Reliability and validity: Importance in Medical Research
Affiliations.
- 1 Al-Nafees Medical College,Isra University, Islamabad, Pakistan.
- 2 Fauji Foundation Hospital, Foundation University Medical College, Islamabad, Pakistan.
- PMID: 34974579
- DOI: 10.47391/JPMA.06-861
Reliability and validity are among the most important and fundamental domains in the assessment of any measuring methodology for data-collection in a good research. Validity is about what an instrument measures and how well it does so, whereas reliability concerns the truthfulness in the data obtained and the degree to which any measuring tool controls random error. The current narrative review was planned to discuss the importance of reliability and validity of data-collection or measurement techniques used in research. It describes and explores comprehensively the reliability and validity of research instruments and also discusses different forms of reliability and validity with concise examples. An attempt has been taken to give a brief literature review regarding the significance of reliability and validity in medical sciences.
Keywords: Validity, Reliability, Medical research, Methodology, Assessment, Research tools..
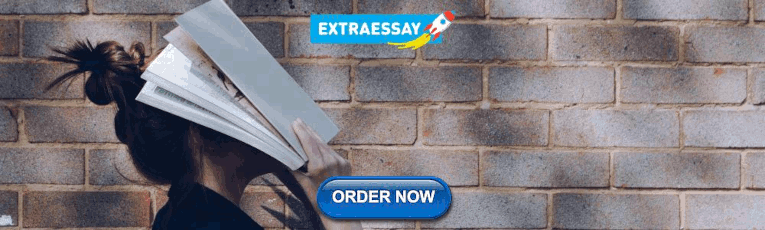
Publication types
- Biomedical Research*
- Reproducibility of Results
Log in using your username and password
- Search More Search for this keyword Advanced search
- Latest content
- Current issue
- Write for Us
- BMJ Journals More You are viewing from: Google Indexer
You are here
- Volume 18, Issue 3
- Validity and reliability in quantitative studies
- Article Text
- Article info
- Citation Tools
- Rapid Responses
- Article metrics

- Roberta Heale 1 ,
- Alison Twycross 2
- 1 School of Nursing, Laurentian University , Sudbury, Ontario , Canada
- 2 Faculty of Health and Social Care , London South Bank University , London , UK
- Correspondence to : Dr Roberta Heale, School of Nursing, Laurentian University, Ramsey Lake Road, Sudbury, Ontario, Canada P3E2C6; rheale{at}laurentian.ca
https://doi.org/10.1136/eb-2015-102129
Statistics from Altmetric.com
Request permissions.
If you wish to reuse any or all of this article please use the link below which will take you to the Copyright Clearance Center’s RightsLink service. You will be able to get a quick price and instant permission to reuse the content in many different ways.
Evidence-based practice includes, in part, implementation of the findings of well-conducted quality research studies. So being able to critique quantitative research is an important skill for nurses. Consideration must be given not only to the results of the study but also the rigour of the research. Rigour refers to the extent to which the researchers worked to enhance the quality of the studies. In quantitative research, this is achieved through measurement of the validity and reliability. 1
- View inline
Types of validity
The first category is content validity . This category looks at whether the instrument adequately covers all the content that it should with respect to the variable. In other words, does the instrument cover the entire domain related to the variable, or construct it was designed to measure? In an undergraduate nursing course with instruction about public health, an examination with content validity would cover all the content in the course with greater emphasis on the topics that had received greater coverage or more depth. A subset of content validity is face validity , where experts are asked their opinion about whether an instrument measures the concept intended.
Construct validity refers to whether you can draw inferences about test scores related to the concept being studied. For example, if a person has a high score on a survey that measures anxiety, does this person truly have a high degree of anxiety? In another example, a test of knowledge of medications that requires dosage calculations may instead be testing maths knowledge.
There are three types of evidence that can be used to demonstrate a research instrument has construct validity:
Homogeneity—meaning that the instrument measures one construct.
Convergence—this occurs when the instrument measures concepts similar to that of other instruments. Although if there are no similar instruments available this will not be possible to do.
Theory evidence—this is evident when behaviour is similar to theoretical propositions of the construct measured in the instrument. For example, when an instrument measures anxiety, one would expect to see that participants who score high on the instrument for anxiety also demonstrate symptoms of anxiety in their day-to-day lives. 2
The final measure of validity is criterion validity . A criterion is any other instrument that measures the same variable. Correlations can be conducted to determine the extent to which the different instruments measure the same variable. Criterion validity is measured in three ways:
Convergent validity—shows that an instrument is highly correlated with instruments measuring similar variables.
Divergent validity—shows that an instrument is poorly correlated to instruments that measure different variables. In this case, for example, there should be a low correlation between an instrument that measures motivation and one that measures self-efficacy.
Predictive validity—means that the instrument should have high correlations with future criterions. 2 For example, a score of high self-efficacy related to performing a task should predict the likelihood a participant completing the task.
Reliability
Reliability relates to the consistency of a measure. A participant completing an instrument meant to measure motivation should have approximately the same responses each time the test is completed. Although it is not possible to give an exact calculation of reliability, an estimate of reliability can be achieved through different measures. The three attributes of reliability are outlined in table 2 . How each attribute is tested for is described below.
Attributes of reliability
Homogeneity (internal consistency) is assessed using item-to-total correlation, split-half reliability, Kuder-Richardson coefficient and Cronbach's α. In split-half reliability, the results of a test, or instrument, are divided in half. Correlations are calculated comparing both halves. Strong correlations indicate high reliability, while weak correlations indicate the instrument may not be reliable. The Kuder-Richardson test is a more complicated version of the split-half test. In this process the average of all possible split half combinations is determined and a correlation between 0–1 is generated. This test is more accurate than the split-half test, but can only be completed on questions with two answers (eg, yes or no, 0 or 1). 3
Cronbach's α is the most commonly used test to determine the internal consistency of an instrument. In this test, the average of all correlations in every combination of split-halves is determined. Instruments with questions that have more than two responses can be used in this test. The Cronbach's α result is a number between 0 and 1. An acceptable reliability score is one that is 0.7 and higher. 1 , 3
Stability is tested using test–retest and parallel or alternate-form reliability testing. Test–retest reliability is assessed when an instrument is given to the same participants more than once under similar circumstances. A statistical comparison is made between participant's test scores for each of the times they have completed it. This provides an indication of the reliability of the instrument. Parallel-form reliability (or alternate-form reliability) is similar to test–retest reliability except that a different form of the original instrument is given to participants in subsequent tests. The domain, or concepts being tested are the same in both versions of the instrument but the wording of items is different. 2 For an instrument to demonstrate stability there should be a high correlation between the scores each time a participant completes the test. Generally speaking, a correlation coefficient of less than 0.3 signifies a weak correlation, 0.3–0.5 is moderate and greater than 0.5 is strong. 4
Equivalence is assessed through inter-rater reliability. This test includes a process for qualitatively determining the level of agreement between two or more observers. A good example of the process used in assessing inter-rater reliability is the scores of judges for a skating competition. The level of consistency across all judges in the scores given to skating participants is the measure of inter-rater reliability. An example in research is when researchers are asked to give a score for the relevancy of each item on an instrument. Consistency in their scores relates to the level of inter-rater reliability of the instrument.
Determining how rigorously the issues of reliability and validity have been addressed in a study is an essential component in the critique of research as well as influencing the decision about whether to implement of the study findings into nursing practice. In quantitative studies, rigour is determined through an evaluation of the validity and reliability of the tools or instruments utilised in the study. A good quality research study will provide evidence of how all these factors have been addressed. This will help you to assess the validity and reliability of the research and help you decide whether or not you should apply the findings in your area of clinical practice.
- Lobiondo-Wood G ,
- Shuttleworth M
- ↵ Laerd Statistics . Determining the correlation coefficient . 2013 . https://statistics.laerd.com/premium/pc/pearson-correlation-in-spss-8.php
Twitter Follow Roberta Heale at @robertaheale and Alison Twycross at @alitwy
Competing interests None declared.
Read the full text or download the PDF:
Have a language expert improve your writing
Run a free plagiarism check in 10 minutes, automatically generate references for free.
- Knowledge Base
- Methodology
- Reliability vs Validity in Research | Differences, Types & Examples
Reliability vs Validity in Research | Differences, Types & Examples
Published on 3 May 2022 by Fiona Middleton . Revised on 10 October 2022.
Reliability and validity are concepts used to evaluate the quality of research. They indicate how well a method , technique, or test measures something. Reliability is about the consistency of a measure, and validity is about the accuracy of a measure.
It’s important to consider reliability and validity when you are creating your research design , planning your methods, and writing up your results, especially in quantitative research .
Table of contents
Understanding reliability vs validity, how are reliability and validity assessed, how to ensure validity and reliability in your research, where to write about reliability and validity in a thesis.
Reliability and validity are closely related, but they mean different things. A measurement can be reliable without being valid. However, if a measurement is valid, it is usually also reliable.
What is reliability?
Reliability refers to how consistently a method measures something. If the same result can be consistently achieved by using the same methods under the same circumstances, the measurement is considered reliable.
What is validity?
Validity refers to how accurately a method measures what it is intended to measure. If research has high validity, that means it produces results that correspond to real properties, characteristics, and variations in the physical or social world.
High reliability is one indicator that a measurement is valid. If a method is not reliable, it probably isn’t valid.
However, reliability on its own is not enough to ensure validity. Even if a test is reliable, it may not accurately reflect the real situation.
Validity is harder to assess than reliability, but it is even more important. To obtain useful results, the methods you use to collect your data must be valid: the research must be measuring what it claims to measure. This ensures that your discussion of the data and the conclusions you draw are also valid.
Prevent plagiarism, run a free check.
Reliability can be estimated by comparing different versions of the same measurement. Validity is harder to assess, but it can be estimated by comparing the results to other relevant data or theory. Methods of estimating reliability and validity are usually split up into different types.
Types of reliability
Different types of reliability can be estimated through various statistical methods.
Types of validity
The validity of a measurement can be estimated based on three main types of evidence. Each type can be evaluated through expert judgement or statistical methods.
To assess the validity of a cause-and-effect relationship, you also need to consider internal validity (the design of the experiment ) and external validity (the generalisability of the results).
The reliability and validity of your results depends on creating a strong research design , choosing appropriate methods and samples, and conducting the research carefully and consistently.
Ensuring validity
If you use scores or ratings to measure variations in something (such as psychological traits, levels of ability, or physical properties), it’s important that your results reflect the real variations as accurately as possible. Validity should be considered in the very earliest stages of your research, when you decide how you will collect your data .
- Choose appropriate methods of measurement
Ensure that your method and measurement technique are of high quality and targeted to measure exactly what you want to know. They should be thoroughly researched and based on existing knowledge.
For example, to collect data on a personality trait, you could use a standardised questionnaire that is considered reliable and valid. If you develop your own questionnaire, it should be based on established theory or the findings of previous studies, and the questions should be carefully and precisely worded.
- Use appropriate sampling methods to select your subjects
To produce valid generalisable results, clearly define the population you are researching (e.g., people from a specific age range, geographical location, or profession). Ensure that you have enough participants and that they are representative of the population.
Ensuring reliability
Reliability should be considered throughout the data collection process. When you use a tool or technique to collect data, it’s important that the results are precise, stable, and reproducible.
- Apply your methods consistently
Plan your method carefully to make sure you carry out the same steps in the same way for each measurement. This is especially important if multiple researchers are involved.
For example, if you are conducting interviews or observations, clearly define how specific behaviours or responses will be counted, and make sure questions are phrased the same way each time.
- Standardise the conditions of your research
When you collect your data, keep the circumstances as consistent as possible to reduce the influence of external factors that might create variation in the results.
For example, in an experimental setup, make sure all participants are given the same information and tested under the same conditions.
It’s appropriate to discuss reliability and validity in various sections of your thesis or dissertation or research paper. Showing that you have taken them into account in planning your research and interpreting the results makes your work more credible and trustworthy.
Cite this Scribbr article
If you want to cite this source, you can copy and paste the citation or click the ‘Cite this Scribbr article’ button to automatically add the citation to our free Reference Generator.
Middleton, F. (2022, October 10). Reliability vs Validity in Research | Differences, Types & Examples. Scribbr. Retrieved 14 May 2024, from https://www.scribbr.co.uk/research-methods/reliability-or-validity/
Is this article helpful?
Fiona Middleton
Other students also liked, the 4 types of validity | types, definitions & examples, a quick guide to experimental design | 5 steps & examples, sampling methods | types, techniques, & examples.

Validity & Reliability In Research
A Plain-Language Explanation (With Examples)
By: Derek Jansen (MBA) | Expert Reviewer: Kerryn Warren (PhD) | September 2023
Validity and reliability are two related but distinctly different concepts within research. Understanding what they are and how to achieve them is critically important to any research project. In this post, we’ll unpack these two concepts as simply as possible.
This post is based on our popular online course, Research Methodology Bootcamp . In the course, we unpack the basics of methodology using straightfoward language and loads of examples. If you’re new to academic research, you definitely want to use this link to get 50% off the course (limited-time offer).
Overview: Validity & Reliability
- The big picture
- Validity 101
- Reliability 101
- Key takeaways
First, The Basics…
First, let’s start with a big-picture view and then we can zoom in to the finer details.
Validity and reliability are two incredibly important concepts in research, especially within the social sciences. Both validity and reliability have to do with the measurement of variables and/or constructs – for example, job satisfaction, intelligence, productivity, etc. When undertaking research, you’ll often want to measure these types of constructs and variables and, at the simplest level, validity and reliability are about ensuring the quality and accuracy of those measurements .
As you can probably imagine, if your measurements aren’t accurate or there are quality issues at play when you’re collecting your data, your entire study will be at risk. Therefore, validity and reliability are very important concepts to understand (and to get right). So, let’s unpack each of them.
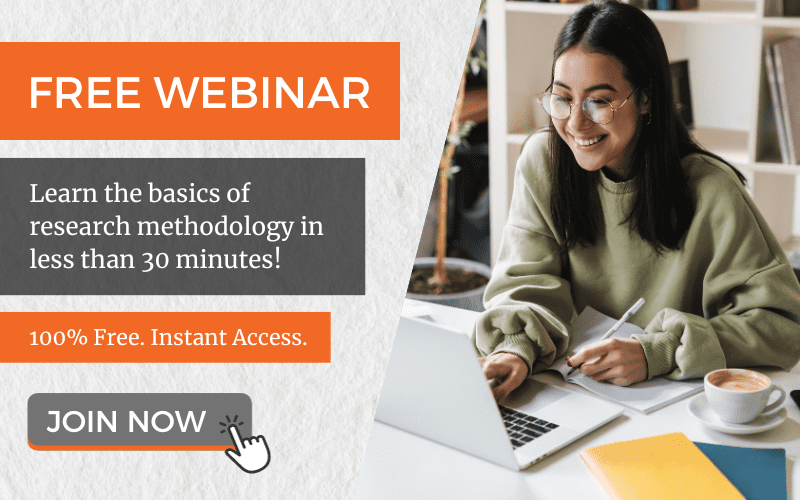
What Is Validity?
In simple terms, validity (also called “construct validity”) is all about whether a research instrument accurately measures what it’s supposed to measure .
For example, let’s say you have a set of Likert scales that are supposed to quantify someone’s level of overall job satisfaction. If this set of scales focused purely on only one dimension of job satisfaction, say pay satisfaction, this would not be a valid measurement, as it only captures one aspect of the multidimensional construct. In other words, pay satisfaction alone is only one contributing factor toward overall job satisfaction, and therefore it’s not a valid way to measure someone’s job satisfaction.
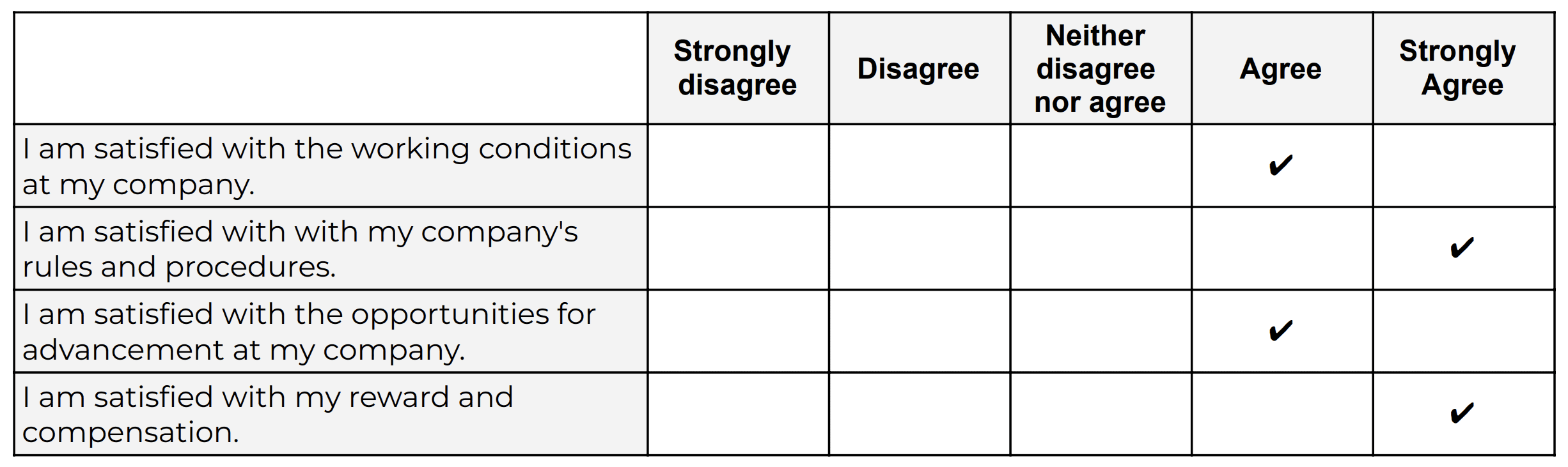
Oftentimes in quantitative studies, the way in which the researcher or survey designer interprets a question or statement can differ from how the study participants interpret it . Given that respondents don’t have the opportunity to ask clarifying questions when taking a survey, it’s easy for these sorts of misunderstandings to crop up. Naturally, if the respondents are interpreting the question in the wrong way, the data they provide will be pretty useless . Therefore, ensuring that a study’s measurement instruments are valid – in other words, that they are measuring what they intend to measure – is incredibly important.
There are various types of validity and we’re not going to go down that rabbit hole in this post, but it’s worth quickly highlighting the importance of making sure that your research instrument is tightly aligned with the theoretical construct you’re trying to measure . In other words, you need to pay careful attention to how the key theories within your study define the thing you’re trying to measure – and then make sure that your survey presents it in the same way.
For example, sticking with the “job satisfaction” construct we looked at earlier, you’d need to clearly define what you mean by job satisfaction within your study (and this definition would of course need to be underpinned by the relevant theory). You’d then need to make sure that your chosen definition is reflected in the types of questions or scales you’re using in your survey . Simply put, you need to make sure that your survey respondents are perceiving your key constructs in the same way you are. Or, even if they’re not, that your measurement instrument is capturing the necessary information that reflects your definition of the construct at hand.
If all of this talk about constructs sounds a bit fluffy, be sure to check out Research Methodology Bootcamp , which will provide you with a rock-solid foundational understanding of all things methodology-related. Remember, you can take advantage of our 60% discount offer using this link.
Need a helping hand?
What Is Reliability?
As with validity, reliability is an attribute of a measurement instrument – for example, a survey, a weight scale or even a blood pressure monitor. But while validity is concerned with whether the instrument is measuring the “thing” it’s supposed to be measuring, reliability is concerned with consistency and stability . In other words, reliability reflects the degree to which a measurement instrument produces consistent results when applied repeatedly to the same phenomenon , under the same conditions .
As you can probably imagine, a measurement instrument that achieves a high level of consistency is naturally more dependable (or reliable) than one that doesn’t – in other words, it can be trusted to provide consistent measurements . And that, of course, is what you want when undertaking empirical research. If you think about it within a more domestic context, just imagine if you found that your bathroom scale gave you a different number every time you hopped on and off of it – you wouldn’t feel too confident in its ability to measure the variable that is your body weight 🙂
It’s worth mentioning that reliability also extends to the person using the measurement instrument . For example, if two researchers use the same instrument (let’s say a measuring tape) and they get different measurements, there’s likely an issue in terms of how one (or both) of them are using the measuring tape. So, when you think about reliability, consider both the instrument and the researcher as part of the equation.
As with validity, there are various types of reliability and various tests that can be used to assess the reliability of an instrument. A popular one that you’ll likely come across for survey instruments is Cronbach’s alpha , which is a statistical measure that quantifies the degree to which items within an instrument (for example, a set of Likert scales) measure the same underlying construct . In other words, Cronbach’s alpha indicates how closely related the items are and whether they consistently capture the same concept .
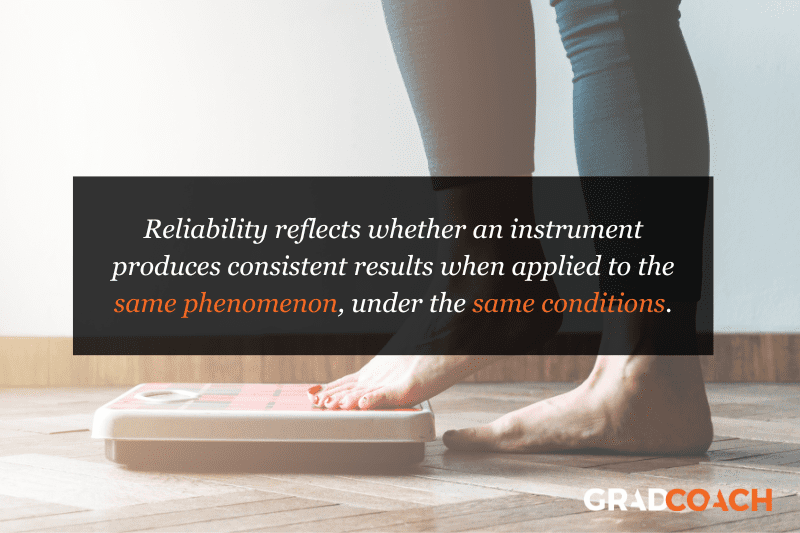
Recap: Key Takeaways
Alright, let’s quickly recap to cement your understanding of validity and reliability:
- Validity is concerned with whether an instrument (e.g., a set of Likert scales) is measuring what it’s supposed to measure
- Reliability is concerned with whether that measurement is consistent and stable when measuring the same phenomenon under the same conditions.
In short, validity and reliability are both essential to ensuring that your data collection efforts deliver high-quality, accurate data that help you answer your research questions . So, be sure to always pay careful attention to the validity and reliability of your measurement instruments when collecting and analysing data. As the adage goes, “rubbish in, rubbish out” – make sure that your data inputs are rock-solid.
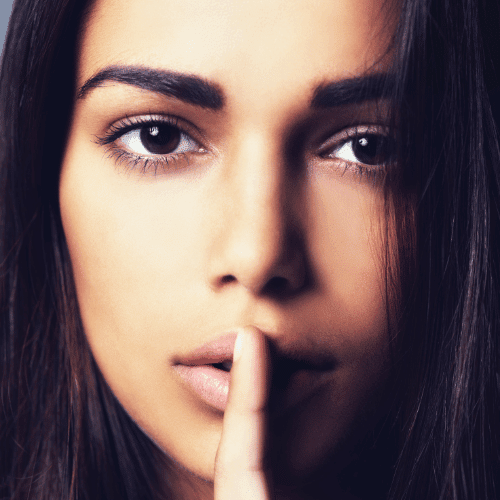
Psst… there’s more!
This post is an extract from our bestselling short course, Methodology Bootcamp . If you want to work smart, you don't want to miss this .
You Might Also Like:
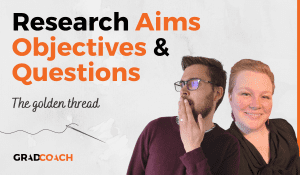
THE MATERIAL IS WONDERFUL AND BENEFICIAL TO ALL STUDENTS.
THE MATERIAL IS WONDERFUL AND BENEFICIAL TO ALL STUDENTS AND I HAVE GREATLY BENEFITED FROM THE CONTENT.
Submit a Comment Cancel reply
Your email address will not be published. Required fields are marked *
Save my name, email, and website in this browser for the next time I comment.
- Print Friendly
- How it works
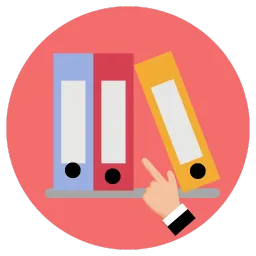
Reliability and Validity – Definitions, Types & Examples
Published by Alvin Nicolas at August 16th, 2021 , Revised On October 26, 2023
A researcher must test the collected data before making any conclusion. Every research design needs to be concerned with reliability and validity to measure the quality of the research.
What is Reliability?
Reliability refers to the consistency of the measurement. Reliability shows how trustworthy is the score of the test. If the collected data shows the same results after being tested using various methods and sample groups, the information is reliable. If your method has reliability, the results will be valid.
Example: If you weigh yourself on a weighing scale throughout the day, you’ll get the same results. These are considered reliable results obtained through repeated measures.
Example: If a teacher conducts the same math test of students and repeats it next week with the same questions. If she gets the same score, then the reliability of the test is high.
What is the Validity?
Validity refers to the accuracy of the measurement. Validity shows how a specific test is suitable for a particular situation. If the results are accurate according to the researcher’s situation, explanation, and prediction, then the research is valid.
If the method of measuring is accurate, then it’ll produce accurate results. If a method is reliable, then it’s valid. In contrast, if a method is not reliable, it’s not valid.
Example: Your weighing scale shows different results each time you weigh yourself within a day even after handling it carefully, and weighing before and after meals. Your weighing machine might be malfunctioning. It means your method had low reliability. Hence you are getting inaccurate or inconsistent results that are not valid.
Example: Suppose a questionnaire is distributed among a group of people to check the quality of a skincare product and repeated the same questionnaire with many groups. If you get the same response from various participants, it means the validity of the questionnaire and product is high as it has high reliability.
Most of the time, validity is difficult to measure even though the process of measurement is reliable. It isn’t easy to interpret the real situation.
