based user studies were conducted with 17.83 users. Table 9 holds information on the number of participants for all studies as well as the composition of groups in terms of seniority.
For all observed works with user studies we list their number of participants (# P) and their composition
NA indicates that #P or compositions were not described in a specific user study
For offline evaluation, they can either be ones with an explicit ground truth given by a dataset containing user rankings, implicit ones by deriving user interactions such as liked or cited papers or expert ones with manually collected expert ratings [ 17 ]. We found 22 explicit offline evaluations (40.74%) corresponding to ones using datasets to estimate relevance (see Table 6 ) and 21 implicit offline evaluations (38.89%) corresponding to ones using paper information to identify relevant recommendations (see Table 6 ). We did not find any expert offline evaluations.
Changes compared to 2016
This chapter briefly summarises some of the changes in the set of papers we observed when compared to the study by Beel et al. [ 16 ]. Before we start the comparison, we want to point to the fact that we observed papers from two years in which the publication process could have been massively affected by the COVID-19 pandemic.
Number of papers per year and publication medium
Beel et al. [ 16 ] studied works between and including 1998 and 2013 while we observed works which appeared between January 2019 and October 2021. While the previous study did include all 185 papers (of which 96 were paper recommendation approaches) in their discussion of papers per year which were published in the area of the topic paper or citation recommendation but later on only studied 62 papers for an in-depth review, we generally only studied 65 publications which present novel paper recommendation approaches (see Sect. 3.5 ) in this aspect. Compared to the time frame observed in this previous literature review, we encountered fewer papers being published on the actual topic of scientific paper recommendation per year. In the former work, the published number of papers was rising and hitting 40 in 2013. We found this number being stuck on a constant level between 21 and 23 in the three years we observed. This could hint at differing interest in this topic over time, with a current demise or the trend to work in this area having surpassed its zenith.
While Beel et al. [ 16 ] found 59% of conference papers and 16% of journal articles, we found 54.85% of conference papers and 41.54% of journal articles. The shift to journal articles could stem from a general shift towards journal articles in computer science 27 .
Classification
While Beel et al. [ 16 ] found 55% of their studied 62 papers applying methods from content-based filtering, we found only found 7.69% (5) of our 65 papers identifying as content-based approaches. Beel et al. [ 16 ] report 18% of approaches applied collaborative filtering. We encountered 4.62% (three) having this component as part of their self-defined classification. As for graph-based recommendation approach, Beel et al. [ 16 ] found 16% while we only encountered 7.69% (five) of papers with this description. In terms of hybrid approaches, Beel et al. [ 16 ] encountered five (8.06%) truly hybrid ones. In our study, we found 18 approaches (27.69%) labelling themselves as hybrid recommendation systems. 28
Table 10 shows the comparison of the distributions of the different types of evaluations between our study observing 54 papers with evaluations and the one conducted by Beel et al. [ 16 ], which regards 75 papers for this aspect. The percentage of quantitative user studies (User quant) is comparable for both studies. A peculiar difference is the percentage of offline evaluations, which is much higher in our current time frame.
Percentage of studies using the different methods. Some studies utilised multiple methods, thus the percentages do not add up to 100%
When observing the evaluation measures, we found some differences compared to the previous study. While 48.15% of papers with an evaluation report precision in our case, in Beel et al.’s [ 16 ] 72% of approaches with an evaluation report this value. As a contrast, we found 50% of papers reporting F1 while only 11% of papers reported this measure according to Beel et al. [ 16 ]. This might hint at a shift away from precision (which Beel et al. [ 16 ] did describe as a problematic measure) to focus more on also incorporating recall into the quality assessment of recommendation systems.
In general, the two reviews regard different time frames. We encounter non-marginal differences in the three dimensions discussed in this Section. A more concise comparison could be made if a time slice would be regarded for both studies, such that the research output and shape could be observed from three years each. We cannot clearly identify emerging trends (as with the offline evaluation) as we do not know if it has been conducted in this percentage of papers since the 2010s or if it only just picked up to be a more wide-spread evaluation form.
Open challenges and objectives
All paper recommendation approaches which were considered in this survey could have been improved in some way or another. Some papers did not conduct evaluations which would satisfy a critical reader, others could be more convincing if they compared their methods to appropriate competitors. The possible problems we encountered within the papers can be summarised in different open challenges, which papers should strive to overcome. We separate our analysis and discussion of open challenges in those which have already been described by previous literature reviews (see Sect. 7.1 ) and ones we identify as new or emerging problems (see Sect. 7.2 ). Lastly we briefly discuss the presented challenges (see Sect. 7.3 ).
Challenges highlighted in previous works
In the following we will explain possible shortcomings which were already explicitly discussed in previous literature reviews [ 9 , 16 , 92 ]. We regard these challenges in light of current paper recommendation systems to identify problems which are nowadays still encountered.
Neglect of user modelling
Neglect of user modelling has been described by Beel et al. [ 16 ] as identification of target audiences’ information needs. They describe the trade-off between specifying keywords which brings recommendation systems closer to search engines and utilising user profiles as input.
Currently only some approaches consider users of systems to influence the recommendation outcome, as seen with Table 3 users are not always part of the input to systems. Instead many paper recommendation systems assume that users do not state their information needs explicitly but only enter keywords or a paper. With paper recommendation systems where users are not considered, the problem of neglecting user modelling still holds.
Focus on accuracy
Focus on accuracy as a problem is described by Beel et al. [ 16 ]. They state putting users’ satisfaction with recommendations on a level with accuracy of approaches does not depict reality. More factors should be considered.
Only over one fourth of current approaches do not only report precision or accuracy but also observe more diversity focused measures such as MMR. We also found usage of less widespread measures to capture different aspects such as popularity, serendipity or click-through-rate.
Translating research into practice
The missing translation of research into practice is described by Beel et al. [ 16 ]. They mention the small percentage of approaches which are available as prototype as well as the discrepancy between real world systems and methods described in scientific papers.
Only five of our observed approaches definitively must have been available online at any point in time [ 28 , 45 , 65 , 84 , 119 ]. We did not encounter any of the more complex approaches being used in widespread paper recommendation systems.
Persistence and authority
Beel et al. [ 16 ] describe the lack of persistence and authority in the field of paper recommendation systems as one of the main reasons why research is not adapted in practice.
The analysis of this possible shortcoming of current work could be highly affected by the short time period from which we observed works. We found several groups publishing multiple papers as seen in Table 11 which corresponds to 29.69% of approaches. The most papers a group published was three so this amount still cannot fully mark a research group as authority in the area.
Overview of research groups with multiple papers
Cooperation
Problems with cooperation are described by Beel et al. [ 16 ]. They state even though approaches have been proposed by multiple authors building upon prior work is rare. Corporations between different research groups are also only encountered sporadically.
Here again we want to point to the fact that our observed time frame of less than three years might be too short to make substantive claims regarding this aspect. Table 12 holds information on the different numbers of authors for papers and the percentage of papers out of the 64 observed ones which are authored by groups of this size. We only encountered little cooperation between different co-author groups (see Haruna et al. [ 39 ] and Sakib et al. [ 88 ] for an exception). There were several groups not extending their previous work [ 121 , 122 ]. We refrain from analysing citations of related previous approaches as our considered period of less than three years is too short for all publications to have been able to be recognised by the wider scientific community.
Percentage of the 64 considered papers with different numbers of authors (#). Publications with 1 and 10 authors were encountered only once (1.56% each)
Information scarcity
Information scarcity is described by Beel et al. [ 16 ] as researchers’ tendency to only provide insufficient detail to re-implement their approaches. This leads to problems with reproducibility.
Many of the approaches we encountered did not provide sufficient information to make a re-implementation possible: with Afsar et al. [ 1 ] it is unclear how the knowledge graph and categories were formed, Collins and Beel [ 28 ] do not describe their Doc2Vec enough, Liu et al. [ 61 ] do not specify the extraction of keywords for papers in the graph and Tang et al. [ 104 ] do not clearly describe their utilisation of Word2Vec. In general oftentimes details are missing [ 3 , 4 , 60 , 117 ]. Exceptions to these observations are e.g. found with Bereczki [ 19 ], Nishioka et al. [ 74 – 76 ] and Sakib et al. [ 88 ].
We did not find a single paper’s code e.g. provided as a link to GitHub.
Pure collaborative filtering systems encounter the cold start problem as described by Bai et al. [ 9 ] and Shahid et al. [ 92 ]. If new users are considered, no historical data is available, they cannot be compared to other users to find relevant recommendations.
While this problem still persists, most current approaches are no pure collaborative filtering based recommendation systems (see Sect. 3.3.1 ). Systems using deep learning could overcome this issue [ 58 ]. There are approaches specifically targeting this problem [ 59 , 96 ], some [ 59 ] also introduced specific evaluation measures (totNP_EU and avgNP_EU) to quantify systems’ ability to overcome the cold start problem.
Sparsity or reduce coverage
Bai et al. [ 9 ] state the user-paper-matrix being sparse for collaborative filtering based approaches. Shahid et al. [ 92 ] also mention this problem as the reduce coverage problem . This trait makes it hard for approaches to learn relevancy of infrequently rated papers.
Again, while this problem is still encountered, current approaches mostly are no longer pure collaborative filtering-based systems but instead utilise more information (see Sect. 3.3.1 ). Using deep learning in the recommendation process might reduce the impact of this problem [ 58 ].
Scalability
The problem of scalability was described by Bai et al. [ 9 ]. They state paper recommendation systems should be able to work in huge, ever expanding environments where new users and papers are added regularly.
A few approaches [ 38 , 46 , 88 , 109 ] contain a web crawling step which directly tackles challenges related to outdated or missing data. Some approaches [ 26 , 61 ] evaluate the time it takes to compute paper recommendations which also indicates their focus on this general problem. But most times scalability is not explicitly mentioned by current paper recommendation systems. There are several works [ 42 , 45 , 96 , 108 , 116 ] evaluating on bigger datasets with over 1 million papers and which thus are able to handle big amounts of data. Sizes of current relevant real-world data collections exceed this threshold many times over (see, e.g. PubMed with over 33 million papers 29 or SemanticScholar with over 203 million papers 30 ). Kanakia et al. [ 45 ] explicitly state scalability as a problem their approach is able to overcome. Instead of comparing each paper to all other papers they utilise clustering to reduce the number of required computations. They present the only approach running on several hundred million publications. Nair et al. [ 71 ] mention scalability issues they encountered even when only considering around 25,000 publications and their citation relations.
The problem of privacy in personalised paper recommendation is described by Bai et al. [ 9 ]. Shahid et al. [ 92 ] also mention this as a problem occurring in collaborative filtering approaches. An issue is encountered when sensitive information such as habits or weaknesses that users might not want to disclose is used by a system. This leads to users’ having negative impressions of systems. Keeping sensitive information private should therefore be a main goal.
In the current approaches, we did not find a discussion of privacy concerns. Some approach even explicitly utilise likes [ 84 ] or association rules [ 3 ] of other users while failing to mention privacy altogether. In approaches not incorporating any user data, this issue does not arise at all.
Serendipity
Serendipity is described by Bai et al. [ 9 ] as an attribute often encountered in collaborative filtering [ 16 ]. Usually paper recommender systems focus on identification of relevant papers even though also including not obviously relevant ones might enhance the overall recommendation. Junior researchers could profit from stray recommendations to broaden their horizon, senior researchers might be able to gain knowledge to enhance their research. The ratio between clearly relevant and serendipitous papers is crucial to prevent users from losing trust in the recommender system.
A main objective of the works of Nishioka et al. [ 74 – 76 ] is serendipity. Other approaches do not mention this aspect.
Unified scholarly data standards
Different data formats of data collections is mentioned as a problem by Bai et al. [ 9 ]. They mention digital libraries containing relevant information which needs to be unified in order to use the data in a paper recommendation system. Additionally the combination of datasets could also lead to problems.
Many of the approaches we observe do not consider data collection or preparation as part of the approach, they often only mention the combination of different datasets as part of the evaluation (see e.g. Du et al. [ 29 ], Li et al. [ 56 ] or Xie et al. [ 115 ]). An exception to this general rule are systems which contain a web crawling step for data (see e.g. Ahmad and Afzal [ 2 ] or Sakib et al. [ 88 ]). Even with this type of approaches the combination of datasets and their diverse data formats is not identified as a problem.
Shahid et al. [ 92 ] describe the problem of synonymy encountered in collaborative filtering approaches. They define this problem as different words having the same meaning.
Even though there are still approaches (not necessarily CF ones) utilising basic TF-IDF representations of papers [ 2 , 42 , 86 , 95 ], nowadays this problem can be bypassed by using a text embedding method such as Doc2Vec or BERT.
Gray sheep is a problem described by Shahid et al. [ 92 ] as an issue encountered in collaborative filtering approaches. They describe it as some users not consistently (dis)agreeing with any reference group.
We did not find any current approach mentioning this problem.
Black sheep
Black sheep is a problem described by Shahid et al. [ 92 ] as an issue encountered in collaborative filtering approaches. They describe it as some users not (dis)agree-ing with any reference group.
Shilling attack
Shilling attacks are described by Shahid et al. [ 92 ] as a problem encountered in collaborative filtering approaches. They define this problem as users being able to manually enhance visibility of their own research by rating authored papers as relevant while negatively rating any other recommendations.
Although we did not find any current approach mentioning this problem we assume maybe it is no longer highly relevant as most approaches are no longer pure collaborative filtering ones. Additionally from the considered collaborative filtering approaches no one explicitly stated to feed relevance ratings back into the system.
Emerging challenges
In addition to the open challenges discussed in former literature reviews by Bai et al. [ 9 ], Beel et al. [ 16 ] and Shahid et al. [ 92 ] we identified the following problems and derive desirable goals for future approaches from them.
User evaluation
Paper recommendation is always targeted at human users. But oftentimes an evaluation with real users to quantify users’ satisfaction with recommended publications is simply not conducted [ 84 ]. Conducting huge user studies is not feasible [ 38 ]. So sometimes user data to evaluate with is fetched from the presented datasets [ 39 , 88 ] or user behaviour is artificially emulated [ 1 , 19 , 57 ]. Noteworthy counter-examples 31 are the studies by Bulut et al. [ 22 ] who emailed 50 researchers to rate relevancy of recommended articles or Chaudhuri et al. [ 26 ] who asked 45 participants to rate their acceptance of recommended publications. Another option to overcome this issue is utilisation of living labs as seen with ArXivDigest [ 36 ], Mr. DLib’s living lab [ 14 ] or LiLAS for the related tasks of dataset recommendation for scientific publications and multi-lingual document retrieval [ 91 ].
Desirable goal Paper recommendation systems targeted at users should always contain a user evaluation with a description of the composition of participants.
Target audience
Current works mostly fail to clearly characterise the intended users of a system altogether and the varying interests of different types of users are not examined in their evaluations. There are some noteworthy counter-examples: Afsar et al. [ 1 ] mention cancer patients and their close relatives as intended target audience. Bereczki [ 19 ] identifies new users as a special group they want to recommend papers to. Hua et al. [ 42 ] consider users who start diving into a topic which they have not yet researched before. Sharma et al. [ 95 ] name subject matter experts incorporating articles into a medical knowledge base as their target audience. Shi et al. [ 96 ] clearly state use cases for their approach which always target users which are unaware of a topic but already have one interesting paper from the area. They strive to recommend more papers similar to the first one.
