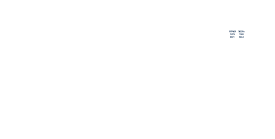
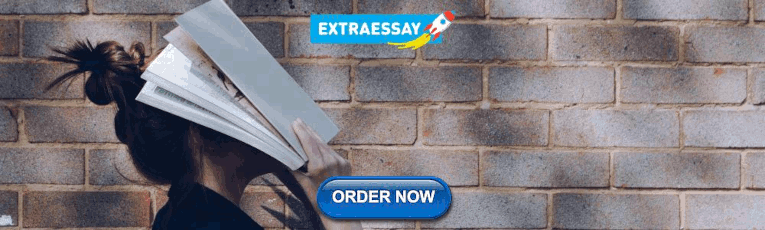
Economic Inequality by Gender
How big are the inequalities in pay, jobs, and wealth between men and women? What causes these differences?
By Esteban Ortiz-Ospina, Joe Hasell and Max Roser
This page was first published in March 2018 and last revised in March 2024.
On this page, you can find writing, visualizations, and data on how big the inequalities in pay, jobs, and wealth are between men and women, how they have changed over time, and what may be causing them
Although economic gender inequalities remain common and large, they are today smaller than they used to be some decades ago.
Related topics
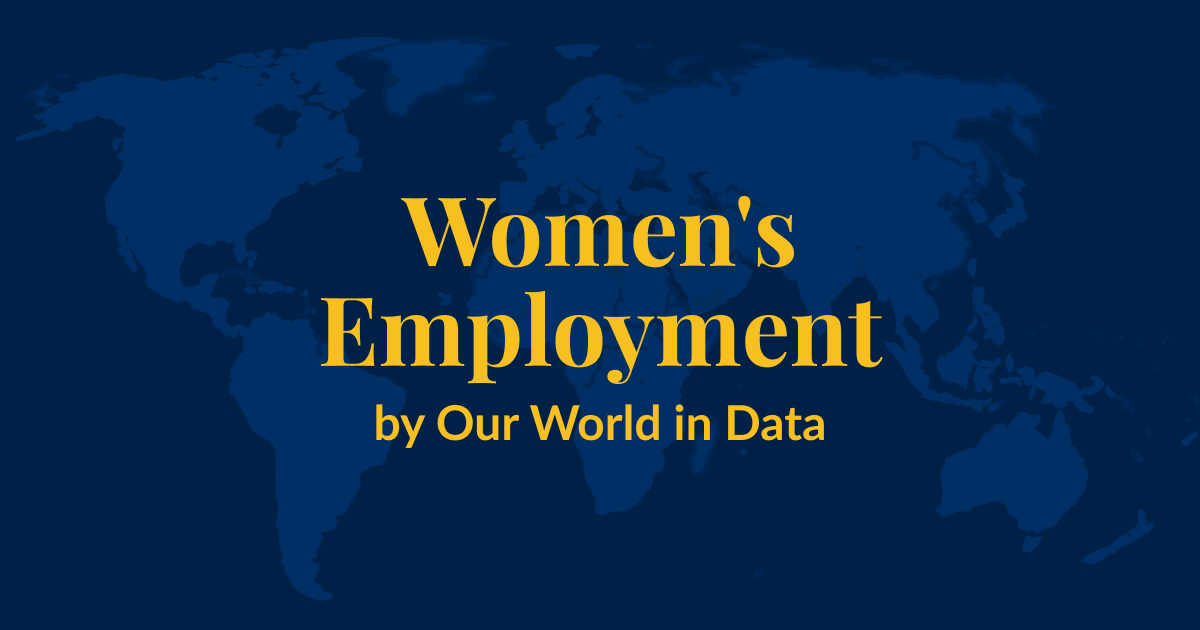
Women's Employment
How does women’s labor force participation differ across countries? How has it changed over time? What is behind these differences and changes?
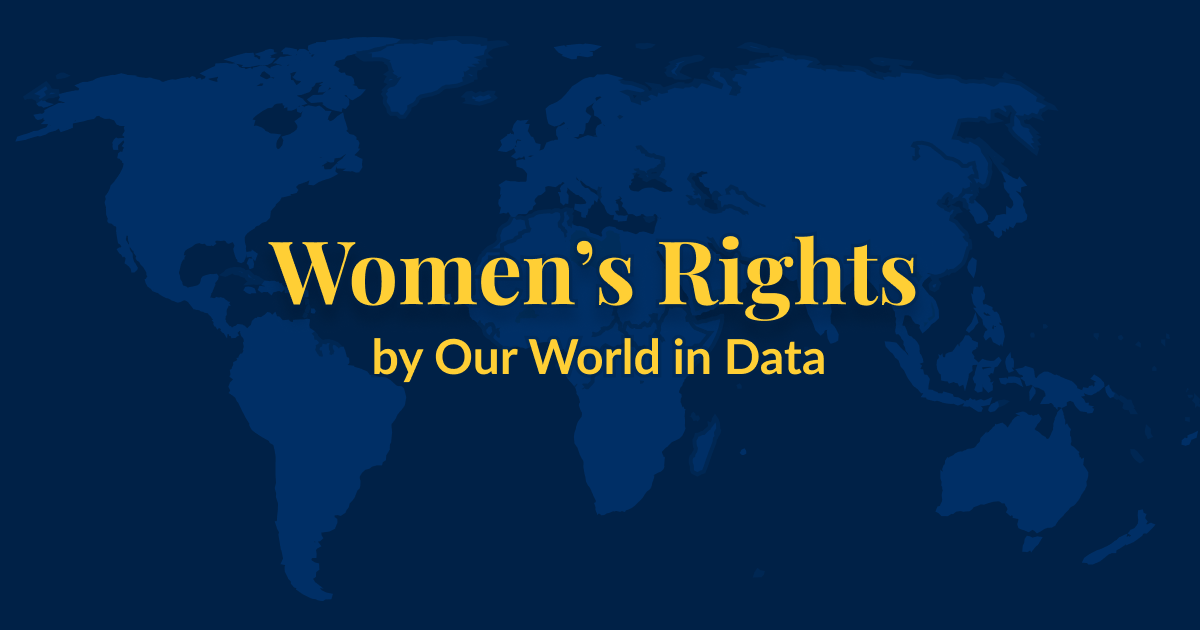
Women’s Rights
How has the protection of women’s rights changed over time? How does it differ across countries? Explore global data and research on women’s rights.
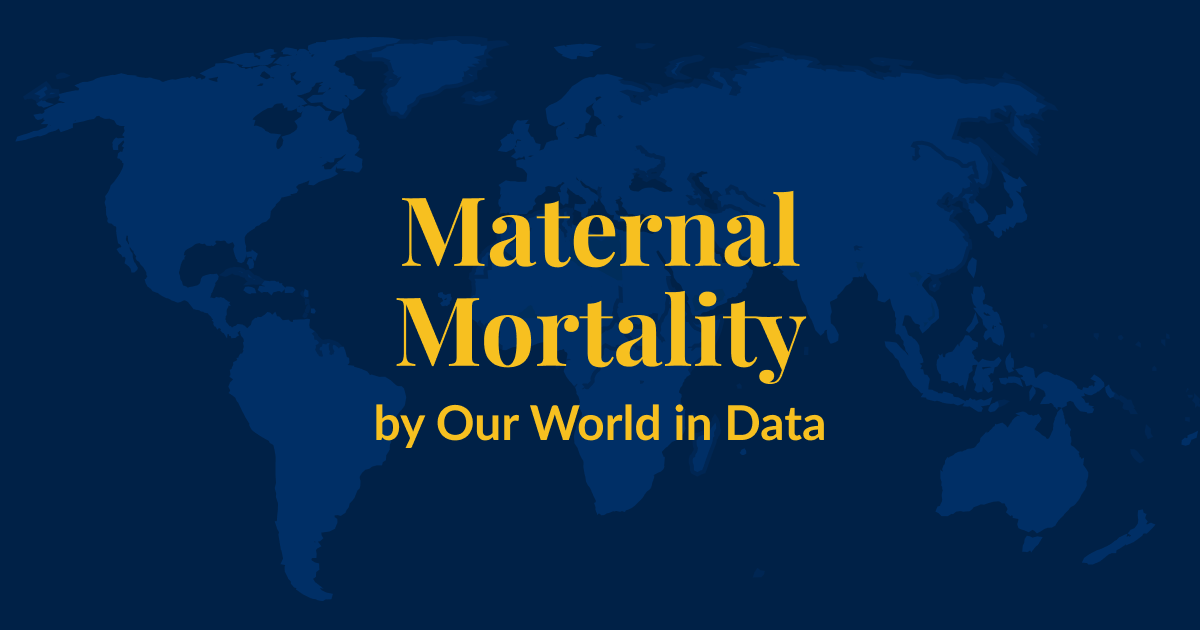
Maternal Mortality
What could be more tragic than a mother losing her life in the moment that she is giving birth to her newborn? Why are mothers dying and what can be done to prevent these deaths?
See all interactive charts on economic inequality by gender ↓
How does the gender pay gap look like across countries and over time?
The 'gender pay gap' comes up often in political debates , policy reports , and everyday news . But what is it? What does it tell us? Is it different from country to country? How does it change over time?
Here we try to answer these questions, providing an empirical overview of the gender pay gap across countries and over time.
The gender pay gap measures inequality but not necessarily discrimination
The gender pay gap (or the gender wage gap) is a metric that tells us the difference in pay (or wages, or income) between women and men. It's a measure of inequality and captures a concept that is broader than the concept of equal pay for equal work.
Differences in pay between men and women capture differences along many possible dimensions, including worker education, experience, and occupation. When the gender pay gap is calculated by comparing all male workers to all female workers – irrespective of differences along these additional dimensions – the result is the 'raw' or 'unadjusted' pay gap. On the contrary, when the gap is calculated after accounting for underlying differences in education, experience, etc., then the result is the 'adjusted' pay gap.
Discrimination in hiring practices can exist in the absence of pay gaps – for example, if women know they will be treated unfairly and hence choose not to participate in the labor market. Similarly, it is possible to observe large pay gaps in the absence of discrimination in hiring practices – for example, if women get fair treatment but apply for lower-paid jobs.
The implication is that observing differences in pay between men and women is neither necessary nor sufficient to prove discrimination in the workplace. Both discrimination and inequality are important. But they are not the same.
In most countries, there is a substantial gender pay gap
Cross-country data on the gender pay gap is patchy, but the most complete source in terms of coverage is the United Nation's International Labour Organization (ILO). The visualization here presents this data. You can add observations by clicking on the option 'add country' at the bottom of the chart.
The estimates shown here correspond to differences between the average hourly earnings of men and women (expressed as a percentage of average hourly earnings of men), and cover all workers irrespective of whether they work full-time or part-time. 1
As we can see: (i) in most countries the gap is positive – women earn less than men, and (ii) there are large differences in the size of this gap across countries. 2
In most countries, the gender pay gap has decreased in the last couple of decades
How is the gender pay gap changing over time? To answer this question, let's consider this chart showing available estimates from the OECD. These estimates include OECD member states, as well as some other non-member countries, and they are the longest available series of cross-country data on the gender pay gap that we are aware of.
Here we see that the gap is large in most OECD countries, but it has been going down in the last couple of decades. In some cases the reduction is remarkable. In the United States, for example, the gap declined by more than half.
These estimates are not directly comparable to those from the ILO, because the pay gap is measured slightly differently here: The OECD estimates refer to percent differences in median earnings (i.e. the gap here captures differences between men and women in the middle of the earnings distribution), and they cover only full-time employees and self-employed workers (i.e. the gap here excludes disparities that arise from differences in hourly wages for part-time and full-time workers).
However, the ILO data shows similar trends.
The conclusion is that in most countries with available data, the gender pay gap has decreased in the last couple of decades.
The gender pay gap is larger for older workers
The United States Census Bureau defines the pay gap as the ratio between median wages – that is, they measure the gap by calculating the wages of men and women at the middle of the earnings distribution, and dividing them.
By this measure, the gender wage gap is expressed as a percent (median earnings of women as a share of median earnings of men) and it is always positive. Here, values below 100% mean that women earn less than men, while values above 100% mean that women earn more. Values closer to 100% reflect a lower gap.
The next chart shows available estimates of this metric for full-time workers in the US, by age group.
First, we see that the series trends upwards, meaning the gap has been shrinking in the last couple of decades. Secondly, we see that there are important differences by age.
The second point is crucial to understanding the gender pay gap: the gap is a statistic that changes during the life of a worker. In most rich countries, it’s small when formal education ends and employment begins, and it increases with age. As we discuss in our analysis of the determinants below, the gender pay gap tends to increase when women marry and when/if they have children.
The gender pay gap is smaller in middle-income countries – which tend to be countries with low labor force participation of women
The chart here plots available ILO estimates on the gender pay gap against GDP per capita. As we can see there is a weak positive correlation between GDP per capita and the gender pay gap. However, the chart shows that the relationship is not really linear. Actually, middle-income countries tend to have the smallest pay gap.
The fact that middle-income countries have low gender wage gaps is, to a large extent, the result of selection of women into employment . Olivetti and Petrongolo (2008) explain it as follows: “[I]f women who are employed tend to have relatively high‐wage characteristics, low female employment rates may become consistent with low gender wage gaps simply because low‐wage women would not feature in the observed wage distribution.” 3
Olivetti and Petrongolo (2008) show that this pattern holds in the data: unadjusted gender wage gaps across countries tend to be negatively correlated with gender employment gaps. That is, the gender pay gaps tend to be smaller where relatively fewer women participate in the labor force .
So, rather than reflect greater equality, the lower wage gaps observed in some countries could indicate that only women with certain characteristics – for instance, with no husband or children – are entering the workforce.
Why is there a gender pay gap?
In almost all countries, if you compare the wages of men and women you find that women tend to earn less than men. These inequalities have been narrowing across the world. In particular, most high-income countries have seen sizeable reductions in the gender pay gap over the last couple of decades.
How did these reductions come about and why do substantial gaps remain?
Before we get into the details, here is a preview of the main points.
- An important part of the reduction in the gender pay gap in rich countries over the last decades is due to a historical narrowing, and often even reversal of the education gap between men and women.
- Today, education is relatively unimportant in explaining the remaining gender pay gap in rich countries. In contrast, the characteristics of the jobs that women tend to do, remain important contributing factors.
- The gender pay gap is not a direct metric of discrimination. However, evidence from different contexts suggests discrimination is indeed important to understand the gender pay gap. Similarly, social norms affecting the gender distribution of labor are important determinants of wage inequality.
- On the other hand, the available evidence suggests differences in psychological attributes and non-cognitive skills are at best modest factors contributing to the gender pay gap.
Differences in human capital
The adjusted pay gap.
Differences in earnings between men and women capture differences across many possible dimensions, including education, experience, and occupation.
For example, if we consider that more educated people tend to have higher earnings, it is natural to expect that the narrowing of the pay gap across the world can be partly explained by the fact that women have been catching up with men in terms of educational attainment, in particular years of schooling.
Indeed, since differences in education partly contribute to explaining differences in wages, it is common to distinguish between 'unadjusted' and 'adjusted' pay differences.
When the gender pay gap is calculated by comparing all male and female workers, irrespective of differences in worker characteristics, the result is the raw or unadjusted pay gap. In contrast to this, when the gap is calculated after accounting for underlying differences in education, experience, and other factors that matter for the pay gap, then the result is the adjusted pay gap.
The idea of the adjusted pay gap is to make comparisons within groups of workers with roughly similar jobs, tenure, and education. This allows us to tease out the extent to which different factors contribute to observed inequalities.
The chart here, from Blau and Kahn (2017) shows the evolution of the adjusted and unadjusted gender pay gap in the US. 4
More precisely, the chart shows the evolution of female-to-male wage ratios in three different scenarios: (i) Unadjusted; (ii) Adjusted, controlling for gender differences in human capital, i.e. education and experience; and (iii) Adjusted, controlling for a full range of covariates, including education, experience, job industry, and occupation, among others. The difference between 100% and the full specification (the green bars) is the “unexplained” residual. 5
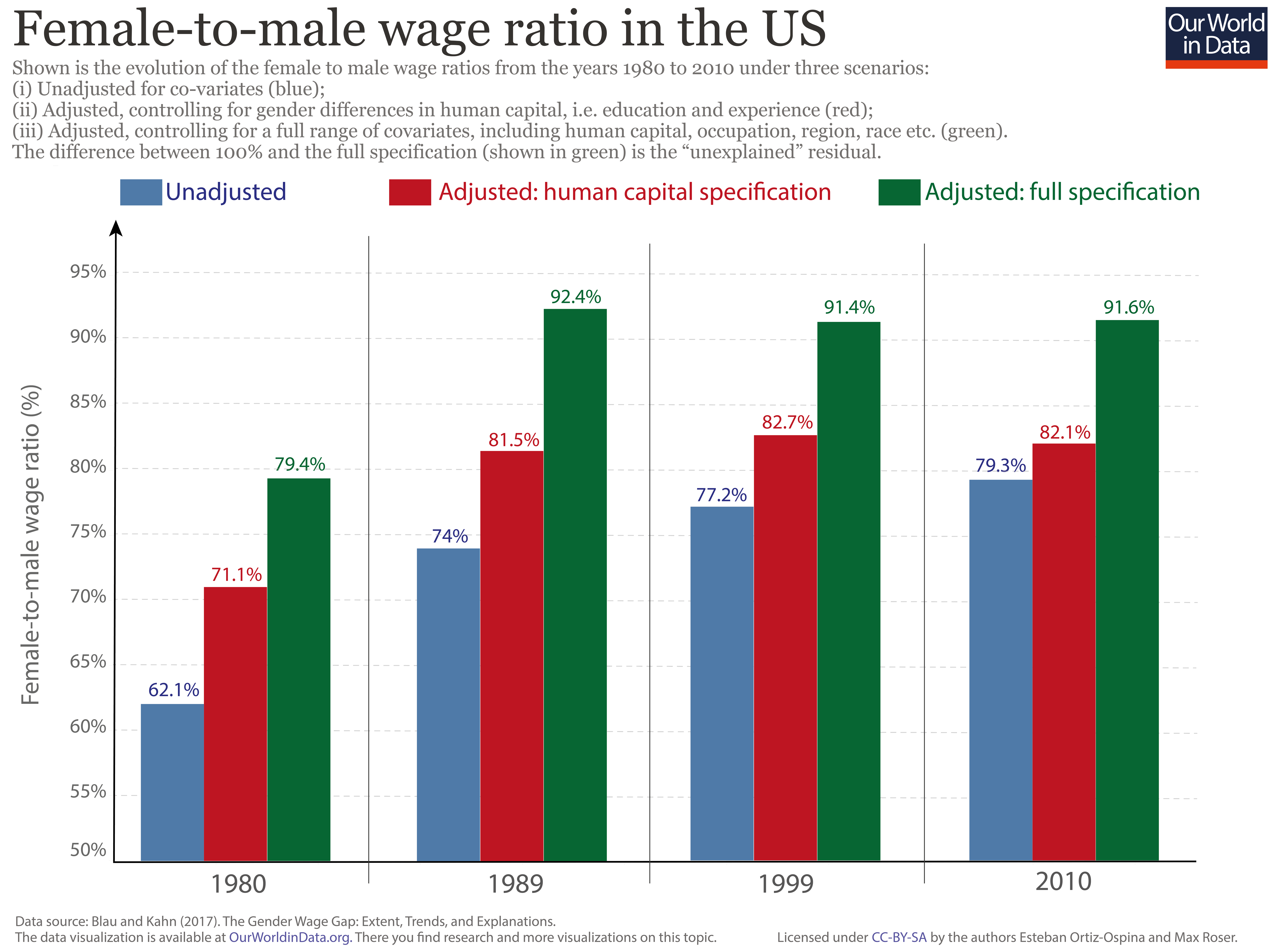
Several points stand out here.
- First, the unadjusted gender pay gap in the US shrunk over this period. This is evident from the fact that the blue bars are closer to 100% in 2010 than in 1980.
- Second, if we focus on groups of workers with roughly similar jobs, tenure, and education, we also see a narrowing. The adjusted gender pay gap has shrunk.
- Third, we can see that education and experience used to help explain a very large part of the pay gap in 1980, but this changed substantially in the decades that followed. This third point follows from the fact that the difference between the blue and red bars was much larger in 1980 than in 2010.
- And fourth, the green bars grew substantially in the 1980s, but stayed fairly constant thereafter. In other words: Most of the convergence in earnings occurred during the 1980s, a decade in which the "unexplained" gap shrunk substantially.
Education and experience have become much less important in explaining gender differences in wages in the US
The next chart shows a breakdown of the adjusted gender pay gaps in the US, factor by factor, in 1980 and 2010.
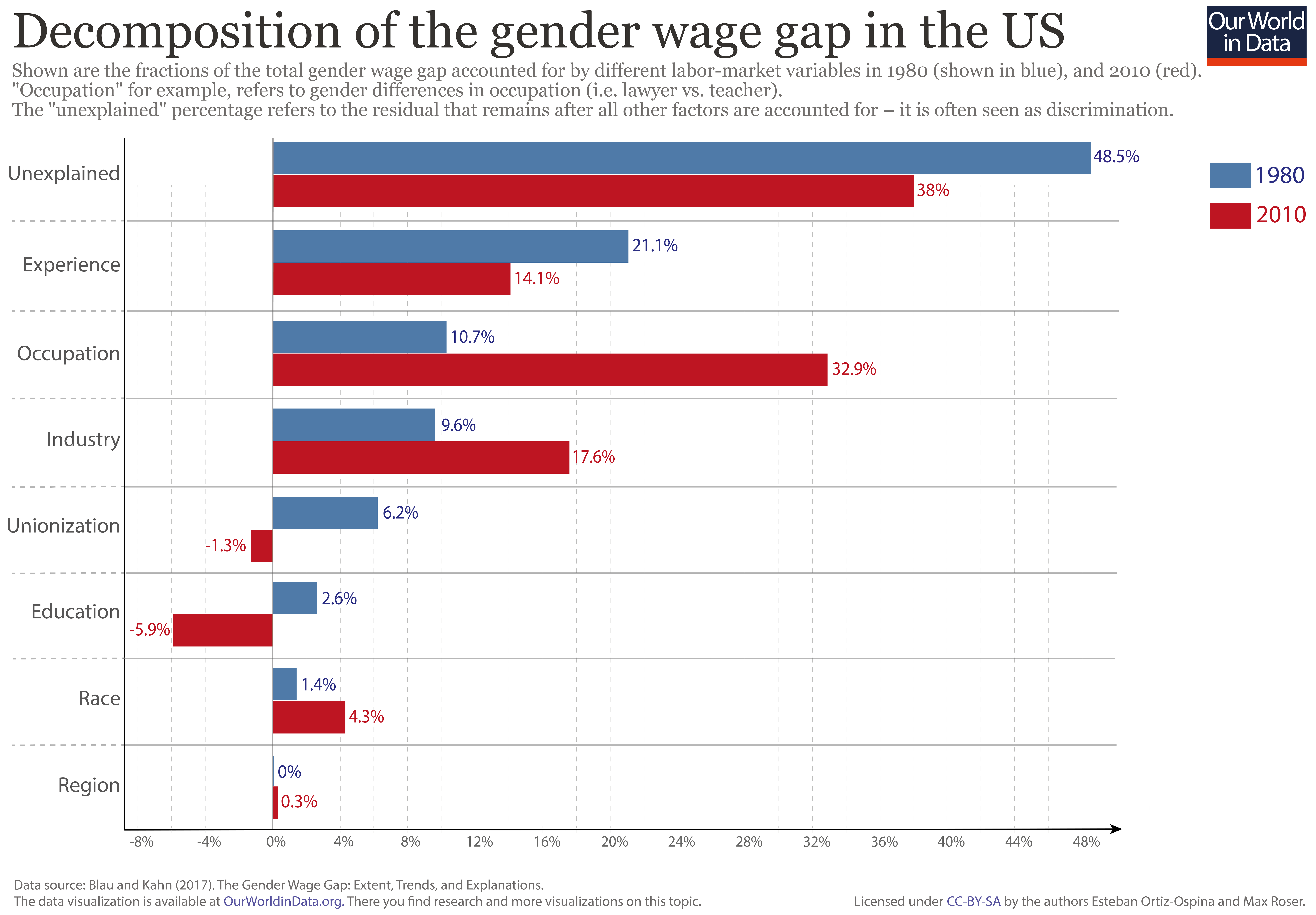
When comparing the contributing factors in 1980 and 2010, we see that education and work experience have become much less important in explaining gender differences in wages over time, while occupation and industry have become more important. 6
In this chart we can also see that the 'unexplained' residual has gone down. This means the observable characteristics of workers and their jobs explain wage differences better today than a couple of decades ago. At first sight, this seems like good news – it suggests that today there is less discrimination, in the sense that differences in earnings are today much more readily explained by differences in 'productivity' factors. But is this really the case?
The unexplained residual may include aspects of unmeasured productivity (i.e. unobservable worker characteristics that could not be accounted for in the study), while the "explained" factors may themselves be vehicles of discrimination.
For example, suppose that women are indeed discriminated against, and they find it hard to get hired for certain jobs simply because of their sex. This would mean that in the adjusted specification, we would see that occupation and industry are important contributing factors – but that is precisely because discrimination is embedded in occupational differences!
Hence, while the unexplained residual gives us a first-order approximation of what is going on, we need much more detailed data and analysis in order to say something definitive about the role of discrimination in observed pay differences.
Gender pay differences around the world are better explained by occupation than by education
The set of three maps here, taken from the World Development Report (2012) , shows that today gender pay differences are much better explained by occupation than by education. This is consistent with the point already made above using data for the US: as education expanded radically over the last few decades, human capital has become much less important in explaining gender differences in wages.
Justin Sandefur at the Center for Global Development shows that education also fails to explain wage gaps if we include workers with zero income (i.e. if we decompose the wage gap after including people who are not employed).
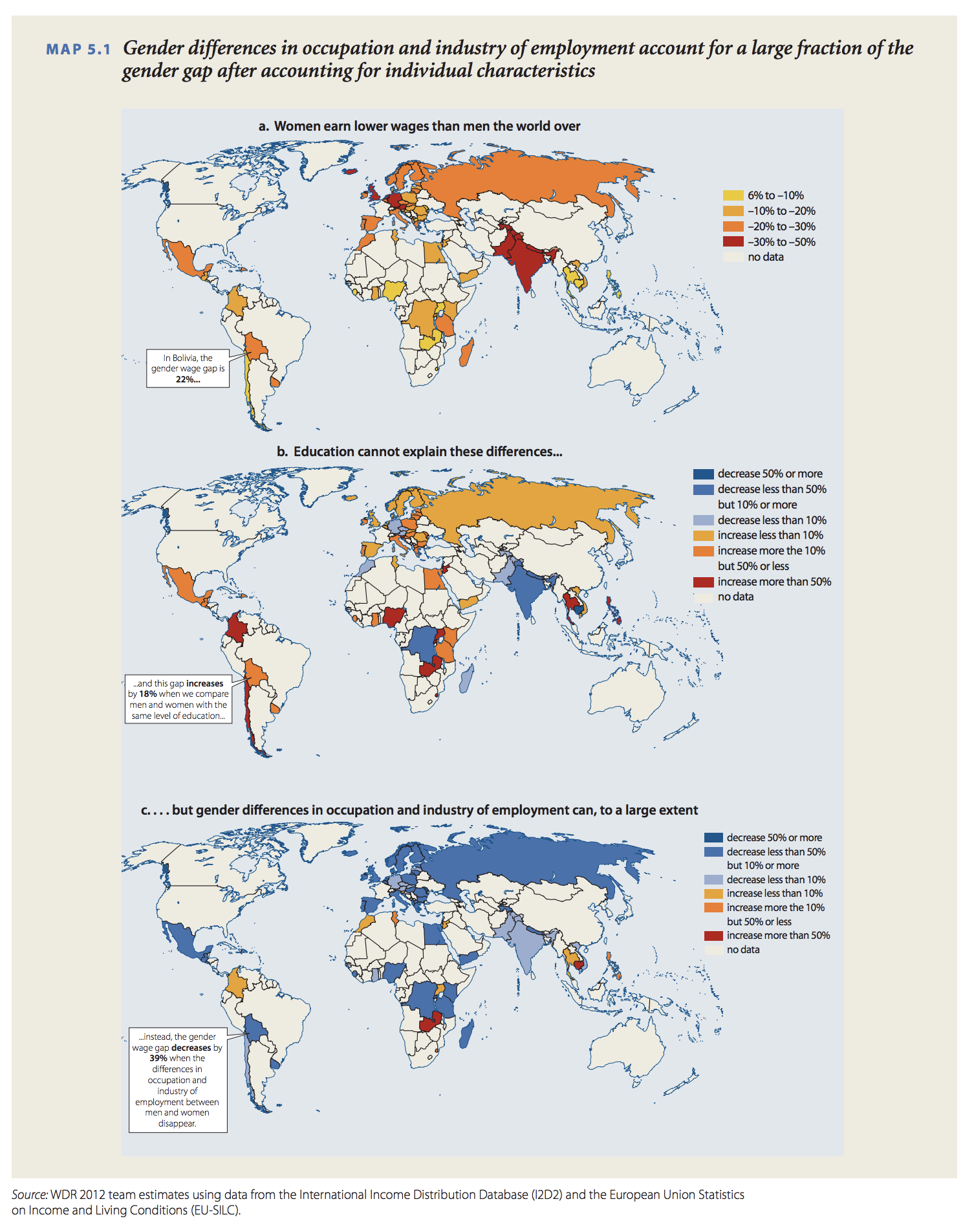
Looking beyond worker characteristics
Job flexibility.
All over the world women tend to do more unpaid care work at home than men – and women tend to be overrepresented in low-paying jobs where they have the flexibility required to attend to these additional responsibilities.
The most important evidence regarding this link between the gender pay gap and job flexibility is presented and discussed by Claudia Goldin in the article ' A Grand Gender Convergence: Its Last Chapter ', where she digs deep into the data from the US. 8 There are some key lessons that apply both to rich and non-rich countries.
Goldin shows that when one looks at the data on occupational choice in some detail, it becomes clear that women disproportionately seek jobs, including full-time jobs, that tend to be compatible with childrearing and other family responsibilities. In other words, women, more than men, are expected to have temporal flexibility in their jobs. Things like shifting hours of work and rearranging shifts to accommodate emergencies at home. And these are jobs with lower earnings per hour, even when the total number of hours worked is the same.
The importance of job flexibility in this context is very clearly illustrated by the fact that, over the last couple of decades, women in the US increased their participation and remuneration in only some fields. In a recent paper, Goldin and Katz (2016) show that pharmacy became a highly remunerated female-majority profession with a small gender earnings gap in the US, at the same time as pharmacies went through substantial technological changes that made flexible jobs in the field more productive (e.g. computer systems that increased the substitutability among pharmacists). 9
The chart here shows how quickly female wages increased in pharmacy, relative to other professions, over the last few decades in the US.
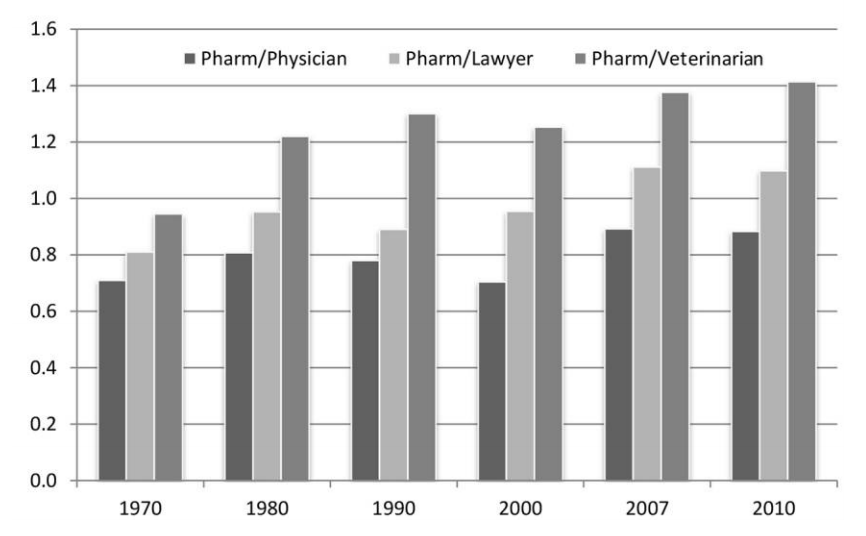
The motherhood penalty
Closely related to job flexibility and occupational choice is the issue of work interruptions due to motherhood. On this front, there is again a great deal of evidence in support of the so-called 'motherhood penalty'.
Lundborg, Plug, and Rasmussen (2017) provide evidence from Denmark – more specifically, Danish women who sought medical help in achieving pregnancy. 10
By tracking women’s fertility and employment status through detailed periodic surveys, these researchers were able to establish that women who had a successful in vitro fertilization treatment, ended up having lower earnings down the line than similar women who, by chance, were unsuccessfully treated.
Lundborg, Plug, and Rasmussen summarise their findings as follows: "Our main finding is that women who are successfully treated by [in vitro fertilization] earn persistently less because of having children. We explain the decline in annual earnings by women working less when children are young and getting paid less when children are older. We explain the decline in hourly earnings, which is often referred to as the motherhood penalty, by women moving to lower-paid jobs that are closer to home."
The fact that the motherhood penalty is indeed about ‘motherhood’ and not ‘parenthood’, is supported by further evidence.
A recent study , also from Denmark, tracked men and women over the period 1980-2013 and found that after the first child, women’s earnings sharply dropped and never fully recovered. But this was not the case for men with children, nor the case for women without children.
These patterns are shown in the chart here. The first panel shows the trend in earnings for Danish women with and without children. The second panel shows the same comparison for Danish men.
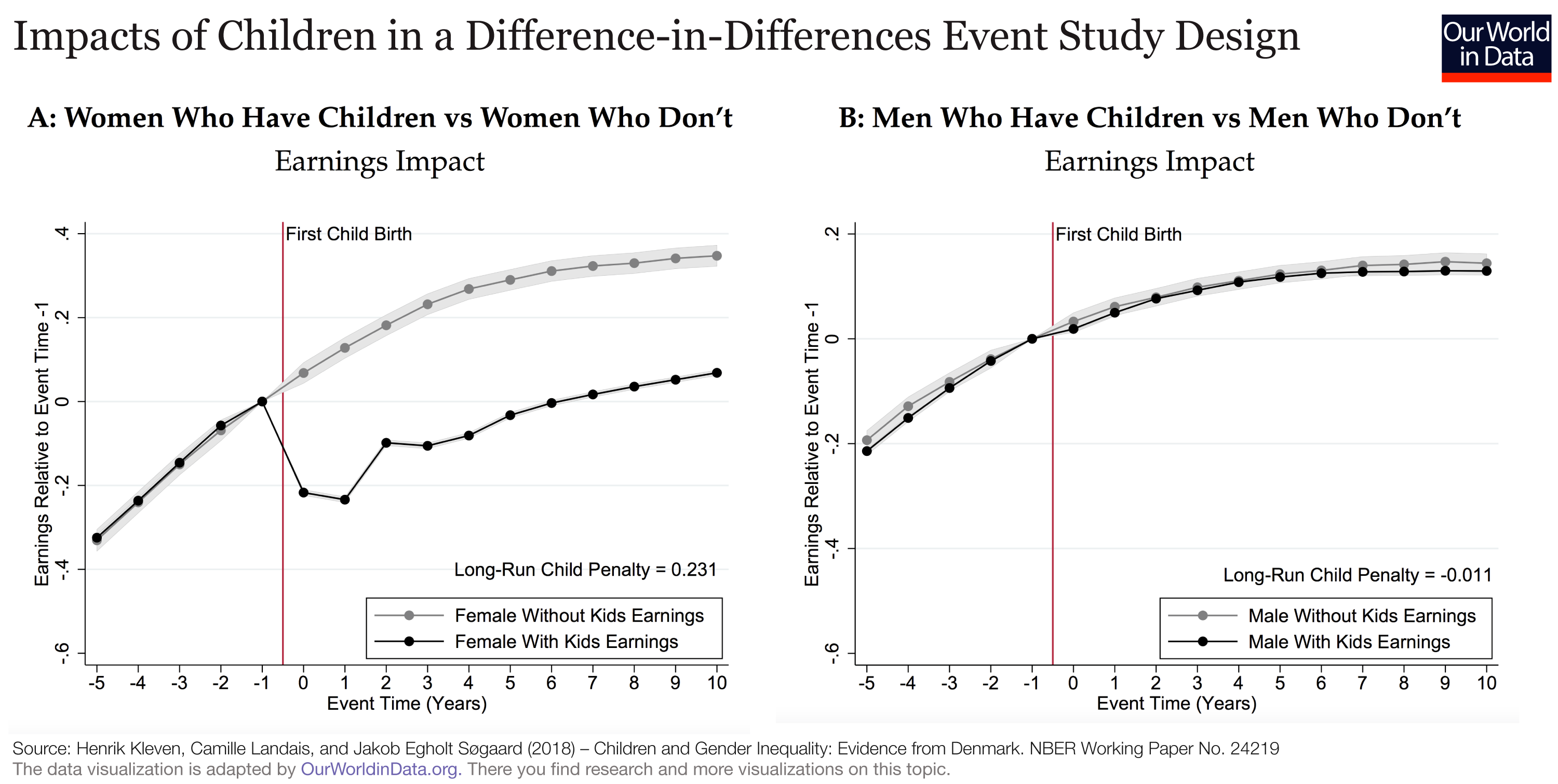
Note that these two examples are from Denmark – a country that ranks high on gender equality measures and where there are legal guarantees requiring that a woman can return to the same job after taking time to give birth.
This shows that, although family-friendly policies contribute to improving female labor force participation and reducing the gender pay gap , they are only part of the solution. Even when there is generous paid leave and subsidized childcare, as long as mothers disproportionately take additional work at home after having children, inequities in pay are likely to remain.
Ability, personality, and social norms
The discussion so far has emphasized the importance of job characteristics and occupational choice in explaining the gender pay gap. This leads to obvious questions: What determines the systematic gender differences in occupational choice? What makes women seek job flexibility and take a disproportionate amount of unpaid care work?
One argument usually put forward is that, to the extent that biological differences in preferences and abilities underpin gender roles, they are the main factors explaining the gender pay gap. In their review of the evidence, Francine Blau and Lawrence Kahn (2017) show that there is limited empirical support for this argument. 11
To be clear, yes, there is evidence supporting the fact that men and women differ in some key attributes that may affect labor market outcomes. For example, standardized tests show that there are statistical gender gaps in maths scores in some countries ; and experiments show that women avoid more salary negotiations , and they often show particular predisposition to accept and receive requests for tasks with low promotion potential . However, these observed differences are far from being biologically fixed – 'gendering' begins early in life and the evidence shows that preferences and skills are highly malleable. You can influence tastes, and you can certainly teach people to tolerate risk, to do maths, or to negotiate salaries.
What's more, independently of where they come from, Blau and Kahn show that these empirically observed differences can typically only account for a modest portion of the gender pay gap.
In contrast, the evidence does suggest that social norms and culture, which in turn affect preferences, behavior, and incentives to foster specific skills, are key factors in understanding gender differences in labor force participation and wages. You can read more about this farther below.
Discrimination and bias
Independently of the exact origin of the unequal distribution of gender roles, it is clear that our recent and even current practices show that these roles persist with the help of institutional enforcement. Goldin (1988), for instance, examines past prohibitions against the training and employment of married women in the US. She touches on some well-known restrictions, such as those against the training and employment of women as doctors and lawyers, before focusing on the lesser known but even more impactful 'marriage bars' that arose in the late 1800s and early 1900s. These work prohibitions are important because they applied to teaching and clerical jobs – occupations that would become the most commonly held among married women after 1950. Around the time the US entered World War II, it is estimated that 87% of all school boards would not hire a married woman and 70% would not retain an unmarried woman who married. 12
The map here highlights that to this day, explicit barriers limit the extent to which women are allowed to do the same jobs as men in some countries. 13
However, even after explicit barriers are lifted and legal protections put in place, discrimination and bias can persist in less overt ways. Goldin and Rouse (2000), for example, look at the adoption of "blind" auditions by orchestras and show that by using a screen to conceal the identity of a candidate, impartial hiring practices increased the number of women in orchestras by 25% between 1970 and 1996. 14
Many other studies have found similar evidence of bias in different labor market contexts. Biases also operate in other spheres of life with strong knock-on effects on labor market outcomes. For example, at the end of World War II only 18% of people in the US thought that a wife should work if her husband was able to support her . This obviously circles back to our earlier point about social norms. 15
Strategies for reducing the gender pay gap
In many countries wage inequality between men and women can be reduced by improving the education of women. However, in many countries, gender gaps in education have been closed and we still have large gender inequalities in the workforce. What else can be done?
An obvious alternative is fighting discrimination. But the evidence presented above shows that this is not enough. Public policy and management changes on the firm level matter too: Family-friendly labor-market policies may help. For example, maternity leave coverage can contribute by raising women’s retention over the period of childbirth, which in turn raises women’s wages through the maintenance of work experience and job tenure. 16
Similarly, early education and childcare can increase the labor force participation of women — and reduce gender pay gaps — by alleviating the unpaid care work undertaken by mothers. 17
Additionally, the experience of women's historical advance in specific professions (e.g. pharmacists in the US), suggests that the gender pay gap could also be considerably reduced if firms did not have the incentive to disproportionately reward workers who work long hours, and fixed, non-flexible schedules. 18
Changing these incentives is of course difficult because it requires reorganizing the workplace. But it is likely to have a large impact on gender inequality, particularly in countries where other measures are already in place. 19
Implementing these strategies can have a positive self-reinforcing effect. For example, family-friendly labor-market policies that lead to higher labor-force attachment and salaries for women will raise the returns on women's investment in education – so women in future generations will be more likely to invest in education, which will also help narrow gender gaps in labor market outcomes down the line. 20
Nevertheless, powerful as these strategies may be, they are only part of the solution. Social norms and culture remain at the heart of family choices and the gender distribution of labor. Achieving equality in opportunities requires ensuring that we change the norms and stereotypes that limit the set of choices available both to men and women. It is difficult, but as the next section shows, social norms can be changed, too.
How well do biological differences explain the gender pay gap?
Across the world, women tend to take on more family responsibilities than men. As a result, women tend to be overrepresented in low-paying jobs where they are more likely to have the flexibility required to attend to these additional responsibilities.
These two facts – documented above – are often used to claim that, since men and women tend to be endowed with different tastes and talents, it follows that most of the observed gender differences in wages stem from biological sex differences. But what’s the broader evidence for these claims?
In a nutshell, here's what the research and data shows:
- There is evidence supporting the fact that statistically speaking, men and women tend to differ in some key aspects, including psychological attributes that may affect labor-market outcomes.
- There is no consensus on the exact weight that nurture and nature have in determining these differences, but whatever the exact weight, the evidence does show that these attributes are strongly malleable.
- Regardless of the origin, these differences can only explain a modest part of the gender pay gap.
Some context regarding the gender distribution of labor
Before we get into the discussion of whether biological attributes explain wage differences via gender roles, let's get some perspective on the gender distribution of work.
The following chart shows, by country, the female-to-male ratio of time devoted to unpaid care work, including tasks like taking care of children at home, housework, or doing community work. As can be seen, all over the world there is a radical unbalance in the gender distribution of labor – everywhere women take a disproportionate amount of unpaid work.
This is of course closely related to the fact that in most countries there are gender gaps in labor force participation and wages .
“Boys are better at maths”
Differences in biological attributes that determine our ability to develop 'hard skills', such as maths, are often argued to be at the heart of the gender pay gap. 21 Do large gender differences in maths skills really exist? If so, is this because of differences in the attributes we are born with?
Let's look at the data.
Are boys better in the mathematics section of the PISA standardized test ? One could argue that looking at top scores is more relevant here since top scores are more likely to determine gaps in future professional trajectories – for example, gaps in access to 'STEM degrees' at the university level.
The chart shows the share of male and female test-takers scoring at the highest level on the PISA test (that's level 6). As we can see, most countries lie above the diagonal line marking gender parity; so yes, achieving high scores in maths tends to be more common among boys than girls. However, there is huge cross-country variation – the differences between countries are much larger than the differences between the sexes. And in many countries, the gap is effectively inexistent. 22
Similarly, researchers have found that within countries there is also large geographic variation in gender gaps in test scores. So clearly these gaps in mathematical ability do not seem to be fully determined by biological endowments. 23
Indeed, research looking at the PISA cross-country results suggests that improved social conditions for women are related to improved math performance by girls. 24
Not only do statistical gaps in test scores vary substantially across societies – they also vary substantially across time. This suggests that social factors play a large role in explaining differences between the sexes.
In the US, for example, the gender gap in mathematics has narrowed in recent decades. 25 And this narrowing took place as high school curricula of boys and girls became more similar. The following chart shows this: In the US boys in 1957 took far more math and science courses than did girls; but by 1992 there was virtual parity in almost all science and math courses.
More importantly for the question at hand, gender gaps in 'hard skills' are not large enough to explain the gender gaps in earnings. In their review of the evidence, Blau and Kahn (2017) concludes that gaps in test scores in the US are too small to explain much of the gender pay at any point in time. 26
So, taken together, the evidence suggests that statistical gaps in maths test scores are both relatively small and heavily influenced by social and environmental factors.
“It’s about personality”
Biological differences in tastes (e.g. preferences for 'people' over 'things'), psychological attributes (e.g. 'risk aversion'), and soft skills (e.g. the ability to get along with others) are also often argued to be at the heart of the gender pay gap.
There are hundreds of studies trying to establish whether there are gender differences in preferences, personality traits, and 'soft skills'. The quality and general relevance (i.e. the internal and external validity) of these studies is the subject of much discussion, as illustrated in the recent debate that ensued from the Google Memo affair .
A recent article from the 'Heterodox Academy ', which was produced specifically in the context of the Google Memo, provides a fantastic overview of the evidence on this topic and the key points of contention among scholars.
For the purpose of this blog post, let's focus on the review of the evidence presented in Blau and Kahn (2017) – their review is particularly helpful because they focus on gender differences in the context of labor markets.
Blau and Kahn point out that, yes, researchers have found statistical differences between men and women that are important in the context of labor-market outcomes. For example, studies have found statistical gender differences in 'people skills' (i.e. ability to listen, communicate, and relate to others). Similarly, experimental studies have found that women more often avoid salary negotiations , and they often show a particular predisposition to accept and receive requests for tasks with low promotability. But are the origins of these differences mainly biological or are they social? And are they strong enough to explain pay gaps?
The available evidence here suggests these factors can only explain a relatively small fraction of the observed differences in wages. 27 And they are anyway far from being purely biological – preferences and skills are highly malleable and 'gendering' begins early in life. 28
Here is a concrete example: Leibbrandt and List (2015) did an experiment in which they assessed how men and women reacted to job advertisements. 29 They found that although men were more likely to negotiate than women when there was no explicit statement that wages were negotiable, the gender difference disappeared and even reversed when it was explicitly stated that wages were negotiable. This suggests that it is not as much about 'talent', as it is about norms and rules.
“A man should earn more than his wife”
The experiment in which researchers found that gender differences in negotiation attitudes disappeared when it was explicitly stated that wages were negotiable, emphasizes the important role that social norms and culture play in labor-market outcomes.
These concepts may seem abstract: What do social norms and culture actually look like in the context of the gender pay gap?
The reproduction of stereotypes through everyday positive enforcement can be seen in a range of aspects: A study analyzing 124 prime-time television programs in the US found that female characters continue to inhabit interpersonal roles with romance, family, and friends, while male characters enact work-related roles. 30 In the realm of children’s books, a study of 5,618 books found that compared to females, males are represented nearly twice as often in titles and 1.6 times as often as central characters. 31 Qualitative research shows that even in the home, parents are often enforcers of gender norms – especially when it comes to fathers endorsing masculinity in male children. 32
Of particular relevance in the context of labor markets, social norms also often take the form of specific behavioral prescriptions such as "a man should earn more than his wife".
The following chart depicts the distribution of the share of the household income earned by the wife, across married couples in the US.
Consistent with the idea that "a man should earn more than his wife", the data shows a sharp drop at 0.5, the point where the wife starts to earn more than the husband.
Distribution of income share earned by the wife across married couples in the US – Bertrand, Kamenica, and Pan (2015) 33
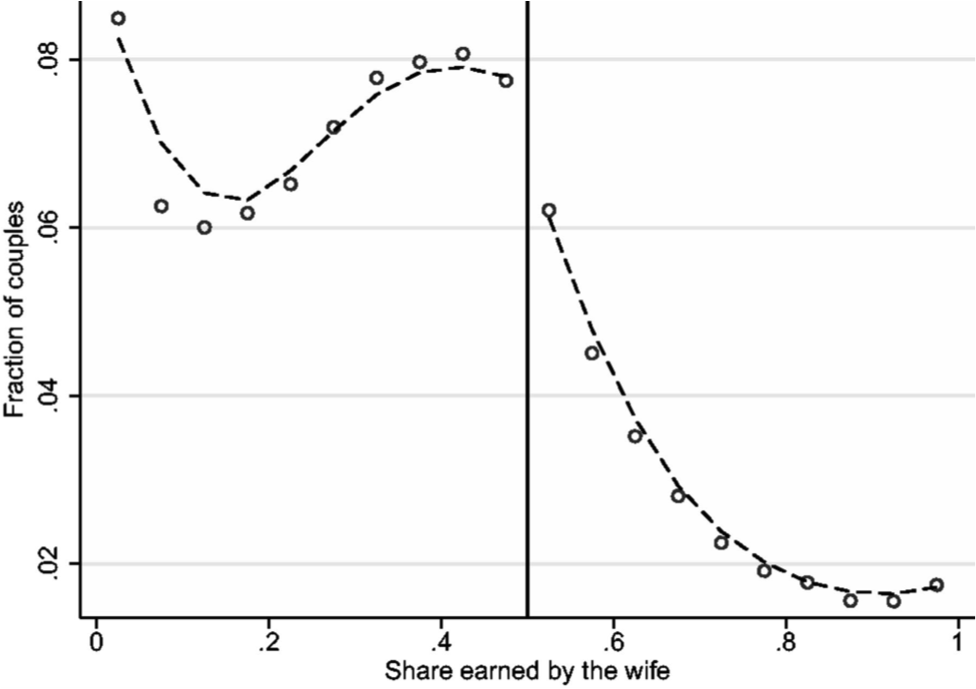
This is the result of two factors. First, it is about the matching of men and women before they marry – 'matches' in which the woman has higher earning potential are less common. Second, it is a result of choices after marriage – the researchers show that married women with higher earning potential than their husbands often stay out of the labor force, or take 'below-potential' jobs. 34
The authors of the study from which this chart is taken explored the data in more detail and found that in couples where the wife earns more than the husband, the wife spends more time on household chores, so the gender gap in unpaid care work is even larger; and these couples are also less satisfied with their marriage and are more likely to divorce than couples where the wife earns less than the husband.