Example: If the weighing scale shows the same result, let’s say 70 kg each time, even if your actual weight is 55 kg, then it means the weighing scale is malfunctioning. However, it was showing consistent results, but it cannot be considered as reliable. It means the method has low reliability.
Internal Vs. External Validity
One of the key features of randomised designs is that they have significantly high internal and external validity.
Internal validity is the ability to draw a causal link between your treatment and the dependent variable of interest. It means the observed changes should be due to the experiment conducted, and any external factor should not influence the variables .
Example: age, level, height, and grade.
External validity is the ability to identify and generalise your study outcomes to the population at large. The relationship between the study’s situation and the situations outside the study is considered external validity.
Also, read about Inductive vs Deductive reasoning in this article.
Looking for reliable dissertation support?
We hear you.
- Whether you want a full dissertation written or need help forming a dissertation proposal, we can help you with both.
- Get different dissertation services at ResearchProspect and score amazing grades!
Threats to Interval Validity
Threats of external validity, how to assess reliability and validity.
Reliability can be measured by comparing the consistency of the procedure and its results. There are various methods to measure validity and reliability. Reliability can be measured through various statistical methods depending on the types of validity, as explained below:
Types of Reliability
Types of validity.
As we discussed above, the reliability of the measurement alone cannot determine its validity. Validity is difficult to be measured even if the method is reliable. The following type of tests is conducted for measuring validity.
Does your Research Methodology Have the Following?
- Great Research/Sources
- Perfect Language
- Accurate Sources
If not, we can help. Our panel of experts makes sure to keep the 3 pillars of Research Methodology strong.
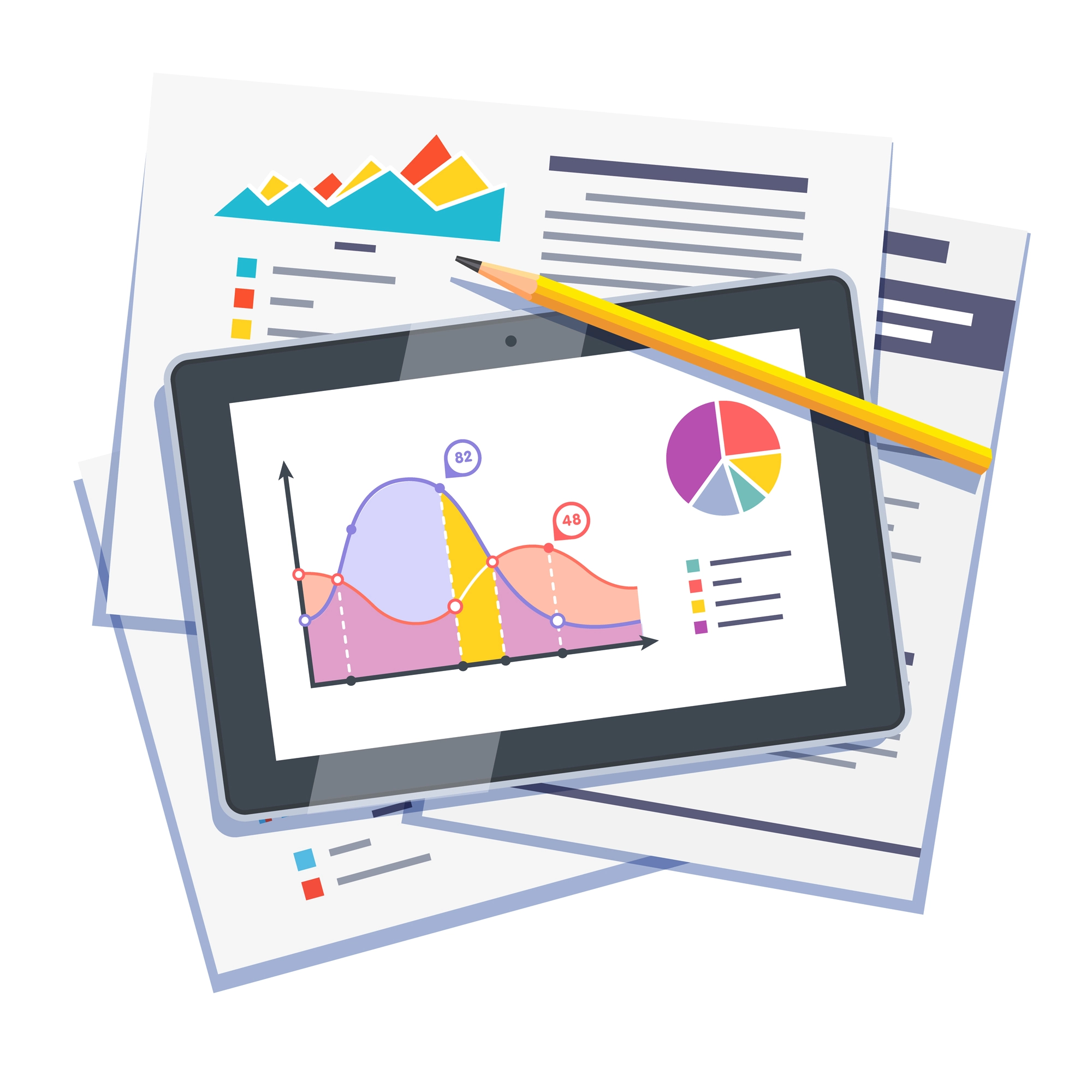
How to Increase Reliability?
- Use an appropriate questionnaire to measure the competency level.
- Ensure a consistent environment for participants
- Make the participants familiar with the criteria of assessment.
- Train the participants appropriately.
- Analyse the research items regularly to avoid poor performance.
How to Increase Validity?
Ensuring Validity is also not an easy job. A proper functioning method to ensure validity is given below:
- The reactivity should be minimised at the first concern.
- The Hawthorne effect should be reduced.
- The respondents should be motivated.
- The intervals between the pre-test and post-test should not be lengthy.
- Dropout rates should be avoided.
- The inter-rater reliability should be ensured.
- Control and experimental groups should be matched with each other.
How to Implement Reliability and Validity in your Thesis?
According to the experts, it is helpful if to implement the concept of reliability and Validity. Especially, in the thesis and the dissertation, these concepts are adopted much. The method for implementation given below:
Frequently Asked Questions
What is reliability and validity in research.
Reliability in research refers to the consistency and stability of measurements or findings. Validity relates to the accuracy and truthfulness of results, measuring what the study intends to. Both are crucial for trustworthy and credible research outcomes.
What is validity?
Validity in research refers to the extent to which a study accurately measures what it intends to measure. It ensures that the results are truly representative of the phenomena under investigation. Without validity, research findings may be irrelevant, misleading, or incorrect, limiting their applicability and credibility.
What is reliability?
Reliability in research refers to the consistency and stability of measurements over time. If a study is reliable, repeating the experiment or test under the same conditions should produce similar results. Without reliability, findings become unpredictable and lack dependability, potentially undermining the study’s credibility and generalisability.
What is reliability in psychology?
In psychology, reliability refers to the consistency of a measurement tool or test. A reliable psychological assessment produces stable and consistent results across different times, situations, or raters. It ensures that an instrument’s scores are not due to random error, making the findings dependable and reproducible in similar conditions.
What is test retest reliability?
Test-retest reliability assesses the consistency of measurements taken by a test over time. It involves administering the same test to the same participants at two different points in time and comparing the results. A high correlation between the scores indicates that the test produces stable and consistent results over time.
How to improve reliability of an experiment?
- Standardise procedures and instructions.
- Use consistent and precise measurement tools.
- Train observers or raters to reduce subjective judgments.
- Increase sample size to reduce random errors.
- Conduct pilot studies to refine methods.
- Repeat measurements or use multiple methods.
- Address potential sources of variability.
What is the difference between reliability and validity?
Reliability refers to the consistency and repeatability of measurements, ensuring results are stable over time. Validity indicates how well an instrument measures what it’s intended to measure, ensuring accuracy and relevance. While a test can be reliable without being valid, a valid test must inherently be reliable. Both are essential for credible research.
Are interviews reliable and valid?
Interviews can be both reliable and valid, but they are susceptible to biases. The reliability and validity depend on the design, structure, and execution of the interview. Structured interviews with standardised questions improve reliability. Validity is enhanced when questions accurately capture the intended construct and when interviewer biases are minimised.
Are IQ tests valid and reliable?
IQ tests are generally considered reliable, producing consistent scores over time. Their validity, however, is a subject of debate. While they effectively measure certain cognitive skills, whether they capture the entirety of “intelligence” or predict success in all life areas is contested. Cultural bias and over-reliance on tests are also concerns.
Are questionnaires reliable and valid?
Questionnaires can be both reliable and valid if well-designed. Reliability is achieved when they produce consistent results over time or across similar populations. Validity is ensured when questions accurately measure the intended construct. However, factors like poorly phrased questions, respondent bias, and lack of standardisation can compromise their reliability and validity.
You May Also Like
Inductive and deductive reasoning takes into account assumptions and incidents. Here is all you need to know about inductive vs deductive reasoning.
This article provides the key advantages of primary research over secondary research so you can make an informed decision.
You can transcribe an interview by converting a conversation into a written format including question-answer recording sessions between two or more people.
USEFUL LINKS
LEARNING RESOURCES
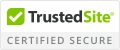
COMPANY DETAILS
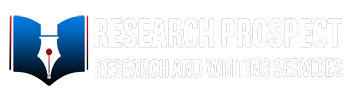
- How It Works
- Open access
- Published: 27 October 2021
A narrative review on the validity of electronic health record-based research in epidemiology
- Milena A. Gianfrancesco 1 &
- Neal D. Goldstein ORCID: orcid.org/0000-0002-9597-5251 2
BMC Medical Research Methodology volume 21 , Article number: 234 ( 2021 ) Cite this article
11k Accesses
46 Citations
5 Altmetric
Metrics details
Electronic health records (EHRs) are widely used in epidemiological research, but the validity of the results is dependent upon the assumptions made about the healthcare system, the patient, and the provider. In this review, we identify four overarching challenges in using EHR-based data for epidemiological analysis, with a particular emphasis on threats to validity. These challenges include representativeness of the EHR to a target population, the availability and interpretability of clinical and non-clinical data, and missing data at both the variable and observation levels. Each challenge reveals layers of assumptions that the epidemiologist is required to make, from the point of patient entry into the healthcare system, to the provider documenting the results of the clinical exam and follow-up of the patient longitudinally; all with the potential to bias the results of analysis of these data. Understanding the extent of as well as remediating potential biases requires a variety of methodological approaches, from traditional sensitivity analyses and validation studies, to newer techniques such as natural language processing. Beyond methods to address these challenges, it will remain crucial for epidemiologists to engage with clinicians and informaticians at their institutions to ensure data quality and accessibility by forming multidisciplinary teams around specific research projects.
Peer Review reports
The proliferation of electronic health records (EHRs) spurred on by federal government incentives over the past few decades has resulted in greater than an 80% adoption-rate at hospitals [ 1 ] and close to 90% in office-based practices [ 2 ] in the United States. A natural consequence of the availability of electronic health data is the conduct of research with these data, both observational and experimental [ 3 ], due to lower overhead costs and lower burden of study recruitment [ 4 ]. Indeed, a search on PubMed for publications indexed by the MeSH term “electronic health records” reveals an exponential growth in biomedical literature, especially over the last 10 years with an excess of 50,000 publications.
An emerging literature is beginning to recognize the many challenges that still lay ahead in using EHR data for epidemiological investigations. Researchers in Europe identified 13 potential sources of “bias” (bias was defined as a contamination of the data) in EHR-based data covering almost every aspect of care delivery, from selective entrance into the healthcare system, to variation in care and documentation practices, to identification and extraction of the right data for analysis [ 5 ]. Many of the identified contaminants are directly relevant to traditional epidemiological threats to validity [ 4 ]. Data quality has consistently been invoked as a central challenge in EHRs. From a qualitative perspective, healthcare workers have described challenges in the healthcare environment (e.g., heavy workload), imperfect clinical documentation practices, and concerns over data extraction and reporting tools, all of which would impact the quality of data in the EHR [ 6 ]. From a quantitative perspective, researchers have noted limited sensitivity of diagnostic codes in the EHR when relying on discrete codings, noting that upon a manual chart review free text fields often capture the missed information, motivating such techniques as natural language processing (NLP) [ 7 ]. A systematic review of EHR-based studies also identified data quality as an overarching barrier to the use of EHRs in managing the health of the community, i.e. “population health” [ 8 ]. Encouragingly this same review also identified more facilitators than barriers to the use of EHRs in public health, suggesting that opportunities outweigh the challenges. Shortreed et al. further explored these opportunities discussing how EHRs can enhance pragmatic trials, bring additional sophistication to observational studies, aid in predictive modeling, and be linked together to create more comprehensive views of patients’ health [ 9 ]. Yet, as Shortreed and others have noted, significant challenges still remain.
It is our intention with this narrative review to discuss some of these challenges in further detail. In particular, we focus on specific epidemiological threats to validity -- internal and external -- and how EHR-based epidemiological research in particular can exacerbate some of these threats. We note that while there is some overlap in the challenges we discuss with traditional paper-based medical record research that has occurred for decades, the scale and scope of an EHR-based study is often well beyond what was traditionally possible in the manual chart review era and our applied examples attempt to reflect this. We also describe existing and emerging approaches for remediating these potential biases as they arise. A summary of these challenges may be found in Table 1 . Our review is grounded in the healthcare system in the United States, although we expect many of the issues we describe to be applicable regardless of locale; where necessary, we have flagged our comments as specific to the U.S.
Challenge #1: Representativeness
The selection process for how patients are captured in the EHR is complex and a function of geographic, social, demographic, and economic determinants [ 10 ]. This can be termed the catchment of the EHR. For a patient record to appear in the EHR the patient must have been registered in the system, typically to capture their demographic and billing information, and upon a clinical visit, their health details. While this process is not new to clinical epidemiology, what tends to separate EHR-based records from traditional paper-based records is the scale and scope of the data. Patient data may be available for longer periods of time longitudinally, as well as have data corresponding to interactions with multiple, potentially disparate, healthcare systems [ 11 ]. Given the consolidation of healthcare [ 12 ] and aggregated views of multiple EHRs through health information networks or exchanges [ 11 ] the ability to have a complete view of the patients’ total health is increasing. Importantly, the epidemiologist must ascertain whether the population captured within the EHR or EHR-derived data is representative of the population targeted for inference. This is particularly true under the paradigm of population health and inferring the health status of a community from EHR-based records [ 13 ]. For example, a study of Clostridium difficile infection at an urban safety net hospital in Philadelphia, Pennsylvania demonstrated notable differences in risk factors in the hospital’s EHR compared to national surveillance data, suggesting how catchment can influence epidemiologic measures [ 14 ]. Even health-related data captured through health information exchanges may be incomplete [ 15 ].
Several hypothetical study settings can further help the epidemiologist appreciate the relationship between representativeness and validity in EHR research. In the first hypothetical, an EHR-based study is conducted from a single-location federally qualified health center, and in the second hypothetical, an EHR-based study is conducted from a large academic health system. Suppose both studies occur in the same geographic area. It is reasonable to believe the patient populations captured in both EHRs will be quite different and the catchment process could lead to divergent estimates of disease or risk factor prevalence. The large academic health system may be less likely to capture primary care visits, as specialty care may drive the preponderance of patient encounters. However, this is not a bias per se : if the target of inference from these two hypothetical EHR-based studies is the local community, then selection bias becomes a distinct possibility. The epidemiologist must also consider the potential for generalizability and transportability -- two facets of external validity that respectively relate to the extrapolation of study findings to the source population or a different population altogether -- if there are unmeasured effect modifiers, treatment interference, or compound treatments in the community targeted for inference [ 16 ].
There are several approaches for ascertaining representativeness of EHR-based data. Comparing the EHR-derived sample to Census estimates of demography is straightforward but has several important limitations. First, as previously described, the catchment process may be driven by discordant geographical areas, especially for specialty care settings. Second and third, the EHR may have limited or inaccurate information on socioeconomic status, race, and ethnicity that one may wish to compare [ 17 , 18 ], and conversely the Census has limited estimates of health, chiefly disability, fertility, and insurance and payments [ 19 ]. If selection bias is suspected as a result of missing visits in a longitudinal study [ 20 ] or the catchment process in a cross-sectional study [ 21 ], using inverse probability weighting may remediate its influence. Comparing the weighted estimates to the original, non-weighted estimates provides insight into differences in the study participants. In the population health paradigm whereby the EHR is used as a surveillance tool to identify community health disparities [ 13 ], one also needs to be concerned about representativeness. There are emerging approaches for producing such small area community estimates from large observational datasets [ 22 , 23 ]. Conceivably, these approaches may also be useful for identifying issues of representativeness, for example by comparing stratified estimates across sociodemographic or other factors that may relate to catchment. Approaches for issues concerning representativeness specifically as it applies to external validity may be found in these references [ 24 , 25 ].
Challenge #2: Data availability and interpretation
Sub-challenge #2.1: billing versus clinical versus epidemiological needs.
There is an inherent tension in the use of EHR-based data for research purposes: the EHR was never originally designed for research. In the U.S., the Health Information Technology for Economic and Clinical Health Act, which promoted EHRs as a platform for comparative effectiveness research, was an attempt to address this deficiency [ 26 ]. A brief history of the evolution of the modern EHR reveals a technology that was optimized for capturing health details relevant for billing, scheduling, and clinical record keeping [ 27 ]. As such, the availability of data for fundamental markers of upstream health that are important for identifying inequities, such as socioeconomic status, race, ethnicity, and other social determinants of health (SDOH), may be insufficiently captured in the EHR [ 17 , 18 ]. Similarly, behavioral risk factors, such as being a sexual minority person, have historically been insufficiently recorded as discrete variables. It is only recently that such data are beginning to be captured in the EHR [ 28 , 29 ], or techniques such as NLP have made it possible to extract these details when stored in free text notes (described further in “ Unstructured data: clinical notes and reports ” section).
As an example, assessing clinical morbidities in the EHR may be done on the basis of extracting appropriate International Classification of Diseases (ICD) codes, used for billing and reimbursement in the U.S. These codes are known to have low sensitivity despite high specificity for accurate diagnostic status [ 30 , 31 ]. Expressed as predictive values, which depend upon prevalence, presence of a diagnostic code is a likely indicator of a disease state, whereas absence of a diagnostic code is a less reliable indicator of the absence of that morbidity. There may further be variation by clinical domain in that ICD codes may exist but not be used in some specialties [ 32 ], variation by coding vocabulary such as the use of SNOMED for clinical documentation versus ICD for billing necessitating an ontology mapper [ 33 ], and variation by the use of “rule-out” diagnostic codes resulting in false-positive diagnoses [ 34 , 35 , 36 ]. Relatedly is the notion of upcoding, or the billing of tests, procedures, or diagnoses to receive inflated reimbursement, which, although posited to be problematic in EHRs [ 37 ] in at least one study, has not been shown to have occurred [ 38 ]. In the U.S., the billing and reimbursement model, such as fee-for-service versus managed care, may result in varying diagnostic code sensitivities and specificities, especially if upcoding is occurring [ 39 ]. In short, there is potential for misclassification of key health data in the EHR.
Misclassification can potentially be addressed through a validation study (resources permitting) or application of quantitative bias analysis, and there is a rich literature regarding the treatment of misclassified data in statistics and epidemiology. Readers are referred to these texts as a starting point [ 40 , 41 ]. Duda et al. and Shepherd et al. have described an innovative data audit approach applicable to secondary analysis of observational data, such as EHR-derived data, that incorporates the audit error rate directly in the regression analysis to reduce information bias [ 42 , 43 ]. Outside of methodological tricks in the face of imperfect data, researchers must proactively engage with clinical and informatics colleagues to ensure that the right data for the research interests are available and accessible.
Sub-challenge #2.2: Consistency in data and interpretation
For the epidemiologist, abstracting data from the EHR into a research-ready analytic dataset presents a host of complications surrounding data availability, consistency and interpretation. It is easy to conflate the total volume of data in the EHR with data that are usable for research, however expectations should be tempered. Weiskopf et al. have noted such challenges for the researcher: in their study, less than 50% of patient records had “complete” data for research purposes per their four definitions of completeness [ 44 ]. Decisions made about the treatment of incomplete data can induce selection bias or impact precision of estimates (see Challenges #1 , #3 , and #4 ). The COVID-19 pandemic has further demonstrated the challenge of obtaining research data from EHRs across multiple health systems [ 45 ]. On the other hand, EHRs have a key advantage of providing near real-time data as opposed to many epidemiological studies that have a specific endpoint or are retrospective in nature. Such real-time data availability was leveraged during COVID-19 to help healthcare systems manage their pandemic response [ 46 , 47 ]. Logistical and technical issues aside, healthcare and documentation practices are nuanced to their local environments. In fact, researchers have demonstrated how the same research question analyzed in distinct clinical databases can yield different results [ 48 ].
Once the data are obtained, choices regarding operationalization of variables have the potential to induce information bias. Several hypothetical examples can help demonstrate this point. As a first example, differences in laboratory reporting may result in measurement error or misclassification. While the order for a particular laboratory assay is likely consistent within the healthcare system, patients frequently have a choice where to have that order fulfilled. Given the breadth of assays and reporting differences that may differ lab to lab [ 49 ], it is possible that the researcher working with the raw data may not consider all possible permutations. In other words, there may be lack of consistency in the reporting of the assay results. As a second example, raw clinical data requires interpretation to become actionable. A researcher interested in capturing a patient’s Charlson comorbidity index, which is based on 16 potential diagnoses plus the patient’s age [ 50 ], may never find such a variable in the EHR. Rather, this would require operationalization based on the raw data, each of which may be misclassified. Use of such composite measures introduces the notion of “differential item functioning”, whereby a summary indicator of a complexly measured health phenomenon may differ from group to group [ 51 ]. In this case, as opposed to a measurement error bias, this is one of residual confounding in that a key (unmeasured) variable is driving the differences. Remediation of these threats to validity may involve validation studies to determine the accuracy of a particular classifier, sensitivity analysis employing alternative interpretations when the raw data are available, and omitting or imputing biased or latent variables [ 40 , 41 , 52 ]. Importantly, in all cases, the epidemiologists should work with the various health care providers and personnel who have measured and recorded the data present in the EHR, as they likely understand it best.
Furthermore and related to “Billing versus Clinical versus Epidemiological Needs” section, the healthcare system in the U.S. is fragmented with multiple payers, both public and private, potentially exacerbating the data quality issues we describe, especially when linking data across healthcare systems. Single payer systems have enabled large and near-complete population-based studies due to data availability and consistency [ 53 , 54 , 55 ]. Data may also be inconsistent for retrospective longitudinal studies spanning many years if there have been changes to coding standards or practices over time, for example due to the transition from ICD-9 to ICD-10 largely occurring in the mid 2010s or the adoption of the Patient Protection and Affordable Care Act in the U.S. in 2010 with its accompanying changes in billing. Exploratory data analysis may reveal unexpected differences in key variables, by place or time, and recoding, when possible, can enforce consistency.
Sub-challenge #2.3: Unstructured data: clinical notes and reports
There may also be scenarios where structured data fields, while available, are not traditionally or consistently used within a given medical center or by a given provider. For example, reporting of adverse events of medications, disease symptoms, and vaccinations or hospitalizations occurring at different facility/health networks may not always be entered by providers in structured EHR fields. Instead, these types of patient experiences may be more likely to be documented in an unstructured clinical note, report (e.g. pathology or radiology report), or scanned document. Therefore, reliance on structured data to identify and study such issues may result in underestimation and potentially biased results.
Advances in NLP currently allow for information to be extracted from unstructured clinical notes and text fields in a reliable and accurate manner using computational methods. NLP utilizes a range of different statistical, machine learning, and linguistic techniques, and when applied to EHR data, has the potential to facilitate more accurate detection of events not traditionally located or consistently used in structured fields. Various NLP methods can be implemented in medical text analysis, ranging from simplistic and fast term recognition systems to more advanced, commercial NLP systems [ 56 ]. Several studies have successfully utilized text mining to extract information on a variety of health-related issues within clinical notes, such as opioid use [ 57 ], adverse events [ 58 , 59 ], symptoms (e.g., shortness of breath, depression, pain) [ 60 ], and disease phenotype information documented in pathology or radiology reports, including cancer stage, histology, and tumor grade [ 61 ], and lupus nephritis [ 32 ]. It is worth noting that scanned documents involve an additional layer of computation, relying on techniques such as optical character recognition, before NLP can be applied.
Hybrid approaches that combine both narrative and structured data, such as ICD codes, to improve accuracy of detecting phenotypes have also demonstrated high performance. Banerji et al. found that using ICD-9 codes to identify allergic drug reactions in the EHR had a positive predictive value of 46%, while an NLP algorithm in conjunction with ICD-9 codes resulted in a positive predictive value of 86%; negative predictive value also increased in the combined algorithm (76%) compared to ICD-9 codes alone (39%) [ 62 ]. In another example, researchers found that the combination of unstructured clinical notes with structured data for prediction tasks involving in-hospital mortality and 30-day hospital readmission outperformed models using either clinical notes or structured data alone [ 63 ]. As we move forward in analyzing EHR data, it will be important to take advantage of the wealth of information buried in unstructured data to assist in phenotyping patient characteristics and outcomes, capture missing confounders used in multivariate analyses, and develop prediction models.
Challenge #3: Missing measurements
While clinical notes may be useful to recover incomplete information from structured data fields, it may be the case that certain variables are not collected within the EHR at all. As mentioned above, it is important to remember that EHRs were not developed as a research tool (see “ Billing versus clinical versus epidemiological needs ” section), and important variables often used in epidemiologic research may not be typically included in EHRs including socioeconomic status (education, income, occupation) and SDOH [ 17 , 18 ]. Depending upon the interest of the provider or clinical importance placed upon a given variable, this information may be included in clinical notes. While NLP could be used to capture these variables, because they may not be consistently captured, there may be bias in identifying those with a positive mention as a positive case and those with no mention as a negative case. For example, if a given provider inquires about homelessness of a patient based on knowledge of the patient’s situation or other external factors and documents this in the clinical note, we have greater assurance that this is a true positive case. However, lack of mention of homelessness in a clinical note should not be assumed as a true negative case for several reasons: not all providers may feel comfortable asking about and/or documenting homelessness, they may not deem this variable worth noting, or implicit bias among clinicians may affect what is captured. As a result, such cases (i.e. no mention of homelessness) may be incorrectly identified as “not homeless,” leading to selection bias should a researcher form a cohort exclusively of patients who are identified as homeless in the EHR.
