- Search Menu
- Browse content in Arts and Humanities
- Browse content in Archaeology
- Anglo-Saxon and Medieval Archaeology
- Archaeological Methodology and Techniques
- Archaeology by Region
- Archaeology of Religion
- Archaeology of Trade and Exchange
- Biblical Archaeology
- Contemporary and Public Archaeology
- Environmental Archaeology
- Historical Archaeology
- History and Theory of Archaeology
- Industrial Archaeology
- Landscape Archaeology
- Mortuary Archaeology
- Prehistoric Archaeology
- Underwater Archaeology
- Urban Archaeology
- Zooarchaeology
- Browse content in Architecture
- Architectural Structure and Design
- History of Architecture
- Residential and Domestic Buildings
- Theory of Architecture
- Browse content in Art
- Art Subjects and Themes
- History of Art
- Industrial and Commercial Art
- Theory of Art
- Biographical Studies
- Byzantine Studies
- Browse content in Classical Studies
- Classical History
- Classical Philosophy
- Classical Mythology
- Classical Literature
- Classical Reception
- Classical Art and Architecture
- Classical Oratory and Rhetoric
- Greek and Roman Epigraphy
- Greek and Roman Law
- Greek and Roman Papyrology
- Greek and Roman Archaeology
- Late Antiquity
- Religion in the Ancient World
- Digital Humanities
- Browse content in History
- Colonialism and Imperialism
- Diplomatic History
- Environmental History
- Genealogy, Heraldry, Names, and Honours
- Genocide and Ethnic Cleansing
- Historical Geography
- History by Period
- History of Emotions
- History of Agriculture
- History of Education
- History of Gender and Sexuality
- Industrial History
- Intellectual History
- International History
- Labour History
- Legal and Constitutional History
- Local and Family History
- Maritime History
- Military History
- National Liberation and Post-Colonialism
- Oral History
- Political History
- Public History
- Regional and National History
- Revolutions and Rebellions
- Slavery and Abolition of Slavery
- Social and Cultural History
- Theory, Methods, and Historiography
- Urban History
- World History
- Browse content in Language Teaching and Learning
- Language Learning (Specific Skills)
- Language Teaching Theory and Methods
- Browse content in Linguistics
- Applied Linguistics
- Cognitive Linguistics
- Computational Linguistics
- Forensic Linguistics
- Grammar, Syntax and Morphology
- Historical and Diachronic Linguistics
- History of English
- Language Acquisition
- Language Evolution
- Language Reference
- Language Variation
- Language Families
- Lexicography
- Linguistic Anthropology
- Linguistic Theories
- Linguistic Typology
- Phonetics and Phonology
- Psycholinguistics
- Sociolinguistics
- Translation and Interpretation
- Writing Systems
- Browse content in Literature
- Bibliography
- Children's Literature Studies
- Literary Studies (Asian)
- Literary Studies (European)
- Literary Studies (Eco-criticism)
- Literary Studies (Romanticism)
- Literary Studies (American)
- Literary Studies (Modernism)
- Literary Studies - World
- Literary Studies (1500 to 1800)
- Literary Studies (19th Century)
- Literary Studies (20th Century onwards)
- Literary Studies (African American Literature)
- Literary Studies (British and Irish)
- Literary Studies (Early and Medieval)
- Literary Studies (Fiction, Novelists, and Prose Writers)
- Literary Studies (Gender Studies)
- Literary Studies (Graphic Novels)
- Literary Studies (History of the Book)
- Literary Studies (Plays and Playwrights)
- Literary Studies (Poetry and Poets)
- Literary Studies (Postcolonial Literature)
- Literary Studies (Queer Studies)
- Literary Studies (Science Fiction)
- Literary Studies (Travel Literature)
- Literary Studies (War Literature)
- Literary Studies (Women's Writing)
- Literary Theory and Cultural Studies
- Mythology and Folklore
- Shakespeare Studies and Criticism
- Browse content in Media Studies
- Browse content in Music
- Applied Music
- Dance and Music
- Ethics in Music
- Ethnomusicology
- Gender and Sexuality in Music
- Medicine and Music
- Music Cultures
- Music and Religion
- Music and Media
- Music and Culture
- Music Education and Pedagogy
- Music Theory and Analysis
- Musical Scores, Lyrics, and Libretti
- Musical Structures, Styles, and Techniques
- Musicology and Music History
- Performance Practice and Studies
- Race and Ethnicity in Music
- Sound Studies
- Browse content in Performing Arts
- Browse content in Philosophy
- Aesthetics and Philosophy of Art
- Epistemology
- Feminist Philosophy
- History of Western Philosophy
- Metaphysics
- Moral Philosophy
- Non-Western Philosophy
- Philosophy of Science
- Philosophy of Language
- Philosophy of Mind
- Philosophy of Perception
- Philosophy of Action
- Philosophy of Law
- Philosophy of Religion
- Philosophy of Mathematics and Logic
- Practical Ethics
- Social and Political Philosophy
- Browse content in Religion
- Biblical Studies
- Christianity
- East Asian Religions
- History of Religion
- Judaism and Jewish Studies
