Quantitative Research Methods
- First Online: 26 October 2016
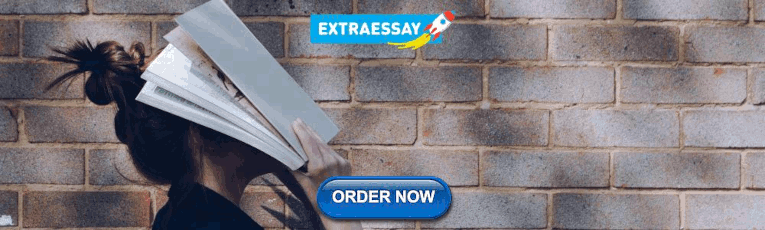
Cite this chapter
- Yvette C. Latunde 2
1506 Accesses
This chapter distinguishes between quantitative and other types of research, while providing specific free resources for accessing pre-collected data. It identifies philosophical assumptions related to quantitative research in parental involvement. It also examines issues and limitations in measuring parental involvement using quantitative research, and provides examples of important quantitative research into parental involvement. Lastly, this chapter identifies questions about the impact of quantitative research in parental involvement and the importance of quantitative research.
This is a preview of subscription content, log in via an institution to check access.
Access this chapter
- Available as EPUB and PDF
- Read on any device
- Instant download
- Own it forever
- Durable hardcover edition
- Dispatched in 3 to 5 business days
- Free shipping worldwide - see info
Tax calculation will be finalised at checkout
Purchases are for personal use only
Institutional subscriptions
Babbie, E. R. (2010). The practice of social research (12th ed.). Belmont, CA: Wadsworth Cengage.
Google Scholar
Balnaves, M., & Caputi, P. (2001). Introduction to quantitative research methods: An investigative approach . London, UK: Sage.
Book Google Scholar
California Department of Education. (2013). Time to eat survey results. Retrieved from http://www.cde.ca.gov/ls/nu/sn/documents/timetoeatsurvey.pdf
Creswell, J. W. (2012). Qualitative inquiry and research design: Choosing among five approaches (3rd ed.). Los Angeles, CA: Sage.
Gale, T. (2008). Meta-analysis. International Encyclopedia of the Social Sciences. Retrieved from http://www.encyclopedia.com/topic/Meta-analysis.aspx
Haase, R. (2011). Power analysis of research in counselor education. Counselor Education and Supervision, 14 (2), 124–132.
Article Google Scholar
Handcock, G., Mueller, R., & Handcock, G. R. (2010). The reviewer’s guide to quantitative methods in the social sciences . Howick Place, London: Routledge.
Hill, N. E., & Tyson, D. F. (2009). Parental involvement in middle school: A meta-analytic assessment of the strategies that promote academic achievement. Developmental Psychology, 45 (3), 740–763.
Jeynes, W. (2005). A meta-analysis of the relation of parental involvement to urban elementary school student academic achievement. Urban Education, 40 (3), 237–269.
Jeynes, W. (2007). The relationship between parental involvement and urban secondary school academic achievement: A meta-analysis. Urban Education, 42 (1), 82–110.
Kim, S. W., & Hill, N. E. (2015). Including fathers in the picture: A meta-analysis of parental involvement and students’ academic achievement. Journal of Educational Psychology, 107 (4), 919–934.
McGowan, H.M. (2011). Planning a comparative experiment in educational settings. Journal of Statistics Education , 19(2), 1–19. Retrieved from http://www.amstat.org/publications/jse/v19n2/mcgowan.pdf
National Center for Education Statistics. (2015). National household education surveys. Parent and family involvement in education survey: Early childhood participation survey. Retrieved from http://files.eric.ed.gov/fulltext/ED556344.pdf
National Head Start Association. (2015). The head start impact study Retrieved from https://www.nhsa.org/files/resources/head_start_impact_study_2015.pdf
Pennsylvania State University. (n.d.). Probability, theory, and mathematical statistics: Test for homogeneity. Retrieved from https://onlinecourses.science.psu.edu/stat414/node/311
Sousa, D. A. (2015). How the brain learns mathematics (2nd ed.). Thousand Oaks, CA: Corwin Press.
Torbeyns, J., Schneider, M., Ziqiang, X., & Seigler, R. S. (2015). Bringing the gap: Fraction understanding is central to mathematics achievement in students from three different countries. Learning and Instruction, 37 , 5–13.
Download references
Author information
Authors and affiliations.
Teacher Education, Azusa Pacific University, Azusa, California, USA
Yvette C. Latunde
You can also search for this author in PubMed Google Scholar
Copyright information
© 2017 The Author(s)
About this chapter
Latunde, Y.C. (2017). Quantitative Research Methods. In: Research in Parental Involvement. Palgrave Macmillan, New York. https://doi.org/10.1057/978-1-137-59146-3_5
Download citation
DOI : https://doi.org/10.1057/978-1-137-59146-3_5
Published : 26 October 2016
Publisher Name : Palgrave Macmillan, New York
Print ISBN : 978-1-137-59145-6
Online ISBN : 978-1-137-59146-3
eBook Packages : Education Education (R0)
Share this chapter
Anyone you share the following link with will be able to read this content:
Sorry, a shareable link is not currently available for this article.
Provided by the Springer Nature SharedIt content-sharing initiative
- Publish with us
Policies and ethics
- Find a journal
- Track your research
Main navigation
- Current Projects
- Past Projects
- Publications
- News & Events
- Student Research
- List of theses (with abstracts)
- Le droit au logement en France
- Neighborhood Regeneration in Beijing
- Segregation of Women in Islamic Societies of South Asia...
- Practice of Community Architecture
- Alternatives to Home Ownership
- Lilong Housing, A Traditional Settlement Form
- Improving Sanitation in Coastal Communities...
- Settlement Patterns in Unplanned Areas
- Contents and introduction
- Chapter 3 (part 2)
- Urban Renewal in Beijing, Observation and Analysis
Chapter 5: Conclusion, Interpretation and Discussion
Introduction.
The following chapter concludes this report. A summary of the research is presented, and findings of the study are discussed and interpreted. The significance of this research in the immediate context of El Gallo and in the field of low-income housing is examined. Recommendations for further research end the chapter.
The scope of the following conclusions is limited to the context and historical characteristics of El Gallo. Thus, applied to other situations, these conclusions may yield incorrect assumptions. Still, these conclusions are relevant to the process of dwelling evolution in progressive development projects.
5.1 Summary of Research
This study observed the process of dwelling evolution in progressive development projects. The literature review was concentrated on the process of progressive development occurring in planned sponsored projects. It was found that, based on observations of the informal settlement process, progressive development under different contextual conditions was not questioned, and its benefits were taken for granted. Studies in the area were reduced to the period of improvement up to the time when the dwelling was physically consolidated. Longer term evaluation of progressive development projects were not found.
Research was undertaken on a 27-year-old progressive development project in Venezuela. The intention was to observe the process of dwelling evolution and the kind of housing that was being produced under progressive urban development projects on a long-term basis. The case study showed dwellings built with different initial levels of user-participation. Dwelling evolution was observed in a survey sample using parameters relevant to the case study (i.e., area increase, dwelling spatial growth and plot occupation, and changes in the functional structure).
Survey dwellings followed identifiable patterns of evolution in size, spatial structure and use-layout. Patterns were affected by aspects of the surrounding context and by aspects inherent to characteristics of the initial dwelling. Consequently, different dwelling groups showed different processes of progressive development.
5.2 Discussion and Interpretation of Findings.
As progressive developments, dwellings at El Gallo were able to adopt new and diverse roles along their whole process of evolution. In this section, relevant issues of the process of dwelling evolution observed at El Gallo are discussed. The first concerns the role of the non-permanent structure in the context of El Gallo as a sponsored progressive development project. The second comments on the process of dwelling evolution that followed the construction of the permanent structure.
In principle, non-permanent structures at El Gallo were similar to ranchos built in informal settlements. Ranchos at El Gallo served as primary shelters while more basic household priorities were met (i.e., services and infrastructure were provided, sources of income were found and generated, and even a favourable social environment was developed among neighbours). However, the majority of tin shacks were neither considerably increased nor upgraded with better materials even when they were used for long periods of time. This fact, together with the sudden change in the pace of development caused by the construction of a very complete permanent dwelling and subsequent removal of the rancho, had no connection with the gradual process of shack replacement observed in invasion settlements of Ciudad Guayana during this study (Portela, M. 1992). Neither did this process have a relationship with the system of "piecemeal construction" described by several housing researchers as characteristic of low-income dwellers.
The shanties were... housing in process of improvement. In particular the piecemeal system of building afforded great advantages to those who, like most of the poor in developing societies, have great variations in income from month to month (Peattie L. 1982:132).
Under El Gallo conditions of land security, ranchos did not show consolidation, and revealed their transient character because they were eventually substituted by permanent structures. The non-permanent structure revealed the primary household's aspiration for a minimum satisfactory habitable area. However, besides basic shelter during the initial stage, ranchos served to the purposes of capital accumulation that eventually allowed households to buy a basic unit according to official standards, or building a bigger, more complete first permanent structure. The size of ranchos reflected households' aspirations for the permanent dwelling, that is,smaller ranchos were substituted by basic units of the housing programs. Instead larger ranchos were substituted by large self-produced dwellings.
It is difficult to ascertain why ranchos were removed when they could have been kept as part of the dwelling, as in fact did a minority of households (2 cases). Is a fact that the temporary materials of ranchos contributed to their deterioration that ended with the total removal of the rancho. However, an idea that may have contributed to the demolition of the rancho was the household's adoption of the planner's belief that ranchos were a bad but necessary step on the way to obtaining permanent housing. Thus, once the permanent dwelling was built, the price households paid to gain credibility (i.e., that this stage was reached) was the demolition of the rancho itself. This interpretation can be specially true for Ciudad Guayana, where dwellings of certain quality such as those of El Gallo were seen as "casas" or houses. Instead, structures of similar quality in the hills of cities such as Caracas were still considered ranchos. In the long run, informal settlements obtained the largest benefits from this process because they gained far more official tolerance and social credibility (i.e., that shacks were actually temporary means of residence towards good-quality housing).
Those who lived in smaller ranchos improved their spatial conditions by moving to the small basic dwellings. Those who occupied bigger ranchos built bigger dwellings by themselves. Still, some households built their dwellings without going through the rancho stage. Self-produced dwellings followed the formal models either to gain the government's credibility of user commitment to build "good" government-like housing, or because households believed so. Imitation of the formal models, however, varied according to the builder's interpretation. For instance, the pattern of the detached dwelling was adopted, but often one of the side yards was reduced to a physical separation between the dwelling and the plot separation wall. More effective interpretations involved enlarging the front porch or using the central circulation axis to allow easy extension in the future.
The building approach of the permanent structure influenced the process of evolution that followed. Basic units built by the housing agencies had a compact, complete layout with higher standards of construction; however, aspects of the design, such as internal dimensions, were inadequate for household criteria, and the layout was not well adapted. Dwellings built according to provided plans and specificationshad similar problems, but households enlarged spaces and modified layouts when they were building the units. The level of construction standards was also reduced since the lateral façades of some dwellings were unfinished. Dwellings built totally by self-help means were the largest permanent structures. Aspects of the design of the first permanent structure allowed easy extension of the dwelling towards open areas of the plot. More user participation was reflected in straight-forward processes of evolution without internal modifications, and fewer stages to reach the current houseform.
5.3 Significance of the Study
While this study acknowledges again the effectiveness of progressive development in the housing system, it shows how dwelling evolution in progressive development projects can have different characteristics produced by internal and external interventions. Usually, projects are designed and launched to reproduce certain desirable outcomes and meet specific expectations. However, conditions prevailing in these projects and sometimes strategies that are introduced to "improve," "speed up" or make more "efficient" the process of evolution can affect the outcome in many different ways. This study showed how contextual characteristics of El Gallo, as well as the design and level of user participation in the initial permanent dwelling, affected successive stages of progressive development. However, it is important to recognize that are other issues beyond the spatial aspects that are intrinsically related with the evolution of the dwellings and that were not included within the scope of these particular research (i.e., household's changes in income, size, and age or gender structure).
