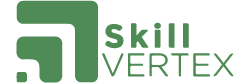
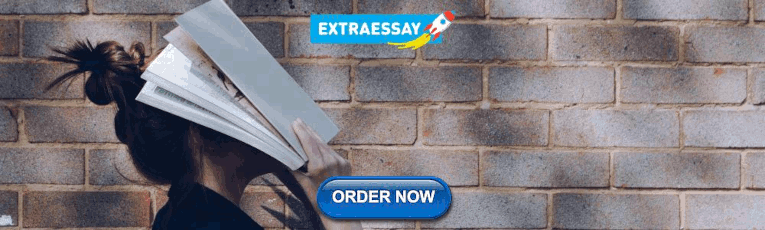
Problem-Solving Agents In Artificial Intelligence
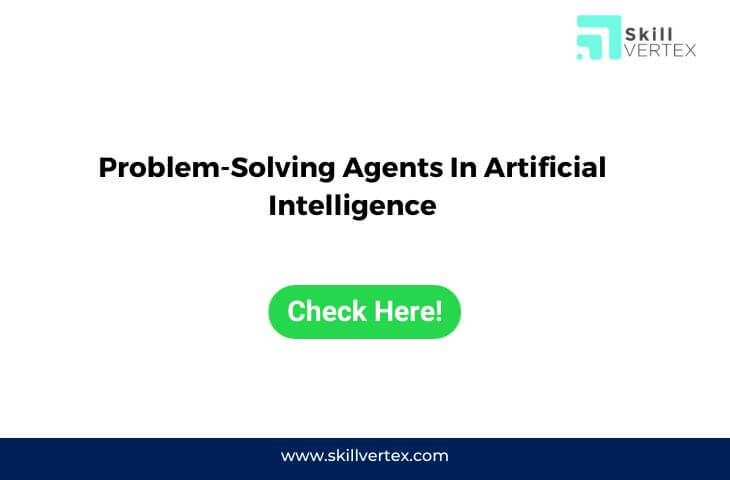
In artificial intelligence, a problem-solving agent refers to a type of intelligent agent designed to address and solve complex problems or tasks in its environment. These agents are a fundamental concept in AI and are used in various applications, from game-playing algorithms to robotics and decision-making systems. Here are some key characteristics and components of a problem-solving agent:
- Perception : Problem-solving agents typically have the ability to perceive or sense their environment. They can gather information about the current state of the world, often through sensors, cameras, or other data sources.
- Knowledge Base : These agents often possess some form of knowledge or representation of the problem domain. This knowledge can be encoded in various ways, such as rules, facts, or models, depending on the specific problem.
- Reasoning : Problem-solving agents employ reasoning mechanisms to make decisions and select actions based on their perception and knowledge. This involves processing information, making inferences, and selecting the best course of action.
- Planning : For many complex problems, problem-solving agents engage in planning. They consider different sequences of actions to achieve their goals and decide on the most suitable action plan.
- Actuation : After determining the best course of action, problem-solving agents take actions to interact with their environment. This can involve physical actions in the case of robotics or making decisions in more abstract problem-solving domains.
- Feedback : Problem-solving agents often receive feedback from their environment, which they use to adjust their actions and refine their problem-solving strategies. This feedback loop helps them adapt to changing conditions and improve their performance.
- Learning : Some problem-solving agents incorporate machine learning techniques to improve their performance over time. They can learn from experience, adapt their strategies, and become more efficient at solving similar problems in the future.
Problem-solving agents can vary greatly in complexity, from simple algorithms that solve straightforward puzzles to highly sophisticated AI systems that tackle complex, real-world problems. The design and implementation of problem-solving agents depend on the specific problem domain and the goals of the AI application.
Hello, I’m Hridhya Manoj. I’m passionate about technology and its ever-evolving landscape. With a deep love for writing and a curious mind, I enjoy translating complex concepts into understandable, engaging content. Let’s explore the world of tech together
Which Of The Following Is A Privilege In SQL Standard
Implicit Return Type Int In C
Leave a Comment Cancel reply
Save my name, email, and website in this browser for the next time I comment.
Reach Out to Us for Any Query
SkillVertex is an edtech organization that aims to provide upskilling and training to students as well as working professionals by delivering a diverse range of programs in accordance with their needs and future aspirations.
© 2024 Skill Vertex
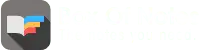
Problem Solving Agents in Artificial Intelligence
In this post, we will talk about Problem Solving agents in Artificial Intelligence, which are sort of goal-based agents. Because the straight mapping from states to actions of a basic reflex agent is too vast to retain for a complex environment, we utilize goal-based agents that may consider future actions and the desirability of outcomes.
You Will Learn
Problem Solving Agents
Problem Solving Agents decide what to do by finding a sequence of actions that leads to a desirable state or solution.
An agent may need to plan when the best course of action is not immediately visible. They may need to think through a series of moves that will lead them to their goal state. Such an agent is known as a problem solving agent , and the computation it does is known as a search .
The problem solving agent follows this four phase problem solving process:
- Goal Formulation: This is the first and most basic phase in problem solving. It arranges specific steps to establish a target/goal that demands some activity to reach it. AI agents are now used to formulate goals.
- Problem Formulation: It is one of the fundamental steps in problem-solving that determines what action should be taken to reach the goal.
- Search: After the Goal and Problem Formulation, the agent simulates sequences of actions and has to look for a sequence of actions that reaches the goal. This process is called search, and the sequence is called a solution . The agent might have to simulate multiple sequences that do not reach the goal, but eventually, it will find a solution, or it will find that no solution is possible. A search algorithm takes a problem as input and outputs a sequence of actions.
- Execution: After the search phase, the agent can now execute the actions that are recommended by the search algorithm, one at a time. This final stage is known as the execution phase.
Problems and Solution
Before we move into the problem formulation phase, we must first define a problem in terms of problem solving agents.
A formal definition of a problem consists of five components:
Initial State
Transition model.
It is the agent’s starting state or initial step towards its goal. For example, if a taxi agent needs to travel to a location(B), but the taxi is already at location(A), the problem’s initial state would be the location (A).
It is a description of the possible actions that the agent can take. Given a state s, Actions ( s ) returns the actions that can be executed in s. Each of these actions is said to be appropriate in s.
It describes what each action does. It is specified by a function Result ( s, a ) that returns the state that results from doing action an in state s.
The initial state, actions, and transition model together define the state space of a problem, a set of all states reachable from the initial state by any sequence of actions. The state space forms a graph in which the nodes are states, and the links between the nodes are actions.
It determines if the given state is a goal state. Sometimes there is an explicit list of potential goal states, and the test merely verifies whether the provided state is one of them. The goal is sometimes expressed via an abstract attribute rather than an explicitly enumerated set of conditions.
It assigns a numerical cost to each path that leads to the goal. The problem solving agents choose a cost function that matches its performance measure. Remember that the optimal solution has the lowest path cost of all the solutions .
Example Problems
The problem solving approach has been used in a wide range of work contexts. There are two kinds of problem approaches
- Standardized/ Toy Problem: Its purpose is to demonstrate or practice various problem solving techniques. It can be described concisely and precisely, making it appropriate as a benchmark for academics to compare the performance of algorithms.
- Real-world Problems: It is real-world problems that need solutions. It does not rely on descriptions, unlike a toy problem, yet we can have a basic description of the issue.
Some Standardized/Toy Problems
Vacuum world problem.
Let us take a vacuum cleaner agent and it can move left or right and its jump is to suck up the dirt from the floor.
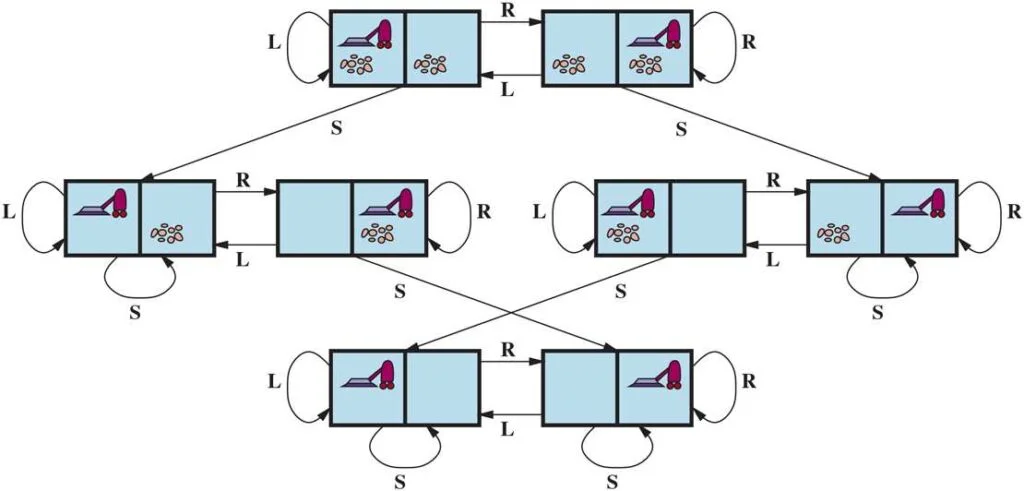
The vacuum world’s problem can be stated as follows:
States: A world state specifies which objects are housed in which cells. The objects in the vacuum world are the agent and any dirt. The agent can be in either of the two cells in the simple two-cell version, and each call can include dirt or not, therefore there are 2×2×2 = 8 states. A vacuum environment with n cells has n×2 n states in general.
Initial State: Any state can be specified as the starting point.
Actions: We defined three actions in the two-cell world: sucking, moving left, and moving right. More movement activities are required in a two-dimensional multi-cell world.
Transition Model: Suck cleans the agent’s cell of any filth; Forward moves the agent one cell forward in the direction it is facing unless it meets a wall, in which case the action has no effect. Backward moves the agent in the opposite direction, whilst TurnRight and TurnLeft rotate it by 90°.
Goal States: The states in which every cell is clean.
Action Cost: Each action costs 1.
8 Puzzle Problem
In a sliding-tile puzzle , a number of tiles (sometimes called blocks or pieces) are arranged in a grid with one or more blank spaces so that some of the tiles can slide into the blank space. One variant is the Rush Hour puzzle, in which cars and trucks slide around a 6 x 6 grid in an attempt to free a car from the traffic jam. Perhaps the best-known variant is the 8- puzzle (see Figure below ), which consists of a 3 x 3 grid with eight numbered tiles and one blank space, and the 15-puzzle on a 4 x 4 grid. The object is to reach a specified goal state, such as the one shown on the right of the figure. The standard formulation of the 8 puzzles is as follows:
STATES : A state description specifies the location of each of the tiles.
INITIAL STATE : Any state can be designated as the initial state. (Note that a parity property partitions the state space—any given goal can be reached from exactly half of the possible initial states.)
ACTIONS : While in the physical world it is a tile that slides, the simplest way of describing action is to think of the blank space moving Left , Right , Up , or Down . If the blank is at an edge or corner then not all actions will be applicable.
TRANSITION MODEL : Maps a state and action to a resulting state; for example, if we apply Left to the start state in the Figure below, the resulting state has the 5 and the blank switched.
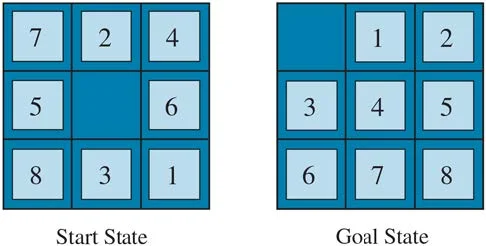
GOAL STATE : It identifies whether we have reached the correct goal state. Although any state could be the goal, we typically specify a state with the numbers in order, as in the Figure above.
ACTION COST : Each action costs 1.
You Might Like:
- Agents in Artificial Intelligence
Types of Environments in Artificial Intelligence
- Understanding PEAS in Artificial Intelligence
- River Crossing Puzzle | Farmer, Wolf, Goat and Cabbage
Share Article:
Digital image processing: all you need to know.
- Speakers & Mentors
- AI services
Examples of Problem Solving Agents in Artificial Intelligence
In the field of artificial intelligence, problem-solving agents play a vital role in finding solutions to complex tasks and challenges. These agents are designed to mimic human intelligence and utilize a range of algorithms and techniques to tackle various problems. By analyzing data, making predictions, and finding optimal solutions, problem-solving agents demonstrate the power and potential of artificial intelligence.
One example of a problem-solving agent in artificial intelligence is a chess-playing program. These agents are capable of evaluating millions of possible moves and predicting the best one to make based on a wide array of factors. By utilizing advanced algorithms and machine learning techniques, these agents can analyze the current state of the game, anticipate future moves, and make strategic decisions to outplay even the most skilled human opponents.
Another example of problem-solving agents in artificial intelligence is autonomous driving systems. These agents are designed to navigate complex road networks, make split-second decisions, and ensure the safety of both passengers and pedestrians. By continuously analyzing sensor data, identifying obstacles, and calculating optimal paths, these agents can effectively solve problems related to navigation, traffic congestion, and collision avoidance.
Definition and Importance of Problem Solving Agents
A problem solving agent is a type of artificial intelligence agent that is designed to identify and solve problems. These agents are programmed to analyze information, develop potential solutions, and select the best course of action to solve a given problem.
Problem solving agents are an essential aspect of artificial intelligence, as they have the ability to tackle complex problems that humans may find difficult or time-consuming to solve. These agents can handle large amounts of data and perform calculations and analysis at a much faster rate than humans.
Problem solving agents can be found in various domains, including healthcare, finance, manufacturing, and transportation. For example, in healthcare, problem solving agents can analyze patient data and medical records to diagnose diseases and recommend treatment plans. In finance, these agents can analyze market trends and make investment decisions.
The importance of problem solving agents in artificial intelligence lies in their ability to automate and streamline processes, improve efficiency, and reduce human error. These agents can also handle repetitive tasks, freeing up human resources for more complex and strategic work.
In addition, problem solving agents can learn and adapt from past experiences, making them even more effective over time. They can continuously analyze and optimize their problem-solving strategies, resulting in better decision-making and outcomes.
In conclusion, problem solving agents are a fundamental component of artificial intelligence. Their ability to analyze information, develop solutions, and make decisions has a significant impact on various industries and fields. Through their automation and optimization capabilities, problem solving agents contribute to improving efficiency, reducing errors, and enhancing decision-making processes.
Problem Solving Agent Architecture
A problem-solving agent is a central component in the field of artificial intelligence that is designed to tackle complex problems and find solutions. The architecture of a problem-solving agent consists of several key components that work together to achieve intelligent problem-solving.
One of the main components of a problem-solving agent is the knowledge base. This is where the agent stores relevant information and data that it can use to solve problems. The knowledge base can include facts, rules, and heuristics that the agent has acquired through learning or from experts in the domain.
Another important component of a problem-solving agent is the inference engine. This is the part of the agent that is responsible for reasoning and making logical deductions. The inference engine uses the knowledge base to generate possible solutions to a problem by applying various reasoning techniques, such as deduction, induction, and abduction.
Furthermore, a problem-solving agent often includes a search algorithm or strategy. This is used to systematically explore possible solutions and search for the best one. The search algorithm can be guided by various heuristics or constraints to efficiently navigate through the solution space.
In addition to these components, a problem-solving agent may also have a learning component. This allows the agent to improve its problem-solving capabilities over time through experience. The learning component can help the agent adapt its knowledge base, refine its inference engine, or adjust its search strategy based on feedback or new information.
Overall, the architecture of a problem-solving agent is designed to enable intelligent problem-solving by combining knowledge representation, reasoning, search, and learning. By utilizing these components, problem-solving agents can tackle a wide range of problems and find effective solutions in various domains.
Uninformed Search Algorithms
In the field of artificial intelligence, problem-solving agents are often designed to navigate a large search space in order to find a solution to a given problem. Uninformed search algorithms, also known as blind search algorithms, are a class of algorithms that do not use any additional information about the problem to guide their search.