The empirical exploration in this study highlights the remarkable power that gender norms and identity have on labor-market outcomes.
Why do gender norms and identity matter?
Does it actually matter if social norms and culture are important determinants of gender roles and labor-market outcomes? Are social norms in our contemporary societies really less fixed than biological traits?
The available research suggests that the answers to these questions are yes and yes. There is evidence that social norms can be actively and rapidly changed.
Here is a concrete example: Jensen and Oster (2009) find that the introduction of cable television in India led to a significant decrease in the reported acceptability of domestic violence towards women and son preference, as well as increases in women’s autonomy and decreases in fertility. 35
Of course, TV is a small aspect of all the big things that matter for social norms. But this study is important for the discussion because it is hard to study how social norms can be changed. TV introduction is a rare opportunity to see how a group that is exposed to a driver of social change actually changes.
As Jensen and Oster point out, most popular cable TV shows in India feature urban settings where lifestyles differ radically from those in rural areas. For example, many female characters on popular soap operas have more education, marry later, and have smaller families than most women in rural areas. And, similarly, many female characters in these tv shows are featured working outside the home as professionals, running businesses, or are shown in other positions of authority.
The bar chart below shows how cable access changed attitudes toward the self-reported preference for their child to be a son. As the authors note, "reported desire for the next child to be a son is relatively unchanged in areas with no change in cable status, but it decreases sharply between 2001 and 2002 for villages that get cable in 2002, and between 2002 and 2003 (but notably not between 2001 and 2002) for those that get cable in 2003. For both measures of attitudes, the changes are large and striking, and correspond closely to the timing of introduction of cable."
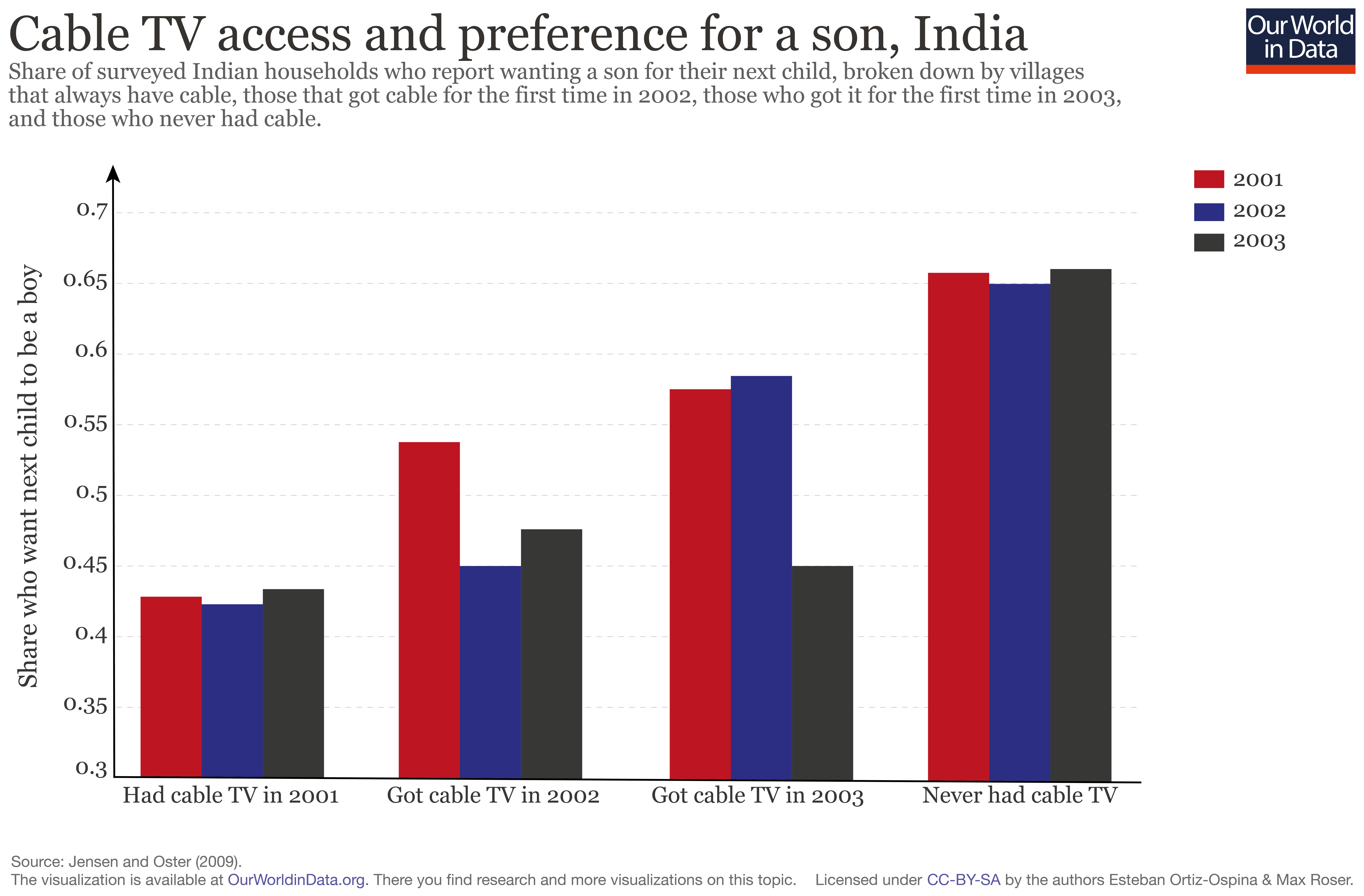
To conclude: The evidence suggests that biological differences are not a key driver of gender inequality in labor-market outcomes; while social norms and culture – which in turn affect preferences, behavior, and incentives to foster specific skills – are very important.
This matters for policy because social norms are not fixed – they can be influenced in a number of ways, including through intergenerational learning processes, exposure to alternative norms, and activism such as that which propelled the women's movement. 36
How are women represented across jobs?
Representation of women at the top of the income distribution.
Despite having fallen in recent decades, there remains a substantial pay gap between the average wages of men and women .
But what does gender inequality look like if we focus on the very top of the income distribution? Do we find any evidence of the so-called 'glass ceiling' preventing women from reaching the top? How did this change over time?
Answers to these questions are found in the work of Atkinson, Casarico and Voitchovsky (2018). Using tax records, they investigated the incomes of women and men separately across nine high-income countries. As such, they were restricted to those countries in which taxes are collected on an individual basis, rather than as couples. 37
In addition to wages they also take into account income from investments and self-employment.
Whilst investment income tends to make up a larger share of the total income of rich individuals in general, the authors found this to be particularly marked in the case of women in top-income groups.
The two charts present the key figures from the study.
One chart shows the proportion of women out of all individuals falling into the top 10%, 1%, and 0.1% of the income distribution. The open circle represents the share of women in the top income brackets back in 2000; the closed circle shows the latest data, which is from 2013.
The other chart shows the data over time for individual countries. You can explore data for other countries using the 'Change country' button on the chart.
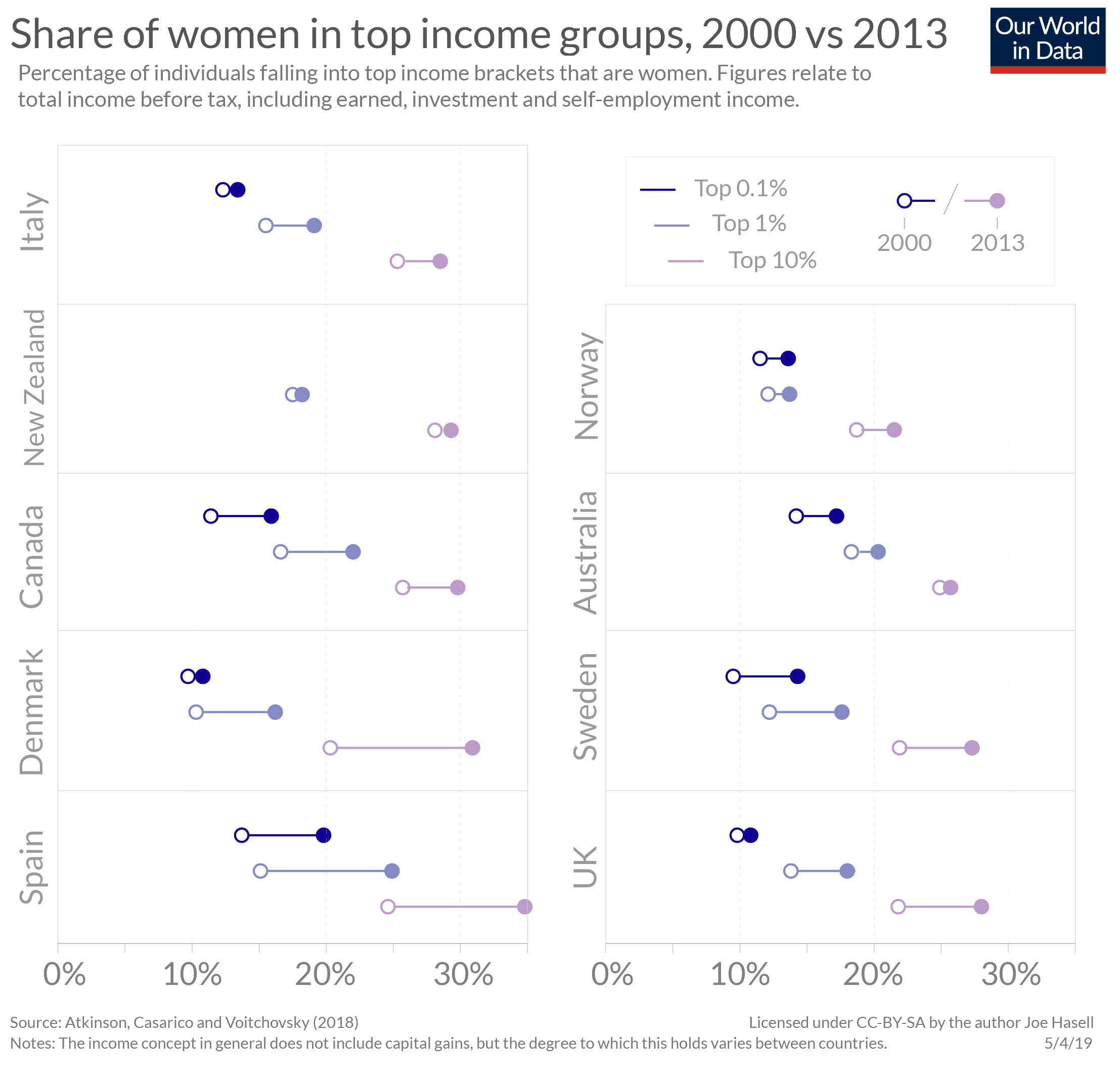
The two charts allow us to answer the initial questions:
- Women are greatly under-represented in top income groups – they make up much less than 50% across each of the nine countries. Within the top 1% women account for around 20% and there is surprisingly little variation across countries.
- The proportion of women is lower the higher you look up the income distribution. In the top 10% up to every third income-earner is a woman; in the top 0.1% only every fifth or tenth person is a woman.
- The trend is the same in all countries of this study: Women are now better represented in all top-income groups than they were in 2000.
- But improvements have generally been more limited at the very top. With the exception of Australia, we see a much smaller increase in the share of women amongst the top 0.1% than amongst the top 10%.
Overall, despite recent inroads, we continue to see remarkably few women making it to the top of the income distribution today.
Representation of women in management positions
The chart here plots the proportion of women in senior and middle management positions around the world. It shows that women all over the world are underrepresented in high-profile jobs, which tend to be better paid.
The next chart provides an alternative perspective on the same issue. Here we show the share of firms that have a woman as manager. We highlight world regions by default, but you can remove them and add specific countries.
As we can see, all over the world firms tend to be managed by men. And, globally, only about 18% of firms have a female manager.
Firms with female managers tend to be different to firms with male managers. For example, firms with female managers tend to also be firms with more female workers .
Representation of women in low-paying jobs
Above we show that women all over the world are underrepresented in high-profile jobs, which tend to be better paid. As it turns out, in many countries women are at the same time overrepresented in low-paying jobs.
This is shown in the chart here, where 'low-pay' refers to workers earning less than two-thirds of the median (i.e. the middle) of the earnings distribution.
A share above 50% implies that women are 'overrepresented', in the sense that among those with low wages, there are more women than men.
The fact that women in rich countries are overrepresented in the bottom of the income distribution goes together with the fact that working women in these countries are overrepresented in low-paying occupations. The chart shows this for the US.
How much control do women have over household resources?
Women often have no control over their personal earned income.
The next chart plots cross-country estimates of the share of women who are not involved in decisions about their own income. The line shows national averages, while the dots show averages for rich and poor households (i.e. averages for women in households within the top and bottom quintiles of the corresponding national income distribution).
As we can see, in many countries, particularly in Sub-Saharan Africa and Asia, a large fraction of women are not involved in household decisions about spending their personal earned income. And this pattern is stronger among low-income households within low-income countries.
Percentage of women not involved in decisions about their own income – World Development Report (2012) 39
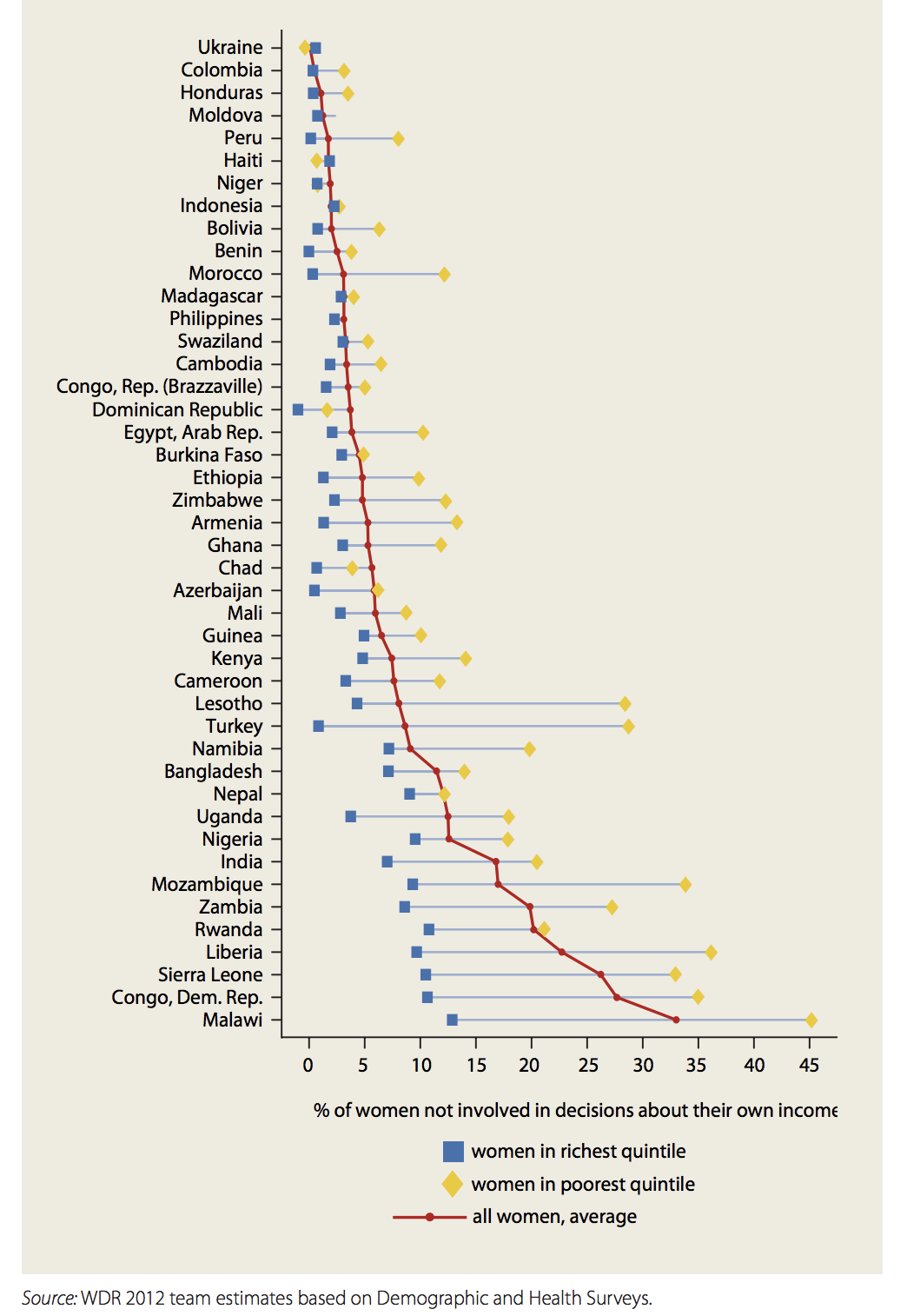
In many countries, women have limited influence over important household decisions
Above we focus on whether women get to choose how their own personal income is spent. Now we look at women's influence over total household income.
In this chart, we plot the share of currently married women who report having a say in major household purchase decisions, against national GDP per capita.
We see that in many countries, notably in Sub-Saharan Africa and Asia, an important number of women have limited influence over major spending decisions.
The chart above shows that women’s control over household spending tends to be greater in richer countries. In the next chart, we show that this correlation also holds within countries: Women’s control is greater in wealthier households. Household wealth is shown by the quintile in the wealth distribution on the x-axis – the poorest households are in the lowest quintiles (Q1) on the left.
There are many factors at play here, and it's important to bear in mind that this correlation partly captures the fact that richer households enjoy greater discretionary income beyond levels required to cover basic expenditure, while at the same time, in richer households women often have greater agency via access to broader networks as well as higher personal assets and incomes.
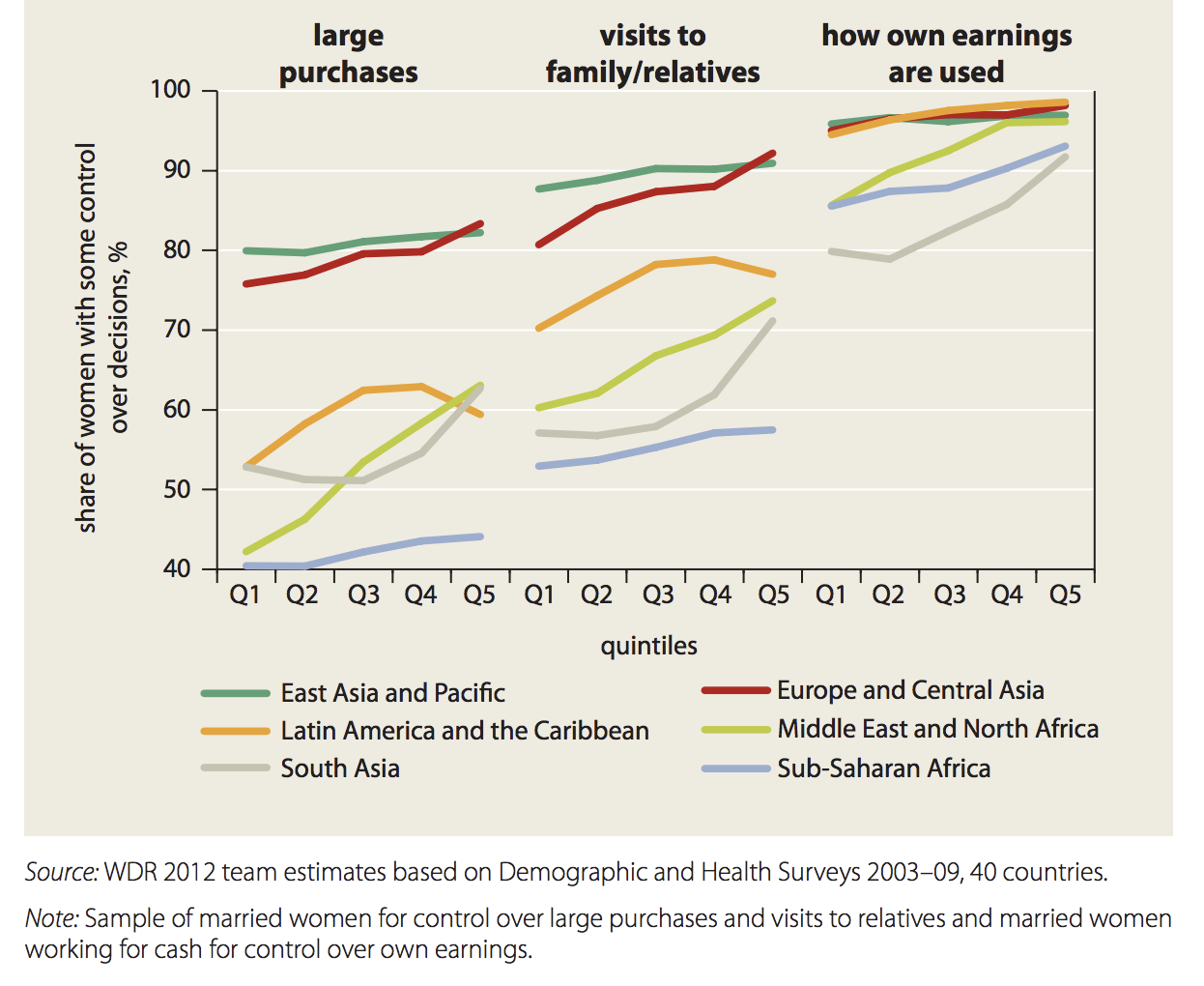
Land ownership is more often in the hands of men
Economic inequalities between men and women manifest themselves not only in terms of wages earned but also in terms of assets owned. For example, as the chart shows, in nearly all low and middle-income countries with data, men are more likely to own land than women.
Women's lack of control over important household assets, such as land, can be a critical problem in case of divorce or the husband’s death.
Closely related to the issue of land ownership is the fact that in several countries women do not have the same rights to property as men. These countries are highlighted in the map. 40
Gender-equal inheritance systems have been adopted in most, but not all countries
Inheritance is one of the main mechanisms for the accumulation of assets. In the map, we provide an overview of the countries that do and do not have gender-equal inheritance systems.
If you move the slider to 1920, you will see that while gender-equal inheritance systems were very rare in the early 20th century, today they are much more common. And still, despite the progress achieved, in many countries, notably in North Africa and the Middle East, women and girls still have fewer inheritance rights than men and boys.
Gender differences in access to productive inputs are often large
Above we show that there are large gender gaps in land ownership across low-income countries. Here we show that there are also large gaps in terms of access to borrowed capital.
The chart shows the percentage of men and women who report borrowing any money in the past 12 months to start, operate, or expand a farm or business.
As we can see, almost everywhere, including in many rich countries, women are less likely to obtain borrowed capital for productive purposes.
This can have large knock-on effects: in agriculture and entrepreneurship, gender differences in access to productive inputs, including land and credit, can lead to gaps in earnings via lower productivity.
Indeed, studies have found that, when statistical gender differences in agricultural productivity exist, they often disappear when access to and use of productive inputs are taken into account. 41
Interactive Charts on Economic Inequality by Gender
Acknowledgements.
We thank Sandra Tzvetkova and Diana Beltekian for their great research assistance.
There are some exceptions to this definition. In particular, sometimes self-employed workers, or part-time workers are excluded.
This measure can also be negative. This means that, on an hourly basis, men earn on average less than women. It is the case for some countries, such as Malaysia.
Olivetti, C., & Petrongolo, B. (2008). Unequal pay or unequal employment? A cross-country analysis of gender gaps. Journal of Labor Economics, 26(4), 621-654.
Blau, Francine D., and Lawrence M. Kahn. 2017. " The Gender Wage Gap: Extent, Trends, and Explanations. " Journal of Economic Literature, 55(3): 789-865.
For each specification, Blau and Kahn (2017) perform regression analyses on data from the PSID (the Michigan Panel Study of Income Dynamics), which includes information on labor-market experience and considers men and women ages 25-64 who were full-time, non-farm, wage and salary workers.
In 2010, unionization and education show negative values; this reflects the fact that women have surpassed men in educational attainment, and unionization in the US has been in general decline with a greater effect on men.
The full source is: World Development Report (2012) Gender Equality and Development , World Bank.
Goldin, C. (2014). A grand gender convergence: Its last chapter. The American Economic Review, 104(4), 1091-1119.
Goldin, C., & Katz, L. F. (2016). A most egalitarian profession: pharmacy and the evolution of a family-friendly occupation. Journal of Labor Economics, 34(3), 705-746.
Lundborg, P., Plug, E., & Rasmussen, A. W. (2017). Can Women Have Children and a Career? IV Evidence from IVF Treatments. American Economic Review, 107(6), 1611-1637.
Blau, Francine D., and Lawrence M. Kahn. 2017. " The Gender Wage Gap: Extent, Trends, and Explanations. " Journal of Economic Literature, 55(3): 789-865
Goldin, C. (1988). Marriage bars: Discrimination against married women workers, 1920's to 1950's .
The data in this map, which comes from the World Bank's World Development Indicators, provides a measure of whether there are any specific jobs that women are not allowed to perform. So, for example, a country might be coded as "No" if women are only allowed to work in certain jobs within the mining industry, such as health care professionals within mines, but not as miners.
Goldin, C., & Rouse, C. (2000). Orchestrating impartiality: The impact of" blind" auditions on female musicians. American Economic Review , 90(4), 715-741.
Blau and Kahn (2017) provide a whole list of experimental studies that have found labor-market discrimination. Another early example is from Neumark et al. (1996), who look at discrimination in restaurants. In this case, male and female pseudo-job-seekers were given similar CVs to apply for jobs waiting on tables at the same set of restaurants in Philadelphia. The results showed discrimination against women in high-priced restaurants.
The full reference of this study is Neumark, D., Bank, R. J., & Van Nort, K. D. (1996). Sex discrimination in restaurant hiring: An audit study. The Quarterly Journal of Economics, 111(3), 915-941.
Waldfogel, J. (1998). Understanding the "family gap" in pay for women with children. The Journal of Economic Perspectives, 12(1), 137-156.
Olivetti, C., & Petrongolo, B. (2017). The economic consequences of family policies: lessons from a century of legislation in high-income countries. The Journal of Economic Perspectives, 31(1), 205-230.
As we show above, in several nations, such as Sweden and Denmark, a “motherhood penalty” in earnings exists, even though these nations have generous family policies, including paid family leave and subsidized child care.
For a discussion of this mechanism, see page 814, Blau, Francine D., and Lawrence M. Kahn. 2017. The Gender Wage Gap: Extent, Trends, and Explanations. Journal of Economic Literature, 55(3): 789-865.
Hard skills are abilities that can be defined and measured, such as writing, reading, or doing maths. By contrast, soft skills are less tangible and harder to measure and quantify.
Also importantly: If we focus on gender differences for average , rather than top students, we find that there is not even a clear tendency in favor of boys. ( This interactive chart compares PISA average math scores for boys and girls ).
For more on this see Pope, D. G., & Sydnor, J. R. (2010). Geographic variation in the gender differences in test scores. Journal of Economic Perspectives, 24(2), 95-108.
Guiso, L., Monte, F., Sapienza, P., & Zingales, L. (2008). Culture, gender, and math. SCIENCE-NEW YORK THEN WASHINGTON-, 320(5880), 1164.
A number of papers have documented the narrowing of gender gaps in test scores. See, for example, Hyde, J. S., Lindberg, S. M., Linn, M. C., Ellis, A. B., & Williams, C. C. (2008). Gender similarities characterize math performance . Science, 321(5888), 494-495.
Blau, Francine D., and Lawrence M. Kahn. 2017. The Gender Wage Gap: Extent, Trends, and Explanations. Journal of Economic Literature, 55(3): 789-865.
Blau and Kahn write: "While findings such as those in table 7 ['Selected Studies Assessing the Role of Psychological Traits in Accounting for the Gender Pay Gap'] are informative in elucidating some of the possible omitted factors that lie behind gender differences in wages as well as the unexplained gap in traditional wage regressions, in general, the results suggest that these factors do not account for a large portion of either the raw or unexplained gender gap."
For a discussion of 'gendering' see West, C., & Zimmerman, D. H. (1987). Doing gender. Gender & Society, 1(2), 125-151.
Leibbrandt, A., & List, J. A. (2014). Do women avoid salary negotiations? Evidence from a large-scale natural field experiment. Management Science, 61(9), 2016-2024.
Lauzen, M. M., Dozier, D. M., & Horan, N. (2008). Constructing gender stereotypes through social roles in prime-time television. Journal of Broadcasting & Electronic Media, 52(2), 200-214.
McCabe, J., Fairchild, E., Grauerholz, L., Pescosolido, B. A., & Tope, D. (2011). Gender in twentieth-century children’s books: Patterns of disparity in titles and central characters. Gender & Society, 25(2), 197-226.
Kane, E. W. (2006). “No way my boys are going to be like that!” Parents’ responses to children’s gender nonconformity. Gender & Society, 20(2), 149-176.
Bertrand, M., Kamenica, E., & Pan, J. (2015). Gender identity and relative income within households. The Quarterly Journal of Economics, 130(2), 571-614.
More precisely, the authors find that in couples where the wife’s potential income is likely to exceed her husband’s (based on the income that would be predicted for her observed characteristics), the wife is less likely to be in the labor force, and if she does work, her income is lower than predicted.
Jensen, R., & Oster, E. (2009). The power of TV: Cable television and women's status in India . In The Quarterly Journal of Economics , 124(3), 1057-1094.
Regarding intergenerational transmission of gender roles, see Fernández, R. (2013). Cultural change as learning: The evolution of female labor force participation over a century. The American Economic Review, 103(1), 472-500.
For a discussion regarding social activism and its link to the determinants of female labor supply, see for example this study by Heer and Grossbard-Shechtman (1981).
Atkinson, A.B., Casarico, A. & Voitchovsky, S. Top incomes and the gender divide . J Econ Inequal (2018) 16: 225.
The authors produced results for 8 countries, and included earlier results for Sweden from Boschini, A., Gunnarsson, K., Roine, J.: Women in Top Incomes: Evidence from Sweden 1974-2013, IZA Discussion paper 10979, August (2017).
World Bank. (2011). World development report 2012: gender equality and development . World Bank Publications.
The map from The World Development Report (2012) provides a more fine-grained overview of different property regimes operating in different countries.
For more discussion of the evidence see page 20 in World Bank (2011) World Development Report 2012: Gender Equality and Development. World Bank Publications.
Cite this work
Our articles and data visualizations rely on work from many different people and organizations. When citing this topic page, please also cite the underlying data sources. This topic page can be cited as:
BibTeX citation
Reuse this work freely
All visualizations, data, and code produced by Our World in Data are completely open access under the Creative Commons BY license . You have the permission to use, distribute, and reproduce these in any medium, provided the source and authors are credited.
The data produced by third parties and made available by Our World in Data is subject to the license terms from the original third-party authors. We will always indicate the original source of the data in our documentation, so you should always check the license of any such third-party data before use and redistribution.
All of our charts can be embedded in any site.
Our World in Data is free and accessible for everyone.
Help us do this work by making a donation.
Report | Wages
What is the gender pay gap and is it real? : The complete guide to how women are paid less than men and why it can’t be explained away
Report • By Elise Gould , Jessica Schieder , and Kathleen Geier • October 20, 2016
Download PDF
Press release
Share this page:
Working women are paid less than working men. A large body of research accounts for, diagnoses, and investigates this “gender pay gap.” But this literature often becomes unwieldy for lay readers, and because pay gaps are political topics, ideological agendas often seep quickly into discussions.
This primer examines the evidence surrounding the gender pay gap, both in the literature and through our own data analyses. We will begin by explaining the different ways the gap is measured, and then go deeper into the data using hourly wages for our analyses, 1 culling from extensive national and regional surveys of wages, educational attainment, and occupational employment.
Why different measures don’t mean the data are unreliable
A number of figures are commonly used to describe the gender wage gap. One often-cited statistic comes from the Census Bureau, which looks at annual pay of full-time workers. By that measure, women are paid 80 cents for every dollar men are paid. Another measure looks at hourly pay and does not exclude part-time workers. It finds that, relative to men, typical women are paid 83 cents on the dollar. 2 Other, less-cited measures show different gaps because they examine the gap at different parts of the wage distribution, or for different demographic subgroups, or are adjusted for factors such as education level and occupation.
The presence of alternative ways to measure the gap can create a misconception that data on the gender wage gap are unreliable. However, the data on the gender wage gap are remarkably clear and (unfortunately) consistent about the scale of the gap. In simple terms, no matter how you measure it, there is a gap. And, different gaps answer different questions. By discussing the data and the rationale behind these seemingly contradictory measures of the wage gap, we hope to improve the discourse around the gender wage gap.
Why adjusted measures can’t gauge the full effects of discrimination
The most common analytical mistake people make when discussing the gender wage gap is to assume that as long as it is measured “correctly,” it will tell us precisely how much gender-based discrimination affects what women are paid.
Specifically, some people note that the commonly cited measures of the gender wage gap do not control for workers’ demographic characteristics (such measures are often labeled unadjusted). They speculate that the “unadjusted” gender wage gap could simply be reflecting other influences, such as levels of education, labor market experiences, and occupations. And because gender wage gaps that are “adjusted” for workers’ characteristics (through multivariate regression) are often smaller than unadjusted measures, people commonly infer that gender discrimination is a smaller problem in the American economy than thought.
However, the adjusted gender wage gap really only narrows the analysis to the potential role of gender discrimination along one dimension : to differential pay for equivalent work. But this simple adjustment misses all of the potential differences in opportunities for men and women that affect and constrain the choices they make before they ever bargain with an employer over a wage. While multivariate regression can be used to distill the role of discrimination in the narrowest sense, it cannot capture how discrimination affects differences in opportunity.
In short, one should have a very precise question that he or she hopes to answer using the data on the wage differences between men and women workers. We hope to provide this careful thinking in the questions we address in this primer.
A summary of some key questions and answers in this primer
Given that gender wage gaps are strikingly persistent in economic data, it is natural to then ask, “What causes these gaps?” And, further, “Do women’s own choices and labor force characteristics drive the gender wage gap, or are women’s opportunities for higher pay constricted relative to men?” Although this paper will largely focus on empirical data to answer questions about the size and scope of the gaps for different groups of women, we will use the data to shed light on some of these “why” questions.
- How much do women make relative to men? A typical, or median, woman working full time is paid 80 cents for every dollar a typical man working full time is paid. When evaluated by wages per hour, a typical woman is paid 83 cents for every dollar a man is paid. Both of these measures are correct, but examining women’s earnings per hour is our preferred way of looking at the wage gap. 3
- Is the wage gap the same whether you are a front-line worker or a high-level executive? There is much greater parity at the lower end of the wage distribution, likely because minimum wages and other labor market policies create a wage floor. At the 10th percentile, women are paid 92 cents on the male dollar, whereas women at the 95th percentile are paid 74 cents relative to the dollar of their male counterparts’ hourly wages.
- Does a woman’s race or ethnicity affect how much she makes relative to a man? Asian and white women at the median actually experience the biggest gaps relative to Asian and white men, respectively. But that is due, in part, to the fact that Asian and white men make much more than black or Hispanic men. Relative to white non-Hispanic men, black and Hispanic women workers are paid only 65 cents and 58 cents on the dollar, respectively, compared with 81 cents for white, non-Hispanic women workers and 90 cents for Asian women.
- Can women close the wage gap by getting more education? It appears not. Women are paid less than similarly educated men at every level of education. And the wage gap tends to rise with education level. This, again, in part likely reflects labor market policies that foster more-equal outcomes for workers in the lower tier of the wage distribution. It also may be affected by certain challenges that disproportionately affect women’s ability to secure jobs at the top of the wage distribution, such as earnings penalties for time out of the workforce, excessive work hours, domestic gender roles, and pay and promotion discrimination.
- Men constitute greater shares of certain types of jobs, or occupations, and women greater shares in others. Some say that these differences in how men and women are distributed across occupations explain much of the gender wage gap. In truth, it explains some of the gap, but not nearly as much as is assumed. And even when we reduce the size of the measured gap by controlling for occupational distributions, that does not mean that the remaining gap provides a complete view of the role of discrimination on women’s wages. Gender discrimination doesn’t happen only in the pay-setting practices of employers making wage offers to nearly identical workers of different genders. It can happen at every stage of a woman’s life, from steering her away from science and technology education to shouldering her with home responsibilities that impede her capacity to work the long hours of demanding professions.
- Women who work in male-dominated occupations are paid significantly less than similarly educated males in those occupations. So even recommending that women choose better-paying occupations does not solve the problem.
- Are women in unions, relative to their male peers, better or worse off? Working women in unions are paid 89 cents for every dollar paid to unionized working men; nonunionized working women are paid 82 cents for every dollar paid to nonunionized working men.
- After giving birth, women’s pay lags behind pay of similarly educated and experienced men and of women without children. There is no corresponding “fatherhood penalty” for men. 4
- Outside the labor market, mothers are also charged a time penalty. For example, among married full-time working parents of children under the age of 18, women still spend 50 percent more time than men engaging in care activities within the home. Among child-rearing couples that include a woman either working part time or staying at home to parent, the burden of caring for family members is even more disproportionately borne by women. This higher share of domestic and care work performed by women suggests that cultural norms and expectations strongly condition (and often restrict) the labor market opportunities of women. Indeed, it likely plays a role in the lower labor force participation of mothers relative to men or women without children.
- The higher share of domestic and care work performed by women is also a disadvantage for women in high-prestige, high-wage jobs in which employers demand very long hours as a condition of work.
- Does a shrinking wage gap unequivocally indicate a good thing—that women are catching up to men? Unfortunately no. Because the gender pay gap has both a numerator (women’s wages) and a denominator (men’s wages), one cannot make firm normative judgments about whether a given fall (or rise) in the gender pay gap is welcome news. For example, about 30 percent of the reduction of the gender wage gap between the median male and female worker since 1979 is due to the decline in men’s wages during this period.
- If we counted benefits, would women be doing less bad relative to men? The gender pay gap in cash wages would not disappear by factoring in other employee benefits because women are less likely than men to have employer-provided health insurance and have fewer retirement resources than men.
The gender pay gap is a fraught topic. Discussions about it would benefit greatly from a thorough review of the empirical evidence. The data can answer only precise questions, but the answers can help us work toward the broader questions. This paper aims to provide this precision in search of broader answers. Readers can access the data we analyze and report in this paper in the EPI State of Working America Data Library . By making the data publicly available and usable, we hope to advance constructive discussions of the gender pay gap.
The gender wage gap 101: The basics
The gender wage gap is a measure of pay disparity between men and women. While it can be measured different ways, the data are clear: women are still paid much less relative to men (about 83 cents per dollar, by our measure), and progress on closing the gap has stalled.
What is the gender wage gap?
The wage gap means women are paid:.
82.7 percent of men’s wages. This translates to 83 cents per dollar. The “typical” (median) woman is paid 83 cents per every dollar the typical man is paid.
In dollar terms this means women bring home:
$3.27 less per hour than men. The median hourly wage is $15.67 for women and $18.94 for men.
The gender wage gap is a measure of what women are paid relative to men. It is commonly calculated by dividing women’s wages by men’s wages, and this ratio is often expressed as a percent, or in dollar terms. This tells us how much a woman is paid for each dollar paid to a man. This gender pay ratio is often measured for year-round, full-time workers and compares the annual wages (of hourly wage and salaried workers) of the median (“typical”) man with that of the median (“typical”) woman; measured this way, the current gender pay ratio is 0.796, or, expressed as a percent, it is 79.6 percent (U.S. Census Bureau 2016). In other words, for every dollar a man makes, a woman makes about 80 cents.
The difference in earnings between men and women is also sometimes described in terms of how much less women make than men. To calculate this gap from the ratio as defined above, simply subtract the ratio from 1. So, if the gender pay ratio is about 80 percent (or 80 cents on the dollar), this means that women are paid 20 percent less (or 20 cents less per dollar) than men. A larger difference between men’s and women’s earnings translates into a lower ratio but a larger gap in their earnings.
We keep with this convention of using median wages of wage and salary workers rather than average wages of wage and salary workers because averages can be skewed by a handful of people making much more or much less than the rest of workers in a sample. However, we examine median wages on an hourly basis and include all workers reporting a positive number of work hours. This hourly measure constitutes a limited “adjustment” in research methodology in that it accounts for the fact that men work more hours on average during the year, and that more women work part time. 5 This limited adjustment allows us to compare women’s and men’s wages without assuming that women, who still shoulder a disproportionate amount of responsibilities at home, would be able or willing to work as many hours as their male counterparts.
Computed this way using data from the federal government’s Current Population Survey Outgoing Rotation Group, or CPS ORG in shorthand, the typical woman is paid 82.7 percent of what the typical man is paid (CPS ORG 2015). Or in common terms, women are paid 83 cents on the male dollar.
Notwithstanding our limited adjustment, this is basically the “raw” or “unadjusted” gap that we explore throughout this report when we consider the ways a large basket of factors interact and create the wage gap women experience when they cash their paychecks.
Would adjusting the raw gender wage gap to include factors such as education help explain the gap? Maybe it is not as big of a problem as it seems?
Adjustments can help round out our understanding but unfortunately, as we explain here, they don’t explain away the gap. It is important to understand why.
The gender wage gap described above and referred to in this primer has the virtue of being clear and simple. It provides a good overview of what is going on with typical women’s earnings relative to men’s. But it does not tell us what the wage gap is between men and women doing similar work, and whether the size of the gap derives in part from differences in education levels, experience levels, and other characteristics of working men and women. To round out our understanding of the disparity between men’s and women’s pay, we also consider “adjusted” measures of the gender wage gap—with the caveat that the adjusted measures may understate the wage disparities.
Adjusted wage gap estimates control for characteristics such as race and ethnicity, level of education, potential work experience, and geographic division. These estimate are made using average wages rather than median because it requires standard regression techniques. Again, using the Current Population Survey data from the CPS Outgoing Rotation Group, but making these adjustments, we find that the wage gap grows, with women on average paid 21.7 percent less than men. 6 The unadjusted penalty for the average woman is 17.9 percent. 7 The measured penalty actually increases when accounting for these influences because women workers, on average, have higher levels of education than men. 8
Models that control for a much larger set of variables—such as occupation, industry, or work hours—are sometimes used to isolate the role of discrimination in setting wages for specific jobs and workers. The notion is that if we can control for these factors, the wage gap will shrink, and what is left can be attributed to discrimination. Think of a man and woman with identical education and years of experience working side-by-side in cubicles but who are paid different wages because of discriminatory pay-setting practices. We also run a model with more of these controls, and find that the wage gap shrinks slightly from the unadjusted measure, from 17.9 percent to 13.5 percent. 9 Researchers have used more extensive datasets to examine these differences. For instance, Blau and Kahn (2016) find an unadjusted penalty of 20.7 percent, a partially adjusted penalty of 17.9 percent, and a fully adjusted penalty of 8.4 percent. 10
But switching to a fully adjusted model of the gender wage gap actually can radically understate the effect of gender discrimination on women’s earnings. This is because gender discrimination doesn’t happen only in the pay-setting practices of employers making wage offers to nearly identical workers of different genders. Instead, it can potentially happen at every stage of a woman’s life, from girlhood to moving through the labor market. By the time she completes her education and embarks on her career, a woman’s occupational choice is the culmination of years of education, guidance by mentors, expectations of parents and other influential adults, hiring practices of firms, and widespread norms and expectations about work/family balance held by employers, co-workers, and society (Gould and Schieder 2016). So it would not be accurate to assume that discrimination explains only the gender wage gap that remains after adjusting for education, occupational choice, and all these other factors. Put another way, we cannot look at our adjusted model and say that discrimination explains at most 13.5 percent of the gender wage gap. Why? Because, for example, by controlling for occupation, this adjusted wage gap no longer includes the discrimination that can influence a woman’s occupational choice.
How much does the gender pay gap cost women over a lifetime?
The average woman worker loses more than $530,000 over the course of her lifetime because of the gender wage gap, and the average college-educated woman loses even more—nearly $800,000 (IWPR 2016). It’s worth noting that each woman’s losses will vary significantly based on a variety of factors—including the health of the economy at various points in her life, her education, and duration of periods out of the labor force—but this estimate demonstrates the significance of the cumulative impact. And, as explained later, the gap may play a role in the retirement insecurity of older American women.
Yes, but isn’t the gender pay gap smaller than it used to be?
Over the past three and a half decades, substantial progress has been made to narrow the pay gap. Women’s wages are now significantly closer to men’s, but in recent years, that progress has stalled.
From 1979 to the early 1990s, the ratio of women’s median hourly earnings to men’s hourly median earnings grew partly because women made disproportionate gains in education and labor force participation. After that, convergence slowed, and over the past two decades, it has stalled. According to the most recent data, as of 2015, women’s hourly wages are 82.7 percent of men’s hourly wages at the median ( Figure A ), with the median woman paid an hourly wage of $15.67, compared with $18.94 for men ( Figure B ).
Progress in closing the gender pay gap has largely stalled : Women's hourly wages as a share of men's at the median, 1979–2015
The data below can be saved or copied directly into Excel.
The data underlying the figure.
Source: EPI analysis of Current Population Survey microdata. For more information on the data sample see EPI's State of Working America Data Library .
Copy the code below to embed this chart on your website.
Women earn less than men at every wage level : Hourly wages by gender and wage percentile, 2015
Source : EPI analysis of Current Population Survey Outgoing Rotation Group microdata. For more information on the data sample see EPI's State of Working America Data Library .
It’s not entirely clear why women have stopped gaining on men. But as discussed later in the section on the “motherhood penalty,” the tendency for women with children to receive systematically lower pay has stubbornly persisted, suggesting that the gender pay gap is not going away anytime soon. Economist Claudia Goldin’s research supports this conclusion. According to Goldin, current trends indicate that women’s wages will still be pulled down over the course of their working lifetimes, even after controlling for education and work time (Goldin 2014).
How much of the narrowing of the gender pay gap is due to women’s earnings rising, and how much is due to men’s earnings falling?
Since 1979, median men’s wages have stagnated, falling 6.7 percent in real terms from $20.30 per hour to $18.94 ( Figure C ). At the same time, women’s real median hourly wages have increased. In 1979, they were equal to roughly 62.4 percent of men’s real median hourly wages. By 2015, they were equal to 82.7 percent of men’s real wages at the median—a substantial reduction in the wage gap. Unfortunately, this means that about 30 percent of the reduction was due to the decline in men’s wages. The stagnation and decline of median men’s wages has played a significant role in the decline in the unadjusted gender wage gap. Women’s wages increased as more women had increased their participation in the labor force, increased their educational attainment, and entered higher-paying occupations. (Davis and Gould 2015). At the same time, for most workers, wages no longer increased with increases in economy-wide productivity. Had workers’ wages continued to keep pace with productivity, both men and women would be earning much more today.
The gender wage gap persists, but has narrowed since 1979 : Median hourly wages, by gender, 1979–2015
Source: EPI analysis of Current Population Survey Outgoing Rotation Group microdata. For more information on the data sample see EPI's State of Working America Data Library .
Does a woman’s race, age, or pay level affect the gender gap she experiences?
Belonging to a certain race or age group does not immunize women from experiencing the gender wage gap. It affects women across the board, though higher-earning women and middle-age women are at a greater disadvantage relative to their male counterparts. And relative to white male wages, black and Hispanic women are the most disadvantaged.
Is the gender wage gap a problem for low- or high-earning women?
The gender wage gap is a problem for women at every wage level. At each and every point in the wage distribution, men significantly out-earn women, although by different amounts, to be sure (Figures B and C).
In 2015, the gap between men’s and women’s hourly wages was smallest among the lowest-earning workers, with 10th percentile women earning 92.0 percent of men’s wages. The minimum wage is partially responsible for this greater equality among the lowest earners. It sets a wage floor that applies to everyone, which means that people near the bottom of the distribution are likely to make more equal wages, even though those wages are very low ( Figure D ).
The gender wage gap is still widest among top earners : Women's hourly wages as a share of men's at various wage percentiles, 1979 and 2015
Notes: The xth-percentile wage is the wage at which x% of wage earners earn less and (100-x)% earn more.
Source: EPI analysis of Current Population Survey Outgoing Rotation group microdata
At the median, women’s hourly wages are equal to 82.7 percent of men’s wages.
The gender wage gap is largest at the top of the wage distribution, with women at the 95th percentile getting paid 73.8 percent of wages at the male 95th percentile. Economist Claudia Goldin argues that women in high-wage professions experience a wider gender gap because they are penalized for not working long, inflexible hours (Goldin 2014). Such rigorous work schedules tend to weigh disproportionately heavily on women, who are still responsible for more housework and child/elder care than men.
It is interesting to note that the wage gap between median men and women workers has narrowed noticeably over the past four decades (Hegewisch and DuMonthier 2016). At the low end, the gap has not closed as much, but the existence of the minimum wage likely kept wages of low-paid men and women closer together even in the 1970s. And the relatively fast growth of men’s wages at the 95th percentile has kept this gap from closing as much as the median gap (Economic Policy Institute 2016).
How do women of different races and ethnicities experience the gender wage gap?
Relative wage gaps are larger for high-wage white and Asian women but black and Hispanic women are paid least relative to white men.
