Qualitative case study data analysis: an example from practice
Affiliation.
- 1 School of Nursing and Midwifery, National University of Ireland, Galway, Republic of Ireland.
- PMID: 25976531
- DOI: 10.7748/nr.22.5.8.e1307
Aim: To illustrate an approach to data analysis in qualitative case study methodology.
Background: There is often little detail in case study research about how data were analysed. However, it is important that comprehensive analysis procedures are used because there are often large sets of data from multiple sources of evidence. Furthermore, the ability to describe in detail how the analysis was conducted ensures rigour in reporting qualitative research.
Data sources: The research example used is a multiple case study that explored the role of the clinical skills laboratory in preparing students for the real world of practice. Data analysis was conducted using a framework guided by the four stages of analysis outlined by Morse ( 1994 ): comprehending, synthesising, theorising and recontextualising. The specific strategies for analysis in these stages centred on the work of Miles and Huberman ( 1994 ), which has been successfully used in case study research. The data were managed using NVivo software.
Review methods: Literature examining qualitative data analysis was reviewed and strategies illustrated by the case study example provided. Discussion Each stage of the analysis framework is described with illustration from the research example for the purpose of highlighting the benefits of a systematic approach to handling large data sets from multiple sources.
Conclusion: By providing an example of how each stage of the analysis was conducted, it is hoped that researchers will be able to consider the benefits of such an approach to their own case study analysis.
Implications for research/practice: This paper illustrates specific strategies that can be employed when conducting data analysis in case study research and other qualitative research designs.
Keywords: Case study data analysis; case study research methodology; clinical skills research; qualitative case study methodology; qualitative data analysis; qualitative research.
- Case-Control Studies*
- Data Interpretation, Statistical*
- Nursing Research / methods*
- Qualitative Research*
- Research Design
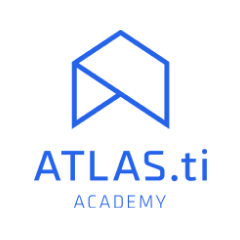
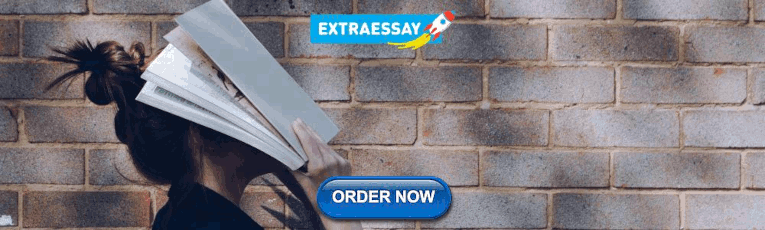
The Ultimate Guide to Qualitative Research - Part 1: The Basics
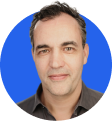
- Introduction and overview
- What is qualitative research?
- What is qualitative data?
- Examples of qualitative data
- Qualitative vs. quantitative research
- Mixed methods
- Qualitative research preparation
- Theoretical perspective
- Theoretical framework
- Literature reviews
Research question
- Conceptual framework
- Conceptual vs. theoretical framework
Data collection
- Qualitative research methods
- Focus groups
- Observational research
What is a case study?
Applications for case study research, what is a good case study, process of case study design, benefits and limitations of case studies.
- Ethnographical research
- Ethical considerations
- Confidentiality and privacy
- Power dynamics
- Reflexivity
Case studies
Case studies are essential to qualitative research , offering a lens through which researchers can investigate complex phenomena within their real-life contexts. This chapter explores the concept, purpose, applications, examples, and types of case studies and provides guidance on how to conduct case study research effectively.
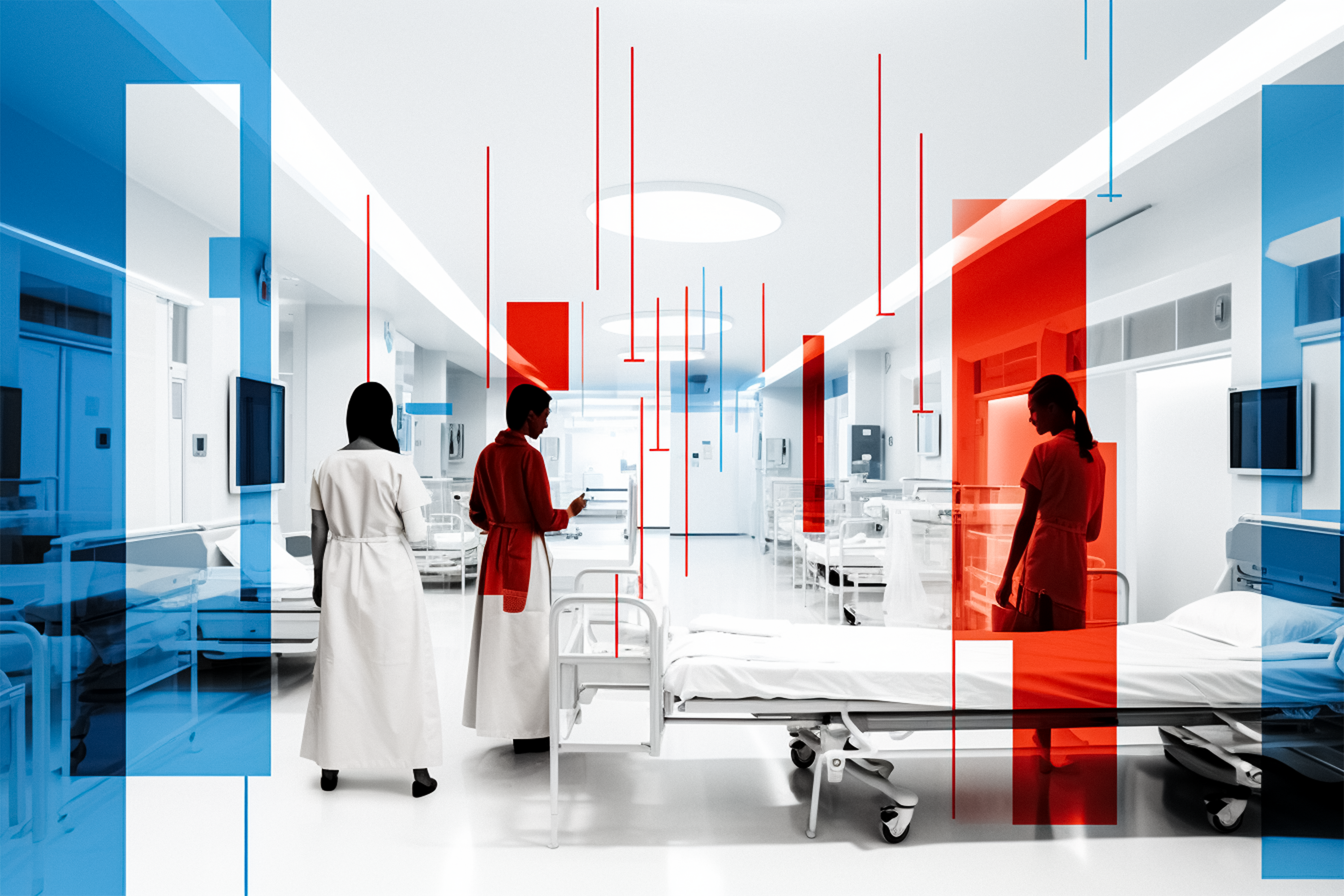
Whereas quantitative methods look at phenomena at scale, case study research looks at a concept or phenomenon in considerable detail. While analyzing a single case can help understand one perspective regarding the object of research inquiry, analyzing multiple cases can help obtain a more holistic sense of the topic or issue. Let's provide a basic definition of a case study, then explore its characteristics and role in the qualitative research process.
Definition of a case study
A case study in qualitative research is a strategy of inquiry that involves an in-depth investigation of a phenomenon within its real-world context. It provides researchers with the opportunity to acquire an in-depth understanding of intricate details that might not be as apparent or accessible through other methods of research. The specific case or cases being studied can be a single person, group, or organization – demarcating what constitutes a relevant case worth studying depends on the researcher and their research question .
Among qualitative research methods , a case study relies on multiple sources of evidence, such as documents, artifacts, interviews , or observations , to present a complete and nuanced understanding of the phenomenon under investigation. The objective is to illuminate the readers' understanding of the phenomenon beyond its abstract statistical or theoretical explanations.
Characteristics of case studies
Case studies typically possess a number of distinct characteristics that set them apart from other research methods. These characteristics include a focus on holistic description and explanation, flexibility in the design and data collection methods, reliance on multiple sources of evidence, and emphasis on the context in which the phenomenon occurs.
Furthermore, case studies can often involve a longitudinal examination of the case, meaning they study the case over a period of time. These characteristics allow case studies to yield comprehensive, in-depth, and richly contextualized insights about the phenomenon of interest.
The role of case studies in research
Case studies hold a unique position in the broader landscape of research methods aimed at theory development. They are instrumental when the primary research interest is to gain an intensive, detailed understanding of a phenomenon in its real-life context.
In addition, case studies can serve different purposes within research - they can be used for exploratory, descriptive, or explanatory purposes, depending on the research question and objectives. This flexibility and depth make case studies a valuable tool in the toolkit of qualitative researchers.
Remember, a well-conducted case study can offer a rich, insightful contribution to both academic and practical knowledge through theory development or theory verification, thus enhancing our understanding of complex phenomena in their real-world contexts.
What is the purpose of a case study?
Case study research aims for a more comprehensive understanding of phenomena, requiring various research methods to gather information for qualitative analysis . Ultimately, a case study can allow the researcher to gain insight into a particular object of inquiry and develop a theoretical framework relevant to the research inquiry.
Why use case studies in qualitative research?
Using case studies as a research strategy depends mainly on the nature of the research question and the researcher's access to the data.
Conducting case study research provides a level of detail and contextual richness that other research methods might not offer. They are beneficial when there's a need to understand complex social phenomena within their natural contexts.
The explanatory, exploratory, and descriptive roles of case studies
Case studies can take on various roles depending on the research objectives. They can be exploratory when the research aims to discover new phenomena or define new research questions; they are descriptive when the objective is to depict a phenomenon within its context in a detailed manner; and they can be explanatory if the goal is to understand specific relationships within the studied context. Thus, the versatility of case studies allows researchers to approach their topic from different angles, offering multiple ways to uncover and interpret the data .
The impact of case studies on knowledge development
Case studies play a significant role in knowledge development across various disciplines. Analysis of cases provides an avenue for researchers to explore phenomena within their context based on the collected data.
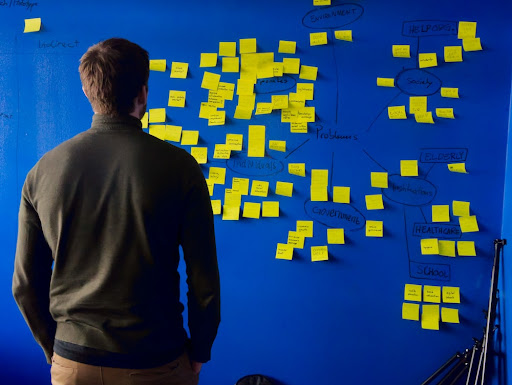
This can result in the production of rich, practical insights that can be instrumental in both theory-building and practice. Case studies allow researchers to delve into the intricacies and complexities of real-life situations, uncovering insights that might otherwise remain hidden.
Types of case studies
In qualitative research , a case study is not a one-size-fits-all approach. Depending on the nature of the research question and the specific objectives of the study, researchers might choose to use different types of case studies. These types differ in their focus, methodology, and the level of detail they provide about the phenomenon under investigation.
Understanding these types is crucial for selecting the most appropriate approach for your research project and effectively achieving your research goals. Let's briefly look at the main types of case studies.
Exploratory case studies
Exploratory case studies are typically conducted to develop a theory or framework around an understudied phenomenon. They can also serve as a precursor to a larger-scale research project. Exploratory case studies are useful when a researcher wants to identify the key issues or questions which can spur more extensive study or be used to develop propositions for further research. These case studies are characterized by flexibility, allowing researchers to explore various aspects of a phenomenon as they emerge, which can also form the foundation for subsequent studies.
Descriptive case studies
Descriptive case studies aim to provide a complete and accurate representation of a phenomenon or event within its context. These case studies are often based on an established theoretical framework, which guides how data is collected and analyzed. The researcher is concerned with describing the phenomenon in detail, as it occurs naturally, without trying to influence or manipulate it.
Explanatory case studies
Explanatory case studies are focused on explanation - they seek to clarify how or why certain phenomena occur. Often used in complex, real-life situations, they can be particularly valuable in clarifying causal relationships among concepts and understanding the interplay between different factors within a specific context.
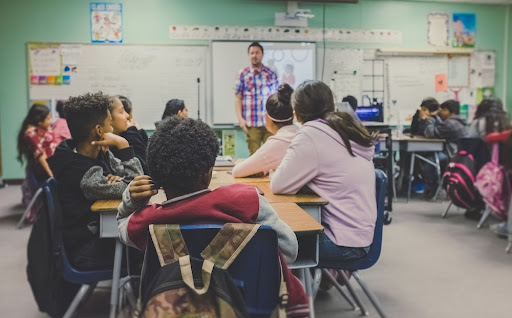
Intrinsic, instrumental, and collective case studies
These three categories of case studies focus on the nature and purpose of the study. An intrinsic case study is conducted when a researcher has an inherent interest in the case itself. Instrumental case studies are employed when the case is used to provide insight into a particular issue or phenomenon. A collective case study, on the other hand, involves studying multiple cases simultaneously to investigate some general phenomena.
Each type of case study serves a different purpose and has its own strengths and challenges. The selection of the type should be guided by the research question and objectives, as well as the context and constraints of the research.
The flexibility, depth, and contextual richness offered by case studies make this approach an excellent research method for various fields of study. They enable researchers to investigate real-world phenomena within their specific contexts, capturing nuances that other research methods might miss. Across numerous fields, case studies provide valuable insights into complex issues.
Critical information systems research
Case studies provide a detailed understanding of the role and impact of information systems in different contexts. They offer a platform to explore how information systems are designed, implemented, and used and how they interact with various social, economic, and political factors. Case studies in this field often focus on examining the intricate relationship between technology, organizational processes, and user behavior, helping to uncover insights that can inform better system design and implementation.
Health research
Health research is another field where case studies are highly valuable. They offer a way to explore patient experiences, healthcare delivery processes, and the impact of various interventions in a real-world context.
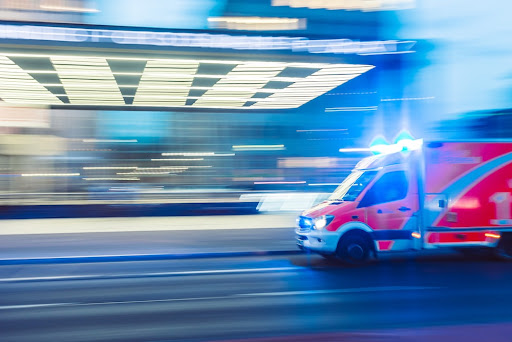
Case studies can provide a deep understanding of a patient's journey, giving insights into the intricacies of disease progression, treatment effects, and the psychosocial aspects of health and illness.
Asthma research studies
Specifically within medical research, studies on asthma often employ case studies to explore the individual and environmental factors that influence asthma development, management, and outcomes. A case study can provide rich, detailed data about individual patients' experiences, from the triggers and symptoms they experience to the effectiveness of various management strategies. This can be crucial for developing patient-centered asthma care approaches.
Other fields
Apart from the fields mentioned, case studies are also extensively used in business and management research, education research, and political sciences, among many others. They provide an opportunity to delve into the intricacies of real-world situations, allowing for a comprehensive understanding of various phenomena.
Case studies, with their depth and contextual focus, offer unique insights across these varied fields. They allow researchers to illuminate the complexities of real-life situations, contributing to both theory and practice.
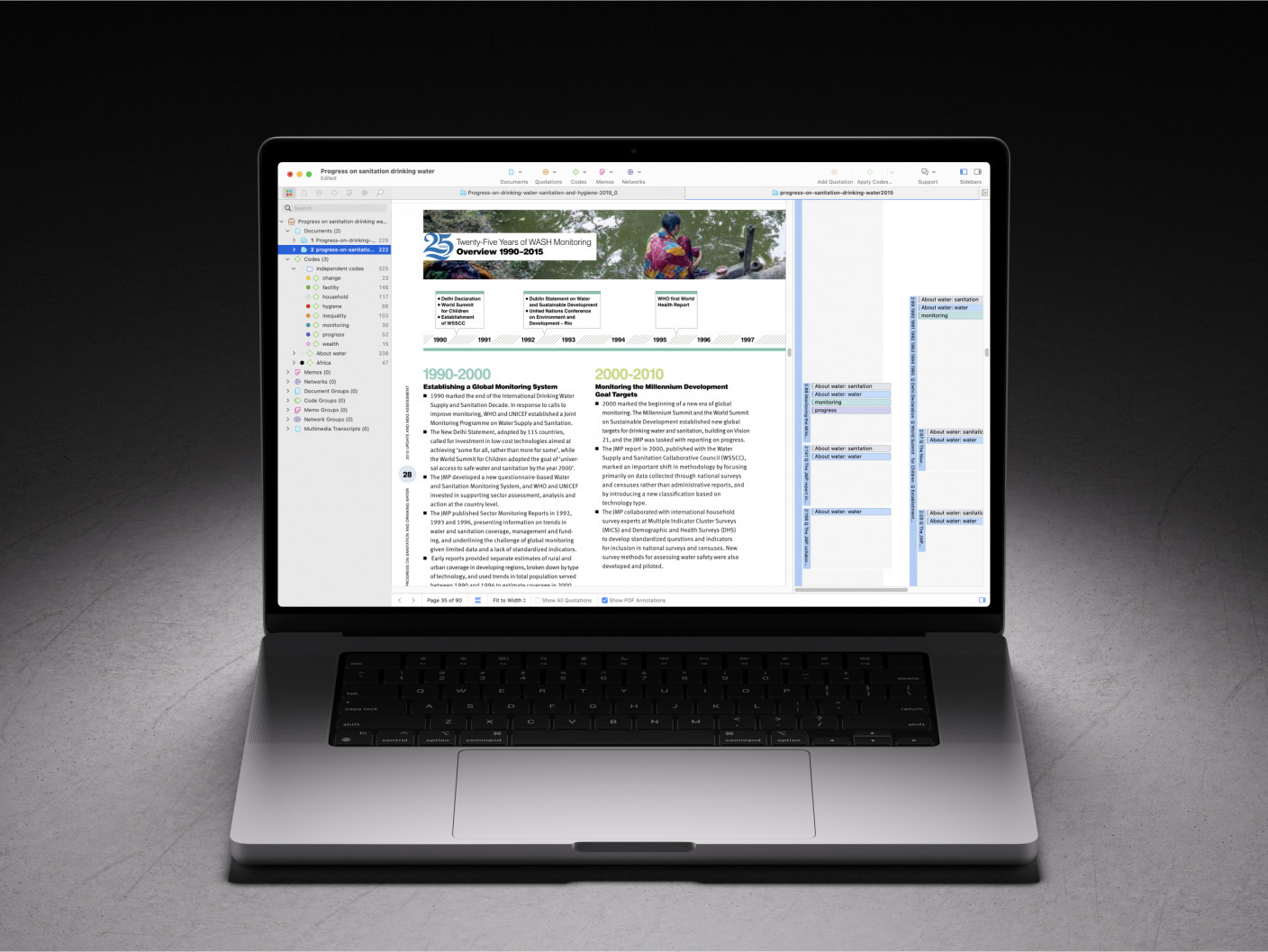
Whatever field you're in, ATLAS.ti puts your data to work for you
Download a free trial of ATLAS.ti to turn your data into insights.
Understanding the key elements of case study design is crucial for conducting rigorous and impactful case study research. A well-structured design guides the researcher through the process, ensuring that the study is methodologically sound and its findings are reliable and valid. The main elements of case study design include the research question , propositions, units of analysis, and the logic linking the data to the propositions.
The research question is the foundation of any research study. A good research question guides the direction of the study and informs the selection of the case, the methods of collecting data, and the analysis techniques. A well-formulated research question in case study research is typically clear, focused, and complex enough to merit further detailed examination of the relevant case(s).
Propositions
Propositions, though not necessary in every case study, provide a direction by stating what we might expect to find in the data collected. They guide how data is collected and analyzed by helping researchers focus on specific aspects of the case. They are particularly important in explanatory case studies, which seek to understand the relationships among concepts within the studied phenomenon.
Units of analysis
The unit of analysis refers to the case, or the main entity or entities that are being analyzed in the study. In case study research, the unit of analysis can be an individual, a group, an organization, a decision, an event, or even a time period. It's crucial to clearly define the unit of analysis, as it shapes the qualitative data analysis process by allowing the researcher to analyze a particular case and synthesize analysis across multiple case studies to draw conclusions.
Argumentation
This refers to the inferential model that allows researchers to draw conclusions from the data. The researcher needs to ensure that there is a clear link between the data, the propositions (if any), and the conclusions drawn. This argumentation is what enables the researcher to make valid and credible inferences about the phenomenon under study.
Understanding and carefully considering these elements in the design phase of a case study can significantly enhance the quality of the research. It can help ensure that the study is methodologically sound and its findings contribute meaningful insights about the case.
Ready to jumpstart your research with ATLAS.ti?
Conceptualize your research project with our intuitive data analysis interface. Download a free trial today.
Conducting a case study involves several steps, from defining the research question and selecting the case to collecting and analyzing data . This section outlines these key stages, providing a practical guide on how to conduct case study research.
Defining the research question
The first step in case study research is defining a clear, focused research question. This question should guide the entire research process, from case selection to analysis. It's crucial to ensure that the research question is suitable for a case study approach. Typically, such questions are exploratory or descriptive in nature and focus on understanding a phenomenon within its real-life context.
Selecting and defining the case
The selection of the case should be based on the research question and the objectives of the study. It involves choosing a unique example or a set of examples that provide rich, in-depth data about the phenomenon under investigation. After selecting the case, it's crucial to define it clearly, setting the boundaries of the case, including the time period and the specific context.
Previous research can help guide the case study design. When considering a case study, an example of a case could be taken from previous case study research and used to define cases in a new research inquiry. Considering recently published examples can help understand how to select and define cases effectively.
Developing a detailed case study protocol
A case study protocol outlines the procedures and general rules to be followed during the case study. This includes the data collection methods to be used, the sources of data, and the procedures for analysis. Having a detailed case study protocol ensures consistency and reliability in the study.
The protocol should also consider how to work with the people involved in the research context to grant the research team access to collecting data. As mentioned in previous sections of this guide, establishing rapport is an essential component of qualitative research as it shapes the overall potential for collecting and analyzing data.
Collecting data
Gathering data in case study research often involves multiple sources of evidence, including documents, archival records, interviews, observations, and physical artifacts. This allows for a comprehensive understanding of the case. The process for gathering data should be systematic and carefully documented to ensure the reliability and validity of the study.
Analyzing and interpreting data
The next step is analyzing the data. This involves organizing the data , categorizing it into themes or patterns , and interpreting these patterns to answer the research question. The analysis might also involve comparing the findings with prior research or theoretical propositions.
Writing the case study report
The final step is writing the case study report . This should provide a detailed description of the case, the data, the analysis process, and the findings. The report should be clear, organized, and carefully written to ensure that the reader can understand the case and the conclusions drawn from it.
Each of these steps is crucial in ensuring that the case study research is rigorous, reliable, and provides valuable insights about the case.
The type, depth, and quality of data in your study can significantly influence the validity and utility of the study. In case study research, data is usually collected from multiple sources to provide a comprehensive and nuanced understanding of the case. This section will outline the various methods of collecting data used in case study research and discuss considerations for ensuring the quality of the data.
Interviews are a common method of gathering data in case study research. They can provide rich, in-depth data about the perspectives, experiences, and interpretations of the individuals involved in the case. Interviews can be structured , semi-structured , or unstructured , depending on the research question and the degree of flexibility needed.
Observations
Observations involve the researcher observing the case in its natural setting, providing first-hand information about the case and its context. Observations can provide data that might not be revealed in interviews or documents, such as non-verbal cues or contextual information.
Documents and artifacts
Documents and archival records provide a valuable source of data in case study research. They can include reports, letters, memos, meeting minutes, email correspondence, and various public and private documents related to the case.
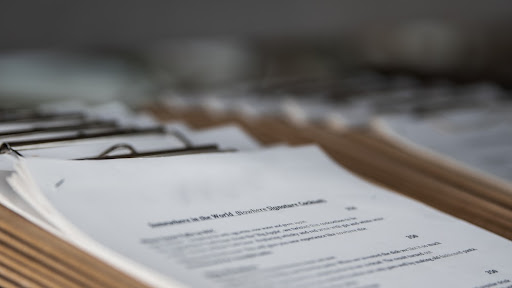
These records can provide historical context, corroborate evidence from other sources, and offer insights into the case that might not be apparent from interviews or observations.
Physical artifacts refer to any physical evidence related to the case, such as tools, products, or physical environments. These artifacts can provide tangible insights into the case, complementing the data gathered from other sources.
Ensuring the quality of data collection
Determining the quality of data in case study research requires careful planning and execution. It's crucial to ensure that the data is reliable, accurate, and relevant to the research question. This involves selecting appropriate methods of collecting data, properly training interviewers or observers, and systematically recording and storing the data. It also includes considering ethical issues related to collecting and handling data, such as obtaining informed consent and ensuring the privacy and confidentiality of the participants.
Data analysis
Analyzing case study research involves making sense of the rich, detailed data to answer the research question. This process can be challenging due to the volume and complexity of case study data. However, a systematic and rigorous approach to analysis can ensure that the findings are credible and meaningful. This section outlines the main steps and considerations in analyzing data in case study research.
Organizing the data
The first step in the analysis is organizing the data. This involves sorting the data into manageable sections, often according to the data source or the theme. This step can also involve transcribing interviews, digitizing physical artifacts, or organizing observational data.
Categorizing and coding the data
Once the data is organized, the next step is to categorize or code the data. This involves identifying common themes, patterns, or concepts in the data and assigning codes to relevant data segments. Coding can be done manually or with the help of software tools, and in either case, qualitative analysis software can greatly facilitate the entire coding process. Coding helps to reduce the data to a set of themes or categories that can be more easily analyzed.
Identifying patterns and themes
After coding the data, the researcher looks for patterns or themes in the coded data. This involves comparing and contrasting the codes and looking for relationships or patterns among them. The identified patterns and themes should help answer the research question.
Interpreting the data
Once patterns and themes have been identified, the next step is to interpret these findings. This involves explaining what the patterns or themes mean in the context of the research question and the case. This interpretation should be grounded in the data, but it can also involve drawing on theoretical concepts or prior research.
Verification of the data
The last step in the analysis is verification. This involves checking the accuracy and consistency of the analysis process and confirming that the findings are supported by the data. This can involve re-checking the original data, checking the consistency of codes, or seeking feedback from research participants or peers.
Like any research method , case study research has its strengths and limitations. Researchers must be aware of these, as they can influence the design, conduct, and interpretation of the study.
Understanding the strengths and limitations of case study research can also guide researchers in deciding whether this approach is suitable for their research question . This section outlines some of the key strengths and limitations of case study research.
Benefits include the following:
- Rich, detailed data: One of the main strengths of case study research is that it can generate rich, detailed data about the case. This can provide a deep understanding of the case and its context, which can be valuable in exploring complex phenomena.
- Flexibility: Case study research is flexible in terms of design , data collection , and analysis . A sufficient degree of flexibility allows the researcher to adapt the study according to the case and the emerging findings.
- Real-world context: Case study research involves studying the case in its real-world context, which can provide valuable insights into the interplay between the case and its context.
- Multiple sources of evidence: Case study research often involves collecting data from multiple sources , which can enhance the robustness and validity of the findings.
On the other hand, researchers should consider the following limitations:
- Generalizability: A common criticism of case study research is that its findings might not be generalizable to other cases due to the specificity and uniqueness of each case.
- Time and resource intensive: Case study research can be time and resource intensive due to the depth of the investigation and the amount of collected data.
- Complexity of analysis: The rich, detailed data generated in case study research can make analyzing the data challenging.
- Subjectivity: Given the nature of case study research, there may be a higher degree of subjectivity in interpreting the data , so researchers need to reflect on this and transparently convey to audiences how the research was conducted.
Being aware of these strengths and limitations can help researchers design and conduct case study research effectively and interpret and report the findings appropriately.
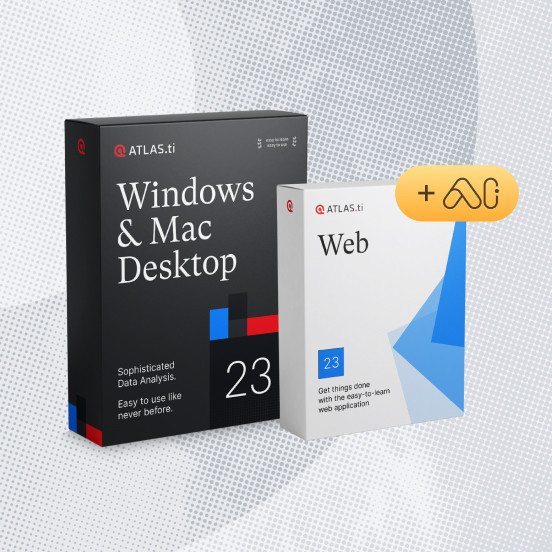
Ready to analyze your data with ATLAS.ti?
See how our intuitive software can draw key insights from your data with a free trial today.
- AI & NLP
- Churn & Loyalty
- Customer Experience
- Customer Journeys
- Customer Metrics
- Feedback Analysis
- Product Experience
- Product Updates
- Sentiment Analysis
- Surveys & Feedback Collection
- Try Thematic
Welcome to the community
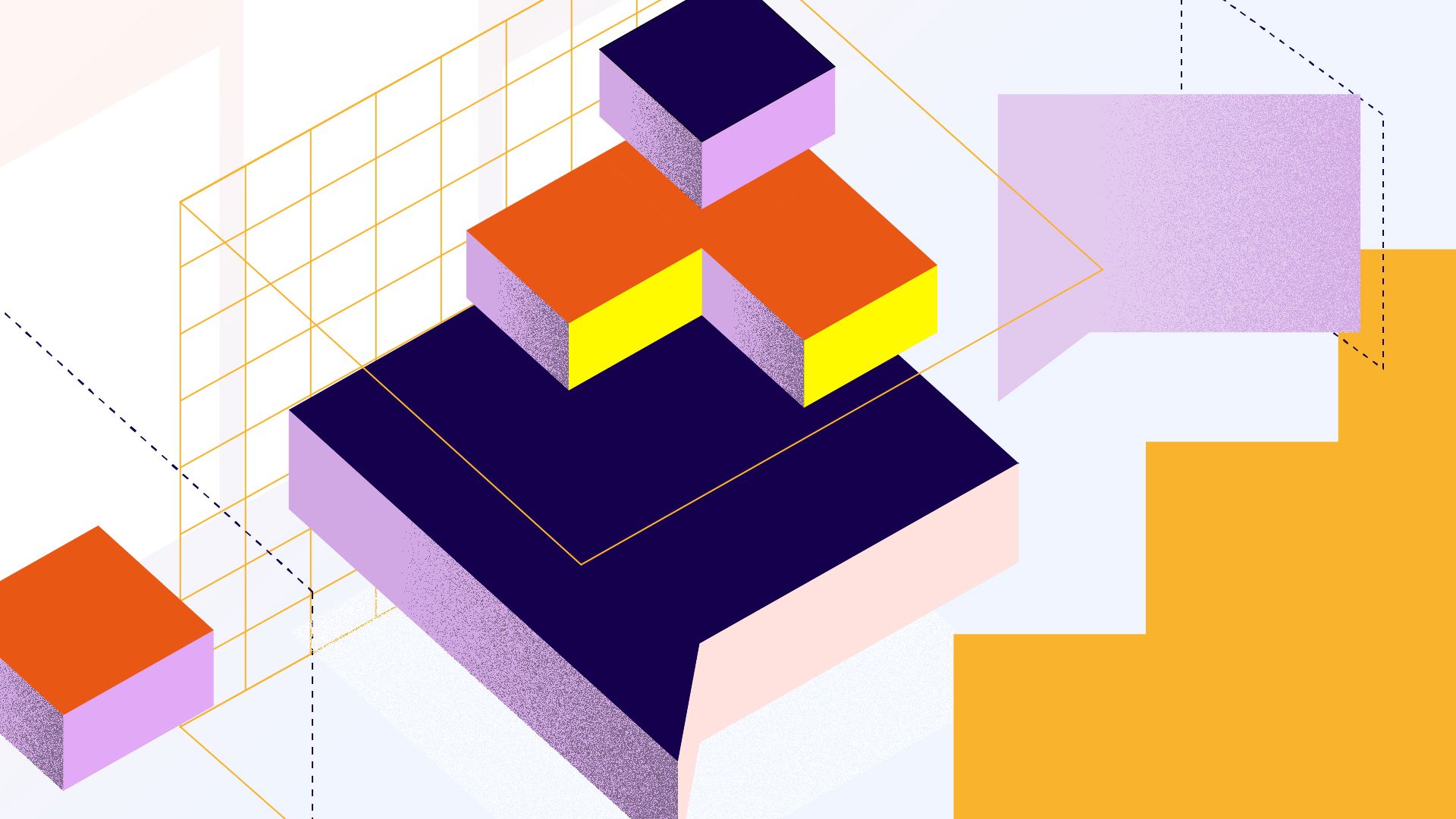
Qualitative Data Analysis: Step-by-Step Guide (Manual vs. Automatic)
When we conduct qualitative methods of research, need to explain changes in metrics or understand people's opinions, we always turn to qualitative data. Qualitative data is typically generated through:
- Interview transcripts
- Surveys with open-ended questions
- Contact center transcripts
- Texts and documents
- Audio and video recordings
- Observational notes
Compared to quantitative data, which captures structured information, qualitative data is unstructured and has more depth. It can answer our questions, can help formulate hypotheses and build understanding.