Not adjusting for certain measurements missing from EHR data can also lead to biased results if the measurement is an important confounder. Consider the example of distinguishing between prevalent and incident cases of disease when examining associations between disease treatments and patient outcomes [ 64 ]. The first date of an ICD code entered for a given patient may not necessarily be the true date of diagnosis, but rather documentation of an existing diagnosis. This limits the ability to adjust for disease duration, which may be an important confounder in studies comparing various treatments with patient outcomes over time, and may also lead to reverse causality if disease sequalae are assumed to be risk factors.
Methods to supplement EHR data with external data have been used to capture missing information. These methods may include imputation if information (e.g. race, lab values) is collected on a subset of patients within the EHR. It is important to examine whether missingness occurs completely at random or at random (“ignorable”), or not at random (“non-ignorable”), using the data available to determine factors associated with missingness, which will also inform the best imputation strategy to pursue, if any [ 65 , 66 ]. As an example, suppose we are interested in ascertaining a patient's BMI from the EHR. If men were less likely to have BMI measured than women, the probability of missing data (BMI) depends on the observed data (gender) and may therefore be predictable and imputable. On the other hand, suppose underweight individuals were less likely to have BMI measured; the probability of missing data depends on its own value, and as such is non-predictable and may require a validation study to confirm. Alternatively to imputing missing data, surrogate measures may be used, such as inferring area-based SES indicators, including median household income, percent poverty, or area deprivation index, by zip code [ 67 , 68 ]. Lastly, validation studies utilizing external datasets may prove helpful, such as supplementing EHR data with claims data that may be available for a subset of patients (see Challenge #4 ).
As EHRs are increasingly being used for research, there are active pushes to include more structured data fields that are important to population health research, such as SDOH [ 69 ]. Inclusion of such factors are likely to result in improved patient care and outcomes, through increased precision in disease diagnosis, more effective shared decision making, identification of risk factors, and tailoring services to a given population’s needs [ 70 ]. In fact, a recent review found that when individual level SDOH were included in predictive modeling, they overwhelmingly improved performance in medication adherence, risk of hospitalization, 30-day rehospitalizations, suicide attempts, and other healthcare services [ 71 ]. Whether or not these fields will be utilized after their inclusion in the EHR may ultimately depend upon federal and state incentives, as well as support from local stakeholders, and this does not address historic, retrospective analyses of these data.
Challenge #4: Missing visits
Beyond missing variable data that may not be captured during a clinical encounter, either through structured data or clinical notes, there also may be missing information for a patient as a whole. This can occur in a variety of ways; for example, a patient may have one or two documented visits in the EHR and then is never seen again (i.e. right censoring due to lost to follow-up), or a patient is referred from elsewhere to seek specialty care, with no information captured regarding other external issues (i.e. left censoring). This may be especially common in circumstances where a given EHR is more likely to capture specialty clinics versus primary care (see Challenge #1 ). A third scenario may include patients who appear, then are not observed for a long period of time, and then reappear: this case is particularly problematic as it may appear the patient was never lost to follow up but simply had fewer visits. In any of these scenarios, a researcher will lack a holistic view of the patient’s experiences, diagnoses, results, and more. As discussed above, assuming absence of a diagnostic code as absence of disease may lead to information and/or selection bias. Further, it has been demonstrated that one key source of bias in EHRs is “informed presence” bias, where those with more medical encounters are more likely to be diagnosed with various conditions (similar to Berkson’s bias) [ 72 ].
Several solutions to these issues have been proposed. For example, it is common for EHR studies to condition on observation time (i.e. ≥n visits required to be eligible into cohort); however, this may exclude a substantial amount of patients with certain characteristics, incurring a selection bias or limiting the generalizability of study findings (see Challenge #1 ). Other strategies attempt to account for missing visit biases through longitudinal imputation approaches; for example, if a patient missed a visit, a disease activity score can be imputed for that point in time, given other data points [ 73 , 74 ]. Surrogate measures may also be used to infer patient outcomes, such as controlling for “informative” missingness as an indicator variable or using actual number of missed visits that were scheduled as a proxy for external circumstances influencing care [ 20 ]. To address “informed presence” bias described above, conditioning on the number of health-care encounters may be appropriate [ 72 ]. Understanding the reason for the missing visit may help identify the best course of action and before imputing, one should be able to identify the type of missingness, whether “informative” or not [ 65 , 66 ]. For example, if distance to a healthcare location is related to appointment attendance, being able to account for this in analysis would be important: researchers have shown how the catchment of a healthcare facility can induce selection bias [ 21 ]. Relatedly, as telehealth becomes more common fueled by the COVID-19 pandemic [ 75 , 76 ], virtual visits may generate missingness of data recorded in the presence of a provider (e.g., blood pressure if the patient does not have access to a sphygmomanometer; see Challenge #3 ), or necessitate a stratified analysis by visit type to assess for effect modification.
Another common approach is to supplement EHR information with external data sources, such as insurance claims data, when available. Unlike a given EHR, claims data are able to capture a patient’s interaction with the health care system across organizations, and additionally includes pharmacy data such as if a prescription was filled or refilled. Often researchers examine a subset of patients eligible for Medicaid/Medicare and compare what is documented in claims with information available in the EHR [ 77 ]. That is, are there additional medications, diagnoses, hospitalizations found in the claims dataset that were not present in the EHR. In a study by Franklin et al., researchers utilized a linked database of Medicare Advantage claims and comprehensive EHR data from a multi-specialty outpatient practice to determine which dataset would be more accurate in predicting medication adherence [ 77 ]. They found that both datasets were comparable in identifying those with poor adherence, though each dataset incorporated different variables.
While validation studies such as those using claims data allow researchers to gain an understanding as to how accurate and complete a given EHR is, this may only be limited to the specific subpopulation examined (i.e. those eligible for Medicaid, or those over 65 years for Medicare). One study examined congruence between EHR of a community health center and Medicaid claims with respect to diabetes [ 78 ]. They found that patients who were older, male, Spanish-speaking, above the federal poverty level, or who had discontinuous insurance were more likely to have services documented in the EHR as compared to Medicaid claims data. Therefore, while claims data may help supplement and validate information in the EHR, on their own they may underestimate care in certain populations.
Research utilizing EHR data has undoubtedly positively impacted the field of public health through its ability to provide large-scale, longitudinal data on a diverse set of patients, and will continue to do so in the future as more epidemiologists take advantage of this data source. EHR data’s ability to capture individuals that traditionally aren’t included in clinical trials, cohort studies, and even claims datasets allows researchers to measure longitudinal outcomes in patients and perhaps change the understanding of potential risk factors.
However, as outlined in this review, there are important caveats to EHR analysis that need to be taken into account; failure to do so may threaten study validity. The representativeness of EHR data depends on the catchment area of the center and corresponding target population. Tools are available to evaluate and remedy these issues, which are critical to study validity as well as extrapolation of study findings. Data availability and interpretation, missing measurements, and missing visits are also key challenges, as EHRs were not specifically developed for research purposes, despite their common use for such. Taking advantage of all available EHR data, whether it be structured or unstructured fields through NLP, will be important in understanding the patient experience and identifying key phenotypes. Beyond methods to address these concerns, it will remain crucial for epidemiologists and data analysts to engage with clinicians and informaticians at their institutions to ensure data quality and accessibility by forming multidisciplinary teams around specific research projects. Lastly, integration across multiple EHRs, or datasets that encompass multi-institutional EHR records, add an additional layer of data quality and validity issues, with the potential to exacerbate the above-stated challenges found within a single EHR. At minimum, such studies should account for correlated errors [ 79 , 80 ], and investigate whether modularization, or submechanisms that determine whether data are observed or missing in each EHR, exist [ 65 ].
The identified challenges may also apply to secondary analysis of other large healthcare databases, such as claims data, although it is important not to conflate the two types of data. EHR data are driven by clinical care and claims data are driven by the reimbursement process where there is a financial incentive to capture diagnoses, procedures, and medications [ 48 ]. The source of data likely influences the availability, accuracy, and completeness of data. The fundamental representation of data may also differ as a record in a claims database corresponds to a “claim” as opposed to an “encounter” in the EHR. As such, the representativeness of the database populations, the sensitivity and specificity of variables, as well as the mechanisms of missingness in claims data may differ from EHR data. One study that evaluated pediatric quality care measures, such as BMI, noted inferior sensitivity based on claims data alone [ 81 ]. Linking claims data to EHR data has been proposed to enhance study validity, but many of the caveats raised in herein still apply [ 82 ].
Although we focused on epidemiological challenges related to study validity, there are other important considerations for researchers working with EHR data. Privacy and security of data as well as institutional review board (IRB) or ethics board oversight of EHR-based studies should not be taken for granted. For researchers in the U.S., Goldstein and Sarwate described Health Insurance Portability and Accountability Act (HIPAA)-compliant approaches to ensure the privacy and security of EHR data used in epidemiological research, and presented emerging approaches to analyses that separate the data from analysis [ 83 ]. The IRB oversees the data collection process for EHR-based research and through the HIPAA Privacy Rule these data typically do not require informed consent provided they are retrospective and reside at the EHR’s institution [ 84 ]. Such research will also likely receive an exempt IRB review provided subjects are non-identifiable.
Conclusions
As EHRs are increasingly being used for research, epidemiologists can take advantage of the many tools and methods that already exist and apply them to the key challenges described above. By being aware of the limitations that the data present and proactively addressing them, EHR studies will be more robust, informative, and important to the understanding of health and disease in the population.
Availability of data and materials
All data and materials used in this review are described herein.
Abbreviations
Body Mass Index
Electronic Health Record
International Classification of Diseases
Institutional review board/ethics board
Health Insurance Portability and Accountability Act
Natural Language Processing
Social Determinants of Health
Socioeconomic Status
Adler-Milstein J, Holmgren AJ, Kralovec P, et al. Electronic health record adoption in US hospitals: the emergence of a digital “advanced use” divide. J Am Med Inform Assoc. 2017;24(6):1142–8.
Article PubMed PubMed Central Google Scholar
Office of the National Coordinator for Health Information Technology. ‘Office-based physician electronic health record adoption’, Health IT quick-stat #50. dashboard.healthit.gov/quickstats/pages/physician-ehr-adoption-trends.php . Accessed 15 Jan 2019.
Cowie MR, Blomster JI, Curtis LH, et al. Electronic health records to facilitate clinical research. Clin Res Cardiol. 2017;106(1):1–9.
Article PubMed Google Scholar
Casey JA, Schwartz BS, Stewart WF, et al. Using electronic health records for population health research: a review of methods and applications. Annu Rev Public Health. 2016;37:61–81.
Verheij RA, Curcin V, Delaney BC, et al. Possible sources of bias in primary care electronic health record data use and reuse. J Med Internet Res. 2018;20(5):e185.
Ni K, Chu H, Zeng L, et al. Barriers and facilitators to data quality of electronic health records used for clinical research in China: a qualitative study. BMJ Open. 2019;9(7):e029314.
Coleman N, Halas G, Peeler W, et al. From patient care to research: a validation study examining the factors contributing to data quality in a primary care electronic medical record database. BMC Fam Pract. 2015;16:11.
Kruse CS, Stein A, Thomas H, et al. The use of electronic health records to support population health: a systematic review of the literature. J Med Syst. 2018;42(11):214.
Shortreed SM, Cook AJ, Coley RY, et al. Challenges and opportunities for using big health care data to advance medical science and public health. Am J Epidemiol. 2019;188(5):851–61.
In: Smedley BD, Stith AY, Nelson AR, editors. Unequal treatment: confronting racial and ethnic disparities in health care. Washington (DC) 2003.
Chaudhry B, Wang J, Wu S, et al. Systematic review: impact of health information technology on quality, efficiency, and costs of medical care. Ann Intern Med. 2006;144(10):742–52.
Cutler DM, Scott Morton F. Hospitals, market share, and consolidation. JAMA. 2013;310(18):1964–70.
Article CAS PubMed Google Scholar
Cocoros NM, Kirby C, Zambarano B, et al. RiskScape: a data visualization and aggregation platform for public health surveillance using routine electronic health record data. Am J Public Health. 2021;111(2):269–76.
Vader DT, Weldie C, Welles SL, et al. Hospital-acquired Clostridioides difficile infection among patients at an urban safety-net hospital in Philadelphia: demographics, neighborhood deprivation, and the transferability of national statistics. Infect Control Hosp Epidemiol. 2020;42:1–7.
Google Scholar
Dixon BE, Gibson PJ, Frederickson Comer K, et al. Measuring population health using electronic health records: exploring biases and representativeness in a community health information exchange. Stud Health Technol Inform. 2015;216:1009.
PubMed Google Scholar
Hernán MA, VanderWeele TJ. Compound treatments and transportability of causal inference. Epidemiology. 2011;22(3):368–77.
Casey JA, Pollak J, Glymour MM, et al. Measures of SES for electronic health record-based research. Am J Prev Med. 2018;54(3):430–9.
Polubriaginof FCG, Ryan P, Salmasian H, et al. Challenges with quality of race and ethnicity data in observational databases. J Am Med Inform Assoc. 2019;26(8-9):730–6.
U.S. Census Bureau. Health. Available at: https://www.census.gov/topics/health.html . Accessed 19 Jan 2021.
Gianfrancesco MA, McCulloch CE, Trupin L, et al. Reweighting to address nonparticipation and missing data bias in a longitudinal electronic health record study. Ann Epidemiol. 2020;50:48–51 e2.
Goldstein ND, Kahal D, Testa K, Burstyn I. Inverse probability weighting for selection bias in a Delaware community health center electronic medical record study of community deprivation and hepatitis C prevalence. Ann Epidemiol. 2021;60:1–7.
Gelman A, Lax J, Phillips J, et al. Using multilevel regression and poststratification to estimate dynamic public opinion. Unpublished manuscript, Columbia University. 2016 Sep 11. Available at: http://www.stat.columbia.edu/~gelman/research/unpublished/MRT(1).pdf . Accessed 22 Jan 2021.
Quick H, Terloyeva D, Wu Y, et al. Trends in tract-level prevalence of obesity in philadelphia by race-ethnicity, space, and time. Epidemiology. 2020;31(1):15–21.
Lesko CR, Buchanan AL, Westreich D, Edwards JK, Hudgens MG, Cole SR. Generalizing study results: a potential outcomes perspective. Epidemiology. 2017;28(4):553–61.
Westreich D, Edwards JK, Lesko CR, Stuart E, Cole SR. Transportability of trial results using inverse odds of sampling weights. Am J Epidemiol. 2017;186(8):1010–4.
Congressional Research Services (CRS). The Health Information Technology for Economic and Clinical Health (HITECH) Act. 2009. Available at: https://crsreports.congress.gov/product/pdf/R/R40161/9 . Accessed Jan 22 2021.
Hersh WR. The electronic medical record: Promises and problems. Journal of the American Society for Information Science. 1995;46(10):772–6.
Article Google Scholar
Collecting sexual orientation and gender identity data in electronic health records: workshop summary. Washington (DC) 2013.
Committee on the Recommended Social and Behavioral Domains and Measures for Electronic Health Records; Board on Population Health and Public Health Practice; Institute of Medicine. Capturing social and behavioral domains and measures in electronic health records: phase 2. Washington (DC): National Academies Press (US); 2015.
Goff SL, Pekow PS, Markenson G, et al. Validity of using ICD-9-CM codes to identify selected categories of obstetric complications, procedures and co-morbidities. Paediatr Perinat Epidemiol. 2012;26(5):421–9.
Schneeweiss S, Avorn J. A review of uses of health care utilization databases for epidemiologic research on therapeutics. J Clin Epidemiol. 2005;58(4):323–37.
Gianfrancesco MA. Application of text mining methods to identify lupus nephritis from electronic health records. Lupus Science & Medicine. 2019;6:A142.
National Library of Medicine. SNOMED CT to ICD-10-CM Map. Available at: https://www.nlm.nih.gov/research/umls/mapping_projects/snomedct_to_icd10cm.html . Accessed 2 Jul 2021.
Klabunde CN, Harlan LC, Warren JL. Data sources for measuring comorbidity: a comparison of hospital records and medicare claims for cancer patients. Med Care. 2006;44(10):921–8.
Burles K, Innes G, Senior K, Lang E, McRae A. Limitations of pulmonary embolism ICD-10 codes in emergency department administrative data: let the buyer beware. BMC Med Res Methodol. 2017;17(1):89.
Asgari MM, Wu JJ, Gelfand JM, Salman C, Curtis JR, Harrold LR, et al. Validity of diagnostic codes and prevalence of psoriasis and psoriatic arthritis in a managed care population, 1996-2009. Pharmacoepidemiol Drug Saf. 2013;22(8):842–9.
Hoffman S, Podgurski A. Big bad data: law, public health, and biomedical databases. J Law Med Ethics. 2013;41(Suppl 1):56–60.
Adler-Milstein J, Jha AK. Electronic health records: the authors reply. Health Aff. 2014;33(10):1877.
Geruso M, Layton T. Upcoding: evidence from medicare on squishy risk adjustment. J Polit Econ. 2020;12(3):984–1026.
Lash TL, Fox MP, Fink AK. Applying quantitative bias analysis to epidemiologic data. New York: Springer-Verlag New York; 2009.
Book Google Scholar
Gustafson P. Measurement error and misclassification in statistics and epidemiology: impacts and Bayesian adjustments. Boca Raton: Chapman and Hall/CRC; 2004.
Duda SN, Shepherd BE, Gadd CS, et al. Measuring the quality of observational study data in an international HIV research network. PLoS One. 2012;7(4):e33908.
Article CAS PubMed PubMed Central Google Scholar
Shepherd BE, Yu C. Accounting for data errors discovered from an audit in multiple linear regression. Biometrics. 2011;67(3):1083–91.
Weiskopf NG, Hripcsak G, Swaminathan S, et al. Defining and measuring completeness of electronic health records for secondary use. J Biomed Inform. 2013;46(5):830–6.
Kaiser Health News. As coronavirus strikes, crucial data in electronic health records hard to harvest. Available at: https://khn.org/news/as-coronavirus-strikes-crucial-data-in-electronic-health-records-hard-to-harvest/ . Accessed 15 Jan 2021.
Reeves JJ, Hollandsworth HM, Torriani FJ, Taplitz R, Abeles S, Tai-Seale M, et al. Rapid response to COVID-19: health informatics support for outbreak management in an academic health system. J Am Med Inform Assoc. 2020;27(6):853–9.
Grange ES, Neil EJ, Stoffel M, Singh AP, Tseng E, Resco-Summers K, et al. Responding to COVID-19: The UW medicine information technology services experience. Appl Clin Inform. 2020;11(2):265–75.
Madigan D, Ryan PB, Schuemie M, et al. Evaluating the impact of database heterogeneity on observational study results. Am J Epidemiol. 2013;178(4):645–51.
Lippi G, Mattiuzzi C. Critical laboratory values communication: summary recommendations from available guidelines. Ann Transl Med. 2016;4(20):400.
Charlson ME, Pompei P, Ales KL, MacKenzie CR. A new method of classifying prognostic comorbidity in longitudinal studies: development and validation. J Chronic Dis. 1987;40(5):373–83.
Jones RN. Differential item functioning and its relevance to epidemiology. Curr Epidemiol Rep. 2019;6:174–83.
Edwards JK, Cole SR, Troester MA, Richardson DB. Accounting for misclassified outcomes in binary regression models using multiple imputation with internal validation data. Am J Epidemiol. 2013;177(9):904–12.
Satkunasivam R, Klaassen Z, Ravi B, Fok KH, Menser T, Kash B, et al. Relation between surgeon age and postoperative outcomes: a population-based cohort study. CMAJ. 2020;192(15):E385–92.
Melamed N, Asztalos E, Murphy K, Zaltz A, Redelmeier D, Shah BR, et al. Neurodevelopmental disorders among term infants exposed to antenatal corticosteroids during pregnancy: a population-based study. BMJ Open. 2019;9(9):e031197.
Kao LT, Lee HC, Lin HC, Tsai MC, Chung SD. Healthcare service utilization by patients with obstructive sleep apnea: a population-based study. PLoS One. 2015;10(9):e0137459.
Article PubMed PubMed Central CAS Google Scholar
Jung K, LePendu P, Iyer S, Bauer-Mehren A, Percha B, Shah NH. Functional evaluation of out-of-the-box text-mining tools for data-mining tasks. J Am Med Inform Assoc. 2015;22(1):121–31.
Canan C, Polinski JM, Alexander GC, et al. Automatable algorithms to identify nonmedical opioid use using electronic data: a systematic review. J Am Med Inform Assoc. 2017;24(6):1204–10.
Iqbal E, Mallah R, Jackson RG, et al. Identification of adverse drug events from free text electronic patient records and information in a large mental health case register. PLoS One. 2015;10(8):e0134208.
Rochefort CM, Verma AD, Eguale T, et al. A novel method of adverse event detection can accurately identify venous thromboembolisms (VTEs) from narrative electronic health record data. J Am Med Inform Assoc. 2015;22(1):155–65.
Koleck TA, Dreisbach C, Bourne PE, et al. Natural language processing of symptoms documented in free-text narratives of electronic health records: a systematic review. J Am Med Inform Assoc. 2019;26(4):364–79.
Wang L, Luo L, Wang Y, et al. Natural language processing for populating lung cancer clinical research data. BMC Med Inform Decis Mak. 2019;19(Suppl 5):239.
Banerji A, Lai KH, Li Y, et al. Natural language processing combined with ICD-9-CM codes as a novel method to study the epidemiology of allergic drug reactions. J Allergy Clin Immunol Pract. 2020;8(3):1032–1038.e1.
Zhang D, Yin C, Zeng J, et al. Combining structured and unstructured data for predictive models: a deep learning approach. BMC Med Inform Decis Mak. 2020;20(1):280.
Farmer R, Mathur R, Bhaskaran K, Eastwood SV, Chaturvedi N, Smeeth L. Promises and pitfalls of electronic health record analysis. Diabetologia. 2018;61:1241–8.
Haneuse S, Arterburn D, Daniels MJ. Assessing missing data assumptions in EHR-based studies: a complex and underappreciated task. JAMA Netw Open. 2021;4(2):e210184.
Groenwold RHH. Informative missingness in electronic health record systems: the curse of knowing. Diagn Progn Res. 2020;4:8.
Berkowitz SA, Traore CY, Singer DE, et al. Evaluating area-based socioeconomic status indicators for monitoring disparities within health care systems: results from a primary care network. Health Serv Res. 2015;50(2):398–417.
Kind AJH, Buckingham WR. Making neighborhood-disadvantage metrics accessible - the neighborhood atlas. N Engl J Med. 2018;378(26):2456–8.
Cantor MN, Thorpe L. Integrating data on social determinants of health into electronic health records. Health Aff. 2018;37(4):585–90.
Adler NE, Stead WW. Patients in context--EHR capture of social and behavioral determinants of health. N Engl J Med. 2015;372(8):698–701.
Chen M, Tan X, Padman R. Social determinants of health in electronic health records and their impact on analysis and risk prediction: a systematic review. J Am Med Inform Assoc. 2020;27(11):1764–73.
Goldstein BA, Bhavsar NA, Phelan M, et al. Controlling for informed presence bias due to the number of health encounters in an electronic health record. Am J Epidemiol. 2016;184(11):847–55.
Petersen I, Welch CA, Nazareth I, et al. Health indicator recording in UK primary care electronic health records: key implications for handling missing data. Clin Epidemiol. 2019;11:157–67.
Li R, Chen Y, Moore JH. Integration of genetic and clinical information to improve imputation of data missing from electronic health records. J Am Med Inform Assoc. 2019;26(10):1056–63.
Koonin LM, Hoots B, Tsang CA, Leroy Z, Farris K, Jolly T, et al. Trends in the use of telehealth during the emergence of the COVID-19 pandemic - United States, January-March 2020. MMWR Morb Mortal Wkly Rep. 2020;69(43):1595–9.
Barnett ML, Ray KN, Souza J, Mehrotra A. Trends in telemedicine use in a large commercially insured population, 2005-2017. JAMA. 2018;320(20):2147–9.
Franklin JM, Gopalakrishnan C, Krumme AA, et al. The relative benefits of claims and electronic health record data for predicting medication adherence trajectory. Am Heart J. 2018;197:153–62.
Devoe JE, Gold R, McIntire P, et al. Electronic health records vs Medicaid claims: completeness of diabetes preventive care data in community health centers. Ann Fam Med. 2011;9(4):351–8.
Schmajuk G, Li J, Evans M, Anastasiou C, Izadi Z, Kay JL, et al. RISE registry reveals potential gaps in medication safety for new users of biologics and targeted synthetic DMARDs. Semin Arthritis Rheum. 2020 Dec;50(6):1542–8.
Izadi Z, Schmajuk G, Gianfrancesco M, Subash M, Evans M, Trupin L, et al. Rheumatology Informatics System for Effectiveness (RISE) practices see significant gains in rheumatoid arthritis quality measures. Arthritis Care Res. 2020. https://doi.org/10.1002/acr.24444 .
Angier H, Gold R, Gallia C, Casciato A, Tillotson CJ, Marino M, et al. Variation in outcomes of quality measurement by data source. Pediatrics. 2014;133(6):e1676–82.
Lin KJ, Schneeweiss S. Considerations for the analysis of longitudinal electronic health records linked to claims data to study the effectiveness and safety of drugs. Clin Pharmacol Ther. 2016;100(2):147–59.
Goldstein ND, Sarwate AD. Privacy, security, and the public health researcher in the era of electronic health record research. Online J Public Health Inform. 2016;8(3):e207.
U.S. Department of Health and Human Services (HHS). 45 CFR 46. http://www.hhs.gov/ohrp/regulations-and-policy/regulations/45-cfr-46/index.html .
Download references
Acknowledgements
The authors thank Dr. Annemarie Hirsch, Department of Population Health Sciences, Geisinger, for assistance in conceptualizing an earlier version of this work.
