
An official website of the United States government
The .gov means it’s official. Federal government websites often end in .gov or .mil. Before sharing sensitive information, make sure you’re on a federal government site.
The site is secure. The https:// ensures that you are connecting to the official website and that any information you provide is encrypted and transmitted securely.
- Publications
- Account settings
Preview improvements coming to the PMC website in October 2024. Learn More or Try it out now .
- Advanced Search
- Journal List
- Entropy (Basel)

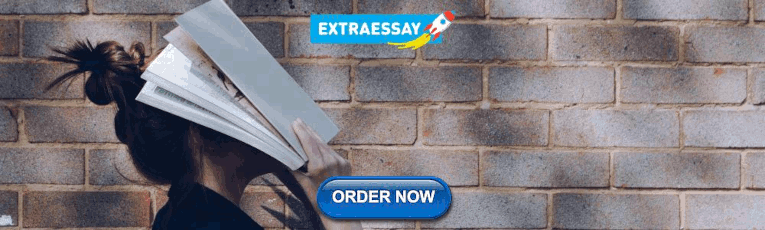
Stock Market Volatility and Return Analysis: A Systematic Literature Review
Roni bhowmik.
1 School of Economics and Management, Jiujiang University, Jiujiang 322227, China
2 Department of Business Administration, Daffodil International University, Dhaka 1207, Bangladesh
Shouyang Wang
3 Academy of Mathematics and Systems Science, Chinese Academy of Sciences, Beijing 100080, China; nc.ca.ssma@gnawys
In the field of business research method, a literature review is more relevant than ever. Even though there has been lack of integrity and inflexibility in traditional literature reviews with questions being raised about the quality and trustworthiness of these types of reviews. This research provides a literature review using a systematic database to examine and cross-reference snowballing. In this paper, previous studies featuring a generalized autoregressive conditional heteroskedastic (GARCH) family-based model stock market return and volatility have also been reviewed. The stock market plays a pivotal role in today’s world economic activities, named a “barometer” and “alarm” for economic and financial activities in a country or region. In order to prevent uncertainty and risk in the stock market, it is particularly important to measure effectively the volatility of stock index returns. However, the main purpose of this review is to examine effective GARCH models recommended for performing market returns and volatilities analysis. The secondary purpose of this review study is to conduct a content analysis of return and volatility literature reviews over a period of 12 years (2008–2019) and in 50 different papers. The study found that there has been a significant change in research work within the past 10 years and most of researchers have worked for developing stock markets.
1. Introduction
In the context of economic globalization, especially after the impact of the contemporary international financial crisis, the stock market has experienced unprecedented fluctuations. This volatility increases the uncertainty and risk of the stock market and is detrimental to the normal operation of the stock market. To reduce this uncertainty, it is particularly important to measure accurately the volatility of stock index returns. At the same time, due to the important position of the stock market in the global economy, the beneficial development of the stock market has become the focus. Therefore, the knowledge of theoretical and literature significance of volatility are needed to measure the volatility of stock index returns.
Volatility is a hot issue in economic and financial research. Volatility is one of the most important characteristics of financial markets. It is directly related to market uncertainty and affects the investment behavior of enterprises and individuals. A study of the volatility of financial asset returns is also one of the core issues in modern financial research and this volatility is often described and measured by the variance of the rate of return. However, forecasting perfect market volatility is difficult work and despite the availability of various models and techniques, not all of them work equally for all stock markets. It is for this reason that researchers and financial analysts face such a complexity in market returns and volatilities forecasting.
The traditional econometric model often assumes that the variance is constant, that is, the variance is kept constant at different times. An accurate measurement of the rate of return’s fluctuation is directly related to the correctness of portfolio selection, the effectiveness of risk management, and the rationality of asset pricing. However, with the development of financial theory and the deepening of empirical research, it was found that this assumption is not reasonable. Additionally, the volatility of asset prices is one of the most puzzling phenomena in financial economics. It is a great challenge for investors to get a pure understanding of volatility.
A literature reviews act as a significant part of all kinds of research work. Literature reviews serve as a foundation for knowledge progress, make guidelines for plan and practice, provide grounds of an effect, and, if well guided, have the capacity to create new ideas and directions for a particular area [ 1 ]. Similarly, they carry out as the basis for future research and theory work. This paper conducts a literature review of stock returns and volatility analysis based on generalized autoregressive conditional heteroskedastic (GARCH) family models. Volatility refers to the degree of dispersion of random variables.
Financial market volatility is mainly reflected in the deviation of the expected future value of assets. The possibility, that is, volatility, represents the uncertainty of the future price of an asset. This uncertainty is usually characterized by variance or standard deviation. There are currently two main explanations in the academic world for the relationship between these two: The leverage effect and the volatility feedback hypothesis. Leverage often means that unfavorable news appears, stock price falls, leading to an increase in the leverage factor, and thus the degree of stock volatility increases. Conversely, the degree of volatility weakens; volatility feedback can be simply described as unpredictable stock volatility that will inevitably lead to higher risk in the future.
There are many factors that affect price movements in the stock market. Firstly, there is the impact of monetary policy on the stock market, which is extremely substantial. If a loose monetary policy is implemented in a year, the probability of a stock market index rise will increase. On the other hand, if a relatively tight monetary policy is implemented in a year, the probability of a stock market index decline will increase. Secondly, there is the impact of interest rate liberalization on risk-free interest rates. Looking at the major global capital markets, the change in risk-free interest rates has a greater correlation with the current stock market. In general, when interest rates continue to rise, the risk-free interest rate will rise, and the cost of capital invested in the stock market will rise simultaneously. As a result, the economy is expected to gradually pick up during the release of the reform dividend, and the stock market is expected to achieve a higher return on investment.
Volatility is the tendency for prices to change unexpectedly [ 2 ], however, all kinds of volatility is not bad. At the same time, financial market volatility has also a direct impact on macroeconomic and financial stability. Important economic risk factors are generally highly valued by governments around the world. Therefore, research on the volatility of financial markets has always been the focus of financial economists and financial practitioners. Nowadays, a large part of the literature has studied some characteristics of the stock market, such as the leverage effect of volatility, the short-term memory of volatility, and the GARCH effect, etc., but some researchers show that when adopting short-term memory by the GARCH model, there is usually a confusing phenomenon, as the sampling interval tends to zero. The characterization of the tail of the yield generally assumes an ideal situation, that is, obeys the normal distribution, but this perfect situation is usually not established.
Researchers have proposed different distributed models in order to better describe the thick tail of the daily rate of return. Engle [ 3 ] first proposed an autoregressive conditional heteroscedasticity model (ARCH model) to characterize some possible correlations of the conditional variance of the prediction error. Bollerslev [ 4 ] has been extended it to form a generalized autoregressive conditional heteroskedastic model (GARCH model). Later, the GARCH model rapidly expanded and a GARCH family model was created.
When employing GARCH family models to analyze and forecast return volatility, selection of input variables for forecasting is crucial as the appropriate and essential condition will be given for the method to have a stationary solution and perfect matching [ 5 ]. It has been shown in several findings that the unchanged model can produce suggestively different results when it is consumed with different inputs. Thus, another key purpose of this literature review is to observe studies which use directional prediction accuracy model as a yardstick from a realistic point of understanding and has the core objective of the forecast of financial time series in stock market return. Researchers estimate little forecast error, namely measured as mean absolute deviation (MAD), root mean squared error (RMSE), mean absolute error (MAE), and mean squared error (MSE) which do not essentially interpret into capital gain [ 6 , 7 ]. Some others mention that the predictions are not required to be precise in terms of NMSE (normalized mean squared error) [ 8 ]. It means that finding the low rate of root mean squared error does not feed high returns, in another words, the relationship is not linear between two.
In this manuscript, it is proposed to categorize the studies not only by their model selection standards but also for the inputs used for the return volatility as well as how precise it is spending them in terms of return directions. In this investigation, the authors repute studies which use percentage of success trades benchmark procedures for analyzing the researchers’ proposed models. From this theme, this study’s authentic approach is compared with earlier models in the literature review for input variables used for forecasting volatility and how precise they are in analyzing the direction of the related time series. There are other review studies on return and volatility analysis and GARCH-family based financial forecasting methods done by a number of researchers [ 9 , 10 , 11 , 12 , 13 ]. Consequently, the aim of this manuscript is to put forward the importance of sufficient and necessary conditions for model selection and contribute for the better understanding of academic researchers and financial practitioners.
Systematic reviews have most notable been expanded by medical science as a way to synthesize research recognition in a systematic, transparent, and reproducible process. Despite the opportunity of this technique, its exercise has not been overly widespread in business research, but it is expanding day by day. In this paper, the authors have used the systematic review process because the target of a systematic review is to determine all empirical indication that fits the pre-decided inclusion criteria or standard of response to a certain research question. Researchers proved that GARCH is the most suitable model to use when one has to analysis the volatility of the returns of stocks with big volumes of observations [ 3 , 4 , 6 , 9 , 13 ]. Researchers observe keenly all the selected literature to answer the following research question: What are the effective GARCH models to recommend for performing market volatility and return analysis?
The main contribution of this paper is found in the following four aspects: (1) The best GARCH models can be recommended for stock market returns and volatilities evaluation. (2) The manuscript considers recent papers, 2008 to 2019, which have not been covered in previous studies. (3) In this study, both qualitative and quantitative processes have been used to examine the literature involving stock returns and volatilities. (4) The manuscript provides a study based on journals that will help academics and researchers recognize important journals that they can denote for a literature review, recognize factors motivating analysis stock returns and volatilities, and can publish their worth study manuscripts.
2. Methodology
A systematic literature examination of databases should recognize as complete a list as possible of relevant literature while keeping the number of irrelevant knocks small. The study is conducted by a systematic based literature review, following suggestions from scholars [ 14 , 15 ]. This manuscript was led by a systematic database search, surveyed by cross-reference snowballing, as demonstrated in Figure 1 , which was adapted from Geissdoerfer et al. [ 16 ]. Two databases were selected for the literature search: Scopus and Web-of-Science. These databases were preferred as they have some major depositories of research and are usually used in literature reviews for business research [ 17 ].

Literature review method.
At first stage, a systematic literature search is managed. The keywords that were too broad or likely to be recognized in literature-related keywords with other research areas are specified below. As shown in Table 1 , the search string “market return” in ‘Title‘ respectively “stock market return”, “stock market volatility”, “stock market return volatility”, “GARCH family model* for stock return”, “forecasting stock return”, and GARCH model*, “financial market return and volatility” in ‘Topic’ separately ‘Article title, Abstract, Keywords’ were used to search for reviews of articles in English on the Elsevier Scopus and Thomson Reuters Web-of-Science databases. The asterisk (*) is a commonly used wildcard symbol that broadens a search by finding words that start with the same letters.
Literature search strings for database.
At second stage, suitable cross-references were recognized in this primary sample by first examining the publications’ title in the reference portion and their context and cited content in the text. The abstracts of the recognized further publications were examined to determine whether the paper was appropriate or not. Appropriate references were consequently added to the sample and analogously scanned for appropriate cross-references. This method was continual until no additional appropriate cross-references could be recognized.
At the third stage, the ultimate sample was assimilated, synthesized, and compiled into the literature review presented in the subsequent section. The method was revised a few days before the submission.
Additionally, the list of affiliation criteria in Table 2 , which is formed on discussions of the authors, with the summaries of all research papers were independently checked in a blind system method. Evaluations were established on the content of the abstract, with any extra information unseen, and were comprehensive rather than exclusive. In order to check for inter-coder dependability, an initial sample of 30 abstracts were studied for affiliation by the authors. If the abstract was not satisfactorily enough, the whole paper was studied. Simply, 4.61 percent of the abstract resulted in variance between the researchers. The above-mentioned stages reduced the subsequent number of full papers for examination and synthesis to 50. In order to recognize magnitudes, backgrounds, and moderators, these residual research papers were reviewed in two rounds of reading.
Affiliation criteria.
3. Review of Different Studies
In this paper, a large amount of articles were studied but only a few were well thought out to gather the quality developed earlier. For every published article, three groups were specified. Those groups were considered as index and forecast time period, input elements, econometric models, and study results. The first group namely “index and forecast time period with input elements” was considered since market situation like emerging, frontier, and developed markets which are important parameters of forecast and also the length of evaluation is a necessary characteristic for examining the robustness of the model. Furthermore, input elements are comparatively essential parameters for a forecast model because the analytical and diagnostic ability of the model is mainly supported on the inputs that a variable uses. In the second group, “model” was considered forecast models proposed by authors and other models for assessment. The last group is important to our examination for comparing studies in relationships of proper guiding return and volatility, acquired by using recommended estimate models, named the “study results” group.
Measuring the stock market volatility is an incredibly complex job for researchers. Since volatility tends to cluster, if today’s volatility is high, it is likely to be high tomorrow but they have also had an attractive high hit rate with major disasters [ 4 , 7 , 11 , 12 ]. GARCH models have a strong background, recently having crossed 30 years of the fast progress of GARCH-type models for investigating the volatility of market data. Literature of eligible papers were clustered in two sub groups, the first group containing GARCH and its variations model, and the second group containing bivariate and other multivariate GARCH models, summarized in a table format for future studies. Table 3 explains the review of GARCH and its variations models. The univariate GARCH model is for a single time series. It is a statistical model that is used to analyze a number of different kinds of financial data. Financial institutions and researchers usually use this model to estimate the volatility of returns for stocks, bonds, and market indices. In the GARCH model, current volatility is influenced by past innovation to volatility. GARCH models are used to model for forecast volatility of one time series. The most widely used GARCH form is GARCH (1, 1) and this has some extensions.
Different literature studies based on generalized autoregressive conditional heteroskedastic (GARCH) and its variations models.
Notes: APARCH (Asymmetric Power ARCH), AIC (Akaike Information Criterion), OHLC (Open-High-Low-Close Chart), NSE (National Stock Exchange of India), EWMA (Exponentially Weighted Moving Average), CGARCH (Component GARCH), BDS (Brock, Dechert & Scheinkman) Test, ARCH-LM (ARCH-Lagrange Multiplier) test, VAR (Vector Autoregression) model, VEC (Vector Error Correction) model, ARFIMA (Autoregressive Fractional Integral Moving Average), FIGARCH (Fractionally Integrated GARCH), SHCI (Shanghai Stock Exchange Composite Index), SZCI (Shenzhen Stock Exchange Component Index), ADF (Augmented Dickey–Fuller) test, BSE (Bombay Stock Exchange), and PGARCH (Periodic GARCH) are discussed.
In a simple GARCH model, the squared volatility σ t 2 is allowed to change on previous squared volatilities, as well as previous squared values of the process. The conditional variance satisfies the following form: σ t 2 = α 0 + α 1 ϵ t − 1 2 + … + α q ϵ t − q 2 + β 1 σ t − 1 2 + … + β p σ t − p 2 where, α i > 0 and β i > 0 . For the GARCH model, residuals’ lags can substitute by a limited number of lags of conditional variances, which abridges the lag structure and in addition the estimation method of coefficients. The most often used GARCH model is the GARCH (1, 1) model. The GARCH (1, 1) process is a covariance-stationary white noise process if and only if α 1 + β < 1 . The variance of the covariance-stationary process is given by α 1 / ( 1 − α 1 − β ) . It specifies that σ n 2 is based on the most recent observation of φ t 2 and the most recent variance rate σ n − 1 2 . The GARCH (1, 1) model can be written as σ n 2 = ω + α φ n − 1 2 + β σ n − 1 2 and this is usually used for the estimation of parameters in the univariate case.