It's important to understand the differences between quantitative data & qualitative data . But unfortunately, analyzing qualitative data is difficult. While tools like Excel, Tableau and PowerBI crunch and visualize quantitative data with ease, there are a limited number of mainstream tools for analyzing qualitative data . The majority of qualitative data analysis still happens manually.
That said, there are two new trends that are changing this. First, there are advances in natural language processing (NLP) which is focused on understanding human language. Second, there is an explosion of user-friendly software designed for both researchers and businesses. Both help automate the qualitative data analysis process.
In this post we want to teach you how to conduct a successful qualitative data analysis. There are two primary qualitative data analysis methods; manual & automatic. We will teach you how to conduct the analysis manually, and also, automatically using software solutions powered by NLP. We’ll guide you through the steps to conduct a manual analysis, and look at what is involved and the role technology can play in automating this process.
More businesses are switching to fully-automated analysis of qualitative customer data because it is cheaper, faster, and just as accurate. Primarily, businesses purchase subscriptions to feedback analytics platforms so that they can understand customer pain points and sentiment.
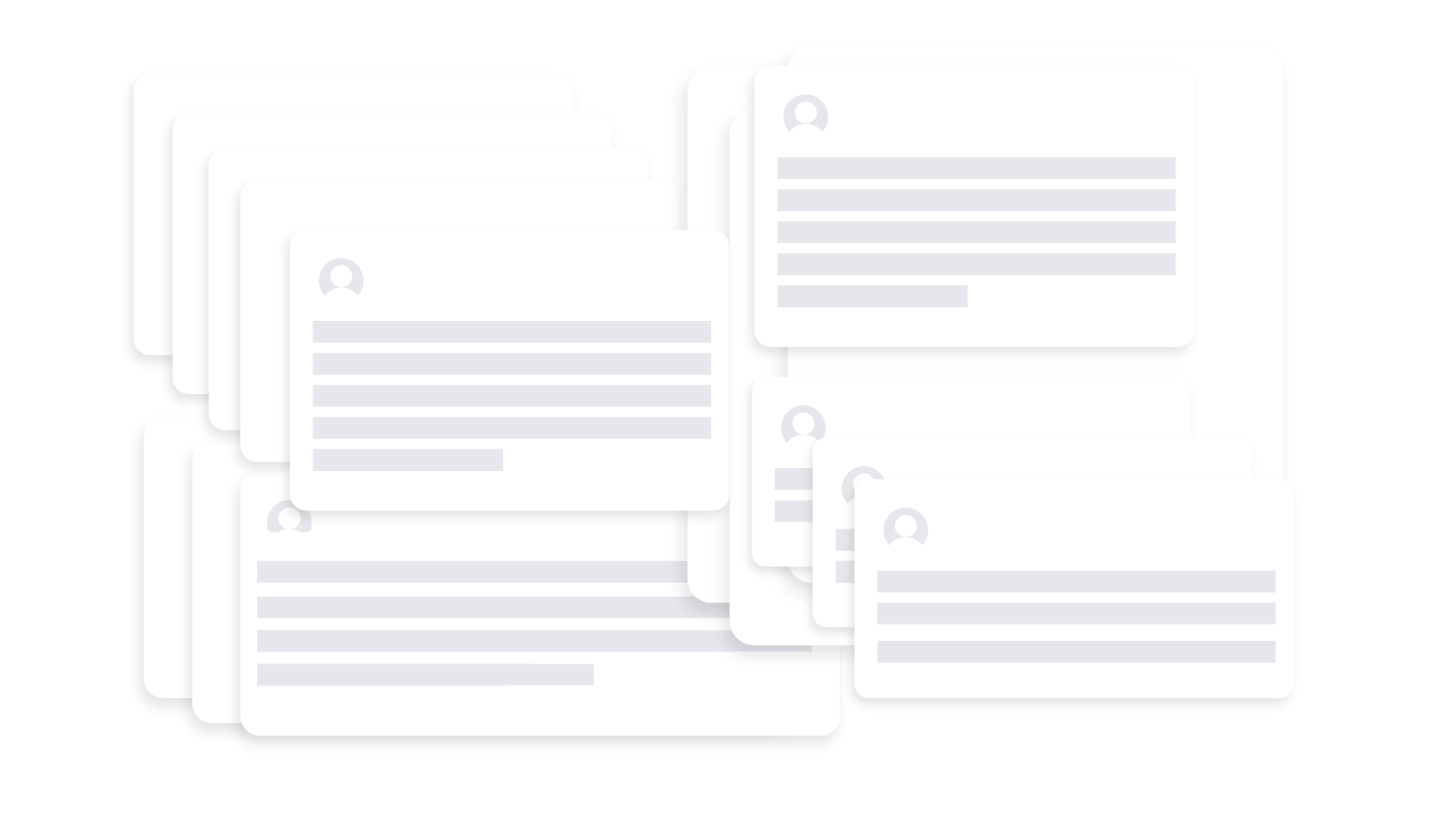
We’ll take you through 5 steps to conduct a successful qualitative data analysis. Within each step we will highlight the key difference between the manual, and automated approach of qualitative researchers. Here's an overview of the steps:
The 5 steps to doing qualitative data analysis
- Gathering and collecting your qualitative data
- Organizing and connecting into your qualitative data
- Coding your qualitative data
- Analyzing the qualitative data for insights
- Reporting on the insights derived from your analysis
What is Qualitative Data Analysis?
Qualitative data analysis is a process of gathering, structuring and interpreting qualitative data to understand what it represents.
Qualitative data is non-numerical and unstructured. Qualitative data generally refers to text, such as open-ended responses to survey questions or user interviews, but also includes audio, photos and video.
Businesses often perform qualitative data analysis on customer feedback. And within this context, qualitative data generally refers to verbatim text data collected from sources such as reviews, complaints, chat messages, support centre interactions, customer interviews, case notes or social media comments.
How is qualitative data analysis different from quantitative data analysis?
Understanding the differences between quantitative & qualitative data is important. When it comes to analyzing data, Qualitative Data Analysis serves a very different role to Quantitative Data Analysis. But what sets them apart?
Qualitative Data Analysis dives into the stories hidden in non-numerical data such as interviews, open-ended survey answers, or notes from observations. It uncovers the ‘whys’ and ‘hows’ giving a deep understanding of people’s experiences and emotions.
Quantitative Data Analysis on the other hand deals with numerical data, using statistics to measure differences, identify preferred options, and pinpoint root causes of issues. It steps back to address questions like "how many" or "what percentage" to offer broad insights we can apply to larger groups.
In short, Qualitative Data Analysis is like a microscope, helping us understand specific detail. Quantitative Data Analysis is like the telescope, giving us a broader perspective. Both are important, working together to decode data for different objectives.
Qualitative Data Analysis methods
Once all the data has been captured, there are a variety of analysis techniques available and the choice is determined by your specific research objectives and the kind of data you’ve gathered. Common qualitative data analysis methods include:
Content Analysis
This is a popular approach to qualitative data analysis. Other qualitative analysis techniques may fit within the broad scope of content analysis. Thematic analysis is a part of the content analysis. Content analysis is used to identify the patterns that emerge from text, by grouping content into words, concepts, and themes. Content analysis is useful to quantify the relationship between all of the grouped content. The Columbia School of Public Health has a detailed breakdown of content analysis .
Narrative Analysis
Narrative analysis focuses on the stories people tell and the language they use to make sense of them. It is particularly useful in qualitative research methods where customer stories are used to get a deep understanding of customers’ perspectives on a specific issue. A narrative analysis might enable us to summarize the outcomes of a focused case study.
Discourse Analysis
Discourse analysis is used to get a thorough understanding of the political, cultural and power dynamics that exist in specific situations. The focus of discourse analysis here is on the way people express themselves in different social contexts. Discourse analysis is commonly used by brand strategists who hope to understand why a group of people feel the way they do about a brand or product.
Thematic Analysis
Thematic analysis is used to deduce the meaning behind the words people use. This is accomplished by discovering repeating themes in text. These meaningful themes reveal key insights into data and can be quantified, particularly when paired with sentiment analysis . Often, the outcome of thematic analysis is a code frame that captures themes in terms of codes, also called categories. So the process of thematic analysis is also referred to as “coding”. A common use-case for thematic analysis in companies is analysis of customer feedback.
Grounded Theory
Grounded theory is a useful approach when little is known about a subject. Grounded theory starts by formulating a theory around a single data case. This means that the theory is “grounded”. Grounded theory analysis is based on actual data, and not entirely speculative. Then additional cases can be examined to see if they are relevant and can add to the original grounded theory.
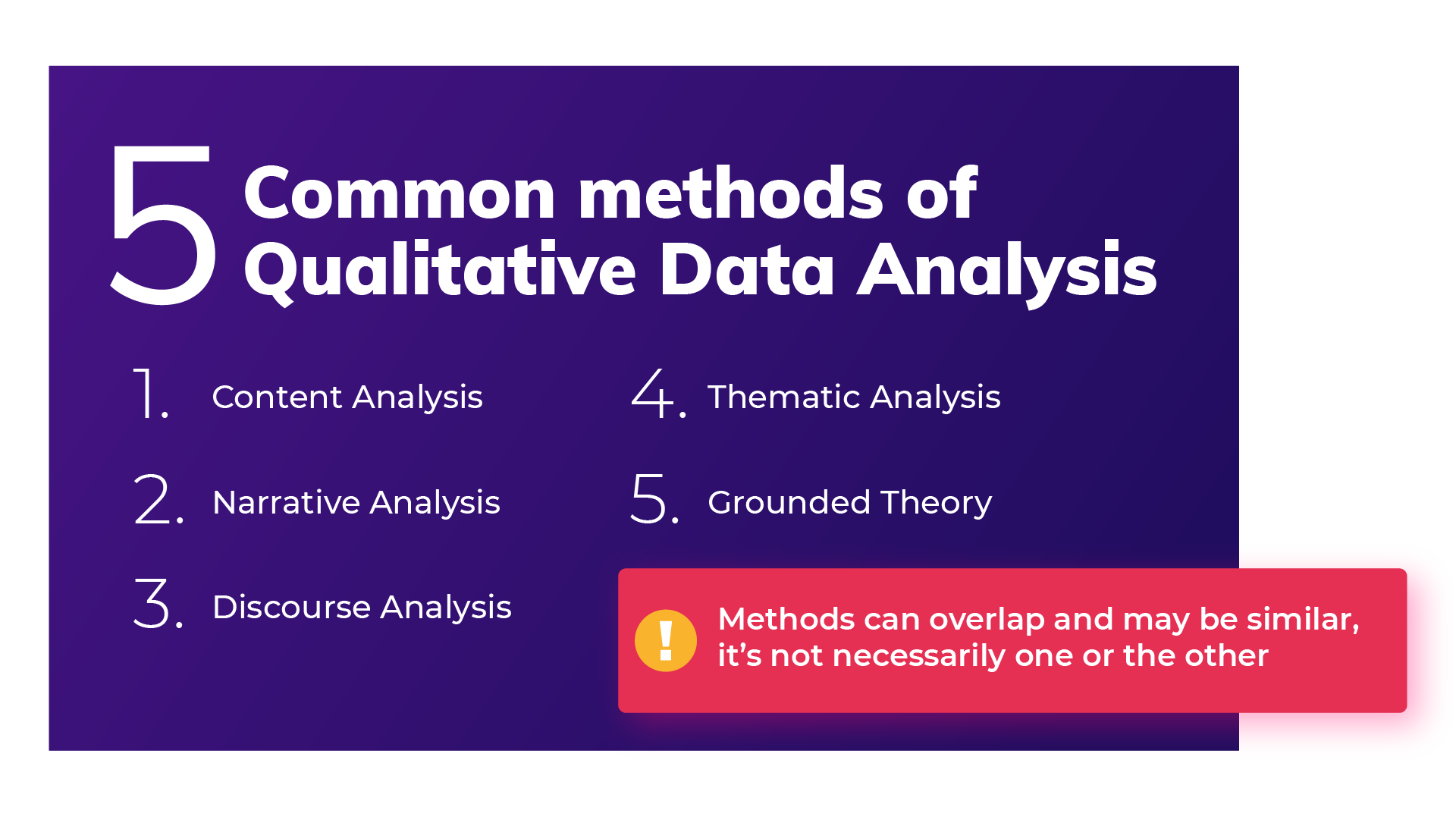
Challenges of Qualitative Data Analysis
While Qualitative Data Analysis offers rich insights, it comes with its challenges. Each unique QDA method has its unique hurdles. Let’s take a look at the challenges researchers and analysts might face, depending on the chosen method.
- Time and Effort (Narrative Analysis): Narrative analysis, which focuses on personal stories, demands patience. Sifting through lengthy narratives to find meaningful insights can be time-consuming, requires dedicated effort.
- Being Objective (Grounded Theory): Grounded theory, building theories from data, faces the challenges of personal biases. Staying objective while interpreting data is crucial, ensuring conclusions are rooted in the data itself.
- Complexity (Thematic Analysis): Thematic analysis involves identifying themes within data, a process that can be intricate. Categorizing and understanding themes can be complex, especially when each piece of data varies in context and structure. Thematic Analysis software can simplify this process.
- Generalizing Findings (Narrative Analysis): Narrative analysis, dealing with individual stories, makes drawing broad challenging. Extending findings from a single narrative to a broader context requires careful consideration.
- Managing Data (Thematic Analysis): Thematic analysis involves organizing and managing vast amounts of unstructured data, like interview transcripts. Managing this can be a hefty task, requiring effective data management strategies.
- Skill Level (Grounded Theory): Grounded theory demands specific skills to build theories from the ground up. Finding or training analysts with these skills poses a challenge, requiring investment in building expertise.
Benefits of qualitative data analysis
Qualitative Data Analysis (QDA) is like a versatile toolkit, offering a tailored approach to understanding your data. The benefits it offers are as diverse as the methods. Let’s explore why choosing the right method matters.
- Tailored Methods for Specific Needs: QDA isn't one-size-fits-all. Depending on your research objectives and the type of data at hand, different methods offer unique benefits. If you want emotive customer stories, narrative analysis paints a strong picture. When you want to explain a score, thematic analysis reveals insightful patterns
- Flexibility with Thematic Analysis: thematic analysis is like a chameleon in the toolkit of QDA. It adapts well to different types of data and research objectives, making it a top choice for any qualitative analysis.
- Deeper Understanding, Better Products: QDA helps you dive into people's thoughts and feelings. This deep understanding helps you build products and services that truly matches what people want, ensuring satisfied customers
- Finding the Unexpected: Qualitative data often reveals surprises that we miss in quantitative data. QDA offers us new ideas and perspectives, for insights we might otherwise miss.
- Building Effective Strategies: Insights from QDA are like strategic guides. They help businesses in crafting plans that match people’s desires.
- Creating Genuine Connections: Understanding people’s experiences lets businesses connect on a real level. This genuine connection helps build trust and loyalty, priceless for any business.
How to do Qualitative Data Analysis: 5 steps
Now we are going to show how you can do your own qualitative data analysis. We will guide you through this process step by step. As mentioned earlier, you will learn how to do qualitative data analysis manually , and also automatically using modern qualitative data and thematic analysis software.
To get best value from the analysis process and research process, it’s important to be super clear about the nature and scope of the question that’s being researched. This will help you select the research collection channels that are most likely to help you answer your question.
Depending on if you are a business looking to understand customer sentiment, or an academic surveying a school, your approach to qualitative data analysis will be unique.
Once you’re clear, there’s a sequence to follow. And, though there are differences in the manual and automatic approaches, the process steps are mostly the same.
The use case for our step-by-step guide is a company looking to collect data (customer feedback data), and analyze the customer feedback - in order to improve customer experience. By analyzing the customer feedback the company derives insights about their business and their customers. You can follow these same steps regardless of the nature of your research. Let’s get started.
Step 1: Gather your qualitative data and conduct research (Conduct qualitative research)
The first step of qualitative research is to do data collection. Put simply, data collection is gathering all of your data for analysis. A common situation is when qualitative data is spread across various sources.
Classic methods of gathering qualitative data
Most companies use traditional methods for gathering qualitative data: conducting interviews with research participants, running surveys, and running focus groups. This data is typically stored in documents, CRMs, databases and knowledge bases. It’s important to examine which data is available and needs to be included in your research project, based on its scope.
Using your existing qualitative feedback
As it becomes easier for customers to engage across a range of different channels, companies are gathering increasingly large amounts of both solicited and unsolicited qualitative feedback.
Most organizations have now invested in Voice of Customer programs , support ticketing systems, chatbot and support conversations, emails and even customer Slack chats.
These new channels provide companies with new ways of getting feedback, and also allow the collection of unstructured feedback data at scale.
The great thing about this data is that it contains a wealth of valubale insights and that it’s already there! When you have a new question about user behavior or your customers, you don’t need to create a new research study or set up a focus group. You can find most answers in the data you already have.
Typically, this data is stored in third-party solutions or a central database, but there are ways to export it or connect to a feedback analysis solution through integrations or an API.
Utilize untapped qualitative data channels
There are many online qualitative data sources you may not have considered. For example, you can find useful qualitative data in social media channels like Twitter or Facebook. Online forums, review sites, and online communities such as Discourse or Reddit also contain valuable data about your customers, or research questions.
If you are considering performing a qualitative benchmark analysis against competitors - the internet is your best friend. Gathering feedback in competitor reviews on sites like Trustpilot, G2, Capterra, Better Business Bureau or on app stores is a great way to perform a competitor benchmark analysis.
Customer feedback analysis software often has integrations into social media and review sites, or you could use a solution like DataMiner to scrape the reviews.
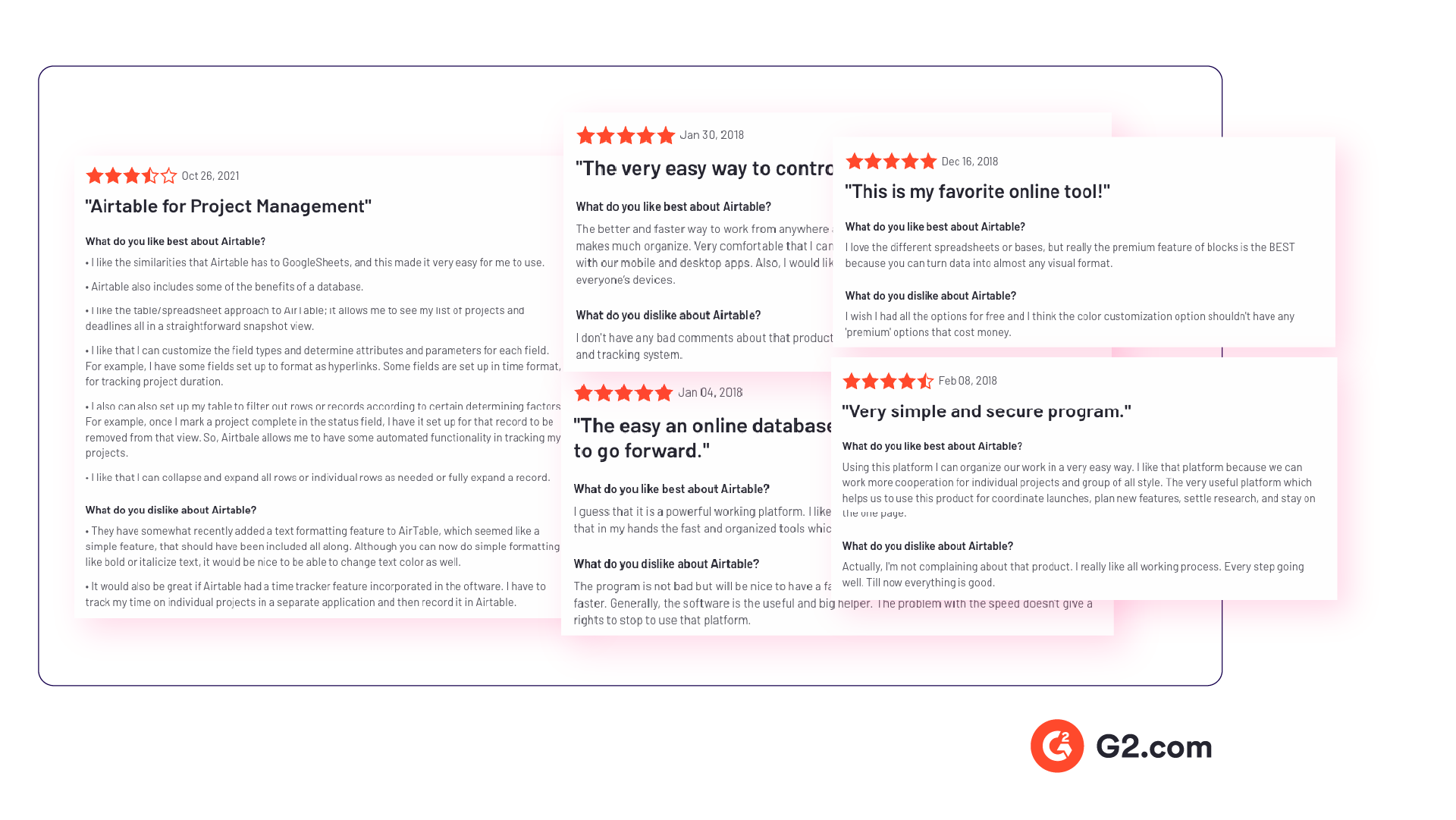
Step 2: Connect & organize all your qualitative data
Now you all have this qualitative data but there’s a problem, the data is unstructured. Before feedback can be analyzed and assigned any value, it needs to be organized in a single place. Why is this important? Consistency!
If all data is easily accessible in one place and analyzed in a consistent manner, you will have an easier time summarizing and making decisions based on this data.
The manual approach to organizing your data
The classic method of structuring qualitative data is to plot all the raw data you’ve gathered into a spreadsheet.
Typically, research and support teams would share large Excel sheets and different business units would make sense of the qualitative feedback data on their own. Each team collects and organizes the data in a way that best suits them, which means the feedback tends to be kept in separate silos.
An alternative and a more robust solution is to store feedback in a central database, like Snowflake or Amazon Redshift .
Keep in mind that when you organize your data in this way, you are often preparing it to be imported into another software. If you go the route of a database, you would need to use an API to push the feedback into a third-party software.
Computer-assisted qualitative data analysis software (CAQDAS)
Traditionally within the manual analysis approach (but not always), qualitative data is imported into CAQDAS software for coding.
In the early 2000s, CAQDAS software was popularised by developers such as ATLAS.ti, NVivo and MAXQDA and eagerly adopted by researchers to assist with the organizing and coding of data.
The benefits of using computer-assisted qualitative data analysis software:
- Assists in the organizing of your data
- Opens you up to exploring different interpretations of your data analysis
- Allows you to share your dataset easier and allows group collaboration (allows for secondary analysis)
However you still need to code the data, uncover the themes and do the analysis yourself. Therefore it is still a manual approach.
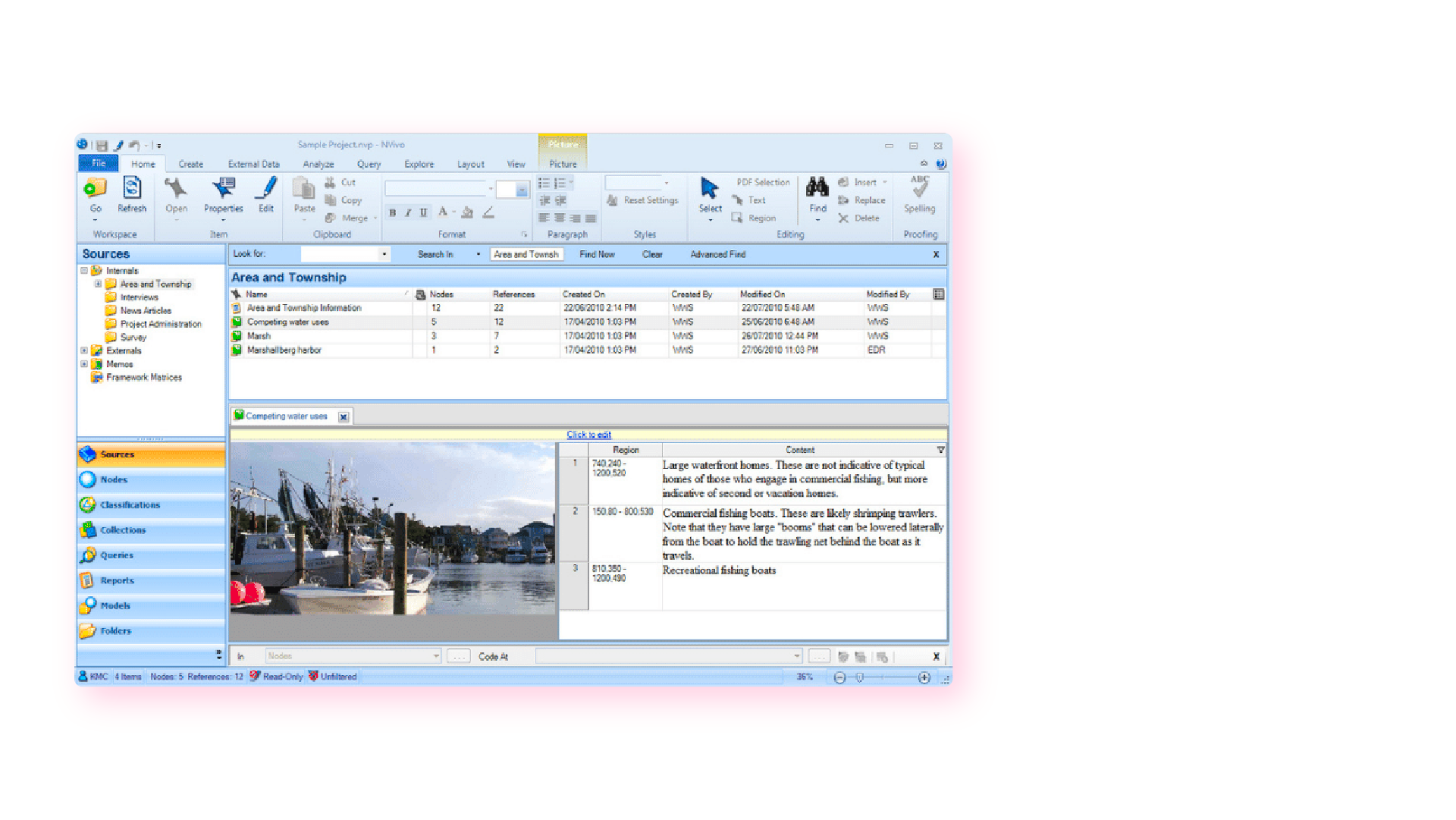
Organizing your qualitative data in a feedback repository
Another solution to organizing your qualitative data is to upload it into a feedback repository where it can be unified with your other data , and easily searchable and taggable. There are a number of software solutions that act as a central repository for your qualitative research data. Here are a couple solutions that you could investigate:
- Dovetail: Dovetail is a research repository with a focus on video and audio transcriptions. You can tag your transcriptions within the platform for theme analysis. You can also upload your other qualitative data such as research reports, survey responses, support conversations, and customer interviews. Dovetail acts as a single, searchable repository. And makes it easier to collaborate with other people around your qualitative research.
- EnjoyHQ: EnjoyHQ is another research repository with similar functionality to Dovetail. It boasts a more sophisticated search engine, but it has a higher starting subscription cost.
Organizing your qualitative data in a feedback analytics platform
If you have a lot of qualitative customer or employee feedback, from the likes of customer surveys or employee surveys, you will benefit from a feedback analytics platform. A feedback analytics platform is a software that automates the process of both sentiment analysis and thematic analysis . Companies use the integrations offered by these platforms to directly tap into their qualitative data sources (review sites, social media, survey responses, etc.). The data collected is then organized and analyzed consistently within the platform.
If you have data prepared in a spreadsheet, it can also be imported into feedback analytics platforms.
Once all this rich data has been organized within the feedback analytics platform, it is ready to be coded and themed, within the same platform. Thematic is a feedback analytics platform that offers one of the largest libraries of integrations with qualitative data sources.
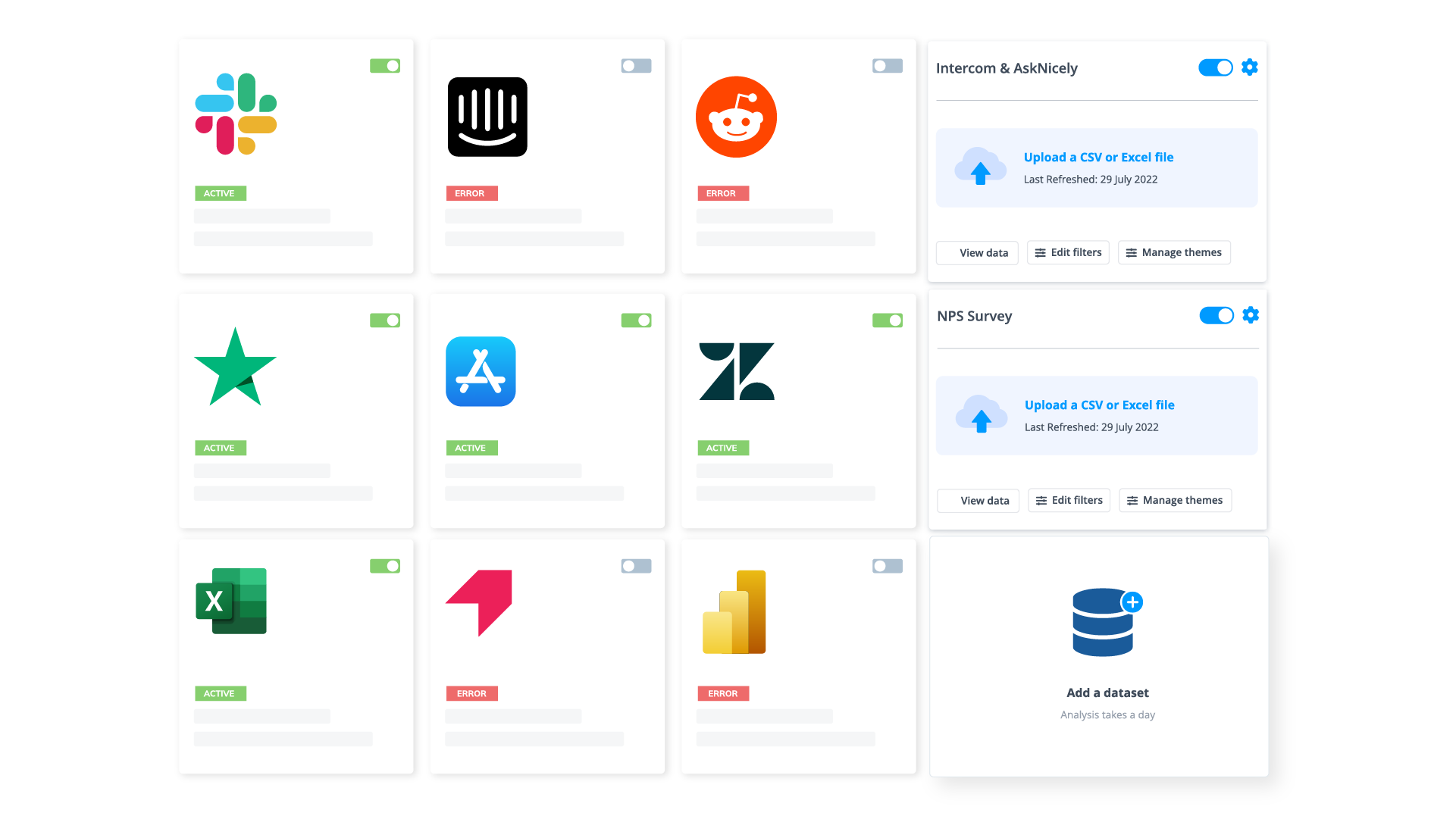
Step 3: Coding your qualitative data
Your feedback data is now organized in one place. Either within your spreadsheet, CAQDAS, feedback repository or within your feedback analytics platform. The next step is to code your feedback data so we can extract meaningful insights in the next step.