Figure E looks at low-, middle-, and high-wage women and compares their wages with those of men within their same racial and ethnic group. Here higher-wage white and Asian women are paid the least relative to their male peers, i.e., the gender wage gap is largest among high-earning whites and Asians.
Women of every race and ethnicity make less than their male counterparts : Women's hourly wages as a percentage of men's hourly wages of the same race, by wages percentile, 2015
Source: EPI analysis of Current Population Survey microdata
When we compare the wages of white women and women of color with wages of white men, white and Asian women fare better than their black and Hispanic counterparts ( Figure F ). White non-Hispanic women are paid 81.0 percent and Asian women 89.8 percent, of what non-Hispanic white men make. But the shares are much lower for black and Hispanic women, at 65.3 percent and 57.6 percent, respectively (CPS ORG 2011–2015).
Black and Hispanic women experience the biggest pay gaps : Women's median hourly wages as a share of white men's and their per hour wage penalties, by race and ethnicity, 2015
Notes: Values displayed above columns represent the difference between women's median hourly wages and median hourly wages of white men.
Source: EPI analysis of Current Population Survey microdata, 2015
In terms of the impact on women’s paychecks, this means that relative to the typical white man, the typical white woman takes home $4.00 less per hour, black women take home $7.31 less per hour, Hispanic women take home $8.91 less per hour, and Asian women take home $2.15 less per hour.
What is the gender wage gap for immigrant women?
Native-born workers of either gender are paid more per hour than (non-naturalized) foreign-born workers ( Figure G ). However, non-naturalized foreign-born women—like their native-born counterparts—experience a wage gap that further reduces their earnings. The typical non-naturalized foreign-born woman is paid 80 cents per dollar of what a foreign-born man is paid ($11.26 as a share of $14.02). Among undocumented Mexican immigrants, the gender wage gap is wider: for every dollar a man is paid, a woman is paid 71 cents (Garcia and Oakford 2013).
Foreign-born women are dually disadvantaged : Median hourly wages by immigration status and gender, 2015
Notes: Includes individuals older than 16. The category native born includes individuals born in the United States, Puerto Rico, and U.S. outlying areas, as well as individuals born abroad of American parents. The category foreign born includes foreign-born individuals who are not citizens of the United States.
So while foreign-born workers overall are disadvantaged in terms of wages, non-naturalized foreign-born women are additionally disadvantaged by the gender wage gap. Compared with native-born men, the average foreign-born woman is paid 58.4 cents on the dollar. Foreign-born naturalized workers not only earn higher wages than their non-naturalized and native-born counterparts, but have a slightly smaller gender wage gap.
Does the gender wage gap get bigger or smaller as women age?
The gender wage gap is quite small for workers in their teens and early 20s, but the gap grows with age ( Figure H ). For typical working men, hourly wages rise until around the age of 45 and then plateau, but for typical working women, hourly wages top off earlier (in the 35 to 44 age range). After around 40, women’s wage growth plateaus and then drops off earlier than men’s. This holds true when measuring the gap using median weekly earnings of full-time wage and salary workers (Hill 2016). The growth in the gender wage gap during this time of life reflects the disproportionate impact of family responsibilities on women’s careers. Other research shows that from the beginning of their working lives, women experience a gender wage gap that is still expected to swell significantly over the course of their careers, regardless of education or work experience (Goldin 2014).
Women's hourly wages plateau and then begin to decline earlier than men's : Median hourly wage gap by age and gender, 2015
How do work experience, schedules, and motherhood affect the gender wage gap.
Women’s experience levels and work schedules do factor into the gender wage gap. Rather than disproving the role of discrimination, work experience, hours, and schedules in part reflect the social expectations that still disadvantage women. These influences all play a role in the “motherhood wage penalty” evident in the data.
Are women paid less because they have less experience?
On average, women have less work experience than men, and this contributes to the gender wage gap. But it would not be correct to conclude that this helps disprove the role of discrimination, because the lack of experience itself is a function of social expectations and norms that disadvantage women in the workplace. Women are more likely to temporarily exit the labor force—most often to raise children, although increasingly to care for an older relative—which leaves them with less work experience. One study of workers with MBAs showed that a year after receiving the degree, only 4 percent of men had experienced a career interruption of six months or more, compared with 9 percent of women (Goldin 2014). Further out from their schooling the gap grows: after 10 years, 10 percent of men had experienced a career interruption, compared with 32 percent of women experiencing a career interruption nine years out. And in the 10 to 16 years following graduation with an MBA, 40 percent of women had experienced a career interruption. (Bertrand, Goldin, and Katz 2009)
Do women’s work schedules affect the gender wage gap?
Women tend to work different hours than men, which affects their earnings. However, the story is different depending on wage level. Women are more likely than men to work low-wage jobs, and low-wage workers are more likely to experience irregular work schedules, such as irregular shift times or on-call shifts, than are other workers (Golden 2015; Davis and Gould 2015). For low-wage parents especially, irregular schedules—often associated with pay that changes from paycheck to paycheck—can be paralyzing as they try to coordinate childcare and meet basic household needs.
Among higher-wage workers, firms tend to disproportionately reward those who work long and particular hours, and those individuals are more likely to be men, which creates a wider wage gap for higher-wage women (Hersch and Stratton 2002; Goldin 2014). But when workers have more temporal flexibility—that is, more choice as to the schedules and number of hours they work—the gender gap narrows. In fact, Goldin (2014) finds that temporal in flexibility is an important contributor to the gender gap. Long, inflexible work schedules tend to weigh disproportionately heavily on women, who are still responsible for more housework and child/elder care than men.
Women are also roughly twice as likely to work part time as men; 24.5 percent of women work part time versus 12.4 percent of men (Golden 2016). The biggest disadvantage part-time workers face is their relatively lower rates of pay and benefits coverage relative to full-time workers. When adjusting for differences in personal, educational, locational, industrial, and occupational characteristics of the workers, women who work part time earn 9 percent less than full-time working women. Disadvantages are compounded when women work part time involuntarily—they are willing and able to work full time but can only obtain part-time work. Women of color are disproportionately involuntarily part time.
What we do know is that, in recent decades, women have been working substantially more hours. Between 1979 and 2012, the median annual hours worked by women increased by 739 hours (Appelbaum, Boushey, and Schmitt 2014). Median annual hours of work by mothers increased even more dramatically, rising 960 hours from 1979 to 2012 (Appelbaum, Boushey, and Schmitt 2014). For mothers and for women overall, all of the increase in work hours took place by 2000 (Appelbaum, Boushey, and Schmitt 2014).
Despite these advances, women still work fewer paid hours than men (OECD 2016).
How does the gender wage gap change after a woman has children?
Research has consistently shown that women with children are paid less than women without children and men with or without children. 11 In short, there does seem to be a motherhood penalty for earnings. Even after researchers control for variables such as education and experience, they find that mothers are paid approximately 4.6 percent less on an hourly basis than women who are not mothers (Budig 2014). Compared with their counterparts 40 years ago, first-time mothers today are older and have more education and work experience; after giving birth, they are less likely to leave the labor force and more likely to return to work quickly (Laughlin 2011). Despite women’s greater experience, education, and attachment to the labor force, the motherhood pay penalty persists (Budig 2014).
Our research on the work hours of parents finds that women with children under the age of 6 work 5.5 hours less per week (13.4 percent fewer weekly hours) than the average working man, while women without children work 4.1 hours less per week (10.1 percent fewer hours) than the average man ( Figure I ).
After the birth of a child, fathers spend more time at the office, whereas mothers spend less : Average weekly hours worked, by gender and household type, 2014
Notes: Sample is limited to prime-age workers (workers age 25–54) with positive average weekly hours worked.
Source: EPI analysis of the March Current Population Survey
Our research also looks at labor force participation, which is generally defined as the share of a given population that is in the labor force (i.e., that is working or looking for work). Because of social norms and home responsibility, women, in general, are less likely to work than men. As shown in Figure J , 71.0 percent of all mothers are in the labor force, as are 73.8 percent of all prime-age women and 88.3 percent of all prime-age men. 12 It’s particularly striking that labor force attachment of parents differs for men and women: fathers are more likely to be in the labor force than are men without children, but mothers are less likely to be in the labor force than are women without children.
Parenthood has opposite effects on mothers' and fathers' labor force participation : Labor force participation by gender and parental status, 2013–2015
Note: Sample limited to people ages 25–54. Children are defined as being less than 18 years old. The labor force participation rate is the percentage of people who either have a job or are actively looking for a job, and are not on active duty in the Armed Forces or living in institutions (such as correctional facilities or nursing homes).
How do education and job and occupational characteristics affect the gender wage gap?
Some have suggested that women could narrow the wage gap if they made different educational or occupational choices. The data suggest it’s not that simple.
Does education level affect the gender wage gap?
One thing the data clearly show is that women have not been able to educate themselves out of the gender wage gap, at least in terms of broad formal credentials. While women are more likely to graduate from college than men, and are more likely to receive a graduate degree than men (Gould and Schieder 2016), at every education level, women are paid less than men ( Figure K ).
Women earn less than men at every education level : Average hourly wages, by gender and education, 2015
Among workers who have not completed high school, women are paid 78.2 percent of what men are paid. Among workers who have a college degree, the share is 75.2 percent; and among workers who have an advanced degree, it is 73.4 percent. Women with advanced degrees still make less per hour than men with college degrees. Even straight out of college, women with a college degree make $4 less per hour than their male peers—a gap that has grown since 2000 (Kroeger, Cooke, and Gould 2016).
Does the choice of college major affect the gender wage gap?
Part of the gender wage gap can be attributed to college major. Women are more likely to major in subjects such as education and the humanities, and these majors are associated with lower-paying jobs after graduation. At the same time, fewer women major in the STEM (science, technology, engineering, and math) subjects, which are associated with the most lucrative jobs (Corbett and Hill 2015).
Although college major doesn’t always determine occupation after graduation, there is a link between major and salary in the workforce. Figure L shows that people with college degrees in majors favored by women are making less 10 years after graduation. For example, engineering majors are paid on average nearly twice as much as education majors 10 years after graduation.
Undergraduate majors favored by women pay less 10 years after graduation : Undergraduate major by gender and salary 10 years after graduation
Notes: Salaries are based on the current or most recent salary of college graduates of the class of 1993 10 years after graduation in 2003. The salaries are then inflated to 2015 dollars using the CPI-U for easier comparison with today's wages. The percentage of graduates who are female by major is based on a survey of college students graduating in 1993 for consistency.
Source: U.S. Department of Education, National Center for Education Statistics, B&B: 93/03 Baccalaureate and Beyond Longitudinal Study
Contrary to what some may believe, educational choices remain gendered today. For example, male seniors graduating in 2008 were more than five times as likely as their female counterparts to have majored in engineering and engineering technology, while women in that same year were three times as likely as their male counterparts to have studied education (NCES 2011–2015).
These choices of college majors, however, should not be seen as completely unconstrained. Women’s experiences before college strongly influence their college trajectories. For example, women arrive in college less interested in STEM fields than their male counterparts. Only 14 percent of first-time college women chose science-related fields in 2012, compared with 39 percent of first-time college men (OECD 2015). Among STEM majors, women are disproportionately in the biological and life sciences, while men dominate engineering and computer science (Corbett and Hill 2015).
How much of the wage gap is due to lower pay in women-dominated occupations versus wage disparities among men and women in the same occupation?
A gender pay gap exists both within and between industries and occupations (Goldin 2014). This means that occupations that have more women in them tend to pay less (the “between occupation” wage gap), and that within each occupation, whether male- or female-dominated, men tend to be paid more than women (the “within-occupation” gap). This within-occupation gap means that even when men and women work in the same occupation—whether as hairdressers, cosmetologists, nurses, teachers, computer engineers, mechanical engineers, or construction workers—men make more, on average, than women (CPS ORG 2011-2015).
Some have argued that the gender wage gap mostly reflects choices women make about career paths—and choices about occupation in particular. But as it turns out the within-occupation gender wage gap plays a larger role in the occupational gender wage gap than the between-occupation wage gap (the fact that both men and women in occupations with higher shares of women are paid less). As a thought experiment, imagine all women are picked out of their jobs and dropped into jobs to mirror how men are distributed throughout the occupational labor market. For example, if 1.22 percent of men are currently software developers, suppose 1.22 percent of women (instead of today’s 0.33 percent of women) became software developers. 13 What would this occupational reassignment of women do to the wage gap? Claudia Goldin imagines this scenario in a 2014 paper (Goldin 2014). After controlling for differences in education and preferences for full-time work, she finds that only 32 percent of the gender pay gap for college graduates would be closed by redistributing women and men across occupations. On the other hand, as much as 68 percent of the gender pay gap by occupation for college graduates is due to the within-occupation gap (Goldin 2014). 14 This means if you left women in their current occupations and just closed the gaps between women and their male counterparts within occupations (e.g., if male and female civil engineers, and male and female teachers, made the same per hour), that would close a whopping 68 percent of the gap.
Furthermore, evidence shows that as women’s participation in a particular occupation rises, pay within that occupation falls (Miller 2016; Oldenziel 1999). Some researchers attribute this phenomenon to “devaluation,” in which employers ascribe a lower value to work done in female-dominated occupations and thus pay them less (Levanon, England, and Allison 2009).
Therefore, changing which occupations women are in will only partially close the gender wage gap. If we want to equalize earnings between men and women, we need to pay as much attention to the fact that women in the same job make less than men as we do to the fact that female-dominated professions pay less.
Has the gender wage gap shrunk as more men and women blaze paths into “nontraditional” occupations?
This is a trick question. From the early 1960s to the 1990s, more men and women moved into “nontraditional” occupations. (An occupation is considered “nontraditional” for a particular gender if that gender constitutes less than 25 percent of employees in the occupation [Carl D. Perkins Career and Technical Education Improvement Act of 2006]). 15 So for example more women found jobs in recreation and more men became nurses (Landivar 2013; Miller 2016). But the movement toward gender integration in occupations slowed down after the 1990s and came to a complete halt during the 2000s (Hegewisch and Hartmann 2014). Gen Xers, who reached their mid-40s mainly in the 2010s, saw an increase in occupational segregation between ages 25 and 45. In fact virtually all cohorts of workers all saw a small increase in occupational segregation in the 2000s and 2010s (Hegewisch and Hartmann 2014).
For all our progress, as of recent years, only about 6 percent of women are employed in nontraditional (i.e., traditionally male) occupations. These same sets of occupations employ 45 percent of all men. At the same time, only about 5 percent of men are in traditionally female occupations, while these occupations employ 40 percent of all female workers.
Figure M shows more simply how gender segregated our occupations still are in the United States. More than 40 percent of workers are in occupations in which more than three-fourths of workers are of one gender.
43 percent of workers are in highly gendered occupations : Percentage of workers in occupations in which more than 75 percent of workers are of a single gender, 2011–2015
Notes: We define gendered-occupations as occupations in which more than 75 percent of workers are of one gender. This definition is based on the definition of "traditional" occupations included in the Carl D. Perkins Vocational and Technical Education Act of 1998 S.250-6. Employment counts are averaged over the time period, 2011-2015.
Source: EPI analysis of Current Population Survey Outgoing Rotation group data
And this segregated distribution of men and women across jobs matters to the gender wage gap. Occupation and industry (taken together) account for about half of the overall gender wage gap (Blau and Kahn 2016).
Finally, it is important to note that the distribution of men and women across occupations is not a simple matter of unconstrained choice. Much research suggests that many women are driven out of nontraditional occupations by hostile work environments. For example, 63 percent of women working in science, engineering, and technology experience sexual harassment (Hewlett et al. 2008). Over time, 52 percent of women in science, engineering, and technology quit their jobs, half of whom end up leaving these fields altogether (Hewlett et al. 2008).
Does union membership close the gender pay gap?
Unions not only raise wages for male and female workers alike, but also reduce the size of the gender wage gap. Women in unions are paid 31 percent more than their nonunionized sisters. Among racial and ethnic subgroups, black, Hispanic, and white women in unions make 34, 42, and 31 percent more than their nonunion counterparts (Anderson, Hegewisch, and Hayes 2015). 16 Unionization raises women’s wages by 11.2 percent, compared with nonunion women who have similar characteristics (Schmitt 2008).
Women in unions also experience a smaller gender pay gap than their nonunionized counterparts ( Figure N ). Women workers in unions are paid 88.7 percent of what their male counterparts are paid, while for nonunionized women the share is 81.8 percent (Anderson, Hegewisch, and Hayes 2015).
Women generally experience a smaller pay gap when their workplace is unionized : Women's median weekly earnings for full-time wage and salary employees as a percent of men's, by race and ethnicity, 2014
Notes: The values represent the difference between the median weekly earnings of full-time wage and salary workers who are union members or are covered by a union contract and those who are not.
Source: EPI analysis of Anderson, Hegewisch, and Hayes, 2015
Does the gender wage gap depend on where you live?
Yes. The gender wage gap varies widely by state. The gender wage gap, as measured by women’s share of men’s hourly wages at the median, ranges from 74.8 percent (in Wyoming) to 92.9 percent (in Washington, D.C.; Figure O ) . Typical female workers in Washington, D.C., and Vermont make more than 90 percent of the wages of their male counterparts. In nine states, women are paid less than 80 percent of their male counterparts’ wages. Similarly, the gender gap in annual earnings ranges from 65.3 percent in Louisiana to 89.5 percent in Washington, D.C. (NWLC 2015).
The difference between men's and women's pay varies greatly by state : Median hourly women's wages as a share of men's by state, 2013–2015
Notes: Values represent averages 2013-2015.
A number of factors may be contributing to these differences, such as the mix of predominant industries or cultural differences. For example, after holding other factors constant, states with a higher score of “religiosity”—including higher frequency of prayer, worship service attendance, and expressed belief in prayer among other measures—experience a wider gender wage gap (Wiseman and Dutta 2016). According to the researchers, the reason for this is that religiosity is often associated with more traditional views about gender roles.
The raw gender wage gap is larger in rural areas than in urban areas . In metropolitan areas, the gender gap in median hourly wages is 83.2 percent, while in nonmetropolitan areas, it is 81.7 percent.
The gender pay gap in the United States is bigger than the gap in many other developed countries. The gender pay gap in the United States is larger than the Organization for Economic Cooperation and Development (OECD) average when considering the difference between the wages of full-time annual median male and female wages. Within the OECD, the United States has the 12th largest gender gap overall, and the U.S. gap is bigger than the gap in most European countries. That said, making direct international comparisons is often difficult. For example, part-time work by one parent is more common in Europe, as is substantial use of parental leave and paid vacations, while single parenthood is more common in the United States (Ruhm 2011).
A common thread in these data is that the burden of parenthood is distributed differently in various countries. This means that policies meant to address the motherhood penalty likely need to be tailored differently across these countries as well. For example, the availability of parental leave might make a woman in Europe less likely to leave her employer following her pregnancy, whereas in the United States, taking any significant amount of time off at all following childbirth might lead to her losing her job. On the other hand, in many of the OECD countries, women are less likely to work full time and less likely to attain high-level positions than are women in the United States, suggesting that flexibility comes at a cost (Blau and Kahn 2013a).
There is another way in which geography might affect the gender wage gap. Women are more willing to move for a husband’s employment than vice versa (Abraham, Auspurg, and Hinz 2010). This suggests that women are less able to widen the geographic net over which to search for good job opportunities.
How might the gender wage gap affect the retirement security of America’s working women?
It is hard to isolate the effect of the gender wage gap on American women’s retirement security. According to the U.S. Department of Labor, women’s lower lifetime earnings means that they receive lower Social Security payments and experience fewer opportunities to save for retirement. Average annual Social Security benefits for women are only $13,392, and the annual median income in retirement for women is only $14,000, well below the $19,000 to $29,000 that a single person needs to live in retirement, depending on geographic area (DOL 2015).
That may be a key reason why elderly women are more likely than elderly men to be economically vulnerable (defined as earning less than twice what they would need to earn to be above the supplemental poverty measure). As shown in Figure P, over half (52.5 percent) of American women age 65 or older are economically vulnerable, compared with 41.9 percent of same-aged men.
Elderly women are more likely than elderly men to be economically vulnerable : Share of the elderly at various income levels, expressed as multiples of the supplemental poverty measure (SPM) threshold, by gender
Source: EPI analysis of pooled 2010–2012 Current Population Survey Annual Social and Economic Supplement microdata
But the gender wage gap is not the only factor that contributes to women’s lower lifetime earnings. Women’s caregiving responsibilities often push them into working fewer hours, and working part time often limits opportunities for advancement. Women’s time out of the workforce translates into lower earnings and can often erode women’s early and mid-life savings. Further, caregiving costs women $274,044 ($142,693 in lost wages and $131,351 in lost Social Security) over their lifetime, plus an additional $50,000 in lost pension income (DOL 2015).
In addition to their lower Social Security and retirement earnings, older women also have limited opportunities to earn money in the labor force. Not only is the gender pay gap highest among workers age 55 to 64, but recent studies suggest that women face “robust” age discrimination in the labor market, and that age discrimination against women is worse than it is for men (Neumark, Burn, and Button 2015; Farber, Silverman, and von Wachter 2015). Since working longer later in life yields less than it would for a man (DOL 2015), this leaves less room for women to catch up on retirement savings. It also means that when older women are given a choice between staying home to care for family or staying in the workplace, the latter option is relatively less advantageous. In a recent survey, one-fifth of all women ages 45 to 74 reported that they had taken time off work within the past five years to act as caregivers (DOL 2015). Older women’s caregiving responsibilities extend not just to their children but also to their parents. About 9.7 million Americans over age 50 care for their parents, and women are the majority of the caregivers.
The labor force participation rate of older women has grown in the past two decades, but it is still lower than older men’s (DOL 2015). In 2012, 35.1 percent of women ages 55 and older were in the labor force, compared with 46.8 percent of their male counterparts (DOL 2015). In 1992, those figures for older women and older men were 22.8 percent and 38.4 percent, respectively (DOL 2015).
What role do “unobservables” like discrimination and productivity play in the wage gap?
The unexplained, or residual, portion of the pay gap is the difference in pay between men and women who are observationally identical. It is what is left when researchers control for all observable characteristics. It is due to factors that are otherwise difficult to measure—which could include not only discrimination but also differences in productivity that are unrelated to influences such as educational level and experience. What can the size and trajectory of this residual gap tell us about the scope of discrimination in the workplace?
Is discrimination an expanding or shrinking factor in gender wage gaps?
Even when researchers control for all observable characteristics, a portion of the gender wage gap is left unexplained. Economists often argue that this unexplained portion, while not synonymous with discrimination, may tell us how much gender discrimination could be affecting wages.
By this measure, discrimination is either stable or increasing. In a 2016 study, economists Francine Blau and Lawrence Kahn found that the unexplained portion of the gender wage gap narrowed dramatically in the 1980s, shrinking from between 21 and 29 percent of the gap in 1980 to between 8 and 18 percent of the gap in 1989. However, after 1989, the unexplained portion of the gap did not narrow any further, and it has remained stable ever since.
In a 2014 study, economist Claudia Goldin found that the unexplained, or “residual,” gap makes up more of the gap today than it did in the 1980s. Women today have more education and work experience, which has whittled away the influence of those factors on the gap. Human capital factors such as education and experience made up about 25 percent of the wage gap in 1979, but only 8 percent in 1998.
This residual gap is not uniform across occupations. Goldin argues that some professions disproportionately reward those who work very long hours, and this might explain why she finds a larger residual gap in business occupations than in science and technology fields. Also some high-wage firms have adopted pay-setting practices that disproportionately reward individuals who work very long and very particular hours, including weekends or late nights. This means that—even if men and women are equally productive per hour—individuals in these firms who are more likely to work a very high number of weekly hours and be available at particular off hours are paid more. This reward of long and nonstandard hours for highly credentialed employees works to men’s advantage (Hersch and Stratton 2002; Goldin 2014).
But expansion or contraction of the residual gap does not mean that discrimination is expanding or contracting to the same degree because the residual wage gap only captures discrimination in pay-setting between similar workers. It does not capture the range of factors that influence the different labor market experience of men and women before employers make hourly pay offers, and discrimination—in the form of society-wide constraints on choices—can certainly enter into these factors. For example, women’s choice of college major or occupation is conditioned on how well educated in science and math they were in college and even before. If gender differences in teachers’ attention or perception of academic excellence influence these choices about college major and occupation, then it will affect pay outcomes. Therefore, controlling for current occupation disguises how discrimination can filter men and women differently into high- or low-paying occupations.
While we can’t precisely measure how big the role of discrimination is, or set a ceiling on its impact, we do know that it exists. Empirical evidence of outright discrimination in hiring, promotions, and even wage-setting is strong and includes the following:
- One famous study found that switching to blind auditions led to a significantly higher proportion of female musicians in orchestras (Goldin and Rouse 1997).
- An experimental study of résumés submitted for job openings found bias against women and mothers and a preference for male applicants (Steinpreis, Anders, and Ritzke 1999). Another résumé study showed discrimination against women in the sciences (Moss-Racusin et al. 2012).
- Researchers have also found that women are viewed as less competent than men, and that mothers are judged as even less competent than childless women (Ridgeway and Correll 2004).
- In her book, Selling Women Short: The Landmark Battle for Workers’ Rights at Wal-Mart (2004), Liza Featherstone reported that “women make up 72 percent of Wal-Mart’s hourly workforce (nonsalaried workers), but only 34 percent of its managers are women. Women also earn less than their male counterparts in nearly every position at the company.”
Is the gender gap a result of men being “better” or more productive workers than women?
As noted, the unexplained, or residual, portion of the pay gap is the difference in pay between men and women who are observationally identical. Some argue that one of the difficult-to-measure factors is differences in productivity that are unrelated to influences such as educational level and experience. Some argue that women’s disproportionate childcare responsibilities may make them less productive.
Studies that have directly explored worker productivity show little evidence of a motherhood penalty on productivity. Recent research by the Federal Reserve Bank of St. Louis that examined productivity among academic economists found that, over the course of a career, women with children were more productive than women without children (Krapf, Ursprung, and Zimmerman 2014). Additionally, women with two children were more productive than women with one child. Another study of blue-collar workers, a group chosen because of the belief that there would likely be productivity differences by gender, found that women were generally as productive as men (Petersen, Snartland, and Milgrom 2006).
In fact, research on impressions of women in the workplace suggests women’s productivity might in fact be systematically underestimated (Burgess 2013). Researchers have noted that women are caught in a paradox between appearing too feminine (not qualified) and not feminine enough (lacking in social skills), which often causes their performance to be evaluated much more strictly than men’s (Burgess 2013). The same study found that mothers were seen as less competent than childless women (Burgess 2013). For men, parenthood status had no effect on their perceived competency.
Another study found both men and women were conflicted by the notion that they should put work before family and other personal affairs (Reid 2015). Women, however, were much less likely to be perceived as putting work first.
Framing the question of pay fairness (as this question does) implies that men’s pay is very closely aligned with their productivity. But in fact, for decades, the wages of the vast majority of both men and women have not kept pace with economy-wide productivity as productivity continued to increase but wages largely stagnated. This contrasts with the decades before about 1980, when wage growth and productivity growth were closely linked.
If wages had continued to grow with productivity, the vast majority of both women and men would be better off today ( Figure Q ). For example, Davis and Gould (2015) have shown that had the gender wage gap closed and had wages grown with productivity since 1979, the median woman’s wages would be nearly 70 percent higher today.
Eliminating the gender and inequality wage gap could raise women’s wages by 69% : Median hourly wages for men and women, compared with wages for all workers had they increased in tandem with productivity, 1979–2015
Source: Reproduced from Figure G in Alyssa Davis and Elise Gould, Closing the Pay Gap and Beyond: A Comprehensive Strategy for Improving Economic Security for Women and Families , EPI Briefing Paper #412, November 18, 2015
EPI analysis of unpublished Total Economy Productivity data from Bureau of Labor Statistics Labor Productivity and Costs program, wage data from the Current Population Survey Outgoing Rotation Group
How might discrimination—in the form of norms and expectations—be affecting the wage gap by constraining women’s choices?
Women do indeed make choices, but those choices do not occur in a vacuum. Our society’s institutions and norms exert a powerful influence on what choices are available and what form they take.
How well do grade school test scores measure aptitude?
One study found that parents are more likely to expect their sons, rather than their daughters, to work in STEM fields, even when their daughters performed at the same level in mathematics (OECD 2015). This suggests that cultural norms influence girls’ confidence which in turn influences their success (Herbert and Stipek 2005).
Though girls are underrepresented among students with the highest math test scores, research shows that this gap differs geographically. In areas where people were more likely to say “women [are] better suited for home” and “math is for boys,” girls were more likely to have lower math scores and higher scores on reading tests (Pope and Syndor 2010). In the same states where girls had stereotypically gender-normative test scores, boys scored higher in math than girls but also lower in reading. More evidence that children’s disparate test scores may be the result of cultural factors, not innate differences, is found in the fact that in some states girls scored better at subjects in which cultural cues might have suggested they should be more gifted, and the same was true for boys.
Other research shows that gender bias among teachers negatively affects girls, with the worst effects for girls in less well-off families and girls whose fathers have more years of schooling than their mothers (Lavy and Sands 2015).
Cultural attitudes also affect girls’ confidence, which in turn affects their math performance (OECD 2015). One study found that girls are more likely to express feelings of anxiety over mathematics, and on average their math scores were lower. But among girls who reported similar levels of confidence as boys, the gender gap in performance disappeared (OECD 2015).
Cultural stereotypes appear to have a direct impact on academic performance (OECD 2015). Asians, for example, are stereotyped as being good at math. When Asian girls were told they were taking a quantitative skills test to assess ethnic differences in performance, they scored higher than a control group, which was given no explanation for why they were taking the test. By contrast, Asian girls scored worse when they were told they were taking a quantitative assessment to determine gender differences.
How does the cultural steering of girls away from math and science affect occupational choice?
In college, girls are less likely to major in STEM subjects than men and are less likely to major in STEM than in other subjects. Yet STEM majors are associated with the highest earnings. But even though they are not studying the subjects that lead to the most lucrative jobs, women’s level of education continues to increase. Today, women earn more than half of all associate degrees, bachelor’s degrees, master’s degrees, and Ph.D.s (although in this last category, they make up only 51 percent of recipients).
One obstacle to increasing women’s share of employment in lucrative fields is the attrition rate of highly qualified women working in science, engineering, and technology (SET) fields. One study found that as many as half of highly qualified female SET professionals left their jobs because of hostile work environments and job pressures at odds with traditionally gendered domestic roles (Hewlett et al. 2008). Yet the gender wage gap persists even among recent graduates (Gould and Kroeger 2016).
Do work scheduling practices disadvantage women?
In some fields—particularly among highly credentialed workers in very well-paid occupations—employees are disproportionately rewarded for working very long hours and/or at inconvenient times, with short notice. There seems to be little compelling evidence that this reflects smart economic thinking by employers. For example, productivity suffers for employees in medical fields who work long hours (Lockley et al. 2007). Yet these practices persist and affect women. As noted earlier, women in high-wage professions experience a wider gender gap because they are penalized for not working long, inflexible hours. Such rigorous work schedules tend to weigh disproportionately heavily on women, who are still responsible for more housework and child/elder care than men.
But in the United States and around the world, when unpaid work is accounted for, women do more work than men, reflecting again the social expectation that women disproportionately undertake nonmarket work. This trend holds even for children: Although girls spend more time doing chores than boys, they are less likely than boys to be paid an allowance (University of Michigan 2007).
Does sex segregation in occupations affect women’s salary expectations?
Sex segregation in occupations is a reality; women dominate some occupations, just as men dominated others. However, when women enter male-dominated occupations, they have similar or lower expected wages than their female counterparts who go into female-dominated occupations (Pitts 2002). This suggests that when women enter female-dominated occupations, they are rationally situating themselves to be paid higher wages once discrimination is taken into account . Another study (Hwang and Polachek 2004) found that women “choose female jobs to earn a relatively greater amenity package than they would have received elsewhere. Similarly, men choose male jobs to earn relatively more.”
How do family and child-rearing roles affect women’s choices?
It is often suggested that women who are planning to have children seek out “mother-friendly” occupations, sacrificing higher pay for work environments that are more conducive to balancing professional and family responsibilities whether because they are lower stress or offer greater flexibility. But Budig and England (2001) find little support in the data for this. They find motherhood does not impact mothers’ pay through the types of jobs women with children choose (except when it comes to choosing part-time jobs, which does partially account for the motherhood penalty). Instead, they find that it is mothers’ breaks in employment, as opposed to the jobs they take, that lead to a discrepancy in pay between mothers and women without children.
Goldin (2014) argues that women’s labor market choices are strongly conditioned by social norms and expectations regarding who bears the burden of domestic work as well as employer indifference toward this burden. “The observed patterns of decreased labor supply and earnings substantially reflect women’s choices given family constraints and the inflexibility of work schedules in many corporate and finance sector jobs, ” the report explains.
Finally, the perception that women with children choose to work less is often false. Instead, mothers in the workplace are simply judged more harshly in regard to their employer commitment than women without children. Correll, Benard, and Paik (2011) find that mothers are seen as less committed to the workplace than women without children in comparable jobs. For men, it’s the opposite: fathers are seen as more committed than childless men.
Do salary and incentive pay setting practices affect the gender wage gap?
Gender differences in salary negotiation explain a portion of the gender gap. Men are more likely to negotiate their salary, which increases their earnings (Babcock and Laschever 2007). However, men and women face different social incentives for negotiation, and there is evidence that women are more likely to be penalized when they negotiate (Bowles, Babcock, and Lei 2006). The constraints on negotiation clearly have an impact: women who consistently negotiate their salary are paid over $1 million more across their lifetime than women who do not negotiate (Babcock and Laschever 2007).
Evidence also shows that men benefit disproportionately from incentive pay (Albanesi, Olivetti, and Prados 2015). Female executives receive a lower share of incentive pay relative to their male counterparts, and this difference accounts for 93 percent of the gender gap in total pay (Albanesi, Olivetti, and Prados 2015). Performance pay also disproportionately rewards male executives. Researchers found that every $1 million increase in firm value generates a $17,150 increase in firm-specific wealth for male executives, but only a $1,670 increase for their female counterparts (Albanesi, Olivetti, and Prados 2015). This research suggests that women are hurt by incentive pay at the top of the earnings spectrum in two ways: (1) women are less likely to be rewarded using incentive pay when they are in high-ranking managerial positions, and (2) they are less likely to reach those commanding heights of the economy where they would receive more of their pay through an incentive-based structure.
Is there a gender gap in other forms of worker compensation, such as health insurance, paid sick leave, and retirement benefits?
Only 60 percent of men and 62 percent of women have access to paid sick days (Williams and Gault 2014). Although there doesn’t appear to be an overall gender gap in paid sick time, Hispanic women are less likely than men to have access to paid sick time; 49 percent of Latinas lack such a benefit. Two disproportionately female groups, low-wage workers and part-time workers, are also less likely to have paid sick leave than their higher wage and full-time counterparts (BLS 2015; Figure R).
Low-wage workers are less likely to have access to paid sick days : Percent of private industry workers with access to paid sick days, by wage group, 2016
Source: Bureau of Labor Statistics' National Compensation Survey--Employee Benefits in the United States, July 2016 (Table 6)
Women are less likely than men to receive health insurance through their own job. In 2015, 34 percent of women had employer-provided health insurance, compared with 43 percent of men ( KFF 2016 ).
However, men’s and women’s overall participation rates in employer retirement plans are about the same, despite the fact that, as of 2012, women were slightly more likely than men to work for employers that offered retirement plans. The equal participation rate is due to a gap in eligibility that limits women’s participation (Brown et al.).
But equal participation does not mean equal retirement security. Because of their care responsibilities, women are more likely to move in and out of the workforce. This weakens their earnings power, and as a result, women have less retirement wealth than men, both in traditional pensions and employer savings accounts such as 401(k)s. In 2010, women’s income from defined-benefit employer pensions was about 33 percent less than men’s (Brown et al.). And an analysis of 3 million participants in money manager Vanguard’s fund showed that the median amount accumulated in defined-contribution retirement accounts (i.e., 401(k)s and the like) was 34 percent less for women than for men (Brown et al.).
Women age 65 and older are 80 percent more likely than their male counterparts to be living in poverty (Brown et al.). And widowed women are twice as likely as widowed men to be living below the poverty line (Brown et al.).
Acknowledgments
This publication was made possible by a grant from the Peter G. Peterson Foundation. The statements made and views expressed are solely the responsibility of the authors. The authors would also like to acknowledge the tireless work of Jin Dai, data programmer, and overall guidance of Josh Bivens, research director.
About the authors
Elise Gould , senior economist, joined EPI in 2003. Her research areas include wages, poverty, economic mobility, and health care. She is a co-author of The State of Working America, 12th Edition . In the past, she has authored a chapter on health in The State of Working America 2008/09 ; co-authored a book on health insurance coverage in retirement; published in venues such as The Chronicle of Higher Education , Challenge Magazine , and Tax Notes ; and written for academic journals including Health Economics , Health Affairs , Journal of Aging and Social Policy , Risk Management & Insurance Review , Environmental Health Perspectives , and International Journal of Health Services . She holds a master’s in public affairs from the University of Texas at Austin and a Ph.D. in economics from the University of Wisconsin at Madison.
Jessica Schieder joined EPI in 2015. As a research assistant, she supports the research of EPI’s economists on topics such as the labor market, wage trends, executive compensation, and inequality. Prior to joining EPI, Jessica worked at the Center for Effective Government (formerly OMB Watch) as a revenue and spending policies analyst, where she examined how budget and tax policy decisions impact working families. She holds a bachelor’s degree in international political economy from Georgetown University.
Kathleen Geier is a Chicago-based writer and researcher. She has written for The Nation , The New Republic , The Baffler , Washington Monthly , and other publications.
1. Wages here refers to the hourly wages of all wage and salary workers between 18 and 64 years old. Throughout we use wage gap and pay gap interchangeably to refer to the wage gap.
2. The typical woman (or man) referred to here and throughout is the median female (or male) worker.
3. Unless otherwise specified, the EPI analyses throughout this piece use data on hourly wages of all workers, not just full-time workers. Technically, this is an adjusted gender wage gap measure because the weekly or annual gender wage gap would allow hours of work to differ. An hourly wage gap will not capture the direct effect of differences in hours or weeks worked, but it will capture the indirect effect of wage differences due to the effect of hours on hourly wages. This limited adjustment allows us to compare women’s and men’s wages without assuming that women, who still shoulder a disproportionate amount of responsibilities at home, would be able or willing to work as many hours as their male counterparts. Examining this “raw” gap, we hope to have a more thorough conversation about the ways a large basket of factors interact and create the wage gap women experience when they cash their paychecks. Of course, our answers to questions about the wage gap also draw on the work of other researchers, who may use different measures. Claudia Goldin for example uses earnings of full-time full-year workers.
4. While there is no fatherhood penalty in the data, there is evidence that fathers who take leave are punished as well (Bertrand, Goldin, and Katz 2009).
5. The median is the value you get if you take a set of numbers, arrange them from highest to lowest, and choose the number that is exactly in the middle. Technically, the median hourly wage is an adjusted gender wage gap measure because the weekly or annual gender wage gap would allow hours of work to differ. For details on the methodology used, see the “Documentation” section of the Economic Policy Institute’s State of Working America Data Library (epi.org/data/).
6. The regression-based gap is based on average wages and controls for gender, race and ethnicity, education, experience, and geographic division. The log of the hourly wage is the dependent variable.
7. Economic Policy Institute (EPI) analysis of CPS ORG hourly wage data for workers age 18 to 64 using a simple weighted regression model with only a gender control variable.
8. Here education is measured on a mutually exclusive five-point scale: workers who have less than a high school diploma, those who have completed high school but no further schooling, those who have some college experience but have not earned a college degree, those who have earned a college degree, and those with advanced degrees.
9. Here we add in controls for major industry category, detailed occupation (four digit), and full-time status.
10. Blau and Kahn’s modified model includes controls for education, experience, race/ethnicity, region, and metropolitan area residence. Their more fully specified model adds in a series of industry, occupation, and union coverage dummy variables.
11. For our purposes, parents are those with children under age 18.
12. Women and men are limited in these comparisons to individuals between ages 25 and 54. Children are defined as under age 18.
13. EPI analysis of CPS ORG data by 532 occupation categories averaged 2011–2015.
14. Using female weights gives a lower share of 58 percent. Using female weights would mean you would move men out of their occupations.
15. Nontraditional occupations are defined by the Carl D. Perkins Career and Technical Education Improvement Act of 2006 (as well as preceding legislation) as “occupations or fields of work, including careers in computer science, technology, and other current and emerging high skill occupations, for which individuals from one gender comprise less than 25 percent of the individuals employed in each such occupation or field of work.”
16. Median weekly earnings for full-time wage and salary workers.
Abraham, Martin, Katrin Auspurg, and Thomas Hinz. 2010. “Migration Decisions within Dual-Earner Partnerships: A Test of Bargaining Theory.” Journal of Marriage and Family, vol. 72, no. 4, 876–892.
Albanesi, Stefania, Claudia Olivetti, and Maria José Prados. 2015. Gender and Dynamic Agency: Theory and Evidence on the Compensation of Top Executives . Federal Reserve Bank of New York Staff Reports, no. 718.
Anderson, Julie, Ariane Hegewisch, and Jeff Hayes. 2015. The Union Advantage for Women . Institute for Women’s Policy Research.
Appelbaum, Eileen, Heather Boushey, and John Schmitt. 2014. The Economic Importance of Women’s Rising Hours of Work: Time to Update Employment Standards . Center for American Progress and the Center for Economic and Policy Research.
Arons, Jessica. 2008. Lifetime Losses: The Career Wage Gap . Center for American Progress.
Babcock, Linda, and Sara Laschever. 2007. Women Don’t Ask: The High Cost of Avoiding Negotiation – And Positive Strategies for Change . New York, NY: Bantam Dell.
Bertrand, Marianne, Claudia Goldin, and Lawrence Katz. 2009. Dynamics of the Gender Gap for Young Professionals in the Corporate and Financial Sectors . National Bureau of Economic Research Working Paper No. 14681.
Blau, Francine D., and Lawrence M. Kahn. 2013. “ Female Labor Supply: Why Is the US Falling Behind? ” American Economic Review , vol. 103, no. 3, 251–256.
Blau, Francine D., and Lawrence M. Kahn. 2016. The Gender Wage Gap: Extent, Trends, and Explanations . National Bureau of Economic Research, Working Paper No. 21913.
Bowles, Hannah Riley, Linda Babcock, and Lei Lai. 2006. “ Social Incentives for Gender Differences in the Propensity to Initiate Negotiations: Sometimes It Does Hurt to Ask. ” Organizational Behavior and Human Decision Processes, vol. 103, 84–103.
Brown, Jennifer Erin, Nari Rhee, Joelle Saad-Lessler, and Diane Oakley. 2016. Shortchanged in Retirement: Continuing Challenges to Women’s Financial Future . National Institute for Retirement Security.
Budig, Michelle J. 2014. The Fatherhood Bonus and the Motherhood Penalty: Parenthood and the Gender Gap in Pay . Third Way Next.
Budig, Michelle J., and Paula England. 2001. “ The Wage Penalty for Motherhood .” American Sociological Review , vol. 66, 204–225.
Bureau of Labor Statistics (BLS). 2015. American Time Use Survey Public Data Series . U.S. Census Bureau.
Burgess, Noël. 2013. The Motherhood Penalty: How Gender and Parental Status Influence Judgments of Job-Related Competence and Organizational Commitment . University of Rhode Island.
Carl D. Perkins Career and Technical Education Improvement Act of 2006 . 2006. 20 U.S.C. 2301 et seq.
Corbett, Christianne, and Catherine Hill. 2015. Solving the Equation: The Variables for Women’s Success in Engineering and Computing . American Association of University Women.
Correll, Shelley J., Stephen Benard, and In Paik. 2011. “ Getting a Job: Is There a Motherhood Penalty? ” American Journal of Sociology , vol. 112, no. 5, 1297–1339.
Current Population Survey Outgoing Rotation Group microdata (CPS ORG). 2011–2015. Survey conducted by the Bureau of the Census for the Bureau of Labor Statistics [ machine-readable microdata file ]. Washington, D.C.: U.S. Census Bureau.
Davis, Alyssa, and Elise Gould. 2015. Closing the Pay Gap and Beyond: A Comprehensive Strategy for Improving Economic Security for Women and Families . Economic Policy Institute, Briefing Paper No. 412.
Economic Policy Institute. 2016. State of Working America Data Library .
Farber, Henry S., Dan Silverman, and Till von Wachter. 2015. Factors Determining Callbacks to Job Applications by the Unemployed: An Audit Study . National Bureau of Economic Research Working Paper No. 21689.
Featherstone, Liza. 2004. Selling Women Short: The Landmark Battle for Workers’ Rights at Wal-Mart . New York: Basic Books.
Garcia, Ann, and Patrick Oakford. 2013. Unequal Pay Day for Immigrant Women . Center for American Progress.
Golden, Lonnie. 2015. Irregular Work Scheduling and Its Consequences . Economic Policy Institute Briefing Paper No. 394.
Golden, Lonnie. 2016 (forthcoming). Still Falling Short: The Persistence of Involuntary Part-time Work in the US Economy—Trends, Sources, Consequences, and Solutions (Working title). Economic Policy Institute report.
Goldin, Claudia. 2014. “ A Grand Gender Convergence: Its Last Chapter .” American Economic Review, vol. 104, no. 4, 1091–1119.
Goldin, Claudia, and Cecilia Rouse. 1997. Orchestrating Impartiality: The Impact of “Blind” Auditions on Female Musicians . National Bureau of Research Working Paper No. 5903.
Gould, Elise, and Teresa Kroeger. 2016. “ Straight Out of College, Women Make $4 Less per Hour than Men—and the Gap Is Getting Wider .” Working Economics (Economic Policy Institute blog), April 3.
Gould, Elise, and Jessica Schieder. 2016. “Women’s Work” and the Gender Pay Gap: How Discrimination, Societal Norms, and Other Forces Affect Women’s Occupational Choices—and Their Pay . Economic Policy Institute.
Hegewisch, Ariane, and Asha DuMonthier. 2016. The Gender Wage Gap: 2015; Earnings Differences by Race and Ethnicity . Institute for Women’s Policy Research.
Hegewisch, Ariane, and Heidi Hartmann. 2014. Occupational Segregation and the Gender Wage Gap: A Job Half Done . Institute for Women’s Policy Research.
Herbert, Jennifer, and Deborah Stipek. 2005. “The Emergence of Gender Difference in Children’s Perceptions of Their Academic Competence.” Journal of Applied Developmental Psychology , vol. 26, no. 3, 276–295.
Hersch, Joni, and Leslie S. Stratton. 2002. “ Housework and Wages .” The Journal of Human Resources , vol. 37, no. 1, 217–229.
Hewlett, Sylvia Ann, Carolyn Buck Luce, Lisa J. Servon, Laura Sherbin, Peggy Shiller, Eytan Sosnovich, and Karen Sumberg. 2008. The Athena Factor: Reversing the Brain Drain in Science, Engineering, and Technology . Harvard Business Review.
Hill, Catherine. 2016. The Simple Truth about the Gender Pay Gap (Spring 2016) . Association of American University Women.
Hwang, Soo Kyeong, and Solomon William Polachek. 2004. Occupational Self-Selection and the Gender Wage Gap: Evidence From Korea and United States . Binghamton University.
Institute for Women’s Policy Research (IWPR). 2016. Status of Women in the States (online database). Accessed October 2016.
The Henry J. Kaiser Family Foundation (KFF). 2016. Women’s Health Insurance Coverage .
Krapf, Mattihas, Heinrich W. Ursprung, and Christian Zimmerman. 2014. Parenthood and Productivity of Highly Skilled Labor: Evidence from the Groves of Academe . Institute for the Study of Labor (IZA) Discussion Paper No. 7904.
Kroeger, Teresa, Tanyell Cooke, and Elise Gould. 2016. The Class of 2016: The Labor Market Is Still Far from Ideal for Young Graduates . Economic Policy Institute.
Landivar, Liana Christin. 2013. Men in Nursing Occupations: American Community Survey Highlight Report . U.S. Census Bureau.
Laughlin, Lynda, 2011. Maternity Leave and Employment Patterns: 2006–2008 . Current Population Report, P70-128. U.S. Census Bureau, Washington, DC.
Lavy, Victor, and Edith Sands. 2015. On the Origins of Gender Human Capital Gaps: Short and Long Term Consequences of Teachers’ Stereotypical Biases . Warwick Economics Research Paper Series No. 1085.
Levanon, Asaf, Paula England, and Paul Allison. 2009. “Occupational Feminization and Pay: Assessing Causal Dynamics Using 1950-2000 U.S. Census Data.” Social Forces, vol. 88, no. 2, 865–892.
Lockley, Steven W., Laura K. Barger, Najib T. Ayas, Jeffrey M. Rothschild, Charles A. Czeisler, and Christopher P. Landrigan. 2007. “ Effects of Health Care Provider Work Hours and Sleep Deprivation on Safety and Performance .” The Joint Commission Journal on Quality and Patient Safety , vol. 33, no. 11, supplement.
Miller, Claire Cain. 2016. “ As Women Take Over a Male-Dominated Field, the Pay Drops .” New York Times , March 18.
Moss-Racusin, Corinne A., John F. Dovidio, Victoria L. Brescoll, Mark J. Graham, and Jo Handelsman. 2012. “ Science Faculty’s Subtle Gender Biases Favor Male Students .” Proceedings of the National Academy of Sciences of the United States of America, 2012 vol. 109, no. 41, 16474–16479.
National Center for Education Statistics (NCES). 2011–2015. B&B: 93/03 Baccalaureate and Beyond Longitudinal Study. U.S. Department of Education. Computation by NCES PowerStats on February 26, 2016.
National Women’s Law Center (NWLC). 2015. “ The Wage Gap by State for Women Overall 2014 .”
