A literature review of emotional intelligence and nursing education
Affiliations.
- 1 Université Rouen Normandie, 76130 Mont Saint Aignan, France; EA3832 Centre d'Etudes des Transformations des Activités Physiques et Sportives, 76821 Mont Saint Aignan, France. Electronic address: [email protected].
- 2 Université Rouen Normandie, 76130 Mont Saint Aignan, France; EA3832 Centre d'Etudes des Transformations des Activités Physiques et Sportives, 76821 Mont Saint Aignan, France.
- 3 Université Caen Normandie, UMR-S 1075, 14032 Caen, France; INSERM, UMR-S 1075 COMETE, 14032 Caen, France.
- PMID: 34175653
- DOI: 10.1016/j.nepr.2021.103124
Aim: The purpose of this study is to investigate the current state of knowledge about emotional intelligence (EI) in nursing education BACKGROUND: Human relationships and emotions are an integral part of nursing care and contribute to care quality. This part of the profession must be addressed in nursing education to prepare students and deal with their emotions and the patients's. Research on emotional intelligence (EI) in nursing has been particularly developed recently. A broad understanding of many benefits of EI is crucial to include EI in this training program.
Design: Systematic literature review METHODS: Undertaken using electronic database (Cairn; PubMed; Science Direct; Wiley online library) and specific search terms. This research covered articles published in English and French between 2007 and 2021. The Preferred Reporting Items for Systematic Reviews and Meta-Analysis guidelines (PRISMA) directed the systematic review process.
Results: 57 articles are included in this systematic review. The literature focuses on EI and nursing education. The findings categorized into four themes: EI and performances; EI and Physical and mental health; EI and Social Relationship; and EI program.
Conclusions: This literature review reveals that EI has many benefits in nursing students. Several training programs aimed at developing this concept have proven to be effective in nursing education. We believe that a synthesis of this knowledge will then allow us to better understand the benefits of EI for a further implementation in nursing education. Perspectives and recommendations will also be formulated to enable the implementation of emotional skill development programs in such training.
Keywords: Emotional intelligence; Health; Nursing education; Performances; Social relationships; Training.
Copyright © 2021 Elsevier Ltd. All rights reserved.
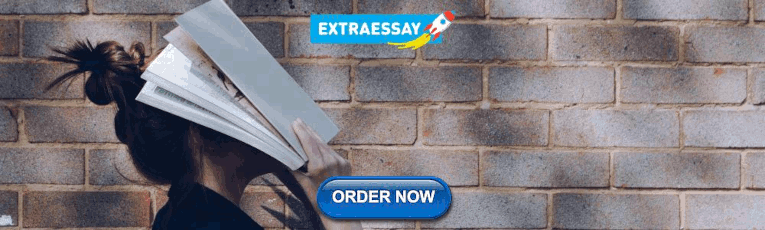
Publication types
- Systematic Review
- Education, Nursing*
- Emotional Intelligence
- Mental Health
- Students, Nursing*
This paper is in the following e-collection/theme issue:
Published on 29.4.2024 in Vol 26 (2024)
The Applications of Artificial Intelligence for Assessing Fall Risk: Systematic Review
Authors of this article:

- Ana González-Castro 1 , PT, MSc ;
- Raquel Leirós-Rodríguez 2 , PT, PhD ;
- Camino Prada-García 3 , MD, PhD ;
- José Alberto Benítez-Andrades 4 , PhD
1 Nursing and Physical Therapy Department, Universidad de León, Ponferrada, Spain
2 SALBIS Research Group, Nursing and Physical Therapy Department, Universidad de León, Ponferrada, Spain
3 Department of Preventive Medicine and Public Health, Universidad de Valladolid, Valladolid, Spain
4 SALBIS Research Group, Department of Electric, Systems and Automatics Engineering, Universidad de León, León, Spain
Corresponding Author:
Ana González-Castro, PT, MSc
Nursing and Physical Therapy Department
Universidad de León
Astorga Ave
Ponferrada, 24401
Phone: 34 987442000
Email: [email protected]
Background: Falls and their consequences are a serious public health problem worldwide. Each year, 37.3 million falls requiring medical attention occur. Therefore, the analysis of fall risk is of great importance for prevention. Artificial intelligence (AI) represents an innovative tool for creating predictive statistical models of fall risk through data analysis.
Objective: The aim of this review was to analyze the available evidence on the applications of AI in the analysis of data related to postural control and fall risk.
Methods: A literature search was conducted in 6 databases with the following inclusion criteria: the articles had to be published within the last 5 years (from 2018 to 2024), they had to apply some method of AI, AI analyses had to be applied to data from samples consisting of humans, and the analyzed sample had to consist of individuals with independent walking with or without the assistance of external orthopedic devices.
Results: We obtained a total of 3858 articles, of which 22 were finally selected. Data extraction for subsequent analysis varied in the different studies: 82% (18/22) of them extracted data through tests or functional assessments, and the remaining 18% (4/22) of them extracted through existing medical records. Different AI techniques were used throughout the articles. All the research included in the review obtained accuracy values of >70% in the predictive models obtained through AI.
Conclusions: The use of AI proves to be a valuable tool for creating predictive models of fall risk. The use of this tool could have a significant socioeconomic impact as it enables the development of low-cost predictive models with a high level of accuracy.
Trial Registration: PROSPERO CRD42023443277; https://tinyurl.com/4sb72ssv
Introduction
According to alarming figures reported by the World Health Organization in 2021, falls cause 37.3 million injuries annually that require medical attention and result in 684,000 deaths [ 1 ]. These figures indicate a significant impact of falls on the health care system and on society, both directly and indirectly [ 2 , 3 ].
Life expectancy has progressively increased over the years, leading to an aging population [ 4 ]. By 2050, it is estimated that 16% of the population will be >65 years of age. In this group, the incidence of falls has steadily risen, becoming the leading cause of accidental injury and death (accounting for 55.8% of such deaths, according to some research) [ 5 , 6 ]. It is estimated that 30% of this population falls at least once a year, negatively impacting their physical and psychological well-being [ 7 , 8 ].
Physically, falls are often associated with severe complications that can lead to extended hospitalizations [ 9 ]. These hospitalizations are usually due to serious injuries, often cranioencephalic trauma, fractures, or soft tissue injuries [ 10 , 11 ]. Psychologically, falls among the older adult population tend to result in self-imposed limitations due to the fear of falling again [ 10 , 12 ]. These limitations lead to social isolation as individuals avoid participating in activities or even individual mobility [ 13 ]. Consequently, falls can lead to psychological conditions such as anxiety and depression [ 14 , 15 ]. Numerous research studies on the risk of falls are currently underway, with ongoing investigations into various innovations and intervention ideas [ 16 - 19 ]. These studies encompass the identification of fall risk factors [ 20 , 21 ], strategies for prevention [ 22 , 23 ], and the outcomes following rehabilitation [ 23 , 24 ].
In the health care field, artificial intelligence (AI) is characterized by data management and processing, offering new possibilities to the health care paradigm [ 24 ]. Some applications of AI in the health care domain include assessing tumor interaction processes [ 25 ], serving as a tool for image-based diagnostics [ 26 , 27 ], participating in virus detection [ 28 ], and, most importantly, as a statistical and predictive method [ 29 - 32 ].
Several publications have combined AI techniques to address health care issues [ 33 - 35 ]. Within the field of predictive models, it is important to understand certain differentiations. In AI, we have machine learning and deep learning [ 36 - 38 ]. Machine learning encompasses a set of techniques applied to data and can be done in a supervised or unsupervised manner [ 39 , 40 ]. On the other hand, deep learning is typically used to work with larger data sets compared to machine learning, and its computational cost is higher [ 41 , 42 ].
Some examples of AI techniques include the gradient boosting machine [ 43 ], learning method, and the long short-term memory (LSTM) [ 44 ] and the convolutional neural network (CNN) [ 45 ], all of them are deep learning methods.
For all the reasons mentioned in the preceding section, it was considered necessary to conduct a systematic review to analyze the scientific evidence of AI applications in the analysis of data related to postural control and the risk of falls.
Data Sources and Searches
This systematic review and meta-analysis were prospectively registered on PROSPERO (ID CRD42023443277) and followed the Meta-Analyses of Observational Studies in Epidemiology checklist [ 46 ] and the recommendations of the Cochrane Collaboration [ 47 ].
The search was conducted in January 2024 on the following databases: PubMed, Scopus, ScienceDirect, Web of Science, CINAHL, and Cochrane Library. The Medical Subject Headings (MeSH) terms used for the search included machine learning , artificial intelligent , accidental falls , rehabilitation , and physical therapy specialty . The terms “predictive model” and “algorithms” were also used. These terms were combined using the Boolean operators AND and OR ( Textbox 1 ).
- (“machine learning”[MeSH Terms] OR “artificial intelligent”[MeSH Terms]) AND “accidental falls”[MeSH Terms]
- (“machine learning”[MeSH Terms] OR “artificial intelligent”) AND (“rehabilitation”[MeSH Terms] OR “physical therapy specialty”[MeSH Terms])
- “accidental falls” [Title/Abstract] AND “algorithms” [Title/Abstract]
- “accidental falls”[Title/Abstract] AND “predictive model” [Title/Abstract]
- TITLE-ABS-KEY (“machine learning” OR “artificial intelligent”) AND TITLE-ABS-KEY (“accidental falls”)
- TITLE-ABS-KEY (“machine learning” OR “artificial intelligent”) AND TITLE-ABS-KEY (“rehabilitation” OR “physical therapy specialty”)
- TITLE-ABS-KEY (“accidental falls” AND “algorithms”)
- TITLE-ABS-KEY (“accidental falls” AND “predictive model”)
ScienceDirect
- Title, abstract, keywords: (“machine learning” OR “artificial intelligent”) AND “accidental falls”
- Title, abstract, keywords: (“machine learning” OR “artificial intelligent”) AND (“rehabilitation” OR “physical therapy specialty”)
- Title, abstract, keywords: (“accidental falls” AND “algorithms”)
- Title, abstract, keywords: (“accidental falls” AND “predictive model”)
Web of Science
- TS=(“machine learning” OR “artificial intelligent”) AND TS=“accidental falls”
- TS=(“machine learning” OR “artificial intelligent”) AND TS= (“rehabilitation” OR “physical therapy specialty”)
- AB= (“accidental falls” AND “algorithms”)
- AB= (“accidental falls” AND “predictive model”)
- (MH “machine learning” OR MH “artificial intelligent”) AND MH “accidental falls”
- (MH “machine learning” OR MH “artificial intelligent”) AND (MH “rehabilitation” OR MH “physical therapy specialty”)
- (AB “accidental falls”) AND (AB “algorithms”)
- (AB “accidental falls”) AND (AB “predictive model”)
Cochrane Library
- (“machine learning” OR “artificial intelligent”) in Title Abstract Keyword AND “accidental falls” in Title Abstract Keyword
- (“machine learning” OR “artificial intelligent”) in Title Abstract Keyword AND (“rehabilitation” OR “physical therapy specialty”) in Title Abstract Keyword
- “accidental falls” in Title Abstract Keyword AND “algorithms” in Title Abstract Keyword
- “accidental falls” in Title Abstract Keyword AND “predictive model” in Title Abstract Keyword
Study Selection
After removing duplicates, 2 reviewers (AGC and RLR) independently screened articles for eligibility. In the case of disagreement, a third reviewer (JABA) finally decided whether the study should be included or not. We calculated the κ coefficient and percentage agreement scores to assess reliability before any consensus and estimated the interrater reliability using κ. Interrater reliability was estimated using κ>0.7 indicating a high level of agreement between the reviewers, κ of 0.5 to 0.7 indicating a moderate level of agreement, and κ<0.5 indicating a low level of agreement [ 48 ].
For the selection of results, the inclusion criteria were established as follows: (1) articles should have been published in the last 5 years (from 2018 to the present); (2) they must apply some AI method; (3) AI analyses should be applied to data from samples of humans; and (4) the sample analyzed should consist of people with independent walking, with or without the use of external orthopedic devices.
After screening the data, extracting, obtaining, and screening the titles and abstracts for inclusion criteria, the selected abstracts were obtained in full texts. Titles and abstracts lacking sufficient information regarding inclusion criteria were also obtained as full texts. Full-text articles were selected in case of compliance with inclusion criteria by the 2 reviewers using a data extraction form.
Data Extraction and Quality Assessment
The 2 reviewers mentioned independently extracting data from the included studies using a customized data extraction table in Excel (Microsoft Corporation). In case of disagreement, both reviewers debated until an agreement was reached.
The data extracted from the included articles for further analysis were: demographic information (title, authors, journal, and year), characteristics of the sample (age, inclusion and exclusion criteria, and number of participants), study-specific parameters (study type, AI techniques applied, and data analyzed), and the results obtained. Tables were used to describe both the studies’ characteristics and the extracted data.
Assessment of Risk of Bias
The methodological quality of the selected articles was evaluated using the Critical Review Form for Quantitative Studies [ 49 ]. The ROBINS-E (Risk of Bias in Nonrandomized Studies of Exposures) tool was used to evaluate the risk of bias [ 50 ].
Characteristics of the Selected Studies
A total of 3858 articles were initially retrieved, with 1563 duplicates removed. From the remaining 2295 articles, 2271 were excluded based on the initial selection criteria, leaving 24 articles for the subsequent analysis. In this second analysis, 2 articles were removed as they were systematic reviews, and 22 articles were finally selected [ 51 - 72 ] ( Figure 1 ). After the first reading of all candidate full texts, the kappa score for inclusion of the results of reviewers 1 and 2 was 0.98, indicating a very high level of agreement.
The methodological quality of the 22 analyzed studies (Table S1 in Multimedia Appendix 1 [ 51 , 52 , 54 , 56 , 58 , 59 , 61 , 63 , 64 , 69 , 70 , 72 ]) ranged from 11 points in 2 (9.1%) studies [ 52 , 65 ] to 16 points in 7 (32%) studies [ 53 , 54 , 56 , 63 , 69 - 71 ].

Study Characteristics and Risk of Bias
All the selected articles were cross-sectional observational studies ( Table 1 ).
In total, 34 characteristics affecting the risk of falls were extracted and classified into high fall-risk and low fall-risk groups with the largest sample sizes significantly differing from the rest. Studies based on data collected from various health care systems had larger sample sizes, ranging from 22,515 to 265,225 participants [ 60 , 65 , 67 ]. In contrast, studies that applied some form of evaluation test had sample sizes ranging from 8 participants [ 56 ] to 746 participants [ 55 ].
It is worth noting the various studies conducted by Dubois et al [ 54 , 72 ], whose publications on fall risk and machine learning started in 2018 and progressed until 2021. A total of 9.1% (2/22) of the articles by this author were included in the final selection [ 54 , 72 ]. Both articles used samples with the same characteristics, even though the first one was composed of 43 participants [ 54 ] and the last one had 30 participants [ 72 ]. All 86.4% (19/22) of the articles used samples of individuals aged ≥65 years [ 51 - 60 , 62 - 65 , 68 - 72 ]. In the remaining 13.6% (3/22) of the articles, the ages ranged between 16 and 62 years [ 61 , 66 , 67 ].
Althobaiti et al [ 61 ] used a sample of participants between the ages of 19 and 35 years for their research, where these participants had to reproduce examples of falls for subsequent analysis. In 2022, Ladios-Martin et al [ 67 ] extracted medical data from participants aged >16 years for their research. Finally, in 2023, the study by Maray et al [ 66 ] used 3 types of samples, with ages ranging from 21 to 62 years. Among the 22 selected articles, only 1 (4.5%) of them did not describe the characteristics of its sample [ 52 ].
Finally, regarding the sex of the samples, 13.6% (3/22) of the articles specified in the characteristics of their samples that only female individuals were included among their participants [ 53 , 59 , 70 ].
a AI: artificial intelligence.
b ML: machine learning.
c nd: none described.
d ADL: activities of daily living.
e TUG: Timed Up and Go.
f BBS: Berg Balance Scale.
g ASM: associative skill memories.
h CNN: convolutional neural network.
i FP: fall prevention.
j IMU: inertial measurement unit.
k AUROC: area under the receiver operating characteristic curve.
l AUPR: area under the precision-recall curve.
m MFS: Morse Fall Scale.
n XGB: extreme gradient boosting.
o MCT: motor control test.
p GBM: gradient boosting machine.
q RF: random forest.
r LOOCV: leave-one-out cross-validation.
s LSTM: long short-term memory.
Applied Assessment Procedures
All articles initially analyzed the characteristics of their samples to subsequently create a predictive model of the risk of falls. However, they did not all follow the same evaluation process.
Regarding the applied assessment procedures, 3 main options stood out: studies with tests or assessments accompanied by sensors or accelerometers [ 51 - 57 , 59 , 61 - 63 , 66 , 70 - 72 ], studies with tests or assessments accompanied by cameras [ 68 , 69 ], or studies based on medical records [ 58 , 60 , 65 , 67 ] ( Figure 2 ). Guillan et al [ 64 ] performed a physical and functional evaluation of the participants. In their study, they evaluated parameters such as walking speed, stride frequency and length, and the minimum space between the toes. Afterward, they asked them to record the fall events they had during the past 2 years in a personal diary.

In total, 22.7% (5/22) of the studies used the Timed Up and Go test [ 53 , 54 , 69 , 71 , 72 ]. In 18.2% (4/22) of them, the participants performed the test while wearing a sensor to collect data [ 53 , 54 , 71 , 72 ]. In 1 (4.5%) study, the test was recorded with a camera for later analysis [ 69 ]. Another commonly used method in studies was to ask participants to perform everyday tasks or activities of daily living while a sensor collected data for subsequent analysis. Specifically, 18.2% (4/22) of the studies used this method to gather data [ 51 , 56 , 61 , 62 ].
A total of 22.7% (5/22) of the studies asked participants to simulate falls and nonfalls while a sensor collected data [ 52 , 61 - 63 , 66 ]. In this way, the data obtained were used to create the predictive model of falls. As for the tests used, Eichler et al [ 68 ] asked participants to perform the Berg Balance Scale while a camera recorded their performance.
Finally, other authors created their own battery of tests for data extraction [ 55 , 59 , 64 , 70 ]. Gillain et al [ 64 ] used gait records to analyze speed, stride length, frequency, symmetry, regularity, and foot separation. Hu et al [ 59 ] asked their participants to perform normal walking, the postural reflexive response test, and the motor control test. In the study by Noh et al [ 55 ], gait tests were conducted, involving walking 20 m at different speeds. Finally, Greene et al [ 70 ] created a 12-question questionnaire and asked their participants to maintain balance while holding a mobile phone in their hand.
AI Techniques
The selected articles used various techniques within AI. They all had the same objective in applying these techniques, which was to achieve a predictive and classification model for the risk of falls [ 51 - 72 ].
In chronological order, in 2018, Nait Aicha et al [ 51 ] compared single-task learning models with multitask learning, obtaining better evaluation results through multitask learning. In the same year, Dubois et al [ 54 ] applied AI techniques that analyzed multiple parameters to classify the risk of falls in their sample. Qiu et al [ 53 ], also in the same year, used 6 machine learning models (logistic regression, naïve Bayes, decision tree, RF, boosted tree, and support vector machine) in their research.
In contrast, in 2019, Ferrete et al [ 52 ] compared the applicability of 2 different deep learning models: the classifier based on associative skill memories and a CNN classifier. In the same year, after confirming the applicability of AI as a predictive method for the risk of falls, various authors investigated through methods such as the RF to identify factors that can predict and quantify the risk of falls [ 63 , 65 ].
Among the selected articles, 5 (22.7%) were published in 2020 [ 58 - 62 ]. The research conducted by Tunca et al [ 62 ] compared the applicability of deep learning LSTM networks with traditional machine learning applied to the risk of falls. Hu et al [ 59 ] first used cross-validation, where algorithms were trained randomly, and then used the gradient boosting machine algorithm to classify participants as high or low risk. Ye et al [ 60 ] and Hsu et al [ 58 ] both used the extreme gradient boosting (XGBoost) algorithm based on machine learning to create their predictive model. In the same year, Althobaiti et al [ 61 ] trained machine learning models for their research.
In 2021, Lockhart et al [ 57 ] started using 3 deep learning techniques simultaneously with the same goal as before: to create a predictive model for the risk of falls. Specifically, they used the RF, RF with feature engineering, and RF with feature engineering and linear and nonlinear variables. Noh et al [ 55 ], again in the same year, used the XGBoost algorithm, while Roshdibenam et al [ 71 ], on the other hand, used the CNN algorithm for each location of the wearable sensors used in their research. Various machine learning techniques were used for classifying the risk of falls and for balance loss events in the research by Hauth et al [ 56 ]. Dubois et al [ 72 ] used the following algorithms: decision tree, adaptive boosting, neural net, naïve Bayes, k-nearest neighbors, linear support vector machine, radial basis function support vector machine, RF, and quadratic discriminant analysis. Hauth et al [ 56 ], on the other hand, used regularized logistic regression and bidirectional LSTM networks. In the research conducted by Greene et al [ 70 ], AI was used, but the specific procedure that they followed is not described.
Tang et al [ 69 ] published their research with innovation up to that point. In their study, they used a smart gait analyzer with the help of deep learning techniques to assess the diagnostic accuracy of fall risk through vision. Months later, in August 2022, Ladios-Martin et al [ 67 ] published their research, in which they compared 2 deep learning models to achieve the best results in terms of specificity and sensitivity in detecting fall risk. The first model used the Bayesian Point Machine algorithm with a fall prevention variable, and the second one did not use the variable. They obtained better results when using that variable, a mitigating factor defined as a set of care interventions carried out by professionals to prevent the patient from experiencing a fall during hospitalization. Particularly controversial, as its exclusion could obscure the model’s performance. Eichler et al [ 68 ], on the other hand, used machine learning–based classifier training and later tested the performance of RFs in score predictions.
Finally, in January 2023, Maray et al [ 66 ] published their research, linking the previously mentioned terms (AI and fall risk) with 3 wearable devices that are commonly used today. They collected data through these devices and applied transfer learning to generalize the model across heterogeneous devices.
The results of the 22 articles provided promising data, and all of them agreed on the feasibility of applying various AI techniques as a method for predicting and classifying the risk of falls. Specifically, the accuracy values obtained in the studies exceed 70%. Noh et al [ 55 ] achieved the “lowest” accuracy among the studies conducted, with a 70% accuracy rate. Ribeiro et al [ 52 ] obtained an accuracy of 92.7% when using CNN to differentiate between normal gait and fall events. Hsu et al [ 58 ] further demonstrated that the XGBoost model is more sensitive than the Morse Fall Scale. Similarly, in their comparative study, Nait Aicha et al [ 51 ] also showed that a predictive model created from accelerometer data with AI is comparable to conventional models for assessing the risk of falls. More specifically, Dubois et al [ 54 ] concluded that using 1 gait-related parameter (excluding velocity) in combination with another parameter related to seated position allowed for the correct classification of individuals according to their risk of falls.
