
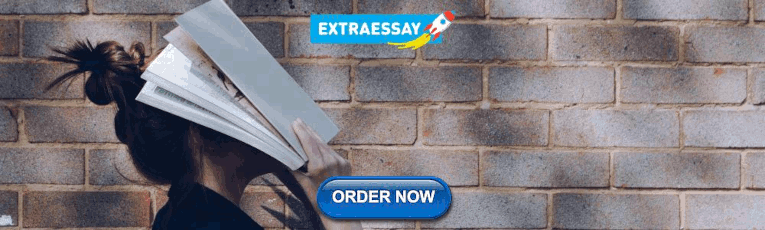
Theoretical vs Conceptual Framework
What they are & how they’re different (with examples)
By: Derek Jansen (MBA) | Reviewed By: Eunice Rautenbach (DTech) | March 2023
If you’re new to academic research, sooner or later you’re bound to run into the terms theoretical framework and conceptual framework . These are closely related but distinctly different things (despite some people using them interchangeably) and it’s important to understand what each means. In this post, we’ll unpack both theoretical and conceptual frameworks in plain language along with practical examples , so that you can approach your research with confidence.
Overview: Theoretical vs Conceptual
What is a theoretical framework, example of a theoretical framework, what is a conceptual framework, example of a conceptual framework.
- Theoretical vs conceptual: which one should I use?
A theoretical framework (also sometimes referred to as a foundation of theory) is essentially a set of concepts, definitions, and propositions that together form a structured, comprehensive view of a specific phenomenon.
In other words, a theoretical framework is a collection of existing theories, models and frameworks that provides a foundation of core knowledge – a “lay of the land”, so to speak, from which you can build a research study. For this reason, it’s usually presented fairly early within the literature review section of a dissertation, thesis or research paper .
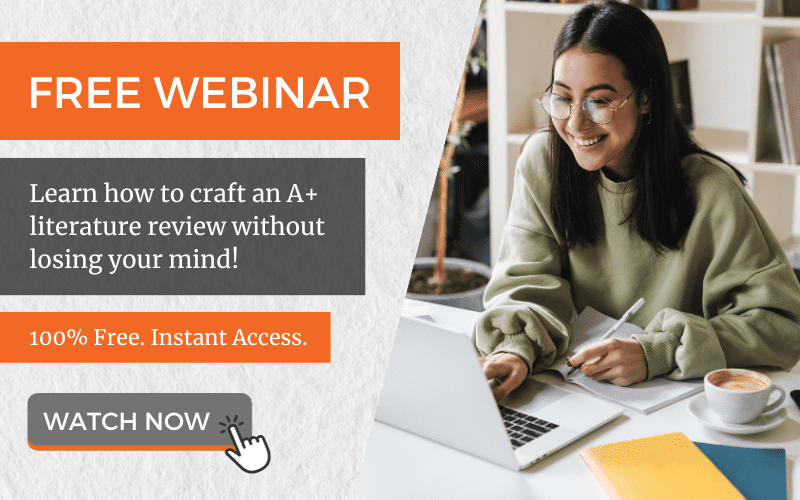
Let’s look at an example to make the theoretical framework a little more tangible.
If your research aims involve understanding what factors contributed toward people trusting investment brokers, you’d need to first lay down some theory so that it’s crystal clear what exactly you mean by this. For example, you would need to define what you mean by “trust”, as there are many potential definitions of this concept. The same would be true for any other constructs or variables of interest.
You’d also need to identify what existing theories have to say in relation to your research aim. In this case, you could discuss some of the key literature in relation to organisational trust. A quick search on Google Scholar using some well-considered keywords generally provides a good starting point.
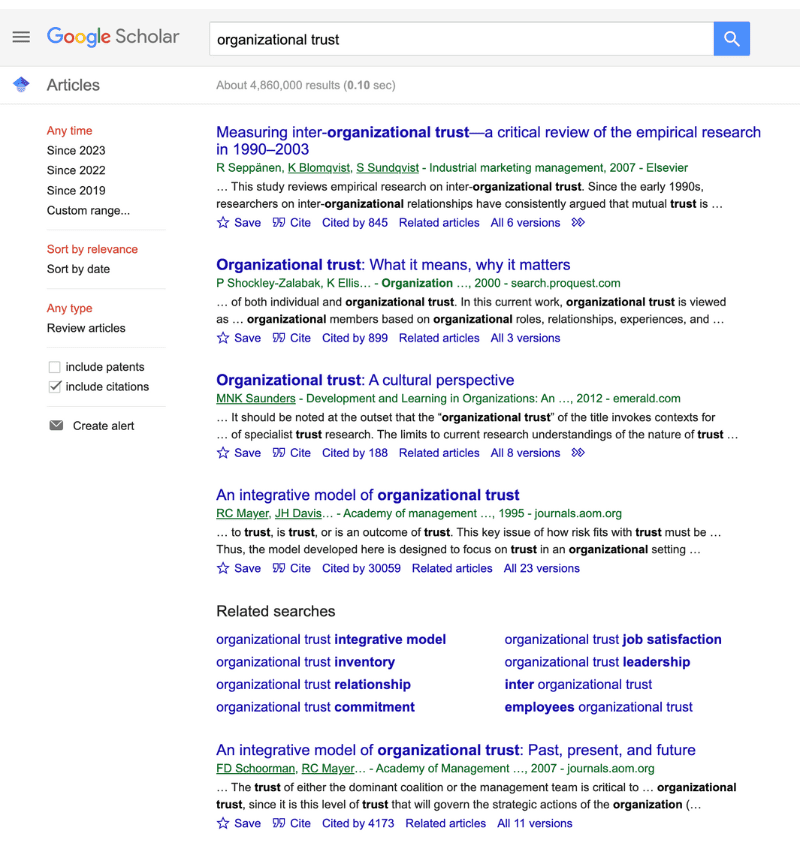
Typically, you’ll present your theoretical framework in written form , although sometimes it will make sense to utilise some visuals to show how different theories relate to each other. Your theoretical framework may revolve around just one major theory , or it could comprise a collection of different interrelated theories and models. In some cases, there will be a lot to cover and in some cases, not. Regardless of size, the theoretical framework is a critical ingredient in any study.
Simply put, the theoretical framework is the core foundation of theory that you’ll build your research upon. As we’ve mentioned many times on the blog, good research is developed by standing on the shoulders of giants . It’s extremely unlikely that your research topic will be completely novel and that there’ll be absolutely no existing theory that relates to it. If that’s the case, the most likely explanation is that you just haven’t reviewed enough literature yet! So, make sure that you take the time to review and digest the seminal sources.
Need a helping hand?
A conceptual framework is typically a visual representation (although it can also be written out) of the expected relationships and connections between various concepts, constructs or variables. In other words, a conceptual framework visualises how the researcher views and organises the various concepts and variables within their study. This is typically based on aspects drawn from the theoretical framework, so there is a relationship between the two.
Quite commonly, conceptual frameworks are used to visualise the potential causal relationships and pathways that the researcher expects to find, based on their understanding of both the theoretical literature and the existing empirical research . Therefore, the conceptual framework is often used to develop research questions and hypotheses .
Let’s look at an example of a conceptual framework to make it a little more tangible. You’ll notice that in this specific conceptual framework, the hypotheses are integrated into the visual, helping to connect the rest of the document to the framework.
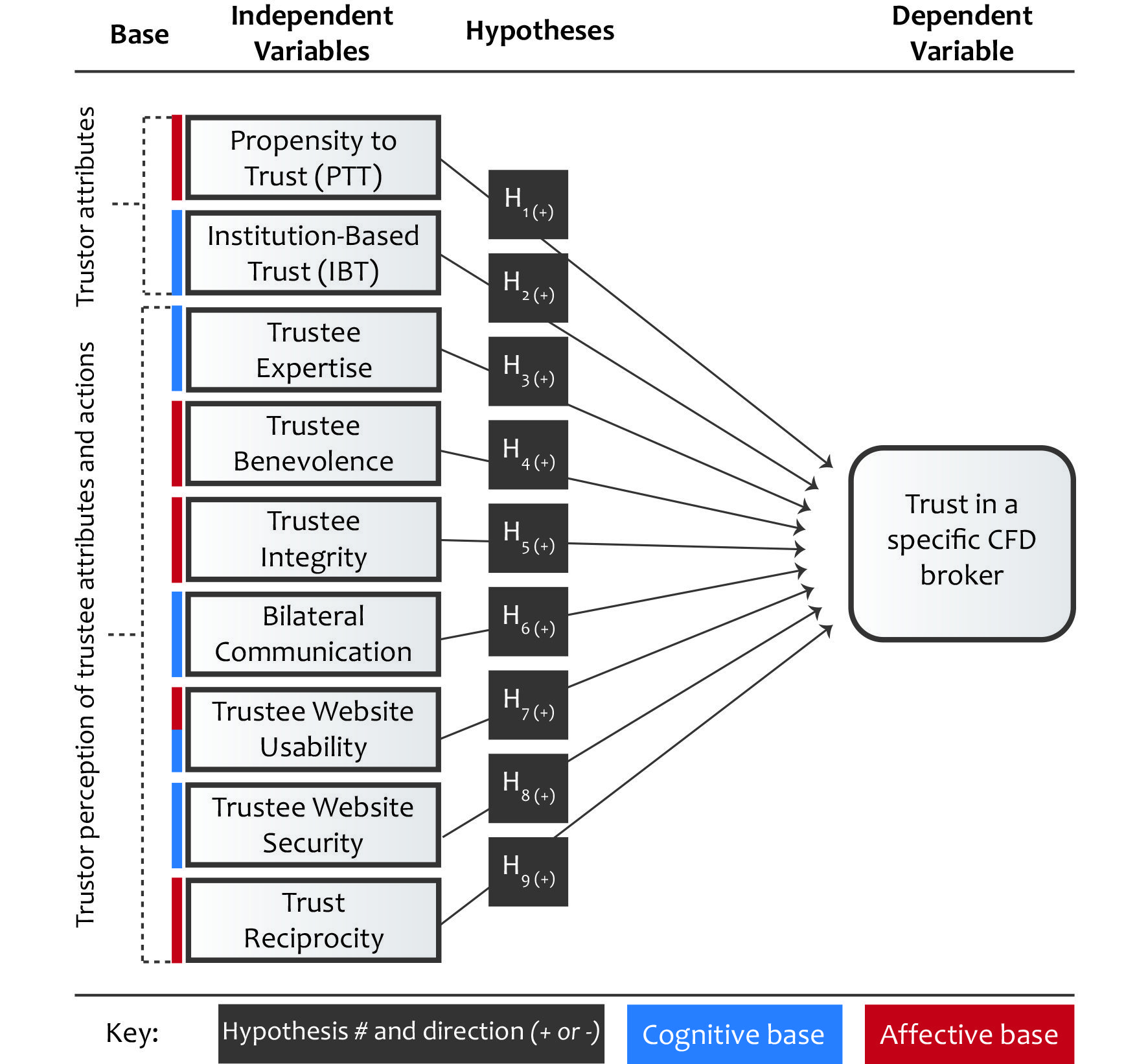
As you can see, conceptual frameworks often make use of different shapes , lines and arrows to visualise the connections and relationships between different components and/or variables. Ultimately, the conceptual framework provides an opportunity for you to make explicit your understanding of how everything is connected . So, be sure to make use of all the visual aids you can – clean design, well-considered colours and concise text are your friends.
Theoretical framework vs conceptual framework
As you can see, the theoretical framework and the conceptual framework are closely related concepts, but they differ in terms of focus and purpose. The theoretical framework is used to lay down a foundation of theory on which your study will be built, whereas the conceptual framework visualises what you anticipate the relationships between concepts, constructs and variables may be, based on your understanding of the existing literature and the specific context and focus of your research. In other words, they’re different tools for different jobs , but they’re neighbours in the toolbox.
Naturally, the theoretical framework and the conceptual framework are not mutually exclusive . In fact, it’s quite likely that you’ll include both in your dissertation or thesis, especially if your research aims involve investigating relationships between variables. Of course, every research project is different and universities differ in terms of their expectations for dissertations and theses, so it’s always a good idea to have a look at past projects to get a feel for what the norms and expectations are at your specific institution.
Want to learn more about research terminology, methods and techniques? Be sure to check out the rest of the Grad Coach blog . Alternatively, if you’re looking for hands-on help, have a look at our private coaching service , where we hold your hand through the research process, step by step.
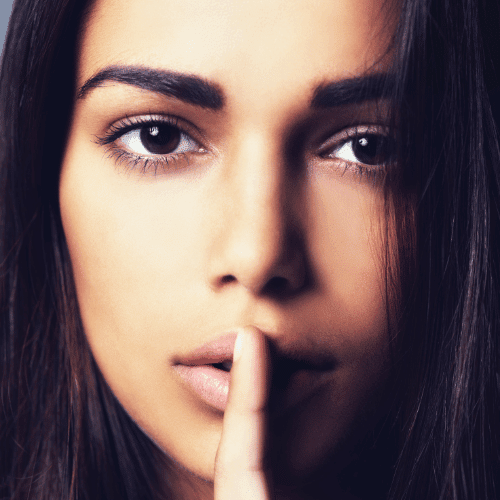
Psst... there’s more!
This post was based on one of our popular Research Bootcamps . If you're working on a research project, you'll definitely want to check this out ...
20 Comments
Thank you for giving a valuable lesson
good thanks!
VERY INSIGHTFUL
thanks for given very interested understand about both theoritical and conceptual framework
I am researching teacher beliefs about inclusive education but not using a theoretical framework just conceptual frame using teacher beliefs, inclusive education and inclusive practices as my concepts
good, fantastic
great! thanks for the clarification. I am planning to use both for my implementation evaluation of EmONC service at primary health care facility level. its theoretical foundation rooted from the principles of implementation science.
This is a good one…now have a better understanding of Theoretical and Conceptual frameworks. Highly grateful
Very educating and fantastic,good to be part of you guys,I appreciate your enlightened concern.
Thanks for shedding light on these two t opics. Much clearer in my head now.
Simple and clear!
The differences between the two topics was well explained, thank you very much!
Thank you great insight
Superb. Thank you so much.
Hello Gradcoach! I’m excited with your fantastic educational videos which mainly focused on all over research process. I’m a student, I kindly ask and need your support. So, if it’s possible please send me the PDF format of all topic provided here, I put my email below, thank you!
I am really grateful I found this website. This is very helpful for an MPA student like myself.
I’m clear with these two terminologies now. Useful information. I appreciate it. Thank you
I’m well inform about these two concepts in research. Thanks
I found this really helpful. It is well explained. Thank you.
very clear and useful. information important at start of research!!
Submit a Comment Cancel reply
Your email address will not be published. Required fields are marked *
Save my name, email, and website in this browser for the next time I comment.
- Print Friendly
Literature Reviews, Theoretical Frameworks, and Conceptual Frameworks: An Introduction for New Biology Education Researchers
Affiliations.
- 1 Department of Mathematics, Social Studies, and Science Education, Mary Frances Early College of Education, University of Georgia, Athens, GA 30602-7124.
- 2 Department of Teaching & Learning, College of Education & Human Ecology, Ohio State University, Columbus, OH 43210.
- 3 Department of Biology, Eastern Washington University, Cheney, WA 99004.
- 4 Department of Biology, Middle Tennessee State University, Murfreesboro, TN 37132.
- PMID: 35759629
- PMCID: PMC9582830
- DOI: 10.1187/cbe.21-05-0134
To frame their work, biology education researchers need to consider the role of literature reviews, theoretical frameworks, and conceptual frameworks as critical elements of the research and writing process. However, these elements can be confusing for scholars new to education research. This Research Methods article is designed to provide an overview of each of these elements and delineate the purpose of each in the educational research process. We describe what biology education researchers should consider as they conduct literature reviews, identify theoretical frameworks, and construct conceptual frameworks. Clarifying these different components of educational research studies can be helpful to new biology education researchers and the biology education research community at large in situating their work in the broader scholarly literature.
Publication types
- Publications
- Research Design
- Research Personnel*
- Review Literature as Topic
Have a language expert improve your writing
Run a free plagiarism check in 10 minutes, automatically generate references for free.
- Knowledge Base
- Dissertation
What is a Theoretical Framework? | A Step-by-Step Guide
Published on 14 February 2020 by Shona McCombes . Revised on 10 October 2022.
A theoretical framework is a foundational review of existing theories that serves as a roadmap for developing the arguments you will use in your own work.
Theories are developed by researchers to explain phenomena, draw connections, and make predictions. In a theoretical framework, you explain the existing theories that support your research, showing that your work is grounded in established ideas.
In other words, your theoretical framework justifies and contextualises your later research, and it’s a crucial first step for your research paper , thesis, or dissertation . A well-rounded theoretical framework sets you up for success later on in your research and writing process.
Instantly correct all language mistakes in your text
Be assured that you'll submit flawless writing. Upload your document to correct all your mistakes.
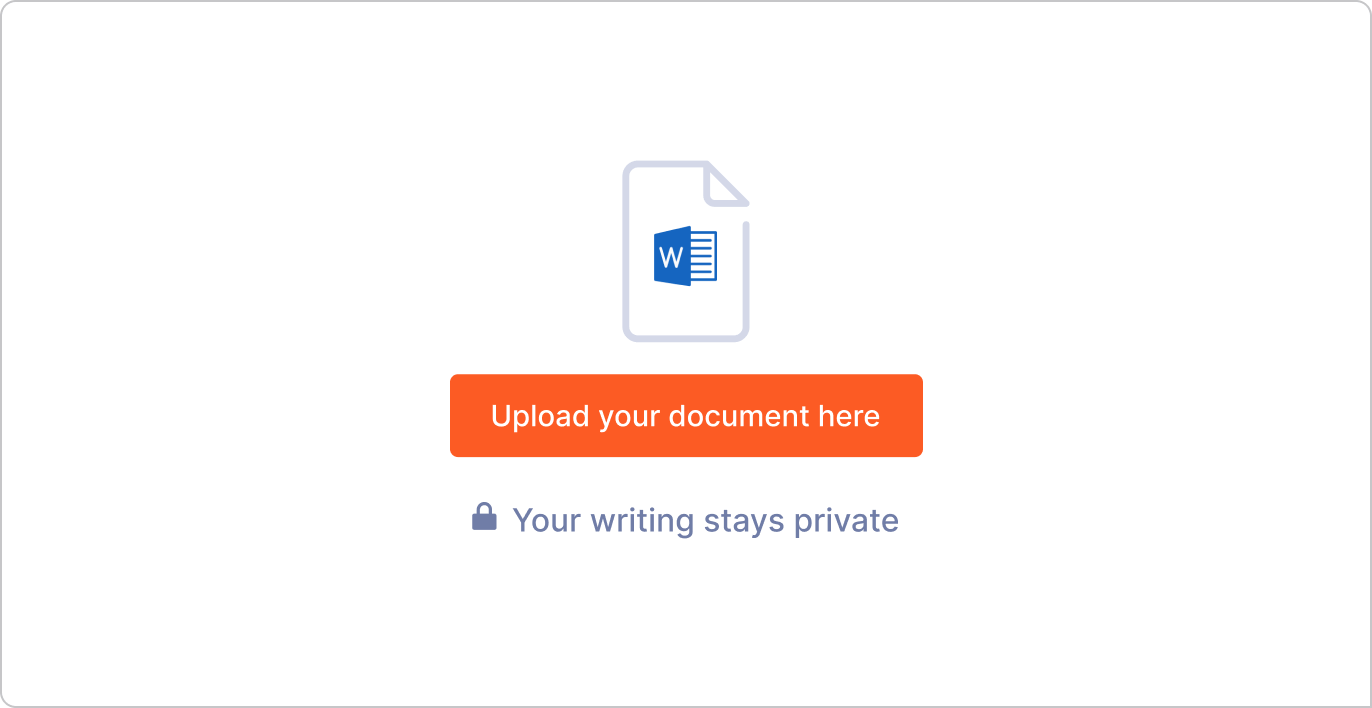
Table of contents
Why do you need a theoretical framework, how to write a theoretical framework, structuring your theoretical framework, example of a theoretical framework, frequently asked questions about theoretical frameworks.
Before you start your own research, it’s crucial to familiarise yourself with the theories and models that other researchers have already developed. Your theoretical framework is your opportunity to present and explain what you’ve learned, situated within your future research topic.
There’s a good chance that many different theories about your topic already exist, especially if the topic is broad. In your theoretical framework, you will evaluate, compare, and select the most relevant ones.
By “framing” your research within a clearly defined field, you make the reader aware of the assumptions that inform your approach, showing the rationale behind your choices for later sections, like methodology and discussion . This part of your dissertation lays the foundations that will support your analysis, helping you interpret your results and make broader generalisations .
- In literature , a scholar using postmodernist literary theory would analyse The Great Gatsby differently than a scholar using Marxist literary theory.
- In psychology , a behaviourist approach to depression would involve different research methods and assumptions than a psychoanalytic approach.
- In economics , wealth inequality would be explained and interpreted differently based on a classical economics approach than based on a Keynesian economics one.
Prevent plagiarism, run a free check.
To create your own theoretical framework, you can follow these three steps:
- Identifying your key concepts
- Evaluating and explaining relevant theories
- Showing how your research fits into existing research
1. Identify your key concepts
The first step is to pick out the key terms from your problem statement and research questions . Concepts often have multiple definitions, so your theoretical framework should also clearly define what you mean by each term.
To investigate this problem, you have identified and plan to focus on the following problem statement, objective, and research questions:
Problem : Many online customers do not return to make subsequent purchases.
Objective : To increase the quantity of return customers.
Research question : How can the satisfaction of company X’s online customers be improved in order to increase the quantity of return customers?
2. Evaluate and explain relevant theories
By conducting a thorough literature review , you can determine how other researchers have defined these key concepts and drawn connections between them. As you write your theoretical framework, your aim is to compare and critically evaluate the approaches that different authors have taken.
After discussing different models and theories, you can establish the definitions that best fit your research and justify why. You can even combine theories from different fields to build your own unique framework if this better suits your topic.
Make sure to at least briefly mention each of the most important theories related to your key concepts. If there is a well-established theory that you don’t want to apply to your own research, explain why it isn’t suitable for your purposes.
3. Show how your research fits into existing research
Apart from summarising and discussing existing theories, your theoretical framework should show how your project will make use of these ideas and take them a step further.
You might aim to do one or more of the following:
- Test whether a theory holds in a specific, previously unexamined context
- Use an existing theory as a basis for interpreting your results
- Critique or challenge a theory
- Combine different theories in a new or unique way
A theoretical framework can sometimes be integrated into a literature review chapter , but it can also be included as its own chapter or section in your dissertation. As a rule of thumb, if your research involves dealing with a lot of complex theories, it’s a good idea to include a separate theoretical framework chapter.
There are no fixed rules for structuring your theoretical framework, but it’s best to double-check with your department or institution to make sure they don’t have any formatting guidelines. The most important thing is to create a clear, logical structure. There are a few ways to do this:
- Draw on your research questions, structuring each section around a question or key concept
- Organise by theory cluster
- Organise by date
As in all other parts of your research paper , thesis, or dissertation , make sure to properly cite your sources to avoid plagiarism .
To get a sense of what this part of your thesis or dissertation might look like, take a look at our full example .
While a theoretical framework describes the theoretical underpinnings of your work based on existing research, a conceptual framework allows you to draw your own conclusions, mapping out the variables you may use in your study and the interplay between them.
A literature review and a theoretical framework are not the same thing and cannot be used interchangeably. While a theoretical framework describes the theoretical underpinnings of your work, a literature review critically evaluates existing research relating to your topic. You’ll likely need both in your dissertation .
A theoretical framework can sometimes be integrated into a literature review chapter , but it can also be included as its own chapter or section in your dissertation . As a rule of thumb, if your research involves dealing with a lot of complex theories, it’s a good idea to include a separate theoretical framework chapter.
A literature review is a survey of scholarly sources (such as books, journal articles, and theses) related to a specific topic or research question .
It is often written as part of a dissertation , thesis, research paper , or proposal .
Cite this Scribbr article
If you want to cite this source, you can copy and paste the citation or click the ‘Cite this Scribbr article’ button to automatically add the citation to our free Reference Generator.
McCombes, S. (2022, October 10). What is a Theoretical Framework? | A Step-by-Step Guide. Scribbr. Retrieved 27 May 2024, from https://www.scribbr.co.uk/thesis-dissertation/the-theoretical-framework/
Is this article helpful?
Shona McCombes
Other students also liked, what is a literature review | guide, template, & examples, how to write a results section | tips & examples, how to write a discussion section | tips & examples.

Home » Education » What is the Difference Between Literature Review and Theoretical Framework
What is the Difference Between Literature Review and Theoretical Framework
The main difference between literature review and theoretical framework is their function. The literature review explores what has already been written about the topic under study in order to highlight a gap, whereas the theoretical framework is the conceptual and analytical approach the researcher is going to take to fill that gap.
Literature review and theoretical framework are two indispensable components of research . Both are equally important for the foundation of a research study.
Key Areas Covered
1. What is Literature Review – Definition, Features 2. What is Theoretical Framework – Definition, Features 3. Difference Between Literature Review and Theoretical Framework – Comparison of Key Differences

What is a Literature Review
A literature review is a vital component of a research study. A literature review is a discussion on the already existing material in the subject area. Thus, this will require a collection of published (in print or online) work concerning the selected research area. In other words, a literature review is a review of the literature in the related subject area. A literature review makes a case for the research study. It analyzes the existing literature in order to identify and highlight a gap in the literature.

Moreover, a good literature review is a critical discussion, displaying the writer’s knowledge of relevant theories and approaches and awareness of contrasting arguments. A literature review should have the following features (Caulley, 1992)
- Compare and contrast different researchers’ views
- Identify areas in which researchers are in disagreement
- Group researchers who have similar conclusions
- Criticize the methodology
- Highlight exemplary studies
- Highlight gaps in research
- Indicate the connection between your study and previous studies
- Indicate how your study will contribute to the literature in general
- Conclude by summarizing what the literature indicates
Furthermore, the structure of a literature review is similar to that of an article or essay . Overall, literature reviews help researchers to evaluate the existing literature, identify a gap in the research area, place their study in the existing research and identify future research.
What is a Theoretical Framework
The theoretical framework is the research component that introduces and describes the theory that explains why the research problem under study exists. It is also the conceptual and analytical approach the researcher is going to take to fill the research gap identified by the literature review. Moreover, it is the structure that holds the structure of the research theory.
The researcher may not easily find the theoretical framework within the literature. Therefore, he or she may have to go through many research studies and course readings for theories and models relevant to the research problem under investigation. In addition, the theory must be selected based on its relevance, ease of application, and explanatory power.
Difference Between Literature Review and Theoretical Framework
A literature review is a critical evaluation of the existing published work in a selected research area, while a theoretical framework is a component in research that introduces and describes the theory behind the research problem.
Moreover, the literature review explores what has already been written about the topic under investigation in order to highlight a gap, whereas the theoretical framework is the conceptual and analytical approach the researcher is going to take to fill that gap. Therefore, a literature review is backwards-looking while theory framework is forward-looking.
In conclusion, the main difference between literature review and theoretical framework is their function. The literature review explores what has already been written about the topic under study in order to highlight a gap, whereas the theoretical framework is the conceptual and analytical approach the researcher is going to take to fill that gap.
1. Caulley, D. N. “Writing a critical review of the literature.” La Trobe University: Bundoora (1992). 2. “ Organizing Your Social Sciences Research Paper: Theoretical Framework .” Research Guide.
Image Courtesy:
About the Author: Hasa
Hasanthi is a seasoned content writer and editor with over 8 years of experience. Armed with a BA degree in English and a knack for digital marketing, she explores her passions for literature, history, culture, and food through her engaging and informative writing.
You May Also Like These
Leave a reply cancel reply.
Ohio State nav bar
The Ohio State University
- BuckeyeLink
- Find People
- Search Ohio State
Literature Review, Theoretical Review or Conceptual Review?
I was researching and refreshing what I learned about literature reviews and research methodology in the 6625 ESLTECH class and came across this post in ResearchGate:
While I was interested in finding out how to conduct a lit review, and what methodologies fit with my “Begin With the End in mind” framework, I remembered that I was actually conducting a conceptual analysis of the Hackathon through two instruments: the opportunistic interviews and my observations. Applying Backward Design as research methodology has been used in the biological sciences. It is called Backward Design for Education Research (BDER) and basically it instructs how to apply teaching-as-research (based on the Scholarship of Teaching and Learning(SoTL) model) to see what works in “providing evidence-based pedagogy and a deeper understanding of causal mechanisms for the broader education community.” (Jenkins, Bailey, Kummer & Weber, 2017). My approach was more like “Backward Market Research,” which consists of eight steps that ultimately resemble Wiggins and McTighe’s Backward Design for curriculum development. The key to backward market research lies in identifying the desired outcome (i.e., what data would answer the question you are asking) before embarking on the project …” (Jenkins, Bailey, Kummer & Weber, 2017). My question is broad – what insights come out of the Hackathon – and the results are formative – data reports generate more questions and identify the need to code certain responses, or to add a new question next year (as with the multitude of comments about Judging from 2017) – but a body or knowledge is being formed.
Click through the PLOS taxonomy to find articles in your field.
For more information about PLOS Subject Areas, click here .
Loading metrics
Open Access
Peer-reviewed
Research Article
Development of a conceptual framework for defining trial efficiency
Roles Conceptualization, Data curation, Formal analysis, Funding acquisition, Investigation, Methodology, Project administration, Resources, Software, Visualization, Writing – original draft, Writing – review & editing
* E-mail: [email protected]
Affiliation Wolfson Institute of Population Health, Queen Mary University of London, London, England, United Kingdom

Roles Conceptualization, Methodology, Supervision, Validation
Affiliation Usher Institute, Asthma UK Centre for Applied Research, The University of Edinburgh, Edinburgh, Scotland, United Kingdom
- Charis Xuan Xie,
- Anna De Simoni,
- Sandra Eldridge,
- Hilary Pinnock,
- Clare Relton
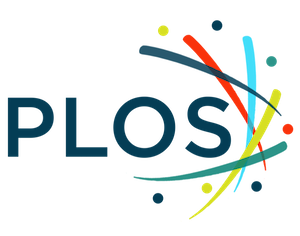
- Published: May 23, 2024
- https://doi.org/10.1371/journal.pone.0304187
- Peer Review
- Reader Comments
Globally, there is a growing focus on efficient trials, yet numerous interpretations have emerged, suggesting a significant heterogeneity in understanding “efficiency” within the trial context. Therefore in this study, we aimed to dissect the multifaceted nature of trial efficiency by establishing a comprehensive conceptual framework for its definition.
To collate diverse perspectives regarding trial efficiency and to achieve consensus on a conceptual framework for defining trial efficiency.
From July 2022 to July 2023, we undertook a literature review to identify various terms that have been used to define trial efficiency. We then conducted a modified e-Delphi study, comprising an exploratory open round and a subsequent scoring round to refine and validate the identified items. We recruited a wide range of experts in the global trial community including trialists, funders, sponsors, journal editors and members of the public. Consensus was defined as items rated “without disagreement”, measured by the inter-percentile range adjusted for symmetry through the UCLA/RAND approach.
Seventy-eight studies were identified from a literature review, from which we extracted nine terms related to trial efficiency. We then used review findings as exemplars in the Delphi open round. Forty-nine international experts were recruited to the e-Delphi panel. Open round responses resulted in the refinement of the initial nine terms, which were consequently included in the scoring round. We obtained consensus on all nine items: 1) four constructs that collectively define trial efficiency containing scientific efficiency, operational efficiency, statistical efficiency and economic efficiency; and 2) five essential building blocks for efficient trial comprising trial design, trial process, infrastructure, superstructure, and stakeholders.
Conclusions
This is the first attempt to dissect the concept of trial efficiency into theoretical constructs. Having an agreed definition will allow better trial implementation and facilitate effective communication and decision-making across stakeholders. We also identified essential building blocks that are the cornerstones of an efficient trial. In this pursuit of understanding, we are not only unravelling the complexities of trial efficiency but also laying the groundwork for evaluating the efficiency of an individual trial or a trial system in the future.
Citation: Xie CX, De Simoni A, Eldridge S, Pinnock H, Relton C (2024) Development of a conceptual framework for defining trial efficiency. PLoS ONE 19(5): e0304187. https://doi.org/10.1371/journal.pone.0304187
Editor: Germain Honvo, University of Liege: Universite de Liege, BELGIUM
Received: December 4, 2023; Accepted: May 7, 2024; Published: May 23, 2024
Copyright: © 2024 Xie et al. This is an open access article distributed under the terms of the Creative Commons Attribution License , which permits unrestricted use, distribution, and reproduction in any medium, provided the original author and source are credited.
Data Availability: All relevant data are within the manuscript and its supporting information files.
Funding: CX is funded by the Wellcome Trust (224863/Z/21/Z). URL: https://wellcome.org/ . For the purpose of Open Access, the author has applied a CC BY public copyright licence to any Author Accepted Manuscript version arising from this submission. The funder does not play any role in the study design, data collection and analysis, decision to publish, and preparation of the manuscript.
Competing interests: The authors have declared that no competing interests exist.
Introduction
Worldwide, trial efficiency is a longstanding priority for the pharmaceutical industry [ 1 ], academia and funding bodies [ 2 , 3 ]. In 2004 in the US, the Clinical Trials Working Group of the National Cancer Advisory Board set the goal of improving operational efficiency to facilitate timely and cost-effective trial execution [ 4 ]. In the UK, the National Institute for Health and Care Research offers additional funding to support clinical trial units to advance the design and execution of efficient, innovative research, aiming to provide robust evidence to inform clinical practice and policy [ 5 ]. A recent article in The Lancet Global Health examined the challenges faced by current clinical trial research in low- and middle-income countries, and argued that efficient trials are needed to address research questions related to the increasing burden of non-communicable diseases in a timely and affordable way [ 6 ].
Currently, the concept of efficiency in healthcare trials has been used to refer to accelerated ethical approval [ 6 ], addressing multiple complex questions in a single trial [ 7 ] and with a minimised sample size [ 6 ], trials conducted with shorter duration [ 7 , 8 ], lower costs [ 9 ], and reduced resource requirements [ 10 ]. In addition, existing literature has discussed trial efficiency in terms of operational efficiency [ 11 – 13 ], scientific efficiency [ 11 ], statistical efficiency [ 13 , 14 ], and economic efficiency [ 15 ]. There is significant heterogeneity as to what is meant by efficiency in the context of trials, which may hinder effective communication and decision-making between stakeholders, and compromise the comparability of studies. Therefore, in this study we aimed to develop a conceptual framework for defining trial efficiency and to achieve expert consensus on the framework constructs.
Study design
We undertook a literature review to identify items that define and comprise trial efficiency. We then conducted an e-Delphi study to refine and validate those items and to achieve consensus on the constructs and the building blocks of trial efficiency. The ethics approval was obtained from Queen Mary University of London research ethics committee (QMERC22.316). This study follows the Guidance on Conducting and Reporting Delphi Studies (CREDES) [ 16 ].
Literature review for generating items
Our goal in the literature review was to collate existing discussions on efficiency in the context of trials, including definitions and attributes described as constituting an efficient trial. As discussions specifically focused on this subject are scarce, we included a broad range of study types, such as full trial papers or protocols, editorials, and opinion pieces that discussed trial efficiency. We considered all types of human trials evaluating medical, surgical, or behavioural interventions, including efficacy trials, effectiveness trials, and implementation trials. The search was limited to English-language articles, and there was no restriction on publication dates. To carry out the review, we searched MEDLINE (via Ovid) database, for terms such as ’trial’ and ’efficien*’ in article titles and keywords. As ’efficiency’ is a common word in literature, we searched for these two keywords only within article titles (rather than within the abstracts) ensuring the results’ relevance to the discussion of trial efficiency. The detailed inclusion and exclusion criteria are listed in S1 Table .
Panel selection and recruitment.
The aim was to recruit a diverse panel of experts from the trial community, encompassing a range of roles and perspectives. This included international researchers identified through the literature review, colleagues who are part of professional trial networks such as UK trial managers’ network, representatives from funding bodies, journal editors, and members of the public who have been involved in trials. Purposive sampling and snowball sampling methods were then used to identify additional participants. We approached those participants with known contact details by individual emails generated through Clinvivo [ 17 ], while for colleagues within professional networks, where we didn’t have individual contact details, we sent a generic recruitment email to the network’s mailing list. Recruitment began in November 2022 and continued until March 2023. Written informed consent was obtained online through the Clinvivo Delphi system.
Data collection.
