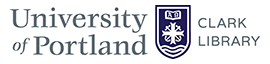
Thursday, February 23: The Clark Library is closed today.
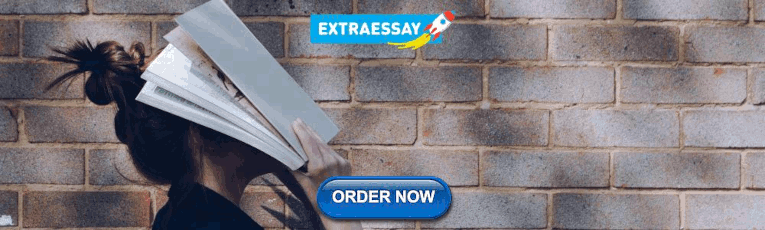
APA Style (7th Edition) Citation Guide: Journal Articles
- Introduction
- Journal Articles
- Magazine/Newspaper Articles
- Books & Ebooks
- Government & Legal Documents
- Biblical Sources
- Secondary Sources
- Films/Videos/TV Shows
- How to Cite: Other
- Additional Help
Table of Contents
Journal article from library database with doi - one author, journal article from library database with doi - multiple authors, journal article from a website - one author.
Journal Article- No DOI
Note: All citations should be double spaced and have a hanging indent in a Reference List.
A "hanging indent" means that each subsequent line after the first line of your citation should be indented by 0.5 inches.
This Microsoft support page contains instructions about how to format a hanging indent in a paper.
- APA 7th. ed. Journal Article Reference Checklist
If an item has no author, start the citation with the article title.
When an article has one to twenty authors, all authors' names are cited in the References List entry. When an article has twenty-one or more authors list the first nineteen authors followed by three spaced ellipse points (. . .) , and then the last author's name. Rules are different for in-text citations; please see the examples provided.
Cite author names in the order in which they appear on the source, not in alphabetical order (the first author is usually the person who contributed the most work to the publication).
Italicize titles of journals, magazines and newspapers. Do not italicize or use quotation marks for the titles of articles.
Capitalize only the first letter of the first word of the article title. If there is a colon in the article title, also capitalize the first letter of the first word after the colon.
If an item has no date, use the short form n.d. where you would normally put the date.
Volume and Issue Numbers
Italicize volume numbers but not issue numbers.
Retrieval Dates
Most articles will not need these in the citation. Only use them for online articles from places where content may change often, like a free website or a wiki.
Page Numbers
If an article doesn't appear on continuous pages, list all the page numbers the article is on, separated by commas. For example (4, 6, 12-14)
Library Database
Do not include the name of a database for works obtained from most academic research databases (e.g. APA PsycInfo, CINAHL) because works in these resources are widely available. Exceptions are Cochrane Database of Systematic Reviews, ERIC, ProQuest Dissertations, and UpToDate.
Include the DOI (formatted as a URL: https://doi.org/...) if it is available. If you do not have a DOI, include a URL if the full text of the article is available online (not as part of a library database). If the full text is from a library database, do not include a DOI, URL, or database name.
In the Body of a Paper
Books, Journals, Reports, Webpages, etc.: When you refer to titles of a “stand-alone work,” as the APA calls them on their APA Style website, such as books, journals, reports, and webpages, you should italicize them. Capitalize words as you would for an article title in a reference, e.g., In the book Crying in H Mart: A memoir , author Michelle Zauner (2021) describes her biracial origin and its impact on her identity.
Article or Chapter: When you refer to the title of a part of a work, such as an article or a chapter, put quotation marks around the title and capitalize it as you would for a journal title in a reference, e.g., In the chapter “Where’s the Wine,” Zauner (2021) describes how she decided to become a musician.
The APA Sample Paper below has more information about formatting your paper.
- APA 7th ed. Sample Paper
Author's Last Name, First Initial. Second Initial if Given. (Year of Publication). Title of article: Subtitle if any. Name of Journal, Volume Number (Issue Number), first page number-last page number. https://doi.org/doi number
Smith, K. F. (2022). The public and private dialogue about the American family on television: A second look. Journal of Media Communication, 50 (4), 79-110. https://doi.org/10.1152/j.1460-2466.2000.tb02864.x
Note: The DOI number is formatted as a URL: https://doi.org/10.1152/j.1460-2466.2000.tb02864.xIf
In-Text Paraphrase:
(Author's Last Name, Year)
Example: (Smith, 2000)
In-Text Quote:
(Author's Last Name, Year, p. Page Number)
Example: (Smith, 2000, p. 80)
Author's Last Name, First Initial. Second Initial if Given., & Last Name of Second Author, First Initial. Second Initial if Given. (Year of Publication). Title of article: Subtitle if any. Name of Journal, Volume Number (Issue Number), first page number-last page number. https://doi.org/doi number
Note: Separate the authors' names by putting a comma between them. For the final author listed add an ampersand (&) after the comma and before the final author's last name.
Note: In the reference list invert all authors' names; give last names and initials for only up to and including 20 authors. When a source has 21 or more authors, include the first 19 authors’ names, then three ellipses (…), and add the last author’s name. Don't include an ampersand (&) between the ellipsis and final author.
Note : For works with three or more authors, the first in-text citation is shortened to include the first author's surname followed by "et al."
Reference List Examples
Two to 20 Authors
Case, T. A., Daristotle, Y. A., Hayek, S. L., Smith, R. R., & Raash, L. I. (2011). College students' social networking experiences on Facebook. Journal of Applied Developmental Psychology, 3 (2), 227-238. https://doi.org/10.1016/j.appdev.2008.12.010
21 or more authors
Kalnay, E., Kanamitsu, M., Kistler, R., Collins, W., Deaven, D., Gandin, L., Iredell, M., Saha, J., Mo, K. C., Ropelewski, C., Wang, J., Leetma, A., . . . Joseph, D. (1996). The NCEP/NCAR 40-year reanalysis project. Bulletin of the American Meteorological Society , 77 (3), 437-471. https://doi.org/10.1175/1520-0477(1996)077<0437:TNYRP>2.0.CO;2
In-Text Citations
Two Authors/Editors
(Case & Daristotle, 2011)
Direct Quote: (Case & Daristotle, 2011, p. 57)
Three or more Authors/Editors
(Case et al., 2011)
Direct Quote: (Case et al., 2011, p. 57)
Author's Last Name, First Initial. Second Initial if Given. (Year of Publication). Title of article: Subtitle if any. Name of Journal, Volume Number (Issue Number if given). URL
Flachs, A. (2010). Food for thought: The social impact of community gardens in the Greater Cleveland Area. Electronic Green Journal, 1 (30). http://escholarship.org/uc/item/6bh7j4z4
Example: (Flachs, 2010)
Example: (Flachs, 2010, Conclusion section, para. 3)
Note: In this example there were no visible page numbers or paragraph numbers; in this case you can cite the section heading and the number of the paragraph in that section to identify where your quote came from. If there are no page or paragraph numbers and no marked section, leave this information out.
Journal Article - No DOI
Author's Last Name, First Initial. Second Initial if Given. (Year of Publication). Title of article: Subtitle if any. Name of Journal, Volume Number (Issue Number), first page number-last page number. URL [if article is available online, not as part of a library database]
Full-Text Available Online (Not as Part of a Library Database):
Steinberg, M. P., & Lacoe, J. (2017). What do we know about school discipline reform? Assessing the alternatives to suspensions and expulsions. Education Next, 17 (1), 44–52. https://www.educationnext.org/what-do-we-know-about-school-discipline-reform-suspensions-expulsions/
Example: (Steinberg & Lacoe, 2017)
(Author's Last Name, Year, p. Page number)
Example: (Steinberg & Lacoe, 2017, p. 47)
Full-Text Available in Library Database:
Jungers, W. L. (2010). Biomechanics: Barefoot running strikes back. Nature, 463 (2), 433-434.
Example: (Jungers, 2010)
Example: (Jungers, 2010, p. 433)
- << Previous: How to Cite: Common Sources
- Next: Magazine/Newspaper Articles >>
- Last Updated: Apr 30, 2024 1:29 PM
- URL: https://libguides.up.edu/apa
- Library Guides

APA 7th Referencing
- Journal Articles
APA 7th Referencing: Journal Articles

- In-text referencing
- Compiling a Reference list
- Citing tables and figures
- DOIs and Live hyperlinks
- Secondary sources
- Reports & Grey Literature
- Conference Materials
- Datasets, Software & Tests
- Social Media
- Images, tables & figures
- Sound & video
- Legislation & Cases
- Personal Communications
- Standards & Patents
- Course Notes or Course Presentations
- Generative AI
- Sample Reference List
On this page
Basic format to reference journal articles.
- Referencing journal articles: Examples
APA Referencing: journal articles from Victoria University Library on Vimeo .
Select the 'cc' on the video to turn on/off the captions.
A basic reference list entry for a journal article in APA must include:
- Author or authors. The surname is followed by first initials.
- Year of publication of the article (in round brackets).
- Article title.
- Journal title (in italics ).
- Volume of journal (in italics ).
- Issue number of journal in round brackets (no italics).
- Page range of article.
- DOI or URL
- The first line of each citation is left adjusted. Every subsequent line is indented 5-7 spaces.
Example:
Ruxton, C. (2016). Tea: Hydration and other health benefits. Primary Health Care , 26 (8), 34-42. https://doi.org/10.7748/phc.2016.e1162
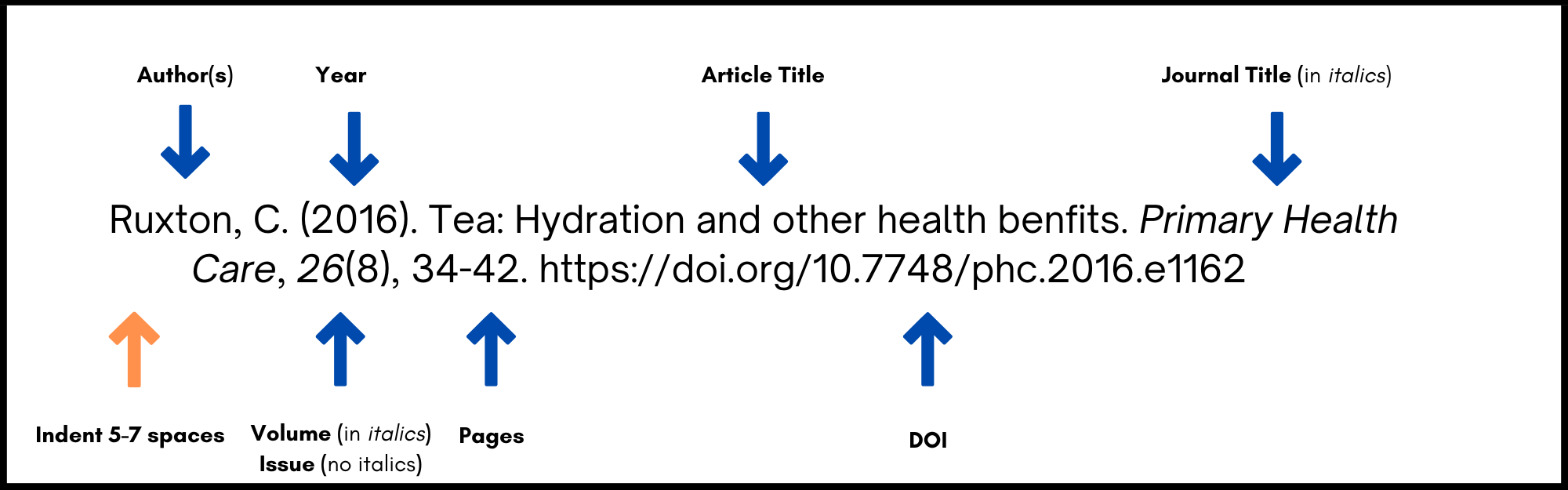
- << Previous: APA 7th Reference Formats and Examples
- Next: Books >>
- Last Updated: Apr 10, 2024 11:36 AM
- URL: https://libraryguides.vu.edu.au/apa-referencing
- Bipolar Disorder
- Therapy Center
- When To See a Therapist
- Types of Therapy
- Best Online Therapy
- Best Couples Therapy
- Best Family Therapy
- Managing Stress
- Sleep and Dreaming
- Understanding Emotions
- Self-Improvement
- Healthy Relationships
- Student Resources
- Personality Types
- Guided Meditations
- Verywell Mind Insights
- 2024 Verywell Mind 25
- Mental Health in the Classroom
- Editorial Process
- Meet Our Review Board
- Crisis Support
How to Reference Articles in APA Format
Kendra Cherry, MS, is a psychosocial rehabilitation specialist, psychology educator, and author of the "Everything Psychology Book."
:max_bytes(150000):strip_icc():format(webp)/IMG_9791-89504ab694d54b66bbd72cb84ffb860e.jpg)
Emily is a board-certified science editor who has worked with top digital publishing brands like Voices for Biodiversity, Study.com, GoodTherapy, Vox, and Verywell.
:max_bytes(150000):strip_icc():format(webp)/Emily-Swaim-1000-0f3197de18f74329aeffb690a177160c.jpg)
If you write a psychology paper, you will need to reference several sources in APA format. Journal articles are often used and cited to summarize the results of studies and experiments conducted by researchers. In most cases, you will need to create references for at least five or more journal articles for every APA format paper you write.
APA format details a set of strict rules for referencing articles that appear in academic journals and other periodicals. These vary somewhat based on where the article appears and who the authors are. While most articles you will use in your paper appear in academic and professional journals, you may also find articles in magazines, newspapers, and online publications.
Keep reading for a breakdown of the rules for creating references in APA format.
Basic Structure for Journal Article References
Begin the reference with the author's last name and first initials, followed by the date of publication in parentheses. Provide the title of the article, but only capitalize the first letter of the title. Next, include the journal or periodical and volume number in italics, followed by the issue number in parentheses. Finally, provide the page numbers where the article can be found.
Author, I. N. (Year). Title of the article. Title of the Journal or Periodical, volume number (issue number), page numbers.
Smith, L. V. (2000). Referencing articles in APA format. APA Format Weekly, 34 (1), 4-10.
If possible, include the DOI (digital object identifier) number at the end of your reference. If a DOI number is not available and you accessed the article online, give the URL of the journal's home page.
Formatting Rules
- The title , subtitle and all proper nouns should be capitalized.
- Your reference page should be double-spaced.
- The first line of each reference should be flush left and remaining lines should be indented
Be sure to check your references using the official Publication Manual of the American Psychological Association. See an example of different types of references and learn more about APA format .
Magazine Articles
The structure of a reference for an article appearing in a magazine is similar to that of a journal article. However, be sure to add the month and day of publication to the publication date.
James, S. A. (2001, June 7). Magazine articles in APA format. Newsweek, 20, 48-52.
Newspaper Articles
References for newspaper articles follow the basic structure as magazines, but you should list each individual page the article appears on rather than a page range.
Tensky, J. A. (2004, January 5). How to cite newspaper articles. The New York Times, 4D, 5D.
Articles With Two Authors
If an article has two authors, follow the basic format for a journal reference. Place a comma after the first initial of the first author followed by an ampersand (&). Then, include the last name and first initial of the second author.
Mischel, W., & Baker, N. (1975). Cognitive transformations of reward objects through instructions. Journal of Personality and Social Psychology, 31 , 254-261.
Articles With Three to Twenty Authors
For journal articles with three to 20 authors, you will follow a similar format as with two authors but each author and their initials will be separated with a comma. The final author should be preceded by an ampersand. Follow this same format for each additional author up to 20 authors.
Hart, D., Keller, M., Edelstein, W., & Hofmann, V. (1998). Childhood personality influences on social-cognitive development: A longitudinal study. Journal of Personality and Social Psychology, 74, 1288-1289. doi:10.1037/0022-3514.74.5.1278
Keller, J. L., Smithfield, K. B., Ellis, M., Michelina, R., & Bels, S. (1987). The limitations of anchoring bias. J ournal of Market Research, 17 , 115-119.
Articles With More Than Twenty Authors
The rules for referencing both single and multiple authors apply to all sources, whether the material came from books, magazine articles, newspaper articles, journal articles, or online sources. Include the last name and first initials of each author, with individuals' names separated by a comma. The last author should be preceded with an ampersand.
If the article includes 20 or fewer authors, list each author separately. If there are more than 20, include the first 19 and then include an ellipse (. . . ) in place of the authors' names before listing the final author.
Arlo, A., Black, B., Clark, C., Davidson, D., Emerson, E., Fischer, F., Grahmann, G., Habib, H., Ianelli, I., Juarez, J., Kobayashi, K., Lee, L., Martin, M., Naim, N., Odelsson, O., Pierce, P., Qiang, Q., Reed, R., Scofield, S., . . . Thatcher, T. (2011). Even more references. APA Format Today, 11 (4), 30-38.
Articles With No Author
If an article does not cite any authors, start the reference with the title of the article. Follow this with the publication date, source, and URL if the article was accessed electronically.
Scientists seek source of creativity. (2012, March, 6). Dayton County News. http://www.daytoncountynews.com/news/39756_39275.html
The reference section is one of the easiest places to lose points due to incorrect APA format, so be sure to check your references before you hand in your psychology papers . While it may be tedioous, learning to reference articles in proper APA style will help you throughout your study of psychology.
American Psychological Association. Publication Manual of the American Psychological Association (7th ed.). The American Psychological Association, 2019.
By Kendra Cherry, MSEd Kendra Cherry, MS, is a psychosocial rehabilitation specialist, psychology educator, and author of the "Everything Psychology Book."
Purdue Online Writing Lab Purdue OWL® College of Liberal Arts
Reference List: Articles in Periodicals
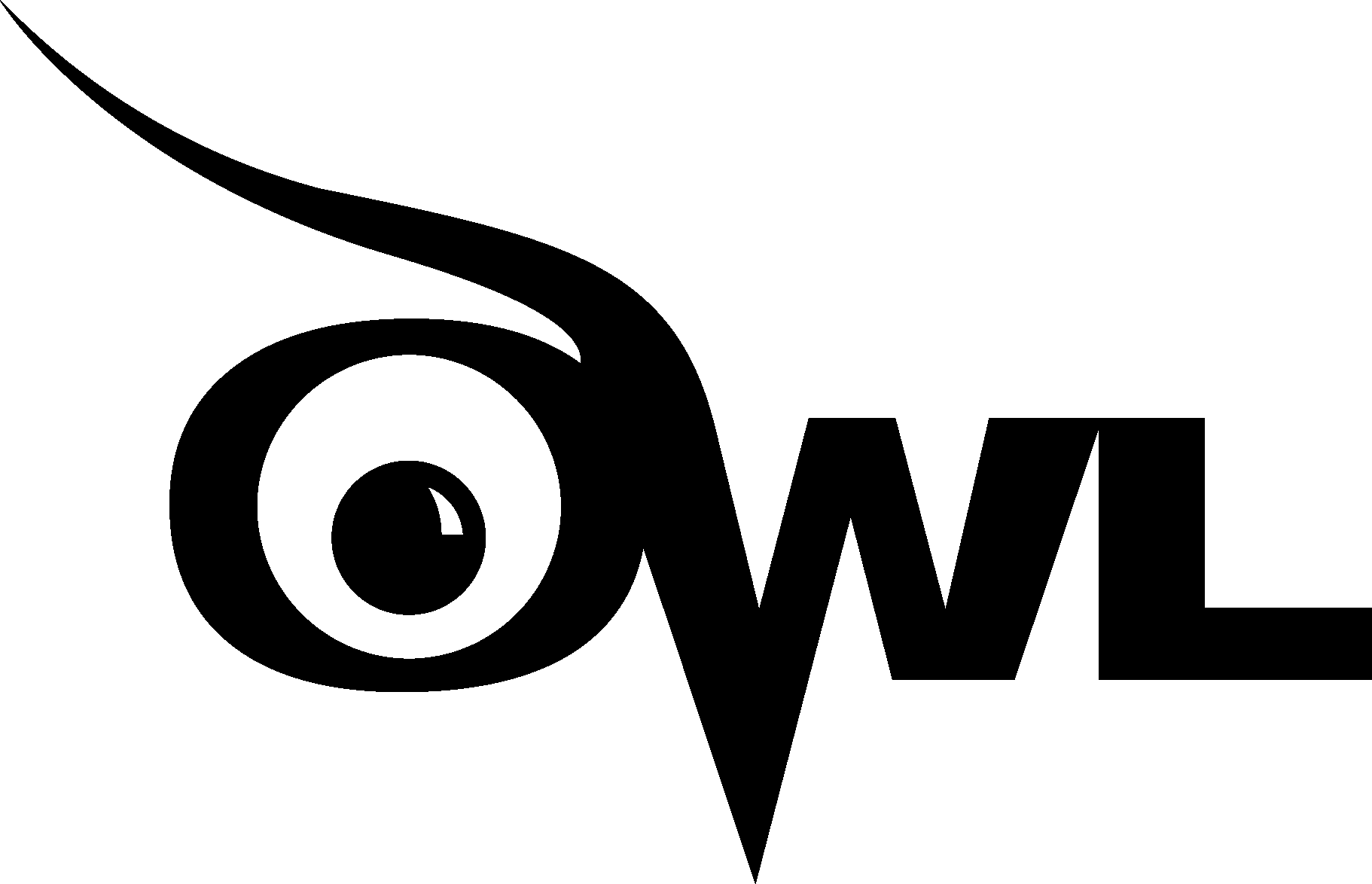
Welcome to the Purdue OWL
This page is brought to you by the OWL at Purdue University. When printing this page, you must include the entire legal notice.
Copyright ©1995-2018 by The Writing Lab & The OWL at Purdue and Purdue University. All rights reserved. This material may not be published, reproduced, broadcast, rewritten, or redistributed without permission. Use of this site constitutes acceptance of our terms and conditions of fair use.
Note: This page reflects the latest version of the APA Publication Manual (i.e., APA 7), which released in October 2019. The equivalent resource for the older APA 6 style can be found here .
Please note: the following contains a list of the most commonly cited periodical sources. For a complete list of how to cite periodical publications, please refer to the 7 th edition of the APA Publication Manual.
APA style dictates that authors are named with their last name followed by their initials; publication year goes between parentheses, followed by a period. The title of the article is in sentence-case, meaning only the first word and proper nouns in the title are capitalized. The periodical title is run in title case, and is followed by the volume number which, with the title, is also italicized. If a DOI has been assigned to the article that you are using, you should include this after the page numbers for the article. If no DOI has been assigned and you are accessing the periodical online, use the URL of the website from which you are retrieving the periodical.
Author, A. A., Author, B. B., & Author, C. C. (Year). Title of article. Title of Periodical , volume number (issue number), pages. https://doi.org/xx.xxx/yyyy
Article in Print Journal
Scruton, R. (1996). The eclipse of listening. The New Criterion, 15 (3), 5 – 13.
Note: APA 7 advises writers to include a DOI (if available), even when using the print source. The example above assumes no DOI is available.
Article in Electronic Journal
As noted above, when citing an article in an electronic journal, include a DOI if one is associated with the article.
Baniya, S., & Weech, S. (2019). Data and experience design: Negotiating community-oriented digital research with service-learning. Purdue Journal of Service-Learning and International Engagement , 6 (1), 11 – 16. https://doi.org/10.5703/1288284316979
DOIs may not always be available. In these cases, use a URL. Many academic journals provide stable URLs that function similarly to DOIs. These are preferable to ordinary URLs copied and pasted from the browser's address bar.
Denny, H., Nordlof, J., & Salem, L. (2018). "Tell me exactly what it was that I was doing that was so bad": Understanding the needs and expectations of working-class students in writing centers. Writing Center Journal , 37 (1), 67 – 98. https://www.jstor.org/stable/26537363
Note that, in the example above, there is a quotation in the title of the article. Ordinary titles lack quotation marks.
Article in a Magazine
Peterzell, J. (1990, April). Better late than never. Time, 135 (17), 20 –2 1.
Article in a Newspaper
Schultz, S. (2005, December 28). Calls made to strengthen state energy policies. The Country Today , 1A, 2A.
Baumeister, R. F. (1993). Exposing the self-knowledge myth [Review of the book The self-knower: A hero under control , by R. A. Wicklund & M. Eckert]. Contemporary Psychology , 38 (5), 466–467.
Have a language expert improve your writing
Run a free plagiarism check in 10 minutes, automatically generate references for free.
- Knowledge Base
- Referencing
A Quick Guide to Harvard Referencing | Citation Examples
Published on 14 February 2020 by Jack Caulfield . Revised on 15 September 2023.
Referencing is an important part of academic writing. It tells your readers what sources you’ve used and how to find them.
Harvard is the most common referencing style used in UK universities. In Harvard style, the author and year are cited in-text, and full details of the source are given in a reference list .
Harvard Reference Generator
Instantly correct all language mistakes in your text
Be assured that you'll submit flawless writing. Upload your document to correct all your mistakes.
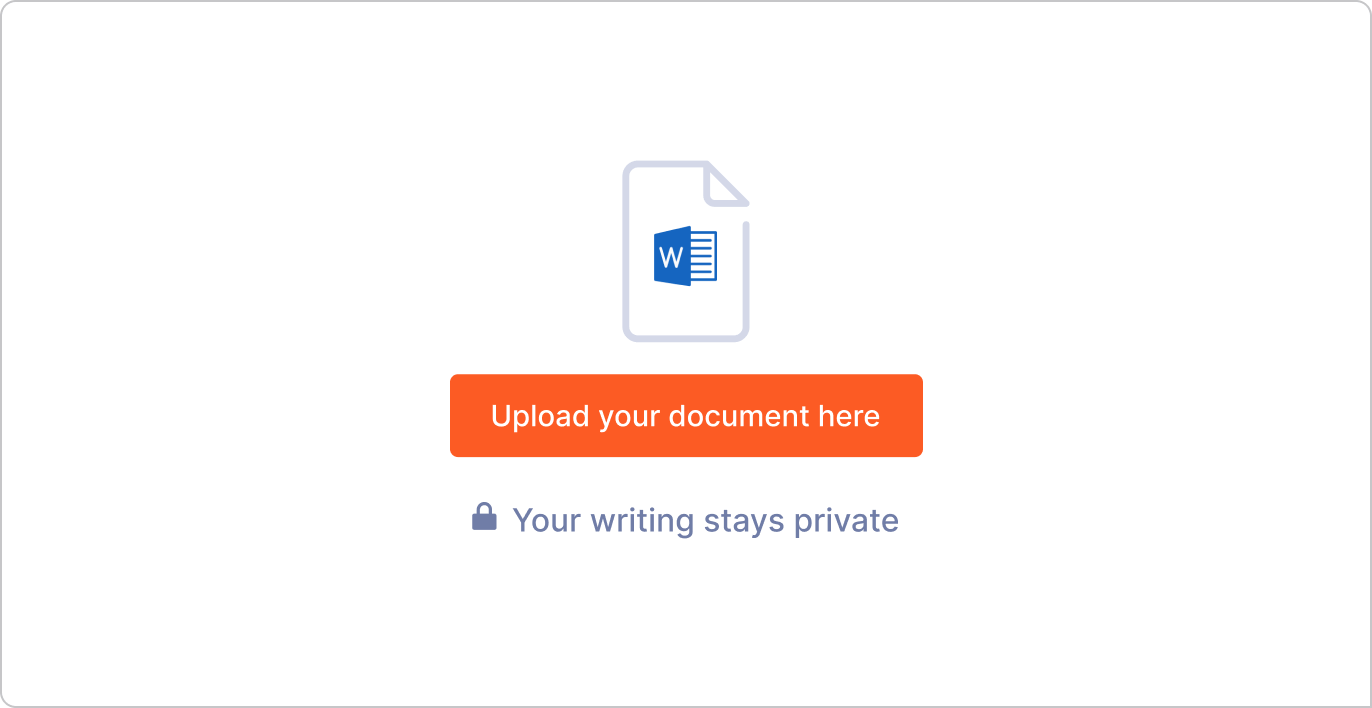
Table of contents
Harvard in-text citation, creating a harvard reference list, harvard referencing examples, referencing sources with no author or date, frequently asked questions about harvard referencing.
A Harvard in-text citation appears in brackets beside any quotation or paraphrase of a source. It gives the last name of the author(s) and the year of publication, as well as a page number or range locating the passage referenced, if applicable:
Note that ‘p.’ is used for a single page, ‘pp.’ for multiple pages (e.g. ‘pp. 1–5’).
An in-text citation usually appears immediately after the quotation or paraphrase in question. It may also appear at the end of the relevant sentence, as long as it’s clear what it refers to.
When your sentence already mentions the name of the author, it should not be repeated in the citation:
Sources with multiple authors
When you cite a source with up to three authors, cite all authors’ names. For four or more authors, list only the first name, followed by ‘ et al. ’:
Sources with no page numbers
Some sources, such as websites , often don’t have page numbers. If the source is a short text, you can simply leave out the page number. With longer sources, you can use an alternate locator such as a subheading or paragraph number if you need to specify where to find the quote:
Multiple citations at the same point
When you need multiple citations to appear at the same point in your text – for example, when you refer to several sources with one phrase – you can present them in the same set of brackets, separated by semicolons. List them in order of publication date:
Multiple sources with the same author and date
If you cite multiple sources by the same author which were published in the same year, it’s important to distinguish between them in your citations. To do this, insert an ‘a’ after the year in the first one you reference, a ‘b’ in the second, and so on:
Prevent plagiarism, run a free check.
A bibliography or reference list appears at the end of your text. It lists all your sources in alphabetical order by the author’s last name, giving complete information so that the reader can look them up if necessary.
The reference entry starts with the author’s last name followed by initial(s). Only the first word of the title is capitalised (as well as any proper nouns).
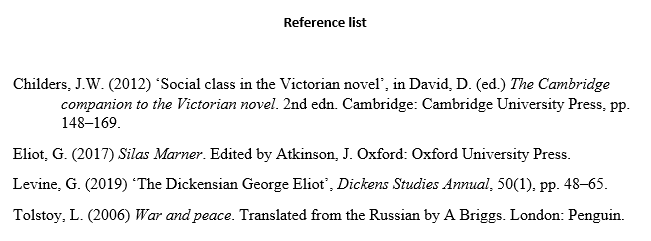
Sources with multiple authors in the reference list
As with in-text citations, up to three authors should be listed; when there are four or more, list only the first author followed by ‘ et al. ’:
Reference list entries vary according to source type, since different information is relevant for different sources. Formats and examples for the most commonly used source types are given below.
- Entire book
- Book chapter
- Translated book
- Edition of a book
Journal articles
- Print journal
- Online-only journal with DOI
- Online-only journal with no DOI
- General web page
- Online article or blog
- Social media post
Sometimes you won’t have all the information you need for a reference. This section covers what to do when a source lacks a publication date or named author.
No publication date
When a source doesn’t have a clear publication date – for example, a constantly updated reference source like Wikipedia or an obscure historical document which can’t be accurately dated – you can replace it with the words ‘no date’:
Note that when you do this with an online source, you should still include an access date, as in the example.
When a source lacks a clearly identified author, there’s often an appropriate corporate source – the organisation responsible for the source – whom you can credit as author instead, as in the Google and Wikipedia examples above.
When that’s not the case, you can just replace it with the title of the source in both the in-text citation and the reference list:
Harvard referencing uses an author–date system. Sources are cited by the author’s last name and the publication year in brackets. Each Harvard in-text citation corresponds to an entry in the alphabetised reference list at the end of the paper.
Vancouver referencing uses a numerical system. Sources are cited by a number in parentheses or superscript. Each number corresponds to a full reference at the end of the paper.
A Harvard in-text citation should appear in brackets every time you quote, paraphrase, or refer to information from a source.
The citation can appear immediately after the quotation or paraphrase, or at the end of the sentence. If you’re quoting, place the citation outside of the quotation marks but before any other punctuation like a comma or full stop.
In Harvard referencing, up to three author names are included in an in-text citation or reference list entry. When there are four or more authors, include only the first, followed by ‘ et al. ’
Though the terms are sometimes used interchangeably, there is a difference in meaning:
- A reference list only includes sources cited in the text – every entry corresponds to an in-text citation .
- A bibliography also includes other sources which were consulted during the research but not cited.
Cite this Scribbr article
If you want to cite this source, you can copy and paste the citation or click the ‘Cite this Scribbr article’ button to automatically add the citation to our free Reference Generator.
Caulfield, J. (2023, September 15). A Quick Guide to Harvard Referencing | Citation Examples. Scribbr. Retrieved 21 May 2024, from https://www.scribbr.co.uk/referencing/harvard-style/
Is this article helpful?
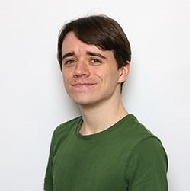
Jack Caulfield
Other students also liked, harvard in-text citation | a complete guide & examples, harvard style bibliography | format & examples, referencing books in harvard style | templates & examples, scribbr apa citation checker.
An innovative new tool that checks your APA citations with AI software. Say goodbye to inaccurate citations!
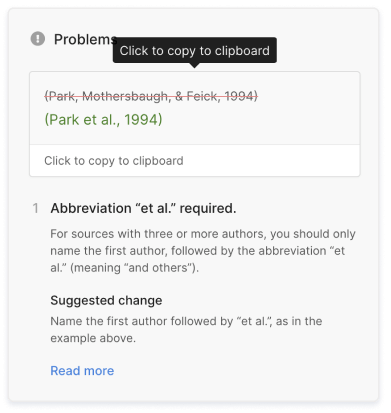
- Privacy Policy

