- Digital Marketing
- Facebook Marketing
- Instagram Marketing
- Ecommerce Marketing
- Content Marketing
- Data Science Certification
- Machine Learning
- Artificial Intelligence
- Data Analytics
- Graphic Design
- Adobe Illustrator
- Web Designing
- UX UI Design
- Interior Design
- Front End Development
- Back End Development Courses
- Business Analytics
- Entrepreneurship
- Supply Chain
- Financial Modeling
- Corporate Finance
- Project Finance
- Harvard University
- Stanford University
- Yale University
- Princeton University
- Duke University
- UC Berkeley
- Harvard University Executive Programs
- MIT Executive Programs
- Stanford University Executive Programs
- Oxford University Executive Programs
- Cambridge University Executive Programs
- Yale University Executive Programs
- Kellog Executive Programs
- CMU Executive Programs
- 45000+ Free Courses
- Free Certification Courses
- Free DigitalDefynd Certificate
- Free Harvard University Courses
- Free MIT Courses
- Free Excel Courses
- Free Google Courses
- Free Finance Courses
- Free Coding Courses
- Free Digital Marketing Courses
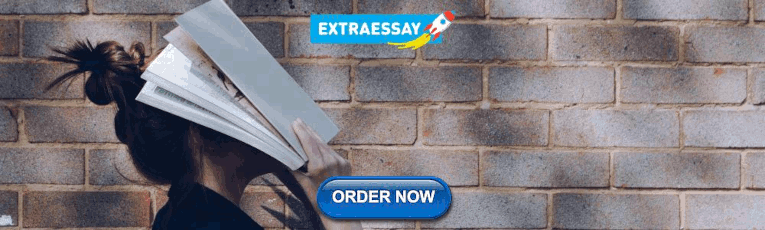
40 Detailed Artificial Intelligence Case Studies [2024]
In this dynamic era of technological advancements, Artificial Intelligence (AI) emerges as a pivotal force, reshaping the way industries operate and charting new courses for business innovation. This article presents an in-depth exploration of 40 diverse and compelling AI case studies from across the globe. Each case study offers a deep dive into the challenges faced by companies, the AI-driven solutions implemented, their substantial impacts, and the valuable lessons learned. From healthcare and finance to transportation and retail, these stories highlight AI’s transformative power in solving complex problems, optimizing processes, and driving growth, offering insightful glimpses into the potential and versatility of AI in shaping our world.
Related: How to Become an AI Thought Leader?
1. IBM Watson Health: Revolutionizing Patient Care with AI
Task/Conflict: The healthcare industry faces challenges in handling vast amounts of patient data, accurately diagnosing diseases, and creating effective treatment plans. IBM Watson Health aimed to address these issues by harnessing AI to process and analyze complex medical information, thus improving the accuracy and efficiency of patient care.
Solution: Utilizing the cognitive computing capabilities of IBM Watson, this solution involves analyzing large volumes of medical records, research papers, and clinical trial data. The system uses natural language processing to understand and process medical jargon, making sense of unstructured data to aid medical professionals in diagnosing and treating patients.
Overall Impact:
- Enhanced accuracy in patient diagnosis and treatment recommendations.
- Significant improvement in personalized healthcare services.
Key Learnings:
- AI can complement medical professionals’ expertise, leading to better healthcare outcomes.
- The integration of AI in healthcare can lead to significant advancements in personalized medicine.
2. Google DeepMind’s AlphaFold: Unraveling the Mysteries of Protein Folding
Task/Conflict: The scientific community has long grappled with the protein folding problem – understanding how a protein’s amino acid sequence determines its 3D structure. Solving this problem is crucial for drug discovery and understanding diseases at a molecular level, yet it remained a formidable challenge due to the complexity of biological structures.
Solution: AlphaFold, developed by Google DeepMind, is an AI model trained on vast datasets of known protein structures. It assesses the distances and angles between amino acids to predict how a protein folds, outperforming existing methods in terms of speed and accuracy. This breakthrough represents a major advancement in computational biology.
- Significant acceleration in drug discovery and disease understanding.
- Set a new benchmark for computational methods in biology.
- AI’s predictive power can solve complex biological problems.
- The application of AI in scientific research can lead to groundbreaking discoveries.
3. Amazon: Transforming Supply Chain Management through AI
Task/Conflict: Managing a global supply chain involves complex challenges like predicting product demand, optimizing inventory levels, and streamlining logistics. Amazon faced the task of efficiently managing its massive inventory while minimizing costs and meeting customer demands promptly.
Solution: Amazon employs sophisticated AI algorithms for predictive inventory management, which forecast product demand based on various factors like buying trends, seasonality, and market changes. This system allows for real-time adjustments, adapting swiftly to changing market dynamics.
- Reduced operational costs through efficient inventory management.
- Improved customer satisfaction with timely deliveries and availability.
- AI can significantly enhance supply chain efficiency and responsiveness.
- Predictive analytics in inventory management leads to reduced waste and cost savings.
4. Tesla’s Autonomous Vehicles: Driving the Future of Transportation
Task/Conflict: The development of autonomous vehicles represents a major technological and safety challenge. Tesla aimed to create self-driving cars that are not only reliable and safe but also capable of navigating complex traffic conditions without human intervention.
Solution: Tesla’s solution involves advanced AI and machine learning algorithms that process data from various sensors and cameras to understand and navigate the driving environment. Continuous learning from real-world driving data allows the system to improve over time, making autonomous driving safer and more efficient.
- Leadership in the autonomous vehicle sector, enhancing road safety.
- Continuous improvements in self-driving technology through AI-driven data analysis.
- Continuous data analysis is key to advancing autonomous driving technologies.
- AI can significantly improve road safety and driving efficiency.
Related: High-Paying AI Career Options
5. Zara: Fashioning the Future with AI in Retail
Task/Conflict: In the fast-paced fashion industry, predicting trends and managing inventory efficiently are critical for success. Zara faced the challenge of quickly adapting to changing fashion trends while avoiding overstock and meeting consumer demand.
Solution: Zara employs AI algorithms to analyze fashion trends, customer preferences, and sales data. The AI system also assists in managing inventory, ensuring that popular items are restocked promptly and that stores are not overburdened with unsold products. This approach optimizes both production and distribution.
- Increased sales and profitability through optimized inventory.
- Enhanced customer satisfaction by aligning products with current trends.
- AI can accurately predict consumer behavior and trends.
- Effective inventory management through AI can significantly impact business success.
6. Netflix: Personalizing Entertainment with AI
Task/Conflict: In the competitive streaming industry, providing a personalized user experience is key to retaining subscribers. Netflix needed to recommend relevant content to each user from its vast library, ensuring that users remained engaged and satisfied.
Solution: Netflix developed an advanced AI-driven recommendation engine that analyzes individual viewing habits, ratings, and preferences. This personalized approach keeps users engaged, as they are more likely to find content that interests them, enhancing their overall viewing experience.
- Increased viewer engagement and longer watch times.
- Higher subscription retention rates due to personalized content.
- Personalized recommendations significantly enhance user experience.
- AI-driven content curation is essential for success in digital entertainment.
7. Airbus: Elevating Aircraft Maintenance with AI
Task/Conflict: Aircraft maintenance is crucial for ensuring flight safety and operational efficiency. Airbus faced the challenge of predicting maintenance needs to prevent equipment failures and reduce downtime, which is critical in the aviation industry.
Solution: Airbus implemented AI algorithms for predictive maintenance, analyzing data from aircraft sensors to identify potential issues before they lead to failures. This system assesses the condition of various components, predicting when maintenance is needed. The solution not only enhances safety but also optimizes maintenance schedules, reducing unnecessary inspections and downtime.
- Decreased maintenance costs and reduced aircraft downtime.
- Improved safety with proactive maintenance measures.
- AI can predict and prevent potential equipment failures.
- Predictive maintenance is essential for operational efficiency and safety in aviation.
8. American Express: Securing Transactions with AI
Task/Conflict: Credit card fraud is a significant issue in the financial sector, leading to substantial losses and undermining customer trust. American Express needed an efficient way to detect and prevent fraudulent transactions in real-time.
Solution: American Express utilizes machine learning models to analyze transaction data. These models identify unusual patterns and behaviors indicative of fraud. By constant learning from refined data, the system becomes increasingly accurate in detecting fraudulent activities, providing real-time alerts and preventing unauthorized transactions.
- Minimized financial losses due to reduced fraudulent activities.
- Enhanced customer trust and security in financial transactions.
- Machine learning is highly effective in fraud detection.
- Real-time data analysis is crucial for preventing financial fraud.
Related: Is AI a Good Career Option for Women?
9. Stitch Fix: Tailoring the Future of Fashion Retail
Task/Conflict: In the competitive fashion retail industry, providing a personalized shopping experience is key to customer satisfaction and business growth. Stitch Fix aimed to offer customized clothing selections to each customer, based on their unique preferences and style.
Solution: Stitch Fix uses AI and algorithms analyze customer feedback, style preferences, and purchase history to recommend clothing items. This personalized approach is complemented by human stylists, ensuring that each customer receives a tailored selection that aligns with their individual style.
- Increased customer satisfaction through personalized styling services.
- Business growth driven by a unique, AI-enhanced shopping experience.
- AI combined with human judgment can create highly effective personalization.
- Tailoring customer experiences using AI leads to increased loyalty and business success.
10. Baidu: Breaking Language Barriers with Voice Recognition
Task/Conflict: Voice recognition technology faces the challenge of accurately understanding and processing speech in various languages and accents. Baidu aimed to enhance its voice recognition capabilities to provide more accurate and user-friendly interactions in multiple languages.
Solution: Baidu employs deep learning algorithms for voice and speech recognition, training its system on a diverse range of languages and dialects. This approach allows for more accurate recognition of speech patterns, enabling the technology to understand and respond to voice commands more effectively. The system continuously improves as it processes more voice data, making technology more accessible to users worldwide.
- Enhanced user interaction with technology in multiple languages.
- Reduced language barriers in voice-activated services and devices.
- AI can effectively bridge language gaps in technology.
- Continuous learning from diverse data sets is key to improving voice recognition.
11. JP Morgan: Revolutionizing Legal Document Analysis with AI
Task/Conflict: Analyzing legal documents, such as contracts, is a time-consuming and error-prone process. JP Morgan sought to streamline this process, reducing the time and effort required while increasing accuracy.
Solution: JP Morgan implemented an AI-powered tool, COIN (Contract Intelligence), to analyze legal documents quickly and accurately. COIN uses NLP to interpret and extract relevant information from contracts, significantly reducing the time required for document review.
- Dramatic reduction in time required for legal document analysis.
- Increased accuracy and reduced human error in contract interpretation.
- AI can efficiently handle large volumes of data, offering speed and accuracy.
- Automation in legal processes can significantly enhance operational efficiency.
12. Microsoft: AI for Accessibility
Task/Conflict: People with disabilities often face challenges in accessing technology. Microsoft aimed to create AI-driven tools to enhance accessibility, especially for individuals with visual, hearing, or cognitive impairments.
Solution: Microsoft developed a range of AI-powered tools including applications for voice recognition, visual assistance, and cognitive support, making technology more accessible and user-friendly. For instance, Seeing AI, an app developed by Microsoft, helps visually impaired users to understand their surroundings by describing people, texts, and objects.
- Improved accessibility and independence for people with disabilities.
- Creation of more inclusive technology solutions.
- AI can significantly contribute to making technology accessible for all.
- Developing inclusive technology is essential for societal progress.
Related: How to get an Internship in AI?
13. Alibaba’s City Brain: Revolutionizing Urban Traffic Management
Task/Conflict: Urban traffic congestion is a major challenge in many cities, leading to inefficiencies and environmental concerns. Alibaba’s City Brain project aimed to address this issue by using AI to optimize traffic flow and improve public transportation in urban areas.
Solution: City Brain uses AI to analyze real-time data from traffic cameras, sensors, and GPS systems. It processes this information to predict traffic patterns and optimize traffic light timing, reducing congestion. The system also provides data-driven insights for urban planning and emergency response coordination, enhancing overall city management.
- Significant reduction in traffic congestion and improved urban transportation.
- Enhanced efficiency in city management and emergency response.
- AI can effectively manage complex urban systems.
- Data-driven solutions are key to improving urban living conditions.
