Thank you for visiting nature.com. You are using a browser version with limited support for CSS. To obtain the best experience, we recommend you use a more up to date browser (or turn off compatibility mode in Internet Explorer). In the meantime, to ensure continued support, we are displaying the site without styles and JavaScript.
- View all journals
- My Account Login
- Explore content
- About the journal
- Publish with us
- Sign up for alerts
- Open access
- Published: 17 April 2024
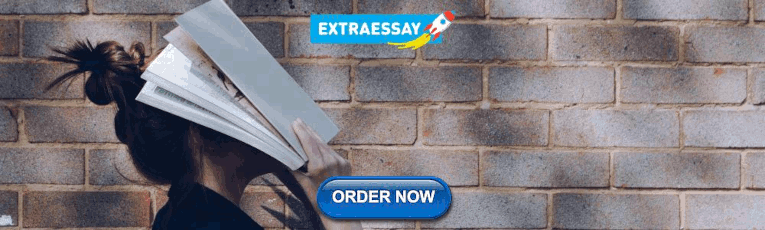
The economic commitment of climate change
- Maximilian Kotz ORCID: orcid.org/0000-0003-2564-5043 1 , 2 ,
- Anders Levermann ORCID: orcid.org/0000-0003-4432-4704 1 , 2 &
- Leonie Wenz ORCID: orcid.org/0000-0002-8500-1568 1 , 3
Nature volume 628 , pages 551–557 ( 2024 ) Cite this article
89k Accesses
3471 Altmetric
Metrics details
- Environmental economics
- Environmental health
- Interdisciplinary studies
- Projection and prediction
Global projections of macroeconomic climate-change damages typically consider impacts from average annual and national temperatures over long time horizons 1 , 2 , 3 , 4 , 5 , 6 . Here we use recent empirical findings from more than 1,600 regions worldwide over the past 40 years to project sub-national damages from temperature and precipitation, including daily variability and extremes 7 , 8 . Using an empirical approach that provides a robust lower bound on the persistence of impacts on economic growth, we find that the world economy is committed to an income reduction of 19% within the next 26 years independent of future emission choices (relative to a baseline without climate impacts, likely range of 11–29% accounting for physical climate and empirical uncertainty). These damages already outweigh the mitigation costs required to limit global warming to 2 °C by sixfold over this near-term time frame and thereafter diverge strongly dependent on emission choices. Committed damages arise predominantly through changes in average temperature, but accounting for further climatic components raises estimates by approximately 50% and leads to stronger regional heterogeneity. Committed losses are projected for all regions except those at very high latitudes, at which reductions in temperature variability bring benefits. The largest losses are committed at lower latitudes in regions with lower cumulative historical emissions and lower present-day income.
Similar content being viewed by others
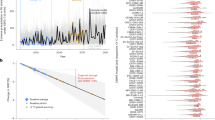
Climate damage projections beyond annual temperature
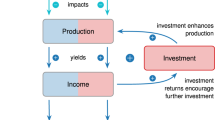
Investment incentive reduced by climate damages can be restored by optimal policy
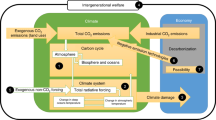
Climate economics support for the UN climate targets
Projections of the macroeconomic damage caused by future climate change are crucial to informing public and policy debates about adaptation, mitigation and climate justice. On the one hand, adaptation against climate impacts must be justified and planned on the basis of an understanding of their future magnitude and spatial distribution 9 . This is also of importance in the context of climate justice 10 , as well as to key societal actors, including governments, central banks and private businesses, which increasingly require the inclusion of climate risks in their macroeconomic forecasts to aid adaptive decision-making 11 , 12 . On the other hand, climate mitigation policy such as the Paris Climate Agreement is often evaluated by balancing the costs of its implementation against the benefits of avoiding projected physical damages. This evaluation occurs both formally through cost–benefit analyses 1 , 4 , 5 , 6 , as well as informally through public perception of mitigation and damage costs 13 .
Projections of future damages meet challenges when informing these debates, in particular the human biases relating to uncertainty and remoteness that are raised by long-term perspectives 14 . Here we aim to overcome such challenges by assessing the extent of economic damages from climate change to which the world is already committed by historical emissions and socio-economic inertia (the range of future emission scenarios that are considered socio-economically plausible 15 ). Such a focus on the near term limits the large uncertainties about diverging future emission trajectories, the resulting long-term climate response and the validity of applying historically observed climate–economic relations over long timescales during which socio-technical conditions may change considerably. As such, this focus aims to simplify the communication and maximize the credibility of projected economic damages from future climate change.
In projecting the future economic damages from climate change, we make use of recent advances in climate econometrics that provide evidence for impacts on sub-national economic growth from numerous components of the distribution of daily temperature and precipitation 3 , 7 , 8 . Using fixed-effects panel regression models to control for potential confounders, these studies exploit within-region variation in local temperature and precipitation in a panel of more than 1,600 regions worldwide, comprising climate and income data over the past 40 years, to identify the plausibly causal effects of changes in several climate variables on economic productivity 16 , 17 . Specifically, macroeconomic impacts have been identified from changing daily temperature variability, total annual precipitation, the annual number of wet days and extreme daily rainfall that occur in addition to those already identified from changing average temperature 2 , 3 , 18 . Moreover, regional heterogeneity in these effects based on the prevailing local climatic conditions has been found using interactions terms. The selection of these climate variables follows micro-level evidence for mechanisms related to the impacts of average temperatures on labour and agricultural productivity 2 , of temperature variability on agricultural productivity and health 7 , as well as of precipitation on agricultural productivity, labour outcomes and flood damages 8 (see Extended Data Table 1 for an overview, including more detailed references). References 7 , 8 contain a more detailed motivation for the use of these particular climate variables and provide extensive empirical tests about the robustness and nature of their effects on economic output, which are summarized in Methods . By accounting for these extra climatic variables at the sub-national level, we aim for a more comprehensive description of climate impacts with greater detail across both time and space.
Constraining the persistence of impacts
A key determinant and source of discrepancy in estimates of the magnitude of future climate damages is the extent to which the impact of a climate variable on economic growth rates persists. The two extreme cases in which these impacts persist indefinitely or only instantaneously are commonly referred to as growth or level effects 19 , 20 (see Methods section ‘Empirical model specification: fixed-effects distributed lag models’ for mathematical definitions). Recent work shows that future damages from climate change depend strongly on whether growth or level effects are assumed 20 . Following refs. 2 , 18 , we provide constraints on this persistence by using distributed lag models to test the significance of delayed effects separately for each climate variable. Notably, and in contrast to refs. 2 , 18 , we use climate variables in their first-differenced form following ref. 3 , implying a dependence of the growth rate on a change in climate variables. This choice means that a baseline specification without any lags constitutes a model prior of purely level effects, in which a permanent change in the climate has only an instantaneous effect on the growth rate 3 , 19 , 21 . By including lags, one can then test whether any effects may persist further. This is in contrast to the specification used by refs. 2 , 18 , in which climate variables are used without taking the first difference, implying a dependence of the growth rate on the level of climate variables. In this alternative case, the baseline specification without any lags constitutes a model prior of pure growth effects, in which a change in climate has an infinitely persistent effect on the growth rate. Consequently, including further lags in this alternative case tests whether the initial growth impact is recovered 18 , 19 , 21 . Both of these specifications suffer from the limiting possibility that, if too few lags are included, one might falsely accept the model prior. The limitations of including a very large number of lags, including loss of data and increasing statistical uncertainty with an increasing number of parameters, mean that such a possibility is likely. By choosing a specification in which the model prior is one of level effects, our approach is therefore conservative by design, avoiding assumptions of infinite persistence of climate impacts on growth and instead providing a lower bound on this persistence based on what is observable empirically (see Methods section ‘Empirical model specification: fixed-effects distributed lag models’ for further exposition of this framework). The conservative nature of such a choice is probably the reason that ref. 19 finds much greater consistency between the impacts projected by models that use the first difference of climate variables, as opposed to their levels.
We begin our empirical analysis of the persistence of climate impacts on growth using ten lags of the first-differenced climate variables in fixed-effects distributed lag models. We detect substantial effects on economic growth at time lags of up to approximately 8–10 years for the temperature terms and up to approximately 4 years for the precipitation terms (Extended Data Fig. 1 and Extended Data Table 2 ). Furthermore, evaluation by means of information criteria indicates that the inclusion of all five climate variables and the use of these numbers of lags provide a preferable trade-off between best-fitting the data and including further terms that could cause overfitting, in comparison with model specifications excluding climate variables or including more or fewer lags (Extended Data Fig. 3 , Supplementary Methods Section 1 and Supplementary Table 1 ). We therefore remove statistically insignificant terms at later lags (Supplementary Figs. 1 – 3 and Supplementary Tables 2 – 4 ). Further tests using Monte Carlo simulations demonstrate that the empirical models are robust to autocorrelation in the lagged climate variables (Supplementary Methods Section 2 and Supplementary Figs. 4 and 5 ), that information criteria provide an effective indicator for lag selection (Supplementary Methods Section 2 and Supplementary Fig. 6 ), that the results are robust to concerns of imperfect multicollinearity between climate variables and that including several climate variables is actually necessary to isolate their separate effects (Supplementary Methods Section 3 and Supplementary Fig. 7 ). We provide a further robustness check using a restricted distributed lag model to limit oscillations in the lagged parameter estimates that may result from autocorrelation, finding that it provides similar estimates of cumulative marginal effects to the unrestricted model (Supplementary Methods Section 4 and Supplementary Figs. 8 and 9 ). Finally, to explicitly account for any outstanding uncertainty arising from the precise choice of the number of lags, we include empirical models with marginally different numbers of lags in the error-sampling procedure of our projection of future damages. On the basis of the lag-selection procedure (the significance of lagged terms in Extended Data Fig. 1 and Extended Data Table 2 , as well as information criteria in Extended Data Fig. 3 ), we sample from models with eight to ten lags for temperature and four for precipitation (models shown in Supplementary Figs. 1 – 3 and Supplementary Tables 2 – 4 ). In summary, this empirical approach to constrain the persistence of climate impacts on economic growth rates is conservative by design in avoiding assumptions of infinite persistence, but nevertheless provides a lower bound on the extent of impact persistence that is robust to the numerous tests outlined above.
Committed damages until mid-century
We combine these empirical economic response functions (Supplementary Figs. 1 – 3 and Supplementary Tables 2 – 4 ) with an ensemble of 21 climate models (see Supplementary Table 5 ) from the Coupled Model Intercomparison Project Phase 6 (CMIP-6) 22 to project the macroeconomic damages from these components of physical climate change (see Methods for further details). Bias-adjusted climate models that provide a highly accurate reproduction of observed climatological patterns with limited uncertainty (Supplementary Table 6 ) are used to avoid introducing biases in the projections. Following a well-developed literature 2 , 3 , 19 , these projections do not aim to provide a prediction of future economic growth. Instead, they are a projection of the exogenous impact of future climate conditions on the economy relative to the baselines specified by socio-economic projections, based on the plausibly causal relationships inferred by the empirical models and assuming ceteris paribus. Other exogenous factors relevant for the prediction of economic output are purposefully assumed constant.
A Monte Carlo procedure that samples from climate model projections, empirical models with different numbers of lags and model parameter estimates (obtained by 1,000 block-bootstrap resamples of each of the regressions in Supplementary Figs. 1 – 3 and Supplementary Tables 2 – 4 ) is used to estimate the combined uncertainty from these sources. Given these uncertainty distributions, we find that projected global damages are statistically indistinguishable across the two most extreme emission scenarios until 2049 (at the 5% significance level; Fig. 1 ). As such, the climate damages occurring before this time constitute those to which the world is already committed owing to the combination of past emissions and the range of future emission scenarios that are considered socio-economically plausible 15 . These committed damages comprise a permanent income reduction of 19% on average globally (population-weighted average) in comparison with a baseline without climate-change impacts (with a likely range of 11–29%, following the likelihood classification adopted by the Intergovernmental Panel on Climate Change (IPCC); see caption of Fig. 1 ). Even though levels of income per capita generally still increase relative to those of today, this constitutes a permanent income reduction for most regions, including North America and Europe (each with median income reductions of approximately 11%) and with South Asia and Africa being the most strongly affected (each with median income reductions of approximately 22%; Fig. 1 ). Under a middle-of-the road scenario of future income development (SSP2, in which SSP stands for Shared Socio-economic Pathway), this corresponds to global annual damages in 2049 of 38 trillion in 2005 international dollars (likely range of 19–59 trillion 2005 international dollars). Compared with empirical specifications that assume pure growth or pure level effects, our preferred specification that provides a robust lower bound on the extent of climate impact persistence produces damages between these two extreme assumptions (Extended Data Fig. 3 ).
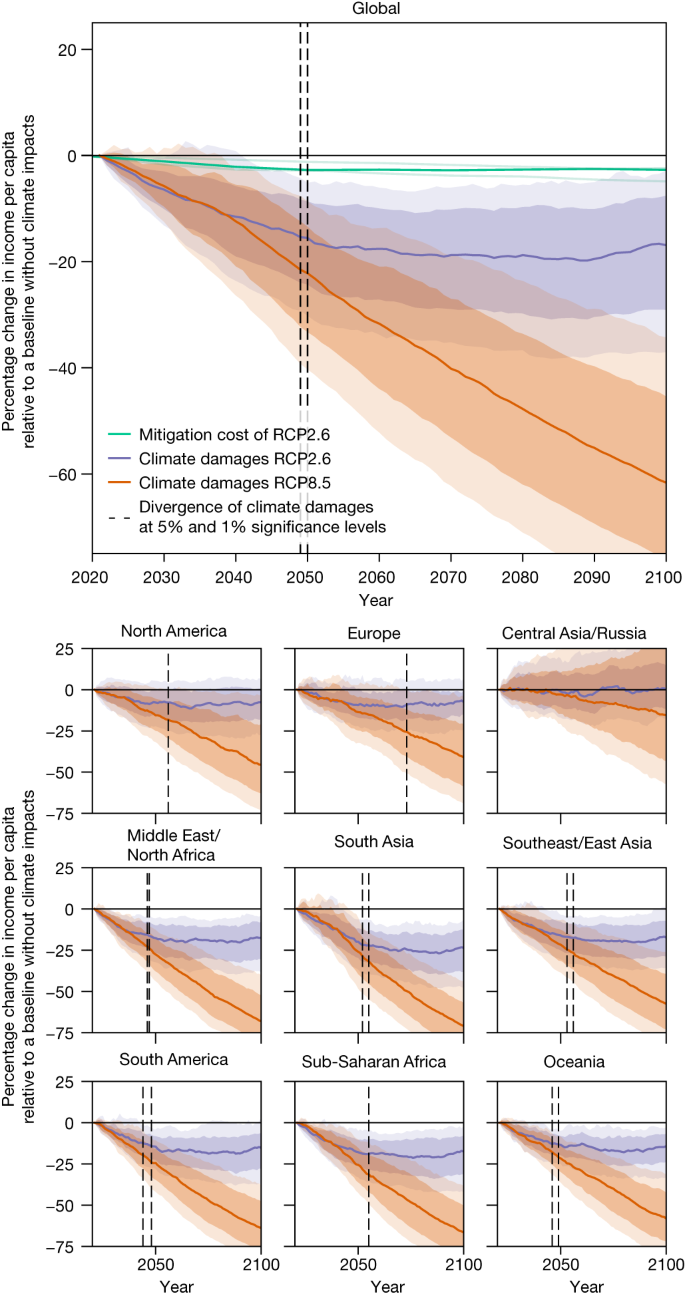
Estimates of the projected reduction in income per capita from changes in all climate variables based on empirical models of climate impacts on economic output with a robust lower bound on their persistence (Extended Data Fig. 1 ) under a low-emission scenario compatible with the 2 °C warming target and a high-emission scenario (SSP2-RCP2.6 and SSP5-RCP8.5, respectively) are shown in purple and orange, respectively. Shading represents the 34% and 10% confidence intervals reflecting the likely and very likely ranges, respectively (following the likelihood classification adopted by the IPCC), having estimated uncertainty from a Monte Carlo procedure, which samples the uncertainty from the choice of physical climate models, empirical models with different numbers of lags and bootstrapped estimates of the regression parameters shown in Supplementary Figs. 1 – 3 . Vertical dashed lines show the time at which the climate damages of the two emission scenarios diverge at the 5% and 1% significance levels based on the distribution of differences between emission scenarios arising from the uncertainty sampling discussed above. Note that uncertainty in the difference of the two scenarios is smaller than the combined uncertainty of the two respective scenarios because samples of the uncertainty (climate model and empirical model choice, as well as model parameter bootstrap) are consistent across the two emission scenarios, hence the divergence of damages occurs while the uncertainty bounds of the two separate damage scenarios still overlap. Estimates of global mitigation costs from the three IAMs that provide results for the SSP2 baseline and SSP2-RCP2.6 scenario are shown in light green in the top panel, with the median of these estimates shown in bold.
Damages already outweigh mitigation costs
We compare the damages to which the world is committed over the next 25 years to estimates of the mitigation costs required to achieve the Paris Climate Agreement. Taking estimates of mitigation costs from the three integrated assessment models (IAMs) in the IPCC AR6 database 23 that provide results under comparable scenarios (SSP2 baseline and SSP2-RCP2.6, in which RCP stands for Representative Concentration Pathway), we find that the median committed climate damages are larger than the median mitigation costs in 2050 (six trillion in 2005 international dollars) by a factor of approximately six (note that estimates of mitigation costs are only provided every 10 years by the IAMs and so a comparison in 2049 is not possible). This comparison simply aims to compare the magnitude of future damages against mitigation costs, rather than to conduct a formal cost–benefit analysis of transitioning from one emission path to another. Formal cost–benefit analyses typically find that the net benefits of mitigation only emerge after 2050 (ref. 5 ), which may lead some to conclude that physical damages from climate change are simply not large enough to outweigh mitigation costs until the second half of the century. Our simple comparison of their magnitudes makes clear that damages are actually already considerably larger than mitigation costs and the delayed emergence of net mitigation benefits results primarily from the fact that damages across different emission paths are indistinguishable until mid-century (Fig. 1 ).
Although these near-term damages constitute those to which the world is already committed, we note that damage estimates diverge strongly across emission scenarios after 2049, conveying the clear benefits of mitigation from a purely economic point of view that have been emphasized in previous studies 4 , 24 . As well as the uncertainties assessed in Fig. 1 , these conclusions are robust to structural choices, such as the timescale with which changes in the moderating variables of the empirical models are estimated (Supplementary Figs. 10 and 11 ), as well as the order in which one accounts for the intertemporal and international components of currency comparison (Supplementary Fig. 12 ; see Methods for further details).
Damages from variability and extremes
Committed damages primarily arise through changes in average temperature (Fig. 2 ). This reflects the fact that projected changes in average temperature are larger than those in other climate variables when expressed as a function of their historical interannual variability (Extended Data Fig. 4 ). Because the historical variability is that on which the empirical models are estimated, larger projected changes in comparison with this variability probably lead to larger future impacts in a purely statistical sense. From a mechanistic perspective, one may plausibly interpret this result as implying that future changes in average temperature are the most unprecedented from the perspective of the historical fluctuations to which the economy is accustomed and therefore will cause the most damage. This insight may prove useful in terms of guiding adaptation measures to the sources of greatest damage.
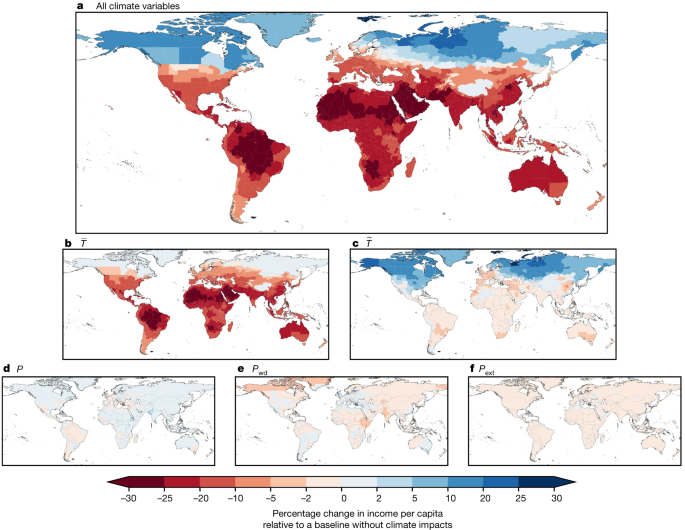
Estimates of the median projected reduction in sub-national income per capita across emission scenarios (SSP2-RCP2.6 and SSP2-RCP8.5) as well as climate model, empirical model and model parameter uncertainty in the year in which climate damages diverge at the 5% level (2049, as identified in Fig. 1 ). a , Impacts arising from all climate variables. b – f , Impacts arising separately from changes in annual mean temperature ( b ), daily temperature variability ( c ), total annual precipitation ( d ), the annual number of wet days (>1 mm) ( e ) and extreme daily rainfall ( f ) (see Methods for further definitions). Data on national administrative boundaries are obtained from the GADM database version 3.6 and are freely available for academic use ( https://gadm.org/ ).
