Accessibility Links
- Skip to content
- Skip to search IOPscience
- Skip to Journals list
- Accessibility help
- Accessibility Help
Click here to close this panel.
Purpose-led Publishing is a coalition of three not-for-profit publishers in the field of physical sciences: AIP Publishing, the American Physical Society and IOP Publishing.
Together, as publishers that will always put purpose above profit, we have defined a set of industry standards that underpin high-quality, ethical scholarly communications.
We are proudly declaring that science is our only shareholder.
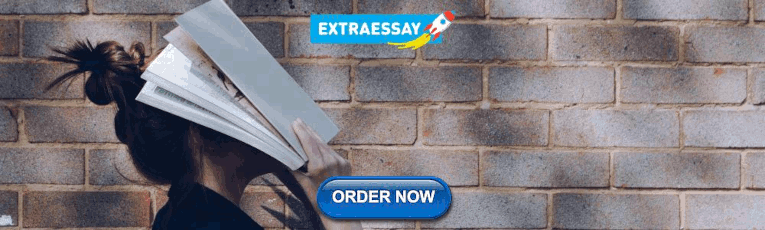
Modelling and Simulation Based on Matlab/Simulink: A Press Mechanism
R Halicioglu 1 , L C Dulger 1 and A T Bozdana 1
Published under licence by IOP Publishing Ltd Journal of Physics: Conference Series , Volume 490 , 2nd International Conference on Mathematical Modeling in Physical Sciences 2013 (IC-MSQUARE 2013) 1–5 September 2013, Prague, Czech Republic Citation R Halicioglu et al 2014 J. Phys.: Conf. Ser. 490 012053 DOI 10.1088/1742-6596/490/1/012053
Article metrics
7393 Total downloads
Share this article
Author affiliations.
1 Gaziantep University, Faculty of Engineering, Mechanical Engineering Department, Gaziantep 27310, Turkey
Buy this article in print
In this study, design and kinematic analysis of a crank-slider mechanism for a crank press is studied. The crank-slider mechanism is the commonly applied one as direct and indirect drive alternatives in practice. Since inexpensiveness, flexibility and controllability are getting more and more important in many industrial applications especially in automotive industry, a crank press with servo actuator (servo crank press) is taken as an application. Design and kinematic analysis of representative mechanism is presented with geometrical analysis for the inverse kinematic of the mechanism by using desired motion concept of slider. The mechanism is modelled in MATLAB/Simulink platform. The simulation results are presented herein.
Export citation and abstract BibTeX RIS
Content from this work may be used under the terms of the Creative Commons Attribution 3.0 licence . Any further distribution of this work must maintain attribution to the author(s) and the title of the work, journal citation and DOI.
- MATLAB Answers
- File Exchange
- AI Chat Playground
- Discussions
- Communities
- Treasure Hunt
- Community Advisors
- Virtual Badges

R-squared. Is Bigger Better?

Celebrating Spring: MATLAB Tulip

What’s New in Simulink R2024a?

Community Q&A – Zhaoxu Liu

Data Type Conversion Between MATLAB and Python: What’s New in R2024a

Dependency-based Test Selection

Example of Using the Profiler to Help Reduce the Execution Time of a Function

Three favorites from TIME Magazine’s “Best Innovations of 2023”

Celebrating Pi Day with cool visualizations

An interview with MATLAB playground: Build your IoT Analysis and Plots for ThingSpeak

Where are they now? – Daniel Tran, BE Semiconductor Industries (Besi Switzerland)

初心者のための Embedded Coder : 設定Tipsとワークフローをまとめて解説

스타트업 스포트라이트: 원격 주행 차량 공유의 안전성 보장

How NASA Langley is Using MATLAB and Simulink to Accelerate Development and Testing of Multirotor Flight Control

Deep Learning in Quantitative Finance: Multiagent Reinforcement Learning for Financial Trading

Build Simulink Apps with App Designer
- Posts (feed)

Mike Croucher is a Customer Success Engineer for Research and Education who previously spent 20 years working in academic research computing. He writes about MATLAB, MathWorks, and intersections with other technologies for those who are changing the world.
The MATLAB Blog Practical Advice for People on the Leading Edge
Open science and reusable research with matlab online and github.
Posted by Mike Croucher , July 20, 2023
- Step 1: Put your MATLAB code onto GitHub
- Step 2: From the repo, c reate a link that lets us open up directly in MATLAB Online and share it anywhere. One way to do this is to add a badge to the repo. Like this one:
Put your MATLAB and Simulink -based research code on GitHub
Add an ‘open in matlab online’ badge to the repo..
- GitHub URL: https://github.com/slevin48/liveapp
- URL to open in MATLAB Online (created with this webpage ): https://matlab.mathworks.com/open/github/v1?repo=slevin48/liveapp&file=SolarPanelEstimator.mlx
Any public r epo, any time , one-click clone
No matlab license no problem., add ‘open in matlab online ’ to your repo now, you are now following this blog post.
You will see updates in your activity feed .
You may receive emails, depending on your notification preferences .

Guy on Simulink Material on GitHub and MATLAB Online

MATLAB and Git

PyTorch Models and Git in MATLAB Online

gatsby-ecommerce-theme

CI with MATLAB and GitHub Actions Workshop

learningMatlab
To leave a comment, please click here to sign in to your MathWorks Account or create a new one.
Research with MATLAB and Simulink
Perform research, collaborate, and publish with matlab and simulink across a wide range of areas., access the tools you need when and where you need them.
Run the latest versions of MATLAB and Simulink. Share and collaborate using cloud-based storage. Generate code, access apps, and integrate MATLAB with several online platforms.
Find Resources for Your Application
Deep learning.
Add deep learning to your research with just a few lines of MATLAB code.
Deploy ROS nodes generated with MATLAB and Simulink to existing ROS networks.
Optimize research in wireless and 6G applications using deep learning.
Collaborate with Other Researchers
Use MATLAB and Simulink on joint projects, on data and compute portals, and in partnership with MathWorks.
Share Your Research
Contribute to the broader scientific community by building standalone applications, sharing code, and inspiring others.
Integrate MATLAB code and Simulink models with other languages, such as C/C++ and Python .
Publish your code on File Exchange and GitHub .
Discover how you can engage in open science with MATLAB (3:33) .
Learn How Researchers Worldwide Are Using MATLAB and Simulink
Climate research and development with matlab and simulink, featured research collaborations with mathworks, deep learning helps detect gravitational waves, sandia national laboratories simulates hawaii microgrid and photovoltaic systems.
“For our group, the main advantages of MATLAB include the ability to rapidly prototype our algorithms, debug them using great visualization tools, and then easily share the code with others in the vision research community.”
— Michael Rubinstein, MIT Computer Science and Artificial Intelligence Laboratory
Select a Web Site
Choose a web site to get translated content where available and see local events and offers. Based on your location, we recommend that you select: .
You can also select a web site from the following list
How to Get Best Site Performance
Select the China site (in Chinese or English) for best site performance. Other MathWorks country sites are not optimized for visits from your location.
- América Latina (Español)
- Canada (English)
- United States (English)
- Belgium (English)
- Denmark (English)
- Deutschland (Deutsch)
- España (Español)
- Finland (English)
- France (Français)
- Ireland (English)
- Italia (Italiano)
- Luxembourg (English)
- Netherlands (English)
- Norway (English)
- Österreich (Deutsch)
- Portugal (English)
- Sweden (English)
- United Kingdom (English)
Asia Pacific
- Australia (English)
- India (English)
- New Zealand (English)
- 简体中文 Chinese
- 日本 Japanese (日本語)
- 한국 Korean (한국어)
Contact your local office
Academia.edu no longer supports Internet Explorer.
To browse Academia.edu and the wider internet faster and more securely, please take a few seconds to upgrade your browser .
Enter the email address you signed up with and we'll email you a reset link.
- We're Hiring!
- Help Center
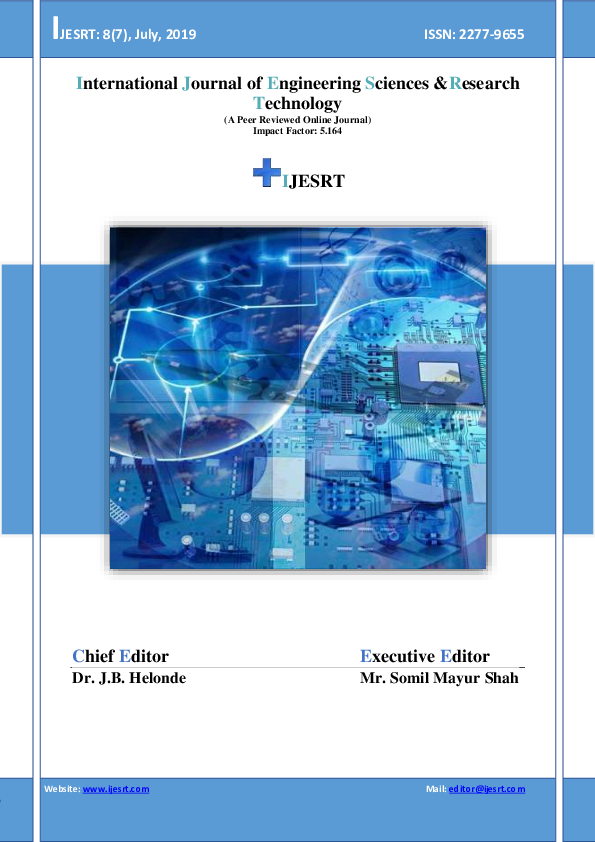
MODELING AND SIMULATION OF HYBRID ELECTRIC VEHICLE BASED ON MATLAB/SIMULINK

