
An official website of the United States government
The .gov means it’s official. Federal government websites often end in .gov or .mil. Before sharing sensitive information, make sure you’re on a federal government site.
The site is secure. The https:// ensures that you are connecting to the official website and that any information you provide is encrypted and transmitted securely.
- Publications
- Account settings
Preview improvements coming to the PMC website in October 2024. Learn More or Try it out now .
- Advanced Search
- Journal List
- PLoS Comput Biol
- v.11(6); 2015 Jun

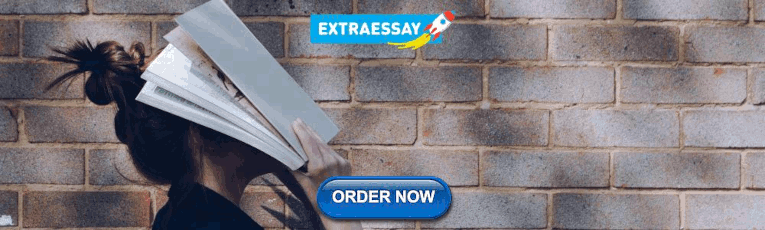
Computational Biology: Moving into the Future One Click at a Time
Christiana n. fogg.
1 Freelance Science Writer, Kensington, Maryland, United States of America
Diane E. Kovats
2 Executive Director, International Society for Computational Biology, La Jolla, California, United States of America
Computational biology has grown and matured into a discipline at the heart of biological research. In honor of the tenth anniversary of PLOS Computational Biology , Phil Bourne, Win Hide, Janet Kelso, Scott Markel, Ruth Nussinov, and Janet Thornton shared their memories of the heady beginnings of computational biology and their thoughts on the field’s promising and provocative future.
Philip E. Bourne
Philip Bourne ( Fig 1 ) began his scientific career in the wet lab, like many of his computational biology contemporaries. He earned his PhD in physical chemistry from the Flinders University of South Australia and pursued postdoctoral training at the University of Sheffield, where he began studying protein structure. Bourne accepted his first academic position in 1995 in the Department of Pharmacology at the University of California, San Diego (UCSD), rose to the rank of professor, and was associate vice chancellor for Innovation and Industry Alliances of the Office of Research Affairs. During his time at UCSD, he built a broad research program that used bioinformatics and systems biology to examine protein structure and function, evolution, drug discovery, disease, and immunology. Bourne also developed the Research Collaboratory for Structural Bioinformatics (RCSB) Protein Data Bank (PDB) and Immune Epitope Database (IEDB), which have become valuable data resources for the research community. In 2014, Bourne accepted the newly created position of associate director for data science (ADDS) at the National Institutes of Health (NIH), and he has been tasked with leading an NIH-wide initiative to better utilize the vast and growing collections of biomedical data in more effective and innovative ways.

Associate director for data science, NIH.
Bourne has been deeply involved with the International Society for Computational Biology (ISCB) throughout his career and is the founding editor-in-chief (EIC) of PLOS Computational Biology , an official journal of ISCB. He has been a firm believer in open access to scientific literature and the effective dissemination of data and results, for which PLOS Computational Biology is an exemplary model. Bourne believes that open access is more than just the ability to read free articles, and he said, “The future is using this content in really effective ways.” He referenced an article he cowrote with J. Lynn Fink and Mark Gerstein in 2008, titled “Open Access: Taking Full Advantage of the Content,” which argued that the full potential of open access has not been realized, as “no killer apps” exist that troll the literature and cross-reference other databases to come up with new discoveries [ 1 ]. Bourne believes that the scientific literature will become more and more open in this digital age and that new tools will be developed to harness the potential of this expansive information treasure chest.
Beyond scientific literature, Bourne sees data as a powerful catalyst that is often trapped in individual servers or hardware from defunct projects. As ADDS, he is steering the development of the Commons that he sees as a virtual space in which data sets, tools, and results can be stored, indexed, and accessed. Bourne anticipates that the Commons can be a way for tools and data to live on and benefit other scientists or, in other cases, act as a way to flag problematic or unusable contributions. He is hopeful this new approach can change the way biomedical research data is used, believing that “it offers the opportunity for serendipitous discovery.” Software tools that scientists store in the Commons will have better exposure and offer new opportunities for their use and attribution.
Bourne was in attendance as President Obama launched the President’s Precision Medicine Initiative on January 30, 2015, at the White House. This initiative aims to revolutionize medicine by harnessing information about an individual’s genome, environment, and lifestyle, and it will support research projects focused on transforming cancer treatment [ 2 ]. A bold long-term goal of the initiative is to create a cohort of 1 million American volunteers who will share their genetic and health information. Bourne sees this potentially revolutionary project as a powerful way to promote collaboration between computational biologists working on basic research problems and medical information scientists focused more on clinical and electronic health record information analysis.
Collaboration will be paramount to the success of projects like the Precision Medicine Initiative, and Bourne has observed how scientists are taking novel approaches to form collaborations in this increasingly connected world. He sees communities self-organizing and springing up spontaneously, and some have become influential and respected advocates for research and data sharing, like the Global Alliance for Genomics and Health and the Research Data Alliance. He said, “These are groups of volunteers [who are] funded to do something else but see [the] value of doing things together.” These communities and alliances offer new ways for scientists with shared research interests to come together both in person and virtually and may offer valuable lessons to scientific societies wanting to remain relevant and useful to their members.
Scientific communities are often grounded in shared ideas, and these ideas can be captured in a community’s specialized publications. During his tenure as EIC of PLOS Computational Biology , Bourne began the “ Ten Simple Rules ” collection, which has become one of the most-viewed article collections in any journal, with over 1 million views. This collection has become a treasured source of ideas and information for the computational biology community and has been relevant and helpful to biomedical scientists and trainees from many disciplines. Bourne considers the popularity of these articles an indicator of the information trainees and scientists are seeking but don’t get during their training. He thinks of the “Ten Simple Rules” articles as starting points and hopes that they genuinely help readers find information or guidance. If not, their entertainment value is remarkably therapeutic for the beleaguered scientific masses.
Bourne is looking forward to the next ten years and beyond as computational biology becomes ever more entwined with biomedical research and medicine.
Winston (Win) Hide
Win Hide ( Fig 2 ) has witnessed the transformation of biological research firsthand, from his early wet-lab training in molecular genetics to his present-day research using computational approaches to understand neurodegenerative diseases. Hide graduated from Temple University with a PhD in molecular genetics, and after his postdoctoral training and time spent in Silicon Valley, he founded the South African National Bioinformatics Institute in 1996. He accepted a position at the Harvard School of Public Health in 2008 and became director of the Harvard Stem Cell Institute Center for Stem Cell Bioinformatics. In 2014, Hide accepted a position at the Sheffield Institute for Translational Neuroscience, University of Sheffield, and became a professor of Computational Biology and Bioinformatics.

Professor of Computational Biology and Bioinformatics, Sheffield Institute for Translational Neuroscience, the University of Sheffield, United Kingdom.
Hide considers the drive to sequence the human genome in the 1990s as a major point at which computational biology transformed into a research field. He recalls remarks made around that time, by Lee Hood of the Institute for Systems Biology, contending that biology was becoming a data science. By 1998, SmithKline Beecham had organized the largest corporate bioinformatics department, headed by computational linguist David Searls, and this investment brought new attention to the power and scale of computational biology [ 3 ].
Biomedical researchers are beginning to acknowledge that biology is changing from a hypothesis-driven science to a data-driven science. But Hide thinks this shift is causing an uncomfortable and unsustainable tension between scientists working in these different realms. He has witnessed the tendency of hypothesis-driven experimentalists to pick the data they want to use, and these choices work against the innate objectivity of data-driven analysis. “People choose the pieces of data-driven science that make sense to them,” he said. “We haven’t reached the point in computational biology to judge how right we are. We’ve reached the probability but not summation of how good a model is in data-driven science. So we leave it to the biologists to decide on the pieces they know might be right and move forward.”
Hide sees a significant and urgent need for the convergence of the computational biology domains, including text mining, crowdsourcing, algorithmics, systems biology, and large surveys, to arrive at how correct models are by using machine learning. He acknowledges that these sorts of projects are difficult to take on but are likely the only way to arrive at models that are actionable.
Biologists across the board need to become more comfortable with data analysis and coding. Hide highlighted a talk given by Sean Eddy of the Howard Hughes Medical Institute at the “High Throughput Sequencing for Neuroscience” meeting in 2014 that was a gentle but compelling challenge to experimental biologists to reclaim their data analysis [ 4 ]. Eddy said, “We are not confident in our ability to analyze our own data. Biology is struggling to deal with the volume and complexity of data that sequencing generates. So far our solution has been to outsource our analysis to bioinformaticians.” He spoke about the widespread outsourcing of sequencing analysis to bioinformatics core facilities. “It is true that sequencing generates a lot of data, and it is currently true that the skills needed to do sequencing data analysis are specialized and in short supply,” he said. “What I want to tell you, though, is that those data analysis skills are easily acquired by biologists, that they must be acquired by biologists, and that that they will be. We need to rethink how we’re doing bioinformatics.” He urged biologists to learn scripting, saying “The most important thing I want you to take away from this talk tonight is that writing scripts in Perl or Python is both essential and easy, like learning to pipette. Writing a script is not software programming. To write scripts, you do not need to take courses in computer science or computer engineering. Any biologist can write a Perl script.”
Hide also sees a great need for computational biologists to be trained to collaborate better. He has witnessed the increasingly collaborative and multidisciplinary nature of biological and biomedical research and contends that computational approaches are becoming a fundamental part of collaborations. In the future, Hide expects that some of the strongest and most successful computational biologists will be specialists in particular fields (e.g., machine learning, semantic web) or domains (e.g., cancer, neuroscience) that excel at reaching across disciplines in nonthreatening and productive ways.
Many experimental biologists first became acquainted with computational biology and bioinformatics through collaborations with researchers running core facilities. Most computational biologists recognize that providing service work is an unavoidable part of their job, but this work is often not appropriately recognized or attributed. Hide believes that computational biologists, collaborators, administrators, and funding agencies must better differentiate between work done for research or as a form of service. Recognition of service work is critical to ensuring that core facilities remain a vibrant part of the research infrastructure and can attract highly skilled computational biologists.
Janet Kelso
Janet Kelso ( Fig 3 ) is working at the cutting edge of computational biology with some of the world’s most ancient DNA. Her research interests include human genetics and genome evolution, with a particular interest in the ancestry of modern Homo sapiens and their ancestors, including the extinct Neanderthal species.

Bioinformatics research group leader, Max-Planck Institute for Evolutionary Anthropology, Leipzig, Germany.
Kelso pursued her PhD studies in bioinformatics in the early 2000s under the guidance of Winston Hide at the South African National Bioinformatics Institute. She has watched firsthand as the era of personal genome sequencing has become a reality. “It has become possible in the last eight years to sequence whole genomes so rapidly and inexpensively that the sequencing of the genomes of individual people is now possible,” she said. Kelso thinks the lowered cost and rapid speed of whole genome sequencing will transform our knowledge of human genetics and change the way that medicine is practiced. She sees a prodigious job ahead for computational biologists when it comes to what we do with this data and how we interpret it. “Reference databases created by computational biologists, with information like sequence variants, will capture the effects of ordinary variation in our genomes on our phenotype—how we get sick, how we age, how we metabolize pharmaceutical drugs,” she explained. “This information will be used for diagnosis and will be important for tailoring treatments to individuals.” Kelso expects there will be insight into how genetic variations impact the effect of different treatments, even though these variations may have nothing to do with the disease itself.
As personal genomics becomes a reality, Kelso thinks computational biologists will have to consider that the public will want access to their tools and resources. “The computational biologist’s role is to provide good resources and tools that allow both biomedical researchers and ordinary people to understand and interpret their genome sequence data. It’s a really hard problem, to go from sequence variants in a genome of 3 billion bases to understanding the effects they may have on how long you live or if you develop a disease.”
Kelso sees that computational biology tools will also have immense value in fields other than human genetics. “Many of the tools we develop can be applied in other domains such as agriculture,” she explained. “For example, how do variations in a plant genome allow it to respond to environment, and what additional nutrients do you need to provide to optimize crop production? Similar computational biology tools can be applied in these different systems.”
Kelso considers many of the technical improvements in the field to have been among the major developments in computational biology over the last decade. She explained, “To me, the biggest contributions of computational biology are developments in how to store and annotate data, how to mine that data and to visualize large quantities of biological data. Our ability to integrate large volumes of data and to extract meaningful information and knowledge from that is a huge contribution and has moved the field forward substantially.”
From Kelso’s perspective, she thinks students are now more comfortable with integrating computation into their training and research. “Compared with ten years ago, lab-oriented students are becoming more skilled in bioinformatics. There will always be a place for specialists in both computational and molecular biology, but there is a larger zone in the middle now where people from these different disciplines understand each other.” Kelso has observed that many students now realize that you can’t be a molecular biologist and not know anything about informatics. “Students who come into our program now spend a lot of time learning bioinformatics and are able to work on reasonably sized data sets.”
Kelso is optimistic about the future of computational biology: “Computational biology is now a mature discipline that has cemented itself as integral to modern biology. As we enter a period of unparalleled data accumulation and analysis, computational biology will undoubtedly continue to contribute to important advances in our understanding of molecular systems.”
Scott Markel
Scott Markel ( Fig 4 ) has spent most of his career working as a software developer in industry. He pursued his PhD in mathematics at the University of Wisconsin–Madison, and like many of his contemporaries, he discovered that he could apply his degree to bioinformatics software development. He said, “I have probably made more of a career in industry than others have by leveraging open source tools, giving back to that community where and when I can.” Indeed, it was the culture of open source software supported by ISCB, especially members of the Bioinformatics Open Source Conference (BOSC) community, which drew Markel to the Society.

Secretary, ISCB. BIOVIA principal bioinformatics architect at Dassault Systèmes.
Markel, like many of his ISCB colleagues, considers the sequencing of the human genome as a major research landmark for computational biology and a powerful driver of the technologies and software developed over the last two decades for sequencing and genomics. Sequencing technology continues to become cheaper, faster, and more portable. Next-generation sequencing (NGS) technology has been adapted widely over the last five years, but Markel also sees increasing use of newer technologies, like those being developed by Oxford Nanopore, which will offer longer sequence reads. Markel has observed that researchers and bioinformatics information technology (IT) support staff are faced with the challenges of storing vast amounts of digital data and are shifting their mind-set in this era of technological flux. He said, “As sequencing gets cheaper, it’s better not to save all the data—just run the sequence analysis again.”
As an industry-oriented computational biologist, Markel has a different view of how software is developed and used. Markel has learned how to listen to clients’ needs while also balancing out what kind of product can be built, sold, and maintained. “Customers don’t want something like BLAST [Basic Local Alignment Search Tool]; they want BLAST. As team sizes get smaller and broader, it’s not worth building something the equivalent of BLAST, which will need maintenance, need to be sold as a product, and users will have to be convinced scientifically…is as good as BLAST.” Markel’s primary product, Pipeline Pilot, is a graphic scientific authoring application that supports data management and analysis. He has observed that clients in biotechnology and pharmaceutical research are working more on biologics, like therapeutic antibodies, and they are handling an increasingly diverse spectrum of data types. Markel has noticed that clients are drawn to software like the new Biotherapeutics Workbench, built for antibody researchers using Pipeline Pilot, because it provides decision support, and he explained, “Lab time is more expensive than doing things computationally. This type of application can identify subsets of candidates that you can take forward.”
Markel’s software development experiences highlight how computational biology is transforming research in industry settings, and he suspects that industry will continue to invest in computational biology-driven technologies. “If you make the programming part easier,” he said, “like being able to modify the workflow by changing settings or deploying a program through a web interface, users are thrilled to be self-enabled. For some people, especially those without a programming background, this is a revelation.”
Computational biology is likely to become a part of routine health care in the future, and Markel suspects that one area we will see this change in is the “internet of things.” Computational biology applications are not limited to research and drug discovery but are already being adapted for clinical use, like implantable devices, home health monitoring, and diagnostics. Markel took notice of Apple’s venture into the clinical trial sector through the launch of the ResearchKit platform [ 5 ], which provides clinical researchers with tools to build clinical trial apps that can be accessed by iPhone users. Markel sees this type of technology as potentially transformative, and he took note of a comment made by Alan Yeung, medical director of Stanford Cardiovascular Heath and an investigator involved with the ResearchKit cardiovascular app. 11,000 iPhone users signed up for this app within the first 24 hours of its launch, and Yueng said to Bloomberg News, “To get 10,000 people enrolled in a medical study, it would take a year and 50 medical centers around the country. That’s the power of the phone” [ 6 ]. This approach to clinical research is not without controversy, as observers are concerned iPhone-based apps can result in a biased selection of users. Others have reservations about the privacy of clinical trial data collected from these sources.
Personal health data are being collected and shared at record volumes in this era of smart phones and wearable devices. Although the openness of this data is up for debate, more intimate and personal data have caused even greater contention in recent years. Open Humans is an open online platform that asks users to share their genomes and other personal information, which can be accessed by anyone who signs into the website, and is intended to make more data available to researchers [ 7 ]. Markel sees this sort of platform as a powerful and rich source of data for computational biologists, but it’s not without controversy. Although users can share their data using an anonymous profile, the data may contain enough unique information to reveal an individual’s identity, which could have unintended consequences.
The success and wide acceptance of these open data projects will impact how the general public sees computational biology as a field, and it may take decades for the public to decide how data should be shared. Nonetheless, it’s an exciting time for computational biology according to Markel, as he sees aspects of the field coming into daily life more and has witnessed how researchers in industry labs have leveraged the power of computation.
Ruth Nussinov
Ruth Nussinov ( Fig 5 ) heads the computational structural biology group in the Laboratory of Experimental Immunology at the National Cancer Institute (NCI)/NIH and is editor-in-chief of PLOS Computational Biology . She earned a PhD in biochemistry from Rutgers University and did her postdoctoral training at the Weizmann Institute. She has spent her career working as a computational biologist and is a pioneer of DNA sequence analysis and RNA structure prediction. Nussinov began her training at a time when the term “computational biology” was poorly understood by biologists and mathematicians and no formal training programs that combined computer science, mathematics, and biology existed. In 1985, Nussinov accepted a position as an associate professor at the Tel Aviv University Medical School, where she began an independent research program. She recalled a conversation with a dean at the school. He said, “Ruth, what are you? A mathematician?” To his chagrin and befuddlement, she replied, “No, I’m a biologist, a computational biologist.” Now computational biology is one of the hottest and fastest growing fields in biology, and training programs are in high demand.

