- TheFreeDictionary
- Word / Article
- Starts with
- Free toolbar & extensions
- Word of the Day
- Free content
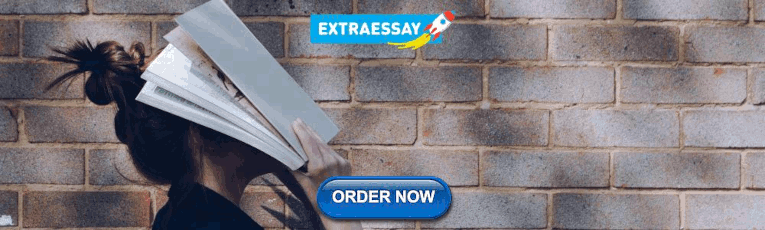
hy·poth·e·sis
Significance .
- ad hoc hypothesis
- alpha error
- alternative hypothesis
- APUD hypothesis
- Avogadro law
- Avogadro number
- Avogadro, Amadeo
- background level
- Bayes theorem
- Bayesian hypothesis
- Beadle, George Wells
- bread mould
- capillarity
- chi squared test
- cohesion-tension hypothesis
- hypotensive
- hypotensive anesthesia
- hypothalamic amenorrhea
- hypothalamic dysfunction
- hypothalamic fever
- hypothalamic infundibulum
- hypothalamic obesity
- hypothalamic sulcus
- hypothalamic-pituitary axis
- hypothalamic-pituitary-adrenal axis
- hypothalamocerebellar fibers
- hypothalamo-hypophyseal portal system
- hypothalamohypophysial
- hypothalamospinal fibers
- Hypothalamus
- hypothenar eminence
- hypothenar fascia
- hypothermal
- hypothermia
- hypothermia therapy
- hypothermia treatment
- hypothermic anesthesia
- hypothermic circulatory arrest
- hypothesis test
- hypothesis to test
- hypothetic mean organism
- hypothetic mean strain
- hypothetical mean organism
- hypothrombinemia
- hypothromboplastinemia
- hypothymism
- hypothyroid
- hypothyroid dwarf
- hypothyroidism
- hypothyroxinemia
- hypotonia-cystinuria syndrome
- Hypotonic Duodenography
- hypotonic labor
- hypotonic saline
- hypotonicity
- hypotoxicity
- hypotransferrinemia
- hypotrichiasis
- Hypothermia After Cardiac Arrest
- Hypothermia After Cardiac Arrest Registry
- hypothermic
- hypothermicly
- Hypothèse Extraterrestre
- Hypothesis Driven Lexical Adaptation
- Hypothesis test
- Hypothesis testing
- hypothesis testing sampling
- Hypothesis-Based Testing
- Hypothesis-Oriented Algorithm for Clinicians
- hypothesise
- hypothesised
- hypothesiser
- hypothesisers
- hypothesises
- Facebook Share
- Bipolar Disorder
- Therapy Center
- When To See a Therapist
- Types of Therapy
- Best Online Therapy
- Best Couples Therapy
- Best Family Therapy
- Managing Stress
- Sleep and Dreaming
- Understanding Emotions
- Self-Improvement
- Healthy Relationships
- Student Resources
- Personality Types
- Guided Meditations
- Verywell Mind Insights
- 2024 Verywell Mind 25
- Mental Health in the Classroom
- Editorial Process
- Meet Our Review Board
- Crisis Support
How to Write a Great Hypothesis
Hypothesis Definition, Format, Examples, and Tips
Kendra Cherry, MS, is a psychosocial rehabilitation specialist, psychology educator, and author of the "Everything Psychology Book."
:max_bytes(150000):strip_icc():format(webp)/IMG_9791-89504ab694d54b66bbd72cb84ffb860e.jpg)
Amy Morin, LCSW, is a psychotherapist and international bestselling author. Her books, including "13 Things Mentally Strong People Don't Do," have been translated into more than 40 languages. Her TEDx talk, "The Secret of Becoming Mentally Strong," is one of the most viewed talks of all time.
:max_bytes(150000):strip_icc():format(webp)/VW-MIND-Amy-2b338105f1ee493f94d7e333e410fa76.jpg)
Verywell / Alex Dos Diaz
- The Scientific Method
Hypothesis Format
Falsifiability of a hypothesis.
- Operationalization
Hypothesis Types
Hypotheses examples.
- Collecting Data
A hypothesis is a tentative statement about the relationship between two or more variables. It is a specific, testable prediction about what you expect to happen in a study. It is a preliminary answer to your question that helps guide the research process.
Consider a study designed to examine the relationship between sleep deprivation and test performance. The hypothesis might be: "This study is designed to assess the hypothesis that sleep-deprived people will perform worse on a test than individuals who are not sleep-deprived."
At a Glance
A hypothesis is crucial to scientific research because it offers a clear direction for what the researchers are looking to find. This allows them to design experiments to test their predictions and add to our scientific knowledge about the world. This article explores how a hypothesis is used in psychology research, how to write a good hypothesis, and the different types of hypotheses you might use.
The Hypothesis in the Scientific Method
In the scientific method , whether it involves research in psychology, biology, or some other area, a hypothesis represents what the researchers think will happen in an experiment. The scientific method involves the following steps:
- Forming a question
- Performing background research
- Creating a hypothesis
- Designing an experiment
- Collecting data
- Analyzing the results
- Drawing conclusions
- Communicating the results
The hypothesis is a prediction, but it involves more than a guess. Most of the time, the hypothesis begins with a question which is then explored through background research. At this point, researchers then begin to develop a testable hypothesis.
Unless you are creating an exploratory study, your hypothesis should always explain what you expect to happen.
In a study exploring the effects of a particular drug, the hypothesis might be that researchers expect the drug to have some type of effect on the symptoms of a specific illness. In psychology, the hypothesis might focus on how a certain aspect of the environment might influence a particular behavior.
Remember, a hypothesis does not have to be correct. While the hypothesis predicts what the researchers expect to see, the goal of the research is to determine whether this guess is right or wrong. When conducting an experiment, researchers might explore numerous factors to determine which ones might contribute to the ultimate outcome.
In many cases, researchers may find that the results of an experiment do not support the original hypothesis. When writing up these results, the researchers might suggest other options that should be explored in future studies.
In many cases, researchers might draw a hypothesis from a specific theory or build on previous research. For example, prior research has shown that stress can impact the immune system. So a researcher might hypothesize: "People with high-stress levels will be more likely to contract a common cold after being exposed to the virus than people who have low-stress levels."
In other instances, researchers might look at commonly held beliefs or folk wisdom. "Birds of a feather flock together" is one example of folk adage that a psychologist might try to investigate. The researcher might pose a specific hypothesis that "People tend to select romantic partners who are similar to them in interests and educational level."
Elements of a Good Hypothesis
So how do you write a good hypothesis? When trying to come up with a hypothesis for your research or experiments, ask yourself the following questions:
- Is your hypothesis based on your research on a topic?
- Can your hypothesis be tested?
- Does your hypothesis include independent and dependent variables?
Before you come up with a specific hypothesis, spend some time doing background research. Once you have completed a literature review, start thinking about potential questions you still have. Pay attention to the discussion section in the journal articles you read . Many authors will suggest questions that still need to be explored.
How to Formulate a Good Hypothesis
To form a hypothesis, you should take these steps:
- Collect as many observations about a topic or problem as you can.
- Evaluate these observations and look for possible causes of the problem.
- Create a list of possible explanations that you might want to explore.
- After you have developed some possible hypotheses, think of ways that you could confirm or disprove each hypothesis through experimentation. This is known as falsifiability.
In the scientific method , falsifiability is an important part of any valid hypothesis. In order to test a claim scientifically, it must be possible that the claim could be proven false.
Students sometimes confuse the idea of falsifiability with the idea that it means that something is false, which is not the case. What falsifiability means is that if something was false, then it is possible to demonstrate that it is false.
One of the hallmarks of pseudoscience is that it makes claims that cannot be refuted or proven false.
The Importance of Operational Definitions
A variable is a factor or element that can be changed and manipulated in ways that are observable and measurable. However, the researcher must also define how the variable will be manipulated and measured in the study.
Operational definitions are specific definitions for all relevant factors in a study. This process helps make vague or ambiguous concepts detailed and measurable.
For example, a researcher might operationally define the variable " test anxiety " as the results of a self-report measure of anxiety experienced during an exam. A "study habits" variable might be defined by the amount of studying that actually occurs as measured by time.
These precise descriptions are important because many things can be measured in various ways. Clearly defining these variables and how they are measured helps ensure that other researchers can replicate your results.
Replicability
One of the basic principles of any type of scientific research is that the results must be replicable.
Replication means repeating an experiment in the same way to produce the same results. By clearly detailing the specifics of how the variables were measured and manipulated, other researchers can better understand the results and repeat the study if needed.
Some variables are more difficult than others to define. For example, how would you operationally define a variable such as aggression ? For obvious ethical reasons, researchers cannot create a situation in which a person behaves aggressively toward others.
To measure this variable, the researcher must devise a measurement that assesses aggressive behavior without harming others. The researcher might utilize a simulated task to measure aggressiveness in this situation.
Hypothesis Checklist
- Does your hypothesis focus on something that you can actually test?
- Does your hypothesis include both an independent and dependent variable?
- Can you manipulate the variables?
- Can your hypothesis be tested without violating ethical standards?
The hypothesis you use will depend on what you are investigating and hoping to find. Some of the main types of hypotheses that you might use include:
- Simple hypothesis : This type of hypothesis suggests there is a relationship between one independent variable and one dependent variable.
- Complex hypothesis : This type suggests a relationship between three or more variables, such as two independent and dependent variables.
- Null hypothesis : This hypothesis suggests no relationship exists between two or more variables.
- Alternative hypothesis : This hypothesis states the opposite of the null hypothesis.
- Statistical hypothesis : This hypothesis uses statistical analysis to evaluate a representative population sample and then generalizes the findings to the larger group.
- Logical hypothesis : This hypothesis assumes a relationship between variables without collecting data or evidence.
A hypothesis often follows a basic format of "If {this happens} then {this will happen}." One way to structure your hypothesis is to describe what will happen to the dependent variable if you change the independent variable .
The basic format might be: "If {these changes are made to a certain independent variable}, then we will observe {a change in a specific dependent variable}."
A few examples of simple hypotheses:
- "Students who eat breakfast will perform better on a math exam than students who do not eat breakfast."
- "Students who experience test anxiety before an English exam will get lower scores than students who do not experience test anxiety."
- "Motorists who talk on the phone while driving will be more likely to make errors on a driving course than those who do not talk on the phone."
- "Children who receive a new reading intervention will have higher reading scores than students who do not receive the intervention."
Examples of a complex hypothesis include:
- "People with high-sugar diets and sedentary activity levels are more likely to develop depression."
- "Younger people who are regularly exposed to green, outdoor areas have better subjective well-being than older adults who have limited exposure to green spaces."
Examples of a null hypothesis include:
- "There is no difference in anxiety levels between people who take St. John's wort supplements and those who do not."
- "There is no difference in scores on a memory recall task between children and adults."
- "There is no difference in aggression levels between children who play first-person shooter games and those who do not."
Examples of an alternative hypothesis:
- "People who take St. John's wort supplements will have less anxiety than those who do not."
- "Adults will perform better on a memory task than children."
- "Children who play first-person shooter games will show higher levels of aggression than children who do not."
Collecting Data on Your Hypothesis
Once a researcher has formed a testable hypothesis, the next step is to select a research design and start collecting data. The research method depends largely on exactly what they are studying. There are two basic types of research methods: descriptive research and experimental research.
Descriptive Research Methods
Descriptive research such as case studies , naturalistic observations , and surveys are often used when conducting an experiment is difficult or impossible. These methods are best used to describe different aspects of a behavior or psychological phenomenon.
Once a researcher has collected data using descriptive methods, a correlational study can examine how the variables are related. This research method might be used to investigate a hypothesis that is difficult to test experimentally.
Experimental Research Methods
Experimental methods are used to demonstrate causal relationships between variables. In an experiment, the researcher systematically manipulates a variable of interest (known as the independent variable) and measures the effect on another variable (known as the dependent variable).
Unlike correlational studies, which can only be used to determine if there is a relationship between two variables, experimental methods can be used to determine the actual nature of the relationship—whether changes in one variable actually cause another to change.
The hypothesis is a critical part of any scientific exploration. It represents what researchers expect to find in a study or experiment. In situations where the hypothesis is unsupported by the research, the research still has value. Such research helps us better understand how different aspects of the natural world relate to one another. It also helps us develop new hypotheses that can then be tested in the future.
Thompson WH, Skau S. On the scope of scientific hypotheses . R Soc Open Sci . 2023;10(8):230607. doi:10.1098/rsos.230607
Taran S, Adhikari NKJ, Fan E. Falsifiability in medicine: what clinicians can learn from Karl Popper [published correction appears in Intensive Care Med. 2021 Jun 17;:]. Intensive Care Med . 2021;47(9):1054-1056. doi:10.1007/s00134-021-06432-z
Eyler AA. Research Methods for Public Health . 1st ed. Springer Publishing Company; 2020. doi:10.1891/9780826182067.0004
Nosek BA, Errington TM. What is replication ? PLoS Biol . 2020;18(3):e3000691. doi:10.1371/journal.pbio.3000691
Aggarwal R, Ranganathan P. Study designs: Part 2 - Descriptive studies . Perspect Clin Res . 2019;10(1):34-36. doi:10.4103/picr.PICR_154_18
Nevid J. Psychology: Concepts and Applications. Wadworth, 2013.
By Kendra Cherry, MSEd Kendra Cherry, MS, is a psychosocial rehabilitation specialist, psychology educator, and author of the "Everything Psychology Book."
- More from M-W
- To save this word, you'll need to log in. Log In
Definition of hypothesis
Did you know.
The Difference Between Hypothesis and Theory
A hypothesis is an assumption, an idea that is proposed for the sake of argument so that it can be tested to see if it might be true.
In the scientific method, the hypothesis is constructed before any applicable research has been done, apart from a basic background review. You ask a question, read up on what has been studied before, and then form a hypothesis.
A hypothesis is usually tentative; it's an assumption or suggestion made strictly for the objective of being tested.
A theory , in contrast, is a principle that has been formed as an attempt to explain things that have already been substantiated by data. It is used in the names of a number of principles accepted in the scientific community, such as the Big Bang Theory . Because of the rigors of experimentation and control, it is understood to be more likely to be true than a hypothesis is.
In non-scientific use, however, hypothesis and theory are often used interchangeably to mean simply an idea, speculation, or hunch, with theory being the more common choice.
Since this casual use does away with the distinctions upheld by the scientific community, hypothesis and theory are prone to being wrongly interpreted even when they are encountered in scientific contexts—or at least, contexts that allude to scientific study without making the critical distinction that scientists employ when weighing hypotheses and theories.
The most common occurrence is when theory is interpreted—and sometimes even gleefully seized upon—to mean something having less truth value than other scientific principles. (The word law applies to principles so firmly established that they are almost never questioned, such as the law of gravity.)
This mistake is one of projection: since we use theory in general to mean something lightly speculated, then it's implied that scientists must be talking about the same level of uncertainty when they use theory to refer to their well-tested and reasoned principles.
The distinction has come to the forefront particularly on occasions when the content of science curricula in schools has been challenged—notably, when a school board in Georgia put stickers on textbooks stating that evolution was "a theory, not a fact, regarding the origin of living things." As Kenneth R. Miller, a cell biologist at Brown University, has said , a theory "doesn’t mean a hunch or a guess. A theory is a system of explanations that ties together a whole bunch of facts. It not only explains those facts, but predicts what you ought to find from other observations and experiments.”
While theories are never completely infallible, they form the basis of scientific reasoning because, as Miller said "to the best of our ability, we’ve tested them, and they’ve held up."
- proposition
- supposition
hypothesis , theory , law mean a formula derived by inference from scientific data that explains a principle operating in nature.
hypothesis implies insufficient evidence to provide more than a tentative explanation.
theory implies a greater range of evidence and greater likelihood of truth.
law implies a statement of order and relation in nature that has been found to be invariable under the same conditions.
Examples of hypothesis in a Sentence
These examples are programmatically compiled from various online sources to illustrate current usage of the word 'hypothesis.' Any opinions expressed in the examples do not represent those of Merriam-Webster or its editors. Send us feedback about these examples.
Word History
Greek, from hypotithenai to put under, suppose, from hypo- + tithenai to put — more at do
1641, in the meaning defined at sense 1a
Phrases Containing hypothesis
- counter - hypothesis
- nebular hypothesis
- null hypothesis
- planetesimal hypothesis
- Whorfian hypothesis
Articles Related to hypothesis
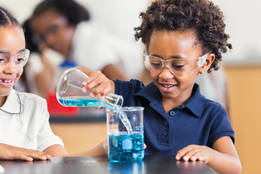
This is the Difference Between a...
This is the Difference Between a Hypothesis and a Theory
In scientific reasoning, they're two completely different things
Dictionary Entries Near hypothesis
hypothermia
hypothesize
Cite this Entry
“Hypothesis.” Merriam-Webster.com Dictionary , Merriam-Webster, https://www.merriam-webster.com/dictionary/hypothesis. Accessed 21 May. 2024.
Kids Definition
Kids definition of hypothesis, medical definition, medical definition of hypothesis, more from merriam-webster on hypothesis.
Nglish: Translation of hypothesis for Spanish Speakers
Britannica English: Translation of hypothesis for Arabic Speakers
Britannica.com: Encyclopedia article about hypothesis
Subscribe to America's largest dictionary and get thousands more definitions and advanced search—ad free!
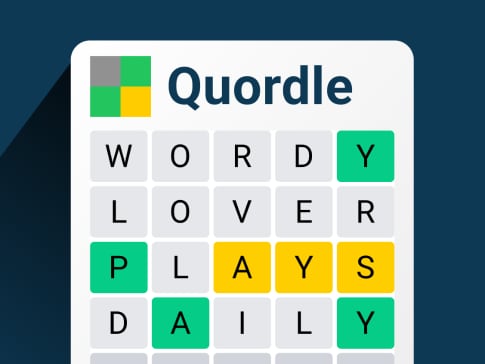
Can you solve 4 words at once?
Word of the day.
See Definitions and Examples »
Get Word of the Day daily email!
Popular in Grammar & Usage
More commonly misspelled words, your vs. you're: how to use them correctly, every letter is silent, sometimes: a-z list of examples, more commonly mispronounced words, how to use em dashes (—), en dashes (–) , and hyphens (-), popular in wordplay, the words of the week - may 17, birds say the darndest things, a great big list of bread words, 10 scrabble words without any vowels, 12 more bird names that sound like insults (and sometimes are), games & quizzes.