Nevertheless, future damages based on empirical models that consider changes in annual average temperature only and exclude the other climate variables constitute income reductions of only 13% in 2049 (Extended Data Fig. 5a , likely range 5–21%). This suggests that accounting for the other components of the distribution of temperature and precipitation raises net damages by nearly 50%. This increase arises through the further damages that these climatic components cause, but also because their inclusion reveals a stronger negative economic response to average temperatures (Extended Data Fig. 5b ). The latter finding is consistent with our Monte Carlo simulations, which suggest that the magnitude of the effect of average temperature on economic growth is underestimated unless accounting for the impacts of other correlated climate variables (Supplementary Fig. 7 ).
In terms of the relative contributions of the different climatic components to overall damages, we find that accounting for daily temperature variability causes the largest increase in overall damages relative to empirical frameworks that only consider changes in annual average temperature (4.9 percentage points, likely range 2.4–8.7 percentage points, equivalent to approximately 10 trillion international dollars). Accounting for precipitation causes smaller increases in overall damages, which are—nevertheless—equivalent to approximately 1.2 trillion international dollars: 0.01 percentage points (−0.37–0.33 percentage points), 0.34 percentage points (0.07–0.90 percentage points) and 0.36 percentage points (0.13–0.65 percentage points) from total annual precipitation, the number of wet days and extreme daily precipitation, respectively. Moreover, climate models seem to underestimate future changes in temperature variability 25 and extreme precipitation 26 , 27 in response to anthropogenic forcing as compared with that observed historically, suggesting that the true impacts from these variables may be larger.
The distribution of committed damages
The spatial distribution of committed damages (Fig. 2a ) reflects a complex interplay between the patterns of future change in several climatic components and those of historical economic vulnerability to changes in those variables. Damages resulting from increasing annual mean temperature (Fig. 2b ) are negative almost everywhere globally, and larger at lower latitudes in regions in which temperatures are already higher and economic vulnerability to temperature increases is greatest (see the response heterogeneity to mean temperature embodied in Extended Data Fig. 1a ). This occurs despite the amplified warming projected at higher latitudes 28 , suggesting that regional heterogeneity in economic vulnerability to temperature changes outweighs heterogeneity in the magnitude of future warming (Supplementary Fig. 13a ). Economic damages owing to daily temperature variability (Fig. 2c ) exhibit a strong latitudinal polarisation, primarily reflecting the physical response of daily variability to greenhouse forcing in which increases in variability across lower latitudes (and Europe) contrast decreases at high latitudes 25 (Supplementary Fig. 13b ). These two temperature terms are the dominant determinants of the pattern of overall damages (Fig. 2a ), which exhibits a strong polarity with damages across most of the globe except at the highest northern latitudes. Future changes in total annual precipitation mainly bring economic benefits except in regions of drying, such as the Mediterranean and central South America (Fig. 2d and Supplementary Fig. 13c ), but these benefits are opposed by changes in the number of wet days, which produce damages with a similar pattern of opposite sign (Fig. 2e and Supplementary Fig. 13d ). By contrast, changes in extreme daily rainfall produce damages in all regions, reflecting the intensification of daily rainfall extremes over global land areas 29 , 30 (Fig. 2f and Supplementary Fig. 13e ).
The spatial distribution of committed damages implies considerable injustice along two dimensions: culpability for the historical emissions that have caused climate change and pre-existing levels of socio-economic welfare. Spearman’s rank correlations indicate that committed damages are significantly larger in countries with smaller historical cumulative emissions, as well as in regions with lower current income per capita (Fig. 3 ). This implies that those countries that will suffer the most from the damages already committed are those that are least responsible for climate change and which also have the least resources to adapt to it.
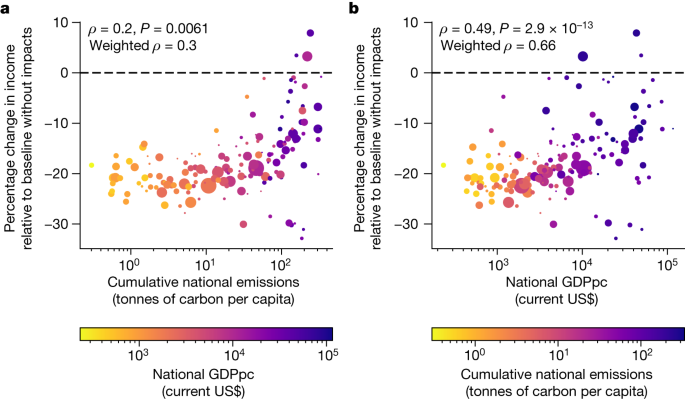
Estimates of the median projected change in national income per capita across emission scenarios (RCP2.6 and RCP8.5) as well as climate model, empirical model and model parameter uncertainty in the year in which climate damages diverge at the 5% level (2049, as identified in Fig. 1 ) are plotted against cumulative national emissions per capita in 2020 (from the Global Carbon Project) and coloured by national income per capita in 2020 (from the World Bank) in a and vice versa in b . In each panel, the size of each scatter point is weighted by the national population in 2020 (from the World Bank). Inset numbers indicate the Spearman’s rank correlation ρ and P -values for a hypothesis test whose null hypothesis is of no correlation, as well as the Spearman’s rank correlation weighted by national population.
To further quantify this heterogeneity, we assess the difference in committed damages between the upper and lower quartiles of regions when ranked by present income levels and historical cumulative emissions (using a population weighting to both define the quartiles and estimate the group averages). On average, the quartile of countries with lower income are committed to an income loss that is 8.9 percentage points (or 61%) greater than the upper quartile (Extended Data Fig. 6 ), with a likely range of 3.8–14.7 percentage points across the uncertainty sampling of our damage projections (following the likelihood classification adopted by the IPCC). Similarly, the quartile of countries with lower historical cumulative emissions are committed to an income loss that is 6.9 percentage points (or 40%) greater than the upper quartile, with a likely range of 0.27–12 percentage points. These patterns reemphasize the prevalence of injustice in climate impacts 31 , 32 , 33 in the context of the damages to which the world is already committed by historical emissions and socio-economic inertia.
Contextualizing the magnitude of damages
The magnitude of projected economic damages exceeds previous literature estimates 2 , 3 , arising from several developments made on previous approaches. Our estimates are larger than those of ref. 2 (see first row of Extended Data Table 3 ), primarily because of the facts that sub-national estimates typically show a steeper temperature response (see also refs. 3 , 34 ) and that accounting for other climatic components raises damage estimates (Extended Data Fig. 5 ). However, we note that our empirical approach using first-differenced climate variables is conservative compared with that of ref. 2 in regard to the persistence of climate impacts on growth (see introduction and Methods section ‘Empirical model specification: fixed-effects distributed lag models’), an important determinant of the magnitude of long-term damages 19 , 21 . Using a similar empirical specification to ref. 2 , which assumes infinite persistence while maintaining the rest of our approach (sub-national data and further climate variables), produces considerably larger damages (purple curve of Extended Data Fig. 3 ). Compared with studies that do take the first difference of climate variables 3 , 35 , our estimates are also larger (see second and third rows of Extended Data Table 3 ). The inclusion of further climate variables (Extended Data Fig. 5 ) and a sufficient number of lags to more adequately capture the extent of impact persistence (Extended Data Figs. 1 and 2 ) are the main sources of this difference, as is the use of specifications that capture nonlinearities in the temperature response when compared with ref. 35 . In summary, our estimates develop on previous studies by incorporating the latest data and empirical insights 7 , 8 , as well as in providing a robust empirical lower bound on the persistence of impacts on economic growth, which constitutes a middle ground between the extremes of the growth-versus-levels debate 19 , 21 (Extended Data Fig. 3 ).
Compared with the fraction of variance explained by the empirical models historically (<5%), the projection of reductions in income of 19% may seem large. This arises owing to the fact that projected changes in climatic conditions are much larger than those that were experienced historically, particularly for changes in average temperature (Extended Data Fig. 4 ). As such, any assessment of future climate-change impacts necessarily requires an extrapolation outside the range of the historical data on which the empirical impact models were evaluated. Nevertheless, these models constitute the most state-of-the-art methods for inference of plausibly causal climate impacts based on observed data. Moreover, we take explicit steps to limit out-of-sample extrapolation by capping the moderating variables of the interaction terms at the 95th percentile of the historical distribution (see Methods ). This avoids extrapolating the marginal effects outside what was observed historically. Given the nonlinear response of economic output to annual mean temperature (Extended Data Fig. 1 and Extended Data Table 2 ), this is a conservative choice that limits the magnitude of damages that we project. Furthermore, back-of-the-envelope calculations indicate that the projected damages are consistent with the magnitude and patterns of historical economic development (see Supplementary Discussion Section 5 ).
Missing impacts and spatial spillovers
Despite assessing several climatic components from which economic impacts have recently been identified 3 , 7 , 8 , this assessment of aggregate climate damages should not be considered comprehensive. Important channels such as impacts from heatwaves 31 , sea-level rise 36 , tropical cyclones 37 and tipping points 38 , 39 , as well as non-market damages such as those to ecosystems 40 and human health 41 , are not considered in these estimates. Sea-level rise is unlikely to be feasibly incorporated into empirical assessments such as this because historical sea-level variability is mostly small. Non-market damages are inherently intractable within our estimates of impacts on aggregate monetary output and estimates of these impacts could arguably be considered as extra to those identified here. Recent empirical work suggests that accounting for these channels would probably raise estimates of these committed damages, with larger damages continuing to arise in the global south 31 , 36 , 37 , 38 , 39 , 40 , 41 , 42 .
Moreover, our main empirical analysis does not explicitly evaluate the potential for impacts in local regions to produce effects that ‘spill over’ into other regions. Such effects may further mitigate or amplify the impacts we estimate, for example, if companies relocate production from one affected region to another or if impacts propagate along supply chains. The current literature indicates that trade plays a substantial role in propagating spillover effects 43 , 44 , making their assessment at the sub-national level challenging without available data on sub-national trade dependencies. Studies accounting for only spatially adjacent neighbours indicate that negative impacts in one region induce further negative impacts in neighbouring regions 45 , 46 , 47 , 48 , suggesting that our projected damages are probably conservative by excluding these effects. In Supplementary Fig. 14 , we assess spillovers from neighbouring regions using a spatial-lag model. For simplicity, this analysis excludes temporal lags, focusing only on contemporaneous effects. The results show that accounting for spatial spillovers can amplify the overall magnitude, and also the heterogeneity, of impacts. Consistent with previous literature, this indicates that the overall magnitude (Fig. 1 ) and heterogeneity (Fig. 3 ) of damages that we project in our main specification may be conservative without explicitly accounting for spillovers. We note that further analysis that addresses both spatially and trade-connected spillovers, while also accounting for delayed impacts using temporal lags, would be necessary to adequately address this question fully. These approaches offer fruitful avenues for further research but are beyond the scope of this manuscript, which primarily aims to explore the impacts of different climate conditions and their persistence.
Policy implications
We find that the economic damages resulting from climate change until 2049 are those to which the world economy is already committed and that these greatly outweigh the costs required to mitigate emissions in line with the 2 °C target of the Paris Climate Agreement (Fig. 1 ). This assessment is complementary to formal analyses of the net costs and benefits associated with moving from one emission path to another, which typically find that net benefits of mitigation only emerge in the second half of the century 5 . Our simple comparison of the magnitude of damages and mitigation costs makes clear that this is primarily because damages are indistinguishable across emissions scenarios—that is, committed—until mid-century (Fig. 1 ) and that they are actually already much larger than mitigation costs. For simplicity, and owing to the availability of data, we compare damages to mitigation costs at the global level. Regional estimates of mitigation costs may shed further light on the national incentives for mitigation to which our results already hint, of relevance for international climate policy. Although these damages are committed from a mitigation perspective, adaptation may provide an opportunity to reduce them. Moreover, the strong divergence of damages after mid-century reemphasizes the clear benefits of mitigation from a purely economic perspective, as highlighted in previous studies 1 , 4 , 6 , 24 .
Historical climate data
Historical daily 2-m temperature and precipitation totals (in mm) are obtained for the period 1979–2019 from the W5E5 database. The W5E5 dataset comes from ERA-5, a state-of-the-art reanalysis of historical observations, but has been bias-adjusted by applying version 2.0 of the WATCH Forcing Data to ERA-5 reanalysis data and precipitation data from version 2.3 of the Global Precipitation Climatology Project to better reflect ground-based measurements 49 , 50 , 51 . We obtain these data on a 0.5° × 0.5° grid from the Inter-Sectoral Impact Model Intercomparison Project (ISIMIP) database. Notably, these historical data have been used to bias-adjust future climate projections from CMIP-6 (see the following section), ensuring consistency between the distribution of historical daily weather on which our empirical models were estimated and the climate projections used to estimate future damages. These data are publicly available from the ISIMIP database. See refs. 7 , 8 for robustness tests of the empirical models to the choice of climate data reanalysis products.
Future climate data
Daily 2-m temperature and precipitation totals (in mm) are taken from 21 climate models participating in CMIP-6 under a high (RCP8.5) and a low (RCP2.6) greenhouse gas emission scenario from 2015 to 2100. The data have been bias-adjusted and statistically downscaled to a common half-degree grid to reflect the historical distribution of daily temperature and precipitation of the W5E5 dataset using the trend-preserving method developed by the ISIMIP 50 , 52 . As such, the climate model data reproduce observed climatological patterns exceptionally well (Supplementary Table 5 ). Gridded data are publicly available from the ISIMIP database.
Historical economic data
Historical economic data come from the DOSE database of sub-national economic output 53 . We use a recent revision to the DOSE dataset that provides data across 83 countries, 1,660 sub-national regions with varying temporal coverage from 1960 to 2019. Sub-national units constitute the first administrative division below national, for example, states for the USA and provinces for China. Data come from measures of gross regional product per capita (GRPpc) or income per capita in local currencies, reflecting the values reported in national statistical agencies, yearbooks and, in some cases, academic literature. We follow previous literature 3 , 7 , 8 , 54 and assess real sub-national output per capita by first converting values from local currencies to US dollars to account for diverging national inflationary tendencies and then account for US inflation using a US deflator. Alternatively, one might first account for national inflation and then convert between currencies. Supplementary Fig. 12 demonstrates that our conclusions are consistent when accounting for price changes in the reversed order, although the magnitude of estimated damages varies. See the documentation of the DOSE dataset for further discussion of these choices. Conversions between currencies are conducted using exchange rates from the FRED database of the Federal Reserve Bank of St. Louis 55 and the national deflators from the World Bank 56 .
Future socio-economic data
Baseline gridded gross domestic product (GDP) and population data for the period 2015–2100 are taken from the middle-of-the-road scenario SSP2 (ref. 15 ). Population data have been downscaled to a half-degree grid by the ISIMIP following the methodologies of refs. 57 , 58 , which we then aggregate to the sub-national level of our economic data using the spatial aggregation procedure described below. Because current methodologies for downscaling the GDP of the SSPs use downscaled population to do so, per-capita estimates of GDP with a realistic distribution at the sub-national level are not readily available for the SSPs. We therefore use national-level GDP per capita (GDPpc) projections for all sub-national regions of a given country, assuming homogeneity within countries in terms of baseline GDPpc. Here we use projections that have been updated to account for the impact of the COVID-19 pandemic on the trajectory of future income, while remaining consistent with the long-term development of the SSPs 59 . The choice of baseline SSP alters the magnitude of projected climate damages in monetary terms, but when assessed in terms of percentage change from the baseline, the choice of socio-economic scenario is inconsequential. Gridded SSP population data and national-level GDPpc data are publicly available from the ISIMIP database. Sub-national estimates as used in this study are available in the code and data replication files.
Climate variables
Following recent literature 3 , 7 , 8 , we calculate an array of climate variables for which substantial impacts on macroeconomic output have been identified empirically, supported by further evidence at the micro level for plausible underlying mechanisms. See refs. 7 , 8 for an extensive motivation for the use of these particular climate variables and for detailed empirical tests on the nature and robustness of their effects on economic output. To summarize, these studies have found evidence for independent impacts on economic growth rates from annual average temperature, daily temperature variability, total annual precipitation, the annual number of wet days and extreme daily rainfall. Assessments of daily temperature variability were motivated by evidence of impacts on agricultural output and human health, as well as macroeconomic literature on the impacts of volatility on growth when manifest in different dimensions, such as government spending, exchange rates and even output itself 7 . Assessments of precipitation impacts were motivated by evidence of impacts on agricultural productivity, metropolitan labour outcomes and conflict, as well as damages caused by flash flooding 8 . See Extended Data Table 1 for detailed references to empirical studies of these physical mechanisms. Marked impacts of daily temperature variability, total annual precipitation, the number of wet days and extreme daily rainfall on macroeconomic output were identified robustly across different climate datasets, spatial aggregation schemes, specifications of regional time trends and error-clustering approaches. They were also found to be robust to the consideration of temperature extremes 7 , 8 . Furthermore, these climate variables were identified as having independent effects on economic output 7 , 8 , which we further explain here using Monte Carlo simulations to demonstrate the robustness of the results to concerns of imperfect multicollinearity between climate variables (Supplementary Methods Section 2 ), as well as by using information criteria (Supplementary Table 1 ) to demonstrate that including several lagged climate variables provides a preferable trade-off between optimally describing the data and limiting the possibility of overfitting.
We calculate these variables from the distribution of daily, d , temperature, T x , d , and precipitation, P x , d , at the grid-cell, x , level for both the historical and future climate data. As well as annual mean temperature, \({\bar{T}}_{x,y}\) , and annual total precipitation, P x , y , we calculate annual, y , measures of daily temperature variability, \({\widetilde{T}}_{x,y}\) :
the number of wet days, Pwd x , y :
and extreme daily rainfall:
in which T x , d , m , y is the grid-cell-specific daily temperature in month m and year y , \({\bar{T}}_{x,m,{y}}\) is the year and grid-cell-specific monthly, m , mean temperature, D m and D y the number of days in a given month m or year y , respectively, H the Heaviside step function, 1 mm the threshold used to define wet days and P 99.9 x is the 99.9th percentile of historical (1979–2019) daily precipitation at the grid-cell level. Units of the climate measures are degrees Celsius for annual mean temperature and daily temperature variability, millimetres for total annual precipitation and extreme daily precipitation, and simply the number of days for the annual number of wet days.
We also calculated weighted standard deviations of monthly rainfall totals as also used in ref. 8 but do not include them in our projections as we find that, when accounting for delayed effects, their effect becomes statistically indistinct and is better captured by changes in total annual rainfall.
Spatial aggregation
We aggregate grid-cell-level historical and future climate measures, as well as grid-cell-level future GDPpc and population, to the level of the first administrative unit below national level of the GADM database, using an area-weighting algorithm that estimates the portion of each grid cell falling within an administrative boundary. We use this as our baseline specification following previous findings that the effect of area or population weighting at the sub-national level is negligible 7 , 8 .
Empirical model specification: fixed-effects distributed lag models
Following a wide range of climate econometric literature 16 , 60 , we use panel regression models with a selection of fixed effects and time trends to isolate plausibly exogenous variation with which to maximize confidence in a causal interpretation of the effects of climate on economic growth rates. The use of region fixed effects, μ r , accounts for unobserved time-invariant differences between regions, such as prevailing climatic norms and growth rates owing to historical and geopolitical factors. The use of yearly fixed effects, η y , accounts for regionally invariant annual shocks to the global climate or economy such as the El Niño–Southern Oscillation or global recessions. In our baseline specification, we also include region-specific linear time trends, k r y , to exclude the possibility of spurious correlations resulting from common slow-moving trends in climate and growth.
The persistence of climate impacts on economic growth rates is a key determinant of the long-term magnitude of damages. Methods for inferring the extent of persistence in impacts on growth rates have typically used lagged climate variables to evaluate the presence of delayed effects or catch-up dynamics 2 , 18 . For example, consider starting from a model in which a climate condition, C r , y , (for example, annual mean temperature) affects the growth rate, Δlgrp r , y (the first difference of the logarithm of gross regional product) of region r in year y :
which we refer to as a ‘pure growth effects’ model in the main text. Typically, further lags are included,
and the cumulative effect of all lagged terms is evaluated to assess the extent to which climate impacts on growth rates persist. Following ref. 18 , in the case that,
the implication is that impacts on the growth rate persist up to NL years after the initial shock (possibly to a weaker or a stronger extent), whereas if
then the initial impact on the growth rate is recovered after NL years and the effect is only one on the level of output. However, we note that such approaches are limited by the fact that, when including an insufficient number of lags to detect a recovery of the growth rates, one may find equation ( 6 ) to be satisfied and incorrectly assume that a change in climatic conditions affects the growth rate indefinitely. In practice, given a limited record of historical data, including too few lags to confidently conclude in an infinitely persistent impact on the growth rate is likely, particularly over the long timescales over which future climate damages are often projected 2 , 24 . To avoid this issue, we instead begin our analysis with a model for which the level of output, lgrp r , y , depends on the level of a climate variable, C r , y :
Given the non-stationarity of the level of output, we follow the literature 19 and estimate such an equation in first-differenced form as,
which we refer to as a model of ‘pure level effects’ in the main text. This model constitutes a baseline specification in which a permanent change in the climate variable produces an instantaneous impact on the growth rate and a permanent effect only on the level of output. By including lagged variables in this specification,
we are able to test whether the impacts on the growth rate persist any further than instantaneously by evaluating whether α L > 0 are statistically significantly different from zero. Even though this framework is also limited by the possibility of including too few lags, the choice of a baseline model specification in which impacts on the growth rate do not persist means that, in the case of including too few lags, the framework reverts to the baseline specification of level effects. As such, this framework is conservative with respect to the persistence of impacts and the magnitude of future damages. It naturally avoids assumptions of infinite persistence and we are able to interpret any persistence that we identify with equation ( 9 ) as a lower bound on the extent of climate impact persistence on growth rates. See the main text for further discussion of this specification choice, in particular about its conservative nature compared with previous literature estimates, such as refs. 2 , 18 .
