
An official website of the United States government
The .gov means it's official. Federal government websites often end in .gov or .mil. Before sharing sensitive information, make sure you're on a federal government site.
The site is secure. The https:// ensures that you are connecting to the official website and that any information you provide is encrypted and transmitted securely.
- Publications
- Account settings
- Browse Titles
NCBI Bookshelf. A service of the National Library of Medicine, National Institutes of Health.
StatPearls [Internet]. Treasure Island (FL): StatPearls Publishing; 2024 Jan-.

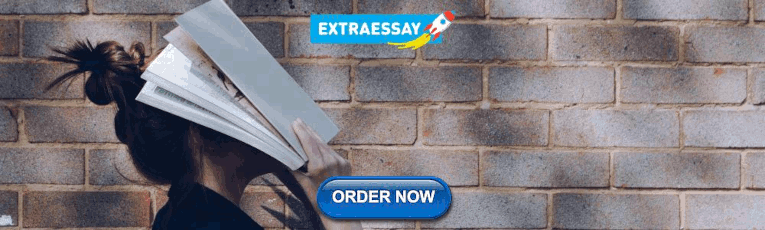
StatPearls [Internet].
Hypothesis testing, p values, confidence intervals, and significance.
Jacob Shreffler ; Martin R. Huecker .
Affiliations
Last Update: March 13, 2023 .
- Definition/Introduction
Medical providers often rely on evidence-based medicine to guide decision-making in practice. Often a research hypothesis is tested with results provided, typically with p values, confidence intervals, or both. Additionally, statistical or research significance is estimated or determined by the investigators. Unfortunately, healthcare providers may have different comfort levels in interpreting these findings, which may affect the adequate application of the data.
- Issues of Concern
Without a foundational understanding of hypothesis testing, p values, confidence intervals, and the difference between statistical and clinical significance, it may affect healthcare providers' ability to make clinical decisions without relying purely on the research investigators deemed level of significance. Therefore, an overview of these concepts is provided to allow medical professionals to use their expertise to determine if results are reported sufficiently and if the study outcomes are clinically appropriate to be applied in healthcare practice.
Hypothesis Testing
Investigators conducting studies need research questions and hypotheses to guide analyses. Starting with broad research questions (RQs), investigators then identify a gap in current clinical practice or research. Any research problem or statement is grounded in a better understanding of relationships between two or more variables. For this article, we will use the following research question example:
Research Question: Is Drug 23 an effective treatment for Disease A?
Research questions do not directly imply specific guesses or predictions; we must formulate research hypotheses. A hypothesis is a predetermined declaration regarding the research question in which the investigator(s) makes a precise, educated guess about a study outcome. This is sometimes called the alternative hypothesis and ultimately allows the researcher to take a stance based on experience or insight from medical literature. An example of a hypothesis is below.
Research Hypothesis: Drug 23 will significantly reduce symptoms associated with Disease A compared to Drug 22.
The null hypothesis states that there is no statistical difference between groups based on the stated research hypothesis.
Researchers should be aware of journal recommendations when considering how to report p values, and manuscripts should remain internally consistent.
Regarding p values, as the number of individuals enrolled in a study (the sample size) increases, the likelihood of finding a statistically significant effect increases. With very large sample sizes, the p-value can be very low significant differences in the reduction of symptoms for Disease A between Drug 23 and Drug 22. The null hypothesis is deemed true until a study presents significant data to support rejecting the null hypothesis. Based on the results, the investigators will either reject the null hypothesis (if they found significant differences or associations) or fail to reject the null hypothesis (they could not provide proof that there were significant differences or associations).
To test a hypothesis, researchers obtain data on a representative sample to determine whether to reject or fail to reject a null hypothesis. In most research studies, it is not feasible to obtain data for an entire population. Using a sampling procedure allows for statistical inference, though this involves a certain possibility of error. [1] When determining whether to reject or fail to reject the null hypothesis, mistakes can be made: Type I and Type II errors. Though it is impossible to ensure that these errors have not occurred, researchers should limit the possibilities of these faults. [2]
Significance
Significance is a term to describe the substantive importance of medical research. Statistical significance is the likelihood of results due to chance. [3] Healthcare providers should always delineate statistical significance from clinical significance, a common error when reviewing biomedical research. [4] When conceptualizing findings reported as either significant or not significant, healthcare providers should not simply accept researchers' results or conclusions without considering the clinical significance. Healthcare professionals should consider the clinical importance of findings and understand both p values and confidence intervals so they do not have to rely on the researchers to determine the level of significance. [5] One criterion often used to determine statistical significance is the utilization of p values.
P values are used in research to determine whether the sample estimate is significantly different from a hypothesized value. The p-value is the probability that the observed effect within the study would have occurred by chance if, in reality, there was no true effect. Conventionally, data yielding a p<0.05 or p<0.01 is considered statistically significant. While some have debated that the 0.05 level should be lowered, it is still universally practiced. [6] Hypothesis testing allows us to determine the size of the effect.
An example of findings reported with p values are below:
Statement: Drug 23 reduced patients' symptoms compared to Drug 22. Patients who received Drug 23 (n=100) were 2.1 times less likely than patients who received Drug 22 (n = 100) to experience symptoms of Disease A, p<0.05.
Statement:Individuals who were prescribed Drug 23 experienced fewer symptoms (M = 1.3, SD = 0.7) compared to individuals who were prescribed Drug 22 (M = 5.3, SD = 1.9). This finding was statistically significant, p= 0.02.
For either statement, if the threshold had been set at 0.05, the null hypothesis (that there was no relationship) should be rejected, and we should conclude significant differences. Noticeably, as can be seen in the two statements above, some researchers will report findings with < or > and others will provide an exact p-value (0.000001) but never zero [6] . When examining research, readers should understand how p values are reported. The best practice is to report all p values for all variables within a study design, rather than only providing p values for variables with significant findings. [7] The inclusion of all p values provides evidence for study validity and limits suspicion for selective reporting/data mining.
While researchers have historically used p values, experts who find p values problematic encourage the use of confidence intervals. [8] . P-values alone do not allow us to understand the size or the extent of the differences or associations. [3] In March 2016, the American Statistical Association (ASA) released a statement on p values, noting that scientific decision-making and conclusions should not be based on a fixed p-value threshold (e.g., 0.05). They recommend focusing on the significance of results in the context of study design, quality of measurements, and validity of data. Ultimately, the ASA statement noted that in isolation, a p-value does not provide strong evidence. [9]
When conceptualizing clinical work, healthcare professionals should consider p values with a concurrent appraisal study design validity. For example, a p-value from a double-blinded randomized clinical trial (designed to minimize bias) should be weighted higher than one from a retrospective observational study [7] . The p-value debate has smoldered since the 1950s [10] , and replacement with confidence intervals has been suggested since the 1980s. [11]
Confidence Intervals
A confidence interval provides a range of values within given confidence (e.g., 95%), including the accurate value of the statistical constraint within a targeted population. [12] Most research uses a 95% CI, but investigators can set any level (e.g., 90% CI, 99% CI). [13] A CI provides a range with the lower bound and upper bound limits of a difference or association that would be plausible for a population. [14] Therefore, a CI of 95% indicates that if a study were to be carried out 100 times, the range would contain the true value in 95, [15] confidence intervals provide more evidence regarding the precision of an estimate compared to p-values. [6]
In consideration of the similar research example provided above, one could make the following statement with 95% CI:
Statement: Individuals who were prescribed Drug 23 had no symptoms after three days, which was significantly faster than those prescribed Drug 22; there was a mean difference between the two groups of days to the recovery of 4.2 days (95% CI: 1.9 – 7.8).
It is important to note that the width of the CI is affected by the standard error and the sample size; reducing a study sample number will result in less precision of the CI (increase the width). [14] A larger width indicates a smaller sample size or a larger variability. [16] A researcher would want to increase the precision of the CI. For example, a 95% CI of 1.43 – 1.47 is much more precise than the one provided in the example above. In research and clinical practice, CIs provide valuable information on whether the interval includes or excludes any clinically significant values. [14]
Null values are sometimes used for differences with CI (zero for differential comparisons and 1 for ratios). However, CIs provide more information than that. [15] Consider this example: A hospital implements a new protocol that reduced wait time for patients in the emergency department by an average of 25 minutes (95% CI: -2.5 – 41 minutes). Because the range crosses zero, implementing this protocol in different populations could result in longer wait times; however, the range is much higher on the positive side. Thus, while the p-value used to detect statistical significance for this may result in "not significant" findings, individuals should examine this range, consider the study design, and weigh whether or not it is still worth piloting in their workplace.
Similarly to p-values, 95% CIs cannot control for researchers' errors (e.g., study bias or improper data analysis). [14] In consideration of whether to report p-values or CIs, researchers should examine journal preferences. When in doubt, reporting both may be beneficial. [13] An example is below:
Reporting both: Individuals who were prescribed Drug 23 had no symptoms after three days, which was significantly faster than those prescribed Drug 22, p = 0.009. There was a mean difference between the two groups of days to the recovery of 4.2 days (95% CI: 1.9 – 7.8).
- Clinical Significance
Recall that clinical significance and statistical significance are two different concepts. Healthcare providers should remember that a study with statistically significant differences and large sample size may be of no interest to clinicians, whereas a study with smaller sample size and statistically non-significant results could impact clinical practice. [14] Additionally, as previously mentioned, a non-significant finding may reflect the study design itself rather than relationships between variables.
Healthcare providers using evidence-based medicine to inform practice should use clinical judgment to determine the practical importance of studies through careful evaluation of the design, sample size, power, likelihood of type I and type II errors, data analysis, and reporting of statistical findings (p values, 95% CI or both). [4] Interestingly, some experts have called for "statistically significant" or "not significant" to be excluded from work as statistical significance never has and will never be equivalent to clinical significance. [17]
The decision on what is clinically significant can be challenging, depending on the providers' experience and especially the severity of the disease. Providers should use their knowledge and experiences to determine the meaningfulness of study results and make inferences based not only on significant or insignificant results by researchers but through their understanding of study limitations and practical implications.
- Nursing, Allied Health, and Interprofessional Team Interventions
All physicians, nurses, pharmacists, and other healthcare professionals should strive to understand the concepts in this chapter. These individuals should maintain the ability to review and incorporate new literature for evidence-based and safe care.
- Review Questions
- Access free multiple choice questions on this topic.
- Comment on this article.
Disclosure: Jacob Shreffler declares no relevant financial relationships with ineligible companies.
Disclosure: Martin Huecker declares no relevant financial relationships with ineligible companies.
This book is distributed under the terms of the Creative Commons Attribution-NonCommercial-NoDerivatives 4.0 International (CC BY-NC-ND 4.0) ( http://creativecommons.org/licenses/by-nc-nd/4.0/ ), which permits others to distribute the work, provided that the article is not altered or used commercially. You are not required to obtain permission to distribute this article, provided that you credit the author and journal.
- Cite this Page Shreffler J, Huecker MR. Hypothesis Testing, P Values, Confidence Intervals, and Significance. [Updated 2023 Mar 13]. In: StatPearls [Internet]. Treasure Island (FL): StatPearls Publishing; 2024 Jan-.
In this Page
Bulk download.
- Bulk download StatPearls data from FTP
Related information
- PMC PubMed Central citations
- PubMed Links to PubMed
Similar articles in PubMed
- The reporting of p values, confidence intervals and statistical significance in Preventive Veterinary Medicine (1997-2017). [PeerJ. 2021] The reporting of p values, confidence intervals and statistical significance in Preventive Veterinary Medicine (1997-2017). Messam LLM, Weng HY, Rosenberger NWY, Tan ZH, Payet SDM, Santbakshsing M. PeerJ. 2021; 9:e12453. Epub 2021 Nov 24.
- Review Clinical versus statistical significance: interpreting P values and confidence intervals related to measures of association to guide decision making. [J Pharm Pract. 2010] Review Clinical versus statistical significance: interpreting P values and confidence intervals related to measures of association to guide decision making. Ferrill MJ, Brown DA, Kyle JA. J Pharm Pract. 2010 Aug; 23(4):344-51. Epub 2010 Apr 13.
- Interpreting "statistical hypothesis testing" results in clinical research. [J Ayurveda Integr Med. 2012] Interpreting "statistical hypothesis testing" results in clinical research. Sarmukaddam SB. J Ayurveda Integr Med. 2012 Apr; 3(2):65-9.
- Confidence intervals in procedural dermatology: an intuitive approach to interpreting data. [Dermatol Surg. 2005] Confidence intervals in procedural dermatology: an intuitive approach to interpreting data. Alam M, Barzilai DA, Wrone DA. Dermatol Surg. 2005 Apr; 31(4):462-6.
- Review Is statistical significance testing useful in interpreting data? [Reprod Toxicol. 1993] Review Is statistical significance testing useful in interpreting data? Savitz DA. Reprod Toxicol. 1993; 7(2):95-100.
Recent Activity
- Hypothesis Testing, P Values, Confidence Intervals, and Significance - StatPearl... Hypothesis Testing, P Values, Confidence Intervals, and Significance - StatPearls
Your browsing activity is empty.
Activity recording is turned off.
Turn recording back on
Connect with NLM
National Library of Medicine 8600 Rockville Pike Bethesda, MD 20894
Web Policies FOIA HHS Vulnerability Disclosure
Help Accessibility Careers
- Bipolar Disorder
- Therapy Center
- When To See a Therapist
- Types of Therapy
- Best Online Therapy
- Best Couples Therapy
- Best Family Therapy
- Managing Stress
- Sleep and Dreaming
- Understanding Emotions
- Self-Improvement
- Healthy Relationships
- Student Resources
- Personality Types
- Guided Meditations
- Verywell Mind Insights
- 2024 Verywell Mind 25
- Mental Health in the Classroom
- Editorial Process
- Meet Our Review Board
- Crisis Support
How to Write a Great Hypothesis
Hypothesis Definition, Format, Examples, and Tips
Kendra Cherry, MS, is a psychosocial rehabilitation specialist, psychology educator, and author of the "Everything Psychology Book."
:max_bytes(150000):strip_icc():format(webp)/IMG_9791-89504ab694d54b66bbd72cb84ffb860e.jpg)
Amy Morin, LCSW, is a psychotherapist and international bestselling author. Her books, including "13 Things Mentally Strong People Don't Do," have been translated into more than 40 languages. Her TEDx talk, "The Secret of Becoming Mentally Strong," is one of the most viewed talks of all time.
:max_bytes(150000):strip_icc():format(webp)/VW-MIND-Amy-2b338105f1ee493f94d7e333e410fa76.jpg)
Verywell / Alex Dos Diaz
- The Scientific Method
Hypothesis Format
Falsifiability of a hypothesis.
- Operationalization
Hypothesis Types
Hypotheses examples.
- Collecting Data
A hypothesis is a tentative statement about the relationship between two or more variables. It is a specific, testable prediction about what you expect to happen in a study. It is a preliminary answer to your question that helps guide the research process.
Consider a study designed to examine the relationship between sleep deprivation and test performance. The hypothesis might be: "This study is designed to assess the hypothesis that sleep-deprived people will perform worse on a test than individuals who are not sleep-deprived."
At a Glance
A hypothesis is crucial to scientific research because it offers a clear direction for what the researchers are looking to find. This allows them to design experiments to test their predictions and add to our scientific knowledge about the world. This article explores how a hypothesis is used in psychology research, how to write a good hypothesis, and the different types of hypotheses you might use.
The Hypothesis in the Scientific Method
In the scientific method , whether it involves research in psychology, biology, or some other area, a hypothesis represents what the researchers think will happen in an experiment. The scientific method involves the following steps:
- Forming a question
- Performing background research
- Creating a hypothesis
- Designing an experiment
- Collecting data
- Analyzing the results
- Drawing conclusions
- Communicating the results
The hypothesis is a prediction, but it involves more than a guess. Most of the time, the hypothesis begins with a question which is then explored through background research. At this point, researchers then begin to develop a testable hypothesis.
Unless you are creating an exploratory study, your hypothesis should always explain what you expect to happen.
In a study exploring the effects of a particular drug, the hypothesis might be that researchers expect the drug to have some type of effect on the symptoms of a specific illness. In psychology, the hypothesis might focus on how a certain aspect of the environment might influence a particular behavior.
Remember, a hypothesis does not have to be correct. While the hypothesis predicts what the researchers expect to see, the goal of the research is to determine whether this guess is right or wrong. When conducting an experiment, researchers might explore numerous factors to determine which ones might contribute to the ultimate outcome.
In many cases, researchers may find that the results of an experiment do not support the original hypothesis. When writing up these results, the researchers might suggest other options that should be explored in future studies.
In many cases, researchers might draw a hypothesis from a specific theory or build on previous research. For example, prior research has shown that stress can impact the immune system. So a researcher might hypothesize: "People with high-stress levels will be more likely to contract a common cold after being exposed to the virus than people who have low-stress levels."
In other instances, researchers might look at commonly held beliefs or folk wisdom. "Birds of a feather flock together" is one example of folk adage that a psychologist might try to investigate. The researcher might pose a specific hypothesis that "People tend to select romantic partners who are similar to them in interests and educational level."
Elements of a Good Hypothesis
So how do you write a good hypothesis? When trying to come up with a hypothesis for your research or experiments, ask yourself the following questions:
- Is your hypothesis based on your research on a topic?
- Can your hypothesis be tested?
- Does your hypothesis include independent and dependent variables?
Before you come up with a specific hypothesis, spend some time doing background research. Once you have completed a literature review, start thinking about potential questions you still have. Pay attention to the discussion section in the journal articles you read . Many authors will suggest questions that still need to be explored.
How to Formulate a Good Hypothesis
To form a hypothesis, you should take these steps:
- Collect as many observations about a topic or problem as you can.
- Evaluate these observations and look for possible causes of the problem.
- Create a list of possible explanations that you might want to explore.
- After you have developed some possible hypotheses, think of ways that you could confirm or disprove each hypothesis through experimentation. This is known as falsifiability.
In the scientific method , falsifiability is an important part of any valid hypothesis. In order to test a claim scientifically, it must be possible that the claim could be proven false.
Students sometimes confuse the idea of falsifiability with the idea that it means that something is false, which is not the case. What falsifiability means is that if something was false, then it is possible to demonstrate that it is false.
One of the hallmarks of pseudoscience is that it makes claims that cannot be refuted or proven false.
The Importance of Operational Definitions
A variable is a factor or element that can be changed and manipulated in ways that are observable and measurable. However, the researcher must also define how the variable will be manipulated and measured in the study.
Operational definitions are specific definitions for all relevant factors in a study. This process helps make vague or ambiguous concepts detailed and measurable.
For example, a researcher might operationally define the variable " test anxiety " as the results of a self-report measure of anxiety experienced during an exam. A "study habits" variable might be defined by the amount of studying that actually occurs as measured by time.
These precise descriptions are important because many things can be measured in various ways. Clearly defining these variables and how they are measured helps ensure that other researchers can replicate your results.
Replicability
One of the basic principles of any type of scientific research is that the results must be replicable.
Replication means repeating an experiment in the same way to produce the same results. By clearly detailing the specifics of how the variables were measured and manipulated, other researchers can better understand the results and repeat the study if needed.
