
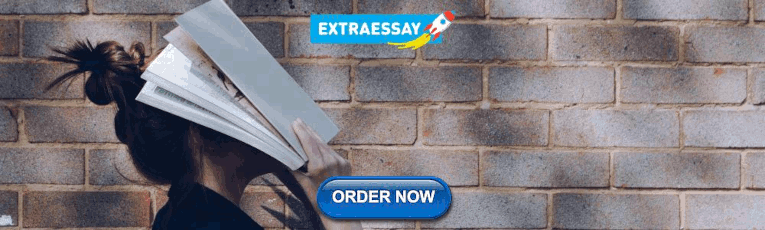
Trusted Market Research Company in Hyderabad
ResearchFox is known to stand out as a premier market research firm located in the capital city Hyderabad, specializing in delivering comprehensive and insightful market research services. We prioritize to support businesses and organizations across diverse industries in gaining valuable perspectives on market trends, consumer behavior, competitive analysis, and other crucial factors.
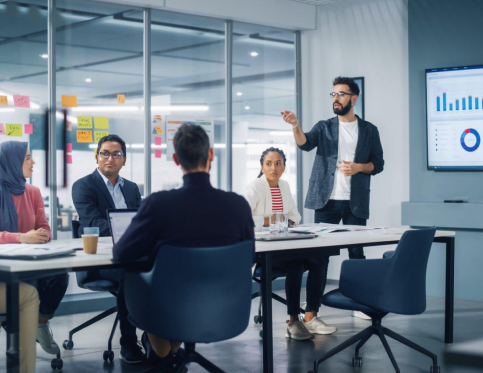
ResearchFox, a market research agency based in Hyderabad, is dedicated to serving businesses of all sizes, including startups and established enterprises. Our team of industry experts excels in providing tailored solutions that meet the unique needs of each client. Partnering with ResearchFox allows entrepreneurs to leverage our extensive expertise, gaining in-depth insights into market demand, target audience identification, and strategic business refinement.
Quantifying Our Success
Years of experience, client engagements, market research report, case studies.
Our mission at ResearchFox is to be your dependable partner, we leverage our unparalleled market research expertise to provide invaluable insights that will empower strategic decision-making.
Standing Out among the Top Market Research Agencies In Hyderabad
ResearchFox, being one of the top market survey companies in Hyderabad has always proved being trustworthy. Researchfox emerges as an unrivaled leader in the realm of market research firms of Hyderabad. Known for its unparalleled services and unmatched industry acumen, Research comprises a team of seasoned researchers and analysts committed to delivering best market insights that propel business growth and triumph. Setting new benchmark in excellence,precision and ingenuinity. We differentiate ourselves from other market research companies in Hyderabad by employing the state of art of methodologies,advanced data analytics techniques and a customer centric approach.

Best Service oriented market Research Company in Hyderabad
Our unwavering focus lies in providing actionable intelligence that yields tangible outcomes for our esteemed clients. We have an impeccable track record,profound market acuity,and an enviable reputation. Researchfox remains top among the market research companies in Hyderabad due to undisputed choice to discerning organizations seeking market research prowess and survey expertise in Hyderabad.Our focus is in providing actionable intelligence that leads to tangible results for our clients.
Quantitative Research:
This service involves the collection and analysis of numerical data to quantify market trends, consumer behaviors, and statistical patterns. It focuses on generating statistical insights to understand the magnitude and relationships between variables.
Qualitative Research:
This service emphasizes gathering non-numerical data through methods such as interviews, focus groups, and observations to explore consumer attitudes, motivations, and perceptions in depth. It provides rich, descriptive insights into consumer experiences and opinions.
Surveys involve the use of questionnaires or structured interviews to collect data from a sample population. This service allows for standardized data collection and analysis, enabling the measurement of opinions, preferences, and behaviours.
Focus Groups Discussion:
Focus groups are interactive group discussions facilitated by a moderator. This service helps gain qualitative insights by exploring participants' perspectives, attitudes, and experiences related to a specific product, service, or topic.
Interviews (Online/offline):
Interviews, whether conducted online or offline, involve one-on-one interactions with participants to gather detailed information, opinions, or experiences. This service allows for in-depth exploration of specific topics or individual perspectives.
Observational Research:
This service involves the collection and analysis of numerical data to quantify market trends, consumer behaviours, and statistical patterns. It focuses on generating statistical insights to understand the magnitude and relationships between variables.
Online Community Research:
Online community research involves studying and engaging with online communities to gather insights, opinions, and trends related to specific markets or consumer segments. It leverages digital platforms to access and analyze consumer discussions and interactions.
Ethnographic Research:
Ethnographic research immerses researchers in the natural environments and daily lives of consumers to gain a deep understanding of their behaviours, cultures, and experiences. This service emphasizes observing and interpreting behaviour within its social and cultural context.
Market Segmentation:
Market segmentation involves dividing a market into distinct groups based on shared characteristics, behaviours, or preferences. This service enables businesses to tailor their marketing strategies and offerings to specific target segments, optimizing their approach.
Competitive Analysis:
Competitive analysis involves researching and analyzing competitors to understand their strengths, weaknesses, strategies, and market positioning. This service provides insights to help businesses gain a competitive advantage and identify opportunities for growth.
Brand Research:
Brand research focuses on assessing brand perception, awareness, loyalty, and associations among consumers. This service helps businesses understand how their brand is perceived in the market and informs brand development strategies.
Concept Testing:
Concept testing involves evaluating new product or service concepts among target consumers to assess market acceptance, demand, and potential success. This service helps refine and optimize concepts before launch.
Customer Satisfaction and Experience Research
This service focuses on measuring and understanding customers' satisfaction levels and overall experience with a product, service, or brand. It helps businesses identify areas for improvement and enhance customer loyalty.
Product Testing and Concept Evaluation:
Product testing involves assessing the performance, functionality, and appeal of a product or service. Concept evaluation aims to understand consumers' perceptions and preferences regarding new product concepts. Both services provide insights to refine and optimize product offerings.
Pricing Research:
Pricing research helps businesses determine the optimal price point for their products or services. It involves analyzing consumer price sensitivity, competitive pricing strategies, and market dynamics to inform pricing decisions.
Market Entry and Expansion Studies:
These studies help businesses assess the feasibility and potential success of entering new markets or expanding existing operations. They involve analyzing market size, competition, consumer behaviours, and regulatory factors to make informed market entry decisions.
Trend Analysis and Forecasting:
Trend analysis involves examining historical data and patterns to identify market trends and anticipate future developments. Forecasting utilizes statistical techniques to predict future market conditions, demand, and consumer behaviour.
Mystery Shopping:
Mystery shopping involves hiring individuals to pose as customers and evaluate the quality of service, compliance with standards, and overall customer experience. It provides objective feedback on staff performance and identifies areas for improvement.
Social Media Listening:
This service involves monitoring and analyzing social media platforms to gain insights into consumer opinions, sentiments, and trends. It helps businesses understand brand perception, identify potential issues, and engage with their target audience.
Neuromarketing:
Neuromarketing combines neuroscience and market research to understand consumer responses at a subconscious level. It leverages techniques such as brain imaging, eye-tracking, and biometrics to study consumer behaviour and inform marketing strategies.
Eye-tracking Studies:
Eye-tracking studies use specialized technology to measure and analyze where and how consumers focus their visual attention. This service helps assess the effectiveness of advertisements, packaging, website design, and other visual stimuli.
A/B Testing:
A/B testing involves comparing two versions of a marketing element (e.g., website design, advertisement) to determine which one performs better. It helps optimize marketing strategies by identifying the most effective option based on consumer response.
Customer Journey Mapping:
Customer journey mapping illustrates brand interactions and touchpoints during a customer's buying journey. It helps businesses identify pain points, optimize customer experiences, and enhance overall satisfaction.
Secondary Research:
Secondary research includes collecting and analyzing existing data and info from diverse sources like industry reports, publications, government databases, and academic studies. It provides a foundation of knowledge and insights to support primary research and inform decision- making.
Net Promoter Score (NPS):
NPS is a metric used to measure customer loyalty and gauge the likelihood of customers recommending a product, service, or brand to others. It helps businesses assess customer satisfaction and loyalty levels to identify promoters, passives, and detractors.
Conjoint Analysis:
Conjoint analysis is a quantitative research technique used to measure and understand how consumers make trade-offs between different product or service attributes. It helps businesses determine the relative importance of product features and optimize their offerings.
Sentiment Analysis:
Sentiment analysis entails examining textual data, like social media posts, customer reviews, and survey answers, to ascertain consumer sentiments. It helps businesses understand public opinion, identify trends, and assess brand perception.
Sensory Research:
Sensory research focuses on understanding how consumers perceive and respond to sensory stimuli such as taste, smell, touch, and sight. It helps businesses develop products that appeal to consumers' sensory preferences and enhance the overall experience.
Experimental Design:
Experimental design involves planning and conducting controlled experiments to test hypotheses and measure the impact of specific variables on consumer behaviour or outcomes. It helps establish causal relationships and provides valuable insights for decision-making.
Geographical Research:
Geographical research examines and analyzes geographic factors that influence consumer behaviour, market dynamics, and business operations. It helps businesses understand regional differences, target specific geographic markets, and optimize distribution strategies.
Mobile Research:
Mobile research focuses on gathering data and insights using mobile devices, such as smartphones and tablets. It leverages mobile technologies to collect real-time data, conduct surveys, and capture consumer behaviours in mobile-centric contexts.
Diaries and Journals:
Diaries and journals involve collecting qualitative data from participants who maintain written records of their experiences, opinions, or behaviours over a specific period. It provides rich, longitudinal insights into consumer behaviours and perceptions.
Co-creation Sessions:
Co-creation sessions involve collaborative workshops or sessions with customers, stakeholders, or experts to generate new ideas, develop concepts, or solve problems. It encourages active participation, fosters creativity, and helps businesses gain insights for product or service innovation.
Car Clinic:
Car clinic research focuses specifically on the automotive industry, involving testing and evaluating new car models, features, or prototypes among target customers. It helps manufacturers understand consumer preferences, assess product performance, and gather feedback for improvement.
Feasibility:
Feasibility research assesses the viability and potential success of a business idea, product, or service. It involves evaluating market demand, competition, financial factors, and other relevant aspects to determine the feasibility and potential risks or challenges.
Catchment Analysis:
Catchment analysis involves analyzing the demographic, geographic, and economic characteristics of a specific area to understand the potential customer base and market opportunities. It helps businesses identify target markets and optimize location-based strategies.
Mystery Audit:
Mystery audits are conducted by undercover auditors who evaluate various aspects of a business's operations, such as customer service, compliance with standards, and overall quality. It provides objective feedback and helps identify areas for improvement.
Brand Understanding:
Brand understanding research focuses on gaining insights into how consumers perceive and understand a brand. It explores brand awareness, associations, personality, and positioning to help businesses develop effective brand strategies.
Brand Perception:
Brand perception research focuses on understanding how consumers perceive and interpret a brand. It delves into consumers' attitudes, emotions, beliefs, and associations with the brand. This research helps businesses assess brand positioning, messaging effectiveness, and brand equity.
Competition Analysis:
Competition analysis involves studying and analyzing the competitive landscape in which a business operates. It examines competitors' strategies, strengths, weaknesses, market share, and customer perceptions. This research helps businesses identify competitive advantages, anticipate market trends, and develop effective differentiation strategies.
MARKET RESEARCH PROCESS

Define Research Objectives
This is the initial step where the purpose and goals of the research project are established. It sets the direction for the entire research process.

Define Research Identify Data Sources
This involves determining the appropriate sources from which to gather data. It could include primary data sources (e.g., surveys, interviews, observations) and secondary data sources (e.g., existing reports, databases, publications).

Gather Data
This stage focuses on collecting data through various methods such as surveys, focus groups, interviews, observational research, online community research, and more.

Organize and Clean Data
Once the data is collected, it needs to be organized and cleaned to ensure accuracy and consistency. This involves tasks such as data entry, data coding, and data validation.

Analyze Data
Data analysis is the process of transforming raw data into meaningful insights. This involves using statistical techniques, qualitative analysis methods, or other analytical approaches to identify patterns, trends, and relationships within the data.

Interpret Findings
Once the data is analyzed, the findings are interpreted to gain a deeper understanding of the research questions or objectives. This involves identifying key insights, exploring correlations, and interpreting the results in the context of the research objectives.

Draw Conclusions
Based on the interpreted findings, conclusions are drawn to summarize the key findings and implications of the research. Conclusions are often supported by statistical analysis or qualitative evidence.

Make Recommendations
Recommendations are formulated based on the conclusions drawn from the research findings. These recommendations provide actionable insights and guidance for decision-making or strategic planning.

Communicate Findings
The final step is to effectively communicate the research findings, conclusions, and recommendations to stakeholders. This could involve preparing reports, presentations, or other forms of communication to ensure the findings are understood and utilized
Why Choose ResearchFox for Market Research Services?
Expertise and experience, objectivity and unbiased approach, access to resources and tools, efficient and timely execution, cost-effectiveness, access to diverse expertise, focus on core competencies, confidentiality and data security, customization and flexibility, long-term partnership, unlock insights,drive success.
Contact us for market research solutions that fuel your business growth.
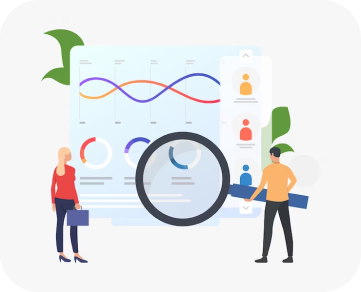
Industries We Are Providing Market Research Services
ResearchFox provides comprehensive market research services in Ahmedabad for the automotive industry, offering insights on market trends, consumer preferences, and emerging technologies. We help automotive companies make data-driven decisions for product development, market expansion, and branding strategies.
ResearchFox conducts research for the insurance industry, offering insights on market dynamics, customer preferences, and emerging trends. We help insurance companies optimize product development, pricing strategies, and customer experience to stay competitive in a rapidly changing market.
ResearchFox offers market research services for the healthcare industry, providing insights on pharmaceuticals, medical devices, healthcare IT, and healthcare services. We help healthcare organizations optimize product development, patient experience, pricing, and healthcare delivery.
Logistics and Supply Chain
ResearchFox provides market research services for the logistics and supply chain industries, offering insights into market trends, customer preferences, and competitive analysis. We help companies optimize their operations, improve efficiency, and identify growth opportunities in the logistics and supply chain sectors.
ResearchFox conducts in-depth research on emerging technologies, market trends, and consumer behaviour for technology companies. We provide insights for informed decisions on product development, market-entry, and strategic partnerships.
Construction
ResearchFox conducts research for the construction industry, providing insights into market trends, customer preferences, and competitive analysis. We help construction companies make data-driven decisions on project selection, market-entry, and strategic partnerships.
ResearchFox analyzes consumer behaviour, market trends, and competitive dynamics to provide insights into the retail industry. We help retailers optimize operations, enhance customer satisfaction, and develop effective marketing strategies.
Beauty and Personal Care
ResearchFox offers market research services for the beauty and personal care industry, providing insights into consumer preferences, product trends, and competitive analysis. We help companies develop innovative products, improve brand positioning, and optimize marketing strategies in the dynamic beauty and personal care market.
Consumer goods
ResearchFox offers tailored market research services for consumer goods companies, helping them meet evolving consumer demands. We provide insights into consumer preferences, product innovation, branding, pricing strategies, and market entry.
Home and Garden
ResearchFox conducts research on the home and garden industry, offering insights on market trends, consumer preferences, and competitive analysis. We help companies develop product strategies, enhance customer experience, and optimize pricing in the home and garden sector.
Financial Services
ResearchFox provides insights on market trends, customer behaviour, and regulatory changes for financial institutions. We help them enhance customer experience, develop new products, and improve risk management.
Sports and Fitness
ResearchFox provides market research services for the sports and fitness industry, offering insights into market trends, consumer behaviour, and competitive analysis. We help companies develop effective marketing strategies, optimize product offerings, and identify growth opportunities in the sports and fitness market.
Food and Beverage
ResearchFox analyzes consumer trends, preferences, and behaviour in the food and beverage industry. We provide insights for developing winning product strategies, optimizing pricing, and improving customer experience.
Fashion and Apparel
ResearchFox conducts research for the fashion and apparel industry, providing insights into consumer trends, brand preferences, and competitive analysis. We help fashion companies stay ahead of the latest trends, optimize product offerings, and enhance brand positioning.
Pharmaceuticals
ResearchFox offers specialized market research services for pharmaceutical companies, providing insights on drug development, market entry strategies, pricing, and patient behaviour.
Pharmaceuticals and Healthcare
ResearchFox offers specialized market research services for the pharmaceuticals and healthcare industry, providing insights into drug development, market dynamics, and patient preferences. We help pharmaceutical companies optimize product strategies, navigate regulatory landscapes, and identify market opportunities.
Energy and Utilities
ResearchFox conducts research on market dynamics, consumer trends, and regulatory landscapes for the energy and utilities sector. We help companies make informed decisions on renewable energy, market-entry, and pricing strategies.
ResearchFox conducts research for the chemicals industry, offering insights on market trends, customer preferences, and competitive analysis. We help companies in sectors such as speciality chemicals, industrial gases, and polymers make informed decisions to drive growth and innovation.
Manufacturing
ResearchFox provides market research services for the manufacturing industry, offering insights into market trends, competitive analysis, and customer preferences. We help manufacturers optimize product development, pricing, and market expansion.
Environmental Services
ResearchFox provides market research services for the environmental services industry, offering insights into market trends, regulatory changes, and customer preferences. We help companies optimize their service offerings, identify emerging opportunities, and drive sustainability initiatives.
Telecommunications
ResearchFox analyzes market trends, consumer behaviour, and technological advancements in the telecommunications industry. We provide insights for companies to improve customer experience, optimize pricing strategies, and drive innovation.
Information Technology
ResearchFox offers market research services for the information technology sector, providing insights on emerging technologies, market trends, and customer preferences. We help technology companies make informed decisions on product development, market-entry, and strategic partnerships.
Media and Entertainment
ResearchFox offers market research services for the media and entertainment industry, providing insights into consumer preferences, content trends, and digital transformation. We help companies make informed decisions on content development, distribution strategies, and audience targeting.
Renewable Energy
ResearchFox conducts research on the renewable energy industry, offering insights into market trends, regulatory landscapes, and customer preferences. We help companies in sectors such as solar, wind, and hydropower optimize their strategies, identify market opportunities, and drive sustainable growth.
Travel and Hospitality
ResearchFox conducts research on travel and hospitality industry trends, customer preferences, and competitive analysis. We provide insights for companies to enhance customer experience, develop effective marketing strategies, and optimize pricing.
Legal Services
ResearchFox provides market research services for the legal services industry, offering insights on market trends, client preferences, and competitive analysis. We help law firms and legal professionals make informed decisions to enhance their service offerings and client satisfaction.
ResearchFox offers market research services for the e-commerce industry, providing insights into consumer behaviour, market trends, and competitive analysis. We help companies optimize their e- commerce strategies, improve customer satisfaction, and drive growth.
Hospitality and Tourism
ResearchFox conducts research for the hospitality and tourism industry, providing insights into market trends, customer preferences, and competitive analysis. We help companies in sectors such as hotels, travel agencies, and attractions optimize their offerings, improve guest experiences, and drive revenue growth.
ResearchFox conducts research on the education sector, providing insights into market trends, student preferences, and technological advancements. We help educational institutions make informed decisions on program development, student recruitment, and online learning strategies.
Government Contracting
ResearchFox provides market research services for government contracting, offering insights on market trends, procurement processes, and competitive analysis. We help companies navigate the complex government landscape, identify contract opportunities, and develop winning strategies to secure government contracts.
Real Estate
ResearchFox offers market research services for the real estate industry, providing insights into market trends, customer preferences, and investment opportunities. We help companies make data-driven decisions on property development, pricing, and market entry.
Transportation and Logistics
ResearchFox conducts research for the transportation and logistics industry, providing insights into market dynamics, customer preferences, and emerging trends. We help companies optimize their supply chain, improve operational efficiency, and identify growth opportunities in the transportation and logistics sector.
Agriculture
ResearchFox conducts research on the agricultural industry, offering insights into market trends, consumer preferences, and technological advancements. We help companies optimize product development, pricing strategies, and market expansion.
Market Research and Consulting
ResearchFox specializes in market research and consulting services, offering insights on market trends, consumer behaviour, and competitive analysis across various industries. We provide custom research solutions to help businesses make informed decisions, optimize strategies, and drive growth in their respective markets.
Non-profit Organizations
ResearchFox provides market research services for non-profit organizations, offering insights on donor behaviour, fundraising strategies, and program effectiveness. We help organizations make informed decisions to achieve their missions effectively.
Human Resources and Staffing
ResearchFox offers market research services for the human resources and staffing industry, providing insights on talent acquisition, employee engagement, and workforce trends. We help companies optimize their HR strategies, improve recruitment and retention efforts, and enhance organizational performance.
Professional Services
ResearchFox offers market research services for professional services firms, providing insights on market trends, client preferences, and competitive analysis. We help businesses in sectors such as consulting, legal services, accounting, and marketing make informed decisions to enhance their service offerings and expand their client base.
Engineering and Architecture
ResearchFox conducts research for the engineering and architecture industry, offering insights on market trends, project opportunities, and competitive analysis. We help companies optimize their project portfolios, identify emerging trends, and make informed decisions to drive innovation and success.
Social Services
ResearchFox provides market research services for the social services sector, offering insights on social issues, program effectiveness, and community needs. We help organizations in areas such as healthcare, education, and non-profit sectors make data-driven decisions to better serve their target communities and fulfil their missions effectively.
Why Choose Researchfox among other Market Research Companies in Hyderabad?
Our journey of pioneering in the market research industry we have developed a deep understanding of Hyderabad’s unique market dynamics. As the leading market research company in Hyderabad, ResearchFox stands out from the competition in several key ways. Our expertise, experience, and dedication to delivering exceptional results set us apart. With a team of seasoned researchers and analysts, we have an in-depth understanding of the local market landscape, enabling us to provide valuable insights and strategic recommendations tailored to our clients' specific needs.
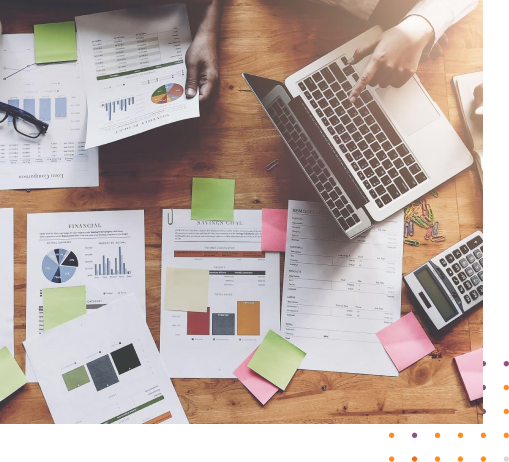
Benefits of Choosing Researchfox as your Market Research firm in Hyderabad
Informed decision making, roi optimization, understanding customer needs, enhanced customer satisfaction, competitive advantage, marketing effectiveness, identifying opportunities, business growth and expansion, risk mitigation, strategic planning, our clients.

