Home â Essay Samples â Information Science and Technology â Data Mining â Data Collection Methods

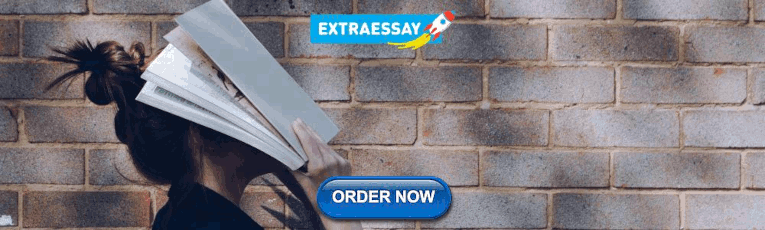
Data Collection Methods
- Categories: Data Mining Information Age
About this sample

Words: 838 |
Published: Aug 16, 2019
Words: 838 | Pages: 2 | 5 min read
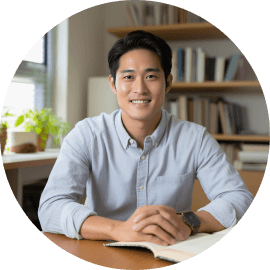
Cite this Essay
Let us write you an essay from scratch
- 450+ experts on 30 subjects ready to help
- Custom essay delivered in as few as 3 hours
Get high-quality help
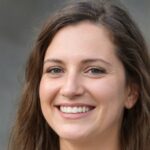
Verified writer
- Expert in: Information Science and Technology
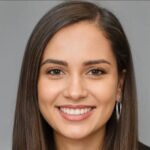
+ 120 experts online
By clicking âCheck Writersâ Offersâ, you agree to our terms of service and privacy policy . Weâll occasionally send you promo and account related email
No need to pay just yet!
Related Essays
6 pages / 2847 words
2 pages / 985 words
3 pages / 1474 words
2 pages / 1094 words
Remember! This is just a sample.
You can get your custom paper by one of our expert writers.
121 writers online
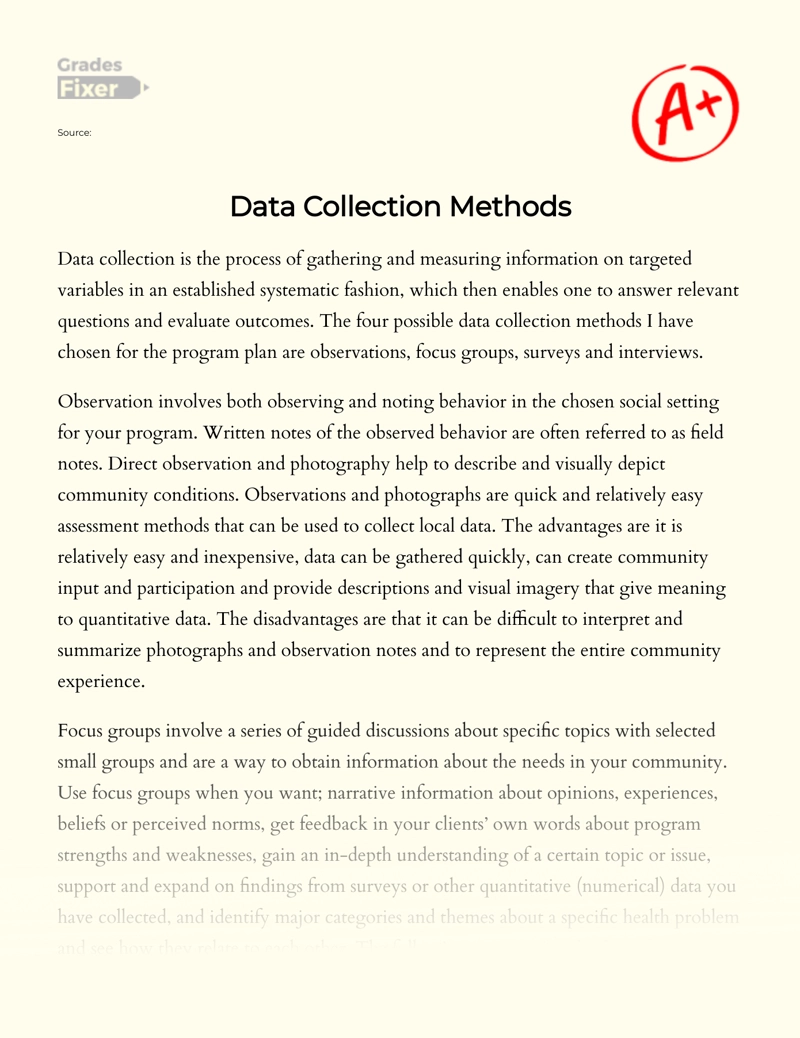
Still canât find what you need?
Browse our vast selection of original essay samples, each expertly formatted and styled
Related Essays on Data Mining
Databases play a critical role in managing and organizing large amounts of information, making them an essential tool in various fields. This essay aims to highlight the importance of databases in managing and organizing large [...]
Data analysis is known as 'analysis of data 'or 'data analytics', is a process of inspecting, cleansing, transforming, and modeling data with the goal of discovering useful information, suggesting conclusions and supporting [...]
Ahmad Aldhafiri CEGR 4802/1/2018 GISA geographic information system (GIS) is a system designed to capture, store, manipulate, analyze, manage, and present all types of geographic data. The key word to this technology is [...]
Podcasting is the process of capturing and posting an audio event in digital sound object online. The word podcast was primarily formed as an amalgam of âiPodâ and âbroadcastâ, so that can give one a general idea of the nature. [...]
In todayâs world it is important for any organization to have business continuity and any loss in data for the company can cost a lost form losing customers to having to fight lawsuits and pay huge fines. All these have to [...]
The paper will first define and describe what is data mining. It will also seek to determine why data mining is useful and show that data mining is concerned with the analysis of data and the use of techniques for finding [...]
Related Topics
By clicking âSendâ, you agree to our Terms of service and Privacy statement . We will occasionally send you account related emails.
Where do you want us to send this sample?
By clicking âContinueâ, you agree to our terms of service and privacy policy.
Be careful. This essay is not unique
This essay was donated by a student and is likely to have been used and submitted before
Download this Sample
Free samples may contain mistakes and not unique parts
Sorry, we could not paraphrase this essay. Our professional writers can rewrite it and get you a unique paper.
Please check your inbox.
We can write you a custom essay that will follow your exact instructions and meet the deadlines. Let's fix your grades together!
Get Your Personalized Essay in 3 Hours or Less!
We use cookies to personalyze your web-site experience. By continuing weâll assume you board with our cookie policy .
- Instructions Followed To The Letter
- Deadlines Met At Every Stage
- Unique And Plagiarism Free
- Privacy Policy

Home » Data Collection – Methods Types and Examples
Data Collection – Methods Types and Examples
Table of Contents

Data Collection
Definition:
Data collection is the process of gathering and collecting information from various sources to analyze and make informed decisions based on the data collected. This can involve various methods, such as surveys, interviews, experiments, and observation.
In order for data collection to be effective, it is important to have a clear understanding of what data is needed and what the purpose of the data collection is. This can involve identifying the population or sample being studied, determining the variables to be measured, and selecting appropriate methods for collecting and recording data.
Types of Data Collection
Types of Data Collection are as follows:
Primary Data Collection
Primary data collection is the process of gathering original and firsthand information directly from the source or target population. This type of data collection involves collecting data that has not been previously gathered, recorded, or published. Primary data can be collected through various methods such as surveys, interviews, observations, experiments, and focus groups. The data collected is usually specific to the research question or objective and can provide valuable insights that cannot be obtained from secondary data sources. Primary data collection is often used in market research, social research, and scientific research.
Secondary Data Collection
Secondary data collection is the process of gathering information from existing sources that have already been collected and analyzed by someone else, rather than conducting new research to collect primary data. Secondary data can be collected from various sources, such as published reports, books, journals, newspapers, websites, government publications, and other documents.
Qualitative Data Collection
Qualitative data collection is used to gather non-numerical data such as opinions, experiences, perceptions, and feelings, through techniques such as interviews, focus groups, observations, and document analysis. It seeks to understand the deeper meaning and context of a phenomenon or situation and is often used in social sciences, psychology, and humanities. Qualitative data collection methods allow for a more in-depth and holistic exploration of research questions and can provide rich and nuanced insights into human behavior and experiences.
Quantitative Data Collection
Quantitative data collection is a used to gather numerical data that can be analyzed using statistical methods. This data is typically collected through surveys, experiments, and other structured data collection methods. Quantitative data collection seeks to quantify and measure variables, such as behaviors, attitudes, and opinions, in a systematic and objective way. This data is often used to test hypotheses, identify patterns, and establish correlations between variables. Quantitative data collection methods allow for precise measurement and generalization of findings to a larger population. It is commonly used in fields such as economics, psychology, and natural sciences.
Data Collection Methods
Data Collection Methods are as follows:
Surveys involve asking questions to a sample of individuals or organizations to collect data. Surveys can be conducted in person, over the phone, or online.
Interviews involve a one-on-one conversation between the interviewer and the respondent. Interviews can be structured or unstructured and can be conducted in person or over the phone.
Focus Groups
Focus groups are group discussions that are moderated by a facilitator. Focus groups are used to collect qualitative data on a specific topic.
Observation
Observation involves watching and recording the behavior of people, objects, or events in their natural setting. Observation can be done overtly or covertly, depending on the research question.
Experiments
Experiments involve manipulating one or more variables and observing the effect on another variable. Experiments are commonly used in scientific research.
Case Studies
Case studies involve in-depth analysis of a single individual, organization, or event. Case studies are used to gain detailed information about a specific phenomenon.
Secondary Data Analysis
Secondary data analysis involves using existing data that was collected for another purpose. Secondary data can come from various sources, such as government agencies, academic institutions, or private companies.
How to Collect Data
The following are some steps to consider when collecting data:
- Define the objective : Before you start collecting data, you need to define the objective of the study. This will help you determine what data you need to collect and how to collect it.
- Identify the data sources : Identify the sources of data that will help you achieve your objective. These sources can be primary sources, such as surveys, interviews, and observations, or secondary sources, such as books, articles, and databases.
- Determine the data collection method : Once you have identified the data sources, you need to determine the data collection method. This could be through online surveys, phone interviews, or face-to-face meetings.
- Develop a data collection plan : Develop a plan that outlines the steps you will take to collect the data. This plan should include the timeline, the tools and equipment needed, and the personnel involved.
- Test the data collection process: Before you start collecting data, test the data collection process to ensure that it is effective and efficient.
- Collect the data: Collect the data according to the plan you developed in step 4. Make sure you record the data accurately and consistently.
- Analyze the data: Once you have collected the data, analyze it to draw conclusions and make recommendations.
- Report the findings: Report the findings of your data analysis to the relevant stakeholders. This could be in the form of a report, a presentation, or a publication.
- Monitor and evaluate the data collection process: After the data collection process is complete, monitor and evaluate the process to identify areas for improvement in future data collection efforts.
- Ensure data quality: Ensure that the collected data is of high quality and free from errors. This can be achieved by validating the data for accuracy, completeness, and consistency.
- Maintain data security: Ensure that the collected data is secure and protected from unauthorized access or disclosure. This can be achieved by implementing data security protocols and using secure storage and transmission methods.
- Follow ethical considerations: Follow ethical considerations when collecting data, such as obtaining informed consent from participants, protecting their privacy and confidentiality, and ensuring that the research does not cause harm to participants.
- Use appropriate data analysis methods : Use appropriate data analysis methods based on the type of data collected and the research objectives. This could include statistical analysis, qualitative analysis, or a combination of both.
- Record and store data properly: Record and store the collected data properly, in a structured and organized format. This will make it easier to retrieve and use the data in future research or analysis.
- Collaborate with other stakeholders : Collaborate with other stakeholders, such as colleagues, experts, or community members, to ensure that the data collected is relevant and useful for the intended purpose.
Applications of Data Collection
Data collection methods are widely used in different fields, including social sciences, healthcare, business, education, and more. Here are some examples of how data collection methods are used in different fields:
- Social sciences : Social scientists often use surveys, questionnaires, and interviews to collect data from individuals or groups. They may also use observation to collect data on social behaviors and interactions. This data is often used to study topics such as human behavior, attitudes, and beliefs.
- Healthcare : Data collection methods are used in healthcare to monitor patient health and track treatment outcomes. Electronic health records and medical charts are commonly used to collect data on patients’ medical history, diagnoses, and treatments. Researchers may also use clinical trials and surveys to collect data on the effectiveness of different treatments.
- Business : Businesses use data collection methods to gather information on consumer behavior, market trends, and competitor activity. They may collect data through customer surveys, sales reports, and market research studies. This data is used to inform business decisions, develop marketing strategies, and improve products and services.
- Education : In education, data collection methods are used to assess student performance and measure the effectiveness of teaching methods. Standardized tests, quizzes, and exams are commonly used to collect data on student learning outcomes. Teachers may also use classroom observation and student feedback to gather data on teaching effectiveness.
- Agriculture : Farmers use data collection methods to monitor crop growth and health. Sensors and remote sensing technology can be used to collect data on soil moisture, temperature, and nutrient levels. This data is used to optimize crop yields and minimize waste.
- Environmental sciences : Environmental scientists use data collection methods to monitor air and water quality, track climate patterns, and measure the impact of human activity on the environment. They may use sensors, satellite imagery, and laboratory analysis to collect data on environmental factors.
- Transportation : Transportation companies use data collection methods to track vehicle performance, optimize routes, and improve safety. GPS systems, on-board sensors, and other tracking technologies are used to collect data on vehicle speed, fuel consumption, and driver behavior.
Examples of Data Collection
Examples of Data Collection are as follows:
- Traffic Monitoring: Cities collect real-time data on traffic patterns and congestion through sensors on roads and cameras at intersections. This information can be used to optimize traffic flow and improve safety.
- Social Media Monitoring : Companies can collect real-time data on social media platforms such as Twitter and Facebook to monitor their brand reputation, track customer sentiment, and respond to customer inquiries and complaints in real-time.
- Weather Monitoring: Weather agencies collect real-time data on temperature, humidity, air pressure, and precipitation through weather stations and satellites. This information is used to provide accurate weather forecasts and warnings.
- Stock Market Monitoring : Financial institutions collect real-time data on stock prices, trading volumes, and other market indicators to make informed investment decisions and respond to market fluctuations in real-time.
- Health Monitoring : Medical devices such as wearable fitness trackers and smartwatches can collect real-time data on a person’s heart rate, blood pressure, and other vital signs. This information can be used to monitor health conditions and detect early warning signs of health issues.
Purpose of Data Collection
The purpose of data collection can vary depending on the context and goals of the study, but generally, it serves to:
- Provide information: Data collection provides information about a particular phenomenon or behavior that can be used to better understand it.
- Measure progress : Data collection can be used to measure the effectiveness of interventions or programs designed to address a particular issue or problem.
- Support decision-making : Data collection provides decision-makers with evidence-based information that can be used to inform policies, strategies, and actions.
- Identify trends : Data collection can help identify trends and patterns over time that may indicate changes in behaviors or outcomes.
- Monitor and evaluate : Data collection can be used to monitor and evaluate the implementation and impact of policies, programs, and initiatives.
When to use Data Collection
Data collection is used when there is a need to gather information or data on a specific topic or phenomenon. It is typically used in research, evaluation, and monitoring and is important for making informed decisions and improving outcomes.
Data collection is particularly useful in the following scenarios:
- Research : When conducting research, data collection is used to gather information on variables of interest to answer research questions and test hypotheses.
- Evaluation : Data collection is used in program evaluation to assess the effectiveness of programs or interventions, and to identify areas for improvement.
- Monitoring : Data collection is used in monitoring to track progress towards achieving goals or targets, and to identify any areas that require attention.
- Decision-making: Data collection is used to provide decision-makers with information that can be used to inform policies, strategies, and actions.
- Quality improvement : Data collection is used in quality improvement efforts to identify areas where improvements can be made and to measure progress towards achieving goals.
Characteristics of Data Collection
Data collection can be characterized by several important characteristics that help to ensure the quality and accuracy of the data gathered. These characteristics include:
- Validity : Validity refers to the accuracy and relevance of the data collected in relation to the research question or objective.
- Reliability : Reliability refers to the consistency and stability of the data collection process, ensuring that the results obtained are consistent over time and across different contexts.
- Objectivity : Objectivity refers to the impartiality of the data collection process, ensuring that the data collected is not influenced by the biases or personal opinions of the data collector.
- Precision : Precision refers to the degree of accuracy and detail in the data collected, ensuring that the data is specific and accurate enough to answer the research question or objective.
- Timeliness : Timeliness refers to the efficiency and speed with which the data is collected, ensuring that the data is collected in a timely manner to meet the needs of the research or evaluation.
- Ethical considerations : Ethical considerations refer to the ethical principles that must be followed when collecting data, such as ensuring confidentiality and obtaining informed consent from participants.
Advantages of Data Collection
There are several advantages of data collection that make it an important process in research, evaluation, and monitoring. These advantages include:
- Better decision-making : Data collection provides decision-makers with evidence-based information that can be used to inform policies, strategies, and actions, leading to better decision-making.
- Improved understanding: Data collection helps to improve our understanding of a particular phenomenon or behavior by providing empirical evidence that can be analyzed and interpreted.
- Evaluation of interventions: Data collection is essential in evaluating the effectiveness of interventions or programs designed to address a particular issue or problem.
- Identifying trends and patterns: Data collection can help identify trends and patterns over time that may indicate changes in behaviors or outcomes.
- Increased accountability: Data collection increases accountability by providing evidence that can be used to monitor and evaluate the implementation and impact of policies, programs, and initiatives.
- Validation of theories: Data collection can be used to test hypotheses and validate theories, leading to a better understanding of the phenomenon being studied.
- Improved quality: Data collection is used in quality improvement efforts to identify areas where improvements can be made and to measure progress towards achieving goals.
Limitations of Data Collection
While data collection has several advantages, it also has some limitations that must be considered. These limitations include:
- Bias : Data collection can be influenced by the biases and personal opinions of the data collector, which can lead to inaccurate or misleading results.
- Sampling bias : Data collection may not be representative of the entire population, resulting in sampling bias and inaccurate results.
- Cost : Data collection can be expensive and time-consuming, particularly for large-scale studies.
- Limited scope: Data collection is limited to the variables being measured, which may not capture the entire picture or context of the phenomenon being studied.
- Ethical considerations : Data collection must follow ethical principles to protect the rights and confidentiality of the participants, which can limit the type of data that can be collected.
- Data quality issues: Data collection may result in data quality issues such as missing or incomplete data, measurement errors, and inconsistencies.
- Limited generalizability : Data collection may not be generalizable to other contexts or populations, limiting the generalizability of the findings.
About the author
Muhammad Hassan
Researcher, Academic Writer, Web developer
You may also like

References in Research – Types, Examples and...

Research Recommendations – Examples and Writing...

Research Contribution – Thesis Guide

Research Project – Definition, Writing Guide and...

Significance of the Study – Examples and Writing...