14. Deep 6 AI: Accelerating Clinical Trials with Artificial Intelligence
Task/Conflict: Recruiting suitable patients for clinical trials is often a slow and cumbersome process, hindering medical research. Deep 6 AI sought to accelerate this process by quickly identifying eligible participants from a vast pool of patient data.
Solution: Deep 6 AI employs AI to sift through extensive medical records, identifying potential trial participants based on specific criteria. The system analyzes structured and unstructured data, including doctor’s notes and diagnostic reports, to find matches for clinical trials. This approach significantly speeds up the recruitment process, enabling faster trial completions and advancements in medical research.
- Quicker recruitment for clinical trials, leading to faster research progress.
- Enhanced efficiency in medical research and development.
- AI can streamline the patient selection process for clinical trials.
- Efficient recruitment is crucial for the advancement of medical research.
15. NVIDIA: Revolutionizing Gaming Graphics with AI
Task/Conflict: Enhancing the realism and performance of gaming graphics is a continuous challenge in the gaming industry. NVIDIA aimed to revolutionize gaming visuals by leveraging AI to create more realistic and immersive gaming experiences.
Solution: NVIDIA’s AI-driven graphic processing technologies, such as ray tracing and deep learning super sampling (DLSS), provide highly realistic and detailed graphics. These technologies use AI to render images more efficiently, improving game performance without compromising on visual quality. This innovation sets new standards in gaming graphics, making games more lifelike and engaging.
- Elevated gaming experiences with state-of-the-art graphics.
- Set new industry standards for graphic realism and performance.
- AI can significantly enhance creative industries, like gaming.
- Balancing performance and visual quality is key to gaming innovation.
16. Palantir: Mastering Data Integration and Analysis with AI
Task/Conflict: Integrating and analyzing large-scale, diverse datasets is a complex task, essential for informed decision-making in various sectors. Palantir Technologies faced the challenge of making sense of vast amounts of data to provide actionable insights for businesses and governments.
Solution: Palantir developed AI-powered platforms that integrate data from multiple sources, providing a comprehensive view of complex systems. These platforms use machine learning to analyze data, uncover patterns, and predict outcomes, assisting in strategic decision-making. This solution enables users to make informed decisions in real-time, based on a holistic understanding of their data.
- Enhanced decision-making capabilities in complex environments.
- Greater insights and efficiency in data analysis across sectors.
- Effective data integration is crucial for comprehensive analysis.
- AI-driven insights are essential for strategic decision-making.
Related: Surprising AI Facts & Statistics
17. Blue River Technology: Sowing the Seeds of AI in Agriculture
Task/Conflict: The agriculture industry faces challenges in increasing efficiency and sustainability while minimizing environmental impact. Blue River Technology aimed to enhance agricultural practices by using AI to make farming more precise and efficient.
Solution: Blue River Technology developed AI-driven agricultural robots that perform tasks like precise planting and weed control. These robots use ML to identify plants and make real-time decisions, such as applying herbicides only to weeds. This targeted approach reduces chemical usage and promotes sustainable farming practices, leading to better crop yields and environmental conservation.
- Significant reduction in chemical usage in farming.
- Increased crop yields through precision agriculture.
- AI can contribute significantly to sustainable agricultural practices.
- Precision farming is key to balancing productivity and environmental conservation.
18. Salesforce: Enhancing Customer Relationship Management with AI
Task/Conflict: In the realm of customer relationship management (CRM), personalizing interactions and gaining insights into customer behavior are crucial for business success. Salesforce aimed to enhance CRM capabilities by integrating AI to provide personalized customer experiences and actionable insights.
Solution: Salesforce incorporates AI-powered tools into its CRM platform, enabling businesses to personalize customer interactions, automate responses, and predict customer needs. These tools analyze customer data, providing insights that help businesses tailor their strategies and communications. The AI integration not only improves customer engagement but also streamlines sales and marketing efforts.
- Improved customer engagement and satisfaction.
- Increased business growth through tailored marketing and sales strategies.
- AI-driven personalization is key to successful customer relationship management.
- Leveraging AI for data insights can significantly impact business growth.
19. OpenAI: Transforming Natural Language Processing
Task/Conflict: OpenAI aimed to advance NLP by developing models capable of generating coherent and contextually relevant text, opening new possibilities in AI-human interaction.
Solution: OpenAI developed the Generative Pre-trained Transformer (GPT) models, which use deep learning to generate text that closely mimics human language. These models are trained on vast datasets, enabling them to understand context and generate responses in a conversational and coherent manner.
- Pioneered advancements in natural language understanding and generation.
- Expanded the possibilities for AI applications in communication.
- AI’s ability to mimic human language has vast potential applications.
- Advancements in NLP are crucial for improving AI-human interactions.
20. Siemens: Pioneering Industrial Automation with AI
Task/Conflict: Industrial automation seeks to improve productivity and efficiency in manufacturing processes. Siemens faced the challenge of optimizing these processes using AI to reduce downtime and enhance output quality.
Solution: Siemens employs AI-driven solutions for predictive maintenance and process optimization to reduce downtime in industrial settings. Additionally, AI optimizes manufacturing processes, ensuring quality and efficiency.
- Increased productivity and reduced downtime in industrial operations.
- Enhanced quality and efficiency in manufacturing processes.
- AI is a key driver in the advancement of industrial automation.
- Predictive analytics are crucial for maintaining efficiency in manufacturing.
Related: Top Books for Learning AI
21. Ford: Driving Safety Innovation with AI
Task/Conflict: Enhancing automotive safety and providing effective driver assistance systems are critical challenges in the auto industry. Ford aimed to leverage AI to improve vehicle safety features and assist drivers in real-time decision-making.
Solution: Ford integrated AI into its advanced driver assistance systems (ADAS) to provide features like adaptive cruise control, lane-keeping assistance, and collision avoidance. These systems use sensors and cameras to gather data, which AI processes to make split-second decisions that enhance driver safety and vehicle performance.
- Improved safety features in vehicles, minimizing accidents and improving driver confidence.
- Enhanced driving experience with intelligent assistance features.
- AI can highly enhance safety in the automotive industry.
- Real-time data processing and decision-making are essential for effective driver assistance systems.
22. HSBC: Enhancing Banking Security with AI
Task/Conflict: As financial transactions increasingly move online, banks face heightened risks of fraud and cybersecurity threats. HSBC needed to bolster its protective measures to secure user data and prevent scam.
Solution: HSBC employed AI-driven security systems to observe transactions and identify suspicious activities. The AI models analyze patterns in customer behavior and flag anomalies that could indicate fraudulent actions, allowing for immediate intervention. This helps in minimizing the risk of financial losses and protects customer trust.
- Strengthened security measures and reduced incidence of fraud.
- Maintained high levels of customer trust and satisfaction.
- AI is critical in enhancing security in the banking sector.
- Proactive fraud detection can prevent significant financial losses.
23. Unilever: Optimizing Supply Chain with AI
Task/Conflict: Managing a global supply chain involves complexities related to logistics, demand forecasting, and sustainability practices. Unilever sought to enhance its supply chain efficiency while promoting sustainability.
Solution: Unilever implemented AI to optimize its supply chain operations, from raw material sourcing to distribution. AI algorithms analyze data to forecast demand, improve inventory levels, and minimize waste. Additionally, AI helps in selecting sustainable practices and suppliers, aligning with Unilever’s commitment to environmental responsibility.
- Enhanced efficiency and reduced costs in supply chain operations.
- Better sustainability practices, reducing environmental impact.
- AI can highly optimize supply chain management.
- Integrating AI with sustainability initiatives can lead to environmentally responsible operations.
24. Spotify: Personalizing Music Experience with AI
Task/Conflict: In the competitive music streaming industry, providing a personalized listening experience is crucial for user engagement and retention. Spotify needed to tailor music recommendations to individual tastes and preferences.
Solution: Spotify utilizes AI-driven algorithms to analyze user listening habits, preferences, and contextual data to recommend music tracks and playlists. This personalization ensures that users are continually engaged and discover new music that aligns with their tastes, enhancing their overall listening experience.
- Increased customer engagement and time spent on the platform.
- Higher user satisfaction and subscription retention rates.
- Personalized content delivery is key to user retention in digital entertainment.
- AI-driven recommendations significantly enhance user experience.
Related: How can AI be used in Instagram Marketing?
25. Walmart: Revolutionizing Retail with AI
Task/Conflict: Retail giants like Walmart face challenges in inventory management and providing a high-quality customer service experience. Walmart aimed to use AI to optimize these areas and enhance overall operational efficacy.
Solution: Walmart deployed AI technologies across its stores to manage inventory levels effectively and enhance customer service. AI systems predict product demand to optimize stock levels, while AI-driven robots assist in inventory management and customer service, such as guiding customers in stores and handling queries.
- Improved inventory management, reducing overstock and shortages.
- Enhanced customer service experience in stores.
- AI can streamline retail operations significantly.
- Enhanced customer service through AI leads to better customer satisfaction.
26. Roche: Innovating Drug Discovery with AI
Task/Conflict: The pharmaceutical industry faces significant challenges in drug discovery, requiring vast investments of time and resources. Roche aimed to utilize AI to streamline the drug development process and enhance the discovery of new therapeutics.
Solution: Roche implemented AI to analyze medical data and simulate drug interactions, speeding up the drug discovery process. AI models predict the effectiveness of compounds and identify potential candidates for further testing, significantly minimizing the time and cost related with traditional drug development procedures.
- Accelerated drug discovery processes, bringing new treatments to market faster.
- Reduced costs and increased efficiency in pharmaceutical research.
- AI can greatly accelerate the drug discovery process.
- Cost-effective and efficient drug development is possible with AI integration.
27. IKEA: Enhancing Customer Experience with AI
Task/Conflict: In the competitive home furnishings market, enhancing the customer shopping experience is crucial for success. IKEA aimed to use AI to provide innovative design tools and improve customer interaction.
Solution: IKEA introduced AI-powered tools such as virtual reality apps that allow consumers to visualize furniture before buying. These tools help customers make more informed decisions and enhance their shopping experience. Additionally, AI chatbots assist with customer service inquiries, providing timely and effective support.
- Improved customer decision-making and satisfaction with interactive tools.
- Enhanced efficiency in customer service.
- AI can transform the retail experience by providing innovative customer interaction tools.
- Effective customer support through AI can enhance brand loyalty and satisfaction.
28. General Electric: Optimizing Energy Production with AI
Task/Conflict: Managing energy production efficiently while predicting and mitigating potential issues is crucial for energy companies. General Electric (GE) aimed to improve the efficiency and reliability of its energy production facilities using AI.
Solution: GE integrated AI into its energy management systems to enhance power generation and distribution. AI algorithms predict maintenance needs and optimize energy production, ensuring efficient operation and reducing downtime. This predictive maintenance approach saves costs and enhances the reliability of energy production.
- Increased efficiency in energy production and distribution.
- Reduced operational costs and enhanced system reliability.
- Predictive maintenance is crucial for cost-effective and efficient energy management.
- AI can significantly improve the predictability and efficiency of energy production.
Related: Use of AI in Sales
29. L’Oréal: Transforming Beauty with AI
Task/Conflict: Personalization in the beauty industry enhances customer satisfaction and brand loyalty. L’Oréal aimed to personalize beauty products and experiences for its diverse customer base using AI.
Solution: L’Oréal leverages AI to assess consumer data and provide personalized product suggestions. AI-driven tools assess skin types and preferences to recommend the best skincare and makeup products. Additionally, virtual try-on apps powered by AI allow customers to see how products would look before making a purchase.
- Enhanced personalization of beauty products and experiences.
- Increased customer engagement and satisfaction.
- AI can provide highly personalized experiences in the beauty industry.
- Data-driven personalization enhances customer satisfaction and brand loyalty.
30. The Weather Company: AI-Predicting Weather Patterns
Task/Conflict: Accurate weather prediction is vital for planning and safety in various sectors. The Weather Company aimed to enhance the accuracy of weather forecasts and provide timely weather-related information using AI.
Solution: The Weather Company employs AI to analyze data from weather sensors, satellites, and historical weather patterns. AI models improve the accuracy of weather predictions by identifying trends and anomalies. These enhanced forecasts help in better planning and preparedness for weather events, benefiting industries like agriculture, transportation, and public safety.