Neumark, David, Ian Burn, and Patrick Button. 2015. Is It Harder for Older Workers to Find Jobs? New and Improved Evidence from a Field Experiment . National Bureau for Economic Research Working Paper No. 21669.
Oldenziel, Ruth. 1999. Making Technology Masculine: Men, Women, and Modern Machines in America, 1870-1945 . Amsterdam: Amsterdam University Press.
Organisation for Economic Co-operation and Development (OECD). 2015. The ABC of Gender Equality in Education: Aptitude, Behavior, Confidence .
Organisation for Economic Co-operation and Development (OECD). 2016. Employment: Time Spent in Paid and Unpaid Work, by Sex .
Petersen, Trond, Vermund Snartland, and Eva M. Meyersson Milgrom. 2006. Are Female Workers Less Productive Than Male Workers? Institute for Research on Labor and Employment (IRLE) Working Paper 136-06.
Pitts, Melissa M. 2002. Why Choose Women’s Work If It Pays Less? A Structural Model of Occupational Choice. Federal Reserve Bank of Atlanta, Working Paper 2002-30.
Pope, Devin G., and Justin R. Sydnor. 2010. “ Geographic Variation in the Gender Differences in Test Scores .” Journal of Economic Perspectives , vol. 24, no. 2, 95–108.
Reid, Erin. 2015. “ Embracing, Passing, Revealing, and the Ideal Worker Image: How People Navigate Expected and Experienced Professional Identities .” Organization Science , vol. 26, no. 4, 997–1017.
Ridgeway, Cecilia L., and Shelley J. Correll. 2004. “ Unpacking the Gender System: A Theoretical Perspective on Gender Beliefs and Social Relations .” Gender and Society, vol. 18, 510.
Ruhm, Christopher J. 2011. “ Policies to Assist Parents with Young Children .” The Future of Children/Center for the Future of Children, the David and Lucile Packard Foundation, vol. 21, no. 2, 37–68.
Schmitt, John. 2008. The Union Advantage for Low-Wage Workers . Center for Economic and Policy Research.
Steinpreis, Rhea E., Katie A. Anders, and Dawn Ritzke. 1999. “ The Impact of Gender on the Review of the Curricula Vitae of Job Applicants and Tenure Candidates: A National Empirical Study .” Sex Roles , vol. 41, nos. 7-8, 509–528.
Williams, Claudia, and Barbara Gault. 2014. Institute for Women’s Policy Research (IWPR). Paid Sick Days Access in the U.S.: Differences by Race/Ethnicity, Occupation, Earnings, and Work Schedule . IWPR Fact Sheet #B328.
Wiseman, Travis, and Nabamita Dutta. 2016. Religion and the Gender Wage Gap: A U.S. State-Level Study .
University of Michigan Institute for Social Research. 2007. Time, Money, and Who Does the Laundry . Research Update No. 4.
U.S. Census Bureau. 2016. Income and Poverty in the United States: 2015: Table A-4. Number and Real Median Earnings of Total Workers and Full-Time, Year-Round Workers by Sex and Female-to-Male Earnings Ratio: 1960 to 2015 .
U.S. Department of Labor Women’s Bureau (DOL). 2015. Older Women Workers and Economic Security . Women’s Bureau Issue Brief.
See related work on Women | Wages
See more work by Elise Gould , Jessica Schieder , and Kathleen Geier

An official website of the United States government
The .gov means it’s official. Federal government websites often end in .gov or .mil. Before sharing sensitive information, make sure you’re on a federal government site.
The site is secure. The https:// ensures that you are connecting to the official website and that any information you provide is encrypted and transmitted securely.
- Publications
- Account settings
Preview improvements coming to the PMC website in October 2024. Learn More or Try it out now .
- Advanced Search
- Journal List
- Front Sociol

The Gender Pay Gap: Income Inequality Over Life Course – A Multilevel Analysis
Lisa toczek.
1 Department of Medical Sociology, Institute of the History, Philosophy and Ethics of Medicine, Faculty of Medicine, University of Ulm, Ulm, Germany
2 Department of Social Medicine, Care and Public Health Research Institute (CAPHRI), Faculty of Health, Medicine and Life Sciences, Maastricht University, Maastricht, Netherlands
Richard Peter
Maria Bohdalova , Comenius University in Bratislava, Slovakia
Associated Data
The datasets presented in this article are not readily available because the study data contain social security information. Due to legal regulations in Germany, it is not permitted to share data with social security information. Requests to access the datasets should be directed to [email protected] .
The gender pay gap has been observed for decades, and still exists. Due to a life course perspective, gender differences in income are analyzed over a period of 24 years. Therefore, this study aims to investigate income trajectories and the differences regarding men and women. Moreover, the study examines how human capital determinants, occupational positions and factors that accumulate disadvantages over time contribute to the explanation of the GPG in Germany. Therefore, this study aims to contribute to a better understanding of the GPG over the life course. The data are based on the German cohort study lidA (living at work), which links survey data individually with employment register data. Based on social security data, the income of men and women over time are analyzed using a multilevel analysis. The results show that the GPG exists in Germany over the life course: men have a higher daily average income per year than women. In addition, the income developments of men rise more sharply than those of women over time. Moreover, even after controlling for factors potentially explaining the GPG like education, work experience, occupational status or unemployment episodes the GPG persists. Concluding, further research is required that covers additional factors like individual behavior or information about the labor market structure for a better understanding of the GPG.
1 Introduction
In the European Union (EU) in 2019, women’s average gross hourly earnings were 14.1% below the earnings of men ( Eurostat, 2021a ). The gender pay gap (GPG) has existed for decades and still remains to date. According to Eurostat GPG statistics, the key priorities of gender policies are to reduce the wage differences between men and women at both the EU and national levels ( Eurostat, 2021a ). Nevertheless, the careers of men and women differ considerably in the labor market, with women being paid less than men ( Arulampalam et al., 2005 ; Radl, 2013 ; Boll et al., 2017 ). A report from the European Parliament in 2015 about gender equality assessed Germany’s performance in that field as mediocre. The federal government in Germany has already improved laws that focus on gender equality ( Botsch, 2015 ). Regarding Germany, in 2019 the earning difference between men and women were found to be 19.2% ( Eurostat, 2021a ). The reasons behind gender income inequality are complex and have multidimensional explanations.
1.1 Determinants of the GPG
The early 1990s represented a turning point for the participation of women in the labor market ( Botsch, 2015 ). In previous years, women’s participation rate in the workforce has strongly increased, from 51.9% in the year 1980 (West Germany) to 74.9% in 2019 ( OECD, 2021 ). This upward trend represents the increase of women working at older ages ( Sackmann, 2018 ). However, the gender income inequality remains. Different explaining factors of the GPG were found in previous research: patterns of employment, access to education and interruptions in the careers of men and women.
Although there are nearly equal numbers of men and women in the labor market, when considering women’s careers, various gender-specific barriers are occurring. The working patterns were found to have a relevant impact on the GPG in previous research. Atypical employment is increasing and this result in an expansion of the low-wage sector, which mainly affects women in Germany ( Botsch, 2015 ). Additionally, labor market integration of women has mainly been in jobs that provide few working hours and low wages ( Botsch, 2015 ). Moreover, part-time employment represents a common employment type in Germany, which is more frequent among women – as various studies have demonstrated – and explains the GPG significantly ( Boll et al., 2017 ; Ponthieux and Meurs, 2015 ; Boll and Leppin, 2015 ). In addition, the part-time employment occurs more often in occupations characterized by a high proportion of women and low wages ( Matteazzi et al., 2018 ; Boll and Leppin, 2015 ; Hasselhorn, 2020 ; Manzoni et al., 2014 ). Another employment type with few working hours and low pay is a special form of part-time work: marginal work. Marginal work is defined as earnings up to 450 Euros per month or up to 5.400 Euros annually. Also, it is also more common among women than among men ( Botsch, 2015 ; Broughton et al., 2016 ). The marginal part-time work has increased in nearly all EU countries, especially in Germany where it can be found to be above the EU average ( Broughton et al., 2016 ). Besides the working time, occupational status influences the wage differences of men and women. Female-dominated occupational sectors are characterized by lower wages compared to male-dominated ones ( Brynin and Perales, 2016 ). Additionally, in women-dominant industries, remunerations are less attractive and it often entails low-status work in sectors like retail, caregiving or education ( Boll and Leppin, 2015 ; Hasselhorn, 2020 ; Matteazzi et al., 2018 ; Brynin and Perales, 2016 ). Hence, working patterns such as the amount of working time or the occupational status are crucial determinants that contribute to explaining the GPG in Germany ( Blau and Kahn, 2017 ; Boll et al., 2017 ).
The access to education and vocational training are important factors, that influence the GPG. Both influence a first access to the labor market and are considered to be ‘door openers’ for the working life ( Manzoni et al., 2014 ). In Germany, education represents a largely stable variable over time, i.e. only few individuals increase their first educational attainment. Education influences the careers of men and women and can be seen as important an determinant of future earnings ( Boll et al., 2017 ; Bovens and Wille, 2017 ). Although women’s educational attainment caught up with those of men’s in recent years, for men, a higher qualification was still rewarded more than for women ( Botsch, 2015 ; Boll et al., 2017 ). Moreover, in previous research the impact of education on the GPG was not found to be consistent with different influences for men than for women ( Aisenbrey and Bruckner, 2008 ; Ponthieux and Meurs, 2015 ). Manzoni et al. (2014) found out, that the effect of education on career developments were dependent of their particular educational levels. In addition, regardless of the women’s educational catching-up in the last years, looking at older cohorts – born between 1950 and 1964 – women had a lower average level of education than men ( Boll et al., 2017 ).
An increasing GPG over time can also be the result of interruptions in careers, which are found more often for women than for men ( Eurostat, 2021a ; Boll and Leppin, 2015 ). Previous research of Boll and Leppin (2015) has identified explanations for the GPG in Germany by analyzing data from the German Socio-Economic Panel (SOEP) in 2011. They demonstrated that the amount of time spent in actual work was lower for women than for men. Therefore, women gain less work experience than their male counterparts ( Boll and Leppin, 2015 ). Career interruptions not only impact the accumulation of work experience but also the scope of future work. Especially in the period of family formation higher rates of part-time employment among women can be observed ( Boll et al., 2017 ; Ponthieux and Meurs, 2015 ). Moreover, work-life interruptions such as raising children or caring for family members have a major impact on the employment development and are more likely to appear for women than for men ( Ponthieux and Meurs, 2015 ). Although the employment rate of mothers has increased in recent years in Germany, it is still considerably lower than that of fathers ( Federal Statistical Office, 2021 ). Hence, taking care of children is still attributed to mothers, to the detriment of their careers ( Botsch, 2015 ). A recent study, however, found sizable wage differences between men and women who were not parents, refuting the assumption that the GPG applies only to parents ( Joshi et al., 2020 ). Other interruptions in the working lives of men and women are caused by unemployment. Azmat et al. (2006) found that in Germany, transition rates from employment to unemployment were higher for women than for men. Career interruptions have lasting negative effects on women’s wages. Therefore, it can be useful to examine unemployment when analyzing gender inequality in the labor market ( Eurostat, 2021b ).
1.2 Theoretical Background
1.2.1 human capital model.
In previous research, economic theories had been applied to explain the income differences of men and women. Two essential factors could be found: qualification and discrimination. The human capital model claims that qualifications with greater investments can be directly related to higher wages of men and women. The earnings are assumed to be based on skills and abilities that are required through education and vocational training, and work experience ( Grybaitė, 2006 ; Lips, 2013 ; Blau and Kahn, 2007 ). Educational attainment of women has caught up in recent years ( Botsch, 2015 ). However, women’s investments in qualifications were still not equally rewarded as those of men. Therefore, the expected narrowing of the GPG was not confirmed in earlier research ( Boll et al., 2017 ; Lips, 2013 ). Another determinant of the human capital model is work experience. Labor market experience contributes to a large extent to the gender inequality in earnings ( Sierminska et al., 2010 ). Hence, work experience influences the wages of men and women. On the one hand, interruptions due to family life lower especially women’s labor market experience compared to men. On the other hand, part-time employment is more frequent among women with fewer working hours and therefore less work experience. The lesser accumulation of work experience leads to lower human capital and lower earnings for women compared with men ( Blau and Kahn, 2007 ; Mincer and Polachek, 1974 ). Nonetheless, the association of work experience and income is more complex. Regarding the wages of men and women the influence of occupation itself also needs to be considered ( Lips, 2013 ). In the paper of Polachek (1981) different occupations over the careers of men and women were explained by different labor force participation over lifetime. Referring to the human capital model, it is argued that women more likely expect discontinuous employment. Therefore, women choose occupations with fewer penalties for interruptions ( Polachek, 1981 ). However, it should be questioned if working in specific occupations can be defined as a simple choice ( Lips, 2013 ). Besides, part-time employment is found to be more frequent among women, which ultimately leads to few working hours and hence low earnings ( Botsch, 2015 ; Ponthieux and Meurs, 2015 ; Boll et al., 2017 ). Though different working hours cannot be defined as a simple choice either ( Lips, 2013 ).
Earlier criticism about the human capital model discussed that the wage differences of men and women cannot only be explained by the qualification and the labor market experience ( Grybaitė, 2006 ; Lips, 2013 ). Another theoretical approach explaining the GPG refers to labor market discriminations, which effect occupations and wages ( Boll et al., 2017 ; Grybaitė, 2006 ). On the one hand, occupational sex segregation can be associated with income differences of men and women. The different occupational allocation in the labor market of men and women are defined as allocative discrimination ( Petersen and Morgan, 1995 ). In addition, occupations in female-dominated sectors are mostly characterized by low-wages compared to more male-dominated occupations ( Brynin and Perales, 2016 ). On the other hand, even with equal occupational positions and skill requirements women mostly earn less than men, this refers to the valuative discrimination ( Petersen and Morgan, 1995 ). Even within female-dominated jobs a certain discrimination exists, with men being paid more than women for the same occupation. Additionally, employment sectors with a large number of female workers are more likely to be associated with less prestige and lower earnings ( Lips, 2013 ). Achatz et al. (2005) analyzed the GPG with an employer-employee database in Germany. The authors examined the discrimination in the allocation of jobs, differences in productivity-, and firm-related characteristics. They found out that in occupational groups within companies, the wages decreased with a higher share of women in a group. Additionally, a higher proportion of women in a groups resulted in a higher wage loss for women than for men ( Achatz et al., 2005 ).
Although relevant criticism of the human capital model exists, its determinants are still found to be important in explaining the wage differences of men and women ( Boll et al., 2017 ). Nonetheless, income differences of men and women can still be found even with the same investments in human capital. The reason for this could be the occupational discrimination of women ( Brynin and Perales, 2016 ; Achatz et al., 2005 ; Lips, 2013 ). Therefore, the occupational positions can be associated as a relevant factor of the GPG.
1.2.2 Life Course Approach
Besides economic theories, there are other theoretical approaches of explaining the GPG. One of them focusses on the accumulation of disadvantages over the life course: the ‘cumulative advantage/disadvantage theory’ by Dannefer (2003) . It also involves social inequalities which can expand over time. The employment histories of men and women evolve over their working lives and during different career stages, advantages and disadvantages can accumulate. First, this life course perspective considers and underlines the dynamic approach of how factors shape each individual life course. Secondly, it can contribute to explain the different income trajectories of men and women over their working lives ( Doren and Lin, 2019 ; Dannefer, 2003 ; Härkönen et al., 2016 ; Manzoni et al., 2014 ; Barone and Schizzerotto, 2011 ).
The importance of the life course perspective was underlined by some earlier studies. They demonstrated that certain conditions in adolescence or early work-life affected future careers of men and women. Visser et al. (2016) found evidence for an accumulation of disadvantages in the labor market over working life, in particular for the lower educated. The cohort study SHARE had assessed economic and social changes over the life course in numerous European countries in several publications ( Börsch-Supan et al., 2013 ). Overall, education and vocational training, occupational positions and income illustrate parts of the social structure which in turn can demonstrate gender inequality in the labor market ( Boll and Leppin, 2015 ; Hasselhorn, 2020 ; Du Prel et al., 2019 ). Moreover, family events and labor market processes repeatedly affect one another over the life course. The work-family trajectories have consequences on employment outcomes such as earnings ( Aisenbrey and Fasang, 2017 ; Jalovaara and Fasang, 2019 ). Furthermore, the income differences of men and women are not steady but tend to be lower at the beginning of employment and increase with age ( Goldin, 2014 ; Eurostat, 2021a ). Therefore, careers should not be analyzed in a single snapshot, but with a more appropriate life course approach that takes into account factors that influences the wages of men and women over time.
1.3 Aim and Hypotheses
The aim of the present study is to examine income trajectories and to investigate the income differences of men and women over their life course. We are interested in how human capital determinants, occupational positions and the accumulation of disadvantages over time contribute to the explanation of the GPG from a life course perspective.
Focusing on older German employees, our study includes 24 years of their careers and considers possible cumulative disadvantages of women in the labor market compared to those of men. In contrast to Polachek (1981) , who analyzed the GPG as a unit over lifetime, we used a life course approach in regard to the theory of cumulative disadvantages of Dannefer (2003) . Accordingly, we analyze explaining factors of the GPG not only in a single snapshot but over the working careers of men and women. Life course data based on register data and characteristics of employment biographies with information on a daily basis are two additional important and valuable advantages of our study. Existing studies rarely have this information in the form of life course data and when they do, the data is either self-reported and retrospective including possible recall bias, or based on register data which was only collected on a yearly basis. We expect to find differences in the income of men and women over a period of time with overall higher, and more increasing earnings of men than of women.
Hypothesis 1 (H1): The differences of income trajectories throughout working life is expected to demonstrate more income over time among men than among women.
Education and vocational training, and work experience are human capital determinants. They have influence on the earnings of men and women. Although previous research estimated additional important factors contributing to the GPG, human capital capabilities continue to be relevant in explaining the wage differences of men and women ( Blau and Kahn, 2007 ; Boll et al., 2017 ). In our life course approach, we control for human capital determinants due to the information about education and vocational training, and work experience via the amount of working time (full-/part-time) for each year. We expect to find a strong influence of both determinants on the wages of men and women in Germany.
Hypothesis 2 (H2): The income differences between men and women can be explained by determinants of the human capital model.
Previous research found out that factors such as occupational status had an impact on the income differences of men and women ( Blau and Kahn, 2007 ; Boll et al., 2017 ). For a better understanding and explanation of the GPG, gender differences regarding occupational positions must be included to human capital determinants ( Boll et al., 2017 ). We assume that men and women can be found in different occupations, measured via occupational status, and these explain a substantial part of the wage differences between men and women.
Hypothesis 3 (H3): The occupational status of men and women can contribute to the explanation of the GPG.
The life-course approach acknowledges time as an important influence on the wages of men and women. Income differences of men and women can change over time and career stages, while the GPG was found to be lower at the beginning of the employment career and widened with age ( Goldin, 2014 ). Hence, the earning differences between men and women tend to be higher for older employees ( Eurostat, 2021a ; Federal Statistical Office, 2016 ). To account for the influence of age, we additionally included the age of each person in our analysis. Another factor that changes over time and contribute to explain the GPG is part-time work. In general, part-time work result in a disadvantage in pay compared to full-time employment ( Ponthieux and Meurs, 2015 ). However, explanations of the GPG due to different amount of part-time work need to include a special form of part-time work: marginal work. Marginal employment conditions are characterized by low wages and high job insecurities. Also discontinuous employment due to unemployment are characterized by job insecurities and affect the low-paid sector – therefore mainly women ( Botsch, 2015 ). Besides the human capital determinants and occupational positions as important factors explaining the GPG, the region of employment influences the wages of men and women and can also change over the career stages. Evidence from the Federal Statistical Office of Germany in 2014 noticed a divergence of the GPG trend in the formerly separated parts of Germany. The GPG among employees was wider in the Western part (24%) compared to the Eastern part of Germany, where it was found to be 9% ( Federal Statistical Office, 2016 ). Therefore, to examine income differences, the amount of less advantaged employment such as marginal work or periods of unemployment throughout the careers of men and women needs to be considered, as well as the region of employment and the age of a person.
Hypothesis 4 (H4): Factors of the living environment such as regional factors, and social disadvantage work conditions such as marginal work or unemployment, contribute to the income difference between men and women.
Our study about the GPG in Germany adds to earlier research in different ways. First, the accumulation of inequalities over the life course of men and women is known, but only few studies exist that focus on income through life course approach. We can analyze factors that influence the GPG over the careers of men and women due to the availability of social security data with daily information of each person. Besides the wages of men and women, the data additionally contains time-varying information about occupational status, working time and unemployment breaks. Therefore, we use longitudinal data of the German baby-boomers which allow us to measure changes of factors explaining the GPG over time. Second, a relevant contribution of our study is that we can consider different factors contributing to the explanation of the GPG through a life course perspective. The few studies focusing on the GPG over life course included either only determinants of the human capital model ( Joshi et al., 2020 ) or factors of occupational careers ( Moore, 2018 ). Some research included both aspects but had other disadvantages, such as Monti et al. (2020) , who could not analyze temporal evolution of the GPG with the data available. Moreover, previous research on the GPG in Germany could not trace vertical occupational segregation due to missing information of part-time workers, included only data of West Germany and used merely accumulated earnings over time ( Boll et al., 2017 ). Nonetheless, previous research demonstrated the need of analyzing the GPG via life course approach with which the accumulation of advantages and disadvantages for both, men and women, can be considered. Third, due to the usage of a multilevel framework we can examine income trajectories simultaneously at an individual and at a time-related level. Moreover, the influences of time-invariant and time-varying factors can be analyzed regarding differences in earnings of men and women. Hence, the multilevel approach examines income changes between and also within individuals. Furthermore, it acknowledges the importance of the life course perspective with including time as a factor in the model. A recent study also used growth curve modelling to explain gender inequality in the US. However, gender inequality measured through gender earnings was analyzed only across education and race without considering other variables explaining the GPG ( Doren and Lin, 2019 ). To our knowledge, there exists no research on the GPG that covers several essential determinants, hence we aim to fill those research gaps with our study.
2 Materials and Methods
The data were obtained from the cohort study lidA (living at work). The lidA sample includes two cohorts of employees (born in 1959 and in 1965) and was drawn randomly from social security data. LidA combines two major sources of information – register data of social insurance and questionnaire data derived from a survey. The survey was conducted in two waves, 2011 (t 0 ) and 2014 (t 1 ) ( Hasselhorn et al., 2014 ). The ethics commission of the University of Wuppertal approved the study.
In Germany, the social insurance system assists people in case of an emergency such as unemployment, illness, retirement, or nursing care. Employees have to make a contribution to the system depending on their income – except of civil servants or self-employed ( Federal Agency for Civic Education, 2021 ). In our analyses, we included men and women in Germany who participated in the baseline (2011) and in the follow-up (2014), were employed during both waves and subjected to social security contributions. We only included persons who agreed via written consent to the linkage of the survey data to their social security data. Thus, our sample for analysis included 3,338 individuals ( Figure 1 ).

Decision tree – inclusion and exclusion criteria in the sample for analysis.
2.2 Measurements
The social security data of the Institute for Employment Research of the German Federal Employment Agency is based on employers’ reports. The so-called “Integrated Employment Biographies” (IEB) or register data comprises information about individual employment; that is, type of employment, occupational status, episodes of unemployment and income with information about age, gender and education and vocational training. The IEB data are retrieved from employers’ yearly reports submitted to the social security authority ( Hasselhorn et al., 2014 ). The information of the register data was available on a daily basis and contained yearly information from 1993 to 2017 for each person. However, the IEB data contain missing details, especially regarding information that is not directly relevant for social security data and therefore, not of the highest priority for employers’ reports. This is particularly true for data on gender and education and vocational training. As our sample participants consented to the linkage of IEB with questionnaire data, we were able to impute the missing information on these variables with the help of the survey data. All time-varying information in the IEB is coded to the day. Our data have a multilevel structure with time of measurements (Level 1) being nested within individuals (Level 2) and defined as follows.
2.2.1 Level 1 Variables
In our analysis the variable time was based on information about the year of measurement. The starting point represents 1993 and was coded with zero. The outcome variable income was calculated from the IEB data as nominal wages in Euros (€). As time-varying variable, it can be defined as the average daily income per year of each person whose work contributes to social security and/or marginal employment. Information about the work experience due to working time was available for jobs that require social security contribution. To draw this information from the IEB data, the time-varying variable working time was computed with three different types: full- and part-time, part-time, and full-time. The data on occupational status were based on the International Standard of Classification of Occupations 2008 (ISCO-08). This time-varying variable contained information on the occupational status of each job that a person has held over the years. For the multilevel analysis, ISCO-08 was transformed from the German classification KldB 2010 (classification of occupations 2010) of the register data. ISCO-08 is structured according to the skill level and specialization of jobs, which are grouped into four hierarchical levels. Occupational status in our study was defined by the 10 major groups (level one of the classifications ISCO-08), without the group of armed forces who did not appear in our data. Therefore, the nine groups were analyzed: elementary occupations; plant and machine operators and assemblers; craft and related trades workers; skilled agricultural, forestry and fishery workers; services and sales workers; clerical support workers; technicians and associate professionals; professionals; and managers ( International Labour Office, 2012 ). Moreover, information about the number of episodes of marginal work could also be drawn from the register data. Marginal work was defined due to having at least one marginal employment per year. The time periods (episodes) of every marginal employment were counted and added up yearly. Furthermore, the duration of unemployment as time-varying variable was calculated due to information of the register data about the days of unemployment per year. In the register data unemployment is defined as being unemployed or unable to work for up to 42 days, excluding those with sickness absence benefits or disability pensions. The IEB data also provided information on the region of employment, which represents the area in which a company is located (East Germany and West Germany). This time-varying variable was available for each person over the years. A description of the Level 1 characteristics of our sample is provided in Table 2 using the last available information (2017) from the IEB data.
Characteristics of Level 1 variables a for men (n = 1,552) and women (n = 1,786).
M mean; SD standard deviation.
* p < 0.05, ** p < 0.01, *** p < 0.001.
2.2.2 Level 2 Variables
Information about the time-invariant variable education and vocational training was assessed from the survey data in 2011 (baseline). Education and vocational achievements of the sample were grouped in: low, intermediate and high education and vocational training (see Supplementary Table S1 ). The time-invariant variable gender had missing values in the register data. Therefore, we imputed the missing data using information of the survey data. The variable was coded 0 = female and 1 = male. Also based on the survey data, we included the time-invariant variable year of birth with measurements of 1959 and 1965 in the analysis. The characteristics of the Level 2 variables are displayed in Table 1 .
Characteristics of the Level 2 variables a for men (n = 1,552) and women (n = 1,786).
2.3 Statistical Analysis
The characteristics of our sample are displayed in Table 1 and Table 2 . Statistical analyses were performed using either Cramer’s V or by unpaired two sample t -test for numeric variables. Regarding the multilevel analysis, we used a so-called growth curve analysis. It demonstrates a multilevel approach for longitudinal data that model growth or decline over time. For this purpose, all daily information in the IEB were transformed into data on a yearly basis. Level 1 (year of measurements) represents the intraindividual change with time-varying variables. Interindividual changes are determined with time-invariant variables on Level 2 (individuals). Therefore, time of measurements predictors was nested within individuals. We applied a random intercept and slope model, which assumed variations in intercept and slope of individuals over time ( Singer and Willett, 2003 ; Rabe-Hesketh and Skrondal, 2012 ; Hosoya et al., 2014 ). Besides the Level 1 and Level 2 predictors, the cross-level interaction of gender*time interaction was constituted to analyze differences in income slopes of men and women over time ( Rabe-Hesketh and Skrondal, 2012 ).
Level 1 of the two-level growth model is presented below ( Eq. (1) ). y i j measures the income trajectory y for individual i at time j . True initial income for each person is represented with β 0 i . The slope of the individual change trajectory demonstrates β i j . T I M E i j stands for the measure of assessment at time j for individual i (Level 1 predictor). The residual or random error, specific to time and the individual is demonstrated by ε i j .
Eq. 2 and 3 represent the submodels of the Level 2. Eq. 2 defines the intercept γ 00 for individual i with the intercept of z i (illustrating a Level 2 predictor) and residual in the intercept v 0 i . The slope at Level 2 is represented in Eq. 3 with γ 10 and the slope error v 1 i . The effect γ 11 provides information on the extent to which the effect of the Level 1 predictor ( T I M E i j ) varies depending on the Level 2 predictor ( z i ).
To test our hypotheses, we calculated the influence of different variables with adjusting various predictors stepwise into the multilevel analysis. First, we estimated an unconditional means model which describes the outcome variation only and not its change over time (model 1). The next preliminary step was calculating the intraclass correlation coefficient (ICC) of this model 1. It identifies and partitions the two components: within- and between-person variance. The ICC estimates the proportion of total variation of the outcome y that lies between persons ( Singer and Willett, 2003 ). In the next model (model 2), we calculated an unconditional growth curve model which included time as predictor on Level 1. In model 3, the GCA was controlled for gender and time as well as the interaction of both variables. Model 4 was additionally adjusted for human capital determinant: education and vocational training, and working time. The GCA of model 5 was controlled for occupational status. The last model included year of birth, number of episodes of marginal work, duration of unemployment and region of employment (model 6 – fully adjusted model).
In Table 5 , the indices of the Akaike’s Information Criterion (AIC) were used to compare models and explore the best model fit ( Singer and Willett, 2003 ; Rabe-Hesketh and Skrondal, 2012 ). The statistical analyses were performed with IBM SPSS 25.
Goodness-of-fit statistics of the GCA.
AIC Akaike’s Information Criterion.
3.1 Descriptive
Characteristics of Level 2 variables stratified by gender are displayed in Table 1 . 1,552 men and 1,786 women were included in the analyses. It is observed that women significantly differ from men in education and vocational training. Women were less likely than men to have both low and high levels of education and vocational training.
The characteristics of Level 1 variable are represented in Table 2 . Men and women differ significantly in their occupational positions. Also, men had a higher average daily income than women. Part-time jobs are more likely among women as compared to men, who are more likely to be represented in full-time jobs. Moreover, the numbers of episodes of marginal work differ significantly between men and women.
Figure 2 displays the income trajectories over the observation period (1993–2017) among men and women. In 24 years, average daily income per year increased for both. However, men have a higher average income over their life course than women. Over time, a steeper growth of the average daily income per year can be observed for men, compared to the income development of women.

Income trajectories of men and women.
3.2 Growth Curve Analysis
Results of the multilevel analyses with average daily income per year as dependent variable concerning H1 are presented in Table 3 . The ICC of the unconditional means model (model 1) demonstrates that 74% of the total variability in income can be attributed to differences between persons and 26% to the differences within persons. Adding time as a predictor in the multilevel analysis (model 2), the variance components on Level 1 become smaller. Concluding that time accounts for 68% (from 607.34 to 197.12) of the within-person variance in average income. On Level 2, time explains 40% of the variance between persons (interindividual). However, there can be still found significant unexplained results in both levels which suggests that predictors on both levels should be further included. The GCA in model 3 was adjusted for gender (with women as reference group) and the interaction gender*time. The results show a significant effect of gender on the average income over time. The starting place (intercept) lies at 41.74€ with an incremental growth per year of 1.76€. However, regarding women as reference group, men have a higher average income. The significant interaction term also indicates different income development of men and women over time – with men having higher average income trajectory than women. As expected, no relevant change can be found in the within-person variance due to the adding of the Level 2 variable: gender. The variance on Level 2, however, become less concluding that gender accounts for 26% of the variance between persons. Overall, we can verify H1 with these results.
Growth curve models 1 to 3: Estimates of average daily income per year.
L1 = Level 1; L2 = Level 2.
Results of the GCA with average daily income per year as the dependent variable controlled by determinants of the human capital model are presented in Table 4 (model 4). In addition to the multilevel analysis of model 3, model 4 is also adjusted for: education and vocational training, and working time. The results show that the average income is found to be significantly higher for full-time workers and higher educated. There is a social gradient for income regarding education and vocational training – with decreasing levels of education, the income also reduces. People who are working full-time have a higher average income than those who work part-time or full- and part-time. The effect of gender is found to be significant with less average income of women compared to men. Moreover, the income development of men and women over time is still significantly different, with more income growth over time for men than for women. The results of the variance components demonstrate that human capital determinants are explaining 16% of the variance within person and 25% of the variance between persons. However, on both levels there can be still found significant variance and additional variables need to be considered. Our hypothesis 2 can be partially confirmed.
Growth curve models 4 to 6: Estimates of average daily income per year.
Model 5 ( Table 4 ) embeds occupational status to the analysis to find out the contribution of the occupational positions on the earning differences of men and women. Significant differences in the daily average income for each occupational group can be identified. The reference group is represented with the highest occupational group ‘manager’. In nearly all other occupations, manager had the highest average income, except of ‘technicians and associate professionals’. Moreover, the effects of occupational status on income are significant for all ISCO groups except for professionals. However, compared to education and vocational training, occupational status trends are less clear, and a social gradient cannot be identified. The estimated of the fixed effect of gender persists and stays the same, concluding that the occupational position of a person could not influence the effect of gender on income. The increase of income over time can be still found to be significant higher for men than for women. Moreover, including the Level 1 variable, occupational position cannot explain a substantial part of the within-person variance. We can identify occupational positions as significant predictor of the income, but a relevant contribution to explain the GPG cannot be observed. Therefore, we cannot approve hypothesis 3.
The results of investigating the influence of factors of the living environment are presented in Table 4 (model 6). Those, who are born earlier (1959) are found to have a higher average daily income, compared to those born in 1965. Having at least one marginal employment per year influences the average daily income negatively, as does having more unemployed days. Furthermore, average income is influenced by the region of employment, being lower in East Germany than in West Germany. The estimate of gender become a little less, but the average income and the development of income over time still substantially differs between men and women. The factors of living environment account for 10% of the variance between persons. We can only partially accept hypothesis 4.
3.3 Goodness of Fit
Table 5 displays the goodness of fit statistics for the different models of the GCA. The AIC is computed to find the best model fit. Considering the different indices of AIC, model 6 has the best fit.
4 Discussion
This study aimed to examine the income differences of men and women over their life course. We investigated how different factors can explain the GPG over time. Even after extensive control for human capital determinants, occupational factors and various factors of the living environment, the effect of gender on the average daily income persisted. Moreover, the average income development was found to be higher for men compared to women.
The accumulation of inequalities over time can be seen in the difference between men’s and women’s wages. Over the period of 24 years, our results showed that the income development of men increased more compared to women – the GPG widened with time. Due to the availability of life course data, we could consider cumulative disadvantages regarding the earnings of men and women. Moreover, the results of the variance componence also showed the importance of including time to explain the GPG ( Table 3 , model 2). Therefore, we can verify our first hypothesis. The steeper incline of income for men compared to women over time substantiates the presence of GPG in Germany. Goldin (2014) also found a small GPG when people enter the labor market and a widening gap with age. Our findings are also in line with information from the Federal Statistical Office (2016) and Eurostat (2021a) who used representative data and not use cohort specific data of the German working population.
The second hypothesis assumed that human capital determinants (education and work experience) can explain the GPG. The effects of education and vocational training on daily average income significantly differed in our results ( Table 4 , model 4). Findings of Bovens and Wille (2017) also demonstrated that the level of a person’s education determines the income level. Our results also support the previous finding, that education is most often a requirement for the achievement of a certain desired financial situation ( Du Prel et al., 2019 ). Our results also showed that the average income significantly differed considering working time. Full-time workers had higher average income, while men were more likely to work full-time compared to women. Earlier research also showed that part-time work was more frequent among women than among men ( Boll and Leppin, 2015 ; Matteazzi et al., 2018 ; Eurostat, 2021a ). After adjusting for human capital determinants, the unexplained variance was still substantial and the effect of gender remained significant. Hence, H2 can only partially be accepted.
In our third hypothesis, we assumed that the gender differences in occupational position can explain the GPG. We demonstrated that the average income differed according to the occupational status of a person. This is in line with previous findings of Blau and Kahn (2001) who assumed occupation to be an important factor of the financial status of a person. After controlling for occupational status, the effect of gender could still be found to be significant. We cannot accept H3 and therefore cannot confirm results of earlier studies ( Blau and Kahn, 2007 ; Boll et al., 2017 ). In contrast to the results of education and vocational training, we did not observe a clear social gradient of occupational status and income in our analyses. One explanation could be the classification of the occupational status. The ISCO classification is structured hierarchically on four levels. The construction is based on skill level and specialization. In our study, we used the major group structure (level one) with 10 different occupational groups. Using ISCO at level one (major groups) cannot be interpreted as a strict hierarchical order of occupations; instead, it can be considered more of a summary information on occupational status regarding skill level. Moreover, we were only able to generate the major groups of the register data and therefore cannot provide more detailed information about the occupational status. However, ISCO is applied in our study for the purpose of international comparability ( International Labour Office, 2012 ).
The accumulation of disadvantages over time could also be found in our results after controlling for factors such as unemployment or marginal employment. Having (at least one) marginal employment per year influenced the income negatively. We found that discontinuities in employment and interruptions such as unemployment also had a significant negative effect. Average income decreased when the number of days per year of unemployment increased. Furthermore, controlling for the region of employment, people in East Germany had lower daily average income compared to those in West Germany. Regarding the difference between men and women, previous findings also suggested a wider GPG in West Germany than in East Germany ( Federal Statistical Office, 2016 ). However, the GPG in West and East Germany should be compared with caution due to different societal models in the past. Moreover, different labour market characteristics and different infrastructure of childcare facilities lead to a lower GPG in East Germany than in West Germany ( Federal Ministry for Family Affairs, Senior Citizens, Women and Youth, 2020 ). The year of birth was included to eliminate cohort effects, and it was found to influence average income. Men and women born earlier (1959) had higher income than those born in 1965. The fact that they are older and have worked longer in the labor market could be an explanation. The significant effects of gender on the average income and the income trajectories remained after adjusting for these factors. Therefore, hypothesis 4 can only be partially confirmed.
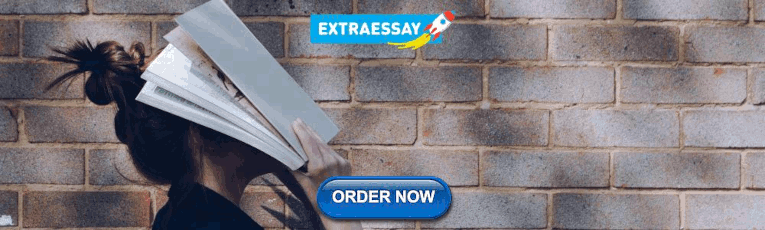
4.1 Strengths and Limitations
Our study has limitations concerning the generalizability of our results due to the database. Our sample includes employees of two age groups (1959 and 1965) in Germany, who are subjected to social security. Thus, the generalizability or extension of the findings to self-employed people, civil servants and other age groups may be limited. The GPG differs considerably between the EU members. The GPG in Germany is one of the widest in the EU, with 19.2% in 2019. Netherlands and Sweden are two EU countries with similar employment rates, but still have lower GPGs with 14.6 and 11.8% ( Eurostat, 2021a ). Efforts to promote gender equality in politics in Germany are limited compared to other EU members. Women are still underrepresented, not only in the political but also in the economic area. Moreover family policy needs to further support full-time employment of women and working mothers ( Andersson et al., 2014 ; Botsch, 2015 ). Therefore, the transfer of our results to other countries should be made with caution. There are some other limitations regarding the IEB data. Information about occupational careers exist from the beginning (1975), but only for persons born in West Germany. Information about people born in East Germany was not available for the period before 1993. Hence, to counteract the systematic bias, we defined 1993 as a cut-off point, when people were either 28 or 34 years old. Additionally, we adjusted our analyses for the region of employment (East/West Germany). Furthermore, information about the marginal work and duration of unemployment were only available from 1999 onwards. Due to the composition of the IEB data, we could not include people who were unwell for long periods of time. Only persons who were unable to work for less than 42 days were included in the data. Regarding the income development of women in our study, Figure 2 shows a decrease between 1997 and 1999. Being in their thirties (32–40 years) and having to raise children at that time can be one possible explanation. Regarding family formation, in 1993 the average age of a mother at birth was 28.4 years ( Federal Statistical Office, 2020 ). At the beginning of our analysis (1993) the average age of both cohorts in the study (28 years; 34 years) is similar to the average age of a mother during that time – especially for the younger cohort. However, our data do not cover information about persons on parental leave or homemakers. Due to the lack of information in the IEB data, implications of family life contributing to a difference in pay for women cannot be included in our analysis. Furthermore, Joshi et al. (2020) could not find a GPG only for parents but also for men and women without children. Therefore, the issue of wage differences between men and women is relevant either way.
Besides these restrictions, our study exhibits several strengths. The study population is highly representative for German employees subject to social insurance contributions, born in 1959 and 1965 and is, therefore, characterized by a high external validity ( Schröder et al., 2013 ). Moreover, the IEB data itself and the nature of the data that the IEB provides, are one important strength of this study. The register data is not subject to possible recall bias. This is a relevant advantage compared to most previous studies that used self-reported data. In addition, the availability of information on a daily basis regarding many variables can be seen as another strength of the study. As a result, income trajectories could be calculated more precisely, compared to many previous studies. Furthermore, in Germany, income is used to calculate the amount of social benefit accruing to each person and therefore represents highly valid information. A further major advantage of our study is represented in our long observation period of 24 years. Only a few studies have applied the life course approach to examine the complexity of the GPG. Our life course data contain various information about employment characteristics which are relevant for the GPG and of high data quality.
Our results showed, even after controlling for relevant factors, that the GPG still persisted. There exist some explanations of the GPG regarding different behaviors of men and women in wage negotiations, which further influence different income developments ( Boll and Leppin, 2015 ). Also, structural disadvantages in the labor market can be a factor explaining the GPG. Individual behavior and labor market structures are not represented in our register data. We can only extract information that is relevant for social security contribution. Nonetheless, previous research of Blau and Kahn (2017) found a larger and more slowly decreasing GPG in the US at the top compared to other levels of the wage distribution. This ‘glass ceiling effect’ describes the reduced career opportunities of women compared to men due to frequent denial of access to leadership positions. Consequently, gender inequality can be found to be greater at the top of the wage distribution. Among European countries, previous studies have found this “glass ceiling effect” in Germany as well ( Arulampalam et al., 2005 ; Boll and Leppin, 2015 ; Huffman et al., 2017 ). However, recent results of Boll et al. (2017) could not confirm the glass ceiling effect in West Germany, thus further research is needed.
5 Conclusion
The gender pay inequalities in the German labor market from a life course perspective exist. Our results demonstrated that human capital determinants continue to be important in explaining the GPG over time. Furthermore, factors of working disadvantages such as marginal work or unemployment are important when trying to explain the income differences of men and women. For further research the availability of more work data over the life course with matching individual data would help to understand the GPG even better.
Acknowledgments
We gratefully acknowledge the support of two staff members of the University Ulm. We would like to thank Gaurav Berry for his support of the data preparation and Diego Montano for his feedback on the statistical analysis.
Data Availability Statement
Ethics statement.
The studies involving human participants were reviewed and approved by the ethics commission of the University of Wuppertal. The patients/participants provided their written informed consent to participate in this study.
Author Contributions
LT substantially contributed to the statistical analysis and interpretation of the data, and wrote the manuscript. HB discussed the results and provided critical comments on the manuscript. RP contributed to the obtaining of the funding, interpreting the data, and critically revised the manuscript for important aspects. All authors read and approved the final manuscript.
This work was supported by the German Research Foundation (DFG), grant number 393153877.
Conflict of Interest
The authors declare that the research was conducted in the absence of any commercial or financial relationships that could be construed as a potential conflict of interest.
Publisher’s Note
All claims expressed in this article are solely those of the authors and do not necessarily represent those of their affiliated organizations, or those of the publisher, the editors and the reviewers. Any product that may be evaluated in this article, or claim that may be made by its manufacturer, is not guaranteed or endorsed by the publisher.
Supplementary Material
The Supplementary Material for this article can be found online at: https://www.frontiersin.org/articles/10.3389/fsoc.2021.815376/full#supplementary-material .
- Achatz J., Gartner H., Glück T. (2005). Bonus Oder Bias? Koelner Z.Soziol.u.Soz.Psychol 57 , 466–493. 10.1007/s11577-005-0185-6 [ CrossRef ] [ Google Scholar ]
- Aisenbrey S., Bruckner H. (2008). Occupational Aspirations and the Gender Gap in Wages . Eur. Sociological Rev. 24 , 633–649. 10.1093/esr/jcn024 [ CrossRef ] [ Google Scholar ]
- Aisenbrey S., Fasang A. (2017). The Interplay of Work and Family Trajectories over the Life Course: Germany and the United States in Comparison . Am. J. Sociol. 122 , 1448–1484. 10.1086/691128 [ CrossRef ] [ Google Scholar ]
- Andersson G., Kreyenfeld M., Mika T. (2014). Welfare State Context, Female Labour-Market Attachment and Childbearing in Germany and Denmark . J. Pop Res. 31 , 287–316. 10.1007/s12546-014-9135-3 [ CrossRef ] [ Google Scholar ]
- Arulampalam W., Booth A. L., Bryan M. L. (2005). Is There a Glass Ceiling over Europe? Exploring the Gender Pay gap across the Wages Distribution . Colchester, EX: ISER Working Paper Series, 25. [ Google Scholar ]
- Azmat G., Güell M., Manning A. (2006). Gender Gaps in Unemployment Rates in OECD Countries . J. Labor Econ. 24 , 1–37. 10.1086/497817 [ CrossRef ] [ Google Scholar ]
- Barone C., Schizzerotto A. (2011). Introduction . Eur. Societies 13 , 331–345. 10.1080/14616696.2011.568248 [ CrossRef ] [ Google Scholar ]
- Blau F. D., Kahn L. M. (2007). The Gender Pay Gap . AMP 21 , 7–23. 10.5465/amp.2007.24286161 [ CrossRef ] [ Google Scholar ]
- Blau F. D., Kahn L. M. (2017). The Gender Wage Gap: Extent, Trends, and Explanations . J. Econ. Lit. 55 , 789–865. 10.1257/jel.20160995 [ CrossRef ] [ Google Scholar ]
- Blau F., Kahn L. (2001). Understanding International Differences in the Gender Pay Gap . Ithaca, NY: NBER Working Paper 8200. Available at: https://www.nber.org/papers/w8200 (Accessed November 30, 2021). [ Google Scholar ]
- Boll C., Jahn M., Lagemann A. (2017). The Gender Lifetime Earnings gap: Exploring Gendered Pay from the Life Course Perspective . Hamburg, Germany: HWWI Research Paper, 179. [ Google Scholar ]
- Boll C., Leppin J. S. (2015). Die geschlechtsspezifische Lohnlücke in Deutschland: Umfang, Ursachen und Interpretation . Wirtschaftsdienst 95 , 249–254. 10.1007/s10273-015-1814-y [ CrossRef ] [ Google Scholar ]
- Börsch-Supan A., Brandt M., Hunkler C., Kneip T., Korbmacher J., Malter F., et al. (2013). Data Resource Profile: the Survey of Health, Ageing and Retirement in Europe (SHARE) . Int. J. Epidemiol. 42 , 992–1001. 10.1093/ije/dyt088 [ PMC free article ] [ PubMed ] [ CrossRef ] [ Google Scholar ]
- Botsch E. (2015). The Policy on Gender Equality in Germany . Available at: https://www.europarl.europa.eu/RegData/etudes/IDAN/2015/510025/IPOL_IDA(2015)510025_EN.pdf (Accessed November 25, 2020).
- Bovens M., Wille A. (2017). Education as a Cleavage . Oxford University Press. [ Google Scholar ]
- Broughton A., Green M., Rickard C., Swift S., Eichhorst W., Tobsch V., et al. (2016). Precarious Employment in Europe: Patterns, Trends and Policy Strategy . Europarl. Available at: https://www.europarl.europa.eu/RegData/etudes/STUD/2016/587285/IPOL_STU(2016)587285_EN.pdf . [ Google Scholar ]
- Brynin M., Perales F. (2016). Gender Wage Inequality: The De-gendering of the Occupational Structure . Eur. Sociol. Rev. 32 , 162–174. 10.1093/esr/jcv092 [ CrossRef ] [ Google Scholar ]
- Dannefer D. (2003). Cumulative Advantage/disadvantage and the Life Course: Cross-Fertilizing Age and Social Science Theory . J. Gerontol. B Psychol. Sci. Soc. Sci. 58 , S327–S337. 10.1093/geronb/58.6.s327 [ PubMed ] [ CrossRef ] [ Google Scholar ]
- Doren C., Lin K. Y. (2019). Diverging Trajectories or Parallel Pathways? an Intersectional and Life Course Approach to the Gender Earnings Gap by Race and Education . Socius 5 , 237802311987381–23. 10.1177/2378023119873816 [ CrossRef ] [ Google Scholar ]
- Du Prel J. B., Schrettenbrunner C., Hasselhorn H. M. (2019). Vertical and Horizontal Social Inequality and Motivation for Early Retirement . Z. Gerontol. Geriatr. 52 , 3–13. 10.1007/s00391-018-1450-4 [ PubMed ] [ CrossRef ] [ Google Scholar ]
- Eurostat (2021a). Statistics Explained: Gender Pay gap Statistics . Available at: https://ec.europa.eu/eurostat/statistics-explained/index.php?title=Gender_pay_gap_statistics (Accessed December 01, 2021).
- Eurostat (2021b). Statistics Explained: Gender Statistics . Available at: https://ec.europa.eu/eurostat/statistics-explained/index.php?title=Gender_statistics#Earnings (Accessed November 30, 2021).
- Federal Agency for Civic Education (2021). Sozialversicherung [Social Insurance] . Available at: https://www.bpb.de/nachschlagen/lexika/das-junge-politik-lexikon/321149/sozialversicherung (Accessed November 30, 2021).
- Federal Ministry for Family Affairs, Senior Citizens, Women and Youth (2020). The Road to Equal Pay for Women and Men . Available at: https://www.bmfsfj.de/bmfsfj/meta/en/publications-en/the-road-to-equal-pay-for-women-and-men-161370 (Accessed December 01, 2021).
