A review of rehabilitation robot
Ieee account.
- Change Username/Password
- Update Address
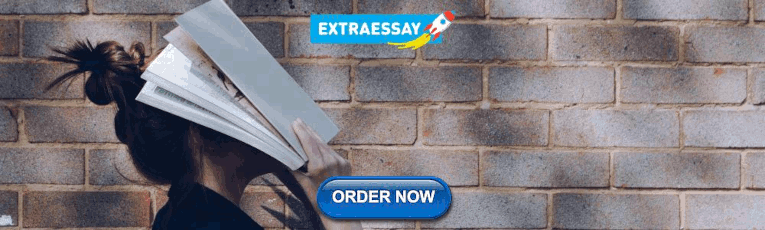
Purchase Details
- Payment Options
- Order History
- View Purchased Documents
Profile Information
- Communications Preferences
- Profession and Education
- Technical Interests
- US & Canada: +1 800 678 4333
- Worldwide: +1 732 981 0060
- Contact & Support
- About IEEE Xplore
- Accessibility
- Terms of Use
- Nondiscrimination Policy
- Privacy & Opting Out of Cookies
A not-for-profit organization, IEEE is the world's largest technical professional organization dedicated to advancing technology for the benefit of humanity. © Copyright 2024 IEEE - All rights reserved. Use of this web site signifies your agreement to the terms and conditions.
Rehabilitation robotics after stroke: a bibliometric literature review
Affiliation.
- 1 Department of Industrial Engineering, University of Padua, Padua, Italy.
- PMID: 35786139
- DOI: 10.1080/17434440.2022.2096438
Introduction: Stroke is the leading cause of long-term disability in developed countries. Due to population aging, the number of people requiring rehabilitation after stroke is going to rise in the coming decades. Robot-mediated neurorehabilitation has the potential to improve clinical outcomes of rehabilitation treatments. A statistical analysis of the literature aims to focus on the main trend of this topic.
Areas covered: A bibliometric survey on post-stroke robotic rehabilitation was performed through a database collection of scientific publications in the field of rehabilitation robotics. By covering the last 20 years, 17,429 sources were collected. Relevant patterns and statistics concerning the main research areas were analyzed. Leading journals and conferences which publish and disseminate knowledge in the field were identified. A detailed nomenclature study was carried out. The time trends of the research field were captured. Opinions and predictions of future trends that are expected to shape the near future of the field were discussed.
Expert opinion: Data analysis reveals the continuous expansion of the research field over the last two decades, which is expected to rise considerably in near future. More attention will be paid to the lower limbs rehabilitation and disease/design specific applications in early-stage patients.
Keywords: Neurorehabilitation; bibliometric study; medical devices; rehabilitation robotics; stroke; upper and lower limb.
Publication types
- Bibliometrics
- Neurological Rehabilitation*
- Stroke Rehabilitation*
- Upper Extremity
Can a Robot Bring Your Life Back? A Systematic Review for Robotics in Rehabilitation
- First Online: 18 February 2020
Cite this chapter
- Esyin Chew 6 &
- David A. Turner 7 , 8
Part of the book series: Advances in Experimental Medicine and Biology ((AEMB,volume 1170))
1153 Accesses
5 Citations
Stroke is a leading cause of disability in the world and the use of robots in rehabilitation has become increasingly common. The Fourth Industrial Revolutions has created a novel and wide range of options for the involvement of computer-guided and artificially intelligent machines to be used in rehabilitation. In this chapter we critically review some of the literature on the use of robots in rehabilitation, and emphasize the diversity of approaches in this burgeoning field. We argue that there is a need to consolidate interdisciplinary evidence on robotics and rehabilitation in a systematic way, as the alternative is to have a literature that continues to grow, following the interests of various specialists, but without offering a synoptic assessment of what is available to medical specialists and patients. A literature review using Scopus and Web of Science, coupled with the Briggs Institute’s Critical Appraisal Tool: Checklist for Case Reports was conducted. The two databases were systematically searched using inter-disciplinary keywords in Feb 2019. An initial search of the databases produced 9894 articles. After rigorous reviews, 35 articles were screened and selected for further interpretation. We examined the current studies on the efficiency and effectiveness of the robot interventions and produced a taxonomy of the review. An original finding of the current robotics in rehabilitation landscaping are critical presented with recommendations and concluding remarks concerning interdisciplinary impact.
This is a preview of subscription content, log in via an institution to check access.
Access this chapter
- Available as PDF
- Read on any device
- Instant download
- Own it forever
- Available as EPUB and PDF
- Durable hardcover edition
- Dispatched in 3 to 5 business days
- Free shipping worldwide - see info
Tax calculation will be finalised at checkout
Purchases are for personal use only
Institutional subscriptions
Similar content being viewed by others
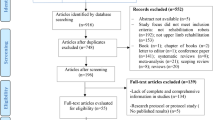
Overview of the role of robots in upper limb disabilities rehabilitation: a scoping review
Tailored, Technological Therapy: Physician and Therapists Point of View on Robotic Rehabilitation
Effects of robot-assisted upper limb rehabilitation in stroke patients: a systematic review with meta-analysis.
Abram HS (1970) The prosthetic man. Compr Psychiatry 11(5):475–481
Article CAS Google Scholar
Agrawal S (2018) The encyclopedia of medical robotics. World Scientific, Singapore, p 280. https://doi.org/10.1142/10770-vol4
Book Google Scholar
Andriella A, GuillemMés AR, Joan FH, Carme T (2018) Deciding the different robot roles for patient cognitive training. Int J Hum Comput Stud Elsevier Ltd 117(March):20–29. https://doi.org/10.1016/j.ijhcs.2018.03.004
Article Google Scholar
Aziz ZA, Lee YY, Ngah BA, Sidek NN, Looi I, Hanip MR, Basri HB (2015) Acute stroke registry Malaysia, 2010–2014: results from the national neurology registry. J Stroke Cerebrovasc Dis 24. https://doi.org/10.1016/j.jstrokecerebrovasdis.2015.07.025
Babaiasl M, Hamed S, Poorya J, Mojtaba Y (2016) A review of technological and clinical aspects of robot-aided rehabilitation of upper-extremity after stroke. Disabil Rehabil Assist Technol Taylor & Francis 11(4):263–280. https://doi.org/10.3109/17483107.2014.1002539
Ballesteros J et al (2017) A biomimetical dynamic window approach to navigation for collaborative control. IEEE Trans Hum Mach Syst 47(6):1123–1133. https://doi.org/10.1109/THMS.2017.2700633
Belda-Lois JM et al (2011) Rehabilitation of gait after stroke: a review towards a top-down approach. J Neuroeng Rehabil BioMed Central Ltd 8(1):66. https://doi.org/10.1186/1743-0003-8-66
Belda-Lois JM et al (2016) Locomotor training through a novel robotic platform for gait rehabilitation in pediatric population: short report. J Neuroeng Rehabil 13(1):1–6. https://doi.org/10.1186/s12984-016-0206-x
Bellingham J et al (2016) Science for robotics and robotics for science. Sci Robot 1(1):eaal2099. https://doi.org/10.1126/scirobotics.aal2099
Bustamante Valles K et al (2016) Technology-assisted stroke rehabilitation in Mexico: a pilot randomized trial comparing traditional therapy to circuit training in a robot/technology-assisted therapy gym. J Neuroeng Rehabil 13(1):1–15. https://doi.org/10.1186/s12984-016-0190-1
Carrillo FM et al (2018) Physiotherapists’ acceptance of a socially assistive robot in ongoing clinical deployment. In: Proceedings of 27th IEEE international symposium on Robot and Human Interactive Communication, RO-MAN’18, Nanjing China, August 27–31, 2018, pp 850–855. https://doi.org/10.1109/ROMAN.2018.8525508
Chen, C.-Y., Huang, Y.-B. and Tzu-Chi Lee, C. (2013) ‘Epidemiology and disease burden of ischemic stroke in Taiwan.Int J Neurosci. England, 123(10), pp. 724–731. doi: https://doi.org/10.3109/00207454.2013.796552
Chen Y, Abel KT, Janecek JT, Chen Y, Zheng K, Cramer SC (2018) Home-based technologies for stroke rehabilitation: a systematic review. Int J Med Inform:11–22. https://doi.org/10.1016/j.ijmedinf.2018.12.001
Galli M et al (2016) Use of the gait profile score for the quantification of the effects of robot-assisted gait training in patients with Parkinson’s disease. In: Proceedings of 2016 IEEE 2nd international forum on Research and Technologies for Society and Industry leveraging a better tomorrow, RTSI 2016, Bologna, Italy, September 7–9, 2016, pp 1–4. https://doi.org/10.1109/RTSI.2016.7740603
Galloway KC et al (2018) Soft robotic glove for combined assistance and rehabilitation during activities of daily living. In: The encyclopedia of medical robotics. World Scientific, pp 135–157. https://doi.org/10.1142/9789813232327_0006
Chapter Google Scholar
Gnjatovic M et al (2018) Pilot corpus of childrobot interaction in therapeutic settings. In: Proceedings of 8th IEEE international conference on Cognitive Infocommunications, CogInfoCom 2018, Debrecen, Hungary, September 11–14, 2018, pp 253–258. https://doi.org/10.1109/CogInfoCom.2017.8268252
Gov.uk (2018) New figures show larger proportion of strokes in the middle aged. UK Government Health and Social Care. https://www.gov.uk/government/news/new-figures-show-larger-proportion-of-strokes-in-the-middle-aged . Accessed 10 Feb 2019
Hansen C et al (2017) Design-validation of a hand exoskeleton using musculoskeletal modeling. Appl Ergon Elsevier 68:283–288. https://doi.org/10.1016/j.apergo.2017.11.015
Hughes CML et al (2016) Community-based neurorehabilitation in underserved populations. In: Proceedings of IEEE Global Humanitarian Technology Conference: technology for the benefit of humanity, GHTC 2016, Seattle, WA, October 13–16, 2016, pp 576–588. https://doi.org/10.1109/GHTC.2016.7857338
Islam MN et al (2013) Burden of stroke in Bangladesh. Int J Stroke 8(3):211–213. https://doi.org/10.1111/j.1747-4949.2012.00885.x
Article PubMed Google Scholar
Jacobs JA (2014) In defense of disciplines: Interdisciplinarity and specialization in the research. University Print, Oxford. https://doi.org/10.7208/chicago/9780226069463.001.0001
Kang J, Agrawal SK (2018) Robot-enhanced walkers for training of children with cerebral palsy: pilot studies. In: The encyclopedia of medical robotics. World Scientific, Singapore, pp 217–240. https://doi.org/10.1142/9789813232327_0009
Khan MJ, Hong K-S (2017) Hybrid EEG–fNIRS-based eight-command decoding for BCI: application to quadcopter control. Front Neurorobot 11:6. Available at: https://www.frontiersin.org/article/10.3389/fnbot.2017.00006
Kim J, Gu GM, Heo P (2016) Robotics for healthcare. In: Jo H et al (eds) Biomedical engineering: frontier research and converging technologies. Springer International Publishing, Cham, pp 489–509. https://doi.org/10.1007/978-3-319-21813-7_21
Kwakkel G, Kollen BJ, Krebs HI (2008) Effects of robot-assisted therapy on upper limb recovery after stroke: a systematic review. Neurorehabil Neural Repair 22(2):111–121
Law P (2015) Social robotics in health-care service: the case of rehabilitation programmes in Hong Kong. In: Vincent J et al (eds) Social robots from a human perspective. Springer International Publishing, Cham, pp 55–65. https://doi.org/10.1007/978-3-319-15672-9_5
Lee HJ et al (2017) Robot-assisted gait training (Lokomat) improves walking function and activity in people with spinal cord injury: a systematic review. J Neuroeng Rehabil 14(1):1–13. https://doi.org/10.1186/s12984-017-0232-3
Li J et al (2014) A pilot study of post-total knee replacement gait rehabilitation using lower limbs robot-assisted training system. Eur J Orthop Surg Traumatol 24(2):203–208. https://doi.org/10.1007/s00590-012-1159-9
Loo KW, Gan SH (2012) Burden of stroke in Malaysia. Int J Stroke 7(2):165–167. https://doi.org/10.1111/j.1747-4949.2011.00767.x
Miao Q et al (2018) Reviewing high-level control techniques on robot-assisted upper-limb rehabilitation. Adv Robot 32(24):1253–1268. https://doi.org/10.1080/01691864.2018.1546617
Naganuma, M., Ohkubo, E. and Kato, N. (2017) ‘Promotion of rehabilitation practice for elderly people using robotic pets’, in van Hoof, J., Demiris, G., and Wouters, E. J. M. (eds) Handbook of smart homes, health care and Well-being. Cham: Springer International Publishing, pp. 543–554. doi: https://doi.org/10.1007/978-3-319-01583-5_65
Google Scholar
Nature (2017) Enabling interdisciplinary research. Nat Hum Behav 1(12):845. https://doi.org/10.1038/s41562-017-0272-5
Niyetkaliyev AS et al (2017) Review on design and control aspects of robotic shoulder rehabilitation orthoses. IEEE Trans Hum Mach Syst 47(6):1134–1145. https://doi.org/10.1109/THMS.2017.2700634
Ochoa-Guaraca M, Pulla-Sánchez D, Robles-Bykbaev V, López-Nores M, Carpio-Moreta M, And García-Duque J (2017) A hybrid system based on robotic assistants and mobile applications to support in speech therapy for children with disabilities and communication disorders. Campus Virtuales 6(1):77–87
Ozaki K et al (2017) Training with a balance exercise assist robot is more effective than conventional training for frail older adults. Geriatr Gerontol Int 17(11):1982–1990. https://doi.org/10.1111/ggi.13009
Palermo E et al (2018) Translational effects of robot-mediated therapy in subacute stroke patients: an experimental evaluation of upper limb motor recovery. PeerJ 6:e5544. https://doi.org/10.7717/peerj.5544
Article PubMed PubMed Central Google Scholar
Piau A et al (2019) Use of a robotic walking aid in rehabilitation to reduce fear of falling is feasible and acceptable from the end user’s perspective: a randomised comparative study. Maturitas Elsevier 120:40–46. https://doi.org/10.1016/j.maturitas.2018.11.008
QS (2019) QS intelligent unit: citations per faculty. http://www.iu.qs.com/university-rankings/indicator-citations-per-faculty/ . Accessed 10 Feb 2019
Rachakorakit M, Charoensuk W (2017) Development of LeHab robot for human lower limb movement rehabilitation. BMEiCON 2017 – 10th biomedical engineering international conference, 2017 January, pp 1–5. https://doi.org/10.1109/BMEiCON.2017.8229148
REF (2014) Research excellence framework, the UK higher education institutions. https://www.ref.ac.uk/2014/about/guidance/citationdata . Accessed 10 Feb 2019
Regen (2019) Rehab programs. http://www.regen.rehab/index.php/what-is-rehabilitation/our-care#rehabprogram . Accessed 25 Feb 2019
Schröder J, Truijen S, Van Criekinge TA, Saeys W (2019) Feasibility and effectiveness of repetitive gait training early after stroke: a systematic review and meta-analysis. J Rehabil Med 51(2):78–88
Scoones (2018) Breaking disciplinary silos to solve real-life puzzles. Institute of Development Studies, Ecosystem Services for Poverty Alleviation. https://www.espa.ac.uk/news-blogs/blog/breaking-disciplinary-silos-solve-real-life-puzzles . Accessed 10 Feb 2019
Shaik MM, Loo KW, Gan SH (2012) Burden of stroke in Nepal. Int J Stroke 7(6):517–520. https://doi.org/10.1111/j.1747-4949.2012.00799.x
Shamsuddin S et al (2017) Preliminary study on the use of therapeutic seal robot for patients with depression. In: Proceedings of IEEE 4th International Symposium on Robotics and Intelligent Sensors: empowering robots with smart sensors, IRIS 2016, Tokyo, Japan, December 17–20, 2016, pp 52–56. https://doi.org/10.1109/IRIS.2016.8066065
Stirling A (2015) Disciplinary dilemma: working across research silos is harder than it looks. Guardian Political Science Blog, 11th June 2014. https://doi.org/10.13140/RG.2.1.1919.3680
Tallis R (2011) Aping mankind: Neuromania, Darwinitis and the misrepresentation of humanity. Acumen, Durham
Tang J, Zhou Z, Yu Y (2017) A hybrid computer interface for robot arm control. In: Proceedings of 8th International Conference on Information Technology in Medicine and Education, ITME 2016, Fuzhou, China, December 23–25, 2016, pp 365–369. https://doi.org/10.1109/ITME.2016.0088
Tang Z, Yang H, Zhang L, Liu P (2018) Effect of shoulder angle variation on sEMG-based elbow joint angle estimation. Int J Ind Ergon 68:280–289
THE (2019) Times Higher Education World University Ranking 2019. https://www.timeshighereducation.com/sites/default/files/the_2019_world_university_rankings_methodology_pwc.pdf . Accessed 10 Feb 2019
The Joanna Briggs Institute (2017) The Joanna Briggs Institute critical appraisal tools for use in JBI systematic review: checklists for case reports. http://joannabriggs.org/assets/docs/critical-appraisal-tools/JBI_Critical_Appraisal-Checklist_for_Case_Reports2017.pdf . Accessed 10 Feb 2019
Tomovic R (1971) Systems approach to skeletal control: concept of the system. Adv Electron Electron Phys 30(C):273–282
Tuisku O et al (2018) Robots do not replace a nurse with a beating heart. Inf Technol People 32(1):47–67. https://doi.org/10.1108/itp-06-2018-0277
UK Parliament (2019) Fourth Industrial Revolution, Education Committee, UK Parliament. https://www.parliament.uk/business/committees/committees-a-z/commons-select/education-committee/inquiries/parliament-2017/fourth-industrial-revolution-inquiry-17-19/
WEF (2016) The fourth industrial revolution: what it means, how to respond. World Economic Forum. https://www.weforum.org/agenda/2016/01/the-fourth-industrial-revolution-what-it-means-and-how-to-respond
Weick KE (1999) That’s moving: theories that matter. J Manag Inq 8(2):134–142
Zhang M, Davies TC, Xie S (2013) Effectiveness of robot-assisted therapy on ankle rehabilitation – a systematic review. J Neuroeng Rehabil 10(1). https://doi.org/10.1186/1743-0003-10-30
Download references
Author information
Authors and affiliations.
EUREKA Robotics Lab, Cardiff School of Technologies, Cardiff Metropolitan University, Cardiff, UK
Institute for International and Comparative Education, Beijing Normal University, Beijing, China
David A. Turner
South Wales University, Wales, UK
You can also search for this author in PubMed Google Scholar
Corresponding author
Correspondence to Esyin Chew .
Editor information
Editors and affiliations.
Institute for Systems and Robotics, Instituto Superior Técnico, Lisbon, Portugal
João Silva Sequeira
1.1.1 Appendix A: Disciplines that Lead in Robotics in Rehabilitation (Figs. 1.11 , 1.12 , 1.13 , 1.14 , 1.15 , 1.16 , 1.17 and 1.18 )
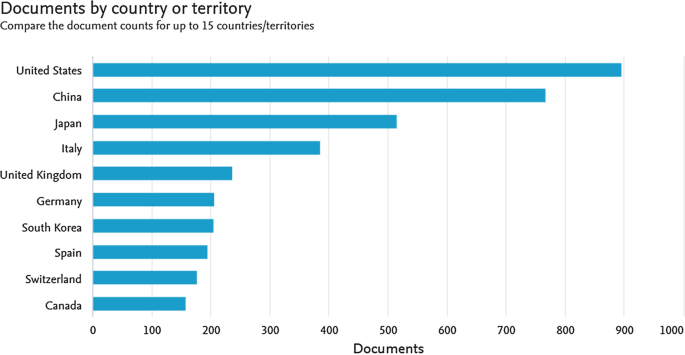
Robotics in Rehab – Engineering Publications
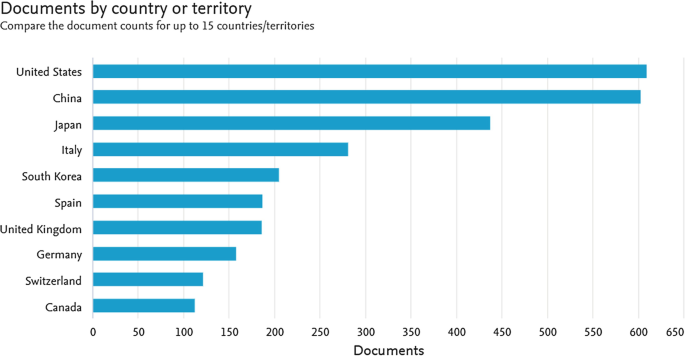
Robotics in Rehab – Computer Science Publications
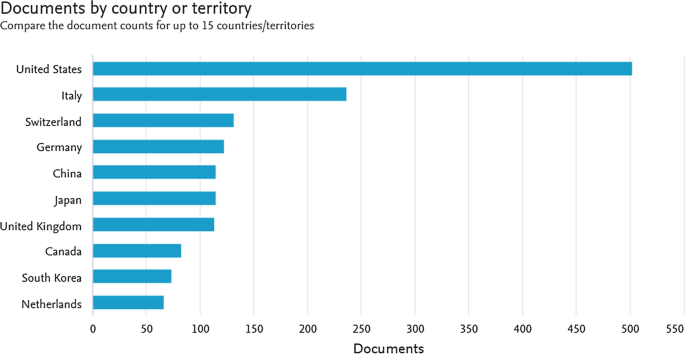
Robotics in Rehab – Medicine Publications
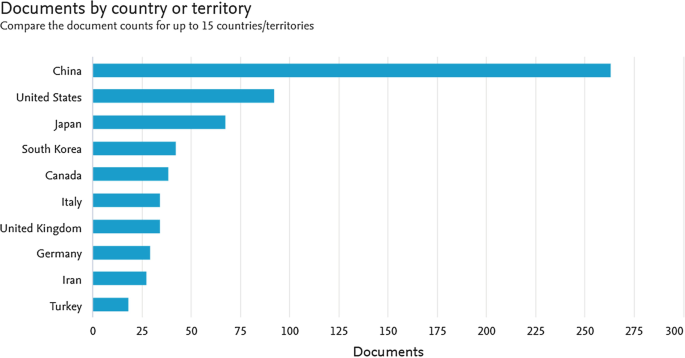
Robotics in Rehab – Maths Publications
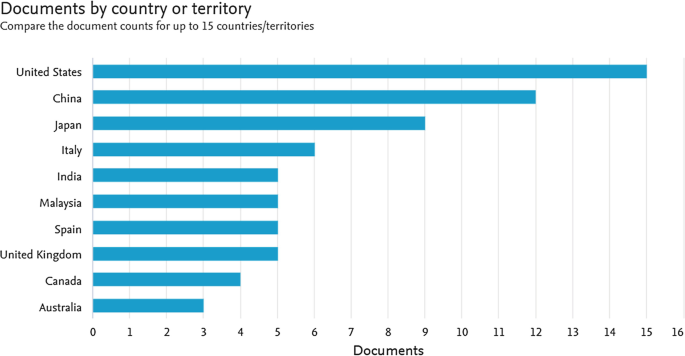
Robotics in Rehab – Social Science and Interdisciplinary Publications
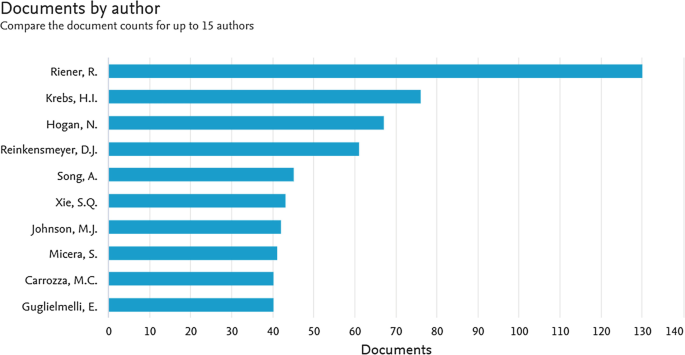
Robotics in Rehab – The Leading Researchers in the Field
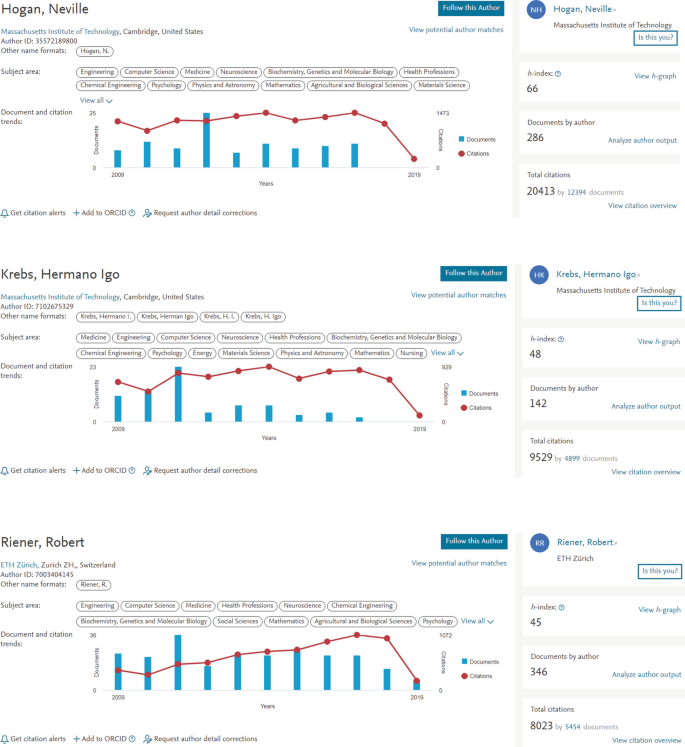
Robotics in Rehab – The Leading Researchers in the Field (Details)
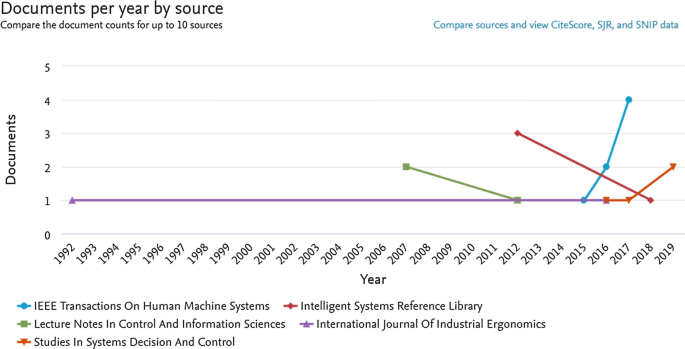
Historical Results by Publication Venues – Social Science and Interdisciplinary Research
1.1.2 Appendix B: Sample of Inclusion Screening Using JBI
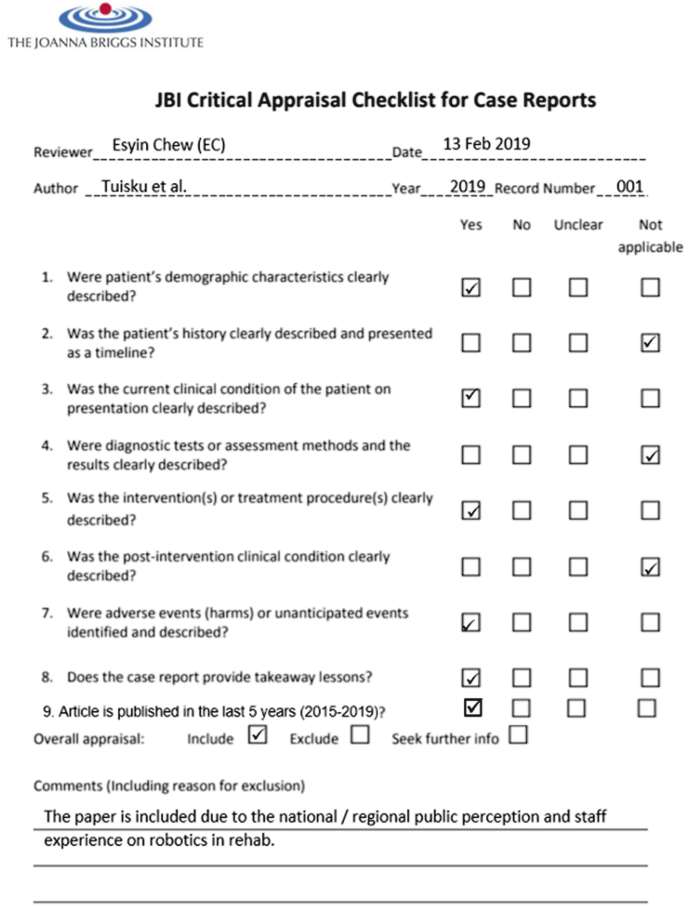
1.1.3 Appendix C: Summary of Included Articles
Rights and permissions.
Reprints and permissions
Copyright information
© 2019 Springer Nature Switzerland AG
About this chapter
Chew, E., Turner, D.A. (2019). Can a Robot Bring Your Life Back? A Systematic Review for Robotics in Rehabilitation. In: Sequeira, J. (eds) Robotics in Healthcare. Advances in Experimental Medicine and Biology, vol 1170. Springer, Cham. https://doi.org/10.1007/978-3-030-24230-5_1
Download citation
DOI : https://doi.org/10.1007/978-3-030-24230-5_1
Published : 18 February 2020
Publisher Name : Springer, Cham
Print ISBN : 978-3-030-24229-9
Online ISBN : 978-3-030-24230-5
eBook Packages : Biomedical and Life Sciences Biomedical and Life Sciences (R0)
Share this chapter
Anyone you share the following link with will be able to read this content:
Sorry, a shareable link is not currently available for this article.
Provided by the Springer Nature SharedIt content-sharing initiative
- Publish with us
Policies and ethics
- Find a journal
- Track your research
- Open access
- Published: 30 August 2019
A Review on Lower Limb Rehabilitation Exoskeleton Robots
- Di Shi 1 , 2 ,
- Wuxiang Zhang ORCID: orcid.org/0000-0001-6141-3368 1 , 2 ,
- Wei Zhang 1 , 2 &
- Xilun Ding 1 , 2
Chinese Journal of Mechanical Engineering volume 32 , Article number: 74 ( 2019 ) Cite this article
251 Citations
Metrics details
Lower limb rehabilitation exoskeleton robots integrate sensing, control, and other technologies and exhibit the characteristics of bionics, robotics, information and control science, medicine, and other interdisciplinary areas. In this review, the typical products and prototypes of lower limb exoskeleton rehabilitation robots are introduced and state-of-the-art techniques are analyzed and summarized. Because the goal of rehabilitation training is to recover patients’ sporting ability to the normal level, studying the human gait is the foundation of lower limb exoskeleton rehabilitation robot research. Therefore, this review critically evaluates research progress in human gait analysis and systematically summarizes developments in the mechanical design and control of lower limb rehabilitation exoskeleton robots. From the performance of typical prototypes, it can be deduced that these robots can be connected to human limbs as wearable forms; further, it is possible to control robot movement at each joint to simulate normal gait and drive the patient’s limb to realize robot-assisted rehabilitation training. Therefore human–robot integration is one of the most important research directions, and in this context, rigid-flexible-soft hybrid structure design, customized personalized gait generation, and multimodal information fusion are three key technologies.
1 Introduction
A rehabilitation robot, which is a robot directly serving humans, has extensive application prospects in rehabilitation therapy with high professional requirements. Therefore, it is of great importance to develop advanced rehabilitation robots.
Research on lower limb rehabilitation robots for patients with limb movement disorders is an important part of rehabilitation robot research. By 2030, 18.2% of China’s population will be over the age of 65. Due to an aging society and improving living standards, the number of people with limb dyskinesia is increasing rapidly. Limb movement disorders can lead to abnormal gait and affect normal walking. For patients with lower limb movement disorder, active rehabilitation training should be started as early as possible. In China, which has the highest stroke rate in the world [ 1 ], there are nearly 15 million disabled people with lower limb motor dysfunctions, such as cerebral palsy, hemiplegia, and paraplegia, and nearly 40 million disabled elderly people who have lost the ability to walk, due to aging. About 350,000 people are in urgent need of rehabilitation technical personnel, but less than 20,000 personnel are available. Therefore, lower limb rehabilitation robots are of great significance. The use of rehabilitation robots can reduce the burden on therapists, realize data detection during training, and aid the quantitative evaluation of recovery in a controllable and repeatable manner [ 2 ].
Lower limb rehabilitation exoskeleton robots, which are a major class of rehabilitation robots, connect with the human body in a wearable way and can control the movement of all joints in the training process. Research on lower limb rehabilitation exoskeleton robots began in the 1960s [ 3 , 4 ]. Due to technical limitations, these early robots failed to reach the expected targets, but laid the foundation for follow-up studies. In recent decades, especially after Lokomat was applied in clinical rehabilitation, lower limb rehabilitation exoskeleton robots have gradually become a major research topic. They are mainly used to provide power assistance and rehabilitation to the elderly and patients with lower limb motor dysfunctions. Exoskeleton robot technology is a comprehensive technology that integrates sensing, control, information and computer science to provide a wearable mechanical device. Many enterprises and research institutions have carried out relevant research work and achieved several milestones in the theory and application of these robots. According to their application, these robots are divided into two types, namely for treadmill-based and overground applications. Patients can receive gait training from treadmill-based exoskeleton robots on a treadmill. In these robots, in addition to the exoskeleton that is used to provide assistance to leg movement [ 3 ], a body weight support (BWS) system is required to reduce gravitational forces acting on the legs, ensure safety, and maintain balance; some examples of such robots include ALEX [ 2 ], Lokomat [ 4 ], and LOPES [ 5 ], as shown in Figure 1 . Overground exoskeleton robots help patients in regaining overground gait, as shown in Figure 2 ; examples include eLEGS (Exoskeleton Lower limb Gait System) [ 6 , 7 ], Indego [ 8 ], ReWalk [ 9 ], MINDWALKER [ 10 , 11 ], and HAL (Hybrid Assistive Limb) [ 12 ].
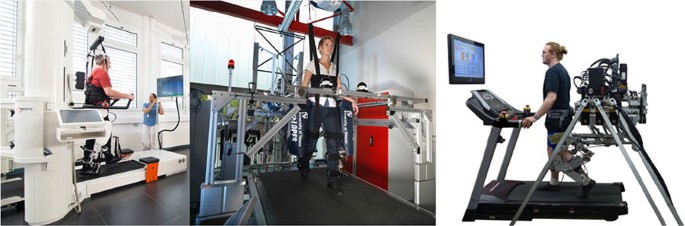
Treadmill-based lower limb rehabilitation exoskeletons
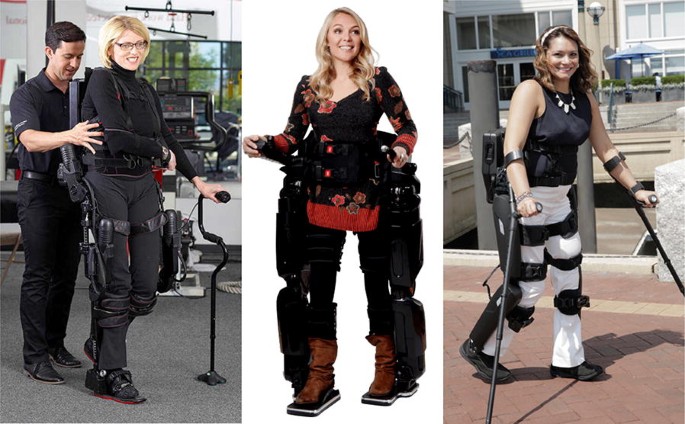
Overground lower limb rehabilitation exoskeletons
At present, a large gap exists in rehabilitation robot technology between China and the developed countries. China urgently requires rehabilitation-assistive devices and has the largest market potential in the world. By 2020, the industrial scale is expected to exceed 700 billion. Universities and institutes in China [ 13 , 14 , 15 , 16 , 17 , 18 , 19 , 20 , 21 , 22 ] have conducted a number of studies and achieved some promising results. However, deep disparities exist between Chinese and overseas research, and no systematic industry has yet been formed. Therefore, China’s lower limb rehabilitation exoskeleton robot research has the potential to produce another revolution in the robot industry.
Hence, in this paper, we present a review on lower limb rehabilitation exoskeleton robots. Research and results in mechanical design and control methods are discussed after collating a summary on human gait analysis. The state-of-the-art research on lower limb rehabilitation exoskeleton robots is described and human–robot integration, which is one of the most important research directions, is discussed.
2 Human Gait Analysis
Wearability is one of the most vital features of lower limb rehabilitation exoskeleton robots and hence such robots must have good human compatibility. Therefore, an illustration of lower limb anatomy and human gait analysis can provide the underlying basis for the design and control of lower limb exoskeleton rehabilitation robots.
2.1 Anatomy of Lower Limbs
The human walking process is mainly accomplished by lower limbs, and hence, analyzing their structure and movement characteristics is necessary.
Walking is achieved by coordination between the pelvis, hip, knee, and ankle. Their ranges of motion (ROM) are illustrated in Table 1 . The pelvis is located between the trunk and thighs. As a ball-and-socket joint, the hip is formed by the head of the femur and pelvic bone and it allows simultaneous movement between the thighs and pelvis [ 23 ]. It allows sagittal flexion/extension, frontal abduction/adduction, and transverse external/internal rotation [ 24 ]. Knee is a joint complex containing tibiofemoral and patellofemoral joints. Their movement occurs in two planes, allowing sagittal flexion/extension and transverse internal/external rotation [ 23 ]. During walking, knees perform important functions. In the swing phase, knees shorten leg length by flexion [ 25 ]. In the stance phase, they remain flexed to absorb shock and transmit forces through legs. The ankle/foot is a complex structure that absorbs this shock and imparts thrust to the body. Ankle movements mainly occur about talocrural and subtalar joints [ 24 ]. The talocrural joint is located between the talus, distal tibia, and fibula to provide plantar/dorsiflexion as a hinge joint, in which the surface of one bone is spool-like and the surface of the other bone is concave. The subtalar joint is located between the calcaneus and talus and allows eversion/inversion and internal/external rotation. The basis for the mechanical design of rehabilitation exoskeleton robots is provided by an analysis of the lower limb structure.
2.2 Analysis on Human Gait
The normal gait pattern of patients cannot be measured directly because of their impaired motor functions. Therefore, it is necessary to conduct rehabilitation training and evaluate normal gait data, which is significant in clinical applications. Patients with hemiplegia or physical disabilities often follow a predetermined trajectory in their rehabilitation. These predetermined trajectories can be obtained from normal gait data collection. Through gait analysis, the relevant characteristics of human gait can be revealed. Step length, width, and speed are all used for human walking gait characterization. Thus, human body movement parameters and structural parameters have a significant influence on human gait characteristics.
Human gait is affected by walking speed [ 26 , 27 ] as confirmed by an analysis of gait parameters [ 28 ] and joint angles [ 29 ], as confirmed by recording and analyze the gait data with different walking speeds on a walkway [ 30 ] or treadmill [ 31 ]. Because most rehabilitation robots use a body support system during rehabilitation training, analyzing human gait on a treadmill is necessary. Additionally, studies have revealed that body height, as a structural parameter, has limited effect on the human gait as compared to walking speed. This was proved by comparing the difference in the correlation between regression models when using speed and normalized speed (normalized to leg length) [ 30 , 32 ] or by using step-wise regression in regression models by including body height [ 31 ] as a parameter. Further, these studies focused on the effect of these parameters on joint angles. Studies have also outlined the relationship between gait parameters and the body mass index (BMI) [ 26 , 33 ]. These studies provide a foundation for control over lower limb rehabilitation exoskeleton robots.
3 Mechanics of Lower Limb Rehabilitation Exoskeleton Robots
Lower limb rehabilitation exoskeleton robots need a mechanical structure matching human lower limbs to realize force and energy transmission through the wearable connection. These can be achieved by designing the appropriate robot mechanism and actuation. An overview of the mechanics involved is presented in Table 2 .
3.1 Anatomy of Human Upper Limbs
The mechanism of lower limb rehabilitation exoskeleton robots should realize movement matching with human lower limbs. The mechanism design of the Berkeley exoskeleton system (BLEEX) laid a foundation for subsequently developed robots. To ensure safety and avoid collisions maximally with users, BLEEX is almost anthropomorphic but does not include all the degrees of freedom available for human legs (Figure 3 ). Additionally, BLEEX joints are purely rotary joints and hence, are different from human joints [ 34 ]. The hip is simplified as three rotatory joints to achieve flexion/extension, abduction/extension, and internal/external rotation. The knee is simplified as a rotating joint to achieve pure sagittal rotation. The ankle is simplified into three rotation joints to achieve plantar/dorsiflexion, eversion/inversion and internal/external rotation. The configuration of the current lower limb exoskeleton robots, such as ALEX [ 35 ], Lokomat [ 4 , 36 ], LOPES [ 37 , 38 ], Rewalk [ 9 ], Rex [ 39 ] and HAL [ 40 ], is mainly based on BLEEX.
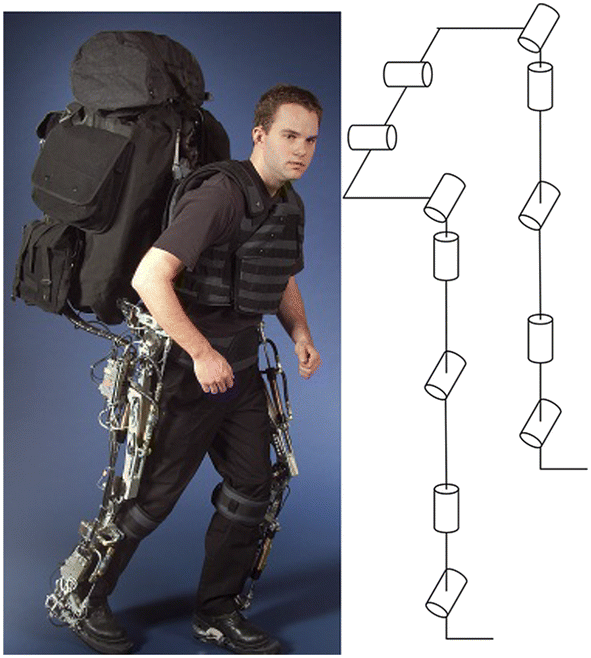
Biomechanical design of BLEEX [ 41 ]
Due to the existence of the BWS system and the fact that the robot body is often connected to a fixed platform, an important feature of treadmill-based exoskeleton robots is that the patient does not need to carry the entire weight of the robot, which complicates the mechanical structure of the robot. At the same time, rehabilitation training on a treadmill requires less room, but there is a difference between gait on the treadmill and natural gait [ 42 , 43 ], which is an important aspect in clinical evaluations [ 44 , 45 , 46 , 47 ]. Additional mechanisms were designed for pelvic movement. Pelvis motion is also being integrated into new robotic devices, such as KineAssist [ 48 , 49 , 50 ]. ALEX III can actively control motions of the pelvis [ 45 , 51 ]. The new version of Lokomat includes an optional FreeD module to improve therapy by allowing for pelvic lateral translation and transverse rotation, as shown in Figure 4 .
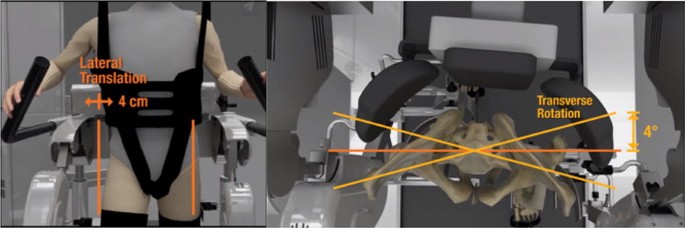
The optional FreeD module
Such simplified design means that there is a motion mismatch between the robot and human, which is manifested in the mismatch between the joint centers of the robot and human. Designing an innovative mechanism can offer a solution to this problem. For hip joints, a parallel structure is adopted to realize three rotational motions and automatic centering with the human hip [ 52 , 53 , 54 ] as shown in Figure 5 . When a 3-UPS parallel mechanism is mounted on the human waist and thigh, the thigh of the human and the mechanism are connected as a whole, which can be considered as a 3-UPS/1-S parallel mechanism [ 52 ] (Figure 5 (a)). A novel metamorphic parallel mechanism was applied for lower limb rehabilitation using two configurations, 3-UPS/S and 2-RPS/UPS/S, by taking into account the human hip joint to satisfy different demands of patients at different phases of rehabilitation therapy [ 53 ] (Figure 5 (b)). An asymmetric fully constrained parallel mechanism prototype is designed for hip joint assistance and rehabilitation and employs pantographs as three-rotation constrained legs instead of using three serial rotation joints-leg to avoid disadvantages such as singularity, uncertainty, or interference with other legs [ 54 ] (Figure 5 (c)).
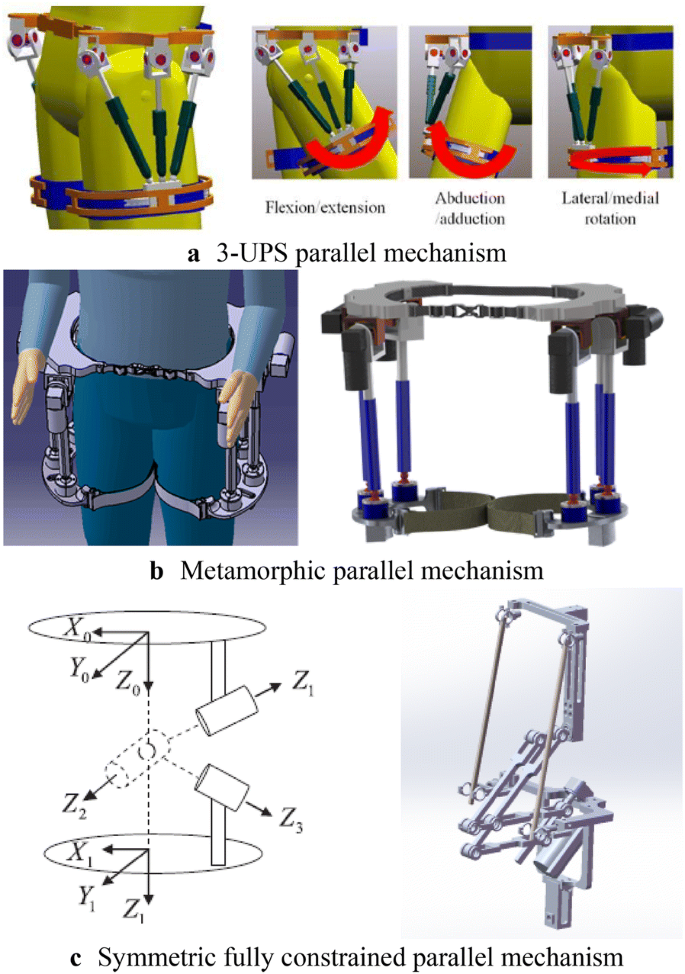
Parallel mechanisms for hip joint assistance
The knee joint is treated as a rotating joint in the simplification process and only the flexion and extension motion are considered. In fact, knee motion is relatively complex and hence different mechanisms have been designed to solve this problem, as shown in Figure 6 . For the knee joint, an adaptive knee was used and it could effectively eliminate negative effects on human knees [ 55 ] (Figure 6 (a)). Based on the knee joint complex, axial translational motion was coupled with rotational motion and a serial-parallel hybrid mechanism was designed for lower limb rehabilitation [ 17 ] (Figure 6 (b)).
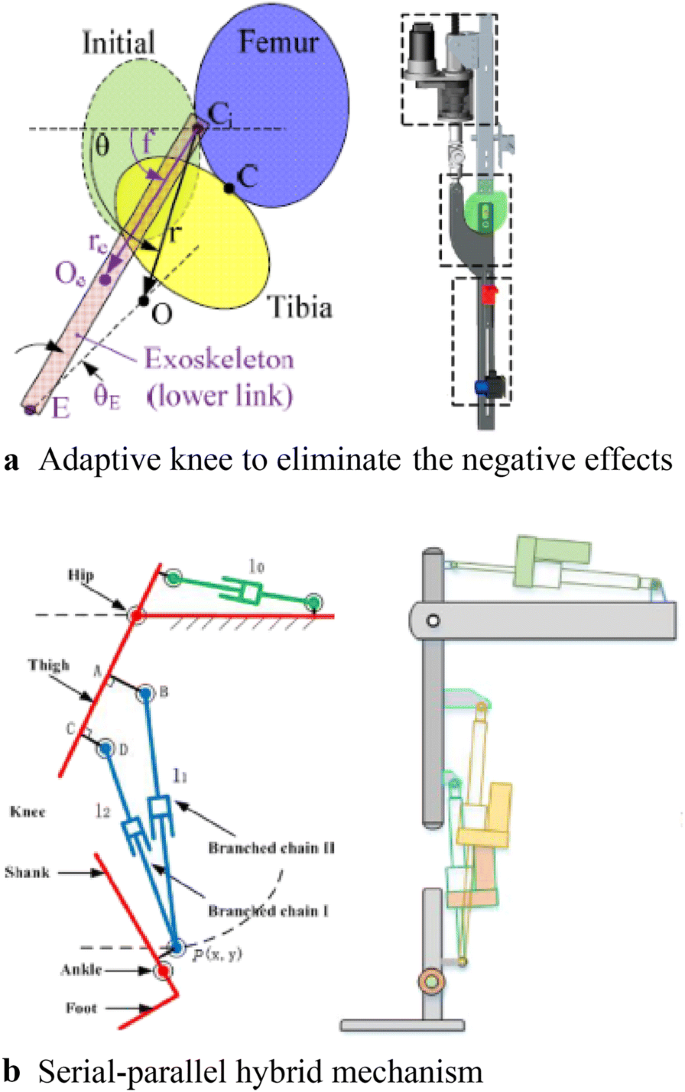
Novel mechanisms for knee joints
The human body is a coupled structure based on bone-muscle tissue structure and characteristics. According to this principle, some scholars have suggested a new kind of structure for lower limb rehabilitation robots. There is no rigid support for the mechanical structure but it includes a soft body and software structure using cables to provide power; this reduces response to muscle contractions and energy consumption of the body. This design for coupled wearable robots is expected to pave a new research direction [ 56 ] (Figure 7 ).
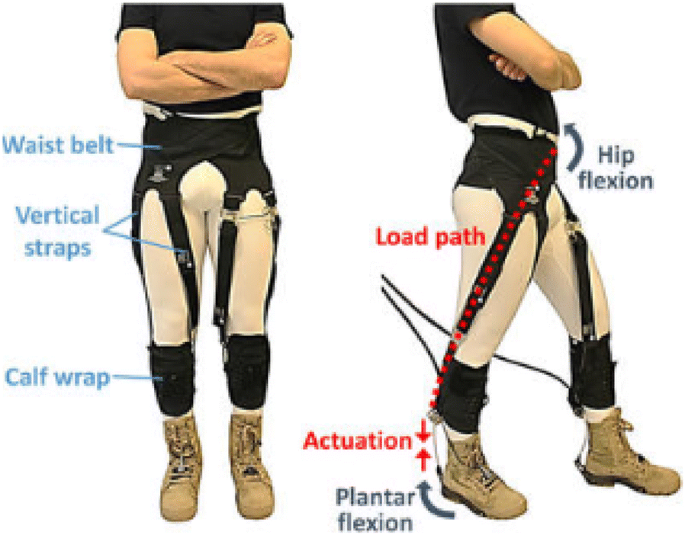
Soft exosuit
3.2 Actuation Design
Most lower limb exoskeleton rehabilitation robots are driven by electric motors. In eLEGS, only sagittal flexion/extension for the hip and knee are actuated using motors while the ankle remains passive [ 57 ]. The hip and knee of Lokomat are actuated by motors with linear ball screws [ 58 ].
A remarkable feature of the above method is that the driver is directly placed on the robot body, which increases its mass and complexity. Therefore, using cable-driven motors can reduce the mass of the exoskeleton robot itself, because the motor and driver are placed on the platform instead of directly on the exoskeleton [ 59 ].
Lower limb rehabilitation exoskeletons are mainly driven by rigid transmission without compliance. This causes a large vibration impact, difficulty in directly controlling the force, and leads to a complicated robot system. Therefore, a series elastic drive (SEA) was designed to achieve force control and enhance drive flexibility in RoboKnee [ 60 ] (Figure 8 (a)). SEA with a combination of cable-driven actuation was applied in LOPES [ 59 ] (Figure 8 (b)). A variable stiffness elastic actuator was designed for lower limb exoskeletons by adjusting the stiffness of the elastic elements driven by series elasticity [ 61 ] (Figure 8 (b)).
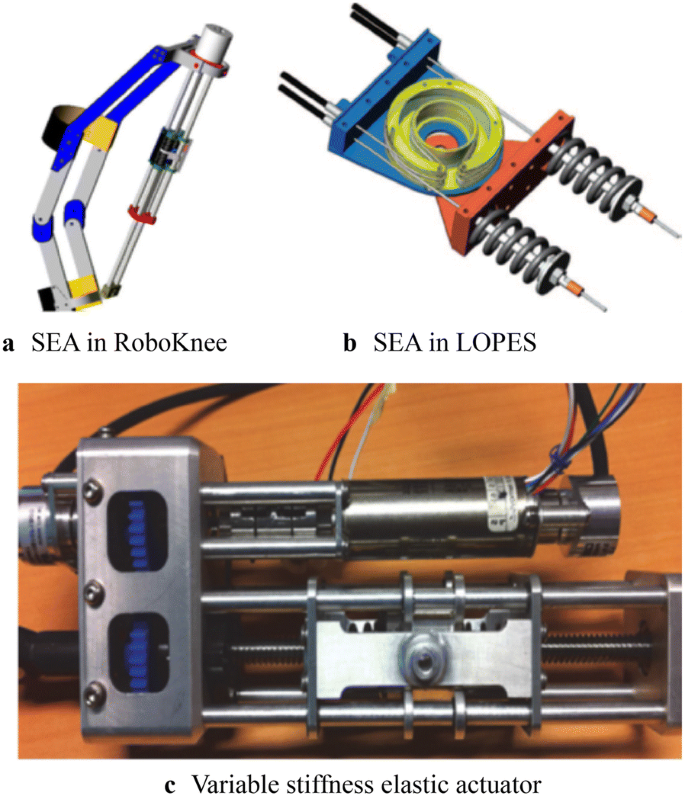
A series elastic actuator
4 Control of Lower Limb Rehabilitation Robots
4.1 trajectory planning.
The main purpose of rehabilitation training is to restore the lower limb motor functions of disabled patients to normal levels [ 62 ]; therefore, in the process of rehabilitation training, a normal gait pattern is required as a reference input to the control system, as a training goal, and as a rehabilitation evaluation standard. For patients with hemiplegia or physical disabilities, a predetermined trajectory is often used. These predetermined trajectories can be obtained from data on normal gait. However, due to the limited amount of data, it is difficult to match the obtained motion data with different human motion characteristics, and hence, a parameterized motion pattern generation method was proposed to predict data not present in the test sample.
The trajectories of Lokomat can be adjusted to a specific patient and step length [ 58 ]. In LOPES, trajectories are generated by a method based on regression analysis to reconstruct the body height and speed-dependent trajectories [ 31 ]; further, “complementary limb motion estimation” may be used to generate reference motion using the motion of healthy limbs [ 63 ]. When the collected number of samples is large enough, statistical learning techniques, such as the radial basis function neural networks (RBFs) [ 64 ] and multi-layer perceptron neural network (MLPNN) [ 65 ], are often used for motion prediction.
There is another type of gait planning that does not rely on specific data; however, it depends on the given gait parameters, such as step length and swing duration to generate gait patterns. The model predictive control (MPC) is used for online trajectory generation based on gait parameters [ 66 ].
4.2 Control System
A hierarchical control strategy is used in most lower limb rehabilitation exoskeleton robots. Generally, the control system is divided into two levels. The upper level is the decision-making layer, which realizes control decisions and trajectory generation, and the lower level is the servo layer, which realizes servo control of the drive system [ 65 ]. As for overground rehabilitation exoskeletons, they are divided into three layers due to the large number of movements involved (Figure 9 ). In particular, a human–robot interaction layer is added to adapt to the training needs of a variety of movements [ 6 ]. An overview of the control method of lower limb exoskeletons is shown in Table 3 .
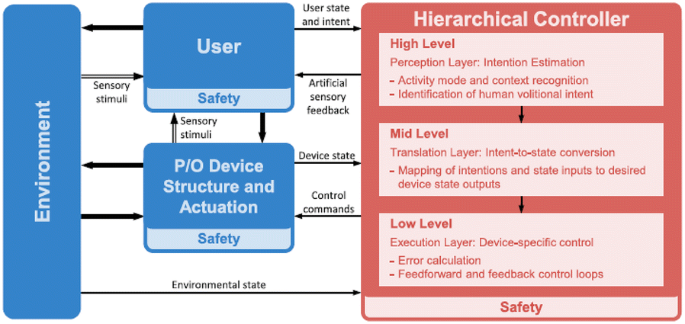
Generalized control framework for lower limbs [ 67 ]
In early rehabilitation stages, the lower limbs of patients are dragged for continuous passive motion (CPM) for passive training, which can effectively keep joints flexible for a long time. Correspondingly, position control ensures that the robot can accurately follow the desired position. In this case, movement information of the robot is measured by sensors, such as linear and rotary potentiometers [ 40 , 68 , 69 ], inertia measurement units (IMUs) [ 70 , 71 ], torque sensors to measure torque [ 70 , 71 ], and foot pressure sensors to measure the ground reaction to detect gait events [ 72 , 73 ]. Control methods widely used in servo control have also been used in lower limb rehabilitation exoskeleton robots. For example, proportional derivative control (PD control) [ 68 ], computed torque control [ 74 ], fuzzy control [ 74 ], robust variable structure control [ 75 ], fuzzy proportional integral derivative control (PID contro), and sliding mode variable structure control [ 76 ] have been used for lower limb exoskeleton robots. Such position control is actually a type of tracking control. Force-position hybrid control was also used to adjust the output force exerted on the patient. This was the first impedance or admittance control strategy ever developed. It has been applied in the Lokomat to guide patients’ legs and supply hip and knee joint torque [ 4 ]. In LOPES, the robotic support was controlled using a virtual model controller (VMC) [ 69 , 77 ].