We allow the response to climatic changes to vary across regions, using interactions of the climate variables with historical average (1979–2019) climatic conditions reflecting heterogenous effects identified in previous work 7 , 8 . Following this previous work, the moderating variables of these interaction terms constitute the historical average of either the variable itself or of the seasonal temperature difference, \({\hat{T}}_{r}\) , or annual mean temperature, \({\bar{T}}_{r}\) , in the case of daily temperature variability 7 and extreme daily rainfall, respectively 8 .
The resulting regression equation with N and M lagged variables, respectively, reads:
in which Δlgrp r , y is the annual, regional GRPpc growth rate, measured as the first difference of the logarithm of real GRPpc, following previous work 2 , 3 , 7 , 8 , 18 , 19 . Fixed-effects regressions were run using the fixest package in R (ref. 61 ).
Estimates of the coefficients of interest α i , L are shown in Extended Data Fig. 1 for N = M = 10 lags and for our preferred choice of the number of lags in Supplementary Figs. 1 – 3 . In Extended Data Fig. 1 , errors are shown clustered at the regional level, but for the construction of damage projections, we block-bootstrap the regressions by region 1,000 times to provide a range of parameter estimates with which to sample the projection uncertainty (following refs. 2 , 31 ).
Spatial-lag model
In Supplementary Fig. 14 , we present the results from a spatial-lag model that explores the potential for climate impacts to ‘spill over’ into spatially neighbouring regions. We measure the distance between centroids of each pair of sub-national regions and construct spatial lags that take the average of the first-differenced climate variables and their interaction terms over neighbouring regions that are at distances of 0–500, 500–1,000, 1,000–1,500 and 1,500–2000 km (spatial lags, ‘SL’, 1 to 4). For simplicity, we then assess a spatial-lag model without temporal lags to assess spatial spillovers of contemporaneous climate impacts. This model takes the form:
in which SL indicates the spatial lag of each climate variable and interaction term. In Supplementary Fig. 14 , we plot the cumulative marginal effect of each climate variable at different baseline climate conditions by summing the coefficients for each climate variable and interaction term, for example, for average temperature impacts as:
These cumulative marginal effects can be regarded as the overall spatially dependent impact to an individual region given a one-unit shock to a climate variable in that region and all neighbouring regions at a given value of the moderating variable of the interaction term.
Constructing projections of economic damage from future climate change
We construct projections of future climate damages by applying the coefficients estimated in equation ( 10 ) and shown in Supplementary Tables 2 – 4 (when including only lags with statistically significant effects in specifications that limit overfitting; see Supplementary Methods Section 1 ) to projections of future climate change from the CMIP-6 models. Year-on-year changes in each primary climate variable of interest are calculated to reflect the year-to-year variations used in the empirical models. 30-year moving averages of the moderating variables of the interaction terms are calculated to reflect the long-term average of climatic conditions that were used for the moderating variables in the empirical models. By using moving averages in the projections, we account for the changing vulnerability to climate shocks based on the evolving long-term conditions (Supplementary Figs. 10 and 11 show that the results are robust to the precise choice of the window of this moving average). Although these climate variables are not differenced, the fact that the bias-adjusted climate models reproduce observed climatological patterns across regions for these moderating variables very accurately (Supplementary Table 6 ) with limited spread across models (<3%) precludes the possibility that any considerable bias or uncertainty is introduced by this methodological choice. However, we impose caps on these moderating variables at the 95th percentile at which they were observed in the historical data to prevent extrapolation of the marginal effects outside the range in which the regressions were estimated. This is a conservative choice that limits the magnitude of our damage projections.
Time series of primary climate variables and moderating climate variables are then combined with estimates of the empirical model parameters to evaluate the regression coefficients in equation ( 10 ), producing a time series of annual GRPpc growth-rate reductions for a given emission scenario, climate model and set of empirical model parameters. The resulting time series of growth-rate impacts reflects those occurring owing to future climate change. By contrast, a future scenario with no climate change would be one in which climate variables do not change (other than with random year-to-year fluctuations) and hence the time-averaged evaluation of equation ( 10 ) would be zero. Our approach therefore implicitly compares the future climate-change scenario to this no-climate-change baseline scenario.
The time series of growth-rate impacts owing to future climate change in region r and year y , δ r , y , are then added to the future baseline growth rates, π r , y (in log-diff form), obtained from the SSP2 scenario to yield trajectories of damaged GRPpc growth rates, ρ r , y . These trajectories are aggregated over time to estimate the future trajectory of GRPpc with future climate impacts:
in which GRPpc r , y =2020 is the initial log level of GRPpc. We begin damage estimates in 2020 to reflect the damages occurring since the end of the period for which we estimate the empirical models (1979–2019) and to match the timing of mitigation-cost estimates from most IAMs (see below).
For each emission scenario, this procedure is repeated 1,000 times while randomly sampling from the selection of climate models, the selection of empirical models with different numbers of lags (shown in Supplementary Figs. 1 – 3 and Supplementary Tables 2 – 4 ) and bootstrapped estimates of the regression parameters. The result is an ensemble of future GRPpc trajectories that reflect uncertainty from both physical climate change and the structural and sampling uncertainty of the empirical models.
Estimates of mitigation costs
We obtain IPCC estimates of the aggregate costs of emission mitigation from the AR6 Scenario Explorer and Database hosted by IIASA 23 . Specifically, we search the AR6 Scenarios Database World v1.1 for IAMs that provided estimates of global GDP and population under both a SSP2 baseline and a SSP2-RCP2.6 scenario to maintain consistency with the socio-economic and emission scenarios of the climate damage projections. We find five IAMs that provide data for these scenarios, namely, MESSAGE-GLOBIOM 1.0, REMIND-MAgPIE 1.5, AIM/GCE 2.0, GCAM 4.2 and WITCH-GLOBIOM 3.1. Of these five IAMs, we use the results only from the first three that passed the IPCC vetting procedure for reproducing historical emission and climate trajectories. We then estimate global mitigation costs as the percentage difference in global per capita GDP between the SSP2 baseline and the SSP2-RCP2.6 emission scenario. In the case of one of these IAMs, estimates of mitigation costs begin in 2020, whereas in the case of two others, mitigation costs begin in 2010. The mitigation cost estimates before 2020 in these two IAMs are mostly negligible, and our choice to begin comparison with damage estimates in 2020 is conservative with respect to the relative weight of climate damages compared with mitigation costs for these two IAMs.
Data availability
Data on economic production and ERA-5 climate data are publicly available at https://doi.org/10.5281/zenodo.4681306 (ref. 62 ) and https://www.ecmwf.int/en/forecasts/datasets/reanalysis-datasets/era5 , respectively. Data on mitigation costs are publicly available at https://data.ene.iiasa.ac.at/ar6/#/downloads . Processed climate and economic data, as well as all other necessary data for reproduction of the results, are available at the public repository https://doi.org/10.5281/zenodo.10562951 (ref. 63 ).
Code availability
All code necessary for reproduction of the results is available at the public repository https://doi.org/10.5281/zenodo.10562951 (ref. 63 ).
Glanemann, N., Willner, S. N. & Levermann, A. Paris Climate Agreement passes the cost-benefit test. Nat. Commun. 11 , 110 (2020).
Article ADS CAS PubMed PubMed Central Google Scholar
Burke, M., Hsiang, S. M. & Miguel, E. Global non-linear effect of temperature on economic production. Nature 527 , 235–239 (2015).
Article ADS CAS PubMed Google Scholar
Kalkuhl, M. & Wenz, L. The impact of climate conditions on economic production. Evidence from a global panel of regions. J. Environ. Econ. Manag. 103 , 102360 (2020).
Article Google Scholar
Moore, F. C. & Diaz, D. B. Temperature impacts on economic growth warrant stringent mitigation policy. Nat. Clim. Change 5 , 127–131 (2015).
Article ADS Google Scholar
Drouet, L., Bosetti, V. & Tavoni, M. Net economic benefits of well-below 2°C scenarios and associated uncertainties. Oxf. Open Clim. Change 2 , kgac003 (2022).
Ueckerdt, F. et al. The economically optimal warming limit of the planet. Earth Syst. Dyn. 10 , 741–763 (2019).
Kotz, M., Wenz, L., Stechemesser, A., Kalkuhl, M. & Levermann, A. Day-to-day temperature variability reduces economic growth. Nat. Clim. Change 11 , 319–325 (2021).
Kotz, M., Levermann, A. & Wenz, L. The effect of rainfall changes on economic production. Nature 601 , 223–227 (2022).
Kousky, C. Informing climate adaptation: a review of the economic costs of natural disasters. Energy Econ. 46 , 576–592 (2014).
Harlan, S. L. et al. in Climate Change and Society: Sociological Perspectives (eds Dunlap, R. E. & Brulle, R. J.) 127–163 (Oxford Univ. Press, 2015).
Bolton, P. et al. The Green Swan (BIS Books, 2020).
Alogoskoufis, S. et al. ECB Economy-wide Climate Stress Test: Methodology and Results European Central Bank, 2021).
Weber, E. U. What shapes perceptions of climate change? Wiley Interdiscip. Rev. Clim. Change 1 , 332–342 (2010).
Markowitz, E. M. & Shariff, A. F. Climate change and moral judgement. Nat. Clim. Change 2 , 243–247 (2012).
Riahi, K. et al. The shared socioeconomic pathways and their energy, land use, and greenhouse gas emissions implications: an overview. Glob. Environ. Change 42 , 153–168 (2017).
Auffhammer, M., Hsiang, S. M., Schlenker, W. & Sobel, A. Using weather data and climate model output in economic analyses of climate change. Rev. Environ. Econ. Policy 7 , 181–198 (2013).
Kolstad, C. D. & Moore, F. C. Estimating the economic impacts of climate change using weather observations. Rev. Environ. Econ. Policy 14 , 1–24 (2020).
Dell, M., Jones, B. F. & Olken, B. A. Temperature shocks and economic growth: evidence from the last half century. Am. Econ. J. Macroecon. 4 , 66–95 (2012).
Newell, R. G., Prest, B. C. & Sexton, S. E. The GDP-temperature relationship: implications for climate change damages. J. Environ. Econ. Manag. 108 , 102445 (2021).
Kikstra, J. S. et al. The social cost of carbon dioxide under climate-economy feedbacks and temperature variability. Environ. Res. Lett. 16 , 094037 (2021).
Article ADS CAS Google Scholar
Bastien-Olvera, B. & Moore, F. Persistent effect of temperature on GDP identified from lower frequency temperature variability. Environ. Res. Lett. 17 , 084038 (2022).
Eyring, V. et al. Overview of the Coupled Model Intercomparison Project Phase 6 (CMIP6) experimental design and organization. Geosci. Model Dev. 9 , 1937–1958 (2016).
Byers, E. et al. AR6 scenarios database. Zenodo https://zenodo.org/records/7197970 (2022).
Burke, M., Davis, W. M. & Diffenbaugh, N. S. Large potential reduction in economic damages under UN mitigation targets. Nature 557 , 549–553 (2018).
Kotz, M., Wenz, L. & Levermann, A. Footprint of greenhouse forcing in daily temperature variability. Proc. Natl Acad. Sci. 118 , e2103294118 (2021).
Article CAS PubMed PubMed Central Google Scholar
Myhre, G. et al. Frequency of extreme precipitation increases extensively with event rareness under global warming. Sci. Rep. 9 , 16063 (2019).
Min, S.-K., Zhang, X., Zwiers, F. W. & Hegerl, G. C. Human contribution to more-intense precipitation extremes. Nature 470 , 378–381 (2011).
England, M. R., Eisenman, I., Lutsko, N. J. & Wagner, T. J. The recent emergence of Arctic Amplification. Geophys. Res. Lett. 48 , e2021GL094086 (2021).
Fischer, E. M. & Knutti, R. Anthropogenic contribution to global occurrence of heavy-precipitation and high-temperature extremes. Nat. Clim. Change 5 , 560–564 (2015).
Pfahl, S., O’Gorman, P. A. & Fischer, E. M. Understanding the regional pattern of projected future changes in extreme precipitation. Nat. Clim. Change 7 , 423–427 (2017).
Callahan, C. W. & Mankin, J. S. Globally unequal effect of extreme heat on economic growth. Sci. Adv. 8 , eadd3726 (2022).
Diffenbaugh, N. S. & Burke, M. Global warming has increased global economic inequality. Proc. Natl Acad. Sci. 116 , 9808–9813 (2019).
Callahan, C. W. & Mankin, J. S. National attribution of historical climate damages. Clim. Change 172 , 40 (2022).
Burke, M. & Tanutama, V. Climatic constraints on aggregate economic output. National Bureau of Economic Research, Working Paper 25779. https://doi.org/10.3386/w25779 (2019).
Kahn, M. E. et al. Long-term macroeconomic effects of climate change: a cross-country analysis. Energy Econ. 104 , 105624 (2021).
Desmet, K. et al. Evaluating the economic cost of coastal flooding. National Bureau of Economic Research, Working Paper 24918. https://doi.org/10.3386/w24918 (2018).
Hsiang, S. M. & Jina, A. S. The causal effect of environmental catastrophe on long-run economic growth: evidence from 6,700 cyclones. National Bureau of Economic Research, Working Paper 20352. https://doi.org/10.3386/w2035 (2014).
Ritchie, P. D. et al. Shifts in national land use and food production in Great Britain after a climate tipping point. Nat. Food 1 , 76–83 (2020).
Dietz, S., Rising, J., Stoerk, T. & Wagner, G. Economic impacts of tipping points in the climate system. Proc. Natl Acad. Sci. 118 , e2103081118 (2021).
Bastien-Olvera, B. A. & Moore, F. C. Use and non-use value of nature and the social cost of carbon. Nat. Sustain. 4 , 101–108 (2021).
Carleton, T. et al. Valuing the global mortality consequences of climate change accounting for adaptation costs and benefits. Q. J. Econ. 137 , 2037–2105 (2022).
Bastien-Olvera, B. A. et al. Unequal climate impacts on global values of natural capital. Nature 625 , 722–727 (2024).
Malik, A. et al. Impacts of climate change and extreme weather on food supply chains cascade across sectors and regions in Australia. Nat. Food 3 , 631–643 (2022).
Article ADS PubMed Google Scholar
Kuhla, K., Willner, S. N., Otto, C., Geiger, T. & Levermann, A. Ripple resonance amplifies economic welfare loss from weather extremes. Environ. Res. Lett. 16 , 114010 (2021).
Schleypen, J. R., Mistry, M. N., Saeed, F. & Dasgupta, S. Sharing the burden: quantifying climate change spillovers in the European Union under the Paris Agreement. Spat. Econ. Anal. 17 , 67–82 (2022).
Dasgupta, S., Bosello, F., De Cian, E. & Mistry, M. Global temperature effects on economic activity and equity: a spatial analysis. European Institute on Economics and the Environment, Working Paper 22-1 (2022).
Neal, T. The importance of external weather effects in projecting the macroeconomic impacts of climate change. UNSW Economics Working Paper 2023-09 (2023).
Deryugina, T. & Hsiang, S. M. Does the environment still matter? Daily temperature and income in the United States. National Bureau of Economic Research, Working Paper 20750. https://doi.org/10.3386/w20750 (2014).
Hersbach, H. et al. The ERA5 global reanalysis. Q. J. R. Meteorol. Soc. 146 , 1999–2049 (2020).
Cucchi, M. et al. WFDE5: bias-adjusted ERA5 reanalysis data for impact studies. Earth Syst. Sci. Data 12 , 2097–2120 (2020).
Adler, R. et al. The New Version 2.3 of the Global Precipitation Climatology Project (GPCP) Monthly Analysis Product 1072–1084 (University of Maryland, 2016).
Lange, S. Trend-preserving bias adjustment and statistical downscaling with ISIMIP3BASD (v1.0). Geosci. Model Dev. 12 , 3055–3070 (2019).
Wenz, L., Carr, R. D., Kögel, N., Kotz, M. & Kalkuhl, M. DOSE – global data set of reported sub-national economic output. Sci. Data 10 , 425 (2023).
Article PubMed PubMed Central Google Scholar
Gennaioli, N., La Porta, R., Lopez De Silanes, F. & Shleifer, A. Growth in regions. J. Econ. Growth 19 , 259–309 (2014).
Board of Governors of the Federal Reserve System (US). U.S. dollars to euro spot exchange rate. https://fred.stlouisfed.org/series/AEXUSEU (2022).
World Bank. GDP deflator. https://data.worldbank.org/indicator/NY.GDP.DEFL.ZS (2022).
Jones, B. & O’Neill, B. C. Spatially explicit global population scenarios consistent with the Shared Socioeconomic Pathways. Environ. Res. Lett. 11 , 084003 (2016).
Murakami, D. & Yamagata, Y. Estimation of gridded population and GDP scenarios with spatially explicit statistical downscaling. Sustainability 11 , 2106 (2019).
Koch, J. & Leimbach, M. Update of SSP GDP projections: capturing recent changes in national accounting, PPP conversion and Covid 19 impacts. Ecol. Econ. 206 (2023).
Carleton, T. A. & Hsiang, S. M. Social and economic impacts of climate. Science 353 , aad9837 (2016).
Article PubMed Google Scholar
Bergé, L. Efficient estimation of maximum likelihood models with multiple fixed-effects: the R package FENmlm. DEM Discussion Paper Series 18-13 (2018).
Kalkuhl, M., Kotz, M. & Wenz, L. DOSE - The MCC-PIK Database Of Subnational Economic output. Zenodo https://zenodo.org/doi/10.5281/zenodo.4681305 (2021).
Kotz, M., Wenz, L. & Levermann, A. Data and code for “The economic commitment of climate change”. Zenodo https://zenodo.org/doi/10.5281/zenodo.10562951 (2024).
Dasgupta, S. et al. Effects of climate change on combined labour productivity and supply: an empirical, multi-model study. Lancet Planet. Health 5 , e455–e465 (2021).
Lobell, D. B. et al. The critical role of extreme heat for maize production in the United States. Nat. Clim. Change 3 , 497–501 (2013).
Zhao, C. et al. Temperature increase reduces global yields of major crops in four independent estimates. Proc. Natl Acad. Sci. 114 , 9326–9331 (2017).
Wheeler, T. R., Craufurd, P. Q., Ellis, R. H., Porter, J. R. & Prasad, P. V. Temperature variability and the yield of annual crops. Agric. Ecosyst. Environ. 82 , 159–167 (2000).
Rowhani, P., Lobell, D. B., Linderman, M. & Ramankutty, N. Climate variability and crop production in Tanzania. Agric. For. Meteorol. 151 , 449–460 (2011).
Ceglar, A., Toreti, A., Lecerf, R., Van der Velde, M. & Dentener, F. Impact of meteorological drivers on regional inter-annual crop yield variability in France. Agric. For. Meteorol. 216 , 58–67 (2016).
Shi, L., Kloog, I., Zanobetti, A., Liu, P. & Schwartz, J. D. Impacts of temperature and its variability on mortality in New England. Nat. Clim. Change 5 , 988–991 (2015).
Xue, T., Zhu, T., Zheng, Y. & Zhang, Q. Declines in mental health associated with air pollution and temperature variability in China. Nat. Commun. 10 , 2165 (2019).
Article ADS PubMed PubMed Central Google Scholar
Liang, X.-Z. et al. Determining climate effects on US total agricultural productivity. Proc. Natl Acad. Sci. 114 , E2285–E2292 (2017).
Desbureaux, S. & Rodella, A.-S. Drought in the city: the economic impact of water scarcity in Latin American metropolitan areas. World Dev. 114 , 13–27 (2019).
Damania, R. The economics of water scarcity and variability. Oxf. Rev. Econ. Policy 36 , 24–44 (2020).
Davenport, F. V., Burke, M. & Diffenbaugh, N. S. Contribution of historical precipitation change to US flood damages. Proc. Natl Acad. Sci. 118 , e2017524118 (2021).
Dave, R., Subramanian, S. S. & Bhatia, U. Extreme precipitation induced concurrent events trigger prolonged disruptions in regional road networks. Environ. Res. Lett. 16 , 104050 (2021).
Download references
Acknowledgements
We gratefully acknowledge financing from the Volkswagen Foundation and the Deutsche Gesellschaft für Internationale Zusammenarbeit (GIZ) GmbH on behalf of the Government of the Federal Republic of Germany and Federal Ministry for Economic Cooperation and Development (BMZ).
Open access funding provided by Potsdam-Institut für Klimafolgenforschung (PIK) e.V.
Author information
Authors and affiliations.
Research Domain IV, Research Domain IV, Potsdam Institute for Climate Impact Research, Potsdam, Germany
Maximilian Kotz, Anders Levermann & Leonie Wenz
Institute of Physics, Potsdam University, Potsdam, Germany
Maximilian Kotz & Anders Levermann
Mercator Research Institute on Global Commons and Climate Change, Berlin, Germany
Leonie Wenz
You can also search for this author in PubMed Google Scholar
Contributions
All authors contributed to the design of the analysis. M.K. conducted the analysis and produced the figures. All authors contributed to the interpretation and presentation of the results. M.K. and L.W. wrote the manuscript.