- Federal Statistical Office (2020). Durchschnittliches Alter der Mutter bei der Geburt ihrer lebend geborenen Kinder: Deutschland, Jahre, Familienstand der Eltern [Average age of the mother at the birth of her children born alive: Germany, years, marital status of parents] . Available at: https://www-genesis.destatis.de/genesis/online (Accessed March 17, 2021).
- Federal Statistical Office (2021). Three in Four Mothers in Germany Were in Employment in 2019 . Available at: https://www.destatis.de/EN/Press/2021/03/PE21_N017_13.html (Accessed March 16, 2021).
- Federal Statistical Office (2016). Unbereinigter Verdienstunterschied nach persönlichen Merkmalen im Jahr 2014 [Gender Pay Gap by personal characteristics in 2014 (unadjusted)] . Available at: https://www.destatis.de/DE/Themen/Arbeit/Verdienste/Verdienste-Verdienstunterschiede/Tabellen/gpg-persoenlich.html (Accessed March 16, 2020).
- Goldin C. (2014). A Grand Gender Convergence: Its Last Chapter . Am. Econ. Rev. 104 , 1091–1119. 10.1257/aer.104.4.1091 [ CrossRef ] [ Google Scholar ]
- Grybaitė V. (2006). Analysis of Theoretical Approaches to Gender Pay gap . J. Business Econ. Manag. 7 , 85–91. 10.3846/16111699.2006.9636127 [ CrossRef ] [ Google Scholar ]
- Härkönen J., Manzoni A., Bihagen E. (2016). Gender Inequalities in Occupational Prestige across the Working Life: An Analysis of the Careers of West Germans and Swedes Born from the 1920s to the 1970s . Adv. Life course Res. 29 , 41–51. 10.1016/j.alcr.2016.01.001 [ CrossRef ] [ Google Scholar ]
- Hasselhorn H. M., Peter R., Rauch A., Schröder H., Swart E., Bender S., et al. (2014). Cohort Profile: the lidA Cohort Study-A German Cohort Study on Work, Age, Health and Work Participation . Int. J. Epidemiol. 43 , 1736–49. 10.1093/ije/dyu021 [ PMC free article ] [ PubMed ] [ CrossRef ] [ Google Scholar ]
- Hasselhorn H. M. (2020). “ Social Inequality in the Transition from Work to Retirement ,” in Handbook of Socioeconomic Determinants of Occupational Health . Editor Theorell T. (Cham: Springer International Publishing; ), 1–26. 10.1007/978-3-030-05031-3_32-1 [ CrossRef ] [ Google Scholar ]
- Hosoya G., Koch T., Eid M. (2014). Längsschnittdaten und Mehrebenenanalyse . Köln Z. Soziol 66 , 189–218. 10.1007/s11577-014-0262-9 [ CrossRef ] [ Google Scholar ]
- Huffman M. L., King J., Reichelt M. (2017). Equality for Whom? Organizational Policies and the Gender Gap across the German Earnings Distribution . ILR Rev. 70 , 16–41. 10.1177/0019793916673974 [ CrossRef ] [ Google Scholar ]
- International Labour Office (2012). International Standard Classification of Occupations: ISCO-08 . Geneva: International Labour Organization. [ Google Scholar ]
- Jalovaara M., Fasang A. E. (2019). Family Life Courses, Gender, and Mid-life Earnings . Eur. Sociol. Rev. 36 , 159–178. 10.1093/esr/jcz057 [ CrossRef ] [ Google Scholar ]
- Joshi H., Bryson A., Wilkinson D., Ward K. (2020). The Gender gap in Wages over the Life Course: Evidence from a British Cohort Born in 1958 . Gend. Work Organ. 28 , 397–415. 10.1111/gwao.12580 [ CrossRef ] [ Google Scholar ]
- Lips H. M. (2013). The Gender Pay Gap: Challenging the Rationalizations. Perceived Equity, Discrimination, and the Limits of Human Capital Models . Sex Roles 68 , 169–185. 10.1007/s11199-012-0165-z [ CrossRef ] [ Google Scholar ]
- Manzoni A., Harkonen J., Mayer K. U. (2014). Moving on? A Growth-Curve Analysis of Occupational Attainment and Career Progression Patterns in West Germany . Social Forces 92 , 1285–1312. 10.1093/sf/sou002 [ CrossRef ] [ Google Scholar ]
- Matteazzi E., Pailhé A., Solaz A. (2018). Part-time Employment, the Gender Wage gap and the Role of Wage-Setting Institutions: Evidence from 11 European Countries . Eur. J. Ind. Relations 24 , 221–241. 10.1177/0959680117738857 [ CrossRef ] [ Google Scholar ]
- Mincer J., Polachek S. (1974). Family Investments in Human Capital: Earnings of Women . J. Polit. Economy 82 , S76–S108. 10.1086/260293 [ CrossRef ] [ Google Scholar ]
- Monti H., Stinson M., Zehr L. (2020). How Long Do Early Career Decisions Follow Women? the Impact of Employer History on the Gender Wage Gap . J. Labor Res. 41 , 189–232. 10.1007/s12122-020-09300-9 [ CrossRef ] [ Google Scholar ]
- Moore T. S. (2018). Occupational Career Change and Gender Wage Inequality . Work and Occupations 45 , 82–121. 10.1177/0730888417742691 [ CrossRef ] [ Google Scholar ]
- OECD (2021). OECD Statistics . Available at: http://stats.oecd.org/ (Accessed March 16, 2021).
- Petersen T., Morgan L. A. (1995). Separate and Unequal: Occupation-Establishment Sex Segregation and the Gender Wage Gap . Am. J. Sociol. 101 , 329–365. 10.1086/230727 [ CrossRef ] [ Google Scholar ]
- Polachek S. W. (1981). Occupational Self-Selection: A Human Capital Approach to Sex Differences in Occupational Structure . Rev. Econ. Stat. 63 , 60. 10.2307/1924218 [ CrossRef ] [ Google Scholar ]
- Ponthieux S., Meurs D. (2015). “ Gender Inequality ,” in Handbook of Income Distribution: Volume 2 . Editors Atkinson A. B., Bourguignon F. (Burlington: Elsevier Science; ), 981–1146. 10.1016/b978-0-444-59428-0.00013-8 [ CrossRef ] [ Google Scholar ]
- Rabe-Hesketh S., Skrondal A. (2012). Multilevel and Longitudinal Modeling Using Stata . 3rd ed.. College Station, Tex: Stata Press. [ Google Scholar ]
- Radl J. (2013). Labour Market Exit and Social Stratification in Western Europe: The Effects of Social Class and Gender on the Timing of Retirement . Eur. Sociological Rev. 29 , 654–668. 10.1093/esr/jcs045 [ CrossRef ] [ Google Scholar ]
- Sackmann R. (2018). “ Demographie als Herausforderung für die Soziologie ,” in Handbuch Soziologie des Alter(n)s . Editors Schroeter K. R., Vogel C., Künemund H. (Wiesbaden: Springer VS; ), 1–23. 10.1007/978-3-658-09630-4_5-1 [ CrossRef ] [ Google Scholar ]
- Schröder H., Kersting A., Gilberg R., Steinwede J. (2013). Methodenbericht zur Haupterhebung lidA - leben in der Arbeit [Methodology Report of the main survey of lidA] . Available at: http://doku.iab.de/fdz/reporte/2013/MR_01-13.pdf (Accessed March 15, 2020).
- Sierminska E. M., Frick J. R., Grabka M. M. (2010). Examining the Gender Wealth gap . Oxford Econ. Pap. 62 , 669–690. 10.1093/oep/gpq007 [ CrossRef ] [ Google Scholar ]
- Singer J. D., Willett J. B. (2003). Applied Longitudinal Data Analysis: Modeling Change and Event Occurrence . Oxford: Oxford Univ. Press. [ Google Scholar ]
- Visser M., Gesthuizen M., Kraaykamp G., Wolbers M. H. J. (2016). Inequality Among Older Workers in the Netherlands: A Life Course and Social Stratification Perspective on Early Retirement . Eur. Sociol. Rev. 32 , 370–382. 10.1093/esr/jcw013 [ CrossRef ] [ Google Scholar ]
Click through the PLOS taxonomy to find articles in your field.
For more information about PLOS Subject Areas, click here .
Loading metrics
Open Access
Peer-reviewed
Research Article
The persistence of pay inequality: The gender pay gap in an anonymous online labor market
Roles Conceptualization, Data curation, Formal analysis, Investigation, Methodology, Project administration, Resources, Supervision, Writing – original draft, Writing – review & editing
* E-mail: [email protected] (LL); [email protected] (LB)
Affiliation Department of Psychology, Lander College, Flushing, New York, United States of America
Roles Conceptualization, Data curation, Formal analysis, Investigation, Methodology, Project administration, Writing – original draft, Writing – review & editing
Affiliation Department of Computer Science, Lander College, Flushing, New York, United States of America
Roles Formal analysis, Writing – original draft, Writing – review & editing
Affiliation Department of Health Policy & Management, Mailman School of Public Health, Columbia University, New York, New York, United States of America
Roles Conceptualization, Writing – review & editing
Affiliation Department of Clinical Psychology, Columbia University, New York, New York, United States of America

Roles Formal analysis
Affiliation Department of Computer Science, Stern College for Women, New York, New York, United States of America
Roles Conceptualization, Methodology, Writing – original draft, Writing – review & editing
Affiliation Department of Epidemiology, Mailman School of Public Health, Columbia University New York, New York, United States of America
- Leib Litman,
- Jonathan Robinson,
- Zohn Rosen,
- Cheskie Rosenzweig,
- Joshua Waxman,
- Lisa M. Bates
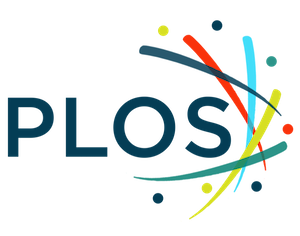
- Published: February 21, 2020
- https://doi.org/10.1371/journal.pone.0229383
- Reader Comments
Studies of the gender pay gap are seldom able to simultaneously account for the range of alternative putative mechanisms underlying it. Using CloudResearch, an online microtask platform connecting employers to workers who perform research-related tasks, we examine whether gender pay discrepancies are still evident in a labor market characterized by anonymity, relatively homogeneous work, and flexibility. For 22,271 Mechanical Turk workers who participated in nearly 5 million tasks, we analyze hourly earnings by gender, controlling for key covariates which have been shown previously to lead to differential pay for men and women. On average, women’s hourly earnings were 10.5% lower than men’s. Several factors contributed to the gender pay gap, including the tendency for women to select tasks that have a lower advertised hourly pay. This study provides evidence that gender pay gaps can arise despite the absence of overt discrimination, labor segregation, and inflexible work arrangements, even after experience, education, and other human capital factors are controlled for. Findings highlight the need to examine other possible causes of the gender pay gap. Potential strategies for reducing the pay gap on online labor markets are also discussed.
Citation: Litman L, Robinson J, Rosen Z, Rosenzweig C, Waxman J, Bates LM (2020) The persistence of pay inequality: The gender pay gap in an anonymous online labor market. PLoS ONE 15(2): e0229383. https://doi.org/10.1371/journal.pone.0229383
Editor: Luís A. Nunes Amaral, Northwestern University, UNITED STATES
Received: March 5, 2019; Accepted: February 5, 2020; Published: February 21, 2020
Copyright: © 2020 Litman et al. This is an open access article distributed under the terms of the Creative Commons Attribution License , which permits unrestricted use, distribution, and reproduction in any medium, provided the original author and source are credited.
Data Availability: Due to the sensitive nature of some of the data, and the terms of service of the websites used during data collection (including CloudResearch and MTurk), CloudResearch cannot release the full data set to make it publically available. The data are on CloudResearch's Sequel servers located at Queens College in the city of New York. CloudResearch makes data available to be accessed by researchers for replication purposes, on the CloudResearch premises, in the same way the data were accessed and analysed by the authors of this manuscript. The contact person at CloudResearch who can help researchers access the data set is Tzvi Abberbock, who can be reached at [email protected] .
Funding: The authors received no specific funding for this work.
Competing interests: We have read the journal's policy and the authors of this manuscript have the following potential competing interest: Several of the authors are employed at Cloud Research (previously TurkPrime), the database from which the data were queried. This does not alter our adherence to PLOS ONE policies on sharing data and materials.
Introduction
The gender pay gap, the disparity in earnings between male and female workers, has been the focus of empirical research in the US for decades, as well as legislative and executive action under the Obama administration [ 1 , 2 ]. Trends dating back to the 1960s show a long period in which women’s earnings were approximately 60% of their male counterparts, followed by increases in women’s earnings starting in the 1980s, which began to narrow, but not close, the gap which persists today [ 3 ]. More recent data from 2014 show that overall, the median weekly earnings of women working full time were 79–83% of what men earned [ 4 – 9 ].
The extensive literature seeking to explain the gender pay gap and its trajectory over time in traditional labor markets suggests it is a function of multiple structural and individual-level processes that reflect both the near-term and cumulative effects of gender relations and roles over the life course. Broadly speaking, the drivers of the gender pay gap can be categorized as: 1) human capital or productivity factors such as education, skills, and workforce experience; 2) industry or occupational segregation, which some estimates suggest accounts for approximately half of the pay gap; 3) gender-specific temporal flexibility constraints which can affect promotions and remuneration; and finally, 4) gender discrimination operating in hiring, promotion, task assignment, and/or compensation. The latter mechanism is often estimated by inference as a function of unexplained residual effects of gender on payment after accounting for other factors, an approach which is most persuasive in studies of narrowly restricted populations of workers such as lawyers [ 10 ] and academics of specific disciplines [ 11 ]. A recent estimate suggests this unexplained gender difference in earnings can account for approximately 40% of the pay gap [ 3 ]. However, more direct estimations of discriminatory processes are also available from experimental evidence, including field audit and lab-based studies [ 12 – 14 ]. Finally, gender pay gaps have also been attributed to differential discrimination encountered by men and women on the basis of parental status, often known as the ‘motherhood penalty’ [ 15 ].
Non-traditional ‘gig economy’ labor markets and the gender pay gap
In recent years there has been a dramatic rise in nontraditional ‘gig economy’ labor markets, which entail independent workers hired for single projects or tasks often on a short-term basis with minimal contractual engagement. “Microtask” platforms such as Amazon Mechanical Turk (MTurk) and Crowdflower have become a major sector of the gig economy, offering a source of easily accessible supplementary income through performance of small tasks online at a time and place convenient to the worker. Available tasks can range from categorizing receipts to transcription and proofreading services, and are posted online by the prospective employer. Workers registered with the platform then elect to perform the advertised tasks and receive compensation upon completion of satisfactory work [ 16 ]. An estimated 0.4% of US adults are currently receiving income from such platforms each month [ 17 ], and microtask work is a growing sector of the service economy in the United States [ 18 ]. Although still relatively small, these emerging labor market environments provide a unique opportunity to investigate the gender pay gap in ways not possible within traditional labor markets, due to features (described below) that allow researchers to simultaneously account for multiple putative mechanisms thought to underlie the pay gap.
The present study utilizes the Amazon Mechanical Turk (MTurk) platform as a case study to examine whether a gender pay gap remains evident when the main causes of the pay gap identified in the literature do not apply or can be accounted for in a single investigation. MTurk is an online microtask platform that connects employers (‘requesters’) to employees (‘workers’) who perform jobs called “Human Intelligence Tasks” (HITs). The platform allows requesters to post tasks on a dashboard with a short description of the HIT, the compensation being offered, and the time the HIT is expected to take. When complete, the requester either approves or rejects the work based on quality. If approved, payment is quickly accessible to workers. The gender of workers who complete these HITs is not known to the requesters, but was accessible to researchers for the present study (along with other sociodemographic information and pay rates) based on metadata collected through CloudResearch (formerly TurkPrime), a platform commonly used to conduct social and behavioral research on MTurk [ 19 ].
Evaluating pay rates of workers on MTurk requires estimating the pay per hour of each task that a worker accepts which can then be averaged together. All HITs posted on MTurk through CloudResearch display how much a HIT pays and an estimated time that it takes for that HIT to be completed. Workers use this information to determine what the corresponding hourly pay rate of a task is likely to be, and much of our analysis of the gender pay gap is based on this advertised pay rate of all completed surveys. We also calculate an estimate of the gender pay gap based on actual completion times to examine potential differences in task completion speed, which we refer to as estimated actual wages (see Methods section for details).
Previous studies have found that both task completion time and the selection of tasks influences the gender pay gap in at least some gig economy markets. For example, a gender pay gap was observed among Uber drivers, with men consistently earning higher pay than women [ 20 ]. Some of the contributing factors to this pay gap include that male Uber drivers selected different tasks than female drivers, including being more willing to work at night and to work in neighborhoods that were perceived to be more dangerous. Male drivers were also likely to drive faster than their female counterparts. These findings show that person-level factors like task selection, and speed can influence the gender pay gap within gig economy markets.
MTurk is uniquely suited to examine the gender pay gap because it is possible to account simultaneously for multiple structural and individual-level factors that have been shown to produce pay gaps. These include discrimination, work heterogeneity (leading to occupational segregation), and job flexibility, as well as human capital factors such as experience and education.
Discrimination.
When employers post their HITs on MTurk they have no way of knowing the demographic characteristics of the workers who accept those tasks, including their gender. While MTurk allows for selective recruitment of specific demographic groups, the MTurk tasks examined in this study are exclusively open to all workers, independent of their gender or other demographic characteristics. Therefore, features of the worker’s identity that might be the basis for discrimination cannot factor into an employer’s decision-making regarding hiring or pay.
Task heterogeneity.
Another factor making MTurk uniquely suited for the examination of the gender pay gap is the relative homogeneity of tasks performed by the workers, minimizing the potential influence of gender differences in the type of work pursued on earnings and the pay gap. Work on the MTurk platform consists mostly of short tasks such as 10–15 minute surveys and categorization tasks. In addition, the only information that workers have available to them to choose tasks, other than pay, is the tasks’ titles and descriptions. We additionally classified tasks based on similarity and accounted for possible task heterogeneity effects in our analyses.
Job flexibility.
MTurk is not characterized by the same inflexibilities as are often encountered in traditional labor markets. Workers can work at any time of the day or day of the week. This increased flexibility may be expected to provide more opportunities for participation in this labor market for those who are otherwise constrained by family or other obligations.
Human capital factors.
It is possible that the more experienced workers could learn over time how to identify higher paying tasks by virtue of, for example, identifying qualities of tasks that can be completed more quickly than the advertised required time estimate. Further, if experience is correlated with gender, it could contribute to a gender pay gap and thus needs to be controlled for. Using CloudResearch metadata, we are able to account for experience on the platform. Additionally, we account for multiple sociodemographic variables, including age, marital status, parental status, education, income (from all sources), and race using the sociodemographic data available through CloudResearch.
Expected gender pay gap findings on MTurk
Due to the aforementioned factors that are unique to the MTurk marketplace–e.g., anonymity, self-selection into tasks, relative homogeneity of the tasks performed, and flexible work scheduling–we did not expect a gender pay gap to be evident on the platform to the same extent as in traditional labor markets. However, potential gender differences in task selection and completion speed, which have implications for earnings, merit further consideration. For example, though we expect the relative homogeneity of the MTurk tasks to minimize gender differences in task selection that could mimic occupational segregation, we do account for potential subtle residual differences in tasks that could differentially attract male and female workers and indirectly lead to pay differentials if those tasks that are preferentially selected by men pay a higher rate. To do this we categorize all tasks based on their descriptions using K-clustering and add the clusters as covariates to our models. In addition, we separately examine the gender pay gap within each topic-cluster.
In addition, if workers who are experienced on the platform are better able to find higher paying HITs, and if experience is correlated with gender, it may lead to gender differences in earnings. Theoretically, other factors that may vary with gender could also influence task selection. Previous studies of the pay gap in traditional markets indicate that reservation wages, defined as the pay threshold at which a person is willing to accept work, may be lower among women with children compared to women without, and to that of men as well [ 21 ]. Thus, if women on MTurk are more likely to have young children than men, they may be more willing to accept available work even if it pays relatively poorly. Other factors such as income, education level, and age may similarly influence reservation wages if they are associated with opportunities to find work outside of microtask platforms. To the extent that these demographics correlate with gender they may give rise to a gender pay gap. Therefore we consider age, experience on MTurk, education, income, marital status, and parental status as covariates in our models.
Task completion speed may vary by gender for several reasons, including potential gender differences in past experience on the platform. We examine the estimated actual pay gap per hour based on HIT payment and estimated actual completion time to examine the effects of completion speed on the wage gap. We also examine the gender pay gap based on advertised pay rates, which are not dependent on completion speed and more directly measure how gender differences in task selection can lead to a pay gap. Below, we explain how these were calculated based on meta-data from CloudResearch.
To summarize, the overall goal of the present study was to explore whether gender pay differentials arise within a unique, non-traditional and anonymous online labor market, where known drivers of the gender pay gap either do not apply or can be accounted for statistically.
Materials and methods
Amazon mechanical turk and cloudresearch..
Started in 2005, the original purpose of the Amazon Mechanical Turk (MTurk) platform was to allow requesters to crowdsource tasks that could not easily be handled by existing technological solutions such as receipt copying, image categorization, and website testing. As of 2010, researchers increasingly began using MTurk for a wide variety of research tasks in the social, behavioral, and medical sciences, and it is currently used by thousands of academic researchers across hundreds of academic departments [ 22 ]. These research-related HITs are typically listed on the platform in generic terms such as, “Ten-minute social science study,” or “A study about public opinion attitudes.”
Because MTurk was not originally designed solely for research purposes, its interface is not optimized for some scientific applications. For this reason, third party add-on toolkits have been created that offer critical research tools for scientific use. One such platform, CloudResearch (formerly TurkPrime), allows requesters to manage multiple research functions, such as applying sampling criteria and facilitating longitudinal studies, through a link to their MTurk account. CloudResearch’s functionality has been described extensively elsewhere [ 19 ]. While the demographic characteristics of workers are not available to MTurk requesters, we were able to retroactively identify the gender and other demographic characteristics of workers through the CloudResearch platform. CloudResearch also facilitates access to data for each HIT, including pay, estimated length, and title.
The study was an analysis of previously collected metadata, which were analyzed anonymously. We complied with the terms of service for all data collected from CloudResearch, and MTurk. The approving institutional review board for this study was IntegReview.
Analytic sample.
We analyzed the nearly 5 million tasks completed during an 18-month period between January 2016 and June 2017 by 12,312 female and 9,959 male workers who had complete data on key demographic characteristics. To be included in the analysis a HIT had to be fully completed, not just accepted, by the worker, and had to be accepted (paid for) by the requester. Although the vast majority of HITs were open to both males and females, a small percentage of HITs are intended for a specific gender. Because our goal was to exclusively analyze HITs for which the requesters did not know the gender of workers, we excluded any HITs using gender-specific inclusion or exclusion criteria from the analyses. In addition, we removed from the analysis any HITs that were part of follow-up studies in which it would be possible for the requester to know the gender of the worker from the prior data collection. Finally, where possible, CloudResearch tracks demographic information on workers across multiple HITs over time. To minimize misclassification of gender, we excluded the 0.3% of assignments for which gender was unknown with at least 95% consistency across HITs.
The main exposure variable is worker gender and the outcome variables are estimated actual hourly pay accrued through completing HITs, and advertised hourly pay for completed HITs. Estimated actual hourly wages are based on the estimated length in minutes and compensation in dollars per HIT as posted on the dashboard by the requester. We refer to actual pay as estimated because sometimes people work multiple assignments at the same time (which is allowed on the platform), or may simultaneously perform other unrelated activities and therefore not work on the HIT the entire time the task is open. We also considered several covariates to approximate human capital factors that could potentially influence earnings on this platform, including marital status, education, household income, number of children, race/ethnicity, age, and experience (number of HITs previously completed). Additional covariates included task length, task cluster (see below), and the serial order with which workers accepted the HIT in order to account for potential differences in HIT acceptance speed that may relate to the pay gap.
Database and analytic approach.
Data were exported from CloudResearch’s database into Stata in long-form format to represent each task on a single row. For the purposes of this paper, we use “HIT” and “study” interchangeably to refer to a study put up on the MTurk dashboard which aims to collect data from multiple participants. A HIT or study consist of multiple “assignments” which is a single task completed by a single participant. Columns represented variables such as demographic information, payment, and estimated HIT length. Column variables also included unique IDs for workers, HITs (a single study posted by a requester), and requesters, allowing for a multi-level modeling analytic approach with assignments nested within workers. Individual assignments (a single task completed by a single worker) were the unit of analysis for all models.
Linear regression models were used to calculate the gender pay gap using two dependent variables 1) women’s estimated actual earnings relative to men’s and 2) women’s selection of tasks based on advertised earnings relative to men’s. We first examined the actual pay model, to see the gender pay gap when including an estimate of task completion speed, and then adjusted this model for advertised hourly pay to determine if and to what extent a propensity for men to select more remunerative tasks was evident and driving any observed gender pay gap. We additionally ran separate models using women’s advertised earnings relative to men’s as the dependent variable to examine task selection effects more directly. The fully adjusted models controlled for the human capital-related covariates, excluding household income and education which were balanced across genders. These models also tested for interactions between gender and each of the covariates by adding individual interaction terms to the adjusted model. To control for within-worker clustering, Huber-White standard error corrections were used in all models.
Cluster analysis.
To explore the potential influence of any residual task heterogeneity and gender preference for specific task type as the cause of the gender pay gap, we use K-means clustering analysis (seed = 0) to categorize the types of tasks into clusters based on the descriptions that workers use to choose the tasks they perform. We excluded from this clustering any tasks which contained certain gendered words (such as “male”, “female”, etc.) and any tasks which had fewer than 30 respondents. We stripped out all punctuation, symbols and digits from the titles, so as to remove any reference to estimated compensation or duration. The features we clustered on were the presence or absence of 5,140 distinct words that appeared across all titles. We then present the distribution of tasks across these clusters as well as average pay by gender and the gender pay gap within each cluster.
The demographics of the analytic sample are presented in Table 1 . Men and women completed comparable numbers of tasks during the study period; 2,396,978 (48.6%) for men and 2,539,229 (51.4%) for women.
- PPT PowerPoint slide
- PNG larger image
- TIFF original image
https://doi.org/10.1371/journal.pone.0229383.t001
In Table 2 we measure the differences in remuneration between genders, and then decompose any observed pay gap into task completion speed, task selection, and then demographic and structural factors. Model 1 shows the unadjusted regression model of gender differences in estimated actual pay, and indicates that, on average, tasks completed by women paid 60 (10.5%) cents less per hour compared to tasks completed by men (t = 17.4, p < .0001), with the mean estimated actual pay across genders being $5.70 per hour.
https://doi.org/10.1371/journal.pone.0229383.t002
In Model 2, adjusting for advertised hourly pay, the gender pay gap dropped to 46 cents indicating that 14 cents of the pay gap is attributable to gender differences in the selection of tasks (t = 8.6, p < .0001). Finally, after the inclusion of covariates and their interactions in Model 3, the gender pay differential was further attenuated to 32 cents (t = 6.7, p < .0001). The remaining 32 cent difference (56.6%) in earnings is inferred to be attributable to gender differences in HIT completion speed.
Task selection analyses
Although completion speed appears to account for a significant portion of the pay gap, of particular interest are gender differences in task selection. Beyond structural factors such as education, household composition and completion speed, task selection accounts for a meaningful portion of the gender pay gap. As a reminder, the pay rate and expected completion time are posted for every HIT, so why women would select less remunerative tasks on average than men do is an important question to explore. In the next section of the paper we perform a set of analyses to examine factors that could account for this observed gender difference in task selection.
Advertised hourly pay.
To examine gender differences in task selection, we used linear regression to directly examine whether the advertised hourly pay differed for tasks accepted by male and female workers. We first ran a simple model ( Table 3 ; Model 3A) on the full dataset of 4.93 million HITs, with gender as the predictor and advertised hourly pay as the outcome including no other covariates. The unadjusted regression results (Model 4) shown in Table 3 , indicates that, summed across all clusters and demographic groups, tasks completed by women were advertised as paying 28 cents (95% CI: $0.25-$0.31) less per hour (5.8%) compared to tasks completed by men (t = 21.8, p < .0001).
https://doi.org/10.1371/journal.pone.0229383.t003
Model 5 examines whether the remuneration differences for tasks selected by men and women remains significant in the presence of multiple covariates included in the previous model and their interactions. The advertised pay differential for tasks selected by women compared to men was attenuated to 21 cents (4.3%), and remained statistically significant (t = 9.9, p < .0001). This estimate closely corresponded to the inferred influence of task selection reported in Table 2 . Tests of gender by covariate interactions were significant only in the cases of age and marital status; the pay differential in tasks selected by men and women decreased with age and was more pronounced among single versus currently or previously married women.
To further examine what factors may account for the observed gender differences in task selection we plotted the observed pay gap within demographic and other covariate groups. Table 4 shows the distribution of tasks completed by men and women, as well as mean earnings and the pay gap across all demographic groups, based on the advertised (not actual) hourly pay for HITs selected (hereafter referred to as “advertised hourly pay” and the “advertised pay gap”). The average task was advertised to pay $4.88 per hour (95% CI $4.69, $5.10).
https://doi.org/10.1371/journal.pone.0229383.t004
The pattern across demographic characteristics shows that the advertised hourly pay gap between genders is pervasive. Notably, a significant advertised gender pay gap is evident in every level of each covariate considered in Table 4 , but more pronounced among some subgroups of workers. For example, the advertised pay gap was highest among the youngest workers ($0.31 per hour for workers age 18–29), and decreased linearly with age, declining to $0.13 per hour among workers age 60+. Advertised houry gender pay gaps were evident across all levels of education and income considered.
To further examine the potential influence of human capital factors on the advertised hourly pay gap, Table 5 presents the average advertised pay for selected tasks by level of experience on the CloudResearch platform. Workers were grouped into 4 experience levels, based on the number of prior HITs completed: Those who completed fewer than 100 HITs, between 100 and 500 HITs, between 500 and 1,000 HITs, and more than 1,000 HITs. A significant gender difference in advertised hourly pay was observed within each of these four experience groups. The advertised hourly pay for tasks selected by both male and female workers increased with experience, while the gender pay gap decreases. There was some evidence that male workers have more cumulative experience with the platform: 43% of male workers had the highest level of experience (previously completing 1,001–10,000 HITs) compared to only 33% of women.
https://doi.org/10.1371/journal.pone.0229383.t005
Table 5 also explores the influence of task heterogeneity upon HIT selection and the gender gap in advertised hourly pay. K-means clustering was used to group HITs into 20 clusters initially based on the presence or absence of 5,140 distinct words appearing in HIT titles. Clusters with fewer than 50,000 completed tasks were then excluded from analysis. This resulted in 13 clusters which accounted for 94.3% of submitted work assignments (HITs).
The themes of all clusters as well as the average hourly advertised pay for men and women within each cluster are presented in the second panel of Table 5 . The clusters included categories such as Games, Decision making, Product evaluation, Psychology studies, and Short Surveys. We did not observe a gender preference for any of the clusters. Specifically, for every cluster, the proportion of males was no smaller than 46.6% (consistent with the slightly lower proportion of males on the platform, see Table 1 ) and no larger than 50.2%. As shown in Table 5 , the gender pay gap was observed within each of the clusters. These results suggest that residual task heterogeneity, a proxy for occupational segregation, is not likely to contribute to a gender pay gap in this market.
Task length was defined as the advertised estimated duration of a HIT. Table 6 presents the advertised hourly gender pay gaps for five categories of HIT length, which ranged from a few minutes to over 1 hour. Again, a significant advertised hourly gender pay gap was observed in each category.
https://doi.org/10.1371/journal.pone.0229383.t006
Finally, we conducted additional supplementary analyses to determine if other plausible factors such as HIT timing could account for the gender pay gap. We explored temporal factors including hour of the day and day of the week. Each completed task was grouped based on the hour and day in which it was completed. A significant advertised gender pay gap was observed within each of the 24 hours of the day and for every day of the week demonstrating that HIT timing could not account for the observed gender gap (results available in Supplementary Materials).
In this study we examined the gender pay gap on an anonymous online platform across an 18-month period, during which close to five million tasks were completed by over 20,000 unique workers. Due to factors that are unique to the Mechanical Turk online marketplace–such as anonymity, self-selection into tasks, relative homogeneity of the tasks performed, and flexible work scheduling–we did not expect earnings to differ by gender on this platform. However, contrary to our expectations, a robust and persistent gender pay gap was observed.
The average estimated actual pay on MTurk over the course of the examined time period was $5.70 per hour, with the gender pay differential being 10.5%. Importantly, gig economy platforms differ from more traditional labor markets in that hourly pay largely depends on the speed with which tasks are completed. For this reason, an analysis of gender differences in actual earned pay will be affected by gender differences in task completion speed. Unfortunately, we were not able to directly measure the speed with which workers complete tasks and account for this factor in our analysis. This is because workers have the ability to accept multiple HITs at the same time and multiple HITs can sit dormant in a queue, waiting for workers to begin to work on them. Therefore, the actual time that many workers spend working on tasks is likely less than what is indicated in the metadata available. For this reason, the estimated average actual hourly rate of $5.70 is likely an underestimate and the gender gap in actual pay cannot be precisely measured. We infer however, by the residual gender pay gap after accounting for other factors, that as much as 57% (or $.32) of the pay differential may be attributable to task completion speed. There are multiple plausible explanations for gender differences in task completion speed. For example, women may be more meticulous at performing tasks and, thus, may take longer at completing them. There may also be a skill factor related to men’s greater experience on the platform (see Table 5 ), such that men may be faster on average at completing tasks than women.
However, our findings also revealed another component of a gender pay gap on this platform–gender differences in the selection of tasks based on their advertised pay. Because the speed with which workers complete tasks does not impact these estimates, we conducted extensive analyses to try to explain this gender gap and the reasons why women appear on average to be selecting tasks that pay less compared to men. These results pertaining to the advertised gender pay gap constitute the main focus of this study and the discussion that follows.
The overall advertised hourly pay was $4.88. The gender pay gap in the advertised hourly pay was $0.28, or 5.8% of the advertised pay. Once a gender earnings differential was observed based on advertised pay, we expected to fully explain it by controlling for key structural and individual-level covariates. The covariates that we examined included experience, age, income, education, family composition, race, number of children, task length, the speed of accepting a task, and thirteen types of subtasks. We additionally examined the time of day and day of the week as potential explanatory factors. Again, contrary to our expectations, we observed that the pay gap persisted even after these potential confounders were controlled for. Indeed, separate analyses that examined the advertised pay gap within each subcategory of the covariates showed that the pay gap is ubiquitous, and persisted within each of the ninety sub-groups examined. These findings allows us to rule out multiple mechanisms that are known drivers of the pay gap in traditional labor markets and other gig economy marketplaces. To our knowledge this is the only study that has observed a pay gap across such diverse categories of workers and conditions, in an anonymous marketplace, while simultaneously controlling for virtually all variables that are traditionally implicated as causes of the gender pay gap.
Individual-level factors
Individual-level factors such as parental status and family composition are a common source of the gender pay gap in traditional labor markets [ 15 ] . Single mothers have previously been shown to have lower reservation wages compared to other men and women [ 21 ]. In traditional labor markets lower reservation wages lead single mothers to be willing to accept lower-paying work, contributing to a larger gender pay gap in this group. This pattern may extend to gig economy markets, in which single mothers may look to online labor markets as a source of supplementary income to help take care of their children, potentially leading them to become less discriminating in their choice of tasks and more willing to work for lower pay. Since female MTurk workers are 20% more likely than men to have children (see Table 1 ), it was critical to examine whether the gender pay gap may be driven by factors associated with family composition.
An examination of the advertised gender pay gap among individuals who differed in their marital and parental status showed that while married workers and those with children are indeed willing to work for lower pay (suggesting that family circumstances do affect reservation wages and may thus affect the willingness of online workers to accept lower-paying online tasks), women’s hourly pay is consistently lower than men’s within both single and married subgroups of workers, and among workers who do and do not have children. Indeed, contrary to expectations, the advertised gender pay gap was highest among those workers who are single, and among those who do not have any children. This observation shows that it is not possible for parental and family status to account for the observed pay gap in the present study, since it is precisely among unmarried individuals and those without children that the largest pay gap is observed.
Age was another factor that we considered to potentially explain the gender pay gap. In the present sample, the hourly pay of older individuals is substantially lower than that of younger workers; and women on the platform are five years older on average compared to men (see Table 1 ). However, having examined the gender pay gap separately within five different age cohorts we found that the largest pay gap occurs in the two youngest cohort groups: those between 18 and 29, and between 30 and 39 years of age. These are also the largest cohorts, responsible for 64% of completed work in total.
Younger workers are also most likely to have never been married or to not have any children. Thus, taken together, the results of the subgroup analyses are consistent in showing that the largest pay gap does not emerge from factors relating to parental, family, or age-related person-level factors. Similar patterns were found for race, education, and income. Specifically, a significant gender pay gap was observed within each subgroup of every one of these variables, showing that person-level factors relating to demographics are not driving the pay gap on this platform.
Experience is a factor that has an influence on the pay gap in both traditional and gig economy labor markets [ 20 ] . As noted above, experienced workers may be faster and more efficient at completing tasks in this platform, but also potentially more savvy at selecting more remunerative tasks compared to less experienced workers if, for example, they are better at selecting tasks that will take less time to complete than estimated on the dashboard [ 20 ]. On MTurk, men are overall more experienced than women. However, experience does not account for the gender gap in advertised pay in the present study. Inexperienced workers comprise the vast majority of the Mechanical Turk workforce, accounting for 67% of all completed tasks (see Table 5 ). Yet within this inexperienced group, there is a consistent male earning advantage based on the advertised pay for tasks performed. Further, controlling for the effect of experience in our models has a minimal effect on attenuating the gender pay gap.
Task heterogeneity
Another important source of the gender pay gap in both traditional and gig economy labor markets is task heterogeneity. In traditional labor markets men are disproportionately represented in lucrative fields, such as those in the tech sector [ 23 ]. While the workspace within MTurk is relatively homogeneous compared to the traditional labor market, there is still some variety in the kinds of tasks that are available, and men and women may have been expected to have preferences that influence choices among these.
To examine whether there is a gender preference for specific tasks, we systematically analyzed the textual descriptions of all tasks included in this study. These textual descriptions were available for all workers to examine on their dashboards, along with information about pay. The clustering algorithm revealed thirteen categories of tasks such as games, decision making, several different kinds of survey tasks, and psychology studies.We did not observe any evidence of gender preference for any of the task types. Within each of the thirteen clusters the distribution of tasks was approximately equally split between men and women. Thus, there is no evidence that women as a group have an overall preference for specific tasks compared to men. Critically, the gender pay gap was also observed within each one of these thirteen clusters.
Another potential source of heterogeneity is task length. Based on traditional labor markets, one plausible hypothesis about what may drive women’s preferences for specific tasks is that women may select tasks that differ in their duration. For example, women may be more likely to use the platform for supplemental income, while men may be more likely to work on HITs as their primary income source. Women may thus select shorter tasks relative to their male counterparts. If the shorter tasks pay less money, this would result in what appears to be a gender pay gap.
However, we did not observe gender differences in task selection based on task duration. For example, having divided tasks into their advertised length, the tasks are preferred equally by men and women. Furthermore, the shorter tasks’ hourly pay is substantially higher on average compared to longer tasks.
Additional evidence that scheduling factors do not drive the gender pay gap is that it was observed within all hourly and daily intervals (See S1 and S2 Tables in Appendix). These data are consistent with the results presented above regarding personal level factors, showing that the majority of male and female Mechanical Turk workers are single, young, and have no children. Thus, while in traditional labor markets task heterogeneity and labor segmentation is often driven by family and other life circumstances, the cohort examined in this study does not appear to be affected by these factors.
Practical implications of a gender pay gap on online platforms for social and behavioral science research
The present findings have important implications for online participant recruitment in the social and behavioral sciences, and also have theoretical implications for understanding the mechanisms that give rise to the gender pay gap. The last ten years have seen a revolution in data collection practices in the social and behavioral sciences, as laboratory-based data collection has slowly and steadily been moving online [ 16 , 24 ]. Mechanical Turk is by far the most widely used source of human participants online, with thousands of published peer-reviewed papers utilizing Mechanical Turk to recruit at least some of their human participants [ 25 ]. The present findings suggest both a challenge and an opportunity for researchers utilizing online platforms for participant recruitment. Our findings clearly reveal for the first time that sampling research participants on anonymous online platforms tends to produce gender pay inequities, and that this happens independent of demographics or type of task. While it is not clear from our findings what the exact cause of this inequity is, what is clear is that the online sampling environment produces similar gender pay inequities as those observed in other more traditional labor markets, after controlling for relevant covariates.
This finding is inherently surprising since many mechanisms that are known to produce the gender pay gap in traditional labor markets are not at play in online microtasks environments. Regardless of what the generative mechanisms of the gender pay gap on online microtask platforms might be, researchers may wish to consider whether changes in their sampling practices may produce more equitable pay outcomes. Unlike traditional labor markets, online data collection platforms have built-in tools that can allow researchers to easily fix gender pay inequities. Researchers can simply utilize gender quotas, for example, to fix the ratio of male and female participants that they recruit. These simple fixes in sampling practices will not only produce more equitable pay outcomes but are also most likely advantageous for reducing sampling bias due to gender being correlated with pay. Thus, while our results point to a ubiquitous discrepancy in pay between men and women on online microtask platforms, such inequities have relatively easy fixes on online gig economy marketplaces such as MTurk, compared to traditional labor markets where gender-based pay inequities have often remained intractable.
Other gig economy markets
As discussed in the introduction, a gender wage gap has been demonstrated on Uber, a gig economy transportation marketplace [ 20 ], where men earn approximately 7% more than women. However, unlike in the present study, the gender wage gap on Uber was fully explained by three factors; a) driving speed predicted higher wages, with men driving faster than women, b) men were more likely than women to drive in congested locations which resulted in better pay, c) experience working for Uber predicted higher wages, with men being more experienced. Thus, contrary to our findings, the gender wage gap in gig economy markets studied thus far are fully explained by task heterogeneity, experience, and task completion speed. To our knowledge, the results presented in the present study are the first to show that the gender wage gap can emerge independent of these factors.
Generalizability
Every labor market is characterized by a unique population of workers that are almost by definition not a representation of the general population outside of that labor market. Likewise, Mechanical Turk is characterized by a unique population of workers that is known to differ from the general population in several ways. Mechanical Turk workers are younger, better educated, less likely to be married or have children, less likely to be religious, and more likely to have a lower income compared to the general United States population [ 24 ]. The goal of the present study was not to uncover universal mechanisms that generate the gender pay gap across all labor markets and demographic groups. Rather, the goal was to examine a highly unique labor environment, characterized by factors that should make this labor market immune to the emergence of a gender pay gap.
Previous theories accounting for the pay gap have identified specific generating mechanisms relating to structural and personal factors, in addition to discrimination, as playing a role in the emergence of the gender pay gap. This study examined the work of over 20,000 individuals completing over 5 million tasks, under conditions where standard mechanisms that generate the gender pay gap have been controlled for. Nevertheless, a gender pay gap emerged in this environment, which cannot be accounted for by structural factors, demographic background, task preferences, or discrimination. Thus, these results reveal that the gender pay gap can emerge—in at least some labor markets—in which discrimination is absent and other key factors are accounted for. These results show that factors which have been identified to date as giving rise to the gender pay gap are not sufficient to explain the pay gap in at least some labor markets.
Potential mechanisms
While we cannot know from the results of this study what the actual mechanism is that generates the gender pay gap on online platforms, we suggest that it may be coming from outside of the platform. The particular characteristics of this labor market—such as anonymity, relative task homogeneity, and flexibility—suggest that, everything else being equal, women working in this platform have a greater propensity to choose less remunerative opportunities relative to men. It may be that these choices are driven by women having a lower reservation wage compared to men [ 21 , 26 ]. Previous research among student populations and in traditional labor markets has shown that women report lower pay or reward expectations than men [ 27 – 29 ]. Lower pay expectations among women are attributed to justifiable anticipation of differential returns to labor due to factors such as gender discrimination and/or a systematic psychological bias toward pessimism relative to an overly optimistic propensity among men [ 30 ].
Our results show that even if the bias of employers is removed by hiding the gender of workers as happens on MTurk, it seems that women may select lower paying opportunities themselves because their lower reservation wage influences the types of tasks they are willing to work on. It may be that women do this because cumulative experiences of pervasive discrimination lead women to undervalue their labor. In turn, women’s experiences with earning lower pay compared to men on traditional labor markets may lower women’s pay expectations on gig economy markets. Thus, consistent with these lowered expectations, women lower their reservation wages and may thus be more likely than men to settle for lower paying tasks.
More broadly, gender norms, psychological attributes, and non-cognitive skills, have recently become the subject of investigation as a potential source for the gender pay gap [ 3 ], and the present findings indicate the importance of such mechanisms being further explored, particularly in the context of task selection. More research will be required to explore the potential psychological and antecedent structural mechanisms underlying differential task selection and expectations of compensation for time spent on microtask platforms, with potential relevance to the gender pay gap in traditional labor markets as well. What these results do show is that pay discrepancies can emerge despite the absence of discrimination in at least some circumstances. These results should be of particular interest for researchers who may wish to see a more equitable online labor market for academic research, and also suggest that novel and heretofore unexplored mechanisms may be at play in generating these pay discrepancies.
A final note about framing: we are aware that explanations of the gender pay gap that invoke elements of women’s agency and, more specifically, “choices” risk both; a) diminishing or distracting from important structural factors, and b) “naturalizing” the status quo of gender inequality [ 30 ] . As Connor and Fiske (2019) argue, causal attributions for the gender pay gap to “unconstrained choices” by women, common as part of human capital explanations, may have the effect, intended or otherwise, of reinforcing system-justifying ideologies that serve to perpetuate inequality. By explicitly locating women’s economic decision making on the MTurk platform in the broader context of inegalitarian gender norms and labor market experiences outside of it (as above), we seek to distance our interpretation of our findings from implicit endorsement of traditional gender roles and economic arrangements and to promote further investigation of how the observed gender pay gap in this niche of the gig economy may reflect both broader gender inequalities and opportunities for structural remedies.
Supporting information
S1 table. distribution of hits, average pays, and gender pay gaps by hour of day..
https://doi.org/10.1371/journal.pone.0229383.s001
S2 Table. Distribution of HITs, average pays, and gender pay gaps by day of the week.
https://doi.org/10.1371/journal.pone.0229383.s002
- 1. United States Equal Employment Opportunity Commission, Lily Ledbetter Fair Pay Act of 2009 (2009), available at https://www.eeoc.gov/eeoc/publications/brochure- equal_pay_and_ledbetter_act.cfm, accessed on 11/12/2018.
- 2. United States Department of Labor (DOL), Office of Federal Contract Compliance Programs (OFCCP), Pay Transparency Nondiscrimination Provision, available at https://www.dol.gov/ofccp/PayTransparencyNondiscrimination.html , accessed on 11/12/2018.
- View Article
- Google Scholar
- 4. United States Department of Labor (DOL), Bureau of Labor Statistics (BLS) (2016) Women’s earning 83 percent of men’s, but vary by occupation. TED Econ Dly , available at https://www.bls.gov/opub/ted/2016/womens-earnings-83-percent-of-mens-but-vary-by-occupation.htm , accessed on 11/12/2018.
- 5. Davis A (2015) Women still earn less than men across the board (Economic Policy Institute, 2015), available at http://www.epi.org/publication/women-still-earn-less-than-men-across-the-board/ , accessed on 11/12/2018.
- 6. “Gender Pay Inequality: Consequences for Women, Families and the Economy” (Joint Economic Committee, 2016). [no author]
- 7. Hartmann H, Hayes J, Clark J (2014) “How Equal Pay for Working Women would Reduce Poverty and Grow the American Economy” (Institute for Women’s Policy Research, 2014).
- 8. OECD (2015) In it together: Why Less Inequality Benefits All (OECD Publishing, Paris) available at http://www.oecd.org/els/soc/OECD2015-In-It-Together-Chapter1-Overview-Inequality.pdf , accessed on 11/12/2018.
- PubMed/NCBI
- 16. Litman L, Robinson J (In Press) Conducting Online Research on Amazon Mechanical Turk and Beyond. Sage Publications.
- 17. Farrell D, Greig F (2016) Paychecks, paydays, and the online platform economy: Big data on income volatility. JP Morgan Chase Institute.
- 18. Kuek SC, Paradi-Guilford C, Fayomi T, Imaizumi S, Ipeirotis P, Pina P, Singh M (2015) The global opportunity in online outsourcing (World Bank Group, 2015) Available at http://documents.worldbank.org/curated/en/138371468000900555/pdf/ACS14228-ESW-white-cover-P149016-Box391478B-PUBLIC-World-Bank-Global-OO-Study-WB-Rpt-FinalS.pdf , accessed on 11/12/2018.
- 23. Bureau of Labor Statistics, U.S. Department of Labor, Labor Force Statistics from the Current Population Survey, Household Data Annual Averages. Employed persons by detailed occupation, sex, race, and Hispanic or Latino ethnicity, on the Internet at https://www.bls.gov/cps/cpsaat11.htm (visited 9/3/18).
