- Technical Note
- Open access
- Published: 22 October 2010
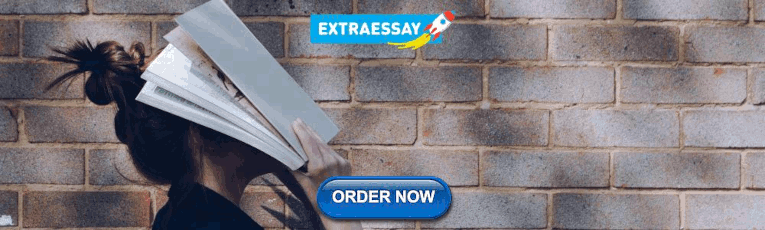
Structural equation modeling in medical research: a primer
- Tanya N Beran 1 &
- Claudio Violato 1
BMC Research Notes volume 3 , Article number: 267 ( 2010 ) Cite this article
99k Accesses
221 Citations
15 Altmetric
Metrics details
Structural equation modeling (SEM) is a set of statistical techniques used to measure and analyze the relationships of observed and latent variables. Similar but more powerful than regression analyses, it examines linear causal relationships among variables, while simultaneously accounting for measurement error. The purpose of the present paper is to explicate SEM to medical and health sciences researchers and exemplify their application.
To facilitate its use we provide a series of steps for applying SEM to research problems. We then present three examples of how SEM has been utilized in medical and health sciences research.
When many considerations are given to research planning, SEM can provide a new perspective on analyzing data and potential for advancing research in medical and health sciences.
Structural equation modeling (SEM) is a powerful multivariate analysis technique that is widely used in the social sciences [ 1 ]. Its applications range from analysis of simple relationships between variables to complex analyses of measurement equivalence for first and higher-order constructs [ 2 ]. It provides a flexible framework for developing and analyzing complex relationships among multiple variables that allow researchers to test the validity of theory using empirical models. Perhaps its greatest advantage is the ability to manage measurement error, which is one of the greatest limitations of most studies. Although its application has been seen in many disciplines, it has yet to be extensively used in medical research and epidemiology.
In a recent paper, we provided a "how to" for medical education researchers [ 3 ]. Specific principles and examples for the field of medical education were utilized. The purpose of the present paper, however, is to introduce structural equation modeling through explanation and demonstration of its methods in an attempt to disseminate it more widely in medical and health sciences research.
The use of SEM has now become widespread across research domains. In psychology, for example, the citation frequency of SEM has steadily increased from 164 in 1994 to 343 in 2000 and then to 742 in the last year (based on the citation frequency of SEM and M[ANOVA] of PsychINFO database 1970-2010) [ 4 , 5 ]. This suggests that researchers recognize its application to a variety of research questions, types of data, and methods of study. An increase in use of sophisticated tools of analysis reflects the increase in complexity of empirical models and theoretical developments seen in the published research over the years.
In a recent (2009) commentary in the International Journal of Epidemiology , Tu expressed concern about the scarcity of SEM models in epidemiological research and urged epidemiologists to use SEM models more frequently [ 6 ]. With its strength as a statistical tool to analyze complex relationships among variables, and even posit and test causal relationships with non-experimental data, it allows researchers to explain the development of phenomena such as disease and health behaviors. The purpose of the present paper is to consider the potential advances that SEM can make in medical and health sciences research and provide a five step approach to implementing SEM research in epidemiology and medical research. First a description of SEM is provided, followed by applications to research. A broad categorization of statistical methods is termed 'latent variable models', which include factor analysis, item response theory, latent class models, and structural equation models [ 7 ]. The focus of the present paper in on structural equation models and the latent variable models that are included in SEM.
1. Description of SEM
Although SEM was developed in the early 1900s as a result of Spearman's (1904) development of factor analysis and Wright's (1918, 1921) invention of path analysis, the first basic introductory textbook on SEM was not published until 1984 [ 8 – 11 ]. With the advances in computer programming such as EQS (EQuationS implementing Structural Equation Modeling), and LISREL (Linear Structural Relationships) researchers began utilizing SEM techniques in their research [ 12 , 13 ]. Indeed, it has become "the preeminent multivariate technique" [ 4 ] and is now accessible on-line at no cost (e.g., http://openmx.psyc.virginia.edu/ ).
There are several integrated analytic techniques within SEM. These include between-group and within-group variance comparisons, which are typically associated with ANOVA. It also includes path analysis (regression analysis) whereby equations representing the effect of one or more variables on others can be solved to estimate their relationships. a Path analysis, thus, represents the hypothesized causal relationships among variables to be tested. Factor analysis is another special case of SEM whereby unobserved variables (factors or latent variables) are calculated from measured variables. These analyses can usually be performed using data in the form of means or correlations and covariances (i.e., unstandardized correlations). These data, moreover, may be obtained from experimental, nonexperimental and observational studies. All of these techniques can be incorporated into the following example.
Several symptoms of a disease are measured and used in a factor model that represents these symptoms. The relationship between the factor(s) and behavioral and/or environmental characteristics are determined through path analysis. The impact of different types of medication on the factor(s) is then compared across the measured behavioral and environmental conditions.
To conduct the above analyses, both a structural (i.e., path) and a measurement model are designed by the researcher. The structural model refers to the relationships among latent variables, and allows the researcher to determine their degree of correlation (calculated as path coefficients). That is, path coefficients were defined by Wright (1920, p. 329) as measuring the importance of a given path of influence from cause to effect [ 14 ]. Each structural equation coefficient is computed while all other variances are taken into account. Thus, coefficients are calculated simultaneously for all endogenous variables rather than sequentially as in regular multiple regression models.
To determine the magnitude of these coefficients, the researcher specifies the structure of the model. This is depicted in Figure 1 . As shown, the researcher may expect that there is a correlation between variables A and B, as shown by the double headed arrow. There may be no expected relationship between variables A and C, so no arrow is drawn. Finally, the researcher may hypothesize that there is a unidirectional relationship of variable C to B, as indicated by an arrow pointing from C to B. The relationships among variables A, B, and C represent the structural model. Researchers detail these relationships by writing a series of equations, hence the term 'structural equation' (referring to the relationships between the variables). The combination of these equations specifies the pattern of relationships [ 12 ].
The second component to be specified is the measurement model. As represented in Figure 1 , it consists of the measured variables (e.g., variables 1-7), which are typically used in research, as well as latent variables. Latent variables are factors like those derived from factor analysis, which consist of at least two inter-related measured variables. They are called latent because they are not directly measured, but rather are represented by the overlapping variance of measured variables. They are said to better represent the research constructs than are measured variables because they contain less measurement error. As indicated in Figure 1 , for example, measurement model A depicts a latent variable A, which is the construct underlying measured variables 1 and 2. To further explicate the process of developing and analyzing a model, the following steps are outlined next.
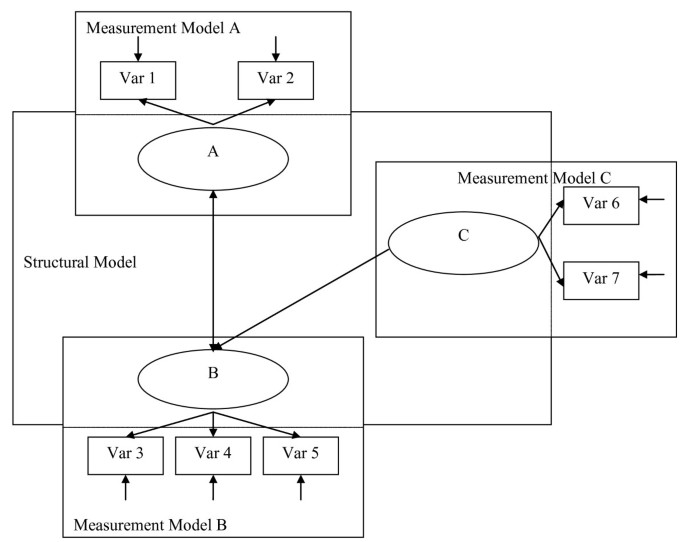
A structural equation model - from Nachtigall C, Kroehne U, Funke F, Steyer R . Why should we use SEM? Pros and cons of structural equation modeling . Meth Psychol Res Online 2003, 8 :1-22.
Step 1: Identify the Research Problem
The researcher develops hypotheses about the relationships among variables that are based on theory, previous empirical findings or both [ 15 ]. These relationships may be direct or indirect whereby intervening variables may mediate the effect of one variable on another. The researcher must also determine if the relationships are unidirectional or bidirectional, by using previous research and theoretical predictions as a guide. The researcher outlines the model by determining the number and relationships of measured and latent variables. Care must be taken in using variables that provide a valid and reliable indicator of the constructs under study. The use of latent variables is not a substitute for poorly measured variables. A path diagram depicting the structural and measurement models will guide the researcher when identifying the model, as described next.
Step 2: Identify the Model
Identifying the model is a crucial step in model development as decisions at this stage will determine whether the model can be feasibly evaluated. For each parameter in the model to be estimated, there must be at least as many values (i.e., variance and covariance values) as model parameters (e.g., path coefficients, measurement error). b A model that has fewer of these values than parameters is referred to as underidentified and impossible to solve mathematically. This problem also occurs when variables are highly intercorrelated (multicollinearity) c , the scales of the variables are not fixed (the path from a latent variable to one of the measured variables must be set as a constant), or there is no unique solution to the equations because the underidentification results in more parameters to be estimated than information provided by the measured variables. In underidentified models there are an infinite number of solutions and therefore no unique one. These problems may be remedied with the addition of independent variables, which requires that the model be conceptualized before data are collected. There are many further issues to consider when managing parameters that cannot be addressed in this primer. For further details on model identification, readers are encouraged to see Kline [ 16 ].
Step 3: Estimate the Model
There are many estimation procedures available to test models, with three primary ones discussed here. ML is set as the default estimator in most SEM software. It is an iterative process that estimates the extent to which the model predicts the values of the sample covariance matrix, with values closer to zero indicating better fit. The name maximum likelihood is based on its calculation. The estimate maximizes the likelihood that the data were drawn from its population. The estimates require large sample sizes, but do not usually depend on the measurement units of the measured variables. It is also robust to non-normal data distributions [ 17 ].
Another widely used estimate is least squares (LS), which minimizes the sum of the squares of the residuals in the model. LS is similar to ML as it also examines patterns of relationships, but does so by determining the optimum solution by minimizing the sum of the squared deviation scores between the hypothesized and observed model. It often performs better with smaller sample sizes and provides more accurate estimates of the model when assumptions of distribution, independence, and asymptotic sample sizes are violated [ 18 ].
The third, asymptotically distribution free (ADF) estimation procedures (also known as Weighted Least Squares) are less often used but may be appropriate if the data are skewed or peaked. ML, however, tends to be more reliable than ADF. This method also requires sample sizes of 200 to 500 to obtain reliable estimates for simple models and may under-estimate model parameters [ 16 , 19 ]. For further details see Hu et al. [ 19 ] and Muthén and Kaplan [ 20 ].
Step 4: Determine the Model's Goodness of Fit
These estimation procedures determine how well the model fits the data. Fitting the latent variable path model involves minimizing the difference between the sample covariances and the covariances predicted by the model. The population model is formally represented as:
Where Σ is the population covariance matrix of observed variables, θ is a vector that contains the model parameters, and Σ (θ) is the covariance matrix written as a function of θ . This simple equation allows the implementation of a general mathematical and statistical approach to the analysis of linear structural equation system through the estimation of parameters and the fitting of models. Estimation can be classified by type of distribution (multinormal, elliptical, arbitrary) assumed of the data and weight matrix used during the computations. The function to be minimized is given by:
where s is the vector of data to be modeled - the variances and covariances of the observed variables - and σ is a model for the data. The model vector σ is a function of more basic parameters θ that are to be estimated so as to minimize Q. W is the weight matrix that can be specified in several ways to yield a number of different estimators that depend on the distribution assumed.
Essentially the researcher attempts to represent the population covariance matrix in the sample variables. Then, an estimation procedure is selected, which runs through an iterative process until the best solution is found.
Another source of information in the output is the fit indices. There are many indices available, with most ranging from 0 to 1 with a high value indicating a great degree of variance in the data accounted for by the model [ 21 ]. The Comparative Fit Index (CFI) is most commonly used and compares the existing model with a null model. A good fit is also represented by low residual values (e.g., .00), which represents the amount of variance not accounted for by the model. These are calculated as indices such as the Root Mean Square Error of Approximation (RMSEA), which is the square root of mean differences between the estimate and the true value. Another goodness-of-fit statistic commonly reported is χ 2 , which assesses the likelihood that the differences between the population covariance matrix and model implied covariance matrix are zero. This statistic, however, varies as a function of sample size, cannot be directly interpreted (because there is no upper bound), and is almost always significant. It is useful, however, when directly comparing models on the same sample. Dahly, Adair, and Bollen [ 22 ], for example, tested various fit indices for different models depicting the relationship between maternal height and arm fat area with fetal growth. When adding and removing variables, as well as specifying varying relationships between variables, each corresponding fit index was calculated. This allowed the researchers to determine factors in the fetal environment that are most significantly related to systolic blood pressure of young adults. In summary, when evaluating fit statistics, CFI values ≥ .90 and RMSEA < .05 are considered adequate [ 23 ].
A comparison of indices was conducted by Hu and Bentler [ 18 ] on data that violated assumptions of normal distribution, independence of observations, and symmetry. Their results indicate that TLI, BL89, RNI, CFI, Mc, Gamma Hat, and RMSEA are able to identify good models. Many of these are provided by standard SEM software packages (e.g., EQS, LISREL, Mplus, AMOS). d
To determine the model's goodness-of-fit, sample size is an important consideration. It must be large enough to obtain stable estimates of the parameters. Many recommendations have been published, suggesting that there is no precise decision rule. Monte Carlo studies provide guidance that sample sizes of 10 for a one-factor, five-observed variable model, and 30 for a two-factor, five-observed variable model provide robust results [ 24 ]. More general guidelines are used in current research with the suggestion that at least 100 but preferably 200 cases are needed to obtain stable results [ 16 ]. Using a large sample reduces the likelihood of random variation that can occur in small samples [ 25 ], but may be difficult to obtain in practice.
Step 5: Re-specify the Model if Necessary
To obtain improved fit results, the above sequence of steps is repeated until the most succinct model is derived (i.e., principle of parsimony). A recommended procedure to improve the model estimations is through examination of the size of the standardized residual values between variables. Large residuals may suggest inadequate model fit. This can be addressed by the addition of a path link, or inclusion of mediating or moderating variables (if theoretically supported). Once the model is re-calculated, its fit may show improvement and residual may be reduced. These results then need to be confirmed on an alternate sample, and through further studies. This replication strengthens confidence in the inferences, and provides implications for theoretical development and practical application.
Further Considerations
Before executing SEM procedures, there are many additional topics to consider. As for any research study, careful planning of design, sampling, and measures is needed to develop valid models. SEM can be used in either cross-sectional or longitudinal studies, whereby the former are identified by links among variables measured at the same point in time, and the latter are specified by the links among variables measured at different points in time. These models often include autoregressive effects where a variable measured at two time points is correlated with itself. This corrects for an over-estimation of the relationship among exogenous (independent) and endogenous (dependent) variables [ 26 ]. While this is a distinct advantage of SEM, it is often disregarded.
Types of measures must also be considered [ 27 ]. Calculations of variances, covariances and product-moment correlations all assume that values are measured on an interval scale. Measures that include, for example, rating scales without equal distances between data points, are not necessarily considered appropriate [ 28 ]. Researchers must be prudent in selecting the appropriate procedures for particular levels of measurement including, for example, dichotomous and polytomous data [ 29 ]. Indeed, another advantage of SEM is the ability to manage continuous and binary data simultaneously.
SEM can be employed for both exploratory and confirmatory models. An exploratory approach is more traditional in that a detailed model specifying the relationships among variables is not made a priori. All latent variables are assumed, therefore, to influence all observed variables so that the number of latent variables are not pre-determined, and measurement errors are not allowed to correlate [ 29 ]. Although both exploratory and confirmatory factor analyses are a subset of SEM involving the measurement model only, the latter is more frequently used to test hypothetical constructs. The following section presents three examples of application of SEM in medical and health sciences research.
2. Examples of SEM
SEM has been applied in psychiatry to understanding patients' experiences of schizophrenia. Loberg and colleagues examined the role of positive symptoms and duration of schizophrenia on dichotic listening of patients [ 30 ]. Dichotic listening tasks are used as a means of assessing functioning within the left temporal lobe language areas. Previous research suggested increased impairment in left temporal lobe language processing among patients with a high number of positive symptoms (e.g., hallucinations and delusions) of schizophrenia.
Loberg, Jorgensen, Green, Rund et al [ 30 ] attempted to replicate these results as well as determine whether duration of illness further decreases language functioning. A total of 129 patients from clinics in Norway and California diagnosed with schizophrenia were included. e All patients were taking haloperidol (an antipsychotic) or an equivalent.
The Extended Brief Psychiatric Rating Scale and Positive and Negative Syndrome scale were completed by blind observers to measure symptoms of schizophrenia, and the duration of the disease was calculated based on initial onset of symptoms. Dichotic listening was measured by patients' responses to consonant and vowel blends spoken through headphones. In one condition patients were told which ear to listen with (attention) and in another they were not (laterality). The theoretical model tested is shown in Figure 2 .
Analysis of this model using SEM indicated it fit the data well. The CFI was 0.986 based on 11 degrees of freedom. Close inspection of the model (Figure 2 ) shows that all the path coefficients to the predicted latent variables are moderate to high (range from .32 to .87). The RMSEA was .048. Positive symptoms were measured by hallucinations, disorganized thoughts, and unusual thought content. Dichotic listening was measured by accuracy of sounds identified in each ear according to the condition in which the patients heard the sounds.
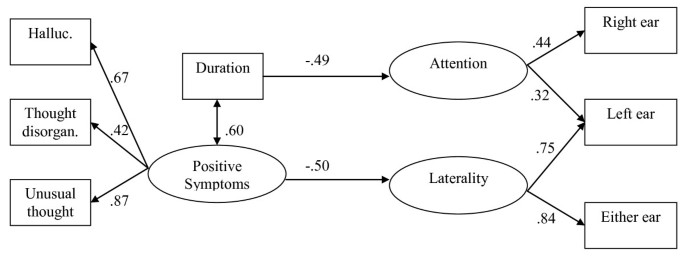
Model of positive symptoms, duration of schizophrenia, and dichotic listening .
In terms of the relationship between dichotic listening and schizophrenia, duration of schizophrenia and number of positive symptoms were related to accuracy of sound detection. That is, patients who have had schizophrenia for a longer duration and experience more positive symptoms, the poorer their identification of vowel-consonant blends. These results support findings from previous research suggesting impaired language processing and structural abnormalities in the left superior temporal gyrus for patients with schizophrenia.
The advantage of this research over other studies is that it examines three types of positive symptoms and duration of schizophrenia simultaneously, rather than separately, in relation to dichotic listening. In other words, the model also suggests that patients with many positive symptoms are likely to have difficulty identifying sounds accurately, especially if the duration of the illness is long. Greater confidence can be placed in these results than other regression models because more than one indicator of the constructs of interest was used in the model. Identifying basic underlying latent variables (positive symptoms and dichotic listening) is another advantage over interpreting simple correlations among measured variables.
Because this is a cross-sectional model, it is unknown whether the language processing deficit existed before, at the same time, or after the onset of schizophrenia. Direction of cause in the model is, thus, unknown. Given that time was an important variable in this model, we can explore the advantages of longitudinal modeling, or measuring variables at more than one point in time. That is, using the same measures of positive symptoms of schizophrenia and language processing taken at Time 1 and Time 2, path coefficients between the two latent variables at both points in time can be simultaneously examined to determine those that are significant. Previously a cross-lagged design would have been used whereby positive symptoms at Time 1 are correlated with language processing at Time 2. This correlation is then compared to the correlation between language processing at Time 1 and positive symptoms at Time 2. This comparison does not account for autoregression, does not include latent variables, and cannot be easily applied to multiple time points or multiple variables. An alternate method is multiple regression analysis whereby the positive symptoms of schizophrenia and language processing measured at Time 1 are used to predict language processing at Time 2. The magnitude of the regression weights would indicate the strength of the relationship between schizophrenia and language processing while controlling for initial language processing. Although this takes autoregression into account and includes multiple measured variables, latent variables cannot be used, and reciprocal patterns (impact of language processing on positive symptoms) cannot be examined.
A second example of SEM is of a model in population health that depicts the relationship between childhood victimization and school achievement. Beran and Lupart postulated that children who are targeted by acts of aggression from their peers may be at risk for poor achievement [ 31 ]. This argument is supported by Eccles' Expectancy-Value theory [ 32 ]. Accordingly, achievement involves the culture, socialization, and the environmental "fit" of schools for students. When children are exposed to positive experiences within this environment they are likely to gain academic and social competence [ 33 ]. Exposure to aggressive initiations from peers, however, may reduce a child's sense of competence for interpersonal interactions. Given that learning at school takes place in a social environment these harmful interactions may reduce learning behaviors such as volunteering answers and asking questions. Rather, children who are targeted may become discouraged and disengaged from peers and classroom learning [ 33 ].
In further developing their model, Beran and Lupart [ 31 ] included several correlates of achievement reported in previous research: impaired peer social skills (helping others), limited friendships (feeling disliked), and disruptive behaviors (aggression towards others, hyperactivity/inattention). All of these factors were simultaneously examined to determine the likelihood of targeted adolescents experiencing poor achievement. The theoretical SEM model is depicted in Figure 3 .
Adolescents between 12-15 years of age (n = 4,111) were drawn from the Canadian National Longitudinal Survey of Children and Youth, which is a stratified random sample of 22,831 households in Canada [ 34 ]. As shown in Figure 3 , harassment was related to disruptive behavior problems and peer interactions, which were related to achievement, χ 2 (32) = 300.00, p < .001, SRMR = .05; CFI = .91. Achievement was measured by four report sources including the language arts and math teachers, who reported on performance in those subjects, and the parent and child's report of overall achievement. Victimization was measured by adolescents' reports of frequency of attack and threats received from peers as well as degree of discomfort they feel among their peers. Victimization and achievement were used as latent variables in the model and were found to be mediated by disruptive behaviors and friendship experiences. This is shown by the arrows and coefficients whereby there is no arrow directly linking victimization with achievement. Rather, harassment was related to friendships and conduct problems, indicating that adolescents who were harassed reported having few or no friends (as shown by the negative sign) and exhibited conduct problems. These conduct problems were related to hyperactivity/inattention and prosocial behaviors such that adolescents with more rule breaking tendencies were likely to demonstrate hyperactive and inattentive behaviors as well as few prosocial, or helping, behaviors. These factors were also related to achievement. These combined results suggest that adolescents who are targeted by their peers are at risk of experiencing poor school achievement if they exhibit disruptive behavior problems and poor peer interactions.
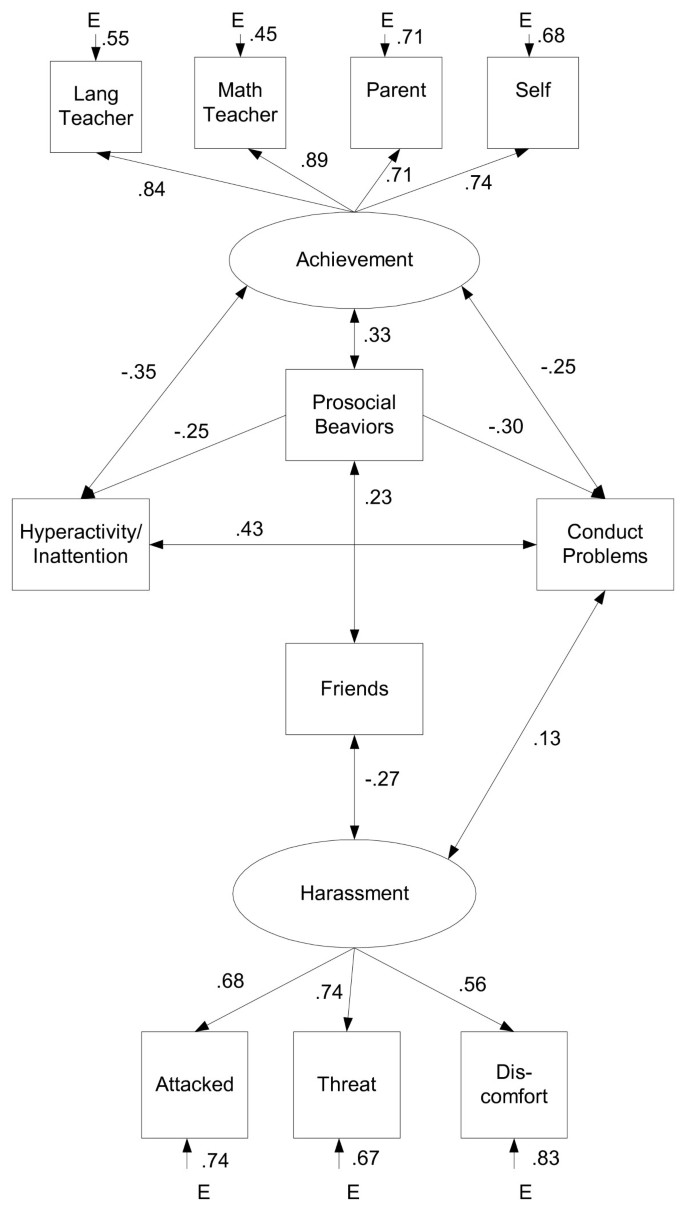
Latent variable path model of harassment and achievement employing maximum likelihood estimation ( n = 613) .
A third example applies SEM within the field of clinical epidemiology by examining how health nutrition behaviors can serve to reduce risk of illness within a senior population. Specifically, Keller [ 35 ] examined behaviors that constitute risk of poor nutrition among seniors as part of a screening intervention. A measurement model of risk factors that constitute poor nutrition was developed a priori based on exploratory results from a previous study that identified four factors from 15 measured variables. A total of 1,218 Canadian seniors were interviewed or self-administered 15 questions about eating behaviors that matched those used previously. Variables such as type and frequency of food eaten created the latent factor food intake; appetite and weight change loaded on the factor adaptation; swallowing and chewing ability loaded on the factor physiologic; and cooking and shopping ability formed the variable functional. These factors were then loaded onto a higher level factor nutritional risk. The model fit the data well according to the CFI (> .90) and the RMSEA (< .05). Factor loadings varied between .15 and .66. It was, thus, concluded that these factors provide a comprehensive and valid indicator of nutritional risk for seniors. This framework was developed from previous research and presents confirmatory evidence for the nutrition behaviors used in the model of nutrition risk.
3. Strengths and Weaknesses of SEM
SEM is a set of statistical methods that allows researchers to test hypotheses based on multiple constructs that may be indirectly or directly related for both linear and nonlinear models [ 36 ]. It is distinguished from other types of analyses in its ability to examine many relationships while simultaneously partialing out measurement error. It can also examine correlated measurement error to determine to what degree unknown factors influence shared error among variables - which may affect the estimated parameters of the model [ 37 ]. It also handles missing data well by fitting raw data instead of summary statistics. SEM, in addition, can be used to analyze dependent observations (e.g., twin and family data). It can, furthermore, manage longitudinal designs such as time series and growth models. For example, Dahly, Adair, and Bollen [ 22 ] developed a longitudinal latent variable medical model showing that maternal characteristics during pregnancy predicted children's blood pressure and weight approximately 20 years later while controlling for child's birth weight. Therefore, SEM can be used for a number of research designs.
A distinct advantage of SEM over conventional multiple regression analyses is that the former has greater statistical power (probability of rejecting a false null hypothesis) than does the latter. This is demonstrated in Budtz-Jørgensen's epidemiological study of benchmark calculations to exposure of environmental toxins [ 38 ]. They were able to show that SEM statistics were more sensitive to changes in toxin exposure than were regression statistics, which resulted in estimates of lower, or safer, exposure levels than did the regression analyses.
SEM has sometimes been referred to as causal modeling; however, caution must be taken when interpreting SEM results as such. Several conditions are deemed necessary, but not sufficient for causation to be determined. There must be an empirical association between the variables - they are significantly correlated. A common cause of the two variables has been ruled out, and the two variables have a theoretical connection. Also, one variable precedes the other, and if the preceding variable changes, the outcome variable also changes (and not vice versa). These requirements are unlikely to be satisfied; thus, causation cannot be definitively demonstrated. Rather, causal inferences are typically made from SEM results. Indeed, researchers argue that even when some of the conditions of causation are not fully met causal inference may still be justifiable [ 39 ].
As with any method, SEM has its limitations. Although a latent variable is a closer approximation of a construct than is a measured variable; it may not be a pure representation of the construct. Its variance may consist of, in addition to true variance of the measured variables, shared error between the measured variables. Also, the advantage of simultaneous examination of multiple variables may be offset by the requirement for larger sample sizes for additional variables to derive a solution to the calculations.
SEM cannot correct for weaknesses inherent in any type of study. Exploration of relationships among variables without a priori specification may result in statistical significance but have little theoretical significance. In addition, poor research planning, unreliable and invalid data, lack of theoretical guidance, and over interpretation of causal relationships can result in misleading conclusions.
With the development of SEM, medical researchers now have powerful analytic tools to examine complex causal models. It is superior over other correlational methods such as regression as multiple variables are analyzed simultaneously, and latent factors reduce measurement error. When used as an exploratory or confirmatory approach within good research design it yields information about the complex nature of disease and health behaviors. It does so by examining both direct and indirect, and unidirectional and bidirectional relationships between measured and latent variables. Despite the valuable contribution of SEM to research methodology, the researcher must be aware of several considerations to develop a legitimate model. These include using an appropriate research design, a necessary sample size, and adequate measures. Nevertheless, the theory and application of SEM and their relevance to understanding human phenomena are well established. In the context of medical research it promises the opportunity of examining multiple symptoms and health behaviors that, with model development and refinement, can be utilized to enhance our research capabilities in medicine and the health sciences.
Authors' information
TNB is an Associate Professor in Medical Education and Research, Faculty of Medicine at the University of Calgary.
CV is a Professor in Medical Education and Research, Faculty of Medicine at the University of Calgary.
a ANOVA and multiple regression analysis are instances of the General Linear Model.
b *p = p (p+1)/2 can be used to determine the number of free parameters (*p) that can be estimated from the number of measured variables (p).
c A model with equal values and parameters is said to be identified, and one with more values than parameters is overidentified; both models can be empirically assessed.
d http://www.mvsoft.com/
http://www.ssicentral.com/lisrel/
http://www.statmodel.com/
http://www.spss.com/amos/
e Diagnoses were based on the criteria listed for classification in both the third revised or fourth edition of the Diagnostic Statistical Manual (DSM-IV), which is an international classification system for mental health disorders in children and adults published by the American Psychiatric Association.
Gonzalez J, de Boeck P, Tuerlinckx F: A double structure structural equation model for three-mode data. Psychol Methods. 2008, 13: 337-53. 10.1037/a0013269.
Article PubMed Google Scholar
Cheung GW: Testing equivalence in the structure, means, and variances of higher-order constructs with structural equation modeling. Organ Res Methods. 2008, 11: 593-613. 10.1177/1094428106298973.
Article Google Scholar
Violato C, Hecker K: How to use structural equation modeling in medical education research: A brief guide. Teach Learn Med. 2007, 19: 362-371.
Hershberger SL: The growth of structural equation modeling: 1994-2001. Struct Equ Model. 2003, 10: 35-46. 10.1207/S15328007SEM1001_2.
Nachtigall C, Kroehne U, Funke F, Steyer R: (Why) should we use SEM? Pros and cons of structural equation modeling. Method Psychol Res. 2003, 8: 1-22.
Google Scholar
Tu YK: Commentary: Is structural equation modeling a step forward for epidemiologists. Int J Epidemiol. 2009, 38: 1-3.
Rabe-Hesketh S, Skrondal A: Classical latent variable models for medical research. Stat Methods Med Res. 2008, 17: 5-32. 10.1177/0962280207081236.
Spearman C: General intelligence, objectively determined and measured. Am J Psychol. 1904, 15: 201-93. 10.2307/1412107.
Wright S: On the nature of size factors. Genetics. 1918, 3: 367-74.
CAS PubMed Central PubMed Google Scholar
Wright S: Correlation and causation. J Agric Res. 1921, 20: 557-85.
Saris WE, Stronkhorst LH: Introduction to Causal Modeling in Nonexperimental Research. 1984, Amsterdam: Sociometric Research Foundation
Bentler PM: EQS Structural Equation Program Manual. 1995, Encino, CA: Multivariate Software
Jöreskog KG, Sörbom D: LISREL 8: User's Reference Guide. 1993, Chicago: Scientific Software International
Wright S: The relative importance of heredity and environment in determining the piebald pattern of guinea-pigs. Proc of the Nat Acad of Sciences. 1920, 6: 320-332. 10.1073/pnas.6.6.320.
Article CAS Google Scholar
Byrne BM: Structural Equation Modeling with EQS and EQS/Windows. 1994, Newbury Park, CA: Sage
Kline RB: Structural Equation Modeling. 1998, New York: Guilford
Muthén B: Goodness of fit with categorical and other nonormal variables. Testing Structural Equation Models. Edited by: Bollen KA, Long JS. 1993, Newbury Park, CA: Sage, 205-234.
Hu L, Bentler PM: Fit indices in covariance structural modeling: Sensitivity to underparameterized model misspecification. Psychol Method. 1998, 3 (4): 424-453. 10.1037/1082-989X.3.4.424.
Hu L, Bentler PM, Kano Y: Can test statistics in covariance structure analysis be trusted?. Psychol Bull. 1992, 112: 351-362. 10.1037/0033-2909.112.2.351.
Article CAS PubMed Google Scholar
Muthén B, Kaplan D: A comparison of some methodologies for the factor analysis of non-normal Likert variables: A note on the size of the model. Brit J Math Stat Psychol. 1992, 45: 19-30.
Tanaka JS: Multifaceted conceptions of fit in structural equation models. Testing Structural Equation Models. Edited by: Bollen KA, Long JS. 1993, Newbury Park, CA: Sage, 10-39.