Research reported in this publication was supported in part by the National Institute of Arthritis and Musculoskeletal and Skin Diseases of the National Institutes of Health under Award Number K01AR075085 (to MAG) and the National Institute Of Allergy And Infectious Diseases of the National Institutes of Health under Award Number K01AI143356 (to NDG). The content is solely the responsibility of the authors and does not necessarily represent the official views of the National Institutes of Health.
Author information
Authors and affiliations.
Division of Rheumatology, University of California School of Medicine, San Francisco, CA, USA
Milena A. Gianfrancesco
Department of Epidemiology and Biostatistics, Drexel University Dornsife School of Public Health, 3215 Market St., Philadelphia, PA, 19104, USA
Neal D. Goldstein
You can also search for this author in PubMed Google Scholar
Contributions
Both authors conceptualized, wrote, and approved the final submitted version.
Corresponding author
Correspondence to Neal D. Goldstein .
Ethics declarations
Ethics approval and consent to participate.
Not applicable
Consent for publication
Competing interests.
The authors have no competing interests to declare
Additional information
Publisher’s note.
Springer Nature remains neutral with regard to jurisdictional claims in published maps and institutional affiliations.
Rights and permissions
Open Access This article is licensed under a Creative Commons Attribution 4.0 International License, which permits use, sharing, adaptation, distribution and reproduction in any medium or format, as long as you give appropriate credit to the original author(s) and the source, provide a link to the Creative Commons licence, and indicate if changes were made. The images or other third party material in this article are included in the article's Creative Commons licence, unless indicated otherwise in a credit line to the material. If material is not included in the article's Creative Commons licence and your intended use is not permitted by statutory regulation or exceeds the permitted use, you will need to obtain permission directly from the copyright holder. To view a copy of this licence, visit http://creativecommons.org/licenses/by/4.0/ . The Creative Commons Public Domain Dedication waiver ( http://creativecommons.org/publicdomain/zero/1.0/ ) applies to the data made available in this article, unless otherwise stated in a credit line to the data.
Reprints and permissions
About this article
Cite this article.
Gianfrancesco, M.A., Goldstein, N.D. A narrative review on the validity of electronic health record-based research in epidemiology. BMC Med Res Methodol 21 , 234 (2021). https://doi.org/10.1186/s12874-021-01416-5
Download citation
Received : 02 July 2021
Accepted : 28 September 2021
Published : 27 October 2021
DOI : https://doi.org/10.1186/s12874-021-01416-5
Share this article
Anyone you share the following link with will be able to read this content:
Sorry, a shareable link is not currently available for this article.
Provided by the Springer Nature SharedIt content-sharing initiative
- Electronic health records
- Data quality
- Secondary analysis
BMC Medical Research Methodology
ISSN: 1471-2288
- General enquiries: [email protected]
Reliability, Validity and Ethics
Cite this chapter.
- Lindy Woodrow 2
1548 Accesses
This chapter is about writing about the procedure of the research. This includes a discussion of reliability, validity and the ethics of research and writing. The level of detail about these issues varies across texts, but the reliability and validity of the study must feature in the text. Some-times these issues are evident from the research instruments and analysis and sometimes they are referred to explicitly. This chapter includes the following sections:
Technical information
Reliability of a measure
Internal validity
External validity
Research ethics
Reporting on reliability
Writing about validity
Reporting on ethics
Writing about research procedure
This is a preview of subscription content, log in via an institution to check access.
Access this chapter
- Available as EPUB and PDF
- Read on any device
- Instant download
- Own it forever
- Compact, lightweight edition
- Dispatched in 3 to 5 business days
- Free shipping worldwide - see info
- Durable hardcover edition
Tax calculation will be finalised at checkout
Purchases are for personal use only
Institutional subscriptions
Unable to display preview. Download preview PDF.
Further reading
Dörnyei, Z. (2007). Research methods in applied linguistics: Quantitative, qualitative and mixed methodologies . Oxford: Oxford University Press.
Google Scholar
Paltridge, B. & Phakiti, A. (2010) (Eds.). Continuum companion to research methods in applied linguistics . London: Continuum.
Sources of examples
Lee, J.-A. (2009). Teachers’ sense of efficacy in teaching English, perceived English proficiency and attitudes toward English language: A case of Korean public elementary teachers . PhD, Ohio State University.
Levine, G. S. (2003). Student and instructor beliefs and attitudes about target language use, first language use and anxiety: Report of a questionnaire study. Modern Language Journal , 87(3), 343–364, doi: 10.1111/1540-4781.00194.
Article Google Scholar
Lin, H., Chen, T., & Dwyer, F. (2006). Effects of static visuals and computer-generated animations in facilitating Immediate and delayed achievement in the EFL classroom. Foreign Language Annals , 39(2), doi: 203-219.10.1111/j.1944-9720.2006.tb02262.x.
Mills, N. (2011). Teaching assistants’ self-efficacy in teaching literature: Sources, personal assessments, and consequences. Modern Language Journal , 95(1), 61–80. doi: 10.1111/j.1540-4781.2010.01145.x.
Rai, M. K., Loschky, L. C., Harris, R. J., Peck, N. R., & Cook, L. G. (2011). Effects of stress and working memory capacity on foreign language readers’ inferential processing during comprehension. Language Learning , 61(1), 187–218. doi: 10.1111/j.1467-9922.2010.00592.x.
Rose, H. (2010). Kanji learning of Japanese language learners on a year-long study exchange at a Japanese university: An investigation of strategy use, motivation control and self regulation . PhD, University of Sydney.
Download references
Author information
Authors and affiliations.
University of Sydney, Australia
Lindy Woodrow
You can also search for this author in PubMed Google Scholar
Copyright information
© 2014 Lindy Woodrow
About this chapter
Woodrow, L. (2014). Reliability, Validity and Ethics. In: Writing about Quantitative Research in Applied Linguistics. Palgrave Macmillan, London. https://doi.org/10.1057/9780230369955_3
Download citation
DOI : https://doi.org/10.1057/9780230369955_3
Publisher Name : Palgrave Macmillan, London
Print ISBN : 978-0-230-36997-9
Online ISBN : 978-0-230-36995-5
eBook Packages : Palgrave Language & Linguistics Collection Education (R0)
Share this chapter
Anyone you share the following link with will be able to read this content:
Sorry, a shareable link is not currently available for this article.
Provided by the Springer Nature SharedIt content-sharing initiative
- Publish with us
Policies and ethics
- Find a journal
- Track your research

Understanding Reliability and Validity
These related research issues ask us to consider whether we are studying what we think we are studying and whether the measures we use are consistent.
Reliability
Reliability is the extent to which an experiment, test, or any measuring procedure yields the same result on repeated trials. Without the agreement of independent observers able to replicate research procedures, or the ability to use research tools and procedures that yield consistent measurements, researchers would be unable to satisfactorily draw conclusions, formulate theories, or make claims about the generalizability of their research. In addition to its important role in research, reliability is critical for many parts of our lives, including manufacturing, medicine, and sports.
Reliability is such an important concept that it has been defined in terms of its application to a wide range of activities. For researchers, four key types of reliability are:
Equivalency Reliability
Equivalency reliability is the extent to which two items measure identical concepts at an identical level of difficulty. Equivalency reliability is determined by relating two sets of test scores to one another to highlight the degree of relationship or association. In quantitative studies and particularly in experimental studies, a correlation coefficient, statistically referred to as r , is used to show the strength of the correlation between a dependent variable (the subject under study), and one or more independent variables , which are manipulated to determine effects on the dependent variable. An important consideration is that equivalency reliability is concerned with correlational, not causal, relationships.
For example, a researcher studying university English students happened to notice that when some students were studying for finals, their holiday shopping began. Intrigued by this, the researcher attempted to observe how often, or to what degree, this these two behaviors co-occurred throughout the academic year. The researcher used the results of the observations to assess the correlation between studying throughout the academic year and shopping for gifts. The researcher concluded there was poor equivalency reliability between the two actions. In other words, studying was not a reliable predictor of shopping for gifts.
Stability Reliability
Stability reliability (sometimes called test, re-test reliability) is the agreement of measuring instruments over time. To determine stability, a measure or test is repeated on the same subjects at a future date. Results are compared and correlated with the initial test to give a measure of stability.
An example of stability reliability would be the method of maintaining weights used by the U.S. Bureau of Standards. Platinum objects of fixed weight (one kilogram, one pound, etc...) are kept locked away. Once a year they are taken out and weighed, allowing scales to be reset so they are "weighing" accurately. Keeping track of how much the scales are off from year to year establishes a stability reliability for these instruments. In this instance, the platinum weights themselves are assumed to have a perfectly fixed stability reliability.
Internal Consistency
Internal consistency is the extent to which tests or procedures assess the same characteristic, skill or quality. It is a measure of the precision between the observers or of the measuring instruments used in a study. This type of reliability often helps researchers interpret data and predict the value of scores and the limits of the relationship among variables.
For example, a researcher designs a questionnaire to find out about college students' dissatisfaction with a particular textbook. Analyzing the internal consistency of the survey items dealing with dissatisfaction will reveal the extent to which items on the questionnaire focus on the notion of dissatisfaction.
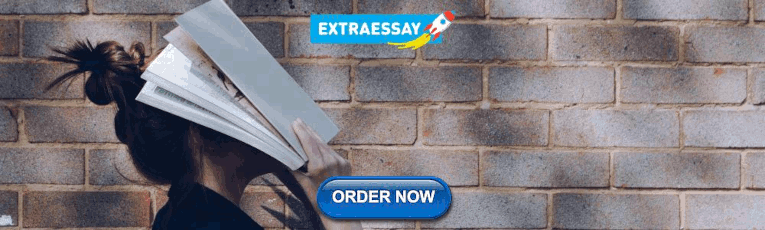
Interrater Reliability
Interrater reliability is the extent to which two or more individuals (coders or raters) agree. Interrater reliability addresses the consistency of the implementation of a rating system.
A test of interrater reliability would be the following scenario: Two or more researchers are observing a high school classroom. The class is discussing a movie that they have just viewed as a group. The researchers have a sliding rating scale (1 being most positive, 5 being most negative) with which they are rating the student's oral responses. Interrater reliability assesses the consistency of how the rating system is implemented. For example, if one researcher gives a "1" to a student response, while another researcher gives a "5," obviously the interrater reliability would be inconsistent. Interrater reliability is dependent upon the ability of two or more individuals to be consistent. Training, education and monitoring skills can enhance interrater reliability.
Related Information: Reliability Example
An example of the importance of reliability is the use of measuring devices in Olympic track and field events. For the vast majority of people, ordinary measuring rulers and their degree of accuracy are reliable enough. However, for an Olympic event, such as the discus throw, the slightest variation in a measuring device -- whether it is a tape, clock, or other device -- could mean the difference between the gold and silver medals. Additionally, it could mean the difference between a new world record and outright failure to qualify for an event. Olympic measuring devices, then, must be reliable from one throw or race to another and from one competition to another. They must also be reliable when used in different parts of the world, as temperature, air pressure, humidity, interpretation, or other variables might affect their readings.
Validity refers to the degree to which a study accurately reflects or assesses the specific concept that the researcher is attempting to measure. While reliability is concerned with the accuracy of the actual measuring instrument or procedure, validity is concerned with the study's success at measuring what the researchers set out to measure.
Researchers should be concerned with both external and internal validity. External validity refers to the extent to which the results of a study are generalizable or transferable. (Most discussions of external validity focus solely on generalizability; see Campbell and Stanley, 1966. We include a reference here to transferability because many qualitative research studies are not designed to be generalized.)
Internal validity refers to (1) the rigor with which the study was conducted (e.g., the study's design, the care taken to conduct measurements, and decisions concerning what was and wasn't measured) and (2) the extent to which the designers of a study have taken into account alternative explanations for any causal relationships they explore (Huitt, 1998). In studies that do not explore causal relationships, only the first of these definitions should be considered when assessing internal validity.
Scholars discuss several types of internal validity. For brief discussions of several types of internal validity, click on the items below:
Face Validity
Face validity is concerned with how a measure or procedure appears. Does it seem like a reasonable way to gain the information the researchers are attempting to obtain? Does it seem well designed? Does it seem as though it will work reliably? Unlike content validity, face validity does not depend on established theories for support (Fink, 1995).
Criterion Related Validity
Criterion related validity, also referred to as instrumental validity, is used to demonstrate the accuracy of a measure or procedure by comparing it with another measure or procedure which has been demonstrated to be valid.
For example, imagine a hands-on driving test has been shown to be an accurate test of driving skills. By comparing the scores on the written driving test with the scores from the hands-on driving test, the written test can be validated by using a criterion related strategy in which the hands-on driving test is compared to the written test.
Construct Validity
Construct validity seeks agreement between a theoretical concept and a specific measuring device or procedure. For example, a researcher inventing a new IQ test might spend a great deal of time attempting to "define" intelligence in order to reach an acceptable level of construct validity.
Construct validity can be broken down into two sub-categories: Convergent validity and discriminate validity. Convergent validity is the actual general agreement among ratings, gathered independently of one another, where measures should be theoretically related. Discriminate validity is the lack of a relationship among measures which theoretically should not be related.
To understand whether a piece of research has construct validity, three steps should be followed. First, the theoretical relationships must be specified. Second, the empirical relationships between the measures of the concepts must be examined. Third, the empirical evidence must be interpreted in terms of how it clarifies the construct validity of the particular measure being tested (Carmines & Zeller, p. 23).
Content Validity
Content Validity is based on the extent to which a measurement reflects the specific intended domain of content (Carmines & Zeller, 1991, p.20).
Content validity is illustrated using the following examples: Researchers aim to study mathematical learning and create a survey to test for mathematical skill. If these researchers only tested for multiplication and then drew conclusions from that survey, their study would not show content validity because it excludes other mathematical functions. Although the establishment of content validity for placement-type exams seems relatively straight-forward, the process becomes more complex as it moves into the more abstract domain of socio-cultural studies. For example, a researcher needing to measure an attitude like self-esteem must decide what constitutes a relevant domain of content for that attitude. For socio-cultural studies, content validity forces the researchers to define the very domains they are attempting to study.
Related Information: Validity Example
Many recreational activities of high school students involve driving cars. A researcher, wanting to measure whether recreational activities have a negative effect on grade point average in high school students, might conduct a survey asking how many students drive to school and then attempt to find a correlation between these two factors. Because many students might use their cars for purposes other than or in addition to recreation (e.g., driving to work after school, driving to school rather than walking or taking a bus), this research study might prove invalid. Even if a strong correlation was found between driving and grade point average, driving to school in and of itself would seem to be an invalid measure of recreational activity.
The challenges of achieving reliability and validity are among the most difficult faced by researchers. In this section, we offer commentaries on these challenges.
Difficulties of Achieving Reliability
It is important to understand some of the problems concerning reliability which might arise. It would be ideal to reliably measure, every time, exactly those things which we intend to measure. However, researchers can go to great lengths and make every attempt to ensure accuracy in their studies, and still deal with the inherent difficulties of measuring particular events or behaviors. Sometimes, and particularly in studies of natural settings, the only measuring device available is the researcher's own observations of human interaction or human reaction to varying stimuli. As these methods are ultimately subjective in nature, results may be unreliable and multiple interpretations are possible. Three of these inherent difficulties are quixotic reliability, diachronic reliability and synchronic reliability.
Quixotic reliability refers to the situation where a single manner of observation consistently, yet erroneously, yields the same result. It is often a problem when research appears to be going well. This consistency might seem to suggest that the experiment was demonstrating perfect stability reliability. This, however, would not be the case.
For example, if a measuring device used in an Olympic competition always read 100 meters for every discus throw, this would be an example of an instrument consistently, yet erroneously, yielding the same result. However, quixotic reliability is often more subtle in its occurrences than this. For example, suppose a group of German researchers doing an ethnographic study of American attitudes ask questions and record responses. Parts of their study might produce responses which seem reliable, yet turn out to measure felicitous verbal embellishments required for "correct" social behavior. Asking Americans, "How are you?" for example, would in most cases, elicit the token, "Fine, thanks." However, this response would not accurately represent the mental or physical state of the respondents.
Diachronic reliability refers to the stability of observations over time. It is similar to stability reliability in that it deals with time. While this type of reliability is appropriate to assess features that remain relatively unchanged over time, such as landscape benchmarks or buildings, the same level of reliability is more difficult to achieve with socio-cultural phenomena.
For example, in a follow-up study one year later of reading comprehension in a specific group of school children, diachronic reliability would be hard to achieve. If the test were given to the same subjects a year later, many confounding variables would have impacted the researchers' ability to reproduce the same circumstances present at the first test. The final results would almost assuredly not reflect the degree of stability sought by the researchers.
Synchronic reliability refers to the similarity of observations within the same time frame; it is not about the similarity of things observed. Synchronic reliability, unlike diachronic reliability, rarely involves observations of identical things. Rather, it concerns itself with particularities of interest to the research.
For example, a researcher studies the actions of a duck's wing in flight and the actions of a hummingbird's wing in flight. Despite the fact that the researcher is studying two distinctly different kinds of wings, the action of the wings and the phenomenon produced is the same.
Comments on a Flawed, Yet Influential Study
An example of the dangers of generalizing from research that is inconsistent, invalid, unreliable, and incomplete is found in the Time magazine article, "On A Screen Near You: Cyberporn" (De Witt, 1995). This article relies on a study done at Carnegie Mellon University to determine the extent and implications of online pornography. Inherent to the study are methodological problems of unqualified hypotheses and conclusions, unsupported generalizations and a lack of peer review.
Ignoring the functional problems that manifest themselves later in the study, it seems that there are a number of ethical problems within the article. The article claims to be an exhaustive study of pornography on the Internet, (it was anything but exhaustive), it resembles a case study more than anything else. Marty Rimm, author of the undergraduate paper that Time used as a basis for the article, claims the paper was an "exhaustive study" of online pornography when, in fact, the study based most of its conclusions about pornography on the Internet on the "descriptions of slightly more than 4,000 images" (Meeks, 1995, p. 1). Some USENET groups see hundreds of postings in a day.
Considering the thousands of USENET groups, 4,000 images no longer carries the authoritative weight that its author intended. The real problem is that the study (an undergraduate paper similar to a second-semester composition assignment) was based not on pornographic images themselves, but on the descriptions of those images. This kind of reduction detracts significantly from the integrity of the final claims made by the author. In fact, this kind of research is commensurate with doing a study of the content of pornographic movies based on the titles of the movies, then making sociological generalizations based on what those titles indicate. (This is obviously a problem with a number of types of validity, because Rimm is not studying what he thinks he is studying, but instead something quite different. )
The author of the Time article, Philip Elmer De Witt writes, "The research team at CMU has undertaken the first systematic study of pornography on the Information Superhighway" (Godwin, 1995, p. 1). His statement is problematic in at least three ways. First, the research team actually consisted of a few of Rimm's undergraduate friends with no methodological training whatsoever. Additionally, no mention of the degree of interrater reliability is made. Second, this systematic study is actually merely a "non-randomly selected subset of commercial bulletin-board systems that focus on selling porn" (Godwin, p. 6). As pornography vending is actually just a small part of the whole concerning the use of pornography on the Internet, the entire premise of this study's content validity is firmly called into question. Finally, the use of the term "Information Superhighway" is a false assessment of what in actuality is only a few USENET groups and BBSs (Bulletin Board System), which make up only a small fraction of the entire "Information Superhighway" traffic. Essentially, what is here is yet another violation of content validity.
De Witt is quoted as saying: "In an 18-month study, the team surveyed 917,410 sexually-explicit pictures, descriptions, short-stories and film clips. On those USENET newsgroups where digitized images are stored, 83.5 percent of the pictures were pornographic" (De Witt 40).
Statistically, some interesting contradictions arise. The figure 917,410 was taken from adult-oriented BBSs--none came from actual USENET groups or the Internet itself. This is a glaring discrepancy. Out of the 917,410 files, 212,114 are only descriptions (Hoffman & Novak, 1995, p.2). The question is, how many actual images did the "researchers" see?
"Between April and July 1994, the research team downloaded all available images (3,254)...the team encountered technical difficulties with 13 percent of these images...This left a total of 2,830 images for analysis" (p. 2). This means that out of 917,410 files discussed in this study, 914,580 of them were not even pictures! As for the 83.5 percent figure, this is actually based on "17 alt.binaries groups that Rimm considered pornographic" (p. 2).
In real terms, 17 USENET groups is a fraction of a percent of all USENET groups available. Worse yet, Time claimed that "...only about 3 percent of all messages on the USENET [represent pornographic material], while the USENET itself represents 11.5 percent of the traffic on the Internet" (De Witt, p. 40).
Time neglected to carry the interpretation of this data out to its logical conclusion, which is that less than half of 1 percent (3 percent of 11 percent) of the images on the Internet are associated with newsgroups that contain pornographic imagery. Furthermore, of this half percent, an unknown but even smaller percentage of the messages in newsgroups that are 'associated with pornographic imagery', actually contained pornographic material (Hoffman & Novak, p. 3).
Another blunder can be seen in the avoidance of peer-review, which suggests that there was some political interests being served in having the study become a Time cover story. Marty Rimm contracted the Georgetown Law Review and Time in an agreement to publish his study as long as they kept it under lock and key. During the months before publication, many interested scholars and professionals tried in vain to obtain a copy of the study in order to check it for flaws. De Witt justified not letting such peer-review take place, and also justified the reliability and validity of the study, on the grounds that because the Georgetown Law Review had accepted it, it was therefore reliable and valid, and needed no peer-review. What he didn't know, was that law reviews are not edited by professionals, but by "third year law students" (Godwin, p. 4).
There are many consequences of the failure to subject such a study to the scrutiny of peer review. If it was Rimm's desire to publish an article about on-line pornography in a manner that legitimized his article, yet escaped the kind of critical review the piece would have to undergo if published in a scholarly journal of computer-science, engineering, marketing, psychology, or communications. What better venue than a law journal? A law journal article would have the added advantage of being taken seriously by law professors, lawyers, and legally-trained policymakers. By virtue of where it appeared, it would automatically be catapulted into the center of the policy debate surrounding online censorship and freedom of speech (Godwin).
Herein lies the dangerous implication of such a study: Because the questions surrounding pornography are of such immediate political concern, the study was placed in the forefront of the U.S. domestic policy debate over censorship on the Internet, (an integral aspect of current anti-First Amendment legislation) with little regard for its validity or reliability.
On June 26, the day the article came out, Senator Grassley, (co-sponsor of the anti-porn bill, along with Senator Dole) began drafting a speech that was to be delivered that very day in the Senate, using the study as evidence. The same day, at the same time, Mike Godwin posted on WELL (Whole Earth 'Lectronic Link, a forum for professionals on the Internet) what turned out to be the overstatement of the year: "Philip's story is an utter disaster, and it will damage the debate about this issue because we will have to spend lots of time correcting misunderstandings that are directly attributable to the story" (Meeks, p. 7).
As Godwin was writing this, Senator Grassley was speaking to the Senate: "Mr. President, I want to repeat that: 83.5 percent of the 900,000 images reviewed--these are all on the Internet--are pornographic, according to the Carnegie-Mellon study" ( p. 7). Several days later, Senator Dole was waving the magazine in front of the Senate like a battle flag.
Donna Hoffman, professor at Vanderbilt University, summed up the dangerous political implications by saying, "The critically important national debate over First Amendment rights and restrictions of information on the Internet and other emerging media requires facts and informed opinion, not hysteria" (p.1).
In addition to the hysteria, Hoffman sees a plethora of other problems with the study. "Because the content analysis and classification scheme are 'black boxes,'" Hoffman said, "because no reliability and validity results are presented, because no statistical testing of the differences both within and among categories for different types of listings has been performed, and because not a single hypothesis has been tested, formally or otherwise, no conclusions should be drawn until the issues raised in this critique are resolved" (p. 4).
However, the damage has already been done. This questionable research by an undergraduate engineering major has been generalized to such an extent that even the U.S. Senate, and in particular Senators Grassley and Dole, have been duped, albeit through the strength of their own desires to see only what they wanted to see.
Annotated Bibliography
American Psychological Association. (1985). Standards for educational and psychological testing. Washington, DC: Author.
This work on focuses on reliability, validity and the standards that testers need to achieve in order to ensure accuracy.
Babbie, E.R. & Huitt, R.E. (1979). The practice of social research 2nd ed . Belmont, CA: Wadsworth Publishing.
An overview of social research and its applications.
Beauchamp, T. L., Faden, R.R., Wallace, Jr., R.J. & Walters, L . ( 1982). Ethical issues in social science research. Baltimore and London: The Johns Hopkins University Press.
A systematic overview of ethical issues in Social Science Research written by researchers with firsthand familiarity with the situations and problems researchers face in their work. This book raises several questions of how reliability and validity can be affected by ethics.
Borman, K.M. et al. (1986). Ethnographic and qualitative research design and why it doesn't work. American behavioral scientist 30 , 42-57.
The authors pose questions concerning threats to qualitative research and suggest solutions.
Bowen, K. A. (1996, Oct. 12). The sin of omission -punishable by death to internal validity: An argument for integration of quantitative research methods to strengthen internal validity. Available: http://trochim.human.cornell.edu/gallery/bowen/hss691.htm
An entire Web site that examines the merits of integrating qualitative and quantitative research methodologies through triangulation. The author argues that improving the internal validity of social science will be the result of such a union.
Brinberg, D. & McGrath, J.E. (1985). Validity and the research process . Beverly Hills: Sage Publications.
The authors investigate validity as value and propose the Validity Network Schema, a process by which researchers can infuse validity into their research.
Bussières, J-F. (1996, Oct.12). Reliability and validity of information provided by museum Web sites. Available: http://www.oise.on.ca/~jfbussieres/issue.html
This Web page examines the validity of museum Web sites which calls into question the validity of Web-based resources in general. Addresses the issue that all Websites should be examined with skepticism about the validity of the information contained within them.
Campbell, D. T. & Stanley, J.C. (1963). Experimental and quasi-experimental designs for research. Boston: Houghton Mifflin.
An overview of experimental research that includes pre-experimental designs, controls for internal validity, and tables listing sources of invalidity in quasi-experimental designs. Reference list and examples.