Computer simulation is an essential means to study the energy management strategy of hybrid electric vehicles. Through computer simulation technology, it can help to reduce unnecessary prototype manufacturing and real vehicle experiments, shorten development time, and save development costs. This paper is written to guide designers to use the "Hybrid Electric Vehicle Simulation System." It is hoped that this article will enable them to reduce unnecessary vehicle maintenance manufacturing and real vehicle experiments. This paper introduces the driver model, energy management strategy model, power source model, transmission system model, and dynamic model of the hybrid electric vehicle. It is convenient for users to match parameters, analyze performance and design energy management strategy for driving simulation hybrid electric vehicle.
RELATED PAPERS
N. Pöllath et alii (eds.), Animals and Humans through Time and Space: Investigating Diverse Relationships. Essays in Honour of Joris Peters. Documenta Archaeobiologiae 16
Carola Metzner-Nebelsick
Acta Botanica Brasilica
Maria das Graças Wanderley
Advances in Mathematics: Scientific Journal
Mridul kanty Dutta
Physical Review A
Mikhail Katsnelson
Annals of the New York Academy of Sciences
Thomas Lallier
ASEAN COMPARATIVE EDUCATION RESEARCH JOURNAL ON ISLAM AND CIVILIZATION (ACER-J). eISSN2600-769X
NATASHA ARIFFIN
shailly barnes
luis melendez
GEORGE ANTWI
Journal of Classics Teaching
Sophia Goula
Journal of Morphology
Alan Kuzirian
Enrique Ruiz
Annals of Physical and Rehabilitation Medicine
Nicolas Vuillerme
Journal of Cellular Biochemistry
Ishtiaq Ahmad
Solid State Communications
Ahmed Hussain
History and Philosophy of Logic
Jan Dejnožka
Revista Brasileira de Ciências do Esporte
jairo morgado
Journal of Industrial Microbiology and Biotechnology
Marcelo Valle de Sousa
Elena Krelja Kurelović
Italian Journal of Food Safety
FREDERIQUE PASQUALI
- We're Hiring!
- Help Center
- Find new research papers in:
- Health Sciences
- Earth Sciences
- Cognitive Science
- Mathematics
- Computer Science
- Academia ©2024
Thank you for visiting nature.com. You are using a browser version with limited support for CSS. To obtain the best experience, we recommend you use a more up to date browser (or turn off compatibility mode in Internet Explorer). In the meantime, to ensure continued support, we are displaying the site without styles and JavaScript.
- View all journals
- Explore content
- About the journal
- Publish with us
- Sign up for alerts
- Published: 08 May 2024
Accurate structure prediction of biomolecular interactions with AlphaFold 3
- Josh Abramson ORCID: orcid.org/0009-0000-3496-6952 1 na1 ,
- Jonas Adler ORCID: orcid.org/0000-0001-9928-3407 1 na1 ,
- Jack Dunger 1 na1 ,
- Richard Evans ORCID: orcid.org/0000-0003-4675-8469 1 na1 ,
- Tim Green ORCID: orcid.org/0000-0002-3227-1505 1 na1 ,
- Alexander Pritzel ORCID: orcid.org/0000-0002-4233-9040 1 na1 ,
- Olaf Ronneberger ORCID: orcid.org/0000-0002-4266-1515 1 na1 ,
- Lindsay Willmore ORCID: orcid.org/0000-0003-4314-0778 1 na1 ,
- Andrew J. Ballard ORCID: orcid.org/0000-0003-4956-5304 1 ,
- Joshua Bambrick ORCID: orcid.org/0009-0003-3908-0722 2 ,
- Sebastian W. Bodenstein 1 ,
- David A. Evans 1 ,
- Chia-Chun Hung ORCID: orcid.org/0000-0002-5264-9165 2 ,
- Michael O’Neill 1 ,
- David Reiman ORCID: orcid.org/0000-0002-1605-7197 1 ,
- Kathryn Tunyasuvunakool ORCID: orcid.org/0000-0002-8594-1074 1 ,
- Zachary Wu ORCID: orcid.org/0000-0003-2429-9812 1 ,
- Akvilė Žemgulytė 1 ,
- Eirini Arvaniti 3 ,
- Charles Beattie ORCID: orcid.org/0000-0003-1840-054X 3 ,
- Ottavia Bertolli ORCID: orcid.org/0000-0001-8578-3216 3 ,
- Alex Bridgland 3 ,
- Alexey Cherepanov ORCID: orcid.org/0000-0002-5227-0622 4 ,
- Miles Congreve 4 ,
- Alexander I. Cowen-Rivers 3 ,
- Andrew Cowie ORCID: orcid.org/0000-0002-4491-1434 3 ,
- Michael Figurnov ORCID: orcid.org/0000-0003-1386-8741 3 ,
- Fabian B. Fuchs 3 ,
- Hannah Gladman 3 ,
- Rishub Jain 3 ,
- Yousuf A. Khan ORCID: orcid.org/0000-0003-0201-2796 3 ,
- Caroline M. R. Low 4 ,
- Kuba Perlin 3 ,
- Anna Potapenko 3 ,
- Pascal Savy 4 ,
- Sukhdeep Singh 3 ,
- Adrian Stecula ORCID: orcid.org/0000-0001-6914-6743 4 ,
- Ashok Thillaisundaram 3 ,
- Catherine Tong ORCID: orcid.org/0000-0001-7570-4801 4 ,
- Sergei Yakneen ORCID: orcid.org/0000-0001-7827-9839 4 ,
- Ellen D. Zhong ORCID: orcid.org/0000-0001-6345-1907 3 ,
- Michal Zielinski 3 ,
- Augustin Žídek ORCID: orcid.org/0000-0002-0748-9684 3 ,
- Victor Bapst 1 na2 ,
- Pushmeet Kohli ORCID: orcid.org/0000-0002-7466-7997 1 na2 ,
- Max Jaderberg ORCID: orcid.org/0000-0002-9033-2695 2 na2 ,
- Demis Hassabis ORCID: orcid.org/0000-0003-2812-9917 1 , 2 na2 &
- John M. Jumper ORCID: orcid.org/0000-0001-6169-6580 1 na2
Nature ( 2024 ) Cite this article
230k Accesses
1 Citations
1186 Altmetric
Metrics details
We are providing an unedited version of this manuscript to give early access to its findings. Before final publication, the manuscript will undergo further editing. Please note there may be errors present which affect the content, and all legal disclaimers apply.
- Drug discovery
- Machine learning
- Protein structure predictions
- Structural biology
The introduction of AlphaFold 2 1 has spurred a revolution in modelling the structure of proteins and their interactions, enabling a huge range of applications in protein modelling and design 2–6 . In this paper, we describe our AlphaFold 3 model with a substantially updated diffusion-based architecture, which is capable of joint structure prediction of complexes including proteins, nucleic acids, small molecules, ions, and modified residues. The new AlphaFold model demonstrates significantly improved accuracy over many previous specialised tools: far greater accuracy on protein-ligand interactions than state of the art docking tools, much higher accuracy on protein-nucleic acid interactions than nucleic-acid-specific predictors, and significantly higher antibody-antigen prediction accuracy than AlphaFold-Multimer v2.3 7,8 . Together these results show that high accuracy modelling across biomolecular space is possible within a single unified deep learning framework.
You have full access to this article via your institution.
Similar content being viewed by others
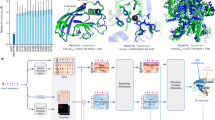
Highly accurate protein structure prediction with AlphaFold
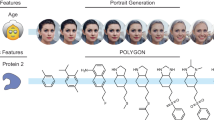
De novo generation of multi-target compounds using deep generative chemistry
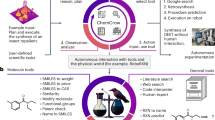
Augmenting large language models with chemistry tools
Author information.
These authors contributed equally: Josh Abramson, Jonas Adler, Jack Dunger, Richard Evans, Tim Green, Alexander Pritzel, Olaf Ronneberger, Lindsay Willmore
These authors jointly supervised this work: Victor Bapst, Pushmeet Kohli, Max Jaderberg, Demis Hassabis, John M. Jumper
Authors and Affiliations
Core Contributor, Google DeepMind, London, UK
Josh Abramson, Jonas Adler, Jack Dunger, Richard Evans, Tim Green, Alexander Pritzel, Olaf Ronneberger, Lindsay Willmore, Andrew J. Ballard, Sebastian W. Bodenstein, David A. Evans, Michael O’Neill, David Reiman, Kathryn Tunyasuvunakool, Zachary Wu, Akvilė Žemgulytė, Victor Bapst, Pushmeet Kohli, Demis Hassabis & John M. Jumper
Core Contributor, Isomorphic Labs, London, UK
Joshua Bambrick, Chia-Chun Hung, Max Jaderberg & Demis Hassabis
Google DeepMind, London, UK
Eirini Arvaniti, Charles Beattie, Ottavia Bertolli, Alex Bridgland, Alexander I. Cowen-Rivers, Andrew Cowie, Michael Figurnov, Fabian B. Fuchs, Hannah Gladman, Rishub Jain, Yousuf A. Khan, Kuba Perlin, Anna Potapenko, Sukhdeep Singh, Ashok Thillaisundaram, Ellen D. Zhong, Michal Zielinski & Augustin Žídek
Isomorphic Labs, London, UK
Alexey Cherepanov, Miles Congreve, Caroline M. R. Low, Pascal Savy, Adrian Stecula, Catherine Tong & Sergei Yakneen
You can also search for this author in PubMed Google Scholar
Corresponding authors
Correspondence to Max Jaderberg , Demis Hassabis or John M. Jumper .
Supplementary information
Supplementary information.
This Supplementary Information file contains the following 9 sections: (1) Notation; (2) Data pipeline; (3) Model architecture; (4) Auxiliary heads; (5) Training and inference; (6) Evaluation; (7) Differences to AlphaFold2 and AlphaFold-Multimer; (8) Supplemental Results; and (9) Appendix: CCD Code and PDB ID tables.
Reporting Summary
Rights and permissions.
Reprints and permissions
About this article
Cite this article.
Abramson, J., Adler, J., Dunger, J. et al. Accurate structure prediction of biomolecular interactions with AlphaFold 3. Nature (2024). https://doi.org/10.1038/s41586-024-07487-w
Download citation
Received : 19 December 2023
Accepted : 29 April 2024
Published : 08 May 2024
DOI : https://doi.org/10.1038/s41586-024-07487-w
Share this article
Anyone you share the following link with will be able to read this content:
Sorry, a shareable link is not currently available for this article.
Provided by the Springer Nature SharedIt content-sharing initiative
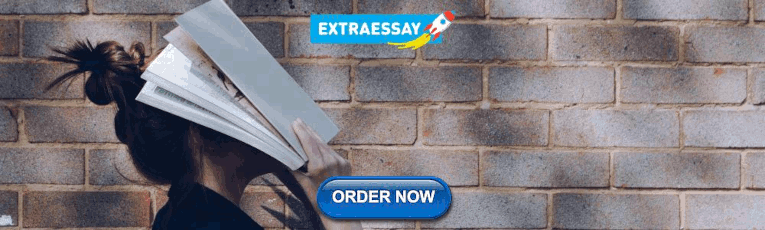
This article is cited by
Major alphafold upgrade offers boost for drug discovery.
- Ewen Callaway
Nature (2024)
By submitting a comment you agree to abide by our Terms and Community Guidelines . If you find something abusive or that does not comply with our terms or guidelines please flag it as inappropriate.
Quick links
- Explore articles by subject
- Guide to authors
- Editorial policies
Sign up for the Nature Briefing: Translational Research newsletter — top stories in biotechnology, drug discovery and pharma.