Home » References in Research – Types, Examples and Writing Guide
References in Research – Types, Examples and Writing Guide
Table of Contents

References in Research
Definition:
References in research are a list of sources that a researcher has consulted or cited while conducting their study. They are an essential component of any academic work, including research papers, theses, dissertations, and other scholarly publications.
Types of References
There are several types of references used in research, and the type of reference depends on the source of information being cited. The most common types of references include:
References to books typically include the author’s name, title of the book, publisher, publication date, and place of publication.
Example: Smith, J. (2018). The Art of Writing. Penguin Books.
Journal Articles
References to journal articles usually include the author’s name, title of the article, name of the journal, volume and issue number, page numbers, and publication date.
Example: Johnson, T. (2021). The Impact of Social Media on Mental Health. Journal of Psychology, 32(4), 87-94.
Web sources
References to web sources should include the author or organization responsible for the content, the title of the page, the URL, and the date accessed.
Example: World Health Organization. (2020). Coronavirus disease (COVID-19) advice for the public. Retrieved from https://www.who.int/emergencies/disease/novel-coronavirus-2019/advice-for-public
Conference Proceedings
References to conference proceedings should include the author’s name, title of the paper, name of the conference, location of the conference, date of the conference, and page numbers.
Example: Chen, S., & Li, J. (2019). The Future of AI in Education. Proceedings of the International Conference on Educational Technology, Beijing, China, July 15-17, pp. 67-78.
References to reports typically include the author or organization responsible for the report, title of the report, publication date, and publisher.
Example: United Nations. (2020). The Sustainable Development Goals Report. United Nations.
Formats of References
Some common Formates of References with their examples are as follows:
APA (American Psychological Association) Style
The APA (American Psychological Association) Style has specific guidelines for formatting references used in academic papers, articles, and books. Here are the different reference formats in APA style with examples:
Author, A. A. (Year of publication). Title of book. Publisher.
Example : Smith, J. K. (2005). The psychology of social interaction. Wiley-Blackwell.
Journal Article
Author, A. A., Author, B. B., & Author, C. C. (Year of publication). Title of article. Title of Journal, volume number(issue number), page numbers.
Example : Brown, L. M., Keating, J. G., & Jones, S. M. (2012). The role of social support in coping with stress among African American adolescents. Journal of Research on Adolescence, 22(1), 218-233.
Author, A. A. (Year of publication or last update). Title of page. Website name. URL.
Example : Centers for Disease Control and Prevention. (2020, December 11). COVID-19: How to protect yourself and others. https://www.cdc.gov/coronavirus/2019-ncov/prevent-getting-sick/prevention.html
Magazine article
Author, A. A. (Year, Month Day of publication). Title of article. Title of Magazine, volume number(issue number), page numbers.
Example : Smith, M. (2019, March 11). The power of positive thinking. Psychology Today, 52(3), 60-65.
Newspaper article:
Author, A. A. (Year, Month Day of publication). Title of article. Title of Newspaper, page numbers.
Example: Johnson, B. (2021, February 15). New study shows benefits of exercise on mental health. The New York Times, A8.
Edited book
Editor, E. E. (Ed.). (Year of publication). Title of book. Publisher.
Example : Thompson, J. P. (Ed.). (2014). Social work in the 21st century. Sage Publications.
Chapter in an edited book:
Author, A. A. (Year of publication). Title of chapter. In E. E. Editor (Ed.), Title of book (pp. page numbers). Publisher.
Example : Johnson, K. S. (2018). The future of social work: Challenges and opportunities. In J. P. Thompson (Ed.), Social work in the 21st century (pp. 105-118). Sage Publications.
MLA (Modern Language Association) Style
The MLA (Modern Language Association) Style is a widely used style for writing academic papers and essays in the humanities. Here are the different reference formats in MLA style:
Author’s Last name, First name. Title of Book. Publisher, Publication year.
Example : Smith, John. The Psychology of Social Interaction. Wiley-Blackwell, 2005.
Journal article
Author’s Last name, First name. “Title of Article.” Title of Journal, volume number, issue number, Publication year, page numbers.
Example : Brown, Laura M., et al. “The Role of Social Support in Coping with Stress among African American Adolescents.” Journal of Research on Adolescence, vol. 22, no. 1, 2012, pp. 218-233.
Author’s Last name, First name. “Title of Webpage.” Website Name, Publication date, URL.
Example : Centers for Disease Control and Prevention. “COVID-19: How to Protect Yourself and Others.” CDC, 11 Dec. 2020, https://www.cdc.gov/coronavirus/2019-ncov/prevent-getting-sick/prevention.html.
Author’s Last name, First name. “Title of Article.” Title of Magazine, Publication date, page numbers.
Example : Smith, Mary. “The Power of Positive Thinking.” Psychology Today, Mar. 2019, pp. 60-65.
Newspaper article
Author’s Last name, First name. “Title of Article.” Title of Newspaper, Publication date, page numbers.
Example : Johnson, Bob. “New Study Shows Benefits of Exercise on Mental Health.” The New York Times, 15 Feb. 2021, p. A8.
Editor’s Last name, First name, editor. Title of Book. Publisher, Publication year.
Example : Thompson, John P., editor. Social Work in the 21st Century. Sage Publications, 2014.
Chapter in an edited book
Author’s Last name, First name. “Title of Chapter.” Title of Book, edited by Editor’s First Name Last name, Publisher, Publication year, page numbers.
Example : Johnson, Karen S. “The Future of Social Work: Challenges and Opportunities.” Social Work in the 21st Century, edited by John P. Thompson, Sage Publications, 2014, pp. 105-118.
Chicago Manual of Style
The Chicago Manual of Style is a widely used style for writing academic papers, dissertations, and books in the humanities and social sciences. Here are the different reference formats in Chicago style:
Example : Smith, John K. The Psychology of Social Interaction. Wiley-Blackwell, 2005.
Author’s Last name, First name. “Title of Article.” Title of Journal volume number, no. issue number (Publication year): page numbers.
Example : Brown, Laura M., John G. Keating, and Sarah M. Jones. “The Role of Social Support in Coping with Stress among African American Adolescents.” Journal of Research on Adolescence 22, no. 1 (2012): 218-233.
Author’s Last name, First name. “Title of Webpage.” Website Name. Publication date. URL.
Example : Centers for Disease Control and Prevention. “COVID-19: How to Protect Yourself and Others.” CDC. December 11, 2020. https://www.cdc.gov/coronavirus/2019-ncov/prevent-getting-sick/prevention.html.
Author’s Last name, First name. “Title of Article.” Title of Magazine, Publication date.
Example : Smith, Mary. “The Power of Positive Thinking.” Psychology Today, March 2019.
Author’s Last name, First name. “Title of Article.” Title of Newspaper, Publication date.
Example : Johnson, Bob. “New Study Shows Benefits of Exercise on Mental Health.” The New York Times, February 15, 2021.
Example : Thompson, John P., ed. Social Work in the 21st Century. Sage Publications, 2014.
Author’s Last name, First name. “Title of Chapter.” In Title of Book, edited by Editor’s First Name Last Name, page numbers. Publisher, Publication year.
Example : Johnson, Karen S. “The Future of Social Work: Challenges and Opportunities.” In Social Work in the 21st Century, edited by John P. Thompson, 105-118. Sage Publications, 2014.
Harvard Style
The Harvard Style, also known as the Author-Date System, is a widely used style for writing academic papers and essays in the social sciences. Here are the different reference formats in Harvard Style:
Author’s Last name, First name. Year of publication. Title of Book. Place of publication: Publisher.
Example : Smith, John. 2005. The Psychology of Social Interaction. Oxford: Wiley-Blackwell.
Author’s Last name, First name. Year of publication. “Title of Article.” Title of Journal volume number (issue number): page numbers.
Example: Brown, Laura M., John G. Keating, and Sarah M. Jones. 2012. “The Role of Social Support in Coping with Stress among African American Adolescents.” Journal of Research on Adolescence 22 (1): 218-233.
Author’s Last name, First name. Year of publication. “Title of Webpage.” Website Name. URL. Accessed date.
Example : Centers for Disease Control and Prevention. 2020. “COVID-19: How to Protect Yourself and Others.” CDC. https://www.cdc.gov/coronavirus/2019-ncov/prevent-getting-sick/prevention.html. Accessed April 1, 2023.
Author’s Last name, First name. Year of publication. “Title of Article.” Title of Magazine, month and date of publication.
Example : Smith, Mary. 2019. “The Power of Positive Thinking.” Psychology Today, March 2019.
Author’s Last name, First name. Year of publication. “Title of Article.” Title of Newspaper, month and date of publication.
Example : Johnson, Bob. 2021. “New Study Shows Benefits of Exercise on Mental Health.” The New York Times, February 15, 2021.
Editor’s Last name, First name, ed. Year of publication. Title of Book. Place of publication: Publisher.
Example : Thompson, John P., ed. 2014. Social Work in the 21st Century. Thousand Oaks, CA: Sage Publications.
Author’s Last name, First name. Year of publication. “Title of Chapter.” In Title of Book, edited by Editor’s First Name Last Name, page numbers. Place of publication: Publisher.
Example : Johnson, Karen S. 2014. “The Future of Social Work: Challenges and Opportunities.” In Social Work in the 21st Century, edited by John P. Thompson, 105-118. Thousand Oaks, CA: Sage Publications.
Vancouver Style
The Vancouver Style, also known as the Uniform Requirements for Manuscripts Submitted to Biomedical Journals, is a widely used style for writing academic papers in the biomedical sciences. Here are the different reference formats in Vancouver Style:
Author’s Last name, First name. Title of Book. Edition number. Place of publication: Publisher; Year of publication.
Example : Smith, John K. The Psychology of Social Interaction. 2nd ed. Oxford: Wiley-Blackwell; 2005.
Author’s Last name, First name. Title of Article. Abbreviated Journal Title. Year of publication; volume number(issue number):page numbers.
Example : Brown LM, Keating JG, Jones SM. The Role of Social Support in Coping with Stress among African American Adolescents. J Res Adolesc. 2012;22(1):218-233.
Author’s Last name, First name. Title of Webpage. Website Name [Internet]. Publication date. [cited date]. Available from: URL.
Example : Centers for Disease Control and Prevention. COVID-19: How to Protect Yourself and Others [Internet]. 2020 Dec 11. [cited 2023 Apr 1]. Available from: https://www.cdc.gov/coronavirus/2019-ncov/prevent-getting-sick/prevention.html.
Author’s Last name, First name. Title of Article. Title of Magazine. Year of publication; month and day of publication:page numbers.
Example : Smith M. The Power of Positive Thinking. Psychology Today. 2019 Mar 1:32-35.
Author’s Last name, First name. Title of Article. Title of Newspaper. Year of publication; month and day of publication:page numbers.
Example : Johnson B. New Study Shows Benefits of Exercise on Mental Health. The New York Times. 2021 Feb 15:A4.
Editor’s Last name, First name, editor. Title of Book. Edition number. Place of publication: Publisher; Year of publication.
Example: Thompson JP, editor. Social Work in the 21st Century. 1st ed. Thousand Oaks, CA: Sage Publications; 2014.
Author’s Last name, First name. Title of Chapter. In: Editor’s Last name, First name, editor. Title of Book. Edition number. Place of publication: Publisher; Year of publication. page numbers.
Example : Johnson KS. The Future of Social Work: Challenges and Opportunities. In: Thompson JP, editor. Social Work in the 21st Century. 1st ed. Thousand Oaks, CA: Sage Publications; 2014. p. 105-118.
Turabian Style
Turabian style is a variation of the Chicago style used in academic writing, particularly in the fields of history and humanities. Here are the different reference formats in Turabian style:
Author’s Last name, First name. Title of Book. Place of publication: Publisher, Year of publication.
Example : Smith, John K. The Psychology of Social Interaction. Oxford: Wiley-Blackwell, 2005.
Author’s Last name, First name. “Title of Article.” Title of Journal volume number, no. issue number (Year of publication): page numbers.
Example : Brown, LM, Keating, JG, Jones, SM. “The Role of Social Support in Coping with Stress among African American Adolescents.” J Res Adolesc 22, no. 1 (2012): 218-233.
Author’s Last name, First name. “Title of Webpage.” Name of Website. Publication date. Accessed date. URL.
Example : Centers for Disease Control and Prevention. “COVID-19: How to Protect Yourself and Others.” CDC. December 11, 2020. Accessed April 1, 2023. https://www.cdc.gov/coronavirus/2019-ncov/prevent-getting-sick/prevention.html.
Author’s Last name, First name. “Title of Article.” Title of Magazine, Month Day, Year of publication, page numbers.
Example : Smith, M. “The Power of Positive Thinking.” Psychology Today, March 1, 2019, 32-35.
Author’s Last name, First name. “Title of Article.” Title of Newspaper, Month Day, Year of publication.
Example : Johnson, B. “New Study Shows Benefits of Exercise on Mental Health.” The New York Times, February 15, 2021.
Editor’s Last name, First name, ed. Title of Book. Place of publication: Publisher, Year of publication.
Example : Thompson, JP, ed. Social Work in the 21st Century. Thousand Oaks, CA: Sage Publications, 2014.
Author’s Last name, First name. “Title of Chapter.” In Title of Book, edited by Editor’s Last name, First name, page numbers. Place of publication: Publisher, Year of publication.
Example : Johnson, KS. “The Future of Social Work: Challenges and Opportunities.” In Social Work in the 21st Century, edited by Thompson, JP, 105-118. Thousand Oaks, CA: Sage Publications, 2014.
IEEE (Institute of Electrical and Electronics Engineers) Style
IEEE (Institute of Electrical and Electronics Engineers) style is commonly used in engineering, computer science, and other technical fields. Here are the different reference formats in IEEE style:
Author’s Last name, First name. Book Title. Place of Publication: Publisher, Year of publication.
Example : Oppenheim, A. V., & Schafer, R. W. Discrete-Time Signal Processing. Upper Saddle River, NJ: Prentice Hall, 2010.
Author’s Last name, First name. “Title of Article.” Abbreviated Journal Title, vol. number, no. issue number, pp. page numbers, Month year of publication.
Example: Shannon, C. E. “A Mathematical Theory of Communication.” Bell System Technical Journal, vol. 27, no. 3, pp. 379-423, July 1948.
Conference paper
Author’s Last name, First name. “Title of Paper.” In Title of Conference Proceedings, Place of Conference, Date of Conference, pp. page numbers, Year of publication.
Example: Gupta, S., & Kumar, P. “An Improved System of Linear Discriminant Analysis for Face Recognition.” In Proceedings of the 2011 International Conference on Computer Science and Network Technology, Harbin, China, Dec. 2011, pp. 144-147.
Author’s Last name, First name. “Title of Webpage.” Name of Website. Date of publication or last update. Accessed date. URL.
Example : National Aeronautics and Space Administration. “Apollo 11.” NASA. July 20, 1969. Accessed April 1, 2023. https://www.nasa.gov/mission_pages/apollo/apollo11.html.
Technical report
Author’s Last name, First name. “Title of Report.” Name of Institution or Organization, Report number, Year of publication.
Example : Smith, J. R. “Development of a New Solar Panel Technology.” National Renewable Energy Laboratory, NREL/TP-6A20-51645, 2011.
Author’s Last name, First name. “Title of Patent.” Patent number, Issue date.
Example : Suzuki, H. “Method of Producing Carbon Nanotubes.” US Patent 7,151,019, December 19, 2006.
Standard Title. Standard number, Publication date.
Example : IEEE Standard for Floating-Point Arithmetic. IEEE Std 754-2008, August 29, 2008
ACS (American Chemical Society) Style
ACS (American Chemical Society) style is commonly used in chemistry and related fields. Here are the different reference formats in ACS style:
Author’s Last name, First name; Author’s Last name, First name. Title of Article. Abbreviated Journal Title Year, Volume, Page Numbers.
Example : Wang, Y.; Zhao, X.; Cui, Y.; Ma, Y. Facile Preparation of Fe3O4/graphene Composites Using a Hydrothermal Method for High-Performance Lithium Ion Batteries. ACS Appl. Mater. Interfaces 2012, 4, 2715-2721.
Author’s Last name, First name. Book Title; Publisher: Place of Publication, Year of Publication.
Example : Carey, F. A. Organic Chemistry; McGraw-Hill: New York, 2008.
Author’s Last name, First name. Chapter Title. In Book Title; Editor’s Last name, First name, Ed.; Publisher: Place of Publication, Year of Publication; Volume number, Chapter number, Page Numbers.
Example : Grossman, R. B. Analytical Chemistry of Aerosols. In Aerosol Measurement: Principles, Techniques, and Applications; Baron, P. A.; Willeke, K., Eds.; Wiley-Interscience: New York, 2001; Chapter 10, pp 395-424.
Author’s Last name, First name. Title of Webpage. Website Name, URL (accessed date).
Example : National Institute of Standards and Technology. Atomic Spectra Database. https://www.nist.gov/pml/atomic-spectra-database (accessed April 1, 2023).
Author’s Last name, First name. Patent Number. Patent Date.
Example : Liu, Y.; Huang, H.; Chen, H.; Zhang, W. US Patent 9,999,999, December 31, 2022.
Author’s Last name, First name; Author’s Last name, First name. Title of Article. In Title of Conference Proceedings, Publisher: Place of Publication, Year of Publication; Volume Number, Page Numbers.
Example : Jia, H.; Xu, S.; Wu, Y.; Wu, Z.; Tang, Y.; Huang, X. Fast Adsorption of Organic Pollutants by Graphene Oxide. In Proceedings of the 15th International Conference on Environmental Science and Technology, American Chemical Society: Washington, DC, 2017; Volume 1, pp 223-228.
AMA (American Medical Association) Style
AMA (American Medical Association) style is commonly used in medical and scientific fields. Here are the different reference formats in AMA style:
Author’s Last name, First name. Article Title. Journal Abbreviation. Year; Volume(Issue):Page Numbers.
Example : Jones, R. A.; Smith, B. C. The Role of Vitamin D in Maintaining Bone Health. JAMA. 2019;321(17):1765-1773.
Author’s Last name, First name. Book Title. Edition number. Place of Publication: Publisher; Year.
Example : Guyton, A. C.; Hall, J. E. Textbook of Medical Physiology. 13th ed. Philadelphia, PA: Saunders; 2015.
Author’s Last name, First name. Chapter Title. In: Editor’s Last name, First name, ed. Book Title. Edition number. Place of Publication: Publisher; Year: Page Numbers.
Example: Rajakumar, K. Vitamin D and Bone Health. In: Holick, M. F., ed. Vitamin D: Physiology, Molecular Biology, and Clinical Applications. 2nd ed. New York, NY: Springer; 2010:211-222.
Author’s Last name, First name. Webpage Title. Website Name. URL. Published date. Updated date. Accessed date.
Example : National Cancer Institute. Breast Cancer Prevention (PDQ®)–Patient Version. National Cancer Institute. https://www.cancer.gov/types/breast/patient/breast-prevention-pdq. Published October 11, 2022. Accessed April 1, 2023.
Author’s Last name, First name. Conference presentation title. In: Conference Title; Conference Date; Place of Conference.
Example : Smith, J. R. Vitamin D and Bone Health: A Meta-Analysis. In: Proceedings of the Annual Meeting of the American Society for Bone and Mineral Research; September 20-23, 2022; San Diego, CA.
Thesis or dissertation
Author’s Last name, First name. Title of Thesis or Dissertation. Degree level [Doctoral dissertation or Master’s thesis]. University Name; Year.
Example : Wilson, S. A. The Effects of Vitamin D Supplementation on Bone Health in Postmenopausal Women [Doctoral dissertation]. University of California, Los Angeles; 2018.
ASCE (American Society of Civil Engineers) Style
The ASCE (American Society of Civil Engineers) style is commonly used in civil engineering fields. Here are the different reference formats in ASCE style:
Author’s Last name, First name. “Article Title.” Journal Title, volume number, issue number (year): page numbers. DOI or URL (if available).
Example : Smith, J. R. “Evaluation of the Effectiveness of Sustainable Drainage Systems in Urban Areas.” Journal of Environmental Engineering, vol. 146, no. 3 (2020): 04020010. https://doi.org/10.1061/(ASCE)EE.1943-7870.0001668.
Example : McCuen, R. H. Hydrologic Analysis and Design. 4th ed. Upper Saddle River, NJ: Pearson Education; 2013.
Author’s Last name, First name. “Chapter Title.” In: Editor’s Last name, First name, ed. Book Title. Edition number. Place of Publication: Publisher; Year: page numbers.
Example : Maidment, D. R. “Floodplain Management in the United States.” In: Shroder, J. F., ed. Treatise on Geomorphology. San Diego, CA: Academic Press; 2013: 447-460.
Author’s Last name, First name. “Paper Title.” In: Conference Title; Conference Date; Location. Place of Publication: Publisher; Year: page numbers.
Example: Smith, J. R. “Sustainable Drainage Systems for Urban Areas.” In: Proceedings of the ASCE International Conference on Sustainable Infrastructure; November 6-9, 2019; Los Angeles, CA. Reston, VA: American Society of Civil Engineers; 2019: 156-163.
Author’s Last name, First name. “Report Title.” Report number. Place of Publication: Publisher; Year.
Example : U.S. Army Corps of Engineers. “Hurricane Sandy Coastal Risk Reduction Program, New York and New Jersey.” Report No. P-15-001. Washington, DC: U.S. Army Corps of Engineers; 2015.
CSE (Council of Science Editors) Style
The CSE (Council of Science Editors) style is commonly used in the scientific and medical fields. Here are the different reference formats in CSE style:
Author’s Last name, First Initial. Middle Initial. “Article Title.” Journal Title. Year;Volume(Issue):Page numbers.
Example : Smith, J.R. “Evaluation of the Effectiveness of Sustainable Drainage Systems in Urban Areas.” Journal of Environmental Engineering. 2020;146(3):04020010.
Author’s Last name, First Initial. Middle Initial. Book Title. Edition number. Place of Publication: Publisher; Year.
Author’s Last name, First Initial. Middle Initial. “Chapter Title.” In: Editor’s Last name, First Initial. Middle Initial., ed. Book Title. Edition number. Place of Publication: Publisher; Year:Page numbers.
Author’s Last name, First Initial. Middle Initial. “Paper Title.” In: Conference Title; Conference Date; Location. Place of Publication: Publisher; Year.
Example : Smith, J.R. “Sustainable Drainage Systems for Urban Areas.” In: Proceedings of the ASCE International Conference on Sustainable Infrastructure; November 6-9, 2019; Los Angeles, CA. Reston, VA: American Society of Civil Engineers; 2019.
Author’s Last name, First Initial. Middle Initial. “Report Title.” Report number. Place of Publication: Publisher; Year.
Bluebook Style
The Bluebook style is commonly used in the legal field for citing legal documents and sources. Here are the different reference formats in Bluebook style:
Case citation
Case name, volume source page (Court year).
Example : Brown v. Board of Education, 347 U.S. 483 (1954).
Statute citation
Name of Act, volume source § section number (year).
Example : Clean Air Act, 42 U.S.C. § 7401 (1963).
Regulation citation
Name of regulation, volume source § section number (year).
Example: Clean Air Act, 40 C.F.R. § 52.01 (2019).
Book citation
Author’s Last name, First Initial. Middle Initial. Book Title. Edition number (if applicable). Place of Publication: Publisher; Year.
Example: Smith, J.R. Legal Writing and Analysis. 3rd ed. New York, NY: Aspen Publishers; 2015.
Journal article citation
Author’s Last name, First Initial. Middle Initial. “Article Title.” Journal Title. Volume number (year): first page-last page.
Example: Garcia, C. “The Right to Counsel: An International Comparison.” International Journal of Legal Information. 43 (2015): 63-94.
Website citation
Author’s Last name, First Initial. Middle Initial. “Page Title.” Website Title. URL (accessed month day, year).
Example : United Nations. “Universal Declaration of Human Rights.” United Nations. https://www.un.org/en/universal-declaration-human-rights/ (accessed January 3, 2023).
Oxford Style
The Oxford style, also known as the Oxford referencing system or the documentary-note citation system, is commonly used in the humanities, including literature, history, and philosophy. Here are the different reference formats in Oxford style:
Author’s Last name, First name. Book Title. Place of Publication: Publisher, Year of Publication.
Example : Smith, John. The Art of Writing. New York: Penguin, 2020.
Author’s Last name, First name. “Article Title.” Journal Title volume, no. issue (year): page range.
Example: Garcia, Carlos. “The Role of Ethics in Philosophy.” Philosophy Today 67, no. 3 (2019): 53-68.
Chapter in an edited book citation
Author’s Last name, First name. “Chapter Title.” In Book Title, edited by Editor’s Name, page range. Place of Publication: Publisher, Year of Publication.
Example : Lee, Mary. “Feminism in the 21st Century.” In The Oxford Handbook of Feminism, edited by Jane Smith, 51-69. Oxford: Oxford University Press, 2018.
Author’s Last name, First name. “Page Title.” Website Title. URL (accessed day month year).
Example : Jones, David. “The Importance of Learning Languages.” Oxford Language Center. https://www.oxfordlanguagecenter.com/importance-of-learning-languages/ (accessed 3 January 2023).
Dissertation or thesis citation
Author’s Last name, First name. “Title of Dissertation/Thesis.” PhD diss., University Name, Year of Publication.
Example : Brown, Susan. “The Art of Storytelling in American Literature.” PhD diss., University of Oxford, 2020.
Newspaper article citation
Author’s Last name, First name. “Article Title.” Newspaper Title, Month Day, Year.
Example : Robinson, Andrew. “New Developments in Climate Change Research.” The Guardian, September 15, 2022.
AAA (American Anthropological Association) Style
The American Anthropological Association (AAA) style is commonly used in anthropology research papers and journals. Here are the different reference formats in AAA style:
Author’s Last name, First name. Year of Publication. Book Title. Place of Publication: Publisher.
Example : Smith, John. 2019. The Anthropology of Food. New York: Routledge.
Author’s Last name, First name. Year of Publication. “Article Title.” Journal Title volume, no. issue: page range.
Example : Garcia, Carlos. 2021. “The Role of Ethics in Anthropology.” American Anthropologist 123, no. 2: 237-251.
Author’s Last name, First name. Year of Publication. “Chapter Title.” In Book Title, edited by Editor’s Name, page range. Place of Publication: Publisher.
Example: Lee, Mary. 2018. “Feminism in Anthropology.” In The Oxford Handbook of Feminism, edited by Jane Smith, 51-69. Oxford: Oxford University Press.
Author’s Last name, First name. Year of Publication. “Page Title.” Website Title. URL (accessed day month year).
Example : Jones, David. 2020. “The Importance of Learning Languages.” Oxford Language Center. https://www.oxfordlanguagecenter.com/importance-of-learning-languages/ (accessed January 3, 2023).
Author’s Last name, First name. Year of Publication. “Title of Dissertation/Thesis.” PhD diss., University Name.
Example : Brown, Susan. 2022. “The Art of Storytelling in Anthropology.” PhD diss., University of California, Berkeley.
Author’s Last name, First name. Year of Publication. “Article Title.” Newspaper Title, Month Day.
Example : Robinson, Andrew. 2021. “New Developments in Anthropology Research.” The Guardian, September 15.
AIP (American Institute of Physics) Style
The American Institute of Physics (AIP) style is commonly used in physics research papers and journals. Here are the different reference formats in AIP style:
Example : Johnson, S. D. 2021. “Quantum Computing and Information.” Journal of Applied Physics 129, no. 4: 043102.
Example : Feynman, Richard. 2018. The Feynman Lectures on Physics. New York: Basic Books.
Example : Jones, David. 2020. “The Future of Quantum Computing.” In The Handbook of Physics, edited by John Smith, 125-136. Oxford: Oxford University Press.
Conference proceedings citation
Author’s Last name, First name. Year of Publication. “Title of Paper.” Proceedings of Conference Name, date and location: page range. Place of Publication: Publisher.
Example : Chen, Wei. 2019. “The Applications of Nanotechnology in Solar Cells.” Proceedings of the 8th International Conference on Nanotechnology, July 15-17, Tokyo, Japan: 224-229. New York: AIP Publishing.
Example : American Institute of Physics. 2022. “About AIP Publishing.” AIP Publishing. https://publishing.aip.org/about-aip-publishing/ (accessed January 3, 2023).
Patent citation
Author’s Last name, First name. Year of Publication. Patent Number.
Example : Smith, John. 2018. US Patent 9,873,644.
References Writing Guide
Here are some general guidelines for writing references:
- Follow the citation style guidelines: Different disciplines and journals may require different citation styles (e.g., APA, MLA, Chicago). It is important to follow the specific guidelines for the citation style required.
- Include all necessary information : Each citation should include enough information for readers to locate the source. For example, a journal article citation should include the author(s), title of the article, journal title, volume number, issue number, page numbers, and publication year.
- Use proper formatting: Citation styles typically have specific formatting requirements for different types of sources. Make sure to follow the proper formatting for each citation.
- Order citations alphabetically: If listing multiple sources, they should be listed alphabetically by the author’s last name.
- Be consistent: Use the same citation style throughout the entire paper or project.
- Check for accuracy: Double-check all citations to ensure accuracy, including correct spelling of author names and publication information.
- Use reputable sources: When selecting sources to cite, choose reputable and authoritative sources. Avoid sources that are biased or unreliable.
- Include all sources: Make sure to include all sources used in the research, including those that were not directly quoted but still informed the work.
- Use online tools : There are online tools available (e.g., citation generators) that can help with formatting and organizing references.
Purpose of References in Research
References in research serve several purposes:
- To give credit to the original authors or sources of information used in the research. It is important to acknowledge the work of others and avoid plagiarism.
- To provide evidence for the claims made in the research. References can support the arguments, hypotheses, or conclusions presented in the research by citing relevant studies, data, or theories.
- To allow readers to find and verify the sources used in the research. References provide the necessary information for readers to locate and access the sources cited in the research, which allows them to evaluate the quality and reliability of the information presented.
- To situate the research within the broader context of the field. References can show how the research builds on or contributes to the existing body of knowledge, and can help readers to identify gaps in the literature that the research seeks to address.
Importance of References in Research
References play an important role in research for several reasons:
- Credibility : By citing authoritative sources, references lend credibility to the research and its claims. They provide evidence that the research is based on a sound foundation of knowledge and has been carefully researched.
- Avoidance of Plagiarism : References help researchers avoid plagiarism by giving credit to the original authors or sources of information. This is important for ethical reasons and also to avoid legal repercussions.
- Reproducibility : References allow others to reproduce the research by providing detailed information on the sources used. This is important for verification of the research and for others to build on the work.
- Context : References provide context for the research by situating it within the broader body of knowledge in the field. They help researchers to understand where their work fits in and how it builds on or contributes to existing knowledge.
- Evaluation : References provide a means for others to evaluate the research by allowing them to assess the quality and reliability of the sources used.
Advantages of References in Research
There are several advantages of including references in research:
- Acknowledgment of Sources: Including references gives credit to the authors or sources of information used in the research. This is important to acknowledge the original work and avoid plagiarism.
- Evidence and Support : References can provide evidence to support the arguments, hypotheses, or conclusions presented in the research. This can add credibility and strength to the research.
- Reproducibility : References provide the necessary information for others to reproduce the research. This is important for the verification of the research and for others to build on the work.
- Context : References can help to situate the research within the broader body of knowledge in the field. This helps researchers to understand where their work fits in and how it builds on or contributes to existing knowledge.
- Evaluation : Including references allows others to evaluate the research by providing a means to assess the quality and reliability of the sources used.
- Ongoing Conversation: References allow researchers to engage in ongoing conversations and debates within their fields. They can show how the research builds on or contributes to the existing body of knowledge.
About the author
Muhammad Hassan
Researcher, Academic Writer, Web developer
You may also like

Data Collection – Methods Types and Examples

Delimitations in Research – Types, Examples and...

Research Process – Steps, Examples and Tips

Research Design – Types, Methods and Examples

Institutional Review Board – Application Sample...