Institutional Review Board – Application Sample...
Table of Contents
What is data collection, why do we need data collection, what are the different data collection methods, data collection tools, the importance of ensuring accurate and appropriate data collection, issues related to maintaining the integrity of data collection, what are common challenges in data collection, what are the key steps in the data collection process, data collection considerations and best practices, choose the right data science program, are you interested in a career in data science, what is data collection: methods, types, tools.
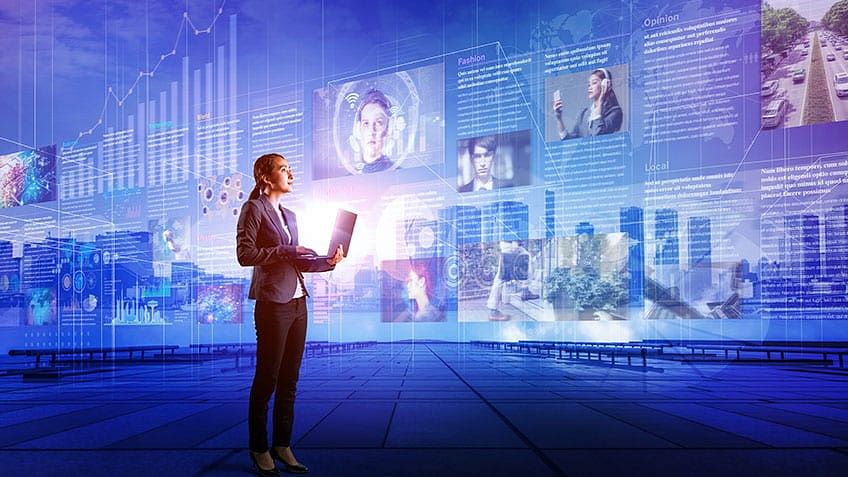
The process of gathering and analyzing accurate data from various sources to find answers to research problems, trends and probabilities, etc., to evaluate possible outcomes is Known as Data Collection. Knowledge is power, information is knowledge, and data is information in digitized form, at least as defined in IT. Hence, data is power. But before you can leverage that data into a successful strategy for your organization or business, you need to gather it. Thatâs your first step.
So, to help you get the process started, we shine a spotlight on data collection. What exactly is it? Believe it or not, itâs more than just doing a Google search! Furthermore, what are the different types of data collection? And what kinds of data collection tools and data collection techniques exist?
If you want to get up to speed about what is data collection process, youâve come to the right place.Â
Transform raw data into captivating visuals with Simplilearn's hands-on Data Visualization Courses and captivate your audience. Also, master the art of data management with Simplilearn's comprehensive data management courses  - unlock new career opportunities today!
Data collection is the process of collecting and evaluating information or data from multiple sources to find answers to research problems, answer questions, evaluate outcomes, and forecast trends and probabilities. It is an essential phase in all types of research, analysis, and decision-making, including that done in the social sciences, business, and healthcare.
Accurate data collection is necessary to make informed business decisions, ensure quality assurance, and keep research integrity.
During data collection, the researchers must identify the data types, the sources of data, and what methods are being used. We will soon see that there are many different data collection methods . There is heavy reliance on data collection in research, commercial, and government fields.
Before an analyst begins collecting data, they must answer three questions first:
- Whatâs the goal or purpose of this research?
- What kinds of data are they planning on gathering?
- What methods and procedures will be used to collect, store, and process the information?
Additionally, we can break up data into qualitative and quantitative types. Qualitative data covers descriptions such as color, size, quality, and appearance. Quantitative data, unsurprisingly, deals with numbers, such as statistics, poll numbers, percentages, etc.
Before a judge makes a ruling in a court case or a general creates a plan of attack, they must have as many relevant facts as possible. The best courses of action come from informed decisions, and information and data are synonymous.
The concept of data collection isnât a new one, as weâll see later, but the world has changed. There is far more data available today, and it exists in forms that were unheard of a century ago. The data collection process has had to change and grow with the times, keeping pace with technology.
Whether youâre in the world of academia, trying to conduct research, or part of the commercial sector, thinking of how to promote a new product, you need data collection to help you make better choices.
Now that you know what is data collection and why we need it, let's take a look at the different methods of data collection. While the phrase âdata collectionâ may sound all high-tech and digital, it doesnât necessarily entail things like computers, big data , and the internet. Data collection could mean a telephone survey, a mail-in comment card, or even some guy with a clipboard asking passersby some questions. But letâs see if we can sort the different data collection methods into a semblance of organized categories.
Primary and secondary methods of data collection are two approaches used to gather information for research or analysis purposes. Let's explore each data collection method in detail:
1. Primary Data Collection:
Primary data collection involves the collection of original data directly from the source or through direct interaction with the respondents. This method allows researchers to obtain firsthand information specifically tailored to their research objectives. There are various techniques for primary data collection, including:
a. Surveys and Questionnaires: Researchers design structured questionnaires or surveys to collect data from individuals or groups. These can be conducted through face-to-face interviews, telephone calls, mail, or online platforms.
b. Interviews: Interviews involve direct interaction between the researcher and the respondent. They can be conducted in person, over the phone, or through video conferencing. Interviews can be structured (with predefined questions), semi-structured (allowing flexibility), or unstructured (more conversational).
c. Observations: Researchers observe and record behaviors, actions, or events in their natural setting. This method is useful for gathering data on human behavior, interactions, or phenomena without direct intervention.
d. Experiments: Experimental studies involve the manipulation of variables to observe their impact on the outcome. Researchers control the conditions and collect data to draw conclusions about cause-and-effect relationships.
e. Focus Groups: Focus groups bring together a small group of individuals who discuss specific topics in a moderated setting. This method helps in understanding opinions, perceptions, and experiences shared by the participants.
2. Secondary Data Collection:
Secondary data collection involves using existing data collected by someone else for a purpose different from the original intent. Researchers analyze and interpret this data to extract relevant information. Secondary data can be obtained from various sources, including:
a. Published Sources: Researchers refer to books, academic journals, magazines, newspapers, government reports, and other published materials that contain relevant data.
b. Online Databases: Numerous online databases provide access to a wide range of secondary data, such as research articles, statistical information, economic data, and social surveys.
c. Government and Institutional Records: Government agencies, research institutions, and organizations often maintain databases or records that can be used for research purposes.
d. Publicly Available Data: Data shared by individuals, organizations, or communities on public platforms, websites, or social media can be accessed and utilized for research.
e. Past Research Studies: Previous research studies and their findings can serve as valuable secondary data sources. Researchers can review and analyze the data to gain insights or build upon existing knowledge.
Now that weâve explained the various techniques, letâs narrow our focus even further by looking at some specific tools. For example, we mentioned interviews as a technique, but we can further break that down into different interview types (or âtoolsâ).
Word Association
The researcher gives the respondent a set of words and asks them what comes to mind when they hear each word.
Sentence Completion
Researchers use sentence completion to understand what kind of ideas the respondent has. This tool involves giving an incomplete sentence and seeing how the interviewee finishes it.
Role-Playing
Respondents are presented with an imaginary situation and asked how they would act or react if it was real.
In-Person Surveys
The researcher asks questions in person.
Online/Web Surveys
These surveys are easy to accomplish, but some users may be unwilling to answer truthfully, if at all.
Mobile Surveys
These surveys take advantage of the increasing proliferation of mobile technology. Mobile collection surveys rely on mobile devices like tablets or smartphones to conduct surveys via SMS or mobile apps.
Phone Surveys
No researcher can call thousands of people at once, so they need a third party to handle the chore. However, many people have call screening and wonât answer.
Observation
Sometimes, the simplest method is the best. Researchers who make direct observations collect data quickly and easily, with little intrusion or third-party bias. Naturally, itâs only effective in small-scale situations.
Accurate data collecting is crucial to preserving the integrity of research, regardless of the subject of study or preferred method for defining data (quantitative, qualitative). Errors are less likely to occur when the right data gathering tools are used (whether they are brand-new ones, updated versions of them, or already available).
Among the effects of data collection done incorrectly, include the following -
- Erroneous conclusions that squander resources
- Decisions that compromise public policy
- Incapacity to correctly respond to research inquiries
- Bringing harm to participants who are humans or animals
- Deceiving other researchers into pursuing futile research avenues
- The study's inability to be replicated and validated
When these study findings are used to support recommendations for public policy, there is the potential to result in disproportionate harm, even if the degree of influence from flawed data collecting may vary by discipline and the type of investigation.
Let us now look at the various issues that we might face while maintaining the integrity of data collection.
In order to assist the errors detection process in the data gathering process, whether they were done purposefully (deliberate falsifications) or not, maintaining data integrity is the main justification (systematic or random errors).
Quality assurance and quality control are two strategies that help protect data integrity and guarantee the scientific validity of study results.
Each strategy is used at various stages of the research timeline:
- Quality control - tasks that are performed both after and during data collecting
- Quality assurance - events that happen before data gathering starts
Let us explore each of them in more detail now.
Quality Assurance
As data collecting comes before quality assurance, its primary goal is "prevention" (i.e., forestalling problems with data collection). The best way to protect the accuracy of data collection is through prevention. The uniformity of protocol created in the thorough and exhaustive procedures manual for data collecting serves as the best example of this proactive step.Â
The likelihood of failing to spot issues and mistakes early in the research attempt increases when guides are written poorly. There are several ways to show these shortcomings:
- Failure to determine the precise subjects and methods for retraining or training staff employees in data collecting
- List of goods to be collected, in part
- There isn't a system in place to track modifications to processes that may occur as the investigation continues.
- Instead of detailed, step-by-step instructions on how to deliver tests, there is a vague description of the data gathering tools that will be employed.
- Uncertainty regarding the date, procedure, and identity of the person or people in charge of examining the data
- Incomprehensible guidelines for using, adjusting, and calibrating the data collection equipment.
Now, let us look at how to ensure Quality Control.
Become a Data Scientist With Real-World Experience
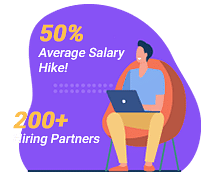
Quality Control
Despite the fact that quality control actions (detection/monitoring and intervention) take place both after and during data collection, the specifics should be meticulously detailed in the procedures manual. Establishing monitoring systems requires a specific communication structure, which is a prerequisite. Following the discovery of data collection problems, there should be no ambiguity regarding the information flow between the primary investigators and staff personnel. A poorly designed communication system promotes slack oversight and reduces opportunities for error detection.
Direct staff observation conference calls, during site visits, or frequent or routine assessments of data reports to spot discrepancies, excessive numbers, or invalid codes can all be used as forms of detection or monitoring. Site visits might not be appropriate for all disciplines. Still, without routine auditing of records, whether qualitative or quantitative, it will be challenging for investigators to confirm that data gathering is taking place in accordance with the manual's defined methods. Additionally, quality control determines the appropriate solutions, or "actions," to fix flawed data gathering procedures and reduce recurrences.
Problems with data collection, for instance, that call for immediate action include:
- Fraud or misbehavior
- Systematic mistakes, procedure violationsÂ
- Individual data items with errors
- Issues with certain staff members or a site's performanceÂ
Researchers are trained to include one or more secondary measures that can be used to verify the quality of information being obtained from the human subject in the social and behavioral sciences where primary data collection entails using human subjects.Â
For instance, a researcher conducting a survey would be interested in learning more about the prevalence of risky behaviors among young adults as well as the social factors that influence these risky behaviors' propensity for and frequency. Let us now explore the common challenges with regard to data collection.
There are some prevalent challenges faced while collecting data, let us explore a few of them to understand them better and avoid them.
Data Quality Issues
The main threat to the broad and successful application of machine learning is poor data quality. Data quality must be your top priority if you want to make technologies like machine learning work for you. Let's talk about some of the most prevalent data quality problems in this blog article and how to fix them.
Inconsistent Data
When working with various data sources, it's conceivable that the same information will have discrepancies between sources. The differences could be in formats, units, or occasionally spellings. The introduction of inconsistent data might also occur during firm mergers or relocations. Inconsistencies in data have a tendency to accumulate and reduce the value of data if they are not continually resolved. Organizations that have heavily focused on data consistency do so because they only want reliable data to support their analytics.
Data Downtime
Data is the driving force behind the decisions and operations of data-driven businesses. However, there may be brief periods when their data is unreliable or not prepared. Customer complaints and subpar analytical outcomes are only two ways that this data unavailability can have a significant impact on businesses. A data engineer spends about 80% of their time updating, maintaining, and guaranteeing the integrity of the data pipeline. In order to ask the next business question, there is a high marginal cost due to the lengthy operational lead time from data capture to insight.
Schema modifications and migration problems are just two examples of the causes of data downtime. Data pipelines can be difficult due to their size and complexity. Data downtime must be continuously monitored, and it must be reduced through automation.
Ambiguous Data
Even with thorough oversight, some errors can still occur in massive databases or data lakes. For data streaming at a fast speed, the issue becomes more overwhelming. Spelling mistakes can go unnoticed, formatting difficulties can occur, and column heads might be deceptive. This unclear data might cause a number of problems for reporting and analytics.
Become a Data Science Expert & Get Your Dream Job
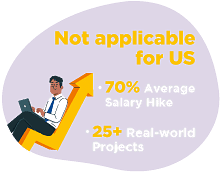
Duplicate Data
Streaming data, local databases, and cloud data lakes are just a few of the sources of data that modern enterprises must contend with. They might also have application and system silos. These sources are likely to duplicate and overlap each other quite a bit. For instance, duplicate contact information has a substantial impact on customer experience. If certain prospects are ignored while others are engaged repeatedly, marketing campaigns suffer. The likelihood of biased analytical outcomes increases when duplicate data are present. It can also result in ML models with biased training data.
Too Much Data
While we emphasize data-driven analytics and its advantages, a data quality problem with excessive data exists. There is a risk of getting lost in an abundance of data when searching for information pertinent to your analytical efforts. Data scientists, data analysts, and business users devote 80% of their work to finding and organizing the appropriate data. With an increase in data volume, other problems with data quality become more serious, particularly when dealing with streaming data and big files or databases.
Inaccurate Data
For highly regulated businesses like healthcare, data accuracy is crucial. Given the current experience, it is more important than ever to increase the data quality for COVID-19 and later pandemics. Inaccurate information does not provide you with a true picture of the situation and cannot be used to plan the best course of action. Personalized customer experiences and marketing strategies underperform if your customer data is inaccurate.
Data inaccuracies can be attributed to a number of things, including data degradation, human mistake, and data drift. Worldwide data decay occurs at a rate of about 3% per month, which is quite concerning. Data integrity can be compromised while being transferred between different systems, and data quality might deteriorate with time.
Hidden Data
The majority of businesses only utilize a portion of their data, with the remainder sometimes being lost in data silos or discarded in data graveyards. For instance, the customer service team might not receive client data from sales, missing an opportunity to build more precise and comprehensive customer profiles. Missing out on possibilities to develop novel products, enhance services, and streamline procedures is caused by hidden data.
Finding Relevant Data
Finding relevant data is not so easy. There are several factors that we need to consider while trying to find relevant data, which include -
- Relevant Domain
- Relevant demographics
- Relevant Time period and so many more factors that we need to consider while trying to find relevant data.
Data that is not relevant to our study in any of the factors render it obsolete and we cannot effectively proceed with its analysis. This could lead to incomplete research or analysis, re-collecting data again and again, or shutting down the study.
Deciding the Data to Collect
Determining what data to collect is one of the most important factors while collecting data and should be one of the first factors while collecting data. We must choose the subjects the data will cover, the sources we will be used to gather it, and the quantity of information we will require. Our responses to these queries will depend on our aims, or what we expect to achieve utilizing your data. As an illustration, we may choose to gather information on the categories of articles that website visitors between the ages of 20 and 50 most frequently access. We can also decide to compile data on the typical age of all the clients who made a purchase from your business over the previous month.
Not addressing this could lead to double work and collection of irrelevant data or ruining your study as a whole.
Dealing With Big Data
Big data refers to exceedingly massive data sets with more intricate and diversified structures. These traits typically result in increased challenges while storing, analyzing, and using additional methods of extracting results. Big data refers especially to data sets that are quite enormous or intricate that conventional data processing tools are insufficient. The overwhelming amount of data, both unstructured and structured, that a business faces on a daily basis.Â
The amount of data produced by healthcare applications, the internet, social networking sites social, sensor networks, and many other businesses are rapidly growing as a result of recent technological advancements. Big data refers to the vast volume of data created from numerous sources in a variety of formats at extremely fast rates. Dealing with this kind of data is one of the many challenges of Data Collection and is a crucial step toward collecting effective data.Â
Low Response and Other Research Issues
Poor design and low response rates were shown to be two issues with data collecting, particularly in health surveys that used questionnaires. This might lead to an insufficient or inadequate supply of data for the study. Creating an incentivized data collection program might be beneficial in this case to get more responses.
Now, let us look at the key steps in the data collection process.
In the Data Collection Process, there are 5 key steps. They are explained briefly below -
1. Decide What Data You Want to Gather
The first thing that we need to do is decide what information we want to gather. We must choose the subjects the data will cover, the sources we will use to gather it, and the quantity of information that we would require. For instance, we may choose to gather information on the categories of products that an average e-commerce website visitor between the ages of 30 and 45 most frequently searches for.Â
2. Establish a Deadline for Data Collection
The process of creating a strategy for data collection can now begin. We should set a deadline for our data collection at the outset of our planning phase. Some forms of data we might want to continuously collect. We might want to build up a technique for tracking transactional data and website visitor statistics over the long term, for instance. However, we will track the data throughout a certain time frame if we are tracking it for a particular campaign. In these situations, we will have a schedule for when we will begin and finish gathering data.Â
3. Select a Data Collection Approach
We will select the data collection technique that will serve as the foundation of our data gathering plan at this stage. We must take into account the type of information that we wish to gather, the time period during which we will receive it, and the other factors we decide on to choose the best gathering strategy.
4. Gather Information
Once our plan is complete, we can put our data collection plan into action and begin gathering data. In our DMP, we can store and arrange our data. We need to be careful to follow our plan and keep an eye on how it's doing. Especially if we are collecting data regularly, setting up a timetable for when we will be checking in on how our data gathering is going may be helpful. As circumstances alter and we learn new details, we might need to amend our plan.
5. Examine the Information and Apply Your Findings
It's time to examine our data and arrange our findings after we have gathered all of our information. The analysis stage is essential because it transforms unprocessed data into insightful knowledge that can be applied to better our marketing plans, goods, and business judgments. The analytics tools included in our DMP can be used to assist with this phase. We can put the discoveries to use to enhance our business once we have discovered the patterns and insights in our data.
Let us now look at some data collection considerations and best practices that one might follow.
We must carefully plan before spending time and money traveling to the field to gather data. While saving time and resources, effective data collection strategies can help us collect richer, more accurate, and richer data.
Below, we will be discussing some of the best practices that we can follow for the best results -
1. Take Into Account the Price of Each Extra Data Point
Once we have decided on the data we want to gather, we need to make sure to take the expense of doing so into account. Our surveyors and respondents will incur additional costs for each additional data point or survey question.
2. Plan How to Gather Each Data Piece
There is a dearth of freely accessible data. Sometimes the data is there, but we may not have access to it. For instance, unless we have a compelling cause, we cannot openly view another person's medical information. It could be challenging to measure several types of information.
Consider how time-consuming and difficult it will be to gather each piece of information while deciding what data to acquire.
3. Think About Your Choices for Data Collecting Using Mobile Devices
Mobile-based data collecting can be divided into three categories -
- IVRS (interactive voice response technology) -Â Will call the respondents and ask them questions that have already been recorded.Â
- SMS data collection - Will send a text message to the respondent, who can then respond to questions by text on their phone.Â
- Field surveyors - Can directly enter data into an interactive questionnaire while speaking to each respondent, thanks to smartphone apps.
We need to make sure to select the appropriate tool for our survey and responders because each one has its own disadvantages and advantages.
4. Carefully Consider the Data You Need to Gather
It's all too easy to get information about anything and everything, but it's crucial to only gather the information that we require.Â
It is helpful to consider these 3 questions:
- What details will be helpful?
- What details are available?
- What specific details do you require?
5. Remember to Consider Identifiers
Identifiers, or details describing the context and source of a survey response, are just as crucial as the information about the subject or program that we are actually researching.
In general, adding more identifiers will enable us to pinpoint our program's successes and failures with greater accuracy, but moderation is the key.
6. Data Collecting Through Mobile Devices is the Way to Go
Although collecting data on paper is still common, modern technology relies heavily on mobile devices. They enable us to gather many various types of data at relatively lower prices and are accurate as well as quick. There aren't many reasons not to pick mobile-based data collecting with the boom of low-cost Android devices that are available nowadays.
The Ultimate Ticket to Top Data Science Job Roles
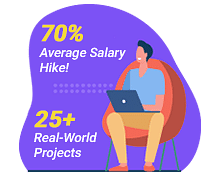
1. What is data collection with example?
Data collection is the process of collecting and analyzing information on relevant variables in a predetermined, methodical way so that one can respond to specific research questions, test hypotheses, and assess results. Data collection can be either qualitative or quantitative. Example: A company collects customer feedback through online surveys and social media monitoring to improve their products and services.
2. What are the primary data collection methods?
As is well known, gathering primary data is costly and time intensive. The main techniques for gathering data are observation, interviews, questionnaires, schedules, and surveys.
3. What are data collection tools?
The term "data collecting tools" refers to the tools/devices used to gather data, such as a paper questionnaire or a system for computer-assisted interviews. Tools used to gather data include case studies, checklists, interviews, occasionally observation, surveys, and questionnaires.
4. Whatâs the difference between quantitative and qualitative methods?
While qualitative research focuses on words and meanings, quantitative research deals with figures and statistics. You can systematically measure variables and test hypotheses using quantitative methods. You can delve deeper into ideas and experiences using qualitative methodologies.
5. What are quantitative data collection methods?
While there are numerous other ways to get quantitative information, the methods indicated aboveâprobability sampling, interviews, questionnaire observation, and document reviewâare the most typical and frequently employed, whether collecting information offline or online.
6. What is mixed methods research?
User research that includes both qualitative and quantitative techniques is known as mixed methods research. For deeper user insights, mixed methods research combines insightful user data with useful statistics.
7. What are the benefits of collecting data?
Collecting data offers several benefits, including:
- Knowledge and Insight
- Evidence-Based Decision Making
- Problem Identification and Solution
- Validation and Evaluation
- Identifying Trends and Predictions
- Support for Research and Development
- Policy Development
- Quality Improvement
- Personalization and Targeting
- Knowledge Sharing and Collaboration
8. Whatâs the difference between reliability and validity?
Reliability is about consistency and stability, while validity is about accuracy and appropriateness. Reliability focuses on the consistency of results, while validity focuses on whether the results are actually measuring what they are intended to measure. Both reliability and validity are crucial considerations in research to ensure the trustworthiness and meaningfulness of the collected data and measurements.
Are you thinking about pursuing a career in the field of data science? Simplilearn's Data Science courses are designed to provide you with the necessary skills and expertise to excel in this rapidly changing field. Here's a detailed comparison for your reference:
Program Name Data Scientist Master's Program Post Graduate Program In Data Science Post Graduate Program In Data Science Geo All Geos All Geos Not Applicable in US University Simplilearn Purdue Caltech Course Duration 11 Months 11 Months 11 Months Coding Experience Required Basic Basic No Skills You Will Learn 10+ skills including data structure, data manipulation, NumPy, Scikit-Learn, Tableau and more 8+ skills including Exploratory Data Analysis, Descriptive Statistics, Inferential Statistics, and more 8+ skills including Supervised & Unsupervised Learning Deep Learning Data Visualization, and more Additional Benefits Applied Learning via Capstone and 25+ Data Science Projects Purdue Alumni Association Membership Free IIMJobs Pro-Membership of 6 months Resume Building Assistance Upto 14 CEU Credits Caltech CTME Circle Membership Cost $$ $$$$ $$$$ Explore Program Explore Program Explore Program
We live in the Data Age, and if you want a career that fully takes advantage of this, you should consider a career in data science. Simplilearn offers a Caltech Post Graduate Program in Data Science  that will train you in everything you need to know to secure the perfect position. This Data Science PG program is ideal for all working professionals, covering job-critical topics like R, Python programming , machine learning algorithms , NLP concepts , and data visualization with Tableau in great detail. This is all provided via our interactive learning model with live sessions by global practitioners, practical labs, and industry projects.
Data Science & Business Analytics Courses Duration and Fees
Data Science & Business Analytics programs typically range from a few weeks to several months, with fees varying based on program and institution.
Recommended Reads
Data Science Career Guide: A Comprehensive Playbook To Becoming A Data Scientist
Difference Between Collection and Collections in Java
An Ultimate One-Stop Solution Guide to Collections in C# Programming With Examples
Managing Data
Capped Collection in MongoDB
What Are Java Collections and How to Implement Them?
Get Affiliated Certifications with Live Class programs
Data scientist.
- Industry-recognized Data Scientist Masterâs certificate from Simplilearn
- Dedicated live sessions by faculty of industry experts
Caltech Data Sciences-Bootcamp
- Exclusive visit to Caltechâs Robotics Lab
Caltech Post Graduate Program in Data Science
- Earn a program completion certificate from Caltech CTME
- Curriculum delivered in live online sessions by industry experts
- PMP, PMI, PMBOK, CAPM, PgMP, PfMP, ACP, PBA, RMP, SP, and OPM3 are registered marks of the Project Management Institute, Inc.
- Skip to main content
- Skip to primary sidebar
- Skip to footer
- QuestionPro

- Solutions Industries Gaming Automotive Sports and events Education Government Travel & Hospitality Financial Services Healthcare Cannabis Technology Use Case NPS+ Communities Audience Contactless surveys Mobile LivePolls Member Experience GDPR Positive People Science 360 Feedback Surveys
- Resources Blog eBooks Survey Templates Case Studies Training Help center