All the control systems described above are passive in nature because the wearer is not considered in the system. By increasing active participation, the dependence of patients on robot assistance can be reduced by improving the effect of rehabilitation training. To achieve this effect, it is important to integrate people into the control system. As mentioned earlier, sensors have been used to measure human movement information and human–robot interaction information to control a robot. In ALEX a force field control is used to guide patients’ legs [ 45 , 70 ].
Another way is to measure human biological signals such as electromyography (EMG) and electroencephalogram (EEG) signals, to perceive body movement. Currently, EMG signals are being used in robots such as HAL [ 78 ]. The EMG signal to be detected is used as a trigger switch to judge the timing of assistance provided by the robot [ 78 , 79 ]. As continuous control, a proportional myoelectric control is used in the robotic ankle exoskeleton [ 80 , 81 ]. However, EMG-based robot-assisted rehabilitation is only suitable for patients who are able to produce a sufficiently high level of muscle activity. Brain-machine interfaces to restore mobility in severely paralyzed patients [ 82 ] have been applied in many lower limb rehabilitation exoskeletons [ 39 , 83 , 84 , 85 ]. However, this research is still in the early stages. All the systems described above rely on the presence of sensors and control the signals measured by sensors. Recently, robots not based on sensors have been developed. In this case, an admittance shaping controller is used [ 86 ].
5 Conclusions and Outlook
5.1 conclusions.
Lower limb rehabilitation exoskeleton robots integrate sensing, control, and other technologies and exhibit characteristics of bionics, robotics, information and control science, medicine, and other interdisciplinary fields and therefore, have become a major research hotspot. In recent years, remarkable progress has been achieved in mechanical design and control system design, based on which, several products have been commercialized. However, there is still a large research gap with respect to human–robot integration. The wearer (patient) should organically combine with the robot to form a whole. Only when true integration of the human body and robot is realized can rehabilitation training be truly effective.
5.2 Outlook
The problem of human–robot integration is a current research hot spot. The National Natural Science Foundation of China (NSFC) has launched the Tri-Co Robot (i.e., the Coexisting-Cooperative-Cognitive Robot), a major research program in 2017, with research themes of robot structure design and control, multi-mode dynamic perception, and natural interaction [ 87 ]. Human–robot integration is a key issue in the design and control of lower limb rehabilitation exoskeleton robots. In summary, such integration should include three components, namely structure, movement, and response.
Humanoid structures and flexible drive systems should be designed to achieve structural integration between robots and patients. Currently, a simplified human movement model and a rigid structural design are adopted, causing movement mismatch between a robot and human and affecting wearability and rehabilitation training. Therefore, SEA is being used to increase the flexibility of the local structure. The addition of flexibility inevitably leads to structural complexity and difficulty in control. An ideal robot structure is a rigid-flexible-soft hybrid structure. However, rigid-soft-soft coupling configurations should be designed to effectively transfer energy from a robot to a human. At the same time, modular designs for exoskeleton mechanism should be explored. In fact, many active orthosis devices can also be referred to as modular single-joint exoskeletons.
Customized and personalized gait patterns should be generated to achieve motion integration between robots and patients. A normal movement mode is often required as a reference and input to the control system as the expected robot movement, training target, and evaluation standard. For patients with hemiplegia or other physical disabilities, a predetermined trajectory is often used in rehabilitation. These predetermined trajectories can be obtained from normal gait data collection. However, due to the limited amount of data collected, it is difficult for the obtained motion data to match different motion characteristics of the human body, and hence, a parameterized motion pattern generation method has been proposed. However, the current planning and gait generation methods based on the biped robot control theory and human natural gait cannot achieve a perfect match; gait generation is mainly focused on the sagittal plane and does not account for human three-dimensional gait characteristics. The mechanism of how human gait is affected by motion and structural parameters is not yet fully understood, and hence, it is difficult to realize a perfect integration of motion levels.
Multimodal information fusion should be used to achieve motion integration between humans and machines. Current research on the design of sensing systems indicates that it is not enough to measure a robot’s movement information; instead, people also should be included in the system, not only to measure human body movement information and biological signals but also to collect interaction information between a human and the force exerted by the robot. However, it can be imagined that more information is not always better as redundant information increases the complexity of a system and affects its practical application. In the process of rehabilitation training, lower limb rehabilitation exoskeleton robots need to participate in effective dynamic interaction with the patient. Such rehabilitation training can effectively improve the level of active participation of the patient and significantly enhance the rehabilitation effect. However, the evaluation method of the robot itself lacks a set of system indicators for the adaptability and degree of matching with the human body. Hence, it is necessary to study multimodal information to realize effective human–robot integration.
G L R O Collaborators. Global, regional, and country-specific lifetime risks of stroke, 1990 and 2016 . New England Journal of Medicine , 2018, 379(25): 2429-2437.
Article Google Scholar
S K Banala, S H Kim, S K Agrawal, et al. Robot assisted gait training with active leg exoskeleton (ALEX) . IEEE Transactions on Neural Systems and Rehabilitation Engineering , 2009, 17(1): 2-8.
G Chen, C K Chan, Z Guo, et al. A review of lower extremity assistive robotic exoskeletons in rehabilitation therapy . Critical Reviews in Biomedical Engineering , 2013, 41(4-5): https://doi.org/10.1615/critrevbiomedeng.2014010453 .
M Bernhardt, M Frey, G Colombo, et al. Hybrid force-position control yields cooperative behaviour of the rehabilitation robot LOKOMAT . International Conference on Rehabilitation Robotics , 2005: 536-539.
Van Asseldonk E H, Van Der Kooij H. Robot-aided gait training with LOPES . Neurorehabilitation Technology, Springer, 2012.
Book Google Scholar
S T Alan. Control and trajectory generation of a wearable mobility exoskeleton for spinal cord injury patients . University of California, Berkeley, 2011.
Google Scholar
S K Ann. Development of a human machine interface for a wearable exoskeleton for users with spinal . University of California, Berkeley, 2011.
R J Farris, H A Quintero, M Goldfarb. Preliminary evaluation of a powered lower limb orthosis to aid walking in paraplegic individuals . IEEE Transactions on Neural Systems and Rehabilitation Engineering , 2011, 19(6): 652-659.
G Zeilig, H Weingarden, M Zwecker, et al. Safety and tolerance of the ReWalk™ exoskeleton suit for ambulation by people with complete spinal cord injury: A pilot study . The Journal of Spinal Cord Medicine , 2012, 35(2): 96-101.
J Gancet, M Ilzkovitz, E Motard, et al. MINDWALKER: going one step further with assistive lower limbs exoskeleton for SCI condition subjects . IEEE RAS & EMBS International Conference on Biomedical Robotics and Biomechatronics , Rome, Italy, June 24-27, 2012: 1794-1800.
J Gancet, M Ilzkovitz, G Cheron, et al. MINDWALKER: a brain controlled lower limbs exoskeleton for rehabilitation. Potential applications to space . 11th Symposium on Advanced Space Technologies in Robotics and Automation , Noordwijk, Netherlands, April 12-15, 2011: 12-14.
H Satoh, T Kawabata, Y Sankai. Bathing care assistance with robot suit HAL . IEEE International Conference on Robotics and Biomimetics , Guilin, China, December 18-22, 2009: 498-503.
H Yan, C Yang. Design and validation of a lower limb exoskeleton employing the recumbent cycling modality for post-stroke rehabilitation . Proceedings of the Institution of Mechanical Engineers, Part C: Journal of Mechanical Engineering Science , 2014, 228(18): 3517-3525.
Y Miao, F Gao, P Dan. Mechanical design of a hybrid leg exoskeleton to augment load-carrying for walking . International Journal of Advanced Robotic Systems , 2013, 10: https://doi.org/10.5772/57238 .
P Pei, Z Pei, Z Shi, et al. Sensorless control for joint drive unit of lower extremity exoskeleton with cascade feedback observer . Mathematical Problems in Engineering , 2018, 3029514: 1-11, https://doi.org/10.1155/2018/3029514 .
Article MathSciNet Google Scholar
D Liu, Z Tang, Z Pei. Variable structure compensation PID control of asymmetrical hydraulic cylinder trajectory tracking . Mathematical Problems in Engineering , 2015, 890704: 1-9. https://doi.org/10.1155/2015/890704 .
Article MathSciNet MATH Google Scholar
M Lyu, W Chen, X Ding, et al. Design of a biologically inspired lower limb exoskeleton for human gait rehabilitation. Review of Scientific Instruments , 2016, 87(10): 104301.
R Huang, H Cheng, H Guo, et al. Hierarchical learning control with physical human-exoskeleton interaction . Information Sciences , 2018, 432: 584-595.
R Huang, H Cheng, Y Chen, et al. Optimisation of reference gait trajectory of a lower limb exoskeleton . International Journal of Social Robotics , 2016, 8(2): 223-235.
D Wang, K Lee, J Ji. A passive gait-based weight-support lower extremity exoskeleton with compliant joints . IEEE Transactions on Robotics , 2016, 32(4): 933-942.
X Wu, D Liu, M Liu, et al. Individualized gait pattern generation for sharing lower limb exoskeleton robot . IEEE Transactions on Automation Science and Engineering , 2018, 15(4): 1459-1470.
C Chen, X Wu, D Liu, et al. Design and voluntary motion intention estimation of a novel wearable full-body flexible exoskeleton robot . Mobile Information Systems , 2017: https://doi.org/10.1155/2017/8682168 .
D A Neumann. Kinesiology of the musculoskeletal system: foundations for rehabilitation . Elsevier Health Sciences, 2013.
J E Muscolino. Kinesiology: The skeletal system and muscle function . Elsevier Medicine, 2011.
A D Kuo. The six determinants of gait and the inverted pendulum analogy: A dynamic walking perspective . Human Movement Science , 2007, 26(4): 617-656.
E F Chehab, T P Andriacchi, J Favre. Speed, age, sex, and body mass index provide a rigorous basis for comparing the kinematic and kinetic profiles of the lower extremity during walking . Journal of Biomechanics , 2017, 58: 11-20.
J W Kwon, S M Son, N K Lee. Changes of kinematic parameters of lower extremities with gait speed: a 3D motion analysis study . Journal of Physical Therapy Science , 2015, 27(2): 477-479.
S Al-Obaidi, J C Wall, A Al-Yaqoub, et al. Basic gait parameters: A comparison of reference data for normal subjects 20 to 29 years of age from Kuwait and Scandinavia . Journal of Rehabilitation Research and Development , 2003, 40(4): 361-366.
T J Cunningham. Three-dimensional quantitative analysis of the trajectory of the foot while running. University of Kentucky, 2007.
J L Lelas, G J Merriman, P O Riley, et al. Predicting peak kinematic and kinetic parameters from gait speed . Gait & Posture , 2003, 17(2): 106-112.
B Koopman, E Van Asseldonk, H Van der Kooij. Speed-dependent reference joint trajectory generation for robotic gait support . Journal of Biomechanics , 2014, 47(6): 1447-1458.
M Hanlon, R Anderson. Prediction methods to account for the effect of gait speed on lower limb angular kinematics . Gait & Posture , 2006, 24(3): 280-287.
S Ko, S Stenholm, L Ferrucci. Characteristic gait patterns in older adults with obesity - results from the baltimore longitudinal study of aging . Journal of Biomechanics , 2010, 43(6): 1104-1110.
A B Zoss, H Kazerooni, et al. Biomechanical design of the Berkeley lower extremity exoskeleton (BLEEX) . IEEE/ASME Transactions on Mechatronics , 2006, 1(2): 128-138.
S K Banala, S K Agrawal, S H Kim, et al. Novel gait adaptation and neuromotor training results using an active leg exoskeleton. Mechatronics , 2010, 15(2): 216-225.
R Riener, L Lunenburger, S Jezernik, et al. Patient-cooperative strategies for robot-aided treadmill training: first experimental results . IEEE Transactions on Neural Systems and Rehabilitation Engineering , 2005, 13(3): 380-394.
J Meuleman, E Van Asseldonk, G Van Oort, et al. LOPES II—design and evaluation of an admittance controlled gait training robot with shadow-leg approach . IEEE Transactions on Neural Systems and Rehabilitation Engineering , 2016, 24(3): 352-363.
R Ekkelenkamp, J Veneman, H Van der Kooij. LOPES: Selective control of gait functions during the gait rehabilitation of CVA patients . International Conference on Rehabilitation Robotics , Chicago, IL, USA, June 28 - July 1, 2005: 361-364.
N Kwak, K Muller, S Lee. Toward exoskeleton control based on steady state visual evoked potentials . International Winter Workshop on Brain-Computer Interface (BCI) , Gangwon Province, Korea (South), February 17-19, 2014: 1-2.
A Tsukahara, Y Hasegawa, K Eguchi, et al. Restoration of gait for spinal cord injury patients using hal with intention estimator for preferable swing speed . IEEE Transactions on Neural Systems and Rehabilitation Engineering , 2015, 23(2): 308-318.
M W Whittle. Gait analysis: an introduction . Butterworth-Heinemann, 2014.
J H Hollman, M K Watkins, A C Imhoff, et al. A comparison of variability in spatiotemporal gait parameters between treadmill and overground walking conditions . Gait & Posture , 2016, 43: 204-209.
S J Lee, J Hidler. Biomechanics of overground vs. treadmill walking in healthy individuals . Journal of Applied Physiology , 2008, 104(3): 747-755.
J Mehrholz, L A Harvey, S Thomas, et al. Is body-weight-supported treadmill training or robotic-assisted gait training superior to overground gait training and other forms of physiotherapy in people with spinal cord injury? A systematic review. Spinal Cord , 2017, 55(8): 722-729.
S Srivastava, P Kao, S H Kim, et al. Assist-as-needed robot-aided gait training improves walking function in individuals following stroke . IEEE Transactions on Neural Systems and Rehabilitation Engineering , 2015, 23(6): 956-963.
T Senthilvelkumar, H Magimairaj, J Fletcher, et al. Comparison of body weight-supported treadmill training versus body weight-supported overground training in people with incomplete tetraplegia: a pilot randomized trial. Clinical Rehabilitation , 2015, 29(1): 42-49.
M Alcobendasmaestro, A Esclarínruz, R M Casadolópez, et al. Lokomat robotic-assisted versus overground training within 3 to 6 months of incomplete spinal cord lesion: randomized controlled trial. Neurorehabilitation & Neural Repair , 2012, 26(9): 1058.
S A Graham, C P Hurt, D A Brown. Minimizing postural demands of walking while still emphasizing locomotor force generation for nonimpaired individuals. IEEE Transactions on Neural Systems and Rehabilitation Engineering: A Publication of the IEEE Engineering in Medicine and Biology Society , 2018, 26(5): 1003-1010.
V C Dionisio, C P Hurt, D A Brown. Effect of forward-directed aiding force on gait mechanics in healthy young adults while walking faster . Gait & Posture , 2018, 64: 12-17.
J Patton, D A Brown, M Peshkin, et al. KineAssist: design and development of a robotic overground gait and balance therapy device . Topics in Stroke Rehabilitation , 2008, 15(2): 131-139.
D Zanotto, P Stegall, S K Agrawal. ALEX III: A novel robotic platform with 12 DOFs for human gait training . IEEE International Conference on Robotics and Automation , Karlsruhe, Germany, 2013, 2013: 3914-3919.
Y Yu, W Liang. Manipulability inclusive principle for hip joint assistive mechanism design optimization. The International Journal of Advanced Manufacturing Technology , 2014, 70(5-8): 929-945.
W Zhang, S Zhang, M Ceccarelli, et al. Design and kinematic analysis of a novel metamorphic mechanism for lower limb rehabilitation . Springer, Cham, 2016.
W Zhang, W Zhang, D Shi, et al. Design of hip joint assistant asymmetric parallel mechanism and optimization of singularity-free workspace . Mechanism and Machine Theory , 2018, 122: 389-403.
D Wang, K Lee, J Guo, et al. Adaptive knee joint exoskeleton based on biological geometries. IEEE/ASME Transactions on Mechatronics , 2014, 19(4): 1268-1278.
B T Quinlivan, S Lee, P Malcolm, et al. Assistance magnitude versus metabolic cost reductions for a tethered multiarticular soft exosuit . Science Robotics , 2017, 2(2): 1-10.
S A Kolakowsky-Hayner, J Crew, S Moran, et al. Safety and feasibility of using the EksoTM bionic exoskeleton to aid ambulation after spinal cord injury . Journal of Spine , 2013, 4: 1-8.
J Hidler, W Wisman, N Neckel. Kinematic trajectories while walking within the Lokomat robotic gait-orthosis . Clinical Biomechanics , 2008, 23(10): 1251-1259.
H Vallery, J Veneman, E Van Asseldonk, et al. Compliant actuation of rehabilitation robots . IEEE Robotics & Automation Magazine , 2008, 15(3): 60-69.
J E Pratt, B T Krupp, C J Morse, et al. The RoboKnee: an exoskeleton for enhancing strength and endurance during walking . Proceedings of IEEE International Conference on Robotics and Automation , New Orleans, LA, USA, April 26 - May 1, 2004: 2430-2435.
H Yu, S Huang, G Chen, et al. Human–robot interaction control of rehabilitation robots with series elastic actuators . Robotics, IEEE Transactions on ., 2015, 31(5): 1089-1100.
E Sariyildiz, G Chen, H Yu. An acceleration-based robust motion controller design for a novel series elastic actuator. IEEE Transactions on Industrial Electronics , 2016, 63(3): 1900-1910.
H Vallery, R Burgkart, C Hartmann, et al. Complementary limb motion estimation for the control of active knee prostheses . Biomedizinische Technik/Biomedical Engineering , 2011, 56(1): 45-51.
K Seo, Y Park, S Yun, et al. Gait pattern generation for robotic gait rehabilitation system on treadmill . 14th International Conference on Control, Automation and Systems , KINTEX, Gyeonggi-do, Korea, October 22-25, 2014: 1090-1094.
H B Lim, T P Luu, K H Hoon, et al. Natural gait parameters prediction for gait rehabilitation via artificial neural network . IEEE/RSJ International Conference on Intelligent Robots and Systems , Taipei, Taiwan, China, October 18-22, 2010: 5398-5403.
L Wang, E H van Asseldonk, H van der Kooij. Model predictive control-based gait pattern generation for wearable exoskeletons . IEEE International Conference on Rehabilitation Robotics , ETH Zurich Science City, Switzerland, June 29 - July 1, 2011: 1-6.
M R Tucker, J Olivier, A Pagel, et al. Control strategies for active lower extremity prosthetics and orthotics: a review . Journal of Neuroengineering and Rehabilitation , 2015, 12(1): 1-30.
S Maggioni, L Lünenburger, R Riener, et al. Robot-aided assessment of walking function based on an adaptive algorithm . IEEE International Conference on Rehabilitation Robotics , Singapore, Aug. 11-14, 2015: 804-809.
B Koopman, E H van Asseldonk, H van der Kooij. Selective control of gait subtasks in robotic gait training: foot clearance support in stroke survivors with a powered exoskeleton . Journal of Neuroengineering and Rehabilitation , 2013, 10(3): 1-21.
X Jin, X Cui, S K Agrawal. Design of a cable-driven active leg exoskeleton (C-alex) and gait training experiments with human subjects . IEEE International Conference on Robotics and Automation , Seattle, Washington, USA, May 26-30, 2015: 5578-5583.
O Harib, A Hereid, A Agrawal, et al. Feedback control of an exoskeleton for paraplegics: toward robustly stable hands-free dynamic walking . IEEE Control Systems Magazine , 2018, 38(6): 61-87.
T Lenzi, M C Carrozza, S K Agrawal. Powered hip exoskeletons can reduce the user’s hip and ankle muscle activations during walking . IEEE Transactions on Neural Systems and Rehabilitation Engineering , 2013, 21(6): 938-948.
D B Fineberg, P Asselin, N Y Harel, et al. Vertical ground reaction force-based analysis of powered exoskeleton-assisted walking in persons with motor-complete paraplegia . The Journal of Spinal Cord Medicine , 2013, 36(4): 313-321.
P K Jamwal, S Q Xie, S Hussain, et al. An adaptive wearable parallel robot for the treatment of ankle injuries . IEEE/ASME Transactions on Mechatronics , 2014, 19(1): 64-75.
S Hussain, S Q Xie, P K Jamwal. Robust nonlinear control of an intrinsically compliant robotic gait training orthosis . IEEE Transactions on Systems, Man, and Cybernetics: Systems , 2013, 43(3): 655-665.
Z Tang, D Shi, D Liu, et al. Electro-hydraulic servo system for Human Lower-limb Exoskeleton based on sliding mode variable structure control . Information and Automation (ICIA), 2013 IEEE International Conference on , 2013: 559-563.
J Pratt, C Chew, A Torres, et al. Virtual model control: An intuitive approach for bipedal locomotion . The International Journal of Robotics Research , 2001, 20(2): 129-143.
T Lenzi, S M De Rossi, N Vitiello, et al. Intention-based EMG control for powered exoskeletons . IEEE Trans. Biomed Eng ., 2012, 59(8): 2180-2190.
H He, K Kiguchi. A study on EMG-based control of exoskeleton robots for human lower-limb motion assist . Information Technology Applications in Biomedicine, 2007. ITAB 2007. 6th International Special Topic Conference on , 2007: 292-295.
P Kao, C L Lewis, D P Ferris. Invariant ankle moment patterns when walking with and without a robotic ankle exoskeleton . Journal of Biomechanics , 2010, 43(2): 203-209.
D P Ferris, K E Gordon, G S Sawicki, et al. An improved powered ankle–foot orthosis using proportional myoelectric control . Gait & Posture , 2006, 23(4): 425-428.
A R Donati, S Shokur, E Morya, et al. Long-term training with a brain-machine interface-based gait protocol induces partial neurological recovery in paraplegic patients . Scientific Reports , 2016, 6: 30383.
D Liu, W Chen, Z Pei, et al. A brain-controlled lower-limb exoskeleton for human gait training . Review of Scientific Instruments , 2017, 88(10): 104302.
N Kwak, K Müller, S Lee. A lower limb exoskeleton control system based on steady state visual evoked potentials . Journal of Neural Engineering , 2015, 12(5): 56009.
R Xu, N Jiang, N Mrachacz-Kersting, et al. A closed-loop brain-computer interface triggering an active ankle-foot orthosis for inducing cortical neural plasticity. IEEE Transactions on Biomedical Engineering , 2014, 61(7): 2092-2101.
G Aguirre-Ollinger, U Nagarajan, A Goswami. An admittance shaping controller for exoskeleton assistance of the lower extremities . Autonomous Robots , 2016, 40(4): 701-728.
H Ding, X Yang, N Zheng, et al. Tri-Co Robot: a Chinese robotic research initiative for enhanced robot interaction capabilities. National Science Review , 2017, 5(6): 799-801.
Download references
Authors’ Contributions
XD was in charge of the whole trial; DS wrote the manuscript; WXZ and WZ assisted with structure and language of the manuscript. All authors read and approved the final manuscript.
Authors’ Information
Di Shi, born in 1988, is currently a PhD candidate at Robotics Institute, Beihang University, China . He received his bachelor degree from Wuhan University of Technology, China , in 2011. And he received his master degree from Beihang University, China , in 2014. His research interests include wearable lower limb rehabilitation robot control.
Wuxiang Zhang, born in 1978, is currently an associate professor at School of Mechanical Engineering and Automation, Beihang University, China . He received his PhD degree from Beihang University, China , in 2009. His research interests include the dynamics of compliant mechanical systems and robots, intelligent device and detection technology.
Wei Zhang, born in 1986, is currently a PhD candidate at Robotics Institute, Beihang University, China . He received his bachelor’s degree from Northwestern Polytechnical University, China , in 2009. His research interests include mechanical design of the wearable robot.
Xilun Ding, born in 1967, is currently a professor and a PhD candidate supervisor at School of Mechanical Engineering and Automation, Beihang University, China . He received his PhD degree from Harbin Institute of Technology, China , in 1997. His research interests include the dynamics of compliant mechanical systems and robots, nonholonomic control of space robots, dynamics and control of aerial robots, and biomimetic robots.
Acknowledgements
The authors sincerely thank Mr. Chong Qi, and Yixin Shao of Beihang University for their critical discussion and reading during manuscript preparation.
Competing Interests
The authors declare that they have no competing interests.
Supported by National Key R&D Program of China (Grant No. 2016YFE0105000) and National Natural Science Foundation of China (Grant No. 91848104).
Author information
Authors and affiliations.
School of Mechanical Engineering and Automation, Beihang University, Beijing, 100191, China
Di Shi, Wuxiang Zhang, Wei Zhang & Xilun Ding
Beijing Advanced Innovation Center for Biomedical Engineering, Beihang University, Beijing, 100191, China
You can also search for this author in PubMed Google Scholar
Corresponding author
Correspondence to Wuxiang Zhang .
Rights and permissions
Open Access This article is distributed under the terms of the Creative Commons Attribution 4.0 International License ( http://creativecommons.org/licenses/by/4.0/ ), which permits unrestricted use, distribution, and reproduction in any medium, provided you give appropriate credit to the original author(s) and the source, provide a link to the Creative Commons license, and indicate if changes were made.
Reprints and permissions
About this article
Cite this article.
Shi, D., Zhang, W., Zhang, W. et al. A Review on Lower Limb Rehabilitation Exoskeleton Robots. Chin. J. Mech. Eng. 32 , 74 (2019). https://doi.org/10.1186/s10033-019-0389-8
Download citation
Received : 05 March 2019
Revised : 30 July 2019
Accepted : 14 August 2019
Published : 30 August 2019
DOI : https://doi.org/10.1186/s10033-019-0389-8
Share this article
Anyone you share the following link with will be able to read this content:
Sorry, a shareable link is not currently available for this article.
Provided by the Springer Nature SharedIt content-sharing initiative
- Control method
- Lower limb exoskeleton
- Mechanical design
- Rehabilitation robot
- Systematic Review
- Open access
- Published: 08 May 2023
Overview of the role of robots in upper limb disabilities rehabilitation: a scoping review
- Khadijeh Moulaei 1 ,
- Kambiz Bahaadinbeigy 1 ,
- Ali Akbar Haghdoostd 2 ,
- Mansour Shahabi Nezhad 3 &
- Abbas Sheikhtaheri 4
Archives of Public Health volume 81 , Article number: 84 ( 2023 ) Cite this article
3961 Accesses
6 Citations
4 Altmetric
Metrics details
Neuromotor rehabilitation and improvement of upper limb functions are necessary to improve the life quality of patients who have experienced injuries or have pathological outcomes. Modern approaches, such as robotic-assisted rehabilitation can help to improve rehabilitation processes and thus improve upper limb functions. Therefore, the aim of this study was to investigate the role of robots in upper limb disability improvement and rehabilitation.
This scoping review was conducted by search in PubMed, Web of Science, Scopus, and IEEE (January 2012– February 2022). Articles related to upper limb rehabilitation robots were selected. The methodological quality of all the included studies will be appraised using the Mixed Methods Appraisal Tool (MMAT). We used an 18-field data extraction form to extract data from articles and extracted the information such as study year, country, type of study, purpose, illness or accident leading to disability, level of disability, assistive technologies, number of participants in the study, sex, age, rehabilitated part of the upper limb using a robot, duration and frequency of treatment, methods of performing rehabilitation exercises, type of evaluation, number of participants in the evaluation process, duration of intervention, study outcomes, and study conclusions. The selection of articles and data extraction was made by three authors based on inclusion and exclusion criteria. Disagreements were resolved through consultation with the fifth author. Inclusion criteria were articles involving upper limb rehabilitation robots, articles about upper limb disability caused by any illness or injury, and articles published in English. Also, articles involving other than upper limb rehabilitation robots, robots related to rehabilitation of diseases other than upper limb, systematic reviews, reviews, and meta-analyses, books, book chapters, letters to the editor, and conference papers were also excluded. Descriptive statistics methods (frequency and percentage) were used to analyses the data.
We finally included 55 relevant articles. Most of the studies were done in Italy (33.82%). Most robots were used to rehabilitate stroke patients (80%). About 60.52% of the studies used games and virtual reality rehabilitate the upper limb disabilities using robots. Among the 14 types of applied evaluation methods, “evaluation and measurement of upper limb function and dexterity” was the most applied evaluation method. “Improvement in musculoskeletal functions”, “no adverse effect on patients”, and “Safe and reliable treatment” were the most cited outcomes, respectively.
Conclusions
Our findings show that robots can improve musculoskeletal functions (musculoskeletal strength, sensation, perception, vibration, muscle coordination, less spasticity, flexibility, and range of motion) and empower people by providing a variety of rehabilitation capabilities.
Peer Review reports
Introduction
Impaired upper limb (UL) functions to restrict the performance of activities of daily living, limit social participation [ 1 ], specifically decrease the independence of affected individuals and reduce patients’ quality of life [ 2 , 3 ]. In upper limb disabilities, patients develop musculoskeletal problems such as paresis, pain, loss of sensation, and spasticity in different parts of the upper limb and so these problems can have manifold consequences in the daily lives of those impacted. These include a decreased capacity to carry out primary self-care tasks and to accomplish life-roles, which can affect emotional, mental, and psychological wellbeing [ 4 ]. Patients with upper limb disabilities need rehabilitation to improve their musculoskeletal status [ 5 ].
Neuromotor rehabilitation and recovery of upper extremity functions are necessary to improve the life quality of individuals who have suffered injuries, disabilities or have pathological outcomes, where it is favorable to raise the development of activities of daily living [ 6 ]. However, the conventional rehabilitations done by one manual-assisted movement training created by physiotherapists suffers from a lot of inherent restrictions, such as heavy labor severity, long-time consumption, lack of repeatability, low patients participation, and their low motivation to perform rehabilitation exercises [ 7 ]. Also, in conventional therapy, the accessibility of therapists, the duration of therapy sessions, and the high cost of rehabilitation tools are all considered parameters that impact on both therapists and patients [ 8 ].
New therapeutic methods have been presented to rehabilitate and improve upper limb function, and such methods are robotic rehabilitation [ 1 ]. Severe repetitions of harmonized motor activities by robots establish an important burden for the therapists who help patients. Moreover and due to economic reasons, the duration of preliminary rehabilitation is getting shorter and shorter [ 9 ]. However, some studies have pointed out that repetitive and high-severity exercises can specifically contribute to the functional recovery of the affected upper limb movement [ 10 ]. Rehabilitation robots are able of decreasing the burden on therapists by replace human intervention and preparing desire therapies that accomplish the following primary principles of upper limb rehabilitation: iteration, high severity, and task particularly [ 11 ]. Moreover, the functional impetus of a patient can be activated to raise strenuous participation and improve cognitive functions. The physical factors and treatment data can be stored and analyzed by the sensing system, and that can provide a realistic basis for optimization training technique and speed up the recovery process [ 7 ]. In addition, major boost has been given by the extension of such devices to clinical care medical and rehabilitation centers [ 1 , 2 ].
Therefore, multiple benefits can be considered for rehabilitation robots : they can produce high-quality repetitive movements and increase rehabilitation strength and intensity; they can offer many types of exercises for therapists to choose from; they supply a man-machine interaction that allows for objective measurement of advancement, which itself can status modifications in interaction by changing control parameters [ 12 ]. On the other hand, task-oriented training is one of the other capabilities of rehabilitation robots, which is known as the dominant and most effective method for motor rehabilitation of upper limb function [ 13 ]. Task-based approaches in which the patient is assisted to perform a specific prescribed movement, such as lifting an object with the hand, show promising results compared to conventional exercises based on passive movement of the impaired joints in the restriction of their range of motion [ 14 ].
Robots can also be used in homes, clinics and medical centers. Patients can do the rehabilitation exercises prescribed by the therapist with the help of the robot at home without having to visit the treatment center frequently. Successful robot-assisted rehabilitation at home can facilitate intensive therapy, facilitate in-person or virtual therapy visits, preferably at low cost, and motivate patients to participate in supervised or unsupervised therapeutic activities at necessary levels for motor learning and generalization to occur [ 15 ]. Moreover, in clinics, rehabilitation robots can decrease the burden of therapists by automating tedious and labor-intensive treatment and by adapting to the particularized needs of targeted individuals [ 16 ]. For example, the hand robot skeleton designed by Wege et al., [ 17 ] can move patients’ fingers skillfully and repeatedly compared to a tired therapist training patients with manual labor.
To our knowledge, no systematic or scoping review has been performed on robots to rehabilitate upper limb disabilities. Only a few systematic reviews have been done in the areas of classification of interactive wearable systems for monitoring body movement and posture during upper body rehabilitation, estimating the wearability of the wearable devices [ 18 ], presenting state of the art in sensor fusion applied in applications for hand rehabilitation [ 19 ], evaluating the role of serious games in upper limb rehabilitation, identifying common procedures and exercises as well as technology patterns [ 20 ], evaluating the effectiveness of upper limb wearable technology to improve activity and engagement in stroke survivors [ 21 ] and determining the effects of robot-assisted treatment on motor-functional improvement in stroke patients [ 22 ]. So, the aim of the present study was to investigate the role of robots in upper limb disability improvement and rehabilitation. In this study, we identify the most common diseases or complications leading to upper limb disability for which robots have been used for rehabilitation. Moreover, we identify the most important technologies that can be used along with rehabilitation robots, the types of evaluation methods of rehabilitation robots and the outcomes of robot use for individuals with upper limb disabilities.
Materials and methods
In the current study, we used the PRISMA scoping reviews checklist for selecting studies and reporting the results [ 23 ].
Search strategy and information sources
To find articles related to the rehabilitation of upper limb disabilities using robots, four databases, PubMed, Web of Science, Scopus, and IEEE were searched. In order to search these databases, the keywords related to the upper limb, rehabilitation, and robots were used. Relevant Medical Subject Heading (MeSH) Keywords, spelling differences and synonyms were included and altered as suitable for each database. Then, the below search strategy was developed by KB, ASH, and KHM and finally approved by AH.
((upper extremity disability OR upper limb disability) AND (rehabilitation OR telerehabilitation) AND (robot OR robotics))
Articles addressing upper limb rehabilitation robots were included in to study. The PRISMA diagram is demonstrated in Fig. 1 .
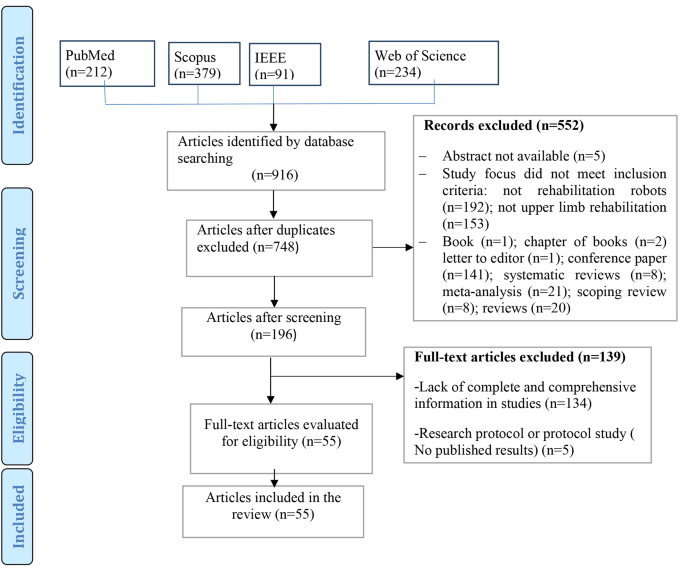
Study selection process
Eligibility criteria.
Inclusion and exclusion criteria are presented below.
Inclusion criteria
Articles involving upper limb rehabilitation robots.
Articles about upper limb disability caused by any illness or injury.
Studies involving rehabilitation robots designed for all ages.
Robotic devices used with any assistive technologies (such as telerehabilitation systems and games based on virtual reality, etc.)
Articles published in English.
Time of publication between January 2012– February 2022.
It should be noted due to the rapid development of technologies and rehabilitation robots, we limited our investigation to the last ten years. We wanted to identify the latest and newest technologies that can be used along with rehabilitation robots, evaluation methods and the outcomes of these robots in recent years. Xue et al. [ 24 ], believed that rehabilitation robotics is a relatively young field growing rapidly with increasing penetration into therapeutic environments. Therefore, the newer dimensions of this rehabilitation tool should always be considered. In addition, the number of articles published in the last ten years was sufficient to answer the questions of this research. Therefore, this scoping review included articles published from January 2012– February 2022.
Exclusion criteria
Articles involving other than upper limb rehabilitation robots.
Robots related to rehabilitation of diseases other than upper limb.
Systematic reviews, reviews, and meta-analysis.
Book chapters.
Letter to the editor.
Conference abstracts.
Research protocol or protocol study.
Study selection
First, abstracts of all related articles were retrieved from four databases: PubMed, Web of Science, Scopus, and IEEE, and imported into EndNote software by KHM. Duplicate articles were removed. After studying the title, and abstract, the relevant articles were selected according to the inclusion and exclusion criteria by KHM and ASH. Then, all studies included in the study were reviewed and finalized by KB and AH. In disagreement, the final decision on each article is decided by discussion among the study team members. Finally, the full text of the articles was studied to extract data and information from KHM and ASH.
Critical appraisal of individual sources of evidence
Critical evaluation of data was done by ASH and KHM independently using Mixed Methods Appraisal Tool (MMAT, version 2018: Hong et al., 2018). Disagreement between authors (n = 4 articles) was resolved by discussion between two other authors (KB and AH) until reaching an agreement. We evaluated studies according to the MMAT criteria according to the selected category. The latest version of the MMAT offers a descriptive quality appraisal instead of a summative numerical score. Answer options in all study categories include “yes”, “no” and “can’t tell”. The answer “can’t tell” indicates that not enough information was reported in the study for a “yes” or “no” answer. A “can’t tell” rating may indicate a need to search for companion studies or contact study authors for more information [ 25 ].
Data charting process and data items
Data were extracted using a predetermined form. The validity of this form was confirmed by two specialists in medical informatics and software engineer, a physiotherapist, and a specialist in health information management. This data extraction form also includes fields such as year, country, type of study, purpose, illness or accident leading to disability (Table 1 ), level of disability (Table 2 ), assistive technologies (telerehabilitation, virtual reality or games) (Table 1 and more details in Appendix B ), number of participants in the study, sex, age (Table 3 and more details in B)), rehabilitated part of the upper limb using a robot (Table 4 ), duration and frequency of treatment, methods of performing rehabilitation exercises, type of evaluation, number of participants in the evaluation process (Table 5 and more details in Appendix B ), study outcomes (Table 6 and more details in Appendix B ) and study conclusions (more details in Appendix B ).
Data collation process
The information extracted from the articles was re-examined by KB and finally approved by AH. In case of disagreement, the consensus was achieved by the review of the members of the study team. It should be noted that for articles with missing data and information, we emailed the corresponding author and asked them to send us the necessary information. Finally, two authors extracted all data from eligible full-text documents through Excel.
Synthesis of results
After the data were stored and managed in MS Excel for processing, to synthesize data, one author (KHM) checks all imported data (e.g., spell check, cell formatting). Then, descriptive statistics (frequency and percentage) were used to summarize the collected data. Descriptive data obtained from the findings of the included articles were organized into tables and figures based on themes to present the findings of this review, which guided the study aims by (KB, AH, and ASH).
Ethical considerations
The protocol of this study was approved by the ethical committee of Kerman University of Medical Sciences) IR.KMU.REC.1400.606).
From the 348 non-duplicate articles found using the search strategy, 55 articles were selected for inclusion (Fig. 1 ). Summarized findings from selected articles are included in Table 1 .
The findings of the quality assessment of studies based on MMAT are presented in Appendix A .
Results of the reviewed studies
Most of the studies were done in Italy (n = 19, 34.55%) [ 26 , 27 , 28 , 31 , 33 , 36 , 37 , 43 , 46 , 47 , 48 , 49 , 57 , 62 , 63 , 68 , 76 , 79 ]. After Italy, 14.24% and 8.9% of the studies were conducted in the USA (n = 8) [ 29 , 42 , 50 , 51 , 56 , 60 , 61 , 72 ] and China (n = 5) [ 32 , 54 , 59 , 65 , 73 ], respectively. (More details in Table 1 ). Also, most of the studies were RCT (n = 26) [ 28 , 29 , 30 , 31 , 35 , 36 , 40 , 41 , 42 , 47 , 48 , 50 , 53 , 54 , 55 , 63 , 66 , 68 , 70 , 72 , 73 , 75 , 76 , 78 , 79 , 80 ].
As shown in Fig. 2 , most articles on robots for upper limb rehabilitation were published in 2017 (n = 9) [ 33 , 34 , 35 , 60 , 61 , 67 , 72 , 79 , 80 ], 2020 (n = 7) [ 46 , 47 , 48 , 49 , 50 , 63 , 64 , 69 ], and 2021 (n = 7) [ 51 , 52 , 53 , 54 , 55 , 65 , 73 ]. (More details in Table 1 )
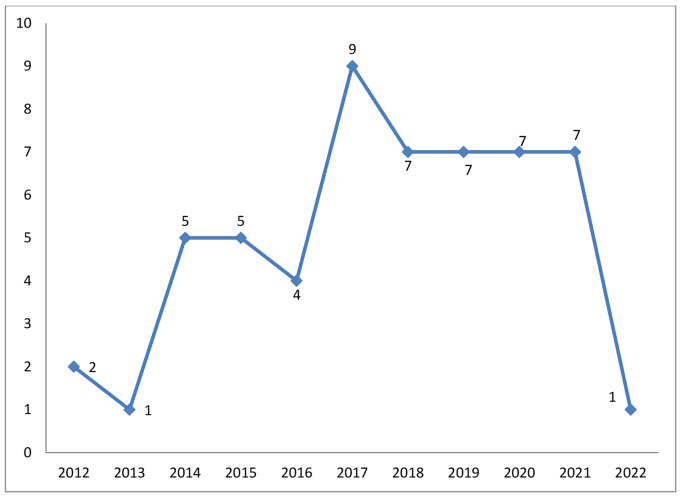
Distribution of the studies in terms of publication year
Robots were used to rehabilitate seven categories of diseases or complications leading to upper limb disability (Fig. 3 ). Most robots were used for patients with stroke (n = 44, 80%) [ 28 , 29 , 31 , 32 , 33 , 34 , 35 , 37 , 38 , 39 , 40 , 41 , 42 , 44 , 45 , 48 , 49 , 50 , 51 , 52 , 53 , 54 , 55 , 56 , 57 , 58 , 59 , 60 , 61 , 62 , 64 , 65 , 66 , 68 , 70 , 72 , 73 , 74 , 75 , 76 , 77 , 78 , 79 , 80 ]. Other diseases with frequencies and percentages are shown in Fig. 3 .
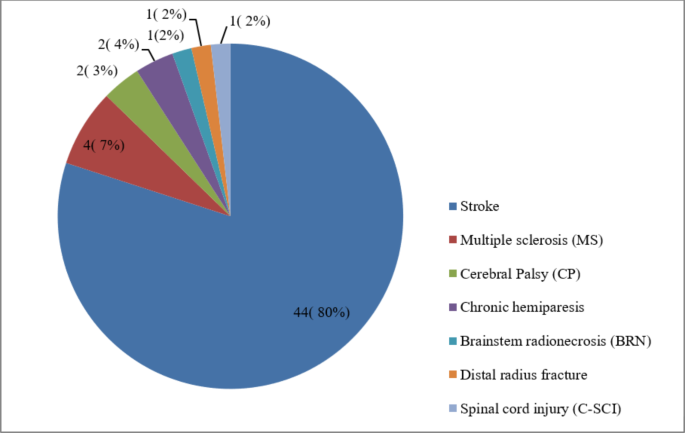
The distribution of the studies based on diseases and injuries leading to upper limb disabilities
Eight different levels of upper limb disability were identified (Table 2 ). The highest frequency of disabilities was related to the entire upper limb (n = 30). Wrist (n = 10), fingers (n = 7) and arm (n = 7) were other parts of the upper limb that had the highest level of disabilities. (More details in Table 1 )
Table 4 shows that some robots were designed to be used to rehabilitate the entire upper limb (n = 15). Then, robots were mostly used for wrist (n = 14), finger (n = 12), and shoulder (n = 11) rehabilitation, respectively. (More details in Appendix B )
Use of telerehabilitation and virtual reality in robots.
60.52% of the studies used games and virtual reality to rehabilitate the upper extremities in robots [ 26 , 30 , 31 , 32 , 36 , 38 , 40 , 42 , 43 , 44 , 45 , 46 , 47 , 48 , 49 , 50 , 51 , 54 , 55 , 57 , 59 , 60 , 61 , 62 , 64 , 66 , 67 , 68 , 69 , 70 , 71 , 72 , 73 , 75 ](n = 34). Moreover, 3.56% of studies had used telerehabilitation in their robots (n = 2) [ 32 , 35 ].
Evaluations in upper limb rehabilitation robots
The number of participants in the evaluation of upper limb rehabilitation robots varied from one [ 43 , 46 , 59 , 64 , 77 ] to 224 [ 48 ]. According to Tables 3 , 89% of the studies used both males and females in the robot evaluation process(n = 49) [ 26 , 27 , 28 , 29 , 31 , 32 , 33 , 34 , 35 , 36 , 37 , 38 , 39 , 40 , 41 , 42 , 44 , 45 , 47 , 48 , 49 , 50 , 51 , 52 , 53 , 54 , 55 , 56 , 57 , 58 , 60 , 61 , 62 , 63 , 65 , 66 , 67 , 68 , 69 , 70 , 71 , 72 , 73 , 74 , 75 , 76 , 78 , 79 , 80 ]. Most of the participants were over 18 years old (n = 47, 85%) [ 26 , 27 , 28 , 29 , 31 , 33 , 34 , 35 , 36 , 37 , 38 , 39 , 40 , 41 , 42 , 43 , 44 , 45 , 46 , 47 , 48 , 49 , 50 , 51 , 53 , 54 , 55 , 56 , 57 , 58 , 59 , 60 , 62 , 63 , 65 , 66 , 67 , 68 , 70 , 71 , 72 , 73 , 75 , 78 , 79 , 80 ]. Moreover, in some studies, the age of the participants was less than or equal to 18 years (n = 4, 7%) [ 30 , 67 , 69 , 76 ]. The minimum and maximum duration of evaluation processes were 30–45 min [ 26 ] to 12 months [ 52 ], respectively. Also, 83% of the studies included both male and female patients in the robot evaluation process. One study included only women [ 43 ], and two studies focused on men [ 46 , 77 ] (More details in Appendix B ).
The duration of treatment of patients in 38% of studies was less than one month (n = 21) [ 26 , 28 , 32 , 33 , 35 , 39 , 47 , 49 , 51 , 54 , 56 , 58 , 60 , 62 , 63 , 64 , 66 , 68 , 72 , 73 , 75 ]. In 41% of the studies (n = 23) [ 29 , 30 , 31 , 34 , 36 , 40 , 43 , 44 , 45 , 46 , 50 , 53 , 55 , 57 , 61 , 65 , 67 , 69 , 71 , 76 , 78 , 79 , 80 ], the duration of treatment of patients was between one and two months. In other studies, the duration of treatment of patients was more than three months [ 27 , 42 , 48 , 52 , 70 ](n = 5, 9%) (More details in Appendix B).
According to Tables 5 , 14 types of evaluation were performed for upper limb rehabilitation robots. “Evaluation and measurement of upper limb function and dexterity” was the most common type of evaluation (n = 52). “Range and motor skills and functional strength of the hand” were ranked next (n = 12). Then, “Neuropsychological assessment” was the most common type of evaluation (n = 11). As shown in Tables 5 , 85 different evaluation methods were used. “Fugl-Meyer Upper Extremity score” was the most used evaluation method in studies (n = 30). Then, “Barthel Index (BI)” (n = 11), “Modified Ashworth Scale (MAS)” (n = 11), and “Box and Block Test (BBT)” (n = 11) were the most widely used evaluation methods.
Outcomes of using upper limb rehabilitation robots
The most important outcomes of upper limb rehabilitation robots were “Improvement in musculoskeletal functions”, “No adverse effect on patients”, and “Safe and reliable treatment”, respectively. Other outcomes are listed in Table 6 . (More details in Appendix B ).
In this scoping review, outcomes of rehabilitation robots for upper limb disabilities and different methods for evaluating rehabilitation robots were identified. Most robots were used for the rehabilitation of patients with stroke. Along with robots, telerehabilitation and virtual reality, technologies were also used for upper limb disabilities rehabilitation. “Evaluation and measurement of upper limb function and dexterity” was the most common type of evaluation among the 14 types performed for upper limb rehabilitation robots. Also, we identified different outcomes of using rehabilitation robots for upper limb disabilities.
As discussed above, telerehabilitation and virtual reality were two types of technology used along with rehabilitation robots. Some studies [ 3 , 82 , 83 ] showed that if special interventions such as game-based virtual reality, and telerehabilitation with rehabilitation robots are used, upper limb function, mental health and patients’ participation in rehabilitation exercises can be improved. Moulaei et al. [ 3 ], showed in a scoping review that telerehabilitation could lead to “improving in musculoskeletal functions”, “increasing patients’ interest and motivation to perform rehabilitation exercises”, “increasing adherence to rehabilitation exercises and more participation in treatment processes”, “remote monitoring and control of patients”, “reducing or relieved pain” and “improving quality of life”. Fernández-Vázquez et al. [ 84 ], also pointed out that virtual reality as a very interesting tool in treating upper limb disorders along with rehabilitation robots can improve the motor function of the upper limb, increase users’ motivation and enjoyment, a large number of repetitions or high intensity rehabilitation. Repetitive and long exercises become easy and reduce the time needed to perform therapeutic exercises. Some studies [ 85 , 86 , 87 ] stated that for telerehabilitation systems and virtual reality to be as effective as robots, they should be easy to use, user-friendly, and have a beautiful and appropriate user interface. Zanatta et al. [ 87 ] pointed out that when telerehabilitation systems, robots and virtual reality are usable and attractive, patients’ motivation to perform rehabilitation exercises increases, their satisfaction with treatment processes increases, and efficiency and effectiveness increase. Therefore, to combine the robot with telerehabilitation or virtual reality to be effective, various factors such as usability, suitable user interface and even technical support should be considered in design, development and implementation.
The findings of this study and other reviews [ 2 , 22 , 88 , 89 ] have shown that rehabilitation robots have been able to improve the musculoskeletal status of patients with upper limb disabilities. FMA-UE scores obtained from different studies showed that robot-based treatments can improve upper limb musculoskeletal function [ 1 , 90 , 91 , 92 ]. In a review of the literature on therapeutic robotics in chronic stroke survivors and individuals with hemiparesis, the FMA total score improved from 2.1 to 15.1 and the ARAT improved to 11.1 [ 1 , 90 , 91 , 92 ]. Milot et al. [ 91 ], tested 20 volunteers with mild to moderate chronic stroke to investigate the robot’s functional outcomes on the upper limb. Their study showed that training with the robot led to significant improvements in FMA, BBT, MAL, WMFT, and quantitative amounts of strength and speed, and these statuses were maintained in the 3-month follow-up [ 91 ]. Cimolin et al., [ 93 ] conducted a clinical trial using the Armeo Spring device on children aged 4 to 17 years with cerebral palsy. The subjects of this study showed promising improvements in different movement patterns and hand functions. It seems that to increase the effect of robots on improving upper limb disabilities, it is better to consider a number of factors. For example, the mechanical characteristics of rehabilitation robots may affect outcomes. Exoskeleton robots can control several joints simultaneously, leading to tight physical interaction between humans and robots, which may increase the patient burden.
Additionally, the exoskeleton robot’s high inertia to its complex structure can intervene with manipulation. Or, in the mechanical dimensions of the robot, the degree of freedom should be considered an important factor in the design. For example, intervention with six degrees of freedom in a three-dimensional space is very challenging for stroke patients with moderate to severe motor impairment [ 1 ]. The term “paradox of reduced number of degrees of freedom” states that to train a patient with a severe movement disorder, one should first use the lowest number of degrees of freedom, then gradually increase the number of degrees of freedom as improvements are achieved [ 94 ]. On the other hand, if the robots are not light, they can put pressure on different organs of patients during exercise. Also, rehabilitation robots can be costly, except for people who might profit from using them for training. As a result, efforts should be made to make rehabilitation robots as cost-effective as possible. Finally, these devices should be easily available to people, and awareness of them as an effective and helpful alternative treatment should also continuously increase.
Although, in this review we observed that rehabilitation robots have no adverse effect on patients, in some studies, the complications of rehabilitation robots have been mentioned [ 89 ]. Bessler et al. [ 95 ], believed that the safety of using rehabilitation robots in clinical trials should be confirmed for monitoring and reporting adverse events. In a systematic literature review, Bessler et al. [ 89 ], gathered information on the type of adverse events associated with the training of static robotic gait trainers. They identified about 17 adverse events per 100 people trained on a stationary robotic gait trainer. The most common types of side effects were classified into two categories: side effects related to soft tissue and musculoskeletal side effects. The third category included physiological adverse events (such as sudden changes in blood pressure) that seem to be unrelated to the mechanical settings of the robotic device in most cases, but are generally considered to be related to the activity engaged. Side effects associated to soft tissue in trainers also included things such as skin redness, skin irritation, open skin lesions and bruising, skin abrasion, as well as hurt and pain in soft tissue areas. Musculoskeletal side effects also included tendinopathy, muscle pain, tibial fracture, back and malleolus pain, and joint discomfort and pain [ 89 ]. Therefore, rehabilitation robots should be designed to minimize the side effects related to all three dimensions, i.e., soft tissue, musculoskeletal, and physiological. First of all, it can be said that the designers of these systems must ensure that there are no side effects before using robots by patients or therapists, so that they can be used safely for rehabilitation. Then, the therapists should set the rehabilitation exercises in terms of dosage and duration in such a way that the side effects caused by the low or high use of the robot do not occur for the patients.
Besides, we should be able to improve the design, development and evaluation of rehabilitation robots so that they have the necessary quality to provide rehabilitation services. Abu-Dakka et al. [ 96 ] believed that rehabilitation robots should be designed in a way that can be easily used by patients, therapists, and clinicians, increase the effectiveness of doctors’ treatments and make patients’ daily activities easier. To achieve these goals, rehabilitation robots must have some functional requirements, including: stability, safety, adaptability to patient needs, accept a wide range of patients, providing a full Range of Motion (ROM), being equipped with the necessary sensors for haptic and visual feedback, etc. [ 97 , 98 ]. Also, in the design and evaluation of rehabilitation robots, we must consider the views and opinions of users (patients and therapists), because these people are the end users of these rehabilitation tools. Considering the perspectives and opinions of patients and therapists in the design process also makes the device develop according to the patients’ needs and preferences. As a result, their level of satisfaction and continued use of this device improves. Zanatta et al., [ 87 ] believed that considering the patient’s perspective when designing or evaluating a rehabilitation device is essential to ensure adequate engagement and adherence to treatment. Or when the usability of a rehabilitation robot is suitable for users, according to Nielsen [ 99 ], learnability, efficiency, memorability and satisfaction of users improve, while the rate of errors also decreases.
Accordingly, a patient who perceives a device as usable is likely also report a positive perception as a concern: ease of learning the system’s function and behavior, effort expended to reach a goal, ease of remembering the system’s performance for any further use, the system’s ability to easily support and recover the case of errors during use and the pleasant design of the system. Thus, assessing such aspects provides important insights into the acceptability and perceived usefulness of devices, thereby allowing an understanding of how to improve patient motivation during treatment. As emphasized in Monardo’s study [ 100 ], motivation plays an essential role during the rehabilitation process because it helps create a sense of patient competence and satisfaction. In addition, patient satisfaction with treatment-rehabilitation processes performed with the help of rehabilitation devices was associated with stronger treatment compliance [ 101 ]. As a result, both factors can be considered key factors for the effectiveness of treatment-rehabilitation processes. On the other hand, the anatomical structure of the upper limb, the severity and degree of the patients’ disabilities, and the age group of the patients (for example, children or the elderly) should also be considered when designing and developing a rehabilitation tool. For example, a rehabilitation robot may only be used by children and not by elderly persons [ 102 ] or a robot may be designed for finger rehabilitation-only [ 103 ].
According to the findings of the studies, we found that robots were a safe and reliable treatment for patients. Therefore, it is necessary to consider a set of factors for the rehabilitation robot to guarantee patient safety and maintain its reliability for patients. Sampson et al. [ 104 ], evaluated the BUiLT + VR system in hemiparetic patients with upper extremity disability and found that this system is reliable for treating patients and can be administered safely. They believed that through Rests between games, ramping of the time spent playing in the early sessions, and adjustments to seating, unit height and angle, it could overcome the challenges related to patient safety and increase its reliability for users. Other studies show that the designed rehabilitation robot should ensure the safety of the patients from the aspects of using the common sensor to monitor and manage the force information of the patients during movement, when the reaction force made by the muscle tension is very high. Also, when the patient experiences a muscle spasm, the robot should automatically stop the current movement so that the muscle is not strained. Therefore, to prevent repeated injury to patients, the space and working environment should be restricted by limiting the switch within a reasonable range. The rehabilitation robot’s speed of displacement and movement should also be limited by the software used to control the robot. The operator or therapists should be able to rationally adjust the parameters of the driving device, and control and monitor the movement status of the robot in real time. Pressing the stop button is very important to avoid accidents. Also, to avoid damage created by the rotation of the joints due to gravity, the rehabilitation robot’s ability to self-lock should be considered [ 105 ].
Reduction or relief of pain was another outcome of this review. Some studies [ 75 , 95 ] have shown that robots can reduce or relieve pain in patients, but factors must be considered when designing or using robots so that the robot can reduce pain more effectively. For example, exceeding forces on the human musculoskeletal system, at the same time misalignment will make high pressure and shear forces via slipping at the cuffs or straps [ 106 , 107 ]. Therefore, forces that are not considered or compensated for in the design of the robot or its interface are easily transmitted to the musculoskeletal systems. On the other hand, if the torques and forces are too high or act in arbitrary directions on the musculoskeletal system, they can create an additional load, resulting in pain and damage to the bones, joints, and muscles [ 95 , 106 ]. Therefore, when designing robots, pain thresholds should be considered for pressures and forces applied during accidental contact such as collisions or closing situations with a robot [ 108 ]. Different limit values for many different parts of the human body are accepted by ISO/TS 15,066 [ 95 ]. The patient’s position to perform therapeutic exercises with the help of the robot is also very effective in reducing pain. The robot developed in Kim et al.‘s [ 75 ] study allows patients to be comfortable and supported in a supine position. This way can easily be linked to other pain therapeutic methods, such as interferential current therapy or hot pack application. For example, installing a friction plate to forbid the compensation of the scapula and to increase the therapy’s effectiveness and adjust the robotic arm’s height using a linear actuator can be very helpful [ 75 ].