Breadth-First Search (BFS)
Breadth-First Search (BFS) is one of the most basic uninformed search algorithms. It explores all the neighbor nodes at the present depth before moving on to the nodes at the next depth level. BFS is implemented using a queue data structure, where the nodes to be explored are added to the back of the queue and the nodes to be explored next are removed from the front of the queue.
For example, BFS can be used to find the shortest path between two cities on a road map, exploring all possible paths in a breadth-first manner to find the optimal solution.
Depth-First Search (DFS)
Depth-First Search (DFS) is another uninformed search algorithm that explores the deepest path first before backtracking. It is implemented using a stack data structure, where nodes are added to the top of the stack and the nodes to be explored next are removed from the top of the stack.
DFS can be used in situations where the goal state is likely to be far from the starting state, as it explores the deepest paths first. However, it may get stuck in an infinite loop if there is a cycle in the search space.
For example, DFS can be used to solve a maze, exploring different paths until the goal state (exit of the maze) is reached.
Overall, uninformed search algorithms provide a foundational approach to problem-solving in artificial intelligence. They do not rely on any additional problem-specific knowledge, making them applicable to a wide range of problems. While they may not always find the optimal solution or have high efficiency, they provide a starting point for more sophisticated search algorithms.
Breadth-First Search
Breadth-First Search is a problem-solving algorithm commonly used in artificial intelligence. It is an uninformed search algorithm that explores all the immediate variations of a problem before moving on to the next level of variations.
Examples of problems that can be solved using Breadth-First Search include finding the shortest path between two points in a graph, solving a sliding puzzle, or searching for a word in a large text document.
How Breadth-First Search Works
The Breadth-First Search algorithm starts at the initial state of the problem and expands all the immediate successor states. It then explores the successor states of the expanded states, continuing this process until a goal state is reached.
At each step of the algorithm, the breadth-first search maintains a queue of states to explore. The algorithm removes a state from the front of the queue, explores its successor states, and adds them to the back of the queue. This ensures that states are explored in the order they were added to the queue, resulting in a breadth-first exploration of the problem space.
The algorithm also keeps track of the visited states to avoid revisiting them in the future, preventing infinite loops in cases where the problem space contains cycles.
Benefits and Limitations
Breadth-First Search guarantees that the shortest path to a goal state is found, if such a path exists. It explores all possible paths of increasing lengths until a goal state is reached, ensuring that shorter paths are explored first.
However, the main limitation of Breadth-First Search is its memory requirements. As it explores all immediate successor states, it needs to keep track of a large number of states in memory. This can become impractical for problems with a large state space. Additionally, Breadth-First Search does not take into account the cost or quality of the paths it explores, making it less suitable for problems with complex cost or objective functions.
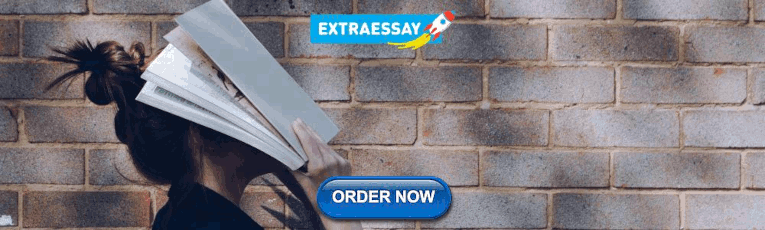
Depth-First Search
Depth-First Search (DFS) is a common algorithm used in the field of artificial intelligence to solve various types of problems. It is a search strategy that explores as far as possible along each branch of a tree-like structure before backtracking.
In the context of problem-solving agents, DFS is often used to traverse graph-based problem spaces in search of a solution. This algorithm starts at an initial state and explores all possible actions from that state until a goal state is found or all possible paths have been exhausted.
One example of using DFS in artificial intelligence is solving mazes. The agent starts at the entrance of the maze and explores one path at a time, prioritizing depth rather than breadth. It keeps track of the visited nodes and backtracks whenever it encounters a dead end, until it reaches the goal state (the exit of the maze).
Another example is solving puzzles, such as the famous Eight Queens Problem. In this problem, the agent needs to place eight queens on a chessboard in such a way that no two queens threaten each other. DFS can be used to explore all possible combinations of queen placements, backtracking whenever a placement is found to be invalid, until a valid solution is found or all possibilities have been exhausted.
DFS has advantages and disadvantages. Its main advantage is its simplicity and low memory usage, as it only needs to store the path from the initial state to the current state. However, it can get stuck in infinite loops if not implemented properly, and it may not always find the optimal solution.
In conclusion, DFS is a useful algorithm for problem-solving agents in artificial intelligence. It can be applied to a wide range of problems and provides a straightforward approach to exploring problem spaces. By understanding its strengths and limitations, developers can effectively utilize DFS to find solutions efficiently.
Iterative Deepening Depth-First Search
Iterative Deepening Depth-First Search (IDDFS) is a popular search algorithm used in problem solving within the field of artificial intelligence. It is a combination of depth-first search and breadth-first search algorithms and is designed to overcome some of the limitations of traditional depth-first search.
IDDFS operates in a similar way to depth-first search by exploring a problem space depth-wise. However, it does not keep track of the visited nodes in the search tree as depth-first search does. Instead, it uses a depth limit, which is gradually increased with each iteration, to restrict the depth to which it explores the search tree. This allows IDDFS to gradually explore the search space, starting from a shallow depth and progressively moving to deeper depths.
- Data Science
- Data Analysis
- Data Visualization
- Machine Learning
- Deep Learning
- Computer Vision
- Artificial Intelligence
- AI ML DS Interview Series
- AI ML DS Projects series
- Data Engineering
- Web Scrapping
- Artificial Intelligence (AI) Algorithms
- AO* algorithm - Artificial intelligence
- What is Artificial General Intelligence (AGI)?
- Turing Test in Artificial Intelligence
- Artificial Intelligence | An Introduction
- Artificial Intelligence - Boon or Bane
- What is Artificial Intelligence?
- Explainable Artificial Intelligence(XAI)
- Game Playing in Artificial Intelligence
- Emergence Of Artificial Intelligence
- Impact and Example of Artificial Intelligence
- Types of Artificial Intelligence
- Advantages and Disadvantage of Artificial Intelligence
- Artificial Intelligence in NASA and DARPA in 2000s
- Problem Solving in Artificial Intelligence
- What is Artificial Narrow Intelligence (ANI)?
- Artificial Intelligence - Terminology
- Chinese Room Argument in Artificial Intelligence
- Artificial Intelligence in Robotics
Agents in Artificial Intelligence
In artificial intelligence, an agent is a computer program or system that is designed to perceive its environment, make decisions and take actions to achieve a specific goal or set of goals. The agent operates autonomously, meaning it is not directly controlled by a human operator.
Agents can be classified into different types based on their characteristics, such as whether they are reactive or proactive, whether they have a fixed or dynamic environment, and whether they are single or multi-agent systems.
- Reactive agents are those that respond to immediate stimuli from their environment and take actions based on those stimuli. Proactive agents, on the other hand, take initiative and plan ahead to achieve their goals. The environment in which an agent operates can also be fixed or dynamic. Fixed environments have a static set of rules that do not change, while dynamic environments are constantly changing and require agents to adapt to new situations.
- Multi-agent systems involve multiple agents working together to achieve a common goal. These agents may have to coordinate their actions and communicate with each other to achieve their objectives. Agents are used in a variety of applications, including robotics, gaming, and intelligent systems. They can be implemented using different programming languages and techniques, including machine learning and natural language processing.
Artificial intelligence is defined as the study of rational agents. A rational agent could be anything that makes decisions, such as a person, firm, machine, or software. It carries out an action with the best outcome after considering past and current percepts(agent’s perceptual inputs at a given instance). An AI system is composed of an agent and its environment . The agents act in their environment. The environment may contain other agents.
An agent is anything that can be viewed as:
- Perceiving its environment through sensors and
- Acting upon that environment through actuators
Note : Every agent can perceive its own actions (but not always the effects).
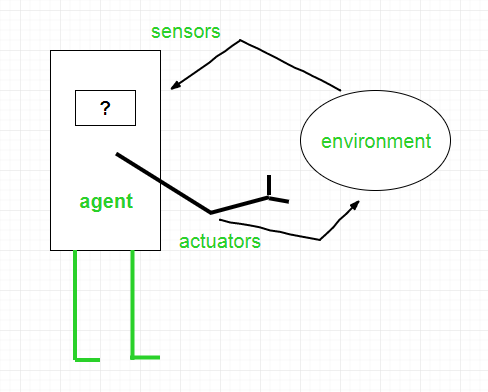
Interaction of Agents with the Environment
Structure of an AI Agent
To understand the structure of Intelligent Agents, we should be familiar with Architecture and Agent programs. Architecture is the machinery that the agent executes on. It is a device with sensors and actuators, for example, a robotic car, a camera, and a PC. An agent program is an implementation of an agent function. An agent function is a map from the percept sequence(history of all that an agent has perceived to date) to an action.
Agent = Architecture + Agent Program
There are many examples of agents in artificial intelligence. Here are a few:
- Intelligent personal assistants: These are agents that are designed to help users with various tasks, such as scheduling appointments, sending messages, and setting reminders. Examples of intelligent personal assistants include Siri, Alexa, and Google Assistant.
- Autonomous robots: These are agents that are designed to operate autonomously in the physical world. They can perform tasks such as cleaning, sorting, and delivering goods. Examples of autonomous robots include the Roomba vacuum cleaner and the Amazon delivery robot.
- Gaming agents: These are agents that are designed to play games, either against human opponents or other agents. Examples of gaming agents include chess-playing agents and poker-playing agents.
- Fraud detection agents: These are agents that are designed to detect fraudulent behavior in financial transactions. They can analyze patterns of behavior to identify suspicious activity and alert authorities. Examples of fraud detection agents include those used by banks and credit card companies.
- Traffic management agents: These are agents that are designed to manage traffic flow in cities. They can monitor traffic patterns, adjust traffic lights, and reroute vehicles to minimize congestion. Examples of traffic management agents include those used in smart cities around the world.
- A software agent has Keystrokes, file contents, received network packages that act as sensors and displays on the screen, files, and sent network packets acting as actuators.
- A Human-agent has eyes, ears, and other organs which act as sensors, and hands, legs, mouth, and other body parts act as actuators.
- A Robotic agent has Cameras and infrared range finders which act as sensors and various motors act as actuators.
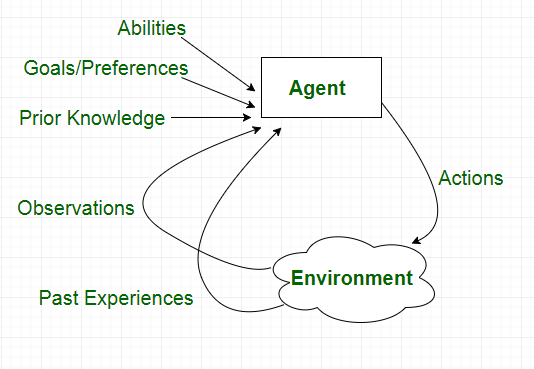
Characteristics of an Agent
Types of Agents
Agents can be grouped into five classes based on their degree of perceived intelligence and capability :
Simple Reflex Agents
Model-Based Reflex Agents
Goal-Based Agents
Utility-Based Agents
Learning Agent
- Multi-agent systems
- Hierarchical agents
Simple reflex agents ignore the rest of the percept history and act only on the basis of the current percept . Percept history is the history of all that an agent has perceived to date. The agent function is based on the condition-action rule . A condition-action rule is a rule that maps a state i.e., a condition to an action. If the condition is true, then the action is taken, else not. This agent function only succeeds when the environment is fully observable. For simple reflex agents operating in partially observable environments, infinite loops are often unavoidable. It may be possible to escape from infinite loops if the agent can randomize its actions.
Problems with Simple reflex agents are :
- Very limited intelligence.
- No knowledge of non-perceptual parts of the state.
- Usually too big to generate and store.
- If there occurs any change in the environment, then the collection of rules needs to be updated.
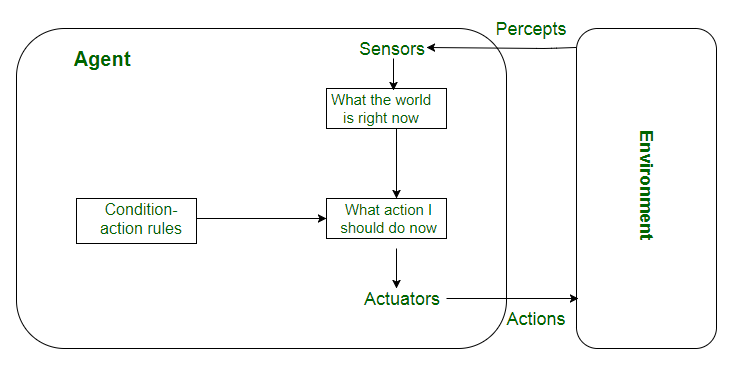
It works by finding a rule whose condition matches the current situation. A model-based agent can handle partially observable environments by the use of a model about the world. The agent has to keep track of the internal state which is adjusted by each percept and that depends on the percept history. The current state is stored inside the agent which maintains some kind of structure describing the part of the world which cannot be seen.
Updating the state requires information about:
- How the world evolves independently from the agent?
- How do the agent’s actions affect the world?
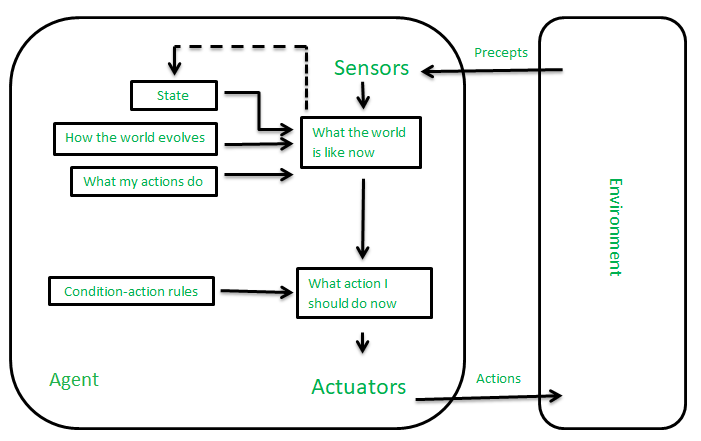
These kinds of agents take decisions based on how far they are currently from their goal (description of desirable situations). Their every action is intended to reduce their distance from the goal. This allows the agent a way to choose among multiple possibilities, selecting the one which reaches a goal state. The knowledge that supports its decisions is represented explicitly and can be modified, which makes these agents more flexible. They usually require search and planning. The goal-based agent’s behavior can easily be changed.
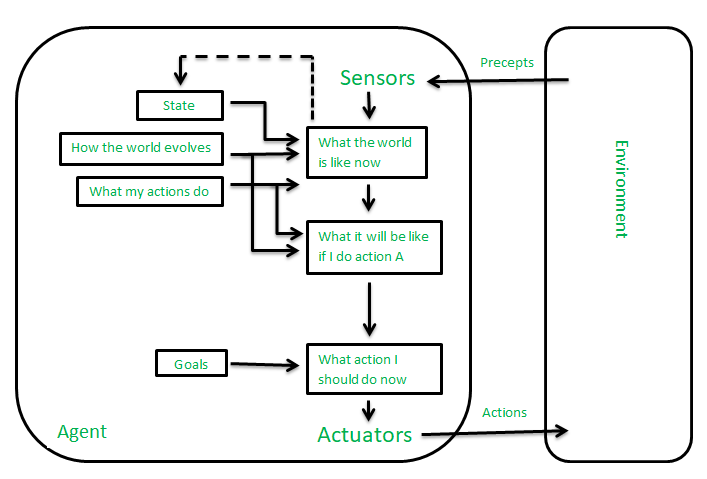
The agents which are developed having their end uses as building blocks are called utility-based agents. When there are multiple possible alternatives, then to decide which one is best, utility-based agents are used. They choose actions based on a preference (utility) for each state. Sometimes achieving the desired goal is not enough. We may look for a quicker, safer, cheaper trip to reach a destination. Agent happiness should be taken into consideration. Utility describes how “happy” the agent is. Because of the uncertainty in the world, a utility agent chooses the action that maximizes the expected utility. A utility function maps a state onto a real number which describes the associated degree of happiness.