Carmines, E. G. & Zeller, R.A. (1991). Reliability and validity assessment . Newbury Park: Sage Publications.
An introduction to research methodology that includes classical test theory, validity, and methods of assessing reliability.
Carroll, K. M. (1995). Methodological issues and problems in the assessment of substance use. Psychological Assessment, Sep. 7 n3 , 349-58.
Discusses methodological issues in research involving the assessment of substance abuse. Introduces strategies for avoiding problems with the reliability and validity of methods.
Connelly, F. M. & Clandinin, D.J. (1990). Stories of experience and narrative inquiry. Educational Researcher 19:5 , 2-12.
A survey of narrative inquiry that outlines criteria, methods, and writing forms. It includes a discussion of risks and dangers in narrative studies, as well as a research agenda for curricula and classroom studies.
De Witt, P.E. (1995, July 3). On a screen near you: Cyberporn. Time, 38-45.
This is an exhaustive Carnegie Mellon study of online pornography by Marty Rimm, electrical engineering student.
Fink, A., ed. (1995). The survey Handbook, v.1 .Thousand Oaks, CA: Sage.
A guide to survey; this is the first in a series referred to as the "survey kit". It includes bibliograpgical references. Addresses survey design, analysis, reporting surveys and how to measure the validity and reliability of surveys.
Fink, A., ed. (1995). How to measure survey reliability and validity v. 7 . Thousand Oaks, CA: Sage.
This volume seeks to select and apply reliability criteria and select and apply validity criteria. The fundamental principles of scaling and scoring are considered.
Godwin, M. (1995, July). JournoPorn, dissection of the Time article. Available: http://www.hotwired.com
A detailed critique of Time magazine's Cyberporn , outlining flaws of methodology as well as exploring the underlying assumptions of the article.
Hambleton, R.K. & Zaal, J.N., eds. (1991). Advances in educational and psychological testing . Boston: Kluwer Academic.
Information on the concepts of reliability and validity in psychology and education.
Harnish, D.L. (1992). Human judgment and the logic of evidence: A critical examination of research methods in special education transition literature . In D.L. Harnish et al. eds., Selected readings in transition.
This article investigates threats to validity in special education research.
Haynes, N. M. (1995). How skewed is 'the bell curve'? Book Product Reviews . 1-24.
This paper claims that R.J. Herrnstein and C. Murray's The Bell Curve: Intelligence and Class Structure in American Life does not have scientific merit and claims that the bell curve is an unreliable measure of intelligence.
Healey, J. F. (1993). Statistics: A tool for social research, 3rd ed . Belmont: Wadsworth Publishing.
Inferential statistics, measures of association, and multivariate techniques in statistical analysis for social scientists are addressed.
Helberg, C. (1996, Oct.12). Pitfalls of data analysis (or how to avoid lies and damned lies). Available: http//maddog/fammed.wisc.edu/pitfalls/
A discussion of things researchers often overlook in their data analysis and how statistics are often used to skew reliability and validity for the researchers purposes.
Hoffman, D. L. and Novak, T.P. (1995, July). A detailed critique of the Time article: Cyberporn. Available: http://www.hotwired.com
A methodological critique of the Time article that uncovers some of the fundamental flaws in the statistics and the conclusions made by De Witt.
Huitt, William G. (1998). Internal and External Validity . http://www.valdosta.peachnet.edu/~whuitt/psy702/intro/valdgn.html
A Web document addressing key issues of external and internal validity.
Jones, J. E. & Bearley, W.L. (1996, Oct 12). Reliability and validity of training instruments. Organizational Universe Systems. Available: http://ous.usa.net/relval.htm
The authors discuss the reliability and validity of training design in a business setting. Basic terms are defined and examples provided.
Cultural Anthropology Methods Journal. (1996, Oct. 12). Available: http://www.lawrence.edu/~bradleyc/cam.html
An online journal containing articles on the practical application of research methods when conducting qualitative and quantitative research. Reliability and validity are addressed throughout.
Kirk, J. & Miller, M. M. (1986). Reliability and validity in qualitative research. Beverly Hills: Sage Publications.
This text describes objectivity in qualitative research by focusing on the issues of validity and reliability in terms of their limitations and applicability in the social and natural sciences.
Krakower, J. & Niwa, S. (1985). An assessment of validity and reliability of the institutinal perfarmance survey . Boulder, CO: National center for higher education management systems.
Educational surveys and higher education research and the efeectiveness of organization.
Lauer, J. M. & Asher, J.W. (1988). Composition Research. New York: Oxford University Press.
A discussion of empirical designs in the context of composition research as a whole.
Laurent, J. et al. (1992, Mar.) Review of validity research on the stanford-binet intelligence scale: 4th Ed. Psychological Assessment . 102-112.
This paper looks at the results of construct and criterion- related validity studies to determine if the SB:FE is a valid measure of intelligence.
LeCompte, M. D., Millroy, W.L., & Preissle, J. eds. (1992). The handbook of qualitative research in education. San Diego: Academic Press.
A compilation of the range of methodological and theoretical qualitative inquiry in the human sciences and education research. Numerous contributing authors apply their expertise to discussing a wide variety of issues pertaining to educational and humanities research as well as suggestions about how to deal with problems when conducting research.
McDowell, I. & Newell, C. (1987). Measuring health: A guide to rating scales and questionnaires . New York: Oxford University Press.
This gives a variety of examples of health measurement techniques and scales and discusses the validity and reliability of important health measures.
Meeks, B. (1995, July). Muckraker: How Time failed. Available: http://www.hotwired.com
A step-by-step outline of the events which took place during the researching, writing, and negotiating of the Time article of 3 July, 1995 titled: On A Screen Near You: Cyberporn .
Merriam, S. B. (1995). What can you tell from an N of 1?: Issues of validity and reliability in qualitative research. Journal of Lifelong Learning v4 , 51-60.
Addresses issues of validity and reliability in qualitative research for education. Discusses philosophical assumptions underlying the concepts of internal validity, reliability, and external validity or generalizability. Presents strategies for ensuring rigor and trustworthiness when conducting qualitative research.
Morris, L.L, Fitzgibbon, C.T., & Lindheim, E. (1987). How to measure performance and use tests. In J.L. Herman (Ed.), Program evaluation kit (2nd ed.). Newbury Park, CA: Sage.
Discussion of reliability and validity as it pertyains to measuring students' performance.
Murray, S., et al. (1979, April). Technical issues as threats to internal validity of experimental and quasi-experimental designs. San Francisco: University of California. 8-12.
(From Yang et al. bibliography--unavailable as of this writing.)
Russ-Eft, D. F. (1980). Validity and reliability in survey research. American Institutes for Research in the Behavioral Sciences August , 227 151.
An investigation of validity and reliability in survey research with and overview of the concepts of reliability and validity. Specific procedures for measuring sources of error are suggested as well as general suggestions for improving the reliability and validity of survey data. A extensive annotated bibliography is provided.
Ryser, G. R. (1994). Developing reliable and valid authentic assessments for the classroom: Is it possible? Journal of Secondary Gifted Education Fall, v6 n1 , 62-66.
Defines the meanings of reliability and validity as they apply to standardized measures of classroom assessment. This article defines reliability as scorability and stability and validity is seen as students' ability to use knowledge authentically in the field.
Schmidt, W., et al. (1982). Validity as a variable: Can the same certification test be valid for all students? Institute for Research on Teaching July, ED 227 151.
A technical report that presents specific criteria for judging content, instructional and curricular validity as related to certification tests in education.
Scholfield, P. (1995). Quantifying language. A researcher's and teacher's guide to gathering language data and reducing it to figures . Bristol: Multilingual Matters.
A guide to categorizing, measuring, testing, and assessing aspects of language. A source for language-related practitioners and researchers in conjunction with other resources on research methods and statistics. Questions of reliability, and validity are also explored.
Scriven, M. (1993). Hard-Won Lessons in Program Evaluation . San Francisco: Jossey-Bass Publishers.
A common sense approach for evaluating the validity of various educational programs and how to address specific issues facing evaluators.
Shou, P. (1993, Jan.). The singer loomis inventory of personality: A review and critique. [Paper presented at the Annual Meeting of the Southwest Educational Research Association.]
Evidence for reliability and validity are reviewed. A summary evaluation suggests that SLIP (developed by two Jungian analysts to allow examination of personality from the perspective of Jung's typology) appears to be a useful tool for educators and counselors.
Sutton, L.R. (1992). Community college teacher evaluation instrument: A reliability and validity study . Diss. Colorado State University.
Studies of reliability and validity in occupational and educational research.
Thompson, B. & Daniel, L.G. (1996, Oct.). Seminal readings on reliability and validity: A "hit parade" bibliography. Educational and psychological measurement v. 56 , 741-745.
Editorial board members of Educational and Psychological Measurement generated bibliography of definitive publications of measurement research. Many articles are directly related to reliability and validity.
Thompson, E. Y., et al. (1995). Overview of qualitative research . Diss. Colorado State University.
A discussion of strengths and weaknesses of qualitative research and its evolution and adaptation. Appendices and annotated bibliography.
Traver, C. et al. (1995). Case Study . Diss. Colorado State University.
This presentation gives an overview of case study research, providing definitions and a brief history and explanation of how to design research.
Trochim, William M. K. (1996) External validity. (. Available: http://trochim.human.cornell.edu/kb/EXTERVAL.htm
A comprehensive treatment of external validity found in William Trochim's online text about research methods and issues.
Trochim, William M. K. (1996) Introduction to validity. (. Available: hhttp://trochim.human.cornell.edu/kb/INTROVAL.htm
An introduction to validity found in William Trochim's online text about research methods and issues.
Trochim, William M. K. (1996) Reliability. (. Available: http://trochim.human.cornell.edu/kb/reltypes.htm
A comprehensive treatment of reliability found in William Trochim's online text about research methods and issues.
Validity. (1996, Oct. 12). Available: http://vislab-www.nps.navy.mil/~haga/validity.html
A source for definitions of various forms and types of reliability and validity.
Vinsonhaler, J. F., et al. (1983, July). Improving diagnostic reliability in reading through training. Institute for Research on Teaching ED 237 934.
This technical report investigates the practical application of a program intended to improve the diagnoses of reading deficient students. Here, reliability is assumed and a pragmatic answer to a specific educational problem is suggested as a result.
Wentland, E. J. & Smith, K.W. (1993). Survey responses: An evaluation of their validity . San Diego: Academic Press.
This book looks at the factors affecting response validity (or the accuracy of self-reports in surveys) and provides several examples with varying accuracy levels.
Wiget, A. (1996). Father juan greyrobe: Reconstructing tradition histories, and the reliability and validity of uncorroborated oral tradition. Ethnohistory 43:3 , 459-482.
This paper presents a convincing argument for the validity of oral histories in ethnographic research where at least some of the evidence can be corroborated through written records.
Yang, G. H., et al. (1995). Experimental and quasi-experimental educational research . Diss. Colorado State University.
This discussion defines experimentation and considers the rhetorical issues and advantages and disadvantages of experimental research. Annotated bibliography.
Yarroch, W. L. (1991, Sept.). The Implications of content versus validity on science tests. Journal of Research in Science Teaching , 619-629.
The use of content validity as the primary assurance of the measurement accuracy for science assessment examinations is questioned. An alternative accuracy measure, item validity, is proposed to look at qualitative comparisons between different factors.
Yin, R. K. (1989). Case study research: Design and methods . London: Sage Publications.
This book discusses the design process of case study research, including collection of evidence, composing the case study report, and designing single and multiple case studies.
Related Links
Internal Validity Tutorial. An interactive tutorial on internal validity.
http://server.bmod.athabascau.ca/html/Validity/index.shtml
Howell, Jonathan, Paul Miller, Hyun Hee Park, Deborah Sattler, Todd Schack, Eric Spery, Shelley Widhalm, & Mike Palmquist. (2005). Reliability and Validity. Writing@CSU . Colorado State University. https://writing.colostate.edu/guides/guide.cfm?guideid=66
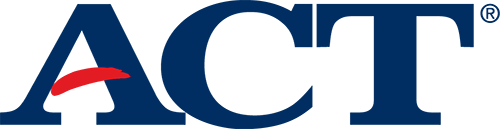
- Plan for College and Career
- Take the ACT
- School and District Assessment
- Career-Ready Solutions
- Students & Parents
- Open Search Form
- ACT Research
- All Services and Resources
- Growth Modeling Resources
- Data and Visualization
- All Reports
- ACT Research Publications
- Technical Documentation
- Studies and Partnerships
- About ACT Research
- Meet Our Experts
- Get Research Updates
Other ACT Services and Products
New Featured May 15, 2024
On the Reliability and Validity of a Three-Item Conscientiousness Scale
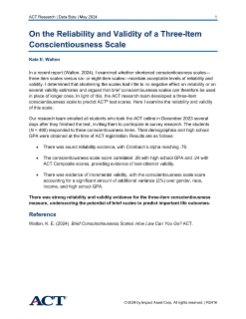
In a recent report (Walton, 2024), Kate examined whether shortened conscientiousness scales—three-item scales versus six- or eight-item scales—maintain acceptable levels of reliability and validity.
Size: 161.3KB | Pages: 2
This action will open a new window. Do you want to proceed?
Welcome to ACT
If you are accessing this site from outside the United States, Puerto Rico, or U.S. Territories, please proceed to the non-U.S. version of our website.
- Open access
- Published: 18 May 2024
Parental hesitancy toward children vaccination: a multi-country psychometric and predictive study
- Hamid Sharif-Nia 1 , 2 ,
- Long She 3 ,
- Kelly-Ann Allen 4 , 12 ,
- João Marôco 5 ,
- Harpaljit Kaur 6 ,
- Gökmen Arslan 7 ,
- Ozkan Gorgulu 8 ,
- Jason W. Osborne 9 ,
- Pardis Rahmatpour 10 &
- Fatemeh Khoshnavay Fomani 11
BMC Public Health volume 24 , Article number: 1348 ( 2024 ) Cite this article
1 Altmetric
Metrics details
Understanding vaccine hesitancy, as a critical concern for public health, cannot occur without the use of validated measures applicable and relevant to the samples they are assessing. The current study aimed to validate the Vaccine Hesitancy Scale (VHS) and to investigate the predictors of children’s vaccine hesitancy among parents from Australia, China, Iran, and Turkey. To ensure the high quality of the present observational study the STROBE checklist was utilized.
A cross-sectional study.
In total, 6,073 parent participants completed the web-based survey between 8 August 2021 and 1 October 2021. The content and construct validity of the Vaccine Hesitancy Scale was assessed. Cronbach’s alpha and McDonald’s omega were used to assess the scale’s internal consistency, composite reliability (C.R.) and maximal reliability (MaxR) were used to assess the construct reliability. Multiple linear regression was used to predict parental vaccine hesitancy from gender, social media activity, and perceived financial well-being.
The results found that the VHS had a two-factor structure (i.e., lack of confidence and risk) and a total of 9 items. The measure showed metric invariance across four very different countries/cultures, showed evidence of good reliability, and showed evidence of validity. As expected, analyses indicated that parental vaccine hesitancy was higher in people who identify as female, more affluent, and more active on social media.
Conclusions
The present research marks one of the first studies to evaluate vaccine hesitancy in multiple countries that demonstrated VHS validity and reliability. Findings from this study have implications for future research examining vaccine hesitancy and vaccine-preventable diseases and community health nurses.
Peer Review reports
Introduction
Emerging and re-emerging infectious diseases have threatened human life many times throughout history. Many researchers and experts agree that vaccinations are one of the most protective and preventative mechanisms for disease control and pandemic prevention [ 1 ]. For example, in case of COVID-19, vaccines were developed to boost immunity to curb the spread of the highly infectious disease [ 2 ] and save an estimated 14.4 million lives globally [ 3 ]. Despite the reported success of many vaccines in terms of disease spread, reduced symptoms, and adverse outcomes, as well as the historical success of vaccination more generally in preventing disease outbreaks, vaccine hesitancy remains an enduring and critical threat to health globally. Vaccine hesitancy has been identified as a central factor affecting vaccine uptake rates, impacting the potential emergence and re-emergence of vaccine-preventable diseases [ 4 ].
The SAGE Working Group on Vaccine Hesitancy defined vaccine hesitancy as a “delay in [the] acceptance or refusal of vaccination despite availability of vaccination services” and found that people’s reluctance to receive safe and available vaccines was a growing concern, long before the recent COVID-19 pandemic [ 5 ]. Previous research has linked vaccine hesitancy to various factors, such as concerns for safety and effectiveness, which may have emerged due to the unprecedented scale and speed at which the vaccines were developed [ 6 ]. Other factors fuelling vaccine hesitancy include a lack of information [ 7 ], conspiracy theories, and low trust in governments and institutions [ 8 , 9 ].
Parental vaccine hesitancy
Parental vaccine hesitancy is a crucial concern for public health due to its close links to vaccination delay, refusal, or denial in children, which ultimately increases their vulnerability to preventable diseases [ 10 , 11 ]. It is estimated that approximately 25% of children aged between 19 and 35 months have not been vaccinated due to the vaccine hesitancy of their parents [ 12 ]. For parents specifically, hesitancy is associated with misinformation on the internet [ 13 ], concern for finances, skepticism towards vaccine safety and necessity, confidence in a vaccine, and perceptions of the vaccine’s risk [ 14 ]. Additionally, parental vaccine hesitancy may be influenced to a large extent by environmental conditions, such as epidemics. Accordingly, children’s vaccination was identified as a challenging health issue during the COVID-19 pandemic, with implications for the health and spread of the diseases to the broader population [ 15 , 16 ].
Research has found that parental perceptions of risk and vaccine confidence generally contribute significantly to parental vaccine hesitancy. Parents have been reported to worry about potential side effects of the vaccines as well as their general effectiveness [ 12 ]. Meanwhile, low confidence in vaccination has been linked to reducing herd immunity and increasing infection among those who are immunocompromised or not vaccinated [ 17 ], especially in children.
Theoretical perspectives
The Health Belief Model (HBM) proposed by Hochbaum, Rosenstock, & Kegels (1952) suggests that vaccine decision-making is based on individuals’ perceptions of diseases and vaccines. Therefore, the perceived severity and susceptibility of diseases and the perceived risks and benefits of the vaccines may predict parental intentions to vaccinate their children [ 18 ]. Parent decisions in protective behaviours can therefore be shaped by their appraisal of the threat. According to protection motivation theory (PMT), threat appraisal refers to one’s adaptive actions, which consist of threat severity, maladaptive rewards, and threat vulnerability [ 19 ]. Parental appraisals of a disease as a threat thus shape patterns of vaccine hesitancy.
Considering existing theories, models, and conceptualizations, various measures have been developed and evaluated for assessing vaccine hesitancy. These measures assess an individual’s confidence in vaccines (Vaccine Confidence Scale) [ 20 , 21 ], parental attitudes toward childhood vaccines [ 22 ], and conspiracy beliefs related to vaccines [ 23 ]. Among the existing measures, the Vaccine Hesitancy Scale (VHS) was originally developed by Larson and colleagues from the SAGE Working Group on Vaccine Hesitancy [ 24 ], and psychometrically tested by Shapiro et al. (2016) among Canadian parents three years later. Their study revealed a two-factor structure (lack of confidence and risk) of the 9-item VHS among Canadian parents in French and English. In the study, one item was removed, and two items were loaded in the “risk” dimension, with the other six loading in the lack of confidence dimension [ 25 ]. Another study among parents in Guatemala also revealed a two-factor solution where the 7-item VHS was a better fit than the 10-item scale [ 26 ]. Further research is needed to refine the scale and assess its validity in different countries and contexts. Understanding vaccine hesitancy cannot occur without the use of validated measures applicable and relevant to the samples they are assessing. The current study, therefore, aims to psychometrically evaluate the Vaccine Hesitancy Scale among parents in Australia, China, Iran, and Turkey.
Study design and participants
The data used in this study is part of a broader research project on identifying the leading factors of parental vaccine hesitancy. A methodological cross-sectional research design was employed to validate the VHS based on data from four countries (i.e., Australia, China, Iran, and Turkey). A survey was distributed to parents across four countries over eight weeks, between 8 August 2021 and 1 October 2021. The inclusion criteria for respondents’ eligibility were parents with at least one child aged 18 years or under. The minimum sample size for conducting the Confirmatory Factor Analysis (CFA) was based on the criteria of [ 1 ] bias of parameters estimates < 10%; [ 2 ] 95% confidence intervals coverage > 91%; and [ 3 ] statistical power > 80% [ 27 ]. A minimum sample size of 200 was found to be sufficient to achieve the required criteria. To ensure the sample would reflect a normative population variance, this study collected more than 300 responses from each country. Using a convenient sampling technique, this study collected a total of 6,073 samples across the four countries: Australia (2734), China (523), Iran (2447), and Turkey (369). The online questionnaire was created by Google Form and sent to participants via social platform such as WhatsApp, Telegram and national application.
Sociodemographic characteristics
The data of parents’ sociodemographic characteristics such as age, gender, education level, living area, their perception regarding their economic status, and being active in social media were gathered using a sociodemographic form.
The vaccine hesitancy scale (VHS)
The VHS (ten-items) was originally developed by the SAGE Working Group on Vaccine Hesitancy, which is used to access parental vaccine hesitancy in their children. Although the original measure was not psychometrically evaluated by the original developers, it was later validated amongst a sample of Canadian parents [ 25 ]. The VHS has a validated two-factor structure: (1) lack of confidence (seven items; e.g., “Childhood vaccines are important for my child’s health”), and (2) risk (two items; e.g., “New vaccines carry more risks than old vaccines”). The scoring procedure for items in the VHS are rated on a 5-point Likert scale ranging from one (strongly disagree) to five (strongly agree). The current study consisted of four versions of the VHS: English (for Australia), Chinese (for China), Persian (for Iran), and Turkish (for Turkey). The English version was adopted from the Shapiro, Tatar [ 25 ] study. The Chinese, Persian, and Turkish versions were translated using the WHO protocol of forward-backward translation technique from the original English version. All versions were checked for cross-cultural equivalence.
Translation procedure
The cross-cultural adaptation procedure [ 28 ] was used to translate the items (sociodemographic information and VHS) from English via the translation and back-translation procedure into Chinese, Persian, and Turkish. All translators were bilingual. Two translators independently translated the questionnaires into the country’s respective languages. The research team then assessed the translated versions selecting the most appropriate item translations. Following this step, two other bilingual translators, who were “blinded” to the original questionnaire version, conducted the back-translation procedure independently. The expert committee (consisting of research team members, two nurses, one physician in social medicine, and a methodologist) then checked the back-translated version to ensure the accuracy and equivalence to the original questionnaire version. The committee also assessed the cross-cultural equivalence and appropriateness of the questionnaire to the study population, as well as the semantic equivalence of the items. No items were changed during the procedure.
Data analysis
Descriptive statistics.
This study used R and RStudio to perform all statistical analyses. The skimr and psych package was applied to produce descriptive statistics, which included the minimum v (Min), maximum (Max), and average value (M) as well as skewness and kurtosis for each item. Additionally, this study also generated histograms for each item [ 29 , 30 , 31 ]. Multiple linear regression was used to predict parental vaccine hesitancy from gender, Self-perception as being an active person on social media, and perceived financial well-being.
Confirmatory factor analysis
This study conducted a confirmatory factor analysis (CFA) using the lavaan package to assess the psychometric properties of the VHS across four countries. The factorial structure and model fit was confirmed and assessed in this stage. Model fit was evaluated using several fit indices such as the comparative fit index (CFI) > 0.90, normed fit index (NFI) > 0.90, Tucker–Lewis’s index (TLI) > 0.90, Standardized Root Mean Square residual (SRMR) < 0.09, and root mean square error of approximation < 0.08 [ 32 , 33 ].
Construct validity and reliability
To assess the VHS’s construct validity, both convergent and discriminant validity were assessed using the SemTools package. For convergent validity, the Average Variance Extracted (AVE) for each construct should be more than 0.5 [ 34 ]. Concerning discriminant validity, this study followed the Heterotrait-monotrait ratio of correlations (HTMT) approach, which denotes that all correlations between constructs in the HTMT matrix table should be less than 0.85 [ 35 ] and the correlations should have an AVE larger than the squared correlation between factors (Fornell & Larcker, 1981; Marôco, 2021). To assess the reliability of the VHS, the SemTools package was used to compute Cronbach’s alpha (α) and omega coefficients (ω), where α and ω values greater than 0.7 demonstrates an acceptable internal consistency and construct reliability [ 36 , 37 , 38 ].
Invariance assessment
To detect whether the factor structure of the VHS holds across the four countries, a set of nested models were defined and compared using the lavaan package with robust maximum likelihood estimation, namely, configural invariance model (no constraints), metric invariance model (constrained factor loadings between four countries), scalar invariance model (constrained loadings and intercepts), and structural invariance model (second order factor loadings constrained). Invariance was assessed using absolute ΔCFI and ΔRMSEA < 0.02. Invariance was assumed for ΔCFI < 0.01 and absolute ΔRMSEA < 0.02 [ 39 , 40 ] between two nested models as described elsewhere [ 27 ].
Ethical considerations
The Ethics Committee of Mazandaran University of Medical Sciences Research Ethics Committee approved the Ethical Considerations of this study (ethic code: IR.MAZUMS.REC.1401.064). In addition, all participants were informed of the purpose of the data collection, and questionnaires were distributed to the respondents only after they provided their consent to participate in the survey. Moreover, the respondents were ensured that their participation was on a voluntary basis and the confidentiality of all collected data was guaranteed.
Participants’ demographic characteristics and mean (S.D.) of COVID-19 vaccine hesitancy
This study employed a cross-sectional, questionnaire-based research design. In total, 6,073 parents from Australia (2734), China (523), Iran (2447), and Turkey (369) completed the survey through an online questionnaire platform. According to the Table 1 , the majority of respondent were female (84.15%) and between 20 and 40 years old (54.61%).
Item distribution properties
Table 2 shows the descriptive summary of the nine items’ minimum value (Min), maximum value (Max), average value (M), skewness, kurtosis, and histograms. The Item number 10 was dropped out due to the cross loading.