Some variables are more difficult than others to define. For example, how would you operationally define a variable such as aggression ? For obvious ethical reasons, researchers cannot create a situation in which a person behaves aggressively toward others.
To measure this variable, the researcher must devise a measurement that assesses aggressive behavior without harming others. The researcher might utilize a simulated task to measure aggressiveness in this situation.
Hypothesis Checklist
- Does your hypothesis focus on something that you can actually test?
- Does your hypothesis include both an independent and dependent variable?
- Can you manipulate the variables?
- Can your hypothesis be tested without violating ethical standards?
The hypothesis you use will depend on what you are investigating and hoping to find. Some of the main types of hypotheses that you might use include:
- Simple hypothesis : This type of hypothesis suggests there is a relationship between one independent variable and one dependent variable.
- Complex hypothesis : This type suggests a relationship between three or more variables, such as two independent and dependent variables.
- Null hypothesis : This hypothesis suggests no relationship exists between two or more variables.
- Alternative hypothesis : This hypothesis states the opposite of the null hypothesis.
- Statistical hypothesis : This hypothesis uses statistical analysis to evaluate a representative population sample and then generalizes the findings to the larger group.
- Logical hypothesis : This hypothesis assumes a relationship between variables without collecting data or evidence.
A hypothesis often follows a basic format of "If {this happens} then {this will happen}." One way to structure your hypothesis is to describe what will happen to the dependent variable if you change the independent variable .
The basic format might be: "If {these changes are made to a certain independent variable}, then we will observe {a change in a specific dependent variable}."
A few examples of simple hypotheses:
- "Students who eat breakfast will perform better on a math exam than students who do not eat breakfast."
- "Students who experience test anxiety before an English exam will get lower scores than students who do not experience test anxiety."
- "Motorists who talk on the phone while driving will be more likely to make errors on a driving course than those who do not talk on the phone."
- "Children who receive a new reading intervention will have higher reading scores than students who do not receive the intervention."
Examples of a complex hypothesis include:
- "People with high-sugar diets and sedentary activity levels are more likely to develop depression."
- "Younger people who are regularly exposed to green, outdoor areas have better subjective well-being than older adults who have limited exposure to green spaces."
Examples of a null hypothesis include:
- "There is no difference in anxiety levels between people who take St. John's wort supplements and those who do not."
- "There is no difference in scores on a memory recall task between children and adults."
- "There is no difference in aggression levels between children who play first-person shooter games and those who do not."
Examples of an alternative hypothesis:
- "People who take St. John's wort supplements will have less anxiety than those who do not."
- "Adults will perform better on a memory task than children."
- "Children who play first-person shooter games will show higher levels of aggression than children who do not."
Collecting Data on Your Hypothesis
Once a researcher has formed a testable hypothesis, the next step is to select a research design and start collecting data. The research method depends largely on exactly what they are studying. There are two basic types of research methods: descriptive research and experimental research.
Descriptive Research Methods
Descriptive research such as case studies , naturalistic observations , and surveys are often used when conducting an experiment is difficult or impossible. These methods are best used to describe different aspects of a behavior or psychological phenomenon.
Once a researcher has collected data using descriptive methods, a correlational study can examine how the variables are related. This research method might be used to investigate a hypothesis that is difficult to test experimentally.
Experimental Research Methods
Experimental methods are used to demonstrate causal relationships between variables. In an experiment, the researcher systematically manipulates a variable of interest (known as the independent variable) and measures the effect on another variable (known as the dependent variable).
Unlike correlational studies, which can only be used to determine if there is a relationship between two variables, experimental methods can be used to determine the actual nature of the relationship—whether changes in one variable actually cause another to change.
The hypothesis is a critical part of any scientific exploration. It represents what researchers expect to find in a study or experiment. In situations where the hypothesis is unsupported by the research, the research still has value. Such research helps us better understand how different aspects of the natural world relate to one another. It also helps us develop new hypotheses that can then be tested in the future.
Thompson WH, Skau S. On the scope of scientific hypotheses . R Soc Open Sci . 2023;10(8):230607. doi:10.1098/rsos.230607
Taran S, Adhikari NKJ, Fan E. Falsifiability in medicine: what clinicians can learn from Karl Popper [published correction appears in Intensive Care Med. 2021 Jun 17;:]. Intensive Care Med . 2021;47(9):1054-1056. doi:10.1007/s00134-021-06432-z
Eyler AA. Research Methods for Public Health . 1st ed. Springer Publishing Company; 2020. doi:10.1891/9780826182067.0004
Nosek BA, Errington TM. What is replication ? PLoS Biol . 2020;18(3):e3000691. doi:10.1371/journal.pbio.3000691
Aggarwal R, Ranganathan P. Study designs: Part 2 - Descriptive studies . Perspect Clin Res . 2019;10(1):34-36. doi:10.4103/picr.PICR_154_18
Nevid J. Psychology: Concepts and Applications. Wadworth, 2013.
By Kendra Cherry, MSEd Kendra Cherry, MS, is a psychosocial rehabilitation specialist, psychology educator, and author of the "Everything Psychology Book."
Research Hypothesis In Psychology: Types, & Examples
Saul Mcleod, PhD
Editor-in-Chief for Simply Psychology
BSc (Hons) Psychology, MRes, PhD, University of Manchester
Saul Mcleod, PhD., is a qualified psychology teacher with over 18 years of experience in further and higher education. He has been published in peer-reviewed journals, including the Journal of Clinical Psychology.
Learn about our Editorial Process
Olivia Guy-Evans, MSc
Associate Editor for Simply Psychology
BSc (Hons) Psychology, MSc Psychology of Education
Olivia Guy-Evans is a writer and associate editor for Simply Psychology. She has previously worked in healthcare and educational sectors.
On This Page:
A research hypothesis, in its plural form “hypotheses,” is a specific, testable prediction about the anticipated results of a study, established at its outset. It is a key component of the scientific method .
Hypotheses connect theory to data and guide the research process towards expanding scientific understanding
Some key points about hypotheses:
- A hypothesis expresses an expected pattern or relationship. It connects the variables under investigation.
- It is stated in clear, precise terms before any data collection or analysis occurs. This makes the hypothesis testable.
- A hypothesis must be falsifiable. It should be possible, even if unlikely in practice, to collect data that disconfirms rather than supports the hypothesis.
- Hypotheses guide research. Scientists design studies to explicitly evaluate hypotheses about how nature works.
- For a hypothesis to be valid, it must be testable against empirical evidence. The evidence can then confirm or disprove the testable predictions.
- Hypotheses are informed by background knowledge and observation, but go beyond what is already known to propose an explanation of how or why something occurs.
Predictions typically arise from a thorough knowledge of the research literature, curiosity about real-world problems or implications, and integrating this to advance theory. They build on existing literature while providing new insight.
Types of Research Hypotheses
Alternative hypothesis.
The research hypothesis is often called the alternative or experimental hypothesis in experimental research.
It typically suggests a potential relationship between two key variables: the independent variable, which the researcher manipulates, and the dependent variable, which is measured based on those changes.
The alternative hypothesis states a relationship exists between the two variables being studied (one variable affects the other).
A hypothesis is a testable statement or prediction about the relationship between two or more variables. It is a key component of the scientific method. Some key points about hypotheses:
- Important hypotheses lead to predictions that can be tested empirically. The evidence can then confirm or disprove the testable predictions.
In summary, a hypothesis is a precise, testable statement of what researchers expect to happen in a study and why. Hypotheses connect theory to data and guide the research process towards expanding scientific understanding.
An experimental hypothesis predicts what change(s) will occur in the dependent variable when the independent variable is manipulated.
It states that the results are not due to chance and are significant in supporting the theory being investigated.
The alternative hypothesis can be directional, indicating a specific direction of the effect, or non-directional, suggesting a difference without specifying its nature. It’s what researchers aim to support or demonstrate through their study.
Null Hypothesis
The null hypothesis states no relationship exists between the two variables being studied (one variable does not affect the other). There will be no changes in the dependent variable due to manipulating the independent variable.
It states results are due to chance and are not significant in supporting the idea being investigated.
The null hypothesis, positing no effect or relationship, is a foundational contrast to the research hypothesis in scientific inquiry. It establishes a baseline for statistical testing, promoting objectivity by initiating research from a neutral stance.
Many statistical methods are tailored to test the null hypothesis, determining the likelihood of observed results if no true effect exists.
This dual-hypothesis approach provides clarity, ensuring that research intentions are explicit, and fosters consistency across scientific studies, enhancing the standardization and interpretability of research outcomes.
Nondirectional Hypothesis
A non-directional hypothesis, also known as a two-tailed hypothesis, predicts that there is a difference or relationship between two variables but does not specify the direction of this relationship.
It merely indicates that a change or effect will occur without predicting which group will have higher or lower values.
For example, “There is a difference in performance between Group A and Group B” is a non-directional hypothesis.
Directional Hypothesis
A directional (one-tailed) hypothesis predicts the nature of the effect of the independent variable on the dependent variable. It predicts in which direction the change will take place. (i.e., greater, smaller, less, more)
It specifies whether one variable is greater, lesser, or different from another, rather than just indicating that there’s a difference without specifying its nature.
For example, “Exercise increases weight loss” is a directional hypothesis.
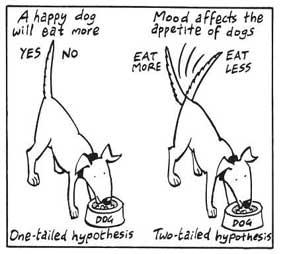
Falsifiability
The Falsification Principle, proposed by Karl Popper , is a way of demarcating science from non-science. It suggests that for a theory or hypothesis to be considered scientific, it must be testable and irrefutable.
Falsifiability emphasizes that scientific claims shouldn’t just be confirmable but should also have the potential to be proven wrong.
It means that there should exist some potential evidence or experiment that could prove the proposition false.
However many confirming instances exist for a theory, it only takes one counter observation to falsify it. For example, the hypothesis that “all swans are white,” can be falsified by observing a black swan.
For Popper, science should attempt to disprove a theory rather than attempt to continually provide evidence to support a research hypothesis.
Can a Hypothesis be Proven?
Hypotheses make probabilistic predictions. They state the expected outcome if a particular relationship exists. However, a study result supporting a hypothesis does not definitively prove it is true.
All studies have limitations. There may be unknown confounding factors or issues that limit the certainty of conclusions. Additional studies may yield different results.
In science, hypotheses can realistically only be supported with some degree of confidence, not proven. The process of science is to incrementally accumulate evidence for and against hypothesized relationships in an ongoing pursuit of better models and explanations that best fit the empirical data. But hypotheses remain open to revision and rejection if that is where the evidence leads.
- Disproving a hypothesis is definitive. Solid disconfirmatory evidence will falsify a hypothesis and require altering or discarding it based on the evidence.
- However, confirming evidence is always open to revision. Other explanations may account for the same results, and additional or contradictory evidence may emerge over time.
We can never 100% prove the alternative hypothesis. Instead, we see if we can disprove, or reject the null hypothesis.
If we reject the null hypothesis, this doesn’t mean that our alternative hypothesis is correct but does support the alternative/experimental hypothesis.
Upon analysis of the results, an alternative hypothesis can be rejected or supported, but it can never be proven to be correct. We must avoid any reference to results proving a theory as this implies 100% certainty, and there is always a chance that evidence may exist which could refute a theory.
How to Write a Hypothesis
- Identify variables . The researcher manipulates the independent variable and the dependent variable is the measured outcome.
- Operationalized the variables being investigated . Operationalization of a hypothesis refers to the process of making the variables physically measurable or testable, e.g. if you are about to study aggression, you might count the number of punches given by participants.
- Decide on a direction for your prediction . If there is evidence in the literature to support a specific effect of the independent variable on the dependent variable, write a directional (one-tailed) hypothesis. If there are limited or ambiguous findings in the literature regarding the effect of the independent variable on the dependent variable, write a non-directional (two-tailed) hypothesis.
- Make it Testable : Ensure your hypothesis can be tested through experimentation or observation. It should be possible to prove it false (principle of falsifiability).
- Clear & concise language . A strong hypothesis is concise (typically one to two sentences long), and formulated using clear and straightforward language, ensuring it’s easily understood and testable.
Consider a hypothesis many teachers might subscribe to: students work better on Monday morning than on Friday afternoon (IV=Day, DV= Standard of work).
Now, if we decide to study this by giving the same group of students a lesson on a Monday morning and a Friday afternoon and then measuring their immediate recall of the material covered in each session, we would end up with the following:
- The alternative hypothesis states that students will recall significantly more information on a Monday morning than on a Friday afternoon.
- The null hypothesis states that there will be no significant difference in the amount recalled on a Monday morning compared to a Friday afternoon. Any difference will be due to chance or confounding factors.
More Examples
- Memory : Participants exposed to classical music during study sessions will recall more items from a list than those who studied in silence.
- Social Psychology : Individuals who frequently engage in social media use will report higher levels of perceived social isolation compared to those who use it infrequently.
- Developmental Psychology : Children who engage in regular imaginative play have better problem-solving skills than those who don’t.
- Clinical Psychology : Cognitive-behavioral therapy will be more effective in reducing symptoms of anxiety over a 6-month period compared to traditional talk therapy.
- Cognitive Psychology : Individuals who multitask between various electronic devices will have shorter attention spans on focused tasks than those who single-task.
- Health Psychology : Patients who practice mindfulness meditation will experience lower levels of chronic pain compared to those who don’t meditate.
- Organizational Psychology : Employees in open-plan offices will report higher levels of stress than those in private offices.
- Behavioral Psychology : Rats rewarded with food after pressing a lever will press it more frequently than rats who receive no reward.

Related Articles
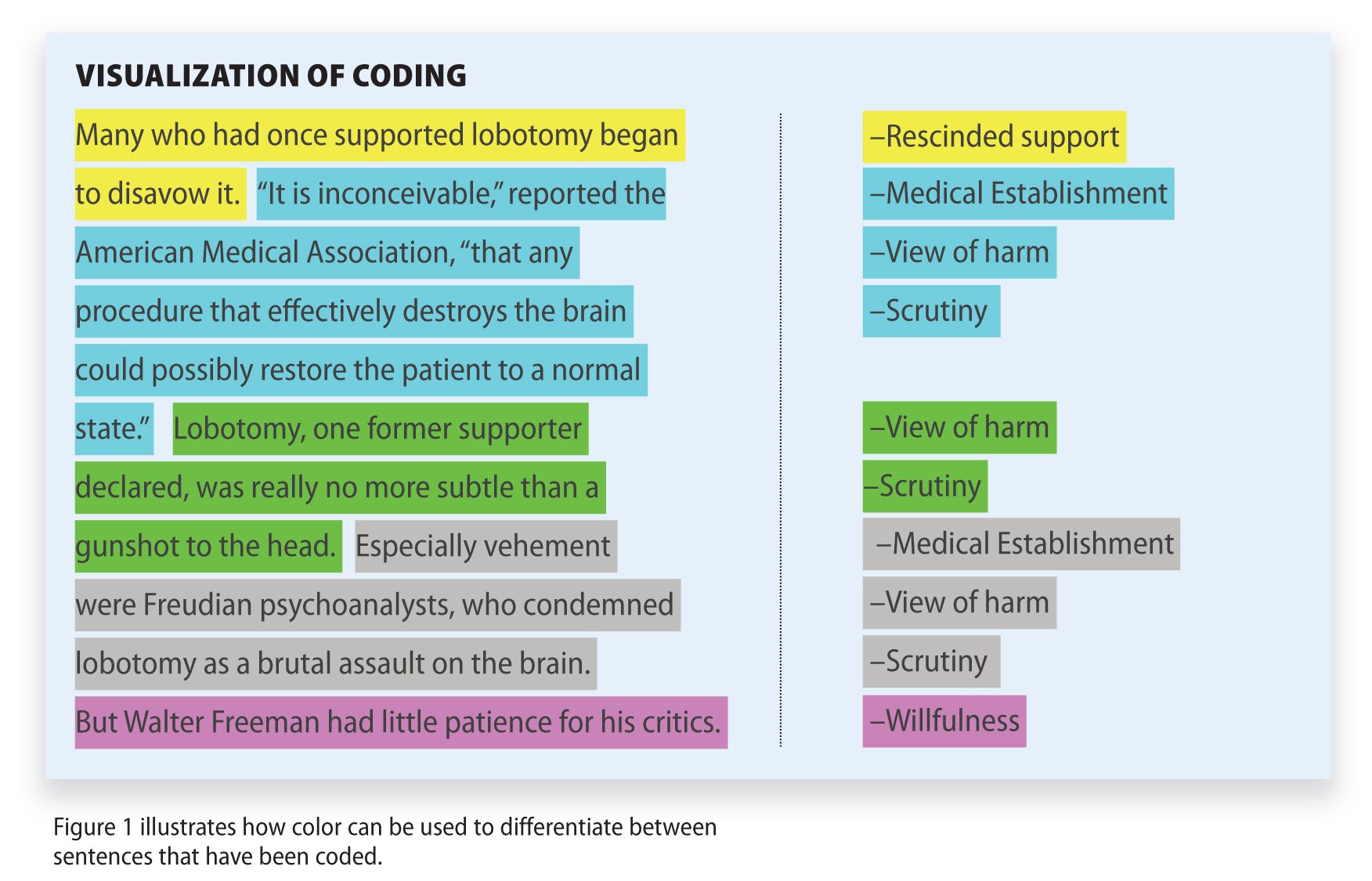
Research Methodology
Qualitative Data Coding
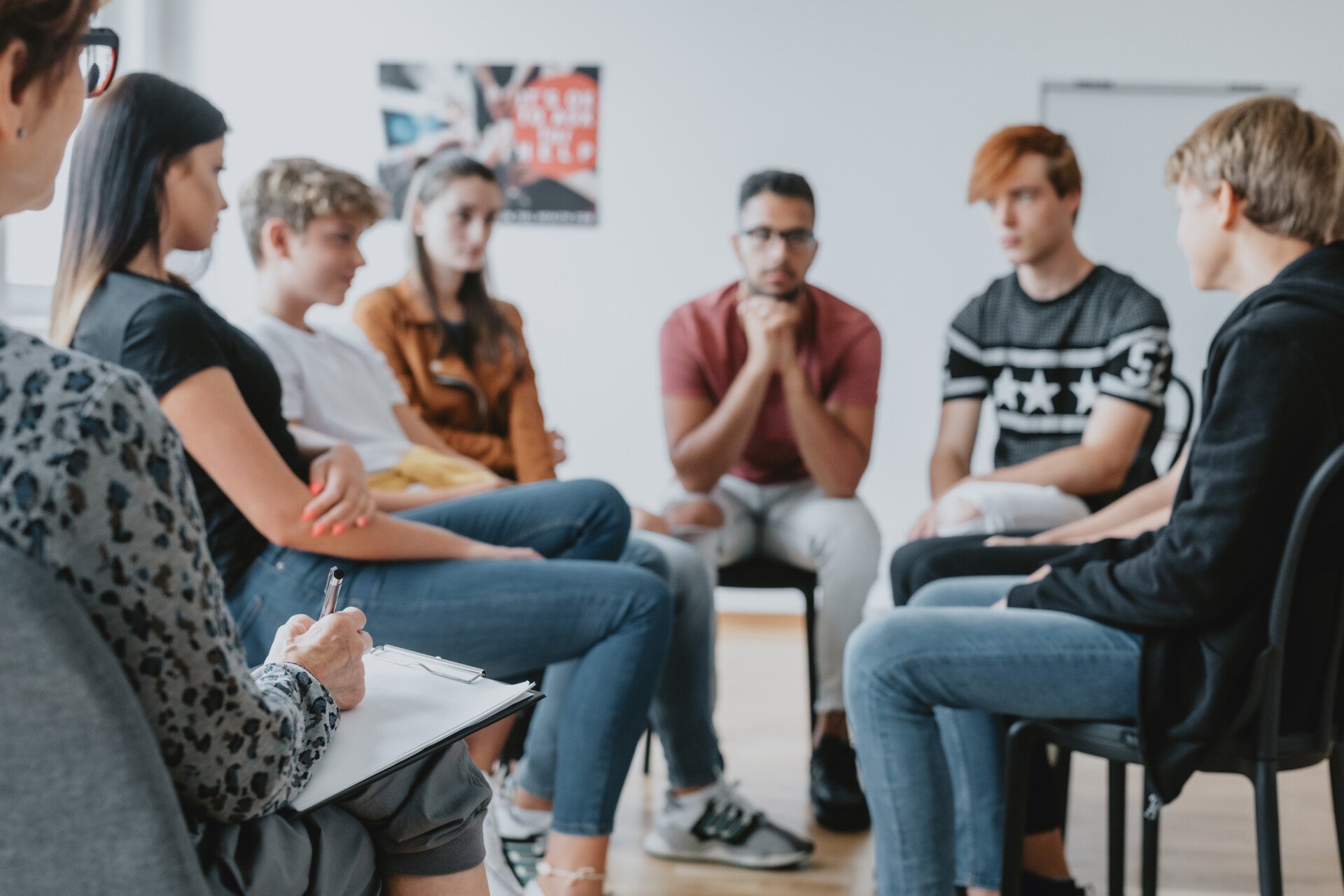
What Is a Focus Group?