Coding is the process of labelling and organizing your data in such a way that you can then identify themes in the data, and the relationships between these themes.
To simplify the coding process, you will take small samples of your customer feedback data, come up with a set of codes, or categories capturing themes, and label each piece of feedback, systematically, for patterns and meaning. Then you will take a larger sample of data, revising and refining the codes for greater accuracy and consistency as you go.
If you choose to use a feedback analytics platform, much of this process will be automated and accomplished for you.
The terms to describe different categories of meaning (‘theme’, ‘code’, ‘tag’, ‘category’ etc) can be confusing as they are often used interchangeably. For clarity, this article will use the term ‘code’.
To code means to identify key words or phrases and assign them to a category of meaning. “I really hate the customer service of this computer software company” would be coded as “poor customer service”.
How to manually code your qualitative data
- Decide whether you will use deductive or inductive coding. Deductive coding is when you create a list of predefined codes, and then assign them to the qualitative data. Inductive coding is the opposite of this, you create codes based on the data itself. Codes arise directly from the data and you label them as you go. You need to weigh up the pros and cons of each coding method and select the most appropriate.
- Read through the feedback data to get a broad sense of what it reveals. Now it’s time to start assigning your first set of codes to statements and sections of text.
- Keep repeating step 2, adding new codes and revising the code description as often as necessary. Once it has all been coded, go through everything again, to be sure there are no inconsistencies and that nothing has been overlooked.
- Create a code frame to group your codes. The coding frame is the organizational structure of all your codes. And there are two commonly used types of coding frames, flat, or hierarchical. A hierarchical code frame will make it easier for you to derive insights from your analysis.
- Based on the number of times a particular code occurs, you can now see the common themes in your feedback data. This is insightful! If ‘bad customer service’ is a common code, it’s time to take action.
We have a detailed guide dedicated to manually coding your qualitative data .
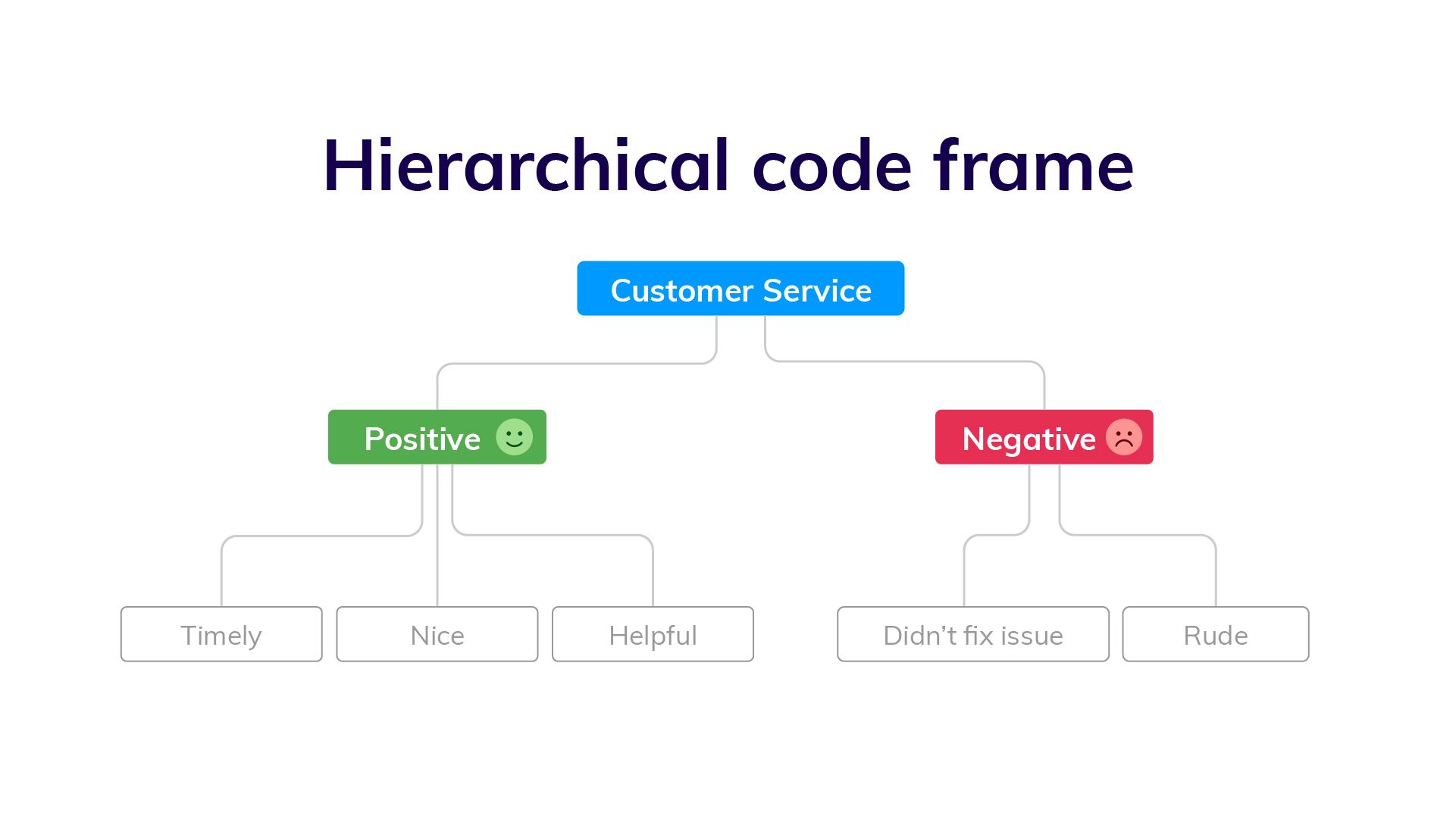
Using software to speed up manual coding of qualitative data
An Excel spreadsheet is still a popular method for coding. But various software solutions can help speed up this process. Here are some examples.
- CAQDAS / NVivo - CAQDAS software has built-in functionality that allows you to code text within their software. You may find the interface the software offers easier for managing codes than a spreadsheet.
- Dovetail/EnjoyHQ - You can tag transcripts and other textual data within these solutions. As they are also repositories you may find it simpler to keep the coding in one platform.
- IBM SPSS - SPSS is a statistical analysis software that may make coding easier than in a spreadsheet.
- Ascribe - Ascribe’s ‘Coder’ is a coding management system. Its user interface will make it easier for you to manage your codes.
Automating the qualitative coding process using thematic analysis software
In solutions which speed up the manual coding process, you still have to come up with valid codes and often apply codes manually to pieces of feedback. But there are also solutions that automate both the discovery and the application of codes.
Advances in machine learning have now made it possible to read, code and structure qualitative data automatically. This type of automated coding is offered by thematic analysis software .
Automation makes it far simpler and faster to code the feedback and group it into themes. By incorporating natural language processing (NLP) into the software, the AI looks across sentences and phrases to identify common themes meaningful statements. Some automated solutions detect repeating patterns and assign codes to them, others make you train the AI by providing examples. You could say that the AI learns the meaning of the feedback on its own.
Thematic automates the coding of qualitative feedback regardless of source. There’s no need to set up themes or categories in advance. Simply upload your data and wait a few minutes. You can also manually edit the codes to further refine their accuracy. Experiments conducted indicate that Thematic’s automated coding is just as accurate as manual coding .
Paired with sentiment analysis and advanced text analytics - these automated solutions become powerful for deriving quality business or research insights.
You could also build your own , if you have the resources!
The key benefits of using an automated coding solution
Automated analysis can often be set up fast and there’s the potential to uncover things that would never have been revealed if you had given the software a prescribed list of themes to look for.
Because the model applies a consistent rule to the data, it captures phrases or statements that a human eye might have missed.
Complete and consistent analysis of customer feedback enables more meaningful findings. Leading us into step 4.
Step 4: Analyze your data: Find meaningful insights
Now we are going to analyze our data to find insights. This is where we start to answer our research questions. Keep in mind that step 4 and step 5 (tell the story) have some overlap . This is because creating visualizations is both part of analysis process and reporting.
The task of uncovering insights is to scour through the codes that emerge from the data and draw meaningful correlations from them. It is also about making sure each insight is distinct and has enough data to support it.
Part of the analysis is to establish how much each code relates to different demographics and customer profiles, and identify whether there’s any relationship between these data points.
Manually create sub-codes to improve the quality of insights
If your code frame only has one level, you may find that your codes are too broad to be able to extract meaningful insights. This is where it is valuable to create sub-codes to your primary codes. This process is sometimes referred to as meta coding.
Note: If you take an inductive coding approach, you can create sub-codes as you are reading through your feedback data and coding it.
While time-consuming, this exercise will improve the quality of your analysis. Here is an example of what sub-codes could look like.
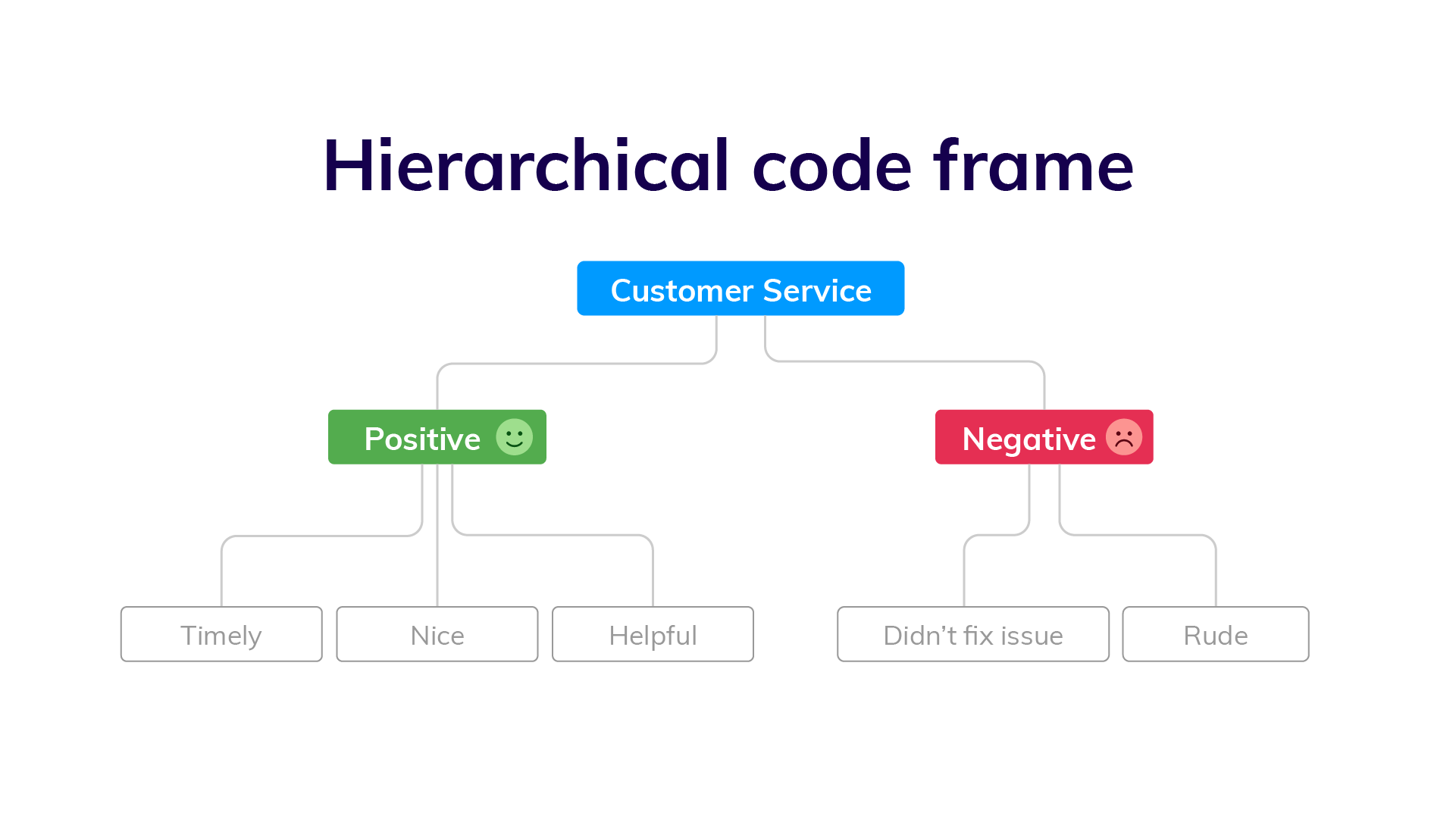
You need to carefully read your qualitative data to create quality sub-codes. But as you can see, the depth of analysis is greatly improved. By calculating the frequency of these sub-codes you can get insight into which customer service problems you can immediately address.
Correlate the frequency of codes to customer segments
Many businesses use customer segmentation . And you may have your own respondent segments that you can apply to your qualitative analysis. Segmentation is the practise of dividing customers or research respondents into subgroups.
Segments can be based on:
- Demographic
- And any other data type that you care to segment by
It is particularly useful to see the occurrence of codes within your segments. If one of your customer segments is considered unimportant to your business, but they are the cause of nearly all customer service complaints, it may be in your best interest to focus attention elsewhere. This is a useful insight!
Manually visualizing coded qualitative data
There are formulas you can use to visualize key insights in your data. The formulas we will suggest are imperative if you are measuring a score alongside your feedback.
If you are collecting a metric alongside your qualitative data this is a key visualization. Impact answers the question: “What’s the impact of a code on my overall score?”. Using Net Promoter Score (NPS) as an example, first you need to:
- Calculate overall NPS
- Calculate NPS in the subset of responses that do not contain that theme
- Subtract B from A
Then you can use this simple formula to calculate code impact on NPS .
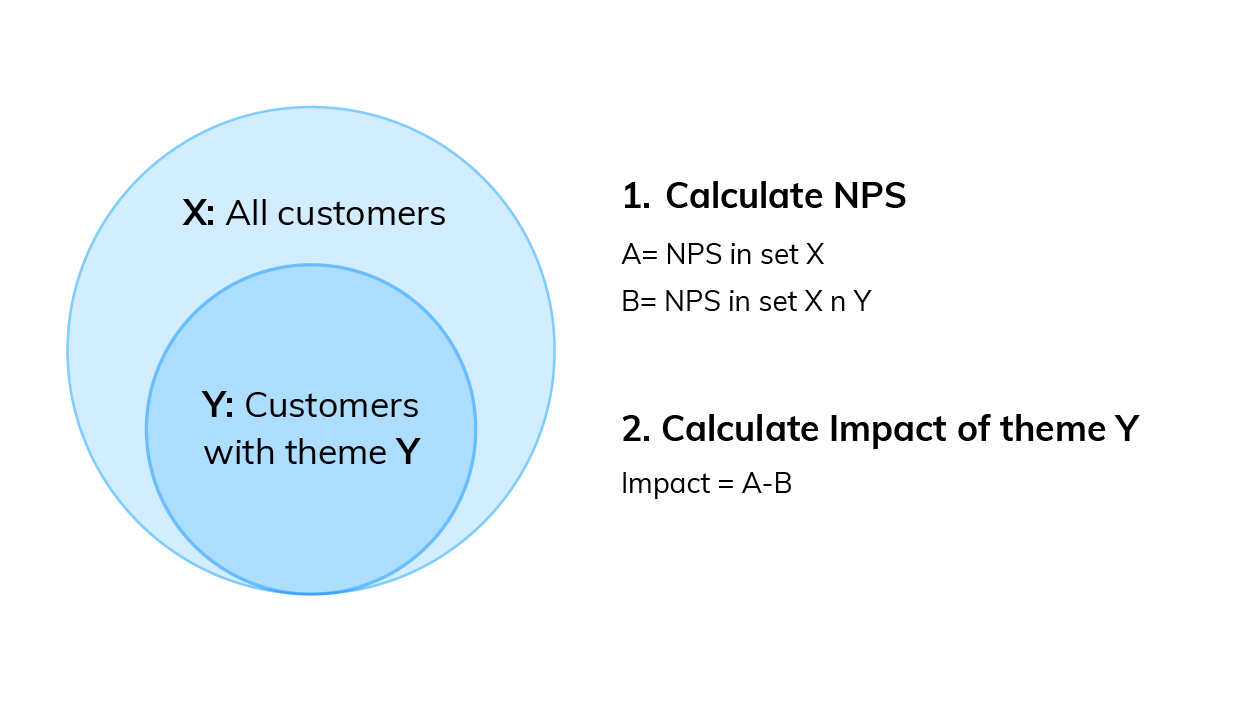
You can then visualize this data using a bar chart.
You can download our CX toolkit - it includes a template to recreate this.
Trends over time
This analysis can help you answer questions like: “Which codes are linked to decreases or increases in my score over time?”
We need to compare two sequences of numbers: NPS over time and code frequency over time . Using Excel, calculate the correlation between the two sequences, which can be either positive (the more codes the higher the NPS, see picture below), or negative (the more codes the lower the NPS).
Now you need to plot code frequency against the absolute value of code correlation with NPS. Here is the formula:
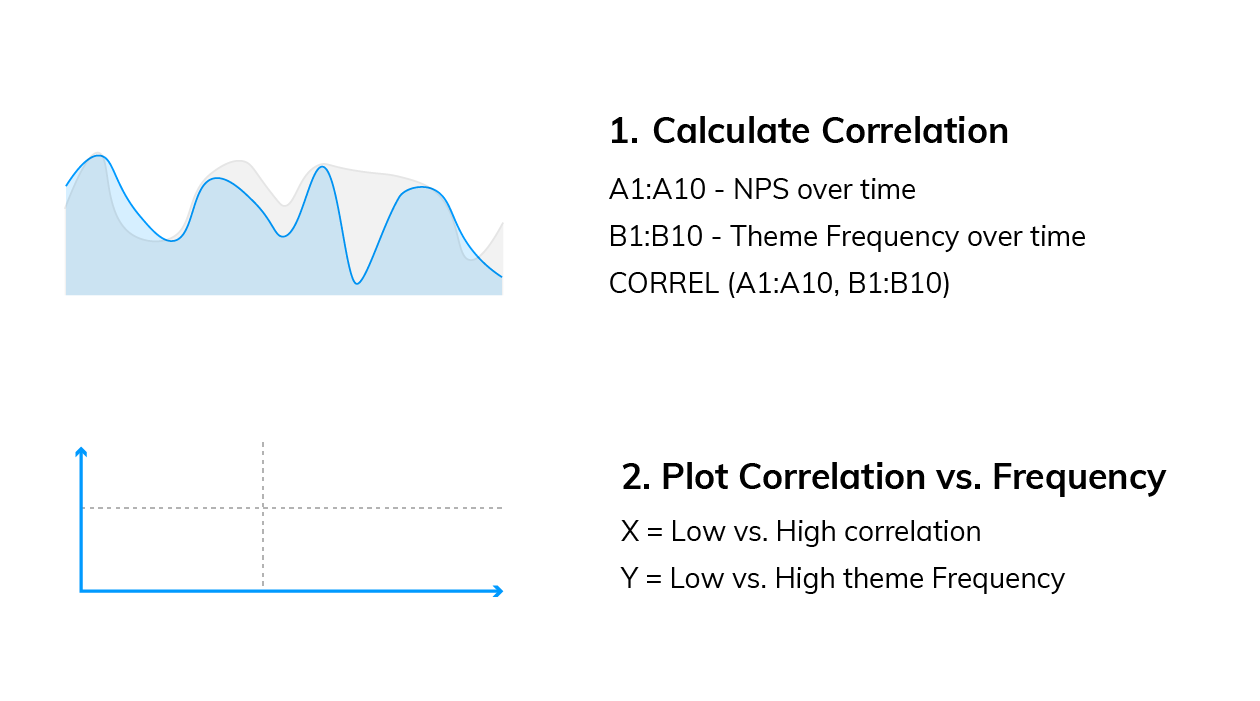
The visualization could look like this:
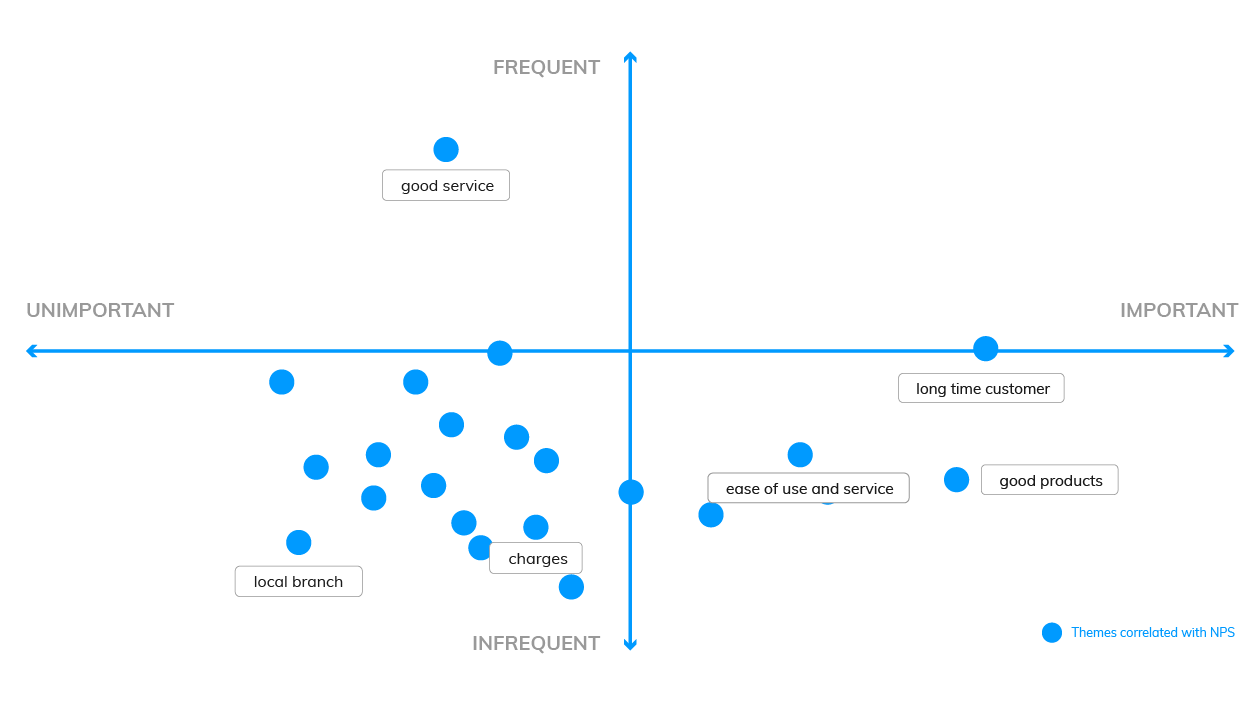
These are two examples, but there are more. For a third manual formula, and to learn why word clouds are not an insightful form of analysis, read our visualizations article .
Using a text analytics solution to automate analysis
Automated text analytics solutions enable codes and sub-codes to be pulled out of the data automatically. This makes it far faster and easier to identify what’s driving negative or positive results. And to pick up emerging trends and find all manner of rich insights in the data.
Another benefit of AI-driven text analytics software is its built-in capability for sentiment analysis, which provides the emotive context behind your feedback and other qualitative textual data therein.
Thematic provides text analytics that goes further by allowing users to apply their expertise on business context to edit or augment the AI-generated outputs.
Since the move away from manual research is generally about reducing the human element, adding human input to the technology might sound counter-intuitive. However, this is mostly to make sure important business nuances in the feedback aren’t missed during coding. The result is a higher accuracy of analysis. This is sometimes referred to as augmented intelligence .
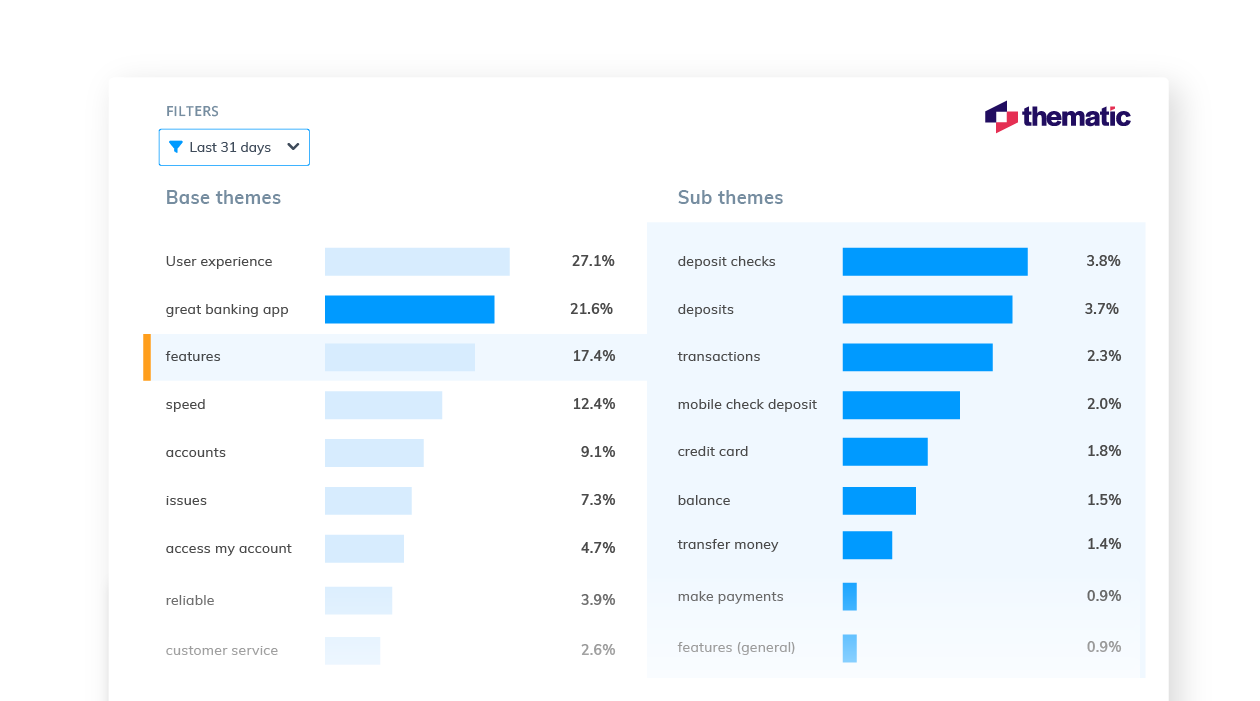
Step 5: Report on your data: Tell the story
The last step of analyzing your qualitative data is to report on it, to tell the story. At this point, the codes are fully developed and the focus is on communicating the narrative to the audience.
A coherent outline of the qualitative research, the findings and the insights is vital for stakeholders to discuss and debate before they can devise a meaningful course of action.
Creating graphs and reporting in Powerpoint
Typically, qualitative researchers take the tried and tested approach of distilling their report into a series of charts, tables and other visuals which are woven into a narrative for presentation in Powerpoint.
Using visualization software for reporting
With data transformation and APIs, the analyzed data can be shared with data visualisation software, such as Power BI or Tableau , Google Studio or Looker. Power BI and Tableau are among the most preferred options.
Visualizing your insights inside a feedback analytics platform
Feedback analytics platforms, like Thematic, incorporate visualisation tools that intuitively turn key data and insights into graphs. This removes the time consuming work of constructing charts to visually identify patterns and creates more time to focus on building a compelling narrative that highlights the insights, in bite-size chunks, for executive teams to review.
Using a feedback analytics platform with visualization tools means you don’t have to use a separate product for visualizations. You can export graphs into Powerpoints straight from the platforms.
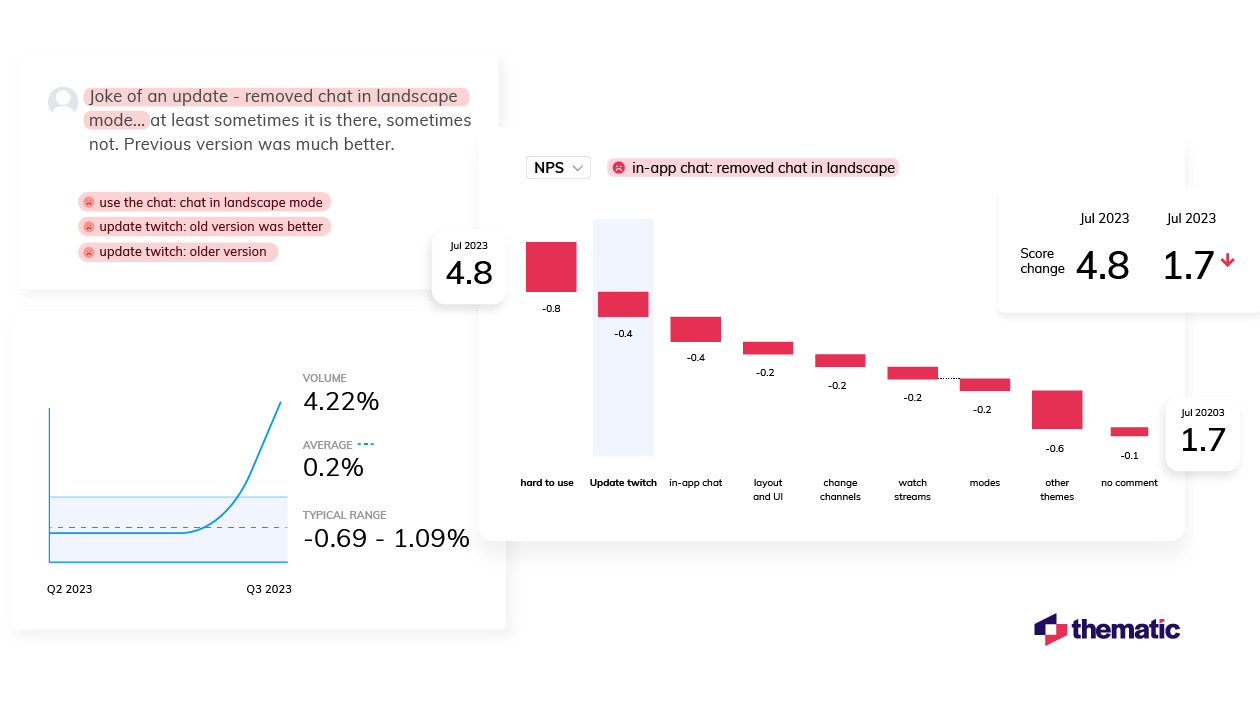
Conclusion - Manual or Automated?
There are those who remain deeply invested in the manual approach - because it’s familiar, because they’re reluctant to spend money and time learning new software, or because they’ve been burned by the overpromises of AI.
For projects that involve small datasets, manual analysis makes sense. For example, if the objective is simply to quantify a simple question like “Do customers prefer X concepts to Y?”. If the findings are being extracted from a small set of focus groups and interviews, sometimes it’s easier to just read them
However, as new generations come into the workplace, it’s technology-driven solutions that feel more comfortable and practical. And the merits are undeniable. Especially if the objective is to go deeper and understand the ‘why’ behind customers’ preference for X or Y. And even more especially if time and money are considerations.
The ability to collect a free flow of qualitative feedback data at the same time as the metric means AI can cost-effectively scan, crunch, score and analyze a ton of feedback from one system in one go. And time-intensive processes like focus groups, or coding, that used to take weeks, can now be completed in a matter of hours or days.
But aside from the ever-present business case to speed things up and keep costs down, there are also powerful research imperatives for automated analysis of qualitative data: namely, accuracy and consistency.
Finding insights hidden in feedback requires consistency, especially in coding. Not to mention catching all the ‘unknown unknowns’ that can skew research findings and steering clear of cognitive bias.
Some say without manual data analysis researchers won’t get an accurate “feel” for the insights. However, the larger data sets are, the harder it is to sort through the feedback and organize feedback that has been pulled from different places. And, the more difficult it is to stay on course, the greater the risk of drawing incorrect, or incomplete, conclusions grows.