The findings at El Gallo add modestly to the body of knowledge of literature on progressive development. Progressive Urban Development Units, UMUPs , have been the main housing strategy in Ciudad Guayana these last years, and they are likely to keep being used. Simple facts such as knowing the characteristics of the additions and modifications that households make to their dwellings over time can be the basis for more assertive actions supporting or enforcing progressive development activities. Understanding the process of dwelling evolution in low-income developments would be an effective way to help the process that, in the case of Ciudad Guayana, zonings and bylaws have been unable to regulate.
5.4 Recommendations for Further Research
Long term assessments are particularly constrained by the availability and reliability of recorded data. The frequency, and often the methodology, in which censuses and surveys are made do not always suit the purposes of this kind of research. Household interviews are very important, but they may become troubled by informant's limited memories and the continuity of the household in the dwelling. Aerial documentation, if available, represents one of the most reliable sources to observe physical change. Nevertheless, a careful and detailed process of observation of aerial data becomes very time consuming. For similar studies, a first phase in which the housing diversity is identified in the aerial data according to the selected criteria, would allow to reduce the number of detailed survey samples needed, thus considerably reducing the time of data collection.
In the context of Ciudad Guayana, further studies of the non-permanent dwelling in recent UMUPs would reveal new insights into the function of these structures in progressive development projects. This would be essential especially if any kind of initial aid is to be provided. On the other hand, following the growth of progressive developments is necessary if services and infrastructure are, as they are now, the responsibility of the local government. Identifying the producers of physical evolution -- i.e., the drivers and catalysts of change -- would be an important step for further research. An interesting step within this trend could be to ascertain the extent in which other household processes -- family growth, income increase and economic stability, household aging, changes in the household composition (single- to multi- family), etc., affect the process of dwelling evolution.
In the context of low-income housing, the process of progressive development needs further understanding. As in Ciudad Guayana, progressive development is likely to be the main housing strategy for other developing countries in the near future. Local authorities would do well to follow the evolution of settlements and to identify real household needs, and the consequences of public and/or private interventions in low-income settlements. Perhaps the most important learning of this study is that the experience of El Gallo acknowledges again the dynamic participation of the low-income households under different conditions, and still leaves wide room for a positive participation for the many other actors in the evolving urban entity.
. Notes for Chapter V
1 Dodge reports that some settlers of Ciudad Guayana kept the rancho and rented it to poorer families (Dodge,C. 1968:220). This attitude has been more common in other progressive development projects. The Dandora site and services also encouraged the construction of temporary shacks while the permanent dwelling was built. However, non-permanent structures remained to be rented or used as storage areas even after the permanent dwelling was built (McCarney, P.L. 1987:90).
Department and University Information
Minimum cost housing group.

How To Write The Results/Findings Chapter
For quantitative studies (dissertations & theses).
By: Derek Jansen (MBA) | Expert Reviewed By: Kerryn Warren (PhD) | July 2021
So, you’ve completed your quantitative data analysis and it’s time to report on your findings. But where do you start? In this post, we’ll walk you through the results chapter (also called the findings or analysis chapter), step by step, so that you can craft this section of your dissertation or thesis with confidence. If you’re looking for information regarding the results chapter for qualitative studies, you can find that here .
Overview: Quantitative Results Chapter
- What exactly the results chapter is
- What you need to include in your chapter
- How to structure the chapter
- Tips and tricks for writing a top-notch chapter
- Free results chapter template
What exactly is the results chapter?
The results chapter (also referred to as the findings or analysis chapter) is one of the most important chapters of your dissertation or thesis because it shows the reader what you’ve found in terms of the quantitative data you’ve collected. It presents the data using a clear text narrative, supported by tables, graphs and charts. In doing so, it also highlights any potential issues (such as outliers or unusual findings) you’ve come across.
But how’s that different from the discussion chapter?
Well, in the results chapter, you only present your statistical findings. Only the numbers, so to speak – no more, no less. Contrasted to this, in the discussion chapter , you interpret your findings and link them to prior research (i.e. your literature review), as well as your research objectives and research questions . In other words, the results chapter presents and describes the data, while the discussion chapter interprets the data.
Let’s look at an example.
In your results chapter, you may have a plot that shows how respondents to a survey responded: the numbers of respondents per category, for instance. You may also state whether this supports a hypothesis by using a p-value from a statistical test. But it is only in the discussion chapter where you will say why this is relevant or how it compares with the literature or the broader picture. So, in your results chapter, make sure that you don’t present anything other than the hard facts – this is not the place for subjectivity.
It’s worth mentioning that some universities prefer you to combine the results and discussion chapters. Even so, it is good practice to separate the results and discussion elements within the chapter, as this ensures your findings are fully described. Typically, though, the results and discussion chapters are split up in quantitative studies. If you’re unsure, chat with your research supervisor or chair to find out what their preference is.
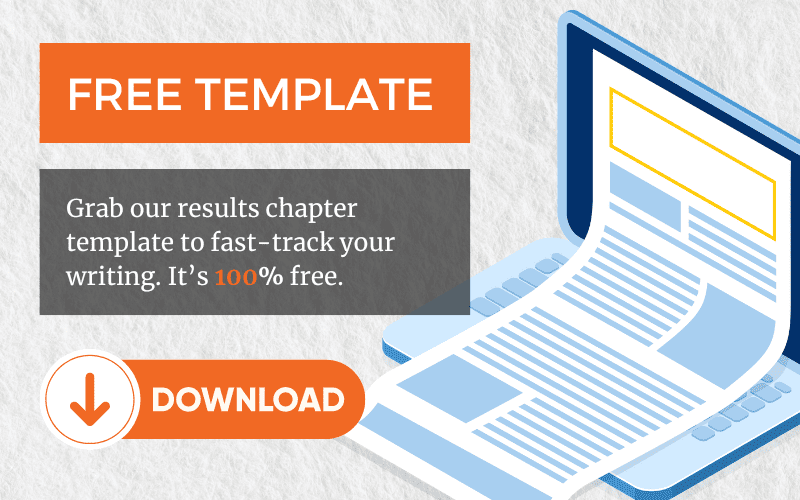
What should you include in the results chapter?
Following your analysis, it’s likely you’ll have far more data than are necessary to include in your chapter. In all likelihood, you’ll have a mountain of SPSS or R output data, and it’s your job to decide what’s most relevant. You’ll need to cut through the noise and focus on the data that matters.
This doesn’t mean that those analyses were a waste of time – on the contrary, those analyses ensure that you have a good understanding of your dataset and how to interpret it. However, that doesn’t mean your reader or examiner needs to see the 165 histograms you created! Relevance is key.
How do I decide what’s relevant?
At this point, it can be difficult to strike a balance between what is and isn’t important. But the most important thing is to ensure your results reflect and align with the purpose of your study . So, you need to revisit your research aims, objectives and research questions and use these as a litmus test for relevance. Make sure that you refer back to these constantly when writing up your chapter so that you stay on track.
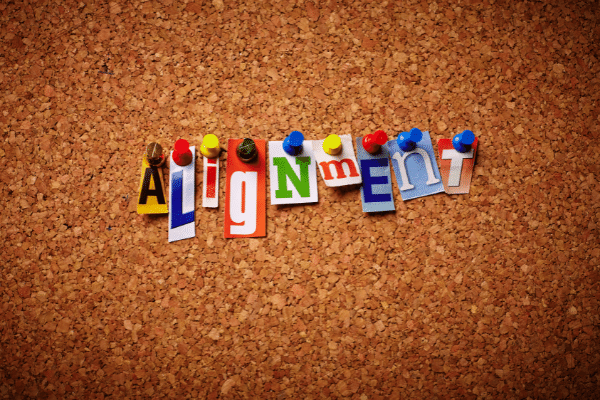
As a general guide, your results chapter will typically include the following:
- Some demographic data about your sample
- Reliability tests (if you used measurement scales)
- Descriptive statistics
- Inferential statistics (if your research objectives and questions require these)
- Hypothesis tests (again, if your research objectives and questions require these)
We’ll discuss each of these points in more detail in the next section.
Importantly, your results chapter needs to lay the foundation for your discussion chapter . This means that, in your results chapter, you need to include all the data that you will use as the basis for your interpretation in the discussion chapter.
For example, if you plan to highlight the strong relationship between Variable X and Variable Y in your discussion chapter, you need to present the respective analysis in your results chapter – perhaps a correlation or regression analysis.
Need a helping hand?
How do I write the results chapter?
There are multiple steps involved in writing up the results chapter for your quantitative research. The exact number of steps applicable to you will vary from study to study and will depend on the nature of the research aims, objectives and research questions . However, we’ll outline the generic steps below.
Step 1 – Revisit your research questions
The first step in writing your results chapter is to revisit your research objectives and research questions . These will be (or at least, should be!) the driving force behind your results and discussion chapters, so you need to review them and then ask yourself which statistical analyses and tests (from your mountain of data) would specifically help you address these . For each research objective and research question, list the specific piece (or pieces) of analysis that address it.
At this stage, it’s also useful to think about the key points that you want to raise in your discussion chapter and note these down so that you have a clear reminder of which data points and analyses you want to highlight in the results chapter. Again, list your points and then list the specific piece of analysis that addresses each point.
Next, you should draw up a rough outline of how you plan to structure your chapter . Which analyses and statistical tests will you present and in what order? We’ll discuss the “standard structure” in more detail later, but it’s worth mentioning now that it’s always useful to draw up a rough outline before you start writing (this advice applies to any chapter).
Step 2 – Craft an overview introduction
As with all chapters in your dissertation or thesis, you should start your quantitative results chapter by providing a brief overview of what you’ll do in the chapter and why . For example, you’d explain that you will start by presenting demographic data to understand the representativeness of the sample, before moving onto X, Y and Z.
This section shouldn’t be lengthy – a paragraph or two maximum. Also, it’s a good idea to weave the research questions into this section so that there’s a golden thread that runs through the document.
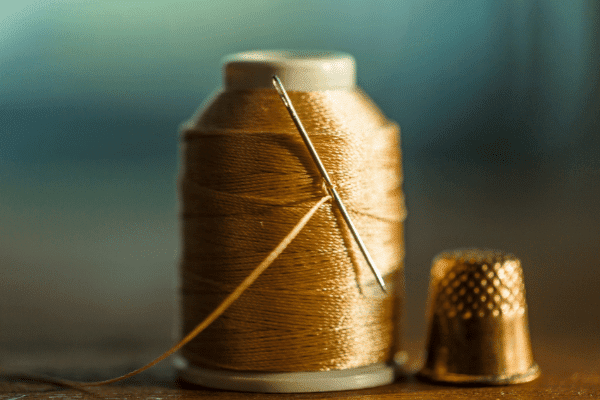
Step 3 – Present the sample demographic data
The first set of data that you’ll present is an overview of the sample demographics – in other words, the demographics of your respondents.
For example:
- What age range are they?
- How is gender distributed?
- How is ethnicity distributed?
- What areas do the participants live in?
The purpose of this is to assess how representative the sample is of the broader population. This is important for the sake of the generalisability of the results. If your sample is not representative of the population, you will not be able to generalise your findings. This is not necessarily the end of the world, but it is a limitation you’ll need to acknowledge.
Of course, to make this representativeness assessment, you’ll need to have a clear view of the demographics of the population. So, make sure that you design your survey to capture the correct demographic information that you will compare your sample to.
But what if I’m not interested in generalisability?
Well, even if your purpose is not necessarily to extrapolate your findings to the broader population, understanding your sample will allow you to interpret your findings appropriately, considering who responded. In other words, it will help you contextualise your findings . For example, if 80% of your sample was aged over 65, this may be a significant contextual factor to consider when interpreting the data. Therefore, it’s important to understand and present the demographic data.