User characteristics such as registration status of users are already mentioned by Beel et al. [ 16 ] as a factor which is disregarded in evaluations. We want to extend on this point and highlight the oftentimes missing or inadequate descriptions of intended users of paper recommendation systems. Traits of users and their information needs are not only important for experiments but should also be regarded in the construction of an approach. The targeted audience of a paper recommendation system should influence its suggestions. Bai et al. [ 9 ] highlight different needs of junior researchers which should be recommended a broad variety of papers as they still have to figure out their direction. They state recommendations for senior researchers should be more in line with their already established interests. Sugiyama and Kan [ 100 ] describe the need to help discover interdisciplinary research for this experienced user group. Most works do not recognise possible different functions of paper recommendation systems for users depending on their level of seniority. If papers include an evaluation with real persons, they e.g. mix Master’s students with professors but do not address their different goals or expectations from paper recommendation [ 74 ]. Chaudhuri et al. [ 26 ] have junior, experienced and expert users as participants of their study and give individual ratings but do not calculate evaluation scores per user group. In some studies the exact composition of test users is not even mentioned (see Table 9 ).
Desirable goal Definition and consideration of a specific target audience for an approach and evaluation with members of this audience. If there is no specific person group a system should suit best, this should be discussed, executed and evaluated accordingly.
Recommendation scenario
Suggested papers from an approach should either be ones to read [ 44 , 109 ], to cite or fulfil another specified information need such as help patients in cancer treatment decision making [ 1 ]. Most work does not clearly state which is the case. Instead recommended papers are only said to be related [ 4 , 28 ], relevant [ 4 , 5 , 26 , 27 , 38 , 42 , 45 , 48 , 56 , 57 , 105 , 115 , 117 ], satisfactory [ 42 , 61 ], suitable [ 21 ], appropriate and useful [ 22 , 88 ] or a description which scenario is tackled is skipped altogether [ 3 , 37 , 39 , 84 ].
In rare cases if the recommendation scenario is mentioned there is the possibility of it not perfectly fitting the evaluated scenario. This can, e.g. be seen in the work of Jing and Yu [ 44 ] where they propose paper recommendation for papers to read but evaluate papers which were cited. Cited papers should always be ones which have been read beforehand but the decision to cite papers can be influenced by multiple aspects [ 34 ].
Desirable goal The clear description of the recommendation scenario is important for comparability of approaches as well as the validity of the evaluation.
Fairness/diversity
Anand et al. [ 8 ] define fairness as the balance between relevance and diversity of recommendation results. Only focusing on fit between the user or input paper and suggestions would lead to highly similar results which might not be vastly different from each other. Having diverse recommendation results can help cover multiple aspects of a user query instead of only satisfying the most prominent feature of the query [ 8 ]. In general more diverse recommendations provide greater utility for users [ 76 ]. Ekstrand et al. [ 31 ] give a detailed overview of current constructs for measuring algorithmic fairness in information access and describe possibly arising problems in this context.
Most of the current paper recommendation systems do not consider fairness but some approaches specifically mention diversity [ 26 , 74 – 76 ] while striving to recommend relevant publications. Thus these systems consider fairness.
Over one fourth of considered approaches with an evaluation report MMR as a measure of their system’s quality. This at least seems to show researchers’ awareness of the general problem of diverse recommendation results.
Desirable Goal Diversification of suggested papers to ensure fairness of the approach.
Paper recommendation systems tend to become more complex, convoluted or composed of multiple parts. We observed this trend by regarding the classification of current systems compared to previous literature reviews (see Sect. 3.3.1 ). While systems’ complexity increases, users’ interaction with the systems should not become more complex. If an approach requires user interaction at all, it should be as simple as possible. Users should not be required to construct sophisticated knowledge graphs [ 109 ] or enter multiple rounds of keywords for an approach to learn their user profile [ 24 ].
Desirable Goal Maintain simplicity of usage even if approaches become more complex.
Explainability
Confidence in the recommendation system has already been mentioned by Beel et al. [ 16 ] as an example of what could enhance users’ satisfaction but what is overlooked in approaches in favour of accuracy. This aspect should be considered with more vigour as the general research area of explainable recommendation has gained immense traction [ 120 ]. Gingstad et al. [ 36 ] regard explainability as a core component of paper recommendation systems. Xie et al. [ 116 ] mention explainability as a key feature of their approach but do not state how they achieve it or if their explanations satisfy users. Suggestions of recommendation systems should be explainable to enhance their trustworthiness and make them more engaging [ 66 ]. Here, different explanation goals such as effectiveness, efficiency, transparency or trust and their influence on each other should be considered [ 10 ]. If an approach uses neural networks [ 24 , 37 , 49 , 56 ] it is oftentimes impossible to explain why the system learned, that a specific suggested paper might be relevant.
Lee et al. [ 51 ] introduce a general approach which could be applied to any paper recommendation system to generate explanations for recommendations. Even though this option seems to help solve the described problem it is not clear how valuable post-hoc explanations are compared to systems which construct them directly.
Desirable Goal The conceptualisation of recommendation systems which comprehensibly explain their users why a specific paper is suggested.
Public dataset
Current approaches utilise many different datasets (see Table 4 ). A large portion of them are built by the authors such that they are not publicly available for others to use as well [ 1 , 30 , 111 ]. Part of the approaches already use open datasets in their evaluation but a large portion still does not seem to regard this as a priority (see Table 5 ). Utilisation of already public data sources or construction of datasets which are also published and remain available thus should be a priority in order to support reproducibility of approaches.
Desirable Goal Utilisation of publicly available datasets in the evaluation of paper recommendation systems.
Comparability
From the approaches we observed, many identified themselves as paper recommendation ones but only evaluated against systems, which are more general recommendation systems or ones utilising some same methodologies but not from the sub-domain of paper recommendation (seen with e.g. Guo et al [ 37 ], Tanner et al. [ 106 ] or Yang et al. [ 117 ]). While some of the works might claim to only be applied on paper recommendation and be of more general applicability (see, e.g. the works by Ahmedi et al. [ 3 ] or Alfarhood and Cheng [ 4 ]) we state that they should still be compared to ones, which mainly identify as paper recommendation systems as seen in the work of Chaudhuri et al. [ 24 ]. Only if a more general approach is compared to a paper recommendation approach, its usefulness for the area of paper recommendation can be fully assessed.
Several times, the baselines to evaluate against are not even other works but artificially constructed ones [ 2 , 38 ] or no other approach at all [ 22 ].
Desirable Goal Evaluation of paper recommendation approaches, even those which are applicable in a wider context, should always be against at least one paper recommendation system to clearly report relevance of the proposed method in the claimed context.
Discussion and outlook
From the already existing problems, several of them are still encountered in current paper recommendation approaches. Users are not always part of the approaches so users are not always modelled but this also prevents privacy issues. Accuracy seems to still be the main focus of recommendation systems. Novel techniques proposed in papers are not available online or applied by existing paper recommendation systems. Approaches do not provide enough details to enable re-implementation. Providing the code online or in a living lab environment could help overcome many of these issues.
Other problems mainly encountered in pure collaborative filtering systems such as the cold start problem, sparsity, synonymy, gray sheep, black sheep and shilling attacks do not seem to be as relevant anymore. We observed a trend towards hybrid models, this recommendation system type can overcome these issues. These hybrid models should also be able to produce serendipitous recommendations.
Unifying data sources is conducted often but nowadays it does not seem to be regarded as a problem. With scalability we encountered the same. Approaches are oftentimes able to handle millions of papers, here they do not specifically mention scalability as a problem they overcome but they also mostly do not consider huge datasets with several hundreds of millions of publications.
Due to the limited scope of our survey we are not able to derive substantive claims regarding cooperation and persistence. We found around 30% of approaches published by groups which authored multiple papers and very few collaborations between different author groups.
As for the newly introduced problems, part of the observed approaches conducted evaluations with users, on publicly available datasets and against other paper recommendation systems. Many works considered a low complexity for users. Even though user evaluations are desirable, they come with high costs. Usage of evaluation datasets with real human annotations could help overcome this issue partially, another straightforward solution would be the incorporation in a living lab. The second option would also help with comparability of approaches. Usage of available datasets can become increasingly complicated if approaches use new data which is currently not contained in existing datasets. 32
Target audiences in general were rarely defined, the recommendation scenario was mostly not described. Diversity was considered by few. Overall the explainability of recommendations was dismissed. The first two of these issues are ones which could be comparatively easily fixed or addressed in the papers without changing the approach. As for diversity and explainability, the approaches would need to be modelled specifically such that these attributes could be satisfied.
To conclude, there are many challenges which are not constantly considered by current approaches. They define the requirements for future works in the area of paper recommendation systems.
This literature review of publications targeting paper recommendation between January 2019 and October 2021 provided comprehensive overviews of their methods, datasets and evaluation measures. We showed the need for a richer multi-dimensional characterisation of paper recommendation as former ones no longer seem sufficient in classifying the increasingly complex approaches. We also revisited known open challenges in the current time frame and highlighted possibly under-observed problems which future works could focus on.
Efforts should be made to standardise or better differentiate between the varying notions of relevancy and recommendation scenarios when it comes to paper recommendation. Future work could try revaluate already existing methods with real humans and against other paper recommendation systems. This could for example be realised in an extendable paper recommendation benchmarking system similar to the in a living lab environments ArXivDigest [ 36 ], Mr. DLib’s living lab [ 14 ] or LiLAS [ 91 ] but with the additional property that it also provides build-in offline evaluations. As fairness and explainability of current paper recommendation systems have not been tackled widely, those aspects should be further explored. Another direction could be the comparison of multiple rare evaluation measures on the same system to help identify those which should be focused on in the future. As we observed a vast variety in datasets utilised for evaluation of the approaches (see Table 4 ), construction of publicly available and widely reusable ones would be worthwhile.
Funding Information
Open Access funding enabled and organized by Projekt DEAL.
1 The most recent surveys [ 9 , 58 , 92 ] focusing on scientific paper recommendation appeared in 2019 such that this time frame is not yet covered.
2 Non-immediate variants allow using methods which require more time to compute recommendations. Temporal patterns of user behaviour could be incorporated in the recommendation process to identify a fitting moment to present new recommendations to a user. The moment a recommendation is presented to a user influences their interest, as the delayed recommendation might no longer be relevant or does not fit the current task of a user.
3 https://dl.acm.org/ .
4 https://dblp.uni-trier.de/ .
5 https://scholar.google.com/ .
6 https://link.springer.com/ .
7 For a survey of current trends in citation recommendation refer to Färber and Jatowt [ 32 ].
8 These papers could either be a demo paper and a later published full paper or the conference and journal version of the same approach, which is then slightly extended by more experiments. These paper clusters are no exact duplicates or fraudulent publications.
9 The number of citations can be regarded both as an input data as well as a method to denote popularity.
10 https://dblp.uni-trier.de/xml/ .
11 https://www.aminer.org/citation .
12 (shortened) http://shorturl.at/cIQR1 .
13 https://github.com/js05212/citeulike-a .
14 https://github.com/js05212/citeulike-t .
15 https://dl.acm.org/ .
16 https://www.scopus.com/home.uri .
17 https://www.aminer.org/ .
18 https://aan.how/download/ .
19 https://citeseerx.ist.psu.edu/index .
20 https://bulkdata.uspto.gov/ .
21 https://snap.stanford.edu/data/cit-HepTh.html .
22 (shortened) http://shorturl.at/orwXY .
23 http://mlg.ucd.ie/datasets/bbc.html .
24 https://sites.google.com/site/tinhuynhuit/dataset .
25 One approach is described in three papers.
26 Shi et al. [ 96 ] also conduct a user study but do not describe their participants.
27 Compare the 99.363 journal articles and 151.617 conference papers published in 2013 to the 187.263 journal articles and 157.460 conference articles in 2021 in dblp.
28 Note that not all approaches classified their type of paper recommendation and several papers did not classify themselves in the wide-spread categorisation (see Sect. 3.3.1 ).
29 https://pubmed.ncbi.nlm.nih.gov/ .
30 https://www.semanticscholar.org/product/api .
31 For a full list of approaches conducting user studies see Table 9 .
32 We did not encounter many papers utilising types of data as part of their approach, which is not typically included in existing datasets; one of the noteworthy exceptions could be the approach by Nishioka et al. [ 74 – 76 ], which utilised Tweets of users.
Publisher's Note
Springer Nature remains neutral with regard to jurisdictional claims in published maps and institutional affiliations.
Contributor Information
Christin Katharina Kreutz, Email: [email protected] .
Ralf Schenkel, Email: ed.reirt-inu@leknehcs .
Log in using your username and password
- Search More Search for this keyword Advanced search
- Latest content
- Current issue
- BMJ Journals More You are viewing from: Google Indexer
You are here
- Volume 33, Issue 5
- Equitable and accessible informed healthcare consent process for people with intellectual disability: a systematic literature review
- Article Text
- Article info
- Citation Tools
- Rapid Responses
- Article metrics

- http://orcid.org/0000-0002-8498-7329 Manjekah Dunn 1 , 2 ,
- Iva Strnadová 3 , 4 , 5 ,
- Jackie Leach Scully 4 ,
- Jennifer Hansen 3 ,
- Julie Loblinzk 3 , 5 ,
- Skie Sarfaraz 5 ,
- Chloe Molnar 1 ,
- Elizabeth Emma Palmer 1 , 2
- 1 Faculty of Medicine & Health , University of New South Wales , Sydney , New South Wales , Australia
- 2 The Sydney Children's Hospitals Network , Sydney , New South Wales , Australia
- 3 School of Education , University of New South Wales , Sydney , New South Wales , Australia
- 4 Disability Innovation Institute , University of New South Wales , Sydney , New South Wales , Australia
- 5 Self Advocacy Sydney , Sydney , New South Wales , Australia
- Correspondence to Dr Manjekah Dunn, Paediatrics & Child Health, University of New South Wales Medicine & Health, Sydney, New South Wales, Australia; manjekah.dunn{at}unsw.edu.au
Objective To identify factors acting as barriers or enablers to the process of healthcare consent for people with intellectual disability and to understand how to make this process equitable and accessible.
Data sources Databases: Embase, MEDLINE, PsychINFO, PubMed, SCOPUS, Web of Science and CINAHL. Additional articles were obtained from an ancestral search and hand-searching three journals.
Eligibility criteria Peer-reviewed original research about the consent process for healthcare interventions, published after 1990, involving adult participants with intellectual disability.
Synthesis of results Inductive thematic analysis was used to identify factors affecting informed consent. The findings were reviewed by co-researchers with intellectual disability to ensure they reflected lived experiences, and an easy read summary was created.
Results Twenty-three studies were included (1999 to 2020), with a mix of qualitative (n=14), quantitative (n=6) and mixed-methods (n=3) studies. Participant numbers ranged from 9 to 604 people (median 21) and included people with intellectual disability, health professionals, carers and support people, and others working with people with intellectual disability. Six themes were identified: (1) health professionals’ attitudes and lack of education, (2) inadequate accessible health information, (3) involvement of support people, (4) systemic constraints, (5) person-centred informed consent and (6) effective communication between health professionals and patients. Themes were barriers (themes 1, 2 and 4), enablers (themes 5 and 6) or both (theme 3).