Principal Findings
The aim of this research was to analyze the scientific evidence regarding the applications of AI in the analysis of data related to postural control and the risk of falls. On the basis of the analysis of results, it can be asserted that the following risk factors were identified in the analyzed studies: age [ 65 ], daily habits [ 65 ], clinical diagnoses [ 65 ], environmental and hygiene factors [ 65 ], sex [ 64 ], stride length [ 55 , 72 ], gait speed [ 55 ], and posture [ 55 ]. This aligns with other research that also identifies sex [ 73 , 74 ], age [ 73 ], and gait speed [ 75 ].
On the other hand, the “fear of falling” has been identified in various studies as a risk factor and a predictor of falls [ 73 , 76 ], but it was not identified in any of the studies included in this review.
As for the characteristics of the analyzed samples, only 9.1% (2/22) of the articles used a sample composed exclusively of women [ 53 , 59 ], and no article used a sample composed exclusively of men. This fact is incongruent with reality, as women have a longer life expectancy than men, and therefore, the number of women aged >65 years is greater than the number of men of the same age [ 77 ]. Furthermore, women experience more falls than men [ 78 ]. The connection between menopause and its consequences, including osteopenia, suggests a higher risk of falls among older women than among men of the same age [ 79 , 80 ].
Within the realm of analysis tools, the most frequently used devices to analyze participants were accelerometers [ 51 - 57 , 59 , 61 - 63 , 66 , 70 - 72 ]. However, only 36.4% (8/22) of the studies provided all the information regarding the characteristics of these devices [ 51 , 53 , 59 , 61 , 63 , 66 , 70 , 72 ]. On the other hand, 18.2% (4/22) of the studies used the term “inertial measurement unit” as the sole description of the devices used [ 55 - 57 , 71 ].
The fact that most of the analyzed procedures involved the use of inertial sensors reflects the current widespread use of these devices for postural control analysis. These sensors, in general (and triaxial accelerometers in particular), have demonstrated great diagnostic capacity for balance [ 81 ]. In addition, they exhibit good sensitivity and reliability, combined with their portability and low economic cost [ 82 ]. Another advantage of triaxial accelerometers is their versatility in both adult and pediatric populations [ 83 - 86 ], although the studies included in this review did not address the pediatric population.
The remaining studies extracted data from cameras [ 68 , 69 ], medical records [ 58 , 60 , 65 , 67 ], and other functional and clinical tests [ 59 , 64 , 70 ]. Regarding the AI techniques used, out of the 18.2% (4/22) of articles that used deep learning techniques [ 52 , 57 , 62 , 71 ], only 4.5% (1/22) did not provide a description of the sample characteristics [ 52 ]. In this case, the authors focused on the AI landscape, while the rest of the articles strike a balance between AI and health sciences.
Regarding the validity of the generated models, only 40.9% (9/22) of the articles assessed this characteristic [ 52 , 53 , 55 , 61 - 64 , 68 , 69 ]. The authors of these 9 (N=22, 40.9%) articles evaluated the validity of the models through accuracy. All the results obtained reflected accuracies exceeding 70%, with Ribeiro et al [ 52 ] achieving a notable accuracy of 92.7% and 100%. Specifically, they obtained a 92.7% accuracy through the CNN model for distinguishing normal gait, the prefall condition, and the falling situation, considering the step before the fall, and 100% when not considering it [ 52 ].
The positive results of sensitivity and specificity can only be compared between the studies of Qiu et al [ 53 ] and Gillain et al [ 64 ], as they were the only ones to take them into account, and in both investigations, they were very high. Similarly, in the case of the F 1 -score, only Althobaiti et al [ 61 ] examined this validity measure. This measure is the result of combining precision and recall into a single figure, and the outcome obtained by these researchers was promising.
Despite these differences, the 22 studies obtained promising results in the health care field [ 51 - 72 ]. Specifically, their outcomes highlight the potential of AI integration into clinical settings. However, further research is necessary to explore how health care professionals can effectively use these predictive models. Consequently, future research should focus on studying the application and integration of the already-developed models. In this context, fall prevention plans could be implemented for the target populations identified by the predictive models. This approach would allow for a retrospective analysis to determine if the combination of predictive models with prevention programs effectively reduces the prevalence of falls in the population.
Limitations
Regarding limitations, the articles showed significant variation in the sample sizes selected. Moreover, even in the study with the largest sample size (with 265,225 participants [ 60 ]), the amount of data analyzed was relatively small. In addition, several of the databases used were not generated specifically for the published research but rather derived from existing medical records [ 58 , 60 , 65 , 67 ]. This could explain the significant variability in the variables analyzed across different studies.
Despite the limitations, this research has strengths, such as being the first systematic review on the use of AI as a tool to analyze postural control and the risk of falls. Furthermore, a total of 6 databases were used for the literature search, and a comprehensive article selection process was carried out by 3 researchers. Finally, only cross-sectional observational studies were selected, and they shared the same objective.
Conclusions
The use of AI in the analysis of data related to postural control and the risk of falls proves to be a valuable tool for creating predictive models of fall risk. It has been identified that most AI studies analyze accelerometer data from sensors, with triaxial accelerometers being the most frequently used.
For future research, it would be beneficial to provide more detailed descriptions of the measurement procedures and the AI techniques used. In addition, exploring larger databases could lead to the development of more robust models.
Conflicts of Interest
None declared.
Quality scores of reviewed studies (Critical Review Form for Quantitative Studies tool results).
PRISMA (Preferred Reporting Items for Systematic Reviews and Meta-Analyses) checklist.
- Step safely: strategies for preventing and managing falls across the life-course. World Health Organization. 2021. URL: https://www.who.int/publications/i/item/978924002191-4 [accessed 2024-04-02]
- Keall MD, Pierse N, Howden-Chapman P, Guria J, Cunningham CW, Baker MG. Cost-benefit analysis of fall injuries prevented by a programme of home modifications: a cluster randomised controlled trial. Inj Prev. Feb 2017;23(1):22-26. [ CrossRef ] [ Medline ]
- Almada M, Brochado P, Portela D, Midão L, Costa E. Prevalence of falls and associated factors among community-dwelling older adults: a cross-sectional study. J Frailty Aging. 2021;10(1):10-16. [ CrossRef ] [ Medline ]
- Menéndez-González L, Izaguirre-Riesgo A, Tranche-Iparraguirre S, Montero-Rodríguez Á, Orts-Cortés MI. [Prevalence and associated factors of frailty in adults over 70 years in the community]. Aten Primaria. Dec 2021;53(10):102128. [ FREE Full text ] [ CrossRef ] [ Medline ]
- Guirguis-Blake JM, Michael YL, Perdue LA, Coppola EL, Beil TL. Interventions to prevent falls in older adults: updated evidence report and systematic review for the US preventive services task force. JAMA. Apr 24, 2018;319(16):1705-1716. [ CrossRef ] [ Medline ]
- Pereira CB, Kanashiro AM. Falls in older adults: a practical approach. Arq Neuropsiquiatr. May 2022;80(5 Suppl 1):313-323. [ FREE Full text ] [ CrossRef ] [ Medline ]
- Byun M, Kim J, Kim M. Physical and psychological factors affecting falls in older patients with arthritis. Int J Environ Res Public Health. Feb 09, 2020;17(3):1098. [ FREE Full text ] [ CrossRef ] [ Medline ]
- Goh HT, Nadarajah M, Hamzah NB, Varadan P, Tan MP. Falls and fear of falling after stroke: a case-control study. PM R. Dec 04, 2016;8(12):1173-1180. [ CrossRef ] [ Medline ]
- Alanazi FK, Lapkin S, Molloy L, Sim J. The impact of safety culture, quality of care, missed care and nurse staffing on patient falls: a multisource association study. J Clin Nurs. Oct 12, 2023;32(19-20):7260-7272. [ CrossRef ] [ Medline ]
- Hossain A, Lall R, Ji C, Bruce J, Underwood M, Lamb SE. Comparison of different statistical models for the analysis of fracture events: findings from the Prevention of Falls Injury Trial (PreFIT). BMC Med Res Methodol. Oct 02, 2023;23(1):216. [ FREE Full text ] [ CrossRef ] [ Medline ]
- Williams CT, Whyman J, Loewenthal J, Chahal K. Managing geriatric patients with falls and fractures. Orthop Clin North Am. Jul 2023;54(3S):e1-12. [ CrossRef ] [ Medline ]
- Gadhvi C, Bean D, Rice D. A systematic review of fear of falling and related constructs after hip fracture: prevalence, measurement, associations with physical function, and interventions. BMC Geriatr. Jun 23, 2023;23(1):385. [ FREE Full text ] [ CrossRef ] [ Medline ]
- Lohman MC, Fallahi A, Mishio Bawa E, Wei J, Merchant AT. Social mediators of the association between depression and falls among older adults. J Aging Health. Aug 12, 2023;35(7-8):593-603. [ CrossRef ] [ Medline ]
- Smith AD, Silva AO, Rodrigues RA, Moreira MA, Nogueira JD, Tura LF. Assessment of risk of falls in elderly living at home. Rev Lat Am Enfermagem. Apr 06, 2017;25:e2754. [ FREE Full text ] [ CrossRef ] [ Medline ]
- Koh V, Matchar DB, Chan A. Physical strength and mental health mediate the association between pain and falls (recurrent and/or injurious) among community-dwelling older adults in Singapore. Arch Gerontol Geriatr. Sep 2023;112:105015. [ CrossRef ] [ Medline ]
- Soh SE, Morgan PE, Hopmans R, Barker AL, Ackerman IN. The feasibility and acceptability of a falls prevention e-learning program for physiotherapists. Physiother Theory Pract. Mar 18, 2023;39(3):631-640. [ CrossRef ] [ Medline ]
- Morat T, Snyders M, Kroeber P, De Luca A, Squeri V, Hochheim M, et al. Evaluation of a novel technology-supported fall prevention intervention - study protocol of a multi-centre randomised controlled trial in older adults at increased risk of falls. BMC Geriatr. Feb 18, 2023;23(1):103. [ FREE Full text ] [ CrossRef ] [ Medline ]
- You T, Koren Y, Butts WJ, Moraes CA, Yeh GY, Wayne PM, et al. Pilot studies of recruitment and feasibility of remote Tai Chi in racially diverse older adults with multisite pain. Contemp Clin Trials. May 2023;128:107164. [ CrossRef ] [ Medline ]
- Aldana-Benítez D, Caicedo-Pareja MJ, Sánchez DP, Ordoñez-Mora LT. Dance as a neurorehabilitation strategy: a systematic review. J Bodyw Mov Ther. Jul 2023;35:348-363. [ FREE Full text ] [ CrossRef ] [ Medline ]
- Jawad A, Baattaiah BA, Alharbi MD, Chevidikunnan MF, Khan F. Factors contributing to falls in people with multiple sclerosis: the exploration of the moderation and mediation effects. Mult Scler Relat Disord. Aug 2023;76:104838. [ CrossRef ] [ Medline ]
- Warren C, Rizo E, Decker E, Hasse A. A comprehensive analysis of risk factors associated with inpatient falls. J Patient Saf. Oct 01, 2023;19(6):396-402. [ CrossRef ] [ Medline ]
- Gross M, Roigk P, Schoene D, Ritter Y, Pauly P, Becker C, et al. Bundesinitiative Sturzprävention. [Update of the recommendations of the federal falls prevention initiative-identification and prevention of the risk of falling in older people living at home]. Z Gerontol Geriatr. Oct 11, 2023;56(6):448-457. [ CrossRef ] [ Medline ]
- Li S, Li Y, Liang Q, Yang WJ, Zi R, Wu X, et al. Effects of tele-exercise rehabilitation intervention on women at high risk of osteoporotic fractures: study protocol for a randomised controlled trial. BMJ Open. Nov 07, 2022;12(11):e064328. [ FREE Full text ] [ CrossRef ] [ Medline ]
- Jiang F, Jiang Y, Zhi H, Dong Y, Li H, Ma S, et al. Artificial intelligence in healthcare: past, present and future. Stroke Vasc Neurol. Dec 2017;2(4):230-243. [ FREE Full text ] [ CrossRef ] [ Medline ]
- Ye Y, Wu X, Wang H, Ye H, Zhao K, Yao S, et al. Artificial intelligence-assisted analysis for tumor-immune interaction within the invasive margin of colorectal cancer. Ann Med. Dec 2023;55(1):2215541. [ FREE Full text ] [ CrossRef ] [ Medline ]
- Kuwahara T, Hara K, Mizuno N, Haba S, Okuno N, Fukui T, et al. Current status of artificial intelligence analysis for the treatment of pancreaticobiliary diseases using endoscopic ultrasonography and endoscopic retrograde cholangiopancreatography. DEN Open. Apr 30, 2024;4(1):e267. [ FREE Full text ] [ CrossRef ] [ Medline ]
- Yokote A, Umeno J, Kawasaki K, Fujioka S, Fuyuno Y, Matsuno Y, et al. Small bowel capsule endoscopy examination and open access database with artificial intelligence: the SEE-artificial intelligence project. DEN Open. Apr 22, 2024;4(1):e258. [ FREE Full text ] [ CrossRef ] [ Medline ]
- Ramalingam M, Jaisankar A, Cheng L, Krishnan S, Lan L, Hassan A, et al. Impact of nanotechnology on conventional and artificial intelligence-based biosensing strategies for the detection of viruses. Discov Nano. Dec 01, 2023;18(1):58. [ FREE Full text ] [ CrossRef ] [ Medline ]
- Yerukala Sathipati S, Tsai MJ, Shukla SK, Ho SY. Artificial intelligence-driven pan-cancer analysis reveals miRNA signatures for cancer stage prediction. HGG Adv. Jul 13, 2023;4(3):100190. [ FREE Full text ] [ CrossRef ] [ Medline ]
- Liu J, Dan W, Liu X, Zhong X, Chen C, He Q, et al. Development and validation of predictive model based on deep learning method for classification of dyslipidemia in Chinese medicine. Health Inf Sci Syst. Dec 06, 2023;11(1):21. [ FREE Full text ] [ CrossRef ] [ Medline ]
- Carou-Senra P, Ong JJ, Castro BM, Seoane-Viaño I, Rodríguez-Pombo L, Cabalar P, et al. Predicting pharmaceutical inkjet printing outcomes using machine learning. Int J Pharm X. Dec 2023;5:100181. [ FREE Full text ] [ CrossRef ] [ Medline ]
- Li X, Zhu Y, Zhao W, Shi R, Wang Z, Pan H, et al. Machine learning algorithm to predict the in-hospital mortality in critically ill patients with chronic kidney disease. Ren Fail. Dec 2023;45(1):2212790. [ FREE Full text ] [ CrossRef ] [ Medline ]
- Bonnin M, Müller-Fouarge F, Estienne T, Bekadar S, Pouchy C, Ait Si Selmi T. Artificial intelligence radiographic analysis tool for total knee arthroplasty. J Arthroplasty. Jul 2023;38(7 Suppl 2):S199-207.e2. [ CrossRef ] [ Medline ]
- Kao DP. Intelligent artificial intelligence: present considerations and future implications of machine learning applied to electrocardiogram interpretation. Circ Cardiovasc Qual Outcomes. Sep 2019;12(9):e006021. [ FREE Full text ] [ CrossRef ] [ Medline ]
- van der Stigchel B, van den Bosch K, van Diggelen J, Haselager P. Intelligent decision support in medical triage: are people robust to biased advice? J Public Health (Oxf). Aug 28, 2023;45(3):689-696. [ FREE Full text ] [ CrossRef ] [ Medline ]
- Jakhar D, Kaur I. Artificial intelligence, machine learning and deep learning: definitions and differences. Clin Exp Dermatol. Jan 09, 2020;45(1):131-132. [ CrossRef ] [ Medline ]
- Ghosh M, Thirugnanam A. Introduction to artificial intelligence. In: Srinivasa KG, Siddesh GM, Sekhar SR, editors. Artificial Intelligence for Information Management: A Healthcare Perspective. Cham, Switzerland. Springer; 2021;88-44.
- Taulli T. Artificial Intelligence Basics: A Non-Technical Introduction. Berkeley, CA. Apress Berkeley; 2019.
- Patil S, Joda T, Soffe B, Awan KH, Fageeh HN, Tovani-Palone MR, et al. Efficacy of artificial intelligence in the detection of periodontal bone loss and classification of periodontal diseases: a systematic review. J Am Dent Assoc. Sep 2023;154(9):795-804.e1. [ CrossRef ] [ Medline ]
- Quek LJ, Heikkonen MR, Lau Y. Use of artificial intelligence techniques for detection of mild cognitive impairment: a systematic scoping review. J Clin Nurs. Sep 10, 2023;32(17-18):5752-5762. [ CrossRef ] [ Medline ]
- Tan D, Mohd Nasir NF, Abdul Manan H, Yahya N. Prediction of toxicity outcomes following radiotherapy using deep learning-based models: a systematic review. Cancer Radiother. Sep 2023;27(5):398-406. [ CrossRef ] [ Medline ]
- Rabilloud N, Allaume P, Acosta O, De Crevoisier R, Bourgade R, Loussouarn D, et al. Deep learning methodologies applied to digital pathology in prostate cancer: a systematic review. Diagnostics (Basel). Aug 14, 2023;13(16):2676. [ FREE Full text ] [ CrossRef ] [ Medline ]
- Li K, Yao S, Zhang Z, Cao B, Wilson C, Kalos D, et al. Efficient gradient boosting for prognostic biomarker discovery. Bioinformatics. Mar 04, 2022;38(6):1631-1638. [ FREE Full text ] [ CrossRef ] [ Medline ]
- Chen T, Chen Y, Li H, Gao T, Tu H, Li S. Driver intent-based intersection autonomous driving collision avoidance reinforcement learning algorithm. Sensors (Basel). Dec 16, 2022;22(24):9943. [ FREE Full text ] [ CrossRef ] [ Medline ]
- Huynh QT, Nguyen PH, Le HX, Ngo LT, Trinh NT, Tran MT, et al. Automatic acne object detection and acne severity grading using smartphone images and artificial intelligence. Diagnostics (Basel). Aug 03, 2022;12(8):1879. [ FREE Full text ] [ CrossRef ] [ Medline ]
- Brooke BS, Schwartz TA, Pawlik TM. MOOSE reporting guidelines for meta-analyses of observational studies. JAMA Surg. Aug 01, 2021;156(8):787-788. [ CrossRef ] [ Medline ]
- Scholten RJ, Clarke M, Hetherington J. The Cochrane collaboration. Eur J Clin Nutr. Aug 28, 2005;59 Suppl 1(S1):S147-S196. [ CrossRef ] [ Medline ]
- Warrens MJ. Kappa coefficients for dichotomous-nominal classifications. Adv Data Anal Classif. Apr 07, 2020;15(1):193-208. [ CrossRef ]
- Law M, Stewart D, Letts L, Pollock N, Bosch J. Guidelines for critical review of qualitative studies. McMaster University Occupational Therapy Evidence-Based Practice Research Group. URL: https://www.canchild.ca/system/tenon/assets/attachments/000/000/360/original/qualguide.pdf [accessed 2024-04-05]
- Higgins JP, Morgan RL, Rooney AA, Taylor KW, Thayer KA, Silva RA, et al. Risk of bias in non-randomized studies - of exposure (ROBINS-E). ROBINS-E tool. URL: https://www.riskofbias.info/welcome/robins-e-tool [accessed 2024-04-02]
- Nait Aicha A, Englebienne G, van Schooten KS, Pijnappels M, Kröse B. Deep learning to predict falls in older adults based on daily-life trunk accelerometry. Sensors (Basel). May 22, 2018;18(5):1654. [ FREE Full text ] [ CrossRef ] [ Medline ]
- Ribeiro NF, André J, Costa L, Santos CP. Development of a strategy to predict and detect falls using wearable sensors. J Med Syst. Apr 04, 2019;43(5):134. [ CrossRef ] [ Medline ]
- Qiu H, Rehman RZ, Yu X, Xiong S. Application of wearable inertial sensors and a new test battery for distinguishing retrospective fallers from non-fallers among community-dwelling older people. Sci Rep. Nov 05, 2018;8(1):16349. [ FREE Full text ] [ CrossRef ] [ Medline ]
- Dubois A, Bihl T, Bresciani JP. Automatic measurement of fall risk indicators in timed up and go test. Inform Health Soc Care. Sep 13, 2019;44(3):237-245. [ CrossRef ] [ Medline ]
- Noh B, Youm C, Goh E, Lee M, Park H, Jeon H, et al. XGBoost based machine learning approach to predict the risk of fall in older adults using gait outcomes. Sci Rep. Jun 09, 2021;11(1):12183. [ CrossRef ] [ Medline ]
- Hauth J, Jabri S, Kamran F, Feleke EW, Nigusie K, Ojeda LV, et al. Automated loss-of-balance event identification in older adults at risk of falls during real-world walking using wearable inertial measurement units. Sensors (Basel). Jul 07, 2021;21(14):4661. [ FREE Full text ] [ CrossRef ] [ Medline ]
- Lockhart TE, Soangra R, Yoon H, Wu T, Frames CW, Weaver R. Prediction of fall risk among community-dwelling older adults using a wearable system. Sci Rep. 2021;11(1):20976. [ CrossRef ]
- Hsu YC, Weng HH, Kuo CY, Chu TP, Tsai YH. Prediction of fall events during admission using eXtreme gradient boosting: a comparative validation study. Sci Rep. Oct 08, 2020;10(1):16777. [ FREE Full text ] [ CrossRef ] [ Medline ]
- Hu Y, Bishnoi A, Kaur R, Sowers R, Hernandez ME. Exploration of machine learning to identify community dwelling older adults with balance dysfunction using short duration accelerometer data. In: Proceedings of the 42nd Annual International Conference of the IEEE Engineering in Medicine & Biology Society. 2020. Presented at: EMBC '20; July 20-24, 2020;812-815; Montreal, QC. URL: https://ieeexplore.ieee.org/document/9175871 [ CrossRef ]
- Ye C, Li J, Hao S, Liu M, Jin H, Zheng L, et al. Identification of elders at higher risk for fall with statewide electronic health records and a machine learning algorithm. Int J Med Inform. May 2020;137:104105. [ FREE Full text ] [ CrossRef ] [ Medline ]
- Althobaiti T, Katsigiannis S, Ramzan N. Triaxial accelerometer-based falls and activities of daily life detection using machine learning. Sensors (Basel). Jul 06, 2020;20(13):3777. [ FREE Full text ] [ CrossRef ] [ Medline ]
- Tunca C, Salur G, Ersoy C. Deep learning for fall risk assessment with inertial sensors: utilizing domain knowledge in spatio-temporal gait parameters. IEEE J Biomed Health Inform. Jul 2020;24(7):1994-2005. [ CrossRef ]
- Kim K, Yun G, Park SK, Kim DH. Fall detection for the elderly based on 3-axis accelerometer and depth sensor fusion with random forest classifier. In: Proceedings of the 41st Annual International Conference of the IEEE Engineering in Medicine and Biology Society. 2019. Presented at: EMBC '19; July 23-27, 2019;4611-4614; Berlin, Germany. URL: https://ieeexplore.ieee.org/document/8856698 [ CrossRef ]
- Gillain S, Boutaayamou M, Schwartz C, Brüls O, Bruyère O, Croisier JL, et al. Using supervised learning machine algorithm to identify future fallers based on gait patterns: a two-year longitudinal study. Exp Gerontol. Nov 2019;127:110730. [ FREE Full text ] [ CrossRef ] [ Medline ]
- Lo Y, Lynch SF, Urbanowicz RJ, Olson RS, Ritter AZ, Whitehouse CR, et al. Using machine learning on home health care assessments to predict fall risk. Stud Health Technol Inform. Aug 21, 2019;264:684-688. [ CrossRef ] [ Medline ]
- Maray N, Ngu AH, Ni J, Debnath M, Wang L. Transfer learning on small datasets for improved fall detection. Sensors (Basel). Jan 18, 2023;23(3):1105. [ FREE Full text ] [ CrossRef ] [ Medline ]
- Ladios-Martin M, Cabañero-Martínez MJ, Fernández-de-Maya J, Ballesta-López FJ, Belso-Garzas A, Zamora-Aznar FM, et al. Development of a predictive inpatient falls risk model using machine learning. J Nurs Manag. Nov 30, 2022;30(8):3777-3786. [ CrossRef ] [ Medline ]
- Eichler N, Raz S, Toledano-Shubi A, Livne D, Shimshoni I, Hel-Or H. Automatic and efficient fall risk assessment based on machine learning. Sensors (Basel). Feb 17, 2022;22(4):1557. [ FREE Full text ] [ CrossRef ] [ Medline ]
- Tang YM, Wang YH, Feng XY, Zou QS, Wang Q, Ding J, et al. Diagnostic value of a vision-based intelligent gait analyzer in screening for gait abnormalities. Gait Posture. Jan 2022;91:205-211. [ CrossRef ] [ Medline ]