We opted for two rounds of data collection because consensus was achieved by the end of the second round. These rounds were preceded by a pilot round to test the feasibility of the open round.
Pilot test . We pilot tested the feasibility of the open round questionnaire amongst colleagues with diverse experience in trial design and conduct at the Pragmatic Clinical Trial Unit of Queen Mary University of London. This provided valuable feedback on the clarity of the questions, the appropriateness of the response options, and the overall structure of the questionnaire. Based on the feedback received during the pilot testing, we made revisions and refinements to the questionnaire to enhance its usability.
Open round . In the open round, we invited panellists to share their thoughts on 1) their understanding of trial efficiency and 2) the most efficient or inefficient aspects they have encountered in the trials they have conducted or in which they have participated. These questions were designed as free-text to encourage detailed, narrative responses. To gain insights into the participants’ backgrounds, we collected information on countries of residence, and roles within the trials (see S1 File for the questionnaire). This open round allowed us to gather diverse viewpoints and experiences related to trial efficiency which contributed to the development of a comprehensive set of items for ranking in the subsequent round. The data collection for this round took place over four weeks, with reminder emails sent to participants after the second and third weeks.
Scoring round . Panel members from open round were emailed a link to the second questionnaire. They were asked to rate the importance of the proposed items on a scale of 1 to 9 (1: not at all important to 9: critically important). At the end of each question, there was a free text space for any comments they wished to share. The scoring round data collection spanned four weeks with weekly reminders to participants.
Data analysis and consensus.
Descriptive statistics were used to analyse quantitative demographics and thematic analysis was used to summarise free text responses from both Delphi rounds. To assess disagreement and appropriateness, we used the Research ANd Development (RAND)/ University of California Los Angeles (UCLA) appropriateness method [ 18 ]. It involves calculating the median score, the inter-percentile range (IPR) (30th and 70th), and the inter-percentile range adjusted for symmetry (IPRAS), for each item being rated. Consensus was defined as items rated “without disagreement”, measured by the IPRAS.
Patient and public involvement.
In this study, members of the public (n = 4) (including two who had participated in trials) were invited to share their thoughts, participate in the ranking process, provided with the outcomes of each round upon completion. They were considered experts due to their lived experience and offered £30 voucher as a compensation for their time.
Delphi participants
Out of 106 international experts approached, and 4 e-mails sent to network mailing lists, forty-nine participants responded to the open round (United Kingdom (n = 37), United States (n = 7), Canada (n = 2), Australia (n = 1), Ireland (n = 1), and Kenya (n = 1)). The panel included a diversity of roles including statisticians (n = 17), trial managers (n = 12), principal investigators (n = 7), funders (n = 4), journal editors (n = 3), member of the public (n = 4), data managers (n = 3), site staff (n = 2), sponsors (n = 2), researchers (n = 2), monitors (n = 2), ethicist (n = 1), clinician (n = 1), CTU manager (n = 1), trial support officer (n = 1), and trial methodologist (n = 1). Many participants had more than one role. See Fig 1 .
- PPT PowerPoint slide
- PNG larger image
- TIFF original image
https://doi.org/10.1371/journal.pone.0304187.g001
Literature review
We included a total of 78 studies for data analysis (see S1 Fig ), including 6 (8%) reviews, 15 (19%) perspectives or commentaries, 1(1%) interview, 2 (3%) case studies, 2 (3%) surveys and 3 (4%) randomised trials, and 49 (63%) methodologies describing new trial designs. Only 8(10%) studies had explicitly defined or explained what ‘efficiency’ meant in the context of their trials (see S2 Table for details). We categorised discussions of efficiency from the literature into nine key items: 1)scientific efficiency [ 11 , 19 , 20 ], 2)operational efficiency [ 11 , 20 , 21 ], 3)statistical efficiency [ 14 , 22 – 24 ] and 4)economic efficiency [ 15 , 25 ], 5)efficiency in trial designs [ 7 , 8 , 23 , 26 – 45 ], 6)trial conduct [ 11 , 20 , 21 , 46 – 66 ], and other aspects such as 7)improving efficiency using information technologies and mobile apps [ 53 , 67 – 70 ]; 8)involving the public and stakeholders [ 20 , 71 ]; and 9)efficient trial reviews and regulatory approvals [ 28 , 66 , 72 – 74 ]. (see Table 1 for details). These results were included as exemplars in the Delphi open round questionnaire. The detailed description of the literature review has previously been made available [ 75 ] to ensure full transparency and to facilitate open scholarly dialogue.
https://doi.org/10.1371/journal.pone.0304187.t001
When asked to define trial efficiency, some participants referred to definitions from the literature review, while other cited similar definitions tailored to their trial context. When asked about the most efficient/inefficient facets of trial efficiency, the responses resonated closely with the findings from our literature review ( Fig 2 ). Specifically, trial design emerged as the facet most frequently cited as enhancing efficiency, whereas data collection was often highlighted as the element that most impeded efficiency.
The x-axis represents the frequency of responses.
https://doi.org/10.1371/journal.pone.0304187.g002
By incorporating findings from this round, we further refined the nine items identified from the literature review and divided them into two groups: 1) theoretical and abstract constructs: scientific efficiency, operational efficiency, statistical efficiency, and economic efficiency; 2) empirical and fundamental building blocks: trial design (including endpoints selection, statistical analysis plan, protocol development, etc.), trial process (including recruitment and retention, data collection and analysis, trial administration, etc.), superstructure (including regulatory approvals, funding application etc.), infrastructure (including financial and physical resources such as cost, information technologies, routine healthcare data, etc.), and stakeholders. This resulted in a total of nine items for rating in the scoring round (see Table 2 ).
https://doi.org/10.1371/journal.pone.0304187.t002
Scoring round and consensus
Forty participants responded (82%) to the scoring round and there was no disagreement on any items ( Table 2 ). We also conducted sub-analyses by five role groups: (1) funders and sponsors (n = 6); (2) statisticians (n = 13); (3) trial managers (n = 10); (4) principal investigators (n = 6); and (5) PPIs (n = 3). Group membership was not mutually exclusive. Stratified results showed widespread agreement that the items were appropriate, with the exception of one of the building blocks–superstructure. The funders and sponsors group disagreed this item was appropriate ( S3 Table ). As a result, no new items were added but we slightly modified the explanation of each proposed item, in line with free-text comments made by the participants.
Theoretical constructs of trial efficiency: Revised definitions incorporating Delphi comments
Scientific efficiency..
Some participants were confused by the provided definition ( Box 1 . quote 1); while some suggested expanding the definition with the inclusion of feasibility and implementation ( Box 1 . quotes 2–3). As such, we refined the definition as the balance of methodological rigour, relevance of the research question, and feasibility of trial design. It prioritises effective use of resources, including data, to minimise research waste, considers the alignment of design and statistical strategies, and underscores the importance of the study’s practical impact on stakeholders and delivering value to end-users.
Operational efficiency.
Some comments suggested the definition should be expanded to consider operation feasibility, bureaucracy, and ongoing evaluation ( Box 1 . quotes 4–6). Therefore, we modified operational efficiency as the optimal management, organisation, execution, and continuous evaluation of trial processes and procedures. It emphasises operational feasibility (such as ensuring there are enough workforce, managing delays, and working effectively with third-party providers), reducing unnecessary bureaucracy and duplication, and continuously assessing the trial for potential improvements.
Statistical efficiency.
The initial definition ( Table 1 ) was expanded based on the participants’ comments (Box1. quotes 7–8), as the application of design and analytical methods that result in more accurate estimates of treatment effects or other parameters of interest. This includes considerations of minimising the amount of data to be collected, accounting for missing data, and managing sources of bias or confounding; its focus is specifically on maximising the accuracy and reliability of results given the data collected.
Economic efficiency.
We increased the clarity of the initial definition according to scoring round feedback ( Box 1 .quotes 9–10): the optimal use of resources in the trial design, implementation and analysis, to ensure immediate and long-term cost-effectiveness of the trial. This focus on value ensures that resources are utilised to their fullest extent without compromising the quality of the research. It emphasises on the cost-effectiveness of conducting the trial.
Box 1. Scoring round exemplar free-text comments related to the construct definitions
Scientific efficiency.
- Quote 1 : “Not sure rigour equates to efficiency” (Participant n. 17, principal trial investigator)
- Quote 2 : “Feasibility of trial design needs to be included here. You could have the perfect trial design but no participants or high withdrawals and lack of site engagement.” (Participant n.2, trial manager)
- Quote 3 : “This may also need to include how important the findings will be to service users and the public and whether there are ways they are expected to be implemented in practice.” (Participant n.28, trial support officer)
Operational efficiency
- Quote 4 : “I’d make particular focus on the bureaucracy ‐ endless paperwork.” (Participant n.3, funder)
- Quote 5 : "Feasibility of operational efficiency. You may have participants and engaged sites but you need operational feasibility to align." (Participant n.2, trial manager)
- Quote 6 : “Would like to see reference to the ongoing assessment of a trial in the descriptor.” (Participant n.39, trial manager)
Statistical efficiency
- Quote 7 : “and accounting for missing data, and sources of bias or confounding” (Participant n.19, principal trial investigator)
- Quote 8 : “Also needs to encompass other aspects of analysis, e.g., health economics.” (Participant n.14, statistician)
Economic efficiency
- Quote 9 : “Allowing for the concept of data sharing beyond the life of the study” (Participant n.37, sponsor)
- Quote 10 : “Need to be clear that this is (I presume) related to the costs of delivering the trial and not the cost of the intervention (i.e. health economic analysis).” (Participant n.26, statistician)
Essential building blocks comprising an efficient trial
Overall, there was a strong consensus on the building blocks; the free-text comments did not suggest significant alterations, but recommended adding some details within each building block. Trial design concerns the planning and organisation of a trial, which may include the trial methodologies, research questions, sample size, interventions, control group, endpoints and outcomes; document development such as funding application; as well as planning feasibility and pilot studies. The trial process involves the setup, execution, and closeout phases of a trial (see S2 Fig for details). Stakeholders are the critical human factor, they are individuals or groups with an interest or concern in the design, execution, and outcomes of a trial. They could be trial participants (e.g. patients, practitioners, health system leaders, public health organisations, etc.), trialists (e.g. investigators, researchers, trial managers, statisticians, etc), funders, sponsors, trial sites and their staff, regulatory authorities, healthcare and clinical practitioners, the scientific community (researchers, academics, and clinicians interested in the trial’s outcomes and its implications for future research) and the general public (the broader population who may ultimately benefit from the knowledge generated by the clinical trial). Infrastructure is the underlying framework, systems, and resources required to design, implement, manage, and analyse a trial, such as resources (human, financial, physical), information systems and technologies, and healthcare data. Superstructure serves as the overarching structure of a trial, including laws, policy, and governance.
With these, we developed a Trial Efficiency Pentagon ( Fig 3 ) to place the five building blocks and to illustrate the multiple connections among them ‐ improvements in one block may potentially lead to trade-offs in one or more other blocks.
https://doi.org/10.1371/journal.pone.0304187.g003
The final conceptual framework for defining trial efficiency
Fig 4 represents the finalised framework. The term trial efficiency is complex and multifaceted, encompassing four conceptual constructs with five essential building blocks.
The outer blue circle outlines theoretical constructs of trial efficiency: Scientific Efficiency, Statistical Efficiency, Operational Efficiency and Economic Efficiency. At its core, the inner pentagon outlines the empirical building blocks: Superstructure, Stakeholders, Infrastructure, Trial Process, and Trial Design. The cyclical arrows indicate the necessity for a balanced consideration of each building block within each construct to optimise trial efficiency.
https://doi.org/10.1371/journal.pone.0304187.g004
Main findings
Consensus was achieved on the four constructs that together define trial efficiency: scientific efficiency, operational efficiency, statistical efficiency and economic efficiency; and the five essential building blocks for considering an efficient trial: trial design, trial process, infrastructure, superstructure, and stakeholder.
The conceptual constructs, empirical building blocks, and interrelationships
Overall there was no disagreement over the constructs that conceptually define trial efficiency. However, some concerns were raised regarding potential overlaps, between scientific efficiency and statistical efficiency, and between operational efficiency and economic efficiency ( S4 Table ). These four constructs share some common elements. However, they are conceptually distinct and each construct brings unique aspects to the concept of trial efficiency. Scientific efficiency, for instance, focuses primarily on the methodological rigour [ 77 ] and feasibility of trial design, while statistical efficiency is concerned with achieving the most accurate results possible with the smallest amount of data collected [ 78 ]. The overlap lies in the fact that both aim to optimize the quality and validity of the trial’s findings, yet their distinct focus underlines their separate roles within the overarching construct of trial efficiency. Similarly, while operational and economic efficiency both aim to make the best use of resources [ 11 ], they do so in different ways and in different contexts. Operational efficiency is about the effective management and organization of trial processes and procedures [ 11 , 13 ], while economic efficiency involves optimizing resource use in relation to the cost of delivering the trial. By maintaining these conceptually distinct constructs, we were able to capture the broad spectrum of abstract factors that define trial efficiency, thus offering a nuanced theoretical framework for its comprehension.
The proposed building blocks create a foundation for the formulation of an efficient trial. In the Delphi scoring round, there was strong consensus regarding the significance of these building blocks, with an average median score of 8.4 on a 1–9 scale. However, some participants perceived hierarchy among the building blocks, suggesting that some (e.g., trial design and process) hold more importance than others. This was reflected in the literature review and responses in the Delphi open round, where certain building blocks ‐ such as trial design ‐ were more frequently discussed as critical determinants of trial efficiency. Despite these observations, we propose that all five building blocks have equal importance and they mutually contribute to the overall efficiency of the trial. These foundational elements are also interconnected, for instance, even the most rigorous and feasible trial design is contingent upon the availability of suitable infrastructure support and requires inputs from stakeholders. Therefore, we advocate for a balanced view where no single building block takes precedence in the trial efficiency pentagon.
There is a layered connection between the constructs and the building blocks: the constructs were conceptualised to provide a broad, overarching view of efficiency within healthcare trials. In contrast, the building blocks were identified as the essential, practical components that operationalise efficiency in real-world settings. In addition to this relationship, we suggest that for a comprehensive understanding, each efficiency construct takes into account all five building blocks. For instance, while it may seem apparent that scientific efficiency is closely linked with trial design, focusing on how the study is conceptualised to ensure methodological soundness; it also intersects with stakeholder involvement, where patient and public engagement can improve the trial design and thus the trial outcomes’ relevance and applicability.
Implications
According to the results from the literature review, few studies explicitly defined efficiency in the context of trials and no effort has been made to develop a unified and agreed definition for trial efficiency. Linguistically, ‘efficiency’ is defined as “the production of the desired effects or results with minimum waste of time, effort, or skill” [ 79 ]. This definition shares similarities with those from the literature ( S2 Table ), wherein the outstanding characteristic corresponds to the balance between the inputs (e.g. resources) and the outputs (e.g. the objectives of the trial). Nevertheless, these interpretations are often narrowly tailored. In this study we hoped to offer a holistic view that captures the nuances and complex aspects of trial efficiency and which may benefit policymakers, funders, and researchers in making informed decisions, leading to improved trial implementation and patient care. Enhancing efficiency was emphasised in the UK Department of Health and Social Care’s 2022–2025 strategic plan for clinical research [ 80 ]. As of the drafting of this paper, the U.S. Food and Drug Administration is announcing the updated recommendations for good clinical practices advocating for greater efficiency in trials by modernising both design and conduct [ 81 ]. Therefore, it is evident that our study is timely, positioning the urgency of comprehensively understanding trial efficiency.
Strengths and limitations
Drawing on both literature review and expert opinion, our study followed a rigorous approach to develop a conceptual framework of trial efficiency. We included a wide range of experts in trial communities including members of the public, enhancing the comprehensiveness and richness of our study. Nevertheless, nine participants did not respond to the scoring round, which could have introduced potential biases in reaching a consensus or perhaps missed subtle distinctions regarding the significance of certain trial elements. However, given the diverse range of participants who did engage, coupled with the triangulation with existing literature, this non-response is not expected to significantly impact the overall validity and comprehensiveness of our Delphi findings.
While we have sought to delineate each construct and building block distinctly, we acknowledge the potential for different interpretations of qualitative data. The interplay between the identified themes is likely to be more intricate, reflecting the complex nature of trial efficiency. Future research could delve deeper into this interplay to unravel the connections.
The ’trial efficiency pentagon’, emerging as a novel concept from this study, is a promising tool for assessing trial efficiency (proactively and retrospectively). For example, it could be developed to support group discussions and/or calibrated as an evaluation instrument to measure the efficiency of a trial. However, it is limited by lacking robust theoretical foundation. To elucidate, while we’ve pieced together insights and perspectives to shape the pentagon, we have not rooted it in any established theory or conceptual model. This could mean that certain fundamental aspects of trial efficiency might be overlooked or not holistically represented. In the future, we aspire to hone the pentagon into an evidence-based, theory-informed tool and we welcome insights from our readers and remain open to potential collaborations to its further development.
This is the first attempt to dissect the concept of trial efficiency into theoretical constructs. In this pursuit of understanding, we are not only unravelling the complexities of trial efficiency but also laying the groundwork for evaluating the efficiency of an individual trial or a trial system in the future.
Supporting information
S1 fig. prisma flowchart..
https://doi.org/10.1371/journal.pone.0304187.s001
S2 Fig. Trial process in general.
https://doi.org/10.1371/journal.pone.0304187.s002
S1 Table. Literature review inclusion and exclusion criteria.
https://doi.org/10.1371/journal.pone.0304187.s003
S2 Table. Efficiency definitions/explanations in the literature.
https://doi.org/10.1371/journal.pone.0304187.s004
S3 Table. Scoring round stratified results.
https://doi.org/10.1371/journal.pone.0304187.s005
S4 Table. Scoring round exemplar quotes related to potential overlaps among the four constructs.
https://doi.org/10.1371/journal.pone.0304187.s006
S1 File. Open round questionnaire.
https://doi.org/10.1371/journal.pone.0304187.s007
Acknowledgments
We thank Prof. Shaun Treweek for his insightful discussion on trial efficiency, which has largely inspired this work. We thank Ann Thomson, Senior Trial Manager at Queen Mary University of London’s Pragmatic Clinical Trials Unit, for her valuable discussions and insights into the trial process. Our thanks also go to the Health Research Board ‐ Trials Methodology Research Network for their assistance in promoting our Delphi study through their email newsletter. We acknowledge the support of the UKCRC Registered CTU Network. The views expressed are those of the author(s) and not of the UKCRC or its members. We are immensely thankful to all participants of the Delphi study rounds for their invaluable contributions and willingness to share their expertise. We have received consent to acknowledge the following participants by name (with no particular order): Monica Taljaard, Lelia Duley, Sarah Markham, Deb Smith, Catey Bunce, Stephen Brealey, Steff Lewis, Laura Miller, Jacqueline French, Fiona Hogarth, Gail Holland, Nikki Totton, Nick Kisengese, Joanne Haviland, Matthew Burns, Richard Hooper, Claire Ayling, Catherine Arundel, Ines Rombach, Seonaidh Cotton, Paula Kareclas. Lastly, we appreciate the reviewer’s comments, which have been instrumental in enhancing the development of the conceptual framework.
- 1. Schulz G. Increasing the Efficiency of Clinical Trials: Tanner Pharma Group; 2023 [Available from: https://tannerpharma.com/increasing-the-efficiency-of-clinical-trials/ .
- 2. CENTRE TF. [Available from: https://www.trialforge.org/trial-forge-centres/ .
- 3. Medicine JH. Improving the Efficiency of Clinical Trials. [Available from: https://clinicalconnection.hopkinsmedicine.org/news/improving-the-efficiency-of-clinical-trials .
- 4. GROUP CTW. Restructuring the National Cancer Clinical Trials Enterprise. National Cancer Institute; 2005.
- 5. Research NIfHaC. Annual Efficient Studies funding calls for CTU projects 2019 [Available from: https://www.nihr.ac.uk/documents/ad-hoc-funding-calls-for-ctu-projects/20141 .
- View Article
- PubMed/NCBI
- Google Scholar
- 17. CLINVIVO. Clinvivo Limited 2015.
- 18. Fitch K BS, Aguilar MD, Burnand B, LaCalle JR, Lazaro P, van het Loo M, McDonnell J, Vader JP, Kahan JP. RAND/UCLA appropriateness method user’s manual. Monica, CA: RAND corporation; 2000.
- 22. Eshima N. Efficiency of Statistical Hypothesis Test Procedures. Statistical Data Analysis and Entropy. Singapore: Springer Singapore; 2020. p. 141–65.
- 78. Efficiency (statistics) Wikipedia [Available from: https://en.wikipedia.org/wiki/Efficiency_(statistics) .
- 79. Medical Dictionary for the Health Professions and Nursing. 2012. efficiency.
- 80. Care DoHaS. The Future of Clinical Research Delivery: 2022 to 2025 implementation plan 2022 [Available from: https://www.gov.uk/government/publications/the-future-of-uk-clinical-research-delivery-2022-to-2025-implementation-plan/the-future-of-clinical-research-delivery-2022-to-2025-implementation-plan .
- 81. Administration tFaD. ICH HARMONISED GUIDELINE GOOD CLINICAL PRACTICE (GCP) E6(R3) 2023.
Synthesizing three decades of digital servitization: a systematic literature review and conceptual framework proposal
- Theoretical article
- Open access
- Published: 08 May 2024
Cite this article
You have full access to this open access article
- Pedro E. Minaya ORCID: orcid.org/0000-0002-1179-9378 1 ,
- Lucía Avella ORCID: orcid.org/0000-0003-2598-7318 2 &
- Juan A. Trespalacios ORCID: orcid.org/0000-0003-0658-4038 2
This study, through a systematic literature review spanning 1990 to 2023, interrogates how servitization, and nowadays digital servitization, enhances manufacturing competitiveness. It introduces the DASOBI (Drivers, Actors, Strategies, Obstacles, Benefits, and Impact) framework for navigating the digital servitization transition, emphasizing strategic adaptability and technological alignment. Analysis of 157 articles reveals a significant increase in research, highlighting digital servitization’s role in competitive enhancement and customer engagement. The DASOBI framework offers manufacturers a novel approach for managing this transition, marking a unique contribution by distilling extensive literature into actionable insights for both theory and practice in the evolving field of digital servitization.
Similar content being viewed by others
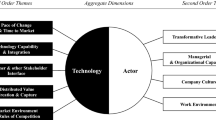
Digital transformation: a review, synthesis and opportunities for future research
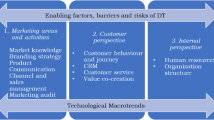
How digital technologies reshape marketing: evidence from a qualitative investigation
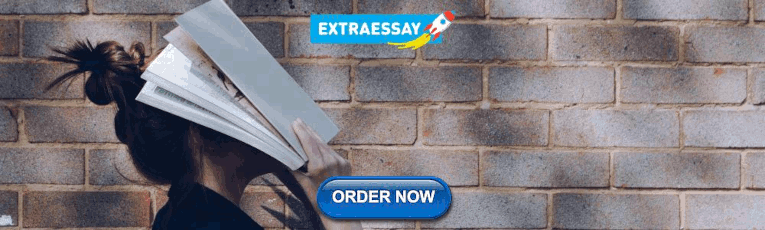
Research Methodology: An Introduction
Avoid common mistakes on your manuscript.
1 Introduction
1.1 context, motivation, and research topic.
In today’s dynamic manufacturing sector, companies are increasingly acknowledging the importance of complementing their product offerings with value-added services. This strategic shift, known as servitization—and more specifically digital servitization—marks a fundamental turn in the contemporary business paradigm. This transformation involves not only a shift from a product-centric to a service-centric focus but also a deep integration of advanced digital technologies. While considerable research has been conducted on individual aspects of servitization, a comprehensive analysis that encompasses all essential facets of this phenomenon, from its motivations to its final outcomes, remains relatively unexplored. This research proposal aims to develop a holistic conceptual framework that synthesizes and extends existing knowledge, thereby providing a more complete and nuanced understanding of digital servitization. This exhaustive review examines this evolving business model, highlighting its key benefits and challenges, its intersection with digital technologies, and its theoretical and practical implications.
The foundational premise, supported by Bustinza et al. ( 2015 ), suggests that manufacturing companies can achieve higher returns by offering services in conjunction with their products, a claim echoed in seminal works by Davies et al. ( 2007 ), Johnstone et al. ( 2009 ), Martín-Peña et al. ( 2017 ), and Leoni and Aria ( 2021 ). These services, ranging from maintenance and support to more sophisticated and customized solutions, expand the revenue streams of these firms. In this context, the contributions of Baines et al. ( 2007 ) and Neely et al. ( 2011 ) are pivotal, as they underscore how transitioning to a service-oriented market is driving strategic transformations in manufacturing firms, emphasizing value creation and differentiation in increasingly competitive markets (Brady et al. 2005 ).
The current market dynamics almost make this shift imperative. As noted by Sandström et al. ( 2008 ) and Tukker ( 2015 ), companies that limit their offerings to products alone face formidable challenges in maintaining profitability, driving them toward business model innovation that incorporates services into their product portfolios, as discussed in the literature by Gebauer and Fleisch ( 2007 ), Visnjic and Van Looy ( 2013 ), and Díaz-Garrido et al. ( 2018 ).
Servitization requires effective coordination among multiple stakeholders. Alghisi and Saccani ( 2015 ) address the critical importance of internal and external alignment, while Ayala et al. ( 2019 ) highlight the essential role of service providers in the successful adoption of servitization strategies. Moreover, Baines et al. ( 2011 ) and Lightfoot et al. ( 2013 ) explore how manufacturing firms can effectively integrate services into their product portfolio, emphasizing the importance of a strategically well-planned approach.
Beyond being a customer-facing strategy, the internal benefits are equally compelling. As delineated by Kamp and Alcalde ( 2014 ), servitization facilitates process optimization and extends the lifespan of machinery. These advantages are further enhanced with the incorporation of digital technologies, particularly in the era of Industry 4.0 (Kamp and Perry 2017 ). This digital servitization, explored in studies by Lee et al. ( 2014 ), Kans and Ingwald ( 2016 ), and Paiola and Gebauer ( 2020 ), offers an enhanced layer of value, encompassing innovative goods and services.
Researchers such as Favoretto et al. ( 2022 ) and Rabetino et al. ( 2023 ) have elucidated how technological advancements act as catalysts for developing differentiated products and services, thereby enhancing competitiveness (Müller et al. 2021 ). This leads to the formulation of hybrid business models, termed Product-Service Systems (PSS), which are economically, socially, and environmentally sustainable. This PSS model provides a more holistic solution, meeting specific customer needs beyond just providing functional products (Barquet et al. 2013 ).
In this process, a demand for specific organizational and technological capabilities is identified. Coreynen et al. ( 2017 ) and Schroeder et al. ( 2022 ) have pinpointed the importance of organizational structure and technological capabilities, particularly in the context of digitalization, as key factors for a successful transition to digital servitization (Parida et al. 2014 ; Kanninen et al. 2017 ).
Implementing servitization, as highlighted by Mathieu ( 2001 ) and Yu and Sung ( 2023 ), is not without its challenges, ranging from internal organizational resistance to external factors, such as customer reluctance. Brax ( 2005 ) and Benedettini et al. ( 2015 ) provide a comprehensive analysis of these risks, emphasizing the importance of effective management to navigate potential obstacles in achieving successful servitization (Windahl and Lakemond 2006 ; Pessôa and Becker 2017 ). The process demands a well-structured and strategically informed approach, incorporating both business and customer perspectives. Proper implementation of servitization can lead to substantial benefits, as demonstrated by Baines et al. ( 2009b , 2017 ) and Wang et al. ( 2018 ), highlighting its potential for long-term value creation (Brady et al. 2005 ).
The phenomenon of servitization, particularly in its digital form, has emerged as a prominent area of study, characterized by its complexity and multidimensionality. Academic literature has thoroughly explored this concept, from underlying motivations to implementation strategies, examining both inherent challenges and potential benefits (Raddats et al. 2016 ; Rabetino et al. 2021 ).
1.2 Research gap
Despite the extensive body of knowledge on servitization amassed by previous studies, there remains a discernible gap characterized by fragmented examinations rather than a consolidated analytical approach. This study pinpoints a need for a unified framework that can effectively guide servitization strategies, addressing this lacuna as a pivotal area for forthcoming research (Calabrese et al. 2019 ; Kohtamäki et al. 2020a ). The advent of the digital era has precipitated transformative shifts, underscoring the servitization concept—the transition from purely selling products to offering integrated product-service solutions. Nevertheless, the interaction between servitization and digital technologies, a realm referred to as digital servitization, remains a relatively uncharted territory. This area lacks a systematic and thorough review spanning the last three decades. This omission highlights the imperative need for an in-depth understanding of how servitization has evolved and the essential development of a framework to adeptly navigate the intricacies involved in implementing these strategies effectively.
1.3 Methodology proposed
To address the identified research gap, our study employs a comprehensive, multi-phased methodology structured as follows: Initially, we conduct an in-depth examination of the literature on servitization and digital servitization. This phase aims to develop an integrative theoretical framework that captures the evolution of servitization over the past three decades, emphasizing the shift toward digital service delivery within the manufacturing sector. Subsequently, the study undertakes a systematic literature review to classify the existing body of work. This review specifically focuses on selecting pertinent studies that encompass both traditional and digital servitization, aiming to identify trends, patterns, and existing research gaps. Following the review, we perform a detailed analysis of the selected articles to explore how various aspects of servitization and digital servitization interact and influence each other. In the final phase, we synthesize the findings from the study to deepen the conceptual understanding of the servitization phenomenon, including its digital components. This synthesis will provide valuable insights into effectively managing the transition toward servitization and digital servitization, highlighting its practical applicability in a business context.
1.4 Expected contributions
The primary goal of this research is to construct an integrative framework that captures the evolution, current state, and future trajectory of servitization and digital servitization. This framework will delineate both the theoretical underpinnings and practical ramifications of servitization, illuminating the challenges and opportunities that have surfaced. Particularly, it will explore the transformative influence of Industry 4.0 technologies—such as the Internet of Things, Big Data analytics, and Artificial Intelligence—on traditional servitization models, steering them toward more advanced digital practices. This examination is crucial for understanding how digital technologies can enhance the competitiveness and value proposition of manufacturing firms engaged in servitization.
The overarching aim of this study is to deepen the comprehension of servitization by exploring its interplay with digitalization, thus broadening its theoretical and managerial relevance. The research intends to offer an integrated perspective that not only advances the academic discourse in this field but also aids manufacturing companies in adeptly navigating the complexities of servitization and digital servitization. Furthermore, this review will articulate a roadmap for manufacturers considering this transition, conceptually enriching a domain that, despite its increasing importance, remains underexplored in scholarly research. By highlighting the enduring interest in adopting servitization correctly and underscoring the necessity for a unified theoretical framework, this study responds to calls for theoretical consolidation and a more comprehensive research agenda (Pettigrew 1988 ; Pye and Pettigrew 2005 ).
In summary, our proposed study aims to provide a detailed analysis that integrates insights from various studies into a cohesive narrative, with a particular focus on the servitization and digital servitization processes within the manufacturing sector. This synthesis will significantly contribute to both academic knowledge and practical applications, emphasizing the complex and evolving nature of servitization in manufacturing, and marking a key conclusion of this thorough examination.
2 Research aims
This study is dedicated to a comprehensive analysis of the servitization phenomenon and its progression toward digital servitization within the manufacturing sector, meticulously examining the most significant research from the past 30 years. The aim is to understand the development and various applications of servitization, along with the challenges and obstacles it entails. The study seeks to identify the motivations driving companies toward servitization, examine the various actors involved in the process and their interplay, and explore the strategies necessary for successful implementation. Furthermore, the organizational and technological capabilities required for transitioning to servitization will be analyzed, as well as the associated risks and challenges, including both internal and external hurdles that companies must overcome to reap the potential benefits of servitization. This analysis is guided by key research in the field (Zhang and Banerji 2017 ; Khanra et al. 2021 ) offering a comprehensive perspective on this significant shift in business dynamics within the manufacturing sector.
Essentially, this study seeks to answer the main research question: To what extent do servitization and digital servitization provide benefits that contribute to enhancing a company’s competitiveness? Alongside this primary question, the study intends to address the following aspects related to the development of servitization and digital servitization:
RQ1. Implementation of a digital servitization strategy. How it should be affected by the company’s business environment? How it should be the co-creation process in an international context? Which new knowledge and new skills need to be developed to be implemented correctly? Which benefits can be obtained by implementing the digital enablers of Industry 4.0? Which changes could it involve in the internal structure of the business? Which changes could it involve in the company’s business environment (relations with suppliers or strategic partners)? How could it face the challenges and obstacles that arise during the transition process?
RQ2. Benefits of developing an effective digital servitization strategy. How it provides greater value to the customer? How can product customization be optimized? How it encourages access to new markets? How it promotes gaining new customers? How it allows innovation in ideas or business models? How it allows the development of goods with novel services? How it effectively allows greater returns to be achieved? How it improves competitiveness?
The focus of this study is not only on analyzing servitization as a strategic shift for manufacturing companies but also on exploring how the integration of digital technologies can enrich and complicate this process. Additionally, the aim is to synthesize existing knowledge to provide a broader and more nuanced understanding of digital servitization, highlighting its key advantages, challenges, and intersection with digital technologies.
Four stages were established for this systematic literature review (Tranfield et al. 2003 ), one for each of the four phases outlined in the first section.
This collection focuses on four fields of research: business administration, marketing, operations management, and administration of services. The studies from the two main databases were examined: Web of Science and Scopus, as they are considered reference sources for the topic being analyzed. Once the information was screened, the most-cited studies were selected, which formed the basis for the present study.
3.1 Review process
In conducting a systematic literature review to gain a profound understanding of servitization and digital servitization within the manufacturing sector, our approach integrated multiple rigorous methodologies (Thomé et al. 2016 ). Initially, following the method proposed by Hertzberg and Rudner ( 1999 ), we conducted a meticulous keyword search in the Web of Science and Scopus databases, aiming to identify pertinent literature using terms like “servitization,” “digital servitization,” and their variants. This was instrumental in capturing the subject’s breadth and depth, allowing for the creation of search strings using the Boolean connector OR. The search strings were incorporated in titles, abstracts, and/or keywords, adhering to the time span of 1990 to 2023 in major databases, thus fulfilling the guidelines set by Tranfield et al. ( 2003 ) for inclusion criteria.
To further refine the search and ensure a robust database, we applied additional parameters and restrictions post-establishing the primary search strings for both databases. We limited our search to open access and hybrid gold journals, focusing on high-quality, readily available research outputs. Additionally, we set a citation threshold to include articles with significant field impact, thereby ensuring the inclusion of seminal works and recent influential studies. This strategy was pivotal in developing a comprehensive, relevant collection of literature, ensuring the inclusion of the most pertinent works in the field of digital servitization.
The approach was enhanced by strictly adhering to three key inclusion criteria: (a) considering publications from 1990 to 2023, to ensure a contemporary and comprehensive review, (b) prioritizing articles from prestigious academic journals within the relevant study areas, thus ensuring source quality and relevance, and (c) selecting articles focusing explicitly on key aspects of servitization and digital servitization. This approach, aligned with the study’s objectives and research questions, ensures a holistic and detailed understanding of the phenomenon, accurately reflecting the dynamics and transformations in the manufacturing sector.
The present study aimed to answer the research question and the various related questions. This was done via the PRISMA method (Preferred Reporting Items for Systemic Reviews and Meta-Analyses). The selection criteria produced 647 articles (from Web of Science) and 630 articles (from Scopus). Once identified, the abstracts of each article were read to screen and select only those in line with the fourth study phase: to help properly understand the concept, how it is managed, and how it is applied. 157 articles were ultimately identified that met all of the inclusion criteria. Figure 1 outlines the PRISMA method used.