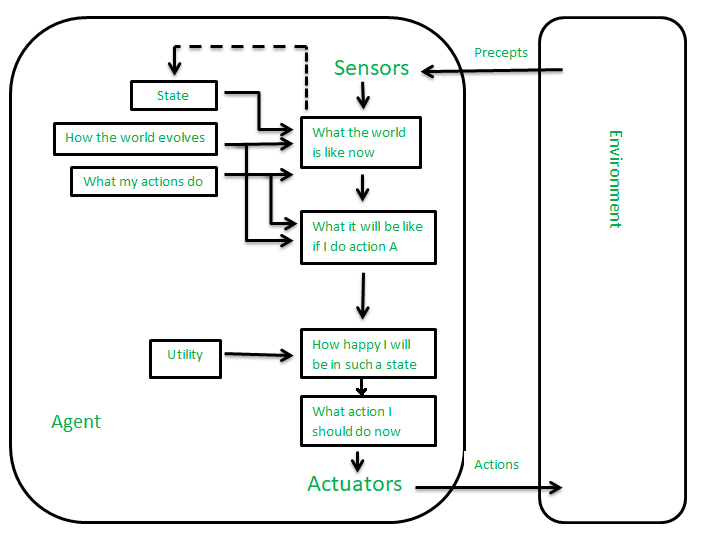
A learning agent in AI is the type of agent that can learn from its past experiences or it has learning capabilities. It starts to act with basic knowledge and then is able to act and adapt automatically through learning. A learning agent has mainly four conceptual components, which are:
- Learning element: It is responsible for making improvements by learning from the environment.
- Critic: The learning element takes feedback from critics which describes how well the agent is doing with respect to a fixed performance standard.
- Performance element: It is responsible for selecting external action.
- Problem Generator: This component is responsible for suggesting actions that will lead to new and informative experiences.
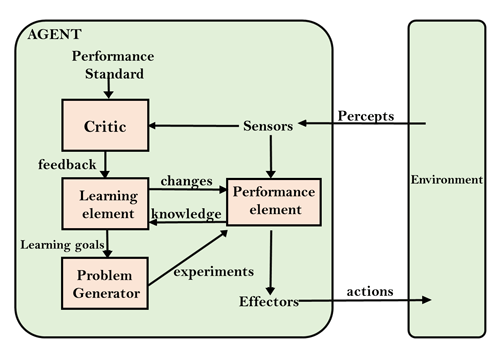
Multi-Agent Systems
These agents interact with other agents to achieve a common goal. They may have to coordinate their actions and communicate with each other to achieve their objective.
A multi-agent system (MAS) is a system composed of multiple interacting agents that are designed to work together to achieve a common goal. These agents may be autonomous or semi-autonomous and are capable of perceiving their environment, making decisions, and taking action to achieve the common objective.
MAS can be used in a variety of applications, including transportation systems, robotics, and social networks. They can help improve efficiency, reduce costs, and increase flexibility in complex systems. MAS can be classified into different types based on their characteristics, such as whether the agents have the same or different goals, whether the agents are cooperative or competitive, and whether the agents are homogeneous or heterogeneous.
- In a homogeneous MAS, all the agents have the same capabilities, goals, and behaviors.
- In contrast, in a heterogeneous MAS, the agents have different capabilities, goals, and behaviors.
This can make coordination more challenging but can also lead to more flexible and robust systems.
Cooperative MAS involves agents working together to achieve a common goal, while competitive MAS involves agents working against each other to achieve their own goals. In some cases, MAS can also involve both cooperative and competitive behavior, where agents must balance their own interests with the interests of the group.
MAS can be implemented using different techniques, such as game theory , machine learning , and agent-based modeling. Game theory is used to analyze strategic interactions between agents and predict their behavior. Machine learning is used to train agents to improve their decision-making capabilities over time. Agent-based modeling is used to simulate complex systems and study the interactions between agents.
Overall, multi-agent systems are a powerful tool in artificial intelligence that can help solve complex problems and improve efficiency in a variety of applications.
Hierarchical Agents
These agents are organized into a hierarchy, with high-level agents overseeing the behavior of lower-level agents. The high-level agents provide goals and constraints, while the low-level agents carry out specific tasks. Hierarchical agents are useful in complex environments with many tasks and sub-tasks.
- Hierarchical agents are agents that are organized into a hierarchy, with high-level agents overseeing the behavior of lower-level agents. The high-level agents provide goals and constraints, while the low-level agents carry out specific tasks. This structure allows for more efficient and organized decision-making in complex environments.
- Hierarchical agents can be implemented in a variety of applications, including robotics, manufacturing, and transportation systems. They are particularly useful in environments where there are many tasks and sub-tasks that need to be coordinated and prioritized.
- In a hierarchical agent system, the high-level agents are responsible for setting goals and constraints for the lower-level agents. These goals and constraints are typically based on the overall objective of the system. For example, in a manufacturing system, the high-level agents might set production targets for the lower-level agents based on customer demand.
- The low-level agents are responsible for carrying out specific tasks to achieve the goals set by the high-level agents. These tasks may be relatively simple or more complex, depending on the specific application. For example, in a transportation system, low-level agents might be responsible for managing traffic flow at specific intersections.
- Hierarchical agents can be organized into different levels, depending on the complexity of the system. In a simple system, there may be only two levels: high-level agents and low-level agents. In a more complex system, there may be multiple levels, with intermediate-level agents responsible for coordinating the activities of lower-level agents.
- One advantage of hierarchical agents is that they allow for more efficient use of resources. By organizing agents into a hierarchy, it is possible to allocate tasks to the agents that are best suited to carry them out, while avoiding duplication of effort. This can lead to faster, more efficient decision-making and better overall performance of the system.
Overall, hierarchical agents are a powerful tool in artificial intelligence that can help solve complex problems and improve efficiency in a variety of applications.
Uses of Agents
Agents are used in a wide range of applications in artificial intelligence, including:
- Robotics: Agents can be used to control robots and automate tasks in manufacturing, transportation, and other industries.
- Smart homes and buildings: Agents can be used to control heating, lighting, and other systems in smart homes and buildings, optimizing energy use and improving comfort.
- Transportation systems: Agents can be used to manage traffic flow, optimize routes for autonomous vehicles, and improve logistics and supply chain management.
- Healthcare: Agents can be used to monitor patients, provide personalized treatment plans, and optimize healthcare resource allocation.
- Finance: Agents can be used for automated trading, fraud detection, and risk management in the financial industry.
- Games: Agents can be used to create intelligent opponents in games and simulations, providing a more challenging and realistic experience for players.
- Natural language processing: Agents can be used for language translation, question answering, and chatbots that can communicate with users in natural language .
- Cybersecurity: Agents can be used for intrusion detection, malware analysis, and network security.
- Environmental monitoring: Agents can be used to monitor and manage natural resources, track climate change, and improve environmental sustainability.
- Social media: Agents can be used to analyze social media data, identify trends and patterns, and provide personalized recommendations to users.
Overall, agents are a versatile and powerful tool in artificial intelligence that can help solve a wide range of problems in different fields.
Please Login to comment...
Similar reads, improve your coding skills with practice.
What kind of Experience do you want to share?
The promise and the reality of gen AI agents in the enterprise
The evolution of generative AI (gen AI) has opened the door to great opportunities across organizations, particularly regarding gen AI agents—AI-powered software entities that plan and perform tasks or aid humans by delivering specific services on their behalf. So far, adoption at scale across businesses has faced difficulties because of data quality, employee distrust, and cost of implementation. In addition, capabilities have raced ahead of leaders’ capacity to imagine how these agents could be used to transform work.
However, as gen AI technologies progress and the next-generation agents emerge, we expect more use cases to be unlocked, deployment costs to decrease, long-tail use cases to become economically viable, and more at-scale automation to take place across a wider range of enterprise processes, employee experiences, and customer interfaces. This evolution will demand investing in strong AI trust and risk management practices and policies as well as platforms for managing and monitoring agent-based systems.
In this interview, McKinsey Digital’s Barr Seitz speaks with senior partners Jorge Amar and Lari Hämäläinen and partner Nicolai von Bismarck to explore the evolution of gen AI agents and how companies can and should implement the technology, where the pools of value lie for the enterprise as a whole. They particularly explore what these developments mean for customer service. An edited transcript of the conversation follows.
Barr Seitz: What exactly is a gen AI agent?

Lari Hämäläinen: When we talk about gen AI agents, we mean software entities that can orchestrate complex workflows, coordinate activities among multiple agents, apply logic, and evaluate answers. These agents can help automate processes in organizations or augment workers and customers as they perform processes. This is valuable because it will not only help humans do their jobs better but also fully digitalize underlying processes and services.
For example, in customer services, recent developments in short- and long-term memory structures enable these agents to personalize interactions with external customers and internal users, and help human agents learn. All of this means that gen AI agents are getting much closer to becoming true virtual workers that can both augment and automate enterprise services in all areas of the business, from HR to finance to customer service. That means we’re well on our way to automating a wide range of tasks in many service functions while also improving service quality.
Barr Seitz: Where do you see the greatest value from gen AI agents?

Jorge Amar: We have estimated that gen AI enterprise use cases could yield $2.6 trillion to $4.4 trillion annually in value across more than 60 use cases. 1 “ The economic potential of generative AI: The next productivity frontier ,” McKinsey, June 14, 2023. But how much of this value is realized as business growth and productivity will depend on how quickly enterprises can reimagine and truly transform work in priority domains—that is, user journeys, processes across an entire chain of activities, or a function.
Gen-AI-enabled agents hold the promise of accelerating the automation of a very long tail of workflows that would otherwise require inordinate amounts of resources to implement. And the potential extends even beyond these use cases: 60 to 70 percent of the work hours in today’s global economy could theoretically be automated by applying a wide variety of existing technology capabilities, including generative AI, but doing so will require a lot in terms of solutions development and enterprise adoption.
Consider customer service. Currently, the value of gen AI agents in the customer service environment is going to come either from a volume reduction or a reduction in average handling times. For example, in work we published earlier this year, we looked at 5,000 customer service agents using gen AI and found that issue resolution increased by 14 percent an hour, while time spent handling issues went down 9 percent. 2 “ The economic potential of generative AI: The next productivity frontier ,” McKinsey, June 14, 2023.
About QuantumBlack, AI by McKinsey
QuantumBlack, McKinsey’s AI arm, helps companies transform using the power of technology, technical expertise, and industry experts. With thousands of practitioners at QuantumBlack (data engineers, data scientists, product managers, designers, and software engineers) and McKinsey (industry and domain experts), we are working to solve the world’s most important AI challenges. QuantumBlack Labs is our center of technology development and client innovation, which has been driving cutting-edge advancements and developments in AI through locations across the globe.
The other area for value is agent training. Typically, we see that it takes somewhere between six to nine months for a new agent to perform at par with the level of more tenured peers. With this technology, we see that time come down to three months, in some cases, because new agents have at their disposal a vast library of interventions and scripts that have worked in other situations.
Over time, as gen AI agents become more proficient, I expect to see them improve customer satisfaction and generate revenue. By supporting human agents and working autonomously, for example, gen AI agents will be critical not just in helping customers with their immediate questions but also beyond, be that selling new services or addressing broader needs. As companies add more gen AI agents, costs are likely to come down, and this will open up a wider array of customer experience options for companies, such as offering more high-touch interactions with human agents as a premium service.
Barr Seitz: What are the opportunities you are already seeing with gen AI agents?
Jorge Amar: Customer care will be one of the first but definitely not the only function with at-scale AI agents. Over the past year, we have seen a lot of successful pilots with gen AI agents helping to improve customer service functions. For example, you could have a customer service agent who is on the phone with a customer and receives help in real time from a dedicated gen AI agent that is, for instance, recommending the best knowledge article to refer to or what the best next steps are for the conversation. The gen AI agent can also give coaching on behavioral elements, such as tone, empathy, and courtesy.
It used to be the case that dedicating an agent to an individual customer at each point of their sales journey was cost-prohibitive. But, as Lari noted, with the latest developments in gen AI agents, now you can do it.

Nicolai von Bismarck: It’s worth emphasizing that gen AI agents not only automate processes but also support human agents. One thing that gen AI agents are so good at, for example, is in helping customer service representatives get personalized coaching not only from a hard-skill perspective but also in soft skills like understanding the context of what is being said. We estimate that applying generative AI to customer care functions could increase productivity by between 30 to 45 percent. 3 “ The economic potential of generative AI: The next productivity frontier ,” McKinsey, June 14, 2023.
Jorge Amar: Yes, and in other cases, gen AI agents assist the customer directly. A digital sales assistant can assist the customer at every point in their decision journey by, for example, retrieving information or providing product specs or cost comparisons—and then remembering the context if the customer visits, leaves, and returns. As those capabilities grow, we can expect these gen AI agents to generate revenue through upselling.
[For more on how companies are using gen AI agents, see the sidebar, “A closer look at gen AI agents: The Lenovo experience.”]
Barr Seitz: Can you clarify why people should believe that gen AI agents are a real opportunity and not just another false technology promise?
A closer look at gen AI agents: The Lenovo experience
Three leaders at Lenovo —Solutions and Services Group chief technology officer Arthur Hu, COO and head of strategy Linda Yao, and Digital Workplace Solutions general manager Raghav Raghunathan—discuss with McKinsey senior partner Lari Hämäläinen and McKinsey Digital’s Barr Seitz how the company uses generative AI (gen AI) agents.
Barr Seitz: What existing gen AI agent applications has Lenovo been running and what sort of impact have you seen from them?

Arthur Hu: We’ve focused on two main areas. One is software engineering. It’s the low-hanging fruit to help our people enhance speed and quality of code production. Our people are already getting 10 percent improvements, and we’re seeing that increase to 15 percent as teams get better at using gen AI agents.
The second one is about support. We have hundreds of millions of interactions with our customers across online, chat, voice, and email. We’re applying LLM [large language model]-enhanced bots to address customer issues across the entire customer journey and are seeing some great improvements already. We believe it’s possible to address as much as 70 to 80 percent of all customer interactions without needing to pull in a human.

Linda Yao: With our gen AI agents helping support customer service, we’re seeing double-digit productivity gains on call handling time. And we’re seeing incredible gains in other places too. We’re finding that marketing teams, for example, are cutting the time it takes to create a great pitch book by 90 percent and also saving on agency fees.
Barr Seitz: How are you getting ready for a world of gen AI agents?
Linda Yao: I was working with our marketing and sales training teams just this morning as part of a program to develop a learning curriculum for our organization, our partners, and our key customers. We’re figuring out what learning should be at all levels of the business and for different roles.
Arthur Hu: On the tech side, employees need to understand what gen AI agents are and how they can help. It’s critical to be able to build trust or they’ll resist adopting it. In many ways, this is a demystification exercise.

Raghav Raghunathan: We see gen AI as a way to level the playing field in new areas. You don’t need a huge talent base now to compete. We’re investing in tools and workflows to allow us to deliver services with much lower labor intensity and better outcomes.
Barr Seitz: What sort of learning programs are you developing to upskill your people?