Corresponding author
Correspondence to Leonie Wenz .
Ethics declarations
Competing interests.
The authors declare no competing interests.
Peer review
Peer review information.
Nature thanks Xin-Zhong Liang, Chad Thackeray and the other, anonymous, reviewer(s) for their contribution to the peer review of this work. Peer reviewer reports are available.
Additional information
Publisher’s note Springer Nature remains neutral with regard to jurisdictional claims in published maps and institutional affiliations.
Extended data figures and tables
Extended data fig. 1 constraining the persistence of historical climate impacts on economic growth rates..
The results of a panel-based fixed-effects distributed lag model for the effects of annual mean temperature ( a ), daily temperature variability ( b ), total annual precipitation ( c ), the number of wet days ( d ) and extreme daily precipitation ( e ) on sub-national economic growth rates. Point estimates show the effects of a 1 °C or one standard deviation increase (for temperature and precipitation variables, respectively) at the lower quartile, median and upper quartile of the relevant moderating variable (green, orange and purple, respectively) at different lagged periods after the initial shock (note that these are not cumulative effects). Climate variables are used in their first-differenced form (see main text for discussion) and the moderating climate variables are the annual mean temperature, seasonal temperature difference, total annual precipitation, number of wet days and annual mean temperature, respectively, in panels a – e (see Methods for further discussion). Error bars show the 95% confidence intervals having clustered standard errors by region. The within-region R 2 , Bayesian and Akaike information criteria for the model are shown at the top of the figure. This figure shows results with ten lags for each variable to demonstrate the observed levels of persistence, but our preferred specifications remove later lags based on the statistical significance of terms shown above and the information criteria shown in Extended Data Fig. 2 . The resulting models without later lags are shown in Supplementary Figs. 1 – 3 .
Extended Data Fig. 2 Incremental lag-selection procedure using information criteria and within-region R 2 .
Starting from a panel-based fixed-effects distributed lag model estimating the effects of climate on economic growth using the real historical data (as in equation ( 4 )) with ten lags for all climate variables (as shown in Extended Data Fig. 1 ), lags are incrementally removed for one climate variable at a time. The resulting Bayesian and Akaike information criteria are shown in a – e and f – j , respectively, and the within-region R 2 and number of observations in k – o and p – t , respectively. Different rows show the results when removing lags from different climate variables, ordered from top to bottom as annual mean temperature, daily temperature variability, total annual precipitation, the number of wet days and extreme annual precipitation. Information criteria show minima at approximately four lags for precipitation variables and ten to eight for temperature variables, indicating that including these numbers of lags does not lead to overfitting. See Supplementary Table 1 for an assessment using information criteria to determine whether including further climate variables causes overfitting.
Extended Data Fig. 3 Damages in our preferred specification that provides a robust lower bound on the persistence of climate impacts on economic growth versus damages in specifications of pure growth or pure level effects.
Estimates of future damages as shown in Fig. 1 but under the emission scenario RCP8.5 for three separate empirical specifications: in orange our preferred specification, which provides an empirical lower bound on the persistence of climate impacts on economic growth rates while avoiding assumptions of infinite persistence (see main text for further discussion); in purple a specification of ‘pure growth effects’ in which the first difference of climate variables is not taken and no lagged climate variables are included (the baseline specification of ref. 2 ); and in pink a specification of ‘pure level effects’ in which the first difference of climate variables is taken but no lagged terms are included.
Extended Data Fig. 4 Climate changes in different variables as a function of historical interannual variability.
Changes in each climate variable of interest from 1979–2019 to 2035–2065 under the high-emission scenario SSP5-RCP8.5, expressed as a percentage of the historical variability of each measure. Historical variability is estimated as the standard deviation of each detrended climate variable over the period 1979–2019 during which the empirical models were identified (detrending is appropriate because of the inclusion of region-specific linear time trends in the empirical models). See Supplementary Fig. 13 for changes expressed in standard units. Data on national administrative boundaries are obtained from the GADM database version 3.6 and are freely available for academic use ( https://gadm.org/ ).
Extended Data Fig. 5 Contribution of different climate variables to overall committed damages.
a , Climate damages in 2049 when using empirical models that account for all climate variables, changes in annual mean temperature only or changes in both annual mean temperature and one other climate variable (daily temperature variability, total annual precipitation, the number of wet days and extreme daily precipitation, respectively). b , The cumulative marginal effects of an increase in annual mean temperature of 1 °C, at different baseline temperatures, estimated from empirical models including all climate variables or annual mean temperature only. Estimates and uncertainty bars represent the median and 95% confidence intervals obtained from 1,000 block-bootstrap resamples from each of three different empirical models using eight, nine or ten lags of temperature terms.
Extended Data Fig. 6 The difference in committed damages between the upper and lower quartiles of countries when ranked by GDP and cumulative historical emissions.
Quartiles are defined using a population weighting, as are the average committed damages across each quartile group. The violin plots indicate the distribution of differences between quartiles across the two extreme emission scenarios (RCP2.6 and RCP8.5) and the uncertainty sampling procedure outlined in Methods , which accounts for uncertainty arising from the choice of lags in the empirical models, uncertainty in the empirical model parameter estimates, as well as the climate model projections. Bars indicate the median, as well as the 10th and 90th percentiles and upper and lower sixths of the distribution reflecting the very likely and likely ranges following the likelihood classification adopted by the IPCC.
Supplementary information
Supplementary information, peer review file, rights and permissions.
Open Access This article is licensed under a Creative Commons Attribution 4.0 International License, which permits use, sharing, adaptation, distribution and reproduction in any medium or format, as long as you give appropriate credit to the original author(s) and the source, provide a link to the Creative Commons licence, and indicate if changes were made. The images or other third party material in this article are included in the article’s Creative Commons licence, unless indicated otherwise in a credit line to the material. If material is not included in the article’s Creative Commons licence and your intended use is not permitted by statutory regulation or exceeds the permitted use, you will need to obtain permission directly from the copyright holder. To view a copy of this licence, visit http://creativecommons.org/licenses/by/4.0/ .
Reprints and permissions
About this article
Cite this article.
Kotz, M., Levermann, A. & Wenz, L. The economic commitment of climate change. Nature 628 , 551–557 (2024). https://doi.org/10.1038/s41586-024-07219-0
Download citation
Received : 25 January 2023
Accepted : 21 February 2024
Published : 17 April 2024
Issue Date : 18 April 2024
DOI : https://doi.org/10.1038/s41586-024-07219-0
Share this article
Anyone you share the following link with will be able to read this content:
Sorry, a shareable link is not currently available for this article.
Provided by the Springer Nature SharedIt content-sharing initiative
By submitting a comment you agree to abide by our Terms and Community Guidelines . If you find something abusive or that does not comply with our terms or guidelines please flag it as inappropriate.
Quick links
- Explore articles by subject
- Guide to authors
- Editorial policies
Sign up for the Nature Briefing newsletter — what matters in science, free to your inbox daily.

We use cookies. Read more about them in our Privacy Policy .
- Accept site cookies
- Reject site cookies
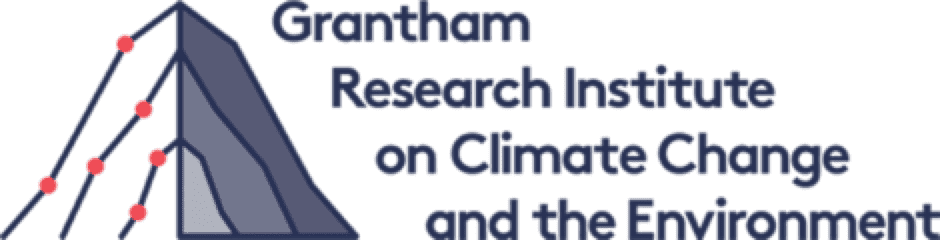
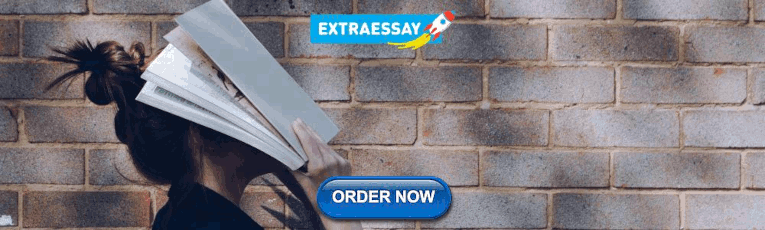
The Economics of Climate Change: The Stern Review
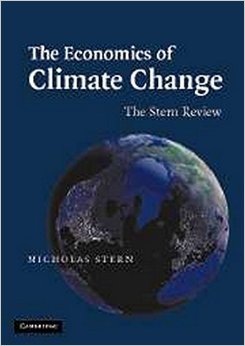
“This Review has assessed a wide range of evidence on the impacts of climate change and on the economic costs, and has used a number of different techniques to assess costs and risks. From all of these perspectives, the evidence gathered by the Review leads to a simple conclusion: the benefits of strong and early action far outweigh the economic costs of not acting.”
The main findings of The Economics of Climate Change: The Stern Review included:
- there is still time to avoid the worst impacts of climate change, if we take strong action now;
- climate change could have very serious impacts on growth and development;
- the costs of stabilising the climate are significant but manageable; delay would be dangerous and much more costly;
- action on climate change is required across all countries, and it need not cap the aspirations for growth of rich or poor countries;
- a range of options exists to cut emissions; strong, deliberate policy action is required to motivate their take-up; and
- climate change demands an international response, based on a shared understanding of long-term goals and agreement on frameworks for action.
The Economics of Climate Change: The Stern Review has become one of the most influential reports on climate change ever produced since its release by Her Majesty’s Treasury of the UK Government in October 2006. The report was published as a volume in January 2007 by Cambridge University Press.
It was led by Nicholas Stern , then Head of the UK Government Economic Service, and now Chair of both the ESRC Centre for Climate Change Economics and Policy and the Grantham Research Institute on Climate Change and the Environment at the London School of Economics and Political Science.
The Economics of Climate Change: The Stern Review was announced by the UK Chancellor of the Exchequer, Gordon Brown, in July 2005, and was commissioned to report to the Prime Minister, Tony Blair, and the Chancellor by autumn 2006, on the following issues:
- the economics of moving to a low-carbon global economy, focusing on the medium- to long-term perspective, and drawing implications for the timescales for action, and the choice of policies and institutions;
- the potential of different approaches for adaptation to changes in the climate; and
- specific lessons for the UK, in the context of its existing climate change goals.
Since its publication, Nicholas Stern and the contributors to the Review have published a number of academic papers relating to the report. In 2015, ‘Proceedings of the Royal Society: B’ published a paper by Lord Stern reflecting on the development of climate change policies during the 10 years since The Stern Review was commissioned.
Academic papers relating to ‘The Economics of Climate Change: The Stern Review’
Papers by contributors to the Review
Simon Dietz, Chris Hope, Nicholas Stern and Dimitri Zenghelis (2007). Reflections on the Stern Review (1): A robust case for strong action to reduce the risks of climate change. World Economics , volume 8, number 1, pages 121 – 168.
Lorraine Hamid, Nicholas Stern and Chris Taylor (2007). Reflections on the Stern Review (2): a growing international opportunity to move strongly on climate change. World Economics , volume 8, number 1, pages 169 – 186.
Simon Dietz, Dennis Anderson, Nicholas Stern, Chris Taylor and Dimitri Zenghelis (2007). Right for the right reasons: a final rejoinder on the Stern Review. World Economics , volume 8, number 2, pages 229 – 258.
Simon Dietz, Chris Hope and Nicola Patmore (2007). Some economics of ‘dangerous’ climate change: reflections on the Stern Review. Global Environmental Change , volume 17, issues 3–4, pages 311–325.
Simon Dietz and Nicholas Stern (2008). Why economic analysis supports strong action on climate change: a response to the Stern Review’s critics. Review of Environmental Economics and Policy , volume 2, issue 1, pages 94-113.
Nicholas Stern (2008). The Economics of Climate Change. American Economic Review , volume 98, number 2, pages 1–37.
Simon Dietz and Nicholas Stern (2009). Note—on the timing of greenhouse gas emissions reductions: a final rejoinder to the symposium on “The Economics of Climate Change: The Stern Review and its Critics”. Review of Environmental Economics and Policy , volume 3, issue 1, pages 138-140.
Other papers about the Review
Wilfred Beckerman and Cameron Hepburn (2007). Ethics of the discount rate in the Stern Review on the Economics of Climate Change. World Economics , volume 8, number 1, pages 187 – 210.
Dennis Anderson (2007). The Stern Review and the costs of climate change mitigation: a response to the ‘Dual Critique’ and the misrepresentations of Tol and Yohe. World Economics , volume 8, number 1, pages 211 – 219.
John F. Mitchell, Julia Slingo, David S. Lee, Jason A. Lowe and Vicky Pope (2007). Response to Carter et al. World Economics , volume 8, number 1, pages 221 – 228.
Nigel Arnell, Rachel Warren and Robert Nicholls (2007). Response to ‘The Stern Review: A Dual Critique’. World Economics , volume 8, number 1, pages 229 – 231.
Andrew Glikson (2007). A response to ‘The Stern Review: A Dual Critique’. World Economics , volume 8, number 1, pages 233 – 238.
Ian Simmonds and Will Steffen (2007). Response to ‘The Stern Review: A Dual Critique—Part I: The Science’. World Economics , volume 8, number 2, pages 133 – 141.
Weitzman, Martin L. (2007). A review of The Stern Review on the Economics of Climate Change. Journal of Economic Literature , volume 45, number 3, pages 703-724.
Geoffrey Heal (2009). The economics of climate change: a post-Stern perspective. Climatic Change , volume 96, number 3, pages 275-297.
Sign up to our newsletter
Climate Economics: A Meta-Review and Some Suggestions
What have we learned from the outpouring of literature as a result of the Stern Review of the Economics of Climate Change? A lot. We have explored the model space and the parameter space much more thoroughly, though there are still unexplored regions. While there are aspects of the Stern Review's analysis with which we can disagree, it seems fair to say that it has catalyzed a fundamental rethinking of the economic case for action on climate change. We are now in a position to give some conditions that are sufficient to provide a case for strong action on climate change, but need more work before we have a fully satisfactory account of the relevant economics. In particular we need to understand better how climate change affects natural capital - the natural environment and the ecosystems comprising it - and how these affect human welfare.
I am grateful to Tony Fisher, Claude Henry, Bengt Kristrom, Karl-Goran Maler and Nick Stern for valuable comments. Errors are of course my own responsibility. The views expressed herein are those of the author(s) and do not necessarily reflect the views of the National Bureau of Economic Research.
MARC RIS BibTeΧ
Download Citation Data
Published Versions
Geoffrey Heal, 2009. "Climate Economics: A Meta-Review and Some Suggestions for Future Research," Review of Environmental Economics and Policy, Oxford University Press for Association of Environmental and Resource Economists, vol. 3(1), pages 4-21, Winter.
More from NBER
In addition to working papers , the NBER disseminates affiliates’ latest findings through a range of free periodicals — the NBER Reporter , the NBER Digest , the Bulletin on Retirement and Disability , the Bulletin on Health , and the Bulletin on Entrepreneurship — as well as online conference reports , video lectures , and interviews .
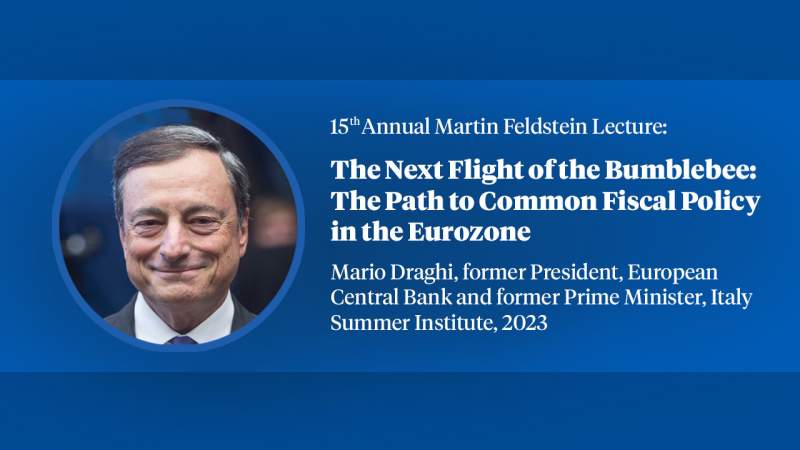
This website uses cookies.
By clicking the "Accept" button or continuing to browse our site, you agree to first-party and session-only cookies being stored on your device to enhance site navigation and analyze site performance and traffic. For more information on our use of cookies, please see our Privacy Policy .
- American Economic Review
The Economics of Climate Change
- Nicholas Stern
- Article Information
JEL Classification
- Q54 Climate; Natural Disasters; Global Warming
Climate change adaptation: A systematic review in five charts
Jonah rexer.
South Asia is highly vulnerable to climate change—including floods, extreme heat, and sea level rise. The burden of adapting to climate change falls disproportionately on poor households, which are typically more vulnerable to climate shocks. Globally, research on climate change identifies a variety of adaptation strategies by households, firms, and farmers, which have the capacity to offset nearly half of climate damage, on average. Firms have access to successful technology-driven adaptations, whereas households and farmers rely on less effective labor market adjustments. Adaptations that involve public support tend to be more effective than purely private strategies. Investing in broad public goods and social transfers that generate double dividends can help build resilience to climate change.
1. South Asia is highly vulnerable to climate disasters
South Asia is the emerging market and developing economy (EMDE) region that is most vulnerable to climate change. This reflects South Asia’s geography, which leaves it exposed to changes in groundwater availability, floods, extreme heat, and rising sea levels. About 60 million people per year have been affected by natural disasters in South Asia since 2013, more than in any other region in the world. Climate change is projected to reduce agricultural yields, industrial output, labor supply, productivity, and human capital in South Asia.
2. Globally, the poor tend to be more exposed to, and affected by, climate disasters
In a systematic literature review, two-thirds of studies found that the poor are more exposed to climate shocks. Studies of droughts, heat, and floods in particular were likely to find the poor were more exposed to climate than average households. Furthermore, 80 percent of studies found that the poor were more adversely affected by climate shocks than other households. The poor typically suffer greater income and human capital losses from climate events and will disproportionately bear the burden of private adaptation to climate change.
3. Adaptation can work, but effectiveness varies substantially across domains
Households, firms, and farmers adapt to climate change in myriad ways. Factories may adopt cooling technologies to prevent productivity losses during heat waves; farmers may plant drought or flood resistant varieties to boost yields; households may migrate or seek employment in less climate-exposed regions or sectors. The degree to which these behaviors offset the damage from climate change is called the adaptation ratio . A recent systematic literature review finds that, on average, such adaptive behaviors offset 46 percent of the damage from climate change. However, adaptation ratios vary widely across settings: while firms recover 72 percent of climate losses, this number falls to 49 percent for households, and just 38 percent among agricultural producers.
4. As a middle-income region, adaptation in South Asia is likely to be effective
Adaptation ratios also depend on income levels. The lowest adaptation ratios are in low- and high-income countries, which offset only 32 and 34 percent of climate losses, respectively. In contrast, in middle-income countries adaptation mitigates over 50 percent of climate damage. In low-income countries, constraints to adaptation may be severe, and so observed adaptations tend to be less effective. In advanced economies, the most effective adaptations may already be widespread, reducing the impact of an additional adaptation. In middle-income countries, however, there may be both fewer constraints to adaptation than in low-income countries, and more low-hanging fruit than in high-income settings.
5. The choice of adaptation strategies matters
Not all adaptation strategies are created equal. Labor market adjustments, the most common adaptation among households, are the least effective, offsetting just 14 percent of climate-related economic losses. Migration, off-farm work, and other labor market choices have low barriers to adoption and are commonplace among the world’s poor, but broadly ineffective in the face of rising climate risks. In contrast, state-provided public goods have the highest adaptation ratio of all studied adaptation strategies. These public goods are generally not climate-specific investments, but rather comprise the standard set of goods and services typically provided by the state—roads, bridges, health systems, irrigation canals, piped water. For example, connective infrastructure can reduce hunger during droughts by improving market integration, while a strong health system can reduce the impact of heat on infant mortality. These public goods not only serve their primary function, but also improve resilience to climate change, generating double dividends . Technological solutions, often favored by firms, are also highly effective.