Though, GARCH model is not a complete model, and thus could be developed, these developments are detected in the form of the alphabet soup that uses GARCH as its key component. There are various additions of the standard GARCH family models. Nonlinear GARCH (NGARCH) was proposed by Engle and Ng [ 18 ]. The conditional covariance equation is in the form: σ t 2 = γ + α ( ε t − 1 − ϑ σ t − 1 ) 2 + β σ t − 1 2 , where α , β , γ > 0 . The integrated GARCH (IGARCH) is a restricted version of the GARCH model, where the sum of all the parameters sum up to one and this model was introduced by Engle and Bollerslev [ 19 ]. Its phenomenon might be caused by random level shifts in volatility. The simple GARCH model fails in describing the “leverage effects” which are detected in the financial time series data. The exponential GARCH (EGARCH) introduced by Nelson [ 5 ] is to model the logarithm of the variance rather than the level and this model accounts for an asymmetric response to a shock. The GARCH-in-mean (GARCH-M) model adds a heteroskedasticity term into the mean equation and was introduced by Engle et al. [ 20 ]. The quadratic GARCH (QGARCH) model can handle asymmetric effects of positive and negative shocks and this model was introduced by Sentana [ 21 ]. The Glosten-Jagannathan-Runkle GARCH (GJR-GARCH) model was introduced by Glosten et al. [ 22 ], its opposite effects of negative and positive shocks taking into account the leverage fact. The threshold GARCH (TGARCH) model was introduced by Zakoian [ 23 ], this model is also commonly used to handle leverage effects of good news and bad news on volatility. The family GARCH (FGARCH) model was introduced by Hentschel [ 24 ] and is an omnibus model that is a mix of other symmetric or asymmetric GARCH models. The COGARCH model was introduced by Klüppelberg et al. [ 25 ] and is actually the stochastic volatility model, being an extension of the GARCH time series concept to continuous time. The power-transformed and threshold GARCH (PTTGARCH) model was introduced by Pan et al. [ 26 ], this model is a very flexible model and, under certain conditions, includes several ARCH/GARCH models.
Based on the researchers’ articles, the symmetric GARCH (1, 1) model has been used widely to forecast the unconditional volatility in the stock market and time series data, and has been able to simulate the asset yield structure and implied volatility structure. Most researchers show that GARCH (1, 1) with a generalized distribution of residual has more advantages in volatility assessment than other models. Conversely, the asymmetry influence in stock market volatility and return analysis was beyond the descriptive power of the asymmetric GARCH models, as the models could capture more specifics. Besides, the asymmetric GARCH models can incompletely measure the effect of positive or negative shocks in stock market return and volatility, and the GARCH (1, 1) comparatively failed to accomplish this fact. In asymmetric effect, the GJR-GARCH model performed better and produced a higher predictable conditional variance during the period of high volatility. In addition, among the asymmetric GARCH models, the reflection of EGARCH model appeared to be superior.
Table 4 has explained the review of bivariate and other multivariate GARCH models. Bivariate model analysis was used to find out if there is a relationship between two different variables. Bivariate model uses one dependent variable and one independent variable. Additionally, the Multivariate GARCH model is a model for two or more time series. Multivariate GARCH models are used to model for forecast volatility of several time series when there are some linkages between them. Multivariate model uses one dependent variable and more than one independent variable. In this case, the current volatility of one time series is influenced not only by its own past innovation, but also by past innovations to volatilities of other time series.
Different literature studies based on bivariate and other multivariate GARCH models.
The most recognizable use of multivariate GARCH models is the analysis of the relations between the volatilities and co-volatilities of several markets. A multivariate model would create a more dependable model than separate univariate models. The vector error correction (VEC) models is the first MGARCH model which was introduced by Bollerslev et al. [ 66 ]. This model is typically related to subsequent formulations. The model can be expressed in the following form: v e c h ( H t ) = ℂ + ∑ j = 1 q X j v e c h ( ϵ t − j ϵ t − j ' ) + ∑ j = 1 p Y j v e c h ( H t − j ) where v e c h is an operator that stacks the columns of the lower triangular part of its argument square matrix and H t is the covariance matrix of the residuals. The regulated version of the VEC model is the DVEC model and was also recommended by Bollerslev et al. [ 66 ]. Compared to the VEC model, the estimation method proceeded far more smoothly in the DVEC model. The Baba-Engle-Kraft-Kroner (BEKK) model was introduced by Baba et al. [ 67 ] and is an innovative parameterization of the conditional variance matrix H t . The BEKK model accomplishes the positive assurance of the conditional covariance by conveying the model in a way that this property is implied by the model structure. The Constant Conditional Correlation (CCC) model was recommended by Bollerslev [ 68 ], to primarily model the conditional covariance matrix circuitously by estimating the conditional correlation matrix. The Dynamic Conditional Correlation (DCC) model was introduced by Engle [ 69 ] and is a nonlinear mixture of univariate GARCH models and also a generalized variety of the CCC model. To overcome the inconveniency of huge number of parameters, the O-GARCH model was recommended by Alexander and Chibumba [ 70 ] and consequently developed by Alexander [ 71 , 72 ]. Furthermore, a multivariate GARCH model GO-GARCH model was introduced by Bauwens et al. [ 73 ].
The bivariate models showed achieve better in most cases, compared with the univariate models [ 85 ]. MGARCH models could be used for forecasting. Multivariate GARCH modeling delivered a realistic but parsimonious measurement of the variance matrix, confirming its positivity. However, by analyzing the relative forecasting accuracy of the two formulations, BEKK and DCC, it could be deduced that the forecasting performance of the MGARCH models was not always satisfactory. By comparing it with the other multivariate GARCH models, BEKK-GARCH model was comparatively better and flexible but it needed too many parameters for multiple time series. Conversely, for the area of forecasting, the DCC-GARCH model was more parsimonious. In this regard, it was significantly essential to balance parsimony and flexibility when modeling multivariate GARCH models.
The current systematic review has identified 50 research articles for studies on significant aspects of stock market return and volatility, review types, and GARCH model analysis. This paper noticed that all the studies in this review used an investigational research method. A literature review is necessary for scholars, academics, and practitioners. However, assessing various kinds of literature reviews can be challenging. There is no use for outstanding and demanding literature review articles, since if they do not provide a sufficient contribution and something that is recent, it will not be published. Too often, literature reviews are fairly descriptive overviews of research carried out among particular years that draw data on the number of articles published, subject matter covered, authors represented, and maybe methods used, without conducting a deeper investigation. However, conducting a literature review and examining its standard can be challenging, for this reason, this article provides some rigorous literature reviews and, in the long run, to provide better research.
4. Conclusions
Working on a literature review is a challenge. This paper presents a comprehensive literature which has mainly focused on studies on return and volatility of stock market using systematic review methods on various financial markets around the world. This review was driven by researchers’ available recommendations for accompanying systematic literature reviews to search, examine, and categorize all existing and accessible literature on market volatility and returns [ 16 ]. Out of the 435 initial research articles located in renowned electronic databases, 50 appropriate research articles were extracted through cross-reference snowballing. These research articles were evaluated for the quality of proof they produced and were further examined. The raw data were offered by the authors from the literature together with explanations of the data and key fundamental concepts. The outcomes, in this research, delivered future magnitudes to research experts for further work on the return and volatility of stock market.
Stock market return and volatility analysis is a relatively important and emerging field of research. There has been plenty of research on financial market volatility and return because of easily increasing accessibility and availability of researchable data and computing capability. The GARCH type models have a good model on stock market volatilities and returns investigation. The popularity of various GARCH family models has increased in recent times. Every model has its specific strengths and weaknesses and has at influence such a large number of GARCH models. To sum up the reviewed papers, many scholars suggest that the GARCH family model provides better results combined with another statistical technique. Based on the study, much of the research showed that with symmetric information, GARCH (1, 1) could precisely explain the volatilities and returns of the data and when under conditions of asymmetric information, the asymmetric GARCH models would be more appropriate [ 7 , 32 , 40 , 47 , 48 ]. Additionally, few researchers have used multivariate GARCH model statistical techniques for analyzing market volatility and returns to show that a more accurate and better results can be found by multivariate GARCH family models. Asymmetric GARCH models, for instance and like, EGARCH, GJR GARCH, and TGARCH, etc. have been introduced to capture the effect of bad news on the change in volatility of stock returns [ 42 , 58 , 62 ]. This study, although short and particular, attempted to give the scholar a concept of different methods found in this systematic literature review.
With respect to assessing scholars’ articles, the finding was that rankings and specifically only one GARCH model was sensitive to the different stock market volatilities and returns analysis, because the stock market does not have similar characteristics. For this reason, the stock market and model choice are little bit difficult and display little sensitivity to the ranking criterion and estimation methodology, additionally applying software is also another matter. The key challenge for researchers is finding the characteristics in stock market summarization using different kinds of local stock market returns, volatility detection, world stock market volatility, returns, and other data. Additional challenges are modeled by differences of expression between different languages. From an investigation perception, it has been detected that different authors and researchers use special datasets for the valuation of their methods, which may put boundary assessments between research papers.
Whenever there is assurance that scholars build on high accuracy, it will be easier to recognize genuine research gaps instead of merely conducting the same research again and again, so as to progress better and create more appropriate hypotheses and research questions, and, consequently, to raise the standard of research for future generation. This study will be beneficial for researchers, scholars, stock exchanges, regulators, governments, investors, and other concerned parties. The current study also contributes to the scope of further research in the area of stock volatility and returns. The content analysis can be executed taking the literature of the last few decades. It determined that a lot of methodologies like GARCH models, Johansen models, VECM, Impulse response functions, and Granger causality tests are practiced broadly in examining stock market volatility and return analysis across countries as well as among sectors with in a country.
Author Contributions
R.B. and S.W. proposed the research framework together. R.B. collected the data, and wrote the document. S.W. provided important guidance and advice during the process of this research. All authors have read and agreed to the published version of the manuscript.
This research received no external funding.
Conflicts of Interest
The authors declare no conflict of interest.
Stock Market Volatility and Return Analysis: A Systematic Literature Review
Affiliations.
- 1 School of Economics and Management, Jiujiang University, Jiujiang 322227, China.
- 2 Department of Business Administration, Daffodil International University, Dhaka 1207, Bangladesh.
- 3 Academy of Mathematics and Systems Science, Chinese Academy of Sciences, Beijing 100080, China.
- PMID: 33286294
- PMCID: PMC7517016
- DOI: 10.3390/e22050522
In the field of business research method, a literature review is more relevant than ever. Even though there has been lack of integrity and inflexibility in traditional literature reviews with questions being raised about the quality and trustworthiness of these types of reviews. This research provides a literature review using a systematic database to examine and cross-reference snowballing. In this paper, previous studies featuring a generalized autoregressive conditional heteroskedastic (GARCH) family-based model stock market return and volatility have also been reviewed. The stock market plays a pivotal role in today's world economic activities, named a "barometer" and "alarm" for economic and financial activities in a country or region. In order to prevent uncertainty and risk in the stock market, it is particularly important to measure effectively the volatility of stock index returns. However, the main purpose of this review is to examine effective GARCH models recommended for performing market returns and volatilities analysis. The secondary purpose of this review study is to conduct a content analysis of return and volatility literature reviews over a period of 12 years (2008-2019) and in 50 different papers. The study found that there has been a significant change in research work within the past 10 years and most of researchers have worked for developing stock markets.
Keywords: GARCH family model; complexity in market volatility forecasting; stock returns; volatility.
Publication types
To read this content please select one of the options below:
Please note you do not have access to teaching notes, stock market volatility: a systematic review.
Journal of Modelling in Management
ISSN : 1746-5664
Article publication date: 14 November 2023
Issue publication date: 13 March 2024
The increasing globalization and technological advancements have increased the information spillover on stock markets from various variables. However, there is a dearth of a comprehensive review of how stock market volatility is influenced by macro and firm-level factors. Therefore, this study aims to fill this gap by systematically reviewing the major factors impacting stock market volatility.
Design/methodology/approach
This study uses a combination of bibliometric and systematic literature review techniques. A data set of 54 articles published in quality journals from the Australian Business Deans Council (ABDC) list is gathered from the Scopus database. This data set is used to determine the leading contributors and contributions. The content analysis of these articles sheds light on the factors influencing market volatility and the potential research directions in this subject area.
The findings show that researchers in this sector are becoming more interested in studying the association of stock markets with “cryptocurrencies” and “bitcoin” during “COVID-19.” The outcomes of this study indicate that most studies found oil prices, policy uncertainty and investor sentiments have a significant impact on market volatility. However, there were mixed results on the impact of institutional flows and algorithmic trading on stock volatility, and a consensus cannot be established. This study also identifies the gaps and paves the way for future research in this subject area.
Originality/value
This paper fills the gap in the existing literature by comprehensively reviewing the articles on major factors impacting stock market volatility highlighting the theoretical relationship and empirical results.
- Volatility spillover
- Stock market
- Diebold Yilmaz
Acknowledgements
Funding : The present research received no specific grant from any of the funding agencies in the public, private or non-profit sectors.
Conflict of interest : The authors declare that there is no conflict of interest.
Dhingra, B. , Batra, S. , Aggarwal, V. , Yadav, M. and Kumar, P. (2024), "Stock market volatility: a systematic review", Journal of Modelling in Management , Vol. 19 No. 3, pp. 925-952. https://doi.org/10.1108/JM2-04-2023-0080
Emerald Publishing Limited
Copyright © 2023, Emerald Publishing Limited
Related articles
We’re listening — tell us what you think, something didn’t work….
Report bugs here
All feedback is valuable
Please share your general feedback
Join us on our journey
Platform update page.
Visit emeraldpublishing.com/platformupdate to discover the latest news and updates
Questions & More Information
Answers to the most commonly asked questions here
- Open access
- Published: 12 October 2020
An empirical examination of investor sentiment and stock market volatility: evidence from India
- Haritha P H 1 &
- Abdul Rishad 2
Financial Innovation volume 6 , Article number: 34 ( 2020 ) Cite this article
27k Accesses
25 Citations
Metrics details
Understanding the irrational sentiments of the market participants is necessary for making good investment decisions. Despite the recent academic effort to examine the role of investors’ sentiments in market dynamics, there is a lack of consensus in delineating the structural aspect of market sentiments. This research is an attempt to address this gap. The study explores the role of irrational investors’ sentiments in determining stock market volatility. By employing monthly data on market-related implicit indices, we constructed an irrational sentiment index using principal component analysis. This sentiment index was modelled in the GARCH and Granger causality framework to analyse its contribution to volatility. The results showed that irrational sentiment significantly causes excess market volatility. Moreover, the study indicates that the asymmetrical aspects of an inefficient market contribute to excess volatility and returns. The findings are crucial for retail investors as well as portfolio managers seeking to make an optimum portfolio to maximise profits.
Introduction
There has been growing academic attention in the past decade on investors’ sentiments and their potential impact on market performance. Investor sentiment is the expectation of market participants about the future cash flows (returns) and investment risk (De Long et al. 1990 ). Because traditional stock market theories comprehended market dynamics under the theoretical framework of the efficient market hypothesis (EMH) and random walk theory, they did not consider investor sentiment as an important aspect. However, they failed to explain the heterogeneous behaviour of investors in the capital market. Investors’ sentiment is a vital aspect of the capital market, as it contributes to frequent fluctuations in the stock price and thus creates uncertainty about future returns on investments. In the past few decades, there have been radical changes in the Indian financial environment, especially in the basic structure—for example, shifting from a savings-oriented economy to an investment-oriented economy. These changes have increased heterogeneity in the composition of participants and impacted investors’ risk-taking behaviour.
As per the EMH in classical financial theory, market participants exhibit rational risk aversion. Moreover, the information efficiency of the market does not allow participants to outperform the market (Fama 1965 ). The classical theory fails to explain the presence of systematic mispricing in the capital markets resulting from sentimental factors. Behavioural financial theories claim that irrational behaviour of noise traders and arbitrators causes a disparity in asset prices from their intrinsic (fundamental) values. Recent theoretical advances in behavioural finance and empirical evidence both have rejected the hypotheses of classical financial theory because of its assumption of rationality of agents in capital markets. In the previous decade, rational participants did not seem to have played a leading role in bringing the value of assets up to the then current value of anticipated cash flows (Baker and Wurgler 2007 ). Behavioural finance offers an alternative model that claims that economic phenomena can be better understood if the investors are accepted to not be entirely rational. In this context, the asset pricing not only includes the risk-related anticipated rates but also the impact of investor expectations on the returns. Behavioural finance explains the relationship between investment and the investor’s psychology. Investor behaviour is reflected in the stock prices, and market fluctuations, which ultimately shape the market, are themselves shaped by the psychology of the investors. Baker and Wurgler ( 2006 ) argued that market sentiment creates a tendency for investors to be optimistic or pessimistic while speculating prices instead of deciding on fundamental factors.