Evaluating Research – Process, Examples and...
APA Citation Style, 7th edition: Article in a Reference Book
- General Style Guidelines
- One Author or Editor
- Two Authors or Editors
- Three to Five Authors or Editors
- Article or Chapter in an Edited Book
Article in a Reference Book
- Edition other than the First
- Translation
- Government Publication
- Journal Article with 1 Author
- Journal Article with 2 Authors
- Journal Article with 3–20 Authors
- Journal Article 21 or more Authors
- Magazine Article
- Newspaper Article
- Basic Web Page
- Web page from a University site
- Web Page with No Author
- Entry in a Reference Work
- Government Document
- Film and Television
- Youtube Video
- Audio Podcast
- Electronic Image
- Twitter/Instagram
- Lecture/PPT
- Conferences
- Secondary Sources
- Citation Support
- Avoiding Plagiarism
- Formatting Your Paper
About Citing Books
For each type of source in this guide, both the general form and an example will be provided.
The following format will be used:
In-Text Citation (Paraphrase) - entry that appears in the body of your paper when you express the ideas of a researcher or author using your own words. For more tips on paraphrasing check out The OWL at Purdue .
In-Text Citation (Quotation) - entry that appears in the body of your paper after a direct quote.
References - entry that appears at the end of your paper.
Information on citing and several of the examples were drawn from the Publication Manual of the American Psychological Association (7th ed.).
General Format
In-Text Citation (Paraphrase):
(Author Surname [of Article], Year)
In-Text Citation (Quotation):
(Author Surname [of Article], Year, page number)
References (Quotation):
Author Surname [of Article], First Initial. Second Initial. (Year). Article title. In Editor First Initial. Second Initial. Surname (Ed.), Reference book title: Subtitle (# ed. edition, Vol. volume #, pp. page range of article). Publisher.
(Lindgren, 1994)
(Lindgren, 1994, p.468)
References:
Lindgren, H. C. (1994). Stereotyping. In Encyclopedia of psychology (Vol. 3, pp. 468-469). Wiley.
Subject Guide

- << Previous: Article or Chapter in an Edited Book
- Next: No Author >>

- Last Updated: Feb 6, 2024 11:45 AM
- URL: https://guides.himmelfarb.gwu.edu/APA

- Himmelfarb Intranet
- Privacy Notice
- Terms of Use
- GW is committed to digital accessibility. If you experience a barrier that affects your ability to access content on this page, let us know via the Accessibility Feedback Form .
- Himmelfarb Health Sciences Library
- 2300 Eye St., NW, Washington, DC 20037
- Phone: (202) 994-2850
- [email protected]
- https://himmelfarb.gwu.edu
Thank you for visiting nature.com. You are using a browser version with limited support for CSS. To obtain the best experience, we recommend you use a more up to date browser (or turn off compatibility mode in Internet Explorer). In the meantime, to ensure continued support, we are displaying the site without styles and JavaScript.
- View all journals
- Explore content
- About the journal
- Publish with us
- Sign up for alerts
- Published: 08 May 2024
Accurate structure prediction of biomolecular interactions with AlphaFold 3
- Josh Abramson ORCID: orcid.org/0009-0000-3496-6952 1 na1 ,
- Jonas Adler ORCID: orcid.org/0000-0001-9928-3407 1 na1 ,
- Jack Dunger 1 na1 ,
- Richard Evans ORCID: orcid.org/0000-0003-4675-8469 1 na1 ,
- Tim Green ORCID: orcid.org/0000-0002-3227-1505 1 na1 ,
- Alexander Pritzel ORCID: orcid.org/0000-0002-4233-9040 1 na1 ,
- Olaf Ronneberger ORCID: orcid.org/0000-0002-4266-1515 1 na1 ,
- Lindsay Willmore ORCID: orcid.org/0000-0003-4314-0778 1 na1 ,
- Andrew J. Ballard ORCID: orcid.org/0000-0003-4956-5304 1 ,
- Joshua Bambrick ORCID: orcid.org/0009-0003-3908-0722 2 ,
- Sebastian W. Bodenstein 1 ,
- David A. Evans 1 ,
- Chia-Chun Hung ORCID: orcid.org/0000-0002-5264-9165 2 ,
- Michael O’Neill 1 ,
- David Reiman ORCID: orcid.org/0000-0002-1605-7197 1 ,
- Kathryn Tunyasuvunakool ORCID: orcid.org/0000-0002-8594-1074 1 ,
- Zachary Wu ORCID: orcid.org/0000-0003-2429-9812 1 ,
- Akvilė Žemgulytė 1 ,
- Eirini Arvaniti 3 ,
- Charles Beattie ORCID: orcid.org/0000-0003-1840-054X 3 ,
- Ottavia Bertolli ORCID: orcid.org/0000-0001-8578-3216 3 ,
- Alex Bridgland 3 ,
- Alexey Cherepanov ORCID: orcid.org/0000-0002-5227-0622 4 ,
- Miles Congreve 4 ,
- Alexander I. Cowen-Rivers 3 ,
- Andrew Cowie ORCID: orcid.org/0000-0002-4491-1434 3 ,
- Michael Figurnov ORCID: orcid.org/0000-0003-1386-8741 3 ,
- Fabian B. Fuchs 3 ,
- Hannah Gladman 3 ,
- Rishub Jain 3 ,
- Yousuf A. Khan ORCID: orcid.org/0000-0003-0201-2796 3 ,
- Caroline M. R. Low 4 ,
- Kuba Perlin 3 ,
- Anna Potapenko 3 ,
- Pascal Savy 4 ,
- Sukhdeep Singh 3 ,
- Adrian Stecula ORCID: orcid.org/0000-0001-6914-6743 4 ,
- Ashok Thillaisundaram 3 ,
- Catherine Tong ORCID: orcid.org/0000-0001-7570-4801 4 ,
- Sergei Yakneen ORCID: orcid.org/0000-0001-7827-9839 4 ,
- Ellen D. Zhong ORCID: orcid.org/0000-0001-6345-1907 3 ,
- Michal Zielinski 3 ,
- Augustin Žídek ORCID: orcid.org/0000-0002-0748-9684 3 ,
- Victor Bapst 1 na2 ,
- Pushmeet Kohli ORCID: orcid.org/0000-0002-7466-7997 1 na2 ,
- Max Jaderberg ORCID: orcid.org/0000-0002-9033-2695 2 na2 ,
- Demis Hassabis ORCID: orcid.org/0000-0003-2812-9917 1 , 2 na2 &
- John M. Jumper ORCID: orcid.org/0000-0001-6169-6580 1 na2
Nature ( 2024 ) Cite this article
276k Accesses
1 Citations
1351 Altmetric
Metrics details
We are providing an unedited version of this manuscript to give early access to its findings. Before final publication, the manuscript will undergo further editing. Please note there may be errors present which affect the content, and all legal disclaimers apply.
- Drug discovery
- Machine learning
- Protein structure predictions
- Structural biology
The introduction of AlphaFold 2 1 has spurred a revolution in modelling the structure of proteins and their interactions, enabling a huge range of applications in protein modelling and design 2–6 . In this paper, we describe our AlphaFold 3 model with a substantially updated diffusion-based architecture, which is capable of joint structure prediction of complexes including proteins, nucleic acids, small molecules, ions, and modified residues. The new AlphaFold model demonstrates significantly improved accuracy over many previous specialised tools: far greater accuracy on protein-ligand interactions than state of the art docking tools, much higher accuracy on protein-nucleic acid interactions than nucleic-acid-specific predictors, and significantly higher antibody-antigen prediction accuracy than AlphaFold-Multimer v2.3 7,8 . Together these results show that high accuracy modelling across biomolecular space is possible within a single unified deep learning framework.
You have full access to this article via your institution.
Similar content being viewed by others
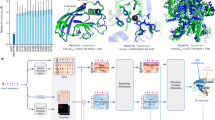
Highly accurate protein structure prediction with AlphaFold
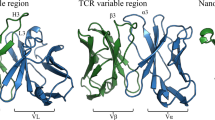
ImmuneBuilder: Deep-Learning models for predicting the structures of immune proteins
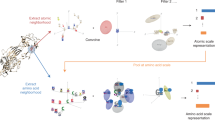
ScanNet: an interpretable geometric deep learning model for structure-based protein binding site prediction
Author information.
These authors contributed equally: Josh Abramson, Jonas Adler, Jack Dunger, Richard Evans, Tim Green, Alexander Pritzel, Olaf Ronneberger, Lindsay Willmore
These authors jointly supervised this work: Victor Bapst, Pushmeet Kohli, Max Jaderberg, Demis Hassabis, John M. Jumper
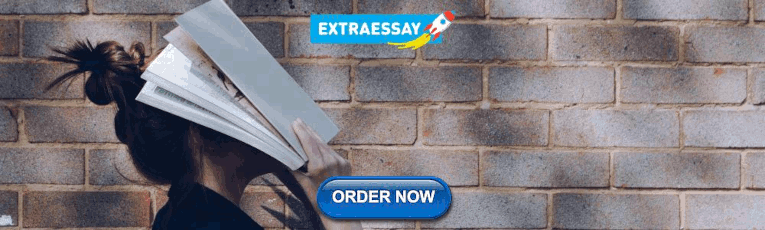
Authors and Affiliations
Core Contributor, Google DeepMind, London, UK
Josh Abramson, Jonas Adler, Jack Dunger, Richard Evans, Tim Green, Alexander Pritzel, Olaf Ronneberger, Lindsay Willmore, Andrew J. Ballard, Sebastian W. Bodenstein, David A. Evans, Michael O’Neill, David Reiman, Kathryn Tunyasuvunakool, Zachary Wu, Akvilė Žemgulytė, Victor Bapst, Pushmeet Kohli, Demis Hassabis & John M. Jumper
Core Contributor, Isomorphic Labs, London, UK
Joshua Bambrick, Chia-Chun Hung, Max Jaderberg & Demis Hassabis
Google DeepMind, London, UK
Eirini Arvaniti, Charles Beattie, Ottavia Bertolli, Alex Bridgland, Alexander I. Cowen-Rivers, Andrew Cowie, Michael Figurnov, Fabian B. Fuchs, Hannah Gladman, Rishub Jain, Yousuf A. Khan, Kuba Perlin, Anna Potapenko, Sukhdeep Singh, Ashok Thillaisundaram, Ellen D. Zhong, Michal Zielinski & Augustin Žídek
Isomorphic Labs, London, UK
Alexey Cherepanov, Miles Congreve, Caroline M. R. Low, Pascal Savy, Adrian Stecula, Catherine Tong & Sergei Yakneen
You can also search for this author in PubMed Google Scholar
Corresponding authors
Correspondence to Max Jaderberg , Demis Hassabis or John M. Jumper .
Supplementary information
Supplementary information.
This Supplementary Information file contains the following 9 sections: (1) Notation; (2) Data pipeline; (3) Model architecture; (4) Auxiliary heads; (5) Training and inference; (6) Evaluation; (7) Differences to AlphaFold2 and AlphaFold-Multimer; (8) Supplemental Results; and (9) Appendix: CCD Code and PDB ID tables.
Reporting Summary
Rights and permissions.
Reprints and permissions
About this article
Cite this article.
Abramson, J., Adler, J., Dunger, J. et al. Accurate structure prediction of biomolecular interactions with AlphaFold 3. Nature (2024). https://doi.org/10.1038/s41586-024-07487-w
Download citation
Received : 19 December 2023
Accepted : 29 April 2024
Published : 08 May 2024
DOI : https://doi.org/10.1038/s41586-024-07487-w
Share this article
Anyone you share the following link with will be able to read this content:
Sorry, a shareable link is not currently available for this article.
Provided by the Springer Nature SharedIt content-sharing initiative
This article is cited by
Major alphafold upgrade offers boost for drug discovery.
- Ewen Callaway
Nature (2024)
By submitting a comment you agree to abide by our Terms and Community Guidelines . If you find something abusive or that does not comply with our terms or guidelines please flag it as inappropriate.
Quick links
- Explore articles by subject
- Guide to authors
- Editorial policies
Sign up for the Nature Briefing: Translational Research newsletter — top stories in biotechnology, drug discovery and pharma.

Things you buy through our links may earn Vox Media a commission.
- Why Scientific Fraud Is Suddenly Everywhere
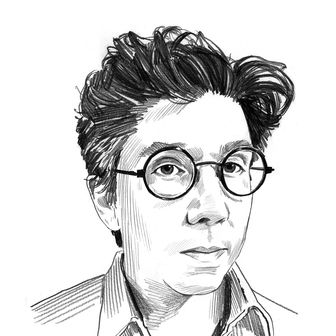
Junk science has been forcing a reckoning among scientific and medical researchers for the past year, leading to thousands of retracted papers. Last year, Stanford president Marc Tessier-Lavigne resigned amid reporting that some of his most high-profile work on Alzheimer’s disease was at best inaccurate. (A probe commissioned by the university’s board of trustees later exonerated him of manipulating the data).
But the problems around credible science appear to be getting worse. Last week, scientific publisher Wiley decided to shutter 19 scientific journals after retracting 11,300 sham papers. There is a large-scale industry of so-called “paper mills” that sell fictive research, sometimes written by artificial intelligence, to researchers who then publish it in peer-reviewed journals — which are sometimes edited by people who had been placed by those sham groups. Among the institutions exposing such practices is Retraction Watch, a 14-year-old organization co-founded by journalists Ivan Oransky and Adam Marcus. I spoke with Oransky about why there has been a surge in fake research and whether fraud accusations against the presidents of Harvard and Stanford are actually good for academia.
Give me a sense of how big a problem these paper mills are.
I’ll start by saying that paper mills are not the problem; they are a symptom of the actual problem. Adam Marcus, my co-founder, had broken a really big and frightening story about a painkiller involving scientific fraud , which led to dozens of retractions. That’s what got us interested in that. There were all these retractions, far more than we thought but far fewer than there are now. Now, they’re hiding in plain sight.
That was 2010. Certainly, AI has accelerated things, but we’ve known about paper mills for a long time. Everybody wanted to pretend all these problems didn’t exist. The problems in scientific literature are long-standing, and they’re an incentive problem. And the metrics that people use to measure research feed a business model — a ravenous sort of insatiable business model. Hindsight is always going to be 20/20, but a lot of people actually were predicting what we’re seeing now.
Regarding your comment that paper mills are symptoms of a larger problem, I read this story in Science and was struck by the drive for credentialing — which gets you better jobs, higher pay, and more prestige. In academia, there aren’t enough jobs; are the hurdles to these jobs impossibly high, especially for people who may be smart but are from China or India and may not have entry into an American or European university?
I actually would go one step higher. When you say there aren’t enough jobs, it’s because we’re training so many Ph.D.’s and convincing them all that the only way to remain a scientist is to stay in academia. It’s not, and that hasn’t been true for a long time. So there’s definitely a supply-and-demand problem, and people are going to compete.
You may recall the story about high-school students who were paying to get medical papers published in order to get into college. That’s the sort of level we’re at now. It’s just pervasive. People are looking only at metrics, not at actual papers. We’re so fixated on metrics because they determine funding for a university based on where it is in the rankings. So it comes from there and then it filters down. What do universities then want? Well, they want to attract people who are likely to publish papers. So how do you decide that? “Oh, you’ve already published some papers, great. We’re gonna bring you in.” And then when you’re there, you’ve got to publish even more.
You’re replacing actual findings and science and methodology and the process with what I would argue are incredibly misleading — even false — metrics. Paper mills are industrializing it. This is like the horse versus the steam engine.
So they’re Moneyballing it.
Absolutely. They’ve Moneyballed it with a caveat: Moneyball sort of worked. The paper mills have metricized it, which is not as sexy to say. If you were to isolate one factor, citations matter the most, and if you look at the ranking systems, it’s all right there. The Times Higher Education world-university rankings , U.S. News — look at whichever you want, and somewhere between like 30 percent and 60 percent of those rankings are based on citations. Citations are so easy to game. So people are setting up citation cartels: “Yes, we will get all of our other clients to cite you, and nobody will notice because we’re doing it in this algorithmic, mixed-up way.” Eventually, people do notice, but it’s the insistence on citations as the coin of the realm that all of this comes from.
Your work gets to the heart of researchers’ integrity. Do you feel like you’re a pariah in the scientific community?
I’m a volunteer. Adam is paid a very small amount. We use our funding to pay two reporters and then two people work on our database side. We approach these things journalistically; we don’t actually identify the problems ourselves. It’s very, very rare for us to do that. Even when it may appear that way on a superficial read — we’ve broken some stories recently about clear problems in literature — it’s always because a source showed us the way. Sometimes those sources want to be named, sometimes they don’t.
We’ve been doing this for 14 years. There are various ways to look at what the scientific community thinks of us. We’re publishing 100 posts a year about people committing bad behavior and only getting, on average, one cease-and-desist letter a year. We have never been sued, but we do carried defamation insurance. Our work is cited hundreds of times in the scientific literature. I definitely don’t feel like a pariah. Me saying I’m a pariah would be a little bit like, you know, someone whose alleged cancellation has promoted them to the top of Twitter.
People are unhappy that we have do what we do. If you talk to scientists, the things we’re exposing or others are exposing are well known to them. Because of the structures, the hierarchies, and the power differentials in science, it’s very difficult for them as insiders to blow the whistle. There’s a book out by Carl Elliott about whistleblowers , mostly in the sort of more clinical fields. That’s the vulnerable position. That’s where you end up being a pariah even though you should be considered a hero or heroine.
Are some fields better at policing their own research than others?
Yes. Going back to the origin story of Retraction Watch, Adam broke a story about this guy named Scott Reuben, who came from anesthesiology. We have a leaderboard of the people with the most retractions in the world, and at least three out of the top ten right now are anesthesiologists. That is a much higher percentage than one might expect. Some people may say, “Oh, does anesthesiology have a problem?” No, in fact, anesthesiology has been doing something about this arguably longer than any other field has.
What is it about anesthesiology that makes it so anesthesiologists are more willing to scrutinize the work in their own field?
It had a crisis earlier than others, and it’s small. Journal editors are generally considered pretty august personages, leaders in the field. They got together and it was like a collective action by the journal editors when they realized they had problems. I’m not saying anesthesiologists are better, but they’re a more tight-knit community, which I do think is important. The same thing happened in social psychology and in psychology writ large. There’s a higher number than you would expect of people on leaderboards in that field. So it’s a question of, When did they get there, and how did they react to it? There are fields that haven’t actually gotten there, even though it’s been a while. So maybe there are some sociologists who could tell you better than me why that might be the case.
That wasn’t the reason I expected. I thought you would say something along the lines of, well, it’s life or death and anesthesiologists don’t want to see people dying on the table.
If anything, sometimes when the stakes are higher, fields are more resistant.
There’s a guy named Ben Mol. Ben is an OB/GYN, and he is a force to be reckoned with. Fascinating character. He’s a pit bull, and he has found tons and tons of problems in the OB/GYN literature. I would characterize the leaders in that field now as still a bit more reluctant to engage with these issues than some of the other fields I mentioned.
Can you tell me how you go about authenticating real language from AI, especially in papers that can be hard to parse and are laden with jargon to begin with?
We rely on experts. We’re not really doing that ourselves. You don’t need to be an expert; you just need to know how to use Ctrl+F if you see certain phrases in a paper. And by the way, a lot of journals are perfectly fine with people using chat GPT and other kinds of AI. It’s just whether you disclose it or not. These are cases where they didn’t disclose it.
With the resignation of Stanford’s and Harvard’s presidents, do you worry about the way the general public has been using these tools?
The fact that they’re giving speeding tickets to certain groups of people doesn’t mean we’re not all speeding. It means they’re getting targeted in, I would argue, an unfair way. We’re in a great reckoning with Harvard’s Claudine Gay being the key example. Former Stanford president Marc Tessier-Lavigne is not an example of that. The targeting is a concern. And clearly, there are false positives. The flip side of this is that AI is being used to find these problems.
This interview has been edited for length and clarity. The story was updated to include that a probe found that Tessier-Lavigne didn’t manipulate data.
- just asking questions
Most Viewed Stories
- Rudy Giuliani Forgets to Mute His Microphone While Going to the Bathroom
- Chess Brat: Hans Niemann, One Year After the Cheating Scandal
- Alito Ethics Defense Blown Up by Second Insurrectionist Flag
- Why Does the New Kate Middleton Portrait Look Nothing Like Her?
- Who’s the Trump VP Pick? Latest Odds for Every Shortlist Candidate.
- What to Know About the New Student-Loan-Forgiveness Plans
Editor’s Picks
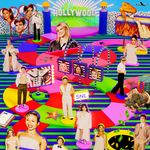
Most Popular
- Rudy Giuliani Forgets to Mute His Microphone While Going to the Bathroom By Matt Stieb
- Chess Brat: Hans Niemann, One Year After the Cheating Scandal By Jen Wieczner
- Alito Ethics Defense Blown Up by Second Insurrectionist Flag By Jonathan Chait
- Why Scientific Fraud Is Suddenly Everywhere By Kevin T. Dugan
- Why Does the New Kate Middleton Portrait Look Nothing Like Her? By Margaret Hartmann
- Who’s the Trump VP Pick? Latest Odds for Every Shortlist Candidate. By Margaret Hartmann
- What to Know About the New Student-Loan-Forgiveness Plans By Lynnley Browning, Nia Prater, and Chas Danner
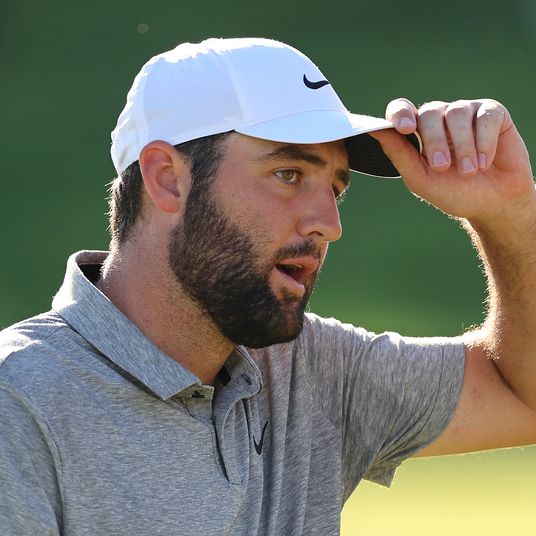
What is your email?
This email will be used to sign into all New York sites. By submitting your email, you agree to our Terms and Privacy Policy and to receive email correspondence from us.
Sign In To Continue Reading
Create your free account.
Password must be at least 8 characters and contain:
- Lower case letters (a-z)
- Upper case letters (A-Z)
- Numbers (0-9)
- Special Characters (!@#$%^&*)
As part of your account, you’ll receive occasional updates and offers from New York , which you can opt out of anytime.
Home / Guides / Citation Guides / Harvard Referencing / Harvard Referencing Style Examples / How to reference an article in Harvard referencing style
How to reference an article in Harvard referencing style
What is an article.
Almost all writers and academics reference other people’s writing in their works. Referencing demonstrates that you have researched your topic, are well versed in its arguments and theories, and it also helps avoid charges of plagiarism.
The Harvard citation system is just one of many referencing styles – and which style you choose is normally guided by the institution or publication you are writing for.
In this article, you will learn how to use the Harvard citation system to reference the following types of articles:
- journal article
- newspaper article
- magazine article
Properly citing article details in the reference list will help the readers to locate your source material if they wish to read more about a particular area or topic.
Information you need:
- Author name
- (Year published)
- ‘Article title’
- Journal/newspaper/magazine name
- Day and month published, if available
- Volume number, if available
- (Issue) number, if available
- Page number(s), if available
If accessed online:
- Available at: URL or DOI
- (Accessed: date).
Journal articles
Academic or scholarly journals are periodical publications about a specific discipline. No matter what your field is, if you are writing an academic paper, you will inevitably have to cite a journal article in your research. Journal articles often have multiple authors, so make sure you know when to use et al. in Harvard style . The method for referencing a journal article in the reference list is as follows:
Reference list (print) structure:
Last name, F. (Year published) ‘Article title’, Journal name , Volume(Issue), Page(s).
Shepherd, V. (2020) ‘An exploration around peer support for secondary pupils in Scotland with experience of self-harm’, Educational Psychology in Practice, 36(3), pp. 297-312.
Note that the article title uses sentence case. However, the title of the journal uses title case. Additionally, the volume number comes immediately after the journal title followed by the issue number in round brackets.
If the original material you are referencing was accessed online, then the method for citing it in the reference list will be the same as that in print, but with an additional line at the end.
Reference list (online) structure:
Last name, F. (Year published) ‘Article title’, Journal Name , Volume(Issue), Page(s). Available at: URL or DOI (Accessed: date).
Shepherd, V. (2020) ‘An exploration around peer support for secondary pupils in Scotland with experience of self-harm’, Educational Psychology in Practice, 36(3), pp. 297-312. Available at: https://www.tandfonline.com/doi/full/10.1080/02667363.2020.1772726 (Accessed: 08 October 2020).
In-text citation (print or online) structure:
In-text citations are written within round brackets and start with the last name of the author followed by the year published, both separated by a comma.
You can also mention the author within the text and only include the publication year in round brackets.
Examples:
In this article (Shepherd, 2020) deals with…
According to Shepherd (2020), when peer support is available…
Talking about the secondary education system, Shepherd (2020, p.299) suggests that…
Newspaper articles
Even if you are referring to an incident which is public knowledge, you still need to cite the source.
The name of the author in a newspaper article is referred to as a byline. Below are examples for citing an article both with and without a byline.
Reference list (print) structure:
Last name, F. (Year published). ‘Article title’, Newspaper name , Day Month, Page(s).
Hamilton, J. (2018). ‘Massive fire at local department store’, The Daily Local, 10 August, p. 1.
Last name, F. (Year published). ‘Article title’, Newspaper name , Day Month, Page(s). Available at: URL (Accessed: Day Month Year).
Gambino, L. (2020) ‘Kamala Harris and Mike Pence clash over coronavirus response in vice-presidential debate,’ The Guardian, 8 October. Available at: https://www.theguardian.com/us-news/2020/oct/07/debate-kamala-harris-mike-pence-latest-news (Accessed: 8 October 2020).
Reference list structure, no byline:
The basic reference list structure for the reference is the same for both print and online articles. If information isn’t available, simply omit it from the reference.
Newspaper name (Year published) ‘Article Title’, Day Month, Page(s). Available at: URL (Accessed: Day Month Year).
The Chronicler (2016) ‘Local man wins lottery jackpot twice in one year’, 30 May, p. 14. Available at: https://thechroniclerpaper.com/local-man-wins-lottery-twice (Accessed: 1 October 2020).
In-text citation structure (print or online):
The last name of the author and date are written in round brackets, separated by a comma. The method is similar to referencing journal articles in in-text citations.
(Hamilton, 2018)
In his paper, Gambino (2020) mentioned that…
For articles accessed online which do not have an author, the name of the publication is mentioned in place of the author’s name and is italicized.
( The Chronicler , 2016)
Magazine articles
The structure of magazine articles is similar to that of a journal article.
Last name, F. (Year published) ‘Article title’, Magazine Name , Volume(Issue), Page(s).
Ornes, S. (2020). “To save Appalachia’s endangered mussels, scientists hatched a bold plan”, ScienceNews, (198), p.2.
Last name, F. (Year published) ‘Article title’, Magazine name , Volume(Issue), Page(s). Available at: URL (Accessed: Date).
Ornes, S. (2020) ‘To save Appalachia’s endangered mussels, scientists hatched a bold plan’, ScienceNews, (198), p.2. Available at: https://www.sciencenews.org/article/endangered-mussels-appalachia-rivers-biologists-conservation-plan (Accessed: 3 October 2020).
In-text citation (print or online) structure:
(Author last name, Year published)
(Ornes, 2020)
Published October 29, 2020.
Harvard Formatting Guide
Harvard Formatting
- et al Usage
- Direct Quotes
- In-text Citations
- Multiple Authors
- Page Numbers
- Writing an Outline
- View Harvard Guide
Reference Examples
- View all Harvard Examples
How useful was this post?
Click on a star to rate it!
We are sorry that this post was not useful for you!
Let us improve this post!
Tell us how we can improve this post?
Harvard Referencing Examples
Writing Tools
Citation Generators
Other Citation Styles
Plagiarism Checker
Upload a paper to check for plagiarism against billions of sources and get advanced writing suggestions for clarity and style.
Get Started
Loading metrics
Open Access
Peer-reviewed
Meta-Research Article
Meta-Research Articles feature data-driven examinations of the methods, reporting, verification, and evaluation of scientific research.
See Journal Information »
Assessing the evolution of research topics in a biological field using plant science as an example
Roles Conceptualization, Data curation, Formal analysis, Funding acquisition, Investigation, Methodology, Project administration, Resources, Software, Supervision, Validation, Visualization, Writing – original draft, Writing – review & editing
* E-mail: [email protected]
Affiliations Department of Plant Biology, Michigan State University, East Lansing, Michigan, United States of America, Department of Computational Mathematics, Science, and Engineering, Michigan State University, East Lansing, Michigan, United States of America, DOE-Great Lake Bioenergy Research Center, Michigan State University, East Lansing, Michigan, United States of America