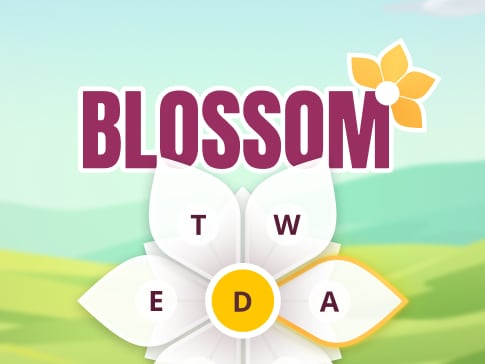
The Research Hypothesis: Role and Construction
- First Online: 01 January 2012
Cite this chapter
- Phyllis G. Supino EdD 3
6020 Accesses
A hypothesis is a logical construct, interposed between a problem and its solution, which represents a proposed answer to a research question. It gives direction to the investigator’s thinking about the problem and, therefore, facilitates a solution. There are three primary modes of inference by which hypotheses are developed: deduction (reasoning from a general propositions to specific instances), induction (reasoning from specific instances to a general proposition), and abduction (formulation/acceptance on probation of a hypothesis to explain a surprising observation).
A research hypothesis should reflect an inference about variables; be stated as a grammatically complete, declarative sentence; be expressed simply and unambiguously; provide an adequate answer to the research problem; and be testable. Hypotheses can be classified as conceptual versus operational, single versus bi- or multivariable, causal or not causal, mechanistic versus nonmechanistic, and null or alternative. Hypotheses most commonly entail statements about “variables” which, in turn, can be classified according to their level of measurement (scaling characteristics) or according to their role in the hypothesis (independent, dependent, moderator, control, or intervening).
A hypothesis is rendered operational when its broadly (conceptually) stated variables are replaced by operational definitions of those variables. Hypotheses stated in this manner are called operational hypotheses, specific hypotheses, or predictions and facilitate testing.
Wrong hypotheses, rightly worked from, have produced more results than unguided observation
—Augustus De Morgan, 1872[ 1 ]—
This is a preview of subscription content, log in via an institution to check access.
Access this chapter
- Available as EPUB and PDF
- Read on any device
- Instant download
- Own it forever
- Compact, lightweight edition
- Dispatched in 3 to 5 business days
- Free shipping worldwide - see info
- Durable hardcover edition
Tax calculation will be finalised at checkout
Purchases are for personal use only
Institutional subscriptions
Similar content being viewed by others
The Nature and Logic of Science: Testing Hypotheses
Abductive Research Methods in Psychological Science
Abductive Research Methods in Psychological Science
De Morgan A, De Morgan S. A budget of paradoxes. London: Longmans Green; 1872.
Google Scholar
Leedy Paul D. Practical research. Planning and design. 2nd ed. New York: Macmillan; 1960.
Bernard C. Introduction to the study of experimental medicine. New York: Dover; 1957.
Erren TC. The quest for questions—on the logical force of science. Med Hypotheses. 2004;62:635–40.
Article PubMed Google Scholar
Peirce CS. Collected papers of Charles Sanders Peirce, vol. 7. In: Hartshorne C, Weiss P, editors. Boston: The Belknap Press of Harvard University Press; 1966.
Aristotle. The complete works of Aristotle: the revised Oxford Translation. In: Barnes J, editor. vol. 2. Princeton/New Jersey: Princeton University Press; 1984.
Polit D, Beck CT. Conceptualizing a study to generate evidence for nursing. In: Polit D, Beck CT, editors. Nursing research: generating and assessing evidence for nursing practice. 8th ed. Philadelphia: Wolters Kluwer/Lippincott Williams and Wilkins; 2008. Chapter 4.
Jenicek M, Hitchcock DL. Evidence-based practice. Logic and critical thinking in medicine. Chicago: AMA Press; 2005.
Bacon F. The novum organon or a true guide to the interpretation of nature. A new translation by the Rev G.W. Kitchin. Oxford: The University Press; 1855.
Popper KR. Objective knowledge: an evolutionary approach (revised edition). New York: Oxford University Press; 1979.
Morgan AJ, Parker S. Translational mini-review series on vaccines: the Edward Jenner Museum and the history of vaccination. Clin Exp Immunol. 2007;147:389–94.
Article PubMed CAS Google Scholar
Pead PJ. Benjamin Jesty: new light in the dawn of vaccination. Lancet. 2003;362:2104–9.
Lee JA. The scientific endeavor: a primer on scientific principles and practice. San Francisco: Addison-Wesley Longman; 2000.
Allchin D. Lawson’s shoehorn, or should the philosophy of science be rated, ‘X’? Science and Education. 2003;12:315–29.
Article Google Scholar
Lawson AE. What is the role of induction and deduction in reasoning and scientific inquiry? J Res Sci Teach. 2005;42:716–40.
Peirce CS. Collected papers of Charles Sanders Peirce, vol. 2. In: Hartshorne C, Weiss P, editors. Boston: The Belknap Press of Harvard University Press; 1965.
Bonfantini MA, Proni G. To guess or not to guess? In: Eco U, Sebeok T, editors. The sign of three: Dupin, Holmes, Peirce. Bloomington: Indiana University Press; 1983. Chapter 5.
Peirce CS. Collected papers of Charles Sanders Peirce, vol. 5. In: Hartshorne C, Weiss P, editors. Boston: The Belknap Press of Harvard University Press; 1965.
Flach PA, Kakas AC. Abductive and inductive reasoning: background issues. In: Flach PA, Kakas AC, editors. Abduction and induction. Essays on their relation and integration. The Netherlands: Klewer; 2000. Chapter 1.
Murray JF. Voltaire, Walpole and Pasteur: variations on the theme of discovery. Am J Respir Crit Care Med. 2005;172:423–6.
Danemark B, Ekstrom M, Jakobsen L, Karlsson JC. Methodological implications, generalization, scientific inference, models (Part II) In: explaining society. Critical realism in the social sciences. New York: Routledge; 2002.
Pasteur L. Inaugural lecture as professor and dean of the faculty of sciences. In: Peterson H, editor. A treasury of the world’s greatest speeches. Douai, France: University of Lille 7 Dec 1954.
Swineburne R. Simplicity as evidence for truth. Milwaukee: Marquette University Press; 1997.
Sakar S, editor. Logical empiricism at its peak: Schlick, Carnap and Neurath. New York: Garland; 1996.
Popper K. The logic of scientific discovery. New York: Basic Books; 1959. 1934, trans. 1959.
Caws P. The philosophy of science. Princeton: D. Van Nostrand Company; 1965.
Popper K. Conjectures and refutations. The growth of scientific knowledge. 4th ed. London: Routledge and Keegan Paul; 1972.
Feyerabend PK. Against method, outline of an anarchistic theory of knowledge. London, UK: Verso; 1978.
Smith PG. Popper: conjectures and refutations (Chapter IV). In: Theory and reality: an introduction to the philosophy of science. Chicago: University of Chicago Press; 2003.
Blystone RV, Blodgett K. WWW: the scientific method. CBE Life Sci Educ. 2006;5:7–11.
Kleinbaum DG, Kupper LL, Morgenstern H. Epidemiological research. Principles and quantitative methods. New York: Van Nostrand Reinhold; 1982.
Fortune AE, Reid WJ. Research in social work. 3rd ed. New York: Columbia University Press; 1999.
Kerlinger FN. Foundations of behavioral research. 1st ed. New York: Hold, Reinhart and Winston; 1970.
Hoskins CN, Mariano C. Research in nursing and health. Understanding and using quantitative and qualitative methods. New York: Springer; 2004.
Tuckman BW. Conducting educational research. New York: Harcourt, Brace, Jovanovich; 1972.
Wang C, Chiari PC, Weihrauch D, Krolikowski JG, Warltier DC, Kersten JR, Pratt Jr PF, Pagel PS. Gender-specificity of delayed preconditioning by isoflurane in rabbits: potential role of endothelial nitric oxide synthase. Anesth Analg. 2006;103:274–80.
Beyer ME, Slesak G, Nerz S, Kazmaier S, Hoffmeister HM. Effects of endothelin-1 and IRL 1620 on myocardial contractility and myocardial energy metabolism. J Cardiovasc Pharmacol. 1995;26(Suppl 3):S150–2.
PubMed CAS Google Scholar
Stone J, Sharpe M. Amnesia for childhood in patients with unexplained neurological symptoms. J Neurol Neurosurg Psychiatry. 2002;72:416–7.
Naughton BJ, Moran M, Ghaly Y, Michalakes C. Computer tomography scanning and delirium in elder patients. Acad Emerg Med. 1997;4:1107–10.
Easterbrook PJ, Berlin JA, Gopalan R, Matthews DR. Publication bias in clinical research. Lancet. 1991;337:867–72.
Stern JM, Simes RJ. Publication bias: evidence of delayed publication in a cohort study of clinical research projects. BMJ. 1997;315:640–5.
Stevens SS. On the theory of scales and measurement. Science. 1946;103:677–80.
Knapp TR. Treating ordinal scales as interval scales: an attempt to resolve the controversy. Nurs Res. 1990;39:121–3.
The Cochrane Collaboration. Open Learning Material. www.cochrane-net.org/openlearning/html/mod14-3.htm . Accessed 12 Oct 2009.
MacCorquodale K, Meehl PE. On a distinction between hypothetical constructs and intervening variables. Psychol Rev. 1948;55:95–107.
Baron RM, Kenny DA. The moderator-mediator variable distinction in social psychological research: conceptual, strategic and statistical considerations. J Pers Soc Psychol. 1986;51:1173–82.
Williamson GM, Schultz R. Activity restriction mediates the association between pain and depressed affect: a study of younger and older adult cancer patients. Psychol Aging. 1995;10:369–78.
Song M, Lee EO. Development of a functional capacity model for the elderly. Res Nurs Health. 1998;21:189–98.
MacKinnon DP. Introduction to statistical mediation analysis. New York: Routledge; 2008.
Download references
Author information
Authors and affiliations.
Department of Medicine, College of Medicine, SUNY Downstate Medical Center, 450 Clarkson Avenue, 1199, Brooklyn, NY, 11203, USA
Phyllis G. Supino EdD
You can also search for this author in PubMed Google Scholar
Corresponding author
Correspondence to Phyllis G. Supino EdD .
Editor information
Editors and affiliations.
, Cardiovascular Medicine, SUNY Downstate Medical Center, Clarkson Avenue, box 1199 450, Brooklyn, 11203, USA
Phyllis G. Supino
, Cardiovascualr Medicine, SUNY Downstate Medical Center, Clarkson Avenue 450, Brooklyn, 11203, USA
Jeffrey S. Borer
Rights and permissions
Reprints and permissions
Copyright information
© 2012 Springer Science+Business Media, LLC
About this chapter
Supino, P.G. (2012). The Research Hypothesis: Role and Construction. In: Supino, P., Borer, J. (eds) Principles of Research Methodology. Springer, New York, NY. https://doi.org/10.1007/978-1-4614-3360-6_3
Download citation
DOI : https://doi.org/10.1007/978-1-4614-3360-6_3
Published : 18 April 2012
Publisher Name : Springer, New York, NY
Print ISBN : 978-1-4614-3359-0
Online ISBN : 978-1-4614-3360-6
eBook Packages : Medicine Medicine (R0)
Share this chapter
Anyone you share the following link with will be able to read this content:
Sorry, a shareable link is not currently available for this article.
Provided by the Springer Nature SharedIt content-sharing initiative
- Publish with us
Policies and ethics
- Find a journal
- Track your research

What Is A Research (Scientific) Hypothesis? A plain-language explainer + examples
By: Derek Jansen (MBA) | Reviewed By: Dr Eunice Rautenbach | June 2020
If you’re new to the world of research, or it’s your first time writing a dissertation or thesis, you’re probably noticing that the words “research hypothesis” and “scientific hypothesis” are used quite a bit, and you’re wondering what they mean in a research context .
“Hypothesis” is one of those words that people use loosely, thinking they understand what it means. However, it has a very specific meaning within academic research. So, it’s important to understand the exact meaning before you start hypothesizing.
Research Hypothesis 101
- What is a hypothesis ?
- What is a research hypothesis (scientific hypothesis)?
- Requirements for a research hypothesis
- Definition of a research hypothesis
- The null hypothesis
What is a hypothesis?
Let’s start with the general definition of a hypothesis (not a research hypothesis or scientific hypothesis), according to the Cambridge Dictionary:
Hypothesis: an idea or explanation for something that is based on known facts but has not yet been proved.
In other words, it’s a statement that provides an explanation for why or how something works, based on facts (or some reasonable assumptions), but that has not yet been specifically tested . For example, a hypothesis might look something like this:
Hypothesis: sleep impacts academic performance.
This statement predicts that academic performance will be influenced by the amount and/or quality of sleep a student engages in – sounds reasonable, right? It’s based on reasonable assumptions , underpinned by what we currently know about sleep and health (from the existing literature). So, loosely speaking, we could call it a hypothesis, at least by the dictionary definition.
But that’s not good enough…
Unfortunately, that’s not quite sophisticated enough to describe a research hypothesis (also sometimes called a scientific hypothesis), and it wouldn’t be acceptable in a dissertation, thesis or research paper . In the world of academic research, a statement needs a few more criteria to constitute a true research hypothesis .
What is a research hypothesis?
A research hypothesis (also called a scientific hypothesis) is a statement about the expected outcome of a study (for example, a dissertation or thesis). To constitute a quality hypothesis, the statement needs to have three attributes – specificity , clarity and testability .
Let’s take a look at these more closely.
Need a helping hand?
Hypothesis Essential #1: Specificity & Clarity
A good research hypothesis needs to be extremely clear and articulate about both what’ s being assessed (who or what variables are involved ) and the expected outcome (for example, a difference between groups, a relationship between variables, etc.).
Let’s stick with our sleepy students example and look at how this statement could be more specific and clear.
Hypothesis: Students who sleep at least 8 hours per night will, on average, achieve higher grades in standardised tests than students who sleep less than 8 hours a night.
As you can see, the statement is very specific as it identifies the variables involved (sleep hours and test grades), the parties involved (two groups of students), as well as the predicted relationship type (a positive relationship). There’s no ambiguity or uncertainty about who or what is involved in the statement, and the expected outcome is clear.
Contrast that to the original hypothesis we looked at – “Sleep impacts academic performance” – and you can see the difference. “Sleep” and “academic performance” are both comparatively vague , and there’s no indication of what the expected relationship direction is (more sleep or less sleep). As you can see, specificity and clarity are key.
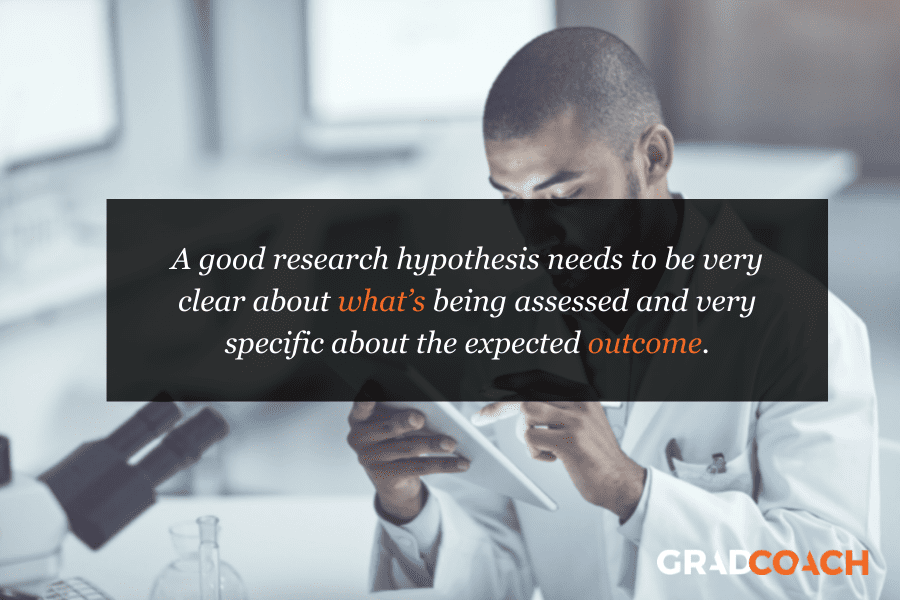
Hypothesis Essential #2: Testability (Provability)
A statement must be testable to qualify as a research hypothesis. In other words, there needs to be a way to prove (or disprove) the statement. If it’s not testable, it’s not a hypothesis – simple as that.
For example, consider the hypothesis we mentioned earlier:
Hypothesis: Students who sleep at least 8 hours per night will, on average, achieve higher grades in standardised tests than students who sleep less than 8 hours a night.
We could test this statement by undertaking a quantitative study involving two groups of students, one that gets 8 or more hours of sleep per night for a fixed period, and one that gets less. We could then compare the standardised test results for both groups to see if there’s a statistically significant difference.
Again, if you compare this to the original hypothesis we looked at – “Sleep impacts academic performance” – you can see that it would be quite difficult to test that statement, primarily because it isn’t specific enough. How much sleep? By who? What type of academic performance?
So, remember the mantra – if you can’t test it, it’s not a hypothesis 🙂
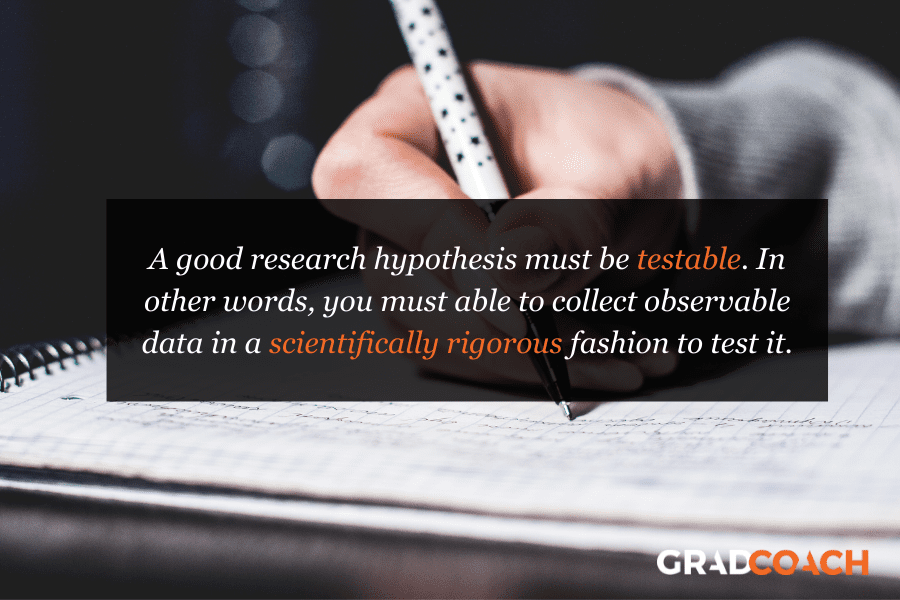
Defining A Research Hypothesis
You’re still with us? Great! Let’s recap and pin down a clear definition of a hypothesis.