Senior investigator and head of computational structural biology group, Laboratory of Experimental Immunology, Cancer and Inflammation Program, NCI, NIH, and professor in the School of Medicine, Tel Aviv University.
As editor-in-chief of PLOS Computational Biology , Nussinov has gained a unique perspective of the field. The breadth of expertise across the journal’s Editorial Board and community of peer reviewers is vast because computational biology as a discipline is so broad; it seems to cover everything.
The vibrancy of the field is clear to Nussinov. “I think we can say it is a field that is very much alive and at the forefront of the sciences,” she said, “It reflects the fact that biology has been shifting from descriptive to a quantitative science.” Nussinov also acknowledges that computation-driven research can’t move forward without a strong relationship with experimental biology. “Computational biology is strongly tied to experiments, and experimental biology is becoming more quantitative. More and more studies provide quantitation, and this type of information is essential for making comparisons across experiments.” As a student, Nussinov recalls reading papers about transcription in which transcription levels were classified as + or ++ and were clearly subjective estimates of transcription levels. Now transcript levels are quantified with exquisite sensitivity using real-time PCR.
In spite of biology’s shift toward quantitation, Nussinov recognizes some of the field’s limitations. She has worked closely with mathematicians throughout her career, and she recalls one conversation with an algorithm development mathematician. He was trying to understand all the parameters of her experiments, and she kept saying, “It depends.” She, like many biologists, is all too familiar with the numerous variables and experimental conditions that come along with seemingly messy biology experiments, and computational biologists spend much of their time contending with this issue. New technologies, especially those based in biophysics, have contributed to improvements in the quality of data used for quantitation, but some variability will always exist.
Nussinov feels that data storage and organization are critical issues facing the future of computational biology. “Data is accumulating fast, and it is extremely diverse.” One of the challenges she sees is how does the community organize the data. She said, “The data relates to populations, disease associations, symptoms, therapeutic drugs, and more. How do you organize it and make it open and shared? By disease, by countries, more isolated or less isolated areas?” These are not easy issues to address and will only become more important as data accumulate. She also considers noise to be a major issue with this data. She said, “How do you overcome the problem of noise, an inevitable problem with vast quantities of data? How do you sift through it and see real trends? You still need cross validation.”
Nussinov believes that some of the major challenges facing computational biologists deal with developing modes of analyses that can validate or negate common beliefs or expectations, uncover unknown trends, obtain insights into fundamental processes, and exploit this information to improve predictions and design. For these, the computational biologist needs data that are openly accessible, shared software, computational power, and importantly, in-depth understanding.
In the end, Nussinov sees immeasurable value in fostering collaborations between experimental and computational biologists. “Experimentalists can’t check all possible models. Computation can provide leads, and experiments can check it. That is the ideal scenario.”
Janet Thornton
Janet Thornton ( Fig 6 ) has spent her research career studying protein structure and is considered a leading researcher in the field of structural bioinformatics. She pursued her PhD in biophysics in the 1970s, when very little information existed on protein structure and nucleotide sequences [ 8 ]. Thornton’s early research career at Oxford included using protein sequences to predict structure, and this type of research marked the earliest beginnings of bioinformatics. She became the director of the European Molecular Biology Laboratory–European Bioinformatics Institute (EMBL-EBI) in 2001, just as genomics and bioinformatics were growing rapidly, and her institution maintained valuable bioinformatics databases with data from throughout Europe. EBI also developed a thriving bioinformatics research community.

Senior scientist and outgoing director of EMBL-EBI.
Thornton’s experiences as a structural biologist and EBI director have given her an exclusive viewpoint on the evolution of computational biology and bioinformatics from its infancy to the present day. She considers developments in five different areas to have been critical to the progress of computational biology:
- Development of new methods for new data-generating technologies (next-generation sequencing , proteomics/metabolomics , genome-wide association studies [GWAS] , and image processing) . Without these methods, the new technologies would have been useless and interpretation of the data impossible.
- Development of methods in systems biology .
- Ontology development and text mining . This area is fundamental to everything in computational biology. Defining the ontologies and the science behind them will ultimately allow for the data integration and comparison needed to understand the biology of life. The opportunities presented by open literature and open data cannot be underestimated, and new methods for text mining are being developed.
- Algorithm development . The effectiveness and efficiency of old algorithms (sequence alignment and protein folding) is constantly being refined, and new algorithms are being developed alongside new technologies.
- Technical development . New methods for handling, validating, transferring, and storing data at all levels are under development, and cloud computing for the biological sciences is emerging.
Thornton reflected on some of the most interesting observations and results to come out of the increasingly diverse corpus of computational biology research—in particular, the use of genomics to identify how microbes evolve during an epidemic, genomic approaches to understanding human evolution, GWAS studies to discern how genetic variants impact disease, the discovery of the breadth of the microbiome and how bacterial populations interact and influence each other, the use of electronic health records to extract clinical data, and the observation that regulatory processes evolve relatively quickly in comparison to protein sequences and structures.
Thornton’s research has changed over time as bioinformatics tools and algorithms improved and protein data flooded the databases. She explained, “The evolution of protein function, especially understanding how the majority of enzyme functions have developed during their evolution from other functions, has been helped by new sequence data for the construction of better [phylogenetic] trees that reveal yet more interesting changes in function.” New algorithms developed in our group have changed the way we compare enzyme functions and have made it quantitative rather than qualitative.” Thornton is also studying how variants affect structure and function, and the 1,000 genomes data have greatly enhanced this work. She said, “The major difference in this area is new data. We can now look at germ-line changes in many individuals. Relationships to diseases are emerging, and many new paradigms will be revealed with 100,000 genomes from individuals with rare diseases.”
The convergence of computational and experimental biology is already underway, and Thornton considers that several pressing biological questions can only be addressed by combining these approaches—in particular, in areas such as building predictive models of the cell, organelles, and organs, understanding aging, designing enzymes, and improving drug design and target validation.
Thornton considers one of the biggest challenges facing computational biology, and potentially hindering these areas of research, is sharing data, especially medical data. She also believes that the computational biology community must make engagement of medical professionals and the public a top priority. She said, “This is really important. At EMBL-EBI, we are training medical professionals in bioinformatics, working on more and more public engagement, which is a huge challenge to do across Europe, especially with limited funds, and we are training scientists to do more public engagement.” It seems clear that computational biology will become a part of everyday life, especially in medicine, and these efforts are critical for gaining support from the medical community and the greater public.
The thoughts shared by these accomplished computational biologists make it clear that biology is becoming a data science, and future breakthroughs will depend on strong collaborations between experimental and computational biologists. Biologists will need to adapt to the data-driven nature of the discipline, and the training of future researchers is likely to reflect these changes as well. Aspects of computational biology are integrating into all levels of medicine and health care. Medical professionals as well as the public need to be well informed and educated about these changes in order to realize the full potential of this new frontier in medicine without fear of the technological advances.
Funding Statement
CNF was paid by ISCB to write this article.

Journal of Computational Biology
Editor-in-Chief: Mona Singh, PhD
Impact Factor: 1.7* *2022 Journal Citation Reports™ (Clarivate, 2023)
Citescore™: 3.2.

The leading peer-reviewed journal in computational biology and bioinformatics, publishing in-depth statistical, mathematical, and computational analysis of methods, as well as their practical impact.
- View Aims & Scope
- Indexing/Abstracting
- Editorial Board
- Featured Content
- About This Publication
- Reprints & Permissions
- News Releases
- Sample Issue
Aims & Scope
Journal of Computational Biology publishes articles whose primary contributions are the development and application of new methods in computational biology, including algorithmic, statistical, mathematical, machine learning and artificial intelligence contributions. The journal welcomes novel methods that tackle established problems within computational biology; novel methods and frameworks that anticipate new problems and data types arising in computational biology; and novel methods that are inspired from studying natural computation. Methods should be tested on real and/or simulated biological data whenever feasible. Papers whose primary contributions are theoretical are also welcome. Available only online, this is an essential journal for scientists and students who want to keep abreast of developments in bioinformatics and computational biology.
Research Articles: Research articles describe new methodology development and application in computational biology. It is recommended that manuscripts should be approximately 3,000 words, excluding tables, figures, legends, abstract, disclosure or references; longer articles can also be submitted. Research articles should include the following sections, in order: abstract, introduction, methods, results, discussion and references.
Software articles: Short 2-4 page articles describing implementations of new or recently developed computational methods for applications in computational biology. The approaches underlying the software should have methodologically interesting components. Software articles can be published as companion articles to primary research articles which describe the main methodological contributions. Software article submissions should be accompanied by a cover letter that concisely states the novel implementation and algorithmic challenges the software tackles.
*Research and software articles should report unique findings not previously published.
Tutorials: These articles highlight important concepts in computational biology. The journal especially welcomes tutorials on algorithms, data structures, machine learning paradigms, and other computational formalisms that are newly being utilized in computational biology. Prospective contributors should contact the journal ( [email protected] ) with brief outlines before proceeding.
Reviews: Brief outlines from prospective contributors are welcome, and these will also be solicited on specific subjects. Articles that benchmark existing approaches are also welcome.
News/Perspectives/Book Reviews: These article types should typically be 2–4 pages long. Contacting the journal before beginning such a paper is suggested.
Conference and other special issues: The Journal of Computational Biology welcomes proposals for special issues related to topics within the scope of the journal.
Journal of Computational Biology coverage includes:
- Algorithms for computational biology
- Mathematical modeling and simulation
- AI / Machine learning
- Statistical formulations
- Software for applied bioinformatics
- Genomics and systems biology
- Evolution and population genomics
- Biomedical applications
- Biocomputing and biology-inspired algorithms
Specific topics of interest include, but are not limited to:
- Molecular sequence analysis
- Sequencing and genotyping technologies
- Regulation and epigenomics
- Transcriptomics, including single-cell
- Metagenomics
- Population and statistical genetics
- Evolutionary, compressive and comparative genomics
- Structure and function of non-coding RNAs
- Computational proteomics and proteogenomics
- Protein structure and function
- Biological networks
- Computational systems biology
- Privacy of biomedical data
Journal of Computational Biology is under the editorial leadership of Editor-in-Chief Mona Singh, PhD , Princeton University; and other leading investigators. View the entire editorial board .
Audience: Computational biologists, bioinformaticians, data scientists, applied mathematicians, and computer scientists, among others.
Indexing/Abstracting:
- PubMed/MEDLINE
- PubMed Central
- Web of Science: Science Citation Index Expanded™ (SCIE)
- Current Contents®/Life Sciences
- Biotechnology Citation Index®
- Biological Abstracts
- BIOSIS Citation Index™
- Journal Citation Reports/Science Edition
- EMBASE/Excerpta Medica
- Chemical Abstracts
- ProQuest databases
- CAB Abstracts
- Global Health
- The DBLP Computer Science Bibliography
Society Affiliations
The Official Journal of:
2022 August
Special Issue: Professor Michael Waterman's 80th Birthday, Part 2
More Special Issues...
Recommended Publications
Genetic Engineering & Biotechnology News
OMICS: A Journal of Integrative Biology
ASSAY and Drug Development Technologies
DNA and Cell Biology
- Search Menu
- Advance articles
- Author Guidelines
- Submission Site
- Open Access
- Why publish with this journal?
- About Bioinformatics
- Journals Career Network
- Editorial Board
- Advertising and Corporate Services
- Self-Archiving Policy
- Dispatch Dates
- Journals on Oxford Academic
- Books on Oxford Academic

Editors-in-Chief
Janet Kelso
Alfonso Valencia
The leading journal in its field, Bioinformatics publishes the highest quality scientific papers and review articles of interest to academic and industrial researchers. Its main focus is on new developments in genome bioinformatics and computational biology.
Learn more about publishing with Bioinformatics here.
Why publish with Bioinformatics ?
Are you looking for a home for your research? Publish with Bioinformatics and enjoy a variety of benefits, including:
- Strong reputation and Impact Factor
- Distinguished and supportive editors
- Fully open access
I'm ready to learn more!
Read our author guidelines to find out more about:
- Manuscript types
- Review processes
- Open access options
- Manuscript preparation
Author guidelines

Format free submissions
At first submission, Bioinformatics authors are no longer required to format their manuscript according to journal guidelines.
Find out more about submitting a manuscript
Bioinformatics is now fully OA
Bioinformatics is now fully open access. Visit the journal's open access page to learn more about the change.
Learn about the change

High-Impact Research Collection
Explore the most read, most cited, and most discussed articles published in Bioinformatics in recent years and discover what has caught the interest of your peers.
Browse the collection

International Society for Computational Biology
Bioinformatics is an official journal of the International Society for Computational Biology, the leading professional society for computational biology and bioinformatics. Members of the society receive a 15% discount on article processing charges when publishing Open Access in the journal.
- Read papers from the ISCB
Find out more
Browse by subject
- Genome analysis
- Sequence analysis
- Phylogenetics
- Structural bioinformatics
- Gene expression
- Genetics and population analysis
- Systems biology
- Data and text mining
- Databases and ontologies
- Bioimage informatics

Bioinformatics and Publons
Bioinformatics is part of a trial with Publons to recognise our expert peer reviewers and raise the status of peer review.
Latest articles

Email alerts
Register to receive table of contents email alerts as soon as new issues of Bioinformatics are published online.

Discover a more complete picture of how readers engage with research in Bioinformatics through Altmetric data. Now available on article pages.

Committee on Publication Ethics (COPE)
This journal is a member of and subscribes to the principles of the Committee on Publication Ethics (COPE)
publicationethics.org

Recommend to your library
Fill out our simple online form to recommend Bioinformatics to your library.
Recommend now
Related Titles
- Recommend to your Library
Affiliations
- Online ISSN 1367-4811
- Copyright © 2024 Oxford University Press
- About Oxford Academic
- Publish journals with us
- University press partners
- What we publish
- New features
- Open access
- Institutional account management
- Rights and permissions
- Get help with access
- Accessibility
- Advertising
- Media enquiries
- Oxford University Press
- Oxford Languages
- University of Oxford
Oxford University Press is a department of the University of Oxford. It furthers the University's objective of excellence in research, scholarship, and education by publishing worldwide
- Copyright © 2024 Oxford University Press
- Cookie settings
- Cookie policy
- Privacy policy
- Legal notice
This Feature Is Available To Subscribers Only
Sign In or Create an Account
This PDF is available to Subscribers Only
For full access to this pdf, sign in to an existing account, or purchase an annual subscription.
Volume 21 Supplement 9
Selected Articles from the 20th International Conference on Bioinformatics & Computational Biology (BIOCOMP 2019)
- Introduction
- Open access
- Published: 03 December 2020
Current trend and development in bioinformatics research
- Yuanyuan Fu 1 ,
- Zhougui Ling 1 , 2 ,
- Hamid Arabnia 3 &
- Youping Deng 1
BMC Bioinformatics volume 21 , Article number: 538 ( 2020 ) Cite this article
10k Accesses
16 Citations
4 Altmetric
Metrics details
This is an editorial report of the supplements to BMC Bioinformatics that includes 6 papers selected from the BIOCOMP’19—The 2019 International Conference on Bioinformatics and Computational Biology. These articles reflect current trend and development in bioinformatics research.
The supplement to BMC Bioinformatics was proposed to launch during the BIOCOMP’19—The 2019 International Conference on Bioinformatics and Computational Biology held from July 29 to August 01, 2019 in Las Vegas, Nevada. In this congress, a variety of research areas was discussed, including bioinformatics which was one of the major focuses due to the rapid development and requirement of using bioinformatics approaches in biological data analysis, especially for omics large datasets. Here, six manuscripts were selected after strict peer review, providing an overview of the bioinformatics research trend and its application for interdisciplinary collaboration.
Cancer is one of the leading causes of morbidity and mortality worldwide. There exists an urgent need to identify new biomarkers or signatures for early detection and prognosis. Mona et al. identified biomarker genes from functional network based on the 407 differential expressed genes between lung cancer and healthy populations from a public Gene Expression Omnibus dataset. The lower expression of sixteen gene signature is associated with favorable lung cancer survival, DNA repair, and cell regulation [ 1 ]. A new class of biomarkers such as alternative splicing variants (ASV) have been studied in recent years. Various platforms and methods, for example, Affymetrix Exon-Exon Junction Array, RNA-seq, and liquid chromatography tandem mass spectrometry (LC–MS/MS), have been developed to explore the role of ASV in human disease. Zhang et al. have developed a bioinformatics workflow to combine LC–MS/MS with RNA-seq which provide new opportunities in biomarker discovery. In their study, they identified twenty-six alternative splicing biomarker peptides with one single intron event and one exon skipping event; further pathways indicated the 26 peptides may be involved in cancer, signaling, metabolism, regulation, immune system and hemostasis pathways which validated by the RNA-seq analysis [ 2 ].
Proteins serve crucial functions in essentially all biological processes and the function directly depends on their three-dimensional structures. Traditional approaches to elucidation of protein structures by NMR spectroscopy are time consuming and expensive, however, the faster and more cost-effective methods are critical in the development of personalized medicine. Cole et al. improved the REDRAFT software package in the important areas of usability, accessibility, and the core methodology which resulted in the ability to fold proteins [ 3 ].
The human microbiome is the aggregation of microorganisms that reside on or within human bodies. Rebecca et al. discussed the tissue-associated microbial detection in cancer using next generation sequencing (NGS). Various computational frameworks could shed light on the role of microbiota in cancer pathogenesis [ 4 ]. How to analyze the human microbiome data efficiently is a huge challenge. Zhang et al. developed a nonparametric test based on inter-point distance to evaluate statistical significance from a Bayesian point of view. The proposed test is more efficient and sensitive to the compositional difference compared with the traditional mean-based method [ 5 ].
Human disease is also considered as the cause of the interaction between genetic and environmental factors. In the last decades, there was a growing interest in the effect of metal toxicity on human health. Evaluating the toxicity of chemical mixture and their possible mechanism of action is still a challenge for humans and other organisms, as traditional methods are very time consuming, inefficient, and expensive, so a limited number of chemicals can be tested. In order to develop efficient and accurate predictive models, Yu et al. compared the results among a classification algorithm and identified 15 gene biomarkers with 100% accuracy for metal toxicant using a microarray classifier analysis [ 6 ].
Currently, there is a growing need to convert biological data into knowledge through a bioinformatics approach. We hope these articles can provide up-to-date information of research development and trend in bioinformatics field.
Availability of data and materials
Not applicable.
Abbreviations
The 2019 International Conference on Bioinformatics and Computational Biology
Liquid chromatography tandem mass spectrometry
Alternative splicing variants
Nuclear Magnetic Resonance
Residual Dipolar Coupling based Residue Assembly and Filter Tool
Next generation sequencing
Mona Maharjan RBT, Chowdhury K, Duan W, Mondal AM. Computational identification of biomarker genes for lung cancer considering treatment and non-treatment studies. 2020. https://doi.org/10.1186/s12859-020-3524-8 .
Zhang F, Deng CK, Wang M, Deng B, Barber R, Huang G. Identification of novel alternative splicing biomarkers for breast cancer with LC/MS/MS and RNA-Seq. Mol Cell Proteomics. 2020;16:1850–63. https://doi.org/10.1186/s12859-020-03824-8 .
Article Google Scholar
Casey Cole CP, Rachele J, Valafar H. Increased usability, algorithmic improvements and incorporation of data mining for structure calculation of proteins with REDCRAFT software package. 2020. https://doi.org/10.1186/s12859-020-3522-x .
Rebecca M, Rodriguez VSK, Menor M, Hernandez BY, Deng Y. Tissue-associated microbial detection in cancer using human sequencing data. 2020. https://doi.org/10.1186/s12859-020-03831-9 .
Qingyang Zhang TD. A distance based multisample test for high-dimensional compositional data with applications to the human microbiome . 2020. https://doi.org/10.1186/s12859-020-3530-x .
Yu Z, Fu Y, Ai J, Zhang J, Huang G, Deng Y. Development of predicitve models to distinguish metals from non-metal toxicants, and individual metal from one another. 2020. https://doi.org/10.1186/s12859-020-3525-7 .
Download references
Acknowledgements
This supplement will not be possible without the support of the International Society of Intelligent Biological Medicine (ISIBM).
About this supplement
This article has been published as part of BMC Bioinformatics Volume 21 Supplement 9, 2020: Selected Articles from the 20th International Conference on Bioinformatics & Computational Biology (BIOCOMP 2019). The full contents of the supplement are available online at https://bmcbioinformatics.biomedcentral.com/articles/supplements/volume-21-supplement-9 .
Publication of this supplement has been supported by NIH grants R01CA223490 and R01 CA230514 to Youping Deng and 5P30GM114737, P20GM103466, 5U54MD007601 and 5P30CA071789.
Author information
Authors and affiliations.
Department of Quantitative Health Sciences, John A. Burns School of Medicine, University of Hawaii at Manoa, Honolulu, HI, 96813, USA
Yuanyuan Fu, Zhougui Ling & Youping Deng
Department of Pulmonary and Critical Care Medicine, The Fourth Affiliated Hospital of Guangxi Medical University, Liuzhou, 545005, China
Zhougui Ling
Department of Computer Science, University of Georgia, Athens, GA, 30602, USA
Hamid Arabnia
You can also search for this author in PubMed Google Scholar
Contributions
YF drafted the manuscript, ZL, HA, and YD revised the manuscript. All authors read and approved the final manuscript.
Corresponding author
Correspondence to Youping Deng .
Ethics declarations
Ethics approval and consent to participate, consent for publication, competing interests.
The authors declare that they have no competing interests.
Additional information
Publisher's note.
Springer Nature remains neutral with regard to jurisdictional claims in published maps and institutional affiliations.
Rights and permissions
Open Access This article is licensed under a Creative Commons Attribution 4.0 International License, which permits use, sharing, adaptation, distribution and reproduction in any medium or format, as long as you give appropriate credit to the original author(s) and the source, provide a link to the Creative Commons licence, and indicate if changes were made. The images or other third party material in this article are included in the article's Creative Commons licence, unless indicated otherwise in a credit line to the material. If material is not included in the article's Creative Commons licence and your intended use is not permitted by statutory regulation or exceeds the permitted use, you will need to obtain permission directly from the copyright holder. To view a copy of this licence, visit http://creativecommons.org/licenses/by/4.0/ . The Creative Commons Public Domain Dedication waiver ( http://creativecommons.org/publicdomain/zero/1.0/ ) applies to the data made available in this article, unless otherwise stated in a credit line to the data.
Reprints and permissions
About this article
Cite this article.
Fu, Y., Ling, Z., Arabnia, H. et al. Current trend and development in bioinformatics research. BMC Bioinformatics 21 (Suppl 9), 538 (2020). https://doi.org/10.1186/s12859-020-03874-y
Download citation
Published : 03 December 2020
DOI : https://doi.org/10.1186/s12859-020-03874-y
Share this article
Anyone you share the following link with will be able to read this content:
Sorry, a shareable link is not currently available for this article.
Provided by the Springer Nature SharedIt content-sharing initiative
- Bioinformatics
- Human disease
BMC Bioinformatics
ISSN: 1471-2105
- General enquiries: [email protected]
Suggestions or feedback?
MIT News | Massachusetts Institute of Technology
- Machine learning
- Social justice
- Black holes
- Classes and programs
Departments
- Aeronautics and Astronautics
- Brain and Cognitive Sciences
- Architecture
- Political Science
- Mechanical Engineering
Centers, Labs, & Programs
- Abdul Latif Jameel Poverty Action Lab (J-PAL)
- Picower Institute for Learning and Memory
- Lincoln Laboratory
- School of Architecture + Planning
- School of Engineering
- School of Humanities, Arts, and Social Sciences
- Sloan School of Management
- School of Science
- MIT Schwarzman College of Computing
Computational biology
Download RSS feed: News Articles / In the Media / Audio
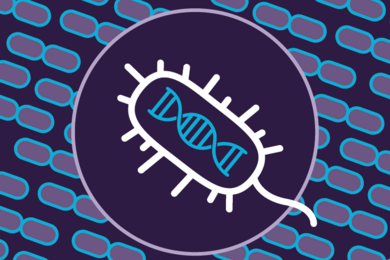
Search algorithm reveals nearly 200 new kinds of CRISPR systems
By analyzing bacterial data, researchers have discovered thousands of rare new CRISPR systems that have a range of functions and could enable gene editing, diagnostics, and more.
November 23, 2023
Read full story →