Dahly DL, Adair LS, Bollen KA: A structural equation model of the developmental origins of blood pressure. Int J Epidemiol. 2008, 38: 538-548. 10.1093/ije/dyn242.
Article PubMed Central PubMed Google Scholar
Hu Lt, Bentler PM: Cutoff criteria for fit indexes in covariance structure analysis: conventional criteria versus new alternatives. Struct Equ Model. 1999, 6: 1-55. 10.1080/10705519909540118.
Geweke JF, Singleton KJ: Interpreting the likelihood ratio statistic in factor models when sample size is small. J Am Stat Assoc. 1980, 75: 133-37. 10.2307/2287400.
Bentler PM, Yuan KH: Structural equation modeling with small samples: Test statistics. Multivar Behav Res. 1999, 34: 181-97. 10.1207/S15327906Mb340203.
Gollob HF, Reichardt CS: Taking account of time lags in causal models. Child Dev. 1987, 58: 80-92. 10.2307/1130293.
Huba GJ, Harlow LL: Robust structural equation models: implications for developmental psychology. Child Dev. 1987, 58: 147-66. 10.2307/1130297.
Biddle BJ, Marlin MM: Causality, confirmation, credulity, and structural equation modeling. Child Dev. 1987, 58: 4-17. 10.2307/1130287.
Bollen KA: Structural equations with latent variables. 1989, NY: Wiley
Chapter Google Scholar
Loberg EM, Jorgensen HA, Green MF, Rund BR, Lund A, Diseth A, Oie M, Hugdahl K: Positive symptoms and duration of illness predict functional laterality and attention modulation in schizophrenia. Acta Psychiatr Scand. 2006, 113: 322-31. 10.1111/j.1600-0447.2005.00627.x.
Beran T, Lupart J: The relationship between school achievement and peer harassment in Canadian adolescents: The importance of mediating factors. School Psychol Int. 2009, 30: 75-91. 10.1177/0143034308101851.
Eccles JS, Adler TF, Futterman R, Goff SB, Kaczala CM, Meece JL: Expectancies, values and academic behaviors. Perspectives on Achievement and Achievement Motives: Psychological and Sociological Approaches. Edited by: Spence J. 1983, San Francisco: Freeman, 75-146.
Eccles JS, Roeser R, Wigfield A, Freedman-Doan C: Academic and motivational pathways through middle childhood. Child Psychology: A Handbook of Contemporary Issues. Edited by: Balter L, Tamler-LeMonda CS. 1999, Philadelphia: Psychology Press, 287-317.
Statistics Canada: National Longitudinal Survey of Children and Youth: Cycle Three Survey Instruments. 1999, Human Resources Development Canada
Keller HH: The SCREEN 1 (Seniors in the Community: Risk Evaluation for Eating and Nutrition) index adequately represents nutritional risk. J Clin Epidemiol. 2006, 59: 836-41. 10.1016/j.jclinepi.2005.06.013.
Cudeck R, Harring JR, duToit SHC: Marginal maximum likelihood estimation of a latent variable model with interaction. J Educ Behav Stat. 2009, 34: 131-144. 10.3102/1076998607313593.
Rifkin RD: Effects of correlated and uncorrelated measurement error on linear regression and correlation in medical method comparison studies. Stat in Med. 1995, 14: 789-798. 10.1002/sim.4780140808.
Budtz-Jørgensen E: Estimation of the benchmark dose by structural equation models. Biostatistics. 2007, 8: 675-688. 10.1093/biostatistics/kxl037.
Jaffe A, Bentler PM: Structural equation modeling and drug use etiology: a historical perspective. Handbook of Drug Use Etiology: Theory, Methods, and Empirical Findings. Edited by: Scheier L. 2010, Washington DC: American Psychological Association, 547-562.
Download references
Author information
Authors and affiliations.
Medical Education and Research Unit Faculty of Medicine University of Calgary 3330 Hospital Dr. N.W. Calgary, AB, T2N 4N1, Canada
Tanya N Beran & Claudio Violato
You can also search for this author in PubMed Google Scholar
Corresponding author
Correspondence to Tanya N Beran .
Additional information
Competing interests.
The authors declare that they have no competing interests.
Authors' contributions
Both authors made substantial intellectual contributions to this paper. They have both read and approved the final manuscript.
Authors’ original submitted files for images
Below are the links to the authors’ original submitted files for images.
Authors’ original file for figure 1
Authors’ original file for figure 2, authors’ original file for figure 3, rights and permissions.
This article is published under license to BioMed Central Ltd. This is an Open Access article distributed under the terms of the Creative Commons Attribution License ( http://creativecommons.org/licenses/by/2.0 ), which permits unrestricted use, distribution, and reproduction in any medium, provided the original work is properly cited.
Reprints and permissions
About this article
Cite this article.
Beran, T.N., Violato, C. Structural equation modeling in medical research: a primer. BMC Res Notes 3 , 267 (2010). https://doi.org/10.1186/1756-0500-3-267
Download citation
Received : 06 September 2010
Accepted : 22 October 2010
Published : 22 October 2010
DOI : https://doi.org/10.1186/1756-0500-3-267
Share this article
Anyone you share the following link with will be able to read this content:
Sorry, a shareable link is not currently available for this article.
Provided by the Springer Nature SharedIt content-sharing initiative
- Schizophrenia
- Latent Variable
- Structural Equation Modeling
- Language Processing
- Positive Symptom
BMC Research Notes
ISSN: 1756-0500
- Submission enquiries: [email protected]
- General enquiries: [email protected]
- Open access
- Published: 22 November 2016
Applications of structural equation modeling (SEM) in ecological studies: an updated review
- Yi Fan ORCID: orcid.org/0000-0001-9412-1791 1 ,
- Jiquan Chen 1 ,
- Gabriela Shirkey 1 ,
- Ranjeet John 1 ,
- Susie R. Wu 1 ,
- Hogeun Park 1 &
- Changliang Shao 1
Ecological Processes volume 5 , Article number: 19 ( 2016 ) Cite this article
229k Accesses
541 Citations
5 Altmetric
Metrics details
This review was developed to introduce the essential components and variants of structural equation modeling (SEM), synthesize the common issues in SEM applications, and share our views on SEM’s future in ecological research.
We searched the Web of Science on SEM applications in ecological studies from 1999 through 2016 and summarized the potential of SEMs, with a special focus on unexplored uses in ecology. We also analyzed and discussed the common issues with SEM applications in previous publications and presented our view for its future applications.
We searched and found 146 relevant publications on SEM applications in ecological studies. We found that five SEM variants had not commenly been applied in ecology, including the latent growth curve model, Bayesian SEM, partial least square SEM, hierarchical SEM, and variable/model selection. We identified ten common issues in SEM applications including strength of causal assumption, specification of feedback loops, selection of models and variables, identification of models, methods of estimation, explanation of latent variables, selection of fit indices, report of results, estimation of sample size, and the fit of model.
Conclusions
In previous ecological studies, measurements of latent variables, explanations of model parameters, and reports of key statistics were commonly overlooked, while several advanced uses of SEM had been ignored overall. With the increasing availability of data, the use of SEM holds immense potential for ecologists in the future.
Introduction
Structural equation modeling (SEM) is a powerful, multivariate technique found increasingly in scientific investigations to test and evaluate multivariate causal relationships. SEMs differ from other modeling approaches as they test the direct and indirect effects on pre-assumed causal relationships. SEM is a nearly 100-year-old statistical method that has progressed over three generations. The first generation of SEMs developed the logic of causal modeling using path analysis (Wright 1918 , 1920 , 1921 ). SEM was then morphed by the social sciences to include factor analysis. By its second generation, SEM expanded its capacity. The third generation of SEM began in 2000 with Judea Pearl’s development of the “structural causal model,” followed by Lee’s ( 2007 ) integration of Bayesian modeling (also see Pearl 2003 ).
Ecologists have enlisted SEM over the past 16 years to test various hypotheses with multiple variables. SEM can analyze the complex networks of causal relationships in ecosystems (Shipley 2002 ; Grace 2006 ). Chang ( 1981 ) and Maddox and Antonovics ( 1983 ) were among the first ecologists who employed SEM in ecological research, clarifying the logical and methodological relationships between correlation and causation. Grace ( 2006 ) provided the first comprehensive book on SEM basics with key examples from a series of ecosystem studies. Now, in the most recent decade, a rapid increase of SEM in ecological sciences has been witnessed (Eisenhauer et al. 2015 ).
SEM is a combination of two statistical methods: confirmatory factor analysis and path analysis. Confirmatory factor analysis, which originated in psychometrics, has an objective to estimate the latent psychological traits, such as attitude and satisfaction (Galton 1888 ; Pearson and Lee 1903 ; Spearman 1904 ). Path analysis, on the other hand, had its beginning in biometrics and aimed to find the causal relationship among variables by creating a path diagram (Wright 1918 , 1920 , 1921 ). The path analysis in earlier econometrics was presented with simultaneous equations (Haavelmo 1943 ). In the early 1970s, SEM combined the two aforementioned methods (Joreskog 1969 , 1970 , 1978 ; Joreskog and Goldberger 1975 ) and became popular in many fields, such as social science, business, medical and health science, and natural science.
This review is an update on Grace et al. ( 2010 ) and Eisenhauer et al. ( 2015 ), who both provided a timely and comprehensive review of SEM applications in ecological studies. This review differs from the above two reviews, which focused on general ecological papers with SEM from 1999 through 2016. More so, Eisenhauer et al. ( 2015 ) only focused on SEM applications in soil ecology before 2012. In this review, we included SEM basic applications—as SEM remains unknown to many ecologists—and summarized the potential applications for SEM models that are often overlooked, including the issues and challenges in applying SEM. We developed our review around three critical questions: (1) is the use of SEM in ecological research statistically sound; (2) what are the common issues facing SEM applications; and (3) what is the future of SEM in ecological studies?
Path analysis
Path analysis was developed to quantify the relationships among multiple variables (Wright 1918 , 1920 , 1921 ). It was the early name for SEM before there were latent variables, and was very powerful in testing and developing the structural hypothesis with both indirect and direct causal effects. However, the two effects have recently been synonymized. Path analysis can explain the causal relationships among variables. A common function of path analysis is mediation, which assumes that a variable can influence an outcome directly and indirectly through another variable. For example, light intensity (PAR), air temperature (Ta), and aboveground temperature (Ts) can influence net ecosystem exchange (NEE) indirectly through respiration (Re); yet PAR and Ts can influence Re directly (Fig. 1 , Shao et al. 2016 ). Santibáñez-Andrade et al. ( 2015 ) applied mediation to evaluate the direct and indirect causes of degradation in the forests of the Magdalena river basin adjacent to Mexico City. The study sought to integrate abiotic controls and disturbance pressure with ecosystem conservation indicators to develop strategies in preserving biodiversity. In another study with SEM, a 23-year field experiment on a plant community in an Alaskan floodplain, found that alder inhibited spruce growth in the drier site directly, while at the wetter site it inhibited growth indirectly through effects mediated by competition with other vegetation and herbivory (Chapin et al. 2016 ).
The basic usage of structural equation modeling (SEM) in path analysis with mediation. The causal relationships include both indirect and direct effects, where Re is a mediator that intervenes with the causal relationships (modified from Shao et al. 2016 ). The acronyms in the models are photosynthetically active radiation ( PAR ), air temperature ( Ta ), soil temperature ( Ts ), net ecosystem exchange ( NEE ), and respiration ( Re )
Latent and observable variables
Measuring an abstract concept, such as “climate change,” “ecosystem structure and/or composition,” “resistance and resilience,” and “ecosystem service,” can pose a problem for ecological research. While direct measurements or units for these abstract concepts may not exist, statistical methods can derive these values from other related variables. SEM applies a confirmatory factor analysis to estimate latent constructs. The latent variable or construct is not in the dataset, as it is a derived common factor of other variables and could indicate a model’s cause or effect (Hoyle 1995 , 2011 ; Grace 2006 ; Kline 2010 ; Byrne 2013 ). For example, latent variables were applied to conclude the natural and social effects on grassland productivity in Mongolia and Inner Mongolia, China (Chen et al. 2015 ). When examining the potential contributions of land use, demographic and economic changes on urban expansion (i.e., green spaces) in the city of Shenzhen, China, Tian et al. ( 2013 ) treated land cover change (LCC), population, and economy as three latent variables, each characterized with two observable variables. Economy was found to play a more important role than population in driving LCC. Liu et al. ( 2016 ) measured the functional traits of trees as a latent variable based on tree height, crown diameter, wood diameter, and hydraulic conductivity. In addition to latent and observable variables, Grace and Bollen ( 2008 ) introduced composite variables for ecological applications of SEM. Composite variables are also unobservable variables, but which assume no error variance among the indicators and is not estimated by factor analysis. Instead of extracting the factors from a set of indicators, compost variable is an exact linear combination of the indicator variables based on given weights. For example, Chaudhary et al. ( 2009 ) conducted a study on the ecological relationship in semiarid scrublands and measured fungal abundance, which is composed of hyphal density and the concentration of Bradford-reactive soil proteins, as a composite variable. Jones et al. ( 2014 ) applied soil minerals as a composite variable to represent the concentrations of zinc, iron, and phosphorus in soil.
Confirmatory factor analysis
Confirmatory factor analysis (CFA) is the method for measuring latent variables (Hoyle 1995 ; 2011 ; Kline 2010 ; Byrne 2013 ). It extracts the latent construct from other variables and shares the most variance with related variables. For example, abiotic stress as a latent variable is measured by the observation of soil changes (i.e., soil salinity, organic matter, flooding height; Fig. 2 , Grace et al. 2010 ). Confirmatory factor analysis estimates latent variables based on the correlated variations of the dataset (e.g., association, causal relationship) and can reduce the data dimensions, standardize the scale of multiple indicators, and account for the correlations inherent in the dataset (Byrne 2013 ). Therefore, to postulate a latent variable, one should be concerned about the reason to use a latent variable. In the abiotic stress example given above, community stress and disturbance are latent variables that account for the correlation in the dataset. Shao et al. ( 2015 ) applied CFA to constrict the soil-nutrition features to one variable that accounted for soil organic carbon, litter total nitrogen, and carbon-to-nitrogen ratio. Also, Capmouteres and Anand ( 2016 ) defined the habitat function as an environmental indicator that explained both plant cover and native bird abundance for the forest ecosystems by using CFA.
Measurements of the latent variables. This SEM measures abstract concepts (i.e., latent variables) in the ovals based on the observed variables (modified from Grace et al. 2010 )
In addition to CFA, there is another type of factor analysis: exploratory factor analysis (EFA). The statistical estimation technique is the same for both. The CFA is applied when the indicators for each latent variable is specified according to the related theories or prior knowledge (Joreskog 1969 ; Brown 2006 ; Harrington 2009 ), whereas EFA is applied to find the underlying latent variables. In practice, EFA is often performed to select the useful underlying latent constructs for CFA when there is little prior knowledge about the latent construct (Browne and Cudeck 1993 ; Cudeck and Odell 1994 ; Tucker and MacCallum 1997 ).
SEM is composed of the measurement model and the structural model. A measurement model measures the latent variables or composite variables (Hoyle 1995 , 2011 ; Kline 2010 ), while the structural model tests all the hypothetical dependencies based on path analysis (Hoyle 1995 , 2011 ; Kline 2010 ).
Performing SEM
There are five logical steps in SEM: model specification, identification, parameter estimation, model evaluation, and model modification (Kline 2010 ; Hoyle 2011 ; Byrne 2013 ). Model specification defines the hypothesized relationships among the variables in an SEM based on one’s knowledge. Model identification is to check if the model is over-identified, just-identified, or under-identified. Model coefficients can be only estimated in the just-identified or over-identified model. Model evaluation assesses model performance or fit, with quantitative indices calculated for the overall goodness of fit. Modification adjusts the model to improve model fit, i.e., the post hoc model modification. Validation is the process to improve the reliability and stability of the model. Popular programs for SEM applications are often equipped with intuitive manuals, such as AMOS, Mplus, LISREI, Lavaan (R-package), piecewiseSEM (R-package), and Matlab (Rosseel 2012 ; Byrne 2013 ; Lefcheck 2015 ). The specific details for SEM applications are complicated, but users can seek help from tutorials provided by Grace ( 2006 ) and Byrne ( 2013 ).
Model evaluation indices
SEM evaluation is based on the fit indices for the test of a single path coefficient (i.e., p value and standard error) and the overall model fit (i.e., χ 2 , RMSEA ). From the literature, the usability of model fit indices appears flexible. Generally, the more fit indices applied to an SEM, the more likely that a miss-specified model will be rejected—suggesting an increase in the probability of good models being rejected. This also suggests that one should use a combination of at least two fit indices (Hu and Bentler 1999 ). There are recommended cutoff values for some indices, though none serve as the golden rule for all applications (Fan et al. 1999 ; Chen et al. 2008 ; Kline 2010 ; Hoyle 2011 ).
Chi-square test (χ 2 ) : χ 2 tests the hypothesis that there is a discrepancy between model-implied covariance matrix and the original covariance matrix. Therefore, the non-significant discrepancy is preferred. For optimal fitting of the chosen SEM, the χ 2 test would be ideal with p > 0.05 (Bentler and Bonett 1980 ; Mulaik et al. 1989 ; Hu and Bentler 1999 ). One should not be overly concerned regarding the χ 2 test because it is very sensitive to the sample size and not comparable among different SEMs (Bentler and Bonett 1980 ; Joreskog and Sorbom 1993 ; Hu and Bentler 1999 ; Curran et al. 2002 ).
Root mean square error of approximation (RMSEA) and standardized root mean square residual (SRMR) : RMSEA is a “badness of fit” index where 0 indicates the perfect fit and higher values indicate the lack of fit (Brown and Cudeck 1993 ; Hu and Bentler 1999 ; Chen et al. 2008 ). It is useful for detecting model misspecification and less sensitive to sample size than the χ 2 test. The acceptable RMSEA should be less than 0.06 (Browne and Cudeck 1993 ; Hu and Bentler 1999 ; Fan et al. 1999 ). SRMR is similar to RMSEA and should be less than 0.09 for a good model fit (Hu and Bentler 1999 ).
Comparative fit index (CFI): CFI represents the amount of variance that has been accounted for in a covariance matrix. It ranges from 0.0 to 1.0. A higher CFI value indicates a better model fit. In practice, the CFI should be close to 0.95 or higher (Hu and Bentler 1999 ). CFI is less affected by sample size than the χ 2 test (Fan et al. 1999 ; Tabachnick and Fidell 2001 ).
Goodness-of-fit index (GFI) : The range of GFI is 0–1.0, with the best fit at 1.0. Because GFI is affected by sample size, it is no longer recommended (MacCallum and Hong 1997 ; Sharma et al. 2005 ).
Normed fit index (NFI) : NFI is highly sensitive to the sample size (Bentler 1990 ). For this reason, NFI is no longer used to assess model fit (Bentler 1990 ; Hoyle 2011 ).
Tucker-Lewis index (TLI) : TLI is a non-normed fit index (NNFI) that partly overcomes the disadvantages of NFI and also proposes a fit index independent of sample size (Bentler and Bonett 1980 ; Bentler 1990 ). A TLI of >0.90 is considered acceptable (Hu and Bentler 1999 ).
Akaike information criterion (AIC) and Bayesian information criterion (BIC) : AIC and BIC are two relative measures from the perspectives of model selection rather than the null hypothesis test. AIC offers a relative estimation of the information lost when the given model is used to generate data (Akaike 1974 ; Kline 2010 ; Hoyle 2011 ). BIC is an estimation of how parsimonious a model is among several candidate models (Schwarz 1978 ; Kline 2010 ; Hoyle 2011 ). AIC and BIC are not useful in testing the null hypothesis but are useful for selecting the model with the least overfitting (Burnham and Anderson 2004 ; Johnson and Omland 2004 ).
Powerful yet unexplored SEMs
Experimental and observational databases in ecological studies are often complex, non-randomly distributed, are hierarchically organized and have spatial and temporal constraints (i.e., potential autocorrelations). While corresponding SEMs exist for each type of unique data, these powerful and flexible SEMs have not yet been widely explored in ecological research. Here we introduce some unexplored SEM uses for future endeavors.
Latent growth curve (LGC) model
LGC models can be used to interpret data with serial changes over time. The LGC model is built on the assumption that there is a structure growing along with the data series. The slope of growth is a latent variable, which represents the change in growth within a specified interval, and the loading factors are a series of growing subjects specified by the user (Kline 2010 ; Hoyle 2011 ; Duncun et al. 2013 ).
There are few ecological publications using LGC models. However, we found a civil engineering study on water quality applying the LGC model to examine the acidic deposition from acid rain in 21 stream sites across the Appalachian Mountain Region from 1980 to 2006 (Chen and Lin 2010 ). This study estimated the time-varying latent variable for each stream as the change of water properties over time by using the LGC model. Because longitudinal data (e.g., time series) is common in ecological research, LGC is especially effective in testing time-varying effects (Duncan et al. 2013 ; Kline 2010 ; Hoyle 2011 ).
In addition to LGC, SEM can be incorporated into a time series analysis (e.g., autoregressive integrated moving average model). For example, Almaraz ( 2005 ) applied a time series SEM to predict the population growth of the purple heron ( Ardea purpurea ). The moving average process was used as a matrix of time-based weights for analyzing the seasonal changes and autocorrelations.
From an ecological perspective, LGC is more plausible than the conventional time series analysis, because an LGC only needs longitudinal data with more than three periods rather than a time series analysis, which requires a larger time series/more observations (e.g., time series of economic or climatic changes). The LGC assumes a stable growth curve of the observation. Therefore, users can weigh the curve based on the time span rather than time series, which requires steady intervals in the series. For further guidance, refer to the book written by Bollen and Curran ( 2006 ).
Bayesian SEM (BSEM)
BSEM assumes theoretical support and that the prior beliefs are strong. One can use new data to update a prior model so that posterior parameters can be estimated (Raftery 1993 ; Lee 2007 ; Kaplan and Depaoli 2012 ). The advantage of BSEM is that it has no requirements on sample size. However, it needs prior knowledge on data distribution and parameters. Arhonditsis et al. ( 2006 ) applied BSEM to explore spatiotemporal phytoplankton dynamics, with a sample size of <60. The estimation of the model parameters’ posterior distribution is based on various Monte Carlo simulations to compute the overall mean and a 95% confidence interval. Due to the Bayesian framework, the model assessment of BSEM is more like a model comparison that is not based on χ 2 , RMSEA, CFI, etc. There are many comparison methods for the Bayesian approach. BIC is widely used, and many statisticians suggest posterior predictive checking to estimate the predictive ability of the model (Raftery 1993 ; Lee 2007 ; Kaplan and Depaoli 2012 ). The SEM analysis, which uses maximum likelihood (ML) and the likelihood ratio χ 2 test, often strictly rejects the substantive theory and unnecessarily utilizes model modification to improve the model fit by chance. Therefore, the Bayesian approach has received escalating attention in SEM applications due to its flexibility and better representation of the theory.
Partial least square SEM (PLS-SEM)
PLS-SEM is the preferred method when the study object does not have a well-developed theoretical base, particularly when there is little prior knowledge on causal relationship. The emphasis here is about the explorations rather than confirmations. PLS-SEM requires neither a large sample size nor a specific assumption on the distribution of the data, or even the missing data. Users with small sample sizes and less theoretical support for their research can apply PLS-SEM to test the causal relationship (Hair et al. 2013 ). The algorithm of PLS-SEM is different from the common SEM, which is based on maximum likelihood. When the sample size and data distribution of research can be hardly used by a common SEM, PLS-SEM has a more functional advantage.
By 2016, no publications on the application of PLS-SEM in ecological studies were found, according to our literature search. We recommend that users at the beginning stage or those who have fewer data apply PLS-SEM to generate the necessary evidence for causal relationship and variable selections. This will allow users to continue collecting long-term data while updating their hypotheses (Monecke and Leisch 2012 ).
Hierarchical SEM
The hierarchical model, also known as multilevel SEM, analyzes hierarchically clustered data. Hierarchical SEM can specify the direct and indirect causal effect between clusters (Curran 2003 ; Mehta and Neale 2005 ; Kline 2010 ). It is common for an experiment to fix some variables constantly, resulting in multiple groups or a nested dataset. The conventional SEM omits the fact that path coefficients and intercepts will potentially vary between hierarchical levels (Curran 2003 ; Mehta and Neale 2005 ; Shipley 2009 ; Kline 2010 ). This method focuses on data generated with a hierarchical structure. Therefore, the sample size needs to be large.
The application of hierarchical SEM is flexible. Take the work by Shipley ( 2009 ), for example, who analyzed the nested effects on plant growth between hierarchies, which included three clusters: site, year, and age. The causal relationship between the levels could be developed by Shipley’s d-sep test. With knowledge of a causal nested system, one can first specify the hierarchies before developing the SEM analysis within each nested structure (Curran 2003 ; Mehta and Neale 2005 ; Kline 2010 , Fig. 3 ). The model in Fig. 3 is a confirmatory factor analysis, with model parameters varying in each hierarchy.
Illustration of a hierarchical SEM. This measurement model has observed variables ( y1 , y2 , y3 ) with three hierarchies ( g1 , g2 , g3 ), which specify the causal effects (i.e., Cluster 1 → Cluster 2 → Cluster 3 ) among the three hierarchies (modified from Rabe-Hesketh et al. 2012 )
SEM models and variable selection
Selecting the appropriate variables and models is the initial step in an SEM application. The selection algorithm can be based on preferable variables and models according to certain statistical criteria (Burnham and Anderson 2002 ; Burnham et al. 2011 ). For example, the selection criterion could be based on fit indexes (e.g., AIC and BIC). Variable selection is also called the feature selection—a process of selecting the most relevant variables to prevent overfitting (Sauerbrei et al. 2007 ; Murtaugh 2009 ; Burnham and Anderson 2002 )—and is also a required procedure for both PLS-SEM and exploratory factor analysis. For example, multiple variables (e.g., water depth, elevation, and zooplankton) were selected to predict the richness of native fish (Murtaugh 2009 ). For other statistical analyses, AIC- or BIC-based models are widely recommended in ecology (Johnson and Omland 2004 ; Sauerbrei et al. 2007 ; Burnham et al. 2011 , Siciliano et al. 2014 ). For both indices, a smaller fit value is sought. Other fit indices can also be used as selection criteria. In a spatially explicit SEM exercise, Lamb ( 2014 ) suggested a preferable model from candidate models of different bin sizes based on χ 2 .
The remaining challenges
Sem applications from 1999 through 2016.
During our literature review, our keyword search included “structural equation modeling” and “ecology” through the Web of Science and Google Scholar. We found and reviewed 146 ecological publications that applied SEM from 1999 through 2016 (Additional file 1 ). The use of SEM in ecological research has rapidly increased in recent years (Eisenhauer et al. 2015 ). It is clear that a major advantage of SEM is that it can visualize data and hypotheses in a graphic model. Almost all of these studies took advantage of this. However, some SEM applications needed to be improved. Some studies did not report the necessary information such as the R 2 or p values of path coefficients (i.e., 22.6% reported R 2 , 65.8% reported p value), model modification/validations, nor an explanation of latent variables in SEMs (i.e., none explained the latent variable estimation, 28.1% did not have an estimation method). More so, 93.2% of the publications did not justify their model selection (Table 1 ).
Issues in SEM applications
Our review of the 146 publications revealed that many SEM applications needed to be improved. We summarized and separated these issues into ten categories (Tables 1 and 2 ).
Evidence of causal relationships
The test of causal relationships is central to SEM. The first step of SEM is to specify the causal relationships and correlations among the variables. Causal relationship and correlations without proper justification or theoretical foundations undermine the causal relationship in the hypotheses (Shipley 2002 ). The majority of the papers (94.2%) provided theoretical bases for their causal and correlation assumptions, while the remaining did not (Table 1 ).
Bollen and Pearl ( 2013 ) stated that strong causal relationships are made by (1) “imposing zero coefficients” and (2) “imposing zero covariance” to the model. They stated that
Strong causal assumptions assume that parameters take specific values. For instance, a claim that one variable has no causal effect on another variable is a strong assumption encoded by setting the coefficient to zero. Or, if one assumes that two disturbances are uncorrelated, then we have another strong assumption that the covariance equals zero.
A hypothesized model is composed of causal relationship and correlation assumptions, both of which should be stated clearly in any research based on design, prior experiences, scientific knowledge, logical arguments, temporal priorities, or other empirical evidence. It is notable that adding a non-zero covariance can improve some of the model fit indices. However, some studies took advantage of this by adding non-zero covariance without theoretical support, making the non-zero covariance less meaningful—even harmful—for a hypothesis testing.
- Feedback loops
Feedback is a basic ecosystem dynamic, which implies a cyclic phenomenon. The feedback loop is a useful function provided by SEM that could be either direct (i.e., V1 ⇄ V2) or indirect (i.e., V1 → V2 → V3 → V1, Fig. 4 ). As useful as this approach may be, there were only a couple studies that applied feedback loops. This is likely because the definition of a feedback loop can easily confuse a new user. Kline ( 2006 ) listed two assumptions for feedback loops:
Illustration of feedback loops in ecosystem analysis. Feedback loops in SEM analysis is flexible and can be direct or indirect
One is that of equilibrium, which means that any changes in the system underlying a feedback relation have already manifested their effects and that the system is in a steady state (Heise 1975 ). The other assumption is that the underlying causal structure does not change over time.
Some data are generated naturally from the ecosystem without artificial manipulation. The specification of the cause and outcome of ecological dynamics is confusing because the underlying mechanisms of data generation are complex and simultaneous. The applications of feedback loops, which specify the causal relationship in a loop, can explain the ecological dynamics in a cyclical perspective. When a research design is based on a loop perspective, the SEM analysis can evaluate if the cycle is virtuous, vicious, or neutral.
Model and variable selection
As argued by Box ( 1976 ), it is difficult to find a completely correct model, but a simple model could represent a complicated phenomenon. Therefore, one needs to select cautiously the model and variables based on the research goal, the statistical foundation, and the theoretical support. In our review, only a few papers applied a model (6.8%) or a variable (8.9%) selection (Table 2 ). The model and variable selection is key to multivariable analysis. One should demonstrate the principle of model postulation in addition to research design. Indeed, there were very few papers discussing the technique and principle of their models. A well-applied principle of parsimony for model users emphasizes the simplicity of a model. According to this principle, the users should justify if a model could present a phenomenon by a few variables. Cover and Thomas ( 2012 ) had proposed other modeling principles .
- Model identification
Model identification was often overlooked, with only 67.8% reporting the model identification, and happened when latent variables were estimated. Kline ( 2010 ) proposed three essential requirements when identifying the appropriate SEM: (1) “the model degrees of freedom must be at least zero to assure the degrees of freedom ( df ) is greater than zero”; (2) “every latent variable (including the residual terms) must be assigned a scale, meaning that either the residual terms’ (disturbance) path coefficient and one of the latent variable’s factor loading should be fixed to 1 or that the variance of a latent variable should be fixed to 1”; and (3) “every latent variable should have at least two indicators.”
Most publications provided the df values in their SEMs and we estimated the df of those that did not report. All publications had a df greater than zero. All the models with CFA met the requirement that each latent variable should have at least two indicators. However, many studies skipped over scaling the latent variables before estimation, resulting in non-robust results. The unscaled latent variable can hardly provide useful information to the causal test. Otherwise, it is likely that the user had just fit the model by chance.
Estimation methods
Many estimation methods in SEM exist, such as maximum likelihood (ML), generalized least squares, weighted least squares, and partial least squares. Maximum likelihood estimation is the default estimation method in many SEM software (Kline 2010 ; Hoyle 2011 ). All of the publications stated the estimation methods were based on ML, which assumes that (1) no skewness or kurtosis in the joint distribution of the variables exists (e.g., multivariate normality); (2) the variables are continuous; and (3) there are very few missing data (i.e., <5%, Kline 2010 ; Hoyle 2011 ). However, very few publications provided this key information about their data. Instead, they simply ignored the data quality or chose not to discuss the raw data. Some papers briefly discussed the multivariate normality of their data, but none discussed the data screening and transformation (i.e., skewness or kurtosis, continuous or discrete, and missing data). We assume that most of their ecological data was continuous, yet one needs to assure the continuity of the data to support their choice of estimation methods. The partial least square method requires neither continuous data nor multi-normality.
Explanations of the measured latent variables
We did not find a publication with sufficient explanation for its CFA in regard to the prior knowledge or preferred function (i.e., unmeasured directly, quantifiable, and necessary to the model) for measuring the latent variable. Factor analysis is a useful tool for dimension reduction. The factor analysis applied in SEM measurement models (CFA or EFA) are used to measure the latent variable, which requires a theoretical basis. The prior knowledge of a measurement model includes two parts: (1) the prior knowledge of indicators for a latent variable and (2) the prior knowledge of the relationships between the latent variable and its indicators (Bentler and Chou 1987 ). For example, the soil fertility of a forest as a latent variable was estimated based on two types of prior knowledge, including (1) the observation of tree density, water resources, and presence of microorganisms and (2) the positive correlations among the three observed variables.
If the estimation of a latent variable is performed without prior knowledge, CFA will become a method only for data dimension reduction. In addition, we did not find any CFAs in the ecological publications explaining the magnitude of the latent variable. Therefore, these latent variables lack a meaningful explanation in regard to the hypothesis of an SEM (Bollen 2002 ; Duncan et al 2013 ). Another issue concerning latent variables in ecological research is that some “observable variables” (e.g., salinity, pH, temperature, and density) are measured as a latent variable. The reasons are very flexible for measuring a latent variable, but they require the user to explain the application of CFA carefully.
SEM requires measurement models to be based on prior knowledge so that latent variables can be interpreted correctly (Bentler and Chou 1987 ). SEM is not a method to only reduce data dimensions. Instead, one should explain the magnitude and importance of indicators and latent variables. Therefore, users should base their explanations on theory when discussing the associated changes between latent variables and indicators. The explanation should include the analysis of the magnitude of the latent variable, indicators, and factor loadings.
