
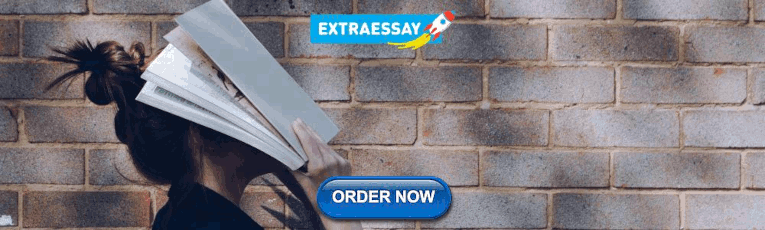
Advancements in Use of Geophysical Methods for Transportation Projects (2020)
Chapter: chapter 5 - conclusions.
Below is the uncorrected machine-read text of this chapter, intended to provide our own search engines and external engines with highly rich, chapter-representative searchable text of each book. Because it is UNCORRECTED material, please consider the following text as a useful but insufficient proxy for the authoritative book pages.
60 This synthesis documents current practices for the application of geophysical methods to transportation projects. Practices were gleaned from a review of literature, a survey of transpor- tation agencies, and case examples of select agencies. This chapter summarizes the most notable findings from each before presenting conclusions and suggestions for future research. Summary of Major Findings The review of the literature on near-surface geophysical methods found a wide range of surface and geophysical techniques available that are applicable to transportation-related problems. This synthesis focused on 15 surface techniques and nine borehole techniques. Brief summaries of each method were provided, as were select applications from the literature. The literature review was also used to assemble an updated matrix of surface and borehole methods and their applications, as well as a summary of relevant training resources on the application, implemen- tation, and fundamentals of geophysical methods. The synthesis survey revealed the vast majority of agencies use geophysical methods, with 43 of 48 respondents (90%) indicating their agency uses geophysical methods. This proportion is approximately the same as that found in the 2006 survey by Sirles. Agency motivations for using geophysical methods varied, with five reasons cited by at least half of the respondents; the most commonly cited was the ability to provide a subsurface image of a large mass of materials. Among the five agencies without geophysical experience, the most commonly cited reasons for not using geophysical methods were technical. All five indicated that agency engineers were unfamiliar with geophysical methods. According to the survey results, the most common estimate for how frequently agencies use geophysical methods is three to five times per year. Seven respondents indicated their agency uses geophysical methods more than 10 times per year. For half of the agencies with geophysical experience, respondents indicated the frequency of use of geophysical methods is increasing. There is not much evidence that the frequency of application has increased since the 2006 survey by Sirles. The estimated frequency of application of geophysical methods was largely the same as reported by Sirles for the 2006 survey, but survey respondent estimates provide some indication that agency spending on geophysical methods may have increased slightly. Eight geophysical methods are reported to have been used by at least one-third of respondents with knowledge of agency geophysical experience. The most common are ground-penetrating radar and seismic refraction, both of which were reported by nearly nine in 10 respondents. These same methods were also found to be the most commonly applied in the 2006 survey. However, active and passive surface wave methods, electrical tomography, microgravity, and optical and acoustic televiewer methods have all experienced a three- to four-fold increase in the C H A P T E R 5 Conclusions
Conclusions 61 proportion of agencies that report experience with those methods. Electrical resistivity imaging (ERI), in particular, was well represented in the case examples provided. Geologic objectives of the geophysical investigations are dominated by those pertaining to rock, especially determina- tion of the depth to bedrock, bedrock topography, and bedrock rippability. The majority of agencies with geophysics experience, 51%, use a mix of in-house capabilities and contractors for performance of geophysical test methods. Forty-four percent perform all geo- physical test methods using contractors. The most common source of funding for geophysical investigations is project design funds, which have been used by more than 90% of respondents. Responses to survey questions about potential new training resources were highly skewed toward a favorable view of such resources. At least 70% of respondents said new training resources would be somewhat likely or very likely to increase agency use of geophysical methods. That result held for three different training formats, with in-person training viewed as the most likely to increase agency use of geophysical methods. Another question asked about the per- ceived usefulness of five different training content areas. The majority of respondents indicated that all five content areas would be very useful, with (1) uses and applications of geophysical methods and (2) interpretation of engineering parameters from geophysical results viewed as the most useful. Five case examples were examined in this survey. The agencies interviewed were all identi- fied as prolific users of geophysical methods. The history of geophysics use among the agencies varied considerably, as did the means of implementation. Three of the agencies performed most geophysical measurements with in-house capabilities. These agencies considered in-house use to be cost saving, allowing them to use the methods for more projects. Two of the agencies per- formed the measurements almost exclusively by external contracting and reported few problems with the contracting process. The agencies provided interesting case examples where geophysical measurements had pro- vided a benefit through cost savings, were the only viable approach for the conditions encoun- tered, allowed for imaging of a large subsurface volume, or allowed for rapid collection of subsurface information. Examples where geophysical results were confirmed by ground truth were also provided. All agencies commented on the need to supplement geophysical investiga- tions with a drilling and sampling program whenever possible. The agencies interviewed also indicated a need for training resources. The primary need identified by the agencies was training for engineers on the capabilities, limitations, and typical applications of geophysical methods. Several commented that a National Highway Institute course on geophysics would be welcomed. Conclusions Comparison of survey results from this synthesis and the 2006 synthesis by Sirles provides a unique opportunity to evaluate how the state of practice for geophysical methods has changed among U.S. transportation agencies. The number of agencies that use geophysical methods and the frequency of geophysical applications do not appear to have changed significantly. Roughly nine of 10 agencies use geophysical methods. Most agencies are relatively casual users with five or fewer geophysical applications per year, though a small handful of agencies implement geo- physical methods routinely. Although the overall frequency of geophysical applications does not appear to have increased since 2006, the survey from this synthesis provides some evidence that agency spending on geophysical methods has increased slightly, and there is strong evidence in the survey results that a variety of methods from across the geophysical spectrum have been implemented by significantly more agencies since 2006.
62 Advancements in Use of Geophysical Methods for Transportation Projects The survey results from this synthesis indicate that agency use of geophysical methods is commonly motivated by a wide variety of factors. Among six potential reasons for using geophysical methods, five were selected by more than half of the agencies with geophysical experience as having motivated their use of geophysical methods. The case example agencies provide strong anecdotal support for these motivators. For example, MnDOT provided a case example where resistivity measurements were used exclusively for foundation condition assess- ment because of site access issues. NJDOT provided an interesting example of opting for a simple and quick passive geophysical method when drilling was too slow and difficult. Caltrans showed an excellent example of imaging a large volume of material between boreholes, and ODOT provided examples of imaging a shallow landslide with resistivity. Last, NJDOT provided an example from a time before it used geophysics, where major cost savings would have been real- ized if geophysical methods had been applied. As evidenced by survey results from this synthesis and the 2006 synthesis, the greatest deter- rent to greater application of geophysical methods is a lack of familiarity and understanding of the methods among agency engineers. Both the 2006 and the present survey indicate broad sup- port for development of new training resources related to geophysical methods. Suggestions for Future Research There are countless avenues for research into geophysical methods that could produce useful and effective results for transportation agencies. For example, there is a need to further develop correlations between geophysical measurements and geotechnical parameters. Toward this end, development of processing routines and interpretation methods that use several geophysical measurements from the same location could be beneficial. It is suggested that research into near-surface applications of cutting-edge methods such as FWI continue to be studied, and that continued documentation of the use of geophysical methods to infer geotechnical parameters and site conditions from case histories is encouraged. Transportation agencies that are able to do so might consider encouraging, supporting, and funding such research. The results of this and of the 2006 (Sirles) synthesis, however, point not to specific research needs but rather to a more basic undertaking that would improve agency use of geophysical methods: development of training resources. That lack of knowledge of geophysical methods among agency engineers impedes the use of geophysical methods, and that agency engineers are eager for new training resources were among the clearest of the survey results. Taken together, the survey findings suggest that development and implementation of training resources would effectively increase agency use of geophysical methods. The survey results also suggest two training content areas would be most useful: (1) appropriate selection of geophysical methods that consider investigation objectives and site characteristics and (2) interpretation of engineering parameters from geophysical results. The case example agency interviews support the survey findings. The most common comment on training was that there was a need to educate engi- neers on the value that geophysical methods can provide to their projects. The experience of case example agencies suggests that agencies could realize significant cost savings, project schedule accelerations, and reliability improvements if training resources were to success- fully move the state of practice toward more frequent and more thoughtful applications of geophysical methods.
Geophysical methods provide a means to rapidly and economically characterize subsurface conditions and infer soil properties over a spatial extent that is not possible with conventional methods.
The TRB National Cooperative Highway Research Program's NCHRP Synthesis 547: Advancements in Use of Geophysical Methods for Transportation Projects evaluates the current state of practice in the use of geophysical methods by state transportation agencies.
Challenges and obstacles remain that must be overcome if routine implementation of geophysical methods for transportation projects is to be realized. Uncertainties associated with insufficient or poor site characterization can lead to overly conservative designs, increased risk of poor performance, cost increases attributable to changed conditions, and project delays.
READ FREE ONLINE
Welcome to OpenBook!
You're looking at OpenBook, NAP.edu's online reading room since 1999. Based on feedback from you, our users, we've made some improvements that make it easier than ever to read thousands of publications on our website.
Do you want to take a quick tour of the OpenBook's features?
Show this book's table of contents , where you can jump to any chapter by name.
...or use these buttons to go back to the previous chapter or skip to the next one.
Jump up to the previous page or down to the next one. Also, you can type in a page number and press Enter to go directly to that page in the book.
To search the entire text of this book, type in your search term here and press Enter .
Share a link to this book page on your preferred social network or via email.
View our suggested citation for this chapter.
Ready to take your reading offline? Click here to buy this book in print or download it as a free PDF, if available.
Get Email Updates
Do you enjoy reading reports from the Academies online for free ? Sign up for email notifications and we'll let you know about new publications in your areas of interest when they're released.
- Open supplemental data
- Reference Manager
- Simple TEXT file
People also looked at
Systematic review article, a systematic review of remotely operated vehicle surveys for visually assessing fish assemblages.
- Institute of Marine and Antarctic Studies, College of Science and Engineering, University of Tasmania, Hobart, TAS, Australia
Anthropogenic activities and greater demands for marine natural resources has led to increases in the spatial extent and duration of pressures on marine ecosystems. Remotely operated vehicles (ROVs) offer a robust survey tool for quantifying these pressures and tracking the success of management intervention while at a range of depths, including those inaccessible to most SCUBA diver-based survey methods (~>30 m). As the strengths, limitations, and biases of ROVs for visually monitoring fish assemblages remain unclear, this review aims to evaluate ROVs as a survey technique and to suggest optimal sampling strategies for use in typical ROV-based studies. Using the search engines Scopus™ and Google Scholar™, 119 publications were identified that used ROVs for visual surveys of fish assemblages. While the sampling strategies and sampling metrics used to annotate the imagery in these publications varied considerably, the total abundance of fish recorded over strip transects of varying dimensions was the most common sampling design. The choice of ROV system appears to be a strong indicator of both the types of surveys available to studies and the success of ROV deployments. For instance, larger, more powerful working-class systems can complete longer and more complex designs (e.g., swath, cloverleaf, and polygonal transects) at greater depths, whereas observation-class systems are less expensive and easier to deploy, but are more susceptible to delays or cancelations of deployments. In more severe sea state conditions, radial transects, or strip transects that employ live-boating or a weight to anchor the tether to the seafloor, can be used to improve the performance of observation-class systems. As these systems often employ shorter tethers, radial transects can also be used to maximize sampling area at greater depths and on large vessels that may rotate substantially while anchored. For highly mobile species, and in survey designs where individuals are likely to be recounted (e.g., transects along oil and gas pipelines), relative abundance (MaxN) may be a more robust sampling metric. By identifying subtle, yet important, differences in the application of ROVs as a tool for visually surveying deep-water marine ecosystems, we identified key areas for improvement for best practice for future studies.
Introduction
In light of global anthropogenic threats, such as climate change, pollution, and overexploitation, an increasing number of marine biological communities require conservation ( Veitch et al., 2012 ). Fundamental to these conservation initiatives is the need for robust monitoring of the focal ecosystems ( Espinoza et al., 2014 ; Addison et al., 2018 ). However, comprehensive monitoring of our marine ecosystems, particularly the deeper regions, is difficult, and has resulted in most of the spatially- and temporally-mature monitoring datasets being constrained to depths shallower than 30 m (e.g., SCUBA-diver based underwater visual census) ( Andaloro et al., 2013 ). While methods do exist for assessing deep-water marine ecosystems, such as longline ( Brooks et al., 2011 ; Santana-Garcon et al., 2014 ; McLean et al., 2015 ), and bottom dredging/trawling ( De Leo et al., 2010 ; D'Onghia et al., 2012 ), ecological concerns encourage the use of non-destructive methods ( Murphy and Jenkins, 2010 ; Lyle et al., 2014 ). The development of robust, non-destructive video-based survey techniques is an emerging field that allows for in situ observations of species, their distributions, behaviors and habitat associations in an array of habitats, including these difficult, deep-water ecosystems ( Mallet and Pelletier, 2014 ). However, some of these mobile video-based methods, including Autonomous Underwater Vehicles (AUVs), underwater towed videos (UTVs) and remotely operated vehicles (ROVs), are in their early stages of development and require proper evaluation and standardization before they can be reliably used for biological monitoring ( Karpov et al., 2012 ; Lauermann, 2014 ).
ROV surveys are novel video-based tool for assessing fish assemblages, yet have a number of strengths that could make them important tools in future biological surveys ( Huvenne et al., 2018 ). These strengths include the ability to deploy high-resolution video (forward and downward looking) along fixed and repeatable transects on targeted seabed features, while maneuvering around complex substrate, and recording the track of the vehicle over base-maps ( Linley et al., 2013 ; Macreadie et al., 2018 ). This combination of strengths allows for quantitative estimates of benthic floral and faunal cover as well as benthic fishes, epi-benthic fishes, pelagic fishes, and ground-truthing of major habitat features ( Quattrini et al., 2017 ). However, the strengths, limitations and biases of ROVs for visually monitoring fish assemblages remain unclear. This review aims to evaluate ROVs as a video-based survey tool and to suggest standardized operating protocols for typical ROV-based studies through trends in the literature.
The Scopus™ and Google Scholar™ databases were accessed in November 2018 to search for peer-reviewed journal publications and gray literature (1965–2018) that used video footage collected by remotely operated vehicles (ROVs) to visually assess fish assemblages ( Figure 1 and Supplementary Material ). The review identified 119 publications that used ROVs to visually survey fish communities by using the keyword combinations “[(remotely AND operated AND vehicle*) OR (ROV OR ROVs) AND fish OR fishes)”] Articles that (i) did not use a video-based approach, and (ii) did not survey fish assemblages, were excluded from this review. The publications from this search provided information on the general trends in ROV survey application and comparisons of ROV surveys with other deep-water survey methodology. As surveys were often conducted over several years, publication years were used to analyze trends. Furthermore, publication years may also better reflect trends in the types of sampling metrics and analyses used for archived video footage from unstandardized industrial ROV surveys on oil and gas structures. ROV system costs were determined by the prices listed online by commercial retailers and inferred for specially-built models owned by research institutions.
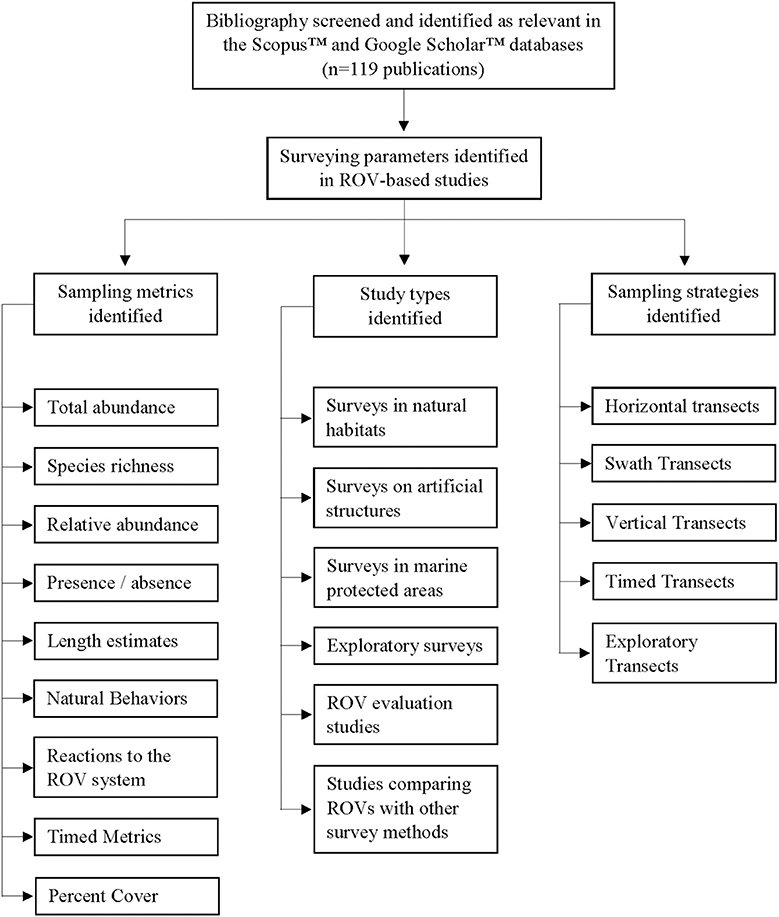
Figure 1 . The sampling parameters identified for visual-based ROV surveys of fish assemblages.
Types of Remotely Operated Vehicles
Remotely Operated Vehicle (ROV) surveys use an unmanned underwater submersible that transmits real-time video observations and environmental readings (e.g., depth, compass heading) via an umbilical tether to the operator at the surface. ROVs are available in a range of systems from smaller observation-class ROVs (~3–20 kg for mini and ~30–120 kg for regular-sized models) to larger working-class systems (100–1500 kg for light and up to 5,000 kg for heavy-duty models), which vary in power, depth rating, accessibility, and additional payload capabilities ( Baker et al., 2012 ; Romano et al., 2017 ; Huvenne et al., 2018 ) ( Table 1 ). Since the first publication in 1996, ROV systems are becoming increasingly used as a deep-water survey method ( Figure 2 ). High-definition video cameras carried as extra payload provide researchers with permanent records of biota and their habitat associations ( Macreadie et al., 2018 ). While some studies used photography to makes these assessments, these studies were typically focused on mega-benthic taxa ( Salvati et al., 2010 ; Thresher et al., 2014 ; Lacharité et al., 2015 ; Cánovas-Molina et al., 2016 ), and may not be ideal for moving targets such as fish.
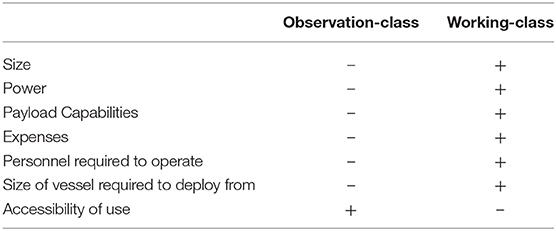
Table 1 . General differences in capabilities between observation-class and working-class ROV systems, with “–” signifying qualities that are less/fewer than and “+” signifying qualities that are more/greater than.
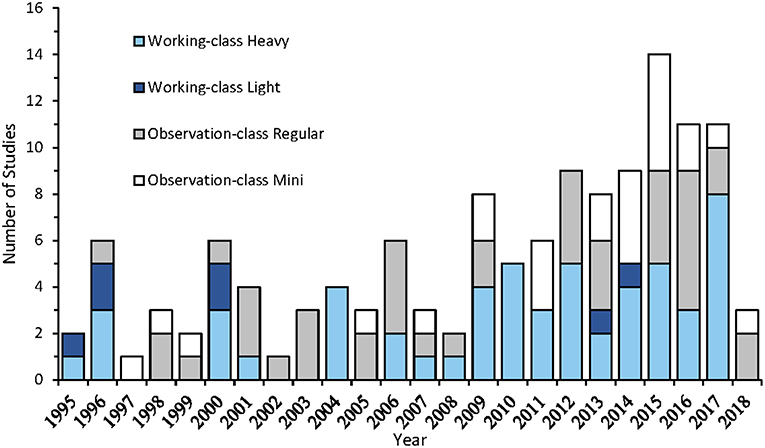
Figure 2 . Temporal trends in ROV publications using observation-class (Mini: White; Regular-sized: Light Gray), and working-class (Light: Dark Blue; Heavy: Light Blue) ROV systems to visually assess fish assemblages.
With advances in technology, a wider range of ROV models are becoming available, including many low-cost models ( Figure 3 ), allowing researchers greater access to deep-water environments. New gear developments have also led to the creation of hybrid ROV designs capable of autonomous deployments without an external energy source ( Huvenne et al., 2018 ). For example, the Boxfish ROV ( https://www.boxfish-research.com/ ) or BlueROV2 ( https://www.bluerobotics.com ) uses battery packs that last between 3 and 6 h that can be periodically exchanged for charged batteries on-board the vessel. As the umbilical tether no longer supplies power to the ROV system, the tether thickness is reduced, improving ROV maneuverability. Open source files on the construction of ROVs from low-cost materials have further increased the accessibility of these systems to researchers and to the public, such as the OpenROV initiative ( https://www.openrov.com ) ( Jessup, 2014 ).
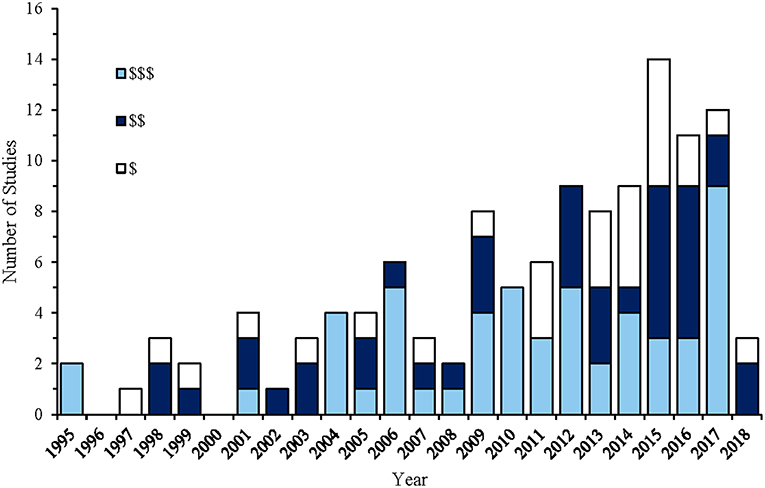
Figure 3 . Temporal trends in the approximate cost of ROV's that have been used in studies to visually assess fish assemblages, with $, Tens of thousands; $$, hundreds of thousands; and $$$, millions of Australian dollars.
Depths/Locations Surveyed
High maneuverability and deep-water capabilities allow ROVs to make fine-scale assessments of fish assemblages over a wide range of habitats (high relief, ledges, crevices) and depth distributions that may not be suitable for other methods. For example, on highly complex substrates in the Caribbean, Quattrini et al. (2017) determined that 42% of fish species were found at greater depths than previously recorded. The specific depth capabilities of each ROV system varies, with smaller observation-class models typically surveying shallower waters than working-class models ( Table 2 ; Figure 4 ). ROV surveys have been conducted off the coast of all continents, with the vast majority of studies having been undertaken off the coast of the United States and Europe ( Figure 5 ). While many studies have taken place in the Mediterranean Sea, the Gulf of Mexico and the north-east and north-west Atlantic Ocean, few studies have been done in the southern hemisphere and Asia ( Figure 5 ).
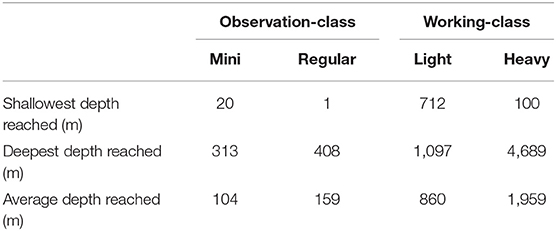
Table 2 . The shallowest, deepest, and average maximum depth (m) achieved by different classes of ROV systems to visually survey fish assemblages.
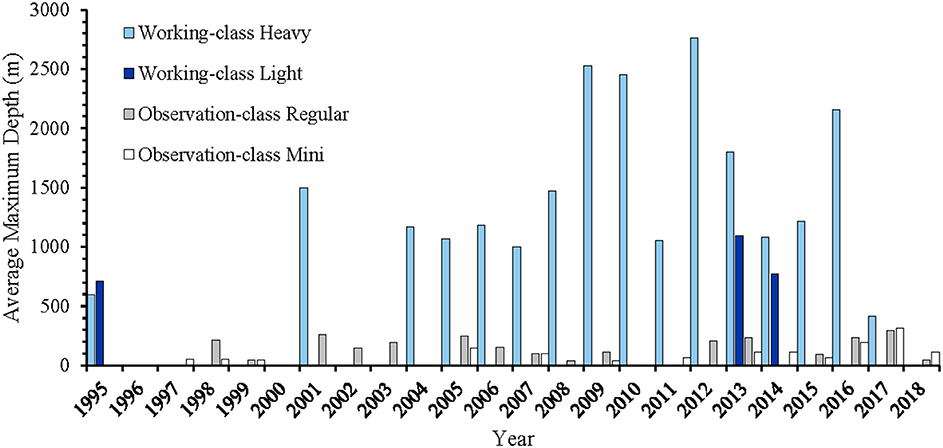
Figure 4 . Temporal trends in the average maximum depth (m) surveyed using mini-sized observation-class (white), regular-sized observation-class (Light Gray), light working-class (Dark Blue), and heavy working-Class (Light Blue) ROV systems.
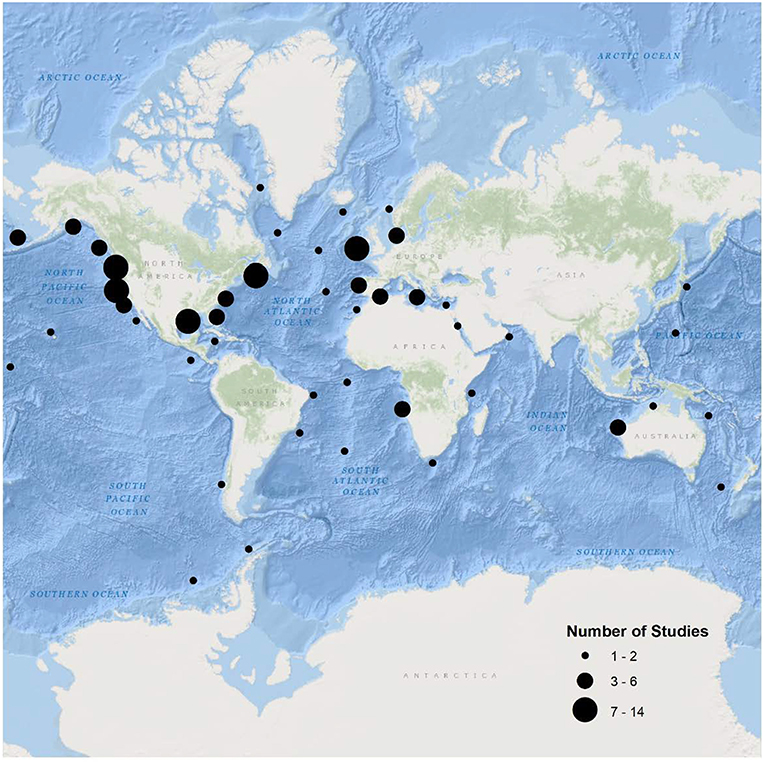
Figure 5 . The location of studies using ROV surveys to visually assess fish assemblages identified using the keywords “remotely operated vehicle*,” “ROV or ROV's,” and “fish or fishes” in the search engines Scopus™ and Google Scholar™.
ROV-Based Surveys
The extensive range of motion capable by ROVs provides new possibilities in surveying methodology not capable by other deep-water (>30 m) or video-based survey techniques. ROV-based sampling strategies often reflected the aims of the study, which could be classified into six major types, (1) surveys in natural habitats, (2) surveys on artificial structures, (3) surveys in marine protected areas (MPAs), (4) opportunistic/exploratory surveys without the use of transects, (5) studies that evaluate the effectiveness of ROVs, and (6) studies that compare ROVs with other survey methods. While ROV surveys have primarily used horizontal strip transects, similar to SCUBA-based underwater visual counts (UVCs), or unstandardized transects ( Figure 6 ), alternative sampling strategies and transect designs (i.e., cloverleaf, radial, and polygonal patterns) are achievable ( Table 3 ; Figure 7 ).
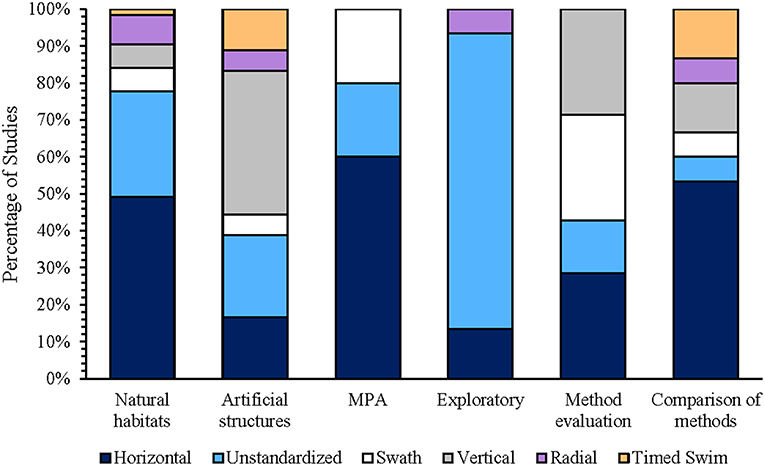
Figure 6 . The percentage of studies using horizontal transects (standardized strip/line transects), exploratory transects (unstandardized transects used to make initial baseline inventories of species and community structure), swath transects (strip transects connected to form a grid-like pattern), vertical transects (mobile point count, continuous roving transect, depth-interval transect, vertical strip transect), radial transects (strip transects radiating from a central point), and timed transects (rapid visual count, timed swim, timed stationary counts, and modified timed swim with timed stationary counts) in surveys in natural habitats ( n = 62 studies), studies on artificial structures ( n = 19 studies), surveys in marine protected areas ( n = 5 studies), exploratory surveys ( n = 15 studies), surveys evaluating the effectiveness of ROV -based san1pling strategies ( n = 7 studies), and surveys comparing ROVs against other surveying methods ( n = 15 studies).
