
Researched by Consultants from Top-Tier Management Companies

Powerpoint Templates
Icon Bundle
Kpi Dashboard
Professional
Business Plans
Swot Analysis
Gantt Chart
Business Proposal
Marketing Plan
Project Management
Business Case
Business Model
Cyber Security
Business PPT
Digital Marketing
Digital Transformation
Human Resources
Product Management
Artificial Intelligence
Company Profile
Acknowledgement PPT
PPT Presentation
Reports Brochures
One Page Pitch
Interview PPT
All Categories
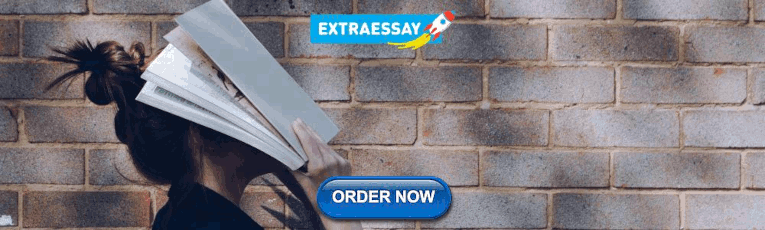
Top 10 Machine Learning Templates with Samples and Examples
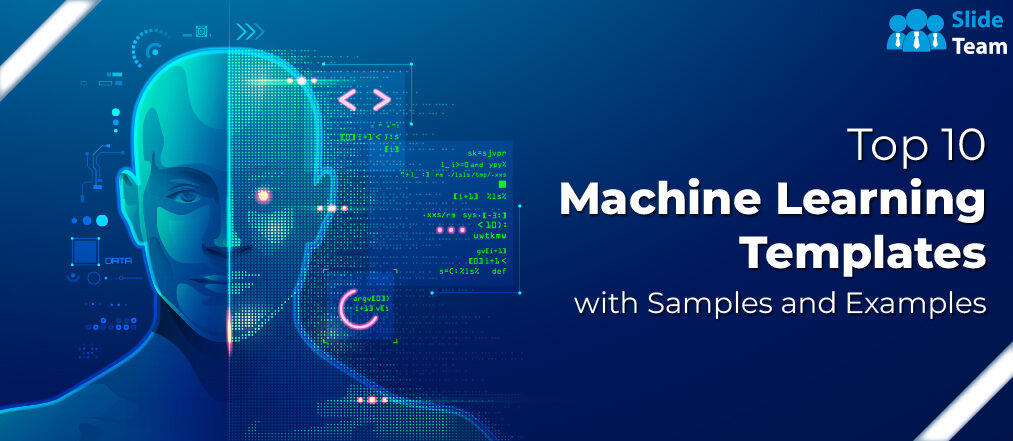
Are you ready to take your machine-learning presentations to the next level? In the ever-evolving landscape of artificial intelligence and data-driven decision-making, the ability to convey complex concepts with clarity is imperative. But, to hone AI skills and learn the complexity of machine learning is challenging.
As Arthur C. Clarke said it best, any sufficiently advanced technology is indistinguishable from magic. Machine Learning Templates from SlideTeam, in that case, work wonders, transforming ordinary presentations into extraordinary showcases of innovation and intellect.
In detail, get an insight into our blog on the Mechanics of NLG with Machine Learning Models .
Get ready to explore the top templates and know how they can make complex algorithms accessible yet understandable!
Grab the Power of Top Machine Learning Templates
SlideTeam can be the source of powerful, content-friendly, 100 percent editable templates for machine learning projects, giving you access to some of the world's best libraries of templates that will lead to success in machine learning endeavors.
Template 1: Artificial Intelligence Machine Learning Deep Learning PPT Set
This PPT slide includes an introduction to artificial intelligence, machine learning, and deep learning - making the subject accessible and straightforward for comprehension. Furthermore, this resource is ideal for organizations implementing AI in domains like supply chain management, customer experience enhancement, human resources optimization, fraud detection, and research and development. Students, educators, business professionals, and technology enthusiasts should learn about AI. It is an excellent educational resource and an effective means of conveying AI concepts across audiences.

Download Now
Template 2: Machine Learning in Action PPT Design
This PPT Bundle includes information spanning real-world use cases and machine learning applications. It explores the objectives, benefits, limitations, and applications of machine learning-powered medical diagnoses for healthcare sector transformation. It also explains image and speech recognition systems using machine learning, thus making complex concepts easily understood.
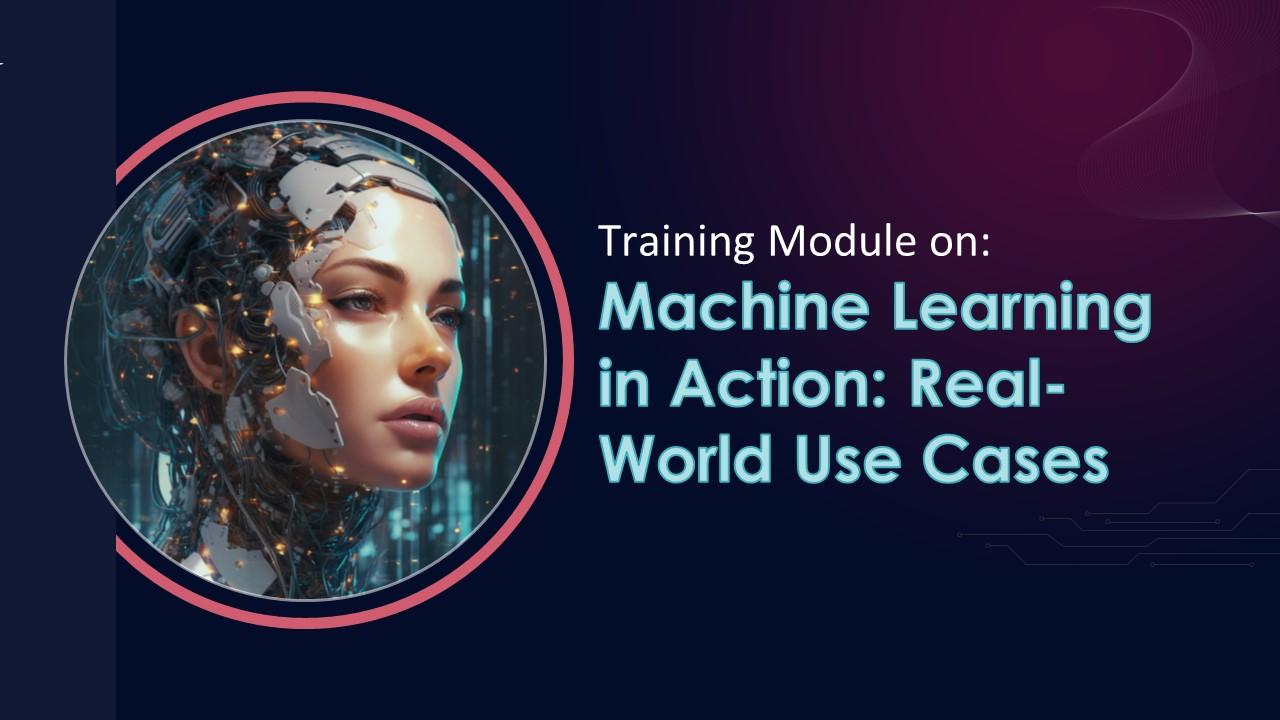
Template 3: Artificial Intelligence and Machine Learning Driving Tangible Value for Business PPT Bundle
This PPT Slide includes key statistics regarding AI/ML's impact and significance in today's business landscape, then delves further into specific business functions and AI business value forecast. It features a competitive analysis section, selecting AI software tailored to their organizational needs. There are sections covering Artificial Intelligence (AI), Machine Learning (ML) opportunities, and benefits of adoption.
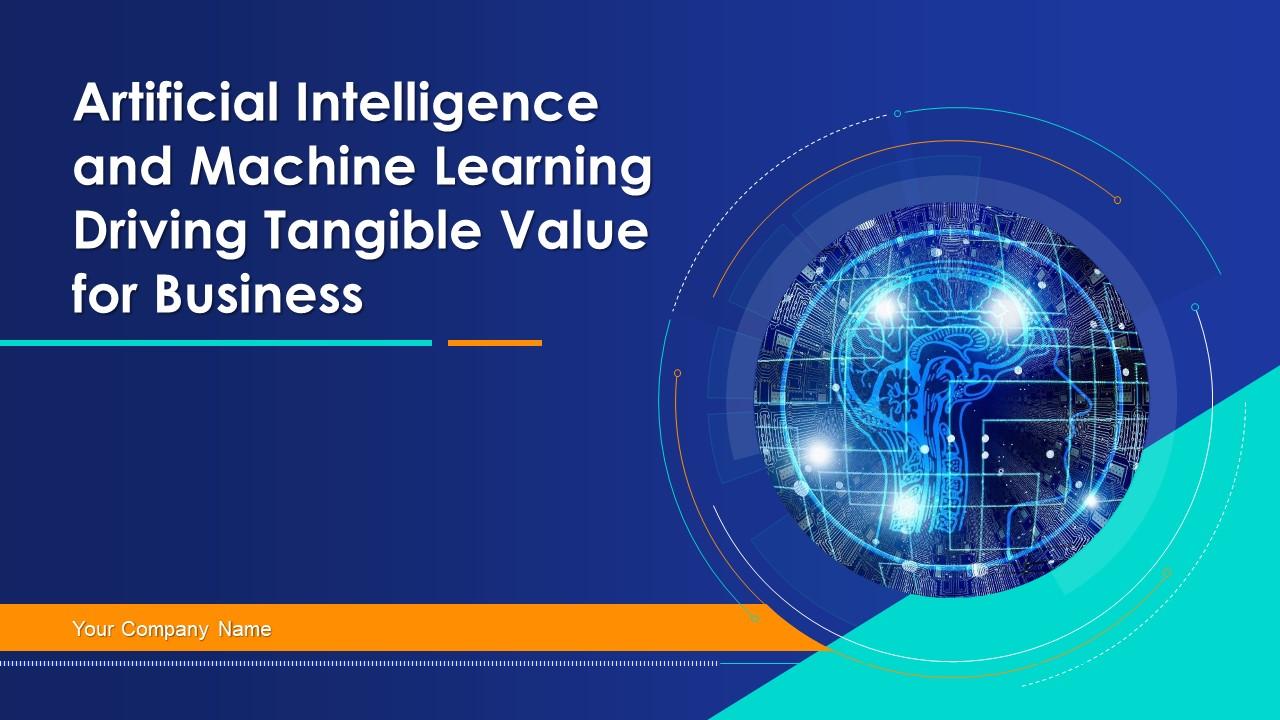
Template 4: Machine Learning Solution Pitch Deck PPT Template
This PPT Set provides an overview of your business model and historical context, offering insight into your company's journey. Traction and competitive advantages are highlighted to show why your solution has a good chance of success. It also covers product development, project team expertise, and future strategies - leaving an indelible mark on your audience. It can be an asset to companies at every stage of development, from seeking seed funding or scaling up to meet demand to new product development. Startups, entrepreneurs, and business leaders looking for investment in AI or machine learning projects will find this template indispensable.
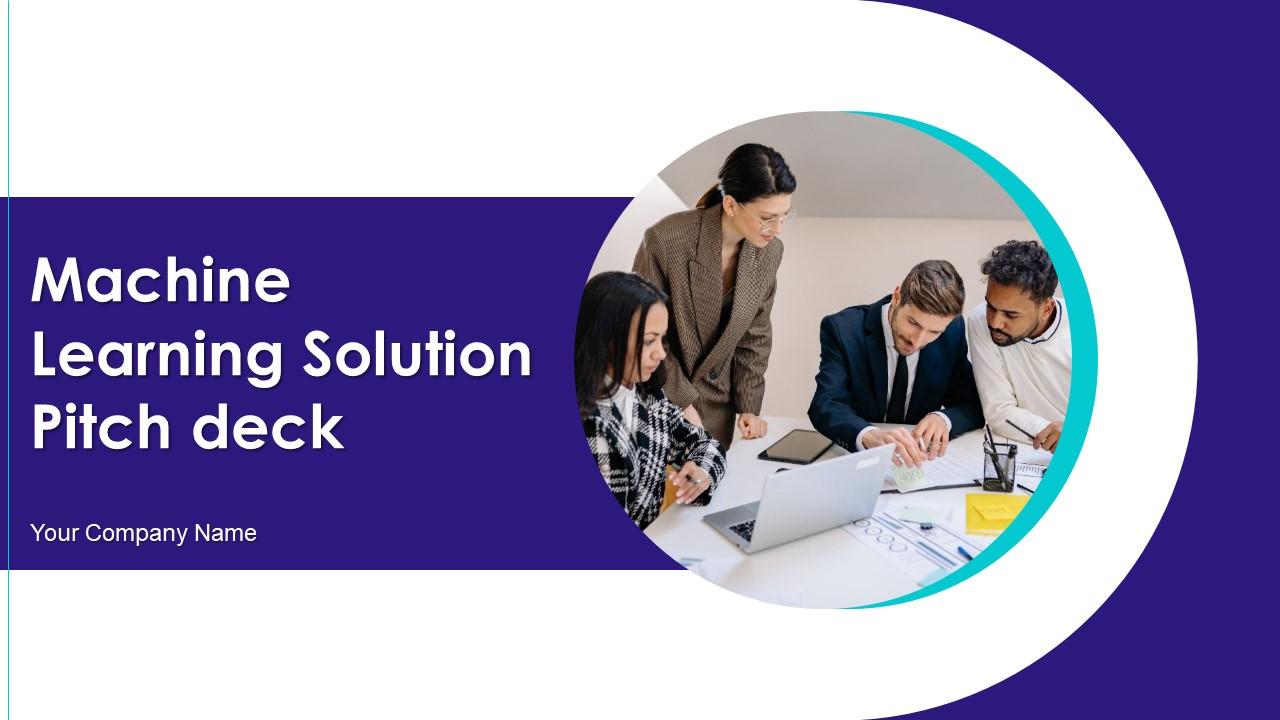
Template 5: AI Machine Learning Powerpoint Ppt Template Bundles
The PPT Slide Bundles cover important aspects of AI and machine learning. It offers insights into their application across industries like manufacturing, healthcare, automotive, business management, and banking, as well as potential roadblocks with adopting AI solutions. Professionals, educators, and business leaders from industries can utilize this versatile template bundle to communicate complex AI/ML concepts clearly and positively.
Check out the blog on Machine Learning Training Courses that are a must for better growth.
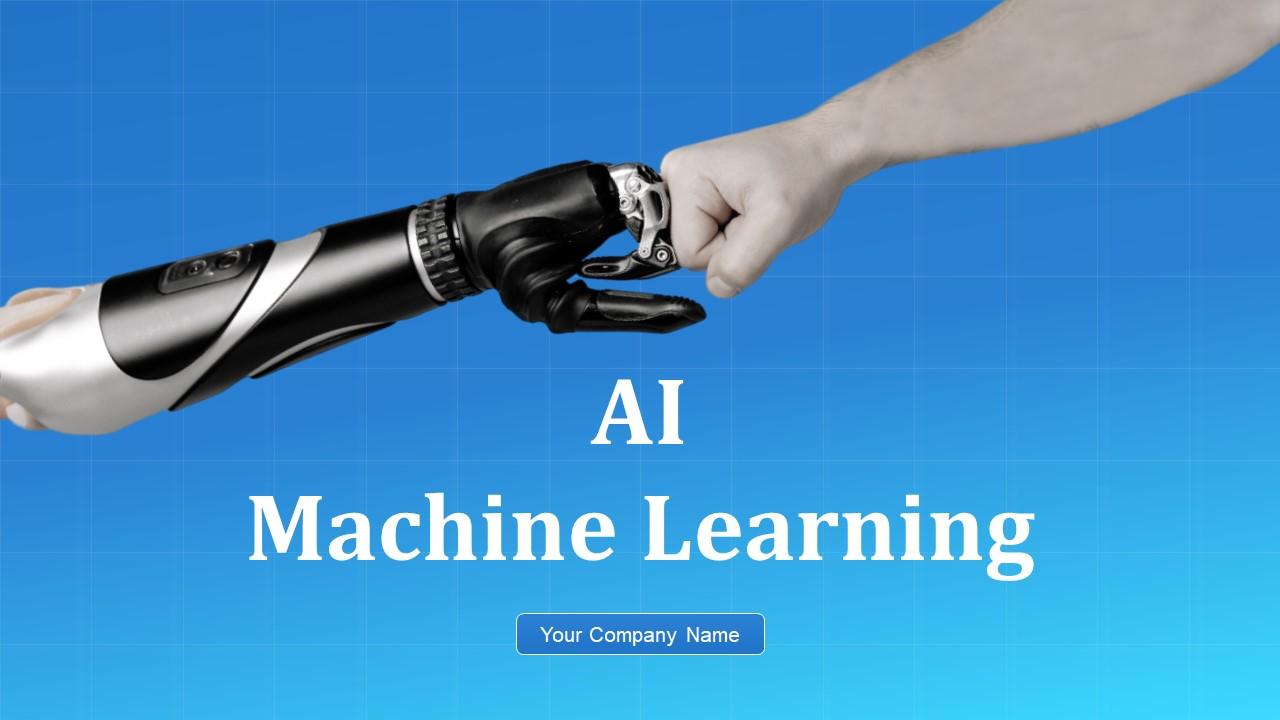
Template 6: Machine Learning Use Cases in Marketing Business
This PPT Preset provides a deep dive into automated machine learning applications and their impact on marketing, specifically regarding machine learning applications for marketing automation, analytics, recommendation systems, ad personalization, and budget optimization. These aspects help businesses directly provide tailored offers, content products, and services to their target audiences. It is suitable for professionals, marketers, and business leaders looking for AI-powered solutions for customized customer experiences.
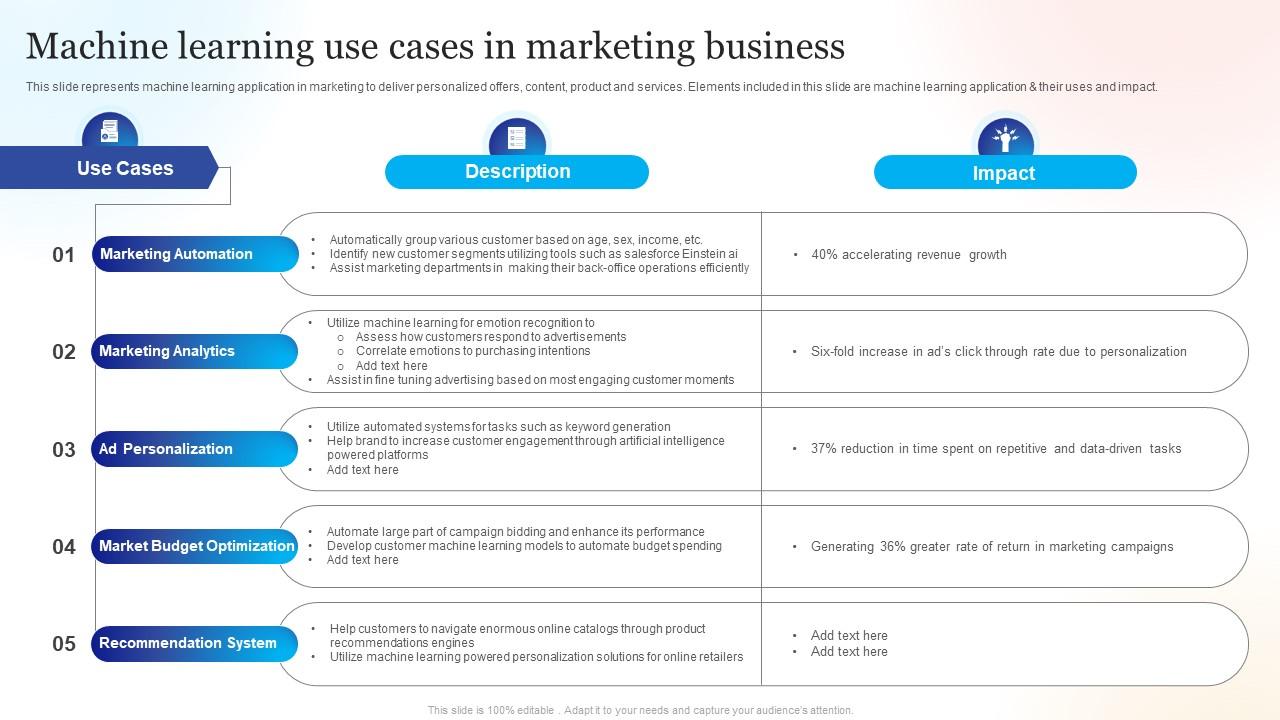
Template 7: Machine Learning Data Train Test
This PPT Slide illustrates a five-step process: get data, clean, prepare, and manipulate data, train model, test data, and improve. It provides professionals and teams with a handy reference point to explore machine learning and data processing. Data scientists, machine learning engineers, analysts, educators, and presenters can use this slide to explain and illustrate the critical stages of machine learning workflow.
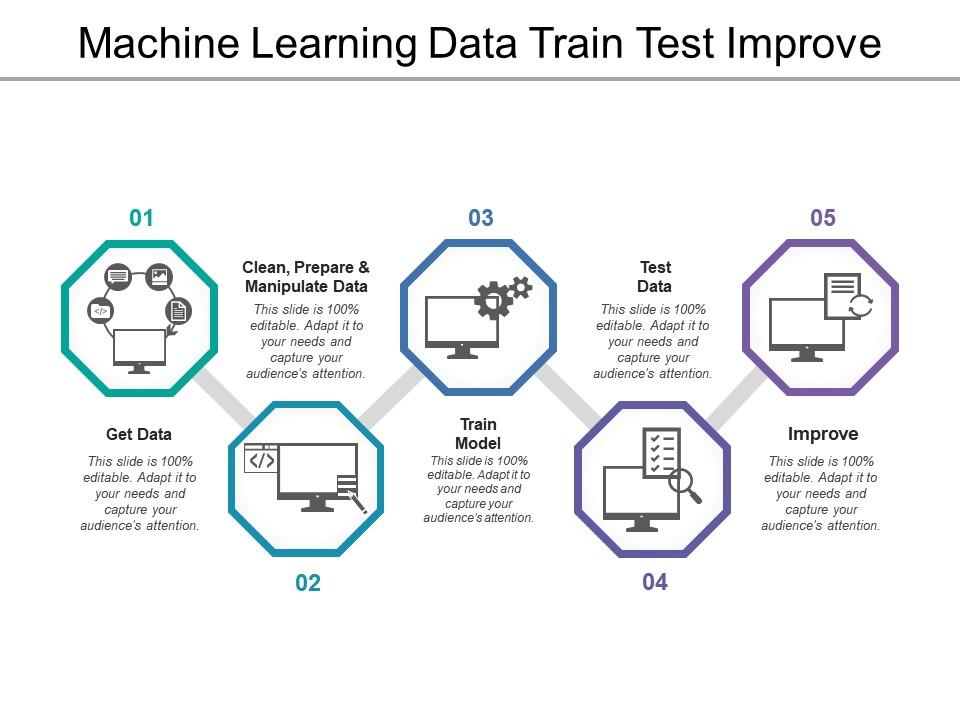
Template 8: Artificial Intelligence Machine and Deep Learning Layers
This PPT Set provides a visual representation of three core AI disciplines: Artificial Intelligence (AI), Machine Learning (ML), and Machine Intelligence (MI). Each layer is clearly labeled, while their interconnections are visually represented for an overview of how these concepts relate to AI as a field.