A research hypothesis (or scientific hypothesis) is a statement about an expected relationship between variables, or explanation of an occurrence, that is clear, specific and testable.
So, when you write up hypotheses for your dissertation or thesis, make sure that they meet all these criteria. If you do, you’ll not only have rock-solid hypotheses but you’ll also ensure a clear focus for your entire research project.
What about the null hypothesis?
You may have also heard the terms null hypothesis , alternative hypothesis, or H-zero thrown around. At a simple level, the null hypothesis is the counter-proposal to the original hypothesis.
For example, if the hypothesis predicts that there is a relationship between two variables (for example, sleep and academic performance), the null hypothesis would predict that there is no relationship between those variables.
At a more technical level, the null hypothesis proposes that no statistical significance exists in a set of given observations and that any differences are due to chance alone.
And there you have it – hypotheses in a nutshell.
If you have any questions, be sure to leave a comment below and we’ll do our best to help you. If you need hands-on help developing and testing your hypotheses, consider our private coaching service , where we hold your hand through the research journey.
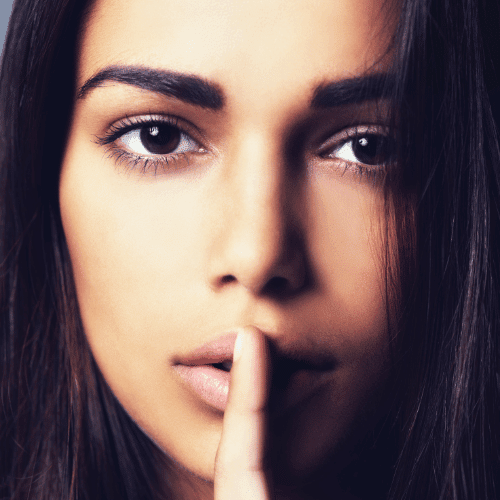
Psst... there’s more!
This post was based on one of our popular Research Bootcamps . If you're working on a research project, you'll definitely want to check this out ...
You Might Also Like:
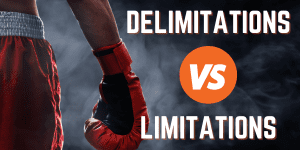
16 Comments
Very useful information. I benefit more from getting more information in this regard.
Very great insight,educative and informative. Please give meet deep critics on many research data of public international Law like human rights, environment, natural resources, law of the sea etc
In a book I read a distinction is made between null, research, and alternative hypothesis. As far as I understand, alternative and research hypotheses are the same. Can you please elaborate? Best Afshin
This is a self explanatory, easy going site. I will recommend this to my friends and colleagues.
Very good definition. How can I cite your definition in my thesis? Thank you. Is nul hypothesis compulsory in a research?
It’s a counter-proposal to be proven as a rejection
Please what is the difference between alternate hypothesis and research hypothesis?
It is a very good explanation. However, it limits hypotheses to statistically tasteable ideas. What about for qualitative researches or other researches that involve quantitative data that don’t need statistical tests?
In qualitative research, one typically uses propositions, not hypotheses.
could you please elaborate it more
I’ve benefited greatly from these notes, thank you.
This is very helpful
well articulated ideas are presented here, thank you for being reliable sources of information
Excellent. Thanks for being clear and sound about the research methodology and hypothesis (quantitative research)
I have only a simple question regarding the null hypothesis. – Is the null hypothesis (Ho) known as the reversible hypothesis of the alternative hypothesis (H1? – How to test it in academic research?
this is very important note help me much more
Trackbacks/Pingbacks
- What Is Research Methodology? Simple Definition (With Examples) - Grad Coach - […] Contrasted to this, a quantitative methodology is typically used when the research aims and objectives are confirmatory in nature. For example,…
Submit a Comment Cancel reply
Your email address will not be published. Required fields are marked *
Save my name, email, and website in this browser for the next time I comment.
- Print Friendly
- Privacy Policy

Home » What is a Hypothesis – Types, Examples and Writing Guide
What is a Hypothesis – Types, Examples and Writing Guide
Table of Contents

Definition:
Hypothesis is an educated guess or proposed explanation for a phenomenon, based on some initial observations or data. It is a tentative statement that can be tested and potentially proven or disproven through further investigation and experimentation.
Hypothesis is often used in scientific research to guide the design of experiments and the collection and analysis of data. It is an essential element of the scientific method, as it allows researchers to make predictions about the outcome of their experiments and to test those predictions to determine their accuracy.
Types of Hypothesis
Types of Hypothesis are as follows:
Research Hypothesis
A research hypothesis is a statement that predicts a relationship between variables. It is usually formulated as a specific statement that can be tested through research, and it is often used in scientific research to guide the design of experiments.
Null Hypothesis
The null hypothesis is a statement that assumes there is no significant difference or relationship between variables. It is often used as a starting point for testing the research hypothesis, and if the results of the study reject the null hypothesis, it suggests that there is a significant difference or relationship between variables.
Alternative Hypothesis
An alternative hypothesis is a statement that assumes there is a significant difference or relationship between variables. It is often used as an alternative to the null hypothesis and is tested against the null hypothesis to determine which statement is more accurate.
Directional Hypothesis
A directional hypothesis is a statement that predicts the direction of the relationship between variables. For example, a researcher might predict that increasing the amount of exercise will result in a decrease in body weight.
Non-directional Hypothesis
A non-directional hypothesis is a statement that predicts the relationship between variables but does not specify the direction. For example, a researcher might predict that there is a relationship between the amount of exercise and body weight, but they do not specify whether increasing or decreasing exercise will affect body weight.
Statistical Hypothesis
A statistical hypothesis is a statement that assumes a particular statistical model or distribution for the data. It is often used in statistical analysis to test the significance of a particular result.
Composite Hypothesis
A composite hypothesis is a statement that assumes more than one condition or outcome. It can be divided into several sub-hypotheses, each of which represents a different possible outcome.
Empirical Hypothesis
An empirical hypothesis is a statement that is based on observed phenomena or data. It is often used in scientific research to develop theories or models that explain the observed phenomena.
Simple Hypothesis
A simple hypothesis is a statement that assumes only one outcome or condition. It is often used in scientific research to test a single variable or factor.
Complex Hypothesis
A complex hypothesis is a statement that assumes multiple outcomes or conditions. It is often used in scientific research to test the effects of multiple variables or factors on a particular outcome.
Applications of Hypothesis
Hypotheses are used in various fields to guide research and make predictions about the outcomes of experiments or observations. Here are some examples of how hypotheses are applied in different fields:
- Science : In scientific research, hypotheses are used to test the validity of theories and models that explain natural phenomena. For example, a hypothesis might be formulated to test the effects of a particular variable on a natural system, such as the effects of climate change on an ecosystem.
- Medicine : In medical research, hypotheses are used to test the effectiveness of treatments and therapies for specific conditions. For example, a hypothesis might be formulated to test the effects of a new drug on a particular disease.
- Psychology : In psychology, hypotheses are used to test theories and models of human behavior and cognition. For example, a hypothesis might be formulated to test the effects of a particular stimulus on the brain or behavior.
- Sociology : In sociology, hypotheses are used to test theories and models of social phenomena, such as the effects of social structures or institutions on human behavior. For example, a hypothesis might be formulated to test the effects of income inequality on crime rates.
- Business : In business research, hypotheses are used to test the validity of theories and models that explain business phenomena, such as consumer behavior or market trends. For example, a hypothesis might be formulated to test the effects of a new marketing campaign on consumer buying behavior.
- Engineering : In engineering, hypotheses are used to test the effectiveness of new technologies or designs. For example, a hypothesis might be formulated to test the efficiency of a new solar panel design.
How to write a Hypothesis
Here are the steps to follow when writing a hypothesis:
Identify the Research Question
The first step is to identify the research question that you want to answer through your study. This question should be clear, specific, and focused. It should be something that can be investigated empirically and that has some relevance or significance in the field.
Conduct a Literature Review
Before writing your hypothesis, it’s essential to conduct a thorough literature review to understand what is already known about the topic. This will help you to identify the research gap and formulate a hypothesis that builds on existing knowledge.
Determine the Variables
The next step is to identify the variables involved in the research question. A variable is any characteristic or factor that can vary or change. There are two types of variables: independent and dependent. The independent variable is the one that is manipulated or changed by the researcher, while the dependent variable is the one that is measured or observed as a result of the independent variable.
Formulate the Hypothesis
Based on the research question and the variables involved, you can now formulate your hypothesis. A hypothesis should be a clear and concise statement that predicts the relationship between the variables. It should be testable through empirical research and based on existing theory or evidence.
Write the Null Hypothesis
The null hypothesis is the opposite of the alternative hypothesis, which is the hypothesis that you are testing. The null hypothesis states that there is no significant difference or relationship between the variables. It is important to write the null hypothesis because it allows you to compare your results with what would be expected by chance.
Refine the Hypothesis
After formulating the hypothesis, it’s important to refine it and make it more precise. This may involve clarifying the variables, specifying the direction of the relationship, or making the hypothesis more testable.
Examples of Hypothesis
Here are a few examples of hypotheses in different fields:
- Psychology : “Increased exposure to violent video games leads to increased aggressive behavior in adolescents.”
- Biology : “Higher levels of carbon dioxide in the atmosphere will lead to increased plant growth.”
- Sociology : “Individuals who grow up in households with higher socioeconomic status will have higher levels of education and income as adults.”
- Education : “Implementing a new teaching method will result in higher student achievement scores.”
- Marketing : “Customers who receive a personalized email will be more likely to make a purchase than those who receive a generic email.”
- Physics : “An increase in temperature will cause an increase in the volume of a gas, assuming all other variables remain constant.”
- Medicine : “Consuming a diet high in saturated fats will increase the risk of developing heart disease.”
Purpose of Hypothesis
The purpose of a hypothesis is to provide a testable explanation for an observed phenomenon or a prediction of a future outcome based on existing knowledge or theories. A hypothesis is an essential part of the scientific method and helps to guide the research process by providing a clear focus for investigation. It enables scientists to design experiments or studies to gather evidence and data that can support or refute the proposed explanation or prediction.
The formulation of a hypothesis is based on existing knowledge, observations, and theories, and it should be specific, testable, and falsifiable. A specific hypothesis helps to define the research question, which is important in the research process as it guides the selection of an appropriate research design and methodology. Testability of the hypothesis means that it can be proven or disproven through empirical data collection and analysis. Falsifiability means that the hypothesis should be formulated in such a way that it can be proven wrong if it is incorrect.
In addition to guiding the research process, the testing of hypotheses can lead to new discoveries and advancements in scientific knowledge. When a hypothesis is supported by the data, it can be used to develop new theories or models to explain the observed phenomenon. When a hypothesis is not supported by the data, it can help to refine existing theories or prompt the development of new hypotheses to explain the phenomenon.
When to use Hypothesis
Here are some common situations in which hypotheses are used:
- In scientific research , hypotheses are used to guide the design of experiments and to help researchers make predictions about the outcomes of those experiments.
- In social science research , hypotheses are used to test theories about human behavior, social relationships, and other phenomena.
- I n business , hypotheses can be used to guide decisions about marketing, product development, and other areas. For example, a hypothesis might be that a new product will sell well in a particular market, and this hypothesis can be tested through market research.
Characteristics of Hypothesis
Here are some common characteristics of a hypothesis:
- Testable : A hypothesis must be able to be tested through observation or experimentation. This means that it must be possible to collect data that will either support or refute the hypothesis.
- Falsifiable : A hypothesis must be able to be proven false if it is not supported by the data. If a hypothesis cannot be falsified, then it is not a scientific hypothesis.
- Clear and concise : A hypothesis should be stated in a clear and concise manner so that it can be easily understood and tested.
- Based on existing knowledge : A hypothesis should be based on existing knowledge and research in the field. It should not be based on personal beliefs or opinions.
- Specific : A hypothesis should be specific in terms of the variables being tested and the predicted outcome. This will help to ensure that the research is focused and well-designed.
- Tentative: A hypothesis is a tentative statement or assumption that requires further testing and evidence to be confirmed or refuted. It is not a final conclusion or assertion.
- Relevant : A hypothesis should be relevant to the research question or problem being studied. It should address a gap in knowledge or provide a new perspective on the issue.
Advantages of Hypothesis
Hypotheses have several advantages in scientific research and experimentation:
- Guides research: A hypothesis provides a clear and specific direction for research. It helps to focus the research question, select appropriate methods and variables, and interpret the results.
- Predictive powe r: A hypothesis makes predictions about the outcome of research, which can be tested through experimentation. This allows researchers to evaluate the validity of the hypothesis and make new discoveries.
- Facilitates communication: A hypothesis provides a common language and framework for scientists to communicate with one another about their research. This helps to facilitate the exchange of ideas and promotes collaboration.
- Efficient use of resources: A hypothesis helps researchers to use their time, resources, and funding efficiently by directing them towards specific research questions and methods that are most likely to yield results.
- Provides a basis for further research: A hypothesis that is supported by data provides a basis for further research and exploration. It can lead to new hypotheses, theories, and discoveries.
- Increases objectivity: A hypothesis can help to increase objectivity in research by providing a clear and specific framework for testing and interpreting results. This can reduce bias and increase the reliability of research findings.
Limitations of Hypothesis
Some Limitations of the Hypothesis are as follows:
- Limited to observable phenomena: Hypotheses are limited to observable phenomena and cannot account for unobservable or intangible factors. This means that some research questions may not be amenable to hypothesis testing.
- May be inaccurate or incomplete: Hypotheses are based on existing knowledge and research, which may be incomplete or inaccurate. This can lead to flawed hypotheses and erroneous conclusions.
- May be biased: Hypotheses may be biased by the researcher’s own beliefs, values, or assumptions. This can lead to selective interpretation of data and a lack of objectivity in research.
- Cannot prove causation: A hypothesis can only show a correlation between variables, but it cannot prove causation. This requires further experimentation and analysis.
- Limited to specific contexts: Hypotheses are limited to specific contexts and may not be generalizable to other situations or populations. This means that results may not be applicable in other contexts or may require further testing.
- May be affected by chance : Hypotheses may be affected by chance or random variation, which can obscure or distort the true relationship between variables.
About the author
Muhammad Hassan
Researcher, Academic Writer, Web developer
You may also like

Data Collection – Methods Types and Examples

Delimitations in Research – Types, Examples and...

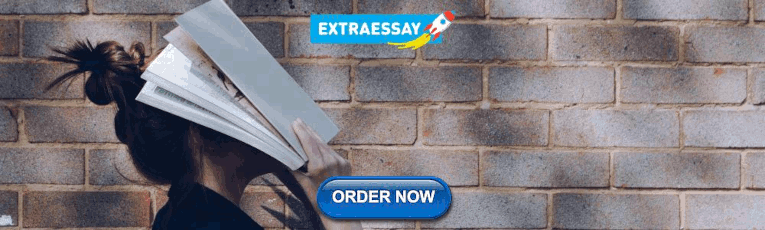
Research Process – Steps, Examples and Tips

Research Design – Types, Methods and Examples

Institutional Review Board – Application Sample...

Evaluating Research – Process, Examples and...
What Is a Hypothesis? (Science)
If...,Then...
Angela Lumsden/Getty Images
- Scientific Method
- Chemical Laws
- Periodic Table
- Projects & Experiments
- Biochemistry
- Physical Chemistry
- Medical Chemistry
- Chemistry In Everyday Life
- Famous Chemists
- Activities for Kids
- Abbreviations & Acronyms
- Weather & Climate
- Ph.D., Biomedical Sciences, University of Tennessee at Knoxville
- B.A., Physics and Mathematics, Hastings College
A hypothesis (plural hypotheses) is a proposed explanation for an observation. The definition depends on the subject.
In science, a hypothesis is part of the scientific method. It is a prediction or explanation that is tested by an experiment. Observations and experiments may disprove a scientific hypothesis, but can never entirely prove one.
In the study of logic, a hypothesis is an if-then proposition, typically written in the form, "If X , then Y ."
In common usage, a hypothesis is simply a proposed explanation or prediction, which may or may not be tested.
Writing a Hypothesis
Most scientific hypotheses are proposed in the if-then format because it's easy to design an experiment to see whether or not a cause and effect relationship exists between the independent variable and the dependent variable . The hypothesis is written as a prediction of the outcome of the experiment.
Null Hypothesis and Alternative Hypothesis
Statistically, it's easier to show there is no relationship between two variables than to support their connection. So, scientists often propose the null hypothesis . The null hypothesis assumes changing the independent variable will have no effect on the dependent variable.
In contrast, the alternative hypothesis suggests changing the independent variable will have an effect on the dependent variable. Designing an experiment to test this hypothesis can be trickier because there are many ways to state an alternative hypothesis.
For example, consider a possible relationship between getting a good night's sleep and getting good grades. The null hypothesis might be stated: "The number of hours of sleep students get is unrelated to their grades" or "There is no correlation between hours of sleep and grades."
An experiment to test this hypothesis might involve collecting data, recording average hours of sleep for each student and grades. If a student who gets eight hours of sleep generally does better than students who get four hours of sleep or 10 hours of sleep, the hypothesis might be rejected.
But the alternative hypothesis is harder to propose and test. The most general statement would be: "The amount of sleep students get affects their grades." The hypothesis might also be stated as "If you get more sleep, your grades will improve" or "Students who get nine hours of sleep have better grades than those who get more or less sleep."
In an experiment, you can collect the same data, but the statistical analysis is less likely to give you a high confidence limit.
Usually, a scientist starts out with the null hypothesis. From there, it may be possible to propose and test an alternative hypothesis, to narrow down the relationship between the variables.
Example of a Hypothesis
Examples of a hypothesis include:
- If you drop a rock and a feather, (then) they will fall at the same rate.
- Plants need sunlight in order to live. (if sunlight, then life)
- Eating sugar gives you energy. (if sugar, then energy)
- White, Jay D. Research in Public Administration . Conn., 1998.
- Schick, Theodore, and Lewis Vaughn. How to Think about Weird Things: Critical Thinking for a New Age . McGraw-Hill Higher Education, 2002.