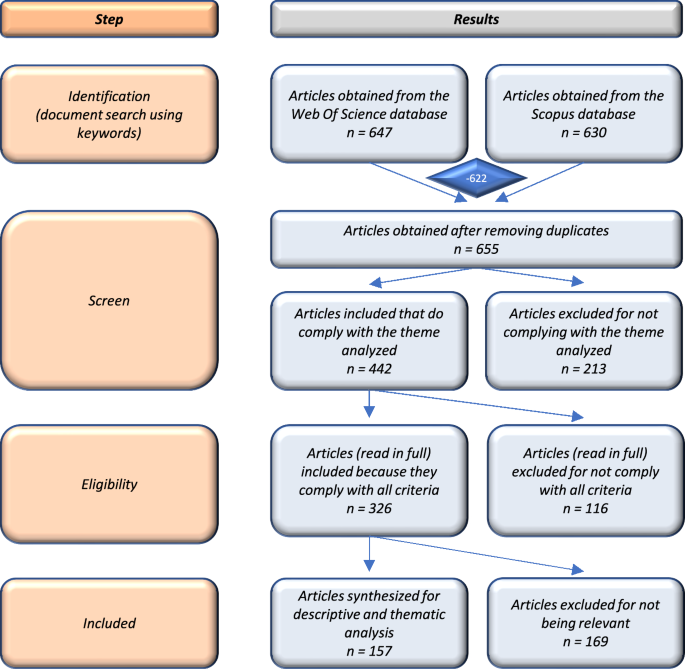
Source: Authors’ own work from Web of Science and Scopus databases
Flow diagram, based on the PRISMA Method, for the selection of relevant documents for the systematic literature review.
3.2 Descriptive analysis
Figure 2 offers an analytical synthesis of the publication trends within the realms of servitization and digital servitization over a span of more than three decades, utilizing data harvested from the Web of Science and Scopus databases. The blue bars across all three charts articulate the volume of literature pertaining to servitization, encompassing its theoretical underpinnings, industry applications, and cross-disciplinary studies. This scholarly corpus embodies the foundational and evolutionary aspects of servitization as a strategic paradigm shift in manufacturing and service industries.
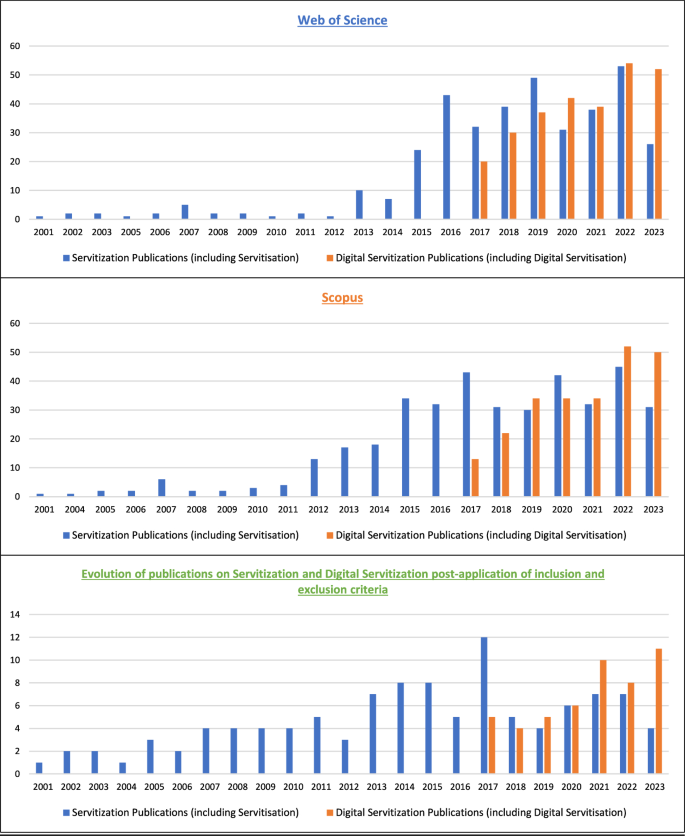
Source: Web of Science and Scopus databases and authors’ own work
Evolution of publications on Servitization and Digital Servitization (1990–2023).
In parallel, the orange bars specifically chart the trajectory of literature focused on digital servitization. This subset of research delves into the intricacies of embedding digital technologies within traditional servitization frameworks. It illuminates the burgeoning intersection of digital innovation and service strategies, reflecting a vibrant and rapidly advancing frontier of research.
The upward trend of both blue and orange bars in the separate charts for Web of Science and Scopus indicates a robust increase in scholarly output. This not only testifies to the growing academic and practical significance of servitization concepts but also their digital counterparts, which are pivotal in today’s technology-driven marketplaces.
The application of inclusion and exclusion criteria to the study of servitization and digital servitization clarifies the focus of academic research, emphasizing the most relevant and impactful studies in these areas. This refined approach highlights the critical and emerging conversations shaping the future of manufacturing industries through servitization and its digital augmentation. The graph reflects the scholarly community’s increasing investment in understanding these concepts and their application, suggesting a dual focus: the persistent importance of servitization in strengthening the interplay between manufacturing and services, and the transformative potential of digital technologies within this framework. Serving both as a retrospective and a forecast, the visualization indicates key areas for future research that promise to advance industrial practices and academic thought.
Regarding the countries in which the identified studies have been carried out, the visual data presented in Fig. 3 captures a comprehensive view of the global research output on servitization and digital servitization from 1990 to 2023, as indexed by the Web of Science and Scopus databases and further refined by the application of inclusion and exclusion criteria. The top section, shown in blue, delineates the Web of Science data, indicating a prominent concentration of scholarly activity within certain countries, possibly linked to their robust research infrastructures, funding provisions, or strong manufacturing sectors that are conducive to studies in servitization.
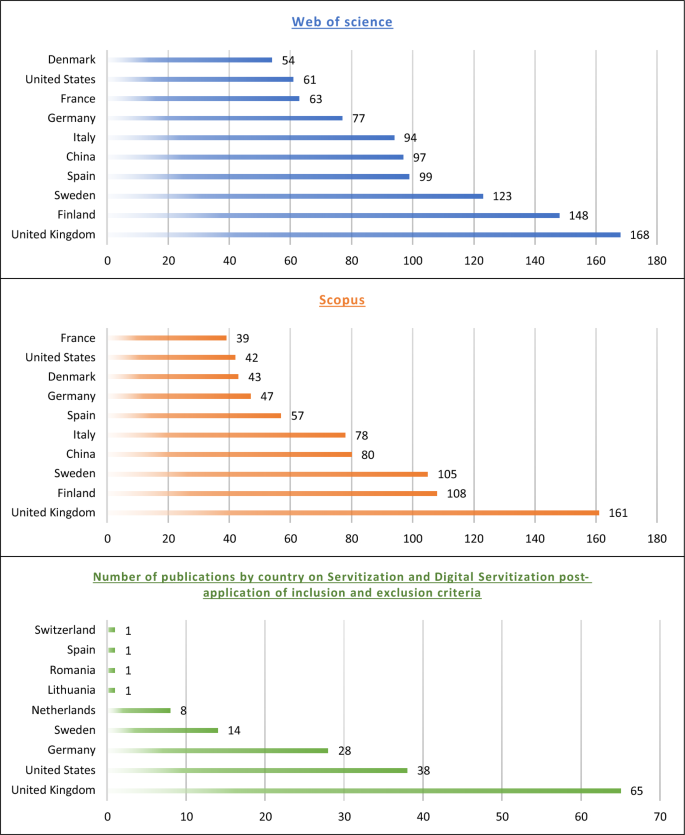
Source: Web of Science and Scopus databases
Number of publications by country on Servitization and Digital Servitization (1990–2023).
The middle section, in orange, portrays the Scopus data, revealing a parallel distribution pattern to that of the Web of Science but with slight variances that may be indicative of the different regional research emphases or variations in the databases’ indexing methodologies. The countries with the highest volume of publications are recognized as potential centers of excellence and innovation in the field of servitization.
The bottom section of the graph, in green, represents the distilled essence of this academic output following the application of the inclusion and exclusion criteria. This section emphasizes the refined and concentrated scholarly work that aligns more closely with the specific nuances and requirements of servitization and digital servitization research as defined by the study. It presents a narrower but more focused spectrum of publications, suggesting a curated body of knowledge that serves as a critical resource for understanding the current state and future directions of servitization in the manufacturing sector.
Together, these three segments of Fig. 3 not only illustrate the quantitative aspects of the research output but also underscore the qualitative focus and depth of scholarly exploration achieved through rigorous selection. This tripartite analysis offers a lens through which to view the international dissemination and development of knowledge in servitization and digital servitization, highlighting established leaders in the field as well as regions with the potential for increased research activity, international collaboration, and contribution to the servitization discourse.
In Fig. 4 , the Web of Science data (represented by the blue graph) lists Oscar Bustinza as the author with the highest number of publications, closely followed by Marko Kohtamäki and Vinit Parida. In contrast, the Scopus data (illustrated by the orange graph) also positions Vinit Parida prominently, yet Marko Kohtamäki’s publication count is lower than that reported in the Web of Science, presenting a notable discrepancy.
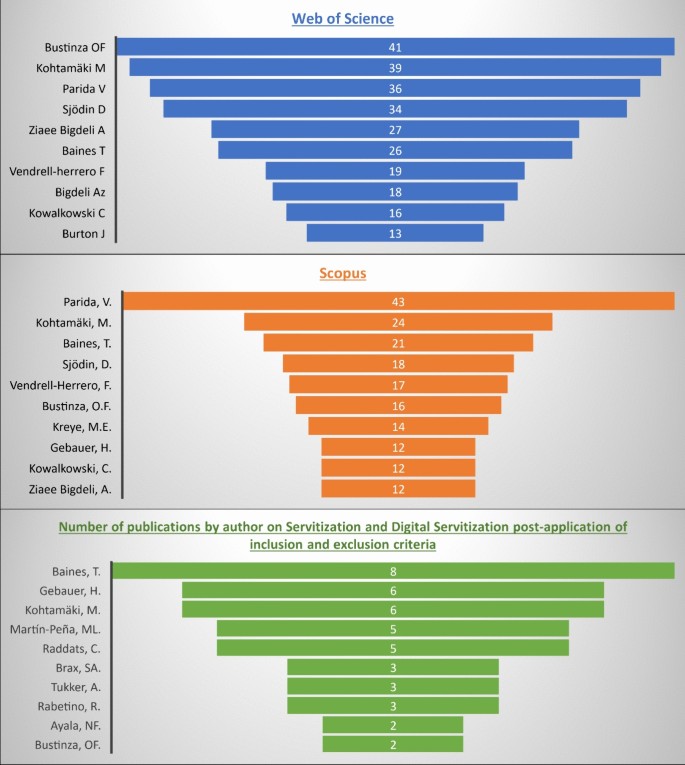
Number of publications by author on Servitization and Digital Servitization (1990–2023).
When the inclusion and exclusion criteria are applied (as shown in the green graph), there is a decrease in the number of publications, which aligns with expectations, given that these criteria aim to omit publications failing to meet the predetermined standards of quality and relevance. Following this filtration, Tim Baines emerges as the author with the most publications, indicating the significant relevance of his research work to the focused aims of this systematic literature review. Consequently, the filtration process underscores those authors whose contributions are particularly central or foundational to the field.
The comparison across the three graphs demonstrates the influence of database selection and methodological rigor on the perceived prominence of authors within the academic community. This analysis goes beyond merely highlighting the leading figures in servitization research; it underscores the importance of thorough evaluation in literature reviews to identify research of substantial impact.
Thus, the filtration process distinctly recognizes authors whose contributions are considered pivotal to the discipline.
Figure 5 provides a succinct overview of journal publication volumes on servitization and digital servitization from 1990 to 2023, based on data from Web of Science and Scopus databases. Prior to applying inclusion and exclusion criteria, the journals listed in the Web of Science (blue) and Scopus (orange) indicate a diverse quantity of publications.
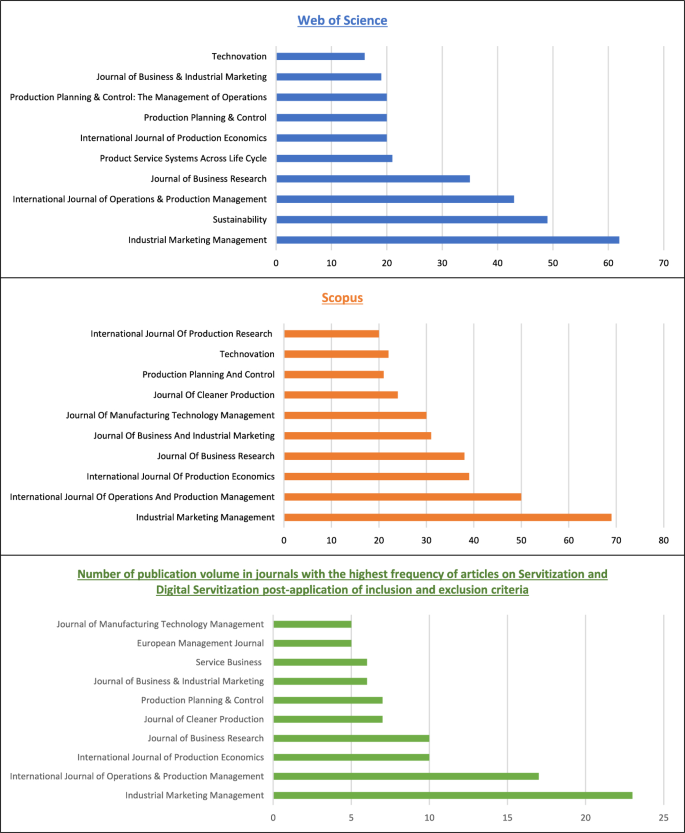
Number of publication volume in journals with the highest frequency of articles on Servitization and Digital Servitization (1990–2023).
Post-application (green), the data are refined to highlight the top ten journals that are most aligned with the research criteria. It is noteworthy that the application of these criteria significantly alters the landscape of the considered literature. Some journals that initially (in the Web of Science or Scopus databases) had a high volume of publications appear to have fewer articles meeting the requirements, which may reflect on the specificity and relevance of their contributions to the field.
The graphic serves as an insightful metric of the research landscape, indicating not only the journals that are most prolific in the domain but also the robustness of articles surviving rigorous scholarly scrutiny. This visual representation is integral to the academic discourse, as it not only informs researchers of the core journals within the field but also reflects the evolving standards and focal areas within the literature on servitization and digital servitization.
The descriptive analyses included in this section serve as a pivotal foundation for the authors’ elaboration, shedding light on the trajectory of academic inquiry into servitization and digital servitization. It encapsulates the dual analysis conducted using the Web of Science and Scopus databases and the meticulous selection process leading to the corpus of papers employed in the systematic literature review. The synthesis of these findings offers valuable insights into the progression of research in this domain, indicating a maturing yet dynamically expanding field of study.
3.3 Classification process
Upon identifying studies that met the established selection criteria, a thorough examination of each was conducted to categorize them according to specific themes. These encompassed the motivations driving companies toward servitization, namely the reasons why manufacturers transition from producing solely goods to combining these with services, including the anticipated benefits of such a transformation. The various actors involved in the servitization process and the nature of their interactions were scrutinized, as well as the strategies necessary for successful implementation, which entailed identifying potential needs for external partners, commonly service providers (Martínez et al. 2010 ; Bastl et al. 2012 ; Spring and Araujo 2013 ; Ziaee et al. 2018 ). The types of services commonly offered were analyzed, categorized as basic, intermediate, or advanced, along with the specific servitization strategies adopted by the companies. Furthermore, the study delved into the organizational and technological capabilities required for an effective transition to servitization (Momeni et al. 2023 ), as well as the potential risks and challenges arising in these transition processes, including both internal and external obstacles that must be overcome to fully capitalize on the potential benefits of servitization (Raddats et al. 2017 ; Reim et al. 2019 ; Minaya et al. 2023 ).
4 Results: theoretical background
4.1 from servitization to digital servitization.
The concept of servitization, which has significantly evolved over the years, has achieved solid recognition in both the academic and industrial spheres. Initially defined by Levitt ( 1972 ) and Vandermerwe and Rada ( 1988 ) as the process of adding value through services (Johnson and Mena 2008 ; Baines et al. 2011 ; Lindman et al. 2016 ; Ruiz-Martín and Díaz-Garrido 2021 ), servitization has expanded to encompass multiple strategic objectives, such as competitive advantage (Baines et al. 2009a ; Raddats et al. 2019 ), financial goals, and marketing benefits (Khanra et al. 2021 ).
The shift toward servitization entails a redefinition of traditional business models, focusing on innovation (Sandström et al. 2008 ; Martín-Peña et al. 2018 ; Qi et al. 2020 ; Xing et al. 2023 ), and transforming manufacturers into service-centric companies (Cusumano 2008 ; Santamaría et al. 2012 ; Mosch et al. 2021 ). In this regard, manufacturing companies are fundamentally reorienting their business models and operational strategies to include value-added services (Gebauer and Kowalkowski 2012 ; Hyun and Kim 2021 ). Baines and Lightfoot ( 2013 ) and Luoto et al. ( 2017 ) highlight the widespread changes this implies in management, marketing, and operations. The change is so substantial that over 50% of a company’s activities and personnel can be involved in providing these newly implemented services, as indicated by multiple studies cited by Martín-Peña and Ziaee ( 2016 ). This is because research has shown that servitization not only adds value but also increases profitability with relatively low asset investments (Davies et al. 2007 ; Kharlamov and Parry 2021 ).
The types of services offered range from basic to advanced (Gebauer et al. 2013 ; Kindström and Kowalkowski 2014 ; Sousa and Da Silveira 2017 ), with advanced services contributing to greater profitability (Eggert et al. 2014 ) and generating higher customer satisfaction (Mont 2002 ; Ostrom et al. 2010 ), leading to improved competitive positioning (Oliva and Kallenberg 2003 ; Durugbo 2014 ). Baines et al. ( 2011 ) argue that servitization involves creating distinctive and sustainable capabilities (Raddats 2011 ; Kimita et al. 2022 ), requiring not just the provision of goods, but also the innovation of value through added services (Tukker and Tischner 2006 ; García Martín et al. 2019 ; Zighan and Abualqumboz 2022 ), enabling companies to maintain their competitive edge (Tuli et al. 2007 ; Brax and Jonsson 2009 ; Nordin and Kowalkowski 2010 ).
While the goal of servitization is to enrich product offerings and drive competitiveness (Neely et al. 2011 ; Gaiardelli et al. 2014 ; Benedettini et al. 2015 ), companies must avoid the “service paradox,” where the focus on new services undermines existing production capabilities (Gebauer et al. 2005 ; Hyun and Kim 2021 ). To this end, various researchers advocate for a comprehensive analysis covering customer needs, pricing strategies, delivery infrastructure, and organizational change (Manzini and Vezzoli 2003 ; Kohtamäki and Partanen 2016 ; Ziaee et al. 2017 ). In summary, moving away from product-centric thinking and engaging in product and servitization logic.
In this context, Santamaría et al. ( 2012 ) and Rabetino et al. ( 2017 ) underscore three fundamental considerations for a successful servitization strategy: the content, process, and context of organizational change. This involves determining what to change, how to change, and why the change is necessary (Kreye et al. 2015 ).
The complexity of servitization also demands internal and external alignments within companies (Gebauer 2008 ; Alghisi and Saccani 2015 ; Kohtamäki et al. 2019a ; Zhang et al. 2023 ). Internally, this involves harmonizing the organization’s strategy with the service portfolio and aligning this strategy throughout the organization (Oliva and Kallenberg 2003 ; Yan et al. 2020 ). Externally, alignment extends to the service provider network and customer expectations (Ceci and Masini 2011 ; Paiola et al. 2013 ). Similarly, servitization applies in B2B and B2C domains, serving as a differentiator and pathway to future alliances and customer loyalty (Baines et al. 2017 ; Pombo and Franco 2023 ).
On the other hand, technological advancements act as significant facilitators in the transition toward servitization, particularly the digital elements of Industry 4.0 (Dalenogare et al. 2018 ; Paschou et al. 2020 ; Opazo-Basáez et al. 2021 ; Tian et al. 2022 ; Le-Dain et al. 2023 ). This involves both internal and external organizational changes, focusing on disruptive innovations and addressing legal and financial challenges (Bustinza et al. 2018 ; Tronvoll et al. 2020 ; Kolagar et al. 2022 ), leading to what is known as digital servitization.
Digital servitization represents the integration of enabling technologies from Industry 4.0 into the servitization process, generating additional benefits and creating value for the customer (Ibarra et al. 2018 ; Grandinetti et al. 2020 ; Ciasullo et al. 2021 ; Bettiol et al. 2022 ). This digital transformation expands the scope of traditional services, allowing for greater customization and efficiency (Frank et al. 2019 ; Chen et al. 2021 ).
Digitalization facilitates data collection and analysis, improving decision-making, and enabling more predictive and proactive services (Lee et al. 2014 ; Chen et al. 2022a ; Rakic et al. 2022 ). Moreover, data-based digital capabilities are fundamental for the success of digital servitization, as they enhance both product support services and customer support services (Chen et al. 2023 ).
Digital servitization also promotes value co-creation and collaboration among manufacturers, suppliers, and customers, optimizing service delivery and strengthening relationships (Coreynen et al. 2017 ; Vendrell-Herrero et al. 2017 ; Kohtamäki et al. 2020b ; Sjödin et al. 2020 ). The business models of digital servitization are also influenced by Industry 4.0 technologies, such as Internet of Things and Big Data, enabling the development of more integrated and customer-centric solutions (Naik et al. 2020 ; Bortoluzzi et al. 2022 ; Minaya et al. 2023 ).
Furthermore, an integral aspect of the servitization landscape, especially in the digital era, is the evolution of Product-Service Systems (PSS). PSS represents a strategic approach that shifts the focus from selling products to offering a combination of products and services designed to fulfill specific customer needs more efficiently (Tukker and Tischner 2006 ; Baines et al. 2017 ). This transition to PSS reflects a broader industry movement toward sustainable and customer-centric business models, where the value proposition extends beyond the physical product to include personalized services. The advent of Industry 4.0 technologies has further propelled this evolution, leading to the development of Smart PSS. Smart PSS integrates digital technologies, such as the Internet of Things, Big Data, and Artificial Intelligence to enhance service delivery, improve customer experience and enable new forms of value creation (Chowdhury et al. 2018 ; Bortoluzzi et al. 2022 ). The adoption of these advanced technologies within PSS frameworks represents a significant leap in how companies’ approach servitization, allowing for greater customization, efficiency, and proactive engagement with customers. Therefore, understanding the role and impact of PSS, particularly Smart PSS, is crucial for comprehending the full scope of digital servitization and its implications for future business strategies.
4.2 Integrating smart product-service systems (smart PSS) into digital servitization: evolution, challenges, and opportunities
Product-Service Systems (PSS) epitomize an evolution in business models, integrating goods and services to fulfill customer needs sustainably and effectively (Galbraith 2002 ; Gebauer et al. 2011 ; Oliveira et al. 2015 ; Haase et al. 2017 ; Gaiardelli et al. 2021 ; Zhou and Song 2021 ). Tukker ( 2004 ) categorizes PSS into product oriented, use oriented, and result oriented, with each type offering distinct benefits, such as improved profit margins and differentiation from competitors (Tukker and Tischner 2006 ; Reim et al. 2015 ; Baines et al. 2017 ; Rabetino et al. 2017 ). Service-oriented PSS prioritize personalized customer experiences, requiring greater customer involvement (Matthyssens and Vandenbempt 2010 ; Cusumano et al. 2014 ; Zighan and Abualqumboz 2022 ).
The advent of Industry 4.0 technologies has given rise to Smart PSS, enhancing traditional PSS frameworks with digital capabilities and aligning with digital servitization’s goals to maximize customer value and competitive advantage (Chowdhury et al. 2018 ; Zheng et al. 2019 ; Wang et al. 2021 ; Bortoluzzi et al. 2022 ; Chen et al. 2023 ). Smart PSS incorporate Internet of Things, Big Data, and Artificial Intelligence to offer tailored services and predictive maintenance, thus improving product reliability and customer experience. However, transitioning to Smart PSS necessitates overcoming internal challenges, such as developing digital capabilities and adapting organizational culture, and external challenges like aligning strategies with customer and supplier expectations (Alghisi and Saccani 2015 ; Baines and Shi 2015 ; Ceci and Masini 2011 ; Mosch et al. 2021 ).
Business models in the context of Smart PSS vary from product centered to service oriented, depending on the company’s servitization maturity and technological capacity, leading to greater competitive differentiation and new market opportunities (Kowalkowski et al. 2017 ; Zheng et al. 2019 ; Baines et al. 2020 ; Chen et al. 2021 ). Implementing Smart PSS calls for a holistic approach, from strategic planning to system design and operational management, with a focus on how digital capabilities enhance PSS offerings and the overall value chain (Coreynen et al. 2017 ; Zheng et al. 2018 ).
In sum, the transition from traditional servitization to digital servitization, through the deployment of Smart PSS, marks a critical shift in value creation and sustaining customer loyalty, propelled by Industry 4.0 innovations (Vandermerwe and Rada 1988 ; Frank et al. 2019 ; Pinillos et al. 2022 ; Raddats et al. 2022 ; Schroeder et al. 2022 ; Chen et al. 2023 ; Martín-Peña et al. 2023 ). Realizing the potential of digital servitization demands an understanding of technological capabilities, fostering innovation, and market adaptability (Kohtamäki et al. 2019b ; Zhang et al. 2023 ). Successful digital servitization and Smart PSS rely on integrating technology with strategic vision and customer centricity, cultivating a business model focused on collaboration, innovation, and value co-creation (Naik et al. 2020 ; Chen et al. 2021 ; Zhou et al. 2021 ; Kolagar et al. 2022 ).
4.3 Digital servitization: crafting superior value in the modern era
As previously noted, servitization, as it evolves into digital servitization, catalyzes a profound and strategic transformation of business models and operational paradigms, emphasizing the importance of both internal and external strategic alignments. This process not only optimizes existing service offerings but also unlocks significant potential for service innovation and market competitiveness. Specifically, the integration of advanced technologies in digital servitization allows companies to create superior and customized value for their customers. This expanded value creation is achieved through a synergistic combination of technological resources and human capabilities, facilitating more predictive, personalized, and proactive services. Thus, digital servitization emerges as an essential and transformative step in business strategy, driving not only efficiency and strategic alignment but also fostering innovation and strengthening competitive positioning in the market.
Digital servitization, a contemporary evolution of traditional servitization, integrates Industry 4.0 technologies into the service domain, creating significant value for the customer. This value manifests in several key dimensions, all driven by digitalization and the emerging capabilities it offers.
Enhanced personalization and customer experience. The ability to collect and analyze large volumes of data using digital technologies enables companies to better understand the needs and preferences of their customers (Tao and Qi 2017 ; Chen et al. 2023 ). This leads to the creation of more personalized service offerings, tailored specifically to individual customer requirements. For instance, data analytics capabilities enhance servitization by enabling service personalization, which is fundamental for improving customer satisfaction and fostering long-term loyalty (Chen et al. 2022b ).
Efficiency and proactivity in service delivery. Digital servitization allows companies to be more efficient and proactive in delivering services. Technologies like the Internet of Things and Artificial Intelligence facilitate remote monitoring and predictive maintenance, anticipating problems before they occur and minimizing downtime (Lee et al. 2014 ; Tao and Qi 2017 ; Raddats et al. 2022 ). This not only improves product reliability but also reduces costs for the customer.
Creation of new opportunities and business models. The integration of digital services opens new avenues for innovative business models. For example, companies can offer usage-based solutions or subscriptions, where customers pay for performance or outcomes rather than the product itself (Vendrell-Herrero et al. 2017 ; Martín-Peña et al. 2020 ; Bortoluzzi et al. 2022 ). This can result in greater flexibility and more attractive cost options for the customer.
Enhanced customer–supplier relationships. Digital servitization fosters greater collaboration and value co-creation between suppliers and customers (Coreynen et al. 2017 ; Sjödin et al. 2020 ; Harrmann et al. 2023 ). This is because digital capabilities enable smoother communication and more transparent information exchange, resulting in stronger and more reliable relationships (Davies et al. 2023 ).
Continuous improvement of products and services. Ongoing feedback and data analysis enable continuous improvement of the products and services offered. Companies can quickly adjust their offerings in response to customer feedback or market changes, ensuring that their services remain relevant and of high quality (Chen et al. 2021 ).
Access to new markets. Digital servitization enables companies to access new markets and customer segments. By offering digital solutions, companies can overcome geographical and logistical barriers, reaching customers who were previously inaccessible (Münch et al. 2022 ; Rakic et al. 2022 ).
In summary, digital servitization not only enhances existing service offerings but also opens new opportunities for service innovation, strategic alignment, and market competitiveness. Its successful implementation is key to creating substantial value for the customer, highlighting the importance of a well-planned and executed strategy in the context of modern servitization.
5 Proposed conceptual framework: guiding the transition to digital servitization
Digital servitization represents a pivotal shift in the business landscape, where manufacturing companies evolve into providers of comprehensive solutions that seamlessly integrate products and services, augmented by digital technologies. This transformation is driven by the need for enhanced competitiveness, customer engagement, and value creation in a rapidly changing digital economy.
The development of our DASOBI conceptual framework, designed to guide the transition to digital servitization, is grounded in a rigorous methodological approach, underpinned by a comprehensive systematic literature review. This review meticulously synthesized three decades of academic research and industry insights, incorporating a total of 157 articles. Our comprehensive review process involved a deep analysis of the most influential and relevant publications in the field, among which notable contributions include Alghisi and Saccani ( 2015 ); Ayala et al. ( 2017 , 2019 ); Coreynen et al. ( 2017 ); Tao and Qi ( 2017 ); Vendrell-Herrero et al. ( 2017 ); Bustinza et al. ( 2018 ); Frank et al. ( 2019 ); Baines et al. ( 2020 ); Martín-Peña et al. ( 2020 ); Naik et al. ( 2020 ); Brax et al. ( 2021 ); Gaiardelli et al. ( 2021 ); Kohtamäki et al. ( 2021 ); Bettiol et al. ( 2022 ); Bortoluzzi et al. ( 2022 ); Marcon et al. ( 2022 ); Münch et al. ( 2022 ); Brekke et al. ( 2023 ); Chen et al. ( 2023 ); Chirumalla et al. ( 2023 ); Shen et al. ( 2023 ). These articles were particularly significant for identifying emerging trends, key challenges, and effective strategies in digital servitization. By systematically analyzing this extensive body of literature, we identified critical themes, challenges, strategies, and outcomes associated with the digital servitization journey. This analysis not only highlighted the multifaceted nature of digital servitization but also emphasized the critical importance of aligning strategic considerations, technological capabilities, and stakeholder roles to successfully navigate this complex transition. The structured framework presented herein not only reflects the evolution of the field but also provides clear guidance for manufacturing companies advancing toward more sophisticated and digitalized servitization practices.
The DASOBI framework, while empirically grounded in a comprehensive literature review, also draws extensively on classical and emerging theories to provide a robust theoretical foundation. For instance, diffusion of innovations theory (Rogers 2003 ) elucidates the “Drivers” and “Obstacles” in the adoption of digital servitization by explaining the rate and process through which new technological innovations spread within industries. Furthermore, the resource-based view (Barney 1991 ) is instrumental in understanding the “Strategies” component of the framework, emphasizing the importance of internal capabilities and resources in gaining a competitive advantage through digital transformation. These theoretical integrations not only enhance the academic rigor of our framework but also offer a deeper understanding of the multifaceted nature of digital servitization.
Therefore, the proposed DASOBI (Drivers, Actors, Strategies, Obstacles, Benefits, and Impact) model emerges as a synthesis of empirical evidence and theoretical insights, designed to offer a coherent and actionable guide for organizations seeking to embrace digital servitization.
This conceptual framework delineates a roadmap for organizations to navigate this complex transition. The framework identifies the core components essential for a successful journey toward digital servitization:
Underlying reasons for the shift (Drivers). Recognizing the strategic imperatives for transitioning toward a digital servitization model is critical. This includes understanding market dynamics, competitive pressures, and technological advancements driving this change.
Key actors involved (Actors). Successful digital servitization necessitates the involvement and alignment of various stakeholders, including internal teams, customers, technology partners, and suppliers. Their roles, expectations, and contributions are pivotal in shaping the servitization journey.
Strategic considerations and tools (Strategies). This encompasses adopting strategic frameworks, methodologies, and digital tools that are conducive to servitization. These tools and strategies should facilitate the integration of digital technologies with traditional product-service offerings, ensuring a seamless transition.
Potential challenges and obstacles (Obstacles). Identifying and addressing challenges such as cultural resistance, skill gaps, technological complexities, and integration issues with existing processes is crucial. Proactive strategies and contingency plans are essential to mitigate these barriers.
Anticipated benefits of the transition (Benefits). The transition to digital servitization should bring about significant benefits, including enhanced customer value, increased revenue streams, and improved competitive positioning. This component focuses on quantifying these benefits and aligning them with organizational goals.
Expected outcomes and impact (Impact). The final component of the framework revolves around the tangible outcomes and impacts of digital servitization. This includes enhanced customer satisfaction, increased market share, and improved operational efficiency.
In the digital servitization framework, the transition toward digital servitization, driven by market dynamics, competitive pressures, and technological advancements, is intrinsically linked to the roles and contributions of key stakeholders, such as internal teams, customers, and technology partners. Strategic considerations and tools must be selected in light of potential challenges, like cultural resistance and skill gaps, ensuring alignment with stakeholder capabilities and expectations for a seamless integration of digital technologies with traditional offerings. This strategic alignment is pivotal in overcoming obstacles and realizing anticipated benefits, such as enhanced customer value and competitive positioning. These benefits, in turn, lead to tangible outcomes, like improved customer satisfaction and operational efficiency, which feedback into the market, influencing ongoing strategic imperatives and shaping the evolution of digital servitization strategies. This dynamic interplay highlights a continuous feedback loop where outcomes inform underlying reasons, reinforcing the need for adaptability and strategic foresight in the digital servitization journey.
The contribution of the DASOBI framework to the existing literature is manifold. By synthesizing empirical findings with theoretical insights from servitization and digital transformation research, this framework addresses identified gaps, such as the integration of digital technologies in traditional servitization models and the management of organizational changes associated with such transitions (Baines and Lightfoot 2013 ; Vargo and Lusch 2008 ). Specifically, the DASOBI framework aids in conceptualizing how companies can strategically navigate the complexities of digital servitization, providing a structured approach that is missing in previous studies. This not only extends the theoretical discourse around servitization but also sets a foundation for future research to explore the dynamic interactions between digital technologies and service strategies in manufacturing sectors.
In conclusion, this conceptual framework serves as a comprehensive guide for firms embarking on the digital servitization journey. It provides a structured approach to understanding and implementing the necessary changes, ensuring a smooth transition and realization of the potential benefits of digital servitization. Figure 6 summarizes this meticulously formulated model (DASOBI), referred to as the Drivers (underlying reasons for the shift), Actors (key actors involved), Strategies (strategic considerations and tools), Obstacles (potential challenges and obstacles), Benefits (anticipated benefits of the transition), and Impact (expected outcomes and impact) of Digital Servitization Strategy, offers a robust framework for scholarly exploration, grounded in an exhaustive review of extant literature.
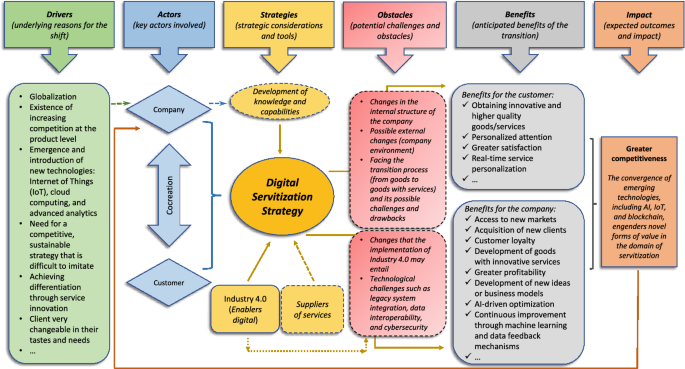
Source: Authors’ own work
Conceptual theoretical model for the analysis of Digital Servitization.
The DASOBI framework orchestrates the shift from traditional service strategies to digitally-enhanced service offerings, underpinned by the alignment of core elements: Drivers, Actors, Strategy, Obstacles, Benefits, and Impact. The model emphasizes a strategic approach, incorporating digital catalytic factors to augment adaptability, customer-centric analytics, and the pursuit of novel revenue streams through digital innovations.
Within this framework, the digital knowledge and capability development are crucial. Firms must harness Big Data to distill customer insights, leverage Artificial Intelligence for identifying opportunities, and increase the flexibility of their service offerings via digital platforms. The role of digital service providers is pivotal, offering expertise to mitigate transition risks, assure service quality, and bolster productivity with cutting-edge technological solutions.
However, the shift is not without its challenges. The resistance to digital transformation and the complexity of measuring profitability in the digital service landscape can impede progress. Moreover, the implications of Industry 4.0 are profound, necessitating organizational restructuring, workforce upskilling, and technological investments to realize the potential of digital servitization.