Previous studies sought to detect the predictability of sentiments as a systematic risk factor valued as per certain conditions in the market. Studies from developed economies like the USA are far ahead in understanding the sentiment-related market dynamics (Barberis et al. 1998 ; Lee et al. 2002 ; Neal and Wheatley 1998 ). Academic study of investor sentiment in developing economies with rapidly growing capital markets is still in infancy. Previous research has mainly focused on the influence of investors’ sentiment on investment returns, whereas the effect of sentiment on the conditional volatility structure of the market is less explored. Also, even among those studies that consider sentiment as a critical factor influencing the time-varying stock return, volatility and potential profitability relating to noise traders were the main aspects of focus. During the periods of high sentiment and low sentiment, noise traders act differently to keep their positions secure. During the high sentiment episodes, their participation and trading is more aggressive compared to that during a low sentiment episode. This is caused by naive and unaware noise traders’ misjudgement of potential risk. Past academic studies about emerging economies have not explored such factors in-depth. The present study is an attempt to address the above-mentioned issues by using a market-oriented sentiment index. We developed an investors’ sentiment index by using multiple sentiment yardsticks mentioned by Baker and Wurgler ( 2006 ). Considering the investors’ sentiments’ contribution to volatility in emerging markets, the current study aimed to establish new empirical evidence to add a more comparative dimension to the existing literature. The findings can help market participants to understand the role of investor sentiment in the determination of conditional volatility of the market and to take decisions to optimise the portfolio.
This study developed the aggregate sentiment index (ASI) from market-oriented sentimental factors such as trading volume, put-call ratio, advance-decline ratio, market turnover, share turnover and number of initial public offers (IPOs) in the period. The use of a constructed sentiment index under the GARCH framework to estimate the association between stock market volatility and investor sentiment makes this study different from existing studies. The findings indicate the persistence of volatility in market indices. Such persistent connection between the sentiment index and stock volatility suggests that investor sentiment is one of the most crucial determinants of Indian stock market volatility.
Theoretical background
According to the conventional theory of ‘market noise’ proposed by the Black ( 1986 ), noise traders operate on noisy signals in financial markets and balance both the systematic and non-systematic risk of an asset. According to this theory, noise makes markets inefficient to some extent and prevents investors from benefitting from inefficiencies. The significance of sentimental factors in asset pricing theories is substantiated by empirical literature from developed economies. The question of how irrational beliefs held by investors affect the market through asset pricing and expected returns is explained in behavioural finance theories. The theoretical model developed by De Long et al. ( 1990 ) explained this phenomenon as ‘Some investors, denominated noise traders, were subject to sentiment – a belief about future cash flows and risks of securities not supported by economic fundamentals of the underlying asset(s) – while other investors were rational arbitrageurs, free of sentiment. The irrational beliefs were caused by noise, interpreted by the irrational traders as information, thus the term noise traders.’
Theoretically, noise is part of irrational behaviour; the irrational traders consider noise as information. Interestingly, proponents of an efficient market claimed that noise traders were exploited by rational arbitrageurs who drove prices towards fundamental equilibrium values. Thus, noise was a reaction of noise traders to the activities of rational arbitrageurs that caused overpricing or underpricing of stocks during periods of high and low sentiment (Lemmon and Portniaguina 2006 ; Baker and Wurgler 2006 ). Researchers have been unable to satisfactorily explain the interaction between rational and irrational investors. The continuing debate on this issue significantly contributes to the literature but concentrates mainly on the role of noise traders in anticipated asset yields and volatility of return. It is not understood how the market reacts to noise, which is caused by a large number of small events. This behaviour can be observed among investors from advanced economies because they believe that systematic risk and return anomaly is associated with irrational investment behaviour (Brown and Cliff 2004 ; Qiu and Welch 2006 ; Lemmon and Portniaguina 2006 ). With this theoretical background, our study examines the role of irrational feelings of investors and their impact on the volatility of the Indian capital market.
Literature review
The development of behavioural finance theories triggered a discussion on the impact of investor sentiment on asset returns in the integrated stock market. According to theoretical and empirical research, investor sentiment strongly influences stock prices with inevitable consequences on portfolio selection and asset management, as psychological differences of heterogeneous investors have implications on the pricing of assets in the market. The influence of investor’s sentiment in asset price volatility is widely described as a combination of investors’ reaction to the current market situation and unjustified expectation of the future cash flows (Baker and Wurgler 2006 , 2007 ).
As a psychological factor, it is not easy to estimate investors’ sentiment because of their subjective and qualitative nature. However, different proxies have been used to measure sentiment. These indicators of the sentiment index are classified as indirect and direct measures. In direct measures, researchers measure the individual investor sentiment via surveys and polling techniques. They are highly sampling-dependent, and the chances of sampling errors are high. Moreover, they may not be able to give a broad picture of the prevailing sentiment. Indirect measures use market-determined sentiment proxies, such as trading volume, turnover volatility ratio, put-call ratio, advance-decline ratio, market turnover and share turnover for measuring the same. They posit that investors’ sentiment are reflected in the structure and breadth of the market and understanding these dynamics helps to capture the irrational aspects of the market. The consistent and theoretically comprehensible nature of the sentiment index has led to its wide adoption (Baker and Wurgler 2006 ; Brown and Cliff 2004 ; Chen et al. 1993 ; Clarke and Statman 1998 ; DeBondt and Thaler 1985 ; Elton et al. 1998 ; Fisher and Statman 2000 ; Lee et al. 2002 ; Neal and Wheatley 1998 ; Sias et al. 2001 ). According to Zhou ( 2018 ), investor sentiment indicates the distance of the asset’s value from its economic bases. This can be measured from different sources, such as official documents, media reports and market surveys. Mushinada and Veluri ( 2018 ) used trading volume and return volatility for understanding the relationship between sentiments and returns. Their findings showed that post-investment analysis was essential to correct errors in previous behavioural estimations. Market participants’ behaviour is heterogeneous because of the risk-return expectation, and it creates noise in the market. These findings contradict with the premises of the efficient market hypothesis that postulate that markets turn information efficient when investors behave rationally.
In the past few decades, empirical studies across the globe have investigated the connection between investors’ sentiment and stock returns for understanding and substantiating theories of market inefficiency (Brown and Cliff 2004 ; Fisher and Statman 2000 ). Chi et al. ( 2012 ) examined the impact of investor sentiment on stock returns and volatility by using mutual fund flows as an investor sentiment proxy in the Chinese stock market. They found that investor sentiment has a great impact on stock returns. The relationship between stock market volatility and investor sentiment has also been reported as statistically significant. Supporting these findings, Zhou and Yang ( 2019 ) stated that the construction of a theoretical model of stochastic investor sentiment influences investor crowdedness and also affects asset prices. Their result indicated that optimistic (pessimistic) expectations of investors can move asset prices above (below) the basic value. By examining the long-term association between investor sentiment in the stock and bond market, Fang et al. ( 2018 ) showed that the index of investor sentiment is positively associated with market volatility. Contradicting the fundamental tenets of the efficient market hypothesis, Shiller ( 1981 ) argued that investors are not completely rational, which could affect market prices aside from fundamental variables. Wang et al. ( 2006 ) noted that the sensitivity of investor sentiments to the information flow affected both market return and volatility. Chiu et al. ( 2018 ) found a positive relationship between investor sentiment, market volatility and macroeconomic variables. Jiang et al. ( 2019 ) constructed the fund manager’s sentiment index as a predictor of aggregate stock market returns. They found that when managers had a high level of sentiment, it caused a reduction in overall income surprises from total investment. Li ( 2014 ) pointed out that the sentiment index has strong predictive power for Chinese stock market returns. Retail investors’ attention will help to mitigate the crash risk, as the retail investors’ attention will not allow any irrational or noise traders to overrun the rational market participants (Wen et al. 2019 ).
Verma and Verma ( 2007 ) studied the role of retail and noise traders in price volatility to yield similar results. Verma and Soydemir’s ( 2009 ) empirical examination of the rational and irrational investors’ impact on market prices also supported the previous finding. They discovered that individual and institutional investors’ feelings influenced the market. Further evidence shows that the response of the market to volatility is not homogeneous; it is heterogeneous depending on the variations in shareholder sentiment. These findings are validated by Gupta ( 2019 ) who found that sentiments of fund managers are a stronger predictor than the returns, when it comes to forecasting volatility. Yang and Copeland ( 2014 ) found that the investor sentiment index has a long-term and short-term asymmetrical impact on volatility. They concluded that bearish sentiment is associated with lower returns than bullish sentiment, which accelerates market return. This shows that the bullish feeling has positive effects on short-term volatility, whereas in the long-term, it has a negative effect on volatility. These findings agree with the findings discussed by Qiang and Shue-e ( 2009 ), namely that positive and negative sentiment create different impacts on stock price variation. Baker et al. ( 2012 ) constructed investor sentiment indicators of six nations but because of the disintegration of different markets owing to the heterogeneous behaviour of investors across the globe, the indicators were not viable. Other studies have reported that investors’ sentiments are driven by overall funding patterns irrespective of the investor being individual or institutional (Baker and Wurgler 2000 ; Henderson et al. 2006 ). Investors’ sentiment has a mutual relationship with the expected return of public bonds and the expected return from the stock market (Bekaert et al. 2010 ).
In the context of the Indian stock market, Sehgal et al. ( 2009 ) discussed the fundamental aspects of investor sentiment and its relationship with market performance. They identified several factors that might act (individually and together) as indicators of market behaviour and investor sentiments’ influence on market behaviour. The authors used macroeconomic factors such as real GDP, corporate profits, inflation, interest rates and liquidity in the economy and market-based factors such as the put-call ratio, advance-decline ratio, earnings surprises, the price to earnings ratio and price to book value as potential factors to explain the underlying investor sentiment at the aggregate market level. They also suggested the development of a sentiment index based on these macroeconomic and market indicators. Using some of these indicators, Dash and Mahakud ( 2013 ) examined the explanatory power of an index of investor sentiment on aggregate returns. They found a significant relationship between the investor sentiment index and stock returns across industries in the Indian stock market. Rahman and Shamsuddin ( 2019 ) studied the excess price ratio and its influence on investor sentiment and found that the price to earnings ratio increased with a rise in investors’ sentiment. Kumari and Mahakud ( 2016 ) and Chandra and Thenmozhi ( 2013 ) studied the impact of investor sentiment in the Indian capital market. They found a positive relationship between investor sentiment and market volatility. Verma and Verma ( 2007 ) showed that investor sentiment has a positive impact on asset return, but it makes an adverse impact on individual and institutional investors owing to market volatility. Aggarwal and Mohanty ( 2018 ) studied the impact of the investor sentiment index on the Indian stock market and found that there is a positive relationship between stock returns and investor sentiments. However, most of these studies focused on the general effect of investors’ sentiment on stock returns. Such an approach restricts our understanding of the phenomenon of investors’ sentiment and its influence on market dynamics to a single dimension. In the present study, we explored the role of investor sentiment in determining excess market returns and volatility.
Data and variables
Being a qualitative factor, it is not easy to quantify the market behaviour of investors. Past studies have used multiple ways to measure investors’ sentiment. Some studies have relied on media reports, events and other publicly available documents to collect information on investor behaviour, and other studies have conducted surveys among investors for the same. Some other researchers have used market-based indicators such as price movements and trading activities for constructing sentiment indexes. A few researchers have used single variables as an indicator of investor sentiment. For instance, Mushinada and Veluri ( 2018 ) used trading volume as an indicator of investor sentiment. Using a single variable may not be sufficient to explain market sentiments because there are multiple factors that cause variation in these single variable proxies. Latest studies have constructed the sentiment index by using multiple market-based indicators that directly reflect the participants’ behaviour. Following Baker et al. ( 2012 ), this study employed multiple market-based indicators for constructing the sentiment index for the period from January 2000 to December 2016. We used the monthly average closing price of the NIFTY 50 (Nifty) stock index to measure market volatility and return. The diversified market representation of the Nifty index over the other benchmark BSE SENSEX (Sensex) motivated us to select the former. The study used monthly data because of the scarcity of high-frequency data on market-related indicators. The data was collected from the official websites and various reports of the National Stock Exchange, Reserve Bank of India and Securities and Exchange Board of India.
The study employed Bollerslev ( 1986 ) generalized autoregressive conditional heteroskedastic model (GARCH) to measure volatility using the conditional variance equation and to capture the dynamics of volatility clustering. This helped us to examine how the investors’ sentiment reacts to market volatility. This model helps verify whether the investors’ shocks are persistent or not. For the serial correlation, this study used the autoregressive conditional heteroskedasticity-Lagrange multiplier (ARCH-LM) of Engle and Ng ( 1993 ), autoregressive conditional heteroskedasticity (ARCH) test of Engle ( 1982 ) and Mcleod and Ll ( 1983 ) tests for the estimation of models. We also employed the Granger causality test to check the direction of causality between sentiment and market volatility.
Construction of investors’ sentiment index
The present study adopted the framework developed by Baker et al. ( 2012 ) to construct the investors’ sentiment index. It considered six variables: trading volume, put-call ratio, advance-decline ratio, market turnover, share turnover and the number of IPOs. The number of IPOs was defined as the total number of IPOs during the period. Baker et al. ( 2012 ) argued that firms try to procure more capital when the market value of the firm is high and repurchase their shares when the market value is low. The intention is to take advantage of the market sentiment until it reaches the fundamental value. In a bullish market, new issue of shares will transfer wealth from new shareholders to the company or to the existing shareholders. This market timing hypothesis suggests that higher (lower) value or number of IPOs means that the market sentiment is bullish (bearish) (Baker and Wurgler 2006 ). The number of IPOs reflects the market pulse; hence, they can be considered as an important component of the sentiment index.
The share turnover ratio is one of the conventional yardsticks for measuring the liquidity position, which reflects the active participation of traders and investors in the market. It is the ratio of the total value of shares traded during the period to the average market capitalization for the period. Turnover is vital in gauging investors’ sentiment in the market. Irrational investors actively participate in the market when they are optimistic and accelerate the volume of turnover (Baker and Stein 2004 ). Theoretically, the relationship between market returns and turnover is expected to be negative (Jones 2001 ). The presence of high turnover ensures liquidity and reduces the chances of abnormal returns.
Market turnover (MT) is the ratio of trading volume to the number of shares listed on the stock exchange. Market sentiment can be sensed from the turnover of the market because turnover will be low in bearish markets and high in bullish markets (Karpoff 1987 ). Small turnover is usually preceded by a price decline, whereas high turnover is associated with an increase in price (Ying 1966 ). Thus, the turnover information is a significant component of measuring the sentiment of market participants.
The advances and declines ratio (ADR) is a market-breadth indicator that analyses the proportion between the number of advancing shares and declining shares. The increasing (decreasing) trends in the ADR confirm the upward (downward) trend in the market (Brown and Cliff 2004 ). Generally, the ADR ratio is expected to be positive because investors’ sentiment makes the market active. Thus, the ADR ratio helps to recognise the recent trend and can be used as an indicator of market performance.
The put-call ratio (PCR) is another indicator to measure the dynamics of the secondary market. This sentiment indicator is measured as the ratio between transactions on all the put options and the call options on Nifty. A higher (lower) ratio indicates a bullish (bearish) sentiment in the market. Incorporating PCR to measure the aggregate sentiment index yields accurate results because it reflects the expectations of market participants. When market participants expect a bearish trend, they try to shield their positions. When trade volumes of put options are higher relative to the trade quantity of call options, the ratio will go up (Brown and Cliff 2004 ; Finter and Ruenzi 2012 ; Wang et al. 2006 ). This derivative market proxy is considered as an indicator of a bullish trend because the bearish market PCR will be small (Brown and Cliff 2004 ).
The trading volume (TV) is a key variable for constructing the sentiment index. It is measured as the monthly average of the Nifty daily trade volume. Frequent trades in an active market increase the volume and create liquidity in the market. Therefore, researchers have used market turnover as a proxy for investor sentiment (Qiang and Shue-e 2009 ; Zhu 2012 ; Li 2014 ; Chuang et al. 2010 ). The present study considered TV as one of the indicators of market sentiment.