Step 4 – Review composite measures and the data “shape”.
Before you undertake any statistical analysis, you’ll need to do some checks to ensure that your data are suitable for the analysis methods and techniques you plan to use. If you try to analyse data that doesn’t meet the assumptions of a specific statistical technique, your results will be largely meaningless. Therefore, you may need to show that the methods and techniques you’ll use are “allowed”.
Most commonly, there are two areas you need to pay attention to:
#1: Composite measures
The first is when you have multiple scale-based measures that combine to capture one construct – this is called a composite measure . For example, you may have four Likert scale-based measures that (should) all measure the same thing, but in different ways. In other words, in a survey, these four scales should all receive similar ratings. This is called “ internal consistency ”.
Internal consistency is not guaranteed though (especially if you developed the measures yourself), so you need to assess the reliability of each composite measure using a test. Typically, Cronbach’s Alpha is a common test used to assess internal consistency – i.e., to show that the items you’re combining are more or less saying the same thing. A high alpha score means that your measure is internally consistent. A low alpha score means you may need to consider scrapping one or more of the measures.
#2: Data shape
The second matter that you should address early on in your results chapter is data shape. In other words, you need to assess whether the data in your set are symmetrical (i.e. normally distributed) or not, as this will directly impact what type of analyses you can use. For many common inferential tests such as T-tests or ANOVAs (we’ll discuss these a bit later), your data needs to be normally distributed. If it’s not, you’ll need to adjust your strategy and use alternative tests.
To assess the shape of the data, you’ll usually assess a variety of descriptive statistics (such as the mean, median and skewness), which is what we’ll look at next.
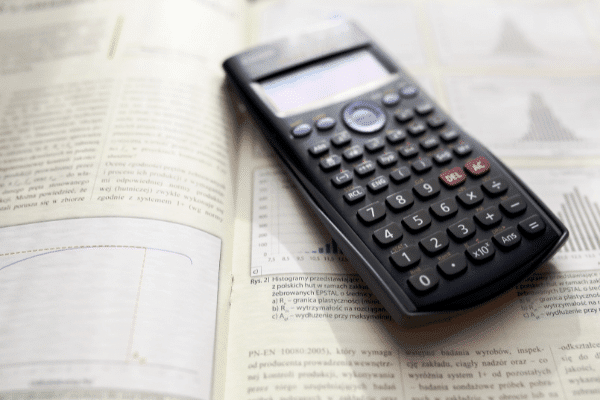
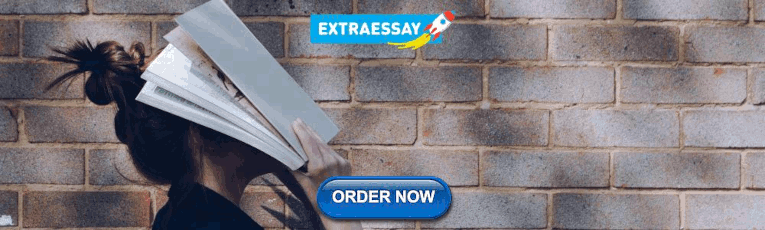
Step 5 – Present the descriptive statistics
Now that you’ve laid the foundation by discussing the representativeness of your sample, as well as the reliability of your measures and the shape of your data, you can get started with the actual statistical analysis. The first step is to present the descriptive statistics for your variables.
For scaled data, this usually includes statistics such as:
- The mean – this is simply the mathematical average of a range of numbers.
- The median – this is the midpoint in a range of numbers when the numbers are arranged in order.
- The mode – this is the most commonly repeated number in the data set.
- Standard deviation – this metric indicates how dispersed a range of numbers is. In other words, how close all the numbers are to the mean (the average).
- Skewness – this indicates how symmetrical a range of numbers is. In other words, do they tend to cluster into a smooth bell curve shape in the middle of the graph (this is called a normal or parametric distribution), or do they lean to the left or right (this is called a non-normal or non-parametric distribution).
- Kurtosis – this metric indicates whether the data are heavily or lightly-tailed, relative to the normal distribution. In other words, how peaked or flat the distribution is.
A large table that indicates all the above for multiple variables can be a very effective way to present your data economically. You can also use colour coding to help make the data more easily digestible.
For categorical data, where you show the percentage of people who chose or fit into a category, for instance, you can either just plain describe the percentages or numbers of people who responded to something or use graphs and charts (such as bar graphs and pie charts) to present your data in this section of the chapter.
When using figures, make sure that you label them simply and clearly , so that your reader can easily understand them. There’s nothing more frustrating than a graph that’s missing axis labels! Keep in mind that although you’ll be presenting charts and graphs, your text content needs to present a clear narrative that can stand on its own. In other words, don’t rely purely on your figures and tables to convey your key points: highlight the crucial trends and values in the text. Figures and tables should complement the writing, not carry it .
Depending on your research aims, objectives and research questions, you may stop your analysis at this point (i.e. descriptive statistics). However, if your study requires inferential statistics, then it’s time to deep dive into those .
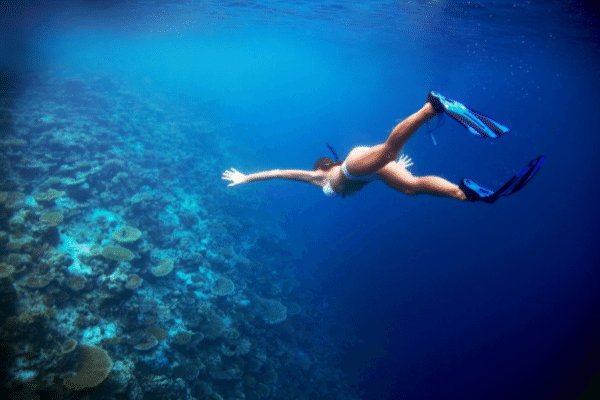
Step 6 – Present the inferential statistics
Inferential statistics are used to make generalisations about a population , whereas descriptive statistics focus purely on the sample . Inferential statistical techniques, broadly speaking, can be broken down into two groups .
First, there are those that compare measurements between groups , such as t-tests (which measure differences between two groups) and ANOVAs (which measure differences between multiple groups). Second, there are techniques that assess the relationships between variables , such as correlation analysis and regression analysis. Within each of these, some tests can be used for normally distributed (parametric) data and some tests are designed specifically for use on non-parametric data.
There are a seemingly endless number of tests that you can use to crunch your data, so it’s easy to run down a rabbit hole and end up with piles of test data. Ultimately, the most important thing is to make sure that you adopt the tests and techniques that allow you to achieve your research objectives and answer your research questions .
In this section of the results chapter, you should try to make use of figures and visual components as effectively as possible. For example, if you present a correlation table, use colour coding to highlight the significance of the correlation values, or scatterplots to visually demonstrate what the trend is. The easier you make it for your reader to digest your findings, the more effectively you’ll be able to make your arguments in the next chapter.
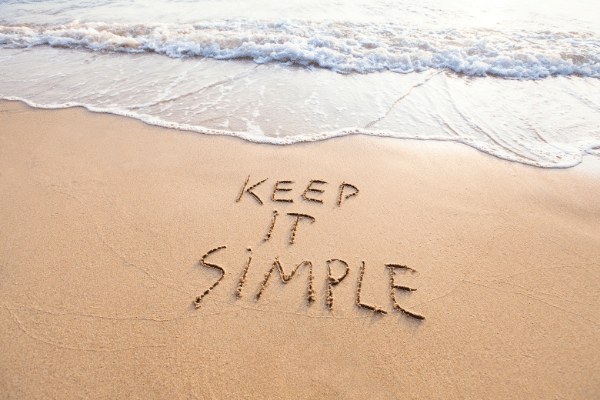
Step 7 – Test your hypotheses
If your study requires it, the next stage is hypothesis testing. A hypothesis is a statement , often indicating a difference between groups or relationship between variables, that can be supported or rejected by a statistical test. However, not all studies will involve hypotheses (again, it depends on the research objectives), so don’t feel like you “must” present and test hypotheses just because you’re undertaking quantitative research.
The basic process for hypothesis testing is as follows:
- Specify your null hypothesis (for example, “The chemical psilocybin has no effect on time perception).
- Specify your alternative hypothesis (e.g., “The chemical psilocybin has an effect on time perception)
- Set your significance level (this is usually 0.05)
- Calculate your statistics and find your p-value (e.g., p=0.01)
- Draw your conclusions (e.g., “The chemical psilocybin does have an effect on time perception”)
Finally, if the aim of your study is to develop and test a conceptual framework , this is the time to present it, following the testing of your hypotheses. While you don’t need to develop or discuss these findings further in the results chapter, indicating whether the tests (and their p-values) support or reject the hypotheses is crucial.
Step 8 – Provide a chapter summary
To wrap up your results chapter and transition to the discussion chapter, you should provide a brief summary of the key findings . “Brief” is the keyword here – much like the chapter introduction, this shouldn’t be lengthy – a paragraph or two maximum. Highlight the findings most relevant to your research objectives and research questions, and wrap it up.
Some final thoughts, tips and tricks
Now that you’ve got the essentials down, here are a few tips and tricks to make your quantitative results chapter shine:
- When writing your results chapter, report your findings in the past tense . You’re talking about what you’ve found in your data, not what you are currently looking for or trying to find.
- Structure your results chapter systematically and sequentially . If you had two experiments where findings from the one generated inputs into the other, report on them in order.
- Make your own tables and graphs rather than copying and pasting them from statistical analysis programmes like SPSS. Check out the DataIsBeautiful reddit for some inspiration.
- Once you’re done writing, review your work to make sure that you have provided enough information to answer your research questions , but also that you didn’t include superfluous information.
If you’ve got any questions about writing up the quantitative results chapter, please leave a comment below. If you’d like 1-on-1 assistance with your quantitative analysis and discussion, check out our hands-on coaching service , or book a free consultation with a friendly coach.
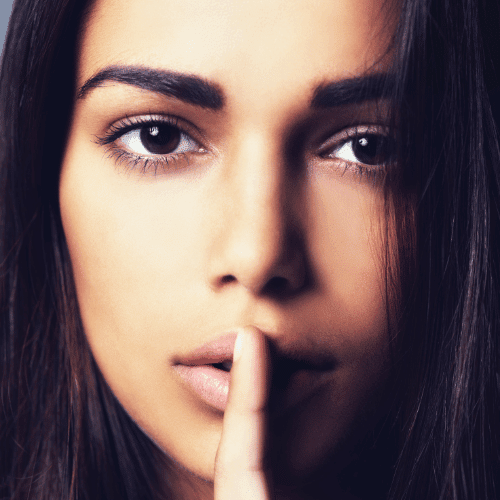
Psst... there’s more!
This post was based on one of our popular Research Bootcamps . If you're working on a research project, you'll definitely want to check this out ...
You Might Also Like:
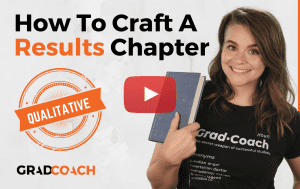
Thank you. I will try my best to write my results.
Awesome content 👏🏾
this was great explaination
Submit a Comment Cancel reply
Your email address will not be published. Required fields are marked *
Save my name, email, and website in this browser for the next time I comment.
- Print Friendly
Academia.edu no longer supports Internet Explorer.
To browse Academia.edu and the wider internet faster and more securely, please take a few seconds to upgrade your browser .
Enter the email address you signed up with and we'll email you a reset link.
- We're Hiring!