Though the process steps for qualitative data analysis have remained pretty much unchanged since psychologist Paul Felix Lazarsfeld paved the path a hundred years ago, the impact digital technology has had on types of qualitative feedback data and the approach to the analysis are profound.
If you want to try an automated feedback analysis solution on your own qualitative data, you can get started with Thematic .
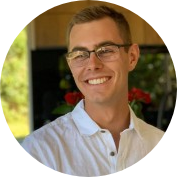
Community & Marketing
Tyler manages our community of CX, insights & analytics professionals. Tyler's goal is to help unite insights professionals around common challenges.
We make it easy to discover the customer and product issues that matter.
Unlock the value of feedback at scale, in one platform. Try it for free now!
- Questions to ask your Feedback Analytics vendor
- How to end customer churn for good
- Scalable analysis of NPS verbatims
- 5 Text analytics approaches
- How to calculate the ROI of CX
Our experts will show you how Thematic works, how to discover pain points and track the ROI of decisions. To access your free trial, book a personal demo today.
Recent posts
When two major storms wreaked havoc on Auckland and Watercare’s infrastructurem the utility went through a CX crisis. With a massive influx of calls to their support center, Thematic helped them get inisghts from this data to forge a new approach to restore services and satisfaction levels.
Become a qualitative theming pro! Creating a perfect code frame is hard, but thematic analysis software makes the process much easier.
Qualtrics is one of the most well-known and powerful Customer Feedback Management platforms. But even so, it has limitations. We recently hosted a live panel where data analysts from two well-known brands shared their experiences with Qualtrics, and how they extended this platform’s capabilities. Below, we’ll share the

Qualitative Data Analysis Methods 101:
The “big 6” methods + examples.
By: Kerryn Warren (PhD) | Reviewed By: Eunice Rautenbach (D.Tech) | May 2020 (Updated April 2023)
Qualitative data analysis methods. Wow, that’s a mouthful.
If you’re new to the world of research, qualitative data analysis can look rather intimidating. So much bulky terminology and so many abstract, fluffy concepts. It certainly can be a minefield!
Don’t worry – in this post, we’ll unpack the most popular analysis methods , one at a time, so that you can approach your analysis with confidence and competence – whether that’s for a dissertation, thesis or really any kind of research project.
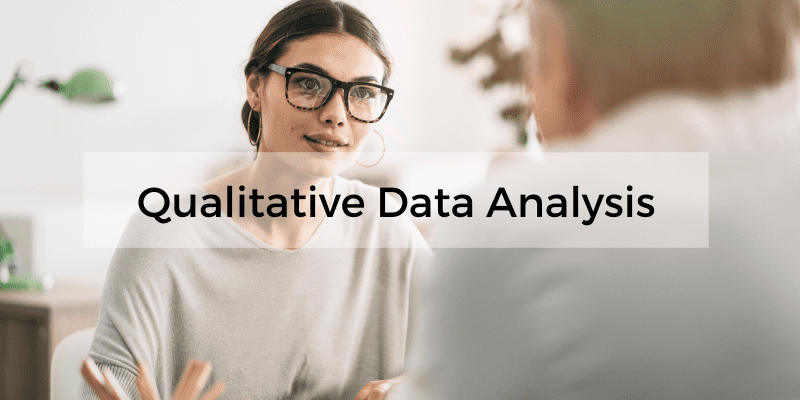
What (exactly) is qualitative data analysis?
To understand qualitative data analysis, we need to first understand qualitative data – so let’s step back and ask the question, “what exactly is qualitative data?”.
Qualitative data refers to pretty much any data that’s “not numbers” . In other words, it’s not the stuff you measure using a fixed scale or complex equipment, nor do you analyse it using complex statistics or mathematics.
So, if it’s not numbers, what is it?
Words, you guessed? Well… sometimes , yes. Qualitative data can, and often does, take the form of interview transcripts, documents and open-ended survey responses – but it can also involve the interpretation of images and videos. In other words, qualitative isn’t just limited to text-based data.
So, how’s that different from quantitative data, you ask?
Simply put, qualitative research focuses on words, descriptions, concepts or ideas – while quantitative research focuses on numbers and statistics . Qualitative research investigates the “softer side” of things to explore and describe , while quantitative research focuses on the “hard numbers”, to measure differences between variables and the relationships between them. If you’re keen to learn more about the differences between qual and quant, we’ve got a detailed post over here .
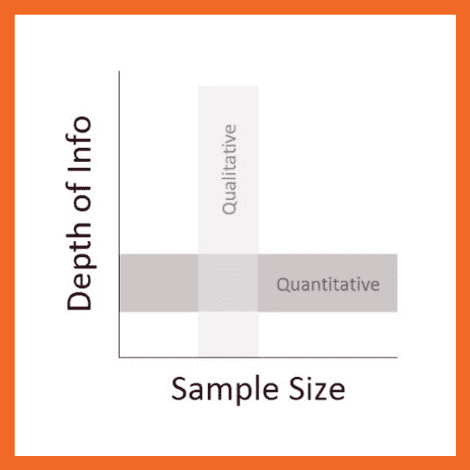
So, qualitative analysis is easier than quantitative, right?
Not quite. In many ways, qualitative data can be challenging and time-consuming to analyse and interpret. At the end of your data collection phase (which itself takes a lot of time), you’ll likely have many pages of text-based data or hours upon hours of audio to work through. You might also have subtle nuances of interactions or discussions that have danced around in your mind, or that you scribbled down in messy field notes. All of this needs to work its way into your analysis.
Making sense of all of this is no small task and you shouldn’t underestimate it. Long story short – qualitative analysis can be a lot of work! Of course, quantitative analysis is no piece of cake either, but it’s important to recognise that qualitative analysis still requires a significant investment in terms of time and effort.
Need a helping hand?
In this post, we’ll explore qualitative data analysis by looking at some of the most common analysis methods we encounter. We’re not going to cover every possible qualitative method and we’re not going to go into heavy detail – we’re just going to give you the big picture. That said, we will of course includes links to loads of extra resources so that you can learn more about whichever analysis method interests you.
Without further delay, let’s get into it.
The “Big 6” Qualitative Analysis Methods
There are many different types of qualitative data analysis, all of which serve different purposes and have unique strengths and weaknesses . We’ll start by outlining the analysis methods and then we’ll dive into the details for each.
The 6 most popular methods (or at least the ones we see at Grad Coach) are:
- Content analysis
- Narrative analysis
- Discourse analysis
- Thematic analysis
- Grounded theory (GT)
- Interpretive phenomenological analysis (IPA)
Let’s take a look at each of them…
QDA Method #1: Qualitative Content Analysis
Content analysis is possibly the most common and straightforward QDA method. At the simplest level, content analysis is used to evaluate patterns within a piece of content (for example, words, phrases or images) or across multiple pieces of content or sources of communication. For example, a collection of newspaper articles or political speeches.
With content analysis, you could, for instance, identify the frequency with which an idea is shared or spoken about – like the number of times a Kardashian is mentioned on Twitter. Or you could identify patterns of deeper underlying interpretations – for instance, by identifying phrases or words in tourist pamphlets that highlight India as an ancient country.
Because content analysis can be used in such a wide variety of ways, it’s important to go into your analysis with a very specific question and goal, or you’ll get lost in the fog. With content analysis, you’ll group large amounts of text into codes , summarise these into categories, and possibly even tabulate the data to calculate the frequency of certain concepts or variables. Because of this, content analysis provides a small splash of quantitative thinking within a qualitative method.
Naturally, while content analysis is widely useful, it’s not without its drawbacks . One of the main issues with content analysis is that it can be very time-consuming , as it requires lots of reading and re-reading of the texts. Also, because of its multidimensional focus on both qualitative and quantitative aspects, it is sometimes accused of losing important nuances in communication.
Content analysis also tends to concentrate on a very specific timeline and doesn’t take into account what happened before or after that timeline. This isn’t necessarily a bad thing though – just something to be aware of. So, keep these factors in mind if you’re considering content analysis. Every analysis method has its limitations , so don’t be put off by these – just be aware of them ! If you’re interested in learning more about content analysis, the video below provides a good starting point.
QDA Method #2: Narrative Analysis
As the name suggests, narrative analysis is all about listening to people telling stories and analysing what that means . Since stories serve a functional purpose of helping us make sense of the world, we can gain insights into the ways that people deal with and make sense of reality by analysing their stories and the ways they’re told.
You could, for example, use narrative analysis to explore whether how something is being said is important. For instance, the narrative of a prisoner trying to justify their crime could provide insight into their view of the world and the justice system. Similarly, analysing the ways entrepreneurs talk about the struggles in their careers or cancer patients telling stories of hope could provide powerful insights into their mindsets and perspectives . Simply put, narrative analysis is about paying attention to the stories that people tell – and more importantly, the way they tell them.
Of course, the narrative approach has its weaknesses , too. Sample sizes are generally quite small due to the time-consuming process of capturing narratives. Because of this, along with the multitude of social and lifestyle factors which can influence a subject, narrative analysis can be quite difficult to reproduce in subsequent research. This means that it’s difficult to test the findings of some of this research.
Similarly, researcher bias can have a strong influence on the results here, so you need to be particularly careful about the potential biases you can bring into your analysis when using this method. Nevertheless, narrative analysis is still a very useful qualitative analysis method – just keep these limitations in mind and be careful not to draw broad conclusions . If you’re keen to learn more about narrative analysis, the video below provides a great introduction to this qualitative analysis method.
QDA Method #3: Discourse Analysis
Discourse is simply a fancy word for written or spoken language or debate . So, discourse analysis is all about analysing language within its social context. In other words, analysing language – such as a conversation, a speech, etc – within the culture and society it takes place. For example, you could analyse how a janitor speaks to a CEO, or how politicians speak about terrorism.
To truly understand these conversations or speeches, the culture and history of those involved in the communication are important factors to consider. For example, a janitor might speak more casually with a CEO in a company that emphasises equality among workers. Similarly, a politician might speak more about terrorism if there was a recent terrorist incident in the country.
So, as you can see, by using discourse analysis, you can identify how culture , history or power dynamics (to name a few) have an effect on the way concepts are spoken about. So, if your research aims and objectives involve understanding culture or power dynamics, discourse analysis can be a powerful method.
Because there are many social influences in terms of how we speak to each other, the potential use of discourse analysis is vast . Of course, this also means it’s important to have a very specific research question (or questions) in mind when analysing your data and looking for patterns and themes, or you might land up going down a winding rabbit hole.
Discourse analysis can also be very time-consuming as you need to sample the data to the point of saturation – in other words, until no new information and insights emerge. But this is, of course, part of what makes discourse analysis such a powerful technique. So, keep these factors in mind when considering this QDA method. Again, if you’re keen to learn more, the video below presents a good starting point.
QDA Method #4: Thematic Analysis
Thematic analysis looks at patterns of meaning in a data set – for example, a set of interviews or focus group transcripts. But what exactly does that… mean? Well, a thematic analysis takes bodies of data (which are often quite large) and groups them according to similarities – in other words, themes . These themes help us make sense of the content and derive meaning from it.
Let’s take a look at an example.
With thematic analysis, you could analyse 100 online reviews of a popular sushi restaurant to find out what patrons think about the place. By reviewing the data, you would then identify the themes that crop up repeatedly within the data – for example, “fresh ingredients” or “friendly wait staff”.
So, as you can see, thematic analysis can be pretty useful for finding out about people’s experiences , views, and opinions . Therefore, if your research aims and objectives involve understanding people’s experience or view of something, thematic analysis can be a great choice.
Since thematic analysis is a bit of an exploratory process, it’s not unusual for your research questions to develop , or even change as you progress through the analysis. While this is somewhat natural in exploratory research, it can also be seen as a disadvantage as it means that data needs to be re-reviewed each time a research question is adjusted. In other words, thematic analysis can be quite time-consuming – but for a good reason. So, keep this in mind if you choose to use thematic analysis for your project and budget extra time for unexpected adjustments.
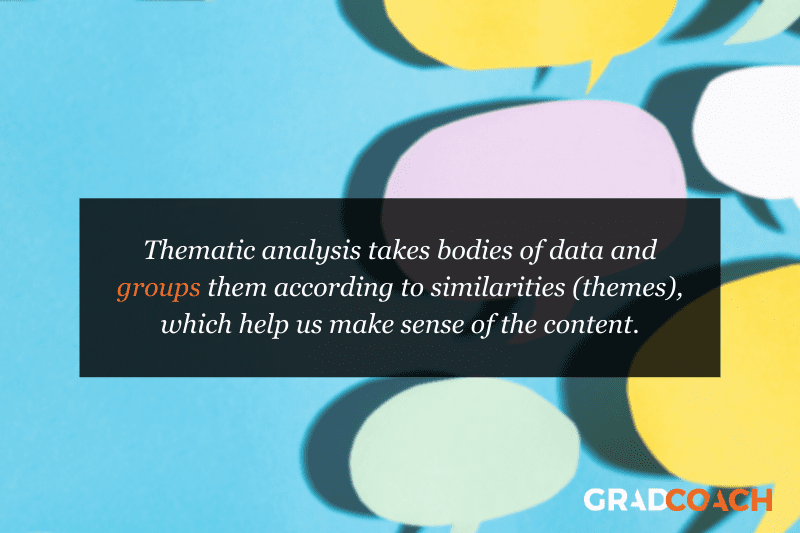
QDA Method #5: Grounded theory (GT)
Grounded theory is a powerful qualitative analysis method where the intention is to create a new theory (or theories) using the data at hand, through a series of “ tests ” and “ revisions ”. Strictly speaking, GT is more a research design type than an analysis method, but we’ve included it here as it’s often referred to as a method.
What’s most important with grounded theory is that you go into the analysis with an open mind and let the data speak for itself – rather than dragging existing hypotheses or theories into your analysis. In other words, your analysis must develop from the ground up (hence the name).
Let’s look at an example of GT in action.
Assume you’re interested in developing a theory about what factors influence students to watch a YouTube video about qualitative analysis. Using Grounded theory , you’d start with this general overarching question about the given population (i.e., graduate students). First, you’d approach a small sample – for example, five graduate students in a department at a university. Ideally, this sample would be reasonably representative of the broader population. You’d interview these students to identify what factors lead them to watch the video.
After analysing the interview data, a general pattern could emerge. For example, you might notice that graduate students are more likely to read a post about qualitative methods if they are just starting on their dissertation journey, or if they have an upcoming test about research methods.
From here, you’ll look for another small sample – for example, five more graduate students in a different department – and see whether this pattern holds true for them. If not, you’ll look for commonalities and adapt your theory accordingly. As this process continues, the theory would develop . As we mentioned earlier, what’s important with grounded theory is that the theory develops from the data – not from some preconceived idea.
So, what are the drawbacks of grounded theory? Well, some argue that there’s a tricky circularity to grounded theory. For it to work, in principle, you should know as little as possible regarding the research question and population, so that you reduce the bias in your interpretation. However, in many circumstances, it’s also thought to be unwise to approach a research question without knowledge of the current literature . In other words, it’s a bit of a “chicken or the egg” situation.
Regardless, grounded theory remains a popular (and powerful) option. Naturally, it’s a very useful method when you’re researching a topic that is completely new or has very little existing research about it, as it allows you to start from scratch and work your way from the ground up .
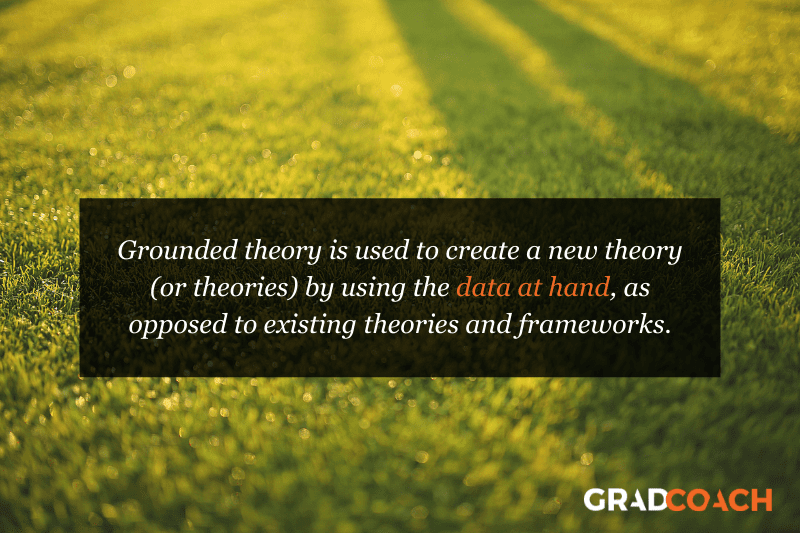
QDA Method #6: Interpretive Phenomenological Analysis (IPA)
Interpretive. Phenomenological. Analysis. IPA . Try saying that three times fast…
Let’s just stick with IPA, okay?
IPA is designed to help you understand the personal experiences of a subject (for example, a person or group of people) concerning a major life event, an experience or a situation . This event or experience is the “phenomenon” that makes up the “P” in IPA. Such phenomena may range from relatively common events – such as motherhood, or being involved in a car accident – to those which are extremely rare – for example, someone’s personal experience in a refugee camp. So, IPA is a great choice if your research involves analysing people’s personal experiences of something that happened to them.
It’s important to remember that IPA is subject – centred . In other words, it’s focused on the experiencer . This means that, while you’ll likely use a coding system to identify commonalities, it’s important not to lose the depth of experience or meaning by trying to reduce everything to codes. Also, keep in mind that since your sample size will generally be very small with IPA, you often won’t be able to draw broad conclusions about the generalisability of your findings. But that’s okay as long as it aligns with your research aims and objectives.
Another thing to be aware of with IPA is personal bias . While researcher bias can creep into all forms of research, self-awareness is critically important with IPA, as it can have a major impact on the results. For example, a researcher who was a victim of a crime himself could insert his own feelings of frustration and anger into the way he interprets the experience of someone who was kidnapped. So, if you’re going to undertake IPA, you need to be very self-aware or you could muddy the analysis.
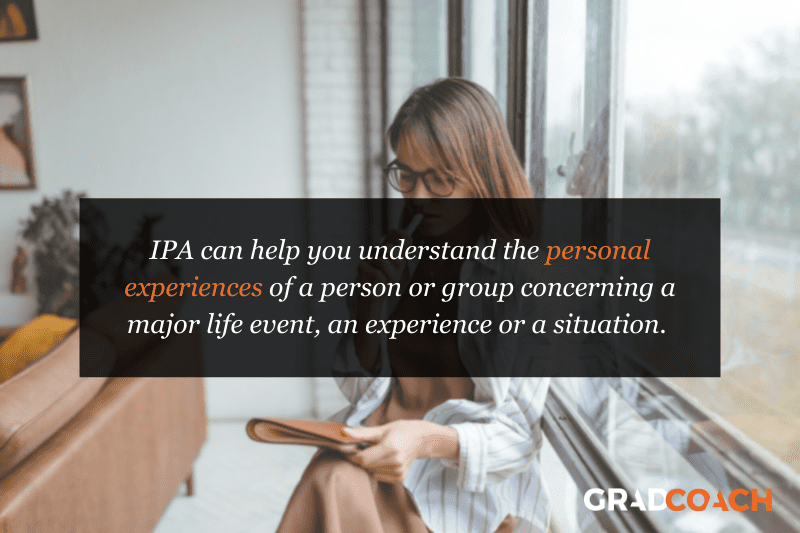
How to choose the right analysis method
In light of all of the qualitative analysis methods we’ve covered so far, you’re probably asking yourself the question, “ How do I choose the right one? ”
Much like all the other methodological decisions you’ll need to make, selecting the right qualitative analysis method largely depends on your research aims, objectives and questions . In other words, the best tool for the job depends on what you’re trying to build. For example:
- Perhaps your research aims to analyse the use of words and what they reveal about the intention of the storyteller and the cultural context of the time.
- Perhaps your research aims to develop an understanding of the unique personal experiences of people that have experienced a certain event, or
- Perhaps your research aims to develop insight regarding the influence of a certain culture on its members.
As you can probably see, each of these research aims are distinctly different , and therefore different analysis methods would be suitable for each one. For example, narrative analysis would likely be a good option for the first aim, while grounded theory wouldn’t be as relevant.
It’s also important to remember that each method has its own set of strengths, weaknesses and general limitations. No single analysis method is perfect . So, depending on the nature of your research, it may make sense to adopt more than one method (this is called triangulation ). Keep in mind though that this will of course be quite time-consuming.
As we’ve seen, all of the qualitative analysis methods we’ve discussed make use of coding and theme-generating techniques, but the intent and approach of each analysis method differ quite substantially. So, it’s very important to come into your research with a clear intention before you decide which analysis method (or methods) to use.
Start by reviewing your research aims , objectives and research questions to assess what exactly you’re trying to find out – then select a qualitative analysis method that fits. Never pick a method just because you like it or have experience using it – your analysis method (or methods) must align with your broader research aims and objectives.
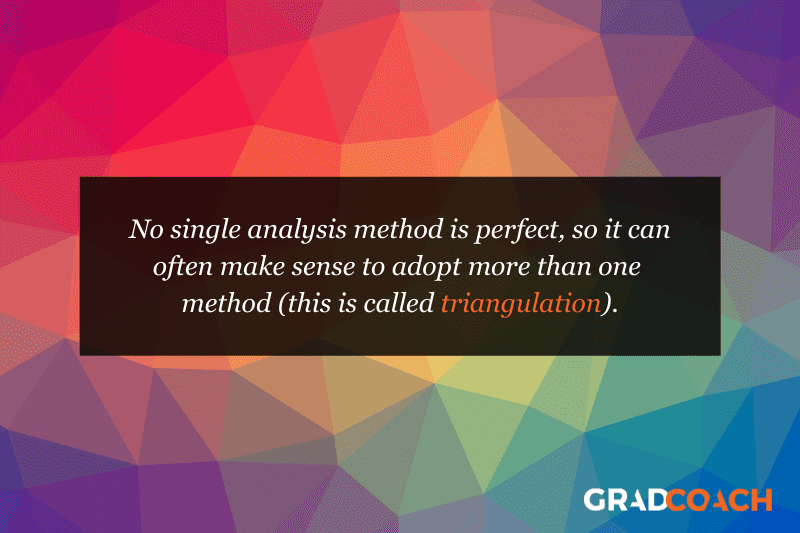
Let’s recap on QDA methods…
In this post, we looked at six popular qualitative data analysis methods:
- First, we looked at content analysis , a straightforward method that blends a little bit of quant into a primarily qualitative analysis.
- Then we looked at narrative analysis , which is about analysing how stories are told.
- Next up was discourse analysis – which is about analysing conversations and interactions.
- Then we moved on to thematic analysis – which is about identifying themes and patterns.
- From there, we went south with grounded theory – which is about starting from scratch with a specific question and using the data alone to build a theory in response to that question.
- And finally, we looked at IPA – which is about understanding people’s unique experiences of a phenomenon.
Of course, these aren’t the only options when it comes to qualitative data analysis, but they’re a great starting point if you’re dipping your toes into qualitative research for the first time.
If you’re still feeling a bit confused, consider our private coaching service , where we hold your hand through the research process to help you develop your best work.
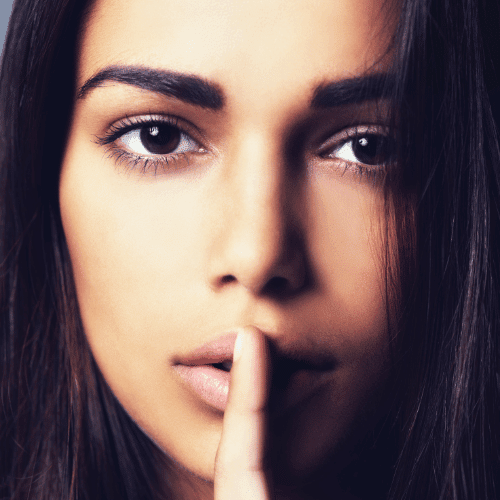
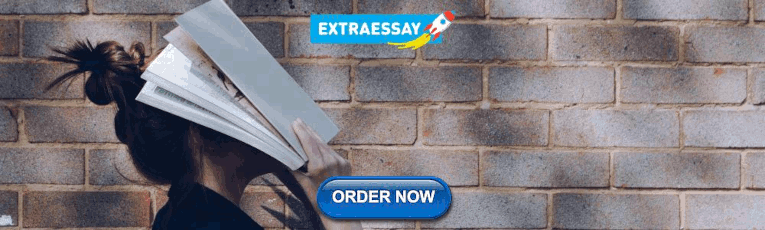
Psst... there’s more!
This post was based on one of our popular Research Bootcamps . If you're working on a research project, you'll definitely want to check this out ...
You Might Also Like:
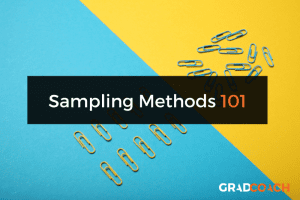
84 Comments
This has been very helpful. Thank you.
Thank you madam,
Thank you so much for this information
I wonder it so clear for understand and good for me. can I ask additional query?
Very insightful and useful
Good work done with clear explanations. Thank you.
Thanks so much for the write-up, it’s really good.
Thanks madam . It is very important .
thank you very good
This has been very well explained in simple language . It is useful even for a new researcher.
Great to hear that. Good luck with your qualitative data analysis, Pramod!
This is very useful information. And it was very a clear language structured presentation. Thanks a lot.
Thank you so much.
very informative sequential presentation
Precise explanation of method.
Hi, may we use 2 data analysis methods in our qualitative research?
Thanks for your comment. Most commonly, one would use one type of analysis method, but it depends on your research aims and objectives.
You explained it in very simple language, everyone can understand it. Thanks so much.
Thank you very much, this is very helpful. It has been explained in a very simple manner that even a layman understands
Thank nicely explained can I ask is Qualitative content analysis the same as thematic analysis?
Thanks for your comment. No, QCA and thematic are two different types of analysis. This article might help clarify – https://onlinelibrary.wiley.com/doi/10.1111/nhs.12048
This is my first time to come across a well explained data analysis. so helpful.
I have thoroughly enjoyed your explanation of the six qualitative analysis methods. This is very helpful. Thank you!
Thank you very much, this is well explained and useful
i need a citation of your book.
Thanks a lot , remarkable indeed, enlighting to the best
Hi Derek, What other theories/methods would you recommend when the data is a whole speech?
Keep writing useful artikel.
It is important concept about QDA and also the way to express is easily understandable, so thanks for all.
Thank you, this is well explained and very useful.
Very helpful .Thanks.
Hi there! Very well explained. Simple but very useful style of writing. Please provide the citation of the text. warm regards
The session was very helpful and insightful. Thank you
This was very helpful and insightful. Easy to read and understand
As a professional academic writer, this has been so informative and educative. Keep up the good work Grad Coach you are unmatched with quality content for sure.
Keep up the good work Grad Coach you are unmatched with quality content for sure.
Its Great and help me the most. A Million Thanks you Dr.
It is a very nice work
Very insightful. Please, which of this approach could be used for a research that one is trying to elicit students’ misconceptions in a particular concept ?
This is Amazing and well explained, thanks
great overview
What do we call a research data analysis method that one use to advise or determining the best accounting tool or techniques that should be adopted in a company.
Informative video, explained in a clear and simple way. Kudos
Waoo! I have chosen method wrong for my data analysis. But I can revise my work according to this guide. Thank you so much for this helpful lecture.
This has been very helpful. It gave me a good view of my research objectives and how to choose the best method. Thematic analysis it is.
Very helpful indeed. Thanku so much for the insight.
This was incredibly helpful.
Very helpful.
very educative
Nicely written especially for novice academic researchers like me! Thank you.
choosing a right method for a paper is always a hard job for a student, this is a useful information, but it would be more useful personally for me, if the author provide me with a little bit more information about the data analysis techniques in type of explanatory research. Can we use qualitative content analysis technique for explanatory research ? or what is the suitable data analysis method for explanatory research in social studies?
that was very helpful for me. because these details are so important to my research. thank you very much
I learnt a lot. Thank you
Relevant and Informative, thanks !
Well-planned and organized, thanks much! 🙂
I have reviewed qualitative data analysis in a simplest way possible. The content will highly be useful for developing my book on qualitative data analysis methods. Cheers!
Clear explanation on qualitative and how about Case study
This was helpful. Thank you
This was really of great assistance, it was just the right information needed. Explanation very clear and follow.
Wow, Thanks for making my life easy
This was helpful thanks .
Very helpful…. clear and written in an easily understandable manner. Thank you.
This was so helpful as it was easy to understand. I’m a new to research thank you so much.
so educative…. but Ijust want to know which method is coding of the qualitative or tallying done?
Thank you for the great content, I have learnt a lot. So helpful
precise and clear presentation with simple language and thank you for that.
very informative content, thank you.
You guys are amazing on YouTube on this platform. Your teachings are great, educative, and informative. kudos!
Brilliant Delivery. You made a complex subject seem so easy. Well done.
Beautifully explained.
Thanks a lot
Is there a video the captures the practical process of coding using automated applications?
Thanks for the comment. We don’t recommend using automated applications for coding, as they are not sufficiently accurate in our experience.
content analysis can be qualitative research?
THANK YOU VERY MUCH.
Thank you very much for such a wonderful content
do you have any material on Data collection
What a powerful explanation of the QDA methods. Thank you.
Great explanation both written and Video. i have been using of it on a day to day working of my thesis project in accounting and finance. Thank you very much for your support.
very helpful, thank you so much
Submit a Comment Cancel reply
Your email address will not be published. Required fields are marked *
Save my name, email, and website in this browser for the next time I comment.
- Print Friendly
Qualitative Data Analysis Methods
In the following, we will discuss basic approaches to analyzing data in all six of the acceptable qualitative designs.
After reviewing the information in this document, you will be able to:
- Recognize the terms for data analysis methods used in the various acceptable designs.
- Recognize the data preparation tasks that precede actual analysis in all the designs.
- Understand the basic analytic methods used by the respective qualitative designs.
- Identify and apply the methods required by your selected design.
Terms Used in Data Analysis by the Six Designs
Each qualitative research approach or design has its own terms for methods of data analysis:
- Ethnography—uses modified thematic analysis and life histories.
- Case study—uses description, categorical aggregation, or direct interpretation.
- Grounded theory—uses open, axial, and selective coding (although recent writers are proposing variations on those basic analysis methods).
- Phenomenology—describes textures and structures of the essential meaning of the lived experience of the phenomenon
- Heuristics—patterns, themes, and creative synthesis along with individual portraits.
- Generic qualitative inquiry—thematic analysis, which is really a foundation for all the other analytic methods. Thematic analysis is the starting point for the other five, and the endpoint for generic qualitative inquiry. Because it is the basic or foundational method, we'll take it first.
Preliminary Tasks in Analysis in all Methods
In all the approaches—case study, grounded theory, generic inquiry, and phenomenology—there are preliminary tasks that must be performed prior to the analysis itself. For each, you will need to:
- Arrange for secure storage of original materials. Storage should be secure and guaranteed to protect the privacy and confidentiality of the participants' information and identities.
- Transcribe interviews or otherwise transform raw data into usable formats.
- Make master copies and working copies of all materials. Master copies should be kept securely with the original data. Working copies will be marked up, torn apart, and used heavily: make plenty.
- Arrange secure passwords or other protection for all electronic data and copies.
- When ready to begin, read all the transcripts repeatedly—at least three times—for a sense of the whole. Don't force it—allow the participants' words to speak to you.
These tasks are done in all forms of qualitative analysis. Now let's look specifically at generic qualitative inquiry.
Data Analysis in Generic Qualitative Inquiry: Thematic Analysis
The primary tool for conducting the analysis of data when using the generic qualitative inquiry approach is thematic analysis, a flexible analytic method for deriving the central themes from verbal data. A thematic analysis can also be used to conduct analysis of the qualitative data in some types of case study.
Thematic analysis essentially creates theme-statements for ideas or categories of ideas (codes) that the researcher extracts from the words of the participants.
There are two main types of thematic analysis:
- Inductive thematic analysis, in which the data are interpreted inductively, that is, without bringing in any preselected theoretical categories.
- Theoretical thematic analysis, in which the participants' words are interpreted according to categories or constructs from the existing literature.
Analytic Steps in Thematic Analysis: Reading
Remember that the last preliminary task listed above was to read the transcripts for a sense of the whole. In this discussion, we'll assume you're working with transcribed data, usually from interviews. You can apply each step, with changes, to any kind of qualitative data. Now, before you start analyzing, take the first transcript and read it once more, as often as necessary, for a sense of what this participant told you about the topic of your study. If you're using other sources of data, spend time with them holistically.
Thematic Analysis: Steps in the Process
When you have a feel for the data,
- Underline any passages (phases, sentences, or paragraphs) that appear meaningful to you. Don't make any interpretations yet! Review the underlined data.