- Null Hypothesis Examples
- Examples of Independent and Dependent Variables
- Difference Between Independent and Dependent Variables
- Null Hypothesis Definition and Examples
- Definition of a Hypothesis
- What Are the Elements of a Good Hypothesis?
- Six Steps of the Scientific Method
- Independent Variable Definition and Examples
- What Are Examples of a Hypothesis?
- Understanding Simple vs Controlled Experiments
- Scientific Method Flow Chart
- Scientific Method Vocabulary Terms
- What Is a Testable Hypothesis?
- What 'Fail to Reject' Means in a Hypothesis Test
- How To Design a Science Fair Experiment
- What Is an Experiment? Definition and Design
- Daily Crossword
- Word Puzzle
- Word Finder
- Word of the Day
- Synonym of the Day
- Word of the Year
- Language stories
- All featured
- Gender and sexuality
- All pop culture
- Writing hub
- Grammar essentials
- Commonly confused
- All writing tips
- Pop culture
- Writing tips
Advertisement
[ hahy- poth - uh -sis , hi- ]
- a proposition, or set of propositions, set forth as an explanation for the occurrence of some specified group of phenomena, either asserted merely as a provisional conjecture to guide investigation working hypothesis or accepted as highly probable in the light of established facts.
- a proposition assumed as a premise in an argument.
- the antecedent of a conditional proposition.
- a mere assumption or guess.
/ haɪˈpɒθɪsɪs /
- a suggested explanation for a group of facts or phenomena, either accepted as a basis for further verification ( working hypothesis ) or accepted as likely to be true Compare theory
- an assumption used in an argument without its being endorsed; a supposition
- an unproved theory; a conjecture
/ hī-pŏth ′ ĭ-sĭs /
, Plural hypotheses hī-pŏth ′ ĭ-sēz′
- A statement that explains or makes generalizations about a set of facts or principles, usually forming a basis for possible experiments to confirm its viability.
- plur. hypotheses (heye- poth -uh-seez) In science, a statement of a possible explanation for some natural phenomenon. A hypothesis is tested by drawing conclusions from it; if observation and experimentation show a conclusion to be false, the hypothesis must be false. ( See scientific method and theory .)
Discover More
Derived forms.
- hyˈpothesist , noun
Other Words From
- hy·pothe·sist noun
- counter·hy·pothe·sis noun plural counterhypotheses
- subhy·pothe·sis noun plural subhypotheses
Word History and Origins
Origin of hypothesis 1
Synonym Study
Example sentences.
Each one is a set of questions we’re fascinated by and hypotheses we’re testing.
Mousa’s research hinges on the “contact hypothesis,” the idea that positive interactions among rival group members can reduce prejudices.
Do more research on it, come up with a hypothesis as to why it underperforms, and try to improve it.
Now is the time to test your hypotheses to figure out what’s changing in your customers’ worlds, and address these topics directly.
Whether computing power alone is enough to fuel continued machine learning breakthroughs is a source of debate, but it seems clear we’ll be able to test the hypothesis.
Though researchers have struggled to understand exactly what contributes to this gender difference, Dr. Rohan has one hypothesis.
The leading hypothesis for the ultimate source of the Ebola virus, and where it retreats in between outbreaks, lies in bats.
In 1996, John Paul II called the Big Bang theory “more than a hypothesis.”
To be clear: There have been no double-blind or controlled studies that conclusively confirm this hair-loss hypothesis.
The bacteria-driven-ritual hypothesis ignores the huge diversity of reasons that could push someone to perform a religious ritual.
And remember it is by our hypothesis the best possible form and arrangement of that lesson.
Taken in connection with what we know of the nebulæ, the proof of Laplace's nebular hypothesis may fairly be regarded as complete.
What has become of the letter from M. de St. Mars, said to have been discovered some years ago, confirming this last hypothesis?
To admit that there had really been any communication between the dead man and the living one is also an hypothesis.
"I consider it highly probable," asserted Aunt Maria, forgetting her Scandinavian hypothesis.
Related Words
- explanation
- interpretation
- proposition
- supposition
More About Hypothesis
What is a hypothesis .
In science, a hypothesis is a statement or proposition that attempts to explain phenomena or facts. Hypotheses are often tested to see if they are accurate.
Crafting a useful hypothesis is one of the early steps in the scientific method , which is central to every field of scientific experimentation. A useful scientific hypothesis is based on current, accepted scientific knowledge and is testable.
Outside of science, the word hypothesis is often used more loosely to mean a guess or prediction.
Why is hypothesis important?
The first records of the term hypothesis come from around 1590. It comes from the Greek term hypóthesis , meaning “basis, supposition.”
Trustworthy science involves experiments and tests. In order to have an experiment, you need to test something. In science, that something is called a hypothesis . It is important to remember that, in science, a verified hypothesis is not actually confirmed to be an absolute truth. Instead, it is accepted to be accurate according to modern knowledge. Science always allows for the possibility that new information could disprove a widely accepted hypothesis .
Related to this, scientists will usually only propose a new hypothesis when new information is discovered because there is no reason to test something that is already accepted as scientifically accurate.
Did you know … ?
It can take a long time and even the discovery of new technology to confirm that a hypothesis is accurate. Physicist Albert Einstein ’s 1916 theory of relativity contained hypotheses about space and time that have only been confirmed recently, thanks to modern technology!
What are real-life examples of hypothesis ?
While in science, hypothesis has a narrow meaning, in general use its meaning is broader.
"This study confirms the hypothesis that individuals who have been infected with COVID-19 have persistent objectively measurable cognitive deficits." (N=81,337) Ventilation subgroup show 7-point reduction in IQ https://t.co/50xrNNHC5E — Claire Lehmann (@clairlemon) July 23, 2021
Not everyone drives. They can walk, cycle, catch a train, tram etc. That’s alternatives. What’s your alternative in your hypothesis? — Barry (@Bazzaboy1982) July 27, 2021
What other words are related to hypothesis ?
- scientific method
- scientific theory
Quiz yourself!
True or False?
In science, a hypothesis must be based on current scientific information and be testable.
Click through the PLOS taxonomy to find articles in your field.
For more information about PLOS Subject Areas, click here .
Loading metrics
Open Access
Peer-reviewed
Research Article
How Doctors Generate Diagnostic Hypotheses: A Study of Radiological Diagnosis with Functional Magnetic Resonance Imaging
* E-mail: [email protected]
Affiliation Laboratory of Medical Informatics (LIM 01), Faculty of Medicine of the University of São Paulo, São Paulo, Brazil
Affiliation Department and Institute of Radiology (LIM 44), Faculty of Medicine of the University of São Paulo, São Paulo, Brazil
Affiliations Department and Institute of Radiology (LIM 44), Faculty of Medicine of the University of São Paulo, São Paulo, Brazil, Center for Mathematics, Computation and Cognition, Federal University of ABC, Santo André, Brazil
Affiliation Wellcome Trust Centre for Neuroimaging, University College London, London, United Kingdom
- Marcio Melo,
- Daniel J. Scarpin,
- Edson Amaro Jr,
- Rodrigo B. D. Passos,
- João R. Sato,
- Karl J. Friston,
- Cathy J. Price
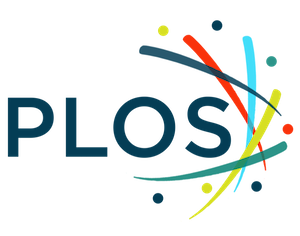
- Published: December 14, 2011
- https://doi.org/10.1371/journal.pone.0028752
- Reader Comments
In medical practice, diagnostic hypotheses are often made by physicians in the first moments of contact with patients; sometimes even before they report their symptoms. We propose that generation of diagnostic hypotheses in this context is the result of cognitive processes subserved by brain mechanisms that are similar to those involved in naming objects or concepts in everyday life.
Methodology and Principal Findings
To test this proposal we developed an experimental paradigm with functional magnetic resonance imaging (fMRI) using radiological diagnosis as a model. Twenty-five radiologists diagnosed lesions in chest X-ray images and named non-medical targets (animals) embedded in chest X-ray images while being scanned in a fMRI session. Images were presented for 1.5 seconds; response times (RTs) and the ensuing cortical activations were assessed. The mean response time for diagnosing lesions was 1.33 (SD ±0.14) seconds and 1.23 (SD ±0.13) seconds for naming animals. 72% of the radiologists reported cogitating differential diagnoses during trials (3.5 seconds). The overall pattern of cortical activations was remarkably similar for both types of targets. However, within the neural systems shared by both stimuli, activation was significantly greater in left inferior frontal sulcus and posterior cingulate cortex for lesions relative to animals.
Conclusions
Generation of diagnostic hypotheses and differential diagnoses made through the immediate visual recognition of clinical signs can be a fast and automatic process. The co-localization of significant brain activation for lesions and animals suggests that generating diagnostic hypotheses for lesions and naming animals are served by the same neuronal systems. Nevertheless, diagnosing lesions was cognitively more demanding and associated with more activation in higher order cortical areas. These results support the hypothesis that medical diagnoses based on prompt visual recognition of clinical signs and naming in everyday life are supported by similar brain systems.
Citation: Melo M, Scarpin DJ, Amaro E Jr, Passos RBD, Sato JR, Friston KJ, et al. (2011) How Doctors Generate Diagnostic Hypotheses: A Study of Radiological Diagnosis with Functional Magnetic Resonance Imaging. PLoS ONE 6(12): e28752. https://doi.org/10.1371/journal.pone.0028752
Editor: André Aleman, University of Groningen, Netherlands
Received: August 15, 2011; Accepted: November 14, 2011; Published: December 14, 2011
Copyright: © 2011 Melo et al. This is an open-access article distributed under the terms of the Creative Commons Attribution License, which permits unrestricted use, distribution, and reproduction in any medium, provided the original author and source are credited.
Funding: This study is part of the Cooperação Interinstitucional de Apoio à Pesquisa sobre o Cerebro (CINAPCE) funded by Fundação de Amparo à Pesquisa do Estado de São Paulo (FAPESP), Brazil. Cathy J. Price and Karl J. Friston are funded by the Wellcome Trust, UK. The funders had no role in study design, data collection and analysis, decision to publish, or preparation of the manuscript.
Competing interests: The authors have declared that no competing interests exist.
Introduction
There is substantial and converging evidence that a significant part of the understanding of the environment that we have in our everyday lives is carried out by brain mechanisms that are fast, automatic, and effortless [1] , [2] , [3] . Possibly as a consequence of these processes, diagnostic hypotheses in medical practice are often made by physicians in the first moments of contact with patients; sometimes even before the report of symptoms [4] , [5] , [6] , [7] , [8] . To exemplify, when a doctor encounters a patient with pronounced jaundice diagnostic hypotheses related to liver diseases immediately and automatically come to her/his awareness. This type of diagnosis has been ascribed to pattern recognition or non-analytical reasoning [9] , [10] .
We propose that the generation of diagnostic hypotheses in such circumstances is the result of neurocognitive processes that are similar to those involved in naming objects or concepts in everyday life. Conversely, recognition of objects in everyday life can be conceptualized as a diagnostic process [11] . A critical test of this proposal would be to compare the brain systems involved in diagnosing lesions with those involved in naming. To explore this hypothesis, radiological diagnosis was used as a model in the visual domain. We developed an experimental paradigm in which radiologists diagnosed lesions in chest X-ray images and named non-medical targets (animals) embedded in chest X-ray images, during functional magnetic resonance imaging (fMRI). We expected to show that diagnosing lesions and naming animals, presented in the same context, would produce a similar pattern of brain activations. Naming letters was introduced as a control task (see Figure 1 ).
- PPT PowerPoint slide
- PNG larger image
- TIFF original image
*Arrows pointing to targets in the image; not present in the original images.
https://doi.org/10.1371/journal.pone.0028752.g001
Mean response times (RTs), error and hesitation rates are shown in Table 1 .
https://doi.org/10.1371/journal.pone.0028752.t001
When naming lesions, subjects reported becoming aware of a greater number of potential names in comparison to animals and letters (as evidenced by the lexical semantic association indices in Table 1 ). The associated words were usually different names, i.e. synonyms, for the same target, e.g. ‘enlarged heart’ while diagnosing cardiomegaly, or the two words name for the lesion, e.g. ‘mediastinal enlargement’. However, 18 (72%) participants reported that while diagnosing some lesions the name of alternative diagnoses came to mind, e.g. ‘bulla’ while diagnosing a cavitation; 15.8% of the lexical semantic associations for lesions were differential diagnoses. Twenty-two (88%) participants reported becoming aware of the name of other animals as alternatives to some of the animals they were naming; e.g. ‘dromedary’ while naming a camel; 64.0% of the lexical semantic associations in this category were the names of other animals.
In 8.00% of the correct responses for lesions, subjects responded with a one-word name other than the name learned during training (e.g. ‘condensation’ in response to pneumonia). Also in 5.13% of lesion trials, the correct responses had more than one word (e.g. ‘aortic elongation’ or ‘pleural effusion’).
The patterns of cortical activations observed when naming each category of stimulus (relative to a control baseline of null events), were strikingly similar in their anatomical deployment ( Figure 2 ). When lexical semantic associations were not controlled, activation was higher for naming lesions than naming animals and letters in the left inferior frontal sulcus and posterior cingulate cortex ( Table 2 and Figure 3 ). Activation in the same areas was also higher for naming animals than naming letters (p<0.001 uncorrected). This decreasing order of activation: lesions>animals>letters ( Figure 3 ) is parallel to a similar order of diminishing lexical semantic association indices ( Table 1 ). Indeed, when lexical semantic associations were co-varied out in the second statistical analysis, there were no areas where activation was significantly higher for lesions than animals and letters. This contrasts to the activation in more posterior regions, posterior fusiform gyrus and posterolateral occipital cortex, that was higher for animals than lesions ( Table 2 and Figure 2 ).
*family wise error rate corrected p<0.05. Statistical parametric maps rendered on an International Consortium of Brain Mapping individual brain.
https://doi.org/10.1371/journal.pone.0028752.g002
* 90% confidence interval. # contrast [lesions>(animals and letters)] inclusively masked with lesions>baseline, lesions>animals, and lesions>letters at p = 0.001.
https://doi.org/10.1371/journal.pone.0028752.g003
https://doi.org/10.1371/journal.pone.0028752.t002
In summary, naming lesions, animals and letters activated the same set of distributed brain regions but to significantly different degrees. In high-order cortical regions (prefrontal and cingulate cortices), activation was proportional to the number of lexical semantic associations (lesions>animals>letters), while in visual cortices activation was higher for animal naming.
This investigation was conducted to test the proposition that generation of diagnostic hypotheses evoked by the immediate visual recognition of clinical signs engages neural systems that are recruited when naming objects in everyday life. The results support this hypothesis by showing very significant and similar activations in a circumscribed set of distributed cortical regions when naming radiological lesions and animals in the same context. However, diagnosing lesions was cognitively more demanding and associated with more activation in higher order cortical areas.
Higher mean RTs, error, and hesitation rates suggest that, on average, diagnosing lesions was more difficult than naming animals in our experimental setting. This could be related to the visual characteristics of lesions and/or the fact that low-frequency (in everyday language) lesion names are more difficult to recall, compared to high-frequency animal and letter names [12] . Lexical semantic associations, i.e. being aware of words/concepts other than that vocalized, were more frequent while diagnosing lesions ( Table 1 ), as compared to the other two categories, also indicating greater cognitive demand related to the selection of appropriate names in this particular task.
A relevant aspect of our results was that generation of diagnostic hypotheses can be very fast; the mean RT to diagnose lesions was 1.33 seconds. It is possible that the training before the fMRI experiment contributed to this performance. But very rapid identification of lesions (<1 second) has already been reported in radiology studies [13] , [14] , [15] .
An important finding was that radiologists were able to cogitate differential diagnoses during the 3.5 seconds of a trial. A similar awareness of alternative names of other animals was reported while naming animals. In a few cases even letters evoked words to subjects ( Table 1 ). Participants were not instructed to make differential diagnoses or to think about alternative names for animals during the task. These results are compatible with a fast and automatic semantic association process, in which the recall of a diagnosis or a name of an animal occurs with the concomitant activation of semantically related concepts [16] , [17] , [18] . Clearly, a formal and definitive diagnosis can not be made in seconds but its core cognitive process, the generation of diagnostic hypotheses which were the names of lesions in our study, is crucial for a final correct diagnosis [4] , [5] , [6] , [7] , [8] .
Diagnosing lesions in radiological images can be conceptualized as a process that is similar to localizing and naming objects in a scene [19] . There are several fMRI studies of location and recognition [20] or naming objects [21] but we are unaware of studies in which all of these tasks are combined. We found very significant brain activations associated with the localization, recognition and naming of targets in radiological images, be they lesions, animals, or letters ( Figure 2 ). Activations were greater in the left inferior frontal sulcus and posterior cingulate cortex for naming lesions than animals and also greater for naming animals than letters ( Table 2 and Figure 3 ). When lexical semantic associations, more frequent for lesions ( Table1 ), were taken into account in an analysis of covariance the difference of activations between those regions was no longer significant (at a corrected level). All the regions activated in the present study have also been reported in object naming in other studies [21] , therefore there was no indication that the participating radiogists were naming animals differently from laypeople.
In agreement with our findings, activation in the left inferior frontal sulcus was reported in two fMRI studies of semantic verbal fluency - generating and vocalizing associated words in response to a word - in which there was greater lexical semantic demand in contrast to the comparison task, reading aloud [22] , [23] . This is consistent with increased cognitive control when it is necessary to make a choice between synonymous or competing concepts, e.g. synonym words or differential diagnoses in our study, respectively [24] .
There were regions more activated by naming animals relative to diagnosing lesions. These regions are usually associated with visual processing and recognition of stimuli; namely, posterior fusiform cortex and posterolateral occipital cortex ( Table 2 and Figure 2 ) [25] . We believe that differences in the visual characteristics of the stimulus categories we used are responsible for the observed differences in activations in these cortical areas.
The cognitive mechanisms underlying medical diagnosis have been studied with different conceptual strategies [10] , [26] , [27] . One important approach relevant to the present study considers it a classification process similar to categorization in everyday life and several authors investigated diagnostic processes with different categorization models [28] , [29] , [30] , [31] , [32] , [33] , [34] . These studies explored several aspects of the cognitive psychology of diagnostic reasoning but did not include a comparison of medical diagnosis tasks with, e.g., categorization of objects.
Interestingly, from a historical point of view, similarities between the classification of diseases and living creatures have been suggested in the past. In the XVII th century Thomas Sydenham in his influential definition of diseases proposed to conceptualize them as specific (ontological) entities similar to plant species [35] . Influenced by Sydenham ideas, Boissier des Sauvages created a classification of diseases, nosology, based on taxonomic principles used in botany and his approach was followed by other phyisicians in the XVIII th century, including Carl Linnaeus [36] .