Advertisement
Gender pay gap perception: a five-country European study
- Original Paper
- Published: 08 November 2021
- Volume 1 , article number 267 , ( 2021 )
Cite this article
- Giulia Lausi ORCID: orcid.org/0000-0002-6676-2230 1 ,
- Jessica Burrai ORCID: orcid.org/0000-0002-3223-4421 1 , 2 ,
- Benedetta Barchielli ORCID: orcid.org/0000-0001-8703-8578 2 ,
- Alessandro Quaglieri ORCID: orcid.org/0000-0003-2341-1876 1 ,
- Emanuela Mari ORCID: orcid.org/0000-0003-2367-3139 1 ,
- Angelo Fraschetti ORCID: orcid.org/0000-0003-1701-5789 1 ,
- Fabrizio Paloni 1 ,
- Pierluigi Cordellieri ORCID: orcid.org/0000-0002-6044-7109 1 ,
- Fabio Ferlazzo ORCID: orcid.org/0000-0003-1083-7624 1 &
- Anna Maria Giannini ORCID: orcid.org/0000-0002-0614-4457 1
891 Accesses
2 Citations
Explore all metrics
Over the past several decades, public administrations have attempted to identify how gender differences affect employment opportunities and social inequalities, which has led to a growing body of literature. However, sufficient and valid conclusions are not yet available to identify the reasons for the gender pay gap (GPG). Building on key theoretical models to explain the wage gap, our research, based on a short survey, aimed to identify which factors could be related to the perception of GPG among employees of small- and medium-sized enterprises in five European countries. Moreover, we investigated the possible relationships between personal characteristics such as gender, age, job satisfaction, gender orientation (which is categorized as ''Negative Gender Orientation'', i.e., sexist beliefs, and ''Positive Gender Orientation'', i.e., perceived gender equality in society), and the GPG and tried to estimate a possible functional relationship between the perceived GPG and the decision-making style. The results revealed differences between personal characteristics and perceptions of the GPG; the findings were discussed in accordance with the present literature on the topic.
This is a preview of subscription content, log in via an institution to check access.
Access this article
Price includes VAT (Russian Federation)
Instant access to the full article PDF.
Rent this article via DeepDyve
Institutional subscriptions
Similar content being viewed by others
Work-life balance: an integrative review.
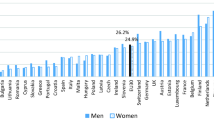
Flexible Working, Work–Life Balance, and Gender Equality: Introduction
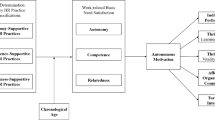
Bringing Self-Determination Theory to the Forefront: Examining How Human Resource Practices Motivate Employees of All Ages to Succeed
Data availability.
The data for this paper are available upon request.
Anderson T, Forth J, Metcalf H, Kirby S (2001) The gender pay gap. Final Report to the Women and Equality Unit, Cabinet Office Women and Equality Unit, London. https://doi.org/10.1177/0950017004040765
Becker GS (1985) Human capital, effort, and the sexual division of labour. J Labour Econ 3(1, Part 2):S33–S58. https://doi.org/10.1086/298075
Article Google Scholar
Becker GS (1991) A treatise on the family, enl. Harvard University Press, Cambridge
Google Scholar
Betsch C (2004) Prafrenz fuer intuition und deliberation. Inventar zur erfassung von affektund kognitionsbasiertem entscheiden [Preference for intuition and deliberation (PID): An inventory for assessing affect- and cognition-based decision-making]. Zeitschrift Fuer Differentielle Und Diagnostische Psychologie 25:179–197. https://doi.org/10.1024/0170-1789.25.4.179
Betsch C, Kuntz JJ (2008) Individual strategy preferences and decisional fit. J Behav Dec Making 21:532–555. https://doi.org/10.1002/bdm.600
Bishu SG, Alkadry MG (2017) A systematic review of the gender pay gap and factors that predict it. Adm Soc 49(1):65–104. https://doi.org/10.1177/0095399716636928
Blau FD, Kahn LM (1997) Swimming upstream: trends in the gender wage differential in the 1980s. J Labor Econ 15(11):1–42. https://doi.org/10.1086/209845
Blau FD, Kahn LM (2003) Understanding international differences in the gender pay gap. J Law Econ 21(1):106–144. https://doi.org/10.1086/344125
Blau FD, Kahn LM (2006) The US gender pay gap in the 1990s: slowing convergence. ILR Rev 60(1):45–66. https://doi.org/10.1177/001979390606000103
Boadway R, Marchand M, Pestieau P, del Mar Racionero M (2002) Optimal redistribution with heterogeneous preferences for leisure. J Public Econ Theory 4(4):475–498. https://doi.org/10.1111/1097-3923.00106
Boll C, Lagemann A (2018) Gender pay gap in EU countries based on SES (2014). Publ Office Eur Union 10:978935
Cappelen AW, Tungodden B (2017) Fairness and the proportionality principle. Soc Choice Welf 49(3):709–719. https://doi.org/10.1007/s00355-016-1016-6
Cappelen AW, Eichele T, Hugdahl K, Specht K, Sørensen EØ, Tungodden B (2014) Equity theory and fair inequality: a neuroeconomic study. Proc Natl Acad Sci 111(43):15368–15372. https://doi.org/10.1073/pnas.1414602111
Činčalová S (2020) Inequalities in social responsibility across Europe focused on work-life balance. Calitatea 21(174):142–146
Clark AE, Oswald AJ (1996) Satisfaction and comparison income. J Public Econ 61(3):359–381. https://doi.org/10.1016/0047-2727(95)01564-7
Cohen GA (1989) On the currency of egalitarian justice. Ethics 99(4):906–944. https://doi.org/10.1086/293126
Corcoran ME (1977) Work experience, labor force withdrawals, and women’s wages: empirical results using the 1976 panel of income dynamicse. In: IIoyd CB, Andrews ES, Gilroy CL (eds) Women in the labor market. Columbia University Press, New York
Croft A, Schmader T, Block K (2015) An underexamined inequality: cultural and psychological barriers to men’s engagement with communal roles. Pers Soc Psychol Rev 19:343–370. https://doi.org/10.1177/1088868314564789
Davis SL (2019) Understanding and improving gender equity in conservation. J Am Inst Conserv 58(4):202–216. https://doi.org/10.1080/01971360.2019.1612723
Duehr E, Bono J (2006) Men, women, and managers: are stereotypes finally changing? Pers Psychol 59(4):815–846. https://doi.org/10.1111/j.1744-6570.2006.00055.x
Dworkin R (1981) What is equality? Part 2: equality of resources 1. Philos Public Aff 10:283–345. https://doi.org/10.1017/S0266267100001930
England P (1992) Comparable worth: theories and evidence. Aldine de Gruyter, New York
Eurostat (2018) Gender pay gap statistics. https://ec.europa.eu/eurostat/statistics-explained/index.php/Gender_pay_gap_statistics
Eurostat (2019) Retribuzioni e costo del lavoro, https://ec.europa.eu/eurostat/statistics-explained/index.php?title=Wages_and_labour_costs/it&oldid=462404#Differenziale_retributivo_di_genere
Evans JSBT (2008) Dual-processing accounts of reasoning, judgment, and social cognition. Annu Rev Psychol 59(1):255–278. https://doi.org/10.1146/annurev.psych.59.103006.093629
Filer RK (1985) Male-female wage differences: the importance of compensating differentials. ILR Rev 38(3):426–437. https://doi.org/10.1177/001979398503800309
Fleurbaey M (1994) On fair compensation. Theory Decis 36(3):277–307. https://doi.org/10.1007/BF01079932
Frohlich N, Oppenheimer J (2004) Self-interest. In: The encyclopedia of public choice. Springer, Boston, pp 842–844. https://doi.org/10.1007/978-0-306-47828-4_185
Gaiaschi C (2019) Same job, different rewards: the gender pay gap among physicians in Italy. Gend Work Organ. https://doi.org/10.1111/gwao.12351
Glick P, Fiske ST (2001) An ambivalent alliance: hostile and benevolent sexism as complementary justifications for gender inequality. Am Psychol 56(2):109
Grimshaw D, Rubery J (2007) Undervaluing women’s work. Equal Opportunities Commission, Manchester
Huffman ML, Cohen PN (2004) Occupational segregation and the gender gap in workplace authority: national versus local labour markets. In: Sociological forum, vol 19(1). Kluwer Academic Publishers-Plenum Publishers, pp 121–147. https://doi.org/10.1023/B:SOFO.0000019650.97510.de
Jamali D, Sidani Y, Kobeissi A (2008) The gender pay gap revisited: insights from a developing country context. Gend Manag 23(4):230–246. https://doi.org/10.1108/17542410810878059
Judge T, Livingston B (2008) Is the gap more than gender? A longitudinal analysis of gender, gender role orientation and earnings. J Appl Psychol 93(5):994–1012. https://doi.org/10.1037/0021-9010.93.5.994
Konow J (2001) Fair and square: the four sides of distributive justice. J Econ Behav Organ 46(2):137–164. https://doi.org/10.1016/S0167-2681(01)00194-9
Kupers TA (2005) Toxic masculinity as a barrier to mental health treatment in prison. J Clin Psychol 61(6):713–724. https://doi.org/10.1002/jclp.20105
Lange T (2008) Communist legacies, gender and the impact on job satisfaction in Central and Eastern Europe. Eur J Ind Relat 14:327–346. https://doi.org/10.1177/0959680108094138
Le Grand C (1991) Explaining the male-female wage gap: job segregation and solidarity wage bargaining in Sweden. Acta Sociologica 34(4):261–277. https://doi.org/10.1177/000169939103400402
Leopold TA, Ratcheva V, Zahidi S (2016) Gender parity and human capital (the global gender gap report 2016). World Economic Forum, Geneva
Lips HM (2013) The gender pay gap: challenging the rationalizations. Perceived equity, discrimination, and the limits of human capital models. Sex Roles 68(3–4):169–185. https://doi.org/10.1007/s11199-012-0165-z
Loo C (2016) Environmental justice as a foundation for a process-based framework for adaptation and mitigation: a Commentary on Brooks. Ethics Policy Environ 19(2):145–149
Lotz S (2015) Spontaneous giving under structural inequality: intuition promotes cooperation in asymmetric social dilemmas. PLoS ONE 10(7):e0131562. https://doi.org/10.1371/journal.pone.0131562
Mincer J, Ofek H (1979) The distribution of lifetime labour force participation of married women: comment. J Polit Econ 87(1):197–201. https://doi.org/10.1086/260748
Mincer J, Polachek S (1974) Family investments in human capital: earnings of women. J Polit Econ 82(2, Part 2):S76–S108. https://doi.org/10.1086/260293
Official Journal of the European Union (2008) Consolidated versions of the Treaty on European Union and the Treaty on the Functioning of the European Union. https://eur-lex.europa.eu/legal-content/EN/TXT/PDF/?uri=OJ:C:2008:215:FULL&from=IT
Olsen W, Walby S (2004) Modelling gender pay gaps, vol 17. Equal Opportunities Commission, Manchester
Oppenheimer J (2012) Principles of politics: a rational choice theory guide to politics and social justice. Cambridge University Press, Cambridge. https://doi.org/10.1017/CBO9781139053334
Book Google Scholar
Paul M (2006) A cross-section analysis of the fairness-of-pay perception of UK employees. J Socio-Econ 35(2):243–267. https://doi.org/10.1016/j.socec.2005.11.005
Plantenga J, Remery C, Figueiredo H, Smith M (2009) Towards a European Union gender equality index. J Eur Soc Policy 19(1):19–33. https://doi.org/10.1177/0958928708098521
Rubery J, Grimshaw D, Figueiredo H (2005) How to close the gender pay gap in Europe: towards the gender mainstreaming of pay policy. Ind Relat J 36(3):184–213. https://doi.org/10.1111/j.1468-2338.2005.00353.x
Salverda W, Nolan B, Smeeding TM (eds) (2009) The Oxford handbook of economic inequality. Oxford University Press, Oxford
Smith RA (2002) Race, gender, and authority in the workplace: theory and research. Ann Rev Sociol 28(1):509–542. https://doi.org/10.1146/annurev.soc.28.110601.141048
Smith M (2012) Social regulation of the gender pay gap in the EU. Eur J Ind Relat 18(4):365–380. https://doi.org/10.1177/0959680112465931
Trattato di Roma (1957) https://eur-lex.europa.eu/legal-content/IT/TXT/?uri=LEGISSUM:xy0023
Vitali F (2009) I luoghi della partecipazione. Una ricerca su donne, lavoro e politica. FrancoAngeli.
Williams CL (2013) The glass escalator, revisited: gender inequality in neoliberal times, SWS feminist lecturer. Gender Soc 27(5):609–629. https://doi.org/10.1177/0891243213490232
Download references
Acknowledgements
This research was part of "Zero Gender Pay Gap Project - Gender e-quality: Innovative tool and awareness raising on GPG”. Project's leading body has been the Department of Psychology of Sapienza University of Rome and the partners have been: NACW (AT); 4 Elements (GR); Equanima (CZ); Gender Project for Bulgaria Foundation (BG); and Fondazione Risorsa Donna (IT).
The authors disclosed receipt of the following financial support for the research, authorship, and/or publication of this article: this research was supported by EC – DG Justice.
Author information
Authors and affiliations.
Department of Psychology, Sapienza University of Rome, Via dei Marsi, 78, 00185, Rome, Italy
Giulia Lausi, Jessica Burrai, Alessandro Quaglieri, Emanuela Mari, Angelo Fraschetti, Fabrizio Paloni, Pierluigi Cordellieri, Fabio Ferlazzo & Anna Maria Giannini
Department of Dynamic and Clinical Psychology, Sapienza University of Rome, Rome, Italy
Jessica Burrai & Benedetta Barchielli
You can also search for this author in PubMed Google Scholar
Contributions
All authors contributed to the collection, analyses, and write-up of the manuscript and have given their consent for submission. All sources used have been duly referenced.
Corresponding author
Correspondence to Giulia Lausi .
Ethics declarations
Conflict of interest.
The authors declared no potential conflicts of interest with respect to the research, authorship, and/or publication of this article.
Rights and permissions
Reprints and permissions
About this article
Lausi, G., Burrai, J., Barchielli, B. et al. Gender pay gap perception: a five-country European study. SN Soc Sci 1 , 267 (2021). https://doi.org/10.1007/s43545-021-00274-8
Download citation
Received : 24 November 2020
Accepted : 07 October 2021
Published : 08 November 2021
DOI : https://doi.org/10.1007/s43545-021-00274-8
Share this article
Anyone you share the following link with will be able to read this content:
Sorry, a shareable link is not currently available for this article.
Provided by the Springer Nature SharedIt content-sharing initiative
- Gender pay gap
- Discrimination
- Find a journal
- Publish with us
- Track your research
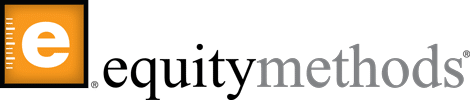
We provide companies of all sizes with financial reporting services for stock-based compensation.
- Reporting Overview
- End-to-End Financial Reporting
- Forecasting & Budgeting
- International Recharge Support
- Tax Reporting
- ASU 2016-09 Services
- ESPP Design and Financial Reporting
- M&A and Spin-Out Transactions
- Small Plans
- Stock Administration System Changes & Financial Reporting Reconciliation
Get fair value measurement and modeling for all sorts of equity-based awards and securities, whether business as usual or special circumstances.
- Valuation Overview
- Total Shareholder Return (TSR) Awards
- Pay vs. Performance Valuation
- Post-Vest Holding Periods
- Award Modifications
Complex Securities Valuation
- Contingent Consideration (Earnouts)
- Contingent Payouts
- Convertible Securities
- Equity Allocation
Stock Options
- Modified Awards
- Assumed Awards
- Private and Newly Public Companies
- Plain Vanilla Options
- Forfeiture Rate Analysis
- Business Valuation
HR Advisory
Our HR advisory services provide the insight you need to improve program design, grant more equity, and tell a cleaner story in your proxy and financial statements.
- HR Advisory Overview
- Modifications, Key Hires & Departures
- Employee Stock Purchase Plan (ESPP) Design
- Proxy Visualization & Quantitative Disclosure
- Award Design & Redesign
- Pay vs. Performance Disclosure
- Private Company Long-Term Incentive Design
- Participant Communication & TSR Tracking
Pay Equity Services
- CEO Pay Ratio
- Pay Ratio Disclosure
- Pay Ratio Analytics
- Compensation Solutions for a Down Market
Dive into our library of publications on a wide range of stock-based compensation topics.
- Tax Reporting for Stock-Based Compensation
- TSR Essentials
- Employee Stock Purchase Plans (ESPPs)
- Modifications
- Pay Versus Performance
- Knowledge Center
We’re dedicated to bringing insight, control, and expanded capability to your compensation strategy.
- Meet the Team
- News & Events
Gender Pay Equity: 15 Questions and Answers for You and Your Compensation Committee
Takis Makridis
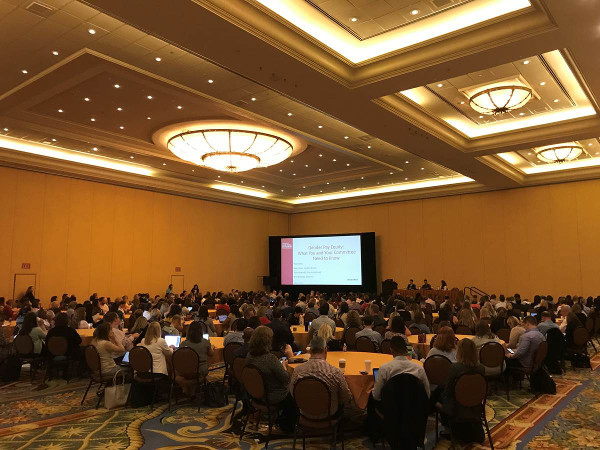
At this year’s WorldatWork Total Rewards Conference in Dallas, I had the opportunity to participate in a panel discussion on gender pay equity. The session drew north of 400 people, showing just how important this topic is in our field.
Although we had a lot to talk about, we wanted to get the audience involved. So, we spent the first 20 minutes of the session polling the room for questions. Then we dedicated the rest of the time to answering them as we walked through a few prepared slides.
By popular demand—from attendees, that is—here’s an FAQ comprised of those questions. During the panel, there was not enough time to go into detail on each question, so this blog also gives me an opportunity to elaborate a bit more. (For a primer on gender pay equity, see this post .)
By the way, last year we published a similar—but much more detailed—FAQ on CEO pay ratio . If you think something like that would be helpful for gender pay equity, please let me know .
Gender Pay Equity at a High Level
1. how do you balance pay equity with performance.
At least in the United States, the idea of pay equity is integrally tied to ideas of performance and meritocracy. When we examine whether there’s a pay equity problem, what we’re really looking to do is validate that compensation is tied to an acceptable reason. Acceptable reasons include factors like tenure, performance, role, and location. Unacceptable reasons include someone’s gender, ethnicity, race, or some other attribute that doesn’t relate to their work and contribution.
Said differently, it’s okay if two people are paid differently because they do different work, or because one outperforms the other, or because they’re in different states and prevailing wages differ between those states. All those things have to do with the work the person is doing and not their identity.
When we study pay equity, we tend to look at performance, since different levels of performance should merit different levels of remuneration. The rub is that if a widespread gender bias exists, then this bias could show up even in performance ratings. Pay equity analyses are largely about disentangling messy cause-and-effect relationships of this nature: Is lower compensation due to genuinely weaker performance, or is a poor performance evaluation a cover, even subconsciously, for underlying biases? The good news is that with statistics and data modeling, you can attack the problem from multiple vantage points and form reasonable hypotheses as to what is actually taking place.
2. What does it mean to close the pay equity gap?
In its strictest sense, closing the pay equity gap means eliminating differences in pay that cannot be explained by appropriate reasons like role, location, performance, or tenure—providing equal pay for equal work. A pay equity gap exists when there are differences in pay not related to these factors, and further, one class of employees, commonly women, are disproportionately affected.
It’s important to understand that the issue centers on pay equity—today. But in the long run, it’s really about human capital. For example, pay levels might be fully explainable by appropriate factors and yet women or minorities are still underrepresented in leadership positions. This could be due to recruiting problems, promotion issues, skewed levels of attrition, or broader and more structural representation issues at the industry level. Either way, it’s worth understanding the gender, race, and ethnicity progression through the organizational hierarchy and where the unexplained failure points might be.
So today the focus is primarily pay equity. That’s a good place to begin, because it has more concrete data that we can study. But plan for the focus to broaden to overall human capital progression, in which pay equity plays a consistent part.
3. What about attributes besides gender, such as race and ethnicity?
Yes! This topic certainly goes beyond gender. Our presentation happened to focus on gender pay equity, but any potential sources of inequity should be studied. For instance, race is the second-most common factor to look at. What holds some companies back is that they don’t collect very much demographic information, so gender is all they’re able to look at.
Side note: There’s a growing school of thought around the idea of “intersectionality,” which looks at the unique challenges that (for example) black women face. The idea behind intersectionality is that there may be an even more nuanced layer of issues when you combine factors and look at them together instead of in isolation. Fortunately, statistical techniques exist to quite easily test whether there is a unique impact associated with intersectionality cases.
Defining the Importance of the Topic
4. why is it important to correct pay equity gaps.
I really like devil’s-advocate questions. I’m sure the individual who asked this thinks pay equity is important, but wanted more specific reasons beyond simply, “It’s the right thing to do.”
In 1997, McKinsey coined the phrase “war for talent” to describe the emerging economy where companies’ abilities to acquire and retain top talent could be the defining factor in their success. Some 20 years later, these predictions are more acute than ever. Companies still compete by creating better semiconductor chips, better advertisements, better supply chains, or better Six Sigma processes. But it all seems secondary to getting their human capital right.
Getting human capital right helps companies improve on a number of dimensions, including not constricting the labor pool you draw from, ensuring growth and advancement for your top talent, and eliminating arbitrary causes of unnecessary turnover. Pay equity is an essential ingredient to keeping employees motivated. In a recent survey by Randstad US, 78% of employees said a workplace where all employees are treated equally is important to them. In short, we think pay equity is a linchpin to winning the war for talent, and as a result, a key to sustainability. And it’s the right thing to do.
5. How do we persuade top management that gender pay equity is important?
Among tech firms on the East or West Coast, pay equity is a hot-button issue to senior executives, investors, and boards. But that’s not universally true in other industries or geographies. Its importance might seem obvious, but I think pay equity needs thoughtful framing to convey the strategic relevance.
So how can you frame it? One way is as a tool in the war for talent. A pay equity study may reveal that your company:
- Doesn’t have a pay equity problem (many companies don’t).
- Doesn’t have a pay equity problem, but does have a related human capital problem (such as women dropping out of the workforce mid-career).
- Does have a pay equity problem, but it’s not widespread or egregious (suggesting that it’s unintentional, solvable, and that overall compensation systems work well).
- Does have a systemic pay equity problem.
- Has poor representation of women or minorities at senior levels, or even in general.
The first finding is good news that you can share in your talent outreach. The second two findings provide an opportunity to address the issues so that they don’t undercut an otherwise highly effective talent strategy. Indeed, the data sets that reveal a problem can also hold clues about how you can address it. (More on that later.) The second to last finding is rare, but in the unlikely case it exists, identifying and managing the issues proactively will have a major talent benefit while reducing risk. The final problem is more common, and presents a distinct challenge for organizations. This is discussed later in this Q&A.
Another way to frame gender pay equity is through a risk management lens. Consider how organizations audit their information security and financial statements. Reasons include preemptively finding problems and being in a position to give positive assurances to external stakeholders. But a third reason is that should something bad happen, an audit can also show that the company made a good-faith effort to prevent it. The same can be true of pay equity. Even if a prior pay equity study had missed a situation in the data, the existence of the study is itself evidence that management took the matter seriously.
Finally, there’s the trend of compensation committees getting involved with human capital management. Companies are increasingly struggling with succession planning and personnel development at all levels of the organization. A robust pay equity analytics effort can equip senior management with answers when the compensation committee comes calling with questions.
To sum up, of course gender pay equity is about doing the right thing. At a very fundamental level, though, I think it’s about being proactive with the human capital assets of the organization and exercising good stewardship.
Measuring Pay Equity
6. how do you actually measure compensation differences to see if there is a bias.
Here’s how we approach the task at Equity Methods. We start with the hypothesis that compensation should be explainable based on factors like role, tenure, location, performance, education, and so on. We use a statistical technique called multiple regression that quantitatively explains the relationship between a dependent variable (pay) and a series of explanatory variables expected to predict compensation (e.g., role, performance, location, etc.). We also include what is called a “dummy variable” indicating gender, race, or any other area of interest. Dummy variable is a statistical term for the fact that it’s a binary (0/1) variable reflecting a certain trait so that we can test for the presence of systemic bias associated with that trait.
If pay equity exists, we will see no discernible impact of these dummy variables, and all of the dependent variability in compensation will be explained by the other variables. If, however, some of the variation is still predicted by our dummy, this indicates the possibility of systematic pay inequity. More often, however, we find there is not systemic bias but biases that are localized to smaller subgroups, such as individual business units or cost centers. The analysis then flags these groups to be looked at more closely.
By deploying this technique, the regression model can also be run to predict what compensation should be for each individual. For example, the model can be run to say that someone who is (for example) a band 9 vice president, working out of Atlanta, who has received above-average performance ratings and sits in the R&D group of the enterprise business unit, should be paid between $105,000 and $120,000. This model prediction is then used to identify whether any people fall outside the predicted band, and patterns in the outliers can be observed and analyzed.
If people or cohorts fall outside the model predictions, this doesn’t necessarily mean a bias exists. In fact, in an appropriate model, some percentage of employees will be paid outside the bands by construction. But it does mean that this particular model doesn’t explain why they’re paid what they are. These employees may be half men and half women, in which case you are probably fine; however, if women are five times as likely as men to be flagged as outliers, then a problem may exist. That’s why it’s necessary to use multiple models to get a more panoramic view of how compensation works. This is also why dialogue with business unit executives and HR generalists is important.
We like to think of models as ways to identify anomalies that need closer study, since not every dimension of pay strategy can be captured in the underlying data. For instance, variables like education, number of direct reports, and financial health of the cost center are not always readily available. But they could explain differences in pay.
7. How should jobs be aggregated for purposes of modeling? Should they be aggregated?
Before I answer this question, first let’s understand the context. Any serious pay equity analysis needs to look at the job or role a person performs. For example, software developers are usually paid more than business analysts. Job level notwithstanding, the underlying labor markets are different, which will result in different types of offers and pay mixes.
We can take that point to the extreme and say that in one sense, every single person has a distinct role. But that would be silly, since an analysis would fall apart without something to compare it to. So, we need a middle ground where we bundle together like employees while not taking it to the point that we’re analyzing fundamentally different roles together.
In general, statistical models work best when they can sift through large amounts of data in order to tease out nuanced relationships among variables. This is also why multivariate regression approaches work much better than calculating average pay for different groups. The regression model can include variables relating to role so that you gain the benefits of a large dataset without erasing key distinctions in the underlying data.
Also, the best pay equity processes are iterative. Modern computing power allows us to run advanced calculations on large datasets in next to no time at all. We develop multiple models, test them, and see how results converge or differ. As we do this, we assess the statistical efficacy of the different models. Where models show less statistical rigor than expected, we iterate to find an alternative specification that works better. Eventually, we have a suite of models that collectively yield the pay equity insight needed to begin forming conclusions. In other words, there isn’t a hard-and-fast answer to how tightly jobs should be aggregated. Plan to try different levels of aggregation in order to find the right balance and what groupings make the most sense.
8. How do the approaches used in the US differ from pay equity reporting in the UK?
The Equality Act 2010 (Gender Pay Gap Information) Regulations 2017 in the United Kingdom require companies in Great Britain with over 250 employees to disclose certain gender pay gap information on their websites and a government website. The results are public and you can peruse them here .
The UK rules are incredibly prescriptive. The average and median male-to-female pay and bonus pay must be reported. The ratio of males to females who received a bonus must also be disclosed. Finally, companies are instructed to organize their workforce into four equal quartiles based on pay, and disclose the number of males and females in each quartile. Relatively specific definitions and protocols must be followed, and perhaps most importantly, this calculation is not at all robust to the fact that women and men perform different jobs within the organization. In fact, the results show that it is in many ways a better measure of the differences in roles than an indicator of equal pay for equal work.
As we’ve explained, pay equity processes in the US are much different because compensation committees and investors are the ones asking the questions. This leads companies to use state-of-the-art statistical techniques to holistically unpack the complexity of pay relationships. Disclosures, such as UK gender pay reporting, must be both formulaic and simplistic in order to apply across a wide range of companies.
Plan for questions as to why UK-reported results differ from those stemming from an analysis done in the home office. Since the home office project will generally be more rigorous and nuanced, part of its focus should be to explain the rationale behind any differences relative to UK-reported results.
Communication and Legal Privilege
9. how should pay equity processes be communicated internally.
So you’ve done a thoughtful pay equity analysis. What do you tell the organization? The right answer depends on your culture.
Some technology companies have such open cultures that the CEO responds to questions personally and is expected to be very open and transparent on even highly sensitive topics. However, in most cases, I’d say you should worry less about internal “marketing” and more on actual problem-solving. In our observations, public statements of the “We did X, which led to Y,” variety make the analysis more discoverable in any future litigation and start to feel like a PR campaign. But in some corporate contexts, this level of clarity is exactly what is needed.
This doesn’t suggest the right answer is pure silence, either, since shareholders and employees may be asking whether pay equity assessment processes are in place. But even then, basic messages like the following work well: “We absolutely look at pay equity and take the topic seriously. We have recurring processes to do that. Further, we also take preventative steps along the lines of anti-bias training for managers, workforce re-entry programs, and college recruiting initiatives to boost the diversity of entry-level hires.” Customize the specifics, but in our experience, phrasing like this seems to have more credibility while preserving the confidentiality of what is being done.
Regarding legal privilege, analyses like these generally should be commissioned by internal or external legal counsel as part of their effort to give legal advice to their client (the CEO, CHRO, or board). The reason maintaining privilege matters is because many cases won’t have black-and-white answers. As a result, organizations may require time to work through what the results mean and how to act on them. Contextually, a process like this is better kept under privilege than open to discovery should an exogenous lawsuit happen.
10. What is legal privilege and how does it play into things?
In our experience, different attorneys give slightly different viewpoints (again, we’re not attorneys). But the textbook explanation is as follows. In litigation, certain communications between a client and the client’s attorney are privileged (i.e., not discoverable by the opposing side) because they entail the client asking for legal advice. However, if the client then takes that privileged communication, forwards it to their colleague and initiates a separate discussion, then that separate discussion is almost certainly taking place outside the bounds of privilege.
In a pay equity study, generally the client asks their attorney to provide employment law support, of which pay equity is just a part. The attorney engages a quantitative specialist to develop robust statistical models and acts as a go-between for the results. The insights from those models help inform the attorney’s legal advice.
To be clear, many companies perform these studies outside the bounds of legal privilege. It’s a business decision to make based on your own organization’s circumstances and prior approaches to similar matters.
With or without legal privilege, some best practices apply. First, be careful about what you put in writing. Perhaps you see something in an analysis that frustrates you. In that moment, resist the temptation to send an email saying, “I can’t believe we did XYZ!” It’s never a bad time to pick up the phone and talk in person.
Second, tie up loose ends in your “work papers” (i.e., the files you keep on the study). A loose end would be an email or document that says something like the following without resolution: “We should probably correct the pay for these 10 people. What do you think?” Close out any hanging questions like that, or set a time to reassess it via an update to the files.
Finally, document the remediation steps you take. In the event of litigation, you need to show how you took the matter seriously by constantly initiating improvements to pay processes, training programs, and so on.
Remediating Pay Equity Problems
11. what happens if we detect a pay equity problem.
Remediation is an important topic. There are three broad approaches and, of course, many shades of gray in between.
The first approach is to communicate openly within the organization. This may come in a statement such as, “We performed a pay equity analysis and found no evidence of systematic bias. Further differences in pay were random between men and women, and most were easily explained by other factors. We made a total of $X in pay adjustments to 100 employees to remediate anomalous pay below expected levels.” This highly visible approach probably fits 15% to 20% of organizations.
Another way is to make pay adjustments so covertly that only a handful of people know the reason. Under this approach, a study yields suggested pay adjustments and those adjustments are woven into the next upcoming merit cycle, but without telling managers or HR leaders why. In most companies, the reasons behind pay adjustments aren’t fully transparent, which means it’s possible to boost pay adjustments without articulating why. This remediation strategy is typically seen in very large organizations.
The third approach, and usually our preferred one, is to take the results of an analysis to senior business line executives (or the HR generalists supporting them) and pull them into the dialogue. We call this the “Study, Consult, and Act” approach. It preserves discretion while yielding two useful benefits. For one thing, there may be factors that are relevant to the analysis but altogether missing from the data. They can help assess whether that’s the case. This outreach also sends a strong signal from the top that pay equity is a CEO-level priority.
We like the third approach because it’s sustainable. It gets people on board with the mission, marries the mathematical models with on-the-ground context, and opens an ongoing dialogue about pay equity. Making pay tweaks here and there is certainly important, but when done in isolation, it addresses symptoms and not causes.
12. How much should we budget for pay adjustments due to a pay equity problem?
This is an important question, since pay equity is important to every organization, but naturally many organizations have fixed budgets and might find it difficult to implement immediate corrective adjustments. Understandably so, there were skeptics in the room thinking: “It’s great that Salesforce.com can shift budget money around. We probably can’t.”
The good news? Our expectation is that most cases won’t turn out to be budget-busters. That’s one reason why we believe more advanced statistical approaches are necessary to navigate the complexity of pay relationships and present a “measure twice, cut once” answer.
A side benefit of the Study, Consult, and Act approach is that senior management gains early indicators of potential pay biases. This way, if it looks like there will need to be pay adjustments, a dialogue can occur that allows affected parties to begin planning.
In terms of the chronology, a study usually takes six to eight weeks, at which point it’s possible to share how numbers are trending. The socialization process with business line executives usually takes another two or three months, since here the goal is showing them the results so that they can discreetly conduct internal research. After that, it’s time for business line executives to share their perspectives and senior management to make their decisions. All in all, the aim is to not let potential issues linger but to drive a methodical process that creates de facto training to business line executives. The byproduct is that the finance function can have time to digest the financial implications and adjust their budgets.
These processes work only when senior management and the board support them.
Nuances in a Pay Equity Study
13. when doing an analysis, how do you address roles that are predominantly occupied by men.
In our opinion, the starting point is a discussion around why these roles are predominantly occupied by men in the first place.
Take software engineering, a field in which studies suggest the percentage of females is 10% to 15%. The question to ask is whether there’s any valid reason for this. Most would say there isn’t.
Many leading companies have taken these statistics and used them to support overhauls to their recruiting procedures. For example, one high-tech company we work with appointed senior officers to forge relationships with local high schools and universities, creating awareness and excitement among women and minorities about careers in technology. In addition to doing good, they also positioned their organization to be at the forefront of future recruiting.
Of course, there is also the topic of self-selection, such as the assertion that women may simply prefer not to work on an oil rig. Be careful here, since one can easily counter-argue that perhaps the entire reason we don’t see many women working in oil rigs is the presence of structural biases that permeate society. Our suggestion is to devote time to internal dialogue on the topic of representation and your human capital strategy. Perhaps the answer is to show up at the local high schools and begin deconstructing stereotypes that lie behind current representation skews. At any rate, we at least want to raise the concept of self-selection as one that merits further discussion.
Smaller organizations may not have the resources to do what this particular organization did, but that doesn’t mean they’re without options. I’ll use Equity Methods as an example (we have just south of 100 professionals). By overhauling our approaches to campus and experienced-hire recruiting, we’ve significantly leapfrogged the male-female ratio seen in most consulting organizations while also achieving strong ethnic diversity.
What about data that shows women or minorities bailing out at higher rates once they hit a certain level in the organization? Such observations can inform improvements to internal mentoring programs, flex-time tracks, and workforce re-entry processes.
The point is, a multivariate regression model or any cohort analysis might perform worse when comparing the pay of hundreds of men to a handful of women. Nicely-sized datasets are the fuel these models run on. But in these cases where the gender imbalance is high and the model robustness is limited, this in and of itself lends insight and helps focus energy on strategies beyond just compensation.
14. When doing an analysis, how do you think about executives and are they treated differently?
Here I need to give the consultant’s notorious “it depends” answer. We like to include everyone in the analysis. Where we go from there depends on the dialogue and the data.
It’s not unheard of to have pay equity challenges even at executive levels, which we would generally define as the firm’s top 10% in terms of compensation. However, there are generally more unique considerations that need to be looked at and which are not in the data. For example, two business unit executives may have the same band level, live in the same state, and have equal performance ratings—but one earns much more because she manages a considerably larger P&L. If that particular fact isn’t in the HRIS data, a regression model won’t pick it up. Further, as there are fewer employees at each level, the models used lack the power to detect systematic bias.
Another factor with executives is that when problems exist, they’re more generally problems of representation. As a result, the study may trigger a more concentrated focus on helping women or minorities to progress through the career track (as I explain above).
Still, the power of modern computing allows analyses to be sliced multiple ways, so we would suggest including the full population and then being sure to cut the analytics by seniority level to see whether the story differs.
15. It’s not a secret that many women exit the workforce when they have children. How are these events handled in an analysis?
It’s important to start by defining the problem. One way of framing it is that talent leaves because they don’t think it’s possible to excel at work and at raising children at the same time. Another is that talent may wish to re-enter at some point, but it’s not clear how to make this easy and seamless.
However you define it, the first step in solving the problem is to study your data to understand what exactly is taking place. That way, conversations about strategy are grounded in facts. Suppose the data shows a clear trend of women exiting at a certain pay band and age level. What’s the right business response? We know some companies have created more part-time and flex-time roles so that they can help people keep one foot in the pond. This approach of course is easier said than done, since you can also end up with pay equity problems in more customized part-time roles.
Other companies have responded by changing their maternity leave policies so that women don’t feel like they’re forced into a choice at such a pivotal life event. Some companies are also extending paternity leave.
Your company may not in be a position to make wholesale changes to parental leave policies. Even so, you could examine the feasibility of part-time or flex-time opportunities. It’s also worth evaluating workforce re-entry programs, given how many women reach a stage where they do want to come back to work (full-time or part-time) and struggle to make that transition. From a human capital perspective, it makes all the sense in the world to understand how pockets of the labor market are being crowded out, making it harder to compete in the war for talent.
I hope you found this discussion helpful. If you’re among those who asked for this writeup, I’ll be sure to follow up personally.
I mentioned before that we published a more in-depth FAQ on CEO pay ratio. In that publication, we examined CEO pay ratio in a fair amount of detail. Do you think that gender pay equity merits a similar type of publication? If so, what would you like to cover? We think the broader topic of pay equity (extending even beyond gender) is considerably more complicated and meaningful than CEO pay ratio, and we’d like to help advance the dialogue in the industry. Please let me know what you think .
Related Content
Worldatwork total rewards 2018 conference roundup.
Equity Methods attended the WorldatWork Total Rewards 2018 Conference in Dallas from May 21 to 23. Here are a few key takeaways from those sessions.
False Positive, False Negative: Beyond the Surface of Gender Pay Equity
Shareholders are demanding it. Governments are legislating it. From Hollywood to Washington, technology to nursing, Millennials to Boomers, equal pay for equal work is now front-page news. Read our Workspan Magazine article about how rigorous analysis is the best way to explain the biases.
Pay Equity Gathers Steam
While CEO pay ratio has dominated discussions in 2017, a much larger emerging issue is pay equity—statistically studying compensation throughout an organization to make sure there are no unexplainable differences linked to gender, race, or ethnicity. Here’s a primer on the topic.
Pay equity is about rewarding people for their work equally based on the value they create, regardless of gender, race or other factors that are unrelated to performance. Companies with…
2018 Executive Compensation Decision Support Survey
In our newest survey report, hear from representatives of 135 companies as they reveal how they make executive compensation and proxy disclosure decisions.
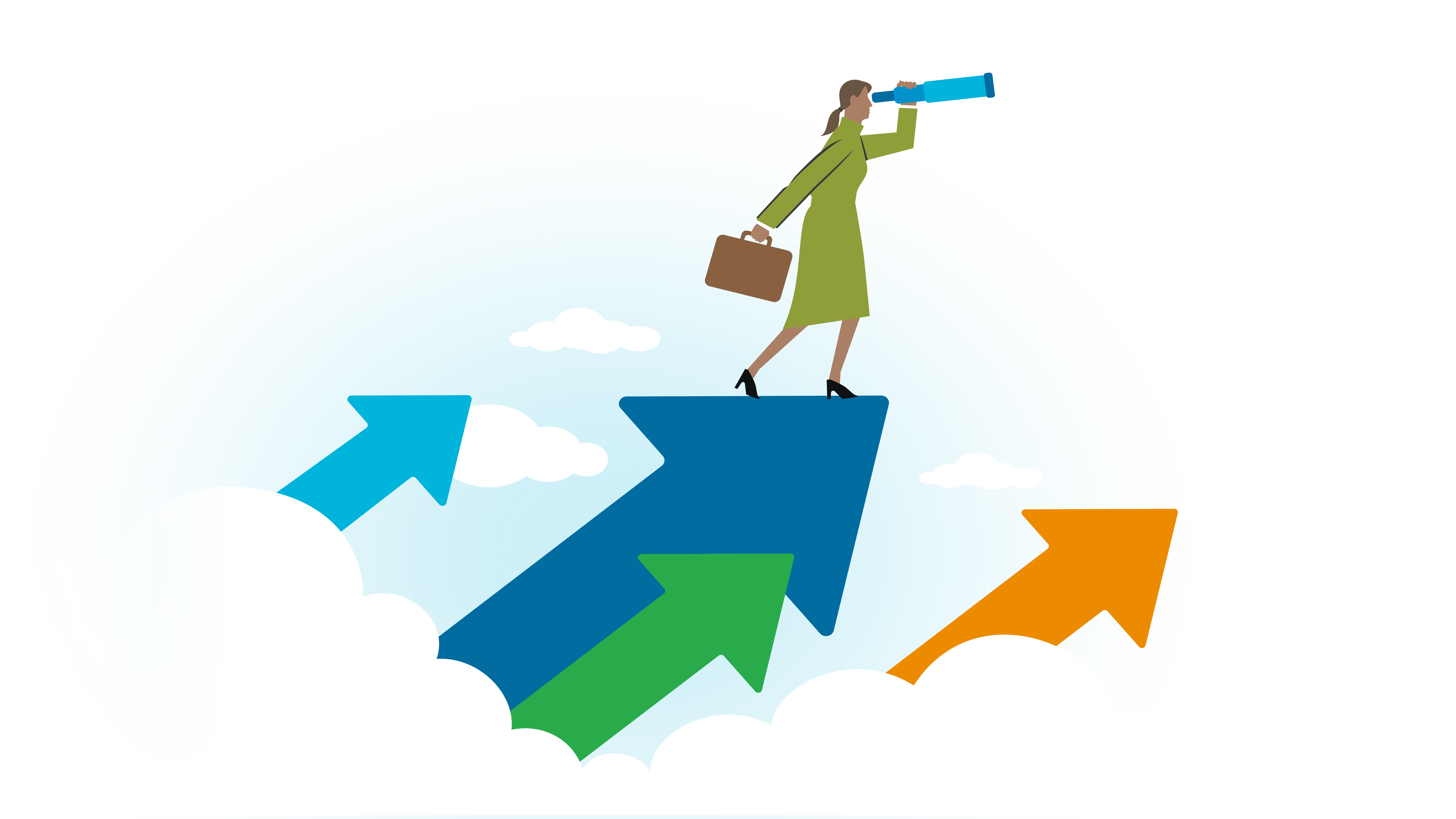
- Search Search Please fill out this field.
Understanding the Gender Wage Gap
How to close the gender wage gap.
- Frequently Asked Questions (FAQs)
The Bottom Line
- Career Advice
How Women Can Break Through the Gender Wage Gap Barrier
:max_bytes(150000):strip_icc():format(webp)/RebeccaRosenberg-a292d8022f1c4cbfa630358be5bb5f57.jpeg)
Investopedia / Joules Garcia
Many traditional gender roles have disappeared. Men and women perform the same jobs, have similar career trajectories, and even take turns as stay-at-home parents. But one gender-based distinction that remains is the wage gap. For every dollar men earn on average, women earn about 84 cents.
While not directly tied to the wage gap, the Investopedia and REAL SIMPLE 2024 Her Money Mindset survey identified areas in which earning more money could benefit women greatly.
For example, 54% of surveyed women said they are struggling to cover at least one monthly expense, and 44% said they have just $250 or less after covering bills each month. That said, 60% of women have never asked for a raise, and fewer than 1 in 4 women who talk with their friends about money are transparent about how much they make.
To help women feel empowered to take control of their income, and support other women doing the same, here's what to know about the wage gap, and how individual actions can build barrier-busting wealth.
Key Takeaways
- The wage gap refers to the disparity in earnings between men and women in the workplace.
- Societal factors play a significant role in perpetuating the wage gap.
- Negotiation skills are crucial for women to achieve fair compensation and benefits.
- Skills development and education can help bridge the wage gap.
- Pay equity depends on improving workplace policies and practices.
The gender wage gap is the difference between what men and women earn. There are many ways to analyze and express the gap, but the most common is to measure the difference in median earnings of women vs. men.
Over the past several decades, Congress and state governments have passed a number of laws to address the gap. For example, the Equal Pay Act of 1963 prohibits employers from paying men and women different wages for performing similar duties under similar conditions. Despite those efforts, the gender pay gap persists.
As of 2022, women earned 84 cents for every dollar men earned in the United States. However, the difference varies widely by region. California and Vermont have the smallest gaps, where women earn 89 cents for every dollar men do. Conversely, women in Louisiana and Utah lag far behind men, earning 75 and 73 cents respectively. There are a multitude of reasons why the gender wage gap exists.
Societal Factors at Play
Society has come a long way in treating men and women fairly. However biases take generations to entirely disappear, and women still face numerous challenges in perception—and compensation—in the workplace, such as:
- Gender discrimination : Discrimination has many forms, but societal biases around job performance and compensation are especially problematic. Women generally have to work harder to gain equal recognition. And women who advocate for more compensation or better treatment are often cast as troublesome or unaccommodating.
- Racial discrimination: The wage gap is worse for many women of color than it is for white women. According to racial wage gap data from the U.S. Bureau of Labor Statistics, Black and Latinx women earn median weekly pay of $887 and $825 respectively, compared with median weekly earnings of $1,040 for white women and $1,254 for white men.
- Motherhood : Having a baby is expensive on its own, but it also has a significant impact on a woman’s earnings. Research from the U.S. Census Bureau indicates that between two years before a child’s birth and one year after, the gender pay gap within a couple doubles. The wage gap continues growing until the child is 10 years old, representing a massive loss of income over the mother’s career.
- Occupational segregation : While unequal compensation for equal work is a core issue, access to equal work is also a primary driver. The National Partnership for Women & Families found that women make up 63.6% of the workforce in the 20 lowest-paying jobs they studied, compared to only 30% of the workforce in the 20 highest-paying jobs.
- Unpaid family care : Women are more likely than men to take career breaks to care for aging or sick family members. Long breaks in employment may raise questions with prospective employers or cause women to lag behind industry trends and new skills, further depressing wages.
How Workplace Policies Impact Earning Disparities
While many federal and state laws prohibit discrimination in pay, certain workplace conditions persist, which keep the gender wage gap from shrinking. These are a few of the most common:
- Salary history on applications : Some states prohibit employers from asking prospective employees about their salary history, and research has found that the ban helped narrow the wage gap. However, many companies work around this by asking applicants for pay expectations, which can lead to a perpetuation of women being underpaid if they don’t feel comfortable asking for a higher salary.
- Pay transparency : The historical secrecy of salaries, and its taboo nature as a topic of discussion, has frequently left women in the dark about how large the gender wage gap was. The National Bureau of Economic Research found that pay transparency laws reduced the gap by 20–40%.
- Individual vs. group salary negotiations : Data also shows women fare better with collectively negotiated compensation. Women in unions earn an average of 89.6% of their male counterparts’ wages, compared to non-unionized women earning 82% of their male counterparts’ wages at the time of the study.
The gender wage gap doesn’t just lead to a smaller paycheck for women every two weeks—it has lifelong consequences. As female workers earn less during any given period, they amass less wealth than their male counterparts and have less financial stability.
Note: Beyond the direct consequences of financial instability, these shortfalls often leave women feeling trapped in jobs where they’re underpaid. When faced with the choice between barely covering bills or not covering bills at all, it’s difficult to risk steady income.
Reducing the gender wage gap is a complex problem. While there’s no single solution, there are some ways women can help themselves and each other reach wage parity with men.
Talk About How Much You Make
Talking about money has historically felt taboo, particularly among women. According to Investopedia and REAL SIMPLE's 2024 Her Money Mindset survey, of the women who talk about money with their friends, only 15% will mention if they are asking for a raise or promotion, and only 24% discuss how much they make.
However, discussing raises and salaries is very beneficial for all parties involved in the conversation and is a powerful tool. By comparing notes, you can learn a lot: if you're making less than market standards, how often and how much people are negotiating for in raises, what sort of salary and bonus structures are out there, and more.
The reality is, most women feel grateful for the topic to be broached and ultimately walk away with more information about the fairness of their wages, inspiring them to put more pressure on their company for better pay.
31% of women who took the Her Money Mindset survey said they think it's important for women to talk to their friends about money.
The hardest part may be breaking the ice, but there are helpful strategies for approaching the topic in a respectful and productive way.
For instance, you can bring up the conversation by casually incorporating a financial topic into a conversation to get a pulse for how your friends feel. It also can be helpful to set ground rules, such as agreeing to confidentiality and actively listening without interruption.
Negotiate Higher Compensation
The 2024 Her Money Mindset survey found 60% of women have never asked for a raise, and 69% have never requested a promotion. Women with higher household income levels were more likely to have asked for a raise or promotion.
Whether it’s a raise at your current job, or a significant bump from moving to a new company, negotiating a higher salary is one of the most important ways women can increase their earning power.
“I don't think people realize the impact the gender wealth gap can have on our lifetime earnings,” says Gloria Carcia Cisnero s, a certified financial planner and wealth manager at Lourd Murray. “When you start with a lower base salary, it means that for all the subsequent pay increases, you are getting less than someone who is getting the same percentage increase, but has negotiated higher pay from the beginning.”