Linda Yao: The learning paths for managers, for example, focus on building up their technical acumen, understanding how to change their KPIs because team outputs are changing quickly. At the executive level, it’s about helping leaders develop a strong understanding of the tech so they can determine what’s a good use case to invest in, and which one isn’t.
Arthur Hu: We’ve found that as our software engineers learn how to work with gen AI agents, they go from basically just chatting with them for code snippets to developing much broader thinking and focus. They start to think about changing the software workflow, such as working with gen AI agents on ideation and other parts of the value chain.
Raghav Raghunathan: Gen AI provides an experiential learning capability that’s much more effective. They can prepare sales people for customer interactions or guide them during sales calls. This approach is having a much greater impact than previous learning approaches. It gives them a safe space to learn. They can practice their pitches ahead of time and learn through feedback in live situations.
Barr Seitz: How do you see the future of gen AI agents evolving?
Linda Yao: In our use cases to date, we’ve refined gen AI agents so they act as a good assistant. As we start improving the technology, gen AI agents will become more like deputies that human agents can deploy to do tasks. We’re hoping to see productivity improvements, but we expect this to be a big improvement for the employee experience. These are tasks people don’t want to do.
Arthur Hu: There are lots of opportunities, but one area we’re exploring is how to use gen AI to capture discussions and interactions, and feed the insights and outputs into our development pipeline. There are dozens of points in the customer interaction journey, which means we have tons of data to mine to understand complex intent and even autogenerate new knowledge to address issues.
Jorge Amar: These are still early days, of course, but the kinds of capabilities we’re seeing from gen AI agents are simply unprecedented. Unlike past technologies, for example, gen AI not only can theoretically handle the hundreds of millions of interactions between employees and customers across various channels but also can generate much higher-quality interactions, such as delivering personalized content. And we know that personalized service is a key driver of better customer service. There is a big opportunity here because we found in a survey of customer care executives we ran that less than 10 percent of respondents in North America reported greater-than-expected satisfaction with their customer service performance. 4 “ Where is customer care in 2024? ,” McKinsey, March 12, 2024.
Lari Hämäläinen: Let me take the technology view. This is the first time where we have a technology that is fitted to the way humans interact and can be deployed at enterprise scale. Take, for example, the IVR [interactive voice response] experiences we’ve all suffered through on calls. That’s not how humans interact. Humans interact in an unstructured way, often with unspoken intent. And if you think about LLMs [large language models], they were basically created from their inception to handle unstructured data and interactions. In a sense, all the technologies we applied so far to places like customer service worked on the premise that the customer is calling with a very structured set of thoughts that fit predefined conceptions.
Barr Seitz: How has the gen AI agent landscape changed in the past 12 months?
Lari Hämäläinen: The development of gen AI has been extremely fast. In the early days of LLMs, some of their shortcomings, like hallucinations and relatively high processing costs, meant that models were used to generate pretty basic outputs, like providing expertise to humans or generating images. More complex options weren’t viable. For example, consider that in the case of an LLM with just 80 percent accuracy applied to a task with ten related steps, the cumulative accuracy rate would be just 11 percent.
Today, LLMs can be applied to a wider variety of use cases and more complex workflows because of multiple recent innovations. These include advances in the LLMs themselves in terms of their accuracy and capabilities, innovations in short- and long-term memory structures, developments in logic structures and answer evaluation, and frameworks to apply agents and models to complex workflows. LLMs can evaluate and correct “wrong” answers so that you can have much higher accuracy. With an experienced human in the loop to handle cases that are identified as tricky, then the joint human-plus-machine outcome can generate great quality and great productivity.
Finally, it’s worth mentioning that a lot of gen AI applications beyond chat have been custom-built in the past year by bringing different components together. What we are now seeing is the standardization and industrialization of frameworks to become closer to “packaged software.” This will speed up implementation and improve cost efficiency, making real-world applications even more viable, including addressing the long-tail use cases in enterprises.
Barr Seitz: What sorts of hurdles are you seeing in adopting the gen AI agent technology for customer service?
Nicolai von Bismarck: One big hurdle we’re seeing is building trust across the organization in gen AI agents. At one bank, for example, they knew they needed to cut down on wrong answers to build trust. So they created an architecture that checks for hallucinations. Only when the check confirms that the answer is correct is it released. And if the answer isn’t right, the chatbot would say that it cannot answer this question and try to rephrase it. The customer is then able to either get an answer to their question quickly or decide that they want to talk to a live agent. That’s really valuable, as we find that customers across all age groups — even Gen Z — still prefer live phone conversations for customer help and support. .
Jorge Amar: We are seeing very promising results, but these are in controlled environments with a small group of customers or agents. To scale these results, change management will be critical. That’s a big hurdle for organizations. It’s much broader than simply rolling out a new set of tools. Companies are going to need to rewire how functions work so they can get the full value from gen AI agents.
Take data, which needs to be in the right format and place for gen AI technologies to use them effectively. Almost 20 percent of most organizations, in fact, see data as the biggest challenge to capturing value with gen AI. 5 “ The state of AI in 2023: Generative AI’s breakout year ,” McKinsey, August 1, 2023. One example of this kind of issue could be a chatbot sourcing outdated information, like a policy that was used during COVID-19, in delivering an answer. The content might be right, but it’s hopelessly out of date. Companies are going to need to invest in cleaning and organizing their data.
In addition, companies need a real commitment to building AI trust and governance capabilities. These are the principles, policies, processes, and platforms that assure companies are not just compliant with fast-evolving regulations—as seen in the recent EU AI law and similar actions in many countries—but also able to keep the kinds of commitments that they make to customers and employees in terms of fairness and lack of bias. This will also require new learning, new levels of collaboration with legal and risk teams, and new technology to manage and monitor systems at scale.
Change needs to happen in other areas as well. Businesses will need to build extensive and tailored learning curricula for all levels of the customer service function—from managers who will need to create new KPIs and performance management protocols to frontline agents who will need to understand different ways to engage with both customers and gen AI agents.
The technology will need to evolve to be more flexible and develop a stronger life cycle capability to support gen AI tools, what we’d call MLOps [machine learning operations] or, increasingly, gen AI Ops [gen AI operations]. The operating model will need to support small teams working iteratively on new service capabilities. And adoption will require sustained effort and new incentives so that people learn to trust the tools and realize the benefits. This is particularly true with more tenured agents, who believe their own skills cannot be augmented or improved on with gen AI agents. For customer operations alone, we’re talking about a broad effort here, but with more than $400 billion of potential value from gen AI at stake, it’s worth it. 6 “ The economic potential of generative AI: The next productivity frontier ,” McKinsey, June 14, 2023.
Barr Seitz: Staying with customer service, how will gen AI agents help enterprises?
Jorge Amar: This is a great question, because we believe the immediate impact comes from augmenting the work that humans do even as broader automation happens. My belief is that gen AI agents can and will transform various corporate services and workflows. It will help us automate a lot of tasks that were not adding value while creating a better experience for both employees and customers. For example, corporate service centers will become more productive and have better outcomes and deliver better experiences.
In fact, we’re seeing this new technology help reduce employee attrition. As gen AI becomes more pervasive, we may see an emergence of more specialization in service work. Some companies and functions will lead adoption and become fully automated, and some may differentiate by building more high-touch interactions.
Nicolai von Bismarck: As an example, we’re seeing this idea in practice at one German company, which is implementing an AI-based learning and coaching engine. And it’s already seeing a significant improvement in the employee experience as measured while it’s rolling this out, both from a supervisor and employee perspective, because the employees feel that they’re finally getting feedback that is relevant to them. They’re feeling valued, they’re progressing in their careers, and they’re also learning new skills. For instance, instead of taking just retention calls, they can now take sales calls. This experience is providing more variety in the work that people do and less dull repetition.
Lari Hämäläinen: Let me take a broader view. We had earlier modeled a midpoint scenario when 50 percent of today’s work activities could be automated to occur around 2055. But the technology is evolving so much more quickly than anyone had expected—just look at the capabilities of some LLMs that are approaching, and even surpassing, in certain cases, average human levels of proficiency. The innovations in gen AI have helped accelerate that midpoint scenario by about a decade. And it’s going to keep getting faster, so we can expect the adoption timeline to shrink even further. That’s a crucial development that every executive needs to understand.
Jorge Amar is a senior partner in McKinsey’s Miami office, Lari Hämäläinen is a senior partner in the Seattle office, and Nicolai von Bismarck is a partner in the Boston office. Barr Seitz is director of global publishing for McKinsey Digital and is based in the New York office.
Explore a career with us
Related articles.

Moving past gen AI’s honeymoon phase: Seven hard truths for CIOs to get from pilot to scale

Creating a European AI unicorn: Interview with Arthur Mensch, CEO of Mistral AI
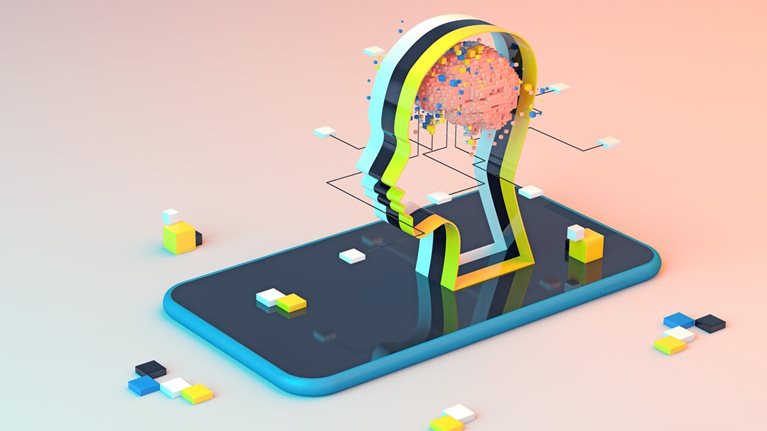
Why AI-enabled customer service is key to scaling telco personalization
Download our App for Study Materials and Placement Preparation 📝✅ | Click Here
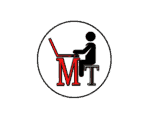
Get Latest Exam Updates, Free Study materials and Tips
Your Branch Computer Engineering IT Engineering EXTC Engineering Mechanical Engineering Civil Engineering Others.. Year Of Engineering First Year Second Year Third Year Final Year
Clear Your Aptitude In Very First Attempt!
What's Included!
What's included, 6+ exercise.

[MCQ’s] Artificial Intelligence
Introduction to intelligent systems and intelligent agents, search techniques, knowledge and reasoning, uncertain knowledge and reasoning, natural language processing.
1. What is the main task of a problem-solving agent? A. Solve the given problem and reach to goal B. To find out which sequence of action will get it to the goal state C. Both A and B D. None of the Above Ans : C Explanation: The problem-solving agents are one of the goal-based agents
2. What is Initial state + Goal state in Search Terminology? A. Problem Space B. Problem Instance C. Problem Space Graph D. Admissibility Ans : B Explanation: Problem Instance : It is Initial state + Goal state.
3. What is Time Complexity of Breadth First search algorithm? A. b B. b^d C. b^2 D. b^b Ans : B Explanation: Time Complexity of Breadth First search algorithm is b^d.
4. Depth-First Search is implemented in recursion with _______ data structure. A. LIFO B. LILO C. FIFO D. FILO Ans : A Explanation: Depth-First Search implemented in recursion with LIFO stack data structure.
5. How many types are available in uninformed search method? A. 2 B. 3 C. 4 D. 5 Ans : D Explanation: The five types of uninformed search method are Breadth-first, Uniform-cost, Depth-first, Depth-limited and Bidirectional search.
6. Which data structure conveniently used to implement BFS? A. Stacks B. Queues C. Priority Queues D. None of the Above Ans : B Explanation: Queue is the most convenient data structure, but memory used to store nodes can be reduced by using circular queues.
7. How many types of informed search method are in artificial intelligence? A. 2 B. 3 C. 4 D. 5 Ans : C Explanation: The four types of informed search method are best-first search, Greedy best-first search, A* search and memory bounded heuristic search.
8. Greedy search strategy chooses the node for expansion in ___________ A. Shallowest B. Deepest C. The one closest to the goal node D. Minimum heuristic cost Ans : C Explanation: Sometimes minimum heuristics can be used, sometimes maximum heuristics function can be used. It depends upon the application on which the algorithm is applied.
9. What is disadvantage of Greedy Best First Search? A. This algorithm is neither complete, nor optimal. B. It can get stuck in loops. It is not optimal. C. There can be multiple long paths with the cost ≤ C* D. may not terminate and go on infinitely on one path Ans : B Explanation: The disadvantage of Greedy Best First Search is that it can get stuck in loops. It is not optimal.
10. Searching using query on Internet is, use of ___________ type of agent. A. Offline agent B. Online Agent C. Goal Based D. Both B and C Ans : D Explanation: Refer to the definitions of both the type of agent.
11. An AI system is composed of? A. agent B. environment C. Both A and B D. None of the Above Ans : C Explanation: An AI system is composed of an agent and its environment.
12. Which instruments are used for perceiving and acting upon the environment? A. Sensors and Actuators B. Sensors C. Perceiver D. Perceiver and Sensor Ans : A Explanation: An agent is anything that can be viewed as perceiving and acting upon the environment through the sensors and actuators.
13. Which of the following is not a type of agents in artificial intelligence? A. Model based B. Utility based C. Simple reflex D. target based Ans : D Explanation: The four types of agents are Simple reflex, Model based, Goal based and Utility based agents.
14. Which is used to improve the agents performance? A. Perceiving B. Observing C. Learning D. Sequence Ans : C Explanation: An agent can improve its performance by storing its previous actions.
15. Rationality of an agent does not depends on? A. performance measures B. Percept Sequence C. reaction D. actions Ans : C Explanation: Rationality of an agent does not depends on reaction
16. Agent’s structure can be viewed as ? A. Architecture B. Agent Program C. Architecture + Agent Program D. None of the Above Ans : C Explanation: Agent’s structure can be viewed as – Agent = Architecture + Agent Program
17. What is the action of task environment in artificial intelligence? A. Problem B. Solution C. Agent D. Observation Ans : A Explanation: Task environments will pose a problem and rational agent will find the solution for the posed problem.
18. What kind of environment is crossword puzzle? A. Dynamic B. Static C. Semi Dynamic D. Continuous Ans : B Explanation: As the problem in crossword puzzle are posed at beginning itself, So it is static.
19. What could possibly be the environment of a Satellite Image Analysis System? A. Computers in space and earth B. Image categorization techniques C. Statistical data on image pixel intensity value and histograms D. All of the above Ans : D Explanation: An environment is something which agent stays in.
20. Which kind of agent architecture should an agent an use? A. Relaxed B. Relational C. Both A and B D. None of the AboveAns : C Explanation: Because an agent may experience any kind of situation, So that an agent should use all kinds of architecture.
21. Which depends on the percepts and actions available to the agent? a) Agent b) Sensor c) Design problem d) None of the mentioned Answer: c Explanation: The design problem depends on the percepts and actions available to the agent, the goals that the agent’s behavior should satisfy.
22. Which were built in such a way that humans had to supply the inputs and interpret the outputs? a) Agents b) AI system c) Sensor d) Actuators Answer: b Explanation: AI systems were built in such a way that humans had to supply the inputs and interpret the outputs.
23. Which technology uses miniaturized accelerometers and gyroscopes? a) Sensors b) Actuators c) MEMS d) None of the mentioned Answer: c Explanation: Micro ElectroMechanical System uses miniaturized accelerometers and gyroscopes and is used to produce actuators.
24. What is used for tracking uncertain events? a) Filtering algorithm b) Sensors c) Actuators d) None of the mentioned Answer: a Explanation: Filtering algorithm is used for tracking uncertain events because in this the real perception is involved.