The anticipated benefits of this digital shift are manifold. Enhanced customer understanding through sophisticated data analytics, improved market positioning through digital innovation, and elevated creative capability with advanced technology are but a few of the advantages. Furthermore, embracing Industry 4.0 technologies within digital servitization amplifies these benefits, leading to superior product quality via smart manufacturing, greater adaptability in production, and increased operational efficiency ensuring timely delivery.
In summary, the DASOBI model meticulously integrates the transition to digital servitization with the digital economy’s imperatives, presenting a coherent roadmap for firms aspiring to harness the full spectrum of benefits offered by Industry 4.0 innovations.
6 Conclusions, limitations, and further research
This study embarked on an exhaustive journey through three decades of literature on servitization and its evolution toward digital servitization within the manufacturing sector. Through a systematic literature review, we explored the strategic transformation that involves integrating advanced services and digital technologies into product offerings, a change driven by the need to enhance competitiveness, customer engagement, and value creation in a rapidly evolving digital economy.
Our research findings have identified key drivers, actors, strategies, challenges, and benefits associated with the transition toward digital servitization. The DASOBI conceptual framework tries to provide a structured guide for understanding and managing this complex transition. This framework emphasizes the importance of recognizing the underlying reasons for adopting digital servitization models, the necessity of aligning and collaborating with diverse stakeholders, and the use of specific strategies to overcome the inherent challenges of this process.
Despite this study’s contribution to the body of knowledge on digital servitization, we acknowledge several limitations. The geographical concentration of the research activity analyzed might limit the generalizability of our findings across diverse cultural and economic contexts. The rapid evolution of digital technologies and business models also suggests that the relevance of our discoveries could be challenged by future developments. Additionally, our research focused primarily on manufacturing firms, which limits the applicability of the findings to other sectors.
These limitations open several avenues for future research. It is imperative to validate and test the generalizability of the DASOBI framework across various organizational and industry contexts. Further research is also needed to develop specific metrics that can measure the impacts of digital servitization. Longitudinal studies could provide a deeper understanding of how servitization strategies influence business outcomes over time.
This study contributes to the academic discussion by clarifying and deepening the concept of servitization and its intersection with digitalization, offering an integrative view that can assist manufacturing firms in navigating the complex landscape of servitization and digital servitization. Although we have tried to establish a solid foundation for future research, it is evident that the field of digital servitization remains dynamic and evolving, requiring ongoing examination to fully comprehend its impact on business strategy and practice.
Data availability
The datasets generated during and/or analyzed during the current study are available from the corresponding author on reasonable request.
Alghisi A, Saccani N (2015) Internal and external alignment in the servitization journey—overcoming the challenges. Prod Plann Control 26:1219–1232. https://doi.org/10.1080/09537287.2015.1033496
Article Google Scholar
Ayala NF, Paslauski CA, Ghezzi A, Frank AG (2017) Knowledge sharing dynamics in service suppliers’ involvement for servitization of manufacturing companies. Int J Prod Econ 193:538–553. https://doi.org/10.1016/j.ijpe.2017.08.019
Ayala NF, Gerstlberger W, Frank AG (2019) Managing servitization in product companies: the moderating role of service suppliers. Int J Oper Prod Manag 39(1):43–74. https://doi.org/10.1108/IJOPM-08-2017-0484
Baines T, Lightfoot H (2013) Servitization of the manufacturing firm: exploring the operations practices and technologies that deliver advanced services. Int J Oper Prod Manag 34(1):2–35. https://doi.org/10.1108/IJOPM-02-2012-0086
Baines T, Shi VG (2015) A Delphi study to explore the adoption of servitization in UK companies. Prod Plann Control 26:1171–1187. https://doi.org/10.1080/09537287.2015.1033490
Baines T, Lightfoot HW, Evans S, Neely A et al (2007) State-of-the-art in product-service systems. J Eng Manuf 221(10):1543–1552. https://doi.org/10.1243/09544054JEM858
Baines T, Lightfoot H, Benedettini O, Kay JM (2009a) The servitization of manufacturing: a review of literature and reflection on future challenges. J Manuf Technol Manag 20(5):547–567. https://doi.org/10.1108/17410380910960984
Baines T, Lightfoot H, Peppard J, Johnson M et al (2009b) Towards an operations strategy for product-centric servitization. Int J Oper Prod Manag 29(5):494–519. https://doi.org/10.1108/01443570910953603
Baines T, Lightfoot H, Smart P (2011) Servitization within manufacturing: exploring the provision of advanced services and their impact on vertical integration. J Manuf Technol Manag 22(7):947–954. https://doi.org/10.1108/17410381111160988
Baines T, Ziaee Bigdeli A, Bustinza OF, Shi VG et al (2017) Servitization: revisiting the state-of-the-art and research priorities. Int J Oper Prod Manag 37(2):256–278. https://doi.org/10.1108/IJOPM-06-2015-0312
Baines T, Ziaee Bigdeli A, Sousa R, Schroeder A (2020) Framing the servitization transformation process: a model to understand and facilitate the servitization journey. Int J Prod Econ 221:1–44. https://doi.org/10.1016/j.ijpe.2019.07.036
Barney J (1991) Firm resources and sustained competitive advantage. J Manag 17(1):99–120
Google Scholar
Barquet APB, De Oliveira MG, Amigo CR, Cunha VP, Rozenfeld H (2013) Employing the business model concept to support the adoption of product-service systems (PSS). Ind Mark Manag 42(5):693–704. https://doi.org/10.1016/j.indmarman.2013.05.003
Bastl M, Johnson M, Lightfoot H, Evans S (2012) Buyer-supplier relationships in a servitized environment. Int J Oper Prod Manag 32(6):650–675. https://doi.org/10.1108/01443571211230916
Benedettini O, Neely A, Swink M (2015) Why do servitized firms fail? A risk-based explanation. Int J Oper Prod Manag 35(6):946–979. https://doi.org/10.1108/IJOPM-02-2014-0052
Bettiol M, Capestro M, Di Maria E, Micelli S (2022) Overcoming pandemic challenges through product innovation: the role of digital technologies and servitization. Eur Manag J 40(5):707–717. https://doi.org/10.1016/j.emj.2022.05.003
Bortoluzzi G, Chiarvesio M, Romanello R, Tabacco R, Veglio V (2022) Servitisation and performance in the business-to-business context: the moderating role of Industry 4.0 technologies. J Manuf Technol Manag 33(9):108–128. https://doi.org/10.1108/JMTM-08-2021-0317
Brady T, Davies A, Gann D (2005) Creating value by delivering integrated solutions. Int J Proj Manag 23(5):360–365. https://doi.org/10.1016/j.ijproman.2005.01.001
Brax SA (2005) A manufacturer becoming service provider—challenges and a paradox. Manag Serv Qual 15(2):142–155. https://doi.org/10.1108/09604520510585334
Brax SA, Jonsson K (2009) Developing integrated solution offerings for remote diagnostics: a comparative case study of two manufacturers. Int J Oper Prod Manag 29(5):539–560. https://doi.org/10.1108/01443570910953621
Brax SA, Calabrese A, Levialdi Ghiron N, Tiburzi L, Gronroos C (2021) Explaining the servitization paradox: a configurational theory and a performance measurement framework. Int J Oper Prod Manag 41(5):517–546. https://doi.org/10.1108/IJOPM-08-2020-0535
Brekke T, Lenka S, Kohtamaki M, Parida V, Solem BAA (2023) Overcoming barriers to transformation in manufacturing firms. A path-dependence perspective of digital servitization. Rev Manag Sci. https://doi.org/10.1007/s11846-023-00641-0
Bustinza OF, Bigdeli AZ, Baines T, Elliot C (2015) Servitization and competitive advantage: the importance of organizational structure and value chain position. Res Technol Manag 58:53–60. https://doi.org/10.5437/08956308X5805354
Bustinza OF, Gomes E, Vendrell-Herrero F, Tarba SY (2018) An organizational change framework for digital servitization: evidence from the Veneto region. Strateg Change 27:111–119. https://doi.org/10.1002/jsc.2186
Calabrese A, Levialdi Ghiron N, Tiburzi L, Baines T, Ziaee Bigdeli A (2019) The measurement of degree of servitization: literature review and recommendations. Prod Plann Control 30:1118–1135. https://doi.org/10.1080/09537287.2019.1592260
Ceci F, Masini A (2011) Balancing specialized and generic capabilities in the provision of integrated solutions. Ind Corp Change 20(1):91–131. https://doi.org/10.1093/icc/dtq069
Chen Y, Visnjic I, Parida V, Zhang Z (2021) On the road to digital servitization—the (dis)continuous interplay between business model and digital technology. Int J Oper Prod Manag 41(5):694–722. https://doi.org/10.1108/IJOPM-08-2020-0544
Chen M, Pu X, Zhang M, Cai Z et al (2022a) Data analytics capability and servitization: the moderated mediation role of bricolage and innovation orientation. Int J Oper Prod Manag 42(4):440–470. https://doi.org/10.1108/IJOPM-10-2021-0663
Chen Y, Wu Z, Yi W, Wang B et al (2022b) Bibliometric method for manufacturing servitization: a review and future research directions. Sustainability 14:1–26. https://doi.org/10.3390/su14148743
Chen L, Dai Y, Ren F, Dong X (2023) Data-driven digital capabilities enable servitization strategy—from service supporting the product to service supporting the client. Technol Forecast Soc Change 197:1–15. https://doi.org/10.1016/j.techfore.2023.122901
Chirumalla K, Leoni L, Oghazi P (2023) Moving from servitization to digital servitization: identifying the required dynamic capabilities and related microfoundations to facilitate the transition. J Bus Res 158:1–23. https://doi.org/10.1016/j.jbusres.2023.113668
Chowdhury S, Haftor D, Pashkevich N (2018) Smart product-service systems (Smart PSS) in industrial firms: a literature review. Procedia CIRP 73:26–31. https://doi.org/10.1016/j.procir.2018.03.333
Ciasullo MV, Polese F, Montera R, Carrubbo L (2021) A digital servitization framework for viable manufacturing companies. J Bus Ind Mark 36(13):142–160. https://doi.org/10.1108/JBIM-07-2020-0349
Coreynen W, Matthyssens P, Van Bockhaven W (2017) Boosting servitization through digitization: pathways and dynamic resource configurations for manufacturers. Ind Mark Manag 60:42–53. https://doi.org/10.1016/j.indmarman.2016.04.012
Cusumano MA (2008) The changing software business: moving from products to services. Computer 41:20–27. https://doi.org/10.1109/MC.2008.29
Cusumano MA, Kahl SJ, Suárez FF (2014) Services, industry evolution, and the competitive strategies of product firms. Strateg Manag J 36:559–575. https://doi.org/10.2139/ssrn.2378868
Dalenogare LS, Benitez GB, Ayala NF, Frank AG (2018) The expected contribution of Industry 4.0 technologies for industrial performance. Int J Prod Econ 204:383–394. https://doi.org/10.1016/j.ijpe.2018.08.019
Davies A, Brady T, Hobday M (2007) Organizing for solutions: systems seller vs. systems integrator. Ind Mark Manag 36(2):183–193. https://doi.org/10.1016/j.indmarman.2006.04.009
Davies P, Bustinza OF, Parry G, Jovanovic M (2023) Unpacking the relationship between digital capabilities, services capabilities, and firm financial performance: a moderated mediation model. Ind Mark Manag 115:1–10. https://doi.org/10.1016/j.indmarman.2023.09.005
Díaz-Garrido E, Pinillos MJ, Soriano-Pinar I, García-Magro C (2018) Changes in the intellectual basis of servitization research: a dynamic analysis. J Eng Technol Manag JET M 48:1–14. https://doi.org/10.1016/j.jengtecman.2018.01.005
Durugbo C (2014) Strategic framework for industrial product-service co-design: findings from the microsystems industry. Int J Prod Res 52:2881–2900. https://doi.org/10.1080/00207543.2013.857054
Eggert A, Hogreve J, Ulaga W, Muenkhoff E (2014) Revenue and profit implications of industrial service strategies. J Serv Res 17:23–39. https://doi.org/10.1177/1094670513485823
Favoretto C, Mendes G, Oliveira M, Cauchick-Miguel P, Coreynen W (2022) From servitization to digital servitization: how digitalization transforms companies’ transition towards services. Ind Mark Manag 102:104–121. https://doi.org/10.1016/j.indmarman.2022.01.003
Frank AG, Mendes GHS, Ayala NF, Ghezzi A (2019) Servitization and Industry 4.0 convergence in the digital transformation of product firms: a business model innovation perspective. Technol Forecast Soc Change 141:341–351. https://doi.org/10.1016/j.techfore.2019.01.014
Gaiardelli P, Songini L, Saccani N (2014) The automotive industry: heading towards servitization in turbulent times. Servitization in Industry. Springer, Cham
Gaiardelli P, Pezzotta G, Rondini A, Romero D et al (2021) Product-service systems evolution in the era of Industry 4.0. Serv Bus 15:177–207. https://doi.org/10.1007/s11628-021-00438-9
Galbraith JR (2002) Organizing to deliver solutions. Organ Dyn 31(2):194–207. https://doi.org/10.1016/S0090-2616(02)00101-8
García Martin PC, Schroeder A, Bigdeli AZ (2019) The value architecture of servitization: expanding the research scope. J Bus Res 104:438–449. https://doi.org/10.1016/j.jbusres.2019.04.010
Gebauer H (2008) Identifying service strategies in product manufacturing companies by exploring environment—strategy configurations. Ind Mark Manage 37(3):278–291. https://doi.org/10.1016/j.indmarman.2007.05.018
Gebauer H, Fleisch E (2007) An investigation of the relationship between behavioral processes, motivation, investments in the service business and service revenue. Ind Mark Manag 36(3):337–348. https://doi.org/10.1016/j.indmarman.2005.09.005
Gebauer H, Kowalkowski C (2012) Customer-focused and service-focused orientation in organizational structures. J Bus Ind Mark 27(7):527–537. https://doi.org/10.1108/08858621211257293
Gebauer H, Elgar F, Thomas F (2005) Overcoming the service paradox in manufacturing companies. Eur Manag J 23:14–26. https://doi.org/10.1016/j.emj.2004.12.006
Gebauer H, Gustafsson A, Witell L (2011) Competitive advantage through service differentiation by manufacturing companies. J Bus Res 64(12):1270–1280. https://doi.org/10.1016/j.jbusres.2011.01.015
Gebauer H, Paiola M, Saccani N (2013) Characterizing service networks for moving from products to solutions. Ind Mark Manag 42:31–46. https://doi.org/10.1016/j.indmarman.2012.11.002
Grandinetti R, Ciasullo MV, Paiola M, Schiavone F (2020) Fourth industrial revolution, digital servitization and relationship quality in Italian B2B manufacturing firms. Explor Study TQM J 32(4):647–671. https://doi.org/10.1108/TQM-01-2020-0006
Haase RP, Pigosso DCA, McAloone TC (2017) Product/service-system origins and trajectories: a systematic literature review of PSS definitions and their characteristics. Procedia CIRP 64:157–162. https://doi.org/10.1016/j.procir.2017.03.053
Harrmann LK, Eggert A, Böhm E (2023) Digital technology usage as a driver of servitization paths in manufacturing industries. Eur J Mark 57(3):834–857. https://doi.org/10.1108/EJM-11-2021-0914
Hertzberg S, Rudner L (1999) Quality of researchers’ searches of the ERIC database. Educ Policy Anal Arch. https://doi.org/10.14507/epaa.v7n25.1999
Hyun M, Kim J (2021) Challenge or opportunity? A case of tire rental servitization from financial and channel perspectives. Serv Bus 15:1–17. https://doi.org/10.1007/s11628-020-00433-6
Ibarra D, Ganzarain J, Igartua JI (2018) Business model innovation through Industry 4.0: a review. Procedia Manuf 22:4–10. https://doi.org/10.1016/J.PROMFG.2018.03.002
Johnson M, Mena C (2008) Supply chain management for servitised products: a multi-industry case study. Int J Prod Econ 114:27–39. https://doi.org/10.1016/j.ijpe.2007.09.011
Johnstone S, Dainty A, Wilkinson A (2009) Integrating products and services through life: an aerospace experience. Int J Oper Prod Manag 29(5):520–538. https://doi.org/10.1108/01443570910953612
Kamp B, Alcalde H (2014) Servitization in the basque economy. Strateg Change 23:359–374. https://doi.org/10.1002/jsc.1982
Kamp B, Parry G (2017) Servitization and advanced business services as levers for competitiveness. Ind Mark Manag 60:11–16. https://doi.org/10.1016/j.indmarman.2016.12.008
Kanninen T, Penttinen E, Tinnilä M, Kaario K (2017) Exploring the dynamic capabilities required for servitization: the case process industry. Bus Process Manag J 23(2):226–247. https://doi.org/10.1108/BPMJ-03-2015-0036
Kans M, Ingwald A (2016) Business model development towards service management 4.0. Procedia CIRP 47:489–494. https://doi.org/10.1016/J.PROCIR.2016.03.228
Khanra S, Dhir A, Parida V, Kohtamäki M (2021) Servitization research: a review and bibliometric analysis of past achievements and future promises. J Bus Res 131:151–166. https://doi.org/10.1016/j.jbusres.2021.03.056
Kharlamov AA, Parry G (2021) The impact of servitization and digitization on productivity and profitability of the firm: a systematic approach. Prod Plann Control 32:185–197. https://doi.org/10.1080/09537287.2020.1718793
Kimita K, McAloone T, Ogata K, Pigosso D (2022) Servitization maturity model: developing distinctive capabilities for successful servitization in manufacturing companies. J Manuf Technol Manag 33(9):61–87. https://doi.org/10.1108/JMTM-07-2021-0248
Kindström D, Kowalkowski C (2014) Service innovation in product-centric firms: a multidimensional business model perspective. J Bus Ind Mark 29(2):96–111. https://doi.org/10.1108/JBIM-08-2013-0165
Kohtamaki M, Henneberg SC, Martinez V, Kimita K, Gebauer H (2019a) A configurational approach to servitization: review and research directions. Serv Sci 11(3):1–29. https://doi.org/10.1287/serv.2019.0245
Kohtamaki M, Rabetino R, Einola S, Parida V, Patel P (2021) Unfolding the digital servitization path from products to product-service-software systems: practicing change through intentional narratives. J Bus Res 137:379–392. https://doi.org/10.1016/j.jbusres.2021.08.027
Kohtamäki M, Partanen J (2016) Co-creating value from knowledge-intensive business services in manufacturing firms: the moderating role of relationship learning in supplier-customer interactions. J Bus Res 69(7):2498–2506. https://doi.org/10.1016/j.jbusres.2016.02.019
Kohtamäki M, Parida V, Oghazi P, Gebauer H, Baines T (2019b) Digital servitization business models in ecosystems: a theory of the firm. J Bus Res 104:380–392. https://doi.org/10.1016/j.jbusres.2019.06.027
Kohtamäki M, Einola S, Rabetino R (2020a) Exploring servitization through the paradox lens: coping practices in servitization. Int J Prod Econ 226:1–15. https://doi.org/10.1016/j.ijpe.2020.107619
Kohtamäki M, Parida V, Patel P, Gebauer H (2020b) The relationship between digitalization and servitization: the role of servitization in capturing the financial potential of digitalization. Technol Forecast Soc Change 151:1–35. https://doi.org/10.1016/j.techfore.2019.119804
Kolagar M, Parida V, Sjödin D (2022) Ecosystem transformation for digital servitization: a systematic review, integrative framework, and future research agenda. J Bus Res 146:176–200. https://doi.org/10.1016/j.jbusres.2022.03.067
Kowalkowski C, Gebauer H, Kamp B, Parry G (2017) Servitization and deservitization: overview, concepts, and definitions. Ind Mark Manag 60:4–10. https://doi.org/10.1016/j.indmarman.2016.12.007
Kreye ME, Roehrich JK, Lewis MA (2015) Servitizing manufacturers: the impact of service complexity and contractual and relational capabilities. Prod Plann Control 26:1233–1246. https://doi.org/10.1080/09537287.2015.1033489
Le-Dain MA, Benhayoun L, Matthews J, Liard M (2023) Barriers and opportunities of digital servitization for SMEs: the effect of smart product-service system business models. Serv Bus 17:359–393. https://doi.org/10.1007/s11628-023-00520-4
Lee J, Kao HA, Yang S (2014) Service innovation and smart analytics for Industry 4.0 and big data environment. Procedia CIRP 16:3–8. https://doi.org/10.1016/j.procir.2014.02.001
Leoni L, Aria M (2021) A thirty-year bibliometric analysis on servitization. Int J Serv Sci Manag Eng Technol 12(3):73–95. https://doi.org/10.4018/IJSSMET.2021050105
Levitt T (1972) Production-line approach to service. Harv Bus Rev 50:41–52
Lightfoot H, Baines T, Smart P (2013) The servitization of manufacturing: a systematic literature review of interdependent trends. Int J Oper Prod Manag 33(11/12):1408–1434. https://doi.org/10.1108/IJOPM-07-2010-0196
Lindman M, Pennanen K, Rothenstei J, Scozzi B, Vincze Z (2016) The value space: how firms facilitate value creation. Bus Process Manag J 22(4):736–762. https://doi.org/10.1108/BPMJ-09-2015-0126
Luoto S, Brax SA, Kohtamäki M (2017) Critical meta-analysis of servitization research: constructing a model-narrative to reveal paradigmatic assumptions. Ind Mark Manag 60:89–100. https://doi.org/10.1016/j.indmarman.2016.04.008
Manzini E, Vezzoli C (2003) A strategic design approach to develop sustainable product service systems: examples taken from the ‘environmentally friendly innovation’ Italian prize. J Clean Prod 11(8):851–857. https://doi.org/10.1016/S0959-6526(02)00153-1
Marcon É, Marcon A, Ayala NF, Frank AG et al (2022) Capabilities supporting digital servitization: a multi-actor perspective. Ind Mark Manag 103:97–116. https://doi.org/10.1016/j.indmarman.2022.03.003
Martínez V, Bastl M, Kingston J, Evans S (2010) Challenges in transforming manufacturing organizations into product-service providers. J Manuf Technol Manag 21(4):449–469. https://doi.org/10.1108/17410381011046571
Martín-Peña ML, Ziaee Bigdeli A (2016) Servitization: academic research and business practice. Univ Bus Rev 49:18–31
Martín-Peña ML, Pinillos MJ, Reyes LE (2017) The intellectual basis of servitization: a bibliometric analysis. J Eng Technol Manag JET M 43:83–97. https://doi.org/10.1016/j.jengtecman.2017.01.005
Martín-Peña ML, Díaz-Garrido E, Sánchez-López JM (2018) The digitalization and servitization of manufacturing: a review on digital business models. Strateg Change 27:91–99. https://doi.org/10.1002/jsc.2184
Martín-Peña ML, Sánchez-López JM, Díaz-Garrido E (2020) Servitization and digitalization in manufacturing: the influence on firm performance. J Bus Ind Mark 35(3):564–574. https://doi.org/10.1108/JBIM-12-2018-0400
Martín-Peña ML, Sanchez-Lopez JM, Kamp B, Gimenez-Fernandez EM (2023) The innovation antecedents behind the servitization-performance relationship. R D Manag 53:1–23. https://doi.org/10.1111/radm.12586
Mathieu V (2001) Service strategies within the manufacturing sector: benefits, costs and partnership. Int J Serv Ind Manag 12(5):451–475. https://doi.org/10.1108/EUM0000000006093
Matthyssens P, Vandenbempt K (2010) Service addition as business market strategy: identification of transition trajectories. J Serv Manag 21(5):693–714. https://doi.org/10.1108/09564231011079101
Minaya PE, Avella L, Trespalacios JA (2023) The effects of digital servitization on business competitiveness: A case study of Spanish manufacturers. J Int Entrep 21:180–213. https://doi.org/10.1007/s10843-023-00333-6
Momeni K, Raddats C, Martinsuo M (2023) Mechanisms for developing operational capabilities in digital servitization. Int J Oper Prod Manag 43(13):101–127. https://doi.org/10.1108/IJOPM-04-2022-0259
Mont O (2002) Clarifying the concept of product-service system. J Clean Prod 10(3):237–245. https://doi.org/10.1016/S0959-6526(01)00039-7
Mosch P, Schweikl S, Obermaier R (2021) Trapped in the supply chain? Digital servitization strategies and power relations in the case of an industrial technology supplier. Int J Prod Econ 236:1–14. https://doi.org/10.1016/j.ijpe.2021.108141
Müller JM, Buliga O, Voigt KI (2021) The role of absorptive capacity and innovation strategy in the design of Industry 4.0 business models—a comparison between SMEs and large enterprises. Eur Manag J 39(3):333–343. https://doi.org/10.1016/j.emj.2020.01.002
Münch C, Marx E, Benz L, Hartmann E, Matzner M (2022) Capabilities of digital servitization: evidence from the socio-technical systems theory. Technol Forecast Soc Change 176:1–17. https://doi.org/10.1016/j.techfore.2021.121361
Naik P, Schroeder A, Kapoor K, Ziaee Bigdeli A (2020) Behind the scenes of digital servitization: actualising IoT-enabled affordances. Ind Mark Manag 89:232–244. https://doi.org/10.1016/j.indmarman.2020.03.010
Neely A, Benedettini O, Visnjic I (2011) The servitization of manufacturing: further evidence. University of Cambridge, Cambridge, pp 1–11
Nordin F, Kowalkowski C (2010) Solutions offerings: a critical review and reconceptualization. J Serv Manag 21(4):441–459. https://doi.org/10.1108/09564231011066105
Oliva R, Kallenberg R (2003) Managing the transition from products to services. Int J Serv Ind Manag 14(2):160–172. https://doi.org/10.1108/09564230310474138
Oliveira MG, Mendes GH, Rozenfeld H (2015) Bibliometric analysis of the product-service system research field. Procedia CIRP 30:114–119. https://doi.org/10.1016/j.procir.2015.02.139
Opazo-Basáez M, Vendrell-Herrero F, Bustinza OF (2021) Digital service innovation: a paradigm shift in technological innovation. J Serv Manag 33:97–120. https://doi.org/10.1108/JOSM-11-2020-0427
Ostrom AL, Bitner MJ, Brown SW, Burkhard KA et al (2010) Moving forward and making a difference: research priorities for the science of service. J Serv Res 13:4–36. https://doi.org/10.1177/1094670509357611
Paiola M, Gebauer H (2020) Internet of things technologies, digital servitization and business model innovation in BtoB manufacturing firms. Ind Mark Manag 89:245–264. https://doi.org/10.1016/j.indmarman.2020.03.009
Paiola M, Saccani N, Perona M, Gebauer H (2013) Moving from products to solutions: strategic approaches for developing capabilities. Eur Manag J 31(4):390–409. https://doi.org/10.1016/j.emj.2012.10.002
Parida V, Sjödin DR, Wincent J, Kohtamäki M (2014) Mastering the transition to product-service provision: insights into business models, learning activities, and capabilities. Res Technol Manag 57:44–52. https://doi.org/10.5437/08956308X5703227
Paschou T, Rapaccini M, Adrodegari F, Saccani N (2020) Digital servitization in manufacturing: a systematic literature review and research agenda. Ind Mark Manag 89:278–292. https://doi.org/10.1016/j.indmarman.2020.02.012
Pessôa MVP, Becker JMJ (2017) Overcoming the product-service model adoption obstacles. Procedia CIRP 64:163–168. https://doi.org/10.1016/j.procir.2017.03.062
Pettigrew AM (1988) The management of strategic change. B. Blackwell, Oxford
Pinillos MJ, Díaz-Garrido E, Martín-Peña ML (2022) The origin and evolution of the concept of servitization: a co-word and network analysis. J Bus Ind Mark 37(7):1497–1514. https://doi.org/10.1108/JBIM-02-2021-0120
Pombo D, Franco M (2023) A qualitative investigation of infusing products with service via strategic alliances among SMEs: a case of servitization. Serv Bus 17:529–555. https://doi.org/10.1007/s11628-023-00530-2
Pye A, Pettigrew A (2005) Studying board context, process and dynamics: some challenges for the future. Brit J Manag 16:27–38. https://doi.org/10.1111/j.1467-8551.2005.00445.x
Qi Y, Mao Z, Zhang M, Guo H (2020) Manufacturing practices and servitization: the role of mass customization and product innovation capabilities. Int J Prod Econ 228:1–10. https://doi.org/10.1016/j.ijpe.2020.107747
Rabetino R, Kohtamäki M, Gebauer H (2017) Strategy map of servitization. Int J Prod Econ 192:144–156. https://doi.org/10.1016/j.ijpe.2016.11.004
Rabetino R, Kohtamäki M, Brax SA, Sihvonen J (2021) The tribes in the field of servitization: discovering latent streams across 30 years of research. Ind Mark Manag 95:70–84. https://doi.org/10.1016/j.indmarman.2021.04.005
Rabetino R, Kohtamäki M, Huikkola T (2023) Digital service innovation (DSI): a multidisciplinary (re)view of its origins and progress using bibliometric and text mining methods. J Serv Manag. https://doi.org/10.1108/JOSM-12-2022-0375
Raddats C (2011) Aligning industrial services with strategies and sources of market differentiation. J Bus Ind Mark 26(5):332–343. https://doi.org/10.1108/08858621111144398
Raddats C, Baines T, Burton J, Story VM, Zolkiewski J (2016) Motivations for servitization: the impact of product complexity. Int J Oper Prod Manag 36(5):572–591. https://doi.org/10.1108/IJOPM-09-2014-0447
Raddats C, Zolkiewski J, Story VM, Burton J et al (2017) Interactively developed capabilities: evidence from dyadic servitization relationships. Int J Oper Prod Manag 37(3):382–400. https://doi.org/10.1108/IJOPM-08-2015-0512
Raddats C, Kowalkowski C, Benedettini O, Burton J, Gebauer H (2019) Servitization: a contemporary thematic review of four major research streams. Ind Mark Manag 83:207–223. https://doi.org/10.1016/j.indmarman.2019.03.015
Raddats C, Naik P, Ziaee Bigdeli A (2022) Creating value in servitization through digital service innovations. Ind Mark Manag 104:1–13. https://doi.org/10.1016/j.indmarman.2022.04.002
Rakic S, Pero M, Sianesi A, Marjanovic U (2022) Digital servitization and firm performance: technology intensity approach. Eng Econ 33(4):398–413. https://doi.org/10.5755/j01.ee.33.4.29649
Reim W, Parida V, Örtqvist D (2015) Product-Service Systems (PSS) business models and tactics—a systematic literature review. J Clean Prod 97:61–75. https://doi.org/10.1016/J.JCLEPRO.2014.07.003
Reim W, Sjödin DR, Parida V (2019) Servitization of global service network actors—a contingency framework for matching challenges and strategies in service transition. J Bus Res 104:461–471. https://doi.org/10.1016/j.jbusres.2019.01.032
Rogers EM (2003) Diffusion of innovations. Free Press, New York
Ruiz-Martín A, Díaz-Garrido E (2021) A review of servitization theoretical foundations. J Ind Eng Manag 14(3):496–519. https://doi.org/10.3926/jiem.3466
Sandström S, Edvardsson B, Kristensson P, Magnusson P (2008) Value in use through service experience. Manag Serv Qual 18(2):112–126. https://doi.org/10.1108/09604520810859184
Santamaría L, Jesús Nieto M, Miles I (2012) Service innovation in manufacturing firms: evidence from Spain. Technovation 32(2):144–155. https://doi.org/10.1016/j.technovation.2011.08.006
Schroeder A, Baines T, Sakao T (2022) Increasing value capture by enhancing manufacturer commitment-managing the servitization process. IEEE Eng Manag Rev 50(3):1–13. https://doi.org/10.1109/EMR.2022.3197075
Shen L, Sun W, Parida V (2023) Consolidating digital servitization research: a systematic review, integrative framework, and future research directions. Technol Forecast Soc Change 191:1–24. https://doi.org/10.1016/j.techfore.2023.122478
Sjödin D, Parida V, Kohtamaki M, Wincent J (2020) An agile co-creation process for digital servitization: a micro-service innovation approach. J Bus Res 112:478–491. https://doi.org/10.1016/j.jbusres.2020.01.009
Sousa R, Da Silveira G (2017) Capability antecedents and performance outcomes of servitization: differences between basic and advanced services. Int J Oper Prod Manag 37(4):444–467. https://doi.org/10.1108/IJOPM-11-2015-0696
Spring M, Araujo L (2013) Beyond the service factory: service innovation in manufacturing supply networks. Ind Mark Manag 42:59–70. https://doi.org/10.1016/j.indmarman.2012.11.006
Tao F, Qi Q (2017) New IT driven service-oriented smart manufacturing: framework and characteristics. IEEE Trans Syst Man Cybern -Syst 49:81–91. https://doi.org/10.1109/TSMC.2017.2723764
Thomé AMT, Scavarda LF, Scavarda AJ (2016) Conducting systematic literature review in operations management. Prod Plann Control 27(5):408–420. https://doi.org/10.1080/09537287.2015.1129464
Tian J, Coreynen W, Matthyssens P, Shen L (2022) Platform-based servitization and business model adaptation by established manufacturers. Technovation 118:1–22. https://doi.org/10.1016/j.technovation.2021.102222
Tranfield D, Denyer D, Smart P (2003) Towards a methodology for developing evidence-informed management knowledge by means of systematic review. Brit J Manag 14:207–222. https://doi.org/10.1111/1467-8551.00375
Tronvoll B, Sklyar A, Sorhammar D, Kowalkowski C (2020) Transformational shifts through digital servitization. Ind Mark Manag 89:293–305. https://doi.org/10.1016/j.indmarman.2020.02.005
Tukker A (2004) Eight types of product-service system: eight ways to sustainability? Experience from SusProNet. Bus Strategy Environ 13:246–260. https://doi.org/10.1002/bse.414
Tukker A (2015) Product services for a resource-efficient and circular economy—a review. J Clean Prod 97:76–91. https://doi.org/10.1016/J.JCLEPRO.2013.11.049
Tukker A, Tischner U (2006) Product-services as a research field: past, present and future. Reflections from a decade of research. J Clean Prod 14(17):1552–1556. https://doi.org/10.1016/j.jclepro.2006.01.022
Tuli KR, Kohli AK, Bharadwaj SG (2007) Rethinking customer solutions: from product bundles to relational processes. J Mark 71(3):1–17. https://doi.org/10.1509/jmkg.71.3.1
Vandermerwe S, Rada J (1988) Servitization of business: adding value by adding services. Eur Manag J 6(4):314–324. https://doi.org/10.1016/0263-2373(88)90033-3
Vargo SL, Lusch RF (2008) Service-dominant logic: continuing the evolution. J Acad Mark Sci 36(1):1–10. https://doi.org/10.1007/s11747-007-0069-6
Vendrell-Herrero F, Bustinza OF, Parry G, Georgantzis N (2017) Servitization, digitization and supply chain interdependency. Ind Mark Manag 60:69–81. https://doi.org/10.1016/j.indmarman.2016.06.013
Visnjic I, Van Looy B (2013) Servitization: disentangling the impact of service business model innovation on manufacturing firm performance. J Oper Manag 31(4):169–180. https://doi.org/10.2139/ssrn.2407380
Wang W, Lai K, Shou Y (2018) The impact of servitization on firm performance: a meta-analysis. Int J Oper Prod Manag 38(7):1562–1588. https://doi.org/10.1108/IJOPM-04-2017-0204
Wang Z, Chen CH, Zheng P, Li X, Khoo LP (2021) A graph-based context-aware requirement elicitation approach in smart product-service systems. Int J Prod Res 59(2):635–651. https://doi.org/10.1080/00207543.2019.1702227
Windahl C, Lakemond N (2006) Developing integrated solutions: the importance of relationships within the network. Ind Mark Manag 35(7):806–818. https://doi.org/10.1016/J.INDMARMAN.2006.05.010
Xing Y, Liu Y, Davies P (2023) Servitization innovation: a systematic review, integrative framework, and future research directions. Technovation 122:1–15. https://doi.org/10.1016/j.technovation.2022.102641
Yan K, Li G, Cheng TCE (2020) The impact of service-oriented organizational design factors on firm performance: the moderating role of service-oriented corporate culture. Int J Prod Econ 228:1–13. https://doi.org/10.1016/j.ijpe.2020.107745
Yu Y, Sung TJ (2023) A value-based view of the smart PSS adoption: a study of smart kitchen appliances. Serv Bus 17:499–527. https://doi.org/10.1007/s11628-023-00529-9
Zhang W, Banerji S (2017) Challenges of servitization: a systematic literature review. Ind Mark Manag 65:217–227. https://doi.org/10.1016/j.indmarman.2017.06.003
Zhang K, Feng L, Wang J, Lin KY, Li Q (2023) Servitization in business ecosystem: a systematic review and implications for business-to-business servitization research. Technol Anal Strateg Manag 35(11):1480–1496. https://doi.org/10.1080/09537325.2021.2010698
Zheng P, Lin T, Chen C, Xu X (2018) A systematic design approach for service innovation of smart product-service systems. J Clean Prod 201:657–667. https://doi.org/10.1016/j.jclepro.2018.08.101
Zheng P, Liu Y, Tao F, Wang Z, Chen C (2019) Smart product-service systems solution design via hybrid crowd sensing approach. IEEE Access 7:1–12. https://doi.org/10.1109/ACCESS.2019.2939828
Zhou C, Song W (2021) Digitalization as a way forward: a bibliometric analysis of 20 years of servitization research. J Clean Prod 300:1–14. https://doi.org/10.1016/j.jclepro.2021.126943
Zhou D, Yan T, Dai W, Feng J (2021) Disentangling the interactions within and between servitization and digitalization strategies: a service-dominant logic. Int J Prod Econ 238:1–16. https://doi.org/10.1016/j.ijpe.2021.108175
Ziaee Bigdeli A, Baines T, Bustinza OF, Guang Shi V (2017) Organisational change towards servitization: a theoretical framework. Compet Rev 27(1):12–39. https://doi.org/10.1108/CR-03-2015-0015
Ziaee Bigdeli A, Baines T, Schroeder A, Brown S (2018) Measuring servitization progress and outcome: the case of ‘advanced services.’ Prod Plann Control 29(4):315–332. https://doi.org/10.1080/09537287.2018.1429029
Zighan S, Abualqumboz M (2022) Dual focus: service-product orientation to manage the change paradox following servitization strategy. Serv Bus 16:29–55. https://doi.org/10.1007/s11628-022-00483-y
Download references
Open Access funding provided thanks to the CRUE-CSIC agreement with Springer Nature.