Duration and intensity of the therapy through the robot are two other factors that effect on the robots in motor control and improving pains. By examining the effectiveness of robots on function and structure in patients with limited upper limb function, Ferreira et al., [ 109 ] showed that when robot-assisted therapy is used with conventional treatment at the same dose and duration, robot-assisted therapy has a significant effect motor control. The group’s analysis also proposed an effect of the number of sessions and duration of treatment on some estimated impacts. The greater number of sessions and the volume of treatment affect motor control [ 109 ]. The more significant treatment dose effect was proposed by Lohse et al. [ 110 ]; however, time as a representation of dose is a rather rough estimate and supplies no evidence of the actual amount of movement or types of movement, nor does it account for periods of inactivity or rest [ 111 ]. In this respect, a previous study showed that, although there is no agreement, the minimum dose should be at least 16 h of exercise [ 112 ]. Poor methodological quality and lower dose and duration of treatment may negatively affect the estimated effects. So, therapists usually consider this approach because it has very few or no side effects [ 109 ]. Moreover, some studies have shown that low or high intensity exercise therapy can increase the risk of injury and worsening pain in the hemiplegic shoulder [ 113 ]. In the study by Kim et al. [ 75 ], participants in the intervention or control groups performed stretching exercises for 30 min per day, five times per week for four weeks; Shoulder pain in the intervention group (they used the robot) was worse than the control group. The authors of this research concluded that this stretching exercise was performed for patients with excessive intensity and more than 30 min of stretching exercise caused pain in some patients even when it was performed with low intensity.
As our study showed, robots can increase patients’ interest and motivation to perform rehabilitation exercises. Therefore, one of the other factors that can be considered to increase patients’ use of robots and their interest and motivation to perform rehabilitation exercises is the use of games and virtual reality. Creating a degree of entertainment through games and virtual reality can improve patients’ adherence to rehabilitation exercises and increase patients’ attention span to spend more time on their rehabilitation program [ 114 ]. Kafri et al. [ 115 ], investigated the effect of virtual reality on patients after a stroke. Patients showed an improvement in activity and reported that they enjoyed the game. Virtual reality also supplies direct visual feedback and can empower patients with a sense and awareness of control over their recovery. Consequently, it is imaginable that people who train with virtual reality and games will enhance their performance when interacting with an avatar presented on the screen [ 114 ]. In another study, Gomez et al., [ 116 ] evaluated patients with multiple sclerosis using games, in which the experimental group received both game sessions and conventional treatment. This study showed that after using the games, interest and motivation to perform rehabilitation exercises improved in the patients.
Efficiency and effectiveness of rehabilitation robots, increasing the patient’s independence in performing rehabilitation exercises, rehabilitation robots’ feasibility for training patients, performing repetitive and long exercises very easily with the help of the robot, reducing the duration of rehabilitation exercises, increasing adherence to rehabilitation exercises and more participation in treatment processes, and improving quality of life and rehabilitation process were other outcomes related to rehabilitation robots identified in this study. But it must be said that these outcomes or other mentioned outcomes will not be created spontaneously due to the use of rehabilitation robots, but a series of main factors or capabilities must be included in the robots so that these outcomes can be created and have a and beneficial effect in rehabilitation. For example, Laut et al. [ 117 ] believed that rehabilitation robots could potentially increase efficiency and access to treatment by having capabilities such as providing continuous training for a long period of time and collecting data to evaluate progress. Furthermore, by pairing rehabilitation robots with health information technologies and presenting assessment and performance data all over the internet to the therapist, different treatment processes can be moved out of specific facilities and into patients’ homes with remote management by a therapist. This can let a therapist treat several patients simultaneously, greatly enhancing the numbers of patients treated and the efficiency of the rehabilitation robot and therapist.
In other words, changing from a hospital to a home rehabilitation program can reduce costs while making rehabilitation more accessible [ 117 ]. Francisco et al. [ 118 ] also stated that by providing frequent and intensive training through rehabilitation robotics, the effectiveness of conventional occupational therapy or physiotherapy can be improved by providing more coherent, accurate, and precise treatment. Therefore, it should be said that for robots to be able to teach patients better or be more efficient, they must be able to provide frequent and intensive training. Also, in order to improve the efficiency and effectiveness of a rehabilitation device, the satisfaction of patients should be maximized. According to Tousignant et al.‘s study [ 119 ], satisfaction is an important indicator and factor for the degree of efficiency and effectiveness. Its high level can increase patient motivation, and adherence to treatment, and improve compliance with treatment. Also, it should be noted that satisfaction is one of the important factors in the quality of health care; it can affect adherence to treatment plans, improve clinical outcomes [ 120 ], and motivation of patients to perform rehabilitation exercises [ 121 ]. In order to maximize satisfaction, the robot must be designed according to the needs and preferences of its users, be usable and easy to use, and have no side effects [ 122 , 123 ].
In addition, concerning adherence to rehabilitation exercises, it can be said that considering technologies such as sensors and computer game technologies for home rehabilitation or mRehab (mobile rehabilitation) systems in robots greatly increases the possibility of objective quantification of adherence [ 124 ]. Also, for patients to engage and be challenged while performing rehabilitation exercises at home in the physical absence of the therapist, there is a need to carefully design scenarios and motivational features that guarantee the patient’s adherence to the treatment plan [ 125 ]. Increasing the quality of life was another important outcome of using rehabilitation robots identified in our study. In the study by Kutner et al. [ 126 ], it was shown that patients who had suffered a stroke experienced a faster increase in quality of life (in physical, mental and social dimensions) after participating in targeted interventions that focused on improving upper limb strength. Mohammed et al. [ 127 ], in their study of patients with upper and lower limb amputation, defined the quality of life in three dimensions physical, mental and social health. Therefore, it should be said that when a rehabilitation robot is designed, in order to increase the quality of life of the patients, it should be focused on all three dimensions of the patients’ health, physical, psychological and social.
In general, it can be said that although the findings of the present study showed that rehabilitation robots could lead to positive outcomes for people with upper limb disabilities with various diseases or injuries, but if the factors that lead to these outcomes in If these robots are not considered, they will never have the necessary efficiency, effectiveness and quality.
Prospects of rehabilitation robots
It is predictable that rehabilitation robots will be applied much more than before in an era where the cost of manual therapy is becoming more expensive. To better popularize and commercialize this goal, future development needs to increase the universality of rehabilitation robots, focusing on portable, lightweight, reconfigurable, smart, and equipment based on artificial intelligence and machine learning techniques, new methods of treatment and thinking to the field of rehabilitation under the hypothesis of guaranteeing safety, mass production and development in a cheap direction [ 24 ]. For example, machine learning techniques may ,allow a robot to independently adapt to the changing needs of each customer over time and perform specific tasks, such as autonomous gaming [ 128 ]. Another example is the portability of the robot, which allows users to carry the robot system for deployment inside homes or medical centers [ 129 ]. People can use portable rehabilitation robots not only at home and in the treatment center, but also in nature and parks. On the other hand, Smart rehabilitation is the future development trend. It should be broken through the human-machine interface barrier, develop multidisciplinary and interdisciplinary collaboration and communication, improve and strengthen cooperation with rehabilitation medicine and artificial intelligence, actively participate in the design, development and evaluation of rehabilitation robots, and improve rehabilitation robots for providing better and more quality service to people.
Moreover, in the future, there should be more focus on “human nature” in the design and development of rehabilitation robots. Human nature means that robots have capabilities such as “perception, thinking, action and cooperation, expression and communication, and learning and adaptability and complete training and operations independently or with the assistance of an automatic machine” [ 24 ]. At present, rehabilitation robots cannot perform all the tasks of professional rehabilitation personnel; especially, the ability of self-learning, adaptability, flexibility and creativity of robots needs many theoretical developments. The most challenging and most difficult thing for rehabilitation robots is the benevolence and moral sense of medical staff [ 130 ]. Since in the future software packages and powerful hardware equipment will be produced, many of these problems can be easily solved.
Limitations of the study
There are a few limitations in this study. In the present study, only articles in English were reviewed; it is better to include articles published in non-English languages in future studies. Also, to find related studies, we searched four databases, Scopus, IEEE, PubMed, and Web of Science. It is suggested that more studies be done in more databases to obtain more comprehensive results.
This scoping review indicates that upper limb rehabilitation robots can improve musculoskeletal functions (Musculoskeletal strength, sensation, perception, vibration, muscle coordination, less spasticity, flexibility, and range of motion); avoid serious side effects or adverse effects on the patient, provide safe and reliable treatment, reduce pain, increase the patient’s independence in performing rehabilitation exercises, reduce the duration of rehabilitation exercises, increase adherence to rehabilitation exercises and treatment processes, and quality of life and rehabilitation processes. The use of rehabilitation robots is growing strongly, especially in developed countries, and it seems that this new rehabilitation technology has had significant effects in helping to improve upper limb disabilities.
Also, rehabilitation robots could provide a platform for motivating people with upper limb disabilities to carry out more rehabilitation exercises without a therapist, which could maximize recovery.
Data Availability
The datasets used and analyzed during the current study are available from the corresponding author on reasonable request.
Abbreviations
Nine-Hole Peg Test
Assisting Hand Assessment
Arm-Hand Function
Arm Motor Ability Test
Action Research Arm Test
Box and Block Test
Barthel Index
Behavioral Inattention Test
Brainstem radionecrosis
Spinal cord injury
Catherine Bergego Scale
Credibility/Expectancy Questionnaire
Cerebral Palsy
Disability Assessment Scale
Disabilities of Arm, Shoulder, and Hand
Douleur Neuropathique 4
Dynamic Surface Electromyography
Quality of Life
Functional Indipendence Measure
Fugl-Meyer Upper Extremity score
Functional magnetic resonance imaging
Functional near infrared spectroscopy
Grooved Pegboard Test
Intrinsic Motivation Inventory
Jebsen-Taylor Hand Function Test
Shoulder Disability Questionnaire
Motor Activity Log
Modified Ashworth Scale
Mental health Composite Score
Medical Subject Heading
Manual Function Test
Motricity Index
Mini-Mental State Examination
Magnetic Resonance Imaging
Modified Rankin Scale
Multiple sclerosis
Multiple Sclerosis Quality of Life-54
Nottingham Extended Activities of Daily Living
National Institutes of Health Stroke Scale
Numeric Rating Pain Scale
Numerical Rating Scale
Oxford Cognitive Screen
Physical health Composite Score
Pediatric Evaluation of Disability Inventory
Performance Oriented Mobility Assessment
Patient-Rated Wrist and Hand Evaluation
Quality of Movement
Quality of Upper Extremity Skills Test
Quick version of the Disabilities of the Arm, Shoulder, and Hand
Reliable Change Index
Randomized controlled trials
Rey Osterrieth complex figure test
Active or Passive Range Of Motion
Stroop Color and Word Test
Symbol Digit Modalities Test
Self-Depression Scale
Short Form Health Survey
Stroke Impact Scale
Total active mobility
Trail making test
Tremor Severity Scale
Usefulness, Satisfaction and Ease-of-use
Visual analog scale
Visual analogue scale
World Health Organization Disability Assessment Schedule
Wolf Motor Function
Lee SH, Park G, Cho DY, Kim HY, Lee J-Y, Kim S, Park S-B, Shin J-H. Comparisons between end-effector and exoskeleton rehabilitation robots regarding upper extremity function among chronic stroke patients with moderate-to-severe upper limb impairment. Sci Rep. 2020;10(1):1806.
Article CAS PubMed PubMed Central Google Scholar
Vélez-Guerrero MA, Callejas-Cuervo M, Mazzoleni S. Artificial Intelligence-Based Wearable Robotic Exoskeletons for Upper Limb Rehabilitation: a review. Sens (Basel). 2021;21(6):2146.
Article Google Scholar
Moulaei K, Sheikhtaheri A, Nezhad MS, Haghdoost A, Gheysari M, Bahaadinbeigy K. Telerehabilitation for upper limb disabilities: a scoping review on functions, outcomes, and evaluation methods. Archives of Public Health. 2022;80(1):196.
Article PubMed PubMed Central Google Scholar
Poltawski L, Allison R, Briscoe S, Freeman J, Kilbride C, Neal D, Turton AJ, Dean SJD. rehabilitation: Assessing the impact of upper limb disability following stroke: a qualitative enquiry using internet-based personal accounts of stroke survivors. 2016, 38(10):945–51.
Maciejasz P, Eschweiler J, Gerlach-Hahn K, Jansen-Troy A, Leonhardt S. A survey on robotic devices for upper limb rehabilitation. J Neuroeng Rehabil. 2014;11(1):3.
Vélez-Guerrero MA, Callejas-Cuervo M, Mazzoleni S. Design, Development, and testing of an Intelligent Wearable Robotic Exoskeleton Prototype for Upper Limb Rehabilitation. Sens (Basel). 2021;21(16):5411.
Wu Q, Wu H. Development, dynamic modeling, and Multi-Modal Control of a therapeutic exoskeleton for Upper Limb Rehabilitation Training. Sens (Basel). 2018;18(11):3611.
Qassim HM, Wan Hasan WZ. A Review on Upper Limb Rehabilitation Robots. Appl Sci 2020, 10(19).
Richards L, Hanson C, Wellborn M, Sethi A. Driving motor recovery after stroke. Top Stroke Rehabil. 2008;15(5):397–411.
Article PubMed Google Scholar
Qian Q, Nam C, Guo Z, Huang Y, Hu X, Ng SC, Zheng Y, Poon W. Distal versus proximal - an investigation on different supportive strategies by robots for upper limb rehabilitation after stroke: a randomized controlled trial. J Neuroeng Rehabil. 2019;16(1):64–4.
Langhorne P, Bernhardt J, Kwakkel GJTL. Stroke rehabilitation. 2011, 377(9778):1693–1702.
Qian Z, Bi ZJAiME. Recent development of rehabilitation robots. 2015, 7(2):563062.
Schweighofer N, Choi Y, Winstein C. Gordon JJAJoPM, Rehabilitation: Task-oriented rehabilitation robotics. 2012, 91(11):S270-S279.
Ennaiem F, Chaker A, Laribi MA, Sandoval J, Bennour S, Mlika A, Romdhane L, Zeghloul SJAS. Task-Based design approach: development of a planar cable-driven parallel robot for upper limb rehabilitation. 2021, 11(12):5635.
Johnson MJ, Loureiro RC, Harwin WSJISR. Collaborative tele-rehabilitation and robot-mediated therapy for stroke rehabilitation at home or clinic. 2008, 1(2):109–21.
Nizamis K, Athanasiou A, Almpani S, Dimitrousis C, Astaras AJS. Converging robotic technologies in targeted neural rehabilitation: A review of emerging solutions and challenges. 2021, 21(6):2084.
Wege A, Zimmermann A. Electromyography sensor based control for a hand exoskeleton. In: 2007 IEEE International Conference on Robotics and Biomimetics (ROBIO) : 2007: IEEE; 2007: 1470–1475.
Wang Q, Markopoulos P, Yu B, Chen W, Timmermans A. Interactive wearable systems for upper body rehabilitation: a systematic review. J Neuroeng Rehabil. 2017;14(1):20.
Herrera-Luna I, Rechy-Ramirez EJ, Rios-Figueroa HV, Marin-Hernandez AJISJ. Sensor fusion used in applications for hand rehabilitation: A systematic review. 2019, 19(10):3581–3592.
Koutsiana E, Ladakis I, Fotopoulos D, Chytas A, Kilintzis V, Chouvarda I. Serious Gaming Technology in Upper Extremity Rehabilitation: scoping review. JMIR Serious Games. 2020;8(4):e19071–1.
Parker J, Powell L, Mawson S. Effectiveness of Upper Limb Wearable Technology for improving activity and participation in adult stroke survivors: systematic review. J Med Internet Res. 2020;22(1):e15981–1.
Kwakkel G, Kollen BJ, Krebs HIJN. repair n: Effects of robot-assisted therapy on upper limb recovery after stroke: a systematic review. 2008, 22(2):111–21.
PRISMA for Scoping Reviews. [ https://prisma-statement.org/Extensions/ScopingReviews ].
Xue X, Yang X, Deng Z, Tu H, Kong D, Li N, Xu F. Global Trends and hotspots in Research on Rehabilitation Robots: a bibliometric analysis from 2010 to 2020. Front Public Health. 2021;9:806723.
Hong QN, Pluye P, Fàbregues S, Bartlett G, Boardman F, Cargo M, Dagenais P, Gagnon M-P, Griffiths F. Nicolau BJRoc: Mixed methods appraisal tool (MMAT), version 2018. 2018, 1148552(10).
Carpinella I, Cattaneo D, Bertoni R, Ferrarin M. Robot training of upper limb in multiple sclerosis: comparing protocols with or without manipulative task components. IEEE Trans neural Syst rehabilitation engineering: publication IEEE Eng Med Biology Soc. 2012;20(3):351–60.
Sale P, Bovolenta F, Agosti M, Clerici P, Franceschini M. Short-term and long-term outcomes of serial robotic training for improving upper limb function in chronic stroke. Int J rehabilitation Res Int Z fur Rehabilitationsforschung Revue Int de recherches de readaptation. 2014;37(1):67–73.
Google Scholar
Pennati GV, Da Re C, Messineo I, Bonaiuti D. How could robotic training and botolinum toxin be combined in chronic post stroke upper limb spasticity? A pilot study. Eur J Phys Rehabil Med. 2015;51(4):381–7.
CAS PubMed Google Scholar
McCabe J, Monkiewicz M, Holcomb J, Pundik S, Daly JJ. Comparison of robotics, functional electrical stimulation, and motor learning methods for treatment of persistent upper extremity dysfunction after stroke: a randomized controlled trial. Arch Phys Med Rehabil. 2015;96(6):981–90.
Gilliaux M, Renders A, Dispa D, Holvoet D, Sapin J, Dehez B, Detrembleur C, Lejeune TM, Stoquart G. Upper Limb Robot-Assisted therapy in cerebral palsy: a single-blind randomized controlled trial. Neurorehabilit Neural Repair. 2015;29(2):183–92.
Taveggia G, Borboni A, Salvi L, Mulé C, Fogliaresi S, Villafañe JH, Casale R. Efficacy of robot-assisted rehabilitation for the functional recovery of the upper limb in post-stroke patients: a randomized controlled study. Eur J Phys Rehabil Med. 2016;52(6):767–73.
PubMed Google Scholar
Song AG, Wu CC, Ni DJ, Li HJ, Qin HY. One-therapist to three-patient Telerehabilitation Robot System for the Upper Limb after Stroke. Int J Social Robot. 2016;8(2):319–29.
Trujillo P, Mastropietro A, Scano A, Chiavenna A, Mrakic-Sposta S, Caimmi M, Molteni F, Rizzo G. Quantitative EEG for Predicting Upper Limb Motor Recovery in Chronic Stroke Robot-Assisted Rehabilitation. IEEE Trans Neural Syst Rehabil Eng. 2017;25(7):1058–67.
Saita K, Morishita T, Hyakutake K, Fukuda H, Shiota E, Sankai Y, Inoue T. Combined therapy using botulinum toxin A and single joint hybrid assistive limb for upper-limb disability due to spastic hemiplegia. J Neurol Sci. 2017;373:182–7.
Article CAS PubMed Google Scholar
Hsieh YW, Wu CY, Wang WE, Lin KC, Chang KC, Chen CC, Liu CT. Bilateral robotic priming before task-oriented approach in subacute stroke rehabilitation: a pilot randomized controlled trial. Clin Rehabil. 2017;31(2):225–33.
Gandolfi M, Vale N, Dimitrova EK, Mazzoleni S, Battini E, Benedetti MD, Gajofatto A, Ferraro F, Castelli M, Camin M et al. Effects of High-intensity Robot-assisted Hand Training on Upper Limb Recovery and Muscle Activity in Individuals With Multiple Sclerosis: A Randomized, Controlled, Single-Blinded Trial. Front Neurol 2018, 9.
Germanotta M, Cruciani A, Pecchioli C, Loreti S, Spedicato A, Meotti M, Mosca R, Speranza G, Cecchi F, Giannarelli G et al. Reliability, validity and discriminant ability of the instrumental indices provided by a novel planar robotic device for upper limb rehabilitation. J Neuroeng Rehabil 2018, 15.
Kim GW, Won YH, Seo JH, Ko MH, EFFECTS OF NEWLY DEVELOPED COMPACT ROBOT-AIDED UPPER EXTREMITY TRAINING SYSTEMS (NEURO-X. (R)) IN PATIENTS WITH STROKE: A PILOT STUDY. J Rehabil Med. 2018;50(7):607–12.
Iwamoto Y, Imura T, Suzukawa T, Fukuyama H, Ishii T, Taki S, Imada N, Shibukawa M, Inagawa T, Araki H, et al. Combination of Exoskeletal Upper Limb Robot and Occupational Therapy improve activities of Daily living function in Acute Stroke Patients. J stroke Cerebrovasc diseases: official J Natl Stroke Association. 2019;28(7):2018–25.
Dehem S, Gilliaux M, Stoquart G, Detrembleur C, Jacquemin G, Palumbo S, Frederick A, Lejeune T. Effectiveness of upper-limb robotic-assisted therapy in the early rehabilitation phase after stroke: a single-blind, randomised, controlled trial. Annals of Physical and Rehabilitation Medicine. 2019;62(5):313–20.
Hung CS, Lin KC, Chang WY, Huang WC, Chang YJ, Chen CL, Grace Yao K, Lee YY. Unilateral vs bilateral hybrid approaches for Upper Limb Rehabilitation in Chronic Stroke: a Randomized Controlled Trial. Arch Phys Med Rehabil. 2019;100(12):2225–32.
Conroy SS, Wittenberg GF, Krebs HI, Zhan M, Bever CT, Whitall J. Robot-Assisted arm training in chronic stroke: addition of transition-to-Task Practice. Neurorehabilit Neural Repair. 2019;33(9):751–61.
Bonanno L, Russo M, Bramanti A, Calabrò RS, Marino S. Functional connectivity in multiple sclerosis after robotic rehabilitative treatment: a case report. Medicine. 2019;98(17):e15047.
Leem MJ, Kim GS, Kim KH, Yi TI, Moon HI. Predictors of functional and motor outcomes following upper limb robot-assisted therapy after stroke. Int J rehabilitation Res Int Z fur Rehabilitationsforschung Revue Int de recherches de readaptation. 2019;42(3):223–8.
Kim DH, Kim KH, Lee SM. The effects of virtual reality training with Upper Limb sensory Exercise Stimulation on the AROM of Upper Limb joints, function, and concentration in chronic stroke patients. Phys Medizin Rehabilitationsmedizin Kurortmedizin. 2020;30(2):86–94.
Tartamella F, Chillura A, Pisano MF, Cacioppo A, Licari S, Caradonna D, Portaro S, Calabr RS, Bramanti P, Naro A. A case report on intensive, robot-assisted rehabilitation program for brainstem radionecrosis. Medicine 2020, 99(10).
Solaro C, Cattaneo D, Basteris A, Carpinella I, De Luca A, Mueller M, Bertoni R, Ferrarin M, Sanguineti V. Haptic vs sensorimotor training in the treatment of upper limb dysfunction in multiple sclerosis: A multi-center, randomised controlled trial. J Neurol Sci 2020, 412.
Aprile I, Germanotta M, Cruciani A, Pecchioli C, Loreti S, Papadopoulou D, Montesano A, Galeri S, Diverio M, Falsini C et al. Poststroke shoulder pain in subacute patients and its correlation with upper limb recovery after robotic or conventional treatment: A secondary analysis of a multicenter randomized controlled trial. Int J stroke: official J Int Stroke Soc 2020:1747493020937192.
Aprile I, Guardati G, Cipollini V, Papadopoulou D, Mastrorosa A, Castelli L, Monteleone S, Redolfi A, Galeri S, Germanotta M. Robotic Rehabilitation: An Opportunity to Improve Cognitive Functions in Subjects With Stroke. An Explorative Study. Front Neurol 2020, 11.
Kim GJ, Chen P. Role of instruction adherence during highly structured robotic arm training on Motor Outcomes for individuals after chronic stroke. Am J Phys Med Rehabil. 2020;99(4):353–6.
Bui KD, Wamsley CA, Shofer FS, Kolson DL, Johnson MJ. Robot-Based Assessment of HIV-Related Motor and Cognitive Impairment for Neurorehabilitation. IEEE Trans Neural Syst Rehabil Eng. 2021;29:576–86.
Flynn N, Froude E, Cooke D, Dennis J, Kuys S. The sustainability of upper limb robotic therapy for stroke survivors in an inpatient rehabilitation setting. Disabil Rehabil 2021.
Terranova TT, Simis M, Santos ACA, Alfieri FM, Imamura M, Fregni F, Battistella LR. Robot-Assisted Therapy and Constraint-Induced Movement Therapy for Motor Recovery in Stroke: Results From a Randomized Clinical Trial. Front Neurorobotics 2021, 15.
Qu QM, Lin YN, He ZJ, Fu JH, Zou F, Jiang ZW, Guo FX, Jia J. The Effect of Applying Robot-Assisted Task-Oriented Training Using Human-Robot Collaborative Interaction Force Control Technology on Upper Limb Function in Stroke Patients: Preliminary Findings. BioMed research international 2021, 2021.
Abd El-Kafy EM, Alshehri MA, El-Fiky AAR, Guermazi MA, Mahmoud HM. The Effect of Robot-Mediated Virtual Reality Gaming on Upper Limb Spasticity Poststroke: A Randomized-Controlled Trial. Games for health journal .
Hu XL, Tong KY, Wei XJ, Rong W, Susanto EA, Ho SK. The effects of post-stroke upper-limb training with an electromyography (EMG)-driven hand robot. J Electromyogr Kinesiol. 2013;23(5):1065–74.
Squeri V, Masia L, Giannoni P, Sandini G, Morasso P. Wrist rehabilitation in chronic stroke patients by means of adaptive, progressive robot-aided therapy. IEEE Trans neural Syst rehabilitation engineering: publication IEEE Eng Med Biology Soc. 2014;22(2):312–25.
Article CAS Google Scholar
Hsieh YW, Lin KC, Wu CY, Lien HY, Chen JL, Chen CC, Chang WH. Predicting clinically significant changes in motor and functional outcomes after robot-assisted stroke rehabilitation. Arch Phys Med Rehabil. 2014;95(2):316–21.
Chen W, Cui X, Zhang J, Wang J. A cable-driven wrist robotic rehabilitator using a novel torque-field controller for human motion training. Rev Sci Instrum. 2015;86(6):065109.
McKenzie A, Dodakian L, See J, Le V, Quinlan EB, Bridgford C, Head D, Han VL, Cramer SC. Validity of Robot-Based assessments of Upper extremity function. Arch Phys Med Rehabil. 2017;98(10):1969–76.
Housley SN, Wu D, Richards K, Belagaje S, Ghovanloo M, Butler AJ. Improving Upper Extremity Function and Quality of Life with a Tongue Driven Exoskeleton: A Pilot Study Quantifying Stroke Rehabilitation. Stroke Res Treat 2017, 2017.
Palermo E, Hayes DR, Russo EF, Calabro RS, Pacilli A, Filoni S. Translational effects of robot-mediated therapy in subacute stroke patients: an experimental evaluation of upper limb motor recovery. Peerj 2018, 6.
Picelli A, Munari D, Modenese A, Filippetti M, Saggioro G, Gandolfi M, Corain M, Smania N. Robot-assisted arm training for treating adult patients with distal radius fracture: a proof-of-concept pilot study. Eur J Phys Rehabil Med. 2020;56(4):444–50.
Bouteraa Y, Ben Abdallah I, Elmogy A. Design and control of an exoskeleton robot with EMG-driven electrical stimulation for upper limb rehabilitation. Industrial Robot-the International Journal of Robotics Research and Application. 2020;47(4):489–501.
Shi XQ, Heung HL, Tang ZQ, Li Z, Tong KY. Effects of a Soft Robotic Hand for Hand Rehabilitation in Chronic Stroke Survivors. J stroke Cerebrovasc diseases: official J Natl Stroke Association. 2021;30(7):105812.
Hwang CH, Seong JW, Son DS. Individual finger synchronized robot-assisted hand rehabilitation in subacute to chronic stroke: a prospective randomized clinical trial of efficacy. Clin Rehabil. 2012;26(8):696–704.
Bishop L, Gordon AM, Kim H. Hand Robotic Therapy in Children with Hemiparesis: a pilot study. Am J Phys Med Rehabil. 2017;96(1):1–7.
Villafañe JH, Taveggia G, Galeri S, Bissolotti L, Mullè C, Imperio G, Valdes K, Borboni A, Negrini S. Efficacy of short-term Robot-Assisted Rehabilitation in patients with Hand Paralysis after Stroke: a Randomized Clinical Trial. Hand (New York NY). 2018;13(1):95–102.
Kuo FL, Lee HC, Hsiao HY, Lin JC. Robotic-assisted hand therapy for improvement of hand function in children with cerebral palsy: a case series study. Eur J Phys Rehabil Med. 2020;56(2):237–42.
Klamroth-Marganska V, Blanco J, Campen K, Curt A, Dietz V, Ettlin T, Felder M, Fellinghauer B, Guidali M, Kollmar A, et al. Three-dimensional, task-specific robot therapy of the arm after stroke: a multicentre, parallel-group randomised trial. Lancet Neurol. 2014;13(2):159–66.
Vanmulken D, Spooren AIF, Bongers HMH, Seelen HAM. Robot-assisted task-oriented upper extremity skill training in cervical spinal cord injury: a feasibility study. Spinal Cord. 2015;53(7):547–51.
Kim GJ, Hinojosa J, Rao AK, Batavia M, O’Dell MW. Randomized Trial on the Effects of Attentional Focus on Motor Training of the Upper Extremity Using Robotics With Individuals After Chronic Stroke. Arch Phys Med Rehabil 2017, 98(10):1924–31.
Chen ZJ, Gu MH, He C, Xiong CH, Xu J, Huang XL. Robot-Assisted Arm Training in Stroke Individuals With Unilateral Spatial Neglect: A Pilot Study. Front Neurol 2021, 12.
Lee KS, Park JH, Beom J, Park HS. Design and Evaluation of Passive Shoulder Joint Tracking Module for Upper-Limb Rehabilitation Robots. Front Neurorobotics 2018, 12.
Kim MS, Kim SH, Noh SE, Bang HJ, Lee KM. Robotic-assisted Shoulder Rehabilitation Therapy effectively improved Poststroke Hemiplegic Shoulder Pain: a Randomized Controlled Trial. Arch Phys Med Rehabil. 2019;100(6):1015–22.
Sale P, Mazzoleni S, Lombardi V, Galafate D, Massimiani MP, Posteraro F, Damiani C, Franceschini M. Recovery of hand function with robot-assisted therapy in acute stroke patients: a randomized-controlled trial. Int J rehabilitation Res Int Z fur Rehabilitationsforschung Revue Int de recherches de readaptation. 2014;37(3):236–42.
Biggar S, Yao W. Design and evaluation of a Soft and Wearable Robotic Glove for Hand Rehabilitation. IEEE Trans neural Syst rehabilitation engineering: publication IEEE Eng Med Biology Soc. 2016;24(10):1071–80.
Orihuela-Espina F, Roldán GF, Sánchez-Villavicencio I, Palafox L, Leder R, Sucar LE, Hernández-Franco J. Robot training for hand motor recovery in subacute stroke patients: a randomized controlled trial. J hand therapy: official J Am Soc Hand Therapists. 2016;29(1):51–7. quiz 57.
Vanoglio F, Bernocchi P, Mule C, Garofali F, Mora C, Taveggia G, Scalvini S, Luisa A. Feasibility and efficacy of a robotic device for hand rehabilitation in hemiplegic stroke patients: a randomized pilot controlled study. Clin Rehabil. 2017;31(3):351–60.
Nam C, Rong W, Li W, Xie Y, Hu X, Zheng Y. The Effects of Upper-Limb Training assisted with an Electromyography-Driven Neuromuscular Electrical Stimulation Robotic Hand on Chronic Stroke. Front Neurol. 2017;8:679.
Aprile I, Germanotta M, Cruciani A, Pecchioli C, Loreti S, Papadopoulou D, Montesano A, Galeri S, Diverio M, Falsini C, et al. Poststroke shoulder pain in subacute patients and its correlation with upper limb recovery after robotic or conventional treatment: a secondary analysis of a multicenter randomized controlled trial. Int J stroke: official J Int Stroke Soc. 2021;16(4):396–405.
Shin JH, Bog Park S, Ho Jang S. Effects of game-based virtual reality on health-related quality of life in chronic stroke patients: a randomized, controlled study. Comput Biol Med. 2015;63:92–8.
Pulman J, Buckley E. Assessing the efficacy of different upper limb hemiparesis interventions on improving health-related quality of life in stroke patients: a systematic review. Top Stroke Rehabil. 2013;20(2):171–88.
Fernández-Vázquez D, Cano-de-la-Cuerda R, Navarro-López V. Haptic Glove Systems in Combination with Semi-Immersive Virtual Reality for Upper Extremity Motor Rehabilitation after Stroke: A Systematic Review and Meta-Analysis. Int J Environ Res Public Health 2022, 19(16).
Wonsick M, Padir TJAS. A systematic review of virtual reality interfaces for controlling and interacting with robots. 2020, 10(24):9051.
Guillén-Climent S, Garzo A, Muñoz-Alcaraz MN, Casado-Adam P, Arcas-Ruiz-Ruano J, Mejías-Ruiz M. Mayordomo-Riera FJJJon, rehabilitation: A usability study in patients with stroke using MERLIN, a robotic system based on serious games for upper limb rehabilitation in the home setting. 2021, 18(1):1–16.
Zanatta F, Giardini A, Pierobon A, D’Addario M, Steca P. A systematic review on the usability of robotic and virtual reality devices in neuromotor rehabilitation: patients’ and healthcare professionals’ perspective. BMC Health Serv Res. 2022;22(1):523.
Babaiasl M, Mahdioun SH, Jaryani P, Yazdani MJD, Technology RA. A review of technological and clinical aspects of robot-aided rehabilitation of upper-extremity after stroke. 2016, 11(4):263–80.
Bessler J, Prange-Lasonder GB, Schulte RV, Schaake L, Prinsen EC, Buurke JH. Occurrence and type of adverse events during the Use of Stationary Gait Robots-A systematic literature review. Front Rob AI. 2020;7:557606.
Housman SJ, Scott KM, Reinkensmeyer DJ. A randomized controlled trial of gravity-supported, computer-enhanced arm exercise for individuals with severe hemiparesis. Neurorehabilit Neural Repair. 2009;23(5):505–14.
Milot MH, Spencer SJ, Chan V, Allington JP, Klein J, Chou C, Bobrow JE, Cramer SC, Reinkensmeyer DJ. A crossover pilot study evaluating the functional outcomes of two different types of robotic movement training in chronic stroke survivors using the arm exoskeleton BONES. J Neuroeng Rehabil. 2013;10:112.
Page SJ, Hill V, White S. Portable upper extremity robotics is as efficacious as upper extremity rehabilitative therapy: a randomized controlled pilot trial. Clin Rehabil. 2013;27(6):494–503.
Cimolin V, Germiniasi C, Galli M, Condoluci C, Beretta E, Piccinini LJJoD, Disabilities P. Robot-Assisted upper limb training for hemiplegic children with cerebral palsy. 2019, 31(1):89–101.
Krebs HI, Saitoh E, Hogan N. Robotic therapy and the Paradox of the diminishing number of degrees of Freedom. Phys Med Rehabil Clin N Am. 2015;26(4):691–702.
Bessler J, Prange-Lasonder GB, Schaake L, Saenz JF, Bidard C, Fassi I, Valori M, Lassen AB, Buurke JH. Safety Assessment of Rehabilitation Robots: a review identifying Safety Skills and current knowledge gaps. Front Rob AI. 2021;8:602878.
Abu-Dakka FJ, Valera A, Escalera JA, Abderrahim M, Page A, Mata V. Passive Exercise Adaptation for Ankle Rehabilitation Based on Learning Control Framework. Sens (Basel) 2020, 20(21).
Martí Carrillo F, Butchart J, Knight S, Scheinberg A, Wise L, Sterling L. McCarthy CJAToH-RI: Adapting a general-purpose social robot for paediatric rehabilitation through in situ design. 2018, 7(1):1–30.
Chisholm KJ, Klumper K, Mullins A, Ahmadi M. A task oriented haptic gait rehabilitation robot. Mechatronics. 2014;24(8):1083–91.
Nielsen J. Usability 101: introduction to usability. Fremont, CA: Nielsen Norman Group. In.; 2012.
Monardo G, Pavese C, Giorgi I, Godi M, Colombo R. Evaluation of patient motivation and satisfaction during technology-assisted Rehabilitation: an Experiential Review. Games for health journal. 2021;10(1):13–27.
Tousignant M, Boissy P, Moffet H, Corriveau H, Cabana F, Marquis F, Simard JJT. e-Health: Patients’ satisfaction of healthcare services and perception with in-home telerehabilitation and physiotherapists’ satisfaction toward technology for post-knee arthroplasty: an embedded study in a randomized trial. 2011, 17(5):376–82.
Andrade RM, Sapienza S, Bonato P. Development of a “transparent operation mode” for a lower-limb exoskeleton designed for children with cerebral palsy. In: 2019 IEEE 16th international conference on rehabilitation robotics (ICORR): 2019 : IEEE; 2019: 512–517.
Wang J, Li J, Zhang Y, Wang S. Design of an exoskeleton for index finger rehabilitation. In: 2009 Annual International Conference of the IEEE Engineering in Medicine and Biology Society: 2009 : IEEE; 2009: 5957–5960.
Sampson M, Shau YW, King MJ. Bilateral upper limb trainer with virtual reality for post-stroke rehabilitation: case series report. Disabil rehabilitation Assist Technol. 2012;7(1):55–62.
Zhang K, Chen X, Liu F, Tang H, Wang J, Wen W. System Framework of Robotics in Upper Limb Rehabilitation on Poststroke Motor Recovery. Behavioural neurology 2018, 2018:6737056.
He Y, Eguren D, Luu TP, Contreras-Vidal JL. Risk management and regulations for lower limb medical exoskeletons: a review. Med devices (Auckland NZ). 2017;10:89–107.
Akiyama Y, Yamada Y, Ito K, Oda S, Okamoto S, Hara S. Test method for contact safety assessment of a wearable robot -analysis of load caused by a misalignment of the knee joint. In: 2012 IEEE RO-MAN: The 21st IEEE International Symposium on Robot and Human Interactive Communication: 9–13 Sept. 2012 2012 ; 2012: 539–544.
Melia M, Geissler B, König J, Ottersbach HJ, Umbreit M, Letzel S, Muttray A. Pressure pain thresholds: subject factors and the meaning of peak pressures. Eur J Pain. 2019;23(1):167–82.
Ferreira F, Chaves MEA, Oliveira VC, Van Petten A, Vimieiro CBS. Effectiveness of robot therapy on body function and structure in people with limited upper limb function: a systematic review and meta-analysis. PLoS ONE. 2018;13(7):e0200330.
Lohse KR, Lang CE, Boyd LA. Is more better? Using metadata to explore dose-response relationships in stroke rehabilitation. Stroke. 2014;45(7):2053–8.
Hayward KS, Brauer SG. Dose of arm activity training during acute and subacute rehabilitation post stroke: a systematic review of the literature. Clin Rehabil. 2015;29(12):1234–43.
Kwakkel, GJp. Intensity of practice after stroke: More is better. 2009, 7:24.
Wilson RD, Chae J. Hemiplegic Shoulder Pain. Phys Med Rehabil Clin North Am. 2015;26(4):641–55.
Tran DA, Pajaro-Blazquez M, Daneault JF, Gallegos JG, Pons J, Fregni F, Bonato P, Zafonte R. Combining Dopaminergic Facilitation with Robot-Assisted Upper Limb Therapy in Stroke Survivors: a focused review. Am J Phys Med Rehabil. 2016;95(6):459–74.
Kafri M, Myslinski MJ, Gade VK, Deutsch JE. Energy expenditure and exercise intensity of interactive video gaming in individuals poststroke. Neurorehabil Neural Repair. 2014;28(1):56–65.
Cuesta-Gómez A, Sánchez-Herrera-Baeza P, Oña-Simbaña ED, Martínez-Medina A, Ortiz-Comino C, Balaguer-Bernaldo-de-Quirós C, Jardón-Huete A. Cano-de-la-Cuerda RJJon, rehabilitation: Effects of virtual reality associated with serious games for upper limb rehabilitation in patients with multiple sclerosis: Randomized controlled trial. 2020, 17(1):1–10.
Laut J, Porfiri M, Raghavan P. The Present and Future of Robotic Technology in Rehabilitation. Curr Phys Med Rehabilitation Rep. 2016;4(4):312–9.
Francisco GE, Yozbatiran N, Berliner J, OʼMalley MK, Pehlivan AU, Kadivar Z, Fitle K, Boake C. Robot-Assisted training of arm and Hand Movement shows functional improvements for incomplete cervical spinal cord Injury. Am J Phys Med Rehabil. 2017;96(10 Suppl 1):171–s177.
Tousignant M, Boissy P, Moffet H, Corriveau H, Cabana F, Marquis F, Simard J. Patients’ satisfaction of healthcare services and perception with in-home telerehabilitation and physiotherapists’ satisfaction toward technology for post-knee arthroplasty: an embedded study in a randomized trial. Telemedicine J e-health: official J Am Telemedicine Association. 2011;17(5):376–82.
Shirley ED, Sanders JO. Patient satisfaction: implications and predictors of success. J bone joint Surg Am volume. 2013;95(10):e69.
Monardo G, Pavese C, Giorgi I, Godi M, Colombo RJGfhj. Evaluation of patient motivation and satisfaction during technology-assisted rehabilitation: an experiential review. 2021, 10(1):13–27.
Kwon SH, Lee BS, Lee HJ, Kim EJ, Lee JA, Yang SP, Kim TY, Pak HR, Kim HK, Kim HYJAoRM. Energy efficiency and patient satisfaction of gait with knee-ankle-foot orthosis and robot (ReWalk)-assisted gait in patients with spinal cord injury. 2020, 44(2):131–41.
Kim DJ, Hazlett-Knudsen R, Culver-Godfrey H, Rucks G, Cunningham T, Portee D, Bricout J, Wang Z, Behal A. How autonomy impacts performance and satisfaction: results from a study with spinal cord injured subjects using an Assistive Robot. IEEE Trans Syst Man Cybernetics - Part A: Syst Hum. 2012;42(1):2–14.
Ramos Muñoz EJ, Swanson VA, Johnson C, Anderson RK, Rabinowitz AR, Zondervan DK, Collier GH, Reinkensmeyer DJ. Using large-scale Sensor Data to test factors predictive of perseverance in Home Movement Rehabilitation: optimal challenge and steady Engagement. Front Neurol. 2022;13:896298.
Akbari A, Haghverd F, Behbahani S. Robotic home-based Rehabilitation Systems Design: from a literature review to a conceptual Framework for Community-Based remote therapy during COVID-19 pandemic. Front Rob AI. 2021;8:612331.
Kutner NG, Zhang R, Butler AJ, Wolf SL, Alberts JL. Quality-of-life change Associated with robotic-assisted therapy to Improve Hand Motor function in patients with Subacute Stroke: a Randomized Clinical Trial. Phys Ther. 2010;90(4):493–504.
Mohammed SA, Shebl AM. Quality of life among egyptian patients with Upper and Lower Limb Amputation: sex differences. Adv Med. 2014;2014:674323.
Rasouli S, Gupta G, Nilsen E, Dautenhahn K. Potential applications of Social Robots in Robot-Assisted interventions for social anxiety. Int J Social Robot. 2022;14(5):1–32.
Kawatsuma S, Mimura R, Asama H. Unitization for portability of emergency response surveillance robot system: experiences and lessons learned from the deployment of the JAEA-3 emergency response robot at the Fukushima Daiichi Nuclear Power plants. ROBOMECH J. 2017;4(1):6.
Treviño LR, Roberge P, Auer ME, Morales A, Torres-Reveron A. Predictors of functional outcome in a cohort of hispanic patients using Exoskeleton Rehabilitation for Cerebrovascular Accidents and Traumatic Brain Injury. Front Neurorobotics. 2021;15:682156.
Download references
Acknowledgements
The authors thank the Central Library and Documentation Center of Kerman University of Medical Sciences for providing access to the knowledge base references required for this study.
This study was supported by the Medical Informatics Research Center of Kerman University of Medical Sciences (Code: 400000856). The funder had no roles in study design, data gathering and analysis.
Author information
Authors and affiliations.
Medical Informatics Research Center, Institute for Futures Studies in Health, Kerman University of Medical Sciences, Kerman, Iran
Khadijeh Moulaei & Kambiz Bahaadinbeigy
HIV/STI Surveillance Research Center, WHO Collaborating Center for HIV Surveillance, Institute for Futures Studies in Health, Kerman University of Medical Sciences, Kerman, Iran
Ali Akbar Haghdoostd
Department of Physical Therapy, Faculty of Allied Medicine, Kerman University of Medical Sciences, Kerman, Iran
Mansour Shahabi Nezhad
Department of Health Information Management, School of Health Management and Information Sciences, Iran University of Medical Sciences, Tehran, Iran
Abbas Sheikhtaheri
You can also search for this author in PubMed Google Scholar
Contributions
Authors’ contributions: KHM and ASH have designed the study, finalized the search strategy and implemented the thematic analysis, AH and KB have searched and screened the articles and extracted the initial codes for data charting. ASH has supervised the whole review process, KHM has contributed in revising the finalizing data analysis and KB and ASH have technically edited and finalized the article. The authors read and approved the final manuscript.
Corresponding author
Correspondence to Abbas Sheikhtaheri .
Ethics declarations
Ethical approval, consent for publication.
Not applicable.
Competing interests
The authors declare that there are no conflicts of interest.
Additional information
Publisher’s note.
Springer Nature remains neutral with regard to jurisdictional claims in published maps and institutional affiliations.
Electronic supplementary material
Below is the link to the electronic supplementary material.
Supplementary Material 1 Aims and scope statement
Supplementary material 2: appendix a, supplementary material 3: appendix b, supplementary material 4: appendix c, rights and permissions.
Open Access This article is licensed under a Creative Commons Attribution 4.0 International License, which permits use, sharing, adaptation, distribution and reproduction in any medium or format, as long as you give appropriate credit to the original author(s) and the source, provide a link to the Creative Commons licence, and indicate if changes were made. The images or other third party material in this article are included in the article’s Creative Commons licence, unless indicated otherwise in a credit line to the material. If material is not included in the article’s Creative Commons licence and your intended use is not permitted by statutory regulation or exceeds the permitted use, you will need to obtain permission directly from the copyright holder. To view a copy of this licence, visit http://creativecommons.org/licenses/by/4.0/ . The Creative Commons Public Domain Dedication waiver ( http://creativecommons.org/publicdomain/zero/1.0/ ) applies to the data made available in this article, unless otherwise stated in a credit line to the data.
Reprints and permissions
About this article
Cite this article.
Moulaei, K., Bahaadinbeigy, K., Haghdoostd, A.A. et al. Overview of the role of robots in upper limb disabilities rehabilitation: a scoping review. Arch Public Health 81 , 84 (2023). https://doi.org/10.1186/s13690-023-01100-8
Download citation
Received : 25 November 2022
Accepted : 29 April 2023
Published : 08 May 2023
DOI : https://doi.org/10.1186/s13690-023-01100-8
Share this article
Anyone you share the following link with will be able to read this content:
Sorry, a shareable link is not currently available for this article.
Provided by the Springer Nature SharedIt content-sharing initiative
- Rehabilitation
- Disabilities
- Digital Health
Archives of Public Health
ISSN: 2049-3258
- General enquiries: [email protected]
- Reference Manager
- Simple TEXT file
People also looked at
Systematic review article, a systematic review of robotic rehabilitation for cognitive training.
- 1 Department of Mechanical, Aerospace, and Biomedical Engineering, University of Tennessee, Knoxville, Knoxville, TN, United States
- 2 School of Nursing, MGH Institute of Health Professions, Boston, MA, United States
A large and increasing number of people around the world experience cognitive disability. Rehabilitation robotics has provided promising training and assistance approaches to mitigate cognitive deficits. In this article, we carried out a systematic review on recent developments in robot-assisted cognitive training. We included 99 articles in this work and described their applications, enabling technologies, experiments, and products. We also conducted a meta analysis on the articles that evaluated robot-assisted cognitive training protocol with primary end users (i.e., people with cognitive disability). We identified major limitations in current robotics rehabilitation for cognitive training, including the small sample size, non-standard measurement of training and uncontrollable factors. There are still multifaceted challenges in this field, including ethical issues, user-centered (or stakeholder-centered) design, the reliability, trust, and cost-effectiveness, personalization of the robot-assisted cognitive training system. Future research shall also take into consideration human-robot collaboration and social cognition to facilitate a natural human-robot interaction.
1. Introduction
It is estimated that ~15% of the world's population, over a billion people, experience some form of disability and a large proportion of this group specifically experience cognitive disability ( WHO, 2011 ). The number of people with disabilities is increasing not only because of the growing aging population who have a higher risk of disability but also due to the global increase in chronic health conditions ( Hajat and Stein, 2018 ). Individuals with cognitive disability, such as Alzheimer's disease (AD) or Autism spectrum disorder (ASD), may have a substantial limitation in their capacity for functional mental tasks, including conceptualizing, planning, sequencing thoughts and actions, remembering, interpreting subtle social cues, and manipulating numbers and symbols ( LoPresti et al., 2008 ). This vulnerable population is usually associated with significant distress or disability in their social, occupational, or other important activities.
With recent advancements of robotics and information and communication technologies (ICTs), rehabilitation robots hold promise in augmenting human healthcare and in aiding exercise and therapy for people with cognitive disabilities. As an augmentation of human caregivers with respect to the substantial healthcare labor shortage and the high burden of caregiving, robots may provide care with high repeatability and without any complaints and fatigue ( Taheri et al., 2015b ). In a meta analysis comparing how people interacted with physical robots and virtual agents, Li (2015) showed that physically present robots were found to be more persuasive, perceived more positively, and result in better user performance compared to virtual agents. Furthermore, robots can facilitate social interaction, communication and positive mood to improve the performance and effectiveness of cognitive training ( Siciliano and Khatib, 2016 ). For example, a recent study ( Pino et al., 2020 ) showed that older adults with mild cognitive impairment (MCI) that received memory training through the humanoid social robot (NAO) achieved more visual gaze, less depression, and better therapeutic behavior. Physically embodied robots hold promise as accessible, effective tools for cognitive training and assistance in future.
There have been a few literature reviews on physical rehabilitation ( Bertani et al., 2017 ; Kim et al., 2017 ; Morone et al., 2017 ; Veerbeek et al., 2017 ), or cognitive rehabilitation for specific user populations, such as children with ASD ( Pennisi et al., 2016 ) and older adults ( Mewborn et al., 2017 ). To the authors' best knowledge, this article presents the first systematic review that focuses on robotic rehabilitation for cognitive training. We present applications, enabling technologies, and products of robotics rehabilitation based on research papers research papers focusing on cognitive training. We also discuss several challenges to the development of robots for cognitive training and present future research directions.
2.1. Search Strategy
We conducted a systematic review in the datasets of Google Scholar, Crossref, PubMed, Scopus, and Web of Science using the key words (“robot” OR “robots” OR “robotics” OR “robotic”) AND (“cognitive training” OR “cognitive rehabilitation” OR “cognitive therapy” OR “cognitive recovery” OR “cognitive restore”). The search was limited to the articles published between 2015 and December 14, 2020. The search in Google Scholar yielded 5,630 articles. Google Scholar ranks sources according to relevance, which takes into account the full text of each source as well as the source's author, the publication in which the source appeared and how often it has been cited in scholarly literature ( University of Minnesota Libraries, 2021 ). We screened the titles of the first 500 articles and excluded the remaining results due to their low relevance. After the analysis of abstracts and written languages, 328 articles were further excluded due to duplication (i.e., exact copy of two works), non-English language, and/or not pertaining to the research topic. Then 172 full articles were reviewed for eligibility. The articles that were aimed for physical rehabilitation or review articles were excluded. Finally, 80 eligible articles were included for further analysis. As illustrated in the PRISMA flow diagram (see Figure 1 ), with the keywords we initially found 200, 31, 106, and 50 articles in the datasets of Crossref, PubMed, Scopus and Web of Science for screening, respectively. These articles were combined with the 80 additional articles from Google Scholar for further screening. After the analysis of titles, abstracts, written languages and types of article, 238 articles were excluded due to duplication, non-English language, non-eligible article types (e.g., book chapter, book, dataset, report, reference entry, editorial and systematic review), and/or not pertaining to the research topic. Then 229 full articles were reviewed for eligibility. The articles that were aimed for physical rehabilitation or review articles were excluded. Finally, 99 eligible articles were included in this systematic review, including journal articles and conference papers presented at conference, symposium and workshop. These papers included the contents of applications, user population, supporting technologies, experimental studies, and/or robot product(s). Moreover, 53 articles that included experimental study of robot-assisted cognitive training with primary end users (i.e., people with cognitive disability) were identified for further meta-analysis.
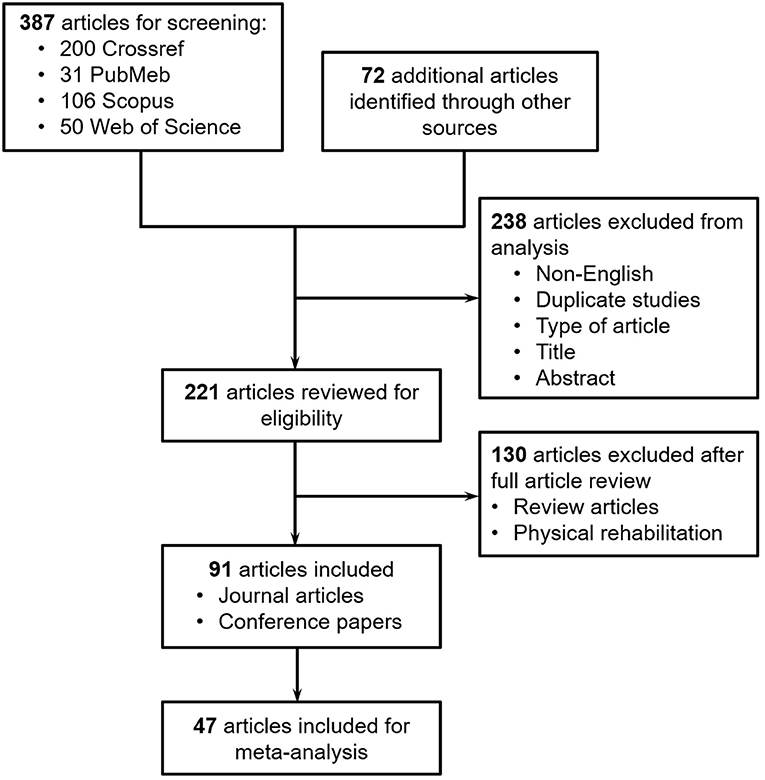
Figure 1 . PRISMA flow diagram illustrating the exclusion criteria and stages of the systematic review.
The literature include cognitive training robots in the forms of companion robots, social robots, assistive robots, social assisted robots, or service robots, which are collectively referred to as “cognitive training robots” in this systematic review. The literature employed different terminologies for cognitive training by rehabilitation robots, such as robot-enhanced therapy ( David et al., 2018 ; Richardson et al., 2018 ), robot-assisted intervention ( Scassellati et al., 2018 ), robot-assisted treatment ( Taheri et al., 2015a ), robot-assisted training ( Tsiakas et al., 2018 ), robot-assisted therapy ( Sandygulova et al., 2019 ), robot-mediated therapy ( Begum et al., 2015 ; Huskens et al., 2015 ). Here, we do not distinguish between these different terms and instead adopt the term of “robot-assisted training” to represent all these different terms.
3.1. Applications
The studies on robot-assisted cognitive training are categorized in terms of their applications and end users in Table 1 . To date, the most researched application (36 out of 98 articles, as shown in Table 1 ) of robots in cognitive training is to improve individual social communication skills, which may include joint attention skills, imitation skills, turn-taking skills and other social interaction skills. For example, Kajopoulos et al. (2015) designed a robot-assisted training protocol based on response to joint attention for children with ASD. The training protocol used a modified attention cueing paradigm, where the robot's head direction cued children's spatial attention to a stimulus presented on one side of the robot. The children were engaged in a game that could be completed only through following the robot's head direction. To aid in the training of imitation skills in children with ASD, Taheri et al. (2020) proposed a reciprocal gross imitation human–robot interaction platform, in which ASD children are expected to imitate the robot body gestures, including a combination of arms, feet, neck, and torso movements. David et al. (2020) developed a robot-enhanced intervention on turn-taking abilities in ASD children. In their protocol, the robot provided instruction (e.g., “Now is my turn”) to the child, checked if the child moved the picture as instructed, and provided feedback (e.g., “Good job”) to the child if the child respected turns by staying with his or her hands still, without interrupting the robot. Robot-assisted cognitive training showed increased cognitive capabilities for people with limited social capabilities, such as children with ASD ( Huijnen et al., 2016 ; Esteban et al., 2017 ; Marino et al., 2019 ) and people with dementia ( Sung et al., 2015 ; Yu et al., 2015 ; Otaki and Otake, 2017 ). Due to cognitive impairment, individuals with dementia may also show deficits in social functioning, such as social withdrawal ( Havins et al., 2012 ; Dickerson, 2015 ). In the pilot study by Sung et al. (2015) about robot-assisted therapy using socially assistive pet robot (PARO), institutionalized older adults showed significantly increased communication and interaction skills and activity participation after receiving 4-week robot-assisted therapy. Another robotic application is to provide intervention to enhance people's impaired cognitive function, such as memory ( Paletta et al., 2018 ), attention ( Lins et al., 2019 ) and concentration ( Tleubayev et al., 2019 ), or reduce their negative psychophysiological feelings, such as stress ( Aminuddin et al., 2016 ) and anxiety ( Ab Aziz et al., 2015 ). Additionally, a few studies adopted the robots to facilitate learning and educational activities for people with cognitive disabilities, such as children with dyslexia ( Andruseac et al., 2015 ) or Traumatic Brain Injury (TBI) ( Barco Martelo and Fosch Villaronga, 2017 ).