- Qumran Studies
- Religion and Education
- Religion and Health
- Religion and Politics
- Religion and Science
- Religion and Law
- Religion and Art, Literature, and Music
- Religious Studies
- Browse content in Society and Culture
- Cookery, Food, and Drink
- Cultural Studies
- Customs and Traditions
- Ethical Issues and Debates
- Hobbies, Games, Arts and Crafts
- Lifestyle, Home, and Garden
- Natural world, Country Life, and Pets
- Popular Beliefs and Controversial Knowledge
- Sports and Outdoor Recreation
- Technology and Society
- Travel and Holiday
- Visual Culture
- Browse content in Law
- Arbitration
- Browse content in Company and Commercial Law
- Commercial Law
- Company Law
- Browse content in Comparative Law
- Systems of Law
- Competition Law
- Browse content in Constitutional and Administrative Law
- Government Powers
- Judicial Review
- Local Government Law
- Military and Defence Law
- Parliamentary and Legislative Practice
- Construction Law
- Contract Law
- Browse content in Criminal Law
- Criminal Procedure
- Criminal Evidence Law
- Sentencing and Punishment
- Employment and Labour Law
- Environment and Energy Law
- Browse content in Financial Law
- Banking Law
- Insolvency Law
- History of Law
- Human Rights and Immigration
- Intellectual Property Law
- Browse content in International Law
- Private International Law and Conflict of Laws
- Public International Law
- IT and Communications Law
- Jurisprudence and Philosophy of Law
- Law and Politics
- Law and Society
- Browse content in Legal System and Practice
- Courts and Procedure
- Legal Skills and Practice
- Primary Sources of Law
- Regulation of Legal Profession
- Medical and Healthcare Law
- Browse content in Policing
- Criminal Investigation and Detection
- Police and Security Services
- Police Procedure and Law
- Police Regional Planning
- Browse content in Property Law
- Personal Property Law
- Study and Revision
- Terrorism and National Security Law
- Browse content in Trusts Law
- Wills and Probate or Succession
- Browse content in Medicine and Health
- Browse content in Allied Health Professions
- Arts Therapies
- Clinical Science
- Dietetics and Nutrition
- Occupational Therapy
- Operating Department Practice
- Physiotherapy
- Radiography
- Speech and Language Therapy
- Browse content in Anaesthetics
- General Anaesthesia
- Neuroanaesthesia
- Browse content in Clinical Medicine
- Acute Medicine
- Cardiovascular Medicine
- Clinical Genetics
- Clinical Pharmacology and Therapeutics
- Dermatology
- Endocrinology and Diabetes
- Gastroenterology
- Genito-urinary Medicine
- Geriatric Medicine
- Infectious Diseases
- Medical Toxicology
- Medical Oncology
- Pain Medicine
- Palliative Medicine
- Rehabilitation Medicine
- Respiratory Medicine and Pulmonology
- Rheumatology
- Sleep Medicine
- Sports and Exercise Medicine
- Clinical Neuroscience
- Community Medical Services
- Critical Care
- Emergency Medicine
- Forensic Medicine
- Haematology
- History of Medicine
- Browse content in Medical Dentistry
- Oral and Maxillofacial Surgery
- Paediatric Dentistry
- Restorative Dentistry and Orthodontics
- Surgical Dentistry
- Browse content in Medical Skills
- Clinical Skills
- Communication Skills
- Nursing Skills
- Surgical Skills
- Medical Ethics
- Medical Statistics and Methodology
- Browse content in Neurology
- Clinical Neurophysiology
- Neuropathology
- Nursing Studies
- Browse content in Obstetrics and Gynaecology
- Gynaecology
- Occupational Medicine
- Ophthalmology
- Otolaryngology (ENT)
- Browse content in Paediatrics
- Neonatology
- Browse content in Pathology
- Chemical Pathology
- Clinical Cytogenetics and Molecular Genetics
- Histopathology
- Medical Microbiology and Virology
- Patient Education and Information
- Browse content in Pharmacology
- Psychopharmacology
- Browse content in Popular Health
- Caring for Others
- Complementary and Alternative Medicine
- Self-help and Personal Development
- Browse content in Preclinical Medicine
- Cell Biology
- Molecular Biology and Genetics
- Reproduction, Growth and Development
- Primary Care
- Professional Development in Medicine
- Browse content in Psychiatry
- Addiction Medicine
- Child and Adolescent Psychiatry
- Forensic Psychiatry
- Learning Disabilities
- Old Age Psychiatry
- Psychotherapy
- Browse content in Public Health and Epidemiology
- Epidemiology
- Public Health
- Browse content in Radiology
- Clinical Radiology
- Interventional Radiology
- Nuclear Medicine
- Radiation Oncology
- Reproductive Medicine
- Browse content in Surgery
- Cardiothoracic Surgery
- Gastro-intestinal and Colorectal Surgery
- General Surgery
- Neurosurgery
- Paediatric Surgery
- Peri-operative Care
- Plastic and Reconstructive Surgery
- Surgical Oncology
- Transplant Surgery
- Trauma and Orthopaedic Surgery
- Vascular Surgery
- Browse content in Science and Mathematics
- Browse content in Biological Sciences
- Aquatic Biology
- Biochemistry
- Bioinformatics and Computational Biology
- Developmental Biology