Author information
Authors and affiliations.
Management and Business Economics Department, University of Leon, Leon, Spain
Pedro E. Minaya
Business Administration Department, University of Oviedo, Oviedo, Spain
Lucía Avella & Juan A. Trespalacios
You can also search for this author in PubMed Google Scholar
Corresponding author
Correspondence to Pedro E. Minaya .
Additional information
Publisher's note.
Springer Nature remains neutral with regard to jurisdictional claims in published maps and institutional affiliations.
Rights and permissions
Open Access This article is licensed under a Creative Commons Attribution 4.0 International License, which permits use, sharing, adaptation, distribution and reproduction in any medium or format, as long as you give appropriate credit to the original author(s) and the source, provide a link to the Creative Commons licence, and indicate if changes were made. The images or other third party material in this article are included in the article's Creative Commons licence, unless indicated otherwise in a credit line to the material. If material is not included in the article's Creative Commons licence and your intended use is not permitted by statutory regulation or exceeds the permitted use, you will need to obtain permission directly from the copyright holder. To view a copy of this licence, visit http://creativecommons.org/licenses/by/4.0/ .
Reprints and permissions
About this article
Minaya, P.E., Avella, L. & Trespalacios, J.A. Synthesizing three decades of digital servitization: a systematic literature review and conceptual framework proposal. Serv Bus (2024). https://doi.org/10.1007/s11628-024-00559-x
Download citation
Received : 28 September 2023
Accepted : 16 April 2024
Published : 08 May 2024
DOI : https://doi.org/10.1007/s11628-024-00559-x
Share this article
Anyone you share the following link with will be able to read this content:
Sorry, a shareable link is not currently available for this article.
Provided by the Springer Nature SharedIt content-sharing initiative
- Digital servitization
- Industry 4.0
- Product-service system
- Systematic literature review
- Business competitiveness
- Find a journal
- Publish with us
- Track your research

An official website of the United States government
The .gov means it’s official. Federal government websites often end in .gov or .mil. Before sharing sensitive information, make sure you’re on a federal government site.
The site is secure. The https:// ensures that you are connecting to the official website and that any information you provide is encrypted and transmitted securely.
- Publications
- Account settings
Preview improvements coming to the PMC website in October 2024. Learn More or Try it out now .
- Advanced Search
- Journal List
- Environ Sci Ecotechnol
- v.19; 2024 May
- PMC10656232
Smarter eco-cities and their leading-edge artificial intelligence of things solutions for environmental sustainability: A comprehensive systematic review
Simon elias bibri.
a School of Architecture, Civil and Environmental Engineering (ENAC), Civil Engineering Institute (IIC), Visual Intelligence for Transportation (VITA), Swiss Federal Institute of Technology Lausanne (EPFL), Lausanne, Switzerland
John Krogstie
b Department of Computer Science, Norwegian University of Science and Technology (NTNU), Trondheim, Norway
Amin Kaboli
c School of Engineering, Institute of Mechanical Engineering, Swiss Federal Institute of Technology Lausanne (EPFL), Lausanne, Switzerland
Alexandre Alahi
The recent advancements made in the realms of Artificial Intelligence (AI) and Artificial Intelligence of Things (AIoT) have unveiled transformative prospects and opportunities to enhance and optimize the environmental performance and efficiency of smart cities. These strides have, in turn, impacted smart eco-cities, catalyzing ongoing improvements and driving solutions to address complex environmental challenges. This aligns with the visionary concept of smarter eco-cities, an emerging paradigm of urbanism characterized by the seamless integration of advanced technologies and environmental strategies. However, there remains a significant gap in thoroughly understanding this new paradigm and the intricate spectrum of its multifaceted underlying dimensions. To bridge this gap, this study provides a comprehensive systematic review of the burgeoning landscape of smarter eco-cities and their leading-edge AI and AIoT solutions for environmental sustainability. To ensure thoroughness, the study employs a unified evidence synthesis framework integrating aggregative, configurative, and narrative synthesis approaches. At the core of this study lie these subsequent research inquiries: What are the foundational underpinnings of emerging smarter eco-cities, and how do they intricately interrelate, particularly urbanism paradigms, environmental solutions, and data-driven technologies? What are the key drivers and enablers propelling the materialization of smarter eco-cities? What are the primary AI and AIoT solutions that can be harnessed in the development of smarter eco-cities? In what ways do AI and AIoT technologies contribute to fostering environmental sustainability practices, and what potential benefits and opportunities do they offer for smarter eco-cities? What challenges and barriers arise in the implementation of AI and AIoT solutions for the development of smarter eco-cities? The findings significantly deepen and broaden our understanding of both the significant potential of AI and AIoT technologies to enhance sustainable urban development practices, as well as the formidable nature of the challenges they pose. Beyond theoretical enrichment, these findings offer invaluable insights and new perspectives poised to empower policymakers, practitioners, and researchers to advance the integration of eco-urbanism and AI- and AIoT-driven urbanism. Through an insightful exploration of the contemporary urban landscape and the identification of successfully applied AI and AIoT solutions, stakeholders gain the necessary groundwork for making well-informed decisions, implementing effective strategies, and designing policies that prioritize environmental well-being.
Graphical abstract

- • AI and AIoT hold significant potential to address complex environmental challenges.
- • AI and AIoT are being applied in smart cities to enhance their performance and efficiency.
- • The concept of smarter eco-cities is based on the groundbreaking convergence of AI and IoT.
- • Smarter eco-cities are leveraging AI and AIoT to advance their environmental sustainability goals.
- • AI and AIoT pose environmental, technical, ethical, social, and regulatory challenges.
1. Introduction
The rapid advancement and groundbreaking convergence of Artificial Intelligence (AI) and the Internet of Things (IoT) has triggered profound transformations across various domains, including environmental sustainability, climate change, and urban development. This surge has led to the emergence of smarter eco-cities, where Artificial Intelligence of Things (AIoT) takes center stage and garners significant attention. In this context, AIoT is poised to offer innovative solutions to the mounting environmental challenges confronting smart cities and, by extension, smart eco-cities. AIoT holds the potential to unlock avenues for improving resource efficiency, reducing energy consumption, streamlining waste management, enhancing transportation management, conserving biodiversity, and mitigating environmental impacts. These advancements hold the power to reshape the urban development landscape in response to the surging wave of urbanization and the increasing complexity of ecological degradation, transforming cities into hubs of intelligence, sustainability, and environmental consciousness.
AI is rapidly reshaping both the technological and urban landscapes, serving as a pivotal driving force behind the advancement of smart cities and smart eco-cities (e.g., Ref. [ [1] , [2] , [3] , [4] ]). Its potential for disruption and innovation (e.g., Ref. [ 5 , 6 ]) has positioned it at the forefront of these developments. This influence profoundly alters the functioning of urban systems and the intricate interactions, behaviors, and responses of their subsystems to the surrounding environment. As a result, urban processes and practices are undergoing significant transformation, marked by their increasing alignment with data-driven scientific urbanism. The impact of AI on urban systems and activities has been consistently expanding [ 7 , 8 ], with its computing capabilities experiencing exponential growth to accommodate the mounting influx of data from diverse sources facilitated by IoT. The potential of IoT lies in its capacity to facilitate data analysis through AI models and algorithms, a synergy poised to catalyze environmentally sustainable urban development.
The centralized infrastructure of IoT is grappling with significant challenges, primarily driven by the extensive strain imposed by the immense volume of data being generated and processed. Harnessing actionable insights from these data necessitates the integration of AI models and algorithms to effectively manage the data flow, storage, and processing inherent in the IoT infrastructure. The emergence of AIoT is underpinned by various factors that set it apart from traditional IoT. To begin with, AIoT capitalizes on the synergies between AI and IoT technologies, facilitating more intelligent and efficient data processing, analysis, and decision-making (e.g., Ref. [ [9] , [10] , [11] ]). Through the integration of AI and Machine Learning (ML)/Deep Learning (DL) capabilities into IoT devices and systems, AIoT empowers real-time data insights, predictive analytics, and adaptive responses, thereby optimizing the overall system performance and efficiency. A key distinction lies in AIoT's ability to overcome the limitations of IoT in managing the copious and diverse data generated by the vast network of interconnected devices. Additionally, AIoT addresses the challenges associated with transmitting the rapid torrent of data from distributed sensor network infrastructure [ 12 , 13 ]. By harnessing the power of AI, AIoT effectively processes and contextualizes intricate and multifaceted data streams, unlocking the potential for advanced applications. Most notably in certain domains, AIoT paves the way for autonomous and intelligent decision-making by IoT devices, enabling them to learn, adapt, and optimize operations in response to shifting environmental conditions and user requirements. In essence, the emergence of AIoT introduces a realm of possibilities for innovation, optimization, and automation across diverse domains, including environmental sustainability, climate change, and smart cities (e.g., Ref. [ 1 , [13] , [14] , [15] , [16] ]).
Similar to AI, AIoT has become integral to the functioning of smart cities and, hence, smarter eco-cities. Notably, it has demonstrated innovative potential in addressing complex environmental challenges. Recent research has concentrated on the practical applications of AI and AIoT across various domains of environmental sustainability and climate change (e.g., Ref. [ [17] , [18] , [19] , [20] , [21] ]). Toward the end of 2020 onward, this focus has expanded to encompass smart cities in terms of their management and planning (e.g., Ref. [ [22] , [23] , [24] , [25] , [26] ]). Fundamentally, however, there is a strong interconnection between smart cities and eco-cities in that they have significantly influenced one another over the last decade, particularly in the domains of environmental sustainability and climate change. Eco-cities have long been associated with these two domains, serving as a well-established paradigm of sustainable urbanism (e.g., Ref. [ [27] , [28] , [29] , [30] , [31] , [32] , [33] ]). However, these two domains have frequently been addressed separately or, more recently, in connection with smart cities, particularly within the context of AI and AIoT, instead of being collectively considered within the framework of smart eco-cities. This suggests that there has been a strong tendency to prioritize one approach over another, specifically the second approach has been given a little attention. Especially, eco-cities and, by extension, smart eco-cities serve as experimental grounds for innovative solutions and environmental transitions (e.g., Ref. [ 27 , [34] , [35] , [36] ]). The experimentation within eco-cities extends beyond climate change to encompass energy transition, resource conservation, transport efficiency, biodiversity conservation, and experimental simulation and modeling [ [37] , [38] , [39] ]. These strategies and principles, in turn, form the fundamental driving forces behind the evolution of smart eco-cities.
Amid a world increasingly beset by uncertainties, compounded by the urgency of the climate crisis and the rapid digital transformation of smart cities and eco-cities, catalyzed by the emergence of AI and AIoT technologies, a compelling trajectory is emerging. This trajectory envisages the integration of these technologies' applied solutions into smart eco-cities to deal with the complexity of environmental degradation and climate disruption — under what can be termed as “smarter eco-cities.” This visionary concept entails the implementation of innovative, forward-looking strategies to reshape the urban landscape of the future. However, it is worth acknowledging that while AI and AIoT technologies hold immense potential yet to unlock, they pose environmental risks and amplify a spectrum of societal, ethical, legal, and regulatory challenges.
The body of literature exploring AI and AIoT solutions within the realm of environmental sustainability, climate change, and smart cities is rapidly expanding. Nonetheless, to the best of our knowledge, no review study has systematically analyzed and synthesized the existing corpus of knowledge regarding the interconnections and synergies between these three domains, coupled with their intersection with smart eco-cities with respect to AI and AIoT technologies and solutions. Furthermore, while a few recent review studies have taken a broader perspective on smart eco-cities, none have ventured into exploring their emerging technological and environmental solutions from an integrative perspective. Bridging these gaps, the present study embarks on a comprehensive systematic review of the burgeoning landscape of smarter eco-cities and their leading-edge AI and AIoT solutions for environmental sustainability. To ensure thoroughness, the study employs a unified evidence synthesis framework that seamlessly integrates configurative, aggregative, and narrative synthesis approaches. To achieve the overarching aim, the study focuses on the following specific objectives:
- • Describe, illustrate, and make meaningful connections between the fundamental concepts underpinning emerging smarter eco-cities.
- • Present the existing work in the field and explain how the present study differs from it and why this is a “step forward” and a new contribution to knowledge.
- • Analyze, synthesize, interpret, and critically evaluate the existing knowledge in the areas of environmental sustainability, climate change, and smart cities to derive comprehensive insights into the role of AI and AIoT solutions in the advancement of emerging smarter eco-cities.
- • Categorize AI and AIoT solutions based on these three areas and further evaluate their impact on advancing environmental sustainability goals.
- • Capture the dynamic landscape of AI and AIoT solutions for these three areas by identifying emerging trends, innovations, and novel approaches within the framework of smarter eco-cities.
- • Identify and discuss the key challenges and barriers that arise when implementing AI and AIoT solutions in the development of emerging smarter eco-cities.
- • Identify existing gaps and explore relevant avenues for future research and areas requiring further investigation.
By pursuing these specific objectives, the study endeavors to provide a holistic and in-depth understanding of the integration of AI and AIoT solutions within emerging smarter eco-cities, ultimately contributing to the advancement of environmental sustainability practices in urban contexts. Toward this end, it pursues the following research questions:
- RQ1: What are the foundational underpinnings of emerging smarter eco-cities, and how do they intricately interrelate, particularly urbanism paradigms, environmental solutions, and data-driven technologies?
- RQ2: What are the key drivers and enablers propelling the materialization of smarter eco-cities?
- RQ3: What are the primary AI and AIoT solutions that can be harnessed in the development of smarter eco-cities?
- RQ4: In what ways do AI and AIoT technologies contribute to fostering environmental sustainability practices, and what potential benefits and opportunities do they offer in the realm of smarter eco-cities?
- RQ5: What challenges and barriers arise when implementing AI and AIoT solutions for the development of smarter eco-cities?
By synthesizing the existing evidence and analyzing the state-of-the-art research to answer these research questions, the systematic review contributes to consolidating, enhancing, and transforming the existing knowledge on smart eco-urbanism by:
- • Uncovering the dynamjc interplay between urbanism paradigms, environmental solutions, and data-driven technologies in emerging smarter eco-cities.
- • Identifying the driving forces behind the materialization of smarter eco-cities, namely technological advancements, environmental concerns, and policy instruments.
- • Examining the multifaceted roles of AI and AIoT in environmental sustainability, climate change, and smart cities and how these technologies can be harnessed in the development of smarter eco-cities.
- • Exploring the specific ways in which AI and AIoT technologies propel sustainable development pracrices and advance environmental goals.
- • Highlighting best practices where AI and AIoT solutions can significantly contribute to improving the environmental sustainability of smarter eco-cities.
- • Identifying and evaluating a spectrum of challenges linked to the implementation of AI and AIoT in smarter eco-cities, aiming to illuminate potential obstacles and devise strategies to mitigate or overcome them.
- • Unveiling uncharted territories and encouraging the exploration of AI and AIoT solutions to drive large-scale implementations of smarter eco-cities, propelling the discourse forward and fostering innovation in sustainable urban development.
- • Providing a visionary outlook by elucidating how AI and AIoT technologies contribute to sustainable urban futures and exploring the transformative potential of these technologies in redefining urban infrastructures and systems.
In essence, the study not only presents a comprehensive overview of the current landscape of smarter eco-cities but also highlights the potential of AI and AIoT technologies in shaping the future of sustainable urban development, in addition to providing a roadmap for advancing the discourse on smarter eco-cities and facilitating interdisciplinary collaborations. Moreover, the applied unified evidence synthesis approach offers a more holistic and nuanced understanding of the research topic addressed by enhancing the thoroughness, depth, and breadth of the systematic review. The insights derived from the systematic review will not only inform researchers and practitioners in the field but also guide policymakers and practitioners in making informed decisions regarding the adoption and implementation of AI and AIoT technologies in sustainable urban management and planning. Overall, by highlighting the solutions, opportunities, benefits, and challenges in the field of smarter eco-cities, the systematic review will further facilitate the advancement of research, policy, and practice in pursuing more sustainable and technologically advanced urban environments.
This study is structured as follows: Section 2 introduces, describes, and illustrates the key conceptual strands of the study. Section 3 addresses the research review related to the study. Section 4 describes and illustrates the methodology applied in the study. Section 5 presents the results of the literature analysis and synthesis. Section 6 provides a detailed discussion, covering key challenges, open issues, and limitations. Section 7 identifies relevant gaps and presents recommendations for potential research directions and areas that require more exploration. This study concludes, in Section 8, with a summary of key findings and implications.
2. Conceptual background
Key relevant concepts need to be clarified together with their integrative and synergistic facets. The value of linking these concepts ( Fig. 1 ) lies in facilitating a better understanding of the foundational underpinnings of emerging smarter eco-cities in terms of urbanism paradigms, environmental solutions, and data-driven technologies.

Smarter eco-cities and their underlying urbanism paradigms, environmental solutions, and data-driven technologies.
2.1. Smarter eco-cities and their underlying urbanism paradigms
2.1.1. smart cities.
Smart cities have gained significant attention as a potential solution to address sustainability, resource management, and urbanization challenges. Numerous attempts have been made to define the concept of smart cities. They suggest many definitions and a plethora of directions to smart city development (e.g., Ref. [ [40] , [41] , [42] ]). The concept has undergone many changes over the past two decades. In this regard, it promotes from a technology-oriented approach, i.e., infrastructures, architectures, platforms, systems, applications, and models, to a people-oriented approach, i.e., stakeholders, citizens, knowledge, services, and related data. Accordingly, it encompasses various dimensions, and there is no universally accepted definition up till now. However, the working definition for this study is justified by its alignment with the research objectives and scope. Accordingly, a smart city denotes an urban environment that leverages advanced technologies and data-driven approaches to conserve resources, minimize its environmental impact, and enhance overall ecological well-being. It prioritizes energy efficiency, sustainable transportation, waste reduction, water conservation, environmental monitoring, and green infrastructure to create a more eco-friendly and livable environment while fostering economic growth for all residents. Smart cities are increasingly emphasizing the role of technological advancements and scalable data-driven solutions to foster sustainable development practices (e.g., Ref. [ [43] , [44] , [45] , [46] ]). By integrating technology with environmental stewardship, smart cities strive to create greener and healthier living environments.
However, smart cities face several challenges that need to be addressed to ensure their successful implementation as well as their integration with other emerging paradigms of urbanism. As mentioned earlier, one of the primary problems in this regard is the lack of a standardized definition for smart cities. This lack of clarity has led to confusion and inconsistency in the planning and implementation of smart city initiatives. Moreover, the existing smart city infrastructures are not designed to support the integration of advanced technologies and data-driven systems. They need to support the connectivity, data collection, and efficient management of resources, which involves scalability and interoperability. As smart cities grow and more devices and sensors are connected, their infrastructure must handle the increasing volume of data and the growing number of users. Integration and seamless communication between different systems and devices are crucial for the smooth functioning of their infrastructure. In addition, with the extensive use of data and connected devices in smart cities, ensuring the security and privacy of their infrastructure becomes paramount. These infrastructures must have robust security measures in place to protect against cyber threats and safeguard the privacy of citizens' data. Several studies (e.g., Ref. [ 47 , 48 ]) highlight the importance of addressing data security and privacy issues and device-level vulnerability in the context of smart cities. Furthermore, coordinating and integrating various domains to create a cohesive and integrated smart city ecosystem is a complex challenge. Smart cities should strategically use networked infrastructure and associated data-driven technologies to produce a smart economy, smart government, smart mobility, smart environment, smart living, and smart people. More so, the social, ethical, political, legal, and regulatory challenges facing smart cities have shown to be difficult to deal with. To address these challenges, a multidimensional approach focusing on technology, citizens, and institutions is necessary. This includes developing robust technology infrastructure, implementing effective governance models, and actively involving citizens in decision-making processes. Overall, while smart cities hold great potential for advancing sustainable urban development, they pose significant challenges that require a comprehensive approach to build successful and inclusive ecosystems.
2.1.2. Eco-cities
The concept of eco-cities refers to “an urban environmental system in which input (of resources) and output (of waste) are minimized,” [ 49 ]. With their ubiquity today, eco-cities vary in the strategies and solutions they prioritize in response to environmental challenges in different urban contexts. They are widely diverse in conceptualization, implementation, and development. Thus, there is no definitive definition of an eco-city but rather a collection of concepts, ideas, and ambitions [ 32 ]. Broadly, eco-cities are urban areas designed and developed with a strong focus on environmental sustainability and ecological balance [ 50 ]. They aim to minimize their ecological footprint and promote a harmonious relationship between humans and nature. They prioritize energy efficiency, renewable energy sources, waste reduction, green spaces, sustainable transportation, and resource conservation. They strive to create a sustainable living environment that supports the well-being of citizens and the surrounding ecosystems while fostering social inclusivity and economic prosperity. The ultimate goal of eco-cities is to create resilient, low-carbon, and environmentally friendly urban spaces that contribute to a more sustainable future ([ 51 , 52 ]).
2.1.3. Smart eco-cities
Eco-cities manifest themselves into different models based mainly on applying the principles of urban ecology or combining the strategies of sustainable cities and the solutions of smart cities. For the latter, the most prominent of those models are smart eco-cities, which integrate IoT and Big Data technologies with environmental technologies for achieving urban sustainability (e.g., Ref. [ 35 , [53] , [54] , [55] , [56] ]). Smart eco-cities refer to urban environments that integrate advanced technologies, data analytics, and intelligent systems to enhance sustainability, efficiency, and quality of life while prioritizing environmental well-being. Accordingly, they leverage data-driven technologies and solutions to promote the use of renewable energy, Biomass Combined Heat Power (BCHP), sustainable transportation (walking, cycling, car sharing, biogas cars), eco-cycle waste management, green infrastructure, urban metabolism, sustainable buildings, smart grids, and sustainable urban planning strategies to minimize environmental impact, conserve resources, and foster sustainable and resilient urban environments (see Refs. [ 53 , 57 ] for illustrative case studies).
Unlike smart cities, smart eco-cities go beyond technology-driven approaches and emphasize environmental sustainability and ecological balance as central pillars. They focus on the integration of sustainable practices and advanced technologies, incorporate nature-based solutions into urban planning and design, and engage communities in environmental stewardship. They aim to create harmonious urban environments that not only leverage technology but also prioritize preserving and enhancing natural resources, biodiversity, and ecosystem services. In essence, they strive for a more holistic and nature-centric approach to urban development, promoting long-term sustainability and resilience and preserving natural resources for future generations to create environmentally friendly and livable urban communities.
2.1.4. Smarter eco-cities
The concept of smarter eco-cities, as specific to this study, describes smart eco-cities that integrate AI and AIoT technologies and solutions with environmental technologies and strategies to maximize the performance of their sustainable systems and integrate them with smart systems given the clear synergies in their operation. This integration is intended to produce combined effects greater than the sum of the separate effects of these systems in terms of boosting the benefits of environmental sustainability. Smart city systems include smart grids, smart traffic lights, smart mobility, smart buildings, smart waste management, and smart environmental monitoring. Smarter eco-cities represent an evolution or advancement of smart eco-cities. They prioritize environmental sustainability, employing cutting-edge technologies and innovative approaches to energy, waste, water, transportation, and urban planning to create more resilient, sustainable, and technologically advanced urban environments. They also emphasize a more holistic and comprehensive approach to urban development by integrating the environmental, social, and economic dimensions of sustainability. Accordingly, they aim to balance environmental preservation, social equity, and economic prosperity. They leverage the advanced technologies and solutions offered by smarter cities, notably AI and AIoT, to optimize urban systems and address complex challenges in a more integrated and intelligent manner. They are characterized by — as derived based on the synthesized studies:
- • The innovative potential of AI and AIoT technologies for sustainability;
- • The enhanced sustainability outcomes enabled by the applied AI and AIoT solutions;
- • The synergies between smart city systems and eco-city systems;
- • The optimized performance and efficiency of smart eco-city systems; and
- • The improved practices of urban management and planning.
In sum, while smart cities focus on technology-driven urban development, smart eco-cities place a stronger emphasis on achieving environmental sustainability through IoT and Big Data technologies. Smarter eco-cities go a step further by incorporating social and economic dimensions, leveraging emerging AI and IoT technologies for a more holistic approach to urban development.
2.2. Data-driven technologies
2.2.1. iot, computing models, and big data.
The term “IoT” describes the collective network of physical objects embedded with sensing, processing, communication, and actuating technologies and capabilities that enable them to exchange data with other devices over the Internet or other networks. These objects, often called “smart devices,” can range from everyday items to complex systems like city infrastructure. IoT allows these devices to communicate and interact with each other, collect data, transfer data, and perform automated tasks, leading to enhanced efficiency, performance, and sustainability in various urban domains. More and more IoT devices are connected worldwide daily and feeding vast amounts of data into analytical systems. It is estimated that 2.5 quintillion bytes of data are being generated globally daily, which will rise to 463 exabytes by 2025 [ 58 ].
Edge, fog, and cloud computing are three interconnected paradigms that play a pivotal role in the IoT ecosystem in smart cities and smart eco-cities in the context of environmental sustainability [ 53 ]. Edge computing involves processing data at or near the data source, often within the device or a nearby gateway. It aims to reduce latency and improve real-time responsiveness by executing computations locally. It is particularly useful for applications that require quick decision-making and low-latency interactions, such as autonomous vehicles. Fog computing extends the concept of edge computing by creating a hierarchical architecture that includes multiple edge devices and gateways. Fog nodes are strategically placed in proximity to data sources to perform intermediate processing, data filtering, and preliminary analytics before transmitting relevant data to the cloud. This approach optimizes bandwidth usage and enhances system performance, making it suitable for scenarios involving distributed data sources and resource-constrained devices. Cloud computing involves using centralized remote servers to store, manage, and process data. It offers vast computational resources and storage capabilities, making it suitable for complex data analytics, machine learning, and large-scale processing. Cloud computing allows data to be accessed and analyzed from anywhere with an internet connection, making it ideal for applications that require extensive computation and storage capabilities. These computing paradigms collaborate to create a holistic IoT ecosystem that efficiently manages data processing and analysis across different levels of the network infrastructure.
Big Data refers to extremely large and complex datasets that cannot be easily managed, processed, or analyzed using traditional data processing techniques. They are huge in volume, high in velocity, diverse in variety, exhaustive in scope, fine-grained in resolution, and relational, among others. Big Data analytics involves using advanced techniques and technologies to extract valuable insights, patterns, and correlations from large and complex datasets. This process typically involves data collection, storage, processing, analysis, and visualization. In summary, IoT and Big Data are interconnected concepts revolutionizing how we collect, analyze, and utilize data. IoT enables the connectivity of smart devices, allowing them to generate vast amounts of data, while Big Data provides the means to manage, analyze, and derive meaningful insights from this data. Together, they have the potential to drive innovation, improve decision-making, and create new opportunities in diverse fields, including urban development [ 59 ].
2.2.2. AI models and techniques
AI is often described as mimicking human intelligent behavior by creating computers or machines capable of its simulation. The working definition for this study describes AI as “any device/system that perceives its environment and takes actions for its goals” [ 60 ]. Broadly, an artificially intelligent machine can learn by acquiring information on the surrounding environment [ 61 ], improving performance with knowledge from experience, and performing complex tasks in a way that is similar to how humans solve problems. The capabilities of AI systems involve data analysis and learning from external data using Natural Computing (NC) and ML (e.g., Ref. [ 62 , 63 ]); emulating human cognitive functions using Computer Vision (CV), Fuzzy Logic (FL), Natural Language Processing (NLP) (e.g., Ref. [ 61 ]); and dealing with the complexities of human thinking and emotion (e.g., [ 64 ]) using decision support, strategic planning, sequential actions [ 65 ], self-learning, and self-improvement [ 66 ]. Concerning NC, it simulates natural phenomena and utilizes natural material as computational media in computers to optimize ML algorithms [ 62 ]. Evolutionary computing (EC) is also used for continuous optimization and in complex optimization problems involving many variables. It is extensively applied to optimize ML models [ 67 ]. For example, evolution and ecology as biological phenomena inspire optimization algorithms [ 68 ]. ML can be based on FL in terms of imitating human reasoning and cognition using 0 and 1 as extreme cases of truth with various intermediate degrees, characterizing the space between black-or-white scenarios, or using fuzzy c-means clustering to separate each data point into different clusters based on probability score attribution. CV applies ML to take information from visual data by recognizing patterns and making meaningful decisions based on that information. In addition, ML overlaps, intersects, or can be used as a tool for different AI models, such as CV, FL, and NLP.
For example, NLP enables computers to understand, analyze, manipulate, and generate human language. NLP plays a significant role in smart cities by enabling efficient and effective communication between humans and smart systems. NLP techniques analyze and understand human language in various forms, allowing for intelligent interactions and decision-making. In smart cities, NLP can be applied in multiple domains, such as smart planning, smart governance, smart mobility, and smart services. By leveraging NLP, smart cities can enhance communication channels, improve service delivery, and gain valuable insights from citizen feedback, leading to more responsive and citizen-centric urban environments. Tyagi and Bhushan [ 69 ] explore the potential of NLP in optimizing Information and Communication Technology (ICT) processes for building smart cities. The study analyzes the architecture, background, and scope of NLP and presents its recent applications in various domains. The authors highlight NLP's role in advancing smart cities and discuss the open challenges in its implementation, aiming to emphasize NLP as a key pillar in building smart cities.
Furthermore, AI involves many techniques that have gained traction over the past few years as part of AI and AIoT applications for environmental sustainability, climate change, and smart cities. These techniques include Artificial Neural Network (ANN), Support Vector Machine (SVM), Linear Regression (LR), Decision Trees (DT), Random Forests (RF), Adaptive Neuro-Fuzzy Inference System (ANFIS), Batch-Normalization (BN), Convolutional Neural Networks (CNNs), Deep Neural Networks (DNNs), and Genetic Algorithm (GA). As regards ML, among the supervised learning techniques used for regression, classification, or both are LR, Generalized Linear Models (LGM), DT, RF, SVM, ANN, and Bayesian Networks (BN). As to DL, it is a biological neural network or brain-inspired type of ML that uses DNN, CNNs, and Recurrent Neural Networks (RNNs) algorithms. Thus, it emulates the way humans gain certain types of knowledge by collecting, analyzing, and interpreting large amounts of data and making decisions in a faster and easier manner. DL techniques leverage neural networks comprising three fundamental layers: the input layer, hidden layers, and the output layer. These layers play a crucial role in acquiring data representation and establishing connections across multiple levels of abstraction.
2.2.3. AIoT and its system pillars: A data science cycle perspective
To manage and analyze the dynamic and relational data generated via IoT increasingly requires powerful computational and analytical capabilities. This has led to the emergence of AIoT, a technological framework that optimizes the efficiency of IoT operations, improves human-machine interactions, advances data management and analytics models, and enhances decision-making processes. AIoT involves connecting and combining IoT devices and sensors with AI models and techniques to enable advanced analysis, enhanced real-time insights, intelligent decision-making, and autonomous behavior. It acts through control and interaction to respond to the dynamic environment, a process where ML/DL has shown value in enhancing control accuracy and facilitating multimodal interactions [ 13 ]. The synergy between AI and IoT through Big Data enables smarter and more efficient applications across various domains, driving innovation and enabling transformative solutions. IoT produces Big Data, which in turn requires “AI to interpret, understand, and make decisions that provide optimal outcomes” [ 70 ] pertaining to a wide variety of practical applications for urban systems in different context of urbanism [ 1 , 71 ]. In other words, IoT enables data-driven AI analytics to optimally accomplish complex tasks and extract useful knowledge in the form of applied intelligence. The resurgence of AI is driven by the abundance and potency of Big Data, thanks to enhanced computing storage capacity and real-time data processing speed.
AIoT enables the utilization of AI to incorporate intelligence and decision-making capabilities into IoT systems and applications. The AI/AIoT-driven system consists of five pillars: (1) sensing, (2) perceiving, (3) learning, (4) visualizing, and (5) acting. This is illustrated in Fig. 2 from a conceptually generic perspective, implying that this system can be tailored to various applications depending on their characteristics, requirements, and objectives. For example, Zhang and Tao [ 13 ] present the progress of AIoT research from four perspectives: (1) perceiving, (2) learning, (3) reasoning, and (4) behaving in connection with smart transportation, smart buildings, and smart grids. This entails empowering smart things with human-like cognitive and behavioral abilities to bring them closer to reality, which is essential to system operation.

The five pillars of an AI/AIoT-driven system: 1-sensing in charge of collecting raw data, 2-perceiving in charge of extracting semantically meaningful information from raw data, 3-learning in charge of learning to predict patterns, 4-visualizing in charge of communicating key insights, and 5-acting in charge of taking action to achieve a certain goal.
An AI/AIoT-driven system is characterized by the ability to process raw data to extract useful insights to enable better decisions and/or take action. This involves different interrelated computational capabilities and processes. Machine perception is the capability of the system to use input data from sensors (e.g., vision, audio, proximity, position, tactile, photoelectric, infrared, light, and ultrasonic) to deduce different facets of the world, e.g., object detection/tracking, action recognition, image classification, semantic segmentation, language recognition, and pose estimation. There are different sensory information that provides patterns to the system for it to generate perceptions. Overall, machine perception aims to translate these data into meaningful information, thereby recognizing and interpreting these data by capturing the sensory information to relate to the real world. The acquisition of sensory data from the surrounding environment and their correct interpretation are key inputs to the learning process. The common state-of-the-art method for learning is based on ML, which allows the system to learn from experience without explicitly being programmed. Learning starts with collecting and preparing data (e.g., sensory, numbers, human pictures, object images, records, texts) to be used as training data, building the ML model to be trained on these data, supplying the data, and letting the system train itself to find patterns or make predictions. The outcome is an ML model that can be used with different sets of data and can use the data for predictive, descriptive, prognostic, and prescriptive functions. For example, the latter function involves suggesting what actions to take.
Further, there are three subcategories of ML: (1) supervised learning (trained with labeled data sets by humans to identify objects or things), (2) unsupervised/semi-unsupervised learning (finding patterns in unlabeled data such as behaviors or trends), and (3) reinforcement learning (trained on trial and error to take the best action based on the right decision such as autonomous driving or automating routine functions). Ullah et al. [ 72 ] illustrate different supervised, unsupervised, and reinforcement learning algorithms. These add to transduction learning, multitasking learning [ 73 ], federated learning [ 74 ], transfer learning [ 75 ], and few-shot learning [ 76 ]. Moreover, to enable the system to learn from the interpreted data and the performed computations to generate repeatable outputs and reliable decisions commonly requires using large datasets to train ML models, thereby, the relevance of DL, which can be leveraged to improve the learning of the system and enable it to adapt to varied situations to enhance its performance. DL has attracted increased attention and has proven useful for improving the intelligence of AIoT applications to handle dynamic and complex environments in the context of unsupervised and reinforcement learning methods [ 13 ].