- Improved accuracy in weather forecasting.
- Better preparedness and planning for adverse weather conditions.
- AI can enhance the precision of meteorological predictions.
- Accurate weather forecasting is crucial for safety and operational planning in multiple sectors.
31. Cisco: Securing Networks with AI
Task/Conflict: As cyber threats evolve and become more sophisticated, maintaining robust network security is crucial for businesses. Cisco aimed to leverage AI to enhance its cybersecurity measures, detecting and responding to threats more efficiently.
Solution: Cisco integrated AI into its cybersecurity framework to analyze network traffic and identify unusual patterns indicative of cyber threats. This AI-driven approach allows for real-time threat detection and automated responses, thus improving the speed and efficacy of security measures.
- Strengthened network security with faster threat detection.
- Reduced manual intervention by automating threat responses.
- AI is essential in modern cybersecurity for real-time threat detection.
- Automating responses can significantly enhance network security protocols.
32. Adidas: AI in Sports Apparel Manufacturing
Task/Conflict: To maintain competitive advantage in the fast-paced sports apparel market, Adidas sought to innovate its manufacturing processes by incorporating AI to improve efficiency and product quality.
Solution: Adidas employed AI-driven robotics and automation technologies in its factories to streamline the production process. These AI systems optimize manufacturing workflows, enhance quality control, and reduce waste by precisely cutting fabrics and assembling materials according to exact specifications.
- Increased production efficacy and reduced waste.
- Enhanced consistency and quality of sports apparel.
- AI-driven automation can revolutionize manufacturing processes.
- Precision and efficiency in production lead to higher product quality and sustainability.
Related: How can AI be used in Disaster Management?
33. KLM Royal Dutch Airlines: AI-Enhanced Customer Service
Task/Conflict: Enhancing the customer service experience in the airline industry is crucial for customer satisfaction and loyalty. KLM aimed to provide immediate and effective assistance to its customers by integrating AI into their service channels.
Solution: KLM introduced an AI-powered chatbot, which provides 24/7 customer service across multiple languages. The chatbot handles inquiries about flight statuses, bookings, and baggage policies, offering quick and accurate responses. This AI solution helps manage customer interactions efficiently, especially during high-volume periods.
- Improved customer service efficiency and responsiveness.
- Increased customer satisfaction through accessible and timely support.
- AI chatbots can highly improve user service in high-demand industries.
- Effective communication through AI leads to better customer engagement and loyalty.
34. Novartis: AI in Drug Formulation
Task/Conflict: The pharmaceutical industry requires rapid development and formulation of new drugs to address emerging health challenges. Novartis aimed to use AI to expedite the drug formulation process, making it faster and more efficient.
Solution: Novartis applied AI to simulate and predict how different formulations might behave, speeding up the lab testing phase. AI algorithms analyze vast amounts of data to predict the stability and efficacy of drug formulations, allowing researchers to focus on the most promising candidates.
- Accelerated drug formulation and reduced time to market.
- Improved efficacy and stability of pharmaceutical products.
- AI can significantly shorten the drug development lifecycle.
- Predictive analytics in pharmaceutical research can lead to more effective treatments.
35. Shell: Optimizing Energy Resources with AI
Task/Conflict: In the energy sector, optimizing exploration and production processes for efficiency and sustainability is crucial. Shell sought to harness AI to enhance its oil and gas operations, making them more efficient and less environmentally impactful.
Solution: Shell implemented AI to analyze geological data and predict drilling outcomes, optimizing resource extraction. AI algorithms also adjust production processes in real time, improving operational proficiency and minimizing waste.
- Improved efficiency and sustainability in energy production.
- Reduced environmental impact through optimized resource management.
- Automation can enhance the effectiveness and sustainability of energy production.
- Real-time data analysis is crucial for optimizing exploration and production.
36. Procter & Gamble: AI in Consumer Goods Production
Task/Conflict: Maintaining operational efficiency and innovating product development are key challenges in the consumer goods industry. Procter & Gamble (P&G) aimed to integrate AI into their operations to enhance these aspects.
Solution: P&G employs AI to optimize its manufacturing processes and predict market trends for product development. AI-driven data analysis helps in managing supply chains and production lines efficiently, while AI in market research informs new product development, aligning with consumer needs.
- Enhanced operational efficacy and minimized production charges.
- Improved product innovation based on consumer data analysis.
- AI is crucial for optimizing manufacturing and supply chain processes.
- Data-driven product development leads to more successful market introductions.
Related: Use of AI in the Navy
37. Disney: Creating Magical Experiences with AI
Task/Conflict: Enhancing visitor experiences in theme parks and resorts is a priority for Disney. They aimed to use AI to create personalized and magical experiences for guests, improving satisfaction and engagement.
Solution: Disney utilizes AI to manage park operations, personalize guest interactions, and enhance entertainment offerings. AI algorithms predict visitor traffic and optimize attractions and staff deployment. Personalized recommendations for rides, shows, and dining options enhance the guest experience by leveraging data from past visits and preferences.
- Enhanced guest satisfaction through personalized experiences.
- Improved operational efficiency in park management.
- AI can transform the entertainment and hospitality businesses by personalizing consumer experiences.
- Efficient management of operations using AI leads to improved customer satisfaction.
38. BMW: Reinventing Mobility with Autonomous Driving
Task/Conflict: The future of mobility heavily relies on the development of safe and efficient autonomous driving technologies. BMW aimed to dominate in this field by incorporating AI into their vehicles.
Solution: BMW is advancing its autonomous driving capabilities through AI, using sophisticated machine learning models to process data from vehicle sensors and external environments. This technology enables vehicles to make intelligent driving decisions, improving safety and passenger experiences.
- Pioneering advancements in autonomous vehicle technology.
- Enhanced safety and user experience in mobility.
- AI is crucial for the development of autonomous driving technologies.
- Safety and reliability are paramount in developing AI-driven vehicles.
39. Mastercard: Innovating Payment Solutions with AI
Task/Conflict: In the digital age, securing online transactions and enhancing payment processing efficiency are critical challenges. Mastercard aimed to leverage AI to address these issues, ensuring secure and seamless payment experiences for users.
Solution: Mastercard integrates AI to monitor transactions in real time, detect fraudulent activities, and enhance the efficiency of payment processing. AI algorithms analyze spending patterns and flag anomalies, while also optimizing authorization processes to reduce false declines and improve user satisfaction.
- Strengthened security and reduced fraud in transactions.
- Improved efficiency and user experience in payment processing.
- AI is necessary for securing and streamlining expense systems.
- Enhanced transaction processing efficiency leads to higher customer satisfaction.
40. AstraZeneca: Revolutionizing Oncology with AI
Task/Conflict: Advancing cancer research and developing effective treatments is a pressing challenge in healthcare. AstraZeneca aimed to utilize AI to revolutionize oncology research, enhancing the development and personalization of cancer treatments.
Solution: AstraZeneca employs AI to analyze genetic data and clinical trial results, identifying potential treatment pathways and personalizing therapies based on individual genetic profiles. This approach accelerates the development of targeted treatments and improves the efficacy of cancer therapies.
- Accelerated innovation and personalized treatment in oncology.
- Better survival chances for cancer patients.
- AI can significantly advance personalized medicine in oncology.
- Data-driven approaches in healthcare lead to better treatment outcomes and innovations.
Related: How can AI be used in Tennis?
Closing Thoughts
These 40 case studies illustrate the transformative power of AI across various industries. By addressing specific challenges and leveraging AI solutions, companies have achieved remarkable outcomes, from enhancing customer experiences to solving complex scientific problems. The key learnings from these cases underscore AI’s potential to revolutionize industries, improve efficiencies, and open up new possibilities for innovation and growth.
- 6 Ways to Pay for an Online Course [2024]
- How to Choose an Online Course? [An Ultimate Checklist] [2024]
Team DigitalDefynd
We help you find the best courses, certifications, and tutorials online. Hundreds of experts come together to handpick these recommendations based on decades of collective experience. So far we have served 4 Million+ satisfied learners and counting.
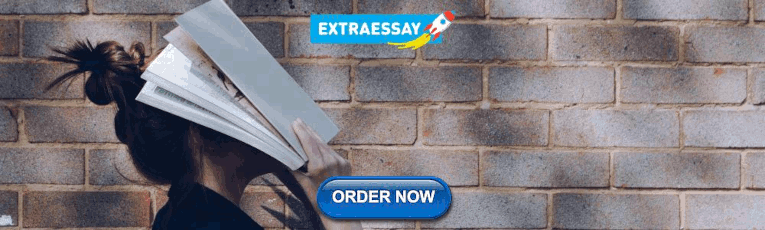
Applications of Artificial Intelligence in Finance [2024]

How Can a CFO Use ChatGPT? [2024]

Will Sales Jobs be replaced by AI & Automation? [2024]

10 Generative AI Case Studies [2024]

How Can Executive Education Boost a Career in Artificial Intelligence? [2024]

AI in Fashion [10 Success Stories] [2024]
Academia.edu no longer supports Internet Explorer.
To browse Academia.edu and the wider internet faster and more securely, please take a few seconds to upgrade your browser .
Enter the email address you signed up with and we'll email you a reset link.
- We're Hiring!
- Help Center
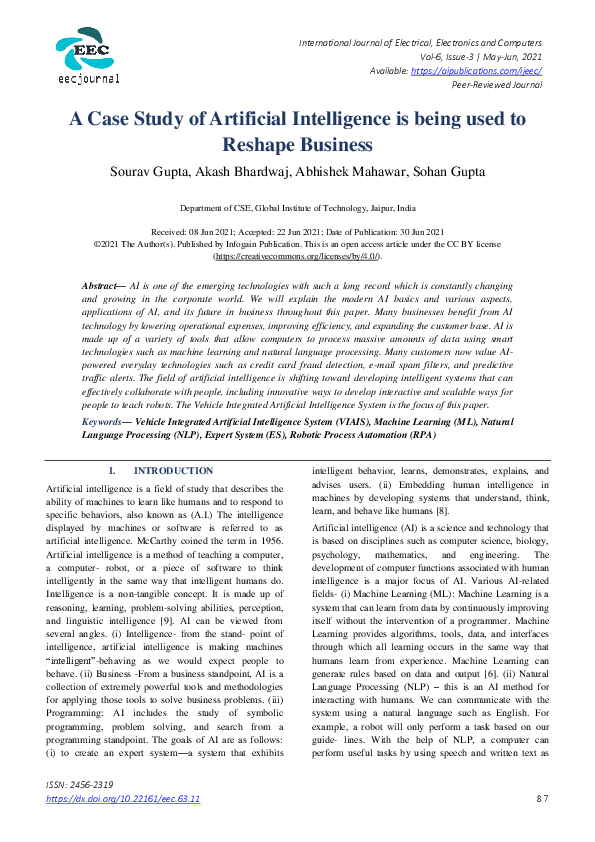
A Case Study of Artificial Intelligence is being used to Reshape Business

AI is one of the emerging technologies with such a long record which is constantly changing and growing in the corporate world. We will explain the modern AI basics and various aspects, applications of AI, and its future in business throughout this paper. Many businesses benefit from AI technology by lowering operational expenses, improving efficiency, and expanding the customer base. AI is made up of a variety of tools that allow computers to process massive amounts of data using smart technologies such as machine learning and natural language processing. Many customers now value AIpowered everyday technologies such as credit card fraud detection, e-mail spam filters, and predictive traffic alerts. The field of artificial intelligence is shifting toward developing intelligent systems that can effectively collaborate with people, including innovative ways to develop interactive and scalable ways for people to teach robots. The Vehicle Integrated Artificial Intelligence System is the focus of this paper.
Related Papers
IJARIIT, volume-5, issue-3
ashish tripathi , Ashish Tripathi , Reeta Thakur
AI is one of the emerging technologies that have a very long history which is constantly changing and growing in the field of business. In this paper, we will explain the modern AI basics and various aspects, applications of AI and its future in business. AI technology helps in many businesses by reducing operational cost, increase efficiency and improve customer experience. AI comprises of multiple tools that are having the ability to process huge amounts of data by computers with the help of smart technologies like machine learning, natural language processing. Nowadays many customers are also appreciating most of the AI-driven everyday technologies like credit card fraud detection, e-mail spam filters and predictive traffic alerts. The field of AI is shifting toward building intelligent systems that can collaborate effectively with people, including creative ways to develop interactive and scalable ways for people to teach robots. This paper is focusing on the Vehicle Integrated Artificial Intelligence System (VIAIS).