- Decide if the underlined data are relevant to the research question and cross out or delete all data unrelated to the research question. Some information in the transcript may be interesting but unrelated to the research question.
- Create a name or "code" for each remaining underlined passage (expressions or meaning units) that focus on one single idea. The code should be:
- Briefer than the passage, should
- Sum up its meaning, and should be
- Supported by the meaning unit (the participant's words).
- Find codes that recur; cluster these together. Now begin the interpretation, but only with the understanding that the codes or patterns may shift and change during the process of analysis.
- After you have developed the clusters or patterns of codes, name each pattern. The pattern name is a theme. Use language supported by the original data in the language of your discipline and field.
- Write a brief description of each theme. Use brief direct quotations from the transcript to show the reader how the patterns emerged from the data.
- Compose a paragraph integrating all the themes you developed from the individual's data.
- Repeat this process for each participant, the "within-participant" analysis.
- Finally, integrate all themes from all participants in "across-participants" analysis, showing what general themes are found across all the data.
Some variation of thematic analysis will appear in most of the other forms of qualitative data analysis, but the other methods tend to be more complex. Let's look at them one at a time. If you are already clear as to which approach or design your study will use, you can skip to the appropriate section below.
Ethnographic Data Analysis
Ethnographic data analysis relies on a modified thematic analysis. It is called modified because it combines standard thematic analysis as previously described for interview data with modified thematic methods applied to artifacts, observational notes, and other non-interview data.
Depending on the kinds of data to be interpreted (for instance pictures and historical documents) Ethnographers devise unique ways to find patterns or themes in the data. Finally, the themes must be integrated across all sources and kinds of data to arrive at a composite thematic picture of the culture.
(Adapted from Bogdan and Taylor, 1975; Taylor and Bogdan, 1998; Aronson, 1994.)
Data Analysis in Grounded Theory
Going beyond the descriptive and interpretive goals of many other qualitative models, grounded theory's goal is building a theory. It seeks explanation, not simply description.
It uses a constant comparison method of data analysis that begins as soon as the researcher starts collecting data. Each data collection event (for example, an interview) is analyzed immediately, and later data collection events can be modified to seek more information on emerging themes.
In other words, analysis goes on during each step of the data collection, not merely after data collection.
The heart of the grounded theory analysis is coding, which is analogous to but more rigorous than coding in thematic analysis.
Coding in Grounded Theory Method
There are three different types of coding used in a sequential manner.
- The first type of coding is open coding, which is like basic coding in thematic analysis. During open coding, the researcher performs:
- A line-by-line analysis (or sentence or paragraph analysis) of the data.
- Labels and categorizes the dimensions or aspects of the phenomenon being studied.
- The researcher also uses memos to describe the categories that are found.
- The second type of coding is axial coding, which involves finding links between categories and subcategories found in the open coding.
- The open codes are examined for their relationships: cause and effect, co-occurrence, and so on.
- The goal here is to picture how the various dimensions or categories of data interact with one another in time and space.
- The third type of coding is selective coding, which identifies a core category and relates the categories subsidiary to this core.
- Selective coding selects the main phenomenon, (core category) around which subsidiary phenomena, (all other categories) are grouped, arranging the groupings, studying the results, and rearranging where the data require it.
The Final Stages of Grounded Theory Analysis, after Coding
From selective coding, the grounded theory researcher develops:
- A model of the process, which is the description of which actions and interactions occur in a sequence or series.
- A transactional system, which is the description of how the interactions of different events explain the phenomenon being investigated.
- Finally, A conditional matrix is diagrammed to help consider the conditions and consequences related to the phenomenon under study.
These three essentially tell the story of the outcome of the research, in other words, the description of the process by which the phenomenon seems to happen, the transactional system supporting it, and the conditional matrix that pictures the explanation of the phenomenon are the findings of a grounded theory study.
(Adapted from Corbin and Strauss, 2008; Strauss and Corbin, 1990, 1998.)
Data Analysis in Qualitative Case Study: Background
There are a few points to consider in analyzing case study data:
- Analysis can be:
- Holistic—the entire case.
- Embedded—a specific aspect of the case.
- Multiple sources and kinds of data must be collected and analyzed.
- Data must be collected, analyzed, and described about both:
- The contexts of the case (its social, political, economic contexts, its affiliations with other organizations or cases, and so on).
- The setting of the case (geography, location, physical grounds, or set-up, business organization, etc.).
Qualitative Case Study Data Analysis Methods
Data analysis is detailed in description and consists of an analysis of themes. Especially for interview or documentary analysis, thematic analysis can be used (see the section on generic qualitative inquiry). A typical format for data analysis in a case study consists of the following phases:
- Description: This entails developing a detailed description of each instance of the case and its setting. The words "instance" and "case" can be confusing. Let's say we're conducting a case study of gay and lesbian members of large urban evangelical Christian congregations in the Southeast. The case would be all such people and their congregations. Instances of the case would be any individual person or congregation. In this phase, all the congregations (the settings) and their larger contexts would be described in detail, along with the individuals who are interviewed or observed.
- Categorical Aggregation: This involves seeking a collection of themes from the data, hoping that relevant meaning about lessons to be learned about the case will emerge. Using our example, a kind of thematic analysis from all the data would be performed, looking for common themes.
- Direct Interpretation: By looking at the single instance or member of the case and drawing meaning from it without looking for multiple instances, direct interpretation pulls the data apart and puts it together in more meaningful ways. Here, the interviews with all the gay and lesbian congregation members would be subjected to thematic analysis or some other form of analysis for themes.
- Within-Case Analysis: This would identify the themes that emerge from the data collected from each instance of the case, including connections between or among the themes. These themes would be further developed using verbatim passages and direct quotation to elucidate each theme. This would serve as the summary of the thematic analysis for each individual participant.
- Cross-Case Analysis: This phase develops a thematic analysis across cases as well as assertions and interpretations of the meaning of the themes emerging from all participants in the study.
- Interpretive Phase: In the final phase, this is the creation of naturalistic generalizations from the data as a whole and reporting on the lesson learned from the case study.
(Adapted from Creswell, 1998; Stake, 1995.)
Data Analysis in Phenomenological Research
There are a few existing models of phenomenological research, and they each propose slightly different methods of data analysis. They all arrive at the same goal, however. The goal of phenomenological analysis is to describe the essence or core structures and textures of some conscious psychological experience. One such model, empirical, was developed at Duquesne University. This method of analysis consists of five essential steps and represents the other variations well. Whichever model is chosen, those wishing to conduct phenomenological research must choose a model and abide by its procedures. Empirical phenomenology is presented as an example.
- Sense of the whole. One reads the entire description in order to get a general sense of the whole statement. This often takes a few readings, which should be approached contemplatively.
- Discrimination of meaning units. Once the sense of the whole has been grasped, the researcher returns to the beginning and reads through the text once more, delineating each transition in meaning.
- The researcher adopts a psychological perspective to do this. This means that the researcher looks for shifts in psychological meaning.
- The researcher focuses on the phenomenon being investigated. This means that the researcher keeps in mind the study's topic and looks for meaningful passages related to it.
- The researcher next eliminates redundancies and unrelated meaning units.
- Transformation of subjects' everyday expressions (meaning units) into psychological language. Once meaning units have been delineated,
- The researcher reflects on each of the meaning units, which are still expressed in the concrete language of the participants, and describes the essence of the statement for the participant.
- The researcher makes these descriptions in the language of psychological science.
- Synthesis of transformed meaning units into a consistent statement of the structure of the experience.
- Using imaginative variation on these transformed meaning units, the researcher discovers what remains unchanged when variations are imaginatively applied, and
- From this develops a consistent statement regarding the structure of the participant's experience.
- The researcher completes this process for each transcript in the study.
- Final synthesis. Finally, the researcher synthesizes all of the statements regarding each participant's experience into one consistent statement that describes and captures [of] the essence of the experience being studied.
(Adapted from Giorgi, 1985, 1997; Giorgi and Giorgi, 2003.)
Data Analysis in Heuristics
Six steps typically characterize the heuristic process of data analysis, consisting of:
- Initial engagement.
- Incubation.
- Illumination.
- Explication.
To start, place all the material drawn from one participant before you (recordings, transcriptions, journals, notes, poems, artwork, and so on). This material may either be data gathered by self-search or by interviews with co-researchers.
- Immerse yourself fully in the material until you are aware of and understand everything that is before you.
- Incubate the material. Put the material aside for a while. Let it settle in you. Live with it but without particular attention or focus. Return to the immersion process. Make notes where they would enable you to remember or classify the material. Continue this rhythm of working with the data and resting until an illumination or essential configuration emerges. From your core or global sense, list the essential components or patterns and themes that characterize the fundamental nature and meaning of the experience. Reflectively study the patterns and themes, dwell inside them, and develop a full depiction of the experience. The depiction must include the essential components of the experience.
- Illustrate the depiction of the experience with verbatim samples, poems, stories, or other materials to highlight and accentuate the person's lived experience.
- Return to the raw material of your co-researcher (participant). Does your depiction of the experience fit the data from which you have developed it? Does it contain all that is essential?
- Develop a full reflective depiction of the experience, one that characterizes the participant's experience reflecting core meanings for the individuals as a whole. Include in the depiction, verbatim samples, poems, stories, and the like to highlight and accentuate the lived nature of the experience. This depiction will serve as the creative synthesis, which will combine the themes and patterns into a representation of the whole in an aesthetically pleasing way. This synthesis will communicate the essence of the lived experience under inquiry. The synthesis is more than a summary: it is like a chemical reaction, a creation anew.
- Return to the data and develop a portrait of the person in such a way that the phenomenon and the person emerge as real.
(Adapted from Douglass and Moustakas, l985; Moustakas, 1990.)
Bogdan, R., & Taylor, S. J. (1975). Introduction to qualitative research methods: A phenomenological approach (3rd ed.). New York, NY: Wiley.
Corbin, J., & Strauss, A. (2008). Basics of qualitative research: Techniques and procedures for developing grounded theory (3rd ed.). Los Angeles, CA: Sage.
Creswell, J. W. (1998). Qualitative inquiry and research design: Choosing among five traditions . Thousand Oaks, CA: Sage.
Douglass, B. G., & Moustakas, C. (1985). Heuristic inquiry: The internal search to know. Journal of Humanistic Psychology , 25(3), 39–55.
Giorgi, A. (Ed.). (1985). Phenomenology and psychological research . Pittsburgh, PA: Duquesne University Press.
Giorgi, A. (1997). The theory, practice and evaluation of phenomenological methods as a qualitative research procedure. Journal of Phenomenological Psychology , 28, 235–260.
Giorgi, A. P., & Giorgi, B. M. (2003). The descriptive phenomenological psychological method. In P. M. Camic, J. E. Rhodes, & L. Yardley (Eds.), Qualitative research in psychology: Expanding perspectives in methodology and design (pp. 243–273). Washington, DC: American Psychological Association.
Moustakas, C. (1990). Heuristic research: Design, methodology, and applications . Newbury Park, CA: Sage.
Stake, R. E. (1995). The art of case study research . Thousand Oaks, CA: Sage.
Strauss, A., & Corbin, J. (1990). Basics of qualitative research: Grounded theory procedures and techniques . Newbury Park, CA: Sage.
Strauss, A., & Corbin, J. (1998). Basics of qualitative research: Techniques and theory for developing grounded theory (2nd ed.). Thousand Oaks, CA: Sage.
Taylor, S. J., & Bogdan, R. (1998). Introduction to qualitative research methods: A guidebook and resource (3rd ed.). New York: Wiley.
Doc. reference: phd_t3_u06s6_qualanalysis.html
- Cancer Nursing Practice
- Emergency Nurse
- Evidence-Based Nursing
- Learning Disability Practice
- Mental Health Practice
- Nurse Researcher
- Nursing Children and Young People
- Nursing Management
- Nursing Older People
- Nursing Standard
- Primary Health Care
- RCN Nursing Awards
- Nursing Live
- Nursing Careers and Job Fairs
- CPD webinars on-demand
- --> Advanced -->

- Clinical articles
- Expert advice
- Career advice
- Revalidation
Data analysis Previous Next
Qualitative case study data analysis: an example from practice, catherine houghton lecturer, school of nursing and midwifery, national university of ireland, galway, republic of ireland, kathy murphy professor of nursing, national university of ireland, galway, ireland, david shaw lecturer, open university, milton keynes, uk, dympna casey senior lecturer, national university of ireland, galway, ireland.
Aim To illustrate an approach to data analysis in qualitative case study methodology.
Background There is often little detail in case study research about how data were analysed. However, it is important that comprehensive analysis procedures are used because there are often large sets of data from multiple sources of evidence. Furthermore, the ability to describe in detail how the analysis was conducted ensures rigour in reporting qualitative research.
Data sources The research example used is a multiple case study that explored the role of the clinical skills laboratory in preparing students for the real world of practice. Data analysis was conducted using a framework guided by the four stages of analysis outlined by Morse ( 1994 ): comprehending, synthesising, theorising and recontextualising. The specific strategies for analysis in these stages centred on the work of Miles and Huberman ( 1994 ), which has been successfully used in case study research. The data were managed using NVivo software.
Review methods Literature examining qualitative data analysis was reviewed and strategies illustrated by the case study example provided.
Discussion Each stage of the analysis framework is described with illustration from the research example for the purpose of highlighting the benefits of a systematic approach to handling large data sets from multiple sources.
Conclusion By providing an example of how each stage of the analysis was conducted, it is hoped that researchers will be able to consider the benefits of such an approach to their own case study analysis.
Implications for research/practice This paper illustrates specific strategies that can be employed when conducting data analysis in case study research and other qualitative research designs.
Nurse Researcher . 22, 5, 8-12. doi: 10.7748/nr.22.5.8.e1307
This article has been subject to double blind peer review
None declared
Received: 02 February 2014
Accepted: 16 April 2014
Case study data analysis - case study research methodology - clinical skills research - qualitative case study methodology - qualitative data analysis - qualitative research
User not found
Want to read more?
Already have access log in, 3-month trial offer for £5.25/month.
- Unlimited access to all 10 RCNi Journals
- RCNi Learning featuring over 175 modules to easily earn CPD time
- NMC-compliant RCNi Revalidation Portfolio to stay on track with your progress
- Personalised newsletters tailored to your interests
- A customisable dashboard with over 200 topics
Alternatively, you can purchase access to this article for the next seven days. Buy now
Are you a student? Our student subscription has content especially for you. Find out more
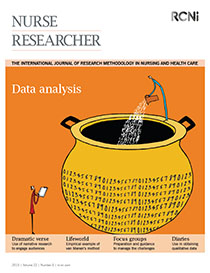
15 May 2015 / Vol 22 issue 5
TABLE OF CONTENTS
DIGITAL EDITION
- LATEST ISSUE
- SIGN UP FOR E-ALERT
- WRITE FOR US
- PERMISSIONS
Share article: Qualitative case study data analysis: an example from practice
We use cookies on this site to enhance your user experience.
By clicking any link on this page you are giving your consent for us to set cookies.
An Interpretive Approach for Data Collection and Analysis
- First Online: 07 July 2017
Cite this chapter
- Hany Elbardan 3 &
- Ahmed Othman Rashwan Kholeif 4
2771 Accesses
2 Citations
This chapter has explained the research design and the methods used in the book. The researcher explicitly articulated the nature of the research problem and determined the ontological stance which led to the epistemological and methodological stances. The study is designed under an interpretive paradigm, which took the form of a multiple-case study and the analysis of a considerable amount of primary and secondary qualitative data. This enabled the researchers to interact closely with the participants and to explore issues in depth. Researchers should be aware that qualitative methodology is more applicable to develop an in-depth understanding of contextual-related problems. Qualitative design is more sensitive to context and flexible to embracing emerging new themes. The richness of data produced through using multiple-case studies helped better to investigate the phenomenon of the IAF adaptation as a response to the ERP systems implementation. The use of case studies provided greater internal and external validity than other quantitative methods would have done. Triangulation of methods was achieved through the use of semi-structured interviews, focus groups and non-participant observation. The qualitative content analysis was found to be the appropriate technique for analysing the data. A qualitative content analysis technique was deemed more desirable for this study as it preserves as much as possible the deep meaning of the qualitative data. Furthermore, it enabled the interpretation of all transcribed interviews, documents and notes of observation and relating each one to the whole in order to gain a holistic picture of the phenomenon. The trustworthiness strategies which were used in this research and the related procedures and techniques were found to be interlinked that fostered the coherence and validity of the research.
This is a preview of subscription content, log in via an institution to check access.
Access this chapter
- Available as EPUB and PDF
- Read on any device
- Instant download
- Own it forever
- Compact, lightweight edition
- Dispatched in 3 to 5 business days
- Free shipping worldwide - see info
- Durable hardcover edition
Tax calculation will be finalised at checkout
Purchases are for personal use only
Institutional subscriptions
Similar content being viewed by others
Qualitative Methods and Analysis
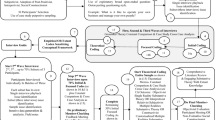
Adapting and blending grounded theory with case study: a practical guide
Depth Interviews and Focus Groups
Al-Twaijry, A., Brierley, J., & Gwilliam, D. (2003). The development of internal audit in Saudi Arabia: An institutional theory perspective. Critical Perspectives on Accounting, 14, 507–531.
Article Google Scholar
American Chamber. (2002). Information technology in Egypt. American Chamber of Commerce in Egypt , Cairo.
Google Scholar
Arena, M., & Azzone, G. (2009). Identifying organizational drivers of internal audit effectiveness. International Journal of Auditing, 13, 43–60.
Avital, M. (2000). Dealing with time in social inquiry: A tension between method and lived experience. Organization Science, 11, 665–673.
Baxter, P., & Jack, S. (2008). Qualitative case study methodology: Study design and implementation for novice researchers. The Qualitative Report, 13 (4), 544–559.
Bell, E., & Bryman, A. (2007) ‘The ethics of management research: An exploratory.
Benbasat, I., Goldstein, D. K., & Mead, M. (1987). The case research strategy in studies of information systems. MIS Quarterly , 396–386.
Berg, B. (2009). Qualitative research methods for the social sciences . London: Allyn and Bacon.
Blumberg, B., Cooper, D., & Schindler, P. (2011). Business research methods . London: McGraw-Hill.
Bryman, A. (1988). Quantity and quality in social research . London: Sage.
Book Google Scholar
Bryman, A. (2004). Social research methods . Oxford: Oxford University Press.
Bryman, A., & Bell, E. (2007). Business research methods . Oxford: Oxford University Press.
Burrell, G., & Morgan, G. (1982). Sociological paradigms and organisational analysis . London: Heinemann.
Caglio, A. (2003). Enterprise resource planning systems and accountants: Towards hybridization? European Accounting Review, 13 (1), 123–153.
Cavaye, A. L. M. (1996). Case study research: A multi-faceted research approach for IS. Information Systems Journal, 6 (3), 227–242.
Collis, J., & Hussey, R. (2009). Business research: A practical guide for undergraduate and post graduate students . Houndmills: Palgrave Macmillan.
Creswell, J. (2009). Qualitative inquiry and research design. Choosing among five approaches . Thousand Oaks: Sage Publications.
Denzin, N. K., & Lincoln, Y. S. (1998). Strategies of qualitative inquiry . Thousand Oaks: Sage.
Díaz Andrade, A. (2009). Interpretive research aiming at theory building: Adopting and adapting the case study design. The Qualitative Report, 14 (1), 42–60.
Doolin, B. (1996). Alternative views of case research in information systems. Australian Journal of Information Systems, 3, 21–29.
Eisenhardt, K. M. (1989). Building theories from case study research. Academy of Management Review, 14 (4), 532–550.
Eisenhardt, K. M. (1991). Better stories and better constructs: the case for rigor and comparative logic. The Academy of Management Review, 16, 620–627.
Eisenhardt, K. M., & Graebner, M. (2007). Theory building from cases: Opportunities and challenges. Academy of Management Journal, 50 (1), 25–32.
El Sayed, H. (2006). ERPs and accountants’ expertise: The construction of relevance. Journal of Enterprise Information Management, 19 (1), 83–96.
Eriksson, P., & Kovalainen A. (2008). Qualitative methods in business research . London: Sage.
Flick, U. (2008). Managing quality in qualitative research . Sage Publications Ltd.
Flick, W. (2009). An introduction to qualitative research . London: Sage Publications.
Guba, E. G., & Lincoln, Y. S. (1994). Competing paradigms in qualitative research. In N. K. Denzin & Y. S. Lincoln (Eds.), Handbook of qualitative research (pp. 105–117). Thousand Oaks: Calif: Sage.
Guba, E. G., & Lincoln, Y. S. (1998). Competing paradigms in qualitative research. In N. Denzin & Y. Lincoln (Eds.), Handbook of qualitative research (pp. 195–220). London: Sage.
Henn, M., Weinstein, M., & Foard, N. (2006). A short introduction to social research . London: Sage.
Hermanowicz, J. C. (2002). The great interview: 25 strategies for studying people in bed. Qualitative Sociology, 25 (4), 479–499.
Hessler, R. M. (1992). Social research methods . New York: West Publishing Company.
Hsieh, H. F., & Shannon, S. E. (2005). Three approaches to qualitative content analysis. Qualitative Health Research, 15 (9), 1277–1288.
Humphrey, C. (2001). Paper prophets and the continuing case for thinking differently about accounting research. British Accounting Review, 33 (1), 91–103.
Irani, Z., Ezingeard, J., Grieve, R., & Race, P. (1999). A case study strategy as part of an information systems research methodology: A critique. The International Journal of Computer Applications in Technology, 12 (2), 190–198.
Johnson, R. B., & Onwuegbuzie, A. J. (2004). Mixed methods research: A research paradigm whose time has come. Educational Researcher, 33 (7), 14–26.
Kaplan, B., & Maxwell, J. A. (1994). Qualitative research methods for evaluating computer information systems’ in evaluating health care information systems: Methods and applications . Thousand Oaks: SAGE Publications.
Kitzinger, J. (1995). Qualitative research: Introducing focus groups. BMJ, 29 (311), 299–302.
Klein, H. K., & Myers, M. D. (1999). A set of principles for conducting and evaluating interpretive field studies in information systems. MIS Quarterly, 23 (1), 67–94.
Krathwohl, D. (1997). Methods of educational and social science research: An integrated approach (2nd ed.). Addison Wesley Longman. ISBN: 0-8013-2029-1.
Krippendorff, K. H. (2004). Content analysis: An introduction to its methodology (2nd ed.). Thousand Oaks, California: Sage Publications.
Kuhn, J., & Sutton, S. (2010). Continuous auditing in ERP system environments: The current state and future directions. Journal of Information Systems, 24 (1), 91–112.
Lee, N., & Lings, I. (2008). Doing business research: A guide to theory and practice . London: Sage Publications.
Lewis, J. (2003). Design issues. In J. Ritchie & J. Lewis (Eds.), Qualitative research practice: A guide for social science students and researchers (pp. 47–76). London: Sage Publications.
Lincoln, Y., & Guba, E. (1994). Naturalistic inquiry . London: Sage Publications.
Lincoln, Y. S., & Guba, E. G. (1986). But is it rigorous? Trustworthiness and authenticity in naturalistic evaluation. In D. D.Williams (Ed.), Naturalistic evaluation (pp. 73–84). New Directions for Program Evaluation, 30. San Francisco, CA: Jossey-Bass.
Maimbo, H., & Pervan, G. (2005). Designing a case study protocol for application in IS research. Proceedings of the 9th Pacific Asia Conference on Information Systems , Bangkok, Thailand, July 10–13.
Miles, M. B., & Huberman, A. M. (1994). Qualitative data analysis: An expanded sourcebook . London: Sage Publications.
Morse, J. (1999). Myth #93: Reliability and validity are not relevant to qualitative inquiry. Qualitative Health Research, 9 (6), 717–718.
Morse, J. M., Barrett, M., Mayan, M., Olson, K., & Spiers, J. (2002). Verification strategies for establishing reliability and validity in qualitative research. International Journal of Qualitative Methods, 1 (2), 1–19.
Myers, D. M., & Avison, D. (2002). Qualitative research in information systems . Sage.
Myers, M. (2009). Qualitative research in business and management . London: Sage Publications.
Myers, M. D., & Newman, M. (2007). The qualitative interview in IS research: Examining the craft. Information and Organization, 17, 2–26.
Neuman, W. (2003). Social research methods: Qualitative and quantitative approaches . London: Pearson Education.
Oates, B. J. (2006). Researching information systems and computing . London: Sage.
Orlikowski, W. J. (1991). Integrated information environment or matrix of control? The contradictory implications of information technology. Accounting, Management and Information Technologies, 1 (1), 9–42.
Orlikowski, W. J. (2009). The sociomateriality of organisational life: Considering technology in management research. Cambridge Journal of Economics, 34, 125–141.
Orlikowski, W. J., & Baroudi, J. J. (1991). Studying information technology in organizations: Research approaches and assumptions. Information Systems Research, 2 (1), 1–28.
Orlikowski, W. J., & Yates, J. (2006). ICT and organizational change: A commentary. Journal of Applied Behavioral Science, 42, 127.
Patton, M. Q. (1990). Qualitative evaluation and research methods . Newbury Park, CA: Sage.
Patton, M. Q. (2002). Qualitative research and evaluation methods . Thousand Oak: Sage Publications.
Payne, G., & Payne, J. (2004). Key concepts in social research . London: Sage Publications.
Perry, C. (1998). Processes of a case study methodology for postgraduate research in marketing. European Journal of Marketing, 32 (9/10), 785–802.
Pettigrew, A., Woodman, R., & Cameron, K. (2001). Studying organizational change and development: Challenges for future research. Academy of Management Journal, 44 (4), 697–713.
Remeniy, D., Williams, B., Money, A., & Swartz, E. (2002). Doing research in business and management . London: Sage.
Romano, C. (1989). Research strategies for small business: A case study. International Small Business Journal, 7 (4), 35–43.
Rowley, J. (2002). Using case studies in research. Management Research News, 25 (1), 16–27.
Saunders, M., Lewis, P., & Thornhill, A. (2009). Research methods for business students . London: Financial Times Prentice Hall.
Sedmak, M., & Longhurst, P. (2010). Methodological choices in enterprise systems research. Business Process Management, 16 (1), 76–92.
Silverman, D. (2010). Doing qualitative research . London: Sage Publications.
Smith, M. (2003). Research methods in accounting . London: Sage Publications.
Stake, R. E. (1995). The art of case study research . Thousand Oaks, CA: Sage.
Taylor, S. J., & Bogdan, R. (1988). Introduction to qualitative research methods: A guidebook and resource . Chichester, New York: Wiley.
Tilley, L., & Woodthorpe, K. (2011). Is it the end for anonymity as we know it?: A critical examination of the ethical principle of anonymity in the context of 21st century demands on the qualitative researcher. Qualitative Research, 11 (2), 197–212.
Voss, C., Tsikriktsis, N., & Frohlich, M. (2002). Case research in operations management. International Journal of Operations & Production Management, 22 (2), 195–219.
Walsham, G. (1993). Interpreting information systems in organisations . Chichester, UK: Wiley.
Walsham, G. (1995a). Interpretive case studies in IS research: Nature and method. European Journal of information Systems, 4, 74–81.
Walsham, G. (1995b). The emergence of interpretivism in IS research. Information Systems Research, 6 (4), 376–394.
Weber, R. P. (1990). Basic content analysis (2nd ed.). Newbury Park, CA: Sage Publications.
Weerakkody, V., Dwivedi, Y., & Irani, Z. (2009). The diffusion and use of institutional theory: A cross-disciplinary longitudinal literature survey. Journal of Information Technology, 24, 354–368.
Yin, R. (2009). Case study research: Design and methods . London: Sage Publications.
Zhang, Y., & Wildemuth, B. (2009). Qualitative analysis of content. In B. Wildemuth, B. (Ed.) Applications of social science research methods to questions in library and information science . Englewood, Co: Libraries Unlimited.
Download references
Author information
Authors and affiliations.
College of Business Administration, American University of the Middle East, Egaila, Kuwait
Hany Elbardan
Business School, Edge Hill University, Ormskirk, UK
Ahmed Othman Rashwan Kholeif
You can also search for this author in PubMed Google Scholar
Corresponding author
Correspondence to Hany Elbardan .
Rights and permissions
Reprints and permissions
Copyright information
© 2017 The Author(s)
About this chapter
Elbardan, H., Kholeif, A.O. (2017). An Interpretive Approach for Data Collection and Analysis. In: Enterprise Resource Planning, Corporate Governance and Internal Auditing. Palgrave Macmillan, Cham. https://doi.org/10.1007/978-3-319-54990-3_5
Download citation
DOI : https://doi.org/10.1007/978-3-319-54990-3_5
Published : 07 July 2017
Publisher Name : Palgrave Macmillan, Cham
Print ISBN : 978-3-319-54989-7
Online ISBN : 978-3-319-54990-3
eBook Packages : Economics and Finance Economics and Finance (R0)
Share this chapter
Anyone you share the following link with will be able to read this content:
Sorry, a shareable link is not currently available for this article.
Provided by the Springer Nature SharedIt content-sharing initiative
- Publish with us
Policies and ethics
- Find a journal
- Track your research
To read this content please select one of the options below:
Please note you do not have access to teaching notes, a systematic qualitative case study: questions, data collection, nvivo analysis and saturation.
Qualitative Research in Organizations and Management
ISSN : 1746-5648
Article publication date: 20 August 2020
Issue publication date: 23 February 2021
The study aims to explore the case study method with the formation of questions, data collection procedures and analysis, followed by how and on which position the saturation is achieved in developing a centralized Shariah governance framework for Islamic banks in Bangladesh.
Design/methodology/approach
Using purposive and snowball sampling procedures, data have been collected from 17 respondents who are working in the central bank and Islamic banks of Bangladesh through face-to-face and semi-structured interviews.
The study claims that researchers can form the research questions by using “what” question mark in qualitative research. Besides, the qualitative research and case study could explore the answers of “what” questions along with the “why” and “how” more broadly, descriptively and extensively about a phenomenon. Similarly, saturation can be considered attaining the ultimate point of data collection by the researchers without adding anything in the databank. Overall, this study proposes three stages of saturation: First, information redundancy. Second, referring the respondents (already considered in the study) without knowing anything about the data collection and their responses. Third, through the NVivo open coding process due to the decrease of reference or quotes in a certain position or in the saturation position as a result of fewer outcomes or insufficient information. The saturation is thus achieved in the diversified positions, i.e. three respondents for regulatory, nine for Shariah scholars and officers and five for the experts concerning the responses and respondents.
Research limitations/implications
The study has potential implications on the qualitative research method, including the case study, saturation process and points, NVivo analysis and qualitative questions formation.
Originality/value
This research defines a case study with the inclusion of “what” and illustrates the saturation process in diverse positions. The qualitative research questions can also be formed with “what” in addition “why” and “how”.
- Qualitative research
Acknowledgements
The author would like to thank both Editors, Associate Editor Dr. Amon Barros and anonymous reviewers for their valuable time, constructive comments and suggestions for the improvement of the manuscript. A special thanks to Dr. Mohd Mursyid Arshad, Senior Lecturer at Faculty of Educational Studies, Universiti Putra Malaysia, Malaysia for his suggestions and unconditional help in the NVivo data analysis process of this research project. The study does not receive any specific fund or research grant.
Alam, M.K. (2021), "A systematic qualitative case study: questions, data collection, NVivo analysis and saturation", Qualitative Research in Organizations and Management , Vol. 16 No. 1, pp. 1-31. https://doi.org/10.1108/QROM-09-2019-1825
Emerald Publishing Limited
Copyright © 2020, Emerald Publishing Limited
Related articles
We’re listening — tell us what you think, something didn’t work….
Report bugs here
All feedback is valuable
Please share your general feedback
Join us on our journey
Platform update page.
Visit emeraldpublishing.com/platformupdate to discover the latest news and updates
Questions & More Information
Answers to the most commonly asked questions here
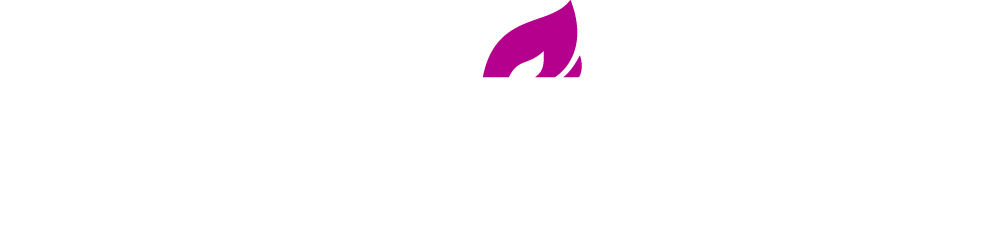
No products in the cart.