- Skip to primary navigation
- Skip to main content
Aggie Research Programs
Texas A&M University
Summer 2024: Dissecting mechanisms of brain excitability in females following traumatic brain injury.

Journal of Materials Chemistry A
Low-temperature tolerant lithium-rich manganese-based cathode enabled by facile sno2 decoration.
Lithium-rich manganese-based cathode materials gain increasing research attention because of their superior specific capacity and high operating voltage. However, it suffers voltage decay and unsatisfied stability during cycling. Meanwhile, the lithium-rich cathode materials could barely be stably operated at low temperatures, which hampers their practical application. Herein, low-temperature tolerant Li[Li0.144Ni0.136Co0.136Mn0.544]O2 cathode material is obtained via facile SnO2 decoration. With the SnO2 modification, LiF-enriched cathode-electrolyte interphase (CEI) is formed on surface of Li[Li0.144Ni0.136Co0.136Mn0.544]O2 at both ambient and low temperatures, which facilitates the Li+ diffusion on the surface. Furthermore, the modified Li[Li0.144Ni0.136Co0.136Mn0.544]O2 demonstrates suppressed voltage decay, high structure stability, as well as low activation energy for Li+ diffusion in CEI layer and charge transfer. Consequently, the SnO2-decorated Li[Li0.144Ni0.136Co0.136Mn0.544]O2 exhibits a high capacity of 221.0 mAh g-1 over 200 cycles, with a high capacity retention of 97.1% at 100 mA g-1 at room temperature. Remarkably, SnO2-decorated Li[Li0.144Ni0.136Co0.136Mn0.544]O2 can be successfully operated at low temperatures with high discharge capacity and outstanding cycle stability. At 0 ℃, a high discharge capacity of 111.5 mAh g-1 with a capacity retention of 79.9% is achieved over 500 cycles at 100 mA g-1. Even at -20 ℃, a high capacity of 109.9 mAh g-1 and a high capacity retention of 84.2% after 400 cycles is obtained at 25 mA g-1. This work provides a facile method to enhance the performance of lithium-rich cathode materials, and would promote the development of batteries based on lithium-rich cathode for broader application.
- This article is part of the themed collection: Journal of Materials Chemistry A HOT Papers
Supplementary files
- Supplementary information PDF (2640K)
Article information
Download citation, permissions.