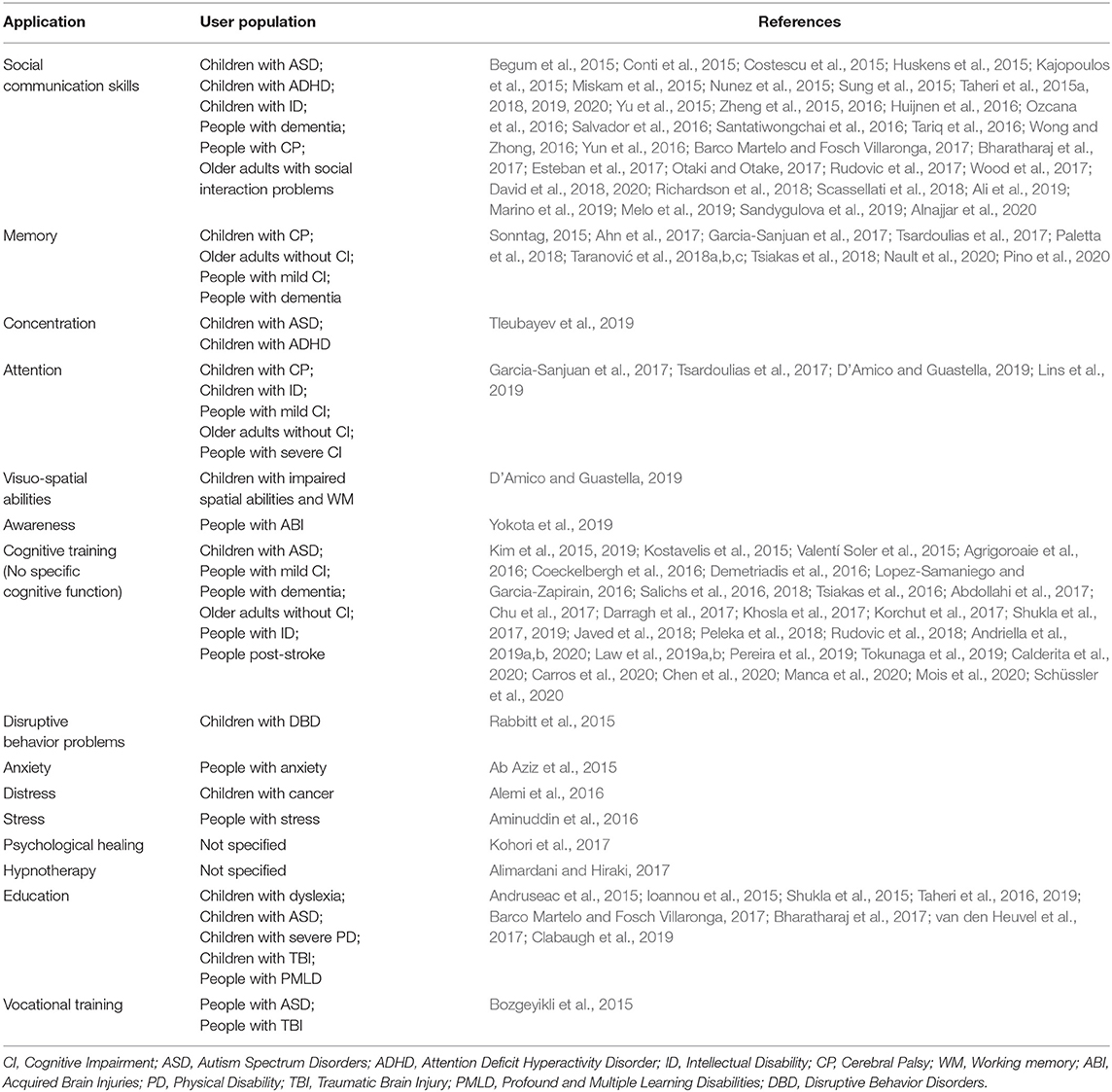
Table 1 . Type of robot-assisted cognitive training and end-user population.
3.2. Enabling Technologies
Recent development in robotics, ICTs, multimodal human-robot interaction, and artificial intelligence leads to significant process in robot-assisted cognitive training and rehabilitation. This section presents a summary on a few important enabling technologies that foster the advancement of robotic rehabilitation for cognitive training, including multimodal perception, multimodal feedback, gamification, virtual and augmented reality, and artificial intelligence.
3.2.1. Design of Physical Appearance
A robot can have a human-like ( Miskam et al., 2015 ; Peleka et al., 2018 ; Taheri et al., 2019 ), animal-like ( Cao et al., 2015 ; Sung et al., 2015 ), or unfamiliar appearance ( Scassellati et al., 2018 ). Besides the appearance, the size, softness, and comfort of the robot can also have an impact on users' perception, affection, cognitive load, and gaze following during interaction ( Kohori et al., 2017 ), and thus the effectiveness of cognitive training. It remains unclear how users' perception is specifically affected by the robot's appearance. On the one hand, the human-like appearance was indicated to significantly positively affect users' perception of anthropomorphism, animacy, likeability, and intelligence toward robots, compared to a snowman-like appearance ( Haring et al., 2016 ). On the other hand, increasing human-like appearance was found not to necessarily increase performance in human-robot interaction. For example, in the survey of expectation about the robots' appearance in robot-assisted ASD therapy, zoomorphic robots were indicated to be less ethically problematic than robots that looked too much like humans ( Coeckelbergh et al., 2016 ). Some of their participants (i.e., parents, therapists, or teachers of children with ASD) worried about the possibility that the robot is perceived by the child as a friend, or that the robot looks too human-like. The relation between robots' human-like appearance and people's reaction to them may relate to the uncanny valley theory ( Mori et al., 2012 ), which describes that people's familiarity (or affinity) with robots increases as the robots appear more human-like but when the robots are almost human, people's response would shift to revulsion. Tung (2016) observed an uncanny valley between the degree of anthropomorphism and children's attitudes toward the robots. In the review paper to study factors affecting social robot acceptability in older adults including people with dementia or cognitive impairment, Whelan et al. (2018) found that there is a lack of consensus regarding to the optimal appearance of social robots and that the uncanny valley concept varies between individuals and groups.
3.2.2. Multimodal Sensing
Having a good understanding of a user's cognitive state, affective state and surrounding environment, which is termed as multimodal perception, is a prerequisite step for robots to provide cognitive training. Usually the concept of multimodal perception involves two stages, multimodal sensing and perception. Further details on these two techniques in previous publications are individually discussed in the following. Various sensors have been adopted to facilitate a robot to achieve multimodal sensing, based on system requirements, end-user population, cost-effectiveness, etc. Among different sensing technologies, visual and auditory sensing are the most popular modalities. We summarize the multiple modalities for sensing in the following aspects.
1. Visual sensing . During human-robot interaction, visual sensors are a very popular, useful and accessible channel for perception. The advancement of technologies, such as manufacturing and ICTs, enabled researchers to integrate small, high-resolution and affordable cameras into their rehabilitation robotic system. Some studies placed cameras in the environment along with the robot ( Melo et al., 2019 ). Other studies included cameras in the robotic mechanical system. For example, in the social robot Pepper, there were 2D and 3D cameras attached to the head ( Paletta et al., 2018 ). With computational approaches, such as computer vision, the robots analyzed the video/images from the cameras and recognized users' critical states, such as their environment, facial expression, body movements, and even emotion and attention ( Paletta et al., 2018 ; Peleka et al., 2018 ; Rudovic et al., 2018 ; Mois et al., 2020 ), which led to a better perception of users ( Johal et al., 2015 ).
2. Auditory sensing . Another popular modality adopted during robot-assisted cognitive training is auditory sensing. Researchers analyzed users' auditory signals in terms of the lexical field, tone and/or volume of voice for speech recognition, emotion detection, and speaker recognition in robots ( Paletta et al., 2018 ; Peleka et al., 2018 ; Rudovic et al., 2018 ). Due to the natural and intuitive nature for users behind the auditory sensing, some end users, such as older adults preferred this sensing channel to the touch input during interaction with the robot ( Zsiga et al., 2018 ).
3. Physiological sensing . Besides visual and auditory sensing, physiological modalities have been incorporated into the robotic system, in order to have a better understanding of users' states (e.g., affective states). Previous studies show that human-robot interaction may be enhanced using physiological signals, such as heart rate, blood pressure, breathing rate, and eye movement ( Sonntag, 2015 ; Lopez-Samaniego and Garcia-Zapirain, 2016 ; Ozcana et al., 2016 ; Ahn et al., 2017 ; Alimardani and Hiraki, 2017 ). For example, Rudovic et al. (2018) employed wearable sensors to detect children's heart-rate, skin-conductance and body temperature during their robot-based therapy for children with ASD, to estimate children's levels of affective states and engagement. When studying robot-assisted training for children with ASD, Nunez et al. (2015) used a wearable device of electromyography (EMG) sensors to detect smiles from children's face.
4. Neural sensing . The inclusion of brain-imaging sensors provides capabilities to measure and/or monitor a user's brain activity and to understand the user's mental states ( Ali et al., 2019 ). This was especially useful when considering the user's cognitive states, such as level of attention and task engagement ( Alimardani and Hiraki, 2017 ; Tsiakas et al., 2018 ). Neural sensing may also be meaningful to users who have difficulty in expressing their intention and feeling because of their physical and/or cognitive limitations, such as the older adults with Alzheimer's disease. Currently, among all candidates of brain-imaging sensors, EEG and functional near-infrared spectroscopy (fNIRS) are two popular modalities due to their advantages of non-invasiveness, portability, and cost-effectiveness. For example, Lins et al. (2019) developed a robot-assisted therapy to stimulate the attention level of children with cerebral palsy and applied electroencephalogram (EEG) sensors to measure children's attention level during the therapy.
3.2.3. Multimodal Feedback
After perceiving its user and environment, a robot shall entail multimodal feedback to interact (e.g., display its behaviors and feedback) with its user in a comfortable, readable, acceptable, and effective way ( Melo et al., 2019 ). Multimodal feedback is particularly meaningful when the end users are unfamiliar with technologies or are limited in cognitive capabilities, such as older adults with dementia. Examples of feedback include voice, video, gesture, and physical appearance, all of which can affect users' perception of the robot during their interaction and thus the effectiveness of cognitive training/rehabilitation ( Ab Aziz et al., 2015 ; Rabbitt et al., 2015 ). The following list shows popular modalities for robotic feedback during interaction with human users.
1. Visual feedback . One of the most widely-used overt feedback modalities is visual feedback or graphical user interface (GUI), displaying two-dimensional information. During robot-assisted cognitive training, visual feedback was delivered through an additional computer screen ( Salvador et al., 2016 ; Taheri et al., 2019 ; Mois et al., 2020 ), or a touchscreen embodied in the robot ( Paletta et al., 2018 ; Peleka et al., 2018 ). Some principles and/or issues on the design of GUI for robot-assisted training were suggested in previous studies. For example, a few studies recommended a larger screen and a simpler interface associated with each function choice for GUI to better facilitate visual feedback during cognitive training ( Ahn et al., 2017 ).
2. Auditory feedback . Another widely used modality for feedback or interaction is auditory output, like speech during human-human interaction. This intuitive auditory communication can reduce the unfamiliarity with robotic technologies and increase the usability of system in the vulnerable population, such as the elderly population ( Zsiga et al., 2018 ). Among previous studies, this feedback was delivered in one or combined form of beep ( Nault et al., 2020 ), speech ( Ab Aziz et al., 2015 ; Miskam et al., 2015 ; Peleka et al., 2018 ; Taheri et al., 2019 ), and music ( Nunez et al., 2015 ). With auditory output, the robot provided daily communication and medication reminder, instructed cognitive training, and made emergent warning (e.g., short of battery) to the user ( Orejana et al., 2015 ).
3. Non-verbal feedback . We refer to non-verbal feedback as all non-verbal communication cues by a robotic body, such as hand gestures and other body movements ( Miskam et al., 2015 ; Taheri et al., 2019 ), eye gaze ( Taheri et al., 2019 ), eye colors ( Miskam et al., 2015 ; Taheri et al., 2019 ), and facial expression. Animation, similar to verbal language, makes a significant contribution to improving robot-assisted cognitive training. Moreover, the robot's animation can particularly introduce social interaction to a user, which is meaningful to individuals with impairments in social interaction skills.
4. Haptic feedback . Haptic feedback, by simulating the sense of touch and motion, may play important role during robot-assisted cognitive training due to the importance of touch in everyday life ( Beckerle et al., 2017 ; Cangelosi and Invitto, 2017 ). Tactile feedback is one type of haptic feedback. During robot-assisted cognitive training, haptic feedback can also be introduced using vibration via wearable devices. For example, Nault et al. (2020) developed a socially assistive robot-facilitated memory game elaborated with audio and/or haptic feedback for older adults with cognitive decline. Although there was no significant difference in participants' game accuracy, preference, and performance in their system pilot study, the results provided insight into future improvements, such as increasing the strength of haptic feedback to increase the ease of being perceived and make the system more engaging. One notable robot, Paro, with the combination of soft, plush surface and additional encouraging haptic feedback (e.g., small vibration) creates a soothing presence. In a pilot study for institutionalized older adults by Sung et al. (2015) , the communication and social skills of participants were improved by the robot-assisted therapy using Paro.
3.2.4. Gamification
Recently, game technology is becoming a popular way to motivate, engage and appeal to users in cognitive tasks, since traditional cognitive tasks are usually effortful, frustrating, repetitive, and disengaging. Serious games and brain training games are a growing research field for cognitive training ( Heins et al., 2017 ). Integration between gaming and robotic technologies has attracted increasing amount of interest in research and application, to further enhance users' engagement in cognitive training. A few types of relationships between robots and games have been developed in the literature. For example, a robot can lead or accompany users through the game for cognitive training by providing instructions on how to perform the task ( Ioannou et al., 2015 ; Chu et al., 2017 ; Tsardoulias et al., 2017 ; Scassellati et al., 2018 ; Sandygulova et al., 2019 ; Taheri et al., 2019 ; Tleubayev et al., 2019 ; Nault et al., 2020 ) or playing a role of an active player in the game ( Tariq et al., 2016 ; Melo et al., 2019 ). Additionally, a robot can provide various types of feedback (see details in section 3.2.3) to encourage users to engage in the game ( Taheri et al., 2015a ; Lopez-Samaniego and Garcia-Zapirain, 2016 ). Often, games associated with cognitive training can be integrated into the robotic systems through a GUI ( Ahn et al., 2017 ; Paletta et al., 2018 ; Peleka et al., 2018 ).
3.2.5. Virtual and Augmented Reality
Combining robot-assisted cognitive training with virtual reality (VR) and/or augmented reality (AR) techniques offers a cost-effective and efficient alternative to traditional training settings. The incorporation of VR/AR allows for replication of the tasks and environments in a more convenient and affordable way. Researchers also explored robotic cognitive training using mixed reality technology in cognitive training. For example, Sonntag (2015) presented an intelligent cognitive enhancement platform for people with dementia, where a mixed reality glass was used to deliver the storyboard (e.g., serious game for active memory training) to the user and a NAO robot served as a cognitive assistant for “daily routine.” Bozgeyikli et al. (2015) used virtual reality in a vocational rehabilitation system, which included six different modules, such as money management in a virtual grocery store, to provide vocational training for persons with ASD and TBI.
3.2.6. Artificial Intelligence
Artificial Intelligence plays a significant role in the field of robot-assisted cognitive training/rehabilitation, including applications in multimodal perception and feedback, personalization, and adaptability ( Ab Aziz et al., 2015 ; Rudovic et al., 2018 ). Given multimodal sensing, a successful multimodal perception further requires robots to integrate signals across multiple modalities of input sensors. To date, a great progress has been made thanks to the advancement of machine learning and deep learning. Multi-modal signals enable the robot with a good interpretation and understanding of its users, including their needs, intention, emotions, and surrounding environment ( Paletta et al., 2018 ). Rudovic et al. (2018) implemented deep learning in a robot for ASD therapy to automatically estimate children's valence, arousal and engagement levels. Javed et al. (2018) utilized multimodal perception, including the analyses of children's motion, speech, and facial expression, to estimate children's emotional states. Using multiple feedback modalities may overload users with redundant information, increase task completion time, and reduce the efficiency of cognitive training ( Taranović et al., 2018c ). Additionally, users may favor certain modalities over others due to personal preference or cognitive disability. Taranović et al. (2018c) designed an experiment of adaptive modality selection (AMS) in robot-assisted sequential memory exercises and applied artificial intelligence to learn the strategy that selects the appropriate combination and amount of feedback modalities tailored to different situations (e.g., environments and users). An appropriate strategy is crucial to successful long-term robot-assisted cognitive intervention. Specifically, reinforcement learning, an area of machine learning, is a promising approach to adapt and personalize the intervention to each individual user as well as to optimize the performance of robot-assisted cognitive training, due to its capability of allowing a robot to learn from its experience of interaction with users ( Sutton and Barto, 2018 ). For example, Tsiakas et al. (2016) used interactive reinforcement learning methods to facilitate the adaptive robot-assisted therapy, that is, adapt the task difficulty level and task duration to users with different skill levels (e.g., expert or novice user), in the context that users need to perform a set of cognitive or physical training tasks. Javed et al. (2018) developed a Hidden Markov model (HMM) in their adaptive framework for child-robot interaction, aiming to enable a child with ASD to engage in robot-assisted ASD therapy over long term. In their HMM, the states took into consideration a child's emotional state or mood, and the actions were the robot's behaviors or other audiovisual feedback. Clabaugh et al. (2019) utilized reinforcement learning to personalize instruction challenge levels and robot feedback based on each child's unique learning patterns for long-term in-home robot interventions. Although reinforcement learning may suffer the problem of sample inefficiency, the slowness of reinforcement learning can be overcome using techniques, such as episodic memory and meta-learning ( Botvinick et al., 2019 ).
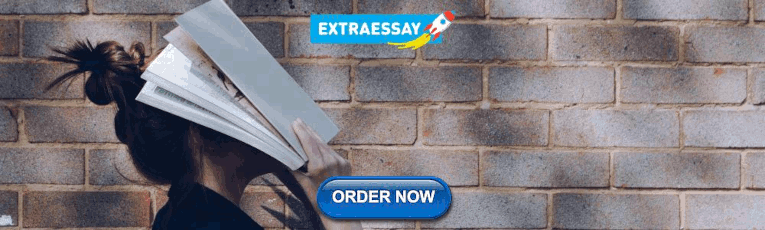
3.3. Experimental Studies
Many experimental studies have been conducted to evaluate the important properties of robotic rehabilitation, such as feasibility, safety, usability, performance, etc. On the one hand, exploratory studies including surveys and interviews with users (e.g., patients, caregivers, and therapists) have been conducted to inform the next stage of study ( Rabbitt et al., 2015 ; Coeckelbergh et al., 2016 ; Salichs et al., 2016 ; Darragh et al., 2017 ; Kohori et al., 2017 ; Korchut et al., 2017 ; Law et al., 2019b ). On the other hand, researchers have conducted experimental studies to verify and/or validate robot-assisted cognitive training systems. Table 2 shows a meta analysis for experimental studies, where the robot-assisted cognitive training was provided to primary end users (i.e., persons with cognitive disabilities). Up to date, majority of the experimental studies were conducted in a controlled lab setting, and only a few studies were conducted in an environment simulating daily activities in real world ( Scassellati et al., 2018 ).

Table 2 . Meta analysis on end-user experiments of robot-assisted cognitive training.
3.3.1. Study Design
Most experimental studies included three phases: pre-training assessment (i.e., baseline assessment), robot-assisted cognitive training, and post-training assessment ( Kajopoulos et al., 2015 ; Kim et al., 2015 ; Sung et al., 2015 ; Yu et al., 2015 ; Alemi et al., 2016 ; Taheri et al., 2016 , 2019 ; van den Heuvel et al., 2017 ; Scassellati et al., 2018 ; Marino et al., 2019 ). The effectiveness of robot-assisted training was evaluated by the comparison of pre- and post-training assessments using machine learning or statistical methods ( Kim et al., 2015 ; Yu et al., 2015 ; Scassellati et al., 2018 ; Marino et al., 2019 ; Taheri et al., 2019 ).
Most studies adopted the group-based design where participants were randomly assigned to control or intervention groups ( Kim et al., 2015 ; Sung et al., 2015 ; Yu et al., 2015 ; Marino et al., 2019 ). Some researchers employed single-case designs (or single-subject designs) to investigate the impact of social robots on cognitive training ( Ioannou et al., 2015 ; Taheri et al., 2015a ; David et al., 2018 ). For example, Ioannou et al. (2015) conducted the single-case study to explore the potential role of co-therapist of humanoid social robot, NAO, during autism therapy session with one child with ASD. In their study, there are four intervention sessions, and one follow-up, post-intervention therapy session to examine the effectiveness of the therapy with NAO.
Sample sizes vary dramatically in the literature ( Ioannou et al., 2015 ; Kajopoulos et al., 2015 ; Kim et al., 2015 ; Sung et al., 2015 ; Chu et al., 2017 ; Khosla et al., 2017 ; Rudovic et al., 2018 ), where some studies were conducted with hundreds of participants whereas some studies included only a few participants. Challenges to recruitment included accessibility of participants and their caregivers, participants' disability, and ethical issues (e.g., privacy).
In terms of the intensity and duration of robot-assisted cognitive training, due to the variety of applications and end users, there was also a great variation in the total number of training sessions as well as the session duration. For example, with respect to one single training session, it took from about 20 min ( Shukla et al., 2015 ; Tleubayev et al., 2019 ) to 90 min ( Kim et al., 2015 ). Corresponding to the total cognitive training period, it varied from a few days ( Bharatharaj et al., 2017 ) to more than 5 years ( Chu et al., 2017 ).
3.3.2. Evaluation
Researchers employed subjective and/or objective evaluation metrics to evaluate the performance of robots in cognitive training. Subjective measurement include qualitative observation, interviews and questionnaires. Objective measurements evaluate the performance from a behavioral or neurophysiological level.
1. Observation . During the experiments or recorded video, the experimenters or professional therapists observed and evaluated participants' behaviors, such as affective feelings, eye contact, communication, and other related interactions, based on their knowledge and experience ( Begum et al., 2015 ; Conti et al., 2015 ; Costescu et al., 2015 ; Ioannou et al., 2015 ; Shukla et al., 2015 ; Taheri et al., 2015a , 2019 ; Yu et al., 2015 ; Tariq et al., 2016 ; Wong and Zhong, 2016 ; Yun et al., 2016 ; Zheng et al., 2016 ; Abdollahi et al., 2017 ; Chu et al., 2017 ; Garcia-Sanjuan et al., 2017 ; Khosla et al., 2017 ; Rudovic et al., 2017 ; David et al., 2018 ; Marino et al., 2019 ; Sandygulova et al., 2019 ; Tleubayev et al., 2019 ). This measurement was a very practical, dominant metric during the study of children with ASD. With the development of ICTs, some studies also applied customized software (instead of human effort) to evaluate user's behaviors, such as smiles and visual attention ( Pino et al., 2020 ).
2. Interview . Interviews were conducted with the primary users (i.e., patients), their caregivers (e.g., parents and other family caregivers), and therapists, to learn about users' opinion and experience, and the performance of the robot-assisted cognitive training ( Yu et al., 2015 ; Bharatharaj et al., 2017 ; Darragh et al., 2017 ; Paletta et al., 2018 ; Sandygulova et al., 2019 ; Taheri et al., 2019 ; Tleubayev et al., 2019 ). As stated in the book on user experience (UX) lifecycle by Hartson and Pyla (2018) , user interview is a useful, practical technique to understand users' needs, design solutions, and evaluate UX, all of which are basic fundamental activities in UX lifecycle. Specifically, interviews can be applied to extract requirements of people with cognitive disability and/or their caregivers, to create the human-robot interaction design concepts, and to verify and refine human-robot interaction design for cognitive training. For example, in the case studies by Orejana et al. (2015) , older adults with chronic health conditions in a rural community used a healthcare robot (iRobi) in their homes for at least 3 months. Then participants were interviewed to learn personal accounts of participants's experience. Through the interview, the authors found that more familiar games may be easier for older people to relate to and therefore increase users' confidence and that a larger screen would make the functions easier to see and use. The interview also revealed that older people sometimes have less dexterity so making the touchscreen less sensitive to long presses may remove accidental triggering of functions.
3. Questionnaire . Most studies utilized questionnaires to evaluate the performance of robot-assisted cognitive training. Researchers adopted questionnaire(s) based on their targeted performance, such as targeted user groups (e.g., patients, caregivers, or therapists), targeted cognitive capabilities (e.g., memory or anxiety), and research goals (e.g., users' perception of robot or effectiveness of robot). A few studies designed their own questionnaires according to their study ( Tariq et al., 2016 ; Abdollahi et al., 2017 ; Ahn et al., 2017 ; Bharatharaj et al., 2017 ; Khosla et al., 2017 ; van den Heuvel et al., 2017 ; Scassellati et al., 2018 ; Lins et al., 2019 ; Tokunaga et al., 2019 ). Table 3 shows a list of common questionnaires in the literature.
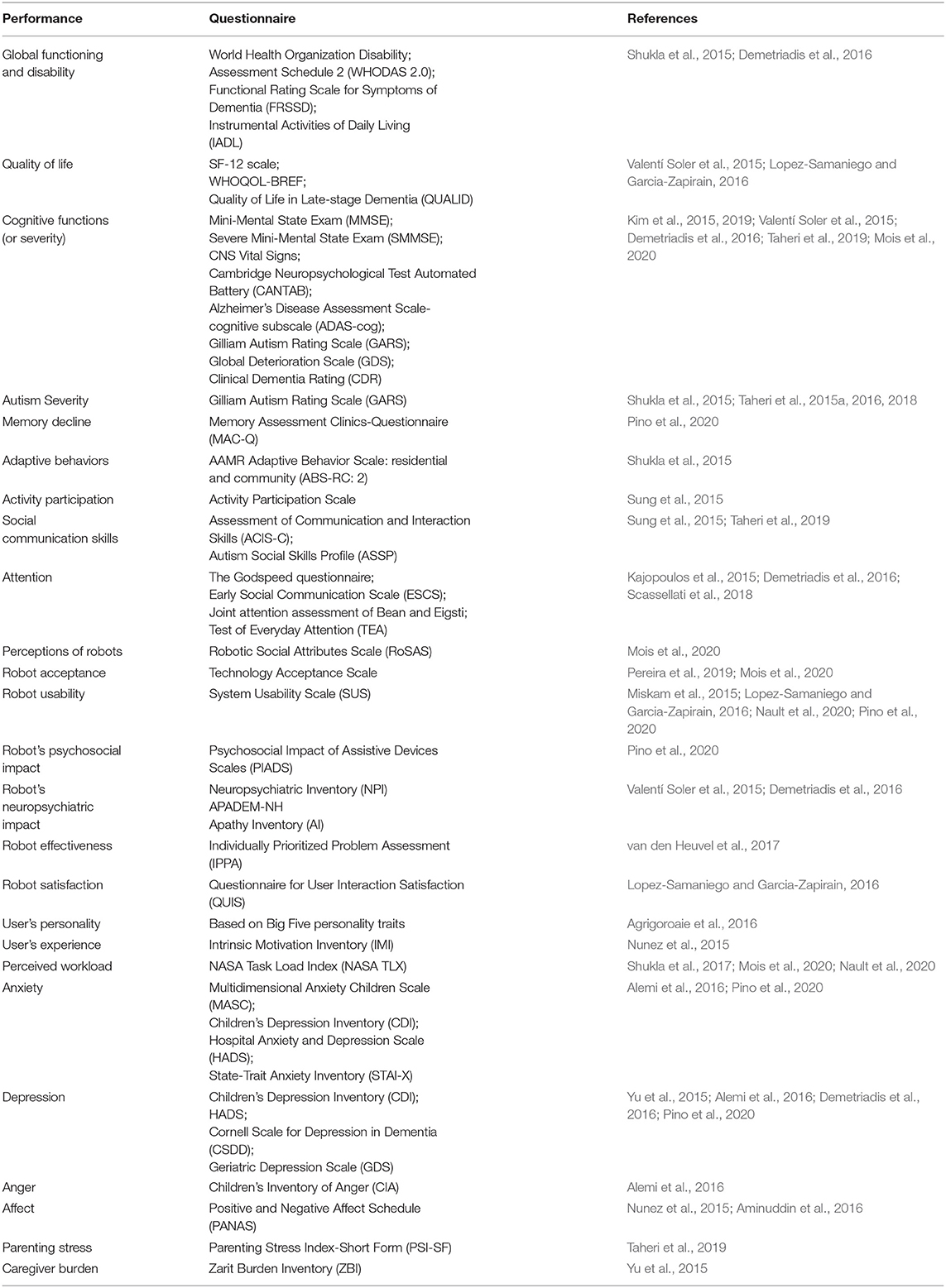
Table 3 . Questionnaires used to evaluate performance of robot-assisted cognitive training.
4. Behavioral measurement . From a behavioral perspective, researchers measured the number of correct/incorrect responses, response time, and/or time to complete the activity by participants to evaluate the performance of robot-assisted training ( Bozgeyikli et al., 2015 ; Costescu et al., 2015 ; Ioannou et al., 2015 ; Kajopoulos et al., 2015 ; Salvador et al., 2016 ; Shukla et al., 2017 ; Lins et al., 2019 ; Nault et al., 2020 ).
5. Neurophysiological measurement . The advancement of brain-imaging technologies and deep learning enables researchers to assess the impact of cognitive training on cognitive capabilities from a neurophysiological perspective, using brain-imaging tools, such as EEG, fNIRS or functional magnetic resonance imaging (fMRI) ( Ansado et al., 2020 ). Researchers also applied such tools to detect changes in the brain associated with participants' cognitive capability as metrics to evaluate the performance of robots in cognitive training ( Kim et al., 2015 ; Alimardani and Hiraki, 2017 ).
3.4. Robot Products
The development of technologies, such as manufacturing and ICTs, has led to the generation of mass-product robots for research, education and therapeutic applications ( Wood et al., 2017 ; Pandey and Gelin, 2018 ). Particularly in the field of cognitive training/rehabilitation, the developed robots are featured with capabilities, such as the aforementioned multimodal perception and multimodal feedback to support the human-robot interaction during cognitive training. Table 4 shows commonly used robot products as well as the important features to enable these robots to assist cognitive training. Their specific applications in cognitive training among previous studies, for example, assisting the intervention for memory and social communication skills, are listed in Table 1 .
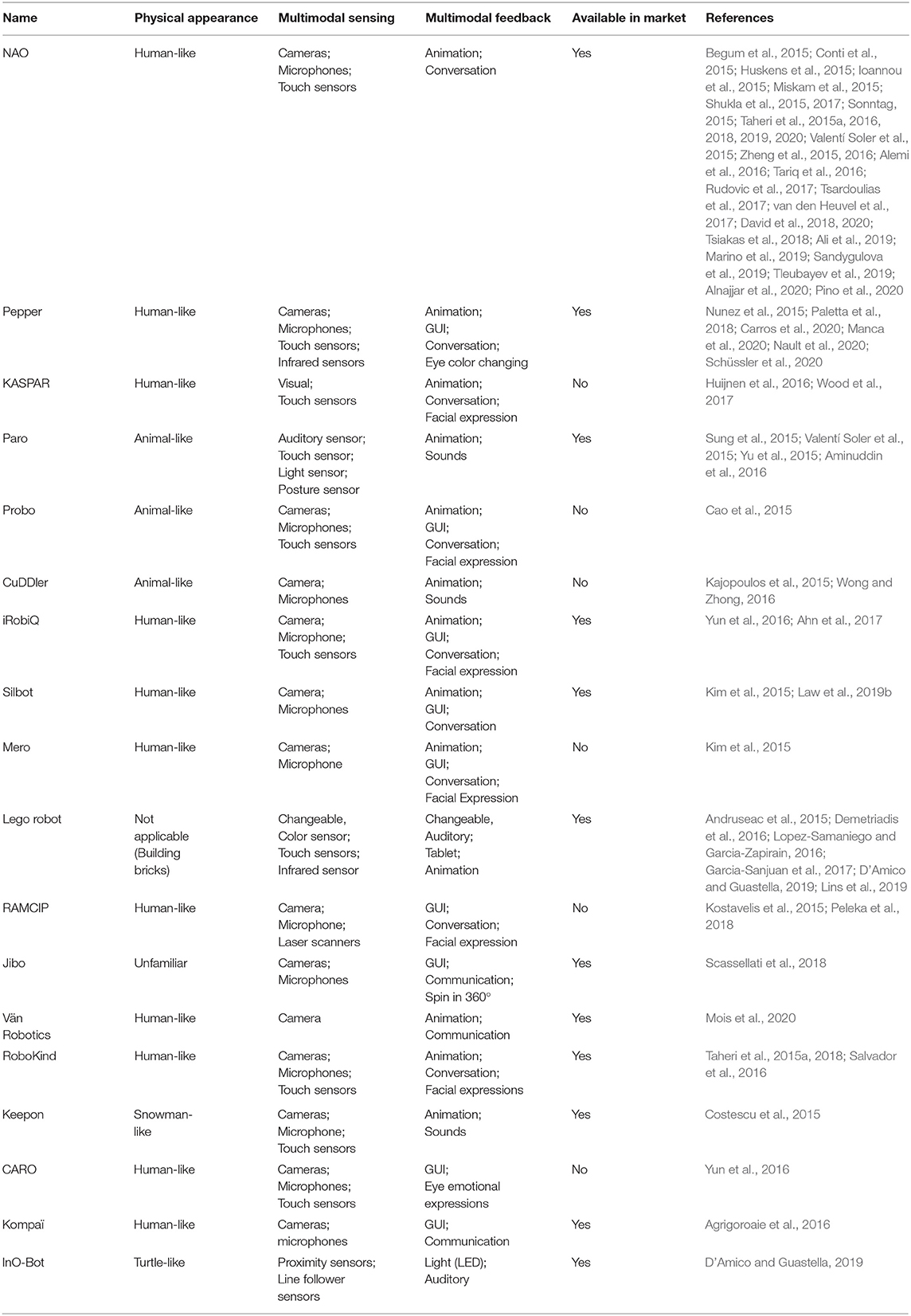
Table 4 . Features of common robot products for cognitive training.
4. Discussions
4.1. limitations, 4.1.1. sample size.
Probably, the most common challenge faced by researchers of cognitive training is a small size of participants. This imposes the generalization and reliability of experimental results in question. The limitation of small sample size was caused by the small number of participants or the limited number of available robots for experiments ( Kajopoulos et al., 2015 ; Shukla et al., 2015 ; Zheng et al., 2015 ; Tariq et al., 2016 ; Bharatharaj et al., 2017 ; Darragh et al., 2017 ; Garcia-Sanjuan et al., 2017 ; Tsardoulias et al., 2017 ; Lins et al., 2019 ; Marino et al., 2019 ; Tleubayev et al., 2019 ). Typically, a research lab has only a few robots. This would be particularly challenging to experimental studies that require multiple sessions for each individual user. In this case, within the same study period, these studies have to control the number of participants to a small number. The recruitment of participants was influenced by the accessibility to participants during the whole study and sometimes the problems associated with their caregivers ( Alemi et al., 2016 ; Taheri et al., 2019 ). For example, in the study ( Alemi et al., 2016 ) of exploring effect of utilizing a robot NAO as a therapy-assistive tool to deal with pediatric distress among children with cancer. In terms of the small sample size, researchers mentioned that due to the novelty of their project and scant number of systematical psychological interventions for patients with cancer or other refractory illness in Iranian hospitals, it was difficult to persuade children's parents to join their study. Also, they mentioned that it was hard for parents to bring their kids to intervention sessions on a regular basis. Moreover, the issue of small sample size also means that the participants in some studies were not general and representative, in terms of factors, such as their severity of cognitive disability, their age and gender ( Begum et al., 2015 ; Yu et al., 2015 ; Chu et al., 2017 ).
4.1.2. Measurement of Training Effectiveness
Another impeding factor in studies of robot-assisted cognitive training is the evaluation of its training effectiveness, which can relate to choosing tools for specific evaluation metrics, identifying relevant evaluation metrics, or designing experiments to facilitate evaluation. In terms of the evaluation metrics, many studies adopted subjective evaluations, which could be biased and inaccurate. To the authors' best knowledge, there is no standardized questionnaire to evaluate robot-assisted cognitive training. As shown in Table 3 , multiple different questionnaires were applied to evaluate the same target (e.g., robot acceptance), which makes it difficult to compare the performance of robot-assisted cognitive training between different studies ( Bharatharaj et al., 2017 ). Often, assessment metrics focus on the impact on the specifically trained cognitive capability, ignoring the potential transfer to other cognitive skills ( Zheng et al., 2016 ) and the long-term performance ( Richardson et al., 2018 ). Moreover, evaluations were frequently conducted for the robot-assisted cognitive training in controlled laboratory settings. The real-world environments are usually noisy and dynamic, which brings greater challenges for a reliable, robust user-robot interaction and a good user experience of the robot ( Salem et al., 2015 ; Trovato et al., 2015 ).
Additionally, the effectiveness of robot-assisted cognitive training may be impacted by users' perceived interaction with the robot. On the one hand, some studies ( Lopez-Samaniego and Garcia-Zapirain, 2016 ; Pereira et al., 2019 ; Mois et al., 2020 ) have evaluated the acceptance, satisfaction and perception of robots for cognitive training. On the other hand, many studies ( Kim et al., 2015 ; Shukla et al., 2015 ; Demetriadis et al., 2016 ; Pino et al., 2020 ) have evaluated effectiveness of robot-assisted cognitive training on participant's cognitive capabilities. However, it is rarely addressed in the literature how acceptance and perception of the robot affects the effectiveness of cognitive training. Moreover, as shown in Table 2 , some studies presented the results of robot-assisted training without comparing to the effectiveness of human-assisted training. For more rigorous evaluation of the effectiveness of a robot-assisted cognitive training approach, it is recommended to compare against human-assisted training and other existing approaches.
4.1.3. Uncontrollable Factors
There always exist uncontrollable factors during the study of robot-assisted cognitive training/rehabilitation. The problem is more noteworthy for multiple-session studies since researchers cannot control participants' daily and social activities outside of the laboratory setting. The topic of uncontrollable factors is relatively less studied. In a study on using a social robot to teach music to children with autism, Taheri et al. (2019) pointed out some improvements observed in music education and/or social skills are attributable to other interventions or education the participants may be receiving. When investigating the influence of robot-assisted training on cortical thickness in the brains of elderly participants, Kim et al. (2015) recognized uncontrollable factors due to participants' daily cognitive activity at home, such as using computers or reading books.
4.2. Challenges and Future Development
4.2.1. ethical challenges.
During the development of robots for cognitive training/rehabilitation, there are some ethical issues with respect to human dignity, safety, legality, and social factors to be considered. For example, during robot-assisted cognitive training, the interaction between the user and the robot happens at both the cognitive (dominant) and physical level ( Villaronga, 2016 ). There could be the issue of perceived safety, or cognitive harm. For example, the user may perceive the robot unsafe or scary ( Salem et al., 2015 ; Coeckelbergh et al., 2016 ). In the study ( Shim and Arkin, 2016 ) exploring the influence of robot deceptive behavior on human-robot interaction, a robot NAO deceptively showed positive feedback to participants' incorrect answers in a motor-cognition dual task. The self-report results revealed that the robot's deceptive feedback positively affected a human's frustration level and task engagement. Even though a robot's deceptive action may lead to positive outcome, Shim and Arkin emphasized that the ethical implications of the robot deception, including those regarding motives for deception, should always be discussed and validated prior to its application. Another arising ethical issue is how responsibility can be allocated, or distribution of responsibility ( Loh, 2019 ; Müller, 2020 ). For example, if a robot acts during cognitive training, will the robot itself, designers or users be responsible, liable or accountable for the robot's actions? We should also pay close attention to ethical issues, such as the affective attachments, dependency on the robot, safety and privacy protection of users' information, and transparency in the use of algorithms in robotic systems ( Kostavelis et al., 2015 ; Casey et al., 2016 ; Richardson et al., 2018 ; Fiske et al., 2019 ). Similarly, designers should accommodate the design of robot to these ethical considerations ( Ozcana et al., 2016 ). To ensure the perceived safety, researchers need to always take end users' perception into account, which can be known through questionnaires and interviews with them (and their caregivers and therapists if needed), and their behavioral and neurophysiological activities. The tendency for humans to form attachments to anthropomorphized robots should be carefully considered during design ( Riek and Howard, 2014 ; Riek, 2016 ). Moreover, for fear that the robot could replace human health care from both patients and the professional caregivers, it should be emphasized that the rehabilitation robots are developed with the aim of supplementing human caregivers, rather than replacing them ( Doraiswamy et al., 2019 ).
4.2.2. User-Centered Design
The goal of robotic cognitive rehabilitation is to provide cost-effective cognitive training to vulnerable people with cognitive disabilities, which can supplement their caregivers and/or therapists ( Doraiswamy et al., 2019 ). Therefore, we encourage the idea of user-driven, instead of technology-driven, robot design and development ( Rehm et al., 2016 ). Emphasis should be given to the primary users (i.e., patients) of the robots and other key stakeholders (e.g., caregivers, therapists, and doctors) to design and shape this robot, including requirement analysis, robot development and evaluation with different stakeholders ( Casey et al., 2016 ; Gerling et al., 2016 ; Leong and Johnston, 2016 ; Rehm et al., 2016 ; Salichs et al., 2016 ; Barco Martelo and Fosch Villaronga, 2017 ; Riek, 2017 ). It is also important to pay attention to potential technical difficulties for vulnerable populations, such as the elderly and children with ASD ( Orejana et al., 2015 ) and the social and contextual environment that the robot will be applied to ( Jones et al., 2015 ). More standardized, unbiased benchmarks and metrics need to be developed for different stakeholders to evaluate the performance of robots from their perspectives. While it is necessary to start pilot studies with healthy participants, it is crucial to relate the developed systems to patients with cognitive impairment at home settings.
Furthermore, robot development is a multidisciplinary study which requires knowledge from multiples fields, such as social cognitive science, engineering, psychology, and health care, such as ASD and dementia. Enhanced collaborations among these fields are needed to improve future technology in robotic rehabilitation.
4.2.3. Reliability, Trust, Transferability, and Cost-Effectiveness
The reliability of the robotic system ensures that the robot can consistently work in noisy, dynamic real-world environment. This makes a significant contribution to a user's confidence and increases positive perception of the robot ( Wood et al., 2017 ). Mistakes made by robots during interaction can cause a loss of trust in human users. More work on human-robot interaction is needed to implement a reliable and robust robot to assist cognitive training. This may cover multimodal sensing technologies, artificial intelligence, and modeling. On the other hand, we need to take into consideration how to effectively restore trust to the robot in case that mistakes are made by the robot during interaction with the user. This may involve how and when the robot apologizes for its mistake for users. Robinette et al. (2015) found that timing is a key to repair robot trust, suggesting that the robot should wait and address the mistake next time a potential trust decision occurs rather than addressing the mistake immediately.
Currently, most studies focus on specific cognitive training tasks and environments, which means that the robot cannot assist in other cognitive tasks. Here, we encourage the implementation of a transferable mechanism in robots for cognitive training. In other words, we should enable more powerful learning algorithms in the robot so that the robot can learn and adapt to more new cognitive training ( Andriella et al., 2019a ). Researchers should also take cost-effectiveness into account during the design of robot ( Wood et al., 2017 ). From the commercial perspective, cost-effectiveness is considered beyond the purchase, maintenance, and training costs for the system ( Riek, 2017 ). Furthermore, from the perspective of time and effort of users, more work is needed to find out the optimal robot-assisted cognitive training strategy (e.g., the frequency and duration of each cognitive session). Therefore, we encourage future studies clearly state the used training strategies, making it easier for the community to compare different strategies.
4.2.4. Personalization
There is no “one-size-fits-all” in health care. To provide a successful cognitive training, the robot needs to be personalized and adaptive in three levels. Firstly, personalization requires the robot to provide appropriate cognitive training and feedback to meet the specific need of groups with different cognitive disabilities (e.g., people with ASD, people with ADRD). Secondly, the robots need to adapt to the diversity existing in the population as well as tailor to each individual user's severity of cognitive impairment, cultural and gender-dependent difference, personality and preference ( Kostavelis et al., 2015 ; Javed et al., 2016 ; Riek, 2016 ; Darragh et al., 2017 ; Rudovic et al., 2017 ; Richardson et al., 2018 ; Sandygulova et al., 2019 ). For example, children with ASD, have a wide range of behavioral, social, and learning difficulties. And each individual may have a different preference to robot's gender and modalities of feedback ( Sandygulova and O'Hare, 2015 ; Nault et al., 2020 ). As a result, we expect that a personalized robot would provide various cognitive training, e.g., a variety of games and adjustable voice, for diverse individual needs and requirements to keep the user engaged and focused over long term ( Scassellati et al., 2018 ; Tleubayev et al., 2019 ). Furthermore, rehabilitation robots should adapt to individually time-changing characters, such as cognitive impairment, task engagement, and even personalities ( Agrigoroaie and Tapus, 2016 ; Tsiakas et al., 2016 , 2018 ). For example, the robot should adjust the cognitive training and feedback if the user feels bored, too difficult, or too easy ( Lins et al., 2019 ). Machine learning methods should also take into consideration of personalization. Existing methods, such as interactive reinforcement learning (IRL) or incremental learning ( Castellini, 2016 ) provide good examples, where one block module is used to specifically model each user's information, such as patient's name, hobbies and personalities related to cognitive training ( Salichs et al., 2016 ). IRL is a variation of reinforcement learning that studies how a human can be included in the agent learning process. Human input play the role of feedback (i.e., reinforcement signal after the selected action) or guidance (i.e., actions to directly intervene/correct current strategy). IRL can also be utilized to enable adaptation and personalization during robot-assisted cognitive training. For example, Tsiakas et al. (2016) proposed an adaptive robot assisted cognitive therapy using IRL, where the primary user feedback input (e.g., engagement levels) were considered as a personalization factor and the guidance input from professional therapist were considered as a safety factor. Their simulation results showed that IRL improved the applied policy and led to a faster convergence to optimal policy. Castellini (2016) proposed an incremental learning model to enforce a true, endless adaptation of the robot to the subject and environment as well as improve the stability and reliability of robot's control. Incremental learning enables an adaptive robot system to update its own model whenever it is required, new information is available, or the prediction is deemed no longer reliable.
4.2.5. Human-Robot Collaboration
Future rehabilitation robots should not only be autonomous but also be collaborative (or co-operative) ( Huijnen et al., 2016 ; Weiss et al., 2017 ). From the perspective of collaboration between the robot and the primary end users (i.e., people with cognitive disability), there is evidence indicating that a fully autonomous robotic system is not the best option for interaction with the vulnerable population ( Peca et al., 2016 ). Instead, a semi-autonomous robot is a more suitable solution ( Wood et al., 2017 ). With the highest-level goal of enhancing user's cognitive capabilities, the robot should “care” about the user's situation, take compensatory reaction as a teammate, engage the user and train/stimulate the user's cognitive capabilities as best as possible. The capability of collaboration may also help to avoid the user's feeling of redundancy and increase their feeling of self-autonomy and long-term engagement in cognitive training ( Orejana et al., 2015 ). The robot should have a good perception of the user's changing situations and an intelligent strategy to engage the user. On the other hand, from the perspective of collaboration among robots, users, and their caregivers (and therapists), more future work is needed to solve the shared control issue. Researchers need to figure out strategies for robots to render the caregivers' and therapists' tasks easier as an assistive tool for cognitive training, instead of totally replacing them ( Kostavelis et al., 2015 ; Coeckelbergh et al., 2016 ). A distribution between autonomy of robots and teleoperation by caregivers/therapists is needed to support the collaboration of robots for cognitive training.
4.2.6. Social Cognition
The knowledge gained in human-human interaction can be applied to foster human-robot interaction and to obtain critical insights for optimizing social encounters between humans and robots ( Henschel et al., 2020 ). Marchesi et al. (2019) conducted a questionnaire study to investigate whether people adopt intentional stance ( Dennett, 1989 ) toward a humanoid robot, iCub. Their results showed that it is possible to sometimes induce adoption of the intentional stance toward humanoid robots. Additionally, non-invasive neuroimaging techniques (e.g., fMRI) in neuroscience enable the possibility of probing social cognitive processing in human brain during interaction with robots. For example, Rauchbauer et al. (2019) observed that neural markers of mentalizing and social motivation were significantly more activated during human-human interaction than human-robot interaction. Klapper et al. (2014) showed that human brain activity within the theory-of-mind network ( Saxe and Wexler, 2005 ; Price, 2012 ; Koster-Hale and Saxe, 2013 ) was reduced when interacting with agents in a form of low human animacy (i.e., physical appearance) compared to high human animacy. These issues become important when we adopt robots for cognitive training for people with cognitive dysfunction ( Frith and Frith, 1999 ; Chevallier et al., 2012 ), as they underline the substantial contrast between human-human and human-agent interactions. Additionally, the advanced non-invasive, portable, and cost-effective neuroimaging techniques (e.g., EEG and fNIRS) hold the promise of evaluating human-robot interaction from controlled laboratory setting to real-world setting. Herein, we encourage to leverage human neuroscience to facilitate the development of robots for cognitive training, such as understanding the effects of robot-assisted cognitive training and learning the extent and contexts at which it can be beneficial from neurophysiological perspective.
4.2.7. Natural Human-Robot Interaction
Similar to human-human interaction during cognitive training by human therapists, robots need to be able to interact with users naturally in robotic rehabilitation. This includes having a good understanding of user's emotions (e.g., happiness, shame, engagement), intentions and personality ( Pettinati and Arkin, 2015 ; Rahbar et al., 2015 ; Vaufreydaz et al., 2016 ; Rudovic et al., 2018 ), being able to provide an emotional response when being shared with personal information ( de Graaf et al., 2015 ; Chumkamon et al., 2016 ), talking day-by-day more to the user on various topics like hobbies, and dealing with novel events ( Dragone et al., 2015 ; Kostavelis et al., 2015 ; Adam et al., 2016 ; Ozcana et al., 2016 ). These natural user-robot interactions require powerful perception, reasoning, acting and learning modules in robots, or in other words, cognitive and social-emotional capabilities. However, from the perception perspective, understanding users' intentions and emotions is still a great challenge for robots ( Matarić, 2017 ). Robots need to interpret multimodal signals (e.g., facial expression, gestures, voice, and speech) simultaneously to understand users' covert intentions and emotions. Similarly, more work is needed for the multimodal feedback in the future. To maximize the benefits of physically present robots and facilitate both the short- and long-term human-robot interaction for cognitive training, we need to develop more embodied communication in robots, not limited in verbal communication ( Paradeda et al., 2016 ; Salichs et al., 2016 ; Matarić, 2017 ). Haptic sensing and feedback should be strongly considered in future research as part of multimodal perception and feedback ( Arnold and Scheutz, 2017 ; Cangelosi and Invitto, 2017 ). More specifically, we need to implement the strategy to enable the robot to associate cognitive assistance and exercise with appropriate multimodal feedback, e.g., spoken words, facial expressions, eye gaze, and other body movements ( Paletta et al., 2018 ). The embodied communication during human-robot interaction is a challenging research area ( Nunez et al., 2015 ). It is still unclear how and how much the embodied communication from the robot can influence user's perception of the robot ( Dubois et al., 2016 ). Moreover, previous studies indicated that users' experience of the robot could also be influenced by the unexpected behaviors ( Lemaignan et al., 2015 ), synchrony and reciprocity ( Lorenz et al., 2016 ), and even cognitive biases ( Biswas and Murray, 2016 , 2017 ) from the robot. A caveat is that there still exist many unknowns for natural human-robot interaction.
In summary, to achieve natural human-robot interaction during cognitive training requires not only multimodal sensing technology and artificial intelligence (e.g., deep learning) ( Jing et al., 2015 ; Lopez-Samaniego and Garcia-Zapirain, 2016 ; Pierson and Gashler, 2017 ) but also the development of related fields ( Wan et al., 2020 ), such as cognitive computing ( Chen et al., 2018 ), social-cognitive mechanisms ( Wiltshire et al., 2017 ), and modeling of cognitive architectures ( Kotseruba et al., 2016 ; Woo et al., 2017 ).
5. Conclusion
Robot-assisted cognitive training is becoming an affordable promise for people with cognitive disabilities. In this review paper, we present a systematic review on the current application, enabling technologies, and main commercial robots in the field of robot-assisted cognitive training. Many studies have been successfully conducted to evaluate the feasibility, safety, usability, and effectiveness of robotic rehabilitation. Existing studies often include a small sample size. Also, the questionnaires need to be standardized both to evaluate the overall experience with the robot and the impact of the robot on the specific cognitive ability that it aims to assess. There are still multifaceted challenges in the application of robots in cognitive training. Firstly, ethical issues, such as human safety and violation of social norms, can arise during robot-assisted cognitive training. Secondly, with respect to the design of a robot-assisted cognitive training system, the developers should have a close collaboration with the end-users and stakeholders from the initial design, implementation, evaluation and improvement. Thirdly, the trust, reliability and the cost-effectiveness should be taken into account. Moreover, the rehabilitation robot should be capable to adapt and personalize to the specific individual need, and also learn to collaborate with users in the future. The recent advancement of social cognition may facilitate the human-robot interaction during cognitive training. Lastly, the rehabilitation robot should be able to interact with users in a natural way, similar to the human-human interaction during cognitive training. Noticeably, these challenges are mutually influencing one another. Cross-disciplinary collaboration is necessary to solve these challenges in future.
Data Availability Statement
The original contributions presented in the study are included in the article/supplementary material, further inquiries can be directed to the corresponding author/s.
Author Contributions
FY, RL, and XZ determined the review scope and review strategies. FY, EK, and ZL conducted the searching and screening of the literature, and reviewing of the selected articles. FY, EK, RL, and XZ wrote the manuscript. All authors contributed to the article and approved the submitted version.
Conflict of Interest
The authors declare that the research was conducted in the absence of any commercial or financial relationships that could be construed as a potential conflict of interest.
Ab Aziz, A., Ahmad, F., Yusof, N., Ahmad, F. K., and Yusof, S. A. M. (2015). “Designing a robot-assisted therapy for individuals with anxiety traits and states,” in 2015 International Symposium on Agents, Multi-Agent Systems and Robotics (ISAMSR) (Putrajaya: IEEE), 98–103. doi: 10.1109/ISAMSR.2015.7379778
CrossRef Full Text | Google Scholar
Abdollahi, H., Mollahosseini, A., Lane, J. T., and Mahoor, M. H. (2017). “A pilot study on using an intelligent life-like robot as a companion for elderly individuals with dementia and depression,” in 2017 IEEE-RAS 17th International Conference on Humanoid Robotics (Humanoids) (Birmingham: IEEE), 541–546. doi: 10.1109/HUMANOIDS.2017.8246925
Adam, C., Johal, W., Pellier, D., Fiorino, H., and Pesty, S. (2016). “Social human-robot interaction: a new cognitive and affective interaction-oriented architecture,” in International Conference on Social Robotics (Springer), 253–263. doi: 10.1007/978-3-319-44884-8
Agrigoroaie, R., Ferland, F., and Tapus, A. (2016). The enrichme project: Lessons learnt from a first interaction with the elderly,” in International Conference on Social Robotics (Kansas City, MO: Springer), 735–745. doi: 10.1007/978-3-319-47437-3_72
Agrigoroaie, R. M., and Tapus, A. (2016). “Developing a healthcare robot with personalized behaviors and social skills for the elderly,” in 2016 11th ACM/IEEE International Conference on Human-Robot Interaction (HRI) (Christchurch: IEEE), 589–590. doi: 10.1109/HRI.2016.7451870
Ahn, H. S., Lee, M. H., Broadbent, E., and MacDonald, B. A. (2017). “Is entertainment services of a healthcare service robot for older people useful to young people?” in 2017 First IEEE International Conference on Robotic Computing (IRC) (Taichung: IEEE), 330–335. doi: 10.1109/IRC.2017.70
Alemi, M., Ghanbarzadeh, A., Meghdari, A., and Moghadam, L. J. (2016). Clinical application of a humanoid robot in pediatric cancer interventions. Int. J. Soc. Robot . 8, 743–759. doi: 10.1007/s12369-015-0294-y
Ali, S., Mehmood, F., Dancey, D., Ayaz, Y., Khan, M. J., Naseer, N., et al. (2019). An adaptive multi-robot therapy for improving joint attention and imitation of asd children. IEEE Access 7, 81808–81825. doi: 10.1109/ACCESS.2019.2923678
Alimardani, M., and Hiraki, K. (2017). Development of a real-time brain-computer interface for interactive robot therapy: an exploration of eeg and emg features during hypnosis. Int. J. Comput. Inform. Eng . 11, 187–195. doi: 10.5281/zenodo.1340062
Alnajjar, F., Cappuccio, M., Renawi, A., Mubin, O., and Loo, C. K. (2020). Personalized robot interventions for autistic children: an automated methodology for attention assessment. Int. J. Soc. Robot . 13, 67–82. doi: 10.1007/s12369-020-00639-8
Aminuddin, R., Sharkey, A., and Levita, L. (2016). “Interaction with the paro robot may reduce psychophysiological stress responses,” in 2016 11th ACM/IEEE International Conference on Human-Robot Interaction (HRI) (Christchurch: IEEE), 593–594. doi: 10.1109/HRI.2016.7451872
Andriella, A., Suárez-Hernández, A., Segovia-Aguas, J., Torras, C., and Alenyà, G. (2019a). “Natural teaching of robot-assisted rearranging exercises for cognitive training,” in International Conference on Social Robotics (Madrid: Springer), 611–621. doi: 10.1007/978-3-030-35888-4_57
Andriella, A., Torras, C., and Alenyà, G. (2019b). “Learning robot policies using a high-level abstraction persona-behaviour simulator,” in 2019 28th IEEE International Conference on Robot and Human Interactive Communication (RO-MAN) (New Delhi: IEEE), 1–8. doi: 10.1109/RO-MAN46459.2019.8956357
Andriella, A., Torras, C., and Alenya, G. (2020). Cognitive system framework for brain-training exercise based on human-robot interaction. Cogn. Comput . 12, 793–810. doi: 10.1007/s12559-019-09696-2
Andruseac, G. G., Adochiei, R. I., Păsărică, A., Adochiei, F. C., Corciovă, C., and Costin, H. (2015). “Training program for dyslexic children using educational robotics,” in 2015 E-Health and Bioengineering Conference (EHB) (Iasi: IEEE), 1–4. doi: 10.1109/EHB.2015.7391547
Ansado, J., Chasen, C., Bouchard, S., Iakimova, G., and Northoff, G. (2020). How brain imaging provides predictive biomarkers for therapeutic success in the context of virtual reality cognitive training. Neurosci. Biobehav. Rev . 120, 583–594. doi: 10.1016/j.neubiorev.2020.05.018
PubMed Abstract | CrossRef Full Text | Google Scholar
Arnold, T., and Scheutz, M. (2017). The tactile ethics of soft robotics: designing wisely for human–robot interaction. Soft Robot . 4, 81–87. doi: 10.1089/soro.2017.0032
Barco Martelo, A., and Fosch Villaronga, E. (2017). “Child-robot interaction studies: from lessons learned to guidelines,” in 3rd Workshop on Child-Robot Interaction, CRI 2017 (Vienna).