Macroeconomic factors that are often flashed in the media tend to influence investor sentiment quite significantly. Factors like the levels of inflation, corporate debt, economic growth rate and foreign exchange rate and reserves tend to affect the behaviour of market participants to a certain extent. Therefore, this study used variables such as the exchange rate, Wholesale Price Index (WPI), Index of Industrial Production (IIP), Net Foreign Institutional Investment (FII) and Term Spread (TS) to measure the intensity of aggregate investor sentiment on market volatility.
Unit root tests
Ensuring the stationarity of the variables is necessary for consistent estimators. This study used the augmented Dickey-Fuller test (ADF) (Dickey and Fuller 1981 ) to analyse the presence of unit roots in the time series properties of each variable. Table 1 shows the results of unit root analysis using the ADF test. Unit root tests were run with the linear trend and at levels and intercept. The result shows that all variables expect MT are stationary at level. MT was converted to stationarity by taking the first difference.
- Principal component analysis
Principal component analysis (PCA) is a multivariate method in which several interconnected quantitative dependent variables describing the observations are analysed. PCA aims to find and extract the most significant information from the data by compressing the size and simplifying the data without losing the important information (Abdi and Williams 2010 ). It consists of several steps for conducting the linear transformations of a large number of correlated variables to obtain a comparatively few unrelated elements. In this way, information is clustered together into narrow sets and multicollinearity is eliminated. The principal goal of PCA is to summarize the indicator data through a common set of variables as efficiently as possible.
First, the six orthogonal sentiment proxies and their first lags were used as factor loadings to calculate the raw sentiment index. The study started with estimating the initial principal component of the six indicators and their lags, which gave a first-stage index with 12 loading factors, namely the six proxies and their lags. Then, we calculated the correlation between the initial index and the current and lagged values of the indicators. Finally, we estimated the sentiment as the first principal component of the correlation matrix of six variables, which were the respective proxy’s lead or lag. We chose whichever had a higher correlation with the first-stage index to rescale the coefficients so that the index had unit variance (Table 2 ). This process yielded a parsimonious index.
Investors’ sentiment and stock market volatility
Following the theoretical and empirical models proposed by Baker and Wurgler ( 2007 ), Brown and Cliff ( 2004 ) and Baker et al. ( 2012 ), this study used market-related indicators for the construction of the investor sentiment index in the initial stage. This study used six indirect proxies to create the sentiment index by considering the first principal component and the lagged components of the variable. The first principal component explains the sample’s variance. Researchers have argued that certain proxies take longer periods to reflect the investors’ sentiment. Therefore, the present study followed the approach of Baker and Wurgler ( 2006 ) and Ding et al. ( 2017 ) to reflect the investors’ sentiment accurately and to assess the PCA with levels as well as their lags to find the main factors.
The GARCH (1,1) model was used to estimate the impact of sentiment on market volatility and stock returns. The GARCH model helps analyse the volatility characteristics of the datasets, especially for financial data, as it has the unique characteristics of heteroscedasticity and volatility clustering (Fig. 1 ). The specific character of financial time series data limits the use of conventional econometrics models to estimate the parameters. The GARCH model helps to capture volatility clustering and to manage issues of heteroskedasticity.
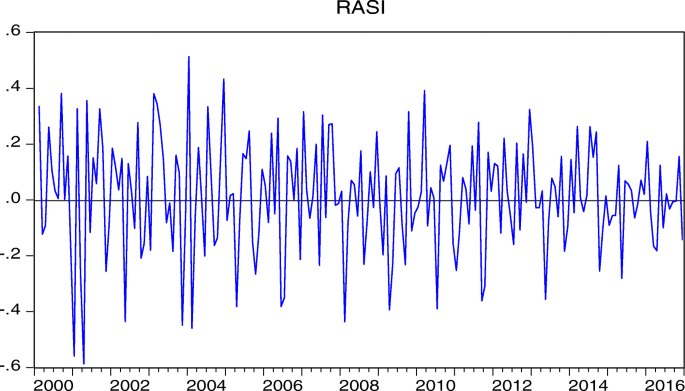
The rational aggregate sentiment index
Stock market volatility can be estimated in two ways: with the help of market-determined option prices or by time series modelling. Non-availability of option prices led us to choose the time-series method. There are multiple indices available to measure the dynamics of the Indian capital market. Among them, Nifty, which consists of 50 companies from different sectors, and Sensex, which covers 30 companies, are prominent. Inclusion of diversified sectors and wider market coverage (market capitalisation) motivated us to select Nifty as the indicator for measuring market volatility. The indicator of market returns and the aggregate sentiment index showed volatility clustering (Fig. 2 ), and the heteroskedastic behaviour was confirmed through the ARCH-LM test. This satisfied the prerequisites for estimating the GARCH model.
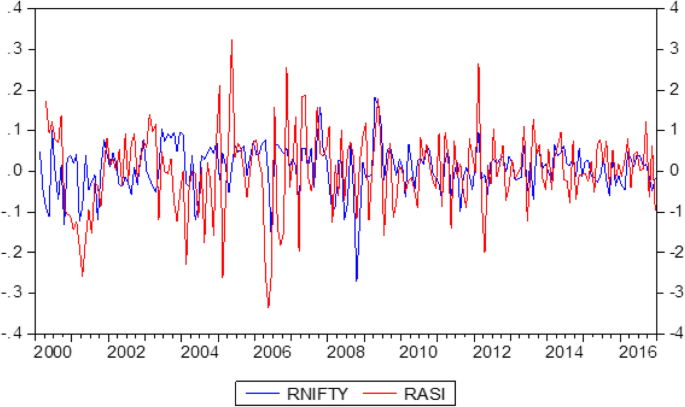
Investors’ sentiment and stock index return
The GARCH ( p, q ) model, introduced by Engle ( 1982 ) and Bollerslev ( 1986 ), can be expressed as follows:
where r t is the log Nifty return (the positive value of r t indicates a bullish trend in the market, and the negative value shows a bearish trend in the market). It is calculated by.
\( {r}_t=\frac{P_1-{P}_0}{P_0} \) , where P 0 and P 1 represent the price at time t-1 and t. γ is the coefficient of the lagged value of the Nifty return ( r t − 1 ). c 0 is the constant of the mean equation; ω is the constant in the variance equations; and ε t is the error term. I t − 1 represents the information available to the market participants. \( {\varepsilon}_{t-1}^2 \) is the ARCH term and \( {\sigma}_{t-1}^2 \) is the GARCH term that explains the instantaneous variance at time t − 1. α +β > 1β ≥ 0, which shows the persistence of volatility. A value close to 1 indicates the persistence of volatility and indicates a low level of mean reversion in the system. By increasing the number of the ARCH and GARCH terms, the model can be generalized to a GARCH (p,q) model. For a well-specified GARCH model, ω > 0, α > 0 and β ≥ 0 should be satisfied.
We modified the basic GARCH model by incorporating a sentiment variable in the equation,
where δ represents the coefficient of the sentiment index.
Empirical results
The estimated result of the GARCH (1,1) model is presented in Table 3 . The coefficients of the ARCH (α) and GARCH terms (β) are statistically significant and different from zero. In addition, the sum of α + β is close to unity. This indicates the high persistence of volatility, that is, the mean reversal process is very slow because of the persistent shocks. The result of the ARCH-LM test indicates the absence of further ARCH effects, which means the model captures the ARCH effects. The statistically significant coefficient of Q and Q 2 at the 20th lag indicates the absence of further autocorrelation in the model.
Sentiment is a crucial element that directly influences market behaviour. The conventional capital asset pricing model theory states that investors should be rewarded according to their risk-taking behaviour. However, the impact of sentiment on market volatility may cause market uncertainty and lead to less returns. If the market participants fail to earn a market risk premium for their expected volatility, they will move away from the market, which further causes volatility in the market. This vicious circle may cause a bearish trend and languid growth and development of the market. The conditional volatility graph shows that the impact of negative sentiment is higher than that of positive sentiment. This indicates that when sentiments are positive, investors actively participate in the market with the expectation of higher returns. However, this causes more speculative activities in the markets and may cause overvaluation of scrips. In contrast, during the dominance of negative sentiments, investors move away from the market because of the negative expectation of market returns. Therefore, it can be theorised that during positive sentiment, companies explore the opportunity to enter the market through IPOs. Similarly, dividend declaration, bonus issue and a rights issue also trigger positive sentiments.
Conditional volatility
The conditional variance graph from the GARCH (1,1) model shows the dynamics of market volatility of the Nifty returns (Fig. 3 ). Up to May 2008, volatility was high, though it can be deemed as moderate when compared to that during the subprime crisis period. During this period, volatility increased exponentially, and this trend continued up to February 2010. Later, the volatility reduced substantially.
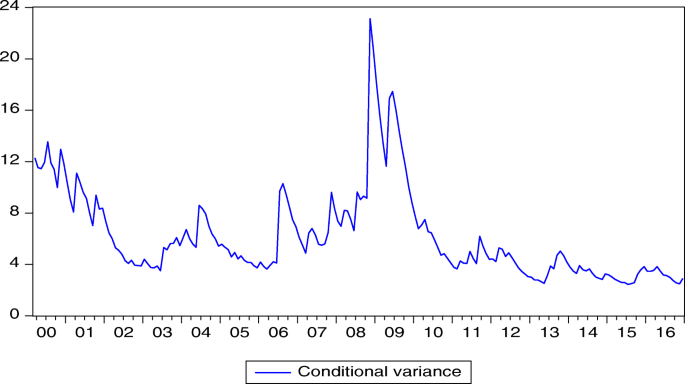
- Granger causality test
The Granger causality test examines the direction of cause among different series (Granger 1969 ). A time series x t Granger-causes another time series y t if series y t can be predicted with better accuracy by using the past values of x t rather than by not doing so. This study examined the causal relationship between the sentiment index (S ent ) and stock market return. Tests between the aggregate sentiment index and stock returns were modelled for understanding the leading and the lagging variables. We found that investors’ sentiment leads to volatility of the market returns. However, volatility in the returns does not cause sentiment (Table 4 ).
De Long et al. ( 1990 ) pointed out that noise traders’ pressure in a market with a strong bullish sentiment on the price to move beyond the fundamental value causes a drop in the expected return. However, if bullish noise traders dominate the market, it causes a rapid upward movement in the market prices because of the upsurge in demand for the high-risk scrips. The expected level of market risk will be higher, creating a ‘hold more’ effect because of the expectation of higher returns. The intensity of sentiments on stock returns closely depends on the effect that dominates the market expectation. The unidirectional causality of sentiments to volatility indicates that the price-pressure effect (noise traders’ pressure on prices reduces the expected return) dominates the market and that noise traders benefit during episodes of a high sentiment index. This way, sentiment leads to volatility. However, once the noise traders start making profit, their expectation on return and risk will increase. Thus, it may not create a reverse causality in a developing market because of information inefficiency. In another way, it can be explained that when investors’ irrational sentiment is positive, their expectation on return is also positive. This may lead to speculative activities on their part to exploit the situation, exciting them to invest more. This leads to volatility in the market. On the other hand, market uncertainty causes withdrawal of market makers and encourages investors to stay inactive because of the uncertain expectation on the return in a risky market. Moreover, in such a situation, investors are always concerned about fundamentally induced equilibrium prices that give the fair value of assets. Following the arguments of Wen et al. ( 2019 ), retail investors should be more attentive in collecting information to minimise their information asymmetry for managing their potential risk.
This research provides a comprehensive examination of the impact of investor sentiment on stock market volatility. The study constructed a sentiment index by using a linear combination of different-market oriented proxies weighted using principal component analysis. The study found an asymmetrical relationship when the sentiment index was decomposed into positive and negative sentiment. The positive sentiment index has a positive effect on excess market return, but the intensity of negative sentiment is less on negative returns. These results imply that when investors are more optimistic about the market generating excess returns, their extreme optimism leads to more speculative activities that tempt them to invest even more. The study also found persistency of market volatility and the sentiment index, which shows the contemporaneous impact on sentiment and excess market returns. The findings reveal that investors consider the market as weak-efficient. This shows that the efficient market hypothesis may not be sufficient in explaining the market behaviour of emerging markets like India. The results indicate the scope for arbitration in the Indian market and thus invalidate the explanation of efficient market volatility in India. This further indicates a deviation from a random walk, but it is difficult to predict the volatility of the market sufficiently to produce excess returns.
The results help to understand the role of non-fundamental factors in driving the Indian equity market away from a fundamentally oriented equilibrium and in influencing the risk-return perception. They also show that sentiment is relatively correlated with unexpected stock returns, and the correlation differs significantly over time. This contradicts with the traditional capital market theories and supports the behavioural theories on capital markets. Proper examination of the market sentiment helps investors and fund managers decide their entry and exit points for investment. By taking the investor sentiment into account as a significant determinant of stock market volatility in asset price models, investors can enhance their portfolio performance. The results can also help policymakers’ efforts to stabilize stock market volatility and uncertainty in order to protect investors’ wealth and attract more investors. Therefore, future research should aim to develop investors’ sentiments from available high-frequency data by incorporating additional comprehensive investor sentiment factors to reflect real-time information.
Availability of data and materials
Not applicable.