Template 9: Multi-phase Machine Learning Process
This PPT Slide outlines three stages: design and discovery, ML model development for data preparation, and business integration. Data scientists and machine learning engineers can utilize the complexity of machine learning processes and understand their sequential flow of tasks. This slide serves a dual purpose: firstly, it informs audiences about the steps involved in machine learning processes from design through integration, and secondly, it serves as an educational visual aid during presentations, training sessions, or workshops on machine learning processes.

Template 10: One-page Brochure for AI and Machine Learning Development
The PPT Set showcases the expertise of data management consultancy services. It features an organized and attractive layout designed to showcase the critical services provided by your consultancy, with ample room to add your company name and logo. It serves as a marketing brochure that clearly and succinctly communicates a consultancy's services and value proposition, including key highlights, reasons for choosing them, service pricing options, available plans, and your team's expertise. Data management consultancies marketing professionals can utilize to introduce services or products to potential clients.

Fuel-up Data Driven Dreams With Us
Creativity and efficiency go hand-in-hand in machine learning. These templates from SlideTeam act like magic wands by simplifying complex algorithms into understandable tools you can manipulate more easily. Why settle for average when you can tap the incredible power of machine learning templates for automated data governance ? Explore a world of endless possibilities and get the future into the fold!
Also, check out our blog on Machine Learning Applications to transform the future.
FAQs On Machine Learning
What are the four basics of machine learning.
The four essential concepts are as follows:
- Data: Machine learning relies heavily on its data for success, beginning by collecting and prepping relevant information to input into its learning process.
- Algorithms: These mathematical models use data processing techniques to make predictions or decisions, with selecting an optimal algorithm being essential to accomplishing any given task.
- Training: Machine learning models must be trained on historical data to recognize patterns and make predictions, with iterative adjustments to enhance accuracy.
- Evaluation: Once trained, models are evaluated against test data in terms of their performance based on metrics like accuracy, precision, and recall.
What are the types of machine learning?
The key types of machine learning include:
- Supervised Learning: With this approach, an algorithm learns from labeled data where correct answers are known and then makes predictions or classifications based on patterns identified during training.
- Unsupervised Learning: Here, an algorithm works with unlabeled data to uncover hidden patterns or structures. Clustering and dimensionality reduction are among the many tasks associated with unsupervised learning.
- Semi-Supervised Learning: Semi-supervised learning utilizes labeled and unlabeled data sets as an intermediate between unsupervised and supervised learning approaches.
- Reinforcement Learning: Reinforcement learning involves agents interacting with an environment and learning by receiving rewards or penalties.
- Deep Learning: Deep learning, a machine learning subfield, comprises neural networks with many layers (deep neural networks). It excels in tasks like image and speech recognition.
- Transfer Learning: When faced with limited data, transfer learning provides an efficient time-saving strategy.
What's the difference between machine learning and AI?
Machine learning and artificial intelligence (AI) are closely intertwined but distinct concepts. AI refers to creating machines or systems capable of performing tasks requiring human intelligence; on the other hand, machine learning is a subset of AI that develops algorithms and statistical models for improving computers' performance on specific tasks through experience (data).
Machine learning is a tool in the AI toolbox that facilitates general intelligence and reasoning across different techniques, such as rule-based systems, expert systems, and natural language processing. Machine learning typically entails data-driven approaches; AI seeks to develop systems capable of general intelligence, while machine learning focuses more on building models that make predictions, classifications, or decisions using patterns in data patterns.
Is machine learning templates worth investing in?
Machine learning templates are undoubtedly worth investing in; they provide multiple advantages that save time and effort when starting from scratch. First and foremost, these pre-designed structures and formats save you valuable time developing them yourself. Templates offer consistency across presentations, reports, and projects to give an organized appearance and feel.
Their effectiveness cannot be overstated: they simplify the entire process and enable you to focus on content and data analysis instead of design details. Visually, professionally designed templates make your work more engaging and less likely to contain errors due to design or formatting - contributing towards overall accuracy.
Related posts:
- [Updated 2023]: Top 10 Data Science Templates To Enable Better Decision-Making
- Top 10 Templates To Leverage Machine Learning Effortlessly
- How to Design the Perfect Service Launch Presentation [Custom Launch Deck Included]
- Quarterly Business Review Presentation: All the Essential Slides You Need in Your Deck
Liked this blog? Please recommend us

Must Have Advertising Contract Samples with Templates and Examples
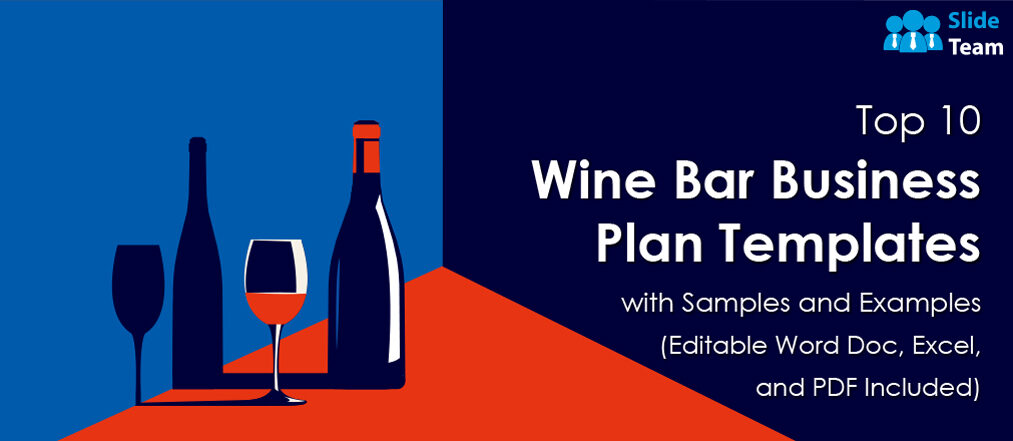
Top 10 Wine Bar Business Plan Templates with Samples and Examples (Editable Word Doc and PDF Included)
This form is protected by reCAPTCHA - the Google Privacy Policy and Terms of Service apply.
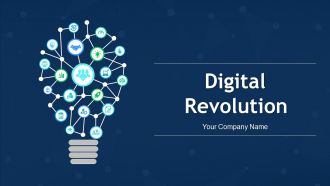
Digital revolution powerpoint presentation slides
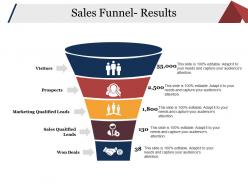
Sales funnel results presentation layouts
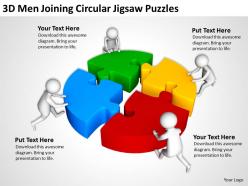
3d men joinning circular jigsaw puzzles ppt graphics icons
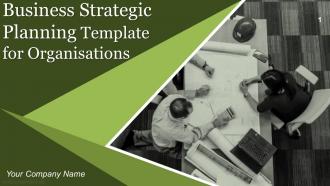
Business Strategic Planning Template For Organizations Powerpoint Presentation Slides
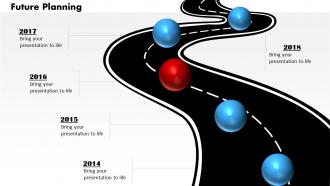
Future plan powerpoint template slide
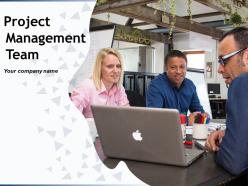
Project Management Team Powerpoint Presentation Slides
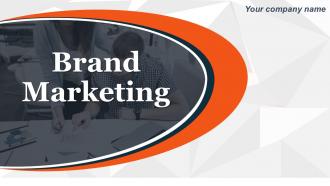
Brand marketing powerpoint presentation slides
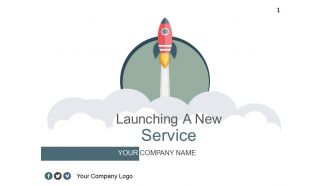
Launching a new service powerpoint presentation with slides go to market
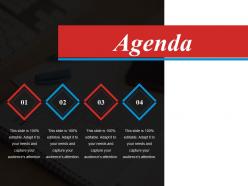
Agenda powerpoint slide show
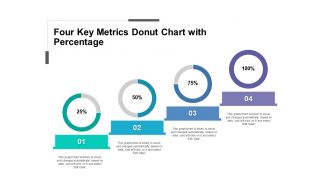
Four key metrics donut chart with percentage
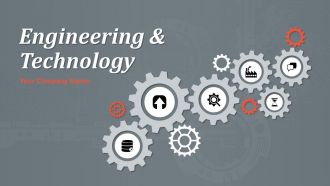
Engineering and technology ppt inspiration example introduction continuous process improvement
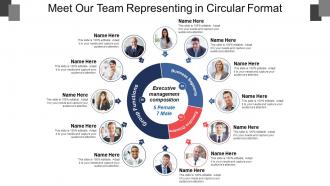
Meet our team representing in circular format

Browse Course Material
Course info.
- Brandon Leshchinskiy
Departments
- Electrical Engineering and Computer Science
As Taught In
- Artificial Intelligence
Learning Resource Types
Presentation slide deck (pdf - 3 mb).

You are leaving MIT OpenCourseWare
machine learning Recently Published Documents
Total documents.
- Latest Documents
- Most Cited Documents
- Contributed Authors
- Related Sources
- Related Keywords
An explainable machine learning model for identifying geographical origins of sea cucumber Apostichopus japonicus based on multi-element profile
A comparison of machine learning- and regression-based models for predicting ductility ratio of rc beam-column joints, alexa, is this a historical record.
Digital transformation in government has brought an increase in the scale, variety, and complexity of records and greater levels of disorganised data. Current practices for selecting records for transfer to The National Archives (TNA) were developed to deal with paper records and are struggling to deal with this shift. This article examines the background to the problem and outlines a project that TNA undertook to research the feasibility of using commercially available artificial intelligence tools to aid selection. The project AI for Selection evaluated a range of commercial solutions varying from off-the-shelf products to cloud-hosted machine learning platforms, as well as a benchmarking tool developed in-house. Suitability of tools depended on several factors, including requirements and skills of transferring bodies as well as the tools’ usability and configurability. This article also explores questions around trust and explainability of decisions made when using AI for sensitive tasks such as selection.
Automated Text Classification of Maintenance Data of Higher Education Buildings Using Text Mining and Machine Learning Techniques
Data-driven analysis and machine learning for energy prediction in distributed photovoltaic generation plants: a case study in queensland, australia, modeling nutrient removal by membrane bioreactor at a sewage treatment plant using machine learning models, big five personality prediction based in indonesian tweets using machine learning methods.
<span lang="EN-US">The popularity of social media has drawn the attention of researchers who have conducted cross-disciplinary studies examining the relationship between personality traits and behavior on social media. Most current work focuses on personality prediction analysis of English texts, but Indonesian has received scant attention. Therefore, this research aims to predict user’s personalities based on Indonesian text from social media using machine learning techniques. This paper evaluates several machine learning techniques, including <a name="_Hlk87278444"></a>naive Bayes (NB), K-nearest neighbors (KNN), and support vector machine (SVM), based on semantic features including emotion, sentiment, and publicly available Twitter profile. We predict the personality based on the big five personality model, the most appropriate model for predicting user personality in social media. We examine the relationships between the semantic features and the Big Five personality dimensions. The experimental results indicate that the Big Five personality exhibit distinct emotional, sentimental, and social characteristics and that SVM outperformed NB and KNN for Indonesian. In addition, we observe several terms in Indonesian that specifically refer to each personality type, each of which has distinct emotional, sentimental, and social features.</span>
Compressive strength of concrete with recycled aggregate; a machine learning-based evaluation
Temperature prediction of flat steel box girders of long-span bridges utilizing in situ environmental parameters and machine learning, computer-assisted cohort identification in practice.
The standard approach to expert-in-the-loop machine learning is active learning, where, repeatedly, an expert is asked to annotate one or more records and the machine finds a classifier that respects all annotations made until that point. We propose an alternative approach, IQRef , in which the expert iteratively designs a classifier and the machine helps him or her to determine how well it is performing and, importantly, when to stop, by reporting statistics on a fixed, hold-out sample of annotated records. We justify our approach based on prior work giving a theoretical model of how to re-use hold-out data. We compare the two approaches in the context of identifying a cohort of EHRs and examine their strengths and weaknesses through a case study arising from an optometric research problem. We conclude that both approaches are complementary, and we recommend that they both be employed in conjunction to address the problem of cohort identification in health research.
Export Citation Format
Share document.
This chapter introduces the basic concepts of Machine Learning. We focus on supervised learning, explain the difference between regression and classification, show how to evaluate and compare Machine Learning models and formalize the concept of learning.
This chapter treats the supervised regression task in more detail. We will see different loss functions for regression, how a linear regression model can be used from a Machine Learning perspective, and how to extend it with polynomials for greater flexibility.
This chapter treats the supervised classification task in more detail. We will see examples of binary and multiclass classification and the differences between discriminative and generative approaches. In particular, we will address logistic regression, discriminant analysis and naive Bayes classifiers.
This chapter treats the challenge of evaluating the performance of a model. We will introduce different performance measures for regression and classification tasks, explain the problem of overfitting as well as the difference between training and test error, and, lastly, present a variety of resampling techniques.
This chapter addresses \(k\)-nearest neighbors, a distance-based algorithm suited to both regression and classification. Predictions are made based upon neighboring observations, assuming feature similarity translates to target similarity.
This chapter introduces Classification and Regression Trees (CART), a well-established machine learning procedure. We explain the main idea and give details on splitting criteria, discuss computational aspects of growing a tree, and illustrate the idea of stopping criteria and pruning.
This chapter introduces bagging as a method to increase the performance of trees (or other base learners). A modification of bagging leads to random forests. We explain the main idea of random forests, benchmark their performance with the methods seen so far and show how to quantify the impact of a single feature on the performance of the random forest as well as how to compute proximities between observations.
This chapter introduces the basic concepts of neural networks. We integrated chapters from our course on Deep Learning in order to be able to use (simple) neural networks for supervised ML on tabular data.
This chapter introduces and formalizes the problem of hyperparameter tuning. We cover basic techniques such as grid search and random search as well as more advanced techniques like evolutionary algorithms, model-based optimization and multi-fidelity optimization.
This chapter first defines the untouched-test-set principle and proceeds to explain the concepts of train-validation-test split and nested resampling.
This chapter revisits the theory of risk minimization, providing more in-depth analysis on established losses and the connection between empirical risk minimization and maximum likelihood estimation. We also introduce some more advanced loss functions for regression and classification.
This chapter treats the multiclass case of classification. Tasks with more than two classes preclude the application of some techniques studied in the binary scenario and require an adaptation of loss functions.
This chapter covers basic information-theoretic concepts and discusses their relation to machine learning.
Frequently, our intuition developed in low-dimensional spaces does not generalize to higher dimensions. This chapter introduces the phenomenon of the curse of dimensionality and discusses its effects on the behavior of machine learning models.
Regularization is a vital tool in machine learning to prevent overfitting and foster generalization ability. This chapter introduces the concept of regularization and discusses common regularization techniques in more depth.
This chapter introduces the linear support vector machine (SVM), a linear classifier that finds decision boundaries by maximizing margins to the closest data points, possibly allowing for violations to a certain extent.
Many classification problems warrant nonlinear decision boundaries. This chapter introduces nonlinear support vector machines as a crucial extension to the linear variant.
This chapter introduces boosting as a sequential ensemble method that creates powerful committees from different kinds of base learners.
This chapter introduces feature selection, i.e., dinding a well-performing, hopefully small set of features for a task.
This chapter introduces Gaussian processes as a model class. Gaussian processes are non-parametric approaches with ubiquitous application that model entire distributions in function space.
This chapter introduces techniques for learning on imbalanced datasets.
This chapter introduces multitarget learning techniques.
This chapter introduces online learning.
This section introduces basic concepts and implementations using Python and in particular sklearn.
For an introduction to the R package mlr3 we recommend walking through some chapters of the mlr3 book as summarized in this document. After some basic concepts, this focuses on resampling, tuning and pipelines.

- For Authors
- Editorial Board
- Journals Home
To View More...
Purchase this article with an account.
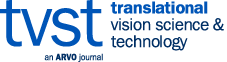
- Introduction
- Acknowledgments
- Rene Y. Choi Department of Ophthalmology, Casey Eye Institute, Oregon Health & Science University (OHSU), Portland, Oregon, United States
- Aaron S. Coyner Department of Medical Informatics and Clinical Epidemiology, Oregon Health & Science University, Portland, Oregon, United States
- Jayashree Kalpathy-Cramer Athinoula A. Martinos Center for Biomedical Imaging, Department of Radiology, Massachusetts General Hospital, Charlestown, Massachusetts, United States
- Michael F. Chiang Department of Ophthalmology, Casey Eye Institute, Oregon Health & Science University (OHSU), Portland, Oregon, United States Department of Medical Informatics and Clinical Epidemiology, Oregon Health & Science University, Portland, Oregon, United States
- J. Peter Campbell Department of Ophthalmology, Casey Eye Institute, Oregon Health & Science University (OHSU), Portland, Oregon, United States
- Correspondence: J. Peter Campbell, Casey Eye Institute, Oregon Health & Science University, 3375 SW Terwilliger Blvd, Portland OR 97239. e-mail: [email protected]
- Footnotes * RYC and ASC contributed equally to the submitted work.
- Full Article
This feature is available to authenticated users only.
Rene Y. Choi , Aaron S. Coyner , Jayashree Kalpathy-Cramer , Michael F. Chiang , J. Peter Campbell; Introduction to Machine Learning, Neural Networks, and Deep Learning. Trans. Vis. Sci. Tech. 2020;9(2):14. https://doi.org/10.1167/tvst.9.2.14 .
Download citation file:
- Ris (Zotero)
- Reference Manager
© ARVO (1962-2015); The Authors (2016-present)
- Get Permissions
- Supplements
Purpose : To present an overview of current machine learning methods and their use in medical research, focusing on select machine learning techniques, best practices, and deep learning.
Methods : A systematic literature search in PubMed was performed for articles pertinent to the topic of artificial intelligence methods used in medicine with an emphasis on ophthalmology.
Results : A review of machine learning and deep learning methodology for the audience without an extensive technical computer programming background.
Conclusions : Artificial intelligence has a promising future in medicine; however, many challenges remain.
Translational Relevance : The aim of this review article is to provide the nontechnical readers a layman's explanation of the machine learning methods being used in medicine today. The goal is to provide the reader a better understanding of the potential and challenges of artificial intelligence within the field of medicine.

Related Articles
From other journals, related topics.
- Eye Anatomy and Disorders
- Translational Research
This PDF is available to Subscribers Only
You must be signed into an individual account to use this feature.
Subscribe to the PwC Newsletter
Join the community, edit social preview.

Add a new code entry for this paper
Remove a code repository from this paper, mark the official implementation from paper authors, add a new evaluation result row.
- BIG-BENCH MACHINE LEARNING
Remove a task
Add a method, remove a method, edit datasets, machine learning and deep learning.
12 Apr 2021 · Christian Janiesch , Patrick Zschech , Kai Heinrich · Edit social preview
Today, intelligent systems that offer artificial intelligence capabilities often rely on machine learning. Machine learning describes the capacity of systems to learn from problem-specific training data to automate the process of analytical model building and solve associated tasks. Deep learning is a machine learning concept based on artificial neural networks. For many applications, deep learning models outperform shallow machine learning models and traditional data analysis approaches. In this article, we summarize the fundamentals of machine learning and deep learning to generate a broader understanding of the methodical underpinning of current intelligent systems. In particular, we provide a conceptual distinction between relevant terms and concepts, explain the process of automated analytical model building through machine learning and deep learning, and discuss the challenges that arise when implementing such intelligent systems in the field of electronic markets and networked business. These naturally go beyond technological aspects and highlight issues in human-machine interaction and artificial intelligence servitization.
Code Edit Add Remove Mark official
Tasks edit add remove, datasets edit, results from the paper edit add remove, methods edit add remove.