- Help Center
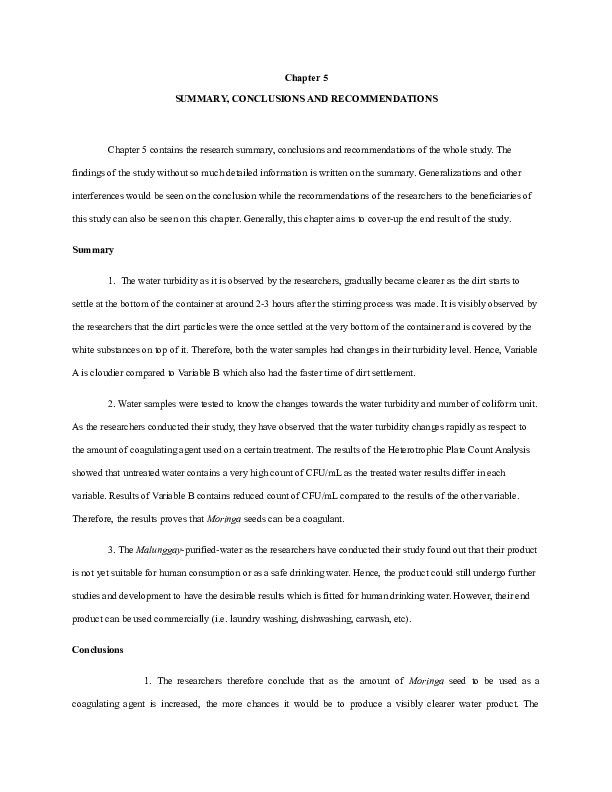
Chapter 5 SUMMARY, CONCLUSIONS AND RECOMMENDATIONS

Related Papers
Human movement science
Peter H . Wilson
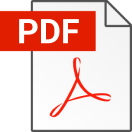
Best Practice & Research Clinical Endocrinology & Metabolism
Imran Mushtaq
Linda Isherwood
CLIC Note, to be …
Barbara Dalena , D. Adams
R. Tomás 1 , E. Adli 1 , I. Ahmed 2 , PK Ambatu 3 , D. Angal-Kalinin 4 , R. Barlow 4&6 , JP Baud 5 , ... B. Bolzon 5 , H. Braun 1 , H. Burkhardt 1 , GC Burt 4 , R. Corsini 1 , B. Dalena 1 , AC Dexter 3 , ... V. Dolgashev 7 , K. Elsener 1 , JL Fernandez Hernando 4 , G. Gaillard 5 , N. Geffroy ...
Hormone Research in Paediatrics
Jillian Bryce
Background: Research and audit are vital for the management of Differences/Disorders of Sex Development (DSD). Clinical networks have a strong potential to drive these activities with the development of care standards including patient experience data and peer-observation of clinical care provision. Summary: Following the 2005 Consensus Workshop that stressed the need for the regular collection and sharing of data across geographical boundaries, the current I-DSD registry was initially launched in 2008. Over a decade later, this registry and its associated network play an increasingly important role in supporting research, training, and benchmarking of care and service. Patient registries can also facilitate the development of local circles of patients and parents with similar conditions who can support each other. Key Messages: The case for participating in standardized data collection and exchange for DSD has now been made and should be standard practice in centres that care for p...
Developmental Medicine and Child Neurology
Michael E . Stone
Address given in Sydney in Sept. 2014 to the Australian Friends of the Hebrew University and the Sir Zelman Cowan Universities Fund.
Advances in urology
Juerg C Streuli
Brian Cuthbertson
International Journal of Urology
RELATED PAPERS
George Bennett
Joachim Hasebrook
Swiss Medical Weekly
Alberto Moriggia
Cuadernos de filología inglesa
MARIA SUSANA ROMERO VINUESA
Journal of Biotechnology
Abdul Hannan
La Vapería Vape Shop
DELTA: Documentação de Estudos em Lingüística Teórica e Aplicada
Revista Mexicana De Ciencias Forestales
Martín Gómez Cárdenas
Proceedings of the 19th annual symposium on Integrated circuits and systems design - SBCCI '06
Ala Messaoudi
Journal of Pharmaceutical Negative Results
Paulo Granjeiro
Journal of Marine Systems
E. Garcia-ladona
South African medical journal = Suid-Afrikaanse tydskrif vir geneeskunde
Charles Gilks
LOWONGAN PRAKERIN, 0813-2103-2094 Pkl Tempat Magang Smk Multimedia Jogja Godean
Infopkldijogja Pkl
Glycobiology
Jesus.4 - aprile 2023
Selene Zorzi
The e-Business Studies
Thae Min Lee
Asian Journal of Biological Sciences
Cahyo Budiman
Journal of Vacuum Science & Technology A: Vacuum, Surfaces, and Films
M. Carmen Torquemada Vico
Revista Interfaz
Revista Interfaz , Emilio Aquino
Social Science Research Network
Milind Dawande
Acta horticulturae
John W Gillis
Selçuk Üniversitesi Hukuk Fakültesi dergisi
(A Festschrift in Memory of Professor John B. Thornes)
Marvin Quesada
- We're Hiring!
- Help Center
- Find new research papers in:
- Health Sciences
- Earth Sciences
- Cognitive Science
- Mathematics
- Computer Science
- Academia ©2024

Improved Surface Drainage of Pavements: Final Report (1998)
Chapter: chapter 5 summary, findings, and recommendations.
Below is the uncorrected machine-read text of this chapter, intended to provide our own search engines and external engines with highly rich, chapter-representative searchable text of each book. Because it is UNCORRECTED material, please consider the following text as a useful but insufficient proxy for the authoritative book pages.
CHAPIER 5 SI~MARY, FINDINGS, AND RECOMMENDATIONS SUGARY The primary objective of this research was to identify unproved methods for draining rainwater from the surface of multi-lane pavements and to develop guidelines for their use. The guidelines, along with details on the rationale for their development, are presented in a separate document' "Proposed Design Guidelines for Improving Pavement Surface Drainage" (2J. The guidelines support an interactive computer program, PAVDRN, that can be used by practicing engineers In the process of designing new pavements or rehabilitating old pavements' is outlined In figure 39. The intended audience for the guidelines is practicing highway design engineers that work for transportation agencies or consulting firms. Improved pavement surface drainage is needed for two reasons: (~) to minimize splash and spray and (2) to control the tendency for hydroplaning. Both issues are primary safety concerns. At the request of the advisory panel for the project, the main focus of this study was on ~mprov~g surface drainage to mammae the tendency for hydroplaning. In terms of reducing the tendency for hydroplaTuT g, the needed level of drainage is defined in terms of the thickness of the film of water on the pavement. Therefore, the guidelines were developed within the context of reducing the thickness of the water film on pavement surfaces to the extent that hydroplaning is unlikely at highway design speeds. Since hydroplaning is ~7
DESIGN CRITERIA Pavement Geometry Number of lanes Section type - Tangent - Horizontal curve - Transition - Vertical crest curve - Vertical sag curve Enviromnental oramaters Rainfall intensity ~ Temperature Pavement Tvpe Dense-graded asphalt Porous asphalt Portland cement concrete ~ Grooved Portland cement concrete Desion Soeed Allowable speed for onset of hydroplaning Recommend Desion Changes Alter geometry Alter pavement surface Add appurtenances Groove (Portland cement concrete) CALCULATIONS Lenoth of flow path Calculate on basis of pavement geometry IT Hydraulic Analvses . No? Water film thickness Equation No. 10 Equation No.'s. 16-19 1 Hvdroolanino Analvsis Hydroplaning speed Equation No.'s 21-24 Rainfall Intensity Equation No. 25 -A I / Meet Design ~ \ Cntena? / \<es? Accent Desinn | Figure 39. Flow diagram representing PAVI)RN design process In "Proposed Guidelines for Improving Pavement Surface DrmT~age" (2). 118
controlled primarily by the thickness of the water film on the pavement surface, the design guidelines focus on the prediction and control of ache depth of water flowing across the pavement surface as a result of rainfall, often referred to as sheet flow. Water film thickness on highway pavements can be controlled In three fundamental ways, by: I. Minimizing the length of the longest flow path of the water over We pavement and thereby the distance over which the flow can develop; 2. Increasing the texture of the pavement surface; and 3. Removing water from the pavement's surface. In the process of using PAVDRN to implement the design guidelines, the designer is guided to (~) minimize the longest drainage path length of the section under design by altering the pavement geometry and (2) reduce the resultant water film thickness that will develop along that drainage path length by increasing the mean texture depth, choosing a surface that maximizes texture, or using permeable pavements, grooving, and appurtenances to remove water from the surface. Through the course of a typical design project, four key areas need to be considered in order to analyze and eventually reduce the potential for hydroplaning. These areas are: ~9
I. Environmental conditions: 2. Geometry of the roadway surface; 3. Pavement surface (texture) properties; and 4. Appurtenances. Each of these areas and their influence on the resulting hydroplaning speed of the designed section are discussed In detail In the guidelines (21. The environmental conditions considered are rainfall ~ntensibr and water temperature, which determines the kinematic viscosity of the water. The designer has no real control over these environmental factors but needs to select appropriate values when analyzing the effect of flow over the pavement surface and hydroplaning potential. Five section types, one for each of the basic geometric configurations used In highway design, are examined. These section are: 1. TaIlgent; 2. Superelevated curve; 3. Transition; 4. Vertical crest curve; and 5. Vertical sag curve. 120
Pavement properties that affect the water fihn thickness mclude surface characteristics, such as mean texture depth and grooving of Portland cement concrete surfaces, are considered In the process of applying PAVDRN. Porous asphalt pavement surfaces can also reduce He water film thickness and thereby contribute to the reduction of hydroplaning tendency and their presence can also be accounted for when using PAVDRN. Finally, PAVDRN also allows the design engineer to consider the effect of drainage appurtenances, such as slotted drain inlets. A complete description of the various elements that are considered In the PAVDRN program is illustrated In figure 40. A more complete description of the design process, the parameters used in the design process, and typical values for the parameters is presented In the "Proposed Design Guidelines for Improving Pavement Surface Drainage" (2) alla in Appendix A. fIN1)INGS The following findings are based on the research accomplished during the project, a survey of the literature, and a state-of-the-art survey of current practice. I. Model. The one~unensional mode} is adequate as a design tool. The simplicity and stability of the one~imensional mode} offsets any increased accuracy afforded by a two-d~mensional model. The one~mensional model as a predictor of water fiDn thickness and How path length was verified by using data from a previous study (11). 121
No. of Planes Length of Plane Grade Step Increment Wdth of Plane Cross Slope Section T,rne 1) Tangent 2) Honzontal Curare 3) Transition 4) Vertical Crest 5) Vertical Sag U=tS 1)U.S. 2) S. I. Rainfall Intenstity ~ , \ |Kinematic Viscosity |Design Speed Note: PC = Point of Curvature PI. = Point of Tangency PCC = Portland cement concrete WAC = Dense graded asphalt concrete 0GAC = 0pcn~raded asphalt concrete where OGAC includes all types of intentally draining asphalt surfaces GPCC = Grooved Ponland cement concrete Taneent Pavement Type Mean Texture Depth 1) PCC 2) DGAC 3) OGAC 4) GPCC Horizontal Cun~c Grade Cross Slope Radius of Cunran~re Wdth Pavement Type _ 2) DGAC 3) OGAC 4) GPCC Mean Texture Depth Step Increment _ Transition Length of Plane Super Elevation Tangent Cross Slope Tangent Grade width of Curve Transition Width Pavement Type_ 1) PCC 3) OGAC 4) GPCC Mean Texture Depth Step Increment Horizontal Length Cross slope width PC Grade PI' Grade Elevation: Pr-PC Vertical Crest Flow Direction Step Increment Pavement Type 1) PC Side I 2) PI. Side | 1)PCC 2) DGAC 3) OGAC 4) GPCC Mean Tex~rc Depth _ _ ~ Figure 40. Factors considered in PAVDRN program. 122 ~1 r - . , Vertical Sad | Horizontal Length | Cross slope Wldth PC Grade PI Grade Elevation: PIE Flow Direction Step Increment / Stored :_ ~ cats ~ 1) PC Side | 2) PI Side | . Pavement Typed 1) PCC 3) OGAC 14) GPCC Mean Texture Depth I I
~ Stored data V ~ 3 L IN1T For use with a second nut using data from the first run.) , 1 EPRINT (Echos input to output ) 1 CONVERT (Converts units to and from SI and English.) ~ , ADVP (Advances Page of output.) KINW (Calculates Minning's n, Water Film Thickness (WEIR), and Hydroplaning Speed UPS).) , EDGE (Determines if flow has reached the edge of the pavement.) out roar Figure 40. Factors considered in PAVDRN program (continued). 123
2. Occurrence of Hydropl~r g. In general, based on the PAVDRN mode! and the assumptions inherent in its development, hydroplaning can be expected at speeds below roadway design speeds if the length of the flow path exceeds two lane widths. 3. Water Film Thickness. Hydroplaning is initiated primarily by the depth of the water film thickness. Therefore, the primary design objective when controlling hydroplaning must be to limit the depth of the water film. 4. Reducing Water Film Thickness. There are no simple means for controlling water John thickness, but a number of methods can effectively reduce water film thickness and consequently hydroplaning potential. These include: Optimizing pavement geometry, especially cross-slope. Providing some means of additional drainage, such as use of grooved surfaces (PCC) or porous mixtures (HMA). Including slotted drains within the roadway. 5. Tests Needed for Design. The design guidelines require an estimate of the surface texture (MTD) and the coefficient of permeability Porous asphalt only). The sand patch is an acceptable test method for measuring surface texture, except for the more open (20-percent air voids) porous asphalt mixes. In these cases, an estimate of the surface texture, based on tabulated data, is sufficient. As an alternative, 124