What is Qualitative Data Analysis Software (QDA Software)?
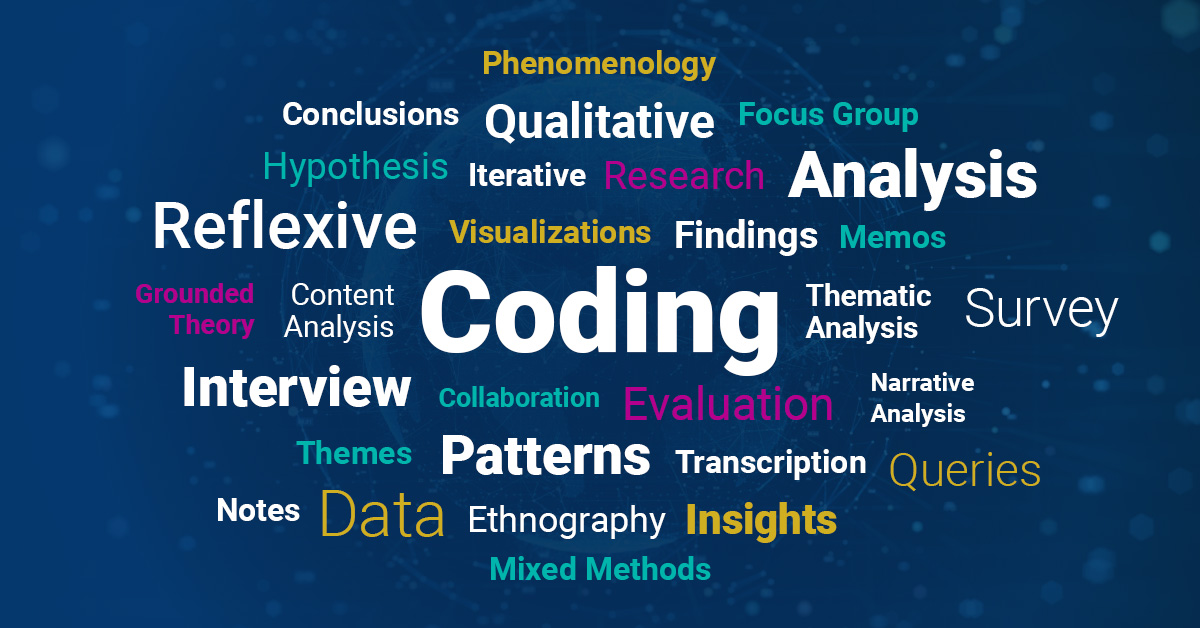
Qualitative Data Analysis Software (QDA software) allows researchers to organize, analyze and visualize their data, finding the patterns in qualitative data or unstructured data: interviews, surveys, field notes, videos, audio files, images, journal articles interviews, web content etc.
Quantitative vs. Qualitative Data Analysis
What is the difference between quantitative and qualitative data analysis. As the name implies, quantitative data analysis has to do with numbers. For example, any time you are doing statistical analysis, you are doing quantitative data analysis. Some examples of quantitative data analysis software are SPSS, STATA, SAS, and Lumivero’s own powerful statistics software, XLSTAT .
In contrast, qualitative analysis "helps you understand people’s perceptions and experiences by systematically coding and analyzing the data", as described in Qualitative vs Quantitative Research 101 . It tends to deal more with words than numbers. It can be useful when working with a lot of rich and deep data and when you aren’t trying to test something very specific. Some examples of qualitative data analysis software are MAXQDA, ATLAS.ti, Quirkos, and Lumivero’s NVivo, the leading tool for qualitative data analysis .
When would you use each one? Well, qualitative data analysis is often used for exploratory research or developing a theory, whereas quantitative is better if you want to test a hypothesis, find averages, and determine relationships between variables. With quantitative research you often want a large sample size to get relevant statistics. In contrast, qualitative research, because so much data in the form of text is involved, can have much smaller sample sizes and still yield valuable insights.
Of course, it’s not always so cut and dry, and many researchers end up taking a «mixed methods» approach, meaning that they combine both types of research. In this case they might use a combination of both types of software programs.
Learn how some qualitative researchers use QDA software for text analysis in the on-demand webinar Twenty-Five Qualitative Researchers Share How-To's for Data Analysis .

How is Qualitative Data Analysis Software Used for Research?
Qualitative Data Analysis Software works with any qualitative research methodology used by a researcher For example, software for qualitative data analysis can be used by a social scientist wanting to develop new concepts or theories may take a ‘grounded theory’ approach. Or a researcher looking for ways to improve health policy or program design might use ‘evaluation methods’. QDA software analysis tools don't favor a particular methodology — they're designed to facilitate common qualitative techniques no matter what method you use.
NVivo can help you to manage, explore and find patterns in your data and conduct thematic and sentiment analysis, but it cannot replace your analytical expertise.
Qualitative Research as an Iterative Process
Handling qualitative and mixed methods data is not usually a step-by-step process. Instead, it tends to be an iterative process where you explore, code, reflect, memo, code some more, query and so on. For example, this picture shows a path you might take to investigate an interesting theme using QDA software, like NVivo, to analyze data:
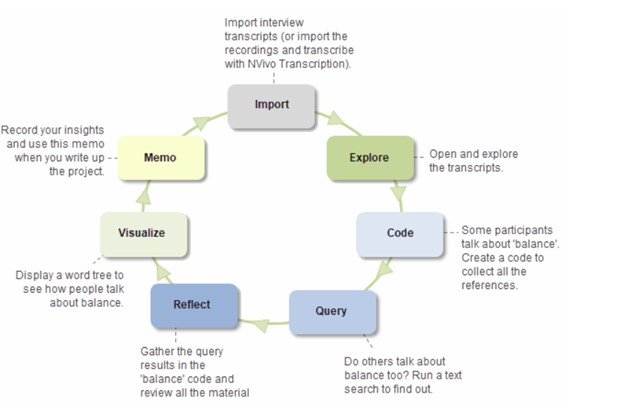
How Do I Choose the Best Approach for My Research Project with QDA Software?
Every research project is unique — the way you organize and analyze the material depends on your methodology, data and research design.
Here are some example scenarios for handling different types of research projects in QDA software — these are just suggestions to get you up and running.
A study with interviews exploring stakeholder perception of a community arts program
Your files consist of unstructured interview documents. You would set up a case for each interview participant, then code to codes and cases. You could then explore your data with simple queries or charts and use memos to record your discoveries.
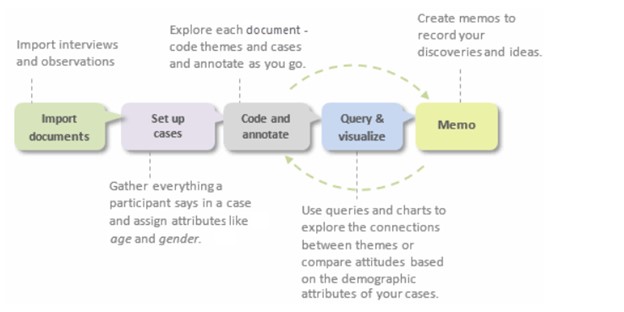
A study exploring community perceptions about climate change using autocoding with AI
Your files consist of structured, consistently formatted interviews (where each participant is asked the same set of questions). With AI, you could autocode the interviews and set up cases for each participant. Then code themes to query and visualize your data.
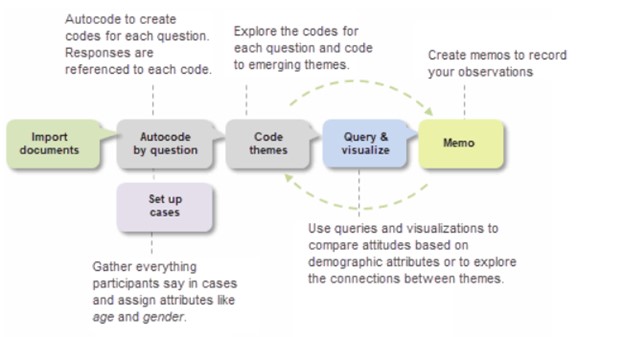
A literature review on adolescent depression
Your files consist of journal articles, books and web pages. You would classify your files before coding and querying them; and then you could critique each file in a memo. With Citavi integration in NVivo, you can import your Citavi references into NVivo.
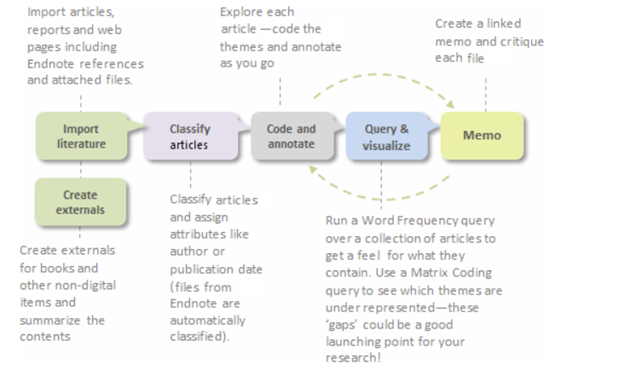
A social media study of the language used by members of an online community
Your files consist of Facebook data captured with NCapture. You would import it as a dataset ready to code and query. Use memos to record your insights.
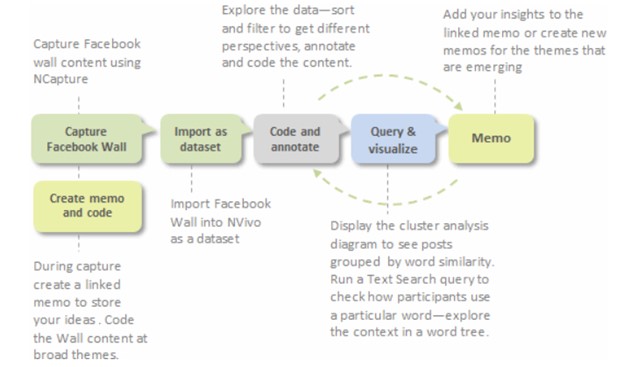
A quick analysis of a local government budget survey
Your file is a large dataset of survey responses. You would import it using the Survey Import Wizard, which prepares your data for analysis. As part of the import, choose to run automated insights with AI to identify and code to themes and sentiment so that you can quickly review results and report broad findings.
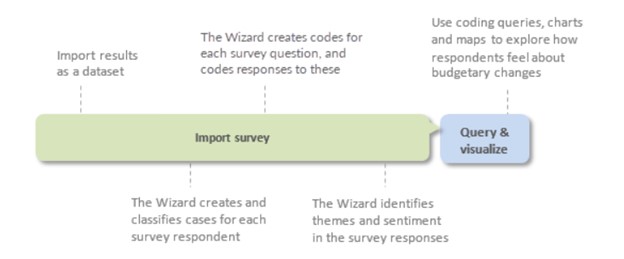
Ways to Get Started with Your Project with Qualitative Analysis Software
Since projects (and researchers) are unique there is no one 'best practice' approach to organizing and analyzing your data but there are some useful strategies to help you get up and running:
- Start now - don't wait until you have collected all the data. Import your research design, grant application or thesis proposal.
- Make a project journa l and state your research questions and record your goals. Why are you doing the project? What is it about? What do you expect to find and why?
- Make a mind map for your preliminary ideas. Show the relationships or patterns you expect to find in your data based on prior experience or preliminary reading.
- Import your interviews, field notes, focus groups —organize these files into folders for easy access.
- Set up an initial code structure based on your early reading and ideas—you could run a Word Frequency query over your data to tease out the common themes for creating your code structure.
- Set up cases for the people, places or other cases in your project.
- Explore your material and code themes as they emerge in your data mining —creating memos and describing your discoveries and interpretations.
- To protect your work, get in the habit of making regular back-ups.
QDA Analysis Tools Help You Work Toward Outcomes that are Robust and Transparent
Using QDA software to organize and analyze your data also increases the 'transparency' of your research outcomes—for example, you can:
- Demonstrate the evolution of your ideas in memos and maps.
- Document your early preconceptions and biases (in a memo or map) and demonstrate how these have been acknowledged and tested.
- Easily find illustrative quotes.
- Always return to the original context of your coded material.
- Save and revisit the queries and visualizations that helped you to arrive at your conclusions.
QDA software, like NVivo, can demonstrate the credibility of your findings in the following ways:
- If you used NVivo for your literature review, run a query or create a chart to demonstrate how your findings compare with the views of other authors.
- Was an issue or theme reported by more than one participant? Run a Matrix Coding query to see how many participants talked about a theme.
- Were multiple methods used to collect the data (interviews, observations, surveys)—and are the findings supported across these text data and video data files? Run a Matrix Coding query to see how often a theme is reported across all your files.

- If multiple researchers analyzed the material — were their findings consistent? Use coding stripes (or filter the contents in a code) to see how various team members have coded the material and run a Coding Comparison query to assess the level of agreement.
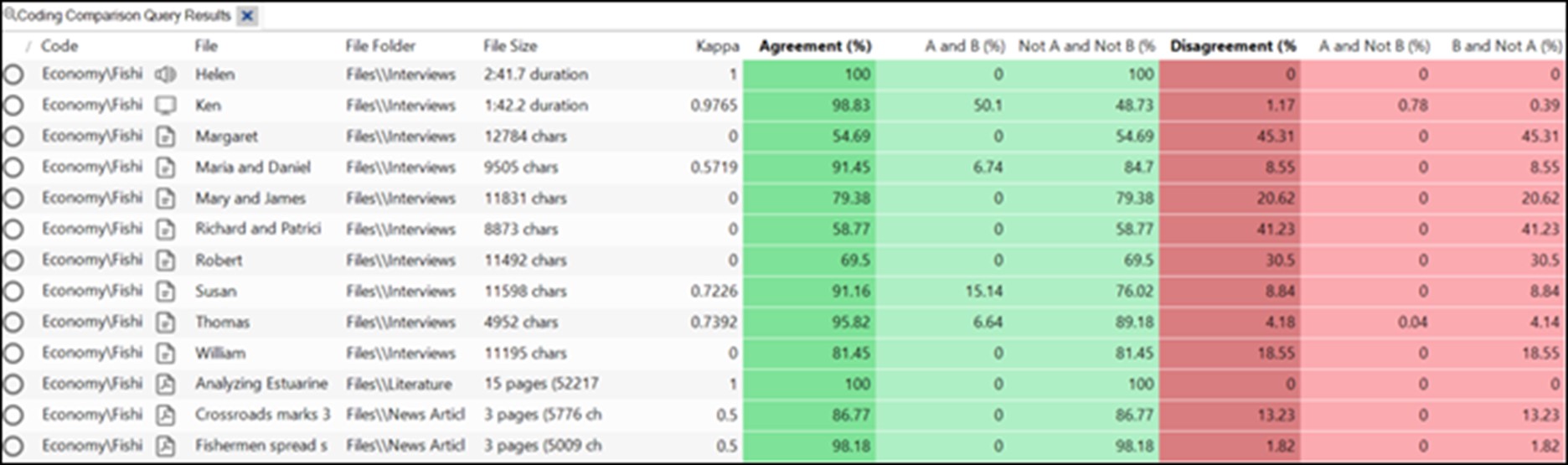
QDA Software Integrations
Many qualitative analysis software options have integration with other software to enhance your research process. NVivo integrates or can be used with the following software:
- NVivo Transcription to save you time and jump start your qualitative data analysis. Learn how in the on-demand webinar Transcription – Go Beyond the Words .
- Reference management software, like Lumivero’s Citavi, for reference management and writing. By combining Citavi and NVivo, you can create complicated searches for certain keywords, terms, and categories and make advanced search syntax, like wildcards, boolean operators, and regular expressions. This integration allows you to take your analyses beyond reference management by developing a central location to collect references and thoughts, analyze literature, and connect empirical data.
- Statistical software, like Lumivero’s XLSTAT , SPSS, or STATA to export your queries from NVivo to run statistical analysis
- Qualtrics, SurveyMonkey to import your survey results into NVivo to start analyzing.
Make Choosing QDA Software Easy — Try NVivo Today!
It's tough choosing QDA software! Test out NVivo, the most cited qualitative data analysis tool, by requesting a free 14-day trial of NVivo to start improving your qualitative and mixed methods research today.
Recent Articles
- Python For Data Analysis
- Data Science
- Data Analysis with R
- Data Analysis with Python
- Data Visualization with Python
- Data Analysis Examples
- Math for Data Analysis
- Data Analysis Interview questions
- Artificial Intelligence
- Data Analysis Projects
- Machine Learning
- Deep Learning
- Computer Vision
- What is Data Analytics?
- What is Statistical Analysis in Data Science?
- What Is Spatial Analysis, and How Does It Work
- What is Data Analysis?
- What is Data Munging in Analysis?
- What is Geospatial Data Analysis?
- What is Exploratory Data Analysis ?
- Qualitative and Quantitative Data
- What are Descriptive Analytics?
- What is Prescriptive Analytics in Data Science?
- Qualitative Data
- Data Analysis Tutorial
- Why Data Visualization Matters in Data Analytics?
- What is Data Lineage?
- Data analysis using R
- What is Data Organization?
- What is a Data Science Platform?
What is Qualitative Data Analysis?
Understanding qualitative information analysis is important for researchers searching for to uncover nuanced insights from non-numerical statistics. By exploring qualitative statistics evaluation, you can still draw close its importance in studies, understand its methodologies, and determine while and the way to apply it successfully to extract meaningful insights from qualitative records.
The article goals to provide a complete manual to expertise qualitative records evaluation, masking its significance, methodologies, steps, advantages, disadvantages, and applications.
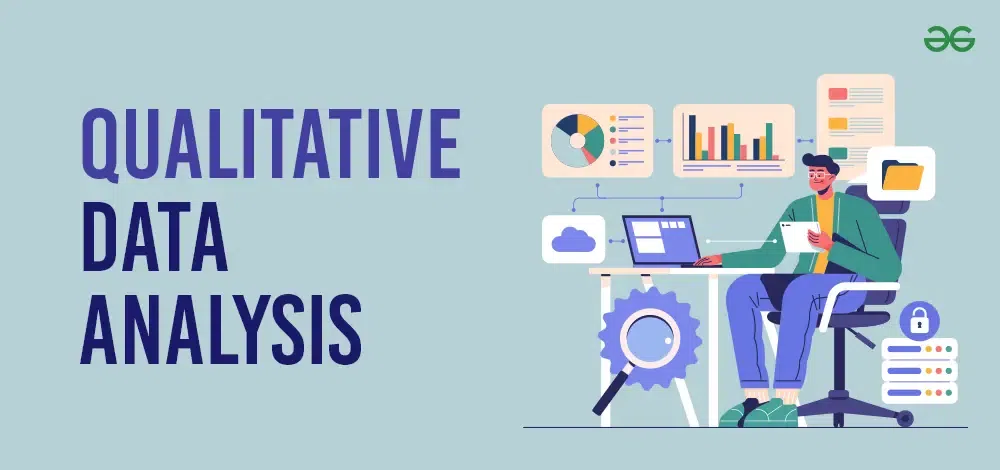
Table of Content
Understanding Qualitative Data Analysis
Importance of qualitative data analysis, steps to perform qualitative data analysis, 1. craft clear research questions, 2. gather rich customer insights, 3. organize and categorize data, 4. uncover themes and patterns : coding, 5. make hypotheses and validating, methodologies in qualitative data analysis, advantages of qualitative data analysis, disadvantages of qualitative data analysis, when qualitative data analysis is used, applications of qualitative data analysis.
Qualitative data analysis is the process of systematically examining and deciphering qualitative facts (such as textual content, pix, motion pictures, or observations) to discover patterns, themes, and meanings inside the statistics· Unlike quantitative statistics evaluation, which focuses on numerical measurements and statistical strategies, qualitative statistics analysis emphasizes know-how the context, nuances, and subjective views embedded inside the information.
Qualitative facts evaluation is crucial because it is going past the bloodless hard information and numbers to provide a richer expertise of why and the way things appear. Qualitative statistics analysis is important for numerous motives:
- Understanding Complexity and unveils the “Why” : Quantitative facts tells you “what” came about (e· g·, sales figures), however qualitative evaluation sheds light on the motives in the back of it (e·g·, consumer comments on product features).
- Contextual Insight : Numbers don’t exist in a vacuum. Qualitative information affords context to quantitative findings, making the bigger photo clearer· Imagine high customer churn – interviews would possibly monitor lacking functionalities or perplexing interfaces.
- Uncovers Emotions and Opinions: Qualitative records faucets into the human element· Surveys with open ended questions or awareness companies can display emotions, critiques, and motivations that can’t be captured by using numbers on my own.
- Informs Better Decisions: By understanding the “why” and the “how” at the back of customer behavior or employee sentiment, companies can make greater knowledgeable decisions about product improvement, advertising techniques, and internal techniques.
- Generates New Ideas : Qualitative analysis can spark clean thoughts and hypotheses· For example, via analyzing consumer interviews, commonplace subject matters may emerge that cause totally new product features.
- Complements Quantitative Data : While each facts sorts are precious, they paintings quality collectively· Imagine combining website site visitors records (quantitative) with person comments (qualitative) to apprehend user revel in on a particular webpage.
In essence, qualitative data evaluation bridges the gap among the what and the why, providing a nuanced know-how that empowers better choice making·
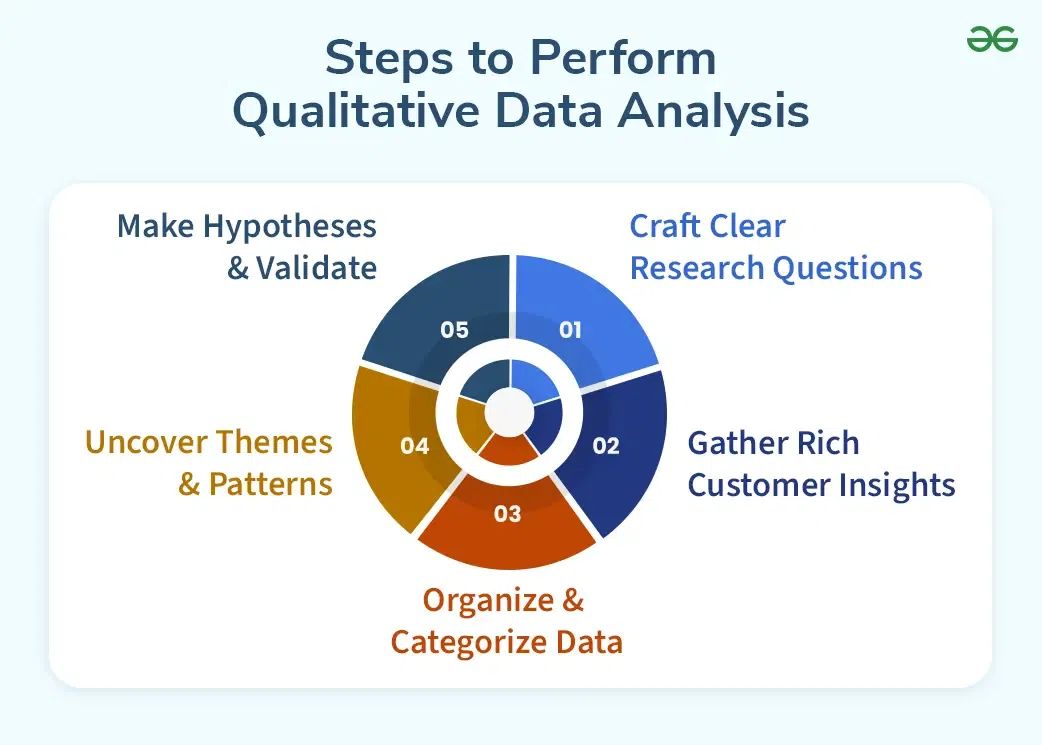
Qualitative data analysis process, follow the structure in below steps:
Qualitative information evaluation procedure, comply with the shape in underneath steps:
Before diving into evaluation, it is critical to outline clear and particular studies questions. These questions ought to articulate what you want to study from the data and manual your analysis towards actionable insights. For instance, asking “How do employees understand the organizational culture inside our agency?” helps makes a speciality of know-how personnel’ perceptions of the organizational subculture inside a selected business enterprise. By exploring employees’ perspectives, attitudes, and stories related to organizational tradition, researchers can find valuable insights into workplace dynamics, communication patterns, management patterns, and worker delight degrees.
There are numerous methods to acquire qualitative information, each offering specific insights into client perceptions and reviews.
- User Feedback: In-app surveys, app rankings, and social media feedback provide direct remarks from users approximately their studies with the products or services.
- In-Depth Interviews : One-on-one interviews allow for deeper exploration of particular topics and offer wealthy, special insights into individuals’ views and behaviors.
- Focus Groups : Facilitating group discussions allows the exploration of numerous viewpoints and permits individuals to construct upon every different’s ideas.
- Review Sites : Analyzing purchaser critiques on systems like Amazon, Yelp, or app shops can monitor not unusual pain points, pride levels, and areas for improvement.
- NPS Follow-Up Questions : Following up on Net Promoter Score (NPS) surveys with open-ended questions allows customers to elaborate on their rankings and provides qualitative context to quantitative ratings.
Efficient facts below is crucial for powerful analysis and interpretation.
- Centralize: Gather all qualitative statistics, along with recordings, notes, and transcripts, right into a valuable repository for smooth get admission to and control.
- Categorize through Research Question : Group facts primarily based at the specific studies questions they deal with. This organizational structure allows maintain consciousness in the course of analysis and guarantees that insights are aligned with the research objectives.
Coding is a scientific manner of assigning labels or categories to segments of qualitative statistics to uncover underlying issues and patterns.
- Theme Identification : Themes are overarching principles or ideas that emerge from the records· During coding, researchers perceive and label segments of statistics that relate to those themes, bearing in mind the identification of vital principles in the dataset.
- Pattern Detection : Patterns seek advice from relationships or connections between exceptional elements in the statistics. By reading coded segments, researchers can locate trends, repetitions, or cause-and-effect relationships, imparting deeper insights into patron perceptions and behaviors.
Based on the identified topics and styles, researchers can formulate hypotheses and draw conclusions about patron experiences and choices.
- Hypothesis Formulation: Hypotheses are tentative causes or predictions based on found styles within the information. Researchers formulate hypotheses to provide an explanation for why certain themes or styles emerge and make predictions approximately their effect on patron behavior.
- Validation : Researchers validate hypotheses by means of segmenting the facts based on one-of-a-kind standards (e.g., demographic elements, usage patterns) and analyzing variations or relationships inside the records. This procedure enables enhance the validity of findings and offers proof to assist conclusions drawn from qualitative evaluation.
There are five common methodologies utilized in Qualitative Data Analysis·
- Thematic Analysis : Thematic Analysis involves systematically figuring out and reading habitual subject matters or styles within qualitative statistics. Researchers begin with the aid of coding the facts, breaking it down into significant segments, and then categorizing these segments based on shared traits. Through iterative analysis, themes are advanced and refined, permitting researchers to benefit insight into the underlying phenomena being studied.
- Content Analysis: Content Analysis focuses on reading textual information to pick out and quantify particular styles or issues. Researchers code the statistics primarily based on predefined classes or subject matters, taking into consideration systematic agency and interpretation of the content. By analyzing how frequently positive themes occur and the way they’re represented inside the data, researchers can draw conclusions and insights relevant to their research objectives.
- Narrative Analysis: Narrative Analysis delves into the narrative or story within qualitative statistics, that specialize in its structure, content, and meaning. Researchers examine the narrative to understand its context and attitude, exploring how individuals assemble and speak their reports thru storytelling. By analyzing the nuances and intricacies of the narrative, researchers can find underlying issues and advantage a deeper know-how of the phenomena being studied.
- Grounded Theory : Grounded Theory is an iterative technique to growing and checking out theoretical frameworks primarily based on empirical facts. Researchers gather, code, and examine information without preconceived hypotheses, permitting theories to emerge from the information itself. Through constant assessment and theoretical sampling, researchers validate and refine theories, main to a deeper knowledge of the phenomenon under investigation.
- Phenomenological Analysis : Phenomenological Analysis objectives to discover and recognize the lived stories and views of people. Researchers analyze and interpret the meanings, essences, and systems of these reviews, figuring out not unusual topics and styles across individual debts. By immersing themselves in members’ subjective stories, researchers advantage perception into the underlying phenomena from the individuals’ perspectives, enriching our expertise of human behavior and phenomena.
- Richness and Depth: Qualitative records evaluation lets in researchers to discover complex phenomena intensive, shooting the richness and complexity of human stories, behaviors, and social processes.
- Flexibility : Qualitative techniques offer flexibility in statistics collection and evaluation, allowing researchers to conform their method based on emergent topics and evolving studies questions.
- Contextual Understanding: Qualitative evaluation presents perception into the context and meaning of information, helping researchers recognize the social, cultural, and historic elements that form human conduct and interactions.
- Subjective Perspectives : Qualitative methods allow researchers to explore subjective perspectives, beliefs, and reviews, offering a nuanced know-how of people’ mind, emotions, and motivations.
- Theory Generation : Qualitative information analysis can cause the generation of recent theories or hypotheses, as researchers uncover patterns, themes, and relationships in the records that might not were formerly recognized.
- Subjectivity: Qualitative records evaluation is inherently subjective, as interpretations can be stimulated with the aid of researchers’ biases, views, and preconceptions .
- Time-Intensive : Qualitative records analysis may be time-consuming, requiring giant data collection, transcription, coding, and interpretation.
- Generalizability: Findings from qualitative studies might not be effortlessly generalizable to larger populations, as the focus is often on know-how unique contexts and reviews in preference to making statistical inferences.
- Validity and Reliability : Ensuring the validity and reliability of qualitative findings may be difficult, as there are fewer standardized methods for assessing and establishing rigor in comparison to quantitative studies.
- Data Management : Managing and organizing qualitative information, together with transcripts, subject notes, and multimedia recordings, can be complicated and require careful documentation and garage.
- Exploratory Research: Qualitative records evaluation is nicely-suited for exploratory studies, wherein the aim is to generate hypotheses, theories, or insights into complex phenomena.
- Understanding Context : Qualitative techniques are precious for knowledge the context and which means of statistics, in particular in studies wherein social, cultural, or ancient factors are vital.
- Subjective Experiences : Qualitative evaluation is good for exploring subjective stories, beliefs, and views, providing a deeper knowledge of people’ mind, feelings, and behaviors.
- Complex Phenomena: Qualitative strategies are effective for studying complex phenomena that can not be effortlessly quantified or measured, allowing researchers to seize the richness and depth of human stories and interactions.
- Complementary to Quantitative Data: Qualitative information analysis can complement quantitative research by means of offering context, intensity, and insight into the meanings at the back of numerical statistics, enriching our knowledge of studies findings.
- Social Sciences: Qualitative information analysis is widely utilized in social sciences to apprehend human conduct, attitudes, and perceptions. Researchers employ qualitative methods to delve into the complexities of social interactions, cultural dynamics, and societal norms. By analyzing qualitative records which include interviews, observations, and textual resources, social scientists benefit insights into the elaborate nuances of human relationships, identity formation, and societal structures.
- Psychology : In psychology, qualitative data evaluation is instrumental in exploring and deciphering person reports, emotions, and motivations. Qualitative methods along with in-depth interviews, cognizance businesses, and narrative evaluation allow psychologists to delve deep into the subjective stories of individuals. This approach facilitates discover underlying meanings, beliefs, and emotions, dropping light on psychological processes, coping mechanisms, and personal narratives.
- Anthropology : Anthropologists use qualitative records evaluation to look at cultural practices, ideals, and social interactions inside various groups and societies. Through ethnographic research strategies such as player statement and interviews, anthropologists immerse themselves within the cultural contexts of different agencies. Qualitative analysis permits them to find the symbolic meanings, rituals, and social systems that form cultural identification and behavior.
- Qualitative Market Research : In the sphere of marketplace research, qualitative statistics analysis is vital for exploring consumer options, perceptions, and behaviors. Qualitative techniques which include consciousness groups, in-depth interviews, and ethnographic research permit marketplace researchers to gain a deeper understanding of customer motivations, choice-making methods, and logo perceptions· By analyzing qualitative facts, entrepreneurs can identify emerging developments, discover unmet wishes, and tell product development and advertising and marketing techniques.
- Healthcare: Qualitative statistics analysis plays a important function in healthcare studies via investigating patient experiences, delight, and healthcare practices. Researchers use qualitative techniques which includes interviews, observations, and patient narratives to explore the subjective reviews of people inside healthcare settings. Qualitative evaluation helps find affected person perspectives on healthcare services, treatment consequences, and pleasant of care, facilitating enhancements in patient-targeted care delivery and healthcare policy.