- Greene BR, McManus K, Ader LG, Caulfield B. Unsupervised assessment of balance and falls risk using a smartphone and machine learning. Sensors (Basel). Jul 13, 2021;21(14):4770. [ FREE Full text ] [ CrossRef ] [ Medline ]
- Roshdibenam V, Jogerst GJ, Butler NR, Baek S. Machine learning prediction of fall risk in older adults using timed up and go test kinematics. Sensors (Basel). May 17, 2021;21(10):3481. [ FREE Full text ] [ CrossRef ] [ Medline ]
- Dubois A, Bihl T, Bresciani JP. Identifying fall risk predictors by monitoring daily activities at home using a depth sensor coupled to machine learning algorithms. Sensors (Basel). Mar 11, 2021;21(6):1957. [ FREE Full text ] [ CrossRef ] [ Medline ]
- Vo MT, Thonglor R, Moncatar TJ, Han TD, Tejativaddhana P, Nakamura K. Fear of falling and associated factors among older adults in Southeast Asia: a systematic review. Public Health. Sep 2023;222:215-228. [ CrossRef ] [ Medline ]
- Torun E, Az A, Akdemir T, Solakoğlu GA, Açiksari K, Güngörer B. Evaluation of the risk factors for falls in the geriatric population presenting to the emergency department. Ulus Travma Acil Cerrahi Derg. Aug 2023;29(8):897-903. [ FREE Full text ] [ CrossRef ] [ Medline ]
- Son NK, Ryu YU, Jeong HW, Jang YH, Kim HD. Comparison of 2 different exercise approaches: Tai Chi versus Otago, in community-dwelling older women. J Geriatr Phys Ther. 2016;39(2):51-57. [ CrossRef ] [ Medline ]
- Sawa R, Doi T, Tsutsumimoto K, Nakakubo S, Kurita S, Kiuchi Y, et al. Overlapping status of frailty and fear of falling: an elevated risk of incident disability in community-dwelling older adults. Aging Clin Exp Res. Sep 11, 2023;35(9):1937-1944. [ FREE Full text ] [ CrossRef ] [ Medline ]
- Calazans JA, Permanyer I. Levels, trends, and determinants of cause-of-death diversity in a global perspective: 1990-2019. BMC Public Health. Apr 05, 2023;23(1):650. [ FREE Full text ] [ CrossRef ] [ Medline ]
- Kakara R, Bergen G, Burns E, Stevens M. Nonfatal and fatal falls among adults aged ≥65 years - United States, 2020-2021. MMWR Morb Mortal Wkly Rep. Sep 01, 2023;72(35):938-943. [ FREE Full text ] [ CrossRef ] [ Medline ]
- Dostan A, Dobson CA, Vanicek N. Relationship between stair ascent gait speed, bone density and gait characteristics of postmenopausal women. PLoS One. Mar 22, 2023;18(3):e0283333. [ FREE Full text ] [ CrossRef ] [ Medline ]
- Zheng Y, Wang X, Zhang ZK, Guo B, Dang L, He B, et al. Bushen Yijing Fang reduces fall risk in late postmenopausal women with osteopenia: a randomized double-blind and placebo-controlled trial. Sci Rep. Feb 14, 2019;9(1):2089. [ FREE Full text ] [ CrossRef ] [ Medline ]
- Woelfle T, Bourguignon L, Lorscheider J, Kappos L, Naegelin Y, Jutzeler CR. Wearable sensor technologies to assess motor functions in people with multiple sclerosis: systematic scoping review and perspective. J Med Internet Res. Jul 27, 2023;25:e44428. [ FREE Full text ] [ CrossRef ] [ Medline ]
- Abdollah V, Dief TN, Ralston J, Ho C, Rouhani H. Investigating the validity of a single tri-axial accelerometer mounted on the head for monitoring the activities of daily living and the timed-up and go test. Gait Posture. Oct 2021;90:137-140. [ CrossRef ] [ Medline ]
- Mielke GI, de Almeida Mendes M, Ekelund U, Rowlands AV, Reichert FF, Crochemore-Silva I. Absolute intensity thresholds for tri-axial wrist and waist accelerometer-measured movement behaviors in adults. Scand J Med Sci Sports. Sep 12, 2023;33(9):1752-1764. [ CrossRef ] [ Medline ]
- Löppönen A, Delecluse C, Suorsa K, Karavirta L, Leskinen T, Meulemans L, et al. Association of sit-to-stand capacity and free-living performance using Thigh-Worn accelerometers among 60- to 90-yr-old adults. Med Sci Sports Exerc. Sep 01, 2023;55(9):1525-1532. [ FREE Full text ] [ CrossRef ] [ Medline ]
- García-Soidán JL, Leirós-Rodríguez R, Romo-Pérez V, García-Liñeira J. Accelerometric assessment of postural balance in children: a systematic review. Diagnostics (Basel). Dec 22, 2020;11(1):8. [ FREE Full text ] [ CrossRef ] [ Medline ]
- Leirós-Rodríguez R, García-Soidán JL, Romo-Pérez V. Analyzing the use of accelerometers as a method of early diagnosis of alterations in balance in elderly people: a systematic review. Sensors (Basel). Sep 09, 2019;19(18):3883. [ FREE Full text ] [ CrossRef ] [ Medline ]
Abbreviations
Edited by A Mavragani; submitted 28.11.23; peer-reviewed by E Andrade, M Behzadifar, A Suárez; comments to author 09.01.24; revised version received 30.01.24; accepted 13.02.24; published 29.04.24.
©Ana González-Castro, Raquel Leirós-Rodríguez, Camino Prada-García, José Alberto Benítez-Andrades. Originally published in the Journal of Medical Internet Research (https://www.jmir.org), 29.04.2024.
This is an open-access article distributed under the terms of the Creative Commons Attribution License (https://creativecommons.org/licenses/by/4.0/), which permits unrestricted use, distribution, and reproduction in any medium, provided the original work, first published in the Journal of Medical Internet Research, is properly cited. The complete bibliographic information, a link to the original publication on https://www.jmir.org/, as well as this copyright and license information must be included.
Thank you for visiting nature.com. You are using a browser version with limited support for CSS. To obtain the best experience, we recommend you use a more up to date browser (or turn off compatibility mode in Internet Explorer). In the meantime, to ensure continued support, we are displaying the site without styles and JavaScript.
- View all journals
- My Account Login
- Explore content
- About the journal
- Publish with us
- Sign up for alerts
- Review Article
- Open access
- Published: 01 May 2024
Digital technologies for construction sustainability: Status quo, challenges, and future prospects
- Weisheng Lu 1 ,
- Jinfeng Lou 1 ,
- Benjamin Kwaku Ababio 1 ,
- Ray Y. Zhong 2 ,
- Zhikang Bao 3 ,
- Xiao Li 4 &
- Fan Xue 1
npj Materials Sustainability volume 2 , Article number: 10 ( 2024 ) Cite this article
Metrics details
- Civil engineering
- Environmental impact
The nexus between digital technologies (DTs) and sustainability in the built environment has attracted increasing research interest in recent years, yet understanding DT utilization and its impact on construction processes remains fragmented. To address this gap, this study conducts a systematic review of the construction sustainability literature to analyze and synthesize research findings on the application of DTs at various stages of the construction lifecycle. We undertake an in-depth content analysis of 72 articles, with findings revealing that prominent DTs for construction sustainability include building information modeling, the Internet of Things, big data, and artificial intelligence. We also identify that the application of DTs for sustainability across the construction lifecycle is clustered in four areas: namely (1) integration and collaboration; (2) optimization, simulation, and decision-making; (3) tracking, monitoring, and control; and (4) training. Based on existing knowledge gaps, future research opportunities are identified, including the development of integrated and interoperable systems, long-term performance and resilience, and advanced simulation and modeling techniques. This study contributes to the literature on construction digitalization by offering a complete overview of research investigations in relation to construction sustainability and identifying research crucial to advancing a DT-enabled sustainable built environment.
Introduction
The construction industry is not just about erecting buildings and infrastructure; it plays a vital role in economic and social development, contributing approximately USD200 billion to global GDP and providing over 220 million jobs 1 . The sector embodies a continuous lifecycle that integrates design, construction, operation & maintenance (O&M), and end of life, where each stage is interlinked and connected to the overall functionality and sustainability of the built environment 2 . The initial design sets the blueprint for sustainable and efficient use of resources 3 , 4 , and this design is materialized in the construction phase 5 . The O&M period extends over the longest phase of a structure’s life, emphasizing energy efficiency and environmental stewardship 6 . Finally, end-of-life is a critical component in the recycling and repurposing of materials 7 , 8 .
The construction industry has long been associated with significant environmental impacts, including resource consumption, waste generation, and carbon emissions 9 , 10 , 11 . It produces 45–65% of the waste dumped in landfills, contributing 35% of the world’s CO 2 emissions 12 . As the focus of the global community on sustainable development intensifies 13 , the construction sector faces an imperative to evolve. Sustainable practices meet the needs of the present without compromising the ability of future generations to meet their own needs 14 . ‘Construction sustainability’, therefore, is the implementation of such practices in construction industry activities 15 , encompassing three core dimensions: environmental protection, social responsibility, and economic viability 16 .
In responding to sustainability challenges, digital technologies (DTs) are a potentially transformative tool. DTs include information and communication technologies (ICTs) that manage information through digital binary computer language. In construction, they range from standalone systems to integrated and web-based technologies, which aid in data capture, storage, processing, display, and communication during various procurement stages. By streamlining processes, reducing waste, and enabling better decision-making, DTs promise to significantly advance the sustainability agenda in construction.
Despite growing recognition of their potential, the academic landscape reveals a fragmented understanding of DTs’ impact on sustainability in construction. Research studies vary in focus, methodology, and findings, making it challenging for industry stakeholders to form a cohesive view of the role of DTs in promoting sustainability. This fragmentation presents a critical barrier to effectively leveraging DTs for sustainable construction practices. To bridge this knowledge gap, this study conducts a systematic review of the construction sustainability literature. The goal is to compile, analyze, and synthesize research findings to present a clear, coherent picture of how DTs are being used to foster sustainability in construction. By critically examining the interplay between DT application and sustainable practices, we aim to identify successful strategies and highlight areas urging further exploration.
Prominent digital technologies in the construction sustainability literature
Reviewing the content of 72 articles in the construction sustainability literature, DTs frequently referenced and thoroughly examined were collected and organized in a Sankey diagram (see Fig. 1 ). Some DTs have received particular attention in the literature, e.g. building information modeling (BIM) and Internet of Things (IoT), while others have remained under-researched in relation to sustainability, e.g. unmanned aerial vehicles (UAV) and cloud computing. The diagram also reveals that DTs are employed for construction sustainability purposes mostly at the design and O&M stages of the construction lifecycle, typically as a standalone technology or paired with one other technology to achieve better performance.
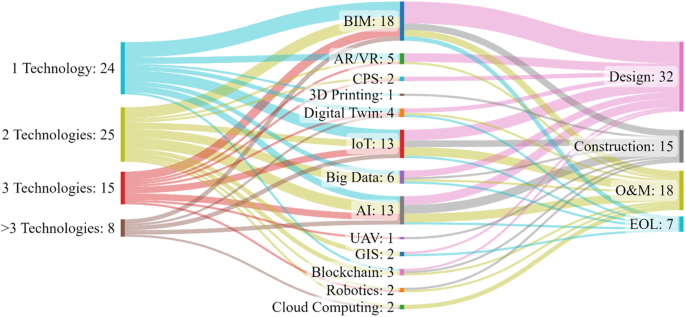
The papers reviewed are categorized according to the number of technologies, the name of the primary technology, and the stage at which the technologies are applied.
Figure 2 presents the distribution in the literature of key DTs for construction sustainability from 2020 to the present. BIM is the most frequently mentioned DT in the sustainability literature, with a peak of 10 research articles published between 2021 and 2022. The results indicate the continuation of a trend, with 17 identifying BIM as a top research theme in the DT-sustainability literature. This is attributable to the function of BIM as a collaborative platform and a means for integrating other DTs at different stages of the construction lifecycle. Studies relating BIM to sustainability in construction processes have seen a steady rise since the inception of BIM, with its application traversing design, construction, and O&M lifecycle stages. This suggests the vital role of BIM in improving sustainability through collaboration, visual representation, and optimization. IoT, with its use of sensors and actuators, has also been extensively discussed in the literature as essential to gathering sustainability-related data. Studies indicate the utilization of IoT in monitoring and controlling energy usage of buildings, optimizing resource use, and enhancing safety on site, all of which enable informed decisions on sustainability-related issues. Big data is also an important DT leveraging the vast amount of data generated throughout the project lifecycle by IoTs and other sources. There is a strong link between big data and IoT in the current body of knowledge, with both concepts often discussed together in optimization studies, environmental assessments, and risk and safety management. Artificial intelligence (AI) and machine learning (ML) in sustainability are gradually gaining momentum. Their ability to process huge amounts of heterogeneous data using computational power is vital for extracting insights into sustainable decision-making that effectively improves construction processes.
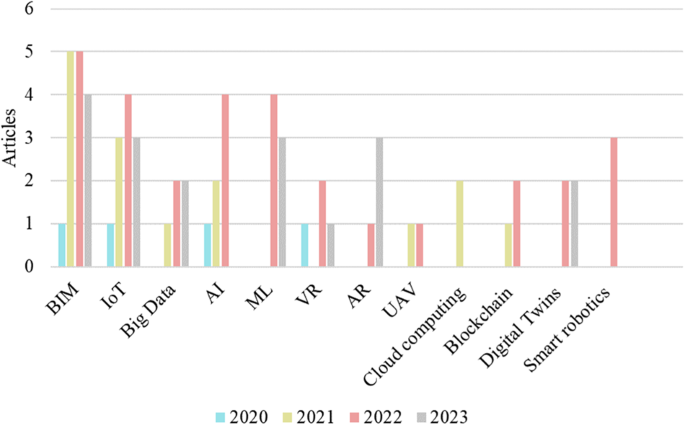
BIM is the most widely used technology for construction sustainability in recent years (2020–2023).
Other prominent DTs in construction sustainability are virtual reality (VR) and augmented reality (AR). Studies involving VR and AR cite their role in construction planning, where these DTs can be used for simulating interactive experiences and reviewing sustainability scenarios such as energy-efficient installations and user comfort. VR and AR technologies have been articulated in existing literature as early design optimization and visualization toolsets promoting a deeper understanding of sustainability features and fostering a sense of commitment towards sustainable outcomes. UAVs, or drones, are another type of DT increasingly used in construction, for surveying, inspection, and monitoring. In the construction sustainability literature, the application of UAVs has been discussed in hazard identification, safe work processes, and waste reduction. Cloud computing has also been identified as relevant to construction sustainability for its potential in providing centralized platforms for information management, communication, and collaboration throughout the project lifecycle. However, many recent studies are shifting their attention to blockchain technology as an enabler of construction sustainability, through providing transparent and immutable records and facilitating the integration of sustainable practices, such as material reuse and tracking. Little attention has been given to digital twins and cyber-physical systems (CPS) in sustainability research, though there has been an increasing trend in recent years for their potential to revolutionize construction sustainability by enabling real-time monitoring, optimization, and performance improvement throughout the project lifecycle.
Based on the results of our review of the literature, the DTs most closely associated with sustainability in the construction industry are BIM, IoT, AI, ML, VR, AR, UAV, robots, blockchain, and CPS. The overall growth in the application of these DTs for construction sustainably is likely to continue as the DT potential progressively permeates the entire lifecycle of construction products and services.
Application of digital technologies in construction sustainability
In examining the application of digital technologies to construction sustainability, it is crucial to analyze their impacts across different stages of the construction lifecycle: design, construction, O&M, and end of life.
In the design stage, recent literature indicates there is a growing nexus of DTs that enhance sustainability. Prominent examples include BIM in its 6D form, AR/VR, and AI for design. Six-dimensional BIM transcends traditional 3D modeling by integrating time (4D), cost (5D), and sustainability (6D) considerations, such as energy performance 18 , resource efficiency 19 , carbon emissions reduction 20 , and retrofit process simulation 21 . The emphasis is on the lifecycle approach 22 , where BIM informs decisions right from the design phase to reduce environmental impact and promote green building practices. AR/VR technologies have evolved to significantly improve design visualization and stakeholder engagement 23 , with discussions extending into the realm of the metaverse, which offers immersive spaces for interaction. The metaverse can benefit sustainability by facilitating the participation of the majority of people in decisions about how to utilize resources more efficiently 24 . AI, particularly in generative design 25 , is leveraged to create multiple sustainable design alternatives based on specific constraints and goals, focusing on material usage reduction 26 and energy efficiency optimization 27 .
The construction stage sees the critical role of blockchain, IoT, radio-frequency identification (RFID), digital twins, and UAVs in advancing sustainability. Blockchain’s potential lies in enhancing transparency and accountability in construction processes 28 , 29 , while smart contracts facilitate complex agreements and compliance with sustainability standards 30 . They can streamline procurement processes 31 , enhance supply chain management 32 , and facilitate more efficient project management 33 , 34 , leading to reduced waste and improved resource allocation. The role of IoT in sustainable construction is growing, with a focus on real-time data collection and monitoring 35 . IoT devices, such as sensors and wearables, are used to track resource utilization and environmental conditions 36 , thus aiding in optimizing resource use and reducing energy consumption 37 . RFID, one of the most powerful IoT technologies, has been extensively employed for effective logistics management, deemed crucial in reducing the carbon footprint of construction activities 38 by enabling accurate tracking of materials, tools, and equipment. RFID systems help in minimizing losses, reducing waste, and ensuring that materials are sourced and used sustainably. In addition, the integration of digital twins in the construction process represents a significant advancement. A digital twin is a virtual replica of a physical building that can be used for monitoring, simulation, and analysis 39 , 40 . Optimized construction efficiency is achievable through digital twins, which help streamline workflows, allocate resources efficiently, and monitor construction progress in real-time. This reduces the risks of delays and material waste by providing active and accurate information 41 . By creating a virtual representation of the construction project, digital twins enable a more dynamic and responsive approach to construction management, further enhancing sustainability. UAVs, commonly known as drones, are increasingly used in construction for various tasks such as site surveying, monitoring, and inspection. They provide a unique vantage point for overseeing construction activities, enabling better management of resources, and ensuring adherence to environmental and safety regulations 42 .
During the O&M phase, human–robot collaboration signifies a shift towards automation, enhancing efficiency and sustainability 43 . In this context, human–robot collaboration is not about replacing human workers, but rather enhancing their capabilities and safety 44 . Robots are used for tasks like cleaning, maintenance, surveillance, and inspection 45 , reducing the workload on human staff, increasing precision, and enhancing safety, particularly in hazardous environments. The concept of digital twins has also gained prominence in the O&M phase 46 . Data management and access post-construction can be complex due to the transition of control and operations among various stakeholders. This technology effectively bridges information gaps, ensuring reliable and convenient project management and facilitating enhanced communication among stakeholders 41 . Moreover, the digital twin provides a dynamic and real-time representation of the building and enables “what-if” analyses in occupant comfort, energy utilization, logistics optimization, asset management, and predictive maintenance, enhancing decision-making capabilities 47 , 48 , 49 . IoT devices and sensors, in addition, are widely used for continuous monitoring and data collection in buildings. This technology trend contributes significantly to the sustainability of facilities by enabling energy efficiency and predictive maintenance. Sensors collect data on aspects such as energy usage, temperature, and occupancy levels 50 , 51 , crucial for optimizing building operations and reducing unnecessary energy consumption 52 . To analyze the vast amount of data generated by IoT devices and other sources, AI and ML algorithms are increasingly applied. These technologies enable predictive maintenance, energy management, and anomaly detection, thereby enhancing the sustainability of building operations 53 , 54 .
At the end-of-life stage, DTs such as big data analytics, computer vision, robotics, and blockchain are key to managing deconstruction and waste processes. Big data analytics assists in material stock assessment, facilitating optimal material reuse and recycling, aligning with circular economy principles 55 , 56 . The role of computer vision in automating waste material sorting, combined with AI algorithms, enhances recycling efficiency 57 , 58 . Robotics in deconstruction focuses on safe material recovery 59 , 60 , while blockchain improves material traceability, ensuring informed decisions for material reuse and recycling 61 .
Prospects for digital technologies in construction sustainability
DTs incorporate several enhancements and modifications to construction value chain processes, increasing efficiency and productivity 19 . The opportunities for adopting these technologies to streamline sustainable production, construction, and operation in the industry are vast. From the analysis of the literature, we find that the driving themes in DT prospects for sustainability are linked with integration and collaboration 62 ; optimization, simulation, and decision-making 63 ; monitoring and control 64 ; and training 24 as presented in Fig. 3 .
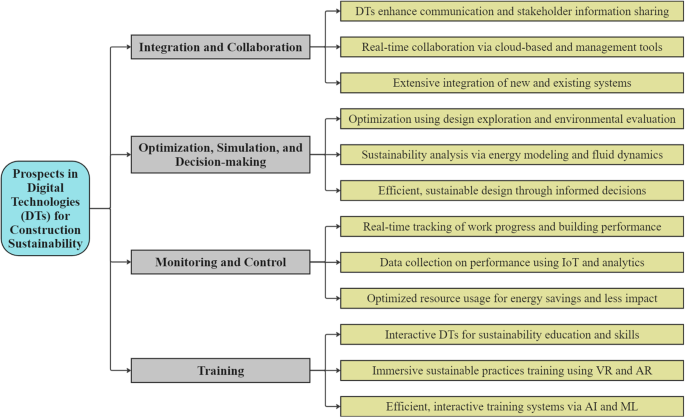
The potential of digital technologies can be explored in four areas, including integration and collaboration, optimization, simulation, and decision-making, monitoring and control, and training.