Making genetic prediction models more inclusive
MIT computer scientists developed a way to calculate polygenic scores that makes them more accurate for people across diverse ancestries.
October 26, 2023
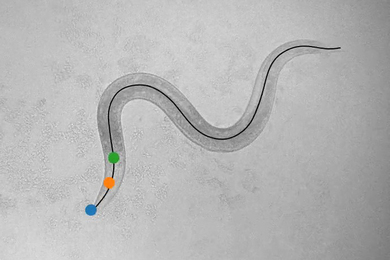
Cracking the code that relates brain and behavior in a simple animal
MIT researchers model and create an atlas for how neurons of the worm C. elegans encode its behaviors, make findings available on their “WormWideWeb.”
August 23, 2023
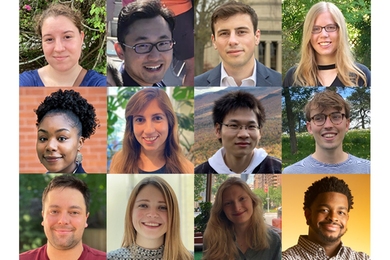
2022-23 Takeda Fellows: Leveraging AI to positively impact human health
New fellows are working on health records, robot control, pandemic preparedness, brain injuries, and more.
January 12, 2023
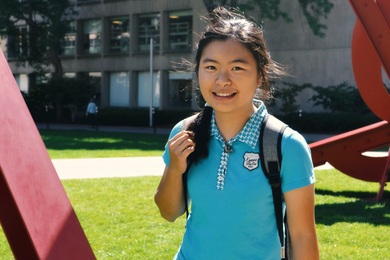
An interdisciplinary journey through living machines
With NEET, Sherry Nyeo is discovering MIT’s undergraduate research community at the intersection of computer science and biological engineering.
October 18, 2022
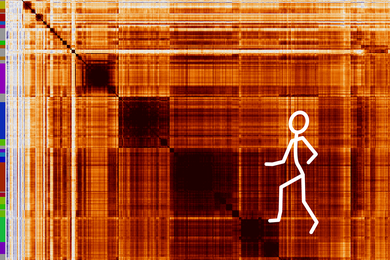
New CRISPR-based map ties every human gene to its function
Jonathan Weissman and collaborators used their single-cell sequencing tool Perturb-seq on every expressed gene in the human genome, linking each to its job in the cell.
June 9, 2022
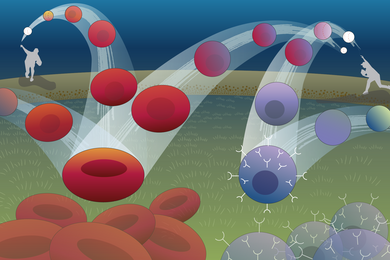
New computational tool predicts cell fates and genetic perturbations
The technique can help predict a cell’s path over time, such as what type of cell it will become.
February 3, 2022
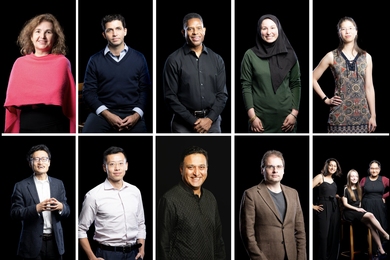
The promise and pitfalls of artificial intelligence explored at TEDxMIT event
MIT scientists discuss the future of AI with applications across many sectors, as a tool that can be both beneficial and harmful.
January 11, 2022
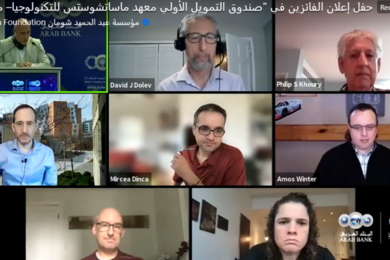
Inaugural fund supports early-stage collaborations between MIT and Jordan
MIT-Jordan Abdul Hameed Shoman Foundation Seed Fund winners announced.
July 29, 2021
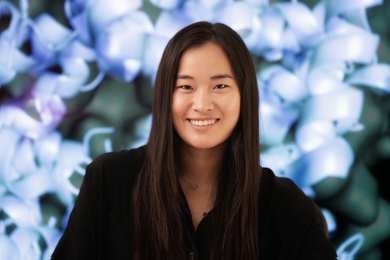
The power of two
Graduate student Ellen Zhong helped biologists and mathematicians reach across departmental lines to address a longstanding problem in electron microscopy.
June 30, 2021
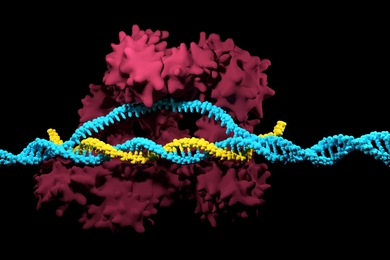
Nature-inspired CRISPR enzymes for expansive genome editing
Applied computational biology discoveries vastly expand the range of CRISPR’s access to DNA sequences.
May 19, 2020
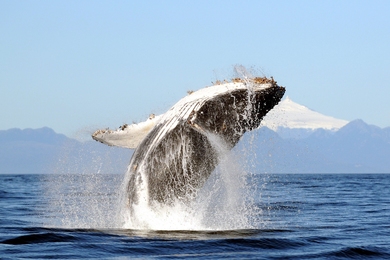
3 Questions: Greg Britten on how marine life can recover by 2050
Committing to aggressive conservation efforts could rebuild ocean habitats and species populations in a few decades.
April 3, 2020
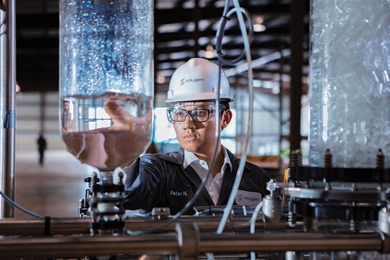
Cleaning up hydrogen peroxide production
Solugen’s engineered enzymes offer a biologically-inspired method for producing the chemical.
September 5, 2019
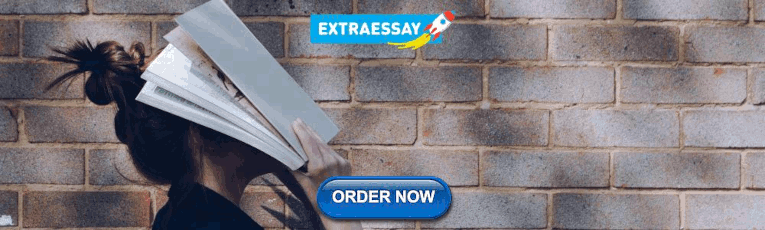
Microbial communities demonstrate high turnover
New research provides insight into the behavior of microbial communities in the ocean.
January 18, 2018
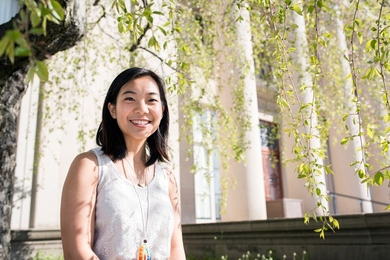
Determined to make a change
Senior Ruth Park draws on her background as she strives for educational reform.
May 12, 2016
Massachusetts Institute of Technology 77 Massachusetts Avenue, Cambridge, MA, USA
- Map (opens in new window)
- Events (opens in new window)
- People (opens in new window)
- Careers (opens in new window)
- Accessibility
- Social Media Hub
- MIT on Facebook
- MIT on YouTube
- MIT on Instagram
- News & Media
- Chemical Biology
- Computational Biology
- Ecosystem Science
- Cancer Biology
- Exposure Science & Pathogen Biology
- Metabolic Inflammatory Diseases
- Advanced Metabolomics
- Mass Spectrometry-Based Measurement Technologies
- Spatial and Single-Cell Proteomics
- Structural Biology
- Biofuels & Bioproducts
- Human Microbiome
- Soil Microbiome
- Synthetic Biology
- Computational Chemistry
- Chemical Separations
- Chemical Physics
- Atmospheric Aerosols
- Human-Earth System Interactions
- Modeling Earth Systems
- Coastal Science
- Plant Science
- Subsurface Science
- Terrestrial Aquatics
- Materials in Extreme Environments
- Precision Materials by Design
- Science of Interfaces
- Friction Stir Welding & Processing
- Dark Matter
- Flavor Physics
- Fusion Energy Science
- Neutrino Physics
- Quantum Information Sciences
- Emergency Response
- AGM Program
- Tools and Capabilities
- Grid Architecture
- Grid Cybersecurity
- Grid Energy Storage
- Earth System Modeling
- Energy System Modeling
- Transmission
- Distribution
- Appliance and Equipment Standards
- Building Energy Codes
- Advanced Building Controls
- Advanced Lighting
- Building-Grid Integration
- Building and Grid Modeling
- Commercial Buildings
- Federal Performance Optimization
- Resilience and Security
- Grid Resilience and Decarbonization
- Building America Solution Center
- Energy Efficient Technology Integration
- Home Energy Score
- Electrochemical Energy Storage
- Flexible Loads and Generation
- Grid Integration, Controls, and Architecture
- Regulation, Policy, and Valuation
- Science Supporting Energy Storage
- Chemical Energy Storage
- Waste Processing
- Radiation Measurement
- Environmental Remediation
- Subsurface Energy Systems
- Carbon Capture
- Carbon Storage
- Carbon Utilization
- Advanced Hydrocarbon Conversion
- Fuel Cycle Research
- Advanced Reactors
- Reactor Operations
- Reactor Licensing
- Solar Energy
- Wind Resource Characterization
- Wildlife and Wind
- Community Values and Ocean Co-Use
- Wind Systems Integration
- Wind Data Management
- Distributed Wind
- Energy Equity & Health
- Environmental Monitoring for Marine Energy
- Marine Biofouling and Corrosion
- Marine Energy Resource Characterization
- Testing for Marine Energy
- The Blue Economy
- Environmental Performance of Hydropower
- Hydropower Cybersecurity and Digitalization
- Hydropower and the Electric Grid
- Materials Science for Hydropower
- Pumped Storage Hydropower
- Water + Hydropower Planning
- Grid Integration of Renewable Energy
- Geothermal Energy
- Algal Biofuels
- Aviation Biofuels
- Waste-to-Energy and Products
- Hydrogen & Fuel Cells
- Emission Control
- Energy-Efficient Mobility Systems
- Lightweight Materials
- Vehicle Electrification
- Vehicle Grid Integration
- Contraband Detection
- Pathogen Science & Detection
- Explosives Detection
- Threat-Agnostic Biodefense
- Discovery and Insight
- Proactive Defense
- Trusted Systems
- Nuclear Material Science
- Radiological & Nuclear Detection
- Nuclear Forensics
- Ultra-Sensitive Nuclear Measurements
- Nuclear Explosion Monitoring
- Global Nuclear & Radiological Security
- Disaster Recovery
- Global Collaborations
- Legislative and Regulatory Analysis
- Technical Training
- Additive Manufacturing
- Deployed Technologies
- Rapid Prototyping
- Systems Engineering
- 5G Security
- RF Signal Detection & Exploitation
- Climate Security
- Internet of Things
- Maritime Security
- Artificial Intelligence
- Graph and Data Analytics
- Software Engineering
- Computational Mathematics & Statistics
- High-Performance Computing
- Visual Analytics
- Lab Objectives
- Publications & Reports
- Featured Research
- Diversity, Equity, Inclusion & Accessibility
- Lab Leadership
- Lab Fellows
- Staff Accomplishments
- Undergraduate Students
- Graduate Students
- Post-graduate Students
- University Faculty
- University Partnerships
- K-12 Educators and Students
- STEM Workforce Development
- STEM Outreach
- Meet the Team
- Internships
- Regional Impact
- Philanthropy
- Volunteering
- Available Technologies
- Industry Partnerships
- Licensing & Technology Transfer
- Entrepreneurial Leave
- Atmospheric Radiation Measurement User Facility
- Electricity Infrastructure Operations Center
- Energy Sciences Center
- Environmental Molecular Sciences Laboratory
- Grid Storage Launchpad
- Institute for Integrated Catalysis
- Interdiction Technology and Integration Laboratory
- PNNL Portland Research Center
- PNNL Seattle Research Center
- PNNL-Sequim (Marine and Coastal Research)
- Radiochemical Processing Laboratory
- Shallow Underground Laboratory
Connecting Computational and Systems Biology for Biodefense
Retooling security with bioagent-agnostic signatures
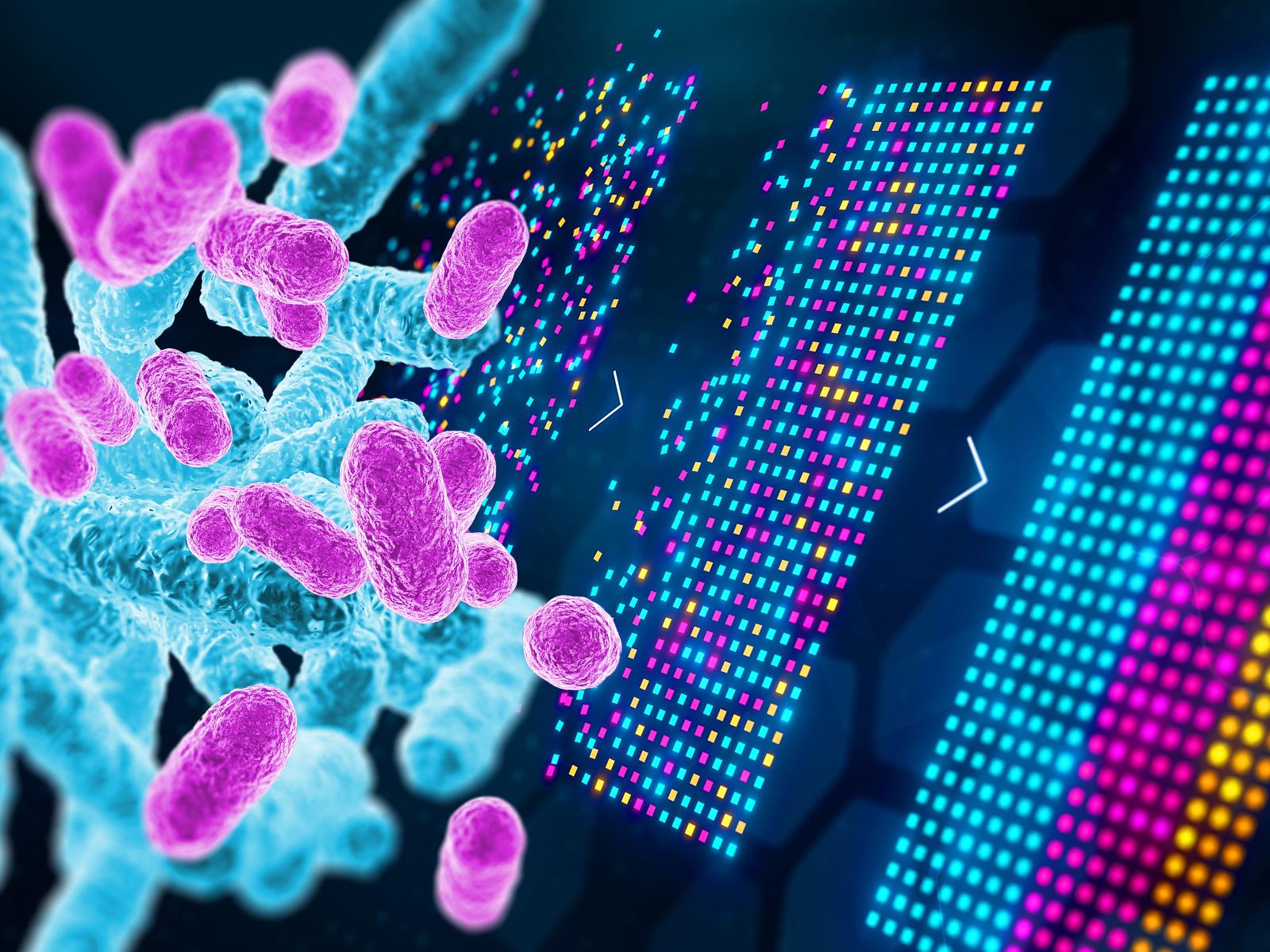
Researchers at Pacific Northwest National Laboratory and the University of Texas at El Paso are exploring the computational challenges of thinking beyond the list and developing bioagent-agnostic signatures to assess threats.
(Composite image by Derek Munson | Pacific Northwest National Laboratory)
Historically, the biodefense community relies on lists of known agents—pathogens and biotoxins like anthrax and ricin—that have been identified and prioritized as threats. In Health Security , a team of researchers at Pacific Northwest National Laboratory (PNNL) and the University of Texas at El Paso (UTEP) discuss the computational challenges of thinking beyond the list and developing bioagent-agnostic signatures to assess threats.
“As biological threats evolve, we face more unknowns and an increased sense of urgency to quickly detect and characterize disease agents to increase our biopreparedness and drive rapid responses. By shifting from an identification-based approach to a characterization-based one—with the right computational and data capabilities—we can accurately and reproducibly assess impacts without prior knowledge of an agent,” said Andy Lin, data scientist at PNNL.
The article “Computational and Systems Biology Advances to Enable Bioagent Agnostic Signatures” explores the computational data challenges of threat-agnostic biodefense , or the ability to characterize an unknown agent’s likely impact to human, animal, and plant health. The researchers discuss how the biodefense community can make the shift to a dual list-based and bioagent-agnostic signatures approach—but it will not be without its challenges. The shift will require policy changes, technological improvements, and improved data analytics.
The research brought together PNNL’s Lin, Errett Hobbs, Karen Taylor , Tony Chiang , and Jay Bardhan , with UTEP’s Cameron Torres, Stephen Aley, and Charles Spencer—convening diverse expertise across data science, computational systems biology, cytometry, immunology, and more. The research showcases the power of a cross-institution collaboration building on PNNL and UTEP’s long-standing partnership and joint appointment program to accelerate the science mission of both institutions. The UTEP team performed experiments that helped demonstrate the challenges discussed in the paper.
The research also builds on PNNL’s previous effort, highlighted in a 2021 Pathogens publication, “ Beyond the List: Bioagent-Agnostic Signatures Could Enable a More Flexible and Resilient Biodefense Posture Than an Approach Based on Priority Agent Lists Alone ,” which addressed how traditional list-based approaches are ill-equipped to accommodate threats posed by emergent, reemergent, or novel pathogens. A threat-agnostic model could present a means to more effectively surveil for and treat known and novel agents alike.
“While the biodefense community may have only just begun to develop technologies for a threat-agnostic approach, we’re highlighting promising new immunological approaches and the data challenges that need to be overcome to build exciting new possible paths forward,” said Lin.
The article was published in the March 2024 issue of Health Security and featured in the March 17 issue of the Global Biodefense headlines .
Published: May 15, 2024
Lin, A., C. M. Torres, E. C. Hobbs, J. Bardhan, S. B. Aley, C. T. Spencer, K. L. Taylor, and T. Chiang. 2024. “Computational and Systems Biology Advances to Enable Bioagent Agnostic Signatures.” Health Security . https://doi.org/10.1089/hs.2023.0076
Research topics
Lab-level communications priority topics.
AlphaFold 3 predicts the structure and interactions of all of life’s molecules
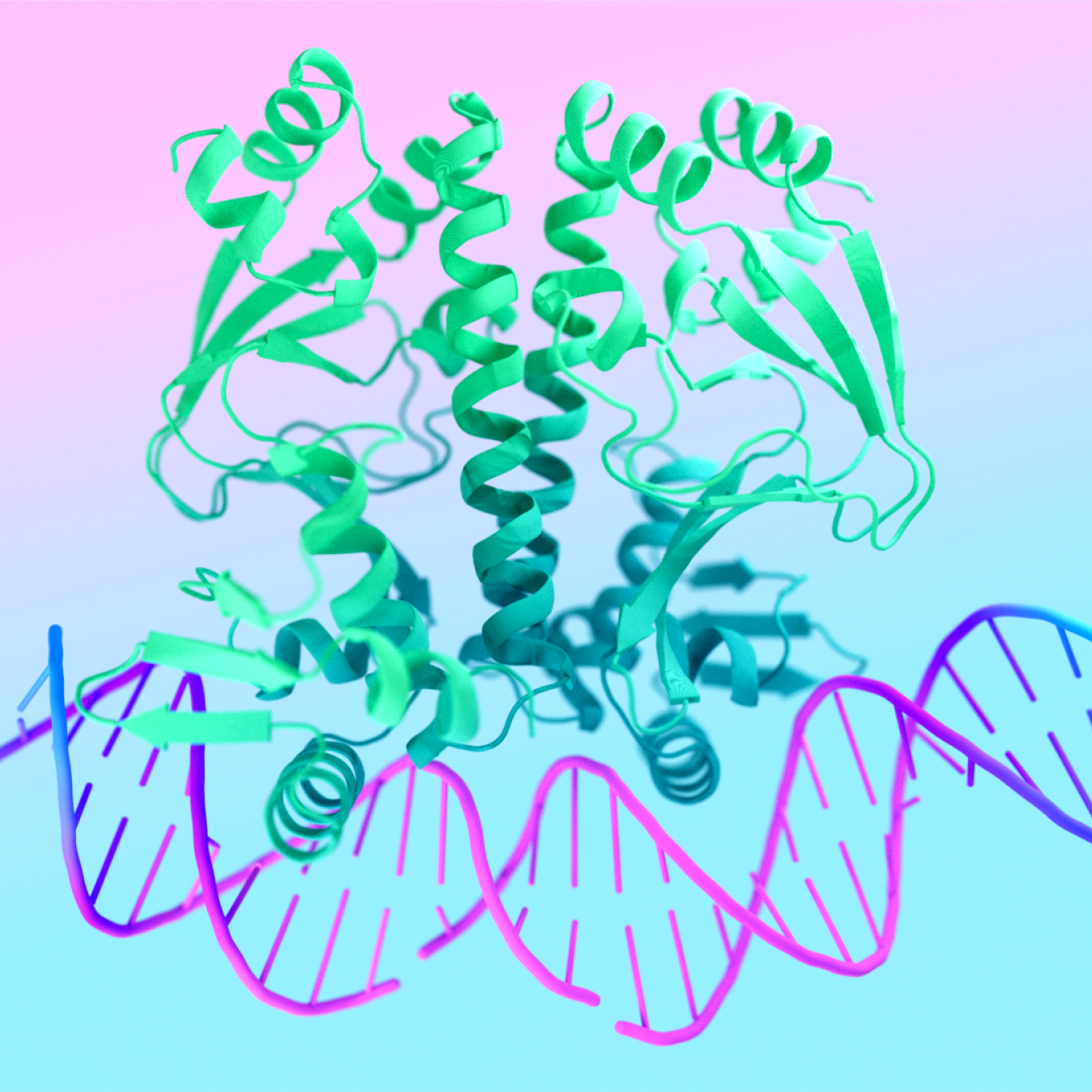
Introducing AlphaFold 3, a new AI model developed by Isomorphic Labs and Google DeepMind. By accurately predicting the structure of proteins, DNA, RNA, ligands and more, and how they interact, we hope it will help to transform our understanding of the biological world and drug discovery.
Inside every plant, animal, and human cell are billions of molecular machines. They’re made up of proteins, DNA, and other molecules, but no single piece works on its own. Only by seeing how they interact together, across millions of types of combinations, can we start to truly understand life’s processes.
In a paper published in Nature , we introduce AlphaFold 3, a revolutionary model that can predict the structure and interactions of all life’s molecules with unprecedented accuracy. For the interactions of proteins with other molecule types we see at least a 50% improvement compared with existing prediction methods, and for some important categories of interaction we have doubled prediction accuracy.
We hope AlphaFold 3 will help transform our understanding of the biological world and drug discovery. Scientists can access the majority of its capabilities, for free, through the newly launched AlphaFold Server , an easy-to-use research tool. To build on AlphaFold 3’s potential for drug design, we at Isomorphic Labs are already collaborating with pharmaceutical companies to apply it to real-world drug design challenges and, ultimately, develop new life-changing treatments for patients.
Our new model builds on the foundations of AlphaFold 2, which in 2020 made a fundamental breakthrough in protein structure prediction . So far, millions of researchers globally have used AlphaFold 2 to make discoveries in areas including malaria vaccines, cancer treatments, and enzyme design. AlphaFold has been cited more than 20,000 times and its scientific impact recognized through many prizes, most recently the Breakthrough Prize in Life Sciences . AlphaFold 3 takes us beyond proteins to a broad spectrum of biomolecules. This leap could unlock more transformative science, from accelerating drug design and genomics research, to developing biorenewable materials and more resilient crops.
7PNM - Spike protein of a common cold virus (Coronavirus OC43) : AlphaFold 3’s structural prediction for a spike protein (blue) of a cold virus as it interacts with antibodies (turquoise) and simple sugars (yellow), accurately matches the true structure (gray). The animation shows the protein interacting with an antibody, then a sugar. Advancing our knowledge of such immune-system processes helps better understand coronaviruses, including COVID-19, raising possibilities for improved treatments.
How AlphaFold 3 reveals life’s molecules
Given an input list of molecules, AlphaFold 3 generates their joint 3D structure, revealing how they all fit together. It models large biomolecules such as proteins, DNA, and RNA, as well as small molecules, also known as ligands - a category encompassing many drugs. Furthermore, AlphaFold 3 can model chemical modifications to these molecules which control the healthy functioning of cells, that when disrupted can lead to disease.
AlphaFold 3’s capabilities come from its next-generation architecture and training that now covers all of life’s molecules. At the core of the model is an improved version of our Evoformer module – a deep learning architecture that underpinned AlphaFold 2’s incredible performance. After processing the inputs, AlphaFold 3 assembles its predictions using a diffusion network, akin to those found in AI image generators. The diffusion process starts with a cloud of atoms, and over many steps converges on its final, most accurate molecular structure.
AlphaFold 3’s predictions of molecular interactions surpass the accuracy of all existing systems. As a single model that computes entire molecular complexes in a holistic way, it’s uniquely able to unify scientific insights.
Read our paper in Nature
7BBV - Enzyme : AlphaFold 3’s prediction for a molecular complex featuring an enzyme protein (blue), an ion (yellow sphere) and simple sugars (yellow), along with the true structure (gray). This enzyme is found in a soil-borne fungus (Verticillium dahliae) that damages a wide range of plants. Insights into how this enzyme interacts with plant cells could help researchers develop healthier, more resilient crops.
Leading drug discovery at Isomorphic Labs
AlphaFold 3 creates capabilities for drug design with predictions for molecules commonly used in drugs, such as ligands and antibodies, that bind to proteins to change how they interact in human health and disease.
AlphaFold 3 achieves unprecedented accuracy in predicting drug-like interactions, including the binding of proteins with ligands and antibodies with their target proteins. AlphaFold 3 is 50% more accurate than the best traditional methods on the PoseBusters benchmark , without needing the input of any structural information, making AlphaFold 3 the first AI system to surpass physics-based tools for biomolecular structure prediction. The ability to predict antibody-protein binding is critical to understanding aspects of the human immune response and the design of new antibodies - a growing class of therapeutics.
Using AlphaFold 3 in combination with a complementary suite of in-house AI models, we are working on drug design for internal projects as well as with pharmaceutical partners. We are using AlphaFold 3 to accelerate and improve the success of drug design - by helping understand how to approach new disease targets, and developing novel ways to pursue existing ones that were previously out of reach.
Read more about how we are using AlphaFold 3 for drug design.
AlphaFold Server: A free and easy-to-use research tool
8AW3 - RNA modifying protein : AlphaFold 3’s prediction for a molecular complex featuring a protein (blue), a strand of RNA (purple), and two ions (yellow) closely matches the true structure (gray). This complex is involved with the creation of other proteins - a cellular process fundamental to life and health.
Google DeepMind’s newly launched AlphaFold Server is the most accurate tool in the world for predicting how proteins interact with other molecules throughout the cell. It is a free platform that scientists around the world can use for non-commercial research. With just a few clicks, biologists can harness the power of AlphaFold 3 to model structures composed of proteins, DNA, RNA, and a selection of ligands, ions, and chemical modifications.
AlphaFold Server helps scientists make novel hypotheses to test in the lab, speeding up workflows and enabling further innovation. This gives researchers an accessible way to generate predictions, regardless of their access to computational resources or their expertise in machine learning.
Experimental protein-structure prediction can take about the length of a PhD and cost hundreds of thousands of dollars. Google DeepMind's previous model, AlphaFold 2, has been used to predict hundreds of millions of structures, which would have taken hundreds of millions of researcher-years at the current rate of experimental structural biology.
"With AlphaFold Server, it’s not only about predicting structures anymore, it’s about generously giving access: allowing researchers to ask daring questions and accelerate discoveries.”
Céline Bouchoux, The Francis Crick Institute
Explore AlphaFold Server
Sharing the power of AlphaFold 3 responsibly
Alongside Google DeepMind, we’ve sought to understand the broad impact of the technology. Working together with the research and safety community to take a science-led approach, we have conducted extensive assessments to mitigate potential risks and share the widespread benefits to biology and humanity.
Building on the external consultations we carried out for AlphaFold 2, Google DeepMind have now engaged with more than 50 domain experts, in addition to specialist third parties, across biosecurity, research, and industry, to understand the capabilities of successive AlphaFold models and any potential risks. We also participated in community-wide forums and discussions ahead of AlphaFold 3’s launch.
AlphaFold Server reflects the ongoing commitment to share the benefits of AlphaFold, including the free database of 200 million protein structures. We’ll continue to work with the scientific community and policy makers to develop and deploy AI technologies responsibly.
Opening up the future of AI-powered cell biology
7R6R - DNA binding protein : AlphaFold 3’s prediction for a molecular complex featuring a protein (blue) bound to a double helix of DNA (pink) is a near-perfect match to the true molecular structure discovered through painstaking experiments (gray).
AlphaFold 3 brings the biological world into high definition. It allows scientists to see cellular systems in all their complexity, across structures, interactions, and modifications. This new window on the molecules of life reveals how they’re all connected and helps understand how those connections affect biological functions – such as the actions of drugs, the production of hormones, and the health-preserving process of DNA repair.
The impacts of AlphaFold 3 and the free AlphaFold Server will be realised through how they empower scientists to accelerate discovery across open questions in biology and new lines of research. We’re just beginning to tap into AlphaFold 3’s potential and can’t wait to see what the future holds.
Learn more:
Read our blog on Rational Drug Design with AlphaFold 3
.png)
Read the Isomorphic Labs blog
Latest from iso.