Conclusions Multiple reasons contribute to poor consent practices for people with intellectual disability in current health systems. Recommendations include addressing health professionals’ attitudes and lack of education in informed consent with clinician training, the co-production of accessible information resources and further inclusive research into informed consent for people with intellectual disability.
PROSPERO registration CRD42021290548.
- Decision making
- Healthcare quality improvement
- Patient-centred care
- Quality improvement
- Standards of care
Data availability statement
Data are available upon reasonable request. Additional data and materials such as data collection forms, data extraction and analysis templates and QualSyst assessment data can be obtained by contacting the corresponding author.
This is an open access article distributed in accordance with the Creative Commons Attribution Non Commercial (CC BY-NC 4.0) license, which permits others to distribute, remix, adapt, build upon this work non-commercially, and license their derivative works on different terms, provided the original work is properly cited, appropriate credit is given, any changes made indicated, and the use is non-commercial. See: http://creativecommons.org/licenses/by-nc/4.0/ .
https://doi.org/10.1136/bmjqs-2023-016113
Statistics from Altmetric.com
Request permissions.
If you wish to reuse any or all of this article please use the link below which will take you to the Copyright Clearance Center’s RightsLink service. You will be able to get a quick price and instant permission to reuse the content in many different ways.
What is already known on this topic
People with intellectual disability are frequently excluded from decision-making processes and not provided equal opportunity for informed consent, despite protections outlined in the United Nations Convention on the Rights of Persons with Disabilities.
People with intellectual disability have the capacity and desire to make informed medical decisions, which can improve their well-being, health satisfaction and health outcomes.
What this review study adds
Health professionals lack adequate training in valid informed consent and making reasonable adjustments for people with intellectual disability, and continue to perpetuate assumptions of incapacity.
Health information provided to people with intellectual disability is often inaccessible and insufficient for them to make informed decisions about healthcare.
The role of support people, systemic constraints, a person-centred approach and ineffective healthcare communication also affect informed consent.
How this review might affect research, practice or policy
Health professionals need additional training on how to provide a valid informed consent process for people with intellectual disability, specifically in using accessible health information, making reasonable adjustments (e.g., longer/multiple appointments, options of a support person attending or not, using plain English), involving the individual in discussions, and communicating effectively with them.
Inclusive research is needed to hear the voices and opinions of people with intellectual disability about healthcare decision-making and about informed consent practices in specific healthcare settings.
Introduction
Approximately 1% of the world’s population have intellectual disability. 1 Intellectual disability is medically defined as a group of neurodevelopmental conditions beginning in childhood, with below average cognitive functioning and adaptive behaviour, including limitations in conceptual, social and practical skills. 2 People with intellectual disability prefer an alternative strength-based definition, reflected in the comment by Robert Strike OAM (Order of Australia Medal): ‘We can learn if the way of teaching matches how the person learns’, 3 reinforcing the importance of providing information tailored to the needs of a person with intellectual disability. A diagnosis of intellectual disability is associated with significant disparities in health outcomes. 4–7 Person-centred decision-making and better communication have been shown to improve patient satisfaction, 8 9 the physician–patient relationship 10 and overall health outcomes 11 for the wider population. Ensuring people with intellectual disability experience informed decision-making and accessible healthcare can help address the ongoing health disparities and facilitate equal access to healthcare.
Bodily autonomy is an individual’s power and agency to make decisions about their own body. 12 Informed consent for healthcare enables a person to practice bodily autonomy and is protected, for example, by the National Safety and Quality Health Service Standards (Australia), 13 Mental Capacity Act (UK) 14 and the Joint Commission Standards (USA). 15 In this article, we define informed consent according to three requirements: (1) the person is provided with information they understand, (2) the decision is free of coercion and (3) the person must have capacity. 16 For informed consent to be valid, this process must be suited to the individual’s needs so that they can understand and communicate effectively. Capacity is the ability to give informed consent for a medical intervention, 17 18 and the Mental Capacity Act outlines that ‘a person must be assumed to have capacity unless it is established that he lacks capacity’ and that incapacity can only be established if ‘all practicable steps’ to support capacity have been attempted without success. 14 These assumptions of capacity are also decision-specific, meaning an individual’s ability to consent can change depending on the situation, the choice itself and other factors. 17
Systemic issues with healthcare delivery systems have resulted in access barriers for people with intellectual disability, 19 despite the disability discrimination legislation in many countries who are signatories to the United Nations (UN) Convention on the Rights of Persons with Disabilities. 20 Patients with intellectual disability are not provided the reasonable adjustments that would enable them to give informed consent for medical procedures or interventions, 21 22 despite evidence that many people with intellectual disability have both the capacity and the desire to make their own healthcare decisions. 21 23
To support people with intellectual disability to make independent health decisions, an equitable and accessible informed consent process is needed. 24 However, current health systems have consistently failed to provide this. 21 25 To address this gap, we must first understand the factors that contribute to inequitable and inaccessible consent. To the best of our knowledge, the only current review of informed consent for people with intellectual disability is an integrative review by Goldsmith et al . 26 Many of the included articles focused on assessment of capacity 27–29 and research consent. 30–32 The review’s conclusion supported the functional approach to assess capacity, with minimal focus on how the informed consent processes can be improved. More recently, there has been a move towards ensuring that the consent process is accessible for all individuals, including elderly patients 33 and people with aphasia. 34 However, there remains a paucity of literature about the informed consent process for people with intellectual disability, with no systematic reviews summarising the factors influencing the healthcare consent process for people with intellectual disability.
To identify barriers to and enablers of the informed healthcare consent process for people with intellectual disability, and to understand how this can be made equitable and accessible.
A systematic literature review was conducted following the Preferred Reporting Items for Systematic Reviews and Meta-Analysis Protocols (PRISMA-P) systematic literature review protocol. 35 The PRISMA 2020 checklist 36 and ENhancing Transparency in REporting the synthesis of Qualitative research (ENTREQ) reporting guidelines were also followed. 37 The full study protocol is included in online supplemental appendix 1 .
Supplemental material
No patients or members of the public were involved in this research for this manuscript.
Search strategy
A search strategy was developed to identify articles about intellectual disability, consent and healthcare interventions, described in online supplemental appendix 2 . Multiple databases were searched for articles published between January 1990 to January 2022 (Embase, MEDLINE, PsychINFO, PubMed, SCOPUS, Web of Science and CINAHL). These databases include healthcare and psychology databases that best capture relevant literature on this topic, including medical, nursing, social sciences and bioethical literature. The search was limited to studies published from 1990 as understandings of consent have changed since then. 38 39 This yielded 4853 unique papers which were imported into Covidence, a specialised programme for conducting systematic reviews. 40
Study selection
Citation screening by abstract and titles was completed by two independent researchers (MD and EEP). Included articles had to:
Examine the informed consent process for a healthcare intervention for people with intellectual disability.
Have collected more than 50% of its data from relevant stakeholders, including adults with intellectual disability, families or carers of a person with intellectual disability, and professionals who engage with people with intellectual disability.
Report empirical data from primary research methodology.
Be published in a peer-reviewed journal after January 1990.
Be available in English.
Full text screening was completed by two independent researchers (MD and EEP). Articles were excluded if consent was only briefly discussed or if it focused on consent for research, capacity assessment, or participant knowledge or comprehension. Any conflicts were resolved through discussion with an independent third researcher (IS).
Additional studies were identified through an ancestral search and by hand-searching three major journals relevant to intellectual disability research. Journals were selected if they had published more than one included article for this review or in previous literature reviews conducted by the research team.
Quality assessment
Two independent researchers (MD and IS) assessed study quality with the QualSyst tool, 41 which can assess both qualitative and quantitative research papers. After evaluating the distribution of scores, a threshold value of 55% was used, as suggested by QualSyst 41 to exclude poor-quality studies but capture enough studies overall. Any conflicts between the quality assessment scores were resolved by a third researcher (EEP). For mixed-method studies, both qualitative and quantitative quality scores were calculated, and the higher value used.
Data collection
Two independent researchers (MD and JH) reviewed each study and extracted relevant details, including study size, participant demographics, year, country of publication, study design, data analysis and major outcomes reported. Researchers used standardised data collection forms designed, with input from senior researchers with expertise in qualitative research (IS and EEP), to extract data relevant to the review’s research aims. The form was piloted on one study, and a second iteration made based on feedback. These forms captured data on study design, methods, participants, any factors affecting the process of informed consent and study limitations. Data included descriptions and paragraphs outlining key findings, the healthcare context, verbatim participant quotes and any quantitative analyses or statistics. Missing or unclear data were noted.
Data analysis
A pilot literature search showed significant heterogeneity in methodology of studies, limiting the applicability of traditional quantitative analysis (ie, meta-analysis). Instead, inductive thematic analysis was chosen as an alternative methodology 42 43 that has been used in recent systematic reviews examining barriers and enablers of other health processes. 44 45 The six-phase approach described by Braun and Clarke was used. 46 47 A researcher (MD) independently coded the extracted data of each study line-by-line, with subsequent data grouped into pre-existing codes or new concepts when necessary. Codes were reviewed iteratively and grouped into categories, subthemes and themes framed around the research question. Another independent researcher (JH) collated and analysed the data on study demographics, methods and limitations. The themes were reviewed by two senior researchers (EEP and IS).
Qualitative methods of effect size calculations have been described in the literature, 48 49 which was captured in this review by the number of studies that identified each subtheme, with an assigned frequency rating to compare their relative significance. Subthemes were given a frequency rating of A, B, C or D if they were identified by >10, 7–9, 4–6 or <3 articles, respectively. The overall significance of each theme was estimated by the number of studies that mentioned it and the GRADE framework, a stepwise approach to quality assessment using a four-tier rating system. Each study was evaluated for risk of bias, inconsistency, indirectness, imprecision and publication bias. 50 51 Study sensitivity was assessed by counting the number of distinct subthemes included. 52 The quality of findings was designated high, moderate or low depending on the frequency ratings, the QualSyst score and the GRADE scores of studies supporting the finding. Finally, the relative contributions of each study were evaluated by the number of subthemes described, guided by previously reported methods for qualitative reviews. 52
Co-research
The findings were reviewed by two co-researchers with intellectual disability (JL and SS), with over 30 years combined experience as members and employees of a self-advocacy organisation. Guidance on the findings and an easy read summary was produced in line with best-practice inclusive research 53 54 over multiple discussions. Input from two health professional researchers (MD and EEP) provided data triangulation and sense-checking of findings.
Twenty-three articles were identified ( figure 1 ): 14 qualitative, 6 quantitative and 3 mixed-methods. Two papers included the same population of study participants: McCarthy 55 and McCarthy, 56 but had different research questions. Fovargue et al 57 was excluded due to a quality score of 35%. Common quality limitations were a lack of verification procedures to establish credibility and limited researcher reflexivity. No studies were excluded due to language requirements (as all were in English) or age restrictions (all studies had majority adult participants).
- Download figure
- Open in new tab
- Download powerpoint
PRISMA 2020 flowchart for the systematic review. 36
Studies were published from 1999 to 2020 and involved participant populations from the UK (n=18), USA (n=3), Sweden (n=1) and Ireland (n=1). Participant numbers ranged from 9 to 604 (median 21), and participants included people with intellectual disability (n=817), health professionals (n=272), carers and support people (n=48), and other professionals that work with people with intellectual disability (n=137, community service agency directors, social workers, administrative staff and care home staff). Ages of participants ranged from 8 to 84 years, though only Aman et al 58 included participants <18 years of age. This study was included as the article states very few children were included. Studies examined consent in different contexts, including contraception and sexual health (6/23 articles), 58–60 medications (5/23 articles), 58–62 emergency healthcare, 63 cervical screening, 64 community referrals, 58–61 65 mental health, 66 hydrotherapy, 64 blood collection 67 and broad decision-making consent without a specific context. 65 68–71 A detailed breakdown of each study is included in online supplemental appendix 3 .
Six major themes were identified from the studies, summarised in figure 2 . An overview of included studies showing study sensitivity, effect size, QualSyst and GRADE scores is given in online supplemental appendix 4 . Studies with higher QualSyst and GRADE scores contributed more to this review’s findings and tended to include more subthemes; specifically, Rogers et al , 66 Sowney and Barr, 63 Höglund and Larsson, 72 and McCarthy 55 and McCarthy. 56 Figure 3 gives the easy read version of theme 1, with the full easy read summary in online supplemental appendix 5 .
Summary of the identified six themes and subthemes.
Theme 1 of the easy read summary.
Theme 1—Health professionals’ attitudes and lack of education about informed consent
Health professionals’ attitudes and practices were frequently (18/21) identified as factors affecting the informed consent process, with substantial evidence supporting this theme. Studies noted the lack of training for health professionals in supporting informed consent for people with intellectual disability, their desire for further education, and stereotypes and discrimination perpetuated by health professionals.
Lack of health professional education on informed consent and disability discrimination legislation
Multiple studies reported inconsistent informed consent practices, for various reasons: some reported that health professionals ‘forgot’ to or ‘did not realise consent was necessary’, 63 73 but inconsistent consent practices were also attributed to healthcare providers’ unfamiliarity with consent guidelines and poor education on this topic. Carlson et al 73 reported that only 44% of general practitioners (GPs) were aware of consent guidelines, and there was the misconception that consent was unnecessary for people with intellectual disability. Similarly, studies of psychologists 66 and nurses 63 found that many were unfamiliar with their obligations to obtain consent, despite the existence of anti-discrimination legislation. People with intellectual disability describe feeling discriminated against by health professionals, reflected in comments such as ‘I can tell, my doctor just thinks I’m stupid – I'm nothing to him’. 74 Poor consent practices by health professionals were observed in Goldsmith et al , 67 while health professionals surveyed by McCarthy 56 were unaware of their responsibility to provide accessible health information to women with intellectual disability. Improving health professional education and training was suggested by multiple studies as a way to remove this barrier. 63 65–67 69 73
Lack of training on best practices for health professions caring for people with intellectual disability
A lack of training in caring for and communicating with people with intellectual disability was also described by midwives, 72 psychologists, 66 nurses, 63 pharmacists 61 and GPs. 56 72 75 Health professionals lacked knowledge about best practice approaches to providing equitable healthcare consent processes through reasonable adjustments such as accessible health information, 56 60 66 longer appointments times, 60 72 simple English 62 67 and flexible approaches to patient needs. 63 72
Health professionals’ stereotyping and assumptions of incapacity
Underlying stereotypes contributed to some health professionals’ (including nurses, 63 GPs 56 and physiotherapists 64 ) belief that people with intellectual disability lack capacity and therefore, do not require opportunities for informed consent. 56 64 In a survey of professionals referring people with intellectual disability to a disability service, the second most common reason for not obtaining consent was ‘patient unable to understand’. 73
Proxy consent as an inappropriate alternative
People with intellectual disability are rarely the final decision-maker in their medical choices, with many health providers seeking proxy consent from carers, support workers and family members, despite its legal invalidity. In McCarthy’s study (2010), 18/23 women with intellectual disability said the decision to start contraception was made by someone else. Many GPs appeared unaware that proxy consent is invalid in the UK. 56 Similar reports came from people with intellectual disability, 55 56 60 64 69 76 health professionals (nurses, doctors, allied health, psychologists), 56 63 64 66 77 support people 64 77 and non-medical professionals, 65 73 and capacity was rarely documented. 56 62 77
Exclusion of people with intellectual disability from decision-making discussions
Studies described instances where health professionals made decisions for their patients with intellectual disability or coerced patients into a choice. 55 72 74 76 77 In Ledger et al 77 , only 62% of women with intellectual disability were involved in the discussion about contraception, and only 38% made the final decision, and others stated in Wiseman and Ferrie 74 : ‘I was not given the opportunity to explore the different options. I was told what one I should take’. Three papers outlined instances where the choices of people with intellectual disability were ignored despite possessing capacity 65 66 69 and when a procedure continued despite them withdrawing consent. 69
Theme 2—Inadequate accessible health information
Lack of accessible health information.