Report of model fit indices
Reporting of fit indices in any SEM is strongly recommended and needed. Approximately 93.8% of the publications provided model fit indices. However, none justified their usage of the chosen fit indices. Those that did not report model fit indices also did not provide the reason for doing so. From these publications, χ 2 , CFI, RMSEA, TLI, GFI, NFI, SRMR, AIC, and BIC were frequently used. The χ 2 was included in almost every paper because it is the robust measure for model fitness. Some publications without significant χ 2 tests reported their SEM results regardless. In addition, GFI and NFI were also used even though they are not recommended as measures for model fit.
Fit indices are important indicators of model performances. Due to their different properties, they are sensitive to many factors, such as data distribution, missing data, model size, and sample size (Hu and Bentler 1999 ; Fan and Sivo 2005 ; Barrett 2007 ). Most fit indices (i.e., χ 2 , CFI, RMSEA, TLI, GFI, NFI, SRMR) are greatly influenced by multivariate normality (i.e., a property of ML method that is applied in SEM). Meanwhile, CFI, RMSEA, and SRMR are useful in detecting model misspecification, and relative fit indices (e.g., AIC and BIC) are mainly used for model selection (Curran et al. 1996 ; Fan and Sivo 2005 ; Ryu 2011 ). Selection of model fit indices in an SEM exercise is key to explaining the model (e.g., type, structure, and hypothesis). Users should at least discuss the usage of fit indices to ensure that they are consistent with their study objectives.
Report of the results
An SEM report should include all the estimation and modeling process reports. However, most publications did not include a full description of the results for their hypothesis tests. Some publications provided their SEMs based on a covariance matrix (Table 1 ), while even fewer studies reported the exact input covariance or correlation matrix. No study reported the multivariate normality, absence, or outliers of their data. The majority of the papers (82.2%) reported the path coefficients, but very few reported both unstandardized and standardized path coefficients. A small percentage (8.9%) of the publications reported the standard error for the path coefficient. The basic statistics (i.e., p value, R 2 , standard errors) are of equal importance as the overall fit indices because they explain the validity and reliability of each path, providing evidence for when the overall fit is poor (Kline 2010 ; Hoyle 2011 ).
Hoyle and Isherwood ( 2013 ) suggested that a publication with an SEM analysis should follow the Journal Article Reporting Standards of the American Psychological Association . The reporting guidelines are comprised of five components (McDonald and Ho 2002 ; Jackson et al. 2009 ; Kline 2010 ; Hoyle and Isherwood 2013 ):
Model specification: Model specification process should be reported, including prior knowledge of the theoretically plausible models, prior knowledge of the positive or negative direct effects among variables, data sampling method, sample size, and model type.
Data preparation: Data processing should be reported, including the assessment of multivariate normality, analysis of missing data, method to address missing data, and data transformations.
Estimation of SEM: The estimation procedure should be reported, including the input matrix, estimation method, software brand and version, and method for fixing the scale of latent variables.
Model evaluation and modification: The model evaluation should be reported, including fit indices with cutoff values and model modification.
Reports of findings: All of the findings from an SEM analysis should be reported, including latent variables, factor loadings, standard errors, p values, R 2 , standardized and unstandardized structure coefficients, and graphic representations of the model.
- Sample size
The estimation of sample size is another issue for the SEM application. So far the estimation of sample size is flexible, and users could refer to several authors’ recommendations (Fan et al. 1999 ; Muthen and Muthen 2002 ; Iacobucci 2010 ). While some (61.0%) studies reported the sample size clearly, none of them provided a justification for the sample size with sound theory (Table 2 ). Technically, sample size for an SEM varies depending on many factors, including fit index, model size, distribution of the variables, amount of missing data, reliability of the variables, and strength of path parameters (Fan et al. 1999 ; Muthen and Muthen 2002 ; Fritz and MacKinnon 2007 ; Iacobucci 2010 ). Some researchers recommend a minimum sample size of 100–200 or five cases per free parameter in the model (Tabachnick and Fidell 2001 ; Kline 2010 ). One should be cautious when applying these general rules, however. Increasingly, use of model-based methods for estimation of sample size is highly recommended, with sound methods based on fit indices or power analysis of the model. Muthen and Muthen ( 2002 ) developed a method based on the Monte Carlo simulation to utilize SEM’s statistical power analysis and calculate sample size (Cohen 2013 ). Kim ( 2005 ) developed equations to compute the sample size based on model fit indices for a given statistical power.
Model validation
We did not find that SEM was validated in the reviewed ecological studies, even though it is a necessary process for quantitative analysis. This is probably because most SEM software is developed without model validation features. The purpose of model validation is to provide more evidence for the hypothetical model. The basic method of model validation is to test a model by two or more random datasets from the same sample. Therefore, the validation requires a large sample size. The principle of the model validation is to assure that the parameters are similar when a model is based on different datasets from the same population. This technique is a required step in many learning models. However, it is still unpopular in SEM applications.
SEM is a powerful multivariate analysis tool that has great potential in ecological research, as data accessibility continues to increase. However, it remains a challenge even though it was introduced to the ecological community decades ago. Regardless of its rapidly increased application in ecological research, well-established models remain rare. In fact, well-established models can serve as a prior model, as this has been extensively used in psychometrics, behavioral science, business, and marketing research. There is an overlooked yet valuable opportunity for ecologists to establish an SEM representing the complex network of any ecosystem.
The future of SEM in ecological studies
Many ecological studies are characterized by large amounts of public data, which need multivariate data analysis. SEM users are provided with this opportunity to look for suitable public data and uncover patterns in research. However, big data will also inevitably bring new issues, such as the uncertainty of data sources. Therefore, improved data preparation protocols for SEM research are urgently needed. Fortunately, the exponential growth of usage in data-driven models, such as machine learning, provides SEM users a promising opportunity to develop creative methods to combine hypothesis-based and data-driven models together.
The growing availability of big data is transforming studies from hypothesis-driven and experiment-based research to more inductive, data-driven, and model-based research. Causal inference derived from data itself with learning algorithms and little prior knowledge has been widely accepted as accurate (Hinton et al. 2006 ; LeCun et al. 2015 ). The original causal foundation of SEM was based on a hypothesis test (Pearl 2003 , 2009 , 2012; Bareinboim and Pearl 2015 ). However, with the advancement of data mining tools, the data-driven and hypothesis-driven models may be mixed in the future. Here, we emphasize the importance of utilizing hypothesis-based models that are from a deductive-scientific stance, with prior knowledge or related theory. Meanwhile, we also agree that new technologies such as machine learning under big data exploration will stimulate new perspectives on ecological systems. On the other hand, the increased data availability and new modeling approaches—as well as their possible marriage with SEM—may skew our attention towards phenomena that deliver easily accessible data, while consequently obscuring other important phenomena (Brommelstroet et al. 2014 ).
Akaike H (1974) A new look at the statistical model identification. IEEE Trans Automat Contr 19(6):716–723
Article Google Scholar
Almaraz P (2005) Ecological time-series analysis through structural modelling with latent constructs: concepts, methods and applications. C R Biol 328(4):301–316
Arhonditsis GB, Stow CA, Steinberg LJ, Kenney MA, Lathrop RC, McBride SJ, Reckhow KH (2006) Exploring ecological patterns with structural equation modeling and Bayesian analysis. Ecol Model 192(3):385–409
Bareinboim E, Pearl J (2015) Causal inference from big data: theoretical foundations and the data-fusion problem. Available via DIALOG. http://oai.dtic.mil/oai/oai?verb=getRecord&metadataPrefix=html&identifier=ADA623167 . Accessed 11 Nov 2016
Barrett P (2007) Structural equation modelling: adjudging model fit. Pers Individ Dif 42(5):815–824
Bentler PM (1990) Comparative fit indexes in structural models. Psychol Bull 107(2):238–246
Article CAS Google Scholar
Bentler PM, Bonett DG (1980) Significance tests and goodness of fit in the analysis of covariance structures. Psychol Bull 88(3):588–606
Bentler PM, Chou CP (1987) Practical issues in structural modeling. Socio Meth Res 16(1):78–117
Bollen KA (2002) Latent variables in psychology and the social sciences. Annu Rev Psychol 53(1):605–634
Bollen KA, Curran PJ (2006) Latent curve models: a structural equation perspective. John Wiley & Sons, New Jersey
Bollen KA, Pearl J (2013) Eight myths about causality and structural equation models. In: Morgan SL (ed) Handbook of causal analysis for social research. Springer, Netherlands
Google Scholar
Box GE (1976) Science and statistics. J Amer Statist Assoc 71(356):791–799
Brommelstroet M, Pelzer P, Geertman S (2014) Forty years after Lee’s Requiem: are we beyond the seven sins. Environ Plann B 41(3):381–387
Brown TA (2006) Confirmatory factor analysis for applied research. Guilford, New York
Browne MW, Cudeck R (1993) Alternative ways of assessing model fit. In: Bollen KA, Long JS (eds) Testing Structural Equation Models. Sage, Newbury Park
Burnham KP, Anderson DR (2002) Model selection and multimodel inference: a practical information-theoretic approach. Springer, New York
Burnham KP, Anderson DR (2004) Multimodel inference understanding AIC and BIC in model selection. Socio Meth Res 33(2):261–304
Burnham KP, Anderson DR, Huyvaert KP (2011) AIC model selection and multimodel inference in behavioral ecology: some background, observations, and comparisons. Behav Ecol Sociobiol 65(1):23–35
Byrne BM (2013) Structural equation modeling with AMOS: basic concepts, applications, and programming. Routledge, New York
Capmourteres V, Anand M (2016) Assessing ecological integrity: a multi-scale structural and functional approach using structural equation modeling. Ecol Indic http://dx.doi.org/ 10.1016/j.ecolind.2016.07.006
Chang WY (1981) Path analysis and factors affecting primary productivity. J Freshwater Ecol 1(1):113–120
Chapin FS, Conway AJ, Johnstone JF, Hollingsworth TN, Hollingsworth J (2016) Absence of net long-term successional facilitation by alder in a boreal Alaska floodplain. Ecology, doi: http://dx.doi.org/ 10.1002/ecy.1529
Chaudhary VB, Bowker MA, O'Dell TE, Grace JB, Redman AE, Rillig MC, Johnson NC (2009) Untangling the biological contributions to soil stability in semiarid shrublands. Ecol Appl 19(1):110–122
Chen Y, Lin L (2010) Structural equation-based latent growth curve modeling of watershed attribute-regulated stream sensitivity to reduced acidic deposition. Ecol Model 221(17):2086–2094
Chen F, Curran PJ, Bollen KA, Kirby J, Paxton P (2008) An empirical evaluation of the use of fixed cutoff points in RMSEA test statistic in structural equation models. Socio Meth Res 36(4):462–494
Chen J, John R, Shao C, Fan Y, Zhang Y, Amarjargal A, Brown DG, Qi J, Han J, Lafortezza R, Dong G (2015) Policy shifts influence the functional changes of the CNH systems on the Mongolian plateau. Environ Res Lett 10(8):085003
Cohen J (2013) Statistical power analysis for the behavioral sciences. Academic Press, New York
Cover TM, Thomas JA (2012) Elements of information theory. John Wiley & Sons, Hoboken, New Jersey
Cudeck R, Odell LL (1994) Applications of standard error estimates in unrestricted factor analysis: significance tests for factor loadings and correlations. Psychol Bull 115(3):475–487
Curran PJ (2003) Have multilevel models been structural equation models all along? Multivar Behav Res 38(4):529–569
Curran PJ, West SG, Finch JF (1996) The robustness of test statistics to nonnormality and specification error in confirmatory factor analysis. Psychol Methods 1(1):16–29
Curran PJ, Bollen KA, Paxton P, Kirby J, Chen F (2002) The noncentral chi-square distribution in misspecified structural equation models: finite sample results from a Monte Carlo simulation. Multivar Behav Res 37(1):1–36
Duncan TE, Duncan SC, Strycker LA (2013) An introduction to latent variable growth curve modeling: concepts, issues, and applications, 2nd edn. Psychology Press, New York
Eisenhauer N, Bowker M, Grace J, Powell J (2015) From patterns to causal understanding: structural equation modeling (SEM) in soil ecology. Pedobiologia 58(2):65–72
Fan X, Sivo SA (2005) Sensitivity of fit indexes to misspecified structural or measurement model components: rationale of two-index strategy revisited. Struct Equ Modeling 12(3):343–367
Fan X, Thompson B, Wang L (1999) Effects of sample size, estimation methods, and model specification on structural equation modeling fit indexes. Struct Equ Modeling 6(1):56–83
Fritz MS, MacKinnon DP (2007) Required sample size to detect the mediated effect. Psychol Sci 18(3):233–239
Galton F (1888) Personal identification and description. Nature 38:173–177
Grace JB (2006) Structural equation modeling and natural systems. Cambridge University Press, New York
Book Google Scholar
Grace JB, Bollen KA (2008) Representing general theoretical concepts in structural equation models: the role of composite variables. Environ Ecol Stat 15(2):191–213
Grace JB, Anderson TM, Olff H, Scheiner SM (2010) On the specification of structural equation models for ecological systems. Ecol Monogr 80(1):67–87
Haavelmo T (1943) The statistical implications of a system of simultaneous equations. Econometrica 11(1):1–12
Hair JF, Hult GT, Ringle C, Sarstedt M (2013) A primer on partial least squares structural equation modeling (PLS-SEM). Sage, Thousand Oak
Harrington D (2009) Confirmatory factor analysis. Oxford University Press, New York
Heise DR (1975) Causal analysis. John Wiley & Sons, Oxford
Hinton GE, Osindero S, Teh YW (2006) A fast learning algorithm for deep belief nets. Neural Comput 18(7):1527–1554
Hoyle RH (1995) Structural equation modeling: concepts, issues, and applications. Sage, Thousand Oak
Hoyle RH (2011) Structural equation modeling for social and personality psychology. Sage, London
Hoyle RH, Isherwood JC (2013) Reporting results from structural equation modeling analyses in Archives of Scientific Psychology. Arch Sci Psychol 1:14–22
Hu LT, Bentler PM (1999) Cutoff criteria for fit indexes in covariance structure analysis: conventional criteria versus new alternatives. Struct Equ Modeling 6(1):1–55
Iacobucci D (2010) Structural equations modeling: fit indices, sample size, and advanced topics. J Consum Psychol doi: http://dx.doi.org/ 10.1016/j.jcps.2009.09.003
Jackson DL, Gillaspy JA, Purc-Stephenson R (2009) Reporting practices in confirmatory factor analysis: an overview and some recommendations. Psychol Methods 14(1):6–23
Johnson JB, Omland KS (2004) Model selection in ecology and evolution. Trends Ecol Evol 19(2):101–108
Jones CM, Spor A, Brennan FP, Breuil MC, Bru D, Lemanceau P, Griffiths B, Hallin S, Philippot L (2014) Recently identified microbial guild mediates soil N 2 O sink capacity. Nat Clim Change 4(9):801–805
Joreskog KG (1969) A general approach to confirmatory maximum likelihood factor analysis. Psychometrika 34(2):183–202
Joreskog KG (1970) A general method for estimating a linear structural equation system. ETS Research Bulletin Series 1970(2):1–41
Joreskog KG (1978) Structural analysis of covariance and correlation matrices. Psychometrika 43(4):443–473
Joreskog KG, Goldberger AS (1975) Estimation of a model with multiple indicators and multiple causes of a single latent variable. JASA 70(351a):631–639
Joreskog K, Sorbom D (1993) LISREL 8: structural equation modeling with the SIMPLIS command language. Scientific Software International, Chicago
Kaplan D, Depaoli S (2012) Bayesian structural equation modeling. In: Hoyle RH (ed) Handbook of structural equation modeling. Guilford, New York
Kim KH (2005) The relation among fit indexes, power, and sample size in structural equation modeling. Struct Equ Modeling 12(3):368–390
Kline RB (2006) Reverse arrow dynamics. Formative measurement and feedback loops. In: Hancock GR, Mueller RO (eds) Structural equation modeling: A second course. Information Age Publishing, Greenwich
Kline RB (2010) Principles and practice of structural equation modeling. Guilford Press, New York
Lamb EG, Mengersen KL, Stewart KJ, Attanayake U, Siciliano SD (2014) Spatially explicit structural equation modeling. Ecology 95(9):2434–2442
LeCun Y, Bengio Y, Hinton G (2015) Deep learning. Nature 521(7553):436–444
Lee SY (2007) Structural equation modeling: a Bayesian approach. John Wiley & Sons, Hong Kong
Lefcheck J (2015) piecewiseSEM: Piecewise structural equation modelling in r for ecology, evolution, and systematics. Methods Ecol Evol, doi: http://dx.doi.org/ 10.1111/2041-210X.12512
Liu X, Swenson NG, Lin D, Mi X, Umaña MN, Schmid B, Ma K (2016) Linking individual-level functional traits to tree growth in a subtropical forest. Ecology, doi: http://dx.doi.org/ 10.1002/ecy.1445
MacCallum RC, Hong S (1997) Power analysis in covariance structure modeling using GFI and AGFI. Multivar Behav Res 32(2):193–210
Maddox GD, Antonovics J (1983) Experimental ecological genetics in Plantago : a structural equation approach to fitness components in P. aristata and P. patagonica . Ecology 64(5):1092–1099
McDonald RP, Ho MH (2002) Principles and practice in reporting structural equation analyses. Psychol Methods 7(1):64–82
Mehta PD, Neale MC (2005) People are variables too: multilevel structural equations modeling. Psychol Methods 10(3):259–284
Monecke A, Leisch F (2012) semPLS: structural equation modeling using partial least squares. J Stat Softw 48(3):1–32
Mulaik SA, James LR, Van Alstine J, Bennett N, Lind S, Stilwell CD (1989) Evaluation of goodness-of-fit indices for structural equation models. Psychol Bull 105(3):430–445
Murtaugh PA (2009) Performance of several variable-selection methods applied to real ecological data. Ecol Lett 12(10):1061–1068
Muthen LK, Muthen BO (2002) How to use a Monte Carlo study to decide on sample size and determine power. Struct Equ Modeling 9(4):599–620
Pearl J (2003) Causality: models, reasoning, and inference. Econometr Theor, doi: : http://dx.doi.org/ 10.1017/S0266466603004109
Pearl J (2009) Causality. Cambridge University Press, New York
Pearl J (2012) The causal foundations of structural equation modeling. In: Hoyle RH (ed) Handbook of Structural Equation Modeling. Guilford, New York, pp 68–91
Pearson K, Lee A (1903) On the laws of inheritance in man. I. Inheritance of physical characters. Biometrika 2(4):357–462
Rabe-Hesketh S, Skrondal A, Zheng X (2012) Multilevel structural equation modeling. In: Hoyle RH (ed) Handbook of Structural Equation Modeling. Guilford, New York, pp 512–531
Raftery AE (1993) Bayesian model selection in structural equation models. In: Bollen KA, Long JS (eds) Testing structural equation models. Sage, Newbury Park
Rosseel Y (2012) lavaan: An R package for structural equation modeling. J Stat Softw 48(2):1–36
Ryu E (2011) Effects of skewness and kurtosis on normal-theory based maximum likelihood test statistic in multilevel structural equation modeling. Behav Res Methods 43(4):1066–1074
Santibáñez-Andrade G, Castillo-Argüero S, Vega-Peña E, Lindig-Cisneros R, Zavala-Hurtado J (2015) Structural equation modeling as a tool to develop conservation strategies using environmental indicators: the case of the forests of the Magdalena river basin in Mexico City. Ecol Indic 54:124–136
Sauerbrei W, Royston P, Binder H (2007) Selection of important variables and determination of functional form for continuous predictors in multivariable model building. Stat Med 26(30):5512–5528
Schwarz G (1978) Estimating the dimension of a model. Ann Stat 6(2):461–464
Shao Y, Bao W, Chen D, Eisenhauer N, Zhang W, Pang X, Xu G, Fu S (2015) Using structural equation modeling to test established theory and develop novel hypotheses for the structuring forces in soil food webs. Pedobiologia 58(4):137–145
Shao J, Zhou X, Luo Y, Li B, Aurela M, Billesbach D, Blanken PD, Bracho R, Chen J, Fischer M, Fu Y, Gu L, Han S, He Y, Kolb T, Li Y, Nagy Z, Niu S, Oechel W, Pinter K, Shi P, Suyker A, Torn M, Varlagin A, Wang H, Yan J, Yu G, Zhang J (2016) Direct and indirect effects of climatic variations on the interannual variability in net ecosystem exchange across terrestrial ecosystems. Tellus B, doi: http://dx.doi.org/ 10.3402/tellusb.v68.30575
Sharma S, Mukherjee S, Kumar A, Dillon WR (2005) A simulation study to investigate the use of cutoff values for assessing model fit in covariance structure models. J Bus Res 58(7):935–943
Shipley B (2002) Cause and correlation in biology: a user's guide to path analysis, structural equations and causal inference. Cambridge University Press, Cambridge
Shipley B (2009) Confirmatory path analysis in a generalized multilevel context. Ecology 90(2):363–368
Siciliano S, Anne P, Tristrom W, Eric L, Andrew B, Mark B, van Josie D, Ji M, Belinda F, Paul G, Chu H, Ian S (2014) Soil fertility is associated with fungal and bacterial richness, whereas pH is associated with community composition in polar soil microbial communities. Soil Biol Biochem 78:10–20
Spearman C (1904) “General Intelligence,” objectively determined and measured. Am J Psychol 15(2):201–292
Tabachnick BG, Fidell LS (2001) Using multivariate statistics. Pearson, New York
Tian T, Chen J, Yu SX (2013) Coupled dynamics of urban landscape pattern and socioeconomic drivers in Shenzhen, China. Landscape Ecol 29(4):715–727
Tucker LR, MacCallum RC (1997) Exploratory factor analysis. Ohio State University, Columbus
Wright S (1918) On the nature of size factors. Genetics 3(4):367–374
CAS Google Scholar
Wright S (1920) The relative importance of heredity and environment in determining the piebald pattern of guinea-pigs. Proc Natl Acad Sci USA 6(6):320–332
Wright S (1921) Correlation and causation. J Agric Res 20(7):557–585
Download references
Acknowledgements
This study is supported by the Sustainable Energy Pathways (CHE) Program (#1230246) and the Dynamics of Coupled Natural and Human Systems (CNH) Program (#1313761) of the US National Science Foundation (NSF). We thank Dr. Zutao Ouyang for the statistical help.
Authors’ contributions
YF designed and carried out the conceptual review of SEM literature. JC constructed the overall structure of the manuscript and revised the content. GS guided and revised the scientific writings. RJ carried out the review of matrices in the relevant literature and revised the manuscript. SW wrote the introduction of PLS-SEM. HP carried out the review of model fit indices in the literature. CS carried out the review of model selection. All authors read and approved the final manuscript.
Authors’ information
Yi Fan is a graduate student of geography with research interests in data mining.
Jiquan Chen is a professor of geography with research interests in ecosystem processes and their interactive feedbacks to biophysical and human changes.
Gabriela Shirkey is a laboratory technician with interests in conservation strategies and community engagement.
Ranjeet John is a research associate with interests in remote sensing and geospatial technology.
Susie R. Wu is a research associate in geography with research interests in sustainable product design.
Hogeun Park is a doctoral student in urban planning with research interests in developing nations and the urbanization process.
Changliang Shao is a research associate with interests in ecosystem carbon, water, and energy fluxes.
All the authors are associated with Center for Global Change and Earth Observations (CGCEO) of Michigan State University.
Competing interests
The authors declare that they have no competing interests.
Author information
Authors and affiliations.
Center for Global Change and Earth Observations (CGCEO)/Department of Geography, Environment, and Spatial Sciences, Michigan State University, East Lansing, MI, 48824, USA
Yi Fan, Jiquan Chen, Gabriela Shirkey, Ranjeet John, Susie R. Wu, Hogeun Park & Changliang Shao
You can also search for this author in PubMed Google Scholar
Corresponding author
Correspondence to Yi Fan .
Additional file
Additional file 1:.
List of reviewed publications. (DOCX 39 kb)
Rights and permissions
Open Access This article is distributed under the terms of the Creative Commons Attribution 4.0 International License ( http://creativecommons.org/licenses/by/4.0/ ), which permits unrestricted use, distribution, and reproduction in any medium, provided you give appropriate credit to the original author(s) and the source, provide a link to the Creative Commons license, and indicate if changes were made.
Reprints and permissions
About this article
Cite this article.
Fan, Y., Chen, J., Shirkey, G. et al. Applications of structural equation modeling (SEM) in ecological studies: an updated review. Ecol Process 5 , 19 (2016). https://doi.org/10.1186/s13717-016-0063-3
Download citation
Received : 30 August 2016
Accepted : 04 November 2016
Published : 22 November 2016
DOI : https://doi.org/10.1186/s13717-016-0063-3
Share this article
Anyone you share the following link with will be able to read this content:
Sorry, a shareable link is not currently available for this article.
Provided by the Springer Nature SharedIt content-sharing initiative
- Model selection
- Latent growth curve

An official website of the United States government
The .gov means it’s official. Federal government websites often end in .gov or .mil. Before sharing sensitive information, make sure you’re on a federal government site.
The site is secure. The https:// ensures that you are connecting to the official website and that any information you provide is encrypted and transmitted securely.
- Publications
- Account settings
Preview improvements coming to the PMC website in October 2024. Learn More or Try it out now .
- Advanced Search
- Journal List

Using structural equation modeling to investigate change and response shift in patient-reported outcomes: practical considerations and recommendations
M. g. e. verdam.
1 Department of Methodology and Statistics, Institute of Psychology, Leiden University, P.O. Box 9555, 2300 RB Leiden, The Netherlands
2 Department of Medical Psychology, Amsterdam University Medical Centre, Amsterdam Public Health Research Institute, Amsterdam, The Netherlands
3 Research Institute Child Development and Education, University of Amsterdam, Amsterdam, The Netherlands
M. A. G. Sprangers
Associated data.
Patient-reported outcomes (PROs) are of increasing importance for health-care evaluations. However, the interpretation of change in PROs may be obfuscated due to changes in the meaning of the self-evaluation, i.e., response shift. Structural equation modeling (SEM) is the most widely used statistical approach for the investigation of response shift. Yet, non-technical descriptions of SEM for response shift investigation are lacking. Moreover, application of SEM is not straightforward and requires sequential decision-making practices that have not received much attention in the literature.
To stimulate appropriate applications and interpretations of SEM for the investigation of response shift, the current paper aims to (1) provide an accessible description of the SEM operationalizations of change that are relevant for response shift investigation; (2) discuss practical considerations in applying SEM; and (3) provide guidelines and recommendations for researchers who want to use SEM for the investigation and interpretation of change and response shift in PROs.
Appropriate applications and interpretations of SEM for the detection of response shift will help to improve our understanding of response shift phenomena and thus change in PROs. Better understanding of patients’ perceived health trajectories will ultimately help to adopt more effective treatments and thus enhance patients’ wellbeing.
Supplementary Information
The online version contains supplementary material available at 10.1007/s11136-020-02742-9.
Introduction
Patient-reported outcomes (PROs) are increasingly recognized as a critical endpoint in health care and medicine, and routine assessment of PROs is becoming standard part of clinical practice [ 1 ]. The importance of measuring PROs, such as health-related quality of life (HRQL), is especially salient in view of aging societies and more powerful health-care interventions, which have led to an increasing number of people living with chronic disease [ 2 ]. That is, the ultimate purpose of health-care interventions may often not be prolonged survival but maintenance or optimization of patients’ quality of life [ 3 ].
Evaluating the impact of disease and treatment on patients’ perceived health trajectories requires longitudinal assessment. However, interpretation of change in PROs is complicated by the fact that the meaning of respondents’ self-evaluations may change too. Sprangers and Schwartz [ 4 ] proposed a theoretical model for change in the meaning of self-evaluations, which they called ‘response shift,’ a term coined by Howard et al. [ 5 ]. Sprangers and Schwartz distinguish three types of response shift: recalibration refers to a change in respondents’ internal criteria with which they assess the construct of interest; reprioritization refers to a change in respondents’ values regarding the relative importance of subdomains; and reconceptualization refers to a change in the meaning of the target construct. Although various refined definitions and theoretical models have subsequently been proposed [ 6 – 8 ], they all share this working definition of response shift. While response shift can often be considered a beneficial treatment or time effect, its effect may lead to an over- or under-estimation of intervention effects, hindering the interpretation of change in HRQL outcomes. It is, thus, important to detect and take into account possible response shift effects.
Structural equation modeling (SEM) is currently the most widely used statistical approach for the investigation of response shift [ 9 ] and has been applied to examine response shift in various patient populations, disease types, and PRO measures. However, application of SEM is relatively complex as it includes many steps that require several decisions regarding, for example, the number and types of response shift to consider. When one is not aware of these different decisions and their consequences, there is a risk of using the SEM method inappropriately. Moreover, there is a lack of non-technical explanations of SEM for response shift detection; the original paper by Oort [ 10 ] is difficult to follow for non-statisticians as it contains many technical specifications, makes an unnecessary distinction between two types of recalibration, and distinguishes several other types of change that are not directly relevant for response shift investigation. The aim of the current paper is therefore to provide an accessible description of the SEM method and its associated sequential decision-making practices, in order to stimulate valid applications and interpretations of SEM for the investigation of response shift and change in HRQL outcomes. Specifically, we describe the operationalization and interpretation of change with SEM addressing only those parameters of interest for the detection of recalibration, reprioritization, and reconceptualization response shift and ‘true’ change in the target construct (i.e., change in the target construct while taking into account response shift), and discuss practical considerations in the application of the SEM approach. In doing so, we provide guidelines and recommendations for the investigation and interpretation of change and response shift.
Our paper is targeted at researchers who are interested in applying SEM for response shift detection and are familiar with latent variable modeling (e.g., see [ 11 ]). Note that SEM can be used to investigate response shift from both a conceptual and a measurement perspective (see [ 9 ] for formal definitions of both perspectives). In the current paper, we address response shift investigation from the measurement perspective, where response shift is defined as a change in the relation between the underlying (latent) target construct (e.g., HRQL) and the observed questionnaire responses. To explain the SEM method, enhance its accessibility, and facilitate the interpretation of its results, we use an example of HRQL measurement over time. However, we would like to emphasize that response shift can occur—and be investigated—in any PRO measure (PROM).
Operationalization and interpretation of change and response shift
Suppose cancer patients are administered a HRQL questionnaire prior to and at the end of chemotherapy. We have their scores on nine different items from a HRQL questionnaire that measures physical (i.e., ‘nausea,’ ‘pain,’ and ‘fatigue’), mental (‘anxiety,’ ‘sadness,’ and ‘happiness’) and social (‘family relations,’ ‘friendships,’ and ‘work relations’) aspects of health. SEM is a statistical technique that can be used to model relationships between observed responses (e.g., patients’ scores on the nine items of the HRQL questionnaire) to be reflective of one or more unobserved latent variables or common factors (e.g., the three domains of the HRQL construct that the items aim to measure) (see Fig. 1 ). Within the SEM framework, the variances and covariances ( Σ , ‘Sigma’) and means ( μ , ‘mu’) of the observed variables ( X ) are given by
where Λ (‘Lambda’) is a matrix of common factor loadings that describes the relationships between the observed variables and underlying common factors (e.g., the relationships between the underlying common factor mental health and the three associated item scores are specified by three common factor loadings), Φ (‘Phi’) is a matrix of common factor variances and covariances that describes the relationships between the underlying factors (e.g., the relations between physical, mental, and social health), Θ (‘Theta’) is a matrix of residual variances and covariances that cannot be explained by the underlying common factors (e.g., the variances of the nine observed item scores that cannot be explained by the three underlying common factors), τ (‘tau’) is a vector of intercepts (e.g., one intercept value for each of the nine item scores), and κ (‘kappa’) is a vector of common factor means (e.g., the means of the underlying common factors physical, mental, and social health). The full matrices of the SEM model for the example from Fig. 1 are provided in Online Appendix A.

A SEM model for physical (P), mental (M), and social (S) health. The squares at the bottom represent nine observed indicators, where p 1 to p 3 refer to the three measures of physical health (i.e., ‘nausea,’ ‘pain,’ and ‘fatigue’), m 1 to m 3 refer to the three measures of mental health (i.e., ‘anxiety,’ ‘sadness,’ and ‘happiness’) and s 1 to s 3 refer to the three measures of social health (i.e., ‘family relations,’ ‘friendships,’ and ‘work relations’). The solid single-headed arrows at the bottom of the squares represent the residual factors of each indicator variable. The circles at the top represent the underlying latent variables that measure everything that the indicators that load on that factor have in common [i.e., a physical (P), mental (M), and social (S) domain of HRQL]. Each arrow from a latent variable to an observed indicator represents a factor loading. The solid double-headed arrows between the latent variables represent common factor covariances
Assessment of different types of change
SEM can be applied to data from multiple measurement occasions to assess change (see Fig. 2 ). Specifically, the SEM method for the investigation of different types of changes in HRQL outcomes [ 10 ] uses change in the pattern of factor loadings, values of factor loadings, and intercepts to operationalize reconceptualization, reprioritization, and recalibration, respectively. In the presence of response shift, the meaning of the construct is not consistent across time. In other words, a comparison of the indicators for which response shift has been detected is compromised, as change in the observed indicators does not (only) reflect change in the underlying variables. A decomposition of change can be used to investigate the impact of response shift on change in the observed indicators [ 12 ]. Moreover, SEM enables the investigation of change in the underlying latent variables, while taking into account possible response shifts. Changes in the common factor means across occasions are indicative of ‘true’ change in the construct of interest. Table Table1 1 provides an overview of the four steps of the SEM approach as proposed by Oort [ 10 ], including examples of the interpretation of response shift.