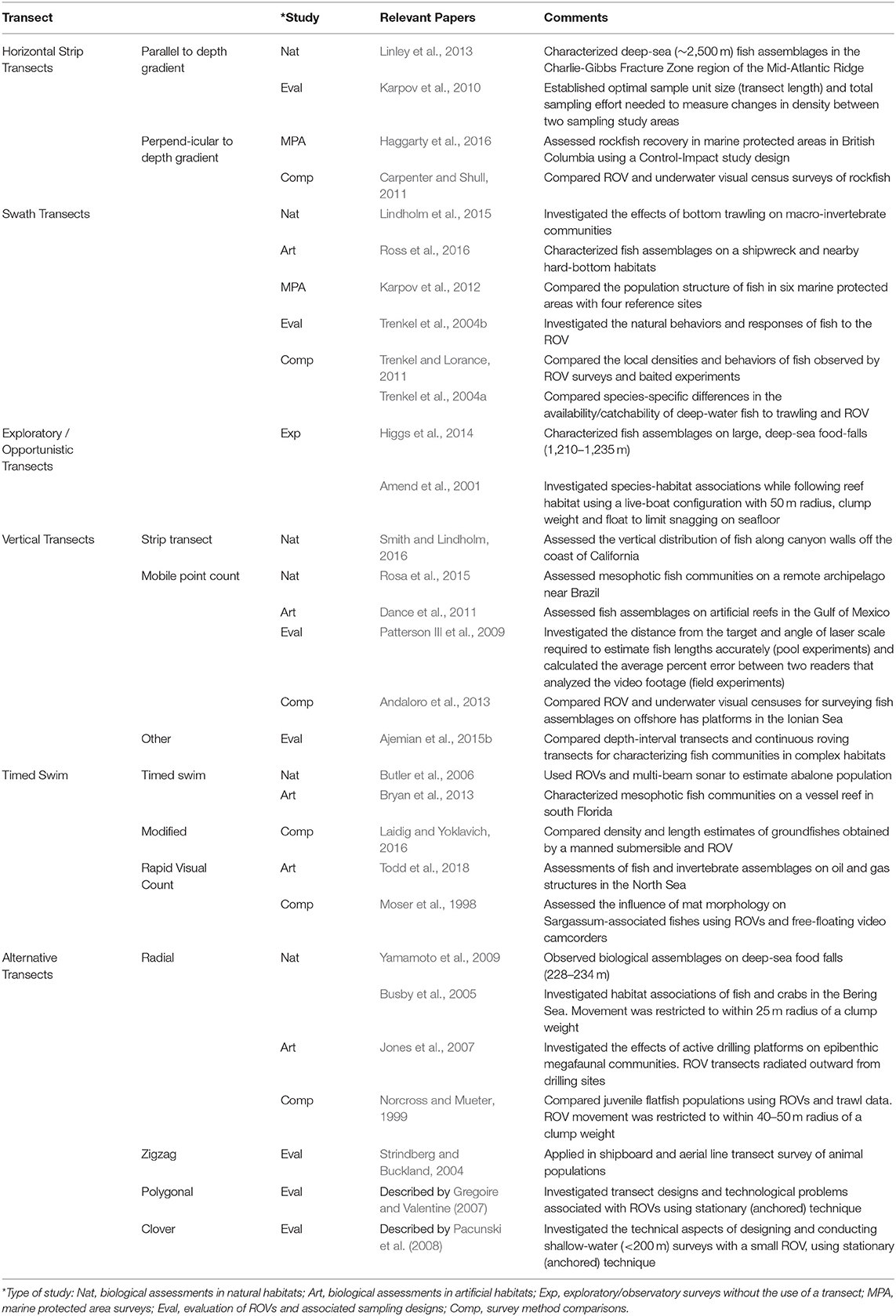
Table 3 . Transect designs used in ROV studies to visually survey fish assemblages.
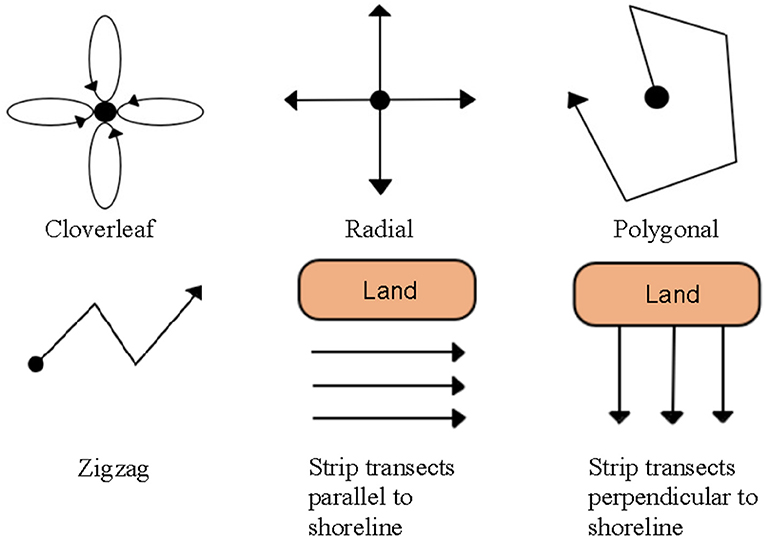
Figure 7 . Different transect designs available for visual surveys of fish assemblages using ROVs.
Metrics Scored From ROV Imagery
Although an extensive array of sampling metrics has been used to analyze ROV surveys, total abundance, diversity, body length estimates, and natural behaviors were consistently used between different study types ( Figure 8A ). While surveys in natural habitats have used each type of sampling metric to annotate the video footage, surveys in marine protected areas have only used total abundance, diversity, body lengths, and natural behaviors of fish communities ( Figure 8B ). Total abundance, the total number of individuals recorded throughout the transect, was the most frequently used metric ( Figure 8B ; 86% of studies), and was often converted into catch-per-unit-effort ( Amend et al., 2001 ) or catch-per-unit-time ( Söffker et al., 2011 ; Bryan et al., 2013 ) to facilitate comparisons across locations and between different survey methods ( Adams et al., 1995 ; Pita et al., 2014 ). As total abundance assumes sampling independence of individuals, fish that can swim faster than the ROV or are attracted to the ROV system itself may result in recounts that could potentially inflate population estimates. Alternative abundance metrics, such as relative abundance (MaxN)—the maximum number of individuals in a deployment ( Ajemian et al., 2015a , b ; McLean et al., 2018 ), and weighted encounters—where scores are assigned based on the order or frequency that species are seen on transect ( Moser et al., 1998 ; Pradella et al., 2014 ), were used to mitigate the effect of individual recounts. This metric was most often used on artificial structures (33% of studies), where multidirectional movement along the structure often resulted in fish overtaking the ROV. Percent cover was the least used sampling metric, accounting for one publication that assessed the distribution of juvenile silver hake ( Auster et al., 1997 ).
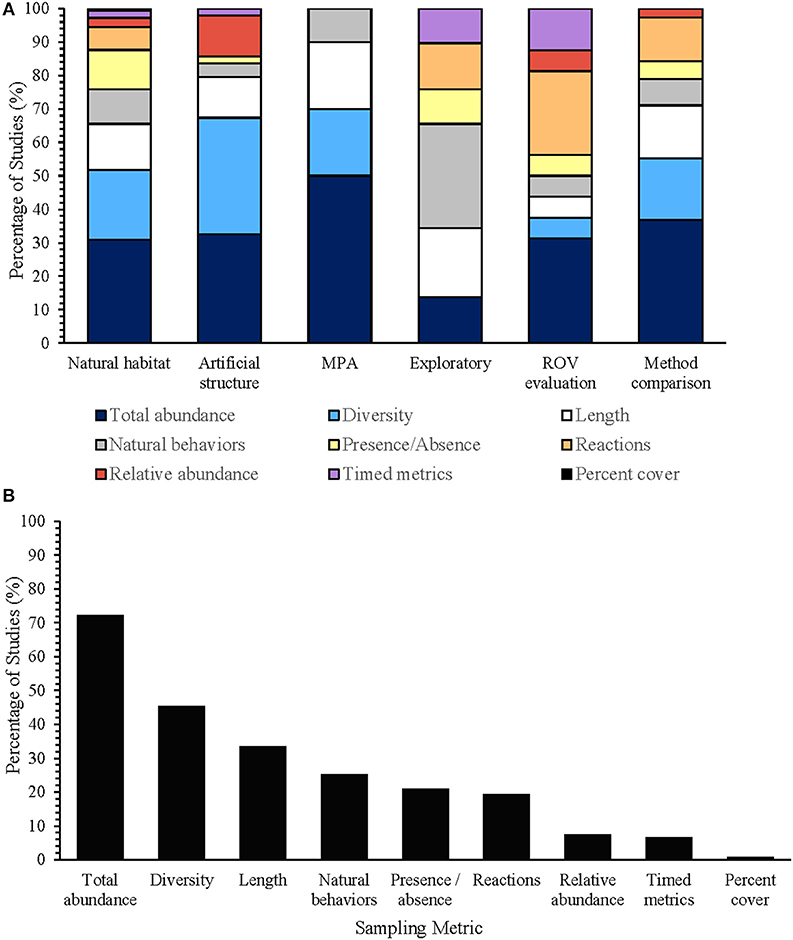
Figure 8 . The percentage of studies using each sampling metric for fish population assessments in natural habitats ( n = 62 publications), for population assessments on artificial structures ( n = 19 publications), for studies in marine protected areas (MPAs) ( n = 5 publications), for exploratory studies without the use of standardized transects ( n = 15 publications), for studies evaluating the effectiveness of ROV methodology ( n = 7 publications), and for studies comparing ROVs against other surveying methodology ( n = 15 publications) (A) , and the percentage of studies using each sampling metric to annotate video footage in surveys ( n = 119 publications) (B) .
Diversity indices were also commonly used for ROV surveys ( Figure 8B , 45% of studies), where they have been used to describe the total number of species recorded per deployment, species richness (S) ( Carpenter and Shull, 2011 ; Consoli et al., 2016 ), and the evenness of each species within the community, Pielou's evenness (J'), Shannon's diversity index (H'), and Simpsons diversity index ( Johnson et al., 2003 ; Harter et al., 2009 ; Ajemian et al., 2015a ; Quattrini et al., 2017 ). Percent occurrence—the sum of transects in which a species was observed, was also used as a measure of diversity across years with different sample sizes ( Auster et al., 1997 ; Pacunski et al., 2013 ). Presence/absence ( Figures 8A,B ) has also been used as a versatile metric for ROV-based studies with unbalanced survey designs ( Duffy et al., 2014 ), where they are effective for inferring distribution ranges and the catchability of species to different survey methods ( Karpov et al., 2004 ).
As a video-based method, ROVs can obtain accurate body length measurements of fish, without the destructiveness of traditional methods, such as trawling or hook, and line. These body length measurements can be used to assess trophic structure ( Auster et al., 2003 ; Dance et al., 2011 ), which is valuable for evaluating the condition of ecosystems and for making comparisons between marine reserves and adjacent habitats. Both calibrated stereo-video and scaling lasers have been used to estimate the fish length. A study by Dunlop et al. (2015) found that while stereo-video was less influenced by the orientation and height of the organism recorded, it took considerably longer than lasers to analyze. Length estimates have also been used to standardize sampling area by limiting population counts to a certain transect width and distance in front of the camera's field-of-view (FOV), while maintaining a constant height above the seafloor ( Mapula et al., 2016 ). Current strength and other weather conditions, however, may interfere with the ROV's ability to maintain a constant position in the water column, leading to inconsistent sampling areas ( Mapula et al., 2016 ). Furthermore, studies in turbid waters and studies that survey small-bodied or cryptic species may require the ROV to be flown closer to the seafloor, decreasing the FOV. Variations in applications have thus led to differences in the height above the seafloor (0.2–3.0 m), the transect width (0.35–6.0 m) and distance recorded in front of the camera (0.5–4.0 m), which may limit comparisons between surveys and locations. Larger, working-class vehicles typically also recorded larger FOVs than with observation-class systems ( Trenkel et al., 2004a , b ; Trenkel and Lorance, 2011 ; Baker et al., 2012 ).
Although initially used for determining substrate composition in deep-water biological assessments as a replacement for manned submersibles ( Koenig et al., 2005 ), advancements in video quality and in ROV technology have allowed ROVs to become a more practical and affordable method for providing assessments for a wide variety of flora and fauna, including elasmobranchs ( Benz et al., 2007 ; Henry et al., 2016 ), teleost fish ( Carpenter and Shull, 2011 ; Haggarty et al., 2016 ), cephalopods ( Smale et al., 2001 ; Zeidberg and Robison, 2007 ), gastropods ( Butler et al., 2006 ; Stierhoff et al., 2012 ), macro-algae ( Spalding et al., 2003 ), corals ( Doughty et al., 2014 ; Etnoyer et al., 2018 ) and other macroinvertebrates ( Grinyó et al., 2016 ; Hemery and Henkel, 2016 ). While ROVs can provide in situ observations of fish, their behaviors and habitat-associations that cannot be determined with traditional methods (i.e., trawls, longline) ( Adams et al., 1995 ; Karpov et al., 2004 ; Linley et al., 2013 ), ROV-based sampling strategies must account for the unique challenges of surveying mobile organisms that are not applicable in surveys of sessile invertebrates and substrate.
Behaviors naturally exhibited by fish ( Figures 8A,B ), such as swimming, feeding, and resting ( Baker et al., 2012 ), may allow for a better understanding of small-scale influences on fish distribution ( Lorance and Trenkel, 2006 ) and indicate behaviors that may result in over-counting ( Ajemian et al., 2015a ). Behavioral reactions of fish to ROVs have been frequently documented ( Figures 8A,B ), and are important for establishing baseline information for species and locations, evaluating the reliability of data generated for this method, and for making comparisons against other survey methods. Baker et al. (2012) used a basic scale of attraction, avoidance and no reaction, whereas Adams et al. (1995) used a scale that differentiated between weak and strong responses of attraction and repulsion. The type and severity of the reaction to the ROV can be influenced by a variety of factors, including the species, trophic position, and the body size and position of the individual relative to the seafloor as well as to different aspects of the ROV system (i.e., artificial lighting, thruster noise, speed) ( Smale et al., 2001 ; Lorance and Trenkel, 2006 ; Stoner et al., 2008 ; Yamamoto et al., 2009 ; Söffker et al., 2011 ; Mapula et al., 2016 ). For example, Laidig et al. (2012) found that more fish reacted to the regular-sized observation-class ROV (57%) than to a larger, manned submersible (11%). The fish that did react to ROV presence were typically smaller-bodied individuals, individuals >1 m above the seafloor and species that aggregate ( Laidig et al., 2012 ). Intuitively, ROVs traveling at greater speeds may increase the frequency and severity of reactions displayed and decrease the probability that cryptic individuals will be detected ( Pacunski et al., 2008 ). However, this review was unable to locate any studies that specifically investigated the effects of different speeds on behavior. Furthermore, while most studies attempted to maintain a relatively constant speed ( Meirelles et al., 2015 ), the actual speed traveled was only calculated for 26% of studies. Speeds reported varied between studies (0.1–1.0 m/s) and within each study ( Quattrini et al., 2017 ), often as a result of current and drag. Standardizing deployment speed, however, is logistically difficult, but may ultimately become more achievable with advancements in thruster technology.
Artificial lighting is another important factor known to influence organism behavior ( Smale et al., 2001 ), but is a critical component of night-time sampling, for surveys beyond the photic zone and in areas of high turbidity, and for improving the detection of small-bodied or cryptic species, such as flatfish ( Norcross and Mueter, 1999 ; Pacunski et al., 2013 ). In an ROV experiment comparing the behavior of sablefish ( Anoplopoma fimbria ) to different lighting conditions around a bait source, Widder et al. (2005) found that more fish avoided white light than red light. While the majority of ROV studies (~67%), did not indicate whether lights were used, the depth surveyed by many of these studies suggests that most would have used some form of artificial lighting. The type and intensity of lighting used for ROV surveys, however, were either unspecified (~16% of studies) or varied considerably. Even though the distribution of many species (e.g., Sebastes spp) are influenced by the time of day ( Hart et al., 2010 ), few studies (~2%) used nocturnal sampling. Timed metrics, such as time at first sighting—the time when a species was first seen ( Norcross and Mueter, 1999 ; Ajemian et al., 2015b ; Smith and Lindholm, 2016 ), and the duration of encounter ( Laurenson et al., 2004 ; Luck and Pietsch, 2008 ; Trenkel and Lorance, 2011 ; Mundy et al., 2018 ), were infrequently used for ROV studies of fish ( Figures 8A,B ; n = 8 studies), but are likely a reflection of species-specific behaviors. Timed metrics have been used primarily in exploratory surveys for obtaining baseline information on species ( Luck and Pietsch, 2008 ; Mundy et al., 2018 ) and in studies evaluating ROVs ( Figure 8A ), where the distance traveled on deployment before first sighting may indicate the sampling power required to survey different species ( Cappo et al., 2007 ).
Use in Natural Habitats
Biological assessments provide crucial information on population dynamics and species-habitat associations necessary for monitoring and conservation. These assessments must employ sampling strategies that are able to survey fish effectively and representatively over major habitat features across large distances ( Trenkel et al., 2004a ). Bathymetric maps created by multibeam echosounder (MBES) can be used to locate and stratify sampling over ecologically important habitat structures, such as reefs or other areas of high relief ( Jones et al., 2012 ; Linley et al., 2013 ). Ultra-short baseline (USBL) or long baseline (LBL) transponders can then be used to track and record the precise deployment path taken over multibeam-derived features ( Stierhoff et al., 2013 ). This allows for accurate estimations of transect length and for the specific location of individual sightings to be determined for a better understanding of microhabitat features ( Ajemian et al., 2015b ).
Horizontal strip transects are a straight-forward and well-established surveying strategy for providing standardized assessments of fish assemblages ( Johnson et al., 2003 ) that are accessible to most ROV systems. Strip transects that are placed parallel to the coastline or isobath allow for greater replication within depth bands, while strip transects placed perpendicular to the coastline or isobath with deployments moving shallower increase the amount of time the seafloor is in view, but may decrease the length of transects on steep topography ( Pacunski et al., 2008 ). Deployments in high wind conditions and current, however, may be limited to traveling down-current from the starting location.
The length of the umbilical tether and the current-induced drag on the tether cord ( Ajemian et al., 2015b ) can influence the distance the ROV can travel from the operating vessel. A “live-boat” technique, in which a clump weight is attached to the umbilical tether a short distance above the seafloor ( Amend et al., 2001 ; Bryan et al., 2013 ; Haggarty et al., 2016 ), can be used to maintain the ROV at depth ( Yamamoto et al., 2009 ) while allowing the unanchored vessel to move freely with the ROV during deployment ( Pacunski et al., 2008 ). For locations that require additional stabilization from the current, particularly for systems with shorter tethers, and are at depth shallow enough to anchor the vessel, clump weights can be used to secure the umbilical tether to the seafloor, where it acts as a central starting point for radial transects. Radial transects, however, can be time-consuming and is not practical for studies using long transect lengths (>100 m) ( Pacunski et al., 2008 ). Although timed swims are an efficient approach, inconsistent sampling effort between surveys and locations indicate that this method should be used sparingly when the survey area cannot be determined or for validating transect lengths generated by the USBL tracking system.
Use on Artificial Structures
Artificial structures, such as decommissioned oil and gas platforms ( Adams et al., 1995 ; Andaloro et al., 2013 ) and artificial reefs ( Patterson III et al., 2009 ; Dance et al., 2011 ) provide substrate for coral reef and sessile invertebrates to attach, creating the habitat complexity necessary for marine communities to prosper. Working-class ROVs have been a long-established tool for inspecting and maintaining underwater pipelines, promoting advancements in these systems as well as the opportunity for researchers to work alongside these companies to collect data on marine communities ( Gates et al., 2017 ). Archived video footage of underwater pipelines can be analyzed into short strip transects to provide fine-scale population assessments of fish biodiversity ( McLean et al., 2017 ). Swath transects can be used to survey fish nearby and over low-relief artificial substrate, such as sunken vessels ( Ross et al., 2016 ), whereas radial transects are effective for assessing the influence of high-relief artificial structures on nearby biological communities ( Taylor et al., 2014 ).
As many artificial structures have complex features and areas of high relief, alternative sampling strategies that incorporate vertical movement while minimizing the possibility of tether entanglement need to be considered. Bryan et al. (2013) used a modified timed swim along the hull of the vessels with a series of timed stationary counts to aid in identifying small-bodied and cryptic species, standardized to within 3 m of the reef. On larger artificial reefs, Ajemian et al. (2015b) , compared continuous roving transects, a UVC-based approach whereby the ROV follows horizontal transects at the bottom and top of the reef, with depth-interval transects, a modified type of mobile point count that replaces 360-degree spins with stationary timed counts at pre-set depth intervals to avoid tether entanglement. This study found that continuous roving transects were more effective at surveying fish with patchy distributions, including several rare species, whereas depth-interval transects were more effective at recording overall fish community composition, were able to estimate fish densities at distinct depth strata and reduced time spent processing video footage. As sampling independence of individuals would be difficult to ensure for many of these approaches, relative abundance would be a more appropriate sampling metric than total abundance for surveys on artificial structures.
Use in Marine Protected Areas
Marine protected areas (MPAs) are a widely recognized tool for conservation that have been shown to increase the overall density and biomass of organisms within the MPA and the surrounding ecosystem ( Barrett et al., 2007 ; Haggarty et al., 2016 ). Exploratory transects are beneficial for characterizing the types and quantities of different habitats and obtaining baseline information on community structure and species distributions to inform proposed MPA designs ( Quattrini and Ross, 2006 ) and to concentrate future sampling efforts ( Butler et al., 2006 ). In studies investigating MPA effects, sites within each reserve (impact) are compared with fished location(s) outside of the reserve that have similar depths and habitat profiles (control) ( Karpov et al., 2012 ). While studies that employ a greater number of control sites are less likely to be influenced by site-specific differences in community, sites nearer to the MPA are more likely to be influenced by spill-over effects ( Karpov et al., 2012 ). Collecting data before and after the establishment of a MPA, as part of a before-after-control-impact (BACI) approach, would account for the natural differences between MPAs and controlled sites, which may more accurately reflect the influence of disturbance events, such as trawling ( Lindholm et al., 2015 ). Since the first publication in 2003, five studies have examined the influence of MPAs on fish communities using ROVs ( Auster et al., 2003 , 2016 ; Harter et al., 2009 ; Karpov et al., 2012 ), with only one employing a BACI sampling design ( Haggarty et al., 2016 ).
Optimal sampling designs must consider the trade-off between transect lengths that are able to detect target species significant quantities and the number of replicates that are required to detect population change within and outside of the MPA. Studies using observation-class ROVs would more effectively survey an area using a greater number of replicate transects, whereas working-class systems can employ large swaths (>500 m segments) to survey marine protected areas ( Karpov et al., 2012 ; Lauermann, 2014 ). Given the few sampling strategies identified, research into alternative sampling designs may provide better insight into strategies that can more effectively detect differences in biological communities between protected and fished sites. However, as gear-selective biases can influence the types and quality of data that are obtained by different survey techniques, one approach should be employed throughout the duration of a BACI study.
Exploratory Surveys
Exploratory studies are important for collecting baseline information in locations with little a priori knowledge, in order to obtain a general understanding of species distributions necessary to inform future research directives ( Hall-Spencer et al., 2002 ). These studies generally maintain a relatively straight-line trajectory but may deviate from the intended route to investigate specific habitat features ( Thresher et al., 2014 ). For rarely documented deep-water species, such as sleeper sharks ( Benz et al., 2007 ) and angler fish ( Ho and McGrouther, 2015 ), as well as unique deep-water communities, such as at whale falls ( Lundsten et al., 2010 ; Higgs et al., 2014 ), collecting information on morphological characteristics and behaviors is crucial for understanding the ecology of organisms in these environments. However, as the FOV is unstandardized, absolute abundances cannot be estimated ( Stein et al., 2005 ; Caldwell et al., 2016 ). As a result, exploratory studies tend to collect qualitative rather than quantitative data ( Söffker et al., 2011 ), which may hamper comparisons between locations and studies.
Method Evaluation Studies
Evaluating the effectiveness of ROVs and ROV-based sampling designs for surveying biological assemblages is essential toward understanding the capabilities of this method. Trenkel et al. (2004a) determined that spatial dispersion of individuals had the greatest effect on between-species variation, with aggregating species more susceptible to ROVs than those that were randomly or uniformly distributed. Optimal sampling units (i.e., transect lengths and number of replicates) are dependent on species distributions within the study location, with longer transects increasing the probability of detecting rare and cryptic species and species with patchy distributions ( Norcross and Mueter, 1999 ; Karpov et al., 2004 , 2010 ; Pacunski et al., 2013 ), but decreasing the overall number of replicates used, due to time constraints ( Trenkel et al., 2004b ). Furthermore, the number of species accumulated with increasing transect length will eventually plateau, decreasing overall sampling efficiency. Karpov et al. (2010) used a power analysis to assess the relationship between rockfish density and variance on strip transects of different sizes (50, 100, 200, 400, and 800 m 2 ) to determine the optimal sampling unit for surveying rockfish off the west coast of the United States. This study evaluated a few sampling strategies, including randomly allocating transects of different lengths across depth and relief strata, and by using long 800 m 2 transects broken up into different-sized transect segments that were either systematically placed parallel to the shoreline or randomly placed within 500 m 2 wide rectangular areas ( Karpov et al., 2010 ). Adams et al. (1995) defined statistical power as the sampling size required to detect a 50% reduction in the transformed mean abundance at a power of 0.8 and an α of 0.05. Karpov et al. (2004) , on the other hand, argued that sample sizes that can detect 1.5 times less than the sample mean may be more practical for detecting depleted species and species that typically undergo large-scale population changes. Furthermore, this study suggested that only species with abundances that can be detected within 3 times the sample mean can be reliably monitored by ROVs ( Karpov et al., 2004 ). The lengths of transects used in ROV surveys of fish, however, varied considerably between studies (0.05–20 km) and even within individual studies ( Du Preez and Tunnicliffe, 2011 ; Baker et al., 2012 ; Duffy et al., 2014 ). While numerous approaches have been used to increase the sampling efficiency of ROV surveys, including towing the ROVs behind vessels ( Pierdomenico et al., 2016 ) or tethering the ROVs to camera sleds ( Quattrini et al., 2017 ), these approaches increase deployment speed at the expense of maneuverability ( Mortensen et al., 2008 ). Sophisticated working-class systems that can maintain a precise deployment path, on the other hand, can reduce time spent deploying and retrieving the ROV for each transect by either dividing long strip transects (>500 m) into separate replicates ( Karpov et al., 2010 ) or by connecting strip transects in a continuous grid-like pattern to form a swath transect.
Investigating how different aspects of the ROV apparatus (i.e., lights, thruster speed, size) affect fish behavior may give insight into species-specific differences in gear-selectivity and allow for specific aspects that minimize behavioral bias to be identified. Spanier et al. (1994) used laboratory and field experiments to investigate how different components of the ROV system influence the boldness and feeding behaviors of American lobsters ( H. americanus ). In laboratory experiments, tanks that had ROVs (treatment) were compared with tanks that did not have ROVs (control). Alternatively, field experiments compared ROVs that had lights, thruster speed set to 50% and a camera flashing every 15 min or whenever the lobster appeared out of its den (treatment) with ROVs that had the lights, thrusters and camera flash turned off (control) ( Spanier et al., 1994 ). This study provided the first scientific evidence of behavioral bias toward ROVs but was unable to identify specific variables that contributed toward changes in lobster behavior as the different components were not individually tested. While Trenkel et al. (2004b) did assess the attraction and repulsion of fish to different intensities of light (1,200 and 2,700 W) and survey speeds (0.25 m/s and 0.5 m/s) of an ROV, this study was unable to generate quantitative estimates of fish abundance.
Method Comparison Studies
Method comparison studies allow researchers to assess the capabilities of each method for surveying fish in different habitats while under the same environmental conditions within the study location as well as the species-specific behaviors and reactions to each survey method that leads to differences in the strengths, limitations, and biases of these methods ( Table 4 ). As a result, comparison studies in different locations or survey different biota may lead to variations in performance. Study designs for method comparison studies must take into consideration the types of sampling metrics used to annotate the imagery. For instance, true abundance estimates obtained by ROV cannot be used to make reliable comparisons to relative abundance estimates collected by stationary methods, such as baited remote underwater video systems (BRUVS). However, studies using these two methods may be able to provide comparisons of community structure and the efficiency and cost of each method. The specific capabilities of ROVs in comparison to other survey methodology should, therefore, be considered when developing appropriate experimental designs ( Table 4 ).
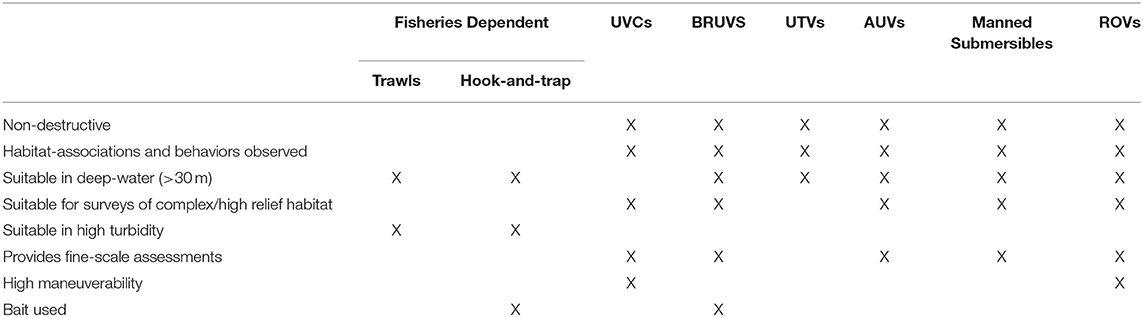
Table 4 . Differences in method application and the advantages and disadvantages of each method when compared with ROVs.
ROV surveys provide direct and non-destructive observations of habitat associations and behaviors not attainable by fisheries-dependent methods, such as longline and bottom trawling ( Busby et al., 2005 ; Bicknell et al., 2016 ; Consoli et al., 2016 ; Mapula et al., 2016 ). Trawls and ROV surveys operate at much different scales, with ROVs intensively sampling a narrow area directly in front and extending up a short height off the bottom, and trawls sampling a much wider area, including a larger area off the bottom, but with greater number of escaped fish ( Adams et al., 1995 ). Consequently, trawls are able to cover large enough areas to compensate for local variability, whereas ROVs must select sampling designs that either employ long strip transect lines or have enough replication selectively placed over targeted habitat features in order to account for patchy distributions ( Norcross and Mueter, 1999 ). The mechanical nature of the sampling gear has resulted in major differences in the type of fish assemblages captured by each method. The catch-per-unit-effort was often higher for ROVs ( Norcross and Mueter, 1999 ) and with lower coefficients of variation than fish obtained by trawls ( Adams et al., 1995 ). While small, benthic, cylindrically-shaped fishes were more susceptible to ROVs ( Adams et al., 1995 ), juveniles under 100 mm ( Norcross and Mueter, 1999 ) and species with larger bodies that were further from the seafloor were more susceptible to trawling ( Trenkel et al., 2004a ). Overall, ROV surveys are better-suited to environmental assessments as higher abundances allowed for smaller population changes to be detected ( Adams et al., 1995 ). In a study comparing the ability of bottom trawls, a stereo-drop camera system (SDC) and ROVs to discriminate between rockfish species, bottom trawl was the most effective, while SDC was the least effective ( Rooper et al., 2012 ). Bottom trawl and SDC, on the other hand, were able to record a larger number of fish measurements. A study by Karpov et al. (2004) comparing hook-and-trap and ROV methods determined that ROVs were more efficient than the hook-and-trap approach, but took considerably longer in post-processing. Furthermore, as hook-and-trap attracts fish from larger areas, rockfish abundances can be overinflated, making these methods less sensitive to actual stock declines ( Karpov et al., 2004 ).
ROVs are able to survey an extensive range of habitats, including deep-water (>40 m) and hazardous environments, that are not attainable by UVCs ( Busby et al., 2005 ; Boavida et al., 2015 ). As a SCUBA-diver based method, UVCs requires training and are limited by the time and the depth divers can spend underwater as well as by the diver's ability to quickly and accurately identify and count fish assemblages ( White et al., 2013 ), which can lead to inconsistent results between divers. While differences between annotators may also lead to discrepancies when processing ROV data, video footage can be reviewed indefinitely with the help of experts if necessary. UVCs were found to be more effective than ROVs at obtaining reliable representations of fish communities at shallow depths (0–6 m and 12–18 m), particularly for crypto-benthic and nekton-benthic species ( Andaloro et al., 2013 ). While Carpenter and Shull (2011) found similar results in a study comparing ROVs with paired-diver surveys of rockfish, the depth that fish densities were highest were below those attainable by conventional SCUBA-diver equipment. Therefore, despite differences in performance, ROVs are still able to access deeper depths not available to UVCs, making it a more robust biological survey tool ( Andaloro et al., 2013 ).