- Ecology and Conservation
- Evolutionary Biology
- Genetics and Genomics
- Microbiology
- Molecular and Cell Biology
- Natural History
- Plant Sciences and Forestry
- Research Methods in Life Sciences
- Structural Biology
- Systems Biology
- Zoology and Animal Sciences
- Browse content in Chemistry
- Analytical Chemistry
- Computational Chemistry
- Crystallography
- Environmental Chemistry
- Industrial Chemistry
- Inorganic Chemistry
- Materials Chemistry
- Medicinal Chemistry
- Mineralogy and Gems
- Organic Chemistry
- Physical Chemistry
- Polymer Chemistry
- Study and Communication Skills in Chemistry
- Theoretical Chemistry
- Browse content in Computer Science
- Artificial Intelligence
- Computer Architecture and Logic Design
- Game Studies
- Human-Computer Interaction
- Mathematical Theory of Computation
- Programming Languages
- Software Engineering
- Systems Analysis and Design
- Virtual Reality
- Browse content in Computing
- Business Applications
- Computer Security
- Computer Games
- Computer Networking and Communications
- Digital Lifestyle
- Graphical and Digital Media Applications
- Operating Systems
- Browse content in Earth Sciences and Geography
- Atmospheric Sciences
- Environmental Geography
- Geology and the Lithosphere
- Maps and Map-making
- Meteorology and Climatology
- Oceanography and Hydrology
- Palaeontology
- Physical Geography and Topography
- Regional Geography
- Soil Science
- Urban Geography
- Browse content in Engineering and Technology
- Agriculture and Farming
- Biological Engineering
- Civil Engineering, Surveying, and Building
- Electronics and Communications Engineering
- Energy Technology
- Engineering (General)
- Environmental Science, Engineering, and Technology
- History of Engineering and Technology
- Mechanical Engineering and Materials
- Technology of Industrial Chemistry
- Transport Technology and Trades
- Browse content in Environmental Science
- Applied Ecology (Environmental Science)
- Conservation of the Environment (Environmental Science)
- Environmental Sustainability
- Environmentalist Thought and Ideology (Environmental Science)
- Management of Land and Natural Resources (Environmental Science)
- Natural Disasters (Environmental Science)
- Nuclear Issues (Environmental Science)
- Pollution and Threats to the Environment (Environmental Science)
- Social Impact of Environmental Issues (Environmental Science)
- History of Science and Technology
- Browse content in Materials Science
- Ceramics and Glasses
- Composite Materials
- Metals, Alloying, and Corrosion
- Nanotechnology
- Browse content in Mathematics
- Applied Mathematics
- Biomathematics and Statistics
- History of Mathematics
- Mathematical Education
- Mathematical Finance
- Mathematical Analysis
- Numerical and Computational Mathematics
- Probability and Statistics
- Pure Mathematics
- Browse content in Neuroscience
- Cognition and Behavioural Neuroscience
- Development of the Nervous System
- Disorders of the Nervous System
- History of Neuroscience
- Invertebrate Neurobiology
- Molecular and Cellular Systems
- Neuroendocrinology and Autonomic Nervous System
- Neuroscientific Techniques
- Sensory and Motor Systems
- Browse content in Physics
- Astronomy and Astrophysics
- Atomic, Molecular, and Optical Physics
- Biological and Medical Physics
- Classical Mechanics
- Computational Physics
- Condensed Matter Physics
- Electromagnetism, Optics, and Acoustics
- History of Physics
- Mathematical and Statistical Physics
- Measurement Science
- Nuclear Physics
- Particles and Fields
- Plasma Physics
- Quantum Physics
- Relativity and Gravitation
- Semiconductor and Mesoscopic Physics
- Browse content in Psychology
- Affective Sciences
- Clinical Psychology
- Cognitive Psychology
- Cognitive Neuroscience
- Criminal and Forensic Psychology
- Developmental Psychology
- Educational Psychology
- Evolutionary Psychology
- Health Psychology
- History and Systems in Psychology
- Music Psychology
- Neuropsychology
- Organizational Psychology
- Psychological Assessment and Testing
- Psychology of Human-Technology Interaction
- Psychology Professional Development and Training
- Research Methods in Psychology
- Social Psychology
- Browse content in Social Sciences
- Browse content in Anthropology
- Anthropology of Religion
- Human Evolution
- Medical Anthropology
- Physical Anthropology
- Regional Anthropology
- Social and Cultural Anthropology
- Theory and Practice of Anthropology
- Browse content in Business and Management
- Business Strategy
- Business Ethics
- Business History
- Business and Government
- Business and Technology
- Business and the Environment
- Comparative Management
- Corporate Governance
- Corporate Social Responsibility
- Entrepreneurship
- Health Management
- Human Resource Management
- Industrial and Employment Relations
- Industry Studies
- Information and Communication Technologies
- International Business
- Knowledge Management
- Management and Management Techniques