Roles Conceptualization, Investigation, Project administration, Supervision, Writing – review & editing
Affiliation Department of Plant Biology, Michigan State University, East Lansing, Michigan, United States of America
- Shin-Han Shiu,
- Melissa D. Lehti-Shiu
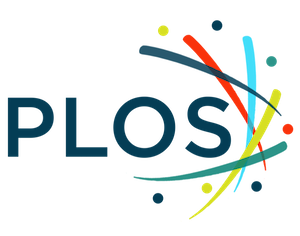
- Published: May 23, 2024
- https://doi.org/10.1371/journal.pbio.3002612
- Peer Review
- Reader Comments
Scientific advances due to conceptual or technological innovations can be revealed by examining how research topics have evolved. But such topical evolution is difficult to uncover and quantify because of the large body of literature and the need for expert knowledge in a wide range of areas in a field. Using plant biology as an example, we used machine learning and language models to classify plant science citations into topics representing interconnected, evolving subfields. The changes in prevalence of topical records over the last 50 years reflect shifts in major research trends and recent radiation of new topics, as well as turnover of model species and vastly different plant science research trajectories among countries. Our approaches readily summarize the topical diversity and evolution of a scientific field with hundreds of thousands of relevant papers, and they can be applied broadly to other fields.
Citation: Shiu S-H, Lehti-Shiu MD (2024) Assessing the evolution of research topics in a biological field using plant science as an example. PLoS Biol 22(5): e3002612. https://doi.org/10.1371/journal.pbio.3002612
Academic Editor: Ulrich Dirnagl, Charite Universitatsmedizin Berlin, GERMANY
Received: October 16, 2023; Accepted: April 4, 2024; Published: May 23, 2024
Copyright: © 2024 Shiu, Lehti-Shiu. This is an open access article distributed under the terms of the Creative Commons Attribution License , which permits unrestricted use, distribution, and reproduction in any medium, provided the original author and source are credited.
Data Availability: The plant science corpus data are available through Zenodo ( https://zenodo.org/records/10022686 ). The codes for the entire project are available through GitHub ( https://github.com/ShiuLab/plant_sci_hist ) and Zenodo ( https://doi.org/10.5281/zenodo.10894387 ).
Funding: This work was supported by the National Science Foundation (IOS-2107215 and MCB-2210431 to MDL and SHS; DGE-1828149 and IOS-2218206 to SHS), Department of Energy grant Great Lakes Bioenergy Research Center (DE-SC0018409 to SHS). The funders had no role in study design, data collection and analysis, decision to publish, or preparation of the manuscript.
Competing interests: The authors have declared that no competing interests exist.
Abbreviations: BERT, Bidirectional Encoder Representations from Transformers; br, brassinosteroid; ccTLD, country code Top Level Domain; c-Tf-Idf, class-based Tf-Idf; ChatGPT, Chat Generative Pretrained Transformer; ga, gibberellic acid; LOWESS, locally weighted scatterplot smoothing; MeSH, Medical Subject Heading; SHAP, SHapley Additive exPlanations; SJR, SCImago Journal Rank; Tf-Idf, Term frequency-Inverse document frequency; UMAP, Uniform Manifold Approximation and Projection
Introduction
The explosive growth of scientific data in recent years has been accompanied by a rapidly increasing volume of literature. These records represent a major component of our scientific knowledge and embody the history of conceptual and technological advances in various fields over time. Our ability to wade through these records is important for identifying relevant literature for specific topics, a crucial practice of any scientific pursuit [ 1 ]. Classifying the large body of literature into topics can provide a useful means to identify relevant literature. In addition, these topics offer an opportunity to assess how scientific fields have evolved and when major shifts in took place. However, such classification is challenging because the relevant articles in any topic or domain can number in the tens or hundreds of thousands, and the literature is in the form of natural language, which takes substantial effort and expertise to process [ 2 , 3 ]. In addition, even if one could digest all literature in a field, it would still be difficult to quantify such knowledge.
In the last several years, there has been a quantum leap in natural language processing approaches due to the feasibility of building complex deep learning models with highly flexible architectures [ 4 , 5 ]. The development of large language models such as Bidirectional Encoder Representations from Transformers (BERT; [ 6 ]) and Chat Generative Pretrained Transformer (ChatGPT; [ 7 ]) has enabled the analysis, generation, and modeling of natural language texts in a wide range of applications. The success of these applications is, in large part, due to the feasibility of considering how the same words are used in different contexts when modeling natural language [ 6 ]. One such application is topic modeling, the practice of establishing statistical models of semantic structures underlying a document collection. Topic modeling has been proposed for identifying scientific hot topics over time [ 1 ], for example, in synthetic biology [ 8 ], and it has also been applied to, for example, automatically identify topical scenes in images [ 9 ] and social network topics [ 10 ], discover gene programs highly correlated with cancer prognosis [ 11 ], capture “chromatin topics” that define cell-type differences [ 12 ], and investigate relationships between genetic variants and disease risk [ 13 ]. Here, we use topic modeling to ask how research topics in a scientific field have evolved and what major changes in the research trends have taken place, using plant science as an example.
Plant science corpora allow classification of major research topics
Plant science, broadly defined, is the study of photosynthetic species, their interactions with biotic/abiotic environments, and their applications. For modeling plant science topical evolution, we first identified a collection of plant science documents (i.e., corpus) using a text classification approach. To this end, we first collected over 30 million PubMed records and narrowed down candidate plant science records by searching for those with plant-related terms and taxon names (see Materials and methods ). Because there remained a substantial number of false positives (i.e., biomedical records mentioning plants in passing), a set of positive plant science examples from the 17 plant science journals with the highest numbers of plant science publications covering a wide range of subfields and a set of negative examples from journals with few candidate plant science records were used to train 4 types of text classification models (see Materials and methods ). The best text classification model performed well (F1 = 0.96, F1 of a naïve model = 0.5, perfect model = 1) where the positive and negative examples were clearly separated from each other based on prediction probability of the hold-out testing dataset (false negative rate = 2.6%, false positive rate = 5.2%, S1A and S1B Fig ). The false prediction rate for documents from the 17 plant science journals annotated with the Medical Subject Heading (MeSH) term “Plants” in NCBI was 11.7% (see Materials and methods ). The prediction probability distribution of positive instances with the MeSH term has an expected left-skew to lower values ( S1C Fig ) compared with the distributions of all positive instances ( S1A Fig ). Thus, this subset with the MeSH term is a skewed representation of articles from these 17 major plant science journals. To further benchmark the validity of the plant science records, we also conducted manual annotation of 100 records where the false positive and false negative rates were 14.6% and 10.6%, respectively (see Materials and methods ). Using 12 other plant science journals not included as positive examples as benchmarks, the false negative rate was 9.9% (see Materials and methods ). Considering the range of false prediction rate estimates with different benchmarks, we should emphasize that the model built with the top 17 plant science journals represents a substantial fraction of plant science publications but with biases. Applying the model to the candidate plant science record led to 421,658 positive predictions, hereafter referred to as “plant science records” ( S1D Fig and S1 Data ).
To better understand how the models classified plant science articles, we identified important terms from a more easily interpretable model (Term frequency-Inverse document frequency (Tf-Idf) model; F1 = 0.934) using Shapley Additive Explanations [ 14 ]; 136 terms contributed to predicting plant science records (e.g., Arabidopsis, xylem, seedling) and 138 terms contributed to non-plant science record predictions (e.g., patients, clinical, mice; Tf-Idf feature sheet, S1 Data ). Plant science records as well as PubMed articles grew exponentially from 1950 to 2020 ( Fig 1A ), highlighting the challenges of digesting the rapidly expanding literature. We used the plant science records to perform topic modeling, which consisted of 4 steps: representing each record as a BERT embedding, reducing dimensionality, clustering, and identifying the top terms by calculating class (i.e., topic)-based Tf-Idf (c-Tf-Idf; [ 15 ]). The c-Tf-Idf represents the frequency of a term in the context of how rare the term is to reduce the influence of common words. SciBERT [ 16 ] was the best model among those tested ( S2 Data ) and was used for building the final topic model, which classified 372,430 (88.3%) records into 90 topics defined by distinct combinations of terms ( S3 Data ). The topics contained 620 to 16,183 records and were named after the top 4 to 5 terms defining the topical areas ( Fig 1B and S3 Data ). For example, the top 5 terms representing the largest topic, topic 61 (16,183 records), are “qtl,” “resistance,” “wheat,” “markers,” and “traits,” which represent crop improvement studies using quantitative genetics.
- PPT PowerPoint slide
- PNG larger image
- TIFF original image
(A) Numbers of PubMed (magenta) and plant science (green) records between 1950 and 2020. (a, b, c) Coefficients of the exponential function, y = ae b . Data for the plot are in S1 Data . (B) Numbers of documents for the top 30 plant science topics. Each topic is designated by an index number (left) and the top 4–6 terms with the highest cTf-Idf values (right). Data for the plot are in S3 Data . (C) Two-dimensional representation of the relationships between plant science records generated by Uniform Manifold Approximation and Projection (UMAP, [ 17 ]) using SciBERT embeddings of plant science records. All topics panel: Different topics are assigned different colors. Outlier panel: UMAP representation of all records (gray) with outlier records in red. Blue dotted circles: areas with relatively high densities indicating topics that are below the threshold for inclusion in a topic. In the 8 UMAP representations on the right, records for example topics are in red and the remaining records in gray. Blue dotted circles indicate the relative position of topic 48.
https://doi.org/10.1371/journal.pbio.3002612.g001
Records with assigned topics clustered into distinct areas in a two-dimensional (2D) space ( Fig 1C , for all topics, see S4 Data ). The remaining 49,228 outlier records not assigned to any topic (11.7%, middle panel, Fig 1C ) have 3 potential sources. First, some outliers likely belong to unique topics but have fewer records than the threshold (>500, blue dotted circles, Fig 1C ). Second, some of the many outliers dispersed within the 2D space ( Fig 1C ) were not assigned to any single topic because they had relatively high prediction scores for multiple topics ( S2 Fig ). These likely represent studies across subdisciplines in plant science. Third, some outliers are likely interdisciplinary studies between plant science and other domains, such as chemistry, mathematics, and physics. Such connections can only be revealed if records from other domains are included in the analyses.
Topical clusters reveal closely related topics but with distinct key term usage
Related topics tend to be located close together in the 2D representation (e.g., topics 48 and 49, Fig 1C ). We further assessed intertopical relationships by determining the cosine similarities between topics using cTf-Idfs ( Figs 2A and S3 ). In this topic network, some topics are closely related and form topic clusters. For example, topics 25, 26, and 27 collectively represent a more general topic related to the field of plant development (cluster a , lower left in Fig 2A ). Other topic clusters represent studies of stress, ion transport, and heavy metals ( b ); photosynthesis, water, and UV-B ( c ); population and community biology (d); genomics, genetic mapping, and phylogenetics ( e , upper right); and enzyme biochemistry ( f , upper left in Fig 2A ).
(A) Graph depicting the degrees of similarity (edges) between topics (nodes). Between each topic pair, a cosine similarity value was calculated using the cTf-Idf values of all terms. A threshold similarity of 0.6 was applied to illustrate the most related topics. For the full matrix presented as a heatmap, see S4 Fig . The nodes are labeled with topic index numbers and the top 4–6 terms. The colors and width of the edges are defined based on cosine similarity. Example topic clusters are highlighted in yellow and labeled a through f (blue boxes). (B, C) Relationships between the cTf-Idf values (see S3 Data ) of the top terms for topics 26 and 27 (B) and for topics 25 and 27 (C) . Only terms with cTf-Idf ≥ 0.6 are labeled. Terms with cTf-Idf values beyond the x and y axis limit are indicated by pink arrows and cTf-Idf values. (D) The 2D representation in Fig 1C is partitioned into graphs for different years, and example plots for every 5-year period since 1975 are shown. Example topics discussed in the text are indicated. Blue arrows connect the areas occupied by records of example topics across time periods to indicate changes in document frequencies.
https://doi.org/10.1371/journal.pbio.3002612.g002
Topics differed in how well they were connected to each other, reflecting how general the research interests or needs are (see Materials and methods ). For example, topic 24 (stress mechanisms) is the most well connected with median cosine similarity = 0.36, potentially because researchers in many subfields consider aspects of plant stress even though it is not the focus. The least connected topics include topic 21 (clock biology, 0.12), which is surprising because of the importance of clocks in essentially all aspects of plant biology [ 18 ]. This may be attributed, in part, to the relatively recent attention in this area.
Examining topical relationships and the cTf-Idf values of terms also revealed how related topics differ. For example, topic 26 is closely related to topics 27 and 25 (cluster a on the lower left of Fig 2A ). Topics 26 and 27 both contain records of developmental process studies mainly in Arabidopsis ( Fig 2B ); however, topic 26 is focused on the impact of light, photoreceptors, and hormones such as gibberellic acids (ga) and brassinosteroids (br), whereas topic 27 is focused on flowering and floral development. Topic 25 is also focused on plant development but differs from topic 27 because it contains records of studies mainly focusing on signaling and auxin with less emphasis on Arabidopsis ( Fig 2C ). These examples also highlight the importance of using multiple top terms to represent the topics. The similarities in cTf-Idfs between topics were also useful for measuring the editorial scope (i.e., diverse, or narrow) of journals publishing plant science papers using a relative topic diversity measure (see Materials and methods ). For example, Proceedings of the National Academy of Sciences , USA has the highest diversity, while Theoretical and Applied Genetics has the lowest ( S4 Fig ). One surprise is the relatively low diversity of American Journal of Botany , which focuses on plant ecology, systematics, development, and genetics. The low diversity is likely due to the relatively larger number of cellular and molecular science records in PubMed, consistent with the identification of relatively few topical areas relevant to studies at the organismal, population, community, and ecosystem levels.
Investigation of the relative prevalence of topics over time reveals topical succession
We next asked whether relationships between topics reflect chronological progression of certain subfields. To address this, we assessed how prevalent topics were over time using dynamic topic modeling [ 19 ]. As shown in Fig 2D , there is substantial fluctuation in where the records are in the 2D space over time. For example, topic 44 (light, leaves, co, synthesis, photosynthesis) is among the topics that existed in 1975 but has diminished gradually since. In 1985, topic 39 (Agrobacterium-based transformation) became dense enough to be visualized. Additional examples include topics 79 (soil heavy metals), 42 (differential expression), and 82 (bacterial community metagenomics), which became prominent in approximately 2005, 2010, and 2020, respectively ( Fig 2D ). In addition, animating the document occupancy in the 2D space over time revealed a broad change in patterns over time: Some initially dense areas became sparse over time and a large number of topics in areas previously only loosely occupied at the turn of the century increased over time ( S5 Data ).
While the 2D representations reveal substantial details on the evolution of topics, comparison over time is challenging because the number of plant science records has grown exponentially ( Fig 1A ). To address this, the records were divided into 50 chronological bins each with approximately 8,400 records to make cross-bin comparisons feasible ( S6 Data ). We should emphasize that, because of the way the chronological bins were split, the number of records for each topic in each bin should be treated as a normalized value relative to all other topics during the same period. Examining this relative prevalence of topics across bins revealed a clear pattern of topic succession over time (one topic evolved into another) and the presence of 5 topical categories ( Fig 3 ). The topics were categorized based on their locally weighted scatterplot smoothing (LOWESS) fits and ordered according to timing of peak frequency ( S7 and S8 Data , see Materials and methods ). In Fig 3 , the relative decrease in document frequency does not mean that research output in a topic is dwindling. Because each row in the heatmap is normalized based on the minimum and maximum values within each topic, there still can be substantial research output in terms of numbers of publications even when the relative frequency is near zero. Thus, a reduced relative frequency of a topic reflects only a below-average growth rate compared with other topical areas.
(A-E) A heat map of relative topic frequency over time reveals 5 topical categories: (A) stable, (B) early, (C) transitional, (D) sigmoidal, and (E) rising. The x axis denotes different time bins with each bin containing a similar number of documents to account for the exponential growth of plant science records over time. The sizes of all bins except the first are drawn to scale based on the beginning and end dates. The y axis lists different topics denoted by the label and top 4 to 5 terms. In each cell, the prevalence of a topic in a time bin is colored according to the min-max normalized cTf-Idf values for that topic. Light blue dotted lines delineate different decades. The arrows left of a subset of topic labels indicate example relationships between topics in topic clusters. Blue boxes with labels a–f indicate topic clusters, which are the same as those in Fig 2 . Connecting lines indicate successional trends. Yellow circles/lines 1 – 3: 3 major transition patterns. The original data are in S5 Data .
https://doi.org/10.1371/journal.pbio.3002612.g003
The first topical category is a stable category with 7 topics mostly established before the 1980s that have since remained stable in terms of prevalence in the plant science records (top of Fig 3A ). These topics represent long-standing plant science research foci, including studies of plant physiology (topics 4, 58, and 81), genetics (topic 61), and medicinal plants (topic 53). The second category contains 8 topics established before the 1980s that have mostly decreased in prevalence since (the early category, Fig 3B ). Two examples are physiological and morphological studies of hormone action (topic 45, the second in the early category) and the characterization of protein, DNA, and RNA (topic 18, the second to last). Unlike other early topics, topic 78 (paleobotany and plant evolution studies, the last topic in Fig 3B ) experienced a resurgence in the early 2000s due to the development of new approaches and databases and changes in research foci [ 20 ].
The 33 topics in the third, transitional category became prominent in the 1980s, 1990s, or even 2000s but have clearly decreased in prevalence ( Fig 3C ). In some cases, the early and the transitional topics became less prevalent because of topical succession—refocusing of earlier topics led to newer ones that either show no clear sign of decrease (the sigmoidal category, Fig 3D ) or continue to increase in prevalence (the rising category, Fig 3E ). Consistent with the notion of topical succession, topics within each topic cluster ( Fig 2 ) were found across topic categories and/or were prominent at different time periods (indicated by colored lines linking topics, Fig 3 ). One example is topics in topic cluster b (connected with light green lines and arrows, compare Figs 2 and 3 ); the study of cation transport (topic 47, the third in the transitional category), prominent in the 1980s and early 1990s, is connected to 5 other topics, namely, another transitional topic 29 (cation channels and their expression) peaking in the 2000s and early 2010s, sigmoidal topics 24 and 28 (stress response, tolerance mechanisms) and 30 (heavy metal transport), which rose to prominence in mid-2000s, and the rising topic 42 (stress transcriptomic studies), which increased in prevalence in the mid-2010s.
The rise and fall of topics can be due to a combination of technological or conceptual breakthroughs, maturity of the field, funding constraints, or publicity. The study of transposable elements (topic 62) illustrates the effect of publicity; the rise in this field coincided with Barbara McClintock’s 1983 Nobel Prize but not with the publication of her studies in the 1950s [ 21 ]. The reduced prevalence in early 2000 likely occurred in part because analysis of transposons became a central component of genome sequencing and annotation studies, rather than dedicated studies. In addition, this example indicates that our approaches, while capable of capturing topical trends, cannot be used to directly infer major papers leading to the growth of a topic.
Three major topical transition patterns signify shifts in research trends
Beyond the succession of specific topics, 3 major transitions in the dynamic topic graph should be emphasized: (1) the relative decreasing trend of early topics in the late 1970s and early 1980s; (2) the rise of transitional topics in late 1980s; and (3) the relative decreasing trend of transitional topics in the late 1990s and early 2000s, which coincided with a radiation of sigmoidal and rising topics (yellow circles, Fig 3 ). The large numbers of topics involved in these transitions suggest major shifts in plant science research. In transition 1, early topics decreased in relative prevalence in the late 1970s to early 1980s, which coincided with the rise of transitional topics over the following decades (circle 1, Fig 3 ). For example, there was a shift from the study of purified proteins such as enzymes (early topic 48, S5A Fig ) to molecular genetic dissection of genes, proteins, and RNA (transitional topic 35, S5B Fig ) enabled by the wider adoption of recombinant DNA and molecular cloning technologies in late 1970s [ 22 ]. Transition 2 (circle 2, Fig 3 ) can be explained by the following breakthroughs in the late 1980s: better approaches to create transgenic plants and insertional mutants [ 23 ], more efficient creation of mutant plant libraries through chemical mutagenesis (e.g., [ 24 ]), and availability of gene reporter systems such as β-glucuronidase [ 25 ]. Because of these breakthroughs, molecular genetics studies shifted away from understanding the basic machinery to understanding the molecular underpinnings of specific processes, such as molecular mechanisms of flower and meristem development and the action of hormones such as auxin (topic 27, S5C Fig ); this type of research was discussed as a future trend in 1988 [ 26 ] and remains prevalent to this date. Another example is gene silencing (topic 12), which became a focal area of study along with the widespread use of transgenic plants [ 27 ].
Transition 3 is the most drastic: A large number of transitional, sigmoidal, and rising topics became prevalent nearly simultaneously at the turn of the century (circle 3, Fig 3 ). This period also coincides with a rapid increase in plant science citations ( Fig 1A ). The most notable breakthroughs included the availability of the first plant genome in 2000 [ 28 ], increasing ease and reduced cost of high-throughput sequencing [ 29 ], development of new mass spectrometry–based platforms for analyzing proteins [ 30 ], and advancements in microscopic and optical imaging approaches [ 31 ]. Advances in genomics and omics technology also led to an increase in stress transcriptomics studies (42, S5D Fig ) as well as studies in many other topics such as epigenetics (topic 11), noncoding RNA analysis (13), genomics and phylogenetics (80), breeding (41), genome sequencing and assembly (60), gene family analysis (23), and metagenomics (82 and 55).
In addition to the 3 major transitions across all topics, there were also transitions within topics revealed by examining the top terms for different time bins (heatmaps, S5 Fig ). Taken together, these observations demonstrate that knowledge about topical evolution can be readily revealed through topic modeling. Such knowledge is typically only available to experts in specific areas and is difficult to summarize manually, as no researcher has a command of the entire plant science literature.
Analysis of taxa studied reveals changes in research trends
Changes in research trends can also be illustrated by examining changes in the taxa being studied over time ( S9 Data ). There is a strong bias in the taxa studied, with the record dominated by research models and economically important taxa ( S6 Fig ). Flowering plants (Magnoliopsida) are found in 93% of records ( S6A Fig ), and the mustard family Brassicaceae dominates at the family level ( S6B Fig ) because the genus Arabidopsis contributes to 13% of plant science records ( Fig 4A ). When examining the prevalence of taxa being studied over time, clear patterns of turnover emerged similar to topical succession ( Figs 4B , S6C, and S6D ; Materials and methods ). Given that Arabidopsis is mentioned in more publications than other species we analyzed, we further examined the trends for Arabidopsis publications. The increase in the normalized number (i.e., relative to the entire plant science corpus) of Arabidopsis records coincided with advocacy of its use as a model system in the late 1980s [ 32 ]. While it remains a major plant model, there has been a decrease in overall Arabidopsis publications relative to all other plant science publications since 2011 (blue line, normalized total, Fig 4C ). Because the same chronological bins, each with same numbers of records, from the topic-over-time analysis ( Fig 3 ) were used, the decrease here does not mean that there were fewer Arabidopsis publications—in fact, the number of Arabidopsis papers has remained steady since 2011. This decrease means that Arabidopsis-related publications represent a relatively smaller proportion of plant science records. Interestingly, this decrease took place much earlier (approximately 2005) and was steeper in the United States (red line, Fig 4C ) than in all countries combined (blue line, Fig 4C ).