Qualitative data evaluation offers intensity, context, and know-how to investigate endeavors, enabling researchers to find wealthy insights and discover complicated phenomena via systematic examination of non-numerical information.
Please Login to comment...
Similar reads.
- Data Analysis
Improve your Coding Skills with Practice
What kind of Experience do you want to share?
University of Minnesota Twin Cities
University digital conservancy, teacher perceptions of the influence of principal instructional leadership on school culture: a case study of the american embassy school in new delhi, india., view/download file, persistent link to this item, journal title, journal issn, volume title, published date, description, collections, series/report number, funding information, isbn identifier, doi identifier, previously published citation, suggested citation.
Content distributed via the University Digital Conservancy may be subject to additional license and use restrictions applied by the depositor. By using these files, users agree to the Terms of Use . Materials in the UDC may contain content that is disturbing and/or harmful. For more information, please see our statement on harmful content in digital repositories .
Thank you for visiting nature.com. You are using a browser version with limited support for CSS. To obtain the best experience, we recommend you use a more up to date browser (or turn off compatibility mode in Internet Explorer). In the meantime, to ensure continued support, we are displaying the site without styles and JavaScript.
- View all journals
- My Account Login
- Explore content
- About the journal
- Publish with us
- Sign up for alerts
- Systematic Review
- Open access
- Published: 17 May 2024
Risk factors and incidence of central venous access device-related thrombosis in hospitalized children: a systematic review and meta-analysis
- Maoling Fu 1 , 2 ,
- Quan Yuan 2 ,
- Qiaoyue Yang 1 , 2 ,
- Yaqi Yu 1 , 2 ,
- Wenshuai Song 1 , 2 ,
- Xiuli Qin 1 ,
- Ying Luo 1 ,
- Xiaoju Xiong 1 &
- Genzhen Yu 1
Pediatric Research ( 2024 ) Cite this article
66 Accesses
Metrics details
The risk factors for central venous access device-related thrombosis (CRT) in children are not fully understood. We used evidence-based medicine to find the risk factors for CRT by pooling current studies reporting risk factors of CRT, aiming to guide clinical diagnosis and treatment.
A systematic search of PubMed, Web of Science, Embase, Cochrane Library, Scopus, CNKI, Sinomed, and Wanfang databases was conducted. RevMan 5.4 was employed for data analysis.
The review included 47 studies evaluating 262,587 children with CVAD placement. Qualitative synthesis and quantitative meta-analysis identified D-dimer, location of insertion, type of catheter, number of lumens, catheter indwelling time, and central line-associated bloodstream infection as the most critical risk factors for CRT. Primarily due to observational design, the quality of evidence was regarded as low certainty for these risk factors according to the GRADE approach.
Because fewer high-quality studies are available, larger sample sizes and well-designed prospective studies are still needed to clarify the risk factors affecting CRT. In the future, developing pediatric-specific CRT risk assessment tools is important. Appropriate stratified preventive strategies for CRT according to risk assessment level will help improve clinical efficiency, avoid the occurrence of CRT, and alleviate unnecessary suffering of children.
This is the latest systematic review of risk factors and incidence of CRT in children.
A total of 47 studies involving 262,587 patients were included in our meta-analysis, according to which the pooled prevalence of CRT was 9.1%.
This study identified several of the most critical risk factors affecting CRT in children, including D-dimer, insertion location, type of catheter, number of lumens, catheter indwelling time, and central line-associated bloodstream infection (CLABSI).
Similar content being viewed by others
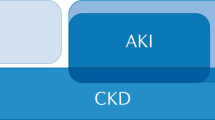
Acute kidney injury
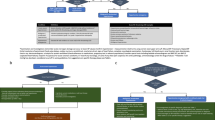
Management of hypertensive crisis: British and Irish Hypertension Society Position document
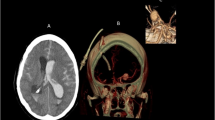
Diagnosis and management of subarachnoid haemorrhage
Introduction.
Central venous access device (CVAD) is an infusion device inserted through different parts to make the tip of the catheter to the vena cava. In the clinic, CVAD is mainly divided into the following four categories: tunneled central venous catheter (CVC), nontunneled CVC, peripherally inserted central catheter (PICC), and totally implantable venous access port (TIVAP). 1 Pediatric patients often require stable, multifunctional, and comfortable long-term vascular access due to factors such as poor puncture cooperation, small vessel diameter, poor peripheral venous visibility and tolerance, high water content in the body leading to easy dehydration, and easy changes in condition after diseases. 2 The application of CVAD can significantly reduce the frequency of venipuncture, relieve the stimulation of drugs on the venous blood vessels, alleviate the pain and fear of the children, improve their medication compliance, ensure the effectiveness of intravenous infusion, and improve the quality of disease treatment. 3 , 4 , 5 Therefore, CVAD is widely used in pediatric clinics and has become an indispensable aspect of complex medical care for children with severe and chronic diseases.
Although CVAD has become an important tool in the pediatric treatment and nursing process, there are also risks of complications related to it, including CVAD-related thrombosis (CRT), phlebitis, fluid and blood leakage at the puncture point, catheter displacement, catheter obstruction, central line-associated bloodstream infection (CLABSI) and so on. 6 , 7 Among these, CRT is one of the most common and serious complications. The prevalence of CRT in children varies significantly by country, age, disease, and medical institution, ranging from 2 to 81%, 4 , 8 , 9 , 10 while in Chinese children without prophylactic treatment ranges from 20 to 66%. 11 , 12 CRT has no obvious clinical symptoms in the early stage, but it may still cause serious side effects, not only increasing the patient pain and medical costs but also delaying treatment timing, affecting prognosis and quality of life, and in severe cases, may even lead to thromboembolism, endangering life. 13 , 14 , 15
Identifying risk factors and incidence of CRT facilitates clinical practitioners in the early identification of high-risk patients, designing specific preventive strategies, treatment regimens, and management plans, thereby effectively reducing the incidence of CRT in hospitalized children and alleviating unnecessary patient suffering. However, most current research on CRT involves only small-scale groups in isolated nursing units or specific disease types. To date, no up-to-date systematic review provides pooled estimates of the risk factors and prevalence of CRT in children. Therefore, this study had a dual purpose: 1. to explore potential risk factors for CRT in children and to determine a pooled level of CRT prevalence; and 2. to provide evidence-based recommendations to improve the recognition, control, and treatment of CRT in children, as well as better nursing management for CRT.
This review was conducted following the Preferred Reporting Items for Systematic Reviews and Meta-Analyses (PRISMA) guidelines. 16 The detailed research protocol can be accessed on the PROSPERO website (registration number: CRD42023421353).
Search strategy
Eight electronic databases were utilized to conduct a thorough literature search: PubMed, Web of Science, Embase, Cochrane Library, Scopus, China National Knowledge Infrastructure (CNKI), Sinomed, and Wanfang. The search in these databases was conducted from the earliest records available up to January 31st, 2024. The search strategy used a combination of Mesh terms and free words. The following Mesh terms and free words were mainly used: “child,” “children,” “adolescent,” “infant,” “pediatrics,” “central venous access device-related thrombosis,” “CRT,” “catheter-related thrombosis,” “catheter-related venous thrombosis,” “CVC-related thrombosis,” “risk factors,” “protective factors,” “predictors,” “causality,” “influencing factors”. The full search strategy for each database is available in the Supplementary Materials. In addition, we screened the reference lists of all included studies for relevant studies that met the criteria. Grey literature was searched as well. Some authors were contacted through email to gather more information or clarify any uncertainties.
Inclusion criteria
The study population was hospitalized children aged ≤18 years.
The primary research objective was to explore the risk factors for CRT.
The study results have at least one statistically significant predictor.
Case-control studies or cohort studies.
Published in English or Chinese.
Exclusion criteria
Catheter-related infection, catheter dysfunction, or other catheter complications as the primary outcome indicators.
Repeated published research.
Case reports, study designs, or clinical trials.
Reviews, editorials, letters, and conference abstracts.
In vitro or animal research.
Data were incomplete and could not be extracted.
Unable to find the original article.
Data extraction
Data from each eligible study were independently extracted by two reviewers using a pre-designed data collection form. Any disagreements were resolved by discussions among all authors. Data on the following characteristics were obtained from all included studies (see Supplementary Table S 1 for details):
Basic information: first author, country, year of publication, study duration, and study design.
Demographic characteristics: study population, sample size, number of CRT, and CRT rate.
Catheter-related features: catheter type, CRT type, and diagnostic method.
Potential risk factors for CRT: odds ratios (OR) or relative risks (RR) values and 95% confidence interval (CI) were extracted for each risk factor. If the study did not provide specific values, it was calculated by constructing a 2 × 2 contingency table.
Quality assessment
Two reviewers evaluated the quality of each study independently using the Risk of Bias Assessment for Nonrandomized Studies tool, 17 with any differences settled via group discussion. The tool assessed six domains of risk of bias: participant selection, confounding variables, exposure measurement, blinding of outcome assessment, incomplete outcome data, and selective outcome reporting. If all six domains were rated as low risk, the overall risk of bias for the study was low. The overall risk of bias was moderate if at least one domain was rated as unclear risk, and no domain was rated as high risk, and high if one or more domains were rated as high risk.
To ensure the accuracy of the assessment results, a third reviewer randomly selected five studies to check the data extraction and quality assessment.
Qualitative synthesis and quantitative meta-analysis
Qualitatively classify each risk factor as definite, likely, unclear, or not a risk factor based on the total number of studies with low and moderate bias risks and the proportion of studies demonstrating positive association (Box 1 in the supplementary material). If a risk factor was reported by more than two studies with low or moderate risk of bias, and the definition and reference range were sufficiently consistent, a quantitative meta-analysis was performed to estimate the combined OR.
Data were analyzed using Revman 5.4 software. In the meta-analysis of risk factors and CRT rate, the generic inverse variance method was applied, which only required effect estimate and standard error (SE). 18 The SE was obtained by inverse transforming the 95% CI applying the standard normal distribution. Heterogeneity tests were performed on the studies included in the Meta-analysis to examine for the combinability of the results of each independent study. P ≥ 0.05 and I-squared ( I 2 ) < 50% considered less heterogeneity between studies and therefore a fixed-effects model was chosen for the analysis, conversely, P < 0.05 or I 2 ≥ 50% considered greater heterogeneity, and a random-effects model was chosen.
Certainty of the evidence
The Grading of Recommendations Assessment, Development, and Evaluation (GRADE) method was used to assess the certainty of the evidence. In this method, observational studies were initially classified as low-quality evidence and then downgraded and upgraded according to five downgrading and three upgrading principles. The 5 downgrading factors included risk of bias, inconsistency, indirectness, imprecision, and publication bias, and the 3 upgrading factors included the magnitude of an effect, dose-response gradient, and effect of plausible residual confounding. Based on these considerations, the overall certainty of each piece of evidence was rated as one of four levels: high, moderate, low, or very low.
The initial search of the databases extracted a total of 4193 articles, of which 1656 were duplicates and removed. The titles and abstracts of the remaining 2537 articles were screened according to the inclusion criteria and 142 were selected for full-text search. After a rigorous eligibility review, 45 articles met the inclusion criteria. In addition, two articles were found to meet the eligibility criteria in a search of the reference lists of the selected articles and grey literature. In the end, a total of 47 articles were included in this review, of which 43 contributed to the qualitative synthesis and quantitative meta-analysis (Fig. 1 ).
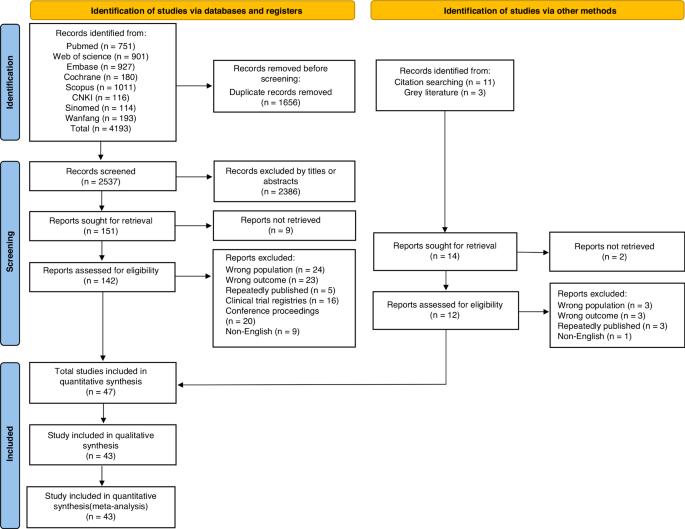
Demonstrate the screening and inclusion process for systematic literature search.
Of the 47 studies, 19 were prospective 4 , 13 , 19 , 20 , 21 , 22 , 23 , 24 , 25 , 26 , 27 , 28 , 29 , 30 , 31 , 32 , 33 , 34 , 35 and the rest were retrospective, 9 , 12 , 36 , 37 , 38 , 39 , 40 , 41 , 42 , 43 , 44 , 45 , 46 , 47 , 48 , 49 , 50 , 51 , 52 , 53 , 54 , 55 , 56 , 57 , 58 , 59 , 60 , 61 of which 10 were multicenter 4 , 9 , 13 , 21 , 23 , 26 , 27 , 28 , 49 , 59 and 37 were single-center. 12 , 19 , 20 , 22 , 24 , 25 , 29 , 30 , 31 , 32 , 33 , 34 , 35 , 36 , 37 , 38 , 39 , 40 , 41 , 42 , 43 , 44 , 45 , 46 , 47 , 48 , 50 , 51 , 52 , 53 , 54 , 55 , 56 , 57 , 58 , 60 , 61 The sample sizes ranged from 47 to 158,299, with the two largest being 71,782 13 and 158,299, 59 respectively. In addition, three studies constructed clinical prediction models. 22 , 28 , 47 Table 1 lists the summary characteristics of the included studies.
Study populations and CRT rates in included studies
These studies investigated a series of hospitalized children of different ages and departments, of which 12 studies with all hospitalized children as the study population, 12 studies with PICU hospitalized children as the study population, six studies with NICU hospitalized children as the study population, one study with all ICU hospitalized children as the study population, four studies with leukemia children as the study population, two studies with infants under 1-year-old as the study population, and the other ten studies with children with a specific disease as the study population.
The combined CRT rate was 9.1% (95% CI : 5.7–14.5%) with a high degree of heterogeneity ( I 2 = 100%). The combined CRT rate was 11.5% (95% CI : 5.7–23.1%; I 2 = 99%) in both male and female children. The frequency of CRT in PICU and NICU was available from 13 articles with 234,464 children and 7 articles with 6093 infants, which combined CRT rates were 10.7% (95% CI : 3.8–23.7%; I 2 = 100%), 2.9% (95% CI : 1.0–6.5%; I 2 = 96%), respectively. The combined CRT rate of children with leukemia was 13.0% (95% CI : 2.9–38.3%; I 2 = 98%) (Supplementary Material Figs. S 1 – 6 )
Quality of the CRT studies
The methodological quality of the included studies varied (Fig. 2 and Supplementary Material Fig. S 7 ). Nine studies had a low overall risk of bias, as all six domains were categorized as low risk. Four studies had a high overall risk of bias, three of which were associated with confounding variables and one to participant selection. The remaining 34 studies had a moderate overall risk of bias, with at least one of the six domains having an unclear risk.
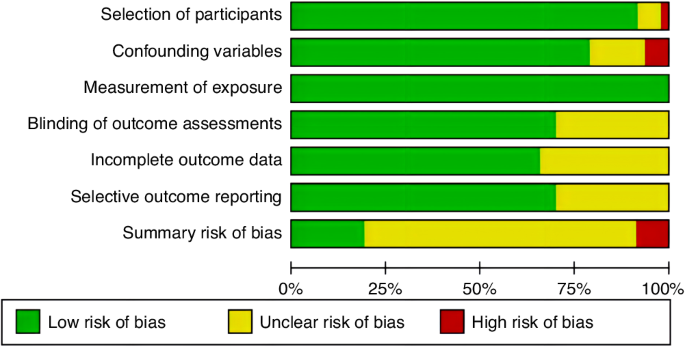
A summary presentation of the assessment results of risk of bias for the 47 studies.
Risk factors of CRT in included studies
The 47 included studies reported 61 statistically significant risk factors for CRT (Table 1 ). These factors were classified into three categories: patient-related risk factors (37.7%, 23/61); CVAD-related risk factors (34.4%, 21/61), and treatment-related risk factors (27.9%, 17/61).
Based on the qualitative synthesis, six variables were considered to be definite risk factors for CRT, including D-dimer, location of insertion, type of catheter, number of lumens, catheter indwelling time, and CLABSI. Eleven variables were considered likely associated with CRT, including gastrointestinal diseases, history of catheterization, thrombophilia, geographic location of line placement, catheter dysfunction, number of catheters, insertion length (cm), catheter to vein ratio, dialysis, hypertonic liquid, and cardiac catheterization. For 42 variables, the relationship with CRT was deemed unclear due to conflicting results from studies assessed as having low and moderate risk of bias, or because they were positively associated in only one study. Additionally, birth weight and gestational age were considered non-risk factors (Table 2 ).
Meta-analyses were implemented for risk factors that were reported by at least two low or moderate risk of bias studies with a consistent definition and reference range (Table 3 and Figs. 3 – 6 ).
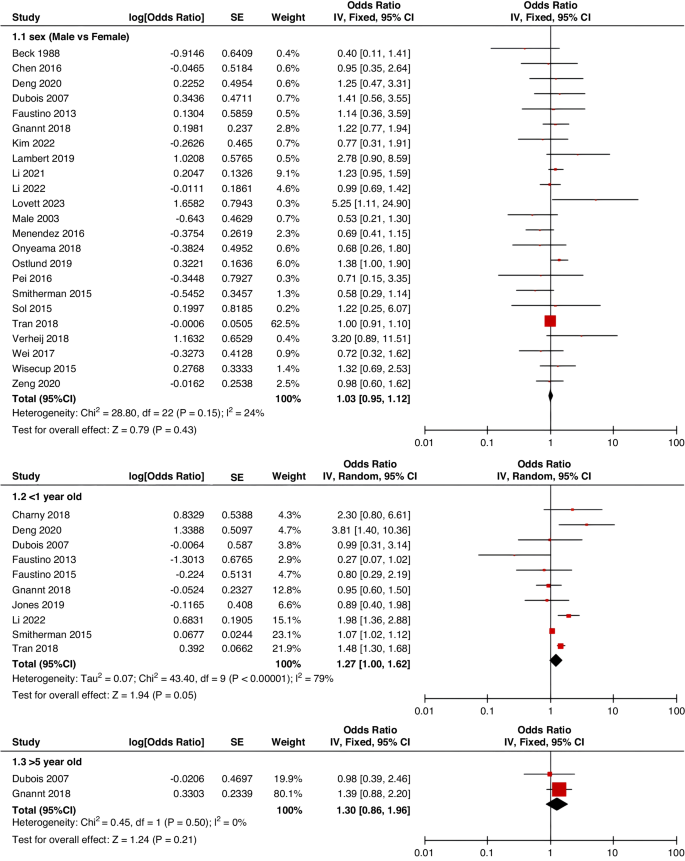
Forest plots of odds ratios (OR) that were included in the quantitative meta-analysis and the associated overall OR. For each OR, the size of the red square region is proportional to the corresponding study weight. Diamond shape intervals represent the overall OR. I 2 represents the fraction of variability among the individual OR that cannot be explained by sampling variability.
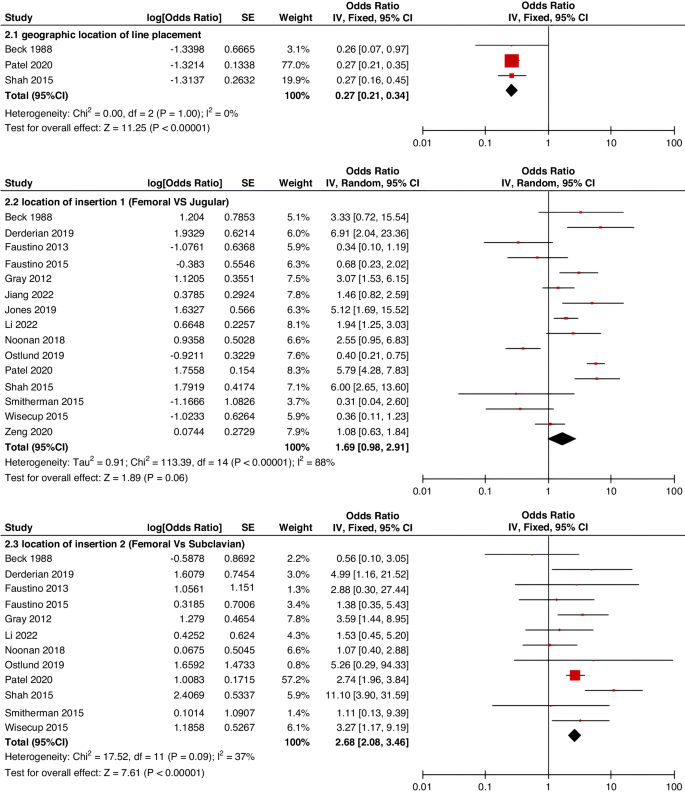
Forest plots of odds ratios (OR) that were included in the quantitative meta-analysis and the associated overall OR. For each OR, the size of the red square region is proportional to the corresponding study weight. Diamond shape intervals represent the overall OR. I 2 represents the fraction of variability among the individual OR that cannot be explained by sampling variability.
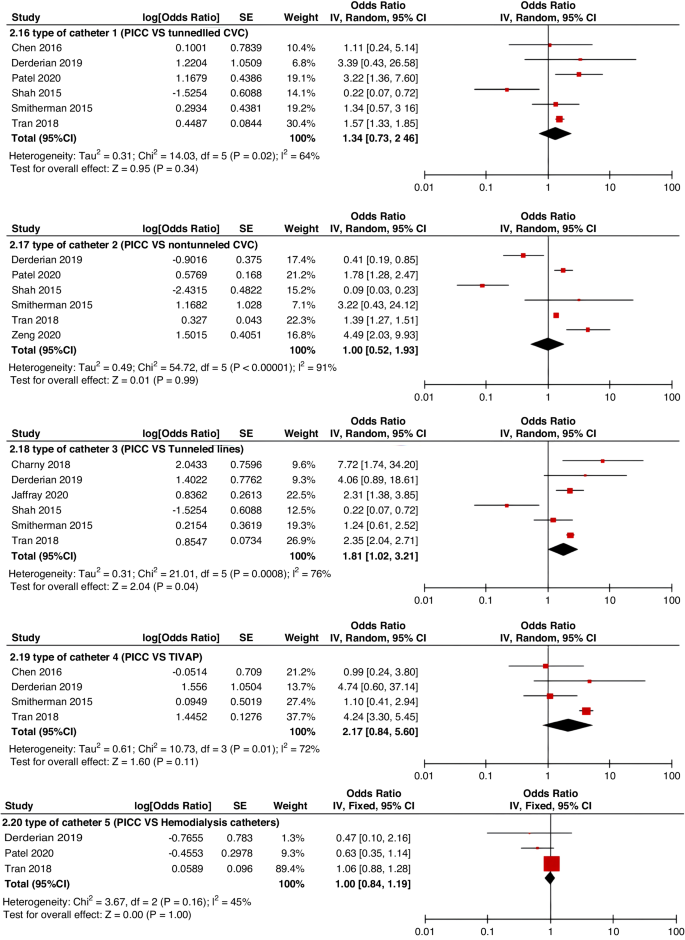
GRADE assessment of evidence
Supplementary Table S 2 shows GRADE assessments for the certainty of evidence. Due to the design of the observational studies, all evidence was initially rated as low certainty. Based on five downgrading and three upgrading principles, 17 pieces of evidence were still rated as low certainty, and the remaining 44 pieces of evidence were downgraded to very low certainty for serious inconsistency and imprecision.
Our study is the latest systematic review of risk factors and the incidence of CRT in hospitalized children. Based on 47 studies included in the current meta-analysis, which involved a total of 262,587 patients, the pooled prevalence of CRT is 9.1%. We conducted a qualitative synthesis analysis of 61 predictive factors and a quantitative meta-analysis of 38 factors, identifying six definite factors, 11 likely factors, and 42 unclear factors associated with CRT. Definite predictors included being of D-dimer, location of insertion, type of catheter, number of lumens, catheter indwelling time and CLABSI. The findings of our systematic review provide the latest comprehensive evidence summary that can inform the early identification of children at risk for CRT and the development of intervention measures to prevent and reduce CRT.
Implantable and temporary medical devices such as CVAD are exposed to blood for weeks to years depending on the type of CVAD in place. Since CVAD is an artificial surface and lacks an endothelial layer that inhibits platelet coagulation and adhesion, it is thought to potentially activate the contact pathways, ultimately leading to thrombosis. Assembly of artificial surface contact systems might be part of the host defense mechanism against foreign substances, but it can lead to kinin and thrombin generation, and complement activation. 62 This eventually promotes thrombosis and inflammation. The presence of CVAD is the most common risk factor for venous thromboembolism (VTE). CRT accounts for 10% of deep vein thrombosis (DVT) in adults and 50–80% in children. 10 , 55 , 63 The incidence of CRT in hospitalized children has increased significantly by 30–70% over the past 20 years, 64 , 65 which may cause serious medical complications besides increasing healthcare expenditures and length of stay.
We discover that a higher level of D-dimer is an independent risk factor for CRT in hospitalized children, consistent with the results of adult studies. 66 D-dimer is a soluble fibrin degradation product deriving from the plasmin-mediated degradation of cross-linked fibrin that is increased or positive in secondary hyperfibrinolysis, such as hypercoagulable states, disseminated intravascular coagulation, and thrombolytic therapy. 67 , 68 Increased D-dimer suggests an association with thrombotic disorders in the body of various origins and an increase in fibrinolytic activity. D-dimer has been extensively investigated for excluding the diagnosis of VTE and is used routinely for this indication. 67 , 69 Therefore, for early recognition and to reduce the incidence of CRT, D-dimer levels should be closely monitored before and after catheterization. However, the elevated D-dimer test results cannot fully explain the cause and location of CRT formation and must be analyzed in conjunction with clinical and other test results. Inherited thrombophilia, caused by genetic defects leading to a deficiency or abnormality in associated proteins, including protein C, protein S, antithrombin, the coagulation factor V Leiden mutation, and factor II mutation G20210A, 70 is considered a potential risk factor for CRT. The prevalence of thrombophilia varies widely among different populations, with a reported prevalence of 10% to 59% in pediatric VTE patients. 71 Children with gastrointestinal diseases like short bowel syndrome (SBS) and inflammatory bowel disease (IBD) have an increased risk of developing CRT during hospitalization. The precise mechanism behind this association is still uncertain according to current research. It may be attributed to the heightened inflammation levels during catheterization, particularly in patients with active IBD episodes or admissions during surgery, which leads to a period of increased inactivity. 55 This suggests that delaying placement during the most active period of inflammation may reduce the rate of thrombosis.
A narrative review pointed out that age is one of the most significant risk factors for VTE. In children, CRT shows a bimodal distribution, with the highest incidence rate in infancy and adolescence. 10 The higher incidence in infancy may be due in part to the smaller diameter of the vein, making insertion difficult and requiring multiple attempts. However, whether age is a risk factor for CRT is still highly controversial. The study by Chojnacka et al. did not find a statistically significant difference, 39 although a trend toward a similar bimodal distribution was found in the study population. Cancer, cardiovascular disease, sepsis, asphyxia, and neurological diseases are also considered unclear factors for CRT. Pediatric patients diagnosed with leukemia have multiple risk factors for VTE formation, such as the presence of hypercoagulable blast cells, the pro-thrombotic nature of the cancer itself, and treatment with steroids and L-asparaginase. Chen et al. 38 and Jaffray et al. 4 concluded that children with leukemia are more likely to develop CRT. Sepsis causes the coagulation mechanism to become fragile, which in turn activates the coagulation system and creates thrombosis. 72 However, a study by Onyeama et al. 52 showed that sepsis was significantly associated with a reduced incidence of CRT, and the exact mechanism is currently unknown.
The location of insertion and type of catheter are critical risk factors for CRT. The incidence of CRT is higher in femoral vein catheterizations compared to subclavian and jugular vein catheterizations in children, which is contrary to findings in adult patients. 73 The femoral location is a larger vessel and allows placement of a larger size catheter. Femoral CVAD is prioritized in urgent and emergency situations. In such cases, the patients tend to be more critically ill and often immobilized, further exacerbating the low-flow state. In addition, there may be vein compression and kinking beneath the inguinal ligament with leg movement, which may increase the risk of CRT. 27 PICC catheters provide a reliable medium to long-term route to intravenous therapy for children, but compared with other types of catheters, the risk of CRT is higher. We speculate that the long tunnel length and relatively large lumen size of the PICC, compared to the diameter of the vessel at the insertion site, may lead to increased blood flow obstruction. 52 Additionally, patients with PICC may be more likely to be diagnosed with symptomatic VTE than tunneled lines (TLs) because PICC is often placed in smaller vessels and journeys through the arm or leg causing limb pain and swelling, whereas TLs are located in the chest.
The risk of CRT increases with the number of lumens. A possible explanation for this finding is that multilumen catheters tend to have larger catheter sizes and thus occupy more area within the vessel lumen, leading to obstruction of normal blood flow within the veins. The relationship between CRT and CLABSI is bidirectional. Following catheter insertion, a fibrin sheath forms around the catheter. Microorganisms, especially staphylococcus aureus, easily adhere to the fibrin sheaths, and may lead to CLABSI. 74 Conversely, CLABSI can trigger inflammatory reactions, leading to further progression of thrombosis. CVAD duration is positively associated with the risk of CRT. Catheter placement may cause mechanical injury to the vein. As the indwelling duration increases, many damaged smooth muscle and endothelial cells become embedded within the fibrin, resulting in thrombus formation. In addition, prolonged indwelling increases the chance of platelet contact with the vessel lining, activating coagulation factors and thrombin, increasing the risk of thrombosis. 22 Therefore, nurses should perform routine maintenance of the catheter in children who require long-term CVAD indwelling. The duration of CVAD should be monitored, the necessity of its indwelling should be assessed daily, and the catheter should be removed as early as possible while ensuring treatment.
As obstruction of venous blood flow from the CVAD is considered an essential causative mechanism for the development of VTE, a high ratio between catheter size and vein diameter could be a risk factor for CRT. The 2012 international guidelines on pediatric CVC insertion recommend that the ratio between the catheter’s external diameter and the cannulated vein’s diameter should not exceed 0.33. 75 However, this suggestion is only based on expert opinions and currently lacks relevant clinical data support. Therefore, further research is still needed to verify it. Catheter dysfunction is mainly caused by small clots or fibrous sheaths wrapping around the tip of the catheter. Prolonged accumulation may lead to incomplete or complete blockage of blood vessels, becoming a gathering point for thrombosis. 74 Journeycake et al. observed that the risk of VTE was highest in pediatric cancer patients with multiple episodes of catheter dysfunction. 76 A study of pediatric brain tumor patients reported that VTE was more common in patients with catheter dysfunction. 77 Thus, these studies and the current data support the need to consider catheter dysfunction as a possible risk factor for CRT and to design further screening and intervention studies for early identification and prevention of catheter dysfunction.
The rationale for studying the relationship between the insertion side of CVAD and the risk of CRT is based on the anatomy of the upper body venous system. The left brachiocephalic vein is longer and courses more horizontally than the right side, thus entering the superior vena cava at a sharper angle. The right jugular vein is the most direct and shortest route for the CVAD to enter the heart. By contrast, the CVAD located in the left jugular vein has a greater distance to the heart and passes through 2 angles in the venous system, which may cause endothelial damage and increase the likelihood of blood flow obstruction and venous wall adhesion. 26 However, our meta-analysis did not find a statistically significant increase in the risk of CRT with left-sided placement compared to right-sided placement. The ideal location for the catheter tip is the junction of the superior vena cava and the right atrium. This location is preferred because of the higher blood flow rate, which may be protective against thrombosis. 43 Currently, the pediatric literature on the effect of optimal tip position on CRT is scarce and inconclusive. In addition, catheter tips do not always remain in that position after initial placement. Therefore, tip movement should be a significant concern in pediatric patients, especially active, growing, and requiring long-term catheter use.