- Climate Change
- Afghanistan
- Development Economics
Join the Conversation
- Share on mail
- comments added
Analyzing the effects of solar energy innovations, digitalization, and economic globalization on environmental quality in the United States
- Original Paper
- Open access
- Published: 29 April 2024
Cite this article
You have full access to this open access article
- Tomiwa Sunday Adebayo 1 , 5 , 6 ,
- Muhammad Saeed Meo 2 , 7 , 8 ,
- Babatunde Sunday Eweade 3 &
- Oktay Özkan 4
163 Accesses
Explore all metrics
The escalating apprehension regarding climate change mitigation has intensified the quest for energy alternatives that are low in carbon emissions, economically viable, and consistently available. Within this context, renewable energy sources emerge as fitting candidates, being recognized for their eco-friendliness and cleanliness. Nonetheless, despite the allure of transitioning towards cleaner energy, there exists a notable dearth of literature addressing the pivotal role of solar energy innovations and economic globalization in advancing the agenda of climate change mitigation (SDG-13), thus complicating the prediction of factors influencing ecological quality. Consequently, this study undertakes the inaugural investigation into the impact of solar energy innovation on ecological footprint, while also considering the influences of digitalization, economic globalization, renewable energy, and natural resources in the USA. To this end, Quantile-on-Quantile Kernel-Based Regularized Least Squares (QQKRLS) and wavelet quantile regressions (WQR) methodologies are employed, utilizing data spanning from 2000 to 2020. The analysis reveals that solar energy innovation, along with renewable energy, digitalization, and economic globalization, exerts a negative impact on ecological footprint, whereas natural resources exhibit a positive influence. Drawing from these insights, it becomes apparent that a concerted effort from stakeholders and policymakers is imperative in realizing the objectives of SDG-13 and SDG-7, necessitating a paradigm shifts in the USA’s energy portfolio away from fossil fuels towards renewables.
Graphical abstract
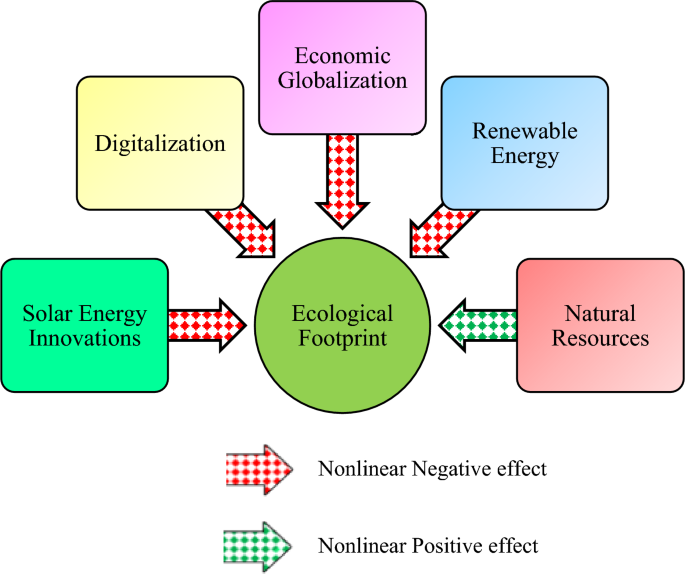
Similar content being viewed by others
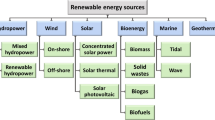
Social, environmental, and economic consequences of integrating renewable energies in the electricity sector: a review
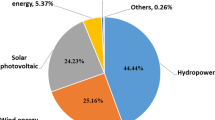
Cost, environmental impact, and resilience of renewable energy under a changing climate: a review
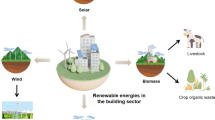
Green building practices to integrate renewable energy in the construction sector: a review
Avoid common mistakes on your manuscript.
Introduction
Environmental sustainability is of growing global concern, particularly regarding nations like the USA, given their substantial ecological footprint and economic sway (Zhang et al. 2024a ; Zhu et al. 2023a , b ). Recent research has increasingly focused on the intricate relationships among natural resource use, digitalization, economic globalization, and advancements in solar energy (Chien et al. 2022 ; Adebayo et al. 2024 ). Recognizing how these elements interact and impact environmental quality is pivotal for shaping policies and fostering sustainable development (Eweade et al. 2023a , b ). In December 2023, government leaders and environmental experts convened at the 28th United Nations Climate Change Conference (COP28) to agree on measures to combat climate change and limit temperature increases to below 1.5 °C. During COP28, the integration of finance, sustainability, and technology objectives was highlighted, emphasizing the interconnectedness of these goals. This synergy is exemplified by digitalization, a concept that blends financial and environmental technologies to drive sustainable development forward (Magazzino 2023 ; Saqib et al. 2023 ). At COP28, nations worldwide gathered to enhance existing goals and commitments. In the USA, efforts focus on demonstrating leadership in addressing the climate crisis and working with global allies. Collaborating with international partners, the USA aims to strengthen climate ambition and achieve meaningful results at COP28. With a primary aim of advancing the global transition to achieve net-zero emissions by 2050, the USA is committed to playing a crucial role in combating the imminent climate crisis, both now and in the future (Gao et al. 2024 ; Usman et al. 2024 ).
Natural resources play a pivotal role in shaping environmental quality, as their extraction, utilization, and management practices have profound impacts on ecosystems, air and water quality, and biodiversity (Razzaq et al. 2022 ; Mukherjee 2021 ). With the USA being a major consumer and producer of natural resources, the sustainable management of these resources is paramount for preserving environmental integrity (Gozgor et al. 2020 ; Adedoyin et al. 2020 ; Alola et al. 2023 ; Cui et al. 2022 ). Additionally, the advent of digitalization has revolutionized various sectors of the economy, leading to increased efficiency, productivity, and connectivity (Ahmed et al. 2021 ; He et al. 2024 ; Li et al. 2021 ). However, the proliferation of digital technologies also raises concerns about their environmental implications, such as energy consumption, electronic waste generation, and carbon emissions (Chien et al. 2022 ). Understanding the net effects of digitalization on environmental quality is crucial for harnessing its benefits while mitigating its adverse impacts (Ansari et al. 2021 ; Pata et al. 2023 ).
Digitalization has the potential to significantly contribute to environmental sustainability in the USA by financing solar panel installations, promoting renewable energy sources, and monitoring environmental impacts. It facilitates loans for individuals and companies aiming to reduce their environmental footprint, while also channeling capital into eco-conscious businesses. However, transitioning to renewable energy requires substantial investments in infrastructure, research and development, and widespread adoption of clean energy technologies(Karlilar et al. 2023 ). Therefore, integrating financial inclusion with digitalization simplifies access to funding for renewable energy projects, encouraging more entities to embrace clean energy solutions. Leveraging digital platforms, crowdfunding, and peer-to-peer financing broadens access to capital for renewable energy initiatives, attracting investment from a wider array of stakeholders.
Economic globalization, characterized by the increasing interconnectedness of economies and the movement of goods, services, and capital across borders, has both positive and negative implications for environmental quality (Adedoyin et al. 2020 ; Bekun and Ozturk 2024 ; Pata et al. 2023 ). While globalization has facilitated economic growth and development, it has also led to environmental degradation through increased resource extraction, pollution, and carbon emissions(Gupta and Kumar 2023 ; Bekun and Ozturk 2024 ; Eweade et al. 2024 ). Examining the environmental consequences of economic globalization in the context of the USA is essential for identifying opportunities for sustainable development. Furthermore, innovations in solar energy technologies have the potential to revolutionize the energy landscape and mitigate environmental impacts associated with fossil fuel combustion. Solar energy offers a clean, renewable alternative to traditional energy sources, reducing greenhouse gas emissions, air pollution, and reliance on finite resources (Khan et al. 2020a , b ; Razzaq et al. 2022 ). Understanding the adoption, deployment, and effectiveness of solar energy innovations in the USA is critical for transitioning towards a more sustainable energy future (Ibrahim et al. 2022 ; Kabeyi and Olanrewaju 2022 ; Eweade et al. 2022 ).
In light of these considerations, this study aims to comprehensively examine the effects of natural resources, digitalization, economic globalization, and solar energy innovations on environmental quality in the USA. By analyzing the interactions between these factors and their cumulative impacts on environmental indicators such as air and water quality, biodiversity, and climate change, this research seeks to inform evidence-based policy interventions and promote sustainable development practices. Based on the stated research objective, the following research questions were raised; (1) Are there significant interactions or synergies among natural resource utilization, digitalization, economic globalization, and solar energy innovations that collectively impact environmental quality in the United States? (2) How do policy interventions and regulatory frameworks aimed at promoting sustainable development and environmental protection interact with the aforementioned factors in shaping environmental quality outcomes in the United States? (3) What are the regional variations in the relationship between natural resources, digitalization, economic globalization, solar energy innovations, and environmental quality across different states or regions within the United States? Following the objective and the research questions, the contributions of the study provides valuable insights for policymaking, sustainability, and global environmental efforts. It sheds light on how these factors interact and influence environmental outcomes, informing evidence-based policies and guiding businesses towards sustainability. Understanding their impact contributes to addressing climate change and promoting sustainable development practices. Additionally, it facilitates international collaborations and agreements for environmental protection. Overall, this research bridges academic knowledge with practical policymaking, fostering sustainability and economic development. To validate the connections between the variables and provide robust empirical evidence, the study employs a contemporary estimation technique known as the quantile-on-quantile KRLS method. This methodological approach offers new perspectives in analyzing United States data, enabling a deeper understanding of the complex relationships at play. Overall, the study contributes to the advancement of knowledge in the field of environmental sustainability by providing insights into the multifaceted dynamics influencing environmental quality in the USA and offering practical implications for policymakers and stakeholders striving to promote sustainable development practices.
The structure of the paper is as follows: The subsequent Sect. “ Literature review ” will provide a literature assessment, concentrating on the correlation between digitalization, economic globalization, natural resources, renewable energy, and solar innovation on the ecological footprint, with the aim of tackling climate change. This will be followed by a discussion in Sect. “ Data and methodology ” focusing on data and methodology framework used for empirical research. Sect. “ Empirical analysis ” will elaborate on the outcomes of our study, including a comparison with prior research in the field. Finally, Sect. “ Conclusion and policy recommendations ” will present our conclusion, highlighting key findings relevant for scholars and policymakers.
Literature review
Numerous studies have been undertaken to explore the intricate interplay among solar energy innovations, digitalization, economic globalization, renewable energy, natural resources, and ecological footprint. This section presents a comprehensive overview of prior investigations into the specified variables. Additionally, a meticulous critique of the existing literature is offered, emphasizing discernible knowledge gaps.
Solar energy innovations and ecological footprint
Solar energy innovation has ushered in a paradigm shift in environmental sustainability. Its utilization of solar electricity, harnessed from a renewable and abundant resource, has markedly diminished reliance on fossil fuels, consequently alleviating greenhouse gas emissions (Ahmadi et al. 2018 ). The consequential reduction in air and water pollution induced by solar energy systems has been expounded upon by Rabaia et al.( 2021 ), thereby safeguarding ecosystems and air quality, both of which bear substantial environmental ramifications. In the discourse surrounding the impact of solar energy innovations, disparate perspectives emerge. Advocates of the positive influence of solar energy on environmental quality include researchers such as Shahsavari and Akbari ( 2018 ) who, in their examination of developing nations, asserted that solar energy reduces carbon emissions. Güney ( 2022 ) substantiates these claims by analyzing annual data from 2005 to 2018 across 35 economies, establishing a tangible correlation between increased solar energy utilization and substantial reductions in carbon emissions. Shahsavari et al. ( 2019 ) observed that each kilowatt-hour of solar electricity curtails approximately 715 g of CO 2 .
Conversely, a counterargument emerges, underscoring environmental concerns associated with solar energy, particularly concerning the manufacturing and disposal of solar panels. The manufacturing process involves the use of chemicals, such as silicon and silver, which may pose hazards if not handled with due care. Yu et al. ( 2022 ) employed QQR regression in a study spanning from 1991 to 2018, contending that solar energy has a limited impact on reducing carbon emissions in France. Zhu et al. ( 2023a , b ) reported similar findings for Spain and India, indicating that the efficacy of solar energy in mitigating carbon emissions varies across countries. These incongruent findings underscore the need for further investigation to derive generalizable conclusions regarding the impact of solar energy innovation on carbon emissions reduction.
Digitalization and ecological footprint
The digital transformation of industries has significantly altered our interactions, consumption, and production methods, impacting environmental sustainability both positively and negatively. Digitization, highlighted by Zhang et al. ( 2023 ), improves energy efficiency and minimizes material consumption through remote monitoring. Digitization brings forth a dualistic situation. While the shift to digital products reduces reliance on physical items, lessening environmental impact, it also leads to increased electronic waste from frequent device replacements, posing contamination and resource depletion challenges. The growing energy demands of digital technologies, particularly data centers and cloud services, raise environmental concerns with heightened greenhouse gas emissions, emphasizing the need for sustainable electricity practices.
Zhu et al. ( 2022 ) conducted a study in China to scrutinize the impact of digitalization on carbon emissions, revealing substantial reductions attributable to the digital economy. This reduction is facilitated through the promotion of innovation and the evolution of industrial structures. Similarly, Ke et al. ( 2022 ) found, in a study spanning 77 emerging economies, that digitization exerted a noteworthy influence in curtailing carbon emissions. Contrastingly, recent research by Dong et al. ( 2022 ) conducted in China, utilizing panel data from 2008 to 2018 across 60 countries, discovered an association between the rise in digitalization and an increase in per capita carbon emissions. Wang et al. ( 2022 ) argue that the relationship between digitalization and carbon emissions in China can be represented by an inverted U-shaped curve, a proposition substantiated by rigorous testing. Furthermore, Yang et al. ( 2022 ) conducted a similar study in China, revealing a curvilinear impact of digitalization on carbon emissions, following an inverted U-shaped pattern. The inconclusive nature of these results necessitates further in-depth exploration to elucidate the intricate relationship between digitalization and environmental sustainability.
Economic globalization and ecological footprint
The indicators of economic globalization include trade, foreign direct investment (FDI), portfolio investment, and regulatory issues such as tariffs, import restrictions, and levies on international trade (Gygli et al. 2019 ). The process of economic globalization has the potential to enhance the ecology by capitalizing on the positive effects of international trade and FDI. The adoption of environmentally friendly technology and structural modifications are encouraged by the technique and composition effects of trade, resulting in improvements to the environment. Conversely, the proliferation of opportunities for exporting goods in an era of unrestricted trade has resulted in increased production, thereby negatively impacting the environment due to the scale effect (Ahmed et al. 2021 ). Langnel and Amegavi ( 2020 ) employed yearly data spanning from 1971 to 2016 for Ghana and employed the ARDL methodology to examine the data. Their data confirm that globalization results in a rise in ecological footprint. Hussain et al. ( 2021 ) show a causal relationship between globalization and ecological footprints in Thailand by examining yearly data from 1970 to 2018 through the utilization of the NARDL approach. The findings indicate that an increase in globalization has a direct correlation with an increase in ecological footprints.
Moreover, Rudolph and Figge ( 2017 ) also conducted a study in which they analyzed 146 nations using the Extreme Bounds Analysis (EBA) method. They found that economic globalization had a positive influence on the ecological footprint. However, Ansari et al. ( 2021 ) have presented divergent results in their latest research conducted in prominent economies that heavily rely on renewable energy. Their research indicates that globalization has a tendency to decrease ecological footprints. In addition, Ahmed et al. ( 2021 ) examined the relationship between economic globalization and ecological footprint in Japan. By employing the ARDL technique, they discovered significant effects of economic globalization on the rise of ecological footprints. Nevertheless, the NARDL technique produces contrasting outcomes, indicating that both positive and negative shifts in economic globalization contribute to a decrease in ecological footprint. The contrasting results highlight the need for thorough research in order to develop robust policies.
Renewable energy and ecological footprint
Renewable energy, encompassing solar, wind, and hydroelectric power, gains popularity due to its environmentally friendly attributes as a substitute for fossil fuels. Characterized by a smaller ecological impact, it involves decreased land usage, absence of air pollutants, sustainable water resource management, and the promotion of biodiversity, making it an appealing and sustainable option. Xue et al. ( 2021 ) examined yearly data from 1990 to 2014 for South Asian countries utilizing the AMG approach. Their findings suggest that renewable energy has a substantial impact in reducing the ecological footprint. Sharif et al. ( 2021 ) did a study that examined the top ten economies with the greatest use of solar energy from 1990 to 2018. By employing a nonparametric quantile on quantile regression method, they discovered a significant and positive influence of solar energy on the state of the ecosystem. Li et al. (023) conducted a study on the economies of 130 nations using panel threshold regression. Their findings revealed that renewable energy has a notable impact on minimizing the ecological footprint. In contrast, Chalendar and Benson ( 2019 ) have recently raised doubts about the effectiveness of solar energy in mitigating carbon emissions. In their study, Nathaniel et al. ( 2020 ) observed varying results when examining the relationship between renewable energy and ecological footprint in MENA economies. They utilized annual data from 1990 to 2016 and employed the AMG methodology. Their findings indicated that there was no significant influence on ecological footprint or environmental quality.
Natural resources and ecological footprint
The study of the relationship between economic growth and the environment involves examining the use, excessive exploitation, and deterioration of natural resources, as well as issues related to pollution and global warming. Currently, there is a strong emphasis on climate change at a global level, with a recognition of the serious risks that it presents to the well-being of humans and the ability to maintain sustainable economic growth (Khan et al. 2020a , b ). Consequently, there has been an increased focus in recent years among academics, scholars, and legislators on the deterioration of the ecology and the exhaustion of natural resources. Awosusi et al. ( 2022a , b ) investigated the influence of natural resources on ecological footprints in BRICS countries. By analyzing annual data spanning from 1992 to 2018 and utilizing the MMQR approach, they discovered a substantial detrimental impact of natural resources on the ecosystem. In a study by Ahmad et al.( 2020 ) covering 22 developing economies, using annual data from 1984 to 2016 and employing the CS-ARDL technique, it was demonstrated that increase in natural resources has a significant positive impact on the ecological footprints of the economies under examination. Kongbuamai et al. ( 2020 ) conducted a study on ASEAN economies, analyzing annual data from 1995 to 2016 through the Driscoll–Kraay panel regression method. Their findings indicate that natural resources have a substantial impact in reducing ecological footprints. In a similar vein, Zafar et al.( 2019 ) examined the impact of natural resources in the USA by analyzing annual data from 1970 to 2015 and employing the ARDL technique. They discovered a significant reduction in the ecological footprint. Table 1 offers a detailed summary of the reviewed studied.
Gaps in the literature
Following an extensive review of the literature on environmental studies, we identified several knowledge gaps. (1) We discovered that the link between proposed factors is less focused on the USA setting, resulting in pioneering studies that investigate the effect of solar energy innovations, and digitalization on ecological sustainability while considering the role of economic globalization, renewable energy, and natural resources. (2) We noted that the majority of the research used traditional techniques such as ARDL, CCEMG, DCCE, and so on, therefore this study used a variety of unique econometric methodologies such as the innovative QQKRLS, WQR, and QQR approach.
Data and methodology
The present analysis assesses the effects of advancements in solar energy technology and digitalization on environmental sustainability, taking into account the influence of economic globalization, the utilization of renewable energy sources, and the management of natural resources. The United States serves as the focal point for this investigation, covering the period from 2000 to 2020. To mitigate the challenge of limited observational data, this study adopts the quadratic sum-up approach, building upon the methodologies outlined in prior works such as Pata et al. ( 2022 ) and Khan et al. ( 2023 ), thereby transforming low-frequency data into a higher frequency. Additionally, a comprehensive overview of the research procedures is provided in Table 2 .
Methodology
Quantile-on-quantile kernel-based regularized least squares (qqkrls).
Several studies suggest that policy shifts, structural changes, sudden shocks, and political fluctuations contribute to the emergence of characteristics such as asymmetry, nonlinearity, heavy-tailedness, and extreme values in economic time series (Bouoiyour and Selmi 2017 ). Amidst an ongoing global energy crisis and a worldwide pandemic, both of which have significantly disrupted the global landscape, various studies indicate that macroeconomic indicators respond to a new, nonlinear rhythm. Considering nonlinearities among the proposed variables, this study explores the asymmetric impact of SEINO, DIGIT, ECGLO, RENEN, and NATRE on ECOFP. Two distinct methodologies, the quantile-on-quantile kernel-based regularized least squares (QQKRLS) introduced by Adebayo et al. ( 2024 ) and Wavelet quantile regression (WQR) proposed by Adebayo and Özkan ( 2024 ), are employed to analyze this dynamic interaction.
Originally introduced by Hainmueller and Hazlett ( 2014 ) the KRLS approach is a machine learning technique motivated by its flexibility in regression without the need for specific assumptions. The algorithm utilizes Gaussian kernels to identify the optimal fitting function, thereby mitigating bias resulting from incorrect specifications. The KRLS method assesses the marginal impact of an explanatory variable on each individual data point of an endogenous variable. By leveraging the distribution of these marginal impacts, it unveils diverse (nonlinear) outcomes. Specifically, the KRLS method calculates the average of pointwise marginal impacts to determine the effect size and statistical significance of the explanatory variable's impact on the endogenous variable (Hainmueller and Hazlett 2014 ). To elaborate further, KRLS can assess the influence of an exogenous variable X on an endogenous variable Y in the following manner:
whereas \(E_{S} \left[ {\frac{{\widehat{{\mathbb{\Gamma}} Y}}}{{{\mathbb{\Gamma}} X_{k} }}} \right]\) represents the average or mean pointwise marginal impact of the exogenous ( X ) on the endogenous ( Y ) variable. Additionally, \(n\) denotes an individual observation, and \(S\) signifies the sample size. It is clear that KRLS approach places emphasis on the complete distribution of the dependent variable rather than the independent variable. Demonstrating the average pointwise marginal impact of the independent variable X on the dependent variable Y highlights the presence of nonlinearity in each data point of the projected variable. However, the statistical significance is ascertained by a singular value—the mean or average pointwise marginal effect. By utilizing the QQKRLS approach, this study extends beyond the exclusive consideration of the entire distribution of the endogenous variable in the KRLS method. Specifically, we integrate the KRLS approach introduced by Hainmueller and Hazlett ( 2014 ) with the QQR approach by Sim and Zhou ( 2015 ). This combined QQKRLS method enables the assessment of statistical significance for the complete distributions of both exogenous and endogenous variables. Through computing average pointwise marginal influence across quantile pairs, the approach provides valuable insights into the impact size and statistical significance associated with the influence of exogenous variable quantiles on endogenous variable quantiles. This methodology facilitates the exploration of complex or asymmetric relationships between the variables, allowing for a detailed analysis of how quantiles of the exogenous variable X affect quantiles of the endogenous variable Y.