CASE STUDIES

IMPACT OF CULTURE ON DIFFERENT SEGMENTS OF ONLINE GROCERY SHOPPERS
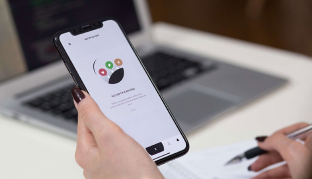
USABILITY TESTING OF MOBILE APP & WEBSITE WITH POTENTIAL ELECTRIC TWO-WHEELER BUYERS
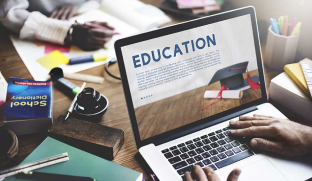
CONSUMER RESEARCH- ONLINE EDUCATION

EVALUATION AND ANALYSIS OF AD CAMPAIGNS

BLIND PRODUCT TESTING - FOOD
ResearchFox differentiates itself through its comprehensive and customized approach to market research. We merge cutting-edge methodologies, advanced data analysis techniques, and industry expertise to deliver actionable insights that drive business growth and success.
Absolutely, ResearchFox is well-equipped to handle large-scale market research projects. We have a dedicated team of researchers, analysts, and project managers who are experienced in managing and executing complex research initiatives efficiently and effectively.
Ensuring the accuracy of collected data is a top priority for ResearchFox. We employ rigorous quality control measures throughout the research process, including careful sampling techniques, data validation checks, and thorough data analysis to ensure the reliability and accuracy of the insights we provide.
Yes, ResearchFox specializes in providing customized market research solutions tailored to the unique needs of each client. We understand that every business has different research objectives, and our team works closely with clients to design research methodologies and deliver insights that align with their specific requirements.
Absolutely, ResearchFox has extensive experience in conducting competitor analysis. We help clients gain a comprehensive understanding of their competitive landscape by analyzing competitors' strengths, weaknesses, market positioning, and strategies. Our insights enable clients to identify opportunities and develop effective strategies to stay ahead of the competition.
Clients can expect a range of deliverables from ResearchFox, including comprehensive research reports, data analysis, charts and graphs, executive summaries, and strategic recommendations. Our deliverables are designed to provide clear and actionable insights that support informed decision-making..
TESTIMONIALS

“I am extremely impressed with the market research services provided by ResearchFox. Their team of experts conducted meticulous research, delivering comprehensive reports and strategic recommendations tailored to our specific needs. Their attention to detail, promptness, and clear communication have been outstanding. ResearchFox has truly helped us gain a competitive edge in our industry, and I wholeheartedly endorse their services to anyone seeking top-quality market research in Gurgaon.”
-Michael Cheek
“ResearchFox has exceeded our expectations in every aspect. Their market research expertise has been instrumental in shaping our business strategy. The team's professionalism, deep industry knowledge, and ability to deliver accurate and actionable insights have been invaluable. ResearchFox has become our trusted partner, and I highly recommend their market research services to any company looking for reliable and insightful guidance in Gurgaon.”
-Neha Gourkar
"ResearchFox has been an exceptional market research company to work with. Their team took the time to understand our specific needs and objectives, conducting in-depth research that provided us with a clear understanding of market trends and customer preferences. The insights and recommendations they provided have greatly influenced our decision-making process. I am truly impressed with their dedication, expertise, and the value they bring to the table. I highly recommend ResearchFox to anyone seeking reliable and professional market research services in Gurgaon."
-Prathiba Singh
# 4 & 5, 1st & 2nd Floor,
1st Cross, Krishna Reddy Colony,
Domlur Layout,
India – 560 071
Contact(IN):+ 91-99864-32408
Contact(IN):+ 91-97410-32859
Email: [email protected]

WE ARE VERY SOCIAL
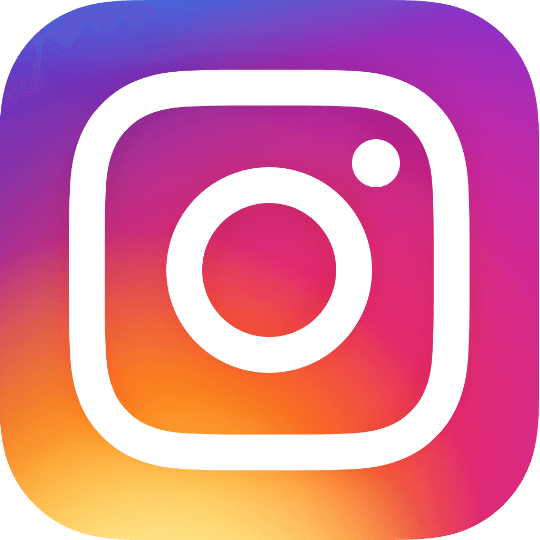
ResearchCafe
EmergingCitiez
Press Releases
OUR SERVICES
Consumer Research
Custom Research
Competitive Intelligence
Content Strategy
Data Management & Analytics
Market Intelligence
Procurement Intelligence
Sales Enablement
SMB Trend Tracker
Specialized Event Support
Startup Ecosystem
Startup Feasibility
OUR PRODUCTS
Subscribe to our newsletter.
When we analyze and discover new insights, we love sharing them with you and keeping you updated through our newsletter.
- Agricultural Sciences
- Astronomy & Space Sciences
- Chemical Sciences
- Cognitive Sciences and Psychology
- Computer Sciences and IT
- COVID-19 Research
- Earth, Atmosphere & Environment Sciences
- Energy Sciences
- Engineering Sciences
- Life Sciences & Biotechnology
- Mathematical Sciences
- Material Sciences
- Medical Sciences
- Pharmaceutical Sciences
- Physical Sciences
- Traditional Knowledge
- Other Areas
- Institutional
- International
- Grants for Seminar and Conferences
- Startup Grants
- Ministries & Departments
- Centres of Excellence
- Thematic Centres
- Centres of Higher Learning
- National Academies
- Statewise S&T Organisations
- Industry Related Associations
- Laboratories
- International Organisations
- Civil Societies
- Science Centres & Planetaria
- All Programmes & Schemes
- Research and Development
- Human Resource and Development
- Women Schemes
- International Programmes
- Societal Development
- Academia Industry Partnerships
- School Students
- Graduate Students
- Post Graduate Students
- PhD Scholars
- Post Doctoral Fellowships
- Scholarships for Women
- Faculty and Scientists
- National Fellows
- Grassroot Innovations
- Industrial Innovations
- COVID-19 Technology
- Earth, Atmosphere & Env. Sciences
- Rural Technologies
Advanced Numerical Research & Analysis Group
Institute State: Telangana Institute Category: Central Sector - Labs & Research Institutions Institute Website: http://www.drdo.gov.in District/Town/City: Kanchanbagh, Hyderabad Contact Info: director[at]anurag[dot]drdo[dot]in Ministry: Defence Research and Development Organisation (DRDO), Govt of India
Hyderabad, India
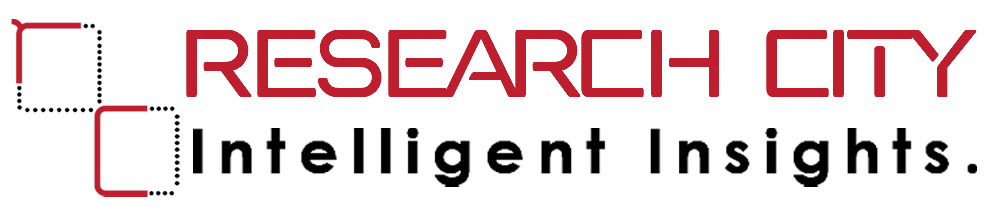
[email protected]
040-40103771.
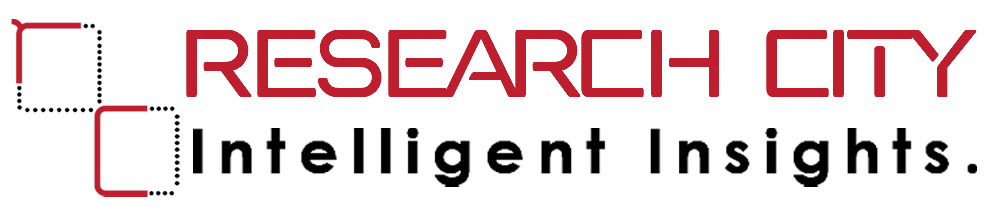
- Case Studies
- Let's Talk
Welcome to Research City
A market research company in Hyderabad, India. We help you in making intelligent and data driven business decisions, we deliver action-oriented research, enabling you to understand market dynamics better and accelerate your organizations success.
Combine, innovate, win.
Being a market research company for more than 10 years, we provide one stop solutions for all your market research needs. Grow your business through real market intelligence.
Why Choose Us
Our B2B services cover most markets in various sectors. For industry-leading companies as well as small and medium-sized businesses, Research city, a b2b agency in hyderabad has expert team with experience in b2b market research.
Research City can understand the data, its applications, its relevance at present and its impact in the future which can be used in innovative marketing, and attentive advertisement, thus providing a special route for reaching the targeted audience to your business.
Research City is more than just a data analytics company, they are your trusted partner in navigating the complex world of marketing and advertising. By harnessing the power of data, they help businesses unlock new possibilities, reach their target audience effectively, and ultimately achieve sustainable success.
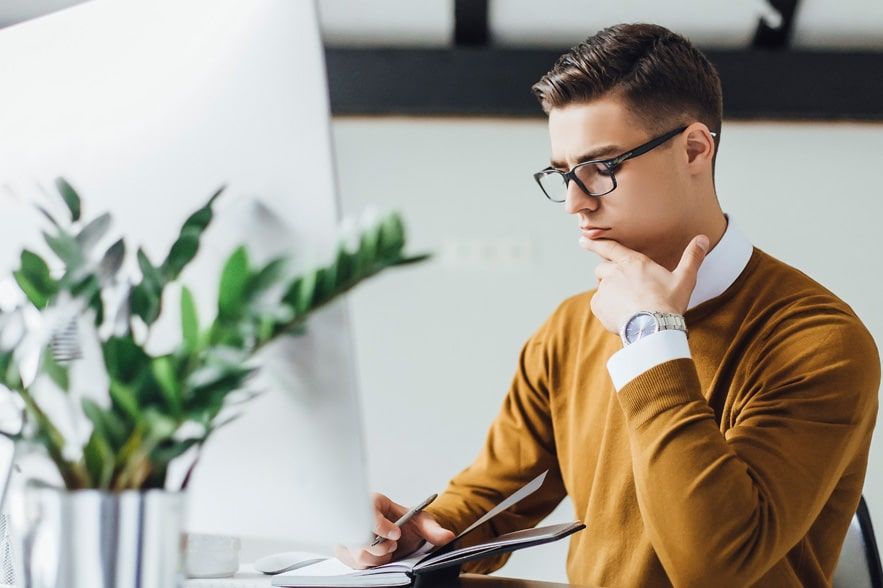
10+ years of experience in a marketing research company, and after working with 500+ clients all over the world, we realized that we compete not with our competitors, but with ourselves and that is how we are becoming better every day.

Market Research
Research means searching for something which has already known. Marketing research is the method of extracting data regarding customer interests, customer taste, likes, dislikes which can be used in finding your target audience and reaching your business towards your future customer. As a market research agency, research city helps to find your target audience within short period of time through suitable marketing research to your business.

Business Consulting & Strategy
Getting a thriving business for your product on the market requires planning, timing, arrangements, and resource management. Research City has professional experts in different departments who are capable of delivering a thriving strategy for your business. A business consultant not only provides advice on successful business but also broadens your business's knowledge and intelligence base, leading to a multiplier effect across different dimensions.
Turbocharge your business strategy.
At our company, we pride ourselves on our ability to provide our clients with the most actionable and data-driven research possible. Our team of experienced professionals deeply understands the market factors that impact your industry, and we utilise the latest tools and technologies to provide you with solutions tailored to your organisational needs. Whether you are looking to implement a new strategic initiative or simply deeper understanding of the market factors that are impacting your business, we are the best market survey companies in India with the experience and expertise to help you achieve your goals.

Get In Touch
Our power of choice is untrammelled and when nothing prevents being able to do what we like best every pleasure.
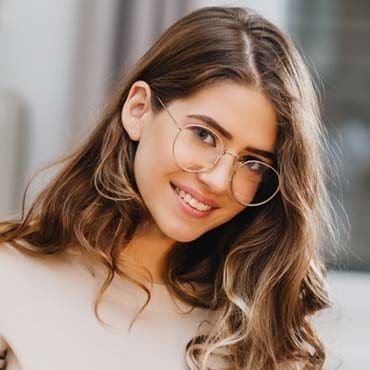
Amelia Margaret