A longitudinal SEM model for the investigation of change and response shift in physical (P), mental (M), and social (S) health. This is the longitudinal SEM model of the same HRQL measurement as depicted in Fig. 1 . The squares at the bottom represent the observed indicators, measuring physical ( p 1 to p 3 ), mental ( m 1 to m 3 ), and social ( s 1 to s 3 ) aspects of health (see Fig. 1 ) at two occasions (T1 and T2). The solid single-headed arrows at the bottom of the squares represent the residual factors of each indicator variable. The dotted double-headed arrows represent the longitudinal relations between the residual factors, where only the residual factors of the same indicator are allowed to correlate. The circles at the top represent the underlying latent variables that measure everything that the indicators that load on that factor have in common [i.e., a physical (P), mental (M), and social (S) domains of HRQL, both at T1 and T2]. Each arrow from a latent variable to an observed indicator represents a factor loading. The solid double-headed arrows between the latent variables represent common factor covariances. The dotted double-headed arrow represents the (nine) longitudinal correlations between the common factors
Illustration of response shift detection using the four-step structural equation modeling (SEM) procedure
Σ is a symmetric matrix that contains the variances and covariances of the observed variables ( X ); Λ is a matrix of common factor loadings, where Λ T1 and Λ T2 contain the factor loadings of baseline (T1) and follow-up (T2), respectively; Φ is a symmetric matrix of common factor (co)variances, where Φ T1 contains the common factor (co)variances at baseline, Φ T2 contains the common factor (co)variances at follow-up, Φ T1,T2 contains the common factor covariances across occasion, and Φ T1,T2 = Φ T2,T1 ; Θ is a matrix of residual (co)variances, where Θ T1 is a diagonal matrix with residual variances at baseline, Θ T2 is a diagonal matrix with residual variances at follow-up, Θ T1,T2 is a diagonal matrix with residual covariances across occasion, and Θ T1,T2 = Θ T2,T1 ; μ is a vector that contains the means of the observed variables; τ is a vector of intercept values, where τ T1 and τ T2 contain the intercepts at baseline and follow-up, respectively; κ is a vector of common factor means, where κ T1 and κ T2 contain the means of the common factors at baseline and follow-up, respectively. In Step 1, the following restrictions apply for identification purposes: diag( Φ T1 ) = I , diag( Φ T2 ) = I , κ T1 = 0, and κ T2 = 0. In Step 2, identification restrictions diag( Φ T1 ) = I , and κ T1 = 0 are sufficient, so that diag( Φ T2 ) and κ T2 are free to be estimated. a Although non-invariance of residual variances can be considered as a type of non-uniform recalibration (see [ 10 ]), the detection of non-uniform recalibration is not important for the investigation of mean change in the common factors and therefore not considered here
Added value of the SEM approach
There are three main advantages of the SEM approach to investigate change in HRQL outcomes. First, it allows for an operationalization of different types of response shift. Second, it can account for the different types of response shift. Third, the flexibility of the SEM framework enables the inclusion of multiple measurements (e.g., analyze more extensive follow-up designs; see [ 13 ]), multiple groups (e.g., compare different patient groups based on disease, treatment, or patient characteristics; see [ 14 ]), multidimensional scales (e.g., include multiple HRQL domains, or other latent variables, simultaneously; see [ 15 ]), or variables with different measurement levels (e.g., continuous subscale scores and categorical item scores; see [ 16 ]), and exogenous variables that possibly explain response shift. For an interpretation of the impact of response shift on the assessment of change, it is also possible to calculate SEM-based effect-size indices [ 12 ].
Practical considerations in application of the SEM approach
As with any method, the validity of the SEM method depends on certain methodological and conceptual assumptions. General discussions about the underlying assumptions of SEM (e.g., [ 11 , 17 ]), and the measurement of latent variables (e.g., [ 18 , 19 ]) can be found elsewhere. Here, we focus on practical issues that are specifically important for valid application and interpretation of the SEM approach for detecting response shift in HRQL outcomes. Table Table2 2 lists these issues and connects them to the four different steps in the SEM procedure.
Overview of practical considerations in application of the SEM approach for the detection of response shift
Know your measures: establishing an appropriate measurement model
The measurement model specifies the relations between the observed variables and underlying latent factor(s) and thus defines the construct that we intend to measure. With longitudinal data, the measurement model includes the specification of the measurement structure at each measurement occasion and also referred to as the longitudinal measurement model (LMM). To arrive at the LMM (i.e., step 1 of the SEM approach), one can establish an appropriate measurement model for each measurement occasion separately and combine all separate measurement models into a single LMM (cf. [ 20 ]). Or, alternatively, one can combine all measurement occasions into a single longitudinal measurement model and establish an appropriate LMM for all measurement occasions simultaneously (cf. [ 21 ]). The only requirements of the specified LMM are that the measurement structure is largely the same (e.g., the same number of underlying common factors) across time, and that it has interpretable common factors. In practice of response shift detection, however, differences in the measurement structure are indicative of reconceptualization response shift. Therefore, the measurement structure is often specified to be the same at each occasion. For example, the LMM of our illustrative example of HRQL is specified by using the three-factor model from Fig. 1 at both baseline and follow-up (see Fig. 2 ).
An appropriate starting point for the specification of a measurement model can be based on the structure of the questionnaire, results from previous research, substantive considerations about the content of the observed measures, exploratory factor analyses, or—more likely—a combination of these approaches. For example, when a HRQL questionnaire is developed based on the idea that the items reflect social, mental, and physical aspects of health, then the measurement model could be specified as a three-factor model, where all items that measure the same domain load on the associated common factor (such as in Fig. 1 ). However, specification of the measurement model can become more complicated in situations where the dimensional structure of a questionnaire is unclear, or where (items of) different questionnaires are combined (cf. [ 14 ]). Moreover, it is often necessary to modify the initially specified measurement model to obtain a well-fitting model. A well-fitting measurement model is necessary, as the measurement model is the baseline model against which all further models (that are used to test for the presence of response shift) will be compared. Thus, the measurement model represents the most parsimonious, the most reasonable or defendable, and the best-fitting model to the data [ 22 ].
To evaluate whether the model fit of the measurement model is appropriate (e.g., assessment of overall model fit) and to guide model specification, when the initial model fit is suboptimal or inadequate (e.g., using differences in model fit), one can use statistical criteria. However, evaluation of statistical criteria for (differences in) model fit is complicated by the fact that there exist many different fit indices, with different decision rules that may be more or less appropriate depending on the context of the study. An overview of the most important fit measures and their (dis)advantages are provided in Table Table3. 3 . As a general recommendation, the researcher could inspect and report several fit indices but should be aware that choice of the specific fit index might depend on the specifics of the data (e.g., sample size), complexity of the model, and/or the hypothesis that is being tested. Detailed discussions on the use of different SEM-based fit indices are provided elsewhere (e.g., see [ 23 – 25 ]).
An overview of SEM-based model-fit indices for the evaluation of overall goodness of model fit and differences in model fit
Making decisions in model (re-)specification also require substantive considerations (i.e., does a model make sense?). For example, statistical indices may indicate that the largest improvement in fit can be achieved by freeing a factor loading of a physical functioning item on a common factor that measures mental health; such a model specification may not make sense substantively. On the other hand, freeing a residual covariance between indicators that share the same item format may be sensible cf. [ 26 ] even though it will not lead to a large improvement in model fit or to a change in interpretation of the common factors. In order to find a substantively reasonable measurement model, it is at least equally—and possibly even more—important to rely on substantive knowledge as on statistical criteria.
Identification of possible response shift
The mere presence of response shift is evaluated by testing whether the equality restrictions on all model parameters associated with response shift are tenable (i.e., Step 2 of the SEM procedure), representing an ‘omnibus test’ for the presence of response shift. This procedure has also been advocated by others [ 27 ] and has been shown to protect against false positives [ 28 ]. However, if there is evidence of the presence of response shift, how does one then accurately locate which observed variable is affected by which type of response shift?
The search for response shift (i.e., step 3 of the SEM approach) requires exploratory model fitting or re-specification, which is referred to as the ‘specification search.’ The specification search can be guided using statistical criteria, such as modification indices, expected parameter changes, Wald tests, inspection of residuals, or differences in model fit [ 29 ]. In order to correctly identify the change in model parameters, it has been recommended to use an iterative procedure [ 30 ], where all model parameters associated with response shift are freed one at a time, and the freely estimated parameter that shows the largest improvement in model fit is incorporated in the model. However, it may be that two different model modifications lead to equivalent improvement in model fit. A decision on which model modification to prioritize can, therefore, not be based on statistical criteria alone. Given the dependence of sequential model re-specification, freeing one model parameter may render freeing the other model parameter unnecessary, i.e., a change to the model can affect other parts of the model too. It may therefore be possible that alternative series of model re-specifications lead to different results. For example, in the search for response shift in our illustrative example of HRQL, it may be that freeing the intercept value of either ‘family relations’ or ‘friendships’ (both indicators of social health; see Fig. 1 ) would lead to an equivalent improvement in model fit, but that freeing one would render freeing the other intercept unnecessary. One thus needs other—substantive—reasons to decide on which response shift effect to include in the model. It may be, for example that recalibration of ‘family relations’ is much more plausible given the type of catalyst (e.g., type of disease or treatment) or prevalence of married patients/marital status in the study population.
Instead of strictly adhering to a procedure where only the modification that leads to the largest model-fit improvement is considered, it may be important to follow different sequences in model re-specification—i.e., choose different modifications that lead to different but more-or-less equivalent model-fit improvement—to investigate whether and to what extent these different sequential decisions lead to different results. This will allow the researcher to see whether detection of response shift is dependent on sequential decision-making practices and to choose among possible differences in these sequences based on a combination of both statistical and substantive considerations. It is this repeated back-and-forth specification search in which one can find confidence in the robustness of results or, alternatively, find that a confident conclusion about the number and types of response shift is not warranted. Clearly, these sequential decision-making practices thus require subjective judgment, and different researchers may make different decisions. This is a necessary consequence of ensuring interpretability of findings. For example, it may be that in different sequences of response shift investigation for our illustrative example of HRQL, the difference in intercepts of ‘family relations’ re-occurs frequently, while the difference in intercepts of ‘friendships’ only occurs sporadically. Such a pattern of results may help to decide between different modifications that lead to similar improvements in model fit.
The specification search for possible response shift effects also requires a decision on when to stop searching. The aim of the specification search is to identify all possible response shift effects (i.e., identify all true positives). Meanwhile, however, one wants to prevent the identification of trivial differences in model parameters across time as being of substantive interest (i.e., identification of false positives, or type 1 errors). In addition to the improvement in model fit for freeing individual parameters, one can rely on the difference in model fit between the measurement model and the model that includes all identified response shift effects. When the overall difference in fit between these models is not significant, this may be taken as an indication that freeing additional model parameters is no longer necessary. Also, one can use the overall model fit of the model to judge whether the model that includes response shift is tenable. These model-fit evaluations may provide more robust stopping criteria. However, it has also been argued that in order to adequately identify all response shift effects, it may be necessary to continue the specification search, even when the established model already shows adequate model fit [ 31 ]. Therefore, model-fit criteria should be used in combination with substantive criteria with regard to the (possible) response shifts. For example, it may be that freeing an additional model parameter will lead to a small, non-significant improvement in model fit, but that the associated response shift has a clear interpretation. For example, when in our illustrative example of HRQL, there is an a-priori hypothesis about the occurrence of reprioritization response shift of ‘nausea’ (see Table Table1), 1 ), it may be informative to report on a small but non-significant effect. As a researcher, one has to find a balance between the goodness of fit and the interpretability of the model. Again, subjective judgment is needed to ensure meaningfulness of the results.
Interpretation of detected response shift and ‘true’ change
With SEM, we do not look at response shifts directly, but at the effects, these response shifts have on the measurement of change in HRQL. This allows us to describe what occurs (i.e., patterns of different types of change), but it does not imply that we also know how it occurs (i.e., what the causes are of the identified response shift). For the substantive interpretation of change, it is therefore important to provide an interpretation and possible explanation of detected response shift. For example, imagine that in our illustrative example, recalibration was detected in the indicator ‘pain’ of physical health, where patients showed a larger decrease in pain as compared to the other indicators of physical health. A possible explanation for this result may be that patients adapted to the experience of pain and therefore rated their pain to be lower at follow-up, even though their actual experience of pain did not change (or changed to a lesser degree), i.e., recalibration response shift (see Table Table1). 1 ). It may also be that patients received treatment or medication that reduced their experienced level of pain. However, one could argue that only the first interpretation coincides with what Sprangers and Schwartz [ 4 ] describe as recalibration response shift. The SEM approach for the detection of response shift does not make such substantive distinctions. Therefore, substantive interpretation of detected response shift is of paramount importance; it is needed both to clarify what is taken as evidence of response shift and to exclude, or make less likely, alternative explanations.
The interpretation of detected response shifts can be based on substantive knowledge of the patient group, the treatment, or disease trajectory. In addition, it is possible to include operationalizations of potential explanations of response shift in the SEM model. If measures of antecedents (e.g., sociodemographic or personality characteristics) or mechanisms (e.g., coping strategies, social comparison) are available, they can be incorporated in the model as possible explanatory variables for response shift effects cf. [ 32 ]. For example, in order to investigate the role of appraisal processes (following [ 7 ]) for the detected recalibration response shift of pain as described above, one could include a direct measure of appraisal in the model and investigate the effect of appraisal on the (change in) scores of the indicator ‘pain.’ Such investigations will help to substantiate whether and how the detected response shifts are influenced by individuals’ cognitive changes in standards, values, or conceptualizations. As such, substantive interpretation and explanation of response shift are necessary to understand both the mechanisms of response shift, and how it affects change in the construct that we intend to measure (i.e., HRQL), which in turn will help to better understand patients’ perceived health trajectories.
Finally, the (clinical) relevance of occurrences of response shift can be evaluated by calculating the impact of response shift on the assessment of change. First, the decomposition of change [ 12 ] can be used to interpret the impact of response shift on change in the observed variables (e.g., change in item scores). The decomposition entails that observed change is decomposed into so-called ‘true’ change (i.e., change due to change in the underlying target construct) and change due to response shift. Second, the impact of response shift on ‘true’ change in the underlying target construct (e.g., HRQL) can be evaluated by comparing estimates of change before and after taking into account response shifts. SEM-based effect-size indices can help to interpret the magnitude of the impact on change assessment [ 12 ]. This is important because substantial and interpretable response shifts do not always exert a considerable impact on ‘true’ change. For example, it may be that the detected recalibration response shift in the indictor ‘pain’ is statistically significant, interpretable (see above), and has substantial impact on the observed change in pain. At the same time, it may be that ‘true’ change in physical health is not influenced by the detected response shift. Then, the detected recalibration response shift has no impact on the interpretation of change in HRQL. Still, the occurrence (and investigation) of response shift is insightful because it shows how change in the target construct is (differentially) related to change in the observed measures. Both types of information regarding the impact of response shift on change assessment can thus be used to better interpret the findings from response shift investigations.
In the current paper, we discuss practical issues that are important for researchers who want to apply SEM for the assessment of change and detection of response shift. We provide general recommendations that can be used for all applications, while acknowledging that decisions are made on a case-by-case basis and require the substantive issues at stake. We wish to emphasize the importance of taking into account substantive considerations in addition to statistical information to guide the sequential decision-making practices. These decisions require subjective judgment and are needed for any statistical modeling procedure to ensure interpretability of findings. Moreover, for a meaningful interpretation of change, it is important to try to substantiate the linkage between detected response shift and patients’ perceived health trajectories, e.g., by using substantive knowledge or direct measures of possible explanatory variables. With the recommendations provided in this paper, we aim to stimulate the appropriate application and interpretation of SEM for the investigation of response shift and assessment of change in PROs and thus improve the scientific stringency of the field. As sound statistical techniques can contribute to a better understanding of patients’ perceived health trajectories, this will ultimately improve the evaluation and interpretation of the effectiveness of health-care interventions and thus improve the quality of patients’ lives.
Below is the link to the electronic supplementary material.
Acknowledgements
Parts of this manuscript are based on Chapters 1 (Introduction) and 9 (General discussion) of the doctoral dissertation of M.G.E. Verdam [ 45 ] that has been published under the Creative Commons License.
Compliance with ethical standards
The authors declare that they have no conflict of interest.
Publisher's Note
Springer Nature remains neutral with regard to jurisdictional claims in published maps and institutional affiliations.
Academia.edu no longer supports Internet Explorer.
To browse Academia.edu and the wider internet faster and more securely, please take a few seconds to upgrade your browser .
Enter the email address you signed up with and we'll email you a reset link.
- We're Hiring!
- Help Center
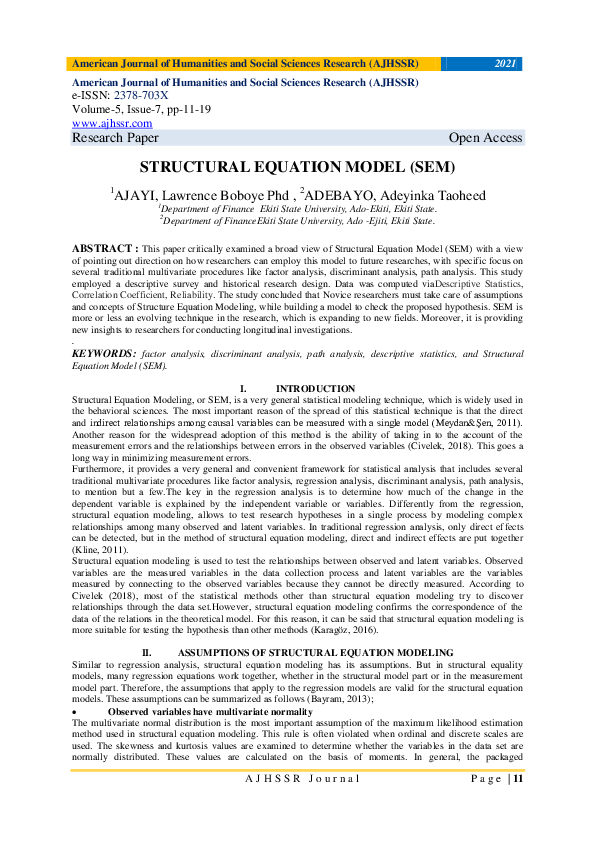
STRUCTURAL EQUATION MODEL (SEM

This paper critically examined a broad view of Structural Equation Model (SEM) with a view of pointing out direction on how researchers can employ this model to future researches, with specific focus on several traditional multivariate procedures like factor analysis, discriminant analysis, path analysis. This study employed a descriptive survey and historical research design. Data was computed viaDescriptive Statistics, Correlation Coefficient, Reliability. The study concluded that Novice researchers must take care of assumptions and concepts of Structure Equation Modeling, while building a model to check the proposed hypothesis. SEM is more or less an evolving technique in the research, which is expanding to new fields. Moreover, it is providing new insights to researchers for conducting longitudinal investigations. .
Related Papers
Journal of Organizational Behavior Review (JOB Review)
Marwan Ghaleb
Structural equation modeling (SEM) is one of the multivariate analyses that is used to test complicated research models, which include several constructs that have a group of independent and dependent variables with a series of hypothesized relations and associations between them. It starts with examining the collected data by conducting a data screening analysis and descriptive statistics. The scale used to measure the variables should be examined by conducting factor analysis (EFA & CFA) to make sure the data fits the research measurement model and to assess the scale's reliability, validity, and its level of fit to the collected data. The analysis of multivariate assumption should be reviewed then path analysis can be done for hypotheses testing and getting the final results. The final results have to be explained and interpreted based on the research's theoretical background and its literature foundation. This review paper explains these steps in detail for quantitative analysis students and other researchers who have basic knowledge of statistics, using simple words without diving deeply into statistics' details and its related formulas.
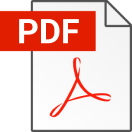
Meliana Lumban Raja
In this article, we provide guidance for substantive researchers on the use of structural equation modeling in practice for theory testing and development. We present a comprehensive, two-step modeling approach that employs a series of nested models and sequential chi-square difference tests. We discuss the comparative advantages of this approach over a one-step approach. Considerations in specification, assessment of fit, and respecification of measurement models using confirmatory factor analysis are reviewed. As background to the two-step approach, the distinction between exploratory and confirmatory analysis, the distinction between complementary approaches for theory testing versus predictive application, and some developments in estimation methods also are discussed. Substantive use of structural equation modeling has been growing in psychology and the social sciences. One reason for this is that these confirmatory methods (e.g., Bentler, 1983; Browne, 1984; Joreskog, 1978)provide researchers withacom-prehensive means for assessing and modifying theoretical models. As such, they offer great potential for furthering theory development. Because of their relative sophistication, however, a number of problems and pitfalls in their application can hinder this potential from being realized. The purpose of this article is to provide some guidance for substantive researchers on the use of structural equation modeling in practice for theory testing and development. We present a comprehensive, two-step modeling approach that provides a basis for making meaningful inferences about theoretical constructs and their interrelations, as well as avoiding some specious inferences. The model-building task can be thought of as the analysis of two conceptually distinct models (Anderson & Gerbing, 1982; Joreskog & Sorbom, 1984). A confirmatory measurement, or factor analysis, model specifies the relations of the observed measures to their posited underlying constructs, with the constructs allowed to intercorrelate freely. A confirmatory structural model then specifies the causal relations of the constructs to one another, as posited by some theory. With full-information estimation methods, such as those provided in the EQS (Bentler, 1985) or LISREL (Joreskog & Sorbom, 1984) programs, the measurement and structural submodels can be estimated simultaneously. The ability to do this in a one-step analysis ap
ELANGOVAN N Christ (Deemed to be University)
The application of Structural Equation Modeling (SEM) in the business research is growing. The second-generation multivariate data analysis technique, SEM is easy to use and provides a high quality statistical analysis. Many visual SEM software programs help in a quick design of the theoretical model and to modify them graphically using simple drawing tools. Further it can estimate the model’s fit, make any modifications and arrive at a final valid model. It is essential to understand how to design the research process appropriate to the SEM analysis. This article describes the steps in SEM analysis, the conventions used in presenting a model, the elements of the SEM technique, the interpretation of the SEM results. The rule of thumb that evaluates the results and the other issues in SEM reporting are discussed.
Youjae Yi , Richard Bagozzi
We provide a comprehensive and user-friendly compendium of standards for the use and interpretation of structural equation models (SEMs). To both read about and do research that employs SEMs, it is necessary to master the art and science of the statistical procedures underpinning SEMs in an integrative way with the substantive concepts, theories, and hypotheses that researchers desire to examine. Our aim is to remove some of the mystery and uncertainty of the use of SEMs, while conveying the spirit of their possibilities.
Dr. Kamran Ahmed Siddiqui
The major objective of this paper is to provide guidelines for using Structural Equation Modeling (SEM) in social research. It provides an abridge version of relevant literature in tabular form. SEM is " a second generation of multivariate analysis " , mainly used for cross-sectional factor analyses, path analyses and regression analyses. It provides guidelines for six mandatory methodological areas (a) disclosure of model building strategy; (b) model specification including measurement models and path models (c) methods of estimation, (d) fit indices with cutoff criteria, (e) model optimization or re-specification, f) sample size requirements for SEM.
Open Journal Nigeria
Olaoye Daniel , Ayandele J . K.
Structural Equation Model (SEM) is a multivariate statistical technique that has been explored to test relationships between variables. The use of SEM to analyze relationship between variables is premised on the weak assumption of path analysis, regression analysis and so on; that variables are measured without error. This review thus sheds light on the meaning of SEM, its assumptions, steps and some of the terms used in SEM. The importance of item parcelling to SEM and its methods were briefly examined. It also dealt on the stages involved in SEM, similarities and differences between SEM and conventional statistical methods, software packages that can be used for SEM. This article employed systematic literature review method because it critically synthesized research studies and findings on structural equation modeling (SEM). It could be concluded that SEM is useful in analyzing a set of relationships between variables using diagrams. SEM can also be useful in minimizing measurement errors and in enhancing reliability of constructs. Based on this, it is recommended that SEM should be employed to test relationship between variables since it can explore complex relationships among variables such as direct, indirect, spurious, hierarchical and non-hierarchical.
Educational Measurement: Issues and Practice
Arthur asda
Structural equation modeling (SEM) is a methodology for representing, estimating, and testing a network of relationships between variables (measured variables and latent constructs). This tutorial provides an introduction to SEM including comparisons between " traditional statistical " and SEM analyses. Examples include path analysis/ regression, repeated measures analysis/latent growth curve modeling, and confirmatory factor analysis. Participants will learn basic skills to analyze data with structural equation modeling. Rationale Analyzing research data and interpreting results can be complex and confusing. Traditional statistical approaches to data analysis specify default models, assume measurement occurs without error, and are somewhat inflexible. However, structural equation modeling requires specification of a model based on theory and research, is a multivariate technique incorporating measured variables and latent constructs, and explicitly specifies measurement error. A model (diagram) allows for specification of relationships between variables. Purpose The purpose of this tutorial is to provide participants with basic knowledge of structural equation modeling methodology. The goals are to present a powerful, flexible and comprehensive technique for investigating relationships between measured variables and latent constructs and to challenge participants to design and plan research where SEM is an appropriate analysis tool. Structural equation modeling (SEM) • is a comprehensive statistical approach to testing hypotheses about relations among observed and latent variables (Hoyle, 1995). • is a methodology for representing, estimating, and testing a theoretical network of (mostly) linear relations between variables (Rigdon, 1998). • tests hypothesized patterns of directional and nondirectional relationships among a set of observed (measured) and unobserved (latent) variables (MacCallum & Austin, 2000). Two goals in SEM are 1) to understand the patterns of correlation/covariance among a set of variables and 2) to explain as much of their variance as possible with the model specified (Kline, 1998). The purpose of the model, in the most common form of SEM, is to account for variation and covariation of the measured variables (MVs). Path analysis (e.g., regression) tests models and relationships among MVs. Confirmatory factor analysis tests models of relationships between latent variables (LVs or common factors) and MVs which are indicators of common factors. Latent growth curve models (LGM) estimate initial level (intercept), rate of change (slope), structural slopes, and variance. Special cases of SEM are regression, canonical correlation, confirmatory factor analysis, and repeated measures analysis of variance (Kline, 1998). Similarities between Traditional Statistical Methods and SEM SEM is similar to traditional methods like correlation, regression and analysis of variance in many ways. First, both traditional methods and SEM are based on linear statistical models. Second, statistical tests associated with both methods are valid if certain assumptions are met. Traditional methods assume a normal distribution and SEM assumes multivariate normality. Third, neither approach offers a test of causality. Differences Between Traditional and SEM Methods Traditional approaches differ from the SEM approach in several areas. First, SEM is a highly flexible and comprehensive methodology. This methodology is appropriate for investigating achievement, economic trends, health issues, family and peer dynamics, self-concept, exercise, self-efficacy, depression, psychotherapy, and other phenomenon. Second, traditional methods specify a default model whereas SEM requires formal specification of a model to be estimated and tested. SEM offers no default model and places few limitations on what types of relations can be specified. SEM model specification requires researchers to support hypothesis with theory or research and specify relations a priori. Third, SEM is a multivariate technique incorporating observed (measured) and unobserved variables (latent constructs) while traditional techniques analyze only measured variables. Multiple, related equations are solved simultaneously to determine parameter estimates with SEM methodology. Fourth, SEM allows researchers to recognize the imperfect nature of their measures. SEM explicitly specifies error while traditional methods assume measurement occurs without error. Fifth, traditional analysis provides straightforward significance tests to determine group differences, relationships between variables, or the amount of variance explained. SEM provides no straightforward tests to determine model fit. Instead, the best strategy for
Journal of Personality Assessment
Jodie Ullman
Nihal Jayamaha , Ishani Soysa , Nigel Grigg
RELATED PAPERS
Eduardo Echeverria
ADRI Occasional Papers
Edwin P . Santiago
Calinic Petcu
History of Psychology
Silvana Vetö
Saryanto SE
pedro ramos diaz
Francisco Hernandez
Master's thesis
Vilja Byström
Babylyn Alcaraz
Craig Michoski
Dinda Putri
ALEKSANDRO C NOGUEIRA
Molecular vision
Amal Gharib
Open Agriculture
Mieke Rochimi Setiawati
Trans/Form/Ação
Julia Vieira
alfin komaru zaman
earthlab.uoi.gr
Tassos A. Mikropoulos
Diego Vega Castro
Life Sciences
Michael Maes
Journal of Clinical Pathology
Ignacio Briceño
Transportation Research Record
Paul Bolster
Inovação em Ciência e Tecnologia de Alimentos 2
Milton Luiz da Paz Lima
Jose Geraldo Speciali
Lecture Notes in Computer Science
CARLOS EDUARDO ARECES
Byron Rolando Tovar Gomez
Veterinary Parasitology: Regional Studies and Reports
Harold Salant
Francesca La Sorte
Peripherica: A Journal of Social, Cultural, and Literary History Vol. 2 Issue 2
Luis Othoniel Rosa
yyjugf hfgerfd
Palaeogeography, Palaeoclimatology, Palaeoecology
FRANCISCO J Sierro
- We're Hiring!
- Help Center
- Find new research papers in:
- Health Sciences
- Earth Sciences
- Cognitive Science
- Mathematics
- Computer Science
- Academia ©2024
- Open access
- Published: 08 May 2024
The relationship between childhood adversity and sleep quality among rural older adults in China: the mediating role of anxiety and negative coping
- Yuqin Zhang 1 ,
- Chengwei Lin 2 ,
- Hongwei Li 1 ,
- Xueyan Zhou 4 ,
- Ying Xiong 5 ,
- Jin Yan 1 ,
- Mengxue Xie 1 ,
- Xueli Zhang 6 ,
- Chengchao Zhou 7 &
- Lian Yang 1
BMC Psychiatry volume 24 , Article number: 346 ( 2024 ) Cite this article
248 Accesses
Metrics details
Studies have revealed the effects of childhood adversity, anxiety, and negative coping on sleep quality in older adults, but few studies have focused on the association between childhood adversity and sleep quality in rural older adults and the potential mechanisms of this influence. In this study, we aim to evaluate sleep quality in rural older adults, analyze the impact of adverse early experiences on their sleep quality, and explore whether anxiety and negative coping mediate this relationship.
Data were derived from a large cross-sectional study conducted in Deyang City, China, which recruited 6,318 people aged 65 years and older. After excluding non-agricultural household registration and lack of key information, a total of 3,873 rural older adults were included in the analysis. Structural equation modelling (SEM) was used to analyze the relationship between childhood adversity and sleep quality, and the mediating role of anxiety and negative coping.
Approximately 48.15% of rural older adults had poor sleep quality, and older adults who were women, less educated, widowed, or living alone or had chronic illnesses had poorer sleep quality. Through structural equation model fitting, the total effect value of childhood adversity on sleep quality was 0.208 (95% CI: 0.146, 0.270), with a direct effect value of 0.066 (95% CI: 0.006, 0.130), accounting for 31.73% of the total effect; the total indirect effect value was 0.142 (95% CI: 0.119, 0.170), accounting for 68.27% of the total effect. The mediating effects of childhood adversity on sleep quality through anxiety and negative coping were significant, with effect values of 0.096 (95% CI: 0.078, 0.119) and 0.024 (95% CI: 0.014, 0.037), respectively. The chain mediating effect of anxiety and negative coping between childhood adversity and sleep quality was also significant, with an effect value of 0.022 (95% CI: 0.017, 0.028).
Conclusions
Anxiety and negative coping were important mediating factors for rural older adult’s childhood adversity and sleep quality. This suggests that managing anxiety and negative coping in older adults may mitigate the negative effects of childhood adversity on sleep quality.
Peer Review reports
The global population is entering an aging stage, and China has the fastest rate of population aging in the world. According to China’s seventh national census, in 2020, 191 million individuals were aged 65 years and older, accounting for 13.50% of the total population [ 1 ], and the proportion of people aged 65 and above in rural is 6.6% higher than in urban [ 2 ]. In addition, China’s long-standing urban-rural dual structure has resulted in inequality in economic, medical, and educational development, leading to significant differences in the health status of China’s urban and rural older populations [ 3 , 4 ]. Relevant studies have found that, urban residents have a higher survival rate [ 5 ], better self-assessed health status and better self-assessed self-care ability than rural dwellers [ 6 ]. Therefore, to reduce health inequalities among older adults, the health status of rural older adults is an important focus.
Good quality sleep has been found to be essential for health [ 7 , 8 , 9 ]. However, sleep problems are prevalent among the older population [ 10 , 11 ]. Gulia and Tatineny have reported that the current prevalence of sleep disorders in the global older population is 30–40% [ 12 , 13 ]. In a systematic review, Lu reported that the overall prevalence of poor sleep among the older population in China had reached 35.9% [ 14 ]. In the rural older adults, the prevalence of sleep disorders is more than 40% [ 15 ], even as high as 58.40% [ 16 ].There are various factors that affect sleep quality [ 17 , 18 ]. Adverse childhood experiences (ACEs) are stressful and/or traumatic experiences that occur during childhood [ 19 ]. There is growing evidence that ACEs may lead to sleep problems in adulthood [ 20 , 21 ] and that the influence can last up to 50 years [ 22 ]. For example, emotional abuse and neglect experienced early in life impede the development of individuals’ social relationships later in life and negatively affect the subjective sleep quality of older adults [ 23 ]. A study by Dorji. found that older adults with multiple (≥ 7) ACEs had a higher incidence of insomnia [ 24 ]. Although previous investigations have indicated the relationship between childhood adversity and sleep quality in older adults, they have ignored possible potential mechanisms for this relationship.
Previous studies have found that anxiety negatively affects sleep quality in older adults [ 25 ], whereas a good mental state can improve their sleep quality. Notably, childhood adversity may be associated with increased anxiety symptoms in late adulthood [ 26 ]. Raposo have reported that older adults who experienced childhood adversity were more likely to suffer from anxiety (OR = 1.48; 95%CI = 1.20–1.83) [ 27 ]. Considering the relationships among anxiety, childhood adversity, and sleep quality, one aim of this study was to verify whether anxiety mediates the relationship between childhood adversity and sleep quality.
A coping style refers to a psychological and behavioral strategy adopted by an individual in response to changes in the internal and external environment [ 28 ]. Negative coping is usually positively associated with sleep disorders [ 29 , 30 ]. Coping style usually evolves over time and may be influenced by exposure to childhood adversity; for instance, people exposed to early adverse experiences show predominantly emotion-focused and avoidance coping styles, such as denial and disengagement [ 31 , 32 ]. In addition to childhood adversity, negative emotions or psychological states also can influence individuals’ coping strategies [ 33 ]. For example, Orgeta reported that older adults with high levels of anxiety were more likely to adopt dysfunctional coping [ 34 ]. Therefore, we hypothesized that anxiety affects coping styles in older adults and that negative coping may be a potential mediator between childhood adversity and sleep quality.