- Operations Management
- Organizational Theory and Behaviour
- Pensions and Pension Management
- Public and Nonprofit Management
- Strategic Management
- Supply Chain Management
- Browse content in Criminology and Criminal Justice
- Criminal Justice
- Criminology
- Forms of Crime
- International and Comparative Criminology
- Youth Violence and Juvenile Justice
- Development Studies
- Browse content in Economics
- Agricultural, Environmental, and Natural Resource Economics
- Asian Economics
- Behavioural Finance
- Behavioural Economics and Neuroeconomics
- Econometrics and Mathematical Economics
- Economic Systems
- Economic History
- Economic Methodology
- Economic Development and Growth
- Financial Markets
- Financial Institutions and Services
- General Economics and Teaching
- Health, Education, and Welfare
- History of Economic Thought
- International Economics
- Labour and Demographic Economics
- Law and Economics
- Macroeconomics and Monetary Economics
- Microeconomics
- Public Economics
- Urban, Rural, and Regional Economics
- Welfare Economics
- Browse content in Education
- Adult Education and Continuous Learning
- Care and Counselling of Students
- Early Childhood and Elementary Education
- Educational Equipment and Technology
- Educational Strategies and Policy
- Higher and Further Education
- Organization and Management of Education
- Philosophy and Theory of Education
- Schools Studies
- Secondary Education
- Teaching of a Specific Subject
- Teaching of Specific Groups and Special Educational Needs
- Teaching Skills and Techniques
- Browse content in Environment
- Applied Ecology (Social Science)
- Climate Change
- Conservation of the Environment (Social Science)
- Environmentalist Thought and Ideology (Social Science)
- Natural Disasters (Environment)
- Social Impact of Environmental Issues (Social Science)
- Browse content in Human Geography
- Cultural Geography
- Economic Geography
- Political Geography
- Browse content in Interdisciplinary Studies
- Communication Studies
- Museums, Libraries, and Information Sciences
- Browse content in Politics
- African Politics
- Asian Politics
- Chinese Politics
- Comparative Politics
- Conflict Politics
- Elections and Electoral Studies
- Environmental Politics
- European Union
- Foreign Policy
- Gender and Politics
- Human Rights and Politics
- Indian Politics
- International Relations
- International Organization (Politics)
- International Political Economy
- Irish Politics
- Latin American Politics
- Middle Eastern Politics
- Political Methodology
- Political Communication
- Political Philosophy
- Political Sociology
- Political Behaviour
- Political Economy
- Political Institutions
- Political Theory
- Politics and Law
- Public Administration
- Public Policy
- Quantitative Political Methodology
- Regional Political Studies
- Russian Politics
- Security Studies
- State and Local Government
- UK Politics
- US Politics
- Browse content in Regional and Area Studies
- African Studies
- Asian Studies
- East Asian Studies
- Japanese Studies
- Latin American Studies
- Middle Eastern Studies
- Native American Studies
- Scottish Studies
- Browse content in Research and Information
- Research Methods
- Browse content in Social Work
- Addictions and Substance Misuse
- Adoption and Fostering
- Care of the Elderly
- Child and Adolescent Social Work
- Couple and Family Social Work
- Developmental and Physical Disabilities Social Work
- Direct Practice and Clinical Social Work
- Emergency Services
- Human Behaviour and the Social Environment
- International and Global Issues in Social Work
- Mental and Behavioural Health
- Social Justice and Human Rights
- Social Policy and Advocacy
- Social Work and Crime and Justice
- Social Work Macro Practice
- Social Work Practice Settings
- Social Work Research and Evidence-based Practice
- Welfare and Benefit Systems
- Browse content in Sociology
- Childhood Studies
- Community Development
- Comparative and Historical Sociology
- Economic Sociology
- Gender and Sexuality
- Gerontology and Ageing
- Health, Illness, and Medicine
- Marriage and the Family
- Migration Studies
- Occupations, Professions, and Work
- Organizations
- Population and Demography
- Race and Ethnicity
- Social Theory
- Social Movements and Social Change
- Social Research and Statistics
- Social Stratification, Inequality, and Mobility
- Sociology of Religion
- Sociology of Education
- Sport and Leisure
- Urban and Rural Studies
- Browse content in Warfare and Defence
- Defence Strategy, Planning, and Research
- Land Forces and Warfare
- Military Administration
- Military Life and Institutions
- Naval Forces and Warfare
- Other Warfare and Defence Issues
- Peace Studies and Conflict Resolution
- Weapons and Equipment

- < Previous chapter
- Next chapter >
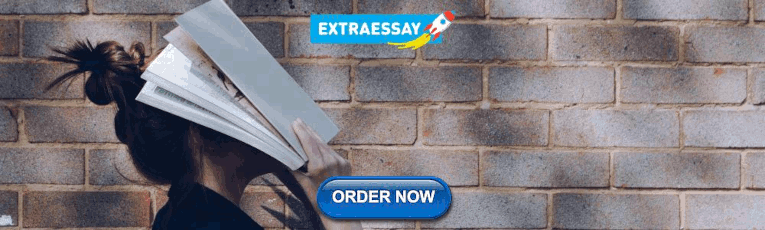
28 Case Selection for Case‐Study Analysis: Qualitative and Quantitative Techniques
John Gerring is Professor of Political Science, Boston University.