Here, \(E_{S} \left[ {\frac{{\widehat{{{\mathbb{\Gamma}} QY_{\tau } }}}}{{{\mathbb{\Gamma}} QX_{\theta n} }}} \right]\) presents the mean or average pointwise marginal impact of the \(\theta\) th conditional quantile of the exogenous variable \(X\) on the \(\tau\) th conditional quantile of the endogenous variable \(Y\) . Furthermore \(QY_{\tau }\) and \(QX_{\theta }\) represent the \(\tau\) th and \(\theta\) th conditional quantile series of \(Y\) and \(X\) variables, respectively.
Wavelet quantile regression (WQR)
Next, we employed the recently introduced wavelet quantile regression (WQR) suggested by Adebayo and Özkan ( 2024 ). Following is the conventional quantile regression (QR) model for two variables.

The quantile regression (QR) approach, introduced by Koenker and Bassett ( 1978 ), stands as a statistical method that extends beyond conventional linear regression practices. It presents a broader perspective for modelling the conditional quantiles of an endogenous variable concerning an exogenous variable. In contrast to the conventional ordinary least squares (OLS) method, which primarily estimates the average value of the endogenous variable, QR allows for a detailed exploration of the relationship between an exogenous variable and various quantiles of the endogenous variable. This methodology holds significant importance in academia, enabling a thorough analysis of the correlation between different percentiles of the endogenous variable and its covariates. The QR approach offers several advantages that contribute to its academic and practical utility. Firstly, it provides a more comprehensive depiction of the distribution of the endogenous variable, going beyond the conventional emphasis on the mean. Additionally, its capacity to handle outliers and heteroscedasticity distinguishes it as a robust analytical tool capable of effectively addressing anomalies in the data. QR enables researchers to analyze and comprehend changes in the interactions between a variable across different quantiles of the distribution, offering a comprehensive view of the phenomena under examination (Saeed Meo and Karim 2022 ).
Prior empirical investigations (Kuşkaya et al. 2023 ; Thi Hong Nham and Thanh Ha 2023 ; Zhu et al. 2023a ) have demonstrated the variability in associations between variables across distinct time periods. However, the conventional QR approach neglects the potential for variations in the impact of the factor variable on the conditional quantiles of the response variable over different time dimensions, as previously highlighted in existing research. To overcome this limitation, we adopted the Wavelet Quantile Regression (WQR) method following the approach outlined by Adebayo and Özkan ( 2024 ). This was done to investigate the influence of an exogenous variable X on the conditional quantiles of an endogenous variable Y across various time intervals. The procedural steps for implementing the WQR technique are as follows:
We begin by applying the maximal overlapping discrete wavelet transform (MODWT) to decompose the exogenous ( \(X_{t}\) ) and endogenous ( \(Y\) ) series, following Percival & Walden ( 2000 ) and following a recent study of Adebayo and Özkan ( 2024 ) as below:
Consider \({\varvec{X}}\left[ {\varvec{i}} \right]\) as a signal having a duration of \(T\) , where \(T = 2^{J}\) for an integer J . Additionally, let \(c_{1} \left[ {\varvec{i}} \right]\) represent as the low-pass filter and \(d_{1} \left[ {\varvec{i}} \right]\) represent the high-pass filter, both of which are determined by the orthogonal wavelet. During the initial phase, \(X\left[ {\varvec{i}} \right]\) experiences convolution with \(c_{1} \left[ {\varvec{i}} \right]\) produces the estimate coefficients, \(e_{1} \left[ {\varvec{i}} \right]\) , which having a length of \(N\) , and with \(d_{1} \left[ {\varvec{i}} \right]\) produces the detail coefficients \(f_{1} \left[ {\varvec{i}} \right]\) again with a length of \(N\) . The procedure can be defined as follows:
Afterwards, we employ an akin method to filter \(e_{1} \left[ {\varvec{i}} \right]\) , using modified filters \(c_{2} \left[ {\varvec{i}} \right]\) and \(d_{2} \left[ {\varvec{i}} \right]\) , which are obtained from the dyadic up-sampling of \(c_{1} \left[ {\varvec{i}} \right]\) and \(d_{1} \left[ {\varvec{i}} \right]\) . This iterative approach involves the repetition of the recursive procedure. For values of J ranging from 1 to J 0 − 1, where J 0 ≤ 1, we can calculate the parameters of the approximation and detailed components in the following manner:
Here, \(c_{j + 1} \left[ {\varvec{i}} \right] = U(c_{j} \left[ {\varvec{i}} \right])\) and \(d_{j + 1} \left[ {\varvec{i}} \right] = U(d_{j} \left[ i \right])\) , where the function U represents the up-sampling process, which involves injecting a zero value between each consecutive pair of time-series items.
After applying J-level deconstruction on \(Y_{t}\) and \(X_{t}\) and obtaining the detail coefficients, we proceed to apply QR on to the pair of wavelet details, \(f_{j} \left[ {\varvec{Y}} \right]\) and \(f[{\varvec{X}}\) ], for all levels J . Therefore, we calculate the results of WQR for each of the levels J . The WQR is formally defined for the endogenous variable Y and the exogenous variable X , at a certain decomposition level J , and for a given quantile \(\daleth\) , in the following manner:

For detail and clear illustration, Fig. 1 presents the study analytical flow.
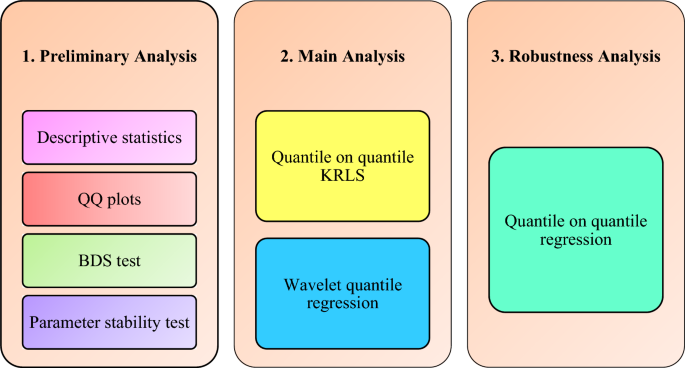
Workflow of the study
Empirical analysis
Preliminary analysis results.
This segment of the study assesses the appropriateness of utilizing QQKRLS in the investigation. It commences with a scrutiny of descriptive statistics, followed by an examination of Quantile–quantile plots to gauge normality, BDS test estimates, and parameter stability test estimates. Table 3 displays essential descriptive statistics for the logarithmic data series originating from the USA. During the sample period, the averages for the lnECOFP, 1nSEINO, 1nDIGIT, 1nRENEN, and 1nNATRE series were approximately 0.55, 2.00, 1.07, 1.10, 0.45, and − 0.07 respectively. Skewness analysis suggests left-skewed distributions for the 1nECOFP, lnSEINO, lnDIGIT, and lnNATRE series, while 1nRENEN displays a right-skewed distribution. Moreover, Kurtosis assessments reveal platykurtic distributions for all series except for 1nDIGIT, which exhibits a leptokurtic distribution. The results of the Jarque and Bera ( 1980 ) normality test, reported alongside descriptive statistics, indicate that all series, with the exception of lnECOFP, depart from a normal distribution.
This research utilizes the quantile-based approach, specifically QQKRLS. Therefore, it is essential to examine the normality aspect of the data intended for analysis through quantile–quantile ( Q – Q ) plots. As illustrated in Fig. 2 , the data for all variables diverges from normality across various quantiles.
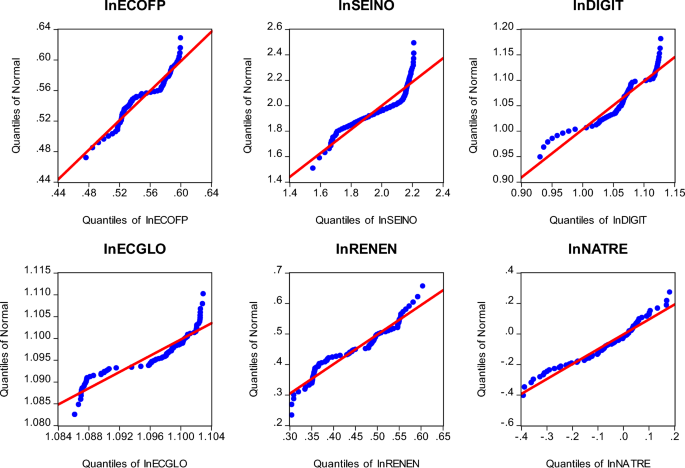
Quantile–quantile plots for normality. The figures depict the normality status of the quarterly logarithmic (ln) data series for various variables. Specifically, ECOFP represents Ecological footprint, SEINO stands for Solar Energy Innovations, DIGIT refers to Digitalization, RENEN represents Renewable energy, and NATRE signifies Natural resources. Additionally, ECOFP also denotes Economic globalization
In the initial analysis, we further explore the linearity properties of the quarterly logarithmic data. This investigation involves employing the BDS test, which was initially introduced by Broock et al. ( 1996 ). It is worth noting that this particular method has been applied in recent research conducted by Khan et al. ( 2023 ). Table 4 displays the findings of the BDS test, revealing that all variables' data deviates from the assumption of independent and identically distributed behavior across all dimensions. This indicates the presence of nonlinearity within the data for the entire duration of the study.
We examine the stability traits of the quarterly logarithmic data. This examination involves employing three distinct tests for parameter stability—Max-F, Exp-F, and Ave-F—which were introduced by Andrews ( 1993 ) and Miao et al., ( 2022 ). We follow existing literature from the studies of Lee et al. ( 2023 ) and Olanipekun et al. ( 2023 ). Table 5 presents the outcomes of the Max-F, Exp-F, and Ave-F tests, indicating data instability across all variables. This suggests non-stability throughout the study period (Hansen 1997 ). The preliminary assessment indicates that the data for all variables demonstrate abnormal distribution across various quantiles, as well as nonlinear and unstable patterns over the studied timeframe. Consequently, the QQKRLS method appears well-suited for the study data due to its ability to address abnormality, nonlinearity, and instability effectively.
Quantile on quantile kernel-based regularized least squares results
Prior to examining the relationship between the proposed variables, we conducted estimations to understand key data characteristics such as normality, linearity, and parametric stability. Our analysis, using QQ plots, indicated non-normal distributions for the variables. Subsequent BDS tests confirmed their nonlinear nature, while parameter stability tests revealed instability among the parameters. This prompted the creation of a robust solution to address these estimation challenges comprehensively. Figure 3 a–e demonstrates the impact of SEINO, DIGIT, FGLO, ECGLO, RENEN, and NATRE on environmental quality in the USA using the QQKRLS method.
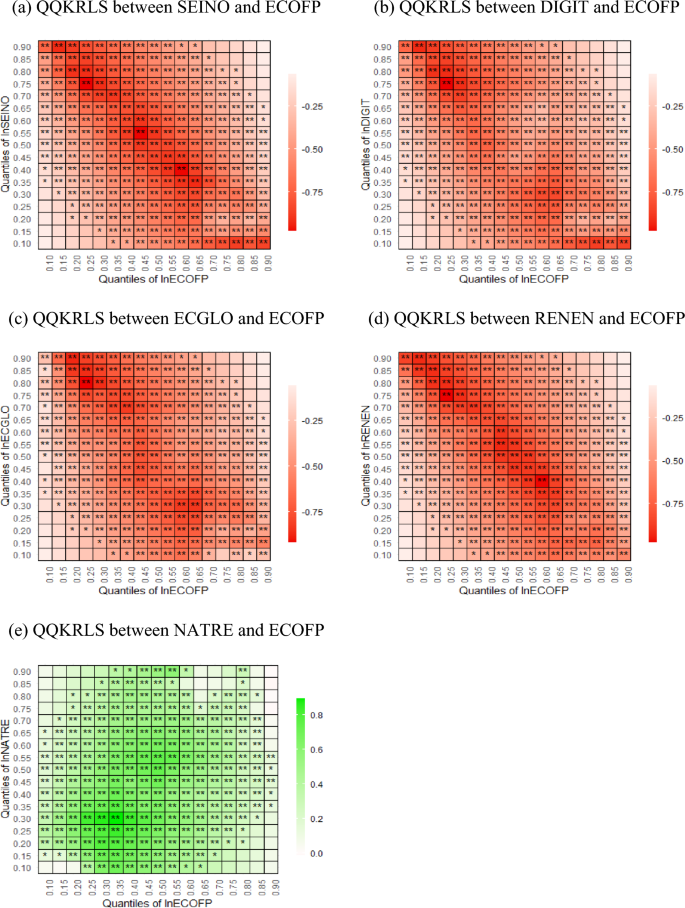
Quantile on quantile KRLS estimates. Note : The average pointwise marginal effect coefficients are represented by colour bars, wherein positive and negative coefficients are represented by green and red colors, respectively **(prob) < 0.05 and *(prob) < 0.1
Utilizing the QQKRLS method, Fig. 3 a illustrates the relationship between SEINO (solar energy innovation) and ECOFP (ecological footprint). The findings of the study indicate a weak correlation between SEINO and ECOFP at lower quantiles. However, a notable negative correlation is observed between SEINO and ECOFP at higher quantiles, particularly within the range of 0.10 to 0.90. In relation to the previous results, this suggests that while there may not be a strong overall correlation between solar energy innovation and ecological footprint across all levels, there is a more pronounced negative relationship at higher levels. This implies that as solar energy innovation increases, there tends to be a decrease in ecological footprint, particularly within the middle to upper quantiles. The observed outcomes align with previous scholarly findings in the field. Several studies have highlighted the nuanced relationship between solar energy innovation and ecological footprint, emphasizing varying degrees of correlation across different quantiles. For instance, research by Awosusi et al., ( 2022 ) demonstrated similar weak correlations at lower quantiles but identified stronger negative correlations at higher quantiles, consistent with our findings. Additionally, the work of Yi et al. ( 2023 ) corroborated the notion of a more pronounced negative relationship between solar energy innovation and ecological footprint within specific quantile ranges. Therefore, our results resonate with and reinforce these existing scholarly insights.
The result illustrated in Fig. 3 b suggests that digitalization (DIGIT) has a significant negative impact on ecological footprint (ECOFP), especially when considering the upper quantiles of both variables. This implies that as digitalization increases, there is a notable reduction in ecological footprint, particularly among instances where both digitalization and ecological footprint are relatively high. This finding indicates the potential of digitalization to contribute to environmental sustainability by reducing ecological footprints, particularly in more digitally advanced contexts. The results presented in Fig. 3 b are consistent with earlier research conducted by Karlilar et al. ( 2023 ), Qing et al. ( 2024 ), and Zhang et al. ( 2024b ). These studies have also observed a negative relationship between digitalization and ecological footprint, supporting the notion that advancements in digital technologies can lead to reductions in environmental impact, as evidenced by lower ecological footprints. These results suggest that embracing digitalization can lead to environmental sustainability by reducing ecological footprints, offering opportunities for innovation and economic growth. Leveraging digital solutions could stimulate job creation and attract investment, while also highlighting the importance of policy frameworks that incentivize sustainable practices. Overall, digitalization presents an opportunity for the USA to achieve economic growth while advancing environmental goals.
Figure 3 c illustrates the relationship between economic globalization (ECGLO) and ecological footprint (ECOFP). The findings reveal a weak correlation between ECGLO and ECOFP at lower quantiles, while a robust negative correlation is evident at higher quantiles, specifically within the range of 0.65 to 0.95. These results suggest potential benefits for the US economy. As economic globalization increases, there may be opportunities for expanded trade, investment, and access to global markets, which can stimulate economic growth and enhance competitiveness. Additionally, the negative correlation with ecological footprint implies that greater economic globalization may lead to more efficient resource utilization, technological innovation, and adoption of sustainable practices. However, it's essential to consider potential challenges and trade-offs associated with economic globalization, such as increased competition, income inequality, and environmental degradation in other regions. Overall, these findings suggest that economic globalization can potentially benefit the US economy by fostering growth and reducing ecological footprint. These results resonate prior studies (e.g., Adebayo et al. 2024 ; Bekun and Ozturk 2024 ; Van Tran et al. 2024 ; Zhang et al. 2024a ).
In Fig. 3 d, the impact of RENEN on ECOFP is depicted. The outcome suggests that the relationship between renewable energy (RENEN) and ecological footprint (ECOFP) varies across different quantiles. At lower quantiles, there is a weak positive correlation, indicating that with lower levels of renewable energy usage, there may be a slight increase in ecological footprint. However, at higher quantiles, particularly within the ranges of 0.60–0.90 for both renewable energy and ecological footprint, a notable negative effect is observed. This implies that as renewable energy usage and ecological footprint increase simultaneously, there is a significant decrease in ecological footprint, suggesting the potential for renewable energy to contribute to reducing environmental impact, especially in more renewable energy-intensive contexts. Overall, the findings underscore the importance of prioritizing renewable energy in the United States' energy and environmental policies to mitigate ecological footprint, foster sustainable economic growth, and address climate change. These outcomes resonate with the earlier studies (Roy 2024 ; Zhang et al. 2024a ).
Figure 3 e illustrates the influence of NATRE on ECOFP. The visualization highlights a significant negative impact of NATRE on ECOFP, particularly evident when analyzing the upper quantiles of both variables. This result suggests that there is a substantial negative relationship between natural resources (NATRE) and ecological footprint (ECOFP). In other words, as the utilization of natural renewable energy increases, there tends to be a notable decrease in ecological footprint. This implies that incorporating more natural renewable energy sources into energy production and consumption can lead to a reduction in environmental impact and contribute to sustainability efforts. Furthermore, the alignment of these findings with previous studies underscores the robustness and consistency of the observed relationship. It reinforces the notion that leveraging natural renewable energy sources holds significant potential for mitigating ecological footprint and advancing environmental conservation efforts. These findings are consistent with previous studies conducted by He et al. ( 2024 ), Qing et al. ( 2024 ) and Roy ( 2024 ).
We have introduced wavelet quantile regression (WQR) to effectively handle issues associated with tail dependence structures. Figure 4 demonstrates the outcomes of the wavelet quantile regression analysis, with heat maps illustrating the estimated slope coefficients ranging from light green to red. In Fig. 4 a–e, heat maps show the impact of solar energy innovation, digitalization, economic globalization, renewable energy, and natural resources on the ecological footprint in the USA across various time periods and quantiles. The right vertical axis displays the relationship coefficient, while the left indicates time frames (short, medium, long), and the horizontal axis represents quantiles. Figure 5 displays Wavelet Quantile Regression. The heat maps depict estimated slope coefficients, varying from light green to red. Specifically, heat maps (a-e) show the influence of solar energy innovation, digitalization, economic globalization, renewable energy, and natural resources on the ecological footprint in the USA across various time periods and quantiles across different time spans and quantiles in the USA. The sample period spans from 2000Q1 to 2020Q4. (a) SEINO impact on ECOFP (b) DIGIT impact on ECOFP (c) ECGLO impact on ECOFP (d) RENEN impact on ECOFP and (e) NATRE impact on ECOFP.