Andrew Cameron

Sofia Charlotte
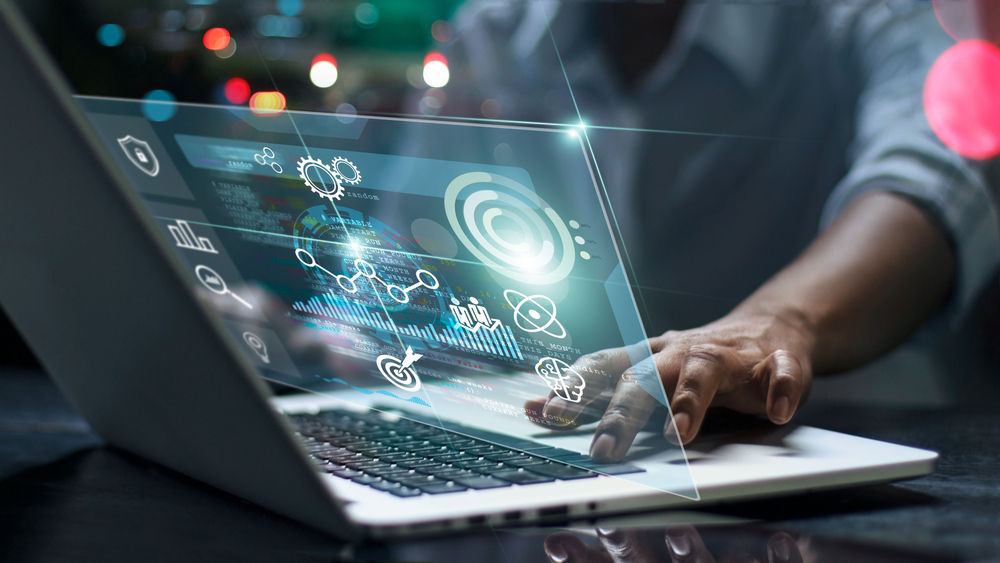
Data Processing & Analysis
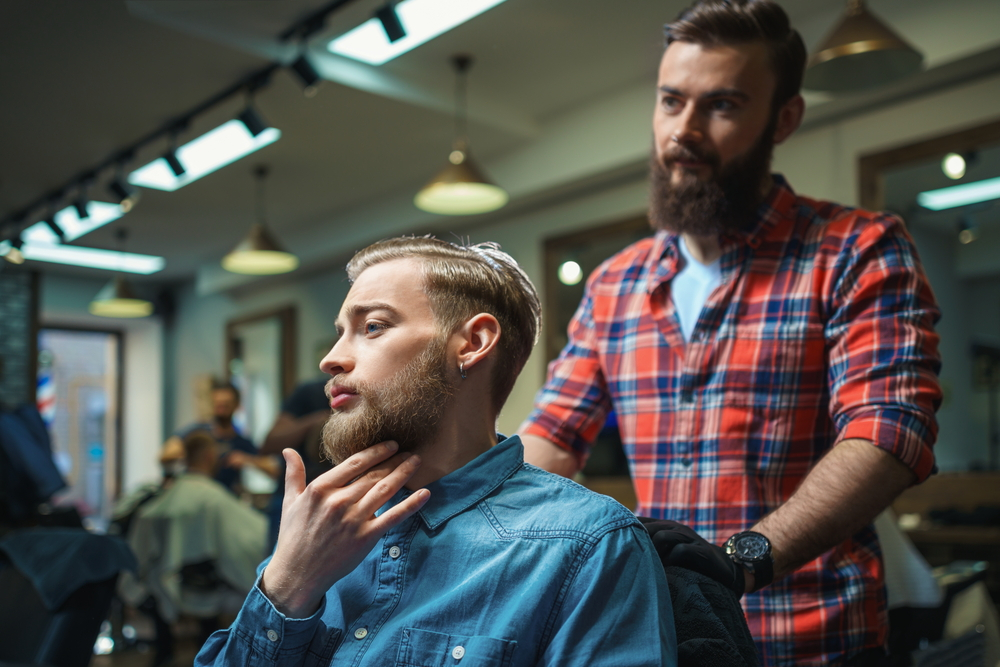
Men’s Luxury Barber Shop
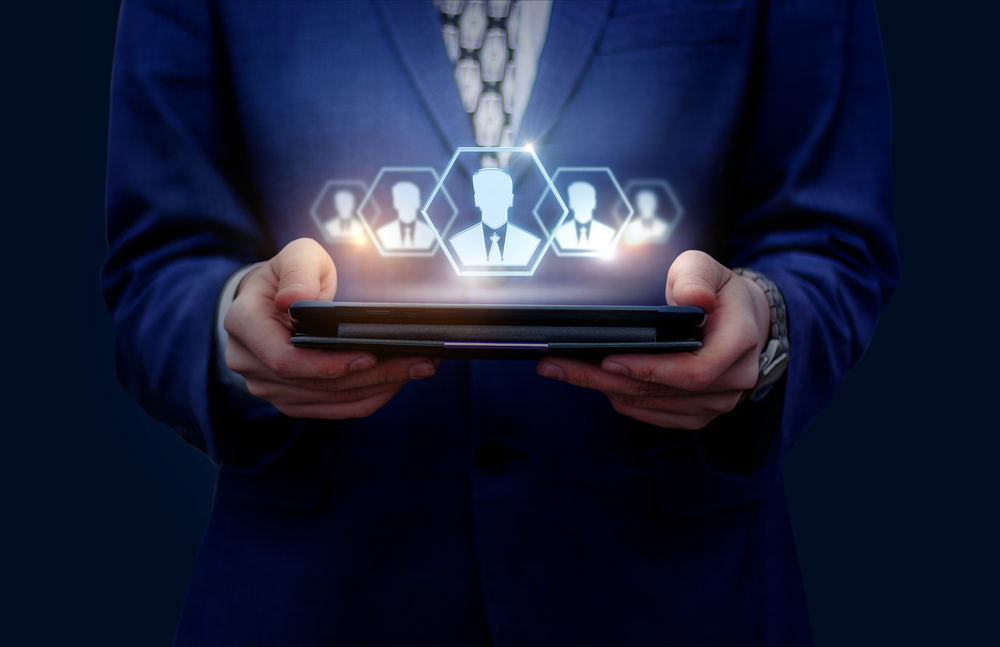
Segmentation of Clients Customer
WHAT WE OFFER
Get the best solutions for your business with us! Our 10+ years of experience in marketing & research and working with 500+ clients from all over the world have given us the expertise to provide top-notch services. We are a committed market research company in Hyderabad, India providing the best solutions that keep you ahead of the competition. Maximise your potential and let us help you reach success!
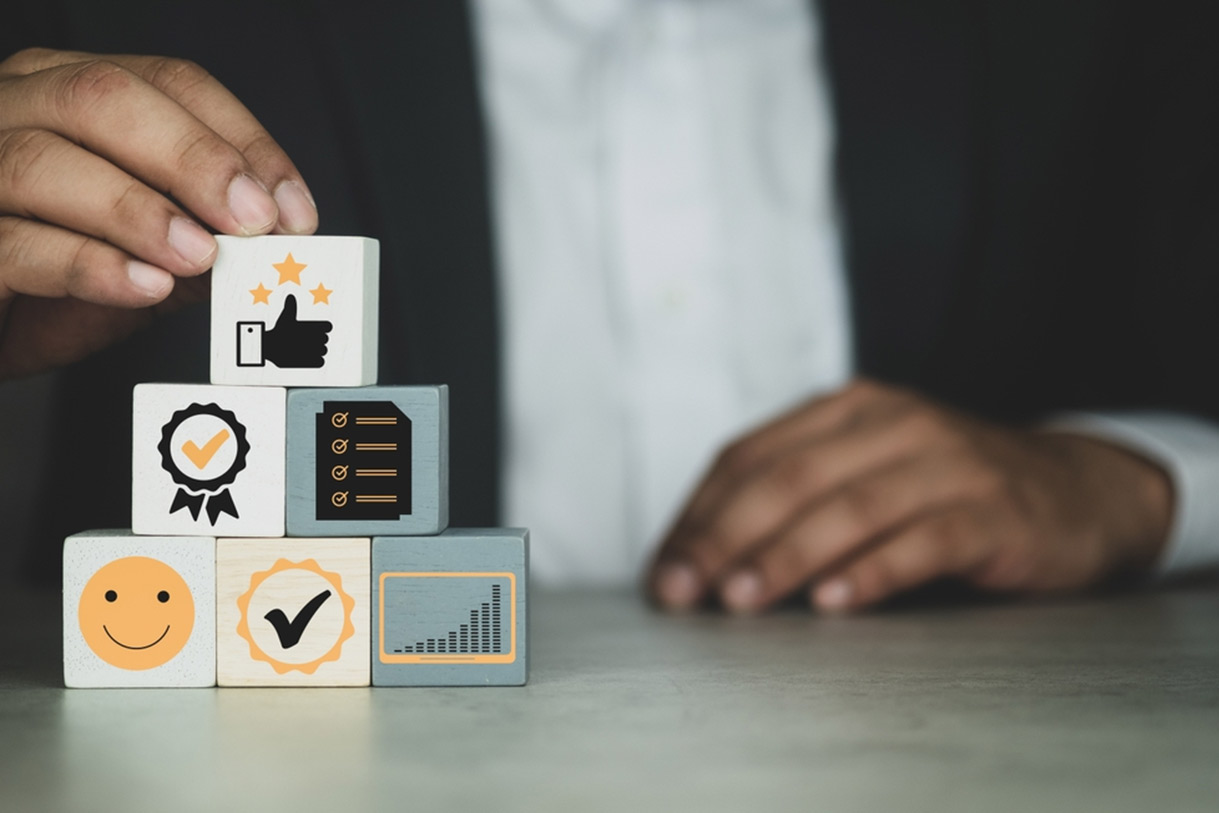
City of Calgary
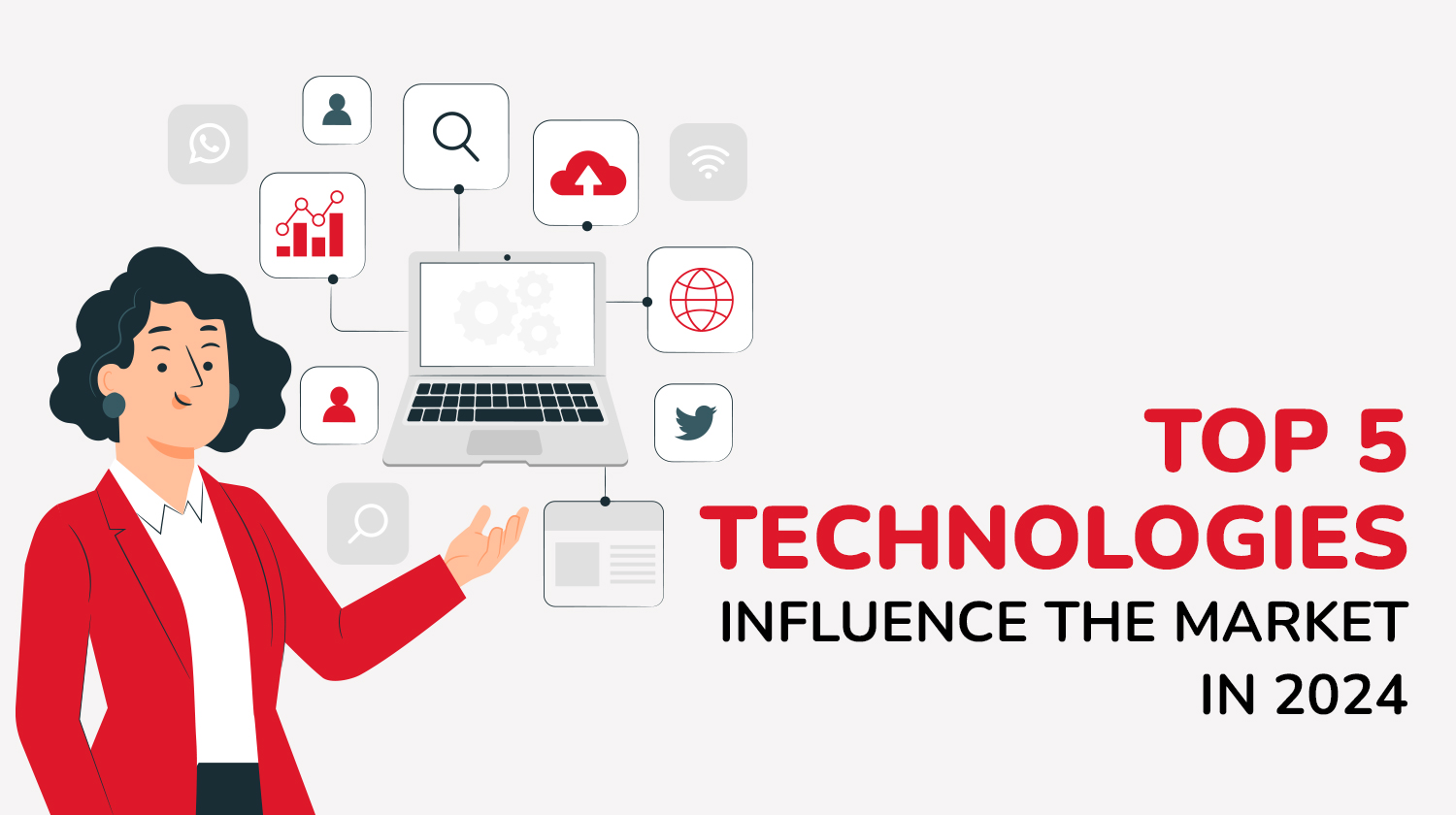
2024 Market Trends Shaped by Emerging Technologies
Research City
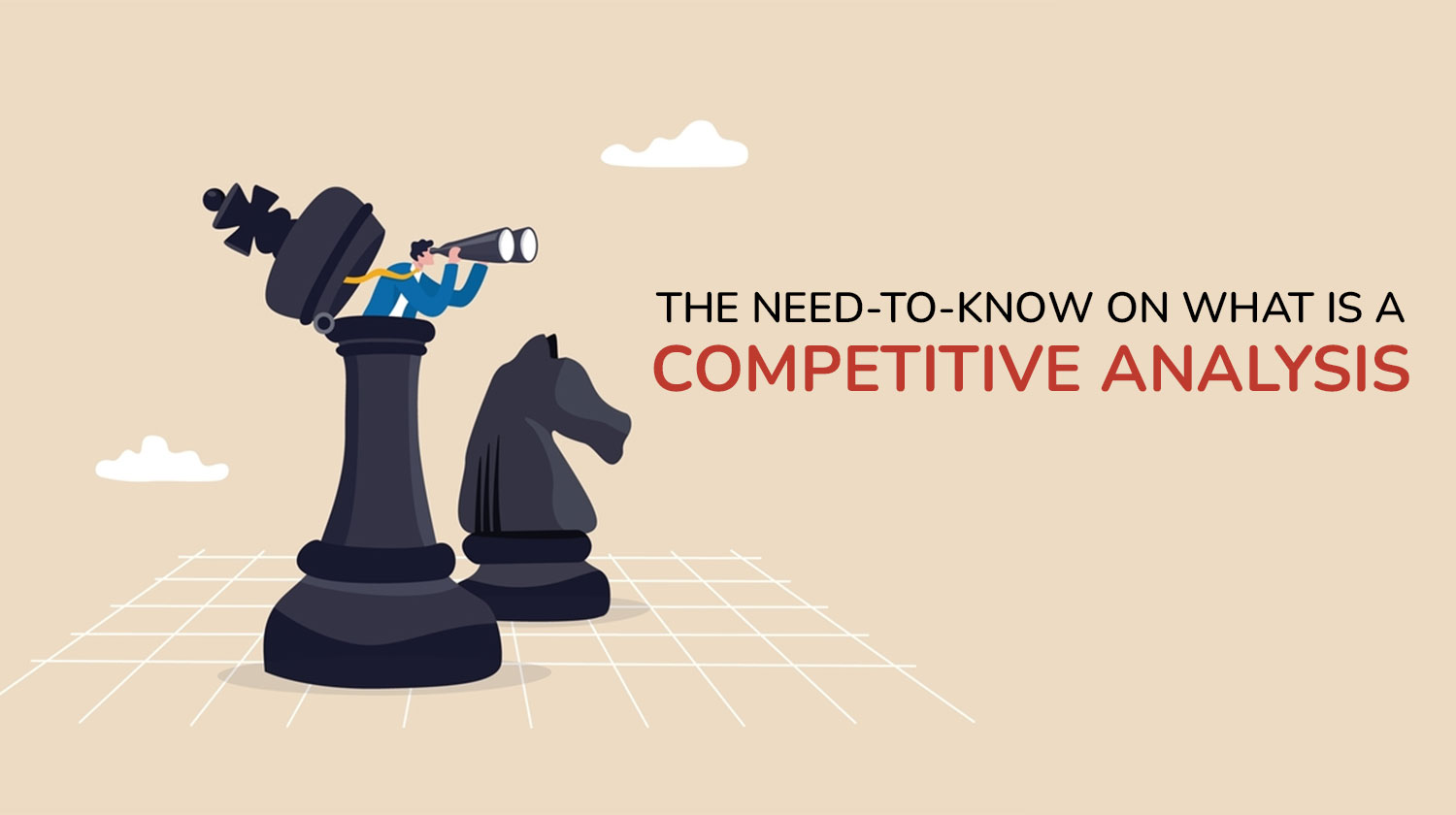
Competitive Analysis
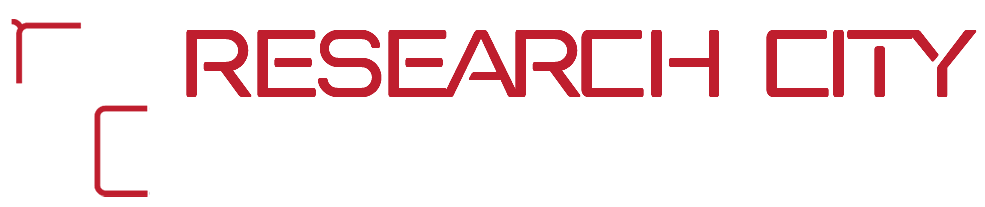
We help you make intelligent and data-driven business decisions. Our problem-solving approach delivers action-oriented research, enabling a deeper understanding of market factors and accelerates your organization to rise to the next opportunity.
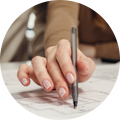
Free Consultation
Hyderabad, India.
Quick Links
Your message (optional)
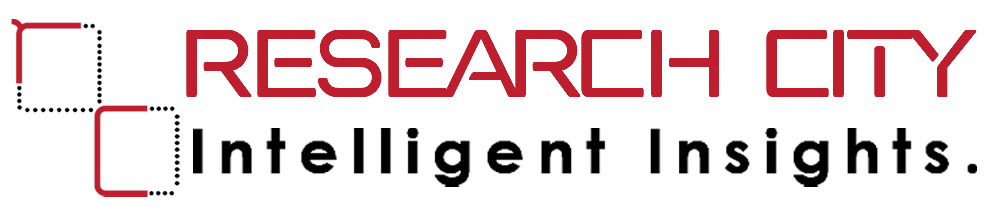
We help you make intelligent and data-driven business decisions. Our problem-solving approach delivers action-oriented research, enabling a deeper understanding of market factors and accelerating your organization to rise to the next opportunity.
Latest Blog
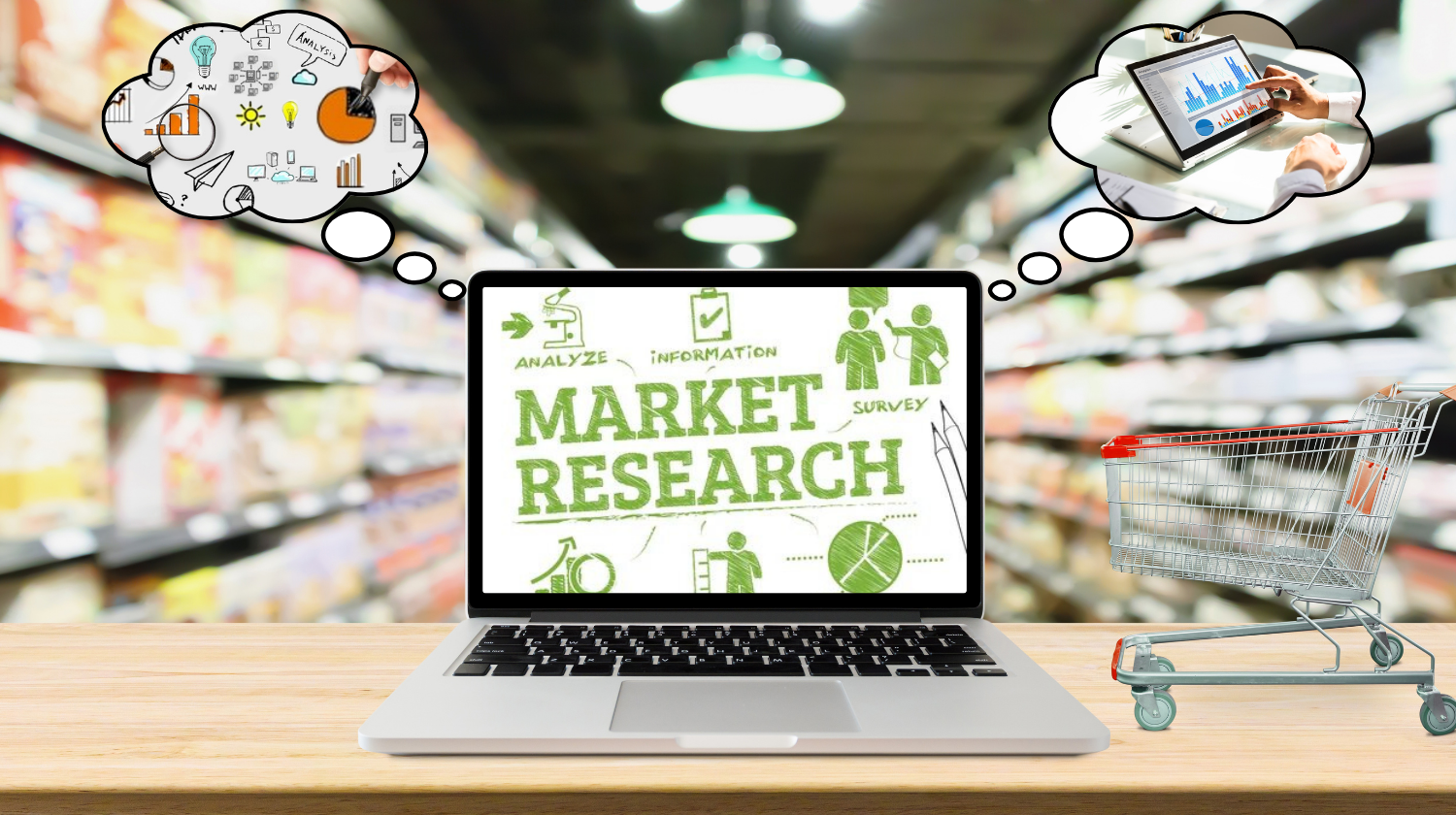
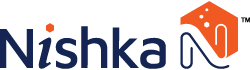
Scanning Electron Microscopy
Scanning electron microscopy / sem testing lab.
Scanning Electron Microscopy is a test process that produces a magnified image of a sample using an electron beam for analysis. It is highly effective in micro-analysis and failure mode analysis of materials and composites.
SEM analysis can facilitate the analysis of microscopic and nanoscopic materials and objects with great precision. scanning electron microscopy technique. There are two methods of electron detection when using SEM. Backscattered electrons can help with the analysis of the elemental composition of the sample and the secondary electrons emitted close to the surface can reveal relevant information about the topography and morphology of the sample’s surface. Combined with EDX, it can facilitate better analysis of the chemical and elemental composition of the samples.
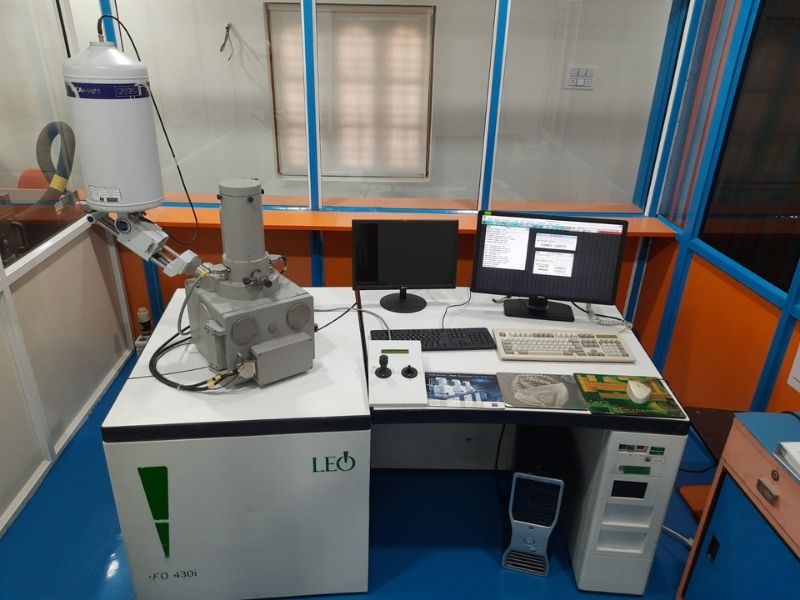
At our labs and research centers, we make sure to use only the best equipment pieces so that no error can appear in the final results we will provide. Several precautions are maintained so that the sample under consideration does not get damaged during the study.
We ensure that during each new sample test, a sterile and disinfected slide is used for better results. Nishka professionals ensure that all the arrangement is perfect and no single object is out of the place so that the final data recorded can have the least flaws. The entire material is studied thoroughly with different samples belonging to the same category until the optima studies are obtained.
Failure mode effects Investigation
Risk and Failure analysis, Mitigation and Corrective services.
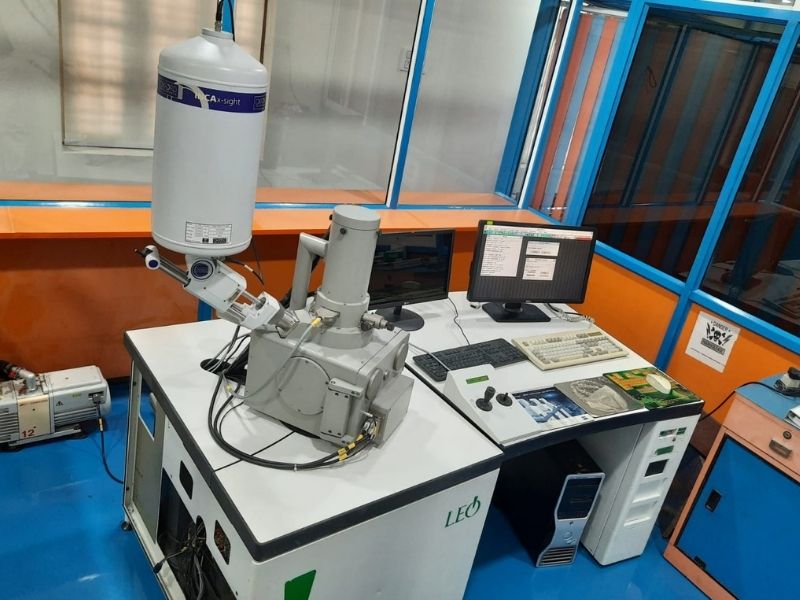
SEM testing plays a critical role in the characterization of materials by producing a 2-D High-quality image of the sample. SEM can be used to analyze traits like external morphology, texture, topography and can be used to measure the thickness of the layered structures with the help of cross-sectional imaging. SEM, coupled with EDS, can reveal the chemical composition (Elemental Analysis) of a material. Particulate and contamination analysis gets accelerated by SEM coupled with Energy Dispersive X-ray spectroscopy (EDAX / EDS). It can facilitate and accelerate the investigation and study of interactions between substances and their substrates.
SEM testing is indispensable to mitigate contamination issues, perform failure mode and effects analysis and Identification & characterization of foreign particulates contamination. With the appropriate sampling methods, SEM becomes a powerful tool for an in-depth analysis.
Why Nishka?
NISHKA’s team of experts has vast experience with in-depth knowledge across various industries such as manufacturing, construction, power, chemicals, medicine and healthcare, electrical, electronics and polymers. This expertise can ensure Total Quality Assurance and can assist in mitigating issues, maintain regulatory standards, and study various materials with ease.
SEM analysis is critical for performing particle composition analysis. The high magnification imaging from our SEM analysis allows clients to understand the wear and tear properties of their material. Our SEM testing services provide analysis of the size, quantity and morphology of small particles. Using these analytical techniques, our team of experts can provide you with valuable insights to best optimize your product cycle.
Remote View SEM facilitates a real-time interaction for a customer with the microscopist allowing direct failure mode analysis , surface feature analysis, particle morphology analysis, and chemical composition analysis to accelerate quality inspections or production speed. This mode enables a client to interact in real-time with a microscopist as they analyze a sample. Remote View SEM accelerates the failure mode analysis process and the production cycle highly. Remote-view SEM Imaging allows the client to execute better decision making with time efficiency and facilitates a better understanding of the features, morphology, and the elemental compositions of the samples by collaborating directly with the microscopist. This service is a game-changer in the failure analysis process.
Nishka routinely extends support for research, failure mode analysis , troubleshooting and quality control requirements to a multitude of clients across various industries, making us an ideal provider for your SEM analysis needs.
FAQs of Scanning Electron Microscope testing (SEM Analysis)
What is SEM analysis?
SEM analysis refers to the process of using a Scanning Electron Microscope (SEM) to examine the surface morphology of a sample at high magnification. The SEM uses an electron beam to scan the surface of the sample, producing high-resolution images that can reveal details about the sample's surface structure and by attaching EDAX we can be identify the composition.
What are the applications of SEM testing?
SEM testing is used in a variety of fields, including Pharmaceuticals, Materials science, Engineering, Biology and electronics. It is often used to study the surface morphology of materials, to identify the composition of substances, and to analyze the microstructure of materials.
How does SEM work?
In SEM testing, the sample is placed in a vacuum chamber and bombarded with a beam of electrons. These electrons will interact with the atoms in the sample, producing secondary electrons, backscattered electrons and X-rays. The secondary electrons are detected by the detector. These interactions provide information about the sample's structure and composition, which is used to create high-resolution 2D images of the sample.
What are the advantages of SEM?
SEM offers several advantages, including high magnification and resolution, the ability to observe both conductive and non-conductive materials (Sputter coated to make it conductive), and the ability to analyze a wide range of samples, from large objects to small particles.
What is sputter coating in SEM analysis?
Sputter coating is a process used in Scanning Electron Microscopy (SEM) to prepare non-conductive samples for analysis. The process involves depositing a thin layer of a conductive material, such as gold or platinum, onto the surface of the sample to make it conductive.
The sputter coating process works by bombarding the surface of the sample with a beam of positively charged particles, known as ions, which are emitted from a sputter source. The ions dislodge atoms from the surface of the sample, and the conductive material is deposited onto the sample to form a thin, uniform layer.
Sputter coating is important in SEM analysis because it enables the examination of non-conductive samples, which would otherwise not be visible in the SEM. By making the sample conductive, the electrons emitted by the SEM can interact with the sample and produce a high-resolution image.
It is important to note that sputter coating can alter the surface of the sample and introduce artefacts into the image. Therefore, it is important to choose a sputter coating material that is compatible with the sample and to carefully control the conditions of the sputter coating process to minimize the risk of sample damage and artefacts.
What are the limitations of SEM?
Here are few limitations of Scanning Electron Microscopy (SEM):
- Conductivity of sample: SEM requires that the sample being examined must be electro conductive or coated with a conductive material. This can limit the types of materials that can be analyzed using SEM.
- Vacuum essential: SEM operates in a vacuum environment, which can limit the types of samples that can be examined and the conditions under which they can be examined. Wet (Moistened) samples cannot be used in SEM.
- Sample preparation: SEM requires that samples be carefully prepared and mounted, which can be time-consuming and complex.
- Sample damage: There is a risk of sample damage during the SEM imaging process, especially for delicate or sensitive samples.
- Cost: SEM equipment and instrumentation can be expensive, and the process can be complex, requiring specialized knowledge and training.
- Resolution: While SEM provides high-resolution images, it may not be as sensitive as other imaging techniques, such as transmission electron microscopy (Even a nano particles can be examined), in terms of detecting subtle differences in sample structure and composition.
Please fill the required information for Downloading White Paper.
Need help or Have a question?
Analytical Techniques
- Materials Testing
- Polymorphic Characterization
- Pharmaceutical Testing
- Food Testing
- Contamination Testing
- Reverse Engineering
- Pharmaceutical Consulting
- Glass Delamination
- Failure Investigation
- Counterfeit Analysis
- Contamination Analysis
- Analytical & Chemical Consulting
- Electronics
- Agriculture
- Oil & Gas
Case Studies
Nishka research, quick links, our services, get in touch.
Nishka Research Pvt. Ltd.
Regus Business Center, 4th floor, Gumidelli Commercial Complex, 1-10-39 to 44, Old Airport Road, Begumpet, Hyderabad-500016 India
Phone : +91 40 29303155
Mobile : +91 7842798518
Left us a 5 star review
Submitted the samples for my phd work . Great work and reports submited with 2 days of time . Also the image clarity and all data are clear .
Thank you for your services.. Looking forward for more collaboration with your labs for my future projects..
A very good lab delivering acurate results. I must also mention about the very cordial staff that they have.
I am a PhD scholar from Kaunas University at Lithuania, Europe. I have given a sample to perform SEM analysis of the organic tissue. The results are highly satisfactory and impressive. The service was really excellent, on time( even before the time schedule), consumer friendly. cost to the academic samples are very less comparing with others. Once again thanks to Nishka for providing me the service ontime as I am really in urgency...
Great work done by nishka research. Thank you so much for your kind Co operation
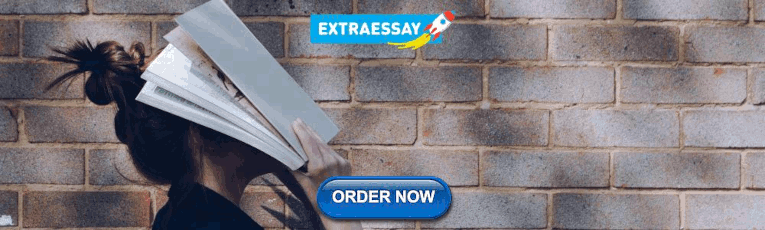
The Effect of Inequality on Redistribution: An Econometric Analysis
Using data on U.S. state and federal taxes and transfers over a quarter century, we estimate a regression model that yields the marginal effect of any shift of market income share from one quintile to another on the entire post tax, post-transfer income distribution. We identify exogenous income distribution changes and account for reverse causality using instruments based on exposure to international trade shocks, international commodity price shocks and national industry demand shocks, as well as lagged endogenous variables, with controls for the level of income, the business cycle and demographics. We find the degree of attenuation of market income shifts initially increases in quintile rank, peaks at the middle quintile and then falls for higher income quintiles, consistent with median voter political economy theory and what Stigler called Director’s Law. We also provide evidence of considerable and systematic spillover effects on quintiles neither gaining nor losing in the “experiments,” also favoring the middle quintile, what we label the greedy median voter. “Voting” and “income insurance” coalition analyses are presented. We find a strong negative relationship between average real income and redistribution and a modest effect of two year led inequality.
We are grateful to Tom MaCurdy, Han Hong, and especially Frank Wolak for helpful econometric suggestions. We thank Daron Acemoglu, Mordecai Kurz and Roger Myerson for providing helpful game theory insights and encouragement at an early stage. We also thank Jesse Davis, Kenneth Kletzer, Erik Loualiche, and participants in the Hoover Economic Policy Seminar for valuable feedback. This research was supported by the Bradley Graduate and Post Graduate Fellowship Program through a grant to the Stanford Institute for Economic Policy Research. The views expressed herein are those of the authors and do not necessarily reflect the views of the National Bureau of Economic Research.
MARC RIS BibTeΧ
Download Citation Data
- data appendix
More from NBER
In addition to working papers , the NBER disseminates affiliates’ latest findings through a range of free periodicals — the NBER Reporter , the NBER Digest , the Bulletin on Retirement and Disability , the Bulletin on Health , and the Bulletin on Entrepreneurship — as well as online conference reports , video lectures , and interviews .
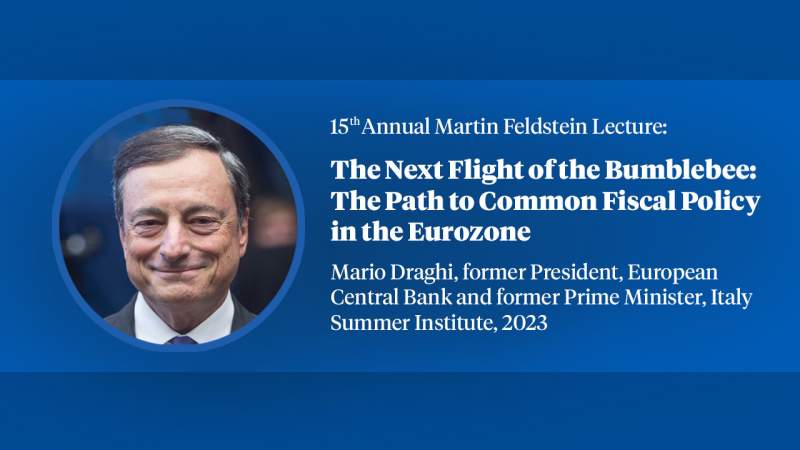
Thank you for visiting nature.com. You are using a browser version with limited support for CSS. To obtain the best experience, we recommend you use a more up to date browser (or turn off compatibility mode in Internet Explorer). In the meantime, to ensure continued support, we are displaying the site without styles and JavaScript.
- View all journals
- My Account Login
- Explore content
- About the journal
- Publish with us
- Sign up for alerts
- Open access
- Published: 17 October 2023
The impact of founder personalities on startup success
- Paul X. McCarthy 1 , 2 ,
- Xian Gong 3 ,
- Fabian Braesemann 4 , 5 ,
- Fabian Stephany 4 , 5 ,
- Marian-Andrei Rizoiu 3 &
- Margaret L. Kern 6
Scientific Reports volume 13 , Article number: 17200 ( 2023 ) Cite this article
59k Accesses
2 Citations
305 Altmetric
Metrics details
- Human behaviour
- Information technology
An Author Correction to this article was published on 07 May 2024
This article has been updated
Startup companies solve many of today’s most challenging problems, such as the decarbonisation of the economy or the development of novel life-saving vaccines. Startups are a vital source of innovation, yet the most innovative are also the least likely to survive. The probability of success of startups has been shown to relate to several firm-level factors such as industry, location and the economy of the day. Still, attention has increasingly considered internal factors relating to the firm’s founding team, including their previous experiences and failures, their centrality in a global network of other founders and investors, as well as the team’s size. The effects of founders’ personalities on the success of new ventures are, however, mainly unknown. Here, we show that founder personality traits are a significant feature of a firm’s ultimate success. We draw upon detailed data about the success of a large-scale global sample of startups (n = 21,187). We find that the Big Five personality traits of startup founders across 30 dimensions significantly differ from that of the population at large. Key personality facets that distinguish successful entrepreneurs include a preference for variety, novelty and starting new things (openness to adventure), like being the centre of attention (lower levels of modesty) and being exuberant (higher activity levels). We do not find one ’Founder-type’ personality; instead, six different personality types appear. Our results also demonstrate the benefits of larger, personality-diverse teams in startups, which show an increased likelihood of success. The findings emphasise the role of the diversity of personality types as a novel dimension of team diversity that influences performance and success.
Similar content being viewed by others
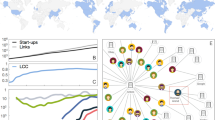
Predicting success in the worldwide start-up network
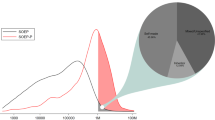
The personality traits of self-made and inherited millionaires
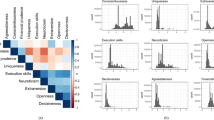
The nexus of top executives’ attributes, firm strategies, and outcomes: Large firms versus SMEs
Introduction.
The success of startups is vital to economic growth and renewal, with a small number of young, high-growth firms creating a disproportionately large share of all new jobs 1 , 2 . Startups create jobs and drive economic growth, and they are also an essential vehicle for solving some of society’s most pressing challenges.
As a poignant example, six centuries ago, the German city of Mainz was abuzz as the birthplace of the world’s first moveable-type press created by Johannes Gutenberg. However, in the early part of this century, it faced several economic challenges, including rising unemployment and a significant and growing municipal debt. Then in 2008, two Turkish immigrants formed the company BioNTech in Mainz with another university research colleague. Together they pioneered new mRNA-based technologies. In 2020, BioNTech partnered with US pharmaceutical giant Pfizer to create one of only a handful of vaccines worldwide for Covid-19, saving an estimated six million lives 3 . The economic benefit to Europe and, in particular, the German city where the vaccine was developed has been significant, with windfall tax receipts to the government clearing Mainz’s €1.3bn debt and enabling tax rates to be reduced, attracting other businesses to the region as well as inspiring a whole new generation of startups 4 .
While stories such as the success of BioNTech are often retold and remembered, their success is the exception rather than the rule. The overwhelming majority of startups ultimately fail. One study of 775 startups in Canada that successfully attracted external investment found only 35% were still operating seven years later 5 .
But what determines the success of these ‘lucky few’? When assessing the success factors of startups, especially in the early-stage unproven phase, venture capitalists and other investors offer valuable insights. Three different schools of thought characterise their perspectives: first, supply-side or product investors : those who prioritise investing in firms they consider to have novel and superior products and services, investing in companies with intellectual property such as patents and trademarks. Secondly, demand-side or market-based investors : those who prioritise investing in areas of highest market interest, such as in hot areas of technology like quantum computing or recurrent or emerging large-scale social and economic challenges such as the decarbonisation of the economy. Thirdly, talent investors : those who prioritise the foundation team above the startup’s initial products or what industry or problem it is looking to address.