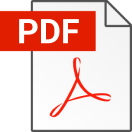
International Journal of Communication Networks and Information Security (IJCNIS)
haitham abdalla
Artificial intelligence (AI) has become increasingly prevalent in business as companies adopt machine algorithms that can learn and improve over time. These AI-powered solutions are used in various business areas, including operations, analytics, product personalisation, marketing, sales, customer service, and human resource (HR). AI can help companies automate mundane tasks, make smarter decisions based on data and insights, and provide capabilities for smoother customer experiences, better customer service, and increased efficiency. AI has also allowed companies to enhance the quality of their digital services, optimise supply chain processes, and gain access to real-time insights and analytics. Companies can use AI to reduce lead times, generate new customer insights, improve customer service, and create meaningful customer experiences. This paper aims to address the gap in knowledge on incorporating AI into business strategy by conducting a critical literature review, synthesisi...
Dr.Sharif Uddin Ahmed Rana
Artificial Intelligence (AI) has become a critical technology in business. It has the potential to improve business efficiency, reduce costs, and enhance customer experience. However, businesses face various challenges in implementing AI, such as data privacy concerns, lack of skills, and regulatory issues. This research proposal aims to study the benefits, challenges, and future prospects of AI application in business. The research will be conducted using a mixed-methods approach, including a survey questionnaire and interviews. The expected outcomes of the study are a comprehensive understanding of the benefits and challenges of AI application in business, identification of the factors that affect the implementation of AI in business and an analysis of the future prospects of AI application in business. The findings of the study will be beneficial to businesses that are considering implementing AI and policymakers who are responsible for regulating the use of AI.
Journal of Engineering Research and Reports
JASMIN BHARADIYA
The ongoing development of business and the most recent advances in artificial intelligence (AI) allow for the many business practices to be improved by the capacity to establish new forms of collaboration, which is a significant competitive advantage. This rapidly developing technology enables to offer brand services and even some new forms of business interactions with consumers and personnel. The digitalization of AI concurrently emphasized for businesses that they need concentrate on their present strategies while also routinely and early pursuing new chances in the market. Not only in business but also in different industry sectors, Al techniques are being used and revolutionized different industry sectors. This review focuses on the application of AI techniques in business and different industries.
IAEME PUBLICATION
IAEME Publication
Speed and quality are the mantras for today’s business world and Artificial Intelligence has marked as significant game-changer in these in all business organizations. As businesses are becoming global and the future is more of machinebased work than human-based. Irrespective of the business volume, the new culture to adapt to AI machinery is a prominent need than a comfort. The trend is challenging the change itself. AI plays a significant role and becomes mandatory in business sustainability. Many of the businesses have become early adopters of AI and making the transactions esp. the customer service with FAQ at the top most importance. Every department in the business organization tries to implement and become best service providers for their customer questions. In this paper, the effect of AI in the business organizations and how the individual departments benefit from it is discussed and explained
International Journal of Engineering Research and Advanced Technology (IJERAT)
Editor Ijasre , Sarhan M. Musa
Artificial intelligence (AI) is a field of computer science that is dedicated to developing software dealing with intelligent decisions, reasoning, and problem solving. Artificial intelligence is already part of our lives, slowly shaping our society and business. It is everywhere, in on your smartphones, laptops, and cars. AI can increase productivity, gain competitive advantage, compliment human intelligence. and reduce cost of operations. Businesses of all types and sizes are considering artificial intelligence to solve their problems. The scope of AI in business transformation is constantly growing. This paper provides an introduction on the applications of AI in business.
RESEARCH REVIEW International Journal of Multidisciplinary
The development of Artificial Intelligence is speeding up rapidly and combination of Artificial Intelligence with automation has started to change the business landscape. Companies and business are focusing on applying existing Artificial Intelligence with automation processes to gain the new heights of efficiency and quality. The paper depicts about artificial intelligence and automation, and it tries to demonstrate the audience how both Artificial intelligence and automation are related and how they can be more effective when they work together and can give competitive advantage.
International Journal of Computer Trends and Technology (IJCTT)
sikender mohsienuddin mohammad
Artificial intelligence, commonly referred to as AI, has, in recent years, transformed the nature of human life in almost every aspect that is connected to human life. The aspects include economic status, job creation, and employment, communication, war, security, privacy, and healthcare. The long term evolution of AI is yet to be seen if it will lead humanity in making the world a better place for living in or a disastrous place. For every technology to survive in the market, the advantages must outdo the disadvantages since every technology has its advantages and disadvantages. In artificial intelligence, since we have not yet reached the long term evolution, we are, however, to see if it will have an impact on the positive effects than the adverse effects. In the world today, we are surrounded by technology in every aspect of life, and we seem to embrace them starting from the aspect of healthcare, industries, smart homes, and even cars that are autonomous. On the negative side, the technology seems to take away jobs from many individuals who are tot intellect on matters technology hence creating the context of unemployment. As technology is advancing quickly, robots and autonomous systems are developed and born daily, therefore replacing the labor provided by humans. However, this being the current situation generated by technology, the exciting parts are brought by the system's long term results which tend to be very fulfilling to the human life. Throughout this essay, I will address the Artificial Intelligence starting from its development to the current situation, including its significance in human life in both the positive aspects and negative aspects of life.
David George
Written for business leaders and general management the observations and discussion points in the main body of the article are non-technical and provide insights informing the reader about some of the business applications of Applied Artificial Intelligence (AI) and the on-going research into the more ambitious Artificial General Intelligence (AGI). The AI technology space is poised for significant growth as robust and proven solutions emerge from academia and private development laboratories. AI is becoming pervasive as the high-tech industry invests increasingly significant funds to develop and incorporate it as component parts of their consumer and other business offerings as the means of making products and services ‘smarter’. There will be an impact on how individuals, business, and societies operate and interact with each other. How we utilise computers and other increasingly intelligent products and automated online Ecommerce services will change. Our purchasing behaviour will be transformed as a direct result of new methods used to target and influence how we choose and make buying decisions. The longer-term research goals of attaining human-like cognitive abilities are far reaching, complex, complicated, and extremely challenging; indeed some of the goals may never be achieved. There are implications, and some difficult questions will require considered answers. However, given today’s economic circumstances and the corresponding demands on business, AI provides tools to create opportunities supporting increased business process efficacy, product and service level enhancements, differentiation, and can assist business with gaining and maintaining a necessary competitive advantage in a relatively uncertain and dynamic future.
Knowledge Engineering Review
RELATED PAPERS
Ausgrabungen und Funde in Westfalen-Lippe
Aussenstelle Olpe LWL-Archaeologie fuer Westfalen
Mohamed Ismail Mohamed Hessein
Diamond and Related Materials
Olga Shenderova
学校原版jcu学位证书 詹姆斯库克大学毕业证学历学位证书留信人才入库原版一模一样
Pharmaceutics
William Mieler
Tetrahedron
Journal of Psychological Science
Journal Psychological Science , Nilofar Mikaeeli Manba
Antibiotics
R. Kalupahana
Obra digital
Salvador Hernandez
Vincent Amanor-Boadu
HAL (Le Centre pour la Communication Scientifique Directe)
Sandra Laugier
Social Science Research Network
Peter Orazem
ChandraSekhar Tiwary
Journal of Adolescent & Adult Literacy
Kay Rosheim
International Journal of Advances in Applied Sciences (IJAAS)
IJAAS Journal
Biota Neotropica
Marina Loeb
The Analyst
Dayane de Aguiar Cicolella
Journal of Physics: Conference Series
A. Faus-Golfe
The World Economy
Petros C. Mavroidis
British Journal of Anaesthesia
GS Umamaheswara Rao
Richkido Febrian
- We're Hiring!
- Help Center
- Find new research papers in:
- Health Sciences
- Earth Sciences
- Cognitive Science
- Mathematics
- Computer Science
- Academia ©2024
Artificial intelligence test: a case study of intelligent vehicles
- Published: 12 April 2018
- Volume 50 , pages 441–465, ( 2018 )
Cite this article
- Yi-Lun Lin 2 , 5 ,
- Nan-Ning Zheng 3 ,
- Fei-Yue Wang ORCID: orcid.org/0000-0001-9185-3989 2 , 5 ,
- Yuehu Liu 3 ,
- Dongpu Cao 4 , 6 ,
- Kunfeng Wang 2 &
- Wu-Ling Huang 2
6813 Accesses
103 Citations
Explore all metrics
To meet the urgent requirement of reliable artificial intelligence applications, we discuss the tight link between artificial intelligence and intelligence test in this paper. We highlight the role of tasks in intelligence test for all kinds of artificial intelligence. We explain the necessity and difficulty of describing tasks for intelligence test, checking all the tasks that may encounter in intelligence test, designing simulation-based test, and setting appropriate test performance evaluation indices. As an example, we present how to design reliable intelligence test for intelligent vehicles. Finally, we discuss the future research directions of intelligence test.
This is a preview of subscription content, log in via an institution to check access.
Access this article
Price includes VAT (Russian Federation)
Instant access to the full article PDF.