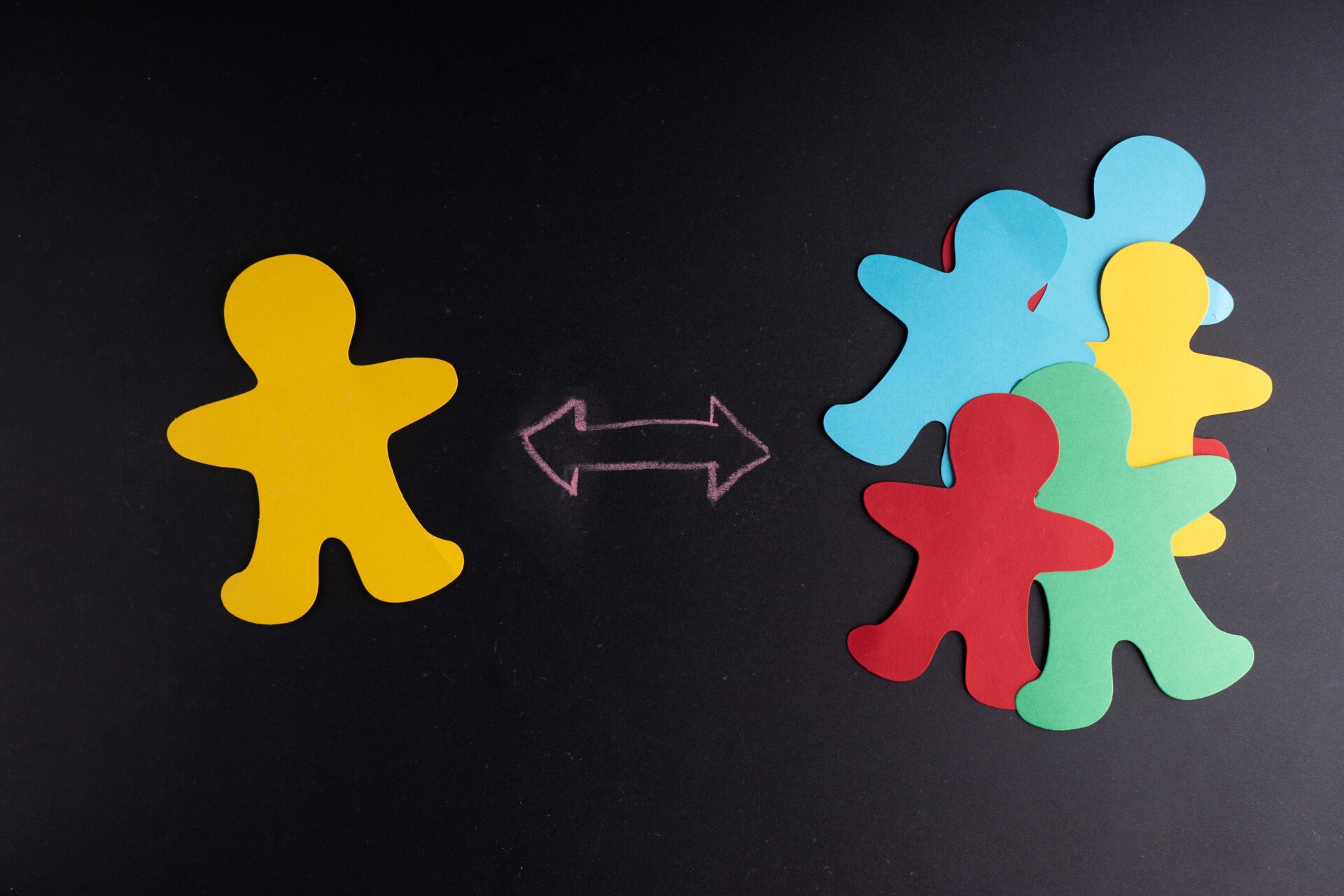
Cross-Cultural Research Methodology In Psychology
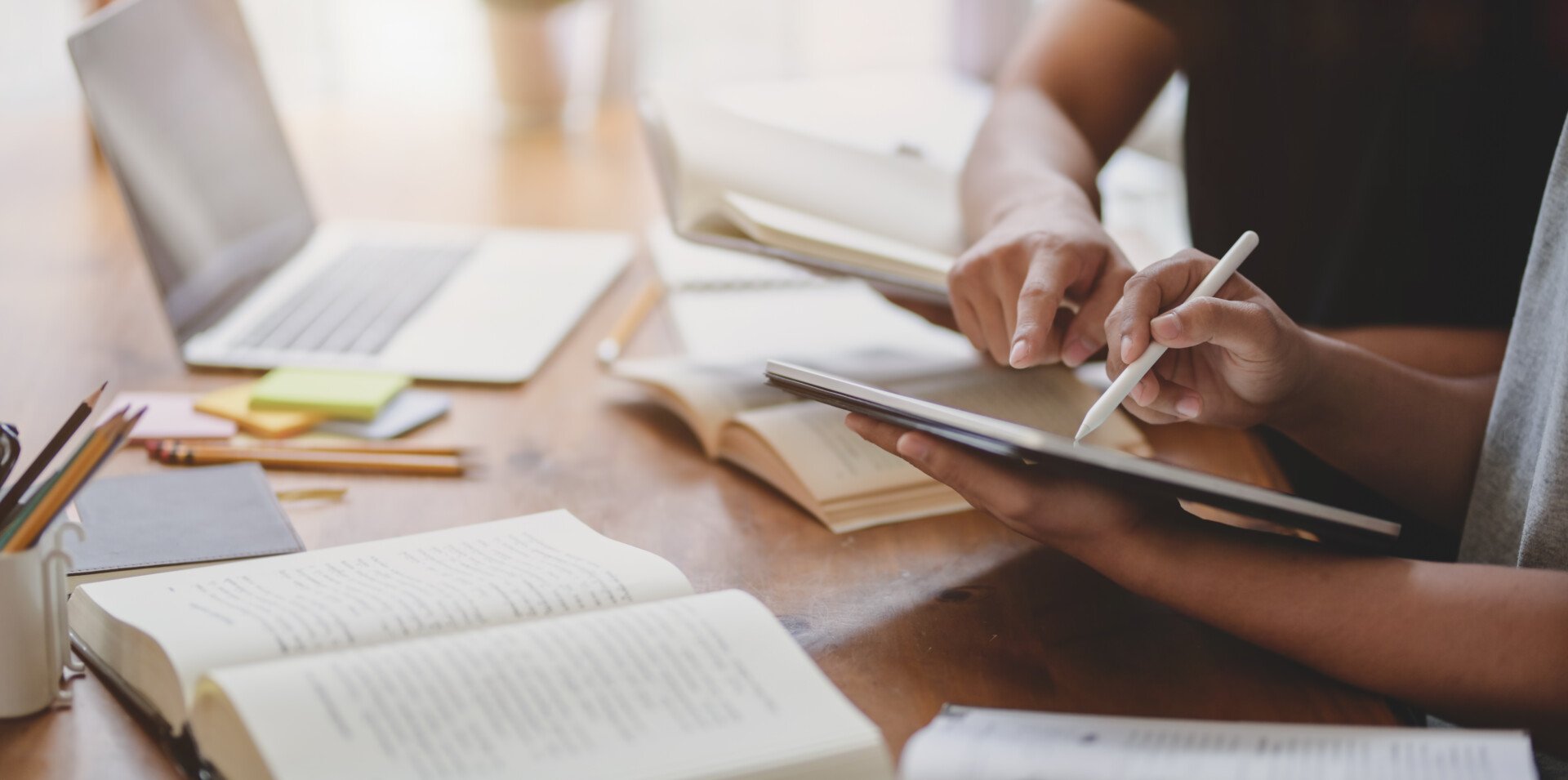
What Is Internal Validity In Research?
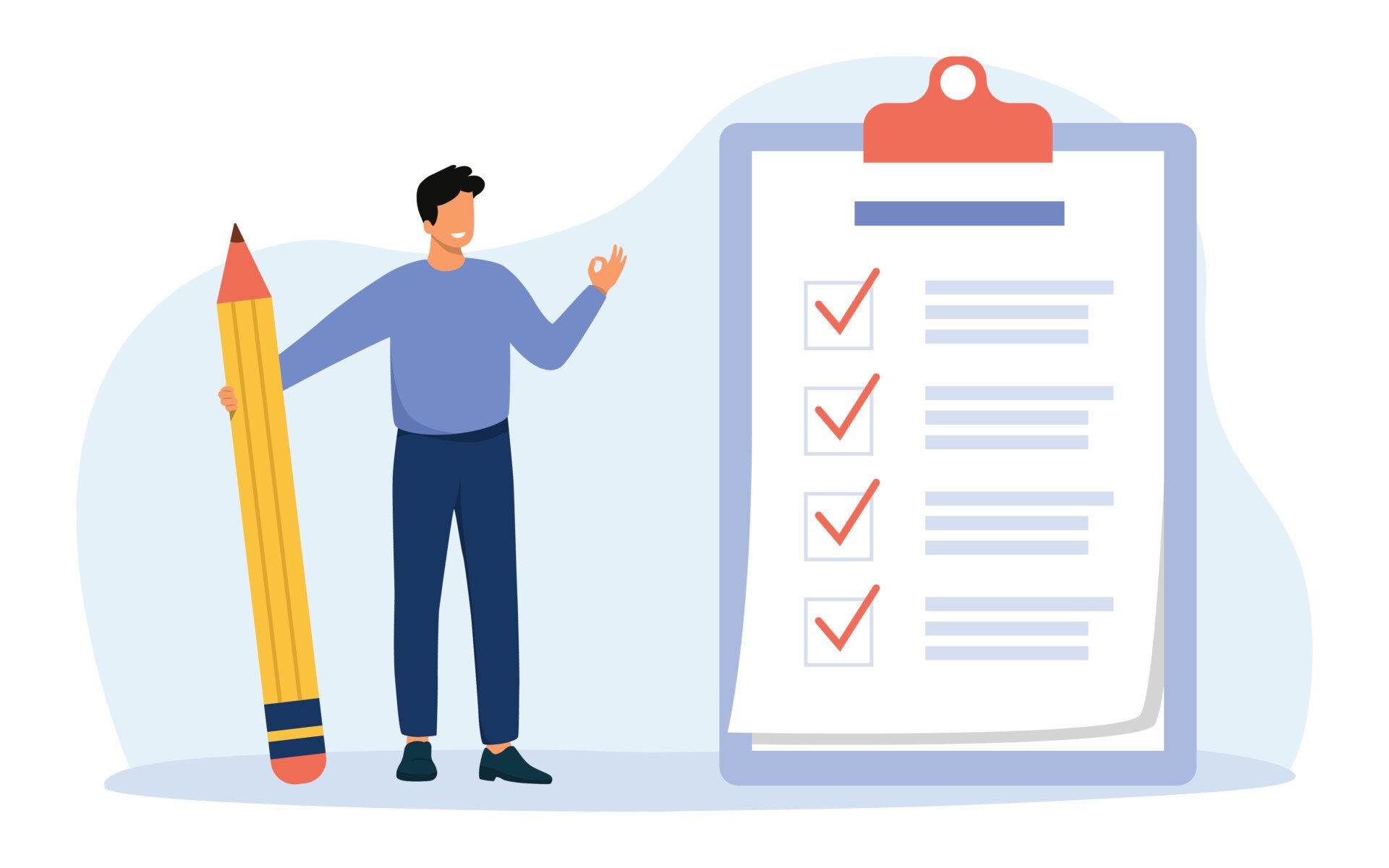
Research Methodology , Statistics
What Is Face Validity In Research? Importance & How To Measure
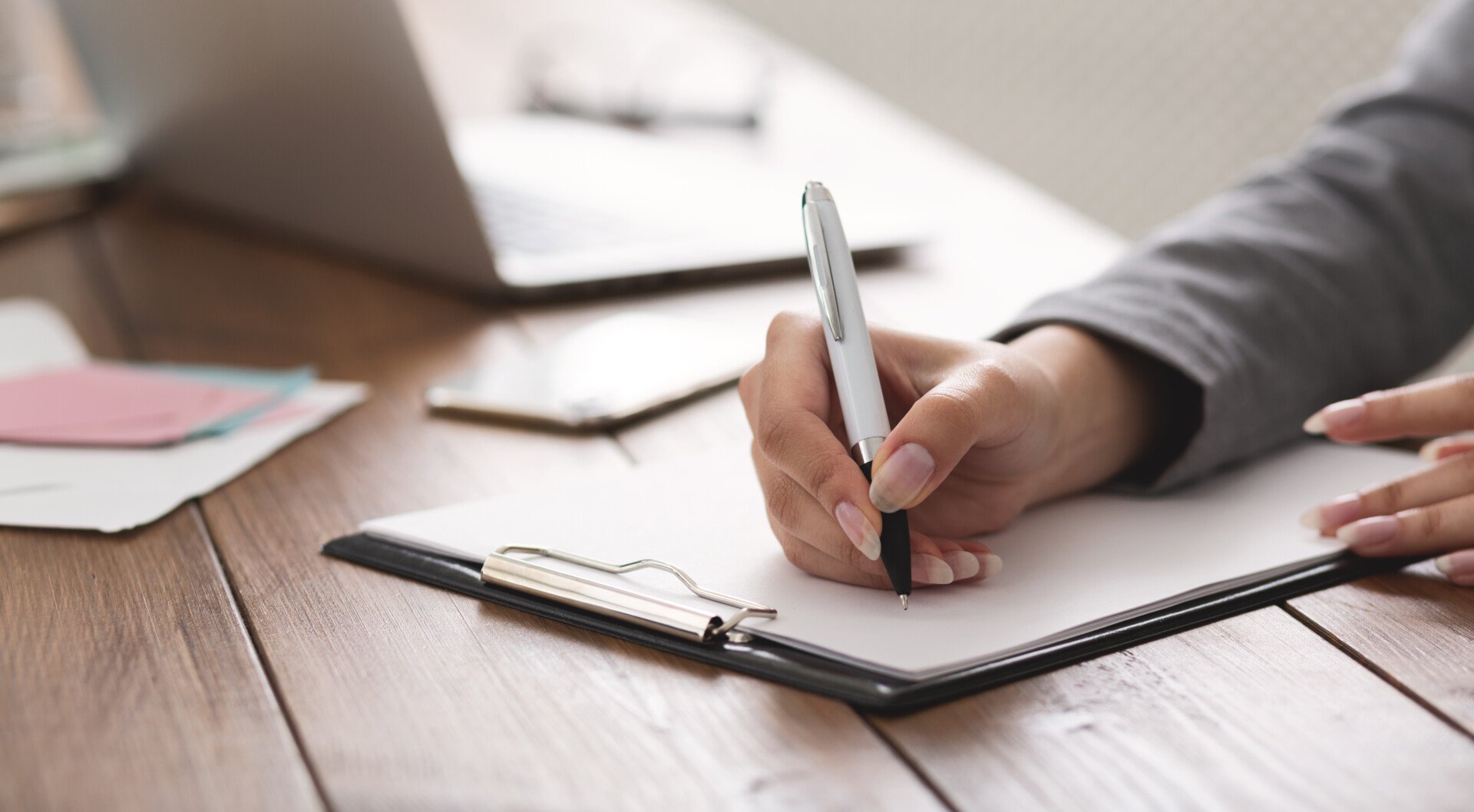
Criterion Validity: Definition & Examples
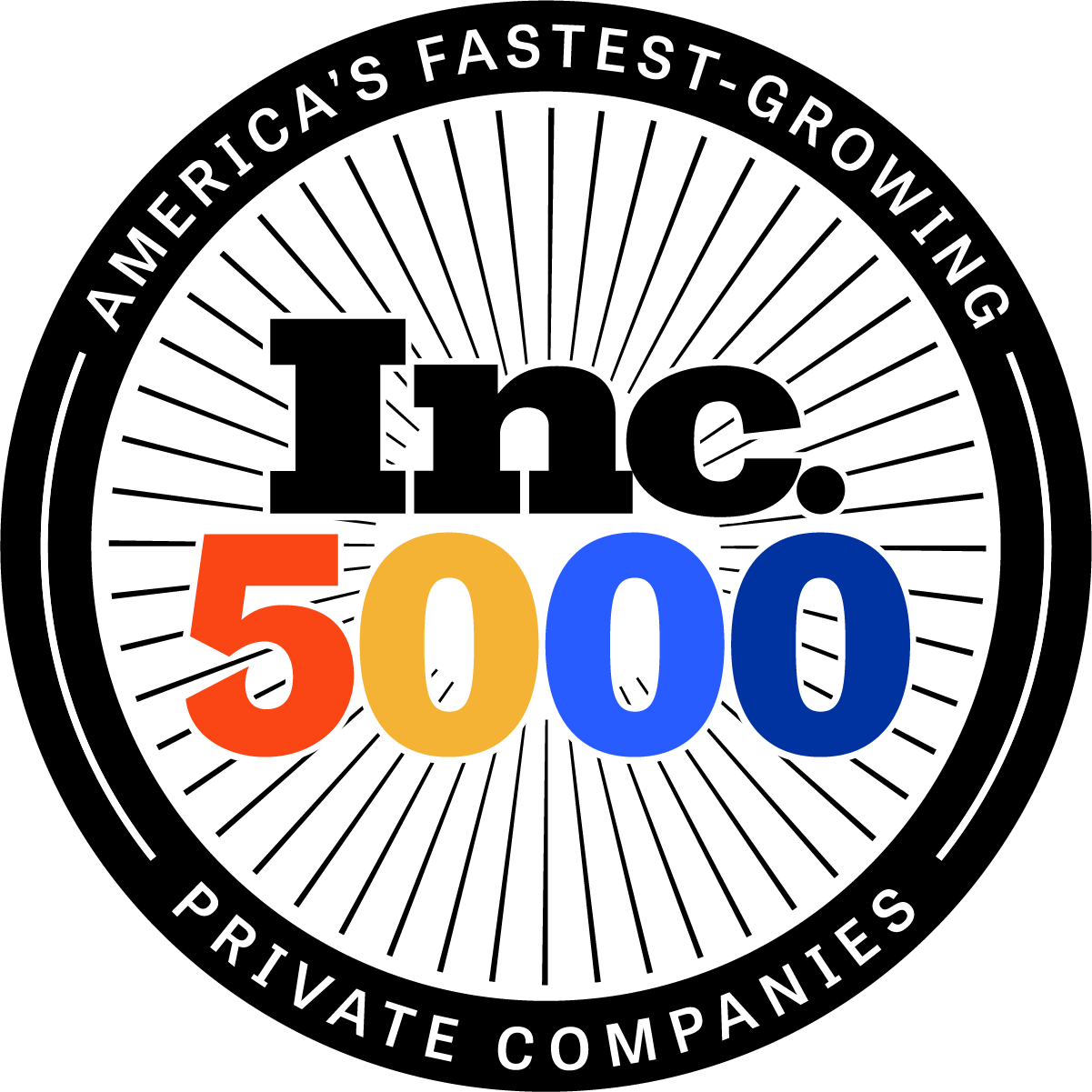
- Physician Physician Board Reviews Physician Associate Board Reviews CME Lifetime CME Free CME MATE and DEA Compliance
- Student USMLE Step 1 USMLE Step 2 USMLE Step 3 COMLEX Level 1 COMLEX Level 2 COMLEX Level 3 96 Medical School Exams Student Resource Center NCLEX - RN NCLEX - LPN/LVN/PN 24 Nursing Exams
- Nurse Practitioner APRN/NP Board Reviews CNS Certification Reviews CE - Nurse Practitioner FREE CE
- Nurse RN Certification Reviews CE - Nurse FREE CE
- Pharmacist Pharmacy Board Exam Prep CE - Pharmacist
- Allied Allied Health Exam Prep Dentist Exams CE - Social Worker CE - Dentist
- Point of Care
- Free CME/CE
Hypothesis Testing, P Values, Confidence Intervals, and Significance
Definition/introduction.