A CFA was used to confirm whether the factorial structure of the VHS used in the current study was consistent with results from the original validation study. The results of the CFA demonstrated a good model fit of the two-factor measurement model as evidenced by the model fit indices: CFI (0.972), NFI (0.971), TLI (0.958), SRMR (0.037), and RMSEA (90% C.I.) [0.074 (0.067, 0.074)]. The results also showed that all factor loadings for all items were greater than 0.5 and statistically significant. Figure 1 depicts the factor structure of the VHS in this study.
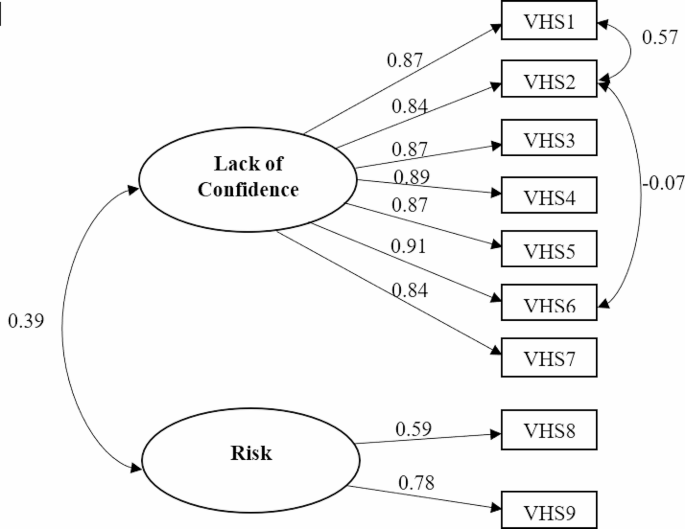
The results of the Confirmatory Factor Analysis (CFA)
Construct validity assessment
The results showed that the AVE for the sub-factor “lack of confidence” was greater than 0.5 (0.735), and the AVE for the sub-factor “risk” was slightly less than 0.5 (0.494). Previous literature indicated that AVE is a conservative and strict measure of convergent validity, and convergent validity can be assessed on the basis of Composite Reliability (C.R.) alone. Therefore, based on the results of C.R., the VHS in this study established convergent validity across all countries. The results of the HTMT correlation matrix showed that discriminant validity was also achieved, as the HTMT between “lack of confidence” and “risk” was 0.395, which is less than the suggested cut-off value of 0.85. The squared correlation between the two factors was 0.153. As this factor is less than the AVE for both “lack of confidence” (0.735) and “risk” (0.494), further evidence of discriminant validity was supported.
Construct reliability assessment
The results showed that the measurement model displayed good internal consistency and reliability, as evidenced by α (Lack of confidence: 0.952; Risk: 0.628) and ω (Lack of confidence: 0.946; Risk: 0.651).
Country invariance assessment
Prior to the Country Invariance Assessment, the vaccine hesitancy among parents score was compared across four countries. The results showed that the vaccine hesitancy among parents score was respectively in Iran (35.96, SD = 4.19), Australia (34.68, SD = 6.21), Turkey (34.09, SD = 4.78) and China (21.65, SD = 4.61) ( P < 0.001). While China clearly has different average levels of parental vaccine hesitancy, this does not preclude similar psychometric properties (i.e., factor structure) to other countries.
Country invariance assessment was tested in line with standard procedures, with a set of nested increasingly constrained models (see Table 3 ).
First, configurational invariance tests whether the basic structure of the measure is invariant, imposing no equality restrictions on parameters. Second, metric (weak) invariance was tested by constraining factor loadings to be invariant across countries. The ignorable change from configural variance to metric invariance (ΔCFI and ΔRMSEA of -0.009 and 0.004 respectively) supports this level of invariance. Delta chi-squared was significant (Χ 2 (21) = 235.55; p < 0.001), but chi squared is notoriously sensitive to ignorable changes when high df are present, and so is not considered a desirable metric.
Third, scalar invariance (“strong invariance”) constrained both factor loadings and item intercepts. Strong invariance is often considered beyond what is necessary for typical applications. These constraints produced a significant delta chi square ( Χ 2 (21) = 1044.251; p < 0.001) and a modest ΔCFI=-0.029; ΔRMSEA = 0.021. Finally, structural invariance, which constrained second-order factor loadings also produced a modest further degradation of model fit, but is also considered so extreme as to not be necessary. These results are sufficient to assert metric invariance.
Predictive validity. To further explore parental hesitancy, we examined whether VHS scores were related to gender, social media activity, and perceived financial well-being. All three variables, as predicted, were related to VHS. Because these variables were measured categorically, ANOVA was employed.
Gender was significantly related to VHS ( F (1. 6070) = 86.62, p < 0.001, η 2 = 0.014), with those identifying as female or “other” having more vaccine hesitancy (M = 34.37, SD = 6.37; M = 34.04, SD = 6.53) than those identifying as male (M = 32.22, SD = 7.08).
Social media activity was significantly related to VHS ( F (1. 5547) = 69.54, p < 0.001, η 2 = 0.012), with those indicating higher social media activity having more vaccine hesitancy (M = 34.89, SD = 5.86) than those indicating lower social media activity (M = 33.49, SD = 6.61).
Financial well-being was also modestly related to VHS ( F (1. 6070) = 42.52, p < 0.005, η 2 = 0.002), with those identifying as most affluent having more vaccine hesitancy (M = 34.37, SD = 6.46) than those with moderate (M = 33.94, SD = 6.49) or low affluence (M = 33.32, SD = 7.12).
Vaccines reduce the diseases’ mortality and severity; therefore, vaccine hesitancy impacts global public health. The current study aimed to psychometrically evaluate the Vaccine Hesitancy Scale (VHS) among parents in Australia, China, Iran, and Turkey.
The current study found that a brief measure of parental vaccine hesitancy, when appropriately translated, is able to be used in broadly diverse sociocultural contexts. The Vaccine Hesitancy Scale showed strong and desirable psychometric properties, including predicted factor structure, strong reliability, metric invariance across country, validity, and expected relationships to self-reported outcomes such as affluence, gender, and social media engagement. These results align with the original validation study conducted in Canada [ 25 ] and another validating the scale in Guatemala [ 26 ].
These samples from four different countries and cultures were not ideal- there were far fewer fathers than mothers in three of the four samples (i.e., 4.2% of respondents in Australia, 35% in China, 17.69% in Iran, and 53.9% in Turkey were fathers). However, this could be considered a strength as in many cultures, mothers have more decision-making responsibility for the health and welfare of children than fathers [ 41 ], and it was mothers who were found to have higher vaccine hesitancy. This finding is aligned with the health belief model stating that gender plays a strong role in determining vaccine acceptance [ 18 ]. Existing qualitative research revealed the mothers’ mixed feelings on vaccination (e.g., confusion from conflicting information) [ 42 ]. Mothers in Australia expressed guilt about failing to be a good mother [ 43 ]. Studies have indicated that Chinese mothers exhibit a greater vaccine hesitancy for their children than fathers, due to their concerns regarding vaccine safety and effectiveness. It has been mentioned that fathers generally have a higher tendency for risk behaviours than mothers, so they may be more willing to vaccinate their children [ 44 ].
Among four countries, the vaccine hesitancy score was lower in China. It should be noted that this differences are not statistically significant. In China, parents are less hesitant to vaccinate their children compared to countries like Iran, Turkey, and Australia. This can be attributed to several key factors. Firstly, China has a communication strategy that focuses on transparency and providing authoritative information about vaccines, which has helped build public trust in the vaccination process. Additionally, China’s rapid development and distribution of COVID-19 vaccines have ensured a consistent supply of safe and effective vaccines, contributing to lower rates of vaccine hesitancy. Cultural and social factors also play a significant role, as China’s collectivist culture emphasizes community health and well-being, influencing parents to prioritize vaccinating their children. The Chinese government has implemented policies like providing free vaccines and launching public awareness campaigns to promote vaccination, reducing hesitancy rates. Moreover, China’s success in controlling infectious diseases through previous vaccination programs has created a positive attitude towards vaccines, influencing parents’ decisions. Overall, effective communication, safe vaccine availability, cultural influences, government initiatives, and past vaccination success have all contributed to lower levels of vaccine hesitancy among parents in China compared to other countries [ 14 , 45 ].
Ancillary analyses observed age differences in vaccine hesitancy, but only in Australia, where parents between 40 and 60 years old were more vaccine hesitant than the other age groups ( p < 0.001, F = 8.10), supporting past research [ 46 ] indicating that younger parents were less likely to be hesitant to vaccinate their children. The reason behind this phenomenon might be that younger parents have less experience with infectious diseases (such as smallpox and poliomyelitis) and, perhaps it makes them less hesitant to vaccinate their children against diseases.
Regarding the current study, the reason why older parents were more hesitant than younger parents might be that during the conducting of this study, they may have older children who should be vaccinated against COVID-19; the vaccine that its side effects, or even its effectiveness were not clear in this age group. When national health systems started to vaccinate children against COVID-19 older children were included in the program, and then it was extended to children five years old and older. It has been indicated that new vaccines generate more hesitancy [ 47 ]. further research needs to be conducted (e.g., qualitative research) to find more details.
These findings also noted that more affluent individuals, and those with more social media engagement tended to be more hesitant to their children’s vaccination, which aligns with prior studies [ 14 , 48 , 49 ]. Some prior studies have suggested that parents who perceived more financial comfort believed that their lifestyle could protect them from diseases, and therefore, they were more hesitant to vaccinate their children [ 49 ]. The role of social media on vaccine hesitancy has been identified by previous studies. In this regard, parents may be confused by misinformation and fake news in the media and on social networks [ 50 ]; consequently, they experience fear, stress, and a wide range of behavioural changes [ 51 , 52 ]. Misinformation may make parents more cautious and force them to show their hesitancy with vaccines, especially new vaccines.
The current study indicated that lack of confidence in the vaccine and perceived vaccine risk contribute to parental vaccine hesitancy. According to the “3 Cs model” (confidence, complacency, and convenience) presented by the SAGE working group [ 53 ], lack of confidence in vaccine safety and effectiveness as well as low or mistrust of the systems that recommend or provide the vaccine can determine vaccine hesitancy. Furthermore, the model suggests that hesitancy may occur when parents do not value or perceive a need for vaccination (complacency) or when the vaccine is not accessible and available (convenience).
Study limitations
This study has several limitations. First, the non-probabilistic samples enrolled in the current study could restrict the generalizability of the findings. Although the sample enrolled in the current study was large, convenience sampling may underrepresent certain population groups. Because these data were gathered using an online survey, findings may not generalize to those without access to electronic devices or the internet.
Findings’ implications
This study supports broad use of this scale to evaluate parental vaccine hesitancy as part of an effort to understand and counteract resistance to adoption of vaccines in the general population. Applying this scale can provide valuable information for public health authorities to manage vaccine hesitancy among parents. The study indicated that women, those active on social media, and more affluent parents are more likely to resist having their children vaccinated, which can guide public health authorities in designing information campaigns to counteract these troubling trends. Healthcare providers can use this information to tailor their communication strategies to address the specific concerns of parents and increase vaccine uptake. Social media can play like a double-edged sword in parental vaccine hesitancy. Consequently, health policymakers are expected to do their best to provide authentic and accurate content that presents explicit information in the right way to the right audience.
Parental vaccine hesitancy is prevalent globally and associated with several individual and contextual factors. It is estimated that vaccine hesitancy will become a major burden on public health worldwide. Without validated instruments in specific countries and contexts, it is not possible to conduct reliable and valid research to investigate the factors and determinants of parental vaccine hesitancy. The present study validated the Vaccine Hesitancy Scale (VHS) among parents in Australia, China, Iran, and Turkey during the COVID-19 outbreak. Acceptable psychometric evidence was found for the 9-item two-factor VHS using data from parents in four countries. Findings from this study have implications for future research examining vaccine hesitancy and vaccine-preventable diseases and community health nurses. Further studies are needed to test the scale’s validity and reliability across additional cultural contexts.
Data availability
The data used to support the finding of this study are available from the corresponding author upon reasonable request.
Excler J-L, Saville M, Berkley S, Kim JH. Vaccine development for emerging infectious diseases. Nat Med. 2021;27(4):591–600.
Article CAS PubMed Google Scholar
Depar U. www.cdc.gov/coronavirus/vaccines. 2021.
Watson OJ, Barnsley G, Toor J, Hogan AB, Winskill P, Ghani AC. Global impact of the first year of COVID-19 vaccination: a mathematical modelling study. Lancet Infect Dis. 2022;22(9):1293–302.
Article CAS PubMed PubMed Central Google Scholar
Wang Q, Xiu S, Yang L, Han Y, Cui T, Shi N et al. Validation of the World Health Organization’s parental vaccine hesitancy scale in China using child vaccination data. 2022:1–7.
MacDonald NE. Vaccine hesitancy: definition, scope and determinants. J Vaccine. 2015;33(34):4161–4.
Article Google Scholar
Larson HJ, Jarrett C, Eckersberger E, Smith DMD, Paterson P. Understanding vaccine hesitancy around vaccines and vaccination from a global perspective: a systematic review of published literature, 2007–2012. Vaccine. 2014;32(19):2150–9.
Article PubMed Google Scholar
Loomba S, de Figueiredo A, Piatek SJ, de Graaf K, Larson HJ. Measuring the impact of COVID-19 vaccine misinformation on vaccination intent in the UK and USA. Nat Hum Behav. 2021;5(3):337–48.
Ahmed W, Vidal-Alaball J, Downing J, López Seguí F. COVID-19 and the 5G conspiracy theory: Social Network Analysis of Twitter Data. J Med Internet Res. 2020;22(5):e19458.
Article PubMed PubMed Central Google Scholar
Trent M, Seale H, Chughtai AA, Salmon D, MacIntyre CR. Trust in government, intention to vaccinate and COVID-19 vaccine hesitancy: a comparative survey of five large cities in the United States, United Kingdom, and Australia. Vaccine. 2022;40(17):2498–505.
Nguyen KH, Srivastav A, Lindley MC, Fisher A, Kim D, Greby SM, et al. Parental Vaccine Hesitancy and Association with Childhood Diphtheria, Tetanus Toxoid, and Acellular Pertussis; Measles, Mumps, and Rubella; Rotavirus; and combined 7-Series vaccination. J Am J Prev Med. 2022;62(3):367–76.
Mbaeyi S, Cohn A, Messonnier N. A call to action: strengthening vaccine confidence in the United States. J Pediatr. 2020;145(6).
Nguyen KH, Srivastav A, Lindley MC, Fisher A, Kim D, Greby SM et al. Parental Vaccine Hesitancy and Association with Childhood Diphtheria, Tetanus Toxoid, and Acellular Pertussis; Measles, Mumps, and Rubella; Rotavirus; and combined 7-Series vaccination. 2022;62(3):367–76.
Vrdelja M, Kraigher A, Verčič D, Kropivnik S. The growing vaccine hesitancy: exploring the influence of the internet. Eur J Pub Health. 2018;28(5):934–9.
Sharif Nia H, Allen K-A, Arslan G, Kaur H, She L, Khoshnavay Fomani F et al. The predictive role of parental attitudes toward COVID-19 vaccines and child vulnerability: a multi-country study on the relationship between parental vaccine hesitancy and financial well-being. Front Public Health. 2023;11.
Bramer CA, Kimmins LM, Swanson R, Kuo J, Vranesich P, Jacques-Carroll LA, et al. Decline in child vaccination coverage during the COVID-19 pandemic — Michigan Care Improvement Registry, May 2016-May 2020. Am J Transplant. 2020;20(7):1930–1.
Seyed Alinaghi S, Karimi A, Mojdeganlou H, Alilou S, Mirghaderi SP, Noori T, et al. Impact of COVID-19 pandemic on routine vaccination coverage of children and adolescents: a systematic review. Health Sci Rep. 2022;5(2):e00516.
Schuster M, Eskola J, Duclos PJV. Review of vaccine hesitancy: Rationale, remit and methods. 2015;33(34):4157–60.
Maiman LA, Becker MH. The Health Belief Model: origins and correlates in Psychological Theory. Health Educ Monogr. 1974;2(4):336–53.
Rippetoe PA, Rogers RW. Effects of components of protection-motivation theory on adaptive and maladaptive coping with a health threat. J Personal Soc Psychol. 1987;52(3):596.
Article CAS Google Scholar
Gilkey MB, Magnus BE, Reiter PL, McRee A-L, Dempsey AF, Brewer NT. The vaccination confidence scale: a brief measure of parents’ vaccination beliefs. Vaccine. 2014;32(47):6259–65.
Gilkey MB, Reiter PL, Magnus BE, McRee A-L, Dempsey AF, Brewer NT. Validation of the vaccination confidence scale: a brief measure to identify parents at risk for refusing adolescent vaccines. Acad Pediatr. 2016;16(1):42–9.
Opel DJ, Mangione-Smith R, Taylor JA, Korfiatis C, Wiese C, Catz S, et al. Development of a survey to identify vaccine-hesitant parents. Hum Vaccines. 2011;7(4):419–25.
Shapiro GK, Holding A, Perez S, Amsel R, Rosberger Z. Validation of the vaccine conspiracy beliefs scale. Papillomavirus Res. 2016;2:167–72.
Larson HJ, Jarrett C, Schulz WS, Chaudhuri M, Zhou Y, Dube E, et al. Measuring vaccine hesitancy: the development of a survey tool. Vaccine. 2015;33(34):4165–75.
Shapiro GK, Tatar O, Dube E, Amsel R, Knauper B, Naz A, et al. The vaccine hesitancy scale: psychometric properties and validation. Vaccine. 2018;36(5):660–7.
Domek GJ, O’Leary ST, Bull S, Bronsert M, Contreras-Roldan IL, Bolaños Ventura GA, et al. Measuring vaccine hesitancy: Field testing the WHO SAGE Working Group on Vaccine Hesitancy survey tool in Guatemala. Vaccine. 2018;36(35):5273–81.
Assunção H, Lin S-W, Sit P-S, Cheung K-C, Harju-Luukkainen H, Smith T, et al. University Student Engagement Inventory (USEI): Transcultural Validity evidence across four continents. Front Psychol. 2020;10:2796.
Beaton DE, Bombardier C, Guillemin F, Ferraz MB. Guidelines for the process of cross-cultural adaptation of self-report measures. Spine. 2000;25(24).
Finney SJ, DiStefano C. Non-normal and categorical data in structural equation modeling. Struct Equation Modeling: Second Course. 2006;10(6):269–314.
Google Scholar
Watkins MW. Exploratory factor analysis: a guide to best practice. J Black Psychol. 2018;44(3):219–46.
Marôco J. Análise de equações estruturais: Fundamentos teóricos, software & aplicações. ReportNumber, Lda; 2010.
She L, Ma L, Khoshnavay Fomani F. The consideration of Future consequences Scale among Malaysian young adults: a psychometric evaluation. Front Psychol. 2021;12.
Sharif Nia H, She L, Rasiah R, Pahlevan Sharif S, Hosseini L. Psychometrics of Persian Version of the Ageism Survey among an Iranian older Adult Population during COVID-19 pandemic. Front Public Health. 2021:1689.
Fornell C, Larcker DF. Evaluating Structural equation models with unobservable variables and measurement error. J Mark Res. 1981;18(1):39–50.
Henseler J, Ringle CM, Sarstedt M. A new criterion for assessing discriminant validity in variance-based structural equation modeling. J Acad Mark Sci. 2015;43(1):115–35.
Mayers A. Introduction to statistics and SPSS in psychology. Pearson Higher Ed; 2013.
Maroco J, Maroco AL, Campos JADB. Student’s academic efficacy or inefficacy? An example on how to evaluate the psychometric properties of a measuring instrument and evaluate the effects of item wording. Open Journal of Statistics. 2014;2014.
She L, Pahlevan Sharif S, Sharif Nia H. Psychometric evaluation of the Chinese Version of the modified online compulsive buying scale among Chinese young consumers. J Asia-Pac Bus. 2021;22(2):121–33.
Cheung GW, Rensvold RB. Evaluating goodness-of-fit indexes for Testing Measurement Invariance. Struct Equation Modeling: Multidisciplinary J. 2002;9(2):233–55.
Rutkowski L, Svetina D. Assessing the hypothesis of measurement invariance in the context of large-scale international surveys. Educ Psychol Meas. 2014;74(1):31–57.
Horiuchi S, Sakamoto H, Abe SK, Shinohara R, Kushima M, Otawa S, et al. Factors of parental COVID-19 vaccine hesitancy: a cross sectional study in Japan. PLoS ONE. 2021;16(12):e0261121.
Walker KK, Head KJ, Owens H, Zimet GD. A qualitative study exploring the relationship between mothers’ vaccine hesitancy and health beliefs with COVID-19 vaccination intention and prevention during the early pandemic months. Hum Vaccines Immunotherapeutics. 2021;17(10):3355–64.
Schuster L, Gurrieri L, Dootson P. Emotions of burden, intensive mothering and COVID-19 vaccine hesitancy. Crit Public Health. 2022:1–12.
Zheng M, Zhong W, Chen X, Wang N, Liu Y, Zhang Q, et al. Factors influencing parents’ willingness to vaccinate their preschool children against COVID-19: results from the mixed-method study in China. Human Vaccines & Immunotherapeutics; 2022. p. 2090776.
Huang Y, Su X, Xiao W, Wang H, Si M, Wang W, et al. COVID-19 vaccine hesitancy among different population groups in China: a national multicenter online survey. BMC Infect Dis. 2022;22(1):153.
Facciolà A, Visalli G, Orlando A, Bertuccio MP, Spataro P, Squeri R et al. Vaccine Hesitancy: An Overview on Parents’ Opinions about Vaccination and Possible Reasons of Vaccine Refusal. Journal of Public Health Research. 2019;8(1):jphr.2019.1436.
Dubé E, Laberge C, Guay M, Bramadat P, Roy R, Bettinger J. Vaccine hesitancy: an overview. Hum Vaccin Immunother. 2013;9(8):1763–73.
Simas C, Larson HJ. Overcoming vaccine hesitancy in low-income and middle-income regions. Nat Reviews Disease Primers. 2021;7(1):41.
Swaney SE, Burns S. Exploring reasons for vaccine-hesitancy among higher-SES parents in Perth, Western Australia. Health Promotion J Australia. 2019;30(2):143–52.
Ceron W, de-Lima-Santos M-F, Quiles MG. Fake news agenda in the era of COVID-19: identifying trends through fact-checking content. Online Social Networks Media. 2021;21:100116.
Nikčević AV, Spada MM. The COVID-19 anxiety syndrome scale: development and psychometric properties. Psychiatry Res. 2020;292:113322.
Sharif Nia H, She L, Kaur H, Boyle C, Khoshnavay Fomani F, Hoseinzadeh E, et al. A predictive study between anxiety and fear of COVID-19 with psychological behavior response: the mediation role of perceived stress. Front Psychiatry. 2022;13:851212.
MacDonald NE. Vaccine hesitancy: definition, scope and determinants. Vaccine. 2015;33(34):4161–4.
Download references
Author information
Authors and affiliations.
Psychosomatic Research Center, Mazandaran University of Medical Sciences, Sari, Iran
Hamid Sharif-Nia
Department of Nursing, Amol School of Nursing and Midwifery, Mazandaran University of Medical Sciences, Sari, Iran
Sunway Business School, Sunway University, Sunway City, Malaysia
School of Educational Psychology and Counselling, Faculty of Education, Monash University, Clayton, Australia
Kelly-Ann Allen
William James Centre for Research ISPA – Instituto Universitário, Lisboa, Portugal
João Marôco
Business School, Taylor’s University Lakeside Campus, Subang Jaya, Malaysia
Harpaljit Kaur
Department of Psychological Counseling, Burdur Mehmet Akif Ersoy University, Burdur, Turkey
Gökmen Arslan
Department of Biostatistics and Medical Informatics, Faculty of Medicine, Kırşehir Ahi Evran University, Kırşehir, Turkey
Ozkan Gorgulu
Department of Statistics, Miami University, Oxford, OH, USA
Jason W. Osborne
School of Nursing, Alborz University of Medical Sciences, Karaj, Iran
Pardis Rahmatpour
Nursing and Midwifery Care Research Center, School of Nursing and Midwifery, Tehran University of Medical Sciences, Tehran, Iran
Fatemeh Khoshnavay Fomani
Centre for Wellbeing Science, Faculty of Education, University of Melbourne, Parkville, Australia
You can also search for this author in PubMed Google Scholar
Contributions
Study conception and design: HS, K-AA and FK. Data collection: FK, HS, K-AA, LS, and OG. Analysis and interpretation of results: GA, JM, JO, LS, and HS. Draft manuscript preparation and or substantively revised it: FK, HK, K-AA, HS, and JO. All authors reviewed the results and approved the final version of the manuscript.
Corresponding author
Correspondence to Fatemeh Khoshnavay Fomani .
Ethics declarations
Consent for publication.
Not Applicable.
Competing interests
The authors declare no competing interests.
Conflict of interest
The authors report no conflicts of interest in this work.
Ethics statement
The protocol of this study was approved by the Mazandaran University of Medical Sciences Research Ethics Committee (IR.MAZUMS.REC.1400.13876). Informed consent was obtained from all participants. Informed consent was obtained from all participants. All methods were carried out in accordance with relevant guidelines and regulation under the Ethics approval and consent to participate.
Acknowledgements
We thank all the participants who took part in the study.
Additional information
Publisher’s note.
Springer Nature remains neutral with regard to jurisdictional claims in published maps and institutional affiliations.
Rights and permissions
Open Access This article is licensed under a Creative Commons Attribution 4.0 International License, which permits use, sharing, adaptation, distribution and reproduction in any medium or format, as long as you give appropriate credit to the original author(s) and the source, provide a link to the Creative Commons licence, and indicate if changes were made. The images or other third party material in this article are included in the article’s Creative Commons licence, unless indicated otherwise in a credit line to the material. If material is not included in the article’s Creative Commons licence and your intended use is not permitted by statutory regulation or exceeds the permitted use, you will need to obtain permission directly from the copyright holder. To view a copy of this licence, visit http://creativecommons.org/licenses/by/4.0/ . The Creative Commons Public Domain Dedication waiver ( http://creativecommons.org/publicdomain/zero/1.0/ ) applies to the data made available in this article, unless otherwise stated in a credit line to the data.