AI advancements make the leap into 3D pathology possible
Human tissue is intricate, complex and, of course, three dimensional. But the thin slices of tissue that pathologists most often use to diagnose disease are two dimensional, offering only a limited glimpse at the tissue's true complexity. There is a growing push in the field of pathology toward examining tissue in its three-dimensional form. But 3D pathology datasets can contain hundreds of times more data than their 2D counterparts, making manual examination infeasible.
In a new study, researchers from Mass General Brigham and their collaborators present Tripath: new, deep learning models that can use 3D pathology datasets to make clinical outcome predictions. In collaboration with the University of Washington, the research team imaged curated prostate cancer specimens, using two 3D high-resolution imaging techniques. The models were then trained to predict prostate cancer recurrence risk on volumetric human tissue biopsies. By comprehensively capturing 3D morphologies from the entire tissue volume, Tripath performed better than pathologists and outperformed deep learning models that rely on 2D morphology and thin tissue slices. Results are published in Cell .
While the new approach needs to be validated in larger datasets before it can be further developed for clinical use, the researchers are optimistic about its potential to help inform clinical decision making.
"Our approach underscores the importance of comprehensively analyzing the whole volume of a tissue sample for accurate patient risk prediction, which is the hallmark of the models we developed and only possible with the 3D pathology paradigm," said lead author Andrew H. Song, PhD, of the Division of Computational Pathology in the Department of Pathology at Mass General Brigham.
"Using advancements in AI and 3D spatial biology techniques, Tripath provides a framework for clinical decision support and may help reveal novel biomarkers for prognosis and therapeutic response," said co-corresponding author Faisal Mahmood, PhD, of the Division of Computational Pathology in the Department of Pathology at Mass General Brigham.
"In our prior work in computational 3D pathology, we looked at specific structures such as the prostate gland network, but Tripath is our first attempt to use deep learning to extract sub-visual 3D features for risk stratification, which shows promising potential for guiding critical treatment decisions," said co-corresponding author Jonathan Liu, PhD, at the University of Washington.
Disclosures: Song and Mahmood are inventors on a provisional patent that corresponds to the technical and methodological aspects of this study. Liu is a co-founder and board member of Alpenglow Biosciences, Inc., which has licensed the OTLS microscopy portfolio developed in his lab at the University of Washington.
Funding: Authors report funding support from the Brigham and Women's Hospital (BWH) President's Fund, Mass General Hospital (MGH) Pathology, the National Institute of General Medical Sciences (R35GM138216), Department of Defense (DoD) Prostate Cancer Research Program (W81WH-18-10358 and W81XWH-20-1-0851), the National Cancer Institute (R01CA268207), the National Institute of Biomedical Imaging and Bioengineering (R01EB031002), the Canary Foundation, the NCI Ruth L. Kirschstein National Service Award (T32CA251062), the Leon Troper Professorship in Computational Pathology at Johns Hopkins University, UKRI, mdxhealth, NHSX, and Clarendon Fund.
- Men's Health
- Prostate Cancer
- Today's Healthcare
- Computer Modeling
- Computational Biology
- Mathematical Modeling
- Computer Graphics
- Prostate cancer
- Computational neuroscience
- Breast cancer
- Echocardiography
- Cervical cancer
Story Source:
Materials provided by Mass General Brigham . Note: Content may be edited for style and length.
Journal Reference :
- Andrew H. Song, Mane Williams, Drew F.K. Williamson, Sarah S.L. Chow, Guillaume Jaume, Gan Gao, Andrew Zhang, Bowen Chen, Alexander S. Baras, Robert Serafin, Richard Colling, Michelle R. Downes, Xavier Farré, Peter Humphrey, Clare Verrill, Lawrence D. True, Anil V. Parwani, Jonathan T.C. Liu, Faisal Mahmood. Analysis of 3D pathology samples using weakly supervised AI . Cell , 2024; 187 (10): 2502 DOI: 10.1016/j.cell.2024.03.035
Cite This Page :
Explore More
- Why Do We Overindulge?
- Jelly Sea Creatures and Underwater Robotics
- Teeth of Sabre-Toothed Tigers
- Robotic 'SuperLimbs' Could Help Moonwalkers
- Solving Quantum Many-Body Problems
- When 'Please' Is More Strategic Than Magic
- Autonomous Drones With Animal-Like 'Brains'
- How Practice Forms New Memory Pathways
- Reversing Brain Damage Caused by Ischemic Stroke
- Earth-Sized Planet Orbiting Ultra-Cool Dwarf
Trending Topics
Strange & offbeat.
Loading metrics
Open Access
Peer-reviewed
Artificial neural networks for model identification and parameter estimation in computational cognitive models
Roles Conceptualization, Data curation, Formal analysis, Investigation, Methodology, Software, Validation, Visualization, Writing – original draft, Writing – review & editing
* E-mail: [email protected]
Affiliation Department of Psychology, University of California, Berkeley, Berkeley, California, United States of America