Stress is defined as the process of adaptive and coping responses when an individual faces or perceives threatening or challenging environmental changes [ 35 ]. People respond to stress with either problem-focused coping or emotion-focused coping [ 36 ]. Stress can be caused by many factors, such as early adversities, and the result of stress is adaptive or maladaptive psychosomatic responses. Based on the above, we constructed a structural equation model of a large cross-sectional dataset to explore the effects of childhood adversity on sleep quality, with childhood adversity as the stressor and anxiety and negative coping as mediators.
Research methods
Research population.
The data were sourced from a large-scale cross-sectional study conducted in 2022 that recruited older adults aged 65 years and older living in 6 districts and counties in Deyang City, Sichuan Province. Using a multistage stratified random cluster sampling method, townships (streets) were randomly selected from six county (districts), administrative villages (communities) were randomly selected from each sample township (streets), finally, people over 65 years old were selected randomly in each chosen village or community. The inclusion criteria were as follows: (1) individuals aged ≥ 65 years; (2) permanent residents in the survey area (those who have lived in the area for 6 months or more); (3) those who signed an informed consent form and agreed to take the questionnaire survey. The exclusion criteria were as follows: (1) unwilling to participate in research; (2) individuals identified by local village doctors who are unable to answer questions independently and have a history of dementia;3) other reasons for not participating in the study. The household registration system is a very important factor affecting the unequal social welfare rights and privileges of urban and rural residents in China [ 37 ], which is associated with poor health [ 38 ]. In this study, rural means that residents with agricultural household registration. A total of 6318 respondents were recruited, excluding non-agricultural household registration (2345) and missing main information (100), and finally included 3873 for analysis. The study was approved by the Medical Ethics Committee of the Affiliated Hospital of Chengdu University of Chinese Medicine, and all participants signed an informed consent form before taking the survey.
Measurement tools
General information.
This includes the age, gender, education level, marital status, chronic disease status, and exercise status of the participating older adults.
Childhood adversity
Childhood adversity was measured using the Adverse Childhood Experiences Scale developed by the Centers for Disease Control and Prevention (USA). The scale contains three major dimensions (abuse, neglect, and household dysfunction) and ten subdimensions including emotional abuse, physical abuse, sexual abuse, and emotional neglect. Higher ACE scores indicate more severe ACE exposure [ 19 , 39 ]. The internal consistency coefficients of the abuse, neglect, and household dysfunction subscales in this study were 0.790, 0.732, and 0.778, respectively.
- Sleep quality
Sleep quality was evaluated using the revised Chinese-version Pittsburgh Sleep Quality Index (PSQI). The scale consists of seven dimensions including subjective sleep quality, sleep latency, sleep duration, sleep efficiency, sleep disturbance, use of sleep medication, and daytime dysfunction. A PSQI score of ≥ 7 is generally considered to indicate poor sleep quality [ 15 , 40 , 41 ]. The internal consistency coefficient of the scale in this study was 0.754.
Anxiety in older adults was measured using the Self-Rating Anxiety Scale (SAS). The scale consists of 20 items and is rated on a 4-point scale. An SAS score of 50 or more is considered to be indicative of anxiety symptoms [ 42 ]. The internal consistency coefficient of this scale in this study was 0.831.
Trait coping style
Negative coping was measured using the Trait Coping Style Questionnaire (TCSQ). The scale consists of 20 questions in 2 dimensions—negative coping and positive coping—and is rated on a 5-point scale. The negative coping and positive coping scores are the sum of the scores for each item in the corresponding dimensions. A positive total score indicates a predominantly positive attitude toward coping with events, whereas a negative score indicates a predominantly negative coping style [ 43 , 44 ]. Only the negative coping dimension of the scale, which has an internal consistency coefficient of 0.929, was selected in this study.
Statistical analysis
The variables in the study were descriptively analyzed using the mean, standard deviation, frequency (n), and constituent ratio (%), and difference tests were conducted using t-tests and the Kruskal-Wallis H test. Spearman’s correlation was used to analyze whether there were correlations between sleep quality and the variables. Finally, a multiple-mediator structural equation model was constructed to analyze the effects of anxiety and negative coping on the relationship between sleep quality and childhood adversity, and the bootstrap method was applied to verify the mediating effect. After the initial establishment of the model, we evaluated the fit degree of the structural equation model and adjusted the model via calculating indicators such as standardized root-mean-square residual (SRMR ≤ 0.08), root-mean-square error of approximation (RMSEA ≤ 0.08), goodness of fit index (GFI ≥ 0.90), comparative fit index (CFI ≥ 0.90), normed fit index (NFI ≥ 0.90) according to the studies by Wen and Kang [ 45 , 46 ]. Data were analyzed using SPSS 25.0 and AMOS 24.0 software, and a P value < 0.05 was considered to be statistically significant. The bootstrap CI was set to 95%, and the bootstrap sample size was 5000. If the 95% CI interval does not contain 0, it indicates a significant mediating effect.
Research results
Comparison of the general information and sleep quality scores of the study participants.
A total of 3,873 older adults were included in this study. The mean participant age was 72.84 ± 6.13 years, ranging from a minimum of 65 years to a maximum of 99 years. The mean PISQ score was 6.94 ± 3.88, and older adults with poor sleep quality (PSQI score ≥ 7) accounted for 48.15%. The mean ACE score was 2.09 ± 1.16, the mean SAS score was 44.13 ± 9.84, and the mean TCSQ negative coping score was 21.88 ± 8.23.
The results of univariate analysis showed that among the different gender populations, women had poorer sleep quality and a statistically significantly higher PSQI score than men at 7.44 ± 3.98 (t = 8.845, p < 0.001). The PSQI score increased with age: that of adults aged 80 years and older was 7.32 ± 4.01, and the difference was statistically significant (H = 11.125, p = 0.004). Regarding the groups with different educational levels, the highest PSQI score was found among illiteracy individuals (7.39 ± 4.01), with a statistically significant difference (H = 39.885, p < 0.001). Sleep quality varied among older adults with different marital statuses, and the worst sleep quality was found in widowed older adults, with a PSQI score of 7.52 ± 4.00, which presented a statistically significant difference (H = 39.582, p < 0.001). Older adults living alone had the worst sleep quality with a statistically significantly different PSQI score of 7.46 ± 3.90 (H = 20.904, p < 0.001). Older adults with chronic diseases had poor sleep quality with a statistically significantly different PSQI score of 7.4 ± 3.95 (t=-8.83, p < 0.001) (Table 1 ).
Association of sleep quality with childhood adversity, anxiety, and negative coping in rural older adults
The relevant analysis results indicated that the PSQI score was positively correlated with the ACE score ( r = 0.092, P < 0.01). The PSQI score was positively correlated with the SAS score and negative coping score ( r = 0.279 and r = 0.239, respectively; both P < 0.01). The ACE score was positively correlated with the SAS score and negative coping score ( r = 0.217 and r = 0.133, respectively; both P < 0.01). There was also a positive correlation between the SAS score and negative coping score ( r = 0.351, P < 0.01) (Table 2 ).
Analysis of mediating effects
Goodness-of-fit indices and path coefficients for the theoretical model of older adults’ sleep quality.
Based on the results of the above analyses, a structural equation model was constructed with childhood adversity as the independent variable, anxiety and negative coping as the mediating variables, and sleep quality as the dependent variable. The final model was screened according to the following model fitting indices: SRMR = 0.05, RMSEA = 0.06, GFI = 0.97, CFI = 0.90 and NFI = 0.89. The results of the fitting indices indicated that the model was well fitted. The differences in each of the standardized path coefficients in the model were statistically significant (all P < 0.05) (Fig. 1 ).
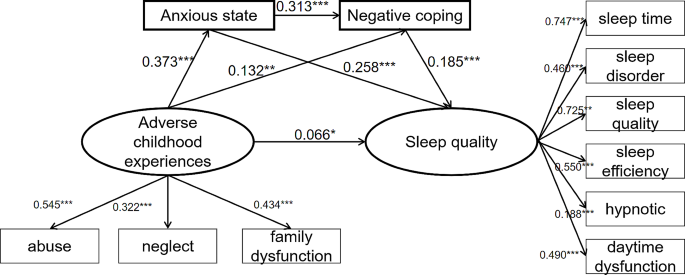
Serial mediation models for childhood adversity, anxiety, negative coping and sleep quality
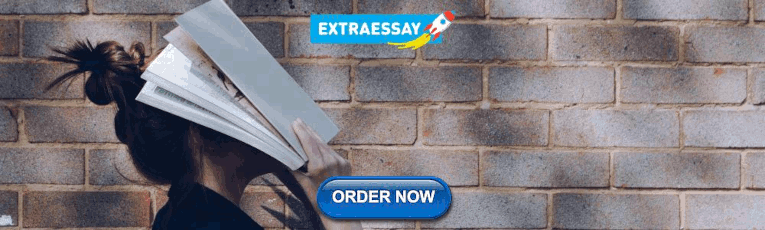
Bootstrap test of the theoretical model of older adults’ sleep quality
Table 3 demonstrates the results of structural modeling: (1) The total effect value of childhood adversity on sleep quality was 0.208 (95% CI: 0.146, 0.270), with a direct effect value of 0.066 (95% CI: 0.006, 0.130), accounting for 31.73% of the total effect, and a total indirect effect value of 0.142 (95% CI: 0.119, 0.170), accounting for 68.27% of the total effect. (2) The mediating effect of anxiety on the association between childhood adversity and sleep quality was significant, with a path effect value of 0.096 (95% CI: 0.078, 0.119), accounting for 46.15% of the total effect. (3) The mediating effect of negative coping on the association between childhood adversity on sleep quality was significant, with a path effect value of 0.024 (95% CI: 0.014, 0.037), accounting for 11.54% of the total effect. (4) The multiple mediating effects of anxiety and negative coping on the association between childhood adversity on sleep quality were also significant, with a pathway effect value of 0.022 (95% CI: 0.017, 0.028), accounting for 10.58% of the total effect (Table 3 ).
Current status and influencing factors of sleep quality in older adults
The proportion of older adults with poor sleep quality (PSQI score ≥ 7) was 48.15%, which is similar to the results of previous studies [ 15 , 16 ]. Due to gradual aging, the sleep-wake cycle of the older adults is disordered, and the efficiency of the circadian rhythm mechanism is reduced, which leads to changes in their sleep duration, sleep architecture, and sleep depth [ 12 ]. Furthermore, the occurrence of a variety of sleep problems such as sleep disruption, early sleep onset, and early awakening [ 47 , 48 , 49 ], result in a general decline in the sleep quality of older adults. We also found that gender, educational level, marital status, residency status, and chronic diseases were influencing factors of sleep quality. First, women have poorer sleep quality than men, which is in accordance with the established viewpoint [ 50 , 51 ]. Poor sleep quality and an increased risk of sleep disorders in older women may be due to the following reasons: (1) women are at a disadvantage in terms of socioeconomic factors, such as education and personal income [ 52 ]; (2) women are more susceptible to somatic [ 53 ] and psychiatric [ 54 , 55 ] disorders than men; and (3) women experience changes in secreted reproductive hormones [ 56 ]. Second, differences in sleep quality among older adults with different educational levels may be due to the fact that well-educated older adults have a higher sense of wellness and are more likely to access healthcare knowledge, which in turn leads to a better sleep state [ 57 ]. Third, the poorer sleep quality in widowed older adults and those living alone than in others may be related to loneliness and lack of social support leading to mood disorders, which in turn may cause reduced sleep efficiency and quality [ 58 ]. Finally, having a chronic disease is also a risk factor for poor sleep quality in older adults, which may be related to the physical discomfort caused by chronic diseases, the side effects of medications, and the associated financial pressure and psychological burden [ 59 ].
Direct effect of childhood adversity on sleep quality in older adults
The present study found that childhood adversity had a direct effect on sleep quality. Early life experiences, such as abuse, poverty, or the death of a parent, can affect sleep not only in childhood and adolescence but also in adulthood [ 60 , 61 ]. Childhood is an important phase for significant development of the hypothalamic-pituitary-adrenal (HPA) axis and the brain [ 58 ], and adverse events experienced during childhood can lead to long-term changes in the HPA axis response to stress (e.g., hyperactivity) and interfere with normal neurodevelopment in childhood and adolescence [ 62 ], increasing the risk of developing psychiatric disorders such as depression and post-traumatic stress disorder, which indirectly affect sleep in adulthood [ 63 ]. In addition, people exposed to ACEs are more likely to adopt unhealthy lifestyles and behaviors [ 64 , 65 ], and these changes may directly affect the sleep-wake cycle and lead to sleep problems.
Mediating effect of anxiety between childhood adversity and sleep quality in older adults
Sleep problems are not only a precursor but also a consequence of mental illness [ 66 , 67 ]. Our study found that anxiety could partially explain the relationship between childhood adversity and sleep disorders. Extensive studies have confirmed that exposure to adverse experiences in early life can increase an individual’s risk of developing psychiatric disorders such as anxiety and depression [ 68 , 69 ]. Anxiety is thus associated with a variety of sleep problems, with higher levels of anxiety corresponding to more severe sleep disorders [ 25 , 70 , 71 ]. Furthermore, anxiety has been found to mediate the effects of childhood adversity on sleep quality. For example, Amarneh found that elevated levels of anxiety sensitivity may explain the relationship between child maltreatment and adult sleep disorders among psychiatric hospitalizations [ 72 ]. Haimov found that COVID-19-related anxiety mediated the association between the number of childhood adversities and adult sleep quality [ 73 ]. The findings of our study further support the mediating role of anxiety on the effects of childhood adversity on sleep quality in older adults, suggesting that actively intervening in older adults’ anxiety states may mitigate the effects of childhood adversity on their sleep quality.
Mediating effect of negative coping between childhood adversity and sleep quality in older adults
Our results also identified a significant mediating effect of negative coping in the action of childhood adversity on sleep quality. Individuals’ exposure to environmental stressors early in life can compromise their adaptive coping strategies [ 74 ] and thus further affect sleep [ 75 ]. This result can be explained by the theory of stress. This theory states that when facing stressful events, people may take measures to disengage from threatening stimuli and generate associated thoughts and emotions (i.e., reducing activity and sleeping longer to minimize exposure to the stressor and the associated maladaptive emotions and thoughts) as well as adopt emotion-focused coping (i.e., regulating emotional responses to problems). However, such approaches may increase alertness and thus produce physiological arousal, disrupting or reducing sleep, which in turn affects sleep quality [ 76 ].
Finally, we founded that childhood adversity affected sleep quality in older adults through anxiety and negative coping. As mentioned above, stressful life events in childhood are associated with an increased risk of anxiety disorders in adulthood. Under the influence of such negative emotions, individuals are more inclined to adopt negative coping, which in turn affects the sleep quality in older adults. The above results facilitate a deeper understanding of the relationships among childhood adversity, anxiety, negative coping, and sleep quality and provide clues for exploring the potential mechanisms of how childhood adversity affects sleep quality in older adults.
Research limitations
In this study, the theoretical structural equation model fit the data well and provided an epidemiologic basis for the associations among childhood adversity, anxiety, negative coping, and sleep quality. However, there are several limitations. First, the results for the main variables in this study were obtained via self-report from the respondents and thus may be subject to unavoidable recall bias. Second, this study utilized a cross-sectional research design, which does not allow for a more precise determination of the causal relationship between variables. Third, this study explored the relationship between ACEs and PSQI scores but did not determine a dose-response relationship or whether different types of childhood adversities have different effects on sleep quality. Finally, the effects of drugs (such as antidepressants and anti-inflammatory drugs) on sleep quality were ignored in this study.
To sum up, anxiety and negative coping not only had direct effects on sleep quality but also played mediating roles in the association between childhood adversity and sleep quality, with a chained multiple mediating effect. These findings suggest that timely intervention for anxiety symptoms and negative coping states in older adults may mitigate the negative impact of childhood adversity on sleep quality.
Data availability
The datasets used and analyzed during the current study are available from the corresponding author on reasonable request.
Abbreviations
Adverse Childhood Experiences
the revised Chinese-version Pittsburgh Sleep Quality Index
Self-Rating Anxiety Scale
Trait Coping Style Questionnaire
Structural equation modelling
confidence interval
root mean square error of approximation
the hypo-thalamic pituitary adrenal axis
Bulletin of the Seventh National. Census (No. 5) - National Bureau of Statistics. https://www.stats.gov.cn/sj/tjgb/rkpcgb/qgrkpcgb/202302/t20230206_1902005.html . Accessed 2 Jan 2024.
China’s Population Aging and Regional Variation | SpringerLink. https://link.springer.com/chapter/10.1007/978-3-030-98032-0_2 . Accessed 2 Jan 2024.
Yuan L, Yu B, Gao L, Du M, Lv Y, Liu X, et al. Decomposition analysis of health inequalities between the urban and rural oldest-old populations in China: evidence from a national survey. SSM Popul Health. 2023;21:101325.
Article PubMed Google Scholar
Zhang J, Li D, Gao J. Health disparities between the Rural and Urban Elderly in China: a cross-sectional study. Int J Environ Res Public Health. 2021;18:8056.
Article PubMed PubMed Central Google Scholar
Yu P, Song X, Shi J, Mitnitski A, Tang Z, Fang X, et al. Frailty and survival of older Chinese adults in urban and rural areas: results from the Beijing Longitudinal Study of Aging. Arch Gerontol Geriatr. 2012;54:3–8.
Yang Y, Wang S, Chen L, Luo M, Xue L, Cui D, et al. Socioeconomic status, social capital, health risk behaviors, and health-related quality of life among Chinese older adults. Health Qual Life Outcomes. 2020;18:291.
Ramar K, Malhotra RK, Carden KA, Martin JL, Abbasi-Feinberg F, Aurora RN, et al. Sleep is essential to health: an American Academy of Sleep Medicine position statement. J Clin Sleep Med. 2021;17:2115–9.
Luyster FS, Strollo PJ, Zee PC, Walsh JK. Boards of directors of the American Academy of Sleep Medicine and the Sleep Research Society. Sleep: a health imperative. Sleep. 2012;35:727–34.
Hall MH, Brindle RC, Buysse DJ. Sleep and cardiovascular disease: emerging opportunities for psychology. Am Psychol. 2018;73:994–1006.
Besedovsky L, Lange T, Haack M. The Sleep-Immune Crosstalk in Health and Disease. Physiol Rev. 2019;99:1325–80.
Liu E, Feng Y, Yue Z, Zhang Q, Han T. Differences in the health behaviors of elderly individuals and influencing factors: evidence from the Chinese longitudinal healthy longevity survey. Int J Health Plann Manage. 2019;34:e1520–32.
Gulia KK, Kumar VM. Sleep disorders in the elderly: a growing challenge. Psychogeriatrics. 2018;18:155–65.
Tatineny P, Shafi F, Gohar A, Bhat A. Sleep in the Elderly. Mo Med. 2020;117:490–5.
PubMed PubMed Central Google Scholar
Lu L, Wang S-B, Rao W, Zhang Q, Ungvari GS, Ng CH, et al. The prevalence of Sleep disturbances and Sleep Quality in older Chinese adults: a Comprehensive Meta-Analysis. Behav Sleep Med. 2019;17:683–97.
Jia G, Yuan P. The association between sleep quality and loneliness in rural older individuals: a cross-sectional study in Shandong Province, China. BMC Geriatr. 2020;20:180.
Li G, Zhu Z, Hu M, He J, Yang W, Zhu J, et al. Effects of carbon dioxide and green space on sleep quality of the elderly in rural areas of Anhui Province, China. Environ Sci Pollut Res Int. 2022;29:21107–18.
Dong X, Wang Y, Chen Y, Wang X, Zhu J, Wang N, et al. Poor sleep quality and influencing factors among rural adults in Deqing, China. Sleep Breath. 2018;22:1213–20.
Gamaldo AA, Wright RS, Aiken-Morgan AT, Allaire JC, Thorpe RJ, Whitfield KE. The Association between Subjective Memory complaints and Sleep within older African American adults. J Gerontol B Psychol Sci Soc Sci. 2019;74:202–11.
Dube SR, Anda RF, Felitti VJ, Chapman DP, Williamson DF, Giles WH. Childhood abuse, household dysfunction, and the risk of attempted suicide throughout the life span: findings from the adverse childhood experiences Study. JAMA. 2001;286:3089–96.
Article CAS PubMed Google Scholar
Sheehan CM, Li L, Friedman EM. Quantity, timing, and type of childhood adversity and sleep quality in adulthood. Sleep Health. 2020;6:246–52.
Kajeepeta S, Gelaye B, Jackson CL, Williams MA. Adverse childhood experiences are associated with adult sleep disorders: a systematic review. Sleep Med. 2015;16:320–30.
Sullivan K, Rochani H, Huang L-T, Donley DK, Zhang J. Adverse childhood experiences affect sleep duration for up to 50 years later. Sleep. 2019;42:zsz087.
Poon CYM, Knight BG. Impact of childhood parental abuse and neglect on sleep problems in old age. J Gerontol B Psychol Sci Soc Sci. 2011;66:307–10.
Dorji N, Dunne M, Deb S. Adverse childhood experiences: association with physical and mental health conditions among older adults in Bhutan. Public Health. 2020;182:173–8.
Yu J, Rawtaer I, Fam J, Jiang M-J, Feng L, Kua EH, et al. Sleep correlates of depression and anxiety in an elderly Asian population. Psychogeriatrics. 2016;16:191–5.
Lähdepuro A, Savolainen K, Lahti-Pulkkinen M, Eriksson JG, Lahti J, Tuovinen S, et al. The impact of early life stress on anxiety symptoms in late adulthood. Sci Rep. 2019;9:4395.
Raposo SM, Mackenzie CS, Henriksen CA, Afifi TO. Time does not heal all wounds: older adults who experienced childhood adversities have higher odds of mood, anxiety, and personality disorders. Am J Geriatr Psychiatry. 2014;22:1241–50.
Zhao X, Li J, Huang Y, Jin Q, Ma H, Wang Y, et al. Genetic variation of FYN contributes to the molecular mechanisms of coping styles in healthy chinese-Han participants. Psychiatr Genet. 2013;23:214–6.
Li Y, Cong X, Chen S, Li Y. Relationships of coping styles and psychological distress among patients with insomnia disorder. BMC Psychiatry. 2021;21:255.
Article CAS PubMed PubMed Central Google Scholar
Otsuka Y, Kaneita Y, Itani O, Nakagome S, Jike M, Ohida T. Relationship between stress coping and sleep disorders among the general Japanese population: a nationwide representative survey. Sleep Med. 2017;37:38–45.
Solberg MA, Peters RM, Resko SM, Templin TN. Does coping mediate the relationship between adverse childhood experiences and Health outcomes in Young adults? J Child Adolesc Trauma. 2023;16:1–13.
Sheffler JL, Piazza JR, Quinn JM, Sachs-Ericsson NJ, Stanley IH. Adverse childhood experiences and coping strategies: identifying pathways to resiliency in adulthood. Anxiety Stress Coping. 2019;32:594–609.
Bjørkløf GH, Engedal K, Selbæk G, Kouwenhoven SE, Helvik A-S. Coping and depression in old age: a literature review. Dement Geriatr Cogn Disord. 2013;35:121–54.
Orgeta V, Orrell M. Coping styles for anxiety and depressive symptoms in Community-Dwelling older adults. Clin Gerontologist. 2014;37:406–17.
Article Google Scholar
Cohen S, Gianaros PJ, Manuck SB. A stage model of stress and disease. Perspect Psychol Sci. 2016;11:456–63.
Folkman S, Stress. Appraisal and Coping. In: Gellman MD, Turner JR, editors. Encyclopedia of behavioral medicine. New York, NY: Springer New York; 2013. pp. 1913–5.
Chapter Google Scholar
Wu X. The Household Registration System and Rural-Urban Educational Inequality in Contemporary China. Chin Sociol Rev. 2011;44:31–51.
Zhang Z, Treiman DJ. Social origins, hukou conversion, and the wellbeing of urban residents in contemporary China. Soc Sci Res. 2013;42:71–89.
Su S, Wang X, Pollock JS, Treiber FA, Xu X, Snieder H, et al. Adverse childhood experiences and blood pressure trajectories from childhood to Young Adulthood: the Georgia stress and heart study. Circulation. 2015;131:1674–81.
Li J, Yao Y, Dong Q, Dong Y, Liu J, Yang L, et al. Characterization and factors associated with sleep quality among rural elderly in China. Arch Gerontol Geriatr. 2013;56:237–43.
Nyqvist F, Cattan M, Conradsson M, Näsman M, Gustafsson Y. Prevalence of loneliness over ten years among the oldest old. Scand J Public Health. 2017;45:411–8.
Yue T, Li Q, Wang R, Liu Z, Guo M, Bai F, et al. Comparison of hospital anxiety and Depression Scale (HADS) and Zung Self-Rating Anxiety/Depression scale (SAS/SDS) in evaluating anxiety and depression in patients with psoriatic arthritis. Dermatology. 2019;236:170–8.
Luo Y, Wang H. Correlation research on psychological health impact on nursing students against stress, coping way and social support. Nurse Educ Today. 2009;29:5–8.
Shao R, He P, Ling B, Tan L, Xu L, Hou Y, et al. Prevalence of depression and anxiety and correlations between depression, anxiety, family functioning, social support and coping styles among Chinese medical students. BMC Psychol. 2020;8:38.
Wen Zhonglin H, Jietai M. Structural equation model testing cutoff criterion for goodness of fit indices and Chi-square test. Acta Physiol Sinica. 2004;36:186–94.
Google Scholar
Kang H, Ahn J-W. Model setting and interpretation of results in Research using Structural equation modeling: a checklist with guiding questions for reporting. Asian Nurs Res (Korean Soc Nurs Sci). 2021;15:157–62.
PubMed Google Scholar
Moraes W, Piovezan R, Poyares D, Bittencourt LR, Santos-Silva R, Tufik S. Effects of aging on sleep structure throughout adulthood: a population-based study. Sleep Med. 2014;15:401–9.
Feinsilver SH. Normal and abnormal sleep in the Elderly. Clin Geriatr Med. 2021;37:377–86.
Ohayon MM, Carskadon MA, Guilleminault C, Vitiello MV. Meta-analysis of quantitative sleep parameters from childhood to old age in healthy individuals: developing normative sleep values across the human lifespan. Sleep. 2004;27:1255–73.
Madrid-Valero JJ, Martínez-Selva JM, Ribeiro do Couto B, Sánchez-Romera JF, Ordoñana JR. Age and gender effects on the prevalence of poor sleep quality in the adult population. Gac Sanit. 2017;31:18–22.
Hwang H, Kim KM, Yun C-H, Yang KI, Chu MK, Kim W-J. Sleep state of the elderly population in Korea: Nationwide cross-sectional population-based study. Front Neurol. 2022;13:1095404.
Lallukka T, Sares-Jäske L, Kronholm E, Sääksjärvi K, Lundqvist A, Partonen T, et al. Sociodemographic and socioeconomic differences in sleep duration and insomnia-related symptoms in Finnish adults. BMC Public Health. 2012;12:565.
Murtagh KN, Hubert HB. Gender differences in physical disability among an elderly cohort. Am J Public Health. 2004;94:1406–11.
Moieni M, Irwin MR, Jevtic I, Olmstead R, Breen EC, Eisenberger NI. Sex differences in depressive and socioemotional responses to an inflammatory challenge: implications for sex differences in depression. Neuropsychopharmacology. 2015;40:1709–16.
Asher M, Aderka IM. Gender differences in social anxiety disorder. J Clin Psychol. 2018;74:1730–41.
Lin CM, Davidson TM, Ancoli-Israel S. Gender differences in obstructive sleep apnea and treatment implications. Sleep Med Rev. 2008;12:481–96.
Li N, Xu G, Chen G, Zheng X. Sleep quality among Chinese elderly people: a population-based study. Arch Gerontol Geriatr. 2020;87:103968.
Yue Z, Zhang Y, Cheng X, Zhang J. Sleep quality among the Elderly in 21st Century Shandong Province, China: a ten-year comparative study. Int J Environ Res Public Health. 2022;19:14296.
Suzuki K, Miyamoto M, Hirata K. Sleep disorders in the elderly: diagnosis and management. J Gen Fam Med. 2017;18:61–71.
Counts CJ, Grubin FC, John-Henderson NA. Childhood socioeconomic status and risk in early family environments: predictors of global sleep quality in college students. Sleep Health. 2018;4:301–6.
Hall Brown TS, Belcher HME, Accardo J, Minhas R, Briggs EC. Trauma exposure and sleep disturbance in a sample of youth from the National Child Traumatic Stress Network Core Data Set. Sleep Health. 2016;2:123–8.
Hulme PA. Childhood sexual abuse, HPA axis regulation, and mental health: an integrative review. West J Nurs Res. 2011;33:1069–97.
Berens AE, Jensen SKG, Nelson CA. Biological embedding of childhood adversity: from physiological mechanisms to clinical implications. BMC Med. 2017;15:135.
Shonkoff JP, Garner AS, Committee on Psychosocial Aspects of Child and Family Health. Committee on Early Childhood, Adoption, and Dependent Care, section on developmental and behavioral pediatrics. The lifelong effects of early childhood adversity and toxic stress. Pediatrics. 2012;129:e232–246.
Lim SS, Vos T, Flaxman AD, Danaei G, Shibuya K, Adair-Rohani H, et al. A comparative risk assessment of burden of disease and injury attributable to 67 risk factors and risk factor clusters in 21 regions, 1990–2010: a systematic analysis for the global burden of Disease Study 2010. Lancet. 2012;380:2224–60.
Asarnow LD, Soehner AM, Harvey AG. Circadian rhythms and psychiatric illness. Curr Opin Psychiatry. 2013;26:566–71.
Jansson-Fröjmark M, Lindblom K. A bidirectional relationship between anxiety and depression, and insomnia? A prospective study in the general population. J Psychosom Res. 2008;64:443–9.
McKay MT, Cannon M, Chambers D, Conroy RM, Coughlan H, Dodd P, et al. Childhood trauma and adult mental disorder: a systematic review and meta-analysis of longitudinal cohort studies. Acta Psychiatr Scand. 2021;143:189–205.
Matheson SL, Shepherd AM, Pinchbeck RM, Laurens KR, Carr VJ. Childhood adversity in schizophrenia: a systematic meta-analysis. Psychol Med. 2013;43:225–38.
Chang KJ, Son SJ, Lee Y, Back JH, Lee KS, Lee SJ, et al. Perceived sleep quality is associated with depression in a Korean elderly population. Arch Gerontol Geriatr. 2014;59:468–73.
Zhu X, Hu Z, Nie Y, Zhu T, Chiwanda Kaminga A, Yu Y, et al. The prevalence of poor sleep quality and associated risk factors among Chinese elderly adults in nursing homes: a cross-sectional study. PLoS ONE. 2020;15:e0232834.
Amarneh D, Lebeaut A, Viana AG, Alfano CA, Vujanovic AA. The role of anxiety sensitivity in the Association between Childhood Maltreatment and Sleep disturbance among adults in Psychiatric Inpatient Treatment. J Nerv Ment Dis. 2023;211:306–13.
Haimov I, Szepsenwol O, Cohen A. Associations between Childhood stressors, COVID-19-Related anxiety, and sleep quality of adults during the Third Wave of the COVID-19 pandemic in Israel. Nat Sci Sleep. 2022;14:1665–75.
Mayo D, Corey S, Kelly LH, Yohannes S, Youngquist AL, Stuart BK, et al. The role of Trauma and Stressful Life events among individuals at Clinical High Risk for psychosis: a review. Front Psychiatry. 2017;8:55.
Ren Z, Zhang X, Shen Y, Li X, He M, Shi H, et al. Associations of negative life events and coping styles with sleep quality among Chinese adolescents: a cross-sectional study. Environ Health Prev Med. 2021;26:85.
Sadeh A, Gruber R. Stress and sleep in adolescence: a clinical-developmental perspective. Adolescent sleep patterns: Biological, social, and psychological influences. New York, NY, US: Cambridge University Press; 2002. pp. 236–53.
Download references
Acknowledgements
We thank the responsible person of local health work, all participants and the staff of data reduction for their cooperation.
This work was funded by the research projects of “Investigation on health status and risk factors of the elderly over 65 years old in Deyang City” (No.301021062) of Chengdu University of Traditional Chinese Medicine.
Author information
Authors and affiliations.
School of Public Health, Chengdu University of Traditional Chinese Medicine, Chengdu, 610075, Sichuan, China
Yuqin Zhang, Hongwei Li, Jin Yan, Mengxue Xie & Lian Yang
Sichuan Provincial Center for Disease Control and Prevention, No.6, Zhongxue Road, Wuhou District, Chengdu, 610041, China
Chengwei Lin
Hospital of Chengdu University of Traditional Chinese Medicine, Deyang Integrated Traditional Chinese and Western Medicine Hospital, Deyang, 618000, China
Centre for Aging Health Service of Deyang City, Deyang, 618000, China
Xueyan Zhou
Health Commission of Deyang City, Deyang, 618000, China
Sichuan Provincial Health Information Center, Chengdu, 610015, Sichuan, China
Xueli Zhang
Centre for Health Management and Policy Research,School of Public Health, Cheeloo College of Medicine,Shandong University, NHC Key Lab of Health Economics and Policy Research, Shandong University, Jinan, 250012, China
Chengchao Zhou
You can also search for this author in PubMed Google Scholar
Contributions
YQ Z, CW L and HW L were responsible for conception and design of the study. L L, XY Z and Y X were involved in recruiting the participants. YQ Z and CW L did the statistical analysis and were involved in manuscript preparation and drafting the article.J Y , MX X, and XL Z were involved in editing and revising the manuscript. CC Z and L Y were responsible for the critical revision of the manuscript. All authors have contributed to and have approved the final manuscript.
Corresponding authors
Correspondence to Chengchao Zhou or Lian Yang .
Ethics declarations
Ethics approval and consent to participate.
The current study was conducted according to the guidelines of the Declaration of Helsinki, approved by the Medical Ethics Committee of the Affiliated Hospital of Chengdu University of Chinese Medicine (Approval no.2023KL-011). All the participants completed informed consent forms before recruitment to the study. For illiterate participants their guardians (usually immediate family members, for example, son, daughter, son and daughter in law etc.) gave written informed consent for participation in the study. The ethics committee had approved the methods of giving consent.
Consent for publication
Not applicable.