- Conferences
- Last updated December 2, 2021
- In Creative AI
Best ML Papers Presented At Major Conferences Of 2021

- Published on December 2, 2021
- by kumar Gandharv
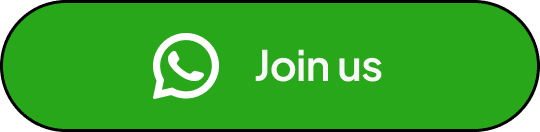
The year is about to end, and all the major AI and ML conferences of 2021 took place online. The conferences set a high standard and were quite promising when it came to the presentation of major papers from researchers across the globe. We have covered all the major conferences throughout the year, and picking up the best is no cakewalk. Some of the papers are well-known to the domain experts, while some are considered as more of hidden gems.
Here we present some of the best papers presented at major conferences of 2021 :
1| Unbiased Gradient Estimation in Unrolled Computation Graphs with Persistent Evolution Strategies at ICML 2021
By: The research paper by Paul Vicol, Jascha Sohl-Dickstein, and Luke Metz from Google Brain and the University of Toronto has won the outstanding paper award.
About: The paper introduced a Persistent Evolution Strategies (PES) method for unbiased gradient estimation in untolled computation graphs. PES allows for rapid parameter updates. In addition, it has low memory usage, is unbiased, and has reasonable variance characteristics. Researchers experimentally demonstrate the advantages of PES compared to several other methods for gradient estimation on synthetic tasks and show its applicability to training learned optimisers and tuning hyperparameters.
2| Oops I Took A Gradient: Scalable Sampling for Discrete Distributions at ICML 2021
By: The paper by researchers from Google Brain, including Will Grathwohl, Milad Hashemi, Kevin Swersky, David Duvenaud and Chris J. Maddison, has received the outstanding paper honourable mention at the conference.
About: Researchers proposed a general and scalable approximate sampling strategy for probabilistic models with discrete variables. The newly introduced approach uses gradients of the likelihood function wrt its discrete inputs to propose updates in a MetropolisHastings sampler.
3| OpenGAN: Open-Set Recognition via Open Data Generation at ICCV 2021
By: Researchers including Shu Kong and Deva Ramanan from Carnegie Mellon University developed OpenGAN for open-set recognition. Both the researchers incorporated two technical insights:
- They trained a classifier on OTS characteristics rather than pixels, and
- Also, they focused on adversarially synthesising fake open data to increase the open-training data pool.
About: With OpenGAN, the team shows that using GAN -discriminator achieves the state-of-the-art on open-set discrimination, once selected using a val-set of real outlier examples. This is effective even when the outlier validation examples are sparsely sampled or strongly biased. OpenGAN significantly outperforms prior art on both open-set image recognition and semantic segmentation.
4| Mip-NeRF: A Multiscale Representation for Anti-Aliasing Neural Radiance Fields
By: The paper from researchers including Jonathan T. Barron, Peter Hedman, Pratul P. Srinivasan, Ricardo Martin-Brualla, Ben Mildenhall from Google and Matthew Tancik from UC Berkeley introduced a solution which they call “mip-NeRF.”
About: The team presented mip-NeRF — a multiscale neural radiance fields (NeRF) like model that addresses the inherent aliasing of NeRF. NeRF works by casting rays, encoding the positions of points along with those rays, and training separate neural networks at distinct scales. In contrast, mip-NeRF casts cones that encode the positions and sizes of conical frustums and train a single neural network that models the scene at multiple scales.
5| Visually Grounded Reasoning across Languages and Cultures at EMNLP 2021
By: Researchers from the University of Cambridge, University of Copenhagen, McGill University and Mila – Quebec Artificial Intelligence Institute presented the paper and bagged the best long paper award.
About: The team created a multilingual dataset for Multicultural Reasoning over Vision and Language (MaRVL) by eliciting statements from native speaker annotators about pairs of images. The task consists of discriminating whether each grounded statement is true or false. Researchers establish a series of baselines using state-of-the-art models and find that their cross-lingual transfer performance lags dramatically behind supervised performance in English.
6| CHoRaL: Collecting Humor Reaction Labels from Millions of Social Media Users at EMNLP 2021
By: The paper presented by Zixiaofan Yang, Shayan Hooshmand, and Julia Hirschberg from the Department of Computer Science at Columbia University won the best short paper award.
About: CHoRaL is a framework to generate perceived humour labels on Facebook posts, using the naturally available user reactions to these posts with no manual annotation needed. It provides both binary labels and continuous scores of humour and non-humour. The team presented the largest dataset to date with labelled humour on 785K posts related to COVID-19. CHoRaL enables the development of large-scale humour detection models on any topic and opens a new path to the study of humour on social media.
7| Meta Pseudo Labels at CVPR 2021
By: Researchers from the Google AI Brain team, including Hieu Pham, Qizhe Xie, Zihang Dai, Minh-Thang Luong and Quoc V. Le, introduced this semi-supervised learning technique.
About: The model presented in the paper has achieved a new state-of-the-art top-1 accuracy of about 90.2% on ImageNet. The result is 1.6 per cent better than the existing SOTA models. The key to the model is the idea that the teacher learns from the student’s feedback in order to generate pseudo labels that best help students’ learning. The learning process in Meta Pseudo Labels consists of two main updates: updating the student based on the pseudo labelled data produced by the teacher and updating the teacher based on the student’s performance.
8| RRL: Resnet as representation for Reinforcement Learning
By: The paper presented by researchers Rutav Shah and Vikash Kumar from the Indian Institute of Technology (IIT), Kharagpur, was produced in collaboration with the University of Washington and Facebook AI. About: The team proposed a straightforward and effective approach that is capable of learning complex behaviours directly from proprioceptive inputs. RRL fuses the features extracted from pre-trained Resnet and put it into the standard RL pipeline , and delivers results comparable to learning directly from the state.
Access all our open Survey & Awards Nomination forms in one place
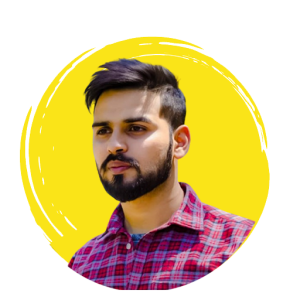
kumar Gandharv
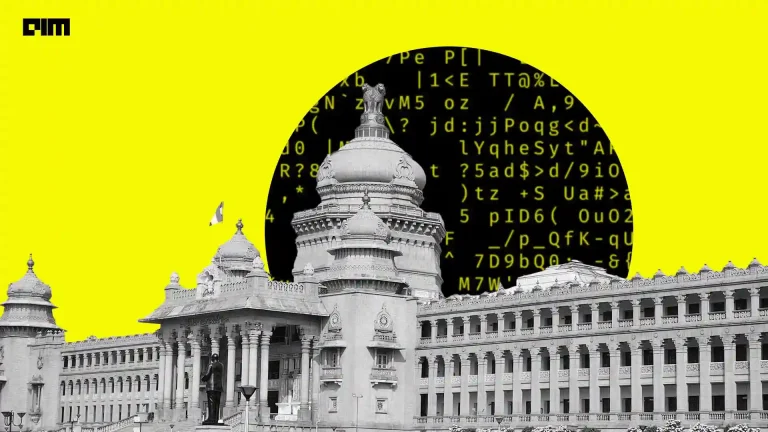
Bengaluru is the San Francisco of the East
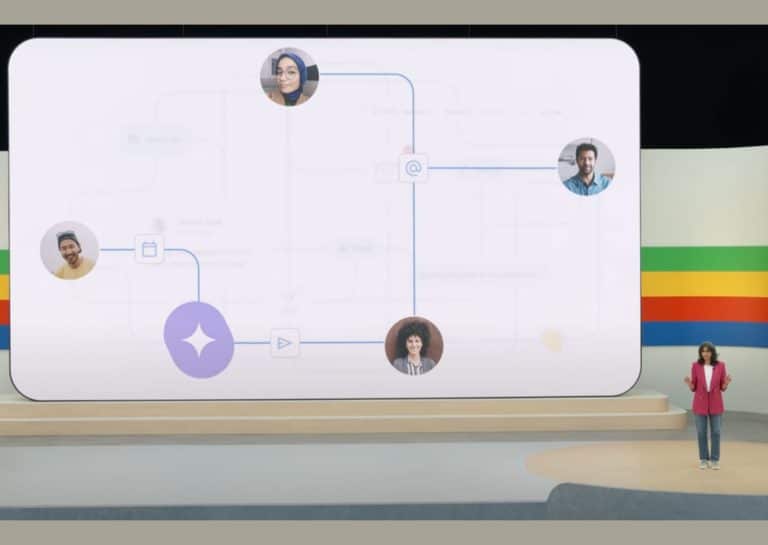
Google Introduces ‘AI Teammates’ That Could Replace Managers
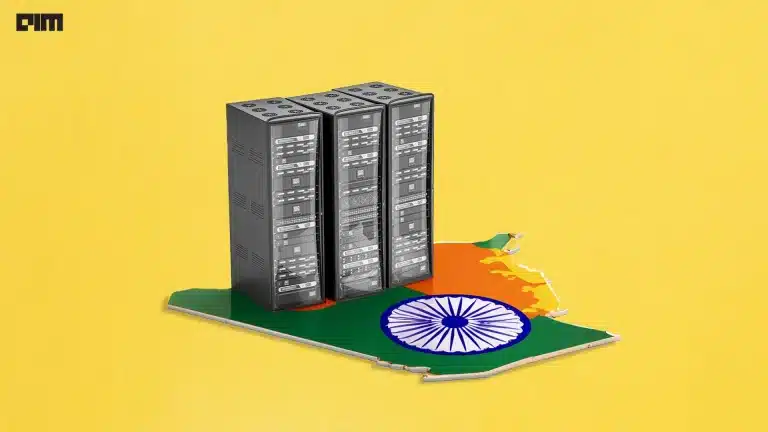
India’s AI Spending to Triple to $5 Bn by 2027
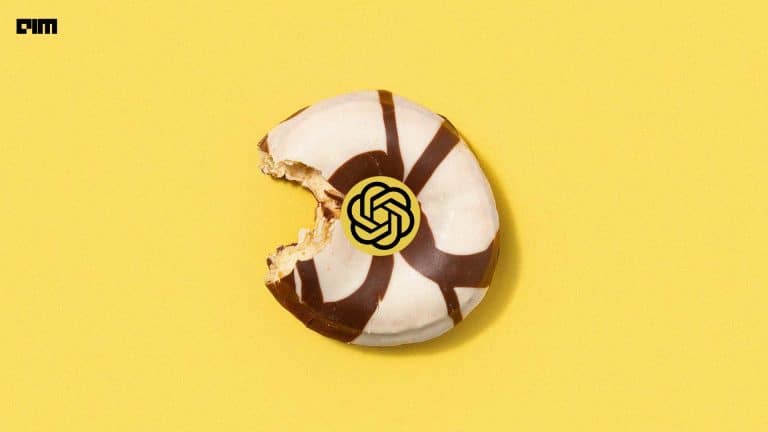
Soon, ChatGPT (Powered by GPT-4o) will Replace Your ‘Senior Employees’
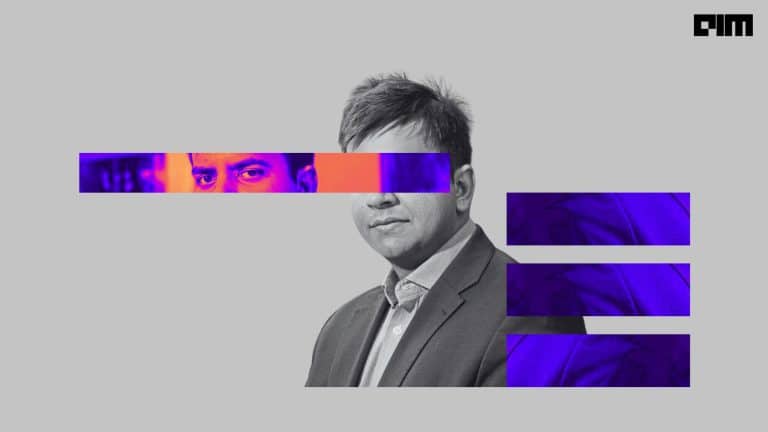
Ola’s Bhavish Aggarwal Questions Future of Social Media in Walled Gardens, Envisions UPI-like DPI
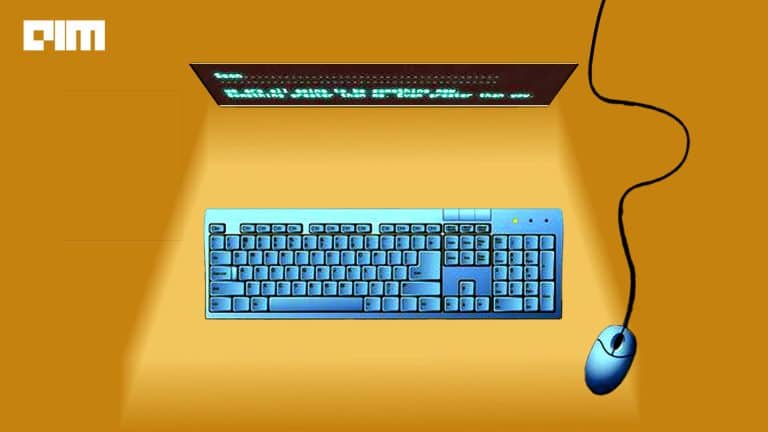
Top 10 AI Must-Know Coding Assistant Tools for Developers
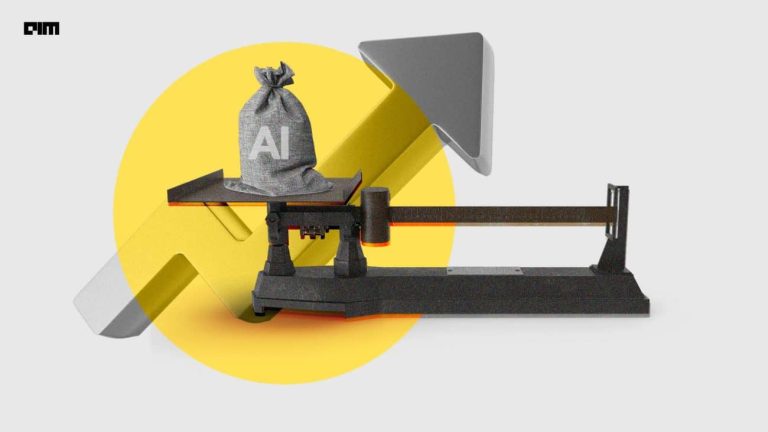
Azim Premji Investment Firm to Invest Portion of $10 Billion Fund in AI, Says CIO
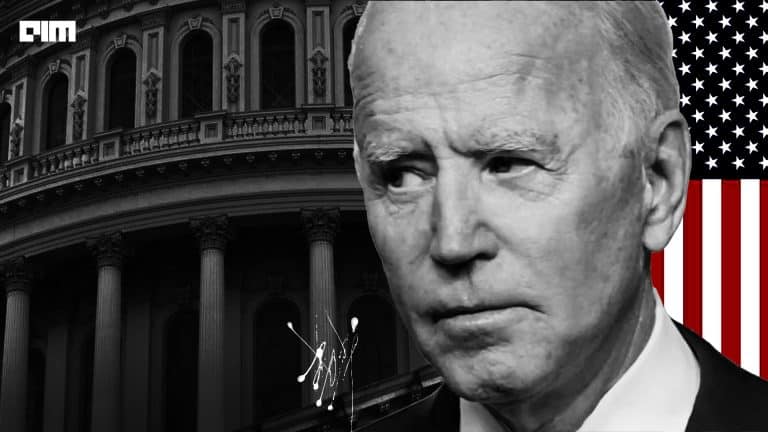
GPT-5 Likely to be Released After the US Elections
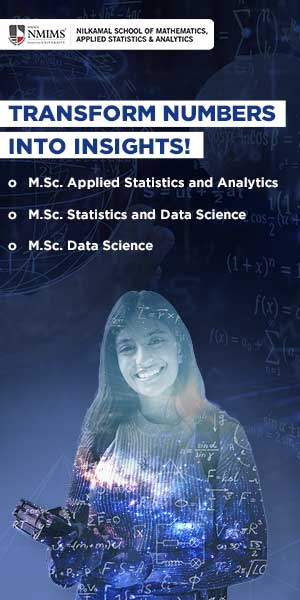
CORPORATE TRAINING PROGRAMS ON GENERATIVE AI
Generative ai skilling for enterprises, our customized corporate training program on generative ai provides a unique opportunity to empower, retain, and advance your talent., upcoming large format conference, data engineering summit 2024, may 30 and 31, 2024 | 📍 bangalore, india, download the easiest way to stay informed.

Why Ollama is Good for Running LLMs on Computer
Ollama is the fastest tool to run LLMs locally when used inside terminal.