Home Market Research
Data Collection: What It Is, Methods & Tools + Examples
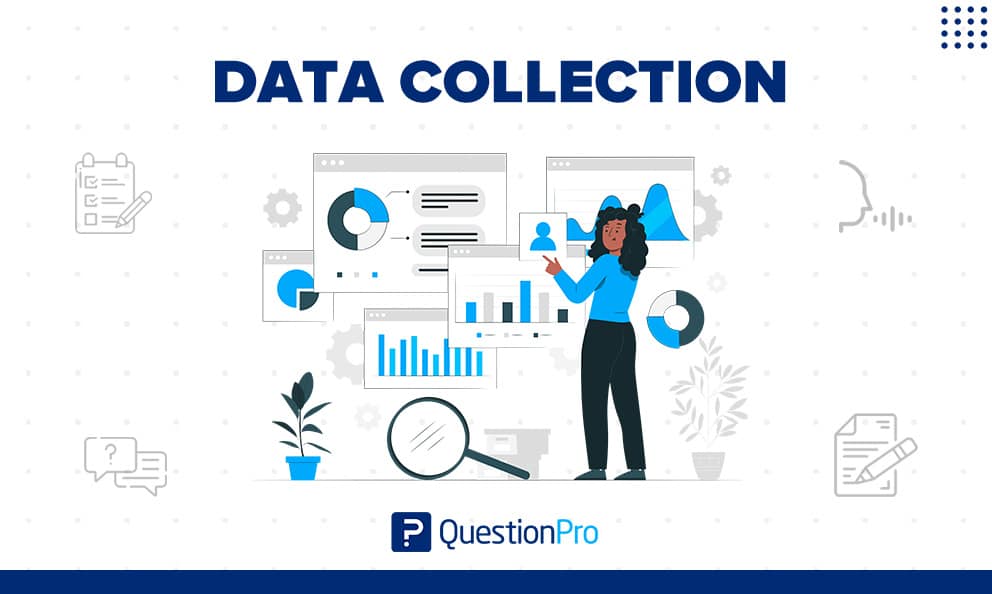
Letâs face it, no one wants to make decisions based on guesswork or gut feelings. The most important objective of data collection is to ensure that the data gathered is reliable and packed to the brim with juicy insights that can be analyzed and turned into data-driven decisions. Thereâs nothing better than good statistical analysis .
LEARN ABOUT: Level of Analysis
Collecting high-quality data is essential for conducting market research, analyzing user behavior, or just trying to get a handle on business operations. With the right approach and a few handy tools, gathering reliable and informative data.
So, letâs get ready to collect some data because when it comes to data collection, itâs all about the details.
Content Index
What is Data Collection?
Data collection methods, data collection examples, reasons to conduct online research and data collection, conducting customer surveys for data collection to multiply sales, steps to effectively conduct an online survey for data collection, survey design for data collection.
Data collection is the procedure of collecting, measuring, and analyzing accurate insights for research using standard validated techniques.
Put simply, data collection is the process of gathering information for a specific purpose. It can be used to answer research questions, make informed business decisions, or improve products and services.
To collect data, we must first identify what information we need and how we will collect it. We can also evaluate a hypothesis based on collected data. In most cases, data collection is the primary and most important step for research. The approach to data collection is different for different fields of study, depending on the required information.
LEARN ABOUT: Action Research
There are many ways to collect information when doing research. The data collection methods that the researcher chooses will depend on the research question posed. Some data collection methods include surveys, interviews, tests, physiological evaluations, observations, reviews of existing records, and biological samples. Letâs explore them.
LEARN ABOUT: Best Data Collection Tools
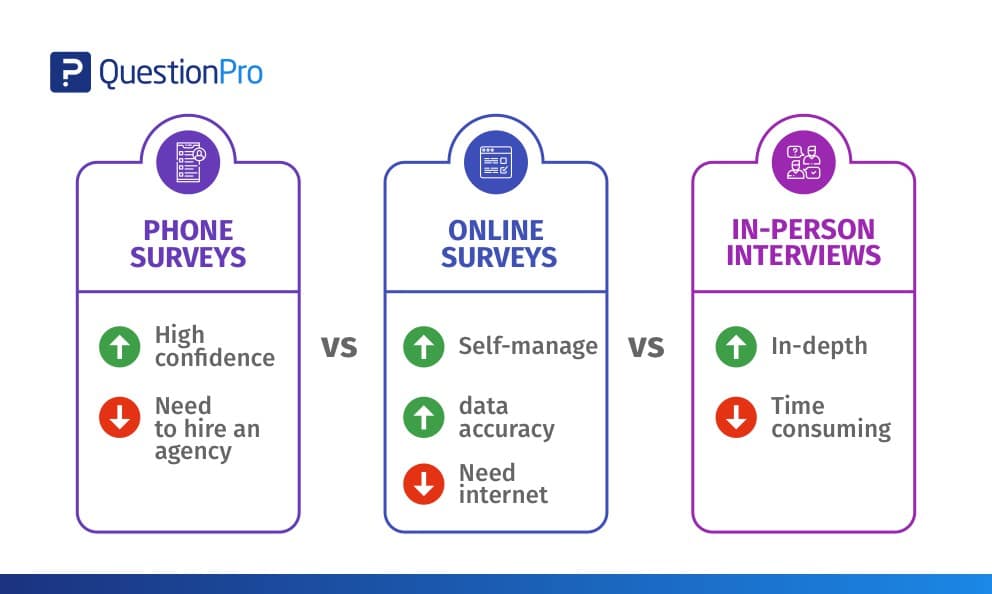
Phone vs. Online vs. In-Person Interviews
Essentially there are four choices for data collection â in-person interviews, mail, phone, and online. There are pros and cons to each of these modes.
- Pros: In-depth and a high degree of confidence in the data
- Cons: Time-consuming, expensive, and can be dismissed as anecdotal
- Pros: Can reach anyone and everyone â no barrier
- Cons: Expensive, data collection errors, lag time
- Pros: High degree of confidence in the data collected, reach almost anyone
- Cons: Expensive, cannot self-administer, need to hire an agency
- Pros: Cheap, can self-administer, very low probability of data errors
- Cons: Not all your customers might have an email address/be on the internet, customers may be wary of divulging information online.
In-person interviews always are better, but the big drawback is the trap you might fall into if you donât do them regularly. It is expensive to regularly conduct interviews and not conducting enough interviews might give you false positives. Validating your research is almost as important as designing and conducting it.
Weâve seen many instances where after the research is conducted â if the results do not match up with the âgut-feelâ of upper management, it has been dismissed off as anecdotal and a âone-timeâ phenomenon. To avoid such traps, we strongly recommend that data-collection be done on an âongoing and regularâ basis.
LEARN ABOUT: Research Process Steps
This will help you compare and analyze the change in perceptions according to marketing for your products/services. The other issue here is sample size. To be confident with your research, you must interview enough people to weed out the fringe elements.
A couple of years ago there was a lot of discussion about online surveys and their statistical analysis plan . The fact that not every customer had internet connectivity was one of the main concerns.
LEARN ABOUT: Statistical Analysis Methods
Although some of the discussions are still valid, the reach of the internet as a means of communication has become vital in the majority of customer interactions. According to the US Census Bureau, the number of households with computers has doubled between 1997 and 2001.
Learn more: Quantitative Market Research
In 2001 nearly 50% of households had a computer. Nearly 55% of all households with an income of more than 35,000 have internet access, which jumps to 70% for households with an annual income of 50,000. This data is from the US Census Bureau for 2001.
There are primarily three modes of data collection that can be employed to gather feedback â Mail, Phone, and Online. The method actually used for data collection is really a cost-benefit analysis. There is no slam-dunk solution but you can use the table below to understand the risks and advantages associated with each of the mediums:
Keep in mind, the reach here is defined as âAll U.S. Households.â In most cases, you need to look at how many of your customers are online and determine. If all your customers have email addresses, you have a 100% reach of your customers.
Another important thing to keep in mind is the ever-increasing dominance of cellular phones over landline phones. United States FCC rules prevent automated dialing and calling cellular phone numbers and there is a noticeable trend towards people having cellular phones as the only voice communication device.
This introduces the inability to reach cellular phone customers who are dropping home phone lines in favor of going entirely wireless. Even if automated dialing is not used, another FCC rule prohibits from phoning anyone who would have to pay for the call.
Learn more: Qualitative Market Research
Multi-Mode Surveys
Surveys, where the data is collected via different modes (online, paper, phone etc.), is also another way of going. It is fairly straightforward and easy to have an online survey and have data-entry operators to enter in data (from the phone as well as paper surveys) into the system. The same system can also be used to collect data directly from the respondents.
Learn more: Survey Research
Data collection is an important aspect of research. Letâs consider an example of a mobile manufacturer, company X, which is launching a new product variant. To conduct research about features, price range, target market, competitor analysis, etc. data has to be collected from appropriate sources.
The marketing team can conduct various data collection activities such as online surveys or focus groups .
The survey should have all the right questions about features and pricing, such as âWhat are the top 3 features expected from an upcoming product?â or âHow much are your likely to spend on this product?â or âWhich competitors provide similar products?â etc.
For conducting a focus group, the marketing team should decide the participants and the mediator. The topic of discussion and objective behind conducting a focus group should be clarified beforehand to conduct a conclusive discussion.
Data collection methods are chosen depending on the available resources. For example, conducting questionnaires and surveys would require the least resources, while focus groups require moderately high resources.
Feedback is a vital part of any organizationâs growth. Whether you conduct regular focus groups to elicit information from key players or, your account manager calls up all your marquee accounts to find out how things are going â essentially they are all processes to find out from your customersâ eyes â How are we doing? What can we do better?
Online surveys are just another medium to collect feedback from your customers , employees and anyone your business interacts with. With the advent of Do-It-Yourself tools for online surveys, data collection on the internet has become really easy, cheap and effective.
Learn more: Online Research
It is a well-established marketing fact that acquiring a new customer is 10 times more difficult and expensive than retaining an existing one. This is one of the fundamental driving forces behind the extensive adoption and interest in CRM and related customer retention tactics.
In a research study conducted by Rice University Professor Dr. Paul Dholakia and Dr. Vicki Morwitz, published in Harvard Business Review, the experiment inferred that the simple fact of asking customers how an organization was performing by itself to deliver results proved to be an effective customer retention strategy.
In the research study, conducted over the course of a year, one set of customers were sent out a satisfaction and opinion survey and the other set was not surveyed. In the next one year, the group that took the survey saw twice the number of people continuing and renewing their loyalty towards the organization data .
Learn more: Research Design
The research study provided a couple of interesting reasons on the basis of consumer psychology, behind this phenomenon:
- Satisfaction surveys boost the customersâ desire to be coddled and induce positive feelings. This crops from a section of the human psychology that intends to âappreciateâ a product or service they already like or prefer. The survey feedback collection method is solely a medium to convey this. The survey is a vehicle to âinteractâ with the company and reinforces the customerâs commitment to the company.
- Surveys may increase awareness of auxiliary products and services. Surveys can be considered modes of both inbound as well as outbound communication. Surveys are generally considered to be a data collection and analysis source. Most people are unaware of the fact that consumer surveys can also serve as a medium for distributing data. It is important to note a few caveats here.
- In most countries, including the US, âselling under the guise of researchâ is illegal. b. However, we all know that information is distributed while collecting information. c. Other disclaimers may be included in the survey to ensure users are aware of this fact. For example: âWe will collect your opinion and inform you about products and services that have come online in the last yearâŚâ
- Induced Judgments: The entire procedure of asking people for their feedback can prompt them to build an opinion on something they otherwise would not have thought about. This is a very underlying yet powerful argument that can be compared to the âProduct Placementâ strategy currently used for marketing products in mass media like movies and television shows. One example is the extensive and exclusive use of the âmini-Cooperâ in the blockbuster movie âItalian Job.â This strategy is questionable and should be used with great caution.
Surveys should be considered as a critical tool in the customer journey dialog. The best thing about surveys is its ability to carry âbi-directionalâ information. The research conducted by Paul Dholakia and Vicki Morwitz shows that surveys not only get you the information that is critical for your business, but also enhances and builds upon the established relationship you have with your customers.
Recent technological advances have made it incredibly easy to conduct real-time surveys and opinion polls . Online tools make it easy to frame questions and answers and create surveys on the Web. Distributing surveys via email, website links or even integration with online CRM tools like Salesforce.com have made online surveying a quick-win solution.
So, youâve decided to conduct an online survey. There are a few questions in your mind that you would like answered, and you are looking for a fast and inexpensive way to find out more about your customers, clients, etc.
First and foremost thing you need to decide what the smart objectives of the study are. Ensure that you can phrase these objectives as questions or measurements. If you canât, you are better off looking at other data sources like focus groups and other qualitative methods . The data collected via online surveys is dominantly quantitative in nature.
Review the basic objectives of the study. What are you trying to discover? What actions do you want to take as a result of the survey? â Answers to these questions help in validating collected data. Online surveys are just one way of collecting and quantifying data .
Learn more: Qualitative Data & Qualitative Data Collection Methods
- Visualize all of the relevant information items you would like to have. What will the output survey research report look like? What charts and graphs will be prepared? What information do you need to be assured that action is warranted?
- Assign ranks to each topic (1 and 2) according to their priority, including the most important topics first. Revisit these items again to ensure that the objectives, topics, and information you need are appropriate. Remember, you canât solve the research problem if you ask the wrong questions.
- How easy or difficult is it for the respondent to provide information on each topic? If it is difficult, is there an alternative medium to gain insights by asking a different question? This is probably the most important step. Online surveys have to be Precise, Clear and Concise. Due to the nature of the internet and the fluctuations involved, if your questions are too difficult to understand, the survey dropout rate will be high.
- Create a sequence for the topics that are unbiased. Make sure that the questions asked first do not bias the results of the next questions. Sometimes providing too much information, or disclosing purpose of the study can create bias. Once you have a series of decided topics, you can have a basic structure of a survey. It is always advisable to add an âIntroductoryâ paragraph before the survey to explain the project objective and what is expected of the respondent. It is also sensible to have a âThank Youâ text as well as information about where to find the results of the survey when they are published.
- Page Breaks â The attention span of respondents can be very low when it comes to a long scrolling survey. Add page breaks as wherever possible. Having said that, a single question per page can also hamper response rates as it increases the time to complete the survey as well as increases the chances for dropouts.
- Branching â Create smart and effective surveys with the implementation of branching wherever required. Eliminate the use of text such as, âIf you answered No to Q1 then Answer Q4â â this leads to annoyance amongst respondents which result in increase survey dropout rates. Design online surveys using the branching logic so that appropriate questions are automatically routed based on previous responses.
- Write the questions . Initially, write a significant number of survey questions out of which you can use the one which is best suited for the survey. Divide the survey into sections so that respondents do not get confused seeing a long list of questions.
- Sequence the questions so that they are unbiased.
- Repeat all of the steps above to find any major holes. Are the questions really answered? Have someone review it for you.
- Time the length of the survey. A survey should take less than five minutes. At three to four research questions per minute, you are limited to about 15 questions. One open end text question counts for three multiple choice questions. Most online software tools will record the time taken for the respondents to answer questions.
- Include a few open-ended survey questions that support your survey object. This will be a type of feedback survey.
- Send an email to the project survey to your test group and then email the feedback survey afterward.
- This way, you can have your test group provide their opinion about the functionality as well as usability of your project survey by using the feedback survey.
- Make changes to your questionnaire based on the received feedback.
- Send the survey out to all your respondents!
Online surveys have, over the course of time, evolved into an effective alternative to expensive mail or telephone surveys. However, you must be aware of a few conditions that need to be met for online surveys. If you are trying to survey a sample representing the target population, please remember that not everyone is online.
Moreover, not everyone is receptive to an online survey also. Generally, the demographic segmentation of younger individuals is inclined toward responding to an online survey.
Learn More: Examples of Qualitarive Data in Education
Good survey design is crucial for accurate data collection. From question-wording to response options, letâs explore how to create effective surveys that yield valuable insights with our tips to survey design.
- Writing Great Questions for data collection
Writing great questions can be considered an art. Art always requires a significant amount of hard work, practice, and help from others.
The questions in a survey need to be clear, concise, and unbiased. A poorly worded question or a question with leading language can result in inaccurate or irrelevant responses, ultimately impacting the dataâs validity.
Moreover, the questions should be relevant and specific to the research objectives. Questions that are irrelevant or do not capture the necessary information can lead to incomplete or inconsistent responses too.
- Avoid loaded or leading words or questions
A small change in content can produce effective results. Words such as could , should and might are all used for almost the same purpose, but may produce a 20% difference in agreement to a question. For example, âThe management could.. should.. might.. have shut the factoryâ.
Intense words such as â prohibit or action, representing control or action, produce similar results. For example, âDo you believe Donald Trump should prohibit insurance companies from raising rates?â.
Sometimes the content is just biased. For instance, âYou wouldnât want to go to Rudolphoâs Restaurant for the organizationâs annual party, would you?â
- Misplaced questions
Questions should always reference the intended context, and questions placed out of order or without its requirement should be avoided. Generally, a funnel approach should be implemented â generic questions should be included in the initial section of the questionnaire as a warm-up and specific ones should follow. Toward the end, demographic or geographic questions should be included.
- Mutually non-overlapping response categories
Multiple-choice answers should be mutually unique to provide distinct choices. Overlapping answer options frustrate the respondent and make interpretation difficult at best. Also, the questions should always be precise.
For example: âDo you like water juice?â
This question is vague. In which terms is the liking for orange juice is to be rated? â Sweetness, texture, price, nutrition etc.
- Avoid the use of confusing/unfamiliar words
Asking about industry-related terms such as caloric content, bits, bytes, MBS , as well as other terms and acronyms can confuse respondents . Ensure that the audience understands your language level, terminology, and, above all, the question you ask.
- Non-directed questions give respondents excessive leeway
In survey design for data collection, non-directed questions can give respondents excessive leeway, which can lead to vague and unreliable data. These types of questions are also known as open-ended questions, and they do not provide any structure for the respondent to follow.
For instance, a non-directed question like â What suggestions do you have for improving our shoes?â can elicit a wide range of answers, some of which may not be relevant to the research objectives. Some respondents may give short answers, while others may provide lengthy and detailed responses, making comparing and analyzing the data challenging.
To avoid these issues, itâs essential to ask direct questions that are specific and have a clear structure. Closed-ended questions, for example, offer structured response options and can be easier to analyze as they provide a quantitative measure of respondentsâ opinions.
- Never force questions
There will always be certain questions that cross certain privacy rules. Since privacy is an important issue for most people, these questions should either be eliminated from the survey or not be kept as mandatory. Survey questions about income, family income, status, religious and political beliefs, etc., should always be avoided as they are considered to be intruding, and respondents can choose not to answer them.
- Unbalanced answer options in scales
Unbalanced answer options in scales such as Likert Scale and Semantic Scale may be appropriate for some situations and biased in others. When analyzing a pattern in eating habits, a study used a quantity scale that made obese people appear in the middle of the scale with the polar ends reflecting a state where people starve and an irrational amount to consume. There are cases where we usually do not expect poor service, such as hospitals.
- Questions that cover two points
In survey design for data collection, questions that cover two points can be problematic for several reasons. These types of questions are often called âdouble-barreledâ questions and can cause confusion for respondents, leading to inaccurate or irrelevant data.
For instance, a question like âDo you like the food and the service at the restaurant?â covers two points, the food and the service, and it assumes that the respondent has the same opinion about both. If the respondent only liked the food, their opinion of the service could affect their answer.
Itâs important to ask one question at a time to avoid confusion and ensure that the respondentâs answer is focused and accurate. This also applies to questions with multiple concepts or ideas. In these cases, itâs best to break down the question into multiple questions that address each concept or idea separately.
- Dichotomous questions
Dichotomous questions are used in case you want a distinct answer, such as: Yes/No or Male/Female . For example, the question âDo you think this candidate will win the election?â can be Yes or No.
- Avoid the use of long questions
The use of long questions will definitely increase the time taken for completion, which will generally lead to an increase in the survey dropout rate. Multiple-choice questions are the longest and most complex, and open-ended questions are the shortest and easiest to answer.
Data collection is an essential part of the research process, whether youâre conducting scientific experiments, market research, or surveys. The methods and tools used for data collection will vary depending on the research type, the sample size required, and the resources available.
Several data collection methods include surveys, observations, interviews, and focus groups. We learn each method has advantages and disadvantages, and choosing the one that best suits the research goals is important.
With the rise of technology, many tools are now available to facilitate data collection, including online survey software and data visualization tools. These tools can help researchers collect, store, and analyze data more efficiently, providing greater results and accuracy.
By understanding the various methods and tools available for data collection, we can develop a solid foundation for conducting research. With these research skills , we can make informed decisions, solve problems, and contribute to advancing our understanding of the world around us.
Analyze your survey data to gauge in-depth market drivers, including competitive intelligence, purchasing behavior, and price sensitivity, with QuestionPro.
You will obtain accurate insights with various techniques, including conjoint analysis, MaxDiff analysis, sentiment analysis, TURF analysis, heatmap analysis, etc. Export quality data to external in-depth analysis tools such as SPSS and R Software, and integrate your research with external business applications. Everything you need for your data collection. Start today for free!
LEARN MORE FREE TRIAL
MORE LIKE THIS
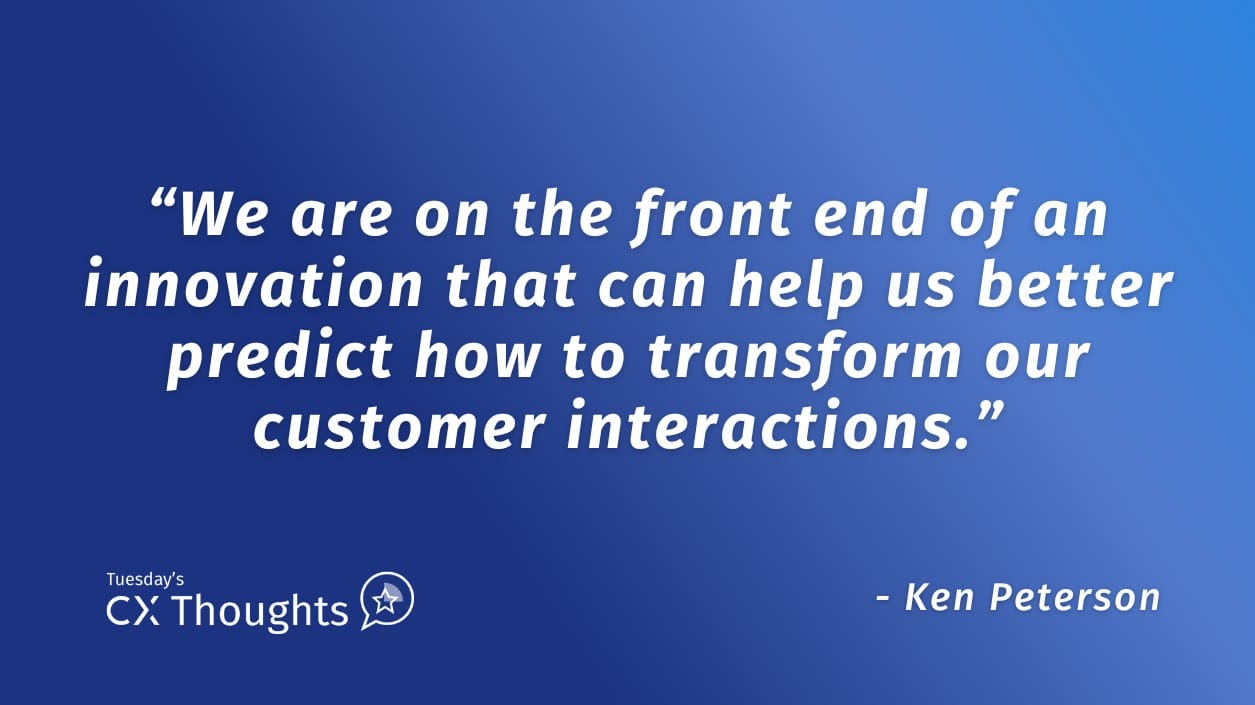
How Can I Help You? â Tuesday CX Thoughts
Jun 5, 2024
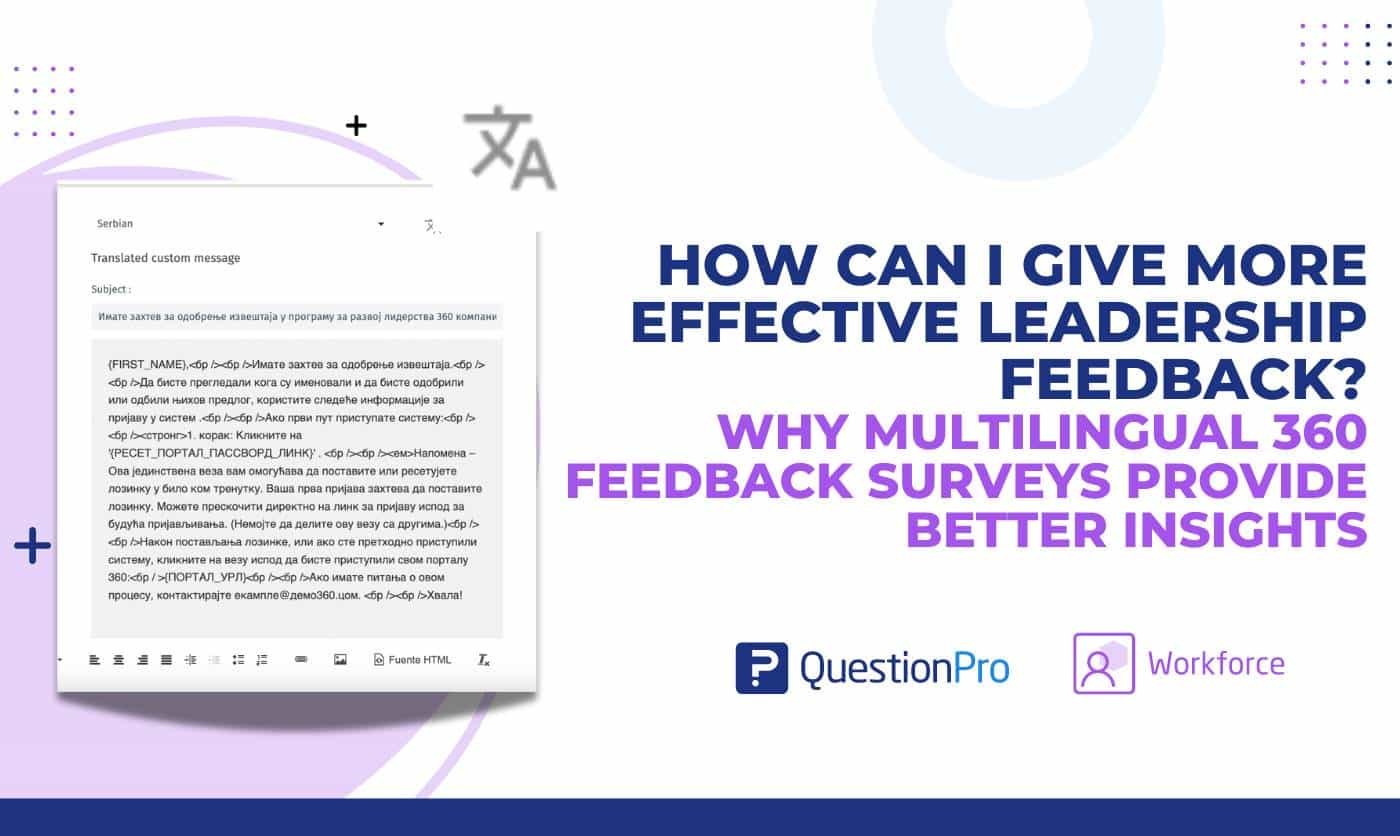
Why Multilingual 360 Feedback Surveys Provide Better Insights
Jun 3, 2024
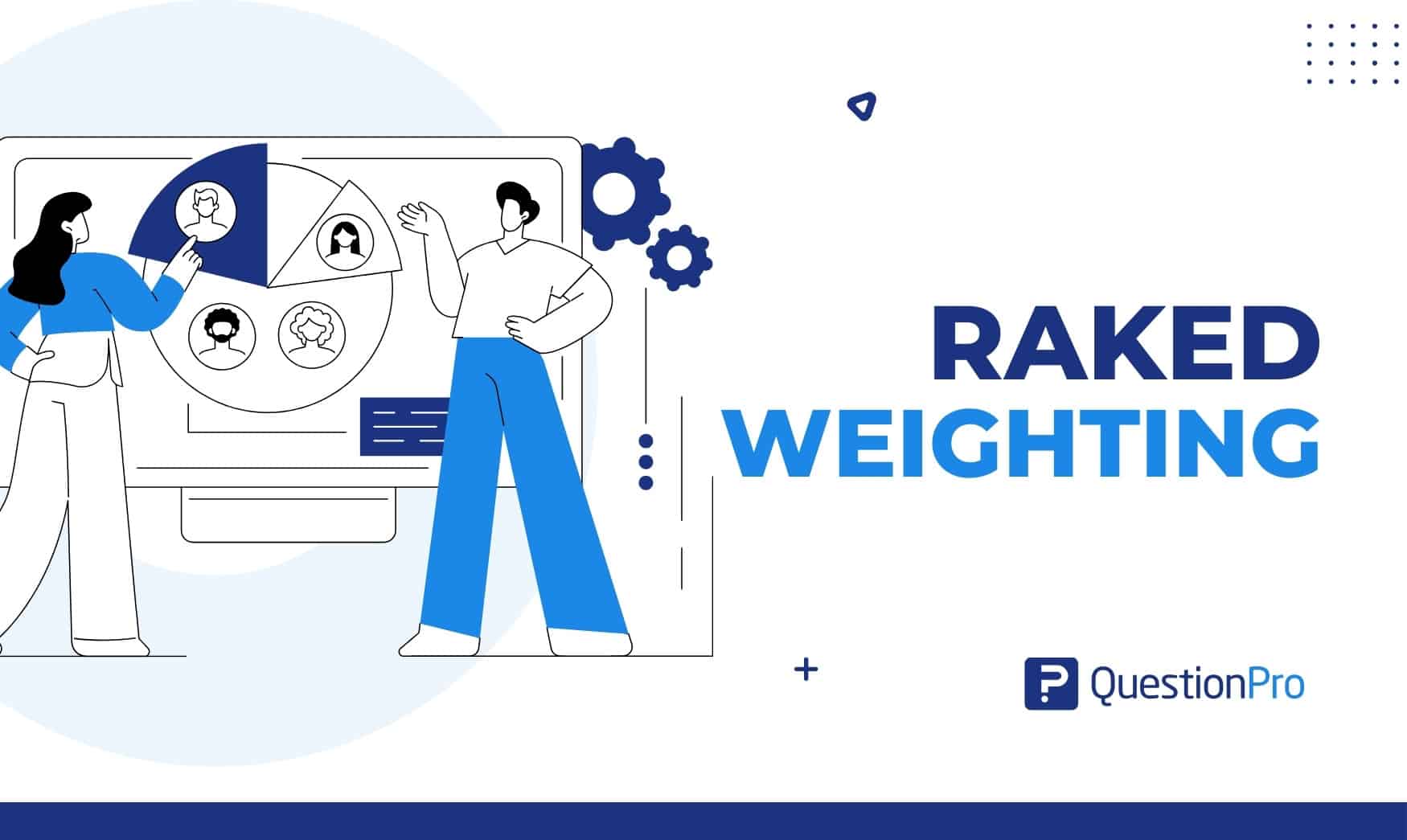
Raked Weighting: A Key Tool for Accurate Survey Results
May 31, 2024
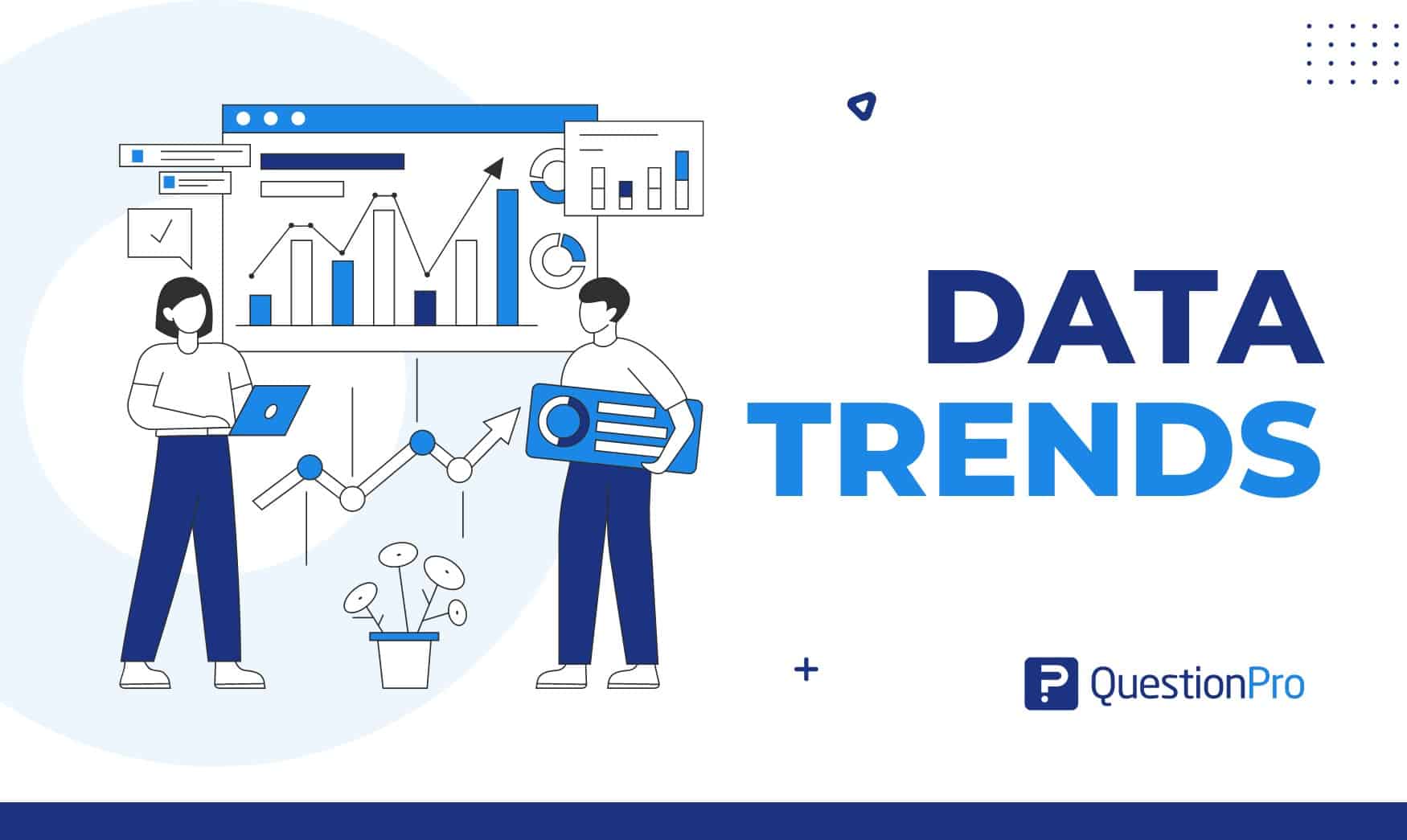
Top 8 Data Trends to Understand the Future of Data
May 30, 2024
Other categories
- Academic Research
- Artificial Intelligence
- Assessments
- Brand Awareness
- Case Studies
- Communities
- Consumer Insights
- Customer effort score
- Customer Engagement
- Customer Experience
- Customer Loyalty
- Customer Research
- Customer Satisfaction
- Employee Benefits
- Employee Engagement
- Employee Retention
- Friday Five
- General Data Protection Regulation
- Insights Hub
- Life@QuestionPro
- Market Research
- Mobile diaries
- Mobile Surveys
- New Features
- Online Communities
- Question Types
- Questionnaire
- QuestionPro Products
- Release Notes
- Research Tools and Apps
- Revenue at Risk
- Survey Templates
- Training Tips
- Uncategorized
- Video Learning Series
- Whatâs Coming Up
- Workforce Intelligence