Instead of using categorization, a concept with different meanings and diverse and conflicting models [37] , [38] , [39] , we chose naming as a conceptually more prudent and descriptive approach. Picture naming has been a model extensively used in cognitive psychology [40] , [41] , [42] , [43] , and functional neuroimaging [21] to investigate how objects are recognized and named.
Some cognitive processes underlying medical skills have been studied with fMRI: one study investigated the neural substrate of visuo-spatial skills in surgery residents [44] and the other compared brain activations in radiologists versus lay participants while viewing X-ray images [45] . However, the neural basis of the medical diagnostic processes per se has not been investigated before.
Our experimental design was not planned to assess the generation of differential diagnostic hypotheses. Taking into account the limitations of the cued retrospective recall employed in the study, the conclusions resulting from the lexical semantic associations data need to be replicated using other methodological approaches.
In contrast to naturalistic and observational studies, planned experiments are by definition artificial and reductionistic due to the need to limit and control independent variables. Radiologists in their usual practice do not usually vocalize their diagnostic hypotheses as they come to their awareness. Conversely, we do not habitually vocalize the names of objects as we recognize them in our everyday life.
Radiologists customarily verbalize their diagnosis using more than one word, e.g. pleural effusion in right hemithorax, in contrast to one-word names for animals. This difference in length of responses could introduce an important confound variable [46] , [47] . To circumvent it, we trained the participants to preferentially use one-word names to diagnose lesions (see Methods and Appendix S1 for details). Probably as a consequence of this experimental stratagem the complete names of the lesions were reported to came to the awareness of participants and were considered competing lexical-semantic associations (see Results ).
Under the blanket rubric ‘medical diagnosis’ there are different cognitive tasks and processes. Considering the case in point of radiological diagnosis: The immediate recognition and diagnosis of an obvious lesion probably recruits different neurocognitive processes as compared to the diagnosis of a subtle and ambiguous alteration with complex differential diagnoses requiring a detailed examination of the radiological image. To create our experimental design we had to limit the scope of the investigation and the conclusions of our study are restricted to the diagnosis of lesions that are immediately identified and diagnosed. It will be important to replicate these results with other approaches, e.g. electrophysiological methods such as electroencephalography or magnetoencephalography. The conceptual hypothesis also needs to be tested in other medical specialties in which diagnosis is strongly based on visual clinical data, e.g. dermatology.
This study is an attempt to investigate the brain mechanisms subserving medical diagnosis. We have demonstrated that differential diagnoses can be automatically elicited in a time frame of seconds in response to clinical signs. Our results support the hypothesis that a process similar to naming things in everyday life occurs when a physician promptly recognizes a characteristic and previously known lesion. In our experimental model, the diagnostic task was cognitively more taxing; more activation in higher order cortical areas was plausibly associated with demands related to the selection of appropriate names as compared to the control task.
The importance of non-analytical reasoning in medical diagnosis has been increasingly stressed [9] , [10] , [27] , [48] . Our study is a contribution to the understanding of its mechanisms. There are recent reviews proposing the application of the knowledge acquired in neuroscience to improve medical education methods [49] , [50] , [51] . An implication of our results is that information obtained from cognitive neuroscience studies on the recognition and naming of objects can be brought to bear on the improvement of diagnostic expertise in the visual domain. In addition, the conceptual hypothesis and the methodological approach described in the present investigation may open new ways to develop studies in medical diagnosis.
Materials and Methods
Participants.
Twenty-six radiologists participated in the investigation. One subject was excluded because the responses were not recorded due to technical problems. Inclusion criteria were completion of radiology residency, right-handedness (as assessed by a modified version of the Edinburgh Handedness Inventory [52] ), and Portuguese as the native language; exclusion criteria were neurological and psychiatric disorders. Sixteen participants were male. The mean age of subjects was 35.9 years (range: 27–55), with a mean of 11.6 years of radiological practice (range: 4–30).
Ethics statement
The protocol was approved by the research ethics committee of the Clinics Hospital, Faculty of Medicine of the University of São Paulo, Brazil. All participants gave written informed consent. They did not receive monetary compensation for their participation.
Radiological images
Our experimental design required the radiological images to have just one circumscribed visual target that could be named. Since many thoracic radiological lesions co-occur, e.g. cardiomegaly is commonly associated with radiological signs of pulmonary venous congestion, we embedded lesions in normal X-ray images using image editing software.
Twenty different types of thoracic radiological lesions, with six different exemplars of each, were created. We used clearly identifiable and easily diagnosable lesions to minimize expertise confounds at the between-subject level and to ensure ceiling performance (to preclude performance confounds). The face validity [53] of radiological images with lesions was assessed by two senior thoracic radiologists. To create non-medical targets line drawings of animals were superimposed on the radiological images. These targets were selected from the database of the International Picture Naming Project [54] . Each type of animal, with six different exemplars, was paired with one type of lesion. Finally, 20 different consonant letters, each with six exemplars from different fonts, were paired with each type of lesion. The resulting radiological images comprised six sets of 60 different stimuli: 20 with lesions, 20 with animals, and 20 with letters.
Longer words or naming targets with more than one word might be associated with longer response times and different patterns of brain activations in regions involved in language processing [46] , [47] . To control for this confounder, we created a list of one-word names to diagnose lesions, e.g. ‘effusion’ for pleural effusion, and asked subjects to use these terms. In additon the duration of vocalization of the radiological lesion names was paired to that of the animal names. Searching for lesions, animals, and letters with the accompanying eye movements was an important variable. We controlled for it matching the locations of the three types of alterations in the chest X-ray images. The methodology used to create the radiological images is detailed in Appendix S1 .
There are many subtleties in a veridical radiological lesion that can not be reproduced with image editing software. For this reason the lesions we created can be considered caricatures of true lesions in the same way that line drawings are an iconic representation of animals.
The key differences between the three categories of stimuli comprised: 1- the visual attributes of lesions where most lesions had simpler and more heterogeneous forms compared to animals and letters which had more defined and homogeneous contours (see figure 1 ); 2- despite the absence of quantitative data on word frequency in medical domain, probably medical terms have in general a lower frequency in daily language and an older age of acquisition in comparison to animals and letters.
The experiment
The creation of images with just one target and the short viewing time of the stimulus images were critical points of our experimental strategy; they were intended to block a more careful scanning of the radiological images, which normally occurs in radiological practice. The neurocognitive processes involved in detailed scanning of the image and images with different numbers of targets to name would be important experimental confounders.
Radiological stimuli were projected through a magnetic shielded glass window to a screen inside the scanner room using a Dell 2400MP digital slide projector. The stimuli subtended 12.5° horizontal and 9.4° vertical visual angles. Each image was presented for 1.5 seconds. Radiologists were oriented just to name the target (lesion, animal or letter) as soon as it was recognized. The task implicitly involved localizing the target, recognizing it, retrieving its name and articulating the response [21] , [55] , [56] . Every image presentation was followed by a black screen with a white (central) fixation cross for 2.0 seconds (i.e., 3.5 seconds per trial). This design was optimized during pilot testing to minimize trial duration, while preserving near-ceiling performance. Participants were trained immediately before the scanning session with three different sets of images.
We used an event-related design: In each session, there were 60 trials (20 lesions, 20 animals, and 20 letters) and 20 null events (with just a fixation cross). There were three sessions per participant with three different sets of images to preclude perceptual learning, repetition suppression and other adaptation effects confounding the naming related responses. Three different sequences of stimuli presentation were optimized in terms of the efficiency to disclose fMRI responses using a genetic algorithm [57] . Each of the three sets of images was presented with one of the three optimal sequences. The order of the sequences was counterbalanced over subjects. The image sets and the order of their presentation for training and scanning were also counterbalanced between participants.
There were two control conditions: 1- naming letters, a high-level baseline, with all cognitive components of the task in diagnosing lesions and naming animals except word retrieval; 2- null events intermixed with stimuli, with a fixation cross during 3.5 seconds, during which participants had no task to execute.
Response time was defined as the elapsed time between the stimulus onset (image presentation) to the beginning of vocalization of the response. Error was defined as error proper or no response. Hesitations were considered as: 1- beginning to vocalize a word and changing to another or 2- stammering on the beginning of the vocalization.
Words and concepts may have different numbers of other words and concepts semantically associated to them [18] . There are indications that the number of potential associates to a word may influence the pattern of brain activations in tasks involving word production [58] . To control for this variable subjects were debriefed immediately after the experiment to assess lexical semantic associations - words other than that vocalized that came to their awareness while naming the different types of stimuli - in each type of stimulus following a standardized protocol (see Appendix S1 ). Participants were not informed beforehand of the debriefing protocol.
Retrospective recall has limitations [59] but we could not monitor those associations during fMRI data acquisition. To instruct subjects to report them after each stimulus could induce participants to actively search for associated words and concepts and create an important confounder in the experimental design. However, classical memory studies investigating cued recall found very high recall rates in tasks more demanding than in our investigation in particular when there was semantic processing while encoding the stimuli [60] , [61] , [62] . Also, retrospective recall methods have been used as reliable assessments in the context of fMRI experiments [63] , [64] .
Data acquisition
MR images were acquired in a 3 Tesla Philips Achieva system with an 8-channel head coil. Blood oxygenation level-dependent (BOLD) sensitive T2*-weighted images were obtained using an SENSE gradient-echo echo-planar imaging pulse sequence with the following parameters: repetition time: 2500 ms, echo time: 30 ms, flip angle: 90°, field of view: 240 mm 2 , in-plane voxel resolution: 3 mm 2 . Fifty 3 mm axial slices were acquired, with a slice gap of 0.3 mm and a +30° image plan tilt to reduce artifacts in inferior temporal lobe [65] . Functional sessions were preceded by 10.0 s of dummy scans to ensure steady-state magnetization. A T1-weighted structural image (voxel size: 1 mm 3 ) was acquired after the functional sessions for coregistration with the fMRI data.
Stimulus presentation and response recording were performed with E-Prime 2.0 software (Psychology Software Tools). A plastic mouthpiece was anatomically adjusted to the mouth of the participants enabling to isolate the sound of the vocalization of responses from the scanner's noise. The voice sound was conducted through a pneumatic system to a high-sensitivity microphone outside the scanner room, pre-amplified, and recorded. Barch et al. described a similar approach to record overt verbal responses [66] . Response times were measured following a standardized protocol using Praat 5.1, a software for phonetic analysis [67] , after filtration of the background noise.
Statistical and data analyses
Data processing and statistical analyses were conducted using SPM8 software (Wellcome Trust Centre for Neuroimaging) [68] . Functional volumes were realigned, un-warped, coregistered to the structural image, normalized to the MNI space, and smoothed with an isotropic Gaussian kernel with 6 mm FWHM.
After preprocessing, a first-level analysis was conducted at the individual level to estimate category-specific activations at each voxel. Time-series from each voxel were high-pass filtered with a cut-off period of 1/128 Hz to remove signal drift and low-frequency noise. A gray-matter image resulting from the segmentation of the structural image was used as a mask in the analysis of functional activations. All category-specific (lesion, animal or letter) trials were modeled as a stick-function and convolved with a canonical hemodynamic response function. Trials with errors, hesitations, and outlying RT's (>2 standard deviations of the mean RT for the respective target type) were modeled as events of no interest. RT's were also included as nuisance variables to remove response time effects within condition.
Category-specific activations were estimated in the usual way using the appropriate t-contrast. The resulting subject-specific contrast images were then entered into a second-level analysis of covariance (ANCOVA). This (random effects) between-subject analysis was conducted without and with lexical semantic associations (the mean number of lexical semantic associations for each category made by every participant) as a nuisance variable. The differences in category-specific activation were then assessed using statistical parametric maps (SPMs) with a criterion of p <0.05 (corrected for multiple comparisons using random field theory).
To limit head movements during the experiment participants were trained before the scanning session. The un-warping of the functional images during preprocessing with SPM8 was an additional measure to compensate for movements during the vocalization of responses [69] . Head movements during fMRI sessions were generally small, with intra-session translation and rotation movements of less than 1.5 mm and 1.5° respectively.
Supporting information.
The methodology for the creation of radiological images with embedded targets, training and debriefing protocols are detailed in Appendix S1 .
Supporting Information
Appendix s1..
https://doi.org/10.1371/journal.pone.0028752.s001
Acknowledgments
We are grateful for the cooperation of the radiologists that participated in all stages of the study and to the staff of the Magnetic Resonance Service of the Institute of Radiology, Clinics Hospital of the Faculty of Medicine, University of São Paulo. We are also thankful for the collaboration of the following people: Maria da Graça Morais Martin contributed to the experimental design suggesting the insertion of objects in the radiological images; Claudio Lucarelli and Marcelo Buarque de Gusmão Funari were responsible for the validation of the lesion images. José Jamelão Macedo de Medeiros, Fernando Araujo Del Lama, Claudia da Costa Leite, and Eduardo Massad assisted the project in several ways.
Data sharing . The complete set of radiological images used in the study are available on request from the corresponding author.
Author Contributions
Conceived and designed the experiments: MM DJS EA RBDP KJF CJP. Performed the experiments: MM. Analyzed the data: MM CJP JRS EA KJF. Contributed reagents/materials/analysis tools: KJF. Wrote the paper: MM KJF CJP. Development of the conceptual model: MM. Creation of the stimuli (radiological images): DJS RBDP MM.
- View Article
- Google Scholar
- 4. Elstein AS, Shulman LS, Sprafka SA (1978) Medical Problem Solving: An Analysis of Clinical Reasoning. Cambridge, MA: Harvard University Press.
- 17. Anderson JR (1983) The Architecture of Cognition. Cambridge, MA: Harvard University Press.
- 25. Grill-Spector K (2009) What has fMRI taught us about object recognition? In: Dickinson SJ, Leonardis A, Schiele B, Tarr MJ, editors. Object Categorization: Computer and Human Vision Perspectives. Cambridge, U.K.: Cambridge University Press. pp. 102–128.
- 27. Kassirer JP, Wong J, Kopelman RI (2010) Learning Clinical Reasoning. Baltimore, MD: Wolters Kluwer.
- 35. Faber K (1930) Nosography: The Evolution of Clinical Medicine in Modern Times. New York: Paul B. Hoeber Inc.
- 36. Bynum WF (1997) Nosology. In: Bynum WF, Porter R, editors. Companion Encyclopedia of the History of Medicine. London: Routledge. pp. 335–356.
- 37. Smith EE, Medin DL (1981) Categories and Concepts. Cambridge, MA: Harvard University Press.
- 39. Murphy GL (2004) The Big Book of Concepts. Cambridge, MA: MIT Press.
- 53. Kerlinger FN, Lee HB (2000) Validity. In: Kerlinger FN, Lee HB, editors. Foundations of Behavioral Research. 4th ed. Belmont, CA: Cengage. pp. 665–688.
- 59. Lockhart RS (2000) Methods of memory research. In: Tulving E, Craik FIM, editors. The Oxford Handbook of Memory. Oxford, U.K.: Oxford University Press. pp. 45–57.
- 68. Friston KJ, Ashburner JT, Kiebel SJ, Nichols TE, Penny WD (2007) Statistical Parametric Mapping: the Analysis of Functional Brain Images. London: Academic Press.
Define Hypothesis: Unveiling the First Step in Scientific Inquiry
Master Scientific Data Visualization. Learn how to make data easier, unlock insights and captivate audiences effectively.
Welcome to the world of research, where you’ll journey through a universe brimming with questions and curiosity. In this cosmos, a hypothesis is one celestial object you can’t miss! Today’s expedition invites you on board an exploration to ‘Define Hypothesis.’ Hop in; it wouldn’t be hyperbole to state we’re about to unlock the nucleus behind every ever scientific theory and inquiry!
Definition of Hypothesis
Introduction to the concept of hypothesis.
Picture yourself as a detective solving a case. Right from inspecting clues, formulating potential theories on whodunit, putting these theories under rigorous tests until finally reaching that elusive conclusive evidence – exciting, isn’t it?
Now replace detective with researcher and voila – here comes our heavyweight term: Hypothesis. Much like how any plausible theory drives detectives’ investigations, scientific hypotheses are vital navigational compasses guiding researchers in their quest for scientific evolutions.
Explanation of What a Hypothesis Is in The Context of Research and Scientific Inquiry
A hypothesis – popularly known as an educated guess or predictive statement – represents an initial supposition or proposed explanation made on limited information but founded on validation-grounded knowledge. It forms the basis for preliminary exploration into a specific set of circumstances or natural phenomena beyond.
Formulated prior to conducting research, scientists employ hypotheses as testable conjectures to explain an observed behavior or event. Confused? Fret not. To put it simply and by example: “If I increase the frequency of watering my plants twice daily (instead of solely relying upon weather conditions), then they will grow faster.” Now that’s what we call an everyday-life hypothesis!
Remember, hypotheses are not wild guesses plucked out of thin air but rather preconceived assertions open to empirical verification. They mark the inception point for any scientific investigation and serve as cornerstones for further experiments.
Characteristics and Components of a Hypothesis
Key characteristics of a hypothesis.
Before plunging into the deep end to define a hypothesis, let’s brush up on the features that contribute to effective hypotheses. For starters, a strong hypothesis is testable. This means it must be possible for empirical evidence to either support the word hypothesis or contradict it. The proposal should also be logically consistent and grounded firmly in existing knowledge.
Further down the line, another salient feature is specificity. Good hypotheses are not broad statements but instead focus on a specific aspect or phenomenon within the intended research field. Moreover, they are typically succinct and easily understandable ensuring information isn’t lost in translation among researchers.
Moreover, any well-structured hypothesis connects the independent and dependent variables together – typically, there’s at least one independent and one dependent variable involved. These elements form a relationship where changes instigated in the independent variable affect the values observed for the dependent variable.
Lastly but importantly, a solid hypothesis often carries potential implications for future research areas and can potentially lead to further tests and studies if verified.
Elements that make up a well-formulated hypothesis
Delving deeper into what shapes up a robust hypothesis, we realize that certain crucial components determine its effectiveness.
Firstly, every good hypothesis or test has clear variables which essentially refer to specific aspects of the study subject matter being measured or manipulated during research. These aspects are segregated as:
- Independent Variable (IV): This component relates directly to what you have control over in your study.
- Dependent Variable (DV): This component consists of outcomes affected by alterations made in IV
Next comes ‘Predicted Outcome’ – what you anticipate happening as repercussions due to modification of two or more variables under scrutiny.
The ‘Testability’ factor also holds veritable importance comprising experimental procedures capable enough to refute or accept your claims.