D. wang, W. Wang, K. Li, J. Song, X. Yuan, Q. Huang, Z. Tang, L. Fu and Y. Wu, J. Mater. Chem. A , 2024, Accepted Manuscript , DOI: 10.1039/D4TA01971G
To request permission to reproduce material from this article, please go to the Copyright Clearance Center request page .
If you are an author contributing to an RSC publication, you do not need to request permission provided correct acknowledgement is given.
If you are the author of this article, you do not need to request permission to reproduce figures and diagrams provided correct acknowledgement is given. If you want to reproduce the whole article in a third-party publication (excluding your thesis/dissertation for which permission is not required) please go to the Copyright Clearance Center request page .
Read more about how to correctly acknowledge RSC content .
Social activity
Search articles by author.
This article has not yet been cited.
Advertisements
Regression Analysis Model Based on Data Processing and MATLAB Numerical Simulation
Ieee account.
- Change Username/Password
- Update Address
Purchase Details
- Payment Options
- Order History
- View Purchased Documents
Profile Information
- Communications Preferences
- Profession and Education
- Technical Interests
- US & Canada: +1 800 678 4333
- Worldwide: +1 732 981 0060
- Contact & Support
- About IEEE Xplore
- Accessibility
- Terms of Use
- Nondiscrimination Policy
- Privacy & Opting Out of Cookies
A not-for-profit organization, IEEE is the world's largest technical professional organization dedicated to advancing technology for the benefit of humanity. © Copyright 2024 IEEE - All rights reserved. Use of this web site signifies your agreement to the terms and conditions.
Research on pipeline leakage localization method based on CZIM algorithm
- Original Article
- Open access
- Published: 18 May 2024
- Volume 14 , article number 135 , ( 2024 )
Cite this article
You have full access to this open access article
- Yuan Yue 1 ,
- Yang Qu 1 ,
- Lei Yan 2 ,
- Zhao Wang 1 &
- Jun Tang ORCID: orcid.org/0000-0002-8657-9388 1
The time difference of arrival is a common method to find the leakage point of water pipeline. The leakage point localization is achieved by calculating the time delay between the signals reaching different sensors. Mainstream time delay estimation algorithms based on signal correlation analysis are susceptible to the introduction of noise signals, low sampling rates, and signal clipping, resulting in inaccurate localization results. The article analyzed the impact of different interference factors and proposed a new time delay estimation algorithm based on signal cross-zero information modulation (CZIM) to address these problems. By normalizing the amplitude of the two signals at the detection points on both sides of the pipeline leakage position, two sets of sparse signal sequences with only two eigenvalues of 0 and 1 are obtained. The error coefficient function is calculated by a similar traversal method to finally index the time delay. In this paper, the principle and characteristics of the algorithm are analyzed and compared with the most commonly used GCC method. In both numerical simulations and actual pipe leakage localization experiments, the CZIM algorithm has shown its wide applicability, low impact by low sampling rate, and adaptability to low signal-to-noise ratios, etc. At the same time, the algorithm is simple in design and has a small amount of calculation and can meet the demand for real-time data processing, providing a new idea for the development of acoustic localization technology.
Avoid common mistakes on your manuscript.
Introduction
Water wastage due to leaking pipes is a problem in many countries (Grant et al. 2012 ); the situation is particularly serious in developing countries, with an estimated annual global economic loss of $39 billion (Iwanaga et al. 2022 ). It is important to locate the pipe leak to reduce water costs and protect public safety (Li et al. 2015 ).
The TDOA (the time difference of arrival) method for pipe leakage localization is one of the most widely used method that using acoustic/vibration sensors to collect leak signals from different locations (Martini et al. 2015 ; Knapp and Carter 1976 ; Ahadi and Bakhtiar 2010 ; Bentoumi et al. 2017 ), estimate the time difference (time delay) of the signal from the source to the different monitoring units through signal processing, and then mathematically calculate the coordinates of the leaks in combination with the known location of the monitoring units (Nesta and Omologo 2011 ), of which time delay estimation is a key part (Wang and Zhao 2020 ).
Due to the complexity of the occurrence of fluid vibration in the pipe, and the signal itself attenuation, reflection, scattering, and other factors during the transmission process, the collected signal tends to be distorted to a certain degree (Li et al. 2020 ). In order to reduce the errors caused by these factors, scholars from various countries have conducted research from multiple angles over years and proposed methods such as the use of the generalized cross-correlation (GCC) algorithm and the least mean square adaptive (LMS) algorithm (Knapp and Carter 1976 ; Zhou and Tian 2020 ), which can improve the accuracy of time delay estimation to a certain extent, but most of these studies are limited by the integrity of the signal. For some complex distorted signals, how to achieve accurate and stable time delay estimation requires further research.
For example, since van Fleck completed a pioneering work on the influence of signal clipping on correlation and proposed the arcsine law (Vleck and Middleton 1966 ), much research has been conducted on the effect of quantization on signal correlation (Watts 1962 ; Cooper 1970 ), finding that loss of amplitude information affects the results of time delay estimation, or although many techniques are effective in general leakage localization, problems may often occur when applied to pipes under conditions of high acoustic signal attenuation (Gao et al. 2004 ).
In response to these issues, this paper analyzes and quantifies the main causes of time delay estimation errors and proposes a time delay estimation method based on sparse cross-zero information. This method does not rely on specific information about the signal amplitude and can achieve accurate localization only by retaining a certain amount of cross-zero information. Therefore, in addition to being suitable for more common scenarios, it also performs well in situations with severe signal amplitude distortion.
In this paper, we first validate the feasibility of the signal cross-zero information modulation (CZIM) algorithm by using simulated signals and further design pipe leakage localization detection experiments. The most commonly acceleration sensors are used to collect leak signals (Choi et al. 2017 ); the reliability of the CZIM algorithm for pipe leakage localization applications was evaluated under several signal distortion conditions. The results show that the CZIM algorithm is a time delay estimation algorithm with high precision and high adaptability. The algorithm gets rid of the dependence of traditional algorithms on signal integrity, and the calculation is also effective in the case of low-signal feature.
Forms and the effects of signal distortion
With the application of acoustic techniques in detecting leaks in fluid-filled pipes proved effective (Gao et al. 2006 ; Khulief et al. 2012 ; Brennan et al. 2007 ), the TDOA method based on generalized cross-correlation (GCC) is widely used in pipe leakage localization (Gao et al. 2017 ; Li and Zhang 2020 ), which was proposed by Knapp and Carter in 1976 using a maximum likelihood estimator and the algorithm flow is shown in Fig. 1 (Knapp and Carter 1976 ; Kothandaraman et al. 2020 ).
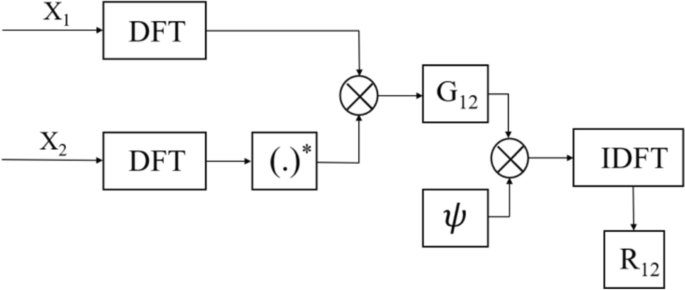
Schematic flow diagram of the GCC algorithm
Two channels of signals \(x_{1} \left( t \right)\) and \(x_{2} \left( t \right)\) are given by
where \(a_{1}\) and \(a_{2}\) are the attenuation coefficients of the signal (it is usually related to the spatial propagation path of the signal. The farther the signal transmission distance, the greater the attenuation, and the greater the value of ‘ \(a\) ’), \(s\left( t \right)\) is the source signal, \(n_{1} \left( t \right)\) and \(n_{2} \left( t \right)\) correspond to the two noise signals.
Substitute the weight function \(\psi\) and then the cross-correlation function of two channel signals
where \(D\) is the time delay between the two signals.
Substituting Eq. ( 1 ) into Eq. ( 2 ), this gives
where \(R_{ss}\) is the auto-correlation function of \(s\left( t \right)\) , \(R_{{sn_{i} }} \left( {i = 1,2} \right)\) is the cross-correlation function of \(s\left( t \right)\) and n \(\left( t \right)\) , and \(R_{{n_{1} n_{2} }}\) is the cross-correlation function of \(n_{1} \left( t \right)\) and \(n_{2} \left( t \right)\) .
From Eq. ( 3 ) it can be found that the mixing of interference noise \(n\left( t \right)\) , the lower sample rate-to-signal dominant frequency ratio and the large amount of distortion of the source signal \(s\left( n \right)\) amplitude information are the main factors that cause the time delay estimation error.
Interference noise
When the signal is collected to locate the leaks of the pipe, due to the complexity of the vibration environment, interference signals are inevitably mixed in during the process of collecting signals, and as the signal-to-noise ratio of the collected signals decreases, the time delay estimation results will be disturbed obviously. And the interference noise is usually not completely independent of each other, which is also a factor that needs to be considered.