UTVs and BRUVS are well-established methods of obtaining non-destructive assessments of fish communities ( Karpov et al., 2004 ; Trenkel et al., 2004a ). UTVs provide rapid assessments of biodiversity over large distances ( Assis et al., 2007 ), whereas the multidirectional thruster-power capabilities of ROV systems allow for greater maneuverability around complex and high relief environments, such as rocky reefs, resulting in more detailed observations of biodiversity and improving the detection of cryptic and rare species ( Consoli et al., 2016 ). BRUVS, on the other hand, have been demonstrated as an effective monitoring tool for monitoring carnivorous, omnivorous, and herbivorous fishes throughout Australia ( Harvey et al., 2007 ; Watson et al., 2010 ; Caldwell et al., 2016 ). BRUVS use bait to attract fish in large abundances to the sampling area, avoiding many of the problems associated with zero-inflated datasets and increasing the statistical power to detect change ( Cappo et al., 2003 ; Watson et al., 2005 ; Malcolm et al., 2011 ; Dorman et al., 2012 ). However, individuals attracted to the bait from the surrounding environment may bias habitat associations toward the site being surveyed. Given that BRUVS are unable to be deployed over highly complex environments, species associations may be further skewed toward more accessible habitat types. Additionally, bait plume variability can also influence the sampling area of attraction, making standardizing survey effort logistically difficult ( Heagney et al., 2007 ; Wraith et al., 2013 ). While ROVs can survey a larger area over a greater range of habitats, BRUVS often record greater species densities in the smaller area sampled.
Few studies have compared ROV with Autonomous underwater vehicles (AUVs) and manned submersibles, despite employing similar methodology. In a study comparing the abundances and lengths of fish collected by ROV and manned submersible surveys across different habitats and depths in California, manned submersibles were able to record a greater number of species, body length estimates, and abundances of species that were found closer to the seafloor ( Laidig and Yoklavich, 2016 ). While the number of studies comparing these methods are limited, ROVs are a more practical and inexpensive tool for monitoring ( Koenig et al., 2005 ), and possess greater maneuverability than AUVs and manned submersibles.
The use of ROVs as a non-destructive method for visually surveying fish assemblages is a rapidly growing field with over 100 publications since 1995, and 65% of these studies coming from the last decade. Evaluation of the ROV as a survey method has been undertaken, with several publications finding that ROV-based surveys are comparable to more established survey techniques such as BRUVS or UVC. In the age of globally standardized datasets, this review identified the need for standardization of sampling protocols for ROV-based surveys. While some consistency was identified, with (for example) the majority of studies using heavy working-class ROVs, it is clear that not all researchers have access to these expensive units. Recent technological advancements, however, are improving the performance and practicality of observation-class ROVs, with some models meeting or surpassing the performance of larger-sized models ( Pacunski et al., 2008 ). Optimal transect design need to be selected with consideration of species-specific distributions and characteristics, with patchily distributed or rare/low abundance species requiring either long transect lengths or greater numbers of replicate transects ( Trenkel et al., 2004b ; Perkins et al., 2016 ), noting the latter provides greater statistical power. Finite structures, such as artificial reefs, sunken vessels and oil pipelines, require transect designs that sample fish more intensively within a smaller area. While we have identified a number of commonalities between studies, transect design often appeared to be arbitrarily chosen, with transect type and length, in particular, varying considerably between studies. We suggest further research is required to better guide researchers in how to select the most appropriate transect design for their particular study. For example, one benefit that is almost never applied when using ROVs is the ability to alter transect designs as required. Meaning, for example, strip transects could be altered to include multiple timed stationary counts at specific features of interest should they occur (such as caves). It should be noted that strict protocols need to be implemented around such a sampling strategy to reduce operator bias.
We identified nine different metrics that were extracted from imagery. Clearly, the suitability of some metrics is likely to change depending on the focus of the study (e.g., high relief artificial structures), with species-specific mobility and reaction to the ROV system (i.e., attraction and repulsion) influencing the catchability of individuals as well as the potential that individuals will be recounted during surveys. For highly mobile species and in survey designs where individuals are likely to be recounted (e.g., vertical transects on oil and gas pipelines), relative abundance (MaxN) may be a more robust sampling metric. However, the inherent variation in metrics between studies will restrict or preclude the ability of the datasets being combined in the future to look a larger-scale patterns (noting that video imagery can be reanalyzed if required). Given the extensive range of lights, thrusters, speeds, and ROV sizes available, investigation into behavioral reactions may provide further insight into each of these different aspects, which could then be used toward standardizing more effective survey strategies.
The choice of ROV system appears to be a strong indicator of both the types of surveys available to studies and the success of ROV deployments ( Table 5 ). For instance, larger, more powerful working-class systems are able to complete longer and more complex designs (e.g., swath, cloverleaf, and polygonal transects) at greater depths while maintaining a more standardized deployment route ( Norcross and Mueter, 1999 ), but are more expensive and difficult to deploy. Studies on highly mobile species should also be cognizant of the distance between study sites and the distance between adjacent sections of complex, multi-directional transect designs. Observation-class systems, on the other hand, are typically flown down-current on strip transects and are more susceptible to delays or cancelations of deployments, with some researchers having to modify their intended sampling strategies ( Ruhl et al., 2003 ; Trenkel et al., 2004b ; Bryan et al., 2013 ; Ajemian et al., 2015b ; Rosa et al., 2015 ). Radial transects, or strip transects that employ live-boating or a weight that anchors the tether to the seafloor, can be used to improve the performance of observation-class systems under severe current and swell conditions. As observation-class systems often employ shorter tethers (< 150 m for mini-sized and < 300 m for regular-sized models), radial transects may be used to maximize sampling area at deeper depths and on large vessels that may rotate substantially while anchored. Further research is clearly needed to provide researchers with the guidance needed to strategically choose between transect designs and lengths as well as the type of metrics that should be annotated from imagery. This will ultimately lead to a more rigorous understanding of ROVs to visually survey the distribution and abundance of deep-water marine fish.
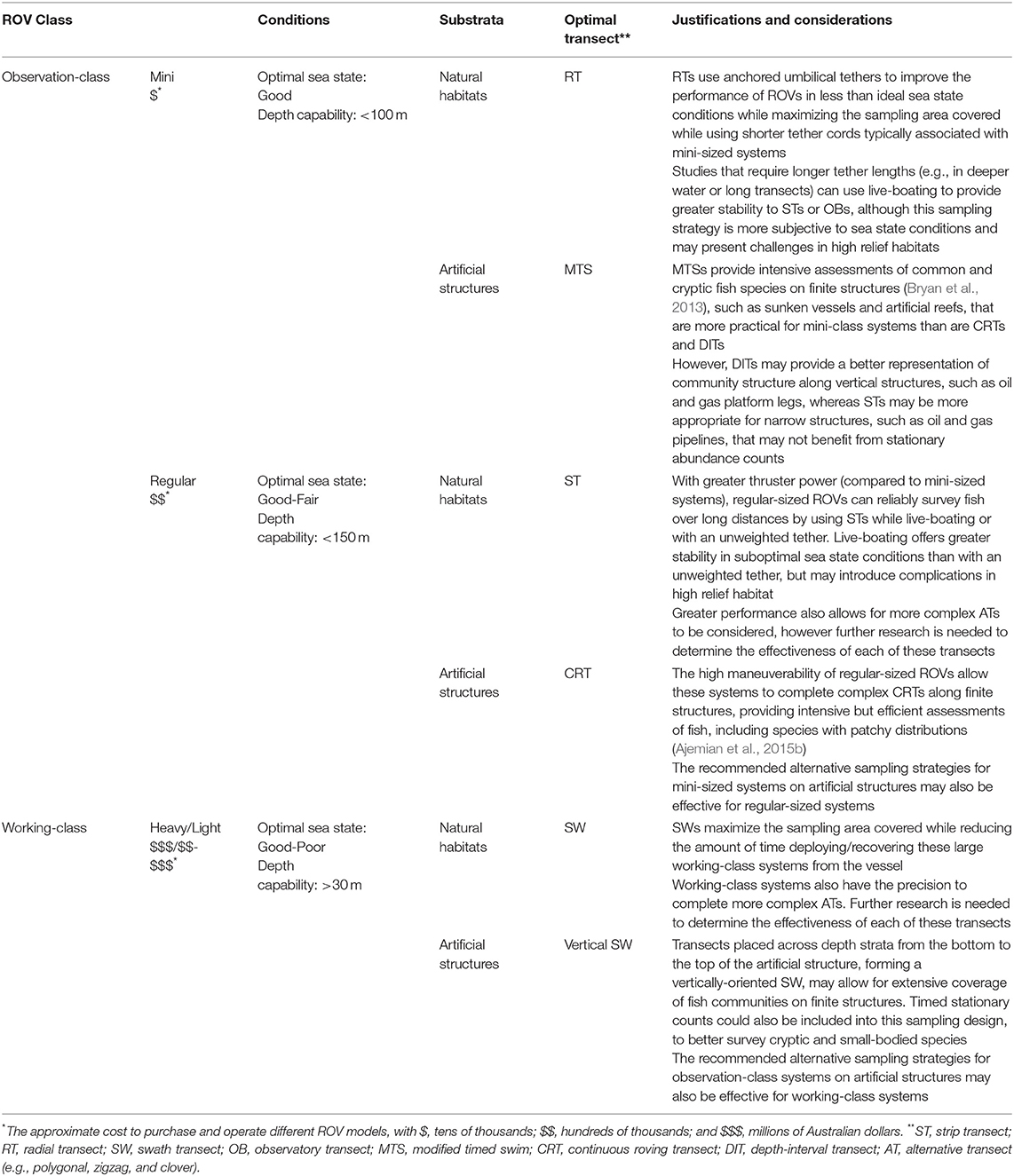
Table 5 . Surveying strategies recommended for each ROV class in different types of studies and environmental conditions.
Author Contributions
DS has provided much of the research and writing into this review. NB and JM provided edits, their expertise, and suggestions for publications to be included.
Conflict of Interest Statement
The authors declare that the research was conducted in the absence of any commercial or financial relationships that could be construed as a potential conflict of interest.
Acknowledgments
This work was undertaken for the Marine Biodiversity Hub, a collaborative partnership supported through funding from the Australian Government's National Environmental Science Programme. NESP Marine Biodiversity Hub partners include the Institute for Marine and Antarctic Studies, University of Tasmania; CSIRO, Geoscience Australia, Australian Institute of Marine Science, Museum Victoria, Charles Darwin University, University of Western Australia, NSW Office of Environment and Heritage, NSW Department of Primary Industries and the Integrated Marine Observing System. DS would like to thank the Oppermans for their encouragement and support of my higher degree education.
Supplementary Material
The Supplementary Material for this article can be found online at: https://www.frontiersin.org/articles/10.3389/fmars.2019.00134/full#supplementary-material
Adams, P. B., Butler, J. L., Baxter, C. H., Laidig, T. E., Dahlin, K. A., and Wakefield, W. W. (1995). Population estimates of pacific coast groundfishes from video transects and swept-area trawls. Fish. Bull. 93, 446–455.
Google Scholar
Addison, P. F. E., Collins, D. J., Trebilco, R., Howe, S., Bax, N., Hedge, P., et al. (2018). A new wave of marine evidence-based management: emerging challenges and solutions to transform monitoring, evaluating, and reporting. ICES J. Mar. Sci. 75, 941–952. doi: 10.1093/icesjms/fsx216
CrossRef Full Text | Google Scholar
Ajemian, M. J., Wetz, J. J., Shipley-Lozano, B., Dale Shively, J., and Stunz, G. W. (2015a). An analysis of artificial reef fish community structure along the northwestern gulf of Mexico shelf: potential impacts of “rigs-to-reefs” programs. PLoS ONE 10:126354. doi: 10.1371/journal.pone.0126354
PubMed Abstract | CrossRef Full Text | Google Scholar
Ajemian, M. J., Wetz, J. J., Shipley-Lozano, B., and Stunz, G. W. (2015b). Rapid assessment of fish communities on submerged oil and gas platform reefs using remotely operated vehicles. Fish. Res. 167, 143–155. doi: 10.1016/j.fishres.2015.02.011
Amend, M., Fox, D., and Romsos, C. (2001). “2001 Nearshore Rocky Reef Assessment ROV Survey,” in Final Report for 2001 Grant Cooperative Agreement PS01053. (Oregon: Oregon Department of Fish and Wildlife, Marine Habitat Project, Marine Program).
Andaloro, F., Ferraro, M., Mostarda, E., Romeo, T., and Consoli, P. (2013). Assessing the suitability of a remotely operated vehicle (ROV) to study the fish community associated with offshore gas platforms in the Ionian Sea: a comparative analysis with underwater visual censuses (UVCs). Helgol. Mar. Res. 67, 241–250. doi: 10.1007/s10152-012-0319-y
Assis, J., Narváez, K., and Haroun, R. (2007). Underwater towed video: a useful tool to rapidly assess elasmobranch populations in large marine protected areas. J. Coast. Conserv. 11, 153–157. doi: 10.1007/s11852-008-0015-x
Auster, P. J., Lindholm, J., and Valentine, P. C. (2003). Variation in habitat use by juvenile Acadian redfish, Sebastes fasciatus. Environ. Biol. Fishes 68, 381–389. doi: 10.1023/B:EBFI.0000005751.30906.d5
Auster, P. J., Malatesta, R. J., and Donaldson, C. L. S. (1997). Distributional responses to small-scale habitat variability by early juvenile silver hake, Merluccius bilinearis . Environ. Biol. Fishes 50, 195–200. doi: 10.1023/A:1007305628035
Auster, P. J., Sánchez-Jiménez, A., Rodríguez-Arrieta, J. A., Quesada, A. J., Pérez, C., Naranjo-Elizondo, B., et al. (2016). Facilitative behavioral interactions between deepwater piscivores at Isla del Coco National Park and Las Gemelas Seamount, Costa Rica. Rev. Biol. Trop. 64, S187–S196. doi: 10.15517/rbt.v64i1.23425
Baker, K. D., Haedrich, R. L., Snelgrove, P. V. R., Wareham, V. E., Edinger, E. N., and Gilkinson, K. D. (2012). Small-scale patterns of deep-sea fish distributions and assemblages of the Grand Banks, Newfoundland continental slope. Deep Sea Res. Part I 65, 171–188. doi: 10.1016/j.dsr.2012.03.012
Barrett, N. S., Edgar, G. J., Buxton, C. D., and Haddon, M. (2007). Changes in fish assemblages following 10 years of protection in Tasmanian marine protected areas. J. Exp. Mar. Biol. Ecol. 345, 141–157. doi: 10.1016/j.jembe.2007.02.007
Benz, G. W., Hoffmayer, E. R., Driggers, W. B., Allen, D., Bishop, L. E., and Brown, D. A. (2007). First record of a sleeper shark in the western Gulf of Mexico and comments on taxonomic uncertainty within Somniosus ( Somniosus ). Bull. Mar. Sci. 80, 343–351.
Bicknell, A. W. J., Godley, B. J., Sheehan, E. V., Votier, S. C., and Witt, M. J. (2016). Camera technology for monitoring marine biodiversity and human impact. Front. Ecol. Environ. 14, 424–432. doi: 10.1002/fee.1322
Boavida, J., Assis, J., Reed, J., Serrão, E. A., and Gonçalves, J. M. S. (2015). Comparison of small remotely operated vehicles and diver-operated video of circalittoral benthos. Hydrobiologia 766, 247–260. doi: 10.1007/s10750-015-2459-y
Brooks, E. J., Sloman, K. A., Sims, D. W., and Danylchuk, A. J. (2011). Validating the use of baited remote underwater video surveys for assessing the diversity, distribution and abundance of sharks in the Bahamas. Endanger. Species Res. 13, 231–243. doi: 10.3354/esr00331
Bryan, D. R., Kilfoyle, K., Gilmore, R. G. Jr., and Spieler, R.E. (2013). Characterization of the mesophotic reef fish community in south Florida, USA. J. Appl. Ichthyol. 29, 108–117. doi: 10.1111/j.1439-0426.2012.02055.x
Busby, M. S., Mier, K. L., and Brodeur, R. D. (2005). Habitat associations of demersal fishes and crabs in the Pribilof Islands region of the Bering Sea. Fish. Res. 75, 15–28. doi: 10.1016/j.fishres.2005.05.012
Butler, J., Neuman, M., Pinkard, D., Kvitek, R., and Cochrane, G. (2006). The use of multibeam sonar mapping techniques to refine population estimates of the endangered white abalone ( Haliotis sorenseni ). Fishery Bull. 104, 521–532.
Caldwell, Z. R., Zgliczynski, B. J., Williams, G. J., and Sandin, S. A. (2016). Reef fish survey techniques: assessing the potential for standardizing methodologies. PLoS ONE 11:e0153066. doi: 10.1371/journal.pone.0153066
Cánovas-Molina, A., Montefalcone, M., Bavestrello, G., Cau, A., Bianchi, C. N., Morri, C., et al. (2016). A new ecological index for the status of mesophotic megabenthic assemblages in the mediterranean based on ROV photography and video footage. Cont. Shelf Res. 121, 13–20. doi: 10.1016/j.csr.2016.01.008
Cappo, M., Harvey, E., Malcolm, H., and Speare, P. (2003). “Potential of video techniques to monitor diversity, abundance and size of fish in studies of marine protected areas,” in Aquatic Protected Areas - What Works Best and How Do We Know? World Congress on Aquatic Protected Areas , eds J. P. Beumer, A. Grant, and D. C. Smith (Cairns, QLD: Australian Society for Fish Biology), 455–464.
Cappo, M., Harvey, E., and Shortis, M. (2007). “Counting and measuring fish with baited video techniques - an overview,” in Australian Society for Fish Biology Workshop Proceedings , eds J. M. Lyle, D. M. Furlani, and C. D. Buxton (Hobart, TAS), 101–114.
Carpenter, B. M., and Shull, D. H. (2011). “A comparison of two methods, paired-diver surveys and remotely operated vehicle surveys, for determining rockfish abundance,” in Rockfish Technical Report, Task 5 (Whatcom County: NOAA).
Consoli, P., Esposito, V., Battaglia, P., Altobelli, C., Perzia, P., Romeo, T., et al. (2016). Fish distribution and habitat complexity on banks of the Strait of Sicily (central Mediterranean Sea) from remotely-operated vehicle (ROV) exploration. PLoS ONE 11:e0167809. doi: 10.1371/journal.pone.0167809
Dance, M. A., Patterson, W. F. III, and Addis, D. T. (2011). Fish community and trophic structure at artificial reef sites in the Northeastern Gulf of Mexico. Bull. Mar. Sci. 87, 301–324. doi: 10.5343/bms.2010.1040
De Leo, F. C., Smith, C. R., Rowden, A. A., Bowden, D. A., and Clark, M. R. (2010). Submarine canyons: hotspots of benthic biomass and productivity in the deep sea. Proc. R. Soc. B Biol. Sci. 277, 2783–2792. doi: 10.1098/rspb.2010.0462
D'Onghia, G., Maiorano, P., Carlucci, R., Capezzuto, F., Carluccio, A., Tursi, A., et al. (2012). Comparing deep-sea fish fauna between coral and non-coral “megahabitats” in the Santa Maria di Leuca cold-water coral province (Mediterranean Sea). PLoS ONE 7:e44509. doi: 10.1371/journal.pone.0044509
Dorman, S. R., Harvey, E. S., and Newman, S. J. (2012). Bait effects in sampling coral reef fish asseblages with stereo-BRUVs. PLoS ONE 7:e41538. doi: 10.1371/journal.pone.0041538
Doughty, C. L., Quattrini, A. M., and Cordes, E. E. (2014). Insights into the population dynamics of the deep-sea coral genus Paramuricea in the Gulf of Mexico. Deep Sea Res. Part II 99, 71–82. doi: 10.1016/j.dsr2.2013.05.023
Du Preez, C., and Tunnicliffe, V. (2011). Shortspine thornyhead and rockfish (Scorpaenidae) distribution in response to substratum, biogenic structures and trawling. Mar. Ecol. Prog. Ser. 425, 217–231. doi: 10.3354/meps09005
Duffy, G. A., Lundsten, L., Kuhnz, L. A., and Paull, C. K. (2014). A comparison of megafaunal communities in five submarine canyons off Southern California, USA. Deep Sea Res. Part II 104, 259–266. doi: 10.1016/j.dsr2.2013.06.002
Dunlop, K. M., Kuhnz, L. A., Ruhl, H. A., Huffard, C. L., Caress, D. W., Henthorn, R. G., et al. (2015). Instruments and Methods: an evaluation of deep-sea benthic megafauna length measurements obtained with laser and stereo camera methods. Deep Sea Res. Part I 96, 38–48. doi: 10.1016/j.dsr.2014.11.003
Espinoza, M., Cappo, M., Heupel, M. R., Tobin, A. J., and Simpfendorfer, C. A. (2014). Quantifying shark distribution patterns and species-habitat associations: implications of marine park zoning. PLoS ONE 9:e106885. doi: 10.1371/journal.pone.0106885
Etnoyer, P. J., Wagner, D., Fowle, H. A., Poti, M., Kinlan, B., Georgian, S. E., et al. (2018). Models of habitat suitability, size, and age-class structure for the deep-sea black coral Leiopathes glaberrima in the Gulf of Mexico. Deep Sea Res. Part II. 150, 218–228. doi: 10.1016/j.dsr2.2017.10.008
Gates, A. R., Benfield, M. C., Booth, D. J., Fowler, A. M., Skropeta, D., and Jones, D. O. B. (2017). Deep-sea observations at hydrocarbon drilling locations: contributions from the SERPENT Project after 120 field visits. Deep Sea Res. II 137, 463–479. doi: 10.1016/j.dsr2.2016.07.011
Gregoire, T. G., and Valentine, H. T. (2007). Sampling Strategies for Natural Resources and the Environment . Boca Raton, FL: Taylor & Francis Group. doi: 10.1201/9780203498880
Grinyó, J., Gori, A., Ambroso, S., Purroy, A., Calatayud, C., Dominguez-Carrió, C., et al. (2016). Diversity, distribution and population size structure of deep Mediterranean gorgonian assemblages (Menorca Channel, Western Mediterranean Sea). Prog. Oceanogr. 145, 42–56. doi: 10.1016/j.pocean.2016.05.001
Haggarty, D. R., Shurin, J. B., and Yamanaka, K. L. (2016). Assessing population recovery inside british columbia's rockfish conservation areas with a remotely operated vehicle. Fish. Res. 183, 165–179. doi: 10.1016/j.fishres.2016.06.001
Hall-Spencer, J., Allain, V., and Fossa, J. H. (2002). Trawling damage to Northeast Atlantic ancient coral reefs. Proc. R. Soc. B Biol. Sci. 269, 507–511. doi: 10.1098/rspb.2001.1910
PubMed Abstract | CrossRef Full Text
Hart, T. D., Clemons, J. E. R., Wakefield, W. W., and Heppell, S. S. (2010). Day and night abundance, distribution, and activity patterns of demersal fishes on Heceta Bank, Oregon. Fishery Bull. 108, 466–477.
Harter, S. L., Ribera, M. M., Shepard, A. N., and Reed, J. K. (2009). Assessment of fish populations and habitat on Oculina bank, a deep-sea coral marine protected area off eastern Florida. Fishery Bull. 107, 195–206.
Harvey, E. S., Cappo, M., Butler, J. J., Hall, N., and Kendrick, G. A. (2007). Bait attraction affects the performance of remote underwater video stations in assessment of demersal fish community structure. Mar. Ecol. Prog. Ser. 350, 245–254. doi: 10.3354/meps07192
Heagney, E. C., Lynch, T. P., Babcock, R. C., and Suthers, I. M. (2007). Pelagic fish assemblages assessed using mid-water baited video: standardising fish counts using bait plume size. Mar. Ecol. Prog. Ser. 350, 255–266. doi: 10.3354/meps07193
Hemery, L., and Henkel, S. (2016). Patterns of benthic mega-invertebrate habitat associations in the Pacific Northwest continental shelf waters: a reassessment. Biodiv. Conserv. 25, 1761–1772. doi: 10.1007/s10531-016-1158-y
Henry, L. A., Stehmann, M. F., De Clippele, L., Findlay, H. S., Golding, N., and Roberts, J. M. (2016). Seamount egg-laying grounds of the deep-water skate Bathyraja richardsoni . J. Fish Biol. 89, 1473–1481. doi: 10.1111/jfb.13041
Higgs, N. D., Gates, A. R., and Jones, D. O. (2014). Fish food in the deep sea: revisiting the role of large food-falls. PLoS ONE 9:96016. doi: 10.1371/journal.pone.0096016
Ho, H. C., and McGrouther, M. (2015). A new anglerfish from eastern Australia and New Caledonia (Lophiiformes: Chaunacidae: Chaunacops), with new data and submersible observation of Chaunacops melanostomus . J. Fish Biol. 86, 940–951. doi: 10.1111/jfb.12607
Huvenne, V. A. I., Robert, K., Marsh, L., Lo Iacono, C., Le Bas, T., and Wynn, R. B. (2018). “ROVs and AUVs,” in Submarine Geomorphology , ed A. Micallef (Southampton: Springer International Publishing AG), 93–108. doi: 10.1007/978-3-319-57852-1_7
Jessup, M. E. (2014). “Mesophotic coral ecosystems: tools and techniques for scientific exploration,” in Diving for Science 2014: Proceedings of the American Academy of Underwater Sciences 33rd Scientific Symposium , eds G. L. Eckert, S. Keller and S. L. Tamone (Sitka, AK; Dauphin Island, AL: American Academy of Underwater Sciences).
Johnson, S. W., Murphy, M. L., and Csepp, D. J. (2003). Distribution, habitat, and behavior of rockfishes, Sebastes spp., in nearshore waters of southeastern Alaska: observations from a remotely operated vehicle. Environ. Biol. Fishes 66, 259–270. doi: 10.1023/A:1023981908146
Jones, D., Wigham, B., Hudson, I., and Bett, B. (2007). Anthropogenic disturbance of deep-sea megabenthic assemblages: a study with remotely operated vehicles in the Faroe-Shetland Channel, NE Atlantic. Mar. Biol. 151, 1731–1741. doi: 10.1007/s00227-007-0606-3
Jones, D. T., Wilson, C. D., De Robertis, A., Rooper, C. N., Weber, T. C., and Butler, J. L. (2012). Evaluation of rockfish abundance in untrawlable habitat: combining acoustic and complementary sampling tools. Fishery Bull. 110, 332–343.
Karpov, K., Bergen, M., Geibel, J. J., Valle, C. F., et al. (2010). Prospective ( a priori ) power analysis for detecting changes in density when sampling with strip transects. Calif. Fish Game 96, 69–81.
Karpov, K., Lauermann, A., Cailliet, G., and Prall, M. (2004). Comparing Fisherman-Directed Hook and Trap Sampling of Groundfish to ROV-Based Assessment: Phase I – Methods Development in Collaboration Between Fisherman, FDFG, and University Scientists . San Jose, CA: California Department of Fish and Game and Moss Landing Marine Laboratories.
Karpov, K. A., Bergen, M., and Geibel, J. J. (2012). Monitoring fish in California Channel Islands marine protected areas with a remotely operated vehicle: the first five years. Mar. Ecol. Prog. Ser. 453, 159–172. doi: 10.3354/meps09629
Koenig, C. C., Shepard, A. N., Reed, J. K., Coleman, F. C., Brooke, S. D., Brusher, J., et al. (2005). Habitat and fish populations in the deep-sea Oculina coral ecosystem of the Western Atlantic. Am. Fish. Soc. Symp. 41, 795–805.
Lacharité, M., Metaxas, A., and Lawton, P. (2015). Using object-based image analysis to determine seafloor fine-scale features and complexity. Limnol. Oceanogr. Methods 13, 553–567. doi: 10.1002/lom3.10047
Laidig, T. E., Krigsman, L. M., and Yoklavich, M. M. (2012). Reactions of fishes to two underwater survey tools, a manned submersible and a remotely operated vehicle. Fishery Bull. 111, 54–67. doi: 10.7755/FB.111.1.5
Laidig, T. E., and Yoklavich, M. M. (2016). A comparison of density and length of Pacific groundfishes observed from 2 survey vehicles: a manned submersible and a remotely operated vehicle. Fishery Bull. 114, 386–396. doi: 10.7755/FB.114.4.2
Lauermann (2014). “South coast MPA study region ROV deployment 1,” in January 2014 Interim Report (Marine Applied Research and Exploration), 1–31. Available online at: http://nrm.dfg.ca.gov/FileHandler.ashx?DocumentID=84543
Laurenson, C. H., Hudson, I. R., Jones, D. O. B., and Priede, I. G. (2004). Deep water observations of Lophius piscatorius in the north-eastern Atlantic Ocean by means of a remotely operated vehicle. J. Fish Biol. 65, 947–960. doi: 10.1111/j.0022-1112.2004.00496.x
Lindholm, J., Gleason, M., Kline, D., Clary, L., Rienecke, S., Cramer, A., et al. (2015). Ecological effects of bottom trawling on the structural attributes of fish habitat in unconsolidated sediments along the central California outer continental shelf. Fishery Bull. 113, 82–96. doi: 10.7755/FB.113.1.8
Linley, T. D., Alt, C. H. S., Jones, D. O. B., and Priede, I. G. (2013). Bathyal demersal fishes of the Charlie-Gibbs Fracture Zone region (49°-54°N) of the Mid-Atlantic Ridge: III. Results from remotely operated vehicle (ROV) video transects. Deep Sea Res. Part II Top. Stud. Oceanogr. 98, 407–411. doi: 10.1016/j.dsr2.2013.08.013
Lorance, P., and Trenkel, V. M. (2006). Variability in natural behaviour, and observed reactions to an ROV, by mid-slope fish species. J. Exp. Mar. Biol. Ecol. 332, 106–119. doi: 10.1016/j.jembe.2005.11.007
Luck, D. G., and Pietsch, T. W. (2008). In-situ observations of a deep-sea ceratioid anglerfish of the Genus Oneirodes (Lophiiformes: Oneirodidae). Copeia 2008, 446–451. doi: 10.1643/CE-07-075
Lundsten, L., Schlining, K. L., Frasier, K., Johnson, S. B., Kuhnz, L. A., Harvey, J. B. J., et al. (2010). Time-series analysis of six whale-fall communities in Monterey Canyon, California, USA. Deep Sea Res. Part I 57, 1573–1584. doi: 10.1016/j.dsr.2010.09.003
Lyle, J. M., Bell, J. D., Chuwen, B. M., Barrett, N., Tracey, S. R., and Buxton, C. D. (2014). “Assessing the impacts of gillnetting in Tasmania: implications for by-catch and biodiversity,” in Fisheries Research & Development Corporation (FRDC) Report . Hobart, TAS: Institute for Marine and Antarctic Studies, University of Tasmania.