Medical providers often rely on evidence-based medicine to guide decision-making in practice. Often a research hypothesis is tested with results provided, typically with p values, confidence intervals, or both. Additionally, statistical or research significance is estimated or determined by the investigators. Unfortunately, healthcare providers may have different comfort levels in interpreting these findings, which may affect the adequate application of the data.
Issues of Concern
Register for free and read the full article, learn more about a subscription to statpearls point-of-care.
Without a foundational understanding of hypothesis testing, p values, confidence intervals, and the difference between statistical and clinical significance, it may affect healthcare providers' ability to make clinical decisions without relying purely on the research investigators deemed level of significance. Therefore, an overview of these concepts is provided to allow medical professionals to use their expertise to determine if results are reported sufficiently and if the study outcomes are clinically appropriate to be applied in healthcare practice.
Hypothesis Testing
Investigators conducting studies need research questions and hypotheses to guide analyses. Starting with broad research questions (RQs), investigators then identify a gap in current clinical practice or research. Any research problem or statement is grounded in a better understanding of relationships between two or more variables. For this article, we will use the following research question example:
Research Question: Is Drug 23 an effective treatment for Disease A?
Research questions do not directly imply specific guesses or predictions; we must formulate research hypotheses. A hypothesis is a predetermined declaration regarding the research question in which the investigator(s) makes a precise, educated guess about a study outcome. This is sometimes called the alternative hypothesis and ultimately allows the researcher to take a stance based on experience or insight from medical literature. An example of a hypothesis is below.
Research Hypothesis: Drug 23 will significantly reduce symptoms associated with Disease A compared to Drug 22.
The null hypothesis states that there is no statistical difference between groups based on the stated research hypothesis.
Researchers should be aware of journal recommendations when considering how to report p values, and manuscripts should remain internally consistent.
Regarding p values, as the number of individuals enrolled in a study (the sample size) increases, the likelihood of finding a statistically significant effect increases. With very large sample sizes, the p-value can be very low significant differences in the reduction of symptoms for Disease A between Drug 23 and Drug 22. The null hypothesis is deemed true until a study presents significant data to support rejecting the null hypothesis. Based on the results, the investigators will either reject the null hypothesis (if they found significant differences or associations) or fail to reject the null hypothesis (they could not provide proof that there were significant differences or associations).
To test a hypothesis, researchers obtain data on a representative sample to determine whether to reject or fail to reject a null hypothesis. In most research studies, it is not feasible to obtain data for an entire population. Using a sampling procedure allows for statistical inference, though this involves a certain possibility of error. [1] When determining whether to reject or fail to reject the null hypothesis, mistakes can be made: Type I and Type II errors. Though it is impossible to ensure that these errors have not occurred, researchers should limit the possibilities of these faults. [2]
Significance
Significance is a term to describe the substantive importance of medical research. Statistical significance is the likelihood of results due to chance. [3] Healthcare providers should always delineate statistical significance from clinical significance, a common error when reviewing biomedical research. [4] When conceptualizing findings reported as either significant or not significant, healthcare providers should not simply accept researchers' results or conclusions without considering the clinical significance. Healthcare professionals should consider the clinical importance of findings and understand both p values and confidence intervals so they do not have to rely on the researchers to determine the level of significance. [5] One criterion often used to determine statistical significance is the utilization of p values.
P values are used in research to determine whether the sample estimate is significantly different from a hypothesized value. The p-value is the probability that the observed effect within the study would have occurred by chance if, in reality, there was no true effect. Conventionally, data yielding a p<0.05 or p<0.01 is considered statistically significant. While some have debated that the 0.05 level should be lowered, it is still universally practiced. [6] Hypothesis testing allows us to determine the size of the effect.
An example of findings reported with p values are below:
Statement: Drug 23 reduced patients' symptoms compared to Drug 22. Patients who received Drug 23 (n=100) were 2.1 times less likely than patients who received Drug 22 (n = 100) to experience symptoms of Disease A, p<0.05.
Statement:Individuals who were prescribed Drug 23 experienced fewer symptoms (M = 1.3, SD = 0.7) compared to individuals who were prescribed Drug 22 (M = 5.3, SD = 1.9). This finding was statistically significant, p= 0.02.
For either statement, if the threshold had been set at 0.05, the null hypothesis (that there was no relationship) should be rejected, and we should conclude significant differences. Noticeably, as can be seen in the two statements above, some researchers will report findings with < or > and others will provide an exact p-value (0.000001) but never zero [6] . When examining research, readers should understand how p values are reported. The best practice is to report all p values for all variables within a study design, rather than only providing p values for variables with significant findings. [7] The inclusion of all p values provides evidence for study validity and limits suspicion for selective reporting/data mining.
While researchers have historically used p values, experts who find p values problematic encourage the use of confidence intervals. [8] . P-values alone do not allow us to understand the size or the extent of the differences or associations. [3] In March 2016, the American Statistical Association (ASA) released a statement on p values, noting that scientific decision-making and conclusions should not be based on a fixed p-value threshold (e.g., 0.05). They recommend focusing on the significance of results in the context of study design, quality of measurements, and validity of data. Ultimately, the ASA statement noted that in isolation, a p-value does not provide strong evidence. [9]
When conceptualizing clinical work, healthcare professionals should consider p values with a concurrent appraisal study design validity. For example, a p-value from a double-blinded randomized clinical trial (designed to minimize bias) should be weighted higher than one from a retrospective observational study [7] . The p-value debate has smoldered since the 1950s [10] , and replacement with confidence intervals has been suggested since the 1980s. [11]
Confidence Intervals
A confidence interval provides a range of values within given confidence (e.g., 95%), including the accurate value of the statistical constraint within a targeted population. [12] Most research uses a 95% CI, but investigators can set any level (e.g., 90% CI, 99% CI). [13] A CI provides a range with the lower bound and upper bound limits of a difference or association that would be plausible for a population. [14] Therefore, a CI of 95% indicates that if a study were to be carried out 100 times, the range would contain the true value in 95, [15] confidence intervals provide more evidence regarding the precision of an estimate compared to p-values. [6]
In consideration of the similar research example provided above, one could make the following statement with 95% CI:
Statement: Individuals who were prescribed Drug 23 had no symptoms after three days, which was significantly faster than those prescribed Drug 22; there was a mean difference between the two groups of days to the recovery of 4.2 days (95% CI: 1.9 – 7.8).
It is important to note that the width of the CI is affected by the standard error and the sample size; reducing a study sample number will result in less precision of the CI (increase the width). [14] A larger width indicates a smaller sample size or a larger variability. [16] A researcher would want to increase the precision of the CI. For example, a 95% CI of 1.43 – 1.47 is much more precise than the one provided in the example above. In research and clinical practice, CIs provide valuable information on whether the interval includes or excludes any clinically significant values. [14]
Null values are sometimes used for differences with CI (zero for differential comparisons and 1 for ratios). However, CIs provide more information than that. [15] Consider this example: A hospital implements a new protocol that reduced wait time for patients in the emergency department by an average of 25 minutes (95% CI: -2.5 – 41 minutes). Because the range crosses zero, implementing this protocol in different populations could result in longer wait times; however, the range is much higher on the positive side. Thus, while the p-value used to detect statistical significance for this may result in "not significant" findings, individuals should examine this range, consider the study design, and weigh whether or not it is still worth piloting in their workplace.
Similarly to p-values, 95% CIs cannot control for researchers' errors (e.g., study bias or improper data analysis). [14] In consideration of whether to report p-values or CIs, researchers should examine journal preferences. When in doubt, reporting both may be beneficial. [13] An example is below:
Reporting both: Individuals who were prescribed Drug 23 had no symptoms after three days, which was significantly faster than those prescribed Drug 22, p = 0.009. There was a mean difference between the two groups of days to the recovery of 4.2 days (95% CI: 1.9 – 7.8).
Clinical Significance
Recall that clinical significance and statistical significance are two different concepts. Healthcare providers should remember that a study with statistically significant differences and large sample size may be of no interest to clinicians, whereas a study with smaller sample size and statistically non-significant results could impact clinical practice. [14] Additionally, as previously mentioned, a non-significant finding may reflect the study design itself rather than relationships between variables.
Healthcare providers using evidence-based medicine to inform practice should use clinical judgment to determine the practical importance of studies through careful evaluation of the design, sample size, power, likelihood of type I and type II errors, data analysis, and reporting of statistical findings (p values, 95% CI or both). [4] Interestingly, some experts have called for "statistically significant" or "not significant" to be excluded from work as statistical significance never has and will never be equivalent to clinical significance. [17]
The decision on what is clinically significant can be challenging, depending on the providers' experience and especially the severity of the disease. Providers should use their knowledge and experiences to determine the meaningfulness of study results and make inferences based not only on significant or insignificant results by researchers but through their understanding of study limitations and practical implications.
Nursing, Allied Health, and Interprofessional Team Interventions
All physicians, nurses, pharmacists, and other healthcare professionals should strive to understand the concepts in this chapter. These individuals should maintain the ability to review and incorporate new literature for evidence-based and safe care.
Jones M, Gebski V, Onslow M, Packman A. Statistical power in stuttering research: a tutorial. Journal of speech, language, and hearing research : JSLHR. 2002 Apr:45(2):243-55 [PubMed PMID: 12003508]
Sedgwick P. Pitfalls of statistical hypothesis testing: type I and type II errors. BMJ (Clinical research ed.). 2014 Jul 3:349():g4287. doi: 10.1136/bmj.g4287. Epub 2014 Jul 3 [PubMed PMID: 24994622]
Fethney J. Statistical and clinical significance, and how to use confidence intervals to help interpret both. Australian critical care : official journal of the Confederation of Australian Critical Care Nurses. 2010 May:23(2):93-7. doi: 10.1016/j.aucc.2010.03.001. Epub 2010 Mar 29 [PubMed PMID: 20347326]
Hayat MJ. Understanding statistical significance. Nursing research. 2010 May-Jun:59(3):219-23. doi: 10.1097/NNR.0b013e3181dbb2cc. Epub [PubMed PMID: 20445438]
Ferrill MJ, Brown DA, Kyle JA. Clinical versus statistical significance: interpreting P values and confidence intervals related to measures of association to guide decision making. Journal of pharmacy practice. 2010 Aug:23(4):344-51. doi: 10.1177/0897190009358774. Epub 2010 Apr 13 [PubMed PMID: 21507834]
Infanger D, Schmidt-Trucksäss A. P value functions: An underused method to present research results and to promote quantitative reasoning. Statistics in medicine. 2019 Sep 20:38(21):4189-4197. doi: 10.1002/sim.8293. Epub 2019 Jul 3 [PubMed PMID: 31270842]
Dorey F. Statistics in brief: Interpretation and use of p values: all p values are not equal. Clinical orthopaedics and related research. 2011 Nov:469(11):3259-61. doi: 10.1007/s11999-011-2053-1. Epub [PubMed PMID: 21918804]
Liu XS. Implications of statistical power for confidence intervals. The British journal of mathematical and statistical psychology. 2012 Nov:65(3):427-37. doi: 10.1111/j.2044-8317.2011.02035.x. Epub 2011 Oct 25 [PubMed PMID: 22026811]
Tijssen JG, Kolm P. Demystifying the New Statistical Recommendations: The Use and Reporting of p Values. Journal of the American College of Cardiology. 2016 Jul 12:68(2):231-3. doi: 10.1016/j.jacc.2016.05.026. Epub [PubMed PMID: 27386779]
Spanos A. Recurring controversies about P values and confidence intervals revisited. Ecology. 2014 Mar:95(3):645-51 [PubMed PMID: 24804448]
Freire APCF, Elkins MR, Ramos EMC, Moseley AM. Use of 95% confidence intervals in the reporting of between-group differences in randomized controlled trials: analysis of a representative sample of 200 physical therapy trials. Brazilian journal of physical therapy. 2019 Jul-Aug:23(4):302-310. doi: 10.1016/j.bjpt.2018.10.004. Epub 2018 Oct 16 [PubMed PMID: 30366845]
Dorey FJ. In brief: statistics in brief: Confidence intervals: what is the real result in the target population? Clinical orthopaedics and related research. 2010 Nov:468(11):3137-8. doi: 10.1007/s11999-010-1407-4. Epub [PubMed PMID: 20532716]
Porcher R. Reporting results of orthopaedic research: confidence intervals and p values. Clinical orthopaedics and related research. 2009 Oct:467(10):2736-7. doi: 10.1007/s11999-009-0952-1. Epub 2009 Jun 30 [PubMed PMID: 19565303]
Gardner MJ, Altman DG. Confidence intervals rather than P values: estimation rather than hypothesis testing. British medical journal (Clinical research ed.). 1986 Mar 15:292(6522):746-50 [PubMed PMID: 3082422]
Cooper RJ, Wears RL, Schriger DL. Reporting research results: recommendations for improving communication. Annals of emergency medicine. 2003 Apr:41(4):561-4 [PubMed PMID: 12658257]
Doll H, Carney S. Statistical approaches to uncertainty: P values and confidence intervals unpacked. Equine veterinary journal. 2007 May:39(3):275-6 [PubMed PMID: 17520981]
Colquhoun D. The reproducibility of research and the misinterpretation of p-values. Royal Society open science. 2017 Dec:4(12):171085. doi: 10.1098/rsos.171085. Epub 2017 Dec 6 [PubMed PMID: 29308247]
Use the mouse wheel to zoom in and out, click and drag to pan the image
- Research article
- Open access
- Published: 19 May 2010
The null hypothesis significance test in health sciences research (1995-2006): statistical analysis and interpretation
- Luis Carlos Silva-Ayçaguer 1 ,
- Patricio Suárez-Gil 2 &
- Ana Fernández-Somoano 3
BMC Medical Research Methodology volume 10 , Article number: 44 ( 2010 ) Cite this article
38k Accesses
23 Citations
18 Altmetric
Metrics details
The null hypothesis significance test (NHST) is the most frequently used statistical method, although its inferential validity has been widely criticized since its introduction. In 1988, the International Committee of Medical Journal Editors (ICMJE) warned against sole reliance on NHST to substantiate study conclusions and suggested supplementary use of confidence intervals (CI). Our objective was to evaluate the extent and quality in the use of NHST and CI, both in English and Spanish language biomedical publications between 1995 and 2006, taking into account the International Committee of Medical Journal Editors recommendations, with particular focus on the accuracy of the interpretation of statistical significance and the validity of conclusions.
Original articles published in three English and three Spanish biomedical journals in three fields (General Medicine, Clinical Specialties and Epidemiology - Public Health) were considered for this study. Papers published in 1995-1996, 2000-2001, and 2005-2006 were selected through a systematic sampling method. After excluding the purely descriptive and theoretical articles, analytic studies were evaluated for their use of NHST with P-values and/or CI for interpretation of statistical "significance" and "relevance" in study conclusions.
Among 1,043 original papers, 874 were selected for detailed review. The exclusive use of P-values was less frequent in English language publications as well as in Public Health journals; overall such use decreased from 41% in 1995-1996 to 21% in 2005-2006. While the use of CI increased over time, the "significance fallacy" (to equate statistical and substantive significance) appeared very often, mainly in journals devoted to clinical specialties (81%). In papers originally written in English and Spanish, 15% and 10%, respectively, mentioned statistical significance in their conclusions.
Conclusions
Overall, results of our review show some improvements in statistical management of statistical results, but further efforts by scholars and journal editors are clearly required to move the communication toward ICMJE advices, especially in the clinical setting, which seems to be imperative among publications in Spanish.
Peer Review reports
The null hypothesis statistical testing (NHST) has been the most widely used statistical approach in health research over the past 80 years. Its origins dates back to 1279 [ 1 ] although it was in the second decade of the twentieth century when the statistician Ronald Fisher formally introduced the concept of "null hypothesis" H 0 - which, generally speaking, establishes that certain parameters do not differ from each other. He was the inventor of the "P-value" through which it could be assessed [ 2 ]. Fisher's P-value is defined as a conditional probability calculated using the results of a study. Specifically, the P-value is the probability of obtaining a result at least as extreme as the one that was actually observed, assuming that the null hypothesis is true. The Fisherian significance testing theory considered the p-value as an index to measure the strength of evidence against the null hypothesis in a single experiment. The father of NHST never endorsed, however, the inflexible application of the ultimately subjective threshold levels almost universally adopted later on (although the introduction of the 0.05 has his paternity also).