The last element circles the argument around presenting a capacity called ‘Relationship’ correlating IV with DV believed to either causing some effect or showcasing an association.
Hence, these prime facets further accentuate your endeavor to adequately define the hypothesis.
Importance and Purpose of a Hypothesis
Understanding the Role of a Hypothesis in Research
First, let’s delve into the overarching role that hypothesis plays within research scenarios. As we define the hypothesis, you should view this as an underlying pillar or guiding star for your investigation. A well-articulated hypothesis steers your exploration by providing clarity on what specifically you aim to examine.
A meaningful analogy would be considering a hypothesis as a compass during a voyage. If research is the vast ocean where confusing whirlpools of data and evidence abound, then it can guide us in our direction rather than letting us drift aimlessly. Furthermore, the formulation of a quality hypothesis inherently demands clarity about your objectives upfront – this essentially sets your research vessel on course bearing towards effective outcomes.
Exploring Why Formulating A Hypothesis is Crucial in Scientific Investigations
So why precisely is nurturing such a detailed forecast vital?
- Structural Advantage: By proposing potential answers to posed questions via hypotheses, researchers streamline their methods and techniques. The approach undertaken depends significantly on what the suggested outcome or phenomenon might be.
- Generate Preliminary Expectations: Even if they’re proven wrong, making observations and developing models based on hypotheses often lead to more interesting inquiries or turn up unexpected findings.
- Quantifiable Predictions: More than simple conjectures, strong hypotheses are testable; they propose results expressed in measurable terms.
In essence, remember that formulating hypotheses smoothes the path towards solid conclusions by being the architect’s blueprints of robust investigations. Never underestimate the forward thrust they provide for progress within scientific inquiry!
Types of Hypotheses
Once we understand to define a hypothesis, we’ll find that hypotheses come in several types. Different classifications of plural hypotheses depend on their formulations and the nature of predictions or assumptions they lead towards – simple, complex, directional, non-directional, null, associative and causal. Let’s explore some of these.
Simple Hypothesis: Definition and Examples
A simple hypothesis is a type of prediction or an educated guess that carries one independent variable and one dependent variable. In essence, it creates a relationship between two singular entities; for instance, ‘Exercise improves memory.’ This suggests that there’s an impact (of improvement) on the ‘memory’ (dependent variable) by ‘exercise’ (independent variable).
Complex Hypothesis: Definition and Examples
On the contrary to its name mate – a simple hypothesis – a complex hypothesis involves more than just two variables. It points out multiple variables and how they interlink with each other. The effects aren’t just limited to cause-and-effect but can be interactive or combined impact-dependent variables too – for instance,’Diet and exercise affect weight loss and heart health.’ Here, diet and exercise are your independent factors influencing multifold aspects like weight loss (a dependent variable) alongside heart health(another dependent variable).
Directional Hypothesis: Definition and Examples
One might argue that the path laid by a directional hypothesis is less twisted as it predicts the directionality of an effect – whether one variable will increase or decrease another variable. An example here could be “Cutting down on alcohol will reduce liver disorders.” Here a reduction in ‘drinking alcohol’ implicitly identifies fewer occurrences of ‘liver disorders.’
Non-directional Hypothesis: Definition and Examples
Sometimes science requires open-ended answers; henceforth comes into play our non-directional hypothesis which merely stipulates that there’s going to be an impact without specifying its course – good, bad or otherwise. For example, “Exposure to secondhand smoke influences lung health.” It infers that there’s an effect on ‘lung health’ due to ‘secondhand smoke,’ without indicating if it’s an improvement or deterioration.
Null Hypothesis: Definition and Examples
The null hypothesis, often symbolized as H0, makes things pretty straight with assumptions; basically, it purports no existence of a relationship between the variables. Researchers utilize this hypothesis chiefly for statistical testing. In lay terms – “Smoking is not linked to lung cancer.” Here a nonexistence of association is suggested between ‘smoking’ and ‘lung cancer.’
Associative and Causal Hypothesis: Explanation and Examples
Now leaving the train station named Null-ville we enter into quite associative terrain where the associative hypothesis foretells ‘relationships’ but are shy when it comes to cause-effects. An instance could be “Students scoring high also tend to play chess.” These fellows here don’t claim that playing chess outrightly shoots up scores yet suggests a specific pattern.
On another spectrum brightful cause-effect claims jump in bravely shouting out not just relationships but boldly stating their causes too – “Consumption of fast food leads to obesity” is being so certain about fast food consumption (cause) escalating obesity levels(effect).
Navigating through these alternative hypotheses and variants allows us to step into researchers’ shoes better while also helps defining complex constructions bit by bit, making them simple outcomes anyone can interpret.
Developing and Testing a Hypothesis
In the world of research, it’s not uncommon to hear someone say “Let’s define hypothesis!” This term may seem complex at first glance, but its essence falls within our natural instinct to question and learn. To give structure to this innate curiosity, we form hypotheses and navigate through the rigorous process of testing them.
Process of Formulating a Hypothesis
Forming an effective hypothesis is both an art and a science. It involves finding a perfect blend between creativity and logical reasoning. Here are some simple yet essential steps you’d want to follow:
- Identify Your Research Question – The first step towards formulating a hypothesis is defining your research question based on preliminary observations or literature review.
- Conduct Thorough Literature Review – Once your question is in place, an extensive read about what has already been studied can help refine it further.
- Create Tentative Explanation – Develop a preliminary answer based on your knowledge and understanding which will serve as your tentative explanation or hypothesis.
- Refine Your Hypothesis : Refine this initial guess considering available resources for empirical testing, ethical implications, and potential outcomes.
Remember that the key is formation clarity in statement-making; overly complex language might obscure rather than clarify your central idea.
Importance of Testing a Hypothesis Through Empirical Research Methods
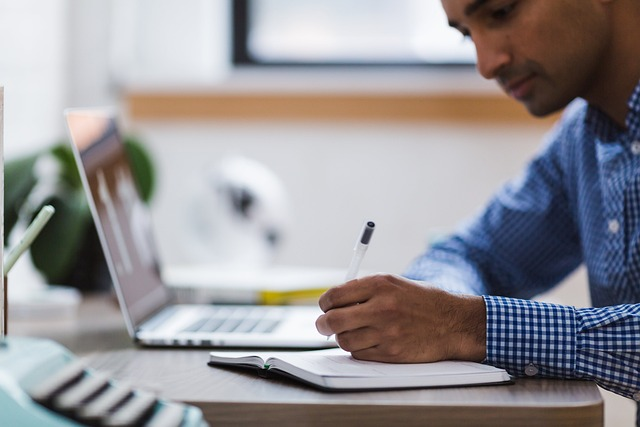
Testing a hypothesis isn’t simply about proving it right or wrong; it’s much more refined than that – it’s about validation and advancement of human knowledge. By applying empirical methods such as observation or experimentation, logic meets practice in real-world scenarios.
These hands-on approaches afford us precious insights into how our theories hold up under scrutiny outside the confines of abstract thought alone.
- Validity Confirmation : Empirical testing helps confirm if our predictions were correct or not, providing validation for our presumptions.
- Understanding Relationships : Testing allows us to assess the relational dynamics between variables under investigation.
- Promotes Scientific Inquiry : Empirical testing encourages a systematic and objective approach to understanding phenomena, which lies at the heart of scientific inquiry.
Consider this: hypotheses are our best-educated guesses – smart hunches rooted in what we know so far. To move beyond guessing and into knowledgeable assertion, we define hypothesis structure as one that can be empirically tested. Only then do we truly start to shape our understanding with any level of certainty.
Examples of Hypotheses in Different Fields
Indeed, it’s fundamental to understand that hypotheses are not confined to a single discipline but span across numerous fields. To better illuminate this, let’s delve into various examples.
Examples of Hypotheses in Scientific Research Studies
In the realm of scientific research studies, hypotheses play a pivotal role in shaping the basis for investigations research hypotheses and experiments. Let’s consider an elementary example: studying plant growth. A researcher might formulate the hypothesis – “If a specific type of fertilizer is used, then plants will grow more rapidly.” This hypothesis aims to validate or refute the assumption that given fertilizer perceptibly affects plant growth rate.
Another common example arises from investigating causal relationships between physical activity and heart health. The scientist may hypothesize that “Regular aerobic exercise decreases the risk of heart disease.”
Examples of Hypotheses in Social Sciences
When we transition towards social sciences, which deals with human behavior and its relation to societal constructs, our formative definitions undergo a change as well.
Imagine researchers examining how socioeconomic status influences educational attainment rates. They could pose a hypothesis saying, “High socioeconomic status positively correlates with higher levels of formal education.” This hypothesis attempts to tie economic background directly to education outcomes.
The correlation between gender diversity within workplace teams and improved business performance presents another illustration. A possible hypothesis could be – “Teams comprising diverse genders exhibit superior business performance than homogenous teams.”
Examples of Hypotheses in Psychology
Within psychology – the study dedicated to how individuals think, feel, and behave; clearly stated hypotheses serve as essential stepping stones for meaningful findings and insights.
Take, for instance, predicting performance under pressure: psychologists may propose an assumption like – “Stress triggers increased errors on complex tasks”. Or when researching cognitive development in children – they may hypothesize – “Language acquisition accelerates once children start attending school”.
Examples of Hypotheses in Medical Research
Lastly but importantly, in medical research, well-articulated hypotheses help probe pressing healthcare questions and identify effective treatments.
For instance: “Patients receiving chemotherapy experience significant weight loss”. Or regarding disease transmission during pandemics – they might propose “Regular hand sanitation reduces the risk of COVID-19 infection.”
In conclusion, these examples hopefully underline the importance and versatility of a hypothesis in scientific inquiry. Irrespective of its utilization within various research fields, a scientific hypothesis still essentially remains an educated assumption that offers direction and purpose to the investigation. Interestingly enough, each study’s defined hypothesis sets forth a path leading towards a better comprehension of our world and life within it.
Common Mistakes to Avoid when Formulating a Hypothesis
Identifying errors that researchers often make when developing a hypothesis.
Many researchers, especially those new in the field, may sometimes falter while crafting their hypotheses. Here are some frequently observed mistakes:
- Framing Vague Hypotheses : Clarity is vital when defining your hypothesis. A common pitfall involves creating an ambiguous statement which leaves room for multiple interpretations. This hinders precise data collection and analysis.
- Formulating Unfalsifiable Hypotheses : These are statements that cannot be proven false because they don’t connect to observable or measurable variables.
- Targeting Unachievable Results : Often, there is an inclination to develop complex hypotheses expecting groundbreaking findings. However, it’s crucial to limit the scope according to practical constraints and possibilities.
- Ignoring Null Hypothesis : The null hypothesis provides a means of contradiction to the alternative hypothesis being tested, making it essential for any research study.
Tips for avoiding these mistakes
After identifying the commonly made errors when forming a hypothesis, let’s now consider some proactive measures you can adopt:
- Crystallize Your Thoughts : Before you articulate your hypothesis, refine and clarify your ideas first. Define the parameters of your study clearly and ensure your proposition directly aligns with them.
- Keep It Simple : Stick with simplicity as much as possible in describing expected relationships or patterns in your research subject area. Remember: A simpler hypothesis often leads to effective testing.
- Embrace Falsifiability . To avoid making unfalsifiable claims, learn how to craft ‘If – Then’ statements articulately in your define hypothesis process.
- Remember the Null Hypothesis : Always formulate and account for a null hypothesis—a statement that negates the relationship between variables—for robust results validation.
In truth, it takes practice to strike the right balance and formulate a solid, practical hypothesis for your research. With these tips in mind, you’re better equipped to avoid common pitfalls that can compromise the quality of your investigation as they guide your approach when you define hypotheses.
Evaluating and Refining a Hypothesis
Laying out a hypothesis is merely the first stage of an intricate journey. Testing and refining this conjecture is equally pivotal in perfecting your next scientific method of undertaking. This pathway comprises evaluation for validity, and relevance, followed by refinement through research findings.
Methods for Assessing the Validity and Relevance of a Hypothesis
To define a hypothesis of meticulosity, we need to subject it to rigorous scrutiny. Utilizing statistical tests enables you to judge the validity of your hypothesis. Here’s a brief look at some key methods that can assist in assessing your theory:
- Empirical Testing : Conduct experiments or surveys as per the requirements of your study.
- Consistency Check : The hypothesis should remain consistent with other established theories and laws within its field.
- Falsifiability principle : Proposed by Karl Popper, a valid hypothesis must be capable of being proven wrong.
Let me reemphasize here, that relevance plays an integral part too especially when defining hypotheses linked with pragmatics like social sciences or business studies.
A relevant hypothesis will hold significance to not just existing knowledge but also pave the way for future work within the particular area of expertise. It should address gaps in current scientific theories while shedding light on possible solutions.
Ways to Refine and Modify a Hypothesis Based on Research Findings
Our job doesn’t end up on developing an initial proposition; it’s crucial to use findings from our research to refine that preliminary conception further. This essential process breathes life into what was once purely speculative.
While refining your conjecture can sound daunting initially, I assure you it’s nothing more complicated than diagnosing any missing links between your original theory and novel evidence you’ve discovered along this research journey.
If H0 (null hypothesis) contradicts your empirical results, then getting back onto the drafting board becomes necessary for crafting H1 (alternative hypothesis). This scientific cycle of formulating, testing then reformulating the hypotheses can continue till we eventually reach statistically significant results.
Remember, it’s important to be open-minded and responsive towards indications from your research findings. They will guide you intuitively in tweaking your working hypothesis in sync with your target goals.
Hence we must embrace this intricate art of defining a hypothesis while simultaneously embracing its dynamic nature which requires periodic refinement based upon insightful feedback from meticulous research.
Summarizing the Key Points About the Definition and Characteristics of a Hypothesis
Having delved into the concept extensively, we can confidently define a hypothesis as an informed and testable guess or prediction that acts as a guiding light in research studies and scientific investigations. When formulated correctly, it comprises two essential elements: clarity and specificity. It should be free from ambiguity, allowing other researchers to easily understand its proposed idea and the direction the study is heading.
In addition, a robust hypothesis exhibits predictability. As a researcher, you’re not only stating what you think will happen but also defining the variables in your experiment – your assumption confines your investigation’s parameters to make it manageable. Lastly, remember that any meaningful hypothesis must be verifiable — capable of being supported or refuted through data collection and analysis.
Reiterating the Importance of Hypotheses in Scientific Inquiry and Research
This discourse wouldn’t be complete without reaffirming how indispensable hypotheses are within scientific explorations and research inquiries. A conceptualized hypothesis serves as a foundational block upon which every aspect of a research project is built. It directs your observations along assumed patterns, thereby saving time during investigations.
We also need to note that formulating hypotheses promotes critical thinking skills among researchers because they require logical reasoning backed by empirical evidence rather than just empty conjectures.
Henceforth, whether you’re treading through unchartered waters of complex scientific endeavors or conducting social science research with less strict rules for predictions – keeping these insights on “define hypothesis” at hand would surely enhance your journey towards revealing valuable truths.
In essence, cultivating a comprehensive understanding of what constitutes a well-formed hypothesis not only lends credibility to our investigative ventures but also enables us to bring precision, focus, and relevance to our chosen field of exploration. The power lies in its simplistic yet profound ability to guide us from uncertainty towards concrete evidential findings – truly embodying scientific inquiry’s spirit!
Unlock the Power of Visualization with Mind the Graph: Elevate Your Hypothesis to New Heights
As a scientist, your hypothesis is the cornerstone of your research journey. But what if you could take it beyond mere words and equations, and transform it into a visual masterpiece that captivates your audience? Enter Mind the Graph , your ultimate ally in scientific visualization. With our intuitive platform, you can seamlessly translate complex hypotheses into stunning graphs, charts, and illustrations that speak volumes. Whether you are presenting at a conference, publishing a paper, or simply sharing your findings with the world, Mind the Graph empowers you to convey your hypotheses with clarity, precision, and undeniable impact. Join the scientific revolution today and let your hypotheses shine like never before with Mind the Graph.
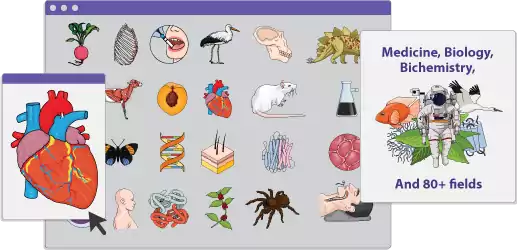
Subscribe to our newsletter
Exclusive high quality content about effective visual communication in science.
Unlock Your Creativity
Create infographics, presentations and other scientifically-accurate designs without hassle — absolutely free for 7 days!
About Fabricio Pamplona
Fabricio Pamplona is the founder of Mind the Graph - a tool used by over 400K users in 60 countries. He has a Ph.D. and solid scientific background in Psychopharmacology and experience as a Guest Researcher at the Max Planck Institute of Psychiatry (Germany) and Researcher in D'Or Institute for Research and Education (IDOR, Brazil). Fabricio holds over 2500 citations in Google Scholar. He has 10 years of experience in small innovative businesses, with relevant experience in product design and innovation management. Connect with him on LinkedIn - Fabricio Pamplona .
Content tags

- ONWARD Medical B.V. Registered Shs-stock
- News for ONWARD Medical B.V. Registered Shs
Nature Medicine Highlights Significant Improvement in Hand and Arm Function After Spinal Cord Injury with Use of ONWARD® ARC-EX® Therapy
ARC Therapy™ shown to be safe and effective in global pivotal trial*
90% of participants improved either strength or function of upper limbs*
Improvement demonstrated in participants up to 34 years post-injury*
87% of participants also reported improvement in overall quality of life*
EINDHOVEN, the Netherlands, May 20, 2024 (GLOBE NEWSWIRE) -- ONWARD Medical N.V. (Euronext: ONWD), the medical technology company creating innovative spinal cord stimulation therapies to restore movement, function, and independence in people with spinal cord injury (SCI), today announces the publication of global Up-LIFT pivotal trial results in Nature Medicine . The study achieved all primary safety and effectiveness endpoints, and ARC-EX Therapy demonstrated significant improvements in upper limb strength, function, and sensation among people with chronic tetraplegia due to cervical SCI.
At the end of the trial, 72% of trial participants were considered responders to non-invasive ARC-EX Therapy* based on a conservative definition requiring responders to meet improvement criteria in both strength and functional domains vs. standard of care rehabilitation alone. Notably, the number of responders increased to 90% when the definition included participants with improvements in at least one strength or functional outcome*.