The lack of accessible health information was the most frequently identified subtheme (16/23 studies). Some studies reported that health professionals provided information to carers instead, 60 avoided providing easy read information due to concerns about ‘offending’ patients 75 or only provided verbal information. 56 67 Informed consent was supported when health professionals recognised the importance of providing medical information 64 and when it was provided in an accessible format. 60 Alternative approaches to health information were explored, including virtual reality 68 and in-person education sessions, 59 with varying results. Overall, the need to provide information in different formats tailored to an individual’s communication needs, rather than a ‘one size fits all’ approach, was emphasised by both people with intellectual disability 60 and health professionals. 66
Insufficient information provided
Studies described situations where insufficient information was provided to people with intellectual disability to make informed decisions. For example, some people felt the information from their GP was often too basic to be helpful (Fish et al 60 ) and wanted additional information on consent forms (Rose et al 78 ).
Theme 3—The involvement of support people
Support people (including carers, family members and group home staff) were identified in 11 articles as both enablers of and barriers to informed consent. The antagonistic nature of these findings and lower frequency of subthemes are reflected in the lower quality assessments of evidence.
Support people facilitated communication with health professionals
Some studies reported carers bridging communication barriers with health to support informed consent. 63 64 McCarthy 56 found 21/23 of women with intellectual disability preferred to see doctors with a support person due to perceived benefits: ‘Sometimes I don’t understand it, so they have to explain it to my carer, so they can explain it to me easier’. Most GPs in this study (93%) also agreed that support people aided communication.
Support people helped people with intellectual disability make decisions
By advocating for people with intellectual disability, carers encouraged decision-making, 64 74 provided health information, 74 77 emotional support 76 and assisted with reading or remembering health information. 55 58 76 Some people with intellectual disability explicitly appreciated their support person’s involvement, 60 such as in McCarthy’s 55 study where 18/23 participants felt supported and safer when a support person was involved.
Support people impeded individual autonomy
The study by Wiseman and Ferrie 74 found that while younger participants with intellectual disability felt family members empowered their decision-making, older women felt family members impaired their ability to give informed consent. This was reflected in interviews with carers who questioned the capacity of the person with intellectual disability they supported and stated they would guide them to pick the ‘best choice’ or even over-ride their choices. 64 Studies of psychologists and community service directors described instances where the decision of family or carers was prioritised over the wishes of the person with intellectual disability. 65 66 Some women with intellectual disability in McCarthy’s studies (2010, 2009) 55 56 appeared to have been coerced into using contraception by parental pressures or fear of losing group home support.
Theme 4—Systemic constraints within healthcare systems
Time restraints affect informed consent and accessible healthcare.
Resource limitations create time constraints that impair the consent process and have been identified as a barrier by psychologists, 66 GPs, 56 hospital nurses 63 and community disability workers. 73 Rogers et al 66 highlighted that a personalised approach that could improve informed decision-making is restricted by inflexible medical models. Only two studies described flexible patient-centred approaches to consent. 60 72 A survey of primary care practices in 2007 reported that most did not modify their cervical screening information for patients with intellectual disability because it was not practical. 75
Inflexible models of consent
Both people with intellectual disability 76 and health professionals 66 recognised that consent is traditionally obtained through one-off interactions prior to an intervention. Yet, for people with intellectual disability, consent should ideally be an ongoing process that begins before an appointment and continues between subsequent ones. Other studies have tended to describe one-off interactions where decision-making was not revisited at subsequent appointments. 56 60 72 76
Lack of systemic supports
In one survey, self-advocates highlighted a lack of information on medication for people with intellectual disability and suggested a telephone helpline and a centralised source of information to support consent. 60 Health professionals also want greater systemic support, such as a health professional specialised in intellectual disability care to support other staff, 72 or a pharmacist specifically to help patients with intellectual disability. 61 Studies highlighted a lack of guidelines about healthcare needs of people with intellectual disabilities such as contraceptive counselling 72 or primary care. 75
Theme 5—Person-centred informed consent
Ten studies identified factors related to a person-centred approach to informed consent, grouped below into three subthemes. Health professionals should tailor their practice when obtaining informed consent from people with intellectual disability by considering how these subthemes relate to the individual. Each subtheme was described five times in the literature with a relative frequency rating of ‘C’, contributing to overall lower quality scores.
Previous experience with decision-making
Arscott et al 71 found that the ability of people with intellectual disability to consent changed with their verbal and memory skills and in different clinical vignettes, supporting the view of ‘functional’ capacity specific to the context of the medical decision. Although previous experiences with decision-making did not influence informed consent in this paper, other studies suggest that people with intellectual disability accustomed to independent decision-making were more able to make informed medical decisions, 66 70 and those who live independently were more likely to make independent healthcare decisions. 56 Health professionals should be aware that their patients with intellectual disability will have variable experience with decision-making and provide individualised support to meet their needs.
Variable awareness about healthcare rights
Consent processes should be tailored to the health literacy of patients, including emphasising available choices and the option to refuse treatment. In some studies, medical decisions were not presented to people with intellectual disability as a choice, 64 and people with intellectual disability were not informed of their legal right to accessible health information. 56
Power differences and acquiescence
Acquiescence by people with intellectual disability due to common and repeated experiences of trauma—that is, their tendency to agree with suggestions made by carers and health professionals, often to avoid upsetting others—was identified as an ongoing barrier. In McCarthy’s (2009) interviews with women with intellectual disability, some participants implicitly rejected the idea that they might make their own healthcare decisions: ‘They’re the carers, they have responsibility for me’. Others appeared to have made decisions to appease their carers: ‘I have the jab (contraceptive injection) so I can’t be blamed for getting pregnant’. 55 Two studies highlighted that health professionals need to be mindful of power imbalances when discussing consent with people with intellectual disability to ensure the choices are truly autonomous. 61 66
Theme 6—Effective communication between health professionals and patients
Implementation of reasonable adjustments for verbal and written information.
Simple language was always preferred by people with intellectual disability. 60 67 Other communication aids used in decision-making included repetition, short sentences, models, pictures and easy read brochures. 72 Another reasonable adjustment is providing the opportunity to ask questions, which women with intellectual disability in McCarthy’s (2009) study reported did not occur. 55
Tailored communication methods including non-verbal communication
Midwives noted that continuity of care allows them to develop rapport and understand the communication preferences of people with intellectual disability. 72 This is not always possible; for emergency nurses, the lack of background information about patients with intellectual disability made it challenging to understand their communication preferences. 63 The use of non-verbal communication, such as body language, was noted as underutilised 62 66 and people with intellectual disability supported the use of hearing loops, braille and sign language. 60
To the best of our knowledge, this is the first systematic review investigating the barriers and enablers of the informed consent process for healthcare procedures for people with intellectual disability. The integrative review by Goldsmith et al 26 examined capacity assessment and shares only three articles with this systematic review. 69 71 73 Since the 2000s, there has been a paradigm shift in which capacity is no longer considered a fixed ability that only some individuals possess 38 39 but instead as ‘functional’: a flexible ability that changes over time and in different contexts, 79 reflected in Goldsmith’s review. An individual’s capacity can be supported through various measures, including how information is communicated and how the decision-making process is approached. 18 80 By recognising the barriers and enablers identified in this review, physicians can help ensure the consent process for their patients with intellectual disability is both valid and truly informed. This review has highlighted the problems of inaccessible health information, insufficient clinical education on how to make reasonable adjustments and lack of person-centred trauma-informed care.
Recommendations
Health professionals require training in the informed consent process for people with intellectual disability, particularly in effective and respectful communication, reasonable adjustments and trauma-informed care. Reasonable adjustments include offering longer or multiple appointments, using accessible resources (such as easy read information or shared decision-making tools) and allowing patient choices (such as to record a consultation or involve a support person). Co-researchers reported that many people with intellectual disability prefer to go without a support person because they find it difficult to challenge their decisions and feel ignored if the health professional only talks to the support person. People with intellectual disability also feel they cannot seek second opinions before making medical decisions or feel pressured to provide consent, raising the possibility of coercion. These experiences contribute to healthcare trauma. Co-researchers raised the importance of building rapport with the person with intellectual disability and of making reasonable adjustments, such as actively advocating for the person’s autonomy, clearly stating all options including the choice to refuse treatment, providing opportunities to contribute to discussions and multiple appointments to ask questions and understand information. They felt that without these efforts to support consent, health professionals can reinforce traumatic healthcare experiences for people with intellectual disability. Co-researchers noted instances where choices were made by doctors without discussion and where they were only given a choice after requesting one and expressed concern that these barriers are greater for those with higher support needs.
Co-researchers showed how these experiences contributed to mistrust of health professionals and poorer health outcomes. In one situation, a co-researcher was not informed of a medication’s withdrawal effects, resulting in significant side-effects when it was ceased. Many people with intellectual disability describe a poor relationship with their health professionals, finding it difficult to trust health information provided due to previous traumatic experiences of disrespect, coercion, lack of choice and inadequate support. Many feel they cannot speak up due to the power imbalance and fear of retaliation. Poor consent practices and lack of reasonable adjustments directly harm therapeutic alliances by reducing trust, contribute to healthcare trauma and lead to poorer health outcomes for people with intellectual disability.
Additional education and training for health professionals is urgently needed in the areas of informed consent, reasonable adjustments and effective communication with people with intellectual disability. The experiences of health professionals within the research team confirmed that there is limited training in providing high-quality healthcare for people with intellectual disability, including reasonable adjustments and accessible health information. Co-researchers also suggested that education should be provided to carers and support people to help them better advocate for people with intellectual disability.
Health information should be provided in a multimodal format, including written easy read information. Many countries have regulation protecting the right to accessible health information and communication support to make an informed choice, such as UK’s Accessible Information Standard, 81 and Australia’s Charter of Health Care Rights, 24 yet these are rarely observed. Steps to facilitate this include routinely asking patients about information requirements, system alerts for an individual’s needs or routinely providing reasonable adjustments. 82 Co-researchers agreed that there is a lack of accessible health information, particularly about medications, and that diagrams and illustrations are underutilised. There is a critical need for more inclusive and accessible resources to help health professionals support informed consent in a safe and high-quality health system. These resources should be created through methods of inclusive research, such as co-production, actively involving people with intellectual disability in the planning, creation, and feedback process. 53
Strengths and limitations
This systematic review involved two co-researchers with intellectual disability in sense-checking findings and co-creating the easy read summary. Two co-authors who are health professionals provided additional sense-checking of findings from a different stakeholder perspective. In future research, this could be extended by involving people with intellectual disability in the design and planning of the study as per recommendations for best-practice inclusive research. 53 83
The current literature is limited by low use of inclusive research practices in research involving people with intellectual disability, increasing vulnerability to external biases (eg, inaccessible questionnaires, involvement of carers in data collection, overcompliance or acquiescence and absence of researcher reflexivity). Advisory groups or co-research with people with intellectual disability were only used in five studies. 58 60 68 74 76 Other limitations include unclear selection criteria, low sample sizes, missing data, using gatekeepers in patient selection and predominance of UK-based studies—increasing the risk of bias and reducing transferability. Nine studies (out of 15 involving people with intellectual disability) explicitly excluded those with severe or profound intellectual disability, reflecting a selection bias; only one study specifically focused on people with intellectual disability with higher support needs. Studies were limited to a few healthcare contexts, with a focus on consent about sexual health, contraception and medications.
The heterogeneity and qualitative nature of studies made it challenging to apply traditional meta-analysis. However, to promote consistency in qualitative research, the PRISMA and ENTREQ guidelines were followed. 36 37 Although no meta-analyses occurred, the duplication of study populations in McCarthy 2009 and 2010 likely contributed to increased significance of findings reported in both studies. Most included studies (13/23) were published over 10 years ago, reducing the current relevance of this review’s findings. Nonetheless, the major findings reflect underlying systemic issues within the health system, which are unlikely to have been resolved since the articles were published, as the just-released final report of the Australian Royal Commission into Violence, Abuse, Neglect and Exploitation of People with Disability highlights. 84 There is an urgent need for more inclusive studies to explore the recommendations and preferences of people with intellectual disability about healthcare choices.
Informed consent processes for people with intellectual disability should include accessible information and reasonable adjustments, be tailored to individuals’ needs and comply with consent and disability legislation. Resources, guidelines and healthcare education are needed and should cover how to involve carers and support people, address systemic healthcare problems, promote a person-centred approach and ensure effective communication. These resources and future research must use principles of inclusive co-production—involving people with intellectual disability at all stages. Additionally, research is needed on people with higher support needs and in specific contexts where informed consent is vital but under-researched, such as cancer screening, palliative care, prenatal and newborn screening, surgical procedures, genetic medicine and advanced therapeutics such as gene-based therapies.
Ethics statements
Patient consent for publication.
Not applicable.
Ethics approval
- Maulik PK ,
- Mascarenhas MN ,
- Mathers CD , et al
- World Health Organisation
- Council for Intellectual Disability
- Emerson E ,
- Shogren KA ,
- Wehmeyer ML ,
- Reese RM , et al
- Cordasco KM
- Hallock JL ,
- Jordens CFC ,
- McGrath C , et al
- Brenner LH ,
- Brenner AT ,
- United Nations Population Fund
- Australian Commission on Safety and Quality in Health Care
- The Joint Commission
- Beauchamp TL ,
- Childress JF
- New South Wales Attorney General
- United Nations General Assembly
- Strnadová I ,
- Loblinzk J ,
- Scully JL , et al
- MacPhail C ,
- McKay K , et al
- Keywood K ,
- Fovargue S ,
- Goldsmith L ,
- Skirton H ,
- Cash J , et al
- Morris CD ,
- Niederbuhl JM ,
- Arscott K ,
- Fisher CB ,
- Davidson PW , et al
- Giampieri M
- Shamseer L ,
- Clarke M , et al
- McKenzie JE ,
- Bossuyt PM , et al
- Flemming K ,
- McInnes E , et al
- Appelbaum PS
- ↵ Covidence systematic review software . Melbourne, Australia ,
- Proudfoot K
- Papadopoulos I ,
- Koulouglioti C ,
- Lazzarino R , et al
- Onwuegbuzie AJ
- BMJ Best Practice
- Guyatt GH ,
- Vist GE , et al
- Garcia-Lee B
- Brimblecombe J , et al
- Benson BA ,
- Farmer CA , et al
- Ferguson L ,
- Graham YNH ,
- Gerrard D ,
- Laight S , et al
- Huneke NTM ,
- Halder N , et al
- Ferguson M ,
- Jarrett D ,
- McGuire BE , et al
- Woodward V ,
- Jackson L , et al
- Conboy-Hill S ,
- Leafman J ,
- Nehrenz GM , et al
- Höglund B ,
- Carlson T ,
- English S , et al
- Wiseman P ,
- Walmsley J ,
- Tilley E , et al
- Khatkar HS , et al
- Holland AJ , et al
- Beauchamp TL
- England National Health Service
- National Health Service England
- Royal Commission into Violence, Abuse, Neglect and Exploitation of People with Disability
Supplementary materials
Supplementary data.