ML is about leveraging data to improve performance on complex tasks by building decision-making models (e.g., Ref. [ 14 , 72 , 77 , 78 ]). Speaking of tasks, Mitchell [ 79 ] conceives of ML as “a computer program learning from experience ‘E’ with respect to some class of tasks ‘T’ and performance measure ‘P,’ if its performance at tasks in ‘T’ as measured by ‘P,’ improves with experience E.’” The workhorses of the decision-making process are ML models and algorithms, given their role in systematically extracting useful knowledge from data (patterns, correlations, predictions, forecasts, etc.) that can support decision-making. For example, by using DL algorithms, the system can conduct real-time analysis of video streams, identify objects, and detect events with absolute precision using CV models for real-time traffic monitoring or analysis of traffic conditions. The recent development of ML is associated with its ability to apply complex mathematical calculations to colossal amounts of data in a repetitive and faster manner. Accordingly, the system renders the decision-making process more data-driven, accurate, clearer, and faster thanks to ML. It makes decisions based on the perceived patterns in the data it receives. Examples of decisions in this regard may include automating a waste management process, enhancing an energy operation, optimizing a planning function, improving an environmental strategy, adjusting a policy, and evaluating risk.
As the decision-making process is based on data-driven insights, data visualization becomes important in terms of conveying complex data in such a way as to make it easier for humans to better comprehend and react to these data. Data visualization entails using specialized algorithms to generate graphical representations or visual displays of data using such elements as charts, infographics, maps, images, animations, and other metaphors. It enables decision-makers to gain and pull insights more rapidly by exploring, monitoring, and interpreting data. Examples of data visualization include city dashboards, cityScore, city metabolism, and situation rooms.
Finally, the last process of the system is acting to maximize a certain goal. To interact with the environment and humans, the system should be able to reason/make inferences and behave. Acting is associated with actuation mechanisms, which enable communication in a smarter eco-city environment and perform output functions. A wide range of actuators constitutes an integral part of the sub-systems of smarter eco-cities for operations, functions, and services. Actuation aims to execute actions to optimize different smart systems, such as power grid, building, transport, traffic, street lighting, waste management, and water distribution. The optimization occurs through adding, minimizing, adjusting, and transferring resources. In this respect, actuation is central to AIoT applications for monitoring things, controlling things, ranging things, operating things, repairing things, evaluating things, and assigning things, to name a few. Functions in smart cities “enable the actuation mechanisms to be employed directly on the IoT-enabled smart devices” [ 80 ]. For a detailed review of smart city actuators, the reader might be directed to Ref. [ 81 ]. However, developing more response systems and actuators is required to improve the engineering applications of AI and AIoT and enable their implementation in emerging smarter eco-cities concerning the performance and behavior of their physical systems.
The rationale for adopting a data science type of cycle as to the pillars of the AI/AIoT system is to emphasize the iterative and data-driven nature of the system in line with most of the synthesized studies reporting on the relationship between AIoT environmental sustainability, climate change, and smart cities. By incorporating data science principles, we seek to highlight the importance of leveraging large volumes of heterogeneous data in AIoT applications. This approach enables the extraction of meaningful insights and the development of predictive models, leading to enhanced decision-making processes and improved system performance. Highlighting the data science cycle provides a holistic AI/AIoT ecosystem perspective. It emphasizes the continuous feedback loop, where data are collected, analyzed, and fed back into the system to optimize its functionality and adaptability. This iterative process aligns with the dynamic nature of AI and AIoT applications, where data from various sources continuously flow and contribute to the intelligence and effectiveness of the overall system.
3. A review of related literature studies
In this section, we present a survey of the existing work conducted in the field of emerging smarter eco-cities and their applied AI and AIoT solutions. This survey aims to provide a comprehensive overview of the current state of research, highlighting the key findings, contributions, and trends in the field. By examining the existing literature, we aim to identify gaps and opportunities for further exploration in developing and implementing smarter eco-cities. This survey serves as a foundation for our comprehensive systematic review, enabling us to synthesize and analyze the findings in a structured and rigorous manner.
It was not until more recently that the literature on AI and AIoT applications for environmental sustainability and climate change started to grow and extend across many domains and disciplines. Several reviews have been performed on AI and AIoT in improving or advancing the different areas of environmental sustainability and climate change ( Table 1 ).
Table 1
A set of literature review studies on AI solutions for environmental sustainability and climate change.
3.1. AI for environmental sustainability and climate change
The review studies on energy conservation and renewable energy contribute to understanding various approaches and strategies for achieving energy efficiency and promoting renewable energy sources in different contexts. These studies highlight the importance of technological advancements, policy frameworks, and behavioral changes in achieving sustainable energy practices. In the field of water resources conservation, review studies contribute to the knowledge on water management practices, including efficient water use, water conservation strategies, and the impact of climate change on water resources. These studies emphasize the need for integrated water resource management and sustainable water use practices to address water scarcity and ensure long-term water sustainability. The research review on waste management demonstrates the significant contributions of AI in waste management, ranging from enhanced waste sorting to optimized collection routes, predictive maintenance, and Decision Support Systems (DSS). These advancements can improve resource efficiency, reduce environmental impact, and promote sustainable waste management practices. The review studies on biodiversity and ecosystem services provide insights into the importance of biodiversity conservation and the role of ecosystem services in sustaining human well-being. These studies emphasize the need for conservation measures, habitat restoration, and the integration of ecosystem services into decision-making processes for sustainable development. In the context of sustainable transportation, studies shed light on various aspects of sustainable transportation, including electric vehicles, intelligent transportation systems, and multimodal transportation options. These studies highlight the potential of sustainable transportation solutions in reducing CO 2 emissions, improving air quality, and enhancing urban mobility. Finally, in the domain of climate change adaptation and mitigation, review studies contribute to understanding the challenges and opportunities in addressing climate change impacts. These studies explore adaptation strategies, mitigation measures, and policy frameworks to reduce GHG emissions and build resilience to climate change. Collectively, these review studies provide valuable insights into various aspects of environmental sustainability and climate change and contribute to the knowledge base in their respective fields. They highlight the importance of adopting holistic approaches, integrating multiple disciplines, and considering technological and policy dimensions to address environmental challenges and promote sustainable practices.
3.2. AI for smart cities
Some reviews have been conducted on the link between AI, IoT, and Big Data in smart cities from a more general perspective (e.g., Ref. [ 1 , 106 , 107 ]), broadly addressing different domains beyond environmental sustainability without providing technical details. Allam and Dhunny [ 106 ] focus mainly on the role of AI in building smart cities, addressing metabolism, governance, and culture, and identifying the strengths and weaknesses of AI. The study contributes to understanding the intersection between Big Data, AI, and smart cities. It explores the potential of utilizing these technologies in the context of smart cities, highlighting their impact on various aspects, such as resource optimization, urban planning, and transportation. It provides insights into the challenges and opportunities associated with integrating Big Data and AI in smart city initiatives. Bibri et al. [ 1 ] examine the research trends and driving factors of environmentally sustainable smart cities and their converging AI, IoT, and Big Data technologies. The authors show that environmentally sustainable smart cities have experienced rapid growth during 2016–2022, driven by both the digitalization and decarbonization agenda and the rapid advancement of data-driven technologies. The study highlights the importance of addressing the environmental costs and ethical risks associated with these technologies. The findings provide insights for scholars, practitioners, and policymakers developing data-driven technology solutions and implementing environmental policies for smart cities. Navarathna and Malagi [ 107 ] focus on the role of AI in smart city analysis. The study examines the application of AI techniques in analyzing the vast amount of data generated by smart city systems. It explores how AI can enhance decision-making processes, optimize resource allocation, and improve the overall efficiency and sustainability of smart cities. It contributes to understanding how AI can be leveraged to address smart city development challenges and complexities.
3.3. AIoT: theoretical foundations and practical applications
Few review studies have been carried out on AIoT as an emerging technological area. Mukhopadhyay et al. [ 108 ] highlight the importance of sensors in IoT systems and their integration with AI. The authors emphasize the need for efficient, intelligent, and connected sensors to make smart decisions and communicate collaboratively. They also mention the emergence of advanced AI technologies that enable sensors to detect performance degradation, identify patterns, and promote innovation. The focus is on sensors, smart data processing, communication protocols, and AI to enable the deployment of AI-based sensors for future IoT applications. Shi et al. [ 11 ] focus on the convergence of IoT and AI. The study compares two AI forms, knowledge-enabled AI and data-driven AI, highlighting their respective advantages and disadvantages. It surveys recent progress in the integration of AI throughout the IoT architecture, covering the sensing, network, and application layers. Zhang and Tao [ 13 ] explore the concept of AIoT and its potential to empower IoT. The study presents a comprehensive survey on AIoT, showcasing how AI techniques, particularly DL, can enhance IoT speed, intelligence, sustainability, and safety. It discusses the AIoT architecture within the context of cloud computing, fog computing, and edge computing. It also highlights promising applications of AIoT and outlines the challenges and research opportunities in this field. Both studies contribute to the understanding of the convergence of IoT and AI, with the first study focusing on the general significance and progress in the integration and the second study specifically exploring the concept of AIoT and its implications for IoT. Mastorakis et al. [ 12 ] take a broader perspective on the convergence of AI and IoT, covering various topics related to AI methods in IoT, including research trends, industry needs, and practical implementation. Their work balances theoretical concepts and real-world applications through case studies and best practices. It serves as a comprehensive resource for researchers and practitioners interested in the integration of AI and IoT. Both contributions provide insights into the integration of AI and IoT technologies and the understanding of AIoT and its applications in specific contexts and the broader IoT landscape. Together, these studies provide valuable insights into the advancements, challenges, and potential applications of AIoT, offering a foundation for further research and development in this area.
The previous review studies on AI and environmental sustainability, AI and climate change, AI and smart cities, and AIoT have laid a strong foundation for understanding the opportunities, benefits, and challenges in creating environmentally sustainable and technologically advanced urban environments. However, with the rapid advancement of AI and AIoT technologies, there is a need to explore the specific intersection of these technologies and existing smart eco-cities and their underlying multifaceted dimensions. The emerging field of AI and AIoT presents new possibilities for tackling the complexity of ecological degradation and the climate crisis in urban areas. By conducting a comprehensive systematic review of emerging smarter eco-cities and their leading-edge AI and AIoT solutions, we can bridge the gap between these solutions and the existing research on environmental sustainability, climate change, and smart cities within the defining context of smarter eco-cities. Overall, this review is the first of its kind and seeks to bring new insights into the flourishing field of smart eco-urbanism and extend the knowledge of its diverse domains by synthesizing a plethora of studies from multiple sources and disciplines.
4. Materials and methods
A systematic literature review addressed the three questions guiding the study and, hence, achieved its specific objectives. It involves retrieving, mapping, aggregating, configuring, and critically evaluating studies published to address and discuss the research topic of smarter eco-cities as an interdisciplinary field [ 109 ]. It allows for the mining of relevant information from the continuously expanding corpus of publications [ 110 ]. As illustrated in Fig. 3 , the study consists of nine key stages: (1) research focus and scope definition, (2) literature search, (3) screening and selection, (4) data extraction, (5) critical evaluation, (6) synthesis and analysis, (7) interpretation and narration, (8) existing gaps and areas requiring further investigation, and (9) summary and manuscript preparation.

Flow diagram outlining the process of conducting the systematic review.
Regarding stages 2 and 3, we followed the Preferred Reporting Items for Systematic Reviews and Meta-analyses (PRISMA) approach for literature search and selection [ [111] , [112] ]. Fig. 4 shows the four-phase flowchart literature search and selection process related to this approach. Among the available pool of academic research databases, SCOPUS was selected given its broad coverage of 455 high-quality peer-reviewed studies related to the topic on focus that meets strict standards for rigor. This online platform is one of the most reliable and trustworthy academic literature sources. To retrieve the scholarly literature, we developed a broad-based search string covering the different topics of the study and the associated links. Accordingly, the search string included: “smart eco-cities,” “smart cities AND internet of things,” “smart cities AND artificial intelligence OR machine learning OR deep learning,” “smart cities AND environmental sustainability,” “environmental sustainability AND artificial intelligence OR machine learning OR deep learning,” “climate change AND artificial intelligence OR machine learning,” “artificial intelligence of things AND environmental sustainability”, “artificial intelligence of things AND smart cities,” “artificial intelligence of things AND climate change,” “artificial intelligence AND smart eco-cities,” “blockchain AND environmental sustainability,” and “blockchain AND artificial intelligence.” These were used to search against the title, abstract, and keywords of articles to produce initial insights. We then refined and narrowed the reading scope, focusing on the documents providing definitive primary information. Accordingly, titles and abstracts from these documents were screened to select those focused on the relationships between smart cities, smart eco-cities, environmental sustainability, climate change, AI and AIoT technologies, and IoT and Big Data technologies. After excluding overlaps, 230 documents remained in the database. These were checked, and 12 papers were excluded as they did not include information on the relationships in question. Afterward, we explored citation tracking or reference chaining techniques to uncover additional relevant sources. Accordingly, the reference sections of the remaining papers were checked, and 17 other relevant papers were added to the final database, which included 235 documents in total. This was considered reliable when conducting a systematic review [ 113 ].

The PRISMA flowchart for literature search and selection. Adapted from [ [111] , [112] ].
The reviewed articles were published in prominent journals and conferences in urban planning, sustainable urban development, computing, and emerging technologies. Among these outlets were “Sustainable Cities and Society,” “International Conference on Smart Sustainable Cities,” “IEEE Transactions on Sustainable Computing,” “Environmental Modeling and Software,” “Cleaner Production,” “Environment and Urban Systems,” “Renewable and Sustainable Energy Reviews,” “Applied Energy,” “Sustainability,” and “Technological Forecasting and Social Change.” These outlets showcased the relevance and significance of the research in advancing the understanding and implementation of AI and AIoT solutions in the context of smart eco-cities.
The literature search was conducted in late March 2023 and returned 455 documents covering 2015 to 2023. The starting year was selected because it marked the approval of the 2030 Agenda for Sustainable Development by the United Nations General Assembly as an international policy framework for the 17 SDGs. The full period, 2015–2023, captures the multifaceted nature of the topic of smarter eco-cities from the perspective of AI and AIoT technologies concerning environmental sustainability, climate change, and smart cities. This is determined by an earlier bibliometric study conducted by Bibri et al. [ 1 ], highlighting several urban trends and events highly relevant to the current study.
Data extraction and synthesis are crucial steps in conducting a systematic review. These steps involve extracting relevant information from the included studies and synthesizing the data to identify themes and patterns. Concerning stage 4, we developed a structured Excel spreadsheet that specifies what information needs to be extracted from these studies, following a deductive approach to content analysis. This information included study characteristics, methodological approaches, technological and sectoral domains, AI models and techniques applied in environmental sustainability and climate change, applied AI and AIoT solutions in smart cities, linkages between smart cities and smart eco-cities, use cases and applications, and knowledge gaps, among others. In terms of methodological approaches, for example, studies were qualitative based on descriptive analysis and literature review, mixed-methods, and quantitative approaches based on modeling and simulation regarding AI and AIoT. Moreover, we evaluated the quality and relevance of the selected documents through a critical appraisal process. This involved analyzing the methodologies used in these documents to assess the strengths and weaknesses of the research approaches. During data extraction, we carefully read and analyzed each included study to identify and record the relevant information.
As regards stage 5 and 6, the primary focus of this study was to identify, make, establish, and project interconnections between the different dimensions of smarter eco-cities based on the synthesized studies. The synthesized analysis involved integrating the extracted data from multiple studies to identify patterns, trends, similarities, and differences across studies to generate findings or conclusions. The synthesis approach applied seamlessly integrated configurative, aggregative, and narrative synthesis as qualitative analytical approaches. Themes were derived from the research objectives and findings from the reviewed studies. The synthesis was performed based on these themes using an integrated approach. Specific to this study, this approach attempted to strike a balance between theoretical, empirical, and practical issues. Accordingly, it included evidence from case studies, exploratory studies, observational studies, experimental studies, theory-building studies, statistical modeling studies, and review studies. The findings of the synthesized studies were merged based on a set of specified conceptual and descriptive categories. This process entailed integrating and fusing information from the multiple studies reporting on the different dimensions of emerging smarter eco-cities and related direct and indirect linkages. This categorization evolved as more precise themes were identified and revised (combined, separated, refined, or discarded). From the identified categories, themes were organized to offer new interpretations beyond the synthesized studies' findings using three different — yet complementary — approaches to synthesis ( Fig. 5 ).

A framework for unified evidence synthesis and its characteristics.
Configurative synthesis involves interpretation during the synthesis process to identify the big picture and construct the overall meaning, i.e., thematic synthesis [ 114 ]. It identifies common themes across the studies and developing a conceptual framework to explain the interconnections and variations observed in the findings. It aims to provide a deeper understanding of the research evidence by exploring the relationships and context in which the findings occur. On the other hand, aggregative synthesis involves summarizing and combining multiple research studies to produce an overall summary of the findings [ 115 ], where the interpretation is performed after the synthesis process to frame the findings, i.e., thematic summary. It aims to provide a comprehensive overview and evaluation of the research evidence. In other words, it adds and leverages evidence to make statements based on particular conceptual positions [ 114 ]. Overall, configurative synthesis goes beyond the aggregation of the research findings and focuses on understanding the underlying patterns, arrangements, or relationships among the research findings [ 116 ]. As a form of storytelling, narrative synthesis involves summarizing, explaining, and integrating the research findings from individual studies through a narrative approach. It focuses on providing a descriptive and interpretive account of the research evidence, often using textual descriptions [ 117 ]. It allows for investigating the similarities and differences between multiple studies and exploring their relationships [ 118 ]. It aims to combine diverse perspectives and findings from multiple studies to generate a coherent narrative highlighting the key concepts, themes, and implications emerging from the research. In this respect, it may also involve identifying common themes or patterns across the studies and providing an overall narrative of the findings.
To present the results, we consolidate all pertinent information concerning emerging smarter eco-cities as an interdisciplinary field. This consolidation encompasses a wide spectrum of theoretical, empirical, and practical evidence and pertains to the various dimensions of smarter eco-cities, highlighting their synergies in producing and enhancing environmental sustainability benefits. Fig. 6 provides a structured and navigable representation of the results.

An overview of the main conceptual categories identified and their relationships.
5.1. The relationship between data-driven technologies, environmental sustainability, smart cities, and smart eco-cities
5.1.1. on the early adoption of iot and big data technologies in smart cities in the field of environmental sustainability.
To become environmentally smarter and more sustainable, both smart cities and eco-cities have undergone large-scale digital transformation enabled by the convergence of IoT, Big Data, and AI technologies. This has occurred at varying degrees and in different periods, given the specific focus of these two paradigms of urbanism in terms of strategies, solutions, and policies. Accordingly, in the early 2010s, numerous studies addressed the role of ICT in tackling the challenges of environmental sustainability in the realm of smart cities in various domains, notably:
- • Power grids: to deliver energy and manage its production, consumption, and distribution to reduce costs and increase the reliability of energy supply (e.g., Ref. [ 119 , 120 ]).
- • Environmental management: to manage natural resources and related infrastructure to improve environmental sustainability (e.g., Ref. [ 119 , 121 , 122 ]).
- • Transportation management: to optimize transport efficiency and manage mobility by taking into account traffic conditions and energy usage (e.g., Ref. [ 121 , [123] , [124] , [125] ]).
- • Waste management: to collect, recycle, reuse, recover, and dispose of different types of waste (e.g., Ref. [ 126 , 127 ]).
ICT enables the implementation of smart grids, which use advanced sensors, communication networks, and data analytics to optimize energy production, consumption, and distribution. This helps reduce costs, improve energy efficiency, integrate renewable energy sources, and enhance the reliability and resilience of the power grid. Moreover, ICT tools and systems facilitate the monitoring, analyzing, and managing of natural resources and related infrastructure. With sensors, remote sensing technologies, and data analytics, environmental parameters such as air quality, water quality, and waste management can be monitored and controlled in real time, enabling more effective environmental sustainability practices. Also, ICT applications contribute to optimizing transport efficiency and managing mobility in smart cities. Intelligent transportation systems, traffic management systems, and real-time data analysis help monitor traffic conditions, optimize routes, and improve energy usage. This leads to reduced congestion, better transportation planning, and reduced energy consumption and GHG emissions. Furthermore, ICT solutions are utilized to improve waste management processes. These include systems for waste collection, recycling, and disposal and technologies for waste monitoring, sorting, and tracking. By optimizing waste management operations and promoting recycling and resource recovery, ICT enables more sustainable and efficient waste management practices. ICT has played a vital role in transforming cities into smart and sustainable environments by enabling key advancements to achieve greater environmental sustainability and resilience in urban areas.
Concurrently, smart cities started to focus on embedding the next–generation of ICT into everyday objects and city structures and systems as part of the early deployment of IoT (e.g., Ref. [ 128 , 129 ]), paving the way for merging digital technologies with urban infrastructures and coordinating and integrating these through digital instrumentation and hyper-connectivity. One of the comprehensive theoretical and empirical studies on smart cities and IoT and Big Data conducted by Batty et al. [ 128 ] defined some goals concerning the development of a new understanding of environmental issues and the identification of critical problems relating to transport, energy, mobility, risks, and hazards. The authors additionally identified some challenges in using management, control, and optimization processes to connect smart city infrastructures to their operational functioning and planning.
During the period 2012–2015, smart cities gained traction as a model for sustainable urban development, with great potential to improve environmental sustainability based on IoT and Big Data Technologies (e.g., Ref. [ [130] , [131] , [132] , [133] , [134] , [135] , [136] ]). This traction stimulated a debate on how innovative data-driven IoT technologies could efficiently manage natural resources and mitigate environmental impacts in response to the rapid pace of urbanization and its potential effects on jeopardizing the sustainability of smart cities. Consequently, IoT and Big Data technologies gained further momentum in the pursuit of environmental sustainability across the different domains of smart cities, especially transport, mobility, energy, waste, pollution control, air quality, and planning (e.g., Ref. [ [137] , [138] , [139] , [140] ]). Subsequently, they became essential to the functioning of smart cities (e.g., Ref. [ 40 , [141] , [142] , [143] , [144] , [145] ]). This was manifested in the processes and practices of smart cities becoming “highly responsive to a form of data-driven urbanism” [ 146 ]. IoT and Big Data technologies provide the ability to monitor urban operations, functions, and structures using advanced forms of decision-making in urban intelligence functions for design and planning to improve environmental sustainability.
5.1.2. The influence of the IoT and Big Data Technologies of smart cities on the materialization of smart eco-cities
It is until around 2014 that smart cities started to have a significant impact on eco-cities for environmental sustainability (e.g., Ref. [ 57 , [147] , [148] , [149] , [150] , [151] ) thanks to the adoption of IoT and Big Data technologies as advanced forms of ICT. [ 54 ]) traces the evolution of smart cities and eco-cities over the past two decades regarding how their conceptual trajectories have converged under “smart eco-cities” from the mid-2010s onwards. The author highlights how this new paradigm of smart, sustainable urbanism is set to leverage the potential of IoT, Big Data, and digital infrastructures to integrate urban and green visions and policies. Smart eco-cities became widespread around 2016/2017 (e.g., Ref. [ 35 , 152 ]) as “a potential niche where environmental and economic reforms can be tested and introduced in areas which are both spatially proximate … and in an international context … through networks of knowledge, technology and policy transfer and learning” ([ 56 ], p. 1). Ever since, IoT and Big Data technologies and their applied solutions have become instrumental in the functioning of smart eco-cities concerning transport, mobility, energy, waste, pollution control, air quality, and planning.
To expand on the relationship between smart cities and eco-cities from an empirical perspective, Bibri and Krogstie [ 53 ] examine and compare the eco-city of Stockholm and the smart city of Barcelona, focusing on the innovative potential of IoT and Big Data technologies for advancing the goals of environmental sustainability. The authors show that smart grids, smart meters, smart buildings, smart environmental monitoring, and smart urban metabolism are the main data-driven IoT solutions adopted to enhance the performance of data-driven smart cities and smart-eco-cities under what they term “environmentally data-driven smart sustainable cities.” They also demonstrate the clear synergy between the eco-city and smart city solutions as to producing “combined effects greater than the sum of their separate effects — concerning energy efficiency and conservation improvement, environmental pollution reduction, renewable energy adoption, and real-time feedback on energy and material flows.” Pasichnyi et al. [ 153 ] propose, as part of case study research, a novel data-driven smart approach to the strategic planning of retrofitting building energy that allows a holistic city-level analysis and assesses change in total energy demand from large-scale retrofitting. Similar to Stockholm City [ 53 ], the energy transition model of Barcelona as one of the leading smart cities in Europe, aims to produce a 100 % certified renewable energy supply plan through smart energy [ 154 ]. Many other eco-cities, mainly from Europe and China, have adopted IoT and Big Data technologies in the domains of energy, transport, waste, water, and planning (e.g., Refs. [ 35 , 54 , [54] , [55] , [56] ]). A recent comprehensive state-of-the-art review conducted by Bibri [ 27 ] on smart eco-cities reveals that the newly planned and ongoing eco-city project developments are increasingly trialing innovative smart technologies to improve several aspects of environmental sustainability and climate change. This entails leveraging the advantages of eco-cities and smart cities and capitalizing on the synergies between their approaches and solutions, ultimately empowering eco-cities to enhance their environmental performance. Besides, IoT and Big Data technologies will fundamentally and irrevocably transform the landscape of eco-urbanism in terms of how eco-cities will be monitored, understood, analyzed, managed, planned, and governed.
5.1.3. The influence of policy instruments and government initiatives on the materialization of smart eco-cities
The materialization of smart eco-cities is strongly influenced by, in addition to technological advancements and environmental concerns, policy instruments. Technological advancements provide the tools and capabilities for these cities to integrate and optimize various urban systems for sustainability. Environmental concerns drive the need for these cities to adopt smart and eco-friendly solutions. However, policy instruments play a crucial role in shaping and guiding the development of smart eco-cities. Policy frameworks, regulations, and incentives create an enabling environment for implementing sustainable practices and innovative technologies and ensure the effective integration of smart solutions into urban environments. The effective use of policy instruments is essential in harnessing the full potential of technological advancements and addressing environmental challenges to realize the vision of smart eco-cities. Indeed, governance and smart eco-cities are deeply intertwined in a self-reinforcing relationship. To put it differently, governance is at the core of smart eco-cities (e.g., Refs. [ 35 , 53 , 56 , 57 , 155 ]), and its key function is to make and implement policy. One of the key roles of urban policy — as a set of plans, laws, rules, regulations, and actions — lies in aligning and mobilizing the stakeholders involved in the governance of smart eco-cities. Smart eco-cities require targeted policies to drive progress in various environmental sustainability areas and effectively implement innovative data-driven solutions. Overall, policy instruments play a crucial role in the materialization of smart eco-cities by providing a framework for planning, implementing, and regulating various initiatives. Among the policy instruments that facilitate the materialization of smart eco-cities [ 35 , 55 , 56 , [154] , [155] , [156] ] are:
- • Government regulations: The government enacts regulations that mandate specific sustainability standards and requirements for smart eco-city development, e.g., setting energy efficiency targets for buildings, enforcing waste management practices, and promoting renewable energy integration.
- • Financial incentives: Governments and local authorities provide financial incentives to encourage the adoption of smart technologies and sustainable practices, including tax incentives, grants, and subsidies for smart eco-city projects that incorporate smart solutions.
- • Public-private partnerships: Governments can partner with technology and energy companies, research institutions, and industry stakeholders to develop and implement sustainable solutions. These partnerships leverage expertise, resources, and funding to implement smart applications successfully.
- • Open data initiatives: Governments can promote sharing data collected from various sources, such as sensors and IoT devices, to foster innovation and facilitate evidence-based decision-making. These initiatives enable researchers, businesses, and policymakers to access and analyze information to develop smart solutions and monitor the performance of smart eco-city projects.
- • Standards and certification programs: Establishment of these programs ensures the interoperability, reliability, and safety of smart solutions in smart eco-cities. Standards organizations and certification bodies can define technical requirements, data privacy guidelines, and cybersecurity protocols to promote trust and facilitate the adoption of advanced technologies in smart eco-cities.
These policy instruments create a supportive environment for the materialization of smart eco-cities. They provide guidance, incentives, and regulations that drive sustainable development, promote technological innovation, and enhance the overall quality of life for residents. As revealed by Joss and Cowley [ 304 ], based on a comparative case study analysis, policy is found to exercise a strong shaping role in what sustainable development for cities is understood to be, which helps explain the considerable differences in priorities and approaches across countries.
5.2. The rise of AI and AIoT in environmental sustainability, climate change, and smart cities: solutions, use cases, and applications
This subsection is concerned with the solutions, applications, and use cases in the realm of AI and AIoT technologies and innovations. In this context, a solution represents a comprehensive approach encompassing software, hardware, processes, and strategies to solve a particular problem. It is implemented in real-world use cases, which depict practical scenarios illustrating how data-driven technologies work in action. On the other hand, an application refers to specific software designed to perform tasks, often utilizing both AI and AIoT models and algorithms. While these terms can overlap, they offer distinct perspectives: applications focus on software functionality, solutions provide holistic problem-solving approaches, and use cases offer practical insights into real-world implementations, collectively advancing data-driven technologies and innovations.
The use and application of AI and AIoT in environmental sustainability, climate change, and smart cities have dramatically increased from 2016 onward. This is seen as a sequel to the wide adoption of IoT and Big Data technologies and solutions in the various domains of smart cities for advancing environmental sustainability, as documented by many studies (e.g., Ref. [ [137] , [138] , [139] , [140] , [143] , [157] ]). As presented below, the empirical, theoretical, and literature research on AI and AIoT solutions, applications, and use cases involve the different areas of environmental sustainability, climate change, and smart cities. These areas are organized into two main periods: 2016–2019 and 2020–2023, which were identified based on an earlier bibliometric study conducted by Bibri et al. [ 1 ]. This study explores the key research trends and driving factors behind the emergence of environmentally sustainable smart cities and maps their thematic evolution over time. It demonstrates the rapidly growing trend of this emerging paradigm of urbanism that markedly escalated during 2016–2022 due to the accelerated digitalization and decarbonization agendas — due to COVID-19 and the rapid advancement of data-driven technologies. Accordingly, the two periods were derived based on the accelerated digitalization of smart cities prompted by COVID-19 in early 2020 and what this entails in terms of harnessing digital technologies for addressing environmental issues (e.g., Ref. [ [158] , [159] , [160] ]), thereby the relevance of subdividing the full period into two distinct sub-periods. Also, several other studies in early 2020, as mentioned earlier, emphasized the increasing recognition of the complexity of environment degradation and climate change challenges and the growing need for more innovative, advanced, and immediate solutions based on emerging data-driven technologies to tackle them. As to the starting year of 2016, it has marked the materialization of smart eco-cities (e.g., Ref. [ 27 , 35 , 152 ]), as discussed in Section 4 .
5.2.1. The first period: 2016–2019
5.2.1.1. environmental sustainability, empirical ai research.
The following empirical studies have significantly contributed to the advancement of emerging smarter eco-cities by applying AI and ML models and techniques to various aspects of environmental sustainability.
- • Water resources conservation: ML techniques, such as ANN, SVM, FL, ANFIS (an ANN-based on FL), LR, and Key-Nearest Neighbors (KNN), have been applied to predict stream flow and examine water quality parameters (e.g., Ref. [ 161 , 162 ]). Some studies have adopted ML techniques and DSS, such as ANN, DT, GA, FL, and ANFIS, to analyze water chemistry and assess water quality (e.g., Ref. [ 163 , 164 ]). These models and techniques have also been applied to hydro-meteorological forecasting and leak detection [ [165] , [166] , [167] ].
- • Energy conservation and renewable energy: Energy-related studies have harnessed ML and DSS with techniques such as ANN, FL, SVM, DT, Evolution Strategies (ES), Evolutionary Computing (EC), BN (an algorithmic method that makes the training of DNN faster and more stable), and ANFIS for tasks including energy operation, production, distribution, maintenance, and planning (e.g., Ref. [ 92 , 161 , [168] , [169] , [170] ]).
- • Sustainable transportation: ML has been applied to traffic forecasting using ANN, DT, and time series models [ 171 , 172 ]. Additionally, transport-related studies have employed ML and DSS with ANN, DT, NC, FL, SVM, LR, and time series models (e.g., Ref. [ 173 , 174 ]).
- • Biodiversity conservation and ecosystem services: ML techniques, including FL, GA, ARIES, and BN (an algorithmic method used for modeling networks in ecosystems), have been used to address different aspects of biodiversity conservation and assess ecosystem services (e.g., Ref. [ 98 , [175] , [176] , [177] , [178] , [179] ]).
The insights gained from the studies conducted on water resources conservation can inform effective water resources management strategies, aiding in the conservation and sustainable use of water in existing smart eco-cities. By utilizing AI algorithms, energy conservation and renewable energy studies have contributed to energy conservation efforts and the integration of renewable energy sources within existing smart eco-cities. As to sustainable transportation, related studies have provided valuable insights for better transportation planning and management, facilitating more efficient and sustainable mobility within existing smart eco-cities. The research on biodiversity conservation and ecosystem services has contributed to a deeper understanding of the relationships between biodiversity, ecosystem services, and the sustainability of existing smart eco-cities. The findings and insights from these studies can inform evidence-based decision-making and promote sustainable practices in smart eco-city development.
IoT's role within the AIoT framework
IoT, as a fundamental component of AIoT, significantly contributes to the above areas of environmental sustainability by providing a robust technical framework for data collection, analysis, and decision-making.
- • Data acquisition: IoT devices equipped with sensors and actuators collect real-time data on environmental parameters. This extensive data acquisition network forms the foundation for environmental monitoring.
- • Data transmission: IoT enables seamless data transmission to centralized platforms, such as cloud computing, ensuring that data from distributed sources is readily available for processing and analysis. Connectivity protocols ensure efficient and secure data transfer.
- • Big data handling: IoT generates vast amounts of data and AI algorithms, including ML and DL, process these data to identify patterns, anomalies, and trends, providing insights into environmental conditions.
- • Predictive analytics: AI-driven predictive models utilize historical and real-time IoT-driven data to forecast environmental changes, which enables proactive decision-making and timely responses.
- • Automation and control: IoT's integration with AI allows for autonomous control of systems and processes. For instance, smart grids can adjust energy distribution based on real-time demand and renewable energy availability, promoting energy efficiency and conservation.
- • Resource optimization: AI algorithms optimize resource allocation and utilization, ensuring minimal waste and maximum efficiency in such areas as energy consumption, water usage, and transportation.
- • Remote monitoring: IoT-enabled devices can be remotely controlled and monitored, reducing the need for physical interventions and minimizing human impact on sensitive ecosystems.
Overall, IoT's technical capabilities within the AIoT framework enable comprehensive data collection, analysis, and automation, fostering environmentally sustainable practices and informed decision-making across various domains.
Theoretical and literature AI research
Similarly, a range of theoretical and literature review studies have made notable contributions in the context of emerging smarter eco-cities across various domains:
- • Water resources conservation: Several studies have focused on water resources conservation by applying ML and DSS, employing techniques such as ANN, SVM, FL, GA, and ANFIS. Key studies in this area include those by Mehr et al. [ 180 ], Oyebode and Stretch [ 90 ], Sahoo et al. [ 181 ], and Valizadeh et al. [ 182 ].
- • Energy conservation and renewable energy: Efforts to conserve energy and promote renewable sources have been supported by ML techniques such as LR, ANN, SVM, NC, and EC. Researchers and scholars like Akhter et al. [ 82 ], Alsadi and Khatib [ 183 ], Das et al. [ 83 ], Dawoud, Lin, and Okba [ 184 ], Khan et al. [ 84 ], Youssef, El-Telbany, and Zekry [ 185 ], and Wang and Srinivasan [ 186 ] have made contributions in this field of study.
- • Sustainable transportation: Related initiatives have been advanced by applying ML, CV, and DSS utilizing ANN, DT, NC, SVM, FL, and time series models. Key studies in this area include those by Jiang and Zhang [ 187 ] and Liyanage et al. [ 101 ].
- • Biodiversity and ecosystem services: Biodiversity-related studies have explored modeling competition and population dynamics, often employing ML techniques like cellular automata [ 188 ]. Additionally, species conservation efforts have benefited from ML and DSS involving SVM, ANN, GA, and FL, as demonstrated by the work of Salcedo-Sanz, Cuadra, and Vermeij [ 97 ]. On the other hand, ecosystem services assessment has leveraged ML methodologies such as ARIES, as exemplified in the research by Ochoa and Urbina-Cardona [ 189 ].