Integration and collaboration
The task of maintaining profitability while achieving cleaner, low-carbon construction processes, and greener and safer job sites, requires extensive stakeholder buy-in, involvement, and support 65 . To achieve this, there is a need to improve collaboration within and across different building lifecycles. DTs facilitate collaboration among stakeholders involved in the construction process by enabling effective communication and information sharing. According to 66 , cloud-based platforms, project management software, and collaborative tools allow real-time collaboration, document sharing, and coordination among architects, engineers, contractors, and sustainability consultants, among others. This collaboration enhances the integration of sustainability considerations into the project, leading to better-informed decisions and improved sustainability outcomes. Furthermore, DTs facilitate the integration of new and existing systems and processes to enhance sustainability outcomes. Integrating BIM models with energy modeling software, for instance, enables automated energy analysis and interference detection 21 . Integration with supply chain management systems allows for tracking and optimizing material usage, reducing waste, and promoting sustainable sourcing practices 67 . DTs provide a comprehensive approach to sustainability over the whole project lifecycle by combining various data sources and platforms.
Optimization, simulation and decision-making
Making informed decisions as early as feasible assists in efficient and cost-effective sustainable design and construction. Sustainability analysis tools, for example, enable better-informed decisions by analyzing numerous design options and identifying sustainable alternatives 68 , 69 . These evaluations assist professionals at various stages in determining the consequences of their building designs for environmental performance and efficiency. To improve sustainability, DTs enable optimization through the exploration of several design possibilities and evaluate their societal, environmental, and energy performance using BIM, ML, and parametric modeling. This aids in the identification of the most sustainable design solutions, decreasing material waste, energy usage, and environmental impacts 70 . Moreover, DTs provide simulation capabilities to assess the performance of buildings and infrastructure systems. For example, studies have incorporated energy modeling software to simulate and analyze energy consumption, daylighting, and thermal performance 18 . Others such as 71 have used computational fluid dynamics simulations to evaluate indoor air quality, ventilation effectiveness, and natural ventilation strategies. These simulations enable designers to make informed decisions, optimize sustainability performance, and identify potential issues before construction.
Monitoring and control
Some studies have explored mechanisms for monitoring sustainable aspects such as construction-related emissions using DTs that enable real-time tracking and monitoring of work progress and building performance 72 . Building management systems, IoT sensors, digital twins, and data analytics platforms collect data on energy consumption, indoor environmental quality, occupancy patterns, and equipment performance. The data helps identify areas of inefficiencies, pinpoint maintenance needs, and optimize resource usage, leading to energy savings, improved occupant comfort, and reduced environmental impact 73 .
For sustainability education and skill development, DTs provide interactive and immersive training alternatives. Immersive training experiences are made possible by VR, AR, and mixed-reality technologies, which allow users to simulate and implement sustainable construction practices, safety regulations, and energy-efficient operations 24 . The deployment of DTs enables safe demonstration and experimentation, promoting knowledge transfer and capacity building. Additionally, AI and ML platforms enable easy access to more efficient, responsive, and interactive train systems linked to sustainability 74 .
Digital technology application bottlenecks in construction sustainability
Deployment of DTs for construction sustainability faces several challenges across different stages of the project lifecycle (Fig. 4 ). First, accurate data and information are essential for conducting sustainability analysis during the design and planning stages of projects 75 . However, the present challenge of data quality and availability of construction products and services makes it difficult to fully benefit from the prospects of DT application. Data on material properties, energy consumption, and environmental impacts may not be readily available or standardized, making it difficult to assess sustainability performance accurately 76 . Standardized protocols, formats, and data exchange mechanisms that ensure consistent data quality and compatibility across different digital tools and platforms are still largely underdeveloped and unable to fully grasp all aspects of sustainability considerations in the construction industry. Interoperability of various DTs for holistic construction sustainability is missing within and across different lifecycle stages 77 . Within the same lifecycle stage, various digital tools and applications such as BIM, geographic information systems, energy modeling software, and sustainability analysis tools generate different types of data in varying formats and qualities 78 . Across different lifecycle stages, gathering real-time data from various sources, such as sensors and equipment, and integrating it into a unified system can be complex 79 . posit that the challenge lies in integrating these diverse datasets into a unified system that allows for comprehensive analysis and decision-making. Thus, ensuring data accuracy, reliability, and interoperability in different platforms and systems is crucial for enabling construction sustainability.
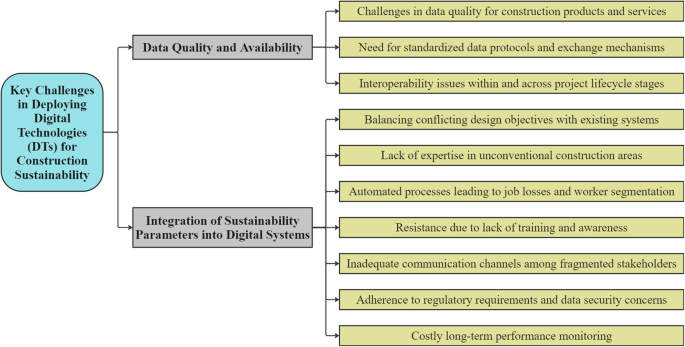
Two categories of challenges are identified: data quality and availability, and integration of sustainability parameters into digital systems.
Another key challenge is the integration of sustainability parameters into digital systems, including balancing conflicting design objectives and ensuring compatibility with already existing systems 80 . The deployment of DTs for sustainable construction requires expertise in certain unconventional areas of construction and given present inadequacies in the construction workforce, such expertise is hard to find 81 . point to many benefits of deploying DTs for construction sustainability, including lowered employment costs for organizations through the application of automated work processes. However, this can lead to significant job losses and worker segmentation, which may in turn result in resistance to change stemming from a lack of training, awareness, and involvement. There is a need to develop innovative and varied instructional approaches, but it is unclear how these challenges faced are being addressed in the present literature 80 . The introduction of both DTs and sustainable practices is nascent in the construction industry but is gaining momentum. Therefore, more conscious planning and capital investment are needed to enhance industry skills and manage behavior change with necessary measures that may completely deviate from existing supply chain operations. Moreover, at the core of DT for construction sustainability is the need for stakeholder collaboration and engagement 82 . Yet, given the vast and fragmented nature of stakeholders along a construction project’s lifecycle, including designers, contractors, facility managers, maintenance personnel, and occupants, it can be challenging to track and foster effective collaboration. This challenge stems from the absence of efficient communication channels, unwillingness to exchange and transmit knowledge and information, and failure by the relevant parties to cultivate proactive sustainability initiatives 83 . Other challenges are linked to adherence to regulatory requirements and data confidence, security, protection, and issues associated with the deployment of DTs 84 . Given the long-term performance monitoring of sustainability features and systems over a building or infrastructure’s lifespan, the need to build trust and efficient systems is critical. Ensuring that digital systems continue to operate optimally, maintaining modern sustainable practices during renovations or retrofits, and monitoring changes in building performance require continuous data collection, analysis, and stakeholder involvement, which can be onerous and costly 85 .
Future research trends and directions
The findings from this critical review of trending topics, prospects, challenges, and application of DTs in promoting construction sustainability offer a full picture of current research activities and reveal relevant gaps and future research needs. These future research needs represent the untapped potential identified from the analysis and evaluation of future works proposed in the existing literature and are explained below.
Limited attention has been paid to the integration of DTs for sustainability in construction 86 . Further research is required to delve into the development of standardized protocols, formats, and data exchange mechanisms to ensure consistent data quality and compatibility across different digital tools and platforms. This includes establishing guidelines for data collection, verification, and validation to enhance the accuracy and reliability of sustainability-related data.
An evolving trend receiving considerable attention has been the utilization of advanced simulation and modeling techniques 21 . Additional study is required to enhance simulation and modeling methodologies in order to achieve more accurate forecasting and analysis of sustainability results. This encompasses the enhancement of energy modeling software, the utilization of computational fluid dynamics simulations, and the implementation of multi-scale modeling techniques to accurately represent intricate interactions and maximize the utilization of resources.
The application of advanced DTs including ML and AI has stimulated conversations on their anticipated threat to social aspects of sustainability. Presently, AI/ML models are used to predict sustainability performance, optimize resource allocation, and identify patterns and trends to support informed decision-making 87 . Though the potential of AI/ML models is much greater, the present utilization of such DTs for sustainable construction is limited. Thus, future research needs to pay attention to their impact at full potential and how risk will be managed among participants in the construction value chain.
The circular economy is an emerging trend in sustainability in construction and would benefit immensely from the application of DT. There is a need to develop digital tools and frameworks that support the principles of the circular economy and material optimization 88 . This includes exploring methods for tracking and managing material flows, assessing the environmental impact of material choices, and optimizing material usage throughout the construction lifecycle 89 . Present research in such areas is still nascent.
An obvious and reoccurring problem in sustainability research is long-term performance and assessment 90 . Despite the application of DTs for sustainable construction, certain inherent challenges such as system resilience and adaptability persist. Therefore, future research may focus on the extended performance and resilience of sustainable construction projects supported by DTs. This could include studying the durability and adaptability of sustainable alternatives, evaluating the performance of digital monitoring systems over extended periods, and exploring the impact of climate change and other external factors on sustainability outcomes.
Studies that look into knowledge-sharing mechanisms and collaboration among stakeholders are crucial and should be promoted. As suggested by Balasubramanian et al. 91 , future research is needed to identify effective mechanisms for sharing best practices, case studies, and lessons learned in the application of DTs for construction sustainability. This can facilitate collective learning, foster innovation, and accelerate the adoption of sustainable practices.
Implications and conclusions
This study provides an overview of current applications of DTs in promoting construction sustainability and highlights opportunities for future research and innovation. It does so by adopting a systematic literature review and content analysis to identify DTs used in relation to construction sustainability. The findings reveal BIM, IoT, and big data to have become prominent technologies over the past 5 years, with digital twins and smart robotics emerging trends in DTs for construction sustainability. The major application areas of the identified DTs are integration and collaboration; optimization, simulation, and decision-making; monitoring and control; and training. Among these application areas, DTs have been extensively researched in optimization and monitoring construction infrastructure for sustainable outcomes. The study also identifies challenges associated with DTs for construction sustainability. Key among these are technical issues of data accuracy, security, and confidence; behavioral and cultural challenges such as resistance to change among stakeholders; and financial barriers due to the high cost of implementation.
The study’s contribution lies in the provision of a holistic perspective on DTs for construction sustainability across the entire construction lifecycle. Its theoretical contribution to the sustainable construction technologies framework is to identify gaps in the existing literature and propose new directions to enable efficient deployment and implementation. It also advances knowledge of how DTs can be applied in different construction scenarios to achieve sustainable outcomes. For industry and practice, the study provides insights into challenges faced in deploying DTs for construction sustainability, enabling practitioners to anticipate and manage risks ahead of implementation. Policymakers can be informed of the landscape of DTs and their application at various stages of construction. This can guide the development of tailored policies and regulations on the efficient advancement of DTs for achieving sustainability in the industry.
Despite these contributions, the study has a few limitations. The analysis is limited to a dataset obtained only from journals in the Web of Science (WoS) database, while the selection criteria and focus on only technical articles present some limitations. While these limitations present opportunities for future research to expand the study’s findings, further studies could also examine the evolution of DTs in the built environment and their connections with sustainable development.
Bibliographic materials collection and processing
Building on the practice of conducting literature reviews within the field of construction research 75 , 92 , this study seeks to elucidate the intersections of DT and construction sustainability through a systematic literature review (SLR), designed to ensure an objective and replicable synthesis of existing research 93 . Such a structured approach mitigates the reliance on simplistic judgment calls, curtails the incidence of subjective inclinations and errors, and upholds the integrity of scholarly research 94 .
The SLR uses the consolidated steps shown in Fig. 5 95 , 96 . First, it defines research objectives and conceptual boundaries to ensure a focused review; specifically, it targets the current and emergent trends of DTs in construction sustainability. Concurrently, conceptual boundaries are defined to delineate the scope of ‘digital technologies’, ‘sustainability’, and the ‘construction industry’. This dual step guarantees that the literature review remains focused and relevant. The second step involves a comprehensive search strategy and its execution. It encompasses selecting a suitable database, which, considering the nascent and interdisciplinary nature of the field, must be expansive. The WoS is apt for this purpose, encompassing a diverse range of scholarly works, including journal articles, conference proceedings, and more 97 . We use the WoS Core Collection, including SCl, SCI-Expanded, SSCl, A&HCI, and ESCI indices. Given the evolving terminology within DTs and sustainability in construction, a detailed search string with diverse terms is formulated and applied to the WoS database. The search is temporally bound to include articles published up to October 2023, ensuring contemporary relevance. It yields 790 articles for further processing. Subsequently, a manual exclusion process is applied, where articles are meticulously screened against predefined criteria. For instance, non-English papers, review articles, and studies that do not synergize sustainability with DT are excluded. A special plan of our SLR is the discussion of excluded papers, where an independent initial assessment by two researchers with at least 7 years of research experience is followed by a mutual discussion to reach a consensus on each paper’s exclusion. This phase ultimately narrowed down the selection to 72 articles. Following this, an independent data encoding and comparative analysis is conducted. To ensure the integrity and impartiality of data extraction, the two researchers independently read articles thoroughly and encoded data from each study. This data encompasses a variety of details, such as bibliographic information, methodologies, DTs examined, stages of concern, and their sustainability implications in construction. The independently derived datasets are compared to resolve discrepancies and to build a consensus on the themes and findings. This comparison fosters a rigorous synthesis of the literature, ensuring that the results are replicable and verifiable. Finally, an analytical synthesis is performed to summarize the current and potential future state of DT in construction sustainability.
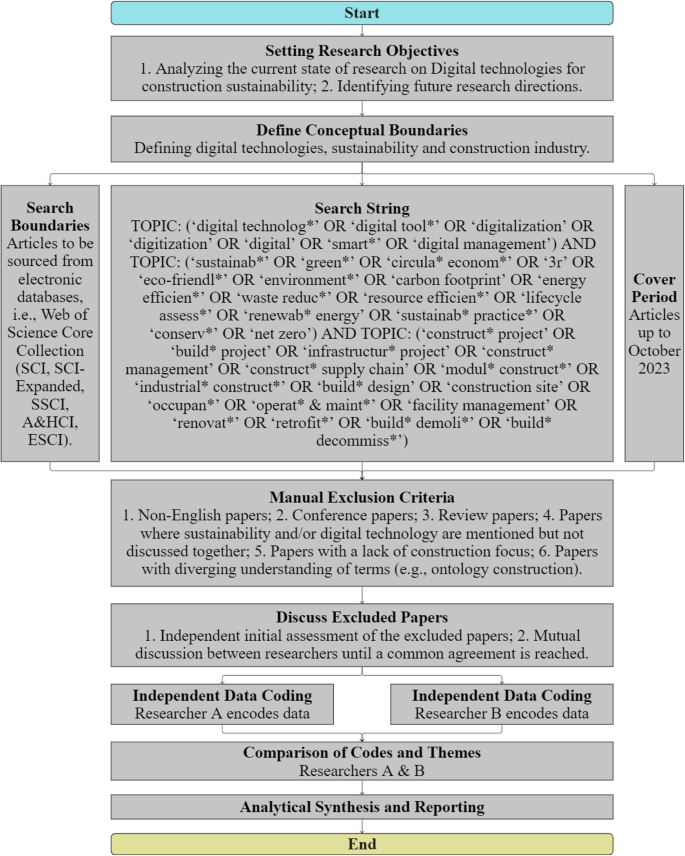
The review process follows a systematic approach.
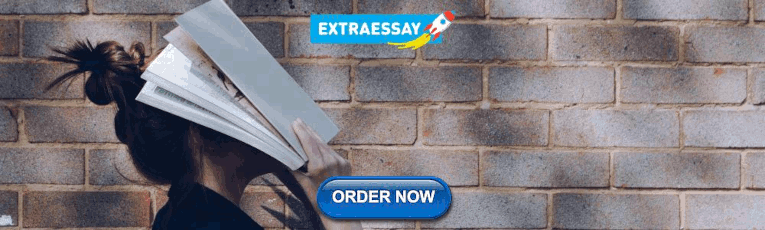
Data availability
The datasets used and/or analyzed during the current study are available from the corresponding author on reasonable request.
Barbosa, F. et al. Reinventing Construction through a Productivity Revolution (McKinsey Global Institute, 2017).
Zhang, A., Yang, J. & Wang, F. Application and enabling technologies of digital twin in operation and maintenance stage of the AEC industry: a literature review. J. Build. Eng. 80 , 107859 (2023).
Article Google Scholar
Wang, J., Li, Z. & Tam, V. W. Critical factors in effective construction waste minimization at the design stage: a Shenzhen case study, China. Resour. Conserv. Recycl. 82 , 1–7 (2014).
Bao, Z., Laovisutthichai, V., Tan, T., Wang, Q. & Lu, W. Design for manufacture and assembly (DfMA) enablers for offsite interior design and construction. Build. Res. Inf. 50 , 325–338 (2022).
Sánchez-Garrido, A. J., Navarro, I. J. & Yepes, V. Multi-criteria decision-making applied to the sustainability of building structures based on modern methods of construction. J. Cleaner Prod. 330 , 129724 (2022).
Beccali, M., Bonomolo, M., Ciulla, G., Galatioto, A. & Brano, V. L. Improvement of energy efficiency and quality of street lighting in South Italy as an action of Sustainable Energy Action Plans. The case study of Comiso (RG). Energy 92 , 394–408 (2015).
Blengini, G. A. Life cycle of buildings, demolition and recycling potential: a case study in Turin, Italy. Build. Environ. 44 , 319–330 (2009).
Bao, Z. & Lu, W. A decision-support framework for planning construction waste recycling: a case study of Shenzhen, China. J. Clean. Prod. 309 , 127449 (2021).
Bao, Z., Lee, W. M. & Lu, W. Implementing on-site construction waste recycling in Hong Kong: barriers and facilitators. Sci. Total Environ. 747 , 141091 (2020).
Article CAS PubMed PubMed Central Google Scholar
Lu, W. et al. Estimating construction waste generation in the Greater Bay Area, China using machine learning. Waste Manag. 134 , 78–88 (2021).
Article PubMed Google Scholar
Sev, A. How can the construction industry contribute to sustainable development? A conceptual framework. Sustain. Dev. 17 , 161–173 (2009).
Lima, L., Trindade, E., Alencar, L., Alencar, M. & Silva, L. Sustainability in the construction industry: a systematic review of the literature. J. Clean. Prod. 289 , 125730 (2021).
Vinuesa, R. et al. The role of artificial intelligence in achieving the Sustainable Development Goals. Nat. Commun. 11 , 1–10 (2020).
Kuhlman, T. & Farrington, J. What is sustainability? Sustainability 2 , 3436–3448 (2010).
Shelbourn, M. et al. Managing knowledge in the context of sustainable construction. J. Inf. Technol. Constr. 11 , 57–71 (2006).
Google Scholar
Zavadskas, E. K., Šaparauskas, J. & Antucheviciene, J. Sustainability in construction engineering. Sustainability 10 , 2236 (2018).
Jin, R., Zou, Y., Gidado, K., Ashton, P. & Painting, N. Scientometric analysis of BIM-based research in construction engineering and management. Eng. Constr. Archit. Manag. 26 , 1750–1776 (2019).
Gnecco, V. M., Vittori, F. & Pisello, A. L. Digital twins for decoding human-building interaction in multi-domain test-rooms for environmental comfort and energy saving via graph representation. Energy Build. 279 , 112652 (2023).
Wong, J. K. W. & Zhou, J. Enhancing environmental sustainability over building life cycles through green BIM: aA review. Autom. Constr. 57 , 156–165 (2015).
Gan, V. J. et al. Holistic BIM framework for sustainable low carbon design of high-rise buildings. J. Clean. Prod. 195 , 1091–1104 (2018).
Mirpanahi, M. V. & Noorzai, E. Modeling the relationship between critical BIM attributes and environmental sustainability criteria using PLS-SEM technique. J. Archit. Eng. 27 , 04021037 (2021).
Motalebi, M., Rashidi, A. & Nasiri, M. M. Optimization and BIM-based lifecycle assessment integration for energy efficiency retrofit of buildings. J. Build. Eng. 49 , 104022 (2022).
Hajirasouli, A., Banihashemi, S., Drogemuller, R., Fazeli, A. & Mohandes, S. R. Augmented reality in design and construction: thematic analysis and conceptual frameworks. Constr. Innov. 22 , 412–443 (2022).
Allam, Z., Sharifi, A., Bibri, S. E., Jones, D. S. & Krogstie, J. The metaverse as a virtual form of smart cities: opportunities and challenges for environmental, economic, and social sustainability in urban futures. Smart Cities 5 , 771–801 (2022).
Buonamici, F., Carfagni, M., Furferi, R., Volpe, Y. & Governi, L. Generative design: an explorative study. Comput.-Aided Des. Appl. 18 , 144–155 (2020).
Alsakka, F. et al. Generative design for more economical and environmentally sustainable reinforced concrete structures. J. Clean. Prod. 387 , 135829 (2023).
Caldas, L. Generation of energy-efficient architecture solutions applying GENE_ARCH: an evolution-based generative design system. Adv. Eng. Inform. 22 , 59–70 (2008).
Xu, J., Lou, J., Lu, W., Wu, L. & Chen, C. Ensuring construction material provenance using Internet of Things and blockchain: learning from the food industry. J. Ind. Inf. Integr. 33 , 100455 (2023).
Lou, J. & Lu, W. Construction information authentication and integrity using blockchain-oriented watermarking techniques. Autom. Constr. 143 , 104570 (2022).
Salmerón-Manzano, E. & Manzano-Agugliaro, F. The role of smart contracts in sustainability: worldwide research trends. Sustainability 11 , 3049 (2019).
Li, J. & Kassem, M. Applications of distributed ledger technology (DLT) and blockchain-enabled smart contracts in construction. Autom. Constr. 132 , 103955 (2021).
Li, X. et al. Blockchain-enabled IoT-BIM platform for supply chain management in modular construction. J. Constr. Eng. Manag. 148 , 04021195 (2022).
Xu, J., Lu, W., Wu, L., Lou, J. & Li, X. Balancing privacy and occupational safety and health in construction: a blockchain-enabled P-OSH deployment framework. Saf. Sci. 154 , 105860 (2022).
Zhao, R., Chen, Z. & Xue, F. A blockchain 3.0 paradigm for digital twins in construction project management. Autom. Constr. 145 , 104645 (2023).
Rao, A. S. et al. Real-time monitoring of construction sites: Sensors, methods, and applications. Automation in Construction 136 , 104099 (2022).