Extended Essay: Conducting Primary Research
- Extended Essay- The Basics
- Step 1. Choose a Subject
- Step 2. Educate yourself!
- Using Brainstorming and Mind Maps
- Identify Keywords
- Do Background Reading
- Define Your Topic
- Conduct Research in a Specific Discipline
- Step 5. Draft a Research Question
- Step 6. Create a Timeline
- Find Articles
- Find Primary Sources
- Get Help from Experts
- Search Engines, Repositories, & Directories
- Databases and Websites by Subject Area
- Create an Annotated Bibliography
- Advice (and Warnings) from the IB
- Chicago Citation Syle
- MLA Works Cited & In-Text Citations
- Step 9. Set Deadlines for Yourself
- Step 10. Plan a structure for your essay
- Evaluate & Select: the CRAAP Test
- Conducting Secondary Research
- Conducting Primary Research
- Formal vs. Informal Writing
- Presentation Requirements
- Evaluating Your Work
Primary Research - a Definition
Primary research is the collecting of original data.
Not all subjects permit the use of primary methods as part of the research process for the EE, so it is important to carefully check your subject guidelines before starting.
On this page, you can find information on:
Primary Research - What is Its Purpose? Primary Research - Preparation Before You Begin Quantitative vs. Qualitative Research Research Methods Considerations for Primary Research Ways That Primary Research Can Fail Video Tutorials
Primary Research - Purpose

After the literature review or secondary research is completed, you must analyze your findings and:
- determine to what extent they answer your research question
- find agreement between authors
- find disagreement, where the evidence reveals differences in points of view or findings.
At this point you may decide to investigate further by carrying out your own primary research , in other words by collecting your own data .
You will have to choose a method or methods that are appropriate to the research question and commonly used in the particular Diploma Programme subject. NOTE: although the method may be appropriate for the subject, in the instance of the EE it may not be permitted, so check the subject guide!
Possible data collection methods include:
- experiments
- investigations
- interviews
The details of how the data was collected are crucial to the validity of any argument based on the findings. You must put in the main body of your essay the details of any primary research you carry out. These include:
- the methods used
- the persons involved
- how and why these were selected
- the relevant results
- any limitations and biases that may have influenced the results
Primary Research - Preparation Before You Begin
Students must follow the accepted process for carrying out their chosen method of research—how the data is recorded, analysed and presented. Otherwise, their data will have little value.
Students need to plan carefully how to carry out their research. In most cases, there will be only one opportunity to collect primary data from a particular source.
It is extremely important that students approach their research in an ethical and legal manner. See:

Quantitative vs. Qualitative Research

Quantitative research follows a well-defined process that yields data that can be analyzed statistically. For example:
- outcomes from experiments
- data collected from surveys where responses involve closed or multiple-choice responses
Qualitative research collects information that may reflect opinions or personal perspectives on a particular situation. The data that is collected gives an overall impression and generally cannot be analyzed statistically. For example:
- responses in interviews
- open-ended questions in surveys
Videos: Primary Data, Conducting Surveys, Primary Research Methods
B2Bwhiteboard. What is primary data? 3 January 2012. YouTube, https://youtu.be/yZgCam-sjCw. Accessed January 2017.
Learn how to conduct an online survey. Topics include: Developing research questions; designing a good questionnaire; choosing the right online survey tool (Google Forms, Sosci Survey, Survey Monkey).
Ebster, Claus. How to conduct an online survey. 5 August 2014. YouTube, https://youtu.be/uTIWl76_klI. Accessed January 2017.
âThis video from Ivory Research provides information on the top 3 primary research methods for graduate students writing a dissertation - including questionnaires, interviews and focus groups: how to collect research and use it for maximum effectiveness.
IvoryResearch. Dissertation tutorial: Top 3 primary research methods. 12 August 2013. YouTube., https://youtu.be/_lR7oCjutZE. Accessed May 2015.
Research Methods

Experiments can be conducted and their data collected using systematic methods and well-defined processes. For example:
- using the scientific method in conducting science experiments
- simulating an experiment on using mobile phone technology to determine the location of a person.
Experiments usually involve quantitative processes to collect and analyze the resulting data.
Investigations
Types of investigation include:
- photographs
- historical documents
- company policies
- original art.
Investigations of original source material can reveal whether a particular situation exists or to what extent it does. Investigations can also extract and analyze data from original sources to demonstrate that a particular outcome does occur.

Interviews can be with one person or a small group, also called a focus group. Face-to-face interviews have the advantage of allowing the interviewer to ask follow-up questions during the interview.
- â face-to-face
- by online chat
- developing questions related to the research question and the findings from secondary research
- doing a trial or test of the questions before the actual interview
- deciding on the best methodology for conducting and recording the interview
- making the purpose and use of the data collected well known in advance
- in some cases, providing the interviewee with the questions in advance
- assuring the anonymity of the interviewee if requested
Question design
Questions should be designed to elicit the responses required to help answer the overall research question.
Recording the interview
Another consideration is how to record the response. You must ask the permission of the interviewee if you wish to use any audio-visual equipment during an interview. Please note that electronic submissions are not acceptable, and so an example of a transcript will need to be attached in the appendix. Refer to the section of this guide on appendices for further information.
Surveys can be carried out:
- using a printed form distributed to a defined group of people under controlled conditions
The researcher is better able to control who is responding to the questions when using paper surveys than online.
Successful surveys require:
- knowing from the secondary research what areas need to be investigated
- forming well-stated questions that yield data that can be analyzed
- ttesting the questions before conducting the survey—this is called a pilot study
- assuring anonymity as requested by the participants
- conducting the survey and collecting the data in a well-defined manner
Quantitative vs qualitative data
To collect quantitative data the survey must ask closed or multiple-choice questions. These:
- have a limited number of responses or
- have scale choices or
- require the respondent to prioritize items
To collect qualitative data , the survey must ask open-ended questions, which allow the respondents to write their own answer.
Sample size and selection
When collecting data from groups of people, you must make certain that:
- the sample is large enough to generate meaningful data
- it is clear how and why she or he selected the participants
Considerations for Primary Research
Whichever method is used, your primary research must be well structured and collect data relating to people, events or objects.
The data collected must be:
- measurable or observable
Questions for you to consider are:
- What do you want to find out from your primary research?
- How will this relate to the findings from your secondary research?
- How will the data collected relate to the research question you have posed?
- What is the best method to collect relevant and reliable data and from where?
- Are there any ethical or legal considerations to using a primary method that must be taken into account?
The analysis of primary research includes:
- the analysis of the data collected
- the connections you will make between the different sources of information used—for example, your secondary and primary research
You time should be spent researching:
- what the different primary data collection methods are
- how to use the different methods to obtain reliable results
- how to use the results as evidence to support your essay’s argument.
Ways That Primary Research Can Fail
A badly designed or implemented experiment or investigation will lead to flawed results . The following list indicates some ways that primary research can fail.
- The survey sample is too small or badly controlled so you cannot reach any conclusions relevant to the research question.
- The survey/interview questions do not take proper account of the secondary research findings or the research question, and so do not lead to relevant conclusions.
- using a recording device without the interviewee being aware that the interview was being recorded
- taking photos in prohibited areas
- downloading copyrighted music to demonstrate how it is done.
- A student has used a research method that is not permitted or not appropriate for the subject in which they are submitting their EE.
- << Previous: Conducting Secondary Research
- Next: Writing Your Essay >>
- Last Updated: May 8, 2024 3:48 PM
- URL: https://libguides.westsoundacademy.org/ee
Have a language expert improve your writing
Run a free plagiarism check in 10 minutes, automatically generate references for free.
- Knowledge Base
- Methodology
- Data Collection Methods | Step-by-Step Guide & Examples
Data Collection Methods | Step-by-Step Guide & Examples
Published on 4 May 2022 by Pritha Bhandari .
Data collection is a systematic process of gathering observations or measurements. Whether you are performing research for business, governmental, or academic purposes, data collection allows you to gain first-hand knowledge and original insights into your research problem .
While methods and aims may differ between fields, the overall process of data collection remains largely the same. Before you begin collecting data, you need to consider:
- The aim of the research
- The type of data that you will collect
- The methods and procedures you will use to collect, store, and process the data
To collect high-quality data that is relevant to your purposes, follow these four steps.
Table of contents
Step 1: define the aim of your research, step 2: choose your data collection method, step 3: plan your data collection procedures, step 4: collect the data, frequently asked questions about data collection.
Before you start the process of data collection, you need to identify exactly what you want to achieve. You can start by writing a problem statement : what is the practical or scientific issue that you want to address, and why does it matter?
Next, formulate one or more research questions that precisely define what you want to find out. Depending on your research questions, you might need to collect quantitative or qualitative data :
- Quantitative data is expressed in numbers and graphs and is analysed through statistical methods .
- Qualitative data is expressed in words and analysed through interpretations and categorisations.
If your aim is to test a hypothesis , measure something precisely, or gain large-scale statistical insights, collect quantitative data. If your aim is to explore ideas, understand experiences, or gain detailed insights into a specific context, collect qualitative data.
If you have several aims, you can use a mixed methods approach that collects both types of data.
- Your first aim is to assess whether there are significant differences in perceptions of managers across different departments and office locations.
- Your second aim is to gather meaningful feedback from employees to explore new ideas for how managers can improve.
Prevent plagiarism, run a free check.
Based on the data you want to collect, decide which method is best suited for your research.
- Experimental research is primarily a quantitative method.
- Interviews , focus groups , and ethnographies are qualitative methods.
- Surveys , observations, archival research, and secondary data collection can be quantitative or qualitative methods.
Carefully consider what method you will use to gather data that helps you directly answer your research questions.
When you know which method(s) you are using, you need to plan exactly how you will implement them. What procedures will you follow to make accurate observations or measurements of the variables you are interested in?
For instance, if you’re conducting surveys or interviews, decide what form the questions will take; if you’re conducting an experiment, make decisions about your experimental design .
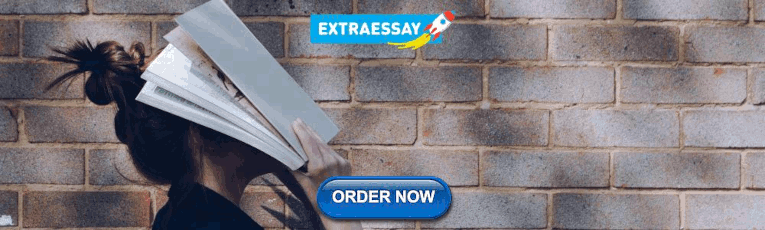
Operationalisation
Sometimes your variables can be measured directly: for example, you can collect data on the average age of employees simply by asking for dates of birth. However, often youâll be interested in collecting data on more abstract concepts or variables that canât be directly observed.
Operationalisation means turning abstract conceptual ideas into measurable observations. When planning how you will collect data, you need to translate the conceptual definition of what you want to study into the operational definition of what you will actually measure.
- You ask managers to rate their own leadership skills on 5-point scales assessing the ability to delegate, decisiveness, and dependability.
- You ask their direct employees to provide anonymous feedback on the managers regarding the same topics.
You may need to develop a sampling plan to obtain data systematically. This involves defining a population , the group you want to draw conclusions about, and a sample, the group you will actually collect data from.
Your sampling method will determine how you recruit participants or obtain measurements for your study. To decide on a sampling method you will need to consider factors like the required sample size, accessibility of the sample, and time frame of the data collection.
Standardising procedures
If multiple researchers are involved, write a detailed manual to standardise data collection procedures in your study.
This means laying out specific step-by-step instructions so that everyone in your research team collects data in a consistent way â for example, by conducting experiments under the same conditions and using objective criteria to record and categorise observations.
This helps ensure the reliability of your data, and you can also use it to replicate the study in the future.
Creating a data management plan
Before beginning data collection, you should also decide how you will organise and store your data.
- If you are collecting data from people, you will likely need to anonymise and safeguard the data to prevent leaks of sensitive information (e.g. names or identity numbers).
- If you are collecting data via interviews or pencil-and-paper formats, you will need to perform transcriptions or data entry in systematic ways to minimise distortion.
- You can prevent loss of data by having an organisation system that is routinely backed up.
Finally, you can implement your chosen methods to measure or observe the variables you are interested in.
The closed-ended questions ask participants to rate their managerâs leadership skills on scales from 1 to 5. The data produced is numerical and can be statistically analysed for averages and patterns.
To ensure that high-quality data is recorded in a systematic way, here are some best practices:
- Record all relevant information as and when you obtain data. For example, note down whether or how lab equipment is recalibrated during an experimental study.
- Double-check manual data entry for errors.
- If you collect quantitative data, you can assess the reliability and validity to get an indication of your data quality.
Data collection is the systematic process by which observations or measurements are gathered in research. It is used in many different contexts by academics, governments, businesses, and other organisations.
When conducting research, collecting original data has significant advantages:
- You can tailor data collection to your specific research aims (e.g., understanding the needs of your consumers or user testing your website).
- You can control and standardise the process for high reliability and validity (e.g., choosing appropriate measurements and sampling methods ).
However, there are also some drawbacks: data collection can be time-consuming, labour-intensive, and expensive. In some cases, it’s more efficient to use secondary data that has already been collected by someone else, but the data might be less reliable.
Quantitative research deals with numbers and statistics, while qualitative research deals with words and meanings.
Quantitative methods allow you to test a hypothesis by systematically collecting and analysing data, while qualitative methods allow you to explore ideas and experiences in depth.
Reliability and validity are both about how well a method measures something:
- Reliability refers to the consistency of a measure (whether the results can be reproduced under the same conditions).
- Validity  refers to the accuracy of a measure (whether the results really do represent what they are supposed to measure).
If you are doing experimental research , you also have to consider the internal and external validity of your experiment.
In mixed methods research , you use both qualitative and quantitative data collection and analysis methods to answer your research question .
Operationalisation means turning abstract conceptual ideas into measurable observations.
For example, the concept of social anxiety isn’t directly observable, but it can be operationally defined in terms of self-rating scores, behavioural avoidance of crowded places, or physical anxiety symptoms in social situations.
Before collecting data , it’s important to consider how you will operationalise the variables that you want to measure.
Cite this Scribbr article
If you want to cite this source, you can copy and paste the citation or click the âCite this Scribbr articleâ button to automatically add the citation to our free Reference Generator.
Bhandari, P. (2022, May 04). Data Collection Methods | Step-by-Step Guide & Examples. Scribbr. Retrieved 3 June 2024, from https://www.scribbr.co.uk/research-methods/data-collection-guide/
Is this article helpful?
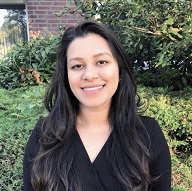
Pritha Bhandari
Other students also liked, qualitative vs quantitative research | examples & methods, triangulation in research | guide, types, examples, what is a conceptual framework | tips & examples.
Academia.edu no longer supports Internet Explorer.
To browse Academia.edu and the wider internet faster and more securely, please take a few seconds to upgrade your browser .
Enter the email address you signed up with and we'll email you a reset link.
- We're Hiring!
- Help Center
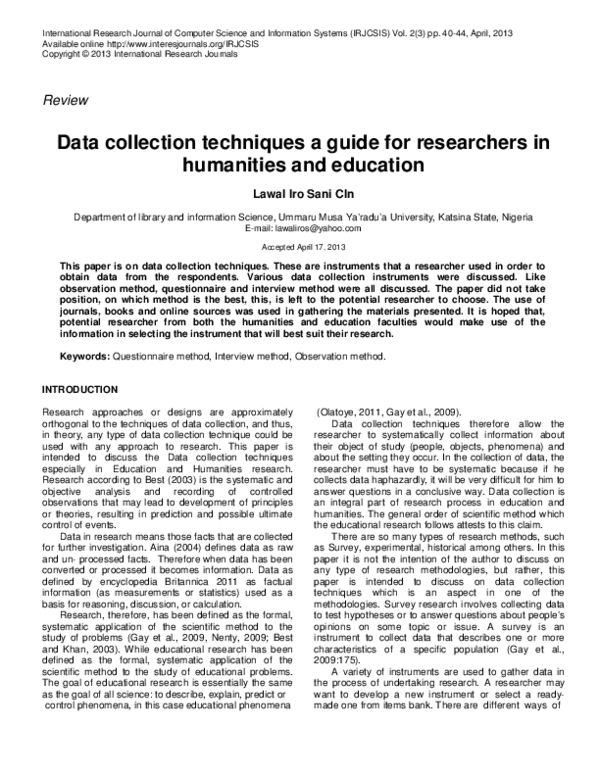
Data collection techniques a guide for researchers in humanities and education