A few years later, Jerzy Neyman and Egon Pearson considered the Fisherian approach inefficient, and in 1928 they published an article [ 3 ] that would provide the theoretical basis of what they called hypothesis statistical testing . The Neyman-Pearson approach is based on the notion that one out of two choices has to be taken: accept the null hypothesis taking the information as a reference based on the information provided, or reject it in favor of an alternative one. Thus, one can incur one of two types of errors: a Type I error, if the null hypothesis is rejected when it is actually true, and a Type II error, if the null hypothesis is accepted when it is actually false. They established a rule to optimize the decision process, using the p-value introduced by Fisher, by setting the maximum frequency of errors that would be admissible.
The null hypothesis statistical testing, as applied today, is a hybrid coming from the amalgamation of the two methods [ 4 ]. As a matter of fact, some 15 years later, both procedures were combined to give rise to the nowadays widespread use of an inferential tool that would satisfy none of the statisticians involved in the original controversy. The present method essentially goes as follows: given a null hypothesis, an estimate of the parameter (or parameters) is obtained and used to create statistics whose distribution, under H 0 , is known. With these data the P-value is computed. Finally, the null hypothesis is rejected when the obtained P-value is smaller than a certain comparative threshold (usually 0.05) and it is not rejected if P is larger than the threshold.
The first reservations about the validity of the method began to appear around 1940, when some statisticians censured the logical roots and practical convenience of Fisher's P-value [ 5 ]. Significance tests and P-values have repeatedly drawn the attention and criticism of many authors over the past 70 years, who have kept questioning its epistemological legitimacy as well as its practical value. What remains in spite of these criticisms is the lasting legacy of researchers' unwillingness to eradicate or reform these methods.
Although there are very comprehensive works on the topic [ 6 ], we list below some of the criticisms most universally accepted by specialists.
The P-values are used as a tool to make decisions in favor of or against a hypothesis. What really may be relevant, however, is to get an effect size estimate (often the difference between two values) rather than rendering dichotomous true/false verdicts [ 7 – 11 ].
The P-value is a conditional probability of the data, provided that some assumptions are met, but what really interests the investigator is the inverse probability: what degree of validity can be attributed to each of several competing hypotheses, once that certain data have been observed [ 12 ].
The two elements that affect the results, namely the sample size and the magnitude of the effect, are inextricably linked in the value of p and we can always get a lower P-value by increasing the sample size. Thus, the conclusions depend on a factor completely unrelated to the reality studied (i.e. the available resources, which in turn determine the sample size) [ 13 , 14 ].
Those who defend the NHST often assert the objective nature of that test, but the process is actually far from being so. NHST does not ensure objectivity. This is reflected in the fact that we generally operate with thresholds that are ultimately no more than conventions, such as 0.01 or 0.05. What is more, for many years their use has unequivocally demonstrated the inherent subjectivity that goes with the concept of P, regardless of how it will be used later [ 15 – 17 ].
In practice, the NHST is limited to a binary response sorting hypotheses into "true" and "false" or declaring "rejection" or "no rejection", without demanding a reasonable interpretation of the results, as has been noted time and again for decades. This binary orthodoxy validates categorical thinking, which results in a very simplistic view of scientific activity that induces researchers not to test theories about the magnitude of effect sizes [ 18 – 20 ].
Despite the weakness and shortcomings of the NHST, they are frequently taught as if they were the key inferential statistical method or the most appropriate, or even the sole unquestioned one. The statistical textbooks, with only some exceptions, do not even mention the NHST controversy. Instead, the myth is spread that NHST is the "natural" final action of scientific inference and the only procedure for testing hypotheses. However, relevant specialists and important regulators of the scientific world advocate avoiding them.
Taking especially into account that NHST does not offer the most important information (i.e. the magnitude of an effect of interest, and the precision of the estimate of the magnitude of that effect), many experts recommend the reporting of point estimates of effect sizes with confidence intervals as the appropriate representation of the inherent uncertainty linked to empirical studies [ 21 – 25 ]. Since 1988, the International Committee of Medical Journal Editors (ICMJE, known as the Vancouver Group ) incorporates the following recommendation to authors of manuscripts submitted to medical journals: "When possible, quantify findings and present them with appropriate indicators of measurement error or uncertainty (such as confidence intervals). Avoid relying solely on statistical hypothesis testing, such as P-values, which fail to convey important information about effect size" [ 26 ].
As will be shown, the use of confidence intervals (CI), occasionally accompanied by P-values, is recommended as a more appropriate method for reporting results. Some authors have noted several shortcomings of CI long ago [ 27 ]. In spite of the fact that calculating CI could be complicated indeed, and that their interpretation is far from simple [ 28 , 29 ], authors are urged to use them because they provide much more information than the NHST and do not merit most of its criticisms of NHST [ 30 ]. While some have proposed different options (for instance, likelihood-based information theoretic methods [ 31 ], and the Bayesian inferential paradigm [ 32 ]), confidence interval estimation of effect sizes is clearly the most widespread alternative approach.
Although twenty years have passed since the ICMJE began to disseminate such recommendations, systematically ignored by the vast majority of textbooks and hardly incorporated in medical publications [ 33 ], it is interesting to examine the extent to which the NHST is used in articles published in medical journals during recent years, in order to identify what is still lacking in the process of eradicating the widespread ceremonial use that is made of statistics in health research [ 34 ]. Furthermore, it is enlightening in this context to examine whether these patterns differ between English- and Spanish-speaking worlds and, if so, to see if the changes in paradigms are occurring more slowly in Spanish-language publications. In such a case we would offer various suggestions.
In addition to assessing the adherence to the above cited statistical recommendation proposed by ICMJE relative to the use of P-values, we consider it of particular interest to estimate the extent to which the significance fallacy is present, an inertial deficiency that consists of attributing -- explicitly or not -- qualitative importance or practical relevance to the found differences simply because statistical significance was obtained.
Many authors produce misleading statements such as "a significant effect was (or was not) found" when it should be said that "a statistically significant difference was (or was not) found". A detrimental consequence of this equivalence is that some authors believe that finding out whether there is "statistical significance" or not is the aim, so that this term is then mentioned in the conclusions [ 35 ]. This means virtually nothing, except that it indicates that the author is letting a computer do the thinking. Since the real research questions are never statistical ones, the answers cannot be statistical either. Accordingly, the conversion of the dichotomous outcome produced by a NHST into a conclusion is another manifestation of the mentioned fallacy.
The general objective of the present study is to evaluate the extent and quality of use of NHST and CI, both in English- and in Spanish-language biomedical publications, between 1995 and 2006 taking into account the International Committee of Medical Journal Editors recommendations, with particular focus on accuracy regarding interpretation of statistical significance and the validity of conclusions.
We reviewed the original articles from six journals, three in English and three in Spanish, over three disjoint periods sufficiently separated from each other (1995-1996, 2000-2001, 2005-2006) as to properly describe the evolution in prevalence of the target features along the selected periods.
The selection of journals was intended to get representation for each of the following three thematic areas: clinical specialties ( Obstetrics & Gynecology and Revista Española de Cardiología) ; Public Health and Epidemiology ( International Journal of Epidemiology and Atención Primaria) and the area of general and internal medicine ( British Medical Journal and Medicina Clínica ). Five of the selected journals formally endorsed ICMJE guidelines; the remaining one ( Revista Española de Cardiología ) suggests observing ICMJE demands in relation with specific issues. We attempted to capture journal diversity in the sample by selecting general and specialty journals with different degrees of influence, resulting from their impact factors in 2007, which oscillated between 1.337 (MC) and 9.723 (BMJ). No special reasons guided us to choose these specific journals, but we opted for journals with rather large paid circulations. For instance, the Spanish Cardiology Journal is the one with the largest impact factor among the fourteen Spanish Journals devoted to clinical specialties that have impact factor and Obstetrics & Gynecology has an outstanding impact factor among the huge number of journals available for selection.
It was decided to take around 60 papers for each biennium and journal, which means a total of around 1,000 papers. As recently suggested [ 36 , 37 ], this number was not established using a conventional method, but by means of a purposive and pragmatic approach in choosing the maximum sample size that was feasible.
Systematic sampling in phases [ 38 ] was used in applying a sampling fraction equal to 60/N, where N is the number of articles, in each of the 18 subgroups defined by crossing the six journals and the three time periods. Table 1 lists the population size and the sample size for each subgroup. While the sample within each subgroup was selected with equal probability, estimates based on other subsets of articles (defined across time periods, areas, or languages) are based on samples with various selection probabilities. Proper weights were used to take into account the stratified nature of the sampling in these cases.
Forty-nine of the 1,092 selected papers were eliminated because, although the section of the article in which they were assigned could suggest they were originals, detailed scrutiny revealed that in some cases they were not. The sample, therefore, consisted of 1,043 papers. Each of them was classified into one of three categories: (1) purely descriptive papers, those designed to review or characterize the state of affairs as it exists at present, (2) analytical papers, or (3) articles that address theoretical, methodological or conceptual issues. An article was regarded as analytical if it seeks to explain the reasons behind a particular occurrence by discovering causal relationships or, even if self-classified as descriptive, it was carried out to assess cause-effect associations among variables. We classify as theoretical or methodological those articles that do not handle empirical data as such, and focus instead on proposing or assessing research methods. We identified 169 papers as purely descriptive or theoretical, which were therefore excluded from the sample. Figure 1 presents a flow chart showing the process for determining eligibility for inclusion in the sample.
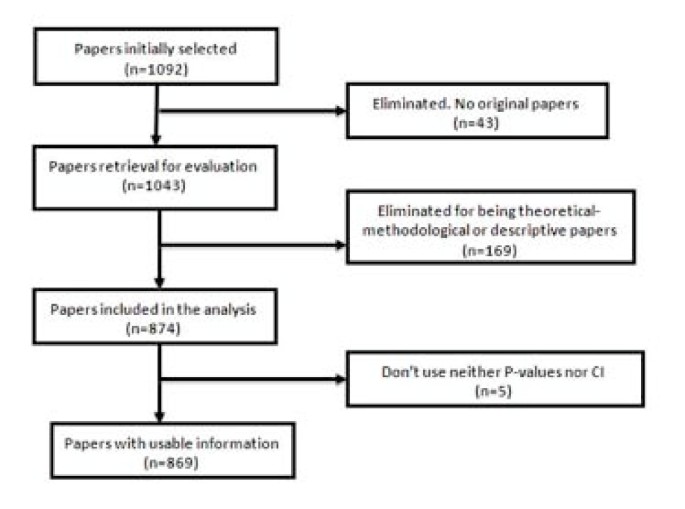
Flow chart of the selection process for eligible papers .
To estimate the adherence to ICMJE recommendations, we considered whether the papers used P-values, confidence intervals, and both simultaneously. By "the use of P-values" we mean that the article contains at least one P-value, explicitly mentioned in the text or at the bottom of a table, or that it reports that an effect was considered as statistically significant . It was deemed that an article uses CI if it explicitly contained at least one confidence interval, but not when it only provides information that could allow its computation (usually by presenting both the estimate and the standard error). Probability intervals provided in Bayesian analysis were classified as confidence intervals (although conceptually they are not the same) since what is really of interest here is whether or not the authors quantify the findings and present them with appropriate indicators of the margin of error or uncertainty.
In addition we determined whether the "Results" section of each article attributed the status of "significant" to an effect on the sole basis of the outcome of a NHST (i.e., without clarifying that it is strictly statistical significance). Similarly, we examined whether the term "significant" (applied to a test) was mistakenly used as synonymous with substantive , relevant or important . The use of the term "significant effect" when it is only appropriate as a reference to a "statistically significant difference," can be considered a direct expression of the significance fallacy [ 39 ] and, as such, constitutes one way to detect the problem in a specific paper.
We also assessed whether the "Conclusions," which sometimes appear as a separate section in the paper or otherwise in the last paragraphs of the "Discussion" section mentioned statistical significance and, if so, whether any of such mentions were no more than an allusion to results.
To perform these analyses we considered both the abstract and the body of the article. To assess the handling of the significance issue, however, only the body of the manuscript was taken into account.
The information was collected by four trained observers. Every paper was assigned to two reviewers. Disagreements were discussed and, if no agreement was reached, a third reviewer was consulted to break the tie and so moderate the effect of subjectivity in the assessment.
In order to assess the reliability of the criteria used for the evaluation of articles and to effect a convergence of criteria among the reviewers, a pilot study of 20 papers from each of three journals ( Clinical Medicine , Primary Care , and International Journal of Epidemiology) was performed. The results of this pilot study were satisfactory. Our results are reported using percentages together with their corresponding confidence intervals. For sampling errors estimations, used to obtain confidence intervals, we weighted the data using the inverse of the probability of selection of each paper, and we took into account the complex nature of the sample design. These analyses were carried out with EPIDAT [ 40 ], a specialized computer program that is readily available.
A total of 1,043 articles were reviewed, of which 874 (84%) were found to be analytic, while the remainders were purely descriptive or of a theoretical and methodological nature. Five of them did not employ either P-values or CI. Consequently, the analysis was made using the remaining 869 articles.
Use of NHST and confidence intervals
The percentage of articles that use only P-values, without even mentioning confidence intervals, to report their results has declined steadily throughout the period analyzed (Table 2 ). The percentage decreased from approximately 41% in 1995-1996 to 21% in 2005-2006. However, it does not differ notably among journals of different languages, as shown by the estimates and confidence intervals of the respective percentages. Concerning thematic areas, it is highly surprising that most of the clinical articles ignore the recommendations of ICMJE, while for general and internal medicine papers such a problem is only present in one in five papers, and in the area of Public Health and Epidemiology it occurs only in one out of six. The use of CI alone (without P-values) has increased slightly across the studied periods (from 9% to 13%), but it is five times more prevalent in Public Health and Epidemiology journals than in Clinical ones, where it reached a scanty 3%.
Ambivalent handling of the significance
While the percentage of articles referring implicitly or explicitly to significance in an ambiguous or incorrect way - that is, incurring the significance fallacy -- seems to decline steadily, the prevalence of this problem exceeds 69%, even in the most recent period. This percentage was almost the same for articles written in Spanish and in English, but it was notably higher in the Clinical journals (81%) compared to the other journals, where the problem occurs in approximately 7 out of 10 papers (Table 3 ). The kappa coefficient for measuring agreement between observers concerning the presence of the "significance fallacy" was 0.78 (CI95%: 0.62 to 0.93), which is considered acceptable in the scale of Landis and Koch [ 41 ].
Reference to numerical results or statistical significance in Conclusions
The percentage of papers mentioning a numerical finding as a conclusion is similar in the three periods analyzed (Table 4 ). Concerning languages, this percentage is nearly twice as large for Spanish journals as for those published in English (approximately 21% versus 12%). And, again, the highest percentage (16%) corresponded to clinical journals.
A similar pattern is observed, although with less pronounced differences, in references to the outcome of the NHST (significant or not) in the conclusions (Table 5 ). The percentage of articles that introduce the term in the "Conclusions" does not appreciably differ between articles written in Spanish and in English. Again, the area where this insufficiency is more often present (more than 15% of articles) is the Clinical area.
There are some previous studies addressing the degree to which researchers have moved beyond the ritualistic use of NHST to assess their hypotheses. This has been examined for areas such as biology [ 42 ], organizational research [ 43 ], or psychology [ 44 – 47 ]. However, to our knowledge, no recent research has explored the pattern of use P-values and CI in medical literature and, in any case, no efforts have been made to study this problem in a way that takes into account different languages and specialties.
At first glance it is puzzling that, after decades of questioning and technical warnings, and after twenty years since the inception of ICMJE recommendation to avoid NHST, they continue being applied ritualistically and mindlessly as the dominant doctrine. Not long ago, when researchers did not observe statistically significant effects, they were unlikely to write them up and to report "negative" findings, since they knew there was a high probability that the paper would be rejected. This has changed a bit: editors are more prone to judge all findings as potentially eloquent. This is probably the frequent denunciations of the tendency for those papers presenting a significant positive result to receive more favorable publication decisions than equally well-conducted ones that report a negative or null result, the so-called publication bias [ 48 – 50 ]. This new openness is consistent with the fact that if the substantive question addressed is really relevant, the answer (whether positive or negative) will also be relevant.
Consequently, even though it was not an aim of our study, we found many examples in which statistical significance was not obtained. However, many of those negative results were reported with a comment of this type: " The results did not show a significant difference between groups; however, with a larger sample size, this difference would have probably proved to be significant ". The problem with this statement is that it is true; more specifically, it will always be true and it is, therefore, sterile. It is not fortuitous that one never encounters the opposite, and equally tautological, statement: " A significant difference between groups has been detected; however, perhaps with a smaller sample size, this difference would have proved to be not significant" . Such a double standard is itself an unequivocal sign of the ritual application of NHST.
Although the declining rates of NHST usage show that, gradually, ICMJE and similar recommendations are having a positive impact, most of the articles in the clinical setting still considered NHST as the final arbiter of the research process. Moreover, it appears that the improvement in the situation is mostly formal, and the percentage of articles that fall into the significance fallacy is huge.
The contradiction between what has been conceptually recommended and the common practice is sensibly less acute in the area of Epidemiology and Public Health, but the same pattern was evident everywhere in the mechanical way of applying significance tests. Nevertheless, the clinical journals remain the most unmoved by the recommendations.
The ICMJE recommendations are not cosmetic statements but substantial ones, and the vigorous exhortations made by outstanding authorities [ 51 ] are not mere intellectual exercises due to ingenious and inopportune methodologists, but rather they are very serious epistemological warnings.
In some cases, the role of CI is not as clearly suitable (e.g. when estimating multiple regression coefficients or because effect sizes are not available for some research designs [ 43 , 52 ]), but when it comes to estimating, for example, an odds ratio or a rates difference, the advantage of using CI instead of P values is very clear, since in such cases it is obvious that the goal is to assess what has been called the "effect size."
The inherent resistance to change old paradigms and practices that have been entrenched for decades is always high. Old habits die hard. The estimates and trends outlined are entirely consistent with Alvan Feinstein's warning 25 years ago: "Because the history of medical research also shows a long tradition of maintaining loyalty to established doctrines long after the doctrines had been discredited, or shown to be valueless, we cannot expect a sudden change in this medical policy merely because it has been denounced by leading connoisseurs of statistics [ 53 ]".
It is possible, however, that the nature of the problem has an external explanation: it is likely that some editors prefer to "avoid troubles" with the authors and vice versa, thus resorting to the most conventional procedures. Many junior researchers believe that it is wise to avoid long back-and-forth discussions with reviewers and editors. In general, researchers who want to appear in print and survive in a publish-or-perish environment are motivated by force, fear, and expedience in their use of NHST [ 54 ]. Furthermore, it is relatively natural that simple researchers use NHST when they take into account that some theoretical objectors have used this statistical analysis in empirical studies, published after the appearance of their own critiques [ 55 ].