Roles Data curation, Formal analysis, Methodology, Software, Validation, Visualization, Writing – original draft, Writing – review & editing
Roles Conceptualization, Investigation, Methodology, Software, Writing – original draft, Writing – review & editing
Affiliation Department of Mathematics, University of California, Berkeley, Berkeley, California, United States of America
Roles Conceptualization, Funding acquisition, Investigation, Project administration, Supervision, Writing – original draft, Writing – review & editing
Affiliations Department of Psychology, University of California, Berkeley, Berkeley, California, United States of America, Helen Wills Neuroscience Institute, University of California, Berkeley, Berkeley, California, United States of America
- Milena Rmus,
- Ti-Fen Pan,
- Liyu Xia,
- Anne G. E. Collins
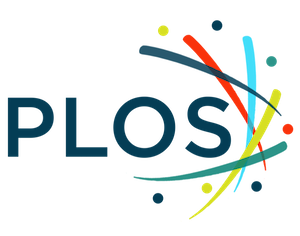
- Published: May 15, 2024
- https://doi.org/10.1371/journal.pcbi.1012119
- Peer Review
- Reader Comments
This is an uncorrected proof.
Computational cognitive models have been used extensively to formalize cognitive processes. Model parameters offer a simple way to quantify individual differences in how humans process information. Similarly, model comparison allows researchers to identify which theories, embedded in different models, provide the best accounts of the data. Cognitive modeling uses statistical tools to quantitatively relate models to data that often rely on computing/estimating the likelihood of the data under the model. However, this likelihood is computationally intractable for a substantial number of models. These relevant models may embody reasonable theories of cognition, but are often under-explored due to the limited range of tools available to relate them to data. We contribute to filling this gap in a simple way using artificial neural networks (ANNs) to map data directly onto model identity and parameters, bypassing the likelihood estimation. We test our instantiation of an ANN as a cognitive model fitting tool on classes of cognitive models with strong inter-trial dependencies (such as reinforcement learning models), which offer unique challenges to most methods. We show that we can adequately perform both parameter estimation and model identification using our ANN approach, including for models that cannot be fit using traditional likelihood-based methods. We further discuss our work in the context of the ongoing research leveraging simulation-based approaches to parameter estimation and model identification, and how these approaches broaden the class of cognitive models researchers can quantitatively investigate.
Author summary
Computational cognitive models occupy an important position in cognitive science research, as they offer a simple way of quantifying cognitive processes (such as how fast someone learns, or how noisy they are in choice selection), and testing which cognitive theories offer a better explanation of the behavior. To relate cognitive models to the behavioral data, researchers rely on statistical tools that require estimating the likelihood of observed data under the assumptions of the cognitive model. This is, however, not possible to do for all models as some models present significant challenges to likelihood computation. In this work, we use artificial neural networks (ANNs) to bypass likelihood computation and approximation altogether, and demonstrate the success of this approach applied to model parameter estimation and model comparison. The proposed method is a contribution to ongoing development of modeling tools which will enable cognitive researchers to test a broader range of theories of cognition.
Citation: Rmus M, Pan T-F, Xia L, Collins AGE (2024) Artificial neural networks for model identification and parameter estimation in computational cognitive models. PLoS Comput Biol 20(5): e1012119. https://doi.org/10.1371/journal.pcbi.1012119
Editor: Ming Bo Cai, University of Miami, UNITED STATES
Received: September 22, 2023; Accepted: April 27, 2024; Published: May 15, 2024
Copyright: © 2024 Rmus et al. This is an open access article distributed under the terms of the Creative Commons Attribution License , which permits unrestricted use, distribution, and reproduction in any medium, provided the original author and source are credited.
Data Availability: The data in this manuscript is simulated using the code stored in the referenced GitHub repository, and can be generated by running the simulation code. Accessible at: https://github.com/MilenaCCNlab/MI-PEstNets.git .
Funding: This work was supported by the National Institutes of Health (NIH R21MH132974 to AGEC). The funders had no role in study design, data collection and analysis, decision to publish, or preparation of the manuscript.
Competing interests: The authors have declared that no competing interests exist.
This is a PLOS Computational Biology Methods paper.
Introduction
Computational modeling is an important tool for studying behavior, cognition, and neural processes. Computational cognitive models translate scientific theories into algorithms using simple equations with a small number of interpretable parameters to make predictions about the cognitive or neural processes that underlie observable behavioral or neural measures. These models have been widely used to test different theories about cognitive processes that shape behavior and relate to neural mechanisms [ 1 – 4 ]. By specifying model equations, researchers can inject different theoretical assumptions into most models, and simulate synthetic data to make predictions and compare against observed behavior. Researchers can quantitatively arbitrate between different theories by comparing goodness of fit [ 5 , 6 ], across different models. Furthermore, by estimating model parameters that quantify underlying cognitive processes, researchers have been able to characterize important individual differences (e.g. developmental: [ 7 – 10 ]; clinical: [ 11 – 15 ]) as well as condition effects [ 16 , 17 ].
Researchers’ ability to benefit from computational modeling crucially depends on the availability of methods for model fitting and comparison. Such tools are available for a large group of cognitive models (such as, for example, reinforcement learning and drift diffusion models). Examples of commonly used model parameter fitting tools include maximum likelihood estimation (MLE, [ 18 ]), maximum a-posteriori (MAP, [ 19 ]), and sampling approaches ([ 20 , 21 ]). Examples of model comparison tools include information criteria such as AIC and BIC [ 5 , 22 ], and Bayesian group level approaches, including protected exceedance probability [ 23 , 24 ]. These methods all have one important thing in common—they necessitate computing the likelihood of the data conditioned on models and parameters, thus limiting their use to models with tractable likelihood. However, many models do not have a tractable likelihood. This severely limits the types of inferences researchers can make about cognitive processes, as many models with intractable likelihood might offer better theoretical accounts of the observed data. Examples of such models include cases where observed data (e.g. choices) might depend on latent variables—such as the unobserved rules that govern the choices [ 25 – 27 ], or a latent state of engagement (e.g. attentive/distracted, [ 28 , 29 ]) a participant/agent might be in during the task. In these cases, computing the likelihood of the data often demands integrating over the latent variables (rules/states) across all trials, which grows exponentially and thus is computationally intractable. This highlights an important challenge—computing likelihoods is essential for estimating model parameters, and performing fitness comparison/model identification, and alternative models are less likely to be considered or taken advantage of to a greater extent.
Some existing techniques attempt to bridge this gap. For example, Inverse Binomial Sampling [ 30 ], particle filtering [ 31 ], and assumed density estimation [ 32 ] provide approximate solutions to the Bayesian inference process in specific cases. Many of these methods, however, require advanced mathematical expertise for effective use and adaptation beyond specific cases they were developed for, making them less accessible many researchers. Approximate Bayesian Computation (ABC, [ 33 – 37 ]) offers a more accessible avenue for estimating parameters in models limited by intractable likelihoods. More widely employed in cognitive modeling, the approach of basic ABC rejection algorithms involves translating trial-level data into summary statistics. Parameter values of the candidate model are then selected based on their ability to produce simulated data that is closely aligned with summarized data, guided by some predefined rejection criterion.
While ABC rejection algorithms provide a useful workaround solution, it’s important to acknowledge their inherent limitations. Specifically, ABC results are sensitive to the choice of summary statistics (and rejection criteria) and sample efficiency of ABC demonstrates scales poorly in cases of high-dimensional data [ 33 , 38 , 39 ]. Recent strides in the field of simulation-based inference/likelihood-free inference have addressed these limitations by using artificial neural network(ANN) structures designed to optimize summary statistics, and consequently infer parameters. These methods enable automated (or semi-automated) construction of summary statistics, minimizing the effect the choice of summary statistics may have on the accuracy of parameter estimation [ 38 , 40 – 44 ]. This innovative approach serves to amortize the computational cost of simulation-based inference, opening new frontiers in terms of scalability and performance [ 40 , 41 , 45 – 50 ].
Here, we test a related, general approach that leverage advances in artificial neural networks (ANNs) to estimate parameters and perform model identification for models with and without tractable likelihood, entirely bypassing the likelihood estimation (or approximation) step. ANNs have been successfully used to fit intractable models in different fields, including weather models [ 51 ] and econometric models [ 6 ], and more recently cognitive models of decision making [ 40 , 41 ]. We develop similar approaches to specifically target the intractability estimation problem in the field of computational cognitive science, including both parameter estimation and model identification, and thoroughly test it in a challenging class of models where there are strong dependencies between trials (e.g. learning experiments).
Our approach relies on the property of ANNs as universal function approximators. The ANN structure we implemented was a recurrent neural network (RNN) with feed-forward layers inspired by [ 52 ] ( Fig 1 ) that is trained to estimate model parameters, or identify which model most likely generated the data based on input data sequences simulated by the cognitive model. Our approach is similar to previous work in the domain of simulation-based inference [ 40 , 41 ], with a difference that such architectures are specifically designed to optimize explicit summary statistics that describe the data patterns (e.g. invertible networks). Here, rather than emphasizing steps involving the reduction of data dimensionality through the creation (and selection) of summary statistic vectors and subsequent inference based on parameter value samples, our focus is on the direct translation of raw data sequences into precise parameter estimates or the identification of the source model (via implicit summary statistics in network layers).
- PPT PowerPoint slide
- PNG larger image
- TIFF original image
A) Traditional methods rely on computing log-likelihood (LLH) of the data under the given model, and optimizing the likelihood to derive model parameter estimates. B) The ANN is trained to map parameter values onto data sequences using a large simulated data set; the trained network can then be used to estimate cognitive model parameters based on new data without the need to compute or approximate likelihood. C) The ANN structure inspired by [ 52 ] is suitable for data with strong inter-trial dependencies: it consists of an RNN and fully connected feed-forward network, with an output containing ANN estimates of parameter values the data was simulated from for each agent. D) As in parameter estimation, traditional tools for model identification rely on likelihood to derive model comparison metrics (e.g. AIC, BIC) that are used to determine which model likely generated the data. E) ANN is instead trained to learn the mapping between data sequences and respective cognitive models the data was simulated from. F) Structure of the ANN follows the structure introduced for parameter estimation, with the key difference of final layer containing the probability distribution over classes representing model candidates, with highest probability class corresponding to the model the network identified as the one that likely generated the agent’s data.
https://doi.org/10.1371/journal.pcbi.1012119.g001
To validate and benchmark our approach, we first compared it against standard model parameter fitting methods most commonly used by cognitive researchers (MLE, MAP, rejection ABC) in cognitive models from different families (reinforcement learning, Bayesian Inference) with tractable likelihood. Next, we demonstrated that neural networks can be used for parameter estimation of models with intractable likelihood, and compared it to standard approximation method (ABC). Finally, we showed that our approach can also be used for model identification.
Our results showed that our method is highly successful and robust at parameter and model identification while remaining technically lightweight and accessible. We highlight the fact that our method can be applied to standard cognitive data sets (i.e. with arbitrarily small number of participants, and normal number of trials per participant), as the ANN training is fully done on a large simulated data set. Our work contributes to the ongoing research focusing on leveraging artificial neural networks to advance the field of computational modeling, and provides multiple new avenues for maximizing the utility of computational cognitive models.
We focused on two distinct artificial neural network (ANNs) applications in cognitive modeling: parameter estimation and model identification. Specifically, we built a network with a structure suitable for sequential data/data with time dependencies (e.g. recurrent neural network (RNN); [ 52 ]). Training deep ANNs requires large training data sets. We generated such a data set at minimal cost by simulating a cognitive computational model on a cognitive task a large number of times. Model behavior in the cognitive task (e.g. a few hundred trials of stimulus-action pairs or stimulus-action-outcome triplets (depending on the task) for each simulated agent) constituted ANN’s training input; true known parameter values (or identity of the model) from which the data was simulated constituted ANNs’ training targets. We evaluated the network’s training performance in predicting parameter values/identity of the model in a separate validation set, and tested the trained network on a held out test set. We tested RNN variants and compared their accuracy against traditional likelihood-based model fitting/identification methods using both likelihood-tractable and likelihood-intractable cognitive models. See Methods section for details on the ANN training and testing process.
Parameter recovery
Benchmark comparison..
First, we sought to validate our ANN method and compare its performance to existing methods by testing it on standard likelihood-tractable cognitive models of different levels of complexity in the same task: 2-parameter (2 P − RL ) and 4-parameter (4 P − RL ) reinforcement learning models commonly used to model behavior on reversal tasks [ 7 , 14 , 53 , 54 ], as well as Bayesian Inference model ( BI ) and Bayesian Inference with Stickiness ( S − BI ) as an alternative model family that has been found to outperform RL in some cases [ 55 – 57 ]. We estimated model parameters using multiple traditional methods for computing (maximum likelihood and maximum a-posteriori estimation; MLE and MAP) and approximating (Approximate Bayesian Computation; ABC) likelihood. We used the results of these tools as a benchmark for evaluating the neural network approach. Next, we estimated parameters of these models using two variants of RNNs: with gated recurrent units (GRUs) or Long-Short-Term-Memory units (LSTM).
We used the same held out data set to evaluate all methods (the test set the ANN has not observed yet, see simulation details). For each of the methods we extracted the best fit parameters, and then quantitatively estimated the method’s performance as the mean squared error (MSE) between estimated and true parameters across all agents. Methods with lower MSE indicated better relative performance. All of the parameters were scaled for the purpose of loss computation, to ensure comparable contribution to loss across different parameters. To quantify overall loss for a cognitive model we averaged across all individual parameter MSE scores; to calculate fitting method’s MSE score for a class of cognitive models (e.g. likelihood tractable models) we averaged across respective method’s MSE scores for those models (See Methods for details about method evaluation).
First, we examined the performance of standard model-fitting tools (MLE, MAP and ABC). The standard tools yielded a pattern of results that are expected based on noisy, realistic-size data sets (with several-hundred trials per agent). Specifically, we found that MAP outperformed MLE ( Fig 2A , average MSEs: MLE =.67, MAP =.35), since the parameter prior applied in MAP regularizes the fitting process. ABC was also worse compared to MAP ( Fig 2A , average MSE: ABC =.53). While fitting process is also regularized in ABC, worse performance in some models can be attributed to signal loss that arises from approximation to the likelihood. Next, we focused on the ANN performance; our results showed that for each of the models, ANN performed better than or just as well as the traditional methods ( Fig 2A , average MSEs for different RNN variants: GRU =.32, LSTM =.35). Better network performance was more evident for parameter estimation in more complex models (e.g. models with higher number of parameters such as 4P-RL and S-BI; average MSE across these 2 models: MLE =.95, MAP =.43, ABC =.71, GRU =.38, LSTM =.44).
A) Parameter recovery loss from the held out test set for the tractable-likelihood models (2P-RL, 4P-RL, BI, S-BI) using each of the tested methods. Loss is quantified as the mean squared error (MSE) based on the discrepancy between true and estimated parameters. Bars represent loss average for each parameter across all agents, with errorbars representing standard error across agents. B) Parameter recovery from the 4P-RL model using MAP and GRU. ρ values represent Spearman ρ correlation between true and estimated parameters. Red line represents a unity line ( x = y ) and black line represents a least squares regression line. All correlations were significant at p <.001.
https://doi.org/10.1371/journal.pcbi.1012119.g002
Next, we visualized parameter recovery. We found that for each of the cognitive models the parameter recovery was largely successful (Spearman ρ correlations between true parameter values and estimated values: β ρ MAP , ρ GRU = [.90, .91], α + ρ MAP , ρ GRU = [.53, .52], α − ρ MAP , ρ GRU = [.88, .89], κ : ρ MAP , ρ GRU = [.78, .79], Fig 2B ; all correlations were significant at p <.001). For conciseness, we only show recovery of the more complex model parameters from the RL model family (and only MAP method as it performed better compared to ABC and MLE, as well as only GRU since it performed better than LSTM), as we would expect a more complex model to emphasize superiority of a fitting method more clearly compared to simpler models. Recovery plots of the remaining models (and respective fitting methods) can be found in S2 – S5 Figs. Our results suggest that 1) ANN performed as well as traditional methods in parameter estimation based on the MSE loss; 2) more complex models may limit accuracy of parameter estimation in traditional methods that neural networks appear to be more robust against. We note that for the 4 P − RL model, parameter recovery was noisy for all methods, with some parameters being less recoverable than others (e.g. α + , Fig 2B ). This is an expected property of cognitive models applied to realistic-sized experimental data as found in most human experiments (i.e. a few hundred trials per participant). To check whether the limited recovery can be attributed to parameter identifiability rather than pitfalls of any specific method, we looked at the correlation between parameter estimates obtained using the standard model fitting method (MAP) and the ANN (GRU) ( S10 Fig )—with parameters that are not well recovered (e.g. α + in 4P-RL model) being of particular interest. High correlation between estimated parameters obtained via 2 methods imply systematic errors in parameter identification that apply to both methods—thus suggesting that the weaker correlation between true and fit parameters for some parameters is more likely due to limitations in the model applied to the data set than method specifications such as poor optimization performance. We further discuss the implications in discussion section—highlighting that computational models should be carefully crafted and specified regardless of the tools used for model fitting.
Testing in cognitive models with intractable likelihood.
Next, we tested our method in two examples of computational models with intractable likelihood. As a comparison method, we implemented Approximate Bayesian Computation (ABC), alongside our ANN approach to estimate parameters. The two example likelihood-intractable models we used had in common the presence of a latent state which conditioned sequential updates: RL with latent attentive state ( RL − LAS ), and a form of non-temporal hierarchical reinforcement learning ( HRL , [ 27 ]). Since we cannot fit these models using MAP or MLE we used only ABC as a benchmark. Because we found LSTM RNN to be more challenging to train and achieve similar results when compared to GRU, we focused on GRU for the remainder of comparisons. We found that average MSE was much lower for the neural network compared to ABC for both RL-LAS ( Fig 3A , average MSEs: ABC =.62, GRU =.21) and HRL ( Fig 3A , average MSEs: ABC =.28, GRU =.19). Spearman correlations were noisier for ABC compared to GRU in both models ( Fig 3B , RL-LAS : β ρ ABC , ρ GRU = [.72, .91], α ρ ABC , ρ GRU = [.83, .95], T ρ ABC , ρ GRU = [.5, .81]; HRL : β ρ ABC , ρ GRU = [.86, .89], α ρ ABC , ρ GRU = [.85, .9]; all correlations were significant at p <.001). Furthermore, some parameters were less recoverable than others (e.g. the T parameter in RL-LAS model, which indexed how long participants remained in an inattentive state); this might be in part due to less straightforward effect of T on behavior ( S6 Fig ). Note that in order to obtain our ABC results we had to perform an extensive exploration procedure to select summary statistics—ensuring reasonable ABC results. Indeed, the choice of summary statistics is not trivial and represents an important difficulty of applying basic rejection ABC [ 33 , 38 ], that we can entirely bypass using our new neural network approach. We acknowledge that recent methods that rely on ANNs replaced standard ABC methods by automating (or semi-automating) construction of summary statistics [ 38 , 40 – 44 , 51 ]. However, we aimed to explore an alternative approach, independent of explicit optimization of summary statistics, and focused on the ABC instantiation that has been most frequently implemented in the field of cognitive science as a benchmark [ 33 – 35 ].