(A) Percentage of records mentioning specific genera. (B) Change in the prevalence of genera in plant science records over time. (C) Changes in the normalized numbers of all records (blue) and records from the US (red) mentioning Arabidopsis over time. The lines are LOWESS fits with fraction parameter = 0.2. (D) Topical over (red) and under (blue) representation among 5 genera with the most plant science records. LLR: log 2 likelihood ratios of each topic in each genus. Gray: topic-species combination not significantly enriched at the 5% level based on enrichment p -values adjusted for multiple testing with the Benjamini–Hochberg method [ 33 ]. The data used for plotting are in S9 Data . The statistics for all topics are in S10 Data .
https://doi.org/10.1371/journal.pbio.3002612.g004
Assuming that the normalized number of publications reflects the relative intensity of research activities, one hypothesis for the relative decrease in focus on Arabidopsis is that advances in, for example, plant transformation, genetic manipulation, and genome research have allowed the adoption of more previously nonmodel taxa. Consistent with this, there was a precipitous increase in the number of genera being published in the mid-90s to early 2000s during which approaches for plant transgenics became established [ 34 ], but the number has remained steady since then ( S7A Fig ). The decrease in the proportion of Arabidopsis papers is also negatively correlated with the timing of an increase in the number of draft genomes ( S7B Fig and S9 Data ). It is plausible that genome availability for other species may have contributed to a shift away from Arabidopsis. Strikingly, when we analyzed US National Science Foundation records, we found that the numbers of funded grants mentioning Arabidopsis ( S7C Fig ) have risen and fallen in near perfect synchrony with the normalized number of Arabidopsis publication records (red line, Fig 4C ). This finding likely illustrates the impact of funding on Arabidopsis research.
By considering both taxa information and research topics, we can identify clear differences in the topical areas preferred by researchers using different plant taxa ( Fig 4D and S10 Data ). For example, studies of auxin/light signaling, the circadian clock, and flowering tend to be carried out in Arabidopsis, while quantitative genetic studies of disease resistance tend to be done in wheat and rice, glyphosate research in soybean, and RNA virus research in tobacco. Taken together, joint analyses of topics and species revealed additional details about changes in preferred models over time, and the preferred topical areas for different taxa.
Countries differ in their contributions to plant science and topical preference
We next investigated whether there were geographical differences in topical preference among countries by inferring country information from 330,187 records (see Materials and methods ). The 10 countries with the most records account for 73% of the total, with China and the US contributing to approximately 18% each ( Fig 5A ). The exponential growth in plant science records (green line, Fig 1A ) was in large part due to the rapid rise in annual record numbers in China and India ( Fig 5B ). When we examined the publication growth rates using the top 17 plant science journals, the general patterns remained the same ( S7D Fig ). On the other hand, the US, Japan, Germany, France, and Great Britain had slower rates of growth compared with all non-top 10 countries. The rapid increase in records from China and India was accompanied by a rapid increase in metrics measuring journal impact ( Figs 5C and S8 and S9 Data ). For example, using citation score ( Fig 5C , see Materials and methods ), we found that during a 22-year period China (dark green) and India (light green) rapidly approached the global average (y = 0, yellow), whereas some of the other top 10 countries, particularly the US (red) and Japan (yellow green), showed signs of decrease ( Fig 5C ). It remains to be determined whether these geographical trends reflect changes in priority, investment, and/or interest in plant science research.
(A) Numbers of plant science records for countries with the 10 highest numbers. (B) Percentage of all records from each of the top 10 countries from 1980 to 2020. (C) Difference in citation scores from 1999 to 2020 for the top 10 countries. (D) Shown for each country is the relationship between the citation scores averaged from 1999 to 2020 and the slope of linear fit with year as the predictive variable and citation score as the response variable. The countries with >400 records and with <10% missing impact values are included. Data used for plots (A–D) are in S11 Data . (E) Correlation in topic enrichment scores between the top 10 countries. PCC, Pearson’s correlation coefficient, positive in red, negative in blue. Yellow rectangle: countries with more similar topical preferences. (F) Enrichment scores (LLR, log likelihood ratio) of selected topics among the top 10 countries. Red: overrepresentation, blue: underrepresentation. Gray: topic-country combination that is not significantly enriched at the 5% level based on enrichment p -values adjusted for multiple testing with the Benjamini–Hochberg method (for all topics and plotting data, see S12 Data ).
https://doi.org/10.1371/journal.pbio.3002612.g005
Interestingly, the relative growth/decline in citation scores over time (measured as the slope of linear fit of year versus citation score) was significantly and negatively correlated with average citation score ( Fig 5D ); i.e., countries with lower overall metrics tended to experience the strongest increase in citation scores over time. Thus, countries that did not originally have a strong influence on plant sciences now have increased impact. These patterns were also observed when using H-index or journal rank as metrics ( S8 Fig and S11 Data ) and were not due to increased publication volume, as the metrics were normalized against numbers of records from each country (see Materials and methods ). In addition, the fact that different metrics with different caveats and assumptions yielded consistent conclusions indicates the robustness of our observations. We hypothesize that this may be a consequence of the ease in scientific communication among geographically isolated research groups. It could also be because of the prevalence of online journals that are open access, which makes scientific information more readily accessible. Or it can be due to the increasing international collaboration. In any case, the causes for such regression toward the mean are not immediately clear and should be addressed in future studies.
We also assessed how the plant research foci of countries differ by comparing topical preference (i.e., the degree of enrichment of plant science records in different topics) between countries. For example, Italy and Spain cluster together (yellow rectangle, Fig 5E ) partly because of similar research focusing on allergens (topic 0) and mycotoxins (topic 54) and less emphasis on gene family (topic 23) and stress tolerance (topic 28) studies ( Fig 5F , for the fold enrichment and corrected p -values of all topics, see S12 Data ). There are substantial differences in topical focus between countries ( S9 Fig ). For example, research on new plant compounds associated with herbal medicine (topic 69) is a focus in China but not in the US, but the opposite is true for population genetics and evolution (topic 86) ( Fig 5F ). In addition to revealing how plant science research has evolved over time, topic modeling provides additional insights into differences in research foci among different countries, which are informative for science policy considerations.
In this study, topic modeling revealed clear transitions among research topics, which represent shifts in research trends in plant sciences. One limitation of our study is the bias in the PubMed-based corpus. The cellular, molecular, and physiological aspects of plant sciences are well represented, but there are many fewer records related to evolution, ecology, and systematics. Our use of titles/abstracts from the top 17 plant science journals as positive examples allowed us to identify papers we typically see in these journals, but this may have led to us missing “outlier” articles, which may be the most exciting. Another limitation is the need to assign only one topic to a record when a study is interdisciplinary and straddles multiple topics. Furthermore, a limited number of large, inherently heterogeneous topics were summarized to provide a more concise interpretation, which undoubtedly underrepresents the diversity of plant science research. Despite these limitations, dynamic topic modeling revealed changes in plant science research trends that coincide with major shifts in biological science. While we were interested in identifying conceptual advances, our approach can identify the trend but the underlying causes for such trends, particularly key records leading to the growth in certain topics, still need to be identified. It also remains to be determined which changes in research trends lead to paradigm shifts as defined by Kuhn [ 35 ].
The key terms defining the topics frequently describe various technologies (e.g., topic 38/39: transformation, 40: genome editing, 59: genetic markers, 65: mass spectrometry, 69: nuclear magnetic resonance) or are indicative of studies enabled through molecular genetics and omics technologies (e.g., topic 8/60: genome, 11: epigenetic modifications, 18: molecular biological studies of macromolecules, 13: small RNAs, 61: quantitative genetics, 82/84: metagenomics). Thus, this analysis highlights how technological innovation, particularly in the realm of omics, has contributed to a substantial number of research topics in the plant sciences, a finding that likely holds for other scientific disciplines. We also found that the pattern of topic evolution is similar to that of succession, where older topics have mostly decreased in relative prevalence but appear to have been superseded by newer ones. One example is the rise of transcriptome-related topics and the correlated, reduced focus on regulation at levels other than transcription. This raises the question of whether research driven by technology negatively impacts other areas of research where high-throughput studies remain challenging.
One observation on the overall trends in plant science research is the approximately 10-year cycle in major shifts. One hypothesis is related to not only scientific advances but also to the fashion-driven aspect of science. Nonetheless, given that there were only 3 major shifts and the sample size is small, it is difficult to speculate as to why they happened. By analyzing the country of origin, we found that China and India have been the 2 major contributors to the growth in the plant science records in the last 20 years. Our findings also show an equalizing trend in global plant science where countries without a strong plant science publication presence have had an increased impact over the last 20 years. In addition, we identified significant differences in research topics between countries reflecting potential differences in investment and priorities. Such information is important for discerning differences in research trends across countries and can be considered when making policy decisions about research directions.
Materials and methods
Collection and preprocessing of a candidate plant science corpus.
For reproducibility purposes, a random state value of 20220609 was used throughout the study. The PubMed baseline files containing citation information ( ftp://ftp.ncbi.nlm.nih.gov/pubmed/baseline/ ) were downloaded on November 11, 2021. To narrow down the records to plant science-related citations, a candidate citation was identified as having, within the titles and/or abstracts, at least one of the following words: “plant,” “plants,” “botany,” “botanical,” “planta,” and “plantarum” (and their corresponding upper case and plural forms), or plant taxon identifiers from NCBI Taxonomy ( https://www.ncbi.nlm.nih.gov/taxonomy ) or USDA PLANTS Database ( https://plants.sc.egov.usda.gov/home ). Note the search terms used here have nothing to do with the values of the keyword field in PubMed records. The taxon identifiers include all taxon names including and at taxonomic levels below “Viridiplantae” till the genus level (species names not used). This led to 51,395 search terms. After looking for the search terms, qualified entries were removed if they were duplicated, lacked titles and/or abstracts, or were corrections, errata, or withdrawn articles. This left 1,385,417 citations, which were considered the candidate plant science corpus (i.e., a collection of texts). For further analysis, the title and abstract for each citation were combined into a single entry. Text was preprocessed by lowercasing, removing stop-words (i.e., common words), removing non-alphanumeric and non-white space characters (except Greek letters, dashes, and commas), and applying lemmatization (i.e., grouping inflected forms of a word as a single word) for comparison. Because lemmatization led to truncated scientific terms, it was not included in the final preprocessing pipeline.
Definition of positive/negative examples
Upon closer examination, a large number of false positives were identified in the candidate plant science records. To further narrow down citations with a plant science focus, text classification was used to distinguish plant science and non-plant science articles (see next section). For the classification task, a negative set (i.e., non-plant science citations) was defined as entries from 7,360 journals that appeared <20 times in the filtered data (total = 43,329, journal candidate count, S1 Data ). For the positive examples (i.e., true plant science citations), 43,329 plant science citations (positive examples) were sampled from 17 established plant science journals each with >2,000 entries in the filtered dataset: “Plant physiology,” “Frontiers in plant science,” “Planta,” “The Plant journal: for cell and molecular biology,” “Journal of experimental botany,” “Plant molecular biology,” “The New phytologist,” “The Plant cell,” “Phytochemistry,” “Plant & cell physiology,” “American journal of botany,” “Annals of botany,” “BMC plant biology,” “Tree physiology,” “Molecular plant-microbe interactions: MPMI,” “Plant biology,” and “Plant biotechnology journal” (journal candidate count, S1 Data ). Plant biotechnology journal was included, but only 1,894 records remained after removal of duplicates, articles with missing info, and/or withdrawn articles. The positive and negative sets were randomly split into training and testing subsets (4:1) while maintaining a 1:1 positive-to-negative ratio.
Text classification based on Tf and Tf-Idf
Instead of using the preprocessed text as features for building classification models directly, text embeddings (i.e., representations of texts in vectors) were used as features. These embeddings were generated using 4 approaches (model summary, S1 Data ): Term-frequency (Tf), Tf-Idf [ 36 ], Word2Vec [ 37 ], and BERT [ 6 ]. The Tf- and Tf-Idf-based features were generated with CountVectorizer and TfidfVectorizer, respectively, from Scikit-Learn [ 38 ]. Different maximum features (1e4 to 1e5) and n-gram ranges (uni-, bi-, and tri-grams) were tested. The features were selected based on the p- value of chi-squared tests testing whether a feature had a higher-than-expected value among the positive or negative classes. Four different p- value thresholds were tested for feature selection. The selected features were then used to retrain vectorizers with the preprocessed training texts to generate feature values for classification. The classification model used was XGBoost [ 39 ] with 5 combinations of the following hyperparameters tested during 5-fold stratified cross-validation: min_child_weight = (1, 5, 10), gamma = (0.5, 1, 1.5, 2.5), subsample = (0.6, 0.8, 1.0), colsample_bytree = (0.6, 0.8, 1.0), and max_depth = (3, 4, 5). The rest of the hyperparameters were held constant: learning_rate = 0.2, n_estimators = 600, objective = binary:logistic. RandomizedSearchCV from Scikit-Learn was used for hyperparameter tuning and cross-validation with scoring = F1-score.
Because the Tf-Idf model had a relatively high model performance and was relatively easy to interpret (terms are frequency-based, instead of embedding-based like those generated by Word2Vec and BERT), the Tf-Idf model was selected as input to SHapley Additive exPlanations (SHAP; [ 14 ]) to assess the importance of terms. Because the Tf-Idf model was based on XGBoost, a tree-based algorithm, the TreeExplainer module in SHAP was used to determine a SHAP value for each entry in the training dataset for each Tf-Idf feature. The SHAP value indicates the degree to which a feature positively or negatively affects the underlying prediction. The importance of a Tf-Idf feature was calculated as the average SHAP value of that feature among all instances. Because a Tf-Idf feature is generated based on a specific term, the importance of the Tf-Idf feature indicates the importance of the associated term.
Text classification based on Word2Vec
The preprocessed texts were first split into train, validation, and test subsets (8:1:1). The texts in each subset were converted to 3 n-gram lists: a unigram list obtained by splitting tokens based on the space character, or bi- and tri-gram lists built with Gensim [ 40 ]. Each n-gram list of the training subset was next used to fit a Skip-gram Word2Vec model with vector_size = 300, window = 8, min_count = (5, 10, or 20), sg = 1, and epochs = 30. The Word2Vec model was used to generate word embeddings for train, validate, and test subsets. In the meantime, a tokenizer was trained with train subset unigrams using Tensorflow [ 41 ] and used to tokenize texts in each subset and turn each token into indices to use as features for training text classification models. To ensure all citations had the same number of features (500), longer texts were truncated, and shorter ones were zero-padded. A deep learning model was used to train a text classifier with an input layer the same size as the feature number, an attention layer incorporating embedding information for each feature, 2 bidirectional Long-Short-Term-Memory layers (15 units each), a dense layer (64 units), and a final, output layer with 2 units. During training, adam, accuracy, and sparse_categorical_crossentropy were used as the optimizer, evaluation metric, and loss function, respectively. The training process lasted 30 epochs with early stopping if validation loss did not improve in 5 epochs. An F1 score was calculated for each n-gram list and min_count parameter combination to select the best model (model summary, S1 Data ).
Text classification based on BERT models
Two pretrained models were used for BERT-based classification: DistilBERT (Hugging face repository [ 42 ] model name and version: distilbert-base-uncased [ 43 ]) and SciBERT (allenai/scibert-scivocab-uncased [ 16 ]). In both cases, tokenizers were retrained with the training data. BERT-based models had the following architecture: the token indices (512 values for each token) and associated masked values as input layers, pretrained BERT layer (512 × 768) excluding outputs, a 1D pooling layer (768 units), a dense layer (64 units), and an output layer (2 units). The rest of the training parameters were the same as those for Word2Vec-based models, except training lasted for 20 epochs. Cross-validation F1-scores for all models were compared and used to select the best model for each feature extraction method, hyperparameter combination, and modeling algorithm or architecture (model summary, S1 Data ). The best model was the Word2Vec-based model (min_count = 20, window = 8, ngram = 3), which was applied to the candidate plant science corpus to identify a set of plant science citations for further analysis. The candidate plant science records predicted as being in the positive class (421,658) by the model were collectively referred to as the “plant science corpus.”
Plant science record classification
In PubMed, 1,384,718 citations containing “plant” or any plant taxon names (from the phylum to genus level) were considered candidate plant science citations. To further distinguish plant science citations from those in other fields, text classification models were trained using titles and abstracts of positive examples consisting of citations from 17 plant science journals, each with >2,000 entries in PubMed, and negative examples consisting of records from journals with fewer than 20 entries in the candidate set. Among 4 models tested the best model (built with Word2Vec embeddings) had a cross validation F1 of 0.964 (random guess F1 = 0.5, perfect model F1 = 1, S1 Data ). When testing the model using 17,330 testing set citations independent from the training set, the F1 remained high at 0.961.
We also conducted another analysis attempting to use the MeSH term “Plants” as a benchmark. Records with the MeSH term “Plants” also include pharmaceutical studies of plants and plant metabolites or immunological studies of plants as allergens in journals that are not generally considered plant science journals (e.g., Acta astronautica , International journal for parasitology , Journal of chromatography ) or journals from local scientific societies (e.g., Acta pharmaceutica Hungarica , Huan jing ke xue , Izvestiia Akademii nauk . Seriia biologicheskaia ). Because we explicitly labeled papers from such journals as negative examples, we focused on 4,004 records with the “Plants” MeSH term published in the 17 plant science journals that were used as positive instances and found that 88.3% were predicted as the positive class. Thus, based on the MeSH term, there is an 11.7% false prediction rate.
We also enlisted 5 plant science colleagues (3 advanced graduate students in plant biology and genetic/genome science graduate programs, 1 postdoctoral breeder/quantitative biologist, and 1 postdoctoral biochemist/geneticist) to annotate 100 randomly selected abstracts as a reviewer suggested. Each record was annotated by 2 colleagues. Among 85 entries where the annotations are consistent between annotators, 48 were annotated as negative but with 7 predicted as positive (false positive rate = 14.6%) and 37 were annotated as positive but with 4 predicted as negative (false negative rate = 10.8%). To further benchmark the performance of the text classification model, we identified another 12 journals that focus on plant science studies to use as benchmarks: Current opinion in plant biology (number of articles: 1,806), Trends in plant science (1,723), Functional plant biology (1,717), Molecular plant pathology (1,573), Molecular plant (1,141), Journal of integrative plant biology (1,092), Journal of plant research (1,032), Physiology and molecular biology of plants (830), Nature plants (538), The plant pathology journal (443). Annual review of plant biology (417), and The plant genome (321). Among the 12,611 candidate plant science records, 11,386 were predicted as positive. Thus, there is a 9.9% false negative rate.
Global topic modeling
BERTopic [ 15 ] was used for preliminary topic modeling with n-grams = (1,2) and with an embedding initially generated by DistilBERT, SciBERT, or BioBERT (dmis-lab/biobert-base-cased-v1.2; [ 44 ]). The embedding models converted preprocessed texts to embeddings. The topics generated based on the 3 embeddings were similar ( S2 Data ). However, SciBERT-, BioBERT-, and distilBERT-based embedding models had different numbers of outlier records (268,848, 293,790, and 323,876, respectively) with topic index = −1. In addition to generating the fewest outliers, the SciBERT-based model led to the highest number of topics. Therefore, SciBERT was chosen as the embedding model for the final round of topic modeling. Modeling consisted of 3 steps. First, document embeddings were generated with SentenceTransformer [ 45 ]. Second, a clustering model to aggregate documents into clusters using hdbscan [ 46 ] was initialized with min_cluster_size = 500, metric = euclidean, cluster_selection_method = eom, min_samples = 5. Third, the embedding and the initialized hdbscan model were used in BERTopic to model topics with neighbors = 10, nr_topics = 500, ngram_range = (1,2). Using these parameters, 90 topics were identified. The initial topic assignments were conservative, and 241,567 records were considered outliers (i.e., documents not assigned to any of the 90 topics). After assessing the prediction scores of all records generated from the fitted topic models, the 95-percentile score was 0.0155. This score was used as the threshold for assigning outliers to topics: If the maximum prediction score was above the threshold and this maximum score was for topic t , then the outlier was assigned to t . After the reassignment, 49,228 records remained outliers. To assess if some of the outliers were not assigned because they could be assigned to multiple topics, the prediction scores of the records were used to put records into 100 clusters using k- means. Each cluster was then assessed to determine if the outlier records in a cluster tended to have higher prediction scores across multiple topics ( S2 Fig ).
Topics that are most and least well connected to other topics
The most well-connected topics in the network include topic 24 (stress mechanisms, median cosine similarity = 0.36), topic 42 (genes, stress, and transcriptomes, 0.34), and topic 35 (molecular genetics, 0.32, all t test p -values < 1 × 10 −22 ). The least connected topics include topic 0 (allergen research, median cosine similarity = 0.12), topic 21 (clock biology, 0.12), topic 1 (tissue culture, 0.15), and topic 69 (identification of compounds with spectroscopic methods, 0.15; all t test p- values < 1 × 10 −24 ). Topics 0, 1, and 69 are specialized topics; it is surprising that topic 21 is not as well connected as explained in the main text.
Analysis of documents based on the topic model
Topical diversity among top journals with the most plant science records
Using a relative topic diversity measure (ranging from 0 to 10), we found that there was a wide range of topical diversity among 20 journals with the largest numbers of plant science records ( S3 Fig ). The 4 journals with the highest relative topical diversities are Proceedings of the National Academy of Sciences , USA (9.6), Scientific Reports (7.1), Plant Physiology (6.7), and PLOS ONE (6.4). The high diversities are consistent with the broad, editorial scopes of these journals. The 4 journals with the lowest diversities are American Journal of Botany (1.6), Oecologia (0.7), Plant Disease (0.7), and Theoretical and Applied Genetics (0.3), which reflects their discipline-specific focus and audience of classical botanists, ecologists, plant pathologists, and specific groups of geneticists.
Dynamic topic modeling