- Published: 02 September 2009
- Cite Icon Cite
- Permissions Icon Permissions
This article presents some guidance by cataloging nine different techniques for case selection: typical, diverse, extreme, deviant, influential, crucial, pathway, most similar, and most different. It also indicates that if the researcher is starting from a quantitative database, then methods for finding influential outliers can be used. In particular, the article clarifies the general principles that might guide the process of case selection in case-study research. Cases are more or less representative of some broader phenomenon and, on that score, may be considered better or worse subjects for intensive analysis. The article then draws attention to two ambiguities in case-selection strategies in case-study research. The first concerns the admixture of several case-selection strategies. The second concerns the changing status of a case as a study proceeds. Some case studies follow only one strategy of case selection.
Case ‐study analysis focuses on one or several cases that are expected to provide insight into a larger population. This presents the researcher with a formidable problem of case selection: Which cases should she or he choose?
In large‐sample research, the task of case selection is usually handled by some version of randomization. However, in case‐study research the sample is small (by definition) and this makes random sampling problematic, for any given sample may be wildly unrepresentative. Moreover, there is no guarantee that a few cases, chosen randomly, will provide leverage into the research question of interest.
In order to isolate a sample of cases that both reproduces the relevant causal features of a larger universe (representativeness) and provides variation along the dimensions of theoretical interest (causal leverage), case selection for very small samples must employ purposive (nonrandom) selection procedures. Nine such methods are discussed in this chapter, each of which may be identified with a distinct case‐study “type:” typical, diverse, extreme, deviant, influential, crucial, pathway, most‐similar , and most‐different . Table 28.1 summarizes each type, including its general definition, a technique for locating it within a population of potential cases, its uses, and its probable representativeness.
While each of these techniques is normally practiced on one or several cases (the diverse, most‐similar, and most‐different methods require at least two), all may employ additional cases—with the proviso that, at some point, they will no longer offer an opportunity for in‐depth analysis and will thus no longer be “case studies” in the usual sense ( Gerring 2007 , ch. 2 ). It will also be seen that small‐ N case‐selection procedures rest, at least implicitly, upon an analysis of a larger population of potential cases (as does randomization). The case(s) identified for intensive study is chosen from a population and the reasons for this choice hinge upon the way in which it is situated within that population. This is the origin of the terminology—typical, diverse, extreme, et al. It follows that case‐selection procedures in case‐study research may build upon prior cross‐case analysis and that they depend, at the very least, upon certain assumptions about the broader population.
In certain circumstances, the case‐selection procedure may be structured by a quantitative analysis of the larger population. Here, several caveats must be satisfied. First, the inference must pertain to more than a few dozen cases; otherwise, statistical analysis is problematic. Second, relevant data must be available for that population, or a significant sample of that population, on key variables, and the researcher must feel reasonably confident in the accuracy and conceptual validity of these variables. Third, all the standard assumptions of statistical research (e.g. identification, specification, robustness) must be carefully considered, and wherever possible, tested. I shall not dilate further on these familiar issues except to warn the researcher against the unreflective use of statistical techniques. 1 When these requirements are not met, the researcher must employ a qualitative approach to case selection.
The point of this chapter is to elucidate general principles that might guide the process of case selection in case‐study research, building upon earlier work by Harry Eckstein, Arend Lijphart, and others. Sometimes, these principles can be applied in a quantitative framework and sometimes they are limited to a qualitative framework. In either case, the logic of case selection remains quite similar, whether practiced in small‐ N or large‐ N contexts.
Before we begin, a bit of notation is necessary. In this chapter “ N ” refers to cases, not observations. Here, I am concerned primarily with causal inference, rather than inferences that are descriptive or predictive in nature. Thus, all hypotheses involve at least one independent variable ( X ) and one dependent variable ( Y ). For convenience, I shall label the causal factor of special theoretical interest X 1 , and the control variable, or vector of controls (if there are any), X 2 . If the writer is concerned to explain a puzzling outcome, but has no preconceptions about its causes, then the research will be described as Y‐centered . If a researcher is concerned to investigate the effects of a particular cause, with no preconceptions about what these effects might be, the research will be described as X‐centered . If a researcher is concerned to investigate a particular causal relationship, the research will be described as X 1 / Y‐centered , for it connects a particular cause with a particular outcome. 2 X ‐ or Y ‐centered research is exploratory; its purpose is to generate new hypotheses. X 1 / Y‐centered research, by contrast, is confirmatory/disconfirmatory; its purpose is to test an existing hypothesis.
1 Typical Case
In order for a focused case study to provide insight into a broader phenomenon it must be representative of a broader set of cases. It is in this context that one may speak of a typical‐case approach to case selection. The typical case exemplifies what is considered to be a typical set of values, given some general understanding of a phenomenon. By construction, the typical case is also a representative case.