Lu, W., Lou, J. & Wu, L. Combining smart construction objects-enabled blockchain oracles and signature techniques to ensure information authentication and integrity in construction. J. Comput. Civil Eng. 37 , 04023031 (2023).
Sajjad, M. et al. Evaluation of the success of industry 4.0 digitalization practices for sustainable construction management: Chinese construction industry. Buildings 13 , 1668 (2023).
Tao, X., Mao, C., Xie, F., Liu, G. & Xu, P. Greenhouse gas emission monitoring system for manufacturing prefabricated components. Autom. Constr. 93 , 361–374 (2018).
Zhao, R. et al. Comparison of BIM collaboration paradigms for digital twin readiness: centralized files, decentralized clouds and distributed blockchains. In Proc. Conference on CIB W78 (eds Kubicki, S. & Rezgui, Y.). 11–15 (International Council for Research and Innovation in Building and Construction (CIB), 2021).
Long, W., Bao, Z., Chen, K., Ng, S. T. & Wuni, I. Y. Developing an integrative framework for digital twin applications in the building construction industry: a systematic literature review. Adv. Eng. Inform. 59 , 102346 (2024).
Opoku, D. G. J., Perera, S., Osei-Kyei, R. & Rashidi, M. Digital twin application in the construction industry: a literature review. J. Build. Eng. 40 , 102726 (2021).
Hammad, A. W., da Costa, B. B., Soares, C. A. & Haddad, A. N. The use of unmanned aerial vehicles for dynamic site layout planning in large-scale construction projects. Buildings 11 , 602 (2021).
Wu, M., Lin, J. R. & Zhang, X. H. How human-robot collaboration impacts construction productivity: an agent-based multi-fidelity modeling approach. Adv. Eng. Inform. 52 , 101589 (2022).
Villani, V., Pini, F., Leali, F. & Secchi, C. Survey on human–robot collaboration in industrial settings: safety, intuitive interfaces and applications. Mechatronics 55 , 248–266 (2018).
Halder, S. & Afsari, K. Robots in inspection and monitoring of buildings and infrastructure: a systematic review. Appl. Sci. 13 , 2304 (2023).
Article CAS Google Scholar
Lu, Q., Xie, X., Parlikad, A. K., Schooling, J. M. & Konstantinou, E. Moving from building information models to digital twins for operation and maintenance. Proc. Institution of Civil Engineers-Smart Infrastructure and Construction. 174 , 46–56 (2020).
Yitmen, I., Alizadehsalehi, S., Akıner, İ. & Akıner, M. E. An adapted model of cognitive digital twins for building lifecycle management. Appl. Sci. 11 , 4276 (2021).
Petri, I., Rezgui, Y., Ghoroghi, A. & Alzahrani, A. Digital twins for performance management in the built environment. J. Ind. Inf. Integr. 33 , 100445 (2023).
Chen, J., Lu, W. & Lou, J. Automatic concrete defect detection and reconstruction by aligning aerial images onto semantic‐rich building information model. Comput.‐Aided Civil and Infrastruct. Eng. 38 , 1079–1098 (2023).
Wang, C. et al. Integrated sensor data processing for occupancy detection in residential buildings. Energy Build. 237 , 110810 (2021).
Floris, A., Porcu, S., Girau, R. & Atzori, L. An IoT-based smart building solution for indoor environment management and occupants prediction. Energies 14 , 2959 (2021).
Nesa, N. & Banerjee, I. IoT-based sensor data fusion for occupancy sensing using Dempster–Shafer evidence theory for smart buildings. IEEE Internet Things J. 4 , 1563–1570 (2017).
Tien, P. W., Wei, S., Darkwa, J., Wood, C. & Calautit, J. K. Machine learning and deep learning methods for enhancing building energy efficiency and indoor environmental quality—a review. Energy AI 10 , 100198 (2022).
Sanzana, M. R., Maul, T., Wong, J. Y., Abdulrazic, M. O. M. & Yip, C. C. Application of deep learning in facility management and maintenance for heating, ventilation, and air conditioning. Autom. Constr. 141 , 104445 (2022).
Yuan, L., Lu, W., Xue, F. & Li, M. Building feature‐based machine learning regression to quantify urban material stocks: a Hong Kong study. J. Ind. Ecol. 27 , 336–349 (2023).
Lu, W., Long, W. & Yuan, L. A machine learning regression approach for pre-renovation construction waste auditing. J. Clean. Prod. 397 , 136596 (2023).
Lu, W., Chen, J. & Xue, F. Using computer vision to recognize composition of construction waste mixtures: a semantic segmentation approach. Resour. Conserv. Recycl. 178 , 106022 (2022).
Chen, J., Lu, W. & Xue, F. “Looking beneath the surface”: a visual-physical feature hybrid approach for unattended gauging of construction waste composition. J. Environ. Manag. 286 , 112233 (2021).
Lublasser, E., Hildebrand, L., Vollpracht, A. & Brell-Cokcan, S. Robot assisted deconstruction of multi-layered façade constructions on the example of external thermal insulation composite systems. Constr. Robot. 1 , 39–47 (2017).
Lee, S., Pan, W., Linner, T. & Bock, T. A framework for robot assisted deconstruction: process, sub-systems and modeling. In 32 nd ISARC: Proc. International Symposium on Automation and Robotics in Construction (ed. Fraser, S. J). (International Association for Automation and Robotics in Construction (IAARC), 2015).
Wu, L., Lu, W., Peng, Z. & Webster, C. A blockchain non-fungible token-enabled ‘passport’ for construction waste material cross-jurisdictional trading. Autom. Constr. 149 , 104783 (2023).
Li, L. et al. Sustainability assessment of intelligent manufacturing supported by digital twin. IEEE Access 8 , 174988–175008 (2020).
Akbarzadeh, O. et al. Heating–cooling monitoring and power consumption forecasting using LSTM for energy-efficient smart management of buildings: a computational intelligence solution for smart homes. Tsinghua Sci.Technol. 29 , 143–157 (2023).
Elnour, M. et al. Performance and energy optimization of building automation and management systems: towards smart sustainable carbon-neutral sports facilities. Renew. Sustain. Energy Rev. 162 , 112401 (2022).
Ababio, B. K., Lu, W. & Ghansah, F. A. Transitioning from green to circular procurement in developing countries: a conceptual framework for Ghana’s construction sector. Build. Res. Inf. 51 , 798–815 (2023).
Oke, A. E., Kineber, A. F., Alsolami, B. & Kingsley, C. Adoption of cloud computing tools for sustainable construction: a structural equation modeling approach. J. Facil. Manag. 21 , 334–351 (2023).
Charef, R. & Lu, W. Factor dynamics to facilitate circular economy adoption in construction. J. Clean. Prod. 319 , 128639 (2021).
Kavitha, R. J. et al. Improved Harris Hawks optimization with hybrid deep learning based heating and cooling load prediction on residential buildings. Chemosphere 309 , 136525 (2022).
Article CAS PubMed Google Scholar
Lou, J., Wang, B., Yuan, Z. & Lu, W. Willingness to pay for well-being housing attributes driven by design layout: evidence from Hong Kong. Build. Environ. 251 , 111227 (2024).
Qiu, J. et al. Design of an evaluation system for disruptive technologies to benefit smart cities. Sustainability 15 , 9109 (2023).
Bay, E., Martinez-Molina, A. & Dupont, W. A. Assessment of natural ventilation strategies in historical buildings in a hot and humid climate using energy and CFD simulations. J. Build. Eng. 51 , 104287 (2022).
Zhang, E., He, X. & Xiao, P. Does smart city construction decrease urban carbon emission intensity? Evidence from a difference-in-difference estimation in China. Sustainability 14 , 16097 (2022).
Wei, Y., Lei, Z. & Altaf, S. An off-site construction digital twin assessment framework using wood panelized construction as a case study. Buildings 12 , 566 (2022).
Chen, Y., Huang, D., Liu, Z., Osmani, M. & Demian, P. Construction 4.0, Industry 4.0, and building information modeling (BIM) for sustainable building development within the smart city. Sustainability 14 , 10028 (2022).
Qi, B., Razkenari, M., Costin, A., Kibert, C. & Fu, M. A systematic review of emerging technologies in industrialized construction. J. Build. Eng. 39 , 102265 (2021).
Petrov, I. & Hakimov, A. Digital technologies in construction monitoring and construction control. In IOP Conference Series: Materials Science and Engineering , (ed. Sergeev, V.) 497, 012016 (IOP Publishing, 2019).
Yevu, S. K., Ann, T. W. & Darko, A. Digitalization of construction supply chain and procurement in the built environment: emerging technologies and opportunities for sustainable processes. J. Clean. Prod. 322 , 129093 (2021).
Meng, Q. et al. A review of integrated applications of BIM and related technologies in whole building life cycle. Eng. Constr. Archit. Manag. 27 , 1647–1677 (2020).
Malagnino, A. et al. Building information modeling and Internet of Things integration for smart and sustainable environments: a review. J. Clean. Prod. 312 , 127716 (2021).
Wang, M., Wang, C. C., Sepasgozar, S. & Zlatanova, S. A systematic review of digital technology adoption in off-site construction: current status and future direction towards industry 4.0. Buildings 10 , 204 (2020).
Love, P. E. & Matthews, J. The ‘how’ of benefits management for digital technology: from engineering to asset management. Autom. Constr. 107 , 102930 (2019).
Liao, L. & Teo, E. A. L. Managing critical drivers for building information modeling implementation in the Singapore construction industry: an organizational change perspective. Int. J. Constr. Manag. 19 , 240–256 (2019).
Durdyev, S., Ismail, S., Ihtiyar, A., Bakar, N. F. S. A. & Darko, A. A partial least squares structural equation modeling (PLS-SEM) of barriers to sustainable construction in Malaysia. J. Clean. Prod. 204 , 564–572 (2018).
Das, M., Luo, H. & Cheng, J. C. Securing interim payments in construction projects through a blockchain-based framework. Autom. Constr. 118 , 103284 (2020).
Aghimien, D. et al. Barriers to digital technology deployment in value management practice. Buildings 12 , 731 (2022).
Moshood, T. D., Adeleke, A. Q., Nawanir, G., Ajibike, W. A. & Shittu, R. A. Emerging challenges and sustainability of industry 4.0 era in the Malaysian construction industry. Emerg. Chall. Sustain. Ind. 4 , 1627–1634 (2020).
Pan, Y. & Zhang, L. Roles of artificial intelligence in construction engineering and management: a critical review and future trends. Autom. Constr. 122 , 103517 (2021).
Antwi-Afari, P. et al. Enhancing life cycle assessment for circular economy measurement of different case scenarios of modular steel slab. Build. Environ. 239 , 110411 (2023).
Bao, Z., Lu, W. & Hao, J. Tackling the “last mile” problem in renovation waste management: a case study in China. Sci. Total Environ. 790 , 148261 (2021).
Zhang, Y. et al. A survey of the status and challenges of green building development in various countries. Sustainability 11 , 5385 (2019).
Balasubramanian, S., Shukla, V., Islam, N. & Manghat, S. Construction industry 4.0 and sustainability: an enabling framework. IEEE Trans. Eng. Manag. 71 , 1–19 (2021).
Ibem, E. O. & Laryea, S. Survey of digital technologies in procurement of construction projects. Autom. Constr. 46 , 11–21 (2014).
Tranfield, D., Denyer, D. & Smart, P. Towards a methodology for developing evidence‐informed management knowledge by means of systematic review. Br. J. Manag. 14 , 207–222 (2003).
Petticrew, M. & Roberts, H. Systematic Reviews in the Social Sciences: A Practical Guide (John Wiley & Sons, 2008).
Holzmann, P. & Gregori, P. The promise of digital technologies for sustainable entrepreneurship: a systematic literature review and research agenda. Int. J. Inf. Manag. 68 , 102593 (2023).
Okoli, C. A guide to conducting a standalone systematic literature review. Commun. Assoc. Inf. Syst. 37 , 43 (2015).
Gusenbauer, M. & Haddaway, N. R. Which academic search systems are suitable for systematic reviews or meta‐analyses? Evaluating retrieval qualities of Google Scholar, PubMed, and 26 other resources. Res. Synth. Methods 11 , 181–217 (2020).
Article PubMed PubMed Central Google Scholar
Download references
Author information
Authors and affiliations.
Department of Real Estate and Construction, Faculty of Architecture, The University of Hong Kong, Pokfulam, Hong Kong
Weisheng Lu, Jinfeng Lou, Benjamin Kwaku Ababio & Fan Xue
Department of Industrial and Manufacturing Systems Engineering, The University of Hong Kong, Pokfulam, Hong Kong
Ray Y. Zhong
School of Energy, Geoscience, Infrastructure and Society, Heriot-Watt University, Edinburgh, UK
Zhikang Bao
Department of Civil Engineering, The University of Hong Kong, Pokfulam, Hong Kong
You can also search for this author in PubMed Google Scholar
Contributions
W.L., J.L., and B.A. conducted the review and wrote the manuscript. R.Z., Z.B., X.L., and F.X. made suggestions and revised the manuscript. All authors read and approved the final manuscript.
Corresponding authors
Correspondence to Jinfeng Lou or Benjamin Kwaku Ababio .
Ethics declarations
Competing interests.
The authors declare no competing interests.
Additional information
Publisher’s note Springer Nature remains neutral with regard to jurisdictional claims in published maps and institutional affiliations.
Rights and permissions
Open Access This article is licensed under a Creative Commons Attribution 4.0 International License, which permits use, sharing, adaptation, distribution and reproduction in any medium or format, as long as you give appropriate credit to the original author(s) and the source, provide a link to the Creative Commons licence, and indicate if changes were made. The images or other third party material in this article are included in the article’s Creative Commons licence, unless indicated otherwise in a credit line to the material. If material is not included in the article’s Creative Commons licence and your intended use is not permitted by statutory regulation or exceeds the permitted use, you will need to obtain permission directly from the copyright holder. To view a copy of this licence, visit http://creativecommons.org/licenses/by/4.0/ .
Reprints and permissions
About this article
Cite this article.
Lu, W., Lou, J., Ababio, B.K. et al. Digital technologies for construction sustainability: Status quo, challenges, and future prospects. npj Mater. Sustain. 2 , 10 (2024). https://doi.org/10.1038/s44296-024-00010-2
Download citation
Received : 18 December 2023
Accepted : 04 February 2024
Published : 01 May 2024
DOI : https://doi.org/10.1038/s44296-024-00010-2
Share this article
Anyone you share the following link with will be able to read this content:
Sorry, a shareable link is not currently available for this article.
Provided by the Springer Nature SharedIt content-sharing initiative
Quick links
- Explore articles by subject
- Guide to authors
- Editorial policies
Sign up for the Nature Briefing: Anthropocene newsletter — what matters in anthropocene research, free to your inbox weekly.

SYSTEMATIC REVIEW article
Predicting histologic grades for pancreatic neuroendocrine tumors by radiologic image-based artificial intelligence: a systematic review and meta-analysis.
- 1 Department of General Surgery, Guangdong Provincial People’s Hospital (Guangdong Academy of Medical Sciences), Southern Medical University, Guangzhou, China
- 2 School of Medicine, South China University of Technology, Guangzhou, China
- 3 Department of General Surgery, Heyuan People’s Hospital, Heyuan, China
Background: Accurate detection of the histological grade of pancreatic neuroendocrine tumors (PNETs) is important for patients’ prognoses and treatment. Here, we investigated the performance of radiological image-based artificial intelligence (AI) models in predicting histological grades using meta-analysis.
Method: A systematic literature search was performed for studies published before September 2023. Study characteristics and diagnostic measures were extracted. Estimates were pooled using random-effects meta-analysis. Evaluation of risk of bias was performed by the QUADAS-2 tool.
Results: A total of 26 studies were included, 20 of which met the meta-analysis criteria. We found that the AI-based models had high area under the curve (AUC) values and showed moderate predictive value. The pooled distinguishing abilities between different grades of PNETs were 0.89 [0.84-0.90]. By performing subgroup analysis, we found that the radiomics feature-only models had a predictive value of 0.90 [0.87-0.92] with I 2 = 89.91%, while the pooled AUC value of the combined group was 0.81 [0.77-0.84] with I 2 = 41.54%. The validation group had a pooled AUC of 0.84 [0.81-0.87] without heterogenicity, whereas the validation-free group had high heterogenicity (I 2 = 91.65%, P=0.000). The machine learning group had a pooled AUC of 0.83 [0.80-0.86] with I 2 = 82.28%.
Conclusion: AI can be considered as a potential tool to detect histological PNETs grades. Sample diversity, lack of external validation, imaging modalities, inconsistent radiomics feature extraction across platforms, different modeling algorithms and software choices were sources of heterogeneity. Standardized imaging, transparent statistical methodologies for feature selection and model development are still needed in the future to achieve the transformation of radiomics results into clinical applications.
Systematic Review Registration: https://www.crd.york.ac.uk/prospero/ , identifier CRD42022341852.
Introduction
Pancreatic neuroendocrine tumors (PNETs), which account for 3–5% of all pancreatic tumors, are a heterogeneous group of tumors derived from pluripotent stem cells of the neuroendocrine system ( 1 – 3 ). In the past 10 years, the incidence and prevalence of PNETs have steadily increased ( 4 – 6 ). Unlike malignant tumors, PNETs are heterogeneous: they range from indolent to aggressive ( 7 , 8 ). The World Health Organization (WHO) histological grading system is used to evaluate the features of PNETs, and a treatment strategy is developed accordingly ( 9 , 10 ). Therefore, accurate evaluation of the histological grade is crucial for patients with PNETs; non-invasive methods are helpful, especially for tumors that are difficult to biopsy.
The application of artificial intelligence (AI) to medicine is becoming more common; it is useful in areas such as radiology, pathology, genomics, and proteomics ( 11 – 14 ), with broad applications in disease diagnosis and treatment ( 15 – 18 ). Owing to developments in AI technology, radiomic analysis can now be used to predict PNETs grade, with promising results ( 19 , 20 ). A study by Guo et al. ( 21 ), which included 37 patients with PNETs, showed that the portal enhancement ratio, arterial enhancement ratio, mean grey-level intensity, kurtosis, entropy, and uniformity were significant predictors of histological grade. Luo et al. ( 22 ) found that by using specific computed tomography (CT) images, the deep learning (DL) algorithm achieved a higher accuracy rate than radiologists (73.12% vs. 58.1%) from G3 to G1/G2. Despite promising results, other studies with different methodologies have produced different findings. Thus, quantitative analysis will be valuable for comparing study efficacy and assessing the overall predictive power of AI in detecting the histological grade for PNETs.
In this review, we aimed to systematically summarize the latest literature on AI histological grade prediction for PNETs. By performing a meta-analysis, we aimed to evaluate AI accuracy and provide evidence for its clinical application and role in decision making.
Materials and methods
This combined systematic review and meta-analysis was based on the Preferred Reporting Items for Systematic reviews and Meta-Analyses (PRISMA) guidelines. The study was registered in the Prospective Register of Systematic Reviews (PROSPERO ID: CRD42022341852).
Search strategy
Primary publications were extracted from multiple electronic databases (PubMed, MEDLINE, Cocorane and Web of Science) in September 2023 using radiomics/DL/machine learning (ML)/AI on CT/magnetic resonance imaging (MRI) examinations of PNETs grade. The search terms consisted of ML, AI, radiomics, or DL, along with PNETs grade. The detail of search string was as follows: (radiomics or machine learning or deep learning or artificial intelligence)and (PNETs or pancreatic neuroendocrine tumors). The reference lists of generated studies were then screened for eligibility.
Study selection
Two researchers determined the eligibility of each article by title and abstract evaluation and removed the duplicates. Case reports, non-original investigations (e.g., editorials, letters, and reviews), and studies that did not focus on the topic of interest were excluded. Based on the “PICOS” principle, the following inclusion criteria were designed. 1) All studies about PNETs grading which trained the models using only histology (and not biopsy) as gold standard were selected; 2) All PNETs grading predictive models built by AI were included. 3) Compared with physicians or models obtained from clinical and traditional imaging characteristics; 4) The main research purposes of the included studies were to differentiate the grades of PNETs; 5) Research types: case-control studies, cohort studies, nested case-control studies, and case-cohort studies; 6) English language. Exclusion criteria were: 1) Only the influencing factors were analyzed and a complete risk model was not built; 2) guides, case reports and non-original investigations (e.g., editorials, letters, meta-analyses and reviews); 3) other than English and animal studies. Any disagreements were resolved by consensus arbitrated by a third author.
Data extraction
Data extraction was performed independently by two reviewers, and any discrepancies were resolved by a third reviewer. The extracted data included first author, country, year of publication, study aim, study type, number of patients, sample size, validation, treatment, reference standard, imaging modality and features, methodology, model features and algorithm, software segmentation, and use of clinical information (e.g., age, tumor stage, and expression biomarkers). A detailed description of the true positive (TP), false positive (FP), true negative (TN), false negative (FN), sensitivity, and specificity were recorded. The AUC value of the validation group along with the 95% confidence interval (CI) or standard error (SE) of the model was also collected if reported.
Quality assessment
All included studies were independently assessed using the radiomics quality score (RQS), for image acquisition, radiomics feature extraction, data modeling, model validation, and data sharing. Each of the sixteen items was scored within a range of -8–36. Subsequently, the score was converted to a percentage, where -8 to 0 was defined as 0% and 36 as 100% ( 23 ).
The methodological quality of the included studies was accessed by the Quality Assessment of Diagnostic Accuracy Studies 2 (QUADAS-2) criteria ( 24 ). Two reviewers independently performed data extraction and quality assessment. Disagreements between the two reviewers were discussed at a research meeting until a consensus was reached.
Statistical analysis
Three software packages, Stata, version 12.0, MedCalc for Windows, version 16.4.3 (MedCalc Software, Ostend, Belgium), and RevMan, version 5.3.21 were used for statistical analysis. A bivariate meta-analysis model was employed to calculate the pooled sensitivity, specificity, positive likelihood ratio (PLR), negative likelihood ratio (NLR), and diagnostic odds ratio (DOR), respectively. The symmetric receiver operating characteristic (SROC) curve was generated. The I 2 value was used to assess statistical heterogeneity and estimate the percentage of variability among the included studies. An I 2 value >50% indicated substantial heterogeneity, and a random-effects model was used to analyze the differences within and between studies. In contrast, if the value was <50%, it signified less heterogeneity and a fixed-effects model was used ( 25 ). Meta-regression and subgroup analysis were conducted to explore the sources of heterogeneity. Moreover, the sensitivity analysis was also performed to evaluate the stability. Deeks’ funnel plot was used to examine publication bias. A p value less than 0.05 was considered significant. Fagan’s nomogram was employed to examine the post-test probability.
Literature selection
We retrieved 260 articles from PubMed and 156 from Web of Science; 137 were duplicates and were eliminated, resulting in 343 studies. After screening titles and abstracts, 85 potentially eligible articles were identified. After full-text review, six articles were excluded because of insufficient information; thus, 26 articles were included in this systematic review ( 21 , 22 , 26 – 49 ). Among them, six studies lacked information on positive and negative diagnosis values; therefore, only 20 articles were eligible for the meta-analysis. The results of the literature search are shown in Figure 1 .