A) Parameter recovery loss from the held out test set for the intractable-likelihood models (RL-LAS, HRL) using ABC and GRU network. Loss is quantified as the mean squared error (MSE) based on the discrepancy between true and estimated parameters. Bars represent MSE average for each parameter across all agents, with errorbars representing standard error across agents ( S17 Fig ) shows variability across seeds). B) Parameter recovery from the RL-LAS and HRL models using ABC (green) and GRU network (yellow). ρ values represent Spearman ρ correlation between true and estimated parameters. Red line represents a unity line ( x = y ) and black line represents a least squares regression line.All correlations were significant at p <.001.
https://doi.org/10.1371/journal.pcbi.1012119.g003
Uncertainty of parameter estimates.
Thus far, we have outlined a method that provides point estimates of parameters based on input data sequences, as is typically the use for much lightweight cognitive modeling (e.g. maximum likelihood estimation or MAP). However, it is sometimes also valuable to compute the uncertainty associated with these estimates [ 21 ]. It is possible to extend our approach to add this capability. While there are various alternative ways to do so (e.g. Bayesian neural networks), the approach we have opted for is incorporating evidential learning into our method [ 58 ]. Evidential learning differs from Bayesian networks in that it places priors over likelihood function, rather than network weights. The network leverages this property to learn both statistical (aleatoric) and systematic (epistemic) uncertainty during the process of estimating a continuous target based on the input data sequences. This marks a shift from optimizing a network to minimize errors based on average prediction, without considering uncertainty.
We applied our method with integrated evidential learning to tractable and intractable versions of the RL models (2P-RL and RL-LAS, Fig 4 ). We found that incorporating this modification did not compromise the point estimate parameter recovery (e.g. compared to our baseline method focused only on maximizing the accuracy of the predictions). Additionally, it enabled the estimation of the uncertainty around the point estimate, as demonstrated by [ 58 ]. This extension appears to be more computationally expensive (with longer training periods) than our original method, but not to a prohibitive extent.
Using evidential learning to evaluate uncertainty of parameter estimates for A) 2-parameter RL model (tractable likelihood) and B) RL model with latent attention states (intractable likelihood). Vertical lines around point estimates illustrate model uncertainty. We are showing only 100 data points for the purpose of cleaner visualization, Spearman ρ values are computed based on the total number of agents in the held-out test data (3k).
https://doi.org/10.1371/journal.pcbi.1012119.g004
Model identification
We also tested the use of our ANN approach for model identification. Specifically, we simulated data from different cognitive models, and trained the network to make a prediction regarding which model most likely generated the data out of all model candidates. The network architecture was identical to the network used for parameter estimation, except that the last layer became a classification layer (with one output unit per model category) instead of a regression layer (with one output unit per target parameter).
For models with tractable likelihood, we performed the same model identification process using AIC [ 5 ] that relies on likelihood computation, penalized by number of parameters, to quantify model fitness as a benchmark. We note that another common criterion, BIC [ 6 ], performed more poorly than AIC in our case. The best fitting model is identified based on the lowest AIC score—a successful model recovery would indicate that the true model has the lowest AIC score compared to other models fit to that data. To construct the confusion matrix, we computed best AIC score proportions for all models, across all agents, for data sets simulated from each cognitive model ( Fig 5 ; see Methods ).
A) Confusion matrix of likelihood-tractable models from PRL task based on 1) likelihood/AIC metric, and 2) ANN identification. AIC confusion matrix revealed a much higher degree of misclassification (e.g. true simulated model being incorrectly identified as a different model). B) Confusion matrix of likelihood-intractable models using ANN (2P-RL and RL-LAS models were simulated on the PRL task; HRL, BI and S-BI models were simulated on the HRL task).
https://doi.org/10.1371/journal.pcbi.1012119.g005
As shown in Fig 5A , model identification performed using our ANN approach was better compared to the AIC confusion matrix, with less “confusion”—lower off-diagonal proportions compared to diagonal proportions (correct identification). Model identification using AIC is likely in part less successful due to some models being nested in others (e.g. 2 P − RL in 4 P − RL , BI in S − BI ). Specifically, since AIC score represents a combination of likelihood and penalty incurred by the number of parameters it is possible that the data from more complex models is incorrectly identified as better fit by a simpler version of that model (e.g. the model with fewer parameters; an issue which would be more pronounced if we used a BIC confusion matrix). The same phenomenon is observed with the network, but to a much lesser extent, showing better identification out of sample—even for nested models. Furthermore, the higher degree of ANN misclassification observed for BI / S − BI was driven by S − BI simulations with stickiness parameter close to 0, which would render the BI and S − BI non-distinguishable ( S7 Fig ).
Because we cannot compute the likelihood for our likelihood-intractable models based on closed-form solutions via MAP, we only report the confusion matrices obtained from our ANN approach In the first confusion matrix we performed model identification for 2 P − RL and RL − LAS , as we reasoned these two models differ by only one mechanism (occasional inattentive state), and thus could potentially pose the biggest challenge to model identification. In the second confusion matrix, we included all models used to simulate data on the HRL task ( HRL model , Bayesian inference model , Bayesian inference with stickiness model ). In both cases, the network successfully identified the correct models as true models, with a very small degree of misidentification, mostly in the nested models. Based on our benchmark comparison to AIC, and the proof of concept identification for likelihood intractable models, our results indicate that the ANN can be leveraged as a valuable tool in model identification.
Robustness tests
Robustness tests: influence of different input trial sequence lengths..
ANNs are sometimes known to fail catastrophically when data is different from the training distribution in minor ways [ 59 – 62 ]. Thus, we investigated the robustness of our method to differences in data format we might expect in empirical data, such as different numbers of trials across participants. Specifically, we conducted robustness experiments by varying the number of trials in each individual simulation contributing to training or test sets, fixing the number of agents in the training set.
To evaluate the quality of parameter recovery, we used the coefficient of determination score ( R 2 ) which normalizes different parameter ranges. We found that the ANNs trained with a higher trial number reach high R 2 scores in long test trials. However, their performance suffers significantly with smaller number of test trials. The results also show a similar trend in model identification tasks except that training with higher trial number doesn’t guarantee a better performance. For instance, the classification accuracy between HRL task models of the ANN trained with 300 trials reaches 87% while the ANN trained with 500 trials is 84%.
Data-augmentation practices in machine learning increase robustness of models during training [ 63 ] by introducing different types of variability in the training data set (e.g. adding noise, different data sizes). Specifically, slicing time-series data into sub-series is a data-augmentation practice that increases accuracy [ 64 ]. Thus, we trained our ANN with the fixed number of simulations of different trial numbers. As predicted, we found that the ANNs trained with a mixture of trial sequence lengths across simulations (purple line) consistently yielded better performance across different numbers of test trials for both parameter recovery and model identification ( Fig 6A and 6B ).
A) Parameter estimation in both RL-LAS and HRL show that training with a mixture of trial sequence lengths (purple line) yields more robust out-of-sample parameter value prediction compared to fixed trial sequence lengths. B) Best model identification results, performed on different combinations of model candidates, were also yielded by mixed trial sequence length training. The number of agents/simulations used for training was kept constant across all the tests (N agents = 30k).
https://doi.org/10.1371/journal.pcbi.1012119.g006
Robustness tests: Prior parameter assumptions.
We also tested the effects of incorrect prior assumptions about the parameter range on method performance. Specifically we 1) trained the network using data simulated from a narrow range of parameters (theoretically informed) and 2) trained the network based on broader range of parameter values. Next, we tested both networks in making out-of-sample predictions for test data sets that were simulated from narrow and broad parameter ranges respectively. The network trained using a narrow parameter range made large errors at estimating parameters for data simulated outside of the range it was trained on; training the network on a broader range overall resulted in smaller error, with some loss of precision for the parameter values in range of most interest (e.g. the narrow range of parameters the alternative network is trained on). We observed similar results with MAP, where we specified narrow/broad prior (where narrow prior would place high density on a specific parameter range). Notably, training the network using a broader range of parameters while oversampling from a range of interest yielded more accurate parameter estimation compared to MAP with broad priors (Approach described in S9 Fig ).
Robustness tests: Model misspecification.
In addition to testing the effects of incorrect priors, we also tested the effect of model misspecification on standard method and ANN performance (focusing on MAP and GRU network, as they performed the best in parameter recovery tests on benchmark models). We fit the Bayesian inference model (without stickiness) to the data simulated from the Bayesian inference model with stickiness using MAP. For the ANN, we trained the neural network to estimate parameters of the Bayesian inference model, and tested it on the separate test set data simulated from the Bayesian inference model with stickiness. For each method, we looked at the correlation between the ground truth Bayesian inference with stickiness model parameters, and the method’s parameter estimates ( S13 Fig ). Our results suggest that the parameters shared between the 2 models are reasonably recoverable using both MAP and ANN (e.g. the recovery is noisier but comparable to that of parameters in Bayesian models without model misspecification ( S4 and S5 Figs); furthermore, the correlation between ground truth and estimated values is similar for the two methods.
To make the model misspecification more extreme, we additionally simulated data from a Bayesian inference model, and estimated RL model parameters from the simulated data. We did this using standard methods (MAP) and ANN, and repeated the same process in reverse (simulating data from an RL model, and fitting Bayesian inference model parameters). We found that both MAP and ANN exhibited similar patterns. That is, in the case of simulating Bayesian inference model and fitting RL model parameters, the estimated β captured the variance from the true β and p switch , while the estimated α parameter captured the variance driven by the Bayesian updating parameters p reward and p switch ( S14 Fig ). In the case of simulating RL model and fitting Bayesian inference model parameters, p switch parameter captured the noise in the simulated data coming from the β parameter, and the variance from the α parameter was attributed to the p reward parameter ( S15 Fig ). We also correlated parameter estimates generated by the two methods. High correlation implies that MAP and GRU generate similar parameter estimates, suggesting that they are impacted by model misspecification in a similar way ( S11 Fig ).
Our results demonstrate that artificial neural networks (ANNs) can be successfully and efficiently used to estimate best fitting free parameters of likelihood-intractable cognitive models, in a way that is independent of likelihood approximation. ANNs also show remarkable promise in successfully arbitrating between competing cognitive models. While our method leverages “big data” techniques, it does not require large experimental data sets: indeed, the large training set used to train the ANNs is obtained purely through efficient and fast model simulation. Thus, our method is applicable to any standard cognitive data set with a normal number of participants and trials per participants. Furthermore, while our method requires some ability to work with ANNs, it does not require any advanced mathematical skills, making it largely accessible to the broad computational cognitive modeling community.
Our method adds to a family of approaches from other attempts at using neural networks for fitting computational cognitive models. Specifically, previous work leveraging amortized inference has focused on taking advantage of large-scale simulations and invertible networks. This approach involves training the summary segment of the network to adeptly learn relevant summary statistic vectors, while concurrently training the inference segment of the network to approximate the posterior distribution of model parameters based on the outputs generated by the summary network [ 40 , 41 , 46 ]. This method has successfully been applied to both parameter estimation and model identification (and performs in a similar range as our method when applied to intractable models we implemented in this paper), bypassing many issues of ABC. In parallel, work by [ 47 ] showcased Likelihood Approximation Networks (LANs) as a method that approximates likelihood of sequential sampling models (but requires ABC-like approaches for training), and recovers posterior parameter distributions with high accuracy for a specific class of models (e.g. drift diffusion models); more recently, [ 48 ] used a similar approach with higher training data efficiency. Work by [ 65 ] used Approximate Bayesian Computation (ABC) in conjunction with mixture density networks to map data to parameter posterior distributions. Unlike most of these approaches our architecture is not dependent on [ 47 , 48 ] or explicitly designed to optimize [ 40 , 41 , 46 ] summary statistics. By necessity, hidden layers of our network do implicitly compute a form of summary statistic that are translated into estimated parameters/model class in the output layer; however, we do not optimize for such statistics explicitly, beyond their ability to support parameter/model recovery.
Other approaches have used ANNs for different purposes than fitting cognitive models [ 66 ]. For example, [ 52 ] leveraged flexibility of RNNs (which inspired our network design) to map data sequences onto separable latent dimensions that have different effects on decision-making behavior of agents, as an alternative to cognitive models that make more restrictive assumptions. Similarly, work by [ 67 ] also used RNNs to estimate RL parameters and make predictions about behavior of RL agents. Our work goes further than this approach in that it focuses on both parameter recovery and model identification of models with intractable likelihood, without relying on likelihood approximation. Furthermore, multiple recent papers [ 68 , 69 ] use ANNs as a replacement for cognitive models, rather than as a tool for supporting cognitive modeling as we do, demonstrating the number of different ways ANNs are taking a place in computational cognitive science.
It is important to note that while ANNs may prove to be a useful tool for cognitive modeling, one should not expect that their use immediately fixes or overrides all issues that may arise in parameter estimation and model identification. For instance, we have observed that while ANNs outperformed many of the traditional likelihood-based methods, recovery for some model parameters was still noisy (e.g. learning rate α in the 4P-RL model, Fig 2 ). This is a property of cognitive models when applied to experimental applied to data sets that range in hundreds of trials. Standard methods (e.g. MAP) fail in a similar way—as shown by the high correlation between MAP and ANN parameter estimates ( S10 Fig ), which suggests that parameter recovery issues have more to do with identifiability limitations of the data and model, rather than other issues such as optimization method. Similarly, often times model parameters are not meaningful in certain numerical ranges, and sometimes model parameters trade off in how they impact behavior through mathematical equations that define the models—making the parameter recovery more challenging. Furthermore, when it comes to model identification, particularly with nested models, the specific parameter ranges can influence the outcome of model identification, favoring simpler models over more complex ones (or vice versa). This was evident in our observations regarding the confusion between Bayesian inference models with and without stickiness, wherein the ground truth values of stickiness played a decisive role in the model identification. This is to say ANNs should be treated as a useful tool that is only useful if the researchers apply significant forethought to developing appropriate, identifiable cognitive models.
In a similar vein, it is important to recognize that the potential negative implications of model misspecification extend to neural networks, much like they impact traditional model-fitting approaches. For instance, our estimation of parameters may be conducted under the assumption of model X, whereas, in reality, model Y might be the most suitable for explaining the data—leading to poor parameter estimation and model predictions. Our test of the systematic effects of model misspecification involved utilizing a network trained to estimate parameters from one model (e.g. Bayesian Inference) to predict parameters for the held-out test set data simulated from a different model (e.g. Bayesian Inference with stickiness, or RL). We compared this to model misspecification with a standard MAP approach. Notably, neither method exhibited significant adverse effects. When models were nested, the parameters shared between the two models were reasonably well recovered. When the model misspecificpation was more extreme (with models from different families), we again observed similar effects on the two methods, where variance driven by one parameter tended to be recovered similarly. Thus, our approach appears equally (but not worse) subject to the risk of model misspecification as other fitting methods. In light of these findings, our key takeaway is to exercise caution against assuming that the use of a neural network remedies all issues typically associated with modeling. Instead, we advocate for the application of conventional diagnostics (e.g., model comparison, predictive checks) that are commonly employed in standard methods to ensure robust and accurate results.
Relatedly, we have shown that the parameter estimation accuracy varies greatly as a function of the parameter range the network was trained on, along with whether the underlying parameter distribution of the held out test-set is included in that range or not. This is an expected property of ANNs that are known to underperform when the test data systematically differs from training examples [ 59 – 61 ]. As such, the range of parameters/models used for inputs constitutes a form of prior that constrains the fit, and it is important to carefully specify it with informed priors (as is done with other methods, such as MAP). We found that training the network using a broader parameter range, while heavily sampling from a range of interest (e.g. plausible parameter values based on previous research) affords both accurate prediction for data generated outside of the main expected range, with limited loss of precision within the range of interest ( S9 Fig ). This kind of practice is also consistent with practices in computational cognitive modeling, where a researcher might specify (e.g. using a prior) that parameter might range between two values, with most falling within a certain, more narrow range.
One factor that is specific to ANN-based methods (as opposed to standard methods) is the effect different hyperparameters (e.g. size of the neural network, choice of the learning rate, dropout values, etc.) may have on network performance—commonly resulting in overfitting or underfitting. We observed that the network performance, particularly in parameter recovery, is most significantly influenced by the number of units in the GRU layer and the chosen dropout rate. A suitable range for the number of GRU units is typically between 90 and 256, covering the needs of most cognitive models. A dropout rate within the range of 0.1 to 0.2 is generally sufficient. We have outlined the details of parameter ranges we tested in the table ( S1 Table ). To address this challenge, we employed an automated hyperparameter tuning approach, as outlined by Bergstra, Yamins, and Cox (2013). This Bayesian optimization for tuning hyper-parameters helps reduce the time required to obtain an optimal parameter set by learning from previous iterations. Additionally, in the process of training a neural network, the initialized random weights play a significant role in determining the network’s convergence and the final performance. Different random seeds can result in different initializations of the network weights, which may affect the optimization process downstream, and potentially yield different final solutions. It is important to be mindful of this; we have inspected effects of setting different seeds on our network performance ( S17 Fig ), and found that overall network performance was stable across different seeds, with slight variations (1 seed) for both parameter estimation and model identification—showcasing the need for cautious practice of inspecting network’s performance under multiple seeds.