25. What is not represented by using propositional logic? a) Objects b) Relations c) Both Objects & Relations d) None of the mentioned Answer: c Explanation: Objects and relations are not represented by using propositional logic explicitly.
26. Which functions are used as preferences over state history? a) Award b) Reward c) Explicit d) Implicit Answer: b Explanation: Reward functions may be that preferences over states are really compared from preferences over state histories.
27. Which kind of agent architecture should an agent an use? a) Relaxed b) Logic c) Relational d) All of the mentioned Answer: d Explanation: Because an agent may experience any kind of situation, So that an agent should use all kinds of architecture.
28. Specify the agent architecture name that is used to capture all kinds of actions. a) Complex b) Relational c) Hybrid d) None of the mentioned Answer: c Explanation: A complete agent must be able to do anything by using hybrid architecture.
29. Which agent enables the deliberation about the computational entities and actions? a) Hybrid b) Reflective c) Relational d) None of the mentioned Answer: b Explanation: Because it enables the agent to capture within itself.
30. What can operate over the joint state space? a) Decision-making algorithm b) Learning algorithm c) Complex algorithm d) Both Decision-making & Learning algorithm
31. What is the action of task environment in artificial intelligence? a) Problem b) Solution c) Agent d) Observation Answer: a Explanation: Task environments will pose a problem and rational agent will find the solution for the posed problem.
32. What is the expansion if PEAS in task environment? a) Peer, Environment, Actuators, Sense b) Perceiving, Environment, Actuators, Sensors c) Performance, Environment, Actuators, Sensors d) None of the mentioned Answer: c Explanation: Task environment will contain PEAS which is used to perform the action independently.
33. What kind of observing environments are present in artificial intelligence? a) Partial b) Fully c) Learning d) Both Partial & Fully Answer: d Explanation: Partial and fully observable environments are present in artificial intelligence.
34. What kind of environment is strategic in artificial intelligence? a) Deterministic b) Rational c) Partial d) Stochastic Answer: a Explanation: If the environment is deterministic except for the action of other agent is called deterministic.
35. What kind of environment is crossword puzzle? a) Static b) Dynamic c) Semi Dynamic d) None of the mentioned Answer: a Explanation: As the problem in crossword puzzle are posed at beginning itself, So it is static.
36. What kind of behavior does the stochastic environment posses? a) Local b) Deterministic c) Rational d) Primary Answer: a Explanation: Stochastic behavior are rational because it avoids the pitfall of predictability.
37. Which is used to select the particular environment to run the agent? a) Environment creator b) Environment Generator c) Both Environment creator & Generator d) None of the mentioned Answer: b Explanation: None.
38. Which environment is called as semi dynamic? a) Environment does not change with the passage of time b) Agent performance changes c) Environment will be changed d) Environment does not change with the passage of time, but Agent performance changes Answer: d Explanation: If the environment does not change with the passage of time, but the agent performance changes by time.
39. Where does the performance measure is included? a) Rational agent b) Task environment c) Actuators d) Sensor Answer: b Explanation: In PEAS, Where P stands for performance measure which is always included in task environment.
1. Which search strategy is also called as blind search? a) Uninformed search b) Informed search c) Simple reflex search d) All of the mentioned Answer: a Explanation: In blind search, We can search the states without having any additional information. So uninformed search method is blind search.
2. How many types are available in uninformed search method? a) 3 b) 4 c) 5 d) 6 Answer: c Explanation: The five types of uninformed search method are Breadth-first, Uniform-cost, Depth-first, Depth-limited and Bidirectional search.
3. Which search is implemented with an empty first-in-first-out queue? a) Depth-first search b) Breadth-first search c) Bidirectional search d) None of the mentioned Answer: b Explanation: Because of FIFO queue, it will assure that the nodes that are visited first will be expanded first.
4. When is breadth-first search is optimal? a) When there is less number of nodes b) When all step costs are equal c) When all step costs are unequal d) None of the mentioned Answer: b Explanation: Because it always expands the shallowest unexpanded node.
5. How many successors are generated in backtracking search? a) 1 b) 2 c) 3 d) 4 Answer: a Explanation: Each partially expanded node remembers which successor to generate next because of these conditions, it uses less memory.
6. What is the space complexity of Depth-first search? a) O(b) b) O(bl) c) O(m) d) O(bm) Answer: d Explanation: O(bm) is the space complexity where b is the branching factor and m is the maximum depth of the search tree.
7. How many parts does a problem consists of? a) 1 b) 2 c) 3 d) 4 Answer: d Explanation: The four parts of the problem are initial state, set of actions, goal test and path cost.
8. Which algorithm is used to solve any kind of problem? a) Breadth-first algorithm b) Tree algorithm c) Bidirectional search algorithm d) None of the mentioned Answer: b Explanation: Tree algorithm is used because specific variants of the algorithm embed different strategies.
9. Which search algorithm imposes a fixed depth limit on nodes? a) Depth-limited search b) Depth-first search c) Iterative deepening search d) Bidirectional search Answer: a Explanation: None.
10. Which search implements stack operation for searching the states? a) Depth-limited search b) Depth-first search c) Breadth-first search d) None of the mentioned Answer: b
11. What is the other name of informed search strategy? a) Simple search b) Heuristic search c) Online search d) None of the mentioned Answer: b Explanation: A key point of informed search strategy is heuristic function, So it is called as heuristic function.
12. How many types of informed search method are in artificial intelligence? a) 1 b) 2 c) 3 d) 4 Answer: d Explanation: The four types of informed search method are best-first search, Greedy best-first search, A* search and memory bounded heuristic search.
13. Which search uses the problem specific knowledge beyond the definition of the problem? a) Informed search b) Depth-first search c) Breadth-first search d) Uninformed search Answer: a Explanation: Informed search can solve the problem beyond the function definition, So does it can find the solution more efficiently.
14. Which function will select the lowest expansion node at first for evaluation? a) Greedy best-first search b) Best-first search c) Depth-first search d) None of the mentioned Answer: b Explanation: The lowest expansion node is selected because the evaluation measures distance to the goal.
15. What is the heuristic function of greedy best-first search? a) f(n) != h(n) b) f(n) < h(n) c) f(n) = h(n) d) f(n) > h(n) Answer: c Explanation: None.
16. Which search uses only the linear space for searching? a) Best-first search b) Recursive best-first search c) Depth-first search d) None of the mentioned Answer: b Explanation: Recursive best-first search will mimic the operation of standard best-first search, but using only the linear space.
17. Which method is used to search better by learning? a) Best-first search b) Depth-first search c) Metalevel state space d) None of the mentioned Answer: c Explanation: This search strategy will help to problem solving efficiency by using learning.
18. Which search is complete and optimal when h(n) is consistent? a) Best-first search b) Depth-first search c) Both Best-first & Depth-first search d) A* search Answer: d Explanation: None.
19. Which is used to improve the performance of heuristic search? a) Quality of nodes b) Quality of heuristic function c) Simple form of nodes d) None of the mentioned Answer: b Explanation: Good heuristic can be constructed by relaxing the problem, So the performance of heuristic search can be improved.
20. Which search method will expand the node that is closest to the goal? a) Best-first search b) Greedy best-first search c) A* search d) None of the mentioned Answer: b Explanation: Because of using greedy best-first search, It will quickly lead to the solution of the problem.
21. In many problems the path to goal is irrelevant, this class of problems can be solved using ____________ a) Informed Search Techniques b) Uninformed Search Techniques c) Local Search Techniques d) Informed & Uninformed Search Techniques Answer: c Explanation: If the path to the goal does not matter, we might consider a different class of algorithms, ones that do not worry about paths at all. Local search algorithms operate using a single current state (rather than multiple paths) and generally move only to neighbors of that state.
22. Though local search algorithms are not systematic, key advantages would include __________ a) Less memory b) More time c) Finds a solution in large infinite space d) Less memory & Finds a solution in large infinite space Answer: d Explanation: Two advantages: (1) they use very little memory-usually a constant amount; and (2) they can often find reasonable solutions in large or infinite (continuous) state spaces for which systematic algorithms are unsuitable.
23. A complete, local search algorithm always finds goal if one exists, an optimal algorithm always finds a global minimum/maximum. a) True b) False Answer: a Explanation: An algorithm is complete if it finds a solution if exists and optimal if finds optimal goal (minimum or maximum).
24. _______________ Is an algorithm, a loop that continually moves in the direction of increasing value – that is uphill. a) Up-Hill Search b) Hill-Climbing c) Hill algorithm d) Reverse-Down-Hill search Answer: b Explanation: Refer the definition of Hill-Climbing approach.
25. When will Hill-Climbing algorithm terminate? a) Stopping criterion met b) Global Min/Max is achieved c) No neighbor has higher value d) All of the mentioned Answer: c Explanation: When no neighbor is having higher value, algorithm terminates fetching local min/max.
26. What are the main cons of hill-climbing search? a) Terminates at local optimum & Does not find optimum solution b) Terminates at global optimum & Does not find optimum solution c) Does not find optimum solution & Fail to find a solution d) Fail to find a solution View Answer Answer: a Explanation: Algorithm terminates at local optimum values, hence fails to find optimum solution.
27. Stochastic hill climbing chooses at random from among the uphill moves; the probability of selection can vary with the steepness of the uphil1 move. a) True b) False Answer: a Explanation: Refer to the definition of variants of hill-climbing search.
28. Hill climbing sometimes called ____________ because it grabs a good neighbor state without thinking ahead about where to go next. a) Needy local search b) Heuristic local search c) Greedy local search d) Optimal local search Answer: c Explanation: None.
29. Hill-Climbing approach stuck for which of the following reasons? a) Local maxima b) Ridges c) Plateaux d) All of the mentioned Answer: d Explanation: Local maxima: a local maximum is a peak that is higher than each of its neighboring states, but lower than the global maximum. Ridges: Ridges result in a sequence of local maxima that is very difficult for greedy algorithms to navigate. Plateaux: a plateau is an area of the state space landscape where the evaluation function is flat.
30. ___________ algorithm keeps track of k states rather than just one. a) Hill-Climbing search b) Local Beam search c) Stochastic hill-climbing search d) Random restart hill-climbing search Answer: b Explanation: Refer to the definition of Local Beam Search algorithm.
31. A genetic algorithm (or GA) is a variant of stochastic beam search in which successor states are generated by combining two parent states, rather than by modifying a single state. a) True b) False Answer: a Explanation: Stochastic beam search, analogous to stochastic hill climbing, helps to alleviate this problem. Instead of choosing the best k from the pool of candidate successors, stochastic beam search chooses k successors at random, with the probability of choosing a given successor being an increasing function of its value.
32. What are the two main features of Genetic Algorithm? a) Fitness function & Crossover techniques b) Crossover techniques & Random mutation c) Individuals among the population & Random mutation d) Random mutation & Fitness function Answer: a Explanation: Fitness function helps choosing individuals from the population and Crossover techniques defines the offspring generated.
33. Searching using query on Internet is, use of ___________ type of agent. a) Offline agent b) Online agent c) Both Offline & Online agent d) Goal Based & Online agent Answer: d Explanation: Refer to the definitions of both the type of agent.
34. In how many directions do queens attack each other? a) 1 b) 2 c) 3 d) 4
Answer: c Explanation: Queens attack each other in three directions- vertical, horizontal and diagonal.
35. Placing n-queens so that no two queens attack each other is called? a) n-queen’s problem b) 8-queen’s problem c) Hamiltonian circuit problem d) subset sum problem
Answer: a Explanation: Placing n queens so that no two queens attack each other is n-queens problem. If n=8, it is called as 8-queens problem.
36. Where is the n-queens problem implemented? a) carom b) chess c) ludo d) cards
Answer: b Explanation: N-queens problem occurs in chess. It is the problem of placing n- queens in a n*n chess board.
37. Not more than 2 queens can occur in an n-queens problem. a) true b) false
Answer: b Explanation: Unlike a real chess game, n-queens occur in a n-queen problem since it is the problem of dealing with n-queens.
38. In n-queen problem, how many values of n does not provide an optimal solution? a) 1 b) 2 c) 3 d) 4
Answer: b Explanation: N-queen problem does not provide an optimal solution of only three values of n (i.e.) n=2,3.
39. Which of the following methods can be used to solve n-queen’s problem? a) greedy algorithm b) divide and conquer c) iterative improvement d) backtracking
Answer: d Explanation: Of the following given approaches, n-queens problem can be solved using backtracking. It can also be solved using branch and bound.
40. Of the following given options, which one of the following is a correct option that provides an optimal solution for 4-queens problem? a) (3,1,4,2) b) (2,3,1,4) c) (4,3,2,1) d) (4,2,3,1)
Answer: a Explanation: Of the following given options for optimal solutions of 4-queens problem, (3, 1, 4, 2) is the right option.
41. How many possible solutions exist for an 8-queen problem? a) 100 b) 98 c) 92 d) 88
Answer: c Explanation: For an 8-queen problem, there are 92 possible combinations of optimal solutions.
42. How many possible solutions occur for a 10-queen problem? a) 850 b) 742 c) 842 d) 724
Answer: d Explanation: For a 10-queen problem, 724 possible combinations of optimal solutions are available.
43. If n=1, an imaginary solution for the problem exists. a) true b) false

44. What is the domination number for 8-queen’s problem? a) 8 b) 7 c) 6 d) 5
Answer: d Explanation: The minimum number of queens needed to occupy every square in n-queens problem is called domination number. While n=8, the domination number is 5.
45. Of the following given options, which one of the following does not provides an optimal solution for 8-queens problem? a) (5,3,8,4,7,1,6,2) b) (1,6,3,8,3,2,4,7) c) (4,1,5,8,6,3,7,2) d) (6,2,7,1,4,8,5,3)
Answer: b Explanation: The following given options for optimal solutions of 8-queens problem, (1,6,3,8,3,2,4,7) does not provide an optimal solution.
46. General games involves ____________ a) Single-agent b) Multi-agent c) Neither Single-agent nor Multi-agent d) Only Single-agent and M0ulti-agent
Answer: d Explanation: Depending upon games it could be single agent (Sudoku) or multi-agent (Chess).
47. Adversarial search problems uses ____________ a) Competitive Environment b) Cooperative Environment c) Neither Competitive nor Cooperative Environment d) Only Competitive and Cooperative Environment
Answer: a Explanation: Since in cooperative environment agents’ goals are I conflicts. They compete for goal.
48. Mathematical game theory, a branch of economics, views any multi-agent environment as a game provided that the impact of each agent on the others is “significant,” regardless of whether the agents are cooperative or competitive. a) True b) False
Answer: a Explanation: None.
49. Zero sum games are the one in which there are two agents whose actions must alternate and in which the utility values at the end of the game are always the same. a) True b) False
Answer: b Explanation: Utility values are always same and opposite.
50. Zero sum game has to be a ______ game. a) Single player b) Two player c) Multiplayer d) Three player
Answer: c Explanation: Zero sum games could be multiplayer games as long as the condition for zero sum game is satisfied.
51. A game can be formally defined as a kind of search problem with the following components. a) Initial State b) Successor Function c) Terminal Test d) All of the mentioned
Answer: d Explanation: The initial state includes the board position and identifies the player to move. A successor function returns a list of (move, state) pairs, each indicating a legal move and the resulting state. A terminal test determines when the game is over. States where the game has ended are called terminal states. A utility function (also called an objective function or payoff function), which gives a numeric value for the terminal states. In chess, the outcome is a win, lose, or draw, with values +1, -1, or 0.
52. The initial state and the legal moves for each side define the __________ for the game. a) Search Tree b) Game Tree c) State Space Search d) Forest
Answer: b Explanation: An example of game tree for Tic-Tac-Toe game.