Investors who adopt the third perspective and prioritise talent often recognise that a good team can overcome many challenges in the lead-up to product-market fit. And while the initial products of a startup may or may not work a successful and well-functioning team has the potential to pivot to new markets and new products, even if the initial ones prove untenable. Not surprisingly, an industry ‘autopsy’ into 101 tech startup failures found 23% were due to not having the right team—the number three cause of failure ahead of running out of cash or not having a product that meets the market need 6 .
Accordingly, early entrepreneurship research was focused on the personality of founders, but the focus shifted away in the mid-1980s onwards towards more environmental factors such as venture capital financing 7 , 8 , 9 , networks 10 , location 11 and due to a range of issues and challenges identified with the early entrepreneurship personality research 12 , 13 . At the turn of the 21st century, some scholars began exploring ways to combine context and personality and reconcile entrepreneurs’ individual traits with features of their environment. In her influential work ’The Sociology of Entrepreneurship’, Patricia H. Thornton 14 discusses two perspectives on entrepreneurship: the supply-side perspective (personality theory) and the demand-side perspective (environmental approach). The supply-side perspective focuses on the individual traits of entrepreneurs. In contrast, the demand-side perspective focuses on the context in which entrepreneurship occurs, with factors such as finance, industry and geography each playing their part. In the past two decades, there has been a revival of interest and research that explores how entrepreneurs’ personality relates to the success of their ventures. This new and growing body of research includes several reviews and meta-studies, which show that personality traits play an important role in both career success and entrepreneurship 15 , 16 , 17 , 18 , 19 , that there is heterogeneity in definitions and samples used in research on entrepreneurship 16 , 18 , and that founder personality plays an important role in overall startup outcomes 17 , 19 .
Motivated by the pivotal role of the personality of founders on startup success outlined in these recent contributions, we investigate two main research questions:
Which personality features characterise founders?
Do their personalities, particularly the diversity of personality types in founder teams, play a role in startup success?
We aim to understand whether certain founder personalities and their combinations relate to startup success, defined as whether their company has been acquired, acquired another company or listed on a public stock exchange. For the quantitative analysis, we draw on a previously published methodology 20 , which matches people to their ‘ideal’ jobs based on social media-inferred personality traits.
We find that personality traits matter for startup success. In addition to firm-level factors of location, industry and company age, we show that founders’ specific Big Five personality traits, such as adventurousness and openness, are significantly more widespread among successful startups. As we find that companies with multi-founder teams are more likely to succeed, we cluster founders in six different and distinct personality groups to underline the relevance of the complementarity in personality traits among founder teams. Startups with diverse and specific combinations of founder types (e. g., an adventurous ‘Leader’, a conscientious ‘Accomplisher’, and an extroverted ‘Developer’) have significantly higher odds of success.
We organise the rest of this paper as follows. In the Section " Results ", we introduce the data used and the methods applied to relate founders’ psychological traits with their startups’ success. We introduce the natural language processing method to derive individual and team personality characteristics and the clustering technique to identify personality groups. Then, we present the result for multi-variate regression analysis that allows us to relate firm success with external and personality features. Subsequently, the Section " Discussion " mentions limitations and opportunities for future research in this domain. In the Section " Methods ", we describe the data, the variables in use, and the clustering in greater detail. Robustness checks and additional analyses can be found in the Supplementary Information.
Our analysis relies on two datasets. We infer individual personality facets via a previously published methodology 20 from Twitter user profiles. Here, we restrict our analysis to founders with a Crunchbase profile. Crunchbase is the world’s largest directory on startups. It provides information about more than one million companies, primarily focused on funding and investors. A company’s public Crunchbase profile can be considered a digital business card of an early-stage venture. As such, the founding teams tend to provide information about themselves, including their educational background or a link to their Twitter account.
We infer the personality profiles of the founding teams of early-stage ventures from their publicly available Twitter profiles, using the methodology described by Kern et al. 20 . Then, we correlate this information to data from Crunchbase to determine whether particular combinations of personality traits correspond to the success of early-stage ventures. The final dataset used in the success prediction model contains n = 21,187 startup companies (for more details on the data see the Methods section and SI section A.5 ).
Revisions of Crunchbase as a data source for investigations on a firm and industry level confirm the platform to be a useful and valuable source of data for startups research, as comparisons with other sources at micro-level, e.g., VentureXpert or PwC, also suggest that the platform’s coverage is very comprehensive, especially for start-ups located in the United States 21 . Moreover, aggregate statistics on funding rounds by country and year are quite similar to those produced with other established sources, going to validate the use of Crunchbase as a reliable source in terms of coverage of funded ventures. For instance, Crunchbase covers about the same number of investment rounds in the analogous sectors as collected by the National Venture Capital Association 22 . However, we acknowledge that the data source might suffer from registration latency (a certain delay between the foundation of the company and its actual registration on Crunchbase) and success bias in company status (the likeliness that failed companies decide to delete their profile from the database).
The definition of startup success
The success of startups is uncertain, dependent on many factors and can be measured in various ways. Due to the likelihood of failure in startups, some large-scale studies have looked at which features predict startup survival rates 23 , and others focus on fundraising from external investors at various stages 24 . Success for startups can be measured in multiple ways, such as the amount of external investment attracted, the number of new products shipped or the annual growth in revenue. But sometimes external investments are misguided, revenue growth can be short-lived, and new products may fail to find traction.
Success in a startup is typically staged and can appear in different forms and times. For example, a startup may be seen to be successful when it finds a clear solution to a widely recognised problem, such as developing a successful vaccine. On the other hand, it could be achieving some measure of commercial success, such as rapidly accelerating sales or becoming profitable or at least cash positive. Or it could be reaching an exit for foundation investors via a trade sale, acquisition or listing of its shares for sale on a public stock exchange via an Initial Public Offering (IPO).
For our study, we focused on the startup’s extrinsic success rather than the founders’ intrinsic success per se, as its more visible, objective and measurable. A frequently considered measure of success is the attraction of external investment by venture capitalists 25 . However, this is not in and of itself a good measure of clear, incontrovertible success, particularly for early-stage ventures. This is because it reflects investors’ expectations of a startup’s success potential rather than actual business success. Similarly, we considered other measures like revenue growth 26 , liquidity events 27 , 28 , 29 , profitability 30 and social impact 31 , all of which have benefits as they capture incremental success, but each also comes with operational measurement challenges.
Therefore, we apply the success definition initially introduced by Bonaventura et al. 32 , namely that a startup is acquired, acquires another company or has an initial public offering (IPO). We consider any of these major capital liquidation events as a clear threshold signal that the company has matured from an early-stage venture to becoming or is on its way to becoming a mature company with clear and often significant business growth prospects. Together these three major liquidity events capture the primary forms of exit for external investors (an acquisition or trade sale and an IPO). For companies with a longer autonomous growth runway, acquiring another company marks a similar milestone of scale, maturity and capability.
Using multifactor analysis and a binary classification prediction model of startup success, we looked at many variables together and their relative influence on the probability of the success of startups. We looked at seven categories of factors through three lenses of firm-level factors: (1) location, (2) industry, (3) age of the startup; founder-level factors: (4) number of founders, (5) gender of founders, (6) personality characteristics of founders and; lastly team-level factors: (7) founder-team personality combinations. The model performance and relative impacts on the probability of startup success of each of these categories of founders are illustrated in more detail in section A.6 of the Supplementary Information (in particular Extended Data Fig. 19 and Extended Data Fig. 20 ). In total, we considered over three hundred variables (n = 323) and their relative significant associations with success.
The personality of founders
Besides product-market, industry, and firm-level factors (see SI section A.1 ), research suggests that the personalities of founders play a crucial role in startup success 19 . Therefore, we examine the personality characteristics of individual startup founders and teams of founders in relationship to their firm’s success by applying the success definition used by Bonaventura et al. 32 .
Employing established methods 33 , 34 , 35 , we inferred the personality traits across 30 dimensions (Big Five facets) of a large global sample of startup founders. The startup founders cohort was created from a subset of founders from the global startup industry directory Crunchbase, who are also active on the social media platform Twitter.
To measure the personality of the founders, we used the Big Five, a popular model of personality which includes five core traits: Openness to Experience, Conscientiousness, Extraversion, Agreeableness, and Emotional stability. Each of these traits can be further broken down into thirty distinct facets. Studies have found that the Big Five predict meaningful life outcomes, such as physical and mental health, longevity, social relationships, health-related behaviours, antisocial behaviour, and social contribution, at levels on par with intelligence and socioeconomic status 36 Using machine learning to infer personality traits by analysing the use of language and activity on social media has been shown to be more accurate than predictions of coworkers, friends and family and similar in accuracy to the judgement of spouses 37 . Further, as other research has shown, we assume that personality traits remain stable in adulthood even through significant life events 38 , 39 , 40 . Personality traits have been shown to emerge continuously from those already evident in adolescence 41 and are not significantly influenced by external life events such as becoming divorced or unemployed 42 . This suggests that the direction of any measurable effect goes from founder personalities to startup success and not vice versa.
As a first investigation to what extent personality traits might relate to entrepreneurship, we use the personality characteristics of individuals to predict whether they were an entrepreneur or an employee. We trained and tested a machine-learning random forest classifier to distinguish and classify entrepreneurs from employees and vice-versa using inferred personality vectors alone. As a result, we found we could correctly predict entrepreneurs with 77% accuracy and employees with 88% accuracy (Fig. 1 A). Thus, based on personality information alone, we correctly predict all unseen new samples with 82.5% accuracy (See SI section A.2 for more details on this analysis, the classification modelling and prediction accuracy).
We explored in greater detail which personality features are most prominent among entrepreneurs. We found that the subdomain or facet of Adventurousness within the Big Five Domain of Openness was significant and had the largest effect size. The facet of Modesty within the Big Five Domain of Agreeableness and Activity Level within the Big Five Domain of Extraversion was the subsequent most considerable effect (Fig. 1 B). Adventurousness in the Big Five framework is defined as the preference for variety, novelty and starting new things—which are consistent with the role of a startup founder whose role, especially in the early life of the company, is to explore things that do not scale easily 43 and is about developing and testing new products, services and business models with the market.
Once we derived and tested the Big Five personality features for each entrepreneur in our data set, we examined whether there is evidence indicating that startup founders naturally cluster according to their personality features using a Hopkins test (see Extended Data Figure 6 ). We discovered clear clustering tendencies in the data compared with other renowned reference data sets known to have clusters. Then, once we established the founder data clusters, we used agglomerative hierarchical clustering. This ‘bottom-up’ clustering technique initially treats each observation as an individual cluster. Then it merges them to create a hierarchy of possible cluster schemes with differing numbers of groups (See Extended Data Fig. 7 ). And lastly, we identified the optimum number of clusters based on the outcome of four different clustering performance measurements: Davies-Bouldin Index, Silhouette coefficients, Calinski-Harabas Index and Dunn Index (see Extended Data Figure 8 ). We find that the optimum number of clusters of startup founders based on their personality features is six (labelled #0 through to #5), as shown in Fig. 1 C.
To better understand the context of different founder types, we positioned each of the six types of founders within an occupation-personality matrix established from previous research 44 . This research showed that ‘each job has its own personality’ using a substantial sample of employees across various jobs. Utilising the methodology employed in this study, we assigned labels to the cluster names #0 to #5, which correspond to the identified occupation tribes that best describe the personality facets represented by the clusters (see Extended Data Fig. 9 for an overview of these tribes, as identified by McCarthy et al. 44 ).
Utilising this approach, we identify three ’purebred’ clusters: #0, #2 and #5, whose members are dominated by a single tribe (larger than 60% of all individuals in each cluster are characterised by one tribe). Thus, these clusters represent and share personality attributes of these previously identified occupation-personality tribes 44 , which have the following known distinctive personality attributes (see also Table 1 ):
Accomplishers (#0) —Organised & outgoing. confident, down-to-earth, content, accommodating, mild-tempered & self-assured.
Leaders (#2) —Adventurous, persistent, dispassionate, assertive, self-controlled, calm under pressure, philosophical, excitement-seeking & confident.
Fighters (#5) —Spontaneous and impulsive, tough, sceptical, and uncompromising.
We labelled these clusters with the tribe names, acknowledging that labels are somewhat arbitrary, based on our best interpretation of the data (See SI section A.3 for more details).
For the remaining three clusters #1, #3 and #4, we can see they are ‘hybrids’, meaning that the founders within them come from a mix of different tribes, with no one tribe representing more than 50% of the members of that cluster. However, the tribes with the largest share were noted as #1 Experts/Engineers, #3 Fighters, and #4 Operators.
To label these three hybrid clusters, we examined the closest occupations to the median personality features of each cluster. We selected a name that reflected the common themes of these occupations, namely:
Experts/Engineers (#1) as the closest roles included Materials Engineers and Chemical Engineers. This is consistent with this cluster’s personality footprint, which is highest in openness in the facets of imagination and intellect.
Developers (#3) as the closest roles include Application Developers and related technology roles such as Business Systems Analysts and Product Managers.
Operators (#4) as the closest roles include service, maintenance and operations functions, including Bicycle Mechanic, Mechanic and Service Manager. This is also consistent with one of the key personality traits of high conscientiousness in the facet of orderliness and high agreeableness in the facet of humility for founders in this cluster.
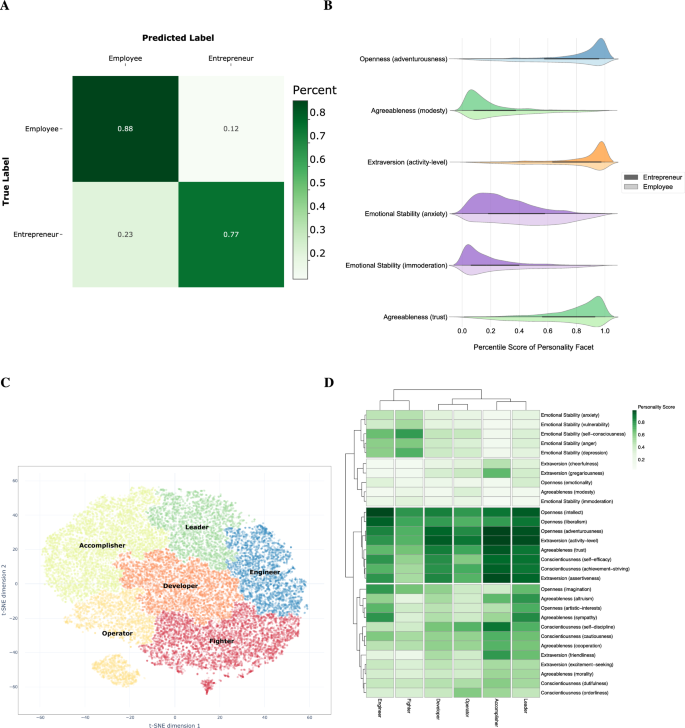
Founder-Level Factors of Startup Success. ( A ), Successful entrepreneurs differ from successful employees. They can be accurately distinguished using a classifier with personality information alone. ( B ), Successful entrepreneurs have different Big Five facet distributions, especially on adventurousness, modesty and activity level. ( C ), Founders come in six different types: Fighters, Operators, Accomplishers, Leaders, Engineers and Developers (FOALED) ( D ), Each founder Personality-Type has its distinct facet.
Together, these six different types of startup founders (Fig. 1 C) represent a framework we call the FOALED model of founder types—an acronym of Fighters, Operators, Accomplishers, Leaders, Engineers and D evelopers.