We compared our artificial neural network approach against existing methods that are commonly used to estimate parameters of likelihood-intractable models (e.g. ABC, [ 33 , 70 ]). While traditional rejection ABC provides a workaround solution, it also imposes certain constraints. Specifically, it is more suitable for data with no sequential-dependencies, and the accuracy of parameter recovery is largely contingent on selection of appropriate summary statistics, which is not always a straightforward problem. More recent advances in the domain of simulation-based inference [ 38 , 40 , 42 , 44 ] solve many ABC-issues by automating the process of construction of summary statistics. For the purpose of this project we have focused on the methods that are most commonly used in cognitive modeling (e.g. maximum likelihood/maximum a posteriori), but future work should extend to conducting the same benchmarking procedure involving these inference methods.
Alternative approximation methods (e.g. particle filtering [ 31 ]; density estimation [ 32 ]); inverse binomial sampling [ 30 ] may prove to be more robust, but frequently require more advanced mathematical knowledge and model case-based adaptations, or are more computationally expensive; indeed, some of them may not be usable or tractable in our type of data and models where there are sequential dependencies between trials [ 30 , 71 ]. ANN-based methods such as ours or others’ [ 40 , 41 , 49 ], on the other hand, offers a more straightforward and time-efficient path to both parameter estimation and model identification. Developing more accessible and robust methods is critical for advances in computational modeling and cognitive science, and the rising popularity of deep learning puts neural networks forward as useful tools for this purpose. Our method also offers an advantage of requiring very little computational power. The aim of the project at its current state was not to optimize our ANN training in terms of time and computing resources; nevertheless, we used Nvidia V100 GPUs with 25 GB memory and required at most 1 hour for model training and predictions. This makes the ANN tool useful, as it requires a low amount of computing resources and can be done fast and inexpensively. All of our code is shared on GitHub .
We primarily focused on extensive tests using synthetic data, in particular in the context of learning experiments that present important challenges for some methods (such as BADS [ 71 ] or ABC [ 33 – 35 ]) due to the dependency between trials, and have not been thoroughly investigated with other ANN-based approaches. A critical next step will be to further validate our approach using empirical data (e.g. participant data from the tasks). Similarly, we relied on RNNs due to their flexibility and capacity to handle sequential data. However, it will be important to explore different structures, such as transformers [ 72 ], for potentially improved accuracy in parameter recovery/model identification, as well as alternative uses in cognitive modeling.
In addition, our baseline approach lacks the capability to quantify the complete uncertainty in parameter estimation, offering only point estimates. This is similar to many lightweight cognitive modeling approaches (such as MAP and LLH), but stands in contrast to other methods that integrate simulation-based inference with neural network structures [ 40 , 41 , 45 , 47 , 48 ], where the ability to capture full uncertainty represents a notable strength. Nevertheless, we have showcased that our method can easily be extended to provide uncertainty estimates by incorporating evidential learning techniques [ 58 ], at a slight computational cost, but minimal impact on point estimates’ accuracy. Furthermore, we included both RL and Bayesian inference models to demonstrate our approach can work with different classes of computational models. Future work will include additional models (e.g. sequential decision making models) to further test robustness of our approach.
In conclusion, we propose an accessible ANN-based method to perform parameter and model identification across a broad class of computational cognitive models for which application of existing methods is challenging. Our work should contribute to a growing literature focused on developing new methods that will allow researchers to quantitatively test a broader family of theories than previously possible.
Materials and methods
Probabilistic reversal learning task..
We have simulated data from different models (see the Models section) on a simple probabilistic reversal learning task (PRL; [ 73 , 74 ]). In the task, an agent chooses between two actions on each trial, and receives binary outcome ( r = 1 [reward] or r = 0 [no reward]). One of the two actions is correct for a number of trials; a correct action is defined as the action that gets rewarded with higher probability (e.g. p ( r = 1| action = correct ) = 0.80), with 1 − p probability of getting no reward if selected. After a certain number of trials, the correct action reverses; thus the action that was previously rewarded with low probability becomes the more frequently rewarded one ( S1 Fig ). This simple task (and its variants) have been extensively used to provide mechanistic insights into learning from reinforcement, inferring probabilistic structure of the environment, and people’s ability (or failure) to update the representation of a correct choice.
Hierarchical reinforcement learning task.
We developed a novel task environment that can be solved using a simple but plausible model with intractable likelihood. In this task, an agent observes N arrows (in different colors), each pointing at either left or right direction. The agent needs to learn which arrow is the correct one, by selecting an action that corresponds to either left or right side (consistent with the direction the arrow is pointing at) in order to get rewarded. Selecting the side the correct arrow is pointing at rewards the agent with high probability ( p =.9); choosing an action by following direction of other arrows leads to no reward ( r = 0) with same high probability. The correct arrow changes unpredictably in the task, which means that the agent must keep track of which arrow most reliably leads to the reward, and update accordingly upon the change. We refer to this task structure as hierarchical because the choice policy (left/right) depends on the higher-level rule (color) agents choose to follow.
Cognitive models
Prl task models..
We implemented multiple models of the PRL task to test the artificial neural network (ANN) approach to parameter estimation. First, we cover the benchmark models; these are the models that we can fit using traditional methods (MLE, MAP), as well as the ANN, to ensure that we can justify using the ANN if it performs at least just as well as (or better than) the traditional methods.
Reinforcement learning models family.
Two-parameter reinforcement learning model . We simulated artificial data on the PRL task using a simple 2-parameter reinforcement learning model (2P-RL). The model assumes that the agent tracks the value of each action contingent on the reward history, and uses these values to inform the action selection on each trial.
The 2p-RL model contained following free parameters: learning rate ( α ) and softmax beta ( β ).
Like in the 2P-RL we also included counterfactual updating of values for non-selected actions. The 4P-RL model included following free parameters: positive learning rate ( α + ), negative learning rate ( α − ), softmax beta ( β ) and stickiness ( κ ).
Bayesian models family.
The BI model included following parameters: inferred probability of reward given the action determined by the current belief ( p reward ), likelihood of the correct action reversing ( p switch ) and softmax beta ( β ).
Intractable likelihood.
As a proof of concept, we implemented a simple model that assumes a latent state of agent’s attention (engaged/disengaged). This model can’t be fit using methods that rely on computing likelihood. While models can have intractable likelihood for a variety of reasons, we focused on leveraging latent variables (e.g. attention state), that are not readily observable in the data. Thus, in the data that is being modeled, only the choices are observed—but not the state the agent was in while executing the choices. The learned choice value which affects the choice likelihood depends on the trial history, including which state the agent was in. Thus, if there are 2 such states, there are 2 N possible sequences that may result in different choice value estimates after N trials. To estimate choice values and likelihood on any given trial one must integrate over the uncertainty of an exponentially increasing latent variable—thus making the likelihood intractable.
RL and latent engagement state.
We simulated a version of a 2p-RL model for a probabilistic reversal learning (PRL) task that also assumes that an agent might occupy two of the latent attention states—engaged or disengaged— during the task (RL-LAS). The model assumes that in the engaged state an agent behaves in accordance with the task structure (e.g. tracks and updates values of actions, and uses action values to inform action selection). In the disengaged state, an agent behaves in a noisy way, in that 1) it does not update the Q value of actions, and 2) chooses between the two actions randomly (e.g. uniform policy) instead of based on their value (e.g. through softmax). Note that assumption 1) is different from a previous version of the model our group considered [ 78 , 79 ], and is the core assumption that renders the likelihood intractable. The agent can shift between different engagement states at any point throughout the task, and the transition between the states is controlled by a parameter τ . Specifically, for each agent we initialized a random value T between 10 and 30 (which roughly maps onto approximately how many trials an agent spends in a latent attention state), and then used a non-linear transformation to compute τ : 1-(1/T). The value of τ , thus quantifies the probability of transitioning between the two states. The agent was initialized to be in an attentive state at the onset of trials.
Cognitive models of the HRL task.
Bayesian models of the HRL task . Bayesian models of the HRL task assume an inference process over the latent variable of which arrow is currently the valid arrow, and thus which side (R/L) (given the current trial’s set of arrows) is most likely to result in positive outcome. The inference relies on the generative model of the task determined by parameters p switch and p reward , history of trial observations O t , set of arrows and stochastic choice based on this inference. Initial prior belief over arrows is initialized uniformly prior = 1/ nA , where nA corresponds to the number of arrows.
This belief is subsequently used to inform arrow choices on the next trial. This model differs from the Bayesian Inference model for the probabilistic task in that 1) p reward and p switch parameters are not free/inferred and 2) the choice of the side is stochastic, allowing for a potential lapse in selecting the side that is not consistent with the selected arrow. This model, thus has following free parameters: decision parameter β and noise parameter ϵ . Like in the in Bayesian inference model for the PRL task, we also tested the model variant with stickiness κ parameter that biases beliefs associated with the arrow/side chosen on the previous trial. Both models have tractable likelihoods.
Hierarchical reinforcement learning.
Likelihood-dependent methods
Maximum likelihood and maximum a posteriori estimation..
As a prior for the MAP approach, we used an empirical prior derived from the true simulating distribution of parameters. We note that this gives an advantage to the MAP method above what would be available for empirical data, allowing MAP to provide a ceiling performance on the test set.
Because MAP and MLE rely on likelihood computation, their use is essentially limited to models with tractable likelihood. We used MAP and MLE to estimate parameters of tractable-likelihood models as one of the benchmarks against which we compared our ANN approach. Specifically, we fit the models to the test-set data used to compute the MSE of the ANN, and compared fit using the same metric across methods (see main text).
Likelihood approximation methods.
Because models with tractable likelihood comprise only a small subset of all possible (and likely more plausible) models, researchers have handled the issue of likelihood intractability by implementing various likelihood approximation methods. While there are different likelihood approximation tools, such as particle filtering [ 31 ] and assumed density estimation [ 32 ], we focus on Approximate Bayesian Computation (ABC; [ 33 , 36 , 37 , 70 ]), as it is more widely accessible and does not require more extensive mathematical expertise. ABC leverages large scale model simulation to approximate likelihood. Specifically, a large synthetic data set is simulated from a model, with parameters randomly sampled from a specific range for each agent. Summary statistics that describe the data (e.g average accuracy or variance in accuracy) are used to construct the empirical likelihood that can be used in combination with classic methods.
The distance metric, like the rejection criterion, is determined by the researcher. The samples that are accepted are the samples with distance to the real data smaller than the criterion, resulting in the conclusion that parameters used to generate the sample data set can plausibly be the ones that capture the target data. Thus, the result of the ABC for each data set is a distribution of plausible parameter values which can be used to obtain point estimates via the mean, median, etc.
ABC is a valuable tool,but standard ABC has serious limitations [ 33 ]. For instance, the choice of summary statistics is not a trivial problem, and different summary statistics can yield significantly different results. Similarly, in the case of rejection algorithm ABC, researchers must choose the rejection criterion which can also affect the parameter estimates. A possible way to address this is using cross validation to determine which rejection criterion is the best, but this also requires specification of the set of possible criteria values for the cross validation algorithm to choose from. Furthermore, one of ABC assumptions is independence of data points, which is violated in many sequential decision making models (e.g. reinforcement learning).
To compare our approach to ABC, we used network training set data as a large scale simulation data set, and then estimated parameters of the held out test set also used to evaluate the ANN.
To apply ABC in our case, we needed to select summary statistics that adequately describe performance on the task. We used the following summary statistics to quantify the agent for the models simulated on the PRL task:
- Learning curves: We computed agents’ probability of selecting the correct action, aligned to the number of trials with reference to the reversal point. Specifically, for each agent we computed an average proportion of trials where a correct action was selected N trials before and N trials after the correct action reversal point, for all reversal points throughout the task. This summary statistic should capture learning dynamics, as the agent learns to select the correct action, and then experiences dip in accuracy once the correct actions switch, subsequently learning to adjust based on feedback after several trials.
- 3-back feedback integration: The 3-back analysis quantifies learning as well; however, instead of aligning the performance to reversal points, it allowed us to examine agents’ tendency to repeat action selection from the previous trial contingent on reward history—specifically the outcome they observed on the most recent 3 trials. Higher probability of repeating the same action following more positive feedback indicates learning/sensitivity to reward as reported in [ 11 ]
- Ab-analysis: The Ab-analysis allowed us to quantify probability of selecting an action at trial t ,contingent both on previous reward and action selection history (trials t − 2 and t − 1, [ 11 , 80 ]).
For the models simulated on a hierarchical task we used the learning curves as summary statistics (same as for the PRL), where reversal points were defined as the switch of the correct rule/arrow to follow. In addition, we quantified agent’s propensity to stick with the previously correct rule/arrow, where the agent should be increasingly less likely to select the side consistent with the arrow that was correct before the switch as the number of trials since the switch increases. Similarly, we used a version of the 3-back analysis where the probability of staying contingent on the reward history referred to the probability of potentially selecting the same cue across the trial window, based on observed choices of the agent. All summary statistics are visualized in S8 Fig .
Model comparison.
We used AIC score as it outperformed BIC model comparison, and thus provided us with ceiling benchmark to evaluate the ANN.
To perform proper model comparison, it is essential to not only evaluate the model fitness (overall AIC/BIC score), but also to test how reliably the true models (that generated the data) can be identified/successfully distinguished from others. To do so, we constructed a confusion matrix based on the AIC score ( Fig 5A ). We used the test set data simulated from each model, and then fit all candidate models to each of the data sets while also computing the AIC score for each fit. If the models are identifiable, we should observe that AIC scores for true models (e.g. the models the data was simulated from) should be the lowest for that model when it’s fit to the data compared to other model candidates.
Artificial neural network-based method
Parameter recovery..
To implement ANNs for parameter estimation we have used the relatively simple neural network structure inspired by the previous work [ 52 ]. In all experiments, we used 1 recurrent GRU layer followed by 3 fully connected dense layers with 2000 dimensional input embeddings ( S1 Table ). To train the network, we simulated a training data set using known parameters. For each model, we used 30000 training samples, 3000 validation samples, and 3000 test samples that are generated from simulations separately. For probabilistic RL, the input sequence consisted of rewards and actions. For hierarchical RL, the sides (left/right) of three arrow stimuli are added to the rewards and actions sequences. The network output dimension was proportional to the number of model parameters. We used a tanh activation in the GRU layer, reLu activations in 2 dense layers, and a linear activation at the final output. Additional training details are given below:
- We used He normal initialization to initialize GRU parameters [ 81 ].
- We used the Adam optimizer with mean square error (MSE) loss and a fixed learning rate of 0.003. Early stopping (e.g. network training was terminated if validation loss failed to decrease after 10 epochs) was applied with a maximum of 200 epochs.
- We selected network hyperparameters with Bayesian optimization algorithms [ 82 ] applied on a validation set. Details of the selected values are shown in S1 Table .
All of the training/validation was run using TensorFlow [ 83 ]. The training was performed on Nvidia V100 GPUs with 25 GB memory.
Network evaluation.
The network predicted the values of parameter on the test set that is unseen in the training and validation. We also conducted robustness tests by varying trial numbers (input size).
To evaluate the output of both ANN and traditional tools we used the following metrics (ensuring our results are robust to the choice of performance quantification):
- Mean squared error (MSE): To evaluate parameter estimation accuracy we calculated a mean squared error between true and estimated model parameter across all agents. Prior to calculating MSE all parameters were normalized, to ensure comparable contribution to MSE across all parameters. Overall loss for a cognitive model (across all parameters) was an average of individual parameter MSE scores. Overall loss for a class of models (e.g. likelihood-tractable models) was an average across all model MSE scores.
- Spearman correlation ( ρ ): We used Spearman correlation as an additional metric for examining how estimated parameter values relate to true parameter values, with higher Spearman ρ values indicating higher accuracy. We paired Spearman correlations with scatter plots, to visualize patterns in parameter recoverability (e.g. whether there are specific parameter ranges where parameters are more/less recoverable).
- R-Squared ( R 2 or the coefficient of determination): R-Squared represents the proportion of variance in true parameters that can be explained by a linear regression between true and predicted values. It thus indicates the goodness of fit of an ANN model. We calculated an R-Squared score for each individual parameters across all agents and used it as an additional evaluation for how well the data fit the regression model.
Uncertainty estimation.
To compute uncertainty of parameter estimates we have incorporated evidential learning into our method [ 58 ]. In the application of evidential learning to continuous regression [ 58 ] observed targets follow a Gaussian distribution, characterized by its mean and variance. Conjugate Gaussian prior, normal inverse-gamma distribution, is created by placing a prior on both the mean (Gaussian distribution) and the variance (inverse-gamma distribution). By sampling from this distribution, specific instance of the likelihood function is obtained (based on both mean and the variance). This approach not only aims for accurate target predictions but also takes into account the uncertainty (quantified by the variance term). For more insights and details into evidential learning, refer to the work by [ 58 ]. Their research also introduces a regularization term, which is useful for penalizing incorrect evidence and data that falls outside the expected distribution.
For the purpose of visualization ( Fig 4 ) we have created upper and lower bounds of targets by adding/subtracting variance from the predicted target values. We then re-scaled these values by applying the inverse scaler (e.g. from the scaler applied to normalize parameters for network training). This provides a scale-appropriate and more interpretable visualization of parameter recovery and uncertainty for each parameter.