Related Papers
LangLit An International Peer-Reviewed Open Access Journal
nagina S mali
Data collection is most important step in research. The Bloomsbury English Dictionary defines data as âinformation, often in the form of facts or figures obtained from experiments or surveys, used as a basis for making calculations or drawing conclusionsâ. So, we can understand that collection of data is a main task of getting result and conclusions as per the objectives of the research topic. Data collection is the process of gathering data for use in decisionmaking, strategic planning, research and other purposes. For research in science, medicine, higher education and other fields, data collection is often a more specialized process, in which researchers create and implement measures to collect specific sets of data. In both the business and research contexts, though, the collected data must be accurate to ensure that analytics findings and research results are valid. During data collection, the researchers must identify the data types, the sources of data, and what methods are being used. (https://www.techtarget.com/searchcio/definition/data-collection) This data can be collected from educational organization, society, medical science, engineering, experimentation etc. But during collection a data researcher can face some problems and similarly researcher has tried to focus it in this research paper. The third chapter Research methodology/Pan and Procedure of the research study is devoted to the method of research tools and techniques for data collection, population and sample. During this process researcher has to follow ethics in data collection. Objectives of the research paper are 1.To find out the tools being used by B.Ed.-M.Ed.(Integrated) and M.Ed. student teachers for their dissertation research .2.To find out Problems in Data Collection for Research among B.Ed.-M.Ed.(Integrated) and M.Ed. student teachers. Researcher have been collected a data from B.Ed.-M.Ed.(Integrated) and M.Ed. student teachers by survey method with the help of researcher made questionnaire. Collected data is analyzed qualitatively and conclusions are drawn as per the objectives.
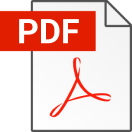
CENTRAL ASIAN JOURNAL OF LITERATURE, PHILOSOPHY AND CULTURE
Urvashi Kaushal
Burcu Akhun
In this article, as data collecting tools interviews and questionnaries used in social science and educational researches were examined. Firstly, the definitions and types of both techiques were discussed. then interwies and questionnaries were compared in terms of various aspects. these comparisions were made regarding information, bias, anonimity and confidentialty, response rate, validity, reliability and data analysis. Finally, the advantages and disadvantages of both techniques were summarised in a table.
Lucky International
Ranjan Kumar Sahoo
Research tools play a most significant role in carrying out research. It helps researchers in the gathering of data with which they are able to test the hypotheses or answer the research questions. There are numerous tools for data collection in educational research, and each tool employs distinctive ways of describing and quantifying the data. One of the widely employed tools for data collection is the interview. It is a purposeful interaction in which one person obtains information from one or more individuals. It is essentially the oral, in-person administration of a questionnaire to each member of the sample. There are many situations in which an interview is the most appropriate as it allows for greater depth of information which is not possible by any other means. In the light of the above, this paper aims to familiarize the researcher with the interview as a data-gathering technique and attempt to develop skills in their use and sensitivity to their effectiveness in specific situations. The intention is also to enable the researchers to choose the most suitable case in which to use Tools fo Data Collection interviews as an instrument for data collection. Furthermore, certain key ideas such as the role of interview along with its various types; guidelines that a researcher should follow while conducting an interview; reliability and validity of an interview; and some of the strengths and limitations of the interview as a data-gathering technique are also set out, so that decisions on their suitability do not become arbitrary and the criterion of fitness for purpose is held high.
Cristina Ispas
The pedagogical research constitutes a critical, dynamic, intentional, active process oriented towards the investigation of the educaional reality under all its aspects. The present paper brings in the forefront the effort of pedagogy to crystalize its own methodology of research in its demarche of affirmation and consolidation of its scientifical status. The complexity and the high number of inter-depedent variables present in the study of the human condition foiled the effort to apply a model of scientific research underlain on the method of natural sciences in the field of social-human sciences. In this context, the pedagogy was bound to shade its methods of research in order to surprise as truthful as possible the complexity and diversity of the educational processes. Thus, besides the empirical-experimental method , the pedagogy was enriched with new methods of research such as the hermeneutic method, the historical method, the rhetorical method, the descriptive method, the...
Seda Khadimally
In the process of designing a solid research study, it is imperative that researchers be aware of the data collection methods within which they are to conduct their study. Sound data collection cannot be performed without the choice of a particular research design best suited for the study, as well as the research questions of the study that need to be answered. In order to conduct a sound research, researchers need to ask right research questions that need to correspond to the problem of their study. Data collection is driven by the design based on which researchers prefer to conduct their study. According to Creswell (2013), this process comprises a âseries of interrelated activities aimed at gathering good information to answer emerging research questionsâ (p. 146). There is an array of data collection methods and whatever research design researchers choose to work with, they should keep in mind that they cannot gather their data in solitude. This means that their study participantsâ role plays a crucial part during data collection. There are also other factors pivotal to collecting data. Creswell (2013) described the entire process as a circle of activities which, when researchers are engaged in, need to be considered through multiple phases such as âlocating the site/individual, gaining access and making rapport, purposefully sampling, collecting data, recording information, resolving field issues, and storing dataâ (p. 146). Researchers should be informed prior to gathering their data that data collection extends beyond conducting interviews with their participants or observing the site, individuals, or groups of people. They should particularly be cognizant of the fact that some of the data collection methods they choose overlap with each other while some considerably differ from one another. With this in mind, the purpose of this paper is to delineate similarities and differences among methods of data collection employed in three different research designs: ethnographic studies, phenomenological studies, and narrative histories. Issues that would lend themselves to these three study types will be addressed, and the challenges that researchers encounter when collecting their data using each type of design will also be discussed.
ASHRAFALSADAT HAKIM
ribwar aljaf
Research, critical thinkers, science philosophy
H. Afandi, Afandi
ABSTRACT Education is the most important item in life. Education could make a change for people. Because education is a toll for opening the world with give mindset and behavior. Education is an activity to take and give about science of the world of science. The activity of education is useful for disseminating scientific information, both research information using trusted references, as well as popular scientific application knowledge information. Education is related to the research. If telling about education it will relate to tell about research. Because they are linking each other. Research is the systematic investigation into and study of materials and sources in order to establish facts and reach new conclusions. Research tool used to collect, measure, and analyze data related object that interest to research. Therefore, many students are surprised to learn and study of research in many fields inquiry. Particularly education is far more conceptual than technical. Educational Research means the collection and analysis of information as entailing systematic, critical and self-critical enquiry which aims to contribute to the advancement of knowledge.
RELATED PAPERS
e-Ciencias de la InformaciĂłn
Omar Antonio Vega
Mario De Beni Arrigoni
Olatunde Durowoju
Health Communication
Aleksandar Mikic
Biochemistry and Molecular Biology Education
Panagiotis Stasinakis
Saudi Journal of Biological Sciences
Zaheer Ul-haq
Collegium antropologicum
İbrahim CicioÄlu
RSC Advances
Mohamed Yusuf , MELISSA LOW PHEY PHEY MKK203014
Revista mĂŠdica de Chile
Ruben Gonzalez
Environmental Health Perspectives
Valerie Brown
Lecture Notes in Computer Science
Alejandro Moreo
Journal of Functional Foods
Francesca Algieri
Familial Cancer
N. Hoogerbrugge
german gordillo
BMC genomics
Rachid Serraj
Cell & Tissue Research
Gordon Warren
Rev Chil Obstet Ginecol
Omar Nazzal
Facial Plastic Surgery
fatih savran
RELATED TOPICS
- We're Hiring!
- Help Center
- Find new research papers in:
- Health Sciences
- Earth Sciences
- Cognitive Science
- Mathematics
- Computer Science
- Academia ©2024

An official website of the United States government
The .gov means itâs official. Federal government websites often end in .gov or .mil. Before sharing sensitive information, make sure youâre on a federal government site.
The site is secure. The https:// ensures that you are connecting to the official website and that any information you provide is encrypted and transmitted securely.
- Publications
- Account settings
Preview improvements coming to the PMC website in October 2024. Learn More or Try it out now .
- Advanced Search
- Journal List
- Can J Hosp Pharm
- v.68(3); May-Jun 2015

Qualitative Research: Data Collection, Analysis, and Management
Introduction.
In an earlier paper, 1 we presented an introduction to using qualitative research methods in pharmacy practice. In this article, we review some principles of the collection, analysis, and management of qualitative data to help pharmacists interested in doing research in their practice to continue their learning in this area. Qualitative research can help researchers to access the thoughts and feelings of research participants, which can enable development of an understanding of the meaning that people ascribe to their experiences. Whereas quantitative research methods can be used to determine how many people undertake particular behaviours, qualitative methods can help researchers to understand how and why such behaviours take place. Within the context of pharmacy practice research, qualitative approaches have been used to examine a diverse array of topics, including the perceptions of key stakeholders regarding prescribing by pharmacists and the postgraduation employment experiences of young pharmacists (see “Further Reading” section at the end of this article).
In the previous paper, 1 we outlined 3 commonly used methodologies: ethnography 2 , grounded theory 3 , and phenomenology. 4 Briefly, ethnography involves researchers using direct observation to study participants in their “real life” environment, sometimes over extended periods. Grounded theory and its later modified versions (e.g., Strauss and Corbin 5 ) use face-to-face interviews and interactions such as focus groups to explore a particular research phenomenon and may help in clarifying a less-well-understood problem, situation, or context. Phenomenology shares some features with grounded theory (such as an exploration of participants’ behaviour) and uses similar techniques to collect data, but it focuses on understanding how human beings experience their world. It gives researchers the opportunity to put themselves in another person’s shoes and to understand the subjective experiences of participants. 6 Some researchers use qualitative methodologies but adopt a different standpoint, and an example of this appears in the work of Thurston and others, 7 discussed later in this paper.
Qualitative work requires reflection on the part of researchers, both before and during the research process, as a way of providing context and understanding for readers. When being reflexive, researchers should not try to simply ignore or avoid their own biases (as this would likely be impossible); instead, reflexivity requires researchers to reflect upon and clearly articulate their position and subjectivities (world view, perspectives, biases), so that readers can better understand the filters through which questions were asked, data were gathered and analyzed, and findings were reported. From this perspective, bias and subjectivity are not inherently negative but they are unavoidable; as a result, it is best that they be articulated up-front in a manner that is clear and coherent for readers.
THE PARTICIPANT’S VIEWPOINT
What qualitative study seeks to convey is why people have thoughts and feelings that might affect the way they behave. Such study may occur in any number of contexts, but here, we focus on pharmacy practice and the way people behave with regard to medicines use (e.g., to understand patients’ reasons for nonadherence with medication therapy or to explore physicians’ resistance to pharmacists’ clinical suggestions). As we suggested in our earlier article, 1 an important point about qualitative research is that there is no attempt to generalize the findings to a wider population. Qualitative research is used to gain insights into people’s feelings and thoughts, which may provide the basis for a future stand-alone qualitative study or may help researchers to map out survey instruments for use in a quantitative study. It is also possible to use different types of research in the same study, an approach known as “mixed methods” research, and further reading on this topic may be found at the end of this paper.
The role of the researcher in qualitative research is to attempt to access the thoughts and feelings of study participants. This is not an easy task, as it involves asking people to talk about things that may be very personal to them. Sometimes the experiences being explored are fresh in the participant’s mind, whereas on other occasions reliving past experiences may be difficult. However the data are being collected, a primary responsibility of the researcher is to safeguard participants and their data. Mechanisms for such safeguarding must be clearly articulated to participants and must be approved by a relevant research ethics review board before the research begins. Researchers and practitioners new to qualitative research should seek advice from an experienced qualitative researcher before embarking on their project.
DATA COLLECTION
Whatever philosophical standpoint the researcher is taking and whatever the data collection method (e.g., focus group, one-to-one interviews), the process will involve the generation of large amounts of data. In addition to the variety of study methodologies available, there are also different ways of making a record of what is said and done during an interview or focus group, such as taking handwritten notes or video-recording. If the researcher is audio- or video-recording data collection, then the recordings must be transcribed verbatim before data analysis can begin. As a rough guide, it can take an experienced researcher/transcriber 8 hours to transcribe one 45-minute audio-recorded interview, a process than will generate 20–30 pages of written dialogue.
Many researchers will also maintain a folder of “field notes” to complement audio-taped interviews. Field notes allow the researcher to maintain and comment upon impressions, environmental contexts, behaviours, and nonverbal cues that may not be adequately captured through the audio-recording; they are typically handwritten in a small notebook at the same time the interview takes place. Field notes can provide important context to the interpretation of audio-taped data and can help remind the researcher of situational factors that may be important during data analysis. Such notes need not be formal, but they should be maintained and secured in a similar manner to audio tapes and transcripts, as they contain sensitive information and are relevant to the research. For more information about collecting qualitative data, please see the “Further Reading” section at the end of this paper.
DATA ANALYSIS AND MANAGEMENT
If, as suggested earlier, doing qualitative research is about putting oneself in another person’s shoes and seeing the world from that person’s perspective, the most important part of data analysis and management is to be true to the participants. It is their voices that the researcher is trying to hear, so that they can be interpreted and reported on for others to read and learn from. To illustrate this point, consider the anonymized transcript excerpt presented in Appendix 1 , which is taken from a research interview conducted by one of the authors (J.S.). We refer to this excerpt throughout the remainder of this paper to illustrate how data can be managed, analyzed, and presented.
Interpretation of Data
Interpretation of the data will depend on the theoretical standpoint taken by researchers. For example, the title of the research report by Thurston and others, 7 “Discordant indigenous and provider frames explain challenges in improving access to arthritis care: a qualitative study using constructivist grounded theory,” indicates at least 2 theoretical standpoints. The first is the culture of the indigenous population of Canada and the place of this population in society, and the second is the social constructivist theory used in the constructivist grounded theory method. With regard to the first standpoint, it can be surmised that, to have decided to conduct the research, the researchers must have felt that there was anecdotal evidence of differences in access to arthritis care for patients from indigenous and non-indigenous backgrounds. With regard to the second standpoint, it can be surmised that the researchers used social constructivist theory because it assumes that behaviour is socially constructed; in other words, people do things because of the expectations of those in their personal world or in the wider society in which they live. (Please see the “Further Reading” section for resources providing more information about social constructivist theory and reflexivity.) Thus, these 2 standpoints (and there may have been others relevant to the research of Thurston and others 7 ) will have affected the way in which these researchers interpreted the experiences of the indigenous population participants and those providing their care. Another standpoint is feminist standpoint theory which, among other things, focuses on marginalized groups in society. Such theories are helpful to researchers, as they enable us to think about things from a different perspective. Being aware of the standpoints you are taking in your own research is one of the foundations of qualitative work. Without such awareness, it is easy to slip into interpreting other people’s narratives from your own viewpoint, rather than that of the participants.
To analyze the example in Appendix 1 , we will adopt a phenomenological approach because we want to understand how the participant experienced the illness and we want to try to see the experience from that person’s perspective. It is important for the researcher to reflect upon and articulate his or her starting point for such analysis; for example, in the example, the coder could reflect upon her own experience as a female of a majority ethnocultural group who has lived within middle class and upper middle class settings. This personal history therefore forms the filter through which the data will be examined. This filter does not diminish the quality or significance of the analysis, since every researcher has his or her own filters; however, by explicitly stating and acknowledging what these filters are, the researcher makes it easer for readers to contextualize the work.
Transcribing and Checking
For the purposes of this paper it is assumed that interviews or focus groups have been audio-recorded. As mentioned above, transcribing is an arduous process, even for the most experienced transcribers, but it must be done to convert the spoken word to the written word to facilitate analysis. For anyone new to conducting qualitative research, it is beneficial to transcribe at least one interview and one focus group. It is only by doing this that researchers realize how difficult the task is, and this realization affects their expectations when asking others to transcribe. If the research project has sufficient funding, then a professional transcriber can be hired to do the work. If this is the case, then it is a good idea to sit down with the transcriber, if possible, and talk through the research and what the participants were talking about. This background knowledge for the transcriber is especially important in research in which people are using jargon or medical terms (as in pharmacy practice). Involving your transcriber in this way makes the work both easier and more rewarding, as he or she will feel part of the team. Transcription editing software is also available, but it is expensive. For example, ELAN (more formally known as EUDICO Linguistic Annotator, developed at the Technical University of Berlin) 8 is a tool that can help keep data organized by linking media and data files (particularly valuable if, for example, video-taping of interviews is complemented by transcriptions). It can also be helpful in searching complex data sets. Products such as ELAN do not actually automatically transcribe interviews or complete analyses, and they do require some time and effort to learn; nonetheless, for some research applications, it may be a valuable to consider such software tools.
All audio recordings should be transcribed verbatim, regardless of how intelligible the transcript may be when it is read back. Lines of text should be numbered. Once the transcription is complete, the researcher should read it while listening to the recording and do the following: correct any spelling or other errors; anonymize the transcript so that the participant cannot be identified from anything that is said (e.g., names, places, significant events); insert notations for pauses, laughter, looks of discomfort; insert any punctuation, such as commas and full stops (periods) (see Appendix 1 for examples of inserted punctuation), and include any other contextual information that might have affected the participant (e.g., temperature or comfort of the room).
Dealing with the transcription of a focus group is slightly more difficult, as multiple voices are involved. One way of transcribing such data is to “tag” each voice (e.g., Voice A, Voice B). In addition, the focus group will usually have 2 facilitators, whose respective roles will help in making sense of the data. While one facilitator guides participants through the topic, the other can make notes about context and group dynamics. More information about group dynamics and focus groups can be found in resources listed in the “Further Reading” section.
Reading between the Lines
During the process outlined above, the researcher can begin to get a feel for the participant’s experience of the phenomenon in question and can start to think about things that could be pursued in subsequent interviews or focus groups (if appropriate). In this way, one participant’s narrative informs the next, and the researcher can continue to interview until nothing new is being heard or, as it says in the text books, “saturation is reached”. While continuing with the processes of coding and theming (described in the next 2 sections), it is important to consider not just what the person is saying but also what they are not saying. For example, is a lengthy pause an indication that the participant is finding the subject difficult, or is the person simply deciding what to say? The aim of the whole process from data collection to presentation is to tell the participants’ stories using exemplars from their own narratives, thus grounding the research findings in the participants’ lived experiences.
Smith 9 suggested a qualitative research method known as interpretative phenomenological analysis, which has 2 basic tenets: first, that it is rooted in phenomenology, attempting to understand the meaning that individuals ascribe to their lived experiences, and second, that the researcher must attempt to interpret this meaning in the context of the research. That the researcher has some knowledge and expertise in the subject of the research means that he or she can have considerable scope in interpreting the participant’s experiences. Larkin and others 10 discussed the importance of not just providing a description of what participants say. Rather, interpretative phenomenological analysis is about getting underneath what a person is saying to try to truly understand the world from his or her perspective.
Once all of the research interviews have been transcribed and checked, it is time to begin coding. Field notes compiled during an interview can be a useful complementary source of information to facilitate this process, as the gap in time between an interview, transcribing, and coding can result in memory bias regarding nonverbal or environmental context issues that may affect interpretation of data.
Coding refers to the identification of topics, issues, similarities, and differences that are revealed through the participants’ narratives and interpreted by the researcher. This process enables the researcher to begin to understand the world from each participant’s perspective. Coding can be done by hand on a hard copy of the transcript, by making notes in the margin or by highlighting and naming sections of text. More commonly, researchers use qualitative research software (e.g., NVivo, QSR International Pty Ltd; www.qsrinternational.com/products_nvivo.aspx ) to help manage their transcriptions. It is advised that researchers undertake a formal course in the use of such software or seek supervision from a researcher experienced in these tools.
Returning to Appendix 1 and reading from lines 8–11, a code for this section might be “diagnosis of mental health condition”, but this would just be a description of what the participant is talking about at that point. If we read a little more deeply, we can ask ourselves how the participant might have come to feel that the doctor assumed he or she was aware of the diagnosis or indeed that they had only just been told the diagnosis. There are a number of pauses in the narrative that might suggest the participant is finding it difficult to recall that experience. Later in the text, the participant says “nobody asked me any questions about my life” (line 19). This could be coded simply as “health care professionals’ consultation skills”, but that would not reflect how the participant must have felt never to be asked anything about his or her personal life, about the participant as a human being. At the end of this excerpt, the participant just trails off, recalling that no-one showed any interest, which makes for very moving reading. For practitioners in pharmacy, it might also be pertinent to explore the participant’s experience of akathisia and why this was left untreated for 20 years.
One of the questions that arises about qualitative research relates to the reliability of the interpretation and representation of the participants’ narratives. There are no statistical tests that can be used to check reliability and validity as there are in quantitative research. However, work by Lincoln and Guba 11 suggests that there are other ways to “establish confidence in the ‘truth’ of the findings” (p. 218). They call this confidence “trustworthiness” and suggest that there are 4 criteria of trustworthiness: credibility (confidence in the “truth” of the findings), transferability (showing that the findings have applicability in other contexts), dependability (showing that the findings are consistent and could be repeated), and confirmability (the extent to which the findings of a study are shaped by the respondents and not researcher bias, motivation, or interest).
One way of establishing the “credibility” of the coding is to ask another researcher to code the same transcript and then to discuss any similarities and differences in the 2 resulting sets of codes. This simple act can result in revisions to the codes and can help to clarify and confirm the research findings.
Theming refers to the drawing together of codes from one or more transcripts to present the findings of qualitative research in a coherent and meaningful way. For example, there may be examples across participants’ narratives of the way in which they were treated in hospital, such as “not being listened to” or “lack of interest in personal experiences” (see Appendix 1 ). These may be drawn together as a theme running through the narratives that could be named “the patient’s experience of hospital care”. The importance of going through this process is that at its conclusion, it will be possible to present the data from the interviews using quotations from the individual transcripts to illustrate the source of the researchers’ interpretations. Thus, when the findings are organized for presentation, each theme can become the heading of a section in the report or presentation. Underneath each theme will be the codes, examples from the transcripts, and the researcher’s own interpretation of what the themes mean. Implications for real life (e.g., the treatment of people with chronic mental health problems) should also be given.
DATA SYNTHESIS
In this final section of this paper, we describe some ways of drawing together or “synthesizing” research findings to represent, as faithfully as possible, the meaning that participants ascribe to their life experiences. This synthesis is the aim of the final stage of qualitative research. For most readers, the synthesis of data presented by the researcher is of crucial significance—this is usually where “the story” of the participants can be distilled, summarized, and told in a manner that is both respectful to those participants and meaningful to readers. There are a number of ways in which researchers can synthesize and present their findings, but any conclusions drawn by the researchers must be supported by direct quotations from the participants. In this way, it is made clear to the reader that the themes under discussion have emerged from the participants’ interviews and not the mind of the researcher. The work of Latif and others 12 gives an example of how qualitative research findings might be presented.
Planning and Writing the Report
As has been suggested above, if researchers code and theme their material appropriately, they will naturally find the headings for sections of their report. Qualitative researchers tend to report “findings” rather than “results”, as the latter term typically implies that the data have come from a quantitative source. The final presentation of the research will usually be in the form of a report or a paper and so should follow accepted academic guidelines. In particular, the article should begin with an introduction, including a literature review and rationale for the research. There should be a section on the chosen methodology and a brief discussion about why qualitative methodology was most appropriate for the study question and why one particular methodology (e.g., interpretative phenomenological analysis rather than grounded theory) was selected to guide the research. The method itself should then be described, including ethics approval, choice of participants, mode of recruitment, and method of data collection (e.g., semistructured interviews or focus groups), followed by the research findings, which will be the main body of the report or paper. The findings should be written as if a story is being told; as such, it is not necessary to have a lengthy discussion section at the end. This is because much of the discussion will take place around the participants’ quotes, such that all that is needed to close the report or paper is a summary, limitations of the research, and the implications that the research has for practice. As stated earlier, it is not the intention of qualitative research to allow the findings to be generalized, and therefore this is not, in itself, a limitation.
Planning out the way that findings are to be presented is helpful. It is useful to insert the headings of the sections (the themes) and then make a note of the codes that exemplify the thoughts and feelings of your participants. It is generally advisable to put in the quotations that you want to use for each theme, using each quotation only once. After all this is done, the telling of the story can begin as you give your voice to the experiences of the participants, writing around their quotations. Do not be afraid to draw assumptions from the participants’ narratives, as this is necessary to give an in-depth account of the phenomena in question. Discuss these assumptions, drawing on your participants’ words to support you as you move from one code to another and from one theme to the next. Finally, as appropriate, it is possible to include examples from literature or policy documents that add support for your findings. As an exercise, you may wish to code and theme the sample excerpt in Appendix 1 and tell the participant’s story in your own way. Further reading about “doing” qualitative research can be found at the end of this paper.
CONCLUSIONS
Qualitative research can help researchers to access the thoughts and feelings of research participants, which can enable development of an understanding of the meaning that people ascribe to their experiences. It can be used in pharmacy practice research to explore how patients feel about their health and their treatment. Qualitative research has been used by pharmacists to explore a variety of questions and problems (see the “Further Reading” section for examples). An understanding of these issues can help pharmacists and other health care professionals to tailor health care to match the individual needs of patients and to develop a concordant relationship. Doing qualitative research is not easy and may require a complete rethink of how research is conducted, particularly for researchers who are more familiar with quantitative approaches. There are many ways of conducting qualitative research, and this paper has covered some of the practical issues regarding data collection, analysis, and management. Further reading around the subject will be essential to truly understand this method of accessing peoples’ thoughts and feelings to enable researchers to tell participants’ stories.
Appendix 1.âExcerpt from a sample transcript
The participant (age late 50s) had suffered from a chronic mental health illness for 30 years. The participant had become a “revolving door patient,” someone who is frequently in and out of hospital. As the participant talked about past experiences, the researcher asked:
- What was treatment like 30 years ago?
- Umm—well it was pretty much they could do what they wanted with you because I was put into the er, the er kind of system er, I was just on
- endless section threes.
- Really…
- But what I didn’t realize until later was that if you haven’t actually posed a threat to someone or yourself they can’t really do that but I didn’t know
- that. So wh-when I first went into hospital they put me on the forensic ward ’cause they said, “We don’t think you’ll stay here we think you’ll just
- run-run away.” So they put me then onto the acute admissions ward and – er – I can remember one of the first things I recall when I got onto that
- ward was sitting down with a er a Dr XXX. He had a book this thick [gestures] and on each page it was like three questions and he went through
- all these questions and I answered all these questions. So we’re there for I don’t maybe two hours doing all that and he asked me he said “well
- when did somebody tell you then that you have schizophrenia” I said “well nobody’s told me that” so he seemed very surprised but nobody had
- actually [pause] whe-when I first went up there under police escort erm the senior kind of consultants people I’d been to where I was staying and
- ermm so er [pause] I . . . the, I can remember the very first night that I was there and given this injection in this muscle here [gestures] and just
- having dreadful side effects the next day I woke up [pause]
- . . . and I suffered that akathesia I swear to you, every minute of every day for about 20 years.
- Oh how awful.
- And that side of it just makes life impossible so the care on the wards [pause] umm I don’t know it’s kind of, it’s kind of hard to put into words
- [pause]. Because I’m not saying they were sort of like not friendly or interested but then nobody ever seemed to want to talk about your life [pause]
- nobody asked me any questions about my life. The only questions that came into was they asked me if I’d be a volunteer for these student exams
- and things and I said “yeah” so all the questions were like “oh what jobs have you done,” er about your relationships and things and er but
- nobody actually sat down and had a talk and showed some interest in you as a person you were just there basically [pause] um labelled and you
- know there was there was [pause] but umm [pause] yeah . . .
This article is the 10th in the CJHP Research Primer Series, an initiative of the CJHP Editorial Board and the CSHP Research Committee. The planned 2-year series is intended to appeal to relatively inexperienced researchers, with the goal of building research capacity among practising pharmacists. The articles, presenting simple but rigorous guidance to encourage and support novice researchers, are being solicited from authors with appropriate expertise.
Previous articles in this series:
Bond CM. The research jigsaw: how to get started. Can J Hosp Pharm . 2014;67(1):28–30.
Tully MP. Research: articulating questions, generating hypotheses, and choosing study designs. Can J Hosp Pharm . 2014;67(1):31–4.
Loewen P. Ethical issues in pharmacy practice research: an introductory guide. Can J Hosp Pharm. 2014;67(2):133–7.
Tsuyuki RT. Designing pharmacy practice research trials. Can J Hosp Pharm . 2014;67(3):226–9.
Bresee LC. An introduction to developing surveys for pharmacy practice research. Can J Hosp Pharm . 2014;67(4):286–91.
Gamble JM. An introduction to the fundamentals of cohort and case–control studies. Can J Hosp Pharm . 2014;67(5):366–72.
Austin Z, Sutton J. Qualitative research: getting started. C an J Hosp Pharm . 2014;67(6):436–40.
Houle S. An introduction to the fundamentals of randomized controlled trials in pharmacy research. Can J Hosp Pharm . 2014; 68(1):28–32.
Charrois TL. Systematic reviews: What do you need to know to get started? Can J Hosp Pharm . 2014;68(2):144–8.
Competing interests: None declared.
Further Reading
Examples of qualitative research in pharmacy practice.
- Farrell B, Pottie K, Woodend K, Yao V, Dolovich L, Kennie N, et al. Shifts in expectations: evaluating physicians’ perceptions as pharmacists integrated into family practice. J Interprof Care. 2010; 24 (1):80â9. [ PubMed ] [ Google Scholar ]
- Gregory P, Austin Z. Postgraduation employment experiences of new pharmacists in Ontario in 2012–2013. Can Pharm J. 2014; 147 (5):290â9. [ PMC free article ] [ PubMed ] [ Google Scholar ]
- Marks PZ, Jennnings B, Farrell B, Kennie-Kaulbach N, Jorgenson D, Pearson-Sharpe J, et al. “I gained a skill and a change in attitude”: a case study describing how an online continuing professional education course for pharmacists supported achievement of its transfer to practice outcomes. Can J Univ Contin Educ. 2014; 40 (2):1â18. [ Google Scholar ]
- Nair KM, Dolovich L, Brazil K, Raina P. It’s all about relationships: a qualitative study of health researchers’ perspectives on interdisciplinary research. BMC Health Serv Res. 2008; 8 :110. [ PMC free article ] [ PubMed ] [ Google Scholar ]
- Pojskic N, MacKeigan L, Boon H, Austin Z. Initial perceptions of key stakeholders in Ontario regarding independent prescriptive authority for pharmacists. Res Soc Adm Pharm. 2014; 10 (2):341â54. [ PubMed ] [ Google Scholar ]
Qualitative Research in General
- Breakwell GM, Hammond S, Fife-Schaw C. Research methods in psychology. Thousand Oaks (CA): Sage Publications; 1995. [ Google Scholar ]
- Given LM. 100 questions (and answers) about qualitative research. Thousand Oaks (CA): Sage Publications; 2015. [ Google Scholar ]
- Miles B, Huberman AM. Qualitative data analysis. Thousand Oaks (CA): Sage Publications; 2009. [ Google Scholar ]
- Patton M. Qualitative research and evaluation methods. Thousand Oaks (CA): Sage Publications; 2002. [ Google Scholar ]
- Willig C. Introducing qualitative research in psychology. Buckingham (UK): Open University Press; 2001. [ Google Scholar ]
Group Dynamics in Focus Groups
- Farnsworth J, Boon B. Analysing group dynamics within the focus group. Qual Res. 2010; 10 (5):605â24. [ Google Scholar ]
Social Constructivism
- Social constructivism. Berkeley (CA): University of California, Berkeley, Berkeley Graduate Division, Graduate Student Instruction Teaching & Resource Center; [cited 2015 June 4]. Available from: http://gsi.berkeley.edu/gsi-guide-contents/learning-theory-research/social-constructivism/ [ Google Scholar ]
Mixed Methods
- Creswell J. Research design: qualitative, quantitative, and mixed methods approaches. Thousand Oaks (CA): Sage Publications; 2009. [ Google Scholar ]
Collecting Qualitative Data
- Arksey H, Knight P. Interviewing for social scientists: an introductory resource with examples. Thousand Oaks (CA): Sage Publications; 1999. [ Google Scholar ]
- Guest G, Namey EE, Mitchel ML. Collecting qualitative data: a field manual for applied research. Thousand Oaks (CA): Sage Publications; 2013. [ Google Scholar ]
Constructivist Grounded Theory
- Charmaz K. Grounded theory: objectivist and constructivist methods. In: Denzin N, Lincoln Y, editors. Handbook of qualitative research. 2nd ed. Thousand Oaks (CA): Sage Publications; 2000. pp. 509â35. [ Google Scholar ]
- Undergraduate
- High School
- Architecture
- American History
- Asian History
- Antique Literature
- American Literature
- Asian Literature
- Classic English Literature
- World Literature
- Creative Writing
- Linguistics
- Criminal Justice
- Legal Issues
- Anthropology
- Archaeology
- Political Science
- World Affairs
- African-American Studies
- East European Studies
- Latin-American Studies
- Native-American Studies
- West European Studies
- Family and Consumer Science
- Social Issues
- Women and Gender Studies
- Social Work
- Natural Sciences
- Pharmacology
- Earth science
- Agriculture
- Agricultural Studies
- Computer Science
- IT Management
- Mathematics
- Investments
- Engineering and Technology
- Engineering
- Aeronautics
- Medicine and Health
- Alternative Medicine
- Communications and Media
- Advertising
- Communication Strategies
- Public Relations
- Educational Theories
- Teacher's Career
- Chicago/Turabian
- Company Analysis
- Education Theories
- Shakespeare
- Canadian Studies
- Food Safety
- Relation of Global Warming and Extreme Weather Condition
- Movie Review
- Admission Essay
- Annotated Bibliography
- Application Essay
- Article Critique
- Article Review
- Article Writing
- Book Review
- Business Plan
- Business Proposal
- Capstone Project
- Cover Letter
- Creative Essay
- Dissertation
- Dissertation - Abstract
- Dissertation - Conclusion
- Dissertation - Discussion
- Dissertation - Hypothesis
- Dissertation - Introduction
- Dissertation - Literature
- Dissertation - Methodology
- Dissertation - Results
- GCSE Coursework
- Grant Proposal
- Marketing Plan
- Multiple Choice Quiz
- Personal Statement
- Power Point Presentation
- Power Point Presentation With Speaker Notes
- Questionnaire
- Reaction Paper
Research Paper
- Research Proposal
- SWOT analysis
- Thesis Paper
- Online Quiz
- Literature Review
- Movie Analysis
- Statistics problem
- Math Problem
- All papers examples
- How It Works
- Money Back Policy
- Terms of Use
- Privacy Policy
- We Are Hiring
Data Collection, Essay Example
Pages: 1
Words: 293
Hire a Writer for Custom Essay
Use 10% Off Discount: "custom10" in 1 Click đ
You are free to use it as an inspiration or a source for your own work.
We will use multiple methods to collect the information we need for the project. We have already interviewed a member of the technology department involved in implementing the change. We have already procured a document outlining the strategy to be used in the State Street from the technology department. Besides, we have collected background information from State Street official website and Google website. Moreover, we searched various articles and some videos on TED website. We will create a survey for the employees who will be implementing the changes; management has already agreed to facilitate this. The survey may be in the form of online or in person.This research will focus on three aspects, strategic, cultural and political lens.
Through this particular procedure, the collection of important information would specifically define the consistency of situations that define the current effect of the website in relation to condition of the perception of the employees towards the major operations that are supposed to be achieved by the organization through this online portal of communication. The three aspects that define the research shall distinctively indicate a more descriptive approach in preparing the data that could identify the capacity of the website to perform the function it is expected to complete. It shall relatively measure the strategic points of the organization as they are accepted and absorbed by the pubic whom the organization hopes to serve. The cultural lens shall also be observed and accounted for relating to how the conditions of the company is developed through reconstructing the operational culture of its members [which directly affects how the external stakeholders of the organization are served]. Constructively, this aspect shall also provide a distinct indication on how the political structure of the organization is affected based on general approach.
Stuck with your Essay?
Get in touch with one of our experts for instant help!
Accounting of Coach Company, Research Paper Example
The Poem âPowerâ by Audre Lorde, Essay Example
Time is precious
donât waste it!
Plagiarism-free guarantee
Privacy guarantee
Secure checkout
Money back guarantee
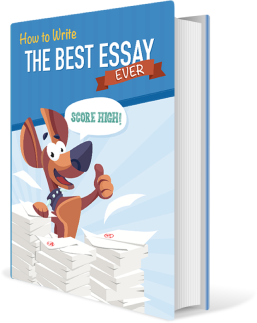
Related Essay Samples & Examples
Voting as a civic responsibility, essay example.
Words: 287
Utilitarianism and Its Applications, Essay Example
Words: 356
The Age-Related Changes of the Older Person, Essay Example
Pages: 2
Words: 448
The Problems ESOL Teachers Face, Essay Example
Pages: 8
Words: 2293
Should English Be the Primary Language? Essay Example
Pages: 4
Words: 999
The Term âSocial Construction of Realityâ, Essay Example
Words: 371
What is Data Collection? self.__wrap_n!=1&&self.__wrap_b(":Rhj6daiukm:",1)