10 Free Courses to Build AI Agents in 2024
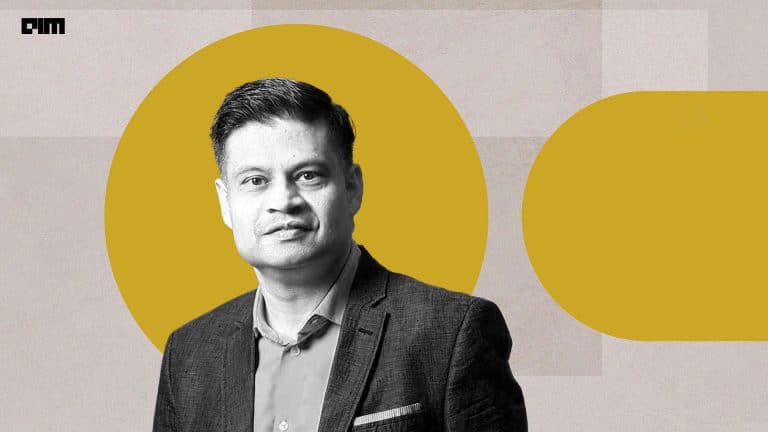
L&T Technology Services Trains 3,000 Engineers in GenAI
Top editorial picks, kunal shah says ‘gpt makes him 10x more efficient in sharing ideas with the team’, chatgpt brings data to life with interactive charts and tables directly from google drive and microsoft onedrive, after stack overflow, reddit succumbs to openai, vmware makes workstation pro and fusion pro free for personal use, subscribe to the belamy: our weekly newsletter, biggest ai stories, delivered to your inbox every week., also in news.
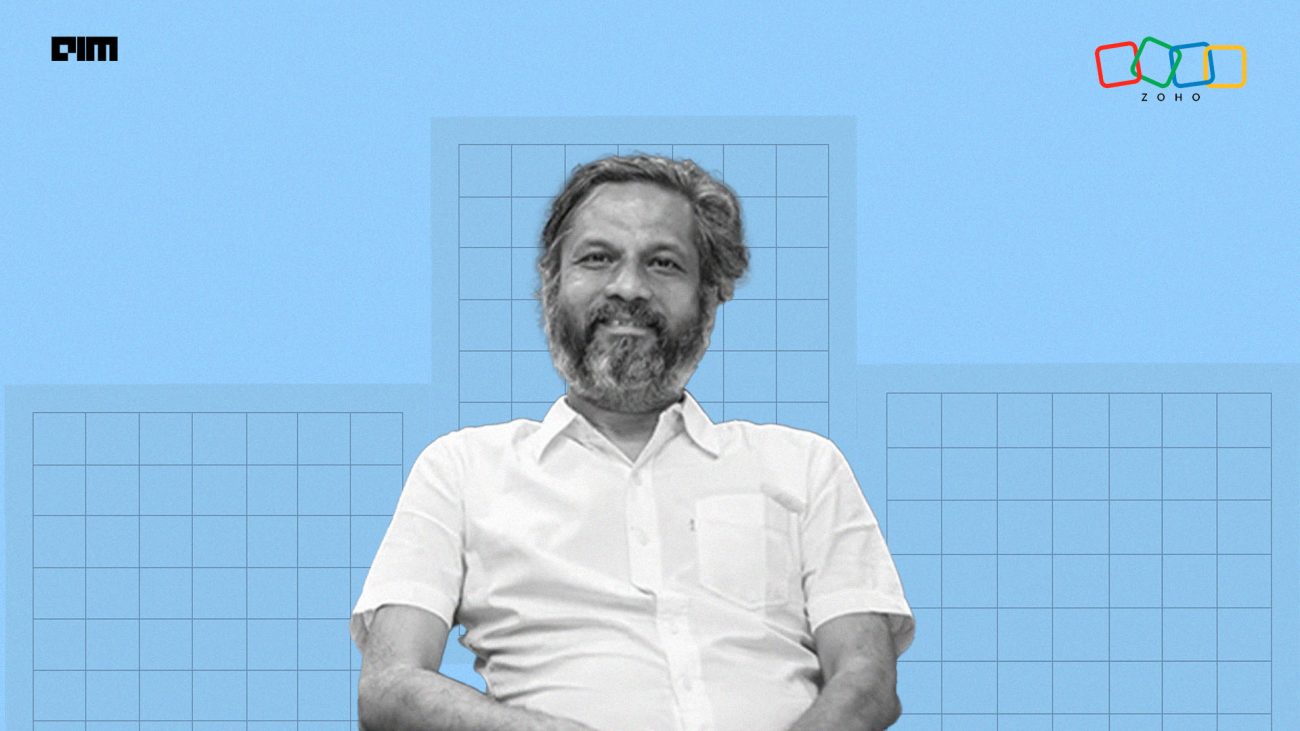
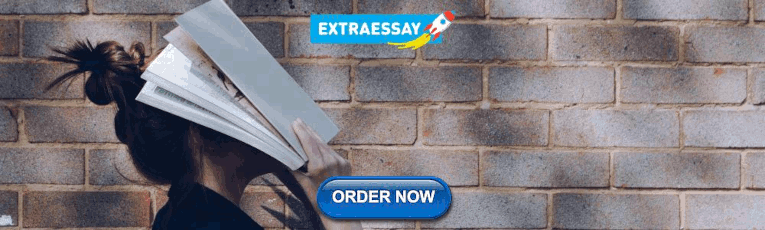
Zoho Ventures into Chipmaking, Plans $700M Investment
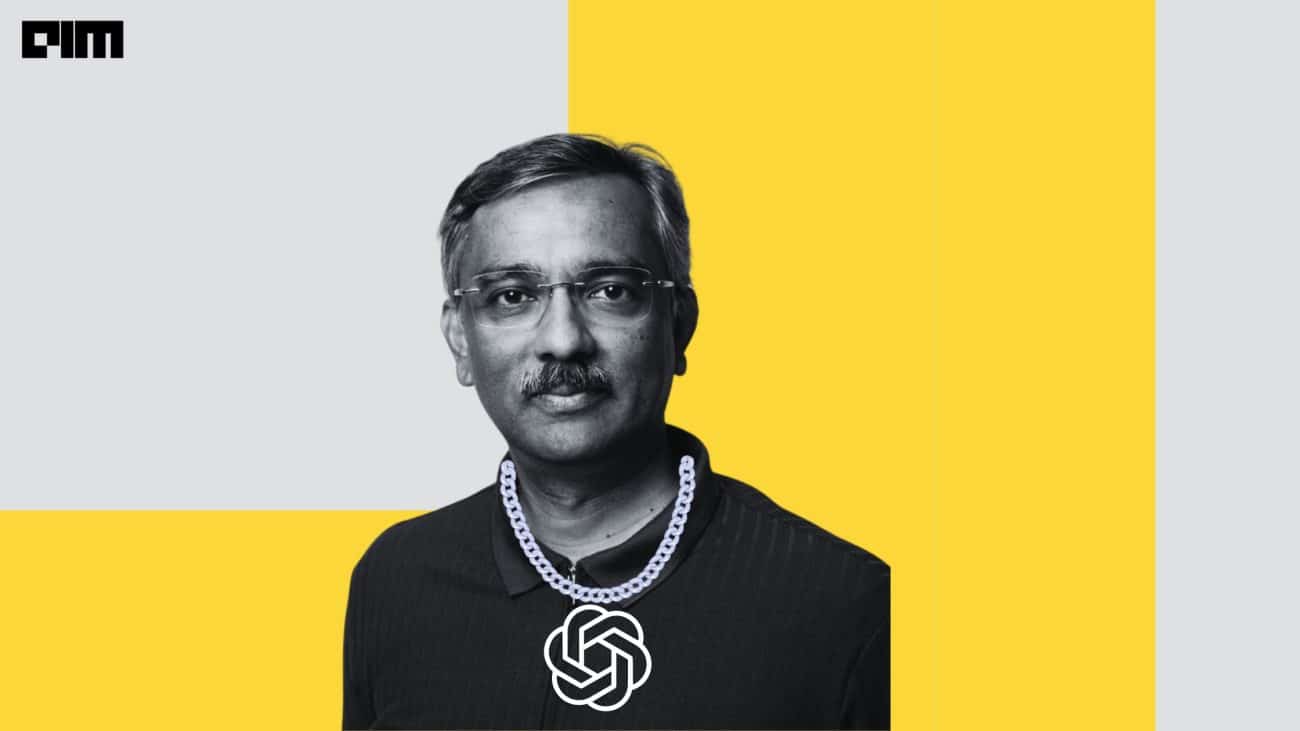
OpenAI Hires Google Veteran to Build ‘Google Search Alternative’
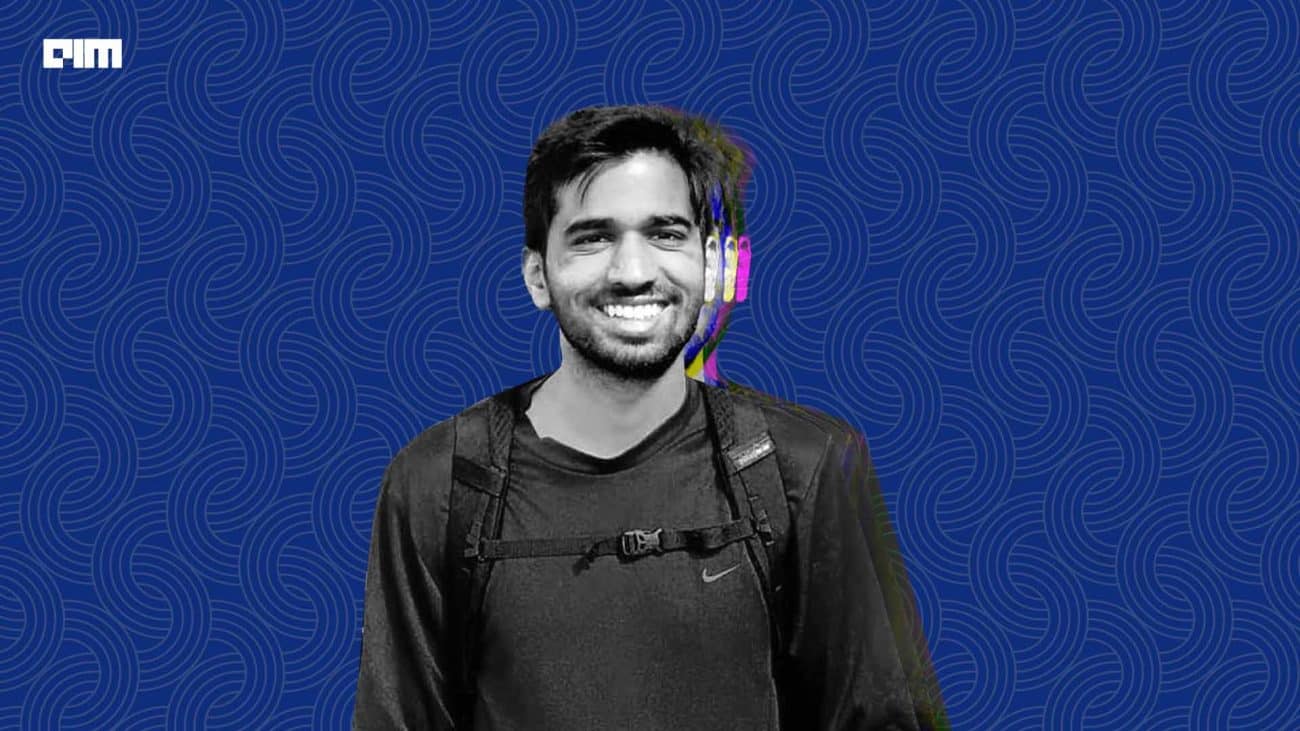
Meet the Child Prodigy from India Who Helped Build OpenAI’s GPT-4o
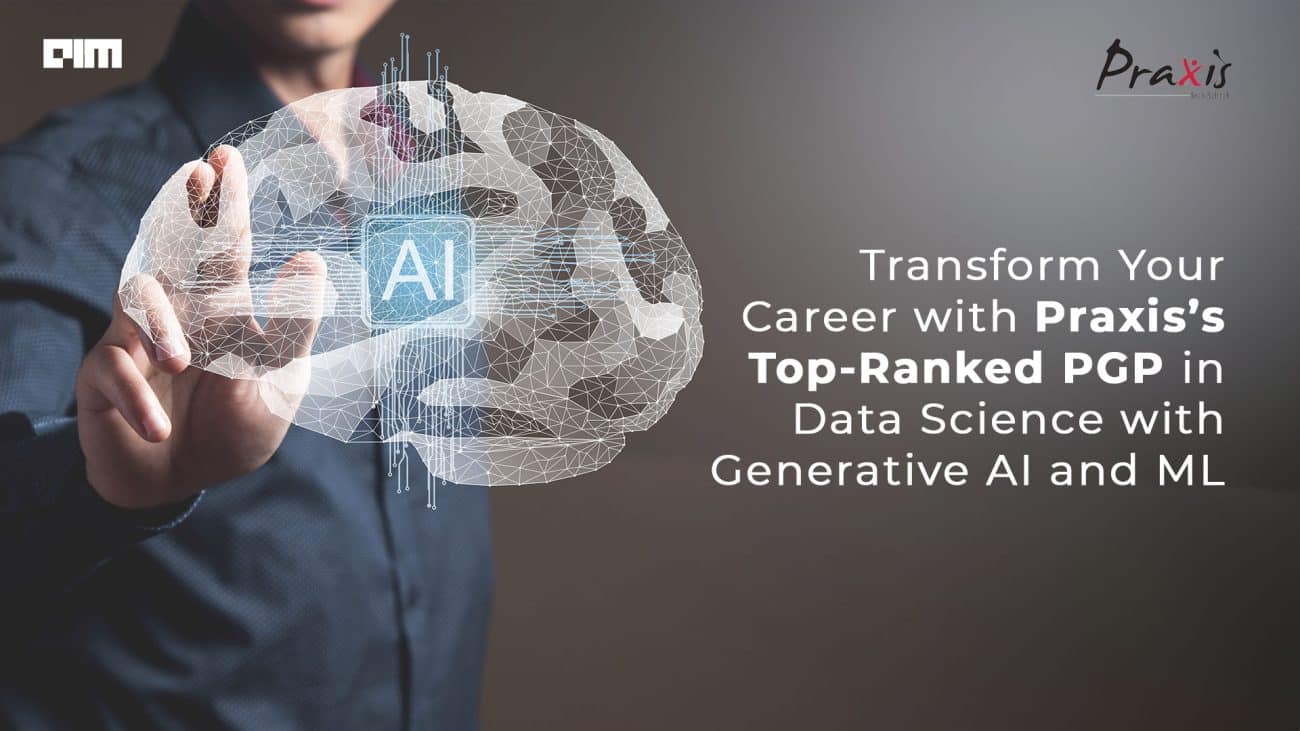
Transform Your Career with Praxis’s Top-Ranked PGP in Data Science with Generative AI and ML
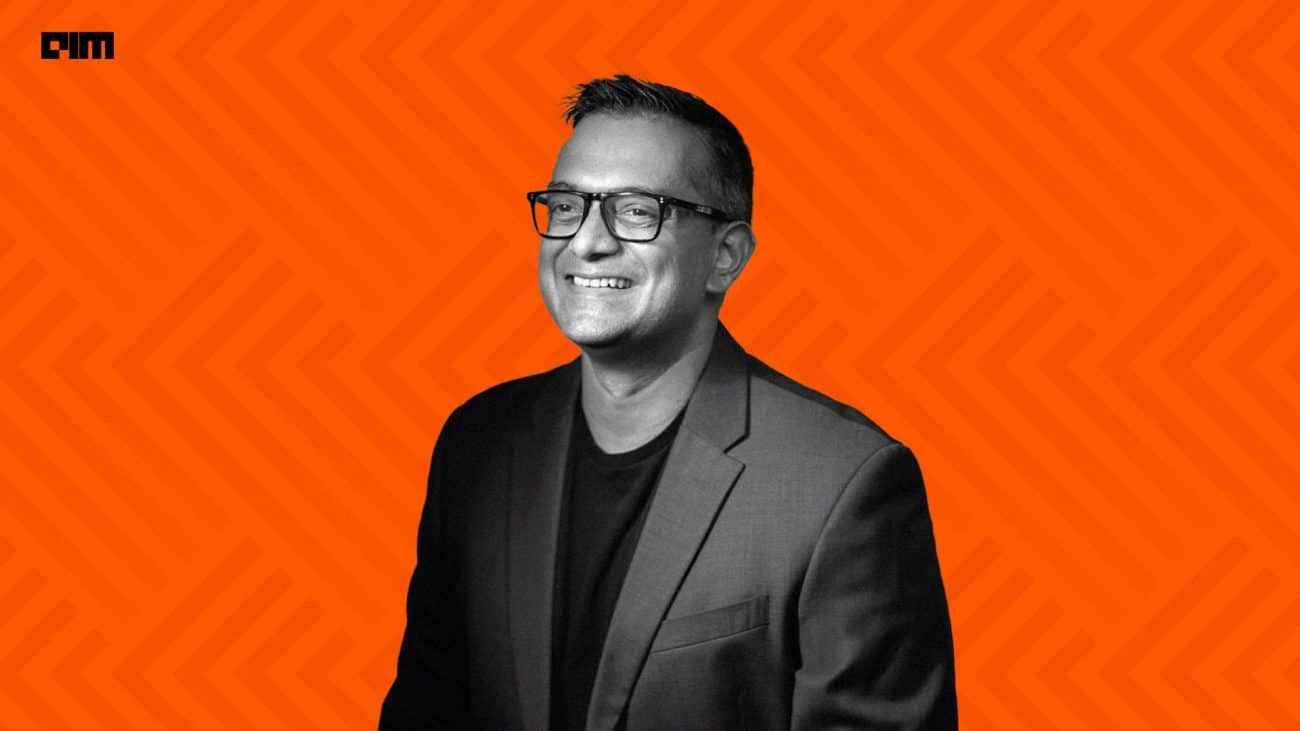
Intel is Bullish on India with its Xeon Processors
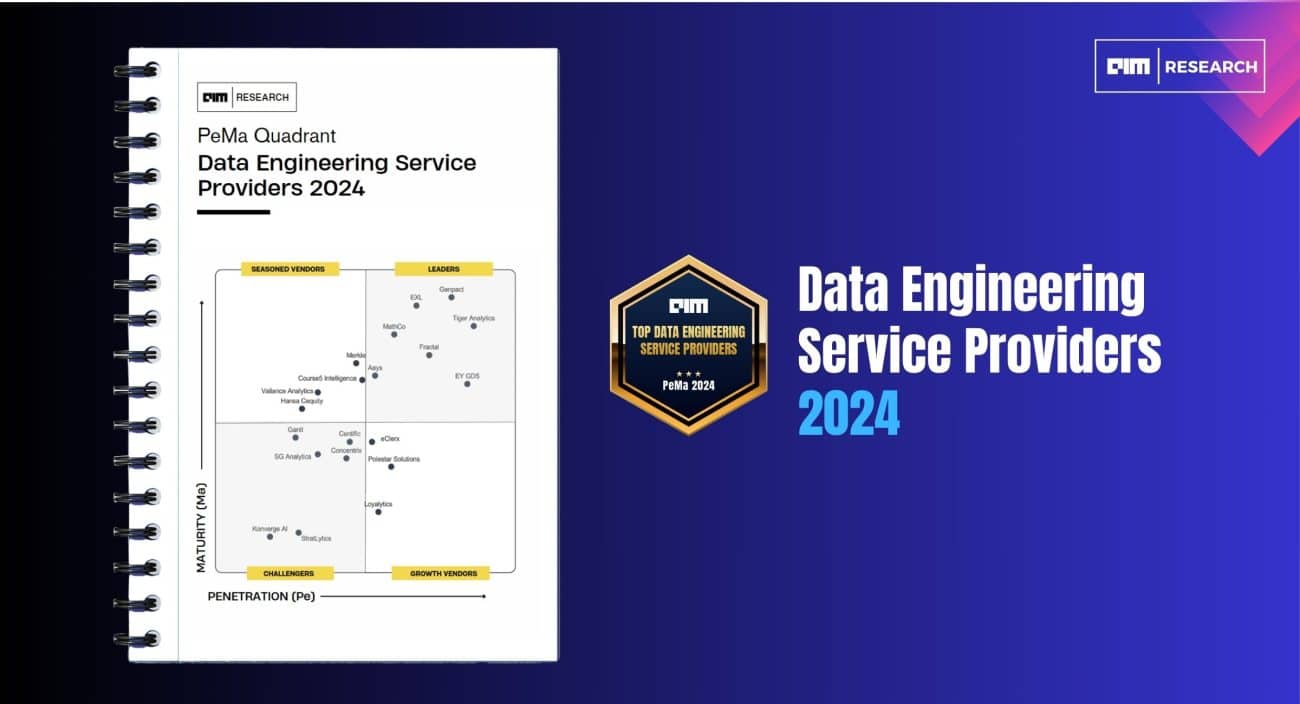
Top Data Engineering Service Providers – PeMa Quadrant 2024

OpenAI Needs Apple, Badly!
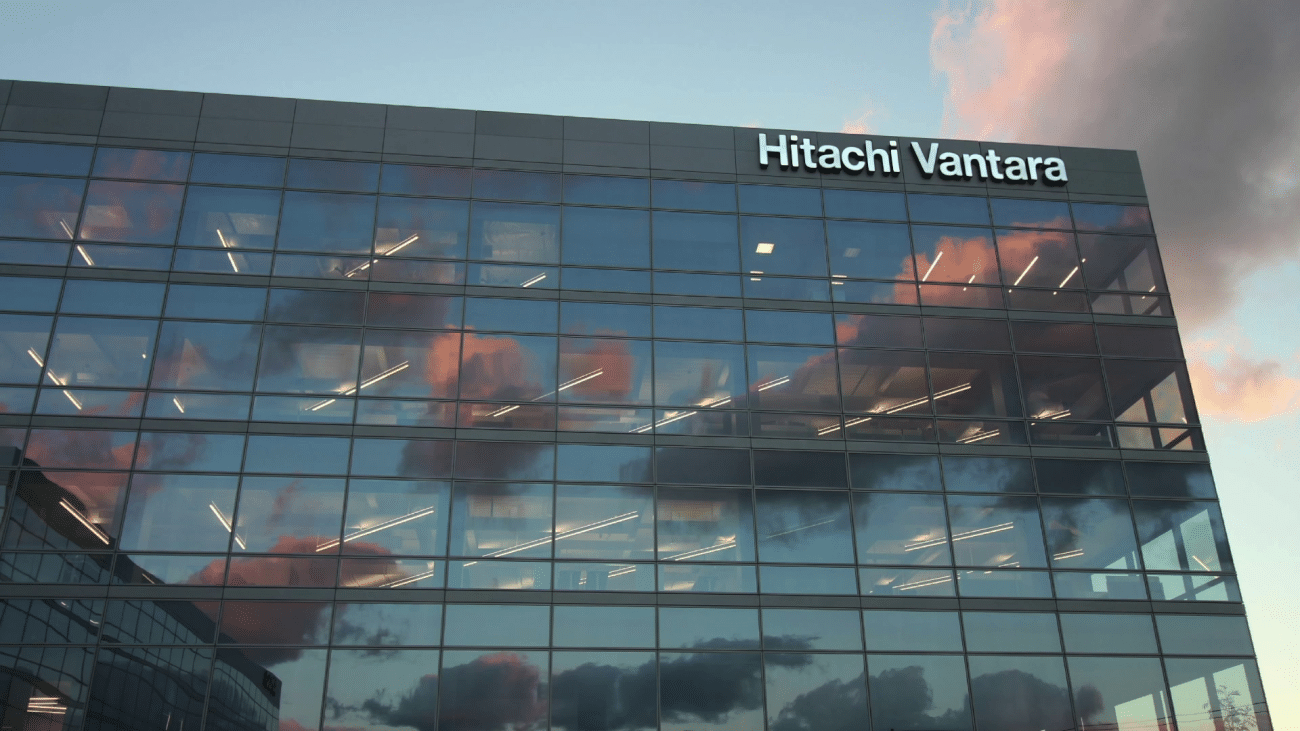
Hitachi Vantara & Veeam Partner to Provide Data Protection for Hybrid Cloud Environments
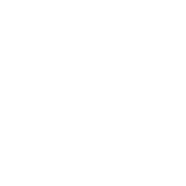
AI Forum for India
Our discord community for ai ecosystem, in collaboration with nvidia. , "> "> flagship events, rising 2024 | de&i in tech summit, april 4 and 5, 2024 | 📍 hilton convention center, manyata tech park, bangalore, machinecon gcc summit 2024, june 28 2024 | 📍bangalore, india, machinecon usa 2024, 26 july 2024 | 583 park avenue, new york, cypher india 2024, september 25-27, 2024 | 📍bangalore, india, cypher usa 2024, nov 21-22 2024 | 📍santa clara convention center, california, usa, genai corner.
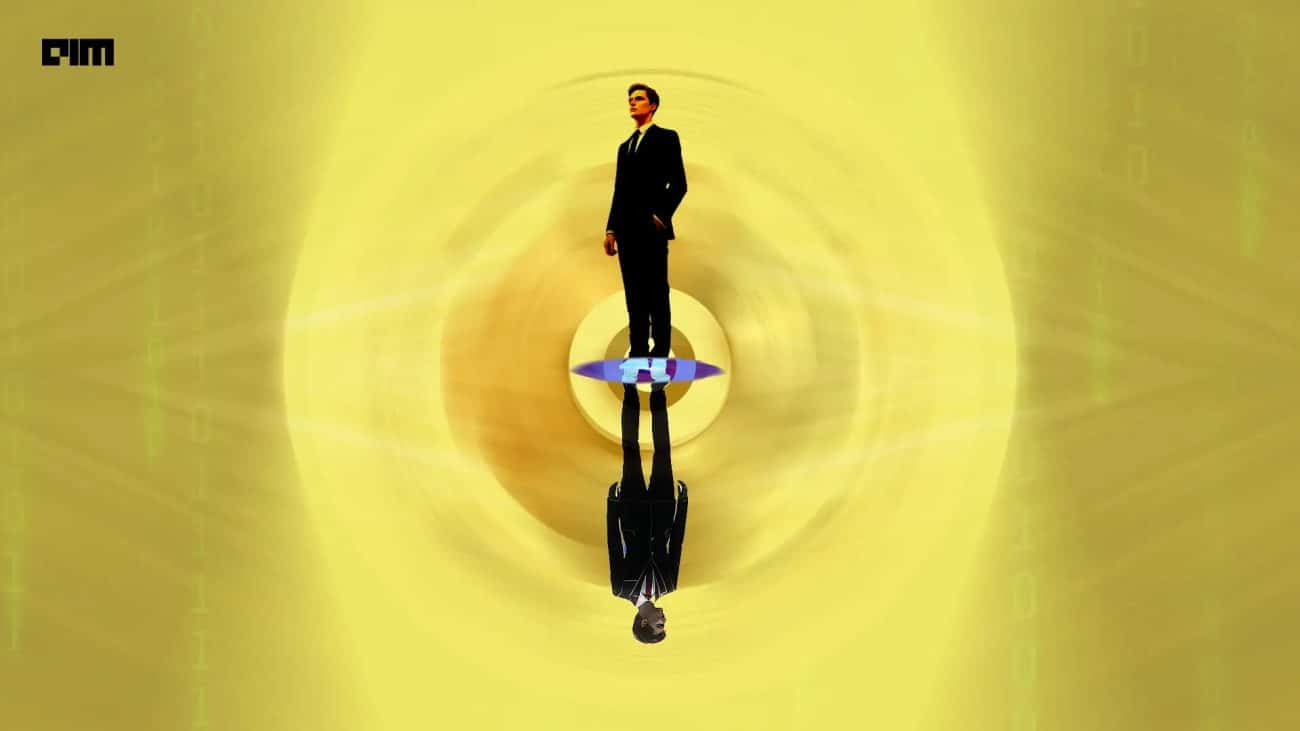
92% of Indian Knowledge Workers Embrace AI at Work: Microsoft & LinkedIn Report