Macreadie, P. I., McLean, D. L., Thomson, P. G., Partridge, J. C., Jones, D. O. B., Gates, A. R., et al. (2018). Eyes in the sea: unlocking the mysteries of the ocean using industrial, remotely operated vehicles (ROVs). Sci. Tot. Environ. 634, 1077–1091. doi: 10.1016/j.scitotenv.2018.04.049
Malcolm, H. A., Jordan, A., and Smith, S. D. A. (2011). Testing a depth-based habitat classification system against reef fish assemblage patterns in a subtropical marine park. Aquat. Conserv. 21, 173–185. doi: 10.1002/aqc.1165
Mallet, D., and Pelletier, D. (2014). Underwater video techniques for observing coastal marine biodiversity: a review of sixty years of publications (1952–2012). Fish. Res. 154, 44–62. doi: 10.1016/j.fishres.2014.01.019
Mapula, S. M., Sven, E. K., Albrecht, G., Kerry, S., Toufiek, S., and Christopher, G. W. (2016). Notes on a remotely operated vehicle survey to describe reef ichthyofauna and habitats–Agulhas Bank, South Africa. Bothalia 46, e1–e7. doi: 10.4102/abc.v46i1.2108
McLean, D. L., Green, M., Harvey, E. S., Williams, A., Daley, R., and Graham, K. J. (2015). Comparison of baited longlines and baited underwater cameras for assessing the composition of continental slope deepwater fish assemblages off southeast Australia. Deep Sea Res. Part I 98, 10–20. doi: 10.1016/j.dsr.2014.11.013
McLean, D. L., Partridge, J. C., Bond, T., Birt, M. J., Bornt, K. R., and Langlois, T. J. (2017). Using industry ROV videos to assess fish associations with subsea pipelines. Cont. Shelf Res. 141, 76–97. doi: 10.1016/j.csr.2017.05.006
McLean, D. L., Taylor, M. D., Partridge, J. C., Gibbons, B., Langlois, T. J., Malseed, B. E., et al. (2018). Fish and habitats on well-head infrastructure on the north west shelf of Western Australia. Cont. Shelf Res. 164, 10–27. doi: 10.1016/j.csr.2018.05.007
Meirelles, P. M., Amado-Filho, G. M., Pereira-Filho, G. H., Pinheiro, H. T., de Moura, R. L., Joyeux, J. C., et al. (2015). Baseline assessment of mesophotic reefs of the vitoria-trindade seamount chain based on water quality, microbial diversity, benthic cover and fish biomass data. PLoS ONE 10:e0130084. doi: 10.1371/journal.pone.0130084
Mortensen, P. B., Buhl-Mortensen, L., Gebruk, A. V., and Krylova, E. M. (2008). Occurrence of deep-water corals on the Mid-Atlantic Ridge based on MAR-ECO data. Deep Sea Res. Part II 55, 142–152. doi: 10.1016/j.dsr2.2007.09.018
Moser, M. L., Auster, P. J., and Bichy, J. B. (1998). Effects of mat morphology on large Sargassum-associated fishes: observations from a remotely operated vehicle (ROV) and free-floating video camcorders. Environ. Biol. Fishes 51, 391–398. doi: 10.1023/A:1007493412854
Mundy, B. C., Gerringer, M. E., Nielsen, J. G., Fryer, P., and Leitner, A. (2018). First in situ observation of an aphyonid fish (Teleostei, Ophidiiformes, Bythitidae). Deep Sea Res. Part II. 150, 164–169. doi: 10.1016/j.dsr2.2017.09.009
Murphy, H. M., and Jenkins, G. P. (2010). Observational methods used in marine spatial monitoring of fishes and associated habitats: a review. Mar. Freshw. Res. 61, 236–252. doi: 10.1071/MF09068
Norcross, B. L., and Mueter, F.-J. (1999). The use of an ROV in the study of juvenile flatfish. Fish. Res. 39, 241–251. doi: 10.1016/S0165-7836(98)00200-8
Pacunski, R. E., Palsson, W. A., and Greene, H. G. (2013). Estimating Fish Abundance and Community Composition on Rocky Habitats in the San Juan Islands Using a Small Remotely Operated Vehicle. Washington, DC: Washington Department of Fish and Wildlife, Fish Program, Fish Management Division.
Pacunski, R. E., Paulsson, W. A., Greene, H. G., and Gunderson, D. (2008). “Conducting visual surveys with a small ROV in shallow water,” in Marine Habitat Mapping Technology for Alaska , eds J. R. Reynolds and H. G. Greene (Fairbanks, AK), 109–128. doi: 10.4027/mhmta.2008.08
Patterson, W. F. III, Dance, M. A., and Addis, D. T. (2009). Development of a remotely operated vehicle based methodology to estimate fish community structure at artificial reef sites in the Northern Gulf of Mexico. Proc. Gulf. Caribb. Fish. Inst. 61, 263–270.
Perkins, N. R., Foster, S. D., Hill, N. A., and Barrett, N. S. (2016). Image subsampling and point scoring approaches for large-scale marine benthic monitoring programs. Estuar. Coast. Shelf Sci. 176, 36–46. doi: 10.1016/j.ecss.2016.04.005
Pierdomenico, M., Martorelli, E., Dominguez-Carrió, C., Gili, J. M., and Chiocci, F. L. (2016). Seafloor characterization and benthic megafaunal distribution of an active submarine canyon and surrounding sectors: the case of Gioia Canyon (Southern Tyrrhenian Sea). J. Mar. Syst. 157, 101–117. doi: 10.1016/j.jmarsys.2016.01.005
Pita, P., Fernández-Márquez, D., and Freire, J. (2014). Short-term performance of three underwater sampling techniques for assessing differences in the absolute abundances and in the inventories of the coastal fish communities of the Northeast Atlantic Ocean. Mar. Freshw. Res. 65, 105–113. doi: 10.1071/MF12301
Pradella, N., Fowler, A. M., Booth, D. J., and Macreadie, P. I. (2014). Fish assemblages associated with oil industry structures on the continental shelf of north-western Australia. J. Fish Biol. 84, 247–255. doi: 10.1111/jfb.12274
Quattrini, A. M., Demopoulos, A. W. J., Singer, R., Roa-Varon, A., and Chaytor, J. D. (2017). Demersal fish assemblages on seamounts and other rugged features in the northeastern Caribbean. Deep Sea Res. Part I 123, 90–104. doi: 10.1016/j.dsr.2017.03.009
Quattrini, A. M., and Ross, S. W. (2006). Fishes associated with North Carolina shelf-edge hardbottoms and initial assessment of a proposed marine protected area. Bull. Mar. Sci. 79, 137–163.
Romano, C., Gerard, D., Edin, O., Joseph, C., Thomas, N., and Daniel, T. (2017). Inspection-class remotely operated vehicles—a review. J. Mar. Sci. Eng. 5:3. doi: 10.3390/jmse5010013
Rooper, C. N., Martin, M. H., Butler, J. L., Jones, D. T., and Zimmerman, M. (2012). Estimating species and size composition of rockfishes to verify targets in acoustic surveys of untrawlable areas. Fishery Bull. 110, 317–331.
Rosa, M. R., Alves, A. C., Medeiros, D. V., Coni, E. O. C., Ferreira, C. M., Ferreira, B. P., et al. (2015). Mesophotic reef fish assemblages of the remote St. Peter and St. Paul's Archipelago, Mid-Atlantic Ridge, Brazil. Coral Reefs 35, 113–123. doi: 10.1007/s00338-015-1368-x
Ross, S. W., Rhode, M., Viada, S. T., and Mather, R. (2016). Fish species associated with shipwreck and natural hard-bottom habitats from the middle to outer continental shelf of the Middle Atlantic Bight near Norfolk Canyon. Fishery Bull. 114, 45–57. doi: 10.7755/FB.114.1.4
Ruhl, H. A., Hastings, P. A., Zarubick, L. A., Jensen, R. M., and Zdzitowiecki, K. (2003). Fish populations of Port Foster, Deception Island, Antarctica and vicinity. Deep Sea Res. Part II 50, 1843–1858. doi: 10.1016/S0967-0645(03)00094-8
Salvati, E., Angiolillo, M., Bo, M., Bavestrello, G., Giusti, M., Cardinali, A., et al. (2010). The population of Errina aspera (Hydrozoa: Stylasteridae) of the Messina Strait (Mediterranean Sea). J. Mar. Biol. Assoc. U. K. 90, 1331–1336. doi: 10.1017/S0025315410000950
Santana-Garcon, J., Braccini, M., Langlois, T. J., Newman, S. J., McAuley, R. B., and Harvey, E. S. (2014). Calibration of pelagic stereo-BRUVs and scientific longline survey for sampling sharks. Methods Ecol. Evolut. 5, 824–833. doi: 10.1111/2041-210X.12216
Smale, M. J., Sauer, W. H. H., and Roberts, M. J. (2001). Behavioural interactions of predators and spawning chokka squid off South Africa: towards quantification. Mar. Biol. 139, 1095–1105. doi: 10.1007/s002270100664
Smith, J. G., and Lindholm, J. (2016). Vertical stratification in the distribution of demersal fishes along the walls of the La Jolla and Scripps submarine canyons, California, USA. Cont. Shelf Res. 125, 61–70. doi: 10.1016/j.csr.2016.07.001
Söffker, M., Sloman, K. A., and Hall-Spencer, J. M. (2011). In situ observations of fish associated with coral reefs off Ireland. Deep Sea Res. Part I 58, 818–825. doi: 10.1016/j.dsr.2011.06.002
Spalding, H. L., Foster, M. S., and Heine, J. N. (2003). Compostion, distribution and abundance of deep-water (>30 m) macroalgae in central California. J. Phycol. 39, 273–284. doi: 10.1046/j.1529-8817.2003.02010.x
Spanier, E., Cobb, J. S., and Clancy, M. (1994). Impacts of Remotely Operated Vehicles (ROVs) on the behavior of marine animals: an example using American lobsters. Mar. Ecol. Prog. Ser. 104, 257–266. doi: 10.3354/meps104257
Stein, D. L., Felley, J. D., and Vecchione, M. (2005). ROV observations of benthic fishes in the Northwind and Canada Basins, Arctic Ocean. Polar Biol. 28, 232–237. doi: 10.1007/s00300-004-0696-z
Stierhoff, K. L., Butler, J. L., Mau, S. A., and Murfin, D. W. (2013). “Abundance and biomass estimates of demersal fishes at the Footprint and Piggy Bank from optical surveys using a remotely operated vehicle (ROV),” in NOAA Technical Memorandum NMFS (La Jolla, CA: U. S. Department of Commerce, National Oceanic and Atmospheric Administration).
Stierhoff, K. L., Neuman, M., and Butler, J. L. (2012). On the road to extinction? Population declines of the endangered white abalone, Haliotis sorenseni. Biol. Conserv. 152, 46–52. doi: 10.1016/j.biocon.2012.03.013
Stoner, A. W., Clifford, H. R., Parker, S. J., Auster, P. J., and Wakefield, W. W. (2008). Evaluating the role of fish behavior in surveys conducted with underwater vehicles. Can. J. Fish. Aquat. Sci. 65, 1230–1243. doi: 10.1139/F08-032
Strindberg, S., and Buckland, S. T. (2004). Zigzag survey designs in line transect sampling. J. Agric. Biol. Environ. Stat. 9, 443–461. doi: 10.1198/108571104X15601
Taylor, J. R., DeVogelaere, A. P., Burton, E. J., Frey, O., Lundsten, L., Kuhnz, L. A., et al. (2014). Deep-sea faunal communities associated with a lost intermodal shipping container in the Monterey Bay National Marine Sanctuary, CA. Mar. Pollut. Bull. 83, 92–106. doi: 10.1016/j.marpolbul.2014.04.014
Thresher, R., Althaus, F., Adkins, J., Gowlett-Holmes, K., Alderslade, P., Dowdney, J., et al. (2014). Strong depth-related zonation of megabenthos on a rocky continental margin (approximately 700-4000 m) off southern Tasmania, Australia. PLoS ONE 9:e85872. doi: 10.1371/journal.pone.0085872
Todd, V. L. G., Lavallin, E. W., and Macreadie, P. I. (2018). Quantitative analysis of fish and invertebrate assemblage dynamics in association with a North Sea oil and gas installation complex. Mar. Environ. Res. 142, 69–79. doi: 10.1016/j.marenvres.2018.09.018
Trenkel, V. M., Chris Francis, R. I. C., Lorance, P., Mahévas, S., Rochet, M.-J., and Tracey, D. M. (2004a). Availability of deep-water fish to trawling and visual observation from a remotely operated vehicle (ROV). Mar. Ecol. Prog. Ser. 284, 293–303. doi: 10.3354/meps284293
Trenkel, V. M., and Lorance, P. (2011). Estimating Synaphobranchus kaupii densities: contribution of fish behaviour to differences between bait experiments and visual strip transects. Deep Sea Res. Part I 58, 63–71. doi: 10.1016/j.dsr.2010.11.006
Trenkel, V. M., Lorance, P., and Mahévas, S. (2004b). Do visual transects provide true population density estimates for deepwater fish? ICES J. Mar. Sci. 61, 1050–1056. doi: 10.1016/j.icesjms.2004.06.002
Veitch, L., Dulvy, N. K., Koldewey, H., Lieberman, S., Pauly, D., Roberts, C. M., et al. (2012). Avoiding empty ocean commitments at Rio+20. Science 336, 1383–1385. doi: 10.1126/science.1223009
Watson, D. L., Harvey, E. S., Anderson, M. J., and Kendrick, G. A. (2005). A comparison of temperate reef fish assemblages recorded by three underwater stereo-video techniques. Mar. Biol. 148, 415–425. doi: 10.1007/s00227-005-0090-6
Watson, D. L., Harvey, E. S., Fitzpatrick, B. M., Langlois, T. J., and Shedrawi, G. (2010). Assessing reef fish assemblage structure: how do different stereo-video techniques compare? Mar. Biol. 157, 1237–1250. doi: 10.1007/s00227-010-1404-x
White, J., Simpfendorfer, C. A., Tobin, A. J., and Heupel, M. R. (2013). Application of baited remote underwater video surveys to quantify spatial distribution of elasmobranchs at an ecosystem scale. J. Exp. Mar. Biol. Ecol. 448, 281–288. doi: 10.1016/j.jembe.2013.08.004
Widder, E. A., Robison, B. H., Reisenbichler, K. R., and Haddock, S. H. D. (2005). Using red light for in situ observations of deep-sea fishes. Deep Sea Res. Part I 52, 2077–2085. doi: 10.1016/j.dsr.2005.06.007
Wraith, J., Lynch, T., Minchinton, T. E., Broad, A., and Davis, A. R. (2013). Bait type affects fish assemblages and feeding guilds observed at baited remote underwater video stations. Mar. Ecol. Prog. Ser. 477, 189–199. doi: 10.3354/meps10137
Yamamoto, J., Nobetsu, T., Iwamori, T., and Sakurai, Y. (2009). Observations of food falls off the Shiretoko Peninsula, Japan, using a remotely operated vehicle. Fish. Sci. 75, 513–515. doi: 10.1007/s12562-008-0055-z
Zeidberg, L. D., and Robison, B. H. (2007). Invasive range expansion by the Humboldt squid, Dosidicus gigas, in the eastern North Pacific. Proc. Natl. Acad. Sci. U.S.A. 104, 12948–12950. doi: 10.1073/pnas.0702043104
Keywords: video survey method, working-class ROV, observation-class ROV, non-destructive, autonomous observing, comparison of methods, standardized operating protocols
Citation: Sward D, Monk J and Barrett N (2019) A Systematic Review of Remotely Operated Vehicle Surveys for Visually Assessing Fish Assemblages. Front. Mar. Sci . 6:134. doi: 10.3389/fmars.2019.00134
Received: 24 August 2018; Accepted: 04 March 2019; Published: 03 April 2019.
Reviewed by:
Copyright © 2019 Sward, Monk and Barrett. This is an open-access article distributed under the terms of the Creative Commons Attribution License (CC BY) . The use, distribution or reproduction in other forums is permitted, provided the original author(s) and the copyright owner(s) are credited and that the original publication in this journal is cited, in accordance with accepted academic practice. No use, distribution or reproduction is permitted which does not comply with these terms.
*Correspondence: Darryn Sward, [email protected]
- International
- Today’s Paper
- Premium Stories
- 🗳️ Elections 2024
- Express Shorts
- Maharashtra HSC Result
- Brand Solutions
Accelerating Development review: State-led growth is key
Are indian states ready to embark on a journey to being viksit economist karthik muralidharan seeks to answer the question in his new book.

Is the Indian state, or rather, are Indian states, ready to embark on the journey to being viksit ? If not, how can they prepare themselves? This is the question author Karthik Muralidharan seeks to answer in his magnum opus, Accelerating India’s Development. With 130 pages of endnotes and 60 pages of references , to supplement 600 pages of text, there is certainly much in this book.
The book is worth reading for the first half of most chapters alone — they are richly researched, lucidly written and are a very accessible introduction to the state of developmental policy in India, with global cross-references. The sweep is broad, from politicians, bureaucrats and businesspersons to civil and talent management, from taxation and choosing between the Union and the states to the tussle between the state and market. Of course, the ingredients of development — health, education, security, jobs and the economy, as well as safety nets and trampolines — are all there. It enables a practitioner to quickly get a lay of the land, and helps a researcher put literature into context.

However, the author is not writing a textbook. The first half of chapters is just the motivation for the second half that outlines many evidence-based ideas for implementation — on how states can accelerate development.
Reading the book, one is frequently reminded of the author’s quote from Rukmini Banerji — asliyat se lagao — and the analogous Chinese proverb popularised by Deng Xiaoping — seeking truth from facts. Muralidharan consistently advocates for systems that generate validated data and argues for processes that draw upon data, even suggesting linking compensation to outcomes measured by district surveys.
Another feature of this book is that ideas are directed at state governments, not the Union government, except to suggest more freedom for states that are delivering good outcomes, e.g., by providing more flexibility in central schemes or accessing data from states through APIs, rather than mandating multiple apps, which gobble frontline time. The focus on states creates multiple sites for ideas to be adopted; a revenue-raising idea here, a health-improving idea there, and so on. Muralidharan is not expecting that the adoption will be top-down, with states in lock-step. Instead, the reliance is on a process of (hopefully rapid) diffusion, in a manner that is in sync with the political culture of a state.

The third characteristic is a claim that “there is often no trade-off between equity and efficiency”, because few, if any, of our states operate on the frontier where such a trade-off is necessary. Instead, it’s argued that there are many actions that can improve both. However, the examples provided, e.g., replacing free electricity to farmers with “income transfers… up to a landholding cap” can face resistance from elites, and, as Muralidharan notes, there is “a pattern of elite bias in every sector discussed in this book”. The book is unclear as to how to overcome this bias . Without that we will get outcomes such as much smaller income transfers, along with free electricity. Other ideas, for example, offering a choice between direct benefit transfers (DBT) or grain from PDS, may be more implementable, though, if the DBT option catches on, could FCI procurement as a mechanism to back minimum support prices continue?
For the most part, Muralidharan’s reform ideas are not just substantive, but also radical. A running theme in the book is the practicum model, which perhaps builds on the author’s own research experience with the cost-effectiveness of additional temporary teachers in schools. Practicum involves mixing classroom instruction with concurrent training in a government department, with some preference in future recruitment to its graduates. This model is fleshed out in degrees of detail for administration, education, health, judiciary, and even the police. Implementing it would, of course, entail a wholesale change in staffing and recruitment practices, adding to abovementioned changes in compensation policy.
At a recent event in Delhi, the author said: “Real power comes not from sharing solutions but distributing agency” — an insight that is hard to appreciate and harder to implement. Not all the reform ideas in the book may pass this test, but many do, though they remain hard to implement. Some green shoots are, however, visible. Tamil Nadu recently established a Human Resources Reforms Committee, which has since submitted its report. Andhra Pradesh has established an in-house data analysis unit serving multiple departments, and recruited over 1,00,000 contractual (recently regularised) frontline workers. It will be interesting to see if Telangana ’s district level KPI surveys survives the change in political regime . All these are in alignment with some of the author’s reform ideas. Others, such as changing voting systems, are certainly not for the faint-hearted, but maybe one can visualise starting with local elections in one state, the drumbeat towards ‘one nation, one election’ notwithstanding.
Throughout the book, the author seems to hope that the reform path will be lit at many places by someone, somewhere, trying some idea, and that successful initiatives will be rapidly replicated. Time will tell if that will happen, but if the lucidity of analysis and provocativeness of the book’s ideas combine to inspire enough states to experiment, the author should consider the labour well-recompensed. Nonetheless, regardless of what happens, the book remains an engaging and thought-provoking read, deserving its place on the shelf.
The writer is a senior fellow at the Centre for Policy Research, New Delhi
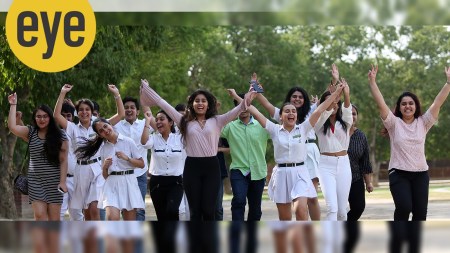
Children can be subversive change-makers Subscriber Only
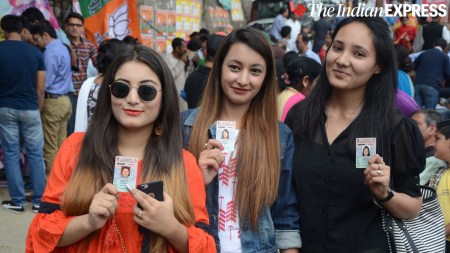
Love at first vote: Gen Z is no longer apolitical
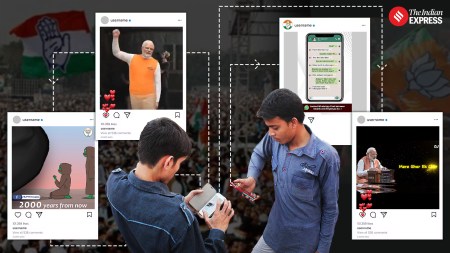
Journey of a political meme: From digital war room to Subscriber Only
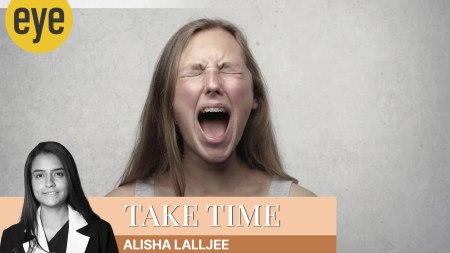
Managing teen anger
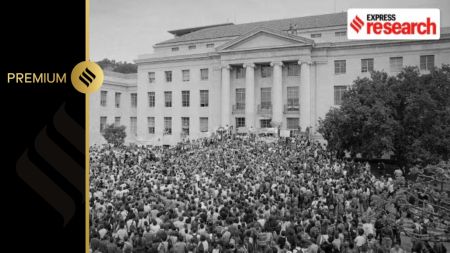
Great Butter Rebellion to Gaza: History of student protests in Subscriber Only
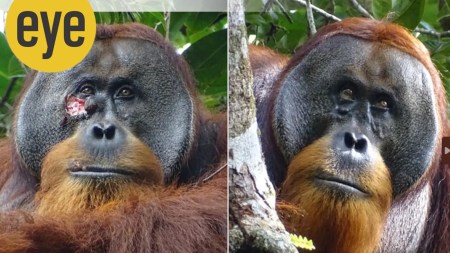
Without vets or hospitals, how do wild animals treat themselves Subscriber Only
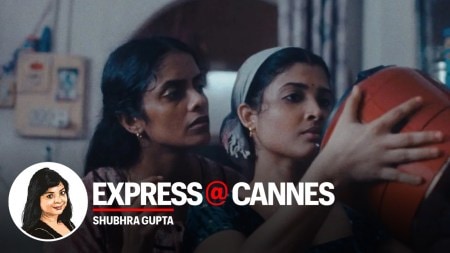
All We Imagine As Light is a meditative soliloquy on
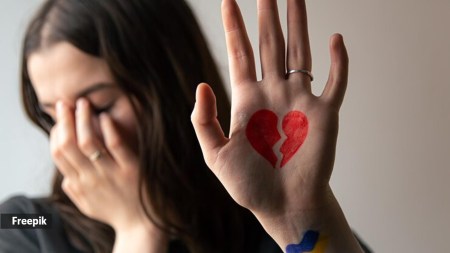
Why breakups in the 30s feel different for women
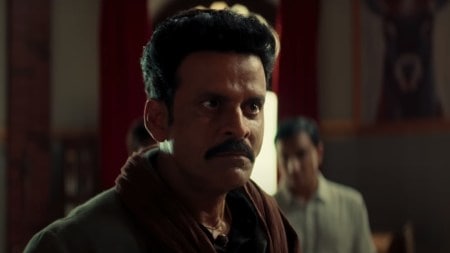
Bhaiyya Ji does Manoj Bajpayee no credit
- Express Premium
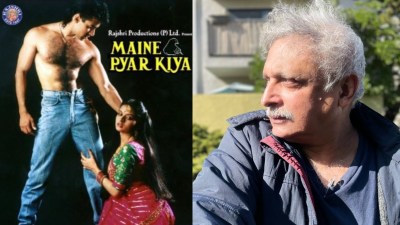
Actor Piyush Mishra shared that he was approached for the lead role in Maine Pyar Kiya, but it wasn't a big deal as he was still a student at National School of Drama and was merely "considered" for the part, which was played by Salman Khan. He believes he wouldn't have been able to handle the stardom at that time and is happy things didn't work out.
- Rajkot Fire Live Updates: 27, including children, killed in massive fire at game zone; SIT formed 3 hours ago
- Delhi Lok Sabha Election 2024 Live Updates: Capital records voter turnout of nearly 57% in Phase 6 of polling 6 hours ago
- India General Elections 2024 Live Updates: Voter turnout recorded 59.6% till 9pm in Phase 6 of polling 8 hours ago
- Cyclone Remal Live Updates: Low-pressure system over Bay of Bengal intensifies into cyclonic storm 'Remal', says IMD 8 hours ago
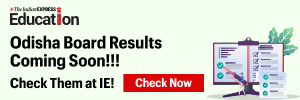
Best of Express
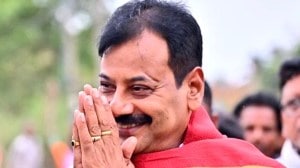
Buzzing Now
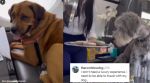
May 25: Latest News
- 01 Bengaluru’s annual Mango Mela begins at Lalbagh but sellers worried over low crop yield
- 02 Defense spending measure would bar Chinese lidar sensors in US military systems
- 03 SRH vs RR Qualifier 2: Sunrisers thrive at home away from home, Heinrich Klaasen’s masterful stroke and other talking points
- 04 SC stays Uttarakhand HC order for its relocation out of Nainital
- 05 BMC appeals to residents to shift from landslide-prone locations ahead of monsoon
- Elections 2024
- Political Pulse
- Entertainment
- Movie Review
- Newsletters
- Web Stories
Solving partial differential equations using large-data models: a literature review
- Open access
- Published: 24 May 2024
- Volume 57 , article number 152 , ( 2024 )
Cite this article
You have full access to this open access article
- Abdul Mueed Hafiz 1 ,
- Irfan Faiq 2 &
- M. Hassaballah 3 , 4
1 Altmetric
Explore all metrics
Mathematics lies at the heart of engineering science and is very important for capturing and modeling of diverse processes. These processes may be naturally-occurring or man-made. One important engineering problem in this regard is the modeling of advanced mathematical problems and their analysis. Partial differential equations (PDEs) are important and useful tools to this end. However, solving complex PDEs for advanced problems requires extensive computational resources and complex techniques. Neural networks provide a way to solve complex PDEs reliably. In this regard, large-data models are new generation of techniques, which have large dependency capturing capabilities. Hence, they can richly model and accurately solve such complex PDEs. Some common large-data models include Convolutional neural networks (CNNs) and their derivatives, transformers, etc. In this literature survey, the mathematical background is introduced. A gentle introduction to the area of solving PDEs using large-data models is given. Various state-of-the-art large-data models for solving PDEs are discussed. Also, the major issues and future scope of the area are identified. Through this literature survey, it is hoped that readers will gain an insight into the area of solving PDEs using large-data models and pursue future research in this interesting area.
Similar content being viewed by others
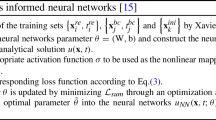
Improved Deep Neural Networks with Domain Decomposition in Solving Partial Differential Equations
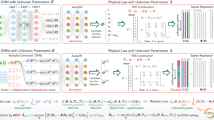
Physics-informed learning of governing equations from scarce data
A Short Note on Solving Partial Differential Equations Using Convolutional Neural Networks
Avoid common mistakes on your manuscript.
1 Introduction
For scientific computing, differential equations (DEs) are efficient for description of various engineering problems (Boussange et al. 2023 ; Mallikarjunaiah 2023 ). Differential equations are first-order or higher derivatives of anonymous functions and are classified accordingly as ordinary differential equations (ODEs) or partial differential equations (PDEs) (Taylor et al. 2023 ; Farlow 2006 ). Unlike algebraic techniques, the approach establishes an equational relationship between unknown expressions and the related derivatives. Hence, the solutions should conform to the relationship. Practically, DEs are very important for depiction of complex problems occurring daily in nature (Namaki et al. 2023 ; Soldatenko and Yusupov 2017 ; Rodkina and Kelly 2011 ). Since PDEs are a very important type of DEs which relate to the issues addressed in this paper, we focus on PDEs. Some simplified PDEs have solutions using common operators (Melchers et al. 2023 ; Farlow 2006 ). Some popular techniques for PDE solving are the Finite element method (FEM) (Zienkiewicz and Taylor 2000 ), the Finite volume method (FVM) (Versteeg and Malalasekera 2011 ), Particle-based methods (Oñate and Owen 2014 ), and the Finite cell method (FCM) (Kollmannsberger 2019 ).
For higher order PDEs, a finite element method (FEM) may be conveniently used for PDE solving and can give an accurate solution using extensive computational resources (Innerberger and Praetorius 2023 ). Also, the multi-iteration solution limits practicality. As of now, solving PDEs is chiefly used for advanced applications like design of aircrafts using fluid dynamics, forecast of weather,... etc. For improving the PDE solving capability, some spline functions have been used (Kolman et al. 2017 ; Qin et al. 2019 ). On similar lines, FEM basically discretizes and approximates the PDE solution numerically. Hence, introducing Fourier transforms or Laplace transforms with higher efficiency for expressing PDEs has better feasibility (Mugler and Scott 1988 ; El-Ajou 2021 ). Large-data models have robust fitting abilities for functions which are multi-variate and high-dimensional, and have been developed for light-weight and rapid solving of PDEs. Before proceeding further, we will give a brief introduction to FEM, and discuss briefly the relevance and importance of neural network techniques in this regard. Next, we will enumerate the large-data models used for PDE solving. These models will be discussed in detail in the paper subsequently.
1.1 Finite element method (FEM)
The numerical approximation of the continuous field u of any PDE can be given by Eq. 1 on a certain domain and can be solved by different techniques including the Finite element method (FEM) (Zienkiewicz and Taylor 2000 ). FEM is discussed here with emphasis on the Galerkin-based FEM.
Let the PDE be given by Eq. 1 , where \(L(\cdot )\) is an arbitrary function of the continuous field u , and let it be defined on the domain \(\Omega \in \mathbb {R}^n\) , which is a set of all possible inputs for the PDE equation, along with boundary conditions (BCs) given by Eqs. 2 and 3 . Let \(y_d\) and g be the Dirichlet and Neumann BCs, respectively. The Dirichlet BC gives the numerical value that the variable u at the domain boundary assumes when solving the PDE. The Neumann BC assumes the derivative value of the variable u applied at the domain boundary \(\omega\) , as against to the variable u itself as in the Dirichlet BC. The Finite element formulation of Eq. 1 on a discrete domain having m elements and n nodes, including the BCs, will give the next system Eq. 4 .
In Eq. 4 , \(K(u^h)\) is the left-hand side matrix and is non-linear, and is known as the stiffness matrix . The stiffness matrix is a matrix that gives the system of linear equations to be solved for ascertaining the approximate solution to the PDE. \(u^h\) is the discretized solution field, and \(F \in \mathbb {R}^n\) is the right-hand side vector giving the forces applied, where \(F_i\) is the force at the \(i^{th}\) node. The equation system can be reduced to be as:
For obtaining the solution \(u^h\) , the Newton-Ralphson method can be used by linearizing \(r(u^h)\) and its tangent. This technique needs solving per iteration, an equation-system which is linear. The iterations keep proceeding till the residual norm \(||r||_n\) adjusts to the tolerance. For a linear operator, convergence is achieved in only one iteration. For excessive elements and nodes, the most computationally expensive FEM step is the one for finding the linear equation-system solution. For applications with critical computational efficiency like real-time models, digital twins, etc. This step needs to be avoided at all costs. Applications of techniques like model-order reduction, build a surrogate to significantly reduce the computational cost. Large-data based techniques like deep networks can do away with this cost completely. Large-data models like Convolutional neural networks have some notable merits for solving PDEs (Willard et al. 2022 ).