Google Scholar
Beckerle, P., Salvietti, G., Unal, R., Prattichizzo, D., Rossi, S., Castellini, C., et al. (2017). A human-robot interaction perspective on assistive and rehabilitation robotics. Front. Neurorobot . 11:24. doi: 10.3389/fnbot.2017.00024
Begum, M., Serna, R. W., Kontak, D., Allspaw, J., Kuczynski, J., Yanco, H. A., et al. (2015). “Measuring the efficacy of robots in autism therapy: how informative are standard HRI metrics?” in Proceedings of the Tenth Annual ACM/IEEE International Conference on Human-Robot Interaction (Portland, OR), 335–342. doi: 10.1145/2696454.2696480
Bertani, R., Melegari, C., Maria, C., Bramanti, A., Bramanti, P., and Calabrò, R. S. (2017). Effects of robot-assisted upper limb rehabilitation in stroke patients: a systematic review with meta-analysis. Neurol. Sci . 38, 1561–1569. doi: 10.1007/s10072-017-2995-5
Bharatharaj, J., Huang, L., Mohan, R. E., Al-Jumaily, A., and Krägeloh, C. (2017). Robot-assisted therapy for learning and social interaction of children with autism spectrum disorder. Robotics 6:4. doi: 10.3390/robotics6010004
Biswas, M., and Murray, J. (2016). “The effects of cognitive biases in long-term human-robot interactions: case studies using three cognitive biases on marc the humanoid robot,” in International Conference on Social Robotics (Kansas City, MO: Springer), 148–158. doi: 10.1007/978-3-319-47437-3_15
Biswas, M., and Murray, J. (2017). The effects of cognitive biases and imperfectness in long-term robot-human interactions: case studies using five cognitive biases on three robots. Cogn. Syst. Res . 43, 266–290. doi: 10.1016/j.cogsys.2016.07.007
Botvinick, M., Ritter, S., Wang, J. X., Kurth-Nelson, Z., Blundell, C., and Hassabis, D. (2019). Reinforcement learning, fast and slow. Trends Cogn. Sci . 23, 408–422. doi: 10.1016/j.tics.2019.02.006
Bozgeyikli, L., Bozgeyikli, E., Clevenger, M., Raij, A., Alqasemi, R., Sundarrao, S., et al. (2015). “Vr4vr: vocational rehabilitation of individuals with disabilities in immersive virtual reality environments,” in Proceedings of the 8th ACM International Conference on PErvasive Technologies Related to Assistive Environments (Corfu), 1–4. doi: 10.1145/2769493.2769592
Calderita, L. V., Vega, A., Barroso-Ramírez, S., Bustos, P., and Núñez, P. (2020). Designing a cyber-physical system for ambient assisted living: a use-case analysis for social robot navigation in caregiving centers. Sensors 20:4005. doi: 10.3390/s20144005
Cangelosi, A., and Invitto, S. (2017). Human-robot interaction and neuroprosthetics: a review of new technologies. IEEE Consum. Electron. Mag . 6, 24–33. doi: 10.1109/MCE.2016.2614423
Cao, H. L., Pop, C., Simut, R., Furnemónt, R., De Beir, A., Van de Perre, G., et al. (2015). “Probolino: a portable low-cost social device for home-based autism therapy,” in International Conference on Social Robotics (Paris: Springer), 93–102. doi: 10.1007/978-3-319-25554-5_10
Carros, F., Meurer, J., Löffler, D., Unbehaun, D., Matthies, S., Koch, I., et al. (2020). “Exploring human-robot interaction with the elderly: results from a ten-week case study in a care home,” in Proceedings of the 2020 CHI Conference on Human Factors in Computing Systems (Honolulu, HI), 1–12. doi: 10.1145/3313831.3376402
Casey, D., Felzmann, H., Pegman, G., Kouroupetroglou, C., Murphy, K., Koumpis, A., et al. (2016). “What people with dementia want: designing mario an acceptable robot companion,” in International Conference on Computers Helping People With Special Needs , (Linz: Springer), 318–325. doi: 10.1007/978-3-319-41264-1_44
Castellini, C. (2016). “Incremental learning of muscle synergies: from calibration to interaction,” in Human and Robot Hands (Cham: Springer), 171–193. doi: 10.1007/978-3-319-26706-7_11
Chen, H., Zhu, H., Teng, Z., and Zhao, P. (2020). Design of a robotic rehabilitation system for mild cognitive impairment based on computer vision. J. Eng. Sci. Med. Diagn. Ther . 3:021108. doi: 10.1115/1.4046396
Chen, M., Herrera, F., and Hwang, K. (2018). Cognitive computing: architecture, technologies and intelligent applications. IEEE Access 6, 19774–19783. doi: 10.1109/ACCESS.2018.2791469
Chevallier, C., Kohls, G., Troiani, V., Brodkin, E. S., and Schultz, R. T. (2012). The social motivation theory of autism. Trends Cogn. Sci . 16, 231–239. doi: 10.1016/j.tics.2012.02.007
Chu, M. T., Khosla, R., Khaksar, S. M. S., and Nguyen, K. (2017). Service innovation through social robot engagement to improve dementia care quality. Assist. Technol . 29, 8–18. doi: 10.1080/10400435.2016.1171807
Chumkamon, S., Hayashi, E., and Koike, M. (2016). Intelligent emotion and behavior based on topological consciousness and adaptive resonance theory in a companion robot. Biol. Inspir. Cogn. Architect . 18, 51–67. doi: 10.1016/j.bica.2016.09.004
Clabaugh, C. E., Mahajan, K., Jain, S., Pakkar, R., Becerra, D., Shi, Z., et al. (2019). Long-term personalization of an in-home socially assistive robot for children with autism spectrum disorders. Front. Robot. AI 6:110. doi: 10.3389/frobt.2019.00110
Coeckelbergh, M., Pop, C., Simut, R., Peca, A., Pintea, S., David, D., et al. (2016). A survey of expectations about the role of robots in robot-assisted therapy for children with ASD: ethical acceptability, trust, sociability, appearance, and attachment. Sci. Eng. Ethics 22, 47–65. doi: 10.1007/s11948-015-9649-x
Conti, D., Di Nuovo, S., Buono, S., Trubia, G., and Di Nuovo, A. (2015). “Use of robotics to stimulate imitation in children with autism spectrum disorder: A pilot study in a clinical setting,” in 2015 24th IEEE International Symposium on Robot and Human Interactive Communication (RO-MAN) (Kobe: IEEE), 1–6. doi: 10.1109/ROMAN.2015.7333589
Costescu, C. A., Vanderborght, B., and David, D. O. (2015). Reversal learning task in children with autism spectrum disorder: a robot-based approach. J. Autism Dev. Disord . 45, 3715–3725. doi: 10.1007/s10803-014-2319-z
D'Amico, A., and Guastella, D. (2019). The robotic construction kit as a tool for cognitive stimulation in children and adolescents: the re4bes protocol. Robotics 8:8. doi: 10.3390/robotics8010008
Darragh, M., Ahn, H. S., MacDonald, B., Liang, A., Peri, K., Kerse, N., et al. (2017). Homecare robots to improve health and well-being in mild cognitive impairment and early stage dementia: results from a scoping study. J. Ame. Med. Direc. Assoc . 18:1099-e1. doi: 10.1016/j.jamda.2017.08.019
David, D. O., Costescu, C. A., Matu, S., Szentagotai, A., and Dobrean, A. (2018). Developing joint attention for children with autism in robot-enhanced therapy. Int. J. Soc. Robot . 10, 595–605. doi: 10.1007/s12369-017-0457-0
David, D. O., Costescu, C. A., Matu, S., Szentagotai, A., and Dobrean, A. (2020). Effects of a robot-enhanced intervention for children with ASD on teaching turn-taking skills. J. Educ. Comput. Res . 58, 29–62. doi: 10.1177/0735633119830344
de Graaf, M. M., Allouch, S. B., and Van Dijk, J. (2015). “What makes robots social? A user's perspective on characteristics for social human-robot interaction,” in International Conference on Social Robotics (Paris: Springer), 184–193. doi: 10.1007/978-3-319-25554-5_19
Demetriadis, S., Tsiatsos, T., Sapounidis, T., Tsolaki, M., and Gerontidis, A. (2016). “Exploring the potential of programming tasks to benefit patients with mild cognitive impairment,” in Proceedings of the 9th ACM International Conference on PErvasive Technologies Related to Assistive Environments (Corfu Island Greece), 1–4. doi: 10.1145/2910674.2935850
Dennett, D. C. (1989). The Intentional Stance . Cambridge, MA: MIT Press.
Dickerson, B. C. (2015). Dysfunction of social cognition and behavior. Continuum 21:660. doi: 10.1212/01.CON.0000466659.05156.1d
Doraiswamy, P. M., London, E., Varnum, P., Harvey, B., Saxena, S., Tottman, S., et al. (2019). Empowering 8 Billion Minds: Enabling Better Mental Health for All via the Ethical Adoption of Technologies . Washington, DC: NAM Perspectives.
Dragone, M., Amato, G., Bacciu, D., Chessa, S., Coleman, S., Di Rocco, M., et al. (2015). A cognitive robotic ecology approach to self-configuring and evolving aal systems. Eng. Appl. Artif. Intell . 45, 269–280. doi: 10.1016/j.engappai.2015.07.004
Dubois, M., Claret, J. A., Basañez, L., and Venture, G. (2016). “Influence of emotional motions in human-robot interactions,” in International Symposium on Experimental Robotics (Tokyo: Springer), 799–808. doi: 10.1007/978-3-319-50115-4_69
Esteban, P. G., Baxter, P., Belpaeme, T., Billing, E., Cai, H., Cao, H. L., et al. (2017). How to build a supervised autonomous system for robot-enhanced therapy for children with autism spectrum disorder. Paladyn J. Behav. Robot . 8, 18–38. doi: 10.1515/pjbr-2017-0002
CrossRef Full Text
Fiske, A., Henningsen, P., and Buyx, A. (2019). Your robot therapist will see you now: ethical implications of embodied artificial intelligence in psychiatry, psychology, and psychotherapy. J. Med. Internet Res . 21:e13216. doi: 10.2196/13216
Frith, C. D., and Frith, U. (1999). Interacting minds-a biological basis. Science 286, 1692–1695. doi: 10.1126/science.286.5445.1692
Garcia-Sanjuan, F., Jaen, J., and Nacher, V. (2017). Tangibot: a tangible-mediated robot to support cognitive games for ageing people—a usability study. Perv. Mob. Comput . 34, 91–105. doi: 10.1016/j.pmcj.2016.08.007
Gerling, K., Hebesberger, D., Dondrup, C., Körtner, T., and Hanheide, M. (2016). Robot deployment in long-term care. Z. Gerontol. Geriatr . 49, 288–297. doi: 10.1007/s00391-016-1065-6
Hajat, C., and Stein, E. (2018). The global burden of multiple chronic conditions: a narrative review. Prev. Med. Rep . 12, 284–293. doi: 10.1016/j.pmedr.2018.10.008
Haring, K. S., Silvera-Tawil, D., Watanabe, K., and Velonaki, M. (2016). “The influence of robot appearance and interactive ability in HRI: a cross-cultural study,” in International Conference on Social Robotics (Kansas City, MO: Springer), 392–401. doi: 10.1007/978-3-319-47437-3_38
Hartson, R., and Pyla, P. S. (2018). The UX Book: Agile UX Design for a Quality User Experience . Cambridge, MA: Morgan Kaufmann.
Havins, W. N., Massman, P. J., and Doody, R. (2012). Factor structure of the geriatric depression scale and relationships with cognition and function in Alzheimer's disease. Dement. Geriatr. Cogn. Disord . 34, 360–372. doi: 10.1159/000345787
Heins, S., Dehem, S., Montedoro, V., Dehez, B., Edwards, M., Stoquart, G., et al. (2017). “Robotic-assisted serious game for motor and cognitive post-stroke rehabilitation,” in 2017 IEEE 5th International Conference on Serious Games and Applications for Health (SeGAH) (Perth, WA: IEEE), 1–8. doi: 10.1109/SeGAH.2017.7939262
Henschel, A., Hortensius, R., and Cross, E. S. (2020). Social cognition in the age of human-robot interaction. Trends Neurosci . 43, 373–384. doi: 10.1016/j.tins.2020.03.013
Huijnen, C. A., Lexis, M. A., and de Witte, L. P. (2016). Matching robot kaspar to autism spectrum disorder (ASD) therapy and educational goals. Int. J. Soc. Robot . 8, 445–455. doi: 10.1007/s12369-016-0369-4
Huskens, B., Palmen, A., Van der Werff, M., Lourens, T., and Barakova, E. (2015). Improving collaborative play between children with autism spectrum disorders and their siblings: the effectiveness of a robot-mediated intervention based on Lego ® therapy. J. Autism Dev. Disord . 45, 3746–3755. doi: 10.1007/s10803-014-2326-0
Ioannou, A., Kartapanis, I., and Zaphiris, P. (2015). “Social robots as co-therapists in autism therapy sessions: a single-case study,” in International Conference on Social Robotics (Paris: Springer), 255–263. doi: 10.1007/978-3-319-25554-5_26
Javed, H., Cabibihan, J. J., Aldosari, M., and Al-Attiyah, A. (2016). “Culture as a driver for the design of social robots for autism spectrum disorder interventions in the Middle East,” in International Conference on Social Robotics (Kansas City, MO: Springer), 591–599. doi: 10.1007/978-3-319-47437-3_58
Javed, H., Jeon, M., and Park, C. H. (2018). “Adaptive framework for emotional engagement in child-robot interactions for autism interventions,” in 2018 15th International Conference on Ubiquitous Robots (UR) (Honolulu, HI: IEEE), 396–400. doi: 10.1109/URAI.2018.8441775
Jing, H., Lun, X., Dan, L., Zhijie, H., and Zhiliang, W. (2015). Cognitive emotion model for eldercare robot in smart home. China Commun . 12, 32–41. doi: 10.1109/CC.2015.7114067
Johal, W., Calvary, G., and Pesty, S. (2015). “Non-verbal signals in HRI: interference in human perception,” in International Conference on Social Robotics (Paris: Springer), 275–284. doi: 10.1007/978-3-319-25554-5_28
Jones, A., Küster, D., Basedow, C. A., Alves-Oliveira, P., Serholt, S., Hastie, H., et al. (2015). “Empathic robotic tutors for personalised learning: a multidisciplinary approach,” in International Conference on Social Robotics (Paris: Springer), 285–295. doi: 10.1007/978-3-319-25554-5_29
Kajopoulos, J., Wong, A. H. Y., Yuen, A. W. C., Dung, T. A., Kee, T. Y., and Wykowska, A. (2015). “Robot-assisted training of joint attention skills in children diagnosed with autism,” in International Conference on Social Robotics (Paris: Springer), 296–305. doi: 10.1007/978-3-319-25554-5_30
Khosla, R., Nguyen, K., and Chu, M. T. (2017). Human robot engagement and acceptability in residential aged care. Int. J. Hum. Comput. Interact . 33, 510–522. doi: 10.1080/10447318.2016.1275435
Kim, G., Lim, S., Kim, H., Lee, B., Seo, S., Cho, K., et al. (2017). Is robot-assisted therapy effective in upper extremity recovery in early stage stroke?—A systematic literature review. J. Phys. Ther. Sci . 29, 1108–1112. doi: 10.1589/jpts.29.1108
Kim, G. H., Jeon, S., Im, K., Kwon, H., Lee, B. H., Kim, G. Y., et al. (2015). Structural brain changes after traditional and robot-assisted multi-domain cognitive training in community-dwelling healthy elderly. PLoS ONE 10:e0123251. doi: 10.1371/journal.pone.0123251
Kim, G. H., Kim, B., and Jeong, J. H. (2019). The effects of home-based cognitive intervention with robots on cognitive performance in patients with mild cognitive impairment. Alzheimers Dement . 15:P154. doi: 10.1016/j.jalz.2019.06.4314
Klapper, A., Ramsey, R., Wigboldus, D., and Cross, E. S. (2014). The control of automatic imitation based on bottom-up and top-down cues to animacy: insights from brain and behavior. J. Cogn. Neurosci . 26, 2503–2513. doi: 10.1162/jocn_a_00651
Kohori, T., Hirayama, S., Hara, T., Muramatsu, M., Naganuma, H., Yamano, M., et al. (2017). “Development and evaluation of an interactive therapy robot,” in International Conference on Advances in Computer Entertainment (London: Springer), 66–83. doi: 10.1007/978-3-319-76270-8_6
Korchut, A., Szklener, S., Abdelnour, C., Tantinya, N., Hernández-Farigola, J., Ribes, J. C., et al. (2017). challenges for service robots—requirements of elderly adults with cognitive impairments. Front. Neurol . 8:228. doi: 10.3389/fneur.2017.00228
Kostavelis, I., Giakoumis, D., Malasiotis, S., and Tzovaras, D. (2015). “Ramcip: towards a robotic assistant to support elderly with mild cognitive impairments at home,” in International Symposium on Pervasive Computing Paradigms for Mental Health (Milan: Springer), 186–195. doi: 10.1007/978-3-319-32270-4_19
Koster-Hale, J., and Saxe, R. (2013). Theory of mind: a neural prediction problem. Neuron 79, 836–848. doi: 10.1016/j.neuron.2013.08.020
Kotseruba, I., Gonzalez, O. J. A., and Tsotsos, J. K. (2016). A review of 40 years of cognitive architecture research: focus on perception, attention, learning and applications. arXiv 1610.08602.
Law, M., Ahn, H. S., MacDonald, B., Vasjakovic, D. S., Lim, J., Lee, M. H., et al. (2019a). “User testing of cognitive training games for people with mild cognitive impairment: design implications,” in International Conference on Social Robotics (Madrid: Springer), 464–473. doi: 10.1007/978-3-030-35888-4_43
Law, M., Sutherland, C., Ahn, H. S., MacDonald, B. A., Peri, K., Johanson, D. L., et al. (2019b). Developing assistive robots for people with mild cognitive impairment and mild dementia: a qualitative study with older adults and experts in aged care. BMJ Open 9:e031937. doi: 10.1136/bmjopen-2019-031937
Lemaignan, S., Fink, J., Mondada, F., and Dillenbourg, P. (2015). “You're doing it wrong! studying unexpected behaviors in child-robot interaction,” in International Conference on Social Robotics (Paris: Springer), 390–400. doi: 10.1007/978-3-319-25554-5_39
Leong, T. W., and Johnston, B. (2016). Co-design and robots: a case study of a robot dog for aging people,” in International Conference on Social Robotics (Kansas City, MO: Springer), 702–711. doi: 10.1007/978-3-319-47437-3_69
Li, J. (2015). The benefit of being physically present: a survey of experimental works comparing copresent robots, telepresent robots and virtual agents. Int. J. Hum. Comput. Stud . 77, 23–37. doi: 10.1016/j.ijhcs.2015.01.001
Lins, A. A., de Oliveira, J. M., Rodrigues, J. J., and de Albuquerque, V. H. C. (2019). Robot-assisted therapy for rehabilitation of children with cerebral palsy-a complementary and alternative approach. Comput. Hum. Behav . 100, 152–167. doi: 10.1016/j.chb.2018.05.012
Loh, J. (2019). Responsibility and robot ethics: a critical overview. Philosophies 4:58. doi: 10.3390/philosophies4040058
Lopez-Samaniego, L., and Garcia-Zapirain, B. (2016). A robot-based tool for physical and cognitive rehabilitation of elderly people using biofeedback. Int. J. Environ. Res. Public Health 13:1176. doi: 10.3390/ijerph13121176
LoPresti, E. F., Bodine, C., and Lewis, C. (2008). Assistive technology for cognition [understanding the needs of persons with disabilities]. IEEE Eng. Med. Biol. Mag . 27, 29–39. doi: 10.1109/EMB.2007.907396
Lorenz, T., Weiss, A., and Hirche, S. (2016). Synchrony and reciprocity: key mechanisms for social companion robots in therapy and care. Int. J. Soc. Robot . 8, 125–143. doi: 10.1007/s12369-015-0325-8
Manca, M., Paternò, F., Santoro, C., Zedda, E., Braschi, C., Franco, R., et al. (2020). The impact of serious games with humanoid robots on mild cognitive impairment older adults. Int. J. Hum. Comput. Stud . 145:102509. doi: 10.1016/j.ijhcs.2020.102509
Marchesi, S., Ghiglino, D., Ciardo, F., Perez-Osorio, J., Baykara, E., and Wykowska, A. (2019). Do we adopt the intentional stance toward humanoid robots? Front. Psychol . 10:450. doi: 10.3389/fpsyg.2019.00450
Marino, F., Chilà, P., Sfrazzetto, S. T., Carrozza, C., Crimi, I., Failla, C., et al. (2019). Outcomes of a robot-assisted social-emotional understanding intervention for young children with autism spectrum disorders. J. Autism Dev. Disord . 50, 1973–1987. doi: 10.1007/s10803-019-03953-x
Matarić, M. J. (2017). Socially assistive robotics: human augmentation versus automation. Sci. Robot . 2:eaam5410. doi: 10.1126/scirobotics.aam5410
Melo, F. S., Sardinha, A., Belo, D., Couto, M., Faria, M., Farias, A., et al. (2019). Project inside: towards autonomous semi-unstructured human-robot social interaction in autism therapy. Artif. Intell. Med . 96, 198–216. doi: 10.1016/j.artmed.2018.12.003
Mewborn, C. M., Lindbergh, C. A., and Miller, L. S. (2017). Cognitive interventions for cognitively healthy, mildly impaired, and mixed samples of older adults: a systematic review and meta-analysis of randomized-controlled trials. Neuropsychol. Rev . 27, 403–439. doi: 10.1007/s11065-017-9350-8
Miskam, M. A., Shamsuddin, S., Yussof, H., Ariffin, I. M., and Omar, A. R. (2015). “A questionnaire-based survey: therapist's response on emotions gestures using humanoid robot for autism,” in 2015 International Symposium on Micro-NanoMechatronics and Human Science (MHS) (Nagoya: IEEE), 1–7. doi: 10.1109/MHS.2015.7438298
Mois, G., Collete, B. A., Renzi-Hammond, L. M., Boccanfuso, L., Ramachandran, A., Gibson, P., et al. (2020). “Understanding robots' potential to facilitate piano cognitive training in older adults with mild cognitive impairment,” in Companion of the 2020 ACM/IEEE International Conference on Human-Robot Interaction (Cambridge), 363–365. doi: 10.1145/3371382.3378299
Mori, M., MacDorman, K. F., and Kageki, N. (2012). The uncanny valley [from the field]. IEEE Robot. Autom. Mag . 19, 98–100. doi: 10.1109/MRA.2012.2192811
Morone, G., Paolucci, S., Cherubini, A., De Angelis, D., Venturiero, V., Coiro, P., et al. (2017). Robot-assisted gait training for stroke patients: current state of the art and perspectives of robotics. Neuropsychiatr. Dis. Treat . 13:1303. doi: 10.2147/NDT.S114102
Müller, V. C. (2020). “Ethics of artificial intelligence and robotics,” in The Stanford Encyclopedia of Philosophy, Winter 2020 Edn ., ed. E. N. Zalta (Stanford, CA: Metaphysics Research Lab, Stanford University).
PubMed Abstract | Google Scholar
Nault, E., Baillie, L., and Broz, F. (2020). “Auditory and haptic feedback in a socially assistive robot memory game,” in Companion of the 2020 ACM/IEEE International Conference on Human-Robot Interaction (Cambridge), 369–371. doi: 10.1145/3371382.3378375
Nunez, E., Matsuda, S., Hirokawa, M., and Suzuki, K. (2015). “Humanoid robot assisted training for facial expressions recognition based on affective feedback,” in International Conference on Social Robotics (Paris: Springer), 492–501. doi: 10.1007/978-3-319-25554-5_49
Orejana, J. R., MacDonald, B. A., Ahn, H. S., Peri, K., and Broadbent, E. (2015). “Healthcare robots in homes of rural older adults,” in International Conference on Social Robotics (Paris: Springer), 512–521. doi: 10.1007/978-3-319-25554-5_51
Otaki, H., and Otake, M. (2017). “Interactive robotic system assisting image based dialogue for the purpose of cognitive training of older adults,” in 2017 AAAI Spring Symposium Series (Stanford, CA).
Ozcana, B., Caligiorea, D., Fosch-Villarongab, E., Speratia, V., Morettac, T., and Baldassarrea, G. (2016). “Designing socially assistive robot (sar) for cognitive child-robot interaction (CCRI) with children with autism spectrum disorder-the case of “+ me,”” in 2nd International Conference on Social Robots in Therapy & Education (Barcelona).
Paletta, L., Fellner, M., Schüssler, S., Zuschnegg, J., Steiner, J., Lerch, A., et al. (2018). “Amigo: towards social robot based motivation for playful multimodal intervention in dementia,” in Proceedings of the 11th PErvasive Technologies Related to Assistive Environments Conference (Corfu), 421–427. doi: 10.1145/3197768.3203182
Pandey, A. K., and Gelin, R. (2018). A mass-produced sociable humanoid robot: pepper: the first machine of its kind. IEEE Robot. Autom. Mag . 25, 40–48. doi: 10.1109/MRA.2018.2833157
Paradeda, R. B., Hashemian, M., Rodrigues, R. A., and Paiva, A. (2016). “How facial expressions and small talk may influence trust in a robot,” in International Conference on Social Robotics (Kansas City, MO: Springer), 169–178. doi: 10.1007/978-3-319-47437-3_17
Peca, A., Coeckelbergh, M., Simut, R., Costescu, C., Pintea, S., David, D., et al. (2016). Robot enhanced therapy for children with autism disorders: measuring ethical acceptability. IEEE Technol. Soc. Mag . 35, 54–66. doi: 10.1109/MTS.2016.2554701
Peleka, G., Kargakos, A., Skartados, E., Kostavelis, I., Giakoumis, D., Sarantopoulos, I., et al. (2018). “Ramcip-a service robot for MCI patients at home,” in 2018 IEEE/RSJ International Conference on Intelligent Robots and Systems (IROS) (Madrid: IEEE), 1–9. doi: 10.1109/IROS.2018.8594214
Pennisi, P., Tonacci, A., Tartarisco, G., Billeci, L., Ruta, L., Gangemi, S., et al. (2016). Autism and social robotics: a systematic review. Autism Res . 9, 165–183. doi: 10.1002/aur.1527
Pereira, J., de Melo, M., Franco, N., Rodrigues, F., Coelho, A., and Fidalgo, R. (2019). “Using assistive robotics for aphasia rehabilitation,” in 2019 Latin American Robotics Symposium (LARS) 2019 Brazilian Symposium on Robotics (SBR) and 2019 Workshop on Robotics in Education (WRE) (Rio Grande: IEEE), 387–392. doi: 10.1109/LARS-SBR-WRE48964.2019.00074
Pettinati, M. J., and Arkin, R. C. (2015). “Towards a robot computational model to preserve dignity in stigmatizing patient-caregiver relationships,” in International Conference on Social Robotics (Paris: Springer), 532–542. doi: 10.1007/978-3-319-25554-5_53
Pierson, H. A., and Gashler, M. S. (2017). Deep learning in robotics: a review of recent research. Adv. Robot . 31, 821–835. doi: 10.1080/01691864.2017.1365009
Pino, O., Palestra, G., Trevino, R., and De Carolis, B. (2020). The humanoid robot NAO as trainer in a memory program for elderly people with mild cognitive impairment. Int. J. Soc. Robot . 12, 21–33. doi: 10.1007/s12369-019-00533-y
Price, C. J. (2012). A review and synthesis of the first 20 years of pet and fmri studies of heard speech, spoken language and reading. Neuroimage 62, 816–847. doi: 10.1016/j.neuroimage.2012.04.062
Rabbitt, S. M., Kazdin, A. E., and Hong, J. H. (2015). Acceptability of robot-assisted therapy for disruptive behavior problems in children. Archiv. Sci. Psychol . 3:101. doi: 10.1037/arc0000017
Rahbar, F., Anzalone, S. M., Varni, G., Zibetti, E., Ivaldi, S., and Chetouani, M. (2015). “Predicting extraversion from non-verbal features during a face-to-face human-robot interaction,” in International Conference on Social Robotics (Paris: Springer), 543–553. doi: 10.1007/978-3-319-25554-5_54
Rauchbauer, B., Nazarian, B., Bourhis, M., Ochs, M., Prévot, L., and Chaminade, T. (2019). Brain activity during reciprocal social interaction investigated using conversational robots as control condition. Philos. Trans. R. Soc. B 374:20180033. doi: 10.1098/rstb.2018.0033
Rehm, M., Krummheuer, A. L., Rodil, K., Nguyen, M., and Thorlacius, B. (2016). From social practices to social robots-user-driven robot development in elder care,” in International Conference on Social Robotics (Kansas City, MO: Springer), 692–701. doi: 10.1007/978-3-319-47437-3_68
Richardson, K., Coeckelbergh, M., Wakunuma, K., Billing, E., Ziemke, T., Gomez, P., et al. (2018). Robot enhanced therapy for children with autism (dream): a social model of autism. IEEE Technol. Soc. Mag . 37, 30–39. doi: 10.1109/MTS.2018.2795096
Riek, L., and Howard, D. (2014). “A code of ethics for the human-robot interaction profession,” in Proceedings of We Robot (Coral Gables, FL).
Riek, L. D. (2016). “Robotics technology in mental health care,” in Artificial Intelligence in Behavioral and Mental Health Care (San Diego, CA: Elsevier), 185–203. doi: 10.1016/B978-0-12-420248-1.00008-8
Riek, L. D. (2017). Healthcare robotics. Commun. ACM 60, 68–78. doi: 10.1145/3127874
Robinette, P., Howard, A. M., and Wagner, A. R. (2015). “Timing is key for robot trust repair,” in International Conference on Social Robotics (Paris: Springer), 574–583. doi: 10.1007/978-3-319-25554-5_57
Rudovic, O., Lee, J., Dai, M., Schuller, B., and Picard, R. W. (2018). Personalized machine learning for robot perception of affect and engagement in autism therapy. Sci. Robot . 3:eaao6760. doi: 10.1126/scirobotics.aao6760
Rudovic, O., Lee, J., Mascarell-Maricic, L., Schuller, B. W., and Picard, R. W. (2017). Measuring engagement in robot-assisted autism therapy: a cross-cultural study. Front. Robot. AI 4:36. doi: 10.3389/frobt.2017.00036
Salem, M., Lakatos, G., Amirabdollahian, F., and Dautenhahn, K. (2015). “Towards safe and trustworthy social robots: ethical challenges and practical issues,” in International Conference on Social Robotics (Paris: Springer), 584–593. doi: 10.1007/978-3-319-25554-5_58
Salichs, E., Fernández-Rodicio, E., Castillo, J. C., Castro-González, Á., Malfaz, M., and Salichs, M. Á. (2018). “A social robot assisting in cognitive stimulation therapy,” in International Conference on Practical Applications of Agents and Multi-Agent Systems (Toledo: Springer), 344–347. doi: 10.1007/978-3-319-94580-4_35
Salichs, M. A., Encinar, I. P., Salichs, E., Castro-González, Á., and Malfaz, M. (2016). Study of scenarios and technical requirements of a social assistive robot for Alzheimer's disease patients and their caregivers. Int. J. Soc. Robot . 8, 85–102. doi: 10.1007/s12369-015-0319-6
Salvador, M., Marsh, A. S., Gutierrez, A., and Mahoor, M. H. (2016). “Development of an aba autism intervention delivered by a humanoid robot,” in International Conference on Social Robotics (Kansas City, MO: Springer), 551–560. doi: 10.1007/978-3-319-47437-3_54
Sandygulova, A., and O'Hare, G. M. (2015). “Children's perception of synthesized voice: robot's gender, age and accent,” in International Conference on Social Robotics (Paris: Springer), 594–602. doi: 10.1007/978-3-319-25554-5_59
Sandygulova, A., Zhexenova, Z., Tleubayev, B., Nurakhmetova, A., Zhumabekova, D., Assylgali, I., et al. (2019). Interaction design and methodology of robot-assisted therapy for children with severe ASD and ADHD. Paladyn J. Behav. Robot . 10, 330–345. doi: 10.1515/pjbr-2019-0027
Santatiwongchai, S., Kaewkamnerdpong, B., Jutharee, W., and Ounjai, K. (2016). “Bliss: using robot in learning intervention to promote social skills for autism therapy,” in Proceedings of the international Convention on Rehabilitation Engineering & Assistive Technology, i-CREATe 2016 (Midview City: Singapore Therapeutic, Assistive & Rehabilitative Technologies [START] Centre), 1–4.
Saxe, R., and Wexler, A. (2005). Making sense of another mind: the role of the right temporo-parietal junction. Neuropsychologia 43, 1391–1399. doi: 10.1016/j.neuropsychologia.2005.02.013
Scassellati, B., Boccanfuso, L., Huang, C. M., Mademtzi, M., Qin, M., Salomons, N., et al. (2018). Improving social skills in children with asd using a long-term, in-home social robot. Sci. Robot . 3:eaat7544. doi: 10.1126/scirobotics.aat7544
Schüssler, S., Zuschnegg, J., Paletta, L., Fellner, M., Lodron, G., Steiner, J., et al. (2020). The effects of a humanoid socially assistive robot versus tablet training on psychosocial and physical outcomes of persons with dementia: protocol for a mixed methods study. JMIR Res. Protoc . 9:e14927. doi: 10.2196/14927
Shim, J., and Arkin, R. C. (2016). “Other-oriented robot deception: how can a robot's deceptive feedback help humans in HRI?” in International Conference on Social Robotics (Kansas City, MO: Springer), 222–232. doi: 10.1007/978-3-319-47437-3_22
Shukla, J., Barreda-Ángeles, M., Oliver, J., and Puig, D. (2017). “Effectiveness of socially assistive robotics during cognitive stimulation interventions: impact on caregivers,” in 2017 26th IEEE International Symposium on Robot and Human Interactive Communication (RO-MAN) (Lisbon: IEEE), 62–67. doi: 10.1109/ROMAN.2017.8172281
Shukla, J., Cristiano, J., Amela, D., Anguera, L., Vergés-Llahí, J., and Puig, D. (2015). “A case study of robot interaction among individuals with profound and multiple learning disabilities,” in International Conference on Social Robotics (Paris: Springer), 613–622. doi: 10.1007/978-3-319-25554-5_61
Shukla, J., Suri, V. R., Garg, J., Verma, K., and Kansal, P. (2019). “Mapping robotic affordances with pre-requisite learning interventions for children with autism spectrum disorder,” in 2019 28th IEEE International Conference on Robot and Human Interactive Communication (RO-MAN) (New Delhi: IEEE), 1–8. doi: 10.1109/RO-MAN46459.2019.8956247
Siciliano, B., and Khatib, O. (2016). Springer Handbook of Robotics . Cham: Springer.
Sonntag, D. (2015). “Kognit: Intelligent cognitive enhancement technology by cognitive models and mixed reality for dementia patients,” in 2015 AAAI Fall Symposium Series (Arlington, VA).
Sung, H. C., Chang, S. M., Chin, M. Y., and Lee, W. L. (2015). Robot-assisted therapy for improving social interactions and activity participation among institutionalized older adults: a pilot study. Asia Pac. Psychiatry 7, 1–6. doi: 10.1111/appy.12131
Sutton, R. S., and Barto, A. G. (2018). Reinforcement Learning: An Introduction . Cambridge, MA: MIT Press.
Taheri, A., Alemi, M., Meghdari, A., Pouretemad, H., Basiri, N. M., and Poorgoldooz, P. (2015a). “Impact of humanoid social robots on treatment of a pair of iranian autistic twins,” in International Conference on Social Robotics (Paris: Springer), 623–632. doi: 10.1007/978-3-319-25554-5_62
Taheri, A., Alemi, M., Meghdari, A., Pouretemad, H., and Holderread, S. (2015b). Clinical application of humanoid robots in playing imitation games for autistic children in Iran. Proc. Soc. Behav. Sci . 176, 898–906. doi: 10.1016/j.sbspro.2015.01.556
Taheri, A., Meghdari, A., Alemi, M., and Pouretemad, H. (2018). Clinical interventions of social humanoid robots in the treatment of a set of high-and low-functioning autistic iranian twins. Sci. Iranica 25, 1197–1214. doi: 10.24200/SCI.2017.4337
Taheri, A., Meghdari, A., Alemi, M., and Pouretemad, H. (2019). Teaching music to children with autism: a social robotics challenge. Sci. Iranica 26, 40–58. doi: 10.24200/sci.2017.4608
Taheri, A., Meghdari, A., Alemi, M., Pouretemad, H., Poorgoldooz, P., and Roohbakhsh, M. (2016). “Social robots and teaching music to autistic children: myth or reality?” in International Conference on Social Robotics (Kansas City, MO: Springer), 541–550. doi: 10.1007/978-3-319-47437-3_53
Taheri, A., Meghdari, A., and Mahoor, M. H. (2020). A close look at the imitation performance of children with autism and typically developing children using a robotic system. Int. J. Soc. Robot . doi: 10.1007/s12369-020-00704-2. [Epub ahead of print].
Taranović, A., Jevtić, A., Hernández-Farigola, J., Tantinyà, N., Abdelnour, C., and Torras, C. (2018a). “Resource-based modality selection in robot-assisted cognitive training,” in Companion of the 2018 ACM/IEEE International Conference on Human-Robot Interaction (Chicago, IL), 255–256. doi: 10.1145/3173386.3176961
Taranović, A., Jevtić, A., and Torras, C. (2018b). “Adaptable multimodal interaction framework for robot-assisted cognitive training,” in Companion of the 2018 ACM/IEEE International Conference on Human-Robot Interaction (Chicago, IL), 327–328. doi: 10.1145/3173386.3176911
Taranović, A., Jevtić, A., and Torras, C. (2018c). “Adaptive modality selection algorithm in robot-assisted cognitive training,” in 2018 IEEE/RSJ International Conference on Intelligent Robots and Systems (IROS) (Madrid: IEEE), 4456–4461. doi: 10.1109/IROS.2018.8593730
Tariq, S., Baber, S., Ashfaq, A., Ayaz, Y., Naveed, M., and Mohsin, S. (2016). “Interactive therapy approach through collaborative physical play between a socially assistive humanoid robot and children with autism spectrum disorder,” in International Conference on Social Robotics (Kansas City, MO: Springer), 561–570. doi: 10.1007/978-3-319-47437-3_55
Tleubayev, B., Zhexenova, Z., Zhakenova, A., and Sandygulova, A. (2019). “Robot-assisted therapy for children with adhd and asd: a pilot study,” in Proceedings of the 2019 2nd International Conference on Service Robotics Technologies (Beijing), 58–62. doi: 10.1145/3325693.3325703
Tokunaga, S., Seaborn, K., Tamura, K., and Otake-Matsuura, M. (2019). “Cognitive training for older adults with a dialogue-based, robot-facilitated storytelling system,” in International Conference on Interactive Digital Storytelling (Little Cottonwood Canyon, UT: Springer), 405–409. doi: 10.1007/978-3-030-33894-7_43
Trovato, G., Galeazzi, J., Torta, E., Ham, J. R., and Cuijpers, R. H. (2015). “Study on adaptation of robot communication strategies in changing situations,” in International Conference on Social Robotics (Paris: Springer), 654–663. doi: 10.1007/978-3-319-25554-5_65
Tsardoulias, E. G., Kintsakis, A. M., Panayiotou, K., Thallas, A. G., Reppou, S. E., Karagiannis, G. G., et al. (2017). Towards an integrated robotics architecture for social inclusion-the rapp paradigm. Cogn. Syst. Res . 43, 157–173. doi: 10.1016/j.cogsys.2016.08.004
Tsiakas, K., Abujelala, M., and Makedon, F. (2018). Task engagement as personalization feedback for socially-assistive robots and cognitive training. Technologies 6:49. doi: 10.3390/technologies6020049
Tsiakas, K., Dagioglou, M., Karkaletsis, V., and Makedon, F. (2016). “Adaptive robot assisted therapy using interactive reinforcement learning,” in International Conference on Social Robotics (Kansas City, MO: Springer), 11–21. doi: 10.1007/978-3-319-47437-3_2
Tung, F. W. (2016). Child perception of humanoid robot appearance and behavior. Int. J. Hum. Comput. Interact . 32, 493–502. doi: 10.1080/10447318.2016.1172808
University of Minnesota Libraries (2021). How Does Google Scholar Work? Minneapolis, MN: University of Minnesota Libraries.
Valentí Soler, M., Agüera-Ortiz, L., Olazarán Rodríguez, J., Mendoza Rebolledo, C., Pérez Muñoz, A., Rodríguez Pérez, I., et al. (2015). Social robots in advanced dementia. Front. Aging Neurosci . 7:133. doi: 10.3389/fnagi.2015.00133
van den Heuvel, R. J., Lexis, M. A., and de Witte, L. P. (2017). Robot zora in rehabilitation and special education for children with severe physical disabilities: a pilot study. Int. J. Rehabil. Res . 40:353. doi: 10.1097/MRR.0000000000000248
Vaufreydaz, D., Johal, W., and Combe, C. (2016). Starting engagement detection towards a companion robot using multimodal features. Robot. Auton. Syst . 75, 4–16. doi: 10.1016/j.robot.2015.01.004
Veerbeek, J. M., Langbroek-Amersfoort, A. C., Van Wegen, E. E., Meskers, C. G., and Kwakkel, G. (2017). Effects of robot-assisted therapy for the upper limb after stroke: a systematic review and meta-analysis. Neurorehabil. Neural Repair 31, 107–121. doi: 10.1177/1545968316666957
Villaronga, E. F. (2016). “What do roboticists need to know about the future of robot law,” in New Friends Conference Proceedings, New Friends: 2nd International Conference on Social Robots in Therapy and Education (Barcelona).
Wan, S., Gu, Z., and Ni, Q. (2020). Cognitive computing and wireless communications on the edge for healthcare service robots. Comput. Commun . 149, 99–106. doi: 10.1016/j.comcom.2019.10.012
Weiss, W., Fuhrmann, F., Zeiner, H., and Unterberger, R. (2017). “Towards an architecture for collaborative human robot interaction in physiotherapeutic applications,” in Proceedings of the Companion of the 2017 ACM/IEEE International Conference on Human-Robot Interaction (Vienna), 319–320. doi: 10.1145/3029798.3038393
Whelan, S., Murphy, K., Barrett, E., Krusche, C., Santorelli, A., and Casey, D. (2018). Factors affecting the acceptability of social robots by older adults including people with dementia or cognitive impairment: a literature review. Int. J. Soc. Robot . 10, 643–668. doi: 10.1007/s12369-018-0471-x
WHO (2011). World Report on Disability 2011 . Geneva: World Health Organization.
Wiltshire, T. J., Warta, S. F., Barber, D., and Fiore, S. M. (2017). Enabling robotic social intelligence by engineering human social-cognitive mechanisms. Cogn. Syst. Res . 43, 190–207. doi: 10.1016/j.cogsys.2016.09.005
Wong, H., and Zhong, Z. (2016). “Assessment of robot training for social cognitive learning,” in 2016 16th International Conference on Control, Automation and Systems (ICCAS) (Gyeongju: IEEE), 893–898. doi: 10.1109/ICCAS.2016.7832420
Woo, J., Botzheim, J., and Kubota, N. (2017). System integration for cognitive model of a robot partner. Intell. Autom. Soft Comput . 4, 1–14. doi: 10.1080/10798587.2017.1364919
Wood, L. J., Zaraki, A., Walters, M. L., Novanda, O., Robins, B., and Dautenhahn, K. (2017). “The iterative development of the humanoid robot Kaspar: an assistive robot for children with autism,” in International Conference on Social Robotics (Tsukuba: Springer), 53–63. doi: 10.1007/978-3-319-70022-9_6
Yokota, K., Ooi, S., and Sano, M. (2019). “A method of generating a dialogue pattern to induce awareness based on a reflection support agent,” in International Conference on Human-Computer Interaction (Orlando, FL: Springer), 421–438. doi: 10.1007/978-3-030-22219-2_32
Yu, R., Hui, E., Lee, J., Poon, D., Ng, A., Sit, K., et al. (2015). Use of a therapeutic, socially assistive pet robot (Paro) in improving mood and stimulating social interaction and communication for people with dementia: study protocol for a randomized controlled trial. JMIR Res. Protoc . 4:e45. doi: 10.2196/resprot.4189
Yun, S. S., Kim, H., Choi, J., and Park, S. K. (2016). A robot-assisted behavioral intervention system for children with autism spectrum disorders. Robot. Auton. Syst . 76, 58–67. doi: 10.1016/j.robot.2015.11.004
Zheng, Z., Nie, G., Swanson, A., Weitlauf, A., Warren, Z., and Sarkar, N. (2016). “Longitudinal impact of autonomous robot-mediated joint attention intervention for young children with ASD,” in International Conference on Social Robotics (Kansas City, MO: Springer), 581–590. doi: 10.1007/978-3-319-47437-3_57
Zheng, Z., Young, E. M., Swanson, A. R., Weitlauf, A. S., Warren, Z. E., and Sarkar, N. (2015). Robot-mediated imitation skill training for children with autism. IEEE Trans. Neural Syst. Rehabil. Eng . 24, 682–691. doi: 10.1109/TNSRE.2015.2475724
Zsiga, K., Tóth, A., Pilissy, T., Péter, O., Dénes, Z., and Fazekas, G. (2018). Evaluation of a companion robot based on field tests with single older adults in their homes. Assist. Technol . 30, 259–266. doi: 10.1080/10400435.2017.1322158
Keywords: rehabilitation robotics, human-robot interaction, robot-assisted cognitive training, socially assistive robotics, multimodal interaction, cognitive disability
Citation: Yuan F, Klavon E, Liu Z, Lopez RP and Zhao X (2021) A Systematic Review of Robotic Rehabilitation for Cognitive Training. Front. Robot. AI 8:605715. doi: 10.3389/frobt.2021.605715
Received: 13 September 2020; Accepted: 06 April 2021; Published: 11 May 2021.
Reviewed by:
Copyright © 2021 Yuan, Klavon, Liu, Lopez and Zhao. This is an open-access article distributed under the terms of the Creative Commons Attribution License (CC BY) . The use, distribution or reproduction in other forums is permitted, provided the original author(s) and the copyright owner(s) are credited and that the original publication in this journal is cited, in accordance with accepted academic practice. No use, distribution or reproduction is permitted which does not comply with these terms.
*Correspondence: Xiaopeng Zhao, xzhao9@utk.edu
This paper is in the following e-collection/theme issue:
Published on 27.5.2024 in Vol 26 (2024)
Advances in the Application of AI Robots in Critical Care: Scoping Review
Authors of this article:

- Yun Li 1, 2 * , MD ;
- Min Wang 1, 2 * , MPH ;
- Lu Wang 1, 2 * , MD ;
- Yuan Cao 3 * , MPH ;
- Yuyan Liu 1, 2 , MPH ;
- Yan Zhao 2 , MPH ;
- Rui Yuan 1, 2 , MD ;
- Mengmeng Yang 2 , MPH ;
- Siqian Lu 4 , DSC ;
- Zhichao Sun 4 , DSC ;
- Feihu Zhou 2 , PhD ;
- Zhirong Qian 4, 5, 6 , PhD ;
- Hongjun Kang 2 , PhD
1 Medical School of Chinese PLA, Beijing, China
2 The First Medical Centre, Chinese PLA General Hospital, Beijing, China
3 The Second Hospital, Hebei Medical University, Hebei, China
4 Beidou Academic & Research Center, Beidou Life Science, Guangzhou, China
5 Department of Radiation Oncology, Fujian Medical University Union Hospital, Fujian, China
6 The Seventh Affiliated Hospital, Sun Yat-sen University, Shenzhen, China
*these authors contributed equally
Corresponding Author:
Hongjun Kang, PhD
The First Medical Centre
Chinese PLA General Hospital
28 Fuxing Road
Haidian District,
Beijing, 100853
Phone: 86 13811989878
Email: [email protected]
Background: In recent epochs, the field of critical medicine has experienced significant advancements due to the integration of artificial intelligence (AI). Specifically, AI robots have evolved from theoretical concepts to being actively implemented in clinical trials and applications. The intensive care unit (ICU), known for its reliance on a vast amount of medical information, presents a promising avenue for the deployment of robotic AI, anticipated to bring substantial improvements to patient care.
Objective: This review aims to comprehensively summarize the current state of AI robots in the field of critical care by searching for previous studies, developments, and applications of AI robots related to ICU wards. In addition, it seeks to address the ethical challenges arising from their use, including concerns related to safety, patient privacy, responsibility delineation, and cost-benefit analysis.
Methods: Following the scoping review framework proposed by Arksey and O’Malley and the PRISMA (Preferred Reporting Items for Systematic Reviews and Meta-Analyses) guidelines, we conducted a scoping review to delineate the breadth of research in this field of AI robots in ICU and reported the findings. The literature search was carried out on May 1, 2023, across 3 databases: PubMed, Embase, and the IEEE Xplore Digital Library. Eligible publications were initially screened based on their titles and abstracts. Publications that passed the preliminary screening underwent a comprehensive review. Various research characteristics were extracted, summarized, and analyzed from the final publications.
Results: Of the 5908 publications screened, 77 (1.3%) underwent a full review. These studies collectively spanned 21 ICU robotics projects, encompassing their system development and testing, clinical trials, and approval processes. Upon an expert-reviewed classification framework, these were categorized into 5 main types: therapeutic assistance robots, nursing assistance robots, rehabilitation assistance robots, telepresence robots, and logistics and disinfection robots. Most of these are already widely deployed and commercialized in ICUs, although a select few remain under testing. All robotic systems and tools are engineered to deliver more personalized, convenient, and intelligent medical services to patients in the ICU, concurrently aiming to reduce the substantial workload on ICU medical staff and promote therapeutic and care procedures. This review further explored the prevailing challenges, particularly focusing on ethical and safety concerns, proposing viable solutions or methodologies, and illustrating the prospective capabilities and potential of AI-driven robotic technologies in the ICU environment. Ultimately, we foresee a pivotal role for robots in a future scenario of a fully automated continuum from admission to discharge within the ICU.
Conclusions: This review highlights the potential of AI robots to transform ICU care by improving patient treatment, support, and rehabilitation processes. However, it also recognizes the ethical complexities and operational challenges that come with their implementation, offering possible solutions for future development and optimization.
Introduction
Artificial intelligence (AI) and robotics are 2 distinct yet interconnected concepts ubiquitous in contemporary media and digital platforms. The term artificial intelligence was first introduced as a Medical Subject Heading in the US National Library of Medicine’s PubMed database in 1986, defined as “Theory and development of computer systems which perform tasks that normally require human intelligence” [ 1 ]. The hallmarks of AI encompass autonomous thinking, learning, recognition, reasoning, judgment, and inference. Medicine has long been considered a promising application field for AI, where it can augment clinical diagnostics and decision-making capacities [ 2 ]. Robotics, as defined by the Medical Subject Headings of the US National Library of Medicine, pertains to “the application of electronic, computerized control systems to mechanical devices designed to perform human functions” [ 3 ]. Presently, a standardized definition for “AI robots” remains elusive. However, they can be perceived as “physical devices inheriting electronic, computerized, and mechanical control systems, capable of perception, reasoning, learning, decision-making, and task execution without direct human control, able to mimic and execute various tasks of human intelligence” [ 4 ]. The crux of this review is the discussion of AI robot applications within the intensive care unit (ICU). While AI technology encompasses machine learning, deep learning, predictive modeling, and natural language processing, this review did not delve into these distinct technologies. Rather, it concentrated on the practical products and application cases where these AI technologies are integrated into robotic systems.
The past few decades have witnessed an exponential proliferation of research into AI robots, particularly in the health care domain. However, most of these developments have remained confined to the stages of product development and testing, with few achieving large-scale clinical implementation. The year 2020 sparked an unforeseen public health incident that catalyzed intense interest in AI robots. The outbreak of COVID-19 expedited the transformative revolution of “Healthcare + AI + Robotics” technologies and their applications [ 5 , 6 ]. The deployment of AI robots significantly curtailed the infection risk for medical personnel in contagion hot spots, and AI robots stood on the front lines in the battle against COVID-19 transmission [ 7 ]. Serving as a technology that improves performance, precision, and time efficiency as well as reducing costs, AI robots have promoted the upgrade and development of modern industry and are being rapidly adopted by many industries [ 8 , 9 ]. The applicability of robots in society is already evident and growing significantly [ 10 ].
Despite the rapid advancements in this field across military, security, transportation, and manufacturing sectors, our focus remains on intensive care scenarios such as COVID-19. ICUs are specialized settings designed to provide systematic, high-quality medical care and life-saving treatment to patients with single- or multiorgan dysfunction, life-threatening illnesses, or potential high-risk factors [ 11 ]. As scientific and technological advancements accelerate, the contradiction between the high demand for quality care for patients who are critically ill and the chronic shortage of medical resources becomes increasingly pronounced [ 12 ]. In 1995, Hanson and Marshall [ 13 ] postulated that AI could reduce care costs for patients in the ICU and improve their prognosis. “There are plenty of areas in critical care where it would be extremely helpful to have efficacious, fair, and transparent AI systems,” notes Gary Weissman, professor of pulmonary and critical care medicine [ 14 ]. The full potential of AI will be realized once it becomes a trusted clinical assistant for intensivists. After all, ICUs, which routinely collect a significant volume of data, provide an ideal setting for the deployment of machine learning technologies [ 15 ].
Currently, the application of AI in intensive care predominantly focuses on “assisting” health care professionals. This review targeted AI robots in ICUs, primarily discussing relevant advancements in recent years and presenting and analyzing challenges faced in ICUs and potential solutions, as well as strategies for health care professionals to handle AI robotic technologies, for the reference of health care professionals and system developers.
We followed the scoping review methodology proposed by Arksey and O’Malley [ 16 ], which includes (1) identifying the research question; (2) identifying relevant studies; (3) study selection; (4) charting the data; and (5) collating, summarizing, and reporting the results. In addition, to ensure the rigor of the scoping review, we adhered to the PRISMA-ScR (Preferred Reporting Items for Systematic Reviews and Meta-Analyses extension for Scoping Reviews) guidelines ( Multimedia Appendix 1 [ 17 ]).
Upon establishing the focus on the application of AI robots in ICUs, we embarked on a keyword search encompassing terms related to robots and intensive care. Due to the novelty and complexity of the research topic, the experimental designs and results presentation vary significantly across the literature, making traditional methods of literature quality assessment inadequate. Consequently, we relied on the profound knowledge and practical experience of domain experts to provide essential perspectives for understanding the complexities presented in the literature. We engaged 4 experts—2 from the IT sector and 2 medical professionals—to devise a search strategy and select appropriate databases. Given the interdisciplinary nature of our research, spanning medicine, robotics engineering, and human-computer interaction design, our search was not confined to medical databases; we also included databases from the engineering field. Our literature search encompassed 3 electronic databases: PubMed, Embase, and the IEEE Xplore Digital Library. We restricted our search to publications in English, imposing no limits on the year of publication. The search was conducted over a brief period, from May 1, 2023, to May 5, 2023. Textbox 1 provides detailed insights into our search methodology.
Search strings
- PubMed: (“Artificial Intelligence” [Medical Subject Heading (MeSH)] OR “AI” OR “Robotics” [MeSH] OR “Robots”) AND (“Intensive Care Units” [MeSH] OR “ICU” OR “Critical Care Units” OR “CCU”)
- Embase: (“artificial intelligence”/exp OR “ai” OR “robotics”/exp OR “robots”) AND (“intensive care unit”/exp OR “icu” OR “critical care unit” OR “ccu”)
- IEEE Xplore Digital Library: (“Artificial Intelligence” OR “AI” OR “Robotics” OR “Robots”) AND (“Intensive Care Units” OR “ICU” OR “Critical Care Units” OR “CCU”)
During our initial search across the 3 databases, we retrieved a total of 5908 articles. These search results were first imported into EndNote (Clarivate Analytics), a literature management tool, to facilitate the deduplication process. Subsequently, LW and YC independently conducted a screening of titles and abstracts to weed out articles that were clearly irrelevant. Given the market’s saturation with robot products of similar functionalities and the constraints on this review’s length, we opted to focus on the most representative or widely adopted technologies among robots offering the same functions. This selection process led to the inclusion of 77 articles in our review. These studies encompassed multiple phases, including the design, development, and validation of robots, collectively covering 21 different AI robotic products, detailing their development, evolution, and application examples. Figure 1 shows a comprehensive view of our study selection process.