For example, Journal of the American Medical Association published a bibliometric study [ 56 ] discussing the impact of statisticians' co-authorship of medical papers on publication decisions by two major high-impact journals: British Medical Journal and Annals of Internal Medicine . The data analysis is characterized by methodological orthodoxy. The authors just use chi-square tests without any reference to CI, although the NHST had been repeatedly criticized over the years by two of the authors:
Douglas Altman, an early promoter of confidence intervals as an alternative [ 57 ], and Steve Goodman, a critic of NHST from a Bayesian perspective [ 58 ]. Individual authors, however, cannot be blamed for broader institutional problems and systemic forces opposed to change.
The present effort is certainly partial in at least two ways: it is limited to only six specific journals and to three biennia. It would be therefore highly desirable to improve it by studying the problem in a more detailed way (especially by reviewing more journals with different profiles), and continuing the review of prevailing patterns and trends.
Curran-Everett D: Explorations in statistics: hypothesis tests and P values. Adv Physiol Educ. 2009, 33: 81-86. 10.1152/advan.90218.2008.
Article PubMed Google Scholar
Fisher RA: Statistical Methods for Research Workers. 1925, Edinburgh: Oliver & Boyd
Google Scholar
Neyman J, Pearson E: On the use and interpretation of certain test criteria for purposes of statistical inference. Biometrika. 1928, 20: 175-240.
Silva LC: Los laberintos de la investigación biomédica. En defensa de la racionalidad para la ciencia del siglo XXI. 2009, Madrid: Díaz de Santos
Berkson J: Test of significance considered as evidence. J Am Stat Assoc. 1942, 37: 325-335. 10.2307/2279000.
Article Google Scholar
Nickerson RS: Null hypothesis significance testing: A review of an old and continuing controversy. Psychol Methods. 2000, 5: 241-301. 10.1037/1082-989X.5.2.241.
Article CAS PubMed Google Scholar
Rozeboom WW: The fallacy of the null hypothesissignificance test. Psychol Bull. 1960, 57: 418-428. 10.1037/h0042040.
Callahan JL, Reio TG: Making subjective judgments in quantitative studies: The importance of using effect sizes and confidenceintervals. HRD Quarterly. 2006, 17: 159-173.
Nakagawa S, Cuthill IC: Effect size, confidence interval and statistical significance: a practical guide for biologists. Biol Rev. 2007, 82: 591-605. 10.1111/j.1469-185X.2007.00027.x.
Breaugh JA: Effect size estimation: factors to consider and mistakes to avoid. J Manage. 2003, 29: 79-97. 10.1177/014920630302900106.
Thompson B: What future quantitative social science research could look like: confidence intervals for effect sizes. Educ Res. 2002, 31: 25-32.
Matthews RA: Significance levels for the assessment of anomalous phenomena. Journal of Scientific Exploration. 1999, 13: 1-7.
Savage IR: Nonparametric statistics. J Am Stat Assoc. 1957, 52: 332-333.
Silva LC, Benavides A, Almenara J: El péndulo bayesiano: Crónica de una polémica estadística. Llull. 2002, 25: 109-128.
Goodman SN, Royall R: Evidence and scientific research. Am J Public Health. 1988, 78: 1568-1574. 10.2105/AJPH.78.12.1568.
Article CAS PubMed PubMed Central Google Scholar
Berger JO, Berry DA: Statistical analysis and the illusion of objectivity. Am Sci. 1988, 76: 159-165.
Hurlbert SH, Lombardi CM: Final collapse of the Neyman-Pearson decision theoretic framework and rise of the neoFisherian. Ann Zool Fenn. 2009, 46: 311-349.
Fidler F, Thomason N, Cumming G, Finch S, Leeman J: Editors can lead researchers to confidence intervals but they can't make them think: Statistical reform lessons from Medicine. Psychol Sci. 2004, 15: 119-126. 10.1111/j.0963-7214.2004.01502008.x.
Balluerka N, Vergara AI, Arnau J: Calculating the main alternatives to null-hypothesis-significance testing in between-subject experimental designs. Psicothema. 2009, 21: 141-151.
Cumming G, Fidler F: Confidence intervals: Better answers to better questions. J Psychol. 2009, 217: 15-26.
Jones LV, Tukey JW: A sensible formulation of the significance test. Psychol Methods. 2000, 5: 411-414. 10.1037/1082-989X.5.4.411.
Dixon P: The p-value fallacy and how to avoid it. Can J Exp Psychol. 2003, 57: 189-202.
Nakagawa S, Cuthill IC: Effect size, confidence interval and statistical significance: a practical guide for biologists. Biol Rev Camb Philos Soc. 2007, 82: 591-605. 10.1111/j.1469-185X.2007.00027.x.
Brandstaetter E: Confidence intervals as an alternative to significance testing. MPR-Online. 2001, 4: 33-46.
Masson ME, Loftus GR: Using confidence intervals for graphically based data interpretation. Can J Exp Psychol. 2003, 57: 203-220.
International Committee of Medical Journal Editors: Uniform requirements for manuscripts submitted to biomedical journals. Update October 2008. Accessed July 11, 2009, [ http://www.icmje.org ]
Feinstein AR: P-Values and Confidence Intervals: two sides of the same unsatisfactory coin. J Clin Epidemiol. 1998, 51: 355-360. 10.1016/S0895-4356(97)00295-3.
Haller H, Kraus S: Misinterpretations of significance: A problem students share with their teachers?. MRP-Online. 2002, 7: 1-20.
Gigerenzer G, Krauss S, Vitouch O: The null ritual: What you always wanted to know about significance testing but were afraid to ask. The Handbook of Methodology for the Social Sciences. Edited by: Kaplan D. 2004, Thousand Oaks, CA: Sage Publications, Chapter 21: 391-408.
Curran-Everett D, Taylor S, Kafadar K: Fundamental concepts in statistics: elucidation and illustration. J Appl Physiol. 1998, 85: 775-786.
CAS PubMed Google Scholar
Royall RM: Statistical evidence: a likelihood paradigm. 1997, Boca Raton: Chapman & Hall/CRC
Goodman SN: Of P values and Bayes: A modest proposal. Epidemiology. 2001, 12: 295-297. 10.1097/00001648-200105000-00006.
Sarria M, Silva LC: Tests of statistical significance in three biomedical journals: a critical review. Rev Panam Salud Publica. 2004, 15: 300-306.
Silva LC: Una ceremonia estadística para identificar factores de riesgo. Salud Colectiva. 2005, 1: 322-329.
Goodman SN: Toward Evidence-Based Medical Statistics 1: The p Value Fallacy. Ann Intern Med. 1999, 130: 995-1004.
Schulz KF, Grimes DA: Sample size calculations in randomised clinical trials: mandatory and mystical. Lancet. 2005, 365: 1348-1353. 10.1016/S0140-6736(05)61034-3.
Bacchetti P: Current sample size conventions: Flaws, harms, and alternatives. BMC Med. 2010, 8: 17-10.1186/1741-7015-8-17.
Article PubMed PubMed Central Google Scholar
Silva LC: Diseño razonado de muestras para la investigación sanitaria. 2000, Madrid: Díaz de Santos
Barnett ML, Mathisen A: Tyranny of the p-value: The conflict between statistical significance and common sense. J Dent Res. 1997, 76: 534-536. 10.1177/00220345970760010201.
Santiago MI, Hervada X, Naveira G, Silva LC, Fariñas H, Vázquez E, Bacallao J, Mújica OJ: [The Epidat program: uses and perspectives] [letter]. Pan Am J Public Health. 2010, 27: 80-82. Spanish.
Landis JR, Koch GG: The measurement of observer agreement for categorical data. Biometrics. 1977, 33: 159-74. 10.2307/2529310.
Fidler F, Burgman MA, Cumming G, Buttrose R, Thomason N: Impact of criticism of null-hypothesis significance testing on statistical reporting practices in conservation biology. Conserv Biol. 2005, 20: 1539-1544. 10.1111/j.1523-1739.2006.00525.x.
Kline RB: Beyond significance testing: Reforming data analysis methods in behavioral research. 2004, Washington, DC: American Psychological Association
Book Google Scholar
Curran-Everett D, Benos DJ: Guidelines for reporting statistics in journals published by the American Physiological Society: the sequel. Adv Physiol Educ. 2007, 31: 295-298. 10.1152/advan.00022.2007.
Hubbard R, Parsa AR, Luthy MR: The spread of statistical significance testing: The case of the Journal of Applied Psychology. Theor Psychol. 1997, 7: 545-554. 10.1177/0959354397074006.
Vacha-Haase T, Nilsson JE, Reetz DR, Lance TS, Thompson B: Reporting practices and APA editorial policies regarding statistical significance and effect size. Theor Psychol. 2000, 10: 413-425. 10.1177/0959354300103006.
Krueger J: Null hypothesis significance testing: On the survival of a flawed method. Am Psychol. 2001, 56: 16-26. 10.1037/0003-066X.56.1.16.
Rising K, Bacchetti P, Bero L: Reporting Bias in Drug Trials Submitted to the Food and Drug Administration: Review of Publication and Presentation. PLoS Med. 2008, 5: e217-10.1371/journal.pmed.0050217. doi:10.1371/journal.pmed.0050217
Sridharan L, Greenland L: Editorial policies and publication bias the importance of negative studies. Arch Intern Med. 2009, 169: 1022-1023. 10.1001/archinternmed.2009.100.
Falagas ME, Alexiou VG: The top-ten in journal impact factor manipulation. Arch Immunol Ther Exp (Warsz). 2008, 56: 223-226. 10.1007/s00005-008-0024-5.
Rothman K: Writing for Epidemiology. Epidemiology. 1998, 9: 98-104. 10.1097/00001648-199805000-00019.
Fidler F: The fifth edition of the APA publication manual: Why its statistics recommendations are so controversial. Educ Psychol Meas. 2002, 62: 749-770. 10.1177/001316402236876.
Feinstein AR: Clinical epidemiology: The architecture of clinical research. 1985, Philadelphia: W.B. Saunders Company
Orlitzky M: Institutionalized dualism: statistical significance testing as myth and ceremony. Accessed Feb 8, 2010, [ http://ssrn.com/abstract=1415926 ]
Greenwald AG, González R, Harris RJ, Guthrie D: Effect sizes and p-value. What should be reported and what should be replicated?. Psychophysiology. 1996, 33: 175-183. 10.1111/j.1469-8986.1996.tb02121.x.
Altman DG, Goodman SN, Schroter S: How statistical expertise is used in medical research. J Am Med Assoc. 2002, 287: 2817-2820. 10.1001/jama.287.21.2817.
Gardner MJ, Altman DJ: Statistics with confidence. Confidence intervals and statistical guidelines. 1992, London: BMJ
Goodman SN: P Values, Hypothesis Tests and Likelihood: implications for epidemiology of a neglected historical debate. Am J Epidemiol. 1993, 137: 485-496.
Pre-publication history
The pre-publication history for this paper can be accessed here: http://www.biomedcentral.com/1471-2288/10/44/prepub
Download references
Acknowledgements
The authors would like to thank Tania Iglesias-Cabo and Vanesa Alvarez-González for their help with the collection of empirical data and their participation in an earlier version of the paper. The manuscript has benefited greatly from thoughtful, constructive feedback by Carlos Campillo-Artero, Tom Piazza and Ann Séror.
Author information
Authors and affiliations.
Centro Nacional de Investigación de Ciencias Médicas, La Habana, Cuba
Luis Carlos Silva-Ayçaguer
Unidad de Investigación. Hospital de Cabueñes, Servicio de Salud del Principado de Asturias (SESPA), Gijón, Spain
Patricio Suárez-Gil
CIBER Epidemiología y Salud Pública (CIBERESP), Spain and Departamento de Medicina, Unidad de Epidemiología Molecular del Instituto Universitario de Oncología, Universidad de Oviedo, Spain
Ana Fernández-Somoano
You can also search for this author in PubMed Google Scholar
Corresponding author
Correspondence to Patricio Suárez-Gil .
Additional information
Competing interests.
The authors declare that they have no competing interests.
Authors' contributions
LCSA designed the study, wrote the paper and supervised the whole process; PSG coordinated the data extraction and carried out statistical analysis, as well as participated in the editing process; AFS extracted the data and participated in the first stage of statistical analysis; all authors contributed to and revised the final manuscript.
Authors’ original submitted files for images
Below are the links to the authors’ original submitted files for images.
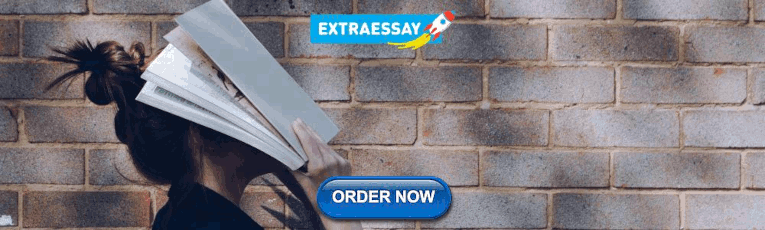
Authors’ original file for figure 1
Rights and permissions.
Open Access This article is published under license to BioMed Central Ltd. This is an Open Access article is distributed under the terms of the Creative Commons Attribution License ( https://creativecommons.org/licenses/by/2.0 ), which permits unrestricted use, distribution, and reproduction in any medium, provided the original work is properly cited.
Reprints and permissions
About this article
Cite this article.
Silva-Ayçaguer, L.C., Suárez-Gil, P. & Fernández-Somoano, A. The null hypothesis significance test in health sciences research (1995-2006): statistical analysis and interpretation. BMC Med Res Methodol 10 , 44 (2010). https://doi.org/10.1186/1471-2288-10-44
Download citation
Received : 29 December 2009
Accepted : 19 May 2010
Published : 19 May 2010
DOI : https://doi.org/10.1186/1471-2288-10-44
Share this article
Anyone you share the following link with will be able to read this content:
Sorry, a shareable link is not currently available for this article.
Provided by the Springer Nature SharedIt content-sharing initiative
- Clinical Specialty
- Significance Fallacy
- Null Hypothesis Statistical Testing
- Medical Journal Editor
- Clinical Journal
BMC Medical Research Methodology
ISSN: 1471-2288
- General enquiries: [email protected]

Statistics Made Easy
The Importance of Statistics in Healthcare (With Examples)
The field of statistics is concerned with collecting, analyzing, interpreting, and presenting data.
In the field of healthcare, statistics is important for the following reasons:
Reason 1 : Statistics allows healthcare professionals to monitor the health of individuals using descriptive statistics.
Reason 2 : Statistics allows healthcare professionals to quantify the relationship between variables using regression models.
Reason 3 : Statistics allows healthcare professionals to compare the effectiveness of different medical procedures using hypothesis tests.
Reason 4 : Statistics allows healthcare professionals to understand the effect of lifestyle choices on health using incidence rate ratio.
In the rest of this article, we elaborate on each of these reasons.
Reason 1: Monitor the Health of Individuals Using Descriptive Statistics
Descriptive statistics are used to describe data.
Healthcare professionals often calculate the following descriptive statistics for a given individual:
- Mean resting heart rate.
- Mean blood pressure.
- Fluctuation in weight during a certain time period.
Using these metrics, healthcare professionals can gain a better understanding of the overall health of individuals.
They can then use these metrics to inform individuals on ways they can improve their health or even prescribe specific medications based on the health of the individual.
Reason 2: Quantify Relationship Between Variables Using Regression Models
Another way that statistics is used in healthcare is in the form of regression models .
These are models that allow healthcare professionals to quantify the relationship between one or more predictor variables and a response variable .
For example, a healthcare professional may have access to data on total hours spent exercising per day, total time spent sitting per day, and overall weight of individuals.
They might then build the following multiple linear regression model:
Weight = 124.33 – 15.33(hours spent exercising per day) + 1.04(hours spent sitting per day)
Here’s how to interpret the regression coefficients in this model:
- For each additional hour spent exercising per day, total weight decreases by an average of 15.33 pounds (assuming hours spent sitting is held constant).
- For each additional hour spent sitting per day, total weight increases by an average of 1.04 pounds (assuming hours spent exercising is held constant).
Using this model, a healthcare professional can quickly understand that more time spent exercising is associated with lower weight and more time spent sitting is associated with higher weight.
They can also quantify exactly how much exercise and sitting affect weight.
Reason 3: Compare Medical Procedures Using Hypothesis Tests
Another way that statistics is used in healthcare is in the form of hypothesis tests .
These are tests that healthcare professionals can use to determine if there is a statistical significance between different medical procedures or treatments.
For example, suppose a doctor believes that a new drug is able to reduce blood pressure in obese patients. To test this, he may measure the blood pressure of 40 patients before and after using the new drug for one month.
He then performs a paired samples t- test using the following hypotheses:
- H 0 : μ after = μ before (the mean blood pressure is the same before and after using the drug)
- H A : μ after < μ before (the mean blood pressure is less after using the drug)
If the p-value of the test is less than some significance level (e.g. α = .05), then he can reject the null hypothesis and conclude that the new drug leads to reduced blood pressure.
Note : This is just one example of a hypothesis test that is used in healthcare. Other common tests include a one sample t-test , two sample t-test , one-way ANOVA , and two-way ANOVA .
Reason 4: Understand Effects of Lifestyle Choices on Health Using Incidence Rate Ratio
An incidence rate ratio allows healthcare professionals to compare the incident rate between two different groups.
For example, suppose it’s known that people who smoke develop lung cancer at a rate of 7 per 100 person-years.
Conversely, suppose it’s known that people who do not smoke develop lung cancer at a rate of 1.5 per 100 person-years.
We would calculate the incidence rate ratio (often abbreviated IRR) as:
- IRR = Incidence rate among smokers / Incidence rate among non-smokers
- IRR = (7/100) / (1.5/100)
Here’s how a healthcare professional would interpret this value: The lung cancer rate among smokers is 4.67 times as high as the rate among non-smokers.
Using this simple calculation, healthcare professionals can gain a good understanding of how different lifestyle choices (like smoking) affect health in individuals.
Additional Resources
The following articles explain the importance of statistics in other fields:
Why is Statistics Important? (10 Reasons Statistics Matters!) The Importance of Statistics in Nursing The Importance of Statistics in Business The Importance of Statistics in Economics The Importance of Statistics in Education
Featured Posts

Hey there. My name is Zach Bobbitt. I have a Masters of Science degree in Applied Statistics and I’ve worked on machine learning algorithms for professional businesses in both healthcare and retail. I’m passionate about statistics, machine learning, and data visualization and I created Statology to be a resource for both students and teachers alike. My goal with this site is to help you learn statistics through using simple terms, plenty of real-world examples, and helpful illustrations.
Leave a Reply Cancel reply
Your email address will not be published. Required fields are marked *
Join the Statology Community
Sign up to receive Statology's exclusive study resource: 100 practice problems with step-by-step solutions. Plus, get our latest insights, tutorials, and data analysis tips straight to your inbox!
By subscribing you accept Statology's Privacy Policy.