1.2 Large-data models for solving PDEs
With time, the popular large-data models like CNNs (Lecun et al. 1998 ; Krizhevsky et al. 2012 ; Hafiz et al. 2021 ) used in deep learning (Hassaballah and Awad 2020 ; Minaee et al. 2023 ; Xu et al. 2023 ; Xiang et al. 2023 ; Hafiz et al. 2022 ; Hafiz and Hassaballah 2021 ), Recurrent neural networks (RNNs) (Ren et al. 2022 ), Long short term memory (LSTM) neural networks (José et al. 2021 ), Generative adversarial networks (GANs) (Gao and Ng 2022 ; Yang 2019 ), and the attention-based Transformers (Cao 2021 ) have also been applied for solving PDEs. Deep learning is an area wherein neural networks with large number of layers are used for classification, regression, etc. Introduced by Lecun et al. ( 1998 ), Convolutional neural networks (CNNs) rose to popularity with Krizhevsky et al. ( 2012 ). AlexNet was a CNN that gave outstanding performance on the ImageNet dataset classification challenge (Vakalopoulou et al. 2023 ; Deng et al. 2009 ). At that time, obtaining a high classification accuracy on the ImageNet images dataset was considered a tough computer vision task. Since then, CNNs and deep learning have shattered many records on applications like computer vision (Hassaballah and Awad 2020 ; Hafiz and Bhat 2020 ; Hafiz et al. 2020 , 2023 ), speech recognition (Jean et al. 2022 ; Bhangale and Kothandaraman 2022 ), financial market forecasting (Zhao and Yang 2023 ; Ashtiani and Raahemi 2023 ), and for developing intelligent chatbots like the popular ChatGPT (Gordijn and ten Have 2023 ). Given the prowess of CNNs, it was only a matter of time before they were applied to tasks like solving PDEs, and demonstrated promising results. This success of CNN based PDE solving was due to their unique strengths like implementation simplicity for supervised learning, and consistency (Smets et al. 2023 ; Alt et al. 2023 ; Jiang et al. 2023 ).
CNNs have both strengths as well as weaknesses for solving PDEs (Michoski et al. 2020 ; Peng et al. 2023 ; Choi et al. 2023 ). The strengths of CNNs in this regard are:
Significant ease of implementing PDEs.
Convenience of using large data.
Consistent solutions over the full space of parameters.
As for (1), it can be said that highly complicated PDEs systems with a very large number of parameters and high dimensionality, can be implemented in Python Language using TensorFlow, and PyTorch in hundreds of code lines in a couple of days (Yiqi and Ng 2023 ; Quan and Huynh 2023 ). TensorFlow and PyTorch are CNN based Python Language code-libraries offering a rich set of functions encapsulating the state-of-the-art CNNs and their required data processing related programming sub-routines. This ease of implementation of CNNs as of now offers a convenient and accurate solution for PDEs. This is much easier than using many legacy solvers for PDEs (Kiyani et al. 2022 ). For (2), using data in the PDEs in supervised learning for CNNs is simple and so is the empirical integration (Jiagang et al. 2022 ; Fang et al. 2023 ; Fuhg et al. 2023 ). As for (3), one more important advantage is that the exploration of the parameter space , requires basic solution-domain augmentation (Ren et al. 2022 ) (i.e., the space-time parameter space, denoting the possible parameter values of: i) the active PDE variable, and ii) the time parameter) with more parameters like \((x,t, p_1, \cdots , p_n)\) , followed by optimization of the CNN for solving the PDE as a function of the input parameters. By augmenting the input-space, much lesser computational complexity is added to the algorithm as compared to solving the PDE in space-time ( x , t ) parameter values at only one parameter point \((p_1, \cdots , p_n)\) which is much easier than the sequential exploration of the parameter space points (Boussif et al. 2022 ; Tanyu et al. 2023 ). It must be noted that space-time refers to the ( x , t ) values, where x may be a PDE input variable like displacement, and t refers to the input time variable.
On the other side, some of the contemporary weaknesses of CNN-based PDE solvers are:
Absence of a guarantee for theoretical convergence of residuals for non-convex PDE minimization.
Overall slower run-time for each forward-solve.
Weak grounding of theoretical methods in analysis of PDEs.
As for (1), there is the challenge of optimization convergence in non-convex domains, wherein the solution may be trapped inside local minima (Shaban et al. 2023 ; Mowlavi and Nabi 2023 ). It may be noted that for a convex function, the global minimum is unique and any local minimum is also the global minimum. However, a non-convex function can have multiple local minima, which are function solutions where the function reaches a low value but this minimum may not be the globally lowest value. Hence, other minima or potentially better solutions may exist. As for (2) it is much more subtle as compared to its appearance and strongly depends on the different aspects of the CNN architecture used, such as hyperparameters optimization, ultimate simulation goal, etc (Tang et al. 2023b ; Grohs et al. 2023 ). With respect to (3), it merely indicates that CNNs have only now been seriously used for solving PDEs, and hence are theoretically untouched at large (Chen et al. 2023 ). In spite of the above weaknesses, having been inspired by the strengths of deep learning, large-data models have also been used for solving PDEs (Hou et al. 2023 ). Examples of CNNs (Lagaris et al. 1998 ) used for solving PDEs are Physics-informed neural networks (PINNs) (Raissi et al. 2019 ; Baydin et al. 2018 ), DeepONet (Lu et al. 2021b ), etc. Examples of other variants of large-data neural networks used are RNNs like PhyCRNet (Ren et al. 2022 ), LSTMs (José et al. 2021 ), GANs (Gao and Ng 2022 ), etc. Also, recently developed large-data models like Transformers (Cao 2021 ), and Deep reinforcement learning neural networks (DRLNNs) (Han et al. 2018 ) have also been used for solving PDEs. Through this literature survey it is hoped that the readers will get some insight into the area of using state-of-the-art large-data models and that they will be encouraged to engage in research in this interesting field. It is also hoped that by this discussion, future inroads into the merger of high-level mathematical modeling and large-data model based simulation and prediction will be laid.
The main contributions of this paper are summarized as follows.
A comprehensive survey paper is presented in the domain of solving PDEs, to help researchers review, summarize, solve challenges, and plan for future.
An overview of current trends and related techniques for solving PDEs using large-data models is given.
The major issues and future scope of using large-data models are also discussed.
The rest of the paper is organized as follows. Section 2 discusses the works related to using large-data models. Section 3 presents the current trends in the area. Section 4 provides the issues and future directions. Finally, the conclusion is given in Sect. 5 .
2 Related work
Solving differential equations with neural networks has been going on for some time (Huang et al. 2022 ). Traditionally, shallow neural networks were used. Shallow neural networks are the older generation of neural networks which have a few layers, and approximate a small number of parameters. One of the first works can be traced to the year 1990 (Hyuk Lee and In Seok 1990 ). In the same work, the first- and higher-order DEs were quantified by finitesimal approaches followed by using an energy function for the transformed algebraic functions. This energy function was subsequently minimized by using Hopfield networks. Since then there has been a lot of research on solving DEs using various models like neural networks, CNNs and recently other large-data models (Boussange et al. 2023 ). As reported by the dimensions online database (Hook et al. 2018 ) the total number of publications is 337 till date for the phrase search: ‘ PDE solving using neural networks OR CNNs OR deep learning OR RNN OR LSTM OR GANs OR Transformers OR DRL’ . The year-wise breakup of the number of publications obtained from the Dimensions online database, for the same phrase, is shown in Fig. 1 . Out of the search results, the important and ground-breaking works with notable impact, and citations, were considered for the current work. Also, those works were included in the current paper which had novelty, and a significant contribution to the field of PDE solving.
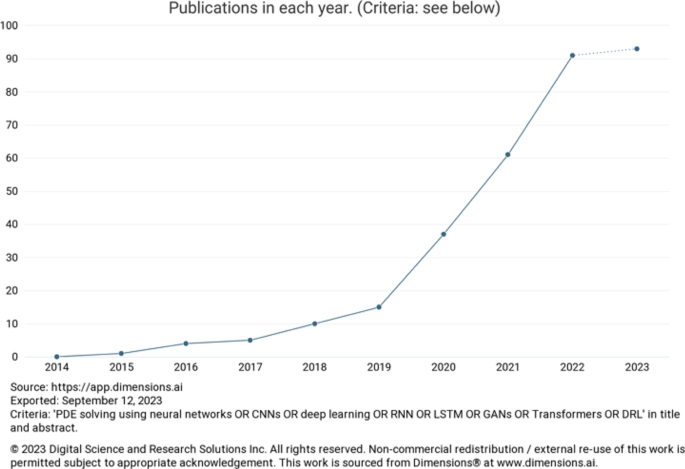
Total number of publications year-wise till date for the phrase search: ’PDE solving using neural networks OR CNNs OR deep learning OR RNN OR LSTM OR GANs OR Transformers OR DRL’ on the dimensions online database (Hook et al. 2018 )
2.1 Shallow neural networks for PDE solving
The mathematical modeling of physical problems can be efficiently incorporated by neural networks. The works of Meade Jr and Fernandez ( 1994a , b ) proved to be important for solving PDEs. In the work (Gobovic and Zaghloul 1993 ), a technique using local connections of neurons was proposed for solving PDEs of heat flow processes. Energy functions are formulated for the PDEs having constant parameters. The energy functions are minimized by using Very large scale integrated (VLSI) Complementary metal oxide (CMOS) circuits. The design of the CMOS circuits was implemented as a neural network with each neuron representing a CMOS cell. Later works like (Gobovic and Zaghloul 1994 ; Yentis and Zaghloul 1994 , 1996 ) used local neural networks for obtaining solutions of the PDEs by parallelization, and by Neural integrated circuits (NICs). The shallow neural networks initially paved the way for more work in the area of PDE solving. However, their weak approximation capabilities due to lesser number of hidden layers, led to the use of CNNs whose capability was better at capturing the numerous dependencies in PDEs. Hence the CNNs performed better than their shallow counterparts in PDE solving.
2.2 Deep neural networks for PDE solving
Deep learning using CNNs is a popular technique for computer vision tasks (Hassaballah and Awad 2020 ; Girshick et al. 2014 ; Girshick 2015 ; Ren et al. 2017 ; Sajid et al. 2021 ; Shelhamer et al. 2017 ; Chen et al. 2018 ; Zhao et al. 2017 ; Hafiz and Bhat 2020 ; Vinyals et al. 2015 ). Deep learning has also been used for tasks like Natural Language Processing (NLP) (Amanat et al. 2022 ). CNNs pre-trained on large datasets like ImageNet (Deng et al. 2009 ) are used after fine-tuning for two notable reasons (Jing and Tian 2021 ). First, the feature maps learned by CNNs from the large datasets help them to generalize better and faster. Second, pre-trained CNNs are adept at avoiding over-fitting during fine-tuning for smaller down-stream applications.
The accuracy of CNNs depends on their architecture (Hafiz et al. 2022 ; Hafiz and Hassaballah 2021 ) and the training technique (Hafiz et al. 2021 ). Many CNNs have been developed with huge numbers of parameters. For training these parameters, huge datasets are required. Some popular CNNs include AlexNet (Krizhevsky et al. 2012 ), VGG (Simonyan and Zisserman 2014 ), GoogLeNet (Szegedy et al. 2015 ), ResNet (He et al. 2016 ), and DenseNet (Huang et al. 2017 ). Popular CNN training datasets for computer vision include ImageNet (Deng et al. 2009 ) and OpenImage (Kuznetsova 2020 ). CNNs have achieved state-of-the-art classification performance for many computer vision tasks (Girshick et al. 2014 ; Shelhamer et al. 2017 ; Vinyals et al. 2015 ; Hassaballah and Hosny 2019 ; Ledig et al. 2017 ; Tran et al. 2015 ; Hafiz et al. 2020 , 2023 ).
2.2.1 CNNs for solving non-linear equations
As per the work of Lagaris et al. ( 1998 ), the Differential Equations can be broken down into sub-components using the Dirichlet and Neumann expressions. By using neural networks (Lagaris et al. 1998 ), non-linear equations were solved up to the seventh decimal digit. Since (Lagaris et al. 1998 ) is the first work to use CNNs for solving PDEs, it is worthy of being explained briefly. Considering a general differential equation given by Eq. 6 which needs a solution:
Here \({x} = (x_1, x_2, ..., x_n) \in \mathbb {R}^n\) for certain boundary conditions as per an arbitrary boundary S , \(B \subset \mathbb {R}^n\) is the defining domain and y ( x , t ) is the solution needed. It should be noted that we do not define the boundary conditions here because we are defining a general equation above.
For obtaining a solution to Eq. 6 , first the domain B has to be discretized into a set of points \(\hat{B}\) . Also the arbitrary boundary S (given here) of the general equation has to discretized into a set of points \(\hat{S}\) . Then, the DE may be expressed as a system which has constraints of the generally defined boundary conditions as per Eq. 7 :
Here y ( x , t ) is the solution. It can be obtained from two components given in Eq. 8 :
Here A ( x ) has fixed parameters, p is the parameter set, and N ( x , p ) is the neural network for minimization.
Although the initial CNNs used for solving PDEs gave promising results, they had certain issues. These included a lack of interpretability, weak adaptation of their structure to problems, and average performance (Ruthotto and Haber 2020 ; Uriarte et al. 2023 ).
2.2.2 Physics-informed neural networks (PINNs) for PDE solving
After taking inspiration from the works of Lagaris et al. ( 1998 ) and Hornik ( 1991 ), wherein CNNs were used for universal approximation, a new genre of CNNs was introduced wherein the physical constraints in the form of PDEs were added to the loss function, hence the name Physics-informed neural networks (PINNs) (Raissi et al. 2019 ; Baydin et al. 2018 ). More specifically, the technique involved applying the laws of physics expressed by PDEs as CNN loss functions. And in turn, the loss functions could be optimized for finding solutions (Maziar and George 2018 ). PINNs do not need discretization of the domains. They are also quite practical as the heavy computation is avoided. PINNs use minimization techniques for non-linear parametric PDEs of the form given by Eq. 9 :
Here y ( x , t ) is the solution which is hidden. \(N[\cdot ; \lambda ]\) is an operator of \(\lambda\) . \(\Omega\) belongs to \({\mathbb {R}^D}\) where D is the number of dimensions.
Using PINNs, Raissi et al. ( 2019 ) extensively studied complex dynamic processes like post-cylindrical flow, and aneurysm in Raissi et al. ( 2019 ),Raissi et al. ( 2020 ). Figure 2 shows the schematic for the PINN. Although PINN was a PDE-dedicated CNN model which led to better performance, it suffered from issues of other CNNs like lack of interpretability, need for large data and long training times (Mowlavi and Nabi 2023 ; Meng et al. 2023 ). In spite of these, it was partly successful as an expert system for solving PDEs. This was due to its PDE-dedicated framework (Tang et al. 2023a ; Jia et al. 2022 ).
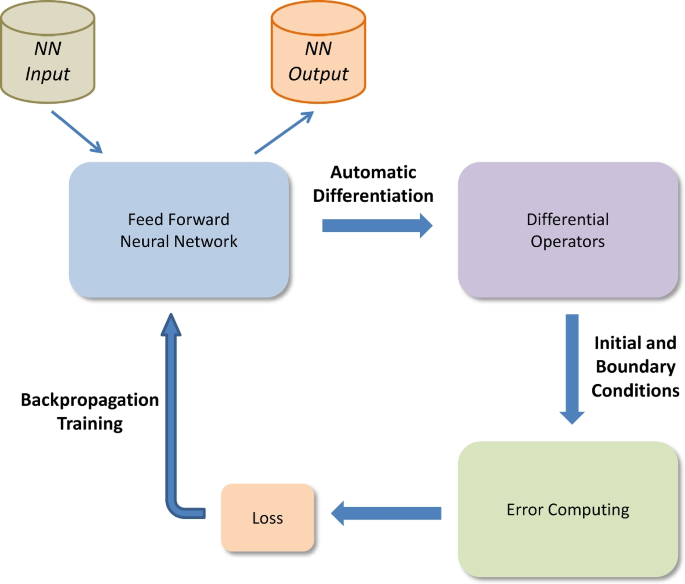
Schematic of the Physics-informed neural network (PINN) used for solving PDEs. Huang et al. ( 2022 )
2.2.3 DeepONet CNN for PDE solving
Due to improved computing capability and availability of high-performance computing (HPC) systems, CNN implementation and training became convenient. Subsequently, different techniques which were previously difficult to implement, were implemented. Lu et al. considered Chen et al.’s non-linear operators (Chen and Chen 1995a , b ) as a theoretical basis for justifying the use of neural networks for operator learning. They came up with a robust CNN called DeepONet (Lu et al. 2021b ). As CNNs have superior expressibility and many special advantages e.g. in computer vision and in sequential analysis respectively, the authors used many CNNs in the DeepONet model for diversifying and targeting various net assemblies. Also, Lu et al. exploited a bifurcated parallel structure in their proposed CNN. Figure 3 shows the schematic for the DeepONet model. FEA-Net (Yao et al. 2019 ), Hierarchical deep-learning neural network (Lei et al. 2021 ), etc. are other examples of CNNs used for PDE solving.
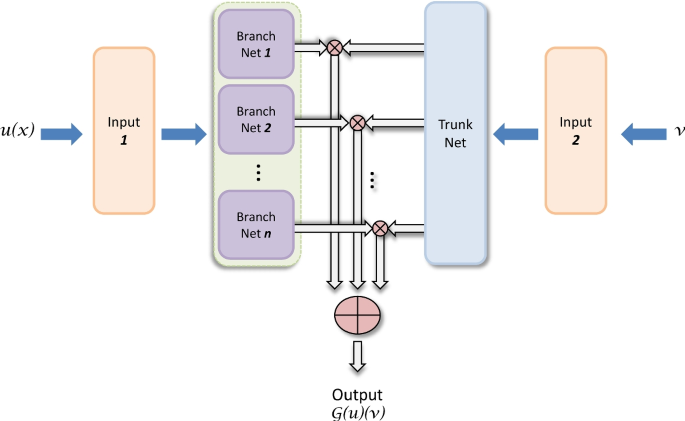
Schematic of the DeepONet CNN model for approximation of the nonlinear operator G ( u )( v ) where u ( x ) and v are the variables of the PDE to be solved (Huang et al. 2022 )
2.2.4 Recurrent neural networks (RNNs) for PDE solving
Ren et al. ( 2022 ) proposed a PINN called physics-informed convolutional recurrent network (PhyCRNet) for solving PDEs. They used a convolutional encoder-decoder long short-term memory (LSTM) network. This network was used for low dimensional feature extraction and evolutional learning. Next, PDE residuals are used for the PDE solution estimation. The residual is a value which is obtained when we substitute the current estimate into the discretized PDE. If the PDE solution depends on time, then the residuals have to converge with every time step. They (Ren et al. 2022 ) used the PDE residual R(x,y,t, \(\theta\) ) given by Eq. 10 :
Here \({y \in \mathbb {R}^n}\) is the solution of the PDE in the temporal domain \(t \in\) [0,T], and in the physical domain \(\Omega\) . \(\textit{u}_t\) is the first order derivative. \(\nabla _x\) is the gradient for x and F is the non-linear function having parameter \(\lambda\) . It must be noted that F plays an important role in Eq. 10 because the residual is obtained by substituting the iterative input parameters in the PDE function.
The loss function L is the sum of squares of the residuals of the PDEs. For a 2D PDE system, L is given by Eq. 11 :
Here n and m are the height and the width in the spatial domain respectively. T is the total of time-steps and \(||\cdot ||_2\) is the \(l_2\) Norm.
The loss function was based on PDE residuals. The initial- and boundary-conditions were fixed inside the network. The model was enhanced by regressive as well as residual links which simulated time flow. They solved three types of PDEs using their model viz. 2D Burgers’ equation, the \(\lambda -\omega\) equation and the FitzHugh Nagumo equation. Promising results were obtained. Figure 4 gives the overview of the PhyCRNet architecture.
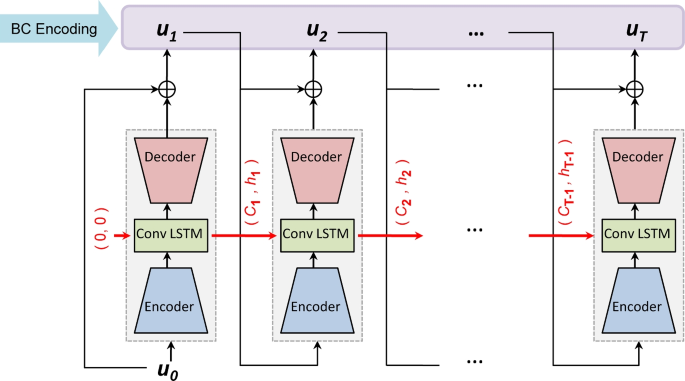
Schematic of the PhyCRNet model for solving PDEs. Each RNN cell consists of an encoder unit, a Long short term memory (LSTM) unit and a decoder unit. C and h are the cell and hidden states of the Convolutional long short term memory (ConvLSTM) units respectively. BC Encoding refers to the Boundary condition (BC) encoding wherein the BCs are enforced on the output variables. \({u_0}\) is the Initial condition (IC) and \({u_i}\) refers to the state variable for time-step \({t \in [1,T]}\) . Ren et al. ( 2022 )
RNNs are usually used for prediction of time-series problems. Their application to PDE solving in forms like PhyCRNet, marked a shift of technique and gave promising results. However, the performance of RNNs for solving PDEs is affected by issues like high complexity, narrow scope, long-training times, etc.
2.3 Long short term memory (LSTM) neural networks for PDE solving
In their work (José et al. 2021 ), Ferrandis et al. proposed prediction of naval vessel motion by PDEs using Long short term memory (LSTM) neural networks. The input to their LSTM model was the stochastic wave elevation for a particular sea state and the output of their LSTM model comprised of the vessel motions viz. pitch, heave and roll. Promising prediction results were obtained for the vessel motion for arbitrary wave elevations. They trained their LSTM neural networks using offline simulation and extended the prediction to online mode. Their objective function for minimization during training was the Mean squared error (MSE) given by Eq. 12 :
Here X is the observed value vector and \(\hat{X_i}\) is the predicted value vector.
Their work was modelled by the universal approximation theorem for functional problems. They claimed that their work was the first to implement such a model for real engineering processes. A schematic of the general LSTM neural network model is shown in Fig. 5 .
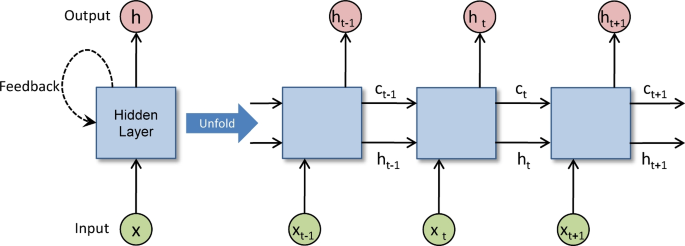
Schematic of the general Long-short term menory (LSTM) neural network illustrating the unfolding of the feedback loop which makes it suitable for sequential data processing. In the unfolded model \(x_i\) is input to the \(i^{th}\) cell, \(c_i\) is cell-state for \(i^{th}\) cell and \(h_i\) is the hidden-state of the \(i^{th}\) cell. The subscripts \(t-1\) , t and \(t+1\) represent three successive time-steps.Song et al. ( 2020 )
As is evident from the above, LSTM NNs are complex in nature. In spite of this, LSTM NNs are suitable for regression problems like time-series prediction tasks, due to their recurrent nature. However, LSTM NNs are used less in PDE solving. And as of now, few works of PDE solving using LSTM NNs are found. This issue is due to the complexity of modeling PDEs with LSTM NNs. In spite of this, some unique applications of LSTM NN based PDE solving have been proposed and promising results are being obtained.
2.4 Generative adversarial networks (GANs) for PDE solving
Generative adversarial networks (GAN) (Goodfellow et al. 2014 ; Gui et al. 2021 ; Yang 2019 ; Gao and Ng 2022 ) are machine learning algorithms that use deep learning for generation of new data. A GAN is made up of two NNs i.e., a generator and a discriminator. The generator and discriminator are trained together. The generator generates artificial data which imitates the real data, while the discriminator separates the data generated by the generator from the real data. GANs have become popular with tasks like image-morphing (Gui et al. 2021 ). In their work (Gao and Ng 2022 ), Gao and Ng proposed the physics informed GAN called Wassertein Generative Adversarial Network (WGAN), which was used for solving PDEs. These GANs use a unique function known as the Wassertein function for convergence. Wassertein function is suitable for PDE solving, and leads to convenient convergence for the latter. The usage of this function has paved the way for using GANs for PDE solving. They stated that GANs could be formulated in the general form given by Eq. 13 :
In Eq. 13 , G and F are the classes of the generator and the discriminator respectively. \(\pi\) is the distribution of the source and v is the distribution used for approximation. \(g_\theta\) and \(f_\alpha\) are the generator and discriminator functions respectively. x , z , \(\alpha\) , and \(\theta\) are the inputs to the PDE function to be solved. They form the input parameter-space to be explored for solving the PDE. \(\mathbb {E}\) is the energy function to be minimized for solving the PDE.
The authors of Gao and Ng ( 2022 ) showed that the generalization error to be minimized for obtaining the PDE solution, converged to the approximation error of the GAN model for large data, and they obtained promising results. Schematic for the WGAN used in their work is shown in Fig. 6 .
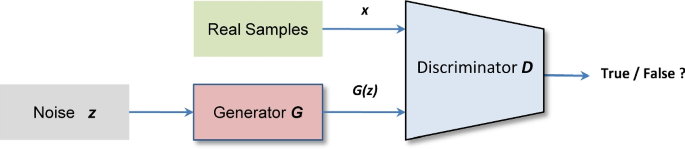
Schematic of the GAN. The GAN is trained till the discriminator which compares the real data x and the generated data G ( z ) cannot distinguish between the two (Wang et al. 2017 )
There are many types of GANs found in literature which have been applied diversely to engineering problems. The most popular application of GANs is image-morphing, though the former is not limited to the latter. Using GANs for solving PDEs comes as a unique application. This is because of the complexity in adapting GANs to solve specific framework problems like those of PDE solving. However as seen with WGANs, progress in this area is being made, and promising results have been obtained.
2.5 Transformers for PDE solving
Inspired by the initial work on Transformers (Vaswani et al. 2017 ), Shuhao Cao applied self-attention based Transformers to data driven learning for PDEs (Cao 2021 ). He used Hilbert space approximation for operators. He showed that soft-max normalization was not needed for the scaled dot product in attention mechanisms. He introduced a novel normalization layer which mimicked the Petrov-Galerkin projection for scalable propagation through the attention-based layers in Transformers. The Galerkin attention operator uses the best approximation of f in the \(l_2\) norm \(||\cdot ||_H\) as given by Eq. 14 :
Here H is the Hilbert space, and \(f \in H\) . \((\mathbb {Q},\mathbb {V})\) refer to the Query and Value subspaces used in the attention maps respectively. \(g_\theta (\cdot )\) is a learnable map of the Galerkin attention operator. \(f_h \in \mathbb {Q}_h\) is the best approximation of f in \(|| \cdot ||_H\) , \(b(\cdot , \cdot ):V \times Q \rightarrow \mathbb {R}\) is the continuous bilinear form and c is the boundary condition limit. \(y \in \mathbb {R}^{n \times d}\) is the current latent representation.
This novel technique helped the Transformer model to obtain a good accuracy in learning operators for un-normalized data tasks. The three PDEs he used for experimentation purposes were the Burgers’ equation, the Darcy flow interface process and a coefficient identification process. He called his improved Transformer as the Galerkin Transformer, which demonstrated a better cost of training and a better performance over the conventional counterparts. A schematic of Galerkin attention mechanism is shown in Fig. 7 .
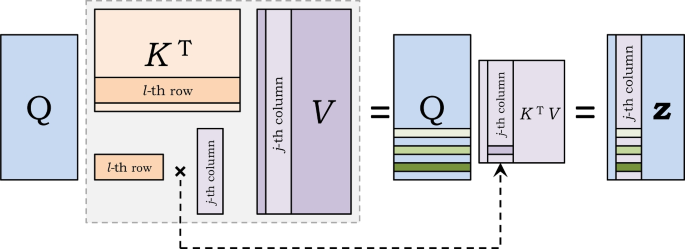
Schematic of the Galerkin attention module used in the novel Transformer which is used to solve PDEs. Here ( Q , K , V ) are the (Query, Key and Value) matrices of the input to the attention module. \(K^T\) denotes the transpose of the Key matrix K . z is the attention-value matrix which is the output of the attention module. Cao ( 2021 )
Transformers were initially developed for Natural language processing (NLP) and were later on adapted to computer vision in the form of Visual transformers (ViTs). Their recent application to PDE solving is an encouraging step. This is because the huge number of training parameters in Transformers can effectively absorb the large number of dependencies in PDEs. However, Transformers have their own issues e.g. need for very large amount of training data, long training times, and those performances which still are not able to rival that of CNNs. Now, as more training data for semi-supervised, un-supervised and formula-driven supervised learning are becoming available, these issues are being addressed.
2.6 Deep reinforcement learning neural networks (DRLNNs) for solving PDEs
Deep reinforcement learning neural networks (DRLNNs) (Hafiz 2023 ; Hafiz et al. 2023 ) are deep networks using Reinforcement learning (RL) (Hafiz et al. 2021 ). DRLNNs have also been used for solving PDEs (Han et al. 2018 ). Han et al. ( 2018 ) proposed a deep learning based technique which was capable of solving high dimensional PDEs. They reformulated the PDEs using stochastic DEs and the solution gradient was approximated by deep neural networks using RL. Their backward stochastic differential equation (BSDE) played the role of model based RL and the solution gradient played the role of the policy function (Han et al. 2018 ). Considering the PDE given by Eq. 15 , its model showed promising results as shown in Table 1 . The PDE used for experimentation was a high dimensional (d = 100) Gobet and Tukedjiev equation from the work (Gobet and Turkedjiev 2017 ) given by Eq. 15 as:
where ( t , x ) are the temporal and spatial variables of the oscillating solution \(y^{*}(t,x)\) given by Eq. 16 as:
Here D is the dimensionality of the system and T is the time. In the above equations k =1.6, \(\lambda\) =0.1 and T =1.
It is observed from Table 1 that the SD is quite low and both the Mean error (%) and SD decrease with the increase in the number of layers in the deep network. This is testimony to the fact that large-data models like DRLNNs are quite capable of solving complex high dimensional PDEs. RL was initially limited to basic algorithms. Now it has developed into a substantial field of research having numerous techniques for many applications. With the development of DRLNNs, the fields of deep learning and RL were merged. However, there is not a perfect merger due to their respective unique natures. Also with RL there is the potential issue of RL systems going ‘rogue’ due to greed and harming their frameworks and environments. Nevertheless, DRLNNs offer solutions to many important modern day problems and are generally controlled (Raissi 2024 ; Siegel et al. 2023 ).
A graphical abstract of the above models for PDE solving is given in Fig. 8 in the form of a timeline. It can be observed from the timeline that the majority of large-data models for solving PDEs are neural networks. To summarize the main large-data models discussed in this work, the PDEs they solve, and the pros and cons of these models, we highlight the same in Table 2 .
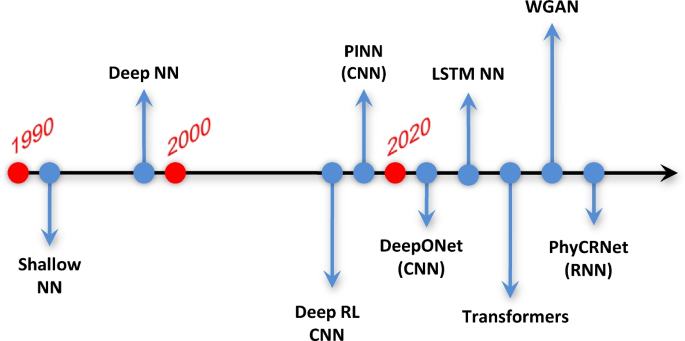
Timeline for using large-data models
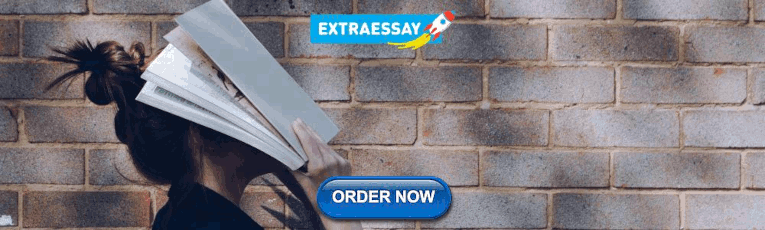
3 Current trends
There has been significant research on analyzing the generalization errors using techniques like PINNs (Mishra and Molinaro 2022 ; Penwarden et al. 2023 ) and DeepONet (Kovachki et al. 2021a ; Lanthaler et al. 2022 ). These techniques have very good performance. Lu et al. ( 2022a ) compared the efficiency of these two techniques. There is often a desire for incremental research based on existing methods like PINNs. The model architecture for neural network training for solving PDEs consists of input, neural net approximation, and the network loss function (Huang et al. 2022 ). A three pronged approach has been used for improving performance as discussed below.