“Trial results far exceeded our hypothesis of a 50% response rate to ARC-EX Therapy, giving new hope to people with SCI and to rehabilitation providers,” said Chet Moritz, Ph.D., publication lead author, co-Principal Investigator, and Professor of Electrical & Computer Engineering and Rehabilitation Medicine at the University of Washington. “After only two months, more than half of Up-LIFT participants achieved average improvements in grasp force greater than that required to lift filled cups and in pinch force equivalent to that required to pick up an item with a fork or insert a key. 1 , 2 This indicates not only improved strength and function, but also the potential for greater independence with ARC-EX Therapy.”
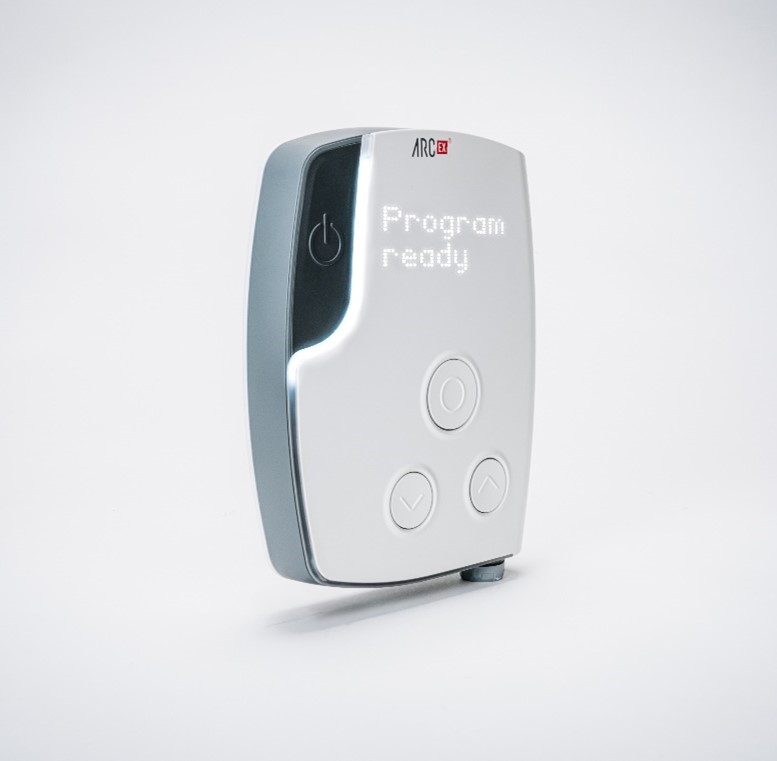
Study participants also reported reduced spasm frequency, improved sleep, and improved upper body sensation, including the sense of touch*. 87% of participants reported that ARC-EX Therapy delivered improvements in overall quality of life*. Self-care, a key component of independence after SCI, also improved significantly*.
“Improvement in arm and hand function is among the highest priorities for people with tetraplegia 3 who have endured far too long without effective therapies for functional recovery,” said Dave Marver, CEO, ONWARD Medical. “The findings published in Nature Medicine provide critical and compelling evidence that ARC-EX has the potential to restore independence in daily activities and improve quality of life. We are laser-focused on our commitment to bring this first-of-its-kind technology to the SCI Community as soon as possible.”
“We are proud that ONWARD has developed this first-ever therapy shown to improve strength and function after chronic incomplete tetraplegia,” said ONWARD Medical co-founders Grégoire Courtine, PhD, Professor of Neuroscience and Neurotechnology at the Swiss Federal Institute of Technology Lausanne (EPFL), and Jocelyne Bloch, MD, Head of Functional Neurosurgery at Lausanne University Hospital and Professor of Neurosurgery at EPFL. “We commend the outstanding researchers and study participants who contributed to this seminal trial.”
ARC-EX Therapy is external, programmed electrical stimulation that targets the spinal cord non-invasively and is designed to aid in functional recovery after SCI. The Up-LIFT study was a prospective, single-arm pivotal study designed to evaluate the safety and effectiveness of the ARC-EX System to treat upper extremity functional deficits in people with chronic tetraplegia. The global study was conducted with 65 participants at 14 leading SCI centers in the United States, Europe, and Canada.
At the end of Q1, ONWARD Medical submitted a De Novo application to the US Food and Drug Administration for market clearance of the ARC-EX System. The Company plans to follow the US application with an application for market approval in Europe.
On Thursday, May 23 rd at 2:30pm CET/8:30am ET following a brief Q1 Business Update, CEO Dave Marver will host a discussion of Up-LIFT pivotal trial results with a panel of principal investigators and study participants.
To join the webcast via Zoom, please register using this link.
Participants may also join by phone:
+32 2 290 9360 (Belgium)
+33 1 7037 2246 (France)
+49 69 3807 9884 (Germany)
+31 20 794 0854 (Netherlands)
+41 22 591 0156 (Switzerland)
+44 203 481 5240 (United Kingdom)
+1 346 248 7799 (US)
Additional telephone numbers available
Meeting ID: 879 5741 2479
A recording of the webcast will be available on the Company’s website following the live event.
Note: All ONWARD ® Medical devices and therapies, including but not limited to ARC-IM ® , ARC-EX ® , ARC-BCI™, and ARC Therapy™, alone or in combination with a brain-computer interface (BCI), are investigational and not available for commercial use.
About ONWARD Medical
ONWARD ® Medical is a medical technology company creating therapies to restore movement, function, and independence in people with spinal cord injury (SCI) and movement disabilities. Building on more than a decade of scientific discovery, preclinical, and clinical research conducted at leading hospitals, rehabilitation clinics, and neuroscience laboratories, the Company has developed ARC Therapy™, which has been awarded ten Breakthrough Device Designations from the US Food and Drug Administration (FDA).
ONWARD ARC Therapy is targeted, programmed spinal cord stimulation designed to be delivered by the Company’s external ARC-EX ® or implantable ARC-IM ® platforms. ARC Therapy can also be delivered by the Company’s ARC-BCI™ platform, which pairs the ARC-IM System with brain-computer interface (BCI) technology to restore movement after SCI with thought-driven control.
Use of non-invasive ARC-EX Therapy significantly improved upper limb function after SCI in the global pivotal Up-LIFT trial, with results published by Nature Medicine in May 2024. The Company has submitted its regulatory application to the FDA for clearance of the ARC-EX System in the US and is preparing for regulatory submission in Europe. In parallel, the Company is conducting clinical studies with its ARC-IM Therapy, which demonstrated positive interim clinical outcomes for improved blood pressure regulation following SCI. Other ongoing clinical studies focus on using ARC-IM Therapy to address mobility after SCI and gait challenges in Parkinson’s disease as well as using the ARC-BCI platform to restore thought-driven movement of both upper and lower limbs after SCI.
Headquartered in Eindhoven, the Netherlands, ONWARD Medical has a Science and Engineering Center in Lausanne, Switzerland and a US office in Boston, Massachusetts. The Company is listed on Euronext Brussels and Amsterdam (ticker: ONWD).
For more information, visit ONWD.com, and connect with us on LinkedIn and YouTube.
For Media Inquiries: Aditi Roy, VP Communications [email protected]
For Investor Inquiries: Amori Fraser, Finance Director [email protected]
Disclaimer
Certain statements, beliefs, and opinions in this press release are forward-looking, which reflect the Company’s or, as appropriate, the Company directors’ current expectations and projections about future events. By their nature, forward-looking statements involve several risks, uncertainties, and assumptions that could cause actual results or events to differ materially from those expressed or implied by the forward-looking statements. These risks, uncertainties, and assumptions could adversely affect the outcome and financial effects of the plans and events described herein. A multitude of factors including, but not limited to, changes in demand, competition, and technology, can cause actual events, performance, or results to differ significantly from any anticipated development. Forward-looking statements contained in this press release regarding past trends or activities should not be taken as a representation that such trends or activities will continue in the future. As a result, the Company expressly disclaims any obligation or undertaking to release any update or revisions to any forward-looking statements in this press release as a result of any change in expectations or any change in events, conditions, assumptions, or circumstances on which these forward-looking statements are based. Neither the Company nor its advisers or representatives nor any of its subsidiary undertakings or any such person’s officers or employees guarantees that the assumptions underlying such forward-looking statements are free from errors nor does either accept any responsibility for the future accuracy of the forward-looking statements contained in this press release or the actual occurrence of the forecasted developments. You should not place undue reliance on forward-looking statements, which speak only as of the date of this press release. All ONWARD Medical devices and therapies referenced here, including but not limited to ARC-IM ® , ARC-EX ® , ARC-BCI™ and ARC Therapy™, are investigational and not available for commercial use.
_________________________________
* Moritz, Chet, et al. “Non-invasive spinal cord stimulation for arm and hand function in chronic tetraplegia: a safety and efficacy trial.” Nature Medicine. 2024. 1 Feingold-Polak, Ronit, et al. “The effects of an object's height and weight on force calibration and kinematics when post-stroke and healthy individuals reach and grasp.” Scientific Reports . 2021. 2 Smaby, Niels, et al. “Identification of key pinch forces required to complete functional tasks.” Journal of Rehabilitation Research & Development . 2004.
3 Anderson, KD. “Targeting recovery: priorities of the spinal cord-injured population.” J ournal of Neurotrauma . 2004.
A photo accompanying this announcement is available at https://www.globenewswire.com/NewsRoom/AttachmentNg/c9a3a475-7e21-41aa-ad6d-ea15e8cc7dae

ONWARD Medical B.V. Registered Shs News MORE
Related stocks.

An official website of the United States government
The .gov means it’s official. Federal government websites often end in .gov or .mil. Before sharing sensitive information, make sure you’re on a federal government site.
The site is secure. The https:// ensures that you are connecting to the official website and that any information you provide is encrypted and transmitted securely.
- Publications
- Account settings
Preview improvements coming to the PMC website in October 2024. Learn More or Try it out now .
- Advanced Search
- Journal List
- v.19(7); 2019 Jul
Hypothesis tests
Associated data.
- • Hypothesis tests are used to assess whether a difference between two samples represents a real difference between the populations from which the samples were taken.
- • A null hypothesis of ‘no difference’ is taken as a starting point, and we calculate the probability that both sets of data came from the same population. This probability is expressed as a p -value.
- • When the null hypothesis is false, p- values tend to be small. When the null hypothesis is true, any p- value is equally likely.
Learning objectives
By reading this article, you should be able to:
- • Explain why hypothesis testing is used.
- • Use a table to determine which hypothesis test should be used for a particular situation.
- • Interpret a p- value.
A hypothesis test is a procedure used in statistics to assess whether a particular viewpoint is likely to be true. They follow a strict protocol, and they generate a ‘ p- value’, on the basis of which a decision is made about the truth of the hypothesis under investigation. All of the routine statistical ‘tests’ used in research— t- tests, χ 2 tests, Mann–Whitney tests, etc.—are all hypothesis tests, and in spite of their differences they are all used in essentially the same way. But why do we use them at all?
Comparing the heights of two individuals is easy: we can measure their height in a standardised way and compare them. When we want to compare the heights of two small well-defined groups (for example two groups of children), we need to use a summary statistic that we can calculate for each group. Such summaries (means, medians, etc.) form the basis of descriptive statistics, and are well described elsewhere. 1 However, a problem arises when we try to compare very large groups or populations: it may be impractical or even impossible to take a measurement from everyone in the population, and by the time you do so, the population itself will have changed. A similar problem arises when we try to describe the effects of drugs—for example by how much on average does a particular vasopressor increase MAP?
To solve this problem, we use random samples to estimate values for populations. By convention, the values we calculate from samples are referred to as statistics and denoted by Latin letters ( x ¯ for sample mean; SD for sample standard deviation) while the unknown population values are called parameters , and denoted by Greek letters (μ for population mean, σ for population standard deviation).
Inferential statistics describes the methods we use to estimate population parameters from random samples; how we can quantify the level of inaccuracy in a sample statistic; and how we can go on to use these estimates to compare populations.
Sampling error
There are many reasons why a sample may give an inaccurate picture of the population it represents: it may be biased, it may not be big enough, and it may not be truly random. However, even if we have been careful to avoid these pitfalls, there is an inherent difference between the sample and the population at large. To illustrate this, let us imagine that the actual average height of males in London is 174 cm. If I were to sample 100 male Londoners and take a mean of their heights, I would be very unlikely to get exactly 174 cm. Furthermore, if somebody else were to perform the same exercise, it would be unlikely that they would get the same answer as I did. The sample mean is different each time it is taken, and the way it differs from the actual mean of the population is described by the standard error of the mean (standard error, or SEM ). The standard error is larger if there is a lot of variation in the population, and becomes smaller as the sample size increases. It is calculated thus:
where SD is the sample standard deviation, and n is the sample size.
As errors are normally distributed, we can use this to estimate a 95% confidence interval on our sample mean as follows:
We can interpret this as meaning ‘We are 95% confident that the actual mean is within this range.’
Some confusion arises at this point between the SD and the standard error. The SD is a measure of variation in the sample. The range x ¯ ± ( 1.96 × SD ) will normally contain 95% of all your data. It can be used to illustrate the spread of the data and shows what values are likely. In contrast, standard error tells you about the precision of the mean and is used to calculate confidence intervals.
One straightforward way to compare two samples is to use confidence intervals. If we calculate the mean height of two groups and find that the 95% confidence intervals do not overlap, this can be taken as evidence of a difference between the two means. This method of statistical inference is reasonably intuitive and can be used in many situations. 2 Many journals, however, prefer to report inferential statistics using p -values.
Inference testing using a null hypothesis
In 1925, the British statistician R.A. Fisher described a technique for comparing groups using a null hypothesis , a method which has dominated statistical comparison ever since. The technique itself is rather straightforward, but often gets lost in the mechanics of how it is done. To illustrate, imagine we want to compare the HR of two different groups of people. We take a random sample from each group, which we call our data. Then:
- (i) Assume that both samples came from the same group. This is our ‘null hypothesis’.
- (ii) Calculate the probability that an experiment would give us these data, assuming that the null hypothesis is true. We express this probability as a p- value, a number between 0 and 1, where 0 is ‘impossible’ and 1 is ‘certain’.
- (iii) If the probability of the data is low, we reject the null hypothesis and conclude that there must be a difference between the two groups.
Formally, we can define a p- value as ‘the probability of finding the observed result or a more extreme result, if the null hypothesis were true.’ Standard practice is to set a cut-off at p <0.05 (this cut-off is termed the alpha value). If the null hypothesis were true, a result such as this would only occur 5% of the time or less; this in turn would indicate that the null hypothesis itself is unlikely. Fisher described the process as follows: ‘Set a low standard of significance at the 5 per cent point, and ignore entirely all results which fail to reach this level. A scientific fact should be regarded as experimentally established only if a properly designed experiment rarely fails to give this level of significance.’ 3 This probably remains the most succinct description of the procedure.
A question which often arises at this point is ‘Why do we use a null hypothesis?’ The simple answer is that it is easy: we can readily describe what we would expect of our data under a null hypothesis, we know how data would behave, and we can readily work out the probability of getting the result that we did. It therefore makes a very simple starting point for our probability assessment. All probabilities require a set of starting conditions, in much the same way that measuring the distance to London needs a starting point. The null hypothesis can be thought of as an easy place to put the start of your ruler.
If a null hypothesis is rejected, an alternate hypothesis must be adopted in its place. The null and alternate hypotheses must be mutually exclusive, but must also between them describe all situations. If a null hypothesis is ‘no difference exists’ then the alternate should be simply ‘a difference exists’.
Hypothesis testing in practice
The components of a hypothesis test can be readily described using the acronym GOST: identify the Groups you wish to compare; define the Outcome to be measured; collect and Summarise the data; then evaluate the likelihood of the null hypothesis, using a Test statistic .
When considering groups, think first about how many. Is there just one group being compared against an audit standard, or are you comparing one group with another? Some studies may wish to compare more than two groups. Another situation may involve a single group measured at different points in time, for example before or after a particular treatment. In this situation each participant is compared with themselves, and this is often referred to as a ‘paired’ or a ‘repeated measures’ design. It is possible to combine these types of groups—for example a researcher may measure arterial BP on a number of different occasions in five different groups of patients. Such studies can be difficult, both to analyse and interpret.
In other studies we may want to see how a continuous variable (such as age or height) affects the outcomes. These techniques involve regression analysis, and are beyond the scope of this article.
The outcome measures are the data being collected. This may be a continuous measure, such as temperature or BMI, or it may be a categorical measure, such as ASA status or surgical specialty. Often, inexperienced researchers will strive to collect lots of outcome measures in an attempt to find something that differs between the groups of interest; if this is done, a ‘primary outcome measure’ should be identified before the research begins. In addition, the results of any hypothesis tests will need to be corrected for multiple measures.
The summary and the test statistic will be defined by the type of data that have been collected. The test statistic is calculated then transformed into a p- value using tables or software. It is worth looking at two common tests in a little more detail: the χ 2 test, and the t -test.
Categorical data: the χ 2 test
The χ 2 test of independence is a test for comparing categorical outcomes in two or more groups. For example, a number of trials have compared surgical site infections in patients who have been given different concentrations of oxygen perioperatively. In the PROXI trial, 4 685 patients received oxygen 80%, and 701 patients received oxygen 30%. In the 80% group there were 131 infections, while in the 30% group there were 141 infections. In this study, the groups were oxygen 80% and oxygen 30%, and the outcome measure was the presence of a surgical site infection.
The summary is a table ( Table 1 ), and the hypothesis test compares this table (the ‘observed’ table) with the table that would be expected if the proportion of infections in each group was the same (the ‘expected’ table). The test statistic is χ 2 , from which a p- value is calculated. In this instance the p -value is 0.64, which means that results like this would occur 64% of the time if the null hypothesis were true. We thus have no evidence to reject the null hypothesis; the observed difference probably results from sampling variation rather than from an inherent difference between the two groups.
Table 1
Summary of the results of the PROXI trial. Figures are numbers of patients.
Continuous data: the t- test
The t- test is a statistical method for comparing means, and is one of the most widely used hypothesis tests. Imagine a study where we try to see if there is a difference in the onset time of a new neuromuscular blocking agent compared with suxamethonium. We could enlist 100 volunteers, give them a general anaesthetic, and randomise 50 of them to receive the new drug and 50 of them to receive suxamethonium. We then time how long it takes (in seconds) to have ideal intubation conditions, as measured by a quantitative nerve stimulator. Our data are therefore a list of times. In this case, the groups are ‘new drug’ and suxamethonium, and the outcome is time, measured in seconds. This can be summarised by using means; the hypothesis test will compare the means of the two groups, using a p- value calculated from a ‘ t statistic’. Hopefully it is becoming obvious at this point that the test statistic is usually identified by a letter, and this letter is often cited in the name of the test.