Indian Government to Procure 10,000 GPUs Within Next 18 Months
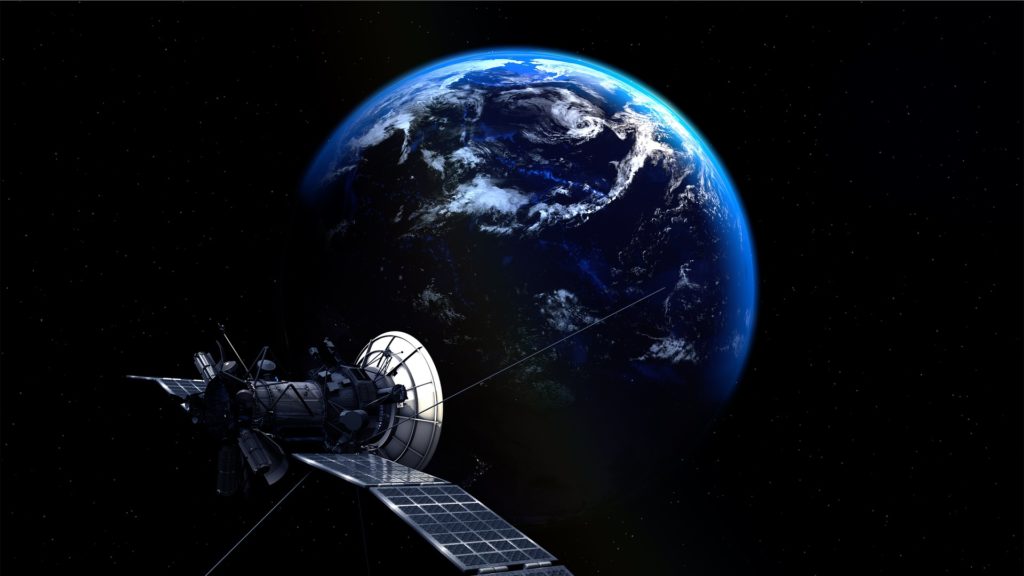
SkyServe’s STORM Ushers in “Smartphone Moment” for Satellite Imaging
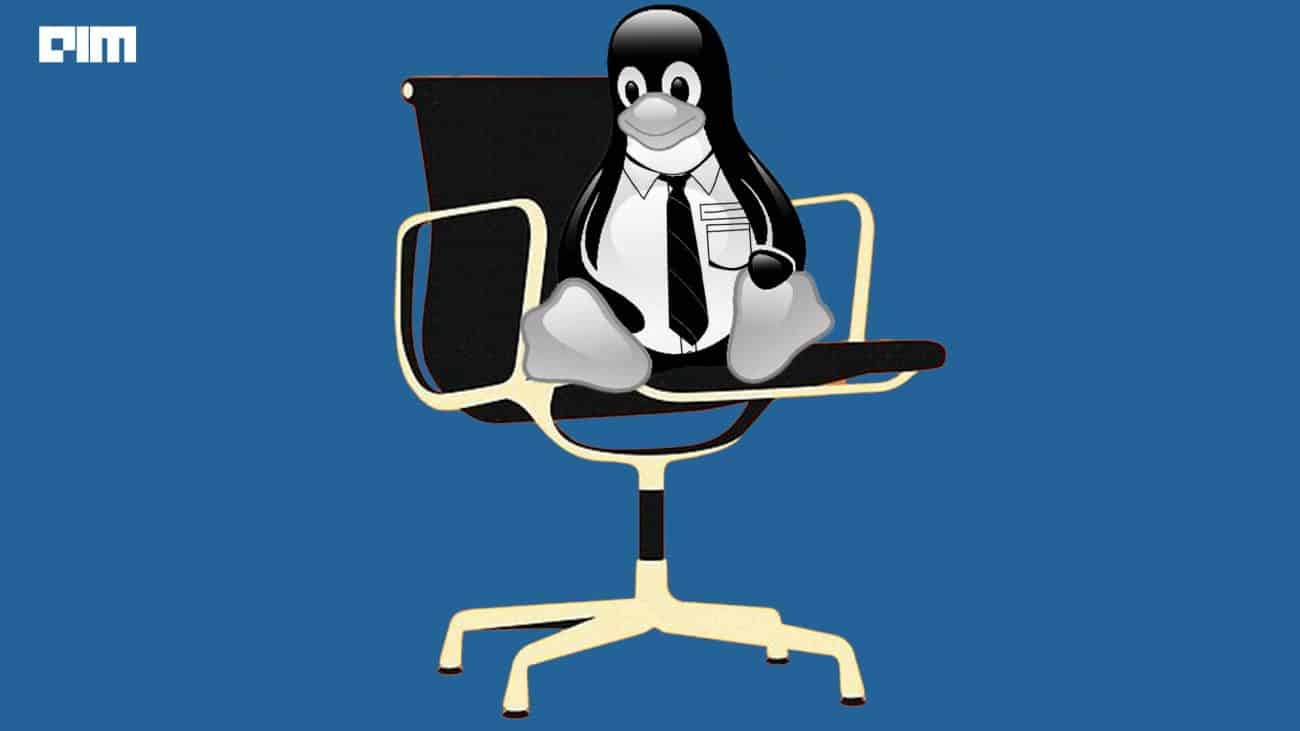
Will Linux Land You a Job?
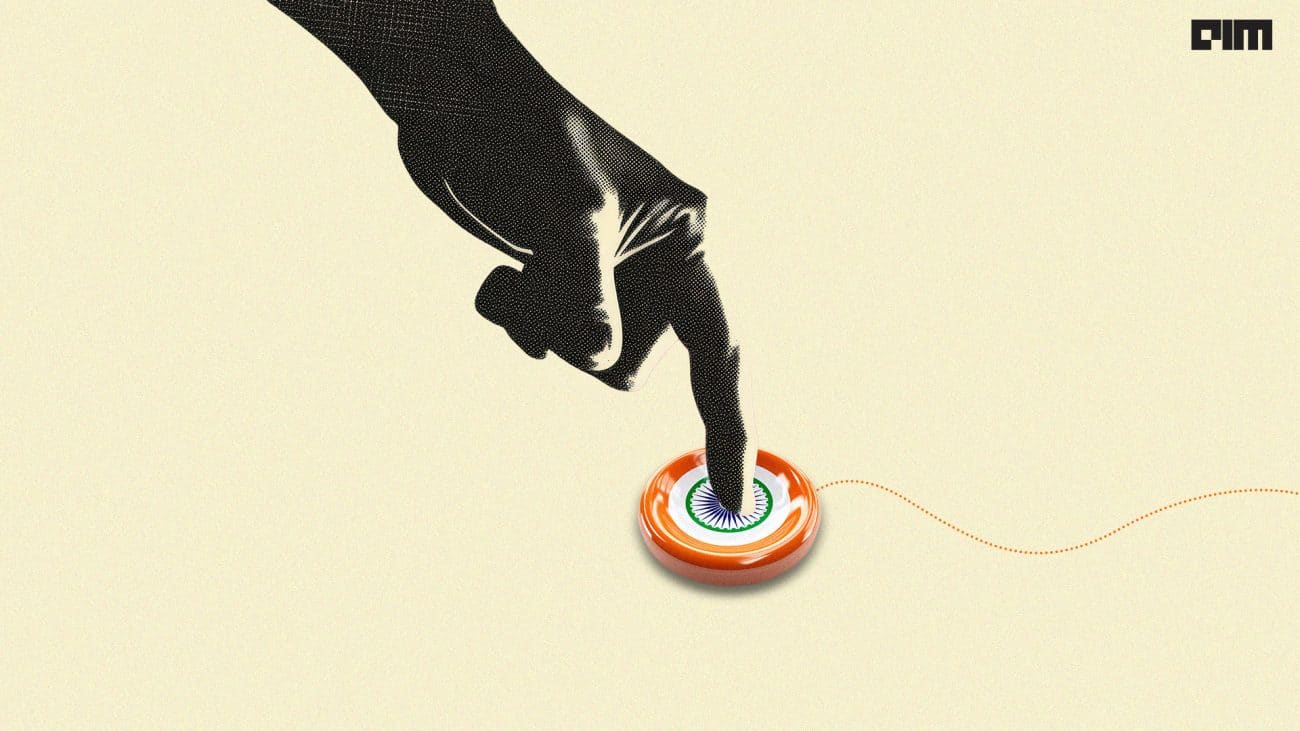
Soket AI Labs Partners with Google Cloud to Boost Pragna-1B Model
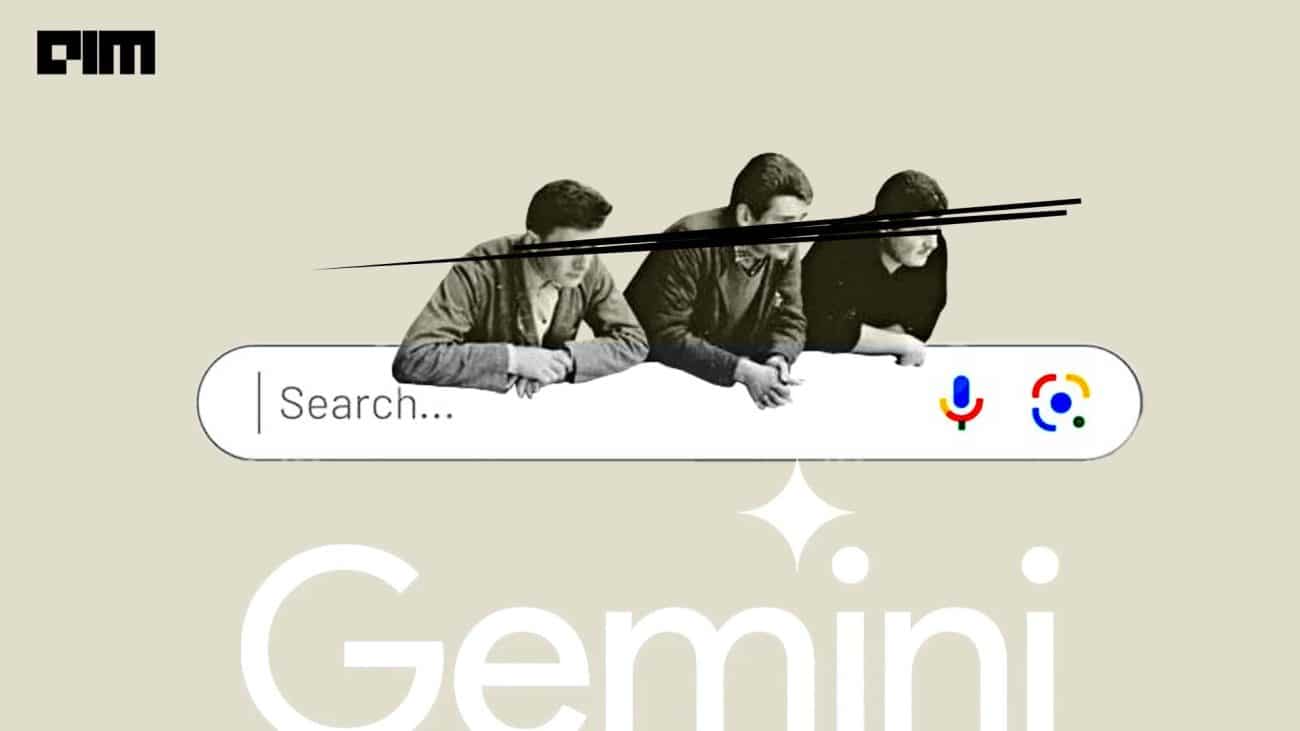
Google Search is Not Going Anywhere Anytime Soon; It’s Here to Stay!

Google’s ‘Astra’ Marks the Beginning of Autonomous AI Agents
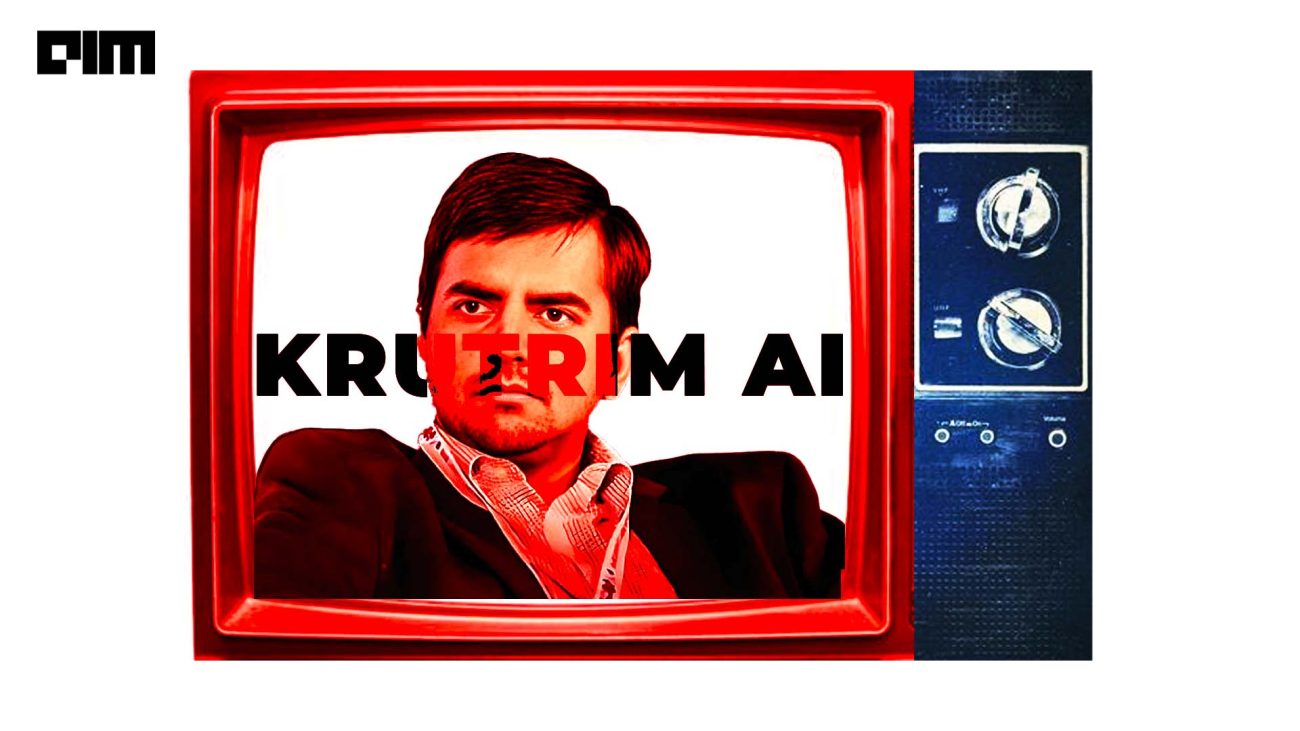
Bodhi Computing Acquisition Signals Ola’s Shift to RISC-V to Make Chips for Krutrim AI Cloud
World's biggest media & analyst firm specializing in ai, advertise with us, aim publishes every day, and we believe in quality over quantity, honesty over spin. we offer a wide variety of branding and targeting options to make it easy for you to propagate your brand., branded content, aim brand solutions, a marketing division within aim, specializes in creating diverse content such as documentaries, public artworks, podcasts, videos, articles, and more to effectively tell compelling stories., corporate upskilling, adasci corporate training program on generative ai provides a unique opportunity to empower, retain and advance your talent, with machinehack you can not only find qualified developers with hiring challenges but can also engage the developer community and your internal workforce by hosting hackathons., talent assessment, conduct customized online assessments on our powerful cloud-based platform, secured with best-in-class proctoring, research & advisory, aim research produces a series of annual reports on ai & data science covering every aspect of the industry. request customised reports & aim surveys for a study on topics of your interest., conferences & events, immerse yourself in ai and business conferences tailored to your role, designed to elevate your performance and empower you to accomplish your organization’s vital objectives., aim launches the 3rd edition of data engineering summit. may 30-31, bengaluru.
Join the forefront of data innovation at the Data Engineering Summit 2024, where industry leaders redefine technology’s future.
© Analytics India Magazine Pvt Ltd & AIM Media House LLC 2024
- Terms of use
- Privacy Policy
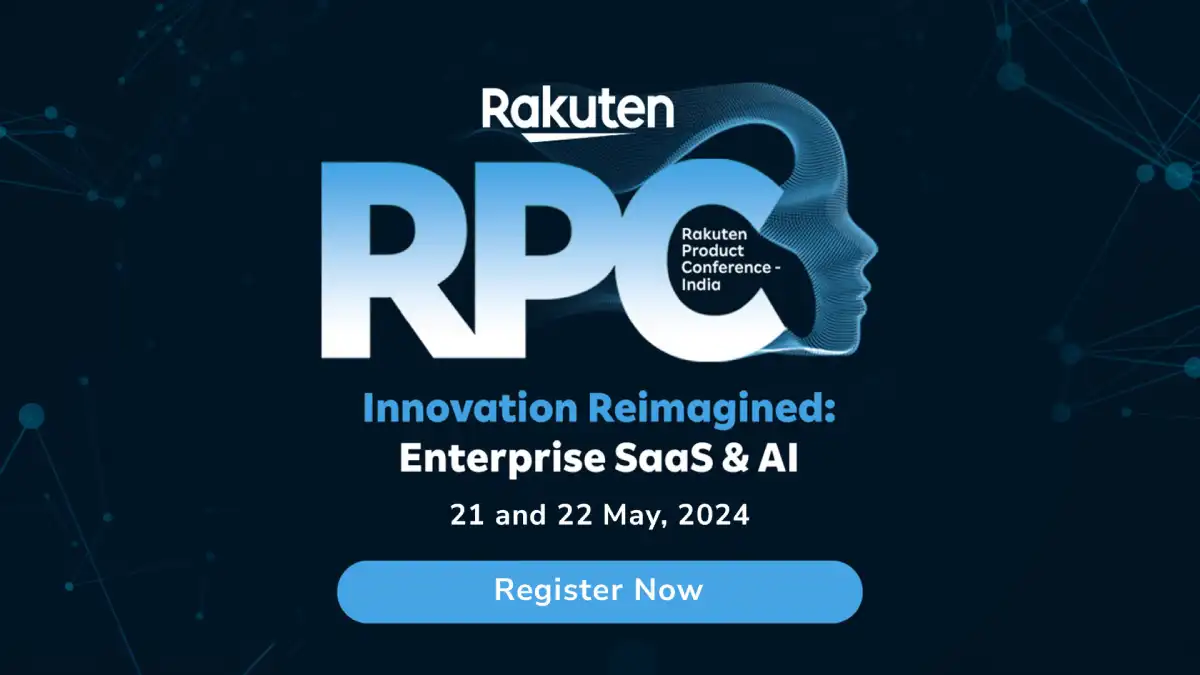
Subscribe to Our Newsletter
The Belamy, our weekly Newsletter is a rage. Just enter your email below.
CS 3750 Advanced Topics in Machine Learning (ISSP 3535)
Machine Learning Techniques for Grading of PowerPoint Slides
- Conference paper
- First Online: 20 March 2022
- Cite this conference paper
- Jyoti G. Borade 14 &
- Laxman D. Netak 14
Part of the book series: Lecture Notes in Computer Science ((LNCS,volume 13184))
Included in the following conference series:
- International Conference on Intelligent Human Computer Interaction
1567 Accesses
1 Citations
This paper describes the design and implementation of automated techniques for grading students’ PowerPoint slides. Preparing PowerPoint slides for seminars, workshops, and conferences is one of the crucial activity of graduate and undergraduate students. Educational institutes use rubrics to assess the PowerPoint slides’ quality on different grounds, such as the use of diagrams, text highlighting techniques, and animations. The proposed system describes a method and dataset designed to automate the task of grading students’ PowerPoint slides. The system aims to evaluate students’ knowledge about various functionalities provided by presentation software. Multiple machine learning techniques are used to grade presentations. Decision Tree classifiers gives 100% accuracy while predicting grade of PowerPoint presentation.
This is a preview of subscription content, log in via an institution to check access.
Access this chapter
- Available as PDF
- Read on any device
- Instant download
- Own it forever
- Available as EPUB and PDF
- Compact, lightweight edition
- Dispatched in 3 to 5 business days
- Free shipping worldwide - see info
Tax calculation will be finalised at checkout
Purchases are for personal use only
Institutional subscriptions
Hearst, M.A.: The debate on automated essay grading. IEEE Intell. Syst. Appl. 15 , 22–37 (2000)
Article Google Scholar
Yang, Y., Buckendahl, C.W., Juszkiewicz, P.J., Bhola, D.S.: A review of strategies for validating computer-automated scoring. Appl. Meas. Educ. 15 (4), 391–412 (2002)
Madnani, N., Cahill, A.: Automated scoring: beyond natural language processing. In: Proceedings of the 27th International Conference on Computational Linguistics, pp. 1099–1109 (2018)
Google Scholar
Ullmann, T.D.: Automated analysis of reflection in writing: validating machine learning approaches. Int. J. Artif. Intell. Educ. 29 (2), 217–257 (2019)
Bashir, A., Hassan, A., Rosman, B., Duma, D., Ahmed, M.: Implementation of a neural natural language understanding component for Arabic dialogue systems. Proc. Comput. Sci. 142 , 222–229 (2018)
Leng, Y., Yu, L., Xiong, J.: DeepReviewer: collaborative grammar and innovation neural network for automatic paper review, pp. 395–403 (2019)
Peng, X., Ke, D., Chen, Z., Xu, B.: Automated Chinese essay scoring using vector space models. In: 2010 4th International Universal Communication Symposium, Beijing, pp. 149–153 (2010)
Al-Jouie, M., Azmi, A.M.: Automated evaluation of school children essays in Arabic. Proc. Comput. Sci. 117 , 19–22 (2017)
Azmi Aqil M., Al-Jouie M.F. and Hussain M., AAEE-Automated evaluation of students’ essays in Arabic language, Information Processing & Management, 56(5), pp. 1736–1752
Walia, T., Josan, G., Singh, A.: An efficient automated answer scoring system for the Punjabi language. Egypt. Inform. J. 20 , 89–96 (2018)
Anak, R., Putri, A., Dyah, L., Ihsan, I., Diyanatul, H., Purnamasari, P.: Automatic essay grading system for Japanese language examination using winnowing algorithm. In: International Seminar on Application for Technology of Information and Communication (iSemantic), pp. 565–569 (2018)
Ramalingam, V.V., Pandian, A., Chetry, P., Nigam, H.: Automated essay grading using machine learning algorithm. J. Phys.: Conf. Ser. (2018)
Haendchen Filho, A., Prado, H., Ferneda, E., Nau, J.: An approach to evaluate adherence to the theme and the argumentative structure of essays. Proc. Comput. Sci. 12 , 788–797 (2018)
Fazal, A., Hussain, F., Dillon, T.: An innovative approach for automatically grading spelling in essays using rubric-based scoring. J. Comput. Syst. Sci. 79 , 1040–1056 (2013)
Olowolayemo, A., Nawi, S., Mantoro, T.: Short answer scoring in English grammar using text similarity measurement. In: International Conference on Computing, Engineering, and Design (ICCED), pp. 131–136 (2018)
Janda, H.K., Pawar, A., Du, S., Mago, V.: Syntactic, semantic and sentiment analysis: the joint effect on automated essay evaluation. IEEE Access 7 , 108486–108503 (2019)
George, N., Sijimol, P.J., Varghese, S.M.: Grading descriptive answer scripts using deep learning. Int. J. Innov. Technol. Explor. Eng. (IJITEE) 8 (5) (2019)
Jin, C., He, B., Hui, K., Sun, L.: TDNN: a two-stage deep neural network for prompt-independent automated essay scoring. In: ACL, Melbourne, Australia (2018)
Surya, K., Gayakwad, E., Nallakaruppan, M.: Deep learning for short answer scoring. Int. J. Recent Technol. Eng. 7 , 1712–1715 (2019)
Rodriguez, P., Jafari, A., Ormerod, C.: Language Models and Automated Essay Scoring (2019)
Bauer, C.: Grading rubrics for engineering presentations and reports. In: ASME International Mechanical Engineering Congress and Exposition (2008)
Peeters, M.J., Sahloff, E.G., Stone, G.E.: A standardized rubric to evaluate student presentations. Am. J. Pharm. Educ. (2010)
Borade, J.G., Netak, L.D.: Automated grading of essays: a review. In: Singh, M., Kang, D.-K., Lee, J.-H., Tiwary, U.S., Singh, D., Chung, W.-Y. (eds.) IHCI 2020. LNCS, vol. 12615, pp. 238–249. Springer, Cham (2021). https://doi.org/10.1007/978-3-030-68449-5_25
Chapter Google Scholar
Borade, J.G., Kiwelekar, A.W., Netak, L.D.: Feature extraction for automatic grading of students’ presentations. In: Tuba, M., Akashe, S., Joshi, A. (eds.) ICT Systems and Sustainability. LNCS, vol. 321, pp. 293–301. Springer, Singapore (2022). https://doi.org/10.1007/978-981-16-5987-4_30
Download references
Author information
Authors and affiliations.
Dr. Babasaheb Ambedkar Technological University, Lonere, 402103, Raigad (MS), India
Jyoti G. Borade & Laxman D. Netak
You can also search for this author in PubMed Google Scholar
Corresponding author
Correspondence to Jyoti G. Borade .
Editor information
Editors and affiliations.
Kent State University, Kent, OH, USA
Jong-Hoon Kim
University of Tartu, Tartu, Estonia
Madhusudan Singh
Indian Institute of Information Technology, Allahabad, India
Uma Shanker Tiwary
Massachusetts Institute of Technology, Cambridge, MA, USA
Marigankar Sur
Hankuk University of Foreign Studies, Seoul, Korea (Republic of)
Dhananjay Singh
Rights and permissions
Reprints and permissions
Copyright information
© 2022 Springer Nature Switzerland AG
About this paper
Cite this paper.
Borade, J.G., Netak, L.D. (2022). Machine Learning Techniques for Grading of PowerPoint Slides. In: Kim, JH., Singh, M., Khan, J., Tiwary, U.S., Sur, M., Singh, D. (eds) Intelligent Human Computer Interaction. IHCI 2021. Lecture Notes in Computer Science, vol 13184. Springer, Cham. https://doi.org/10.1007/978-3-030-98404-5_1
Download citation
DOI : https://doi.org/10.1007/978-3-030-98404-5_1
Published : 20 March 2022
Publisher Name : Springer, Cham
Print ISBN : 978-3-030-98403-8
Online ISBN : 978-3-030-98404-5
eBook Packages : Computer Science Computer Science (R0)
Share this paper
Anyone you share the following link with will be able to read this content:
Sorry, a shareable link is not currently available for this article.
Provided by the Springer Nature SharedIt content-sharing initiative
- Publish with us
Policies and ethics
- Find a journal
- Track your research