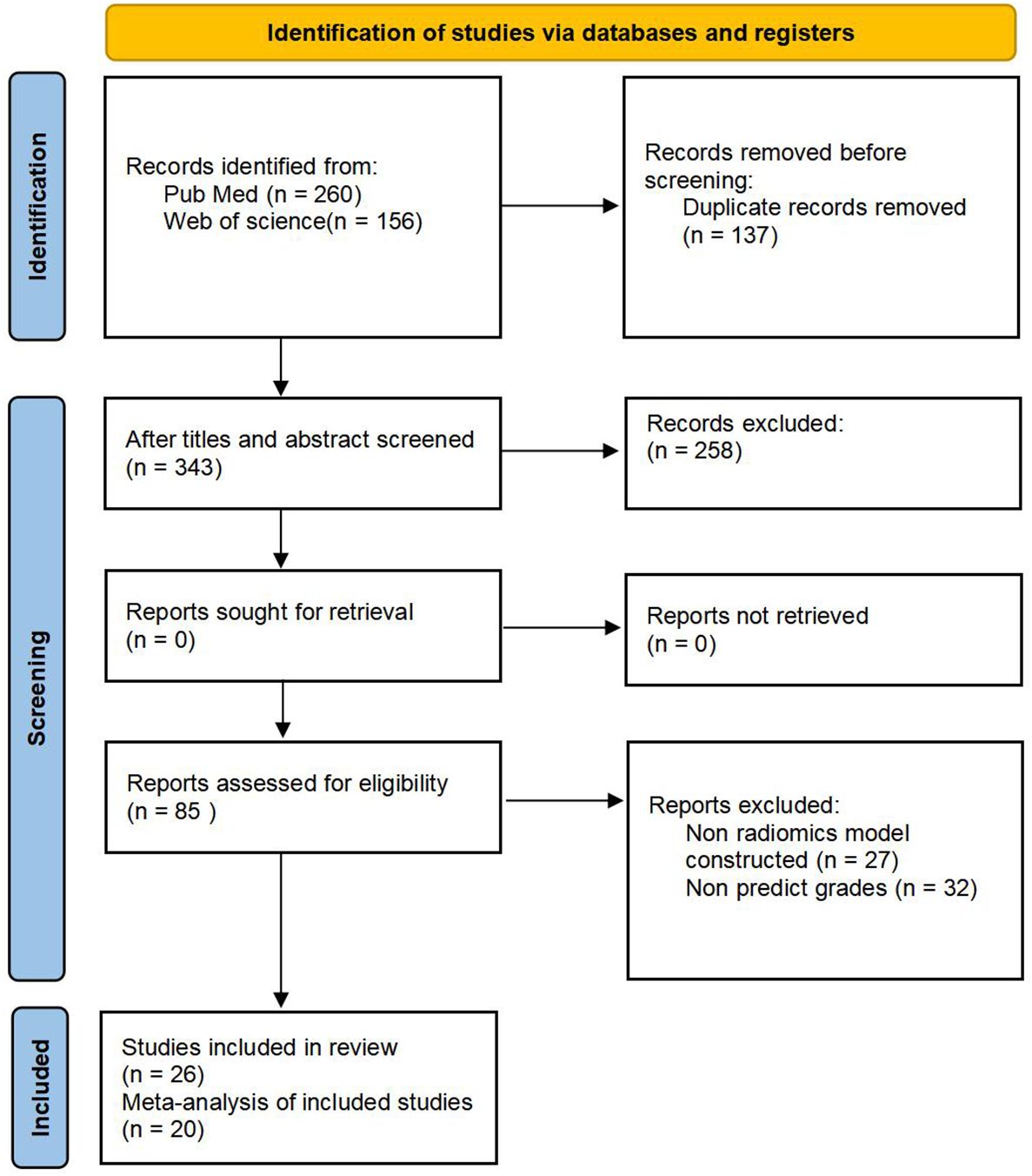
Figure 1 Flowchart of the article selection.
Quality and risk bias assessment
As shown in Table 1 , the selected articles were published between 2018 and 2023. The RQS average total and relative scores were 9.58 (2–20) and 26.60% (5.56–55.56%), respectively. No validation group in 13 studies, and five were based on two datasets from more than two distinct institutes. Due to the lack of prospective studies, deficiency of phantom studies on all scanners, absence of imaging at multiple time points, shortness of cost-effectiveness analysis, and unavailable open science and data, all the 11 included studies obtained the point of zero in these items. A detailed report of the RQS allocated by the expert reader is presented in Supplementary Table S1 .
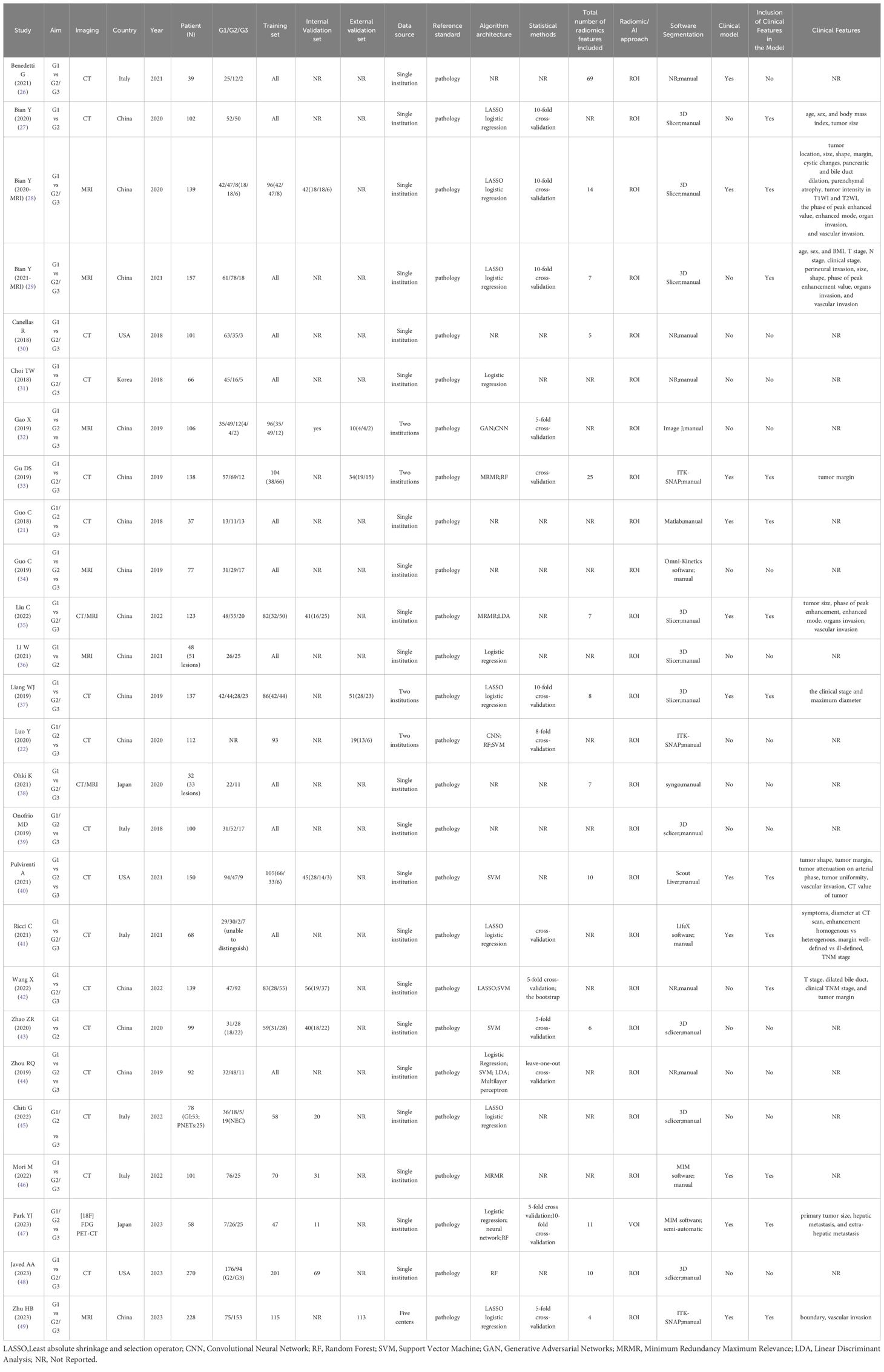
Table 1 Characteristic of all included studies.
Study quality and risk of bias were assessed using the QUADAS-2 criteria; the details are presented in Supplementary Figure S1 . A majority of studies showed a low or unclear risk of bias in each domain. In the Patient Selection domain, one study is at high risk, 25 studies are at moderate risk, and this risk mainly arises from “discontinuous patient inclusion”. In the Index Test domain, 9 studies are at moderate risk due to the insufficient information provided to make a judgment, while others were at low risk. In the Reference Standard domain, only one study is at high risk because some patients cannot be accurately categorized to the specific grading in this study. In the Flow and Timing domain, all were at low risk.
Publication bias
Deeks’ funnel plot asymmetry test was adopted to detect publication bias: no bias was detected within the meta-analysis (p=0.347, Figure 2 ).
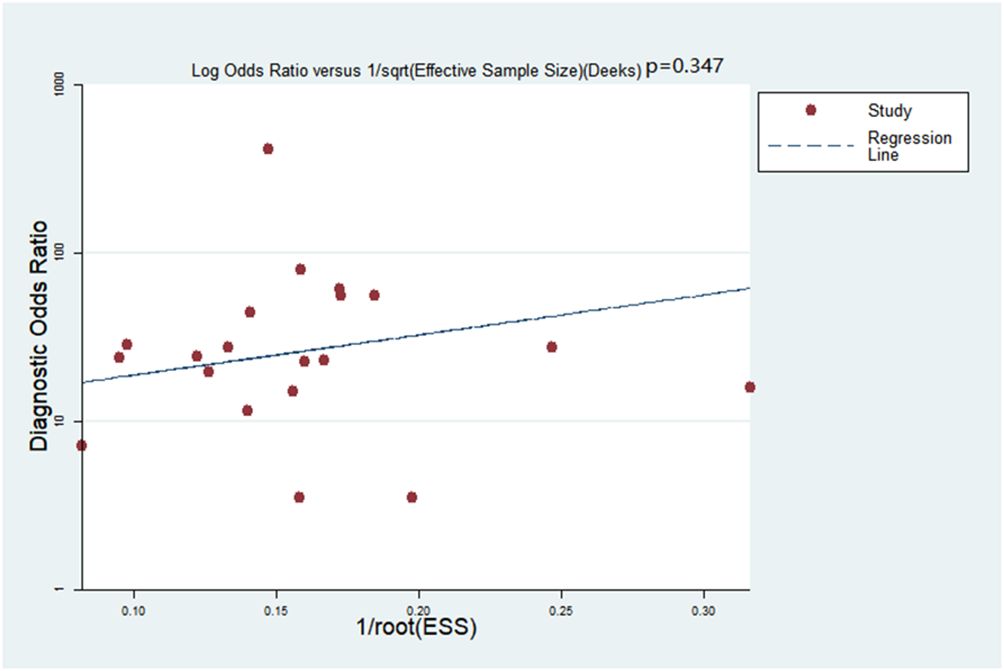
Figure 2 Deeks’ funnel plot evaluating the potential publication bias (p=0.034).
Clinical diagnostic value of grading PNETs
As shown in Figure 3 , Fagan’s nomogram was useful for evaluating the diagnostic value of PNETs grade, and clinical application. The results showed an increase of post-test probability of the positive result (at 50%) to 81%, and a decrease of the negative result to 4%.
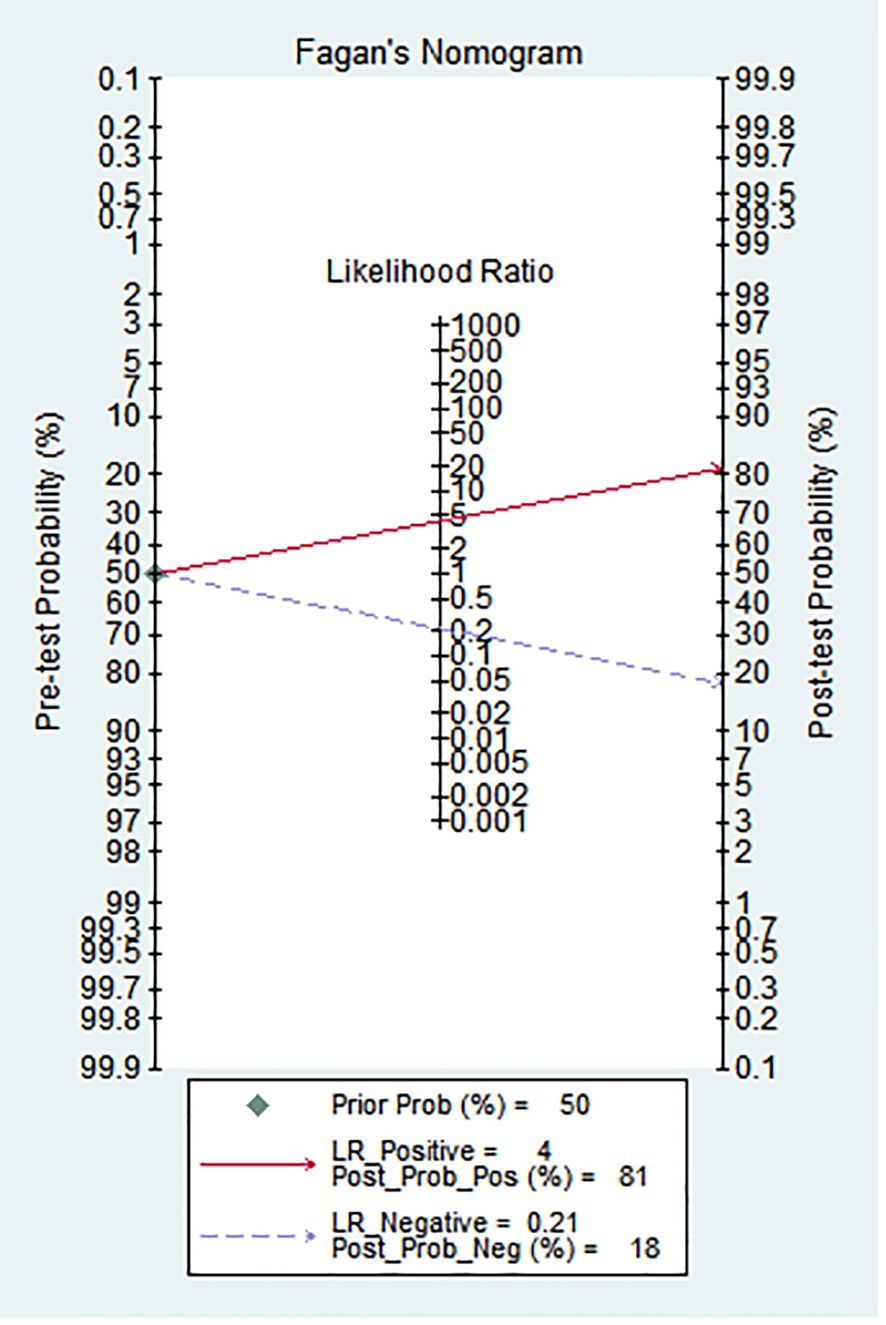
Figure 3 Fagan’s nomogram assessing the clinical diagnostic value of PNETs.
Study characteristics
Study characteristics are summarized in Table 1 . All studies employed a retrospective design, were published between 2018 and 2023, and the number of included patients was 32–270. Among the 26 studies, China was the main publication country (15 studies), followed by Italy (5 studies), the USA (3 studies), Korea (2 study), and Japan (1 study). Nineteen studies were based on CT and eight on MRI images, while two combined images from CT and MRI, and one applied for PET-CT. Thirteen of the 26 studies had validation sets; five were externally validated using data from another institute. The majority (20/26) used different kinds of ML classifications (such as Randon Rorest (RF); Support Vector Machine (SVM); Least absolute shrinkage and selection operator (LASSO) logistic regression), and two of them adopted Convolutional Neural Network (CNN). About half of the included studies (11/21) used models combined with clinical features (such as tumor size, tumor margin, TMN stage, etc.), while others used only radiomics features. Thirteen studies applied cross-validation to select stable features between observers.
The details of TP/FP/FN/TN and the models’ sensitivity and specificity are shown in Table 2 . The highest area under the curve (AUC) value of the AI-based validation model was 0.99 (95% CI: 0.97–1.00). Six studies offered no details regarding TP/FP/FN/TN, and the AUC value of four studies was incomplete; thus, all of these six studies were excluded in meta-analysis.
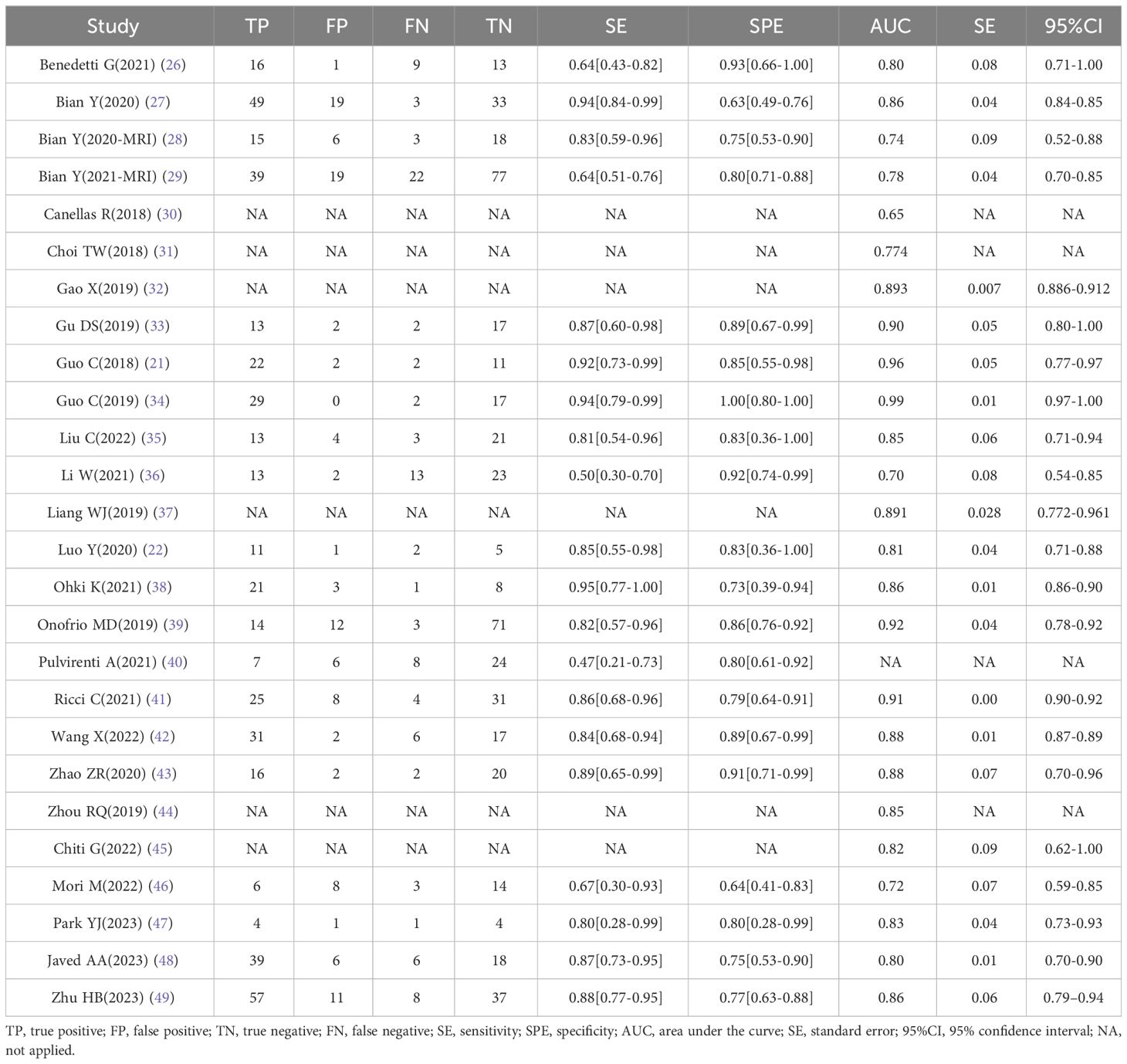
Table 2 Results for accuracy to predict grade of PNETs.
Meta-analysis
Overall performance of the ai models.
Twenty studies with 2639 patients were included in the meta-analysis, which provided data on TP/FP/FN/TN and model sensitivity and specificity, and 19 studies offered the AUC with 95% CI of the models. The results are reported in Tables 2 and 3 and Figure 4 . The AI models for PNETs showed an overall pooled sensitivity of 0.826 [0.759, 0.877], a pooled specificity of 0.812 [0.765, 0.851] and the pooled PLR and NLR were 4.382 [3.509, 5.472] and 0.215 [0.155, 0.298], respectively. Moreover, the pooled DOR was 20.387 [13.108, 31.706], and the AUC of the SROC curve was 0.89 [0.84-0.90] with I 2 = 90.42% [81.10-99.73], P=0.000.