Some typical cases serve an exploratory role. Here, the author chooses a case based upon a set of descriptive characteristics and then probes for causal relationships. Robert and Helen Lynd (1929/1956) selected a single city “to be as representative as possible of contemporary American life.” Specifically, they were looking for a city with
1) a temperate climate; 2) a sufficiently rapid rate of growth to ensure the presence of a plentiful assortment of the growing pains accompanying contemporary social change; 3) an industrial culture with modern, high‐speed machine production; 4) the absence of dominance of the city's industry by a single plant (i.e., not a one‐industry town); 5) a substantial local artistic life to balance its industrial activity …; and 6) the absence of any outstanding peculiarities or acute local problems which would mark the city off from the midchannel sort of American community. ( Lynd and Lynd 1929/1956 , quoted in Yin 2004 , 29–30)
After examining a number of options the Lynds decided that Muncie, Indiana, was more representative than, or at least as representative as, other midsized cities in America, thus qualifying as a typical case.
This is an inductive approach to case selection. Note that typicality may be understood according to the mean, median, or mode on a particular dimension; there may be multiple dimensions (as in the foregoing example); and each may be differently weighted (some dimensions may be more important than others). Where the selection criteria are multidimensional and a large sample of potential cases is in play, some form of factor analysis may be useful in identifying the most‐typical case(s).
However, the more common employment of the typical‐case method involves a causal model of some phenomenon of theoretical interest. Here, the researcher has identified a particular outcome ( Y ), and perhaps a specific X 1 / Y hypothesis, which she wishes to investigate. In order to do so, she looks for a typical example of that causal relationship. Intuitively, one imagines that a case selected according to the mean values of all parameters must be a typical case relative to some causal relationship. However, this is by no means assured.
Suppose that the Lynds were primarily interested in explaining feelings of trust/distrust among members of different social classes (one of the implicit research goals of the Middletown study). This outcome is likely to be affected by many factors, only some of which are included in their six selection criteria. So choosing cases with respect to a causal hypothesis involves, first of all, identifying the relevant parameters. It involves, secondly, the selection of a case that has a “typical” value relative to the overall causal model; it is well explained. Cases with untypical scores on a particular dimension (e.g. very high or very low) may still be typical examples of a causal relationship. Indeed, they may be more typical than cases whose values lie close to the mean. Thus, a descriptive understanding of typicality is quite different from a causal understanding of typicality. Since it is the latter version that is more common, I shall adopt this understanding of typicality in the remainder of the discussion.
From a qualitative perspective, causal typicality involves the selection of a case that conforms to expectations about some general causal relationship. It performs as expected. In a quantitative setting, this notion is measured by the size of a case's residual in a large‐ N cross‐case model. Typical cases lie on or near the regression line; their residuals are small. Insofar as the model is correctly specified, the size of a case's residual (i.e. the number of standard deviations that separate the actual value from the fitted value) provides a helpful clue to how representative that case is likely to be. “Outliers” are unlikely to be representative of the target population.
Of course, just because a case has a low residual does not necessarily mean that it is a representative case (with respect to the causal relationship of interest). Indeed, the issue of case representativeness is an issue that can never be definitively settled. When one refers to a “typical case” one is saying, in effect, that the probability of a case's representativeness is high, relative to other cases. This test of typicality is misleading if the statistical model is mis‐specified. And it provides little insurance against errors that are purely stochastic. A case may lie directly on the regression line but still be, in some important respect, atypical. For example, it might have an odd combination of values; the interaction of variables might be different from other cases; or additional causal mechanisms might be at work. For this reason, it is important to supplement a statistical analysis of cases with evidence drawn from the case in question (the case study itself) and with our deductive knowledge of the world. One should never judge a case solely by its residual. Yet, all other things being equal, a case with a low residual is less likely to be unusual than a case with a high residual, and to this extent the method of case selection outlined here may be a helpful guide to case‐study researchers faced with a large number of potential cases.
By way of conclusion, it should be noted that because the typical case embodies a typical value on some set of causally relevant dimensions, the variance of interest to the researcher must lie within that case. Specifically, the typical case of some phenomenon may be helpful in exploring causal mechanisms and in solving identification problems (e.g. endogeneity between X 1 and Y , an omitted variable that may account for X 1 and Y , or some other spurious causal association). Depending upon the results of the case study, the author may confirm an existing hypothesis, disconfirm that hypothesis, or reframe it in a way that is consistent with the findings of the case study. These are the uses of the typical‐case study.
2 Diverse Cases
A second case‐selection strategy has as its primary objective the achievement of maximum variance along relevant dimensions. I refer to this as a diverse‐case method. For obvious reasons, this method requires the selection of a set of cases—at minimum, two—which are intended to represent the full range of values characterizing X 1 , Y , or some particular X 1 / Y relationship. 3
Where the individual variable of interest is categorical (on/off, red/black/blue, Jewish/Protestant/Catholic), the identification of diversity is readily apparent. The investigator simply chooses one case from each category. For a continuous variable, the choices are not so obvious. However, the researcher usually chooses both extreme values (high and low), and perhaps the mean or median as well. The researcher may also look for break‐points in the distribution that seem to correspond to categorical differences among cases. Or she may follow a theoretical hunch about which threshold values count, i.e. which are likely to produce different values on Y .