She also notes that companies expect candidates to negotiate. “The earlier the better, make sure you start negotiating in your 20s and 30s to take advantage of the exponential growth.”
Investopedia and REAL SIMPLE's survey found that 30% of women in the millennial generation and younger have a goal to get a raise and/or promotion in the next three years.
One negotiating tip from Michelle Kruger , certified financial planner and senior financial planner at Gratus Capital, is using benefits as a tool for negotiating salary.
“Bring cost differences like an increased health insurance premium or a lower 401(k) match to your potential new employer’s attention,” Kruger says. “Calculate the value of the lost benefits to you, and ask for a commensurate increase in the offer.”
Consider these key strategies when asking for a raise:
- Prepare in advance : Collect data for comparable jobs at your company and industry. Plan out key points you want to make and be ready for pushback.
- Be assertive : No one cares more about your welfare than you. Treat your boss with respect, but ensure that it’s mutual and advocate for yourself.
- Practice : Ask a friend to roleplay your boss and rehearse your talking points. Make sure they throw you some curveball questions.
- Negotiate : Bosses often have budget constraints that limit how flexible they can be. Push for your desired salary, but be ready to take concessions elsewhere, such as additional paid time off or other benefits.
- Time your request : Set yourself up for success by asking for a raise when it’s likely you’ll get a positive reaction. If your company just went through a round of layoffs or the economy is trending down, you’ll face more resistance.
Enhance Your Skills and Education
If negotiating a raise isn’t going as well as you hoped, the next step is to bolster what you can do at work. The best way to do this is by enhancing your skills or seeking further education.
In many cases, learning new skills can help break down occupational segregation. For example, office managers and executive assistants are often women, many of whom have extensive business experience and a wide range of abilities. But they end up pigeonholed into administrative jobs, limiting their earning potential. By developing specialized skills such as accounting, human resources, or project management, they can advance their careers and shrink the wage gap.
Women have a range of options for building their skills and resumes:
- Professional certifications : Professional certifications offer a concrete way to increase earning potential by demonstrating specific skills and qualifications to prospective employers.
- Company-sponsored development : Many companies cover some or all of the costs for professional development courses. Everyone benefits, as employees learn more skills and employers gain access to those skills.
- Returning to school : Going back to college might seem daunting. But with the proliferation of online learning, ongoing education can fit any schedule. Finishing a degree or getting a new one can open up opportunities to earn more.
Build Wealth By Investing
Setting money aside for investments can be hard when you’re juggling multiple financial goals, or just trying to make ends meet. However, investing helps women close the wage gap in two ways:
- Increased financial stability : As women amass more wealth, it allows them more freedom to make career choices. When not tied to a job just to keep bills paid, women can explore more lucrative opportunities and advance professionally.
- Source of passive income : Many types of investments don’t just grow over time, they also generate income. Whether it’s dividends from stocks or rent from real estate, passive income supplements wages from employers and increases overall wealth.
If you’re just starting out, a high-yield savings account can be a good option. You’ll see compounding gains from interest as time goes on. The money is FDIC-insured, so there’s no risk of loss. And it’s easy to access if an emergency comes up and you need access to the funds.
As time goes on, you can diversify into other investments:
- Index funds spread your money across groups of different stocks, insulating you from the risk of one company hurting your portfolio.
- Individual stocks can perform well but require more research and active management.
- Bonds are a relatively stable investment, but have smaller payoffs and take time to mature.
- Crypto has the potential for large gains, but the lack of regulation introduces significant risk.
- Real estate often has a high cost of entry, but generates income over time.
Financial advisors generally recommend a diverse portfolio based on the investor’s age. Younger investors can afford more risk, like stocks and index funds, while older investors tend to move towards more stable choices, like bonds.
Support Women in Leadership Positions
As of 2024, women made up 46.9% of the American workforce. But they remain underrepresented in the upper echelons of business. Only 10.6% of CEOs and 30.4% of board members at Fortune 500 companies are female.
Breaking through the glass ceiling isn’t easy, but it has an immense payoff.
Elise Awwad , who currently serves as DeVry University's president and CEO, started her career with the company as an admissions advisor. While working her way up in the company, Awwad says “I recognized the need to support other women in the workplace.” and “The male-dominated culture is still prevalent in many tech companies and can make women feel like they don’t belong.”
In 2019, she established EDGE (Empowerment, Diversity, Growth, and Excellence), a network of leadership scholars and professionals who promote the enhanced career experience and advancement of women in leadership roles at DeVry, and in the broader community. She also spearheaded DeVry’s Women+Tech Scholars program, created to “empower women through mentorship, job search resources, credentialing, and scholarships, encouraging them to take the first step toward a tech-focused career.”
Debbie Sanders , COO of Visory Health, also notes the importance of advocacy and support for career advancement. “Look for a mentor, and look for positions and jobs where you feel supported and will be respected and compensated for the great ideas and hard work you put forth,” Sanders commented. “Getting places in your career usually means not only do you need to excel at what you do, but also have someone in an executive position there to support you as internal politics increase.”
In the long term, putting women in leadership positions will foster a culture of equality and—hopefully—reduce the gender wage gap. Better representation in C-suites and board rooms will lead to more balanced policies and help break down implicit cultural biases that persist within some companies.
Frequently Asked Questions (FAQs)
What country has the highest gender wage gap.
Not every country has reliable wage data available. But according to the Organisation for Economic Cooperation and Development (OECD), Korea has the highest gender pay gap at 31.2% in 2022. The only other country above 25% is Israel, at 25.4%.
Which Countries Have the Lowest Gender Wage Gap?
Based on the same OECD data, Belgium’s gender wage gap of 1.2% is the smallest in the world. It's joined by four other countries under 5%—Costa Rica (1.4%), Colombia (1.9%), Bulgaria (2.5%), and Norway (4.5%).
How Has the Gender Pay Gap Changed Over Time?
Women made significant gains in the later part of the 20th century. Pew Research found that between 1982 and 2002, women’s earnings relative to men’s rose from 65% to 80%. But in the following 20 years, the gap remained relatively stable, hovering between 80% and 85%. Researchers have not found evidence of any single factor causing the stagnation. Many of the topics this article has discussed contribute, including discrimination and occupational segregation.
Gender disparities can vary widely by industry and job type. At one end of the spectrum, there are a handful of jobs where women earn more on average. Tutors top this list, where women earn 35% more than men. Conversely, women lag far behind males in jobs like finance and manual trades. Financial services sales agents have the largest pay gap, where women earn 55.1% of what men do.
There’s no quick solution to closing the gender wage gap. And unfortunately, much of the struggle involves deeply rooted cultural biases. As individuals, every woman needs to advocate for herself by negotiating higher pay and building personal wealth through investing. Collectively, women can fight to empower their peers in leadership and lift each other up. Systemic change takes years. But through continued efforts for equality, we can build a workforce where our daughters and granddaughters receive equal pay with our sons and grandsons.
:max_bytes(150000):strip_icc():format(webp)/AmandaMorelli-689cb2ec372149cfad914c01bc4e683c.png)
U.S Bureau of Labor Statistics. " Women's Earnings Were 83.6% of Men's in 2023 ."
U.S. Equal Employment Opportunity Commission. “ The Equal Pay Act of 1963 .”
National Women's Law Center. " The Wage Gap, State by State ."
U.S. Bureau of Labor Statistics. " Usual Weekly Earnings of Wage and Salary Workers First Quarter 2024 ."
U.S. Census Bureau. " The Parental Gender Earnings Gap in the United States ." Page 12.
The National Partnership for Women & Families. " Women's Work Is Undervalued, and It's Costing Us Billions ." Page 2.
Paycor. " States with Salary History Bans ."
U.S. Office of Personnel Management. " RELEASE: OPM Finalizes Regulation to Prohibit Use of Non-Federal Salary History ."
Baker, Michael and et al. " Pay Transparency and the Gender Gap ." National Bureau of Economic Research , Working Paper 25834, December 2021, pp. 17.
Institute for Women's Policy Research. " The Facts Are Clear: Unions Help Women Close the Pay Gap ."
Federal Deposit Insurance Corporation. " Understanding Deposit Insurance ."
Pew Research Center. " Women Make Up Nearly Half of the Labor Force; Share Will Remain Steady Over The Next Decade ."
Pew Research Center. " The Data on Women Leaders ."
Organisation for Economic Cooperation and Development. " Gender Wage Gap ."
Pew Research Center. " The Enduring Grip of the Gender Pay Gap ."
U.S. Department of Labor Women's Bureau. " Occupations With the Smallest Gender Earnings Gap ."
U.S. Department of Labor Women's Bureau. " Occupations With the Largest Gender Earnings Gap ."
:max_bytes(150000):strip_icc():format(webp)/INV-HER-MONEY-MINDSET-Spotlight-Recirc-308b00b726ad49b2928526cafb85b0a7.jpg)
- Terms of Service
- Editorial Policy
- Privacy Policy
- Your Privacy Choices
Female labor force participation
Across the globe, women face inferior income opportunities compared with men. Women are less likely to work for income or actively seek work. The global labor force participation rate for women is just over 50% compared to 80% for men. Women are less likely to work in formal employment and have fewer opportunities for business expansion or career progression. When women do work, they earn less. Emerging evidence from recent household survey data suggests that these gender gaps are heightened due to the COVID-19 pandemic.
Women’s work and GDP
Women’s work is posited to be related to development through the process of economic transformation.
Levels of female labor force participation are high for the poorest economies generally, where agriculture is the dominant sector and women often participate in small-holder agricultural work. Women’s participation in the workforce is lower in middle-income economies which have much smaller shares of agricultural activities. Finally, among high-income economies, female labor force participation is again higher, accompanied by a shift towards a service sector-based economy and higher education levels among women.
This describes the posited U-shaped relationship between development (proxied by GDP per capita) and female labor force participation where women’s work participation is high for the poorest economies, lower for middle income economies, and then rises again among high income economies.
This theory of the U-shape is observed globally across economies of different income levels. But this global picture may be misleading. As more recent studies have found, this pattern does not hold within regions or when looking within a specific economy over time as their income levels rise.
In no region do we observe a U-shape pattern in female participation and GDP per capita over the past three decades.
Structural transformation, declining fertility, and increasing female education in many parts of the world have not resulted in significant increases in women’s participation as was theorized. Rather, rigid historic, economic, and social structures and norms factor into stagnant female labor force participation.
Historical view of women’s participation and GDP
Taking a historical view of female participation and GDP, we ask another question: Do lower income economies today have levels of participation that mirror levels that high-income economies had decades earlier?
The answer is no.
This suggests that the relationship of female labor force participation to GDP for lower-income economies today is different than was the case decades past. This could be driven by numerous factors -- changing social norms, demographics, technology, urbanization, to name a few possible drivers.
Gendered patterns in type of employment
Gender equality is not just about equal access to jobs but also equal access for men and women to good jobs. The type of work that women do can be very different from the type of work that men do. Here we divide work into two broad categories: vulnerable work and wage work.
The Gender gap in vulnerable and wage work by GDP per capita
Vulnerable employment is closely related to GDP per capita. Economies with high rates of vulnerable employment are low-income contexts with a large agricultural sector. In these economies, women tend to make up the higher share of the vulnerably employed. As economy income levels rise, the gender gap also flips, with men being more likely to be in vulnerable work when they have a job than women.
From COVID-19 crisis to recovery
The COVID-19 crisis has exacerbated these gender gaps in employment. Although comprehensive official statistics from labor force surveys are not yet available for all economies, emerging studies have consistently documented that working women are taking a harder hit from the crisis. Different patterns by sector and vulnerable work do not explain this. That is, this result is not driven by the sectors in which women work or their higher rates of vulnerable work—within specific work categories, women fared worse than men in terms of COVID-19 impacts on jobs.
Among other explanations is that women have borne the brunt of the increase in the demand for care work (especially for children). A strong and inclusive recovery will require efforts which address this and other underlying drivers of gender gaps in employment opportunities.
- Research and Publications
- Projects and Operations
- Privacy Notice
- Site Accessibility
- Access to Information
- REPORT FRAUD OR CORRUPTION
Numbers, Facts and Trends Shaping Your World
Read our research on:
Full Topic List
Regions & Countries
- Publications
- Our Methods
- Short Reads
- Tools & Resources
Read Our Research On:
Racial, gender wage gaps persist in U.S. despite some progress
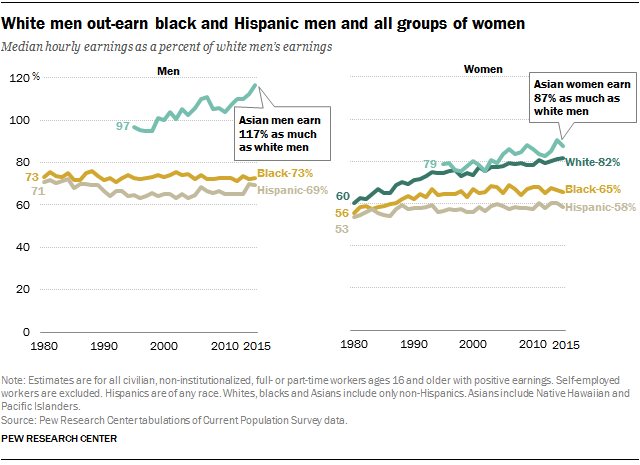
Large racial and gender wage gaps in the U.S. remain, even as they have narrowed in some cases over the years. Among full- and part-time workers in the U.S., blacks in 2015 earned just 75% as much as whites in median hourly earnings and women earned 83% as much as men.
Looking at gender, race and ethnicity combined, all groups, with the exception of Asian men, lag behind white men in terms of median hourly earnings , according to a new Pew Research Center analysis of Bureau of Labor Statistics data. White men are often used in comparisons such as this because they are the largest demographic group in the workforce – 33% in 2015.
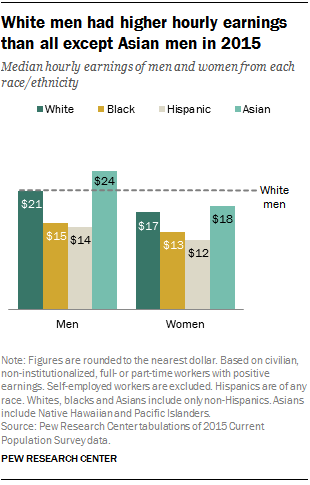
In 2015, average hourly wages for black and Hispanic men were $15 and $14, respectively, compared with $21 for white men. Only the hourly earnings of Asian men ($24) outpaced those of white men.
Among women across all races and ethnicities, hourly earnings lag behind those of white men and men in their own racial or ethnic group. But the hourly earnings of Asian and white women ($18 and $17, respectively) are higher than those of black and Hispanic women ($13 and $12, respectively) – and also higher than those of black and Hispanic men.
While the hourly earnings of white men continue to outpace those of women, all groups of women have made progress in narrowing this wage gap since 1980, reflecting at least in part a significant increase in the education levels and workforce experience of women over time.
White and Asian women have narrowed the wage gap with white men to a much greater degree than black and Hispanic women. For example, white women narrowed the wage gap in median hourly earnings by 22 cents from 1980 (when they earned, on average, 60 cents for every dollar earned by a white man) to 2015 (when they earned 82 cents). By comparison, black women only narrowed that gap by 9 cents, from earning 56 cents for every dollar earned by a white man in 1980 to 65 cents today. Asian women followed roughly the trajectory of white women (but earned a slightly higher 87 cents per dollar earned by a white man in 2015), whereas Hispanic women fared even worse than black women, narrowing the gap by just 5 cents (earning 58 cents on the dollar in 2015).
Black and Hispanic men, for their part, have made no progress in narrowing the wage gap with white men since 1980, in part because there have been no improvements in the hourly earnings of white, black or Hispanic men over this 35-year period. As a result, black men earned the same 73% share of white men’s hourly earnings in 1980 as they did in 2015, and Hispanic men earned 69% of white men’s earnings in 2015 compared with 71% in 1980.
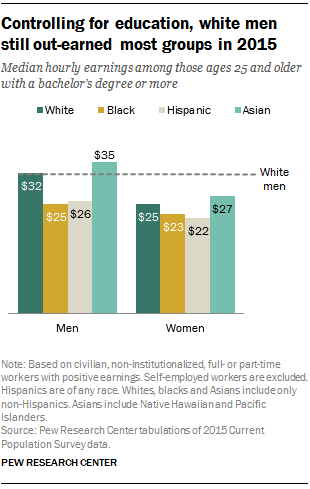
To be sure, some of these wage gaps can be attributed to the fact that lower shares of blacks and Hispanics are college educated . U.S. workers with a four-year college degree earn significantly more than those who have not completed college. Among adults ages 25 and older, 23% of blacks and 15% of Hispanics have a bachelor’s degree or more education, compared with 36% of whites and 53% of Asians.
However, looking just at those with a bachelor’s degree or more education, wage gaps by gender, race and ethnicity persist. College-educated black and Hispanic men earn roughly 80% the hourly wages of white college educated men ($25 and $26 vs. $32, respectively). White and Asian college-educated women also earn roughly 80% the hourly wages of white college-educated men ($25 and $27, respectively). However, black and Hispanic women with a college degree earn only about 70% the hourly wages of similarly educated white men ($23 and $22, respectively). As with workers overall, college-educated Asian men out-earn college-educated white men by about $3 per hour of work.
What contributes to these persistent wage gaps? Research shows that a majority of each of these gaps can be explained by differences in education, labor force experience, occupation or industry and other measurable factors.
For example, NBER researchers Francine Blau and Lawerence Kahn found that education and workforce experience accounted for 8% of the total gender wage gap in 2010, while industry and occupation explained 51% of the difference. When it comes to race, sociologists Eric Grodsky and Devah Pager found that education and workforce experience accounted for 52% of the wage gap between black and white men working in the public sector in 1990, and that adding occupational differences explained approximately 20% of the wage gap. And NBER researcher Roland Fryer found that for one group of adults in their 40s, controlling for standardized-test scores reduced the wage gap between black men and white men in 2006 by roughly 70%.
The remaining gaps not explained by these concrete factors are often attributed, at least in part, to discrimination. Blau and Kahn point out, however, that there are both portions of this “unmeasured” difference that could be due to factors other than discrimination (e.g., gender differences in behaviors like risk aversion or negotiation) as well as portions of the “measured” difference that may in fact be due to discrimination (e.g., a woman or minority not entering a high-paying STEM field because of experiences that may be rooted in prejudice, such as greater encouragement for men than women to pursue these studies).
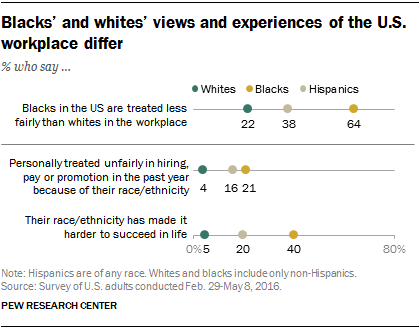
When it comes to racial discrimination in the workplace, most Americans (60%) say blacks and whites are treated about equally, but opinions on this vary considerably across racial and ethnic groups. A new Pew Research Center report finds that roughly two-thirds (64%) of blacks say black people in the U.S. are generally treated less fairly than whites in the workplace; just 22% of whites and 38% of Hispanics agree.
About two-in-ten black adults (21%) and 16% of Hispanics say that in the past year they have been treated unfairly in hiring, pay or promotion because of their race or ethnicity; just 4% of white adults say the same. And while 40% of blacks say their race or ethnicity has made it harder for them to succeed in life, just 5% of whites – and 20% of Hispanics – say this. Some 31% of whites say their race or ethnicity has eased the way toward their success. At least six-in-ten whites (62%) and Hispanics (65%), and about half of blacks (51%), say their race or ethnicity hasn’t made much of a difference.
For their part, about a quarter of women (27%) say their gender has made it harder for them to succeed in life, compared with just 7% of men. About six-in-ten men and women say their gender hasn’t made much difference, but men are much more likely than women to say their gender has made it easier to succeed (30% vs. 8%). In addition, a 2013 Pew Research Center survey found that about one-in-five women (18%) say they have faced gender discrimination at work , including 12% who say they have earned less than a man doing the same job because of their gender. By comparison, one-in-ten men say they have faced gender-based workplace discrimination, including 3% who say their gender has been a factor in earning lower wages.
- Business & Workplace
- Economic Inequality
- Economics, Work & Gender
- Gender & LGBTQ
- Gender & Work
- Gender Equality & Discrimination
- Gender Pay Gap
- Income & Wages
- Race & Ethnicity
Eileen Patten is a former research analyst focusing on Hispanic, social and demographic trends at Pew Research Center .
Half of Latinas Say Hispanic Women’s Situation Has Improved in the Past Decade and Expect More Gains
A majority of latinas feel pressure to support their families or to succeed at work, a look at small businesses in the u.s., majorities of adults see decline of union membership as bad for the u.s. and working people, a look at black-owned businesses in the u.s., most popular.
1615 L St. NW, Suite 800 Washington, DC 20036 USA (+1) 202-419-4300 | Main (+1) 202-857-8562 | Fax (+1) 202-419-4372 | Media Inquiries
Research Topics
- Age & Generations
- Coronavirus (COVID-19)
- Economy & Work
- Family & Relationships
- Immigration & Migration
- International Affairs
- Internet & Technology
- Methodological Research
- News Habits & Media
- Non-U.S. Governments
- Other Topics
- Politics & Policy
- Email Newsletters
ABOUT PEW RESEARCH CENTER Pew Research Center is a nonpartisan fact tank that informs the public about the issues, attitudes and trends shaping the world. It conducts public opinion polling, demographic research, media content analysis and other empirical social science research. Pew Research Center does not take policy positions. It is a subsidiary of The Pew Charitable Trusts .
Copyright 2024 Pew Research Center
More From Forbes
Inequality doubles in gender pay gap for women, new survey shows.
- Share to Facebook
- Share to Twitter
- Share to Linkedin
Facing inequality: the wage gap for women has doubled.
The gender pay gap - the difference between what men and women are paid for the same role - has doubled from 2.9% in 2022 to 6.0% in April 2024. According to data pulled from over 1 million resumes in the US, new research commissioned by Adzuna reveals that, on average, women continue to make a fraction of what men are paid for the same (or similar) roles. For some career paths, the disparity is even greater than the average 6% deficit.
Careers in STEM (Science, Technology, Engineering and Math) have the widest gender pay gaps, according to the survey. In March 2023, the gender pay gap in science was 13.1% - meaning that women are paid 87% of the wages their male counterparts receive. In engineering, the gap is 9.5%, for women in the workforce. The legal sector has seen the pay gap increase significantly, where the gender pay gap is now 11%. That’s a significant reversal from 2022 and 2023, where women in the legal profession outearned men by 5%.
“Despite ongoing endeavors to enhance female participation and representation within STEM fields, the scales remain overwhelmingly tilted in favor of men, with women in science still earning a staggering 13% less than their male counterparts on average. Our data underscores a pressing need for better efforts to advance gender parity in the workplace," says James Neave , Head of Data Science at job search engine Adzuna.
Inequality for Women: Reversed in Some Sectors
The survey revealed the one sector where women out-earn their male counterparts. While women in IT earn 7% less than their male colleagues (note that this figure is actually an increase in earnings of about 5% vs. last year), accounting is the place where wages for women have actually exceeded what men are paid. In accounting, the survey says, women earn $1.04 for every dollar earned by men. Close behind is the field of banking and finance, where women are earning 99 cents for every dollar paid to their male counterparts. In finance and accounting, the gender pay gap is more equitable - even favorable - for women.
Will Education Help with Inequality in Wages?
Does a college degree help to even the odds, when it comes to wage inequality? According to Pew Research, about 62% of US adults over the age of 25 don’t have a college degree. (Perhaps that’s why so many are interested in high-income skills you can learn without a degree). Indeed, education takes many forms in 2024 - and college isn’t the answer for everybody. But, for those enrolled in undergraduate and graduate programs, the data shows that the future is female.
Fallout Dethroned In Amazon Prime Video s Top 10 List By A New Offering
Google chrome gets second emergency update in a week as new exploit confirmed, metallica makes history with their new no 1 single.
Since February 2023, the labor force participation for prime-age women (those between the ages of 25 and 54) has exceeded its all-time high. In other words, women are entering the workforce in greater numbers, with a participation rate of 77.8%. Research from the Hamilton Project, a division of the Brookings Institute dedicated to opportunity, prosperity and growth (based on the initial work of Alexander Hamilton), shows that women have contributed most to the overall labor force since the pandemic.
Meanwhile, Pew Research shows that women are outpacing men in college graduation rates. For adults age 25-34, women surpass men as a percentage of the population with a bachelor’s degree: 46% of women versus 36% for men. According to the National Student Clearinghouse Research Center, women are more likely to graduate in four years and less likely to drop out: just over 51% of women who enrolled in college in 2014 finished in four years (versus 41% of men). Statista projects that there will be 36% more women than men enrolled in college in 2031. While universities are not the only source of education and experience, these statistics point to a potential shift in the workforce, as women have a greater propensity for enrolling (and completing) college programs.
According to BeyondCollegeAccess.com, societal shifts are driving the desire for more education. And by extension, a desire for equal pay. “One significant factor is the changing societal and cultural norms that now encourage and support women in their educational endeavors. As gender roles evolve and traditional barriers are dismantled, women are more empowered to pursue their academic and professional aspirations,” the website shares.
While progress is shown in educational institutions, there’s still room for improvement - which is simply another way of saying, “equality” - when it comes to the wage gap for women. The key to the job search conversation is knowing your worth, and knowing what a position pays, so that you can ask for what you deserve (not just what you think you can get). While systemic change is needed, on a micro level every job interview is a negotiation - an opportunity to share your story and your value in a way that creates fairness, inclusion and equality. Does that sound like a wish for sunshine and peppermints, or a strategy that’s just good business? The answer depends on how you negotiate - and who is on the other side of the table.
While one person can’t change national statistics, change always happens one person (and one conversation) at a time. As the workplace conversation evolves, and the workforce continues to shift, equal pay for equal work won’t be a matter of negotiation. It will be a matter of necessity.
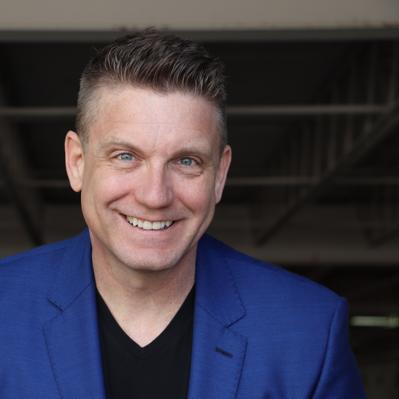
- Editorial Standards
- Reprints & Permissions
Join The Conversation
One Community. Many Voices. Create a free account to share your thoughts.
Forbes Community Guidelines
Our community is about connecting people through open and thoughtful conversations. We want our readers to share their views and exchange ideas and facts in a safe space.
In order to do so, please follow the posting rules in our site's Terms of Service. We've summarized some of those key rules below. Simply put, keep it civil.
Your post will be rejected if we notice that it seems to contain:
- False or intentionally out-of-context or misleading information
- Insults, profanity, incoherent, obscene or inflammatory language or threats of any kind
- Attacks on the identity of other commenters or the article's author
- Content that otherwise violates our site's terms.
User accounts will be blocked if we notice or believe that users are engaged in:
- Continuous attempts to re-post comments that have been previously moderated/rejected
- Racist, sexist, homophobic or other discriminatory comments
- Attempts or tactics that put the site security at risk
- Actions that otherwise violate our site's terms.
So, how can you be a power user?
- Stay on topic and share your insights
- Feel free to be clear and thoughtful to get your point across
- ‘Like’ or ‘Dislike’ to show your point of view.
- Protect your community.
- Use the report tool to alert us when someone breaks the rules.
Thanks for reading our community guidelines. Please read the full list of posting rules found in our site's Terms of Service.
The Gender Pay Gap Is Even Bigger for Freelancers, New Survey Shows
D espite decades of effort to achieve better pay equity and equal opportunity in the workplace, the gender pay gap remains a complex and ongoing challenge in the United States. This is one of the most pernicious problems in the American economy because it's forcing half the population to be underpaid at work and under-resourced in investing for the future .
According to recent data from the Pew Research Center, American women get paid about $0.82 for every $1 that men get paid, or about 18% less. But the gender pay gap is not just an issue for big corporations. It's rearing its head in the world of small business and self-employment, too.
A new survey from OnDeck, a small business lender, found that freelancers and independent professionals are also vulnerable to a freelance gender pay gap. OnDeck's study shows that male freelancers charge about 26% more per hour than female freelancers do.
Let's look at a few hard facts about the freelance gender pay gap between male and female freelancers.
Male freelancers charge 26.4% more than female freelancers
OnDeck did a survey on the Freelancer Pay Gap 2024 , which included analysis of more than 9,000 freelancers on Upwork (a freelance talent platform) to compare hourly billing rates. OnDeck's research discovered that men tend to charge significantly more than women for their professional freelance services.
According to OnDeck's research, male freelancers charge an average of $75.44 per hour, while female freelancers charge an average of $59.70 per hour. This amounts to a difference of $15.74 per hour, or about 26.4%. That makes the freelance gender pay gap even bigger than the national average of 18%.
Freelance gender pay gaps are bigger for some professions
There's a wide range of services in the gig economy that count as "freelance" or "consulting." Not every freelancer is a creative professional like a writer or graphic designer; some have other business expertise or technical skills. The OnDeck survey examined the gender pay gap for a range of service categories and professions.
Here are a few professional fields that have the biggest freelance gender pay gaps, based on average billable rates per hour:
- Legal: Men charge $144.78 per hour vs. $68.19 for women (112% gap)
- Accounting & consulting: Men charge $92.17 per hour vs. $66.62 for women (38% gap)
- Engineering & architecture: Men charge $67.99 per hour vs. $55.25 for women (23% gap)
- Data science & analytics: Men charge $90.42 per hour vs. $77.11 for women (17% gap)
There's not always a clear cause for the freelance gender pay gap. But in general, the fact that women tend to charge lower freelance rates than men in certain technical professions like consulting, engineering, and legal work could be a sign of other systemic and cultural biases against women's labor and talent .
Women are often most underrepresented in the same professions that have the biggest freelance pay gaps. If a career field is unwelcoming to women, women might feel less valued and less confident charging as much as men charge for their skills (or men might feel confident charging more). The same gender disparities that appear in full-time jobs could also be showing up in the freelance pay gap.
Some good news on freelance gender pay gaps
Not all categories of freelance work have a big gender pay gap. The OnDeck study also found that women charge more than men do in a few categories of freelance services:
- Design & creative: Women charge an average of $62.86 per hour vs. $62.15 for men
- Customer service: Women charge an average of $36.07 per hour vs. $33.90 for men
- Admin support: Women charge an average of $57.95 per hour vs. $49.67 for men
This data shows some categories of freelance work that are more equitable -- and where women might even have a slight advantage in pricing their services. But these categories tend to be the lowest-paid types of freelance work. This is another reason why the gender pay gap persists: It's not always a matter of women getting paid less for the same work; it's that the market doesn't value the types of work that women are more likely to do.
Bottom line
The gender pay gap makes it harder for women to get ahead, no matter how clever they are at budgeting . Unfortunately, gender-based wage disparities happen not only in traditional employment but also with freelancers who can set their own hourly rates. If you're a freelancer, you have the right to negotiate your own pay on a project-by-project basis -- and you can decline to work for clients who don't pay your desired rates.
Freelancers also need to create a spirit of pay transparency within their industries. By networking with other freelancers and doing research into what "competitive" market pay rates truly are for your professional skill set, you can get more of what you deserve as a small business owner -- and get more cash in your business checking account .
Alert: highest cash back card we've seen now has 0% intro APR until 2025
This credit card is not just good – it's so exceptional that our experts use it personally. It features a 0% intro APR for 15 months, a cash back rate of up to 5%, and all somehow for no annual fee!
Click here to read our full review for free and apply in just 2 minutes.
We're firm believers in the Golden Rule, which is why editorial opinions are ours alone and have not been previously reviewed, approved, or endorsed by included advertisers. The Ascent does not cover all offers on the market. Editorial content from The Ascent is separate from The Motley Fool editorial content and is created by a different analyst team.Discover Financial Services is an advertising partner of The Ascent, a Motley Fool company. The Motley Fool recommends Discover Financial Services. The Motley Fool has a disclosure policy .
- Reference Manager
- Simple TEXT file
People also looked at
Systematic review article, stem and gender gap: a systematic review in wos, scopus, and eric databases (2012–2022).
- 1 Universidad Metropolitana de Ciencias de la Educación, Ñuñoa, Chile
- 2 Universidad de Chile, Santiago, Chile
- 3 Centro de Investigación en Psicología, Educación y Familia (CIPEF), Universidad Finis Terrae, Santiago, Chile
Introduction: This article offers a thorough examination of relevant literature in the WoS, Scopus, and Eric databases for the period 2012–2022, utilizing the PRISMA model (2020) to address STEM and gender gap factors.
Methods: A comprehensive search of the Web of Science, Scopus, and Eric databases spanning the years 2012 to 2022 was conducted. Employing the PRISMA (2020) model, inclusion and exclusion criteria were applied to identify pertinent studies that examined the relationship between STEM education and the gender gap. After rigorous evaluation, 24 articles that adhered to the established criteria were selected. These articles were thoroughly analyzed to extract relevant information pertaining to the factors contributing to the gender gap in STEM fields and educational interventions designed to alleviate these disparities.
Results: This analysis hinges on two fundamental dimensions. The first addresses the factors that contribute to the gender gap in STEM fields, while the second focuses on educational interventions crafted to mitigate bias. These interventions include activities aimed at enhancing skills in science, mathematics, engineering, and technology as well as fostering a growth mindset. The findings of this review suggest that research on gender and STEM predominantly emphasizes key issues using quantitative methodologies; however, it is recommended to explore other methodologies as well.
Discussion: The practical implications of this research relate to identifying critical areas in need of attention to address the identified gap and recognizing the necessity of diversifying the methods and tools used for gathering information to explore new factors that could account for gender biases in scientific fields. The study’s limitations lie in its exclusive focus on the binary gender gap between women and men without considering other relevant factors. Future analyses should incorporate the intersectionality perspective.
Introduction
The promotion gender equality in education is an ongoing global challenge in the twenty-first century, due to persistent disparities between men and women ( Fernandez et al., 2023 ). Unfortunately, a gender-based culture that differentiates expectations, skills, and life projects continues to prevail. One area of particular concern is the limited access given to women in scientific disciplines such as science, technology, engineering, and mathematics, collectively referred to as STEM ( OECD, 2016 ). This situation reveals a troubling scenario of social inequality that educators and policymakers worldwide must address.
According to a recent report by UN Women, at the current pace of progress, it may take 286 years to eliminate existing gaps ( United Nations Women, 2022 ). Advancing toward achieving these goals is crucial because providing education for all increases social resilience, mobility, and economic progress. For this reason, the agenda 2030, approved by the United Nations General Assembly in September 2015, is of utmost relevance. It includes 17 Sustainable Development Goals (SDGs), among which Goal 4 stands out, referring to quality, inclusive, and equitable education that promotes lifelong learning opportunities for all. Additionally, SDG 5 addresses gender equality, empowering women, and ending all forms of violence ( Economic Commission for Latin America and the Caribbean, 2020 ). In this regard, according to OECD Indicators (2021) , men strongly dominate STEM-related fields, highlighting the need to focus on this issue as it demonstrates the loss of talent by not effectively including women.
STEM is an interdisciplinary approach that emerged in the United States after World War II, driven by the need for technological progress. Its development was further propelled by the historical context of the Cold War, particularly with the launch of the R-7 rocket carrying the Sputnik 1 satellite, which had a significant impact on American politics ( Razi and Zhou, 2022 ). Its educational orientation was established by the National Science Foundation (NSF) and subsequently promoted and adopted worldwide. Its objectives are to provide students with critical thinking skills for creative problem-solving and, ultimately, to make them more marketable in the workforce ( White, 2014 ). In this way, the STEM approach contributes to higher-order skills and provides a foundation for innovation, influencing the economic well-being of nations ( Barakos et al., 2012 ).
However, women have been relegated from STEM objectives due to societal gender stereotypes, which shape a shared set of beliefs about the attributes that are characteristic of members of a social category ( Greenwald and Banaji, 2017 ). These beliefs can be implicit or explicit in individuals. By implicit we state thoughts and beliefs that are not commonly recognized but influence our explicit actions toward these objectives. This situation influences the expectations that humans have about their own capabilities. Lippmann (1922) defined stereotypes as mental images of different social groups, with their utility lying in simplifying perception and cognition. One of the most widely accepted definitions is provided by Ashmore and Del Boca (1981) , who conceive stereotypes as a set of beliefs about the personal attributes of a group of individuals.
Human beings develop generalized stereotypes related to specific disciplinary areas from an early age, which become an integral part of their developmental system ( Fine, 2018 ). These stereotypes influence their identity, that is, what they believe about themselves, their future, their interests, and their motivation to learn ( Meltzoff and Cvencek, 2019 ). In this regard, women encounter stereotypes with negative consequences for their interest and academic performance, necessitating efforts to attract and retain them in the STEM workforce to maximize innovation, creativity, and competitiveness ( Hill et al., 2010 ; Gaweł and Krstić, 2021 ). Thus, the educational space, as a socializing agent, is a structure that gives rise to interactions contributing to the reinforcement of stereotypes, which leads to the reproduction of symbolic violence ( Bourdieu and Passeron, 2018 ). Stereotypes describe and proscribe, inducing behavior as individuals conform to the norms attributed to them by society, which are further reinforced through educational institutions. The issue of the STEM education gap presents significant challenges for women, as they are hindered by stereotypes imposed by educational contexts regarding their own creative abilities ( Rippon, 2019 ), thereby limiting their access to careers in these fields.
When investigating the potential causes of the gender gap and biases in STEM, one can explore the most relevant theories and approaches that explain behaviors related to gender stereotypes, providing a suitable theoretical and empirical foundation for the study. These are summarized in Figure 1 .
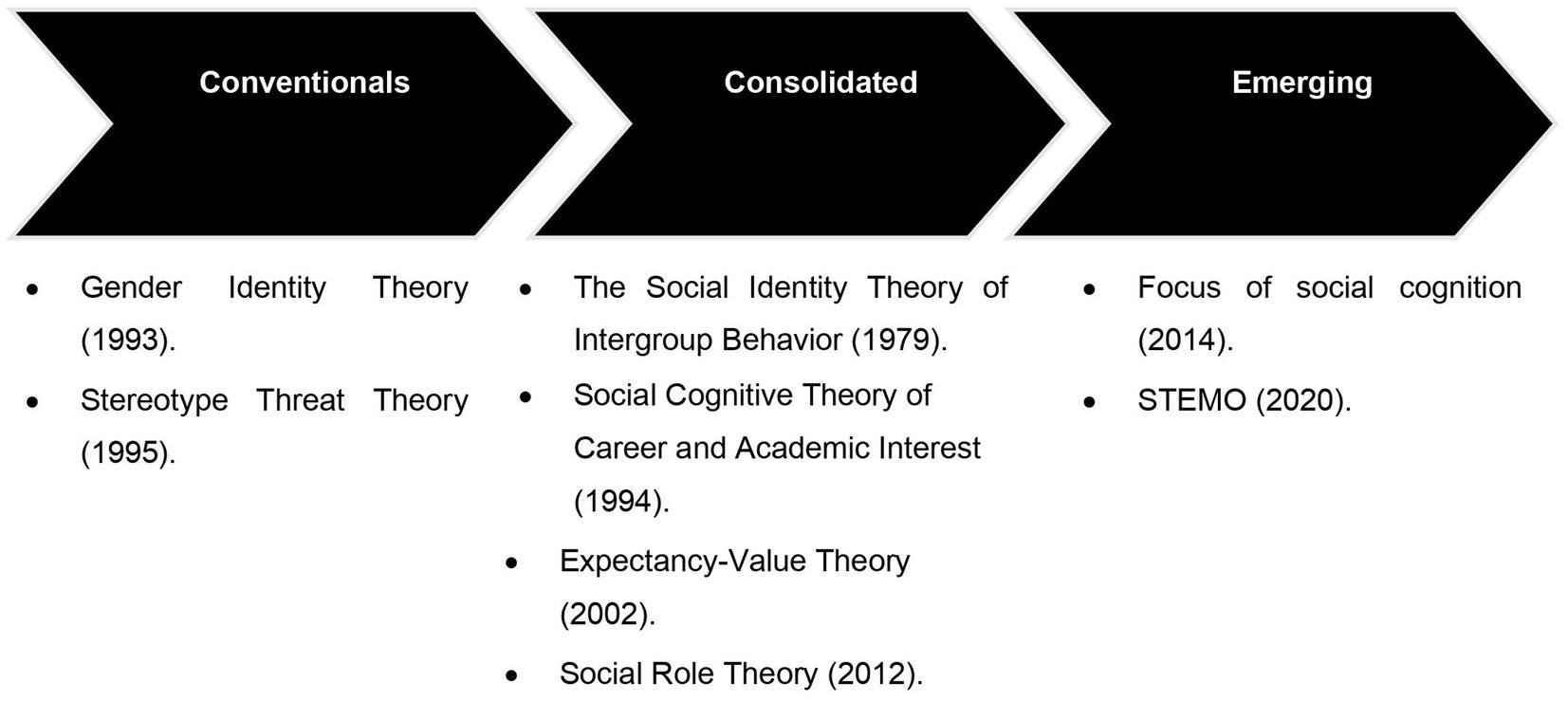
Figure 1 . Theoretical models explaining stereotyped behaviors.
In the first group of the so-called “conventional” theories, we have Spence’s gender identity theory ( Spence, 1993 ), which presents a multifactorial approach to gender-associated attributes. Individuals adopt interests and behaviors expected of their gender, conforming to conventional roles. Additionally, the stereotype threat theory by Steele and Aronson (1995) reinforces and introduces new elements. It highlights the risk of confirming a negative stereotype about one’s own group, which can affect performance on specific tasks. In line with this, women are often associated with weaker mathematical skills compared to men, which influences their performance and interest. However, this does not align with their actual level of ability, as studies indicate that women achieve higher grades in this area ( Perez-Felkner et al., 2017 ; Lundberg, 2020 ).
In the group of established theories, we have Tajfel and Turner’s social identity theory of intergroup behavior ( Tajfel and Turner, 1979 ), which highlights the impact on individuals’ behavior when they perceive themselves as members of social categories. This theory involves three psychological processes: social categorization, identification, and social comparisons. Another theory in this group is the social cognitive career and academic interest theory (SCCT) proposed by Lent et al. (1994) . SCCT focuses on self-efficacy, outcome expectations, goal mechanisms, and how they can interact with gender, contextual support systems, and experiential learning factors ( Lent et al., 1994 ). Eccles and Wigfield’s expectancy-value theory ( Eccles and Wigfield, 2002 ) is a theoretical model that introduces other relevant variables. It addresses the theoretical pathway through which stereotypes affect students’ academic outcomes, choices, and the role of motivation as a change agent ( Wigfield et al., 2015 ). While it emphasizes the role of motivation in addressing the gender gap, it overlooks contextual barriers. It is not only through motivation and elevated expectations that individuals achieve their goals, but social, economic, and personal interferences also play a role. In this regard, identity emerges as a crucial aspect. Finally, we have Eagly and Wood (2012) , which aims to explain the behavior of women and men, as well as the relevant stereotypes, ideologies, and attitudes related to sex and gender.
In the emerging approaches, the contributions of Greenwald and Banaji (1995) are noteworthy. They had already introduced the concept of implicit social cognition to address the influences of stimuli that impact individuals outside of conscious control. Later, Baron et al. (2014) incorporated this concept and focused on social cognition to address stereotypes, emphasizing that it is a cognitive process that develops throughout an individual’s life, including elements such as social association, the assigned gender identity, and self-concept ( Meltzoff and Cvencek, 2019 ). In a similar vein, the nascent proposal of Master and Meltzoff (2020) , called STEMO (Stereotypes, Motivation, and Outcomes), integrates aspects of educational research, human development, and social psychology to understand the mechanisms contributing to gender gaps. Their hypothesis describes the ways in which female STEM students encounter negative stereotypes, leading to biased self-representations regarding their group membership and consequent effects on their interest and academic performance.
The current debate in empirical studies on gender stereotypes in STEM revolves around the differences in preferences and interests between men and women. In this regard, findings indicate that stereotypical images persist and apply to all areas. Given the limited number of studies, it is still risky to make comparisons or inferences ( Master and Meltzoff, 2020 ). On the other hand, some lines of research have focused on the preschool stage and the effect of math stereotypes on teacher-student interaction networks ( Ortega et al., 2021 ). Similarly, research on the role of implicit and explicit beliefs related to mathematics in primary school students, in connection with the influence of parents on their behavior, is noteworthy ( Siani and Dacin, 2018 ). From another perspective, studies have focused on considering the socioeconomic and cultural profiles of female high school students who intend to pursue STEM careers ( Kızılay et al., 2020 ). Meanwhile, recent research addresses the experiences of female graduate students in STEM careers regarding gender gaps and the challenges they face ( Lim et al., 2021 ).
Studies on STEM education have progressively increased in recent years, delineating different scientific trends ( Bogdan and García-Carmona, 2021 ). Therefore, it is essential to identify the approaches that have been developed and envision research gaps for the advancement of new perspectives. Hence, this systematic review follows the PRISMA 2020 guidelines ( Page et al., 2021 ) and aims to describe the scope of research on STEM and the gender gap in primary, secondary, and tertiary education between the years 2012 and 2022. The following questions will help us address this objective:
• What are the most frequently addressed research topics? (Q1).
• What are the most widely used theories that guide research? (Q2).
• How are the topics addressed in the studies? (Q3).
• What is the most developed research method in the studies? (Q4).
The following section delineates the methodological criteria and search strategies employed in this study. After this, the systematic review’s findings are elucidated, leading into discussions, conclusions, and the acknowledgment of limitations, ultimately culminating in a comprehensive compilation of bibliographic references utilized in this study.
This Systematic Review follows the PRISMA (2020) criteria. The research incorporates empirical scientific articles published in open access journals from 2012 to 2022, using the search engines Scopus, Eric, and WoS, recognized as those that compile the highest number of multidisciplinary scientific publications. The purpose is to gather knowledge advancement in a specific subject. The search date for this review was June 21, 2022, and the following English keyword combinations were used: STEM Education AND gender gap, STEM AND gender gap, and STEM AND education AND gap AND gender.
This study is structured based on pre-established criteria for selection/exclusion on this matter, such as the temporal dimension and the object of study. Additionally, the scientific mapping considered five stages: (1) study design, (2) data collection, (3) data analysis, (4) data visualization, and (5) interpretation. In the study design stage, the guiding question was: “What were the results of publications on STEM education and the gender gap indexed in the Scopus, Eric, and WoS databases for the period 2012–2022. The data collection stage consists of three sub-stages: (1) data gathering, (2) data screening, and (3) data cleaning. Table 1 condenses the research protocol criteria that represent the search filters.
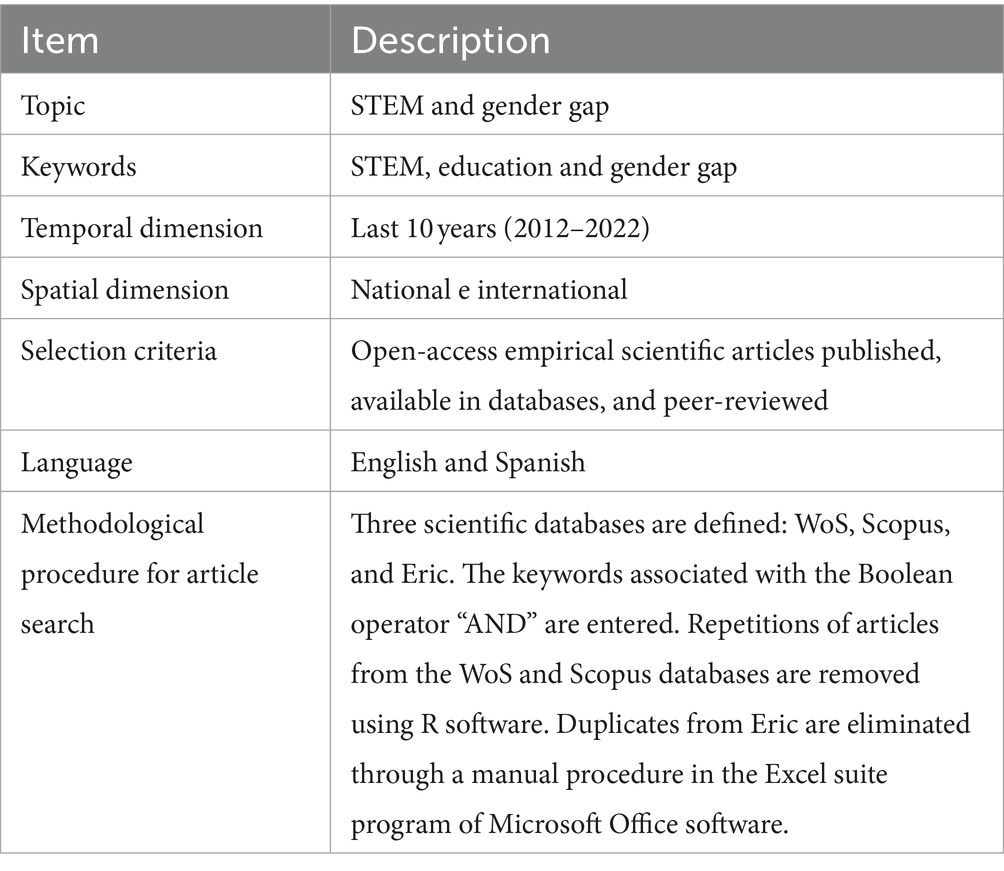
Table 1 . Research protocol summary.
Before proceeding with the selection of articles, the following inclusion and exclusion criteria were defined:
Inclusion criteria
1. Open-access empirical scientific articles that are available in databases, and peer-reviewed, published in the last 10 years.