Craig Dennis
May 22, 2024

This is just one example of using data to power your business, where analysis is used to inform decisions made within a business. However, you need to collect data before using it. Accenture reports that 57% of marketing executives donât have the necessary data. This is because companies use inefficient ways to collect data, preventing them from getting insights into downstream tools.
In this article, weâll discuss:
- Why is data collection important
- What type of data can you collect
- Data collection tools
- Data collection use cases
- How to unlock these use cases
What is Data Collection?
Data collection is the process of gathering customer data from internal or external data sources and storing it in a single location, such as a data warehouse or data lake to unlock and uncover critical insights about your customers. You can collect data through customer surveys, an event collection tool, or extract it from existing tools like a Customer Relationship Management (CRM) or an Email Service Provider (ESP). Storing this data in a single location makes it easy to perform analytics and use your data to drive meaningful outcomes that positively impact your bottom line.
Why is Data Collection Important?
The simple act of collecting data is just one piece of the puzzle; it's the outcomes you can power from your data that make data collection valuable. Data collection is the underpinning that powers all data use cases, specifically analytics and activation, so that you can unlock value for your business.
Analytics aims to provide high-level insights into your business, helping you understand performance within different initiatives and make decisions. Based on the aspect being focused on, you can split analytics into different categories.
- Business intelligence (BI) helps you understand your business's health. It can reveal what products are selling, your geographic revenue growth, and your KPIs. It can also show where certain aspects of your business might be failing or need attention and strategize ways to improve them. For example, you could run a report to see how well your product lines sell in specific regions and run targeted promotions for those underperforming regions.
- Consumer insights uncover consumer trends and shifts in the competitive landscape. They help predict future market trends, understand competitors' strengths and weaknesses, and understand consumers' purchasing decisions. Market analytics provides you with the information to stay current with your customers' needs and even predict what they might be. For example, you can monitor trends in technology improvements and consumer preference for new features, then prioritize research and development on the most in-demand features to capture this customer interest.
- Product analytics analyzes behavioral data of users interacting with your product so you can drive users to get the maximum value from your product. These analytics help to inform product decisions that optimize the user experience. For example, through product analytics, you might notice that many users drop off during the onboarding process. You could use analytics to identify any friction points and implement changes to streamline the process.
Activation is taking action on the insights learned from your analytics by sending relevant data into your marketing tools. This step often involves manual work and can be a sticking point for businesses. However, itâs the one true way to get value from your data and analytics. Here are some of the use cases that are possible through activation.
- Personalization : By gaining a clear understanding of your customers through data like browsing history, purchases, and predication-based data science models, you can use that data to personalize your campaigns or website and create a more relevant experience for customers that will increase conversions. For example, you could use a customerâs browsing history to personalize your homepage with items similar to what theyâve been browsing.
- Advertising : Knowing which of your customers are of greatest value to you means creating audiences you can leverage for different advertising campaigns, such as lookalike , retargeting, and suppression audiences. For example, you could create an audience of high-value users and send that to an ad platform to create a lookalike audience with similar traits to increase the quality of your user acquisitions.
- Automation : Most teams use specific tools that can be isolated from other data within your business. Activation can automate sending relevant data to your sales, customer service, and finance teams through activation. For example, you could send customer lead scores to Salesforce so the sales team can prioritize those high-value customers.
What Types of Data Can You Collect?
You can collect three types of data through data collection. They are categorized as:
- First-party is data youâve collected directly from the source. You can collect this data through website activity, survey results, or customer interactions.
- Second-party is someone elseâs first-party data, like a partner company, that has been shared with you. This data is typically shared through a data clean room .
- Third-party is data youâve sourced from an external provider. It could be data from a data enrichment provider or public data sources.
How Does Data Collection Work?
Much goes into data collection, but fundamentally, the framework is the same across organizations, regardless of size or data volume. You need to employ four critical steps to collect data: identify your data sources, collect or extract data from your sources, govern the data youâre collecting, and centralize your data in a single, secure, and accessible location.
1. Identify Your Data Sources
The first step before you start data collection is to identify all of your data sources. There are a range of different sources from which you can collect data, but you can group them into the following two categories: offline data and online data. The difference between online and offline data is that you collect online data through internet-connected devices. In contrast, offline data is collected physically and doesnât involve the internet.
2. Collect or Extract Data From Your Data Sources
The second step is collecting or extracting the data from your data sources. There are three major ways you can do this.
- Event Tracking : You need a form of event tracking to capture users' behavior on your website or mobile app. Event tracking can help you track events such as page views, button clicks, add-to-carts, or user logins. It can help you understand your users' behaviors and unlock use cases like audience targeting, personalization, analytics, experimentation, and attribution.
- Manual Collection : This method involves interacting directly with customers and collecting their data. You can do this through surveys and questionnaires, which gives you better control over the data you collect because you create the questions yourself. Itâs a great way of gathering customer feedback and insights using surveys like Net Promoter Score (NPS) or Customer Satisfaction Score (CSAT).
- ETL : ETL (Extract, Transform, and Load) is a process that data engineers use to extract data from a source and push it to a warehouse for analytics and modeling. A more modern approach to ETL companies adopt is ELT, which is similar, but data is transformed in the warehouse. You can either build your own pipelines through API integrations or use a tool like Fivetran.
3. Govern the Data Youâre Collecting
The third step is to be conscious of your data quality. Bad data quality can introduce multiple problems in your business: incorrect analysis, bad personalization, inaccurate recommendations, failed AI models, and more.
Using data contracts is one method to ensure youâre collecting the right data. Data contracts are formal agreements that can help you structure your data before sending it to other systems. You specify the data format you will capture and declare its properties, such as whether the user_id should be a string or the value should be empty. Any data that doesn't fit gets flagged with your enforcement rules and dealt with accordingly. Data contracts help you to be more confident in your data as you know it's being captured at the source correctly. It can also save you time by not having to retroactively amend any event data that's been collected due to an error in the event collection setup.
4. Centralize Your Data in a Single Source of Truth
The last step is to use a data warehouse, where the single source of truth should live. It's one of the most cost-effective and flexible places to store data. Data storage is inexpensive, so it can handle terabytes of data at a considerable cost. It also allows you to model your data however you want, regardless of its form. Itâs the only true place to store all your data and use it to power your analytics and activation use cases.
Data Collection Tools
Itâs possible to create your own solutions for collecting data, however, unless your business has unique challenges, itâs more worthwhile to use a tool instead. Here are a selection of data collection tools you could use.
Behavioral Data Tools
Behavioral data tools help you to collect and analyze user interactions across various platforms, such as websites, mobile apps, and IoT devices. They help you understand user behavior and track events.
- Hightouch Events gathers and stores event data directly in your data warehouse. The tool tracks Software Development Kits (SDKs), which you can install across web, mobile, and server-side languages. Itâs easy to rip and replace with other leading event collection tools and provides better data quality through Data Contracts.
- Snowplow is an open-source analytics and data collection platform that enables clickstream data collection. The platform's open-source nature means it's more customizable and extensible, and you can get started for free. Snowplow offers many capabilities but can be difficult to implement and maintain.
ETL tools help you integrate and manage data from multiple sources into a centralized data warehouse. These tools automate the process of extracting data, transforming it into a suitable format, and loading it into a data warehouse.
- Fivetran provides fully managed pipelines that automatically handle the ETL tasks and ensure data is up-to-date. The platform is designed to be easy to use, focusing on ease of maintenance with automated schema migrations.
- Stitch is a simple and scalable ETL service that focuses on data extraction and loading and lacks the most data transformation options.
- Matillion is an ELT tool designed specifically for cloud data warehouses like Snowflake, Amazon Redshift, Google BigQuery, and Microsoft Azure Synapse. The platform provides a visual interface for designing and managing complex data transformations within the data warehouse environment.
Surveys and Questionnaires
Surveys and questionnaires help you collect structured data directly from users. These tools let you design and distribute customized forms and gather real-time responses.
- Typeform is an online form builder known for its interactive and well-designed surveys. The tool offers a designable and customizable welcome screen and thank you page. Itâs advanced form builder lets you sync multiple surveys, remembering usersâ past responses to tailor their journey. Typeform also supports campaigns and UTM tracking to provide deeper response analysis.
- SurveyMonkey is a leading survey platform that offers robust tools for creating, distributing, and analyzing surveys. The platform offers 250+ ready-to-use survey templates, thousands of pre-written questions by experts, and AI-powered personalized recommendations.
Data Collection Use Cases
Once youâve collected all your data and have it in your data warehouse, you can power four major use cases: analytics, AI, machine learning, and activation.
- Analytics helps you have a greater range of insights into your business, which enables you to make decisions based on facts that lead to a positive outcome. You'd see insights such as what campaigns are performing the best or where you're spending the most money in your business so you can investigate possible cost reductions. For example, if you are a retailer like Target, you can use analytics to understand, through buying habits, when they need to order inventory to the shelves stock and deal with future customer demand.
- AI can be used with your data to streamline your business processes. You could utilize AI to assist with customer service by providing relevant insights for dealing with complex customer queries or automating repetitive daily tasks that support agents do. A tool like Hightouch Campaign Intelligence , where you could ask questions about your data, and it responds by providing you with appropriate reports. The data you've collected is the foundation AI can build off to enhance different departments throughout your business. For example, customer support tools like Intercom use AI to help you generate answers to customer tickets by reviewing similar past responses and supporting documentation to help reduce customer resolution times.
- Machine Learning helps you to predict the future by using your data. By analyzing data from previously churned customers, you can calculate a churn score for current customers, enabling you to proactively address those with high scores. You could also use it to analyze customer shopping habits and then power a recommendation engine to show products that customers are more likely to be interested in. For example, if you are a car manufacturer like Tesla, you can use machine learning algorithms to help their cars operate faster and increase response times in the real world based on product data from other Teslas.
- Activation works alongside all three of the use cases. Itâs one thing to get provided with insights from your machine-learning algorithms or analytical reports, but you need to take action on that information. Activation is key. It can help move the data you need to power these insights. If you have a list of products you want to recommend to a certain subset of customers, you can gather that data from your warehouse and send it to an email service provider to personalize a sales email. For example, PetSmart uses activation to get data out of its warehouse to serve 4 billion personalized emails for its loyalty program to help pet wonders take better care of their pets through products, offers, and recommendations.
How to Unlock These Use Cases
Data collection isnât just a single process. Itâs part of a sequence within your business, a step towards getting the most value out of your data. Why wouldnât you want to produce the best experience possible for your customers, which will positively impact your bottom line?
With all the data collected in your data warehouse, one of the best methods of getting value for your data is using a Composble CDP. A Composable CDP harnesses your current investment in your data infrastructure to grow your business and power your most complex personalization use cases. Book a demo with a solutions engineer if you want to know more about how Hightouch!
More on the blog

What is Reverse ETL? The Definitive Guide
Learn how Reverse ETL works, why it's different from traditional ETL, and how you can use it to activate your data.

Friends Donât Let Friends Buy a CDP
How spending the first half of his professional career at Segment drove Tejas Manohar to disrupt the 3.5 billion dollar CDP category.

What is a Composable CDP?
Learn why Composable CDPs are seeing such rapid adoption, how they work, and why they're replacing traditional CDPs.
Recognized as an industry leader .css-ozv6cb{display:none;}@media screen and (min-width: 48em){.css-ozv6cb{display:block;}} by industry leaders