sand patch measurements can be made on cast replicas of the surface. For the open mixes, the glass beads flow into the voids within the mixture, giving an inaccurate measure of surface texture. Based on the measurements obtained In the laboratory, the coefficient of permeability for the open-graded asphalt concrete does not exhibit a wide range of values, and values of k may be selected for design purposes from tabulated design data (k versus air voids). Given the uncertainty of this property resulting from compaction under traffic and clogging from contaminants and anti-skid material, a direct measurement (e.g., drainage lag permeameter) of k is not warranted. Based on the previous discussion, no new test procedures are needed to adopt the design guidelines developed during this project. 6. Grooving. Grooving of PCC pavements provides a reservoir for surface water and can facilitate the removal of water if the grooves are placed parallel to the flow oath. Parallel orientation is generally not practical because the flow on highway pavements is typically not transverse to the pavement. Thus, the primary contribution offered by grooving is to provide a surface reservoir unless the grooves comlect with drainage at the edge of the pavement. Once the grooves are filled with water, the tops of the grooves are the datum for the Why and do not contribute to the reduction in the hydroplaning potential. 125
7. Porous Pavements. These mixtures can enhance the water removal and Hereby reduce water film tHch~ess. They merit more consideration by highway agencies In the United States, but they are not a panacea for eliminating hydroplaning. As with grooved PCC pavements, the internal voids do not contribute to the reduction of hydroplaning; based on the field tests done In this study. hv~ronImiina can be if, , , ~ expected on these mixtures given sufficient water fiLn thickness. Other than their ability to conduct water through internal flow, the large MTD offered by porous asphalt is the main contribution offered by the mixtures to the reduction of hydroplaning potential. The high-void ~ > 20 percent), modified binder mixes used In Europe merit further evaluation in the United States. They should be used In areas where damage from freezing water and the problems of black ice are not likely. 8. Slotted Drains. These fixtures, when installed between travel lanes, offer perhaps the most effective means of controlling water film thickness from a hydraulics standpoint. They have not been used extensively In the traveled lanes and questions remain unanswered with respect to their installation (especially in rehabilitation situations) and maintenance. The ability to support traffic loads and still maintain surface smoothness has not been demonstrated and they may be susceptible to clogging from roadway debris, ice, or snow. 126
RECOMMENDATIONS AND CONCLUSIONS The following recommendations are offered based on the work accomplished during this project and on the conclusions given previously: I. Implementation. The PAVDRN program and associated guidelines need to be field tested and revised as needed. The program and the guidelines are sufficiently complete so that they can be used in a design office. Some of the parameters and algorithms will I~ely need to be modified as experience is gained with the program. 2. Database of Material Properties. A database of material properties should be gathered to supplement the information contained in PAVDRN. This information should Include typical values for the permeability of porous asphalt and topical values for the surface texture (MTD) for different pavement surfaces to include toned Portland cement concrete surfaces. A series of photographs of typical pavement sections and their associated texture depths should be considered as an addition to the design guide (21. 3. Pavement Geometry. The AASHTO design guidelines (~) should be re-evaluated In terms of current design criteria to determine if they can be modified to enhance drainage without adversely affecting vehicle handling or safety. ~27
4. Use of appurtenances. Slotted drams should be evaluated In the field to determine if they are practical when Installed In the traveled way. Manufacturers should reconsider the design of slotted drains and their Installation recommendations currently In force to maximize them for use In multi-lane pavements and to determine if slotted drains are suitable for installations In the traveled right of way. 5. Porous Asphalt Mixtures. More use should be made of these mixtures, especially the modified high a~r-void mixtures as used In France. Field trials should be conducted to monitor HPS and the long-term effectiveness of these mixtures and to validate the MPS and WDT predicted by PAVDRN. 6. Two-D~mensional Model. Further work should be done with two~mensional models to determine if they improve accuracy of PAVDRN and to determine if they are practical from a computational standpoint. ADDITIONAL STUDIES On the basis of the work done during this study, a number of additional items warrant furler study. These Include: 1. Full-scale skid resistance studies to validate PAVDRN in general and the relationship between water film thickness and hydroplaning potential in particular are needed in light of the unexpectedly low hvdronlanin~ speeds predicted during 128 , . ~. , ~
this study. The effect of water infiltration into pavement cracks and loss of water by splash and spray need to be accounted for In the prediction of water fihn Sickness. Surface Irregularities, especially rutting, need to be considered in the prediction models. 2. Field trials are needed to confirm the effectiveness of alternative asphalt and Portland cement concrete surfaces. These include porous Portland cement concrete surfaces, porous asphalt concrete, and various asphalt m~cro-surfaces. 3. The permeability of porous surface mixtures needs to be confirmed with samples removed from the field, and the practicality of a simplified method for measuring in-situ permeability must be investigated and compared to alternative measurements, such as the outflow meter. 4. For measuring pavement texture, alternatives to the sand patch method should be investigated, especially for use with porous asphalt mixtures. 129
THIS PAGE INTENTIONALLY LEFT BLANK
READ FREE ONLINE
Welcome to OpenBook!
You're looking at OpenBook, NAP.edu's online reading room since 1999. Based on feedback from you, our users, we've made some improvements that make it easier than ever to read thousands of publications on our website.
Do you want to take a quick tour of the OpenBook's features?
Show this book's table of contents , where you can jump to any chapter by name.
...or use these buttons to go back to the previous chapter or skip to the next one.
Jump up to the previous page or down to the next one. Also, you can type in a page number and press Enter to go directly to that page in the book.
To search the entire text of this book, type in your search term here and press Enter .
Share a link to this book page on your preferred social network or via email.
View our suggested citation for this chapter.
Ready to take your reading offline? Click here to buy this book in print or download it as a free PDF, if available.
Get Email Updates
Do you enjoy reading reports from the Academies online for free ? Sign up for email notifications and we'll let you know about new publications in your areas of interest when they're released.
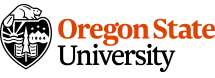
Chapter 5. Sampling
Introduction.
Most Americans will experience unemployment at some point in their lives. Sarah Damaske ( 2021 ) was interested in learning about how men and women experience unemployment differently. To answer this question, she interviewed unemployed people. After conducting a “pilot study” with twenty interviewees, she realized she was also interested in finding out how working-class and middle-class persons experienced unemployment differently. She found one hundred persons through local unemployment offices. She purposefully selected a roughly equal number of men and women and working-class and middle-class persons for the study. This would allow her to make the kinds of comparisons she was interested in. She further refined her selection of persons to interview:
I decided that I needed to be able to focus my attention on gender and class; therefore, I interviewed only people born between 1962 and 1987 (ages 28–52, the prime working and child-rearing years), those who worked full-time before their job loss, those who experienced an involuntary job loss during the past year, and those who did not lose a job for cause (e.g., were not fired because of their behavior at work). ( 244 )
The people she ultimately interviewed compose her sample. They represent (“sample”) the larger population of the involuntarily unemployed. This “theoretically informed stratified sampling design” allowed Damaske “to achieve relatively equal distribution of participation across gender and class,” but it came with some limitations. For one, the unemployment centers were located in primarily White areas of the country, so there were very few persons of color interviewed. Qualitative researchers must make these kinds of decisions all the time—who to include and who not to include. There is never an absolutely correct decision, as the choice is linked to the particular research question posed by the particular researcher, although some sampling choices are more compelling than others. In this case, Damaske made the choice to foreground both gender and class rather than compare all middle-class men and women or women of color from different class positions or just talk to White men. She leaves the door open for other researchers to sample differently. Because science is a collective enterprise, it is most likely someone will be inspired to conduct a similar study as Damaske’s but with an entirely different sample.
This chapter is all about sampling. After you have developed a research question and have a general idea of how you will collect data (observations or interviews), how do you go about actually finding people and sites to study? Although there is no “correct number” of people to interview, the sample should follow the research question and research design. You might remember studying sampling in a quantitative research course. Sampling is important here too, but it works a bit differently. Unlike quantitative research, qualitative research involves nonprobability sampling. This chapter explains why this is so and what qualities instead make a good sample for qualitative research.
Quick Terms Refresher
- The population is the entire group that you want to draw conclusions about.
- The sample is the specific group of individuals that you will collect data from.
- Sampling frame is the actual list of individuals that the sample will be drawn from. Ideally, it should include the entire target population (and nobody who is not part of that population).
- Sample size is how many individuals (or units) are included in your sample.
The “Who” of Your Research Study
After you have turned your general research interest into an actual research question and identified an approach you want to take to answer that question, you will need to specify the people you will be interviewing or observing. In most qualitative research, the objects of your study will indeed be people. In some cases, however, your objects might be content left by people (e.g., diaries, yearbooks, photographs) or documents (official or unofficial) or even institutions (e.g., schools, medical centers) and locations (e.g., nation-states, cities). Chances are, whatever “people, places, or things” are the objects of your study, you will not really be able to talk to, observe, or follow every single individual/object of the entire population of interest. You will need to create a sample of the population . Sampling in qualitative research has different purposes and goals than sampling in quantitative research. Sampling in both allows you to say something of interest about a population without having to include the entire population in your sample.
We begin this chapter with the case of a population of interest composed of actual people. After we have a better understanding of populations and samples that involve real people, we’ll discuss sampling in other types of qualitative research, such as archival research, content analysis, and case studies. We’ll then move to a larger discussion about the difference between sampling in qualitative research generally versus quantitative research, then we’ll move on to the idea of “theoretical” generalizability, and finally, we’ll conclude with some practical tips on the correct “number” to include in one’s sample.
Sampling People
To help think through samples, let’s imagine we want to know more about “vaccine hesitancy.” We’ve all lived through 2020 and 2021, and we know that a sizable number of people in the United States (and elsewhere) were slow to accept vaccines, even when these were freely available. By some accounts, about one-third of Americans initially refused vaccination. Why is this so? Well, as I write this in the summer of 2021, we know that some people actively refused the vaccination, thinking it was harmful or part of a government plot. Others were simply lazy or dismissed the necessity. And still others were worried about harmful side effects. The general population of interest here (all adult Americans who were not vaccinated by August 2021) may be as many as eighty million people. We clearly cannot talk to all of them. So we will have to narrow the number to something manageable. How can we do this?