Competing interests
The authors declare that they have no conflict of interest.
Additional information
Publisher’s note.
Springer Nature remains neutral with regard to jurisdictional claims in published maps and institutional affiliations.
Rights and permissions
Open Access This article is licensed under a Creative Commons Attribution 4.0 International License, which permits use, sharing, adaptation, distribution and reproduction in any medium or format, as long as you give appropriate credit to the original author(s) and the source, provide a link to the Creative Commons licence, and indicate if changes were made. The images or other third party material in this article are included in the article’s Creative Commons licence, unless indicated otherwise in a credit line to the material. If material is not included in the article’s Creative Commons licence and your intended use is not permitted by statutory regulation or exceeds the permitted use, you will need to obtain permission directly from the copyright holder. To view a copy of this licence, visit http://creativecommons.org/licenses/by/4.0/ . The Creative Commons Public Domain Dedication waiver ( http://creativecommons.org/publicdomain/zero/1.0/ ) applies to the data made available in this article, unless otherwise stated in a credit line to the data.
Reprints and permissions
About this article
Cite this article.
Zhang, Y., Lin, C., Li, H. et al. The relationship between childhood adversity and sleep quality among rural older adults in China: the mediating role of anxiety and negative coping. BMC Psychiatry 24 , 346 (2024). https://doi.org/10.1186/s12888-024-05792-2
Download citation
Received : 03 January 2024
Accepted : 25 April 2024
Published : 08 May 2024
DOI : https://doi.org/10.1186/s12888-024-05792-2
Share this article
Anyone you share the following link with will be able to read this content:
Sorry, a shareable link is not currently available for this article.
Provided by the Springer Nature SharedIt content-sharing initiative
- Rural older adults
- Childhood adversity experiences
- Negative coping
- Chain mediation
BMC Psychiatry
ISSN: 1471-244X
- Submission enquiries: [email protected]
- General enquiries: [email protected]
Thank you for visiting nature.com. You are using a browser version with limited support for CSS. To obtain the best experience, we recommend you use a more up to date browser (or turn off compatibility mode in Internet Explorer). In the meantime, to ensure continued support, we are displaying the site without styles and JavaScript.
- View all journals
- My Account Login
- Explore content
- About the journal
- Publish with us
- Sign up for alerts
- Open access
- Published: 08 May 2024
Exploring the dynamics of consumer engagement in social media influencer marketing: from the self-determination theory perspective
- Chenyu Gu ORCID: orcid.org/0000-0001-6059-0573 1 &
- Qiuting Duan 2
Humanities and Social Sciences Communications volume 11 , Article number: 587 ( 2024 ) Cite this article
404 Accesses
2 Altmetric
Metrics details
- Business and management
- Cultural and media studies
Influencer advertising has emerged as an integral part of social media marketing. Within this realm, consumer engagement is a critical indicator for gauging the impact of influencer advertisements, as it encompasses the proactive involvement of consumers in spreading advertisements and creating value. Therefore, investigating the mechanisms behind consumer engagement holds significant relevance for formulating effective influencer advertising strategies. The current study, grounded in self-determination theory and employing a stimulus-organism-response framework, constructs a general model to assess the impact of influencer factors, advertisement information, and social factors on consumer engagement. Analyzing data from 522 samples using structural equation modeling, the findings reveal: (1) Social media influencers are effective at generating initial online traffic but have limited influence on deeper levels of consumer engagement, cautioning advertisers against overestimating their impact; (2) The essence of higher-level engagement lies in the ad information factor, affirming that in the new media era, content remains ‘king’; (3) Interpersonal factors should also be given importance, as influencing the surrounding social groups of consumers is one of the effective ways to enhance the impact of advertising. Theoretically, current research broadens the scope of both social media and advertising effectiveness studies, forming a bridge between influencer marketing and consumer engagement. Practically, the findings offer macro-level strategic insights for influencer marketing.
Similar content being viewed by others
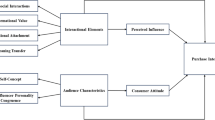
Exploring the effects of audience and strategies used by beauty vloggers on behavioural intention towards endorsed brands
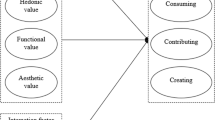
COBRAs and virality: viral campaign values on consumer behaviour
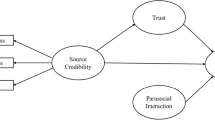
Exploring the impact of beauty vloggers’ credible attributes, parasocial interaction, and trust on consumer purchase intention in influencer marketing
Introduction.
Recent studies have highlighted an escalating aversion among audiences towards traditional online ads, leading to a diminishing effectiveness of traditional online advertising methods (Lou et al., 2019 ). In an effort to overcome these challenges, an increasing number of brands are turning to influencers as their spokespersons for advertising. Utilizing influencers not only capitalizes on their significant influence over their fan base but also allows for the dissemination of advertising messages in a more native and organic manner. Consequently, influencer-endorsed advertising has become a pivotal component and a growing trend in social media advertising (Gräve & Bartsch, 2022 ). Although the topic of influencer-endorsed advertising has garnered increasing attention from scholars, the field is still in its infancy, offering ample opportunities for in-depth research and exploration (Barta et al., 2023 ).
Presently, social media influencers—individuals with substantial follower bases—have emerged as the new vanguard in advertising (Hudders & Lou, 2023 ). Their tweets and videos possess the remarkable potential to sway the purchasing decisions of thousands if not millions. This influence largely hinges on consumer engagement behaviors, implying that the impact of advertising can proliferate throughout a consumer’s entire social network (Abbasi et al., 2023 ). Consequently, exploring ways to enhance consumer engagement is of paramount theoretical and practical significance for advertising effectiveness research (Xiao et al., 2023 ). This necessitates researchers to delve deeper into the exploration of the stimulating factors and psychological mechanisms influencing consumer engagement behaviors (Vander Schee et al., 2020 ), which is the gap this study seeks to address.
The Stimulus-Organism-Response (S-O-R) framework has been extensively applied in the study of consumer engagement behaviors (Tak & Gupta, 2021 ) and has been shown to integrate effectively with self-determination theory (Yang et al., 2019 ). Therefore, employing the S-O-R framework to investigate consumer engagement behaviors in the context of influencer advertising is considered a rational approach. The current study embarks on an in-depth analysis of the transformation process from three distinct dimensions. In the Stimulus (S) phase, we focus on how influencer factors, advertising message factors, and social influence factors act as external stimuli. This phase scrutinizes the external environment’s role in triggering consumer reactions. During the Organism (O) phase, the research explores the intrinsic psychological motivations affecting individual behavior as posited in self-determination theory. This includes the willingness for self-disclosure, the desire for innovation, and trust in advertising messages. The investigation in this phase aims to understand how these internal motivations shape consumer attitudes and perceptions in the context of influencer marketing. Finally, in the Response (R) phase, the study examines how these psychological factors influence consumer engagement behavior. This part of the research seeks to understand the transition from internal psychological states to actual consumer behavior, particularly how these states drive the consumers’ deep integration and interaction with the influencer content.
Despite the inherent limitations of cross-sectional analysis in capturing the full temporal dynamics of consumer engagement, this study seeks to unveil the dynamic interplay between consumers’ psychological needs—autonomy, competence, and relatedness—and their varying engagement levels in social media influencer marketing, grounded in self-determination theory. Through this lens, by analyzing factors related to influencers, content, and social context, we aim to infer potential dynamic shifts in engagement behaviors as psychological needs evolve. This approach allows us to offer a snapshot of the complex, multi-dimensional nature of consumer engagement dynamics, providing valuable insights for both theoretical exploration and practical application in the constantly evolving domain of social media marketing. Moreover, the current study underscores the significance of adapting to the dynamic digital environment and highlights the evolving nature of consumer engagement in the realm of digital marketing.
Literature review
Stimulus-organism-response (s-o-r) model.
The Stimulus-Response (S-R) model, originating from behaviorist psychology and introduced by psychologist Watson ( 1917 ), posits that individual behaviors are directly induced by external environmental stimuli. However, this model overlooks internal personal factors, complicating the explanation of psychological states. Mehrabian and Russell ( 1974 ) expanded this by incorporating the individual’s cognitive component (organism) into the model, creating the Stimulus-Organism-Response (S-O-R) framework. This model has become a crucial theoretical framework in consumer psychology as it interprets internal psychological cognitions as mediators between stimuli and responses. Integrating with psychological theories, the S-O-R model effectively analyzes and explains the significant impact of internal psychological factors on behavior (Koay et al., 2020 ; Zhang et al., 2021 ), and is extensively applied in investigating user behavior on social media platforms (Hewei & Youngsook, 2022 ). This study combines the S-O-R framework with self-determination theory to examine consumer engagement behaviors in the context of social media influencer advertising, a logic also supported by some studies (Yang et al., 2021 ).
Self-determination theory
Self-determination theory, proposed by Richard and Edward (2000), is a theoretical framework exploring human behavioral motivation and personality. The theory emphasizes motivational processes, positing that individual behaviors are developed based on factors satisfying their psychological needs. It suggests that individual behavioral tendencies are influenced by the needs for competence, relatedness, and autonomy. Furthermore, self-determination theory, along with organic integration theory, indicates that individual behavioral tendencies are also affected by internal psychological motivations and external situational factors.
Self-determination theory has been validated by scholars in the study of online user behaviors. For example, Sweet applied the theory to the investigation of community building in online networks, analyzing knowledge-sharing behaviors among online community members (Sweet et al., 2020 ). Further literature review reveals the applicability of self-determination theory to consumer engagement behaviors, particularly in the context of influencer marketing advertisements. Firstly, self-determination theory is widely applied in studying the psychological motivations behind online behaviors, suggesting that the internal and external motivations outlined within the theory might also apply to exploring consumer behaviors in influencer marketing scenarios (Itani et al., 2022 ). Secondly, although research on consumer engagement in the social media influencer advertising context is still in its early stages, some studies have utilized SDT to explore behaviors such as information sharing and electronic word-of-mouth dissemination (Astuti & Hariyawan, 2021 ). These behaviors, which are part of the content contribution and creation dimensions of consumer engagement, may share similarities in the underlying psychological motivational mechanisms. Thus, this study will build upon these foundations to construct the Organism (O) component of the S-O-R model, integrating insights from SDT to further understand consumer engagement in influencer marketing.
Consumer engagement
Although scholars generally agree at a macro level to define consumer engagement as the creation of additional value by consumers or customers beyond purchasing products, the specific categorization of consumer engagement varies in different studies. For instance, Simon and Tossan interpret consumer engagement as a psychological willingness to interact with influencers (Simon & Tossan, 2018 ). However, such a broad definition lacks precision in describing various levels of engagement. Other scholars directly use tangible metrics on social media platforms, such as likes, saves, comments, and shares, to represent consumer engagement (Lee et al., 2018 ). While this quantitative approach is not flawed and can be highly effective in practical applications, it overlooks the content aspect of engagement, contradicting the “content is king” principle of advertising and marketing. We advocate for combining consumer engagement with the content aspect, as content engagement not only generates more traces of consumer online behavior (Oestreicher-Singer & Zalmanson, 2013 ) but, more importantly, content contribution and creation are central to social media advertising and marketing, going beyond mere content consumption (Qiu & Kumar, 2017 ). Meanwhile, we also need to emphasize that engagement is not a fixed state but a fluctuating process influenced by ongoing interactions between consumers and influencers, mediated by the evolving nature of social media platforms and the shifting sands of consumer preferences (Pradhan et al., 2023 ). Consumer engagement in digital environments undergoes continuous change, reflecting a journey rather than a destination (Viswanathan et al., 2017 ).
The current study adopts a widely accepted definition of consumer engagement from existing research, offering operational feasibility and aligning well with the research objectives of this paper. Consumer engagement behaviors in the context of this study encompass three dimensions: content consumption, content contribution, and content creation (Muntinga et al., 2011 ). These dimensions reflect a spectrum of digital engagement behaviors ranging from low to high levels (Schivinski et al., 2016 ). Specifically, content consumption on social media platforms represents a lower level of engagement, where consumers merely click and read the information but do not actively contribute or create user-generated content. Some studies consider this level of engagement as less significant for in-depth exploration because content consumption, compared to other forms, generates fewer visible traces of consumer behavior (Brodie et al., 2013 ). Even in a study by Qiu and Kumar, it was noted that the conversion rate of content consumption is low, contributing minimally to the success of social media marketing (Qiu & Kumar, 2017 ).
On the other hand, content contribution, especially content creation, is central to social media marketing. When consumers comment on influencer content or share information with their network nodes, it is termed content contribution, representing a medium level of online consumer engagement (Piehler et al., 2019 ). Furthermore, when consumers actively upload and post brand-related content on social media, this higher level of behavior is referred to as content creation. Content creation represents the highest level of consumer engagement (Cheung et al., 2021 ). Although medium and high levels of consumer engagement are more valuable for social media advertising and marketing, this exploratory study still retains the content consumption dimension of consumer engagement behaviors.
Theoretical framework
Internal organism factors: self-disclosure willingness, innovativeness, and information trust.
In existing research based on self-determination theory that focuses on online behavior, competence, relatedness, and autonomy are commonly considered as internal factors influencing users’ online behaviors. However, this approach sometimes strays from the context of online consumption. Therefore, in studies related to online consumption, scholars often use self-disclosure willingness as an overt representation of autonomy, innovativeness as a representation of competence, and trust as a representation of relatedness (Mahmood et al., 2019 ).
The use of these overt variables can be logically explained as follows: According to self-determination theory, individuals with a higher level of self-determination are more likely to adopt compensatory mechanisms to facilitate behavior compared to those with lower self-determination (Wehmeyer, 1999 ). Self-disclosure, a voluntary act of sharing personal information with others, is considered a key behavior in the development of interpersonal relationships. In social environments, self-disclosure can effectively alleviate stress and build social connections, while also seeking societal validation of personal ideas (Altman & Taylor, 1973 ). Social networks, as para-social entities, possess the interactive attributes of real societies and are likely to exhibit similar mechanisms. In consumer contexts, personal disclosures can include voluntary sharing of product interests, consumption experiences, and future purchase intentions (Robertshaw & Marr, 2006 ). While material incentives can prompt personal information disclosure, many consumers disclose personal information online voluntarily, which can be traced back to an intrinsic need for autonomy (Stutzman et al., 2011 ). Thus, in this study, we consider the self-disclosure willingness as a representation of high autonomy.
Innovativeness refers to an individual’s internal level of seeking novelty and represents their personality and tendency for novelty (Okazaki, 2009 ). Often used in consumer research, innovative consumers are inclined to try new technologies and possess an intrinsic motivation to use new products. Previous studies have shown that consumers with high innovativeness are more likely to search for information on new products and share their experiences and expertise with others, reflecting a recognition of their own competence (Kaushik & Rahman, 2014 ). Therefore, in consumer contexts, innovativeness is often regarded as the competence dimension within the intrinsic factors of self-determination (Wang et al., 2016 ), with external motivations like information novelty enhancing this intrinsic motivation (Lee et al., 2015 ).
Trust refers to an individual’s willingness to rely on the opinions of others they believe in. From a social psychological perspective, trust indicates the willingness to assume the risk of being harmed by another party (McAllister, 1995 ). Widely applied in social media contexts for relational marketing, information trust has been proven to positively influence the exchange and dissemination of consumer information, representing a close and advanced relationship between consumers and businesses, brands, or advertising endorsers (Steinhoff et al., 2019 ). Consumers who trust brands or social media influencers are more willing to share information without fear of exploitation (Pop et al., 2022 ), making trust a commonly used representation of the relatedness dimension in self-determination within consumer contexts.
Construction of the path from organism to response: self-determination internal factors and consumer engagement behavior
Following the logic outlined above, the current study represents the internal factors of self-determination theory through three variables: self-disclosure willingness, innovativeness, and information trust. Next, the study explores the association between these self-determination internal factors and consumer engagement behavior, thereby constructing the link between Organism (O) and Response (R).
Self-disclosure willingness and consumer engagement behavior
In the realm of social sciences, the concept of self-disclosure willingness has been thoroughly examined from diverse disciplinary perspectives, encompassing communication studies, sociology, and psychology. Viewing from the lens of social interaction dynamics, self-disclosure is acknowledged as a fundamental precondition for the initiation and development of online social relationships and interactive engagements (Luo & Hancock, 2020 ). It constitutes an indispensable component within the spectrum of interactive behaviors and the evolution of interpersonal connections. Voluntary self-disclosure is characterized by individuals divulging information about themselves, which typically remains unknown to others and is inaccessible through alternative sources. This concept aligns with the tenets of uncertainty reduction theory, which argues that during interpersonal engagements, individuals seek information about their counterparts as a means to mitigate uncertainties inherent in social interactions (Lee et al., 2008 ). Self-disclosure allows others to gain more personal information, thereby helping to reduce the uncertainty in interpersonal relationships. Such disclosure is voluntary rather than coerced, and this sharing of information can facilitate the development of relationships between individuals (Towner et al., 2022 ). Furthermore, individuals who actively engage in social media interactions (such as liking, sharing, and commenting on others’ content) often exhibit higher levels of self-disclosure (Chu et al., 2023 ); additional research indicates a positive correlation between self-disclosure and online engagement behaviors (Lee et al., 2023 ). Taking the context of the current study, the autonomous self-disclosure willingness can incline social media users to read advertising content more attentively and share information with others, and even create evaluative content. Therefore, this paper proposes the following research hypothesis:
H1a: The self-disclosure willingness is positively correlated with content consumption in consumer engagement behavior.
H1b: The self-disclosure willingness is positively correlated with content contribution in consumer engagement behavior.
H1c: The self-disclosure willingness is positively correlated with content creation in consumer engagement behavior.
Innovativeness and consumer engagement behavior
Innovativeness represents an individual’s propensity to favor new technologies and the motivation to use new products, associated with the cognitive perception of one’s self-competence. Individuals with a need for self-competence recognition often exhibit higher innovativeness (Kelley & Alden, 2016 ). Existing research indicates that users with higher levels of innovativeness are more inclined to accept new product information and share their experiences and discoveries with others in their social networks (Yusuf & Busalim, 2018 ). Similarly, in the context of this study, individuals, as followers of influencers, signify an endorsement of the influencer. Driven by innovativeness, they may be more eager to actively receive information from influencers. If they find the information valuable, they are likely to share it and even engage in active content re-creation to meet their expectations of self-image. Therefore, this paper proposes the following research hypotheses:
H2a: The innovativeness of social media users is positively correlated with content consumption in consumer engagement behavior.
H2b: The innovativeness of social media users is positively correlated with content contribution in consumer engagement behavior.
H2c: The innovativeness of social media users is positively correlated with content creation in consumer engagement behavior.
Information trust and consumer engagement
Trust refers to an individual’s willingness to rely on the statements and opinions of a target object (Moorman et al., 1993 ). Extensive research indicates that trust positively impacts information dissemination and content sharing in interpersonal communication environments (Majerczak & Strzelecki, 2022 ); when trust is established, individuals are more willing to share their resources and less suspicious of being exploited. Trust has also been shown to influence consumers’ participation in community building and content sharing on social media, demonstrating cross-cultural universality (Anaya-Sánchez et al., 2020 ).
Trust in influencer advertising information is also a key predictor of consumers’ information exchange online. With many social media users now operating under real-name policies, there is an increased inclination to trust information shared on social media over that posted by corporate accounts or anonymously. Additionally, as users’ social networks partially overlap with their real-life interpersonal networks, extensive research shows that more consumers increasingly rely on information posted and shared on social networks when making purchase decisions (Wang et al., 2016 ). This aligns with the effectiveness goals of influencer marketing advertisements and the characteristics of consumer engagement. Trust in the content posted by influencers is considered a manifestation of a strong relationship between fans and influencers, central to relationship marketing (Kim & Kim, 2021 ). Based on trust in the influencer, which then extends to trust in their content, people are more inclined to browse information posted by influencers, share this information with others, and even create their own content without fear of exploitation or negative consequences. Therefore, this paper proposes the following research hypotheses:
H3a: Information trust is positively correlated with content consumption in consumer engagement behavior.
H3b: Information trust is positively correlated with content contribution in consumer engagement behavior.
H3c: Information trust is positively correlated with content creation in consumer engagement behavior.
Construction of the path from stimulus to organism: influencer factors, advertising information factors, social factors, and self-determination internal factors
Having established the logical connection from Organism (O) to Response (R), we further construct the influence path from Stimulus (S) to Organism (O). Revisiting the definition of influencer advertising in social media, companies, and brands leverage influencers on social media platforms to disseminate advertising content, utilizing the influencers’ relationships and influence over consumers for marketing purposes. In addition to consumer’s internal factors, elements such as companies, brands, influencers, and the advertisements themselves also impact consumer behavior. Although factors like the brand image perception of companies may influence consumer behavior, considering that in influencer marketing, companies and brands do not directly interact with consumers, this study prioritizes the dimensions of influencers and advertisements. Furthermore, the impact of social factors on individual cognition and behavior is significant, thus, the current study integrates influencers, advertisements, and social dimensions as the Stimulus (S) component.
Influencer factors: parasocial identification
Self-determination theory posits that relationships are one of the key motivators influencing individual behavior. In the context of social media research, users anticipate establishing a parasocial relationship with influencers, resembling real-life relationships. Hence, we consider the parasocial identification arising from users’ parasocial interactions with influencers as the relational motivator. Parasocial interaction refers to the one-sided personal relationship that individuals develop with media characters (Donald & Richard, 1956 ). During this process, individuals believe that the media character is directly communicating with them, creating a sense of positive intimacy (Giles, 2002 ). Over time, through repeated unilateral interactions with media characters, individuals develop a parasocial relationship, leading to parasocial identification. However, parasocial identification should not be directly equated with the concept of social identification in social identity theory. Social identification occurs when individuals psychologically de-individualize themselves, perceiving the characteristics of their social group as their own, upon identifying themselves as part of that group. In contrast, parasocial identification refers to the one-sided interactional identification with media characters (such as celebrities or influencers) over time (Chen et al., 2021 ). Particularly when individuals’ needs for interpersonal interaction are not met in their daily lives, they turn to parasocial interactions to fulfill these needs (Shan et al., 2020 ). Especially on social media, which is characterized by its high visibility and interactivity, users can easily develop a strong parasocial identification with the influencers they follow (Wei et al., 2022 ).
Parasocial identification and self-disclosure willingness
Theories like uncertainty reduction, personal construct, and social exchange are often applied to explain the emergence of parasocial identification. Social media, with its convenient and interactive modes of information dissemination, enables consumers to easily follow influencers on media platforms. They can perceive the personality of influencers through their online content, viewing them as familiar individuals or even friends. Once parasocial identification develops, this pleasurable experience can significantly influence consumers’ cognitions and thus their behavioral responses. Research has explored the impact of parasocial identification on consumer behavior. For instance, Bond et al. found that on Twitter, the intensity of users’ parasocial identification with influencers positively correlates with their continuous monitoring of these influencers’ activities (Bond, 2016 ). Analogous to real life, where we tend to pay more attention to our friends in our social networks, a similar phenomenon occurs in the relationship between consumers and brands. This type of parasocial identification not only makes consumers willing to follow brand pages but also more inclined to voluntarily provide personal information (Chen et al., 2021 ). Based on this logic, we speculate that a similar relationship may exist between social media influencers and their fans. Fans develop parasocial identification with influencers through social media interactions, making them more willing to disclose their information, opinions, and views in the comment sections of the influencers they follow, engaging in more frequent social interactions (Chung & Cho, 2017 ), even if the content at times may be brand or company-embedded marketing advertisements. In other words, in the presence of influencers with whom they have established parasocial relationships, they are more inclined to disclose personal information, thereby promoting consumer engagement behavior. Therefore, we propose the following research hypotheses:
H4: Parasocial identification is positively correlated with consumer self-disclosure willingness.
H4a: Self-disclosure willingness mediates the impact of parasocial identification on content consumption in consumer engagement behavior.
H4b: Self-disclosure willingness mediates the impact of parasocial identification on content contribution in consumer engagement behavior.
H4c: Self-disclosure willingness mediates the impact of parasocial identification on content creation in consumer engagement behavior.
Parasocial identification and information trust
Information Trust refers to consumers’ willingness to trust the information contained in advertisements and to place themselves at risk. These risks include purchasing products inconsistent with the advertised information and the negative social consequences of erroneously spreading this information to others, leading to unpleasant consumption experiences (Minton, 2015 ). In advertising marketing, gaining consumers’ trust in advertising information is crucial. In the context of influencer marketing on social media, companies, and brands leverage the social connection between influencers and their fans. According to cognitive empathy theory, consumers project their trust in influencers onto the products endorsed, explaining the phenomenon of ‘loving the house for the crow on its roof.’ Research indicates that parasocial identification with influencers is a necessary condition for trust development. Consumers engage in parasocial interactions with influencers on social media, leading to parasocial identification (Jin et al., 2021 ). Consumers tend to reduce their cognitive load and simplify their decision-making processes, thus naturally adopting a positive attitude and trust towards advertising information disseminated by influencers with whom they have established parasocial identification. This forms the core logic behind the success of influencer marketing advertisements (Breves et al., 2021 ); furthermore, as mentioned earlier, because consumers trust these advertisements, they are also willing to share this information with friends and family and even engage in content re-creation. Therefore, we propose the following research hypotheses:
H5: Parasocial identification is positively correlated with information trust.
H5a: Information trust mediates the impact of parasocial identification on content consumption in consumer engagement behavior.
H5b: Information trust mediates the impact of parasocial identification on content contribution in consumer engagement behavior.
H5c: Information trust mediates the impact of parasocial identification on content creation in consumer engagement behavior.
Influencer factors: source credibility
Source credibility refers to the degree of trust consumers place in the influencer as a source, based on the influencer’s reliability and expertise. Numerous studies have validated the effectiveness of the endorsement effect in advertising (Schouten et al., 2021 ). The Source Credibility Model, proposed by the renowned American communication scholar Hovland and the “Yale School,” posits that in the process of information dissemination, the credibility of the source can influence the audience’s decision to accept the information. The credibility of the information is determined by two aspects of the source: reliability and expertise. Reliability refers to the audience’s trust in the “communicator’s objective and honest approach to providing information,” while expertise refers to the audience’s trust in the “communicator being perceived as an effective source of information” (Hovland et al., 1953 ). Hovland’s definitions reveal that the interpretation of source credibility is not about the inherent traits of the source itself but rather the audience’s perception of the source (Jang et al., 2021 ). This differs from trust and serves as a precursor to the development of trust. Specifically, reliability and expertise are based on the audience’s perception; thus, this aligns closely with the audience’s perception of influencers (Kim & Kim, 2021 ). This credibility is a cognitive statement about the source of information.
Source credibility and self-disclosure willingness
Some studies have confirmed the positive impact of an influencer’s self-disclosure on their credibility as a source (Leite & Baptista, 2022 ). However, few have explored the impact of an influencer’s credibility, as a source, on consumers’ self-disclosure willingness. Undoubtedly, an impact exists; self-disclosure is considered a method to attempt to increase intimacy with others (Leite et al., 2022 ). According to social exchange theory, people promote relationships through the exchange of information in interpersonal communication to gain benefits (Cropanzano & Mitchell, 2005 ). Credibility, deriving from an influencer’s expertise and reliability, means that a highly credible influencer may provide more valuable information to consumers. Therefore, based on the social exchange theory’s logic of reciprocal benefits, consumers might be more willing to disclose their information to trustworthy influencers, potentially even expanding social interactions through further consumer engagement behaviors. Thus, we propose the following research hypotheses:
H6: Source credibility is positively correlated with self-disclosure willingness.
H6a: Self-disclosure willingness mediates the impact of Source credibility on content consumption in consumer engagement behavior.
H6b: Self-disclosure willingness mediates the impact of Source credibility on content contribution in consumer engagement behavior.
H6c: Self-disclosure willingness mediates the impact of Source credibility on content creation in consumer engagement behavior.
Source credibility and information trust
Based on the Source Credibility Model, the credibility of an endorser as an information source can significantly influence consumers’ acceptance of the information (Shan et al., 2020 ). Existing research has demonstrated the positive impact of source credibility on consumers. Djafarova, in a study based on Instagram, noted through in-depth interviews with 18 users that an influencer’s credibility significantly affects respondents’ trust in the information they post. This credibility is composed of expertise and relevance to consumers, and influencers on social media are considered more trustworthy than traditional celebrities (Djafarova & Rushworth, 2017 ). Subsequently, Bao and colleagues validated in the Chinese consumer context, based on the ELM model and commitment-trust theory, that the credibility of brand pages on Weibo effectively fosters consumer trust in the brand, encouraging participation in marketing activities (Bao & Wang, 2021 ). Moreover, Hsieh et al. found that in e-commerce contexts, the credibility of the source is a significant factor influencing consumers’ trust in advertising information (Hsieh & Li, 2020 ). In summary, existing research has proven that the credibility of the source can promote consumer trust. Influencer credibility is a significant antecedent affecting consumers’ trust in the advertised content they publish. In brand communities, trust can foster consumer engagement behaviors (Habibi et al., 2014 ). Specifically, consumers are more likely to trust the advertising content published by influencers with higher credibility (more expertise and reliability), and as previously mentioned, consumer engagement behavior is more likely to occur. Based on this, the study proposes the following research hypotheses:
H7: Source credibility is positively correlated with information trust.
H7a: Information trust mediates the impact of source credibility on content consumption in consumer engagement behavior.
H7b: Information trust mediates the impact of source credibility on content contribution in consumer engagement behavior.
H7c: Information trust mediates the impact of source credibility on content creation in consumer engagement behavior.
Advertising information factors: informative value
Advertising value refers to “the relative utility value of advertising information to consumers and is a subjective evaluation by consumers.” In his research, Ducoffe pointed out that in the context of online advertising, the informative value of advertising is a significant component of advertising value (Ducoffe, 1995 ). Subsequent studies have proven that consumers’ perception of advertising value can effectively promote their behavioral response to advertisements (Van-Tien Dao et al., 2014 ). Informative value of advertising refers to “the information about products needed by consumers provided by the advertisement and its ability to enhance consumer purchase satisfaction.” From the perspective of information dissemination, valuable advertising information should help consumers make better purchasing decisions and reduce the effort spent searching for product information. The informational aspect of advertising has been proven to effectively influence consumers’ cognition and, in turn, their behavior (Haida & Rahim, 2015 ).
Informative value and innovativeness
As previously discussed, consumers’ innovativeness refers to their psychological trait of favoring new things. Studies have shown that consumers with high innovativeness prefer novel and valuable product information, as it satisfies their need for newness and information about new products, making it an important factor in social media advertising engagement (Shi, 2018 ). This paper also hypothesizes that advertisements with high informative value can activate consumers’ innovativeness, as the novelty of information is one of the measures of informative value (León et al., 2009 ). Acquiring valuable information can make individuals feel good about themselves and fulfill their perception of a “novel image.” According to social exchange theory, consumers can gain social capital in interpersonal interactions (such as social recognition) by sharing information about these new products they perceive as valuable. Therefore, the current study proposes the following research hypothesis:
H8: Informative value is positively correlated with innovativeness.
H8a: Innovativeness mediates the impact of informative value on content consumption in consumer engagement behavior.
H8b: Innovativeness mediates the impact of informative value on content contribution in consumer engagement behavior.
H8c: Innovativeness mediates the impact of informative value on content creation in consumer engagement behavior.
Informative value and information trust
Trust is a multi-layered concept explored across various disciplines, including communication, marketing, sociology, and psychology. For the purposes of this paper, a deep analysis of different levels of trust is not undertaken. Here, trust specifically refers to the trust in influencer advertising information within the context of social media marketing, denoting consumers’ belief in and reliance on the advertising information endorsed by influencers. Racherla et al. investigated the factors influencing consumers’ trust in online reviews, suggesting that information quality and value contribute to increasing trust (Racherla et al., 2012 ). Similarly, Luo and Yuan, in a study based on social media marketing, also confirmed that the value of advertising information posted on brand pages can foster consumer trust in the content (Lou & Yuan, 2019 ). Therefore, by analogy, this paper posits that the informative value of influencer-endorsed advertising can also promote consumer trust in that advertising information. The relationship between trust in advertising information and consumer engagement behavior has been discussed earlier. Thus, the current study proposes the following research hypotheses:
H9: Informative value is positively correlated with information trust.
H9a: Information trust mediates the impact of informative value on content consumption in consumer engagement behavior.
H9b: Information trust mediates the impact of informative value on content contribution in consumer engagement behavior.
H9c: Information trust mediates the impact of informative value on content creation in consumer engagement behavior.
Advertising information factors: ad targeting accuracy
Ad targeting accuracy refers to the degree of match between the substantive information contained in advertising content and consumer needs. Advertisements containing precise information often yield good advertising outcomes. In marketing practice, advertisers frequently use information technology to analyze the characteristics of different consumer groups in the target market and then target their advertisements accordingly to achieve precise dissemination and, consequently, effective advertising results. The utility of ad targeting accuracy has been confirmed by many studies. For instance, in the research by Qiu and Chen, using a modified UTAUT model, it was demonstrated that the accuracy of advertising effectively promotes consumer acceptance of advertisements in WeChat Moments (Qiu & Chen, 2018 ). Although some studies on targeted advertising also indicate that overly precise ads may raise concerns about personal privacy (Zhang et al., 2019 ), overall, the accuracy of advertising information is effective in enhancing advertising outcomes and is a key element in the success of targeted advertising.
Ad targeting accuracy and information trust
In influencer marketing advertisements, due to the special relationship recognition between consumers and influencers, the privacy concerns associated with ad targeting accuracy are alleviated (Vrontis et al., 2021 ). Meanwhile, the informative value brought by targeting accuracy is highlighted. More precise advertising content implies higher informative value and also signifies that the advertising content is more worthy of consumer trust (Della Vigna, Gentzkow, 2010 ). As previously discussed, people are more inclined to read and engage with advertising content they trust and recognize. Therefore, the current study proposes the following research hypotheses:
H10: Ad targeting accuracy is positively correlated with information trust.
H10a: Information trust mediates the impact of ad targeting accuracy on content consumption in consumer engagement behavior.
H10b: Information trust mediates the impact of ad targeting accuracy on content contribution in consumer engagement behavior.