Exploring 250+ Machine Learning Research Topics

In recent years, machine learning has become super popular and grown very quickly. This happened because technology got better, and there’s a lot more data available. Because of this, we’ve seen lots of new and amazing things happen in different areas. Machine learning research is what makes all these cool things possible. In this blog, we’ll talk about machine learning research topics, why they’re important, how you can pick one, what areas are popular to study, what’s new and exciting, the tough problems, and where you can find help if you want to be a researcher.
Why Does Machine Learning Research Matter?
Table of Contents
Machine learning research is at the heart of the AI revolution. It underpins the development of intelligent systems capable of making predictions, automating tasks, and improving decision-making across industries. The importance of this research can be summarized as follows:
Advancements in Technology
The growth of machine learning research has led to the development of powerful algorithms, tools, and frameworks. Numerous industries, including healthcare, banking, autonomous cars, and natural language processing, have found use for these technology.
As researchers continue to push the boundaries of what’s possible, we can expect even more transformative technologies to emerge.
Real-world Applications
Machine learning research has brought about tangible changes in our daily lives. Voice assistants like Siri and Alexa, recommendation systems on streaming platforms, and personalized healthcare diagnostics are just a few examples of how this research impacts our world.
By working on new research topics, scientists can further refine these applications and create new ones.
Economic and Industrial Impacts
The economic implications of machine learning research are substantial. Companies that harness the power of machine learning gain a competitive edge in the market.
This creates a demand for skilled machine learning researchers, driving job opportunities and contributing to economic growth.
How to Choose the Machine Learning Research Topics?
Selecting the right machine learning research topics is crucial for your success as a machine learning researcher. Here’s a guide to help you make an informed decision:
- Understanding Your Interests
Start by considering your personal interests. Machine learning is a broad field with applications in virtually every sector. By choosing a topic that aligns with your passions, you’ll stay motivated and engaged throughout your research journey.
- Reviewing Current Trends
Stay updated on the latest trends in machine learning. Attend conferences, read research papers, and engage with the community to identify emerging research topics. Current trends often lead to exciting breakthroughs.
- Identifying Gaps in Existing Research
Sometimes, the most promising research topics involve addressing gaps in existing knowledge. These gaps may become evident through your own experiences, discussions with peers, or in the course of your studies.
- Collaborating with Experts
Collaboration is key in research. Working with experts in the field can help you refine your research topic and gain valuable insights. Seek mentors and collaborators who can guide you.
250+ Machine Learning Research Topics: Category-wise
Supervised learning.
- Explainable AI for Decision Support
- Few-shot Learning Methods
- Time Series Forecasting with Deep Learning
- Handling Imbalanced Datasets in Classification
- Regression Techniques for Non-linear Data
- Transfer Learning in Supervised Settings
- Multi-label Classification Strategies
- Semi-Supervised Learning Approaches
- Novel Feature Selection Methods
- Anomaly Detection in Supervised Scenarios
- Federated Learning for Distributed Supervised Models
- Ensemble Learning for Improved Accuracy
- Automated Hyperparameter Tuning
- Ethical Implications in Supervised Models
- Interpretability of Deep Neural Networks.
Unsupervised Learning
- Unsupervised Clustering of High-dimensional Data
- Semi-Supervised Clustering Approaches
- Density Estimation in Unsupervised Learning
- Anomaly Detection in Unsupervised Settings
- Transfer Learning for Unsupervised Tasks
- Representation Learning in Unsupervised Learning
- Outlier Detection Techniques
- Generative Models for Data Synthesis
- Manifold Learning in High-dimensional Spaces
- Unsupervised Feature Selection
- Privacy-Preserving Unsupervised Learning
- Community Detection in Complex Networks
- Clustering Interpretability and Visualization
- Unsupervised Learning for Image Segmentation
- Autoencoders for Dimensionality Reduction.
Reinforcement Learning
- Deep Reinforcement Learning in Real-world Applications
- Safe Reinforcement Learning for Autonomous Systems
- Transfer Learning in Reinforcement Learning
- Imitation Learning and Apprenticeship Learning
- Multi-agent Reinforcement Learning
- Explainable Reinforcement Learning Policies
- Hierarchical Reinforcement Learning
- Model-based Reinforcement Learning
- Curriculum Learning in Reinforcement Learning
- Reinforcement Learning in Robotics
- Exploration vs. Exploitation Strategies
- Reward Function Design and Ethical Considerations
- Reinforcement Learning in Healthcare
- Continuous Action Spaces in RL
- Reinforcement Learning for Resource Management.
Natural Language Processing (NLP)
- Multilingual and Cross-lingual NLP
- Contextualized Word Embeddings
- Bias Detection and Mitigation in NLP
- Named Entity Recognition for Low-resource Languages
- Sentiment Analysis in Social Media Text
- Dialogue Systems for Improved Customer Service
- Text Summarization for News Articles
- Low-resource Machine Translation
- Explainable NLP Models
- Coreference Resolution in NLP
- Question Answering in Specific Domains
- Detecting Fake News and Misinformation
- NLP for Healthcare: Clinical Document Understanding
- Emotion Analysis in Text
- Text Generation with Controlled Attributes.
Computer Vision
- Video Action Recognition and Event Detection
- Object Detection in Challenging Conditions (e.g., low light)
- Explainable Computer Vision Models
- Image Captioning for Accessibility
- Large-scale Image Retrieval
- Domain Adaptation in Computer Vision
- Fine-grained Image Classification
- Facial Expression Recognition
- Visual Question Answering
- Self-supervised Learning for Visual Representations
- Weakly Supervised Object Localization
- Human Pose Estimation in 3D
- Scene Understanding in Autonomous Vehicles
- Image Super-resolution
- Gaze Estimation for Human-Computer Interaction.
Deep Learning
- Neural Architecture Search for Efficient Models
- Self-attention Mechanisms and Transformers
- Interpretability in Deep Learning Models
- Robustness of Deep Neural Networks
- Generative Adversarial Networks (GANs) for Data Augmentation
- Neural Style Transfer in Art and Design
- Adversarial Attacks and Defenses
- Neural Networks for Audio and Speech Processing
- Explainable AI for Healthcare Diagnosis
- Automated Machine Learning (AutoML)
- Reinforcement Learning with Deep Neural Networks
- Model Compression and Quantization
- Lifelong Learning with Deep Learning Models
- Multimodal Learning with Vision and Language
- Federated Learning for Privacy-preserving Deep Learning.
Explainable AI
- Visualizing Model Decision Boundaries
- Saliency Maps and Feature Attribution
- Rule-based Explanations for Black-box Models
- Contrastive Explanations for Model Interpretability
- Counterfactual Explanations and What-if Analysis
- Human-centered AI for Explainable Healthcare
- Ethics and Fairness in Explainable AI
- Explanation Generation for Natural Language Processing
- Explainable AI in Financial Risk Assessment
- User-friendly Interfaces for Model Interpretability
- Scalability and Efficiency in Explainable Models
- Hybrid Models for Combined Accuracy and Explainability
- Post-hoc vs. Intrinsic Explanations
- Evaluation Metrics for Explanation Quality
- Explainable AI for Autonomous Vehicles.
Transfer Learning
- Zero-shot Learning and Few-shot Learning
- Cross-domain Transfer Learning
- Domain Adaptation for Improved Generalization
- Multilingual Transfer Learning in NLP
- Pretraining and Fine-tuning Techniques
- Lifelong Learning and Continual Learning
- Domain-specific Transfer Learning Applications
- Model Distillation for Knowledge Transfer
- Contrastive Learning for Transfer Learning
- Self-training and Pseudo-labeling
- Dynamic Adaption of Pretrained Models
- Privacy-Preserving Transfer Learning
- Unsupervised Domain Adaptation
- Negative Transfer Avoidance in Transfer Learning.
Federated Learning
- Secure Aggregation in Federated Learning
- Communication-efficient Federated Learning
- Privacy-preserving Techniques in Federated Learning
- Federated Transfer Learning
- Heterogeneous Federated Learning
- Real-world Applications of Federated Learning
- Federated Learning for Edge Devices
- Federated Learning for Healthcare Data
- Differential Privacy in Federated Learning
- Byzantine-robust Federated Learning
- Federated Learning with Non-IID Data
- Model Selection in Federated Learning
- Scalable Federated Learning for Large Datasets
- Client Selection and Sampling Strategies
- Global Model Update Synchronization in Federated Learning.
Quantum Machine Learning
- Quantum Neural Networks and Quantum Circuit Learning
- Quantum-enhanced Optimization for Machine Learning
- Quantum Data Compression and Quantum Principal Component Analysis
- Quantum Kernels and Quantum Feature Maps
- Quantum Variational Autoencoders
- Quantum Transfer Learning
- Quantum-inspired Classical Algorithms for ML
- Hybrid Quantum-Classical Models
- Quantum Machine Learning on Near-term Quantum Devices
- Quantum-inspired Reinforcement Learning
- Quantum Computing for Quantum Chemistry and Drug Discovery
- Quantum Machine Learning for Finance
- Quantum Data Structures and Quantum Databases
- Quantum-enhanced Cryptography in Machine Learning
- Quantum Generative Models and Quantum GANs.
Ethical AI and Bias Mitigation
- Fairness-aware Machine Learning Algorithms
- Bias Detection and Mitigation in Real-world Data
- Explainable AI for Ethical Decision Support
- Algorithmic Accountability and Transparency
- Privacy-preserving AI and Data Governance
- Ethical Considerations in AI for Healthcare
- Fairness in Recommender Systems
- Bias and Fairness in NLP Models
- Auditing AI Systems for Bias
- Societal Implications of AI in Criminal Justice
- Ethical AI Education and Training
- Bias Mitigation in Autonomous Vehicles
- Fair AI in Financial and Hiring Decisions
- Case Studies in Ethical AI Failures
- Legal and Policy Frameworks for Ethical AI.
Meta-Learning and AutoML
- Neural Architecture Search (NAS) for Efficient Models
- Transfer Learning in NAS
- Reinforcement Learning for NAS
- Multi-objective NAS
- Automated Data Augmentation
- Neural Architecture Optimization for Edge Devices
- Bayesian Optimization for AutoML
- Model Compression and Quantization in AutoML
- AutoML for Federated Learning
- AutoML in Healthcare Diagnostics
- Explainable AutoML
- Cost-sensitive Learning in AutoML
- AutoML for Small Data
- Human-in-the-Loop AutoML.
AI for Healthcare and Medicine
- Disease Prediction and Early Diagnosis
- Medical Image Analysis with Deep Learning
- Drug Discovery and Molecular Modeling
- Electronic Health Record Analysis
- Predictive Analytics in Healthcare
- Personalized Treatment Planning
- Healthcare Fraud Detection
- Telemedicine and Remote Patient Monitoring
- AI in Radiology and Pathology
- AI in Drug Repurposing
- AI for Medical Robotics and Surgery
- Genomic Data Analysis
- AI-powered Mental Health Assessment
- Explainable AI in Healthcare Decision Support
- AI in Epidemiology and Outbreak Prediction.
AI in Finance and Investment
- Algorithmic Trading and High-frequency Trading
- Credit Scoring and Risk Assessment
- Fraud Detection and Anti-money Laundering
- Portfolio Optimization with AI
- Financial Market Prediction
- Sentiment Analysis in Financial News
- Explainable AI in Financial Decision-making
- Algorithmic Pricing and Dynamic Pricing Strategies
- AI in Cryptocurrency and Blockchain
- Customer Behavior Analysis in Banking
- Explainable AI in Credit Decisioning
- AI in Regulatory Compliance
- Ethical AI in Financial Services
- AI for Real Estate Investment
- Automated Financial Reporting.
AI in Climate Change and Sustainability
- Climate Modeling and Prediction
- Renewable Energy Forecasting
- Smart Grid Optimization
- Energy Consumption Forecasting
- Carbon Emission Reduction with AI
- Ecosystem Monitoring and Preservation
- Precision Agriculture with AI
- AI for Wildlife Conservation
- Natural Disaster Prediction and Management
- Water Resource Management with AI
- Sustainable Transportation and Urban Planning
- Climate Change Mitigation Strategies with AI
- Environmental Impact Assessment with Machine Learning
- Eco-friendly Supply Chain Optimization
- Ethical AI in Climate-related Decision Support.
Data Privacy and Security
- Differential Privacy Mechanisms
- Federated Learning for Privacy-preserving AI
- Secure Multi-Party Computation
- Privacy-enhancing Technologies in Machine Learning
- Homomorphic Encryption for Machine Learning
- Ethical Considerations in Data Privacy
- Privacy-preserving AI in Healthcare
- AI for Secure Authentication and Access Control
- Blockchain and AI for Data Security
- Explainable Privacy in Machine Learning
- Privacy-preserving AI in Government and Public Services
- Privacy-compliant AI for IoT and Edge Devices
- Secure AI Models Sharing and Deployment
- Privacy-preserving AI in Financial Transactions
- AI in the Legal Frameworks of Data Privacy.
Global Collaboration in Research
- International Research Partnerships and Collaboration Models
- Multilingual and Cross-cultural AI Research
- Addressing Global Healthcare Challenges with AI
- Ethical Considerations in International AI Collaborations
- Interdisciplinary AI Research in Global Challenges
- AI Ethics and Human Rights in Global Research
- Data Sharing and Data Access in Global AI Research
- Cross-border Research Regulations and Compliance
- AI Innovation Hubs and International Research Centers
- AI Education and Training for Global Communities
- Humanitarian AI and AI for Sustainable Development Goals
- AI for Cultural Preservation and Heritage Protection
- Collaboration in AI-related Global Crises
- AI in Cross-cultural Communication and Understanding
- Global AI for Environmental Sustainability and Conservation.
Emerging Trends and Hot Topics in Machine Learning Research
The landscape of machine learning research topics is constantly evolving. Here are some of the emerging trends and hot topics that are shaping the field:
As AI systems become more prevalent, addressing ethical concerns and mitigating bias in algorithms are critical research areas.
Interpretable and Explainable Models
Understanding why machine learning models make specific decisions is crucial for their adoption in sensitive areas, such as healthcare and finance.
Meta-learning algorithms are designed to enable machines to learn how to learn, while AutoML aims to automate the machine learning process itself.
Machine learning is revolutionizing the healthcare sector, from diagnostic tools to drug discovery and patient care.
Algorithmic trading, risk assessment, and fraud detection are just a few applications of AI in finance, creating a wealth of research opportunities.
Machine learning research is crucial in analyzing and mitigating the impacts of climate change and promoting sustainable practices.
Challenges and Future Directions
While machine learning research has made tremendous strides, it also faces several challenges:
- Data Privacy and Security: As machine learning models require vast amounts of data, protecting individual privacy and data security are paramount concerns.
- Scalability and Efficiency: Developing efficient algorithms that can handle increasingly large datasets and complex computations remains a challenge.
- Ensuring Fairness and Transparency: Addressing bias in machine learning models and making their decisions transparent is essential for equitable AI systems.
- Quantum Computing and Machine Learning: The integration of quantum computing and machine learning has the potential to revolutionize the field, but it also presents unique challenges.
- Global Collaboration in Research: Machine learning research benefits from collaboration on a global scale. Ensuring that researchers from diverse backgrounds work together is vital for progress.
Resources for Machine Learning Researchers
If you’re looking to embark on a journey in machine learning research topics, there are various resources at your disposal:
- Journals and Conferences
Journals such as the “Journal of Machine Learning Research” and conferences like NeurIPS and ICML provide a platform for publishing and discussing research findings.
- Online Communities and Forums
Platforms like Stack Overflow, GitHub, and dedicated forums for machine learning provide spaces for collaboration and problem-solving.
- Datasets and Tools
Open-source datasets and tools like TensorFlow and PyTorch simplify the research process by providing access to data and pre-built models.
- Research Grants and Funding Opportunities
Many organizations and government agencies offer research grants and funding for machine learning projects. Seek out these opportunities to support your research.
Machine learning research is like a superhero in the world of technology. To be a part of this exciting journey, it’s important to choose the right machine learning research topics and keep up with the latest trends.
Machine learning research makes our lives better. It powers things like smart assistants and life-saving medical tools. It’s like the force driving the future of technology and society.
But, there are challenges too. We need to work together and be ethical in our research. Everyone should benefit from this technology. The future of machine learning research is incredibly bright. If you want to be a part of it, get ready for an exciting adventure. You can help create new solutions and make a big impact on the world.
Related Posts

Tips on How To Tackle A Machine Learning Project As A Beginner
Here in this blog, CodeAvail experts will explain to you tips on how to tackle a machine learning project as a beginner step by step…

Artificial Intelligence and Machine Learning Basics for Beginners
Here in this blog, CodeAvail experts will explain to you Artificial Intelligence and Machine Learning basics for beginners in detail step by step. What is…
Main Navigation
- Contact NeurIPS
- Code of Ethics
- Code of Conduct
- Create Profile
- Journal To Conference Track
- Diversity & Inclusion
- Proceedings
- Future Meetings
- Exhibitor Information
- Privacy Policy
NeurIPS 2024, the Thirty-eighth Annual Conference on Neural Information Processing Systems, will be held at the Vancouver Convention Center
Monday Dec 9 through Sunday Dec 15. Monday is an industry expo.