Abbreviations
Indian Aggregate Sentiment Index
Gross domestic product
Price to earnings ratio
Generalized autoregressive conditional heteroskedastic model
Autoregressive conditional heteroscedasticity-Lagrange multiplier
Put-call ratio
Initial public offer
Abdi H, Williams LJ (2010) Principal component analysis. Wiley Interdiscip Rev Comput Stat 2(4):433–459
Article Google Scholar
Aggarwal D, Mohanty P (2018) Do Indian stock market sentiments impact contemporaneous returns? S Asian J Bus Stud 7(3):332–346
Baker M, Stein JC (2004) Market liquidity as a sentiment indicator. J Financ Mark 7(3):271–299
Baker M, Wurgler J (2000) The equity shares in new issues and aggregate stock returns. J Financ 55:2219–2257
Baker M, Wurgler J (2006) Investor sentiment and cross-section of stock returns. J Financ 61(4):1645–1980
Baker M, Wurgler J (2007) Investor sentiment in the stock market. J Econ Perspect 21:129–152
Baker M, Wurgler J, Yuan Y (2012) Global, local, and contagious investor sentiment. J Financ Econ 104(2):272–287
Barberis N, Shleifer A, Vishny R (1998) A model of investor sentiment. J Financ Econ 49(3):307–343
Bekaert G, Baele L, Inghelbrecht K (2010) The determinants of stock and bond return comovements. Rev Financ Stud 23:2374–2428
Black F (1986) Noise. J Financ 41(2):529–543
Bollerslev T (1986) Generalized autoregressive conditional heteroskedasticity. J Econ 31:307–327
Brown GW, Cliff MT (2004) Investor sentiment and the near-term stock market. J Empir Financ 11(1):1–27
Chandra A, Thenmozhi M (2013) Investor sentiment, volatility and stock return comovements. Volatility and Stock Return Comovements
Google Scholar
Chen N, Kan R, Miller M (1993) Are the discounts on closed-end funds a sentiment? index? J Financ 48:795–800
Chi L, Zhuang X, Song D (2012) Investor sentiment in the Chinese stock market: an empirical analysis. Appl Econ Lett 19(4):345–348
Chiu CWJ, Harris RD, Stoja E, Chin M (2018) Financial market volatility, macroeconomic fundamentals and investor sentiment. J Bank Financ 92:130–145
Chuang W-J, Ouyang L-Y, Lo W-C (2010) The impact of investor sentiment on excess returns: a Taiwan market cases. Int J Inf Manag Sci 21:13–28
Clarke RG, Statman M (1998) Bullish or bearish? Financ Anal J 54:63–72
Dash SR, Mahakud J (2013) Investor sentiment and stock returns: do industries matter? Margin J Appl Econ Res 7:315–349
De Long JB, Shleifer A, Summers LH, Waldmann RJ (1990) Noise trader risk in financial markets. J Polit Econ 98(4):703–738
DeBondt WF, Thaler R (1985) Does the stock market overreact? J Finance XL(3):793–805
Dickey DA, Fuller WA (1981) Likelihood ratio statistics for autoregressive time-series with a unit-root. Econometrica 49:1057–1072
Ding Z, Liu Z, Zhang Y, Long R (2017) The contagion effect of international crude oil price fluctuations on Chinese stock market investor sentiment. Appl Energy 187:27–36
Elton EJ, Gruber MJ, Busse JA (1998) Do investors care about sentiment? J Bus 71:477–500
Engle RF (1982) Autoregressive conditional heteroskedasticity with estimates of the variance of United Kingdom inflation. Econometrica 50:987–1007
Engle RF, Ng VK (1993) Measuring and testing the impact of news on volatility. J Financ 48:1749–1778
Fama EF (1965) The Behavior of Stock-Market Prices. J Bus 38(1):34–105
Fang L, Yu H, Huang Y (2018) The role of investor sentiment in the long-term correlation between US stock and bond markets. Int Rev Econ Financ 58:127–139
Finter P, Ruenzi S (2012) The impact of investor sentiment on the German stock market. Z Betriebswirt 82(2):133–163
Fisher KL, Statman M (2000) Investor sentiment and stock returns. Financ Anal J 56:16–23
Granger CWJ (1969) Inves~ga~g causal relations by econometric models and cross-spectral models. Econometrica 37:424–438
Gupta R (2019) Manager sentiment and stock market volatility. Journal of Manag Inf Decis Sci 22(1):11–21
Henderson BJ, Jegdeesh N, Weisbach MS (2006) World markets for raising new capital. J Financ Econ 82:63–101
Jiang F, Lee J, Martin X, Zhou G (2019) Manager sentiment and stock returns. J Financ Econ 132(1):126–149
Jones C (2001) A century of stock market liquidity and trading costs. Columbia University Mimeo, New York
Karpoff JM (1987) The relation between price changes and trading volume: a survey. J Financ Quant Anal 22(1):109–126
Kumari J, Mahakud J (2016) Investor sentiment and stock market volatility: evidence from India. J Asia Pac Bus 17(2):173–202
Lee WY, Jiang CX, Indro DC (2002) Stock market volatility, excess returns, and the role of investor sentiments. J Bank Financ 26:2277–2299
Lemmon M, Portniaguina E (2006) Consumer confidence and asset prices: some empirical evidence. Rev Financ Stud 19(4):1499–1529
Li BH (2014) Does investor sentiment predict stock returns? The evidence from the Chinese stock market. J Syst Sci Complex 27:130–143
Mcleod AI, Ll WK (1983) Diagnostic checking ARMA time series models using squared residual autocorrelations. J Time Ser Anal 4:269–273
Mushinada VNC, Veluri VSS (2018) Investors overconfidence behaviour at the Bombay Stock Exchange. Int J Manag Financ 14(5):613–632
Neal R, Wheatley SM (1998) Do measures of investor sentiment predict returns? J Financ Quant Anal 33(04):523–547
Qiang Z, Shue-e Y (2009) Noise trading, investor sentiment volatility, and stock returns. Syst Eng Theory Pract 29(3):40–47
Qiu L, Welch I (2006) Investor sentiment measures. In: Schmeling M (ed) Investor sentiment, herd-like behavior and stock returns http://ssrn.com/abstract=589641
Rahman ML, Shamsuddin A (2019) Investor sentiment and the price-earnings ratio in the G7 stock markets. Pac Basin Financ J 55:46–62
Sehgal S, Sood GS, Rajput N (2009) Investor sentiment in India: a survey. Vision: J Bus Perspect 13(2):13–23
Shiller RJ (1981) Do stock prices move too much to be justified by subsequent changes in dividends? Am Econ Rev 71(3):421–436
Sias RW, Starks LT, Tinic SM (2001) Is noise trader risk priced? J Financ Res 24:311–329
Verma R, Soydemir G (2009) The impact of individual and institutional investor sentiment on the market price of risk. Q Rev Econ Finance 49(3):1129–1145
Verma R, Verma P (2007) Noise trading and stock market volatility. J Multinatl Financ Manag 17:231–243
Wang YH, Keswani A, Taylor SJ (2006) The relationships between sentiment, returns and volatility. Int J Forecasting 22:109–123
Wen F, Xu L, Ouyang G, Kou G (2019) Retail investor attention and stock price crash risk: Evidence from China. Int Rev Financ Anal 65:101376
Yang Y, Copeland L (2014) The effects of sentiment on market return and volatility and the cross-sectional risk premium of sentiment-affected volatility, Cardiff economics working papers, no. E2014/12. Cardiff Business School, Cardiff
Ying CC (1966) Stock market prices and volumes of sales. Econometrica 34:676–685
Zhou G (2018) Measuring investor sentiment. Ann Rev Financ Econ 10:239–259
Zhou L, Yang C (2019) Stochastic investor sentiment, crowdedness and deviation of asset prices from fundamentals. Econ Model 79:130–140
Zhu X (2012) Investor sentiment and volatility of stock index an empirical analysis from the perspective of behavioural finance. Adv Appl Econ Finance 3(4):627–629
Download references
Acknowledgements
Author information, authors and affiliations.
Central University of Kerala, Kasaragod, India
Haritha P H
Central University of Himachal Pradesh, Dharamsala, Himachal Pradesh, India
Abdul Rishad
You can also search for this author in PubMed Google Scholar
Contributions
The authors analyze the role of irrational investor sentiment in determining Indian stock market volatility using monthly information from India’s National Stock Exchange between June 2000 and December 2016. Sentiment index with the assistance of principal component analysis was developed using market-related implicit indices. Further, this sentiment index was modelled in the GARCH and Granger Causality framework to analyses its contribution to volatility. The results show that irrational sentiment significantly causes excess market volatility. Moreover, the study reveals that the asymmetrical aspects of an inefficient market contribute to excess volatility and returns. The findings reveal that investors consider the market as weak-efficient. This shows that the efficient market hypothesis may not be sufficient in explaining the market behaviour of emerging markets like India. The author(s) read and approved the final manuscript.
Corresponding author
Correspondence to Haritha P H .
Ethics declarations
Competing interests, additional information, publisher’s note.
Springer Nature remains neutral with regard to jurisdictional claims in published maps and institutional affiliations.
Rights and permissions
Open Access This article is licensed under a Creative Commons Attribution 4.0 International License, which permits use, sharing, adaptation, distribution and reproduction in any medium or format, as long as you give appropriate credit to the original author(s) and the source, provide a link to the Creative Commons licence, and indicate if changes were made. The images or other third party material in this article are included in the article's Creative Commons licence, unless indicated otherwise in a credit line to the material. If material is not included in the article's Creative Commons licence and your intended use is not permitted by statutory regulation or exceeds the permitted use, you will need to obtain permission directly from the copyright holder. To view a copy of this licence, visit http://creativecommons.org/licenses/by/4.0/ .
Reprints and permissions
About this article
Cite this article.
P H, H., Rishad, A. An empirical examination of investor sentiment and stock market volatility: evidence from India. Financ Innov 6 , 34 (2020). https://doi.org/10.1186/s40854-020-00198-x
Download citation
Received : 29 August 2019
Accepted : 18 August 2020
Published : 12 October 2020
DOI : https://doi.org/10.1186/s40854-020-00198-x
Share this article
Anyone you share the following link with will be able to read this content:
Sorry, a shareable link is not currently available for this article.
Provided by the Springer Nature SharedIt content-sharing initiative
- Investor sentiment
- Stock market volatility
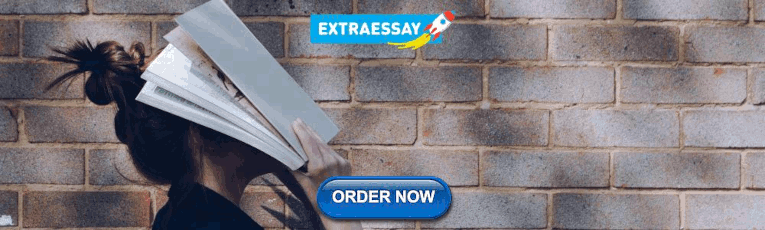
Change your language and LGT location here.
- Our company
- Sustainability
- Our engagement
- LGT worldwide
- Investment solutions
- UHNWI services
- LGT as an employer
- Career opportunities
- Market view
- LGT SmartBanking
- Publications
- Financial intermediaries
Our Insights provide informative, inspiring, surprising, and entertaining insights behind the scenes of finance and economics, as well as society or art. The monthly newsletter keeps you up to date.
Demystifying volatility
Market fluctuations are normal and largely unavoidable, but are they also something to fear?
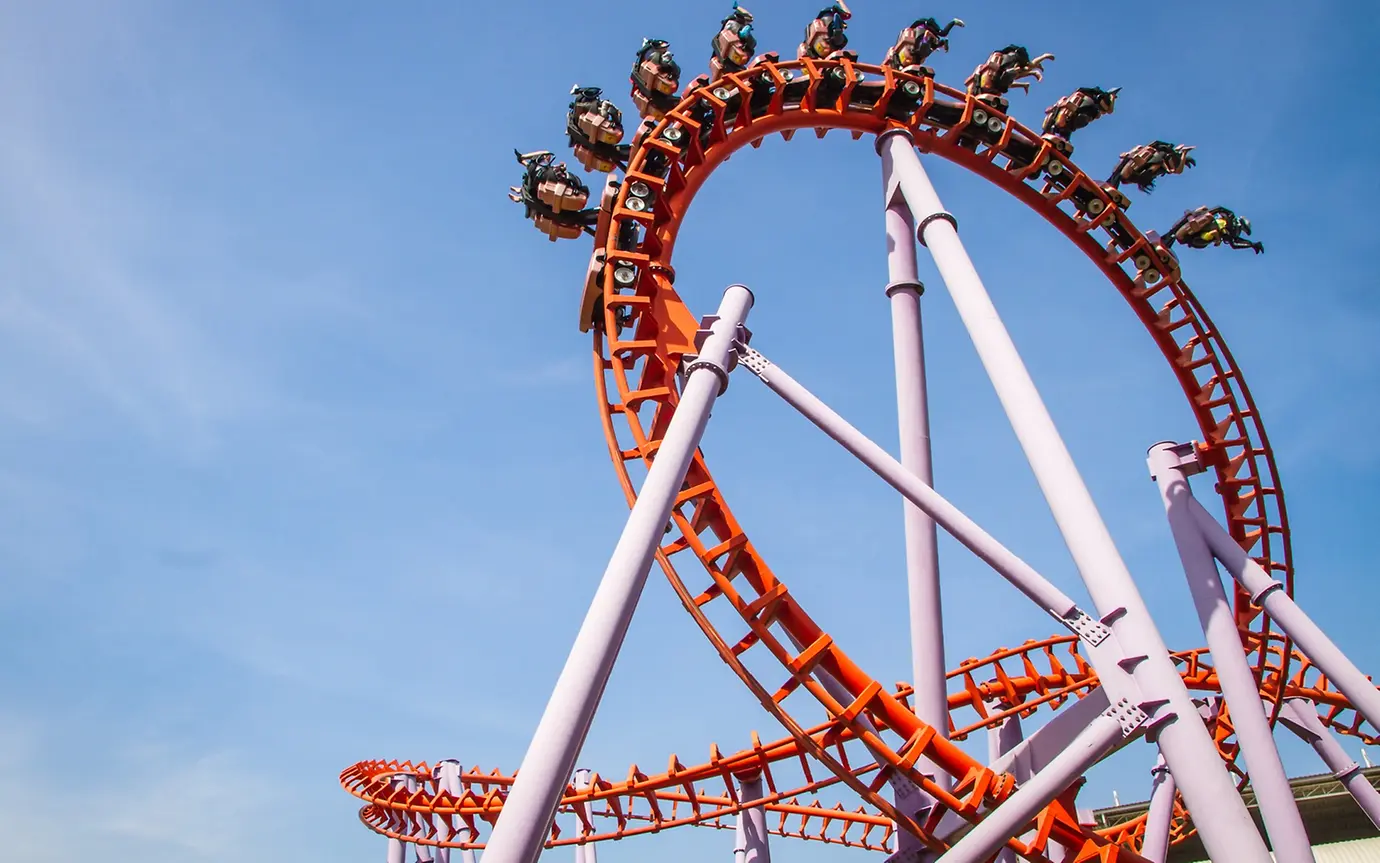
Given the turbulence of the stock markets over the last few years, starting with the pandemic, there's been increasing interest in how volatility affects investment portfolios. While volatility can be nerve-wracking, it is an unavoidable feature of markets and not necessarily something to avoid. So what exactly is volatility?
Measuring volatility
In finance, volatility refers to how much trading prices vary over time. It's commonly assessed using standard deviation, which measures the amount of variation in a data set relative to the mean. When the standard deviation is low, there is little deviation from the mean. When it's high, there's more deviation and more volatility.
Volatility in and of itself doesn't tell you anything about the direction of the returns. Derek Horstmeyer, Professor at George Mason University School of Business
Another important measurement of volatility is the CBOE Volatility Index, often called by its ticker symbol, VIX. This index measures the market's expectations for volatility over the next 30 days, and is sometimes known as the fear index. When there is more uncertainty and more volatility, the VIX is higher. When market sentiment is more optimistic, with less anxiety, values are lower.
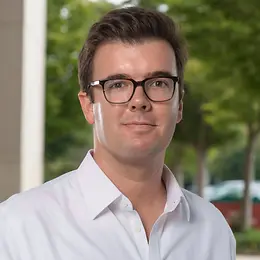
Unlike standard deviation, which looks at past data, the VIX looks forward and measures volatility by tracking S&P 500 index options trading. Options are contracts that give investors the right, but not the obligation, to buy or sell stock at a specific price by a certain date. By tracking the prices of options, the index provides a glimpse into market sentiment regarding the near future. The option prices are weighted, giving prominent, market-moving options more heft, to get a more accurate picture of overall sentiment.
Notably, volatility doesn't measure whether markets are heading up or down, but how much they've been fluctuating, or might fluctuate, in the near future.
"It can be an up market or a down market when you have bouts of volatility. Volatility in and of itself doesn't tell you anything about the direction of the returns," says Derek Horstmeyer, a professor at George Mason University School of Business, who specialises in the performance of exchange-traded funds and mutual funds.
High-volatility funds yield higher returns
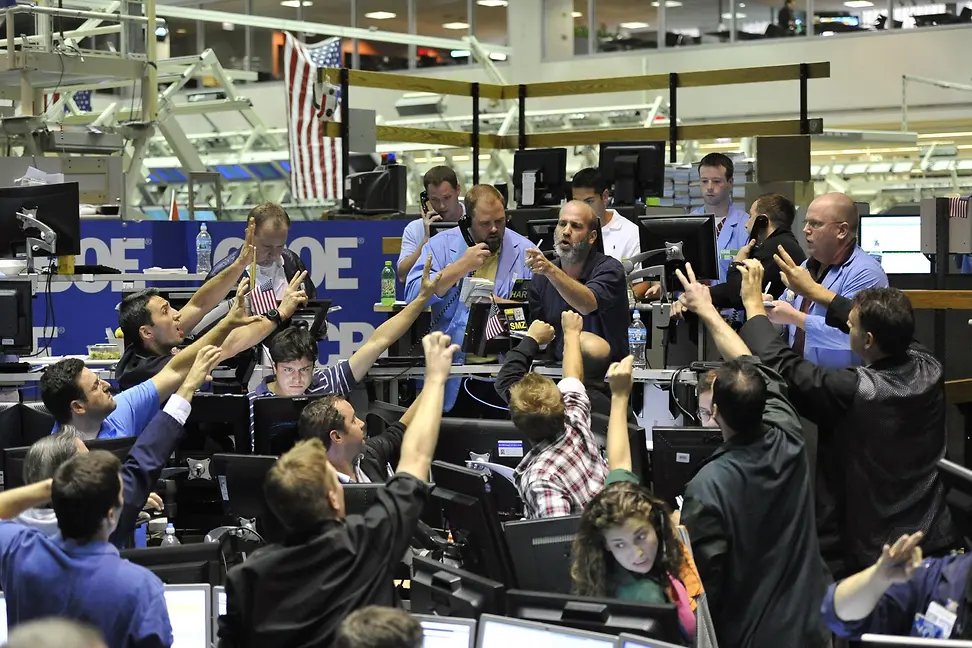
Volatility plays an important role in assessing potential returns and investment risk. In a recent study, Horstmeyer set out to determine if high- or low-volatility strategies yield higher equity returns. Drawing on data from Morningstar Direct on low- and high-volatility mutual funds and ETFs over the past decade, he found "clear and unequivocal" evidence that high-volatility funds yielded much higher returns than low-volatility funds.