53. General algorithm applied on game tree for making decision of win/lose is ____________ a) DFS/BFS Search Algorithms b) Heuristic Search Algorithms c) Greedy Search Algorithms d) MIN/MAX Algorithms
Answer: d Explanation: Given a game tree, the optimal strategy can be determined by examining the min/max value of each node, which we write as MINIMAX- VALUE(n). The min/max value of a node is the utility (for MAX) of being in the corresponding state, assuming that both players play optimally from there to the end of the game. Obviously, the min/max value of a terminal state is just its utility. Furthermore, given a choice, MAX will prefer to move to a state of maximum value, whereas MIN prefers a state of minimum value.
54. The minimax algorithm computes the minimax decision from the current state. It uses a simple recursive computation of the minimax values of each successor state, directly implementing the defining equations. The recursion proceeds all the way down to the leaves of the tree, and then the minimax values are backed up through the tree as the recursion unwinds. a) True b) False
Answer: a Explanation: Refer definition of minimax algorithm.
55. What is the complexity of minimax algorithm? a) Same as of DFS b) Space – bm and time – bm c) Time – bm and space – bm d) Same as BFS
Answer: a Explanation: Same as DFS.
56. Which search is equal to minimax search but eliminates the branches that can’t influence the final decision? a) Depth-first search b) Breadth-first search c) Alpha-beta pruning d) None of the mentioned
Answer: c Explanation: The alpha-beta search computes the same optimal moves as minimax, but eliminates the branches that can’t influence the final decision.
57. Which values are independant in minimax search algorithm? a) Pruned leaves x and y b) Every states are dependant c) Root is independant d) None of the mentioned
Answer: a Explanation: The minimax decision are independant of the values of the pruned values x and y because of the root values.
58. To which depth does the alpha-beta pruning can be applied? a) 10 states b) 8 States c) 6 States d) Any depth
Answer: d Explanation: Alpha–beta pruning can be applied to trees of any depth and it is possible to prune entire subtree rather than leaves.
59. Which search is similar to minimax search? a) Hill-climbing search b) Depth-first search c) Breadth-first search d) All of the mentioned
Answer: b Explanation: The minimax search is depth-first search, So at one time we just have to consider the nodes along a single path in the tree.
60. Which value is assigned to alpha and beta in the alpha-beta pruning? a) Alpha = max b) Beta = min c) Beta = max d) Both Alpha = max & Beta = min
Answer: d Explanation: Alpha and beta are the values of the best choice we have found so far at any choice point along the path for MAX and MIN.
61. Where does the values of alpha-beta search get updated? a) Along the path of search b) Initial state itself c) At the end d) None of the mentioned
Answer: a Explanation: Alpha-beta search updates the value of alpha and beta as it gets along and prunes the remaining branches at node.
62. How the effectiveness of the alpha-beta pruning gets increased? a) Depends on the nodes b) Depends on the order in which they are executed c) All of the mentioned d) None of the mentioned
63. What is called as transposition table? a) Hash table of next seen positions b) Hash table of previously seen positions c) Next value in the search d) None of the mentioned
Answer: b Explanation: Transposition is the occurrence of repeated states frequently in the search.
64. Which is identical to the closed list in Graph search? a) Hill climbing search algorithm b) Depth-first search c) Transposition table d) None of the mentioned
65. Which function is used to calculate the feasibility of whole game tree? a) Evaluation function b) Transposition c) Alpha-beta pruning d) All of the mentioned
Answer: a Explanation: Because we need to cut the search off at some point and apply an evaluation function that gives an estimate of the utility of the state.
1. There exist only two types of quantifiers, Universal Quantification and Existential Quantification. a) True b) False Answer: a Explanation: None.
2. Translate the following statement into FOL. “For every a, if a is a philosopher, then a is a scholar” a) ∀ a philosopher(a) scholar(a) b) ∃ a philosopher(a) scholar(a) c) All of the mentioned d) None of the mentioned Answer: a Explanation: None.
3. A _________ is used to demonstrate, on a purely syntactic basis, that one formula is a logical consequence of another formula. a) Deductive Systems b) Inductive Systems c) Reasoning with Knowledge Based Systems d) Search Based Systems Answer: a Explanation: Refer the definition of Deductive based systems.
4. The statement comprising the limitations of FOL is/are ____________ a) Expressiveness b) Formalizing Natural Languages c) Many-sorted Logic d) All of the mentioned Answer: d Explanation: The Löwenheim–Skolem theorem shows that if a first-order theory has any infinite model, then it has infinite models of every cardinality. In particular, no first-order theory with an infinite model can be categorical. Thus there is no first-order theory whose only model has the set of natural numbers as its domain, or whose only model has the set of real numbers as its domain. Many extensions of first-order logic, including infinitely logics and higher-order logics, are more expressive in the sense that they do permit categorical axiomatizations of the natural numbers or real numbers. This expressiveness comes at a meta-logical cost, however: by Lindström’s theorem, the compactness theorem and the downward Löwenheim–Skolem theorem cannot hold in any logic stronger than first-order. Formalizing Natural Languages : First-order logic is able to formalize many simple quantifier constructions in natural language, such as “every person who lives in Perth lives in Australia”. But there are many more complicated features of natural language that cannot be expressed in (single-sorted) first-order logic. Many-sorted Logic: Ordinary first-order interpretations have a single domain of discourse over which all quantifiers range. Many-sorted first-order logic allows variables to have different sorts, which have different domains.
5. A common convention is: • is evaluated first • and are evaluated next • Quantifiers are evaluated next • is evaluated last. a) True b) False Answer: a Explanation: None.
6. A Term is either an individual constant (a 0-ary function), or a variable, or an n-ary function applied to n terms: F(t1 t2 ..tn). a) True b) False Answer: a Explanation: Definition of term in FOL.
7. First Order Logic is also known as ___________ a) First Order Predicate Calculus b) Quantification Theory c) Lower Order Calculus d) All of the mentioned Answer: d Explanation: None.
8. The adjective “first-order” distinguishes first-order logic from ___________ in which there are predicates having predicates or functions as arguments, or in which one or both of predicate quantifiers or function quantifiers are permitted. a) Representational Verification b) Representational Adequacy c) Higher Order Logic d) Inferential Efficiency Answer: c Explanation: None.
9. Which is created by using single propositional symbol? a) Complex sentences b) Atomic sentences c) Composition sentences d) None of the mentioned Answer: b Explanation: Atomic sentences are indivisible syntactic elements consisting of single propositional symbol.
10. Which is used to construct the complex sentences? a) Symbols b) Connectives c) Logical connectives d) All of the mentioned Answer: c Explanation: None.
11. How many proposition symbols are there in artificial intelligence? a) 1 b) 2 c) 3 d) 4 Answer: b Explanation: The two proposition symbols are true and false.
12. How many logical connectives are there in artificial intelligence? a) 2 b) 3 c) 4 d) 5 Answer: d Explanation: The five logical symbols are negation, conjunction, disjunction, implication and biconditional.
13. Which is used to compute the truth of any sentence? a) Semantics of propositional logic b) Alpha-beta pruning c) First-order logic d) Both Semantics of propositional logic & Alpha-beta pruning Answer: a Explanation: Because the meaning of the sentences is really needed to compute the truth.
14. Which are needed to compute the logical inference algorithm? a) Logical equivalence b) Validity c) Satisfiability d) All of the mentioned Answer: d Explanation: Logical inference algorithm can be solved be using logical equivalence, Validity and satisfiability.
15. From which rule does the modus ponens are derived? a) Inference rule b) Module rule c) Both Inference & Module rule d) None of the mentioned Answer: a Explanation: Inference rule contains the standard pattern that leads to desired goal. The best form of inference rule is modus ponens.
16. Which is also called single inference rule? a) Reference b) Resolution c) Reform d) None of the mentioned Answer: b Explanation: Because resolution yields a complete inference rule when coupled with any search algorithm.
17. Which form is called as a conjunction of disjunction of literals? a) Conjunctive normal form b) Disjunctive normal form c) Normal form d) All of the mentioned Answer: a Explanation: None.
18. What can be viewed as a single lateral of disjunction? a) Multiple clause b) Combine clause c) Unit clause d) None of the mentioned Answer: c Explanation: A single literal can be viewed as a disjunction or one literal also, called a unit clause.
19. Which is a refutation complete inference procedure for propositional logic? a) Clauses b) Variables c) Propositional resolution d) Proposition Answer: c Explanation: Propositional resolution is a refutation complete inference procedure for propositional logic.
20. What kind of clauses are available in Conjunctive Normal Form? a) Disjunction of literals b) Disjunction of variables c) Conjunction of literals d) Conjunction of variables Answer: a Explanation: First-order resolution requires the clause to be in disjunction of literals in Conjunctive Normal Form.
21. What is the condition of literals in variables? a) Existentially quantified b) Universally quantified c) Quantified d) None of the mentioned Answer: b Explanation: Literals that contain variables are assumed to be universally quantified.
22. Which can be converted to inferred equivalent CNF sentence? a) Every sentence of propositional logic b) Every sentence of inference c) Every sentence of first-order logic d) All of the mentioned Answer: c Explanation: Every sentence of first-order logic can be converted to inferred equivalent CNF sentence.
23. Which sentence will be unsatisfiable if the CNF sentence is unsatisfiable? a) Search statement b) Reading statement c) Replaced statement d) Original statement Answer: d Explanation: The CNF statement will be unsatisfiable just when the original sentence is unsatisfiable.
24. Which rule is equal to the resolution rule of first-order clauses? a) Propositional resolution rule b) Inference rule c) Resolution rule d) None of the mentioned Answer: a Explanation: The resolution rule for first-order clauses is simply a lifted version of the propositional resolution rule.
25. At which state does the propositional literals are complementary? a) If one variable is less b) If one is the negation of the other c) All of the mentioned d) None of the mentioned Answer: b Explanation: Propositional literals are complementary if one is the negation of the other.
26. What is meant by factoring? a) Removal of redundant variable b) Removal of redundant literal c) Addition of redundant literal d) Addition of redundant variable Answer: b Explanation: None.
27. What will happen if two literals are identical? a) Remains the same b) Added as three c) Reduced to one d) None of the mentioned Answer: c Explanation: Propositional factoring reduces two literals to one if they are identical.
28. When the resolution is called as refutation-complete? a) Sentence is satisfiable b) Sentence is unsatisfiable c) Sentence remains the same d) None of the mentioned Answer: b Explanation: Resolution is refutation-complete, if a set of sentence is unsatisfiable, then resolution will always be able to derive a contradiction.
29. Which condition is used to cease the growth of forward chaining? a) Atomic sentences b) Complex sentences c) No further inference d) All of the mentioned Answer: c Explanation: Forward chain can grow by adding new atomic sentences until no further inference is made.
30. Which closely resembles propositional definite clause? a) Resolution b) Inference c) Conjunction d) First-order definite clauses Answer: d Explanation: Because they are disjunction of literals of which exactly one is positive.
31. What is the condition of variables in first-order literals? a) Existentially quantified b) Universally quantified c) Both Existentially & Universally quantified d) None of the mentioned Answer: b Explanation: First-order literals will accept variables only if they are universally quantified.
32. Which are more suitable normal form to be used with definite clause? a) Positive literal b) Negative literal c) Generalized modus ponens d) Neutral literal Answer: c Explanation: Definite clauses are a suitable normal form for use with generalized modus ponen.
33. Which will be the instance of the class datalog knowledge bases? a) Variables b) No function symbols c) First-order definite clauses d) None of the mentioned Answer: b Explanation: If the knowledge base contains no function symbols means, it is an instance of the class datalog knowledge base.
34. Which knowledge base is called as fixed point? a) First-order definite clause are similar to propositional forward chaining b) First-order definite clause are mismatch to propositional forward chaining c) All of the mentioned d) None of the mentioned Answer: a Explanation: Fixed point reached by forward chaining with first-order definiteclause are similar to those for propositional forward chaining.
35. How to eliminate the redundant rule matching attempts in the forward chaining? a) Decremental forward chaining b) Incremental forward chaining c) Data complexity d) None of the mentioned Answer: b Explanation: We can eliminate the redundant rule matching attempts in the forward chaining by using incremental forward chaining.
36. From where did the new fact inferred on new iteration is derived? a) Old fact b) Narrow fact c) New fact d) All of the mentioned Answer: c Explanation: None.
37. Which will solve the conjuncts of the rule so that the total cost is minimized? a) Constraint variable b) Conjunct ordering c) Data complexity d) All of the mentioned Answer: b Explanation: Conjunct ordering will find an ordering to solve the conjuncts of the rule premise so that the total cost is minimized.
38. How many possible sources of complexity are there in forward chaining? a) 1 b) 2 c) 3 d) 4 Answer: c Explanation: The three possible sources of complexity are an inner loop, algorithm rechecks every rule on every iteration, algorithm might generate many facts irrelevant to the goal.
39. Which algorithm will work backward from the goal to solve a problem? a) Forward chaining b) Backward chaining c) Hill-climb algorithm d) None of the mentioned Answer: b Explanation: Backward chaining algorithm will work backward from the goal and it will chain the known facts that support the proof.
40. Which is mainly used for automated reasoning? a) Backward chaining b) Forward chaining c) Logic programming d) Parallel programming Answer: c Explanation: Logic programming is mainly used to check the working process of the system.
41. What will backward chaining algorithm will return? a) Additional statements b) Substitutes matching the query c) Logical statement d) All of the mentioned Answer: b Explanation: It will contains the list of goals containing a single element and returns the set of all substitutions satisfying the query.
42. How can be the goal is thought of in backward chaining algorithm? a) Queue b) List c) Vector d) Stack View Answer Answer: d Explanation: The goals can be thought of as stack and if all of them us satisfied means, then current branch of proof succeeds.
43. What is used in backward chaining algorithm? a) Conjuncts b) Substitution c) Composition of substitution d) None of the mentioned Answer: c Explanation: None.
44. Which algorithm are in more similar to backward chaining algorithm? a) Depth-first search algorithm b) Breadth-first search algorithm c) Hill-climbing search algorithm d) All of the mentioned Answer: a Explanation: It is depth-first search algorithm because its space requirements are linear in the size of the proof.
45. Which problem can frequently occur in backward chaining algorithm? a) Repeated states b) Incompleteness c) Complexity d) Both Repeated states & Incompleteness Answer: d Explanation: If there is any loop in the chain means, It will lead to incompleteness and repeated states.
46. How the logic programming can be constructed? a) Variables b) Expressing knowledge in a formal language c) Graph d) All of the mentioned Answer: b Explanation: Logic programming can be constructed by expressing knowledge in a formal expression and the problem can be solved by running inference process.
47. What form of negation does the prolog allows? a) Negation as failure b) Proposition c) Substitution d) Negation as success Answer: a Explanation: None.
48. Which is omitted in prolog unification algorithm? a) Variable check b) Occur check c) Proposition check d) Both Occur & Proposition check Answer: b Explanation: Occur check is omitted in prolog unification algorithm because of unsound inferences.
49. Knowledge and reasoning also play a crucial role in dealing with __________________ environment. a) Completely Observable b) Partially Observable c) Neither Completely nor Partially Observable d) Only Completely and Partially Observable Answer: b Explanation: Knowledge and reasoning could aid to reveal other factors that could complete environment.
50. Treatment chosen by doctor for a patient for a disease is based on _____________ a) Only current symptoms b) Current symptoms plus some knowledge from the textbooks c) Current symptoms plus some knowledge from the textbooks plus experience d) All of the mentioned Answer: c Explanation: None.
51. A knowledge-based agent can combine general knowledge with current percepts to infer hidden aspects of the current state prior to selecting actions. a) True b) False Answer: a Explanation: Refer definition of Knowledge based agents.
52. A) Knowledge base (KB) is consists of set of statements. B) Inference is deriving a new sentence from the KB. Choose the correct option. a) A is true, B is true b) A is false, B is false c) A is true, B is false d) A is false, B is true Answer: a Explanation: None.