This web only file has been produced by the BMJ Publishing Group from an electronic file supplied by the author(s) and has not been edited for content.
- Data supplement 1
- Data supplement 2
- Data supplement 3
- Data supplement 4
- Data supplement 5
Contributors MD, EEP and IS conceived the idea for the systematic review. MD drafted the search strategy which was refined by EEP and IS. MD and EEP completed article screening. MD and IS completed quality assessments of included articles. MD and JH completed data extraction. MD drafted the original manuscript. JL and SS were co-researchers who sense-checked findings and were consulted to formulate dissemination plans. JL and SS co-produced the easy read summary with MD, CM, JH, EEP and IS. MD, JLS, EEP and IS reviewed manuscript wording. All authors critically reviewed the manuscript and approved it for publication. The corresponding author attests that all listed authors meet authorship criteria and that no others meeting the criteria have been omitted. MD is the guarantor responsible for the overall content of this manuscript.
Funding This systematic literature review was funded by the National Health & Medical Research Council (NHMRC), Targeted Call for Research (TCR) into Improving health of people with intellectual disability. Research grant title "GeneEQUAL: equitable and accessible genomic healthcare for people with intellectual disability". NHMRC application ID: 2022/GNT2015753.
Competing interests None declared.
Provenance and peer review Not commissioned; externally peer reviewed.
Supplemental material This content has been supplied by the author(s). It has not been vetted by BMJ Publishing Group Limited (BMJ) and may not have been peer-reviewed. Any opinions or recommendations discussed are solely those of the author(s) and are not endorsed by BMJ. BMJ disclaims all liability and responsibility arising from any reliance placed on the content. Where the content includes any translated material, BMJ does not warrant the accuracy and reliability of the translations (including but not limited to local regulations, clinical guidelines, terminology, drug names and drug dosages), and is not responsible for any error and/or omissions arising from translation and adaptation or otherwise.
Linked Articles
- Editorial It is up to healthcare professionals to talk to us in a way that we can understand: informed consent processes in people with an intellectual disability Jonathon Ding Richard Keagan-Bull Irene Tuffrey-Wijne BMJ Quality & Safety 2024; 33 277-279 Published Online First: 30 Jan 2024. doi: 10.1136/bmjqs-2023-016830
Read the full text or download the PDF:
Influence of political tensions on scientific productivity, citation impact, and knowledge combinations
- Published: 23 April 2024
Cite this article
- Moxin Li 1 &
- Yang Wang ORCID: orcid.org/0000-0002-0092-927X 1
49 Accesses
Explore all metrics
Over the past decades, international scientific collaborations have thrived as a vital avenue for generating new knowledge and advancing scientific breakthroughs. However, recent political tensions between the United States and China have raised concerns about potential ramifications on scientific productivity and innovation. While existing research highlighted the adverse effects of these tensions on scientific collaborations, limited attention focused on knowledge combinations. Drawing upon large-scale bibliometric datasets, we conduct a systematic study to examine the effects of the “China Initiative” on Chinese scientists’ productivity, citation impact, and knowledge combinations at the individual level. Firstly, we find the “China Initiative” has shown detrimental effects on scientific productivity and citation impact of Chinese scientists collaborating with U.S. scientists. Moreover, scientists from prestigious Chinese institutions and those with dual affiliations from both countries experienced greater negative impacts from the “China Initiative”. Furthermore, we explore knowledge combination patterns and find that Chinese scientists who collaborated with US scientists published less novel and interdisciplinary papers after the “China Initiative”. Interestingly, we observe a shift in collaborative behaviors, with an increase in the quantity and citations of domestic papers and collaborative papers with countries other than the United States. By shedding light on the influence of the “China Initiative”, our study contributes to the understanding of the complex interplay between political dynamics and scientific progress, highlighting the importance of an open academic environment in an era of geopolitical challenges.
This is a preview of subscription content, log in via an institution to check access.
Access this article
Price includes VAT (Russian Federation)
Instant access to the full article PDF.
Rent this article via DeepDyve
Institutional subscriptions
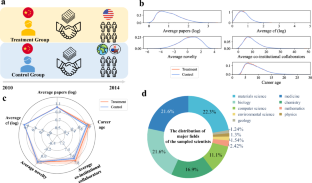
Similar content being viewed by others
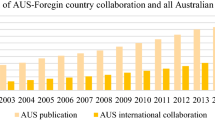
Scientific knowledge production and research collaboration between Australia and South Korea: patterns and dynamics based on co-authorship
Influence of international co-authorship on the research citation impact of young universities.
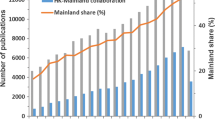
Growing scientific collaboration between Hong Kong and Mainland China since the handover: a 20-year bibliometric analysis
Abramo, G., D’Angelo, C. A., & Di Costa, F. (2019a). The collaboration behavior of top scientists. Scientometrics, 118 (1), 215–232. https://doi.org/10.1007/s11192-018-2970-9
Article Google Scholar
Abramo, G., D’Angelo, C. A., & Di Costa, F. (2019b). A gender analysis of top scientists’ collaboration behavior: Evidence from Italy. Scientometrics, 120 (2), 405–418. https://doi.org/10.1007/s11192-019-03136-6
Abramo, G., D’Angelo, C. A., & Solazzi, M. (2011). The relationship between scientists’ research performance and the degree of internationalization of their research. Scientometrics, 86 , 629–643. https://doi.org/10.1007/s11192-010-0284-7
Adams, J. (2013). The fourth age of research. Nature, 497 (7451), 557–560. https://doi.org/10.1038/497557a
Adams, J. D., Black, G. C., Clemmons, J. R., & Stephan, P. E. (2005). Scientific teams and institutional collaborations: Evidence from U.S. universities, 1981–1999. Research Policy, 34 (3), 259–285. https://doi.org/10.1016/j.respol.2005.01.014
Aghion, P., Antonin, C., Paluskiewicz, L., Stromberg, D., Sun, X., Wargon, R., & Westin, K. (2023). Does Chinese research hinge on US coauthors? Evidence from the China Initiative? CEP discussion papers dp1936 , centre for economic performance, LSE . https://EconPapers.repec.org/RePEc:cep:cepdps:dp1936
Agrawal, A., Kapur, D., McHale, J., & Oettl, A. (2011). Brain drain or brain bank? The impact of skilled emigration on poor-country innovation. Journal of Urban Economics, 69 (1), 43–55. https://doi.org/10.1016/j.jue.2010.06.003
Asubiaro, T. (2019). How collaboration type, publication place, funding and author’s role affect citations received by publications from Africa: A bibliometric study of LIS research from 1996 to 2015. Scientometrics, 120 (3), 1261–1287. https://doi.org/10.1007/s11192-019-03157-1
Bauder, H. (2020). International mobility and social capital in the academic field. Minerva, 58 (3), 367–387. https://doi.org/10.1007/s11024-020-09401-w
Cai, X., Fry, C. V., & Wagner, C. S. (2021). International collaboration during the COVID-19 crisis: Autumn 2020 developments. Scientometrics, 126 (4), 3683–3692. https://doi.org/10.1007/s11192-021-03873-7
Cao, C. (2008). China’s brain drain at the high end: Why government policies have failed to attract first-rate academics to return. Asian Population Studies, 4 (3), 331–345. https://doi.org/10.1080/17441730802496532
Cao, C. (2023). China must draw on internal research strength. Nature, 623 (7986), S14. https://doi.org/10.1038/d41586-023-03445-0
Cao, C., Baas, J., Wagner, C., & Jonkers, K. (2020). Returning scientists and the emergence of China’s science system. Science and Public Policy, 47 , 172–183. https://doi.org/10.1093/scipol/scz056
Chen, K., Zhang, Y., & Fu, X. (2018). International research collaboration: An emerging domain of innovation studies? Research Policy, 48 (1), 149–168. https://doi.org/10.1016/j.respol.2018.08.005
Chinchilla-Rodríguez, Z., Sugimoto, C. R., & Larivière, V. (2019). Follow the leader: On the relationship between leadership and scholarly impact in international collaborations. PLoS ONE, 14 (6), e0218309. https://doi.org/10.1371/journal.pone.0218309
Coccia, M., & Wang, L. (2016). Evolution and convergence of the patterns of international scientific collaboration. Proceedings of the National Academy of Sciences, 113 (8), 2057–2061. https://doi.org/10.1073/pnas.1510820113
Crow, J. M. (2022). US-China partnerships bring strength in numbers to big science projects. Nature, 603 (7900), S6–S8. https://doi.org/10.1038/d41586-022-00570-0
Deem, R., Mok, K. H., & Lucas, L. (2008). Transforming higher education in whose image? Exploring the concept of the ‘World-Class’ university in Europe and Asia. Higher Education Policy, 21 , 83–97. https://doi.org/10.1057/palgrave.hep.8300179
Deichmann, D., Moser, C., Birkholz, J. M., Nerghes, A., Groenewegen, P., & Wang, S. (2020). Ideas with impact: How connectivity shapes idea diffusion. Research Policy, 49 (1), 103881. https://doi.org/10.1016/j.respol.2019.103881
Didegah, F., & Thelwall, M. (2013). Which factors help authors produce the highest impact research? Collaboration, journal and document properties. Journal of Informetrics, 7 , 861–873. https://doi.org/10.1016/j.joi.2013.08.006
Ding, J., Shen, Z., Ahlgren, P., Jeppsson, T., Minguillo, D., & Lyhagen, J. (2021). The link between ethnic diversity and scientific impact: The mediating effect of novelty and audience diversity. Scientometrics, 126 (9), 7759–7810. https://doi.org/10.1007/s11192-021-04071-1
Dusdal, J., & Powell, J. J. W. (2021). Benefits, motivations, and challenges of international collaborative research: A sociology of science case study. Science and Public Policy, 48 (2), 235–245. https://doi.org/10.1093/scipol/scab010
Ellis, P. D., & Zhan, G. (2011). How international are the international business journals? International Business Review, 20 (1), 100–112. https://doi.org/10.1016/j.ibusrev.2010.07.004
Färber, M., & Tampakis, L. (2023). Analyzing the impact of companies on AI research based on publications [Preprint]. arXiv . https://arxiv.org/abs/2310.20444
Fedderke, J. W., & Goldschmidt, M. (2015). Does massive funding support of researchers work?: Evaluating the impact of the South African research chair funding initiative. Research Policy, 44 (2), 467–482. https://doi.org/10.1016/j.respol.2014.09.009
Fleming, L. (2001). Recombinant uncertainty in technological search. Management Science, 47 (1), 117–132. https://doi.org/10.1287/mnsc.47.1.117.10671
Fortunato, S., Bergstrom, C., Borner, K., Evans, J., Helbing, D., Milojevic, S., Petersen, A., Radicchi, F., Sinatra, R., Uzzi, B., Vespignani, A., Waltman, L., Wang, D., & Barabasi, A.-L. (2018). Science of science. Science, 359 (6379), eaao0185. https://doi.org/10.1126/science.aao0185
Foster, J. G., Rzhetsky, A., & Evans, J. A. (2015). Tradition and innovation in scientists’ research strategies. American Sociological Review, 80 (5), 875–908. https://doi.org/10.1177/0003122415601618
Franzoni, C., Giuseppe, S., & Stephan, P. (2011). Changing incentives to publish. Science, 333 (6043), 702–703. https://doi.org/10.1126/science.1197286
Gates, A. J., Ke, Q., Varol, O., & Barabási, A. L. (2019). Nature’s reach: Narrow work has broad impact. Nature, 575 (7781), 32–34. https://doi.org/10.1038/d41586-019-03308-7
Gibson, J., & McKenzie, D. (2014). Scientific mobility and knowledge networks in high emigration countries: Evidence from the Pacific. Research Policy, 43 (9), 1486–1495. https://doi.org/10.1016/j.respol.2014.04.005
Gilbert, N., & Kozlov, M. (2022). The controversial China Initiative is ending—Researchers are relieved. Nature, 603 (7900), 214–215. https://doi.org/10.1038/d41586-022-00555-z
Glänzel, W., & Lange, C. (2002). A distributional approach to multinationality measures of international scientific collaboration. Scientometrics, 54 (1), 75–89. https://doi.org/10.1023/A:1015684505035
Glänzel, W., & Schubert, A. (2001). Double effort = Double impact? A critical view at international co-authorship in chemistry. Scientometrics, 50 (2), 199–214. https://doi.org/10.1023/A:1010561321723
Gök, A., Rigby, J., & Shapira, P. (2015). The impact of research funding on scientific outputs: Evidence from six smaller European countries. Journal of the Association for Information Science and Technology, 67 (3), 715–730. https://doi.org/10.1002/asi.23406
Hainmueller, J. (2012). Entropy balancing for causal effects: A multivariate reweighting method to produce balanced samples in observational studies. Political Analysis, 20 (1), 25–46. https://doi.org/10.1093/pan/mpr025
Herron, P., Mehta, A., Cao, C., & Lenoir, T. (2016). Research diversification and impact: The case of national nanoscience development. Scientometrics, 109 (2), 629–659. https://doi.org/10.1007/s11192-016-2062-7
Hicks, D., Wouters, P., Waltman, L., de Rijcke, S., & Rafols, I. (2015). Bibliometrics: The Leiden Manifesto for research metrics. Nature, 520 (7548), 429–431. https://doi.org/10.1038/520429a
Hottenrott, H., & Lawson, C. (2017). A first look at multiple institutional affiliations: A study of authors in Germany, Japan and the UK. Scientometrics, 111 (1), 285–295. https://doi.org/10.1007/s11192-017-2257-6
Hottenrott, H., & Lawson, C. (2021). What is behind multiple institutional affiliations in academia? Science and Public Policy, 49 (3), 382–402. https://doi.org/10.1093/scipol/scab086
Hottenrott, H., Rose, M., & Lawson, C. (2021). The rise of multiple institutional affiliations in academia. Journal of the Association for Information Science and Technology, 72 (8), 1039–1058. https://doi.org/10.1002/asi.24472
Hug, S., & Brändle, M. (2017). The coverage of Microsoft Academic: Analyzing the publication output of a university. Scientometrics, 113 (3), 1551–1571. https://doi.org/10.1007/s11192-017-2535-3
Jia, R., Roberts, M., Wang, Y., & Yang, E. (2022). The impact of U.S.–China tensions on U.S. science. National Bureau of Economic Research working paper series , no. 29941 . https://doi.org/10.2139/ssrn.4086231
Jiang, L., Zhu, N., Yang, Z., Xu, S., & Jun, M. (2018). The relationships between distance factors and international collaborative research outcomes: A bibliometric examination. Journal of Informetrics, 12 (3), 618–630. https://doi.org/10.1016/j.joi.2018.04.004
Jones, B. F., & Weinberg, B. A. (2011). Age dynamics in scientific creativity. Proceedings of the National Academy of Sciences, 108 (47), 18910–18914. https://doi.org/10.1073/pnas.1102895108
Jones, B. F., Wuchty, S., & Uzzi, B. (2008). Multi-university research teams: Shifting impact, geography, and stratification in science. Science, 322 (5905), 1259–1262. https://doi.org/10.1126/science.1158357