In Fig. 2 , we use Gaussian white noise to simulate the leak signal and carry out a correlation analysis, although this is not strictly true in practice due to the limited bandwidth of the leak noise at the source (Brennan et al. 2019 ), but it can be acceptable for use in simulation. The auto-correlation function of a set of Gaussian signals with a standard deviation of 1.0 is calculated, and the result is shown in Fig. 2 a. The signal is divided into two channels and independent white noise is added as interference noise to make the signal-to-noise ratio of − 5 dB, the cross-correlation function of the two channels is calculated, and the result is shown in Fig. 2 b. If the added white noise is correlated, some corresponding sub-peaks are generated on the image, even though the signal-to-noise ratio remains at − 5 dB, as shown in Fig. 2 c.
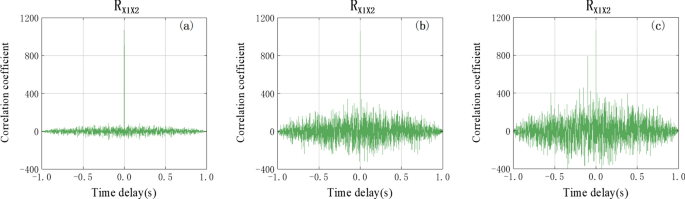
Cross(auto)-correlation function calculation result under different conditions
Limited sampling frequency
During the sampling process, due to the limitation of the data acquisition instrument itself, the signal collected by the system is limited discrete points, while the actual signal is a continuous line, so the only way to obtain a valid signal waveform is to ensure that the number of points is large enough and that the initial phases of the sampling are all integers.
If the sampling frequency is limited in the face of high-frequency signals (Hu et al. 2018 ), such as wireless sensors in use, considering the limitation of transmission bandwidth, high-frequency real-time sampling cannot be realized. Which resulting in a large amount of signal information being lost when the sampling rate to signal dominant frequency ratio is too low, will affect the results of time delay estimation to a greater extent.
As shown in Fig. 3 , using 1280 Hz and 2560 Hz to sample the same set of waveforms, the completeness of the signal information obtained is quite different.
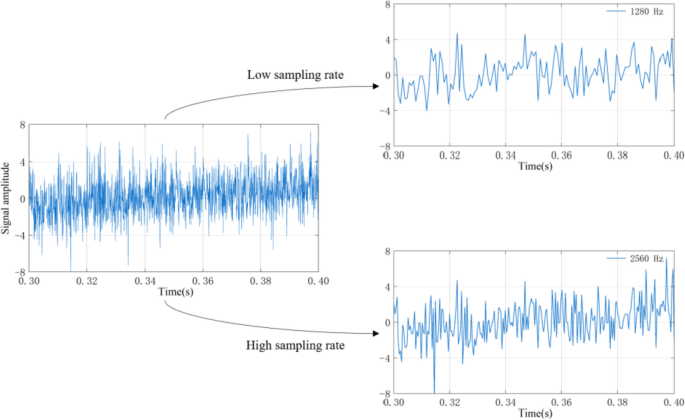
Signals collected at different sampling rates
Taking the sine wave whose maximum amplitude is A as an example, two sets of samples are performed on the same waveform with the sampling rate K ; then the error value E of each sampling point of the two sets of samples satisfies:
Strong interference noise
The strong interference occasionally introduced in the sampling process is also an important factor that causes delay calculation errors. As shown in Fig. 4 , strong interference often has the characteristics of large amplitude and short duration, and it can be found from Eq. ( 3 ) that this kind of signal can easily cause great disturbance to \(R_{{x_{1} x_{2} }}\) .
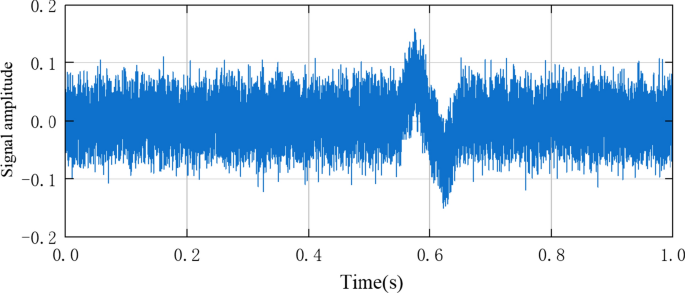
Sudden strong interference during sampling
Furthermore, if both detection units that need to perform delay estimation receive a certain strong interference, the correlation between the interference signals will further amplify the influence.
Aiming at the main problems such as signal acquisition mixed with noise, sampling rate limitation, and mixed with strong interference, which cause time delay estimation errors, this research proposes a time delay estimation method based on sparse cross-zero information. The method minimizes the impact of signal distortion by normalizing the signal and using a traversal-like approach to achieve accurate calculation the time delay of signal under various complex conditions.
Cross-zero information modulation (CZIM) algorithm
The flow chart of the time delay estimation algorithm based on signal cross-zero information modulation (CZIM) is shown in Fig. 5 . After the leak occurs, the signal propagates along the pipe wall toward both ends and is collected by sensor 1 for signal \(x_{1} \left( t \right)\) and collected by sensor 2 for signal \(x_{2} \left( t \right)\) , respectively.
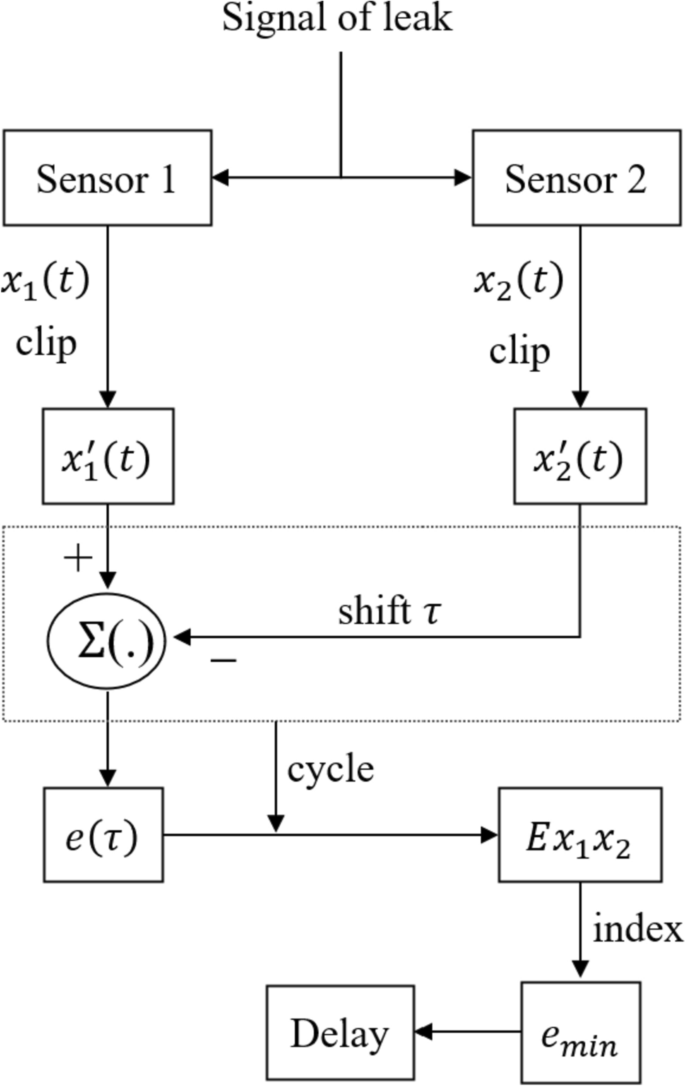
Schematic flow diagram of the CZIM algorithm
Clipping is performed on \(x_{1} \left( t \right)\) and \(x_{2} \left( t \right)\) , and the time–amplitude information of the signal is converted into a sparse signal with only two eigenvalues of 0 and 1. That is for a certain moment t, if the signal amplitude \(y > 0\) , let \(y = 1\) ; if the signal amplitude \(y \le 0\) , let \(y = 0\) . Only the cross-zero information or positive can be retained.
Thus, two sets of clipped rectangular waveform signals \(x_{1}{\prime} \left( t \right)\) and \(x_{2}{\prime} \left( t \right)\) can be obtained. The rectangular waveform signal is a sparse signal containing only two characteristic values of 0 and 1. Taking a segment of the signal actually collected as an example, the signal waveform amplitude information sparse processing is shown in Fig. 6 .
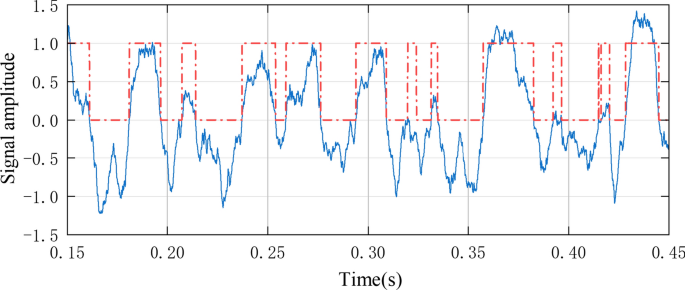
Signal normalization schematic
Add the signals \(x_{1}{\prime} \left( t \right)\) and \(- x_{2}{\prime} \left( t \right)\) correspondingly, define the terms that are not 0 as error points, calculate the number of error points to get \(Y^{\prime}\) , and divide \(Y^{\prime}\) by the total number of terms N to obtain the error coefficient \(e_{0}\) .
Let the signal \(x_{1}{\prime} \left( t \right)\) be misaligned and then add it to \(- x_{2}{\prime} \left( t \right)\) with the misalignment value \(\tau\) . Thus
The misaligned calculation is performed within a certain range, connect each \(e_{\tau }\) to obtain an error curve \(E_{{x_{1} x_{2} }}\) . The curve is indexed to the minimum value to obtain the minimum error factor \(e_{\min }\) . At this time the corresponding misalignment value \(\tau\) is the required time delay.
Taking a set of ideal signal as an example, in the absence of on interference we can get \(e_{\min } = 0\) and the error coefficient curve \(E_{{x_{1} x_{2} }}\) is shown in Fig. 7 .
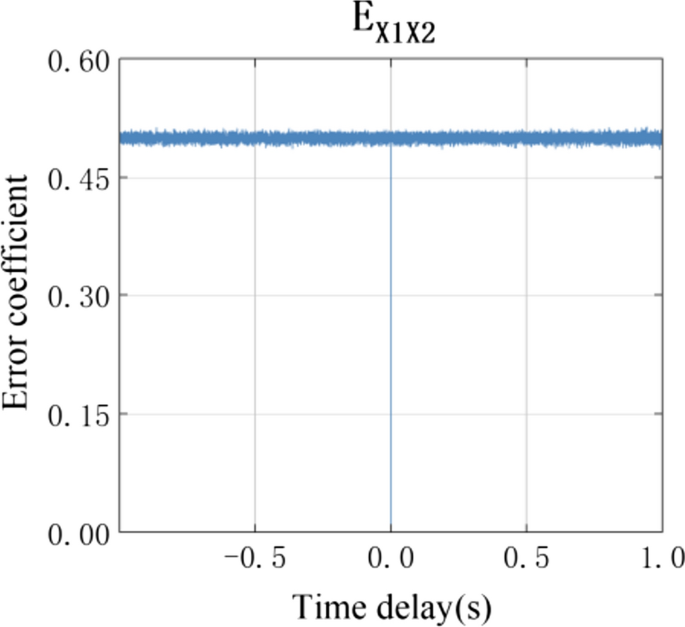
Error coefficient curve without interference
This algorithm first performs rectangular processing on signal \(x_{1}\) and \(x_{2}\) , then makes the two rectangular waves perform offset subtraction calculation, and finally obtains the error coefficient curve \(E_{{x_{1} x_{2} }}\) . The number of error point \(Y^{\prime}\) is represented on the image as the area of the non-overlapping part of the two rectangular waves after the relative translation of the covariate \(\tau\) . The smaller the area, the higher the correlation of the signal corresponding to \(\tau\) .
Compared with the traditional method, the time delay estimation method based on signal cross-zero information is not only applicable to the calculation of time delay estimation under general conditions, but can also meet the demand for time delay estimation under signal distortion conditions. Through rectangular processing, the signal can be converted into a sequence composed of 0 and 1. When small amplitude signal distortion occurs, the amplitude positive and negative information of most points on the signal curve will not change, so there will be almost no disturbance to the 0 and 1 sequence as shown in Fig. 8 . And when the signal has a large-amplitude distortion, such as the introduction of strong burst interference, due to the normalized processing method, the large-amplitude change will be converted into a limited 0, 1 sequence change, thereby effectively limiting the impact of strong interference on the overall curve and obtaining more accurate and stable time delay estimation results.
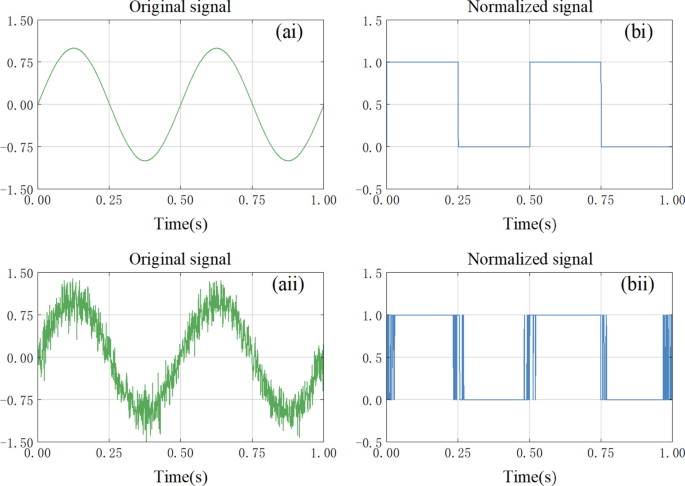
Original signal and normalized signal before/after introduction of interference
Performance evaluation test of CZIM algorithm
Several sets of representative simulation experiments were designed to research the impact of the three main factors on the CZIM algorithm which are mentioned in “ Forms and the effects of signal distortion ” section.
In order to make the simulation results closer to the actual application, the standard sound source of the coupled cavity is used to send out the signal and use standard microphone to collect the signal, we designed time delay estimation experiments under different conditions. The frequency and time delay of the simulated signal are set, the AWA14424S coupled-cavity standard sound source is controlled to emit the set signal, and the 130E22 standard microphone is used to collect the emitted sound signal. In the experiment, the standard sound source of the coupled cavity can be used to avoid environmental interference and can effectively control the signal-to-noise ratio of the signal.
Different signal-to-noise ratios
Gaussian random signal is a random signal with a normal distribution of probability density distribution, which is a common random signal (Lopes 2020 ). In the simulation experiment, two Gaussian random signals with standard deviation of 0.2 are selected as the source signals, and the channel time delay of the two signals is set to 0.5 s with a sampling rate of 10000 Hz. Uncorrelated white noise is mixed in both Gaussian signals as the interference signal, adjust white noise to make the signal-to-noise ratio (SNR) of 5 dB, 0 dB, and − 5 dB, respectively, for testing, and the time delay estimation ability of the CZIM algorithm under different SNR conditions is investigated, and the GCC algorithm is used as the control group for comparison. The results are shown in Figs. 9 and 10 .
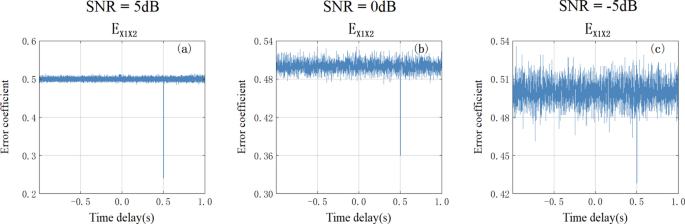
Estimation of time delay under different signal-to-noise conditions by CZIM algorithm
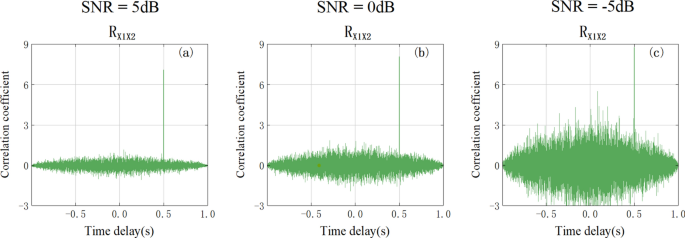
Estimation of time delay under different signal-to-noise conditions by GCC algorithm
It can be found from the experimental results that for common Gaussian random signals mixed with white noise, CZIM algorithm and GCC algorithm can accurately calculate the time delay \(d = 0.5\) under the three signal-to-noise ratio conditions and have relatively similar time delay estimation characteristics.
Lower sampling frequency
Designed time delay estimation experiments under different sampling frequencies to study the impact of high and low sampling frequencies on the CZIM algorithm, and also set the GCC algorithm as a control group. Use Gaussian random signal mixed with white noise as the experimental signal, make the signal-to-noise ratio of the signals input to the two coupled-cavity standard sound sources both − 5 dB, and set the time delay of the two signals to 0.5 s. Microphones are used to collect signals at sampling rates of 1000, 3000, and 10,000 Hz. Based on the acoustic signals collected by the microphones, the CZIM algorithm and GCC algorithm are used to calculate the time delay. The calculation results are shown in Figs. 11 and 12 .