Hypothesis and Theory in Health Services: 2022
Hypothesis and Theory 02 August 2023 Health System Transformation Playbook and Unified Care Model: an integrated design, systems & complexity thinking approach to health system transformation Ken Wah Teo , 5 more and Yeuk Fan Ng 4,240 views 0 citations
Hypothesis and Theory 16 June 2023 Intentional practice: a common language, approach and set of methods to design, adapt and implement contextualised wellbeing solutions Ivan J. Raymond 1,680 views 2 citations
Loading... Hypothesis and Theory 22 July 2022 Understanding dynamic complexity in context—Enriching contextual analysis in implementation science from a constructivist perspective Juliane Mielke , 5 more and Sandra Staudacher 10,325 views 6 citations
Loading... Hypothesis and Theory 09 June 2022 Clinician Maladaptive Anxious Avoidance in the Context of Implementation of Evidence-Based Interventions: A Commentary Emily M. Becker-Haimes , 6 more and Miya L. Barnett 2,196 views 7 citations
Hypothesis and Theory 26 November 2021 A Value-Based Steering Model for Healthcare Laura J. Pitkänen , 2 more and Paulus Torkki 3,744 views 1 citations
Research brief: Making evidence go further in health-science research
May 28, 2024.
Health-science research could benefit from pairing two unique methods of study, argue SFI External Professor Ross Hammond and Sharin Barkin in a May 15 perspective in PNAS . When used together, traditional trials and computational models “offer powerful synergy,” write the authors.
Randomized controlled trials, a longstanding approach in health sciences, help researchers establish clear links between treatments and outcomes. But RCTs have inherent limitations. They can be hard to generalize, may not identify a mechanism of action, and rely on necessary assumptions. In addition, cost, feasibility, and ethics can constrain the tests.
An alternative is agent-based models — computational tools that can simulate an intervention in various dynamic environments and across a diverse and interacting population. But ABMs are also limited; real-world data from experiments or observation are necessary to validate the models.
It’s still rare for health science research to pair these two methods, but recent case studies illustrate their potential. Integrating RCTs and ABMs through iterative work could help health science researchers better understand complex diseases and expand the impact of limited resources.
Read the paper “Making evidence go further: Advancing synergy between agent-based modeling and randomized control trials” in PNAS (May 15, 2024). DOI: 10.1073/pnas.2314993121
- Sign Up For SFI News
News Media Contact
Santa fe institute.
Office of Communications [email protected] 505-984-8800
- SFI News Release
More SFI News
Research brief: hierarchy in dynamic environments, mahzarin banaji receives honorary doctorate from yale, the value of failure in conservation programs, new work extends the thermodynamic theory of computation, new series presents pivotal papers in complexity science, research news brief: random processes shape science and math, sfi receives award from academia film olomouc, in memoriam: daniel c. dennett, new book: the time for complexity economics has come, karen willcox winner of the 2024 theodore von kármán prize, tim kohler to deliver linda s. cordell lecture, to accelerate biosphere science, reconnect three scientific cultures, mirta galesic receives prestigious erc advanced grant, carlo rovelli receives 2024 lewis thomas prize, research news brief: defining a city using cell-phone data, complexity tools for usda nutritional guidelines, quantifying the potential value of data, carlo rovelli joins sfi's fractal faculty, new book offers thoughtful approach to modeling complex social systems.
The Relationship between Locus of Control and Psychotic Experiences: Moderating Effect of past-year cannabis use Frequency among Young Adult Lifetime cannabis Users
- Original Article
- Published: 03 June 2024
Cite this article
- Feten Fekih-Romdhane ORCID: orcid.org/0000-0002-2569-8017 1 , 2 ,
- Ramy Ounis 1 , 2 ,
- Majda Cheour 1 , 2 &
- Souheil Hallit 3 , 4 , 5
Explore all metrics
Studies examining the relationship between locus of control (LoC; i.e., the extent to which individuals believe they have control over their lives) and psychotic symptoms in both clinical and non-clinical samples did not take cannabis use into account. Understanding the role of cannabis in this relationship is important to inform prevention efforts. To contribute to the field, this study aimed to test the hypothesis that past-year cannabis use frequency moderates the association between LoC and psychotic experiences (PEs). This study has a cross-sectional design. A self-administered online questionnaire was adopted to collect data from participants, using the snowball sampling method. The questionnaire included a section on sociodemographic information, and the three following measures: the Prodromal Questionnaire-Brief, the Internal–External Locus of Control Short Scale-4, and a 7-point single-item scale to assess past-year cannabis use frequency. A total of 475 young adult lifetime cannabis users from Tunisia (age range 18–35 years, with a mean age of 26.87 ± 3.90 years and 44.2% females), with no history of psychotic disorders and/or antipsychotics intake, were included. After controlling for potential confounders (age, marital status, education level, living arrangement, region of living, tobacco and alcohol use, household crowding index, and personal history of psychiatric illness), moderation analyses showed that past-year cannabis use moderated the association between external LoC and PEs (beta = .10; t = 2.29; p = .022; 95% CI 01; .18). At moderate (beta = .64; p < .001) and high (beta = .74; p < .001) levels of cannabis use, higher external LoC was significantly associated with more severe PEs. No moderating role was found for internal LoC. Findings suggest that among young adults who have a more externally oriented LoC, moderate- to high-frequency past-year cannabis users are more likely to exhibit greater PEs. Therefore, more attention should be directed towards this group of individuals in the context of early intervention programs. Future longitudinal studies in different cultural contexts and more representative samples are still required to confirm the present findings.
This is a preview of subscription content, log in via an institution to check access.
Access this article
Price includes VAT (Russian Federation)
Instant access to the full article PDF.
Rent this article via DeepDyve
Institutional subscriptions
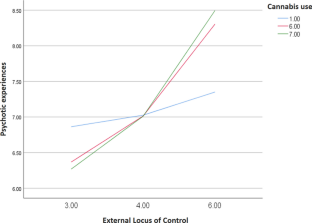
Similar content being viewed by others
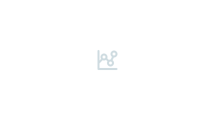
Smoking Mostly Alone as a Risk Factor for Cannabis Use Disorders and Depressive Symptoms
Exploring social context and psychological distress in adult canadians with cannabis use disorder: to what extent do social isolation and negative relationships predict mental health.
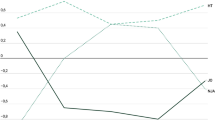
Dispositional Mindfulness Profiles and Cannabis Use in Young Adults
Arciniegas, D. B. (2015). Psychosis. Continuum (Minneap Minn) , 21 (3 Behavioral Neurology and Neuropsychiatry), pp. 715–736. https://doi.org/10.1212/01.CON.0000466662.89908.e7
Aviad, Y., & Cohen-Louck, K. (2021). Locus of Control and Purpose in Life as Protective Factors against the Risk for Suicide in Older Adults. Smith College Studies in Social Work, 91 (4), 295–308. https://doi.org/10.1080/00377317.2021.1968323
Article Google Scholar
Bentall, R., Kinderman, P., & Kaney, S. (1994). The self, attributional process and normal beliefs: Towards a model of persecutory delusions. Behaviour Research and Therapy, 32 (3), 331–341.
Article CAS PubMed Google Scholar
Bentall, R. P. (2019). Cognitive biases and abnormal beliefs: Towards a model of persecutory delusions. The neuropsychology of schizophrenia, 337–360.
Boumparis, N., Loheide-Niesmann, L., Blankers, M., Ebert, D. D., Korf, D., Schaub, M. P., Spijkerman, R., Tait, R. J., & Riper, H. (2019). Short- and long-term effects of digital prevention and treatment interventions for cannabis use reduction: A systematic review and meta-analysis. Drug and Alcohol Dependence, 200 , 82–94. https://doi.org/10.1016/j.drugalcdep.2019.03.016
Article PubMed Google Scholar
Carney, R., Cotter, J., Firth, J., Bradshaw, T., & Yung, A. R. (2017). Cannabis use and symptom severity in individuals at ultra high risk for psychosis: A meta-analysis. Acta Psychiatrica Scand., 136 (1), 5–15. https://doi.org/10.1111/acps.12699
Article CAS Google Scholar
Cheng, C., Jose, P. E., Sheldon, K. M., Singelis, T. M., Cheung, M. W., Tiliouine, H., Alao, A. A., Chio, J. H., Lui, J. Y., & Chun, W. Y. (2011). Sociocultural differences in self-construal and subjective well-being: A test of four cultural models. Journal of Cross-Cultural Psychology, 42 (5), 832–855.
Cheng, C., Cheung, S. F., Chio, J.H.-M., & Chan, M.-P.S. (2013). Cultural meaning of perceived control: A meta-analysis of locus of control and psychological symptoms across 18 cultural regions. Psychological Bulletin, 139 (1), 152.
Cicero, D. C., Martin, E. A., Becker, T. M., & Kerns, J. G. (2016). Decreased self-concept clarity in people with schizophrenia. The Journal of Nervous and Mental Disease, 204 (2), 142–147.
Article PubMed PubMed Central Google Scholar
Davis, M. L., Powers, M. B., Handelsman, P., Medina, J. L., Zvolensky, M., & Smits, J. A. (2015). Behavioral therapies for treatment-seeking cannabis users: A meta-analysis of randomized controlled trials. Evaluation & the Health Professions, 38 (1), 94–114.
Di Luzio, M., Pontillo, M., Di Vincenzo, C., Bellantoni, D., Demaria, F., & Vicari, S. (2022). Hallucinations in the child and adolescent “ultra-high risk” population: A systematic review. Schizophrenia Bulletin Open, 3 (1), sgac068.
Donohoe, G., Spoletini, I., McGlade, N., Behan, C., Hayden, J., O’Donoghue, T., Peel, R., Haq, F., Walker, C., & O’Callaghan, E. (2008). Are relational style and neuropsychological performance predictors of social attributions in chronic schizophrenia? Psychiatry Research, 161 (1), 19–27.
Fekih-Romdhane, F., Hakiri, A., Fadhel, S. B., & Cheour, M. (2019). Cannabis use in subjects at ultra high risk for psychosis. Presse Medicale, 48 (11 Pt 1), 1229–1236.
Fekih-Romdhane, F., Sassi, H., Ennaifer, S., Tira, S., & Cheour, M. (2020). Prevalence and Correlates of Psychotic Like Experiences in a Large Community Sample of Young Adults in Tunisia. Community Mental Health Journal, 56 (6), 991–1003. https://doi.org/10.1007/s10597-019-00542-1
Fekih-Romdhane, F., Alhuwailah, A., Shuwiekh, H. A. M., Stambouli, M., Hakiri, A., Cheour, M., Loch, A. A., & Hallit, S. (2024a). Development and initial validation of the cannabis-related psychosis risk literacy scale (CPRL): A multinational psychometric study. BMC Psychiatry, 24 (1), 298. https://doi.org/10.1186/s12888-024-05727-x
Fekih-Romdhane, F., Ghrissi, F., Stambouli, M., Hakiri, A., Loch, A. A., Cheour, M., & Hallit, S. (2024). Moderating effect of alexithymia between problem gambling and psychotic experiences in university students. BMC Psychiatry, 24 (1), 19.
Fekih-Romdhane, F., Hallit, R., Sallam, M., Malaeb, D., Sakr, F., Dabbous, M., Obeid, S., & Hallit, S. (n.d). Psychometric Properties of an Arabic Translation of the Four-Item Scale Offear of Autonomous Robots and Artificial Intelligence (Farai). Available at SSRN 4584560 .
Fekih-Romdhane, F., Alhuwailah, A., Shuwiekh, H. A. M., Stambouli, M., Hakiri, A., Jaoua, A., Cheour, M., Lloret-Irles, D., & Hallit, S. (2023). Cross-national Validation of the Arabic Version of the Cannabis Use Intention Questionnaire (CUIQ) Among University Students from Egypt, Kuwait, and Tunisia. International Journal of Mental Health and Addiction , 1–16.
Fekih-Romdhane, F., Hallit, R., Malaeb, D., Dabbous, M., Sakr, F., El Khatib, S., Sawma, T., Obeid, S., & Hallit, S. (2023). Psychometric Properties of the Arabic versions of the 3-item General Self-Efficacy Short Scale–3 (GSE-3), and the Internal–External Locus of Control Short Scale–4 (IE-4).
Fekih-Romdhane, F., Jahrami, H., Alhuwailah, A., Fawaz, M., Shuwiekh, H. A. M., Helmy, M., Mohammed Hassan, I. H., Naser, A. Y., Zarrouq, B., & Chebly, M. (2023). Cross-country validation of the Arabic Version of the Prodromal Questionnaire–Brief (PQ‐B) in young adults from the general population of the Middle East and North Africa (MENA) region. International Journal of Mental Health and Addiction , 1–21.
Fekih-Romdhane, F., Malaeb, D., Postigo, A., Sakr, F., Dabbous, M., Khatib, S. E., Obeid, S., & Hallit, S. (2024). The relationship between climate change anxiety and psychotic experiences is mediated by death anxiety. International Journal of Social Psychiatry , 00207640231221102.
Flores, J., Caqueo-Urízar, A., Ramírez, C., Arancio, G., & Cofré, J. P. (2020). Locus of control, self-control, and gender as predictors of internalizing and externalizing problems in children and adolescents in Northern Chile. Frontiers in Psychology, 11 , 2015. https://doi.org/10.3389/fpsyg.2020.02015
Frenkel, E., Kugelmass, S., Nathan, M., & Ingraham, L. J. (1995). Locus of control and mental health in adolescence and adulthood. Schizophrenia Bulletin, 21 (2), 219–226.
Fusar-Poli, P., Raballo, A., & Parnas, J. (2017). What is an attenuated psychotic symptom? on the Importance of the context. Schizophrenia Bulletin, 43 (4), 687–692. https://doi.org/10.1093/schbul/sbw182
Gale, C. R., Batty, G. D., & Deary, I. J. (2008). Locus of control at age 10 years and health outcomes and behaviors at age 30 years: The 1970 British Cohort Study. Psychosomatic Medicine, 70 (4), 397–403. https://doi.org/10.1097/PSY.0b013e31816a719e
Garety, P. A., Bebbington, P., Fowler, D., Freeman, D., & Kuipers, E. (2007). Implications for neurobiological research of cognitive models of psychosis: A theoretical paper. Psychological Medicine, 37 (10), 1377–1391.
Hair Jr, J. F., Hult, G. T. M., Ringle, C. M., Sarstedt, M., Danks, N. P., & Ray, S. (2021). Partial least squares structural equation modeling (PLS-SEM) using R: A workbook . Springer Nature.
Hairaty, K., Sadeghmoghadam, L., Alami, A., & Moshki, M. (2019). Effect of education based on health locus of control theory on health literacy among older adult. Internal Medicine Today, 25 (1), 37–42.
Google Scholar
Harrow, M., Hansford, B. G., & Astrachan-Fletcher, E. B. (2009). Locus of control: Relation to schizophrenia, to recovery, and to depression and psychosis—A 15-year longitudinal study. Psychiatry Research, 168 (3), 186–192.
Heckman, J. J., Stixrud, J., & Urzua, S. (2006). The effects of cognitive and noncognitive abilities on labor market outcomes and social behavior. Journal of Labor Economics, 24 (3), 411–482.
Hibell, B., Guttormsson, U., Ahlström, S., Balakireva, O., Bjarnason, T., Kokkevi, A., & Kraus, L. (2012). The 2011 ESPAD Report, The Swedish Council for Information on Alcohol and other Drugs (CAN). Stockholm: ESPAD .
Jarrett, R. B., Vittengl, J. R., Doyle, K., & Clark, L. A. (2007). Changes in cognitive content during and following cognitive therapy for recurrent depression: Substantial and enduring, but not predictive of change in depressive symptoms. Journal of Consulting and Clinical Psychology, 75 (3), 432.
Jones, S. R., & Fernyhough, C. (2007). The roles of locus of control and self-esteem in hallucination-and delusion-proneness in a non-clinical sample. Personality and Individual Differences, 43 (5), 1087–1097.
Kesavayuth, D., Poyago-Theotoky, J., & Zikos, V. (2020). Locus of control, health and healthcare utilization. Economic Modelling, 86 , 227–238.
Kinderman, P., & Bentall, R. P. (2000). Self-discrepancies and causal attributions: Studies of hypothesized relationships. British Journal of Clinical Psychology, 39 (3), 255–273.
Kinderman, P., Dunbar, R., & Bentall, R. P. (1998). Theory-of-mind deficits and causal attributions. British Journal of Psychology, 89 (2), 191–204.
Kurtović, A., Vuković, I., & Gajić, M. (2018). The effect of locus of control on university students’ mental health: Possible mediation through self-esteem and coping. The Journal of Psychology, 152 (6), 341–357.
Langdon, R., Ward, P. B., & Coltheart, M. (2010). Reasoning anomalies associated with delusions in schizophrenia. Schizophrenia Bulletin, 36 (2), 321–330.
Leeson, V. C., Harrison, I., Ron, M. A., Barnes, T. R., & Joyce, E. M. (2012). The effect of cannabis use and cognitive reserve on age at onset and psychosis outcomes in first-episode schizophrenia. Schizophrenia Bulletin, 38 (4), 873–880. https://doi.org/10.1093/schbul/sbq153
Lefcourt, H. M. (2014). Locus of control: Current trends in theory & research . Psychology Press.
Book Google Scholar
Loewy, R. L., Bearden, C. E., Johnson, J. K., Raine, A., & Cannon, T. D. (2005). The prodromal questionnaire (PQ): Preliminary validation of a self-report screening measure for prodromal and psychotic syndromes. Schizophrenia Research, 79 (1), 117–125.
Loewy, R. L., Pearson, R., Vinogradov, S., Bearden, C. E., & Cannon, T. D. (2011). Psychosis risk screening with the Prodromal Questionnaire—brief version (PQ-B). Schizophrenia Research, 129 (1), 42–46.
Mahfoud, D., Hallit, S., Haddad, C., Fekih-Romdhane, F., & Haddad, G. (2023). The moderating effect of cognitive impairment on the relationship between inner speech and auditory verbal hallucinations among chronic patients with schizophrenia. BMC Psychiatry, 23 (1), 431.
Melo, S. S., Taylor, J. L., & Bentall, R. P. (2006). Poor me versus bad me paranoia and the instability of persecutory ideation. Psychology and Psychotherapy: Theory, Research and Practice, 79 (2), 271–287.
Mendolia, S., & Walker, I. (2014). The effect of noncognitive traits on health behaviours in adolescence. Health Economics, 23 (9), 1146–1158.
Moreira, P., Vaz, J. M., Stevanovic, D., Atilola, O., Dodig-Ćurković, K., Franic, T., Djoric, A., Davidovic, N., Avicenna, M., & Noor, I. M. (2020). Locus of control, negative live events and psychopathological symptoms in collectivist adolescents. Personality and Individual Differences, 154 , 109601.
Mujtaba, A., Malik, F., & Iftikhar, R. (2015). Anger, depression and locus of control among heavy smokers, cannabis users and heroin addicts. The International Journal of Indian Psychology , 3 (1).