Alternative models.
We have also tested the network with long short-term memory (LSTM) units since LSTM units are more complex and expressive than GRU units; nevertheless they achieved the similar performance as GRU units but are more computationally expensive, and thus we mostly focused on the GRU version of the model. Since LSTM worked, but not better than GRU, the LSTM results are reported in S2 – S5 Figs.
Model identification.
The network structure and training process were similar to that of the network used for parameter recovery, with an exception of the output layer that utilized categorical cross-entropy loss and a softmax activation function. The network validation approach was the same as the one we used for parameter recovery (e.g. based on the held-out test set). We also observed a better performance when training with various trial numbers.
Robustness test: Influence of different input trial numbers.
For all robustness experiments, we followed the same training procedures as described previously while varying the training data. The details of training data generation are given below:
We simulated 30,000 training samples with 2000 trials per simulation in the probabilistic reversal learning task. For shorter fixed trial sequence lengths per training samples (e.g 500), we used the same training set truncated to the first 500 trials. To generate the training data with different trial numbers across training samples, we reused the same training set, with sequences of trials truncated to a given number. There were 6000 training samples of 50, 100, 500, 1000, 1500, and 2000 trials, each.
The process of data generation for model identification robustness checks was similar to parameter recovery. However, we only simulated 500 trials for each model because we found no significant increase in accuracy with higher trial numbers.
Supporting information
S1 fig. tasks..
A) Probabilistic reversal learning task. We simulated artificial agents using cognitive models of behavior on a Probabilistic reversal learning (PRL) task, which provides a dynamic context for studying reward-driven learning. In this task, an agent chooses between two actions, where one of the actions gets rewarded with higher probability ( p ( r ) =.80) and one with lower (1 − p ). After a certain number of correct trials, the reward probabilities of the two actions reverse. The task provides an opportunity to observe how agents update their model of the task (e.g. correct actions) based on observed feedback. B) Hierarchical reinforcement learning task. In this task, three differently colored arrows represent three potential rules an agent can follow when selecting one of the two actions (left/right) corresponding to the side the chosen arrow is pointing at. Selecting a side consistent with correct arrow is rewarded with probability p =.90. Correct arrow switches after a certain number of trials. The task provides a possibility to examine how following latent rules may shape agents’ learning behavior.
https://doi.org/10.1371/journal.pcbi.1012119.s001
S2 Fig. 2 Parameter RL (2P-RL) model parameter recovery using different fitting methods.
ρ corresponds to Spearman correlation coefficient, red line represents a unity line (x = y), and black line represents a least squares regression line.
https://doi.org/10.1371/journal.pcbi.1012119.s002
S3 Fig. 4 Parameter RL model (4P-RL) parameter recovery using different fitting methods.
https://doi.org/10.1371/journal.pcbi.1012119.s003
S4 Fig. Bayesian Inference (BI) model parameter recovery using different fitting methods.
https://doi.org/10.1371/journal.pcbi.1012119.s004
S5 Fig. Bayesian Inference with stickiness (S-BI) model parameter recovery using different fitting methods.
https://doi.org/10.1371/journal.pcbi.1012119.s005
S6 Fig. Bayesian Inference with stickiness (S-BI) model parameter recovery using different fitting methods.
Correlation between the average experienced time intervals in attentive state and the τ parameter in RL-LAS model that captures transition between disengaged/engaged attention states estimated by the ANN.
https://doi.org/10.1371/journal.pcbi.1012119.s006
S7 Fig. Misclassification of Bayes and sticky Bayes model.
Misclassification of Bayes and sticky Bayes model is contingent on the value of the stickiness parameter κ . The misclassification percentage is higher at κ values closer to 0.
https://doi.org/10.1371/journal.pcbi.1012119.s007
S8 Fig. Summary statistics for Approximate Bayesian Computation (ABC).
Top row shows summary statistics computed for all models simulated on a probabilistic reversal learning task; the figure only shows agents simulated using a 4-parameter RL model. Bottom row shows summary statistics computed for all models simulated on a hierarchical reversal learning task; the figure only shows performance of HRL model agents. Both rows depict 200 out of 3000 test set agents. Gray lines represent individual agents; black line represents an average across the agents.
https://doi.org/10.1371/journal.pcbi.1012119.s008
S9 Fig. Effect of prior misspecification on parameter estimation in MAP and our ANN approach.
A) Applying too narrow prior specification to the fitting procedure (prior in MAP, training samples in ANN) results in difficulty estimating out-of-range parameters for both MAP and ANN. Broader prior specification addresses this issue, with only a slight loss of precision in specific target ranges. Training the network with a broad range of parameters while oversampling parameters from regions of interest yields most robust results. B) Visualization of fitting with MAP and ANN with a wide prior, tested on a full range/wide range data set—training the network with broader range while oversampling from the most plausible range yields less noisy performance in the range compared to MAP. Red lines delineate the range of the narrow prior, which corresponds to the main text results. C) The broad prior was designed by sampling from the full broader range ( β ∈ [0, 10], α ∈ [0, 1]), with the constraint that 70% of samples are in the expected narrow range ( β ∈ [2, 6], α ∈ [0.5, 1], and 30% outside).
https://doi.org/10.1371/journal.pcbi.1012119.s009
S10 Fig. Consistency between methods for parameter estimation.
A) The correlation between parameter estimates in the 4P-RL model derived using MAP and ANN, is high, and indeed stronger than the correlation between true and derived parameters (see Fig 2 ), showing that both methods systematically misidentify some parameters similarly, likely due to specific data patterns. B) The correlation between parameter estimates in the Bayesian inference model derived using MAP and ANN shows similar results.
https://doi.org/10.1371/journal.pcbi.1012119.s010
S11 Fig. Consistency between methods for parameter estimation in two model misspecification cases.
A) The correlation between MAP and GRU RL model parameter estimates, fit to data simulated from Bayesian Inference model. B) The correlation between MAP and GRU Bayes model parameter estimates, fit to data simulated from the RL model. High correlation would imply similarities in estimates between MAP and GRU, suggesting that ANNs are similarly impacted by model misspecification as traditional methods such as MAP.
https://doi.org/10.1371/journal.pcbi.1012119.s011
S12 Fig. Comparison of model predictions of ground truth simulated behavior (black line) and choices simulated using A) MAP and B) GRU estimated parameters (gray line) of the 4P-RL model.
We randomly sampled 100 agents from the test set, and the respective parameter estimates for each of the methods. We simulated data from the model and compared it to ground truth. Both methods successfully recover choices from the ground truth agents.
https://doi.org/10.1371/journal.pcbi.1012119.s012
S13 Fig. Effect of model misspecification on standard method and ANN performance.
We randomly sampled 100 agents from the test set, and the respective parameter estimates for each of the methods. We simulated data from the model and compared it to ground truth. Both methods successfully recover choices from the ground truth agents. A) We fit the Bayesian Inference model (without stickiness) to the data simulated from the Bayesian Inference Model with stickiness using MAP. We correlated the estimated Bayesian inference model parameters (y axis) with the ground truth parameters from the model with stickiness (x-axis). B) For the ANN, we trained the neural network to estimate parameters of the Bayesian inference model, and tested it on the data simulated from the Bayesian inference model with stickiness. We looked at the correlation between the ground truth parameters (from a separate test set), and the predictions of the network trained on the model without stickiness. Both methods show that parameters shared between the misspecified models can be reasonably and similarly recovered. Both ANN and MAP generated some non-zero estimates of stickiness when data simulated from model without stickiness was fit using the model/network that assumes presence of stickiness in the model; however, these values were closely clustered around 0, to a similar degree between methods ( S16 Fig ).
https://doi.org/10.1371/journal.pcbi.1012119.s013
S14 Fig. Effect of model class misspecification on standard method and ANN performance.
We fit the RL model to the data simulated from the Bayesian Inference model in the probabilistic reversal learning task (see Methods section on Tasks and Cognitive Models) using A) MAP and B) GRU. We correlated the estimated RL model parameters (y axis) with the ground truth parameters from the Bayesian inference model (x-axis). MAP and GRU show similar patterns between estimated and true parameters, such that variance driven by true parameter β p switch are both captured in the fit β parameters, while the fit learning rate parameter α captures behavioral variance driven by the Bayesian update parameters p reward and p switch .
https://doi.org/10.1371/journal.pcbi.1012119.s014
S15 Fig. Effect of model class misspecification on standard method and ANN performance.
We fit the Bayesian Inference model to the data simulated from the RL model in the probabilistic reversal learning task (see Methods section on Tasks and Cognitive Models) using A) MAP and B) GRU. We correlated the estimated Bayesian inference model parameters (y axis) with the ground truth parameters from the RL model (x-axis). MAP and GRU again show similar patterns between estimated and true parameters. In particular, we see that in both cases, noise in behavior due to β in the RL model tends to be attributed to the p switch fit parameter rather than the fit Bayesian model β parameter. Effect of learning rate parameter α are attributed to p reward by both methods.
https://doi.org/10.1371/journal.pcbi.1012119.s015
S16 Fig. Stickiness parameter estimates.
Stickiness parameter estimates from the data simulated from the Bayesian inference model without stickiness from A) fitting the Bayesian inference model with stickiness using MAP, and B) utilizing the ANN trained to estimate parameters of the model with stickiness. Despite both methods producing non-zero estimates of stickiness, they tend to cluster around the value of 0.
https://doi.org/10.1371/journal.pcbi.1012119.s016
S17 Fig. Neural network performance variability by different seeds for model identification and parameter estimation.
For conciseness, we show tests from 10 different seeds on model identification with 4 models simulated on the PRL task (e.g. same as Fig 5 ) and parameter estimation of one of the likelihood-intractable models (e.g. RL-LAS model). We found that overall both model identification and parameter estimation had relatively stable results across different seeds, with an exception of one seed value in both cases.
https://doi.org/10.1371/journal.pcbi.1012119.s017
S1 Table. Summary of hyper-parameter values selected from Bayesian optimization algorithms.
https://doi.org/10.1371/journal.pcbi.1012119.s018
Acknowledgments
We thank Jasmine Collins, Kshitiz Gupta, Yi Liu and Jaeyoung Park for their contributions to the project. We thank Bill Thompson and all CCN lab members for useful feedback on the project.
- View Article
- PubMed/NCBI
- Google Scholar
- 3. Shultz TR. Computational developmental psychology. Mit Press; 2003.
- 32. Minka TP. Expectation propagation for approximate Bayesian inference. arXiv preprint arXiv:13012294. 2013;.
- 36. Palestro JJ, Sederberg PB, Osth AF, Van Zandt T, Turner BM. Likelihood-free methods for cognitive science. Springer; 2018.
- 38. Lavin A, Krakauer D, Zenil H, Gottschlich J, Mattson T, Brehmer J, et al. Simulation intelligence: Towards a new generation of scientific methods. arXiv preprint arXiv:211203235. 2021;.
- 41. Radev ST, Voss A, Wieschen EM, Bürkner PC. Amortized Bayesian Inference for Models of Cognition. arXiv preprint arXiv:200503899. 2020;.
- 43. Chen Y, Zhang D, Gutmann M, Courville A, Zhu Z. Neural approximate sufficient statistics for implicit models. arXiv preprint arXiv:201010079. 2020;.
- 46. Schmitt M, Bürkner PC, Köthe U, Radev ST. Detecting model misspecification in amortized Bayesian inference with neural networks. arXiv preprint arXiv:211208866. 2021;.
- 55. Särkkä S, Svensson L. Bayesian filtering and smoothing. vol. 17. Cambridge university press; 2023.
- 59. Nguyen A, Yosinski J, Clune J. Deep neural networks are easily fooled: High confidence predictions for unrecognizable images. In: Proceedings of the IEEE conference on computer vision and pattern recognition; 2015. p. 427–436.
- 60. Szegedy C, Zaremba W, Sutskever I, Bruna J, Erhan D, Goodfellow I, et al. Intriguing properties of neural networks. arXiv preprint arXiv:13126199. 2013;.
- 61. Liang S, Li Y, Srikant R. Enhancing the reliability of out-of-distribution image detection in neural networks. arXiv preprint arXiv:170602690. 2017;.
- 62. Moosavi-Dezfooli SM, Alhussein Fawzi OF. Pascal Frossard.”. In: Universal adversarial perturbations.” 2017 IEEE Conference on Computer Vision and Pattern Recognition (CVPR); 2017.
- 70. Sisson SA, Fan Y, Beaumont M. Handbook of approximate Bayesian computation. CRC Press; 2018.
- 72. Devlin J, Chang MW, Lee K, Toutanova K. Bert: Pre-training of deep bidirectional transformers for language understanding. arXiv preprint arXiv:181004805. 2018;.
- 75. Sutton RS, Barto AG. Reinforcement learning: An introduction. MIT press; 2018.
- 78. Li JJ, Shi C, Li L, Collins AG. A generalized method for dynamic noise inference in modeling sequential decision-making. In: Proceedings of the Annual Meeting of the Cognitive Science Society. vol. 45; 2023.
- 81. He K, Zhang X, Ren S, Sun J. Delving deep into rectifiers: Surpassing human-level performance on imagenet classification. In: Proceedings of the IEEE international conference on computer vision; 2015. p. 1026–1034.
- 82. Bergstra J, Yamins D, Cox D. Making a science of model search: Hyperparameter optimization in hundreds of dimensions for vision architectures. In: International conference on machine learning. PMLR; 2013. p. 115–123.
- 83. Abadi M, Barham P, Chen J, Chen Z, Davis A, Dean J, et al. {TensorFlow}: a system for {Large-Scale} machine learning. In: 12th USENIX symposium on operating systems design and implementation (OSDI 16); 2016. p. 265–283.
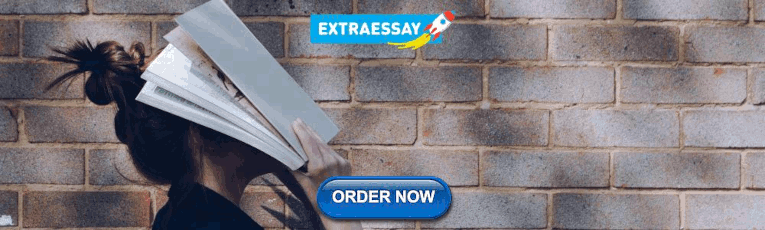
IMAGES
VIDEO
COMMENTS
Computational biology and bioinformatics articles from across Nature Portfolio. Atom; RSS Feed; Definition. Computational biology and bioinformatics is an interdisciplinary field that develops and ...
Research Article. Identifying essential factors for energy-efficient walking control across a wide range of velocities in reflex-based musculoskeletal systems. ... This Collection aims to increase the coverage of computational biology-related topics in Wikipedia by rewarding authors with a citable, PubMed-indexed static version of the living ...
Representative fields of view show the trajectories of individual cells tracked from Control keratinocytes (left) and keratinocytes with enhanced PIEZO1 activity (right) during collective cell migration. Each color-coded trajectory delineates the path of an individual cell. Chen & Holt et al 2024.
Here, sixteen computational biologists around the globe present "A field guide to cultivating computational biology," focusing on solutions. Biology in the digital era requires computation and collaboration. A modern research project may include multiple model systems, use multiple assay technologies, collect varying data types, and require ...
Contents . Outstanding primary research articles on all aspects of computational biology applied to different and integrated biological scales, from molecules and cells to patient populations and ecosystems.; Invited and submitted reviews and perspectives on topics of broad interest to the readership.. Historical reviews and high-quality tutorials (including multimedia presentations) teaching ...
Biological computation involves the design and development of computational techniques inspired by natural biota. On the other hand, computational biology involves the development and application of computational techniques to study biological systems. We present a comprehensive review showcasing how biology and computer science can guide and benefit each other, resulting in improved ...
Computational Biology and Chemistry publishes original research papers and review articles in all areas of computational life sciences.High quality research contributions with a major computational component in the areas of nucleic acid and protein sequence research, molecular evolution, molecular genetics (functional genomics and proteomics), theory and practice of either biology-specific or ...
Overview. Computational biology has grown and matured into a discipline at the heart of biological research. In honor of the tenth anniversary of PLOS Computational Biology, Phil Bourne, Win Hide, Janet Kelso, Scott Markel, Ruth Nussinov, and Janet Thornton shared their memories of the heady beginnings of computational biology and their thoughts on the field's promising and provocative future.
Research articles should include the following sections, in order: abstract, introduction, methods, results, discussion and references. Software articles: Short 2-4 page articles describing implementations of new or recently developed computational methods for applications in computational biology. The approaches underlying the software should ...
Biophysics and Computational Biology. Recent articles Research Article May 15, 2024. Myxococcus xanthus encapsulin cargo protein EncD is a flavin-binding protein with ferric reductase activity. Elif Eren, Norman R. Watts, James F. Conway, Paul T. Wingfield,
Bioinformatics is an official journal of the International Society for Computational Biology, the leading professional society for computational biology and bioinformatics. Members of the society receive a 15% discount on article processing charges when publishing Open Access in the journal. Read papers from the ISCB. Find out more.
Computational biology and bioinformatics. Read the latest research from universities and research institutes around the world. Full text, images, free.
These articles reflect current trend and development in bioinformatics research. The supplement to BMC Bioinformatics was proposed to launch during the BIOCOMP'19—The 2019 International Conference on Bioinformatics and Computational Biology held from July 29 to August 01, 2019 in Las Vegas, Nevada. In this congress, a variety of research ...
Computational biology. Download RSS feed: News Articles / In the Media / Audio. Displaying 1 - 15 of 30 news articles related to this topic. Show: News Articles. In the Media. Audio. Search algorithm reveals nearly 200 new kinds of CRISPR systems ... New research provides insight into the behavior of microbial communities in the ocean.
Some notable examples include Journal of Computational Biology and PLOS Computational Biology, a peer-reviewed open access journal that has many notable research projects in the field of computational biology. They provide reviews on software, tutorials for open source software, and display information on upcoming computational biology conferences.
Here, I argue that computational thinking and techniques are so central to the quest of understanding life that today all biology is computational biology. Computational biology brings order into our understanding of life, it makes biological concepts rigorous and testable, and it provides a reference map that holds together individual insights. The next modern synthesis in biology will be ...
The article "Computational and Systems Biology Advances to Enable Bioagent Agnostic Signatures" explores the computational data challenges of threat-agnostic biodefense, or the ability to characterize an unknown agent's likely impact to human, animal, and plant health. The researchers discuss how the biodefense community can make the ...
Sharing the power of AlphaFold 3 responsibly Alongside Google DeepMind, we've sought to understand the broad impact of the technology. Working together with the research and safety community to take a science-led approach, we have conducted extensive assessments to mitigate potential risks and share the widespread benefits to biology and humanity.
Researchers present Tripath: new, deep learning models that can use 3D pathology datasets to make clinical outcome predictions. The research team imaged curated prostate cancer specimens, using ...
Identifying functionally important cell states and structure within a heterogeneous tumor remains a significant biological and computational challenge. Moreover, current clustering or trajectory-based computational models are ill-equipped to address the notion that cancer cells reside along a phenotypic continuum. To address this, we present Archetypal Analysis network (AAnet), a neural ...
Chemical Biology & Drug Design is a leading journal dedicated to the advancement of science, ... Department of Chemistry & Applied Computational Chemistry Research Unit, School of Science, King Mongkut's Institute of Technology Ladkrabang, Bangkok, Thailand. Search for more papers by this author.
Biology in the digital era requires computation and collaboration. A modern research project may include multiple model systems, use multiple assay technologies, collect varying data types, and require complex computational strategies, which together make effective design and execution difficult or impossible for any individual scientist.
Eran Ophir, Ph.D., Chief Scientific Officer at Compugen added, "Our paper published online today in Cancer Immunology Research provides an advanced understanding of the unique biology of PVRIG and ...
Author summary Computational cognitive models occupy an important position in cognitive science research, as they offer a simple way of quantifying cognitive processes (such as how fast someone learns, or how noisy they are in choice selection), and testing which cognitive theories offer a better explanation of the behavior. To relate cognitive models to the behavioral data, researchers rely ...