US high-volatility funds earned an annualized return of 15.89 per cent on a post-tax basis over the past ten years, compared to 5.16 per cent over the same period for low-volatility funds. The results were similar when looking at global funds, excluding the US. High-volatility funds returned an average of 5.81 per cent annually versus 2.51 per cent for low-volatility funds. For emerging markets, high-volatility funds returned an average of 4.55 per cent annually versus 0.11 per cent for low-volatility funds.
Risk and volatility are often used interchangeably, but they are not the same thing.
Volatility is an important factor to consider when investing, but this doesn't mean it should be avoided. Volatility doesn't indicate if prices are heading up or down. But the more volatile prices are, the more opportunity there is to buy low and sell high. When there is high volatility, "there are more chances to have big differences in the price. You have more chance of buying bargains when prices are volatile. So if you're a long-term investor, volatility should be a positive. But many investors don't look at it that way," says Robert Johnson, a professor of finance at Creighton University's Heider College of Business.
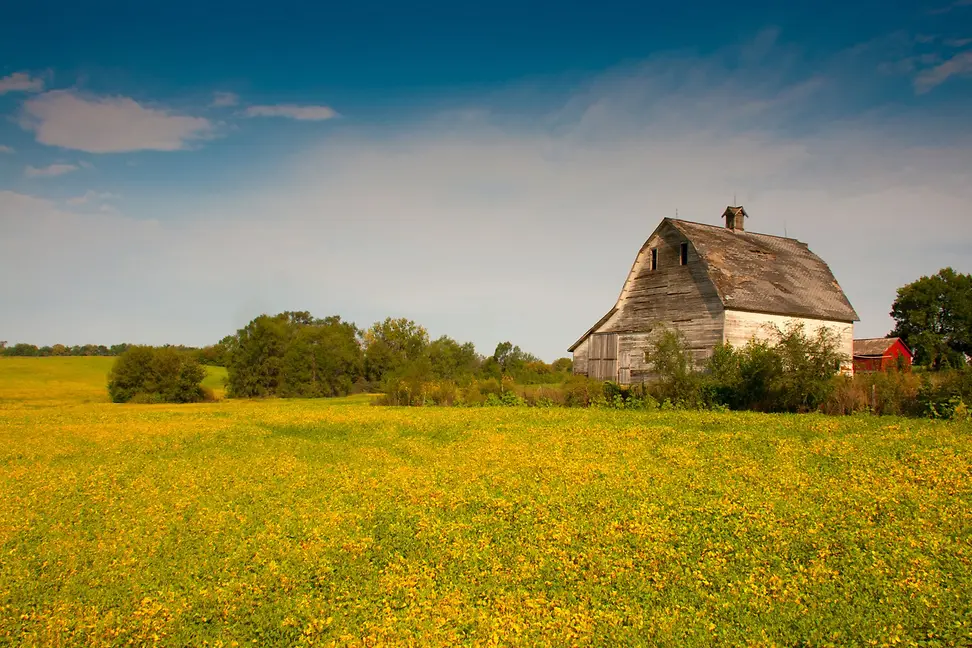
Younger investors interested in capital growth have many years to invest and can afford to have more volatility in their portfolios. Conversely, older investors near to or in retirement, and generally interested in capital preservation, might want to stay away from volatility. That's because if markets decline by more than 30 per cent in a year, as they did during the financial crisis in 2008, there is less time for the investments to recover.
Volatility vs risk
When talking about investing, risk and volatility are often used interchangeably. But they are not the same. Volatility measures how much prices move around. Risk , on the other hand, is the possibility that something bad could happen.
Johnson acknowledges that the two terms are used interchangeably in finance, but it's important to differentiate them. To explain the difference, Johnson likes to cite an example from famed value investor Warren Buffett , who bought farmland when the price dropped to USD 600 an acre from USD 2,000 an acre.
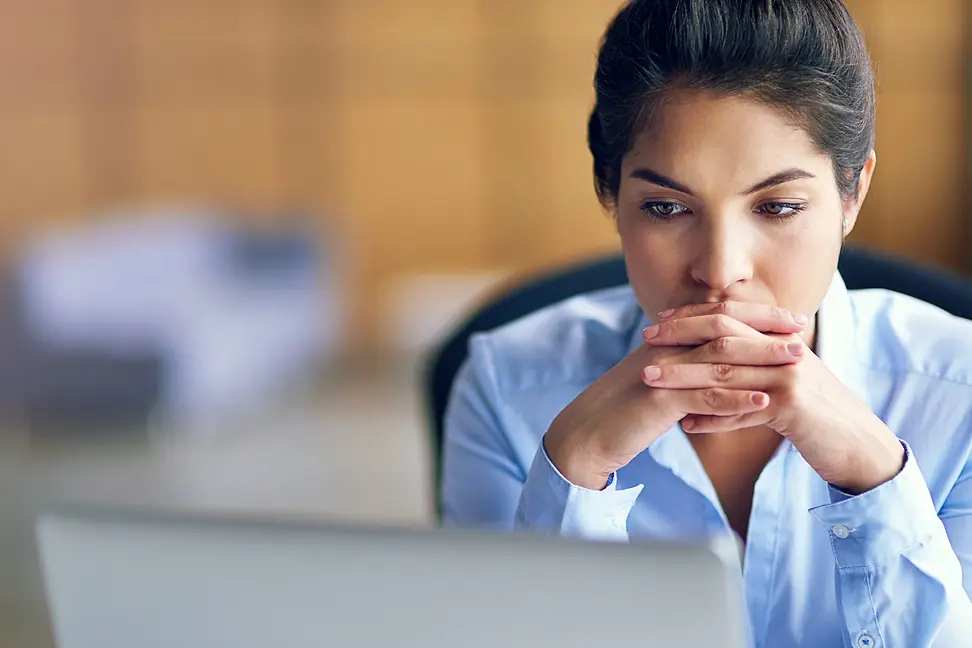
"If volatility equals risk, people would say that buying farmland at USD 600 was more risky than at USD 2,000," Johnson explains. "But the farmland wasn't any more risky than before; it was simply market sentiment about farmland that lowered the price."
Another example that illustrates the difference: certain chip stocks have risen 300 per cent in the last year because of the sudden interest in artificial intelligence. A 300 per cent increase in one year makes a stock volatile, but that doesn't mean it's risky.
Of course, there are times when an investment is truly more risky because the stock price may very well drop further. And that kind of risk is something to look out for when investing. Johnson points to two types of risk: business risk and price risk. Business risk is the risk that internal or external factors could put a dent in a company's financial results. Price risk is the risk that a security or portfolio could decline due to factors like market dynamics, interest rates, or global events, and should not be confused with volatility.
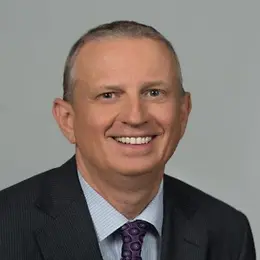
Business risk is crucial when looking at a particular stock for long-term investment. Johnson believes that investors should focus on assessing if the company has a sustainable business model. "That's a better indicator of risk than if the price moved 50 per cent in the last year. This is the fundamental thing about volatility that is mistaken by investors," according to Johnson.
Volatility is an unavoidable part of investing. While seeing wild swings on the market can make some investors uneasy, understanding and accepting that market fluctuations are a normal part of investing is key to staying on track with a long-term wealth management plan.
Related content
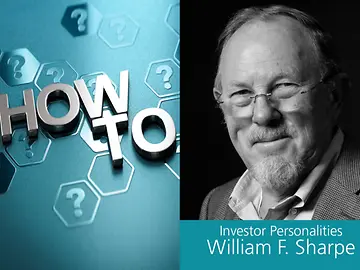
William F. Sharpe: The ivory tower meets the real world
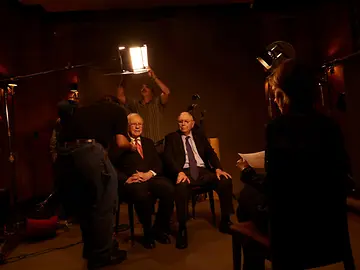
The wit and wisdom of Warren Buffett and Charlie Munger
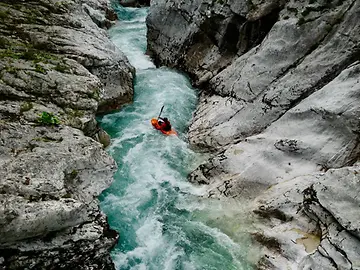
Positive performance in any market?
The LGT newsletters provide you with regular information on insights and news from the fields of business, finance and much more.
The market for carbon offsets: insights from US stock exchanges
- Original Paper
- Published: 15 May 2024
Cite this article
- Stephen F. Diamond 1 &
- Jennifer W. Kuan ORCID: orcid.org/0000-0001-8094-6821 2
Carbon offsets are a critical factor in addressing the harmful effects of climate change. The recent growth in voluntary carbon offsets is a welcome development in a setting dominated by compliance-oriented carbon markets driven by government emissions targets. However, fragmentation, volatile pricing, and low quality offsets have been problematic indications of inefficiency in this market. We argue that the underlying economic theory for compliance-oriented markets is different from that of voluntary offsets. Coase’s ( 1960 ) Problem of Social Cost lays the groundwork for the former while Akerlof’s ( 1970 ) Market for Lemons underpins the latter. We propose a literature on successful responses to the lemons problem, which employ a two-sided market structure (or multi-sided platform, MSP). We suggest that the value chain in the voluntary offset market could be reconfigured using this structure, as one possible response to the lemons problem. This structure has the added advantage of driving innovation and adoption in accounting and other market standards that would be tailored to support the carbon offset market.
This is a preview of subscription content, log in via an institution to check access.
Access this article
Price includes VAT (Russian Federation)
Instant access to the full article PDF.
Rent this article via DeepDyve
Institutional subscriptions
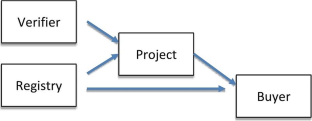
Similar content being viewed by others
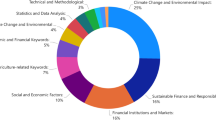
Advancing green finance: a review of sustainable development
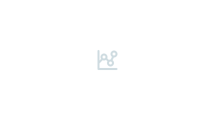
The impact of climate risk on firm performance and financing choices: An international comparison
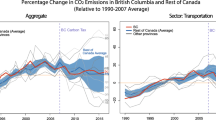
Does a Carbon Tax Reduce CO2 Emissions? Evidence from British Columbia
It took several decades at the end of the 19th and beginning of the 20th centuries for the modern market for stocks, as we know them today, to emerge. Both underwriters and the NYSE played leading roles in establishing the standards that made efficient trading possible (Carosso, 1970 ; Banner, 1998 ).
Ahonen, H., Kessler, J., & Singh, A. (2023). Raising climate ambition with carbon credits, Perspectives, Freiburg.
Akerlof, G. (1970). The Market for Lemons: Qualitative Uncertainty and the Market Mechanism, Q. J. Econ , August, Vol. 84(3), pp. 488–900.
Banner, S. (1998). Anglo-american securities Regulation: Cultural and political roots, 1690–1860 . Cambridge University Press.
Blaufelder, C., Levy, C., Mannion, P., & Pinner, D. (2021). A blueprint for scaling voluntary carbon markets to meet the climate challenge . McKinsey Sustainability.
BloombergNEF, (2023). Carbon Offset Market Could Reach $1 Trillion With Right Rules, January 23.
Broekhoff, D., Gillenwater, M., Colbert-Sangree, T., & Cage, P. (2019). Securing Climate Benefit: A guide to using Carbon offsets . Stockholm Environment Institute & Greenhouse Gas Management Institute.
Carosso, V. P. (1970). Investment Banking in America: A history . Harvard University Pres.
Civillini, M. (2023a). Revealed: How Shell cashed in on dubious carbon offsets from Chinese rice paddies. Climate Home News , March 28 at 3:07 PM.
Civillini, M. (2023b). Verra boss steps down after criticism of its carbon credits. Climate Home News , May 23 at 6:09 PM.
Coase, R. H. (1960). The Problem of Social Cost. Journal of Law and Economics , Vol. 3, October, pp. 31– 44.
Dhanda, K. K., & Laura, P. H. (2011). The ethics of carbon neutrality: A critical examination of voluntary carbon offset providers. Journal of Business Ethics , 100 , 119–149.
Article Google Scholar
Diamond, S. F., & Kuan, J. W. (2018). Are the stock markets “rigged”? An empirical analysis of regulatory change. International Review of Law and Economics , 55 , 33–40.
Ecosystem, M. (2022). The art of Integrity: State of Voluntary Carbon markets, Q3 insights briefing . Forest Trends Association.
Forest Trends Association. State of the Voluntary Carbon Markets 2023. Washington DC: Forest Trends’ Ecosystem Marketplace, Foster, Vivien, & Hahn, R. W. (2023). 1995. Designing More Efficient Markets: Lessons from Los Angeles Smog Control. Journal of Law and Economics 38(1): 19–48.
Goulder, L. H. (2013). Markets for pollution allowances: What are the (new) lessons? Journal of Economics Perspectives , 27 (1), 87–102.
Greenfield, P., & The Guardian (2023). Revealed: more than 90% of rainforest carbon offsets by biggest certifier are worthless, analysis shows: Investigation into Verra Carbon standard finds most are ‘phantom credits’ and may worsen global heating. Jan 18.
Halper, E. Los Angeles Times , 2021. Burned trees and billions in cash: How a California climate program lets companies keep polluting. Sept 8.
Jingle County Sailing Carbon Sink Development Co. LTD (2023). Xinzhou Echeng Afforestation Project Description.
Kaplan, R. S., Ramanna, K., & Roston, M. (2023a). Accounting for carbon offsets—Establishing the foundation for carbon-trading markets. Harvard Business School Working Paper 23–050.
Kaplan, R. S., Ramanna, K., & Roston, M. (2023b). Accounting for carbon offsets . Harvard Business Review.
MacDonagh, J., & Williams, A. (2024). Investigations into voluntary carbon credit quality have prompted increased focus on high-integrity credits, from both buyers and providers. PitchBook .
Mandelbrot, B. (1963). The variation of certain speculative prices. The Journal of Business , 36 (4), 394–419.
Michaelowa, A., Shishlov, I., & Brescia, D. (2019). Evolution of international carbon markets: lessons for the Paris Agreement. Wiley Interdisciplinary Reviews: Climate Change. Vol. 10(6) November.
Nowicki, B. (2021). Resignation letter from California Air Resources Board available at https://ww2.arb.ca.gov/sites/default/files/2021-02/nowicki_brian_offsets_task_force_letter_020821.pdf
Parker, G. G., & Van Alstyne, M. W. (2005). Two-sided network effects: A theory of information product design. Management Science , 51 (1), 1494–1504.
Reuters (2023). Voluntary carbon markets set to become at least five times bigger by 2030-Shell. January 19 at 2:13 am PST.
Rochet, J. C., & Tirole, J. (2003). Platform competition in two-sided markets. Journal of the European Economic Association , 1 (4), 990–1029.
Rochet, J. C., & Tirole, J. (2006). Two-sided markets: A Progress Report. RAND Journal of Economics , 37 (3), 645–667.
Roston, M., Seiger, A., & Heller, T. (2023). What’s next after carbon accounting? Emissions liability management. Stanford working paper.
SourceMaterial (2023). The Carbon Con, Source-Material.org, January 18.