Katz, J., & Martin, B. (1997). What is research collaboration? Research Policy, 26 (1), 1–18. https://doi.org/10.1016/S0048-7333(96)00917-1
Kwiek, M. (2015). The internationalization of research in Europe: A quantitative study of 11 national systems from a micro-level perspective. Journal of Studies in International Education, 19 (4), 341–359. https://doi.org/10.1177/1028315315572898
Lee, J., & Li, X. (2021). Racial profiling among scientists of Chinese descent and consequences for the U.S. Scientific Community . Committee of 100. https://www.committee100.org/wp-content/uploads/2021/10/C100-Lee-Li-White-Paper-FINAL-FINAL-10.28.pdf
Lee, Y. N., Walsh, J., & Wang, J. (2014). Creativity in scientific teams: Unpacking novelty and impact. Research Policy, 44 (3), 684–697. https://doi.org/10.1016/j.respol.2014.10.007
Lester, R., Tsai, L., Berger, S., Fisher, P., Fravel, M., Goldston, D., Huang, Y., & Rus, D. (2023). Managing United States-China university relations and risks. Science, 380 (6642), 246–248. https://doi.org/10.1126/science.adg5619
Leydesdorff, L., & Wagner, C. (2009). International collaboration in science and the formation of a core group. Journal of Informetrics, 2 (4), 317–325. https://doi.org/10.1016/j.joi.2008.07.003
Leydesdorff, L., Wagner, C. S., & Bornmann, L. (2019). Interdisciplinarity as diversity in citation patterns among journals: Rao-Stirling diversity, relative variety, and the Gini coefficient. Journal of Informetrics, 13 (1), 255–269. https://doi.org/10.1016/j.joi.2018.12.006
Li, D., Heimeriks, G., & Alkemade, F. (2020). The emergence of renewable energy technologies at country level: Relatedness, international knowledge spillovers and domestic energy markets. Industry and Innovation, 27 (9), 991–1013. https://doi.org/10.1080/13662716.2020.1713734
Li, W., Zhang, S., Zheng, Z., Cranmer, S., & Clauset, A. (2022). Untangling the network effects of productivity and prominence among scientists. Nature Communications, 13 (1), 4907. https://doi.org/10.1038/s41467-022-32604-6
Lin, Y., Frey, C., & Wu, L. (2022). Remote collaboration fuses fewer breakthrough ideas. Nature, 623 (7989), 987–991. https://doi.org/10.1038/s41586-023-06767-1
Liu, L., Yu, J., Huang, J., Xia, F., & Jia, T. (2020). The dominance of big teams in China’s scientific output. Quantitative Science Studies, 2 (1), 350–362. https://doi.org/10.1162/qss_a_00099
Liu, X., Lu, J., Filatotchev, I., & Buck, T. (2010). Returnee entrepreneurs, knowledge spillovers and innovation in high-tech firms in emerging economies. Journal of International Business Studies, 41 (7), 1183–1197. https://doi.org/10.1057/jibs.2009.50
Luukkonen, T., Persson, O., & Sivertsen, G. (1992). Understanding patterns of international scientific collaboration. Science Technology & Human Values, 17 (1), 101–126. https://doi.org/10.1177/016224399201700106
Lyu, D., Gong, K., Ruan, X., Cheng, Y., & Li, J. (2021). Does research collaboration influence the “disruption” of articles? Evidence from neurosciences. Scientometrics, 126 (1), 287–303. https://doi.org/10.1007/s11192-020-03757-2
Maher, B. S., & Van Noorden, R. (2021). How the COVID pandemic is changing global science collaborations. Nature, 594 (7863), 316–319. https://doi.org/10.1038/d41586-021-01570-2
Manuel, F. E., Merton, R., & Bowen, C. D. (1967). On the shoulders of giants: A Shandean postscript. Political Science Quarterly, 82 , 159. https://doi.org/10.2307/2147342
Matveeva, N., & Ferligoj, A. (2020). Scientific collaboration in Russian universities before and after the excellence initiative Project 5-100. Scientometrics, 124 (3), 2383–2407. https://doi.org/10.1007/s11192-020-03602-6
Morse, R., & Brooks, E. (2021). How U.S. news calculated the 2021 best colleges rankings . https://www.usnews.com/education/best-colleges/articles/how-us-news-calculated-the-rankings
Narin, F., Stevens, K., & Whitlow, E. S. (1991). Scientific co-operation in Europe and the citation of multi-nationally authored papers. Scientometrics, 21 , 313–323. https://doi.org/10.1007/BF02093973
National Science Board. (2020). Science and engineering indicators 2020 (NSB - 2020 - 1) . National Science Foundation. https://www.nsf.gov/pubs/2020/nsb20201/nsb20201.pdf
Netz, N., Hampel, S., & Aman, V. (2020). What effects does international mobility have on scientists’ careers? A systematic review. Research Evaluation, 29 (3), 327–351. https://doi.org/10.1093/reseval/rvaa007
Noorden, R. V. (2022). The number of researchers with dual US-China affiliations is falling. Nature, 606 (7913), 235–236. https://doi.org/10.1038/d41586-022-01492-7
Okamura, K. (2022). A half-century of international research collaboration dynamism: Congregate or disperse? [Preprint]. arXiv . https://arxiv.org/abs/2211.04429
Packalen, M. (2019). Edge factors: Scientific frontier positions of nations. Scientometrics, 118 (3), 787–808. https://doi.org/10.1007/s11192-018-2991-4
Peterson, M. F. (2001). International collaboration in organizational behavior research. Journal of Organizational Behavior, 22 (1), 59–81. https://doi.org/10.1002/job.61
Radicchi, F., Fortunato, S., & Castellano, C. (2008). Universality of citation distributions: Toward an objective measure of scientific impact. Proceedings of the National Academy of Sciences, 105 (45), 17268–17272. https://doi.org/10.1073/pnas.0806977105
Rafols, I., Leydesdorff, L., O’Hare, A., Nightingale, P., & Stirling, A. (2012). How journal rankings can suppress interdisciplinary research: A comparison between Innovation Studies and Business & Management. Research Policy, 41 (7), 1262–1282. https://doi.org/10.1016/j.respol.2012.03.015
Rao, C. R. (1982). Diversity: Its measurement, decomposition, apportionment and analysis. Sankhyā: The Indian Journal of Statistics, Series A, 44 (1), 1–22.
MathSciNet Google Scholar
Reichman, H. (2020). Nobel laureates and science groups demand NIH review decision to kill coronavirus grant . Sciences. https://www.science.org/content/article/preposterous-77-nobel-laureates-blast-nih-decision-cancel-coronavirus-grant-demand
Roberts, B. (2023). Chinese scientists leave United States amid geopolitical tensions . Cato Institute. https://clips.cato.org/sites/default/files/Bier_Fior_Scientists.pdf
Roth, J. (2022). Pretest with caution: Event-study estimates after testing for parallel trends. American Economic Review: Insights, 4 (3), 305–322. https://doi.org/10.1257/aeri.20210236
Sanfilippo, P., Hewitt, A. W., & Mackey, D. A. (2018). Plurality in multi-disciplinary research: Multiple institutional affiliations are associated with increased citations. PeerJ, 6 , e5664. https://doi.org/10.7717/peerj.5664
Scellato, G., Franzoni, C., & Stephan, P. (2015). Migrant scientists and international networks. Research Policy, 44 (1), 108–120. https://doi.org/10.1016/j.respol.2014.07.014
Schumpeter, J. A. (1934). The theory of economic development: An inquiry into profits, capital, credit, interest, and the business cycle . Harvard University Press.
Google Scholar
Shen, Z., Ma, H., & Wang, K. (2018). A Web-scale system for scientific knowledge exploration. In Annual meeting of the Association for Computational Linguistics . https://api.semanticscholar.org/CorpusID:44123741
Shi, D., Liu, W., & Wang, Y. (2023). Has China’s Young Thousand Talents program been successful in recruiting and nurturing top-caliber scientists? Science, 379 (6627), 62–65. https://doi.org/10.1126/science.abq1218
Silver, A. (2020). Scientists in China say US government crackdown is harming collaborations. Nature, 583 (7816), 341–342. https://doi.org/10.1038/d41586-020-02015-y
Simon, D., & Cao, C. (2009). China’s emerging technological edge: Assessing the role of high-end talent . Cambridge University Press. https://doi.org/10.1017/CBO9780511803468
Book Google Scholar
Stirling, A. (1998). On the economics and analysis of diversity. SPRU electronic working papers series ( working paper no. 28 ).
Tang, L., Cao, C., Wang, Z., & Zhou, Z. (2021). Decoupling in science and education: A collateral damage beyond deteriorating US–China relations. Science and Public Policy, 48 (5), 630–634. https://doi.org/10.1093/scipol/scab035
Tang, L., & Shapira, P. (2011). China–US scientific collaboration in nanotechnology: Patterns and dynamics. Scientometrics, 88 (1), 1–16. https://doi.org/10.1007/s11192-011-0376-z
The White House. (2022). Technologies for American innovation and national security . https://www.whitehouse.gov/ostp/news-updates/2022/02/07/technologies-for-american-innovation-and-national-security/
Thorp, H. H. (2022). The China Initiative must end. Science Advances, 8 (8), eabo6563. https://doi.org/10.1126/sciadv.abo6563
Trippl, M. (2013). Scientific mobility and knowledge transfer at the interregional and intraregional level. Regional Studies, 47 (10), 1653–1667. https://doi.org/10.1080/00343404.2010.549119
Turpin, T., Woolley, R., Marceau, J., & Hill, S. (2008). Conduits of knowledge in the Asia Pacific : Research training, networks and country of origin. Asian Population Studies, 4 (3), 247–265. https://doi.org/10.1080/17441730802496490
Uzzi, B., Mukherjee, S., Stringer, M. J., & Jones, B. F. (2013). Atypical combinations and scientific impact. Science, 342 (6157), 468–472. https://doi.org/10.1126/science.1240474
Velez-Estevez, A., García-Sánchez, P., Moral-Munoz, J., & Cobo, M. (2022). Why do papers from international collaborations get more citations? A bibliometric analysis of Library and Information Science papers. Scientometrics, 127 (12), 7517–7555. https://doi.org/10.1007/s11192-022-04486-4
Visser, M., van Eck, N. J., & Waltman, L. (2021). Large-scale comparison of bibliographic data sources: Scopus, Web of Science, Dimensions, Crossref, and Microsoft Academic. Quantitative Science Studies, 2 (1), 20–41. https://doi.org/10.1162/qss_a_00112
Vogel, K., & Ouagrham-Gormley, S. (2023). Scientists as spies? Assessing U.S. claims about the security threat posed by China’s thousand talents program for the U.S. life sciences. Politics and the Life Sciences, 42 (1), 32–64. https://doi.org/10.1017/pls.2022.13
Wagner, C., & Jonkers, K. (2017). Open countries have strong science. Nature, 555 (7698), 580–580. https://doi.org/10.1038/550032a
Wagner, C., Park, H., & Leydesdorff, L. (2015). The continuing growth of global cooperation networks in research: A conundrum for national governments. PLoS ONE, 10 (7), e0131816. https://doi.org/10.1371/journal.pone.0131816
Wagner, C. S., & Cai, X. (2022). Changes in co-publication patterns among China, the European Union (28) and the United States of America, 2016–2021 [Preprint]. arXiv . https://arxiv.org/abs/2202.00453
Wagner, C. S., & Leydesdorff, L. (2005). Network structure, self-organization, and the growth of international collaboration in science. Research Policy, 34 (10), 1608–1618. https://doi.org/10.1016/j.respol.2005.08.002
Wagner, C. S., Whetsell, T. A., & Mukherjee, S. (2019). International research collaboration: Novelty, conventionality, and atypicality in knowledge recombination. Research Policy, 48 (5), 1260–1270. https://doi.org/10.1016/j.respol.2019.01.002
Wang, K., Shen, Z., Huang, C., Wu, C.-H., Dong, Y., & Kanakia, A. (2020). Microsoft Academic Graph: When experts are not enough. Quantitative Science Studies, 1 (1), 396–413. https://doi.org/10.1162/qss_a_00021
Wang, Y., Jones, B. F., & Wang, D. (2019). Early-career setback and future career impact. Nature Communications, 10 (1), 4331. https://doi.org/10.1038/s41467-019-12189-3
Wang, Y., Li, N., Zhang, B., Huang, Q., Wu, J., & Wang, Y. (2023). The effect of structural holes on producing novel and disruptive research in physics. Scientometrics, 128 (3), 1801–1823. https://doi.org/10.1007/s11192-023-04635-3
Way, S., Morgan, A., Larremore, D., & Clauset, A. (2019). Productivity, prominence, and the effects of academic environment. Proceedings of the National Academy of Sciences, 116 (22), 10729–10733. https://doi.org/10.1073/pnas.1817431116
Woolston, C. (2023). Nature Index Annual Tables 2023: China tops natural-science table. Nature . https://doi.org/10.1038/d41586-023-01868-3
Xie, Y., & Killewald, A. (2012). Is American science in decline . Harvard University Press.
Xie, Y., Lin, X., Li, J., He, Q., & Huang, J. (2023). Caught in the crossfire: Fears of Chinese-American scientists. Proceedings of the National Academy of Sciences of the United States of America, 120 (27), e2216248120. https://doi.org/10.1073/pnas.2216248120
Yang, Y., Tian, T. Y., Woodruff, T. K., Jones, B. F., & Uzzi, B. (2022). Gender-diverse teams produce more novel and higher-impact scientific ideas. Proceedings of the National Academy of Sciences, 119 (36), e2200841119. https://doi.org/10.1073/pnas.2200841119
Yegros, A., Capponi, G., & Frenken, K. (2021). A spatial-institutional analysis of researchers with multiple affiliations. PLoS ONE, 16 (6), e0253462. https://doi.org/10.1371/journal.pone.0253462
Yuan, L., Hao, Y., Li, M., Bao, C., Li, J., & Wu, D. (2018). Who are the international research collaboration partners for China? A novel data perspective based on NSFC grants. Scientometrics, 116 (1), 401–422. https://doi.org/10.1007/s11192-018-2753-3
Zha, Q. (2023). Reimagining China-US university relations: A global “ecosystem” perspective. Studies in Higher Education . https://doi.org/10.1080/03075079.2023.2269966
Zhang, C., & Guo, J. (2017). China’s international research collaboration: Evidence from a panel gravity model. Scientometrics, 113 (2), 1129–1139. https://doi.org/10.1007/s11192-017-2513-9
Zheng, H., Li, W., & Wang, D. (2022). Expertise diversity of teams predicts originality and long-term impact in science and technology [Preprint]. arXiv . https://arxiv.org/abs/2210.04422
Zhou, P., & Glänzel, W. (2010). In-depth analysis on China’s international cooperation in science. Scientometrics, 82 (3), 597–612. https://doi.org/10.1007/s11192-010-0174-z
Zhu, W., Jin, C., Ma, Y., & Xu, C. (2023). Earlier recognition of scientific excellence enhances future achievements and promotes persistence. Journal of Informetrics, 17 (2), 101408. https://doi.org/10.1016/j.joi.2023.101408
Zong, X., & Zhang, W. (2019). Establishing world-class universities in China: Deploying a quasi-experimental design to evaluate the net effects of Project 985. Studies in Higher Education, 44 (3), 417–431. https://doi.org/10.1080/03075079.2017.1368475
Article MathSciNet Google Scholar
Zweig, D. (2008). Competing for talent: China’s strategies to reverse the brain drain. International Labour Review, 145 (1–2), 65–90. https://doi.org/10.1111/j.1564-913X.2006.tb00010.x
Zweig, D., Changgui, C., & Rosen, S. (2004). Globalization and transitional human capital: Overseas and returnee scholars to China. The China Quarterly, 179 , 735–757. https://doi.org/10.1017/S0305741004000566
Zweig, D., & Han, D. (2010). “Sea Turtles” or “Seaweed”? The employment of overseas returnees in China. In C. Kuptsch & S. Geneva (Eds.), The internationalization of labour markets (pp. 89–104). International Labour Organization.