Each founder’s personality type has its distinct facet footprint (for more details, see Extended Data Figure 10 in SI section A.3 ). Also, we observe a central core of correlated features that are high for all types of entrepreneurs, including intellect, adventurousness and activity level (Fig. 1 D).To test the robustness of the clustering of the personality facets, we compare the mean scores of the individual facets per cluster with a 20-fold resampling of the data and find that the clusters are, overall, largely robust against resampling (see Extended Data Figure 11 in SI section A.3 for more details).
We also find that the clusters accord with the distribution of founders’ roles in their startups. For example, Accomplishers are often Chief Executive Officers, Chief Financial Officers, or Chief Operating Officers, while Fighters tend to be Chief Technical Officers, Chief Product Officers, or Chief Commercial Officers (see Extended Data Fig. 12 in SI section A.4 for more details).
The ensemble theory of success
While founders’ individual personality traits, such as Adventurousness or Openness, show to be related to their firms’ success, we also hypothesise that the combination, or ensemble, of personality characteristics of a founding team impacts the chances of success. The logic behind this reasoning is complementarity, which is proposed by contemporary research on the functional roles of founder teams. Examples of these clear functional roles have evolved in established industries such as film and television, construction, and advertising 45 . When we subsequently explored the combinations of personality types among founders and their relationship to the probability of startup success, adjusted for a range of other factors in a multi-factorial analysis, we found significantly increased chances of success for mixed foundation teams:
Initially, we find that firms with multiple founders are more likely to succeed, as illustrated in Fig. 2 A, which shows firms with three or more founders are more than twice as likely to succeed than solo-founded startups. This finding is consistent with investors’ advice to founders and previous studies 46 . We also noted that some personality types of founders increase the probability of success more than others, as shown in SI section A.6 (Extended Data Figures 16 and 17 ). Also, we note that gender differences play out in the distribution of personality facets: successful female founders and successful male founders show facet scores that are more similar to each other than are non-successful female founders to non-successful male founders (see Extended Data Figure 18 ).
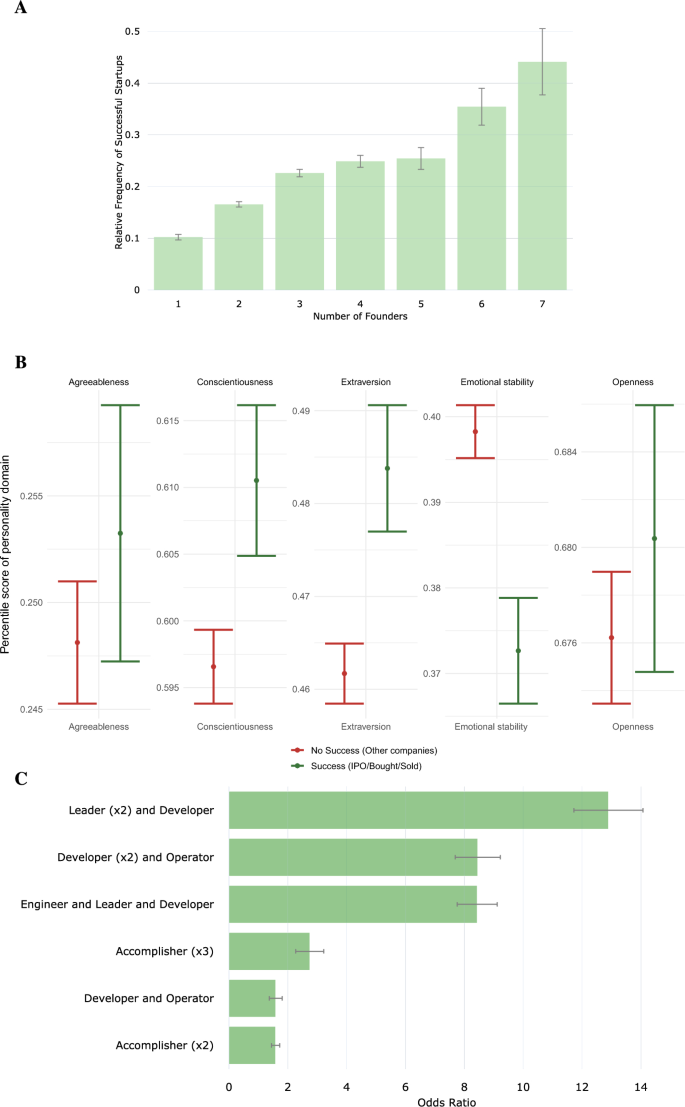
The Ensemble Theory of Team-Level Factors of Startup Success. ( A ) Having a larger founder team elevates the chances of success. This can be due to multiple reasons, e.g., a more extensive network or knowledge base but also personality diversity. ( B ) We show that joint personality combinations of founders are significantly related to higher chances of success. This is because it takes more than one founder to cover all beneficial personality traits that ‘breed’ success. ( C ) In our multifactor model, we show that firms with diverse and specific combinations of types of founders have significantly higher odds of success.
Access to more extensive networks and capital could explain the benefits of having more founders. Still, as we find here, it also offers a greater diversity of combined personalities, naturally providing a broader range of maximum traits. So, for example, one founder may be more open and adventurous, and another could be highly agreeable and trustworthy, thus, potentially complementing each other’s particular strengths associated with startup success.
The benefits of larger and more personality-diverse foundation teams can be seen in the apparent differences between successful and unsuccessful firms based on their combined Big Five personality team footprints, as illustrated in Fig. 2 B. Here, maximum values for each Big Five trait of a startup’s co-founders are mapped; stratified by successful and non-successful companies. Founder teams of successful startups tend to score higher on Openness, Conscientiousness, Extraversion, and Agreeableness.
When examining the combinations of founders with different personality types, we find that some ensembles of personalities were significantly correlated with greater chances of startup success—while controlling for other variables in the model—as shown in Fig. 2 C (for more details on the modelling, the predictive performance and the coefficient estimates of the final model, see Extended Data Figures 19 , 20 , and 21 in SI section A.6 ).
Three combinations of trio-founder companies were more than twice as likely to succeed than other combinations, namely teams with (1) a Leader and two Developers , (2) an Operator and two Developers , and (3) an Expert/Engineer , Leader and Developer . To illustrate the potential mechanisms on how personality traits might influence the success of startups, we provide some examples of well-known, successful startup founders and their characteristic personality traits in Extended Data Figure 22 .
Startups are one of the key mechanisms for brilliant ideas to become solutions to some of the world’s most challenging economic and social problems. Examples include the Google search algorithm, disability technology startup Fingerwork’s touchscreen technology that became the basis of the Apple iPhone, or the Biontech mRNA technology that powered Pfizer’s COVID-19 vaccine.
We have shown that founders’ personalities and the combination of personalities in the founding team of a startup have a material and significant impact on its likelihood of success. We have also shown that successful startup founders’ personality traits are significantly different from those of successful employees—so much so that a simple predictor can be trained to distinguish between employees and entrepreneurs with more than 80% accuracy using personality trait data alone.
Just as occupation-personality maps derived from data can provide career guidance tools, so too can data on successful entrepreneurs’ personality traits help people decide whether becoming a founder may be a good choice for them.
We have learnt through this research that there is not one type of ideal ’entrepreneurial’ personality but six different types. Many successful startups have multiple co-founders with a combination of these different personality types.
To a large extent, founding a startup is a team sport; therefore, diversity and complementarity of personalities matter in the foundation team. It has an outsized impact on the company’s likelihood of success. While all startups are high risk, the risk becomes lower with more founders, particularly if they have distinct personality traits.
Our work demonstrates the benefits of personality diversity among the founding team of startups. Greater awareness of this novel form of diversity may help create more resilient startups capable of more significant innovation and impact.
The data-driven research approach presented here comes with certain methodological limitations. The principal data sources of this study—Crunchbase and Twitter—are extensive and comprehensive, but there are characterised by some known and likely sample biases.
Crunchbase is the principal public chronicle of venture capital funding. So, there is some likely sample bias toward: (1) Startup companies that are funded externally: self-funded or bootstrapped companies are less likely to be represented in Crunchbase; (2) technology companies, as that is Crunchbase’s roots; (3) multi-founder companies; (4) male founders: while the representation of female founders is now double that of the mid-2000s, women still represent less than 25% of the sample; (5) companies that succeed: companies that fail, especially those that fail early, are likely to be less represented in the data.
Samples were also limited to those founders who are active on Twitter, which adds additional selection biases. For example, Twitter users typically are younger, more educated and have a higher median income 47 . Another limitation of our approach is the potentially biased presentation of a person’s digital identity on social media, which is the basis for identifying personality traits. For example, recent research suggests that the language and emotional tone used by entrepreneurs in social media can be affected by events such as business failure 48 , which might complicate the personality trait inference.
In addition to sampling biases within the data, there are also significant historical biases in startup culture. For many aspects of the entrepreneurship ecosystem, women, for example, are at a disadvantage 49 . Male-founded companies have historically dominated most startup ecosystems worldwide, representing the majority of founders and the overwhelming majority of venture capital investors. As a result, startups with women have historically attracted significantly fewer funds 50 , in part due to the male bias among venture investors, although this is now changing, albeit slowly 51 .
The research presented here provides quantitative evidence for the relevance of personality types and the diversity of personalities in startups. At the same time, it brings up other questions on how personality traits are related to other factors associated with success, such as:
Will the recent growing focus on promoting and investing in female founders change the nature, composition and dynamics of startups and their personalities leading to a more diverse personality landscape in startups?
Will the growth of startups outside of the United States change what success looks like to investors and hence the role of different personality traits and their association to diverse success metrics?
Many of today’s most renowned entrepreneurs are either Baby Boomers (such as Gates, Branson, Bloomberg) or Generation Xers (such as Benioff, Cannon-Brookes, Musk). However, as we can see, personality is both a predictor and driver of success in entrepreneurship. Will generation-wide differences in personality and outlook affect startups and their success?
Moreover, the findings shown here have natural extensions and applications beyond startups, such as for new projects within large established companies. While not technically startups, many large enterprises and industries such as construction, engineering and the film industry rely on forming new project-based, cross-functional teams that are often new ventures and share many characteristics of startups.
There is also potential for extending this research in other settings in government, NGOs, and within the research community. In scientific research, for example, team diversity in terms of age, ethnicity and gender has been shown to be predictive of impact, and personality diversity may be another critical dimension 52 .
Another extension of the study could investigate the development of the language used by startup founders on social media over time. Such an extension could investigate whether the language (and inferred psychological characteristics) change as the entrepreneurs’ ventures go through major business events such as foundation, funding, or exit.
Overall, this study demonstrates, first, that startup founders have significantly different personalities than employees. Secondly, besides firm-level factors, which are known to influence firm success, we show that a range of founder-level factors, notably the character traits of its founders, significantly impact a startup’s likelihood of success. Lastly, we looked at team-level factors. We discovered in a multifactor analysis that personality-diverse teams have the most considerable impact on the probability of a startup’s success, underlining the importance of personality diversity as a relevant factor of team performance and success.
Data sources
Entrepreneurs dataset.
Data about the founders of startups were collected from Crunchbase (Table 2 ), an open reference platform for business information about private and public companies, primarily early-stage startups. It is one of the largest and most comprehensive data sets of its kind and has been used in over 100 peer-reviewed research articles about economic and managerial research.
Crunchbase contains data on over two million companies - mainly startup companies and the companies who partner with them, acquire them and invest in them, as well as profiles on well over one million individuals active in the entrepreneurial ecosystem worldwide from over 200 countries and spans. Crunchbase started in the technology startup space, and it now covers all sectors, specifically focusing on entrepreneurship, investment and high-growth companies.
While Crunchbase contains data on over one million individuals in the entrepreneurial ecosystem, some are not entrepreneurs or startup founders but play other roles, such as investors, lawyers or executives at companies that acquire startups. To create a subset of only entrepreneurs, we selected a subset of 32,732 who self-identify as founders and co-founders (by job title) and who are also publicly active on the social media platform Twitter. We also removed those who also are venture capitalists to distinguish between investors and founders.
We selected founders active on Twitter to be able to use natural language processing to infer their Big Five personality features using an open-vocabulary approach shown to be accurate in the previous research by analysing users’ unstructured text, such as Twitter posts in our case. For this project, as with previous research 20 , we employed a commercial service, IBM Watson Personality Insight, to infer personality facets. This service provides raw scores and percentile scores of Big Five Domains (Openness, Conscientiousness, Extraversion, Agreeableness and Emotional Stability) and the corresponding 30 subdomains or facets. In addition, the public content of Twitter posts was collected, and there are 32,732 profiles that each had enough Twitter posts (more than 150 words) to get relatively accurate personality scores (less than 12.7% Average Mean Absolute Error).
The entrepreneurs’ dataset is analysed in combination with other data about the companies they founded to explore questions about the nature and patterns of personality traits of entrepreneurs and the relationships between these patterns and company success.
For the multifactor analysis, we further filtered the data in several preparatory steps for the success prediction modelling (for more details, see SI section A.5 ). In particular, we removed data points with missing values (Extended Data Fig. 13 ) and kept only companies in the data that were founded from 1990 onward to ensure consistency with previous research 32 (see Extended Data Fig. 14 ). After cleaning, filtering and pre-processing the data, we ended up with data from 25,214 founders who founded 21,187 startup companies to be used in the multifactor analysis. Of those, 3442 startups in the data were successful, 2362 in the first seven years after they were founded (see Extended Data Figure 15 for more details).
Entrepreneurs and employees dataset
To investigate whether startup founders show personality traits that are similar or different from the population at large (i. e. the entrepreneurs vs employees sub-analysis shown in Fig. 1 A and B), we filtered the entrepreneurs’ data further: we reduced the sample to those founders of companies, which attracted more than US$100k in investment to create a reference set of successful entrepreneurs (n \(=\) 4400).
To create a control group of employees who are not also entrepreneurs or very unlikely to be of have been entrepreneurs, we leveraged the fact that while some occupational titles like CEO, CTO and Public Speaker are commonly shared by founders and co-founders, some others such as Cashier , Zoologist and Detective very rarely co-occur seem to be founders or co-founders. To illustrate, many company founders also adopt regular occupation titles such as CEO or CTO. Many founders will be Founder and CEO or Co-founder and CTO. While founders are often CEOs or CTOs, the reverse is not necessarily true, as many CEOs are professional executives that were not involved in the establishment or ownership of the firm.
Using data from LinkedIn, we created an Entrepreneurial Occupation Index (EOI) based on the ratio of entrepreneurs for each of the 624 occupations used in a previous study of occupation-personality fit 44 . It was calculated based on the percentage of all people working in the occupation from LinkedIn compared to those who shared the title Founder or Co-founder (See SI section A.2 for more details). A reference set of employees (n=6685) was then selected across the 112 different occupations with the lowest propensity for entrepreneurship (less than 0.5% EOI) from a large corpus of Twitter users with known occupations, which is also drawn from the previous occupational-personality fit study 44 .
These two data sets were used to test whether it may be possible to distinguish successful entrepreneurs from successful employees based on the different patterns of personality traits alone.
Hierarchical clustering
We applied several clustering techniques and tests to the personality vectors of the entrepreneurs’ data set to determine if there are natural clusters and, if so, how many are the optimum number.
Firstly, to determine if there is a natural typology to founder personalities, we applied the Hopkins statistic—a statistical test we used to answer whether the entrepreneurs’ dataset contains inherent clusters. It measures the clustering tendency based on the ratio of the sum of distances of real points within a sample of the entrepreneurs’ dataset to their nearest neighbours and the sum of distances of randomly selected artificial points from a simulated uniform distribution to their nearest neighbours in the real entrepreneurs’ dataset. The ratio measures the difference between the entrepreneurs’ data distribution and the simulated uniform distribution, which tests the randomness of the data. The range of Hopkins statistics is from 0 to 1. The scores are close to 0, 0.5 and 1, respectively, indicating whether the dataset is uniformly distributed, randomly distributed or highly clustered.
To cluster the founders by personality facets, we used Agglomerative Hierarchical Clustering (AHC)—a bottom-up approach that treats an individual data point as a singleton cluster and then iteratively merges pairs of clusters until all data points are included in the single big collection. Ward’s linkage method is used to choose the pair of groups for minimising the increase in the within-cluster variance after combining. AHC was widely applied to clustering analysis since a tree hierarchy output is more informative and interpretable than K-means. Dendrograms were used to visualise the hierarchy to provide the perspective of the optimal number of clusters. The heights of the dendrogram represent the distance between groups, with lower heights representing more similar groups of observations. A horizontal line through the dendrogram was drawn to distinguish the number of significantly different clusters with higher heights. However, as it is not possible to determine the optimum number of clusters from the dendrogram, we applied other clustering performance metrics to analyse the optimal number of groups.