H10c: Information trust mediates the impact of ad targeting accuracy on content creation in consumer engagement behavior.
Social factors: subjective norm
The Theory of Planned Behavior, proposed by Ajzen ( 1991 ), suggests that individuals’ actions are preceded by conscious choices and are underlain by plans. TPB has been widely used by scholars in studying personal online behaviors, these studies collectively validate the applicability of TPB in the context of social media for researching online behaviors (Huang, 2023 ). Additionally, the self-determination theory, which underpins this chapter’s research, also supports the notion that individuals’ behavioral decisions are based on internal cognitions, aligning with TPB’s assertions. Therefore, this paper intends to select subjective norms from TPB as a factor of social influence. Subjective norm refers to an individual’s perception of the expectations of significant others in their social relationships regarding their behavior. Empirical research in the consumption field has demonstrated the significant impact of subjective norms on individual psychological cognition (Yang & Jolly, 2009 ). A meta-analysis by Hagger, Chatzisarantis ( 2009 ) even highlighted the statistically significant association between subjective norms and self-determination factors. Consequently, this study further explores its application in the context of influencer marketing advertisements on social media.
Subjective norm and self-disclosure willingness
In numerous studies on social media privacy, subjective norms significantly influence an individual’s self-disclosure willingness. Wirth et al. ( 2019 ) based on the privacy calculus theory, surveyed 1,466 participants and found that personal self-disclosure on social media is influenced by the behavioral expectations of other significant reference groups around them. Their research confirmed that subjective norms positively influence self-disclosure of information and highlighted that individuals’ cognitions and behaviors cannot ignore social and environmental factors. Heirman et al. ( 2013 ) in an experiment with Instagram users, also noted that subjective norms could promote positive consumer behavioral responses. Specifically, when important family members and friends highly regard social media influencers as trustworthy, we may also be more inclined to disclose our information to influencers and share this information with our surrounding family and friends without fear of disapproval. In our subjective norms, this is considered a positive and valuable interactive behavior, leading us to exhibit engagement behaviors. Based on this logic, we propose the following research hypotheses:
H11: Subjective norms are positively correlated with self-disclosure willingness.
H11a: Self-disclosure willingness mediates the impact of subjective norms on content consumption in consumer engagement behavior.
H11b: Self-disclosure willingness mediates the impact of subjective norms on content contribution in consumer engagement behavior.
H11c: Self-disclosure willingness mediates the impact of subjective norms on content creation in consumer engagement behavior.
Subjective norm and information trust
Numerous studies have indicated that subjective norms significantly influence trust (Roh et al., 2022 ). This can be explained by reference group theory, suggesting people tend to minimize the effort expended in decision-making processes, often looking to the behaviors or attitudes of others as a point of reference; for instance, subjective norms can foster acceptance of technology by enhancing trust (Gupta et al., 2021 ). Analogously, if a consumer’s social network generally holds positive attitudes toward influencer advertising, they are also more likely to trust the endorsed advertisement information, as it conserves the extensive effort required in gathering product information (Chetioui et al., 2020 ). Therefore, this paper proposes the following research hypotheses:
H12: Subjective norms are positively correlated with information trust.
H12a: Information trust mediates the impact of subjective norms on content consumption in consumer engagement behavior.
H12b: Information trust mediates the impact of subjective norms on content contribution in consumer engagement behavior.
H12c: Information trust mediates the impact of subjective norms on content creation in consumer engagement behavior.
Conceptual model
In summary, based on the Stimulus (S)-Organism (O)-Response (R) framework, this study constructs the external stimulus factors (S) from three dimensions: influencer factors (parasocial identification, source credibility), advertising information factors (informative value, Ad targeting accuracy), and social influence factors (subjective norms). This is grounded in social capital theory and the theory of planned behavior. drawing on self-determination theory, the current study constructs the individual psychological factors (O) using self-disclosure willingness, innovativeness, and information trust. Finally, the behavioral response (R) is constructed using consumer engagement, which includes content consumption, content contribution, and content creation, as illustrated in Fig. 1 .
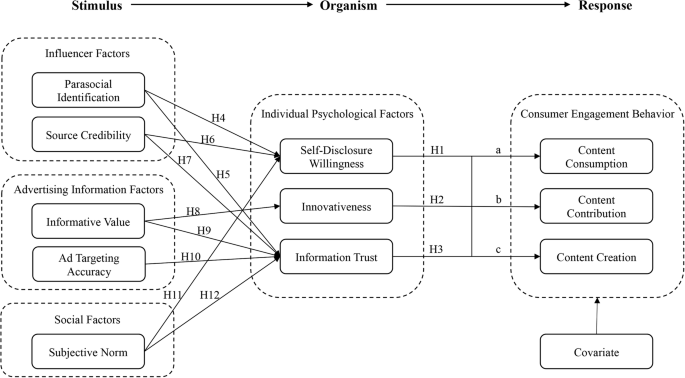
Consumer engagement behavior impact model based on SOR framework.
Materials and methods
Participants and procedures.
The current study conducted a survey through the Wenjuanxing platform to collect data. Participants were recruited through social media platforms such as WeChat, Douyin, Weibo et al., as samples drawn from social media users better align with the research purpose of our research and ensure the validity of the sample. Before the survey commenced, all participants were explicitly informed about the purpose of this study, and it was made clear that volunteers could withdraw from the survey at any time. Initially, 600 questionnaires were collected, with 78 invalid responses excluded. The criteria for valid questionnaires were as follows: (1) Respondents must have answered “Yes” to the question, “Do you follow any influencers (internet celebrities) on social media platforms?” as samples not using social media or not following influencers do not meet the study’s objective, making this question a prerequisite for continuing the survey; (2) Respondents had to correctly answer two hidden screening questions within the questionnaire to ensure that they did not randomly select scores; (3) The total time taken to complete the questionnaire had to exceed one minute, ensuring that respondents had sufficient time to understand and thoughtfully answer each question; (4) Respondents were not allowed to choose the same score for eight consecutive questions. Ultimately, 522 valid questionnaires were obtained, with an effective rate of 87.00%, meeting the basic sample size requirements for research models (Gefen et al., 2011 ). Detailed demographic information of the study participants is presented in Table 1 .
Measurements
To ensure the validity and reliability of the data analysis results in this study, the measurement tools and scales used in this chapter were designed with reference to existing established research. The main variables in the survey questionnaire include parasocial identification, source credibility, informative value, ad targeting accuracy, subjective norms, self-disclosure willingness, innovativeness, information trust, content consumption, content contribution, and content creation. The measurement scale for parasocial identification was adapted from the research of Schramm and Hartmann, comprising 6 items (Schramm & Hartmann, 2008 ). The source credibility scale was combined from the studies of Cheung et al. and Luo & Yuan’s research in the context of social media influencer marketing, including 4 items (Cheung et al., 2009 ; Lou & Yuan, 2019 ). The scale for informative value was modified based on Voss et al.‘s research, consisting of 4 items (Voss et al., 2003 ). The ad targeting accuracy scale was derived from the research by Qiu Aimei et al., 2018 ) including 3 items. The subjective norm scale was adapted from Ajzen’s original scale, comprising 3 items (Ajzen, 2002 ). The self-disclosure willingness scale was developed based on Chu and Kim’s research, including 3 items (Chu & Kim, 2011 ). The innovativeness scale was formulated following the study by Sun et al., comprising 4 items (Sun et al., 2006 ). The information trust scale was created in reference to Chu and Choi’s research, including 3 items (Chu & Choi, 2011 ). The scales for the three components of social media consumer engagement—content consumption, content contribution, and content creation—were sourced from the research by Buzeta et al., encompassing 8 items in total (Buzeta et al., 2020 ).
All scales were appropriately revised for the context of social media influencer marketing. To avoid issues with scoring neutral attitudes, a uniform Likert seven-point scale was used for each measurement item (ranging from 1 to 7, representing a spectrum from ‘strongly disagree’ to ‘strongly agree’). After the overall design of the questionnaire was completed, a pre-test was conducted with 30 social media users to ensure that potential respondents could clearly understand the meaning of each question and that there were no obstacles to answering. This pre-test aimed to prevent any difficulties or misunderstandings in the questionnaire items. The final version of the questionnaire is presented in Table 2 .
Data analysis
Since the model framework of the current study is derived from theoretical deductions of existing research and, while logically constructed, does not originate from an existing research model, this study still falls under the category of exploratory research. According to the analysis suggestions of Hair and other scholars, in cases of exploratory research model frameworks, it is more appropriate to choose Smart PLS for Partial Least Squares Path Analysis (PLS) to conduct data analysis and testing of the research model (Hair et al., 2012 ).
Measurement of model
In this study, careful data collection and management resulted in no missing values in the dataset. This ensured the integrity and reliability of the subsequent data analysis. As shown in Table 3 , after deleting measurement items with factor loadings below 0.5, the final factor loadings of the measurement items in this study range from 0.730 to 0.964. This indicates that all measurement items meet the retention criteria. Additionally, the Cronbach’s α values of the latent variables range from 0.805 to 0.924, and all latent variables have Composite Reliability (CR) values greater than the acceptable value of 0.7, demonstrating that the scales of this study have passed the reliability test requirements (Hair et al., 2019 ). All latent variables in this study have Average Variance Extracted (AVE) values greater than the standard acceptance value of 0.5, indicating that the convergent validity of the variables also meets the standard (Fornell & Larcker, 1981 ). Furthermore, the results show that the Variance Inflation Factor (VIF) values for each factor are below 10, indicating that there are no multicollinearity issues with the scales in this study (Hair, 2009 ).
The current study then further verified the discriminant validity of the variables, with specific results shown in Table 4 . The square roots of the average variance extracted (AVE) values for all variables (bolded on the diagonal) are greater than the Pearson correlation coefficients between the variables, indicating that the discriminant validity of the scales in this study meets the required standards (Fornell & Larcker, 1981 ). Additionally, a single-factor test method was employed to examine common method bias in the data. The first unrotated factor accounted for 29.71% of the variance, which is less than the critical threshold of 40%. Therefore, the study passed the test and did not exhibit serious common method bias (Podsakoff et al., 2003 ).
To ensure the robustness and appropriateness of our structural equation model, we also conducted a thorough evaluation of the model fit. Initially, through PLS Algorithm calculations, the R 2 values of each variable were greater than the standard acceptance value of 0.1, indicating good predictive accuracy of the model. Subsequently, Blindfolding calculations were performed, and the results showed that the Stone-Geisser Q 2 values of each variable were greater than 0, demonstrating that the model of this study effectively predicts the relationships between variables (Dijkstra & Henseler, 2015 ). In addition, through CFA, we also obtained some indicator values, specifically, χ 2 /df = 2.528 < 0.3, RMSEA = 0.059 < 0.06, SRMR = 0.055 < 0.08. Given its sensitivity to sample size, we primarily focused on the CFI, TLI, and NFI values, CFI = 0.953 > 0.9, TLI = 0.942 > 0.9, and NFI = 0.923 > 0.9 indicating a good fit. Additionally, RMSEA values below 0.06 and SRMR values below 0.08 were considered indicative of a good model fit. These indices collectively suggested that our model demonstrates a satisfactory fit with the data, thereby reinforcing the validity of our findings.
Research hypothesis testing
The current study employed a Bootstrapping test with a sample size of 5000 on the collected raw data to explore the coefficients and significance of the paths in the research model. The final test data results of this study’s model are presented in Table 5 .
The current study employs S-O-R model as the framework, grounded in theories such as self-determination theory and theory of planned behavior, to construct an influence model of consumer engagement behavior in the context of social media influencer marketing. It examines how influencer factors, advertisement information factors, and social influence factors affect consumer engagement behavior by impacting consumers’ psychological cognitions. Using structural equation modeling to analyze collected data ( N = 522), it was found that self-disclosure willingness, innovativeness, and information trust positively influence consumer engagement behavior, with innovativeness having the largest impact on higher levels of engagement. Influencer factors, advertisement information factors, and social factors serve as effective external stimuli, influencing psychological motivators and, consequently, consumer engagement behavior. The specific research results are illustrated in Fig. 2 .
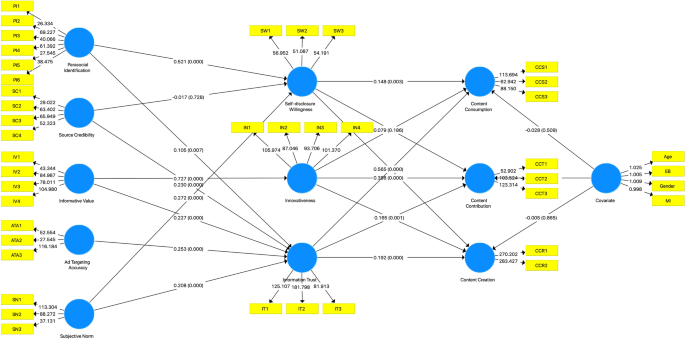
Tested structural model of consumer engagement behavior.
The impact of psychological motivators on different levels of consumer engagement: self-disclosure willingness, innovativeness, and information trust
The research analysis indicates that self-disclosure willingness and information trust are key drivers for content consumption (H1a, H2a validated). This aligns with previous findings that individuals with a higher willingness to disclose themselves show greater levels of engagement behavior (Chu et al., 2023 ); likewise, individuals who trust advertisement information are more inclined to engage with advertisement content (Kim, Kim, 2021 ). Moreover, our study finds that information trust has a stronger impact on content consumption, underscoring the importance of trust in the dissemination of advertisement information. However, no significant association was found between individual innovativeness and content consumption (H3a not validated).
Regarding the dimension of content contribution in consumer engagement, self-disclosure willingness, information trust, and innovativeness all positively impact it (H1b, H2b, and H3b all validated). This is consistent with earlier research findings that individuals with higher self-disclosure willingness are more likely to like, comment on, or share content posted by influencers on social media platforms (Towner et al., 2022 ); the conclusions of this paper also support that innovativeness is an important psychological driver for active participation in social media interactions (Kamboj & Sharma, 2023 ). However, at the level of consumer engagement in content contribution, while information trust also exerts a positive effect, its impact is the weakest, although information trust has the strongest impact on content consumption.
In social media advertising, the ideal outcome is the highest level of consumer engagement, i.e., content creation, meaning consumers actively join in brand content creation, seeing themselves as co-creators with the brand (Nadeem et al., 2021 ). Our findings reveal that self-disclosure willingness, innovativeness, and information trust all positively influence content creation (H1c, H2c, and H3c all validated). The analysis found that similar to the impact on content contribution, innovativeness has the most significant effect on encouraging individual content creation, followed by self-disclosure willingness, with information trust having the least impact.
In summary, while some previous studies have shown that self-disclosure willingness, innovativeness, and information trust are important factors in promoting consumer engagement (Chu et al., 2023 ; Nadeem et al., 2021 ; Geng et al., 2021 ), this study goes further by integrating and comparing all three within the same research framework. It was found that to trigger higher levels of consumer engagement behavior, trust is not the most crucial psychological motivator; rather, the most effective method is to stimulate consumers’ innovativeness, thus complementing previous research. Subsequently, this study further explores the impact of different stimulus factors on various psychological motivators.
The influence of external stimulus factors on psychological motivators: influencer factors, advertisement information factors, and social factors
The current findings indicate that influencer factors, such as parasocial identification and source credibility, effectively enhance consumer engagement by influencing self-disclosure willingness and information trust. This aligns with prior research highlighting the significance of parasocial identification (Shan et al., 2020 ). Studies suggest parasocial identification positively impacts consumer engagement by boosting self-disclosure willingness and information trust (validated H4a, H4b, H4c, and H5a), but not content contribution or creation through information trust (H5b, H5c not validated). Source credibility’s influence on self-disclosure willingness was not significant (H6 not validated), thus negating the mediating effect of self-disclosure willingness (H6a, H6b, H6c not validated). Influencer credibility mainly affects engagement through information trust (H7a, H7b, H7c validated), supporting previous findings (Shan et al., 2020 ).
Advertisement factors (informative value and ad targeting accuracy) promote engagement through innovativeness and information trust. Informative value significantly impacts higher-level content contribution and creation through innovativeness (H8b, H8c validated), while ad targeting accuracy influences consumer engagement at all levels mainly through information trust (H10a, H10b, H10c validated).
Social factors (subjective norms) enhance self-disclosure willingness and information trust, consistent with previous research (Wirth et al., 2019 ; Gupta et al., 2021 ), and further promote consumer engagement across all levels (H11a, H11b, H11c, H12a, H12b, and H12c all validated).
In summary, influencer, advertisement, and social factors impact consumer engagement behavior by influencing psychological motivators, with influencer factors having the greatest effect on content consumption, advertisement content factors significantly raising higher-level consumer engagement through innovativeness, and social factors also influencing engagement through self-disclosure willingness and information trust.
Implication
From a theoretical perspective, current research presents a comprehensive model of consumer engagement within the context of influencer advertising on social media. This model not only expands the research horizon in the fields of social media influencer advertising and consumer engagement but also serves as a bridge between two crucial themes in new media advertising studies. Influencer advertising has become an integral part of social media advertising, and the construction of a macro model aids researchers in understanding consumer psychological processes and behavioral patterns. It also assists advertisers in formulating more effective strategies. Consumer engagement, focusing on the active role of consumers in disseminating information and the long-term impact on advertising effectiveness, aligns more closely with the advertising effectiveness measures in the new media context than traditional advertising metrics. However, the intersection of these two vital themes lacks comprehensive research and a universal model. This study constructs a model that elucidates the effects of various stimuli on consumer psychology and engagement behaviors, exploring the connections and mechanisms through different mediating pathways. By differentiating levels of engagement, the study offers more nuanced conclusions for diverse advertising objectives. Furthermore, this research validates the applicability of self-determination theory in the context of influencer advertising effectiveness. While this psychological theory has been utilized in communication behavior research, its effectiveness in the field of advertising requires further exploration. The current study introduces self-determination theory into the realm of influencer advertising and consumer engagement, thereby expanding its application in the field of advertising communication. It also responds to the call from the advertising and marketing academic community to incorporate more psychological theories to explain the ‘black box’ of consumer psychology. The inclusion of this theory re-emphasizes the people-centric approach of this research and highlights the primary role of individuals in advertising communication studies.
From a practical perspective, this study provides significant insights for adapting marketing strategies to the evolving media landscape and the empowered role of audiences. Firstly, in the face of changes in the communication environment and the empowerment of audience communication capabilities, traditional marketing approaches are becoming inadequate for new media advertising needs. Traditional advertising focuses on direct, point-to-point effects, whereas social media advertising aims for broader, point-to-mass communication, leveraging audience proactivity to facilitate the viral spread of content across online social networks. Secondly, for brands, the general influence model proposed in this study offers guidance for influencer advertising strategy. If the goal is to maximize reach and brand recognition with a substantial advertising budget, partnering with top influencers who have a large following can be an effective strategy. However, if the objective is to maximize cost-effectiveness with a limited budget by leveraging consumer initiative for secondary spread, the focus should be on designing advertising content that stimulates consumer creativity and willingness to innovate. Thirdly, influencers are advised to remain true to their followers. In influencer marketing, influencers attract advertisers through their influence over followers, converting this influence into commercial gain. This influence stems from the trust followers place in the influencer, thus influencers should maintain professional integrity and prioritize the quality of information they share, even when presented with advertising opportunities. Lastly, influencers should assert more control over their relationships with advertisers. In traditional advertising, companies and brands often exert significant control over the content. However, in the social media era, influencers should negotiate more creative freedom in their advertising partnerships, asserting a more equal relationship with advertisers. This approach ensures that content quality remains high, maintaining the trust influencers have built with their followers.
Limitations and future directions
while this study offers valuable insights into the dynamics of influencer marketing and consumer engagement on social media, several limitations should be acknowledged: Firstly, constrained by the research objectives and scope, this study’s proposed general impact model covers three dimensions: influencers, advertisement information, and social factors. However, these dimensions are not limited to the five variables discussed in this paper. Therefore, we call for future research to supplement and explore more crucial factors. Secondly, in the actual communication environment, there may be differences in the impact of communication effectiveness across various social media platforms. Thus, future research could also involve comparative studies and explorations between different social media platforms. Thirdly, the current study primarily examines the direct effects of various factors on consumer engagement. However, the potential interaction effects between these variables (e.g., how influencers’ credibility might interact with advertisement information quality) are not extensively explored. Future research could investigate these complex interrelationships for a more holistic understanding. Lastly, our study, being cross-sectional, offers preliminary insights into the complex and dynamic nature of engagement between social media influencers and consumers, yet it does not incorporate the temporal dimension. The diverse impacts of psychological needs on engagement behaviors hint at an underlying dynamism that merits further investigation. Future research should consider employing longitudinal designs to directly observe how these dynamics evolve over time.
The findings of the current study not only theoretically validate the applicability of self-determination theory in the field of social media influencer marketing advertising research but also broaden the scope of advertising effectiveness research from the perspective of consumer engagement. Moreover, the research framework offers strategic guidance and reference for influencer marketing strategies. The main conclusions of this study can be summarized as follows.
Innovativeness is the key factor in high-level consumer engagement behavior. Content contribution represents a higher level of consumer engagement compared to content consumption, as it not only requires consumers to dedicate attention to viewing advertising content but also to share this information across adjacent nodes within their social networks. This dissemination of information is a pivotal factor in the success of influencer marketing advertisements. Hence, companies and brands prioritize consumers’ content contribution over mere viewing of advertising content (Qiu & Kumar, 2017 ). Compared to content consumption and contribution, content creation is considered the highest level of consumer engagement, where consumers actively create and upload brand-related content, and it represents the most advanced outcome sought by enterprises and brands in advertising campaigns (Cheung et al., 2021 ). The current study posits that to pursue better outcomes in social media influencer advertising marketing, enhancing consumers’ willingness for self-disclosure, innovativeness, and trust in advertising information are effective strategies. However, the crux lies in leveraging the consumer’s subjective initiative, particularly in boosting their innovativeness. If the goal is simply to achieve content consumption rather than higher levels of consumer engagement, the focus should be on fostering trust in advertising information. There is no hierarchy in the efficacy of different strategies; they should align with varying marketing contexts and advertising objectives.
The greatest role of social media influencers lies in attracting online traffic. information trust is the core element driving content consumption, and influencer factors mainly affect consumer engagement behaviors through information trust. Therefore, this study suggests that the primary role of influencers in social media advertising is to attract online traffic, i.e., increase consumer behavior regarding ad content consumption (reducing avoidance of ad content), and help brands achieve the initial goal of making consumers “see and complete ads.” However, their impact on further high-level consumer engagement behaviors is limited. This mechanism serves as a reminder to advertisers not to overestimate the effects of influencers in marketing. Currently, top influencers command a significant portion of the ad budget, which could squeeze the budget for other aspects of advertising, potentially affecting the overall effectiveness of the campaign. Businesses and brands should consider deeper strategic implications when planning their advertising campaigns.
Valuing Advertising Information Factors, Content Remains King. Our study posits that in the social media influencer marketing context, the key to enhancing consumer contribution and creation of advertising content lies primarily in the advertising information factors. In other words, while content consumption is important, advertisers should objectively assess the role influencers play in advertising. In the era of social media, content remains ‘king’ in advertising. This view indirectly echoes the points made in the previous paragraph: influencers effectively perform initial ‘online traffic generation’ tasks in social media, but this role should not be overly romanticized or exaggerated. Whether it’s companies, brands, or influencers, providing consumers with advertisements rich in informational value is crucial to achieving better advertising outcomes and potentially converting consumers into stakeholders.
Subjective norm is an unignorable social influence factor. Social media is characterized by its network structure of information dissemination, where a node’s information is visible to adjacent nodes. For instance, if user A likes a piece of content C from influencer I, A’s follower B, who may not follow influencer I, can still see content C via user A’s page. The aim of marketing in the social media era is to influence a node and then spread the information to adjacent nodes, either secondarily or multiple times (Kumar & Panda, 2020 ). According to the Theory of Planned Behavior, an individual’s actions are influenced by significant others in their lives, such as family and friends. Previous studies have proven the effectiveness of the Theory of Planned Behavior in influencing attitudes toward social media advertising (Ranjbarian et al., 2012 ). Current research further confirms that subjective norms also influence consumer engagement behaviors in influencer marketing on social media. Therefore, in advertising practice, brands should not only focus on individual consumers but also invest efforts in groups that can influence consumer decisions. Changing consumer behavior in the era of social media marketing doesn’t solely rely on the company’s efforts.
As communication technology advances, media platforms will further empower individual communicative capabilities, moving beyond the era of the “magic bullet” theory. The distinction between being a recipient and a transmitter of information is increasingly blurred. In an era where everyone is both an audience and an influencer, research confined to the role of the ‘recipient’ falls short of addressing the dynamics of ‘transmission’. Future research in marketing and advertising should thus focus more on the power of individual transmission. Furthermore, as Marshall McLuhan famously said, “the medium is the extension of man.” The evolution of media technology remains human-centric. Accordingly, future marketing research, while paying heed to media transformations, should emphasize the centrality of the ‘human’ element.
Data availability
The datasets generated and/or analyzed during the current study are not publicly available due to privacy issues. Making the full data set publicly available could potentially breach the privacy that was promised to participants when they agreed to take part, and may breach the ethics approval for the study. The data are available from the corresponding author on reasonable request.