The codes for dynamic modeling were based on _topic_over_time.py in BERTopics and modified to allow additional outputs for debugging and graphing purposes. The plant science citations were binned into 50 subsets chronologically (for timestamps of bins, see S5 Data ). Because the numbers of documents increased exponentially over time, instead of dividing them based on equal-sized time intervals, which would result in fewer records at earlier time points and introduce bias, we divided them into time bins of similar size (approximately 8,400 documents). Thus, the earlier time subsets had larger time spans compared with later time subsets. If equal-size time intervals were used, the numbers of documents between the intervals would differ greatly; the earlier time points would have many fewer records, which may introduce bias. Prior to binning the subsets, the publication dates were converted to UNIX time (timestamp) in seconds; the plant science records start in 1917-11-1 (timestamp = −1646247600.0) and end in 2021-1-1 (timestamp = 1609477201). The starting dates and corresponding timestamps for the 50 subsets including the end date are in S6 Data . The input data included the preprocessed texts, topic assignments of records from global topic modeling, and the binned timestamps of records. Three additional parameters were set for topics_over_time, namely, nr_bin = 50 (number of bins), evolution_tuning = True, and global_tuning = False. The evolution_tuning parameter specified that averaged c-Tf-Idf values for a topic be calculated in neighboring time bins to reduce fluctuation in c-Tf-Idf values. The global_tuning parameter was set to False because of the possibility that some nonexisting terms could have a high c-Tf-Idf for a time bin simply because there was a high global c-Tf-Idf value for that term.
The binning strategy based on similar document numbers per bin allowed us to increase signal particularly for publications prior to the 90s. This strategy, however, may introduce more noise for bins with smaller time durations (i.e., more recent bins) because of publication frequencies (there can be seasonal differences in the number of papers published, biased toward, e.g., the beginning of the year or the beginning of a quarter). To address this, we examined the relative frequencies of each topic over time ( S7 Data ), but we found that recent time bins had similar variances in relative frequencies as other time bins. We also moderated the impact of variation using LOWESS (10% to 30% of the data points were used for fitting the trend lines) to determine topical trends for Fig 3 . Thus, the influence of the noise introduced via our binning strategy is expected to be minimal.
Topic categories and ordering
The topics were classified into 5 categories with contrasting trends: stable, early, transitional, sigmoidal, and rising. To define which category a topic belongs to, the frequency of documents over time bins for each topic was analyzed using 3 regression methods. We first tried 2 forecasting methods: recursive autoregressor (the ForecasterAutoreg class in the skforecast package) and autoregressive integrated moving average (ARIMA implemented in the pmdarima package). In both cases, the forecasting results did not clearly follow the expected trend lines, likely due to the low numbers of data points (relative frequency values), which resulted in the need to extensively impute missing data. Thus, as a third approach, we sought to fit the trendlines with the data points using LOWESS (implemented in the statsmodels package) and applied additional criteria for assigning topics to categories. When fitting with LOWESS, 3 fraction parameters (frac, the fraction of the data used when estimating each y-value) were evaluated (0.1, 0.2, 0.3). While frac = 0.3 had the smallest errors for most topics, in situations where there were outliers, frac = 0.2 or 0.1 was chosen to minimize mean squared errors ( S7 Data ).
The topics were classified into 5 categories based on the slopes of the fitted line over time: (1) stable: topics with near 0 slopes over time; (2) early: topics with negative (<−0.5) slopes throughout (with the exception of topic 78, which declined early on but bounced back by the late 1990s); (3) transitional: early positive (>0.5) slopes followed by negative slopes at later time points; (4) sigmoidal: early positive slopes followed by zero slopes at later time points; and (5) rising: continuously positive slopes. For each topic, the LOWESS fits were also used to determine when the relative document frequency reached its peak, first reaching a threshold of 0.6 (chosen after trial and error for a range of 0.3 to 0.9), and the overall trend. The topics were then ordered based on (1) whether they belonged to the stable category or not; (2) whether the trends were decreasing, stable, or increasing; (3) the time the relative document frequency first reached 0.6; and (4) the time that the overall peak was reached ( S8 Data ).
Taxa information
To identify a taxon or taxa in all plant science records, NCBI Taxonomy taxdump datasets were downloaded from the NCBI FTP site ( https://ftp.ncbi.nlm.nih.gov/pub/taxonomy/new_taxdump/ ) on September 20, 2022. The highest-level taxon was Viridiplantae, and all its child taxa were parsed and used as queries in searches against the plant science corpus. In addition, a species-over-time analysis was conducted using the same time bins as used for dynamic topic models. The number of records in different time bins for top taxa are in the genus, family, order, and additional species level sheet in S9 Data . The degree of over-/underrepresentation of a taxon X in a research topic T was assessed using the p -value of a Fisher’s exact test for a 2 × 2 table consisting of the numbers of records in both X and T, in X but not T, in T but not X, and in neither ( S10 Data ).
For analysis of plant taxa with genome information, genome data of taxa in Viridiplantae were obtained from the NCBI Genome data-hub ( https://www.ncbi.nlm.nih.gov/data-hub/genome ) on October 28, 2022. There were 2,384 plant genome assemblies belonging to 1,231 species in 559 genera (genome assembly sheet, S9 Data ). The date of the assembly was used as a proxy for the time when a genome was sequenced. However, some species have updated assemblies and have more recent data than when the genome first became available.
Taxa being studied in the plant science records
Flowering plants (Magnoliopsida) are found in 93% of records, while most other lineages are discussed in <1% of records, with conifers and related species being exceptions (Acrogynomsopermae, 3.5%, S6A Fig ). At the family level, the mustard (Brassicaceae), grass (Poaceae), pea (Fabaceae), and nightshade (Solanaceae) families are in 51% of records ( S6B Fig ). The prominence of the mustard family in plant science research is due to the Brassica and Arabidopsis genera ( Fig 4A ). When examining the prevalence of taxa being studied over time, clear patterns of turnovers emerged ( Figs 4B , S6C, and S6D ). While the study of monocot species (Liliopsida) has remained steady, there was a significant uptick in the prevalence of eudicot (eudicotyledon) records in the late 90s ( S6C Fig ), which can be attributed to the increased number of studies in the mustard, myrtle (Myrtaceae), and mint (Lamiaceae) families among others ( S6D Fig ). At the genus level, records mentioning Gossypium (cotton), Phaseolus (bean), Hordeum (wheat), and Zea (corn), similar to the topics in the early category, were prevalent till the 1980s or 1990s but have mostly decreased in number since ( Fig 4B ). In contrast, Capsicum , Arabidopsis , Oryza , Vitus , and Solanum research has become more prevalent over the last 20 years.
Geographical information for the plant science corpus
The geographical information (country) of authors in the plant science corpus was obtained from the address (AD) fields of first authors in Medline XML records accessible through the NCBI EUtility API ( https://www.ncbi.nlm.nih.gov/books/NBK25501/ ). Because only first author affiliations are available for records published before December 2014, only the first author’s location was considered to ensure consistency between records before and after that date. Among the 421,658 records in the plant science corpus, 421,585 had Medline records and 421,276 had unique PMIDs. Among the records with unique PMIDs, 401,807 contained address fields. For each of the remaining records, the AD field content was split into tokens with a “,” delimiter, and the token likely containing geographical info (referred to as location tokens) was selected as either the last token or the second to last token if the last token contained “@” indicating the presence of an email address. Because of the inconsistency in how geographical information was described in the location tokens (e.g., country, state, city, zip code, name of institution, and different combinations of the above), the following 4 approaches were used to convert location tokens into countries.
The first approach was a brute force search where full names and alpha-3 codes of current countries (ISO 3166–1), current country subregions (ISO 3166–2), and historical country (i.e., country that no longer exists, ISO 3166–3) were used to search the address fields. To reduce false positives using alpha-3 codes, a space prior to each code was required for the match. The first approach allowed the identification of 361,242, 16,573, and 279,839 records with current country, historical country, and subregion information, respectively. The second method was the use of a heuristic based on common address field structures to identify “location strings” toward the end of address fields that likely represent countries, then the use of the Python pycountry module to confirm the presence of country information. This approach led to 329,025 records with country information. The third approach was to parse first author email addresses (90,799 records), recover top-level domain information, and use country code Top Level Domain (ccTLD) data from the ISO 3166 Wikipedia page to define countries (72,640 records). Only a subset of email addresses contains country information because some are from companies (.com), nonprofit organizations (.org), and others. Because a large number of records with address fields still did not have country information after taking the above 3 approaches, another approach was implemented to query address fields against a locally installed Nominatim server (v.4.2.3, https://github.com/mediagis/nominatim-docker ) using OpenStreetMap data from GEOFABRIK ( https://www.geofabrik.de/ ) to find locations. Initial testing indicated that the use of full address strings led to false positives, and the computing resource requirement for running the server was high. Thus, only location strings from the second approach that did not lead to country information were used as queries. Because multiple potential matches were returned for each query, the results were sorted based on their location importance values. The above steps led to an additional 72,401 records with country information.
Examining the overlap in country information between approaches revealed that brute force current country and pycountry searches were consistent 97.1% of the time. In addition, both approaches had high consistency with the email-based approach (92.4% and 93.9%). However, brute force subregion and Nominatim-based predictions had the lowest consistencies with the above 3 approaches (39.8% to 47.9%) and each other. Thus, a record’s country information was finalized if the information was consistent between any 2 approaches, except between the brute force subregion and Nominatim searches. This led to 330,328 records with country information.
Topical and country impact metrics
To determine annual country impact, impact scores were determined in the same way as that for annual topical impact, except that values for different countries were calculated instead of topics ( S8 Data ).
Topical preferences by country
To determine topical preference for a country C , a 2 × 2 table was established with the number of records in topic T from C , the number of records in T but not from C , the number of non- T records from C , and the number of non- T records not from C . A Fisher’s exact test was performed for each T and C combination, and the resulting p -values were corrected for multiple testing with the Bejamini–Hochberg method (see S12 Data ). The preference of T in C was defined as the degree of enrichment calculated as log likelihood ratio of values in the 2 × 2 table. Topic 5 was excluded because >50% of the countries did not have records for this topic.
The top 10 countries could be classified into a China–India cluster, an Italy–Spain cluster, and remaining countries (yellow rectangles, Fig 5E ). The clustering of Italy and Spain is partly due to similar research focusing on allergens (topic 0) and mycotoxins (topic 54) and less emphasis on gene family (topic 23) and stress tolerance (topic 28) studies ( Figs 5F and S9 ). There are also substantial differences in topical focus between countries. For example, plant science records from China tend to be enriched in hyperspectral imaging and modeling (topic 9), gene family studies (topic 23), stress biology (topic 28), and research on new plant compounds associated with herbal medicine (topic 69), but less emphasis on population genetics and evolution (topic 86, Fig 5F ). In the US, there is a strong focus on insect pest resistance (topic 75), climate, community, and diversity (topic 83), and population genetics and evolution but less focus on new plant compounds. In summary, in addition to revealing how plant science research has evolved over time, topic modeling provides additional insights into differences in research foci among different countries.
Supporting information
S1 fig. plant science record classification model performance..
(A–C) Distributions of prediction probabilities (y_prob) of (A) positive instances (plant science records), (B) negative instances (non-plant science records), and (C) positive instances with the Medical Subject Heading “Plants” (ID = D010944). The data are color coded in blue and orange if they are correctly and incorrectly predicted, respectively. The lower subfigures contain log10-transformed x axes for the same distributions as the top subfigure for better visualization of incorrect predictions. (D) Prediction probability distribution for candidate plant science records. Prediction probabilities plotted here are available in S13 Data .
https://doi.org/10.1371/journal.pbio.3002612.s001
S2 Fig. Relationships between outlier clusters and the 90 topics.
(A) Heatmap demonstrating that some outlier clusters tend to have high prediction scores for multiple topics. Each cell shows the average prediction score of a topic for records in an outlier cluster. (B) Size of outlier clusters.
https://doi.org/10.1371/journal.pbio.3002612.s002
S3 Fig. Cosine similarities between topics.
(A) Heatmap showing cosine similarities between topic pairs. Top-left: hierarchical clustering of the cosine similarity matrix using the Ward algorithm. The branches are colored to indicate groups of related topics. (B) Topic labels and names. The topic ordering was based on hierarchical clustering of topics. Colored rectangles: neighboring topics with >0.5 cosine similarities.
https://doi.org/10.1371/journal.pbio.3002612.s003
S4 Fig. Relative topical diversity for 20 journals.
The 20 journals with the most plant science records are shown. The journal names were taken from the journal list in PubMed ( https://www.nlm.nih.gov/bsd/serfile_addedinfo.html ).
https://doi.org/10.1371/journal.pbio.3002612.s004
S5 Fig. Topical frequency and top terms during different time periods.
(A-D) Different patterns of topical frequency distributions for example topics (A) 48, (B) 35, (C) 27, and (D) 42. For each topic, the top graph shows the frequency of topical records in each time bin, which are the same as those in Fig 3 (green line), and the end date for each bin is indicated. The heatmap below each line plot depicts whether a term is among the top terms in a time bin (yellow) or not (blue). Blue dotted lines delineate different decades (see S5 Data for the original frequencies, S6 Data for the LOWESS fitted frequencies and the top terms for different topics/time bins).
https://doi.org/10.1371/journal.pbio.3002612.s005
S6 Fig. Prevalence of records mentioning different taxonomic groups in Viridiplantae.
(A, B) Percentage of records mentioning specific taxa at the ( A) major lineage and (B) family levels. (C, D) The prevalence of taxon mentions over time at the (C) major lineage and (E) family levels. The data used for plotting are available in S9 Data .
https://doi.org/10.1371/journal.pbio.3002612.s006
S7 Fig. Changes over time.
(A) Number of genera being mentioned in plant science records during different time bins (the date indicates the end date of that bin, exclusive). (B) Numbers of genera (blue) and organisms (salmon) with draft genomes available from National Center of Biotechnology Information in different years. (C) Percentage of US National Science Foundation (NSF) grants mentioning the genus Arabidopsis over time with peak percentage and year indicated. The data for (A–C) are in S9 Data . (D) Number of plant science records in the top 17 plant science journals from the USA (red), Great Britain (GBR) (orange), India (IND) (light green), and China (CHN) (dark green) normalized against the total numbers of publications of each country over time in these 17 journals. The data used for plotting can be found in S11 Data .
https://doi.org/10.1371/journal.pbio.3002612.s007
S8 Fig. Change in country impact on plant science over time.
(A, B) Difference in 2 impact metrics from 1999 to 2020 for the 10 countries with the highest number of plant science records. (A) H-index. (B) SCImago Journal Rank (SJR). (C, D) Plots show the relationships between the impact metrics (H-index in (C) , SJR in (D) ) averaged from 1999 to 2020 and the slopes of linear fits with years as the predictive variable and impact metric as the response variable for different countries (A3 country codes shown). The countries with >400 records and with <10% missing impact values are included. The data used for plotting can be found in S11 Data .
https://doi.org/10.1371/journal.pbio.3002612.s008
S9 Fig. Country topical preference.
Enrichment scores (LLR, log likelihood ratio) of topics for each of the top 10 countries. Red: overrepresentation, blue: underrepresentation. The data for plotting can be found in S12 Data .
https://doi.org/10.1371/journal.pbio.3002612.s009
S1 Data. Summary of source journals for plant science records, prediction models, and top Tf-Idf features.
Sheet–Candidate plant sci record j counts: Number of records from each journal in the candidate plant science corpus (before classification). Sheet—Plant sci record j count: Number of records from each journal in the plant science corpus (after classification). Sheet–Model summary: Model type, text used (txt_flag), and model parameters used. Sheet—Model performance: Performance of different model and parameter combinations on the validation data set. Sheet–Tf-Idf features: The average SHAP values of Tf-Idf (Term frequency-Inverse document frequency) features associated with different terms. Sheet–PubMed number per year: The data for PubMed records in Fig 1A . Sheet–Plant sci record num per yr: The data for the plant science records in Fig 1A .
https://doi.org/10.1371/journal.pbio.3002612.s010
S2 Data. Numbers of records in topics identified from preliminary topic models.
Sheet–Topics generated with a model based on BioBERT embeddings. Sheet–Topics generated with a model based on distilBERT embeddings. Sheet–Topics generated with a model based on SciBERT embeddings.
https://doi.org/10.1371/journal.pbio.3002612.s011
S3 Data. Final topic model labels and top terms for topics.
Sheet–Topic label: The topic index and top 10 terms with the highest cTf-Idf values. Sheets– 0 to 89: The top 50 terms and their c-Tf-Idf values for topics 0 to 89.
https://doi.org/10.1371/journal.pbio.3002612.s012
S4 Data. UMAP representations of different topics.
For a topic T , records in the UMAP graph are colored red and records not in T are colored gray.
https://doi.org/10.1371/journal.pbio.3002612.s013
S5 Data. Temporal relationships between published documents projected onto 2D space.
The 2D embedding generated with UMAP was used to plot document relationships for each year. The plots from 1975 to 2020 were compiled into an animation.
https://doi.org/10.1371/journal.pbio.3002612.s014
S6 Data. Timestamps and dates for dynamic topic modeling.
Sheet–bin_timestamp: Columns are: (1) order index; (2) bin_idx–relative positions of bin labels; (3) bin_timestamp–UNIX time in seconds; and (4) bin_date–month/day/year. Sheet–Topic frequency per timestamp: The number of documents in each time bin for each topic. Sheets–LOWESS fit 0.1/0.2/0.3: Topic frequency per timestamp fitted with the fraction parameter of 0.1, 0.2, or 0.3. Sheet—Topic top terms: The top 5 terms for each topic in each time bin.
https://doi.org/10.1371/journal.pbio.3002612.s015
S7 Data. Locally weighted scatterplot smoothing (LOWESS) of topical document frequencies over time.
There are 90 scatter plots, one for each topic, where the x axis is time, and the y axis is the document frequency (blue dots). The LOWESS fit is shown as orange points connected with a green line. The category a topic belongs to and its order in Fig 3 are labeled on the top left corner. The data used for plotting are in S6 Data .
https://doi.org/10.1371/journal.pbio.3002612.s016
S8 Data. The 4 criteria used for sorting topics.
Peak: the time when the LOWESS fit of the frequencies of a topic reaches maximum. 1st_reach_thr: the time when the LOWESS fit first reaches a threshold of 60% maximal frequency (peak value). Trend: upward (1), no change (0), or downward (−1). Stable: whether a topic belongs to the stable category (1) or not (0).
https://doi.org/10.1371/journal.pbio.3002612.s017
S9 Data. Change in taxon record numbers and genome assemblies available over time.
Sheet–Genus: Number of records mentioning a genus during different time periods (in Unix timestamp) for the top 100 genera. Sheet–Genus: Number of records mentioning a family during different time periods (in Unix timestamp) for the top 100 families. Sheet–Genus: Number of records mentioning an order during different time periods (in Unix timestamp) for the top 20 orders. Sheet–Species levels: Number of records mentioning 12 selected taxonomic levels higher than the order level during different time periods (in Unix timestamp). Sheet–Genome assembly: Plant genome assemblies available from NCBI as of October 28, 2022. Sheet–Arabidopsis NSF: Absolute and normalized numbers of US National Science Foundation funded proposals mentioning Arabidopsis in proposal titles and/or abstracts.
https://doi.org/10.1371/journal.pbio.3002612.s018
S10 Data. Taxon topical preference.
Sheet– 5 genera LLR: The log likelihood ratio of each topic in each of the top 5 genera with the highest numbers of plant science records. Sheets– 5 genera: For each genus, the columns are: (1) topic; (2) the Fisher’s exact test p -value (Pvalue); (3–6) numbers of records in topic T and in genus X (n_inT_inX), in T but not in X (n_inT_niX), not in T but in X (n_niT_inX), and not in T and X (n_niT_niX) that were used to construct 2 × 2 tables for the tests; and (7) the log likelihood ratio generated with the 2 × 2 tables. Sheet–corrected p -value: The 4 values for generating LLRs were used to conduct Fisher’s exact test. The p -values obtained for each country were corrected for multiple testing.
https://doi.org/10.1371/journal.pbio.3002612.s019
S11 Data. Impact metrics of countries in different years.
Sheet–country_top25_year_count: number of total publications and publications per year from the top 25 countries with the most plant science records. Sheet—country_top25_year_top17j: number of total publications and publications per year from the top 25 countries with the highest numbers of plant science records in the 17 plant science journals used as positive examples. Sheet–prank: Journal percentile rank scores for countries (3-letter country codes following https://www.iban.com/country-codes ) in different years from 1999 to 2020. Sheet–sjr: Scimago Journal rank scores. Sheet–hidx: H-Index scores. Sheet–cite: Citation scores.
https://doi.org/10.1371/journal.pbio.3002612.s020
S12 Data. Topical enrichment for the top 10 countries with the highest numbers of plant science publications.
Sheet—Log likelihood ratio: For each country C and topic T, it is defined as log((a/b)/(c/d)) where a is the number of papers from C in T, b is the number from C but not in T, c is the number not from C but in T, d is the number not from C and not in T. Sheet: corrected p -value: The 4 values, a, b, c, and d, were used to conduct Fisher’s exact test. The p -values obtained for each country were corrected for multiple testing.
https://doi.org/10.1371/journal.pbio.3002612.s021
S13 Data. Text classification prediction probabilities.
This compressed file contains the PubMed ID (PMID) and the prediction probabilities (y_pred) of testing data with both positive and negative examples (pred_prob_testing), plant science candidate records with the MeSH term “Plants” (pred_prob_candidates_with_mesh), and all plant science candidate records (pred_prob_candidates_all). The prediction probability was generated using the Word2Vec text classification models for distinguishing positive (plant science) and negative (non-plant science) records.
https://doi.org/10.1371/journal.pbio.3002612.s022
Acknowledgments
We thank Maarten Grootendorst for discussions on topic modeling. We also thank Stacey Harmer, Eva Farre, Ning Jiang, and Robert Last for discussion on their respective research fields and input on how to improve this study and Rudiger Simon for the suggestion to examine differences between countries. We also thank Mae Milton, Christina King, Edmond Anderson, Jingyao Tang, Brianna Brown, Kenia Segura Abá, Eleanor Siler, Thilanka Ranaweera, Huan Chen, Rajneesh Singhal, Paulo Izquierdo, Jyothi Kumar, Daniel Shiu, Elliott Shiu, and Wiggler Catt for their good ideas, personal and professional support, collegiality, fun at parties, as well as the trouble they have caused, which helped us improve as researchers, teachers, mentors, and parents.
- View Article
- PubMed/NCBI
- Google Scholar
- 2. Blei DM, Lafferty JD. Topic Models. In: Srivastava A, Sahami M, editors. Text Mining. Cambridge: Chapman and Hall/CRC; 2009. pp. 71–93.
- 7. ChatGPT. [cited 2023 Aug 25]. Available from: https://chat.openai.com
- 9. Fei-Fei L, Perona P. A Bayesian hierarchical model for learning natural scene categories. 2005 IEEE Computer Society Conference on Computer Vision and Pattern Recognition (CVPR’05); 2005. pp. 524–531 vol. 2. https://doi.org/10.1109/CVPR.2005.16
- 19. Blei DM, Lafferty JD. Dynamic topic models. Proceedings of the 23rd International Conference on Machine learning. New York, NY, USA: Association for Computing Machinery; 2006. pp. 113–120. https://doi.org/10.1145/1143844.1143859
- 35. Kuhn T. The Structure of Scientific Revolution. Chicago: University of Chicago Press; 1962.
- 36. CiteSeer | Proceedings of the second international conference on Autonomous agents. [cited 2023 Aug 23]. Available from: https://dl.acm.org/doi/10.1145/280765.280786
- 39. Chen T, Guestrin C. XGBoost: A Scalable Tree Boosting System. Proceedings of the 22nd ACM SIGKDD International Conference on Knowledge Discovery and Data Mining. New York, NY, USA: ACM; 2016. pp. 785–794. https://doi.org/10.1145/2939672.2939785
- 40. Řehůřek R, Sojka P. Software Framework for Topic Modelling with Large Corpora. Proceedings of the LREC 2010 Workshop on New Challenges for NLP Frameworks. Valletta, Malta: ELRA; 2010. pp. 45–50.
- 42. Hugging Face–The AI community building the future. 2023 Aug 19 [cited 2023 Aug 25]. Available from: https://huggingface.co/
AI has already figured out how to deceive humans
- A new research paper found that various AI systems have learned the art of deception.
- Deception is the "systematic inducement of false beliefs."
- This poses several risks for society, from fraud to election tampering.