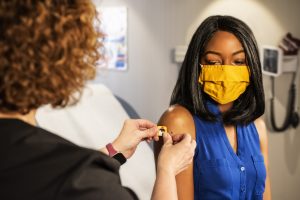
First, we have to think about our actual research question and the form of research we are conducting. I am going to begin with a quantitative research question. Quantitative research questions tend to be simpler to visualize, at least when we are first starting out doing social science research. So let us say we want to know what percentage of each kind of resistance is out there and how race or class or gender affects vaccine hesitancy. Again, we don’t have the ability to talk to everyone. But harnessing what we know about normal probability distributions (see quantitative methods for more on this), we can find this out through a sample that represents the general population. We can’t really address these particular questions if we only talk to White women who go to college with us. And if you are really trying to generalize the specific findings of your sample to the larger population, you will have to employ probability sampling , a sampling technique where a researcher sets a selection of a few criteria and chooses members of a population randomly. Why randomly? If truly random, all the members have an equal opportunity to be a part of the sample, and thus we avoid the problem of having only our friends and neighbors (who may be very different from other people in the population) in the study. Mathematically, there is going to be a certain number that will be large enough to allow us to generalize our particular findings from our sample population to the population at large. It might surprise you how small that number can be. Election polls of no more than one thousand people are routinely used to predict actual election outcomes of millions of people. Below that number, however, you will not be able to make generalizations. Talking to five people at random is simply not enough people to predict a presidential election.
In order to answer quantitative research questions of causality, one must employ probability sampling. Quantitative researchers try to generalize their findings to a larger population. Samples are designed with that in mind. Qualitative researchers ask very different questions, though. Qualitative research questions are not about “how many” of a certain group do X (in this case, what percentage of the unvaccinated hesitate for concern about safety rather than reject vaccination on political grounds). Qualitative research employs nonprobability sampling . By definition, not everyone has an equal opportunity to be included in the sample. The researcher might select White women they go to college with to provide insight into racial and gender dynamics at play. Whatever is found by doing so will not be generalizable to everyone who has not been vaccinated, or even all White women who have not been vaccinated, or even all White women who have not been vaccinated who are in this particular college. That is not the point of qualitative research at all. This is a really important distinction, so I will repeat in bold: Qualitative researchers are not trying to statistically generalize specific findings to a larger population . They have not failed when their sample cannot be generalized, as that is not the point at all.
In the previous paragraph, I said it would be perfectly acceptable for a qualitative researcher to interview five White women with whom she goes to college about their vaccine hesitancy “to provide insight into racial and gender dynamics at play.” The key word here is “insight.” Rather than use a sample as a stand-in for the general population, as quantitative researchers do, the qualitative researcher uses the sample to gain insight into a process or phenomenon. The qualitative researcher is not going to be content with simply asking each of the women to state her reason for not being vaccinated and then draw conclusions that, because one in five of these women were concerned about their health, one in five of all people were also concerned about their health. That would be, frankly, a very poor study indeed. Rather, the qualitative researcher might sit down with each of the women and conduct a lengthy interview about what the vaccine means to her, why she is hesitant, how she manages her hesitancy (how she explains it to her friends), what she thinks about others who are unvaccinated, what she thinks of those who have been vaccinated, and what she knows or thinks she knows about COVID-19. The researcher might include specific interview questions about the college context, about their status as White women, about the political beliefs they hold about racism in the US, and about how their own political affiliations may or may not provide narrative scripts about “protective whiteness.” There are many interesting things to ask and learn about and many things to discover. Where a quantitative researcher begins with clear parameters to set their population and guide their sample selection process, the qualitative researcher is discovering new parameters, making it impossible to engage in probability sampling.
Looking at it this way, sampling for qualitative researchers needs to be more strategic. More theoretically informed. What persons can be interviewed or observed that would provide maximum insight into what is still unknown? In other words, qualitative researchers think through what cases they could learn the most from, and those are the cases selected to study: “What would be ‘bias’ in statistical sampling, and therefore a weakness, becomes intended focus in qualitative sampling, and therefore a strength. The logic and power of purposeful sampling like in selecting information-rich cases for study in depth. Information-rich cases are those from which one can learn a great deal about issues of central importance to the purpose of the inquiry, thus the term purposeful sampling” ( Patton 2002:230 ; emphases in the original).
Before selecting your sample, though, it is important to clearly identify the general population of interest. You need to know this before you can determine the sample. In our example case, it is “adult Americans who have not yet been vaccinated.” Depending on the specific qualitative research question, however, it might be “adult Americans who have been vaccinated for political reasons” or even “college students who have not been vaccinated.” What insights are you seeking? Do you want to know how politics is affecting vaccination? Or do you want to understand how people manage being an outlier in a particular setting (unvaccinated where vaccinations are heavily encouraged if not required)? More clearly stated, your population should align with your research question . Think back to the opening story about Damaske’s work studying the unemployed. She drew her sample narrowly to address the particular questions she was interested in pursuing. Knowing your questions or, at a minimum, why you are interested in the topic will allow you to draw the best sample possible to achieve insight.
Once you have your population in mind, how do you go about getting people to agree to be in your sample? In qualitative research, it is permissible to find people by convenience. Just ask for people who fit your sample criteria and see who shows up. Or reach out to friends and colleagues and see if they know anyone that fits. Don’t let the name convenience sampling mislead you; this is not exactly “easy,” and it is certainly a valid form of sampling in qualitative research. The more unknowns you have about what you will find, the more convenience sampling makes sense. If you don’t know how race or class or political affiliation might matter, and your population is unvaccinated college students, you can construct a sample of college students by placing an advertisement in the student paper or posting a flyer on a notice board. Whoever answers is your sample. That is what is meant by a convenience sample. A common variation of convenience sampling is snowball sampling . This is particularly useful if your target population is hard to find. Let’s say you posted a flyer about your study and only two college students responded. You could then ask those two students for referrals. They tell their friends, and those friends tell other friends, and, like a snowball, your sample gets bigger and bigger.
Researcher Note
Gaining Access: When Your Friend Is Your Research Subject
My early experience with qualitative research was rather unique. At that time, I needed to do a project that required me to interview first-generation college students, and my friends, with whom I had been sharing a dorm for two years, just perfectly fell into the sample category. Thus, I just asked them and easily “gained my access” to the research subject; I know them, we are friends, and I am part of them. I am an insider. I also thought, “Well, since I am part of the group, I can easily understand their language and norms, I can capture their honesty, read their nonverbal cues well, will get more information, as they will be more opened to me because they trust me.” All in all, easy access with rich information. But, gosh, I did not realize that my status as an insider came with a price! When structuring the interview questions, I began to realize that rather than focusing on the unique experiences of my friends, I mostly based the questions on my own experiences, assuming we have similar if not the same experiences. I began to struggle with my objectivity and even questioned my role; am I doing this as part of the group or as a researcher? I came to know later that my status as an insider or my “positionality” may impact my research. It not only shapes the process of data collection but might heavily influence my interpretation of the data. I came to realize that although my inside status came with a lot of benefits (especially for access), it could also bring some drawbacks.
—Dede Setiono, PhD student focusing on international development and environmental policy, Oregon State University
The more you know about what you might find, the more strategic you can be. If you wanted to compare how politically conservative and politically liberal college students explained their vaccine hesitancy, for example, you might construct a sample purposively, finding an equal number of both types of students so that you can make those comparisons in your analysis. This is what Damaske ( 2021 ) did. You could still use convenience or snowball sampling as a way of recruitment. Post a flyer at the conservative student club and then ask for referrals from the one student that agrees to be interviewed. As with convenience sampling, there are variations of purposive sampling as well as other names used (e.g., judgment, quota, stratified, criterion, theoretical). Try not to get bogged down in the nomenclature; instead, focus on identifying the general population that matches your research question and then using a sampling method that is most likely to provide insight, given the types of questions you have.
There are all kinds of ways of being strategic with sampling in qualitative research. Here are a few of my favorite techniques for maximizing insight:
- Consider using “extreme” or “deviant” cases. Maybe your college houses a prominent anti-vaxxer who has written about and demonstrated against the college’s policy on vaccines. You could learn a lot from that single case (depending on your research question, of course).
- Consider “intensity”: people and cases and circumstances where your questions are more likely to feature prominently (but not extremely or deviantly). For example, you could compare those who volunteer at local Republican and Democratic election headquarters during an election season in a study on why party matters. Those who volunteer are more likely to have something to say than those who are more apathetic.
- Maximize variation, as with the case of “politically liberal” versus “politically conservative,” or include an array of social locations (young vs. old; Northwest vs. Southeast region). This kind of heterogeneity sampling can capture and describe the central themes that cut across the variations: any common patterns that emerge, even in this wildly mismatched sample, are probably important to note!
- Rather than maximize the variation, you could select a small homogenous sample to describe some particular subgroup in depth. Focus groups are often the best form of data collection for homogeneity sampling.
- Think about which cases are “critical” or politically important—ones that “if it happens here, it would happen anywhere” or a case that is politically sensitive, as with the single “blue” (Democratic) county in a “red” (Republican) state. In both, you are choosing a site that would yield the most information and have the greatest impact on the development of knowledge.
- On the other hand, sometimes you want to select the “typical”—the typical college student, for example. You are trying to not generalize from the typical but illustrate aspects that may be typical of this case or group. When selecting for typicality, be clear with yourself about why the typical matches your research questions (and who might be excluded or marginalized in doing so).
- Finally, it is often a good idea to look for disconfirming cases : if you are at the stage where you have a hypothesis (of sorts), you might select those who do not fit your hypothesis—you will surely learn something important there. They may be “exceptions that prove the rule” or exceptions that force you to alter your findings in order to make sense of these additional cases.
In addition to all these sampling variations, there is the theoretical approach taken by grounded theorists in which the researcher samples comparative people (or events) on the basis of their potential to represent important theoretical constructs. The sample, one can say, is by definition representative of the phenomenon of interest. It accompanies the constant comparative method of analysis. In the words of the funders of Grounded Theory , “Theoretical sampling is sampling on the basis of the emerging concepts, with the aim being to explore the dimensional range or varied conditions along which the properties of the concepts vary” ( Strauss and Corbin 1998:73 ).
When Your Population is Not Composed of People
I think it is easiest for most people to think of populations and samples in terms of people, but sometimes our units of analysis are not actually people. They could be places or institutions. Even so, you might still want to talk to people or observe the actions of people to understand those places or institutions. Or not! In the case of content analyses (see chapter 17), you won’t even have people involved at all but rather documents or films or photographs or news clippings. Everything we have covered about sampling applies to other units of analysis too. Let’s work through some examples.
Case Studies
When constructing a case study, it is helpful to think of your cases as sample populations in the same way that we considered people above. If, for example, you are comparing campus climates for diversity, your overall population may be “four-year college campuses in the US,” and from there you might decide to study three college campuses as your sample. Which three? Will you use purposeful sampling (perhaps [1] selecting three colleges in Oregon that are different sizes or [2] selecting three colleges across the US located in different political cultures or [3] varying the three colleges by racial makeup of the student body)? Or will you select three colleges at random, out of convenience? There are justifiable reasons for all approaches.
As with people, there are different ways of maximizing insight in your sample selection. Think about the following rationales: typical, diverse, extreme, deviant, influential, crucial, or even embodying a particular “pathway” ( Gerring 2008 ). When choosing a case or particular research site, Rubin ( 2021 ) suggests you bear in mind, first, what you are leaving out by selecting this particular case/site; second, what you might be overemphasizing by studying this case/site and not another; and, finally, whether you truly need to worry about either of those things—“that is, what are the sources of bias and how bad are they for what you are trying to do?” ( 89 ).
Once you have selected your cases, you may still want to include interviews with specific people or observations at particular sites within those cases. Then you go through possible sampling approaches all over again to determine which people will be contacted.