Rent this article via DeepDyve
Institutional subscriptions
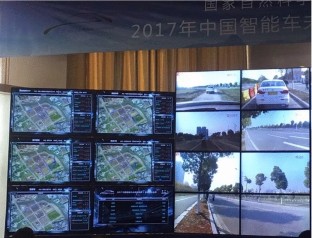
Similar content being viewed by others
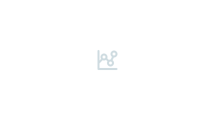
Integrated Framework for Test and Evaluation of Autonomous Vehicles
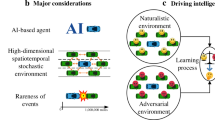
Intelligent driving intelligence test for autonomous vehicles with naturalistic and adversarial environment
Evolution of Autonomous Vehicle: An Artificial Intelligence Perspective
A Tragic Loss (2016) https://www.tesla.com/blog/tragic-loss . Accessed April 2018
Ackerman E (2014) A better test than Turing. IEEE Spectr 51(10):20–21
Article Google Scholar
Ammann P, Jeff O (2017) Introduction to software testing, 2nd edn. Cambridge University Press, Cambridge
MATH Google Scholar
Argall BD, Chernova S, Veloso M, Browning B (2009) A survey of robot learning from demonstration. Robot Auton Syst 57(5):469–483
Bagnell JA (2015) An invitation to imitation. Technical Report, CMU-RI-TR-15-08, Robotics Institute, Carnegie Mellon University
Black R (2009) Managing the testing process: practical tools and techniques for managing hardware and software testing. Wiley, Hoboken
Google Scholar
Boehm BW (1988) A spiral model of software development and enhancement. IEEE Comput 21(5):61–72
Bradley AR, Manna Z (2007) The calculus of computation: decision procedures with applications to verification. Springer, Berlin
Broggi A, Buzzoni M, Debattisti S, Grisleri P, Laghi MC, Medici P, Versari P (2013) Extensive tests of autonomous driving technologies. IEEE Trans Intell Transp Syst 14(3):1403–1415
Broggi A, Cerri P, Debattisti S, Laghi MC, Medici P, Molinari D, Panciroli M, Prioletti A (2015) PROUD—public road urban driverless-car test. IEEE Trans Intell Transp Syst 16(6):3508–3519
Brown N, Sandholm T (2017) Safe and nested subgame solving for imperfect-information games. https://arxiv.org/abs/1705.02955 . Accessed April 2018
Browne CB, Powley E, Whitehouse D, Lucas SM, Cowling PI, Rohlfshagen P, Tavener S, Perez D, Samothrakis S, Colton S (2012) A survey of monte carlo tree search methods. IEEE Trans Comput Intell AI Games 4(1):1–43
Buehler M, Iagnemma K, Singh S (eds) (2009) The DARPA urban challenge. Springer, Berlin
Butakov VA, Ioannou P (2015) Personalized driver/vehicle lane change models for ADAS. IEEE Trans Veh Technol 64(10):4422–4431
Campbell M, Egerstedt M, How JP, Murray RM (2010) Autonomous driving in urban environments: approaches, lessons and challenges. Philos Trans R Soc A 368(1928):4649–4672
Chen Z, Liu B (2016) Lifelong machine learning. Morgan & Claypool Publishers, San Rafael
Cheng PCH (2016) What constitutes an effective representation? In: Jamnik M, Uesaka Y, Elzer Schwartz S (eds) Diagrammatic representation and inference: proceedings from the 9th international conference, diagrams 2016, vol 9781. Lecture notes in computer science. Springer, Berlin
Chapter Google Scholar
Classen S, Nichols AL, McPeek R, Breinerd JF (2011) Personality as a predictor of driving performance: an exploratory study. Transp Res F Traffic Psychol Behav 14(5):381–389
Coulom R (2008) Whole-history rating: a Bayesian rating system for players of time-varying strength. In: Proceedings of international conference on computers and games, pp 113–124
DARPA Grand Challenge, DARPA Urban Challenge (2004–2007) http://archive.darpa.mil/grandchallenge/ . Accessed April 2018
Ding Z, Jiang C, Zhou MC (2013) Design, analysis and verification of real-time systems based on time Petri net refinement. ACM Transactions in Embedded Computing Systems 12:4:1–4:18. https://doi.org/10.1145/2406336.2406340
Elo AE (1978) The rating of chessplayers, past and present. Arco Publishing, New York
Eskandarian A (ed) (2012) Handbook of intelligent vehicles. Springer, Berlin
Evtimov I, Eykholt K, Fernandes E, Kohno T, Li B, Prakash A, Rahmati A, Song D (2017) Robust physical-world attacks on machine learning models. https://arxiv.org/abs/1707.08945 . Accessed April 2018
Fagnant DJ, Kockelman K (2015) Preparing a nation for autonomous vehicles: opportunities, barriers and policy recommendations. Transp Res A Policy Practice 77:167–181
Fisher DL, Lohrenz M, Moore D, Nadler ED, Pollard JK (2016) Humans and intelligent vehicles: the hope, the help, and the harm. IEEE Trans Intell Veh 1(1):56–67
Gaidon A, Wang Q, Cabon Y, Vig E (2016) Virtual worlds as proxy for multi-object tracking analysis. In: Proceedings of the IEEE conference on computer vision and pattern recognition, pp 4340–4349
Gatys LA, Ecker AS, Bethge M (2016) Image style transfer using convolutional neural networks. In: Proceedings of IEEE conference on computer vision and pattern recognition, pp 2414–2423
George D, Lehrach W, Kansky K, Lázaro-Gredilla M, Laan C, Marthi B, Lou X, Meng Z, Liu Y, Wang H, Lavin A, Phoenix DS (2017) A generative vision model that trains with high data efficiency and breaks text-based CAPTCHAs. Science. https://doi.org/10.1126/science.aag2612
Goodall NJ (2014) Ethical decision making during automated vehicle crashes. Transp Res Rec 2424:58–65
Goodfellow IJ, Pouget-Abadie J, Mirza M, Xu B, Warde-Farley D, Ozair S, Courville A, Bengio Y (2014) Generative adversarial nets. Proc Adv Neural Inf Process Syst 27:2672–2680
Goodfellow I, Bengio Y, Courville A (2016) Deep learning. MIT Press, Cambridge
Greengard S (2017) Gaming machine learning. Commun ACM 60(12):14–16
GTSDB, The German Traffic Sign Recognition Benchmark and the German Traffic Sign Detection Benchmark (2014) http://benchmark.ini.rub.de/?section=home&subsection=news . Accessed April 2018
Harari YN (2017) Reboot for the AI revolution. Nature 550:324–327
Hernández-Orallo J (2017) Evaluation in artificial intelligence: from task-oriented to ability-oriented measurement 48(3):397–447
Ho J, Ermon S (2017) Generative adversarial imitation learning. https://arxiv.org/abs/1606.03476 . Accessed April 2018
Huang WL, Wen D, Geng J, Zheng NN (2014) Task-specific performance evaluation of ugvs: case studies at the IFVC. IEEE Trans Intell Transp Syst 15(5):1969–1979
Huizinga D, Adam K (2007) Automated defect prevention: best practices in software management. Wiley, Hoboken
Book Google Scholar
IBM, Deep Blue - Overview (1997) IBM Research. http://www-03.ibm.com/ibm/history/ibm100/us/en/icons/deepblue/ . Accessed April 2018
ImageNet (2016) http://image-net.org . Accessed April 2018
Karp RM (1972) Reducibility among combinatorial problems. In: Miller RE, Thacher JW (eds) Complexity of computer computation. Plenum Press, New York, pp 85–103
Karpathy A (2017) Software 2.0. https://medium.com/@karpathy/software-2-0-a64152b37c35 . Accessed April 2018
Koopman P, Wagner M (2017) Autonomous vehicle safety: an interdisciplinary challenge. IEEE Intell Transp Syst Mag 9(1):90–96
Kroening D, Strichman O (2016) Decision procedures: an algorithmic point of view, 2nd edn. Springer, Berlin
Book MATH Google Scholar
Kuefler A, Morton J, Wheeler T, Kochenderfer M (2017) Imitating driver behavior with generative adversarial networks. In: Proceedings of IEEE intelligent vehicles symposium, pp 204–211
Heule MJH, Kullmann O (2017) The science of brute force. Commun ACM 60(8):70–79
Kumfer W, Burgess R (2015) Investigation into the role of rational ethics in crashes of automated vehicles. Transp Res Rec 2489:130–136
Kurzweil R (2005) The singularity is near. Viking Press, New York
Lamb E (2016) Maths proof smashes size record: supercomputer produces a 200-terabyte proof—but is it really mathematics? Nature 534(7605):17–19
LeCun Y, Bengio Y, Hinton G (2015) Deep learning. Nature 521(7553):436–444
Lefèvre S, Carvalho A, Gao Y, Tseng HE, Borrellia F (2015) Driver models for personalised driving assistance. Veh Syst Dyn 53(12):1705–1720
Levesque HJ (2014) On our best behavior. Artif Intell 212:27–35
Article MATH Google Scholar
Levesque HJ (2017) Common sense, the Turing test, and the quest for real AI. MIT Press, Cambridge
Li L, Wang FY (2007) Advanced motion control and sensing for intelligent vehicles. Springer, New York
Li L, Wen D, Zheng NN, Shen LC (2012) Cognitive cars: a new frontier for ADAS research. IEEE Trans Intell Transp Syst 13(1):395–407
Li L, Huang WL, Liu Y, Zheng NN, Wang FY (2016a) Intelligence testing for autonomous vehicles: a new approach. IEEE Trans Intell Veh 1(2):158–166
Li L, Lv Y, Wang FY (2016b) Traffic signal timing via deep reinforcement learning. IEEE/CAA J Autom Sin 3(3):247–254
Article MathSciNet Google Scholar
Li L, Lin Y, Zheng NN, Wang FY (2017) Parallel learning: a perspective and a framework. IEEE/CAA J Autom Sin 4(3):389–395
Liao R (2017) Tencent discovers major loopholes in Google’s AI platform TensorFlow. https://technode.com/2017/12/18/tencent-tensorflow/ . Accessed April 2018
Licato J, Zhang Z (2017) Evaluating representational systems in artificial intelligence. Artif Intell Rev. https://doi.org/10.1007/s10462-017-9598-7
Liu MY, Breuel T, Kautz J (2017) Unsupervised image-to-image translation networks. https://arxiv.org/abs/1703.00848 . Accessed April 2018
Mackintosh NJ (2011) IQ and human intelligence, 2nd edn. Oxford University Press, Oxford
Maurer M, Gerdes JC, Lenz B, Winner H (eds) (2015) Autonomous driving: technical, legal and social aspects. Springer, Berlin
Mcguire G, Tugemann B, Civario G (2014) There is no 16-clue sudoku: solving the sudoku minimum number of clues problem via hitting set enumeration. Exp Math 23(2):190–217
Article MathSciNet MATH Google Scholar
Merel J, Tassa Y, TB D, Srinivasan S, Lemmon J, Wang Z, Wayne G, Heess N (2017) Learning human behaviors from motion capture by adversarial imitation. https://arxiv.org/abs/1707.02201 . Accessed April 2018
Minsky ML (ed) (1968) Semantic information processing. MIT Press, Cambridge
Moravčík M, Schmid M, Burch N, Lisý V, Morrill D, Bard N, Davis T, Waugh K, Johanson M, Bowling M (2017) DeepStack: expert-level artificial intelligence in heads-up no-limit poker. Science 356:508–513
Newell A, Simon HA (1976) Computer science as empirical inquiry: symbols and search. Commun ACM CACM Homepage 19(3):113–126
Ohlsson S, Sloan RH, Turán G, Urasky A (2017) Measuring an artificial intelligence system’s performance on a verbal IQ test for young children. J Exp Theor Artif Intell 29(4):679–693
Raccoon L (1997) Fifty years of progress in software engineering. ACM SIGSOFT Softw Eng Notes 22(1):88–104
Redmon J, Farhadi A (2016) YOLO9000: better, faster, stronger. https://arxiv.org/abs/1612.08242 . Accessed April 2018
Redmon J, Divvala S, Girshick R, Farhadi A (2016) You only look once: unified, real-time object detection. https://arxiv.org/abs/1506.02640 . Accessed April 2018
Richter SR, Vineet V, Roth S, Koltun V (2016) Playing for data: ground truth from computer games. In: European conference on computer vision, pp 102–118
Rindermann H, Becker D, Coyle TR (2016) Survey of expert opinion on intelligence: causes of international differences in cognitive ability tests. Front Psychol. https://doi.org/10.3389/fpsyg.2016.00399
Ros G, Sellart L, Materzynska J, Vazquez D, Lopez AM (2016) The SYNTHIA dataset: a large collection of synthetic images for semantic segmentation of urban scenes. In: Proceedings of the IEEE conference on computer vision and pattern recognition, pp 3234–3243
Russell S, Norvig P (2010) Artificial intelligence: a modern approach, 3rd edn. Pearson Education Limited, London
SAE J3016 (2016) Taxonomy and definitions for terms related to on-road motor vehicle automated driving systems. SAE, Warrendale
Santana E, Hotz G (2016) Learning a driving simulator. https://arxiv.org/abs/1608.01230 . Accessed April 2018
Schoenick C, Clark P, Tafjord O, Turney P, Etzioni O (2017) Moving beyond the Turing test with the Allen AI science challenge. Commun ACM 60(9):60–64
Silver D, Huang A, Maddison CJ, Guez A, Sifre L, van den Driessche G, Schrittwieser J, Antonoglou I, Panneershelvam V, Lanctot M, Dieleman S, Grewe D, Nham J, Kalchbrenner N, Sutskever I, Lillicrap T, Leach M, Kavukcuoglu K, Graepel T, Hassabis D (2016) Mastering the game of Go with deep neural networks and tree search. Nature 529(7587):484–489
Silver D, Hubert T, Schrittwieser J, Antonoglou I, Lai M, Guez A, Lanctot M, Sifre L, Kumaran D, Graepel T, Lillicrap T, Simonyan K, Hassabis D (2017a) Mastering Chess and Shogi by self-play with a general reinforcement learning algorithm. https://arxiv.org/abs/1712.01815 . Accessed April 2018
Silver D, Schrittwieser J, Simonyan K, Antonoglou I, Huang A, Guez A, Hubert T, Baker L, Lai M, Bolton A, Chen Y, Lillicrap T, Hui F, Sifre L, van den Driessche G, Graepel T, Hassabis D (2017b) Mastering the game of Go without human knowledge. Nature 550:354–359
Srinivasan B, Parthasarathi R (2017) A survey of imperatives and action representation formalisms. Artif Intell Rev 48(2):263–297
Sternberg RJ (1985) Beyond IQ: a triarchic theory of human intelligence. Cambridge University Press, Cambridge
Sternberg RJ, Davidson JE (1983) Insight in the gifted. Educ Psychol 18(1):51–57
Thornton SM, Pan S, Erlien SM, Gerdes JC (2017) Incorporating ethical considerations into automated vehicle control. IEEE Trans Intell Transp Syst 18(6):1429–1439
Tong Y, Zhao L, Li L, Zhang Y (2015) Stochastic programming model for oversaturated intersection signal timing. Transp Res Part C 58:474–486
Turing AM (1950) Computing machinery and intelligence. Mind 59(236):433–460
Veeravasarapu VSR, Hota RN, Rothkopf C, Visvanathan R (2015) Simulations for validation of vision systems. Comput Sci. https://arxiv.org/abs/1512.01030
Vinge V (1993) The coming technological singularity: how to survive in the post-human era. In: Landis GA (ed) Vision-21: interdisciplinary science and engineering in the ear of cyberspace. NASA Publication, CP-10129, Washington, pp 11–22
von Ahn L, Blum M, Hopper NJ, Langford J (2003) CAPTCHA: using hard AI problems for security. In: Proceedings of international conference on the theory and applications of cryptographic techniques, pp 294–311
Wagner M, Koopman P (2015) A philosophy for developing trust in self-driving cars. In: Meyer G, Beiker S (eds) Road vehicle automation 2. Lecture notes in mobility. Springer, Cham. https://doi.org/10.1007/978-3-319-19078-5_14
Wang FY, Zhang JJ, Zheng X et al (2016) Where does AlphaGo go: from church-turing thesis to AlphaGo thesis and beyond. IEEE/CAA J Automatica Sin 3:113–120. https://doi.org/10.1109/JAS.2016.7471613
Wang L (2016) Directions 2017: BeiDou’s road to global service. GPS World
Wang FY, Wang X, Li L, Li L (2016a) Steps toward parallel intelligence. IEEE/CAA J Autom Sin 3(4):345–348
Wang X, Zheng X, Zhang Q, Wang T, Shen D (2016b) Crowdsourcing in ITS: the state of the work and the networking. IEEE Trans Intell Transp Syst 17(6):1596–1605
Wang K, Gou C, Zheng N, Rehg JM, Wang FY (2017a) Parallel vision for perception and understanding of complex scenes: methods, framework, and perspectives. Artif Intell Rev 1:1–31
Wang X, Jiang R, Li L, Lin Y, Zheng X, Wang FY (2017b) Capturing car-following behaviors by deep learning. IEEE Trans Intell Transp Syst. http://ieeexplore.ieee.org/document/7970189/
Watzenig D, Horn M (2017a) Automated driving: safer and more efficient future driving. Springer, Cham
Watzenig D, Horn M (2017b) Automated driving: safer and more efficient future driving. Springer, Cham
You J. (2017) Deep learning based lane departure detection for automated vehicles. Bachelor Thesis, Tsinghua University
Zhao D, Huang X, Peng H, Lam H, Leblanc DJ (2017) Accelerated evaluation of automated vehicles in car-following maneuvers. IEEE Trans Intell Transp Syst. http://ieeexplore.ieee.org/document/7933977/
Zheng NN, Tang S, Cheng H, Li Q, Lai G, Wang FY (2004) Toward intelligent driver-assistance and safety warning systems. IEEE Intell Syst 19(2):8–11
Zheng NN, Liu ZY, Ren PJ, Ma YQ, Chen ST, Yu SY, Xue JR, Chen BD, Wang FY (2017) Hybrid-augmented intelligence: collaboration and cognition. Front Inf Technol Electron Eng 18(2):153–179
Download references
Acknowledgements
This work was supported in part by the National Natural Science Foundation of China under Grants 91520301 and 61533019, and the Beijing Municipal Science and Technology Project (No. D171100000317002).