Stanford Law, S. (2022). Voluntary Carbon Markets Symposium. October 25. https://conferences.law.stanford.edu/carbonmarkets1/
Stavins, R. (2022). The relative merits of carbon pricing instruments: Taxes versus trading. Review of Environmental Economics and Policy , 16 (1), Winter 2022.
Stubbs, M., Hoover, K., & Ramseur, J. L. (2021). Agriculture and Forestry offsets in Carbon markets: Background and selected issues. Congressional Research Service Reports R46956, Nov. 3.
Twidale, S., & Mcfarlane, S. (2023). Carbon credit market confidence ebbs as big names retreat. Reuters Sept . 1.
Verra (2024). https://verra.org/about/overview/ , last checked on Jan. 17, 2024.
West, T. A. P., Sven Wunder, E. O., Sills, J., Börner, S. W., Rifai, Alexandra, N., Neidermeier, & Kontoleon, A. (2023). Action needed to make carbon offsets from tropical forest conservation work for climate change mitigation, January 5, Preprint available at arXiv:2301.03354.
Zelljadt, E. (2022). Carbon markets on a bull run: Emissions trading gains influence. Refinitiv June 29.
Download references
Author information
Authors and affiliations.
School of Law, Santa Clara University, Santa Clara, USA
Stephen F. Diamond
College of Business, California State University Monterey Bay, Seaside, USA
Jennifer W. Kuan
You can also search for this author in PubMed Google Scholar
Corresponding author
Correspondence to Jennifer W. Kuan .
Ethics declarations
Conflict of interest.
The authors have no conflicts of interest related to this research.
Additional information
Publisher’s note.
Springer Nature remains neutral with regard to jurisdictional claims in published maps and institutional affiliations.
About this article
Diamond, S.F., Kuan, J.W. The market for carbon offsets: insights from US stock exchanges. Rev World Econ (2024). https://doi.org/10.1007/s10290-024-00535-7
Download citation
Accepted : 02 March 2024
Published : 15 May 2024
DOI : https://doi.org/10.1007/s10290-024-00535-7
Share this article
Anyone you share the following link with will be able to read this content:
Sorry, a shareable link is not currently available for this article.
Provided by the Springer Nature SharedIt content-sharing initiative
- Carbon market
- Emissions trading
- Carbon offset
- Lemons problem
- Stock exchange
- Find a journal
- Publish with us
- Track your research
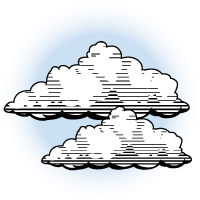
Color Scheme
- Use system setting
- Light theme
Cocoa plunges 19% as rain forecasts stoke market volatility
Cocoa futures in New York saw the largest price drop in records going back more than 60 years, with a forecast of wetter weather for key producers feeding further volatility to the market.
The most-active contract in New York fell as much as 19% on Monday, while London futures fell as much as 18%. Rainfall is boosting the outlook for crops, and low open interest in cocoa markets is amplifying price moves.
Rains “should improve conditions quite a bit” in Ghana, the world’s second-biggest grower, and Indonesia, Donald Keeney, senior meteorologist at Maxar Technologies Inc., said in an email. Top producer Ivory Coast will also benefit from wet weather, said Keeney, but still needs more rainfall to completely end the dryness stressing crops.
In Ghana, the combination of rain and sunshine has been good for cocoa, and growers would like the pattern to continue, said Michael Acheampong, a chief farmer in the town of Kwarbeng about 100 kilometers (62 miles) north of Accra.
Low open interest means that “the tiniest updates to weather forecasts in West Africa or signs of demand destruction will cause these big price swings,” said John Goodwin, a senior commodity analyst at ArrowStream Inc.
A lack of moisture in top West African cocoa producers has weighed on supply in a market already hit by aging trees and disease. Prices saw a 9% recovery last week, with money managers boosting their net-bullish bets to a three-week high. Still, some expect that a record price set in mid-April will mark the peak of the historic rally.
More than 65,000 Spokane-area workers soon will begin building WA Cares benefits
In every community across Washington, families are facing the challenge of accessing and affording long-term care.
Academia.edu no longer supports Internet Explorer.
To browse Academia.edu and the wider internet faster and more securely, please take a few seconds to upgrade your browser .
Enter the email address you signed up with and we'll email you a reset link.
- We're Hiring!
- Help Center
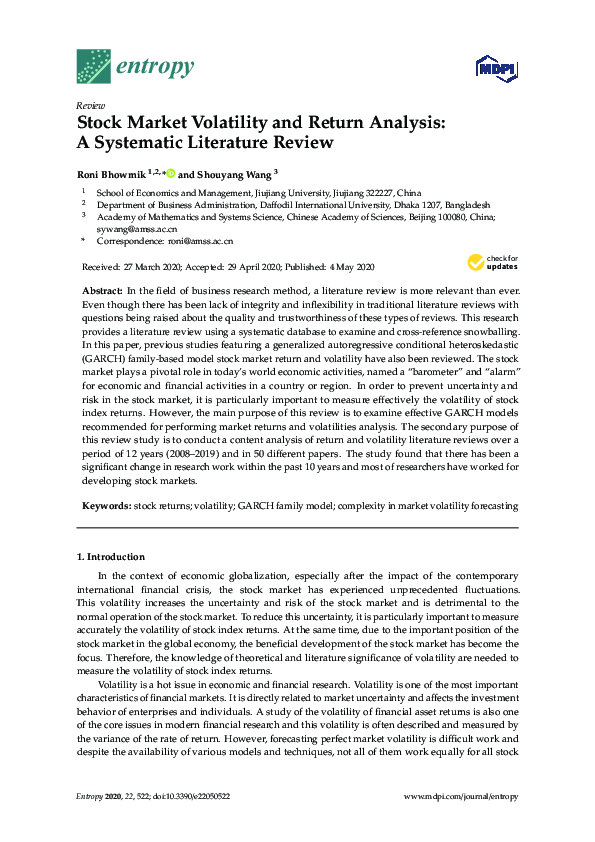
Stock Market Volatility and Return Analysis: A Systematic Literature Review

2020, Entropy
In the field of business research method, a literature review is more relevant than ever. Even though there has been lack of integrity and inflexibility in traditional literature reviews with questions being raised about the quality and trustworthiness of these types of reviews. This research provides a literature review using a systematic database to examine and cross-reference snowballing. In this paper, previous studies featuring a generalized autoregressive conditional heteroskedastic (GARCH) family-based model stock market return and volatility have also been reviewed. The stock market plays a pivotal role in today’s world economic activities, named a “barometer” and “alarm” for economic and financial activities in a country or region. In order to prevent uncertainty and risk in the stock market, it is particularly important to measure effectively the volatility of stock index returns. However, the main purpose of this review is to examine effective GARCH models recommended for...
Related Papers
Econometric Modeling: International Financial Markets - Volatility & Financial Crises eJournal
sartaj hussain
This study aims to gain insights on various issues that surround stock market volatility. For this purpose, more than forty empirical studies have been examined to critically assess issues like, heteroscedasticity, asymmetric effect, risk-return framework, spillovers and forecasting accuracy. With the help of time-series plots, the study demonstrates in layman terms how mean-reversion, clustering and heteroscedasticty exhibits in stock market volatility. This study finds GARCH variants to have a wider applicability in the modelling of volatility persistence despite fearing poorly in evaluation against naive methods like realised volatility, EWMA. The asymmetric effect doesn’t seem to be as strong at firm level as it appears at the broad market index level. Evidence of statistically weak relation between conditional volatility and expected returns raises questions about accuracy of the volatility measures plugged for testing the relation. In case of spillover effects, immunity/propen...
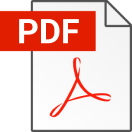
This article aims to highlight a controversial issue of great interest ie the intrinsic structure of emerging capital market behavior. Synthesizing, empirical analysis aims to analyze emerging capital markets volatility. Emerging capital markets establish a separate category in the financial field, with highly dynamic characteristics, especially in times of financial crisis. Emerging capital markets are extremely attractive considering the growth prospects and investment opportunities. However, volatility of returns is significant and represents an undeniable obstacle in attracting investors. Modeling and forecasting volatility of emerging capital markets is still an underexploited area although it has quite interesting research resources. Stock prices volatility can be used as a measure of risk in financial markets, so its importance is even greater in emerging capital markets. A sharp introspection regarding cointegration of emerging stock markets raised significant issues as a direct consequence of international portofolio diversification and financial globalization.
Muhammad Imtiaz Subhani-PhD
A healthy stock market is a sign of sound and healthy economy. Stock market is a volatile market affected, at times directly and most often indirectly, by many micro and macroeconomic players. Of these players interest rates and exchange rates are among the ones undertaken in this study. The rationale behind this study is to ascertain the volatility in stock returns of various stock exchanges in relevance to interest rates and exchange rates over a range of 8 countries for assorted periods. GARCH (1, 1) was deployed for investigating the possible eventualities of volatilities of stock markets. The findings were found varying for Pakistan, India, Hong Kong, Japan, United States, United Kingdom, Spain and Germany. Moreover, almost for all countries GARCH (1, 1) yielded significant results confirming the existence of volatility of stock markets for the current period of outlined countries due to volatility of those stock markets during the previous lags. The findings may help investors know the stock markets’ trends which are also for some cases (nations) affected by interest rates and/or exchange rates and thus to invest accordingly.
Thomas Chiang
Journal of Economics and Finance
Ramon Degennaro
Albert Agyemang-Badu
In this paper, volatility of stock returns in Ghana is modeled from July 4, 2011 to October 3, 2014 using both symmetric and asymmetric univariate Generalized Autoregressive Conditional Heteroscedasticity (GARCH) models under the normal Gaussian distribution assumption. Results show that equity returns exhibit stylized characteristics such as volatility clustering, peakedness, and leverage effect found with most advanced stock markets. Further, results show that shocks to the Ghana equity market are usually transient with minimal instances of persistence. It is further confirmed that EGARCH (1, 1) is superior in modeling the volatility of returns on the equity market for the studied period.
Himanshu Mathur
The National Stock Exchange and Bombay Stock Exchange are the two major stock exchanges in India. The Bombay Stock Exchange is the first stock exchange of Asia and 10th largest stock exchange in the world in the terms of market capitalisation. Stock markets significantly contributes in the economic development of India. The stock markets have volatile character which results into the uncertainty of the returns, volatility is caused by the variability in speculative market prices and the instability of business performance. Volatility plays a significant role in financial decisions of the investors, managers, policy makers and the researchers as it can assess the risk exposures in their investments and the uncertainty in stocks returns. The risk averse investor avoid investment in highly volatile market. The stock return forecasting leads to volatility forecasting. This paper has made an attempt to analyse the volatility with reference to Bombay Stock Exchange. The daily data of S&P ...
Suliman Zakaria Suliman Abdalla
Review of Pacific Basin Financial Markets and Policies
Ravinder Arora
This paper investigates the behavior of stock returns and volatility in 10 emerging markets and compares them with those of developed markets under different measures of frequency (daily, weekly, monthly and annual) over the period January 1, 2002 to December 31, 2006. The ratios of mean return to volatility for emerging markets are found to be higher than those of developed markets. Sample statistics for stock returns of all emerging and developed markets indicate that return distributions are not normal and return volatility shows clustering. In most cases, GARCH (1, 1) specification is adequate to describe the stock return volatility. The significant lag terms in the mean equation of GARCH specification depend on the frequency of the return data. The presence of leverage effect in volatility behavior is examined using the TAR-GARCH model and the evidence indicates that is not present across all markets under all measures of frequency. Its presence in different markets depends on ...
International Journal of Business and Development Studies
This paper investigates the nature of volatility characteristics of stock returns in the Bangladesh stock markets employing daily all share price index return data of Dhaka Stock Exchange (DSE) and Chittagong Stock Exchange (CSE) from 02 January 1993 to 27 January 2013 and 01 January 2004 to 20 August 2015 respectively. Furthermore, the study explores the adequate volatility model for the stock markets in Bangladesh. Results of the estimated MA(1)-GARCH(1,1) model for DSE and GARCH(1,1) model for CSE reveal that the stock markets of Bangladesh capture volatility clustering, while volatility is moderately persistent in DSE and highly persistent in CSE. Estimated MA(1)-EGARCH(1,1) model shows that effect of bad news on stock market volatility is greater than effect induced by good news in DSE, while EGARCH(1,1) model displays that volatility spill over mechanism is not asymmetric in CSE. Therefore, it is concluded that return series of DSE show evidence of three common events, namely volatility clustering, leptokurtosis and the leverage effect, while return series of CSE contains leptokurtosis, volatility clustering and long memory. Finally, this study explores that MA(1)-GARCH(1,1) is the best model for modeling volatility of Dhaka stock market returns, while GARCH models are inadequate for volatility modeling of CSE returns.
RELATED TOPICS
- We're Hiring!
- Help Center
- Find new research papers in:
- Health Sciences
- Earth Sciences
- Cognitive Science
- Mathematics
- Computer Science
- Academia ©2024
- International
- Today’s Paper
- Premium Stories
- ⏪ Election Rewind
- Express Shorts
- Health & Wellness
- Brand Solutions
India VIX Index rallies: What does market volatility mean for investors?
India vix is a measure of the market’s expectation of volatility. it typically rises ahead of events like the lok sabha elections, which could have a major impact on the market trajectory. what is the outlook on india vix at present.

India VIX, which is an indicator of the market’s expectation of volatility over the near term, surged past the 21 mark on Tuesday (May 14). The rise shows that fear among traders or market participants on the expected volatility is more now, as compared to 15 days earlier. Currently, the fear among the market players is coming from the outcome of the ongoing Lok Sabha elections.
What is the Volatility Index?
The Volatility Index, VIX or the Fear Index, is a measure of the market’s expectation of volatility over the near term. Volatility is often described as the ‘rate and magnitude of changes in prices’ and in finance often referred to as risk. Usually, during periods of market volatility, the market moves steeply up or down and the volatility index tends to rise. As volatility subsides, the Volatility Index declines.

The Volatility Index is a measure of the amount by which an underlying index is expected to fluctuate in the near term, (calculated as annualised volatility, denoted in percentage e.g. 20 per cent) based on the order book of the underlying index options.
The Chicago Board of Options Exchange (CBOE) was the first to introduce the volatility index for the US markets in 1993 based on S&P 100 Index option prices. In 2003, the methodology was revised and the new volatility index was based on S&P 500 Index options.
Since its inception, it has become an indicator of how market practitioners think about volatility. Investors use it to gauge market volatility and base their investment decisions accordingly.

What is India VIX?
India VIX is a volatility index computed by the NSE based on the order book of NIFTY Options. For this, the best bid-ask quotes of near and next-month NIFTY options contracts, which are traded on the F&O segment of NSE are used.
India VIX indicates the investor’s perception of the market’s volatility in the near term i.e. it depicts the expected market volatility over the next 30 calendar days. The higher the India VIX values, the higher the expected volatility and vice versa, as per NSE.
‘VIX’ is a trademark of the CBOE, and Standard & Poor’s has granted a license to NSE, with permission from the CBOE, to use such a mark in the name of the India VIX and for purposes relating to the India VIX.
Why has India VIX surged?
In May so far, the India VIX has risen by around 53 per cent to above 20. On Tuesday, the index touched a high of 21.88 in afternoon trades. On Monday, India VIX soared 16 per cent to touch the 21.48 mark during the intraday trades, before ending at 20.6.
On Monday, after rising 998 points from the intraday lows, the BSE Sensex ended 112 points up at 72,776.13. The NSE’s Nifty also ended 48.85 points up at 22,104.05; it declined nearly 1 per cent during the day.
The volatility seen in the benchmark equity indices was amid concerns over the results of the ongoing elections, set to be declared on June 4. The market participants said a lower voter turnout ratio in this election may have some impact on the BJP ’s seat count. Heavy selling by foreign portfolio investors, who have dumped Rs 18,375 crore (till May 13) of Indian equities, have also led to the fall in the domestic market.
“A critical domestic event like the Lok Sabha elections does command a certain level of uncertainty for its outcome. Just like earlier elections in 2014 and 2019, similar fear, or I would say uncertainty, with respect to the outcome remains,” said Sahaj Agarwal, Senior Vice President – Derivatives Research, Kotak Securities.