Reverse ETL Category Leader
Marketplace Partner of the Year

Cool Vendor in Marketing Data & Analytics
Ecosystem Partner of the Year

Best Estimated ROI
One to Watch for Activation & Measurement
CDP Category Leader

Easiest Setup & Fastest Implementation
Activate your data in less than 5 minutes self.__wrap_n!=1&&self.__wrap_b(":R2cqtaiukm:",1)
- Accountancy
- Business Studies
- Organisational Behaviour
- Human Resource Management
- Entrepreneurship
- CBSE Class 11 Statistics for Economics Notes
Chapter 1: Concept of Economics and Significance of Statistics in Economics
- Statistics for Economics | Functions, Importance, and Limitations
Chapter 2: Collection of Data
Methods of data collection.
- Sources of Data Collection | Primary and Secondary Sources
- Direct Personal Investigation: Meaning, Suitability, Merits, Demerits and Precautions
- Indirect Oral Investigation : Suitability, Merits, Demerits and Precautions
- Difference between Direct Personal Investigation and Indirect Oral Investigation
- Information from Local Source or Correspondents: Meaning, Suitability, Merits, and Demerits
- Questionnaires and Schedules Method of Data Collection
- Difference between Questionnaire and Schedule
- Qualities of a Good Questionnaire and Types of Questionnaires
- What are the Published Sources of Collecting Secondary Data?
- What Precautions should be taken before using Secondary Data?
- Two Important Sources of Secondary Data: Census of India and Reports & Publications of NSSO
- What is National Sample Survey Organisation (NSSO)?
- What is Census Method of Collecting Data?
- Sample Method of Collection of Data
- Methods of Sampling
- Father of Indian Census
- What makes a Sampling Data Reliable?
- Difference between Census Method and Sampling Method of Collecting Data
- What are Statistical Errors?
Chapter 3: Organisation of Data
- Organization of Data
- Objectives and Characteristics of Classification of Data
- Classification of Data in Statistics | Meaning and Basis of Classification of Data
- Concept of Variable and Raw Data
- Types of Statistical Series
- Difference between Frequency Array and Frequency Distribution
- Types of Frequency Distribution
Chapter 4: Presentation of Data: Textual and Tabular
- Textual Presentation of Data: Meaning, Suitability, and Drawbacks
- Tabular Presentation of Data: Meaning, Objectives, Features and Merits
- Different Types of Tables
- Classification and Tabulation of Data
Chapter 5: Diagrammatic Presentation of Data
- Diagrammatic Presentation of Data: Meaning , Features, Guidelines, Advantages and Disadvantages
- Types of Diagrams
- Bar Graph | Meaning, Types, and Examples
- Pie Diagrams | Meaning, Example and Steps to Construct
- Histogram | Meaning, Example, Types and Steps to Draw
- Frequency Polygon | Meaning, Steps to Draw and Examples
- Ogive (Cumulative Frequency Curve) and its Types
- What is Arithmetic Line-Graph or Time-Series Graph?
- Diagrammatic and Graphic Presentation of Data
Chapter 6: Measures of Central Tendency: Arithmetic Mean
- Measures of Central Tendency in Statistics
- Arithmetic Mean: Meaning, Example, Types, Merits, and Demerits
- What is Simple Arithmetic Mean?
- Calculation of Mean in Individual Series | Formula of Mean
- Calculation of Mean in Discrete Series | Formula of Mean
- Calculation of Mean in Continuous Series | Formula of Mean
- Calculation of Arithmetic Mean in Special Cases
- Weighted Arithmetic Mean
Chapter 7: Measures of Central Tendency: Median and Mode
- Median(Measures of Central Tendency): Meaning, Formula, Merits, Demerits, and Examples
- Calculation of Median for Different Types of Statistical Series
- Calculation of Median in Individual Series | Formula of Median
- Calculation of Median in Discrete Series | Formula of Median
- Calculation of Median in Continuous Series | Formula of Median
- Graphical determination of Median
- Mode: Meaning, Formula, Merits, Demerits, and Examples
- Calculation of Mode in Individual Series | Formula of Mode
- Calculation of Mode in Discrete Series | Formula of Mode
- Grouping Method of Calculating Mode in Discrete Series | Formula of Mode
- Calculation of Mode in Continuous Series | Formula of Mode
- Calculation of Mode in Special Cases
- Calculation of Mode by Graphical Method
- Mean, Median and Mode| Comparison, Relationship and Calculation
Chapter 8: Measures of Dispersion
- Measures of Dispersion | Meaning, Absolute and Relative Measures of Dispersion
- Range | Meaning, Coefficient of Range, Merits and Demerits, Calculation of Range
- Calculation of Range and Coefficient of Range
- Interquartile Range and Quartile Deviation
- Partition Value | Quartiles, Deciles and Percentiles
- Quartile Deviation and Coefficient of Quartile Deviation: Meaning, Formula, Calculation, and Examples
- Quartile Deviation in Discrete Series | Formula, Calculation and Examples
- Quartile Deviation in Continuous Series | Formula, Calculation and Examples
- Mean Deviation: Coefficient of Mean Deviation, Merits, and Demerits
- Calculation of Mean Deviation for different types of Statistical Series
- Mean Deviation from Mean | Individual, Discrete, and Continuous Series
- Mean Deviation from Median | Individual, Discrete, and Continuous Series
- Standard Deviation: Meaning, Coefficient of Standard Deviation, Merits, and Demerits
- Standard Deviation in Individual Series
- Standard Deviation in Discrete Series
- Standard Deviation in Frequency Distribution Series
- Combined Standard Deviation: Meaning, Formula, and Example
- How to calculate Variance?
- Coefficient of Variation: Meaning, Formula and Examples
- Lorenz Curveb : Meaning, Construction, and Application
Chapter 9: Correlation
- Correlation: Meaning, Significance, Types and Degree of Correlation
- Methods of Measurements of Correlation
- Scatter Diagram Correlation | Meaning, Interpretation, Example
- Spearman's Rank Correlation Coefficient in Statistics
- Karl Pearson's Coefficient of Correlation | Assumptions, Merits and Demerits
- Karl Pearson's Coefficient of Correlation | Methods and Examples
Chapter 10: Index Number
- Index Number | Meaning, Characteristics, Uses and Limitations
- Methods of Construction of Index Number
- Unweighted or Simple Index Numbers: Meaning and Methods
- Methods of calculating Weighted Index Numbers
- Fisher's Index Number as an Ideal Method
- Fisher's Method of calculating Weighted Index Number
- Paasche's Method of calculating Weighted Index Number
- Laspeyre's Method of calculating Weighted Index Number
- Laspeyre's, Paasche's, and Fisher's Methods of Calculating Index Number
- Consumer Price Index (CPI) or Cost of Living Index Number: Construction of Consumer Price Index|Difficulties and Uses of Consumer Price Index
- Methods of Constructing Consumer Price Index (CPI)
- Wholesale Price Index (WPI) | Meaning, Uses, Merits, and Demerits
- Index Number of Industrial Production : Characteristics, Construction & Example
- Inflation and Index Number
Important Formulas in Statistics for Economics
- Important Formulas in Statistics for Economics | Class 11
Data Collection refers to the systematic process of gathering, measuring, and analyzing information from various sources to get a complete and accurate picture of an area of interest. Different methods of collecting data include Direct Personal Investigation, Indirect Oral Investigation, Information from Local Sources or Correspondents, Information through Questionnaires and Schedules, and Published Sources and Unpublished Sources.
What is Data Collection?
Data Collection is the process of collecting information from relevant sources in order to find a solution to the given statistical enquiry. Collection of Data is the first and foremost step in a statistical investigation.Â
Here, statistical enquiry means an investigation made by any agency on a topic in which the investigator collects the relevant quantitative information. In simple terms, a statistical enquiry is a search for truth by using statistical methods of collection, compiling, analysis, interpretation, etc. The basic problem for any statistical enquiry is the collection of facts and figures related to this specific phenomenon that is being studied. Therefore, the basic purpose of data collection is collecting evidence to reach a sound and clear solution to a problem.
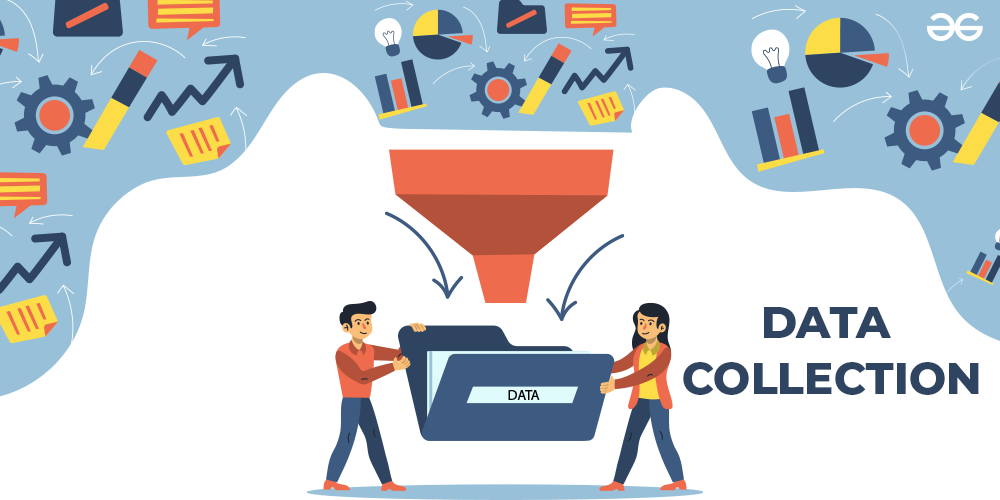
Table of Content
Important Terms related to Data Collection:
Methods of collecting data, a. methods of collecting primary data:, b. methods of collecting secondary data, 1. published sources, 2. unpublished sources, methods of data collection – faqs.
1. Investigator: An investigator is a person who conducts the statistical enquiry.
2. Enumerators: In order to collect information for statistical enquiry, an investigator needs the help of some people. These people are known as enumerators.
3. Respondents: A respondent is a person from whom the statistical information required for the enquiry is collected.
4. Survey: I t is a method of collecting information from individuals. The basic purpose of a survey is to collect data to describe different characteristics such as usefulness, quality, price, kindness, etc. It involves asking questions about a product or service from a large number of people.
Also Read: Sources of Data Collection | Primary and Secondary Sources
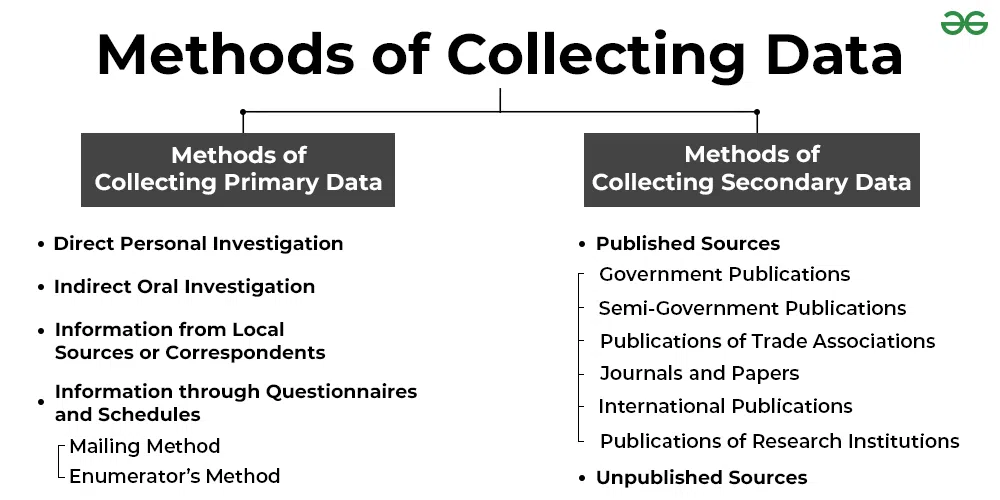
There are two different methods of collecting data: Primary Data Collection and Secondary Data Collection.Â
There are a number of methods of collecting primary data, Some of the common methods are as follows:
1. Direct Personal Investigation : As the name suggests, the method of direct personal investigation involves collecting data personally from the source of origin. In simple words, the investigator makes direct contact with the person from whom he/she wants to obtain information. This method can attain success only when the investigator collecting data is efficient, diligent, tolerant and impartial. For example, direct contact with the household women to obtain information about their daily routine and schedule.
2. Indirect Oral Investigation : In this method of collecting primary data, the investigator does not make direct contact with the person from whom he/she needs information, instead they collect the data orally from some other person who has the necessary required information. For example, collecting data of employees from their superiors or managers.
3. Information from Local Sources or Correspondents : In this method, for the collection of data, the investigator appoints correspondents or local persons at various places, which are then furnished by them to the investigator. With the help of correspondents and local persons, the investigators can cover a wide area.
4. Information through Questionnaires and Schedules : In this method of collecting primary data, the investigator, while keeping in mind the motive of the study, prepares a questionnaire. The investigator can collect data through the questionnaire in two ways:
- Mailing Method: This method involves mailing the questionnaires to the informants for the collection of data. The investigator attaches a letter with the questionnaire in the mail to define the purpose of the study or research. The investigator also assures the informants that their information would be kept secret, and then the informants note the answers to the questionnaire and return the completed file.Â
- Enumeratorâs Method: This method involves the preparation of a questionnaire according to the purpose of the study or research. However, in this case, the enumerator reaches out to the informants himself with the prepared questionnaire. Enumerators are not the investigators themselves; they are the people who help the investigator in the collection of data.
Also Read: Qualities of a Good Questionnaire and Types of Questionnaires Difference between Questionnaire and Schedule
Secondary data can be collected through different published and unpublished sources. Some of them are as follows:
- Government Publications: Government publishes different documents which consists of different varieties of information or data published by the Ministries, Central and State Governments in India as their routine activity. As the government publishes these Statistics, they are fairly reliable to the investigator. Examples of Government publications on Statistics are the Annual Survey of Industries, Statistical Abstract of India, etc.
- Semi-Government Publications: Different Semi-Government bodies also publish data related to health, education, deaths and births. These kinds of data are also reliable and used by different informants. Some examples of semi-government bodies are Metropolitan Councils, Municipalities, etc.
- Publications of Trade Associations: Various big trade associations collect and publish data from their research and statistical divisions of different trading activities and their aspects. For example , data published by Sugar Mills Association regarding different sugar mills in India.
- Journals and Papers: Different newspapers and magazines provide a variety of statistical data in their writings, which are used by different investigators for their studies.
- International Publications: Different international organizations like IMF , UNO , ILO, World Bank, etc., publish a variety of statistical information which are used as secondary data.
- Publications of Research Institutions: Research institutions and universities also publish their research activities and their findings, which are used by different investigators as secondary data. For example National Council of Applied Economics, the Indian Statistical Institute, etc.
Another source of collecting secondary data is unpublished sources. The data in unpublished sources is collected by different government organizations and other organizations. These organizations usually collect data for their self-use and are not published anywhere. For example, research work done by professors, professionals, teachers and records maintained by business and private enterprises .Â
The table below shows the production of rice in India.
The above table contains the production of rice in India in different years. It can be seen that these values vary from one year to another. Therefore, they are known as variable . A variable is a quantity or attribute, the value of which varies from one investigation to another. In general, the variables are represented by letters such as X, Y, or Z. In the above example, years are represented by variable X, and the production of rice is represented by variable Y. The values of variable X and variable Y are data from which an investigator and enumerator collect information regarding the trends of rice production in India.Â
Thus, Data is a tool that helps an investigator in understanding the problem by providing him with the information required. Data can be classified into two types; viz., Primary Data and Secondary Data . Primary Data is the data collected by the investigator from primary sources for the first time from scratch. However, Secondary Data is the data already in existence that has been previously collected by someone else for other purposes. It does not include any real-time data as the research has already been done on that information.
Why is data collection important in economics?
Data collection is crucial in economics because it provides the empirical foundation for analyzing economic phenomena, testing theories, forecasting trends, and informing policy decisions. Accurate data collection ensures the reliability and validity of economic analyses.
What are surveys and questionnaires, and how are they used?
Surveys and questionnaires are tools used to collect data from a large number of respondents. They contain a series of questions designed to gather information on specific topics. Surveys can be conducted online, by phone, by mail, or in person. They are widely used in economics to collect data on consumer behavior, market trends, and economic conditions.
How are interviews conducted, and what types are there?
Interviews involve direct interaction between the interviewer and the respondent to gather detailed information. Types of interviews include: Structured Interviews: Follow a fixed set of questions. Unstructured Interviews: Open-ended, allowing for in-depth exploration. Semi-Structured Interviews: Combination of structured and unstructured formats. Interviews are useful for obtaining qualitative data and understanding complex economic issues.
How do you choose the appropriate data collection method for a study?
Choosing a data collection method depends on several factors: Research Objectives: Define the goals and questions of the study. Nature of Data Required: Determine whether quantitative or qualitative data is needed. Resources Available: Consider budget, time, and personnel constraints. Population and Sample: Assess the accessibility and characteristics of the target population. Ethical Considerations: Ensure ethical standards are met in data collection.
What are the ethical considerations in data collection?
Ethical considerations include obtaining informed consent from participants, ensuring confidentiality and privacy, avoiding harm to respondents, and maintaining data integrity. Ethical practices are essential for the credibility and validity of the research.
Please Login to comment...
Similar reads.
- Commerce - 11th
- Statistics for Economics
Improve your Coding Skills with Practice
What kind of Experience do you want to share?
Ensuring quality data collection at scale: Best practices from a multi-sector multi-respondent survey
IDinsight Field Manager Lead Syed Maqbool (second from right) during data collection in Andhra Pradesh, India ŠIDinsight
Anyone who has been a part of any primary data collection exercise is well aware of the ample challenges practitioners face in order to collect high quality information. From hiring the best field team, to setting up the right systems, to managing incoming data to maintaining quality, one needs to account for each of these activities along the process. While primary data collection by itself can be challenging, conducting a survey on a large scale presents unique challenges that require careful planning and execution.Â
In partnership with the Bill and Melinda Gates Foundation and Niti Aayog, as part of the Aspirational Districts Program (ADP), IDinsight recently conducted primary data collection in 38 districts 1 across eight states 2 in India. The aim of the data collection exercise was to collect and verify administrative data in the health, education, and nutrition sectors, starting from where data originates (beneficiary-level reports in the health facility and school registers) to where itâs aggregated into paper proformas, to where itâs digitised into the portal. As part of the project team, this presented unique challenges for us, given the scale and scope of the exercise.

Given the different sectors and levels of data collection, our respondents ranged from bureaucrats in the local government (Regional Government officials) to frontline workers at health facilities and Anganwadi centres (government-sponsored child care centres in India) to headmasters in schools to program beneficiaries (which primarily included pregnant and lactating women and parents of school-going children). This required us to conduct in-person as well as phone household surveys in addition to facility and school-level surveys. We conducted the data collection across 1100 schools, 1100 anganwadi centres, 4100 health facilities , 500 local government offices and 8300 households. Overall, the scale, varying sectors, and respondents, the different levels of data collection, and the varied modes of the survey warranted substantial planning to mitigate expected challenges.
In this blog post, we share the detailed processes and systems we set up along the data collection pipeline to ensure quality and facilitate this complex data collection. Our experiences offer valuable insights for others looking to conduct primary data collection at scale, and we hope it serves as a useful resource for researchers facing similar challenges.
Key Challenges
1. Timely inter-department collaboration and information exchange: The scope of our survey called for close collaboration with the local administration (district) departments for two main reasons.Â
- Our sampling methodology relied on the list of health facilities, Anganwadi Centers, and schools made available by the respective departments.Â
- Since the objective was to verify administrative data, we required permissions and explicit approval from different bureaucrats to collect data.
However, this collaborative requirement presented a major challenge – the possibility of delays if coordination failed with even one department in one region.
2. Building local teams of expert data collectors from the ground-up: Given that we were hiring survey teams (12 personnel per district) simultaneously across multiple districts, we had to ensure that the processes and tools were standardised. As a result, the ad-hoc traditional tracker, interview questions, etc. used for screening and shortlisting enumerator candidates traditionally would have led to differing candidate quality, mismanagement of personal applicant data and additional resource investment. Secondly, we anticipated encountering a limited pool of qualified candidates in some districts, and the complexity of the survey and its long timelines increased the possibility of attrition. This was going to be a major challenge in about 50% of districts where we were hiring a local survey team for the first time and hence, our team did not have any prior network .
3. Capacity building and management at scale: Training a large number of enumerators can be a daunting task and in the absence of standardisation in delivery, coordination across locations, and maintenance of motivation, our enumerators would have lacked the necessary skills to collect accurate data.Â
4. Creating management systems to ensure quality data collection: Given the complexity of the survey and size of the survey team, we expected challenges in field management and quality monitoring as well when the data collection activity would kick off. We will talk about how building rigorous survey systems and processes helped us mitigate these challenges in our next blog post.
Fostering Effective Frameworks and Building a Strong Team
Liaising with local government departments.
– Building rapport by leveraging communications: Coordinating with different administrative units can be a challenging task, and therefore, we standardised our communication process to ensure effective coordination. We assigned a Point of Contact (PoC) for 4-5 districts who used an email template for the first line of communication. The email introduced our verification process, upcoming activities, and the required support from the local governments. However, we anticipated the need for further follow-ups, which were done by our field coordinators (with support from the PoC) through phone calls or in-person visits to ensure compliance with the requirements. We were able to collate the required datasets and official letters of support from 37 of the 38 districts within a month, without requiring a major in-person presence in most of them. Each PoC used the same naming convention to encrypt and upload the data in a secure folder. The relevant core team members then performed basic checks on these datasets and followed up with the government department via the PoC, wherever necessary.
Hiring Enumerators
Based on calculations of sample size, survey length, nature of the survey, expected daily productivity, and the project budget, we estimated a requirement of about 12 personnel per district for data collection, including 10 enumerators and 2 supervisors. In all, we were looking at hiring 400+ candidates across all districts. In order to efficiently manage this high-volume hiring which would pose a diverse range of challenges, we put in place the following measures.
– Decentralised execution of a standardised hiring process: For screening and selecting the survey team, we followed a three-staged approach across all districts which included shortlisting applications, phone based interviews followed by a thorough assessment through in-person classroom and field training.
The materials required to run the above-mentioned steps (such as the application form, job description, and interview guide) and the tools (such as district hiring trackers and a dashboard) were developed by the central team with inputs and feedback from the field team. Taking into consideration the profile of the intended users, the application form and interview questions were translated into three local languages- Odia, Assamese and Hindi.
However, the execution of the process was highly decentralised with the respective districtâs field team, i.e. Cluster Coordinator (CC) 3 and Regional Coordinator (RC) 4 , leading most of the processes. For instance, while scoring and shortlisting of the applicants across the three stages were mostly automated (as explained below), the coordinators were able to manually override whenever there was a clear rationale as well as change the cut-off scores whenever required. Similarly, depending on the local hiring scenarios, the coordinators also shortlisted a few buffer candidates at every stage. Such provisions proved to be of great benefit to tackle district-specific challenges such as limited availability of candidates, low success rate, and last-minute dropouts.Â
With this arrangement, we were able to give control back to the CC, thus allowing them to make quick hiring decisions with oversight from the central team in the form of standardised processes, materials, and tools as well as regular supervision.
– Hiring a hyper-local survey team: From our experience, we have learned that local enumerators are much more familiar with district local dialects, cultural context, locations, and road networks, and hiring them goes a long way in improving survey productivity and data quality. Moreover, for the current project, we expected facilities and beneficiaries to be spread out across blocks in a district. Thus, adding another layer of localisation- hiring at a block level seemed like a reasonable strategy to optimise budgets and time. But as one would expect, such a hyper-local recruitment strategy was not feasible across all districts. In such cases, exceptions were made allowing the selection of candidates from neighbouring districts and/or blocks.

Through all these efforts we received about 6700 applications, which were assessed and screened through interviews and training – both of which were conducted by the coordinators in the districtâs local language.
– Automating most repetitive and time-consuming tasks:Â For any high-volume recruitment, automation of repetitive admin tasks not only saves the coordinator’s time and allows them to focus on more complex tasks, but also helps with maintaining transparency and consistency across the hiring processes. We were able to achieve this automation through custom-built trackers developed by SurveyStream . 5

With the help of these automated trackers, we were able to hire a survey team of about 430 local candidates across 38 districts in a timespan of about a month . By automating various tasks such as manual data entry, de-duplication, filtering, and publishing district-specific information, we saved a significant amount of time. This streamlined approach allowed us to efficiently manage various user groups, including district and state coordinators and central team members while also reducing the risk of human errors.
Training of Field Team
For our survey, we were looking at training 37 cluster coordinators and 8 regional coordinators, along with a survey team of 430 enumerators across Hindi, Odiya and Assamese-speaking districts. To effectively train such a diverse field team, we followed a cascade model, wherein we trained our RCs-CCs online. The CCs (with support from RCs) then trained the surveyor team in person, in their respective districts.
To maintain the quality of training across 37 districts, we carefully systematised the processes throughout the training pipeline.
- Tailoring material structure and language for effective information uptake : In order to maintain consistency in the content of our training, the central team developed all the training materials which were then translated into regional languages and thoroughly vetted these translations via back translations. For the questionnaire and protocol training sessions, we chose to record videos instead of written documentation so that the coordinators could directly use those during training and pause at regular intervals for detailing or following up with the surveyors. These videos were also shared with the surveyors later to be used as self-learning materials.Â
- Leveraging gamification to improve information uptake and learning behaviour: Given the size of the groups, it was important for us to pay close attention to participant engagement and their technical understanding of the concepts. For this purpose, we administered short quizzes after every module during the coordinatorsâ training as well the surveyor training. Moreover, these graded quizzes helped the trainers assess the performance of the participants and take appropriate measures in the form of corrective feedback or re-training on certain concepts. These quizzes were also used to shortlist the candidates and finalise the enumerators. The quiz scores were directly linked to the hiring tracker, to facilitate easy filtering and selection of candidates.
- Close monitoring and adopting hands-on onboarding feedback simultaneously : We conducted daily debriefs, with a pre-specified agenda with the RC-CCs while surveyor training was ongoing. We used these meetings to troubleshoot issues, answer any open questions, and specifically track district-wise quiz scores to give feedback on what sessions need to be reconducted or which surveyors need targeted attention.
There were 3-4 districts that had to extend their training by a day, to conduct retraining sessions as necessary. However, we had already budgeted for ample buffers throughout the training and data collection period. This was essential to maintain the same training quality across multiple districts. Another thing to note is that since this was a four month long data collection exercise, there would be CCs who would go on leaves, in which case we had RCs substitute for them during the leave period and take up training conduction or data collection management.Â
In our upcoming blog post, we’ll delve into how establishing effective systems and assembling a well-suited team as detailed above helped us overcome field management and quality monitoring challenges during a large-scale survey. Stay tuned for more details!
Acknowledgements
We deeply appreciate the comments and reviews on this blog from our colleagues at IDinsight. Thank you, Emily Coppel, Girish Tripathi, Leonard Tin, Lipika Biswal, Jahnavi Meher, Jasmeet Khanuja, Pramod Kumar, and Puneet Kaur.