In our examination of the 77 articles selected for inclusion, we meticulously identified the key characteristics of each study. These characteristics encompassed the design purpose of the technology, the AI algorithms used, the anticipated application scenarios, and the target user groups. From this analysis, we developed an initial classification framework aimed at organizing technologies based on their primary functions and application contexts. For instance, technologies designed for nursing tasks were classified under nursing assistance robots, whereas those with therapeutic responsibilities were designated as therapeutic assistance robots. Similarly, devices integrated with ventilators capable of remote operation and achieving therapeutic goals were also classified as therapeutic assistance robots.
To validate the accuracy and logic behind our classification, we sought insights from additional experts in the domains of medicine and information engineering. Their feedback prompted adjustments and refinements to our framework, ensuring that it precisely represented the nuances and relationships among the various technologies.
Subsequently, applying the refined classification framework, we categorized the included studies into their respective groups, deriving organized results. This meticulous classification was undertaken with the aim of ensuring clarity and comprehensibility for all potential readers, including professionals (physicians and engineers), patients, and their families. Our goal was to provide a clear understanding of the applications and potential of various technologies in the ICU, making the information accessible and valuable to a broad audience.
Inclusion and Exclusion Criteria
This review included studies that met two main criteria: (1) the studies needed to focus on actual products or application cases that conformed to the definition of AI robots, and (2) the application scenario of the study had to be the ICU or any place for patients discharged from the ICU.
We excluded studies that met any of the following conditions: (1) limited to AI algorithms such as machine learning, deep learning predictive models, or decision support systems; and (2) not published in English.
Application of AI Robots in Intensive Care
Currently, there is a broad array of experimental studies and AI robots applied in ICUs. We categorized these into 5 main types based on their application scenarios and functions in ICUs: therapeutic assistance robots, nursing assistance robots, rehabilitation assistance robots, telepresence robots, and logistics and disinfection robots ( Figure 2 ). The application of robotics in the medical field is extensive, encompassing myriad functions and scenarios, rendering their precise classification a formidable task. Multimedia Appendix 2 [ 6 , 18 - 90 ], which is neither exclusive nor exhaustive, summarizes the representative research groups and commercial suppliers to provide readers with a panoramic view of the field.

Therapeutic Assistance Robots
The ICU is a specialized department dedicated to the treatment of patients who are critically ill. Driven by the necessity to enhance therapeutic outcomes, mitigate medical risks, alleviate the workload of health care personnel, and provide personalized treatment regimens for patients, therapeutic assistance robots in the ICU have become indispensable [ 91 ].
Ventilator-Mounted Cartesian Robot
This can also be viewed as a type of telepresence robot. A teleoperated ventilator controller system has been developed, which consists of a custom robotic patient-side device and a touch-based master console. Computer vision tasks that enable an intuitive user interface and accurate robot control are executed on the master console. The system was initially developed and deployed for the most popular touch screen–controlled ventilator at Johns Hopkins Hospital, the Maquet Servo-u (Getinge AB) [ 18 ]. Different from the traditional ventilators in most ICUs, the robot performs remote control and monitoring outside the ICU room through the network. The actual ventilator settings are controlled and adjusted remotely via physical controls (such as buttons and knobs) and synchronized real-time image transmission video feedback [ 19 ]. It reduces the time staff spend entering the ICU performing simple tasks such as changing ventilator settings, reducing the risk of infection and stress on personal protective clothing resources. Following a qualitative assessment in clinical environments, feedback from respiratory therapists highlighted that the system could significantly empower the respiratory care team by liberating valuable resources. The design’s Cartesian layout enables the robot to approach the operating table in as horizontal a manner as possible. This approach not only minimizes the installation burden but also ensures that the robot’s operation remains closely aligned with conventional manual procedures. Such a configuration facilitates ease of use and integration into existing medical workflows, thereby enhancing efficiency without compromising the quality of patient care [ 18 ]. In a simulated ICU environment, a Cartesian robot reduced the total time required for a respiratory therapist to make typical setup adjustments to a traditional ventilator from 271 to 109 seconds, which was 2.49 times faster [ 18 ]. More recently, Song et al [ 20 ] developed an integrated telemonitoring or operation system with an accurate XY positioner and a 3-df end effector for accurate manipulation (maximum positioning error of 0.695 mm; repeatability is 0.348 mm).
In ICUs where a single brand of ventilator is predominantly used, configuring Cartesian robots proves to be more convenient. Nevertheless, to accommodate a broader array of ICU wards and equipment types such as infusion pumps, it is imperative to expand the variety and range of control interfaces. This expansion aims to enhance the robot system’s capabilities for physical control interactions. However, a significant hurdle to the clinical application of these robotic systems is the challenge associated with their cleaning and disinfection. This issue could be mitigated in the future by enclosing the equipment within acrylic covers, thereby simplifying the process of maintaining hygiene and ensuring the equipment’s safety for patient care [ 6 ]. This solution not only addresses infection control concerns but also aids in the seamless integration of robotic systems into the rigorous and cleanliness-focused environment of the ICU.
McSleepy (Intelligent Technology in Anesthesia research group laboratory, McGill University, Montreal, Quebec, Canada), a real robot for anesthesia, is able to autonomously control hypnosis, analgesia, and neuromuscular block at the same time with regard to induction, maintenance, and emergence [ 21 ]. The anesthesiologist begins by entering patient data, including height, weight, type of surgery, and medication history, on the touch screen. In fully automatic mode, the system will use remifentanil, propofol, and rocuronium or succinylcholine to induce anesthesia. McSleepy assists the anesthesiologist in the same way that automatic transmission assists people when driving. Through a sensor that measures muscle movement, it monitors the patient’s depth of consciousness, pain severity, and muscle movement and injects the corresponding dose of medication intravenously according to a built-in algorithm based on the obtained data. It provides deep or peripheral muscle relaxation in different closed-loop models [ 22 ]. As such, anesthesiologists can focus more on other aspects of direct patient care. An additional feature is that the system can communicate with PDAs, making distant monitoring and anesthetic control possible [ 21 , 23 , 24 ].
In a pilot study in the Department of Anesthesiology and Critical Care of the Bordeaux University Hospital, McSleepy performed automatic anesthesia for cardiac surgery with isoproterenol, remifentanil, and rocuronium without manual control. Automatic cardiac anesthesia was successfully performed in 80% (97.5% CI 53%-95%) of cases. Hypnosis was monitored using the bispectral index, which was <20% of the bispectral index target of 45, showing better control over hypnosis and duration of anesthesia [ 25 ]. A randomized controlled trial investigating a novel closed-loop drug delivery system found that automated delivery achieves superior sedation control over manual methods. This improvement is credited to the closed-loop system’s ability to frequently or continuously monitor control variables and adjust drug delivery rates more often, circumventing the fatigue that can impair manual administration [ 26 ]. To guarantee the safety of this automated system, numerous protective features have been implemented. These include preventing the administration of muscle relaxants when mask ventilation proves difficult and querying any manual actions that breach established safety protocols [ 27 ]. Recent advancements in research highlight the successful application of robotic technology in anesthesiology. Beyond the surgical anesthesia applications that we have detailed, delicate procedures such as endotracheal intubation are also seeing promising developments [ 28 ]. Anesthesiologists are encouraged to engage with robotic systems, leveraging their significant contributions to enhancing medical quality and efficacy, thereby ensuring the highest standard of patient care and treatment.
Cardiopulmonary Resuscitation Robots (Seoul University Medical College, South Korea)
Some reports indicate that failure of cardiopulmonary resuscitation (CPR) is an important limiting factor for life-prolonging treatment of patients in the ICU [ 29 , 30 ]. Robots have enough power to achieve high-quality CPR, which could overcome the shortcomings of manual CPR and mechanical CPR devices. Recently, Jung et al [ 31 ] developed an automated robotic CPR system that performs CPR automatically, analyzes the patient’s condition, and relays the information to the CPR system. In the initial state of the CPR process, the robot manipulator determines the optimal compression position by adjusting the point of pressure and, guided by end-tidal carbon dioxide levels, periodically and repeatedly delivers adequate speed and depth for performing CPR. The combined CPR system can accurately capture the compression condition of patients, overcome the blank periods when medical personnel alternately perform CPR, and increase the probability of cardiac resuscitation [ 31 , 32 ].
In a study using a porcine model of cardiac arrest, the use of a CPR robot did not significantly enhance the success rate of resuscitation efforts compared to manual CPR. However, there was a notable improvement in the neurological deficit scores observed 48 hours after resuscitation, suggesting a potential benefit in postresuscitation neurological outcomes [ 32 ]. Another study indicated that robot-assisted CPR outperformed traditional manual CPR methods, achieving higher resuscitation success rates in patients without specific injuries [ 31 ]. The anticipated benefits of incorporating robot-assisted CPR into clinical settings extend beyond merely improving resuscitation success rates. They also include the potential to reduce labor costs and minimize the instances of ICU staff congregating around a patient’s bedside to alternate performing CPR. This technological advancement suggests a promising direction for enhancing the efficiency and effectiveness of resuscitation efforts, thereby improving patient outcomes while optimizing resource use within critical care environments.
Nursing Assistance Robots
Nursing care robots, designed to assist patients who are bed-ridden with simple long-term care services, have been widely used in hospitals to assist older adults and individuals with disabilities. The many invasive diagnostic procedures and heavy nursing workload (multiple multitasking) in the ICU make medical staff vulnerable and overloaded, with very limited ability to provide timely care to patients. Through the intervention of intelligent robots, heavy physical activity in nursing work is alleviated to a certain extent, and nurses are liberated from it so that they can put their energies into more professional and meticulous nursing work.
First, despite the proliferation of devices currently available on the market to assist with venipuncture, there remain certain limitations. Technologies such as ultrasound, which can easily penetrate human tissue, thereby enabling the high-resolution visualization of both shallow and deep tissue structures for vascular guidance, and devices such as the VeinViewer and AccuVein AV300 [ 33 , 34 ], which use near-infrared spectroscopy technology for vascular imaging, are fairly mature. However, these techniques are still constrained by their inability to provide depth of the vein as well as the fact that imaging technology does not directly aid the insertion of the needle. VenousPro (VascuLogic, LLC), an automated robotic venipuncture device, addresses these issues. It identifies vessels suitable for cannulation and robotically guides an attached needle toward the lumen center, enabling the safe drawing of blood from peripheral forearm veins [ 35 , 36 ]. The device uses 940-nm near-infrared light to enhance the contrast of the subcutaneous peripheral vein, selecting the appropriate vein through real-time imaging and mapping of the 3D spatial coordinates of the subcutaneous vein [ 36 ]. Chen et al [ 36 ] evaluated the system’s cannulation accuracy on a vascular model through tracking, free-space localization, and use of a dark-skinned phlebotomy training model. Early versions of the device successfully demonstrated the feasibility of automatic venous access with a 100% success rate for venipuncture and placing of the needle in the desired position with high precision (mean positioning error 0.21 mm, SD 0.02 mm) [ 35 ]. This effectively reduced the risk of acupuncture injury [ 37 ].
Subsequently, the team developed an improved instrument based on a 9-df image-guided venipuncture robot, which increased radial rotation in response to rolling vein deformation and allowed for real-time adjustments to the pose and orientation of the needle [ 38 ]. Through robust optimization of near-infrared imaging coupled with image analysis and a robotic control system, this venipuncture robot realizes the automation of venipuncture, minimizing the likelihood of needlestick injuries and related bloodborne infections. This not only liberates nursing staff from the repetitive task of venipuncture but also protects the safety of practitioners [ 36 ]. In addition, this device may, in the future, be integrated with diagnostics, facilitating automated blood draws and rapid patient information synchronization. Moreover, the foundational imaging, computer vision, model recognition, and robotic technology developed for this device can be extended to arterial pathways, computer-assisted diagnostics, and miniature robotic surgery, potentially revolutionizing practice in ICUs and other departments.
Second, prompt and effective airway management can prove pivotal to the survival of patients who are critically ill. However, for patients with substantial secretions, sputum aspiration undertaken by nursing staff becomes a frequent necessity, significantly demanding the latter’s time and effort. Therefore, a sputum suction robot (Xi’an Jiaotong University, China) has been developed, which is a simple 6-df manipulator with a steering gear. Exhibiting stable movement, the sputum suction robot is proficient in smoothly performing the clamping, insertion, back-off protection, and removal of the suction tube, thereby achieving effective sputum aspiration [ 39 ]. Naturally, the current iteration of this sputum suction robot faces certain challenges, such as the complexity of the mechanical arm structure and limited mobility that may result in some sputum being left unaspirated. The simplification of the driving mechanism and the optimization of mobility present promising directions for future enhancements. Such improvements aim to realize high-precision, high-quality automated sputum suction within the ICU.
Third, kangaroo care is a nursing method aimed at premature infants. It serves as a nonpharmacological intervention for treating surgical pain in infants. By promoting skin-to-skin contact between the infant and parents, it reduces the adverse effects of repeated surgical pain on the long-term development of the nervous system [ 40 ]. However, a notable challenge within the neonatal ICU (NICU) setting is that parents cannot always be physically present to provide this essential care. This limitation calls for innovative solutions to ensure that premature infants still receive the benefits of kangaroo care, possibly through alternative methods or support systems that can simulate the presence and therapeutic effects of parental contact. Calmer (University of British Columbia, Vancouver, British Columbia, Canada) was developed to manage acute pain effectively for preterm infants in the NICU by simulating key pain-reducing components of human touch–based treatment. The human tactile pain treatment effect is achieved by placing the infant prone on the Calmer, which provides sensory intervention similar to parental skin contact while simulating the heartbeat and respiratory frequency of the parents with customized physiological signal processing software to mitigate the adverse neurodevelopmental effects of early pain exposure in preterm infants [ 41 ]. The effects of this robotic device on pain management during routine blood collection were studied in 10 infants. Calmer reduced physiological pain reactivity during and after painful blood collection procedures [ 34 , 40 ]. This approach not only aligns with the philosophy of nonpharmacological pain management but also potentially saves the NICU approximately US $380,000 per year in nursing time (United States). However, it is crucial to clarify that Calmer does not intend to replace the role of parents or neonatal care. It merely provides an alternative solution when such avenues are not available.
Fourth, older patients consistently represent a predominant segment of ICU admissions, manifesting unique characteristics and frequently presenting with cognitive impairments and mobility limitations. Physical assistive robots are strategically engineered to address the exigencies of daily living activities [ 92 ]. A robot named Paro has been extensively integrated within ICU environments and subsequent post-ICU discharge home care, offering socioemotional and psychological support to older patients in the ICU. With its lifelike appearance and behavior, Paro emulates genuine animal esthetics and movements, enabling it to perceive and respond to human vocalizations, touch, and actions. Recognized as one of the world’s premier nursing assistance robots, Paro has been commercialized and operationalized across diverse care settings, particularly within ICU care, in multiple countries [ 42 ]. While it is designed to provide cognitive stimulation, no definitive evidence has surfaced indicating immediate or long-term cognitive improvement after Paro intervention. However, we cannot overlook its positive psychological impact in several settings. Its widespread application and accessibility also serve as a blueprint for the development of companion robots [ 43 ].
Beyond the 4 nursing assistance robots previously mentioned, a diverse range of technologies is under development aimed at enhancing nursing practice. These technologies are designed to assist with various tasks, including feeding patients, transporting patients, bathing, providing emotional support, administering medication, and facilitating other daily activities. Notable examples include the Care-O-bot, a compact, highly integrated service robot that primarily functions as a household assistant [ 44 ]. Another example is “RI-MAN,” a soft-bodied robot designed to safely lift adults [ 45 ]. While these robots undertake certain nursing responsibilities and are potentially beneficial to the general population, they were not included in the scope of this review. This decision reflects our focus on specific categories of medical robot modules directly applicable to critical care and ICU settings, thereby delineating a clear boundary for our review’s content and objectives.
The extensive research by Locsin [ 93 ] underscores a future where the integration of nursing with AI technology is not just a possibility but an inevitability. This projection implies that, as technology evolves, nurses will need to become proficient in advanced technological tools, ensuring that their participation in clinical practices is grounded in informed consent. In the nursing practices of the future, technology will serve to augment human nursing activities. This includes leveraging technology for predictive interventions to enhance nursing efficiency. However, it is critical to note that human cognition and emotional intelligence will remain at the heart of technological care. This integration suggests a model of care where technology and human elements complement each other, ensuring that patient care remains personalized, empathetic, and efficient, thereby reflecting the intrinsic values of nursing while embracing the advancements of AI technology.
Thus, the future of nursing practice is envisioned as a symbiotic relationship between human nurses and medical robots. This partnership aims not merely at task completion but also at leveraging sophisticated technology to deepen patient understanding and enhance care, thereby elevating the standard of nursing services provided. Human nurses are poised to maintain their pivotal role in health care, with person-centered care continuing to serve as the foundational principle of nursing. Through this fusion of technology and human touch, nursing will evolve, ensuring that care remains empathetic, responsive, and fundamentally human at its core.
Rehabilitation Assistance Robots
Most patients who are critically ill, confined to bed rest for extended periods, exhibit a high incidence of ICU-acquired weakness, significantly influencing patient prognoses [ 94 ]. Rehabilitation therapy, a potential solution to this issue, can notably enhance patient outcomes and quality of life. Numerous studies have attested to the pivotal role of early rehabilitation in improving patient prognoses and ensuring quality of life. Rehabilitation robots are devised to aid the restoration of impaired sensory, motor, and cognitive skills [ 95 ]. The deployment of these robots can relieve physicians of strenuous training tasks, allowing for the analysis of robot-derived data during training sessions to evaluate patient rehabilitation status. It has been established that intensive locomotor training can affect improvements in walking function for patients with movement disorders after stroke or spinal cord injury (SCI) [ 96 - 98 ]. Rehabilitation robots facilitate more extensive and intensive training compared to traditional therapeutic methods. Moreover, rehabilitation robots typically engage and challenge patients to meet objectives interactively. Although their application within the ICU is currently minimal, these robots hold promising potential in assisting early rehabilitation efforts for patients in the ICU. There are presently several commercially available and experimental-stage noncommercial rehabilitation robots reported in the literature. Some cases are presented in the following paragraphs.
First, Lokomat (Hocoma, Inc) is a sophisticated robotic system designed for gait rehabilitation. It targets patients who exhibit locomotor anomalies induced by brain injury, spinal cord damage, neurological disorders, muscular injuries, and orthopedic diseases, facilitating improved mobility in patients who are neurologically compromised [ 46 , 47 ]. The Lokomat system comprises a structural frame affixed to a treadmill, featuring a load-bearing arrangement such as a gait orthosis. When attached to the patient, it modulates hip and knee joint movements to generate a predefined gait pattern [ 48 ]. Regular Lokomat-assisted training has been effectively used in rehabilitating patients who were comatose with cerebral hemorrhage. Following a 4-month therapeutic regimen, patients exhibited remarkable improvements in the severity of their comatose state (as per the Glasgow Coma Scale score of 4), an extension in walking duration from 15 to 32 minutes, and recuperation of eye and joint movement [ 49 ].
In a rigorous evaluation, Chillura et al [ 47 ] used Lokomat in a 6-month intensive conventional rehabilitation therapy. The outcome was a marked improvement in muscle strength (42/60), physical and mental independence (80/126), and 6-minute walk distance (47 m) in patients in the ICU with acquired weakness. A separate prospective study corroborates the potential of Lokomat in ameliorating patient rehabilitation after stroke [ 50 ]. A randomized single-blind, parallel-group clinical trial (40 participants per group) showed that training with the Lokomat system for 3 to 6 months after lesion can improve the walking ability of patients with incomplete SCI. This improvement was manifested in increased walking endurance and enhanced strength in the lower limbs [ 51 ]. Furthermore, 6 additional randomized controlled trials using the Lokomat system corroborated these findings [ 52 - 57 ], underscoring the consistency and reliability of the Lokomat as an effective rehabilitation tool for improving mobility and strength in individuals with incomplete SCI. This body of evidence strongly supports the Lokomat system’s role in advancing the recovery process for patients with SCI, offering them a viable pathway to regain mobility and improve their quality of life.
Currently, advancements in robot-assisted lower-limb rehabilitation have led to the development of 3 main types of devices: exoskeleton, end effector, and portable powered robotic exoskeletons. End-effector devices interact directly with the patient’s lower limbs, applying force, assisting movement, or guiding patients through specific movement patterns, exemplified by the “G-EO-Systems” [ 99 ] and “Haptic Walker” [ 100 ]. Exoskeleton-type devices, exemplified by “LOPES” from Delft University of Technology in the Netherlands [ 101 ], encase the patient’s legs and provide support and movement assistance directly aligned with the limb’s natural biomechanics. Among these, Lokomat stands out due to its superior customization capabilities, safety, comfort, and integration of virtual reality (VR). It also boasts features such as participation detection and motivational elements to engage users more effectively in their rehabilitation process. With 651 institutions worldwide adopting Lokomat, its widespread use underscores its significant advantages and effectiveness in facilitating the rehabilitation of patients with lower-limb impairments [ 58 ].
Such findings hint toward a broad applicability of Lokomat in ICU settings, enabling both early and post-ICU rehabilitation to be personalized and intelligent. As AI and machine learning continue to advance, the Lokomat system’s ability to analyze patients’ gait data and physiological indicators to offer precise rehabilitation strategies and real-time feedback is becoming a reality. Leveraging VR technology may render the rehabilitation training environment for patients in the ICU more captivating and web-based, fostering increased patient engagement and positivity during the recuperative process. Furthermore, integration with biometric sensors and remote monitoring technologies could feasibly enable real-time tracking and remote guidance of patients’ rehabilitation progress, heralding the future direction of Lokomat’s potential applications.
Second, aside from lower-limb or gait assistance rehabilitation robots, research into upper-limb rehabilitation robotics remains an active field of investigation. ArmeoSpring (Hocoma, Inc) is a rehabilitative exoskeleton that stabilizes the arm via a fixed frame. It is a passive exoskeleton that uses adjustable springs to deliver gravitational compensation for the patient, thus allowing the individual undergoing rehabilitation to concentrate solely on executing requisite tasks. Despite its passive nature, the ArmeoSpring exoskeleton is laden with sensors, enabling its application as an evaluation instrument for assessing the capacity and range of the user’s arm [ 59 ]. These sensors also facilitate interactive training and integration with VR, enabling patients to simulate task-oriented motor exercises within a virtual learning environment on a computer screen, providing auditory and visual performance feedback during and after interaction.
A 5-year randomized controlled trial involving a subacute stroke program evaluated 215 patients with stroke with moderate to severe arm injuries who were undergoing rehabilitation therapy [ 60 ]. It appeared that there was no substantial difference in functional upper-extremity improvement with ArmeoSpring robotic intervention, although sensorimotor scores did demonstrate enhancement (13.32 vs 11.78). This equivocal result could be ascribed to a lack of sequencing of early testing interventions [ 61 ]. Conversely, another clinical trial involving a patient with mild to moderate hemiparesis demonstrated significant improvement in upper-extremity arm motion after 4 months of ArmeoSpring treatment [ 62 ]. It remains uncertain whether robotic-assisted rehabilitation definitively surpasses conventional physical therapy; rather, it appears to offer advantages to traditional treatments. In addition, in contrast to conventional physical therapy, careful patient selection is essential for robotic-assisted interventions. Factors such as the degree of functional impairment, age, disease duration, and cognitive levels play significant roles in this selection process. A study focusing on the evaluation of upper-limb movement parameters in patients after a stroke using the ArmeoSpring demonstrated its effectiveness in reliably and sensitively assessing motor impairments and the influence of therapeutic interventions on the motor learning process. The research highlighted the device’s potential as a valuable assessment tool for quantifying sensorimotor disorders in the upper limb [ 59 ].
Another type of end-effector robot, the “MIT-MANUS” (Massachusetts Institute of Technology, United States) [ 63 ], trains the upper limb by applying force at a single point on the patient’s arm. However, due to its linkage mechanism, the robot has a long mechanical arm and low torque output capacity, making it difficult to perform tasks requiring high-load resistance training. In addition, it has a large footprint. It lacks the ability to perform complex movements of the upper-limb joints, similar to the ArmeoSpring. Therefore, we did not include the MIT-MANUS in this review.
Third, patients in the ICU not only grapple with their primary illness but also frequently experience newly acquired long-term physical, psychological, and cognitive impairments, collectively referred to as post–intensive care syndrome [ 102 ]. Given the inconsistency in results from various screening tools, there is an urgent need for an objective, comprehensive, simplified, and unified assessment tool. The Kinesiological Instrument for Normal and Altered Reaching Movements (KINARM; Kingston, Ontario, Canada) is a robotic research tool specifically designed to execute quantitative neurological evaluations of sensorimotor, proprioceptive, and cognitive brain functions. It comprises a wheelchair and an upper-extremity exoskeleton tailored to patients based on their physical specifications. The KINARM permits researchers to gauge the coordination of limbs across multiple joints while also precisely measuring the joint-specific force exerted by the patient during task execution. The precision of this tool eliminates the subjectivity typically intrinsic to physiotherapeutic assessments of neurological status, such as muscle tone, spasticity, proprioception, and others [ 64 ].
A total of 104 patients in the ICU underwent sensorimotor and neurocognitive assessments using the KINARM 3 and 12 months after discharge. The team then performed a series of kinase evaluations on stroke survivors 3 and 12 months after discharge in patients who were critically ill and receiving acute renal replacement therapy and obtained a 0.3 correlation (90% strength in 89 patients) between regional cerebral oxygen saturation (a surrogate marker of cerebral autonomic regulation) and delirium in patients who were critically ill through KINARM scoring [ 65 ]. The tool has also been used to assess the correlation between brain tissue oxygenation (a surrogate marker of brain perfusion) during the acute phase of critical illness (ie, 24 hours) and long-term neurological dysfunction [ 66 , 103 ], as well as to evaluate sensorimotor deficits in patients with stroke and traumatic brain injury [ 67 , 104 ]. The KINARM provides objective and quantifiable data for sensorimotor and neurocognitive functions in ICU survivors. As a diagnostic and assessment tool, it aids rehabilitation and supports ICU survivors in regaining autonomy and independence in their daily lives. Despite its limitations, efforts must continue to enhance mobility and portability, broaden applicability and scope, and reduce cost and operational complexity.
Compared to traditional physiotherapy, robot-assisted rehabilitation based on AI and VR offers patients more intensive, systematic, repetitive, and task-oriented rehabilitation training, which plays a crucial role in promoting the process of functional recovery. Although many studies have shown that robot-assisted rehabilitation can effectively enhance the rehabilitation effect, a review of the clinical application of stroke rehabilitation points out that robot-assisted therapy has not shown obvious advantages in improving the motor function of patients with stroke [ 68 ]. Compared with traditional training or stand-alone training, its effect on the rehabilitation of patients with chronic stroke is still questionable. Similarly, the effectiveness of using exoskeleton devices for upper-limb motor function training also lacks sufficient evidence [ 105 ]. Therefore, at the current stage, robot-assisted rehabilitation therapy should be considered as a supplement to traditional physiotherapy, not a replacement. There is also no clear evidence to show that robot gait training can outperform traditional physical therapy when applied alone to patients with chronic stroke [ 106 ]. On the basis of the existing evidence, we can conclude that robot-assisted rehabilitation therapy can improve the motor function of patients needing rehabilitation and serve as an additional treatment intervention in combination with traditional rehabilitation therapy. However, with the further development of AI and machine learning technology in the future, we expect robot-assisted rehabilitation therapy to have greater development potential.
On the other hand, we must acknowledge that the current rehabilitative assistance robots face 3 core challenges: energy endurance, comfort assurance, and cost control. First, we need to change the existing endurance mode and adopt more effective energy supply methods. Second, we need to solve the comfort issues that may arise during the use of robots, such as blood circulation problems and muscle deformation that may be caused by wearing methods. Finally, we need to focus on controlling costs so that all patients who need rehabilitation can afford it.
Telepresence Robots
Telepresence represents a potential avenue for enhancing information accessibility for providers, encompassing aspects such as patients’ visual and auditory feedback, bedside care, and vital sign data facilitated by remote monitoring or telechecking [ 107 ]. Given that most patients in the ICU are susceptible to unpredictable conditions, there is an acute need for swift identification and prompt response during emergent situations. The significance of telepresence robots lies in their ability to deliver expert health care services over distances, effectively mitigating the need for colocation of physicians and patients. This approach greatly augments the accessibility of health care services for patients in remote areas. Moreover, it potentially eradicates the likelihood of infectious disease transmission between patients and health care professionals [ 108 ]. Using AI and human-machine interaction, these telepresence robots supplement diagnostic and therapeutic processes via medical professionals’ expertise, thereby enhancing the exchange of visual and electronic information between the patient and health care staff [ 109 ]. To illustrate this, we provide the following example.
InTouch Health Remote Presence-7 (RP-7; InTouch Health Systems) is a real-time audiovisual robotic telepresence system that provides communication among patients, hospital staff, and remote physicians [ 33 , 69 , 110 ]. Remote assessors used the RP-7 robot end point to conduct their clinical coma evaluations [ 69 ]. Compared with the total scores on the Glasgow Coma Scale or Full Outline of Unresponsiveness of the remote physician evaluators, the RP-7 robotic system had a similar score (difference in scores of 0.25 and 0.40, respectively), and it can serve as a reliable scoring system to help evaluate patients in a coma [ 69 ]. In an ICU setting, the RP-7 assisted in the assessment of increased efficiency, care coordination, and throughput, with a decrease in patient ICU stay (–0.8 days), an increase in hospital discharges (+11%), and a significant decrease in the number of unexpected events (–1.2 days) [ 70 , 109 ]. Regarding the team cooperation ability (attitude, behavior, and cognition) of clinicians, the use of the RP-7 maintained the cooperation, trust, communication, and psychological safety of the team [ 107 ]. However, the RP-7 does not enhance collaboration between nurses and physicians in patient care decisions as compared to traditional telephone night checks [ 111 ].
A year later, the InTouch Vita, developed by InTouch Health and iRobot, was found to improve the independence of remote clinicians in managing patient care [ 71 , 112 , 113 ]. The Vita has an improved navigation system with an autopilot feature that enables remote service providers to directly control or automatically direct it to a predetermined location for improved efficiency [ 113 ]. In addition, the product provides real-time clinical access to patient data and has been cleared by the Food and Drug Administration for active patient monitoring that may be needed for immediate clinical action [ 72 ]. The Remote Presence Virtual + Independent Telemedicine Assistant (RP-VITA) also has an iPad interface that allows pilots to browse quickly and easily [ 114 ]. Unlike the RP-7, which must be powered using an active joystick, the RP-VITA only needs a mobile phone to log into the system and issue commands verbally to move the robot around to the designated location to complete the task [ 71 ]. To enable the robot to accurately follow the target character and maintain the corresponding safe distance and speed, Long et al [ 73 ] used the improved Gaussian filter algorithm to estimate and correct the centroid of the human body in real time, effectively improving the stability and safety of human tracking.
As a remote, virtual, and independent telemedicine assistant, the RP-VITA enables physicians to directly interact with patients from anywhere in the world. This technological advancement effectively transcends the traditional barriers of physical and biological constraints commonly encountered in ICU settings, where the immediacy of medical services is crucial yet often challenging to maintain. With the RP-VITA, physicians no longer need to undertake urgent commutes to the ward; instead, they can simply access the robot platform from the comfort of their home, offering a solution that is not only more expedient but also significantly more convenient [ 74 ]. This achieves truly meaningful health care services, delivering the right expertise to the right place at the right time to do the right thing at the right price. The RP-VITA exemplifies the future of health care delivery, emphasizing efficiency, accessibility, and the strategic allocation of medical expertise.
Second, Stevie has a stethoscope port and a high-definition pan-tilt-zoom camera, which can relay information during an examination of a patient and help physicians identify illnesses and diseases in the ICU [ 75 ]. It was developed by a research team from Trinity College, Dublin, Ireland, and is currently in use at Steve Biko Academic Hospital, South Africa, in the second design iteration [ 76 ]. It was designed to be neutral so as to avoid perceptions of gender, race, and age [ 115 ]. The upper body of the robot consists of a humanoid head (digital display), trunk, and 2 short arms [ 116 ]. A humanoid form makes robots more acceptable [ 117 ]. The control system gives the user the ability to operate the robot remotely, including controlling the robot voice and media volume as well as motion and motion. In addition, Stevie’s humanoid facial expressions convey clear emotions [ 76 ]. Humanlike limbs can be used to form intuitive gestures, emphasize emotional states, and direct attention, conveying more information than facial expressions [ 116 ]. To provide static stability, the robot is equipped with wheels that support omnidirectional, allowing it to maneuver smoothly in any direction without shifting its base. This feature is essential for navigating complex environments and enhancing operational efficiency. Stevie is known as the “most popular baby” for the ICU team [ 115 ].
Third, MGI Ultrasound System-Remote 3 (MGIUS-R3; MGI Tech Co, Ltd), a robot-assisted teleultrasound diagnostic system, has considerable application value in the ICU [ 77 , 118 ]. It combines a robotic arm, an ultrasound imaging system, and audiovisual communication for remote manipulation, allowing physicians to manipulate the robotic arm and adjust parameters outside the ICU room for remote ultrasound workups via the fifth-generation (5G) network technology for real-time transmission of audio, video, and ultrasound images [ 78 ].
It has been used as a long-range ultrasound device to fight the COVID-19 outbreak in many places in China such as Wuhan [ 79 ]. Powerful cloud data transfer rates (up to 10 GB per second) with management mode enable high-definition image capture and high-quality information transfer for large data set sharing. At a distance of 700 or even 1479 km (between the isolation ward of Zhejiang Provincial People’s Hospital and Central South Hospital of Wuhan University in Hubei Province and between Wuhan city in Hubei Province and Sanya city in Hainan Province), it can successfully conduct remote ultrasound examination of the lung and other parts of the patient with the quality meeting the requirements for clinical diagnosis [ 80 , 119 ]. A double-blind diagnostic trial of COVID-19 in 22 cases showed that the diagnostic accuracy of the MGIUS-R3 ultrasound for positive lesions was good (93%), which could replace the traditional scanning method to some extent (difference: P =.09) [ 77 ]. Another study also successfully performed ultrasound examination of the liver, gallbladder, pancreas, spleen, and kidney in 32 patients, and the high-quality image (average score of 4.73) met the requirements for remote ultrasound diagnosis [ 78 ]. Overall, the MGIUS-R3 is noninvasive and repeatable, reduces the risk of infection for patients and physicians, increases safety, and is highly feasible in the ICU. However, all remote ultrasound procedures and communications for the robot are based on 5G networks, which require stable network support throughout the procedure [ 119 , 120 ].
Through the network, remote ultrasound robots transmit ultrasound images of challenging cases from remote areas to tertiary hospitals, where consulting experts diagnose and analyze them, providing decision feedback. This provides an effective solution for situations with limited medical resources, lack of expertise, and high risk of infection.
Fourth, the intrinsic characteristics of the ICU, despite their provision of the highest level of medical care and reliance on advanced organ-support therapies, inherently limit the ability to facilitate real-time visits and communication between patients and their family members. This restriction not only affects the emotional well-being of patients due to a lack of verbal encouragement and emotional support from loved ones but also poses a challenge to patient recovery processes within the high-stress environment of the ICU. For the safety and emotional needs of patients and their families, as well as to achieve the goal of resource optimization, visiting robots have emerged. In 2021, the first 5G+ medical robot+VR visiting system was officially launched in the stroke ICU ward of West China Hospital of Sichuan University. The system successfully enables remote visits for family members at designated hospital locations equipped with matching VR glasses, allowing family members to interact with patients in the ICU in real-time through 2-way communication, facilitating an immersive visitation experience at the bedside [ 121 ]. The family members only need to wear VR glasses in the designated place of the hospital and operate the robot remotely through a computer, iPad, or mobile phone. The robot will automatically go to the patient bed designated to be visited after receiving the instruction. 5G networks with high-speed and low-latency transmission features (speeds of 10 to 30 Gbps) transmit back real-time 8K (resolutions of up to 7680 × 4320 pixels) ultra-high-definition full-motion video, enabling families to “physically” visit a patient’s bedside [ 81 , 121 ].
The introduction of visiting robots represents a significant step forward in addressing a previously underappreciated aspect of health care—the impact of patient relationships, particularly with family members, on the process of disease recovery. By facilitating connections that were once hindered due to logistical, health, or institutional barriers, these robots enhance the humanization of health care services. They embody an innovative shift in the delivery models of health care services, ensuring that emotional support and the therapeutic benefits of family presence are not overlooked in patient care. This innovation underscores the importance of integrating technological advancements with the core values of empathy, care, and support, thereby enriching the patient experience.
Logistics and Disinfection Robots
The number of medical interventions in ICUs is larger than that in the general ward, and they are more invasive. In addition, the physiological condition of patients who are critical is often fragile, which makes patients in the ICU particularly vulnerable to iatrogenic injury [ 122 , 123 ]. At the same time, ICU health care workers face a high risk of infection [ 124 ]. The presence of logistics and disinfection robots avoids the spread of viruses, ensures clean areas for clinicians and patients, and minimizes the risk of infection for medical staff. Logistics and disinfection robots could have also worked in this application in a hospital setting during the COVID-19 pandemic, focusing on these same tasks and supporting patient management [ 125 ]. The common forms of logistics and disinfection robots are transport robots and infection control robots, which can solve the problem of ICU staff shortages and share heavy and tedious work. A brief description follows.
Transport Robot HelpMate (HelpMate Robotics Inc)
The transportation of goods and patients is an indispensable part of intensive care. However, a large amount of reciprocating work will consume most of the physical strength of nurses, long-term handling of patients will also cause serious lumbar muscle injury in nurses, and unarmed handling may cause secondary injuries to patients. HelpMate is a mature solution that provides autonomous transportation of materials and supplies, with users dispatching tasks through their console interface, and autonomous operations with unsupervised navigation technologies, such as proximity sensors for obstacle avoidance and path planning for navigation [ 48 ]. In the ICU, the use of automated transport vehicles will increase efficiency and avoid the potential for cross-infection, especially during the COVID-19 lockdown [ 82 ].
As early as the 1980s, the object transfer robot HelpMate was used to carry medical supplies, meals, and experimental samples, among other things. HelpMate mainly navigates based on prestored maps and has a certain obstacle avoidance ability [ 83 ]. In 2003, the University of Maryland Medical Center began a pilot program to determine the logistical capabilities and functional utility of the automated pharmacy system II Robot-Rx (McKesson) robotic technology in the delivery of medications from satellite pharmacies to ICU patient care units [ 84 ].
In view of the situation that it is not suitable to change a patient’s posture during the process of transfer, Osaka General Medical Center in Japan found that more than half of nurses were willing to use a robot for patient transfer after using the robot Coupling-Parallel Adaption Merged (bilateral transfer bed, mainly through a conveyor belt to complete patient transfer between the bed and stretcher), and nearly half of the patients showed no discomfort during transfer [ 85 ].
Infection Control Robots
Infection control robots could make ICU wards safer and cleaner than ever before [ 126 , 127 ]. They are a major step up from traditional human cleaning methods, which take more time, are less effective, and often miss crevices that can hide nasty bugs. This is especially important for ICUs and clean rooms for patients who are immunocompromised, and it is possible that automated cleaning will soon become standard practice throughout the health care system. The existing disinfection robots can be mainly divided into 2 groups: UV robots and hydrogen peroxide vapor robots.
The Xenex robots use pulsed xenon to create intense bursts of broad-spectrum UV light that can cut bacterial contamination by a factor of 20 and kill 95% of deadly pathogens. More than 100 hospitals now use Xenex robots [ 86 ].
An “EPS” logistics disinfection robot (Ipsen Smart Health Tech [Shenzhen] Co, Ltd), which has both transport and disinfection functions, has been officially on duty in the ICU of Central South Hospital (Wuhan, China), undertaking the drug distribution work of nurses from the station to the ICU wards of patients with severe COVID-19 [ 87 ]. At the same time, it can customize the disinfection time and route for high-frequency areas of physician-patient activity and can carry out automatic UV disinfection.
The hydrogen peroxide vapor disinfecting robot combines a hydrogen peroxide device with a robot (Shanghai Jiao Tong University and Lingzhi Technology joint research and development, China) [ 88 ]. The disinfecting system inside the robot generates disinfecting gas and can realize autonomous navigation and autonomous movement in an unmanned environment. At present, this disinfection robot has been effectively used in the prevention and control of the COVID-19 pandemic [ 89 ]. It is mainly used in ICUs, negative pressure isolation wards, infectious wards, and other closed spaces requiring sterilization.
Moreover, the literature has reported a novel robot system capable of real-time air pathogen monitoring in ICUs. The system comprises an automatic guided vehicle, an air sample collector, and a pathogen detection system. By autonomously patrolling and collecting air samples, the robot uses biosensor technology to perform real-time detection of airborne pathogens. This detection process includes lysis of pathogen particles, amplification of target sequences, and sensitive detection via the Clustered Regularly Interspaced Short Palindromic Repeats (CRISPR) associate system. This robot system allows for real-time monitoring of airborne pathogens within the ICU, aiding in the prevention of hospital-acquired infections and reducing the burden of ICU management. Endowed with a high degree of autonomy and real-time responsiveness, this system provides a new avenue for infection control within ICUs [ 90 ].
The ICU is a highly specific and complex area for monitoring patients who are critically ill, requiring not only 24-hour continuous care but also more intensive, timely, and coordinated interventions. Although the introduction of AI robots into ICUs is still in the initial stage, their autonomy, ease of training, and strong adaptability enhance their performance and provide substantial assistance to medical staff. In particular, the COVID-19 outbreak has increased the demand for AI robots that are not fatigued or infected, which may reduce the fatigue of ICU medical staff, reduce medical errors, and improve patient safety. However, for example, monitoring robots for single-organ life-support devices, comprehensive cardiopulmonary-monitoring robots for patients with multiple organ failure, and robots that are flexible in dealing with various unexpected tasks arising during patient care have not yet appeared. Therefore, the demand and development potential of AI robots in ICUs is huge.
Challenges and Potential Solutions Related to the Use of AI Robots in ICUs
Despite significant advancements and widespread applications of AI robots across all processes within the ICU, they face a host of challenges due to the complexity and uncertainty of the real world, algorithmic limitations, and ethical and moral considerations. These challenges encompass safety, dignity, privacy, and questions of liability. In addition, the implementation of advanced technologies requires considerable investment, making cost-benefit considerations indispensable. Our objective is to develop AI robots for ICU application that uphold human values, demonstrate ethically sound behavior, and draw robust conclusions [ 128 ]. It is imperative to accurately recognize these extant issues and propose effective solutions.
The “Asilomar AI Principles,” signed at the 2017 Asilomar conference held in Asilomar, California, United States, call on global AI professionals to adhere to these principles to safeguard the interests and safety of humanity in the future [ 129 ]. The principles emphasize ethical standards and values, including privacy, security, fairness, and transparency. Although these principles only provide an ethical framework and guiding principles for AI development and do not offer specific policies, regulations, or standards, they still serve as reference guidelines for the development of the AI field.
Security Issues
With the continuous evolution of AI robotic technology, its capabilities and functions are constantly being enhanced. However, there is a lack of clear standards to define the meaning of safety and accuracy and evaluate its specific programs. Narrow technical approaches are insufficient to ensure the safety of AI robots, which must be considered within the broader sociotechnical context in which they operate [ 130 ]. Therefore, systems with moral and social reasoning capabilities are becoming increasingly important. In some cases, human involvement can serve as a constraint on robot design, especially in decisions involving life and death. However, in the realm of high-speed decision-making, robots require built-in moral and social reasoning capabilities [ 131 ]. In addition, the lack of sample size, heterogeneity of diseases, and complex operations lead to biases in AI algorithms, which cannot ensure the safety and effectiveness of robot treatments. These challenges should be evaluated alongside the risks already familiar to humans, allowing us to set realistic expectations and foresee significant advancements in the realm of robot safety.
In an effort to confront the emerging safety challenges within the AI domain, various countries have embarked on proactive measures aimed at addressing these potential concerns. Initiatives such as the AI Foundation Model working group established by the United Kingdom in April 2023 [ 132 ] and the series of AI white papers updated annually by the Chinese Association for Artificial Intelligence are significant steps toward understanding and addressing these issues [ 133 ]. Moreover, the global AI Safety Summit held in November 2023 marked a pivotal moment, with 28 countries coming together to sign the “Bletchley Declaration.” This declaration represents a unified commitment to scrutinize the risks associated with the frontiers of AI technology, such as natural language processing, computer vision, and reinforcement learning, with a specific focus on the development of large language models by leading companies such as OpenAI, Meta, and Google [ 134 ]. These concerted efforts underline a global recognition of the complexities and challenges posed by AI, as well as a determined move toward collaborative solutions. By focusing on risk assessment, ethical standards, and safety protocols, these initiatives highlight an international resolve to navigate the advancements of AI technology in a manner that not only benefits human society but also safeguards against potential hazards.
Dignity Issues
Medicine has always been a humanistic science where physicians are expected to not only adopt a scientific attitude toward patients but also resonate emotionally with them, embodying empathetic care. Patients in the ICU typically exhibit both physical and psychological fragility, necessitating humanistic care and emotional support from medical staff. This cannot be substituted by a robot due to its “mechanical”; “pre-programmed”; and, thus, “neutral” way of interacting with patients. Emotional recognition technology can be incorporated into AI robotic systems, providing corresponding emotional support by recognizing patient emotions, for instance, through voice, facial expressions, or body language. AI robots often have an unequal communication with patients, leaning more toward 1-way output from the robot. Before engaging in the discourse on equality between humans and AI robots, it is crucial to address a foundational question: should AI be classified as a tool or an agent? This distinction becomes especially pertinent in the context of conversational AI robots. When perceived merely as tools, there is a risk of undervaluing the anthropomorphic attributes and functionalities they embody. Conversely, viewing them as agents presents its own set of challenges as they inherently lack humanlike qualities such as empathy, intentionality, and the capacity to bear responsibility [ 135 ]. This debate is not new but remains central to the evolving conversation around AI’s role in society. Recent technological developments suggest that, by integrating natural language processing and voice recognition technologies, robots can become more anthropomorphic and capable of responding to patients’ language needs, alleviating feelings of loneliness and neglect in patients in the ICU. For example, the integration of the popular ChatGPT with AI robots may enhance their linguistic potential. Intelligent interactive AI robots combining ChatGPT’s linguistic skills with the computer vision and tangible abilities of robots could revolutionize the way humans interact with technology [ 136 ]. They could be better at navigating the subtleties of human interaction, boasting superior natural language generation capabilities.
On the other hand, the narrow technical AI safety field lacks ideological and demographic diversity, leading to a lack of breadth and rigor in knowledge. Moreover, practitioners in this field often come from White, male, and underrepresented groups, which is insufficient to meet the broad participation and shared human care needed for technological development, potentially leading to racial, gender, and other biases in technology application [ 137 , 138 ]. For instance, using Framingham Heart Study data to predict cardiovascular event risk in people of color may lead to both overestimation and underestimation of risk [ 139 ]. Similar racial biases may inadvertently be built into health care algorithms. Therefore, it is necessary to expand the space for broader participation, pursuing equality and common development from the source of technology, to avoid defects in AI robot products [ 140 ].
Privacy Issues
To enhance the monitoring of patients who are critically ill, robots are often equipped with surveillance apparatuses to log pertinent data and wirelessly transmit information. Such actions may infringe upon the privacy rights of patients, jeopardizing their confidentiality. Nonetheless, these features also hold merit in ensuring patient safety [ 141 ]. As AI robots proliferate across various sectors, including health care, concerns over privacy safeguards have been a recurrent topic of critique. It is imperative that AI robots adhere to privacy regulations when handling medical data, such as the General Data Protection Regulation that is prevalent in Europe [ 142 ]. Encryption techniques should be used during data collection and storage, narrowing the scope of data acquisition and ensuring anonymization. Of paramount importance is the establishment of stringent access control mechanisms, ensuring that data are accessible only to authorized personnel. Concurrently, it is vital to instate ethical guidelines and standards focused on privacy protection, which will dictate the conduct of AI robots and their data-handling procedures. All these regulations must ensure that individuals retain a voice over the collection, storage, and use of their information [ 143 ].
Attribution of Liability Issues
The integration of AI into robotic systems has rendered questions of accountability more intricate. Endowing robots with autonomy and decision-making capabilities stands as a primary objective of AI integration into robotics. However, given the current trajectory of technological advancements, intelligent analytics still bear systemic decision-making risks. When malfunctions occur, attributing responsibility for the robot’s actions becomes contentious given that robots lack comprehension of reprimand, sanctions, accountability, or remorse [ 144 , 145 ]. Consequently, the liability arising from erroneous decisions made by robots can pose significant legal conundrums [ 141 ]. Accountability in computer science encompasses a multifaceted domain, probing the responsibility attribution across its creation, dissemination, and use phases [ 146 ]. Thus, devising a legal framework pertinent to AI robots is essential, delineating the responsibilities and obligations of robot manufacturers, operators, and users spanning every facet of robot design, production, operation, and use. Regulatory bodies also play a pivotal role as rigorous supervisory protocols and technical benchmarks are needed to scrutinize and accredit AI robot designs and operations. Penalties and sanctions become indispensable components of this framework. Introducing a robotic liability insurance system might also serve as an efficacious remedy to mitigate the damages and risks incurred by robots. Manufacturers, operators, and users could opt for such liability insurance, necessitating clear demarcations of responsibility. Meanwhile, both the United States and the European Union advocate for a focus on algorithm transparency and accountability, aiming to make their decision-making processes more transparent and comprehensible. This will facilitate a better assessment of responsibility and serve as a warning against outsourcing moral responsibility to algorithms [ 147 ]. This calls for collaboration between manufacturers and developers, with the National Institute for Health and Care Excellence in the United States requiring developers to program code in an environment of technological feasibility and respect for intellectual property rights, ensuring reproducibility and error checking [ 148 ].
Addressing the issue of robotic accountability necessitates a holistic perspective, incorporating technical, legal, ethical, and societal dimensions; fostering collaborative mechanisms with multistakeholder participation to collectively bear the decision-making risks and responsibilities; and ensuring the utmost protection of the interests and safety of robot users and beneficiaries.
The United Kingdom has introduced the Code of Conduct for Data-Driven Health and Care Technology, adopting a “Regulation as a Service” model. This innovative approach ensures that regulatory checks are embedded at various stages throughout the AI development cycle, aiming to uphold high standards of safety, efficacy, and ethics in the creation and implementation of AI technologies in health care [ 149 ]. In June 2021, the US Government Accountability Office released an AI accountability framework covering 4 aspects: governance (promoting accountability by establishing processes to manage, operate, and oversee implementation), data (ensuring quality, reliability, and representativeness of data sources and processing), performance (producing results consistent with program objectives), and monitoring (ensuring reliability and relevance over time). This accountability framework sets principles and directions for future legislation and policy making and also serves as a model for the advancement of accountability systems in other countries [ 150 ].
Cost-Benefit Issues
Economic viability remains a pivotal facet in the societal integration of any nascent technology, with the deployment of AI robots in ICUs presenting intricate cost-benefit deliberations. Being an avant-garde technology, the initial research and development expenditures for AI robots are considerable. Once successfully developed and incorporated into ICU settings, they are further subjected to financial burdens, encompassing but not confined to the costs of system enhancements and upgrades; resource use during staff training and acclimatization; and investments necessitated by data security, personal dignity, privacy safeguards, and responsibility allocation issues. However, when observed from a utility standpoint, the application of AI robots bears significant value, especially for populous nations such as China. In the face of scarce grassroots medical resources, AI robots serve as catalysts, facilitating the dispersion of health care provision to underserved and remote locales, meeting the medical needs of a vast populace—this aligns with the principal objective underpinning this technological advancement. In addition, the integration of AI robots can effectively bridge disparities in health care accessibility between high- and low-income nations, substantially augmenting the societal benefits of this technology [ 151 ]. Therefore, despite the steep initial investments and continual operational expenses, in the broader spectrum of health care service provision and societal equity realization, AI robots undoubtedly offer pronounced advantages and value.
Principal Findings
AI robots have firmly established their significance within intensive care, with their integration into the ICU regimen continually deepening. This paper delineates 5 distinct application domains of AI robotic systems, be it experimental or commercial, within the ICU, addressing both technical impediments and prospective research avenues while proposing potential remedial strategies. The trajectory for AI robots within the ICU setting is promising. Currently at the nascent phase of AI robot technological deployment, there remains an extensive scope of endeavors to be pursued and myriad challenges to be surmounted. As the propagation of pertinent technologies ensues, health care professionals should welcome such intelligent implementations with optimism, recognizing the present-day confines of AI apparatuses synergistically amalgamating human and system intellect, thereby maximizing the data analysis, promptings, and recommendation proficiencies of intelligent robots. Today’s robotic entities coalesce seamlessly with AI, where sophisticated robotic systems, characterized by safety and flexibility melded with augmented computational proficiencies, yield invaluable big data insights. Simultaneously, anthropomorphic designs provide patients in the ICU with a more comforting medical experience.
“There are plenty of areas in critical care where it would be extremely helpful to have efficacious, fair, and transparent AI systems,” notes Gary Weissman, assistant professor in pulmonary and critical care medicine at the University of Pennsylvania Perelman School of Medicine [ 152 ]. Similarly, Dr Brijesh Patel, an intensivist at the Royal Brompton Hospital in London, emphasizes that “intensive care is a specialty with special prospects for AI. After all, the ICU is a space where a large amount of data is routinely collected, making it an ideal place for deploying machine learning techniques” [ 14 ]. Dr Patel, who dedicates a considerable portion of his ward rounds to adjusting ventilator settings, points out that the continuous advancement in AI technology could automate such repetitive tasks.
However, there is a consensus among experts that AI is not poised to replace physicians entirely. Instead, it is seen as a tool to streamline certain tasks, enhancing efficiency where it is most needed. Aldo Faisal, a professor of AI and neuroscience at Imperial College London, emphasizes a balanced perspective on AI’s role within health care teams. He advocates for a realistic understanding of AI’s capabilities and limitations, suggesting that neither undue fear nor excessive reverence is helpful [ 153 ].
This paragraph presents a futuristic scenario representing the potential evolution and future blueprint of AI robotics in the ICU ward. Envision a scenario where a patient who is critically injured is admitted to the ICU and greeted by “IntelliGreet”—an intelligent reception robot that collates the patient’s fundamental data and medical history, seamlessly completing admission formalities. The therapeutic reins are taken over by “MediBot,” a state-of-the-art medical robot that executes an array of treatment procedures (ranging from drug administration and wound care to life support) predicated on the patient’s condition and physician’s directives. The patient’s nursing needs are catered to by “CareCompanion,” an omnipresent nursing robot that meticulously monitors vital signs, offering essential care services (such as cleaning, feeding, and movement) while concurrently assuming the responsibility of reporting to physicians. As the patient recuperates under their meticulous care and nears discharge, “DischargeDuty” steps in, formulating discharge plans and subsequent treatment regimens based on physician prescriptions, facilitating communication with both the patient and their kin, and overseeing the discharge processes. Finally, the domestic care robot “HomeCareHelper” persists in its caregiving, administering medication reminders, monitoring patient health, and even offering rudimentary domestic aid for solitary individuals. All AI robots in this envisioned setting are interlinked via a cloud data platform, enabling real-time sharing of patient medical data, achieving cohesive and synergized medical service delivery. Reflecting upon the advancements in AI robotic technology over recent decades, it is undeniable that this vision is poised to materialize.
Limitations of This Review
This review acknowledges a number of limitations that could affect the interpretation and applicability of its findings. One significant concern is the potential for publication bias, a common issue in scientific literature where studies with negative results are less likely to be published. This could lead to an overrepresentation of positive findings in this review. In addition, despite efforts to mitigate bias by involving interdisciplinary experts and employing dual reviewers during the literature search and data collection phases, subjective biases could still influence the selection and interpretation of the studies.
Another challenge is the absence of a universally accepted classification system for ICU robotic systems. In response, our classification framework was developed based on expert opinions and existing literature, striving for as comprehensive and rational an approach as possible. Nevertheless, the potential for omissions exists given the rapidly evolving nature of technology and the diverse applications of robotics in critical care settings. These limitations highlight the need for ongoing research and critical evaluation of emerging technologies in health care, emphasizing the importance of transparency and methodological rigor in scientific reviews.
Conclusions
This scoping review comprehensively covered AI robots in the ICU, detailing the most widely used or newly developed robotic devices on the market. Robots in ICU wards are becoming valuable assistants to physicians and nurses. Although ethical and safety concerns remain unresolved in this field, these challenges are inevitable in the development of new technologies, and experts and developers are focusing on addressing them. Future research should focus on developing policies and regulations to prevent or resolve these issues, making AI robots an integral part of ICUs and other hospital wards.
Acknowledgments
This research received no specific grant from any funding agency in the public, commercial, or not-for-profit sectors.
Authors' Contributions
HK was responsible for hypothesis generation. HK and ZQ were responsible for the conception of this study. Y Li, MW, LW, YC, Y Liu, YZ, RY, MY, SL, and ZS contributed substantially to all aspects of the work, including literature review and manuscript drafting. FZ revised the manuscript. All authors contributed to the manuscript and approved the submitted version.
Conflicts of Interest
None declared.
PRISMA-ScR (Preferred Reporting Items for Systematic Reviews and Meta-Analyses extension for Scoping Reviews) checklist.
Classification of intensive care unit robots.