Zweig, D., & Kang, S. (2020). America challenges China’s National Talent Programs. Center for Strategic and International Studies. http://www.jstor.org/stable/resrep24782
Zweig, D., Siqin, K., & Huiyao, W. (2020). ‘The Best are yet to Come:’ State programs, domestic resistance and reverse migration of high-level talent to China. Journal of Contemporary China, 29 (125), 1–16. https://doi.org/10.1080/10670564.2019.1705003
Download references
Acknowledgements
We thank the referees for their valuable comments. This work was supported by the National Natural Science Foundation of China under Grant Nos. 72004177, L2324122, the Shaanxi Provincial Project of the Soft Science Fund (2024ZC-YBXM-100), and the Fundamental Research Funds for the Central Universities.
Author information
Authors and affiliations.
School of Public Policy and Administration, Xi’an Jiaotong University, Xi’an, 710049, China
Moxin Li & Yang Wang
You can also search for this author in PubMed Google Scholar
Corresponding author
Correspondence to Yang Wang .
Ethics declarations
Competing interests.
The authors declare no competing interests.
Additional information
Publisher's note.
Springer Nature remains neutral with regard to jurisdictional claims in published maps and institutional affiliations.
See Figs. 8 , 9 , and 10 .
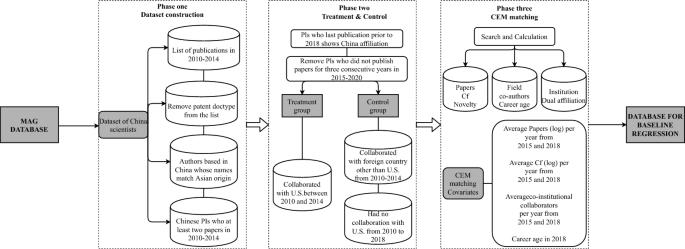
Data processing flow chart
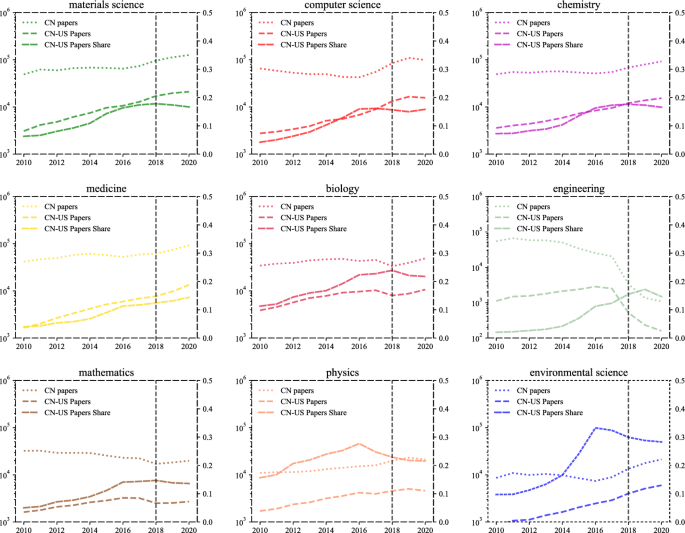
International collaborations between China and the U.S. for various scientific domains
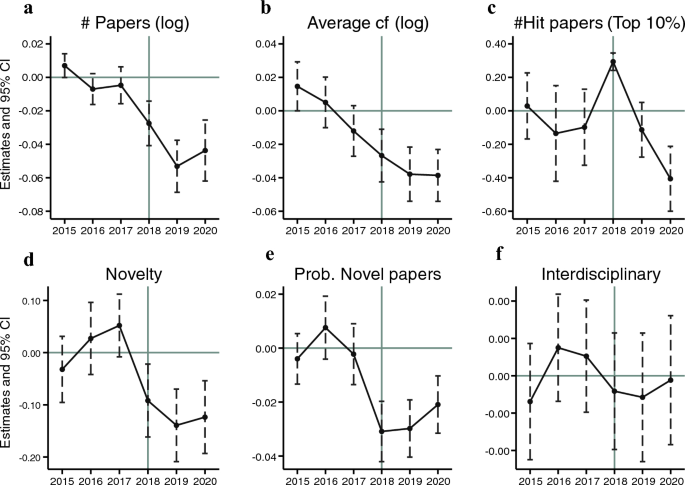
The impact of political tensions on Chinese scientists: Results from the Event Study using the Entropy Balancing method. Regression coefficients and their corresponding 95% confidence intervals for a the number of papers, b average normalized citation impact, c the number of hit papers (defined as being top 10% highly cited papers), d average novelty, e the probability to publish novel papers, and f average interdisciplinarity levels
Rights and permissions
Springer Nature or its licensor (e.g. a society or other partner) holds exclusive rights to this article under a publishing agreement with the author(s) or other rightsholder(s); author self-archiving of the accepted manuscript version of this article is solely governed by the terms of such publishing agreement and applicable law.
Reprints and permissions
About this article
Li, M., Wang, Y. Influence of political tensions on scientific productivity, citation impact, and knowledge combinations. Scientometrics (2024). https://doi.org/10.1007/s11192-024-04973-w
Download citation
Received : 05 August 2023
Accepted : 15 February 2024
Published : 23 April 2024
DOI : https://doi.org/10.1007/s11192-024-04973-w
Share this article
Anyone you share the following link with will be able to read this content:
Sorry, a shareable link is not currently available for this article.
Provided by the Springer Nature SharedIt content-sharing initiative
- International scientific collaboration
- Political tensions
- Citation impact
- Interdisciplinary research
- Find a journal
- Publish with us
- Track your research
Featured Clinical Reviews
- Screening for Atrial Fibrillation: US Preventive Services Task Force Recommendation Statement JAMA Recommendation Statement January 25, 2022
- Evaluating the Patient With a Pulmonary Nodule: A Review JAMA Review January 18, 2022
Select Your Interests
Customize your JAMA Network experience by selecting one or more topics from the list below.
- Academic Medicine
- Acid Base, Electrolytes, Fluids
- Allergy and Clinical Immunology
- American Indian or Alaska Natives
- Anesthesiology
- Anticoagulation
- Art and Images in Psychiatry
- Artificial Intelligence
- Assisted Reproduction
- Bleeding and Transfusion
- Caring for the Critically Ill Patient
- Challenges in Clinical Electrocardiography
- Climate and Health
- Climate Change
- Clinical Challenge
- Clinical Decision Support
- Clinical Implications of Basic Neuroscience
- Clinical Pharmacy and Pharmacology
- Complementary and Alternative Medicine
- Consensus Statements
- Coronavirus (COVID-19)
- Critical Care Medicine
- Cultural Competency
- Dental Medicine
- Dermatology
- Diabetes and Endocrinology
- Diagnostic Test Interpretation
- Drug Development
- Electronic Health Records
- Emergency Medicine
- End of Life, Hospice, Palliative Care
- Environmental Health
- Equity, Diversity, and Inclusion
- Facial Plastic Surgery
- Gastroenterology and Hepatology
- Genetics and Genomics
- Genomics and Precision Health
- Global Health
- Guide to Statistics and Methods
- Hair Disorders
- Health Care Delivery Models
- Health Care Economics, Insurance, Payment
- Health Care Quality
- Health Care Reform
- Health Care Safety
- Health Care Workforce
- Health Disparities
- Health Inequities
- Health Policy
- Health Systems Science
- History of Medicine
- Hypertension
- Images in Neurology
- Implementation Science
- Infectious Diseases
- Innovations in Health Care Delivery
- JAMA Infographic
- Law and Medicine
- Leading Change
- Less is More
- LGBTQIA Medicine
- Lifestyle Behaviors
- Medical Coding
- Medical Devices and Equipment
- Medical Education
- Medical Education and Training
- Medical Journals and Publishing
- Mobile Health and Telemedicine
- Narrative Medicine
- Neuroscience and Psychiatry
- Notable Notes
- Nutrition, Obesity, Exercise
- Obstetrics and Gynecology
- Occupational Health
- Ophthalmology
- Orthopedics
- Otolaryngology
- Pain Medicine
- Palliative Care
- Pathology and Laboratory Medicine
- Patient Care
- Patient Information
- Performance Improvement
- Performance Measures
- Perioperative Care and Consultation
- Pharmacoeconomics
- Pharmacoepidemiology
- Pharmacogenetics
- Pharmacy and Clinical Pharmacology
- Physical Medicine and Rehabilitation
- Physical Therapy
- Physician Leadership
- Population Health
- Primary Care
- Professional Well-being
- Professionalism
- Psychiatry and Behavioral Health
- Public Health
- Pulmonary Medicine
- Regulatory Agencies
- Reproductive Health
- Research, Methods, Statistics
- Resuscitation
- Rheumatology
- Risk Management
- Scientific Discovery and the Future of Medicine
- Shared Decision Making and Communication
- Sleep Medicine
- Sports Medicine
- Stem Cell Transplantation
- Substance Use and Addiction Medicine
- Surgical Innovation
- Surgical Pearls
- Teachable Moment
- Technology and Finance
- The Art of JAMA
- The Arts and Medicine
- The Rational Clinical Examination
- Tobacco and e-Cigarettes
- Translational Medicine
- Trauma and Injury
- Treatment Adherence
- Ultrasonography
- Users' Guide to the Medical Literature
- Vaccination
- Venous Thromboembolism
- Veterans Health
- Women's Health
- Workflow and Process
- Wound Care, Infection, Healing
- Download PDF
- Share X Facebook Email LinkedIn
- Permissions
Peer Review and Scientific Publication at a Crossroads : Call for Research for the 10th International Congress on Peer Review and Scientific Publication
- 1 Meta-Research Innovation Center at Stanford (METRICS), Stanford University, Stanford, California
- 2 Department of Medicine, Stanford University School of Medicine, Stanford, California
- 3 JAMA and the JAMA Network, Chicago, Illinois
- 4 The BMJ , London, England
- Editorial Three Decades of Peer Review Congresses Drummond Rennie, MD; Annette Flanagin, RN, MA JAMA
The way science is assessed, published, and disseminated has markedly changed since 1986, when the launch of a new Congress focused on the science of peer review was first announced. There have been 9 International Peer Review Congresses since 1989, typically running on an every-4-year cycle, and most recently in 2022 after a 1-year delay due to the COVID-19 pandemic. 1 Here, we announce that the 10th International Congress on Peer Review and Scientific Publication will be held in Chicago, Illinois, on September 3-5, 2025.
The congresses have been enormously productive, incentivizing and publicizing important empirical work into how science is produced, evaluated, published, and disseminated. 2 - 4 However, peer review and scientific publication are currently at a crossroads and their future more difficult than ever to predict. After decades of experience and research in these fields, we have learned a lot about a wide range of aspects of peer review and scientific publication. 2 - 5 We have accumulated a large body of empirical evidence on how systems function and how they can malfunction. There is also growing evidence on how to make peer review, publication, and dissemination processes more efficient, fair, open, transparent, reliable, and equitable. 6 - 15 Experimental randomized evaluations of peer review practices are only a small part of the literature, but their numbers have been growing since the early trials of anonymized peer review. 16 - 22 Research has revealed a rapidly growing list of biases, inefficiencies, and threats to the trustworthiness of published research, some now well recognized, others deserving of more attention. 2 , 3 Moreover, practices continue to change and diversify in response to new needs, tools, and technologies as well as the persistent “publish or perish” pressures on scientists-as-authors.
With the continued evolution of electronic platforms and tools—most recently the emergence and use of large language models and artificial intelligence (AI)—peer review and scientific publication are rapidly evolving to address new opportunities and threats. 23 , 24 Moreover, a lot of money is at stake; scientific publishing is a huge market with one of the highest profit margins among all business enterprises, and it supports a massive biomedical and broader science economy. Many stakeholders try to profit from or influence the scientific literature in ways that do not necessarily serve science or enhance its benefits to society. The number of science journal titles and articles is steadily increasing 25 ; many millions of scientists coauthor scientific papers, and perverse reward systems do not help improve the quality of this burgeoning corpus. Furthermore, principled mandates for immediate and open access to research and data may not be fully understood, accepted, or funded. Many other new, often disruptive, ideas abound on how to improve dissemination of and access to science, some more speculative, utopian, or self-serving than others. In addition, deceptive, rogue actors, such as predatory and pirate publishers, fake reviewers, and paper mills continue to threaten the integrity of peer review and scientific publication. Careful testing of the many proposals to improve peer review and publication and of interventions and processes to address threats to their integrity in a rigorous and timely manner are essential to the future of science and the scholarly publishing enterprise.
Proposed remedies for several of the problems and biases have been evaluated, 4 but many are untested or have inconclusive evidence for or against their use. New biases continue to appear (or at least to be recognized). In addition, there is tension about how exactly to correct the scientific literature, where a large share of what is published may not be replicable or is obviously false. 26 Even outright fraud may be becoming more common—or may simply be recognized and reported more frequently than before. 27 , 28
By their very nature, peer review and scientific publication practices are in a state of flux and may be unstable as they struggle to serve rapidly changing circumstances, technologies, and stakeholder needs and goals. Therefore, some unease would exist even in the absence of major perturbations, even if all the main stakeholders (authors, journals, publishers, funders) simply wanted to continue business as usual. However, the emergence of additional rapid changes further exacerbates the challenges, while also providing opportunities to improve the system at large. The COVID-19 crisis was one major quake that shook the way research is designed, conducted, evaluated, published, disseminated, and accessed. 29 , 30 Advances in AI and large language models may be another, potentially even larger, seismic force, with some viewing the challenge posed by these new developments as another hyped tempest in a teapot and others believing them to be an existential threat to truth and all of humanity. Scientific publication should fruitfully absorb this energy. 23 , 24 Research has never been needed more urgently to properly examine, test, and correct (in essence: peer review) scientific and nonscientific claims for the sake of humanity’s best interests. The premise of all Peer Review Congresses is that peer review and scientific publication must be properly examined, tested, and corrected in the same way the scientific method and its products are applied, vetted, weighted, and interpreted. 2
The range of topics on which we encourage research to be conducted, presented, and discussed at the 10th International Congress on Peer Review and Scientific Publication expands what was covered by the 9 previous iterations of the congress ( Box ). 1 , 2 , 4 We understand that new topics may yet emerge; 2 years until September 2025 is a relatively long period, during which major changes are possible, and even likely. Therefore, we encourage research in any area of work that may be relevant to peer review and scientific publication, including novel empirical investigations of processes, biases, policies, and innovations. The congress has the ambitious goal to cover all branches and disciplines of science. It is increasingly recognized that much can be learned by comparing experiences in research and review practices across different disciplines. While biomedical sciences have had the lion’s share in empirical contributions to research on peer review in the past, we want to help correct this imbalance. Therefore, we strongly encourage the contribution of work from all scientific disciplines, including the natural and physical sciences, social sciences, psychological sciences, economics, computer science, mathematics, and new emerging disciplines. Interdisciplinary work is particularly welcome.