A range of Clustering performance metrics were used to help determine the optimal number of clusters in the dataset after an apparent clustering tendency was confirmed. The following metrics were implemented to evaluate the differences between within-cluster and between-cluster distances comprehensively: Dunn Index, Calinski-Harabasz Index, Davies-Bouldin Index and Silhouette Index. The Dunn Index measures the ratio of the minimum inter-cluster separation and the maximum intra-cluster diameter. At the same time, the Calinski-Harabasz Index improves the measurement of the Dunn Index by calculating the ratio of the average sum of squared dispersion of inter-cluster and intra-cluster. The Davies-Bouldin Index simplifies the process by treating each cluster individually. It compares the sum of the average distance among intra-cluster data points to the cluster centre of two separate groups with the distance between their centre points. Finally, the Silhouette Index is the overall average of the silhouette coefficients for each sample. The coefficient measures the similarity of the data point to its cluster compared with the other groups. Higher scores of the Dunn, Calinski-Harabasz and Silhouette Index and a lower score of the Davies-Bouldin Index indicate better clustering configuration.
Classification modelling
Classification algorithms.
To obtain a comprehensive and robust conclusion in the analysis predicting whether a given set of personality traits corresponds to an entrepreneur or an employee, we explored the following classifiers: Naïve Bayes, Elastic Net regularisation, Support Vector Machine, Random Forest, Gradient Boosting and Stacked Ensemble. The Naïve Bayes classifier is a probabilistic algorithm based on Bayes’ theorem with assumptions of independent features and equiprobable classes. Compared with other more complex classifiers, it saves computing time for large datasets and performs better if the assumptions hold. However, in the real world, those assumptions are generally violated. Elastic Net regularisation combines the penalties of Lasso and Ridge to regularise the Logistic classifier. It eliminates the limitation of multicollinearity in the Lasso method and improves the limitation of feature selection in the Ridge method. Even though Elastic Net is as simple as the Naïve Bayes classifier, it is more time-consuming. The Support Vector Machine (SVM) aims to find the ideal line or hyperplane to separate successful entrepreneurs and employees in this study. The dividing line can be non-linear based on a non-linear kernel, such as the Radial Basis Function Kernel. Therefore, it performs well on high-dimensional data while the ’right’ kernel selection needs to be tuned. Random Forest (RF) and Gradient Boosting Trees (GBT) are ensembles of decision trees. All trees are trained independently and simultaneously in RF, while a new tree is trained each time and corrected by previously trained trees in GBT. RF is a more robust and straightforward model since it does not have many hyperparameters to tune. GBT optimises the objective function and learns a more accurate model since there is a successive learning and correction process. Stacked Ensemble combines all existing classifiers through a Logistic Regression. Better than bagging with only variance reduction and boosting with only bias reduction, the ensemble leverages the benefit of model diversity with both lower variance and bias. All the above classification algorithms distinguish successful entrepreneurs and employees based on the personality matrix.
Evaluation metrics
A range of evaluation metrics comprehensively explains the performance of a classification prediction. The most straightforward metric is accuracy, which measures the overall portion of correct predictions. It will mislead the performance of an imbalanced dataset. The F1 score is better than accuracy by combining precision and recall and considering the False Negatives and False Positives. Specificity measures the proportion of detecting the true negative rate that correctly identifies employees, while Positive Predictive Value (PPV) calculates the probability of accurately predicting successful entrepreneurs. Area Under the Receiver Operating Characteristic Curve (AUROC) determines the capability of the algorithm to distinguish between successful entrepreneurs and employees. A higher value means the classifier performs better on separating the classes.
Feature importance
To further understand and interpret the classifier, it is critical to identify variables with significant predictive power on the target. Feature importance of tree-based models measures Gini importance scores for all predictors, which evaluate the overall impact of the model after cutting off the specific feature. The measurements consider all interactions among features. However, it does not provide insights into the directions of impacts since the importance only indicates the ability to distinguish different classes.
Statistical analysis
T-test, Cohen’s D and two-sample Kolmogorov-Smirnov test are introduced to explore how the mean values and distributions of personality facets between entrepreneurs and employees differ. The T-test is applied to determine whether the mean of personality facets of two group samples are significantly different from one another or not. The facets with significant differences detected by the hypothesis testing are critical to separate the two groups. Cohen’s d is to measure the effect size of the results of the previous t-test, which is the ratio of the mean difference to the pooled standard deviation. A larger Cohen’s d score indicates that the mean difference is greater than the variability of the whole sample. Moreover, it is interesting to check whether the two groups’ personality facets’ probability distributions are from the same distribution through the two-sample Kolmogorov-Smirnov test. There is no assumption about the distributions, but the test is sensitive to deviations near the centre rather than the tail.
Privacy and ethics
The focus of this research is to provide high-level insights about groups of startups, founders and types of founder teams rather than on specific individuals or companies. While we used unit record data from the publicly available data of company profiles from Crunchbase , we removed all identifiers from the underlying data on individual companies and founders and generated aggregate results, which formed the basis for our analysis and conclusions.
Data availability
A dataset which includes only aggregated statistics about the success of startups and the factors that influence is released as part of this research. Underlying data for all figures and the code to reproduce them are available on GitHub: https://github.com/Braesemann/FounderPersonalities . Please contact Fabian Braesemann ( [email protected] ) in case you have any further questions.
Change history
07 may 2024.
A Correction to this paper has been published: https://doi.org/10.1038/s41598-024-61082-7
Henrekson, M. & Johansson, D. Gazelles as job creators: A survey and interpretation of the evidence. Small Bus. Econ. 35 , 227–244 (2010).
Article Google Scholar
Davila, A., Foster, G., He, X. & Shimizu, C. The rise and fall of startups: Creation and destruction of revenue and jobs by young companies. Aust. J. Manag. 40 , 6–35 (2015).
Which vaccine saved the most lives in 2021?: Covid-19. The Economist (Online) (2022). noteName - AstraZeneca; Pfizer Inc; BioNTech SE; Copyright - Copyright The Economist Newspaper NA, Inc. Jul 14, 2022; Last updated - 2022-11-29.
Oltermann, P. Pfizer/biontech tax windfall brings mainz an early christmas present (2021). noteName - Pfizer Inc; BioNTech SE; Copyright - Copyright Guardian News & Media Limited Dec 27, 2021; Last updated - 2021-12-28.
Grant, K. A., Croteau, M. & Aziz, O. The survival rate of startups funded by angel investors. I-INC WHITE PAPER SER.: MAR 2019 , 1–21 (2019).
Google Scholar
Top 20 reasons start-ups fail - cb insights version (2019). noteCopyright - Copyright Newstex Oct 21, 2019; Last updated - 2022-10-25.
Hochberg, Y. V., Ljungqvist, A. & Lu, Y. Whom you know matters: Venture capital networks and investment performance. J. Financ. 62 , 251–301 (2007).
Fracassi, C., Garmaise, M. J., Kogan, S. & Natividad, G. Business microloans for us subprime borrowers. J. Financ. Quantitative Ana. 51 , 55–83 (2016).
Davila, A., Foster, G. & Gupta, M. Venture capital financing and the growth of startup firms. J. Bus. Ventur. 18 , 689–708 (2003).
Nann, S. et al. Comparing the structure of virtual entrepreneur networks with business effectiveness. Proc. Soc. Behav. Sci. 2 , 6483–6496 (2010).
Guzman, J. & Stern, S. Where is silicon valley?. Science 347 , 606–609 (2015).
Article ADS CAS PubMed Google Scholar
Aldrich, H. E. & Wiedenmayer, G. From traits to rates: An ecological perspective on organizational foundings. 61–97 (2019).
Gartner, W. B. Who is an entrepreneur? is the wrong question. Am. J. Small Bus. 12 , 11–32 (1988).
Thornton, P. H. The sociology of entrepreneurship. Ann. Rev. Sociol. 25 , 19–46 (1999).
Eikelboom, M. E., Gelderman, C. & Semeijn, J. Sustainable innovation in public procurement: The decisive role of the individual. J. Public Procure. 18 , 190–201 (2018).
Kerr, S. P. et al. Personality traits of entrepreneurs: A review of recent literature. Found. Trends Entrep. 14 , 279–356 (2018).
Hamilton, B. H., Papageorge, N. W. & Pande, N. The right stuff? Personality and entrepreneurship. Quant. Econ. 10 , 643–691 (2019).
Salmony, F. U. & Kanbach, D. K. Personality trait differences across types of entrepreneurs: A systematic literature review. RMS 16 , 713–749 (2022).
Freiberg, B. & Matz, S. C. Founder personality and entrepreneurial outcomes: A large-scale field study of technology startups. Proc. Natl. Acad. Sci. 120 , e2215829120 (2023).
Article CAS PubMed PubMed Central Google Scholar
Kern, M. L., McCarthy, P. X., Chakrabarty, D. & Rizoiu, M.-A. Social media-predicted personality traits and values can help match people to their ideal jobs. Proc. Natl. Acad. Sci. 116 , 26459–26464 (2019).
Article ADS CAS PubMed PubMed Central Google Scholar
Dalle, J.-M., Den Besten, M. & Menon, C. Using crunchbase for economic and managerial research. (2017).
Block, J. & Sandner, P. What is the effect of the financial crisis on venture capital financing? Empirical evidence from us internet start-ups. Ventur. Cap. 11 , 295–309 (2009).
Antretter, T., Blohm, I. & Grichnik, D. Predicting startup survival from digital traces: Towards a procedure for early stage investors (2018).
Dworak, D. Analysis of founder background as a predictor for start-up success in achieving successive fundraising rounds. (2022).
Hsu, D. H. Venture capitalists and cooperative start-up commercialization strategy. Manage. Sci. 52 , 204–219 (2006).
Blank, S. Why the lean start-up changes everything (2018).
Kaplan, S. N. & Lerner, J. It ain’t broke: The past, present, and future of venture capital. J. Appl. Corp. Financ. 22 , 36–47 (2010).
Hallen, B. L. & Eisenhardt, K. M. Catalyzing strategies and efficient tie formation: How entrepreneurial firms obtain investment ties. Acad. Manag. J. 55 , 35–70 (2012).
Gompers, P. A. & Lerner, J. The Venture Capital Cycle (MIT Press, 2004).
Shane, S. & Venkataraman, S. The promise of entrepreneurship as a field of research. Acad. Manag. Rev. 25 , 217–226 (2000).
Zahra, S. A. & Wright, M. Understanding the social role of entrepreneurship. J. Manage. Stud. 53 , 610–629 (2016).
Bonaventura, M. et al. Predicting success in the worldwide start-up network. Sci. Rep. 10 , 1–6 (2020).
Schwartz, H. A. et al. Personality, gender, and age in the language of social media: The open-vocabulary approach. PLoS ONE 8 , e73791 (2013).
Plank, B. & Hovy, D. Personality traits on twitter-or-how to get 1,500 personality tests in a week. In Proceedings of the 6th workshop on computational approaches to subjectivity, sentiment and social media analysis , pp 92–98 (2015).
Arnoux, P.-H. et al. 25 tweets to know you: A new model to predict personality with social media. In booktitleEleventh international AAAI conference on web and social media (2017).
Roberts, B. W., Kuncel, N. R., Shiner, R., Caspi, A. & Goldberg, L. R. The power of personality: The comparative validity of personality traits, socioeconomic status, and cognitive ability for predicting important life outcomes. Perspect. Psychol. Sci. 2 , 313–345 (2007).
Article PubMed PubMed Central Google Scholar
Youyou, W., Kosinski, M. & Stillwell, D. Computer-based personality judgments are more accurate than those made by humans. Proc. Natl. Acad. Sci. 112 , 1036–1040 (2015).
Soldz, S. & Vaillant, G. E. The big five personality traits and the life course: A 45-year longitudinal study. J. Res. Pers. 33 , 208–232 (1999).
Damian, R. I., Spengler, M., Sutu, A. & Roberts, B. W. Sixteen going on sixty-six: A longitudinal study of personality stability and change across 50 years. J. Pers. Soc. Psychol. 117 , 674 (2019).
Article PubMed Google Scholar
Rantanen, J., Metsäpelto, R.-L., Feldt, T., Pulkkinen, L. & Kokko, K. Long-term stability in the big five personality traits in adulthood. Scand. J. Psychol. 48 , 511–518 (2007).
Roberts, B. W., Caspi, A. & Moffitt, T. E. The kids are alright: Growth and stability in personality development from adolescence to adulthood. J. Pers. Soc. Psychol. 81 , 670 (2001).
Article CAS PubMed Google Scholar
Cobb-Clark, D. A. & Schurer, S. The stability of big-five personality traits. Econ. Lett. 115 , 11–15 (2012).
Graham, P. Do Things that Don’t Scale (Paul Graham, 2013).
McCarthy, P. X., Kern, M. L., Gong, X., Parker, M. & Rizoiu, M.-A. Occupation-personality fit is associated with higher employee engagement and happiness. (2022).
Pratt, A. C. Advertising and creativity, a governance approach: A case study of creative agencies in London. Environ. Plan A 38 , 1883–1899 (2006).
Klotz, A. C., Hmieleski, K. M., Bradley, B. H. & Busenitz, L. W. New venture teams: A review of the literature and roadmap for future research. J. Manag. 40 , 226–255 (2014).
Duggan, M., Ellison, N. B., Lampe, C., Lenhart, A. & Madden, M. Demographics of key social networking platforms. Pew Res. Center 9 (2015).
Fisch, C. & Block, J. H. How does entrepreneurial failure change an entrepreneur’s digital identity? Evidence from twitter data. J. Bus. Ventur. 36 , 106015 (2021).
Brush, C., Edelman, L. F., Manolova, T. & Welter, F. A gendered look at entrepreneurship ecosystems. Small Bus. Econ. 53 , 393–408 (2019).
Kanze, D., Huang, L., Conley, M. A. & Higgins, E. T. We ask men to win and women not to lose: Closing the gender gap in startup funding. Acad. Manag. J. 61 , 586–614 (2018).
Fan, J. S. Startup biases. UC Davis Law Review (2022).
AlShebli, B. K., Rahwan, T. & Woon, W. L. The preeminence of ethnic diversity in scientific collaboration. Nat. Commun. 9 , 1–10 (2018).
Article CAS Google Scholar
Żbikowski, K. & Antosiuk, P. A machine learning, bias-free approach for predicting business success using crunchbase data. Inf. Process. Manag. 58 , 102555 (2021).
Corea, F., Bertinetti, G. & Cervellati, E. M. Hacking the venture industry: An early-stage startups investment framework for data-driven investors. Mach. Learn. Appl. 5 , 100062 (2021).
Chapman, G. & Hottenrott, H. Founder personality and start-up subsidies. Founder Personality and Start-up Subsidies (2021).
Antoncic, B., Bratkovicregar, T., Singh, G. & DeNoble, A. F. The big five personality-entrepreneurship relationship: Evidence from slovenia. J. Small Bus. Manage. 53 , 819–841 (2015).
Download references
Acknowledgements
We thank Gary Brewer from BuiltWith ; Leni Mayo from Influx , Rachel Slattery from TeamSlatts and Daniel Petre from AirTree Ventures for their ongoing generosity and insights about startups, founders and venture investments. We also thank Tim Li from Crunchbase for advice and liaison regarding data on startups and Richard Slatter for advice and referrals in Twitter .
Author information
Authors and affiliations.
The Data Science Institute, University of Technology Sydney, Sydney, NSW, Australia
Paul X. McCarthy
School of Computer Science and Engineering, UNSW Sydney, Sydney, NSW, Australia
Faculty of Engineering and Information Technology, University of Technology Sydney, Sydney, Australia
Xian Gong & Marian-Andrei Rizoiu
Oxford Internet Institute, University of Oxford, Oxford, UK
Fabian Braesemann & Fabian Stephany
DWG Datenwissenschaftliche Gesellschaft Berlin, Berlin, Germany
Melbourne Graduate School of Education, The University of Melbourne, Parkville, VIC, Australia
Margaret L. Kern
You can also search for this author in PubMed Google Scholar
Contributions
All authors designed research; All authors analysed data and undertook investigation; F.B. and F.S. led multi-factor analysis; P.M., X.G. and M.A.R. led the founder/employee prediction; M.L.K. led personality insights; X.G. collected and tabulated the data; X.G., F.B., and F.S. created figures; X.G. created final art, and all authors wrote the paper.
Corresponding author
Correspondence to Fabian Braesemann .
Ethics declarations
Competing interests.
The authors declare no competing interests.
Additional information
Publisher's note.
Springer Nature remains neutral with regard to jurisdictional claims in published maps and institutional affiliations.
The original online version of this Article was revised: The Data Availability section in the original version of this Article was incomplete, the link to the GitHub repository was omitted. Full information regarding the corrections made can be found in the correction for this Article.
Supplementary Information
Supplementary information., rights and permissions.
Open Access This article is licensed under a Creative Commons Attribution 4.0 International License, which permits use, sharing, adaptation, distribution and reproduction in any medium or format, as long as you give appropriate credit to the original author(s) and the source, provide a link to the Creative Commons licence, and indicate if changes were made. The images or other third party material in this article are included in the article's Creative Commons licence, unless indicated otherwise in a credit line to the material. If material is not included in the article's Creative Commons licence and your intended use is not permitted by statutory regulation or exceeds the permitted use, you will need to obtain permission directly from the copyright holder. To view a copy of this licence, visit http://creativecommons.org/licenses/by/4.0/ .
Reprints and permissions
About this article
Cite this article.
McCarthy, P.X., Gong, X., Braesemann, F. et al. The impact of founder personalities on startup success. Sci Rep 13 , 17200 (2023). https://doi.org/10.1038/s41598-023-41980-y
Download citation
Received : 15 February 2023
Accepted : 04 September 2023
Published : 17 October 2023
DOI : https://doi.org/10.1038/s41598-023-41980-y
Share this article
Anyone you share the following link with will be able to read this content:
Sorry, a shareable link is not currently available for this article.
Provided by the Springer Nature SharedIt content-sharing initiative
By submitting a comment you agree to abide by our Terms and Community Guidelines . If you find something abusive or that does not comply with our terms or guidelines please flag it as inappropriate.
Quick links
- Explore articles by subject
- Guide to authors
- Editorial policies
Sign up for the Nature Briefing: AI and Robotics newsletter — what matters in AI and robotics research, free to your inbox weekly.