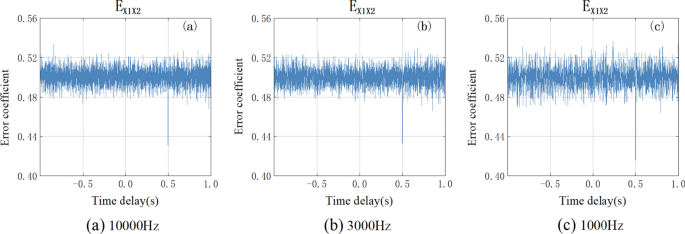
Estimation of time delay at different sampling frequencies by CZIM algorithm
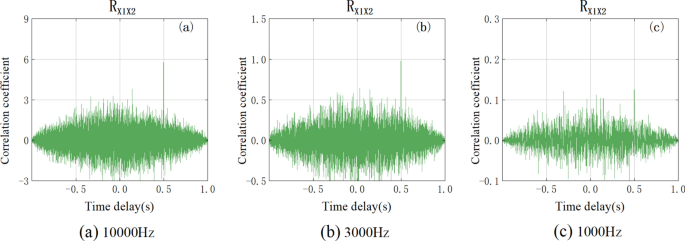
Estimation of time delay at different sampling frequencies by GCC algorithm
From Fig. 12 , it can be found that the generalized cross-correlation algorithm is more obviously affected by the sampling frequency. As the sampling frequency increases, the signal information becomes more accurate and the obtained time delay estimation results are more accurate, while at lower sampling frequencies, the GCC algorithm has difficulty in obtaining the required time delay estimation results.
Under the same conditions, the time delay estimation results of the CZIM algorithm are shown in Fig. 11 . It can be found that the CZIM algorithm is not sensitive to changes in sampling frequency and can still calculate accurate time delay even at low sampling frequencies compared to the GCC algorithm.
The low sampling rate will lead to the loss of part of the signal information, as shown in Fig. 13 , which is one of the important reasons for the error in the time delay estimation using the GCC algorithm under the condition of low sampling rate. For the CZIM algorithm, the cross-zero information of the signal is always easier to retain than the exact amplitude information under the low sampling rate, as shown in Fig. 14 , so the CZIM algorithm can perform better time delay estimation characteristics.
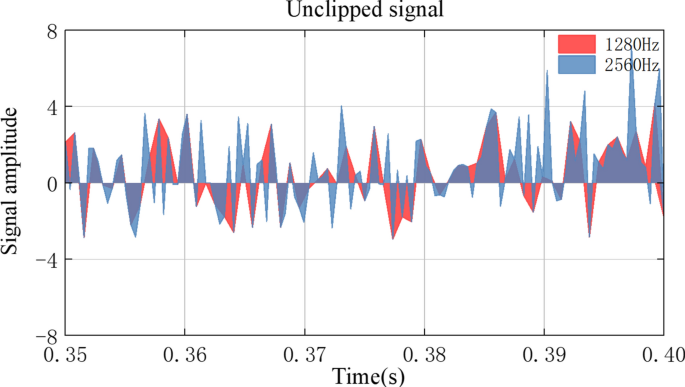
Completeness of signal information at different sampling rates
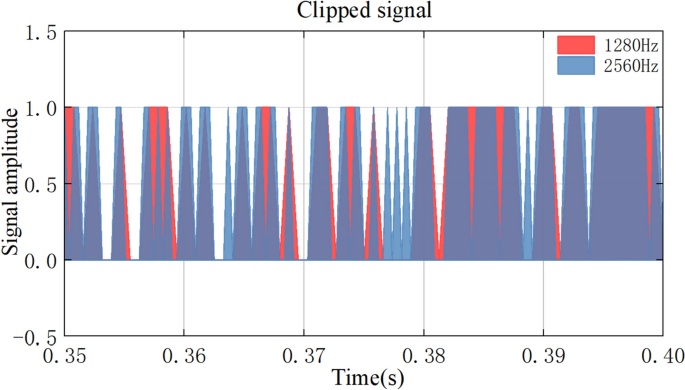
Strong interference
As mentioned in “ Strong interference noise ” section, the introduction of strong interference noise in the signal sampling process can have a significant impact on the time delay estimation results of traditional algorithms such as the GCC algorithm. As mentioned above, the CZIM algorithm will normalize the signal amplitude to reduce such effects.
In order to verify the stability of the CZIM algorithm under the condition of strong interference resistance, a simulation experiment is designed: The Gaussian random signal mixed with white noise is used as the source signal, set the signal-to-noise ratio to − 5 dB, the time delay of the two signals is 0.5 s, and the sampling rate is 10000 Hz; a triangular wave with larger amplitude is added to one of the signals to simulate strong interference noise as shown in Fig. 15 a and then use the CZIM and GCC algorithms to calculate the time delay for the two signals.
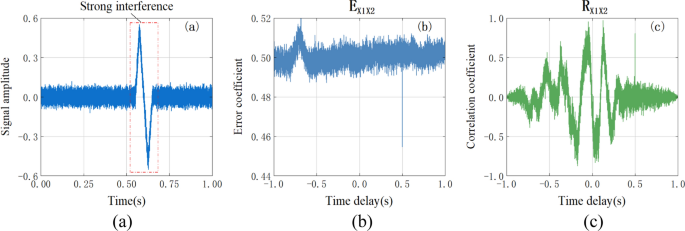
Time delay estimation under strong interference noise: a the strong interference noise; b time delay estimation result using CZIM algorithm; c time delay estimation result using GCC algorithm
The results in Fig. 15 c show that when the introduced strong interference noise amplitude reaches about 5 times the source signal amplitude, the result obtained by the GCC algorithm is completely distorted, while the CZIM algorithm is almost unaffected and the calculated time delay is very clear (shown in Fig. 15 b).
In practical applications, the distance between the detection units is generally limited so in most cases both units will be affected by strong interference noise. We need to be aware that the correlation of the strong interference signals themselves can also have a significant impact on the time delay estimation. In order to verify the applicability of the CZIM algorithm under such conditions, further simulation experiments were set up: Using the same source signals as above, strong interference noise with the characteristics shown in Fig. 16 a was added to the both signals, and the time delay between the two sets of strong interference signals was − 0.25 s. The time delay between the two channels was calculated using the CZIM algorithm and the GCC algorithm.
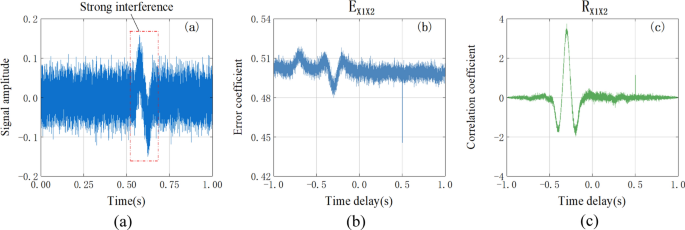
Time delay estimation under strong interference noise with correlation: a the strong interference noise; b time delay estimation result using CZIM algorithm; c time delay estimation result using GCC algorithm
It can be found that in the case where the strong interference noises are correlated, even if the amplitude of the strong interference noise is only about 1.5 times that of the source signal, the peak at d = − 0.25 s in Fig. 16 c has far exceeded the peak d = 0.5 s. In this interference situation, GCC algorithm cannot get accurate delay estimation results. The correlation of the strong interference noises just forming only a small peak in Fig. 16 b does not affect the correctness of the time delay calculation with CZIM algorithm.
When strong disturbances are introduced, both methods show different degrees of deviation, while the GCC algorithm shows a larger deviation due to the fact that the magnitude of the correlation coefficient is in a kind of proportional relationship with the amplitude; a strong interference noise with a large amplitude will cause the delay estimation result of the GCC algorithm to have a large deviation to it; for the CZIM algorithm, due to its different computing mechanism, large-amplitude variation is converted into a limited number of 0 and 1 sequence variation during normalization processing, which greatly reduces the influence of strong interference noise, and the final result is shown as a small deviation.
Leakage localization of pipe
In the pipe leakage localization research, due to the complexity of the pipe fluid acoustic system, the vibration signal collected by the sensor is often distorted, which makes the location results unstable or inaccurate, while the CZIM algorithm can reduce the impact of signal distortion to a certain extent. In order to verify the practical effectiveness of the CZIM algorithm, we built a test system for pipe leakage localization and conducted experiments. Due to the particularity of the fuel pipeline, this paper replaces the fluid medium in the pipeline with water to carry out simulation experiments.
In the experiment, the water pressure in the pressure storage tank was controlled to be constant at 0.15 MPa, and the valve at a certain position on the pipe was opened to simulate the occurrence of pipe leak. The acceleration signal was collected by the 1A315E piezoelectric acceleration sensor, and the test system is shown in Fig. 17 .
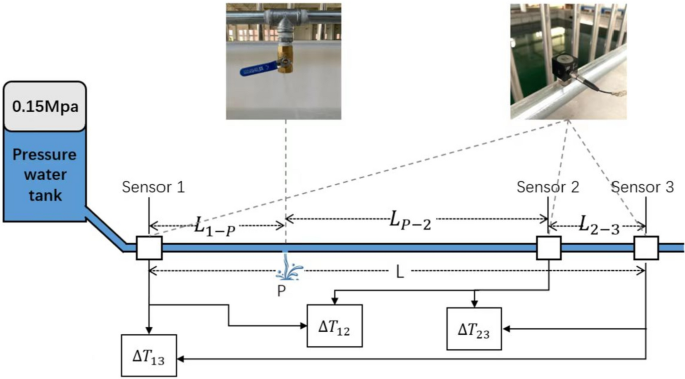
Schematic diagram of pipe leakage localization system
The three acceleration sensors are placed at points 1, 2, and 3, and the mutual distance between the three points is known. After the leak occurs at point \(p\) , the vibration signal propagates along the pipe wall to both sides and is collected by the three sensors successively, with the leak point \(p\) between points 1 and 2 as an example.
Since \(L_{2 - 3}\) (the length between point 1 and point 2) is known, the time delay \(\Delta T_{23}\) between the signals collected by sensor 2 and sensor 3 can be used to calculate the vibration signal transmission speed by
In the experiment, the speed of transmission of the vibration signal through the pipe is considered to be constant. Therefore, the time delay difference \(\Delta T_{12}\) between sensors 1 and 2 can be used to calculate the distance difference between the leak point \(p\) and the sensor sampling points 1 and 2 as follows
The locations of points 1 and 2 are known. Thus
Similarly, \(L_{2 - 3}\) can also be calculated according to the time delay \(\Delta T_{13}\) between sensor 1 and sensor 3. Since the distance difference corresponds to
Thus the verification formula can be obtained
Obviously, the key part of the leakage localization is the accurate solution of the time delay between the arrival of the leak signal at each sensor.
The power spectrum analysis of the vibration signals collected at sensor 1 and sensor 3 is shown in Fig. 18 . Sensor 1 is closer to the leak position, and sensor 3 is far away. From the peak change of the power spectrum function, it can be found that the vibration signal attenuates significantly as the distance gets farther away, but the main frequency band of the leak signal is always concentrated in 3600–4600 Hz and does not change significantly.
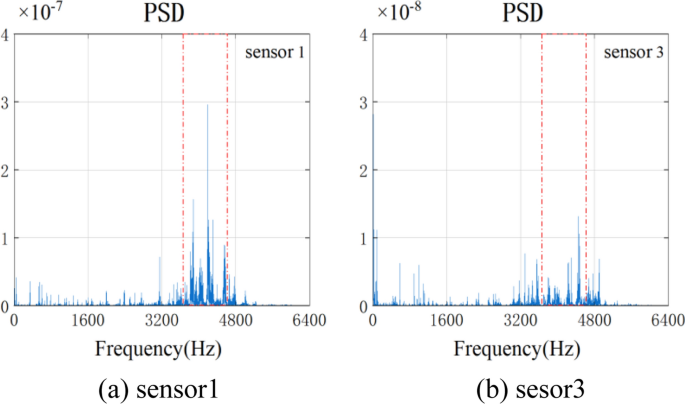
Power spectrum of signal
In order to study the time delay estimation ability of CZIM algorithm under extreme working conditions, the sampling rate is set to 12800 Hz, so that the sampling rate-to-signal dominant frequency is at a low level. The time delay is estimated using the CZIM algorithm and GCC algorithm for the signals collected by the three sensors in such operating conditions, and the experimental results are shown in Fig. 19 .
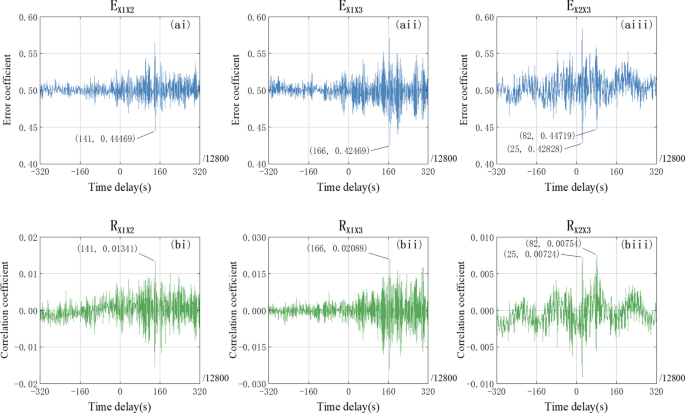
Time delay estimation results of CZIM algorithm and GCC algorithm
From the results in Fig. 19 , it can be found that the results of the CZIM algorithm and the GCC algorithm for time delays \(\Delta T_{12}\) and \(\Delta T_{13}\) are the same. But for \(\Delta T_{23}\) , the estimated time delay of the CZIM algorithm is 1.953125 × 10 −3 s (25/12800), while the estimated time delay of the GCC algorithm is 6.40625 × 10 −3 s (82/12800). By verifying Eq. ( 10 ), it can be determined that the GCC algorithm has made an error in the calculation of \(\Delta T_{23}\) , the GCC algorithm has a 69.5% ( \(\frac{82 - 25}{{82}} \times 100\%\) ) higher error rate than the CZIM algorithm, and this error would affect the solution of the vibration transfer velocity \(v\) and render subsequent calculations meaningless. Table 1 lists the leakage localization results of the CZIM algorithm and the relative error between the calculation results and the experimental setting values.