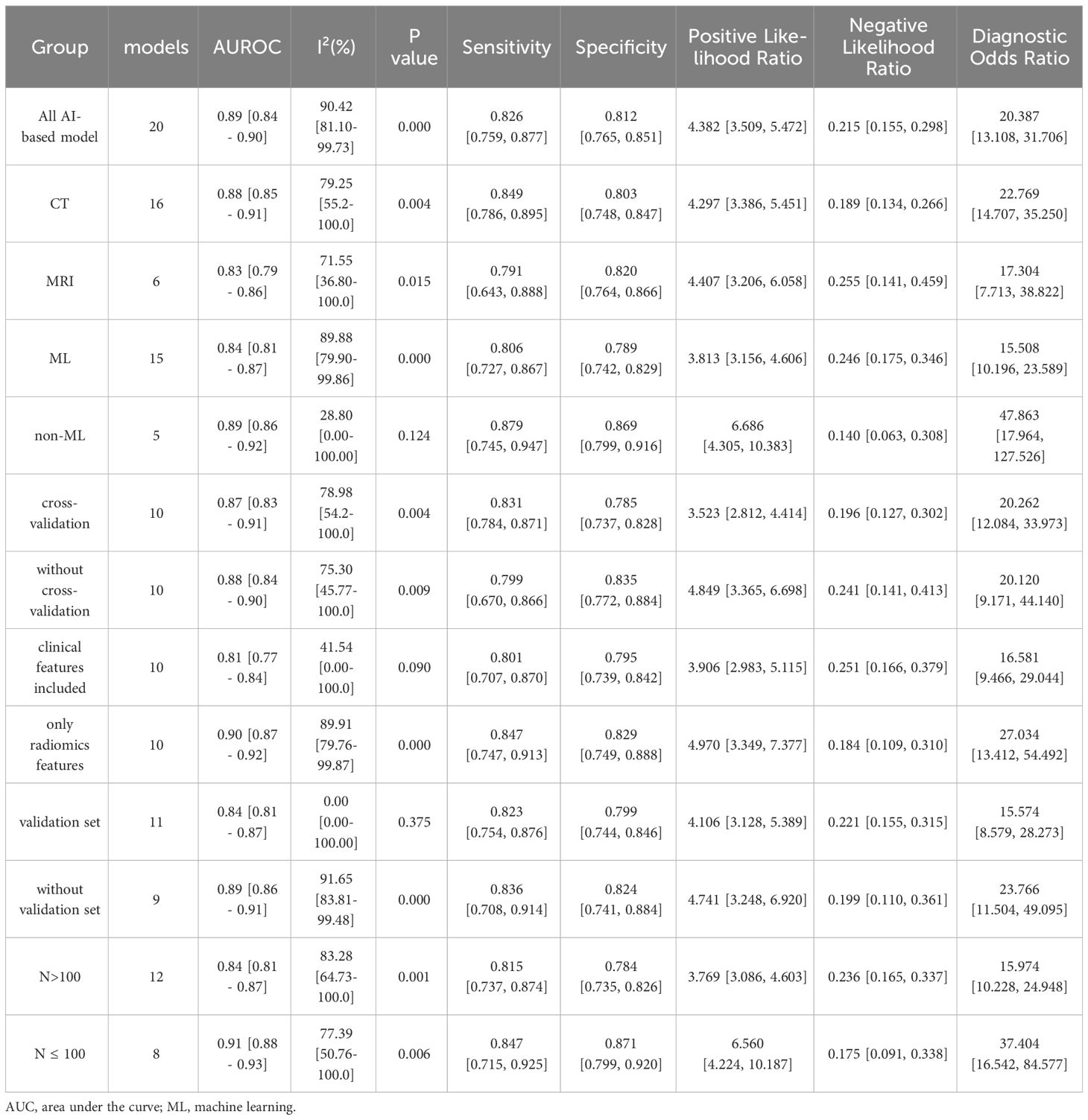
Table 3 Subgroup analysis and estimates pooled of PNETs.
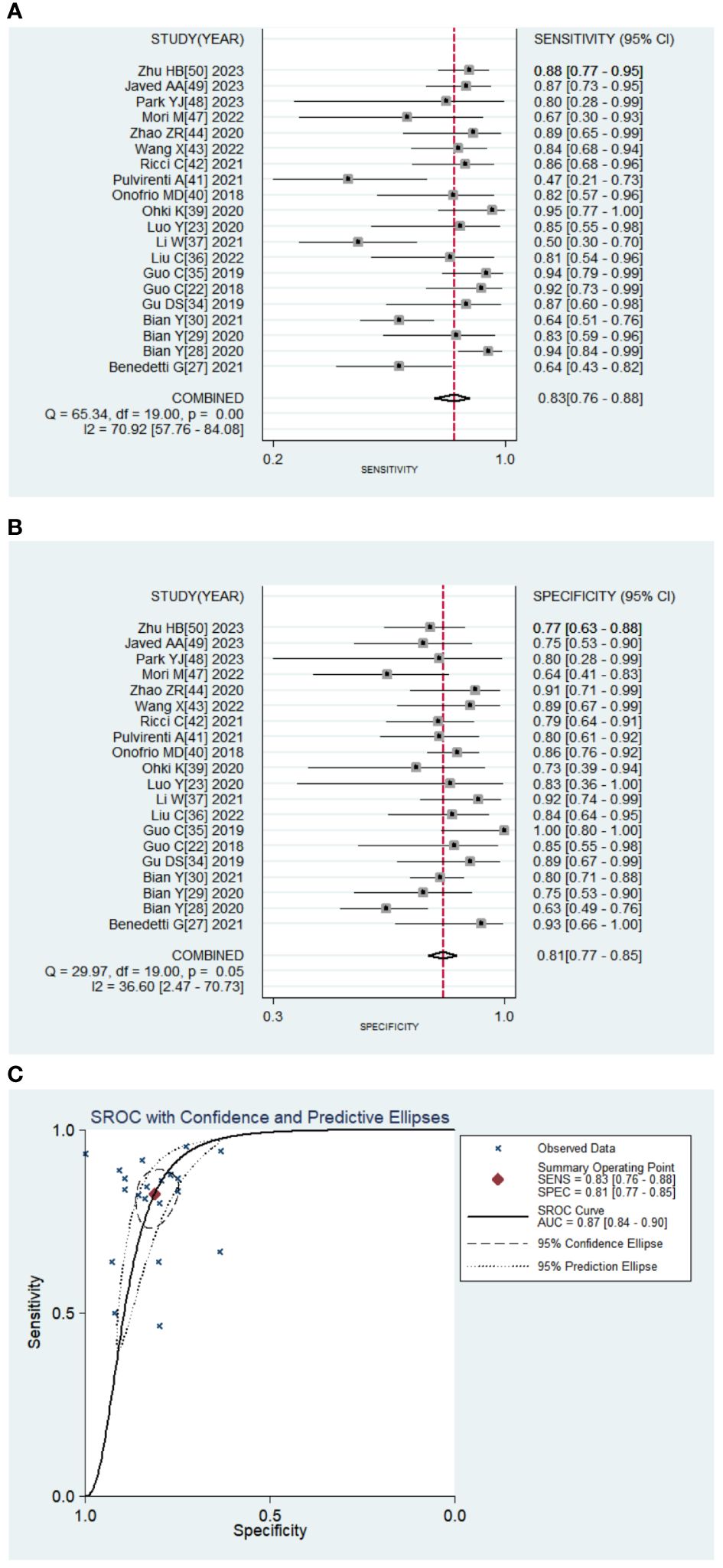
Figure 4 Pooled diagnostic accuracy of PNETs. (A, B) Forest plots of sensitivity, specificity; (C) . Summary receiver operator characteristic curve.
Subgroup analysis based on the image source and AI methodology
Meta-regression was conducted and found there was no significant differences between groups ( Supplementary Table S2 ). Then subgroup analysis was performed to compare studies evaluating the performance of different image sources: CT and MRI. Two models used both CT and MRI images; thus, 16 models extracted radiomic features from CT images and six models from MRI. The pooled SE, SP, PLR, and NLR were 0.849 [0.786, 0.895], 0.803 [0.748, 0.847], 4.297 [3.386, 5.451], and 0.189 [0.134, 0.266], respectively for CT models, and 0.791 [0.643, 0.888], 0.820 [0.764, 0.866], 4.407 [3.206, 6.058], 0.255 [0.141, 0.459], respectively for MRI models. The pooled DOR was 22.769 [14.707, 35.250] and 17.304 [7.713, 38.822] for CT and MRI models, respectively. The AUC of the SROC curve was 0.88 [0.85-0.91] with heterogeneity (I 2 = 79.25% [55.20-100.00], P=0.004) on CT images compared with MRI (AUC=0.83 [0.79-0.86], I 2 = 71.55%[36.80-100.00], P=0.015).
Subgroup analysis of different AI methodologies was used to compare algorithm architecture; most models not only applied ML classifiers, but more than one classifier. In total, 15 models were conducted using ML for PNETs. The pooled SE, SP, PLR, and NLR were 0.806 [0.727, 0.867, 0.789 [0.742, 0.829], 3.813 [3.156, 4.606], and 0.246 [0.175, 0.346], respectively. The pooled DOR was 15.508 [10.196, 23.589] and the AUC of the SROC curve was 0.84[0.81-0.87] with heterogenicity, I 2 = 89.88%[79.90-99.86]. Of the remaining three models for non-ML, the pooled AUC value was 0.89 [0.86-0.92] with I 2 = 28.80[0.00-100.00] ( Table 3 ).
There were ten models using cross-validation to select the best features and models. The group with cross-validation had a pooled AUC of 0.87 [0.83-0.91] with I 2 = 78.98%, while the group without was 0.88 [0.84-0.90] with I 2 = 75.30%. The pooled SE, SP, PLR, and NLR were 0.831 [0.784, 0.871], 0.785 [0.737, 0.828], 3.523 [2.812, 4.414] and 0.196 [0.127, 0.302], respectively for the cross-validation group, and 0.799 [0.670, 0.866], 0.835 [0.772, 0.884], 4.849 [3.365, 6.698], and 0.241 [0.141, 0.413], respectively for the group without ICC. The pooled DOR were 20.262 [12.084, 33.973] and 20.120 [9.171, 44.140] for the groups with and without ICC, respectively.
Subgroup analysis based on dataset characteristics
We also compared the models that included clinical data and by utilizing radiomics features only, and found that clinical features reduced heterogenicity. The pooled SE, SP, PLR, and NLR were 0.801 [0.707, 0.870], 0.795 [0.739, 0.842], 3.906 [2.983, 5.115], and 0.251 [0.166, 0.379], respectively for the group including clinical data, and 0.847 [0.747, 0.913], 0.829 [0.749, 0.888], 4.970 [3.349, 7.377], and 0.184 [0.109, 0.310], respectively for the radiomics-only group. The pooled DOR for the radiomics group was 27.034 [13.412, 54.492], and the AUC of the SROC curve was 0.81 [0.77-0.84] with I 2 = 41.54%, which was a little higher than that of the included clinical data group (DOR: 16.581 [9.466, 29.044]); AUC: 0.90 [0.87-0.92]) ( Table 3 ).
Moreover, 11 models were validated, while nine models were not. The pooled SE, SP, PLR, and NLR were 0.823 [0.754, 0.876], 0.799 [0.744, 0.846], 4.106 [3.128, 5.389], and 0.221 [0.155, 0.315], respectively for the validated group, and 0.836 [0.708, 0.914], 0.824 [0.741, 0.884], 4.741 [3.248, 6.920], and 0.199 [0.110,0.361], respectively for the control group. The pooled DOR for the validated group was 15.574 [8.579, 28.273] and 23.766 [11.504, 49.095] for the control group. The AUC of the SROC curve was 0.84 [0.81-0.87] without heterogeneity for the validation group and 0.89 [0.86-0.91] with I 2 = 91.65% for the control group.
In a subgroup analysis based on the number of patients, the pooled results of 12 models, which included >100 patients, were 0.815 [0.737, 0.874], 0.784 [0.735, 0.826], 3.769 [3.086, 4.603], and 0.236 [0.165, 0.337] for the pooled SE, SP, PLR, and NLR, respectively. For the remaining eight models, the pooled SE, SP, PLR, and NLR were 0.847 [0.715, 0.925], 0.871 [0.799, 0.920], 6.560 [4.224, 10.187], and 0.175 [0.091, 0.338], respectively. The pooled DOR and the AUC values for the two groups were 15.974 [10.228, 24.948] and 0.84 [0.81-0.87] vs. 37.404 [16.542, 84.577] and 0.91 [0.88-0.93] ( Table 3 ).
PNETs are a heterogeneous group of malignancies: they can be grouped into grades G1, G2, and G3 according to mitotic count and Ki-67 index ( 1 – 3 ). Accurate classification of PNETs grades is important for treatment selection, prognosis, and follow-up. However, due to the heterogeneity of PNETs, tumor grading may not be uniform within a single lesion or between different lesions in the same patient ( 7 , 8 ). Moreover, histology is currently the only validated tool to grade tumors and describe their characteristics; surgery and endoscopic biopsy are used clinically to analyze the histological grade of PNETs. However, it is difficult to perform a satisfactory biopsy for PNETs located around major vessels, or small tumors—especially using fine-needle aspiration biopsy ( 50 – 53 ). Therefore, the detection of histological grades based on radiological images is also an important diagnostic tool. With increasing AI application in medical fields, we believe that AI-based models can enhance the prediction value of tumor grading. To the best of our knowledge, we are only aware of few and insufficiently updated systematic review on this topic that has evaluated the diagnostic accuracy of radiomics.
In our study, we investigated the ability of imaging-based AI to detect PNETs histologic grading. Our results showed that AI-based grading of PNETs with an AUC of 0.89 [0.84 - 0.90] exhibited good performance but high heterogeneity (I 2 = 90.42% [81.10-99.73], P = 0.000). Among the included studies, we found considerable heterogeneity in pooled sensitivity and specificity. Moreover, according to our sensitive analysis, 3 articles ( 29 , 40 , 46 ) had poor robustness and may be one of the sources of heterogeneity ( Figure S2 ). There was no significant publication bias between studies.
The diagnostic performance of the radiomics model varied with the strategies employed. CT and MRI images are the main sources for analyzing PNETs. Because of its high availability and low cost, CT is widely used than MRI. In this study, we found that imaging techniques may be influencing factors of prediction power, but not independently so. CT was more commonly used (16 studies) and showed better performance than MRI (6 studies) in grading PNETs, with an AUC of 0.88 [0.85-0.91] vs. 0.83 [0.79-0.86]. Although unconfirmed, we speculate that CT may be more powerful for obtaining vessel enhancement characteristics and observing the neo-vascular distribution, which is useful in vascularly-rich PNETs ( 54 ). Future studies are needed to validate this finding. We had only one study applied PET-CT grading PNETs and found AUROC of 0.864 in the tumor grade prediction model which showed good forecasting ability ( 47 ). Thus, more investigation into PET-CT will be useful in developing AI models, which showing good predictive performance (AUC = 0.992) and can detect cell surface expression of somatostatin receptors ( 55 , 56 ).
Clinical data such as age, gender, tumor size, tumor shape, tumor margin and CT stage are closely related to the pathogenic process of PNETs and therefore should not be ignored in diagnostic models ( 27 – 29 , 47 , 49 ).,Liang et al. ( 37 ) built a combined model which can improve the performance (0.856, [0.730–0.939] vs. 0.885 [0.765–0.957]). Wang et al. ( 42 ) found that the addition of clinical features can improve the radiomics models (from 0.837 [0.827–0.847] to 0.879 [0.869–0.889]). However, we found that including clinical factors did not always result in better performance but did decrease the heterogenicity (AUC of 0.81 [0.77-0.84] with I 2 = 41.94% vs. 0.90 [0.87-0.92] with I 2 = 89.91%). This may due to the data are processed differently, such as age or other clinical numerical data can be easily quantified by radiomic modeling (i.e., age as a variable in an algorithm or function). And in clinical models, age regarded as risk factors always varied in different situations. Therefore, future radiomics analyses should incorporate clinical features to create more reliable models or add radiomics features to existing diagnostic models to validate their true diagnostic power.
The lack of standardized quality control and reporting throughout the workflow limits the application of radiomics ( 17 , 57 ). For example, validation/testing data must remain completely independent or hidden until validation/testing is performed in order to create generalizable predictive models at each step of a radiomics study. In our study, 11 studies of 20 had validation set and only 3 had external validation. Lack of proper external validation would influence the transportability and generalizability of the models in the studies and also hamper the conclusions of the review. Moreover, according to our findings, lacking validation sets was also one of the main causes of heterogeneity. There should be no direct comparison between the results obtained by studying only the primary cohort and those obtained by studying both the primary and validation cohorts. Validated models should be considered more reliable and promising, even if the reported performance is lower.
As shown in Table 1 , there were also a wide variety of feature extraction and model selection methods, and although AI classifiers did not show outstanding diagnostic performance in our evaluation, it is undeniably a future research direction and trend. Most of the included studies used more than one machine learning or deep learning for feature selection or classification, but the best performing AI classifiers varied from study to study. To date, there are no universal and well-recognized classifiers, and the characteristics of the samples are a key factor affecting the performance of classifiers ( 58 , 59 ). Finding uniform and robust classifiers for specific medical problems has always been a challenge.
Despite the encouraging results of this meta-analysis, the overall methodological quality of the included literature was poor, reducing the reliability and reproducibility of radiomics models for clinical applications. Lack of prospective studies with scanner modeling studies, lack of imaging studies at multiple time points, insufficient validation and calibration validity of the models, short time frame for cost-effectiveness analyses, insufficient cost-effectiveness analyses, and lack of publicly available science and data contributed to the low RQS scores. In addition, only half of the studies were internally validated and less independent external validation. To further standardize the process and improve the quality of radiomics, the RQS should be used not only to assess the methodological quality of radiomics studies, but also to guide the design of radiomics studies ( 17 ).
Diversity of the samples, inconsistencies with radiomics feature extraction across platforms, different modeling algorithms, and simultaneous incorporation of clinical features may all account for the high heterogenicity of the combined models. According to our sub-analysis results, the heterogenicity mainly came from the different imaging materials (CT vs MRI), the algorithm architecture (ML vs non-ML), whether validated or not and clinical features included. Thus, standardized imaging, a standardized independent and robust set of features, as well as validation even external validation are all approaches to lower the heterogenicity and highlights for attention in future research. To sum, the AI method was effective in the preoperative prediction of PNETs grade; this may help with the understanding of tumor behavior, and facilitate vision-making in clinical practice.
Our study has several limitations. First, most included studies were single-center and retrospective, inevitably causing patient selection bias. Second, different methods were investigated, including the type of imaging scans utilized, the type and number of radiological features studied, the choice of software, and the type of analysis/methods implemented, thus leading to the high heterogeneity among studies. Therefore, some pooled estimates of the quantitative results must be interpreted with caution. Further prospective studies could validate these results; a stable method of data extraction and analysis is important for developing a reproducible AI model.
Conclusions
Overall, this meta-analysis demonstrated the value of AI models in predicting PNETs grading. According to our result, diversity of the samples, lack of external validation, imaging modalities, inconsistencies with radiomics feature extraction across platforms, different modeling algorithms and the choice of software all are sources of heterogeneity. Thus, standardized imaging, as well as a standardized, independent and robust set of features will be important for future application. Multi-center, large-sample, randomized clinical trials could be used to confirm the predictive power of image-based AI systems in clinical practice. To sum, AI can be considered as a potential tool to detect histological PNETs grades.
Data availability statement
The original contributions presented in the study are included in the article/ Supplementary Material . Further inquiries can be directed to the corresponding authors.
Author contributions
QY: Conceptualization, Methodology, Visualization, Writing – original draft. YC: Data curation, Methodology, Software, Writing – original draft. CL: Data curation, Investigation, Software, Writing – original draft. HS: Data curation, Investigation, Validation, Writing – original draft. MH: Formal analysis, Software, Writing – original draft. ZW: Investigation, Validation, Writing – original draft. SH: Data curation, Formal analysis, Funding acquisition, Writing – review & editing. CZ: Funding acquisition, Project administration, Supervision, Writing – review & editing. BH: Funding acquisition, Project administration, Supervision, Writing – review & editing.
The author(s) declare that financial support was received for the research, authorship, and/or publication of this article. This study was supported by the Natural Science Foundation of China (82102961, 82072635 and 82072637), High-level Hospital Construction Research Project of Heyuan People's Hospital (YNKT202202), the Science and Technology Program of Heyuan (23051017147335), the Science and Technology Program of Guangzhou (2024A04J10016 and 202201011642).
Conflict of interest
The authors declare that the research was conducted in the absence of any commercial or financial relationships that could be construed as a potential conflict of interest.
Publisher’s note
All claims expressed in this article are solely those of the authors and do not necessarily represent those of their affiliated organizations, or those of the publisher, the editors and the reviewers. Any product that may be evaluated in this article, or claim that may be made by its manufacturer, is not guaranteed or endorsed by the publisher.
Supplementary material
The Supplementary Material for this article can be found online at: https://www.frontiersin.org/articles/10.3389/fonc.2024.1332387/full#supplementary-material
Supplementary Figure 1 | The quality assessment of 26 included studies by QUADAS-2 tool.
Supplementary Figure 2 | The sensitive analysis of 26 included studies.