Zscaler: A Leader in the 2024 Gartner® Magic Quadrant™ for Security Service Edge (SSE)

Figure 1: Attack chain and execution flow for Anatsa campaigns.
Recently, we identified two malicious payloads, associated with Anatsa, that were distributed in the Google Play store by threat actors. This campaign impersonated PDF reader and QR code reader applications, as is often the case, to attract a large number of installations. The high number of installations further aids in deceiving victims into believing that these applications are genuine. At the time of analysis, both applications had already amassed over 70,000 installations.
The figure below displays these PDF and QR code reader applications. However, these applications are actually malicious installers that, if installed, initiate the infection process.

Figure 2: Malicious installers disguised as a legitimate PDF reader and QR code reader in the Google Play store.
Technical Analysis
As mentioned previously, Anatsa utilizes remote payloads retrieved from C2 servers to carry out further malicious activity.
In the figure below, the dropper application is shown with encoded links to remote servers, from which the next stage payload will be downloaded. In addition to downloading the payload, the malware also retrieves a configuration file from the remote server to execute the next stage payload.

Figure 3: Anatsa dropper’s payload and configuration URLs.
In the figure below, the DEX file is downloaded and will be loaded by the parent fake QR code application.

Figure 4: Anatsa dropper’s network request to download the DEX file for the next stage payload.
The application utilizes reflection to invoke code from a loaded DEX file. The necessary configuration to load the DEX file is downloaded from the control server, as depicted in the network response shown below.

Figure 5: Anatsa dropper’s configuration to run the downloaded DEX file.
After the next stage payload is downloaded, Anatsa performs a series of checks for the device environment and device type. This is likely designed to detect analysis environments and malware sandboxes. Upon successful verification, it proceeds to download the third stage and final payload from the remote server, as depicted in the figure below.

Figure 6: Code that checks the device environment and downloads final stage Anatsa payload.
In this particular campaign, the Anatsa malware injected uncompressed raw manifest data into the APK. The threat actors also intentionally corrupted the compression parameters in the manifest file to hinder analysis. The figure below depicts the corrupted ZIP headers.

Figure 7: Anti-analysis technique utilized by Anatsa with malformed ZIP parameters.
In order to statically analyze the payload, the headers of the ZIP file must be fixed alongside the compressed data.
After the APK is loaded, the malware requests various permissions, including the SMS and accessibility options, which are commonly associated with mobile banking trojans. The malware conceals the final DEX payload within the asset files. During runtime, the payload decrypts the DEX file using a static key embedded within the code.

Figure 8: Anatsa malware with the correct manifest.
Upon execution, the malware decodes all encoded strings, including the C2 communication. The malware establishes communication with the C2 server to carry out various activities, such as registering the infected device and retrieving a list of targeted applications for code injections.
In order to steal data from financial applications, Anatsa downloads a target list. The figure below shows the Anatsa configuration request and response.

Figure 9: Anatsa configuration request and response being intercepted.
The figure below shows the request and response data being decoded with an XOR key.

Upon receiving a list of financial application package names, the malware scans the victim's device to check if any of these targeted applications are installed. Once the malware identifies the presence of a targeted application, Anatsa communicates this information to the C2 server. In response, the C2 server provides a fake login page for the banking application. This activity is illustrated in the figure below.
Figure 11: Anatsa injection configuration request based on the presence of a specific financial application.
The fake login page is loaded within a JavaScript Interface (JSI) enabled webview, which is designed to deceive the user into providing their banking credentials. Once the victim enters their credentials that data is sent back to the C2 server.
Based on our analysis, the most commonly leveraged application category for threat actors to spread malware is tools, which accounts for almost 40%. Personalization and photography constitute about 20% and 13%, respectively. The graph below shows which application categories are the most exploited by threat actors attempting to spread malware via the Google Play store.
.png)
Figure 12: A breakdown of the most commonly exploited application types by threat actors.
The choice of using the tools category to distribute malware is likely due to the popularity of these types of applications making them easier to blend into the Google Play store.
During our analysis, we identified several malware families distributed via the Google Play store including:
- Facestealer
The graph below shows which malware families ThreatLabz has observed used by threat actors to orchestrate attacks via the Google Play store.
.png)
Figure 13: Malware family distribution in the Google Play store.
Although they take up the smallest share at 2% and 1% (respectively), Antasa and Coper are well-known and highly impactful banking trojan malware families. Last year, we observed multiple instances of Coper banking malware present in the Google Play store.
The recent campaigns conducted by threat actors deploying the Anatsa banking trojan highlight the risks faced by Android users, in multiple geographic regions, who downloaded these malicious applications from the Google Play store. As the mobile threat landscape continues to evolve, it becomes crucial for organizations to implement proactive security measures to safeguard their systems and sensitive financial information. To enhance the security of your network, we recommend implementing Zscaler's zero trust architecture. This approach focuses on user-centric security and ensures that users are authenticated and authorized before accessing any resources, regardless of their device or location.
Zscaler’s multilayered cloud security platform detects indicators related to Anatsa at various levels with the following threat names:
- Android.Banker.Anatsa
- AND/Agent5.AE
- AndroidOS/Agent.BOI
Mobile matrix
Was this post useful, explore more zscaler blogs.

By submitting the form, you are agreeing to our privacy policy .
- Share full article
Advertisement
Supported by
Fate of Retired Research Chimps Still in Limbo
The National Institutes of Health, which owns the chimps at the Alamogordo Primate Facility in New Mexico, has no plans to move the animals to sanctuary, despite a ruling from a federal judge.

By Emily Anthes
It has been more than two decades since chimpanzee research came to a halt at the Alamogordo Primate Facility in New Mexico. And yet, some two dozen chimps still live there, despite a federal law that requires such retired research chimps to be moved to sanctuary.
In 2022, a judge ruled that the National Institutes of Health, which owns the chimps, was violating the law by refusing to move the animals to a wooded sanctuary in Louisiana. Earlier this year, the agency dropped its appeal of the ruling.
But the N.I.H. says it has no immediate plans to move the animals, citing concerns about the animals’ health — and a legal footnote that may exempt the agency from moving chimps that are “moribund,” a term that typically means near death.
As of last October, 28 chimps remained at Alamogordo, all of whom were moribund, the N.I.H. said in an email. It defined moribund as suffering from “life-threatening, systemic disease that poses a constant threat and could result in abrupt death.”
Some of the animals had previously been diagnosed with advanced cardiovascular disease, which is common in older chimps.
The N.I.H.’s refusal to transfer the chimps has drawn criticism from lawmakers, veterinarians and animal rights advocates.
The Humane Society of the United States, which sued the N.I.H. over its unwillingness to move the chimps, noted that the agency had been describing the chimps as “moribund” for years, making it unlikely that the animals were truly on the brink of death.
“They really need to stop making excuses,” said Kathleen Conlee, vice president of animal research issues at the Humane Society. “A lot of chimpanzees have moved to sanctuary with health conditions and thrived in their new environment.”
Independent veterinary experts, who had not examined the Alamogordo chimps personally, also wondered how animals could be moribund for years.
“‘Moribund’ in human or veterinary medicine is generally understood to mean death is imminent,” said Dr. Felicia Nutter, a wildlife veterinarian at Tufts University. “So any determination that chimps have been moribund for three years — there’s something not right with that.”
Transportation does pose risks, said Dr. Kathryn Gamble, the director of veterinary medicine at Lincoln Park Zoo in Chicago. But even significant cardiac disease does not make such moves impossible, she said.
“We have humans that have anything from mild to severe cardiac disease — many of them still travel,” Dr. Gamble said. And the risks of transport should be weighed against the potential benefits that might come from the change in environment, she said.
The Chimpanzee Health Improvement Maintenance Protection, or CHIMP, Act, which Congress passed in 2000, established a national chimpanzee sanctuary system and stipulated that federally owned chimps that were not needed for research be sent to sanctuary. Chimp Haven, a 200-acre sanctuary in Louisiana, serves as the designated retirement home.
In 2015, the N.I.H. announced that it would no longer support biomedical research on chimpanzees, and many Alamogordo chimps have since moved to Chimp Haven. But in 2019, the N.I.H. said that a veterinary panel had concluded that the 44 chimps remaining at Alamogordo were too sick to leave .
“The physiological and psychological stressors associated with transportation, quarantine, change in social structure and change in human care provider could trigger a fatal cardiac event,” the panel wrote in one case summary. The panel also expressed concern that chimps with disabilities, including cataracts and a leg amputation, might not integrate safely into new social groups and settings.
The Humane Society and other animal rights groups sued over the decision, accusing the N.I.H. of violating the CHIMP Act. In December 2022, a federal judge ruled in their favor, but added, in a footnote, “The parties appear to agree that N.I.H. is not obligated to transfer a ‘moribund’ chimpanzee to sanctuary.”
The Santa Fe New Mexican reported last month that the N.I.H. had no immediate plans to transfer the chimps.
“N.I.H. plans to conduct an annual evaluation of the chimpanzees at Alamogordo to determine if they are moribund, or if they are no longer moribund and can be safely transported to Chimp Haven,” the agency said in an email.
A plan for annual evaluations suggested that the chimps were not on the brink of death, said Rana Smith, the president of Chimp Haven.
Ms. Smith also objected to the agency’s interpretation of the law, noting that the CHIMP Act does not use the word “moribund” or make exceptions for chronically ill chimps. “We have found that chimpanzees are an incredibly resilient species and thrive in the sanctuary environment,” she said.
Several U.S. lawmakers have been urging the N.I.H. to transfer the remaining Alamogordo chimps for years. “I have been advocating for a humane and permanent solution for these chimpanzees, so they can live out the rest of their lives in a non-laboratory sanctuary environment,” Senator Martin Heinrich, Democrat of New Mexico, said in an emailed statement.
The agency’s refusal to move those chimps “stands in direct violation of federal law,” he added. “I am urging them to reconsider.”
Emily Anthes is a science reporter, writing primarily about animal health and science. She also covered the coronavirus pandemic. More about Emily Anthes
Explore the Animal Kingdom
A selection of quirky, intriguing and surprising discoveries about animal life..
The Lord Howe Island stick insect vanished from its home, but an effort at zoos in San Diego and Melbourne highlights the possibilities and challenges of conserving invertebrate animals.
A genetic analysis of the German cockroach explained its rise in southern Asia millenniums ago, and how it eventually turned up in your kitchen .
Scientists say they have found an “alphabet” in the songs of sperm whales , raising the possibility that the animals are communicating in a complex language.
Indigenous rangers in Australia’s Western Desert got a rare close-up with the northern marsupial mole , which is tiny, light-colored and blind, and almost never comes to the surface.
For the first time, scientists observed a primate in the wild treating a wound with a plant that has medicinal properties.
The future of financial analysis: How GPT-4 is disrupting the industry, according to new research
- Share on Facebook
- Share on LinkedIn
Join us in returning to NYC on June 5th to collaborate with executive leaders in exploring comprehensive methods for auditing AI models regarding bias, performance, and ethical compliance across diverse organizations. Find out how you can attend here .
Researchers from the University of Chicago have demonstrated that large language models (LLMs) can conduct financial statement analysis with accuracy rivaling and even surpassing that of professional analysts. The findings, published in a working paper titled “ Financial Statement Analysis with Large Language Models ,” could have major implications for the future of financial analysis and decision-making.
The researchers tested the performance of GPT-4 , a state-of-the-art LLM developed by OpenAI , on the task of analyzing corporate financial statements to predict future earnings growth. Remarkably, even when provided only with standardized, anonymized balance sheets, and income statements devoid of any textual context, GPT-4 was able to outperform human analysts.
“We find that the prediction accuracy of the LLM is on par with the performance of a narrowly trained state-of-the-art ML model,” the authors write. “LLM prediction does not stem from its training memory. Instead, we find that the LLM generates useful narrative insights about a company’s future performance.”

Chain-of-thought prompts emulate human analyst reasoning
A key innovation was the use of “ chain-of-thought ” prompts that guided GPT-4 to emulate the analytical process of a financial analyst, identifying trends, computing ratios, and synthesizing the information to form a prediction. This enhanced version of GPT-4 achieved a 60% accuracy in predicting the direction of future earnings, notably higher than the 53-57% range of human analyst forecasts.
The AI Impact Tour: The AI Audit
Join us as we return to NYC on June 5th to engage with top executive leaders, delving into strategies for auditing AI models to ensure fairness, optimal performance, and ethical compliance across diverse organizations. Secure your attendance for this exclusive invite-only event.
“Taken together, our results suggest that LLMs may take a central role in decision-making,” the researchers conclude. They note that the LLM’s advantage likely stems from its vast knowledge base and ability to recognize patterns and business concepts, allowing it to perform intuitive reasoning even with incomplete information.