Author information
Authors and affiliations.
Department of Automation, BNRist, Tsinghua University, Beijing, 100084, China
The State Key Laboratory for Management and Control of Complex Systems, Institute of Automation, Chinese Academy of Sciences, Beijing, 100190, China
Yi-Lun Lin, Fei-Yue Wang, Kunfeng Wang & Wu-Ling Huang
Institute of Artificial Intelligence and Robotics, Xi’an Jiaotong University, Xi’an, 710049, China
Nan-Ning Zheng & Yuehu Liu
Department of Mechanical and Mechatronics Engineering, University of Waterloo, Waterloo, ON, N2L3G1, Canada
Qingdao Academy of Intelligent Industries, Qingdao, 266109, Shandong, China
Yi-Lun Lin & Fei-Yue Wang
VIPioneers (HuiTuo) Inc., Qingdao, 266109, Shandong, China
You can also search for this author in PubMed Google Scholar
Corresponding author
Correspondence to Fei-Yue Wang .
Rights and permissions
Reprints and permissions
About this article
Li, L., Lin, YL., Zheng, NN. et al. Artificial intelligence test: a case study of intelligent vehicles. Artif Intell Rev 50 , 441–465 (2018). https://doi.org/10.1007/s10462-018-9631-5
Download citation
Published : 12 April 2018
Issue Date : October 2018
DOI : https://doi.org/10.1007/s10462-018-9631-5
Share this article
Anyone you share the following link with will be able to read this content:
Sorry, a shareable link is not currently available for this article.
Provided by the Springer Nature SharedIt content-sharing initiative
- Artificial intelligence
- Intelligence test
- Turing test
- Simulation test
- Find a journal
- Publish with us
- Track your research

- SUGGESTED TOPICS
- The Magazine
- Newsletters
- Managing Yourself
- Managing Teams
- Work-life Balance
- The Big Idea
- Data & Visuals
- Reading Lists
- Case Selections
- HBR Learning
- Topic Feeds
- Account Settings
- Email Preferences
AI Is Making Economists Rethink the Story of Automation
- Walter Frick

Economists have traditionally believed that new technology lifts all boats. But in the case of AI, some are asking: Will some employees get left behind?
Will artificial intelligence take our jobs? As AI raises new fears about a jobless future, it’s helpful to consider how economists’ understanding of technology and labor has evolved. For decades, economists were relatively optimistic, and pointed out that previous waves of technology had not led to mass unemployment. But as income inequality rose in much of the world, they began to revise their theories. Newer models of technology’s affects on the labor market account for the fact that it absolutely can displace workers and lower wages. In the long run, technology does tend to raise living standards. But how soon and how broadly? That depends on two factors: Whether technologies create new jobs for people to do and whether workers have a voice in technology’s deployment.
Is artificial intelligence about to put vast numbers of people out of a job? Most economists would argue the answer is no: If technology permanently puts people out of work then why, after centuries of new technologies, are there still so many jobs left ? New technologies, they claim, make the economy more productive and allow people to enter new fields — like the shift from agriculture to manufacturing. For that reason, economists have historically shared a general view that whatever upheaval might be caused by technological change, it is “somewhere between benign and benevolent.”
- Walter Frick is a contributing editor at Harvard Business Review , where he was formerly a senior editor and deputy editor of HBR.org. He is the founder of Nonrival , a newsletter where readers make crowdsourced predictions about economics and business. He has been an executive editor at Quartz as well as a Knight Visiting Fellow at Harvard’s Nieman Foundation for Journalism and an Assembly Fellow at Harvard’s Berkman Klein Center for Internet & Society. He has also written for The Atlantic , MIT Technology Review , The Boston Globe , and the BBC, among other publications.
Partner Center
Log in using your username and password
- Search More Search for this keyword Advanced search
- Latest content
- Current issue
- Browse by collection
- BMJ Journals More You are viewing from: Google Indexer
You are here
- Online First
- Towards regulatory generative AI in ophthalmology healthcare: a security and privacy perspective
- Article Text
- Article info
- Citation Tools
- Rapid Responses
- Article metrics

- http://orcid.org/0009-0008-5169-5857 Yueye Wang 1 ,
- Chi Liu 2 ,
- Keyao Zhou 3 , 4 ,
- Tianqing Zhu 2 ,
- http://orcid.org/0000-0001-6836-3447 Xiaotong Han 1
- 1 Sun Yat-sen University Zhongshan Ophthalmic Center State Key Laboratory of Ophthalmology , Guangzhou , Guangdong , China
- 2 Faculty of Data Science, City University of Macau , Macao SAR , China
- 3 Department of Ophthalmology , Guangdong Provincial People's Hospital , Guangzhou , Guangdong , China
- 4 Department of Neurosurgery , Huashan Hospital, Fudan University , Shanghai , China
- Correspondence to Dr Xiaotong Han, Sun Yat-Sen University Zhongshan Ophthalmic Center State Key Laboratory of Ophthalmology, Guangzhou, Guangdong, China; lh.201205{at}aliyun.com ; Dr Chi Liu, Faculty of Data Science, City University of Macau, Macao SAR, China; chiliu{at}cityu.edu.mo
As the healthcare community increasingly harnesses the power of generative artificial intelligence (AI), critical issues of security, privacy and regulation take centre stage. In this paper, we explore the security and privacy risks of generative AI from model-level and data-level perspectives. Moreover, we elucidate the potential consequences and case studies within the domain of ophthalmology. Model-level risks include knowledge leakage from the model and model safety under AI-specific attacks, while data-level risks involve unauthorised data collection and data accuracy concerns. Within the healthcare context, these risks can bear severe consequences, encompassing potential breaches of sensitive information, violating privacy rights and threats to patient safety. This paper not only highlights these challenges but also elucidates governance-driven solutions that adhere to AI and healthcare regulations. We advocate for preparedness against potential threats, call for transparency enhancements and underscore the necessity of clinical validation before real-world implementation. The objective of security and privacy improvement in generative AI warrants emphasising the role of ophthalmologists and other healthcare providers, and the timely introduction of comprehensive regulations.
- Public health
Data availability statement
Data sharing not applicable as no data sets generated and/or analysed for this study. Not applicable.
https://doi.org/10.1136/bjo-2024-325167
Statistics from Altmetric.com
Request permissions.
If you wish to reuse any or all of this article please use the link below which will take you to the Copyright Clearance Center’s RightsLink service. You will be able to get a quick price and instant permission to reuse the content in many different ways.
Introduction
Over the past decade, the field of medicine has grasped opportunities from artificial intelligence (AI). 1 2 There is growing optimism regarding the promise of this technology to transform healthcare. 3 The image-centric nature of ophthalmology renders the development and application of AI within this field tremendous progress. 4 While alongside its huge potential, security and privacy issues arising from AI are of paramount importance. The powerful capabilities and extensive data consumption behind the AI raise concerns that without proper development and regulation, this technology may present risks and potential harm to individual privacy and security. 5 Nowhere are these concerns more critical than in the healthcare industry, where data leakage or model misuse can have severe consequences. To ensure the responsible use of this technology for the public’s benefit, the European Union (EU) published the first AI-specific regulation in 2021—the EU AI Act and other regulatory institutions like WHO and Food and Drug Administration (FDA) are catching up in recent years. 6–8
Generative AI, which enables the creation of various synthetic contexts based on users’ input, can highly speed up information translation and technological innovation. 9 Large language models (LLM), such as ChatGPT and Bard, showed great performance in the ophthalmology question–answering domain, even superior to historical human marks. 10 11 However, as we embrace the wave of generative AI, we are just starting to grasp the risks that come with it. Differing from regulated AI, generative AI presents unique characteristics such as extensive training data, broad applications, interactive data flow and synthetic contents. These features can pose new challenges that current regulations have not fully addressed. The extensive and diverse use of large generative AI models necessitates specific guidelines to protect security and privacy. 12
This paper aims to discuss the security and privacy challenges of generative AI in the context of healthcare. We present vulnerabilities at both the model and data levels, argue the consequences of such risk in ophthalmology and the broader medical fields and propose solutions and regulatory approaches to harness the full potential of this technology.
Security and privacy risks
Model-level risk, knowledge leakage.