Another sort of diverse case takes account of the values of multiple variables (i.e. a vector), rather than a single variable. If these variables are categorical, the identification of causal types rests upon the intersection of each category. Two dichotomous variables produce a matrix with four cells. Three trichotomous variables produce a matrix of eight cells. And so forth. If all variables are deemed relevant to the analysis, the selection of diverse cases mandates the selection of one case drawn from within each cell. Let us say that an outcome is thought to be affected by sex, race (black/white), and marital status. Here, a diverse‐case strategy of case selection would identify one case within each of these intersecting cells—a total of eight cases. Things become slightly more complicated when one or more of the factors is continuous, rather than categorical. Here, the diversity of case values do not fall neatly into cells. Rather, these cells must be created by fiat—e.g. high, medium, low.
It will be seen that where multiple variables are under consideration, the logic of diverse‐case analysis rests upon the logic of typological theorizing—where different combinations of variables are assumed to have effects on an outcome that vary across types ( Elman 2005 ; George and Bennett 2005 , 235; Lazarsfeld and Barton 1951 ). George and Smoke, for example, wish to explore different types of deterrence failure—by “fait accompli,” by “limited probe,” and by “controlled pressure.” Consequently, they wish to find cases that exemplify each type of causal mechanism. 4
Diversity may thus refer to a range of variation on X or Y , or to a particular combination of causal factors (with or without a consideration of the outcome). In each instance, the goal of case selection is to capture the full range of variation along the dimension(s) of interest.
Since diversity can mean many things, its employment in a large‐ N setting is necessarily dependent upon how this key term is defined. If it is understood to pertain only to a single variable ( X 1 or Y ), then the task is fairly simple. A categorical variable mandates the choice of at least one case from each category—two if dichotomous, three if trichotomous, and so forth. A continuous variable suggests the choice of at least one “high” and “low” value, and perhaps one drawn from the mean or median. But other choices might also be justified, according to one's hunch about the underlying causal relationship or according to natural thresholds found in the data, which may be grouped into discrete categories. Single‐variable traits are usually easy to discover in a large‐ N setting through descriptive statistics or through visual inspection of the data.
Where diversity refers to particular combinations of variables, the relevant cross‐ case technique is some version of stratified random sampling (in a probabilistic setting) or Qualitative Comparative Analysis (in a deterministic setting) ( Ragin 2000 ). If the researcher suspects that a causal relationship is affected not only by combinations of factors but also by their sequencing , then the technique of analysis must incorporate temporal elements ( Abbott 2001 ; Abbott and Forrest 1986 ; Abbott and Tsay 2000 ). Thus, the method of identifying causal types rests upon whatever method of identifying causal relationships is employed in the large‐ N sample.
Note that the identification of distinct case types is intended to identify groups of cases that are internally homogeneous (in all respects that might affect the causal relationship of interest). Thus, the choice of cases within each group should not be problematic, and may be accomplished through random sampling or purposive case selection. However, if there is suspected diversity within each category, then measures should be taken to assure that the chosen cases are typical of each category. A case study should not focus on an atypical member of a subgroup.
Indeed, considerations of diversity and typicality often go together. Thus, in a study of globalization and social welfare systems, Duane Swank (2002) first identifies three distinctive groups of welfare states: “universalistic” (social democratic), “corporatist conservative,” and “liberal.” Next, he looks within each group to find the most‐typical cases. He decides that the Nordic countries are more typical of the universalistic model than the Netherlands since the latter has “some characteristics of the occupationally based program structure and a political context of Christian Democratic‐led governments typical of the corporatist conservative nations” ( Swank 2002 , 11; see also Esping‐Andersen 1990 ). Thus, the Nordic countries are chosen as representative cases within the universalistic case type, and are accompanied in the case‐study portion of his analysis by other cases chosen to represent the other welfare state types (corporatist conservative and liberal).
Evidently, when a sample encompasses a full range of variation on relevant parameters one is likely to enhance the representativeness of that sample (relative to some population). This is a distinct advantage. Of course, the inclusion of a full range of variation may distort the actual distribution of cases across this spectrum. If there are more “high” cases than “low” cases in a population and the researcher chooses only one high case and one low case, the resulting sample of two is not perfectly representative. Even so, the diverse‐case method probably has stronger claims to representativeness than any other small‐ N sample (including the standalone typical case). The selection of diverse cases has the additional advantage of introducing variation on the key variables of interest. A set of diverse cases is, by definition, a set of cases that encompasses a range of high and low values on relevant dimensions. There is, therefore, much to recommend this method of case selection. I suspect that these advantages are commonly understood and are applied on an intuitive level by case‐study researchers. However, the lack of a recognizable name—and an explicit methodological defense—has made it difficult for case‐study researchers to utilize this method of case selection, and to do so in an explicit and self‐conscious fashion. Neologism has its uses.