- Bobrow DG, Stefik MJ. Perspectives on artificial intelligence programming. Science. Feb 28, 1986;231(4741):951-957. [ CrossRef ] [ Medline ]
- Haug CJ, Drazen JM. Artificial intelligence and machine learning in clinical medicine, 2023. N Engl J Med. Mar 30, 2023;388(13):1201-1208. [ CrossRef ] [ Medline ]
- Georgopoulos AP, Schwartz AB, Kettner RE. Response: neuronal coding and robotics. Science. Jul 17, 1987;237(4812):301. [ CrossRef ] [ Medline ]
- McKendrick M, Yang S, McLeod GA. The use of artificial intelligence and robotics in regional anaesthesia. Anaesthesia. Jan 2021;76 Suppl 1:171-181. [ FREE Full text ] [ CrossRef ] [ Medline ]
- Javaid M, Haleem A, Vaishya R, Bahl S, Suman R, Vaish A. Industry 4.0 technologies and their applications in fighting COVID-19 pandemic. Diabetes Metab Syndr. 2020;14(4):419-422. [ FREE Full text ] [ CrossRef ] [ Medline ]
- Yang GZ, J Nelson B, Murphy RR, Choset H, Christensen H, H Collins S, et al. Combating COVID-19-the role of robotics in managing public health and infectious diseases. Sci Robot. Mar 25, 2020;5(40):eabb5589. [ CrossRef ] [ Medline ]
- Fong SJ, Dey N, Chaki J. Artificial Intelligence for Coronavirus Outbreak. Singapore, Singapore. Springer; 1999.
- Howard J. Artificial intelligence: implications for the future of work. Am J Ind Med. Nov 2019;62(11):917-926. [ CrossRef ] [ Medline ]
- Agarwal S, Punn NS, Sonbhadra SK, Tanveer M, Nagabhushan P, Pandian KK, et al. Unleashing the power of disruptive and emerging technologies amid COVID-19: a detailed review. arXiv.. . Preprint posted online on May 23, 2020.
- Siciliano B, Khatib O. Robotics and the handbook. In: Siciliano B, Khatib O, editors. Springer Handbook of Robotics. Cham, Switzerland. Springer; 2016:1-6.
- Marshall JC, Bosco L, Adhikari NK, Connolly B, Diaz JV, Dorman T, et al. What is an intensive care unit? A report of the task force of the World Federation of Societies of Intensive and Critical Care Medicine. J Crit Care. Feb 2017;37:270-276. [ CrossRef ] [ Medline ]
- Clancy TR. Artificial intelligence and nursing: the future is now. J Nurs Adm. Mar 2020;50(3):125-127. [ CrossRef ] [ Medline ]
- Hanson CWIII, Marshall BE. Artificial intelligence applications in the intensive care unit. Crit Care Med. Feb 2001;29(2):427-435. [ CrossRef ] [ Medline ]
- Burki TK. Artificial intelligence hold promise in the ICU. Lancet Respir Med. Aug 2021;9(8):826-828. [ CrossRef ]
- Gutierrez G. Artificial intelligence in the intensive care unit. Crit Care. Mar 24, 2020;24(1):101. [ FREE Full text ] [ CrossRef ] [ Medline ]
- Arksey H, O'Malley L. Scoping studies: towards a methodological framework. Int J Soc Res Methodol. Feb 2005;8(1):19-32. [ CrossRef ]
- Tricco AC, Lillie E, Zarin W, O'Brien KK, Colquhoun H, Levac D, et al. PRISMA extension for scoping reviews (PRISMA-ScR): checklist and explanation. Ann Intern Med. Oct 02, 2018;169(7):467-473. [ FREE Full text ] [ CrossRef ] [ Medline ]
- Vagvolgyi BP, Khrenov M, Cope J, Deguet A, Kazanzides P, Manzoor S, et al. Telerobotic operation of intensive care unit ventilators. Front Robot AI. Jun 24, 2021;8:612964. [ FREE Full text ] [ CrossRef ] [ Medline ]
- Ehmke C, Kopp P, Karakikes M, Jahn R, Huang Y, Geiger J. A remote controller accessory for ventilators. GitHub. 2021. URL: https://github.com/claasehmke/Remote-Controller-Accessory-for-Ventilators [accessed 2024-04-29]
- Song C, Yang G, Park S, Jang N, Jeon S, Oh SR, et al. On the design of integrated tele-monitoring/ operation system for therapeutic devices in isolation intensive care unit. IEEE Robot Autom Lett. Oct 2022;7(4):8705-8712. [ CrossRef ]
- Hemmerling TM, Arbeid E, Wehbe M, Cyr S, Taddei R, Zaouter C. Evaluation of a novel closed-loop total intravenous anaesthesia drug delivery system: a randomized controlled trial. Br J Anaesth. Jun 2013;110(6):1031-1039. [ FREE Full text ] [ CrossRef ] [ Medline ]
- Hemmerling TM. Automated anesthesia. Curr Opin Anaesthesiol. Dec 2009;22(6):757-763. [ CrossRef ] [ Medline ]
- Wehbe M, Arbeid E, Cyr S, Mathieu PA, Taddei R, Morse J, et al. A technical description of a novel pharmacological anesthesia robot. J Clin Monit Comput. Feb 2014;28(1):27-34. [ CrossRef ] [ Medline ]
- Naidoo S. Understanding artificial intelligence and machine learning in anaesthesia. School of Clinical Medicine, Discipline of Anaesthesiology and Critical Care. 2021. URL: https://anaesthetics.ukzn.ac.za/wp-content/uploads/2021/01/2021-15-Jan-Artificial-Intelligence-in-Anaesthesia-S-Naidoo.pdf [accessed 2024-04-29]
- Zaouter C, Hemmerling TM, Lanchon R, Valoti E, Remy A, Leuillet S, et al. The feasibility of a completely automated total IV anesthesia drug delivery system for cardiac surgery. Anesth Analg. Oct 2016;123(4):885-893. [ CrossRef ] [ Medline ]
- Hemmerling TM, Charabati S, Zaouter C, Minardi C, Mathieu PA. A randomized controlled trial demonstrates that a novel closed-loop propofol system performs better hypnosis control than manual administration. Can J Anaesth. Aug 2010;57(8):725-735. [ CrossRef ] [ Medline ]
- Hemmerling TM, Taddei R, Wehbe M, Morse J, Cyr S, Zaouter C. Robotic anesthesia - a vision for the future of anesthesia. Transl Med UniSa. Sep 2011;1:1-20. [ FREE Full text ] [ Medline ]
- Tighe PJ, Badiyan SJ, Luria I, Lampotang S, Parekattil S. Robot-assisted airway support: a simulated case. Anesth Analg. Oct 2010;111(4):929-931. [ FREE Full text ] [ CrossRef ] [ Medline ]
- Sprung CL, Ricou B, Hartog CS, Maia P, Mentzelopoulos SD, Weiss M, et al. Changes in end-of-life practices in European intensive care units from 1999 to 2016. JAMA. Nov 05, 2019;322(17):1692-1704. [ FREE Full text ] [ CrossRef ] [ Medline ]
- Levitov A, Frankel HL, Blaivas M, Kirkpatrick AW, Su E, Evans D, et al. Guidelines for the appropriate use of bedside general and cardiac ultrasonography in the evaluation of critically ill patients-part II: cardiac ultrasonography. Crit Care Med. Jun 2016;44(6):1206-1227. [ CrossRef ] [ Medline ]
- Jung J, Kim J, Kim S, Kwon WY, Na SH, Kim KS, et al. Application of robot manipulator for cardiopulmonary resuscitation. In: Proceedings of the 2016 International Symposium on Experimental Robotics. 2016. Presented at: ISER '16; October 3-6, 2016:266-274; Tokyo, Japan. URL: https://link.springer.com/chapter/10.1007/978-3-319-50115-4_24 [ CrossRef ]
- Suh GJ, Park J, Lee JC, Na SH, Kwon WY, Kim KS, et al. End-tidal CO-guided automated robot CPR system in the pig. Preliminary communication. Resuscitation. Jun 2018;127:119-124. [ CrossRef ] [ Medline ]
- Garingo A, Friedlich P, Tesoriero L, Patil S, Jackson P, Seri I. The use of mobile robotic telemedicine technology in the neonatal intensive care unit. J Perinatol. Jan 2012;32(1):55-63. [ CrossRef ] [ Medline ]
- Williams N, MacLean K, Guan L, Collet JP, Holsti L. Pilot testing a robot for reducing pain in hospitalized preterm infants. OTJR (Thorofare N J). Apr 2019;39(2):108-115. [ CrossRef ] [ Medline ]
- Balter ML, Chen AI, Maguire TJ, Yarmush ML. The system design and evaluation of a 7-DOF image-guided venipuncture robot. IEEE Trans Robot. Aug 2015;31(4):1044-1053. [ FREE Full text ] [ CrossRef ] [ Medline ]
- Chen A, Nikitczuk K, Nikitczuk J, Maguire T, Yarmush M. Portable robot for autonomous venipuncture using 3D near infrared image guidance. Technology (Singap World Sci). Sep 2013;1(1):72-87. [ FREE Full text ] [ CrossRef ] [ Medline ]
- Lippi G, Cadamuro J. Novel opportunities for improving the quality of preanalytical phase. A glimpse to the future? J Med Biochem. Oct 2017;36(4):293-300. [ FREE Full text ] [ CrossRef ] [ Medline ]
- Chen AI, Balter ML, Maguire TJ, Yarmush ML. Real-time needle steering in response to rolling vein deformation by a 9-DOF image-guided autonomous venipuncture robot. Rep U S. 2015;2015:2633-2638. [ FREE Full text ] [ CrossRef ] [ Medline ]
- Tan W, Shen C, Luo Y, Zhu H, Ma T, Dong D, et al. Development of motion unit of simulated intelligent endotracheal suctioning robot. Zhongguo Yi Liao Qi Xie Za Zhi. Jan 30, 2019;43(1):17-20. [ CrossRef ] [ Medline ]
- Kostandy RR, Ludington-Hoe SM. The evolution of the science of kangaroo (mother) care (skin-to-skin contact). Birth Defects Res. Sep 01, 2019;111(15):1032-1043. [ CrossRef ] [ Medline ]
- Holsti L, MacLean K, Oberlander T, Synnes A, Brant R. Calmer: a robot for managing acute pain effectively in preterm infants in the neonatal intensive care unit. Pain Rep. 2019;4(2):e727. [ FREE Full text ] [ CrossRef ] [ Medline ]
- Yu C, Sommerlad A, Sakure L, Livingston G. Socially assistive robots for people with dementia: systematic review and meta-analysis of feasibility, acceptability and the effect on cognition, neuropsychiatric symptoms and quality of life. Ageing Res Rev. Jun 2022;78:101633. [ FREE Full text ] [ CrossRef ] [ Medline ]
- Demange M, Pino M, Kerhervé H, Rigaud AS, Cantegreil-Kallen I. Management of acute pain in dementia: a feasibility study of a robot-assisted intervention. J Pain Res. 2019;12:1833-1846. [ FREE Full text ] [ CrossRef ] [ Medline ]
- Reiser U, Connette C, Fischer J, Kubacki J, Bubeck A, Weisshardt F. Care-O-bot® 3 - creating a product vision for service robot applications by integrating design and technology. In: Proceedings of the 2009 IEEE/RSJ International Conference on Intelligent Robots and Systems. 2009. Presented at: IROS '09; October 11-15, 2009:1992-1998; St. Louis, MO. URL: https://ieeexplore.ieee.org/document/5354526 [ CrossRef ]
- Odashima T, Onishi M, Tahara K, Takagi K, Asano F, Kato Y. A soft human-interactive robot RI-MAN. In: Proceedings of the 2006 IEEE/RSJ International Conference on Intelligent Robots and Systems. 2006. Presented at: IROS '06; October 9-15, 2006:1; Beijing, China. URL: https://ieeexplore.ieee.org/abstract/document/4058352 [ CrossRef ]
- Riener R, Lünenburger L, Maier I, Colombo G, Dietz V. Locomotor training in subjects with sensori-motor deficits: an overview of the Robotic Gait Orthosis Lokomat. J Healthc Eng. Jun 2010;1(2):197-216. [ CrossRef ]
- Chillura A, Bramanti A, Tartamella F, Pisano MF, Clemente E, Lo Scrudato M, et al. Advances in the rehabilitation of intensive care unit acquired weakness: a case report on the promising use of robotics and virtual reality coupled to physiotherapy. Medicine (Baltimore). Jul 10, 2020;99(28):e20939. [ FREE Full text ] [ CrossRef ] [ Medline ]
- Dahl TS, Kamel Boulos MN. Robots in health and social care: a complementary technology to home care and telehealthcare? Robotics. Dec 30, 2013;3(1):1-21. [ CrossRef ]
- Rusek W, Adamczyk M, Baran J, Leszczak J, Pop T. Feasibility of Using Erigo and Lokomat in Rehabilitation for Patient in Vegetative State: A Case Report. J Clin Case Rep. 2020;10(1318):1-4. [ FREE Full text ]
- Uivarosan D, Tit DM, Iovan C, Nistor-Cseppento DC, Endres L, Lazar L, et al. Effects of combining modern recovery techniques with neurotrophic medication and standard treatment in stroke patients. Sci Total Environ. Aug 20, 2019;679:80-87. [ CrossRef ] [ Medline ]
- Alcobendas-Maestro M, Esclarín-Ruz A, Casado-López RM, Muñoz-González A, Pérez-Mateos G, González-Valdizán E, et al. Lokomat robotic-assisted versus overground training within 3 to 6 months of incomplete spinal cord lesion: randomized controlled trial. Neurorehabil Neural Repair. 2012;26(9):1058-1063. [ CrossRef ] [ Medline ]
- Esclarín-Ruz A, Alcobendas-Maestro M, Casado-Lopez R, Perez-Mateos G, Florido-Sanchez MA, Gonzalez-Valdizan E, et al. A comparison of robotic walking therapy and conventional walking therapy in individuals with upper versus lower motor neuron lesions: a randomized controlled trial. Arch Phys Med Rehabil. Jun 2014;95(6):1023-1031. [ CrossRef ] [ Medline ]
- Labruyère R, van Hedel HJ. Strength training versus robot-assisted gait training after incomplete spinal cord injury: a randomized pilot study in patients depending on walking assistance. J Neuroeng Rehabil. Jan 09, 2014;11:4. [ FREE Full text ] [ CrossRef ] [ Medline ]
- Tang Q, Huang Q, Hu C. Research on design theory and compliant control for underactuated lower-extremity rehabilitation robotic systems code: (51175368); 2012.01-2015.12. J Phys Ther Sci. Oct 2014;26(10):1597-1599. [ FREE Full text ] [ CrossRef ] [ Medline ]
- Shin JC, Kim JY, Park HK, Kim NY. Effect of robotic-assisted gait training in patients with incomplete spinal cord injury. Ann Rehabil Med. Dec 2014;38(6):719-725. [ FREE Full text ] [ CrossRef ] [ Medline ]
- Duffell LD, Niu X, Brown G, Mirbagheri MM. Variability in responsiveness to interventions in people with spinal cord injury: do some respond better than others? Annu Int Conf IEEE Eng Med Biol Soc. 2014;2014:5872-5875. [ CrossRef ] [ Medline ]
- Field-Fote EC, Lindley SD, Sherman AL. Locomotor training approaches for individuals with spinal cord injury: a preliminary report of walking-related outcomes. J Neurol Phys Ther. Sep 2005;29(3):127-137. [ CrossRef ] [ Medline ]
- Home page. Hocoma. URL: https://www.hocoma.com/us/solutions/lokomat/ [accessed 2023-03-05]
- Brihmat N, Loubinoux I, Castel-Lacanal E, Marque P, Gasq D. Kinematic parameters obtained with the ArmeoSpring for upper-limb assessment after stroke: a reliability and learning effect study for guiding parameter use. J Neuroeng Rehabil. Sep 29, 2020;17(1):130. [ FREE Full text ] [ CrossRef ] [ Medline ]
- Rémy-Néris O, Le Jeannic A, Dion A, Médée B, Nowak E, Poiroux É, et al. REM Investigative Team*. Additional, mechanized upper limb self-rehabilitation in patients with subacute stroke: the REM-AVC randomized trial. Stroke. Jun 2021;52(6):1938-1947. [ CrossRef ] [ Medline ]
- Bernhardt J, Hayward KS. What is next after this well-conducted, but neutral, multisite trial testing self-rehabilitation approaches? Stroke. Jun 2021;52(6):1948-1950. [ CrossRef ] [ Medline ]
- Colomer C, Baldoví A, Torromé S, Navarro M, Moliner B, Ferri J, et al. Efficacy of Armeo® Spring during the chronic phase of stroke. Study in mild to moderate cases of hemiparesis. Neurologia. Jun 2013;28(5):261-267. [ FREE Full text ] [ CrossRef ] [ Medline ]
- Krebs HI, Ferraro M, Buerger SP, Newbery MJ, Makiyama A, Sandmann M, et al. Rehabilitation robotics: pilot trial of a spatial extension for MIT-Manus. J Neuroeng Rehabil. Oct 26, 2004;1(1):5. [ FREE Full text ] [ CrossRef ] [ Medline ]
- Wood MD, Maslove DM, Muscedere J, Scott SH, Boyd JG, Canadian Critical Care Trials Group. Robotic technology provides objective and quantifiable metrics of neurocognitive functioning in survivors of critical illness: a feasibility study. J Crit Care. Dec 2018;48:228-236. [ CrossRef ] [ Medline ]
- Jawa NA, Holden RM, Silver SA, Scott SH, Day AG, Norman PA, et al. Identifying neurocognitive outcomes and cerebral oxygenation in critically ill adults on acute kidney replacement therapy in the intensive care unit: the INCOGNITO-AKI study protocol. BMJ Open. Aug 17, 2021;11(8):e049250. [ FREE Full text ] [ CrossRef ] [ Medline ]
- Wood M, Maslove D, Muscedere J, Scott S, Boyd J. Using robotic technology to quantify neurological deficits among survivors of critical illness: do they relate to brain tissue oxygen levels? a pilot study. Intensive Care Med Exp. Oct 1, 2015;3(S1):1-2. [ CrossRef ]
- Debert CT, Herter TM, Scott SH, Dukelow S. Robotic assessment of sensorimotor deficits after traumatic brain injury. J Neurol Phys Ther. Jun 2012;36(2):58-67. [ CrossRef ] [ Medline ]
- Chang WH, Kim YH. Robot-assisted therapy in stroke rehabilitation. J Stroke. Sep 2013;15(3):174-181. [ FREE Full text ] [ CrossRef ] [ Medline ]
- Adcock AK, Kosiorek H, Parikh P, Chauncey A, Wu Q, Demaerschalk BM. Reliability of robotic telemedicine for assessing critically ill patients with the full outline of UnResponsiveness Score and Glasgow Coma Scale. Telemed J E Health. Jul 2017;23(7):555-560. [ FREE Full text ] [ CrossRef ] [ Medline ]
- McNelis J, Schwall GJ, Collins JF. Robotic remote presence technology in the surgical intensive care unit. J Trauma Acute Care Surg. Feb 2012;72(2):527-530. [ CrossRef ] [ Medline ]
- Pransky J. The Pransky interview: Dr Yulun Wang, founder and CEO of InTouch Health. Ind Robot. 2015;42(5):381-385. [ CrossRef ]
- Hand L. FDA clears telemedicine robot for use in hospitals. Medscape. 2013. URL: https://www.medscape.com/viewarticle/778672 [accessed 2023-05-19]
- Long Y, Xu Y, Xiao Z, Shen Z. Kinect-based human body tracking system control of medical care service robot. In: Proceedings of the 2018 WRC Symposium on Advanced Robotics and Automation. 2018. Presented at: WRC SARA '18; August 15-19, 2018:281-288; Beijing, China. URL: https://ieeexplore.ieee.org/document/8584246 [ CrossRef ]
- Ackerman E. iRobot and InTouch health announce RP-VITA telemedicine robot. IEEE Spectrum. 2012. URL: https://spectrum.ieee.org/irobot-and-intouch-health-announce-rpvita-telemedicine-robot [accessed 2024-03-03]
- Swanepoel L. Steve Biko academic hospital has robot for ICU. NuusFlits. 2021. URL: https://find-it.co.za/2021/07/07/steve-biko-academic-hospital-has-robot-for-icu/ [accessed 2024-02-28]
- Taylor L, Downing A, Noury GA, Masala G, Palomino M, McGinn C. Exploring the applicability of the socially assistive robot Stevie in a day center for people with dementia. In: Proceedings of the 30th IEEE International Conference on Robot & Human Interactive Communication. 2021. Presented at: RO-MAN '21; August 8-12, 2021:957-962; Vancouver, BC. URL: https://ieeexplore.ieee.org/document/9515423 [ CrossRef ]
- Wu S, Wu D, Ye R, Li K, Lu Y, Xu J, et al. Pilot study of robot-assisted teleultrasound based on 5G network: a new feasible strategy for early imaging assessment during COVID-19 pandemic. IEEE Trans Ultrason Ferroelectr Freq Control. Nov 2020;67(11):2241-2248. [ CrossRef ]
- Duan S, Liu L, Chen Y, Yang L, Zhang Y, Wang S, et al. A 5G-powered robot-assisted teleultrasound diagnostic system in an intensive care unit. Crit Care. Apr 07, 2021;25(1):134. [ FREE Full text ] [ CrossRef ] [ Medline ]
- Li X, Guo L, Sun L, Yue PW, Xu H. Teleultrasound for the COVID-19 pandemic: a statement from China. Adv Ultrasound Diagn Ther. 2020;4(2):50-56. [ CrossRef ]
- Wang J, Peng C, Zhao Y, Ye R, Hong J, Huang H, et al. Application of a robotic tele-echography system for COVID-19 pneumonia. J Ultrasound Med. Feb 2021;40(2):385-390. [ CrossRef ] [ Medline ]
- Li D. 5G and intelligence medicine-how the next generation of wireless technology will reconstruct healthcare? Precis Clin Med. Dec 2019;2(4):205-208. [ FREE Full text ] [ CrossRef ] [ Medline ]
- Khakharia A, Mehendale N. Robotic motion planning using Probabilistic Collision Hidden Markov Model (PCHMM) for contactless drug delivery. SSRN Journal.. . Preprint posted online on June 15, 2021. [ FREE Full text ] [ CrossRef ]
- Evans J, Krishnamurthy B, Pong W, Croston R, Weiman C, Engelberger G. HelpMate™: a robotic materials transport system. Robot Auton Syst. Nov 1989;5(3):251-256. [ CrossRef ]
- Summerfield MR, Seagull FJ, Vaidya N, Xiao Y. Use of pharmacy delivery robots in intensive care units. Am J Health Syst Pharm. Jan 01, 2011;68(1):77-83. [ CrossRef ] [ Medline ]
- Wang H, Kasagami F. Careful-patient mover used for patient transfer in hospital. In: Proceedings of the 2007 IEEE/ICME International Conference on Complex Medical Engineering, 2007. Presented at: IEEE/ICME '07; May 23-27, 2007:20-26; Beijing, China. URL: https://ieeexplore.ieee.org/document/4381684 [ CrossRef ]
- Stibich M, Stachowiak J. The microbiological impact of pulsed xenon ultraviolet disinfection on resistant bacteria, bacterial spore and fungi and viruses. S Afr J Infect Dis. Mar 31, 2016;31(1):12-15. [ CrossRef ]
- Logistics Disinfection Robot of epsgroup Hospital Put into Anti-Epidemic Work in Central South Hospital of Wuhan University. Tencent QQ. 2020. URL: https://page.om.qq.com/page/OqCoH8_owiYNyNG8UvggcMHg0 [accessed 2024-02-29]
- The "autonomous mobile disinfection robot" produced in Shanghai has arrived and has been used on the front line of the fight against the epidemic. China News Network. 2020. URL: https://baijiahao.baidu.com/s?id=1658226694285619350&wfr=spider&for=pc [accessed 2024-02-11]
- Maclean M, McKenzie K, Moorhead S, Tomb RM, Coia JE, MacGregor SJ, et al. Decontamination of the hospital environment: new technologies for infection control. Curr Treat Options Infect Dis. Jan 30, 2015;7(1):39-51. [ CrossRef ]
- Yu LH. Real-time monitoring of air pathogens in the ICU with biosensors and robots. Crit Care. Nov 14, 2022;26(1):353. [ FREE Full text ] [ CrossRef ] [ Medline ]
- Weil MH, Tang W. From intensive care to critical care medicine: a historical perspective. Am J Respir Crit Care Med. Jun 01, 2011;183(11):1451-1453. [ CrossRef ] [ Medline ]
- Site A, Lohan ES, Jolanki O, Valkama O, Hernandez RR, Latikka R, et al. Managing perceived loneliness and social-isolation levels for older adults: a survey with focus on wearables-based solutions. Sensors (Basel). Feb 01, 2022;22(3):1108. [ FREE Full text ] [ CrossRef ] [ Medline ]
- Locsin RC. The co-existence of technology and caring in the theory of technological competency as caring in nursing. J Med Invest. 2017;64(1.2):160-164. [ FREE Full text ] [ CrossRef ] [ Medline ]
- Hermans G, Van den Berghe G. Clinical review: intensive care unit acquired weakness. Crit Care. Aug 05, 2015;19(1):274. [ FREE Full text ] [ CrossRef ] [ Medline ]
- Calabrò RS, Cacciola A, Bertè F, Manuli A, Leo A, Bramanti A, et al. Robotic gait rehabilitation and substitution devices in neurological disorders: where are we now? Neurol Sci. Apr 2016;37(4):503-514. [ CrossRef ] [ Medline ]
- van Kammen K, Boonstra AM, van der Woude LH, Visscher C, Reinders-Messelink HA, den Otter R. Lokomat guided gait in hemiparetic stroke patients: the effects of training parameters on muscle activity and temporal symmetry. Disabil Rehabil. Oct 2020;42(21):2977-2985. [ CrossRef ] [ Medline ]
- Baronchelli F, Zucchella C, Serrao M, Intiso D, Bartolo M. The effect of robotic assisted gait training with Lokomat® on balance control after stroke: systematic review and meta-analysis. Front Neurol. 2021;12:661815. [ FREE Full text ] [ CrossRef ] [ Medline ]
- Calafiore D, Negrini F, Tottoli N, Ferraro F, Ozyemisci-Taskiran O, de Sire A. Efficacy of robotic exoskeleton for gait rehabilitation in patients with subacute stroke : a systematic review. Eur J Phys Rehabil Med. Feb 2022;58(1):1-8. [ CrossRef ] [ Medline ]
- Louie DR, Eng JJ, Lam T, Spinal Cord Injury Research Evidence (SCIRE) Research Team. Gait speed using powered robotic exoskeletons after spinal cord injury: a systematic review and correlational study. J Neuroeng Rehabil. Oct 14, 2015;12:82. [ FREE Full text ] [ CrossRef ] [ Medline ]
- Mehrholz J, Pohl M. Electromechanical-assisted gait training after stroke: a systematic review comparing end-effector and exoskeleton devices. J Rehabil Med. Mar 2012;44(3):193-199. [ FREE Full text ] [ CrossRef ] [ Medline ]
- Veneman JF, Kruidhof R, Hekman EE, Ekkelenkamp R, Van Asseldonk EH, van der Kooij H. Design and evaluation of the LOPES exoskeleton robot for interactive gait rehabilitation. IEEE Trans Neural Syst Rehabil Eng. Sep 2007;15(3):379-386. [ FREE Full text ] [ CrossRef ] [ Medline ]
- Petrinec AB, Martin BR. Post-intensive care syndrome symptoms and health-related quality of life in family decision-makers of critically ill patients. Palliat Support Care. Dec 26, 2018;16(6):719-724. [ CrossRef ] [ Medline ]
- Wood MD, Maslove D, Muscedere J, Scott SH, Day A, Boyd JG. Assessing the relationship between brain tissue oxygenation and neurological dysfunction in critically ill patients: study protocol. Int J Clin Trials. Aug 06, 2016;3(3):98-105. [ CrossRef ]
- Dukelow SP, Herter TM, Moore KD, Demers MJ, Glasgow JI, Bagg SD, et al. Quantitative assessment of limb position sense following stroke. Neurorehabil Neural Repair. Feb 2010;24(2):178-187. [ CrossRef ] [ Medline ]
- Veerbeek JM, Langbroek-Amersfoort AC, van Wegen EE, Meskers CG, Kwakkel G. Effects of robot-assisted therapy for the upper limb after stroke. Neurorehabil Neural Repair. Feb 24, 2017;31(2):107-121. [ CrossRef ] [ Medline ]
- Schwartz I, Meiner Z. Robotic-assisted gait training in neurological patients: who may benefit? Ann Biomed Eng. May 2015;43(5):1260-1269. [ CrossRef ] [ Medline ]
- Lazzara EH, Benishek LE, Patzer B, Gregory ME, Hughes AM, Heyne K, et al. Utilizing telemedicine in the trauma intensive care unit: does it impact teamwork? Telemed J E Health. Aug 2015;21(8):670-676. [ CrossRef ] [ Medline ]
- Avgousti S, Christoforou EG, Panayides AS, Voskarides S, Novales C, Nouaille L, et al. Medical telerobotic systems: current status and future trends. Biomed Eng Online. Aug 12, 2016;15(1):96. [ FREE Full text ] [ CrossRef ] [ Medline ]
- Vespa PM, Miller C, Hu X, Nenov V, Buxey F, Martin NA. Intensive care unit robotic telepresence facilitates rapid physician response to unstable patients and decreased cost in neurointensive care. Surg Neurol. Apr 2007;67(4):331-337. [ CrossRef ] [ Medline ]
- Chung KK, Grathwohl KW, Poropatich RK, Wolf SE, Holcomb JB. Robotic telepresence: past, present, and future. J Cardiothorac Vasc Anesth. Aug 2007;21(4):593-596. [ CrossRef ] [ Medline ]
- Bettinelli M, Lei Y, Beane M, Mackey C, Liesching TN. Does robotic telerounding enhance nurse-physician collaboration satisfaction about care decisions? Telemed J E Health. Aug 2015;21(8):637-643. [ CrossRef ] [ Medline ]
- Jang SM, Hong YJ, Lee K, Kim S, Chiến BV, Kim J. Assessment of user needs for telemedicine robots in a developing nation hospital setting. Telemed J E Health. Jun 2021;27(6):670-678. [ CrossRef ] [ Medline ]
- Bloss R. Unmanned vehicles while becoming smaller and smarter are addressing new applications in medical, agriculture, in addition to military and security. Ind Robot. 2014;41(1):82-86. [ CrossRef ]
- Kristoffersson A, Coradeschi S, Loutfi A. A Review of Mobile Robotic Telepresence. Adv Hum Comput Interact. 2013;2013:1-17. [ CrossRef ]
- Botha CF. Gender and humanoid robots: a somaesthetic analysis. Filos Theor J Afr Philos Cult Relig. Dec 13, 2021;10(3):119-130. [ CrossRef ]
- McGinn C, Bourke E, Murtagh A, Donovan C, Lynch P, Cullinan MF, et al. Meet Stevie: a socially assistive robot developed through application of a ‘design-thinking’ approach. J Intell Robot Syst. Jul 17, 2019;98(1):39-58. [ CrossRef ]
- Păvăloiu IB, Vasilățeanu A, Popa R, Scurtu D, Hang A, Goga N. Healthcare robotic telepresence. In: Proceedings of the 13th International Conference on Electronics, Computers and Artificial Intelligence. 2021. Presented at: ECAI '21; July 1-3, 2021:1-6; Pitesti, Romania. URL: https://ieeexplore.ieee.org/document/9515025 [ CrossRef ]
- Evans KD, Yang Q, Liu Y, Ye R, Peng C. Sonography of the lungs: diagnosis and surveillance of patients with COVID-19. J Diagn Med Sonogr. Apr 21, 2020;36(4):370-376. [ CrossRef ]
- Sarker S, Jamal L, Ahmed SF, Irtisam N. Robotics and artificial intelligence in healthcare during COVID-19 pandemic: a systematic review. Rob Auton Syst. Dec 2021;146:103902. [ FREE Full text ] [ CrossRef ] [ Medline ]
- Teng R, Ding Y, See KC. Use of robots in critical care: systematic review. J Med Internet Res. May 16, 2022;24(5):e33380. [ FREE Full text ] [ CrossRef ] [ Medline ]
- Sichuan's first "5G+medical robot+VR" visitation system officially launched. China News Network. URL: https://www.chinanews.com.cn/jk/2021/02-08/9408104.shtml [accessed 2023-06-10]
- Kelly FE, Fong K, Hirsch N, Nolan JP. Intensive care medicine is 60 years old: the history and future of the intensive care unit. Clin Med (Lond). Aug 2014;14(4):376-379. [ FREE Full text ] [ CrossRef ] [ Medline ]
- Marshall JC. Critical illness is an iatrogenic disorder. Crit Care Med. Oct 2010;38(10 Suppl):S582-S589. [ CrossRef ] [ Medline ]
- Buetti N, Ruckly S, de Montmollin E, Reignier J, Terzi N, Cohen Y, et al. COVID-19 increased the risk of ICU-acquired bloodstream infections: a case-cohort study from the multicentric OUTCOMEREA network. Intensive Care Med. Feb 2021;47(2):180-187. [ FREE Full text ] [ CrossRef ] [ Medline ]
- Sierra Marín SD, Gomez-Vargas D, Céspedes N, Múnera M, Roberti F, Barria P, et al. Expectations and perceptions of healthcare professionals for robot deployment in hospital environments during the COVID-19 pandemic. Front Robot AI. 2021;8:612746. [ FREE Full text ] [ CrossRef ] [ Medline ]
- Di Lallo A, Murphy R, Krieger A, Zhu J, Taylor RH, Su H. Medical robots for infectious diseases: lessons and challenges from the COVID-19 pandemic. IEEE Robot Autom Mag. Mar 2021;28(1):18-27. [ CrossRef ]
- Tamantini C, di Luzio FS, Cordella F, Pascarella G, Agro FE, Zollo L. A Robotic Health-Care Assistant for COVID-19 Emergency: A Proposed Solution for Logistics and Disinfection in a Hospital Environment. IEEE Robot Autom Mag. Mar 2021;28(1):71-81. [ CrossRef ]
- Curtis N. To ChatGPT or not to ChatGPT? The impact of artificial intelligence on academic publishing. Pediatr Infect Dis J. Apr 01, 2023;42(4):275. [ CrossRef ] [ Medline ]
- The FLI Team. A principled AI discussion in Asilomar. Future of Life Institute. 2017. URL: https://futureoflife.org/2017/01/17/principled-ai-discussion-asilomar/ [accessed 2024-02-28]
- Lazar S, Nelson A. AI safety on whose terms? Science. Jul 14, 2023;381(6654):138. [ CrossRef ] [ Medline ]
- Yang GZ, Bellingham J, Dupont PE, Fischer P, Floridi L, Full R, et al. The grand challenges of science robotics. Sci Robot. Jan 31, 2018;3(14):aar7650. [ CrossRef ] [ Medline ]
- Department for Science, Innovation and Technology, AI Safety Institute, Smith C, Sunak R. Tech entrepreneur Ian Hogarth to lead UK’s AI foundation model taskforce. Government of UK. URL: https://www.gov.uk/government/news/tech-entrepreneur-ian-hogarth-to-lead-uks-ai-foundation-model-taskforce [accessed 2023-05-10]
- Chinese artificial intelligence white paper series. Chinese Association for Artificial Intelligence. URL: https://www.caai.cn/index.php?s=/home/article/detail/id/3188.html [accessed 2024-02-29]
- The Bletchley declaration by countries attending the AI safety summit. Department for Science IT, Government of UK. URL: https://tinyurl.com/2xbn9wcv [accessed 2023-05-10]
- Sedlakova J, Trachsel M. Conversational artificial intelligence in psychotherapy: a new therapeutic tool or agent? Am J Bioeth. May 2023;23(5):4-13. [ FREE Full text ] [ CrossRef ] [ Medline ]
- Tustumi F, Andreollo NA, Aguilar-Nascimento JE. Future of the language models in healthcare: the role of ChatGPT. Arq Bras Cir Dig. 2023;36:e1727. [ FREE Full text ] [ CrossRef ] [ Medline ]
- Crigger E, Reinbold K, Hanson C, Kao A, Blake K, Irons M. Trustworthy augmented intelligence in health care. J Med Syst. Jan 12, 2022;46(2):12. [ FREE Full text ] [ CrossRef ] [ Medline ]
- Zou J, Schiebinger L. Ensuring that biomedical AI benefits diverse populations. EBioMedicine. May 2021;67:103358. [ FREE Full text ] [ CrossRef ] [ Medline ]
- Gijsberts CM, Groenewegen KA, Hoefer IE, Eijkemans MJ, Asselbergs FW, Anderson TJ, et al. Race/ethnic differences in the associations of the Framingham risk factors with carotid IMT and cardiovascular events. PLoS One. 2015;10(7):e0132321. [ FREE Full text ] [ CrossRef ] [ Medline ]
- Kostick-Quenet KM, Gerke S. AI in the hands of imperfect users. NPJ Digit Med. Dec 28, 2022;5(1):197. [ FREE Full text ] [ CrossRef ] [ Medline ]
- Safdar NM, Banja JD, Meltzer CC. Ethical considerations in artificial intelligence. Eur J Radiol. Jan 2020;122:108768. [ CrossRef ] [ Medline ]
- Rumbold JM, Pierscionek BK. A critique of the regulation of data science in healthcare research in the European Union. BMC Med Ethics. Apr 08, 2017;18(1):27. [ FREE Full text ] [ CrossRef ] [ Medline ]
- Sanderson K. GPT-4 is here: what scientists think. Nature. Mar 2023;615(7954):773. [ CrossRef ] [ Medline ]
- O'Sullivan S, Nevejans N, Allen C, Blyth A, Leonard S, Pagallo U, et al. Legal, regulatory, and ethical frameworks for development of standards in artificial intelligence (AI) and autonomous robotic surgery. Int J Med Robot. Feb 09, 2019;15(1):e1968. [ CrossRef ] [ Medline ]
- Da Silva M, Horsley T, Singh D, Da Silva E, Ly V, Thomas B, et al. Legal concerns in health-related artificial intelligence: a scoping review protocol. Syst Rev. Jun 17, 2022;11(1):123. [ FREE Full text ] [ CrossRef ] [ Medline ]
- Liebrenz M, Schleifer R, Buadze A, Bhugra D, Smith A. Generating scholarly content with ChatGPT: ethical challenges for medical publishing. Lancet Digit Health. Mar 2023;5(3):e105-e106. [ FREE Full text ] [ CrossRef ] [ Medline ]
- Feijóo C, Kwon Y, Bauer JM, Bohlin E, Howell B, Jain R, et al. Harnessing artificial intelligence (AI) to increase wellbeing for all: the case for a new technology diplomacy. Telecomm Policy. Jul 2020;44(6):101988. [ FREE Full text ] [ CrossRef ] [ Medline ]
- Challen R, Denny J, Pitt M, Gompels L, Edwards T, Tsaneva-Atanasova K. Artificial intelligence, bias and clinical safety. BMJ Qual Saf. Mar 2019;28(3):231-237. [ FREE Full text ] [ CrossRef ] [ Medline ]
- Greaves F, Joshi I, Campbell M, Roberts S, Patel N, Powell J. What is an appropriate level of evidence for a digital health intervention? Lancet. Dec 22, 2019;392(10165):2665-2667. [ CrossRef ] [ Medline ]
- Artificial intelligence: an accountability framework for federal agencies and other entities. U.S. Government Accountability Office. URL: https://www.gao.gov/products/gao-21-519sp [accessed 2023-05-10]
- Sarfraz Z, Sarfraz A, Iftikar HM, Akhund R. Is COVID-19 pushing us to the fifth industrial revolution (society 5.0)? Pak J Med Sci. 2021;37(2):591-594. [ FREE Full text ] [ CrossRef ] [ Medline ]
- Cosgriff CV, Stone DJ, Weissman G, Pirracchio R, Celi LA. The clinical artificial intelligence department: a prerequisite for success. BMJ Health Care Inform. Jul 2020;27(1):e100183. [ FREE Full text ] [ CrossRef ] [ Medline ]
- Gottesman O, Johansson F, Komorowski M, Faisal A, Sontag D, Doshi-Velez F, et al. Guidelines for reinforcement learning in healthcare. Nat Med. Jan 2019;25(1):16-18. [ FREE Full text ] [ CrossRef ] [ Medline ]
Abbreviations
Edited by S Ma, T Leung; submitted 29.10.23; peer-reviewed by S Wei, TAR Sure; comments to author 26.02.24; revised version received 07.03.24; accepted 22.04.24; published 27.05.24.
©Yun Li, Min Wang, Lu Wang, Yuan Cao, Yuyan Liu, Yan Zhao, Rui Yuan, Mengmeng Yang, Siqian Lu, Zhichao Sun, Feihu Zhou, Zhirong Qian, Hongjun Kang. Originally published in the Journal of Medical Internet Research (https://www.jmir.org), 27.05.2024.
This is an open-access article distributed under the terms of the Creative Commons Attribution License (https://creativecommons.org/licenses/by/4.0/), which permits unrestricted use, distribution, and reproduction in any medium, provided the original work, first published in the Journal of Medical Internet Research, is properly cited. The complete bibliographic information, a link to the original publication on https://www.jmir.org/, as well as this copyright and license information must be included.
- Open access
- Published: 21 May 2024
The impact of early weight-bearing on results following anterior cruciate ligament reconstruction
- Sehmuz Kaya 1 ,
- Yunus Can Unal 1 ,
- Necip Guven 1 ,
- Can Ozcan 2 ,
- Abdulrahim Dundar 3 ,
- Tulin Turkozu 1 ,
- Sezai Ozkan 1 ,
- Cihan Adanas 1 &
- Mehmet Ata Gokalp 1
BMC Musculoskeletal Disorders volume 25 , Article number: 395 ( 2024 ) Cite this article
Metrics details
Introduction
Anterior cruciate ligament (ACL) ruptures are common injuries that typically affect young, physically active individuals and may require surgical reconstruction. Studies have shown that the long time success of ACL reconstruction depends on the surgical technique and the postoperative rehabilitation strategy. However, there is still no consensus on the content of rehabilitation programs. Hence, additional research is required to elucidate the significance of early weight-bearing in the rehabilitation process following ACL reconstruction. The aim of this article is to examine the impact of weight-bearing on the clinical results of ACL reconstruction.
Materials and methods
We retrospectively reviewed patient records who had undergone arthroscopic reconstruction using a semitendinosus-gracilis tendon graft for anterior cruciate ligament rupture between January 2018 and December 2020. The study included the data of 110 patients. The patients were split into two groups: Group 1 underwent early weight-bearing, while Group 2 followed a non-weight-bearing regimen for three weeks. We assessed the patients using the anterior drawer test, Lachman test, range of motion, Lysholm knee scale, Cincinnati scale, Tegner scale, International Knee Documentation Committee (IKDC) form and clinical records. Analytical tests were conducted to compare the results.
The complication rates did not show a significant difference between the groups. Group 1 had higher frequencies of positive anterior drawer and Lachman tests. The Lysholm and Cincinnati knee scores of patients in Group 1 were notably lower than those of patients in Group 2. Additionally, the Tegner activity scores and IKDC scores of patients in Group 1 were also meaningfully lower than those of patients in Group 2. In Group 1 patients, there was no notable relationship observed between body mass index (BMI) and the results of the anterior drawer test (ADT) or Lachman test. However, patients with a BMI of 25 or higher in Group 1 showed a decrease in postoperative IKDC scores. In Group 2 patients, no significant relationship was identified between BMI and either the ADT or the Lachman test outcome.
Based on current literature and current rehabilitation guidelines following ACL reconstruction, the decision to initiate early weight-bearing is based on a limited number of studies with low levels of evidence. In our study, we found that patients who followed a non-weight-bearing regimen for 3 weeks after surgery had better mid-term results than those who were allowed to bear weight early. It appears that further prospective studies on this topic are needed to update rehabilitation guidelines in the next.
Peer Review reports
An anterior cruciate ligament (ACL) tear is a widespread injury that typically affects young, physically active individuals and requires reconstruction [ 1 ]. In the United States alone, it is estimated that more than 200,000 new ACL injuries occur every year [ 2 ]. The long-term effectiveness of anterior cruciate ligament reconstruction depends on the chosen method of reconstruction and the postoperative rehabilitation process. Several factors can influence the success of the surgery and rehabilitation, including the patient’s age, physical activity level, body mass index, extent of cartilage damage, range of motion limitations in the knee joint, muscle atrophy, presence of effusion, pain, time elapsed between injury and surgery, smoking habits, and whether the patient underwent preoperative rehabilitation. These factors can contribute to a more successful postoperative rehabilitation process for patients [ 3 ].
A technically flawless reconstruction may have negative outcomes due to inadequate and inappropriate rehabilitation [ 4 ]. Considering that % 35 of athletes do not return to their preinjury sports level within two years after anterior cruciate ligament reconstruction and that %35 of patients develop symptomatic tibiofemoral osteoarthritis in the 10 years after surgery, effective anterior cruciate ligament reconstruction and postoperative rehabilitation are of the utmost importance [ 3 , 5 ]. However, there is no agreement on the best strategy or assessment method for rehabilitation programs [ 6 ]. This can be confusing for both patients and physiotherapists [ 7 ]. A study reviewing six high-quality publications outlining rehabilitation guidelines after anterior cruciate ligament reconstruction found different results regarding the impact of early full weight-bearing on clinical outcomes. This highlighted the need for clearer definitions of accelerated rehabilitation and early load bearing [ 8 ]. Unfortunately, the rate of failed ACL reconstruction requiring reoperation has been reported to be between % 10 and %15 [ 9 ]. Aggressive rehabilitation protocols performed before biological integration and complete ligamentization can cause bone tunnel widening due to exposure of the graft-bone interface to early stresses [ 10 ]. Based on our clinical observations and literature review, we found that there is no consensus on weight bearing after anterior cruciate ligament reconstruction. Therefore, the aim of our study was to address this confusion by investigating the medium-term effects of early weight-bearing compared to non-weight-bearing on the clinical outcomes of rehabilitation following anterior cruciate ligament reconstruction surgery.
We analysed the records of 353 patients who underwent reconstruction with semitendinosus-gracilis tendon grafts for ACL rupture between January 2018 and December 2020. Patients who used braces in the postoperative period, patients with concomitant injuries and patients who did not attend the follow-up appointment were excluded from the study. Patients with isolated anterior cruciate ligament injury were included in the study. The number of patients included in the study was 110 and all operations were performed by 3 surgeons.
The patients were categorized into two groups: Group 1 consisted of 54 patients who were allowed early weight-bearing on the 1st day of the postoperative follow-up period, while Group 2 consisted of 56 patients who were not allowed to non-weight-bearing for 3 weeks. Neither group received preoperative rehabilitation. The results and scores of the anterior drawer test, Lachman test, range of motion, Lysholm knee scale, Cincinnati scale, Tegner scale and International Knee Documentation Committee (IKDC) form were obtained from the patients’ clinical records and control examinations performed by 2 experienced surgeons (Fig. 1 ). The quadriceps muscle strength of the patients was measured with a hand dynamometer by the same surgeons. In addition, perioperative tibial slope was measured.
Surgical technique, medication, and rehabilitation
All patients underwent arthroscopic ACL reconstruction using a tourniquet. Preoperative antibiotic prophylaxis and postoperative low molecular weight heparin prophylaxis were administered. The knee joint was evaluated by opening standard arthroscopy portals. Gracilis and semitendinosus tendons were removed using a tendon stripper. The femoral tunnel was opened in the appropriate position according to the anatomical side and in the appropriate thickness for the graft. The tibial tunnel was opened at a 55-degree angle to the tibial plateau.
The prepared grafts were fixed using a single bundle fixed-loop device in the femur and absorbable screws and staples in the tibia. Drains were used in all patients and removed on postoperative day 1.
Patients in group 1 were given as much weight as they could tolerate the pain with crutches on postoperative day 1. In the following days, the amount of weight bearing was increased and full weight bearing without support was achieved on postoperative day 5. Patients in group 2 were mobilised with crutches on postoperative day 1 and non weight bearing was performed until postoperative day 20. On the 21st postoperative day, the amount of weight bearing was increased and full weight bearing was achieved on the 25th postoperative day. The same rehabilitation programs were applied for both groups except for the weight-bearing protocol. Swelling management was done with Ice, compression and elevation. In both groups, we started open chain exercises from postoperative day 1, full extension and flexion up to 90 degrees were allowed for the first 3 weeks and full flexion was allowed after the 3rd week. After the 3rd postoperative day, they were allowed to lift 1 kg weight and increase weight by 1 kg every 3 days and we also applied a home exercise program for rehabilitation.
Statistical analysis
All data were analysed using SPSS version 26 after coding. The Kolmogorov‒Smirnov test and histogram graphs were utilized to assess whether normal distribution conditions were met. For variables that exhibited normal distribution, the mean and standard deviation values were taken into consideration. For nonnormally distributed parameters, the median and minimum-maximum values were provided. Percentage and ratio values were calculated for nominal and categorical variables. The relationship between the variable of early load or not and the analysis of complications was performed using the chi-square test. Similarly, the Mann‒WhitneyU test was employed to compare the scores with the variable of giving or not giving early load. Two way ANOVA was used in the comparisons of “group 1-group 2” and “BMI groups”. Following this analysis, “Bonferroni post-hoc test” was used to determine the groups making the difference for each score. A statistical significance level of %5was accepted.
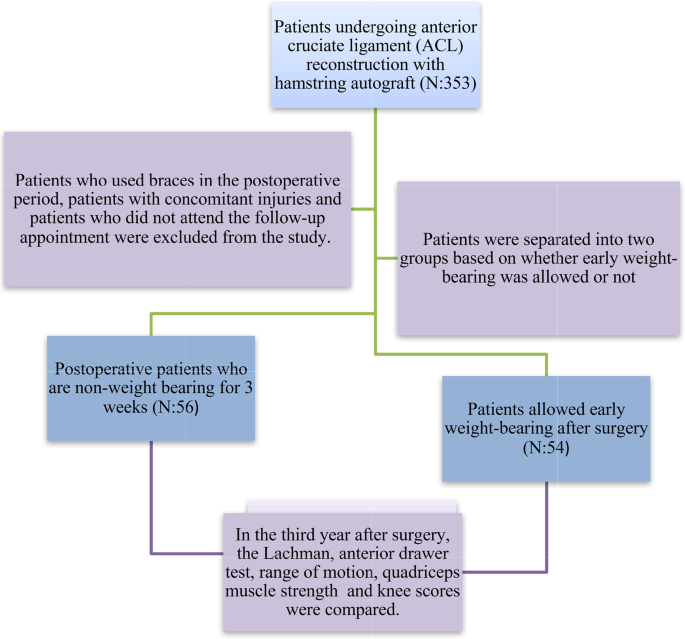
Participant flow chart
The study included 110 adult patients who underwent anterior cruciate ligament reconstruction. The number of patients in group 1 was 54 and 56 in group 2. Of these patients, 109 (% 99.1) were male. The median age of the patients in Group 1 was 31.5 years, while in Group 2, it was 28.8 years. Due to some technical problems, the operation time was prolonged in a few cases, the mean operation time was 75 min (range: 65–110 min). Table 1 presents the demographic data.
Tibial slopes of patients in group 1 7.60 ± 0.80, tibial slopes of patients in group 2 7.80 ± 0.79 and there was no statistically significant difference ( p = 0.782).
There was no notable difference in complication rates between Group 1 and Group 2 ( p = 0.345). These complications included haemarthrosis, rerupture, infection and deep vein thrombosis. In group 1, 4 patients had rerupture, 2 patients had infection and 1 patient had dvt, whereas in group 2, 3 patients had haemarthrosis, 3 patients had rerupture and 1 patient had infection. Comparisons were made between the postoperative results of the anterior drawer tests (ADT) and Lachman tests of patients in Group 1 and Group 2. It was observed that Group 1 patients had positive anterior drawer tests more frequently than Group 2 patients, which was statistically significant ( p = 0.024). Similarly, Group 1 patients had positive Lachman tests more frequently than Group 2 patients, which was also statistically significant ( p = 0.013). When analysing the Lysholm knee scores of patients in both groups, there was no significant difference in the preoperative Lysholm score ( p = 0.811). However, the postoperative Lysholm knee scores of Group 1 patients were significantly lower than those of Group 2 patients. The score was 46.67 in Group 1 and 64.02 in Group 2 ( p = 0.004). The Cincinnati knee scores of patients in both groups were not significantly different preoperatively, but postoperatively, Group 1 patients had significantly lower scores ( p = 0.003). By comparing the postoperative Tegner activity score between the two groups, it was found that Group 1 patients had a significantly lower Tegner score than Group 2 patients ( p < 0.001). Similarly, when comparing the postoperative IKDC score, Group 1 patients had significantly lower scores than Group 2 patients ( p = 0.005). There was no significant difference in range of motion between the two groups. When we looked at the quadriceps muscle strength of the patients, it was 127,78 N ± 11,962 in group 1 and 127,14 N ± 13,172, in group 2 and it was not statistically significant ( p = 0.792).
In Group 1, the ADT and Lachman test results were analysed using chi-square analysis based on BMI categories: <25 and ≥ 25. There was no significant correlation observed between the ADT and BMI ( p = 0.285), as well as between the Lachman test and BMI ( p = 0.458). Similar findings were observed in Group 2, where no difference was found between the BMI and ADT ( p = 1) or between the BMI and Lachman test ( p = 0.573).
When the results of the two-factor comparison were analysed, the interaction effect of early weight bearing and BMI on the Lysholm score was not significant ( p = 0.134). While the interaction was not significant in Cincinati and Tegner scores ( p = 0.093/ p = 272), the interaction was significant only in IKDC score ( p = 0.048).
Anterior cruciate ligament (ACL) reconstruction is a frequently performed procedure to help patients return to their active lifestyle. Proper rehabilitation of the reconstructed knee is crucial for patients to return totheir previous level of physical activity. Many randomized studies have been conducted to assess the effectiveness of early weight-bearing versus non-weight-bearing after ACL reconstruction [ 11 ]. Early studies on early weight-bearing after ACL reconstruction reported that it reduced anterior knee pain and improved Lysholm scores. However, it is important to note that these studies involved patients who were evaluated at an average of 7 months after undergoing reconstruction with a patellar tendon graft, which may explain the difference in our study results [ 12 ]. A systematic review on rehabilitation after ACL reconstruction in 2016 concluded that early weight-bearing reduced anterior knee pain and did not increase the risk of tunnel widening [ 3 ]. According to the rehabilitation guidelines of the Multicentre Orthopaedic Outcomes Network (MOON) group, postoperative early weight-bearing is recommended [ 4 ]. However, it is worth noting that this recommendation is based on a single study conducted in 1998. In our study, we found that early weight-bearing increased knee laxity and decreased knee scores. Based on these findings, it appears that further studies are needed to inform updates to rehabilitation guidelines.
Even studies conducted on living subjects to develop rehabilitation programs that do not strain or restrict the healing of the ACL graft have not yielded conclusive results regarding the impact of loading on ACL healing [ 13 ]. Publications that highlight the positive outcomes of early motion and weight-bearing also acknowledge the uncertainty surrounding the safe threshold of loading. The debate about which rehabilitation approaches are too aggressive and what level of loading is sufficient persists [ 14 ]. This uncertainty may expose patients to anunnecessary risk of reinjury. Our study’s findings, which show superior scores among patients who did not undergo non-weight-bearing, can contribute to resolving this debate. Clinicians can achieve satisfactory clinical outcomes by refraining from applying weight-bearing to patients for 3 weeks and closely monitoring them during the postoperative rehabilitation process, without concern about the patient experiencing a recurrence of ACL insufficiency. In a publication comparing the clinical and radiological outcomes of patients following a non-weight-bearing regimen for 1 week compared with 2 weeks during the postoperative rehabilitation period, no significant differences were found in Tegner activity level, Lysholm score, anterior laxity, or muscle strength values. However, the same study reported that early weight bearing could potentially increase the risk of femoral tunnel enlargement [ 15 ]. In light of these factors, it would be prudent to avoid implementing aggressive rehabilitation protocols that carry the risk of potential reinjury until the literature provides clearer guidance on this matter. Another study demonstrated that aggressive rehabilitation led to increased femoral tunnel enlargement and decreased IKDC scores, emphasizing the importance of a balanced approach to rehabilitation [ 16 ]. This study emphasised the importance of early mobilisation and rehabilitation to restore normal range of motion after surgery and also observed the positive effects of non-weight bearing on outcomes. In the literature, there are inconsistent and conflicting findings about whether the patient’s BMI is a risk factor for the development of anterior cruciate ligament insufficiency after reconstruction [ 17 ]. In our study, we found that one of the scores decreased but the other scores did not change.
In the current literature, it has been observed that according to rehabilitation guidelines after ACL reconstruction, the decision to begin early weight-bearing is based on low level evidence in a limited number of studies. Our study shows that patients who were not allowed to bear weight for 3 weeks after ACL reconstruction had better results in the mid-term compared to those who started early weight-bearing. Additionally, we found that early weight-bearing in overweight patients led to a greater decrease in knee scores. Therefore, it is important to exercise caution when considering early weight-bearing in the overweight patient group. Future updates to rehabilitation guidelines should include evidence from prospective and more comprehensive studies on early weight bearing.
Restrictions
The retrospective nature of our study is our first limitation. Another limitation of our study may be the lack of preoperative whole knee scores, quadriceps strength data and knee alignment for all patient groups. Another factor is that radiographic imaging methods were not used in our study. More powerful studies can be done by investigating the correlation between clinical scores and radiographic findings. A larger number of patients in the groups would have increased the power of the study. Another limitation is that not all operations were performed by a single surgeon. This may create the possibility that surgeons may affect the results by using a different technique.
Data availability
The datasets used and/or analyzed during the current study are available from the corresponding author on reasonable request.
DiMiceli R, Marambio CB, Zati A, Monesi R, Benedetti MG. Do knee bracingand delayed weight-term functional outcome after Anterior Cruciate Ligament. Reconstruction? Joints. 2017;5(4):202–6.