Naegele, H., Betzler, F., Viohl, L., Koslowski, M., Ernst, F., & Petzold, M. B. (2022). Cannabis use, use motives and cannabis use disorder among berlin college students. Journal of Drug Issues, 52 (4), 568–584. https://doi.org/10.1177/00220426221086877
Naegele, H., Petzold, M. B., Viohl, L., Bendau, A., Helmer-Ohlmeier, S., & Betzler, F. (2023). Determinants of Cannabis initiation and cessation among berlin college students - a longitudinal study. Substance Use and Misuse, 58 (10), 1262–1272. https://doi.org/10.1080/10826084.2023.2215320
Nießen, D., Schmidt, I., Groskurth, K., Rammstedt, B., & Lechner, C. M. (2022). The Internal-external locus of control short scale–4 (IE-4): A comprehensive validation of the english-language adaptation. PLoS ONE, 17 (7), e0271289.
Pagnini, F., Bercovitz, K., & Langer, E. (2016). Perceived control and mindfulness: Implications for clinical practice. Journal of Psychotherapy Integration, 26 (2), 91.
Paulbeck, R. (2013). Locus of control and quality of life in individuals with schizotypy.
Phares, E. J. (1976). Locus of control in personality. (No Title) .
Ragazzi, T. C., Shuhama, R., Menezes, P. R., & Del-Ben, C. M. (2018). Cannabis use as a risk factor for psychotic-like experiences: A systematic review of non-clinical populations evaluated with the community assessment of psychic experiences. Early Intervention in Psychiatry, 12 (6), 1013–1023.
Ribolsi, M., Albergo, G., Leonetti, F., Niolu, C., Di Lazzaro, V., Siracusano, A., & Di Lorenzo, G. (2022). Locus of Control behavior in ultra-high risk patients. Rivista Di Psichiatria, 57 (3), 123–126.
PubMed Google Scholar
Rotter, J. B. (1966). Generalized expectancies for internal versus external control of reinforcement. Psychological Monographs: General and Applied, 80 (1), 1.
Spriggens, L., & Hides, L. (2015). Patterns of cannabis use, psychotic-like experiences and personality styles in young cannabis users. Schizophrenia Research, 165 (1), 3–8.
Staines, L., Healy, C., Coughlan, H., Clarke, M., Kelleher, I., Cotter, D., & Cannon, M. (2022). Psychotic experiences in the general population, a review; definition, risk factors, outcomes and interventions. Psychological Medicine, 52 (15), 1–12. https://doi.org/10.1017/s0033291722002550
Sullivan, S. A., Thompson, A., Kounali, D., Lewis, G., & Zammit, S. (2017). The longitudinal association between external locus of control, social cognition and adolescent psychopathology. Social Psychiatry and Psychiatric Epidemiology, 52 , 643–655.
Sullivan, S., Yamasaki, S., Ando, S., Endo, K., & Nishida, A. (2021). The association between locus of control and psychopathology: A cross-cohort comparison between a UK (Avon longitudinal study of parents and children) and a Japanese (Tokyo teen cohort) cohort. Frontiers in Psychology, 12 , 600941.
Thompson, A., Sullivan, S., Lewis, G., Zammit, S., Heron, J., Horwood, J., Thomas, K., Gunnell, D., Hollis, C., & Wolke, D. (2011). Association between locus of control in childhood and psychotic symptoms in early adolescence: Results from a large birth cohort. Cognitive Neuropsychiatry, 16 (5), 385–402.
Verdoux, H., Sorbara, F., Gindre, C., Swendsen, J. D., & Van Os, J. (2003). Cannabis use and dimensions of psychosis in a nonclinical population of female subjects. Schizophrenia Research, 59 (1), 77–84.
Zhang, W., Liu, H., Jiang, X., Wu, D., & Tian, Y. (2014). A longitudinal study of posttraumatic stress disorder symptoms and its relationship with coping skill and locus of control in adolescents after an earthquake in China. PLoS ONE, 9 (2), e88263.
Download references
Author information
Authors and affiliations.
The Tunisian Center of Early Intervention in Psychosis, Department of Psychiatry “Ibn Omrane”, Razi Hospital, 2010, Manouba, Tunisia
Feten Fekih-Romdhane, Ramy Ounis & Majda Cheour
Tunis El Manar University, Faculty of Medicine of Tunis, Tunis, Tunisia
School of Medicine and Medical Sciences, Holy Spirit University of Kaslik, P.O. Box 446, Jounieh, Lebanon
Souheil Hallit
Psychology Department, College of Humanities, Effat University, 21478, Jeddah, Saudi Arabia
Applied Science Research Center, Applied Science Private University, Amman, Jordan
You can also search for this author in PubMed Google Scholar
Corresponding authors
Correspondence to Feten Fekih-Romdhane or Souheil Hallit .
Ethics declarations
Conflict of interest.
Author FFR, Author RO, author MC, and author SH declare that they have no conflict of interest.
Ethics Approval
The protocol was approved by the ethics committee of Razi Hospital, Manouba, Tunisia.
Additional information
Publisher's note.
Springer Nature remains neutral with regard to jurisdictional claims in published maps and institutional affiliations.
Rights and permissions
Springer Nature or its licensor (e.g. a society or other partner) holds exclusive rights to this article under a publishing agreement with the author(s) or other rightsholder(s); author self-archiving of the accepted manuscript version of this article is solely governed by the terms of such publishing agreement and applicable law.
Reprints and permissions
About this article
Fekih-Romdhane, F., Ounis, R., Cheour, M. et al. The Relationship between Locus of Control and Psychotic Experiences: Moderating Effect of past-year cannabis use Frequency among Young Adult Lifetime cannabis Users. Int J Ment Health Addiction (2024). https://doi.org/10.1007/s11469-024-01337-6
Download citation
Accepted : 17 May 2024
Published : 03 June 2024
DOI : https://doi.org/10.1007/s11469-024-01337-6
Share this article
Anyone you share the following link with will be able to read this content:
Sorry, a shareable link is not currently available for this article.
Provided by the Springer Nature SharedIt content-sharing initiative
- Locus of control
- Psychotic experiences
- Cannabis use
- Young adults
- Find a journal
- Publish with us
- Track your research
The Daily Digest
Saunas really can improve your health says new research
Posted: September 4, 2023 | Last updated: April 27, 2024
Was Joe Rogan right all along?
Saunas are great for your cardiovascular system and they’re particularly helpful after exercise according to new research from a doctoral researcher at the University of Jyväskylä.
We have some proof
The health effects of exercise have been well documented over the years but scientists have had little to say about how useful saunas can be for our fitness, at least until now.
Groundbreaking research
In a groundbreaking doctoral dissertation, the University of Jyväskylä’s Earric Lee has shown that "Finnish sauna bathing" can have major health benefits according to a new release.
Spending time in a sauna
Lee found that spending time in a sauna can have positive effects on your cardiovascular system and those effects could be made even more potent if you exercised beforehand.
Findings from the first study
“The first research study we conducted showed that 2x 15 minutes of sauna bathing was able to acutely reduce blood pressures and indices of vascular stiffness,” Lee explained.
"A short bout of aerobic exercise"
"We subsequently found that when a short bout of aerobic exercise was performed prior to 15 minutes of sauna bathing, these effects appear to be prolonged,” Lee continued.
A new hypothesis
Lee used his initial findings to hypothesize that exercising before entering a sauna could work synergistically to improve a person's cardiovascular system more than if they just worked out and didn’t bathe in a sauna afterward.
Exercise is helpful
“It has been well established that after a single bout of exercise, there is an increase in angiogenic factors and insulin sensitivity, together with decreases in blood lipid and blood pressure levels,” Lee said in the University of Jyväskylä’s news release.
Testing the theory
“As such, the final experiment in the dissertation was designed to take advantage of this unique opportunity and put this hypothesis to the test,” Lee continued.
Lee's second study
Lee ran a randomized controlled trial for eight weeks with a group of middle-aged adult participants that had at least one major cardiovascular risk factor and made them follow Finland's national health guidelines on exercise for the whole trial period.
A 15-minute sauna
One group was told to only exercise according to the guidelines and the second group was asked to do the same but follow their workout up with a 15-minute sauna post-exercise.
Discovering what we already knew
Lee’s final dissertation research showed that exercising regularly was able to reduce his study participant’s body mass and improve their fitness, which the news release from the University of Jyväskylä said was to be expected. But it also proved saunas helped.
Improving health outcomes
Based on the research, the group that spent 15 minutes in a sauna after their workout showed better improvements in their cardiovascular fitness than those who only exercised.
Even more benefits
The news release from the University of Jyväskylä also noted that those who spent time in a sauna post-exercise also showed “a decrease in systolic blood pressure and total cholesterol levels.”
Don't drink and sauna
If you do want to hop into a sauna to help your health there are a few key things you should know before you do so. Harvard University recommends that you never get into if you’ve had any alcohol, are on any medications, or are ill.
Other important things to know
Moreover, Harvard says that you should only stay in for 15-20 minutes and that you should drink at least four glasses of cold water after your steam bath, presumably to rehydrate you after sweating out your toxins.
More for You
A Family Affair official trailer
Spanish princess Leonor wears mysterious necklace
Notable People who died in 2024
The most beautiful actresses of all time
China's military unveils machine gun-wielding robot 'war hounds'
Snoring is more dangerous than you think
Fish Falls From the Sky and Slams Into Car
The most beautiful actresses in the world
Too Much Talent: How will Real Madrid play with Mbappe?
The 32 Most Dangerous Animals On Earth (Some Might Surprise You)
Famous Actors In their First Roles Vs How They Look Now
Age gaps in relationships: how much is too much?
Don’t want IBS? Three simple life changes will help make you immune
The simplest cars ever made
Famous musicians who are self-taught
Albert's Lyrebird seen performing exotic mating dance in Australia
The 60 Best Action Movies of All Time
Celebrities Who Are Actually Very Smart
Russia suffered its worst losses of the entire war last month
10 Foods You Should Eat Before Drinking Alcohol
Ancient Civilizations - The Silurian Hypothesis The Reality Revolution Podcast
- Health & Fitness
It's called the Silurian Hypothesis (and lest you think scientists aren't nerds, it's named after a bunch of Doctor Who aliens). Basically, it states that human beings might not be the first intelligent life forms to have evolved on this planet and that if there really were precursors some 100 million years ago, virtually all signs of them would have been lost by now. It might seem obvious that we'd see the signs of such a civilization — after all, there were dinosaurs 100 million years ago, and we know that because we've found their fossils. But they were around for more than 150 million years. That's important because it's not just about how old the ruins of this hypothetical civilization would be, nor how widespread it was. It's also about how long it was around. Humanity has spread across the globe in a remarkably short amount of time — over the course of about 100,000 years. If another species did the same, then our chances of spotting it in the geological record would be a whole lot smaller. For decades the archaeological community labored under the theory that human civilization began after the last Ice Age. The theory conjectured that, prior to that time, humans were no more than primitive hunter-gatherers incapable of communal organization or sophisticated abilities, and it was only after the last glacial period—following the melting of the 10,000 foot thick ice sheets that covered much of the northern portion of the world’s continents—that our human ancestors began to develop agriculture and complex economic and social structures, sometime around 4000 B.C. Archaeologists therefore theorized that the first cities did not develop until about 3500 B.C. in Mesopotamia and Egypt. Contemporary discoveries have dramatically transformed those theories. Modern research has unearthed buried civilizations and discovered submerged cities one after another—archaeology and anthropology now reconstruct an unsuspected antiquity of man—fresh discoveries prove all history false and paint a canvas of stunningly mysterious dimensions. What if ancient civilizations existed. What would it mean? Let's discuss it. 💰Large Sums Of Money Activation Trainings - 12 hours of training on activating the large sums of money reality https://realityrevolutioncon.com/largesumsofmoney 🎨 Buy My Art - Unique Sigil Magic and Energy Activation Through Flow Art and Voyages Through Space and Imagination. https://www.newearth.art/ 📕BUY MY BOOK! https://www.amazon.com/Reality-Revolution-Mind-Blowing-Movement-Hack/dp/154450618X/ 🎧Listen to my book on audible https://www.audible.com/pd/The-Reality-Revolution-Audiobook/B087LV1R5V ✨ Alternate Universe Reality Activation get full access to new meditations, new lectures, recordings from the reality con and the 90 day AURA meditation schedule https://realityrevolutionlive.com/aura45338118 🌎→The New Earth Activation trainings - Immerse yourself in 12 hours of content focused on the new earth with channeling, meditations, advanced training and access to the new earth https://realityrevolutioncon.com/newearth ✨Sign up for my newsletter and email list https://chiefexecutiveprime.activehosted.com/f/1 ✨Sign up for my newsletter and email list https://chiefexecutiveprime.activehosted.com/f/1 🌐Join The Reality Revolution – https://www.therealityrevolution.com ➡Join The Reality Revolution Facebook Group - https://www.facebook.com/groups/523814491927119/ ➡ Follow Us On Facebook https://www.facebook.com/The-Reality-Revolution-Podcast-Hosted-By-Brian-Scott-102555575116999 ➡Join The Reality Revolution Discord https://discord.gg/Xbh6H88D8k ➡ Join our Board On Reddit: https://www.reddit.com/r/TheRealityRevolution/ ➡Instagram: https://www.instagram.com/the_reality_revolution/ ➡Twitter: https://twitter.com/mediaprime ➡Spoutible: https://spoutible.com/BrianScott ➡Tumblr: https://www.tumblr.com/the-reality-revolution ➡Linkedin: https://www.li
- Episode Website
- More Episodes
Top Podcasts In Health & Fitness
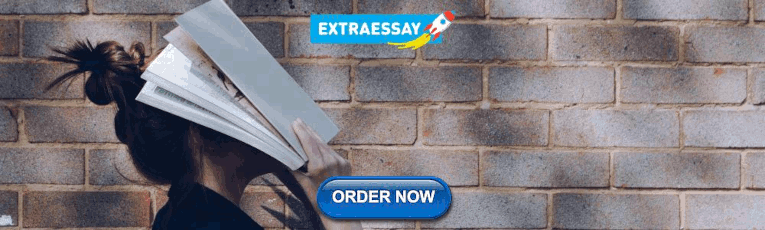
IMAGES
VIDEO
COMMENTS
Medical providers often rely on evidence-based medicine to guide decision-making in practice. Often a research hypothesis is tested with results provided, typically with p values, confidence intervals, or both. Additionally, statistical or research significance is estimated or determined by the investigators. Unfortunately, healthcare providers may have different comfort levels in interpreting ...
What is a hypothesis and how can you write a great one for your research? A hypothesis is a tentative statement about the relationship between two or more variables that can be tested empirically. Find out how to formulate a clear, specific, and testable hypothesis with examples and tips from Verywell Mind, a trusted source of psychology and mental health information.
Hypothesis testing is the process used to evaluate the strength of evidence from the sample and provides a framework for making determinations related to the population, ie, it provides a method for understanding how reliably one can extrapolate observed findings in a sample under study to the larger population from which the sample was drawn ...
Developing a hypothesis (with example) Step 1. Ask a question. Writing a hypothesis begins with a research question that you want to answer. The question should be focused, specific, and researchable within the constraints of your project. Example: Research question.
Examples. A research hypothesis, in its plural form "hypotheses," is a specific, testable prediction about the anticipated results of a study, established at its outset. It is a key component of the scientific method. Hypotheses connect theory to data and guide the research process towards expanding scientific understanding.
Issues of Concern. Without a foundational understanding of hypothesis testing, p values, confidence intervals, and the difference between statistical and clinical significance, it may affect healthcare providers' ability to make clinical decisions without relying purely on the research investigators deemed level of significance.
The relationship between the confidence level and the significance level for a hypothesis test is as follows: Confidence level = 1 - Significance level (alpha) For example, if your significance level is 0.05, the equivalent confidence level is 95%. Both of the following conditions represent statistically significant results: The P-value in a ...
The null hypothesis statistical testing (NHST) has been the most widely used statistical approach in health research over the past 80 years. Its origins dates back to 1279 [] although it was in the second decade of the twentieth century when the statistician Ronald Fisher formally introduced the concept of "null hypothesis" H 0 - which, generally speaking, establishes that certain parameters ...
ABSTRACT. "Hypothesis testing" is an integral and most important component of research methodology, in all researches, whether in. medical sciences, social sciences or any such allied field ...
So, Nurse Jory's research purpose is "The purpose of this research study is to explore barriers to appointment attendance.". After the research problem and purpose statement comes the research hypothesis, by identifying the research variables. Research variables are the concepts that are measured, manipulated, or controlled in a study.
Present the findings in your results and discussion section. Though the specific details might vary, the procedure you will use when testing a hypothesis will always follow some version of these steps. Table of contents. Step 1: State your null and alternate hypothesis. Step 2: Collect data. Step 3: Perform a statistical test.
Reason 3: Compare Medical Procedures Using Hypothesis Tests. Another way that statistics is used in healthcare is in the form of hypothesis tests. These are tests that healthcare professionals can use to determine if there is a statistical significance between different medical procedures or treatments.
In this second course of the Biostatistics in Public Health Specialization, you'll learn to evaluate sample variability and apply statistical hypothesis testing methods. Along the way, you'll perform calculations and interpret real-world data from the published scientific literature. Topics include sample statistics, the central limit theorem ...
Hypothesis testing is one statistical method that can help healthcare professionals make data-driven decisions. Collecting and analyzing data can test hypotheses about patient care and determine ...
This Research Topic includes high-quality Hypothesis and Theory articles published in every section of Frontiers in Health Services in 2022. The mission of the journal is to ultimately contribute to helping health systems improve both experiences and outcomes of patients and make health systems more efficient. Hypothesis and Theory articles aim to highlight a novel argument, interpretation, or ...
Expanding the Mediated Imagined Interaction Hypothesis, this article contributes the development of a scale to test the theoretical construct. Interweaving imagined interactions and media, the mediated imagined interaction hypothesis examines how media impacts how people imagine their real life conversations and interactions with others.
The serotonin deficit hypothesis explanation for major depressive disorder (MDD) has persisted among clinicians and the general public alike despite insufficient supporting evidence. To combat rising mental health crises and eroding public trust in science and medicine, researchers and clinicians must be able to communicate to patients and the ...
Health-science research could benefit from pairing two unique methods of study, argue SFI External Professor Ross Hammond and Sharin Barkin in a May 15 perspective in PNAS. When used together, traditional trials and computational models "offer powerful synergy," write the authors.
To contribute to the field, this study aimed to test the hypothesis that past-year cannabis use frequency moderates the association between LoC and psychotic experiences (PEs). This study has a cross-sectional design. A self-administered online questionnaire was adopted to collect data from participants, using the snowball sampling method ...
The health effects of exercise have been well documented over the years but scientists have had little to say about how useful saunas can be for our fitness, at least until now. ... A new hypothesis.
Health & Fitness It's called the Silurian Hypothesis (and lest you think scientists aren't nerds, it's named after a bunch of Doctor Who aliens). Basically, it states that human beings might not be the first intelligent life forms to have evolved on this planet and that if there really were precursors some 100 million years ago, virtually all ...