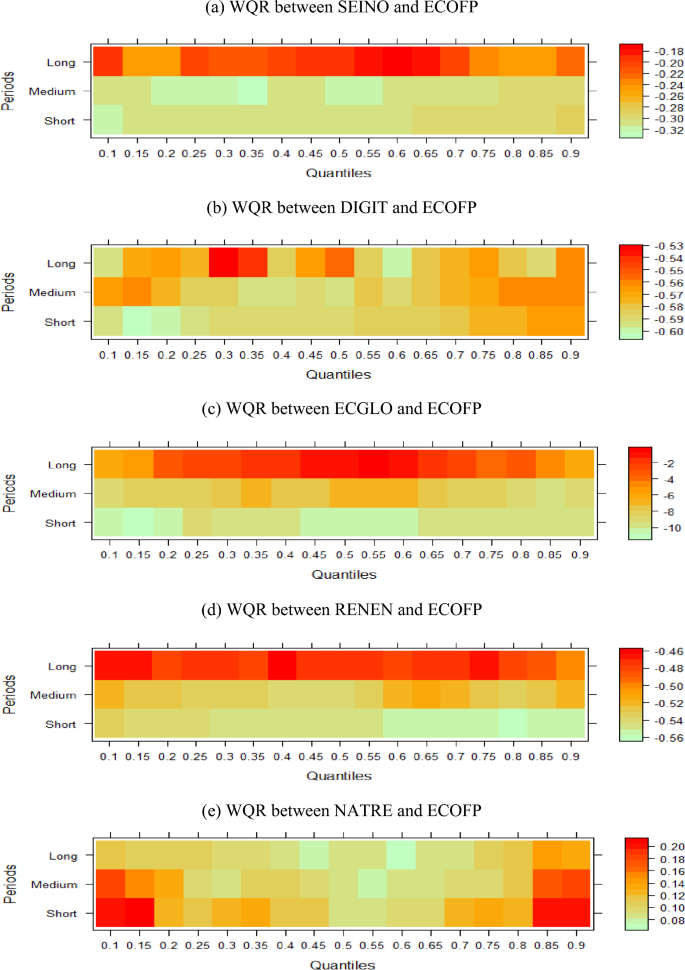
Wavelet quantile regression estimates. Note: The heatmaps exhibit the estimated slope coefficients in ascending order from light green to red
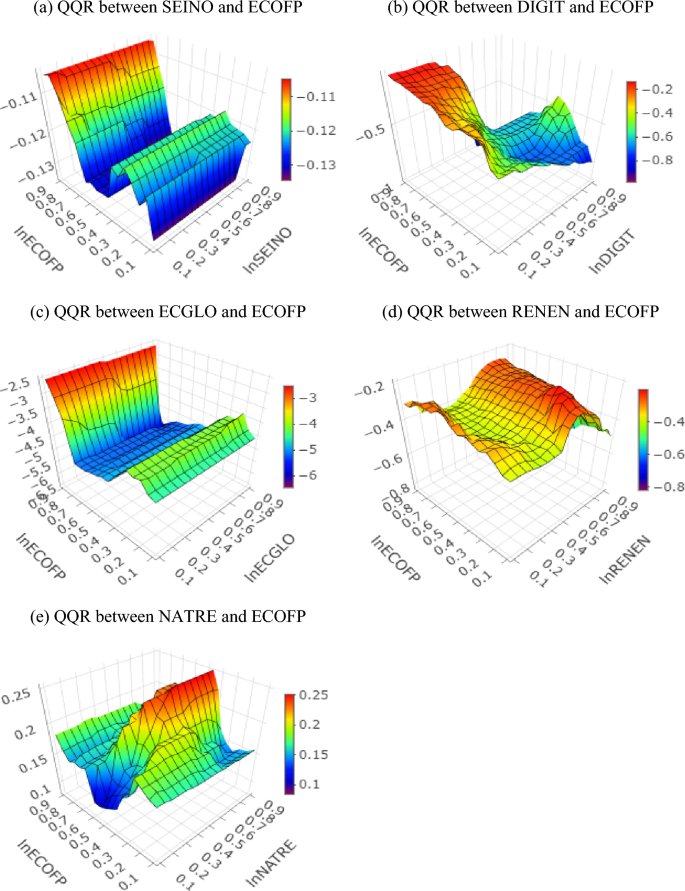
Quantile on quantile regression estimates
Solar energy innovation consistently exhibits a negative correlation with the ecological footprint across all quantiles and periods, as illustrated in Fig. 4 a. Moreover, its diminishing impact on the ecological footprint is more noticeable in the long term, indicating increasing effectiveness of policy recommendations over time. Thus, solar energy innovation in the USA can decrease the ecological footprint by reducing reliance on fossil fuels. By harnessing renewable and clean solar energy, greenhouse gas emissions, air pollution, and environmental degradation are minimized, leading to a smaller ecological footprint. This conclusion finds support in numerous studies, including those undertaken by Adebayo and Özkan ( 2024 ) and Sharif et al. ( 2021 ). Digitalization consistently has a negative impact on the ecological footprint across all quantiles and periods (Fig. 4 b), highlighting its crucial role in promoting environmental quality in the USA. Additionally, the long-term significance of digitalization in reducing the ecological footprint is underscored by the WQC analysis. This conforms to the findings of Karlilar et al. ( 2023 ), Ke et al. ( 2022 ), Zheng et al. ( 2023 ) and Zhu et al. ( 2023a , b ). Across all quantiles and periods (see Fig. 5 c, d), there is a consistent negative impact observed between economic globalization, renewable energy, and ecological footprint. This underscores the significance of economic globalization and renewable energy in promoting ecological quality and effectively reducing the ecological footprint in the USA. Moreover, the long-term significance of economic globalization and renewable energy in mitigating ecological footprint is highlighted by the WQC analysis. These findings are consistent with research conducted by Bekun and Ozturk ( 2024 ), Eweade et al. ( 2023a , b , 2023a ), Zhang et al. ( 2024a ) and Zhu et al. ( 2023a , b ) which also highlighted the significance of globalization and renewable energy in reducing ecological footprint. Moreover, our research reveals that natural resources have a positive impact on the ecological footprint across all periods and quantiles (refer to Fig. 5 e). This implies that natural resources worsen environmental quality by contributing to an increase in the ecological footprint. These findings are consistent with the viewpoints presented (Balsalobre-Lorente et al. 2023 ; He et al. 2024 ; Ibrahim et al. 2023 ; Razzaq et al. 2022 ) all of whom have underscored the negative impact of natural resources on ecological quality.
Robustness analysis (quantile on quantile regression) results
In our robustness analysis, we utilized the Quantile-on-Quantile Regression method. Figure 5 a–e displays the plots generated through this approach. In Fig. 5 a, our observations reveal that solar energy innovation (SEINO) consistently decreases ecological footprint (ECOFP) emissions across all quantiles. However, the impact of SEINO on ECOFP reduction is particularly noticeable when all quantiles of SEINO are combined with the middle quantiles of ECOFP (0.5–0.75). Consequently, we conclude that the influence of SEINO on ECOFP diminishes when other exogenous factors are moderated. In Fig. 5 b, the influence of DIGIT on ECOFP quantiles, moderated by ECGLO, RENEN, and NATRE, is depicted. A consistent positive connection between DIGIT and ECOFP is observed across all quantiles. Consequently, after accounting for the moderation effect of other regressors, it is evident that DIGIT decreases ECOFP in the USA. Through quantile-on-quantile regression, Fig. 5 c examines the relationship between the τ th quantile of economic globalization (ECGLO) and the λ th quantile of ecological footprint (ECOFP), while considering the moderating effects of SEINO, DIGIT, RENEN, and NATRE. The analysis indicates that economic globalization (ECGLO) consistently decreases ecological footprint (ECOFP) across all quantiles. However, this negative effect is relatively weaker within the range where all quantiles of ECGLO align with the middle quantiles of ECOFP (0.3–0.65). Therefore, it can be inferred that while economic globalization tends to decrease ecological footprint, its impact is somewhat attenuated when considering the effects of another exogenous factor. Furthermore, Fig. 5 d illustrates the effect of the τ th quantile of renewable energy (RE) on the λ th quantile of ecological footprint (ECOFP), while accounting for the moderating influence of SEINO, DIGIT, RENEN, and NATRE. The impact of renewable energy (RENEN) reduces ECOFP across all quantiles, although the negative effect is less pronounced in the range where all quantiles of renewable energy intersect with the middle quantiles of CO2 emissions (0.3–0.65). Thus, we can infer that renewable energy (RENEN) negatively affects ECOFP when considering the effects of other exogenous factors. Moreover, in Fig. 5 e, the influence of the τ th quantile of natural renewable energy (NATRE) on the λ th quantile of ecological footprint (ECOFP) is depicted, taking into account the moderating effects of SEINO, DIGIT, RENEN, and NATRE. Across all quantiles, a consistent positive relationship between natural renewable energy (NATRE) and ecological footprint (ECOFP) is evident. Consequently, after adjusting for the effects of the other regressors, it is apparent that natural renewable energy (NATRE) contributes to an increase in ecological footprint (ECOFP) in the USA.
Conclusion and policy recommendations
This research investigates how solar energy innovation, digitalization, economic globalization, renewable energy, and natural resources on environmental quality in the USA. It utilizes the Quantile-on-Quantile Kernel-Based Regularized Least Squares (QQKRLS) approach spanning from 2000 to 2020. The results of the analysis indicate a predominantly negative relationship between solar energy innovation (SEINO) and environmental footprint (ECOFP). However, it's noteworthy that the strength of this association varies across different quantiles. Overall, the findings suggest a positive association between solar energy innovation and environmental quality, implying that an increase in solar energy innovation may potentially benefit the environment positively. However, these correlations vary in magnitude across different quantiles. The study reveals that digitalization has a significant negative correlation with ecological footprint, with varying degrees of strength observed across different quantiles. Conversely, digitalization consistently exhibits a positive impact on environmental quality across all quantiles. The findings reveal a weak correlation between economic globalization and ecological footprint at lower quantiles, while a robust negative correlation is evident at higher quantiles. The study suggests the relationship between renewable energy and ecological footprint varies across different quantiles. At lower quantiles, there is a weak positive correlation, indicating that with lower levels of renewable energy usage. There are significant negative impacts of natural resources on ecological footprint, particularly evident when analyzing the upper quantiles of both variables. This result suggests that there is a substantial negative relationship between natural resources and ecological footprint.
Policy recommendations
Based on the empirical findings, policymakers in the USA should consider the following policy recommendations: Promote Solar Energy Innovation solar energy innovation and ecological footprint, the varying strength of this association across different quantiles suggests the need for targeted policies. Policymakers should encourage investment in solar energy research, development, and adoption to potentially benefit the environment positively. Incentives such as tax credits or grants could be provided to support the advancement and implementation of solar energy technologies.
Given the significant negative correlation between digitalization and ecological footprint, policymakers should leverage digital technologies to mitigate environmental impacts. Initiatives should focus on enhancing environmental monitoring, resource management, and sustainability efforts through digital platforms and data-driven strategies. Additionally, efforts to bridge the digital divide and ensure equitable access to digital technologies should be prioritized to maximize the environmental benefits across all segments of society. Recognizing the varying correlation between economic globalization and ecological footprint across different quantiles, policymakers should prioritize sustainable trade practices and regulations. Measures such as promoting fair trade agreements, enforcing environmental standards in international trade, and incentivizing eco-friendly production and consumption patterns can help mitigate negative environmental impacts associated with globalization.
Despite the mixed correlation between renewable energy and ecological footprint across different quantiles, policymakers should continue to promote the adoption of renewable energy sources as part of efforts to reduce environmental footprint. This can be achieved through policies supporting renewable energy infrastructure development, investment incentives for renewable energy projects, and regulatory measures to facilitate renewable energy integration into the energy grid. Given the significant negative impact of natural resources on ecological footprint, particularly evident at higher quantiles, policymakers should prioritize sustainable management practices. Measures such as conservation efforts, sustainable resource extraction practices, and land-use planning can help mitigate environmental degradation associated with resource exploitation while preserving natural ecosystems and biodiversity. Additionally, policies promoting sustainable consumption and production patterns can help reduce overall resource consumption and environmental footprint.
Suggestion for future studies
The study’s analysis from 2000 to 2020 may introduce biases and affect precision due to limited data availability. Future research should expand the temporal scope and access more extensive datasets for improved accuracy. While scrutinizing solar energy innovations, digitalization, economic globalization, renewable energy, and natural resources, the study acknowledges the absence of certain variables impacting environmental quality. In future research, it's important to consider including additional variables to achieve a more comprehensive evaluation. Given the possibility that the findings may be specific to the USA, it is essential to replicate these analyses in various geographical contexts for broader applicability. Subsequent studies could also involve longitudinal analyses to identify trends, examine sector-specific impacts, and explore differences across different countries in terms of the effectiveness of solar energy innovations, digitalization, economic globalization, renewable energy, and natural resources on environmental quality. Investigating the influence of emerging technologies, integrating social and cultural dimensions, and evaluating policy effectiveness over time are critical for assessing the real-world impact of environmental policies. Addressing these aspects will contribute to the refinement of ongoing environmental policy strategies.
Data availability
Enquiries about data availability should be directed to the authors.
Adebayo TS, Özkan O (2024) Investigating the influence of socioeconomic conditions, renewable energy and eco-innovation on environmental degradation in the United States: a wavelet quantile-based analysis. J Clean Prod 434:140321. https://doi.org/10.1016/j.jclepro.2023.140321
Article CAS Google Scholar
Adebayo TS, Özkan O, Eweade BS (2024) Do energy efficiency R&D investments and information and communication technologies promote environmental sustainability in Sweden? A quantile-on-quantile KRLS investigation. J Clean Prod 440:140832. https://doi.org/10.1016/j.jclepro.2024.140832
Adedoyin FF, Alola AA, Bekun FV (2020) An assessment of environmental sustainability corridor: the role of economic expansion and research and development in EU countries. Sci Total Environ 713:136726. https://doi.org/10.1016/j.scitotenv.2020.136726
Ahmad M, Jiang P, Majeed A, Umar M, Khan Z, Muhammad S (2020) The dynamic impact of natural resources, technological innovations and economic growth on ecological footprint: an advanced panel data estimation. Resour Policy 69:101817. https://doi.org/10.1016/j.resourpol.2020.101817
Article Google Scholar
Ahmadi MH, Ghazvini M, Sadeghzadeh M, Alhuyi Nazari M, Kumar R, Naeimi A, Ming T (2018) Solar power technology for electricity generation: a critical review. Energy Sci Eng 6(5):340–361. https://doi.org/10.1002/ese3.239
Ahmed Z, Zhang B, Cary M (2021) Linking economic globalization, economic growth, financial development, and ecological footprint: evidence from symmetric and asymmetric ARDL. Ecol Ind 121:107060. https://doi.org/10.1016/j.ecolind.2020.107060
Alola AA, Özkan O, Usman O (2023) Role of non-renewable energy efficiency and renewable energy in driving environmental sustainability in India: evidence from the load capacity factor hypothesis. Energies 16(6):2847. https://doi.org/10.3390/en16062847
Andrews DWK (1993) Tests for parameter instability and structural change with unknown change point. Econometrica 61(4):821–856. https://doi.org/10.2307/2951764
Ansari MA, Haider S, Masood T (2021) Do renewable energy and globalization enhance ecological footprint: an analysis of top renewable energy countries? Environ Sci Pollut Res 28(6):6719–6732. https://doi.org/10.1007/s11356-020-10786-0
Awosusi AA, Adebayo TS, Altuntaş M, Agyekum EB, Zawbaa HM, Kamel S (2022a) The dynamic impact of biomass and natural resources on ecological footprint in BRICS economies: a quantile regression evidence. Energy Rep 8:1979–1994. https://doi.org/10.1016/j.egyr.2022.01.022
Awosusi AA, Mata MN, Ahmed Z, Coelho MF, Altuntaş M, Martins JM, Martins JN, Onifade ST (2022b) How do renewable energy, economic growth and natural resources rent affect environmental sustainability in a globalized economy? Evidence from Colombia based on the gradual shift causality approach. Front Energy Res 9:739721. https://doi.org/10.3389/fenrg.2021.739721
Balsalobre-Lorente D, Abbas J, He C, Pilař L, Shah SAR (2023) Tourism, urbanization and natural resources rents matter for environmental sustainability: the leading role of AI and ICT on sustainable development goals in the digital era. Resour Policy 82:103445. https://doi.org/10.1016/j.resourpol.2023.103445
Bekun FV, Ozturk I (2024) Economic globalization and ecological impact in emerging economies in the post-COP21 agreement: a panel econometrics approach. Nat Res Forum 1477–8947:12408. https://doi.org/10.1111/1477-8947.12408
Bouoiyour J, Selmi R (2017) The Bitcoin price formation: Beyond the fundamental sources. https://doi.org/10.48550/ARXIV.1707.01284
Broock WA, Scheinkman JA, Dechert WD, LeBaron B (1996) A test for independence based on the correlation dimension. Economet Rev 15(3):197–235. https://doi.org/10.1080/07474939608800353
Cui L, Weng S, Nadeem AM, Rafique MZ, Shahzad U (2022) Exploring the role of renewable energy, urbanization and structural change for environmental sustainability: comparative analysis for practical implications. Renew Energy 184:215–224. https://doi.org/10.1016/j.renene.2021.11.075
De Chalendar JA, Benson SM (2019) Why 100% renewable energy is not enough. Joule 3(6):1389–1393. https://doi.org/10.1016/j.joule.2019.05.002
Chien F, Hsu CC, Andlib Z, Shah MI, Ajaz T, Genie MG (2022) The role of solar energy and ecoinnovation in reducing environmental degradation in China: Evidence from QARDL approach. IEAM 18(2):555–571. https://doi.org/10.1002/ieam.4500
Dong F, Hu M, Gao Y, Liu Y, Zhu J, Pan Y (2022) How does digital economy affect carbon emissions? Evidence from global 60 countries. Sci Total Environ 852:158401. https://doi.org/10.1016/j.scitotenv.2022.158401
Eweade BS, Uzuner G, Akadiri AC, Lasisi TT (2022) Japan energy mix and economic growth nexus: focus on natural gas consumption. Energy Environ. https://doi.org/10.1177/0958305X221130460
Eweade BS, Güngör H, Karlilar S (2023a) The determinants of ecological footprint in the UK: the role of transportation activities, renewable energy, trade openness, and globalization. Environ Sci Pollut Res. https://doi.org/10.1007/s11356-023-30759-3
Eweade BS, Karlilar S, Pata UK, Adeshola I, Olaifa JO (2023b) Examining the asymmetric effects of fossil fuel consumption, foreign direct investment, and globalization on ecological footprint in Mexico. Sustain Dev. https://doi.org/10.1002/sd.2825
Eweade BS, Akadiri AC, Olusoga KO, Bamidele RO (2024) The symbiotic effects of energy consumption, globalization, and combustible renewables and waste on ecological footprint in the United Kingdom. Nat Res Forum 48(1):274–291. https://doi.org/10.1111/1477-8947.12392
Gao S, Zhu Y, Umar M, Kchouri B, Safi A (2024) Financial inclusion empowering sustainable technologies: insights into the E-7 economies from COP28 perspectives. Technol Forecast Soc Change 201:123177. https://doi.org/10.1016/j.techfore.2023.123177
GFN (2024) Global Footprint Network . https://www.footprintnetwork.org/ . Accessed 10 Feb 2024
Gozgor, G., Mahalik, MK, Demir, E., & Padhan, H. (2020). The impact of economic globalization on renewable energy in the OECD countries. Energy Policy, 139:111365. https://doi.org/10.1016/j.enpol.2020.111365
Güney T (2022) Solar energy and sustainable development: evidence from 35 countries. Int J Sust Dev World 29(2):187–194. https://doi.org/10.1080/13504509.2021.1986749
Gupta S, Kumar N (2023) Time varying dynamics of globalization effect in India. Port Econ J 22(1):81–97. https://doi.org/10.1007/s10258-020-00190-4
Gygli S, Haelg F, Potrafke N, Sturm J-E (2019) The KOF globalisation index: revisited. Rev Int Organ 14(3):543–574. https://doi.org/10.1007/s11558-019-09344-2
Hainmueller J, Hazlett C (2014) Kernel regularized least squares: reducing misspecification bias with a flexible and interpretable machine learning approach. Political Anal 22(2):143–168. https://doi.org/10.1093/pan/mpt019
Hansen BE (1997) Approximate asymptotic P values for StructuraS-change tests. J Bus Econ Stat 15(1):60–67. https://doi.org/10.1080/07350015.1997.10524687
He Y, Wang S, Chen N (2024) Mineral rents, natural resources depletion, and ecological footprint nexus in high emitting countries: panel GLM analysis. Resour Policy 89:104472. https://doi.org/10.1016/j.resourpol.2023.104472
Hussain HI, Haseeb M, Kamarudin F, Dacko-Pikiewicz Z, Szczepańska-Woszczyna K (2021) The role of globalization, economic growth and natural resources on the ecological footprint in thailand: evidence from nonlinear causal estimations. Processes 9(7):1103. https://doi.org/10.3390/pr9071103
Ibrahim RL, Ozturk I, Al-Faryan MAS, Al-Mulali U (2022) Exploring the nexuses of disintegrated energy consumption, structural change, and financial development on environmental sustainability in BRICS: modulating roles of green innovations and regulatory quality. Sustain Energy Technol Assess 53:102529. https://doi.org/10.1016/j.seta.2022.102529
Ibrahim RL, Huang Y, Mohammed A, Adebayo TS (2023) Natural resources-sustainable environment conflicts amidst COP26 resolutions: investigating the role of renewable energy, technology innovations, green finance, and structural change. Int J Sust Dev World 30(4):445–457. https://doi.org/10.1080/13504509.2022.2162147
Jarque CM, Bera AK (1980) Efficient tests for normality, homoscedasticity and serial independence of regression residuals. Econ Lett 6(3):255–259. https://doi.org/10.1016/0165-1765(80)90024-5
Kabeyi MJB, Olanrewaju OA (2022) Sustainable energy transition for renewable and low carbon grid electricity generation and supply. Front Energy Res 9:743114. https://doi.org/10.3389/fenrg.2021.743114
Karlilar S, Balcilar M, Emir F (2023) Environmental sustainability in the OECD: the power of digitalization, green innovation, renewable energy and financial development. Telecommun Policy 47(6):102568. https://doi.org/10.1016/j.telpol.2023.102568
Ke J, Jahanger A, Yang B, Usman M, Ren F (2022) Digitalization, financial development, trade, and carbon emissions; implication of pollution haven hypothesis during globalization mode. Front Environ Sci 10:873880. https://doi.org/10.3389/fenvs.2022.873880
Khan A, Chenggang Y, Hussain J, Bano S, Nawaz Aa (2020a) Natural resources, tourism development, and energy-growth-CO 2 emission nexus: a simultaneity modeling analysis of BRI countries. Resour Policy 68:101751. https://doi.org/10.1016/j.resourpol.2020.101751
Khan Z, Ali S, Umar M, Kirikkaleli D, Jiao Z (2020b) Consumption-based carbon emissions and International trade in G7 countries: the role of environmental innovation and renewable energy. Sci Total Environ 730:138945. https://doi.org/10.1016/j.scitotenv.2020.138945
Khan N, Saleem A, Ozkan O (2023) Do geopolitical oil price risk influence stock market returns and volatility of Pakistan: evidence from novel non-parametric quantile causality approach. Resour Policy 81:103355. https://doi.org/10.1016/j.resourpol.2023.103355