Article Google Scholar
Gianotti SM, Marshall SW, Hume PA, Bunt L. Incidence of anterior cruciate ligament injury and other knee ligament injuries: a national population-based study. J SciMedSport. 2009;12(6):622–7.
Google Scholar
Van Melick N, vanCingel RE, Brooijmans F, Neeter C, vanTienen T, Hullegie W, Nijhuis-van der Sanden MW. Evidence-: practice guidelines for anterior cruciate ligament rehabilitation based on a systematic review and multidisciplinary consensus. Br J Sports Med. 2016;50(24):1506–15.
Article PubMed Google Scholar
Wright RW, Haas AK, Anderson J, Calabrese G, Cavanaugh J, Hewett TE, Lorring D, McKenzie C, Preston E, Williams G, MOON Group. Anterior Cruciate Ligament Reconstruction Rehabilitation: MOON guidelines. Sports Health. 2015;7(3):239–43.
Article PubMed PubMed Central Google Scholar
Lie MM, Risberg MA, Storheim K, Engebretsen L, Øiestad BE. What’sthe rate of knee osteoarthritis 10 years after anterior cruciate ligament injury? An updated systematic review. Br J Sports Med. 2019;53(18):1162–7.
Kim JG, Kim WS, Kim SG, Lee DH. Accelerated Versus non-accelerated Rehabilitation after Primary Anterior Cruciate Ligament Reconstruction using hamstring autografts: a systematic review and Meta-analysis of comparative studies. IndianJOrthop. 2021;55(2):405–15.
Makhni EC, Crump EK, Steinhaus ME, Verma NN, Ahmad CS, Cole BJ, Bach BR Jr. Quality and variability of online available physical therapy protocols from academic orthopaedic surgery programs for Anterior Cruciate Ligament Reconstruction. Arthroscopy. 2016;32(8):16–21.
Andrade R, Pereira R, vanCingel R, Staal JB, Espregueira-Mendes J. How should clinicians rehabilitate patients after ACL reconstruction? A systematic review of clinical practice guidelines (CPGs) with a focus on quality appraisal (AGREE II). Br J Sports Med. 2020;54(9):512–9.
Maak TG, Voos JE, Wickiewicz TL, Warren RF. Tunnelwidening in revision anterior cruciate ligament reconstruction. J AmAcadOrthopSurg. 2010;18(11):695–706.
Yu JK, Paessler HH. Relationship between tunnel widening and different rehabilitation procedures after anterior cruciate ligament reconstruction with quadrupled hamstring tendons. ChinMed J (Engl). 2005;118(4):320–6.
Wright RW, Preston E, Fleming BC, Amendola A, Andrish JT, Bergfeld JA, Dunn WR, Kaeding C, Kuhn JE, Marx RG, McCarty EC, Parker RC, Spindler KP, Wolcott M, Wolf BR, Williams GN. A systematic review of anterior cruciate ligament reconstruction rehabilitation: part I: continuous passive motion, early weight bearing, postoperative bracing, and home-based rehabilitation. J KneeSurg. 2008;21(3):217–24.
Tyler TF, McHugh MP, Gleim GW, Nicholas SJ. Theeffect of immediate weight bearing after anterior cruciate ligamentre construction. ClinOrthopRelatRes. 1998;(357):141–8.
Luque-Seron JA, Medina-Porqueres I. Anterior cruciate ligament strain in vivo: a systematic review. Sports Health. 2016;8(5):451–5.
Jenkins SM, Guzman A, Gardner BB, Bryant SA, Del Sol SR, McGahan P, Chen J. Rehabilitation after Anterior Cruciate Ligament Injury: review of current literature and recommendations. CurrRevMusculoskeletMed. 2022;15(3):170–9.
Tajima T, Yamaguchi N, Nagasawa M, Morita Y, Nakamura Y, Chosa E. Earlyweight-bearing after anterior cruciate ligament reconstruction with hamstring grafts induce femoral bone tunnel enlargement: a prospective clinical and radiographic study. BMC Musculoskelet Disord. 2019;20(1):274.
Zhu W, Wang D, Han Y, Zhang N, Zeng Y. Anteriorcruciateligament (ACL) auto graft reconstruction with hamstring tendons: clinical research among three rehabilitation procedures. Eur J OrthopSurgTraumatol. 2013;23(8):939–43.
Shen X, Qin Y, Zuo J, Liu T, Xiao J. A systematic review of risk factors for Anterior Cruciate Ligament Reconstruction failure. Int J Sports Med. 2021;42(8):682–93.
Download references
Acknowledgements
Author information, authors and affiliations.
Department of Orthopaedics and Traumatology, Yuzuncu Yil University, Van, Turkey
Sehmuz Kaya, Yunus Can Unal, Necip Guven, Tulin Turkozu, Sezai Ozkan, Cihan Adanas & Mehmet Ata Gokalp
Department of Orthopaedics and Traumatology, Orhaneli State Hospital, Bursa, Turkey
Department of Orthopaedics and Traumatology, Hitit University, Corum, Turkey
Abdulrahim Dundar
You can also search for this author in PubMed Google Scholar
Contributions
SK, YCU and MAG designed and directed the article. NG, TT and CO wrote the manuscript. AD, CA and SO revised the manuscript. All authors have read and verifed the underlying data and approved the final version of the manuscript.
Corresponding author
Correspondence to Sehmuz Kaya .
Ethics declarations
Ethics approval and consent to participate.
This retrospective observational study was confirmed by the ethics committee at the local institution. Informed consent forms for surgery were obtained from all subjects before surgery.
Consent for publication
All authors agree to publish this article.
Competing interests
The authors declare no competing interests.
Additional information
Publisher’s note.
Springer Nature remains neutral with regard to jurisdictional claims in published maps and institutional affiliations.
Rights and permissions
Open Access This article is licensed under a Creative Commons Attribution 4.0 International License, which permits use, sharing, adaptation, distribution and reproduction in any medium or format, as long as you give appropriate credit to the original author(s) and the source, provide a link to the Creative Commons licence, and indicate if changes were made. The images or other third party material in this article are included in the article’s Creative Commons licence, unless indicated otherwise in a credit line to the material. If material is not included in the article’s Creative Commons licence and your intended use is not permitted by statutory regulation or exceeds the permitted use, you will need to obtain permission directly from the copyright holder. To view a copy of this licence, visit http://creativecommons.org/licenses/by/4.0/ . The Creative Commons Public Domain Dedication waiver ( http://creativecommons.org/publicdomain/zero/1.0/ ) applies to the data made available in this article, unless otherwise stated in a credit line to the data.
Reprints and permissions
About this article
Cite this article.
Kaya, S., Unal, Y.C., Guven, N. et al. The impact of early weight-bearing on results following anterior cruciate ligament reconstruction. BMC Musculoskelet Disord 25 , 395 (2024). https://doi.org/10.1186/s12891-024-07525-8
Download citation
Received : 05 November 2023
Accepted : 15 May 2024
Published : 21 May 2024
DOI : https://doi.org/10.1186/s12891-024-07525-8
Share this article
Anyone you share the following link with will be able to read this content:
Sorry, a shareable link is not currently available for this article.
Provided by the Springer Nature SharedIt content-sharing initiative
- Anterior cruciate ligament
- Arthroscopic reconstruction
- Rehabilitation
- Early weight bearing
- Body mass index
BMC Musculoskeletal Disorders
ISSN: 1471-2474
- Submission enquiries: [email protected]
- General enquiries: [email protected]

An official website of the United States government
The .gov means it’s official. Federal government websites often end in .gov or .mil. Before sharing sensitive information, make sure you’re on a federal government site.
The site is secure. The https:// ensures that you are connecting to the official website and that any information you provide is encrypted and transmitted securely.
- Publications
- Account settings
Preview improvements coming to the PMC website in October 2024. Learn More or Try it out now .
- Advanced Search
- Journal List
- Biomed Eng Online

Robotic devices for paediatric rehabilitation: a review of design features
Alberto gonzalez.
1 BioDesign Lab, School of Engineering, Computer and Mathematical Sciences, Auckland University of Technology, Auckland, New Zealand
Lorenzo Garcia
Peter mcnair.
2 Health and Rehabilitation Research Institute, Auckland University of Technology, Auckland, New Zealand
Associated Data
Not applicable.
Children with physical disabilities often have limited performance in daily activities, hindering their physical development, social development and mental health. Therefore, rehabilitation is essential to mitigate the adverse effects of the different causes of physical disabilities and improve independence and quality of life. In the last decade, robotic rehabilitation has shown the potential to augment traditional physical rehabilitation. However, to date, most robotic rehabilitation devices are designed for adult patients who differ in their needs compared to paediatric patients, limiting the devices’ potential because the paediatric patients’ needs are not adequately considered. With this in mind, the current work reviews the existing literature on robotic rehabilitation for children with physical disabilities, intending to summarise how the rehabilitation robots could fulfil children’s needs and inspire researchers to develop new devices. A literature search was conducted utilising the Web of Science, PubMed and Scopus databases. Based on the inclusion–exclusion criteria, 206 publications were included, and 58 robotic devices used by children with a physical disability were identified. Different design factors and the treated conditions using robotic technology were compared. Through the analyses, it was identified that weight, safety, operability and motivation were crucial factors to the successful design of devices for children. The majority of the current devices were used for lower limb rehabilitation. Neurological disorders, in particular cerebral palsy, were the most common conditions for which devices were designed. By far, the most common actuator was the electric motor. Usually, the devices present more than one training strategy being the assistive strategy the most used. The admittance/impedance method is the most popular to interface the robot with the children. Currently, there is a trend on developing exoskeletons, as they can assist children with daily life activities outside of the rehabilitation setting, propitiating a wider adoption of the technology. With this shift in focus, it appears likely that new technologies to actuate the system (e.g. serial elastic actuators) and to detect the intention (e.g. physiological signals) of children as they go about their daily activities will be required.
Introduction
Mobility and exploration are essential in children’s development and contribute towards cognitive, physical, social and emotional development. However, children with physical disabilities present limitations when performing activities autonomously, which hinders their typical development [ 1 ]. Ongoing paediatric physical disability arose from many different causes, including neurological disorders like cerebral palsy (CP) [ 2 ], Stroke [ 3 ] and acquired brain injury (ABI) [ 4 ], neuromuscular diseases such as Duchenne muscular dystrophy (DMD) [ 5 ] and spinal muscular atrophy (SMA) [ 6 ], or traumatic injuries [ 7 , 8 ] (Table (Table1 1 ).
Incidence or prevalence of conditions that cause physical disabilities in children
Rehabilitation is essential to help the children recover or maintain functionality when interacting with their environment, improving the quality of life and autonomy [ 9 , 10 ]. Furthermore, early access to rehabilitation is critical for children while they are in the stage of development. The gait pattern and motor abilities are still malleable [ 11 ], intending to reduce the probability of developing more severe levels of disability [ 12 , 13 ].
The standard therapies to manage the musculoskeletal system’s deterioration and improve and maintain physical ability include passive orthoses, surgery, and physiotherapy [ 15 , 16 ]. Physiotherapists prescribe, monitor, and guide exercise, which can prevent an unnecessarily sedentary or immobile lifestyle. The most extensively investigated aspect of physiotherapy is the effect of direct interventions on upper or lower limbs. Such interventions often involve intensive stretching and strengthening exercises facilitated by the physiotherapist [ 2 ] to improve motor skills. These interventions are often highly labour intensive and can be challenging to perform [ 17 ]. Furthermore, the effectiveness of physiotherapy often depends on the experience of the physiotherapist. Thus, it is not easy to achieve optimal consistency and repeatability between rehabilitation sessions [ 18 , 19 ].
There is a growing interest in robots that can support the patient, the family and the medical professional in a wide range of activities used for the care of people with physical disabilities, for example, companion robots [ 20 , 21 ], monitoring robots [ 22 ] and surgery robots [ 23 ], all of them can be considered as healthcare robots.
Healthcare robots can be divided into three main categories, clinical robots, assistive robots and rehabilitation robots [ 24 , 25 ]. Clinical robots are focus on supportive care and cure process (e.g. help in surgery and diagnosis) in clinical environments; assistive robots primary function is to provide assistive help either to carers or directly to patients either in a hospital or in a specialist care facility (e.g. patient lifting and to assist in routine services); rehabilitation robots are robots design towards restoring the functionality and mobility of people with physical disabilities, in that case, the recovery of mobility could be achieved by assisting the patient during ADLs (e.g. walking and grasping objects) [ 17 , 26 ] or with physical training therapy [ 24 , 27 – 30 ], and are the main focus of this study.
Rehabilitation therapy for the recovery of mobility based on robots has been proposed as a new procedure for children with physical disabilities [ 31 ]. This robot-assisted rehabilitation therapy consists of a mechatronic device that provides highly repetitive and task-specific guided movements autonomously [ 32 , 33 ]. The use of robots in rehabilitation therapies bring advantages over traditional therapies, as they allow extensive practice in children with substantial disabilities, reduced effort required of therapists during the exercises, and provide a quantitative assessment of the patient’s motor function (e.g. quantitative feedback of range of motion (ROM) and strength with each repetition) [ 34 – 38 ].
Rehabilitation robots are often classified by their mechanical structure and are generally divided into end-effectors and exoskeletons [ 28 , 39 ]. End-effector devices work by applying forces to the distal segments of limbs, creating what is termed a “mechanical chain” that prompts movements of other parts of the limb generating a pattern of specific activity across different joints. If utilised on a single segment and joint, their simple structure makes it easier to adapt them to many patients and needs less complicated control algorithms. However, it is difficult to isolate specific joints since they produce complex movements that involve the whole limb [ 40 ].
Contrarily, robotic exoskeletons could be termed “wearable machines” that mirror the patient’s skeletal structure; therefore, they only move the joint of the limb where the exoskeleton is worn. This approach allows for independent and concurrent control of specific segments of the limb. However, it is essential to adjust the length of sections of the robot to the lengths of the segments of the patient limb. Moreover, when the joint is in motion, the position of the centre of rotation can change, creating discomfort in the user. Thus, increasing the number of degrees of freedom of the robot increases the control algorithm’s complexity, weight, mechanical complexity, and power requirements, making it unattainable for home use [ 18 , 41 ].
Apart from mechanical structure, robots possess essential elements to ensure the systems’ reliability and robustness [ 42 ]. Actuators, training strategy and the Human–computer interface (HCI) are among these essential elements. The actuators play a crucial role because they determine the torque and movement provided by the robot and influence the total weight and compliance of the system [ 29 , 43 ]. The training strategy and the HCI are an integral part of the robot-assisted rehabilitation since it determines how the patient interacts with the robot and the type of assistance that the robot can provide. Many authors have analysed these last two characteristics as part of the robots control [ 28 , 30 , 44 ]. However, control also involves “low level” considerations that are more related to the internal communication of the components (sensors, structures, microcontrollers, actuators, etc.) at a hardware level rather than how the device interacts with the patient [ 45 – 49 ].
Although multiple devices for the robotic rehabilitation of upper and lower limbs have been developed, at least in a proof-of-concept phase [ 24 , 39 , 42 , 44 , 45 , 50 , 51 ], most presented robots were designed for adult users, impeding their use on the paediatric population. For example, commercial exoskeletons are made for a subject 150 cm tall onwards [ 52 ], while the average height for a 5-year-old child will be around 110 cm [ 53 ].
However, to develop technology planned to be used on the paediatric population is not only a matter of reducing the size of the robots. But it should be tailored to their own capabilities and goals that differ from those of the adults. For instance, a simple downscaling of the robots is not enough as the normalised joint torques on adults are greater than those of a child [ 54 , 55 ], making them potentially dangerous when used on small children. Additionally, in the case of children, as their cognitive abilities are still developing, it could be hard for them to fully understand how the technology works [ 52 , 56 ]. Hence, it is hard to adapt a robot made for adults to be used by children since the robots do not fulfil the children’s needs [ 26 , 57 ].
Consequently, to address the children’s needs adequately, it is essential to include them and other stakeholders (e.g. family members, clinicians, and health care providers) during the development process, providing feedback to identify possible issues of importance [ 1 , 43 ]. Furthermore, it is essential to focus not only on addressing the impairment or limitation in users’ functional abilities, but also on other fundamental needs, like accessibility and aesthetics [ 58 ], to avoid the user abandoning the rehabilitation device due to frustration [ 59 ].
Despite the progressive development of robotic rehabilitation devices, their application to the paediatric population is still scarce. Consequently, the key features to design an optimal robotic rehabilitation device that better enhance children’s abilities with physical disabilities have not been well defined yet. Based on this framework, this review aims to address the following questions: (1) What are the design requirements for paediatric rehabilitation robots? (2) How does the current technology contribute to achieving the paediatric design requirements? And (3) How do the paediatric conditions impact the device design?
An in-depth literature search was performed to conduct the review, following the search strategy of the Preferred Reporting Items for Systematic Reviews and Meta-Analyses (PRISMA) guidelines [ 60 ].
A literature search was conducted to identify literature associated with the topic based on searches in PubMed, Scopus, and Web of Science, using the combination of the following keywords: (pediatric OR kid* OR child*) AND (aid OR assist* OR improve* OR augment* OR enhance* OR reinforce* OR therap* OR rehabilitation) AND (active ortho* OR exoskeleton* OR wearable robot* OR portable robot* OR robot* suit OR robot*) AND (movement OR motion OR walk* OR gait OR grasp* OR handl*). To make our search as complete as possible, a search through the university library databases was also conducted.
After the preliminary search, the following inclusion and exclusion criteria to narrow the literature search were used. The inclusion criteria were:
- Studies involving robotic devices for robot-assisted rehabilitation therapy,
- Studies involving robotic devices for assessment of patients with Physically disabilities,
- Studies involving devices designed for children or utilised with a paediatric population (< 18 years old),
- written in English,
- full-text articles.
And the exclusion criteria were:
- Studies that only present software solutions or simulations,
- Studies involving passive devices (do not have actuators),
- Studies involving postural change,
- Studies involving only the adult population and
- Studies involving robots that do not replace the movement itself (e.g. wheelchairs).
The outcome of this literature review is compiled in the following sections:
- An overview of the literature search,
- the paediatric robotic rehabilitation design requirements,
- an analysis of the type of robots used in paediatric robotic rehabilitation;
- the actuators to drive the robots;
- training strategy of the robots;
- the human–computer interface of the assistive systems, and
- the treated conditions in children with physical disabilities.
Literature search
Based on the keywords mentioned in the methods section, 1604 publications were found, with:
- 811 publications from Web of Science,
- 547 publications from PubMed,
- 241 from Scopus, and
- 5 from a search on the University library.
First, a check was made for duplicated publications. After this process, the abstracts of 1248 publications were screened, and 301 titles were selected for full-text reading. After carefully applying the inclusion–exclusion criteria to the full read papers, 206 publications were selected. Among the chosen publications, 10 were reviews, 42 only discussed a section of the design process of the rehabilitation robot, 138 presented a clinical application, and 16 included the design process plus a clinical application (Fig. 1 shows a flow diagram that illustrates the process of the selection of the papers).

Literature search flow diagram
The ten review articles examined a variety of rehabilitation robots for children with physical disabilities. They were focused on children with neurological problems (e.g. CP, ABI, and Stroke) or SCI and only investigated their use as part of physical therapy. In Fasoli et al. [ 35 ], Meyer et al. [ 61 ], and Bayon et al. [ 62 ], the robot assistive therapies for children with CP were examined. Vova et al. [ 63 ] reviewed the efficacy of functional electrical stimulation and exoskeletons in gait training to improve motor function and gait pattern in children with CP. Zwicker et al. [ 64 ] reviewed the efficiency of robot-assisted treadmill training compared to traditional treadmill training in children with CP. Chen et al. [ 65 ] examined the effectiveness of various devices for upper limb robotic therapy on children with CP. The effects of robotic gait training practices in individuals with CP were investigated in Carvalho et al. [ 31 ]. Falzarano et al. [ 10 ] and Mahamud et al. [ 66 ] investigated upper and lower limb rehabilitation devices for neurological diseases. Dannenberg et al. [ 67 ] compared different locomotor training, including robotic training, in children with SCI. Compared with the previous reviews, this work analyses a broader range of aspects of paediatric rehabilitation robots, focused on the design parameters to fulfil the paediatric needs and how the technology and different conditions affect the robot design.
Paediatric robotic rehabilitation design requirements
Fifteen different requirements were identified (Table (Table2). 2 ). The requirements are based on those proposed by Batavia and Hammer for assistive devices [ 68 ] and expanded by proposed requirements for paediatric rehabilitation devices highlighted by Weightman et al. [ 69 ], Bützer et al. [ 26 ] and Keller et al. [ 57 ]. In paediatric rehabilitation, it was apparent that the stakeholder’s needs related to operability, weight, safety, and motivation factors were relevant.
Paediatric rehabilitation robots’ requirements and examples
In paediatric rehabilitation devices, operability is critical as children are in a continuous development phase during which their bodies, cognitive capabilities and physical abilities (e.g. skill levels) are changing, making them a “heterogeneous population” [ 52 , 70 ]. Consequently, the device must adapt to different children’s abilities and sizes [ 71 ].
An important consideration is that the robot’s weight could obstruct the movement pattern of the limb and increase the child’s energy consumption [ 72 , 73 ]. Furthermore, due to their musculoskeletal system’s immature development, their muscle strength and joint torque generation may not be adequate to assist in the movement being undertaken [ 74 ].
Concerning safety, children often cannot adequately assess the hazards of using complex technological devices [ 56 ]. Therefore, it is crucial to design safety mechanisms that minimise risky situations. These should be able to be activated remotely by adults with the child [ 57 ]. Furthermore, the use of compliant materials with shock-absorbing features (e.g. elastic elements like spring and Bowden cables) would be beneficial [ 17 ].
Finally, motivation is crucial because function recovery is not enough to engage children in the rehabilitation process [ 75 ]. Consequently, researchers have used strategies to engage children, like aesthetic designs attractive to the children [ 26 , 76 ] or a virtual environment where they can interact with virtual objects [ 77 , 78 ].
Type of robots used on paediatric robotic rehabilitation
Fifty-eight different devices were found that at least had a prototype in action. In Tables Tables3, 3 , ,4, 4 , ,5, 5 , ,6, 6 , ,7 7 (Figs. (Figs.2, 2 , ,3, 3 , ,4, 4 , ,5, 5 , ,6), 6 ), the rehabilitation robots are presented chronologically and separated by their mechanical structure (end-effector or exoskeleton) and the anatomical part of the body where they are working (upper limb or lower limb). Furthermore, the tables summarise the characteristic features of the selected devices. This tabulated summary constitutes the reference for information provided in subsequent sections.
Upper limb end-effectors rehabilitation robots
Upper limb exoskeleton rehabilitation robots
Lower limb end-effectors rehabilitation robots
Lower limb exoskeleton rehabilitation robots
End-effectors rehabilitation robots for upper and lower limbs

Picture of upper limb end-effectors rehabilitation robots: a Inmotion2/Mitmanus [ 79 ], b wrist robot [ 80 ], c REAPlan [ 81 ]

Picture of upper limb exoskeletons rehabilitation robots a KINARM [ 82 ], b GLOREHA [ 83 ], c HAL single joint [ 84 ], and d PneuGlove [ 85 ]

Picture of lower limb end-effectors rehabilitation robots: a Innowalk [ 86 ], b UFMG [ 87 ], c 3DCaLT [ 88 ], d Leg Press [ 89 ].
Reprinted from Biomedical Signal Processing and Control, Vol. 38, F. Chrif et al., Control design for a lower-limb paediatric therapy device using linear motor technology, Page 121, Copyright (2017), with permission from Elsevier

Picture of lower limb exoskeletons rehabilitation robots: a Lokomat [ 90 ], b HAL [ 91 ], c CPWalker [ 92 ], d PediAnklebot [ 93 ], e wearable ankle rehabilitation robot developed by the Rehabilitation Institute of Chicago [ 94 ], f P.REX [ 95 ]

Picture of KPT Cycla [ 96 ] an end-effectors rehabilitation robot for both a lower and b upper limbs
In respect of the developmental stage of the devices, the stages were classified into four categories: (1) commercial in the case the robots are available for its commercialisation; (2) clinical trial when the robot undergo a study where the participants were assigned to groups undergoing similar forms of therapy, but at different intensities, using various devices or undergoing various forms of therapy in a different order, aiming to determine the efficiency of therapy [ 28 ]; (3) feasibility study when the experiments conducted with a low number of people, often using the prototype of a device, to evaluate its safety and clinical feasibility without showing the potential benefits of the device [ 28 ]; (4) prototypes when the robots had not performed any test that involves people. It can be observed that 18 of them reached the commercialisation phase, but only 9 of them are certified by the US Food and Drug Administration (FDA). However, only 5 of the commercially available devices present a paediatric version of the rehabilitation system. In the case of clinical studies, 34 systems conduct at least one clinical trial, 9 presented a feasibility study, and 15 are in the prototyping phase.
From the 58 devices, it was apparent that the majority (67%) were designed or had been redesigned for children. When it comes to the type of robot, more than half were an exoskeleton type structure. In the past five years, there is a trend (Tables (Tables3, 3 , ,4, 4 , ,5, 5 , ,6, 6 , ,7) 7 ) for this structure to be more popular with designers than end-effectors for this structure robotic rehabilitation in paediatrics. Additionally, it can be noted that the majority of robots not explicitly designed for children are end-effector devices. In the case of the exoskeletons, the degrees of freedom (DOFs) are related to the number of joints and limbs that are powered. Therefore, it is possible to find exoskeletons with passive DOFs, which means that those joints are not actuated, but allow the free movement of the children´s joint. In contrast, for the end-effectors, the relation of DOFs of the robot and the actuated joints is not linear and depends on the robot’s mechanical design.
Rehabilitation robots are moved by devices called actuators. Actuators convert a source of energy (e.g. electrical, thermal, pneumatic) into mechanical motion. Commonly rehabilitation assisted robots are powered by electrical actuators. Among the compared systems in Tables Tables3, 3 , ,4, 4 , ,5, 5 , ,6, 6 , ,7, 7 , over 93% of the robots used electric motors as the actuator, and about 7% used pneumatic actuators.
Motor actuator
In robotic-assisted rehabilitation, the most common actuators are electrical motors with a rigid power transmission element such as a harmonic drive, ball-screws, timing belts, and chains. Unfortunately, their need for transmission negatively affects the back-drivability, efficiency, safety, size, and mass [ 97 ]. Nevertheless, they were likely chosen since they are efficient and easy to control. Some examples of paediatric robotic rehabilitation devices using electrical motors and rigid transmission are the Pedianklebot that used two brushless dc motors and a Rohlix linear traction device [ 98 ], the electric motor with timing belt used in ChARMin [ 99 ], or the motor with chain transmission used in P.REX [ 100 ].
Some researchers [ 64 ] used a cable-driven transmission to replace the rigid transmission for an elastic cable to improve the power to weight ratio and lower the inertia over the treated body segment. Examples include the ankle exoskeleton designed by The University of Arizona [ 101 ] or TPAD [ 102 ], an end-effector robot for gait rehabilitation that used Bowden cables attached to the hip to generate assistive forces. This change in the transmission brings other advantages like modularity, simple architecture and is convenient for reconfiguration, even though they present some disadvantages being unidirectional and difficult to model and control [ 97 , 103 , 104 ].
Following the concept of adding a flexible element in series with the actuator to improve the electric motors’ compliance, serial elastic actuators (SEAs) incorporate an elastic part in series with the electric actuator. This elastic element helps to decrease the actuator’s impedance and inertia and increases the back-drivability allowing better force control, even though they are limited by a large volume, heavy mass and complicated structure [ 17 , 105 ]. An example of the use of this technology is the ATLAS exoskeleton [ 106 ].
Pneumatic actuators
Some authors considered that the mechanical linkage of the electric actuators is too heavy and can generate resistance at the joints, making them inadequate for rehabilitation applications [ 74 , 107 ]. Instead of electrical motors, they used pneumatic actuators, consisting of a simple air pressurisation mechanism in an expandable chamber, converting the energy from the compressed air to mechanical motion [ 18 , 108 , 109 ]. Their main advantage is improved back-drivability, and they are often lightweight at the site of actuation, have high power density, and can generate fast movements. They are not without limitations; firstly, poor portability because they need external compressors or fluid tanks as the power source. Secondly, it is challenging to create a good model and control strategy due to their nonlinear response to input pressure [ 109 – 111 ]. Among the devices analysed that used pneumatic actuators were the Rutgers ankle platform [ 112 ] for CP children and two gloves for hand rehabilitation PneuGlove [ 113 ] and Gloreha [ 33 ].
Training strategy
Devices for robotic rehabilitation may provide different training strategies depending on the type and severity of the patient’s impairment. These can be divided into passive, active, assistive, or resistive [ 42 ]. In general, the devices can offer more than one type of training.
In passive training, the force/motion is generated by the robot alone to perform the exercise. The advantage of this training is that patients with minimal muscle activity can receive therapy. For instance, through repetition of a movement, ROM can often be maintained with muscles and joint structures (e.g. ligaments) repeatedly stretched, ultimately maintaining their physiological length. Such movement reduces contractures at joints, which can finally be very useful to caregivers making a notable difference to the ease of transfers (e.g. sitting in a wheelchair to lying in bed). Examples of devices using passive training are Innowalk [ 114 ] and Intellistretch [ 115 ].
In the active training mode, the patient’s muscle can still generate activity on the affected limb. The robot does not help, making the patients perform the exercise by themselves at least partially. The active mode provides data concerning torques and the ROM produced, allowing assessments before and after therapy/surgery. For instance, Kinarm [ 116 ] and Lokomat [ 117 ] are devices that can perform active training.
For assistive or active-assistive training, the muscles of the affected body part can still be activated. Therefore, the patient can at least partially perform the exercise or movement without the robot. The assistance will be triggered after a particular event is detected through an HCI, allowing the patients to move further with the robot’s help. Assistive training is relevant as it involves the active participation of the children. Moreover, it improves the physiological responses needed to maintain and increase muscle strength and length, ultimately leading to improved ROM, in which the muscles provide some of the torque required. Due to these advantages, many designers have produced devices that use this training mode. Examples are Pedianklebot [ 118 ] and the wrist-robot [ 119 ].
As the name implies, the robot applies a force opposing the desired movement in resistive training, making the task more challenging. Resistive training is used to enhance muscle strength in the treated limb. This type of training was employed in the ankle device developed by the University of Arizona [ 120 ] and the upper limb end-effector NJIT-RAVR [ 121 ].
Human–computer interface (HCI)
The term HCI refers to methodologies to identify the user’s intent to move in the desired direction from different input sources and translate this intention into a command for the robot to move to facilitate the appropriate actions [ 122 ]. The designers who report upon the use of an HCI have primarily developed assistive training. Two main types of HCI inputs were identified: those associated with physical interactions and physiological signals [ 123 ]. In this aspect, the devices can rely upon only one signal as the input source or use two or more signals as input to start the desired movement.
The main physical interactions used on HCIs to control such robots are Impedance/admittance, body-powered control, and gait phases detectors. Impedance and admittance control are the two most commonly used HCI. They are based on the relation between position and force rather than controlling either force or position explicitly. Impedance control accepts position or velocity as the input and outputs force or torque, and admittance is the opposite of impedance. Hence, force or torque are inputs, and velocity or position the outputs. This method could provide a natural, comfortable, and safe touch interface [ 122 ]. Some examples of devices that used this HCI are the NJIT-RAVR [ 124 ] and Rehaptic [ 125 ] upper limb robots that employed admittance control or the robots for upper and lower limb Inmotion2 [ 126 ] and Pedianklebot [ 118 ] that applied impedance control.
When the children cannot generate an intention to move with the treated limb, body-powered control is applied. It consists of using the movement of a different body part as the trigger signal to initiate the rehabilitation robot. The main drawback of this approach is that it is hard to control many degrees of freedom due to the activation system’s simplicity. An example of this HCI is the Ekso robot, where the activation was made by moving one’s body weight laterally and then forward to trigger the assistance [ 127 ].
In assisted gait, a favoured approach for HCI is the use of gait phase detection. This technique identifies the different gait phases (heel strike, midstance, toe-off, and the swing phase) to apply forces to assist the children’s movement depending on the gait phase. Robots usually perform gait segmentation using inertial measurement units (IMUs) to detect angular velocities of the shank and/or the thigh, or footswitches to detect the foot’s ground reaction forces while the child is walking [ 128 ]. The main advantage is that splitting the gait cycle into discrete phases provides enhanced consistency and robustness to an inherently variable process and allows lower-level controllers’ implementation within each phase. The problem is that gait detection should be characterised for every target group, as the physical disability modifies the gait pattern [ 100 ]. An example of this type of system is the P.REX exoskeleton which utilised a combination of the footswitch and IMUs to detect the different gait phases to provide different levels of assistance within each phase [ 100 ].
Alternatively, for HCI based on physiological signals, Electromyograms (EMG) that measure electrical activity in the muscles and electroencephalograms (EEG), which measure electrical activity in the brain, are the main signals used. They are widely utilised because they can be obtained using non-invasive techniques without the need for medical intervention.
Concerning electromyograms, the primary type is surface electromyography (sEMG), a non-invasive and easy-to-configure procedure in which adhesive electrodes are placed on the skin above the muscle of interest. The benefit of using the EMG signal is that it allows detection of the user’s intent before the movement occurs. The electrical activity can be detected even if it is insufficient to generate movement of a joint. However, sEMG can suffer from contamination of the signal by electromagnetic interference, skin perspiration, movement of electrodes and crosstalk artefacts. Also, for each muscle group of interest, a single EMG channel only shows the activation of that group. So, to perform an activity where many muscles fibres are recruited, it is necessary to use multichannel sEMG. Some examples of this technology in paediatric rehabilitation robots are the lower limb exoskeleton HAL [ 129 ] and the device for upper elbow rehabilitation of the San Juan National University [ 130 ].
The electroencephalogram (EEG) signal is recorded using many small surface electrodes, often configured in a bathing like cap placed over the scalp that detects the underlying electrical signals. The main advantage of the EEG signal is that the physical disability level does not limit it. Even if the patient has lost all their ability to move the limb required for a task, the brain activity thought to be related to the intent to activate the muscles can be recorded. There are two main disadvantages to this system. Firstly, it is unsuitable for children with brain damage as they cannot generate standard brain patterns for limb activation. Secondly, the EEG signal has greater variability within it than the EMG signal, and it is also easily affected by changes in the patient’s mood and attention. Examples of the EEG signal use are the CP walker that used this signal as a part of its HCI to help children with a physical disability move their legs [ 120 ] and the Exohand-2 that used the EEG signal to interact with the exoskeleton [ 131 ].
Treated conditions
The majority of studies and devices were for children with neurological conditions (np = 183, 89%), CP being the most studied condition (np = 129, 63%). In contrast, other neurological disorders included ABI and strokes. Significantly few researchers investigated other conditions such as neuromuscular diseases (np = 15, 8%) and traumatic injuries to limbs and the spine (np = 6, 3%). The results obtained from the studies that perform clinical trials or a feasibility study suggests that robotic rehabilitation could benefit children with physical disabilities.
Neurological disorders
Concerning CP, there was evidence of improvement in physical disability using assisted rehabilitation robots. The benefits include an increase in muscle activity [ 120 , 132 ], endurance for physical activities [ 133 , 134 ], improvements of balance [ 114 ], walking speed [ 134 , 135 ], the strength of the muscles [ 136 , 137 ], ROM of the joints [ 84 , 138 ], upper limb kinematics [ 139 ], and manual dexterity [ 33 , 140 ].
For paediatric ABI, there were reports in the improvement of the walking ability [ 141 ], improvement of the lower limb motor performance [ 94 ], increase in the ROM of the wrist joint and force increase in the hand [ 142 ], improvement in motor function, and gait pattern [ 143 , 144 ].
In children who suffer a stroke, three studies used rehabilitation robots while performing physical therapy. Marini et al. [ 119 ] demonstrated an improvement in wrist motion after the robotic therapy, and Bützer et al. [ 26 ] showed the possibility of using a wearable hand exoskeleton to assist children during task-oriented training could be helpful for rehabilitation therapies or assist children during ADLs.
Neuromuscular diseases
The neuromuscular disease presented a different scenario than neurological disorder due to the degeneration of their muscles as the disease progresses, making hard the use of rehabilitation robot due to stiffness in the robot’s joints, which can harm children’s weak muscles. Hence they require compliant actuation [ 145 , 146 ]. Jansen et al. [ 145 ] found that robotic rehabilitation therapy on upper and lower limbs help prevent functional deterioration in children with DMD. Meanwhile, Ganguly et al. [ 147 ], Garcia et al. [ 148 ] and Sanz-Merodio et al. [ 146 ] showed an improvement in walking ability in children with SMA with the assistance of ATLAS, and the exoskeleton was designed to provide Robotic-assisted gait training for children with SMA. Moreover, Koo et al. [ 149 ] reported improved arm mobility in children with DMD while using a robotic arm device.
Traumatic injuries
Even if traumatic injuries are common in the paediatric population, robot rehabilitation has not been applied widely in injuries that differ from those at the head. Only scarce information was found related to these conditions. A study of hands robotic rehabilitation was found, highlighting the possibility of using robotic devices to treat burns [ 150 ]. Additionally, a study observed a significant improvement in the arm movement and elbowed angle after physical therapy using an upper-limb exoskeleton for 3 months in children that suffer a car accident [ 130 ]. Finally, another case reported improving walking ability after robotic-assisted gait training in a girl with SCI [ 151 ].
It is possible to see that various novel rehabilitation robots have become available to rehabilitation professionals and clients in recent years. And this trend will continue as is possible to incorporate them in activity programs aimed at improving independent function [ 34 – 37 ] where they offer advantages over the traditional rehabilitation therapies, as they reduced the required effort of therapists during the exercises of the therapies, allowed massed practice in children with substantial limitations and provide information of the patient. Furthermore, they have the potential to be used as assistive devices to aid functional performance for users when they are worn. These possibilities will lead to a new variety of ways for assessment and intervention impacting users’ abilities, task demands, or the environment to promote functional performance and participation.
The findings of this review indicate that the design and development of robotic technologies for the physical rehabilitation of children is in a preliminary stage of development, as many of the devices were designed for adult patients. However, there is a trend toward creating robots specifically for children [ 17 , 26 , 57 , 148 ]. Yet less has been done to prove the benefits and constraints of such a system.
Traditionally, rehabilitation robot designers have focused solely on improving physical function [ 58 ], which can lead to rejection of the devices as not all the needs of children with disabilities are considered. Thus, to ensure successful adoption of the technology, the rehabilitation robots should cover these needs of the children. Hence, stakeholders’ cooperation is essential through their integration within the design and production process by providing feedback. Designers can use this feedback to validate that the robot meets the stakeholder’s needs. However, the fulfilment of these needs has strong relationships to the chosen technology, mainly the type of robot, the actuator, the training strategy and the HCI. Thus, it is essential to know the advantages and disadvantages of the technology.
Type of robot
When it comes to the type of robot, we can see a trend to migrate from end-effector to exoskeletons. However, most of the devices that had performed clinical trials were end-effector robots designed for adults. This relation could be because the end-effector robot works on the distal part of the limb, guiding the children limb through a movement [ 152 ]. This property is helpful in the case of operability as it does not require adaptation to match the children limb’s size, making it easy to be used by a diverse group of children. Furthermore, the bulky frames over the patient limbs are avoided, helping to reduce the weight that the children need to handle. These advantages come with the problem of the systems requiring bulky and heavy external structures, reducing the device’s portability, constraining its use to medical facilities or specific spaces inside a building. Thus, limiting the amount of therapy that the children can have [ 153 ]. Additionally, the activity is restricted to a workspace constraining the number of possible movements [ 40 ], which could reduce motivation.
On the other hand, exoskeletons work in parallel to the patient limb to perform the activity. Hence, they can be portable devices with the possibility to provide assistive help during activities of ADLs and robotic-assisted rehabilitation therapy in a single device [ 26 ]. This advantage will help to provide free movement to enhance the subject’s motivation and autonomously practise their movement training for longer periods [ 17 , 154 ]. Furthermore, as technology advance, this freedom in mobility will help to increase the participation of children with physical disabilities in different social activities [ 155 ]. However, as the technology moves from clinical facilities to open spaces and robots interact more closely with the children, designers will face notable challenges (e.g. the irregularities of the surfaces on which one walks and how the robot reacts to perturbations outdoors environment). Consequently, the requirements of weight, comfort, safety, portability and social acceptability for the exoskeletons will be harder to achieve.
The paediatric robotic rehabilitation technology is moving from end-effectors to exoskeletons due to their versatility to be used as a rehabilitation tool or an assistive device [ 26 ]. Consequently, actuation technology starts to be a critical part of the design as it negatively influences the weight and the size of the robot.
For the end-effectors robots, actuators are not as critical as with the exoskeletons because they could be placed in external structures. This advantage makes it possible to use bulky and heavy actuators like electric motors. However, using electric motors is hard to achieve compliance that is an important property to increase safety as it is needed to avoid opposing forces that can injure the children. In end-effector robots, compliance was achieved using sensors and a control strategy [ 146 ] or using a soft material like the Bowden cables [ 103 ].
On the other hand, for exoskeletons, the robot design requirements are hard to enhance with the currently used technology. The actuation system components such as motor and rigid elements are designed for industrial applications not to interact with and to be worn by children. However, they are still the standard as they have the advantages of efficiency, are easy to control, and are readably available in the market. Therefore, the choice of the actuation system is crucial to improve the weight, portability and safety of the exoskeleton.
The first exoskeletons relied on electric motors with rigid transmissions, making them bulky and heavy, reducing their compliance as they generate high resistive torque from the metallic links of the exoskeleton. Therefore, making it difficult to move and less safe can cause non-desirable inertial movements [ 156 , 157 ]. Furthermore, they require external structures to manage the weight of the exoskeleton.
As the rehabilitation robots move from rehabilitation therapies inside a medical facility to assist the children during ADLs, new actuation technology is needed. This challenge led to using SEA actuators and cable transmission since they have the advantage of been intrinsically complaints as they incorporate soft materials, making the device safer.
Using Bowden cables in the transmissions brings other advantages like simple architecture, low weight on the limb’s distal part, and easy to reconfigure. This last advantage is significant in paediatric rehabilitation as it allows to change the motor easily depending on the abilities and size of the children [ 26 , 73 ], even though they present some disadvantages because they become unidirectional and difficult to model and control. Instead, in the case of SEAs, which still require rigid links, they were highlighted on the use for children with neuromuscular diseases, as some children are not only weak on the affected joint but the entire body. Thus the exoskeleton must hold the children, but at the same time being compliant to avoid inertial forces that can harm the weak muscles of the children [ 146 ].
Another type of actuator used on the robots was the pneumatic. Their attributes of low weight and easy-to-manufacture actuators of different shapes and sizes [ 158 , 159 ] make them a desirable technology in this field. They are easy to adapt to children with various conditions. However, their main constraint is that they are typically connected to external mechanisms like compressors and pumps cumbersome and noisy. Thus, reducing their portability and appealing making them impractical to use outside clinical facilities.
In Table Table8, 8 , the advantages and disadvantages of the current actuator technology are presented. It is possible to notice that there is no perfect actuator technology, so more research in this area is needed. Moreover, in the future will be interesting to see devices that use different soft actuators technologies that are inherent compliant and lightweight, such as the already mention SEAs, pneumatic, and Bowden cables. But also new technologies that are under research to be used on rehabilitation robots, like shape memory alloys [ 160 ], dielectric elastomer [ 161 ], or twisted and coiled polymers actuators [ 162 ], as they will reduce the overall weight and increase the compliance. Furthermore, this new technology can be manufactured in different sizes and shapes [ 163 ] that could be easily adapted to robots for children of varying height and ability conditions.
General summary of advantages and drawbacks of each actuation technology
In the case of the training strategy, there is no best strategy, but it rather depends on many factors like the abilities and disease that the children have. For example, passive training is suitable for patients with limited mobility; however, when the children are able to generate movements, it tends to decrease children’s participation during the exercise, thus reducing the efficiency of the training [ 164 ]. That is why most of the research on the training strategy is centred on assistive training, where the children’s participation is needed. This engagement with the therapy increases the motivation of the children to perform the activities, enhancing the benefits from the therapy [ 165 ]. Another advantage of assistive training is that it is used together with video games to increase children’s motivation and social interaction [ 166 ]. In addition, this strategy is required for assistive devices. It needs to provide the required intensity to generate the movement safely, efficiently, and reliably, depending on the applied force by the user [ 167 ]. However, there is no clear which is the best strategy to provide assistive movement, where some examples of different assistive strategies are guidance force, path control, and locomotion strategy [ 57 , 154 , 165 , 166 ].
Alternatively, some researchers suggest that resistive training could be more beneficial for rehabilitation therapy than an assistive force, as it increases the engagement of the children, which can help drive motor learning [ 120 , 168 ]. Hence, further research is required on the optimal training strategy to increase the benefits from the rehabilitation therapies.
Human–computer interface
The HCIs are essential in developing robotic rehabilitation robots, as they are the medium for the interaction between the children and the robot, impacting the functioning of the actuators and training strategies directly. Thus, HICs are a crucial factor for safety and motivation needs, as it is how the children “communicate” their intention to the robot. Consequently, If the HCI is complex to use, it could lead to the rejection of the device [ 52 ]. Furthermore, for safety reasons, the HCI must detect the trigger signal properly and discern between intended movements and involuntary movements, as it can generate undesirable responses [ 41 , 169 ]. For instance, in the case of CP patients presenting increased muscle tone, rapidly occurring muscle spasms, and severe jerks, which can be considered as a deviation of pattern, causing the robot to apply undesired forces to correct for it or turn off the device suddenly [ 170 ].
Consequently, selecting the best HCI for every case needs to be evaluated depending on the capabilities of the children. For example, it could be challenging for patients with advanced muscular dystrophy to use EMG and admittance/impedance interfaces as their muscles progressively deteriorate, turning unable to activate the muscles to generate a movement or a detectable signal [ 149 , 171 ]. On the other hand, EEG could lead to a better motor function recovery for children with CP as it integrates the central nervous system into rehabilitation therapy [ 154 ]. However, this technology could be hard to implement in patients with a cognitive deficit, requiring concentration [ 131 , 172 ]. For HCIs, it would be interesting to see more devices using physiological signals as they can also evaluate the efficiency of the therapy [ 154 ], novel approaches of body-powered control to address children with limited mobility of limbs [ 173 ], and devices that integrate different HCI strategies to make the system more robust and adaptable [ 71 , 167 , 174 ].
Treated condition
To better understand how the technology can positively impact children’s lives, it is essential to analyse how the rehabilitation robot’s technology has addressed the different paediatric conditions that can generate physical disability. Because, even if they share in common the deterioration of the musculoskeletal system, each one of the conditions presents certain specific characteristics that need to be considered.
Most of the research has focused on children with neurological conditions, particularly children with CP. However, it seems likely that many of the designed robots that currently work with neurological conditions could also be utilised in traumatic injury scenarios, especially because they have been designed for rehabilitation therapies that can improve common problems across both neurological and traumatic injuries like ROM deficits and a lack of ability to generate muscle force [ 80 , 150 ]. Contrarily, neuromuscular diseases present a different scenario than neurological disorders and traumatic injuries as the diseases are progressive, making it hard to obtain a permanent improvement on the children skills. Thus, the designs have been focused on design devices capable of assisting with exercise and helping with the ADLs to maintain specific abilities (e.g. walking) for a longer period of time [ 122 , 155 ].
Unfortunately, the outcomes proving the efficiency of rehabilitation robot in children is still scarce, as the pieces of evidence are low and weak. Hence, the information coming from these studies should be asses carefully, as there are very few randomised controlled trials, with small sample sizes and variability in children’s ability, outcomes measures, treatment protocols, and used devices [ 31 , 65 ]. Thus, to better understand if the designed robots fulfil the paediatric needs properly by improving their quality of life and physical ability, more studies and robots designed especially for them are needed. Furthermore, more studies with children presenting different conditions from neurological ones are needed, as it can be noticed that the treated condition impacts the requirements design of the rehabilitation robot.
Limitations
It was apparent that some studies were conducted with participants from a wide range of ages; therefore, it was difficult to target all the articles that include paediatric participants. Another problem was the upper bound on the paediatric population’s age as some papers with the term young adults included paediatric participants.
While robotic rehabilitation is gaining momentum with increasing numbers of devices being produced for adults, there is a lack of well-designed and effective products available for children. Early examples of robots have often been created by scaling downsize to meet the smaller stature of children. Few robots have been specifically designed and produced, with children being the focus of the project/program. It is apparent that children have special needs, and these need to be incorporated into designs early in the development program. And even if the fulfilment of these needs is closely related to the chosen structural and technological components like the actuator, training strategy and HCI, they go beyond them. Consideration must also be given to the aesthetics that appeal to children and the need for the robot’s structure to be as unobtrusive as possible. Without such needs being met, no matter how effective the robot works from an engineering perspective, it will not be utilised well by the child.
It is apparent that there is still a lack of understanding of what the most effective therapy is to improve function and quality of life in specific paediatric conditions (e.g. CP or Stroke). Nevertheless, common impairments (e.g. ROM, strength) must be addressed across numerous clinical conditions if patients improve function in everyday tasks. Hence, there is much opportunity for robots to play a role in assisting paediatric rehabilitation. A much more difficult goal to achieve is the development of robots to assist children. At the same time, they do function-related tasks like walking, sitting, lying, and assisting when the child moves from one posture to another. This demand increased complexity throughout the various engineering systems of the robot. After that, a further challenge lies in the robot being able to assist indoors within a relatively safe environment and outdoors where the “lay of the land” is notably different and less predictable. Hence, exploring new technologies to actuate the system and detect children’s intentions when they want to move is necessary.
Acknowledgements
Not applicable
Abbreviations
Authors' contributions.
AG reviewed the literature, wrote the manuscript, and prepared the illustrations. LG, JK, PM contributed to the basic concept of the paper and critically revised the draft paper. All authors read and approved the manuscript.
Availability of data and materials
Declarations.
The authors declare that they have no competing interests.
Publisher's Note
Springer Nature remains neutral with regard to jurisdictional claims in published maps and institutional affiliations.
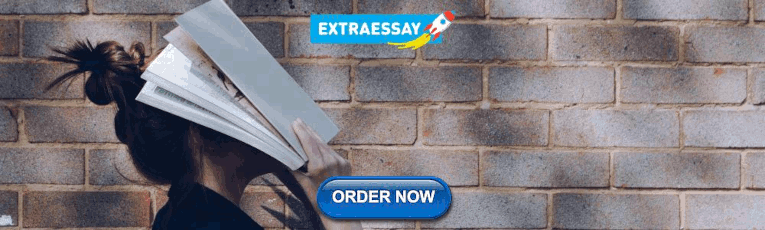
IMAGES
VIDEO
COMMENTS
This review paper outlines the effort devoted to the development and integration of robotic technology for rehabilitation. ... The use of robots in rehabilitation has since grown substantially ... et al. Rehabilitation of arm function after stroke. Literature review. Annals of Physical and Rehabilitation Medicine. 2009; 52 (3):269-293 ...
Its feasibility has been evaluated through several studies using state-of-the-art home robots. The literature surveys indicated the feasibility of self-administered treatment at home using rehabilitation robots in terms of functional outputs, training duration, user acceptance, motivation, and safety concerns.
The literature include cognitive training robots in the forms of companion robots, social robots, assistive robots, social assisted robots, or service robots, which are collectively referred to as "cognitive training robots" in this systematic review. The literature employed different terminologies for cognitive training by rehabilitation ...
Purpose of Review Research in assistive and rehabilitation robotics is a growing, promising, and challenging field emerged due to various social and medical needs such as aging populations, neuromuscular, and musculoskeletal disorders. Such robots can be used in various day-to-day scenarios or to support motor functionality, training, and rehabilitation. This paper reflects on the human-robot ...
This paper gives an overall review of research status in rehabilitation robot technology. In order to study the status of rehabilitation robot technology, they are divided into two categories. One of them is auxiliary and replacement robots and another is training and therapeutic robots. The fixed and mobile robots, intelligent artificial limbs and supporting tools of the auxiliary and ...
This review article presents an in-depth examination of research and development in the fields of rehabilitation, assistive technologies, and humanoid robots. It focuses on parallel robots designed for human body joints with three degrees of freedom, specifically the neck, shoulder, wrist, hip, and ankle. A systematic search was conducted across multiple databases, including Scopus, Web of ...
Robot-assisted rehabilitation is an exciting field which aims to incorporate relevant developments in robotics related to rehabilitation with the intention of defining new methodologies for intervening problems related to muscular, neuromuscular, and osseous diseases. In this study, a systematic and comprehensive literature analysis is conducted to identify the contribution of artificial ...
A comprehensive literature review on rehabilitation robots is carried out to identify the key issues. The main design requirements and development complications are identified and the various approaches used in past robots are reviewed. It begins with a survey of existing human rehabilitation devices designed for use in human assistance and ...
The innovations in the development of the next-generation rehabilitation robots can lead to significant benefits to human beings. In this paper, a critical literature review is conducted to identify the limitations of existing works and clarify the prosperous research directions in the development of assistive robots.
Rehabilitation is the process of treating post-stroke consequences. Impaired limbs are considered the common outcomes of stroke, which require a professional therapist to rehabilitate the impaired limbs and restore fully or partially its function. Due to the shortage in the number of therapists and other considerations, researchers have been working on developing robots that have the ability ...
Rehabilitation robots can be further divided into exoskeletons and orthoses [Citation 12, Citation 13]. In general, both exoskeletons and orthoses refer to external devices worn on the body to provide support, assistance, or rehabilitation, but they have distinct characteristics. ... (PRR) through systematic literature review, and it uses a ...
Various conditions, including traffic accidents, sports injuries, and neurological disorders, can impair human wrist movements, underscoring the importance of effective rehabilitation methods. Robotic devices play a crucial role in this regard, particularly in wrist rehabilitation, given the complexity of the human wrist joint, which encompasses three degrees of freedom: flexion/extension ...
Robot-mediated neurorehabilitation has the potential to improve clinical outcomes of rehabilitation treatments. A statistical analysis of the literature aims to focus on the main trend of this topic. Areas covered: A bibliometric survey on post-stroke robotic rehabilitation was performed through a database collection of scientific publications ...
As interdisciplinary researchers with backgrounds in the social sciences and sciences, we wish to pursue the literature review through the lens of social science and interdisciplinary studies, rather than the dominant science-based field of robotics and rehabilitation. 1.2.2 Robot and Rehabilitation in Social Science Research
In this paper, a comprehensive review on developed home-based rehabilitation technologies of the last 10 years (2011-2020), categorizing them into upper and lower limb devices and considering both commercialized and state-of-the-art realms. ... Its feasibility has been evaluated through several studies using state-of-the-art home robots. The ...
This paper presents a survey of existing robotic systems for lower-limb rehabilitation. It is a general assumption that robotics will play an important role in therapy activities within rehabilitation treatment. In the last decade, the interest in the field has grown exponentially mainly due to the initial success of the early systems and the growing demand caused by increasing numbers of ...
Lower limb rehabilitation exoskeleton robots integrate sensing, control, and other technologies and exhibit the characteristics of bionics, robotics, information and control science, medicine, and other interdisciplinary areas. In this review, the typical products and prototypes of lower limb exoskeleton rehabilitation robots are introduced and state-of-the-art techniques are analyzed and ...
Rehabilitation robots can be further divided into exoskeletons and orthoses [12,13]. In general, both exoskeletons and orthoses refer to external devices worn on the body to provide support, assistance, or rehabilitation, but they have distinct characteristics. ... (PRR) through systematic literature review, and it uses a meta-analysis method ...
Neuromotor rehabilitation and improvement of upper limb functions are necessary to improve the life quality of patients who have experienced injuries or have pathological outcomes. Modern approaches, such as robotic-assisted rehabilitation can help to improve rehabilitation processes and thus improve upper limb functions. Therefore, the aim of this study was to investigate the role of robots ...
Among the methods of hand function rehabilitation after stroke, robot-assisted rehabilitation is widely used, and the use of hand rehabilitation robots can provide functional training of the hand or assist the paralyzed hand with activities of daily living. However, patients with hand disorders consistently report that the needs of some users are not being met. The purpose of this review is to ...
A large and increasing number of people around the world experience cognitive disability. Rehabilitation robotics has provided promising training and assistance approaches to mitigate cognitive deficits. In this article, we carried out a systematic review on recent developments in robot-assisted cognitive training. We included 99 articles in this work and described their applications, enabling ...
Conclusions: This review highlights the potential of AI robots to transform ICU care by improving patient treatment, support, and rehabilitation processes. However, it also recognizes the ethical complexities and operational challenges that come with their implementation, offering possible solutions for future development and optimization.
Abstract. In order to address the problem of functional rehabilitation after ankle fracture surgery, this paper presented a novel ankle fracture rehabilitation robot. The robot adopted R-3RRS-P hybrid structure, which was simple in structure and had two working modes: rehabilitation training and motion axis switching. Compared with the existing ankle rehabilitation robot, the proposed robot ...
The assessment of rehabilitation robot safety is a vital aspect of the development process, which is often experienced as difficult. Safety of rehabilitation robot use in clinical trials, including the monitoring and reporting of adverse events (Mehrholz et al., 2017; Mehrholz et al., 2018), is one important aspect.
A systematic review on rehabilitation after ACL reconstruction in 2016 concluded that early weight-bearing reduced anterior knee pain and did not increase the risk of tunnel widening . According to the rehabilitation guidelines of the Multicentre Orthopaedic Outcomes Network (MOON) group, postoperative early weight-bearing is recommended ...
The findings of this review indicate that the design and development of robotic technologies for the physical rehabilitation of children is in a preliminary stage of development, as many of the devices were designed for adult patients. However, there is a trend toward creating robots specifically for children [ 17, 26, 57, 148 ].
As important auxiliary equipment, rehabilitation robots are widely used in rehabilitation treatment and daily life assistance. The rehabilitation robot proposed in this paper is mainly composed of an omnidirectional mobile platform module, a lower limb exoskeleton module, and a support module. According to the characteristics of the robot's omnidirectional mobility and good stiffness, the ...