A high number indicates participants are getting more cautious and expect volatility as the event unfolds.
India VIX reflects the expected volatility and typically rises ahead of events like elections that could have a major impact on the market’s trajectory. As expectations of outcome keep changing, VIX also keeps rising, said Deepak Jasani, Head of Retail Research, HDFC securities Ltd.
“However this time around though the VIX has risen, it has risen at a pace slower than in the previous elections as there was lower uncertainty about the outcome when the elections began,” he said.
What is the outlook on India VIX?
According to Geojit Financial Services Chief Market Strategist Anand James, the behavior of India VIX now has a lot of similarities to the period shortly before the 2019 electoral results announcement.
Then too, markets had come off peaks in March, and VIX shot up to 28.6. The main difference is that prior to this, VIX was in a 20-14 range during the previous 6 months, pointing to volatility expectations being reasonably high for an extended period.
In stark contrast, VIX’s rise from record lows to above 20 now, has unfolded in just a fortnight’s time, James said.
“So, while recent history points to more room for upside in VIX and thereby volatility, the abruptness in the rate of change of VIX may lead to a cool-off, perhaps even before the electoral results,” he said.
HDFC Securities ’ Jasani said VIX could keep rising and peak out before the 6th or 7th phase of the elections and once the results are out, VIX can fall quite sharply.
What should investors do?
Kotak Securities’ Agarwal said that in isolation, the India VIX does not give a view on the market.
“But considering all the parameters, we believe the markets are in a corrective/consolidation phase. Only a cross above 23,000 changes the undertone to buying on dips for higher targets – till the same does not happen, I would be passive on broader markets and selectively positive,” Agarwal added.
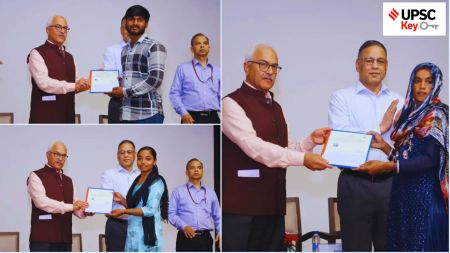
UPSC Key | CAA, UAPA, Chief of Defence Staff and Subscriber Only
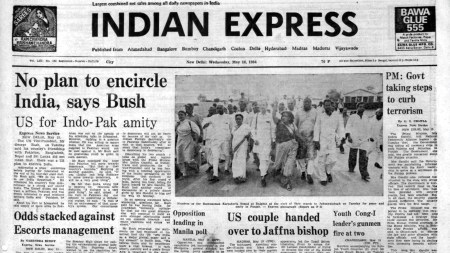
May 16, 1984, Forty Years Ago: Bush Reassures India Subscriber Only
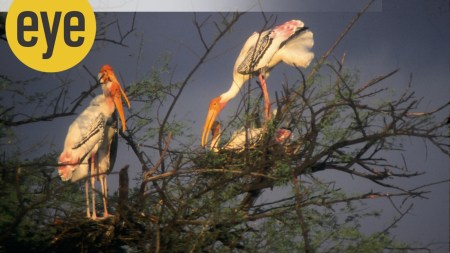
The many types of parents in the animal kingdom Subscriber Only
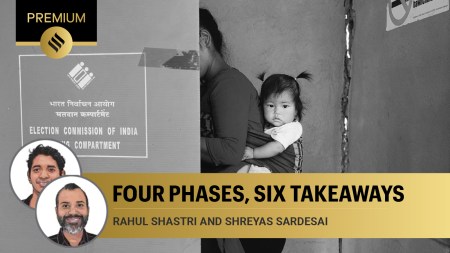
Four phases, six takeaways: Women's voting patterns Subscriber Only
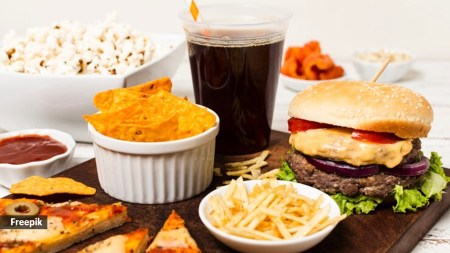
Does adding nutrients make ultra-processed foods healthy?
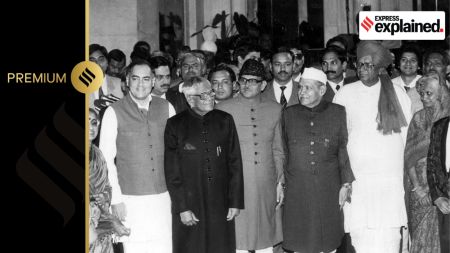
How 1989 elections led to a one-year VP Singh term Subscriber Only
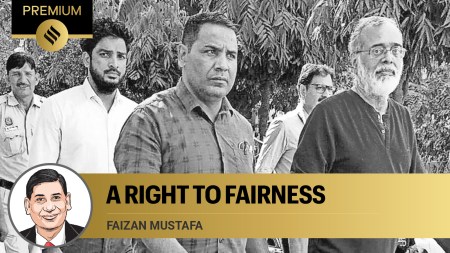
SC verdict on Newsclick shows due process is more than Subscriber Only
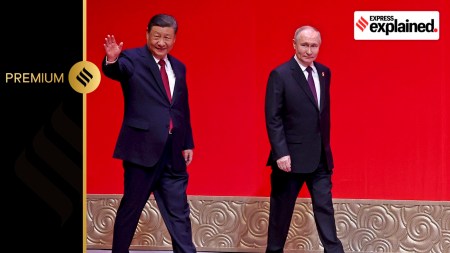
With deepening Russia-China ties, what are the concerns for India? Subscriber Only
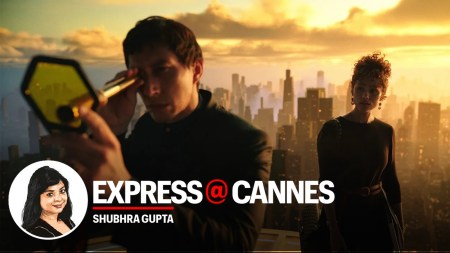
Coppola's Megalopolis is a monumental mess
- Explained Economics
- Express Explained
- Express Premium
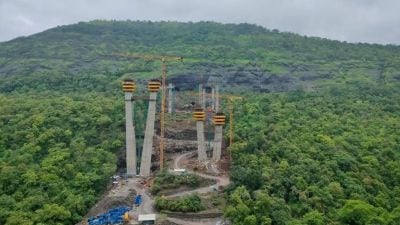
The Maharashtra State Road Development Corporation (MSRDC) will conduct a technical inspection and repair of the gantry on a stretch of the Yashwantrao Chavan Expressway (Pune channel) on May 18 and 19, 2024. During this time, all motorists are advised to use alternate routes.
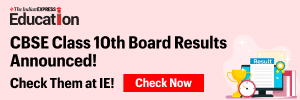
More Explained
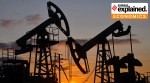
Best of Express
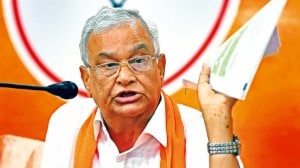
EXPRESS OPINION
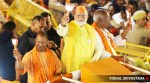
May 18: Latest News
- 01 Gunmen kill three foreign tourists in Afghanistan’s central Bamyan province
- 02 Sahara Group warns of legal action against makers after Scam 2010: The Subrata Roy Saga announcement: ‘An abusive act has been demonstrated….’
- 03 Bhinde says he was not director when hoarding put up; police says he was aware of illegality
- 04 The Great Indian Kapil Show drops mid-season trailer featuring Kartik Aaryan and Janhvi Kapoor; Ed Sheeran says Pushpa’s iconic line. Watch
- 05 EU bans distribution of four Russian news outlets
- Elections 2024
- Political Pulse
- Entertainment
- Movie Review
- Newsletters
- Web Stories
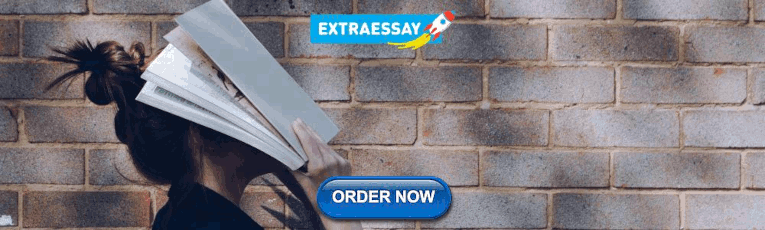
IMAGES
VIDEO
COMMENTS
However, the main purpose of this review is to examine effective GARCH models recommended for performing market returns and volatilities analysis. The secondary purpose of this review study is to conduct a content analysis of return and volatility literature reviews over a period of 12 years (2008-2019) and in 50 different papers.
The current systematic review has identified 50 research articles for studies on significant aspects of stock market return and volatility, review types, and GARCH model analysis. This paper noticed that all the studies in this review used an investigational research method. A literature review is necessary for scholars, academics, and ...
this review study is to conduct a content analysis of return and volatility literature reviews over a. period of 12 years (2008-2019) and in 50 di ff erent papers. The study found that there ...
2. Literature review. Capital flows exert significant effects on stock markets, particularly on market volatilities. As demonstrated by Bennett and Sias (Citation 2001), money flow appears to provide investors with information regarding future returns and can to certain extent explain cross-sectional variation in future returns.Nonetheless, minimal studies test the effect of capital flows on ...
This research provides a literature review using a systematic database to examine and cross-reference snowballing. In this paper, previous studies featuring a generalized autoregressive conditional heteroskedastic (GARCH) family-based model stock market return and volatility have also been reviewed. The stock market plays a pivotal role in ...
Abstract. This study aims to gain insights on various issues that surround stock market volatility. For this purpose, more than forty empirical studies have been examined to critically assess ...
However, there is a dearth of a comprehensive review of how stock market volatility is influenced by macro and firm-level factors. Therefore, this study aims to fill this gap by systematically reviewing the major factors impacting stock market volatility.,This study uses a combination of bibliometric and systematic literature review techniques.
The secondary purpose of this review study is to conduct a content analysis of return and volatility literature reviews over a period of 12 years (2008-2019) and in 50 different papers.
This research provides a literature review using a systematic database to examine and cross-reference snowballing and examines effective GARCH models recommended for performing market returns and volatilities analysis. In the field of business research method, a literature review is more relevant than ever. Even though there has been lack of integrity and inflexibility in traditional ...
Purpose The increasing globalization and technological advancements have increased the information spillover on stock markets from various variables. However, there is a dearth of a comprehensive review of how stock market volatility is influenced by macro and firm-level factors. Therefore, this study aims to fill this gap by systematically reviewing the major factors impacting stock market ...
Stock Market Volatility: A Review of the Empirical Literature. This study aims to gain insights on various issues that surround stock market volatility. For this purpose, more than forty empirical studies have been examined to critically assess issues like, heteroscedasticity, asymmetric effect, risk-return framework, spillovers and forecasting ...
This study aims to gain insights on various issues that surround stock market volatility. For this purpose, more than forty empirical studies have been examine. ... Sartaj and Murthy, K. V. Bhanu and Singh, Amit, Stock Market Volatility: A Review of the Empirical Literature (March 11, 2019). IUJ Journal of Management, Vol.7, Issue 01 ...
However, the main purpose of this review is to examine studies which especially use to analyze return and stock market volatility. The secondary purpose of this review study is to conduct the content analysis of return and volatility literature review over a period of twelve years (2008 - 2019) and in 50 different papers.
This study investigates the impacts of behavioral finance on stock market volatility. The primary aims are to explain the reasons behind changes in the S&P 500 price within the context of behavioral finance and to analyze investor behavior in response to these changes. ... Literature review. Behavioral finance has emerged as a significant field ...
Literature review. The concept of Stock Market Volatility (SMV) is traced back to 1960, when researchers tried to find different market volatility behaviors (e.g., ... Based on the above literature review, the followings research hypotheses are H1: There is a co-integration of SMV, FPI, FXR, IR, and GDP; H2: There is a short-run association ...
The earlier literature review papers on stock market liquidity have considered an inconsistent number of studies from various research databases while some have examined a few studies that highlight different perspectives of stock market liquidity. ... market volatility has been identified as a strong determinant of stock liquidity (Bai & Qin ...
Most of the deep-learning algorithms on stock price volatility prediction in the existing literature use data such as same-frequency market indicators or technical indicators, and less consider mixed-frequency data, such as macro-data. Compared with the traditional model that only inputs the same-frequency data such as technical indicators and market indicators, this study proposes an improved ...
Understanding the irrational sentiments of the market participants is necessary for making good investment decisions. Despite the recent academic effort to examine the role of investors' sentiments in market dynamics, there is a lack of consensus in delineating the structural aspect of market sentiments. This research is an attempt to address this gap. The study explores the role of ...
Specifically, high volatility is responsible for increasing cost of capital, depressing market liquidity and even current consumption and investment. Due to importance of volatility for investment community, there is a vast amount of literature on why stock price volatility changes.
In this paper, we show that industry volatility concentration is a strong predictor for aggregate stock market returns. Our monthly industry volatility concentration (IVC) index displays significant predictive ability, with in-sample and out-of-sample R 2 statistics of 0.686% and 0.712%, respectively, which outperforms a host of prevailing return predictors.
The results were similar when looking at global funds, excluding the US. High-volatility funds returned an average of 5.81 per cent annually versus 2.51 per cent for low-volatility funds. For emerging markets, high-volatility funds returned an average of 4.55 per cent annually versus 0.11 per cent for low-volatility funds.
Even though the reduction in the BI Rate was aimed at providing economic stimulus, the stock market continued to experience significant volatility during the pandemic. Unexpected developments in the infection and uncertainty about the COVID-19 pandemic's long-term economic impact might have a negative influence on investor sentiment and cause ...
1. Introduction. The Black-Scholes-Merton (BSM73, henceforth) formula is one of the most recognized result in modern finance. Within a simple set of assumptions, the formula offers a framework for pricing European options based on just six inputs: the underlying asset's spot price, the option's strike price, the risk-free rate of interest, the dividend yield, the time to maturity of the ...
entropy Review Stock Market Volatility and Return Analysis: A Systematic Literature Review Roni Bhowmik 1,2, * 1 2 3 * and Shouyang Wang 3 School of Economics and Management, Jiujiang University, Jiujiang 322227, China Department of Business Administration, Daffodil International University, Dhaka 1207, Bangladesh Academy of Mathematics and ...
Actually, the existing research literature have shown that there is a significant correlation between the carbon market and the stock market (Creti et al., 2012; Koch et al., 2013 Jiménez-Rodríguez, 2019), and the mechanism of interconnection includes both of return and volatility spillovers. Return spillover, measured by first moment ...
However, the main purpose of this review is to examine e ective GARCH models recommended for performing market returns and volatilities analysis. The secondary purpose of this review study is to conduct a content analysis of return and volatility literature reviews over a period of 12 years (2008-2019) and in 50 di erent papers.
In the context of carbon markets, the literature discusses compliance-driven markets and voluntary markets. Compliance-driven markets are built upon a theory by Coase that gives government a key role in assigning property rights and, in practice, also designing and implementing the market.Voluntary carbon markets have arisen more recently and involve autonomous demand for carbon offsets.
The most-active contract in New York fell as much as 19% on Monday, while London futures fell as much as 18%. Rainfall is boosting the outlook for crops, and low open interest in cocoa markets is ...
entropy Review Stock Market Volatility and Return Analysis: A Systematic Literature Review Roni Bhowmik 1,2, * 1 2 3 * and Shouyang Wang 3 School of Economics and Management, Jiujiang University, Jiujiang 322227, China Department of Business Administration, Daffodil International University, Dhaka 1207, Bangladesh Academy of Mathematics and ...
India VIX is a volatility index computed by the NSE based on the order book of NIFTY Options. For this, the best bid-ask quotes of near and next-month NIFTY options contracts, which are traded on the F&O segment of NSE are used. India VIX indicates the investor's perception of the market's volatility in the near term i.e. it depicts the ...