The research on water resource conservation has provided insights into effective water resource management strategies. Regarding energy conservation and renewable energy, the studies in this area have contributed to optimizing energy operations, promoting energy efficiency, and fostering the adoption of renewable energy technologies. The research on sustainable transportation has provided insights into traffic management, transportation planning, and improving the overall efficiency and sustainability of transportation systems. Concerning the studies in biodiversity conservation they contribute to our understanding of biodiversity and the sustainable management of ecosystems. Overall, these theoretical and literature review studies collectively contribute to the advancement of smarter eco-cities, and their outcomes provide insights into the development of evidence-based approaches for building more sustainable and resilient smart eco-cities.
5.2.1.2. Climate change: empirical AI research
In empirical AI research on climate change, notable attention has been directed toward specific areas, including scenario analysis, marine resources management, and disaster management and resilience. This focus has yielded significant contributions to advancing smarter eco-cities:
- • Scenario analysis: Studies have employed various AI and ML models and techniques. Noteworthy works have performed scenario analysis based on ML, EC, and FL using ANN, BN, SVM, GA, and neuro-fuzzy (e.g., Ref. [ [190] , [191] , [192] ]); analysis across sustainability elements based on ML using ANN (e.g., Ref. [ 193 ]); and CO2 emission [ [194] , [195] , [196] ] and natural disaster (e.g. Ref. [ 197 , 198 ], based on ML and FL using ANN, SVM, EC, and neuro-fuzzy. Studies on ocean and cryosphere (e.g., Ref. [ 199 ]) and atmospheric forecasting (e.g., Ref. [ 200 , 201 ]) have applied ML using ANN. Furthermore, scenario analysis focuses on developing and analyzing different scenarios of future climate conditions based on various parameters, data inputs, and assumptions. It considers a range of potential future outcomes for climate change, including different levels of GHG emissions, temperature increases, sea-level rise, and other climate-related factors. In scenario analysis, various modeling techniques are used, including Generative Adversarial Networks (GANs), DL (CNNs and RNNs) and NLP, to create and analyze these scenarios. The goal is to provide insights into the potential consequences of different climate pathways and to help inform decision-making and policy development. Scenario analysis is valuable for understanding the range of possible future outcomes and their associated risks and impacts. It allows stakeholders to consider various “what-if” scenarios and plan accordingly for a range of potential climate-related challenges.
- • Disaster management and resilience: The domain of disaster resilience has witnessed significant AI-driven contributions in prediction and forecasting and resilient infrastructure and urban planning. The studies carried out by Cheng and Hoang [ 305 ], Choubin et al. [ 202 ], and Ji et al. [ 203 ] have advanced the field by employing different AI models and techniques. Among these are ML in predictive modeling, pattern recognition, and damage assessment; NLP in sentiment analysis and information extraction; CV in image analysis and object recognition; AI-driven Geographic Information Systems (GIS) in spatial analysis and mapping and visualization; reinforcement learning in resource allocation optimization; and DSS in dynamic resource allocation.
- • Marine resources management: AI research has played a pivotal role in marine resources management, encompassing water pollution monitoring, pollutant tracing in water quality, pollution reduction and prevention strategies, acidification mitigation, and habitat and species protection. These endeavors have harnessed various AI models and techniques, as demonstrated by the works of Lu et al. [ 204 ] and Wang et al. [ 205 ], to address challenges related to the sustainable use and conservation of marine ecosystems. Some of these models and techniques include ML, DL (e.g., CNNs and RNNs), GA, and ML-based Species Distribution Models (SDMs), NLP, time series forecasting, Autonomous Underwater Vehicles (AUVs) and Remotely Operated Vehicles (ROVs), and DSS.
This body of research underscores the critical role of AI in addressing climate change challenges, highlighting its potential to inform smarter eco-city initiatives and promote sustainable urban development. Specifically, the research on scenario analysis has provided insights into climate change impacts, vulnerability, and adaptation strategies; the interdependencies and interactions between different aspects of sustainability; understanding and managing CO2 emissions; and enabling the development of strategies for reducing carbon footprints. Regarding natural disaster analysis, the key insights gained pertain to predicting and forecasting natural disasters, resilient infrastructure planning, and environmental hazard detection. Disaster resilience studies have enhanced disaster resilience, enabling effective preparedness, response, and recovery measures. The studies on marine resources management have provided new perspectives on tackling different relevant challenges and enhancing the sustainable management of marine resources. Overall, these empirical studies provide valuable insights and advancements in understanding climate change challenges in the context of emerging smarter eco-cities.
5.2.1.3. Smart cities
During the first period, AI research on environmental sustainability was expanding to include smart cities based on various AI models, including ML, DL, CV, NLP, and robotics (e.g., Ref. [ 107 , [206] , [207] , [208] , [209] ]). It also involved using ML in empirical studies (e.g., Ref. [ 174 , 210 ]). Concerning climate change in the urban context, some studies addressed mitigation concerning urban planning, mobility, and land use (e.g., Ref. [ 211 , 212 ]). For AI-powered IoT architecture, research tended to focus mainly on the theoretical aspects of ML and data analysis (e.g., Ref. [ [213] , [214] , [215] ]), cognitive AI (e.g., Ref. [ 216 , 217 ]), knowledge-based DSS [ 218 ], and knowledge-based AI [ 174 ]. Regarding the latter, expert systems are associated with the capability of AI to solve complex problems, e.g., assessment of climate change impacts, and to aid decision-making by relying on specific knowledge derived from databases [ 17 ]. AIoT has been deployed in multiple ways to help users efficiently manage energy to reduce cost as well as energy producers optimize their equipment for better service delivery [ 219 ]. It also found important applications in vehicles and transportation, especially self-driving or autonomous vehicles. These are embedded with several sensing instruments (e.g., cameras, Light Detection and Ranging (LIDAR), and radar) and thus generate massive amounts of data [ 220 ]. LIDAR is a remote sensing technology that uses laser light to measure distances and generate precise, three-dimensional representations of objects as well as landscapes.
5.2.2. The second period: 2020–2023
During the second period, 2020–2023, the empirical, theoretical, and literature research on AI increased and expanded across environmental sustainability, climate change, and smart cities. This increase and expansion pertain to energy conservation and renewable energy, water resources conservation, sustainable transport and mobility, biodiversity conservation, pollution control, climate adaptation and mitigation, and disaster management and resilience. The rapidly growing body of research in environmental sustainability, climate change, and smart cities has made further valuable contributions and advancements in the context of emerging smarter eco-cities, enhancing and extending the knowledge gained for the strategies and solutions for addressing and overcoming environmental challenges.
5.2.2.1. Environmental sustainability
While the main areas of AI and AIoT research in environmental sustainability continued to attract attention, academic interest slightly decreased compared to the previous period. This is likely attributed to COVID-19 taking priority in research during the second period, inducing scholars in smart cities and environmental sustainability to investigate the link between COVID-19 and CO 2 emissions reduction and air quality improvement. Worth pointing out is that, during 2020–2021, COVID-19-related publications received 20 % of all citations, and 98 of the 100 most-cited publications were associated with COVID-19 [ 1 ]. There was a shift during this period in academic interest from focusing on environmental sustainability challenges toward focusing on the massive deployment and implementation of digital technologies and applied solutions offered by smart cities. However, the areas of environmental sustainability that continued to attract AI research during COVID-19 include:
- • Energy conservation and air quality efforts encompass pollution reduction and prevention, pollution monitoring, pollutant filtering and capture, air quality prediction, and early hazard warning, as well as the promotion of clean and renewable energy sources (e.g., Ref. [ 21 , 86 , 87 , 99 , [221] , [222] , [223] , [224] ]) and environmental quality control [ 225 ].
- • Sustainable transportation initiatives include the evaluation of energy consumption in household transportation through ML models [ 226 ], energy planning, transportation connectivity, urban traffic management, assessment of transport network capacity, urban traffic surveillance, and optimization of commuting corridors and jobs-housing balance using techniques, such as GA, EC, ANN, Spatial DNA, and reinforcement learning (e.g., Ref. [ 17 , 99 , [227] , [228] , [229] , [230] ]).
- • Clean water security and water resource conservation efforts involve various aspects such as water quality management, water supply quantity optimization, water control, water treatment, and sanitation (e.g., Ref. [ 93 , 93 , 231 , 232 ]). Several AI models and techniques have been applied to address challenges related to clean water security and water resource conservation, including ML, DL, ANN, CNNs, RNNs, GA, Particle Swarm Optimization (PSO), NLP, and DSS.
- • Biodiversity and conservation endeavors revolve around enhancing and protecting natural capital, preserving ecosystem health, safeguarding habitats, restoring ecosystems, maintaining forest landscape visual quality, protecting species, conserving biological diversity, preventing marine pollution, and ensuring the preservation of marine resources (e.g., Ref. [ 99 , [233] , [234] , [235] , [236] , [237] , 238 ]). Among the AI models and techniques being used in biodiversity and conservation efforts are ML, DL, FL, SVM, CNNs RNNs, GA, NLP, ARIES, AI-driven GIS, and AI-powered drones.
In the pursuit of creating more intelligent and sustainable urban environments, a wealth of research has emerged to address the unique challenges emerging smarter eco-cities face. These studies, spanning diverse domains, have made further contributions to shaping the trajectory of these cities. The studies on energy conservation and air quality studies significantly enhance their environmental performance and efficiency. The research in sustainable transportation research plays a crucial role in promoting eco-friendly and efficient urban transport systems. Studies on clean water security and resource conservation are pivotal for ensuring sustainable water resource management. The research on biodiversity and conservation is instrumental in supporting the development of eco-friendly and sustainable environments. These advancements can reshape the landscape of urban development models beyond smarter eco-cities in response to the growing wave of urbanization, making cities more intelligent and environmentally conscious.
5.2.2.2. Climate change
Many more studies were conducted during the second period, indicating increased scholarly interest in climate change and its relation to AI and AIoT. The digitalized transformation triggered by COVID-19 significantly contributed to climate actions [ 158 , 159 ]. Consequently, AI and AIoT technologies gained strong traction because of the accelerated digitalization prompted by COVID-19.
Mitigation and adaptation
In the face of escalating environmental challenges, the exploration of innovative technologies has become paramount to addressing the urgent concerns of climate change. This narrative dives into the realm of climate change mitigation and adaptation, shedding light on the pivotal role that AI and AIoT play in reshaping sustainable urban development in this regard. Climate change has accelerated the need for proactive measures, particularly in urban areas where high energy consumption contributes significantly to GHG emissions. Extensive research conducted during the second period highlights the urgency of addressing climate change as cities contribute significantly to CO 2 emissions through high energy consumption. It emphasizes the potential of AI to mitigate climate change by integrating knowledge, design strategies, and innovative technologies. It further discusses AI applications in transportation, urban energy, water use, and waste management, showcasing their impact on reshaping urban planning and design. Overall, it demonstrates that implementing AI-driven solutions can improve the sustainability of future cities and contribute to climate change mitigation efforts. Ivanova, Ivanova, and Medarov [ 239 ] acknowledge the growing influence of AI across various domains and predict its continued expansion in the coming decades. The authors emphasize the prevalence of narrow AI, specialized neural networks designed to solve specific problems, in technical fields. They focus on applying narrow AI to investigate the impact of climate change on transport infrastructure, providing guidelines for data collection and AI modeling. They highlight the controllability and capabilities of narrow AI, underscoring their potential for studying climate change's effects on transportation systems.
Furthermore, in connection with the first period, one of the areas that received more focus in the theoretical and empirical research on AI applications for climate change is disaster resilience and management, including early warning systems, resilience and planning, and simulation and prediction (e.g., Ref. [ 19 , 240 , 241 ]). However, Leal Filho [ 18 ] report on— in a systematic review and survey questionnaire — all studies conducted during 2020–2022 on the relationship between AI and climate change and its opportunities for adaptation and mitigation, covering several areas that have attracted research on AI applications. Among the themes studied by the authors in relevance to the current study while expanding on those mentioned in the first period are:
- • Large-scale urbanization impacts under climate change scenarios.
- • Eco-services and tradeoffs model valuation for ecosystem services quantification.
- • Water utilization management using and combining Blockchain and AI.
- • AI for disaster response, digital response, and disaster management.
- • IoT-Based smart tree management.
- • AI and ML for wildfire evacuations, wildfire prediction and prevention, wildfire susceptibility mapping, human-caused wildfire occurrence, risk-reduction strategies for floods and droughts, conservation planning under climate-changing patterns, and green-roof irrigation optimization.
- • ML for flood prediction and protection.
- • AI for improving resilience and preparedness against flood events impact.
- • ANN for drought tolerance determination.
- • Evolutionary Neural Network (ENN) for forecasting carbon emissions, energy demand, and wind generation.
- • ANFIS for modeling climate change impact on wind power resources.
- • DL for modeling sub-grid processes in climate models.
- • ML for water security improvement and water demand forecasting in cities.
- • ML for adaptation policy.
- • AI for sustainable development.
Some of these themes are linked to smart cities and smart eco-cities for renewable energy, water, biodiversity, climate, planning, and policy within the framework of SDG 11: Sustainable Cities and Communities, SDG 9: Clean and Affordable Energy, and SDG 13: Climate Action. Moreover, AI can be a critical change agent because it enables climate change mitigation through carbon neutrality in energy production, distribution, transportation, buildings, construction, and others [ 242 ]. Some studies proposed benchmark datasets with additional modeling components for better climate change prediction [ 243 ]. For example, Samadi [ 20 ] notes that the convergence of AI and IoT has the potential to accurately predict floods and accelerate the convergence of AI models and techniques to advance flood analytics research. The author discusses the workflow of an AIoT prototype, namely Flood Analytics Information System (FAIS), which integrates ML, NLP, CNNs, and others.
In the context of climate change mitigation and adaptation, IoT as a core component of AIoT brings critical technical aspects to the forefront. As to data sensing and collection, IoT devices equipped with sensors monitor relevant environmental parameters. This continuous data stream forms the basis for understanding climate change patterns and trends. In terms of network connectivity, IoT ensures seamless data transmission and communication between devices and central data repositories. Robust communication protocols and networks, such as 5G/6G, facilitate rapid data sharing. Concerning data analysis, AI algorithms process vast datasets from IoT sensors, identifying climate change patterns and trends. ML/DL models and algorithms enable predictive analytics for anticipating changes and their potential impacts. IoT-connected weather and climate monitoring stations, coupled with AI-driven forecasting models, enable the development of early warning systems for extreme weather events and natural disasters. Moreover, IoT devices track energy consumption in real-time as part of climate change mitigation approaches. AI algorithms analyze these data to optimize energy use, identify areas for conservation, and promote the integration of renewable energy sources to reduce environmental impacts. Also, IoT-enabled infrastructure, such as smart buildings and resilient urban planning, enhances adaptation efforts. In this regard, sensors monitor structural integrity and climate-related risks, facilitating adaptive responses. Furthermore, IoT sensors in ecosystems track changes in flora and fauna behavior and health. AI algorithms aid in assessing the impact of climate change on biodiversity and guiding conservation efforts. Additionally, IoT-connected satellites and drones equipped with AI-enabled remote sensing technology provide critical data for monitoring various environmental changes. Lastly, regarding real-time decision support: IoT systems provide real-time climate data and actionable insights to decision-makers, allowing for adaptive strategies and informed policy development. In sum, IoT's technical capabilities within AIoT are instrumental in climate change mitigation and adaptation efforts. They enable data-driven decision-making, resource optimization, and resilience-building, all crucial components in addressing the challenges posed by climate change.
5.2.2.3. Smart cities: transportation, energy, waste, environmental management, and the SDGs
Compared to the first period, AI, AIoT, and Blockchain technologies have proliferated and expanded, attracting more research interest, especially with their applications in smart cities. This also implies that smart cities are embracing AI and AIoT solutions developed initially for the different areas of environmental sustainability and climate change as separate fields.
AI and AIoT applications
AI and AIoT applications span many domains of smart cities in the field of environmental sustainability. In a systematic literature review on smart cities and AI, Yigitcanlar et al. [ 244 ] found that the key contributions of AI to environmental sustainability areas include:
- • optimizing energy production and consumption via domotics (home automation),
- • predicting the risks of climate change via ML algorithms and climate models,
- • monitoring changes in the natural environment via remote sensing with autonomous drones, and
- • operationalizing transport systems via mobility-as-a-service (MaaS).
AI applications related to the latter theme also include the management of transport systems of cities in terms of shared autonomous mobility-on-demand [ 245 ], autonomous cities [ 246 ], and autonomous vehicles (e.g., Ref. [ [247] , [248] , [249] ]). Concerning the latter, the deployment of AIoT at the network edge and secure trust models offer potential solutions for the real-time processing of sensor data to enable fast response to complex scenarios, such as obstacle avoidance and velocity adaptation [ 10 ]. Moreover, Zhang and Tao [ 13 ] synthesize several studies on the application of AIoT using DL in smart transportation (e.g., traffic participants, traffic infrastructures, connected logistics, and in-car driver behavior monitoring) as well as smart grids (grid fault diagnosis, building management and optimization, load monitoring and scheduling, and cyber-attack detection). To solve the load forecasting problem in energy management, Han et al. [ 250 ] propose a DL–based framework to predict future energy consumption in smart residential homes and industries, where the IoT network is connected to smart grids to maintain energy demand and supply activities effectively. The experimental results demonstrate the ability of the approach to predict energy consumption with high accuracy. El Himer et al. [ 251 ] address the role of AIoT in providing new opportunities in distributed energy resources (DER), focusing on AIoT applications in renewable energy sources, such as solar and wind. An AIoT system developed by Puri et al. [ 16 ] generates energy from different sensors, such as piezoelectric sensors, including from stress caused by human body weight, heat generated by the movement of the human body, and sunlight. The authors built and validated the data collected from the sensors with ANN and ANFIS models to predict generated power output and demonstrated that their system could produce accurate results in predicting the power generated from renewable resources.
Broadly, AIoT can be used in smart cities to analyze and track how different consumers and residents use energy to make decisions on where and what kind of renewable energy sources could be used, as well as where energy is being wasted and how it can be directed for other uses or conserved. Sleem and Elhenawy [ 252 ] discuss the contribution of AIoT to the development of smart buildings and their functionality, as well as its benefits for reducing energy consumption and costs, improving occupant comfort and productivity, and increasing safety and security. The authors also address the challenges associated with deploying AIoT and emphasize the potential of AIoT-empowered smart buildings to contribute to sustainable urban development and improve the quality of life. Furthermore, AIoT applications are converging in smart cities. Seng et al. [ 10 ] review and discuss several dimensions of AIoT applications. These are more relevant to this study — energy and smart grids, industry and smart buildings, vehicles and smart transportation, and robotics and computer vision.
Furthermore, AI and AIoT have been instrumental in developing advanced waste collection systems that optimize several parameters and maximize efficiency. Fang et al. [ 94 ] provide a comprehensive review of the application of AI in waste management, including waste-to-energy, smart bins, waste-sorting robots, waste generation models, waste monitoring and tracking, waste logistics, waste disposal, waste resource recovery, waste process efficiency, waste cost savings, and improving public health. The authors highlight the benefits of AI in waste logistics in terms of reducing transportation distance and time savings, as well as improving waste pyrolysis, carbon emission estimation, and energy conversion. They also emphasize the role of AI in increasing efficiency and reducing waste identification and sorting costs in smart cities. Nasir and Aziz Al-Talib [ 95 ] discuss the challenges in waste classification and the potential of AI and image processing techniques to address them. They acknowledge the limitations of current waste classification models driven by DL and highlight the need for improvements in accuracy and runtime to achieve precise results. They argue that accurate waste classification is crucial for multiple reasons, including enabling recycling and resource recovery, safeguarding the environment and human health, and minimizing waste management costs. The core idea distilled from the study is that waste is the byproduct of various human activities, encompassing domestic, agricultural, and industrial sectors. Different types of waste exist, including non-biodegradable, hazardous, industrial, municipal solid, and agricultural waste. Solid waste can take hundreds of years to decompose, posing environmental risks. Mounaded et al. [ 96 ] focus on applying AI techniques in municipal solid waste (MSW) management. They emphasize the use of ANN in various MSW-related problems and highlight the challenges related to data reliability and the absence of clear performance baselines for assessing AI approaches. Overall, these studies contribute to understanding how AI can revolutionize waste management by improving waste logistics, classification, and treatment processes. They highlight the potential benefits and challenges of implementing AI in the field, providing valuable insights for future research and practical applications.
In particular, the need to overcome the constraints and complexities associated with conventional approaches (e.g., RFID, GPS, GIS), especially the status and waste level in bins, has driven the development and implementation of various advanced techniques. These include PSO (e.g., Ref. [ 253 ]), ANN (e.g., Ref. [ 254 ]), and Backtracking Search Algorithm (BSA) (e.g., Ref. [ 255 ]) for waste collection optimization. GA and nearest neighborhood search algorithms have also been used for waste vehicle routing (e.g., Ref. [ 256 ]). However, these techniques still lack precision and require a long execution time. Therefore, new techniques are needed to deal with cost and emission issues and consider bin capacity, waste weight inside the bin, collection frequency, vehicle capacity and maintenance, and trip rate [ 257 ]. Further, however, AI models can be applied to predict equipment failures in waste management facilities. AI models can identify potential issues in advance by analyzing data patterns, allowing for timely maintenance and minimizing downtime. Also, AI models can enable powerful DSS for waste management. These systems integrate various data sources, including weather conditions, population density, and waste composition, to provide insights and recommendations for effective waste management strategies.
Blockchain and AI and IoT applications
Blockchain technology is gaining widespread popularity across various domains, including energy, environmental conservation, and urban development, owing to its capacity to decentralize data and processes while ensuring robust security measures. In essence, Blockchain is an open-source, peer-to-peer, distributed ledger system that encompasses multiple transactions and their associated data organized within a chain of interconnected blocks within a decentralized, peer-to-peer, and openly accessible network, using technologies such as AI, ML, and Big Data (e.g., Ref. [ 258 ]). These blocks are subject to cryptographic validation by the network itself. According to Parmentola [ 259 ], Blockchain is a rapidly evolving approach that enables the recording, sharing, updating, and synchronizing of information and transactions across multiple data ledgers or databases within a distributed and openly accessible network of diverse participants. Consequently, it fosters enhanced collaboration and interaction among various organizations and individuals participating in the network. Moreover, it is distinguished by its core attributes, including anonymity, transparency, auditability, permanence, persistence, and decentralization, which collectively translate into improved operational performance, efficiency gains, and cost reductions [ 258 , 260 ].
In more recent years, Blockchain has become an innovative technology and solution for smart cities in environmental sustainability. It has been used by many governments to improve environmental sustainability. Integrating blockchain into renewable energy sources can unlock energy sustainability by facilitating the development of a decentralized and democratized energy system while aiding in improved climate governance through its attributes of transparency, global decentralization, and collaborative capabilities [ 261 ]. As demonstrated in a recent bibliometric study on environmentally sustainable smart cities, Blockchain is linked to the challenges, services, and resources of smart cities and AI, IoT, and big data analytics [ 1 ]. For the latter, in a use case of a developed “blockchain-based carbon emission rights verification system,” AI and Big Data are used to learn proven data [ 262 ]. Miao et al. [ 263 ] propose a blockchain and AI-based architecture for the natural gas intelligent IoT to address the supply chain failure of existing centralized energy supply architectures because of their overwhelming numerous requests that could cause pressure, temperature, and natural gas load to exceed safety limits. Also, based on multi-sensor-driven (or IoT-based) AI tools, blockchain platforms can optimize circular economy loops ([ 264 , 265 ]), allowing carbon footprint reduction and solid waste disposal control and thereby contributing to sustainability transitions [ 266 ]. Xiao et al. [ 267 ] propose using Blockchain for intelligent driving edge systems. The approach utilizes a double auction mechanism to optimize the satisfaction of users and service providers for edge computing and shows potential for better performance for resource utilization.
The value of Blockchain technology lies in storing data on green energy production activities related to environmental degradation and air pollution; enabling new means of green energy production, supply chain and logistics, real-time data collection and analysis for timely decision-making pertaining to green and low-carbon processes; and monitoring EV charging systems [ 259 ]. Moreover, Blockchain informs consumers and users about the use of less-efficient appliances. It enables them to improve their consumption behavior and thus reduce their carbon emissions [ 268 ], in addition to monitoring compliance with environmental standards by utilizing product traceability that can decrease resource inefficiencies and losses at different supply chain stages [ 269 ]. Also, Blockchain-based initiatives have been designed to provide credible trading services for polluters. They have also been used to tokenize carbon credits [ 269 ] and monitor carbon emissions. In a recent review of Blockchain applications in sustainable and smart cities, Makani et al. [ 270 ] provide a detailed account of how Blockchain technologies contribute to various urban domains, including transportation, smart grids, smart operational management, and smart homes. As regards the trading of renewable energy by local energy producers based on cryptocurrencies, Blockchain contributes to energy supply diversification, supply disruption risk reduction [ 269 ], and renewable energy promotion through microgrids and other alternative models [ 259 ]. These suggest the potential for innovative approaches that support localized and community-driven renewable energy production. By improving the alignment of energy supply and demand, these approaches can strengthen energy security and resilience, offering new avenues to enhance sustainable energy prospects. Concerning carbon emissions monitoring, the combination of Blockchain and IoT provides reliability for data and establishes measurement criteria homogeneity about registration systems and measurement tools, respectively. These solutions “minimize registration errors and eliminate fraud arising from the accounting and measurement of gas emissions” [ 269 ].
Regarding the role of AI in preventing and reducing marine pollution [ 271 ], Blockchain monitors water pollution changes and preserves marine resources [ 259 ]. It is also used in rewards schemes for residents of coastal areas using tokens of cryptocurrencies, which “can later be redeemed for credit to collect and share data on environmental conditions of water bodies” that can aid in enhancing decisions and designing regulations [ 269 ]. Similar reward schemes can raise public awareness and increase public participation in waste management and recycling by developing models to reward active users. Further, implementing Blockchain and AI as smart city technologies has several co-benefits associated with water management. Contributions in this regard relate to water provision efficiency, wastewater management, ensuring water security, groundwater monitoring, environmental awareness, promoting peer-to-peer trading of water rights, conserving water resources, and tackling the nexus between various natural resources in urban areas [ 259 , 269 , 271 , 272 ]. In particular, Blockchain and AI technologies could optimize water management in water-stressed urban areas by facilitating autonomous water distribution and management systems These minimize loss and control quality.
Overall, Blockchain technology, combined with AI and IoT, is crucial in advancing environmental sustainability. It provides a decentralized and transparent platform for securely recording and verifying transactions, data, and information. Integrating AI and IoT enables efficient data collection, analysis, and decision-making processes, leading to improved resource management, reduced environmental impact, and enhanced sustainability practices. The combination of blockchain, AI, and IoT allows for the development of innovative solutions such as smart grids, decentralized energy systems, waste management, and carbon footprint tracking. By fostering trust, traceability, and accountability, blockchain enhances the implementation of sustainable practices and facilitates the transition toward a greener and more sustainable future.
The sustainable development goals (SDGs)
The potential benefits of smart cities in catalyzing the transition to SDG 11 through advanced technologies and data-driven approaches are evident. Iris-Panagiota and Egleton [ 3 ] explore the role of AI within smart, sustainable cities, emphasizing its contributions to urban planning, management, and development. Zaidi et al. [ 273 ] analyze the trajectory of AI in smart sustainable cities research, pinpointing publication trends and research hotspots, including digital innovation, intelligent data systems, smart energy efficiency, and AI-IoT data analytics nexus. Yigitcanlar and Cugurullo [ 26 ] explore the sustainability of AI within the context of smart, sustainable cities, generating insights into emerging urban AI and the potential symbiosis between AI and smart, sustainable urbanism. The study reveals that AI applications have become integral in urban services, managing various aspects of urban life, such as transport systems, infrastructure, and environmental monitoring. The increasing adoption of AI is expected to continue, impacting the three dimensions of urban sustainability. Vinuesa et al. [ 271 ] reveal the potential of AI to advance 134 targets across all goals while hindering 59 targets. Collectively, these studies enrich the understanding of AI's role within smart, sustainable cities, an overarching umbrella term for smarter eco-cities — its applications in urban planning, the AI research landscape in smart cities, the integration of AI and IoT in urban contexts, the alignment of AI with SDG objectives, and the status of smarter eco-cities. Nonetheless, the trade-offs of smart cities — privacy, cybersecurity, digital divide, technology misuse, and legal frameworks — demand attention considering the use of AI and its integration (e.g., Ref. [ 1 , 3 ]). The imperative lies in devising measures that amplify social and economic priorities in smart city planning and development toward rendering smart eco-cities smarter and more sustainable.
6. Discussion: challenges, open issues, and limitations
6.1. smart city ai, iot, and big data technologies as key factors impacting the dynamics of existing smart eco-cities.
AI, IoT, and Big Data technologies are transforming how smart cities — and hence smart eco-cities — function by optimizing their processes, enhancing their practices, augmenting their solution capabilities, and improving their environmental sustainability performance. Since the mid-2010s, the data-driven technologies and solutions of smart cities as changing elements have gradually impacted the dynamics of eco-cities toward becoming smart in their approach to environmental sustainability by integrating their core domains with smart city domains. This will continue in the same direction as the AI, IoT, and Big Data technologies and solutions of smart cities become more advanced and integrated with sustainable technologies and strategies to provide innovative approaches that can demonstrate the ability to tackle more complex challenges. This, in turn, means making smart eco-cities smarter in their pursuit of achieving environmental sustainability thanks to the increasing use of AI and AIoT applications. The essence of AIoT revolves around the need to harness and leverage the power of smart city technologies and solutions, given the clear synergies in their operation concerning the optimization, efficiency, management, and planning processes of smarter eco-cities. This entails integrating their systems, coordinating their domains, and coupling their networks, creating many new opportunities that could be realized in environmental sustainability.
At the technical level, AI empowers the analysis of the colossal amounts of data generated via the IoT infrastructure in smart cities [ 14 ] and hence smart eco-cities, largely using ML for decision-making processes. Regarding AI-enabled sustainable smart cities, ML models can grow over time, detect invisible anomalies and alterations, exhibit various behaviors on different runs for the same input, and help provide real-time feedback for transport management, pollution control, energy management [ 2 ], and water management systems. Intelligent machines can “learn from experience, adjust to new inputs, and perform human-like tasks” ([ 274 ], p. 63) to “interpret external data correctly, to learn from such data, and to use those learnings to achieve specific goals through flexible adaptation” ([ 63 ], p. 17). Overall, AI can provide unsurpassed ways of automating or autonomizing the repetitive, complex, cognitively demanding, and time-consuming tasks associated with the operational functioning and planning of smarter eco-cities.
Concerning environmentally smart sustainable urbanism as an underlying paradigm of smart eco-cities, it is increasingly becoming a powerful societal framework for the transition toward environmental sustainability. This lies in developing joint actions for preserving the environment based on analyzing large-scale databases, understanding the complexity of climate change and modeling and simulating its potential impacts, improving the health of ecosystems, and enabling high integration of renewable energy and smart energy [ 271 ], enhancing smart renewable energy infrastructures in smart cities [ 275 ], optimizing energy consumption and production, developing more environmentally efficient transport systems, enhancing environmental monitoring (e.g., Ref. [ [245] , [276] , [277] ]), and strengthening low-carbon energy systems by supporting circular economies and smart eco-cities [ 271 ]. In particular, more than 250 studies applied AI to energy conservation and renewable energy during 2015–2019 [ 17 ]. The focus on the potential of AI and AIoT for energy can be justified by its pivotal role in the transition to smart eco-cities. This occurs through integrating large shares of renewable energy with smart energy through additional flexibility and decarbonizing other key emitting sectors, notably manufacturing, industry, transport, and buildings. Addressing the energy crisis and reducing fossil fuels will mitigate the impacts of climate change and make adaptation easier [ 278 ].
However, the dynamics of smarter eco-cities should evaluate AI and AIoT technologies as key components initiating changes in different domains. Investments in large-scale AI and AIoT as digital ecosystems are expected to positively impact smarter eco-cities that may involve feedback mechanisms, resulting in further adoption of these ecosystems and additional future investments. In other words, AI and AIoT technologies are likely to benefit from providing innovative applications in response to the need to overcome environmental sustainability challenges. Accordingly, they may exhibit positive feedback in that the more their solutions are implemented, the more likely they will be further implemented, thanks to network effects, learning, adaptation, and coordination. While the relationship between outcomes, investments, and implementations is expected to advance the transition of smart eco-cities toward environmental sustainability, stating a strong causal relationship resulting from such linkages needs to be more accurate. For this reason, coupled with other complex intertwined internal and external factors, understanding the dynamics of smarter eco-cities remains a daunting and uncertain challenge. This can be justified and elucidated in what remains of this discussion.
6.2. Environmental challenges and costs of AI and AIoT technologies
AI, IoT, and Big Data technologies pose significant challenges when making smart eco-cities environmentally smarter. Therefore, paying attention to both the opportunities and threats of AI and AIoT technologies is necessary. Smarter eco-cities must be environmentally friendly, thereby minimizing the negative impacts resulting from the wide use and increasing adoption of the applied solutions of AI and AIoT technologies. These enabling, integrative, and constitute technologies are embedded into a much wider socio-technical landscape involving a complex set of intertwined and heterogenous factors and actors. There is a risk of a mismatch between the environmental goals of smarter eco-cities and the opportunities offered by AI and AIoT technologies. This is due to their indirect, direct, rebound, and systemic effects, which are generated through their development, design, use, application, and disposal (see Ref. [ 279 ] for a detailed discussion). In particular, the indirect effects are expected to be exacerbated the most due to the increasing demand for AI and AIoT applications. The operation of AI and AIoT applications requires a lot of energy to power the IoT infrastructure, data processing platforms, cloud and edge computing, high-speed wireless networks, and large-scale AI systems. Concerning the latter, large data centers, which provide massive computational resources required by AI research, design, and development, are associated with significant energy consumption and, thus, carbon footprint, compromising the efforts supporting energy reduction and climate action [ 271 ]. According to current estimates, the global electricity demand for advanced ICT could increase to 20 % compared to around 1 % today [ 271 ]. AI involves establishing heavy energy dependency due to the intensive use of innovative technologies, increasing environmental impacts [ [280] , [281] ], extending car traveling distance, and causing urban sprawl [ 249 ]. The latter two relate to exurbanization, a process whereby upper-class or affluent dwellers move from urban areas to rural areas to maintain an urban life or live in high-end housing through advanced technology or long-distance commuting.
However, the high energy requirements for AI and AIoT applications in the case of the use of non-renewable sources of energy will undermine the efforts to achieve the environmental targets of smarter eco-cities. Concerning the direct effects, building smarter eco-cities requires deploying urban operating systems, urban operations centers, and urban dashboards [ 53 ] and thus massive amounts of natural resources for developing, installing, and maintaining AI and AIoT ecosystems. In addition, IoT and AI production, distribution, service, and disposal produce vast amounts of e-waste, unsustainable materials, and toxic pollution [ [63] , [282] ]. Almalki et al. [ 282 ] discuss, in a recent comprehensive review, the capabilities and potentials of IoT to respond to the needs of smart cities while highlighting the challenges for future research on smart city data-driven IoT applications, with a focus on their risks to environmental sustainability in terms of energy consumption, toxic pollution, e-waste, and others. All in all, the applied AI and AIoT solutions for smarter eco-cities are challenged by the effects of high energy-intensive structures, undermining the efforts deployed to avoid the overexploitation of primary resources to achieve carbon neutrality.
The green growth of AI, IoT, Big Data technologies, green computing, and eco-friendly design is critical to mitigating the risks of the mismatch between the environmental goals of smarter eco-cities and the opportunities offered by AI and AIoT technologies. This is consistent with the environment being intrinsic to SDG 11 in terms of recognizing the need to apply the most innovative technologies to make critical urban infrastructure resource-efficient, low-emission, and resilient by reaching the targets related to energy, climate, transport, waste, and water, as well as integrated policy and planning. The positive impacts of adopting sustainable approaches to the development, use, application, and disposal of AI and AIoT technologies lie in creating eco-friendly environments that are healthier and more livable in smarter eco-cities while accelerating their digital transformation. In this respect, Almalki et al. [ 282 ] analyze the various techniques and strategies for enhancing the quality of life and well-being by making cities greener, smarter, safer, and more sustainable. Bibri [ [157] , [279] ] sheds light on the innovative role of advanced ICT as a potential remedy for mitigating its carbon footprint and thus advancing environmental sustainability goals, enabling the transition from smart cities to environmentally smarter cities. In this line of thinking, Almalki et al. [ 282 ] note that the smart things enabled by IoT in smart cities “become smarter to perform their tasks autonomously” while communicating “among themselves and humans with efficient bandwidth utilization, energy efficiency, mitigation of hazardous emissions, and reducing e-waste to make the city eco-friendly and sustainable.” Here comes the role of AI in green computing concerning smarter eco-city sensor integrated transportation systems, energy systems, building systems, waste systems, environmental monitoring systems, and so on. Green computing is key to decreasing carbon emissions and energy consumption to fulfill the environmental goals of sustainability in smart cities.