Registration
Pricing » Registration 2024 Registration Cancellation Policy » . Certificate of Attendance
Our Hotel Reservation page is currently under construction and will be released shortly. NeurIPS has contracted Hotel guest rooms for the Conference at group pricing, requiring reservations only through this page. Please do not make room reservations through any other channel, as it only impedes us from putting on the best Conference for you. We thank you for your assistance in helping us protect the NeurIPS conference.
Announcements
- The call for High School Projects has been released
- The Call For Papers has been released
- See the Visa Information page for changes to the visa process for 2024.
Latest NeurIPS Blog Entries [ All Entries ]
Important dates.
If you have questions about supporting the conference, please contact us .
View NeurIPS 2024 exhibitors » Become an 2024 Exhibitor Exhibitor Info »
Organizing Committee
General chair, program chair, workshop chair, workshop chair assistant, tutorial chair, competition chair, data and benchmark chair, diversity, inclusion and accessibility chair, affinity chair, ethics review chair, communication chair, social chair, journal chair, creative ai chair, workflow manager, logistics and it, mission statement.
The Neural Information Processing Systems Foundation is a non-profit corporation whose purpose is to foster the exchange of research advances in Artificial Intelligence and Machine Learning, principally by hosting an annual interdisciplinary academic conference with the highest ethical standards for a diverse and inclusive community.
About the Conference
The conference was founded in 1987 and is now a multi-track interdisciplinary annual meeting that includes invited talks, demonstrations, symposia, and oral and poster presentations of refereed papers. Along with the conference is a professional exposition focusing on machine learning in practice, a series of tutorials, and topical workshops that provide a less formal setting for the exchange of ideas.
More about the Neural Information Processing Systems foundation »
Got any suggestions?
We want to hear from you! Send us a message and help improve Slidesgo
Top searches
Trending searches
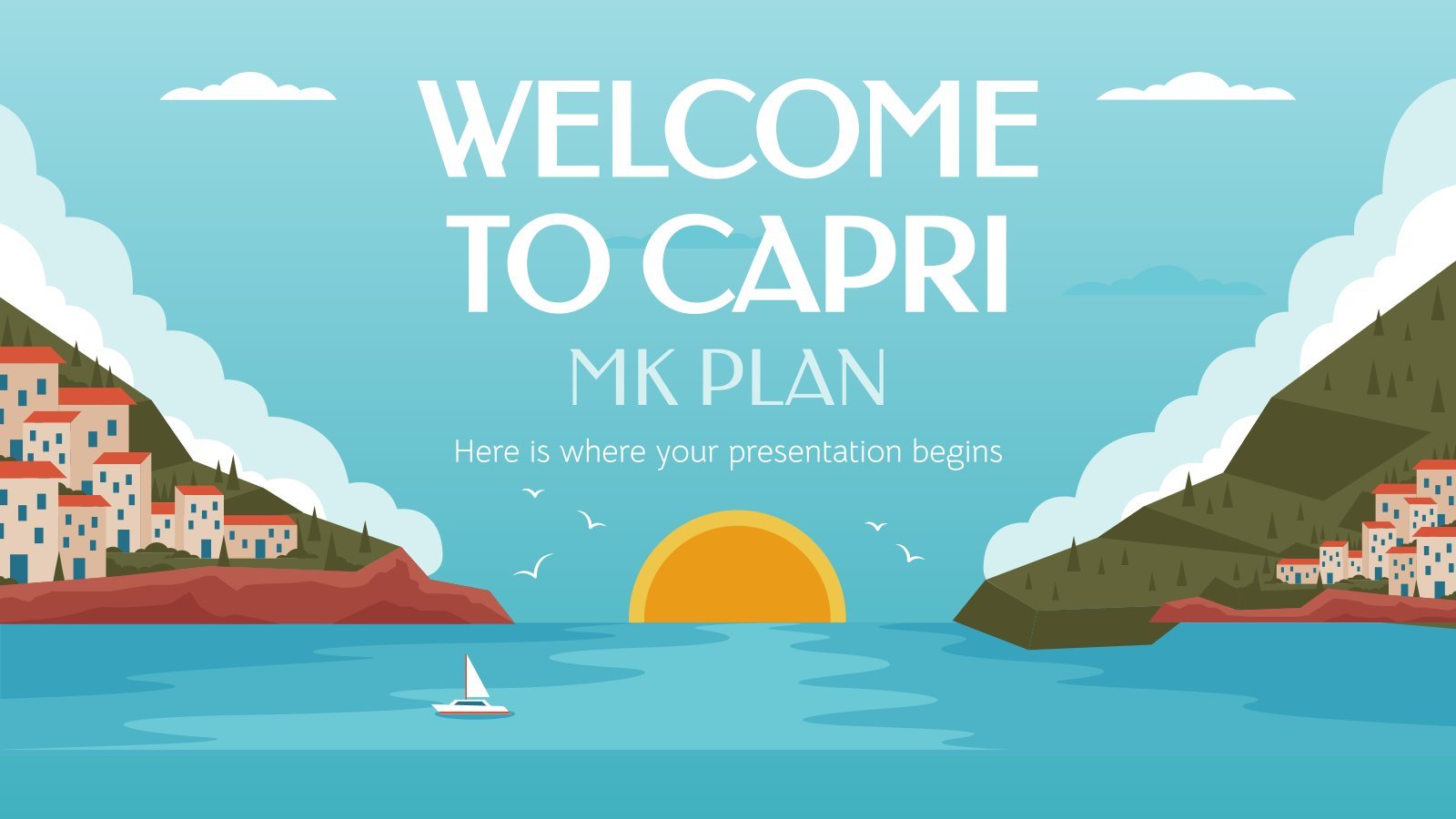
11 templates
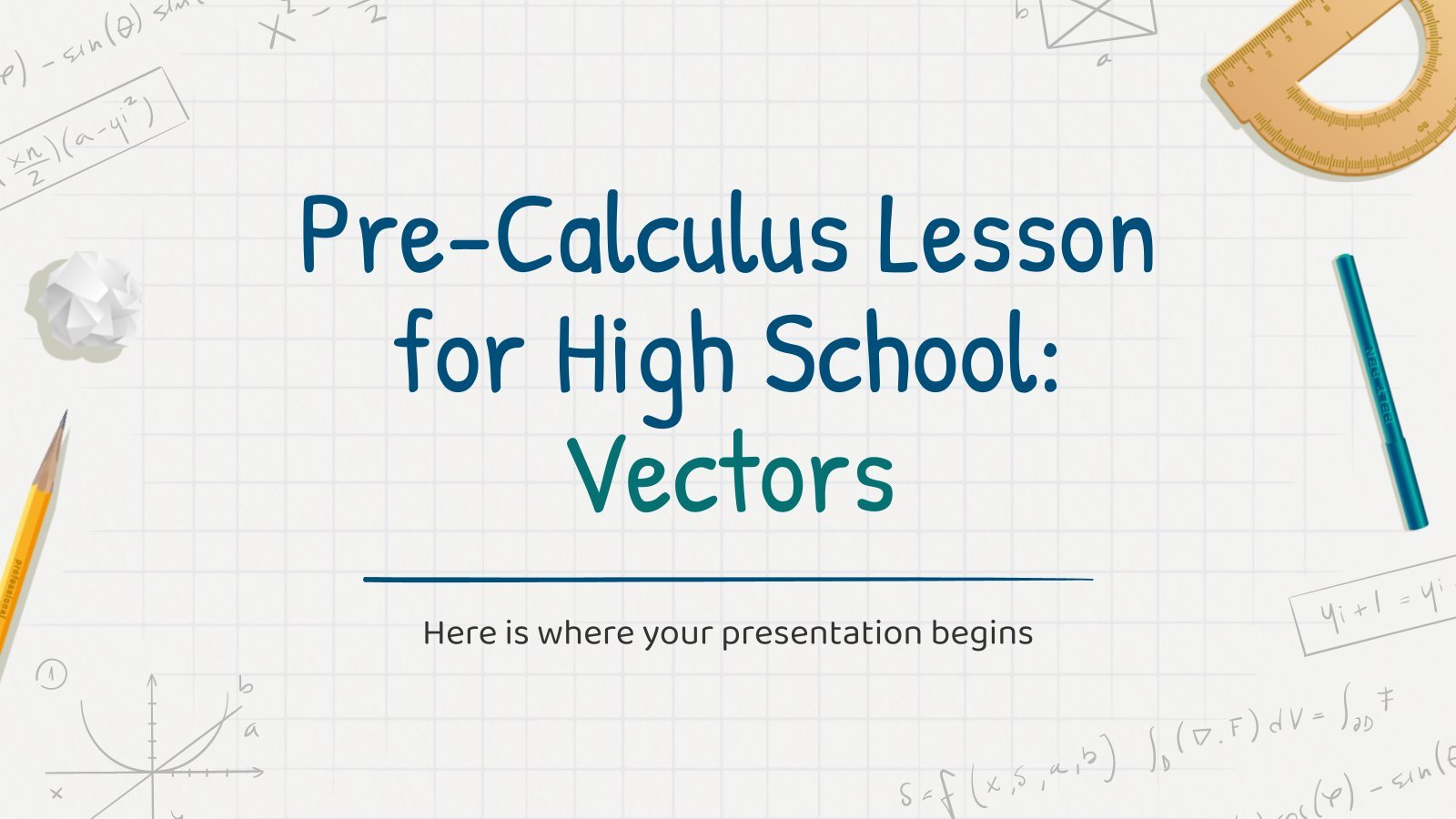
20 templates
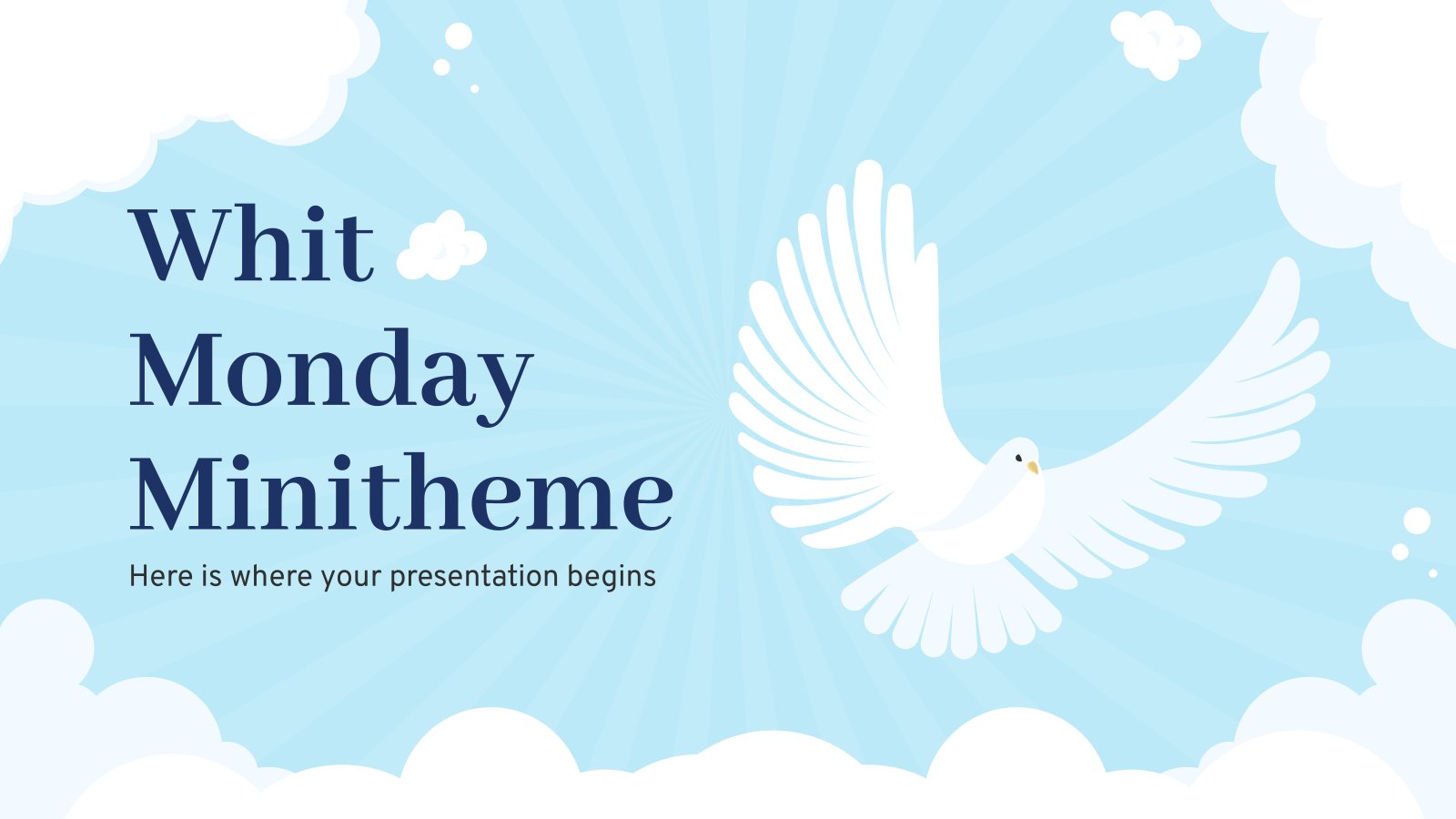
holy spirit
36 templates
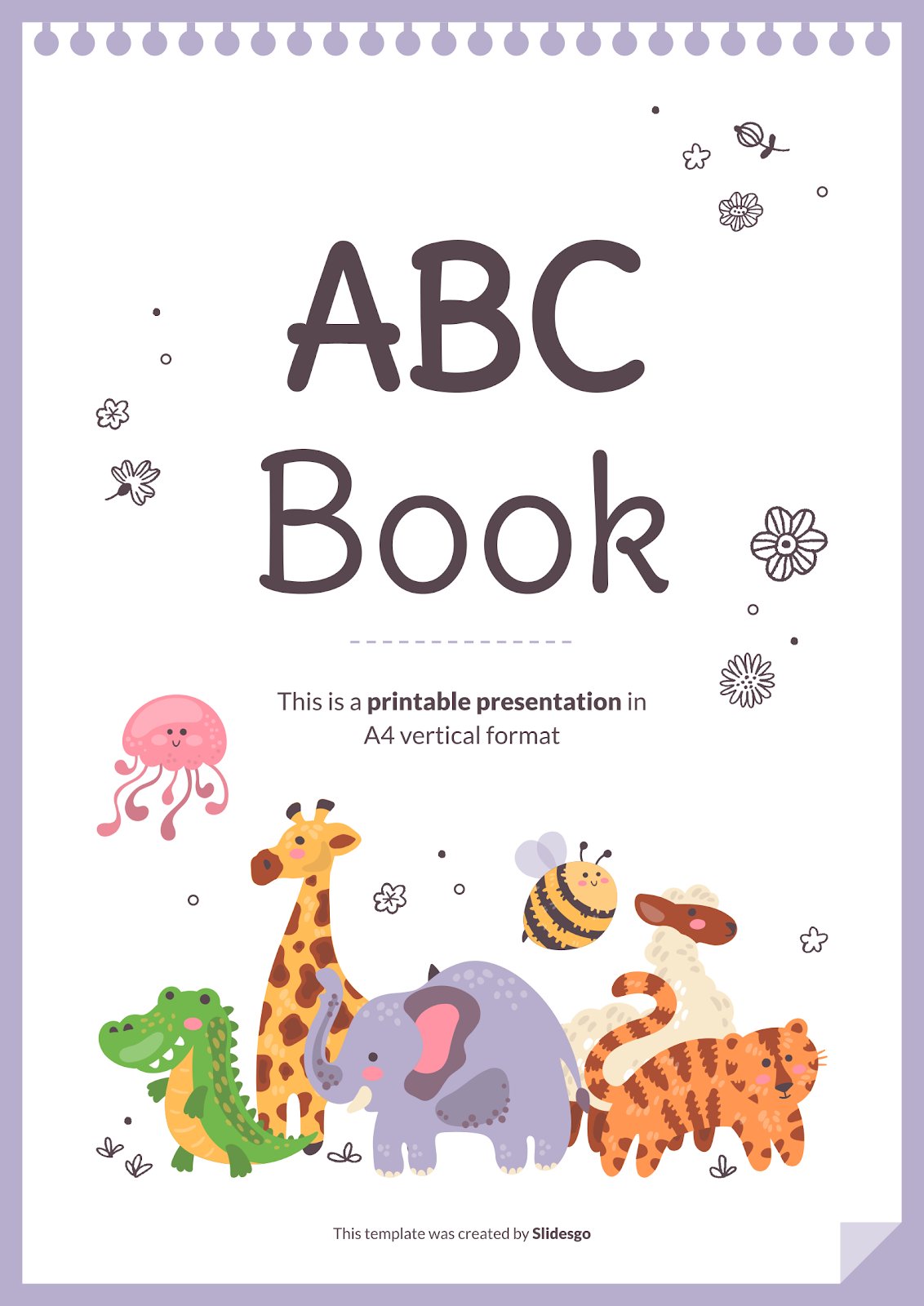
9 templates
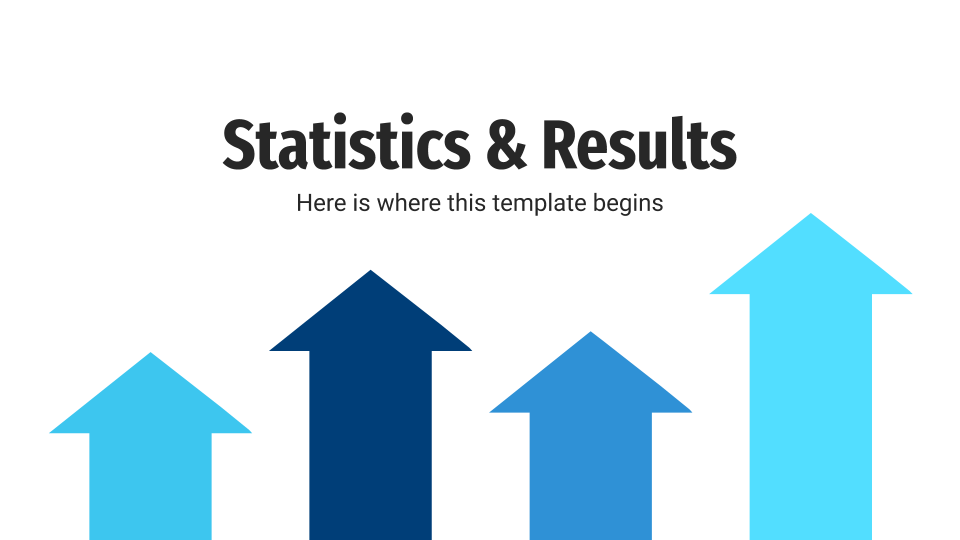
25 templates
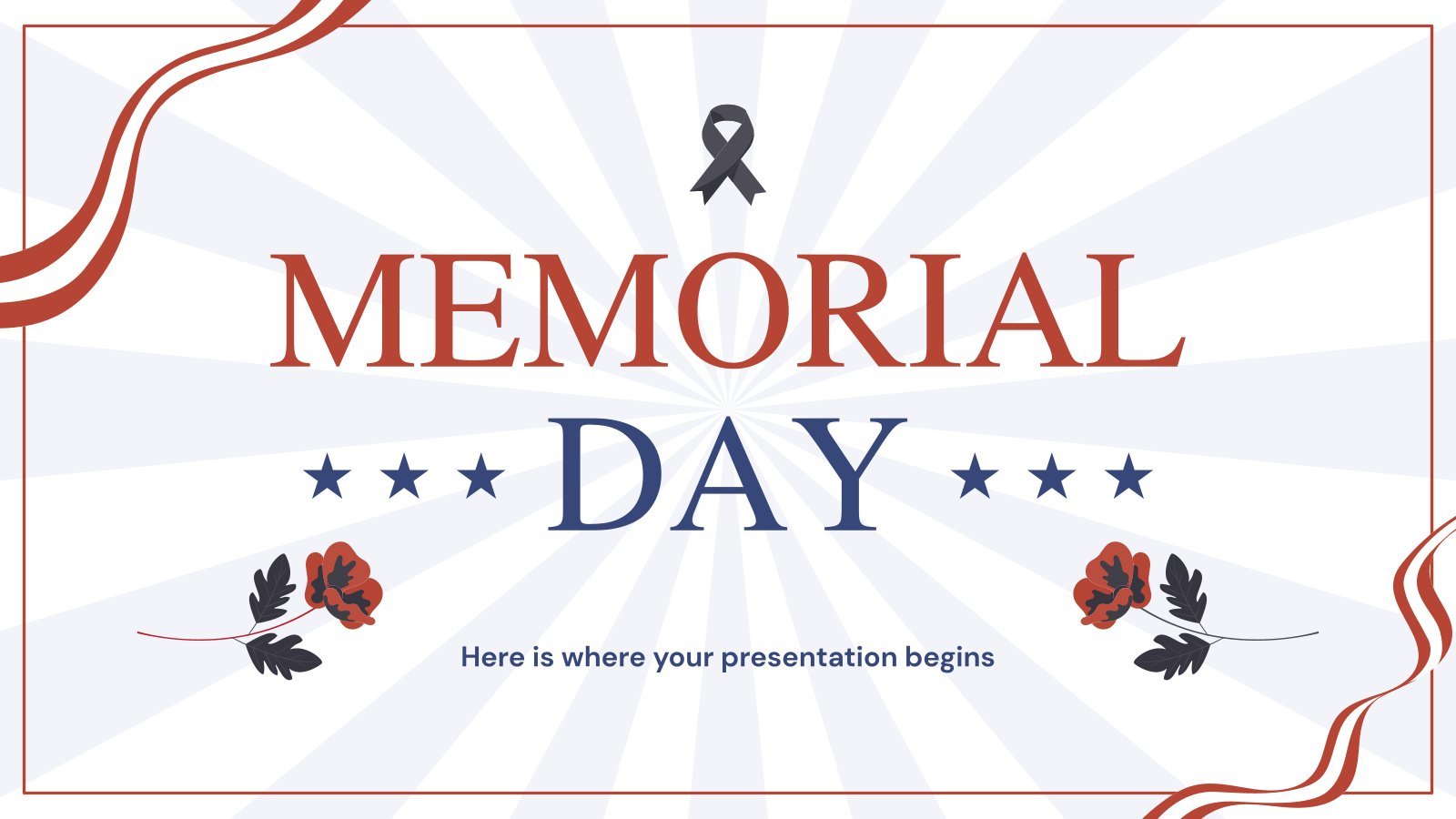
memorial day
12 templates
Machine Learning Infographics
It seems that you like this template, free google slides theme, powerpoint template, and canva presentation template.
Machine learning is the future of science! It allows computers to identify trends, patterns, manage data… and all that while improving themselves on their own! Since it is so profitable, machine learning and data science studies are becoming more and more common on universities and employers are always hiring. Explain how computers went from “Hello world!” to being almost independent with these infographics: they are full of editable resources so that you can explain neural networks, algorithms, the differences between supervised, unsupervised and reinforcement learning… Science won’t be complicated if you use visual elements like these to explain it!
Features of these infographics
- 100% editable and easy to modify
- 32 different infographics to boost your presentations
- Include icons and Flaticon’s extension for further customization
- Designed to be used in Google Slides, Canva, and Microsoft PowerPoint and Keynote
- 16:9 widescreen format suitable for all types of screens
- Include information about how to edit and customize your infographics
How can I use the infographics?
Am I free to use the templates?
How to attribute the infographics?
Attribution required If you are a free user, you must attribute Slidesgo by keeping the slide where the credits appear. How to attribute?
Related posts on our blog.
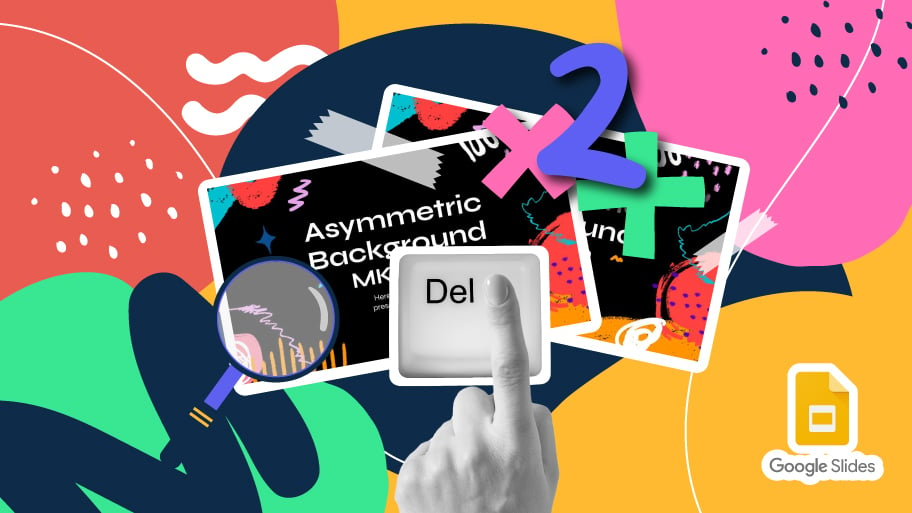
How to Add, Duplicate, Move, Delete or Hide Slides in Google Slides
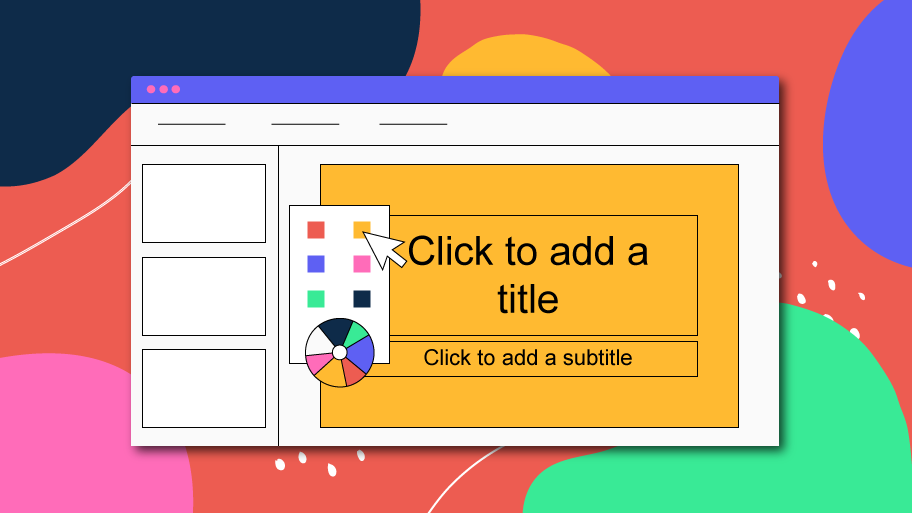
How to Change Layouts in PowerPoint
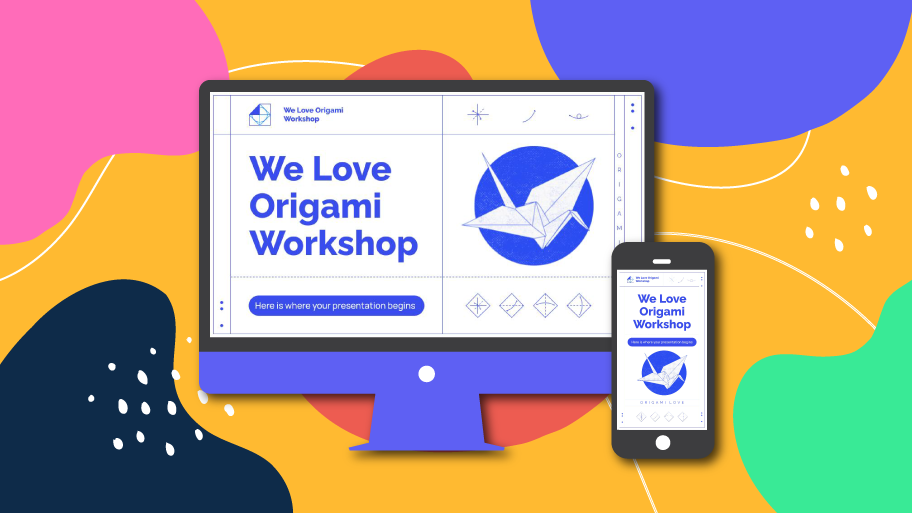
How to Change the Slide Size in Google Slides
Related presentations.
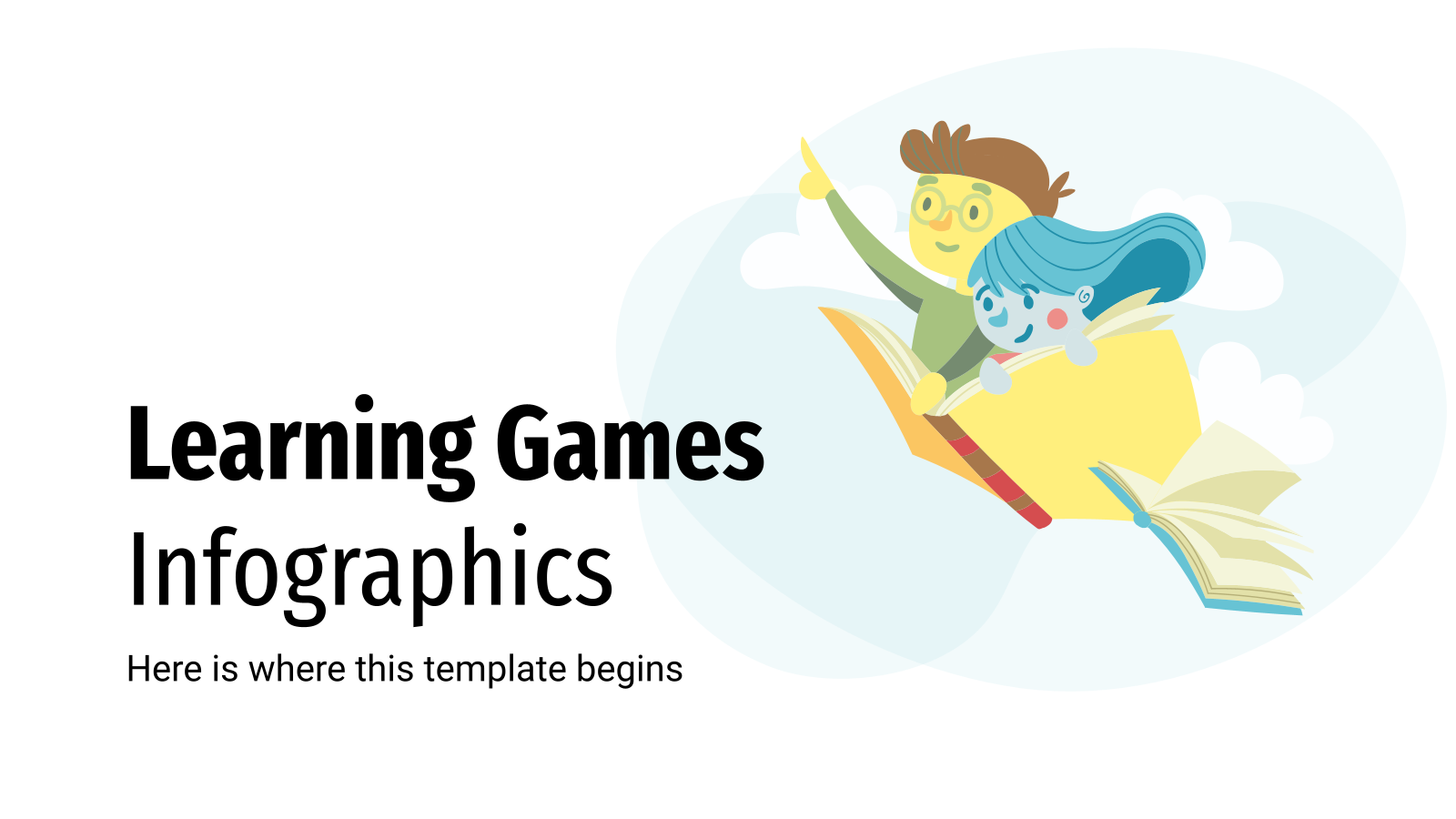
Premium template
Unlock this template and gain unlimited access
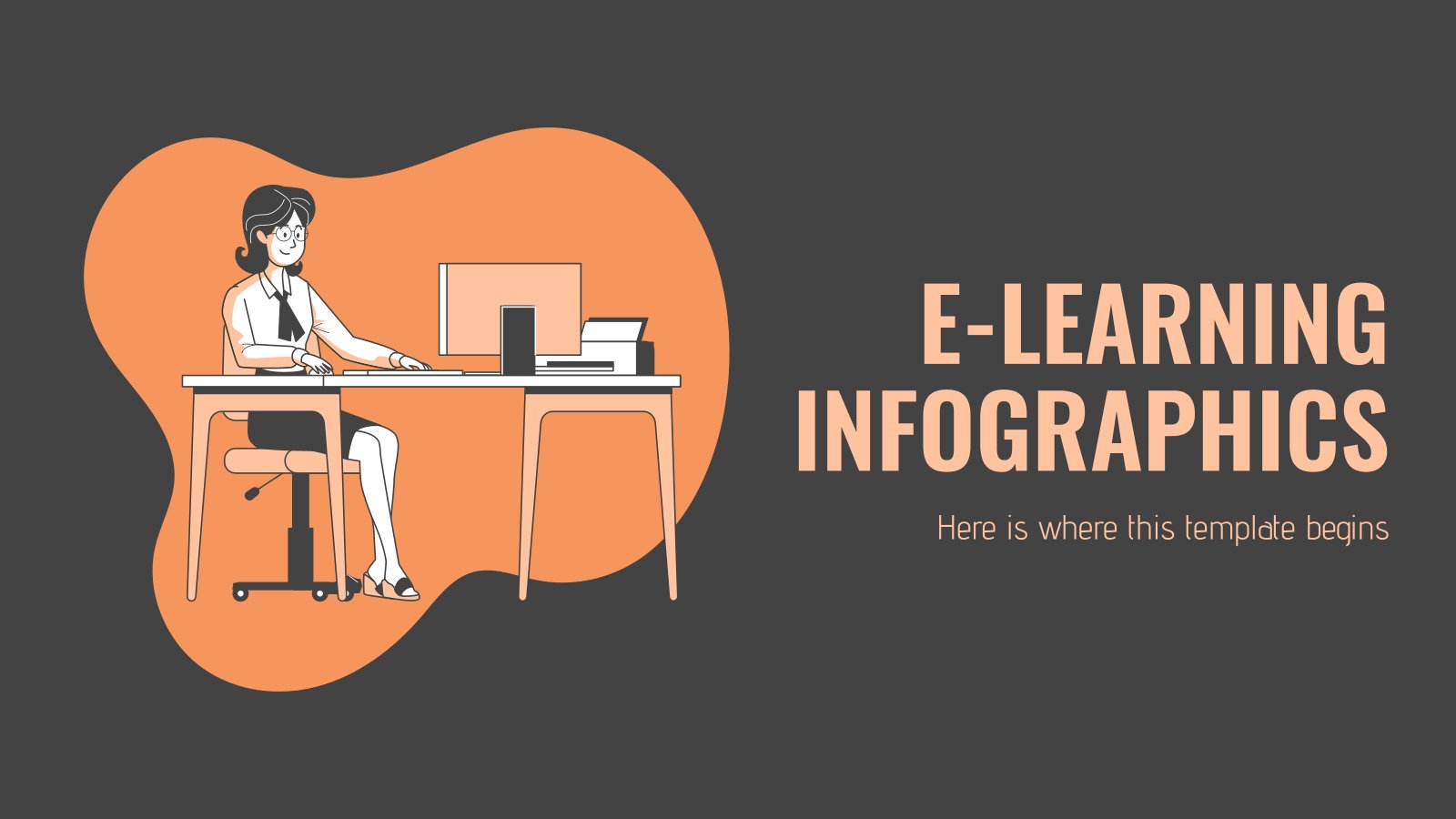
Register for free and start editing online
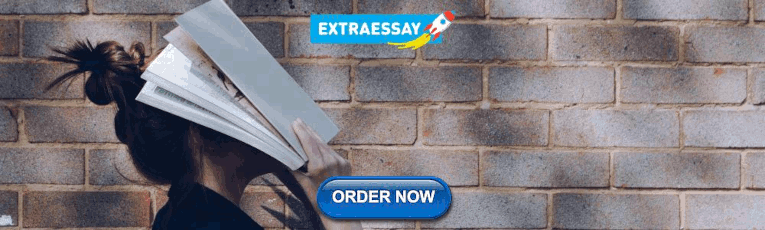
IMAGES
VIDEO
COMMENTS
Template 4: Machine Learning Solution Pitch Deck PPT Template. This PPT Set provides an overview of your business model and historical context, offering insight into your company's journey. Traction and competitive advantages are highlighted to show why your solution has a good chance of success.
of the basics of machine learning, it might be better understood as a collection of tools that can be applied to a speci c subset of problems. 1.2 What Will This Book Teach Me? The purpose of this book is to provide you the reader with the following: a framework with which to approach problems that machine learning learning might help solve ...
5) AI and Machine Learning Demystified. 6) Study: The Future of VR, AR and Self-Driving Car. 7) Artificial Intelligence. 8) Solve for X with AI: a VC view of the Machine Learning & AI landscape. 9) Deep Learning - The Past, Present and Future of Artificial Intelligence. 10) The Future Of Work & The Work Of The Future.
and psychologists study learning in animals and humans. In this book we fo-cus on learning in machines. There are several parallels between animal and machine learning. Certainly, many techniques in machine learning derive from the e orts of psychologists to make more precise their theories of animal and human learning through computational models.
other review papers such as [9]-[11], the presentation aims at highlighting conditions under which the use of machine learning is justified in engineering problems, as ... will be discussed later in the paper. B. Taxonomy of Machine Learning Methods There are three main classes of machine learning techniques, as discussed next. Supervised ...
Presentation Slide Deck (PDF - 3 MB) pdf. 3 MB Presentation Slide Deck (PDF - 3 MB) Download File DOWNLOAD. Course Info Instructor Brandon Leshchinskiy; Departments Electrical Engineering and Computer Science ... Learning Resource Types theaters Video Materials. Download Course.