Providing renal replacement therapy is a lifelong task for pediatric end-stage renal disease (ESRD) patients. Although successful transplantation can be achieved even in young patients, the lifespan of the graft is limited. Consequently, many transplant recipients may be put back on dialysis as part of their ESRD treatment. 78 CVC remains the main vascular access for hemodialysis in children. Long-term reliance on CVC is related to a high incidence of catheter dysfunction and failure. The frequent need for recurrent CVC placement in such patients leads to an elevated risk of central vein stenosis and CRT. Cardiac catheterization is also a possible risk factor for CRT. Appropriate anticoagulation is required during catheterization, without which the risk of thrombosis is up to 40%. However, the use of unfractionated heparin in pediatric patients is challenging because the coagulation system and heparin response are different from that of adults. 79 There’s a need for further research to determine if children are receiving adequate doses of heparin during cardiac catheterization to prevent thrombosis without increasing the risk of bleeding complications. The incidence of VTE in adult patients who are chronically bedridden and braked is 3.59 times higher than in patients with normal activity levels. 80 In critically ill or surgical children, mechanical ventilation is often performed in the early stages, requiring continuous use of multiple sedative or inotropic drugs to reduce cardiac load and protect pulmonary function. During sedation, the child is in a braked state, limb activity is reduced or even inactive, blood flow slows down, and blood stagnates in the veins, increasing the chance of platelet adhesion to the endothelium, which may increase the risk of CRT. Therefore, passive movements such as limb abduction, internal rotation, elbow flexion and elbow extension should be performed appropriately when the child’s condition permits.
Nutritional support is an important part of critical illness treatment, including enteral and parenteral nutrition (PN). CVAD is the supply channel for total parenteral nutrition (TPN), and some children may even need this method to provide calories for a long time. High glucose and calcium concentrations in PN are both possible triggers of CRT, and PN has been shown to upregulate the extrinsic coagulation cascade, especially with long-term use. 60 Diamanti et al. reported that the incidence rate of TPN complicated with CRT was 20%. 81 Mannitol or glycerol fructose are widely used as hypertonic drugs in clinical practice, which can increase plasma osmolality to dehydrate tissues after entering the body. At the same time, it may cause a cellular stress response, induce apoptosis, and can activate inflammatory cytokines and coagulation pathways to induce thrombosis. Jiang et al. 22 found vasoactive drugs to be a risk factor for CRT. The possible reason is that vasoactive drugs can cause strong vasoconstriction, endothelial function damage or impairment, and promote fibrinogen synthesis. However, this is contrary to the findings of Marquez et al. 28 and Faustino et al. 21 Therefore, larger prospective studies are still needed to assess this risk factor more precisely.
The strengths of this study include the systematic identification of all relevant studies of risk factors for CRT in hospitalized children and the classification of risk factors into three categories, patient-related risk factors, CVAD-related risk factors, and treatment-related risk factors, to offer a logical progression of the possible causes of CRT in children. However, several limitations of this systematic review should be stated. Firstly, as most of the studies originate from Western countries, extrapolating these results to Eastern populations is questionable. Second, significant heterogeneity was encountered in our analysis, potentially stemming from variations in regimen, duration, population enrolled, and center setting, among other factors. This diversity necessitates a cautious interpretation of the results. In addition, only a few high-quality studies with a low risk of bias, and many of the studies suffer from significant sources of bias. Furthermore, the effect in many occasions was assessed by very few studies. Therefore, the evidence to support it is low, which needs to be validated in future studies. Finally, risk factors for CRT could not be made causal assertions since the majority of studies were retrospective.
Conclusions
In conclusion, we have identified several critical factors that affect CRT, including D-dimer, location of insertion, type of catheter, number of lumens, catheter indwelling time, and CLABSI. Nevertheless, none of the included studies considered the impact of socio-demographic factors on CRT, such as parental education level, occupation, and family economic status. Therefore, larger sample sizes and well-designed prospective studies are still needed to clarify the predictors affecting CRT in the future. In addition, there is a lack of pediatric-specific CRT risk assessment tools, which need to be further developed and validated. Machine learning (ML), as a method for designing risk assessment models that help to efficiently explore and mine useful information, has been widely used in recent years to solve a variety of challenging medical problems. Likewise, the application of ML in CRT risk diagnosis may contribute to a more precise assessment. In clinical practice, it is necessary to take appropriate stratified preventive measures according to the level of CRT risk assessment of children, to improve the efficiency of clinical work, reduce the burden of clinical work, and minimize the occurrence of CRT under the premise of ensuring the safety of children.
Data availability
The datasets used and/or analyzed during the current study are available from the corresponding author upon reasonable request.
Yeow, M. et al. A systematic review and network meta-analysis of randomized controlled trials on choice of central venous access device for delivery of chemotherapy. J. Vasc. Surg. Venous Lymphat. Disord. 10 , 1184–91.e8 (2022).
Article PubMed Google Scholar
Cellini, M. et al. Guidelines of the Italian Association of Pediatric Hematology and Oncology for the management of the central venous access devices in pediatric patients with onco-hematological disease. J. Vasc. Access 23 , 3–17 (2022).
Ares, G. & Hunter, C. J. Central venous access in children: indications, devices, and risks. Curr. Opin. Pediatr. 29 , 340–346 (2017).
Jaffray, J. et al. Peripherally inserted central catheters lead to a high risk of venous thromboembolism in children. Blood 135 , 220–226 (2020).
Article CAS PubMed Google Scholar
Zhang, J. J. et al. Factors affecting mechanical complications of central venous access devices in children. Pediatr. Surg. Int. 38 , 1067–1073 (2022).
Article PubMed PubMed Central Google Scholar
Akhtar, N. & Lee, L. Utilization and Complications of Central Venous Access Devices in Oncology Patients. Curr. Oncol. 28 , 367–377 (2021).
Ullman, A. J., Marsh, N., Mihala, G., Cooke, M. & Rickard, C. M. Complications of Central Venous Access Devices: A Systematic Review. Pediatrics 136 , e1331–e1344 (2015).
Östlund, Å. et al. Erratum to ‘Incidence of and risk factors for venous thrombosis in children with percutaneous non-tunnelled central venous catheters’ (Br J Anaesth 2019; 123: 316-24). Br. J. Anaesth. 123 , 918 (2019).
McLaughlin, C. M. et al. Symptomatic catheter-associated thrombosis in pediatric trauma patients: Choose your access wisely. Surgery 166 , 1117–1121 (2019).
Citla Sridhar, D., Abou-Ismail, M. Y. & Ahuja, S. P. Central venous catheter-related thrombosis in children and adults. Thromb. Res. 187 , 103–112 (2020).
Zhou, X. et al. A retrospective analysis of risk factors associated with catheter-related thrombosis: a single-center study. Perfusion 35 , 806–813 (2020).
Li, S. et al. Risk factors for central venous catheter-related thrombosis in hospitalized children: a single-center a retrospective cohort study. Transl. Pediatr. 11 , 1840–1851 (2022).
Patel, N., Petersen, T. L., Simpson, P. M., Feng, M. & Hanson, S. J. Rates of Venous Thromboembolism and Central Line-Associated Bloodstream Infections Among Types of Central Venous Access Devices in Critically Ill Children. Crit. Care Med. 48 , 1340–1348 (2020).
Timsit, J. F. et al. A state of the art review on optimal practices to prevent, recognize, and manage complications associated with intravascular devices in the critically ill. Intensive Care Med. 44 , 742–759 (2018).
Ullman, A. J. et al. Pediatric central venous access devices: practice, performance, and costs. Pediatr. Res. 92 , 1381–1390 (2022).
Hutton, B. et al. The PRISMA extension statement for reporting of systematic reviews incorporating network meta-analyses of health care interventions: checklist and explanations. Ann. Intern Med. 162 , 777–784 (2015).
Kim, S. Y. et al. Testing a tool for assessing the risk of bias for nonrandomized studies showed moderate reliability and promising validity. J. Clin. Epidemiol. 66 , 408–414 (2013).
Borenstein, M., Hedges, L. V., Higgins, J. P. & Rothstein, H. R. A basic introduction to fixed-effect and random-effects models for meta-analysis. Res. Synth. Methods 1 , 97–111 (2010).
Beck, C., Dubois, J., Grignon, A., Lacroix, J. & David, M. Incidence and risk factors of catheter-related deep vein thrombosis in a pediatric intensive care unit: a prospective study. J. Pediatr. 133 , 237–241 (1998).
Dubois, J. et al. Incidence of deep vein thrombosis related to peripherally inserted central catheters in children and adolescents. Cmaj 177 , 1185–1190 (2007).
Faustino, E. V. et al. Incidence and acute complications of asymptomatic central venous catheter-related deep venous thrombosis in critically ill children. J. Pediatr. 162 , 387–391 (2013).
Jiang, W. et al. Construction and validation of a risk prediction model for central venous catheter-associated deep venous thromboses in children with congenital heart disease after surgery. Chin. J. Nurs. 57 , 2217–2224 (2022).
Google Scholar
Faustino, E. V. et al. Factor VIII May Predict Catheter-Related Thrombosis in Critically Ill Children: A Preliminary Study. Pediatr. Crit. Care Med. 16 , 497–504 (2015).
Jones, S., Butt, W., Monagle, P., Cain, T. & Newall, F. The natural history of asymptomatic central venous catheter-related thrombosis in critically ill children. Blood 133 , 857–866 (2019).
Kim, E. H. et al. Central venous catheter-related thrombosis in pediatric surgical patients: A prospective observational study. Paediatr. Anaesth. 32 , 563–571 (2022).
Male, C. et al. Central venous line-related thrombosis in children: association with central venous line location and insertion technique. Blood 101 , 4273–4278 (2003).
Male, C., Julian, J. A., Massicotte, P., Gent, M. & Mitchell, L. Significant association with location of central venous line placement and risk of venous thrombosis in children. Thromb. Haemost. 94 , 516–521 (2005).
Marquez, A., Shabanova, V. & Faustino, E. V. Prediction of Catheter-Associated Thrombosis in Critically Ill Children. Pediatr. Crit. Care Med. 17 , e521–e528 (2016).
Menéndez, J. J. et al. Incidence and risk factors of superficial and deep vein thrombosis associated with peripherally inserted central catheters in children. J. Thromb. Haemost. 14 , 2158–2168 (2016).
Rubio Longo, M. C. et al. Catheter-related deep vein thrombosis in newborn infants. Arch. Argent. Pediatr. 119 , 32–38 (2021).
PubMed Google Scholar
Östlund, Å. et al. Incidence of and risk factors for venous thrombosis in children with percutaneous non-tunnelled central venous catheters. Br. J. Anaesth. 123 , 316–324 (2019).
Sol, J. J. et al. Chronic Complications After Femoral Central Venous Catheter-related Thrombosis in Critically Ill Children. J. Pediatr. Hematol. Oncol. 37 , 462–467 (2015).
van Rooden, C. J. et al. Infectious complications of central venous catheters increase the risk of catheter-related thrombosis in hematology patients: a prospective study. J. Clin. Oncol. 23 , 2655–2660 (2005).
Zeng, X., Zhang, C. & Shi, Y. Analysis of risk factors for complicated catheter-related thrombosis in children. Chin. J. Emerg. Med. 29 , 719–723 (2020).
Wei, Y. et al. The incidence and risk factors of catheter-related-thrombosis during induction chemotherapy in acute lymphocytic leukemia children. Chin. J. Hematol. 38 , 313–317 (2017).
CAS Google Scholar
Deng, G. & Liao, Q. Analysis of risk factors for venous thrombosis after PICC placement in critically ill children. Int. I Nurs. 39 , 775–777 (2020).
Badheka, A. V. et al. Catheter related thrombosis in hospitalized infants: A neural network approach to predict risk factors. Thromb. Res. 200 , 34–40 (2021).
Chen, K. et al. Risk factors for central venous catheter-related thrombosis in children: a retrospective analysis. Blood Coagul. Fibrinol. 27 , 384–388 (2016).
Article Google Scholar
Chojnacka, K., Krasiński, Z., Wróblewska-Seniuk, K. & Mazela, J. Catheter-related venous thrombosis in NICU: A case-control retrospective study. J. Vasc. Access 23 , 88–93 (2022).
Derderian, S. C., Good, R., Vuille-Dit-Bille, R. N., Carpenter, T. & Bensard, D. D. Central venous lines in critically ill children: Thrombosis but not infection is site dependent. J. Pediatr. Surg. 54 , 1740–1743 (2019).
Diamond, C. E. et al. Catheter-Related Venous Thrombosis in Hospitalized Pediatric Patients with Inflammatory Bowel Disease: Incidence, Characteristics, and Role of Anticoagulant Thromboprophylaxis with Enoxaparin. J. Pediatr. 198 , 53–59 (2018).
Noailly Charny, P. A. et al. Increased Risk of Thrombosis Associated with Peripherally Inserted Central Catheters Compared with Conventional Central Venous Catheters in Children with Leukemia. J. Pediatr. 198 , 46–52 (2018).
Gnannt, R. et al. Increased risk of symptomatic upper-extremity venous thrombosis with multiple peripherally inserted central catheter insertions in pediatric patients. Pediatr. Radio. 48 , 1013–1020 (2018).
Gray, B. W. et al. Characterization of central venous catheter-associated deep venous thrombosis in infants. J. Pediatr. Surg. 47 , 1159–1166 (2012).
Haddad, H. et al. Routine surveillance ultrasound for the management of central venous catheters in neonates. J. Pediatr. 164 , 118–122 (2014).
Lambert, I., Tarima, S., Uhing, M. & Cohen, S. S. Risk Factors Linked to Central Catheter-Associated Thrombosis in Critically Ill Infants in the Neonatal Intensive Care Unit. Am. J. Perinatol. 36 , 291–295 (2019).
Li, H. et al. Prediction of central venous catheter-associated deep venous thrombosis in pediatric critical care settings. BMC Med. Inf. Decis. Mak. 21 , 332 (2021).
Article CAS Google Scholar
Lovett, M. E. et al. Catheter-associated deep vein thrombosis in children with severe traumatic brain injury: A single-center experience. Pediatr. Blood Cancer 70 , e30044 (2023).
MacLean, J. et al. Need for tissue plasminogen activator for central venous catheter dysfunction is significantly associated with thrombosis in pediatric cancer patients. Pediatr. Blood Cancer 65 , e27015 (2018).
Noonan, P. J., Hanson, S. J., Simpson, P. M., Dasgupta, M. & Petersen, T. L. Comparison of Complication Rates of Central Venous Catheters Versus Peripherally Inserted Central Venous Catheters in Pediatric Patients. Pediatr. Crit. Care Med. 19 , 1097–1105 (2018).
Pei, L. et al. Clinical characteristics and risk factors of symptomatic central venous catheter-related deep vein thrombosis in children. Chin. Pediatr. Emerg. Med. 23 , 450–454 (2016).
Onyeama, S. N. et al. Central Venous Catheter-associated Venous Thromboembolism in Children With Hematologic Malignancy. J. Pediatr. Hematol. Oncol. 40 , e519–e524 (2018).
Shah, S. H. et al. Clinical risk factors for central line-associated venous thrombosis in children. Front. Pediatr. 3 , 35 (2015).
Shin, H. S., Towbin, A. J., Zhang, B., Johnson, N. D. & Goldstein, S. L. Venous thrombosis and stenosis after peripherally inserted central catheter placement in children. Pediatr. Radio. 47 , 1670–1675 (2017).
Smitherman, A. B. et al. The incidence of catheter-associated venous thrombosis in noncritically ill children. Hosp. Pediatr. 5 , 59–66 (2015).
Steen, E. H. et al. Central Venous Catheter-Related Deep Vein Thrombosis in the Pediatric Cardiac Intensive Care Unit. J. Surg. Res 241 , 149–159 (2019).
Wang, J. & Ren, G. Peripherally inserted central catheter related venous thromboembolism in children with acute leukemia: a factorial analysis. Chin. J. Biomed. Eng. 27 , 288–293 (2021).
Dubbink-Verheij, G. H. et al. Femoral Vein Catheter is an Important Risk Factor for Catheter-related Thrombosis in (Near-)term Neonates. J. Pediatr. Hematol. Oncol. 40 , e64–e68 (2018).
Tran, M., Shein, S. L., Ji, X. & Ahuja, S. P. Identification of a “VTE-rich” population in pediatrics - Critically ill children with central venous catheters. Thromb. Res 161 , 73–77 (2018).
Wisecup, S., Eades, S. & Turiy, Y. Characterizing the Risk Factors Associated With Venous Thromboembolism in Pediatric Patients After Central Venous Line Placement. J. Pediatr. Pharm. Ther. 20 , 358–366 (2015).
Zhu, W., Zhang, H., Xing, Y. Clinical Characteristics of Venous Thrombosis Associated with Peripherally Inserted Central Venous Catheter in Premature Infants. Children 9 , https://doi.org/10.3390/children9081126 (2022).
Ekdahl, K. N. et al. Innate immunity activation on biomaterial surfaces: a mechanistic model and coping strategies. Adv. Drug Deliv. Rev. 63 , 1042–1050 (2011).
Article CAS PubMed PubMed Central Google Scholar
Takemoto, C. M. et al. Hospital-associated venous thromboembolism in children: incidence and clinical characteristics. J. Pediatr. 164 , 332–338 (2014).
Boulet, S. L. et al. Trends in venous thromboembolism-related hospitalizations, 1994-2009. Pediatrics 130 , e812–e820 (2012).
Raffini, L., Huang, Y. S., Witmer, C. & Feudtner, C. Dramatic increase in venous thromboembolism in children’s hospitals in the United States from 2001 to 2007. Pediatrics 124 , 1001–1008 (2009).
Lin, S., Zhu, N., YihanZhang, Du, L. & Zhang, S. Development and validation of a prediction model of catheter-related thrombosis in patients with cancer undergoing chemotherapy based on ultrasonography results and clinical information. J. Thromb. Thrombolysis 54 , 480–491 (2022).
Johnson, E. D., Schell, J. C. & Rodgers, G. M. The D-dimer assay. Am. J. Hematol. 94 , 833–839 (2019).
Favresse, J. et al. D-dimer: Preanalytical, analytical, postanalytical variables, and clinical applications. Crit. Rev. Clin. Lab Sci. 55 , 548–577 (2018).
Weitz, J. I., Fredenburgh, J. C. & Eikelboom, J. W. A Test in Context: D-Dimer. J. Am. Coll. Cardiol. 70 , 2411–2420 (2017).
Darlow, J. & Mould, H. Thrombophilia testing in the era of direct oral anticoagulants. Clin. Med. 21 , e487–e491 (2021).
Monagle, P. et al. American Society of Hematology 2018 Guidelines for management of venous thromboembolism: treatment of pediatric venous thromboembolism. Blood Adv. 2 , 3292–3316 (2018).
Meziani, F., Gando, S. & Vincent, J. L. Should all patients with sepsis receive anticoagulation? Yes. Intensive Care Med 43 , 452–454 (2017).
Saber, W. et al. Risk factors for catheter-related thrombosis (CRT) in cancer patients: a patient-level data (IPD) meta-analysis of clinical trials and prospective studies. J. Thromb. Haemost. 9 , 312–319 (2011).
Journeycake, J. M. & Buchanan, G. R. Thrombotic complications of central venous catheters in children. Curr. Opin. Hematol. 10 , 369–374 (2003).
Lamperti, M. et al. International evidence-based recommendations on ultrasound-guided vascular access. Intensive Care Med 38 , 1105–1117 (2012).
Journeycake, J. M. & Buchanan, G. R. Catheter-related deep venous thrombosis and other catheter complications in children with cancer. J. Clin. Oncol. 24 , 4575–4580 (2006).
Deitcher, S. R., Gajjar, A., Kun, L. & Heideman, R. L. Clinically evident venous thromboembolic events in children with brain tumors. J. Pediatr. 145 , 848–850 (2004).
Mandel-Shorer, N., Tzvi-Behr, S., Harvey, E. & Revel-Vilk, S. Central venous catheter-related venous thrombosis in children with end-stage renal disease undergoing hemodialysis. Thromb. Res 172 , 150–157 (2018).
Chen, D., Långström, S., Petäjä, J., Heikinheimo, M. & Pihkala, J. Thrombin formation and effect of unfractionated heparin during pediatric cardiac catheterization. Catheter Cardiovasc Inter. 81 , 1174–1179 (2013).
Reynolds, P. M. et al. Evaluation of Prophylactic Heparin Dosage Strategies and Risk Factors for Venous Thromboembolism in the Critically Ill Patient. Pharmacotherapy 39 , 232–241 (2019).
Diamanti, A. et al. Prevalence of life-threatening complications in pediatric patients affected by intestinal failure. Transpl. Proc. 39 , 1632–1633 (2007).
Download references
This study was supported by the Fundamental Research Funds for the Central Universities [grant numbers YCJJ20230244] and Tongji Hospital, Tongji Medical College, Huazhong University of Science and Technology Research Fund [grant numbers 2022C09].
Author information
Authors and affiliations.
Department of Nursing, Tongji Hospital, Tongji Medical College, Huazhong University of Science and Technology, Wuhan, China
Maoling Fu, Qiaoyue Yang, Yaqi Yu, Wenshuai Song, Xiuli Qin, Ying Luo, Xiaoju Xiong & Genzhen Yu
School of Nursing, Tongji Medical College, Huazhong University of Science and Technology, Wuhan, China
Maoling Fu, Quan Yuan, Qiaoyue Yang, Yaqi Yu & Wenshuai Song
You can also search for this author in PubMed Google Scholar
Contributions
GY and YL framed the review questions on the basis of input from MF and QY. YY and XQ conducted the literature search. MF, WS, and QY screened and evaluated the identified papers. GY and YY performed data extraction and analysis. MF, WS, XQ and QY prepared the initial manuscript with revisions and comments from GY, YL, and XX. All authors approved the final manuscript as submitted and agreed to be accountable for all aspects of the work.
Corresponding author
Correspondence to Genzhen Yu .
Ethics declarations
Competing interests.
The authors declare no competing interests.
Additional information
Publisher’s note Springer Nature remains neutral with regard to jurisdictional claims in published maps and institutional affiliations.
Supplementary information
Supplementary checklist, supplemental digital tables1, supplemental digital tables2, supplemental digital, rights and permissions.
Open Access This article is licensed under a Creative Commons Attribution 4.0 International License, which permits use, sharing, adaptation, distribution and reproduction in any medium or format, as long as you give appropriate credit to the original author(s) and the source, provide a link to the Creative Commons licence, and indicate if changes were made. The images or other third party material in this article are included in the article’s Creative Commons licence, unless indicated otherwise in a credit line to the material. If material is not included in the article’s Creative Commons licence and your intended use is not permitted by statutory regulation or exceeds the permitted use, you will need to obtain permission directly from the copyright holder. To view a copy of this licence, visit http://creativecommons.org/licenses/by/4.0/ .
Reprints and permissions
About this article
Cite this article.
Fu, M., Yuan, Q., Yang, Q. et al. Risk factors and incidence of central venous access device-related thrombosis in hospitalized children: a systematic review and meta-analysis. Pediatr Res (2024). https://doi.org/10.1038/s41390-024-03225-0
Download citation
Received : 06 October 2023
Revised : 18 March 2024
Accepted : 25 March 2024
Published : 17 May 2024
DOI : https://doi.org/10.1038/s41390-024-03225-0
Share this article
Anyone you share the following link with will be able to read this content:
Sorry, a shareable link is not currently available for this article.
Provided by the Springer Nature SharedIt content-sharing initiative
Quick links
- Explore articles by subject
- Guide to authors
- Editorial policies


- Liberty University
- Jerry Falwell Library
- Special Collections
- < Previous
Home > ETD > Doctoral > 5543
Doctoral Dissertations and Projects
Virtual coaching, self-directed learning, and the implementation of evidence-based practices: a single qualitative case study.
Elisabeth Myers , Liberty University Follow
School of Education
Doctor of Philosophy in Education (PhD)
Christine Saba
virtual coaching, self-directed learning, evidence-based practices, self-determination theory, sustained implementation
Disciplines
Education | Educational Leadership
Recommended Citation
Myers, Elisabeth, "Virtual Coaching, Self-Directed Learning, and the Implementation of Evidence-Based Practices: A Single Qualitative Case Study" (2024). Doctoral Dissertations and Projects . 5543. https://digitalcommons.liberty.edu/doctoral/5543
The purpose of this single instrumental case study was to understand how a virtual coaching program provides opportunities for self-directed learning during the implementation of evidence-based practices for adults at Navigator Coaching. The theory guiding this study was Deci and Ryan’s self-determination theory as conceptualizations of self-directed learning described in the literature mirror descriptions of self-determination. The central research question was: How does a virtual coaching program provide opportunities for self-directed learning during the implementation of evidence-based practices? As a single instrumental case, the setting for this study was one virtual life-coaching program in North America. The sample of participants included 12 adults who were currently enrolled in the program for a minimum of 6 months and participated in weekly program activities. Multiple data collection methods were employed to describe and understand the case: observations, audiovisual materials, and individual interviews. Interpretational analysis and a multistep data analysis process including direct interpretation, categorical aggregation, correspondence tables, and interpretive commentaries were utilized to develop the themes and overall synthesis of the case. Opportunities for self-directed learning were provided in weekly live sessions, modules in the program library, and in the Facebook group. Program members utilized instructional opportunities to satisfy their need for autonomy, thus becoming students of self. Participation in a purposeful community that was focused on solutions provided opportunities for program members to satisfy competence and relatedness needs. Program members implemented evidence-based practices and developed skills to create weekly learning plans, which assisted them in becoming agents of their highest selves.
Included in
Educational Leadership Commons
- Collections
- Faculty Expert Gallery
- Theses and Dissertations
- Conferences and Events
- Open Educational Resources (OER)
- Explore Disciplines
Advanced Search
- Notify me via email or RSS .
Faculty Authors
- Submit Research
- Expert Gallery Login
Student Authors
- Undergraduate Submissions
- Graduate Submissions
- Honors Submissions
Home | About | FAQ | My Account | Accessibility Statement
Privacy Copyright
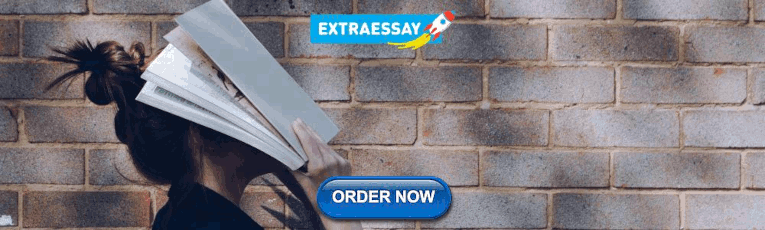
IMAGES
VIDEO
COMMENTS
Furthermore, the ability to describe in detail how the analysis was conducted ensures rigour in reporting qualitative research. Data sources: The research example used is a multiple case study that explored the role of the clinical skills laboratory in preparing students for the real world of practice. Data analysis was conducted using a ...
The types of qualitative research included: 24 case studies, 19 generic qualitative studies, and eight phenomenological studies. Notably, about half of the articles reported analyzing their qualitative data via content analysis and a constant comparative method, which was also commonly referred to as a grounded theory approach and/or inductive ...
A case study is one of the most commonly used methodologies of social research. This article attempts to look into the various dimensions of a case study research strategy, the different epistemological strands which determine the particular case study type and approach adopted in the field, discusses the factors which can enhance the effectiveness of a case study research, and the debate ...
Case study protocol is a formal document capturing the entire set of procedures involved in the collection of empirical material . It extends direction to researchers for gathering evidences, empirical material analysis, and case study reporting . This section includes a step-by-step guide that is used for the execution of the actual study.
Case studies play a significant role in knowledge development across various disciplines. Analysis of cases provides an avenue for researchers to explore phenomena within their context based on the collected data. Analysis of qualitative data from case study research can contribute to knowledge development.
Aims of Qualitative Data Analysis The analysis of qualitative data can have several aims. The first aim may be to describe a phenomenon in some or greater detail. The phenomenon can be the subjective experi-ences of a specific individual or group (e.g. the way people continue to live after a fatal diagnosis). This can focus on the case (indi-
Step 1: Gather your qualitative data and conduct research (Conduct qualitative research) The first step of qualitative research is to do data collection. Put simply, data collection is gathering all of your data for analysis. A common situation is when qualitative data is spread across various sources.
Qualitative case study methodology is an appropriate strategy for exploring phenomena such as lived experiences, events, and the contexts in which they occur (Houghton et al. 2014;Miles and ...
After describing qualitative data and strategies for analysis, this chapter examines five broad classifications of designs: case study, phenomenological, ethnographic, narrative, and mixed methods. These designs require complex collection of data as sources of evidence for claims about the meaning of the data.
McMaster University, West Hamilton, Ontario, Canada. Qualitative case study methodology prov ides tools for researchers to study. complex phenomena within their contexts. When the approach is ...
There are many different research methods you can use to collect data on your subject. Case studies tend to focus on qualitative data using methods such as interviews, observations, and analysis of primary and secondary sources (e.g., newspaper articles, photographs, official records). Sometimes a case study will also collect quantitative data.
Therefore, the purpose of this paper is to provide a concise explanation of four common qualitative approaches, case study, ethnography, narrative, and phenomenology, demonstrating how each approach is linked to specific types of data collection and analysis. ... Qualitative data analysis: conceptual and practical considerations. Health ...
According to the book Understanding Case Study Research, case studies are "small scale research with meaning" that generally involve the following: The study of a particular case, or a number of cases. That the case will be complex and bounded. That it will be studied in its context. That the analysis undertaken will seek to be holistic.
Learn the basics of qualitative data analysis (QDA) plus the most popular approaches, including content, discourse and narrative analysis. About Us; Services. 1-On-1 Coaching. ... Clear explanation on qualitative and how about Case study. Reply. Ogobuchi Otuu on January 31, 2023 at 5:40 pm This was helpful. Thank you. Reply.
Each qualitative research approach or design has its own terms for methods of data analysis: Ethnography—uses modified thematic analysis and life histories. Case study—uses description, categorical aggregation, or direct interpretation. Grounded theory—uses open, axial, and selective coding (although recent writers are proposing ...
Data sources The research example used is a multiple case study that explored the role of the clinical skills laboratory in preparing students for the real world of practice. Data analysis was conducted using a framework guided by the four stages of analysis outlined by Morse ( 1994 ): comprehending, synthesising, theorising and recontextualising.
A case study is a research method that involves an in-depth examination and analysis of a particular phenomenon or case, such as an individual, organization, community, event, or situation. It is a qualitative research approach that aims to provide a detailed and comprehensive understanding of the case being studied.
propose an approach to the analysis of case study data by logically linking the data to a series of propositions and then interpreting the subsequent information. Like the Yin (1994) strategy, the Miles and Huberman (1994) process of analysis of case study data, although quite detailed, may still be insufficient to guide the novice researcher.
The study is designed under an interpretive paradigm , which took the form of a multiple-case study and the analysis of a considerable amount of primary and secondary qualitative data . This enabled the researchers to interact closely with the participants and to explore issues in depth.
The study aims to explore the case study method with the formation of questions, data collection procedures and analysis, followed by how and on which position the saturation is achieved in developing a centralized Shariah governance framework for Islamic banks in Bangladesh.,Using purposive and snowball sampling procedures, data have been ...
Published: Oct. 23, 2023. Qualitative Data Analysis Software (QDA software) allows researchers to organize, analyze and visualize their data, finding the patterns in qualitative data or unstructured data: interviews, surveys, field notes, videos, audio files, images, journal articles interviews, web content etc.
Further, often the contribution of a qualitative case study research (QCSR) emerges from the 'extension of a theory' or 'developing deeper understanding—fresh meaning of a phenomenon'. ... Thematic analysis of qualitative data: AMEE Guide No. 131. Medical Teacher. Crossref. PubMed. Google Scholar. Ryan G. W., & Bernard H. R. (2003 ...
Understanding Qualitative Data Analysis. Qualitative data analysis is the process of systematically examining and deciphering qualitative facts (such as textual content, pix, motion pictures, or observations) to discover patterns, themes, and meanings inside the statistics· Unlike quantitative statistics evaluation, which focuses on numerical measurements and statistical strategies ...
The purpose of this case study is to examine the influence of principal instructional leadership on school culture in the American Embassy School (AES) in New Delhi, India. Using mixed quantitative and qualitative methods, this case study addresses three key research questions. Two existing survey instruments, the Principal Instructional Management Rating Scale developed by Philip Hallinger ...
RevMan 5.4 was employed for data analysis. The review included 47 studies evaluating 262,587 children with CVAD placement. ... Repeated published research. Case reports, study designs, or clinical ...
This article outlines a five-phase process of qualitative analysis that draws on deductive (codes developed a priori) and inductive (codes developed in the course of the analysis) coding strategies...
The purpose of this single instrumental case study was to understand how a virtual coaching program provides opportunities for self-directed learning during the implementation of evidence-based practices for adults at Navigator Coaching. The theory guiding this study was Deci and Ryan's self-determination theory as conceptualizations of self-directed learning described in the literature ...