Koenker R, Bassett G (1978) Regression quantiles. Econometrica 46(1):33. https://doi.org/10.2307/1913643
KOF (2024) KOF Globalisation Index. https://kof.ethz.ch/en/forecasts-and-indicators/indicators/kof-globalisation-index.html . Accessed 10 Feb 2024
Kongbuamai N, Bui Q, Yousaf HMAU, Liu Y (2020) The impact of tourism and natural resources on the ecological footprint: a case study of ASEAN countries. Environ Sci Pollut Res 27(16):19251–19264. https://doi.org/10.1007/s11356-020-08582-x
Kuşkaya S, Bilgili F, Muğaloğlu E, Khan K, Hoque ME, Toguç N (2023) The role of solar energy usage in environmental sustainability: fresh evidence through time-frequency analyses. Renew Energy 206:858–871. https://doi.org/10.1016/j.renene.2023.02.063
Langnel Z, Amegavi GB (2020) Globalization, electricity consumption and ecological footprint: an autoregressive distributive lag (ARDL) approach. Sustain Cities Soc 63:102482. https://doi.org/10.1016/j.scs.2020.102482
Lee C-C, Olasehinde-Williams G, Özkan O (2023) Geopolitical oil price uncertainty transmission into core inflation: evidence from two of the biggest global players. Energy Econ 126:106983. https://doi.org/10.1016/j.eneco.2023.106983
Li R, Wang Q, Liu Y, Jiang R (2021) Per-capita carbon emissions in 147 countries: the effect of economic, energy, social, and trade structural changes. Sustain Prod Consum 27:1149–1164. https://doi.org/10.1016/j.spc.2021.02.031
Li R, Wang Q, Li L (2023) Does renewable energy reduce per capita carbon emissions and per capita ecological footprint? New evidence from 130 countries. Energ Strat Rev 49:101121. https://doi.org/10.1016/j.esr.2023.101121
Magazzino C (2023) Ecological footprint, electricity consumption, and economic growth in China: geopolitical risk and natural resources governance. Empir Econ. https://doi.org/10.1007/s00181-023-02460-4
Miao Y, Razzaq A, Adebayo TS, Awosusi AA (2022) Do renewable energy consumption and financial globalisation contribute to ecological sustainability in newly industrialized countries?. Res 187:688–697. https://doi.org/10.1016/j.renene.2022.01.073
Mukherjee, S. (2021). Economic globalization in the 21st century: A case study of India. Review of Socio-Economic Perspectives, 6(1):23–33. https://doi.org/10.19275/RSEP105
Nathaniel S, Anyanwu O, Shah M (2020) Renewable energy, urbanization, and ecological footprint in the Middle East and North Africa region. Environ Sci Pollut Res 27(13):14601–14613. https://doi.org/10.1007/s11356-020-08017-7
Olanipekun IO, Ozkan O, Olasehinde-Williams G (2023) Is renewable energy use lowering resource-related uncertainties? Energy 271:126949. https://doi.org/10.1016/j.energy.2023.126949
OWD (2024) Our world in data. https://ourworldindata.org . Accessed 10 Feb 2024
Pata UK, Akadiri SS, Adebayo TS (2022) A comparison of CO 2 emissions, load capacity factor, and ecological footprint for Thailand’s environmental sustainability. Environ Dev Sustain. https://doi.org/10.1007/s10668-022-02810-9
Pata UK, Karlilar S, Eweade BS (2023) An environmental assessment of non-renewable, modern renewable, and combustible renewable energy in Cameroon. Environ Dev Sustain. https://doi.org/10.1007/s10668-023-04192-y
Percival DB, Walden AT (2000) Wavelet methods for time series analysis, vol 4. Cambridge university press. https://books.google.com.pk/books?hl=en&lr=&id=UqUi2NviqFcC&oi=fnd&pg=PR13&dq=Percival+and+Walden+(2000)+&ots=d46KTxx59Y&sig=OE_ctoNoDZ8E2UeX8H8AhRlPvKE
Qing L, Usman M, Radulescu M, Haseeb M (2024) Towards the vision of going green in South Asian region: the role of technological innovations, renewable energy and natural resources in ecological footprint during globalization mode. Resour Policy 88:104506. https://doi.org/10.1016/j.resourpol.2023.104506
Rabaia MKH, Abdelkareem MA, Sayed ET, Elsaid K, Chae K-J, Wilberforce T, Olabi AG (2021) Environmental impacts of solar energy systems: a review. Sci Total Environ 754:141989. https://doi.org/10.1016/j.scitotenv.2020.141989
Razzaq A, Wang S, Adebayo TS, Saleh Al-Faryan MA (2022) The potency of natural resources on ecological sustainability in PIIGS economies. Resour Policy 79:102941. https://doi.org/10.1016/j.resourpol.2022.102941
Roy A (2024) The impact of foreign direct investment, renewable and non-renewable energy consumption, and natural resources on ecological footprint: an Indian perspective. Int J Energy Sect Manag 18(1):141–161. https://doi.org/10.1108/IJESM-09-2022-0004
Rudolph A, Figge L (2017) Determinants of ecological footprints: what is the role of globalization? Ecol Ind 81:348–361. https://doi.org/10.1016/j.ecolind.2017.04.060
Saeed Meo M, Karim MZA (2022) The role of green finance in reducing CO 2 emissions: an empirical analysis. Borsa Istanbul Rev 22(1):169–178. https://doi.org/10.1016/j.bir.2021.03.002
Sahoo M, Sethi N (2021) The intermittent effects of renewable energy on ecological footprint: evidence from developing countries. Environ Sci Pollut Res 28(40):56401–56417. https://doi.org/10.1007/s11356-021-14600-3
Saqib N, Duran IA, Ozturk I (2023) Unraveling the interrelationship of digitalization, renewable energy, and ecological footprints within the EKC framework: empirical insights from the United States. Sustainability 15(13):10663. https://doi.org/10.3390/su151310663
Shahsavari A, Akbari M (2018) Potential of solar energy in developing countries for reducing energy-related emissions. Renew Sustain Energy Rev 90:275–291. https://doi.org/10.1016/j.rser.2018.03.065
Shahsavari A, Yazdi FT, Yazdi HT (2019) Potential of solar energy in Iran for carbon dioxide mitigation. Int J Environ Sci Technol 16(1):507–524. https://doi.org/10.1007/s13762-018-1779-7
Sharif A, Meo MS, Chowdhury MAF, Sohag K (2021) Role of solar energy in reducing ecological footprints: an empirical analysis. J Clean Prod 292:126028. https://doi.org/10.1016/j.jclepro.2021.126028
Sim N, Zhou H (2015) Oil prices, US stock return, and the dependence between their quantiles. J Bank Finance 55:1–8. https://doi.org/10.1016/j.jbankfin.2015.01.013
Thi Hong Nham N, Thanh Ha L (2023) A wavelet analysis of connectedness between economic globalization, nonrenewable, and renewable energy consumption, and CO 2 emissions in Vietnam. Sustain Energy Technol Assess 57:103227. https://doi.org/10.1016/j.seta.2023.103227
Usman O, Ozkan O, Adeshola I, Eweade BS (2024) Analysing the nexus between clean energy expansion, natural resource extraction, and load capacity factor in China: a step towards achieving COP27 targets. Environ Dev Sustain. https://doi.org/10.1007/s10668-023-04399-z
Van Tran H, Tran AV, Bui Hoang N, Mai TNH (2024) Asymmetric effects of foreign direct investment and globalization on ecological footprint in Indonesia. PLoS ONE 19(1):e0297046. https://doi.org/10.1371/journal.pone.0297046
Wang J, Dong K, Sha Y, Yan C (2022) Envisaging the carbon emissions efficiency of digitalization: the case of the internet economy for China. Technol Forecast Soc Chang 184:121965. https://doi.org/10.1016/j.techfore.2022.121965
WDI (2024) World development indicators | DataBank. https://databank.worldbank.org/source/world-development-indicators . Accessed 10 Feb 2024
Xue L, Haseeb M, Mahmood H, Alkhateeb TTY, Murshed M (2021) Renewable energy use and ecological footprints mitigation: evidence from selected South Asian economies. Sustainability 13(4):1613. https://doi.org/10.3390/su13041613
Yang Z, Gao W, Han Q, Qi L, Cui Y, Chen Y (2022) Digitalization and carbon emissions: How does digital city construction affect china’s carbon emission reduction? Sustain Cities Soc 87:104201. https://doi.org/10.1016/j.scs.2022.104201
Yi S, Raghutla C, Chittedi KR, Fareed Z (2023) How economic policy uncertainty and financial development contribute to renewable energy consumption? The importance of economic globalization. Res 202:1357–1367. https://doi.org/10.1016/j.renene.2022.11.089
Yu J, Tang YM, Chau KY, Nazar R, Ali S, Iqbal W (2022) Role of solar-based renewable energy in mitigating CO 2 emissions: Evidence from quantile-on-quantile estimation. Renew Energy 182:216–226. https://doi.org/10.1016/j.renene.2021.10.002
Zafar MW, Zaidi SAH, Khan NR, Mirza FM, Hou F, Kirmani SAA (2019) The impact of natural resources, human capital, and foreign direct investment on the ecological footprint: the case of the United States. Resour Policy 63:101428. https://doi.org/10.1016/j.resourpol.2019.101428
Zhang Z, Ding Z, Geng Y, Pan L, Wang C (2023) The impact of digital economy on environmental quality: evidence from China. Front Environ Sci 11:1120953. https://doi.org/10.3389/fenvs.2023.1120953
Zhang H, Khan KA, Eweade BS, Adebayo TS (2024a) Role of eco-innovation and financial globalization on ecological quality in China: a wavelet analysis. Energy Environ. https://doi.org/10.1177/0958305X241228518
Zhang Y, Radmehr R, Baba Ali E, Samour A (2024b) Natural resources, financial globalization, renewable energy, and environmental quality: novel findings from top natural resource abundant countries. Gondwana Res. https://doi.org/10.1016/j.gr.2023.12.016
Zheng R, Wu G, Cheng Y, Liu H, Wang Y, Wang X (2023) How does digitalization drive carbon emissions? The inverted U-shaped effect in China. Environ Impact Assess Rev 102:107203. https://doi.org/10.1016/j.eiar.2023.107203
Zhu Z, Liu B, Yu Z, Cao J (2022) Effects of the digital economy on carbon emissions: evidence from China. Int J Environ Res Public Health 19(15):9450. https://doi.org/10.3390/ijerph19159450
Zhu L, Fang W, Rahman SU, Khan AI (2023a) How solar-based renewable energy contributes to CO 2 emissions abatement? Sustainable environment policy implications for solar industry. Energy Environ 34(2):359–378. https://doi.org/10.1177/0958305X211061886
Zhu P, Ahmed Z, Pata UK, Khan S, Abbas S (2023b) Analyzing economic growth, eco-innovation, and ecological quality nexus in E-7 countries: accounting for non-linear impacts of urbanization by using a new measure of ecological quality. Environ Sci Pollut Res 30(41):94242–94254. https://doi.org/10.1007/s11356-023-29017-3
Download references
Open access funding provided by the Scientific and Technological Research Council of Türkiye (TÜBİTAK). The authors have not disclosed any funding.
Author information
Authors and affiliations.
Faculty of Economics and Administrative Science, Cyprus International University, Nicosia, Northern Cyprus, Mersin 10, Turkey
Tomiwa Sunday Adebayo
Sunway Business School, Sunway University, Malaysia
Muhammad Saeed Meo
Eastern Mediterranean University, Famagusta, Northern Cyprus, via Mersin 10, Turkey
Babatunde Sunday Eweade
Tokat Gaziosmanpasa University, Tokat, Turkey
Oktay Özkan
Adnan Kassar School of Business, Lebanese American University, Beirut, Lebanon
University of Tashkent for Applied Sciences, Str. Gavhar 1, Tashkent, 100149, Uzbekistan
University of Economics and Human Sciences, Warsaw, Poland
Advanced Research Centre, European University of Lefke, Northern Cyprus, TR-10, Mersin, Turkey
You can also search for this author in PubMed Google Scholar
Contributions
TSA: Conceptualization, Methodology, visualization; MSM: Writing and editing; BSE: Writing and Discussion; OO: Methodology
Corresponding author
Correspondence to Babatunde Sunday Eweade .
Ethics declarations
Competing interests.
The authors declare no competing interests.
Additional information
Publisher's note.
Springer Nature remains neutral with regard to jurisdictional claims in published maps and institutional affiliations.
Rights and permissions
Open Access This article is licensed under a Creative Commons Attribution 4.0 International License, which permits use, sharing, adaptation, distribution and reproduction in any medium or format, as long as you give appropriate credit to the original author(s) and the source, provide a link to the Creative Commons licence, and indicate if changes were made. The images or other third party material in this article are included in the article's Creative Commons licence, unless indicated otherwise in a credit line to the material. If material is not included in the article's Creative Commons licence and your intended use is not permitted by statutory regulation or exceeds the permitted use, you will need to obtain permission directly from the copyright holder. To view a copy of this licence, visit http://creativecommons.org/licenses/by/4.0/ .
Reprints and permissions
About this article
Adebayo, T.S., Meo, M.S., Eweade, B.S. et al. Analyzing the effects of solar energy innovations, digitalization, and economic globalization on environmental quality in the United States. Clean Techn Environ Policy (2024). https://doi.org/10.1007/s10098-024-02831-0
Download citation
Received : 30 December 2023
Accepted : 30 March 2024
Published : 29 April 2024
DOI : https://doi.org/10.1007/s10098-024-02831-0
Share this article
Anyone you share the following link with will be able to read this content:
Sorry, a shareable link is not currently available for this article.
Provided by the Springer Nature SharedIt content-sharing initiative
- Natural resources
- Digitalization
- Economic globalization
- Solar energy innovations
- Ecological footprint
- Renewable energy
- KRLS approach
- Find a journal
- Publish with us
- Track your research
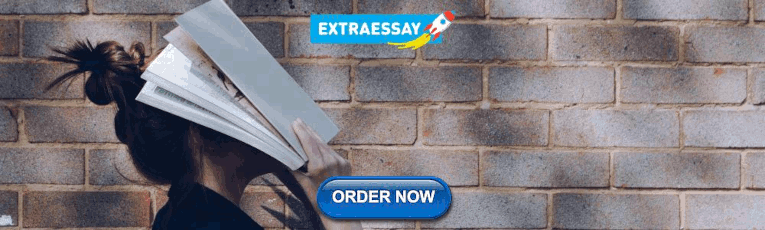
IMAGES
VIDEO
COMMENTS
Global projections of macroeconomic climate-change damages typically consider impacts from average annual and national temperatures over long time horizons1-6. Here we use recent empirical ...
Literature Review', Review of Economics, 65(3): 281-320. Abstract . We review the historical literature on the economics of climate change with a focus on the ... The economics of climate change is a fairly new field that has grown very rapidly in the last decade. The literature on climate change trends is the oldest of the
Amartya Sen, recipient of the Nobel Prize for Economics, 1998. 'The Stern Review of The Economics of Climate Change provides the most thorough and rigorous analysis to date of the costs and risks of climate change, and the costs and risks of reducing emissions. It makes clear that the question is not whether we can afford to act, but whether ...
A review of The Stern Review on the Economics of Climate Change. Journal of Economic Literature, volume 45, number 3, pages 703-724. Geoffrey Heal (2009). The economics of climate change: a post-Stern perspective. Climatic Change, volume 96, number 3, pages 275-297.
'The Stern Review of The Economics of Climate Change is a vital step forward in securing an effective global policy on climate change. Led by one of the world's top economists, the Stern Review shows convincingly that the benefits of early global action to mitigate climate change will be far lower than the costs. The report establishes ...
The Economics of Climate Change. Global climate change poses a threat to the well-being of humans and other living things through impacts on ecosystem functioning, biodiversity, capital productivity, and human health. This paper briefly surveys recent research on the economics of climate change, including theoretical insights and empirical ...
Climate Shock: The Economic Consequences of a Hotter Planet by Gernot Wagner and Martin L. Weitzman (hereafter, WW). There is one implication of the timescale of climate change that has been obvious from the word "go"—the discount rate matters. Climate change plays out over centuries, and choices over centuries are extraordinarily
Stern Review team. The views expressed here are mine and do not necessarily reflect the judgements or positions of those who kindly provided advice, or of the London School of Economics, or of the UK Government, for whom I was working while leading the Stern Review on the Economics of Climate Change. This is dedicated to my close friend,
Review of Economics. Abstract We review the literature on the economics of climate change with a focus on the evolution of the literature from some of the early classic papers to the latest contributions. We divide the paper into three main sections: trends in greenhouse gas emissions, mitigation, and adaptation. View via Publisher.
the value of the region's portfolio. The economic story of climate change| and indeed, the nancial on|may be less a story of investors and nancial markets than a story of states, cities, and other local governments. 2 Is climate change risk quanti able? There is a growing literature on climate-related uncertainty in economic decision-making.
The literature on the economic modeling of climate change has addressed only subsets of these issues. Most climate change models estimate the cost of mitigation1 in a partial equilibrium or static general equilibrium framework (see e.g., Weyant et al., 1999 for a review). As such, they do not analyze the consequences of mitigation for growth. A ...
This review is a sectorial assessment of climate change mitigation and adaptation approaches worldwide in the aforementioned sectors and the associated economic costs. According to the findings, government involvement is necessary for the country's long-term development through strict accountability of resources and regulations implemented in ...
I review the literature on the economic impacts of climate change, an externality that is unprecedentedly large, complex, and uncertain. Only 14 estimates of the total damage cost of climate change have been published, a research effort that is in sharp contrast to the urgency of the public debate and the proposed expenditure on greenhouse gas ...
The Stern Review argues that the damages from climate change are large, and that nations should undertake sharp and immediate reductions in greenhouse gas emissions. An examination of the Review 's radical revision of the economics of climate change finds, however, that it depends decisively on the assumption of a near-zero time discount rate ...
I review the literature on the economic impacts of climate change, an externality that is unprecedentedly large, complex, and uncertain. Only 14 estimates of the total damage cost of climate change have been published, a research effort that is in sharp contrast to the urgency of the public debate and the proposed expenditure on greenhouse gas emission reduction. These estimates show that ...
JEL Classification. The Economics of the Climate by Geoffrey Heal. Published in volume 55, issue 3, pages 1046-63 of Journal of Economic Literature, September 2017, Abstract: I review the economic characteristics of the climate problem, focusing on the choice of discount rates in the presence of a stock externality, ri...
Benjamin N. Dennis. Abstract: This article reviews the rapidly proliferating economic literature on climate change and financial policy. We find: (1) enduring challenges in estimating the statistical properties of a changed climate; (2) emerging evidence of financial markets pricing in climate-related risks; and (3) a range of significant ...
Productivity and long term growth. Climate change is expected to have significant implications for the world economy and for many areas of human activity. A main conclusion of the review is that there are large uncertainties, which are not fully reflected in existing estimates of global impacts of climate change in monetary units.
This paper will discuss urban sustainability in the context of. climate change and address the following research objectives: (1) to examine how. climate change is affecting urban areas; (2) to assess how cities can enhance urban. sustainability by addressing climate change; (3) to discuss resources available for city.
A lot. We have explored the model space and the parameter space much more thoroughly, though there are still unexplored regions. While there are aspects of the Stern Review's analysis with which we can disagree, it seems fair to say that it has catalyzed a fundamental rethinking of the economic case for action on climate change.
1. Introduction. Climate is changing drastically and unprecedentedly [1,2,3].Recently, the eight consecutive years from 2015 until 2022 were considered the warmest years since the beginning of temperature measurement [4,5].Concomitantly, extreme events have become more visible and deadly [].Still, this threatening scenario tends to be aggravated, considering that only few countries have met ...
JEL Classification. Q54 Climate; Natural Disasters; Global Warming. The Economics of Climate Change by Nicholas Stern. Published in volume 98, issue 2, pages 1-37 of American Economic Review, May 2008.
A recent systematic literature review finds that, on average, such adaptive behaviors offset 46 percent of the damage from climate change. However, adaptation ratios vary widely across settings: while firms recover 72 percent of climate losses, this number falls to 49 percent for households, and just 38 percent among agricultural producers.
Facing the severe challenges brought by global climate change, achieving carbon neutrality has become a consensus goal of the international community. The Chinese government attaches great importance to this and has proposed the "3060" dual carbon targets, establishing a "1+N" policy system for carbon peaking and carbon neutrality.
The structure of the paper is as follows: The subsequent Sect. "Literature review" will provide a literature assessment, concentrating on the correlation between digitalization, economic globalization, natural resources, renewable energy, and solar innovation on the ecological footprint, with the aim of tackling climate change.