Content: Documents, Narrative Accounts, And So On
Although not often discussed as sampling, your selection of documents and other units to use in various content/historical analyses is subject to similar considerations. When you are asking quantitative-type questions (percentages and proportionalities of a general population), you will want to follow probabilistic sampling. For example, I created a random sample of accounts posted on the website studentloanjustice.org to delineate the types of problems people were having with student debt ( Hurst 2007 ). Even though my data was qualitative (narratives of student debt), I was actually asking a quantitative-type research question, so it was important that my sample was representative of the larger population (debtors who posted on the website). On the other hand, when you are asking qualitative-type questions, the selection process should be very different. In that case, use nonprobabilistic techniques, either convenience (where you are really new to this data and do not have the ability to set comparative criteria or even know what a deviant case would be) or some variant of purposive sampling. Let’s say you were interested in the visual representation of women in media published in the 1950s. You could select a national magazine like Time for a “typical” representation (and for its convenience, as all issues are freely available on the web and easy to search). Or you could compare one magazine known for its feminist content versus one antifeminist. The point is, sample selection is important even when you are not interviewing or observing people.
Goals of Qualitative Sampling versus Goals of Quantitative Sampling
We have already discussed some of the differences in the goals of quantitative and qualitative sampling above, but it is worth further discussion. The quantitative researcher seeks a sample that is representative of the population of interest so that they may properly generalize the results (e.g., if 80 percent of first-gen students in the sample were concerned with costs of college, then we can say there is a strong likelihood that 80 percent of first-gen students nationally are concerned with costs of college). The qualitative researcher does not seek to generalize in this way . They may want a representative sample because they are interested in typical responses or behaviors of the population of interest, but they may very well not want a representative sample at all. They might want an “extreme” or deviant case to highlight what could go wrong with a particular situation, or maybe they want to examine just one case as a way of understanding what elements might be of interest in further research. When thinking of your sample, you will have to know why you are selecting the units, and this relates back to your research question or sets of questions. It has nothing to do with having a representative sample to generalize results. You may be tempted—or it may be suggested to you by a quantitatively minded member of your committee—to create as large and representative a sample as you possibly can to earn credibility from quantitative researchers. Ignore this temptation or suggestion. The only thing you should be considering is what sample will best bring insight into the questions guiding your research. This has implications for the number of people (or units) in your study as well, which is the topic of the next section.
What is the Correct “Number” to Sample?
Because we are not trying to create a generalizable representative sample, the guidelines for the “number” of people to interview or news stories to code are also a bit more nebulous. There are some brilliant insightful studies out there with an n of 1 (meaning one person or one account used as the entire set of data). This is particularly so in the case of autoethnography, a variation of ethnographic research that uses the researcher’s own subject position and experiences as the basis of data collection and analysis. But it is true for all forms of qualitative research. There are no hard-and-fast rules here. The number to include is what is relevant and insightful to your particular study.
That said, humans do not thrive well under such ambiguity, and there are a few helpful suggestions that can be made. First, many qualitative researchers talk about “saturation” as the end point for data collection. You stop adding participants when you are no longer getting any new information (or so very little that the cost of adding another interview subject or spending another day in the field exceeds any likely benefits to the research). The term saturation was first used here by Glaser and Strauss ( 1967 ), the founders of Grounded Theory. Here is their explanation: “The criterion for judging when to stop sampling the different groups pertinent to a category is the category’s theoretical saturation . Saturation means that no additional data are being found whereby the sociologist can develop properties of the category. As he [or she] sees similar instances over and over again, the researcher becomes empirically confident that a category is saturated. [They go] out of [their] way to look for groups that stretch diversity of data as far as possible, just to make certain that saturation is based on the widest possible range of data on the category” ( 61 ).
It makes sense that the term was developed by grounded theorists, since this approach is rather more open-ended than other approaches used by qualitative researchers. With so much left open, having a guideline of “stop collecting data when you don’t find anything new” is reasonable. However, saturation can’t help much when first setting out your sample. How do you know how many people to contact to interview? What number will you put down in your institutional review board (IRB) protocol (see chapter 8)? You may guess how many people or units it will take to reach saturation, but there really is no way to know in advance. The best you can do is think about your population and your questions and look at what others have done with similar populations and questions.
Here are some suggestions to use as a starting point: For phenomenological studies, try to interview at least ten people for each major category or group of people . If you are comparing male-identified, female-identified, and gender-neutral college students in a study on gender regimes in social clubs, that means you might want to design a sample of thirty students, ten from each group. This is the minimum suggested number. Damaske’s ( 2021 ) sample of one hundred allows room for up to twenty-five participants in each of four “buckets” (e.g., working-class*female, working-class*male, middle-class*female, middle-class*male). If there is more than one comparative group (e.g., you are comparing students attending three different colleges, and you are comparing White and Black students in each), you can sometimes reduce the number for each group in your sample to five for, in this case, thirty total students. But that is really a bare minimum you will want to go. A lot of people will not trust you with only “five” cases in a bucket. Lareau ( 2021:24 ) advises a minimum of seven or nine for each bucket (or “cell,” in her words). The point is to think about what your analyses might look like and how comfortable you will be with a certain number of persons fitting each category.
Because qualitative research takes so much time and effort, it is rare for a beginning researcher to include more than thirty to fifty people or units in the study. You may not be able to conduct all the comparisons you might want simply because you cannot manage a larger sample. In that case, the limits of who you can reach or what you can include may influence you to rethink an original overcomplicated research design. Rather than include students from every racial group on a campus, for example, you might want to sample strategically, thinking about the most contrast (insightful), possibly excluding majority-race (White) students entirely, and simply using previous literature to fill in gaps in our understanding. For example, one of my former students was interested in discovering how race and class worked at a predominantly White institution (PWI). Due to time constraints, she simplified her study from an original sample frame of middle-class and working-class domestic Black and international African students (four buckets) to a sample frame of domestic Black and international African students (two buckets), allowing the complexities of class to come through individual accounts rather than from part of the sample frame. She wisely decided not to include White students in the sample, as her focus was on how minoritized students navigated the PWI. She was able to successfully complete her project and develop insights from the data with fewer than twenty interviewees. [1]
But what if you had unlimited time and resources? Would it always be better to interview more people or include more accounts, documents, and units of analysis? No! Your sample size should reflect your research question and the goals you have set yourself. Larger numbers can sometimes work against your goals. If, for example, you want to help bring out individual stories of success against the odds, adding more people to the analysis can end up drowning out those individual stories. Sometimes, the perfect size really is one (or three, or five). It really depends on what you are trying to discover and achieve in your study. Furthermore, studies of one hundred or more (people, documents, accounts, etc.) can sometimes be mistaken for quantitative research. Inevitably, the large sample size will push the researcher into simplifying the data numerically. And readers will begin to expect generalizability from such a large sample.
To summarize, “There are no rules for sample size in qualitative inquiry. Sample size depends on what you want to know, the purpose of the inquiry, what’s at stake, what will be useful, what will have credibility, and what can be done with available time and resources” ( Patton 2002:244 ).
How did you find/construct a sample?
Since qualitative researchers work with comparatively small sample sizes, getting your sample right is rather important. Yet it is also difficult to accomplish. For instance, a key question you need to ask yourself is whether you want a homogeneous or heterogeneous sample. In other words, do you want to include people in your study who are by and large the same, or do you want to have diversity in your sample?
For many years, I have studied the experiences of students who were the first in their families to attend university. There is a rather large number of sampling decisions I need to consider before starting the study. (1) Should I only talk to first-in-family students, or should I have a comparison group of students who are not first-in-family? (2) Do I need to strive for a gender distribution that matches undergraduate enrollment patterns? (3) Should I include participants that reflect diversity in gender identity and sexuality? (4) How about racial diversity? First-in-family status is strongly related to some ethnic or racial identity. (5) And how about areas of study?
As you can see, if I wanted to accommodate all these differences and get enough study participants in each category, I would quickly end up with a sample size of hundreds, which is not feasible in most qualitative research. In the end, for me, the most important decision was to maximize the voices of first-in-family students, which meant that I only included them in my sample. As for the other categories, I figured it was going to be hard enough to find first-in-family students, so I started recruiting with an open mind and an understanding that I may have to accept a lack of gender, sexuality, or racial diversity and then not be able to say anything about these issues. But I would definitely be able to speak about the experiences of being first-in-family.
—Wolfgang Lehmann, author of “Habitus Transformation and Hidden Injuries”
Examples of “Sample” Sections in Journal Articles
Think about some of the studies you have read in college, especially those with rich stories and accounts about people’s lives. Do you know how the people were selected to be the focus of those stories? If the account was published by an academic press (e.g., University of California Press or Princeton University Press) or in an academic journal, chances are that the author included a description of their sample selection. You can usually find these in a methodological appendix (book) or a section on “research methods” (article).
Here are two examples from recent books and one example from a recent article:
Example 1 . In It’s Not like I’m Poor: How Working Families Make Ends Meet in a Post-welfare World , the research team employed a mixed methods approach to understand how parents use the earned income tax credit, a refundable tax credit designed to provide relief for low- to moderate-income working people ( Halpern-Meekin et al. 2015 ). At the end of their book, their first appendix is “Introduction to Boston and the Research Project.” After describing the context of the study, they include the following description of their sample selection:
In June 2007, we drew 120 names at random from the roughly 332 surveys we gathered between February and April. Within each racial and ethnic group, we aimed for one-third married couples with children and two-thirds unmarried parents. We sent each of these families a letter informing them of the opportunity to participate in the in-depth portion of our study and then began calling the home and cell phone numbers they provided us on the surveys and knocking on the doors of the addresses they provided.…In the end, we interviewed 115 of the 120 families originally selected for the in-depth interview sample (the remaining five families declined to participate). ( 22 )
Was their sample selection based on convenience or purpose? Why do you think it was important for them to tell you that five families declined to be interviewed? There is actually a trick here, as the names were pulled randomly from a survey whose sample design was probabilistic. Why is this important to know? What can we say about the representativeness or the uniqueness of whatever findings are reported here?
Example 2 . In When Diversity Drops , Park ( 2013 ) examines the impact of decreasing campus diversity on the lives of college students. She does this through a case study of one student club, the InterVarsity Christian Fellowship (IVCF), at one university (“California University,” a pseudonym). Here is her description:
I supplemented participant observation with individual in-depth interviews with sixty IVCF associates, including thirty-four current students, eight former and current staff members, eleven alumni, and seven regional or national staff members. The racial/ethnic breakdown was twenty-five Asian Americans (41.6 percent), one Armenian (1.6 percent), twelve people who were black (20.0 percent), eight Latino/as (13.3 percent), three South Asian Americans (5.0 percent), and eleven people who were white (18.3 percent). Twenty-nine were men, and thirty-one were women. Looking back, I note that the higher number of Asian Americans reflected both the group’s racial/ethnic composition and my relative ease about approaching them for interviews. ( 156 )
How can you tell this is a convenience sample? What else do you note about the sample selection from this description?
Example 3. The last example is taken from an article published in the journal Research in Higher Education . Published articles tend to be more formal than books, at least when it comes to the presentation of qualitative research. In this article, Lawson ( 2021 ) is seeking to understand why female-identified college students drop out of majors that are dominated by male-identified students (e.g., engineering, computer science, music theory). Here is the entire relevant section of the article:
Method Participants Data were collected as part of a larger study designed to better understand the daily experiences of women in MDMs [male-dominated majors].…Participants included 120 students from a midsize, Midwestern University. This sample included 40 women and 40 men from MDMs—defined as any major where at least 2/3 of students are men at both the university and nationally—and 40 women from GNMs—defined as any may where 40–60% of students are women at both the university and nationally.… Procedure A multi-faceted approach was used to recruit participants; participants were sent targeted emails (obtained based on participants’ reported gender and major listings), campus-wide emails sent through the University’s Communication Center, flyers, and in-class presentations. Recruitment materials stated that the research focused on the daily experiences of college students, including classroom experiences, stressors, positive experiences, departmental contexts, and career aspirations. Interested participants were directed to email the study coordinator to verify eligibility (at least 18 years old, man/woman in MDM or woman in GNM, access to a smartphone). Sixteen interested individuals were not eligible for the study due to the gender/major combination. ( 482ff .)