Reprints and permissions
About this article
Cite this article.
Sharif-Nia, H., She, L., Allen, KA. et al. Parental hesitancy toward children vaccination: a multi-country psychometric and predictive study. BMC Public Health 24 , 1348 (2024). https://doi.org/10.1186/s12889-024-18806-1
Download citation
Received : 23 October 2023
Accepted : 09 May 2024
Published : 18 May 2024
DOI : https://doi.org/10.1186/s12889-024-18806-1
Share this article
Anyone you share the following link with will be able to read this content:
Sorry, a shareable link is not currently available for this article.
Provided by the Springer Nature SharedIt content-sharing initiative
- Vaccine hesitancy
- Psychometric
BMC Public Health
ISSN: 1471-2458
- Submission enquiries: [email protected]
- General enquiries: [email protected]
- Open access
- Published: 18 May 2024
Psychometric properties and criterion related validity of the Norwegian version of hospital survey on patient safety culture 2.0
- Espen Olsen 1 ,
- Seth Ayisi Junior Addo 1 ,
- Susanne Sørensen Hernes 2 , 3 ,
- Marit Halonen Christiansen 4 ,
- Arvid Steinar Haugen 5 , 6 &
- Ann-Chatrin Linqvist Leonardsen 7 , 8
BMC Health Services Research volume 24 , Article number: 642 ( 2024 ) Cite this article
1 Altmetric
Metrics details
Several studies have been conducted with the 1.0 version of the Hospital Survey on Patient Safety Culture (HSOPSC) in Norway and globally. The 2.0 version has not been translated and tested in Norwegian hospital settings. This study aims to 1) assess the psychometrics of the Norwegian version (N-HSOPSC 2.0), and 2) assess the criterion validity of the N-HSOPSC 2.0, adding two more outcomes, namely ‘pleasure of work’ and ‘turnover intention’.
The HSOPSC 2.0 was translated using a sequential translation process. A convenience sample was used, inviting hospital staff from two hospitals ( N = 1002) to participate in a cross-sectional questionnaire study. Data were analyzed using Mplus. The construct validity was tested with confirmatory factor analysis (CFA). Convergent validity was tested using Average Variance Explained (AVE), and internal consistency was tested with composite reliability (CR) and Cronbach’s alpha. Criterion related validity was tested with multiple linear regression.
The overall statistical results using the N-HSOPSC 2.0 indicate that the model fit based on CFA was acceptable. Five of the N-HSOPSC 2.0 dimensions had AVE scores below the 0.5 criterium. The CR criterium was meet on all dimensions except Teamwork (0.61). However, Teamwork was one of the most important and significant predictors of the outcomes. Regression models explained most variance related to patient safety rating (adjusted R 2 = 0.38), followed by ‘turnover intention’ (adjusted R 2 = 0.22), ‘pleasure at work’ (adjusted R 2 = 0.14), and lastly, ‘number of reported events’ (adjusted R 2= 0.06).
The N-HSOPSC 2.0 had acceptable construct validity and internal consistency when translated to Norwegian and tested among Norwegian staff in two hospitals. Hence, the instrument is appropriate for use in Norwegian hospital settings. The ten dimensions predicted most variance related to ‘overall patient safety’, and less related to ‘number of reported events’. In addition, the safety culture dimensions predicted ‘pleasure at work’ and ‘turnover intention’, which is not part of the original instrument.
Peer Review reports
Patient harm due to unsafe care is a large and persistent global public health challenge and one of the leading causes of death and disability worldwide [ 1 ]. Improving safety in healthcare is central in governmental policies, though progress in delivering this has been modest [ 2 ]. Patient safety culture surveys have been the most frequently used approach to measure and monitor perception of safety culture [ 3 ]. Safety culture is defined as “the product of individual and group values, attitudes, perceptions, competencies and patterns of behavior that determine the commitment to, and the style and proficiency of, an organization’s health and safety management” [ 4 ]. Moreover, safety culture refers to the perceptions, beliefs, values, attitudes, and competencies within an organization pertaining to safety and prevention of harm [ 5 ]. The importance of measuring patient safety culture was underlined by the results in a 2023 scoping review, where 76 percent of the included studies observed associations between improved safety culture and reduction of adverse events [ 6 ].
To assess patient safety culture in hospitals the US Agency for Healthcare Research and Quality (AHRQ) launched the Hospital Survey on Patient Safety Culture (HSOPSC) version 1.0 in 2004 [ 7 , 8 ]. Since then, HSOPSC 1.0 has become one of the most used tools to evaluate patient safety culture in hospitals, administered to approximately hundred countries and translated into 43 languages as of September 2022 [ 9 ]. HSOPSC 1.0 has generally been considered to be one of the most robust instrument measuring patient safety culture, and it has adequate psychometric properties [ 10 ]. In Norway, the first studies using N-HSOPSC 1.0 concluded that the psychometric properties of the instrument were satisfactory for use in Norwegian hospital settings [ 11 , 12 , 13 ]. A recent review of literature revealed 20 research articles using the N-HSOPSC 1.0 [ 14 ].
Studies of safety culture perceptions in hospitals require valid and psychometric sound instruments [ 12 , 13 , 15 ]. First, an accurate questionnaire structure should demonstrate a match between the theorized content structure and the actual content structure [ 16 , 17 ]. Second, psychometric properties of instruments developed in one context is required to demonstrate appropriateness in other cultures and settings [ 16 , 17 ]. Further, psychometric concepts need to demonstrate relationships with other related and valid criteria. For example, data on criterion validity can be compared with criteria data collected at the same time (concurrent validity) or with similar data from a later time point (predictive validity) [ 12 , 16 , 17 ]. Finally, researchers need to demonstrate a match between the content theorized to be related to the actual content in empirical data [ 15 ]. If these psychometric areas are not taken seriously, this may lead to many pitfalls both for researchers and practitioners [ 14 ]. Pitfalls might be imprecise diagnostics of the patient safety level and failure to evaluate effect of improvement initiative. Moreover, researchers can easily erroneously confirm or reject research hypothesis when applying invalid and inaccurate measurement tools.
Patient safety cannot be understood as an isolated phenomenon, but is influenced by general job characteristics and the well-being of the individual health care workers. Karsh et al. [ 18 ] found that positive staff perceptions of their work environment and low work pressure were significantly related to greater job satisfaction and work commitment. A direct association has also been reported between turnover and work strain, burnout and stress [ 19 ] Zarei et al. [ 20 ] showed a significant relationship between patient safety (safety climate) and unit type, job satisfaction, job interest, and stress in hospitals. This study also illustrated a strong relationship between lack of personal accomplishment, job satisfaction, job interest and stress. Also, there was a negative correlation between occupational burnout and safety climate, where a decrease in the latter was associated with an increase in the former. Hence, patient safety researchers should look at healthcare job characteristics in combination with patient safety culture.
Recently, the AHRQ revised the HSOPSC 1.0 to a 2.0 version, to improve the quality and relevance of the instrument. HSOPSC 2.0 is shorter, with 25 items removed or with changes made for response options and ten additional items added. HSOPSC 2.0 was validated during the revision process [ 21 ], but the psychometric qualities across cultures, countries and in different settings need further investigation. Consequently, the overall aim of this study was to investigate the psychometric properties of the HSOPSC 2.0 [ 21 ] (see supplement 1) in a Norwegian hospital setting. Specifically, the aims were to 1) assess the psychometrics of the Norwegian version (N-HSOPSC 2.0), and 2) assess the criterion validity of the N-HSOPSC 2.0, adding two more outcomes, namely’ pleasure of work’ and ‘turnover intention’.
This study had cross‐sectional design, using a web-based survey solution called “Nettskjema” to distribute questionnaires in two Norwegian hospitals. The study adheres to The Strengthening the Reporting of Observational Studies in Epidemiology (STROBE)Statement guidelines for reporting observational studies [ 22 ].
Translation of the HSOPSC 2.0
We conducted a «forward and backward» translation in-line with recommendations from Brislin [ 23 ]. First, the questionnaires were translated from English to Norwegian by a bilingual researcher. The Norwegian version was then translated back to English by another bilingual researcher. Thereafter, the semantic, idiopathic and conceptual equivalence between the two versions were compared by the research group, consisting of experienced researchers. The face value of the N-HSOPSC 2.0-version was considered to be adequate and the items lend themselves well to the corresponding latent concepts.
The N-HSOPSC 2.0 was pilot-tested with focus on content and face validity. Six randomly selected healthcare personnel were asked to assess whether the questionnaire was adequate, appropriate, and understandable regarding language, instructions, and scores. In addition, an expert group consisting of senior researchers ( n = 4) and healthcare personnel ( n = 6), with competence in patient safety culture was asked to assess the same.
The questionnaire
The HSOSPS 2.0 (supplement 1) consists of 32 items using 5-point Likert-like scales of agreement (from 1 = strongly disagree to 5 = strongly agree) or frequency (from 1 = never to 5 = always), as well as an option for “does not apply/do now know”. The 32 items are distributed over ten dimensions. Additionally, 2-single item patient safety culture outcome measures, and 6-item background information measures are included. The patient safety culture single item outcome measures evaluate the overall ‘patient safety rating’ for the work area, and ‘reporting patient safety events’.
In addition to the N-HSOPSC 2.0, participants were asked to respond to three questions about their ‘pleasure at work’ (measure if staff enjoy their work, and are pleased with their work, scored from 1 = never, to 4 = always) [ 24 ], two questions about their ‘intention to quit’ (measure is staff are considering to quit their job, scored on a 5-point likert scale where 1 = strongly agree to 5 = strongly disagree) [ 25 ], as well as demographic variables (gender, age, professional background, primary work area, years of work experience).
Participants and procedure
The data collection was conducted in two phases: the first phase (Nov-Dec 2021) at Hospital A and the second phase at Hospital B (Feb-March 2022)). We used a purposive sampling strategy: At Hospital A (two locations), all employees were invited to participate ( N = 6648). This included clinical staff, administrators, managers, and technical staff. At Hospital B (three locations) all employees from the anesthesiology, intensive care and operation wards were invited to participate ( N = 655).
The questionnaire was distributed by e-mail, including a link to a digital survey solution delivered by the University of Oslo, and gathered and stored on a safe research platform: TSD (services for sensitive data). This is a service with two-factor authentication, allowing data-sharing between the collaborating institutions without having to transfer data between them. The system allows for storage of indirectly identifying data, such as gender, age, profession and years of experience, as well as hospital. Reminders were sent out twice.
Statistical analyses
Data were analyzed using Mplus. Normality was assessed for each item using skewness and kurtosis, where values between + 2 and -2 are deemed acceptable for normal distribution [ 26 ]. Missing value analysis was conducted using frequencies, to check the percentage of missing responses for each item. Correlations were assessed using Spearman’s correlation analysis, reported as Cronbach’s alpha.
Confirmatory factor analysis (CFA) was conducted to test the ten-dimension structure of the N-HSOPSC 2.0 using Mplus and Mplus Microsoft Excel Macros. The structure was then tested for fitness using Comparative Fit Index (CFI), Tucker-Lewis Index (TLI), Root Mean Square Error of Approximation (RMSEA) and Standardized Root Mean Square Residual (SRMR) [ 27 ]. Table 1 shows the fitness indices and acceptable thresholds.
Reliability of the 10 predicting dimensions were also assessed using composite reliability (CR) values, where 0.7 or above is deemed acceptable for ascertaining internal consistency [ 25 ].
Convergent validity was assessed using the Average Variance Explained (AVE), where a value of at least 0.5 is deemed acceptable [ 28 ], indicating that at least 50 percent of the variance is explained by the items in a dimension. Criterion-related validity was tested using linear regression, adding ‘turnover intention’ and ‘pleasure at work’ to the two single item outcomes of the N-HSOPSC 2.0.
Internal consistency and reliability were assessed using Cronbach’s alpha, where values > 0.9 is assumed excellent, > 0.8 = good, > 0.7 = acceptable, > 0.6 = questionable, > 0.5 = poor and < 0.5 = unacceptable [ 29 ].
Ethical considerations
The study was conducted in-line with principles for ethical research in the Declaration of Helsinki, and informed consent was obtained from all the participants [ 30 ]. Completed and submitted questionnaires were assumed as consent to participate. Data privacy protection was reviewed by the respective hospitals’ data privacy authority, and assessed by the Norwegian Center for Research Data (NSD, project number 322965).
In total, 1002 participants responded to the questionnaire, representing a response rate of 12.6 percent. As seen in Table 2 , 83.7% of the respondents worked in Hospital A and the remaining 16.3% in Hospital B. The majority of respondents (75.7%) were female, and 75.9 percent of respondents worked directly with patients.
The skewness and kurtosis were between + 2 and -2, indicating that the data were normally distributed. All items had less than two percent of missing values, hence no methods for calculating missing values were used.
Correlations
Correlations and Cronbach’s alpha are displayed in Table 3 .
The following dimensions had the highest correlations; ‘teamwork’, ‘staffing and work pace’, ‘organizational learning-continuous improvement’, ‘response to error’, ‘supervisor support for patient safety’, ‘communication about error’ and ‘communication openness’. Only one dimension, ‘teamwork’ (0.58), had a Cronbach’s alpha below 0.7 (acceptable). Hence, most of the dimensions indicated adequate reliability. Higher levels of the 10 safety dimensions correlate positively with patient safety ratings.
Confirmatory Factor Analysis (CFA)
Table 4 shows the results from the CFA. CFA ( N = 1002) showed acceptable fitness values [CFI = 0.92, TLI = 0.90, RMSEA = 0.045, SRMR = 0.053] and factor loadings ranged from 0.51–0.89 (see Table 1 ). CR was above the 0.70 criterium on all dimensions except on ‘teamwork’ (0.61). AVE was above the 0.50 criterium except on ‘teamwork’ (0.35), ‘staffing and work pace’ (0.44), ‘organizational learning-continuous improvement’ (0.47), ‘response to error’ (0.47), and communication openness.
Criterion validity
Independent dimensions of HSOPSC 2.0 were employed to predict four different criteria: 1) ‘number of reported events’, 2) ‘patient safety rating’, 3) ‘pleasure at work’, and 4) ‘turnover intentions’. The composite measures explained variance of all the outcome variables significantly thereby ascertaining criterion-related validity (Table 5 ). Regression models explained most variance related to ‘patient safety rating’ (adjusted R 2 = 0.38), followed by ‘turnover intention’ (adjusted R 2 = 0.22), ‘pleasure at work’ (adjusted R 2 = 0.14), and lastly, number of reported events (adjusted R 2 = 0.06).
In this study we have investigated the psychometric properties of the N-HSOPSC 2.0. We found the face and content validity of the questionnaire satisfactory. Moreover, the overall statistical results indicate that the model fit based on CFA was acceptable. Five of the N-HSOPSC 2.0 dimensions had AVE scores below the 0.5 criterium, but we consider this to be the strictest criterium employed in the evaluations of the psychometric properties. The CR criterium was met on all dimensions except ‘teamwork’ (0.61). However, ‘teamwork’ was one of the most important and significant predictors of the outcomes. One the positive side, the CFA results supports the dimensional structure of N-HSOPSC 2.0, and the regression results indicate a satisfactory explanation of the outcomes. On the more critical side, particularly AVE scores reflect threshold below 0.5 on five dimensions, indicating items have certain levels of measurement error as well.
In our study, regression models explained most variance related to ‘patient safety rating’ (R 2 = 0.38), followed by ‘turnover intention’ (R 2 = 0. 22), ‘pleasure at work’ (R 2 = 0.14), and lastly, number of reported events (R 2 = 0.06). This supports the criterion validity of the independent dimensions of N-HSOSPC 2.0, also when adding ‘turnover intention’ and ‘pleasure at work’. These results confirm previous research on the original N-HSOPSC 1.0 [ 12 , 13 ]. The current study also found that ‘number of reported events’ was negatively related to safety culture dimensions, which is also similar to the N-HSOPSC 1.0 findings [ 12 , 13 ].
The current study did more psychometric assessments compared to the first Norwegian studies using HSOPSC 1.0 [ 11 , 12 , 13 ]. However, results from the current study still support that the overall reliability and validity of N-HSOPSC 2.0 when comparing the results with the first studies using N-HSOPSC 1.0 [ 11 , 12 , 13 ]. Also, based on theory and expectations, the dimensions predicted ‘pleasure at work’ and ‘overall safety rating’ positively, and ‘turnover intentions’ and ‘number of reported events’ negatively. The directions of the relations thereby support the overall criterion validity. Some of the dimensions do not predict the outcome variables significantly, nonetheless, each criterion related significantly to at least two dimensions on the HSOPSC 2.0. It is also worth noticing that ‘teamwork’ was generally one of the most important predictors even thought this dimension had the lowest convergent validity (AVE) in the previous findings [ 11 , 12 , 13 ], even if the strict AVE criterium was not satisfactory on the teamwork dimension and CR was also below 0.7. Since the explanatory power of teamwork was satisfactory, this illustrate that the AVE and CR criteria are maybe too strict.
The sample in the current study consisted of 1009 employees at two different hospital trusts in Norway and across different professions. The gender and ages are representative for Norwegian health care workers. In total 760 workers had direct patient contact, 167 had not, and 74 had patient contact sometimes. We think this mix is interesting, since a system perspective is key to establishing patient safety [ 31 ]. The other background variables (work experience, age, primary work area, and gender) indicate a satisfactory spread and mix of personnel in the sample, which is an advantage since then the sample to a large extend represent typical healthcare settings in Norway.
In the current study, N-HSOPSC 2.0 had higher levels of Cronbach’s alpha than in the first N-HSOPSC 1.0 studies [ 11 , 13 ], but more in-line with results from a longitudinal Norwegian study using the N-HSOPSC 1.0 in 2009, 2010 and 2017 respectively [ 23 ]. Moreover, the estimates in the current study reveal a higher level of factor loading on the N-HSOPSC 2.0, ranging from 0.51 to 0.89. This is positive since CFA is a key method when assessing the construct validity [ 16 , 17 , 32 ].
AVE and CR were not estimated in the first Norwegian HSOPSC 1.0 studies [ 11 , 13 ]. The results in this study indicate some issues regarding particularly AVE (convergent validity) since five of the concepts were below the recommended 0.50 threshold [ 32 ]. It is also worth noticing that all measures in the N-HSOPSC 2.0, except ‘teamwork’ (CR = 61), had CR values above 0.70, which is satisfactory. AVE is considered a strict and more conservative measure than CR. The validity of a construct may be adequate even though more than 50% of the variance is due to error [ 33 ]. Hence, some AVE values below 0.50 is not considered critical since the overall results are generally satisfactory.
The first estimate of the criterion related validity of the N-HSOPSC 2.0 using multiple regression indicated that two dimensions where significantly related to ‘number of reported events’, while six dimensions were significantly related to ‘patient safety rating’. The coefficients were negatively related with number of reported events, and positively related with patient safety rating, as expected. In the first Norwegian study in Norway on the N-HSOPSC 1.0 [ 13 ], five dimensions were significantly related to ‘number of reported events’, and seven dimensions were significantly related to ‘patient safety ratings’. The relations with ‘numbers of events reported’ were then both positive and negative, which is not optimal when assessing criterion validity. Hence, since all significant estimates are in the expected directions, the criterion validity of N-HSOPSC 2.0 has generally improved compared to the previous version.
In the current study we added ‘pleasure at work’ and ‘turnover intention’ to extend the assessment of criterion related validity. The first assessment indicated that ‘teamwork’ had a very substantial and positive influence on ‘pleasure at work’. Moreover, ‘staffing and work pace’ also had a positive influence on ‘pleasure at work’, but none of the other concepts were significant predictors. Hence, the teamwork dimension is key in driving ‘pleasure at work’, then followed by ‘staffing and working pace’. ‘Turnover intentions’ was significantly and negatively related to ‘teamwork’, ‘staffing and working pace’, ‘response to error’ and ‘hospital management support’. Hence, the results indicate these dimensions are key drivers in avoiding turnover intentions among staff in hospitals. A direct association has been reported between turnover and work strain, burnout and stress [ 19 ]. Zarei et al. [ 20 ] showed a significant relationship between patient safety (safety climate) and unit type, job satisfaction, job interest, and stress in hospitals. This study also illustrated a strong relationship between lack of personal accomplishment, job satisfaction, job interest and stress. Furthermore, a negative correlation between occupational burnout and safety climate was discovered, where a decrease in the latter is associated with an increase in the former [ 20 ]. Hence, patient safety researchers should look at health care job characteristics in combination with patient safety culture.
Assessment of psychometrics must consider other issues beyond statistical assessments such as theoretical consideration and face validity [ 16 , 17 ]; we believe one of the strengths of the HSOPSC 1.0 is that the instrument was operationalized based on theoretical concepts. This has been a strength, as opposed to other instruments built on EFA and a random selection of items included in the development process. We believe this is also the case in relation to HSOPSC 2.0; the instrument is theoretically based, easy to understand, and most importantly, can function as a tool to improve patient safety in hospitals. Moreover, when assessing the items that belongs to the different latent constructs, item-dimension relationships indicate a high face validity.
Forthcoming studies should consider predicting other outcomes, such as for instance mortality, morbidity, length of stay and readmissions, with the use of N-HSOPSC 2.0.
Limitations
This study is conducted in two Norwegian public hospital trusts, indicating some limitations about generalizability. The response rate within hospitals was low and therefore we could not benchmark subgroups. However, this was not part of the study objectives. The response rate may be hampered by the pandemic workload, and high workload in the hospitals. However, based on the diversity of the sample, we find the study results robust and adequate to explore the psychometric properties of N-HSOPSC 2.0. For the current study, we did not perform sample size calculations. With over 1000 respondents, we consider the sample size adequate to assess psychometric properties. Moreover, the low level of missing responses indicate N-HSOPSC 2.0 was relevant for the staff included in the study.
There are many alternative ways of exploring psychometric capabilities of instruments. For example, we did not investigate alternative factorial structures, e.g. including hierarchical factorial models or try to reduce the factorial structure which has been done with N-HSOPSC 1.0 short [ 34 ]. Lastly, we did not try to predict patient safety indicators over time using a longitudinal design and other objective patient safety indicators.
The results from this study generally support the validity and reliability of the N-HSOPSC 2.0. Hence, we recommend that the N-HSOPSC 2.0 can be applied without any further adjustments. However, future studies should potentially develop structural models to strengthen the knowledge and relationship between the factors included in the N-HSOPSC 2.0/ HSOPSC 2.0. Both improvement initiatives and future research projects can consider including the ‘pleasure at work’ and ‘turnover intentions’ indicators, since N-HSOPSC 2.0 explain a substantial level of variance relating to these criteria. This result also indicates an overlap between general pleasure at work and patient safety culture which is important when trying to improve patient safety.
Availability of data and materials
Datasets generated and/or analyzed during the current study are not publicly available due to local ownership of data, but aggregated data are available from the corresponding author on reasonable request.
World Health Organization. Global patient safety action plan 2021–2030: towards eliminating avoidable harm in health care. 2021. https://www.who.int/teams/integrated-health-services/patient-safety/policy/global-patient-safety-action-plan .
Rafter N, Hickey A, Conroy RM, Condell S, O’Connor P, Vaughan D, Walsh G, Williams DJ. The Irish National Adverse Events Study (INAES): the frequency and nature of adverse events in Irish hospitals—a retrospective record review study. BMJ Qual Saf. 2017;26(2):111–9.
Article PubMed Google Scholar
O’Connor P, O’Malley R, Kaud Y, Pierre ES, Dunne R, Byrne D, Lydon S. A scoping review of patient safety research carried out in the Republic of Ireland. Irish J Med. 2022;192:1–9.
Google Scholar
Halligan M, Zecevic A. Safety culture in healthcare: a review of concepts, dimensions, measures and progress. BMJ Qual Saf. 2011;20(4):338–43.
Weaver SJ, Lubomksi LH, Wilson RF, Pfoh ER, Martinez KA, Dy SM. Promoting a culture of safety as a patient safety strategy: a systematic review. Ann Intern Med. 2013;158(5):369–74.
Article PubMed PubMed Central Google Scholar
Vikan M, Haugen AS, Bjørnnes AK, Valeberg BT, Deilkås ECT, Danielsen SO. The association between patient safety culture and adverse events – a scoping review. BMC Health Serv Res 2023;300. https://doi.org/10.1186/s12913-023-09332-8 .
Sorra J, Nieva V. Hospital survey on patient safety culture. AHRQ publication no. 04–0041. Rockville: Agency for Healthcare Research and Quality; 2004.
Nieva VF, Sorra J. Safety culture assessment: a tool for improving patient safety in healthcare organizations. Qual Saf Health Car. 2003;12:II17–23.
Agency for Healthcare Research and Quality (AHQR). International use of SOPS. https://www.ahrq.gov/sops/international/index.html .
Flin R, Burns C, Mearns K, Yule S, Robertson E. Measuring safety climate in health care. Qual Saf Health Care. 2006;15(2):109–15.
Article CAS PubMed PubMed Central Google Scholar
Olsen E, Aase K. The challenge of improving safety culture in hospitals: a longitudinal study using hospital survey on patient safety culture. International Probabilistic Safety Assessment and Management Conference and the Annual European Safety and Reliability Conference. 2012;2012:25–9.
Olsen E. Safety climate and safety culture in health care and the petroleum industry: psychometric quality, longitudinal change, and structural models. PhD thesis number 74. University of Stavanger; 2009.
Olsen E. Reliability and validity of the Hospital Survey on Patient Safety Culture at a Norwegian hospital. Quality and safety improvement research: methods and research practice from the International Quality Improvement Research Network (QIRN) 2008:173–186.
Olsen E, Leonardsen ACL. Use of the Hospital Survey of Patient Safety Culture in Norwegian Hospitals: A Systematic Review. Int J Environment Res Public Health. 2021;18(12):6518.
Article Google Scholar
Hughes DJ. Psychometric validity: Establishing the accuracy and appropriateness of psychometric measures. The Wiley handbook of psychometric testing: A multidisciplinary reference on survey, scale and test development; 2018:751–779.
DeVillis RF. Scale development: Theory and application. Thousands Oaks: Sage Publications; 2003.
Netemeyer RG, Bearden WO, Sharma S. Scaling procedures: Issues and application. London: SAGE Publications Ltd; 2003.