It can be found from Table 1 that even under the conditions of low delay and obvious signal attenuation, the CZIM algorithm can still accurately solve the signal time delay. And the error of solution result is only 0.04 m, achieving an error rate of only 0.167% on a 24-m class pipe.
Further analysis of the results of the time delay estimation results of the two methods can be found that at low sampling rate-to-signal dominant frequency ratio, the traditional GCC method is more likely to be affected by signal distortion and will form a large number of sub-peaks near the main peak, which affects the stability of the time delay estimation, and as the signal-to-noise ratio further decreases, the main peak will be suppressed by the sub-peaks, resulting in wrong locating results; while the CZIM algorithm proposed in the paper is less affected by signal distortion, in the estimation of time delays \(\Delta T_{12}\) , \(\Delta T_{13}\) , and \(\Delta T_{23}\) , the peaks are more obvious than those of the GCC method, especially in the estimation of time delays \(\Delta T_{23}\) , although the sub-peaks also appear at 6.40625 × 10 −3 s (82/12800), but do not exceed the main peaks and can still achieve accurate localization. Therefore, under complex signal distortion conditions caused by low signal-to-noise ratio and low sampling rate-to-signal dominant frequency ratio, the CZIM algorithm has more stable and accurate localization characteristics than the GCC algorithm.
The time delay estimation of the traditional GCC algorithm is susceptible to signal distortion. The paper analyzes the influence of several main signal distortions on the time delay estimation and proposes a time delay estimation method based on sparse cross-zero information. The method normalizes the signal amplitude, draws the error curve, and finally obtains the time delay, which can effectively reduce the influence of signal distortion.
Simulation experiments are designed to verify the theoretical correctness of the CZIM algorithm according to several major signal distortions mentioned in the paper. At the same time, the CZIM algorithm is compared with the GCC algorithm. The experimental results show that the CZIM algorithm is easy to design, simple to implement, and has a good time delay estimation effect for various use conditions, and the scope of application is relatively wide; it is insensitive to changes in sampling frequency and can be used for experiments on time delay estimation at low sampling frequencies; it can effectively overcome the influence of signal amplitude distortion and can still guarantee good results under more extreme conditions such as mixed with strong interference. The paper applies the CZIM algorithm to the pipe leakage localization experiment in a complex environment. The results show that the CZIM algorithm can still perform more accurate and stable time delay estimation under the conditions of low signal-to-noise ratio, low sampling rate to signal dominant frequency ratio, and significant signal distortion, which has obvious advantages over the traditional GCC algorithm.
In addition, the CZIM algorithm proposed in the paper adopts a new way of thinking and has a lot of room for further research. For example, in addition to the update and improvement of the algorithm, a certain degree of expansion research can also be carried out on the hardware. Up to now, a low-cost, low-power wireless sensor is being considered to be combined with the CZIM algorithm for use in the water pipe leakage localization scheme. With the successful implementation of the algorithm validation experiments, it is foreseen that the algorithm will have long-term potential in the field of acoustic localization.
Ahadi M, Bakhtiar MS (2010) Leak detection in water-filled plastic pipes through the application of tuned wavelet transforms to acoustic emission signals. Appl Acoust 71(7):634–639
Article Google Scholar
Bentoumi M, Chikouche D, Mezache A et al (2017) Wavelet DT method for water leak-detection using a vibration sensor: an experimental analysis. IET Signal Process 11(4):396–405
Brennan MJ, Gao Y, Joseph PF (2007) On the relationship between time and frequency domain methods in time delay estimation for leak detection in water distribution pipes. J Sound Vib 304(1–2):213–223
Brennan MJ, Gao Y, Ayala PC et al (2019) Amplitude distortion of measured leak noise signals caused by instrumentation: effects on leak detection in water pipes using the cross-correlation method. J Sound Vib 461:114905
Choi J, Shin J, Song C et al (2017) Leak detection and location of water pipes using vibration sensors and modified ML prefilter. Sensors 17(9):2104
Cooper BFC (1970) Correlators with two-bit quantization. Aust J Phys 23:521–527
Google Scholar
Gao Y, Brennan MJ, Joseph PF et al (2004) A model of the correlation function of leak noise in buried plastic pipes. J Sound Vib 277(1–2):133–148
Gao Y, Brennan MJ, Joseph PF (2006) A comparison of time delay estimators for the detection of leak noise signals in plastic water distribution pipes. J Sound Vib 292(3–5):552–570
Gao Y, Liu Y, Muggleton JM (2017) Axisymmetric fluid-dominated wave in fluid-filled plastic pipes: loading effects of surrounding elastic medium. Appl Acoust 116:43–49
Grant SB, Saphores JD, Feldman DL et al (2012) Taking the “waste” out of “wastewater” for human water security and ecosystem sustainability. Science 337(6095):681–686
Article CAS Google Scholar
Hu Z, Le C, Zhang Y (2018) Generalized cross-correlation time delay estimation algorithm based on frequency division in reverberation environment. Comput Eng 44(9):269–273 ( in Chinese )
Iwanaga MK, Brennan MJ, Almeida FCL et al (2022) A laboratory-based leak noise simulator for buried water pipes. Appl Acoust 185:108346
Khulief YA, Khalifa A, Mansour RB et al (2012) Acoustic detection of leaks in water pipelines using measurements inside pipe. J Pipeline Syst Eng Pract 3(2):47–54
Knapp C, Carter G (1976) The generalized correlation method for estimation of time delay. IEEE Trans Acoust Speech Signal Process 24(4):320–327
Kothandaraman M, Law Z, Gnanamuthu EMA et al (2020) An adaptive ICA-based cross-correlation techniques for water pipeline leakage localization utilizing acousto-optic sensors. IEEE Sens J 20(17):10021–10031
Li B, Zhang X (2020) Improved microphone array sound source localization method based on generalized mutual correlation. J Nanjing University Nat Sci 56(6):917–922 ( in Chinese )
Li R, Huang H, Xin K et al (2015) A review of methods for burst/leakage detection and location in water distribution systems. Water Sci Technol Water Supply 15(3):429–441
Li J, Chen Y, Qian Z et al (2020) Research on VMD based adaptive denoising method applied to water supply pipeline leakage location. Measurement 151:107153
Lopes PAC (2020) Bayesian step least mean squares algorithm for Gaussian signals. IET Signal Process 14(8):506–512
Martini A, Troncossi M, Rivola A (2015) Automatic leak detection in buried plastic pipes of water supply networks by means of vibration measurements. Shock Vib 2015:1–13
Nesta F, Omologo M (2011) Generalized state coherence transform for multidimensional TDOA estimation of multiple sources. IEEE Trans Audio Speech Lang Process 20(1):246–260
Van Vleck JH, Middleton D (1966) The spectrum of clipped noise. Proc IEEE 54(1):2–19
Wang H, Zhao X (2020) Analysis of accurate time delay extraction method based on endpoint detection and its localization accuracy. Acoust. Technol. https://kns.cnki.net/kcms/detail/31.1449.TB.20200908.0923.002.html (in Chinese)
Watts DG (1962) A general theory of amplitude quantization with applications to correlation determination. Proc IEE Part C Monogr 109(15):209–218
Zhou H, Tian Z (2020) Improvement of time de-lay estimation based on generalized mutual correlation algorithm. In: Proceedings of the 2020 Western China acoustical academic exchange conference. Editorial Board of Acoustics Technology, p 3 (in Chinese)
Download references
Acknowledgements
The authors would like to thank the members of the School of Civil Engineering of Tianjin University. We thank LetPub ( www.letpub.com ) for its linguistic assistance during the preparation of this manuscript. We also thank the associate editor and the reviewers for their useful feedback that improved this paper.
This work was supported by the National Key R&D Program of China (Grant No. 2020YFA040070) and Science and Technology Commission Innovation Fund (Grant No. 22020401023).
Author information
Authors and affiliations.
School of Civil Engineering, Tianjin University, No. 92 Weijin Road, Nankai District, Tianjin, 300072, China
Yuan Yue, Yang Qu, Zhao Wang & Jun Tang
China Academy of Launch Vehicle Technology, Beijing, 100076, China
You can also search for this author in PubMed Google Scholar
Corresponding author
Correspondence to Jun Tang .
Ethics declarations
Conflict of interest.
No conflict of interest exists in this manuscript, and manuscript is approved by all authors for publication. I would like to declare on behalf of my co-authors that the work described was original research that has not been published previously, and not under consideration for publication elsewhere, in whole or in part. All the authors listed have approved the manuscript that is enclosed.
Rights and permissions
Open Access This article is licensed under a Creative Commons Attribution 4.0 International License, which permits use, sharing, adaptation, distribution and reproduction in any medium or format, as long as you give appropriate credit to the original author(s) and the source, provide a link to the Creative Commons licence, and indicate if changes were made. The images or other third party material in this article are included in the article's Creative Commons licence, unless indicated otherwise in a credit line to the material. If material is not included in the article's Creative Commons licence and your intended use is not permitted by statutory regulation or exceeds the permitted use, you will need to obtain permission directly from the copyright holder. To view a copy of this licence, visit http://creativecommons.org/licenses/by/4.0/ .