1. Chang A, Sherman SK, Howe JR, Sahai V. Progress in the management of pancreatic neuroendocrine tumors. Annu Rev Med . (2022) 73:213–29. doi: 10.1146/annurev-med-042320-011248
PubMed Abstract | CrossRef Full Text | Google Scholar
2. Ma ZY, Gong YF, Zhuang HK, Zhou ZX, Huang SZ, Zou YP, et al. Pancreatic neuroendocrine tumors: A review of serum biomarkers, staging, and management. World J Gastroenterol . (2020) 26:2305–22. doi: 10.3748/wjg.v26.i19.2305
3. Cives M, Strosberg JR. Gastroenteropancreatic neuroendocrine tumors. CA Cancer J Clin . (2018) 68:471–87. doi: 10.3322/caac.21493
4. Pulvirenti A, Marchegiani G, Pea A, Allegrini V, Esposito A, Casetti L, et al. Clinical implications of the 2016 international study group on pancreatic surgery definition and grading of postoperative pancreatic fistula on 775 consecutive pancreatic resections. Ann Surg . (2018) 268:1069–75. doi: 10.1097/SLA.0000000000002362
5. Fan JH, Zhang YQ, Shi SS, Chen YJ, Yuan XH, Jiang LM, et al. A nation-wide retrospective epidemiological study of gastroenteropancreatic neuroendocrine neoplasms in China. Oncotarget . (2017) 8:71699–708. doi: 10.18632/oncotarget.17599
6. Yao JC, Hassan M, Phan A, Dagohoy C, Leary C, Mares JE, et al. One hundred years after "carcinoid": epidemiology of and prognostic factors for neuroendocrine tumors in 35,825 cases in the United States. J Clin Oncol . (2008) 26:3063–72. doi: 10.1200/JCO.2007.15.4377
7. Yang Z, Tang LH, Klimstra DS. Effect of tumor heterogeneity on the assessment of Ki67 labeling index in well-differentiated neuroendocrine tumors metastatic to the liver: implications for prognostic stratification. Am J Surg Pathol . (2011) 35:853–60. doi: 10.1097/PAS.0b013e31821a0696
8. Partelli S, Gaujoux S, Boninsegna L, Cherif R, Crippa S, Couvelard A, et al. Pattern and clinical predictors of lymph node involvement in nonfunctioning pancreatic neuroendocrine tumors (NF-PanNETs). JAMA Surg . (2013) 148:932–9. doi: 10.1001/jamasurg.2013.3376
9. Marchegiani G, Landoni L, Andrianello S, Masini G, Cingarlini S, D'Onofrio M, et al. Patterns of recurrence after resection for pancreatic neuroendocrine tumors: who, when, and where? Neuroendocrinology . (2019) 108:161–71. doi: 10.1159/000495774
10. Nagtegaal ID, Odze RD, Klimstra D, Paradis V, Rugge M, Schirmacher P, et al. The 2019 WHO classification of tumours of the digestive system. Histopathology . (2020) 76:182–8. doi: 10.1111/his.13975
11. Hosny A, Parmar C, Quackenbush J, Schwartz LH, Aerts HJWL. Artificial intelligence in radiology. Nat Rev Cancer . (2018) 18:500–10. doi: 10.1038/s41568-018-0016-5
12. Jin P, Ji X, Kang W, Li Y, Liu H, Ma F, et al. Artificial intelligence in gastric cancer: a systematic review. J Cancer Res Clin Oncol . (2020) 146:2339–50. doi: 10.1007/s00432-020-03304-9
13. Yu KH, Beam AL, Kohane IS. Artificial intelligence in healthcare. Nat BioMed Eng . (2018) 2:719–31. doi: 10.1038/s41551-018-0305-z
14. Beam AL, Kohane IS. Big data and machine learning in health care. JAMA . (2018) 319:1317–8. doi: 10.1001/jama.2017.18391
15. Greener JG, Kandathil SM, Moffat L, Jones DT. A guide to machine learning for biologists. Nat Rev Mol Cell Biol . (2022) 23:40–55. doi: 10.1038/s41580-021-00407-0
16. Issa NT, Stathias V, Schürer S, Dakshanamurthy S. Machine and deep learning approaches for cancer drug repurposing. Semin Cancer Biol . (2021) 68:132–42. doi: 10.1016/j.semcancer.2019.12.011
17. Bezzi C, Mapelli P, Presotto L, Neri I, Scifo P, Savi A, et al. Radiomics in pancreatic neuroendocrine tumors: methodological issues and clinical significance. Eur J Nucl Med Mol Imaging . (2021) 48:4002–15. doi: 10.1007/s00259-021-05338-8
18. Rauschecker AM, Rudie JD, Xie L, Wang J, Duong MT, Botzolakis EJ, et al. Artificial intelligence system approaching neuroradiologist-level differential diagnosis accuracy at brain MRI. Radiology . (2020) 295:626–37. doi: 10.1148/radiol.2020190283
19. Caruso D, Polici M, Rinzivillo M, Zerunian M, Nacci I, Marasco M, et al. CT-based radiomics for prediction of therapeutic response to Everolimus in metastatic neuroendocrine tumors. Radiol Med . (2022) 127:691–701. doi: 10.1007/s11547-022-01506-4
20. Yang J, Xu L, Yang P, Wan Y, Luo C, Yen EA, et al. Generalized methodology for radiomic feature selection and modeling in predicting clinical outcomes. Phys Med Biol . (2021) 66:10.1088/1361-6560/ac2ea5. doi: 10.1088/1361-6560/ac2ea5
CrossRef Full Text | Google Scholar
21. Guo C, Zhuge X, Wang Z, Wang Q, Sun K, Feng Z, et al. Textural analysis on contrast-enhanced CT in pancreatic neuroendocrine neoplasms: association with WHO grade. Abdom Radiol (NY) . (2019) 44:576–85. doi: 10.1007/s00261-018-1763-1
22. Luo Y, Chen X, Chen J, Song C, Shen J, Xiao H, et al. Preoperative prediction of pancreatic neuroendocrine neoplasms grading based on enhanced computed tomography imaging: validation of deep learning with a convolutional neural network. Neuroendocrinology . (2020) 110:338–50. doi: 10.1159/000503291
23. Lambin P, Leijenaar RTH, Deist TM. Radiomics: the bridge between medical imaging and personalized medicine. Nat Rev Clin Oncol . (2017) 14:749–62. doi: 10.1038/nrclinonc.2017.141
24. Whiting PF, Rutjes AW, Westwood ME, Mallett S, Deeks JJ, Reitsma JB, et al. QUADAS-2: a revised tool for the quality assessment of diagnostic accuracy studies. Ann Intern Med . (2011) 155:529–36. doi: 10.7326/0003-4819-155-8-201110180-00009
25. Higgins JP, Thompson SG, Deeks JJ, Altman DG. Measuring inconsistency in meta- analyses. BMJ . (2003) 327:557–60. doi: 10.1136/bmj.327.7414.557
26. Benedetti G, Mori M, Panzeri MM, Barbera M, Palumbo D, Sini C, et al. CT-derived radiomic features to discriminate histologic characteristics of pancreatic neuroendocrine tumors. Radiol Med . (2021) 126:745–60. doi: 10.1007/s11547-021-01333-z
27. Bian Y, Jiang H, Ma C, Wang L, Zheng J, Jin G, et al. CT-based radiomics score for distinguishing between grade 1 and grade 2 nonfunctioning pancreatic neuroendocrine tumors. AJR Am J Roentgenol . (2020) 215:852–63. doi: 10.2214/AJR.19.22123
28. Bian Y, Zhao Z, Jiang H, Fang X, Li J, Cao K, et al. Noncontrast radiomics approach for predicting grades of nonfunctional pancreatic neuroendocrine tumors. J Magn Reson Imaging . (2020) 52:1124–36. doi: 10.1002/jmri.27176
29. Bian Y, Li J, Cao K, Fang X, Jiang H, Ma C, et al. Magnetic resonance imaging radiomic analysis can preoperatively predict G1 and G2/3 grades in patients with NF-pNETs. Abdom Radiol (NY) . (2021) 46:667–80. doi: 10.1007/s00261-020-02706-0
30. Canellas R, Burk KS, Parakh A, Sahani DV. Prediction of pancreatic neuroendocrine tumor grade based on CT features and texture analysis. AJR Am J Roentgenol . (2018) 210:341–6. doi: 10.2214/AJR.17.18417
31. Choi TW, Kim JH, Yu MH, Park SJ, Han JK. Pancreatic neuroendocrine tumor: prediction of the tumor grade using CT findings and computerized texture analysis. Acta Radiol . (2018) 59:383–92. doi: 10.1177/0284185117725367
32. Gao X, Wang X. Deep learning for World Health Organization grades of pancreatic neuroendocrine tumors on contrast-enhanced magnetic resonance images: a preliminary study. Int J Comput Assist Radiol Surg . (2019) 14:1981–91. doi: 10.1007/s11548-019-02070-5
33. Gu D, Hu Y, Ding H, Wei J, Chen K, Liu H, et al. CT radiomics may predict the grade of pancreatic neuroendocrine tumors: a multicenter study. Eur Radiol . (2019) 29:6880–90. doi: 10.1007/s00330-019-06176-x
34. Guo CG, Ren S, Chen X, Wang QD, Xiao WB, Zhang JF, et al. Pancreatic neuroendocrine tumor: prediction of the tumor grade using magnetic resonance imaging findings and texture analysis with 3-T magnetic resonance. Cancer Manag Res . (2019) 11:1933–44. doi: 10.2147/CMAR
35. Liu C, Bian Y, Meng Y, Liu F, Cao K, Zhang H, et al. Preoperative prediction of G1 and G2/3 grades in patients with nonfunctional pancreatic neuroendocrine tumors using multimodality imaging. Acad Radiol . (2022) 29:e49–60. doi: 10.1016/j.acra.2021.05.017
36. Li W, Xu C, Ye Z. Prediction of pancreatic neuroendocrine tumor grading risk based on quantitative radiomic analysis of MR. Front Oncol . (2021) 11:758062. doi: 10.3389/fonc.2021.758062
37. Liang W, Yang P, Huang R, Xu L, Wang J, Liu W, et al. A combined nomogram model to preoperatively predict histologic grade in pancreatic neuroendocrine tumors. Clin Cancer Res . (2019) 25:584–94. doi: 10.1158/1078-0432.CCR-18-1305
38. Ohki K, Igarashi T, Ashida H, Takenaga S, Shiraishi M, Nozawa Y, et al. Usefulness of texture analysis for grading pancreatic neuroendocrine tumors on contrast-enhanced computed tomography and apparent diffusion coefficient maps. Jpn J Radiol . (2021) 39:66–75. doi: 10.1007/s11604-020-01038-9
39. D'Onofrio M, Ciaravino V, Cardobi N, De Robertis R, Cingarlini S, Landoni L, et al. CT enhancement and 3D texture analysis of pancreatic neuroendocrine neoplasms. Sci Rep . (2019) 9:2176. doi: 10.1038/s41598-018-38459-6
40. Pulvirenti A, Yamashita R, Chakraborty J, Horvat N, Seier K, McIntyre CA, et al. Quantitative computed tomography image analysis to predict pancreatic neuroendocrine tumor grade. JCO Clin Cancer Inform . (2021) 5:679–94. doi: 10.1200/CCI.20.00121
41. Ricci C, Mosconi C, Ingaldi C, Vara G, Verna M, Pettinari I, et al. The 3-dimensional-computed tomography texture is useful to predict pancreatic neuroendocrine tumor grading. Pancreas . (2021) 50:1392–9. doi: 10.1097/MPA.0000000000001927
42. Wang X, Qiu JJ, Tan CL, Chen YH, Tan QQ, Ren SJ, et al. Development and validation of a novel radiomics-based nomogram with machine learning to preoperatively predict histologic grade in pancreatic neuroendocrine tumors. Front Oncol . (2022) 12:843376. doi: 10.3389/fonc.2022.843376
43. Zhao Z, Bian Y, Jiang H, Fang X, Li J, Cao K, et al. CT-radiomic approach to predict G1/2 nonfunctional pancreatic neuroendocrine tumor. Acad Radiol . (2020) 27:e272–81. doi: 10.1016/j.acra.2020.01.002
44. Zhou RQ, Ji HC, Liu Q, Zhu CY, Liu R. Leveraging machine learning techniques for predicting pancreatic neuroendocrine tumor grades using biochemical and tumor markers. World J Clin Cases . (2019) 7:1611–22. doi: 10.12998/wjcc.v7.i13.1611
45. Chiti G, Grazzini G, Flammia F, Matteuzzi B, Tortoli P, Bettarini S, et al. Gastroenteropancreatic neuroendocrine neoplasms (GEP-NENs): a radiomic model to predict tumor grade. Radiol Med . (2022) 127:928–38. doi: 10.1007/s11547-022-01529-x
46. Mori M, Palumbo D, Muffatti F, Partelli S, Mushtaq J, Andreasi V, et al. Prediction of the characteristics of aggressiveness of pancreatic neuroendocrine neoplasms (PanNENs) based on CT radiomic features. Eur Radiol . (2023) 33:4412–21. doi: 10.1007/s00330-022-09351-9
47. Park YJ, Park YS, Kim ST, Hyun SH. A machine learning approach using [18F]FDG PET-based radiomics for prediction of tumor grade and prognosis in pancreatic neuroendocrine tumor. Mol Imaging Biol . (2023) 25:897–910. doi: 10.1007/s11307-023-01832-7
48. Javed AA, Zhu Z, Kinny-Köster B, Habib JR, Kawamoto S, Hruban RH, et al. Accurate non-invasive grading of nonfunctional pancreatic neuroendocrine tumors with a CT derived radiomics signature. Diagn Interv Imaging . (2024) 105:33–39. doi: 10.1016/j.diii.2023.08.002
49. Zhu HB, Zhu HT, Jiang L, Nie P, Hu J, Tang W, et al. Radiomics analysis from magnetic resonance imaging in predicting the grade of nonfunctioning pancreatic neuroendocrine tumors: a multicenter study. Eur Radiol . (2023) 34:90–102. doi: 10.1007/s00330-023-09957-7
50. Sadula A, Li G, Xiu D, Ye C, Ren S, Guo X, et al. Clinicopathological characteristics of nonfunctional pancreatic neuroendocrine neoplasms and the effect of surgical treatment on the prognosis of patients with liver metastases: A study based on the SEER database. Comput Math Methods Med . (2022) 2022:3689895. doi: 10.1155/2022/3689895
51. Wallace MB, Kennedy T, Durkalski V, Eloubeidi MA, Etamad R, Matsuda K, et al. Randomized controlled trial of EUS-guided fine needle aspiration techniques for the detection of Malignant lymphadenopathy. Gastrointest Endosc . (2001) 54:441–7. doi: 10.1067/mge.2001.117764
52. Canakis A, Lee LS. Current updates and future directions in diagnosis and management of gastroenteropancreatic neuroendocrine neoplasms. World J Gastrointest Endosc . (2022) 14:267–90. doi: 10.4253/wjge.v14.i5.267
53. Sallinen VJ, Le Large TYS, Tieftrunk E, Galeev S, Kovalenko Z, Haugvik SP, et al. Prognosis of sporadic resected small (≤2 cm) nonfunctional pancreatic neuroendocrine tumors—a multiinstitutional study. HPB . (2018) 20:251–9. doi: 10.1016/j.hpb.2017.08.034
54. Liu Y, Shi S, Hua J, Xu J, Zhang B, Liu J, et al. Differentiation of solid-pseudopapillary tumors of the pancreas from pancreatic neuroendocrine tumors by using endoscopic ultrasound. Clin Res Hepatol Gastroenterol . (2020) 44:947–53. doi: 10.1016/j.clinre.2020.02.002
55. Mapelli P, Bezzi C, Palumbo D, Canevari C, Ghezzo S, Samanes Gajate AM, et al. 68Ga-DOTATOC PET/MR imaging and radiomic parameters in predicting histopathological prognostic factors in patients with pancreatic neuroendocrine well-differentiated tumours. Eur J Nucl Med Mol Imaging . (2022) 49:2352–63. doi: 10.1007/s00259-022-05677-0
56. Atkinson C, Ganeshan B, Endozo R, Wan S, Aldridge MD, Groves AM, et al. Radiomics-based texture analysis of 68Ga-DOTATATE positron emission tomography and computed tomography images as a prognostic biomarker in adults with neuroendocrine cancers treated with 177Lu-DOTATATE. Front Oncol . (2021) 11:686235. doi: 10.3389/fonc.2021.686235
57. Jha AK, Mithun S, Sherkhane UB, Dwivedi P, Puts S, Osong B, et al. Emerging role of quantitative imaging (radiomics) and artificial intelligence in precision oncology. Explor Target Antitumor Ther . (2023) 4:569–82. doi: 10.37349/etat
58. Parmar C, Grossmann P, Bussink J, Lambin P, Aerts HJWL. Machine learning methods for quantitative radiomic biomarkers. Sci Rep . (2015) 5:13087. doi: 10.3389/fonc.2015.00272
59. Avanzo M, Wei L, Stancanello J, Vallières M, Rao A, Morin O, et al. Machine and deep learning methods for radiomics. Med Phys . (2020) 47:e185–202. doi: 10.1002/mp.13678
Keywords: pancreatic neuroendocrine tumors, meta-analysis, radiomics, machine learning, deep learning
Citation: Yan Q, Chen Y, Liu C, Shi H, Han M, Wu Z, Huang S, Zhang C and Hou B (2024) Predicting histologic grades for pancreatic neuroendocrine tumors by radiologic image-based artificial intelligence: a systematic review and meta-analysis. Front. Oncol. 14:1332387. doi: 10.3389/fonc.2024.1332387
Received: 02 November 2023; Accepted: 02 April 2024; Published: 23 April 2024.
Reviewed by:
Copyright © 2024 Yan, Chen, Liu, Shi, Han, Wu, Huang, Zhang and Hou. This is an open-access article distributed under the terms of the Creative Commons Attribution License (CC BY) . The use, distribution or reproduction in other forums is permitted, provided the original author(s) and the copyright owner(s) are credited and that the original publication in this journal is cited, in accordance with accepted academic practice. No use, distribution or reproduction is permitted which does not comply with these terms.
*Correspondence: Shanzhou Huang, [email protected] ; Chuanzhao Zhang, [email protected] ; Baohua Hou, [email protected]
† These authors have contributed equally to this work
Disclaimer: All claims expressed in this article are solely those of the authors and do not necessarily represent those of their affiliated organizations, or those of the publisher, the editors and the reviewers. Any product that may be evaluated in this article or claim that may be made by its manufacturer is not guaranteed or endorsed by the publisher.
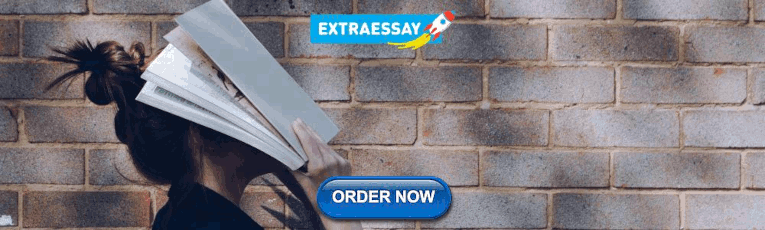
IMAGES
VIDEO
COMMENTS
Emotional intelligence (EI) refers to the ability to perceive, express, understand, and manage emotions. Current research indicates that it may protect against the emotional burden experienced in certain professions. This article aims to provide an updated systematic review of existing instruments to assess EI in professionals, focusing on the ...
A systematic literature review of 29 emotional intelligence (EI) studies was performed, to examine where the studies were undertaken and what were the outcomes. The study further provides insights into what conflicts occur in organizations, what measurement tools have been employed to resolve conflicts, and to identify if EI has been utilized ...
1. ] as an ability-based construct analogous to general intelligence. They argued that. individuals with a high level of EI had certain skills related to the evaluation and regulation. of emotions ...
Emotional intelligence (EI) refers to the ability to perceive, express, understand, and manage emotions. Current research indicates that it may protect against the emotional burden experienced in certain professions. This article aims to provide an updated systematic review of existing instruments to assess EI in professionals, focusing on the description of their characteristics as well as ...
Emotional intelligence is strongly correlated with academic performance, ... The meta-analysis and the literature review suggest that the effect size of EI on academic performance is moderate-high (0.390). ... Preferred reporting items for systematic review and meta-analysis protocols (PRISMA-P) 2015: Elaboration and explanation ...
Arora S., Ashrafian H., Davis R., Athanasiou T., Darzi A., Sevdalis N. (2010). Emotional intelligence in medicine: A systematic review through the context of the ACGME competencies. Medical Education, 44, 749-764. Crossref. PubMed. ISI. ... Systematic literature review on emotional intelligence and conflict ma... Go to citation Crossref ...
Department of Psychology, Université Libre de Bruxelles, Belgium. Abstract. Emotional intelligence (EI) can be defined as the ability to identify, express, understand, manage, and use emotions ...
Emotional intelligence (EI) refers to the ability to perceive, express, understand, and manage emotions. Current research indicates that it may protect against the emotional burden experienced in certain professions. This article aims to provide an updated systematic review of existing instruments to assess EI in professionals, focusing on the ...
Based on our literature review we suggest that a very good, comprehensive measure of trait EI is the Trait Emotional Intelligence Questionnaire, or TEIQue (Petrides and Furnham, 2001). If users are not restricted by time or costs (commercial users need to pay, researchers do not) then the TEIQue is a very good option.
Introduction. Emotional intelligence (EI) is a psychological protective factor that can improve subjective well-being (WB) in adolescents. This study aims to establish the overall relationship between different EI models (performance-based ability model, self-report ability model, and self-report mixed model) and subjective WB in adolescents, analyze the affective WB and cognitive WB ...
Emotional intelligence (EI) can be defined as the ability to identify, express, understand, manage, and use emotions. EI has been shown to have an important impact on health, relationships, and work/academic performance. In this article, we present a systematic review of 46 EI intervention studies on adult populations in order to assess their outcomes. Overall, these findings provide some ...
The development of Emotional Intelligence (EI) has gained importance in the context of goals to spur a new generation of people intent upon improving human health. ... Moher D., Page M. J., Koffel J. B. (2021) PRISMA-S: An extension to the PRISMA statement for reporting literature searches in systematic reviews. Systematic Reviews 10(1): 39 ...
The notion of emotional intelligence and its association with academic outcomes have been a field of intense interest for quite a long time. The current study's aim is to explore the effect level of emotional intelligence on academic achievement by using a meta-analytic approach. To this end, SCOPUS, Education Resources Information Center (ERIC) and Academic Search Ultimate databases were ...
2015) Emotional intelligence (EI) is t he capacity to. recognise, utilise, comprehend, and manage. emotions and emotional inform ation. Emotional. intelligence plays a significant role in the ...
Emotional intelligence (EI) has been widely researched in different fields of knowledge. This paper reviews the literature on emotional intelligence, leadership, and teams in 104 peer-reviewed articles and reviews provided by the Web of Science and Scopus databases from 1998 to 2022. It is a hybrid or mixed review as it uses both quantitative ...
In this narrative, comprehensive, and updated review of the literature, we summarize evidence about the effectiveness of interventions aimed at reducing emotion dysregulation and improving emotion regulation in children, adolescents, and adults. After introducing emotion dysregulation and emotion regulation from a theoretical standpoint, we discuss the factors commonly associated with emotion ...
Results: 57 articles are included in this systematic review. The literature focuses on EI and nursing education. The findings categorized into four themes: EI and performances; EI and Physical and mental health; EI and Social Relationship; and EI program. Conclusions: This literature review reveals that EI has many benefits in nursing students.
systematic review is characterized by having a wide coverage (i.e., studies published in languages other than English) and having as a framework a consensus of description and taxonomy of valid evidence (i.e., "Standards") [23]. 2. Materials and Methods This work contains a systematic review of the scientific literature published to date
ABSTRACT A systematic literature review of 29 emotional intelligence (EI) studies was performed, to examine where the studies were undertaken and what were the outcomes. The study further provides insights into what conflicts occur in organizations, what measurement tools have been employed to resolve conflicts, and to identify if EI has been utilized in conflict resolution.
The present meta-analysis investigated the literature pertaining to emotional intelligence and romantic relationship satisfaction by means of a systematic literature review and meta-analysis, relying on methods that followed current best practices and using cutting-edge data analyses.
Emotional Intelligence (EI), defined as the ability to perceive, identify, understand, and regulate emotional states is related to health outcomes. In this line, some studies examined this personal resource in the cancer area and, specifically, in breast cancer. ... a critical and systematic review of the literature. Scand. J. Psychol., 59 (2 ...
Researchers are leveraging Artificial Intelligence (AI) and Natural Language Processing (NLP) techniques to impart emotional intelligence capabilities in chatbots. This study provides a systematic review of research on developing emotionally intelligent chatbots. ... The systematic literature review guidelines by Kitchenham and Charters outline ...
A systematic literature review of 29 emotional intelligence (EI) studies was performed, to examine where the studies were undertaken and what were the outcomes. The study further provides insights ...
The rapid development of artificial intelligence has driven the transformation of educational evaluation into big data-driven. This study used a systematic literature review method to analyzed 44 empirical research articles on the evaluation of big data education.
A systematic literature review design was used in this study following the guidelines of Paul and Criado (Citation 2020). There are various types of systematic literature reviews, including structured reviews, framework-based reviews, bibliometric reviews, and meta-analysis reviews.
This paper explores the notion of strategic intelligence, namely with respect to leadership and business-oriented areas. Strategic intelligence is a researched concept that primarily concerns the collection and analysis of intelligence for policy and military planning, mainly at national and international levels, whereas this study of management and organizational decision-making application ...
Background: Falls and their consequences are a serious public health problem worldwide. Each year, 37.3 million falls requiring medical attention occur. Therefore, the analysis of fall risk is of great importance for prevention. Artificial intelligence (AI) represents an innovative tool for creating predictive statistical models of fall risk through data analysis.
The systematic literature review has shown the link between emotional intelligence. and organisational culture. Leadership, knowledge dissemination, and corporate decision-making are dimensions ...
To address this gap, this study conducts a systematic review of the construction sustainability literature to analyze and synthesize research findings on the application of DTs at various stages ...
Method: A systematic literature search was performed for studies published before September 2023. Study characteristics and diagnostic measures were extracted. ... Predicting histologic grades for pancreatic neuroendocrine tumors by radiologic image-based artificial intelligence: a systematic review and meta-analysis. Front. Oncol. 14:1332387 ...