2. Studies that link the analysis of standardized assessment databases with other attitudinal, cognitive, and social variables from governmental and non-governmental entities.
3. They must be empirical studies with educational implications and case studies.
4. The studies that address STEM and the gender gap in primary, secondary, or tertiary education.
Exclusion criteria
1. Book chapters, conferences, theoretical articles, systematic reviews, and meta-analyses.
2. Studies only focused on the database analysis published, prior to 2012 of this systematic review, on standardized assessments.
3. Database studies on academic performance analysis without linkage to other attitudinal, cognitive, and social variables from governmental and non-governmental entities.
4. Studies focused on the topics of ethnicity, race, graduate students, and practicing professionals in STEM.
5. Studies that mainly address the implementation of other guidelines, with STEM and gender gap not being their focus of analysis.
6. Once the systematic review protocol outlined in the PRISMA model (2020) proposed by Page et al. (2021) was applied to the WoS, Scopus, and Eric databases, a total of 24 scientific articles that met the inclusion and exclusion criteria were obtained, corresponding to the primary units of analysis for the research. The process is presented in the following flowchart ( Figure 2 ).
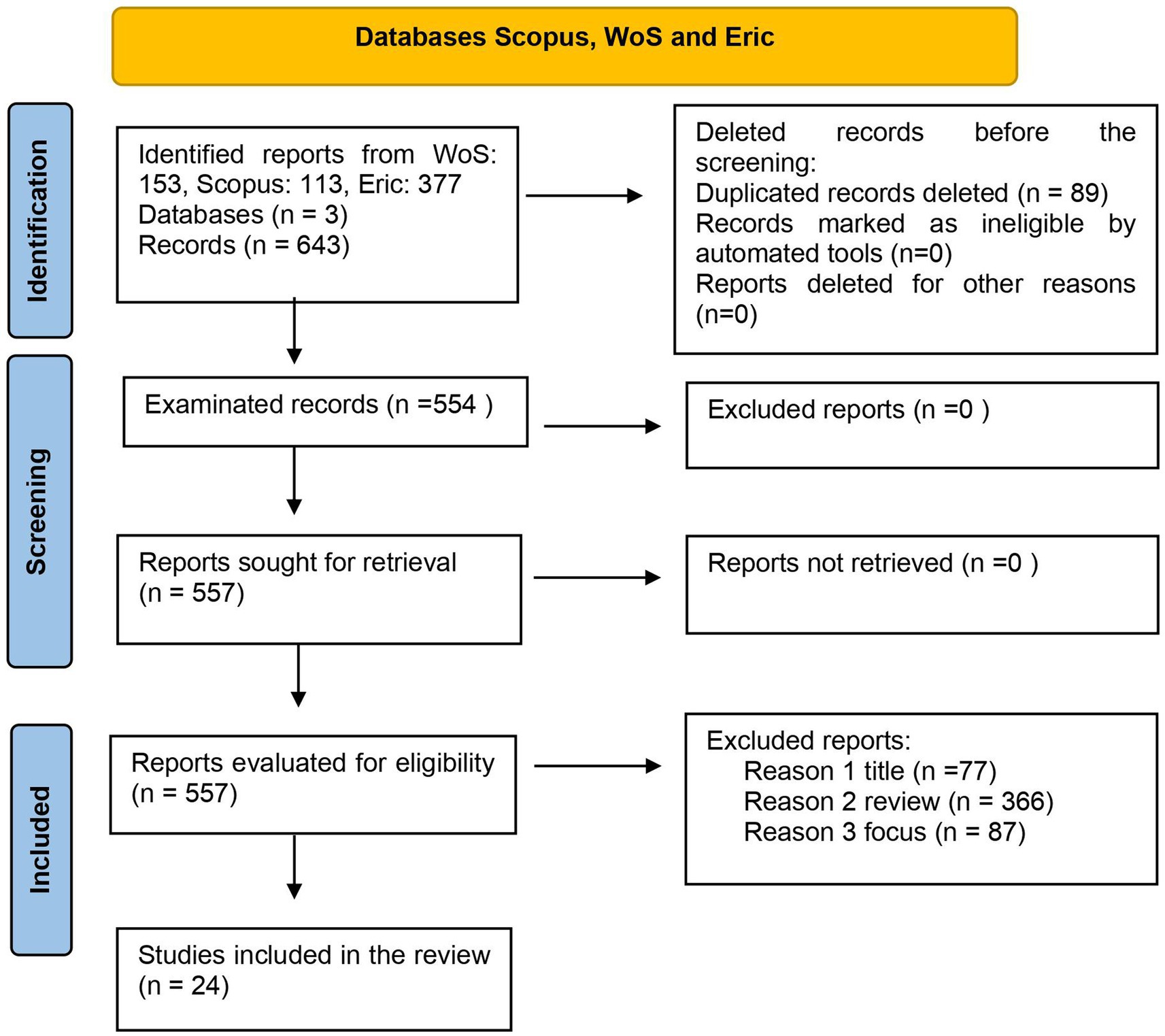
Figure 2 . Flowchart of scientific article search.
A synthesis of the selected research results is presented in the following table organized by authors, theory, sample, methodology, and results. The analysis that will be conducted next follows the projected order that is most relevant for facilitating understanding and integration of the findings. Table 2 shows a summary of the analyzed studies.
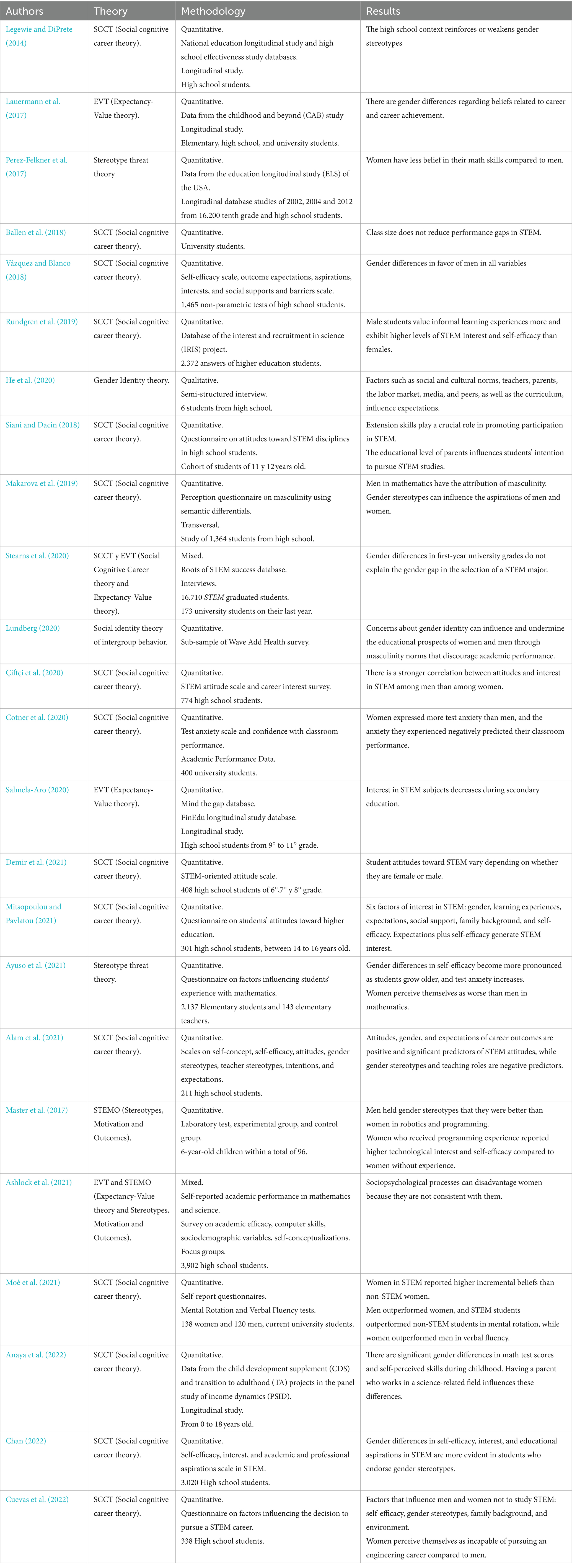
Table 2 . Characteristics of the reviewed studies.
The results have been organized into two dimensions derived from the analysis of the articles found: factors influencing the gender gap in STEM and learning experiences to overcome the gender gap in STEM. Under the factors influencing the gender gap in STEM, categories such as self-efficacy, motivation, expectations, social support, gender roles, identity, teaching roles, anxiety, skills, interests, goals, confidence, stereotype attribution bias, value beliefs, occupational aspirations, burnout, well-being, academic performance, self-perceived skills, influence of the job market, and peer influence emerge. In this regard, four factors that dominated the reviewed research are highlighted: beliefs, self-efficacy, motivation, and expectations. In the realm of learning experiences to overcome the gender gap in STEM , a growth mindset is emphasized.
Similarly, the quantitatively most studied focus has been the discipline of mathematics, even though STEM also encompasses other areas such as chemistry, physics, biology, engineering, astronomy, among others.
Gender gap in STEM is a challenge that involves combined factors such as stereotypical beliefs, self-efficacy, expectations, and motivation. Overcoming biases should be directed toward various aspects, such as university and government support, financial assistance, assertive teacher interaction ( Wang, 2013 ), and, of course, meaningful scientific learning experiences ( Rundgren et al., 2019 ).
The analysis of factors and strategies to address and detect inequalities imposed by biases is limited and should consider other variables. It has mostly focused on studies that gather personal perceptions from each subject, without examining the underlying relationship that governs gender social inequalities between men and women, such as power dynamics and collaborative work. Exploring these aspects would provide alternative perspectives to the issue and contribute to the retention of individuals in STEM fields.
Research has focused on the use of the social cognitive career theory, as there is an interest in understanding the social, cognitive, and psychological factors that influence women’s choices in STEM fields. However, they have not considered the brain structures involved in the adoption of stereotypes.
Furthermore, although significant progress has been made in research on STEM and the gender gap ( Le Thi Thu et al., 2021 ), studies have predominantly focused on the development of quantitative methodologies, highlighting the limited presence of qualitative and mixed methods studies. Moreover, surveys and databases have been commonly used as research tools. The use of database analysis reveals serious issues due to the lack of familiarity and control over the data structure ( Bryman, 2016 ). Therefore, it would be advisable to diversify the methods and tools for gathering information in order to explore new factors that could explain gender biases in scientific fields.
Factors that affect the gender gap in STEM
Gender stereotypes are unconscious and conscious beliefs that underpin the gender gap in STEM and are in line with the status quo , as they depend on who is stereotyping and who is being stereotyped to generate an implicit and explicit response in their cognition that translates into social behavior. The factors influencing the gender gap in STEM education are addressed in various studies and can be grouped into three types: psychological, contextual, and sociocultural. However, the boundaries between these factors are blurred, as there is an interactive and dynamic relationship among them.
Within the most investigated factors, one can identify self-efficacy, motivation, expectations, social support, gender roles, identity, aspirations, family background, attitudes, socioeconomic context, teaching role, anxiety, skills, interests, goals, confidence, stereotype attribution bias, values beliefs, external support, masculinity, occupational aspirations, burnout, well-being, academic performance, self-perceived skills, influence of the job market, and classmates ( Legewie and DiPrete, 2014 ; Lauermann et al., 2017 ; Master et al., 2017 ; Siani and Dacin, 2018 ; Vázquez and Blanco, 2018 ; Makarova et al., 2019 ; Çiftçi et al., 2020 ; Cotner et al., 2020 ; He et al., 2020 ; Lundberg, 2020 ; Salmela-Aro, 2020 ; Stearns et al., 2020 ; Alam et al., 2021 ; Ashlock et al., 2021 ; Ayuso et al., 2021 ; Demir et al., 2021 ; Mitsopoulou and Pavlatou, 2021 ; Moè et al., 2021 ; Anaya et al., 2022 ; Chan, 2022 ; Cuevas et al., 2022 ). In this sense, four gender gap factors stood out from the reviewed research: beliefs, self-efficacy, motivation, and expectations. These factors were quantitatively addressed the most and the study conclusions indicated them as critical elements mediating biases.
Self-efficacy is the mechanism that assist individuals determine their activity and environmental choices ( Bandura, 1982 ). It contributes to the persistence and regulation of emotions, behaviors, and interest in entering or persisting in STEM disciplines ( Rundgren et al., 2019 ). However, self-efficacy is influenced by a multitude of factors, among which learning experiences stand out, whether in primary, secondary, or tertiary education, which, in turn, are influenced by various contextual elements such as social, cultural, and economic factors, and are directly related to stereotypical beliefs ( Chan, 2022 ).
Both motivation and interest in STEM among women are directly related to beliefs about their skills, leading to demotivation and self-doubt, which in turn affects career choices ( Chan, 2022 ). However, what enables women to become interested and motivated in choosing other careers? According to the social cognitive career theory, both supports and barriers influence interests and motivations. Studies indicate that greater interests are expressed by males ( Vázquez and Blanco, 2018 ; Çiftçi et al., 2020 ). In this regard, according to Mitsopoulou and Pavlatou (2021) , the combination of outcome expectations and self-efficacy levels results in interest in STEM. However, beliefs play a crucial role as girls have a lower perception of their skills compared to boys from an early age ( Perez-Felkner et al., 2017 ). Girls may not choose engineering, even if their STEM scores are high ( Cuevas et al., 2022 ), as they are confined to spaces imposed by society through symbolic violence. This situation is further exacerbated by the fact that students’ interest in STEM subjects decreases during secondary education ( Bailey et al., 2017 ; Ballen et al., 2018 ). Therefore, constant opportunities and motivating learning experiences are required.
Learning experiences in overcoming the gender gap in STEM education
Based on the reviewed research, the relevance of learning experiences that foster interest in STEM among women can be concluded. These experiences include extracurricular activities focused on scientific enrichment ( Master et al., 2017 ; Siani and Dacin, 2018 ; Rundgren et al., 2019 ; Demi̇r et al., 2021). Within this framework, the absence of connections with scientific activities in teaching and learning practices in educational institutions is detrimental to overcoming the gap, as it not only hinders engagement in STEM careers but also hampers innovation, creativity, critical thinking, and student autonomy. Studies indicate that learning experiences are essential for entering and persisting in these fields ( Maltese and Tai, 2011 ; Wang, 2013 ). Overall participation in several types of scientific education experiences, including informal, every day, and school-directed experiences ( DeWitt and Archer, 2017 ), is significant for developing scientific capital among female students.
The growth mindset ( Perez-Felkner et al., 2017 ; Moè et al., 2021 ) emerges from successful learning experiences, triggering positive activating emotions such as motivation and interest ( Ayuso et al., 2021 ). In this way, it would facilitate overcoming the gender gap in these fields, which are essential for sustainable development. Implicit and explicit stereotypical beliefs about gender roles must be eradicated to progress in social, economic, and cultural advancement. This is not only beneficial for states but also for non-governmental entities in terms of providing a skilled workforce ( Legewie and DiPrete, 2014 ), thus promoting the reduction of social inequalities.
This systematic review endeavors to uncover the prevailing trends in research conducted between 2012 and 2022, focusing on the factors that contribute to gender disparities in STEM fields. The insights derived from this initial exploration serve as the foundation for a comprehensive examination of our research findings and their implications for fostering gender equity in STEM education and career.
Returning to the research questions, we can indicate that:
• Q1: The most investigated topics are factors influencing the gender gap in STEM as well as educational interventions to promote interest and motivation.
• Q2: The most used theories are the social cognitive career theory, as it provides insights into the determinants that influence career choices.
• Q3: The addressed topics focus on women and are studied by considering variables that should be considered in gender gaps in STEM. Possible solutions are also emerging in the discussions.
• Q4: The most used method is quantitative, and the predominant instruments and techniques for gathering information are databases and surveys.
Ultimately, the space for research growth is evident both at the theoretical and methodological levels, due to the predominance of quantitative studies, with a minority presence of qualitative and mixed methods studies. While quantitative studies are of high quality, as they are longitudinal, experimental, and observational, it is important to explore the reality through other approaches that allow for a deeper understanding of the issue.
On the other hand, the tools used as self-report instruments and databases are insufficient for understanding the phenomenon. Subsequent studies should investigate other factors that influence the gender gap in STEM education and link them with critical perspectives on the underlying causes, rather than just focusing on the consequences or self-perceptions of those stereotypical beliefs. The combined effects and experiences across educational levels must be observed to understand academic and career choices.
The findings of this systematic review offer a comprehensive summary of the empirical research conducted within this field of study. This study aims to promote scientific knowledge from a global perspective. It is of utmost importance for researchers and policymakers to be knowledgeable about the systematization of STEM studies and the gender gap. This knowledge is crucial for understanding the methods developed to advance knowledge, raise awareness about the issue, and propose innovative solutions to address this phenomenon.
Limitations
The present systematic review concentrated exclusively on the gender gap within the binary framework of women and men. Nevertheless, it is crucial to incorporate the perspective of intersectionality in future systematic reviews, as it encompasses various categories of analysis that would significantly contribute to the investigation of disparities in STEM fields.
Data availability statement
The original contributions presented in the study are included in the article/supplementary material, further inquiries can be directed to the corresponding author.
Author contributions
FB-V: Conceptualization, Data curation, Formal analysis, Investigation, Methodology, Project administration, Software, Supervision, Validation, Visualization, Writing – original draft, Writing – review & editing. NS-G: Funding acquisition, Resources, Visualization, Writing – original draft, Writing – review & editing.
The author(s) declare that financial support was received for the research, authorship, and/or publication of this article. This research was funded by the National Doctoral Scholarship Program 2022–2024 of the National Agency for Research and Development of Chile (ANID No. 21220061; GOP No. 242230011); and National Fund for Scientific and Technological Development (FONDECYT), ANID, Chile (No. 1231574).
Conflict of interest
The authors declare that the research was conducted in the absence of any commercial or financial relationships that could be construed as a potential conflict of interest.
Publisher's note
All claims expressed in this article are solely those of the authors and do not necessarily represent those of their affiliated organizations, or those of the publisher, the editors and the reviewers. Any product that may be evaluated in this article, or claim that may be made by its manufacturer, is not guaranteed or endorsed by the publisher.
Alam, M., Sajid, S., Kok, J., Rehman, M., and Amin, A. (2021). Factors that influence high school female students’ intentions to pursue science, technology, engineering and mathematics (STEM) education in Malaysia. Pertanika J. Soc. Sci. Human. 29, 839–867. doi: 10.47836/pjssh.29.2.06
Crossref Full Text | Google Scholar
Anaya, L., Stafford, F., and Zamarro, G. (2022). Gender gaps in math performance, perceived mathematical ability and college STEM education: the role of parental occupation. Educ. Econ. 30, 113–128. doi: 10.1080/09645292.2021.1974344
Ashlock, J., Stojnic, M., and Tufekci, Z. (2021). Gender differences in academic efficacy across STEM fields. Sociol. Perspect. 65, 555–579. doi: 10.1177/07311214211028617
Ashmore, R. D., and Del Boca, F. K. (1981). “Conceptual approaches to stereotypes and stereotyping” in Cognitive processes in stereotyping and intergroup behavior . ed. D. L. Hamilton (Psychology Press), 1–35.
Google Scholar
Ayuso, N., Fillola, E., Masia, B., Murillo, A. C., Trillo-Lado, R., Baldassarri, S., et al. (2021). Gender gap in STEM: a cross-sectional study of primary school students’ self-perception and test anxiety in mathematics. IEEE Trans. Educ. 64, 40–49. doi: 10.1109/TE.2020.3004075
Bailey, D., Duncan, G. J., Odgers, C. L., and Yu, W. (2017). Persistence and fadeout in the impacts of child and adolescent interventions. J. Res. Educ. Effect. 10, 7–39. doi: 10.1080/19345747.2016.1232459
PubMed Abstract | Crossref Full Text | Google Scholar
Ballen, C. J., Aguillon, S. M., Brunelli, R., Drake, A. G., Wassenberg, D., Weiss, S. L., et al. (2018). Do small classes in higher education reduce performance gaps in STEM? Bioscience 68, 593–600. doi: 10.1093/biosci/biy056
Bandura, A. (1982). Self-efficacy mechanism in human agency. Am. Psychol. 37, 122–147. doi: 10.1037/0003-066X.37.2.122
Bandura, A. (1999). Social cognitive theory of personality . Available at: https://www.semanticscholar.org/paper/Social-cognitive-theory-of-personality.-Bandura/e9cc8502ef2ca8e4ca6c7be45e67416f8d25a947
Bandura, A. (2018). Toward a psychology of human agency: pathways and reflections. Perspect. Psychol. Sci. 13, 130–136. doi: 10.1177/1745691617699280
Barakos, L., Lujan, V., and Strang, C. (2012). Science, technology, engineering, mathematics (STEM): catalyzing change amid the confusion. : Center on Instruction Available at: https://eric.ed.gov/?id=ED534119 .
Baron, A. S., Schmader, T., Cvencek, D., and Meltzoff, A. N. (2014). “The gendered self-concept: how implicit gender stereotypes and attitudes shape self-definition” in Gender and development . Eds P. J. Leman and H. R. Tenenbaum (Psychology Press), 109–132.
Blickenstaff, J. (2005). Women and science careers: leaky pipeline or gender filter? Gend. Educ. 17, 369–386. doi: 10.1080/09540250500145072
Bogdan, R., and García-Carmona, A. (2021). «De STEM nos gusta todo menos STEM». Análisis crítico de una tendencia educativa de moda [«of STEM we like everything but STEM». A critical analysis of a buzzing educational trend]. Enseñanza Cienc. 39, 65–80. doi: 10.5565/rev/ensciencias.3093
Bourdieu, P., and Passeron, J.-C. (2018). La reproducción: Elementos para una teoría del sistema educativo . Buenos Aires: Siglo XXI.
Breit, M., Brunner, M., and Preckel, F. (2020). General intelligence and specific cognitive abilities in adolescence: tests of age differentiation, ability differentiation, and their interaction in two large samples. Dev. Psychol. 56, 364–384. doi: 10.1037/dev0000876
Bryman, A. (2016). Social research methods . 5th Edn. Oxford, United Kingdom: Oxford University Press.
Cernas-Ortiz, D., Mercado, P., and Leon-Cazares, F. (2017). La varianza del método común en la relación entre la satisfacción laboral y la satisfacción con la vida. Estudi. Geren. 33, 321–329. doi: 10.1016/j.estger.2017.11.004
Chan, R. C. H. (2022). A social cognitive perspective on gender disparities in self-efficacy, interest, and aspirations in science, technology, engineering, and mathematics (STEM): the influence of cultural and gender norms. Int. J. STEM Educ. 9:37. doi: 10.1186/s40594-022-00352-0
Çiftçi, A., Topcu, M., and Erdogan, I. (2020). Gender gap and career choices in STEM education: Turkey sample. Int. J. Progress. Educ. 16, 53–66. doi: 10.29329/ijpe.2020.248.4
Cotner, S., Jeno, L. M., Walker, J. D., Jørgensen, C., and Vandvik, V. (2020). Gender gaps in the performance of Norwegian biology students: the roles of test anxiety and science confidence. Int. J. STEM Educ. 7:55. doi: 10.1186/s40594-020-00252-1
Cuevas, P., García-Arenas, M., and Rico, N. (2022). Why not STEM? A study case on the influence of gender factors on students’ higher education choice. Mathematics 10:239. doi: 10.3390/math10020239
Cvencek, D., Meltzoff, A. N., and Greenwald, A. G. (2011). Math-gender stereotypes in elementary school children. Child Dev. 82, 766–779. doi: 10.1111/j.1467-8624.2010.01529.x
Cvencek, D., Meltzoff, A. N., and Kapur, M. (2014). Cognitive consistency and math-gender stereotypes in Singaporean children. J. Exp. Child Psychol. 117, 73–91. doi: 10.1016/j.jecp.2013.07.018
Demir, C., Önal, N., and Önal, N. (2021). Investigation of middle school students’ attitudes towards science, technology, engineering and mathematics (STEM) education and determination of the predictors. J. Sci. Learn. 4:101. doi: 10.17509/jsl.v4i2.28859
DeWitt, J., and Archer, L. (2017). Participation in informal science learning experiences: the rich get richer? Int. J. Sci. Educ., Part B 7, 356–373. doi: 10.1080/21548455.2017.1360531
Eagly, A. H., and Wood, W. (2012). “Social role theory” in Handbook of theories of social psychology: volume 2 . Eds. n P. A. M. Van Lange, A. W. Kruglanski and E. T. Higgins (SAGE Publications Ltd.), 458–476.
Eccles, J. (2011). Gendered educational and occupational choices: applying the Eccles et al. model of achievement-related choices. Int. J. Behav. Dev. 35, 195–201. doi: 10.1177/0165025411398185
Eccles, J., and Wigfield, A. (2002). Motivational beliefs, values and goals. Annu. Rev. Psychol. 53, 109–132. doi: 10.1146/annurev.psych.53.100901.135153
Economic Commission for Latin America and the Caribbean (ECLAC) (2020). The 2030 Agenda for Sustainable Development in the new global and regional context: scenarios and projections in the current crisis (LC/PUB.2020/5), Santiago. Available at: https://sustainabledevelopment.un.org/content/documents/26088ECLAC_contribution_2020.pdf
Fernandez, D., White, S., Smith, H. C. M., Connor, P. M., and Ryan, M. (2023). Gender inequality in science, technology, engineering and mathematics: gendered time disparities in perceived and actual time spent in practical laboratory-based activities. Front. Educ. 8:1194968. doi: 10.3389/feduc.2023.1194968
Fine, C. (2018). Testosterona Rex. Mitos sobre el sexo, ciencia y sociedad . Barcelona: Paidos.
Gaweł, A., and Krstić, M. (2021). Gender gaps in entrepreneurship and education levels from the perspective of clusters of european countries. J. Dev. Entrep. 26:2150024. doi: 10.1142/S1084946721500242
Greenwald, A. G., and Banaji, M. R. (1995). Implicit social cognition: attitudes, self-esteem, and stereotypes. Psychol. Rev. 102, 4–27. doi: 10.1037/0033-295x.102.1.4
Greenwald, A. G., and Banaji, M. R. (2017). The implicit revolution: reconceiving the relation between conscious and unconscious. Am. Psychol. 72, 861–871. doi: 10.1037/amp0000238
He, L., Zhou, G., Salinitri, G., and Xu, L. (2020). Female underrepresentation in STEM subjects: an exploratory study of female high school students in China. EURASIA J. Math. Sci. Technol. Educ. 16:em1802. doi: 10.29333/ejmste/109657
Hill, C., Corbett, C., and St. Rose, A. (2010). Why so few? Women in science, technology, engineering, and mathematics : American Association of University Women Available at: https://eric.ed.gov/?id=ED509653 .
Joel, D., Berman, Z., Tavor, I., Wexler, N., Gaber, O., Stein, Y., et al. (2015). Sex beyond the genitalia: the human brain mosaic. Proc. Natl. Acad. Sci. U. S. A. 112, 15468–15473. doi: 10.1073/pnas.1509654112
Kızılay, E., Yamak, H., and Kavak, N. (2020). Development of the STEM career interest scale for high school students. Eur. J. Educ. Sci. 7, 1–15. doi: 10.19044/ejes.v7no3a4
Lauermann, F., Tsai, Y.-M., and Eccles, J. S. (2017). Math-related career aspirations and choices within Eccles et al.’s expectancy–value theory of achievement-related behaviors. Dev. Psychol. 53, 1540–1559. doi: 10.1037/dev0000367
Le Thi Thu, H., Tran, T., Trinh Thi Phuong, T., Le Thi Tuyet, T., Le Huy, H., and Vu Thi, T. (2021). Two decades of STEM education research in middle school: a Bibliometrics analysis in Scopus database (2000–2020). Educ. Sci. 11:353. doi: 10.3390/educsci11070353
Legewie, J., and DiPrete, T. A. (2014). The high school environment and the gender gap in science and engineering. Sociol. Educ. 87, 259–280. doi: 10.1177/0038040714547770
Lent, R. W., Brown, S. D., and Hackett, G. (1994). Toward a unifying social cognitive theory of career and academic interest, choice, and performance. J. Vocat. Behav. 45, 79–122. doi: 10.1006/jvbe.1994.1027
Lim, J. H., Wang, Y., Wu, T., Li, Z., and Sun, T. (2021). Walking on gender tightrope with multiple marginalities: Asian international female students in STEM graduate programs. J. Int. Stud. 11, 647–665. doi: 10.32674/jis.v11i3.2132
Lippmann, W. (1922). Public opinion . New York: Harcourt, Brace and Company.
Lundberg, S. (2020). Educational gender gaps. South. Econ. J. 87, 416–439. doi: 10.1002/soej.12460
Makarova, E., Aeschlimann, B., and Herzog, W. (2019). The gender gap in STEM fields: the impact of the gender stereotype of math and science on secondary students’ career aspirations. Front. Educ. 4:60. doi: 10.3389/feduc.2019.00060
Maltese, A. V., and Tai, R. H. (2011). Pipeline persistence: examining the association of educational experiences with earned degrees in STEM among U.S. students. Sci. Educ. 95, 877–907. doi: 10.1002/sce.20441
Master, A., Cheryan, S., Moscatelli, A., and Meltzoff, A. N. (2017). Programming experience promotes higher STEM motivation among first-grade girls. J. Exp. Child Psychol. 160, 92–106. doi: 10.1016/j.jecp.2017.03.013
Master, A., and Meltzoff, A. (2020). Cultural stereotypes and sense of belonging contribute to gender gaps in STEM. Sci. Technol. 12:47,
Meltzoff, A. N., and Cvencek, D. (2019). How stereotypes shape children’s STEM identity and learning. In: Developing minds in the digital age: Towards a science of learning for 21st century education. Eds. P. Kuhl et al. (Chapter 3, pp. 37–47). OECD Publishing.
Mitsopoulou, A., and Pavlatou, E. (2021). Factors associated with the development of secondary school students’ interest towards STEM studies. Educ. Sci. 11:746. doi: 10.3390/educsci11110746
Moè, A., Hausmann, M., and Hirnstein, M. (2021). Gender stereotypes and incremental beliefs in STEM and non-STEM students in three countries: relationships with performance in cognitive tasks. Psychol. Res. 85, 554–567. Scopus. doi: 10.1007/s00426-019-01285-0
Nix, S., Perez-Felkner, L., and Thomas, K. (2015). Perceived mathematical ability under challenge: a longitudinal perspective on sex segregation among STEM degree fields. Front. Psychol. 6:530. doi: 10.3389/fpsyg.2015.00530
OECD (2016). Mujeres, Gobierno y Diseño de Políticas Públicas en los países de la OCDE: Fomentar la diversidad para el crecimiento incluyente : Organisation for Economic Co-operation and Development Available at: https://www.oecd-ilibrary.org/governance/mujeres-gobierno-y-diseno-de-politicas-publicas-en-los-paises-de-la-ocde_9789264256613-es .
OECD (2021). Education at a glance 2021: OECD indicators : OECD.
Ortega, L., Treviño, E., and Gelber, D. (2021). The inclusion of girls in Chilean mathematics classrooms: gender bias in teacher-student interaction networks (La inclusión de las niñas en las aulas de matemáticas chilenas: sesgo de género en las redes de interacciones profesor-estudiante). J. Study Educ. Dev. 44, 623–674. doi: 10.1080/02103702.2020.1773064
Page, M. J., McKenzie, J. E., Bossuyt, P. M., Boutron, I., Hoffmann, T. C., Mulrow, C. D., et al. (2021). Declaración PRISMA 2020: Una guía actualizada para la publicación de revisiones sistemáticas. Rev. Esp. Cardiol. 74, 790–799. doi: 10.1016/j.recesp.2021.06.016
Perez-Felkner, L., Nix, S., and Thomas, K. (2017). Gendered pathways: how mathematics ability beliefs shape secondary and postsecondary course and degree field choices. Front. Psychol. 8:386. doi: 10.3389/fpsyg.2017.00386
Razi, A., and Zhou, G. (2022). STEM, iSTEM, and STEAM: what is next? Int. J. Technol. Educ. 5, 1–29. doi: 10.46328/ijte.119
Rippon, G. (2019). The gendered brain: the new neuroscience that shatters the myth of the female brain . London: Bodley Head.
Rundgren, S.-N. C., Sun, Y., and Jidesjo, A. (2019). Examining gender differences in students’ entrance into and persistence in STEM programs in Swedish higher education. Eur. J. Educ. Sci. 6, 66–94. doi: 10.19044/ejes.v6no1a5
Salmela-Aro, K. (2020). The role of motivation and academic wellbeing – the transition from secondary to further education in STEM in Finland. Eur. Rev. 28, S121–S134. doi: 10.1017/S1062798720000952
Sanders, M. (2009). STEM, STEM education, STEMmania. Technol. Teach. 68, 20–26,
Schunk, D. H., and DiBenedetto, M. K. (2020). Motivation and social cognitive theory. Contemp. Educ. Psychol. 60:101832. doi: 10.1016/j.cedpsych.2019.101832
Siani, A., and Dacin, C. (2018). An evaluation of gender bias and pupils’ attitude towards STEM disciplines in the transition between compulsory and voluntary schooling. New Direct. Teach. Phys. Sci. doi: 10.29311/ndtps.v0i13.2966
Spence, J. T. (1993). Gender-related traits and gender ideology: evidence for a multifactorial theory. J. Pers. Soc. Psychol. 64, 624–635. doi: 10.1037//0022-3514.64.4.624
Stearns, E., Bottia, M. C., Giersch, J., Mickelson, R. A., Moller, S., Jha, N., et al. (2020). Do relative advantages in STEM grades explain the gender gap in selection of a STEM major in college? A multimethod answer. Am. Educ. Res. J. 57, 218–257. doi: 10.3102/0002831219853533
Steele, C. M., and Aronson, J. (1995). Stereotype threat and the intellectual test performance of African Americans. J. Pers. Soc. Psychol. 69, 797–811. doi: 10.1037/0022-3514.69.5.797
Tajfel, H., and Turner, J. C. (1979). “An integrative theory of intergroup conflict | ALNAP” in The social psychology of intergroup relations (Brooks/Cole), 33–37. Available at: https://www.alnap.org/help-library/an-integrative-theory-of-intergroup-conflict
United Nations Women. (2022). Progress on the sustainable development goals: the gender snapshot 2022 . Available at: https://data.unwomen.org/publications/progress-sustainable-development-goals-gender-snapshot-2022
Vázquez, I. M. V., and Blanco, Á. (2018). Factores sociocognitivos asociados a la elección de estudios científico-matemáticos. Un análisis diferencial por sexo y curso en la Educación Secundaria. Rev. Invest. Educ. 37, 269–286. doi: 10.6018/rie.37.1.303531
Wang, X. (2013). Why students choose STEM majors: motivation, high school learning, and postsecondary context of support. Am. Educ. Res. J. 50, 1081–1121. doi: 10.3102/0002831213488622
White, D. W. (2014). What is STEM education and why is it important? Flor. Assoc. Teach. Educ. J. 1, 1–9,
Wigfield, A., Eccles, J. S., Fredricks, J. A., Simpkins, S., Roeser, R., and Schiefele, U. (2015). Development of achievementmotivation and engagement. In: Handbook of child psychology and developmental science, Vol. 3: socioemotional processes . Eds. R. M. Lerner (Series Ed.), and M. Lamb (Vol. Ed.), (7th ed., pp. 657–700). Hoboken, NJ: Wiley.
Keywords: stereotypes, stem, gender gap, education, academic performance
Citation: Beroíza-Valenzuela F and Salas-Guzmán N (2024) STEM and gender gap: a systematic review in WoS, Scopus, and ERIC databases (2012–2022). Front. Educ . 9:1378640. doi: 10.3389/feduc.2024.1378640
Received: 30 January 2024; Accepted: 23 April 2024; Published: 06 May 2024.
Reviewed by:
Copyright © 2024 Beroíza-Valenzuela and Salas-Guzmán. This is an open-access article distributed under the terms of the Creative Commons Attribution License (CC BY) . The use, distribution or reproduction in other forums is permitted, provided the original author(s) and the copyright owner(s) are credited and that the original publication in this journal is cited, in accordance with accepted academic practice. No use, distribution or reproduction is permitted which does not comply with these terms.
*Correspondence: Natalia Salas-Guzmán, [email protected]
This article is part of the Research Topic
Women's Experience and Gender Bias in Higher Education

Trang web chính thức của chính phủ Hoa Kỳ
Dưới đây là một số thông tin bạn có thể tìm hiểu
.gov có nghĩa là trang web chính thức. Các trang web của chính quyền liên bang thường kết thúc bằng .gov hoặc .mil. Trước khi chia sẻ thông tin nhạy cảm, hãy đảm bảo bạn đang sử dụng trang web của chính quyền liên bang.
Trang web này an toàn. Giao thức https:// đảm bảo rằng bạn đang kết nối với trang web chính thức và mọi thông tin mà bạn cung cấp đều được mã hóa cũng như được truyền đi một cách an toàn.
Hiện tại chưa có bản dịch cho ngôn ngữ bạn đã chọn
5 Fast Facts: The Gender Wage Gap
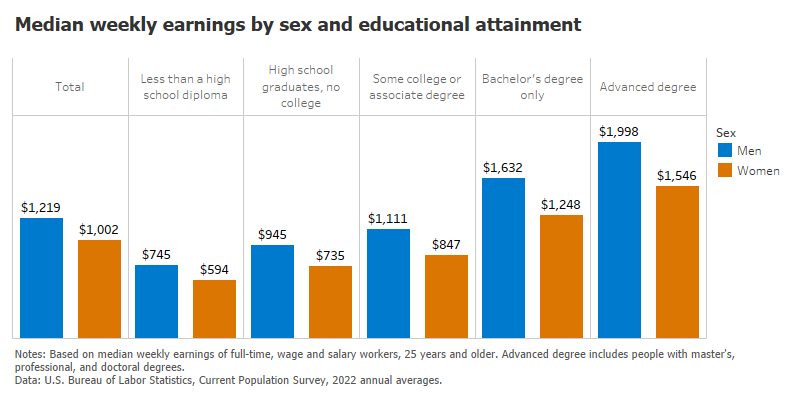
March 14 is Equal Pay Day. Here are five fast facts about the gender wage gap.
- Stats. Overall, women are not paid as much as men, even when working full time and year round. On average, women working full time, year round are paid 83.7% of what men are paid . This inequity is even greater for Black and Hispanic women.
- Causes. Women’s labor is undervalued. Most of the disparity in women and men’s pay cannot be explained by measurable differences between them . Out of the causes of the wage gap that we can measure, the main contributor is that women are more likely than men to work in low-paying jobs that offer fewer benefits .
- Education. Education is not enough to eliminate the gender wage gap. On average, women have more years of education and are more likely than men to have completed Associate’s, Bachelor’s or Master’s degrees. Yet there is a significant gender wage gap at every level of education . Overall, women must complete one additional degree in order to be paid the same wages as a man with less education.
- Age. The gender wage gap does not resolve itself as women age and develop further in their careers. In fact, the wage gap for older women workers is larger than for younger women , and older Black and Hispanic women have the most extreme differences in pay .
- Occupations. The largest identifiable causes of the gender wage gap are differences in the occupations and industries where women and men are most likely to work. Women are 2 out of every 3 full-time workers in occupations that pay less than $30,000 per year, and fewer than 1 in 3 full-time workers in jobs paying an average of $100,000 or more. However, even within the same occupations, women earn less on average than men .
Learn more about equal pay in the United States .
Wendy Chun-Hoon is the director of the U.S. Department of Labor’s Women’s Bureau. Follow the agency on Twitter: @WB_DOL .
Brecha Salarial por Género: 5 Datos
El 14 de marzo es el Día de la Igualdad Salarial. Consulte estos 5 datos sobre la brecha salarial de género.
- Estadísticas: En general, a las mujeres no se les paga tanto como a los hombres, incluso trabajando a tiempo completo todo el año. A las mujeres que trabajan a tiempo completo durante todo el año se les paga como promedio el 83,7% de lo que a los hombres. Esta inequidad es aún mayor para mujeres Negras e Hispanas.
- Causas . El trabajo de la mujer está infravalorado. La mayor parte de la disparidad salarial no puede explicarse por diferencias medibles entre hombres y mujeres. De las causas que sí podemos medir, el principal contribuyente es que las mujeres tienen más probabilidades que los hombres de trabajar en ocupaciones mal pagadas y con menos beneficios.
- Educación . La educación no es suficiente para eliminar la brecha salarial de género. En promedio, las mujeres tienen más años de educación y son más proclives que los hombres a haber completado títulos de Asociado, Licenciatura o Maestría. Sin embargo, existe una significativa brecha salarial por género en todos los niveles educativos. Por lo general, las mujeres deben completar un título adicional para recibir el mismo salario que un hombre con menos educación.
- Edad . La brecha salarial de género no se resuelve por sí sola a medida que avanza la edad de las mujeres y crecen en sus carreras. De hecho, la brecha salarial para trabajadoras mayores es superior que para mujeres más jóvenes. Y las mujeres mayores Negras e Hispanas registran las diferencias salariales más extremas.
- Ocupaciones . Los mayores causantes de la brecha salarial de género son las diferencias en las ocupaciones e industrias en las que es más probable que trabajen mujeres y hombres. Las mujeres representan 2 de cada 3 trabajadores a tiempo completo en ocupaciones que pagan menos de $30,000 al año, y menos de 1 de cada 3 a tiempo completo en trabajos que pagan como promedio $100,000 o más. Sin embargo, incluso en las mismas ocupaciones, las mujeres en promedio ganan menos que los hombres.
Aprenda más sobre la igualdad salarial en Estados Unidos.
Wendy Chun-Hoon es la directora de la Oficina de la Mujer del Departamento de Trabajo de EE.UU. Siga a la agencia por Twitter: @WB_DOL .
- working women
- Equal Pay Day
- Women's Bureau
Chia sẻ Nội dung này:
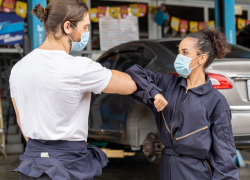
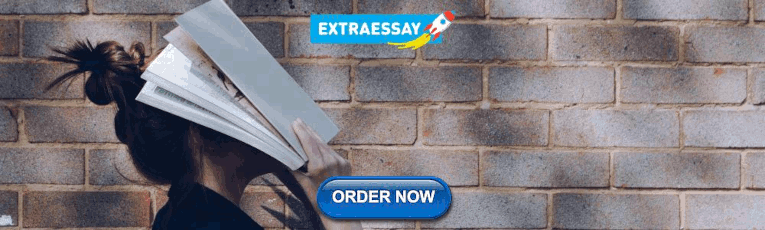
IMAGES
COMMENTS
A good share of the increase in the gender pay gap takes place when women are between the ages of 35 and 44. In 2022, women ages 25 to 34 earned about 92% as much as men of the same ages, but women ages 35 to 44 and 45 to 54 earned 83% as much. The ratio dropped to 79% among those ages 55 to 64.
Introduction. The gender pay gap, the disparity in earnings between male and female workers, has been the focus of empirical research in the US for decades, as well as legislative and executive action under the Obama administration [1, 2].Trends dating back to the 1960s show a long period in which women's earnings were approximately 60% of their male counterparts, followed by increases in ...
Here we try to answer these questions, providing an empirical overview of the gender pay gap across countries and over time. The gender pay gap measures inequality but not necessarily discrimination. The gender pay gap (or the gender wage gap) is a metric that tells us the difference in pay (or wages, or income) between women and men.
The gender gap in pay has remained relatively stable in the United States over the past 20 years or so. In 2022, women earned an average of 82% of what men earned, according to a new Pew Research Center analysis of median hourly earnings of both full- and part-time workers. These results are similar to where the pay gap stood in 2002, when ...
trends in the US gender wage gap and on their sources (in a descriptive sense). Accounting for the sources of the level and changes in the gender pay gap will provide guidance for understanding recent research studying gender and the labor market. Figure 1 shows the long-run trends in the gender pay gap over the 1955-2014 period based on two
To understand how gender pay gaps change with employees' firm tenure, I build on Correll and Benard (2006) and distinguish between information- and status-based theories of pay disparities. Information-based approaches, such as statistical discrimination, emphasize that managers are uncertain of applicants' future productivity (e.g., Akerlof, 1970; Bidwell, 2011; Halaby, 1988; Jovanovic ...
Our main research question is how gender wage transparency affects the GPG. To answer this question, the ideal paper will use variation in gender wage transparency to study outcomes related to employee and firm level variables. ... The gender wage gap refers to the differences between the wages earned by women and men in comparable jobs that ...
Working women are paid less than working men. A large body of research accounts for, diagnoses, and investigates this "gender pay gap." But this literature often becomes unwieldy for lay readers, and because pay gaps are political topics, ideological agendas often seep quickly into discussions. This primer examines the evidence surrounding the gender pay gap,…
Find out with our pay gap calculator. In 2019 women in the United States earned 82% of what men earned, according to a Pew Research Center analysis of median annual earnings of full-time, year-round workers. The gender wage gap varies by age and metropolitan area, and in most places, has narrowed since 2000. See how women's wages compare with ...
Our research question seeks to estimate the effect of the pay transparency intervention on the gender pay gap. To achieve this, we adopt a difference-in-differences like design in which we compare log real annual earnings of men and women to a sharp change around the gender pay publication by THE .
Abstract. The gender pay gap has been observed for decades, and still exists. Due to a life course perspective, gender differences in income are analyzed over a period of 24 years. Therefore, this study aims to investigate income trajectories and the differences regarding men and women. Moreover, the study examines how human capital ...
This brief2 compiles recent research on the impact of equal pay laws and policies on the gender wage gap. It presents studies under five topic areas: (1) salary history bans; (2) pay transparency policies; (3) gender and salary negotiations; (4) gender bias in performance management and performance-related pay; and (5) occupational segregation ...
Studies in this line of research decompose the gender gap to its constituent components, while making a distinction between the 'explained' and the 'unexplained' portions of the gender pay gap (Kunze, 2008). The 'explained' portion refers to the part of the gap that is the consequence of differences between men and women in their ...
The study uses meta-analysis as a research tool to estimate gender pay gap from 263 prior studies that estimate the gender pay gap on the workforce. The study concludes that raw gender pay differential has steadily declined across the globe but the pay gap is still persistent.
Studies of the gender wage gap using data from the late 1980s through the early 2000s consistently identify an unconditional gender wage gap at research universities of about 20%. The unexplained portion of the gap is in the range of 4% to 6% of salaries, or 20% to 30% of the unconditional gap.
The idea that between-firm segregation might play an important role in shaping the gender wage gap is not new (Bayard et al., 2003; Blau, 1977; Groshen, 1991a, 1991b; Tomaskovic-Devey, 1993) and a growing body of research has returned to employer sorting to explain the gender wage gap.Recent research in economics has used employer-employee data to investigate the contribution of between-firm ...
Home | U.S. Department of Labor
Studies of the gender pay gap are seldom able to simultaneously account for the range of alternative putative mechanisms underlying it. Using CloudResearch, an online microtask platform connecting employers to workers who perform research-related tasks, we examine whether gender pay discrepancies are still evident in a labor market characterized by anonymity, relatively homogeneous work, and ...
Research Question 1: identify, using the aggregated data from the 5 countries, the possible relationships between personal characteristics and gender orientation and perceived gender equality in the workplace. ... While the focus of the research is on the gender pay gap, the paper has presented more broadly interesting empirical insights into ...
Research has shown that wage inequality across fields of study can be as great as the average gap between a high school diploma and a bachelor's degree (Kim et al. 2015; Webber 2016), and that gender segregation across majors has contributed to the overall gender wage gap (Blau and Kahn 2017). It follows that field of study segregation, which ...
The Equality Act 2010 (Gender Pay Gap Information) Regulations 2017 in the United Kingdom require companies in Great Britain with over 250 employees to disclose certain gender pay gap information on their websites and a government website. The results are public and you can peruse them here. The UK rules are incredibly prescriptive.
Pay transparency: The historical secrecy of salaries, and its taboo nature as a topic of discussion, has frequently left women in the dark about how large the gender wage gap was. The National ...
The Gender gap in vulnerable and wage work by GDP per capita. Vulnerable employment is closely related to GDP per capita. Economies with high rates of vulnerable employment are low-income contexts with a large agricultural sector. In these economies, women tend to make up the higher share of the vulnerably employed.
White and Asian women have narrowed the wage gap with white men to a much greater degree than black and Hispanic women. For example, white women narrowed the wage gap in median hourly earnings by 22 cents from 1980 (when they earned, on average, 60 cents for every dollar earned by a white man) to 2015 (when they earned 82 cents).
The gender pay gap - the difference between what men and women are paid for the same role - has doubled from 2.9% in 2022 to 6.0% in April 2024.
The idea that women are less likely to ask for higher pay has long been one explanation for the gender pay gap — the difference in earnings between men and women — but new research finds women MBAs are now more likely to negotiate than their male counterparts.. Why it matters: A clear understanding of the reasons women earn less than men (around 16% less according to government data) is ...
According to recent data from the Pew Research Center, American women get paid about $0.82 for every $1 that men get paid, or about 18% less. But the gender pay gap is not just an issue for big ...
IntroductionThis article offers a thorough examination of relevant literature in the WoS, Scopus, and Eric databases for the period 2012-2022, utilizing the PRISMA model (2020) to address STEM and gender gap factors.MethodsA comprehensive search of the Web of Science, Scopus, and Eric databases spanning the years 2012 to 2022 was conducted. Employing the PRISMA (2020) model, inclusion and ...
A greater percentage of U.S.-born Latinas than immigrants say they feel pressure to conform to traditional gender roles, according to a new Pew Research Center survey.. Why it matters: Nearly 1 in 5 women in the U.S. is a Latina, but Hispanic women have staggering wage gaps and are underrepresented in health care, corporate America and other key industries.
With an advanced degree, men are paid $1,998 and women $1,546. March 14 is Equal Pay Day. Here are five fast facts about the gender wage gap. Stats. Overall, women are not paid as much as men, even when working full time and year round. On average, women working full time, year round are paid 83.7% of what men are paid.