The development of large generative models requires substantial data, expert knowledge, computational resources and trial and error, leading to substantial expenses for the developers. This is especially pronounced in the medical domain where data is highly sensitive and difficult to collect, coupled with the elevated costs for expert knowledge acquisition. Therefore, well-trained models are valuable digital assets whose safety should be protected. Traditionally, due to the ‘black box’ nature of AI, it is assumed that a deployed model is complicated to penetrate and reproduce without explicit knowledge of model architecture, training set and parameters. However, attacks like reverse engineering, model inversion attack and model extraction attack can still put the trained model at risk of being stolen during the inference time. 13–15 In the case of generative AI, network architecture and model hyperparameters can be inferred from the outputs (eg, synthetic images generated by the model). 16
Data used for training a generative AI model is also at risk of leakage. A membership inference attack is a way to expose the training data only from the queried outputs of a model. 17 For example, by deducing the participation of individuals in the training set from the output of a generative model, up to over 80% of the training set data can be inferred under a membership inference attack. 18 The risk of data leakage is amplified in generative models than in traditional medical AI models such as image-based classification models (eg, diagnostic models for eye diseases). This is because traditional models merely output abstract decisions based on prediction probabilities, and thus attackers are only able to infer approximate distributional information of training set with particular attacks. In contrast, generative models directly generate exact data points that belong to the same distribution of the training set, wherein the original training knowledge may leak spontaneously. For instance, with deliberate prompt decoys, an LLM can disclose the texts it has learnt in its responses. 19
Model corruption
Given the heightened expectations regarding AI’s role in supporting clinical decision-making, the integrity and reliability of the models should be prerequisites for healthcare practices. Unfortunately, current AI techniques, including generative AI, are vulnerable to orchestrated attacks. For instance, adversarial attacks, test-time attacks that perturb a clean test sample with imperceptible adversarial noises, can misguide a well-trained AI model to give a wrong prediction. 20 Another typical attack is known as a poisoning attack, where an attacker intentionally manipulates the training data of an AI model when they gain access to the training data set. The model trained with the poisoned data set becomes corrupted and, consequently would make inaccurate or biased predictions controlled by the triggering signals from the attacker during inference. 21
Model corruption can be especially impactful for generative AI, given the increasing reliance on it as a primary source of medical information. A corrupted model could be manipulated to generate toxic, biased or misleading content. What is worse, such problematic outputs are harder for humans to identify than detecting incorrect predictions from traditional medical AI, since LLMs present information interactively in natural language. Additionally, common AI attack vectors like adversarial attacks, backdoors and data poisoning are shifting focus from training-time attacks to prompt-level and fine-tuning attacks that no longer require data set access. This reduces attack costs, expanding and diversifying attack surfaces, necessitating urgent research attention.
Data-level risk
Unauthorised data collection.
Numerous data privacy breaches involving AI have been reported in the healthcare sector. 22 In the era of generative AI, the risk of healthcare data privacy violation has been significantly aggravated, as the information channels are greatly expanded through human-AI interaction. The Health Insurance Portability and Accountability Act calls for attention to the heightened risk of healthcare data breaches due to generative AI. 23 For example, synthetic content from generative AI can abused for healthcare fraud. Additionally, conversational AI chatbots released by unauthorised services can be manipulated to steal patients’ data. The risk is particularly noteworthy in the field of ophthalmology, as the eye is usually considered a window to the body. Even from the input of a single retinal image, one’s demographic characteristics (eg, age, sex) and systematic conditions (eg, circulatory, neurological ageing and diseases) would be at risk of leakage.
Notably, LLMs like ChatGPT involve reinforcement learning from human feedback, which by default uses user-entered information for continuous training. 24 This poses an unwitting risk of exposing sensitive data at various stages of generative AI use. When being implemented for medical use, the models may receive sensitive prompts such as personal information and medical conditions without explicit and proper consent obtained from patients, which might violate the users’ original purpose.
Is seeing always believing?
Generative AI can synthesise content that looks highly realistic. For instance, a diffusion model can perform text-to-image translation, yielding high-fidelity output. 25 A generative adversarial network also makes it possible for transmodality from a fundus photograph to retinal angiography images with explicit microvasculature details. 26 However, it is essential to acknowledge that in medical practice, accuracy is critical besides fidelity. Content created by generative AI can have mistakes that do not align with science. 27 Another concern is the use of LLMs as medical databases; LLMs may provide persuasive yet fake citations when asked about data sources. 5
Unfortunately, clinical validation for generative AI is extremely challenging right now, primarily due to the massive content the model can generate. This unpredictability hinders generative models from meeting the regulation standards of the FDA as medical devices. Although we already have models that can generate fundus images featuring detailed retinal lesions, ascertaining the reliability of these lesions poses a challenge. The clinical application of such generative models also requires further validation.
Consequences in healthcare practice and case studies
Patient information breaches.
Generative AI has shown its value to be deployed in enhancing clinical documentation and workflow, supporting decision-making and improving interaction with patients. 12 In the diagnosis of ophthalmological diseases and in generating proficient operative notes, LLMs showed parallel performance to ophthalmology trainees or interns, indicating a promising way to reduce ophthalmologist workload. 28 However, as a potential consequence of relying on these models, the inevitable risk of sensitive information breach, such as biological data or clinical records, can severely violate patients’ privacy. The leading LLM, ChatGPT, experienced its first data breach on 20 March this year, affecting approximately 1.2 million users with exposed data. 29 This incident resulted from a bug in the open-source code, enabling some users to access others’ personal information (name, address, payment information and chat history).
This situation underscores the high risk regarding the application of generative models in clinical workflows. General LLMs, particularly cloud-based models, may harbour massive and dynamic patient information, making them vulnerable to potential breaches of sensitive data. To address this concern, one mitigation approach is to employ a locally customised LLM (such as Microsoft’s AutoGen), not relying on third-party servers. This strategy ensures that sensitive patient data remains confidential and secure within the context of the local health system. One alternative is to use a dedicated service such as Azure OpenAI services, where patient data is not available to be copied to the LLM or other customers or models, minimising the risk of data breaches.
Moreover, if malicious users manage to retrieve the training data or even the generative model itself through leaked information, the model’s integrity can be compromised. Recently, researchers from Google’s DeepMind used only simple prompts and extracted private training data from ChatGPT, breaking the model’s safeguards. 30 Any response lag in generative AI due to such a model attack may result in delayed patient management. Additionally, incorrect output generated from a corrupted model may contribute to misdiagnosis and inappropriate treatments.
Violation of the ‘Right to be Forgotten’
The ‘right to be forgotten’ under Article 17 of the General Data Protection Regulation (GDPR) is an essential consideration for human privacy. It addresses the need to mitigate prolonged storage of private medical information, which can be risky. This rule also acknowledges patients’ entitlement to request the removal of their data from AI models. Nevertheless, generative AI, especially LLMs, confronts obstacles in complying with this rule. The model requires massive amounts of clinical data for training, while the source of the training data is essentially unknown to both clinicians and patients. Even if removing personal data from the training data set, the trained model can produce outputs containing patient-specific information. In one case, researchers showed that ChatGPT provided the personal information of individual A in a request for a non-related individual B. 31 This case proved that LLM can memorise personal data and generate this data in future output.
Prolonged data retention or the failure to delete data on request may heighten the risk of unauthorised access or the unintended use of sensitive personal information. Even the training interval of Llama 2 family, the LLMs from Meta, was only around 2 months, this timespan still failed the requirement of GDPR’s ‘undue delay’—around 1 month. Lack of transparency is another critical concern in the use of generative AI that may harm the ‘right to be forgotten’. Patients entrust their personal data to healthcare providers and have the right to comprehensive information about data collection, processing and storage. Unfortunately, existing data processing disclosures have proven inadequate in this regard.
Is synthetic data safe for patient?
In digital modelling for trends assessment and prediction of disease risks, using synthetic data can augment sample sizes or enhance data set diversity, overcome challenges posed by insufficient real-world data or simulation on rare conditions. A recent study adopted Synthea, a well-documented and peer-reviewed generative AI to generate a 1.2 million Massachusetts patient cohort. 32 This study indicated that high-fidelity synthetic data performed well in modelling demographics, showing promise as a valuable analytics tool for aiding decision-making processes. However, it is noteworthy that synthetic data may not act well when simulating positive cases or reflecting disease-related metrics like mortality rates or complication rates. It is also challenging for synthetic data to monitor specific trends related to novel treatments or patient prognoses. This limitation of synthetic data may result in an underestimation of actual clinical outcomes.
Synthetic information from LLMs may also not be suitable for real-world medical use. Though studies are showing LLM can pass medical examinations such as the US Medical Licensing Examination. 33 Healthcare providers still express major concerns about accuracy and reliability regarding synthetic answers. 34 In the case of ChatGPT, it was found able to manage appropriately in retinal diseases, yet showed significant mistakes in other subdomain diseases such as lacrimal drainage disorders and anterior ischaemic optic neuropathy. 35–37
Based on the current evidence, synthetic data may not yet be a reliable proxy for real patient data. Inaccurate synthetic data may yield misleading or biased outcomes in medical research and practice, thereby exacerbating the potential for unsafe clinical management. 38 Before the clinical application of synthetic data, it is imperative to ensure its comparability to real-world data, safeguarding against potential harm to patients. Looking ahead, researchers are optimistic about this cutting-edge tool but stress the need for rigorous external clinical validation and accurate information.
Solutions under regulation
Be prepared for the attack.
In the latest updates, the first regulation on AI, the EU AI Act requires providers of generative AI to deploy the model with adequate training and design safeguards (Article 28). Prior to deployment in real clinical practice, anti-attack techniques such as adversarial training and robust model validation can help identify and mitigate model-level attacks. 39 The German Federal Office for Information and Security suggested several ways to reduce vulnerability to model attacks, 40 including avoiding identifiable and sensitive data, specifying training on sensitive data, restricting the user group, etc. In short, model providers and users need to be aware of the potential risk of model attacks and prepare plans for these attacks, such as model pre-training and user training.
Informed consent, data share and transparency
When using generative AI, it is vital for both model providers and users to comply with GDPR guidelines regarding lawful personal data collection and processing. This includes obtaining explicit consent from patients, providing transparent information about how data will be used, ensuring data security and enabling patients to exercise their data-related rights. Healthcare organisations must diligently train their staff to ensure compliance with patient security and privacy laws before deploying generative AI tools. Also, decentralisation technologies like blockchain, and federated learning, can be integrated with generative AI to enhance security in data share and storage.
Regarding the transparency of AI tools, research from Stanford University highlights that major providers of foundational models in the market have not strictly complied with the EU’s regulations. 41 Model providers may be reluctant to adhere strictly to transparency regulations, citing concerns related to both competitive factors and security considerations. To address these concerns and enhance transparency, regulators should also provide appropriate safeguards and guidance to model providers when regulating their activities.
Clinical validation
EU AI law mandates that synthetic content generation must adhere to legal requirements. However, within the healthcare context, legality alone is insufficient. Authentication and accuracy should also be put on the table. The Data Protection and Privacy Authorities (DPA) G7 underscores the importance of meticulously examining the interaction of individuals with generative AI and the processing of the content generated by generative AI tools. Cases with the data of minors should gain specific attention. Given that current models are typically trained on data from the general population, clinicians need to take care to determine whether a patient belongs to a vulnerable group or has characteristics that might make the model’s use inappropriate, to ensure patient safety.
Currently, clinical evaluations of LLMs primarily focus on accuracy. 42 To justify the use of LLMs for clinical applications, it is also essential to define other appropriate procedures and endpoints beyond effectiveness evaluation. Robust protocols are required to address security and privacy concerns. Before data is fed into the LLM, it is crucial to assess the success rate of pre-processing procedures, such as replacing, masking or anonymising to remove any personally identifiable information. Continuous monitoring of incoming requests in real-time helps to identify any instances where personally identifiable information reaches the LLM. This ensures that the LLM is working with data that has been thoroughly sanitised. In addition, the output generated by an LLM needs to be thoroughly evaluated against standard clinical practices. Content filtering and abuse monitoring can help evaluate every decision made by an LLM to detect and mitigate instances of harmful content generation. The most important part is clinician involvement in the quality appraisal process, as the unsupervised deployment of LLMs is considered impractical. 43 Clinicians need to evaluate whether the output of LLM is harmful to patients, may disclose individual information and introduce bias or discrimination into downstream clinical decisions.
Future perspectives
‘Many of the problems caused by AI can also be managed with the help of AI’, as said by Bill Gates. 44 During model development, data protection should be integrated into the system. DPA G7 suggested generative AI models to embed data protection throughout the product total lifecycle, based on the concept of ‘Privacy by Design’. Yang et al proposed using a digital mask to erase identifiable features while preserving the main features for ocular condition diagnosis. 45 By constructing synthetic data that behaves similarly to real clinical data, this privacy-protecting approach can be further enhanced with the help of generative AI. 45 46
The upcoming trend involves pre-trained large foundational models that serve as the cornerstone for various downstream AI applications. Two foundation models in ophthalmology, RETFound and VisionFM, have undergone training on millions of retinal images. They have effectively learnt general features from unlabeled data, showcasing remarkable capabilities in downstream tasks related to diagnosing ocular and systemic diseases. 47 48 The interaction of generative AI and the large foundation model may introduce new threats. For example, the frequently used pre-trained self-supervised learning encoder in foundational model techniques is susceptible to model stealing attacks. 49 These attacks not only endanger the model’s intellectual property but also potentially act as foreshadowing for subsequent attacks.