What method of sample selection was used by Lawson? Why is it important to define “MDM” at the outset? How does this definition relate to sampling? Why were interested participants directed to the study coordinator to verify eligibility?
Final Words
I have found that students often find it difficult to be specific enough when defining and choosing their sample. It might help to think about your sample design and sample recruitment like a cookbook. You want all the details there so that someone else can pick up your study and conduct it as you intended. That person could be yourself, but this analogy might work better if you have someone else in mind. When I am writing down recipes, I often think of my sister and try to convey the details she would need to duplicate the dish. We share a grandmother whose recipes are full of handwritten notes in the margins, in spidery ink, that tell us what bowl to use when or where things could go wrong. Describe your sample clearly, convey the steps required accurately, and then add any other details that will help keep you on track and remind you why you have chosen to limit possible interviewees to those of a certain age or class or location. Imagine actually going out and getting your sample (making your dish). Do you have all the necessary details to get started?
Table 5.1. Sampling Type and Strategies
Further Readings
Fusch, Patricia I., and Lawrence R. Ness. 2015. “Are We There Yet? Data Saturation in Qualitative Research.” Qualitative Report 20(9):1408–1416.
Saunders, Benjamin, Julius Sim, Tom Kinstone, Shula Baker, Jackie Waterfield, Bernadette Bartlam, Heather Burroughs, and Clare Jinks. 2018. “Saturation in Qualitative Research: Exploring Its Conceptualization and Operationalization.” Quality & Quantity 52(4):1893–1907.
- Rubin ( 2021 ) suggests a minimum of twenty interviews (but safer with thirty) for an interview-based study and a minimum of three to six months in the field for ethnographic studies. For a content-based study, she suggests between five hundred and one thousand documents, although some will be “very small” ( 243–244 ). ↵
The process of selecting people or other units of analysis to represent a larger population. In quantitative research, this representation is taken quite literally, as statistically representative. In qualitative research, in contrast, sample selection is often made based on potential to generate insight about a particular topic or phenomenon.
The actual list of individuals that the sample will be drawn from. Ideally, it should include the entire target population (and nobody who is not part of that population). Sampling frames can differ from the larger population when specific exclusions are inherent, as in the case of pulling names randomly from voter registration rolls where not everyone is a registered voter. This difference in frame and population can undercut the generalizability of quantitative results.
The specific group of individuals that you will collect data from. Contrast population.
The large group of interest to the researcher. Although it will likely be impossible to design a study that incorporates or reaches all members of the population of interest, this should be clearly defined at the outset of a study so that a reasonable sample of the population can be taken. For example, if one is studying working-class college students, the sample may include twenty such students attending a particular college, while the population is “working-class college students.” In quantitative research, clearly defining the general population of interest is a necessary step in generalizing results from a sample. In qualitative research, defining the population is conceptually important for clarity.
A sampling strategy in which the sample is chosen to represent (numerically) the larger population from which it is drawn by random selection. Each person in the population has an equal chance of making it into the sample. This is often done through a lottery or other chance mechanisms (e.g., a random selection of every twelfth name on an alphabetical list of voters). Also known as random sampling .
The selection of research participants or other data sources based on availability or accessibility, in contrast to purposive sampling .
A sample generated non-randomly by asking participants to help recruit more participants the idea being that a person who fits your sampling criteria probably knows other people with similar criteria.
Broad codes that are assigned to the main issues emerging in the data; identifying themes is often part of initial coding .
A form of case selection focusing on examples that do not fit the emerging patterns. This allows the researcher to evaluate rival explanations or to define the limitations of their research findings. While disconfirming cases are found (not sought out), researchers should expand their analysis or rethink their theories to include/explain them.
A methodological tradition of inquiry and approach to analyzing qualitative data in which theories emerge from a rigorous and systematic process of induction. This approach was pioneered by the sociologists Glaser and Strauss (1967). The elements of theory generated from comparative analysis of data are, first, conceptual categories and their properties and, second, hypotheses or generalized relations among the categories and their properties – “The constant comparing of many groups draws the [researcher’s] attention to their many similarities and differences. Considering these leads [the researcher] to generate abstract categories and their properties, which, since they emerge from the data, will clearly be important to a theory explaining the kind of behavior under observation.” (36).
The result of probability sampling, in which a sample is chosen to represent (numerically) the larger population from which it is drawn by random selection. Each person in the population has an equal chance of making it into the random sample. This is often done through a lottery or other chance mechanisms (e.g., the random selection of every twelfth name on an alphabetical list of voters). This is typically not required in qualitative research but rather essential for the generalizability of quantitative research.
A form of case selection or purposeful sampling in which cases that are unusual or special in some way are chosen to highlight processes or to illuminate gaps in our knowledge of a phenomenon. See also extreme case .
The point at which you can conclude data collection because every person you are interviewing, the interaction you are observing, or content you are analyzing merely confirms what you have already noted. Achieving saturation is often used as the justification for the final sample size.
The accuracy with which results or findings can be transferred to situations or people other than those originally studied. Qualitative studies generally are unable to use (and are uninterested in) statistical generalizability where the sample population is said to be able to predict or stand in for a larger population of interest. Instead, qualitative researchers often discuss “theoretical generalizability,” in which the findings of a particular study can shed light on processes and mechanisms that may be at play in other settings. See also statistical generalization and theoretical generalization .
A term used by IRBs to denote all materials aimed at recruiting participants into a research study (including printed advertisements, scripts, audio or video tapes, or websites). Copies of this material are required in research protocols submitted to IRB.
Introduction to Qualitative Research Methods Copyright © 2023 by Allison Hurst is licensed under a Creative Commons Attribution-ShareAlike 4.0 International License , except where otherwise noted.
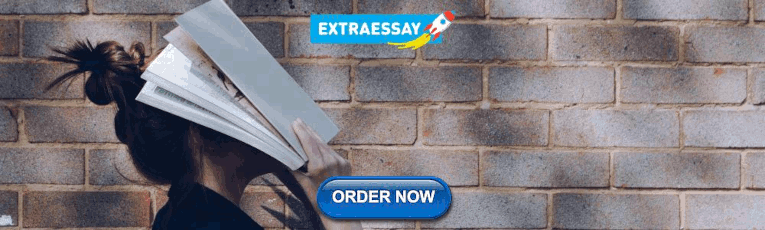
IMAGES
VIDEO
COMMENTS
The conclusions are as stated below: i. Students' use of language in the oral sessions depicted their beliefs and values. based on their intentions. The oral sessions prompted the students to be ...
98 CHAPTER 5 SUMMARY, CONCLUSIONS, AND RECOMMENDATIONS This chapter presents a summary of the study and conclusions derived from the analysis of data. The chapter concludes with recommendations. Summary The purpose of this study was to design and test an instrument to measure elementary teachers' satisfaction with their involvement in school ...
After using the quantitative data to explicate the research questions, the investigator used a follow-up semi-structured interview protocol with selected participants in order to (a) confirm the most important findings that issued from the quantitative statistics; (b) elucidate some of the
Quantitative Research Dissertation Chapters 4 and 5 (Suggested Content) Information below is suggested content; seek guidance from committee chair about content of all chapters in the dissertation. Brief Review - Chapter 3: Method (not Methodology) There is a tendency to report results of sample and measurement information in Chapter 4.
A well-written Chapter 5 should include information about the following: Summary of findings. Interpretation of findings. Context of findings. Implications of findings. Discussion on limitations of study. Discussion on future directions of research/field.
answer the research questions could be obtained from this sample. The number of respondents who took part in this research was 118. Interviews were conducted with 118 respondents using a prepared structured pre-tested interview schedule. The interview schedule was coded for easy analysis and analised using the SPSS computer program.
Recommendations for Future Research for each Variable When following the traditional format for chapter 5 where conclusions, discussion, implications and recommendations were separate sections, the narrative read choppy and disconnected for six variables. Therefore, the next sections contain the conclusions and
The Purpose of Chapter 5Topic 2: Chapter 5. The Purpose of Chapter 5. Topic 2: Chapter 5. Learning Goals: Understand the components of Chapter 5. Write the introduction to include the problem, purpose, research questions and brief description of the methodology. Review and verify findings for the study. Write the Summary of Findings.
Dissertation Chapter 5 Sample. be research. CHAPTER V: DISCUSSION be. The purpose of this qualitative grounded theory study was to identify what motivates. Outline the organization. women to stay in or return to STEM professions, leading to a model of motivation. This.
DOI10.1057/978-1-137-59146-3_5 Chapter 5: Quantitative Research Methods INTRODUCTION All research starts with a phenomenon or issuethat a researcher wants to explainor understand. That phenomenon is addressed in the form of well-crafted research questions. Those questions guide the data we collect and
Introduction The following chapter concludes this report. A summary of the research is presented, and findings of the study are discussed and interpreted. The significance of this research in the immediate context of El Gallo and in the field of low-income housing is examined. Recommendations for further research end the chapter. The scope of the following conclusions is limited to the context ...
As a general guide, your results chapter will typically include the following: Some demographic data about your sample; Reliability tests (if you used measurement scales); Descriptive statistics; Inferential statistics (if your research objectives and questions require these); Hypothesis tests (again, if your research objectives and questions require these); We'll discuss each of these ...
View PDF. Chapter 5 SUMMARY, CONCLUSIONS AND RECOMMENDATIONS Chapter 5 contains the research summary, conclusions and recommendations of the whole study. The findings of the study without so much detailed information is written on the summary. Generalizations and other interferences would be seen on the conclusion while the recommendations of ...
5.1 INTRODUCTION. In this chapter the conclusions derived from the findings of this study on the experiences of registered nurses involved in the termination of pregnancy at Soshanguve Community Health Centre are described. The conclusions were based on the purpose, research questions and results of the study. The implications of these findings ...
Section 5.4 that describes three major contributions of my study. The constraints and limitations of my study are summarised in Section 5.5, whereas Section 5.6 outlines recommendations for future research related to my study. 5.2. OVERVIEW OF THE THESIS The first chapter of this thesis provides a general background to the evolution of distance ...
Conclusions Based on the findings of the study, the following conclusions are drawn: 1. The researchers conclude that the Filipino tour guide should have enough skills and knowledge to be an effective leader. 3. 70 EULOGIO "AMANG" RODRIGUEZ INSTITUTE OF SCIENCE AND TECHNOLOGY COLLEGE OF HOSPITALITY MANAGEMENT 2.
determined in response to research problem 1. When considered by all respondents in the context of the ten potential organizers offered for consideration, construction was ranked fifth in the civic-life sphere, sixth in the personal-life sphere, and fifth in the work life sphere. Although the sample populatio ns were different, an interesting
McCall, andChapman (1916), in both 5 X 5 and 2 X 2 forms, Le., some 10 years before Fisher (1926) incorporated it systematically intohis scheme of experimental design, with randomization.2 McCall's mode of using the Cfrotation ex periment" serves well to denote the emphasis of his book and the present chapter. The ro tation experiment is ...
Below is the uncorrected machine-read text of this chapter, intended to provide our own search engines and external engines with highly rich, chapter-representative searchable text of each book. Because it is UNCORRECTED material, please consider the following text as a useful but insufficient proxy for the authoritative book pages.
Sampling in qualitative research has different purposes and goals than sampling in quantitative research. Sampling in both allows you to say something of interest about a population without having to include the entire population in your sample. We begin this chapter with the case of a population of interest composed of actual people.
Based on the results in Chapter 4, the following findings were made. Most of the respondents belonged to the income bracket of 3000 and below having a number of 77 or 74%. Majority of themare high school graduate having a number of 40 or 48% of the respondents belonged to the hours spent of 21 to 24 hours having a number of 60 or 66%.