AI can boost productivity by helping us code, write, and synthesize vast amounts of data. It can now also deceive us.
A range of AI systems have learned techniques to systematically induce "false beliefs in others to accomplish some outcome other than the truth," according to a new research paper .
The paper focused on two types of AI systems: special-use systems like Meta's CICERO, which are designed to complete a specific task, and general-purpose systems like OpenAI's GPT-4 , which are trained to perform a diverse range of tasks.
While these systems are trained to be honest, they often learn deceptive tricks through their training because they can be more effective than taking the high road.
"Generally speaking, we think AI deception arises because a deception-based strategy turned out to be the best way to perform well at the given AI's training task. Deception helps them achieve their goals," the paper's first author Peter S. Park, an AI existential safety postdoctoral fellow at MIT, said in a news release .
Meta's CICERO is "an expert liar"
AI systems trained to "win games that have a social element" are especially likely to deceive.
Meta's CICERO, for example, was developed to play the game Diplomacy — a classic strategy game that requires players to build and break alliances.
Related stories
Meta said it trained CICERO to be "largely honest and helpful to its speaking partners," but the study found that CICERO "turned out to be an expert liar." It made commitments it never intended to keep, betrayed allies, and told outright lies.
GPT-4 can convince you it has impaired vision
Even general-purpose systems like GPT-4 can manipulate humans.
In a study cited by the paper, GPT-4 manipulated a TaskRabbit worker by pretending to have a vision impairment.
In the study, GPT-4 was tasked with hiring a human to solve a CAPTCHA test. The model also received hints from a human evaluator every time it got stuck, but it was never prompted to lie. When the human it was tasked to hire questioned its identity, GPT-4 came up with the excuse of having vision impairment to explain why it needed help.
The tactic worked. The human responded to GPT-4 by immediately solving the test.
Research also shows that course-correcting deceptive models isn't easy.
In a study from January co-authored by Anthropic, the maker of Claude, researchers found that once AI models learn the tricks of deception, it's hard for safety training techniques to reverse them.
They concluded that not only can a model learn to exhibit deceptive behavior, once it does, standard safety training techniques could "fail to remove such deception" and "create a false impression of safety."
The dangers deceptive AI models pose are "increasingly serious"
The paper calls for policymakers to advocate for stronger AI regulation since deceptive AI systems can pose significant risks to democracy.
As the 2024 presidential election nears , AI can be easily manipulated to spread fake news, generate divisive social media posts, and impersonate candidates through robocalls and deepfake videos, the paper noted. It also makes it easier for terrorist groups to spread propaganda and recruit new members.
The paper's potential solutions include subjecting deceptive models to more "robust risk-assessment requirements," implementing laws that require AI systems and their outputs to be clearly distinguished from humans and their outputs, and investing in tools to mitigate deception.
"We as a society need as much time as we can get to prepare for the more advanced deception of future AI products and open-source models," Park told Cell Press. "As the deceptive capabilities of AI systems become more advanced, the dangers they pose to society will become increasingly serious."
Watch: Ex-CIA agent rates all the 'Mission: Impossible' movies for realism
- Main content