Shreya More
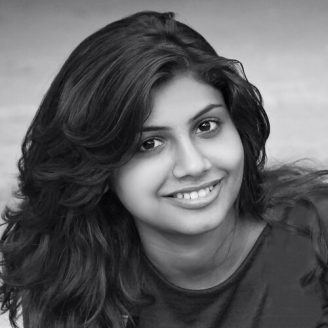
Isha Fuletra
Tom wein launches marketplace dignity book.
3 June 2024
Call for partners â IDinsight Dignity Initiative Randomized Controlled Trial (RCT)
16 May 2024
Request for Partners: What works for more respectful development?
Access to health services: kilifi county case study.
15 May 2024
How DataDelta created a data verification strategy to support local development efforts in India
Idinsight s’associe au millennium challenge account sĂŠnĂŠgal (mca) pour une initiative en faveur de l’ĂŠlectricitĂŠ.
14 May 2024
IDinsight Partners with Millennium Challenge Account Senegal (MCA) for Electricity Initiative
Humanizing artificial intelligence: putting people at the center of data-driven decisions.
13 May 2024
Subsidizing sanitation: Updated evidence on ‘smart’ subsidies for latrines
Q+a: how (and why) policymakers are turning to large-scale, representative surveys.
- 1. A district is an administrative division of the Indian state that is managed by the local government.
- 2. Assam, Bihar, Uttar Pradesh, Jharkhand, Madhya Pradesh, Rajasthan, and Odisha
- 3. A Cluster Coordinator (CC) manages survey teams and on-ground operations for a combination of two or more districts, which are put together as a single unit and referred to as a cluster.
- 4. A Regional Coordinator (RC) supervises and supports the CCs across one or more Indian states and facilitates communication between the field team and central team as needed.
- 5. SurveyStream is an in-house survey management software product built to support data collection activities undertaken by IDinsightâs DataDelta (DD) team.
Related content
Why idinsight is investing in building data systems for primary data collection activities.
25 February 2022
Voter rolls can be cheaper and faster for sampling in India. But are they accurate?
20 October 2020
IDinsight partners with Radiant Earth Foundation
18 January 2022
Stay up-to-date with IDinsight
Research Methodology: Data Collection and Analysis Essay
- To find inspiration for your paper and overcome writerâs block
- As a source of information (ensure proper referencing)
- As a template for you assignment
Research Methodology
Data collection, instrumentation, sampling technique, data analysis.
This chapter discusses the methodological approaches used by the researcher to collect, analyze and present its research findings. In this case, the study followed a positivist and phenomenological paradigm. The selection of the paradigm determines how the study expresses the underlying research perspective toward how things are connected (Brenda, 2003).
The studyâs choice of a positivism and phenomenal approach remain appropriate to the study. This paradigm succeeds in investigating the study scenario in its natural setting to deal with the existing factors affecting children because of the âNo Child left Behind Act.â
The researcher used a mixed research design in the collection, and analysis of data. The study shall collect quantitative data by administering a survey questionnaire. The survey instrument shall enable the researcher to collect and analyze empirical data to investigate the effect of âNo Child left Behindâ Act on children. The collection of quantitative data is appropriate to determining the existence and extent of the relationship between the Act and several strategic effects (Brenda, 2003).
The researcherâs choice of a qualitative research design remains appropriate to the study scenario because it allows a researcher to examine the phenomenon in its natural setting during the study. Phenomenological approach enables the researcher to explain factors influencing the factors in the research topic to enable a plausible explanation of the study theory.
The researcher collected qualitative data by using focused interviews to assess the effect of the Act on disabled children (Brenda, 2003). The interview shall consist of open-ended questions to give a general insight into the possible effects of the programs under the guidance of âNo Child left Behindâ Act.
The use of open-end questions is to help the researcher to win first hand confidence of the respondents, and objectivity of the responses (Merriam, 1998).
The study utilizes a random sampling approach in selecting the sample of the study. This technique ensures that the research cushions its results from errors of biased source of data.
Qualitative Data Analysis
The researcherâs use of thematic analysis to analyze interview findings helps to analyze data drawn from interviews (Merriam, 1998). This approach is based on fundamental trends, patterns of behavior or life exhibited by individuals within their environment. After interview data, the researcher developed patterns related to the data.
Later, data cataloguing was used to identify and formulate themes. Pattern grouping was finally done and relationships between groups determined (Merriam, 1998). This gave room for a general assertion that could explain and clarify practices of âNo Child left Behindâ Act on disabled children.
Quantitative Data Analysis
Quantitative research on the other hand, deals with collecting and analyzing numeric data. This form of research entails the use of relatively large-scale data in order to examine or ascertain a social phenomenon through statistical analysis. In this research, the researcher selected a qualitative research design with the view to answer the research question under review. According to Merriam (1998), qualitative research studies things in their natural way or setting, in which the researcher is an integral tool in data collection.
Under this approach, data collected is in the form of words, or pictures and the outcome are used as a process and not product. The application of qualitative research method in this study was to enable the research to find and develop theories that would explain the relationship between variables through elements of qualitative research (Brenda, 2003).
Brenda, L. (2003). Design research: methods and perspectives . New York: MIT Press.
Merriam, S. B. (1998). Qualitative research and case study applications in education. New York, NY: Jossey-Bass Publishers
- Ultrasound Physics and Instrumentation
- A Phenomenological Study of Families With Drug-Using Children
- Geopolitical and Phenomenological Place and Health
- Cell Phone Usage at Al Ittihad Model School Among Male Students
- Writing and Researching Skills
- Effective and Ineffective Assessment
- The Difference between a Hypothesis and a Research Question
- Documentary Research
- Chicago (A-D)
- Chicago (N-B)
IvyPanda. (2019, May 21). Research Methodology: Data Collection and Analysis. https://ivypanda.com/essays/research-methodology-essay/
"Research Methodology: Data Collection and Analysis." IvyPanda , 21 May 2019, ivypanda.com/essays/research-methodology-essay/.
IvyPanda . (2019) 'Research Methodology: Data Collection and Analysis'. 21 May.
IvyPanda . 2019. "Research Methodology: Data Collection and Analysis." May 21, 2019. https://ivypanda.com/essays/research-methodology-essay/.
1. IvyPanda . "Research Methodology: Data Collection and Analysis." May 21, 2019. https://ivypanda.com/essays/research-methodology-essay/.
Bibliography
IvyPanda . "Research Methodology: Data Collection and Analysis." May 21, 2019. https://ivypanda.com/essays/research-methodology-essay/.

1. Introduction
3. results and discussion, 4. conclusions, 5. related literature, supporting information.

research papers \(\def\hfill{\hskip 5em}\def\hfil{\hskip 3em}\def\eqno#1{\hfil {#1}}\)

Pillar data-acquisition strategies for cryo-electron tomography of beam-sensitive biological samples
a Rosalind Franklin Institute, Harwell Science and Innovation Campus, Didcot OX11 0FA, United Kingdom, b Diamond Light Source, Harwell Science and Innovation Campus, Didcot OX11 0DE, United Kingdom, c Thermo Fisher Scientific, Vlastimila Pecha, Brno, Czechia, d Electron Physical Science Imaging Centre, Diamond Light Source, Harwell Science and Innovation Campus, Didcot OX11 0DE, United Kingdom, e Department of Materials, University of Oxford, Parks Road, Oxford OX1 3PH, United Kingdom, and f Division of Structural Biology, University of Oxford, Roosevelt Drive, Oxford OX3 7BN, United Kingdom * Correspondence e-mail: [email protected]
For cryo-electron tomography (cryo-ET) of beam-sensitive biological specimens, a planar sample geometry is typically used. As the sample is tilted, the effective thickness of the sample along the direction of the electron beam increases and the signal-to-noise ratio concomitantly decreases, limiting the transfer of information at high tilt angles. In addition, the tilt range where data can be collected is limited by a combination of various sample-environment constraints, including the limited space in the objective lens pole piece and the possible use of fixed conductive braids to cool the specimen. Consequently, most tilt series are limited to a maximum of ±70°, leading to the presence of a missing wedge in Fourier space. The acquisition of cryo-ET data without a missing wedge, for example using a cylindrical sample geometry, is hence attractive for volumetric analysis of low-symmetry structures such as organelles or vesicles, lysis events, pore formation or filaments for which the missing information cannot be compensated by averaging techniques. Irrespective of the geometry, electron-beam damage to the specimen is an issue and the first images acquired will transfer more high-resolution information than those acquired last. There is also an inherent trade-off between higher sampling in Fourier space and avoiding beam damage to the sample. Finally, the necessity of using a sufficient electron fluence to align the tilt images means that this fluence needs to be fractionated across a small number of images; therefore, the order of data acquisition is also a factor to consider. Here, an n -helix tilt scheme is described and simulated which uses overlapping and interleaved tilt series to maximize the use of a pillar geometry, allowing the entire pillar volume to be reconstructed as a single unit. Three related tilt schemes are also evaluated that extend the continuous and classic dose-symmetric tilt schemes for cryo-ET to pillar samples to enable the collection of isotropic information across all spatial frequencies. A fourfold dose-symmetric scheme is proposed which provides a practical compromise between uniform information transfer and complexity of data acquisition.
Keywords: cryo-electron tomography ; cryo-ET ; multislice image simulation ; data-collection strategy ; reconstruction ; alignment ; beam-sensitive specimens .
In this paper, we describe and simulate an n -helix data-acquisition scheme for cryo-ET that can be used to both tilt the sample and shift the sample image to reconstruct large volumes. We also perform an analysis of a set of extended tilt schemes with varied angular sampling for pillar samples of beam-sensitive biological specimens. In particular, we consider imaging without any prior knowledge about the specimen with the aim of providing optimal information transfer over the entire angular range. We describe three families of tilt schemes that can be related through simple rules. These families of tilt schemes are referred to as the spiral, swinging and symmetric schemes. The spiral and swinging schemes extend the continuous data-acquisition scheme and the symmetric scheme extends the classic dose-symmetric scheme. Through analysis of the simulated data sets, we assess the information transfer provided by these schemes as well as their suitability for real-world application by examining the data-acquisition time and fluence gradient between adjacent images. We propose a fourfold dose-symmetric scheme for pillar samples that we believe provides a practical and effective compromise between optimal information transfer, data-acquisition time and data-collection complexity.
2.1. Properties of data-acquisition schemes for pillars
The properties of an ideal data-acquisition scheme for cryo-ET of pillar samples are as follows.
(i) Uniform information transfer : the tilt scheme should produce uniform information transfer at all spatial frequencies to obtain isotropic resolution in reconstructions.
(ii) Low total accumulated stage tilt : the tilt scheme should have low total accumulated stage tilt for faster data acquisition.
(iv) Sufficient signal for alignment : there must be sufficient signal in each tilt image to allow their alignment prior to reconstruction. This limits the extent to which the available electron budget can be fractionated across images.
(v) High resolution with a large field of view : in cryo-ET (as in all imaging methods) there is a compromise between the field of view of the data-collection area and the achievable spatial resolution (for a finite detector pixel and array size): to collect higher resolution data, a finer sampling and hence a smaller data-collection area is required. Data acquisition that allows the entire volume to be reconstructed as a single object at high resolution means that rare objects of interest are less likely to be missed and analysis within a larger cellular context can be performed.
2.2. Angular sampling
2.3. n -helix data acquisition, 2.4. simulation of cryo-et images, 2.5. assessment of reconstruction quality, 3.1. alignment of images in pillar and planar samples, 3.2. information transfer as a function of angular sampling.
The angular distribution of the information transfer depends on several factors, including the total incident fluence, the beam sensitivity of the specimen, the fluence and flux for a particular image, the total number of tilt images in the data acquisition and the order of image acquisition. In the following analysis, we assume that each image receives the same number of incident electrons, and that the B factor increases linearly as a function of the number of incident electrons such that B = 8 π 2 D E S E , where D E is the fluence and S E is a sensitivity coefficient. The total fluence across the data sets is assumed to be 140 e − Å −2 , which is typical for that used in cryo-ET, and this is assumed to be evenly distributed across the 40 images in the tilt series. In the following analysis, a value of S E = 0.04 Å 4 /e − was used.
3.3. Anisotropy of information transfer
3.4. accumulated stage tilt, 3.5. accumulated fluence jump at angle.
In many cases, it is necessary to remove tilt images from the data set due to the presence of contamination, due to Bragg diffraction from crystalline material or due to poor tracking during data acquisition. Ideally, tilt schemes need to be robust to the removal of such images. The robustness will be proportional to the relative fluence jump between adjacent images in that if an image is removed from the tilt series then the maximum fluence jump between adjacent images will double. Therefore, robustness to the removal of images is another factor that makes it desirable to have a lower relative fluence jump between adjacent tilt images.
3.6. Fluence distribution as a function of position
To enable the reconstruction of an entire pillar as a single object, the n -helix data-acquisition strategy collects images from overlapping regions on the sample which are subsequently stitched together during reconstruction. The number of overlapping regions is allowed to vary according to the value of n . For example, the one-helix strategy has no overlaps in the data-acquisition area; each sweep position is shifted by a whole field of view. For the two-helix strategy, images at every second angle are shifted by 1/2 of the field of view to allow 50% overlap in the data-acquisition position, thereby allowing the fields of view to be stitched together. For the four-helix and the eight-helix strategies, images at adjacent tilt angles are shifted by 1/4 and 1/8 of the field of view, respectively. Hence, the use of different n -helices will result in different distributions of fluence across the sample.
For a beam size of L , it can be seen that for the one-helix strategy parts of the sample will receive a relative fluence of 1, corresponding to the total fluence of a single tilt series; however, since for this beam size the corners of the detector will not be illuminated, some areas of the sample may receive zero fluence. This means that no information will be transferred about these parts of the sample. However, it should be noted that at commonly used pixel sizes and pillar thicknesses the corners of the detector should typically contain very little sample if the pillar is properly aligned and oriented parallel along a detector edge. Therefore, it is still possible to ensure that only a minimal amount of information is lost.
For a beam size of 2 1/2 L , it can be seen that for the one-helix strategy parts of the sample will receive a relative fluence of 1, corresponding to the total fluence of a single tilt series; however, a significant area of the sample will receive twice this fluence. For those parts of the sample receiving a relative fluence of 2, for every electron that results in useful signal there is another electron that only causes damage to the sample, with no transfer of information. For both beam sizes, as the amount of overlap between the sweeps increases, as defined by the n -helix parameter, the distribution of fluence is increasingly uniformly distributed across the sample, while the mean fluence received by the sample remains constant. This is also illustrated by the histograms of the voxel fluence distributions. As the n -helix parameter increases, the variance of the fluence distribution decreases, with the variance for the eight-helix being much lower than for the one-helix strategy.
For a circular beam of size 2 1/2 L , the mean fluence for higher order n -helix strategies tends to a value of 1.36 across the sample. This means that for this beam size, the n -helix data-acquisition strategy will always result in a higher exposure than a single tilt series, which is a natural consequence of having overlapping regions. In practice, this would mean that to give a final electron fluence of 136 e − Å −2 , each tilt-series acquisition should be configured to have a lower fluence of 100 e − Å −2 . Set against this is that there will always be some damage done to the sample by electrons which do not contribute useful signal during the data collection.
3.7. Reconstruction quality
We have shown that a continuous tilt scheme may result in anisotropic information transfer for beam-sensitive biological samples due to the limited ability to fractionate the fluence across many images. Higher order dose-symmetric schemes can result in a more uniform distribution of fluence at the expense of greater complexity in the data acquisition. In this regard, hardware limitations may hinder the ability to execute arbitrarily complex tilt schemes. However, higher order dose-symmetric schemes are no more demanding on the mechanics of the tilt stage than the classic dose-symmetric scheme which is used regularly to collect high-resolution cryo-ET data. The more uniform information transfer also comes at the cost of a larger jump in fluence between adjacent tilt images, which could result in alignment issues due to fluence-induced sample deformation. The higher the symmetry, the larger the maximum jump in fluence between adjacent images.
The analysis described here focused on an idealized pillar sample which has a constant circular cross section over the length of the pillar. However, in practice, the pillar may have a conical geometry, with a tapered circular cross section which is thinner at the end of the pillar than at the base. In this case, the data-collection strategies described here would be unaltered. In contrast, where the pillar cross section is not circular it may be preferable to match the symmetry of the scheme to the symmetry of the pillar cross section. As an extreme example, for a square cross section the fourfold scheme would be preferred.
The fourfold dose-symmetric scheme is more efficient than the classic dose-symmetric scheme in terms of the total accumulated stage tilt, requiring 75% less total stage rotation, and only has a marginally higher maximum fluence jump between adjacent images. It also produces a much more isotropic transfer of information. A practical approach, therefore, may be to use the continuous tilt scheme when the sample is not beam-sensitive or isotropic information transfer is not necessary and to use the fourfold dose-symmetric scheme if the sample is beam-sensitive and isotropic information transfer is desirable. Future work will focus on experimental validation of these results once the routine production of biological pillar samples is possible.
Supplementary information including Supplementary Figures. DOI: https://doi.org/10.1107/S2059798324004546/ai5012sup1.pdf
Funding information
This work was supported by the Rosalind Franklin Institute, which is an EPSRC core funded institute, and was funded by the Wellcome Trust as part of the Electrifying Life Sciences project (220526/Z/20/Z).
This is an open-access article distributed under the terms of the Creative Commons Attribution (CC-BY) Licence , which permits unrestricted use, distribution, and reproduction in any medium, provided the original authors and source are cited.
NEET Preparation App by RK 4+
Mcqs, mock test, papers & note, piyush korat, designed for ipad.
- Offers In-App Purchases
Screenshots
Description.
Chapter-wise and Year-wise Paper Solutions for NEET is a question bank having questions from past 37 years. MCQs for NEET Exam is a collection of asked questions for the NTA NEET. The App contains questions which were asked in last 37 year ranging from 1988 to 2024. The App covering chapter-wise and topic-wise a total of around 7200+ MCQs followed by answer key, hint and detailed solutions. Chapter-wise & Topic-wise questions gives a clear idea regarding chapter weightage. Once after completing the chapter, attempting questions from previous years exams give a real indication of the efforts required. Key functionality of the application: ⢠Chapter-wise & topic-wise solved papers ⢠Mock test facility ⢠Speed test facility a. Chapter-wise speed test b. Year-wise speed test ⢠Result Analysis ⢠Bookmark important questions ⢠Test result records Content highlights: - Past solved papers of NEET exam ranging from the year 1988 to 2024. - The app contains around 7200+ NEET MCQs. - The Topics have been arranged exactly in accordance to the NCERT syllabus so as to make it 100% convenient to Class 11 and 12 students. - The detailed hint and solutions of all questions are provided at the bottom of each chapter inside Hint button to bring conceptual clarity. The answers have been given in such a manner that a student can easily understand it. - Practice Mock Test and Sample Papers for NEET 2025 Examination - Last Minute Revision Mind maps, Review Notes and Quick Formula of Physics, Chemistry and Biology. The chapters have been divided as per the Class 11th and 12th syllabus followed by the NCERT. Some of the chapters which are split in the class 11th and 12th syllabus in NCERT have been combined. There might be certain topics, chapters which are not covered in NCERT but are a part of NEET syllabus. Source of Information: Our app provides solutions to NEET questions. Our solutions are based on our team's expertise and understanding of the NEET curriculum. We do not claim to represent NEET or any official government entity. Our solutions are intended to assist students in understanding and practicing the material covered in NCERT textbooks and NEET Papers. For official announcements, information, or services related to NCERT and NEET, please refer to their official website or communication channels. Use of NEET Terminology: Our app, app icons or logos, name, subtitle, promotional text, descriptions, app screenshots or in-app elements uses NEET-related terminology for descriptive purposes to assist our users in finding NEET relevant content. This mobile application (App) is not affiliated with or endorsed by the official NEET (National Eligibility cum Entrance Test) authorities. It is an independent application developed by DECMAC SOLUTIONS for informational and educational purposes only. Disclaimer for auto-renew subscription:- ⢠The specified subscription payment will be charged via Apple ID account at purchase confirmation. ⢠Subscription auto-renews unless canceled at least 24 hours prior to the current billing period. ⢠You can manage your subscription and auto-renew can be turned off in App Store Account Settings after purchase. ⢠Prices may vary by location. ⢠Family Sharing is not supported. Privacy policy: https://rktechnology.xyz/Terms/neetpreparation-privacy Terms of Service: https://rktechnology.xyz/Terms/ios-user-agreement
App Privacy
The developer, Piyush Korat , indicated that the appâs privacy practices may include handling of data as described below. For more information, see the developerâs privacy policy .
Data Not Linked to You
The following data may be collected but it is not linked to your identity:
- Identifiers
- Diagnostics
Privacy practices may vary, for example, based on the features you use or your age. Learn More
Information
- Upgrade to premium $4.99
- Upgrade to premium $0.99
- Developer Website
- App Support
- Privacy Policy
More By This Developer
NEET Prep: Solved Papers
Ncert books, solutions & notes
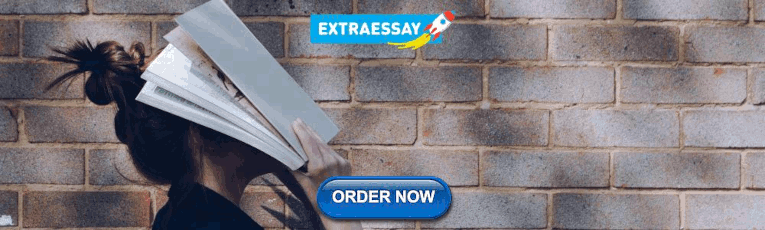
COMMENTS
Data collection is the process of gathering and measuring information on targeted variables in an established systematic fashion, which then enables one to answer relevant questions and evaluate outcomes. The four possible data collection methods I have chosen for the program plan are observations, focus groups, surveys and interviews.
Observation is a data collection method where the researcher uses his eyes and participates in certain activities as he collects data. Observation may be open where the researcher takes data from the occurrence of certain activities or may be closed where the observer takes data from a limited number of people (Axinn & Lisa, 2006). Focus groups.
Data Collection | Definition, Methods & Examples. Published on June 5, 2020 by Pritha Bhandari.Revised on June 21, 2023. Data collection is a systematic process of gathering observations or measurements. Whether you are performing research for business, governmental or academic purposes, data collection allows you to gain first-hand knowledge and original insights into your research problem.
For carrying out the data collection, the appropriate methods should be applied. In the research, the data collection could be done through the interview, for example, face-to-face and telephone interview. To collect the data by using interview technique, the questionnaire is commonly employed as the instrument. 1201 Words. 5 Pages. Good Essays.
PDF | Learn how to choose the best data collection methods and tools for your research project, with examples and tips from ResearchGate experts. | Download and read the full-text PDF.
Introduction. The purpose of the paper is the evaluation of data collection methods and the creation of efficient instruments for the accumulation of information about BI System usage and its effects on organizational performance. It is observed that BI software creates benefits in the data organization and operation (Agiu, Mateescu, & Muntean ...
Data collection is the process of gathering and collecting information from various sources to analyze and make informed decisions based on the data collected. This can involve various methods, such as surveys, interviews, experiments, and observation. In order for data collection to be effective, it is important to have a clear understanding ...
Step2: Select reasons and set objectives. After identification of several reasons and opportunities for data collection, priority is established and goals are set. The step involves choosing one or few critical issues to research. As such, the step assists the organization to be focused on specific issues at a time.
Learn about the concept, types, and issues of data collection methods, with examples and tips from ResearchGate's experts. Download the PDF for free.
Data collection is the process of collecting and evaluating information or data from multiple sources to find answers to research problems, answer questions, evaluate outcomes, and forecast trends and probabilities. It is an essential phase in all types of research, analysis, and decision-making, including that done in the social sciences ...
Therefore, this essay aims to expound on the methods for data collection and founding trust. Concurrent with Salhin et al. (2016), there are myriad quantitative data gathering approaches. To begin is the administration of surveys with closed-ended questions, which might entail face-to-face and telephone interviews or mail questionnaires, is ...
Data collection methods are important, because how the information collected is used and what explanations it can generate are determined by the methodology and analytical approach applied by the researcher. 1, 2 Five key data collection methods are presented here, with their strengths and limitations described in the online supplemental material.
Data collection methods are chosen depending on the available resources. For example, conducting questionnaires and surveys would require the least resources, while focus groups require moderately high resources. Reasons to Conduct Online Research and Data Collection . Feedback is a vital part of any organization's growth.
The paper encompasses a systematic literature review, focusing on core ethical principles in data collection and analytical processes, the roles of consent and privacy, the complexities introduced ...
Possible data collection methods include: experiments ; investigations ; interviews ; surveys; The details of how the data was collected are crucial to the validity of any argument based on the findings. You must put in the main body of your essay the details of any primary research you carry out. These include: the methods used; the persons ...
Table of contents. Step 1: Define the aim of your research. Step 2: Choose your data collection method. Step 3: Plan your data collection procedures. Step 4: Collect the data. Frequently asked questions about data collection.
The survey technique is a data collection-gathering tool used to collect data from large population. The quantitative research often uses a survey technique to collect data, and the descriptive statistics are used for the analysis. The results are presented in tables, graphs and charts.
Data collection is the process of gathering data for use in decisionmaking, strategic planning, research and other purposes. For research in science, medicine, higher education and other fields, data collection is often a more specialized process, in which researchers create and implement measures to collect specific sets of data.
In this article, we review some principles of the collection, analysis, and management of qualitative data to help pharmacists interested in doing research in their practice to continue their learning in this area. Qualitative research can help researchers to access the thoughts and feelings of research participants, which can enable ...
For instance, when people's behaviours, attitudes, and decisions are in the researcher's lens, the meaning is vital. Some of the most common qualitative data collection methods are interviews, observations, focus group discussions and action research (Saunders, Lewis & Thornhill 2009). To identify some factors affecting decision-makers as ...
Data Collection, Essay Example. Pages: 1. Words: 293. Essay. Hire a Writer for Custom Essay. Use 10% Off Discount: "custom10" in 1 Click đ. HIRE A WRITER! You are free to use it as an inspiration or a source for your own work. We will use multiple methods to collect the information we need for the project.
Data collection is the process of gathering customer data from internal or external data sources and storing it in a single location, such as a data warehouse or data lake to unlock and uncover critical insights about your customers. You can collect data through customer surveys, an event collection tool, or extract it from existing tools like ...
Data Collection is the process of collecting information from relevant sources in order to find a solution to the given statistical enquiry. Collection of Data is the first and foremost step in a statistical investigation. ... Journals and Papers: Different newspapers and magazines provide a variety of statistical data in their writings, ...
We conducted the data collection across 1100 schools, 1100 anganwadi centres, 4100 health facilities , 500 local government offices and 8300 households. Overall, the scale, varying sectors, and respondents, the different levels of data collection, and the varied modes of the survey warranted substantial planning to mitigate expected challenges.
Interviewing is one of the most common data collection tools in qualitative research. It is widely discussed in research methods classes and literature and considered as an invaluable tool for ...
In this paper, we study safe data collection for the purpose of policy evaluation in tabular Markov decision processes (MDPs). In policy evaluation, we are given a \textit{target} policy and asked to estimate the expected cumulative reward it will obtain. ... Search 218,823,900 papers from all fields of science. Search. Sign In Create Free ...
The collection of quantitative data is appropriate to determining the existence and extent of the relationship between the Act and several strategic effects (Brenda, 2003). The researcher's choice of a qualitative research design remains appropriate to the study scenario because it allows a researcher to examine the phenomenon in its natural ...
(a) The overlapping data-collection areas as the beam is shifted along the axis of the pillar sample. (b) The order of image acquisition between â90° and â76° is illustrated by image number and colour as light (first image) to dark (last image). The beam is shifted along the pillar to acquire tilt images at each angle before tilting the ...
MCQs for NEET Exam is a collection of asked questions for the NTA NEET. The App contains questions which were asked in last 37 year ranging from 1988 to 2024. The App covering chapter-wise and topic-wise a total of around 7200+ MCQs followed by answer key, hint and detailed solutions. Chapter-wise & Topic-wise questions gives a clear idea ...