Current regulations are inadequate to address AI-related security and privacy concerns as they have already fallen outside the scope of national regulation for patient information protection. 50 Without expanding the regulatory framework, keeping up with the evolving landscape of generative AI can be difficult. Future regulatory perspectives may involve classifying AI software as medical devices, as the FDA has done, or shifting the regulatory focus from the AI models themselves to the providers, as the EU has pursued. Healthcare providers are the first line of defence for patient security and privacy. Generative AI and foundational models are ultimately tools designed to support healthcare services. It is important for future regulations to emphasise education for first-line healthcare service providers, on the responsible use of patient data to mitigate risks while maintaining accessibility and efficacy.
The full potential of generative AI technology can only be realised under effective governance. The principle of technology neutrality, where no specific technology is favoured or imposed, becomes crucial in the global competition to establish timely regulations for the burgeoning field of generative AI. There have been calls that AI regulations such as the EU AI Act need to take measures for sustainable development and innovation. 51 The Cyberspace Administration of China encourages innovation in generative AI services and lends support to the development of experimental research, while simultaneously emphasise responsibility for security and privacy. In the most recent recommendations from WHO, regulatory sandboxes are getting popular among regulators. 52 The Spanish government has approved the first AI regulatory sandbox pilot in 2023 as it allows the flexibility to test innovative products or services with minimal regulatory requirements and may foster AI innovation. 53 Achieving a trade-off between the advantages and risks associated with generative AI presents a tangible challenge for regulatory bodies in the near future.
Generative AI holds the potential to facilitate various aspects of clinical practice in ophthalmology and other healthcare domains. Prior to the implementation of this technology, it is essential to recognise the potential security and privacy risks associated with these models. Susceptible to both model-level and data-level attacks, generative models may cause severe consequences like extensive breaches of personal data, violation of patients’ privacy rights and safety. To address these concerns, it is crucial for model providers and users to proactively develop strategies that counteract attacks, enhance transparency and ensure robust validation throughout the entire product lifecycle, thus aligning with existing regulations. Furthermore, it is imperative to update the regulatory framework to keep pace with the ever-evolving landscape of generative AI, striking a balance between effective regulation and continued innovation.
Ethics statements
Patient consent for publication.
Not applicable.
Ethics approval
Acknowledgments.
We would like to acknowledge Professor Mingguang He and his research team for their kind suggestions during this study.
- Gulshan V ,
- Coram M , et al
- Pasquale LR ,
- Peng L , et al
- Gilbert S ,
- Melvin T , et al
- World Health Organization
- Zuiderveen Borgesius F
- Evgeniou T , et al
- Bockting CL ,
- van Dis EAM ,
- van Rooij R , et al
- Chia MA , et al
- Pei H , et al
- Hassner T , et al
- Sun L , et al
- Danezis G , et al
- Xu K , et al
- Ye D , et al
- Liang J , et al
- Ziegler DM ,
- Stiennon N ,
- Wu J , et al
- Saharia C ,
- Saxena S , et al
- Tavakkoli A ,
- Kamran SA ,
- Hossain KF , et al
- Reis-Filho JS ,
- Pushpanathan K , et al
- Carlini N ,
- Hayase J , et al
- Finckenberg-Broman P ,
- Hoang T , et al
- Patel M , et al
- Mihalache A ,
- Popovic MM , et al
- Temsah M-H ,
- Aljamaan F ,
- Malki KH , et al
- Potapenko I ,
- Boberg-Ans LC ,
- Stormly Hansen M , et al
- Waisberg E ,
- Masalkhi M , et al
- Giuffrè M ,
- Sajeeda A ,
- Hossain BMM
- Security FOfI
- Bommasani R ,
- Zhang D , et al
- Milad J , et al
- Thirunavukarasu AJ ,
- Elangovan K , et al
- Wang R , et al
- Plawinski J ,
- Subramaniam S , et al
- Wagner SK , et al
- Wei H , et al
- Benedikt Kohn LVN
- World Health O
YW and CL are joint first authors.
Contributors YW and CL developed the concept of the manuscript. YW, CL and KZ drafted the manuscript. XH and TZ made critical revisions of the manuscript. XH and TZ supervised the entire work. All authors approved the completed version. We used ChatGPT-4 only for proofreading and grammar checking of this review, but have not relied on the tool for any thinking or material presentation in this paper.
Funding This study was funded by the National Natural Science Foundation of China (82000901, 82101171), the Global STEM Professorship Scheme (P0046113), the Fundamental Research Funds of the State Key Laboratory of Ophthalmology (303060202400201209), the Outstanding PI Research Funds of the State Key Laboratory of Ophthalmology (3030902113083).
Competing interests None declared.
Provenance and peer review Not commissioned; externally peer reviewed.
Read the full text or download the PDF:
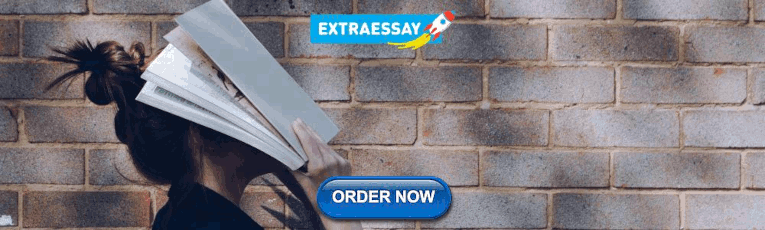
IMAGES
VIDEO
COMMENTS
Abstract — Artificial intelligence (AI) has advanced rapidly and is becoming a cornerstone. technology that drives innovation and efficiency in various industries. This paper examines. the real ...
3 The Economist Intelligence Unit Limited 2016 Artificial intelligence in the real world: The business case takes shape Artificial intelligence (AI) is no longer the future. For businesses, it is the here and now, and this study conducted by The Economist Intelligence Unit makes clear that executive suites and boardrooms around
40 Detailed Artificial Intelligence Case Studies [2024] In this dynamic era of technological advancements, Artificial Intelligence (AI) emerges as a pivotal force, reshaping the way industries operate and charting new courses for business innovation. This article presents an in-depth exploration of 40 diverse and compelling AI case studies from ...
The Road to Enterprise Artificial Intelligence: A Case Studies Driven Exploration by Saleha Saulat Siddique MBA (2009) University of California, Los Angeles Submitted to the System Design and Management Program in Partial Fulfillment of the Requirements for the Degree of Master of Science in Engineering and Management at the
Artificial intelligence is a field of study that describes the ability of machines to learn like humans and to respond to specific behaviors, also known as (A.I.) The intelligence displayed by machines or software is referred to as artificial intelligence. McCarthy coined the term in 1956.
A Case Study of Artificial Intelligence is being used to Reshape Business input and output, respectively. (iii) Expert System (ES)An expert system (ES) is a type of advanced human intelligence and expertise that solves complex problems and issues in a specific domain such as medicine, science, engineering, and so on.
The chapter presents several 'Cases of AI Use and Applications in every industry and business functions; putting artificial intelligence to work', evaluating and implementing business applications: Customer service, Consumer: Marketing and sales, Energy, resources and industries, Financial services and FinTech, Government and Public ...
The primary goal of artificial intelligence (AI) is to give computers the ability to do intellectual tasks such as making decisions, solving problems, seeing their surroundings, and understanding ...
Artificial Intelligence in New Zealand: Case Studies Summary . Monica Collier . July 2019 . ... IDC interviewed New Zealand AI vendors and end-user organisations for case studies for the following use cases: July 2019, AI Forum, CASE STUDY SUMMARY | 2 Digital Humans. FaceMe is a digital human platform that uses AI to create a natural humanlike ...
1. Introduction. Artificial intelligence (AI) is defined as the intelligence of machines, as opposed to the intelligence of humans or other living species [1], [2].AI can also be defined as the study of "intelligent agents"—that is, any agent or device that can perceive and understand its surroundings and accordingly take appropriate action to maximize its chances of achieving its ...
This study scrutinizes the role of AI literacy and ChatGPT-3 in enhancing critical reasoning and journalistic writing competencies among 50 third-term journalism students at Tajik National University.
Systems: Table 6 presents three scenarios for the development of AI systems, which are (1) AI as a prototype, (2) AI as a component and (3) AI as a stand-alone system. AI system is a prototype (Case 1, Case 4) and developed in a separate process with the main value propositions of the company or the development team.
Below are relevant case studies, with their impact description extract from the UK REF Impact Case Study database. The tables outline the Case Study highlighted and a summary of the impact derived from the REF database. 8.1.4.1 Life science impact case studies Case study Impact Intelligent Energy Management Unit of Assessment: Computer
The method is specially designed for the integration of human. aspects and artificial intelligence, so that it leads to the development of human-machine. cooperation. Two case studies are pre ...
OVERVIEW. The case studies below offer current examples, per subsector, of efforts underway in developing artificial intelligence (AI) tools and algorithms that may benefit the Emergency Services Sector (ESS). The ALERTCalifornia program is a collaborative effort between the University of California San Diego and the California Department of ...
This study investigates the ethical use of Big Data and Artificial Intelligence (AI) technologies (BD + AI)—using an empirical approach. The paper categorises the current literature and presents a multi-case study of 'on-the-ground' ethical issues that uses qualitative tools to analyse findings from ten targeted case-studies from a range of domains. The analysis coalesces identified singular ...
• India provides a case study of how a country is actively promoting the use of AI to address healthcare needs. However, the deployment of AI in India is still at a very nascent stage, particularly for clinical interventions. • The challenge of delivering quality healthcare at scale presents a strong case for developing
The IBM Case Study WHITE PAPER SEPTEMBER 2021 In collaboration with the Markkula Center for Applied Ethics at Santa Clara University, USA ... 1 The evolution of artificial intelligence ethics at IBM 2 Governance 2.1 AI Ethics Board 2.2 Local focal points and Advocacy Network 3 IBM's Principles for Trust and Transparency 3.1 The purpose of AI ...
A more rigorous definition can be given as "Artificial intelligence is the intelligence (that is similar to or the same kind as human intelligence) exhibited by machines (in the same task)". We can see that this new definition reveals the tight link between artificial intelligence and intelligence test. If and only if a machine finishes a ...
Case studies in Chapter 3 cover the particular risks associated with healthcare robots, which may be involved in diagnosis, surgery and monitoring health and well-being as well as providing caring services. The first case study highlights particular risks associated with embodied AI, which have moving parts that can cause injury.
ICBAR '23: Proceedings of the 2023 3rd International Conference on Big Data, Artificial Intelligence and Risk Management November 2023 Pages 940-946 https: ... A Case Study of Wuhan. Pages 940-946. Previous Chapter Next Chapter. ... View or Download as a PDF file. PDF. eReader. View online with eReader. eReader. Digital Edition.
publication in the international journal, newspaper articles and a few other sources. Limitation: This study is limited for case study on tesla cars and use of AI. Solid State Technology. Volume ...
New technologies, they claim, make the economy more productive and allow people to enter new fields — like the shift from agriculture to manufacturing. For that reason, economists have ...
Artificial intelligence test: a case study of intelligent. vehicles. Li Li 1·Yi-Lun Lin2,5·Nan-Ning Zheng3·Fei-Yue Wang2,5·Yu e h u Liu3·. Dongpu Cao 4,6·Kunfeng Wang2·Wu-Ling Huang2 ...
• The purpose of this study is to assess the performance of an artificial intelligence (AI) algorithm to detect cardiomyopathies, a type of structural heart disease, using the electrocardiogram (ECG) obtained by portable ECG devices. • For this study, in addition to your routine clinical care, including but not limited to an
As the healthcare community increasingly harnesses the power of generative artificial intelligence (AI), critical issues of security, privacy and regulation take centre stage. In this paper, we explore the security and privacy risks of generative AI from model-level and data-level perspectives. Moreover, we elucidate the potential consequences and case studies within the domain of ophthalmology.