Loss function: Improving the loss function is very useful for obtaining superior performance. Jagtap et al. ( 2020 ) improved the PINNs by using the cPINNs as well as the XPINNs (Jagtap and Karniadakis 2021 ). They used multiple domains and additional constraints. Kutz et al. and Patel et al. did the same by using parsimony (Nathan Kutz and Brunton 2022 ) and spatio-temporal schemes (Patel et al. 2022 ). Other studies have used the initial conditions and the boundary differently. For instance, Li et al. ( 2022 ) who fused partial integration and level set techniques.
Model used: Recurrent Neural Networks (RNNs), Generative Adversarial Networks (GANs), Long short term memory (LSTM) neural networks, and even the attention based Transformers have been recently used and have given better performance (Ren et al. 2022 ; José et al. 2021 ; Cao 2021 ; Gao and Ng 2022 ; Meng et al. 2022 ) in solving PDEs. U-FNO with more U-Fourier layers (Wen et al. 2022 ) and the attention based model viz. FNO (Peng et al. 2022 ) have been developed. Also, research has been done for optimization of activation functions (Wang et al. 2021 ; Yifan and Zaki 2021 ; Venturi and Casey 2023 ).
Input: Kovacs et al. ( 2022 ) used parameters for determination of the eigen-value equation coefficients as additional inputs . Hadorn improved the DeepONet model by allowing the base function to shift and scale the input values (Patrik 2022 ). Lye et al. improved the ML technique accuracy by using a multi-level approach (Lye et al. 2021 ).
The state-of-the-art technologies have led to much better capability. Lu et al. ( 2021a ) crafted DeepXDE using PINNs and DeepONet. In the industry, NVIDIA the popular Graphics Processing Unit (GPU) manufacturing company has used PINNs, DeepONet, and enhanced finite numerical optimization techniques in a toolbox to build the Digital Twin. Also, the advances in quantum-based technology are favoring numerical optimization (Swan et al. 2021 ). In addition to these, more research (Wang et al. 2022 ) is being done on PDE solving by introduction of Gaussian processes (Chen et al. 2021 ), and use of hybrid Finite Element Method - Neural Network models (Mitusch et al. 2021 ). Li et al. have improved their previous works of Finite Numerical Optimization and have come up with a CNN model for operator learning (Kovachki et al. 2021b ). A notable aspect of the newly developed model is its robustness to discretization-invariance, leading to its use for a wider range of applications. As mentioned earlier, like the case of DeepONet (Lu et al. 2021b ), there is an effort to use the state-of-the-art deep learning models in this area. In spite of the fact that there are numerous new techniques, they share a common thing. That is, the boundary between theoretical process mechanism and experimental data is being dissolved. Lastly fusion of these two aspects has profoundly improved (Nelsen and Stuart 2021 ; Kadeethum et al. 2021 ; Gupta and Jaiman 2022 ; Gin et al. 2021 ; Bao et al. 2020 ; Jin et al. 2022 ; Lu et al. 2022b ). Using alternate techniques for developing large-data models like Transformers (Cao 2021 ) is also an interesting pointer to the potential to be unlocked in PDE solving. The diversity of large-data models available today also offers rich choices for solving PDEs and has many potential applications (Antony et al. 2023 ; Li et al. 2023 ; Shen et al. 2023 ).
4 Issues and future scope
The mixing of scientific computing with deep learning is very likely due to the advances in technology and research (Huang et al. 2022 ). However, this trend is reaching a flash point due to abundant computational resources warranting new research directions. Also, the gaps in the theoretical models and the experimental data, pose a problem and their elimination is difficult by conventional means. Further, in spite of the fact that CNNs have robust pattern recognition capabilities, their interpretability and inner working are not extensively researched. New techniques like partial integration and numerical optimization have tried to relate the earlier knowledge of PDEs and the new information of big-data from models like CNNs. Large-data models are often referred to as being ‘data-hungry’ due to the need for extensive training. As such there needs to be research on ways to augment training data without the need for huge amounts of ‘natural’ data. This is an open research area. Again, the interpretability and complexity raise issues, and addressing the same remains an open problem.
Techniques can be coarsely classified into iterative numerical techniques and machine learning based techniques (Psaros et al. 2023 ). Numerical analysis directly depicts the DE mechanism, while as other ML techniques use probabilistic expressions for data characterization. However, for handling specific PDE solving, approximation can also be used. An example is the parameter approximation used in CNNs for PDEs. Also, special CNNs like DeepONet may be used for functional description of the large-data physical models. These techniques are promising for solving PDEs. Hence using different large-data models can also benefit by their respective strengths. In addition to using neural networks and bifurcated structures, different models may be used (Jagtap et al. 2022 ; Sirignano and Spiliopoulos 2018 ; Gupta et al. 2021 ). Also, the reverse path can be used in dynamical system pattern application for model enhancement. This can lead to substantial benefits for model learning interpretability. As mentioned above, one interesting area is the generation of training data for ‘data-hungry’ models without the use of manual collection. An example of this is mathematical formula-based generation of training data, e.g., by Formula-driven supervised learning or FDSL (Hafiz et al. 2023 ).
5 Conclusion
In this review paper, an overview of solving Partial differential equation (PDE) using large-data models was given. An introduction to the area was presented along with its publication trends. This was followed by a discussion of various techniques used for solving PDEs using large-data models. The large-data models discussed included Convolutional neural networks (CNNs), Recurrent neural networks (RNNs), Long-short term memory (LSTM) neural networks, Generative adversarial networks (GANs), attention-based Transformers and the Deep reinforcement learning neural networks (DRLNNs). The pros and cons of these techniques were discussed. A trend timeline for the purpose was also given. Then, the major issues and future scope in the area were discussed. Finally, we hope this literature survey becomes a quick guide for the researchers and motivates them to consider using large-data models to solve significant problems in PDE based mathematical modeling.
Data availibility
Data sharing not applicable to this article as no datasets were generated or analysed during the current study.
Alt T, Schrader K, Augustin M, Peter P, Weickert J (2023) Connections between numerical algorithms for PDEs and neural networks. J Math Imaging Vis 65(1):185–208
Article MathSciNet Google Scholar
Antony ANM, Narisetti N, Gladilin E (2023) FDM data driven U-Net as a 2D Laplace PINN solver. Sci Rep 13(1):9116
Article Google Scholar
Ashtiani MN, Raahemi B (2023) News-based intelligent prediction of financial markets using text mining and machine learning: a systematic literature review. Expert Syst Appl 217:119509
Bao G, Ye X, Zang Y, Zhou H (2020) Numerical solution of inverse problems by weak adversarial networks. Inverse Probl 36(11):115003
Baydin AG, Pearlmutter BA, Radul AA, Siskind JM (2018) Automatic differentiation in machine learning: a survey. J March Learn Res 18:1–43
Google Scholar
Bhangale KB, Kothandaraman M (2022) Survey of deep learning paradigms for speech processing. Wirel Pers Commun 125(2):1913–1949
Boussange V, Becker S, Jentzen A, Kuckuck B, Pellissier L (2023) Deep learning approximations for non-local nonlinear PDEs with Neumann boundary conditions. Partial Differ Equ Appl 4(6):51
Boussif O, Bengio Y, Benabbou L, Assouline D (2022) MAgnet: mesh agnostic neural PDE solver. Adv Neural Inf Process Syst 35:31972–31985
Cao S (2021) Choose a transformer: Fourier or Galerkin. Adv Neural Inf Process Syst 34:24924–24940
Chen T, Chen H (1995a) Approximation capability to functions of several variables, nonlinear functionals, and operators by radial basis function neural networks. IEEE Trans Neural Netw 6(4):904–910
Chen T, Chen H (1995b) Universal approximation to nonlinear operators by neural networks with arbitrary activation functions and its application to dynamical systems. IEEE Trans Neural Netw 6(4):911–917
Chen L-C, Papandreou G, Kokkinos I, Murphy K, Yuille AL (2018) DeepLab: semantic image segmentation with deep convolutional nets, atrous convolution, and fully connected CRFs. IEEE Trans Pattern Anal Mach Intell 40(4):834–848
Chen Y, Hosseini B, Owhadi H, Stuart AM (2021) Solving and learning nonlinear PDEs with gaussian processes. J Comput Phys 447:110668
Chen M, Niu R, Zheng W (2023) Adaptive multi-scale neural network with resnet blocks for solving partial differential equations. Nonlinear Dyn 111(7):6499–6518
Choi J, Kim N, Hong Y (2023) Unsupervised Legendre-Galerkin neural network for solving partial differential equations. IEEE Access 11:23433–23446
El-Ajou A (2021) Adapting the Laplace transform to create solitary solutions for the nonlinear time-fractional dispersive PDEs via a new approach. Eur Phys J Plus 136(229):1–22
Fang X, Qiao L, Zhang F, Sun F (2023) Explore deep network for a class of fractional partial differential equations. Chaos Solitons Fract 172:113528
Farlow SJ (2006) An introduction to differential equations and their applications. Dover Publications, Mineola
Fuhg JN, Karmarkar A, Kadeethum T, Yoon H, Bouklas N (2023) Deep convolutional Ritz method: parametric PDE surrogates without labeled data. Appl Math Mech 44(7):1151–1174
Gao Y, Ng MK (2022) Wasserstein generative adversarial uncertainty quantification in physics-informed neural networks. J Comput Phys 463:111270
Gin CR, Shea DE, Brunton SL, Nathan Kutz J (2021) DeepGreen: deep learning of Green’s functions for nonlinear boundary value problems. Sci Rep 11(1):21614
Gobet E, Turkedjiev P (2017) Adaptive importance sampling in least-squares Monte Carlo algorithms for backward stochastic differential equations. Stoch Process Appl 127(4):1171–1203
Grohs P, Hornung F, Jentzen A, Zimmermann P (2023) Space-time error estimates for deep neural network approximations for differential equations. Adv Comput Math 49(1):4
Gupta R, Jaiman R (2022) A hybrid partitioned deep learning methodology for moving interface and fluid-structure interaction. Comput Fluids 233:105239
Gupta G, Xiao X, Bogdan P (2021) Multiwavelet-based operator learning for differential equations. Adv Neural Inf Process Syst 34:24048–24062
Hafiz AM (2023) A survey of deep Q-networks used for reinforcement learning: state of the art. In: Rajakumar G, Ke-Lin D, Vuppalapati C, Beligiannis GN (eds) Intelligent communication technologies and virtual mobile networks. Springer, Singapore, pp 393–402
Chapter Google Scholar
Hafiz AM, Bhat GM (2020a) A survey on instance segmentation: state of the art. Int J Multimed Inf Retr 9(3):171–189
Hafiz AM, Bhat GM (2020b) A survey of deep learning techniques for medical diagnosis. In: Tuba M, Akashe S, Joshi A (eds) Information and communication technology for sustainable development, Singapore. Springer, Singapore, pp 161–170
Hafiz AM, Hassaballah M (2021) Digit image recognition using an ensemble of one-versus-all deep network classifiers. In: Shamim Kaiser M, Xie J, Rathore VS (eds) Information and communication technology for competitive strategies. Springer, Singapore, pp 445–455
Hafiz AM, Parah SA, Bhat RA (2021) Reinforcement learning applied to machine vision: state of the art. Int J Multimed Inf Retr 10(2):71–82
Hafiz AM, Hassaballah M, Alqahtani A, Alsubai S, Hameed MA (2023) Reinforcement learning with an ensemble of binary action deep Q-networks. Comput Syst Sci Eng 46(3):2651–2666
Hafiz AM, Bhat RUA, Parah SA, Hassaballah M (2023) SE-MD: a single-encoder multiple-decoder deep network for point cloud reconstruction from 2D images. Pattern Anal Appl 26:1291–1302
Han J, Jentzen A, Weinan E (2018) Solving high-dimensional partial differential equations using deep learning. Proc Natl Acad Sci 115(34):8505–8510
Hassaballah M, Awad AI (2020) Deep learning in computer vision: principles and applications. CRC Press, Boca Raton
Book Google Scholar
Hassaballah M, Hosny KM (2019) Recent advances in computer vision: theories and applications. Springer, Berlin
Hornik K (1991) Approximation capabilities of multilayer feedforward networks. Neural Netw 4(2):251–257
Hou J, Li Y, Ying S (2023) Enhancing PINNs for solving PDEs via adaptive collocation point movement and adaptive loss weighting. Nonlinear Dyn 111(16):15233–15261
Hyuk L, In SK (1990) Neural algorithm for solving differential equations. J Comput Phys 91(1):110–131
Innerberger M, Praetorius D (2023) MooAFEM: an object oriented Matlab code for higher-order adaptive FEM for (nonlinear) elliptic PDEs. Appl Math Comput 442:127731
MathSciNet Google Scholar
Jagtap AD, Kharazmi E, Karniadakis GE (2020) Conservative physics-informed neural networks on discrete domains for conservation laws: applications to forward and inverse problems. Comput Methods Appl Mech Eng 365:113028
Jagtap AD, Shin Y, Kawaguchi K, Karniadakis GE (2022) Deep Kronecker neural networks: a general framework for neural networks with adaptive activation functions. Neurocomputing 468:165–180
Jean LK, Fendji E, Tala DCM, Yenke BO, Atemkeng M (2022) Automatic speech recognition using limited vocabulary: a survey. Appl Artif Intell 36(1):2095039
Jia X, Meng D, Zhang X, Feng X (2022) PDNet: progressive denoising network via stochastic supervision on reaction-diffusion-advection equation. Inf Sci 610:345–358
Jiagang Q, Cai W, Zhao Y (2022) Learning time-dependent PDEs with a linear and nonlinear separate convolutional neural network. J Comput Phys 453:110928
Jiang Z, Jiang J, Yao Q, Yang G (2023) A neural network-based PDE solving algorithm with high precision. Sci Rep 13(1):4479
Jin P, Meng S, Lu L (2022) MIONet: learning multiple-input operators via tensor product. SIAM J Sci Comput 44(6):A3490–A3514
Jing L, Tian Y (2021) Self-supervised visual feature learning with deep neural networks: a survey. IEEE Trans Pattern Anal Mach Intell 43(11):4037–4058
José del Águila F, Triantafyllou MS, Chryssostomidis C, Karniadakis GE (2021) Learning functionals via LSTM neural networks for predicting vessel dynamics in extreme sea states. Proc R Soc A 477(2245):20190897
Kadeethum T, O’Malley D, Fuhg JN, Choi Y, Lee J, Viswanathan HS, Bouklas N (2021) A framework for data-driven solution and parameter estimation of PDEs using conditional generative adversarial networks. Nat Comput Sci 1(12):819–829
Kiyani E, Silber S, Kooshkbaghi M, Karttunen M (2022) Machine-learning-based data-driven discovery of nonlinear phase-field dynamics. Phys Rev E 106(6):065303
Kolman R, Okrouhlík M, Berezovski A, Gabriel D, Kopačka J, Plešek J (2017) B-spline based finite element method in one-dimensional discontinuous elastic wave propagation. Appl Math Model 46:382–395
Kovachki N, Lanthaler S, Mishra S (2021a) On universal approximation and error bounds for Fourier neural operators. J Mach Learn Res 22(1):13237–13312
Kovacs A, Exl L, Kornell A, Fischbacher J, Hovorka M, Gusenbauer M, Breth L, Oezelt H, Yano M, Sakuma N et al (2022) Conditional physics informed neural networks. Commun Nonlinear Sci Numer Simul 104:106041
Krizhevsky A, Sutskever I, Hinton GE (2012) ImageNet classification with deep convolutional neural networks. In: Pereira F, Burges CJ, Bottou L, Weinberger KQ (eds) Advances in neural information processing systems, vol 25. Curran Associates Inc, Montreal
Kuznetsova A et al (2020) The open images dataset v4. Int J Comput Vis 128(7):1956–1981
Lagaris IE, Likas A, Fotiadis DI (1998) Artificial neural networks for solving ordinary and partial differential equations. IEEE Trans Neural Netw 9(5):987–1000
Lanthaler S, Mishra S, Karniadakis GE (2022) Error estimates for deeponets: a deep learning framework in infinite dimensions. Trans Math Appl 6(1):tnac001
Lecun Y, Bottou L, Bengio Y, Haffner P (1998) Gradient-based learning applied to document recognition. Proc IEEE 86(11):2278–2324
Li C, Yang Y, Liang H, Boying W (2022) Learning high-order geometric flow based on the level set method. Nonlinear Dyn 107(3):2429–2445
Li S, Zhang C, Zhang Z, Zhao H (2023) A data-driven and model-based accelerated Hamiltonian Monte Carlo method for Bayesian elliptic inverse problems. Stat Comput 33(4):90
Lu L, Meng X, Mao Z, Karniadakis GE (2021a) DeepXDE: a deep learning library for solving differential equations. SIAM Rev 63(1):208–228
Lu L, Jin P, Pang G, Zhang Z, Karniadakis GE (2021b) Learning nonlinear operators via DeepONet based on the universal approximation theorem of operators. Nat Mach Intell 3(3):218–229
Lu L, Meng X, Cai S, Mao Z, Goswami S, Zhang Z, Karniadakis GE (2022a) A comprehensive and fair comparison of two neural operators (with practical extensions) based on fair data. Comput Methods Appl Mech Eng 393:114778
Lu L, Pestourie R, Johnson SG, Romano G (2022b) Multifidelity deep neural operators for efficient learning of partial differential equations with application to fast inverse design of nanoscale heat transport. Phys Rev Res 4(2):023210
Lye KO, Mishra S, Molinaro R (2021) A multi-level procedure for enhancing accuracy of machine learning algorithms. Eur J Appl Math 32(3):436–469
Mallikarjunaiah SM (2023) A deep learning feed-forward neural network framework for the solutions to singularly perturbed delay differential equations. Appl Soft Comput 148:110863
Maziar R, George EK (2018) Hidden physics models: machine learning of nonlinear partial differential equations. J Comput Phys 357:125–141
Meade Jr AJ, Fernandez AA (1994) Solution of nonlinear ordinary differential equations by feedforward neural networks. Math Comput Model 20(9):19–44
Meade Jr AJ, Fernandez AA (1994) The numerical solution of linear ordinary differential equations by feedforward neural networks. Math Comput Model 19(12):1–25
Melchers H, Crommelin D, Koren B, Menkovski V, Sanderse B (2023) Comparison of neural closure models for discretised PDEs. Comput Math Appl 143:94–107
Meng X, Yang L, Mao Z, del Águila FJ, George EK (2022) Learning functional priors and posteriors from data and physics. J Comput Phys 457:111073
Meng Z, Qian Q, Mengqiang X, Bo Yu, Yıldız AR, Mirjalili S (2023) PINN-FORM: a new physics-informed neural network for reliability analysis with partial differential equation. Comput Methods Appl Mech Eng 414:116172
Michoski C, Milosavljević M, Oliver T, Hatch DR (2020) Solving differential equations using deep neural networks. Neurocomputing 399:193–212
Mishra S, Molinaro R (2022) Estimates on the generalization error of physics-informed neural networks for approximating a class of inverse problems for PDEs. IMA J Numer Anal 42(2):981–1022
Mitusch SK, Funke SW, Kuchta M (2021) Hybrid FEM-NN models: combining artificial neural networks with the finite element method. J Comput Phys 446:110651
Mowlavi S, Nabi S (2023) Optimal control of PDEs using physics-informed neural networks. J Comput Phys 473:111731
Mugler DH, Scott RA (1988) Fast Fourier transform method for partial differential equations, case study: the 2-D diffusion equation. Comput Math Appl 16(3):221–228
Namaki N, Eslahchi MR, Salehi R (2023) The use of physics-informed neural network approach to image restoration via nonlinear PDE tools. Comput Math Appl 152:355–363
Nathan Kutz J, Brunton SL (2022) Parsimony as the ultimate regularizer for physics-informed machine learning. Nonlinear Dyn 107(3):1801–1817
Nelsen NH, Stuart AM (2021) The random feature model for input-output maps between Banach spaces. SIAM J Sci Comput 43(5):A3212–A3243
Oñate E, Owen R (2014) Particle-based methods: fundamentals and applications. Computational Methods in Applied Sciences. Springer, Dordrecht
Patel RG, Manickam I, Trask NA, Wood MA, Lee M, Tomas I, Cyr EC (2022) Thermodynamically consistent physics-informed neural networks for hyperbolic systems. J Comput Phys 449:110754
Peng W, Yuan Z, Wang J (2022) Attention-enhanced neural network models for turbulence simulation. Phys Fluids 34(2):025111
Peng Y, Dan H, Zin-Qin John X (2023) A non-gradient method for solving elliptic partial differential equations with deep neural networks. J Comput Phys 472:111690
Penwarden M, Zhe S, Narayan A, Kirby RM (2023) A metalearning approach for physics-informed neural networks (PINNs): application to parameterized PDEs. J Comput Phys 477:111912
Psaros AF, Meng X, Zou Z, Guo L, Karniadakis GE (2023) Uncertainty quantification in scientific machine learning: methods, metrics, and comparisons. J Comput Phys 477:111902
Qin D, Yanwei D, Liu B, Huang W (2019) A B-spline finite element method for nonlinear differential equations describing crystal surface growth with variable coefficient. Adv Differ Equ 2019(1):1–16
Quan HD, Huynh HT (2023) Solving partial differential equation based on extreme learning machine. Math Comput Simul 205:697–708
Raissi M, Perdikaris P, Karniadakis GE (2019) Physics-informed neural networks: a deep learning framework for solving forward and inverse problems involving nonlinear partial differential equations. J Comput Phys 378:686–707
Raissi M, Yazdani A, Karniadakis GE (2020) Hidden fluid mechanics: learning velocity and pressure fields from flow visualizations. Science 367(6481):1026–1030
Ren S, He K, Girshick R, Sun J (2017) Faster R-CNN: towards real-time object detection with region proposal networks. IEEE Trans Pattern Anal Mach Intell 39(6):1137–1149
Ren P, Rao C, Liu Y, Wang J-X, Sun H (2022) PhyCRNet: physics-informed convolutional-recurrent network for solving spatiotemporal PDEs. Comput Methods Appl Mech Eng 389:114399
Ruthotto L, Haber E (2020) Deep neural networks motivated by partial differential equations. J Math Imaging Vis 62:352–364
Sajid F, Javed AR, Basharat A, Kryvinska N, Afzal A, Rizwan M (2021) An efficient deep learning framework for distracted driver detection. IEEE Access 9:169270–169280
Shaban WM, Elbaz K, Zhou A, Shen S-L (2023) Physics-informed deep neural network for modeling the chloride diffusion in concrete. Eng Appl Artif Intell 125:106691
Shelhamer E, Long J, Darrell T (2017) Fully convolutional networks for semantic segmentation. IEEE Trans Pattern Anal Mach Intell 39(4):640–651
Shen C, Appling AP, Gentine P, Bandai T, Gupta H, Tartakovsky A, Baity-Jesi M, Fenicia F, Kifer D, Li L et al (2023) Differentiable modelling to unify machine learning and physical models for geosciences. Nat Rev Earth Environ 4(8):552–567
Siegel JW, Hong Q, Jin X, Hao W, Jinchao X (2023) Greedy training algorithms for neural networks and applications to PDEs. J Comput Phys 484:112084
Sirignano J, Spiliopoulos K (2018) DGM: a deep learning algorithm for solving partial differential equations. J Comput Phys 375:1339–1364
Smets BMN, Portegies J, Bekkers EJ, Duits R (2023) PDE-based group equivariant convolutional neural networks. J Math Imaging Vis 65(1):209–239
Song X, Liu Y, Xue L, Wang J, Zhang J, Wang J, Jiang L, Cheng Z (2020) Time-series well performance prediction based on long short-term memory (LSTM) neural network model. J Pet Sci Eng 186:106682
Swan M, Witte F, dos Santos RP (2021) Quantum information science. IEEE Internet Comput 26(1):7–14
Tang S, Feng X, Wei W, Hui X (2023a) Physics-informed neural networks combined with polynomial interpolation to solve nonlinear partial differential equations. Comput Math Appl 132:48–62
Tang K, Wan X, Yang C (2023b) DAS-PINNs: a deep adaptive sampling method for solving high-dimensional partial differential equations. J Comput Phys 476:111868
Tanyu DN, Ning J, Freudenberg T, Heilenkötter N, Rademacher A, Iben U, Maass P (2023) Deep learning methods for partial differential equations and related parameter identification problems. Inverse Probl 39(10):103001
Taylor JM, Pardo D, Muga I (2023) A deep Fourier residual method for solving PDEs using neural networks. Comput Methods Appl Mech Eng 405:115850
Uriarte C, Pardo D, Muga I, Muñoz-Matute J (2023) A deep double Ritz method (D2RM) for solving partial differential equations using neural networks. Comput Methods Appl Mech Eng 405:115892
Venturi S, Casey T (2023) SVD perspectives for augmenting DeepONet flexibility and interpretability. Comput Methods Appl Mech Eng 403:115718
Versteeg HK, Malalasekera W (2011) An introduction to computational fluid dynamics: the finite, vol Method. Pearson Education, Limited, London
Wang K, Gou C, Duan Y, Lin Y, Zheng X, Wang F-Y (2017) Generative adversarial networks: introduction and outlook. IEEE/CAA J Autom Sin 4(4):588–598
Wang S, Wang H, Perdikaris P (2021) On the eigenvector bias of Fourier feature networks: from regression to solving multi-scale PDEs with physics-informed neural networks. Comput Methods Appl Mech Eng 384:113938
Wang H, Planas R, Chandramowlishwaran A, Bostanabad R (2022) Mosaic flows: a transferable deep learning framework for solving PDEs on unseen domains. Comput Methods Appl Mech Eng 389:114424
Wen G, Li Z, Azizzadenesheli K, Anandkumar A, Benson SM (2022) U-FNO: an enhanced Fourier neural operator-based deep-learning model for multiphase flow. Adv Water Resour 163:104180
Willard J, Jia X, Shaoming X, Steinbach M, Kumar V (2022) Integrating scientific knowledge with machine learning for engineering and environmental systems. ACM Comput Surv 55(4):1–37
Xiang H, Zou Q, Nawaz MA, Huang X, Zhang F, Yu H (2023) Deep learning for image inpainting: a survey. Pattern Recogn 134:109046
Xu M, Yoon S, Fuentes A, Park DS (2023) A comprehensive survey of image augmentation techniques for deep learning. Pattern Recogn 137:109347
Yentis R, Zaghloul ME (1996) VLSI implementation of locally connected neural network for solving partial differential equations. IEEE Trans Circ Syst I: Fundam Theory Appl 43(8):687–690
Yifan D, Zaki TA (2021) Evolutional deep neural network. Phys Rev E 104(4):045303
Yiqi G, Ng MK (2023) Deep neural networks for solving large linear systems arising from high-dimensional problems. SIAM J Sci Comput 45(5):A2356–A2381
Zhang L, Cheng L, Li H, Gao J, Cheng Y, Domel R, Yang Y, Tang S, Liu WK (2021) Hierarchical deep-learning neural networks: finite elements and beyond. Comput Mech 67:207–230
Zhao Y, Yang G (2023) Deep learning-based integrated framework for stock price movement prediction. Appl Soft Comput 133:109921
Zienkiewicz OC, Taylor RL (2000) The finite element method, the basis. The finite element method. Wiley, New York
Amanat A, Rizwan M, Javed AR, Maha A, Alsaqour R, Pandya S, Uddin M (2022) Deep learning for depression detection from textual data. Electronics 11(5)
Deng J, Dong W, Socher R, Li L-J, Li K, Fei-Fei L (2009) ImageNet: a large-scale hierarchical image database. In: IEEE conference on computer vision and pattern recognition, pp 248–255
Girshick R (2015) Fast R-CNN. In: IEEE international conference on computer vision, pp 1440–1448
Girshick R, Donahue J, Darrell T, Malik J (2014) Rich feature hierarchies for accurate object detection and semantic segmentation. In: IEEE conference on computer vision and pattern recognition, pp 580–587
Gobovic D, Zaghloul ME (1993) Design of locally connected CMOS neural cells to solve the steady-state heat flow problem. In: 36th midwest symposium on circuits and systems. IEEE, pp 755–757
Gobovic D, Zaghloul ME (1994) Analog cellular neural network with application to partial differential equations with variable mesh-size. In: IEEE international symposium on circuits and systems. IEEE, vol 6, pp 359–362
Goodfellow IJ, Pouget-Abadie J, Mirza M, Bing X, Warde-Farley D, Ozair S, Courville A, Bengio Y (2014) Generative adversarial networks. arxiv preprint arxiv:1406:2661
Gordijn B, ten Have H (2023) ChatGPT: evolution or revolution? Medicine, health care and philosophy, pp 1–2
Gui J, Sun Z, Wen Y, Tao D, Ye J (2021) A review on generative adversarial networks: algorithms, theory, and applications. IEEE Trans Knowl Data Eng
Hafiz AM, Bhat GM (2021) Fast training of deep networks with one-class CNNs. In: Modern approaches in machine learning and cognitive science: a walkthrough: latest trends in AI. Springer, Berlin, pp 409–421
Hafiz AM, Bhat RA, Hassaballah M (2022) Image classification using convolutional neural network tree ensembles. Multimed Tools Appl, pp 1–18
Hafiz AM, Hassaballah M, Binbusayyis A (2023) Formula-driven supervised learning in computer vision: a literature survey. Appl Sci 13(2)
He K, Zhang X, Ren S, Sun J (2016) Deep residual learning for image recognition. In: IEEE conference on computer vision and pattern recognition, pp 770–778
Hook DW, Porter SJ, Herzog C (2018) Dimensions: building context for search and evaluation. Front Res Metr Anal 3:23. https://www.frontiersin.org/articles/10.3389/frma.2018.00023/pdf
Huang S, Feng W, Tang C, Lv J (2022) Partial differential equations meet deep neural networks: a survey. Preprint arxiv:2211.05567
Huang G, Liu Z, Van Der Maaten L, Weinberger KQ (July 2017) Densely connected convolutional networks. In: IEEE conference on computer vision and pattern recognition, Los Alamitos, CA, USA, pp 2261–2269
Jagtap AD, Karniadakis GE (2021) Extended physics-informed neural networks (XPINNs): a generalized space-time domain decomposition based deep learning framework for nonlinear partial differential equations. In: AAAI spring symposium: MLPS, pp 2002–2041
Kollmannsberger S (2019) The finite cell method: towards engineering applications. Technische Universität München
Kovachki N, Li Z, Liu B, Azizzadenesheli K, Bhattacharya K, Stuart A, Anandkumar A (2021b) Neural operator: learning maps between function spaces. arxiv preprint arxiv:2108.08481
Ledig C, Theis L, Huszar F, Caballero J, Cunningham A, Acosta A, Aitken A, Tejani A, Totz J, Wang Z, Shi W (July 2017) Photo-realistic single image super-resolution using a generative adversarial network. In: IEEE conference on computer vision and pattern recognition
Minaee S, Abdolrashidi A, Su H, Bennamoun M, Zhang D (2023) Biometrics recognition using deep learning: a survey. Artif Intell Rev, pp 1–49
Patrik SH (2022) Shift-DeepONet: extending deep operator networks for discontinuous output functions. ETH Zurich, Seminar for applied mathematics
Raissi M (2024) Forward-backward stochastic neural networks: deep learning of high-dimensional partial differential equations. In Peter Carr Gedenkschrift: research advances in mathematical finance. World Scientific, pp 637–655
Rodkina A, Kelly C (2011) Stochastic difference equations and applications
Simonyan K, Zisserman A (2014) Very deep convolutional networks for large-scale image recognition
Soldatenko S, Yusupov R (2017) Predictability in deterministic dynamical systems with application to weather forecasting and climate modelling. In: Dynamical systems-analytical and computational techniques. IntechOpen, p 101
Szegedy C, Liu W, Jia Y, Sermanet P, Reed S, Anguelov D, Erhan D, Vanhoucke V, Rabinovich A (Jun 2015) Going deeper with convolutions. In: IEEE conference on computer vision and pattern recognition, Los Alamitos, CA, USA. IEEE Computer Society, pp 1–9
Tran D, Bourdev L, Fergus R, Torresani L, Paluri M (December 2015) Learning spatiotemporal features with 3D convolutional networks. In: IEEE international conference on computer vision
Vakalopoulou M, Christodoulidis S, Burgos N, Colliot O, Lepetit V (2023) Deep learning: basics and convolutional neural networks (CNNs). Mach Learn Brain Disord, pp 77–115
Vaswani A, Shazeer N, Parmar N, Uszkoreit J, Jones L, Gomez AN, Kaiser Ł, Polosukhin I (2017) Attention is all you need. In: Advances in neural information processing systems, vol 30
Vinyals O, Toshev A, Bengio S, Erhan D (June 2015) Show and tell: a neural image caption generator. In: IEEE conference on computer vision and pattern recognition, Los Alamitos, CA, USA. IEEE Computer Society, pp 3156–3164
Yang L et al (2019) Highly-scalable, physics-informed GANs for learning solutions of stochastic PDEs. In: IEEE/ACM 3rd workshop on deep learning on supercomputers. IEEE, pp 1–11
Yao H, Ren Y, Liu Y (2019) FEA-Net: a deep convolutional neural network with physicsprior for efficient data driven PDE learning. In: AIAA Scitech 2019 forum, p 0680
Yentis R, Zaghloul ME (1994) CMOS implementation of locally connected neural cells to solve the steady-state heat flow problem. In: 37th midwest symposium on circuits and systems. IEEE, vol 1, pp 503–506
Zhao H, Shi J, Qi X, Wang X, Jia J (2017) Pyramid scene parsing network. In: IEEE conference on computer vision and pattern recognition, pp 6230–6239
Download references
The work is not funded.