53. Wumpus World is a classic problem, best example of _______ a) Single player Game b) Two player Game c) Reasoning with Knowledge d) Knowledge based Game Answer: c Explanation: Refer the definition of Wumpus World Problem.
54. ‘α |= β ‘(to mean that the sentence α entails the sentence β) if and only if, in every model in which α is _____ β is also _____ a) True, true b) True, false c) False, true d) False, false Answer: a Explanation: Refer the definition of law of entailment.
55. Which is not a property of representation of knowledge? a) Representational Verification b) Representational Adequacy c) Inferential Adequacy d) Inferential Efficiency Answer: a Explanation: None.
56. Which is not Familiar Connectives in First Order Logic? a) and b) iff c) or d) not Answer: d Explanation: “not” is coming under propositional logic and is therefore not a connective.
57. Inference algorithm is complete only if _____________ a) It can derive any sentence b) It can derive any sentence that is an entailed version c) It is truth preserving d) It can derive any sentence that is an entailed version & It is truth preserving
58. An inference algorithm that derives only entailed sentences is called sound or truth-preserving. a) True b) False Answer: a Explanation: None.
59. The rule of Universal Instantiation (UI for short) says that we can infer any sentence obtained by substituting a ground term (a term without variables) for the variable. a) True b) False Answer: a Explanation: Rule of universal instantiation.
60. The corresponding Existential Instantiation rule: for the existential quantifier is slightly more complicated. For any sentence a, variable v, and constant symbol k that does not appear elsewhere in the knowledge base. a) True b) False Answer: a Explanation: Rule of existential instantiation.
61. What among the following could the universal instantiation of ___________ For all x King(x) ^ Greedy(x) => Evil(x) a) King(John) ^ Greedy(John) => Evil(John) b) King(y) ^ Greedy(y) => Evil(y) c) King(Richard) ^ Greedy(Richard) => Evil(Richard) d) All of the mentioned Answer: d Explanation: Refer the definition if universal instantiation.
62. Lifted inference rules require finding substitutions that make different logical expressions looks identical. a) Existential Instantiation b) Universal Instantiation c) Unification d) Modus Ponen Answer: c Explanation: None.
63. Which of the following is not the style of inference? a) Forward Chaining b) Backward Chaining c) Resolution Refutation d) Modus Ponen Answer: d Explanation: Modus ponen is a rule for an inference.
64. In order to utilize generalized Modus Ponens, all sentences in the KB must be in the form of Horn sentences. a) True b) False Answer: a Explanation: None.
65. For resolution to apply, all sentences must be in conjunctive normal form, a conjunction of disjunctions of literals. a) True b) False Answer: a Explanation: None.
66. What are the two basic types of inferences? a) Reduction to propositional logic, Manipulate rules directly b) Reduction to propositional logic, Apply modus ponen c) Apply modus ponen, Manipulate rules directly d) Convert every rule to Horn Clause, Reduction to propositional logic Answer: a Explanation: None.
67. Which among the following could the Existential instantiation of ∃x Crown(x) ^ OnHead(x, Johnny)? a) Crown(John) ^ OnHead(John, Jonny) b) Crown(y) ^ OnHead(y, y, x) c) Crown(x) ^ OnHead(x, Jonny) d) None of the mentioned Answer: a Explanation: None.
68. Translate the following statement into FOL. “For every a, if a is a PhD student, then a has a master degree” a) ∀ a PhD(a) -> Master(a) b) ∃ a PhD(a) -> Master(a) c) A is true, B is true d) A is false, B is false Answer: a Explanation: None
69. The rule of Universal Instantiation (UI for short) says that we can infer any sentence obtained by substituting a ground term (a term without variables) for the variable. a) True b) False Answer: a Explanation: Rule of universal instantiation.
70. The corresponding Existential Instantiation rule: for the existential quantifier is slightly more complicated. For any sentence a, variable v, and constant symbol k that does not appear elsewhere in the knowledge base. a) True b) False Answer: a Explanation: Rule of existential instantiation.
1. Which is the most straightforward approach for planning algorithm? a) Best-first search b) State-space search c) Depth-first search d) Hill-climbing search
Answer: b Explanation: The straightforward approach for planning algorithm is state space search because it takes into account of everything for finding a solution.
2. What are taken into account of state-space search? a) Postconditions b) Preconditions c) Effects d) Both Preconditions & Effects
Answer: d Explanation: The state-space search takes both precondition and effects into account for solving a problem.
3. How many ways are available to solve the state-space search? a) 1 b) 2 c) 3 d) 4
Answer: b Explanation: There are two ways available to solve the state-space search. They are forward from the initial state and backward from the goal.
4. What is the other name for forward state-space search? a) Progression planning b) Regression planning c) Test planning d) None of the mentioned
Answer: a Explanation: It is sometimes called as progression planning, because it moves in the forward direction.
5. How many states are available in state-space search? a) 1 b) 2 c) 3 d) 4
Answer: d Explanation: There are four states available in state-space search. They are initial state, actions, goal test and step cost.
6. What is the main advantage of backward state-space search? a) Cost b) Actions c) Relevant actions d) All of the mentioned
Answer: c Explanation: The main advantage of backward search will allow us to consider only relevant actions.
7. What is the other name of the backward state-space search? a) Regression planning b) Progression planning c) State planning d) Test planning
Answer: a Explanation: Backward state-space search will find the solution from goal to the action, So it is called as Regression planning.
8. What is meant by consistent in state-space search? a) Change in the desired literals b) Not any change in the literals c) No change in goal state d) None of the mentioned
Answer: b Explanation: Consistent means that the completed actions will not undo any desired literals.
9. What will happen if a predecessor description is generated that is satisfied by the initial state of the planning problem? a) Success b) Error c) Compilation d) Termination
Answer: d Explanation: None.
10. Which approach is to pretend that a pure divide and conquer algorithm will work? a) Goal independence b) Subgoal independence c) Both Goal & Subgoal independence d) None of the mentioned
Answer: b Explanation: Subgoal independence approach is to pretend that a pure divide and conquer algorithm will work for admissible heuristics.
11. The process by which the brain incrementally orders actions needed to complete a specific task is referred as ______________ a) Planning problem b) Partial order planning c) Total order planning d) Both Planning problem & Partial order planning
Answer: b Explanation: Definition of partial order planning.
12. To complete any task, the brain needs to plan out the sequence by which to execute the behavior. One way the brain does this is with a partial-order plan. a) True b) False
13. In partial order plan. A. Relationships between the actions of the behavior are set prior to the actions B. Relationships between the actions of the behavior are not set until absolutely necessary Choose the correct option. a) A is true b) B is true c) Either A or B can be true depending upon situation d) Neither A nor B is true
Answer: a Explanation: Relationship between behavior and actions is established dynamically.
14. Partial-order planning exhibits the Principle of Least Commitment, which contributes to the efficiency of this planning system as a whole. a) True b) False
15. Following is/are the components of the partial order planning. a) Bindings b) Goal c) Causal Links d) All of the mentioned
Answer: d Explanation: Bindings: The bindings of the algorithm are the connections between specific variables in the action. Bindings, as ordering, only occur when it is absolutely necessary. Causal Links: Causal links in the algorithm are those that categorically order actions. They are not the specific order (1,2,3) of the actions, rather the general order as in Action 2 must come somewhere after Action 1, but before Action 2. Plan Space: The plan space of the algorithm is constrained between its start and finish. The algorithm starts, producing the initial state and finishes when all parts of the goal is been achieved.
16. Partial-order planning is the opposite of total-order planning. a) True b) False
Answer: a Explanation: Partial-order planning is the opposite of total-order planning, in which actions are sequenced all at once and for the entirety of the task at hand.
17. Sussman Anomaly can be easily and efficiently solved by partial order planning. a) True b) False
Answer: a Explanation: http://en.wikipedia.org/wiki/Sussman_Anomaly.
18. Sussman Anomaly illustrates a weakness of interleaved planning algorithm. a) True b) False
Answer: b Explanation: Sussman Anomaly illustrates a weakness of non interleaved planning algorithm.
19. One the main drawback of this type of planning system is that it requires a lot of computational powers at each node. a) True b) False
20. What are you predicating by the logic: ۷x: €y: loyalto(x, y). a) Everyone is loyal to someone b) Everyone is loyal to all c) Everyone is not loyal to someone d) Everyone is loyal
Answer: a Explanation: ۷x denotes Everyone or all, and €y someone and loyal to is the proposition logic making map x to y.
21. A plan that describe how to take actions in levels of increasing refinement and specificity is ____________ a) Problem solving b) Planning c) Non-hierarchical plan d) Hierarchical plan
Answer: d Explanation: A plan that describes how to take actions in levels of increasing refinement and specificity is Hierarchical (e.g., “Do something” becomes the more specific “Go to work,” “Do work,” “Go home.”) Most plans are hierarchical in nature.
22. A constructive approach in which no commitment is made unless it is necessary to do so, is ____________ a) Least commitment approach b) Most commitment approach c) Nonlinear planning d) Opportunistic planning
Answer: a Explanation: Because we are not sure about the outcome.
23. Uncertainty arises in the Wumpus world because the agent’s sensors give only ____________ a) Full & Global information b) Partial & Global Information c) Partial & local Information d) Full & local information
Answer: c Explanation: The Wumpus world is a grid of squares surrounded by walls, where each square can contain agents and objects. The agent (you) always starts in the lower left corner, a square that will be labeled [1, 1]. The agent’s task is to find the gold, return to [1, 1] and climb out of the cave. Therefore, uncertainty is there as the agent gives partial and local information only. Global variable are not goal specific problem solving.
24. Which of the following search belongs to totally ordered plan search? a) Forward state-space search b) Hill-climbing search c) Depth-first search d) Breadth-first search
Answer: a Explanation: Forward and backward state-space search are particular forms of totally ordered plan search.
25. Which cannot be taken as advantage for totally ordered plan search? a) Composition b) State search c) Problem decomposition d) None of the mentioned
Answer: c Explanation: As the search explore only linear sequences of actions, So they cannot take advantage of problem decomposition.
26. What is the advantage of totally ordered plan in constructing the plan? a) Reliability b) Flexibility c) Easy to use d) All of the mentioned
Answer: b Explanation: Totally ordered plan has the advantage of flexibility in the order in which it constructs the plan.
27. Which strategy is used for delaying a choice during search? a) First commitment b) Least commitment c) Both First & Least commitment d) None of the mentioned
Answer: b Explanation: The general strategy of delaying a choice during search is called the least commitment strategy.
28. Which algorithm places two actions into a plan without specifying which should come first? a) Full-order planner b) Total-order planner c) Semi-order planner d) Partial-order planner
Answer: d Explanation: Any planning algorithm that can place two actions into a plan without specifying which should come first is called partial-order planner.
29. How many possible plans are available in partial-order solution? a) 3 b) 4 c) 5 d) 6
Answer: d Explanation: The partial-order solution corresponds to six possible total-order plans.
30. What is the other name of each and every total-order plans? a) Polarization b) Linearization c) Solarization d) None of the mentioned Answer: b Explanation: Each and every total order plan is also called as linearization of the partial-order plan.
31. What are present in the empty plan? a) Start b) Finish c) Modest d) Both Start & Finish View Answer
32. What are not present in start actions? a) Preconditions b) Effect c) Finish d) None of the mentioned Answer: a Explanation: Start has no precondition and has as its effects all the literals in the initial state of the planning problem.
33. What are not present in finish actions? a) Preconditions b) Effect c) Finish d) None of the mentioned Answer: b Explanation: Finish has no effects and has as its preconditions the goal literals of the planning algorithm.
34. Which can be adapted for planning algorithms? a) Most-constrained variable b) Most-constrained literal c) Constrained d) None of the mentioned Answer: a Explanation: The most-constrained variable heuristic from CSPs can be adapted for planning algorithm and seems to work well.
1. Using logic to represent and reason we can represent knowledge about the world with facts and rules. a) True b) False
2. Uncertainty arises in the wumpus world because the agent’s sensors give only ___________ a) Full & Global information b) Partial & Global Information c) Partial & local Information d) Full & local information
Answer: c Explanation: The Wumpus world is a grid of squares surrounded by walls, where each square can contain agents and objects. The agent (you) always starts in the lower left corner, a square that will be labeled [1, 1]. The agent’s task is to find the gold, return to [1, 1] and climb out of the cave. So uncertainty is there as the agent gives partial and local information only. Global variable are not goal specific problem solving.
3. A Hybrid Bayesian network contains ___________ a) Both discrete and continuous variables b) Only Discrete variables c) Only Discontinuous variable d) Both Discrete and Discontinuous variable
Answer: a Explanation: To specify a Hybrid network, we have to specify two new kinds of distributions: the conditional distribution for continuous variables given discrete or continuous parents, and the conditional distribution for a discrete variable given continuous parents.
4. How is Fuzzy Logic different from conventional control methods? a) IF and THEN Approach b) FOR Approach c) WHILE Approach d) DO Approach
Answer: a Explanation: FL incorporates a simple, rule-based IF X AND Y THEN Z approach to a solving control problem rather than attempting to model a system mathematically.
5. If a hypothesis says it should be positive, but in fact it is negative, we call it ___________ a) A consistent hypothesis b) A false negative hypothesis c) A false positive hypothesis d) A specialized hypothesis
Answer: c Explanation: Consistent hypothesis go with examples, If the hypothesis says it should be negative but in fact it is positive, it is false negative. If a hypothesis says it should be positive, but in fact it is negative, it is false positive. In a specialized hypothesis we need to have certain restrict or special conditions.
6. The primitives in probabilistic reasoning are random variables. a) True b) False
Answer: a Explanation: The primitives in probabilistic reasoning are random variables. Just like primitives in Propositional Logic are propositions. A random variable is not in fact a variable, but a function from a sample space S to another space, often the real numbers.
7. Which is true for Decision theory? a) Decision Theory = Probability theory + utility theory b) Decision Theory = Inference theory + utility theory c) Decision Theory = Uncertainty + utility theory d) Decision Theory = Probability theory + preference
8. A constructive approach in which no commitment is made unless it is necessary to do so is ___________ a) Least commitment approach b) Most commitment approach c) Nonlinear planning d) Opportunistic planning
9. What is the extraction of the meaning of utterance? a) Syntactic b) Semantic c) Pragmatic d) None of the mentioned
Answer: b Explanation: Semantic analysis is used to extract the meaning from the group of sentences.
10. What is the process of associating a FOL expression with a phrase? a) Interpretation b) Augmented reality c) Semantic interpretation d) Augmented interpretation
Answer: c Explanation: Semantic interpretation is the process of associating a FOL expression with a phrase.
11. What is meant by compositional semantics? a) Determining the meaning b) Logical connectives c) Semantics d) None of the mentioned
Answer: a Explanation: Compositional semantics is the process of determining the meaning of P*Q from P, Q and *.
12. What is used to augment a grammar for arithmetic expression with semantics? a) Notation b) DCG notation c) Constituent d) All of the mentioned
Answer: b Explanation: DCG notation is used to augment a grammar for arithmetic expression with semantics and it is used to build a parse tree.
13. What can’t be done in the semantic interpretation? a) Logical term b) Complete logical sentence c) Both Logical term & Complete logical sentence d) None of the mentioned
Answer: c Explanation: Some kind of sentence in the semantic interpretation can’t be logical term nor a complete logical sentence.
14. How many verb tenses are there in the English language? a) 1 b) 2 c) 3 d) 4
Answer: c Explanation: There are three types of tenses available in english language are past, present and future.
15. Which is used to mediate between syntax and semantics? a) Form b) Intermediate form c) Grammer d) All of the mentioned
16. What is meant by quasi-logical form? a) Sits between syntactic and logical form b) Logical connectives c) All of the mentioned d) None of the mentioned
Answer: a Explanation: It can be translated into a regular first-order logical sentence, So that it Sits between syntactic and logical form.