Topics of Interest for the 10th International Congress on Peer Review and Scientific Publication
Efforts to avoid, manage, or account for bias in research methods, design, conduct, and reporting and interpretation
Publication and reporting bias
Bias on the part of researchers, authors, reviewers, editors, funders, commentators, influencers, disseminators, and consumers of scientific information
Interventions to address gender, race and ethnicity, geographic location, career stage, and discipline biases in peer review, publication, research dissemination, and impact
Improving and measuring diversity, equity, and inclusion of authors, reviewers, editors, and editorial board members
Motivational factors for bias related to rewards and incentives
New forms of bias introduced by wider use of large language models and other forms of artificial intelligence (AI)
Editorial and Peer Review Decision-Making
Assessment and testing of models of peer review and editorial decision-making and workflows used by journals, publishers, funders, and research disseminators
Evaluations of the quality, validity, and practicality of peer review and editorial decision-making
Challenges, new biases, and opportunities with mega-journals
Assessment of practices related to publication of special issues with guest editors
Economic and systemic evaluations of the peer review machinery and the related publishing business sector
Methods for ascertaining use of large language models and other forms of AI in authoring and peer review of scientific papers
AI in peer review and editorial decision-making
Quality assurance for reviewers, editors, and funders
Editorial policies and responsibilities
Editorial freedom and integrity
Peer review of grant proposals
Peer review of content for meetings
Editorial handling of science journalism
Role of journals as publishing venues vs peer review venues
COVID-19 pandemic and postpandemic effects
Research and Publication Ethics
Ethical concerns for researchers, authors, reviewers, editors, publishers, and funders
Authorship, contributorship, accountability, and responsibility for published material
Conflicts of interest (financial and nonfinancial)
Research and publication misconduct
Editorial nepotism or favoritism
Paper mills
Citation cartels, citejacking, and other manipulation of citations
Conflicts of interest among those who critique or criticize published research and researchers
Ethical review and approval of studies
Confidentiality considerations
Rights of research participants in scientific publication
Effects of funding and sponsorship on research and publication
Influence of external stakeholders: funders, journal owners, advertisers/sponsors, libraries, legal representatives, news media, social media, fact-checkers, technology companies, and others
Tools and software to detect wrongdoing, such as duplication, fraudulent manuscripts and reviewers, image manipulation, and submissions from paper mills
Corrections and retractions
Legal issues in peer review and correction of the literature
Evaluations of censorship in science
Intrusion of political and ideological agendas in scientific publishing
Science and scientific publication under authoritarian regimes
Improving Research Design, Conduct, and Reporting
Effectiveness of guidelines and standards designed to improve the design, conduct, and reporting of scientific studies
Evaluations of the methodological rigor of published information
Data sharing, transparency, reliability, and access
Research reanalysis, reproducibility, and replicability
Approaches for efficient and effective correction of errors
Curtailing citation and continued spread of retracted science
Innovations in best, fit-for-purpose methods and statistics, and ways to improve their appropriate use
Implementations of AI and related tools to improve research design, conduct, and reporting
Innovations to improve data and scientific display
Quality and reliability of data presentation and scientific images
Standards for multimedia and new content models for dissemination of science
Quality and effectiveness of new formats for scientific articles
Fixed articles vs evolving versions and innovations to support updating of scientific articles and reviews
Models for Peer Review and Scientific Publication
Single-anonymous, double-anonymous, collaborative, and open peer review
Pre–study conduct peer review
Open and public access
Preprints and prepublication posting and release of information
Prospective registration of research
Postpublication review, communications, and influence
Engaging statistical and other technical expertise in peer review
Evaluations of reward systems for authors, reviewers, and editors
Approaches to improve diversity, equity, and inclusion in peer review and publication
Innovations to address reviewer fatigue
Scientific information in multimedia and new media
Publication and performance metrics and usage statistics
Financial and economic models of peer-reviewed publication
Quality and influence of advertising and sponsored publication
Quality and effectiveness of content tagging, markup, and linking
Use of AI and software to improve peer review, decision-making, and dissemination of science
Practices of opportunistic, predatory, and pirate operators
Threats to scientific publication
The future of scientific publication
Dissemination of Scientific and Scholarly Information
New technologies and methods for improving the quality and efficiency of, and equitable access to, scientific information
Novel mechanisms, formats, and platforms to disseminate science
Funding and reward systems for science and scientific publication
Use of bibliometrics and alternative metrics to evaluate the quality and equitable dissemination of published science
Best practices for corrections and retracting fraudulent articles
Comparisons of and lessons from various scientific disciplines
Mapping of scientific methods and reporting practices and of meta-research across disciplines
Use and effects of social media
Misinformation and disinformation
Reporting, publishing, disseminating, and accessing science in emergency situations (pandemics, natural disasters, political turmoil, wars)
The congress is organized under the auspices of JAMA and the JAMA Network, The BMJ , and the Meta-research Innovation Center at Stanford (METRICS) and is guided by an international panel of advisors who represent diverse areas of science and of activities relevant to peer review and scientific publication. 4 The abstract submission site is expected to open on December 1, 2024, with an anticipated deadline for abstract submission by January 31, 2025. Announcements will appear on the congress website ( https://peerreviewcongress.org/ ). 4
Corresponding Author: John P. A. Ioannidis, MD, DSc, Stanford Prevention Research Center, Stanford University, 1265 Welch Rd, MSOB X306, Stanford, CA 94305 ( [email protected] ).
Published Online: September 22, 2023. doi:10.1001/jama.2023.17607
Conflict of Interest Disclosures: All authors serve as directors or coordinators of the Peer Review Congress. Ms Flanagin reports serving as an unpaid board member for STM: International Association of Scientific, Technical, and Medical Publishers. Dr Bloom reports being a founder of medRxiv and a member of the Board of Managers of American Institute of Physics Publishing.
Additional Information: Drs Ioannidis and Berkwits are directors; Ms Flanagin, executive director; and Dr Bloom, European director and coordinator for the International Congress on Peer Review and Scientific Publication.
Note: This article is being published simultaneously in The BMJ and JAMA .
See More About
Ioannidis JPA , Berkwits M , Flanagin A , Bloom T. Peer Review and Scientific Publication at a Crossroads : Call for Research for the 10th International Congress on Peer Review and Scientific Publication . JAMA. 2023;330(13):1232–1235. doi:10.1001/jama.2023.17607
Manage citations:
© 2024
Artificial Intelligence Resource Center
Cardiology in JAMA : Read the Latest
Browse and subscribe to JAMA Network podcasts!
Others Also Liked
- Register for email alerts with links to free full-text articles
- Access PDFs of free articles
- Manage your interests
- Save searches and receive search alerts
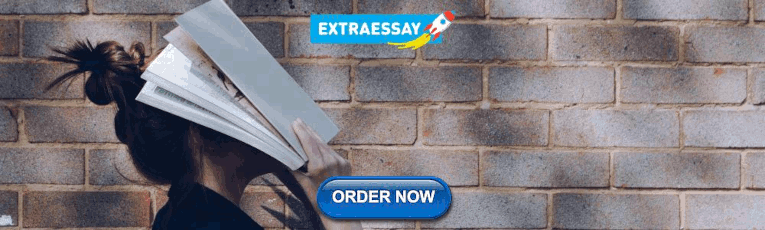
IMAGES
VIDEO
COMMENTS
Literature reviews are in great demand in most scientific fields. Their need stems from the ever-increasing output of scientific publications .For example, compared to 1991, in 2008 three, eight, and forty times more papers were indexed in Web of Science on malaria, obesity, and biodiversity, respectively .Given such mountains of papers, scientists cannot be expected to examine in detail every ...
Examples of literature reviews. Step 1 - Search for relevant literature. Step 2 - Evaluate and select sources. Step 3 - Identify themes, debates, and gaps. Step 4 - Outline your literature review's structure. Step 5 - Write your literature review.
A literature review is a document or section of a document that collects key sources on a topic and discusses those sources in conversation with each other (also called synthesis ). The lit review is an important genre in many disciplines, not just literature (i.e., the study of works of literature such as novels and plays).
A literature review is a critical analysis and synthesis of existing research on a particular topic. It provides an overview of the current state of knowledge, identifies gaps, and highlights key findings in the literature. 1 The purpose of a literature review is to situate your own research within the context of existing scholarship ...
A literature review is a surveys scholarly articles, books and other sources relevant to a particular. issue, area of research, or theory, and by so doing, providing a description, summary, and ...
When this happens, - the ultimate good of science can be realised. A literature review is structured differently from an original research article. It is developed based on themes, rather than stages of the scientific method. ... Note that this is an example using only two papers - most literature reviews would be presenting information on ...
The best proposals are timely and clearly explain why readers should pay attention to the proposed topic. It is not enough for a review to be a summary of the latest growth in the literature: the ...
A literature review may consist of simply a summary of key sources, but in the social sciences, a literature review usually has an organizational pattern and combines both summary and synthesis, often within specific conceptual categories.A summary is a recap of the important information of the source, but a synthesis is a re-organization, or a reshuffling, of that information in a way that ...
A literature review is a survey of scholarly sources on a specific topic. It provides an overview of current knowledge, allowing you to identify relevant theories, methods, and gaps in the existing research. There are five key steps to writing a literature review: Search for relevant literature. Evaluate sources. Identify themes, debates and gaps.
A scientific literature review usually includes a title, abstract, index, introduction, corpus, bibliography, and appendices (if needed). Present the problem clearly. Mention the paper's methodology, research methods, analysis, instruments, etc. Present literature review examples that can help you express your ideas. Remember to cite accurately.
In the narrative or traditional literature review, the available scientific literature is synthesized and no new data are presented. This article first discusses the process of selecting an appropriate topic. ... One article on the topic of scientific reviews suggests that at least 15 to 20 relevant research papers published within the previous ...
The Literature Research Workflow Web of Science The world's largest and highest quality ... Essential Science Indicators Reveals emerging science trends as well as influential individuals, institutions, papers, journals, and countries across 22 categories of research. ... Literature Review A literature review is a survey of scholarly sources ...
A systematic literature review is a method which sets out a series of steps to methodically organize the review. In this paper, we present a guide designed for researchers and in particular early-stage researchers in the computer-science field. The contribution of the article is the following: • Clearly defined strategies to follow for a ...
2. Benefits of Review Articles to the Author. Analysing literature gives an overview of the "WHs": WHat has been reported in a particular field or topic, WHo the key writers are, WHat are the prevailing theories and hypotheses, WHat questions are being asked (and answered), and WHat methods and methodologies are appropriate and useful [].For new or aspiring researchers in a particular ...
This paper discusses literature review as a methodology for conducting research and offers an overview of different types of reviews, as well as some guidelines to how to both conduct and evaluate a literature review paper. It also discusses common pitfalls and how to get literature reviews published. 1. Introduction.
Literature reviews are valuable resources for the scientific community. With research accelerating at an unprecedented speed in recent years and more and more original papers being published, review articles have become increasingly important as a means to keep up-to-date with developments in a particular area of research.
Literature reviews are valuable resources for the scientific community. With research accelerating at an unprecedented speed in recent years and more and more original papers being published, review articles have become increasingly important as a means to keep up to date with developments in a particular area of research.
A literature review is a body of text that aims to review the critical points of current knowledge on a particular topic. Most often associated with science-oriented literature, such as a thesis, the literature review usually proceeds a research proposal, methodology and results section. ... The literature review is the section of your paper in ...
a critical review of the relevant literature and then ensuring that their research design, methods, results, and conclusions follow logically from these objectives (Maier, 2013). There exist a number of papers devoted to instruction on how to write a good review paper. Among the most . useful for scientific reviews, in my estimation, are those by
Literature reviews play a critical role in scholarship because science remains, first and foremost, a cumulative endeavour (vom Brocke et al., 2009). As in any academic discipline, rigorous knowledge syntheses are becoming indispensable in keeping up with an exponentially growing eHealth literature, assisting practitioners, academics, and graduate students in finding, evaluating, and ...
Our Mastering Literature Review with Litmaps course allows instructors to seamlessly bring Litmaps into the classroom to teach fundamental literature review and research concepts. Learn More. Join the 250,000+ researchers, students, and professionals using Litmaps to accelerate their literature review. Find the right papers faster.
Unlock the secrets of academic writing with our guide to the key differences between a literature review and a research paper! 📚 Dive into the world of scholarly exploration as we break down how a literature review illuminates existing knowledge, identifies gaps, and sets the stage for further research. 🌐 Then, gear up for the adventure of crafting a research paper, where you become the ...
Conducting literature reviews for scientific papers is essential for understanding research, its limitations, and building on existing work. It is a tedious task which makes an automatic literature review generator appealing. Unfortunately, many existing works that generate such reviews using Large Language Models (LLMs) have significant ...
A comprehensive search strategy was developed with the assistance of a specialist librarian to access selected databases including: the Cumulative Index to Nursing and Allied Health Literature (CINAHL), Medline, Web of Science and PsychInfo. The review was restricted to original research studies published since 2009, available in English and ...
The promotion and development of healthy cities are vital for enhancing human habitats and fostering sustainable economic growth. Based on the core databases of Web of Science, PubMed, Google Scholar, and PsycINFO, and the knowledge graph software, this paper presents a quantitative analysis of the literature related to attention recovery abroad. It is found that in recent years, the research ...
This paper presents the second part of the two-part survey series on decomposition-based evolutionary multi-objective optimization where we mainly focus on discussing the literature related to multi-objective evolutionary algorithms based on decomposition (MOEA/D). Complementary to the first part, here we employ a series of advanced data mining approaches to provide a comprehensive anatomy of ...
In this literature review we observe papers recently published in the area of scientific paper recommendation between and including January 2019 and October 2021 1. We strive to give comprehensive overviews on their utilised methods as well as their datasets, evaluation measures and open challenges of current approaches.
Objective To identify factors acting as barriers or enablers to the process of healthcare consent for people with intellectual disability and to understand how to make this process equitable and accessible. Data sources Databases: Embase, MEDLINE, PsychINFO, PubMed, SCOPUS, Web of Science and CINAHL. Additional articles were obtained from an ancestral search and hand-searching three journals ...
International scientific collaborations. International scientific collaboration represents a collective effort of scientists working across national boundaries, sharing the common goal of generating new knowledge and advancing science (Katz & Martin, 1997).Over the past few decades, scholarly papers resulting from international collaborations have witnessed a remarkable surge, encompassing ...
The congress is organized under the auspices of JAMA and the JAMA Network, The BMJ, and the Meta-research Innovation Center at Stanford (METRICS) and is guided by an international panel of advisors who represent diverse areas of science and of activities relevant to peer review and scientific publication. 4 The abstract submission site is expected to open on December 1, 2024, with an ...