Author information
Authors and affiliations.
Department of Electronics & Communication Engineering, Institute of Technology, University of Kashmir, Srinagar, J &K, 190006, India
Abdul Mueed Hafiz
Department of Applied Sciences, Institute of Technology, University of Kashmir, Srinagar, J &K, 190006, India
Department of Computer Science, College of Computer Engineering and Sciences, Prince Sattam Bin Abdulaziz University, AlKharj, 16278, Saudi Arabia
M. Hassaballah
Department of Computer Science, Faculty of Computers and Information, South Valley University, Qena, Egypt
You can also search for this author in PubMed Google Scholar
Contributions
All authors wrote, prepared figures and reviewed the manuscript.
Corresponding author
Correspondence to Abdul Mueed Hafiz .
Ethics declarations
Conflict of interest.
The authors declare no conflict of interest.
Additional information
Publisher's note.
Springer Nature remains neutral with regard to jurisdictional claims in published maps and institutional affiliations.
Rights and permissions
Open Access This article is licensed under a Creative Commons Attribution 4.0 International License, which permits use, sharing, adaptation, distribution and reproduction in any medium or format, as long as you give appropriate credit to the original author(s) and the source, provide a link to the Creative Commons licence, and indicate if changes were made. The images or other third party material in this article are included in the article's Creative Commons licence, unless indicated otherwise in a credit line to the material. If material is not included in the article's Creative Commons licence and your intended use is not permitted by statutory regulation or exceeds the permitted use, you will need to obtain permission directly from the copyright holder. To view a copy of this licence, visit http://creativecommons.org/licenses/by/4.0/ .
Reprints and permissions
About this article
Hafiz, A.M., Faiq, I. & Hassaballah, M. Solving partial differential equations using large-data models: a literature review. Artif Intell Rev 57 , 152 (2024). https://doi.org/10.1007/s10462-024-10784-5
Download citation
Accepted : 04 May 2024
Published : 24 May 2024
DOI : https://doi.org/10.1007/s10462-024-10784-5
Share this article
Anyone you share the following link with will be able to read this content:
Sorry, a shareable link is not currently available for this article.
Provided by the Springer Nature SharedIt content-sharing initiative
- Partial differential equations
- Solving PDEs
- Large-data models
- Transformers
- Find a journal
- Publish with us
- Track your research
- Open access
- Published: 16 May 2024
Experiences of UK clinical scientists (Physical Sciences modality) with their regulator, the Health and Care Professions Council: results of a 2022 survey
- Mark McJury 1
BMC Health Services Research volume 24 , Article number: 635 ( 2024 ) Cite this article
995 Accesses
Metrics details
In healthcare, regulation of professions is an important tool to protect the public. With increasing regulation however, professions find themselves under increasing scrutiny. Recently there has also been considerable concern with regulator performance, with high profile reports pointing to cases of inefficiency and bias. Whilst reports have often focused on large staff groups, such as doctors, in the literature there is a dearth of data on the experiences of smaller professional groups such Clinical Scientists with their regulator, the Health and Care Professions Council.
This article reports the findings of a survey from Clinical Scientists (Physical Sciences modality) about their experiences with their regulator, and their perception of the quality and safety of that regulation.
Between July–October 2022, a survey was conducted via the Medical Physics and Engineering mail-base, open to all medical physicists & engineers. Questions covered typical topics of registration, communication, audit and fitness to practice. The questionnaire consisted of open and closed questions. Likert scoring, and thematic analysis were used to assess the quantitative and qualitative data.
Of 146 responses recorded, analysis was based on 143 respondents. Overall survey sentiment was significantly more negative than positive, in terms of regulator performance (negative responses 159; positive 106; significant at p < 0.001). Continuous Professional Development audit was rated median 4; other topics were rated as neutral (fitness to practice, policies & procedures); and some as poor (value).
Conclusions
The Clinical Scientist (Physical Sciences) professional registrants rated the performance of their regulator more negatively than other reported assessments (by the Professional Standards Authority). Survey respondents suggested a variety of performance aspects, such as communication and fitness to practice, would benefit from improvement. Indications from this small dataset, suggest a larger survey of HCPC registrants would be useful.
Peer Review reports
In Healthcare, protection of patients and the public is a core principle. Part the framework of protections, includes regulation of professions [ 1 ]. This aims to mitigate risks such as the risk from bogus practitioners – insufficiently trained people acting as fully-trained professional practitioners, see Fig. 1 .
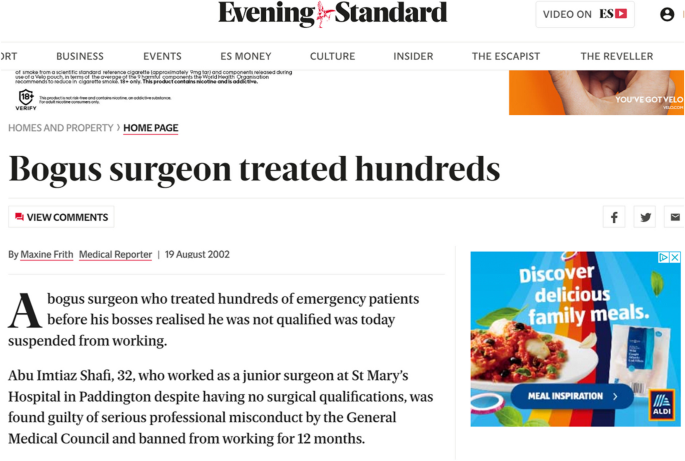
Recent UK media report on a bogus healthcare practitioner [ 2 ]
Regulation of professions ensures that titles (e.g. Doctor, Dentist, Clinical Scientist) are protected in law. The protected title means someone may only use that title, if they are on the national register, managed by the regulator – the Health and Care Professions Council (HCPC). It is a criminal offence to use a protected title if you are not entitled to do so [ 3 ]. There are a large number of regulators in healthcare – see Table 1 . Most of the regulators manage a register for one profession, except the HCPC which regulates 15 professions.
To be included on the register, a candidate must meet the regulators criteria for knowledge and training, and a key element to remain, is to show evidence of continuous professional development (CPD). Being on the register ensures that a practitioner has met the appropriate level of competence and professional practice.
For many healthcare workers, being on the HCPC register is a compulsory requirement to be appointable to a post. They must pay the necessary annual fees, and abide by the policies drawn-up by the regulator, and generally professions have no choice of regulator – these are statutory bodies, setup by government.
Recently, there has been considerable public dissatisfaction with the activity & performance of some regulators, notably Ofwat [ 4 ], and Ofgem [ 5 ]. Healthcare workers should expect a high level of professionalism, efficiency, and integrity from a regulator, as the regulator’s performance directly affects staff and public safety.
In terms of the regulation of UK Clinical Scientists, there is a dearth of data regarding experiences with the HCPC and views on the quality of regulation provided.
Findings are reported here from a 2022 survey of Medical Physicists and Engineers (one of the 16 job roles or ‘modalities’ under the umbrella of Clinical Scientist). The research aim was to assess experiences, and the level of ‘satisfaction’ with the regulator. For the remainder of this report, the term Clinical Scientist will be taken to mean Clinical Scientist (Medical Physicist/Engineer). The survey was designed to gather & explore data about opinions and experiences regarding several key aspects of how the HCPC performs its role, and perception of the quality & safety of regulation delivered.
A short survey questionnaire was developed, with questions aimed to cover the main regulatory processes, including registration & renewal, CPD audit, and fitness-to-practice. There were also questions relating more generally to HCPC’s performance as an organisation, e.g. handling of personal data. Finally, participants were asked to rate the HCPC’s overall performance and what they felt was the ‘value’ of regulation. The survey questions are listed in the Supplementary file along with this article.
Questions were carefully worded and there was a balance of open and closed questions. A five-point Likert score was used to rate closed questions. The survey was anonymous, and the questions were not compulsory, allowing the responders to skip irrelevant or difficult questions. The survey also aimed to be as short & concise as possible, to be a minimal burden to busy clinical staff & hopefully maximise response rate. There were a small number of questions at the start of the survey, to collect basic demographics on the respondents (role, grade, UK nation etc.).
The survey was advertised on the online JISC-hosted UK Medical Physics and Engineering (UKMPE) mail-base. This offered convenient access for the majority of Clinical Scientists. The survey was advertised twice, to allow for potential work absence, holiday/illness etc. It was active from the end of July 2002 until October 2022, when responses appeared to saturate.
The data is a combination of quantitative rating scores, and qualitative text responses. This allows a mixed-methods approach to data analysis, combining quantitative assessment of the Likert scoring, and (recursive) thematic analysis of the free-text answers [ 6 ]. Thematic analysis is a standard tool, and has been reported as a useful & appropriate for assessing experiences, thoughts, or behaviours in a dataset [ 7 ]. The survey questions addressed the main themes, but further themes were identified using an inductive, data-driven approach. Qualitative data analysis (QDA) was performed using NVivo (QSR International).
Two survey questions attempted to obtain an overall perception of HCPC’s performance: the direct one (Q12), and a further question’Would you recommend HCPC as a regulator…?’. This latter question doesn’t perhaps add anything more, and in fact a few respondents suggested it was a slightly awkward question, given professions do not have a choice of regulator – so that has been excluded from the analysis.
Study conduct was performed in accordance with relevant guidelines and regulations [ 8 , 9 ]. Before conducting the survey of Clinical Scientists, the survey was sent to their professional body, the Institute of Physics and Engineering in Medicine (IPEM). The IPEM Professional Standards Committee reviewed the survey questions [ 10 ]. Written informed consent was obtained from participants.
Data analysis
Data was collected via an MS form, in a single excel sheet and stored on a secure network drive. The respondents were anonymised, and the data checked for errors. The data was then imported into NVivo v12.
Qualitative data was manually coded for themes, and auto-coded for sentiment. An inductive approach was used to develop themes.
The sample size of responses allowed the use of simple parametric tests to establish the level of statistical significance.
Survey demographics
A total of 146 responses were collected. Two respondents noted that they worked as an HCPC Partner (a paid role). They were excluded from the analysis due to potential conflict of interest. One respondent’s responses were all blank aside from the demographic data, so they were also excluded from further analysis.
Analysis is based on 143 responses, which represents ~ 6% of the UK profession [ 11 ]. It is arguable whether it is representative of the profession at this proportion of response – but these responses do offer the only sizeable pool of data currently available. The survey was aimed at those who are on the statutory register as they are most likely to have relevant interactions & experiences of the HCPC, but a small number of responses were also received from Clinical Technologists (Medical Technical Officers-MTOs) and Engineers (CEs) and these have been included in the analysis. Figure 2 shows the breakdown in respondents, by nation.
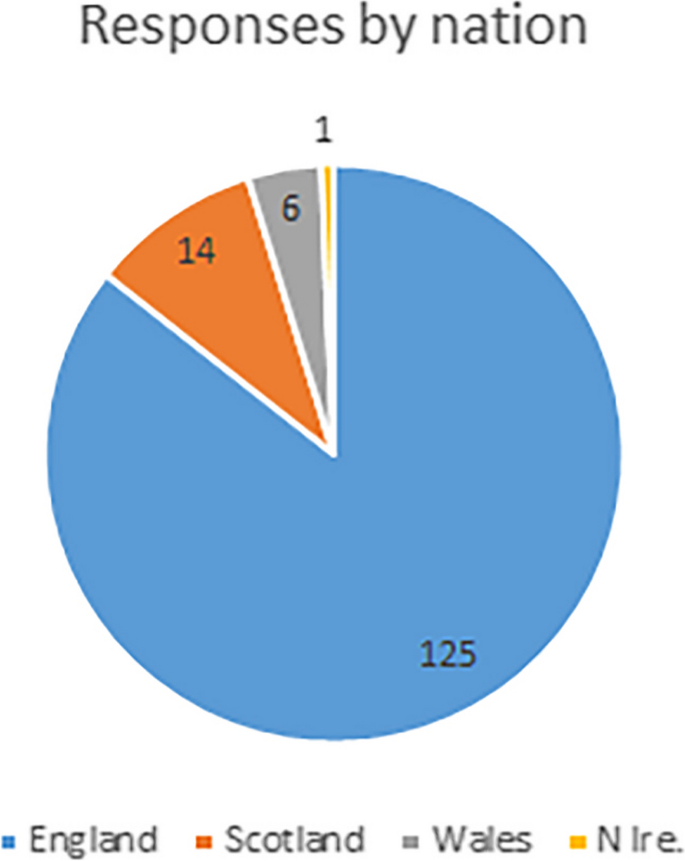
Proportion of respondents, by nation
Of the respondents, 91% are registered Clinical Scientists, and would therefore have a broad range of experience with HCPC and its processes. Mean time on the register was 12 yrs. Respondents show a large range in seniority, and their roles are shown in Fig. 3 (CS-Clinical Scientist; CE-Clinical Engineer; MTO-Medical Technical Officer/Technician; CS-P are those working in private healthcare settings, so not on Agenda for Change (AfC) pay bands).
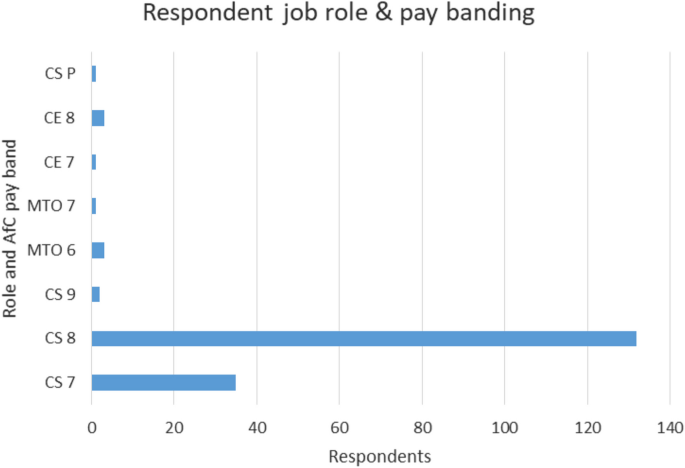
Breakdown in respondents, by role and pay banding
These data can be compared with the most recent HCPC ‘snapshot’ of the CS registrants (find here: Registrants by profession snapshot—1967 to 2019 | ( https://www.hcpc-uk.org/resources/data/2019/registrant-snapshot/ )).
The perception of overall regulator performance, can be assessed in two ways – one interview question directly asked for a rating score, and the overall survey sentiment also offers additional insight.
The score for overall performance was a median of 3 (mean 2.7; response rate 90%) which suggests neutral satisfaction.
Respondents were not asked directly to explain this overall performance rating – themes were extracted from the questionnaire as a whole.
The auto-coded sentiment scores generated in the NVivo software are shown in Table 2 . There is a significantly stronger negative sentiment than positive for HCPC performance – moderate, strong and total sentiment scores are all higher for negative sentiment. The normal test for a single proportion (109), shows the negative and positive sentiment differences have statistical significance with p < 0.001. Whilst the PSA assessment of HCPC performance in 2022–23 shows 100% performance for 4 out of 5 assessment areas, survey data here from regulated professionals suggests considerably less satisfaction with HCPC. This raises associated questions about the relevance and validity of PSA assessment.
A large number of respondents seem to question the value of regulation. Whilst many accepted the value for it in terms of protecting the safety of the public, many questioned its relevance & benefit to themselves. Many respondents also queried the payment model where although the main beneficiaries of regulation are the public & the employer, it is the registrants actually pay the fees for registration. There was very little mention in survey responses, of benefit in terms of protected-title. These issues were amalgamated into Theme 1— Value of regulation , with the two sub-themes Value in monetary terms (value-for-money) and Value in professional terms (benefit and relevance to the individual professional) (see Table 3 ).
In the survey, several aspects of HCPC organisational performance were scored – handling of personal data, registration and renewal, engagement with the profession, audit, and the quality and usefulness of HCPC policies. These formed Theme 2 and its sub-themes.
A third theme Registrant competence and vulnerability , was developed to focus on responses to questions related to the assessment of registrant competence and Fitness To Practice (FTP) processes.
Finally, the survey also directly asked respondents if they could suggest improvements which would have resulted in higher scoring for regulation quality and performance. These were grouped into Theme 4.
Theme 1 – Value of regulation
Value in monetary terms.
The Likert score for value-for-money was a median of 2 (mean 2.3; response rate 100%) which suggests dissatisfaction. This is one of the few survey questions to elicit a 100% response rate – a clear signal of its importance for registrants.
There was a high number of responses suggesting fees are too expensive (and a significantly smaller number suggesting good value). This ties in with some respondents explaining that the ‘benefit’ from registration is mainly for the employer (an assurance of high quality, well-trained staff). Several respondents point to little ‘tangible’ benefit for registrants and query whether the payment model is fair and if the employer should pay registrant fees.
“Expensive fees for what appears to be very little support.” Resp094
“It seems that I pay about £100 per year to have my name written on a list. It is unclear to me what the HCPC actually does in order to justify such a high fee.” Resp014
“I get, quite literally, nothing from it. It’s essentially a tax on work.” Resp008
Several respondents suggested that as registration was mandated by the employer, it was in essence an additional ‘tax’ on their employment, which was highlighted previously by Unison [ 12 ]. A comparator for payment model, are the checks preformed on potential staff who will be working with children and vulnerable adults. In general, these ‘disclosure’ checks are paid for by the employer, however the checks are not recurrent cost for each individual, but done once at recruitment.
Value in professional terms & relevance
This was not a direct question on the questionnaire, but emerged consistently in survey responses. Aside from value-for-money, the value of regulation can also refer to more general benefit and relevance for a professional, for example in protecting a professional title or emphasising the importance of a role. Many respondents commented, in relation to the ‘value’ of regulation, about the relevance of the HCPC to them and their job/role.
The largest number of responses highlighted the lack of clarity about HCPC’s role, and also to note its lack of relevance felt by a significant proportion of respondents.
“Not sure I have seen any value in my registration except that it is a requirement for my role” Resp017
“I really fail to understand what (sic) the benefits of registration.” Resp018
“They do not promote the profession. I see no evidence of supporting the profession. I pay to have the title and I am not aware of any other benefits.” Resp038
Theme 2 – HCPC performance
Communication & handling data.
The survey questionnaire did not have a specific question relating to communication, therefore no specific Likert scores are available. Rather, communication was a sub-theme which emerged in survey responses. The response numbers related to positive (1) and negative experiences (50) clearly suggest an overall experience of poor communication processes (and statistically significant at p < 0.001 for a normal proportion test).
One respondent noted they had ‘given up’ trying to communicate with HCPC electronically. Several respondents also noted issues with conventional communication—letters from HCPC going to old addresses, or being very slow to arrive.
“…I have given up on contacting by electronic means.” Resp134
When trying to renew their registration, communication with HCPC was so difficult that two respondents noted they raised a formal complaint.
A number of respondents noted that when they eventually got through to the HCPC, staff were helpful, so the main communication issue may relate to insufficiently resourced lines of communication (phones & email) or the need for a more focussed first point of contact e.g. some form of helpdesk or triaging system.
“Recently long wait to get through to speak to someone… Once through staff very helpful.” Resp126
This topic overlaps with the next (Processing Registration & renewals) in that both involve online logins, website use etc.
Security & data handling was rated as neutral (median 3, mean 3.4; response rate 91%). Although responses were balanced in terms of satisfaction, a significant number noted a lack of knowledge about HCPC processes. There are almost equal proportions of respondents reporting no issues, some problems with handling of personal data, or insufficient knowledge to express an opinion.
Registration and renewal
The score for processing registrations & renewals, was a median of 4 (mean 3.5; response rate 92%) which suggests modest satisfaction.
The overall rating also suggests that the issues may have been experienced by a comparative minority of registrants and that for most, renewal was straightforward.
“They expected people to call their phone number, which then wasn’t picked up. They didn’t reply to emails except after repeated attempts and finally having to resort to raising a complaint.” Resp023
“Difficult to get a timely response. Difficult to discuss my situation with a human being…” Resp044
Although the Likert score is positive, the themes in responses explaining the rating, are more mixed. Many respondents mentioned either having or knowing others who had issues with registration renewal, and its online processes including payments. A few respondents mentioned that the process was unforgiving of small errors. One respondent, for example, missed ticking a box on the renewal form, was removed from the register and experienced significant difficulties (poor communication with HCPC) getting the issue resolved.
Some respondents noted issues related to a long absence from work (e.g. maternity/illness etc.) causing them to miss registration deadlines – for some, this seems to have resulted in additional fees to renew registration. It seems rather easy for small errors (on either side) to result in registrants being removed from the register. For registrants, this can have very serious consequences and it can then be difficult and slow to resolve this, sometimes whilst on no pay. There have also been other reported instances of renewal payment collection errors [ 13 ].
“I had been off work… and had missed their renewal emails…I was told that there would be no allowances for this situation, and I would have to pay an additional fee to re-register…” Resp139.
Some respondents raised the issue of exclusion – certain staff groups not being included on the register—such as Clinical Technologists and Clinical Engineers. This desire for inclusion, also points to a perception of value in being on the register. One respondent raised an issue of very difficult and slow processing of registration for a candidate from outside the UK.
“Staff member who qualified as medical physicist abroad…has had a dreadful, drawn out and fruitless experience.” Resp135
Overall, many respondents noted difficulties in renewing registration and issues with HCPC’s online processes. Some of these issues (e.g. website renewal problems) may have been temporary and are now resolved, but others (e.g. available routes for registration) remain to be resolved.
Audit process & policies
In the survey, 12% respondents reported having been audited by HCPC regarding their CPD (response rate 97%). This is well above the level of 2.5% of each profession, which HCPC aims to review at each renewal [ 14 ], and similar values reported by some professional bodies [ 15 ]. The participants seem representative, although two respondents mentioned their perception of low audit rates. Data on CPD audit is available here: https://www.hcpc-uk.org/about-us/insights-and-data/cpd/cpd-audit-reports/
Respondents rated the process of being audited as a median of 4 (mean 3.7), which is the joint highest score on the survey, pointing to satisfaction with the process. From the responses, the overall perception could be summed up as straight-forward, but time-consuming. Without regular record-keeping, unfortunately most audits will be time-consuming – the HCPC more so, as it is not an annual audit, but covers the two preceding years.
Some respondents did find the process not only straight-forward, but also useful (related to feedback received). However, responses regarding feedback were mixed, with comments on both good, and poor feedback from HCPC.
“Not difficult but quite long-winded” Resp008
“Very stressful and time consuming” Resp081
“While it was a lot of work the process seemed very thorough and well explained.” Resp114
The HCPC’s policies & procedures were rated as a median of 3 (mean 3.2; response rate 98%). This neutral score could suggest a mixture of confidence in HCPC practise. This score may also reflect the fact that the majority of respondents had either not read, or felt they had no need to read the policies, and so are largely unfamiliar with them.
The reasons for this lack of familiarity are also explained by some respondents – four commented that the policies & procedures are rather too generic/vague. Three respondents noted that they felt the policies were not sufficiently relevant to their clinical roles to be useful. This may be due to the policies being written at a level to be applicable to registrants from all 16 modalities – and perhaps a limitation of the nature of HCPC as a very large regulator. Familiarity seemed mainly to be restricted to policies around registration, and CPD. There were slightly lower response levels for positive sentiment (6), than negative sentiment (9).
“I’ve never had cause to read them.” Resp115
“Detached from the real clinical interface for our professions…” Resp083
HCPC split their policies into ‘corporate’- which relate to organisational issues (e.g. equality & diversity; find them here: Our policies and procedures | ( https://www.hcpc-uk.org/about-us/corporate-governance/freedom-of-information/policies/#:~:text=Our%20main%20policies%20and%20procedures%201%20Customer%20feedback,scheme%20...%207%20Freedom%20of%20Information%20Policy%20 )) and those more relevant to professions (e.g. relating to the register; find them here: Resources | ( https://www.hcpc-uk.org/resources/?Query=&Categories=76 )).
One respondent noted not only that the policies were ‘as you might expect’, but felt the policies were less demanding than those from other similar bodies such as the CQC ( https://www.cqc.org.uk/publications ).
“…Other regulatory bodies (such as the CQC for example) have policies and procedures that are a lot more challenging to comply with.” Resp022
Theme 3 – Registrant competence and vulnerability
In this survey, 3.5% (5/143) of respondents noted some involvement with the HCPC’s Fitness to Practice service. These interactions were rated at a median of 3 (mean 2.8) suggesting neutral sentiment.
Firstly, we can immediately see the level of interaction with the FTP team is very small. CS registrants represent approx. 2% of HCPC registrants, and the level of CS referrals to FTP in 2020–21 was 0.2% [ 16 ].
The data is a very small sample, but responses vary strongly, so it is worth digging a little further into the granularity of individual responses. Response scores were 1, 1, 2, 5, 5 – which are mainly at the extremes of the rating spectrum. The majority of respondents described poor experiences with the FTP team: errors, a process which was ‘extremely prolonged’, involved slow/poor communication, and processes which were ‘entirely opaque’.
“It is slow, the process was badly managed… and the system was entirely opaque,” Resp37
“They were hard to contact and I didn't feel they listened…no explanation, apology or assurance it would not happen again. It left my colleague disillusioned and me very angry on their behalf…” Resp044
Some respondents commented that the team were not only difficult to contact, but also didn’t seem to listen. At the end of a process which involved errors from HCPC, one respondent noted were ‘no explanation, apologies or assurance that it would not happen again’, leaving the registrant ‘disillusioned’. These experiences do not fit with the HCPC’s stated goal to be a compassionate regulator, see Fig. 4 . Arguably it is more difficult to change a culture of behaviour and beliefs, than to publish a corporate goal or statement of vision.
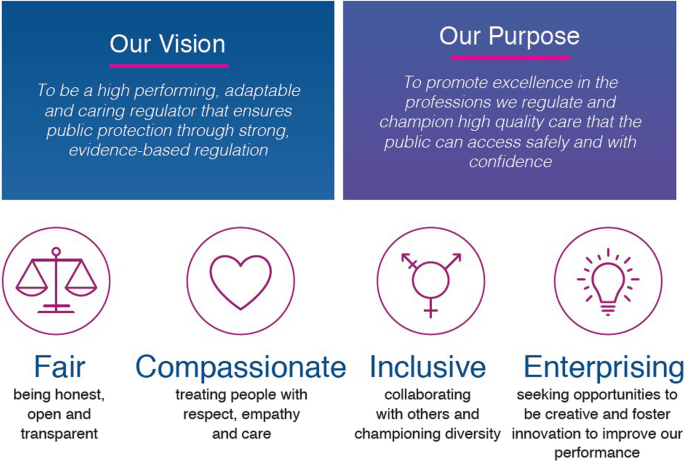
HCPC’s vision statement & purpose [ 17 ]
Some survey respondents have noted the necessity of regulation for our profession.
“Ultimately I am very grateful that I can register as a professional.” Resp024
Theme 4 – Suggestions for improved regulation
Following the question relating to overall performance, respondents were invited to suggest things which might improve their rating for HCPC’s performance and value. These suggestions were also combined with those which appeared in earlier survey responses.
Although we are in a current cost-of-living crisis, responses did not query simply high absolute cost of fees, but also queried the value/benefit of HCPC regulation for registrants. Many responses expressed doubt as to the added value & relevance of HCPC registration for them. They seem to point to a desire for more tangible benefit from their fees. Perhaps, given the costs and levels of scrutiny, registrants want some definite benefit to balance the scales .
“Cost less and do more for the people who are on the register.” Resp089
“Vastly reduced cost. Employer paying registrant fees.” Resp074
A significant number of responses pointed out that the main benefits of registration are for the public, and for employers – but that it is the registrants who pay for registration. Many queries why this should be, and whether there should be a different payment model, where for example employers pay.
Similarly, some respondents felt that the HCPC’s unusual position of regulating a large swathe of healthcare professions was not necessarily helpful for their profession or others.
Communication and response times are obviously an issue of concern for registrants, and improvements are needed based on the low satisfaction levels reported here. This is also linked to a wish for increased engagement with the CS profession.
“Engagement with the workforce, specialism specific development, reduced fees” Resp025
Some responses suggested they would be comforted by increased accountability / governance of HCPC including improved FTP efficiency.
“More accountability to registrants” Resp130
Finally, improvement in terms of additional registration routes for Engineers & Technical staff were also suggested. It may be damaging to work-place moral, if two professionals doing roles of a similar nature are not being governanced is the same way and if there is not parity of their gross salary due to mandatory professional fees & reductions.
Value-for-money : This will vary between individuals depending on many variables, such as upbringing & environment, salary, lifestyle priorities, political persuasion, and so on. However, many of these factors should balance in a large sample. In general, it can be suggestive of satisfaction (or lack of) with a service. The score here suggesting dissatisfaction, echoes with other reports on HCPC’s spending, and financial irregularities [ 18 , 19 ].
In the survey findings, respondents have voiced dissatisfaction with registration value for money. In fact, HCPC’s registration fees are not high when compared to the other healthcare professions regulators. Table 1 shows data from 2021–22 for regulator annual registration fees. However, the HCPC has risen from having the lowest regulator fees in 2014–5, to its current position (9 th of 13) slightly higher in the table. Perhaps more concerning than the absolute level of fees, are when large increases are proposed [ 12 , 20 , 21 , 22 ].
However, fees have regularly increased to current figure of £196.48 for a two-year cycle. During a consultation process in 2018, the Academy for Healthcare Clinical Scientists (AHCS) wrote an open letter to the HCPC, disputing what they felt was a disproportionate fee increase [ 23 ]. Further fee rises have also been well above the level of inflation at the time.