It is essential to focus on “reducing pollution hazards, traffic waste, resource usage, energy consumption, providing public safety, life quality, and sustaining the environment and cost management” to make smart cities eco-friendly [ 282 ]. This, in turn, means that AI and AIoT solutions should be carefully implemented in combination with sustainable and eco-friendly design principles, energy-efficient policy instruments, and other relevant measures. This is to ensure that the efficiency gains enabled by AI and AIoT solutions lead to reducing energy use and carbon footprint. Almalki et al. [ 282 ] provide practical insights into the data-driven IoT-based eco-friendly and sustainable cities research field. Wang and Liao [ 283 ] explore the intersection of eco-design with AI and Big Data. In doing so, they identify automation and control systems and computer science among the leading application disciplines. The authors argue for the necessity of more concerted efforts “to advance both the theoretical and empirical research on the nascent topic among researchers, funding bodies, policy-makers, and industry professionals given that the notion of eco-design of AI and Big Data applications is expected to be pertinent and relevant for designing greener strategies, products, and services for green digital transformation.” As a justification for a more consolidated green approach to AI, most attempts at using AI applications to enhance urban efficiencies have struggled, if not failed, to accomplish the transformative changes to smart cities due to “the short-sighted, technologically determined, and reductionist AI approaches being applied to complex urbanization problems” [ 4 ].
6.3. Technical and computational challenges of AI and AIoT technologies
Inherent to AI models and systems are several technical and analytical challenges. These include, as indicated by Nishant et al. [ 17 ] in a study conducted on AI for environmental sustainability, the overreliance of ML models on historical data, the uncertainty surrounding how humans behave in response to AI-based interventions, and the difficulty in measuring the effects of intervention strategies. Most new AI systems rely on Big Data and fail to demonstrate self-ideation or self-creation. AI must develop new concepts and models of intelligence cognition beyond ML/DL. This could offer novel solutions for environmental sustainability and climate change, feeding into new models of smarter eco-cities that may reduce decision biases due to the incompleteness and uncertainty of the data collected and aggregated in real-time. Concerning the over-reliance on Big (historical) Data in smart cities, Batty et al. (Ref. [ 128 ], p. 507) note that the prospect of real-time data collection and aggregation to deal with urban changes at any spatial or temporal scale “is a long way off and will never be reached … but what it does promise is an ability to have a real-time view of change at different spatial scales and over different time scales. This will change both the models we can build and how these technologies can inform the decision process with simulations and decision support being telescoped across space and time.” While this may be relevant to climate change in modeling and simulation, human-related variables are, to some extent, unpredictable and dynamically changing. This implies that more and varied types of data need to be collected and aggregated, new and more extensive sources of data to be explored, and new and more advanced tools for handling various velocities of data to be created. Significantly, historical datasets tend to be of limited value about climate periods and cycles, which makes it difficult to make precise predictions or decisions. A deterministic approach is difficult to adopt in climate change, as it is impossible to estimate or determine potential outcomes precisely. Non-deterministic ML models are more relevant to transport management, energy management, water management, waste management, and pollution monitoring, where they can detect anomalies and alterations and help provide real-time feedback. Furthermore, the variance-bias tradeoffs associated with ML [ 284 ] have implications for climate change solutions due to the bias and oversimplification inherent in predicting future climate change scenarios.
In addition, Kuguoglu et al. [ 15 ] investigate the reasons behind the failure of many smart city initiatives that rely on AIoT to scale up. Through a combination of literature study and expert interviews, the study identifies various factors contributing to the lack of scalability. These factors include resource and capability constraints, overlooking the importance of comprehensive change, and the influence of different factors at different stages of implementation. Regarding the technical challenges related to DL-based AIoT, they include [ 13 ]:
- • Multimodal heterogeneous data processing, transmission, and storage pertaining to the massive numbers of heterogeneous sensors and the vast data streams of different formats, sizes, and timestamps they generate.
- • Limited computational and storage resources in relation to using DL models for real-time data stream processing and low latency.
- • Computational scheduling in AIoT architecture and related intense computation. This entails meticulous coordination across cloud centers, fog nodes, and edge devices, factoring in variables like data type, volume, network bandwidth, processing latency, performance accuracy, data security, privacy specific to the application scenario, and unbalanced data flow and user demands over time.
- • Labeling unlabeled big data for DL in AIoT in terms of managing the time-consuming and financially demanding nature of this process while ensuring high-quality results.
- • Data monopoly, where access to proprietary data is restricted due to vested interests, poses challenges to achieving equitable access to extensive proprietary datasets.
6.4. Challenges and considerations of explainable AI and interpretable ML
Explainable AI (XAI) and Interpretable ML (IML) encounter significant challenges in the context of AI and AIoT solutions for smart cities, environmental sustainability, and climate change, particularly in the evolving landscape of smarter eco-cities. XAI and IML are interconnected concepts aiming to enhance the transparency, comprehensibility, and credibility of AI models for various stakeholders involved in smarter eco-city development. While XAI focuses on explaining the decision-making process of AI systems, IML specifically concentrates on creating ML models that produce easily interpretable outcomes. XAI encompasses diverse approaches, including IML techniques, to explain AI decisions, aligning with the broader aim of enhancing explainability in AI. Both XAI and IML play pivotal roles in creating AI and AIoT systems that foster accountability, trustworthiness, and effective human-AI interaction, which is vital for making informed decisions in the context of smarter eco-cities. Nonetheless, several challenges and issues arise in this context (e.g., Ref. [ [285] , [286] , [287] , [288] , [289] , [290] , [291] , 292 ]), including, but are not limited to:
- • Complexity and interpretability: Applying AI and AIoT solutions to complex challenges in smarter eco-cities can lead to intricate models, hindering their interpretability. Ensuring these complex systems generate transparent decisions amid intricate environmental and climate data is crucial.
- • Black-box models: Many advanced AI models (e.g., DNN) are considered black boxes, lacking transparency in decision-making. This lack of insight can hinder trust in and adoption of smart city systems, especially when critical decisions are at stake.
- • Bias and fairness: Bias in AI models, derived from biased training data, can perpetuate existing inequalities in resource allocation and exacerbate environmental disparities. Overcoming these biases and ensuring fair outcomes is a daunting task. Biased decision-making becomes evident in real-time and predictive analytics, hindering the pursuit of environmental sustainability.
- • The trade-off between accuracy and interpretability: Striking a balance between accurate predictions and clear explanations is essential, as more complex models might offer better predictions but sacrifice interpretability.
- • Interdisciplinary nature: Addressing environmental sustainability and climate change requires expertise from diverse fields. Ensuring that AI and AIoT solutions are interpretable to domain experts, policymakers, and citizens across various disciplines is a challenge, as technical terminology can create barriers to communication.
- • Data privacy and security: While explaining AI decisions and promoting transparency, care must be taken not to compromise sensitive or private information about individuals, potentially compromising their privacy.
- • Dynamic and evolving environments: Smart cities and smarter eco-cities are dynamically changing environments, necessitating adaptable and robust methods for interpreting AI decisions.
- • Education and adoption: Educating stakeholders, including policymakers, city planners, city managers, and citizens, about the benefits and limitations of AI and AIoT solutions, building trust and confidence, and encouraging adoption are critical factors in realizing smarter eco-cities.
The real challenge of XAI lies in granting substantial power to smarter eco-city systems without simultaneously enabling them to explain the intricate decision-making processes to different groups of domain experts. AI and ML models and algorithms assume control over decision-making by analyzing generated data, predicting outcomes, and maximizing value based on certain criteria. This reduces the rich complexity of urban life and the unpredictability of urban dynamics and systems to narrow quantitative and unitary languages, potentially disregarding the significance of cultural, ethical, social, and political values. As a result, technological advancements may pose difficulties in achieving the status of smarter eco-cities due to the mechanistic way of perceiving these complex systems. Therefore, a recent wave of research has started to focus on XAI to address some of the concerns posed by the application of AI in various domains. Mayuri, Vasile, and Indranath [ 290 ] present several applications of XAI/IML and methods to make AI/ML models explainable/interpretable. Ghonge [ 287 ] addressed several case studies and use cases of XAI as well as its impacts and challenges in smart city applications. Javid et al. [ 288 ] comprehensively delve into the landscape of XAI in smart cities, focusing on current and future developments, trends, enabling factors, use cases, challenges, and solutions. The authors outline research projects, standardization efforts, lessons learned, and technical hurdles.
XAI and IML methods are pivotal for the sustainable advancement of AI and AIoT solutions, allowing society to foster trust in the environmental and social-economic aspects of sustainability. These methods explain accuracy, fairness, transparency, accountability, and human-centeredness outcomes in AI and AIoT-powered decision-making, addressing ethical and governance concerns. These principles hold substantial relevance for data-driven decision-making in smarter eco-cities, thereby the need for creating explainable/interpretable models, techniques, and tools. Collaborative efforts among AI/AIoT experts, environmental scientists, urban planners, and policy-makers are essential to ensure the effective contribution of AI and AIoT technologies to environmental sustainability and climate change mitigation in the evolving landscape of smarter eco-cities. Also, future research endeavors will play a pivotal role in realizing transparent, effective, and ethically sound applications of XAI and IML methods within AI and AIoT solutions, advancing environmental sustainability in smarter eco-cities while ensuring equitable outcomes for all stakeholders.
6.5. Ethical and Societal Challenges of AI and AIoT technologies
AI technology's ethical and humanistic issues and risks are subject to long-standing intellectual and philosophical debates. The development, deployment, and adoption of AI technology raise these concerns, irrespective of the environmental benefits of its applied innovative solutions, depending on the application domain. Against the backdrop of this study, the use of AI involves making biased decisions, exacerbating privacy and cybersecurity, and limiting public trust [ 244 , 293 , 294 ]. Most of these challenges also apply by extension to AIoT, e.g., AIoT security for smart cities, AIoT and intrusion detection, and AIoT and trust recommendation [ 10 ]. Koffka [ 9 ] addresses critical concerns, including security and privacy, interoperability, and ethics, underscoring the importance of a responsible AIoT ecosystem. Furthermore, using AI entails devaluating human abilities, deepening information asymmetries, undermining equal power relations, and causing system failures. Regarding the latter, increasing public awareness of this type of risk is crucial before launching large-scale AI deployments in a society increasingly dependent on AI technology [ 271 ]. This indeed is arcane in that its actual functionalities and mechanisms are understood by only a group of people, despite being already part of the everyday life of many of us [ 6 ]. Therefore, given that AI as a disruptive technology will greatly transform smart eco-cities, it needs to earn public trust regarding how people perceive it. Also, AI technology needs to gain the trust in the minds of government agencies and public organizations investing in it [ 295 ]. The challenges posed by AI generally involve gaps in ethical standards, including safety, fairness, transparency [ 293 , 296 ], socio-economic equality, cultural diversity, and social inclusion. For example, safety is a key topic in ethical and legal debates over autonomous systems [ 297 , 298 ]. Most ethical issues raised by AI and AIoT applications relate to the difficulties in explaining AI models or interpreting ML algorithms, which resemble black boxes, with some being the hardest for humans to comprehend. There is a need for developing new methods for Explainable AI (XAI) or Interpretable ML (IML) that allow humans (designers, engineers, researchers, city planners, city managers, regulators, and policymakers) to understand and trust the decisions or predictions that AI models and systems make in terms of their potential biases and expected impacts. Ghonge [ 287 ] addressed several case studies and use cases of XAI as well as its impacts and open challenges in smart city applications.
The underlying ethical gaps of AI technology call for designing and implementing appropriate regulatory frameworks to address the counterproductive outcomes emanating from the penetrative patterns of AI (e.g., Ref. [ 63 , 244 , 299 , 300 ]) in urban life domains in emerging smarter eco-cities. Especially, early in the decision-making process of its deployment — when the opportunity for effective inputs and informed choices is greatest. This pertains to developing “responsible and ethical AI” before it is too late [ 297 , [301] , [302] ]. There is a warrant for this as the integration of AI with IoT and Big Data is speeding up the pace of advancements and innovations in both AI and AIoT systems, particularly the exponential rise of their computational power, paving the way for them to gain more and more power of the automation and autonomization of smart cities, with profound implications for smarter eco-cities. While it is possible to automate certain urban processes and practices concerning environmental sustainability and climate change, it is necessary to carefully plan and implement them to avoid generating fully automated or autonomous smarter eco-cities based on mechanical decisions. In this regard, it is essential to address and overcome the regulatory challenges pertaining to the use of AI and AIoT applications to advance environmental sustainability. Vinuesa et al. [ 271 ] emphasize the need for regulatory insight and oversight to support the development of AI-based technologies for sustainable development.
The realization of the common good of AI and AIoT technologies remains highly improbable when AI systems operate solely according to the algorithms designed and implemented by powerful corporations driven by ambitions for power, profit, and extensive reach and influence. These tech giants pursue various trajectories and explore uncharted possibilities, raising concerns about the potential consequences of the large-scale implementation of AI and AIoT systems. There is an urgent demand for well-regulated and responsible AI and AIoT systems that prioritize safeguarding public and civic values within the context of smart eco-cities. Such systems must be designed to ensure that the broader benefit to society takes precedence over corporate interests and unchecked advancements. Indeed, civic values and public values play vital roles in the functioning of both civil society and government. These values are the moral compass that guides individuals, communities, and public institutions in their pursuit of a just, inclusive, and prosperous society. In a civil society, civic values, such as social justice, freedom, tolerance, compassion, and tolerance, are the cornerstones of a harmonious and fair community. They inspire individuals to engage in civic activities, advocate for their rights, and work collectively to address societal issues. Civic engagement is fostered by these values, encouraging citizens to participate in public discourse and actively contribute to the betterment of society. At the same time, public values are fundamental to the proper functioning of government. These principles, including accountability, transparency, integrity, inclusivity, public participation, and environmental stewardship, ensure that public institutions operate in the best interests of the people they serve. However, for example, in relation to environmental stewardship, the crucial aspects of environmental protection, justice, and preservation are often sidelined when unregulated economic interests drive urban development.
While technological advancements, such as AI and AIoT systems, have the potential to enhance the efficiency and effectiveness of both civil society and government, it is essential to recognize that certain core functions should not be outsourced to these systems. The decision-making processes guided by civic and public values require the nuanced judgment and ethical considerations that only humans can provide. AI and AioT systems can be valuable tools, but they should support and complement the efforts of individuals and institutions rather than replace or overshadow the importance of these foundational values. The interplay between civic and public values and emerging technologies should be carefully managed to ensure that they continue to serve as the moral and ethical foundations of our society and government. However, Kassens-Noor and Hintze [ 303 ] argue that the adoption rate of AI technology, coupled with policy regulations and unforeseen events, has the potential to transform bustling metropolises into deserted ghost cities. The complete advancement of AI and AIoT may signify a decline in moral and societal values, raising concerns about the potential demise of the human race. Nevertheless, despite the enticing conceptual and discursive benefits (which relate to both ideas, theories, and perceptions, as well as the ways in which they are discussed and communicated) of transitioning cities into eco-cities, formidable obstacles have hindered large-scale implementations since the early 1990s, not to mention the development of smart(er) eco-cities. One of the most significant challenges confronting urban transformations lies in the significant costs, risks, and uncertainties associated with the incorporation of AI and AIoT into the realm of eco-urbanism.
6.6. Methodological limitations
It is important to acknowledge the methodological limitations of this comprehensive systematic review to allow the readers to assess the reliability and validity of the findings and understand the potential implications for future research and practice. These limitations, which arose from the various aspects of the review process, include:
- • Search strategy: Despite efforts to conduct a thorough literature search, some relevant studies may have been missed. Limitations in database selection, search keywords, language restriction, and inclusion/exclusion criteria could influence the breadth and depth of the included studies for synthesis. Moreover, as the study relied mainly on peer-reviewed documents, there is a risk of excluding a large part of grey literature and stakeholder input and not gaining extensive insights on emerging smarter eco-cities. This area of research is still evolving, and most of its existing work drew mainly from the fields of environmental sustainability, climate change, and smart cities with respect to AI and AIoT technologies and solutions. Even these fields are associated with a paucity of knowledge and a sparsity of empirical evidence due to the burgeoning nature of these technologies and solutions.
- • Publication bias: The study relied on published literature, and there is a risk of publication bias, where studies with positive results are more likely to be published, while studies with negative findings may be overlooked. This can affect the comprehensiveness and representativeness of the systematic review. It is common for researchers and journals to preferentially publish studies based on the direction or significance of their findings. This can lead to an overrepresentation of studies with positive results, while studies with negative results may be less likely to get published and thus be included in the systematic review. In addition, there is a language bias in that studies published in English are more accessible and commonly included in systematic reviews, leading to the potential exclusion of valuable evidence. Especially, English language was one of the inclusion criteria applied in the study. Funding sources are another bias regarding the studies funded by industry having a higher likelihood of being published — if they produce favorable results.
- • Data extraction and synthesis pertain to the complexities and challenges of extracting data from selected studies and synthesizing their findings. One of the primary issues posed in the study was the heterogeneity across studies. This included variations in study designs (e.g., methodologies) that complicated the synthesis of findings and reporting formats (differences in the presentation of results) that affected the extraction and synthesis process. These differences can make it difficult to directly compare and combine data from different studies, thus obtaining a comprehensive overview of the evidence and drawing robust conclusions from the systematic review.
7. Suggestions for future research
Smarter eco-city scholars, practitioners, and policymakers have a new opportunity to foster sustainable development practices based on a new paradigm of solution-thinking grounded in a deeper understanding of the interplay between techno-scientific and socio-political solutions. Developing this multi-faceted change process is one of the most critical challenges of sustainable urban development to achieve the status of smarter eco-cities. This emerging area of research is empirically under-researched, theoretically under-developed, and critically under-thought to allow for large-scale implementations. This means that a plethora of problems and questions need to be addressed and answered to guide the development of smarter eco-cities and, hence, large-scale AI and AIoT deployments for the common good. Some gaps in our knowledge of emerging smarter eco-cities follow from our results and discussion. These gaps span a broad set of topics that are significant to investigate or critically engage with and that can be approached from various perspectives in the form of suggestions for future research.
Considering the transformative potential of AI and AIoT technologies in reshaping smarter eco-cities, a comprehensive investigation is warranted to unravel the dimensions, opportunities, benefits, and challenges inherent in this emerging urban paradigm. Given the nascent stage of research at the intersection of environmental sustainability, climate change, and smart cities within the context of AI and AIoT solutions, the following avenues are crucial:
- • Identify key drivers: Delve into the multifaceted drivers that underpin the evolution of smart eco-cities, encompassing social, economic, institutional, and political factors beyond technological and environmental aspects.
- • Evaluate effectiveness: Prioritize assessing the real-world effectiveness and scalability of AI and AIoT applications in smarter eco-cities, determining their actual impact on achieving SDGs.
- • Explore long-term benefits: Probe the long-term benefits and opportunities offered by AI and AIoT technologies in fostering sustainability practices in smarter eco-cities. This includes exploring their potential for resource optimization, energy efficiency, waste reduction, and enhancing the quality of life for citizens.
- • Overcome barriers and risks: Confront challenges and mitigate risks associated with AI and AIoT implementation, such as privacy concerns, data security, and governance frameworks.
- • Promote responsible AI practices: Investigate guidelines and best practices for the responsible design, deployment, and governance of AI and AIoT solutions. Ensuring fairness, transparency, and accountability in decision-making processes is essential.
- • Integrate disciplines: Foster interdisciplinary research merging environmental sustainability, climate change, and smart cities with AI and AIoT. This approach unravels intricate relationships and facilitates a comprehensive understanding of the complex interactions and interdependencies between these domains, and enables the development of integrated and holistic solutions
- • Formulate policies and frameworks: Develop robust policy and governance frameworks that facilitate the ethical and transparent use of AI and AIoT technologies and support their adoption and implementation in sustainable urban development. This includes examining regulatory mechanisms, standards, and guidelines to ensure transparency, accountability, and ethical use of these technologies.
- • Advance XAI and IML methods: Develop solutions for interpretability in intricate models, user-centric model training, tailored solutions for smarter eco-cities, resilience and adaptability enhancement, and ethical implications. This entails developing XAI techniques for elucidating complex AI models in AIoT systems, exploring IML integration to engage users in refining models, utilizing XAI and IML to tackle distinct environmental challenges, fortifying AIoT systems' resilience against dynamic urban scenarios, and scrutinizing the ethical and societal implications of deploying XAI and IML in AIoT solutions to ensure equity and transparency.
- • Promote community engagement: Explore ways to involve local communities, stakeholders, and citizens in the design, implementation, and monitoring of AI and AIoT solutions for smarter eco-cities. Their active participation can lead to more inclusive and effective outcomes.
- • Quantify environmental impact: Develop methodologies to quantify the environmental impact of AI and AIoT solutions in smarter eco-cities. This involves assessing factors like energy consumption, carbon footprint, and resource utilization to understand the overall sustainability gains.
- • Conduct lifecycle analysis: Assess the sustainability of AI and AIoT technologies across their entire lifecycle, from production to disposal. This holistic approach can reveal potential environmental hotspots and guide improvements.
- • Promote citizen participation: Explore ways to empower citizens with AI-augmented information and tools that enable them to participate in environmental conservation and sustainable behaviors actively.
- • Enhance behavioral insights: Investigate how AI can leverage behavioral insights to encourage environmentally friendly behaviors among citizens, such as energy conservation and waste reduction.
- • Perform case studies: Conduct in-depth empirical inquiries and practical applications of AI and AIoT in actual smart eco-city projects. These real-world insights illuminate challenges, best practices, and valuable lessons for effective integration.
By addressing these knowledge gaps and pursuing these research avenues, the field of smarter eco-cities can advance its understanding, implementation, and impact. This will contribute to developing more sustainable and technologically advanced urban environments that benefit society and the environment.
8. Conclusion
As disruptive technologies, AI and AIoT lay the foundational technological infrastructure essential for constructing the digital ecosystem of emerging smarter eco-cities to amplify and sustain their contributions to environmental sustainability goals. This pursuit involves enhancing the efficiency and effectiveness of their operations, functions, strategies, and policies in alignment with the environmental targets of SDG 11. Within this context, it is important to acknowledge the immense potential of AI and AIoT technologies to develop robust intelligent systems generating profound insights for decision-making processes. However, these technologies cannot serve as a universal remedy or panacea for the wicked problems characterizing smarter eco-cities as complex systems. In this study, we aimed to provide a comprehensive systematic review of emerging smarter eco-cities and their leading-edge AI and AIoT solutions for environmental sustainability, employing a unified approach to evidence synthesis. The study's key findings concerning the five research questions are outlined as follows:
Interlinked foundational underpinnings of smarter eco-cities: The study showed that the fundamental concepts underpinning smarter eco-cities are intricately interconnected and build one on another on various scales. The key underlying urbanism paradigms, namely smart cities and eco-cities, serve as the foundation for integrating data-driven technologies and environmental solutions. Data-driven technologies enable real-time monitoring, analysis, and decision-making, while environmental solutions focus on optimizing resource efficiency and minimizing ecological footprint. Data-driven insights enhance the effectiveness of environmental strategies, ultimately contributing to creating more resilient, livable, and environmentally friendly urban environments.
The materialization of smarter eco-cities: The study identified several intertwined factors contributing to the materialization of smarter eco-cities as an emerging paradigm of urbanism, including the growing need for sustainable development, advancements in technology, environmental considerations, policy instruments, and government initiatives, and the recognition of the potential of data-driven technologies in addressing complex environmental challenges.
The primary AI and AIoT solutions harnessed in the development of emerging smarter eco-cities: The study identified many applied solutions of AI and AIoT technologies, demonstrating their role in urban planning, management, and development. These solutions encompass energy conservation and renewable energy, sustainable transportation management, traffic control, water resources conservation, waste management for efficient resource utilization, biodiversity and ecosystem services, environmental monitoring and control, climate change adaptation and mitigation, and disaster resilience and management.
The benefits and opportunities of AI and AIoT technologies in fostering sustainability practices in emerging smarter eco-cities: The study identified the opportunities and benefits offered by AI and AIoT technologies in the context of environmental sustainability. Combined, these opportunities and benefits included optimized resource management, increased energy efficiency, enhanced waste management, improved transportation, and mobility management, reduced environmental impacts, increased resilience to environmental challenges, enhanced decision-making in urban management and planning, and the potential for creating more sustainable and technologically advanced urban environments. Identifying opportunities — favorable circumstances and possibilities — helps understand the potential areas where AI and AIoT solutions can bring about positive changes and contribute to the overall development of smarter eco-cities. Benefits — positive outcomes and advantages — highlight the tangible and intangible gains that can be achieved by adopting AI and AIoT solutions.
Challenges and barriers arising in the implementation of AI and AIoT solutions for the development of emerging smarter eco-cities: The study identified and evaluated the key challenges pertaining to environmental costs, privacy concerns related to data collection and usage, cybersecurity risks related to interconnected systems, public trust, and social acceptance, limited technical expertise and knowledge, the lack of robust regulatory frameworks to ensure ethical and responsible AI and AIoT deployment and the requirement for addressing the social issues to ensure equitable and transparent use of AI and AIoT technologies.
Overall, the study highlighted the significance of AI and AIoT technologies in advancing the transition toward environmental sustainability in smarter eco-cities. While these technologies provide new and largely expanded opportunities to understand better and prevent environmental problems, they pose significant challenges that must be addressed and overcome to successfully implement smarter eco-cities. Therefore, it is important to emphasize the need for interdisciplinary research, policy support, and collaboration among different stakeholders to overcome these challenges and maximize the benefits of these technologies.
The synthesized evidence presented in this study has significant implications for researchers, practitioners, and policymakers involved in designing, managing, and planning smarter eco-cities. It offers valuable insights into the various dimensions of emerging smarter eco-cities and identifies best practices that can inform decision-making processes. The systematic review serves as a knowledge repository, guiding stakeholders in understanding the current state of research, identifying gaps, and shaping future strategies for sustainable urban development. Firstly, by identifying the core conceptual underpinnings of emerging smarter eco-cities and the intricate interconnections between them (RQ1), coupled with the intertwined factors propelling the materialization of smarter eco-cities (RQ2), the study encourages interdisciplinary collaboration among researchers and practitioners from various fields and foster new research avenues and practice pathways, leading to a more thorough understanding and focused improvement of emerging smarter eco-cities. Secondly, by identifying the key applied solutions of AI and AIoT technologies (RQ3), the study can inform researchers, practitioners, and policymakers about the technological advancements and innovative approaches employed in fostering sustainable urban development practices. Furthermore, exploring the potential opportunities and benefits offered by AI and AIoT technologies in this regard (RQ4) can provide valuable insights for decision-makers and urban planners seeking to leverage these technologies for achieving the SDGs, especially SDG 11. Lastly, identifying challenges and barriers in implementing AI and AIoT solutions in emerging smarter eco-cities (RQ5) can inform policymakers and stakeholders about the potential obstacles and open issues that need to be addressed when integrating these technologies into urban development strategies. Overall, the research, practice, and policymaking implications of this study encompass a wide range of areas, including urban planning, technology implementation, sustainability practices, and policy development, facilitating informed decision-making, and promoting the advancement of smarter eco-cities.
Ultimately, the findings of the systematic review contribute to the broader goal of creating smarter eco-cities that prioritize environmental sustainability, resource efficiency, and human well-being. The invaluable insights gained accordingly will empower stakeholders to make strategic choices, implement innovative solutions, and drive positive change in urban planning and management. By leveraging the potential of AI and AIoT, policymakers, urban planners, researchers, and practitioners can work together toward creating smarter, more resilient, more livable, and environmentally conscious cities that meet the needs of present and future generations. To sum up, AI and AIoT technologies will offer unprecedented capabilities to rise to many of the grand environmental challenges, but how these technologies will be used and what other possible directions this use might take is up to all of us, especially the research community, and for the time to tell.
CRediT authorship contribution statement
Simon Elias Bibri : Conceptualization, Methodology, Formal Analysis, Investigation, Data Curation, Visualization, Software, Writing - Original Draft, Writing - Review & Editing. John Krogstie : Conceptualization, Writing - Review & Editing. Alexandre Alahi and Amin Kaboli : Writing - Review & Editing. All authors read and approved the published version of the manuscript.
Declaration of competing interest
The authors declare that they have no known competing financial interests or personal relationships that could have influenced the work reported in this article.
Acknowledgments
This project has received funding from the European Union's Horizon 2020 research and innovation program under the Marie Sklodowska-Curie grant agreement No. 101034260.
Abbreviations
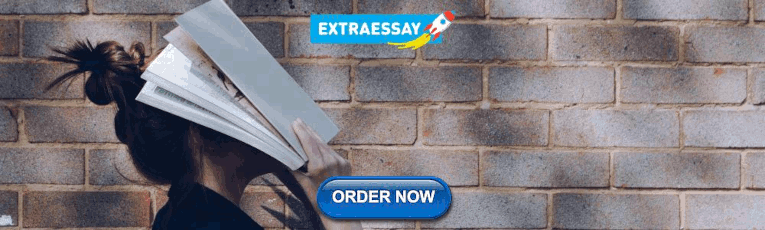
IMAGES
VIDEO
COMMENTS
A literature review may reach beyond BER and include other education research fields. A theoretical framework does not rationalize the need for the study, and a theoretical framework can come from different fields. A conceptual framework articulates the phenomenon under study through written descriptions and/or visual representations.
The theoretical framework is used to lay down a foundation of theory on which your study will be built, whereas the conceptual framework visualises what you anticipate the relationships between concepts, constructs and variables may be, based on your understanding of the existing literature and the specific context and focus of your research.
This essay starts with a discussion of the literature review, theoretical framework, and conceptual framework as components of a manuscript. This discussion includes similarities and distinctions ...
This essay starts with a discussion of the literature review, theoretical framework, and conceptual framework as components of a manuscript. This discussion includes similarities and distinctions among these components and their relation to other sections of a manuscript such as the problem statement, discussion, and implications.
reviews, theoretical frameworks, and conceptual frameworks as critical elements of the research and writing process. However, these elements can be confusing for scholars new ... A literature review is foundational to any research study in edu - cation or science. In education, a well-conceptualized and ...
Developing a conceptual framework in research. A conceptual framework is a representation of the relationship you expect to see between your variables, or the characteristics or properties that you want to study. Conceptual frameworks can be written or visual and are generally developed based on a literature review of existing studies about ...
A theoretical framework is a foundational review of existing theories that serves as a roadmap for developing the arguments you will use in your own work. Theories are developed by researchers to explain phenomena, draw connections, and make predictions. In a theoretical framework, you explain the existing theories that support your research ...
This is generally referred to as the "literature review," "theoretical framework," or "research background." However, for a literature review to become a proper research methodology, as with any other research, follow proper steps need to be followed and action taken to ensure the review is accurate, precise, and trustworthy.
Abstract. While 'conceptual framework' means a researcher's own perceptions about the scope and structure of a problem, the literature review provides others' ideas and work in areas close to that under study. With such a philosophy in mind, this chapter first constructs the author's own thinking as to how the problem in question has ...
This essay starts with a discussion of the literature review, theoretical framework, and conceptual framework as components of a manuscript. This discussion includes similarities and distinctions among these components and their relation to other sections of a manuscript such as the problem statement, discussion, and implications. The essay concludes with an overview of the literature review ...
first section will discuss literature review, theoretical framework, and conceptual framework as components of a manuscript. The second section will discuss literature review, theoretical framework, and conceptual framework as types of manuscripts. The first area to be discussed is that these terms are used interchangeably.
A Review of the Theoretical Literature" (Theoretical literature review about the development of economic migration theory from the 1950s to today.) Example literature review #2: "Literature review as a research methodology: An overview and guidelines" (Methodological literature review about interdisciplinary knowledge acquisition and ...
conceptual and theoretical frameworks. As conceptual defines the key co ncepts, variables, and. relationships in a research study as a roadmap that outlines the researcher's understanding of how ...
To frame their work, biology education researchers need to consider the role of literature reviews, theoretical frameworks, and conceptual frameworks as critical elements of the research and writing process. However, these elements can be confusing for scholars new to education research. This <i>Res</i> …
work. I have also inferred, like many have done, that the basic components of literature review consist of introduction, review of theoretical and empirical literature, implication of the review, and theoretical and/or conceptual framework/s. Its implication is that any research work needs to pave its pathways distinctly for its successful ...
A theoretical framework is a foundational review of existing theories that serves as a roadmap for developing the arguments you will use in your own work. Theories are developed by researchers to explain phenomena, draw connections, and make predictions. In a theoretical framework, you explain the existing theories that support your research ...
Fourthly, the paper explains how a theoretical framework for a research project is developed. Finally, I provide an example of the development of a real theoretical framework and explain how it could be applied in data analysis. 2. Systematic Literature Review Methodology: What Is a Theory? A systematic review of pertinent literature provides ...
Now, let's explore the key differences between literature reviews and theoretical frameworks. Key Differences: Focus: A literature review focuses on summarizing existing research, while a theoretical framework focuses on providing a conceptual foundation for the study. Scope: A literature review covers a broad range of related research, while a ...
Step 2: Build a Literature Review and Identify a Theoretical or Conceptual Framework Written and Compiled by Amanda J. Rockinson-Szapkiw & Anita Knight Introduction Foundations are important. There is a Biblical parable about two builders. One builder builds his house on a solid foundation. When storms come, the house remains standing.
The main difference between literature review and theoretical framework is their function. The literature review explores what has already been written about the topic under study in order to highlight a gap, whereas the theoretical framework is the conceptual and analytical approach the researcher is going to take to fill that gap.
3) the part of a research paper that reviews the literature in an empirical study. I've seen papers contain both "theoretical analysis" and "conceptual analysis" in the title, as in they can be fairly indistinguishable. However, 'theoretical analysis' can refer to an actual analysis of empirical data obtained through experiments ...
A literature review and a theoretical framework are not the same thing and cannot be used interchangeably. While a theoretical framework describes the theoretical underpinnings of your work, a literature review critically evaluates existing research relating to your topic. You'll likely need both in your dissertation.
Drawing on both literature review and expert opinion, our study followed a rigorous approach to develop a conceptual framework of trial efficiency. We included a wide range of experts in trial communities including members of the public, enhancing the comprehensiveness and richness of our study.
Purpose - The authors perform a large-scale literature review and use conceptual theory building to introduce the concept of sustainability to the field of supply chain management and ...
A comprehensive evaluation of theoretical, methodological, and empirical issues pertaining to conceptual approaches, antecedents, dynamics, and results of GEO was conducted on 59 studies. This study contributes several findings to the entrepreneurship literature. These research findings give implications for both theory and practice.
This phase aims to develop an integrative theoretical framework that captures the evolution of servitization over the past three decades, emphasizing the shift toward digital service delivery within the manufacturing sector. Subsequently, the study undertakes a systematic literature review to classify the existing body of work.
THEORETICAL FRAMEWORK Set of interrelated construct, definitions, and prepositions that presents a systematic view of phenomena. Existing and well developed theory Located mainly in quantitative CONCEPTUAL FRAMEWORK Created by the researcher from a variety of conceptual/theoretical perspectives Researcher's own position on a problem after his exposure to various theories (bearing on the problem).
It identifies common themes across the studies and developing a conceptual framework to explain the interconnections and variations observed in the findings. It aims to provide a deeper understanding of the research evidence by exploring the relationships and context in which the findings occur. ... Overall, these theoretical and literature ...
Theoretical Framework Example for a Thesis or Dissertation. Published on October 14, 2015 by Sarah Vinz . Revised on July 18, 2023 by Tegan George. Your theoretical framework defines the key concepts in your research, suggests relationships between them, and discusses relevant theories based on your literature review.
This review covers the diverse range of literature, from 2001 to 2024, conducted using the SPAR‐4‐SLR protocol and proposes the integrated conceptual framework of the concept. The article synthesizes online impulse buying research conducted through a systematic literature review (SLR) approach. The present review covers the diverse range of literature, from 2001 to 2024, conducted using ...