Reprints and permissions
About this article
Yue, Y., Qu, Y., Yan, L. et al. Research on pipeline leakage localization method based on CZIM algorithm. Appl Water Sci 14 , 135 (2024). https://doi.org/10.1007/s13201-024-02112-7
Download citation
Received : 10 March 2023
Accepted : 22 January 2024
Published : 18 May 2024
DOI : https://doi.org/10.1007/s13201-024-02112-7
Share this article
Anyone you share the following link with will be able to read this content:
Sorry, a shareable link is not currently available for this article.
Provided by the Springer Nature SharedIt content-sharing initiative
- Time delay estimation
- Pipeline leak
- Acoustic localization
- Amplitude distortion
- Cross-zero information
- Find a journal
- Publish with us
- Track your research
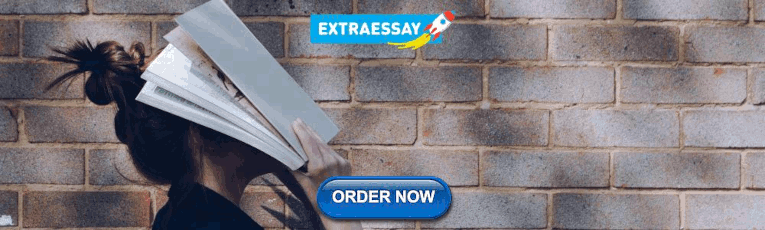
IMAGES
VIDEO
COMMENTS
This paper proposes a data-driven human-like driver model (HDM) based on the analysis and understanding of human drivers' behavior in path-tracking tasks. The proposed model contains a visual ...
This paper presents MATLAB-based programs developed for power system dynamic analysis. The programs can be used for educational purposes and research studies. With the program, time-domain simulation, system linearization, modal analysis, participation factor analysis and visualization, optimal placement of controller, feedback signal selection, frequency response analysis, and control design ...
Overview of the existing course. The existing model used to teach MATLAB was based on the traditional face-to-face lecture/tutorial/lab format. Each engineering discipline was teaching introductory MATLAB material separately within a 10-credit discipline-specific course, e.g. in Mechanical Engineering within the course 'Mechanical Engineering Design 2', and in Civil Engineering within the ...
This paper develops a Matlab/Simulink-based power system simulation toolbox for power system research and education, called MatPSST. It has been used in research and teaching at HUST. A series of achievements about research and education have been obtained based on MatPSST. In this paper, the motivations and design philosophy have been introduced.
This paper presents an open-access Matlab/Simulink-based power system simulation toolbox (MatPSST) for research and education. In MatPSST, dynamic modeling is imple-mented by Simulink. Only the initialization process is coded in Matlab. This structure makes full use of Simulink, enabling users to fully use the rich solvers and built-in tool-
CHAPTER 2 MATLAB FOR SIGNAL PROCESSING 19 2.1 Chapter Objectives 19 2.2 Introduction 19 2.3 What Is MATLAB? 19 2.4 Getting Started 20 2.5 Everything Is a Matrix 20 ... who can make use of signal processing in a research context. The examples given to introduce the topics have been chosen to clearly introduce the motivation behind the topic and ...
In this paper, we investigate to which degree recent research on tools for model-based development of embedded systems meets the requirements for replicability of experimental results. We focus on tools for MATLAB/Simulink (referred to as Simulink, for short), which has emerged as a de facto standard for automatic control and digital signal ...
Image processing and the analysis of images in order to extract relevant data is an ever-growing topic of research. Although there are numerous methods readily available, the task of image preprocessing and feature extraction requires developing specific algorithms for specific problems by combining different functions and tweaking their parameters. This paper proposes a framework that allows ...
The key content of the research in the field of technology and engineering application. ... Based on the constitutive model of concrete material provided by MATLAB, this paper mainly introduces the fixed dispersion crack model used in the concrete tension area. ... Research and implementation of the information management system of construction ...
This paper focuses on the research of simulation model and experiment of a novel energy storage system (ESS). This novel ESS is dedicated to supplying power flow effectively for a new type of linear engine, which is used in alternative energy vehicle firstly. The control strategy has been proposed based on the ESS model, which adopts bidirectional four modes of operation to control the power ...
Matrix Laboratory (MATLAB) is a high-performance interactive software package developed by Math Works Inc. (2009) scientific and engineering computing [ 2 ]. MATLAB allows matrix operations, algorithm implementation, simulation, drawing functions and data, signal and image processing, and image processing toolbox.
University of Massachusetts Amherst. University of Texas at Austin. Researchers worldwide are doing applied research using artificial intelligence with the help of MATLAB and Simulink in collaboration with MathWorks. Explore featured researchers, project topics, and related publications.
Design and kinematic analysis of representative mechanism is presented with geometrical analysis for the inverse kinematic of the mechanism by using desired motion concept of slider. The mechanism is modelled in MATLAB/Simulink platform. The simulation results are presented herein. Export citation and abstract BibTeX RIS.
The developed simulator based on MATLAB's 5G toolbox was then modified to incorporate the functionality of carrier aggregation. Finally, the signal generated with the simulator was loaded into an Arbitrary Waveform Generator (AWG) and propagated to an oscilloscope, first using a back-to-back electrical configuration and then using an optical link.
MATLAB Online helps makes it easier to use. Of course, your users are free to download your code from GitHub or use command line git to clone the rep ository. They can then make use of their desktop version of MATLAB to work with your research.
Share Your Research. Contribute to the broader scientific community by building standalone applications, sharing code, and inspiring others. Integrate MATLAB code and Simulink models with other languages, such as C/C++ and Python. Publish your code on File Exchange and GitHub. Discover how you can engage in open science with MATLAB (3:33).
This paper presents a review of the many simulation tools that have been reported for modeling and managing the energy for Hybrid Electric Vehicle (HEV).In all one hundred and twenty-five simulation tools have been identified by various researchers and among them few tools have been summarized to facilitate selection of the most appropriate tools for specific tasks.
In 1996, he published his first MATLAB book in Chinese, entitled "Computer aided Control System Design with MATLAB Applications" by Tsinghua University Press in China, which was regarded as the first in China, and one of the earliest in the world. In more than 30 years of time, he published 12 books in English plus numerous books in Chinese.
Feature papers represent the most advanced research with significant potential for high impact in the field. ... The software environment used in this experiment is also the MATLAB R2023b under the Windows 11 system. ... Dai, X. Research on Radar Plot Classification Based on Fully Connected Neural Network. In Proceedings of the 2019 3rd Interna ...
In this paper, we describe our AlphaFold 3 model with a substantially updated diffusion-based architecture, which is capable of joint structure prediction of complexes including proteins, nucleic ...
The second phase, dubbed "introduction," is proving that a certain person is connected to a specific face. Then, using a duplicate of the scene, a model for facial image recognition—also known as face recognition—is created. One of the biometrics technologies that has attracted the most research focus is facial recognition.
Satellite formation flying technology currently represents a focal point in space mission research. Traditional spacecraft payload performance and lifespan are often constrained by propellant limitations. Electromagnetic Formation Flying (EMFF), a propellant-free formation flying technique, has garnered widespread attention. Its inherent strong nonlinearity and coupling present challenges for ...
Mentored research program focused on animal models of brain injury, neural-glial interactions and cell edema in the CNS, and sex-differences in brain excitability. ... activity in awake, behaving mice. The candidate will learn 2-photon imaging data processing and analysis using MATLAB, Fiji/ImageJ, and Excel software. In addition, there is an ...
Lithium-rich manganese-based cathode materials gain increasing research attention because of their superior specific capacity and high operating voltage. However, it suffers voltage decay and unsatisfied stability during cycling. Meanwhile, the lithium-rich cathode materials could barely be stably operated a Journal of Materials Chemistry A HOT Papers
Regression analysis is a method to analyze the relationship between a dependent variable and an independent variable. Which is one of the most powerful tools for statistical data analysis. Through mining 14 groups of experimental data, this paper tries to use this method to build the model and calculate the model parameters. Then the significance test is carried out on the analysis results by ...
In the first part, the dynamic model of DC moto r speed control is presented (Fig. 4). The DC motor specifications are as follows: Shaft power of the motor = 1 kw. Va is armature voltage applied ...
The time difference of arrival is a common method to find the leakage point of water pipeline. The leakage point localization is achieved by calculating the time delay between the signals reaching different sensors. Mainstream time delay estimation algorithms based on signal correlation analysis are susceptible to the introduction of noise signals, low sampling rates, and signal clipping ...