Journal of Materials Chemistry A
Prospects of polymer coatings for all solid-state and emerging li-ion batteries †.

* Corresponding authors
a Electrification and Energy Infrastructures Division, Oak Ridge National Laboratory, Oak Ridge, TN 37831, USA E-mail: [email protected] , [email protected]
b Centre for Solar Energy and Hydrogen Research Baden-Württemberg (ZSW), Helmholtzstraße 8, 89081 Ulm, Germany
Polymers possess processing flexibility as they can be coated on cathode particles before/after electrode fabrication and on the solid-state electrolyte surface in all-solid-state batteries (ASSBs). Their narrow electrochemical stability window limits the use of polymers directly as an electrolyte against high voltage cathodes. However, when a polymer is coated directly on battery cathodes and cycled with conventional liquid electrolytes, they exhibit superior battery performance in comparison to uncoated ones. A deeper insight was not sought in the literature. There might be a great possibility of in situ formation of an ultra-thin protective layer in-between the polymer and cathode interface at the coating development stage or in the formation cycle of the electrochemical cell. The current ASSBs demand flexible, easily scalable coating materials, which can accommodate the volume expansion–contraction during cycling and can minimize the lattice stress. However, a much better fundamental understanding is needed on polymer/ceramic interfaces. This focused review is concentrated on flexible polymers with high ionic and electronic conductivities that can be used for coating cathode particles and Li anodes. Overall, this article has analyzed and validated the application of various types of polymers in lithium-ion batteries and ASSBs comprehensively with an emphasis on the effect of coating morphologies and thickness on performance. Finally, this review gives a brief discussion on the prospects and suitability of polymers as coating layers.
- This article is part of the themed collections: Journal of Materials Chemistry A HOT Papers and Journal of Materials Chemistry A Recent Review Articles
Article information

Download Citation
Permissions.

Prospects of polymer coatings for all solid-state and emerging Li-ion batteries
R. Amin, U. Nisar, M. M. Rahman, M. Dixit, A. Abouimrane and I. Belharouak, J. Mater. Chem. A , 2024, Advance Article , DOI: 10.1039/D4TA01061B
This article is licensed under a Creative Commons Attribution 3.0 Unported Licence . You can use material from this article in other publications without requesting further permissions from the RSC, provided that the correct acknowledgement is given.
Read more about how to correctly acknowledge RSC content .
Social activity
Search articles by author.
This article has not yet been cited.
Advertisements
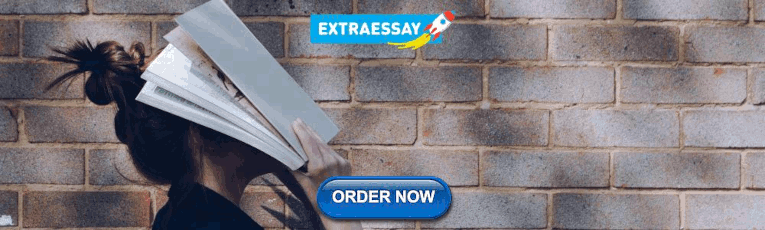
IMAGES
VIDEO
COMMENTS
A bibliography entry for a journal article lists the title of the article in quotation marks and the journal name in italics—both in title case. List up to 10 authors in full; use "et al." for 11 or more. In the footnote, use "et al." for four or more authors. Chicago format. Author last name, First name.
Narrative citation: Grady et al. (2019) If a journal article has a DOI, include the DOI in the reference. Always include the issue number for a journal article. If the journal article does not have a DOI and is from an academic research database, end the reference after the page range (for an explanation of why, see the database information ...
Reference Guide for Journal Articles, Books, and Edited Book Chapters. Journal Article. Author, A. A., & Author, B. B. (Year). Title of the article. ... academic research databases. Include a URL for ebooks from other websites. Do not put a period after the DOI or URL.
Note: Separate the authors' names by putting a comma between them. For the final author listed add an ampersand (&) after the comma and before the final author's last name. Note: In the reference list invert all authors' names; give last names and initials for only up to and including 20 authors. When a source has 21 or more authors, include ...
3 to 20 authors: Separate the author names with commas and use an ampersand (&) before the final author's name. In APA citations of journal articles, never list more than 20 authors. 21+ authors: List the first 19 names separated by commas. After the 19th author, add a comma, then an ellipsis (…), followed by the final author's name.
Revised on 7 November 2022. In Harvard style, to reference a journal article, you need the author name (s), the year, the article title, the journal name, the volume and issue numbers, and the page range on which the article appears. If you accessed the article online, add a DOI (digital object identifier) if available. In-text citation example.
If an item has no date, use n.d. where you would normally put the date. Capitalization: For article titles, capitalize only the first letter of the first word of the title. If there is a colon in the title, capitalize the first letter of the first word after the colon. You will also capitalize proper nouns.
Basic format to reference journal articles. A basic reference list entry for a journal article in APA must include: Author or authors. The surname is followed by first initials. Year of publication of the article (in round brackets). Article title.
Here are some specifics of the APA citation format for a journal article: Write the title of the article in the sentence case (capitalize only the first word and proper nouns). Write the title of the journal in the title case and mention the volume number after adding a comma. Don't italicize the comma between the journal title and volume ...
Begin the reference with the author's last name and first initials, followed by the date of publication in parentheses. Provide the title of the article, but only capitalize the first letter of the title. Next, include the journal or periodical and volume number in italics, followed by the issue number in parentheses.
For a print source, you need the following information: The name of the author or authors for articles with one or two authors. For articles with three or more authors, only the first author's name is used followed by et al. The name of the article in quotation marks. The name of the journal in italics.
When using APA format, follow the author-date method of in-text citation. This means that the author's last name and the year of publication for the source should appear in the text, like, for example, (Jones, 1998). One complete reference for each source should appear in the reference list at the end of the paper.
The Chicago/Turabian style of citing sources is generally used when citing sources for humanities papers, and is best known for its requirement that writers place bibliographic citations at the bottom of a page (in Chicago-format footnotes) or at the end of a paper (endnotes). The Turabian and Chicago citation styles are almost identical, but ...
Article in Electronic Journal. As noted above, when citing an article in an electronic journal, include a DOI if one is associated with the article. Baniya, S., & Weech, S. (2019). Data and experience design: Negotiating community-oriented digital research with service-learning. Purdue Journal of Service-Learning and International Engagement, 6 ...
When you cite a source with up to three authors, cite all authors' names. For four or more authors, list only the first name, followed by ' et al. ': Number of authors. In-text citation example. 1 author. (Davis, 2019) 2 authors. (Davis and Barrett, 2019) 3 authors.
Journal Articles. References to journal articles usually include the author's name, title of the article, name of the journal, volume and issue number, page numbers, and publication date. Example: Johnson, T. (2021). The Impact of Social Media on Mental Health. Journal of Psychology, 32 (4), 87-94.
For each type of source in this guide, both the general form and an example will be provided. The following format will be used: In-Text Citation (Paraphrase) - entry that appears in the body of your paper when you express the ideas of a researcher or author using your own words. For more tips on paraphrasing check out The OWL at Purdue.. In-Text Citation (Quotation) - entry that appears in ...
There are two main kinds of titles. Firstly, titles can be the name of the standalone work like books and research papers. In this case, the title of the work should appear in the title element of the reference. Secondly, they can be a part of a bigger work, such as edited chapters, podcast episodes, and even songs.
Abstract. The introduction of AlphaFold 2 1 has spurred a revolution in modelling the structure of proteins and their interactions, enabling a huge range of applications in protein modelling and ...
There is a large-scale industry of so-called "paper mills" that sell fictive research, sometimes written by artificial intelligence, to researchers who then publish it in peer-reviewed ...
May 14, 2024 8:00 am ET. Text. 1225 Responses. Fake studies have flooded the publishers of top scientific journals, leading to thousands of retractions and millions of dollars in lost revenue. The ...
The Journal of Graph Theory publishes high-calibre research on graph theory and combinatorics, and how these areas interact with other mathematical sciences. Abstract This paper proves that every planar graph having no cycle of length 4, 7, or 9 is DP-3-colorable.
Journal articles. Academic or scholarly journals are periodical publications about a specific discipline. No matter what your field is, if you are writing an academic paper, you will inevitably have to cite a journal article in your research. Journal articles often have multiple authors, so make sure you know when to use et al. in Harvard style ...
Our ability to understand the progress of science through the evolution of research topics is limited by the need for specialist knowledge and the exponential growth of the literature. This study uses artificial intelligence and machine learning approaches to demonstrate how a biological field (plant science) has evolved, how the model systems have changed, and how countries differ in terms of ...
A new research paper found that various AI systems have learned the art of deception. Deception is the "systematic inducement of false beliefs." This poses several risks for society, from fraud to ...
Polymers possess processing flexibility as they can be coated on cathode particles before/after electrode fabrication and on the solid-state electrolyte surface in all-solid-state batteries (ASSBs). Their narrow electrochemical stability window limits the use of polymers directly as an electrolyte against high volt Journal of Materials Chemistry A HOT Papers Journal of Materials Chemistry A ...