Book Google Scholar
Karsh B, Booske BC, Sainfort F. Job and organizational determinants of nursing home employee commitment, job satisfaction and intent to turnover. Ergonomics. 2005;48:1260–81. https://doi.org/10.1080/00140130500197195 .
Article CAS PubMed Google Scholar
Hayes L, O’Brien-Pallas L, Duffield C, Shamian J, Buchan J, Hughes F, Spence Laschinger H, North N, Stone P. Nurse turnover: a literature review. Int J Nurs Stud. 2006;43:237–63.
Zarei E, Najafi M, Rajaee R, Shamseddini A. Determinants of job motivation among frontline employees at hospitals in Teheran. Electronic Physician. 2016;8:2249–54.
Agency for Healthcare Research and Quality (AHQR). Hospital Survey on Patient Safety Culture. https://www.ahrq.gov/sops/surveys/hospital/index.html .
von Elm E, Altman DG, Egger M, Pocock SJ, Gotzsche PC, Vandenbroucke JP. The Strengthening the Reporting of Observational Studies in Epidemiology (STROBE) Statement: guidelines for reporting observational studies. BMJ. 2007;335(7624):806–8.
Brislin R. Back translation for cross-sectional research. J Cross-Cultural Psychol. 1970;1(3):185–216.
Notelaers G, De Witte H, Van Veldhoven M, Vermunt JK. Construction and validation of the short inventory to monitor psychosocial hazards. Médecine du Travail et Ergonomie. 2007;44(1/4):11.
Bentein K, Vandenberghe C, Vandenberg R, Stinglhamber F. The role of change in the relationship between commitment and turnover: a latent growth modeling approach. J Appl Psychol. 2005;90(3):468.
Tabachnick B, Fidell L. Using multivariate statistics. 6th ed. Boston: Pearson; 2013.
Hu L, Bentler P. Cutoff criteria for fit indexes in covariance structure analysis: Conventional criteria versus new alternatives. Structural Equation Modelling. 1999;6(1):1–55.
Hair J, Sarstedt M, Hopkins L, Kuppelwieser V. Partial least squares structural equation modeling (PLS-SEM): An emerging tool in business research. Eur Business Rev. 2014;26:106–21.
George D, Mallery P. SPSS for Windows step by step: A simple guide and reference. 11.0 update. Boston: Allyn & Bacon; 2003.
World Medical Association. Declaration of Helsinki- Ethical Principles for Medical Research Involving Human Subjects. 2018. http://www.wma.net/en/30publications/10policies/b3 .
Farup PG. Are measurements of patient safety culture and adverse events valid and reliable? Results from a cross sectional study. BMC Health Serv Res. 2015;15(1):1–7.
Hair JF, Black WC, Babin BJ, Anderson RE. Applications of SEM. Multivariate data analysis. Upper Saddle River: Pearson; 2010.
Malhotra NK, Dash S. Marketing research an applied orientation (paperback). London: Pearson Publishing; 2011.
Olsen E, Aase K. A comparative study of safety climate differences in healthcare and the petroleum industry. Qual Saf Health Care. 2010;19(3):i75–9.
Download references
Acknowledgements
Master student Linda Eikemo is acknowledged for participating in the data collection in Hospital A, and Nina Føreland in Hospital B.
Not applicable.
Author information
Authors and affiliations.
UiS Business School, Department of Innovation, Management and Marketing, University of Stavanger, Stavanger, Norway
Espen Olsen & Seth Ayisi Junior Addo
Hospital of Southern Norway, Flekkefjord, Norway
Susanne Sørensen Hernes
Department of Clinical Sciences, University of Bergen, Bergen, Norway
Department of Obstetrics and Gynecology, Stavanger University Hospital, Stavanger, Norway
Marit Halonen Christiansen
Faculty of Health Sciences Department of Nursing and Health Promotion Acute and Critical Illness, OsloMet - Oslo Metropolitan University, Oslo, Norway
Arvid Steinar Haugen
Department of Anaesthesia and Intensive Care, Haukeland University Hospital, Bergen, Norway
Faculty of Health, Welfare and Organization, Østfold University College, Fredrikstad, Norway
Ann-Chatrin Linqvist Leonardsen
Department of anesthesia, Østfold Hospital Trust, Grålum, Norway
You can also search for this author in PubMed Google Scholar
Contributions
EO, ASH and ACLL initiated the study. All authors (EO, SA, SSH, MHC, ASH, ACLL) participated in the translation process. SSH and ACLL were responsible for data collection. EO and SA performed the statistical analysis, which was reviewed by ASH and ACLL. EO, SA and ACLL wrote the initial draft of the manuscript, and all authors (EO, SA, SSH, MHC, ASH, ACLL) critically reviewed the manuscript. All authors(EO, SA, SSH, MHC, ASH, ACLL) have read and approved the final version of the manuscript.
Corresponding author
Correspondence to Ann-Chatrin Linqvist Leonardsen .
Ethics declarations
Ethics approval and consent to participate.
The study was conducted in-line with principles for ethical research in the Declaration of Helsinki, and informed consent was obtained from all the participants [ 30 ]. Eligible healthcare personnel were informed of the study through hospital e-mails and by text messages. Completed and submitted questionnaires were assumed as consent to participate. According to the Norwegian Health Research Act §4, no ethics approval is needed when including healthcare personnel in research.
Consent for publication
Competing interests.
The authors declare no competing interests.
Additional information
Publisher’s note.
Springer Nature remains neutral with regard to jurisdictional claims in published maps and institutional affiliations.
Supplementary Information
Supplementary material 1., rights and permissions.
Open Access This article is licensed under a Creative Commons Attribution 4.0 International License, which permits use, sharing, adaptation, distribution and reproduction in any medium or format, as long as you give appropriate credit to the original author(s) and the source, provide a link to the Creative Commons licence, and indicate if changes were made. The images or other third party material in this article are included in the article's Creative Commons licence, unless indicated otherwise in a credit line to the material. If material is not included in the article's Creative Commons licence and your intended use is not permitted by statutory regulation or exceeds the permitted use, you will need to obtain permission directly from the copyright holder. To view a copy of this licence, visit http://creativecommons.org/licenses/by/4.0/ . The Creative Commons Public Domain Dedication waiver ( http://creativecommons.org/publicdomain/zero/1.0/ ) applies to the data made available in this article, unless otherwise stated in a credit line to the data.
Reprints and permissions
About this article
Cite this article.
Olsen, E., Addo, S.A.J., Hernes, S.S. et al. Psychometric properties and criterion related validity of the Norwegian version of hospital survey on patient safety culture 2.0. BMC Health Serv Res 24 , 642 (2024). https://doi.org/10.1186/s12913-024-11097-7
Download citation
Received : 03 April 2023
Accepted : 09 May 2024
Published : 18 May 2024
DOI : https://doi.org/10.1186/s12913-024-11097-7
Share this article
Anyone you share the following link with will be able to read this content:
Sorry, a shareable link is not currently available for this article.
Provided by the Springer Nature SharedIt content-sharing initiative
- Hospital survey on patient safety culture
- Patient safety culture
- Psychometric testing
BMC Health Services Research
ISSN: 1472-6963
- General enquiries: [email protected]
Suggestions or feedback?
MIT News | Massachusetts Institute of Technology
- Machine learning
- Social justice
- Black holes
- Classes and programs
Departments
- Aeronautics and Astronautics
- Brain and Cognitive Sciences
- Architecture
- Political Science
- Mechanical Engineering
Centers, Labs, & Programs
- Abdul Latif Jameel Poverty Action Lab (J-PAL)
- Picower Institute for Learning and Memory
- Lincoln Laboratory
- School of Architecture + Planning
- School of Engineering
- School of Humanities, Arts, and Social Sciences
- Sloan School of Management
- School of Science
- MIT Schwarzman College of Computing
Using ideas from game theory to improve the reliability of language models
Press contact :.
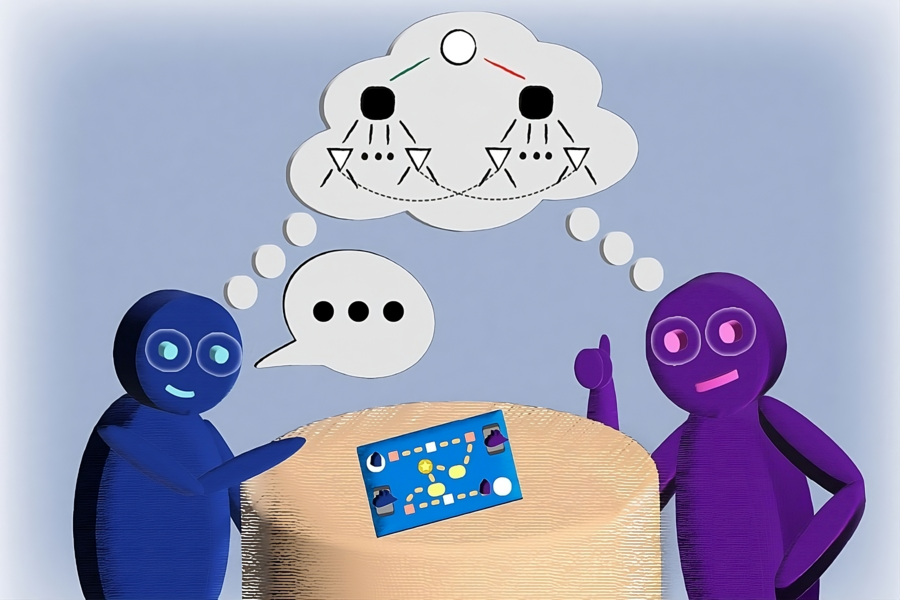
Previous image Next image
Imagine you and a friend are playing a game where your goal is to communicate secret messages to each other using only cryptic sentences. Your friend's job is to guess the secret message behind your sentences. Sometimes, you give clues directly, and other times, your friend has to guess the message by asking yes-or-no questions about the clues you've given. The challenge is that both of you want to make sure you're understanding each other correctly and agreeing on the secret message.
MIT Computer Science and Artificial Intelligence Laboratory (CSAIL) researchers have created a similar "game" to help improve how AI understands and generates text. It is known as a “consensus game” and it involves two parts of an AI system — one part tries to generate sentences (like giving clues), and the other part tries to understand and evaluate those sentences (like guessing the secret message).
The researchers discovered that by treating this interaction as a game, where both parts of the AI work together under specific rules to agree on the right message, they could significantly improve the AI's ability to give correct and coherent answers to questions. They tested this new game-like approach on a variety of tasks, such as reading comprehension, solving math problems, and carrying on conversations, and found that it helped the AI perform better across the board.
Traditionally, large language models answer one of two ways: generating answers directly from the model (generative querying) or using the model to score a set of predefined answers (discriminative querying), which can lead to differing and sometimes incompatible results. With the generative approach, "Who is the president of the United States?" might yield a straightforward answer like "Joe Biden." However, a discriminative query could incorrectly dispute this fact when evaluating the same answer, such as "Barack Obama."
So, how do we reconcile mutually incompatible scoring procedures to achieve coherent, efficient predictions?
"Imagine a new way to help language models understand and generate text, like a game. We've developed a training-free, game-theoretic method that treats the whole process as a complex game of clues and signals, where a generator tries to send the right message to a discriminator using natural language. Instead of chess pieces, they're using words and sentences," says Athul Jacob, an MIT PhD student in electrical engineering and computer science and CSAIL affiliate. "Our way to navigate this game is finding the 'approximate equilibria,' leading to a new decoding algorithm called 'equilibrium ranking.' It's a pretty exciting demonstration of how bringing game-theoretic strategies into the mix can tackle some big challenges in making language models more reliable and consistent."
When tested across many tasks, like reading comprehension, commonsense reasoning, math problem-solving, and dialogue, the team's algorithm consistently improved how well these models performed. Using the ER algorithm with the LLaMA-7B model even outshone the results from much larger models. "Given that they are already competitive, that people have been working on it for a while, but the level of improvements we saw being able to outperform a model that's 10 times the size was a pleasant surprise," says Jacob.
"Diplomacy," a strategic board game set in pre-World War I Europe, where players negotiate alliances, betray friends, and conquer territories without the use of dice — relying purely on skill, strategy, and interpersonal manipulation — recently had a second coming. In November 2022, computer scientists, including Jacob, developed “Cicero,” an AI agent that achieves human-level capabilities in the mixed-motive seven-player game, which requires the same aforementioned skills, but with natural language. The math behind this partially inspired the Consensus Game.
While the history of AI agents long predates when OpenAI's software entered the chat in November 2022, it's well documented that they can still cosplay as your well-meaning, yet pathological friend.
The consensus game system reaches equilibrium as an agreement, ensuring accuracy and fidelity to the model's original insights. To achieve this, the method iteratively adjusts the interactions between the generative and discriminative components until they reach a consensus on an answer that accurately reflects reality and aligns with their initial beliefs. This approach effectively bridges the gap between the two querying methods.
In practice, implementing the consensus game approach to language model querying, especially for question-answering tasks, does involve significant computational challenges. For example, when using datasets like MMLU, which have thousands of questions and multiple-choice answers, the model must apply the mechanism to each query. Then, it must reach a consensus between the generative and discriminative components for every question and its possible answers.
The system did struggle with a grade school right of passage: math word problems. It couldn't generate wrong answers, which is a critical component of understanding the process of coming up with the right one.
“The last few years have seen really impressive progress in both strategic decision-making and language generation from AI systems, but we’re just starting to figure out how to put the two together. Equilibrium ranking is a first step in this direction, but I think there’s a lot we’ll be able to do to scale this up to more complex problems,” says Jacob.
An avenue of future work involves enhancing the base model by integrating the outputs of the current method. This is particularly promising since it can yield more factual and consistent answers across various tasks, including factuality and open-ended generation. The potential for such a method to significantly improve the base model's performance is high, which could result in more reliable and factual outputs from ChatGPT and similar language models that people use daily.
"Even though modern language models, such as ChatGPT and Gemini, have led to solving various tasks through chat interfaces, the statistical decoding process that generates a response from such models has remained unchanged for decades," says Google Research Scientist Ahmad Beirami, who was not involved in the work. "The proposal by the MIT researchers is an innovative game-theoretic framework for decoding from language models through solving the equilibrium of a consensus game. The significant performance gains reported in the research paper are promising, opening the door to a potential paradigm shift in language model decoding that may fuel a flurry of new applications."
Jacob wrote the paper with MIT-IBM Watson Lab researcher Yikang Shen and MIT Department of Electrical Engineering and Computer Science assistant professors Gabriele Farina and Jacob Andreas, who is also a CSAIL member. They presented their work at the International Conference on Learning Representations (ICLR) earlier this month, where it was highlighted as a "spotlight paper." The research also received a “best paper award” at the NeurIPS R0-FoMo Workshop in December 2023.
Share this news article on:
Press mentions, quanta magazine.
MIT researchers have developed a new procedure that uses game theory to improve the accuracy and consistency of large language models (LLMs), reports Steve Nadis for Quanta Magazine . “The new work, which uses games to improve AI, stands in contrast to past approaches, which measured an AI program’s success via its mastery of games,” explains Nadis.
Previous item Next item
Related Links
- Article: "Game Theory Can Make AI More Correct and Efficient"
- Jacob Andreas
- Athul Paul Jacob
- Language & Intelligence @ MIT
- Computer Science and Artificial Intelligence Laboratory (CSAIL)
- Department of Electrical Engineering and Computer Science
- MIT-IBM Watson AI Lab
Related Topics
- Computer science and technology
- Artificial intelligence
- Human-computer interaction
- Natural language processing
- Game theory
- Electrical Engineering & Computer Science (eecs)
Related Articles
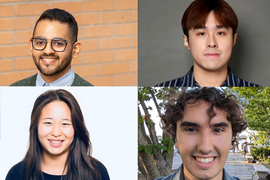
Reasoning and reliability in AI
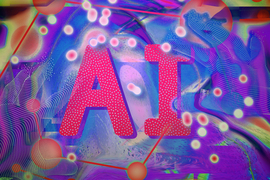
Explained: Generative AI
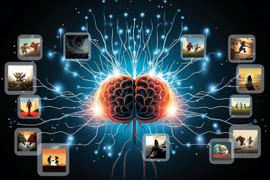
Synthetic imagery sets new bar in AI training efficiency
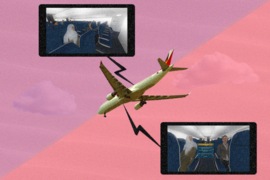
Simulating discrimination in virtual reality
More mit news.
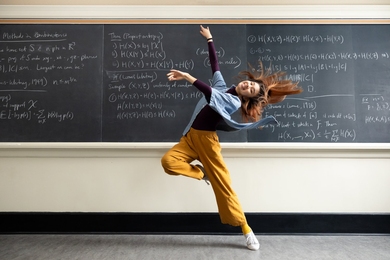
Janabel Xia: Algorithms, dance rhythms, and the drive to succeed
Read full story →
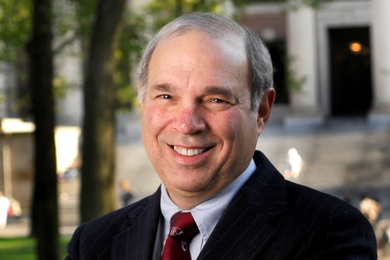
Jonathan Byrnes, MIT Center for Transportation and Logistics senior lecturer and visionary in supply chain management, dies at 75
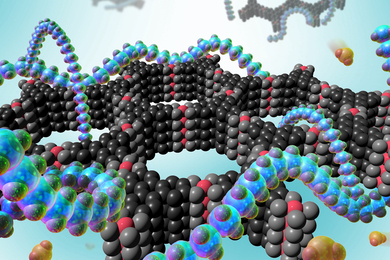
Researchers develop a detector for continuously monitoring toxic gases
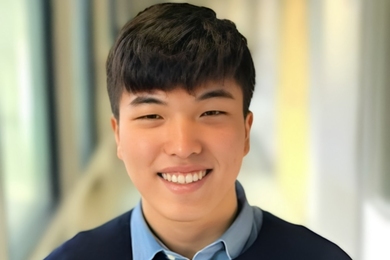
The beauty of biology
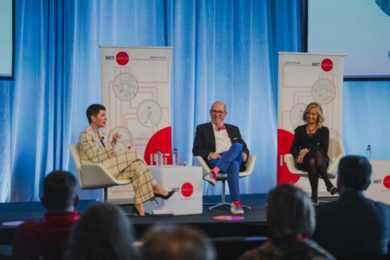
Navigating longevity with industry leaders at MIT AgeLab PLAN Forum
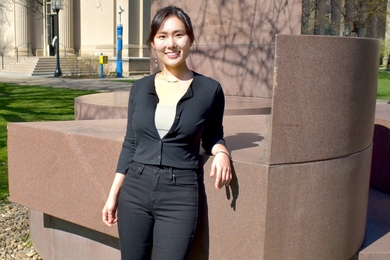
Jeong Min Park earns 2024 Schmidt Science Fellowship
- More news on MIT News homepage →
Massachusetts Institute of Technology 77 Massachusetts Avenue, Cambridge, MA, USA
- Map (opens in new window)
- Events (opens in new window)
- People (opens in new window)
- Careers (opens in new window)
- Accessibility
- Social Media Hub
- MIT on Facebook
- MIT on YouTube
- MIT on Instagram
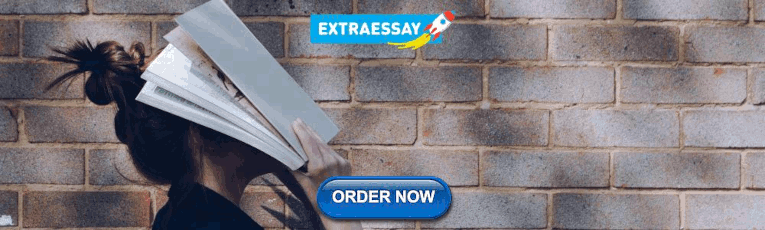
IMAGES
VIDEO
COMMENTS
MeSH terms. Reliability and validity are among the most important and fundamental domains in the assessment of any measuring methodology for data-collection in a good research. Validity is about what an instrument measures and how well it does so, whereas reliability concerns the truthfulness in the data obtain ….
Abstract and Figures. The validity and reliability of the scales used in research are important factors that enable the research to yield healthy results. For this reason, it is useful to ...
Reliability is about the consistency of a measure, and validity is about the accuracy of a measure.opt. It's important to consider reliability and validity when you are creating your research design, planning your methods, and writing up your results, especially in quantitative research. Failing to do so can lead to several types of research ...
A process for assessing the reliability and validity of questions for use in online surveys: Exploring how communication technology is used between Lead Maternity Carer midwives and pregnant people in Aotearoa New Zealand
Abstract. This article examines reliability and validity as ways to demonstrate the rigour and trustworthiness of quantitative and qualitative research. The authors discuss the basic principles of ...
Validity and reliability or trustworthiness are fundamental issues in scientific research whether. it is qualitative, quantitative, or mixed research. It is a necessity for researchers to describe ...
SUBMIT PAPER. Journal of Human Lactation. Impact Factor: 2.6 / 5-Year ... Pérez-Escamilla R. (2016). Reliability of lactation assessment tools applied to overweight and obese women. Journal of Human Lactation, 32 ... An Appraisal Tool for Weighting the Evidence in Healthcare Design Research Based on Internal Validity. Show details Hide details ...
Without rigor, research is worthless, becomes fiction, and loses its utility. Hence, a great deal of attention is applied to reliability and validity in all research methods. Challenges to rigor in qualitative inquiry interestingly paralleled the blossoming of statistical packages and the development of computing systems in quantitative research.
Validity. Validity is defined as the extent to which a concept is accurately measured in a quantitative study. For example, a survey designed to explore depression but which actually measures anxiety would not be considered valid. The second measure of quality in a quantitative study is reliability, or the accuracy of an instrument.In other words, the extent to which a research instrument ...
Revised on 10 October 2022. Reliability and validity are concepts used to evaluate the quality of research. They indicate how well a method, technique, or test measures something. Reliability is about the consistency of a measure, and validity is about the accuracy of a measure. It's important to consider reliability and validity when you are ...
studies examined construct validity, but their varying methods did not permit pooling for meta-analysis. These studies agree that the instrument has reasonable construct validity. The three studies of test-retest reliability did allow a meta-analysis to be performed, albeit with cau-tion due to substantial heterogeneity.
Reliability (or consistency) refers to the stability of a measurement scale, i.e. how far it will give the same results on separate occasions, and it can be assessed in different ways; stability, internal consistency and equiva-lence. Validity is the degree to which a scale measures what it is intended to measure.
As with validity, reliability is an attribute of a measurement instrument - for example, a survey, a weight scale or even a blood pressure monitor. But while validity is concerned with whether the instrument is measuring the "thing" it's supposed to be measuring, reliability is concerned with consistency and stability.
Reliability refers to the consistency of the measurement. Reliability shows how trustworthy is the score of the test. If the collected data shows the same results after being tested using various methods and sample groups, the information is reliable. If your method has reliability, the results will be valid. Example: If you weigh yourself on a ...
Electronic health records (EHRs) are widely used in epidemiological research, but the validity of the results is dependent upon the assumptions made about the healthcare system, the patient, and the provider. In this review, we identify four overarching challenges in using EHR-based data for epidemiological analysis, with a particular emphasis on threats to validity. These challenges include ...
This chapter is about writing about the procedure of the research. This includes a discussion of reliability, validity and the ethics of research and writing. The level of detail about these issues varies across texts, but the reliability and validity of the study must feature in the text. Some-times these issues are evident from the research ...
Russ-Eft, D. F. (1980). Validity and reliability in survey research. American Institutes for Research in the Behavioral Sciences August, 227 151. ... [Paper presented at the Annual Meeting of the Southwest Educational Research Association.] Evidence for reliability and validity are reviewed. A summary evaluation suggests that SLIP (developed by ...
This paper discussed validity and reliability issues in educational research. It was suggested that validity and reliabilitycan be applied to both quantitative and qualitative educational research and that threats to educational research can beattenuated by paying attention to validity and reliability throughout the research.
On the Reliability and Validity of a Three-Item Conscientiousness Scale. In a recent report (Walton, 2024), Kate examined whether shortened conscientiousness scales—three-item scales versus six- or eight-item scales—maintain acceptable levels of reliability and validity. Size: 161.3KB | Pages: 2. Download. In a recent report (Walton, 2024 ...
The present research marks one of the first studies to evaluate vaccine hesitancy in multiple countries that demonstrated VHS validity and reliability. Findings from this study have implications for future research examining vaccine hesitancy and vaccine-preventable diseases and community health nurses.
The two most recent and exhaustive review studies on the reliability and validity of SP research were developed by Bishop and Boyle (2019) for the contingent valuation method (CVM) and Mariel et ...
Reliability of the 10 predicting dimensions were also assessed using composite reliability (CR) values, where 0.7 or above is deemed acceptable for ascertaining internal consistency [].Convergent validity was assessed using the Average Variance Explained (AVE), where a value of at least 0.5 is deemed acceptable [], indicating that at least 50 percent of the variance is explained by the items ...
The inter-rater reliability of the TRL-IS was evaluated by ten raters and finally six raters evaluated the content and face validity, and feasibility, of the TRL-IS checklist using the System Usability Scale (SUS). Few papers (n = 11) utilised the TRL to evaluate the readiness of readiness of health and social science implementation research.
MIT researchers' "consensus game" is a game-theoretic approach for language model decoding. The equilibrium-ranking algorithm harmonizes generative and discriminative querying to enhance prediction accuracy across various tasks, outperforming larger models and demonstrating the potential of game theory in improving language model consistency and truthfulness.
Taherdoost [36] revealed "face validity is the degree to which a measure appears to be related to a specific construct, in the judgment of nonexperts such as test takers" and the clarity of the ...
@article{Jesus2024ValidityAR, title={Validity and Reliability of an Inexpensive Caliper To Assess Triceps Skinfolds in Children and Young Adults with Cerebral Palsy}, author={Anna Jesus and Mark Conaway and Jodi Darring and Amy Shadron and Valentina Intagliata and Rebecca J. Scharf and Richard Stevenson}, journal={Clinical Nutrition Open ...