The t -test comes in a number of guises, depending on the comparison being made. A single sample can be compared with a standard (Is the BMI of school leavers in this town different from the national average?); two samples can be compared with each other, as in the example above; or the same study subjects can be measured at two different times. The latter case is referred to as a paired t- test, because each participant provides a pair of measurements—such as in a pre- or postintervention study.
A large number of methods for testing hypotheses exist; the commonest ones and their uses are described in Table 2 . In each case, the test can be described by detailing the groups being compared ( Table 2 , columns) the outcome measures (rows), the summary, and the test statistic. The decision to use a particular test or method should be made during the planning stages of a trial or experiment. At this stage, an estimate needs to be made of how many test subjects will be needed. Such calculations are described in detail elsewhere. 5
Table 2
The principle types of hypothesis test. Tests comparing more than two samples can indicate that one group differs from the others, but will not identify which. Subsequent ‘post hoc’ testing is required if a difference is found.
Controversies surrounding hypothesis testing
Although hypothesis tests have been the basis of modern science since the middle of the 20th century, they have been plagued by misconceptions from the outset; this has led to what has been described as a crisis in science in the last few years: some journals have gone so far as to ban p -value s outright. 6 This is not because of any flaw in the concept of a p -value, but because of a lack of understanding of what they mean.
Possibly the most pervasive misunderstanding is the belief that the p- value is the chance that the null hypothesis is true, or that the p- value represents the frequency with which you will be wrong if you reject the null hypothesis (i.e. claim to have found a difference). This interpretation has frequently made it into the literature, and is a very easy trap to fall into when discussing hypothesis tests. To avoid this, it is important to remember that the p- value is telling us something about our sample , not about the null hypothesis. Put in simple terms, we would like to know the probability that the null hypothesis is true, given our data. The p- value tells us the probability of getting these data if the null hypothesis were true, which is not the same thing. This fallacy is referred to as ‘flipping the conditional’; the probability of an outcome under certain conditions is not the same as the probability of those conditions given that the outcome has happened.
A useful example is to imagine a magic trick in which you select a card from a normal deck of 52 cards, and the performer reveals your chosen card in a surprising manner. If the performer were relying purely on chance, this would only happen on average once in every 52 attempts. On the basis of this, we conclude that it is unlikely that the magician is simply relying on chance. Although simple, we have just performed an entire hypothesis test. We have declared a null hypothesis (the performer was relying on chance); we have even calculated a p -value (1 in 52, ≈0.02); and on the basis of this low p- value we have rejected our null hypothesis. We would, however, be wrong to suggest that there is a probability of 0.02 that the performer is relying on chance—that is not what our figure of 0.02 is telling us.
To explore this further we can create two populations, and watch what happens when we use simulation to take repeated samples to compare these populations. Computers allow us to do this repeatedly, and to see what p- value s are generated (see Supplementary online material). 7 Fig 1 illustrates the results of 100,000 simulated t -tests, generated in two set of circumstances. In Fig 1 a , we have a situation in which there is a difference between the two populations. The p- value s cluster below the 0.05 cut-off, although there is a small proportion with p >0.05. Interestingly, the proportion of comparisons where p <0.05 is 0.8 or 80%, which is the power of the study (the sample size was specifically calculated to give a power of 80%).

The p- value s generated when 100,000 t -tests are used to compare two samples taken from defined populations. ( a ) The populations have a difference and the p- value s are mostly significant. ( b ) The samples were taken from the same population (i.e. the null hypothesis is true) and the p- value s are distributed uniformly.
Figure 1 b depicts the situation where repeated samples are taken from the same parent population (i.e. the null hypothesis is true). Somewhat surprisingly, all p- value s occur with equal frequency, with p <0.05 occurring exactly 5% of the time. Thus, when the null hypothesis is true, a type I error will occur with a frequency equal to the alpha significance cut-off.
Figure 1 highlights the underlying problem: when presented with a p -value <0.05, is it possible with no further information, to determine whether you are looking at something from Fig 1 a or Fig 1 b ?
Finally, it cannot be stressed enough that although hypothesis testing identifies whether or not a difference is likely, it is up to us as clinicians to decide whether or not a statistically significant difference is also significant clinically.
Hypothesis testing: what next?
As mentioned above, some have suggested moving away from p -values, but it is not entirely clear what we should use instead. Some sources have advocated focussing more on effect size; however, without a measure of significance we have merely returned to our original problem: how do we know that our difference is not just a result of sampling variation?
One solution is to use Bayesian statistics. Up until very recently, these techniques have been considered both too difficult and not sufficiently rigorous. However, recent advances in computing have led to the development of Bayesian equivalents of a number of standard hypothesis tests. 8 These generate a ‘Bayes Factor’ (BF), which tells us how more (or less) likely the alternative hypothesis is after our experiment. A BF of 1.0 indicates that the likelihood of the alternate hypothesis has not changed. A BF of 10 indicates that the alternate hypothesis is 10 times more likely than we originally thought. A number of classifications for BF exist; greater than 10 can be considered ‘strong evidence’, while BF greater than 100 can be classed as ‘decisive’.
Figures such as the BF can be quoted in conjunction with the traditional p- value, but it remains to be seen whether they will become mainstream.
Declaration of interest
The author declares that they have no conflict of interest.
The associated MCQs (to support CME/CPD activity) will be accessible at www.bjaed.org/cme/home by subscribers to BJA Education .
Jason Walker FRCA FRSS BSc (Hons) Math Stat is a consultant anaesthetist at Ysbyty Gwynedd Hospital, Bangor, Wales, and an honorary senior lecturer at Bangor University. He is vice chair of his local research ethics committee, and an examiner for the Primary FRCA.
Matrix codes: 1A03, 2A04, 3J03
Supplementary data to this article can be found online at https://doi.org/10.1016/j.bjae.2019.03.006 .
Supplementary material
The following is the Supplementary data to this article:

A .gov website belongs to an official government organization in the United States.
A lock ( ) or https:// means you've safely connected to the .gov website. Share sensitive information only on official, secure websites.
- Preventing Chronic Diseases: What You Can Do Now
- Living with a Chronic Condition
- Chronic Disease Data
- Community Health Workers
- Workgroup on Translation Tools
- National Center for Chronic Disease Prevention and Health Promotion (NCCDPHP)
About Chronic Diseases
- Chronic diseases are the leading cause of illness, disability, and death in America.
- Most chronic diseases are caused by a short list of risk factors: smoking, poor nutrition, physical inactivity, and excessive alcohol use.
- Some groups are more affected than others because of factors that limit their ability to make healthy choices.
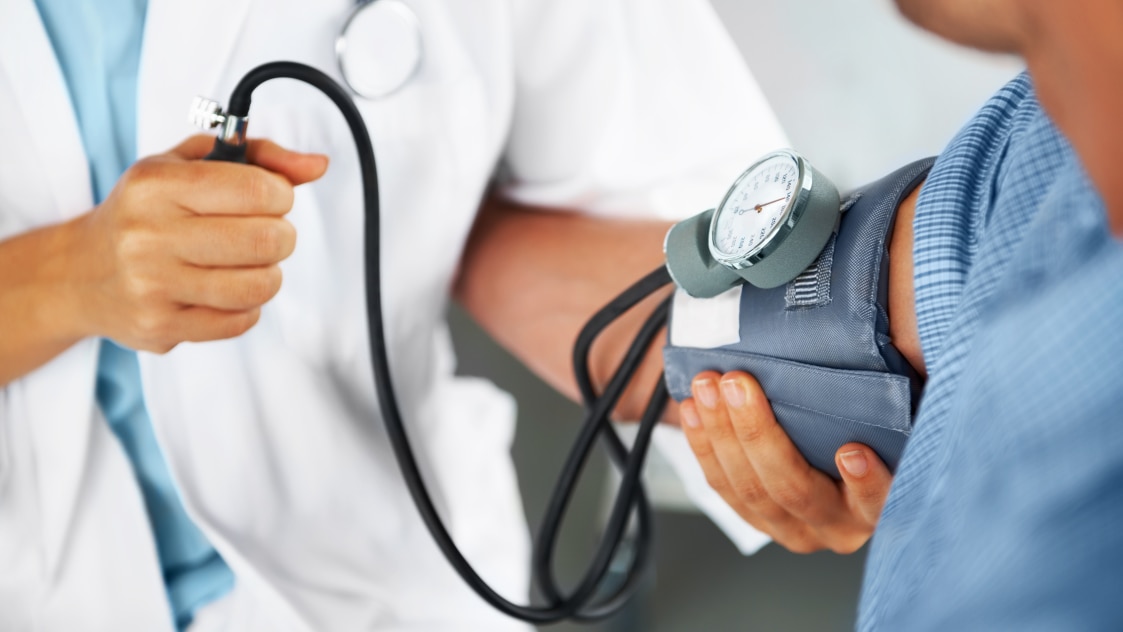
Chronic diseases in America
Definition.
Chronic diseases such as heart disease , cancer , and diabetes are the leading causes of death and disability in the United States. They are also leading drivers of the nation's $4.5 trillion in annual health care costs . 1 2 3
Many preventable chronic diseases are caused by a short list of risk behaviors: smoking, poor nutrition, physical inactivity, and excessive alcohol use.
Risk factors
Cigarette smoking causes more than 480,000 deaths each year in the United States, and over 16 million Americans are living with a disease caused by smoking. Smoking causes cancer, heart disease, stroke, lung diseases, diabetes, and chronic obstructive pulmonary disease (COPD), which includes emphysema and chronic bronchitis.
Poor nutrition and physical inactivity
Poor nutrition and physical inactivity are significant risk factors for obesity and other chronic diseases, such as type 2 diabetes, heart disease, stroke, certain cancers, and depression.
Excessive alcohol use
Over time, excessive alcohol use can lead to serious problems, including alcohol use disorder and problems with learning, memory, and mental health. Chronic health conditions linked to excessive alcohol use include high blood pressure, heart disease, stroke, liver disease, and some kinds of cancer.
Who is at risk
Some groups are at higher risk of chronic diseases because of conditions where they are born, live, work, and age. These nonmedical factors, called social determinants of health, can be positive or negative. When they are negative, they limit the opportunities to make healthy choices and get good medical care.
For example, some communities lack safe spaces like parks for people to be active, or grocery stores that sell fresh fruits and vegetables. In some rural areas, it's hard to get medical care because of doctor shortages, hospital closures, or long distances to care. This makes it challenging to get preventive screenings or specialist follow-up care.
What CDC is doing
CDC's National Center for Chronic Disease Prevention and Health Promotion supports state, local, tribal, and territorial public health organizations to reduce chronic disease risk factors. Funded programs like REACH and Getting Further Faster focus on addressing the social determinants of health, so that everyone can have the same opportunity to live their healthiest life.
- National health expenditure data: historical. Center for Medicare & Medicaid Services. Updated December 13, 2023. Accessed February 6, 2024. https://www.cms.gov/data-research/statistics-trends-and-reports/national-health-expenditure-data/historical
- Buttorff C, Ruder T, Bauman M. Multiple Chronic Conditions in the United States . Rand Corp.; 2017.
- Leading causes of death. Centers for Disease Control and Prevention. Updated January 23, 2023. Accessed November 7, 2023. https://www.cdc.gov/nchs/fastats/leading-causes-of-death.htm
- Chronic disease definition: US Department of Health and Human Services. Multiple Chronic Conditions: A Strategic Framework; 2010.
Chronic Disease
Prevalence, costs, risks, prevention, and management of chronic diseases in the United States
For Everyone
Public health.
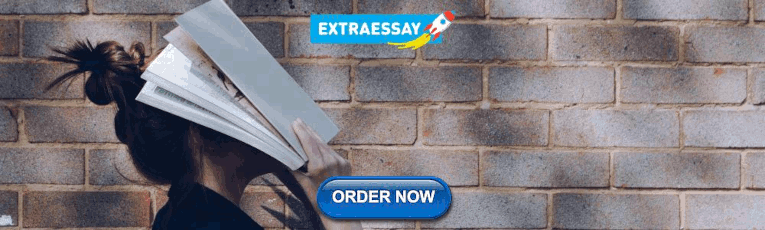
IMAGES
VIDEO
COMMENTS
hypothesis. (hī-pŏth′ĭ-sĭs) n. pl. hypothe·ses (-sēz′) 1. A tentative explanation for an observation, phenomenon, or scientific problem that can be tested by further investigation. 2. Something taken to be true for the purpose of argument or investigation; an assumption. 3. The antecedent of a conditional statement.
A snapshot analysis of citation activity of hypothesis articles may reveal interest of the global scientific community towards their implications across various disciplines and countries. As a prime example, Strachan's hygiene hypothesis, published in 1989,10 is still attracting numerous citations on Scopus, the largest bibliographic database ...
A hypothesis is a tentative statement about the relationship between two or more variables. It is a specific, testable prediction about what you expect to happen in a study. It is a preliminary answer to your question that helps guide the research process. Consider a study designed to examine the relationship between sleep deprivation and test ...
hypothesis: [noun] an assumption or concession made for the sake of argument. an interpretation of a practical situation or condition taken as the ground for action.
Formulating Hypotheses for Different Study Designs. Generating a testable working hypothesis is the first step towards conducting original research. Such research may prove or disprove the proposed hypothesis. Case reports, case series, online surveys and other observational studies, clinical trials, and narrative reviews help to generate ...
A medical hypothesis article has two main aims: to serve as a forum for theoretical work in medicine; and to facilitate the publication of potentially radical ideas. Medical hypotheses are particularly important in a field such as integrative medicine. ... A hypothesis is, by definition, unproven, and for every new hypothesis that proves to be ...
Developing a hypothesis (with example) Step 1. Ask a question. Writing a hypothesis begins with a research question that you want to answer. The question should be focused, specific, and researchable within the constraints of your project. Example: Research question.
A hypothesis (from the Greek, foundation) is a logical construct, interposed between a problem and its solution, which represents a proposed answer to a research question. It gives direction to the investigator's thinking about the problem and, therefore, facilitates a solution. Unlike facts and assumptions (presumed true and, therefore, not ...
Medical Hypotheses is a forum for ideas in medicine and related biomedical sciences. It will publish interesting and important theoretical papers that foster the diversity and debate upon which the scientific process thrives. The Aims and Scope of Medical Hypotheses are no different now from what was proposed by the founder of the journal, the ...
A research hypothesis (also called a scientific hypothesis) is a statement about the expected outcome of a study (for example, a dissertation or thesis). To constitute a quality hypothesis, the statement needs to have three attributes - specificity, clarity and testability. Let's take a look at these more closely.
Medical providers often rely on evidence-based medicine to guide decision-making in practice. Often a research hypothesis is tested with results provided, typically with p values, confidence intervals, or both. Additionally, statistical or research significance is estimated or determined by the investigators. Unfortunately, healthcare providers may have different comfort levels in interpreting ...
Definition: Hypothesis is an educated guess or proposed explanation for a phenomenon, based on some initial observations or data. It is a tentative statement that can be tested and potentially proven or disproven through further investigation and experimentation. Hypothesis is often used in scientific research to guide the design of experiments ...
A hypothesis (plural hypotheses) is a proposed explanation for an observation. The definition depends on the subject. In science, a hypothesis is part of the scientific method. It is a prediction or explanation that is tested by an experiment. Observations and experiments may disprove a scientific hypothesis, but can never entirely prove one.
Hypothesis testing is the process used to evaluate the strength of evidence from the sample and provides a framework for making determinations related to the population, ie, it provides a method for understanding how reliably one can extrapolate observed findings in a sample under study to the larger population from which the sample was drawn ...
Hypothesis definition: a proposition, or set of propositions, set forth as an explanation for the occurrence of some specified group of phenomena, either asserted merely as a provisional conjecture to guide investigation (working hypothesis ) or accepted as highly probable in the light of established facts.. See examples of HYPOTHESIS used in a sentence.
HYPOTHESIS definition: 1. an idea or explanation for something that is based on known facts but has not yet been proved…. Learn more.
HYPOTHESIS TESTING. A clinical trial begins with an assumption or belief, and then proceeds to either prove or disprove this assumption. In statistical terms, this belief or assumption is known as a hypothesis. Counterintuitively, what the researcher believes in (or is trying to prove) is called the "alternate" hypothesis, and the opposite ...
The hypothesis of Andreas Cellarius, showing the planetary motions in eccentric and epicyclical orbits.. A hypothesis (pl.: hypotheses) is a proposed explanation for a phenomenon.For a hypothesis to be a scientific hypothesis, the scientific method requires that one can test it. Scientists generally base scientific hypotheses on previous observations that cannot satisfactorily be explained ...
Background In medical practice, diagnostic hypotheses are often made by physicians in the first moments of contact with patients; sometimes even before they report their symptoms. We propose that generation of diagnostic hypotheses in this context is the result of cognitive processes subserved by brain mechanisms that are similar to those involved in naming objects or concepts in everyday life.
Medical Hypotheses is not, however, a journal for publishing workaday reviews of the literature, nor is it a journal for primary data (except when preliminary data is used to lend support to the main hypothesis presented). Many of the articles submitted do not clearly identify the hypothesis and simply read like reviews.
Examples of Hypotheses in Medical Research. Lastly but importantly, in medical research, well-articulated hypotheses help probe pressing healthcare questions and identify effective treatments. ... To define a hypothesis of meticulosity, we need to subject it to rigorous scrutiny. Utilizing statistical tests enables you to judge the validity of ...
Hypothesis-testing (Quantitative hypothesis-testing research) - Quantitative research uses deductive reasoning. - This involves the formation of a hypothesis, collection of data in the investigation of the problem, analysis and use of the data from the investigation, and drawing of conclusions to validate or nullify the hypotheses.
An electronic health record (EHR) digitizes a patient's paper chart. It collects the patient's history of conditions, tests and treatments and can be used to create a more holistic view of the patient's care. A medical EHR also improves upon paper by making the patient's information available instantly and securely to an authorized user. But for all these advantages, EHRs can create ...
ONWARD ® Medical is a medical technology company creating therapies to restore movement, function, and independence in people with spinal cord injury (SCI) and movement disabilities. Building on ...
A hypothesis test is a procedure used in statistics to assess whether a particular viewpoint is likely to be true. They follow a strict protocol, and they generate a 'p-value', on the basis of which a decision is made about the truth of the hypothesis under investigation.All of the routine statistical 'tests' used in research—t-tests, χ 2 tests, Mann-Whitney tests, etc.—are all ...
Definition. Chronic diseases are defined broadly as conditions that last 1 year or more and require ongoing medical attention or limit activities of daily living or both. Chronic diseases such as heart disease , cancer, and diabetes are the leading causes of death and disability in the United States. They are also leading drivers of the ...