LLMs poised to transform financial analysis despite challenges
The findings are all the more remarkable given that numerical analysis has traditionally been a challenge for language models. “One of the most challenging domains for a language model is the numerical domain, where the model needs to carry out computations, perform human-like interpretations, and make complex judgments,” said Alex Kim, one of the study’s co-authors. “While LLMs are effective at textual tasks, their understanding of numbers typically comes from the narrative context and they lack deep numerical reasoning or the flexibility of a human mind.”
Some experts caution that the “ ANN ” model used as a benchmark in the study may not represent the state-of-the-art in quantitative finance. “That ANN benchmark is nowhere near state of the art,” commented one practitioner on the Hacker News forum . “People didn’t stop working on this in 1989 — they realized they can make lots of money doing it and do it privately.”
Nevertheless, the ability of a general-purpose language model to match the performance of specialized ML models and exceed human experts points to the disruptive potential of LLMs in the financial domain. The authors have also created an interactive web application to showcase GPT-4’s capabilities for curious readers, though they caution that its accuracy should be independently verified.
As AI continues its rapid advance, the role of the financial analyst may be the next to be transformed. While human expertise and judgment are unlikely to be fully replaced anytime soon, powerful tools like GPT-4 could greatly augment and streamline the work of analysts, potentially reshaping the field of financial statement analysis in the years to come.
Stay in the know! Get the latest news in your inbox daily
By subscribing, you agree to VentureBeat's Terms of Service.
Thanks for subscribing. Check out more VB newsletters here .
An error occured.
Return-to-Office Orders: A Survey Analysis of Employment Impacts
How did employers expect return-to-office (RTO) orders to affect employment? Were those expectations correct? We use special questions from the Richmond Fed business surveys to shed light on these questions. Overall, RTO orders were expected to reduce employment, but there was both substantial uncertainty and heterogeneity in expectations. Some employers even expected that RTO would increase employment. Ex post, employers believe RTO orders had a muted effect on employment. We find that the service sector was more likely to both issue RTO orders and expect and experience a reduction in employment.
The COVID-19 pandemic changed the way that both employers and employees think about the location of work. 1 The advent of remote work en masse in 2020 has been followed by a gradual implementation of requiring workers to work from the office, at least for some of their workweek. These forced return-to-office (RTO) orders have come with controversy: Many employers have implemented these policies, while many employees have resisted.
In this article, we attempt to shed light on the effects of RTO by reporting on special questions we asked in the March Richmond Fed business surveys . Specifically, these questions shed light on both the anticipated and realized employment outcomes of RTO orders from the employer's perspective. We find that uncertainty in the decision-making process was prevalent, but also that realized outcomes were generally muted. RTO did have an expected and actual negative effect on employment, but only in some sectors and for some employers. For others, RTO was a means of increasing employment. Our results highlight the large uncertainty in the pandemic, the heterogeneity of firms and the large heterogeneity of workers.
Why Examine the Impacts of RTO Orders?
This survey builds on a recent literature investigating the implications of remote work for workers, businesses and local economies . Uniquely, it attempts to discern how business leaders anticipated RTO policies would impact their firms as well as the actual impact on employment within their firms. Although there is work evaluating the benefits and costs to employers in terms of productivity or labor/non-labor costs, 2 there has been little work to understand the firm-by-firm implication of articulating and enforcing an RTO order.
Research indicates that hybrid options are highly valued by employees , 3 but how many separations can be attributed to an RTO policy? There is evidence that managers value in-person work more than employees, 4 but does that result in actual separations when RTO orders are implemented? Our results suggest the effects of these policies were muted.
There is also evidence of wide variation in employee hybrid-work preferences and in their willingness to pay for the option to work from home 5 as well as evidence that the value workers place on the "amenity" of remote or hybrid work has implications for aggregate wage changes in the macroeconomy. 6 Our work indicates this heterogeneity in preferences may have dampened the effect of RTO orders on employment. Our results are consistent with a literature that is still relatively mixed about the net effect on employers and workers of remote or on-site policies.
Methodology
The Federal Reserve Bank of Richmond has surveyed CEOs and other business leaders across the Fifth Federal Reserve District 7 for almost 30 years, currently gathering around 200-250 responses per month. The survey panel underweights the smallest firms and, due to the history of the survey, manufacturing firms make up about one-third of respondents even though they make up a much smaller share of establishments in the Fifth District or the nation.
In addition to a series of questions about variables such as demand, employment and prices, respondents are commonly asked a set of ad hoc questions. Here, we focus on a set of questions asked in March 2024 regarding the extent to which respondents articulated and enforced a mandatory RTO policy and what they expected upon its implementation. Emily Corcoran reported on employers' on-site general expectations for employees and how those have changed. But here, we focus on business leaders' expectations of RTO policy effects, providing insight into the anticipated and unanticipated employment effects of RTO orders. We begin by assessing whether the establishment implemented RTO. These results are tabulated in Table 1.
Overall, explicit RTO orders were relatively rare, with only 20 percent of respondents articulating RTO orders in the last three years. This small percentage is partly because 37 percent of respondents — many of them manufacturing firms — were fully on-site before the end of 2020, and an additional 26 percent of respondents said RTO wasn't applicable for their companies. 8 Of the remaining companies, there is a roughly equal split between firms that have an explicit RTO policy (20 percent of the full sample) and those that do not (16 percent of the full sample).
We asked these 20 percent of employers about the expected consequences of issuing RTO orders. Did they expect workers to quit because of these policies? Were they sure about the effect on employment? We also asked employers about their assessment of realized outcomes. Did workers quit as anticipated? Did RTO help the firm recruit workers?
What Did Employers Expect, and What Actually Happened?
Perhaps surprisingly, we found two-thirds of employers expected no impact on (net) employment from RTO orders, while 16 percent were too unsure of the impact to answer (Table 2). Among the 18 percent that expected some impact, the anticipated outcome was split between those that expected a decrease in employment (11 percent) and those that expected an increase (7 percent).
Why might employment increase? One possibility derives from employees feeling more connected to their co-workers with greater mentoring opportunities when in the office. 9 This could reduce quitting and improve hiring, as one survey respondent reported that, "...the employees that [formerly] chose to work remotely decided that they were more productive in the office. We are [now] 90+ percent in the office."
Additionally, RTO orders have often been hybrid, 10 potentially allowing the benefits of office culture to be obtained without sacrificing all of the flexibility associated with remote work.
We also asked employers about their evaluation of outcomes, and the results are given in Table 3. Here, a greater percentage reported no impact (82 percent), while 4 percent assessed that RTO had decreased employment, and 4 percent assessed that RTO had actually increased employment. (Nine percent were still unsure.)
Sectoral level analysis reveals employment impacts (both expected and realized) were concentrated in the service sector. In manufacturing, no firms concretely expected a change in employment (though some were unsure), and ex post they believe RTO did not cause them to lose workers. In services, however, only 59 percent expected no impact, while 16 percent expected a negative impact on employment. Ex post, impact on employment was less than expected.
While our analysis is suggestive, there are a few limitations. Foremost, our effective sample size was small, meaning some of these results could be driven by sampling error. Second, it has been years since some employers implemented RTO policies, so their memories of their expectations could be inaccurate. Third, our survey did not control for any other firm changes — such as changes in wages or product demand — that could confound our findings. Fourth, although our findings provide insight into net employment gains and losses, they do not speak to hiring and firing separately. 11
With these caveats in mind, however, our results show that RTO — while still a common topic of conversation — is not necessarily important to employers' and workers' employment decisions. Concerns about employment effects ex ante mostly did not materialize. Employment effects that did materialize were concentrated in services and resulted in a net gain of employees in some cases, rather than a loss.
Grey Gordon is a senior economist and Sonya Ravindranath Waddell is a vice president and economist, both in the Research Department of the Federal Reserve Bank of Richmond. The authors thank Jason Kosakow for helping to develop and execute the survey and for providing the tabulations underlying this analysis and thank RC Balaban, Zach Edwards and Claudia Macaluso for providing feedback on an earlier draft.
See, for example, the 2023 paper " The Evolution of Work From Home " by Jose Maria Barrero, Nicholas Bloom and Steven Davis.
See, for example, the 2024 working paper " The Big Shift in Working Arrangements: Eight Ways Unusual " by Steven Davis.
See, for example, the 2023 working paper " How Hybrid Working From Home Works Out " by Nicholas Bloom, Ruobing Han and James Liang.
See the previously cited paper " How Hybrid Working From Home Works Out ."
See, for example, the 2021 working paper " Why Working From Home Will Stick " by Jose Maria Barrero, Nicholas Bloom and Steven Davis.
See, for example, the 2024 working paper " Job Amenity Shocks and Labor Reallocation (PDF) " by Sadhika Bagga, Lukas Mann, Aysegul Sahin and Giovanni Violante.
The Fifth District comprises the District of Columbia, Maryland, North Carolina, South Carolina, Virginia and most of West Virginia.
Those who answered "not applicable" are presumably firms where work is necessarily done in person.
See, for example, the 2023 article " About a Third of U.S. Workers Who Can Work From Home Now Do So All the Time " by Kim Parker.
The previously cited article by Emily Corcoran noted that 38 percent of firms are in the office in between one and four days a week.
See the 2022 article " Changing Recruiting Practices and Methods in the Tight Labor Market " by Claudia Macaluso and Sonya Ravindranath Waddell for an analysis of how hiring practices have changed in the tight labor market that has prevailed since 2020.
This article may be photocopied or reprinted in its entirety. Please credit the authors, source, and the Federal Reserve Bank of Richmond and include the italicized statement below.
V iews expressed in this article are those of the authors and not necessarily those of the Federal Reserve Bank of Richmond or the Federal Reserve System.
Subscribe to Economic Brief
Receive a notification when Economic Brief is posted online.
By submitting this form you agree to the Bank's Terms & Conditions and Privacy Notice.
Thank you for signing up!
As a new subscriber, you will need to confirm your request to receive email notifications from the Richmond Fed. Please click the confirm subscription link in the email to activate your request.
If you do not receive a confirmation email, check your junk or spam folder as the email may have been diverted.
Phone Icon Contact Us
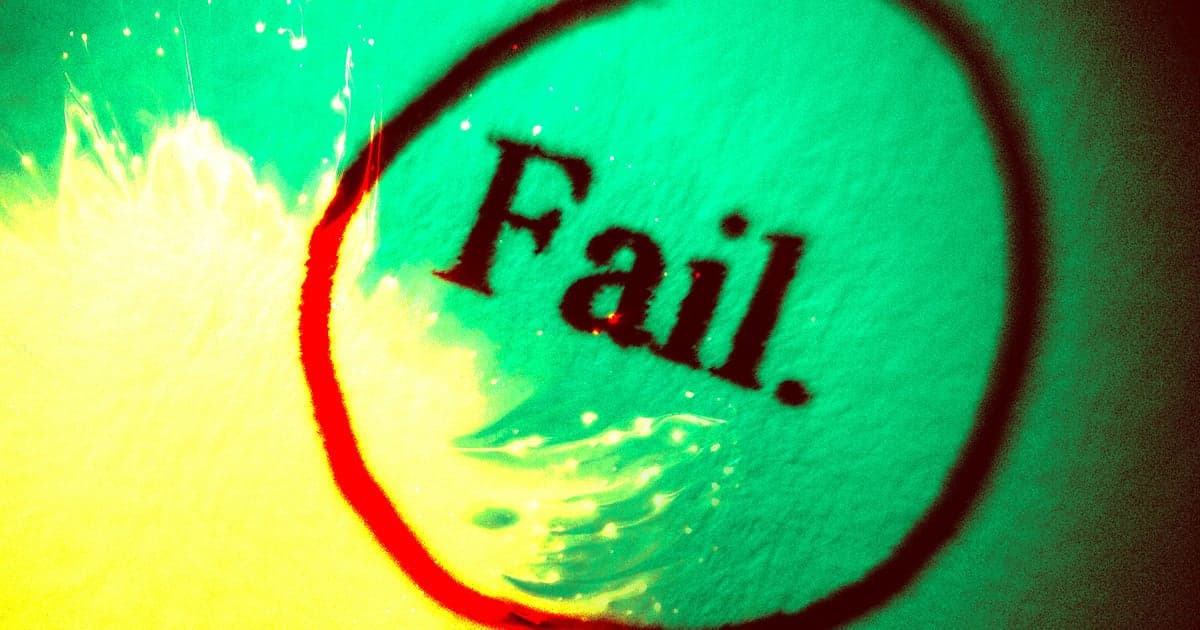
Study Finds That 52 Percent of ChatGPT Answers to Programming Questions Are Wrong
Ah yes. and yet..., not so smart.
In recent years, computer programmers have flocked to chatbots like OpenAI's ChatGPT to help them code, dealing a blow to places like Stack Overflow, which had to lay off nearly 30 percent of its staff last year.
The only problem? A team of researchers from Purdue University presented research this month at the Computer-Human Interaction conference that shows that 52 percent of programming answers generated by ChatGPT are incorrect.
That's a staggeringly large proportion for a program that people are relying on to be accurate and precise, underlining what other end users like writers and teachers are experiencing: AI platforms like ChatGPT often hallucinate totally incorrectly answers out of thin air .
For the study, the researchers looked over 517 questions in Stack Overflow and analyzed ChatGPT's attempt to answer them.
"We found that 52 percent of ChatGPT answers contain misinformation, 77 percent of the answers are more verbose than human answers, and 78 percent of the answers suffer from different degrees of inconsistency to human answers," they wrote.
Robot vs Human
The team also performed a linguistic analysis of 2,000 randomly selected ChatGPT answers and found they were "more formal and analytical" while portraying "less negative sentiment" — the sort of bland and cheery tone AI tends to produce.
What's especially troubling is that many human programmers seem to prefer the ChatGPT answers. The Purdue researchers polled 12 programmers — admittedly a small sample size — and found they preferred ChatGPT at a rate of 35 percent and didn't catch AI-generated mistakes at 39 percent.
Why is this happening? It might just be that ChatGPT is more polite than people online.
"The follow-up semi-structured interviews revealed that the polite language, articulated and text-book style answers, and comprehensiveness are some of the main reasons that made ChatGPT answers look more convincing, so the participants lowered their guard and overlooked some misinformation in ChatGPT answers," the researchers wrote.
The study demonstrates that ChatGPT still has major flaws — but that's cold comfort to people laid off from Stack Overflow or programmers who have to fix AI-generated mistakes in code.
More on OpenAI: Machine Learning Researcher Links OpenAI to Drug-Fueled Sex Parties
Share This Article
Help | Advanced Search
Computer Science > Cryptography and Security
Title: sok: leveraging transformers for malware analysis.
Abstract: The introduction of transformers has been an important breakthrough for AI research and application as transformers are the foundation behind Generative AI. A promising application domain for transformers is cybersecurity, in particular the malware domain analysis. The reason is the flexibility of the transformer models in handling long sequential features and understanding contextual relationships. However, as the use of transformers for malware analysis is still in the infancy stage, it is critical to evaluate, systematize, and contextualize existing literature to foster future research. This Systematization of Knowledge (SoK) paper aims to provide a comprehensive analysis of transformer-based approaches designed for malware analysis. Based on our systematic analysis of existing knowledge, we structure and propose taxonomies based on: (a) how different transformers are adapted, organized, and modified across various use cases; and (b) how diverse feature types and their representation capabilities are reflected. We also provide an inventory of datasets used to explore multiple research avenues in the use of transformers for malware analysis and discuss open challenges with future research directions. We believe that this SoK paper will assist the research community in gaining detailed insights from existing work and will serve as a foundational resource for implementing novel research using transformers for malware analysis.
Submission history
Access paper:.
- HTML (experimental)
- Other Formats

References & Citations
- Google Scholar
- Semantic Scholar
BibTeX formatted citation

Bibliographic and Citation Tools
Code, data and media associated with this article, recommenders and search tools.
- Institution
arXivLabs: experimental projects with community collaborators
arXivLabs is a framework that allows collaborators to develop and share new arXiv features directly on our website.
Both individuals and organizations that work with arXivLabs have embraced and accepted our values of openness, community, excellence, and user data privacy. arXiv is committed to these values and only works with partners that adhere to them.
Have an idea for a project that will add value for arXiv's community? Learn more about arXivLabs .
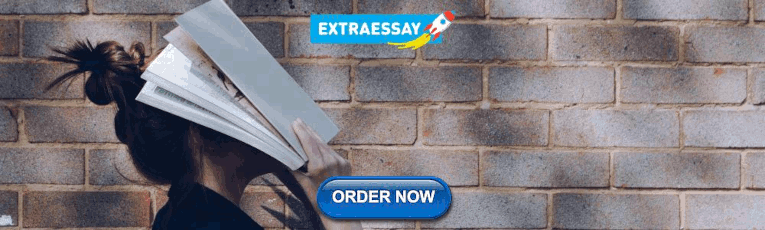
IMAGES
VIDEO
COMMENTS
Orison Solutions, LLC. Hyderabad, Telangana, India 4 weeks ago. Today's top 697 Research Analyst jobs in Hyderabad, Telangana, India. Leverage your professional network, and get hired. New Research Analyst jobs added daily.
3.9. 413 Research Analyst jobs available in Hyderabad, Telangana on Indeed.com.
Planning and executing reach out campaigns - emails, LinkedIn etc. according to the results of the research. 1,261 Research Analysis jobs available in Hyderabad, Telangana on Indeed.com.
173 Market research analyst jobs in Hyderābād. Most relevant. Blackridge Research & Consulting. Market Research Analyst. India. ₹3L - ₹5L (Employer Est.) Easy Apply. In your role as a research analyst, you will receive a competitive salary package and bonus as applicable.
Today's top 482 Research Analysis jobs in Hyderabad, Telangana, India. Leverage your professional network, and get hired. New Research Analysis jobs added daily.
How much does a Research Analyst make in Hyderabad, Telangana? Average base salary Data source tooltip for average base salary. ₹27,036. 8%. below national average. The average salary for a research analyst is ₹27,036 per month in Hyderabad, Telangana. 119 salaries reported, updated at 2 November 2023 ...
Search similar titles. Today's top 30 Financial Research Analyst jobs in Greater Hyderabad Area. Leverage your professional network, and get hired. New Financial Research Analyst jobs added daily.
Advanced Numerical Research & Analysis Group. Advanced Numerical Research and Analysis Group ( ANURAG) is a laboratory of the Defence Research and Development Organisation (DRDO). Located in Kanchanbagh, Hyderabad, it is involved in the development of computing solutions for numerical analysis and their use in other DRDO projects.
Conjoint analysis is a quantitative research technique used to measure and understand how consumers make trade-offs between different product or service attributes. It helps businesses determine the relative importance of product features and optimize their offerings. ... ResearchFox is one of the leading market research firms in Hyderabad ...
The top 3 best-rated Market Research agencies in Hyderabad on Clutch include: UXReactor (4.8 stars, 8 reviews) Studio Pinak (4.5 stars, 1 reviews) User Connect Consultancy (5 stars, 1 reviews) These Market Research agencies have consistently been lauded for their exceptional performance and dedication to client success.
Defence Research and Development Organisation (DRDO), Govt of India. ISTI Portal is a central repository of content generated in and by the Indian STI ecosystem with a major thrust to reach out to students, researchers, scholars, scientists from India and abroad, as to help them choose fellowships, scholarships, funding, and startup ...
Market research companies in Hyderabad conduct surveys, interviews, and data analysis to gather information on healthcare consumer behavior, pricing trends, and market dynamics. This data helps healthcare companies make informed decisions about product development, marketing strategies, and expansion plans.
Get the best solutions for your business with us! Our 10+ years of experience in marketing & research and working with 500+ clients from all over the world have given us the expertise to provide top-notch services. We are a committed market research company in Hyderabad, India providing the best solutions that keep you ahead of the competition.
NISHKA Research is a leader in scientific research and technical consulting services to forge solutions for the major industries. analytical lab. ... materials and components. We collaborate with clients in framing and solving problems through technical analysis. We assist our clients with decision-making and provide high quality results and ...
The average Market Research Analyst I salary in Hyderabad, IN is ₹737,638 as of March 18, 2021, but the range typically falls between ₹426,355 and ₹1,085,878. Salary ranges can vary widely depending on many important factors, including education, certifications, additional skills, the number of years you have spent in your profession.
Hyderabad, Telangana: Reliably commute or planning to relocate before starting work (Preferred). Total work: 1 year (Preferred). Employer Active 17 days ago ... Stay updated on industry trends, competitors, and market conditions through research and analysis ...
Scanning Electron Microscopy is a test process that produces a magnified image of a sample using an electron beam for analysis. Read more about SEM here. ... Nishka routinely extends support for research, failure mode analysis, ... Hyderabad-500016 India. Phone : +91 40 29303155 . Mobile : +91 7842798518 .
Salesforce. Hyderabad, Telangana, India. Actively Hiring. 4 days ago. Today's top 302 Operations Research Analyst jobs in Hyderabad, Telangana, India. Leverage your professional network, and get hired. New Operations Research Analyst jobs added daily.
If you have the questionnaire and the analysis platform available with you, and you just need good quality fieldwork to be done, yes! we take up these work as well. N. ... Modulus Research & Analysis Pvt. Ltd. 44, Shantipally, Sanjay Bhawan, 5th Floor Kolkata-700107 | India. More Location. Get in touch. Name. Email Address. Phone. Purpose. Message.
Using data on U.S. state and federal taxes and transfers over a quarter century, we estimate a regression model that yields the marginal effect of any shift of market income share from one quintile to another on the entire post tax, post-transfer income distribution. We identify exogenous income ...
This new and growing body of research includes several reviews and meta-studies, which show that personality traits play an important role in both career success and entrepreneurship 15,16,17,18 ...
In this particular campaign, the Anatsa malware injected uncompressed raw manifest data into the APK. The threat actors also intentionally corrupted the compression parameters in the manifest file to hinder analysis. The figure below depicts the corrupted ZIP headers. Figure 7: Anti-analysis technique utilized by Anatsa with malformed ZIP ...
The National Institutes of Health, which owns the chimps at the Alamogordo Primate Facility in New Mexico, has no plans to move the animals to sanctuary, despite a ruling from a federal judge.
Search similar titles. Today's top 70 Equity Research Analyst jobs in Hyderabad, Telangana, India. Leverage your professional network, and get hired. New Equity Research Analyst jobs added daily.
University of Chicago researchers demonstrate GPT-4's remarkable ability to predict corporate earnings, outperforming human analysts and transforming the future of financial statement analysis.
The COVID-19 pandemic changed the way that both employers and employees think about the location of work. 1 The advent of remote work en masse in 2020 has been followed by a gradual implementation of requiring workers to work from the office, at least for some of their workweek. These forced return-to-office (RTO) orders have come with controversy: Many employers have implemented these ...
Search similar titles. Today's top 700 Research Analyst jobs in Greater Hyderabad Area. Leverage your professional network, and get hired. New Research Analyst jobs added daily.
A team of researchers from Purdue University presented research this month at the Computer-Human Interaction conference that shows that 52 percent of programming answers generated by ChatGPT are ...
An analysis of data from 2023 found the U.S. Department of Agriculture's annual cost-of-living adjustment had little effect on the generosity of SNAP benefits in 2023. Key Findings In the last quarter of 2023, a 53-cent gap remained between the $3.37 cost of a modestly priced meal, and SNAP's average maximum benefit of $2.84—a shortfall ...
The introduction of transformers has been an important breakthrough for AI research and application as transformers are the foundation behind Generative AI. A promising application domain for transformers is cybersecurity, in particular the malware domain analysis. The reason is the flexibility of the transformer models in handling long sequential features and understanding contextual ...