17. How many types of quantification are available in artificial intelligence? a) 1 b) 2 c) 3 d) 4
Answer: b Explanation: There are two types of quantification available. They are universal and existential.
18. What kind of interpretation is done by adding context-dependant information? a) Semantic b) Syntactic c) Pragmatic d) None of the mentioned
19. How many issues are available in describing degree of belief? a) 1 b) 2 c) 3 d) 4
Answer: b Explanation: The main issues for degree of belief are nature of the sentences and the dependance of degree of the belief.
20. What is used for probability theory sentences? a) Conditional logic b) Logic c) Extension of propositional logic d) None of the mentioned
Answer: c Explanation: The version of probability theory we present uses an extension of propositional logic for its sentences.
21. Where does the dependance of experience is reflected in prior probability sentences? a) Syntactic distinction b) Semantic distinction c) Both Syntactic & Semantic distinction d) None of the mentioned
Answer: a Explanation: The dependance on experience is reflected in the syntactic distinction between prior probability statements.
22. Where does the degree of belief is applied? a) Propositions b) Literals c) Variables d) Statements
23. How many formal languages are used for stating propositions? a) 1 b) 2 c) 3 d) 4
Answer: b Explanation: The two formal languages used for stating propositions are propositional logic and first-order logic.
24. What is the basic element of a language? a) Literal b) Variable c) Random variable d) All of the mentioned
Answer: c Explanation: The basic element for a language is the random variable, which can be thought as a part of world and its status is initially unknown.
25. How many types of random variables are available? a) 1 b) 2 c) 3 d) 4
Answer: c Explanation: The three types of random variables are boolean, discrete and continuous.
26. Which is the complete specification of the state of the world? a) Atomic event b) Complex event c) Simple event d) None of the mentioned
Answer: a Explanation: An atomic event is the complete specification of the state of the world about which the event is uncertain.
27. Which variable cannot be written in entire distribution as a table? a) Discrete b) Continuous c) Both Discrete & Continuous d) None of the mentioned
Answer: b Explanation: For continuous variables, it is not possible to write out the entire distribution as a table.
28. What is meant by probability density function? a) Probability distributions b) Continuous variable c) Discrete variable d) Probability distributions for Continuous variables
29. How many terms are required for building a bayes model? a) 1 b) 2 c) 3 d) 4
Answer: c Explanation: The three required terms are a conditional probability and two unconditional probability.
30. What is needed to make probabilistic systems feasible in the world? a) Reliability b) Crucial robustness c) Feasibility d) None of the mentioned
Answer: b Explanation: On a model-based knowledge provides the crucial robustness needed to make probabilistic system feasible in the real world.
31. Where does the bayes rule can be used? a) Solving queries b) Increasing complexity c) Decreasing complexity d) Answering probabilistic query
Answer: d Explanation: Bayes rule can be used to answer the probabilistic queries conditioned on one piece of evidence.
32. What does the bayesian network provides? a) Complete description of the domain b) Partial description of the domain c) Complete description of the problem d) None of the mentioned
Answer: a Explanation: A Bayesian network provides a complete description of the domain.
33. How the entries in the full joint probability distribution can be calculated? a) Using variables b) Using information c) Both Using variables & information d) None of the mentioned
Answer: b Explanation: Every entry in the full joint probability distribution can be calculated from the information in the network.
34. How the bayesian network can be used to answer any query? a) Full distribution b) Joint distribution c) Partial distribution d) All of the mentioned
Answer: b Explanation: If a bayesian network is a representation of the joint distribution, then it can solve any query, by summing all the relevant joint entries.
35. How the compactness of the bayesian network can be described? a) Locally structured b) Fully structured c) Partial structure d) All of the mentioned
Answer: a Explanation: The compactness of the bayesian network is an example of a very general property of a locally structured system.
36. To which does the local structure is associated? a) Hybrid b) Dependant c) Linear d) None of the mentioned
Answer: c Explanation: Local structure is usually associated with linear rather than exponential growth in complexity.
37. Which condition is used to influence a variable directly by all the others? a) Partially connected b) Fully connected c) Local connected d) None of the mentioned
38. What is the consequence between a node and its predecessors while creating bayesian network? a) Functionally dependent b) Dependant c) Conditionally independent d) Both Conditionally dependant & Dependant
1. What is the field of Natural Language Processing (NLP)? a) Computer Science b) Artificial Intelligence c) Linguistics d) All of the mentioned
2. NLP is concerned with the interactions between computers and human (natural) languages. a) True b) False
Answer: a Explanation: NLP has its focus on understanding the human spoken/written language and converts that interpretation into machine understandable language.
3. What is the main challenge/s of NLP? a) Handling Ambiguity of Sentences b) Handling Tokenization c) Handling POS-Tagging d) All of the mentioned
Answer: a Explanation: There are enormous ambiguity exists when processing natural language.
4. Modern NLP algorithms are based on machine learning, especially statistical machine learning. a) True b) False
5. Choose form the following areas where NLP can be useful. a) Automatic Text Summarization b) Automatic Question-Answering Systems c) Information Retrieval d) All of the mentioned
6. Which of the following includes major tasks of NLP? a) Automatic Summarization b) Discourse Analysis c) Machine Translation d) All of the mentioned
Answer: d Explanation: There is even bigger list of tasks of NLP. http://en.wikipedia.org/wiki/Natural_language_processing#Major_tasks_in_NLP.
7. What is Coreference Resolution? a) Anaphora Resolution b) Given a sentence or larger chunk of text, determine which words (“mentions”) refer to the same objects (“entities”) c) All of the mentioned d) None of the mentioned
Answer: b Explanation: Anaphora resolution is a specific type of coreference resolution.
8. What is Machine Translation? a) Converts one human language to another b) Converts human language to machine language c) Converts any human language to English d) Converts Machine language to human language
Answer: a Explanation: The best known example of machine translation is google translator.
9. The more general task of coreference resolution also includes identifying so-called “bridging relationships” involving referring expressions. a) True b) False
Answer: a Explanation: Refer the definition of Coreference Resolution.
10. What is Morphological Segmentation? a) Does Discourse Analysis b) Separate words into individual morphemes and identify the class of the morphemes c) Is an extension of propositional logic d) None of the mentioned
11. Given a stream of text, Named Entity Recognition determines which pronoun maps to which noun. a) False b) True
Answer: a Explanation: Given a stream of text, Named Entity Recognition determines which items in the text maps to proper names.
12. Natural Language generation is the main task of Natural language processing. a) True b) False
Answer: a Explanation: Natural Language Generation is to Convert information from computer databases into readable human language.
13. OCR (Optical Character Recognition) uses NLP. a) True b) False
Answer: a Explanation: Given an image representing printed text, determines the corresponding text.
14. Parts-of-Speech tagging determines ___________ a) part-of-speech for each word dynamically as per meaning of the sentence b) part-of-speech for each word dynamically as per sentence structure c) all part-of-speech for a specific word given as input d) all of the mentioned
Answer: d Explanation: A Bayesian network provides a complete description of the domain.
15. Parsing determines Parse Trees (Grammatical Analysis) for a given sentence. a) True b) False
Answer: a Explanation: Determine the parse tree (grammatical analysis) of a given sentence. The grammar for natural languages is ambiguous and typical sentences have multiple possible analyses. In fact, perhaps surprisingly, for a typical sentence there may be thousands of potential parses (most of which will seem completely nonsensical to a human).
16. IR (information Retrieval) and IE (Information Extraction) are the two same thing. a) True b) False
Answer: b Explanation: Information retrieval (IR) – This is concerned with storing, searching and retrieving information. It is a separate field within computer science (closer to databases), but IR relies on some NLP methods (for example, stemming). Some current research and applications seek to bridge the gap between IR and NLP. Information extraction (IE) – This is concerned in general with the extraction of semantic information from text. This covers tasks such as named entity recognition, Coreference resolution, relationship extraction, etc.
17. Many words have more than one meaning; we have to select the meaning which makes the most sense in context. This can be resolved by ____________ a) Fuzzy Logic b) Word Sense Disambiguation c) Shallow Semantic Analysis d) All of the mentioned
Answer: b Explanation: Shallow Semantic Analysis doesn’t cover word sense disambiguation.
18. Given a sound clip of a person or people speaking, determine the textual representation of the speech. a) Text-to-speech b) Speech-to-text c) All of the mentioned d) None of the mentioned
Answer: b Explanation: NLP is required to linguistic analysis.
19. Speech Segmentation is a subtask of Speech Recognition. a) True b) False

/ Youtube Channel: https://www.youtube.com/channel/UCGFNZxMqKLsqWERX_N2f08Q
Follow For Latest Updates, Study Tips & More Content!

Login with your site account
Remember Me
Not a member yet? Register now
Register a new account
Are you a member? Login now
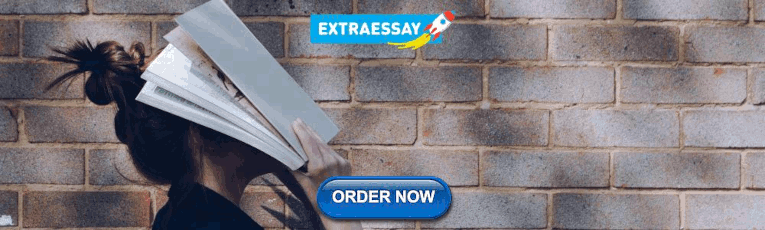
IMAGES
VIDEO
COMMENTS
The problem solving agent chooses a cost function that reflects its own performance measure. The solution to the problem is an action sequence that leads from initial state to goal state and the ...
May 10, 2024. In artificial intelligence, a problem-solving agent refers to a type of intelligent agent designed to address and solve complex problems or tasks in its environment. These agents are a fundamental concept in AI and are used in various applications, from game-playing algorithms to robotics and decision-making systems.
The problem-solving agent performs precisely by defining problems and several solutions. So we can say that problem solving is a part of artificial intelligence that encompasses a number of techniques such as a tree, B-tree, heuristic algorithms to solve a problem. We can also say that a problem-solving agent is a result-driven agent and always ...
Problem-solving agents are a type of artificial intelligence that helps automate problem-solving. They can be used to solve problems in natural language, algebra, calculus, statistics, and machine learning. There are three types of problem-solving agents: propositional, predicate, and automata. Propositional problem-solving agents can ...
Problem-solving agents Restricted form of general agent: function Simple-Problem-Solving-Agent (percept) returns an action static: seq, an action sequence, initially empty state, some description of the current world state goal, a goal, initially null problem, a problem formulation state ← Update-State (state,percept) if seq is empty then ...
- Search algorithms - input is a problem, output is a solution (action sequence) Execute - Given the solution, perform the actions. Problem Solving Agent - Special type of goal based agent. Environment - static - agent assumes that in the time it takes to formulate and solve the problem the environment doesn't change
CPE/CSC 580-S06 Artificial Intelligence - Intelligent Agents Well-Defined Problems exact formulation of problems and solutions initial state current state / set of states, or the state at the beginning of the problem-solving process must be known to the agent operator description of an action state space set of all states reachable from the ...
Chapter3 2. Problem-solving agents. functionSimple-Problem-Solving-Agent(percept) returnsan action static: seq, an action sequence, initially empty state, some description of the current world state goal, a goal, initially null problem, a problem formulation state←Update-State(state,percept) ifseq is empty then goal←Formulate-Goal(state ...
• Chapter 2, R&N: simplifies task of translating agent specification to formal design • First step in problem solving: formulation of goal(s ) - "accept no substitutes" • Chapters 3-4, R&N: goal ≡{world states | goal test is satisfied} Problem-Solving Agents [2]: Preliminary Design Problem Formulation
• define main performance measures for search. cis716-spring2006-parsons-lect06 2 Problem Solving Agents • Lecture 1 introduced rational agents. • Now consider agents as problem solvers: Systems which set themselves goals and find sequences of actions that achieve these goals. • What is a problem? A goal and a means for achieving the ...
The problem solving agent follows this four phase problem solving process: Goal Formulation: This is the first and most basic phase in problem solving. It arranges specific steps to establish a target/goal that demands some activity to reach it. AI agents are now used to formulate goals. Problem Formulation: It is one of the fundamental steps ...
One of the main components of a problem-solving agent is the knowledge base. This is where the agent stores relevant information and data that it can use to solve problems. The knowledge base can include facts, rules, and heuristics that the agent has acquired through learning or from experts in the domain.
The agent's task is to find out which sequence of actions will get it to a goal state. Before it can do this, it needs to decide what sorts of actions and states to consider. ... The problem-solving agent chooses a cost function that reflects its own performance measure. For the agent trying to get to Bucharest, time is of the essence, so ...
The low-level agents are responsible for carrying out specific tasks to achieve the goals set by the high-level agents. These tasks may be relatively simple or more complex, depending on the specific application. ... The reason for manufactured insights is learning, problem-solving, reasoning, and perception. This term may be connected to any ...
The problem-solving agent selects a cost function, which reflects its performance measure. Remember, an optimal solution has the lowest path cost among all the solutions.
What is the main task of a problem-solving agent? a) Solve the given problem and reach to goal b) To find out which sequence of action will get it to the goal state c) All of the mentioned d) None of the mentioned View Answer. Answer: c Explanation: The problem-solving agents are one of the goal-based agents. 2. What is state space?
Study with Quizlet and memorize flashcards containing terms like 1. What is the main task of a problem-solving agent? a. Solve the given problem and reach to goal b. To find out which sequence of action will get it to the goal state c. All the mentioned d. None of the mentioned, 2. What is state space? a. The whole problem b. Your Definition to a problem c. Problem you design d. Representing ...
This chapter describes one kind of goal-based agent called a problem-solving agent, and limits ourselves to the simplest kind of task environment, for which the solution to a problem is always a fixed sequence of actions. In which we see how an agent can find a sequence of actions that achieves its goals, when no single action will do. The simplest agents discussed in Chapter 2 were the reflex ...
The problem-solving agent perfoms precisely by defining problems and its several solutions. According to psychology, "a problem-solving refers to a state where we wish to reach to a definite goal from a present state or condition." According to computer science, a problem-solving is a part of artificial intelligence which
What is the main task of a problem-solving agent? a) Solve the given problem and reach to goal b) To find out which sequence of action will get it to the goal state c) All of the mentioned d) None of the mentioned, 2. ... The problem-solving agent with several immediate options of unknown value can decide what to do by just examining different ...
The evolution of generative AI (gen AI) has opened the door to great opportunities across organizations, particularly regarding gen AI agents—AI-powered software entities that plan and perform tasks or aid humans by delivering specific services on their behalf. So far, adoption at scale across businesses has faced difficulties because of data quality, employee distrust, and cost of ...
What is the main task of a problem-solving agent? A. Solve the given problem and reach to goal B. To find out which sequence of action will get it to the goal state C. Both A and B D. None of the Above Ans : C Explanation: The problem-solving agents are one of the goal-based agents. 2. What is Initial state + Goal state in Search Terminology?
Computer Science. Computer Science questions and answers. 1- What is the main task of a problem-solving agent? Select one: a. Solve the given problem and reach to goal b. To find out which sequence of action will get it to the goal state c. Both A and B d. None of the Above 2- What is disadvantage of Greedy Best First Search? Select one: a.
What is the main task of a problem-solving agent? (a) Solve the given problem and reach to goal (b) To find out which sequence of action will get it to the goal state (c) All of the mentioned (d) None of the mentioned. artificial-intelligence; Share It On Facebook Twitter Email. Play Quiz Game > 1 Answer. 0 votes ...
Heterogeneous image change detection is a very practical and challenging task because the data in the original image have a large distribution difference and the labeled samples of the remote sensing image are usually very few. In this study, we focus on solving the issue of comparing heterogeneous images without supervision. This paper first designs a self-paced multi-scale joint feature ...