Abbasi AZ, Tsiotsou RH, Hussain K, Rather RA, Ting DH (2023) Investigating the impact of social media images’ value, consumer engagement, and involvement on eWOM of a tourism destination: a transmittal mediation approach. J Retail Consum Serv 71:103231. https://doi.org/10.1016/j.jretconser.2022.103231
Article Google Scholar
Ajzen I (2002) Perceived behavioral control, self‐efficacy, locus of control, and the theory of planned behavior 1. J Appl Soc Psychol 32(4):665–683. https://doi.org/10.1111/j.1559-1816.2002.tb00236.x
Ajzen I (1991) The theory of planned behavior. Organ Behav Hum Decis Process 50(2):179–211. https://doi.org/10.1016/0749-5978(91)90020-T
Altman I, Taylor DA (1973) Social penetration: the development of interpersonal relationships. Holt, Rinehart & Winston
Anaya-Sánchez R, Aguilar-Illescas R, Molinillo S, Martínez-López FJ (2020) Trust and loyalty in online brand communities. Span J Mark ESIC 24(2):177–191. https://doi.org/10.1108/SJME-01-2020-0004
Astuti BA, Hariyawan A (2021) Perspectives of social capital and self-determination on e-WOM at millennial generation in Yogyakarta. Integr J Bus Econ 5(1):399475. https://doi.org/10.33019/ijbe.v5i1.338
Bao Z, Wang D (2021) Examining consumer participation on brand microblogs in China: perspectives from elaboration likelihood model, commitment–trust theory and social presence. J Res Interact Mark 15(1):10–29. https://doi.org/10.1108/JRIM-02-2019-0027
Barta S, Belanche D, Fernández A, Flavián M (2023) Influencer marketing on TikTok: the effectiveness of humor and followers’ hedonic experience. J Retail Consum Serv 70:103149. https://doi.org/10.1016/j.jretconser.2022.103149
Bond BJ (2016) Following your “friend”: social media and the strength of adolescents’ parasocial relationships with media personae. Cyberpsych Behav Soc Netw 19(11):656–660. https://doi.org/10.1089/cyber.2016.0355
Breves P, Amrehn J, Heidenreich A, Liebers N, Schramm H (2021) Blind trust? The importance and interplay of parasocial relationships and advertising disclosures in explaining influencers’ persuasive effects on their followers. Int J Advert 40(7):1209–1229. https://doi.org/10.1080/02650487.2021.1881237
Brodie RJ, Ilic A, Juric B, Hollebeek L (2013) Consumer engagement in a virtual brand community: an exploratory analysis. J Bus Res 66(1):105–114. https://doi.org/10.1016/j.jbusres.2011.07.029
Buzeta C, De Pelsmacker P, Dens N (2020) Motivations to use different social media types and their impact on consumers’ online brand-related activities (COBRAs). J Interact Mark 52(1):79–98. https://doi.org/10.1016/j.intmar.2020.04.0
Chen KJ, Lin JS, Shan Y (2021) Influencer marketing in China: The roles of parasocial identification, consumer engagement, and inferences of manipulative intent. J Consum Behav 20(6):1436–1448. https://doi.org/10.1002/cb.1945
Chetioui Y, Benlafqih H, Lebdaoui H (2020) How fashion influencers contribute to consumers’ purchase intention. J Fash Mark Manag 24(3):361–380. https://doi.org/10.1108/JFMM-08-2019-0157
Cheung ML, Pires GD, Rosenberger III PJ, De Oliveira MJ (2021) Driving COBRAs: the power of social media marketing. Mark Intell Plan 39(3):361–376. https://doi.org/10.1108/MIP-11-2019-0583
Cheung MY, Luo C, Sia CL, Chen H (2009) Credibility of electronic word-of-mouth: Informational and normative determinants of on-line consumer recommendations. Int J Electron Comm 13(4):9–38. https://doi.org/10.2753/JEC1086-4415130402
Chung S, Cho H (2017) Fostering parasocial relationships with celebrities on social media: Implications for celebrity endorsement. Psychol Mark 34(4):481–495. https://doi.org/10.1002/mar.21001
Chu SC, Choi SM (2011) Electronic word-of-mouth in social networking sites: a cross-cultural study of the United States and China. J Glob Mark 24(3):263–281. https://doi.org/10.1080/08911762.2011.592461
Chu SC, Kim Y (2011) Determinants of consumer engagement in electronic word-of-mouth (eWOM) in social networking sites. Int J Advert 30(1):47–75. https://doi.org/10.2501/IJA-30-1-047-075
Chu TH, Sun M, Crystal Jiang L (2023) Self-disclosure in social media and psychologicalwell-being: a meta-analysis. J Soc Pers Relat 40(2):576–599. https://doi.org/10.1177/02654075221119429
Cropanzano R, Mitchell MS (2005) Social exchange theory: an interdisciplinary review. J Manag 31(6):874–900. https://doi.org/10.1177/0149206305279602
Della Vigna S, Gentzkow M (2010) Persuasion: empirical evidence. Annu Rev Econ 2(1):643–669. https://doi.org/10.1146/annurev.economics.102308.124309
Dijkstra TK, Henseler J (2015) Consistent and asymptotically normal PLS estimators for linear structural equations. Comput Stat Data 81:10–23. https://doi.org/10.1016/j.csda.2014.07.008
Article MathSciNet Google Scholar
Djafarova E, Rushworth C (2017) Exploring the credibility of online celebrities’ Instagram profiles in influencing the purchase decisions of young female users. Comput Hum Behav 68:1–7. https://doi.org/10.1016/j.chb.2016.11.009
D Horton D, Richard Wohl R (1956) Mass communication and para-social interaction: Observations on intimacy at a distance. Psychiatry 19(3):215–229. https://doi.org/10.1080/00332747.1956.11023049
Ducoffe RH (1995) How consumers assess the value of advertising. J Curr Issues Res Adver 17(1):1–18. https://doi.org/10.1080/10641734.1995.10505022
Fornell C, Larcker DF (1981) Structural equation models with unobservable variables and measurement error: Algebra and statistics. J Mark Res 18(3):382–388. https://doi.org/10.1177/002224378101800313
Gefen D, Straub DW, Rigdon EE (2011) An update and extension to SEM guidelines for administrative and social science research. Mis Quart 35(2):iii–xiv. https://doi.org/10.2307/23044042
Geng S, Yang P, Gao Y, Tan Y, Yang C (2021) The effects of ad social and personal relevance on consumer ad engagement on social media: the moderating role of platform trust. Comput Hum Behav 122:106834. https://doi.org/10.1016/j.chb.2021.106834
Giles DC (2002) Parasocial interaction: a review of the literature and a model for future research. Media Psychol 4(3):279–305. https://doi.org/10.1207/S1532785XMEP0403_04
Gräve JF, Bartsch F (2022) # Instafame: exploring the endorsement effectiveness of influencers compared to celebrities. Int J Advert 41(4):591–622. https://doi.org/10.1080/02650487.2021.1987041
Gupta R, Ranjan S, Gupta A (2021) Consumer’s perceived trust and subjective norms as antecedents of mobile wallets adoption and continuance intention: a technology acceptance approach. Recent Adv Technol Accept Models Theor 211–224. https://doi.org/10.1007/978-3-030-64987-6_13
Habibi MR, Laroche M, Richard MO (2014) The roles of brand community and community engagement in building brand trust on social media. Comput Hum Behav 37:152–161. https://doi.org/10.1016/j.chb.2014.04.016
Hagger MS, Chatzisarantis NL (2009) Integrating the theory of planned behaviour and self‐determination theory in health behaviour: a meta‐analysis. Brit J Health Psych 14(2):275–302. https://doi.org/10.1348/135910708X373959
Haida A, Rahim HL (2015) Social media advertising value: A Study on consumer’s perception. Int Acad Res J Bus Technol 1(1):1–8. https://www.researchgate.net/publication/280325676_Social_Media_Advertising_Value_A_Study_on_Consumer%27s_Perception
Google Scholar
Hair JF (2009) Multivariate data analysis. Prentice Hall, Upper Saddle River
Hair JF, Ringle CM, Gudergan SP, Fischer A, Nitzl C, Menictas C (2019) Partial least squares structural equation modeling-based discrete choice modeling: an illustration in modeling retailer choice. Bus Res 12(1):115–142. https://doi.org/10.1007/s40685-018-0072-4
Hair JF, Sarstedt M, Ringle CM, Mena JA (2012) An assessment of the use of partial least squares structural equation modeling in marketing research. Acad Mark Sci 40:414–433. https://doi.org/10.1007/s11747-011-0261-6
Heirman W, Walrave M, Ponnet K (2013) Predicting adolescents’ disclosure of personal information in exchange for commercial incentives: An application of an extended theory of planned behavior. Cyberpsych Behav Soc Netw16(2):81–87. https://doi.org/10.1089/cyber.2012.0041
Hewei T, Youngsook L (2022) Factors affecting continuous purchase intention of fashion products on social E-commerce: SOR model and the mediating effect. Entertain Comput 41:100474. https://doi.org/10.1016/j.entcom.2021.100474
Hovland CI, Janis IL, Kelley HH (1953) Communication and persuasion. Yale University Press
Hsieh JK, Li YJ (2020) Will you ever trust the review website again? The importance of source credibility. Int J Electron Commerce 24(2):255–275. https://doi.org/10.1080/10864415.2020.1715528
Huang YC (2023) Integrated concepts of the UTAUT and TPB in virtual reality behavioral intention. J Retail Consum Serv 70:103127. https://doi.org/10.1016/j.jretconser.2022.103127
Hudders L, Lou C (2023) The rosy world of influencer marketing? Its bright and dark sides, and future research recommendations. Int J Advert 42(1):151–161. https://doi.org/10.1080/02650487.2022.2137318
Itani OS, Kalra A, Riley J (2022) Complementary effects of CRM and social media on customer co-creation and sales performance in B2B firms: The role of salesperson self-determination needs. Inf Manag 59(3):103621. https://doi.org/10.1016/j.im.2022.103621
Jang W, Kim J, Kim S, Chun JW (2021) The role of engagement in travel influencer marketing: the perspectives of dual process theory and the source credibility model. Curr Issues Tour 24(17):2416–2420. https://doi.org/10.1080/13683500.2020.1845126
Jin SV, Ryu E, Muqaddam A (2021) I trust what she’s# endorsing on Instagram: moderating effects of parasocial interaction and social presence in fashion influencer marketing. J Fash Mark Manag 25(4):665–681. https://doi.org/10.1108/JFMM-04-2020-0059
Kamboj S, Sharma M (2023) Social media adoption behaviour: consumer innovativeness and participation intention. Int J Consum Stud 47(2):523–544. https://doi.org/10.1111/ijcs.12848
Kaushik AK, Rahman Z (2014) Perspectives and dimensions of consumer innovativeness: a literature review and future agenda. J Int Consum Mark 26(3):239–263. https://doi.org/10.1080/08961530.2014.893150
Kelley JB, Alden DL (2016) Online brand community: through the eyes of self-determination theory. Internet Res 26(4):790–808. https://doi.org/10.1108/IntR-01-2015-0017
K Kim DY, Kim HY (2021) Trust me, trust me not: A nuanced view of influencer marketing on social media. J Bus Res 134:223–232. https://doi.org/10.1016/j.jbusres.2021.05.024
Koay KY, Ong DLT, Khoo KL, Yeoh HJ (2020) Perceived social media marketing activities and consumer-based brand equity: Testing a moderated mediation model. Asia Pac J Mark Logist 33(1):53–72. https://doi.org/10.1108/APJML-07-2019-0453
Kumar S, Panda BS (2020) Identifying influential nodes in Social Networks: Neighborhood Coreness based voting approach. Phys A: Stat Mech Appl 553:124215. https://doi.org/10.1016/j.physa.2020.124215
Lee D, Hosanagar K, Nair HS (2018) Advertising content and consumer engagement on social media: evidence from Facebook. Manag Sci 64(11):5105–5131. https://doi.org/10.1287/mnsc.2017.2902
Lee DH, Im S, Taylor CR (2008) Voluntary self‐disclosure of information on the Internet: a multimethod study of the motivations and consequences of disclosing information on blogs. Psychol Mark 25(7):692–710. https://doi.org/10.1002/mar.20232
Lee J, Rajtmajer S, Srivatsavaya E, Wilson S (2023) Online self-disclosure, social support, and user engagement during the COVID-19 pandemic. ACM Trans Soc Comput 6(3-4):1–31. https://doi.org/10.1145/3617654
Lee Y, Lee J, Hwang Y (2015) Relating motivation to information and communication technology acceptance: self-determination theory perspective. Comput Hum Behav 51:418–428. https://doi.org/10.1016/j.chb.2015.05.021
Leite FP, Baptista PDP (2022) The effects of social media influencers’ self-disclosure on behavioral intentions: The role of source credibility, parasocial relationships, and brand trust. J Mark Theory Pr 30(3):295–311. https://doi.org/10.1080/10696679.2021.1935275
Leite FP, Pontes N, de Paula Baptista P (2022) Oops, I’ve overshared! When social media influencers’ self-disclosure damage perceptions of source credibility. Comput Hum Behav 133:107274. https://doi.org/10.1016/j.chb.2022.107274
León SP, Abad MJ, Rosas JM (2009) Giving contexts informative value makes information context-specific. Exp Psychol. https://doi.org/10.1027/1618-3169/a000006
Lou C, Tan SS, Chen X (2019) Investigating consumer engagement with influencer-vs. brand-promoted ads: The roles of source and disclosure. J Interact Advert 19(3):169–186. https://doi.org/10.1080/15252019.2019.1667928
Lou C, Yuan S (2019) Influencer marketing: how message value and credibility affect consumer trust of branded content on social media. J Interact Advert 19(1):58–73. https://doi.org/10.1080/15252019.2018.1533501
Luo M, Hancock JT (2020) Self-disclosure and social media: motivations, mechanisms and psychological well-being. Curr Opin Psychol 31:110–115. https://doi.org/10.1016/j.copsyc.2019.08.019
Article PubMed Google Scholar
Mahmood S, Khwaja MG, Jusoh A (2019) Electronic word of mouth on social media websites: role of social capital theory, self-determination theory, and altruism. Int J Space-Based Situat Comput 9(2):74–89. https://doi.org/10.1504/IJSSC.2019.104217
Majerczak P, Strzelecki A (2022) Trust, media credibility, social ties, and the intention to share towards information verification in an age of fake news. Behav Sci 12(2):51. https://doi.org/10.3390/bs12020051
Article PubMed PubMed Central Google Scholar
McAllister DJ (1995) Affect-and cognition-based trust as foundations for interpersonal cooperation in organizations. Acad Manag J 38(1):24–59. https://doi.org/10.5465/256727
Mehrabian A, Russell JA (1974). An approach to environmental psychology. The MIT Press
Minton EA (2015) In advertising we trust: Religiosity’s influence on marketplace and relational trust. J Advert 44(4):403–414. https://doi.org/10.1080/00913367.2015.1033572
Moorman C, Deshpande R, Zaltman G (1993) Factors affecting trust in market research relationships. J Mark 57(1):81–101. https://doi.org/10.1177/002224299305700106
Muntinga DG, Moorman M, Smit EG (2011) Introducing COBRAs: Exploring motivations for brand-related social media use. Int J Advert 30(1):13–46. https://doi.org/10.2501/IJA-30-1-013-046
Nadeem W, Tan TM, Tajvidi M, Hajli N (2021) How do experiences enhance brand relationship performance and value co-creation in social commerce? The role of consumer engagement and self brand-connection. Technol Forecast Soc 171:120952. https://doi.org/10.1016/j.techfore.2021.120952
Oestreicher-Singer G, Zalmanson L (2013) Content or community? A digital business strategy for content providers in the social age. MIS Quart 37(2):591–616. https://www.jstor.org/stable/43825924
Okazaki S (2009) Social influence model and electronic word of mouth: PC versus mobile internet. Int J Advert 28(3):439–472. https://doi.org/10.2501/S0265048709200692
Piehler R, Schade M, Kleine-Kalmer B, Burmann C (2019) Consumers’ online brand-related activities (COBRAs) on SNS brand pages: an investigation of consuming, contributing and creating behaviours of SNS brand page followers. Eur J Mark 53(9):1833–1853. https://doi.org/10.1108/EJM-10-2017-0722
Podsakoff PM, MacKenzie SB, Lee JY, Podsakoff NP (2003) Common method biases in behavioral research: a critical review of the literature and recommended remedies. J Appl Psychol 88(5):879. https://doi.org/10.1037/0021-9010.88.5.879
Pop RA, Săplăcan Z, Dabija DC, Alt MA (2022) The impact of social media influencers on travel decisions: The role of trust in consumer decision journey. Curr Issues Tour 25(5):823–843. https://doi.org/10.1080/13683500.2021.1895729
Pradhan B, Kishore K, Gokhale N (2023) Social media influencers and consumer engagement: a review and future research agenda. Int J Consum Stud 47(6):2106–2130. https://doi.org/10.1111/ijcs.12901
Qiu A, Chen M (2018) 基于UTAUT修正模型的微信朋友圈广告接受意愿分析 [Analysis of WeChat moments advertising acceptance intention based on a modified UTAUT model]. Stat Decis 34(12):99–102. https://doi.org/10.13546/j.cnki.tjyjc.2018.12.024
Qiu L, Kumar S (2017) Understanding voluntary knowledge provision and content contribution through a social-media-based prediction market: a field experiment. Inf Syst Res 28(3):529–546. https://doi.org/10.1287/isre.2016.0679
Racherla P, Mandviwalla M, Connolly DJ (2012) Factors affecting consumers’ trust in online product reviews. J Consum Behav 11(2):94–104. https://doi.org/10.1002/cb.385
Ranjbarian B, Gharibpoor M, Lari A (2012) Attitude toward SMS advertising and derived behavioral intension, an empirical study using TPB (SEM method). J Am Sci 8(7):297–307. https://www.ceeol.com/search/article-detail?id=466212
Robertshaw GS, Marr NE (2006) The implications of incomplete and spurious personal information disclosures for direct marketing practice. J Database Mark Custom Strategy Manag. 13:186–197. https://doi.org/10.1057/palgrave.dbm.3240296
Roh T, Seok J, Kim Y (2022) Unveiling ways to reach organic purchase: Green perceived value, perceived knowledge, attitude, subjective norm, and trust. J Retail Consum Serv 67:102988. https://doi.org/10.1016/j.jretconser.2022.102988
Schivinski B, Christodoulides G, Dabrowski D (2016) Measuring consumers’ engagement with brand-related social-media content: Development and validation of a scale that identifies levels of social-media engagement with brands. J Advert Res 56(1):64–80. https://doi.org/10.2501/JAR-2016-004
Schouten AP, Janssen L, Verspaget M (2021) Celebrity vs. Influencer endorsements in advertising: the role of identification, credibility, and product-endorser fit. Leveraged marketing communications, Routledge. pp. 208–231
Schramm H, Hartmann T (2008) The PSI-Process Scales. A new measure to assess the intensity and breadth of parasocial processes. Communications. https://doi.org/10.1515/COMM.2008.025
Shan Y, Chen KJ, Lin JS (2020) When social media influencers endorse brands: the effects of self-influencer congruence, parasocial identification, and perceived endorser motive. Int J Advert 39(5):590–610. https://doi.org/10.1080/02650487.2019.1678322
Shi Y (2018) The impact of consumer innovativeness on the intention of clicking on SNS advertising. Mod Econ 9(2):278–285. https://doi.org/10.4236/me.2018.92018
Article CAS Google Scholar
Simon F, Tossan V (2018) Does brand-consumer social sharing matter? A relational framework of customer engagement to brand-hosted social media. J Bus Res 85:175–184. https://doi.org/10.1016/j.jbusres.2017.12.050
Steinhoff L, Arli D, Weaven S, Kozlenkova IV (2019) Online relationship marketing. J Acad Mark Sci 47:369–393. https://doi.org/10.1007/s11747-018-0621-6
Stutzman F, Capra R, Thompson J (2011) Factors mediating disclosure in social network sites. Comput Hum Behav 27(1):590–598. https://doi.org/10.1016/j.chb.2010.10.017
Sun T, Youn S, Wu G, Kuntaraporn M (2006) Online word-of-mouth (or mouse): An exploration of its antecedents and consequences. J Comput-Mediat Comm 11(4):1104–1127. https://doi.org/10.1111/j.1083-6101.2006.00310.x
Sweet KS, LeBlanc JK, Stough LM, Sweany NW (2020) Community building and knowledge sharing by individuals with disabilities using social media. J Comput Assist Lear 36(1):1–11. https://doi.org/10.1111/jcal.12377
Tak P, Gupta M (2021) Examining travel mobile app attributes and its impact on consumer engagement: An application of SOR framework. J Internet Commer 20(3):293–318. https://doi.org/10.1080/15332861.2021.1891517
Towner E, Grint J, Levy T, Blakemore SJ, Tomova L (2022) Revealing the self in a digital world: a systematic review of adolescent online and offline self-disclosure. Curr Opin Psychol 45:101309. https://doi.org/10.1016/j.copsyc.2022.101309
Vander Schee BA, Peltier J, Dahl AJ (2020) Antecedent consumer factors, consequential branding outcomes and measures of online consumer engagement: current research and future directions. J Res Interact Mark 14(2):239–268. https://doi.org/10.1108/JRIM-01-2020-0010
Van-Tien Dao W, Nhat Hanh Le A, Ming-Sung Cheng J, Chao Chen D (2014) Social media advertising value: The case of transitional economies in Southeast Asia. Int J Advert 33(2):271–294. https://doi.org/10.2501/IJA-33-2-271-294
Viswanathan V, Hollebeek LD, Malthouse EC, Maslowska E, Jung Kim S, Xie W (2017) The dynamics of consumer engagement with mobile technologies. Serv Sci 9(1):36–49. https://doi.org/10.1287/serv.2016.0161
Voss KE, Spangenberg ER, Grohmann B (2003) Measuring the hedonic and utilitarian dimensions of consumer attitude. J Mark Res 40(3):310–320. https://doi.org/10.1509/jmkr.40.3.310.19238
Vrontis D, Makrides A, Christofi M, Thrassou A (2021) Social media influencer marketing: A systematic review, integrative framework and future research agenda. Int J Consum Stud 45(4):617–644. https://doi.org/10.1111/ijcs.12647
Wang T, Yeh RKJ, Chen C, Tsydypov Z (2016) What drives electronic word-of-mouth on social networking sites? Perspectives of social capital and self-determination. Telemat Inf 33(4):1034–1047. https://doi.org/10.1016/j.tele.2016.03.005
Watson JB (1917) An Attempted formulation of the scope of behavior psychology. Psychol Rev 24(5):329. https://doi.org/10.1037/h0073044
Wehmeyer ML (1999) A functional model of self-determination: Describing development and implementing instruction. Focus Autism Dev Dis 14(1):53–61. https://www.imdetermined.org/wp-content/uploads/2018/06/SD5_A-Functional-Model-of.pdf
Wei X, Chen H, Ramirez A, Jeon Y, Sun Y (2022) Influencers as endorsers and followers as consumers: exploring the role of parasocial relationship, congruence, and followers’ identifications on consumer–brand engagement. J Interact Advert 22(3):269–288. https://doi.org/10.1080/15252019.2022.2116963
Wirth J, Maier C, Laumer S (2019) Subjective norm and the privacy calculus: explaining self-disclosure on social networking sites. Paper presented at the 27th European Conference on Information Systems (ECIS). Stockholm & Uppsala, Sweden, 8–14, June 2019 https://aisel.aisnet.org/ecis2019_rp
Xiao L, Li X, Zhang Y (2023) Exploring the factors influencing consumer engagement behavior regarding short-form video advertising: a big data perspective. J Retail Consum Serv 70:103170. https://doi.org/10.1016/j.jretconser.2022.103170
Yang J, Peng MYP, Wong S, Chong W (2021) How E-learning environmental stimuli influence determinates of learning engagement in the context of COVID-19? SOR model perspective. Front Psychol 12:584976. https://doi.org/10.3389/fpsyg.2021.584976
Yang K, Jolly LD (2009) The effects of consumer perceived value and subjective norm on mobile data service adoption between American and Korean consumers. J Retail Consum Serv 16(6):502–508. https://doi.org/10.1016/j.jretconser.2009.08.005
Yang S, Zhou S, Cheng X (2019) Why do college students continue to use mobile learning? Learning involvement and self‐determination theory. Brit J Educ Technol 50(2):626–637. https://doi.org/10.1111/bjet.12634
Yusuf AS, Busalim AH (2018) Influence of e-WOM engagement on consumer purchase intention in social commerce. J Serv Mark 32(4):493–504. https://doi.org/10.1108/JSM-01-2017-0031
Zhang G, Yue X, Ye Y, Peng MYP (2021) Understanding the impact of the psychological cognitive process on student learning satisfaction: combination of the social cognitive career theory and SOR model. Front Psychol 12:712323. https://doi.org/10.3389/fpsyg.2021.712323
Zhang J, Liu J, Zhong W (2019) 广告精准度与广告效果:基于隐私关注的现场实验 [Ad targeting accuracy and advertising effectiveness: a field experiment based on privacy concerns]. Manag Sci 32(06):123–132
CAS Google Scholar
Download references
Acknowledgements
The authors thank all the participants of this study. The participants were all informed about the purpose and content of the study and voluntarily agreed to participate. The participants were able to stop participating at any time without penalty. Funding for this study was provided by Minjiang University Research Start-up Funds (No. 324-32404314).
Author information
Authors and affiliations.
School of Journalism and Communication, Minjiang University, Fuzhou, China
School of Journalism and Communication, Shanghai University, Shanghai, China
Qiuting Duan
You can also search for this author in PubMed Google Scholar
Contributions
Conceptualization: CG; methodology: CG and QD; software: CG and QD; validation: CG; formal analysis: CG and QD; investigation: CG and QD; resources: CG; data curation: CG and QD; writing—original draft preparation: CG; writing—review and editing: CG; visualization: CG; project administration: CG. All authors have read and agreed to the published version of the manuscript.
Corresponding author
Correspondence to Chenyu Gu .
Ethics declarations
Competing interests.
The authors declare no competing interests.
Ethical approval
The questionnaire and methodology for this study were approved by the School of Journalism and Communication, Minjiang University, Committee on Ethical Research (No. MJUCER20230621). The procedures used in this study adhere to the tenets of the Declaration of Helsinki.
Informed consent
Informed consent was obtained from all participants and/or their legal guardians.
Additional information
Publisher’s note Springer Nature remains neutral with regard to jurisdictional claims in published maps and institutional affiliations.
Supplementary information
Rights and permissions.
Open Access This article is licensed under a Creative Commons Attribution 4.0 International License, which permits use, sharing, adaptation, distribution and reproduction in any medium or format, as long as you give appropriate credit to the original author(s) and the source, provide a link to the Creative Commons licence, and indicate if changes were made. The images or other third party material in this article are included in the article’s Creative Commons licence, unless indicated otherwise in a credit line to the material. If material is not included in the article’s Creative Commons licence and your intended use is not permitted by statutory regulation or exceeds the permitted use, you will need to obtain permission directly from the copyright holder. To view a copy of this licence, visit http://creativecommons.org/licenses/by/4.0/ .
Reprints and permissions
About this article
Cite this article.
Gu, C., Duan, Q. Exploring the dynamics of consumer engagement in social media influencer marketing: from the self-determination theory perspective. Humanit Soc Sci Commun 11 , 587 (2024). https://doi.org/10.1057/s41599-024-03127-w
Download citation
Received : 17 December 2023
Accepted : 23 April 2024
Published : 08 May 2024
DOI : https://doi.org/10.1057/s41599-024-03127-w
Share this article
Anyone you share the following link with will be able to read this content:
Sorry, a shareable link is not currently available for this article.
Provided by the Springer Nature SharedIt content-sharing initiative
Quick links
- Explore articles by subject
- Guide to authors
- Editorial policies

Determining the Impact of ICT-Based Promotional Initiatives on the Effectiveness of E-Business Using Structural Equation Modeling
- Conference paper
- First Online: 14 May 2024
- Cite this conference paper
- Dipanwita Chakrabarty ORCID: orcid.org/0000-0003-2774-2218 13 ,
- Soumya Kanti Dhara ORCID: orcid.org/0000-0002-2487-7929 13 ,
- Arunangshu Giri ORCID: orcid.org/0000-0003-1937-868X 13 &
- Adrinil Santra ORCID: orcid.org/0000-0003-3360-3345 13
Part of the book series: Lecture Notes in Networks and Systems ((LNNS,volume 916))
Included in the following conference series:
- International Conference on Information and Communication Technology for Competitive Strategies
Purpose : The aim of the study is to determine the effectiveness of e-business impacted through the use of ICT-based promotional initiatives in the retail and service sectors. The conceptual model has been designed from the previous literatures that explained ICT-based promotional activities, viz. advertising, direct marketing, personal selling, resulted benefits in terms of branding, cost-effectiveness, positioning, and customer satisfaction. These in turn enhance the effectiveness of e-business. Research Question : (a) Whether ICT-based promotional activities influence business effectiveness? (b) How are the above said promotional activities influencing business effectiveness? Methodology : Data were collected from 400 respondents for hypothesis testing through a structured questionnaire in 5-point Likert scale. Exploratory factor analysis and structural equation modeling were used for establishing hypotheses used in the research model through SPSS 28.0 and Amos 28.0. Findings of the study : The result ascertains the positive significant influence of all the factors on the effectiveness of e-business. Originality : This paper contributes to test the effectiveness of e-business influenced by ICT-based promotional activities, and no such existing literature of previous research conducted has been found to provide any secondary data. Hence the analysis is based on primary data collected, which justifies originality of the study.
This is a preview of subscription content, log in via an institution to check access.
Access this chapter
- Available as PDF
- Read on any device
- Instant download
- Own it forever
- Available as EPUB and PDF
- Compact, lightweight edition
- Dispatched in 3 to 5 business days
- Free shipping worldwide - see info
Tax calculation will be finalised at checkout
Purchases are for personal use only
Institutional subscriptions
Arslandere M, Tuncer İ, Ada S (2020) The impact of ICT use in promotional activities on export performance: an empirical investigation. Bus Manag Stud Int J 8(2):2384–2413
Google Scholar
Laudon KC, Laudon JP (2012) Management information systems: managing the digital firm, 15th edn. Prentice Hall, New Delhi
Bell J, Loane S (2010) ‘New-wave’ global firms: web 2.0 and SME internationalization. J Market Manag 26(3–4):213–229
Article Google Scholar
Gilmore JH, Pine J (2000) Markets of one: creating customer-unique value through mass customization. J Bus Strat Camb 21(4):46
Setiowati R, Hartoyo Daryanto HK, Arifin B (2015) The effects of ICT adoption on marketing capabilities and business performance of Indonesian SMEs in the fashion industry. J Bus Retail Manag Res (JBRMR) 43:100–115
Luo H, Cheng S, Zhou W, Song W, Yu S, Lin X (2021) Research on the impact of online promotions on consumers’ impulsive online shopping intentions. J Theor Appl Electr Commer Res 16:2386–2404
Bakator M, Đorđević D, Ćoćkalo D (2018) Promotional activities and customer satisfaction: long-term influence or a temporary marketing “mirage”? Marketing 49(2):113–123
Bermeo-Giraldo MC, Valencia-Arias A, Rosas JDR, Benjumea-Arias M, Calderón JAV (2022) Factors influencing the use of digital marketing by small and medium-sized enterprises during COVID-19. Informatics 9(86):1224
Kotler P, Armstrong G (2013) Principles of marketing, 16th edn. Pearson, Harlow
Hoffman KD, Bateson JEG (2011) Services marketing: concepts, strategies, and cases, 4th edn. Cengage Learning
Giri A, Pandey M (2016) Relationship marketing as an effective promotional tool of yoga marketing in the urban Indian market: an empirical study. Indian J Market 46(5):42–54
Chatterjee S, Giri A, Paul P, Bag M (2019) Impact of ‘customer relationship management (CRM) software’ on patient satisfaction in public hospitals of urban West Bengal, India: an empirical analysis. Int J Eng Adv Technol 8(5):521–526
Giri A, Biswas W, Biswas D (2018) The impact of social networking sites on college students: a survey study in West Bengal. Indian J Market 48(8):7–23
Giri A, Gangopadhyay S, Majumder J, Paul P (2019) Model development for employee retention in Indian construction industry using structural equation modeling (SEM). Int J Manag (IJM) 10(4):196–204
Giri A, Chatterjee S, Paul P, Chakraborty S (2019) Determining the impact of artificial intelligence on ‘developing marketing strategies’ in organized retail sector of West Bengal. India. Int J Eng Adv Technol 8(6):3031–3036
Chakraborty S, Giri A, Biswas S, Bag M (2020) Measuring the impact of celebrity endorsement on consumer purchase intention of beauty soap in Indian context. Int J Sci Technol Res 9(2):1019–1022
Giri A, Chatterjee S (2020) Impact of fluid team performance on strategic HRM: an empirical study in the organized retail sector of West Bengal. Prabandhan Indian J Manag 13(4):25–42
Paul P, Giri A, Chatterjee S, Biswas S (2019) Determining the effectiveness of ‘cloud computing’ on human resource management by structural equation modeling (SEM) in manufacturing sector of West Bengal, India. Int J Innovat Technol Explor Eng 8(10):1937–1942
Giri A, Chatterjee S, Biswas S, Aich A (2020) Factors influencing consumer purchase intention of daily groceries through B2C websites in metro-cities of India. Int J Sci Technol Res 9(1):719–722
Chakraborty S, Giri A, Aich A, Biswas S (2020) Evaluating influence of artificial intelligence on human resource management using PLS-SEM (Partial least squares-structural equation modeling). Int J Sci Technol Res 9(3):5876–5880
Kim E, Euh Y, Yoo J, Lee JG, Jo Y, Lee D (2021) Which business strategy improves ICT startup companies’ technical efficiency? Technol Anal Strat Manag 33(7):843–856
Belousova V, Bondarenko O, Chichkanov N, Lebedev D, Miles I (2022) Coping with greenhouse gas emissions: insights from digital business services. Energies 15:2745
Download references
Author information
Authors and affiliations.
Haldia Institute of Technology, Haldia, West Bengal, India
Dipanwita Chakrabarty, Soumya Kanti Dhara, Arunangshu Giri & Adrinil Santra
You can also search for this author in PubMed Google Scholar
Corresponding author
Correspondence to Arunangshu Giri .
Editor information
Editors and affiliations.
Global Knowledge Research Foundation, Ahmedabad, Gujarat, India
Nottingham Trent University, Nottingham, UK
Mufti Mahmud
University of Peradeniya, Delthota, Sri Lanka
Roshan G. Ragel
Department of CSE, SNS College of Technology, Coimbatore, Tamil Nadu, India
Rights and permissions
Reprints and permissions
Copyright information
© 2024 The Author(s), under exclusive license to Springer Nature Singapore Pte Ltd.
About this paper
Cite this paper.
Chakrabarty, D., Dhara, S.K., Giri, A., Santra, A. (2024). Determining the Impact of ICT-Based Promotional Initiatives on the Effectiveness of E-Business Using Structural Equation Modeling. In: Joshi, A., Mahmud, M., Ragel, R.G., Kartik, S. (eds) ICT: Cyber Security and Applications. ICTCS 2022. Lecture Notes in Networks and Systems, vol 916. Springer, Singapore. https://doi.org/10.1007/978-981-97-0744-7_21
Download citation
DOI : https://doi.org/10.1007/978-981-97-0744-7_21
Published : 14 May 2024
Publisher Name : Springer, Singapore
Print ISBN : 978-981-97-0743-0
Online ISBN : 978-981-97-0744-7
eBook Packages : Engineering Engineering (R0)
Share this paper
Anyone you share the following link with will be able to read this content:
Sorry, a shareable link is not currently available for this article.
Provided by the Springer Nature SharedIt content-sharing initiative
- Publish with us
Policies and ethics
- Find a journal
- Track your research
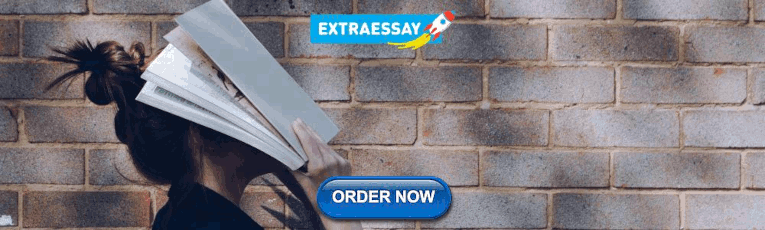
IMAGES
VIDEO
COMMENTS
Structural equation modeling is a multivariate data analysis method for analyzing complex relationships among constructs and indicators. To estimate structural equation models, researchers generally draw on two methods: covariance-based SEM (CB-SEM) and partial least squares SEM (PLS-SEM). Whereas CB-SEM is primarily used to confirm theories ...
This study develops a checklist with guidelines for the methods and important factors to consider in research using structural equation modeling (SEM). Method. The paper discusses the factors to consider in the process across the three stages of 1) model setting, 2) model evaluation and modification, and 3) interpretation and reporting of SEM ...
Structural equation modeling (SEM) is a powerful statistical approach for the testing of networks of direct and indirect theoretical causal relationships in complex data sets with intercorrelated ...
Explore the current issue of Structural Equation Modeling: A Multidisciplinary Journal, Volume 31, Issue 3, 2024. ... News & calls for papers Advertising information; Browse all articles & issues Browse. Latest articles ... Abstract forPenalized Structural Equation Models | Full Text | References | PDF (1.5 MB) | EPUB | Supplemental. 261 Views ...
The focus of the present paper in on structural equation models and the latent variable models that are included in SEM. 1. Description of SEM ... A structural equation model - from Nachtigall C, Kroehne U, Funke F, Steyer R. ... Rabe-Hesketh S, Skrondal A: Classical latent variable models for medical research. Stat Methods Med Res. 2008, 17: 5 ...
This paper is a tribute to researchers who have significantly contributed to improving and advancing structural equation modeling (SEM). It is, therefore, a brief overview of SEM and presents its beginnings, historical development, its usefulness in the social sciences and the statistical and philosophical (theoretical) controversies which have often appeared in the literature pertaining to SEM.
Abstract. Structural equation modeling is a multivariate data analysis method for analyzing complex relationships among constructs and indicators. To estimate structural equation models ...
Structural equation modeling always uses the same general analytic technique (i.e., comparing a hypothesis with an observed covariance matrix), whereas causal DAGs are not associated with any particular statistical model. In structural equation modeling, the model diagram is drawn to reflect a specific model the researcher plans to test.
The focus of the present paper in on structural equation models and the latent variable models that are included in SEM. 1. Description of SEM ... A structural equation model - from Nachtigall C, Kroehne U, Funke F, Steyer R. ... Skrondal A. Classical latent variable models for medical research. Stat Methods Med Res. 2008; 17:5-32. doi: 10. ...
This paper provides a tutorial discussion on analyzing structural equation modelling (SEM). SEM ... Structural Equation Modeling, General Linear Model, Regression ... This feature is a vital ability in the current research in which the model has an essential factor of mediation, i.e., a dependent variable, such as internal operation or ...
Aims This review was developed to introduce the essential components and variants of structural equation modeling (SEM), synthesize the common issues in SEM applications, and share our views on SEM's future in ecological research. Methods We searched the Web of Science on SEM applications in ecological studies from 1999 through 2016 and summarized the potential of SEMs, with a special focus ...
To complement recent articles in this journal on structural equation modeling (SEM) practice and principles by Martens and by Quintana and Maxwell, respectively, the authors offer a consumer's guide to SEM. Using an example derived from theory and research on vocational psychology, the authors outline six steps in SEM: model specification ...
Structural equation modeling (SEM) is a multivariate statistical framework that is used to model complex. relationships between directly and indirectly observed (latent) variables. SEM is a ...
AbstractA large segment of management research in recent years has used structural equation modeling (SEM) as an analytical approach that simultaneously combines factor analysis and linear regression models for theory testing. With this approach, latent variables (factors) represent the concepts of a theory, and data from measures (indicators) are used as input for statistical analyses that ...
The use of structural equation modeling (SEM) has grown substantially over the past 40 years within organizational research and beyond. There have been many different developments in SEM that make it increasingly useful for a variety of data types, research designs, research questions, and research contexts in the organizational sciences. To give researchers a better understanding of how and ...
Aims. To stimulate appropriate applications and interpretations of SEM for the investigation of response shift, the current paper aims to (1) provide an accessible description of the SEM operationalizations of change that are relevant for response shift investigation; (2) discuss practical considerations in applying SEM; and (3) provide guidelines and recommendations for researchers who want ...
An increasing number of studies applying structural equation modelling have been witnessed to the research on teaching and teacher education. This paper reviews 15 of 132 articles that use structural equation modelling as the main strategy of data analysis published in Teaching and Teacher Education from 1985 to 2020. The 15 articles touch on three themes of teaching and teacher education ...
PLS-SEM is a useful approach to estimating structural models in L2 and education research. Considering its features and the research situations that suit its objectives, such as analyzing complex theoretical models, handling non-normal data, achieving statistical power with smaller sample sizes, and focusing on the model's predictive capability ...
Question: How can quantitative research be used to identify causal mechanisms? Kosuke Imai (Princeton) Structural Equation Modeling POL572 Spring 2016 3 / 39. Direct and Indirect Effects Causal mediation analysis: Mediator, M Indrect (Mediation) ... The Linear Structural Equation Model Y i
This paper critically examined a broad view of Structural Equation Model ... Issue-7, pp-11-19 www.ajhssr.com Research Paper Open Access STRUCTURAL EQUATION MODEL (SEM) 1 AJAYI, Lawrence Boboye Phd , 2ADEBAYO, Adeyinka Taoheed 1 Department of Finance Ekiti State University, Ado-Ekiti, Ekiti State. Department of FinanceEkiti State University ...
Analysis of mediating effects Goodness-of-fit indices and path coefficients for the theoretical model of older adults' sleep quality. Based on the results of the above analyses, a structural equation model was constructed with childhood adversity as the independent variable, anxiety and negative coping as the mediating variables, and sleep quality as the dependent variable.
The introduction of AlphaFold 21 has spurred a revolution in modelling the structure of proteins and their interactions, enabling a huge range of applications in protein modelling and design2-6 ...
Abstract and Figures. Purpose-The main objective of this paper is to provide a well-organized guide for the application of partial least squares structural equation modeling (PLS-SEM) in tourism ...
In this paper, the structural stability of seepage system in fractured rock mass is studied by numerical response analysis based on bifurcation theory and nonlinear seepage dynamics equation.
Therefore, this paper proposes the following research hypothesis: ... To ensure the robustness and appropriateness of our structural equation model, we also conducted a thorough evaluation of the ...
The existing ship hull structure stress monitoring can only give the stress state of typical measuring points, but the monitoring point may not be the most dangerous location of the ship hull structure. To solve this problem, this paper carried out the research of ship strength digital twin. Concretely, to meet the real-time requirements for obtaining the structural stress state, this paper ...
Exploratory factor analysis and structural equation modeling were used for establishing hypotheses used in the research model through SPSS 28.0 and Amos 28.0. Findings of the study: The result ascertains the positive significant influence of all the factors on the effectiveness of e-business.