HCPC expenditure (which is linked to registration fees) has arguably been even more controversial than fee increases – noted by several respondents. A freedom of information (FOI) request in 2016 showed HCPC’s spending of £17,000 for their Christmas party [ 18 ] – which amounts to just over £76 per person. This cost was close to the annual registration fee (at that time) for registrants.
In 2019, regulation of social workers in England moved from HCPC, to Social Work England. This resulted in a loss of over 100,000 registrants, and a loss in registration fee income. HCPC raised fees to compensate, but a freedom of information (FoI) request in 2020 [ 18 ] showed that even though there was an associated lowering in workload associated with the loss of 100 k registrants, the HCPC had no redundancies, suggesting the loss of income was compensated mainly by the fees increase.
Inherent value & relevance
One of HCPC’s aims is to promote ‘the value of regulation’ [ 24 ]. However, not only is there dissatisfaction with value-for-money, the second highest response suggests a lack of inherent value (or benefit) from regulation to the individual registrant. In some ways, there is a lack of balance – registrants are under increasing scrutiny, but feel there is little direct benefit, to provide balance.
This also suggests that HCPC’s aim or message is not getting through to the CS profession. It’s not clear what the HCPC 2021–22 achieved milestone – ‘Embedded our registrant experiences research into employee learning and development and inductions’ has actually achieved.
A large number of responses pointed to the lack of clarity about HCPC’s role, and also to note its lack of relevance for respondents. Some of this is understandable – until recently, many CS registrants will have little interaction with HCPC. They would typically get one email reminder each year to renew their registration and pay those fees, and hear little else from the HCPC. That is beginning to change, and HCPC have recently begun to send more regular, direct emails/updates to registrants.
However, for many registrants, the HCPC appears not to be clearly communicating its role, or the relevance/importance of regulation. As mentioned above, this also links in to previous mentions of the lack of any tangible benefit for registrants. Some note little more relevance other than the mandatory aspects of regulation.
Finally, relevance is also queried in relation to the limited access for some professional groups to a professional register. The current situation of gaps in registration for some groups, results in two situations – firstly, for Clinical Scientists and Clinical Engineers/Technologists, one group has to compulsorily pay a fee to be allowed/approved to do their job and the other does not; also, the public are routinely helped and assisted by Clinical Scientists and Clinical Engineers/Technologists – but only one group is regulated to ensure public safety.
HCPC Communication
This was highlighted by respondents as often poor. Recently in the media, there has been a concern raised by The College of Paramedics (CoP) about communications issues with HCPC—changes to the HCPC policy on the use of social media [ 25 ]. They raised particular concerns about the use of social media content and ‘historical content’ in the context of investigations of fitness-to practice.
There have previously been some concerns raised on the UKMPE mail-base regarding handling of personal data, and lack of efficiency in addressing the issue [ 26 ]. Several messages detailed HCPC communicating unencrypted registrant passwords in emails and sending personal data to the incorrect registrant. Some on the forum noted that they had reported this problem over a period of several years to HCPC, suggesting HCPC’s response to these serious issues was extremely slow. Several responses noted these previous issues.
Registration processes
Although responses here show some satisfaction, there have been reports in the media of significant issues with registration (such as removing registrants from the register in error) with associated impact for patients and the public [ 27 , 28 ]. Similarly, there were reports on the UKMPE mail-base of significant issues with registration renewals being problematic [ 26 ]. In Scotland, NHS.net email accounts ceased to be supported in July-Sept 2020 and the associated lack of access to email accounts and messages used for HCPC communication and registration, caused a major issue in registration renewal. This coincided with COVID lockdowns and a period of unusually difficult communication with HCPC. If NHS staff lose registration (irrespective of the reason), respondents noted that some Human Resources (HR) departments were quick to suspend staff from work, and in some cases withhold pay. That spike in difficulties is likely the cause of the most common responses suggesting issues with a complicated process.
In safe-guarding public safety, a key task for a healthcare regulator is assessing the competence of registrants. This is done via a small set of related activities. Registrants must return regular evidence of CPD, and these are audited for 2.5% registrants. This process is simple and routine, and as seen in Theme 2 responses here suggest registrants are reasonably satisfied with this process.
More formal and in-depth competence assessment happens when a complaint is raised against a registrant, either by a work colleague/management, a member of the public or occasionally by the HCPC itself. The process is complex, lengthy and can end in a registrant attending a court hearing [ 29 ].
It is usual for registrants to continue in their normal job during FTP investigations – effectively the public remains at risk from a registrant if their competence is eventually proven to be below the regulators standards, so there is a need for investigations to be efficient both in timeliness, and outcome.
Obviously, being under investigation can be highly stressful, and has the potential for the registrant to be ‘struck off’ the register, and lose their job if registration is mandated (e.g. NHS posts). There are many reports of the process & experience either provoking or increasing underlying mental health challenges [ 30 , 31 , 32 ]. Along with efficiency, a regulator needs to behave compassionately. Investigations of highly-skilled professionals engaging in complex work activities, is also necessarily complex and requires a high degree of knowledge and experience from the regulator’s investigational panel.
The Professional Standards Authority (PSA) regulate the HCPC, and publish annual reviews of their performance ( https://www.professionalstandards.org.uk/publications/performance-reviews ) (see Table 4 ). HCPC performance as reported by PSA, seems to be generally higher than noted by survey respondents here. For 2022–23, aside from one area, the HCPC has scored 100% for performance, which seems at odds with these survey responses [ 33 ]. The FTP team is notable in repeatedly performing very poorly compared to most other sections of the HCPC (even though the majority of the HCPC budget goes to FTP activity, see Fig. 4 ). The HCPC Annual Report 2018–9 [ 34 ] highlighted the completion of the first phase of the Fitness-To-Practice Improvement Plan. This delivered “A root and branch review of this regulatory function… a restructure, tightened roles and processes and the introduction of a new Threshold Policy”, but this seems to have no impact on the performance reported by the PSA for the next few years shown in Table 4 . However, the most recent data does suggest improvement, and HCPC continues to develop FTP team practice [ 17 ].
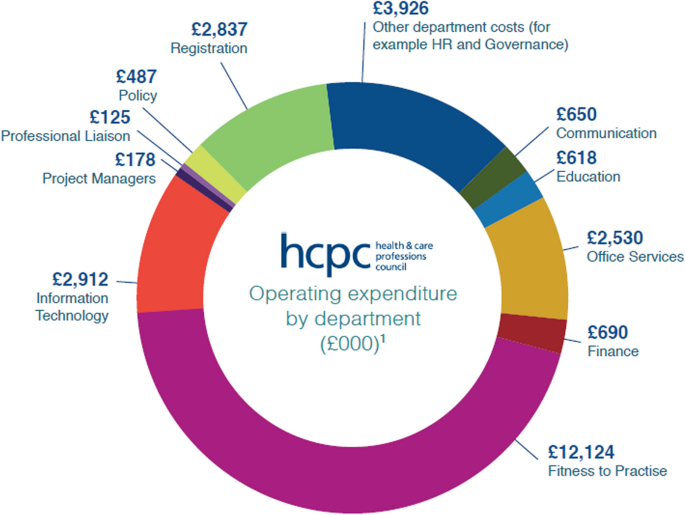
HCPC expenditure for the year 2020–21 [ 17 ]
There are other reports of poor experiences with this team [ 35 , 36 ], and in one report the FTP team’s processes have been noted as being rather inhumane [ 35 ].
Regulation is an important part of public protection, but how effectively it is managed & enforced is also a concern, given it involves increased scrutiny of registrants. A topical comparator is the current dissatisfaction by a large section of the public about several other government regulators allowing seemingly poor performance to go unchecked [ 4 , 5 ].
It is arguable, that registrants remain on the register as long as the HCPC allows them. Several respondents in this survey noted being removed from the register through HCPC administrative error. Removal could also happen through poor judgement/decision-making – the FTP team handle large numbers of very complex investigational cases – 1603 concluded cases for the year 2021–22 and 1024 hearings [ 16 ]. Every justice system is subject to a level of error – guilty parties can be erroneously ‘cleared’, and vice-versa. It is essential therefore, that policies & procedures relating to FTP are fit for purpose—that the FTP team work effectively and humanely, and that there is genuine & effective governance of HCPC to ensure accountability. In this survey, some respondents seem to be saying that currently this seems not to be the case.
It might have been anticipated that the greatest concern is costs, especially in the current cost-of-living crisis. The recent HCPC consultation to increase fees [ 37 ] seems particularly tone-deaf and has caused concern across the professions [ 21 , 22 ].
Above findings show respondents are interested in lower fees, but also increased benefit for their fees. Some respondents pointed out that whilst registrants pay for registration, benefit is mainly for the public and employers. The HCPC is a statutory body, its funding model will have been designed/decided upon by government, and may be unlikely to change. However, there are a variety of potential regulation models [ 38 ], and so change is possible. A review of the financial model for regulation may be welcome.
Regulator size
Some aspects of HCPC performance, policies, and distribution of spending, is related to the nature of it being the largest and only multi-professional regulator in the healthcare sector. Data from the HCPC suggests (see Fig. 5 ) that the majority of spending relates to FTP activity. Data also points to Clinical Scientists having very low levels of FTP investigation compared to others in HCPC [ 16 ]. This suggests that a significant proportion of CS registrant fees are used to investigate other professions. It’s possible (perhaps simplistically) that if, like many other healthcare professions such as doctors & dentists who’s regulator is concerned only with that single profession, if CSs were regulated separately, their registrant fees may be reduced. This model of single-profession regulation may also mitigate against other disadvantages of the HCPC’s practice, such as the ‘generic’ policies aiming to apply to a pool of 15 professions.
Although there is a very low level of data for this topic, the concerned raised by registrants are serious in nature. There also seems to be issues in handling of complaints related to this service and advocacy for registrants. Certainly, there is a clear governance path via PSA, to the Health Secretary. However, this does not offer a route for individual complaints to be raised and addressed. Unlike complaints from the public in other areas, there is no recourse to an ombudsman for registrants. The only option for individual registrants, is the submission of a formal complaint to the HCPC itself, which is dealt with internally. Comments from survey respondents suggest this process does not guarantee satisfaction. Indeed, one of the respondents who mentioned submitting a complaint, made it clear they remained unhappy with HCPC’s response. Overall, there seems to be a lack of clear & effective advocacy for registrants.
“…the HCPC’s stance appeared to be guilty until proven innocent… At no point did I feel the HCPC cared that their (sic) was an individual involved....” Resp044.
FTP processes affect a comparatively small number of CS registrants, compared to other professions. However, it seems clear that the majority of those who have interacted with the FTP team have had poor experiences, and respondents have suggested improvements are needed. The reason for FTP investigations, is protection of staff and the public. If processes are slow, and investigations prolonged, or decisions flawed, the public may be exposed to increased levels of risk, as healthcare practitioners who may be lacking in competence continue to practice. The data in Table 4 shows concerning but improving trends in FTP performance levels.
Limitations
There are two main limitations to this work. Firstly, due to time constraints, there was no pilot work done when designing the survey questionnaire. This may have helped, as noted earlier, a few responses pointed to some awkwardness with one survey question. Although no pilot work was done, the questionnaire was reviewed by the IPEM Professional Standards Committee, as noted in the Acknowledgements section.
The other obvious limitation is the low response rate (~ 6% of UK Medical Physicists). Circulation of the survey was performed via the only online forum for the profession currently available. The survey was advertised multiple times to ensure visibility to staff who may have missed it initially due to leave etc. However, the forum does reach 100% of the profession, and some addressees may have filters set to send specific posts to junk folders etc. The professional body IPEM declined to offer support in circulating the survey (believing the issues involved would affect/be of interest only to a small minority of members.)
The low response rate also has a particular impact on the pool of responses relating to FTP issues, which inherently affect low numbers of registrants.
However, the importance of some of the findings here (e.g. expressed dissatisfaction with regulation in terms of value; the poor experience of some members with the Registration, Communication and FTP teams) and the low sample surveyed, both justify the need for a larger follow-on survey, across all of Clinical Science.
In Healthcare, regulation of professions is a key aspect of protecting the public. However, to be effective, regulation must be performed professionally, impartially, and associated concerns or complaints investigated efficiently and respectfully.
This report presents findings from a survey aimed at collecting a snap-shot of the experiences of Clinical Scientists with their regulator, and their perception of the quality and safety of that regulation performance.
Overall survey sentiment scores showed a significantly more negative responses than positive. Survey comments relate not only to current issues, but to previous problems and controversial issues [ 18 , 26 ]. It seems that some respondents have at some point lost confidence and trust in the HCPC, and survey responses suggest there has not been enough engagement and work done by HCPC to repair and rebuild this trust.
In the midst of a cost of living crisis, costs are a large concern for many. The HCPC fees are neither the highest not lowest amongst the healthcare regulators. Spending is transparent, and details can be found in any of the HCPC’s annual reports.
A repeating sub-theme in responses, was a lack of tangible value for the registrant, and that the employer should pay the costs of registration, where registration is mandated by the job.
Many respondents have suggested that they feel there should be more proactive engagement from HCPC with the profession. Most respondents were not familiar with or felt the HCPC policies are relevant/important to them.
Survey data showed moderate satisfaction with registration processes for the majority of respondents. Some respondents also noted a lack of registration route for engineering & technical healthcare staff. CPD processes also achieved a score indicating registrant satisfaction. This generated the highest ratings in the survey. Communication scored poorly and many respondents suggests there needs to be improved levels of communication in terms of response times and access to support.
The CS profession experiences low levels of interaction with the FTP service. However, those interactions which were recorded in the survey, show some poor experiences for registrants. There also seems to be a lack of advocacy/route for complaints about HCPC from individual registrants. There may need to be more engagement between registrants and their professional body regarding HCPC performance, and more proactivity from the stake-holder, IPEM.
Some of the findings reported here relate to important issues, but the survey data are based on a low response rate. A larger survey across all of Clinical Science is being planned.
Availability of data and materials
To protect confidentiality of survey respondents, the source data is not available publicly, but are available from the author on reasonable request.
Abbreviations
Agenda for Change
Academy for Healthcare Clinical Scientists
Continuous professional development
Clinical Engineer
Clinical Scientist
College of Paramedics
Clinical Technologist
Freedom of Information
Fitness-to-practice
Health and Care Professions Council
Human resources
Institute of Physics and Engineering in Medicine
Joint Information Systems Committee
Medical Technical Officer
Professional Standards Authority
Professional Standards Committee
Qualitative data analysis
UK Medical Physics and Engineering
Professional Standards Authority. Professional healthcare regulation in the UK. https://www.professionalstandards.org.uk/news-and-blog/blog/detail/blog/2018/04/10/professional-healthcare-regulation-explained#:~:text=Regulation%20is%20simply%20a%20way,may%20face%20when%20receiving%20treatment . Accessed 26 Jul 2023
Evening Standard. Bogus surgeon treated hundreds. https://www.standard.co.uk/hp/front/bogus-surgeon-treated-hundreds-6326549.html . Accessed 26 Jul 2023.
HCPC . About registration: protected titles. http://www.hcpc-uk.org/aboutregistration/protectedtitles/ . Accessed 27 Jul 23.
The Guardian. Public patience is wearing thin. Ofwat must wield the big stick | Nils Pratley | https://www.theguardian.com/business/nils-pratley-on-finance/2022/dec/08/public-patience-is-wearing-thin-ofwat-must-wield-the-big-stick . Accessed 19 Jul 2023.
TrustPilot. Reviews of Ofgem. Ofgem Reviews | Read Customer Service Reviews of ofgem.com (trustpilot.com). Accessed 19 Jul 2023.
Braun V, Clarke V. Using thematic analysis in psychology. Qual Res Psychol. 2006;3(2):77–101.
Article Google Scholar
Kiger ME, Varpio L. Thematic analysis of qualitative data: AMEE Guide No. 131. Med Teach. 2020;42(8):846–54.
Article PubMed Google Scholar
Declaration of Helsinki. 2013. https://www.wma.net/policies-post/wma-declaration-of-helsinki-ethical-principles-for-medical-research-involving-human-subjects/ . Accessed 12 Sept 2023.
UK Data Protection Act. 2018. https://www.gov.uk/data-protection . Accessed 15 Sept 2023.
Rowbottom C. Private communication on behalf of the IPEM Professional Standards Committee; 2022.
IPEM Workforce Team. Clinical scientist & engineer workforce data. Personal communication. 2022.
Unison. HCPC fee increase is an unjustified ‘tax on practising.’ https://www.unison.org.uk/news/press-release/2019/02/hcpc-fee-increase-unjustified-tax-practising/ . Accessed 27 Jul 2023.
HCPC. Direct debit collection errors. https://www.hcpc-uk.org/news-and-events/news/2020/early-direct-debit-collections/?dm_i=2NJF,141CO,7C0ZNI,4A8IE,1 . Accessed 27 Jul 23.
HCPC. CPD audit rates. https://www.hcpc-uk.org/cpd/cpd-audits/ . Accessed 21 Jul 2023.
IPEM. CPD audit rates. https://www.ipem.ac.uk/your-career/cpd-career-development/cpd-audit/ . Accessed 21 Jul 2023.
HCPC. Fitness to practice annual report 2020–21. https://www.hcpc-uk.org/about-us/insights-and-data/ftp/fitness-to-practise-annual-report-2020-21/ . Accessed 23 Jul 2023.
HCPC. Annual report and accounts, 2020–21. https://www.hcpc-uk.org/resources/reports/2022/annual-report-and-accounts-2020-21/ . Accessed 19 Jul 2023.
Wikipedia. The health and care professions council. https://en.wikipedia.org/wiki/Health_and_Care_Professions_Council . Accessed 2 Jul 23.
HCPC. Annual report 2005–06. https://www.hcpc-uk.org/resources/reports/2006/annual-report-2005-06/ . Accessed 19 Jul 2023.
British Dental Association. BDA very disappointed by HCPC decision to raise registration fees by 18%. https://www.bda.uk.com/resource/bda-very-disappointed-by-hcpc-decision-to-raise-registration-fees-by-18.html . Accessed 27 Jul 2023.
British Psychological Society. HCPC fees consultation – share your views. https://www.bps.org.uk/news/hcpc-fee-consultation-share-your-views . Accessed 27 Jul 23.
IBMS. IBMS response to the HCPC registration fees consultation. https://www.ibms.org/resources/news/ibms-response-to-hcpc-registration-fees-consultation/ . Accessed 17 Jul 23.
Association of HealthCare Scientists. Open letter to HCPC. https://www.ahcs.ac.uk/wp-content/uploads/2018/11/HCPC-Open-Letter.pdf . Accessed 27 Jul 23.
HCPC. Corporate plan 2022–23. https://www.hcpc-uk.org/resources/reports/2022/hcpc-corporate-plan-2022-23/ . Accessed 23 Jul 2023.
College of Paramedics. Our formal response to the HCPC consultation. https://collegeofparamedics.co.uk/COP/News/2023/Our%20formal%20response%20to%20the%20HCPC%20consultation.aspx . Accessed 27 Jul 23.
JISC Mail - MPE mailbase. JISCMail - Medical-physics-engineering list at www.jiscmail.ac.uk . Accessed 19 July 2023.
The Guardian. Thousands miss out on treatment as physiotherapists are taken off UK register. https://www.theguardian.com/society/2022/may/14/thousands-miss-out-on-treatment-as-physiotherapists-are-struck-off-uk-register . Accessed 27 Jul 2023.
HSJJobs.com. https://www.hsjjobs.com/article/thousands-of-clinicians-unable-to-work-after-registration-blunder . Accessed 27 Jul 2023.
HCPC. How we investigate. https://www.hcpc-uk.org/concerns/how-we-investigate/ . Accessed 21 Nov 2023.
Sirriyeh R, Lawton R, Gardner P, Armitage G. Coping with medical error: a systematic review of papers to assess the effects of involvement in medical errors on healthcare professionals’ psychological well-being. Br Med J Qual Saf. 2010;19:6.
Google Scholar
Bourne T, Wynants L, Peters M, van Audenhove C, Timmerman D, van Calster B, et al. The impact of complaints procedures on the welfare, health and clinical practise of 7926 doctors in the UK: a cross-sectional survey. BMJ Open. 2015;5:e006687.
Article PubMed PubMed Central Google Scholar
Jones-Berry S. Suicide risk for nurses during fitness to practice process. Ment Health Pract. 2016;19:8.
Professional Standards Authority. HCPC performance review 2022–23. https://www.professionalstandards.org.uk/publications/performance-review-detail/periodic-review-hcpc-2022-23 . Accessed 25 Jul 2023
HCPC. Annual report and accounts, 2018–19. https://www.hcpc-uk.org/resources/reports/2019/hcpc-annual-report-and-accounts-2018-19/ . Accessed 19 Jul 2023.
Maben J, Hoinville L, Querstret D, Taylor C, Zasada M, Abrams R. Living life in limbo: experiences of healthcare professionals during the HCPC fitness to practice investigation process in the UK. BMC Health Serv Res. 2021;21:839–54.
Leigh J, Worsley A, Richard C, McLaughlin K. An analysis of HCPC fitness to practise hearings: fit to practise or fit for purpose? Ethics Soc Welfare. 2017;11(4):382–96.
HCPC. Consultation changes to fees. https://www.hcpc-uk.org/news-and-events/consultations/2022/consultation-on-changes-to-fees/ . Accessed 27 Jul 23
Department of Health. Review of the regulation of public health professions. London: DoH; 2010.
Download references
Acknowledgements
The author wishes to kindly acknowledge the input of Dr Carl Rowbottom (IPEM Professional Standards Committee), in reviewing the survey questions. Thanks also to Dr Nina Cockton for helpful advice on ethics and recruitment issues.
There were no sources of funding required for this work.
Author information
Authors and affiliations.
University of Glasgow, Level 2, ICE Building, Queen Elizabeth University Hospital Campus, 1345 Govan Road, Glasgow, G51 4TF, UK
Mark McJury
You can also search for this author in PubMed Google Scholar
Contributions
All work to collect, analyse & publish this survey, are the work of the author Dr Mark McJury.
Corresponding author
Correspondence to Mark McJury .
Ethics declarations
Ethics approval and consent to participate.
As this study relates to low risk, survey data, formal ethics committee approval is not required (exemption obtained from NHSGGC REC04 REC Officer Dr Judith Godden [email protected]). As the survey responses were from members of a professional body (The Institute of Medical Physics and Engineering in Medicine (IPEM) it was consulted. Its Professional Standards Committee (PSC) reviewed the survey and raised no objections. The survey questions were assessed for bias and approved unchanged (acknowledged in the manuscript). Written informed consent was obtained from all participants in the study.
Consent for publication
Not applicable.
Competing interests
The authors declare no competing interests.
Additional information
Publisher’s note.
Springer Nature remains neutral with regard to jurisdictional claims in published maps and institutional affiliations.
Supplementary Information
Additional file 1..
The survey questionnaire has been provided as a supplementary file.
Rights and permissions
Open Access This article is licensed under a Creative Commons Attribution 4.0 International License, which permits use, sharing, adaptation, distribution and reproduction in any medium or format, as long as you give appropriate credit to the original author(s) and the source, provide a link to the Creative Commons licence, and indicate if changes were made. The images or other third party material in this article are included in the article's Creative Commons licence, unless indicated otherwise in a credit line to the material. If material is not included in the article's Creative Commons licence and your intended use is not permitted by statutory regulation or exceeds the permitted use, you will need to obtain permission directly from the copyright holder. To view a copy of this licence, visit http://creativecommons.org/licenses/by/4.0/ . The Creative Commons Public Domain Dedication waiver ( http://creativecommons.org/publicdomain/zero/1.0/ ) applies to the data made available in this article, unless otherwise stated in a credit line to the data.
Reprints and permissions
About this article
Cite this article.
McJury, M. Experiences of UK clinical scientists (Physical Sciences modality) with their regulator, the Health and Care Professions Council: results of a 2022 survey. BMC Health Serv Res 24 , 635 (2024). https://doi.org/10.1186/s12913-024-10956-7
Download citation
Received : 06 September 2023
Accepted : 05 April 2024
Published : 16 May 2024
DOI : https://doi.org/10.1186/s12913-024-10956-7
Share this article
Anyone you share the following link with will be able to read this content:
Sorry, a shareable link is not currently available for this article.
Provided by the Springer Nature SharedIt content-sharing initiative
- Regulation of professions
- Clinical scientists
- Medical physicists
BMC Health Services Research
ISSN: 1472-6963
- General enquiries: [email protected]
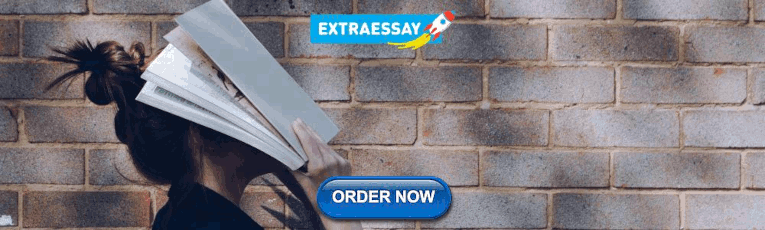
COMMENTS
The present literature review is not exhaustive, and we focus primarily on applications relevant to soil structure characterization. ... Repeated geophysical surveys or semipermanent geophysical monitoring make it possible to deduce influences of soil structure indirectly via its impact on various soil dynamic processes.
Explore the latest full-text research PDFs, articles, conference papers, preprints and more on GEOPHYSICAL SURVEY. Find methods information, sources, references or conduct a literature review on ...
7.1.1 Introduction. Geophysical surveys occupy an important position in the entire gamut of mineral exploration with a well-defined objective in all stages of exploration, thus the role of geophysical surveys is multidimensional. Geophysical surveys are essentially source-response geological studies; source being the natural fields of the earth ...
This chapter discussed briefly about data acquisition, processing and interpretation of airborne geophysical surveys and their integrated interpretation using three examples from three different regions in Norway. First example shows that extent of uranium rich alum shale is detected by airborne radiometry data.
This paper is a literature survey. This review paper provides an overview of recen t . ... geophysical surveys in difficult terrains, such as offshore and mountainous areas. Overall, the .
The synthesis information was acquired from a variety of sources, including a literature review, interviews of agency personnel, and a survey questionnaire. The survey questionnaire was sent to 70 agencies, including transportation agencies for all 50 U.S. states, several federal agencies, and most Canadian provinces.
Survey have developed from those broadly suggested by the Geophysical Sub-Commit tee of the Committee of Civil Research, whose report was published for the Empire Marketing Board by H.M. Stationery Office in 1927. Under the title of the Imperial Geophysical Experimenta l Survey, field parties have
Seabed surveying is the basis of engineering development in shallow waters. At present, geophysical survey methods mainly utilize sonars for qualitative surveying, which requires the calibration of the results found through in situ drilling and sampling. Among them, the parameters required for engineering designs are obtained from either in situ tests or laboratory experiments of soil samples ...
Practices were gleaned from a review of literature, a survey of transpor- tation agencies, and case examples of select agencies. ... Summary of Major Findings The review of the literature on near-surface geophysical methods found a wide range of surface and geophysical techniques available that are applicable to transportation-related problems ...
One of the main conclusions of the review is that in landslide characterization, the geophysical survey design is still a much-debated question, and no unique strategy has arisen from the literature. Reference [ 11 ] is the last review published in an international journal and available online that focused on the advantages and limitations of ...
Performing a reliable stability analysis of a landslide slope requires a good understanding of the internal geometries and an accurate characterisation of the geotechnical parameters of the identified strata. Geotechnical models are commonly based on geomorphological data combined with direct and intrusive geotechnical investigations. However, the existence of numerous empirical correlations ...
This paper summarizes a study of all the geophysical surveys carried out in the northwest of England before 2006. The study assessed the performance of geophysical surveys in archaeological terms and was centred on a detailed analysis of 35 sites for which there is good comparative excavation data or which have particularly illustrative case ...
3 Literature Review. Dams have been played a vital role in the growth of any country. Several dam rehabilitation projects across India, as well as worldwide, have been taken place till now. ... Raji WO, Adedoyin AD (2019) Dam safety assessment using 2D electrical resistivity geophysical survey and geological mapping. J King Soud Univ Sci:1123 ...
A review of the use of unmanned aerial vehicles (UAV) based photogrammetry for the investigation of landslides was also conducted to highlight the latest advancement and discuss the synergy between UAV and geophysical in four possible broader areas: (i) survey planning, (ii) LS investigation, (iii) LS dynamics and (iv) presentation of results ...
The major problem associated with the unproductive wells has been identified to be an inadequate geological and geophysical survey which reveals the proper understanding of the hydrogeological characteristics before drilling of boreholes and hand-dug wells in the area. 1.2. Location and accessibility of the study area. Odo Ayedun-Ekiti, the ...
Groundwater resource plays a central role in every sector of life globally. Unfortunately, the roles of groundwater are often unappreciated in many parts of the world, especially in developing nations. The paper applied a combined geo-electrical method and hydrochemical analysis alongside physical surveys to investigate aquifer promising zones, the quality and the source of water supply in the ...
Examples of literature reviews. Step 1 - Search for relevant literature. Step 2 - Evaluate and select sources. Step 3 - Identify themes, debates, and gaps. Step 4 - Outline your literature review's structure. Step 5 - Write your literature review.
Among anthropogenic activities, marine seismic surveys are a fundamental tool for oil and gas explorations, geophysical research, environmental hazard, and risk analysis. This resulting noise may affect a range of species, eliciting masking, behavioral responses, and changes in acoustic repertoires. There is an urgency to understand in depth the potential effects of seismic surveys on marine ...
Anthropogenic activities and greater demands for marine natural resources has led to increases in the spatial extent and duration of pressures on marine ecosystems. Remotely operated vehicles (ROVs) offer a robust survey tool for quantifying these pressures and tracking the success of management intervention while at a range of depths, including those inaccessible to most SCUBA diver-based ...
The town of Mamre is located on the water-scarce western coast of South Africa, and has been completely dependent on treated (softened and chlorinated) groundwater from the Atlantis wellfield, located about 10 km to the south. Atlantis wellfield water has also been used for livestock watering and irrigation. In an effort to conserve potable water reserves within the Atlantis wellfield and ...
Through this literature survey, it is hoped that readers will gain an insight into the area of solving PDEs using large-data models and pursue future research in this interesting area. Mathematics lies at the heart of engineering science and is very important for capturing and modeling of diverse processes. These processes may be naturally-occurring or man-made. One important engineering ...
British Geological Survey Research Report RR/00/8, 2000. Google Scholar. HERAIL G., FORNARI M., and BERGENDAHL M. H.: ... geophysical and geochemical datasets', British Geological Survey, Mineral Reconnaissance Report, no. 141, 1996. ... Literature review . From Localised Social Structures to Localities as Agents. Show details Hide details.
Reviews of Geophysics. JOURNAL METRICS >. Online ISSN: 1944-9208. Print ISSN: 8755-1209. Reviews of Geophysics is an invitation-only reviews journal that provides overviews of recent research in all areas of the Earth and space sciences. Click here to request an invitation. Challenges in Measuring Aerosol Cloud-Mediated Radiative Forcing.
2,962 likes, 38 comments - william_and_mary on August 12, 2020: "Move-In looks a little different this year, and we know there are mixed emotions right now. We want ...
Employing a systematic literature review and content analysis, the research scrutinizes peer-reviewed articles, reports, and case studies from 2014 to 2024, focusing on the application of AI in ...
It enables a practitioner to quickly get a lay of the land, and helps a researcher put literature into context. However, the author is not writing a textbook. The first half of chapters is just the motivation for the second half that outlines many evidence-based ideas for implementation — on how states can accelerate development.
Through this literature survey, it is hoped that readers will gain an insight into the area of solving PDEs using large-data models and pursue future research in this interesting area. ... News-based intelligent prediction of financial markets using text mining and machine learning: a systematic literature review. Expert Syst Appl 217:119509
Background In healthcare, regulation of professions is an important tool to protect the public. With increasing regulation however, professions find themselves under increasing scrutiny. Recently there has also been considerable concern with regulator performance, with high profile reports pointing to cases of inefficiency and bias. Whilst reports have often focused on large staff groups, such ...