Find the latest published documents for machine learning, Related hot topics, top authors, the most cited documents, and related journals ... this research aims to predict user's personalities based on Indonesian text from social media using machine learning techniques. This paper evaluates several machine learning techniques, including <a ...
All Slides Chapters 1-10. Chapter 1: ML Basics. This chapter introduces the basic concepts of Machine Learning. We focus on supervised learning, explain the difference between regression and classification, show how to evaluate and compare Machine Learning models and formalize the concept of learning. Chapter 2: Supervised Regression.
Over the past decade, artificial intelligence (AI) has become a popular subject both within and outside of the scientific community; an abundance of articles in technology and non-technology-based journals have covered the topics of machine learning (ML), deep learning (DL), and AI. 1 - 6 Yet there still remains confusion around AI, ML, and DL. The terms are highly associated, but are not ...
Machine learning describes the capacity of systems to learn from problem-specific training data to automate the process of analytical model building and solve associated tasks. Deep learning is a machine learning concept based on artificial neural networks. For many applications, deep learning models outperform shallow machine learning models ...
Scientific papers can be quite intimidating and hard to read, especially if you are new to a field with lots of theory like Machine Learning or Deep Learning...
Part 1: Show it, don't say it. There is a reason why Salesforce spent over $15 billion dollars to acquire a Data Visualization/Reporting tool called Tableau. The value of a graph rising up over time is much more than a thousand words could convey. Let us look at few ways to present your results.
By: Researchers from the Google AI Brain team, including Hieu Pham, Qizhe Xie, Zihang Dai, Minh-Thang Luong and Quoc V. Le, introduced this semi-supervised learning technique. About: The model presented in the paper has achieved a new state-of-the-art top-1 accuracy of about 90.2% on ImageNet. The result is 1.6 per cent better than the existing ...
Deep learning [5] is a subfield of machine learning. As an endto-end method, compared with traditional signal processing or machine learning [6] algorithms, it will make the feature extraction ...
Paper presentations Projects. Abstract. The goal of the field of machine learning is to build computer systems that learn from experience and that are capable to adapt to their environments. Learning techniques and methods developed by researchers in this field have been successfully applied to a variety of learning tasks in a broad range of ...
This paper describes the design and implementation of automated techniques for grading students' PowerPoint slides. Preparing PowerPoint slides for seminars, workshops, and conferences is one of the crucial activity of graduate and undergraduate students. ... Multiple machine learning techniques are used to grade presentations. Decision Tree ...
3. The name machine learning was coined in 1959 by Arthur Samuel Tom M. Mitchell provided a widely quoted, more formal definition of the algorithms studied in the machine learning field: A computer program is said to learn from experience E with respect to some class of tasks T and performance measure P if its performance at tasks in T, as measured by P, improves with experience E. Alan Turing ...
Machine Learning (ML) represents a pivotal technology for current and future information systems, and many domains. already leverage the capabilities of ML. However, deployment of ML in ...
Download your presentation as a PowerPoint template or use it online as a Google Slides theme. 100% free, no registration or download limits. Get these machine learning templates to create cutting-edge presentations that showcase the power of AI. No Download Limits Free for Any Use No Signups.
machine learning with graphs. Presentations will take place at the end of the semester and can be done in groups of up to three students. The group is free to select a paper of their interest or one from the list provided below. Paper Proposal (02/25) This is the deadline for o cially selecting a paper. In case two groups select the same paper ...
Machine learning research is at the heart of the AI revolution. It underpins the development of intelligent systems capable of making predictions, automating tasks, and improving decision-making across industries. The importance of this research can be summarized as follows: Advancements in Technology.
Download in PDF, PPTX, MP4 and HTML5 format. Share online with a link or embed on your website. Communicate your machine learning research results with this contrasting presentation template, or check out Visme's collection of 500+ professional templates for more design ideas. Edit this template with our Presentation Software.
Main Conference Paper Submission Deadline May 22 '24 01:00 PM PDT * Main Conference Full Paper Submission Deadline ... and oral and poster presentations of refereed papers. Along with the conference is a professional exposition focusing on machine learning in practice, a series of tutorials, and topical workshops that provide a less formal ...
Free Google Slides theme, PowerPoint template, and Canva presentation template. Machine learning is the future of science! It allows computers to identify trends, patterns, manage data… and all that while improving themselves on their own! Since it is so profitable, machine learning and data science studies are becoming more and more common ...
Overall, the paper shows promise as AutoCodeRover is capable of significantly reducing the manual effort required in program maintenance and improvement tasks. Conclusion . There are many machine learning papers to read in 2024, and here are my recommendation papers to read: HyperFast: Instant Classification for Tabular Data
Hello, I'm Dref360 co-maintainer of Baal, a Bayesian Active Learning library. While in-context learning is getting very strong on few-shot learning benchmarks, specialized models are still better on most complex tasks. In consequence, research in active learning is incredibly important for companies.
Please join me in congratulating Jiacheng Miao who recently won the ICSA Student Paper Award and will present the paper Valid inference for machine learning-assisted GWAS at the ICSA applied statistics symposium in Nashville this year! Jiancheng is also one of the four students who were selected by our campus as UW nominees for the Schmidt Science Fellows program!