3 Extreme Case
The extreme‐case method selects a case because of its extreme value on an independent ( X 1 ) or dependent ( Y ) variable of interest. Thus, studies of domestic violence may choose to focus on extreme instances of abuse ( Browne 1987 ). Studies of altruism may focus on those rare individuals who risked their lives to help others (e.g. Holocaust resisters) ( Monroe 1996 ). Studies of ethnic politics may focus on the most heterogeneous societies (e.g. Papua New Guinea) in order to better understand the role of ethnicity in a democratic setting ( Reilly 2000–1 ). Studies of industrial policy often focus on the most successful countries (i.e. the NICS) ( Deyo 1987 ). And so forth. 5
Often an extreme case corresponds to a case that is considered to be prototypical or paradigmatic of some phenomena of interest. This is because concepts are often defined by their extremes, i.e. their ideal types. Italian Fascism defines the concept of Fascism, in part, because it offered the most extreme example of that phenomenon. However, the methodological value of this case, and others like it, derives from its extremity (along some dimension of interest), not its theoretical status or its status in the literature on a subject.
The notion of “extreme” may now be defined more precisely. An extreme value is an observation that lies far away from the mean of a given distribution. This may be measured (if there are sufficient observations) by a case's “Z score”—the number of standard deviations between a case and the mean value for that sample. Extreme cases have high Z scores, and for this reason may serve as useful subjects for intensive analysis.
For a continuous variable, the distance from the mean may be in either direction (positive or negative). For a dichotomous variable (present/absent), extremeness may be interpreted as unusual . If most cases are positive along a given dimension, then a negative case constitutes an extreme case. If most cases are negative, then a positive case constitutes an extreme case. It should be clear that researchers are not simply concerned with cases where something “happened,” but also with cases where something did not. It is the rareness of the value that makes a case valuable, in this context, not its positive or negative value. 6 Thus, if one is studying state capacity, a case of state failure is probably more informative than a case of state endurance simply because the former is more unusual. Similarly, if one is interested in incest taboos a culture where the incest taboo is absent or weak is probably more useful than a culture where it is present or strong. Fascism is more important than nonfascism. And so forth. There is a good reason, therefore, why case studies of revolution tend to focus on “revolutionary” cases. Theda Skocpol (1979) had much more to learn from France than from Austro‐Hungary since France was more unusual than Austro‐Hungary within the population of nation states that Skocpol was concerned to explain. The reason is quite simple: There are fewer revolutionary cases than nonrevolutionary cases; thus, the variation that we explore as a clue to causal relationships is encapsulated in these cases, against a background of nonrevolutionary cases.
Note that the extreme‐case method of case selection appears to violate the social science folk wisdom warning us not to “select on the dependent variable.” 7 Selecting cases on the dependent variable is indeed problematic if a number of cases are chosen, all of which lie on one end of a variable's spectrum (they are all positive or negative), and if the researcher then subjects this sample to cross‐case analysis as if it were representative of a population. 8 Results for this sort of analysis would almost assuredly be biased. Moreover, there will be little variation to explain since the values of each case are explicitly constrained.
However, this is not the proper employment of the extreme‐case method. (It is more appropriately labeled an extreme‐ sample method.) The extreme‐case method actually refers back to a larger sample of cases that lie in the background of the analysis and provide a full range of variation as well as a more representative picture of the population. It is a self‐conscious attempt to maximize variance on the dimension of interest, not to minimize it. If this population of cases is well understood— either through the author's own cross‐case analysis, through the work of others, or through common sense—then a researcher may justify the selection of a single case exemplifying an extreme value for within‐case analysis. If not, the researcher may be well advised to follow a diverse‐case method, as discussed above.
By way of conclusion, let us return to the problem of representativeness. It will be seen that an extreme case may be typical or deviant. There is simply no way to tell because the researcher has not yet specified an X 1 / Y causal proposition. Once such a causal proposition has been specified one may then ask whether the case in question is similar to some population of cases in all respects that might affect the X 1 / Y relationship of interest (i.e. unit homogeneous). It is at this point that it becomes possible to say, within the context of a cross‐case statistical model, whether a case lies near to, or far from, the regression line. However, this sort of analysis means that the researcher is no longer pursuing an extreme‐case method. The extreme‐case method is purely exploratory—a way of probing possible causes of Y , or possible effects of X , in an open‐ended fashion. If the researcher has some notion of what additional factors might affect the outcome of interest, or of what relationship the causal factor of interest might have with Y , then she ought to pursue one of the other methods explored in this chapter. This also implies that an extreme‐case method may transform into a different kind of approach as a study evolves; that is, as a more specific hypothesis comes to light. Useful extreme cases at the outset of a study may prove less useful at a later stage of analysis.
4 Deviant Case
The deviant‐case method selects that case(s) which, by reference to some general understanding of a topic (either a specific theory or common sense), demonstrates a surprising value. It is thus the contrary of the typical case. Barbara Geddes (2003) notes the importance of deviant cases in medical science, where researchers are habitually focused on that which is “pathological” (according to standard theory and practice). The New England Journal of Medicine , one of the premier journals of the field, carries a regular feature entitled Case Records of the Massachusetts General Hospital. These articles bear titles like the following: “An 80‐Year‐Old Woman with Sudden Unilateral Blindness” or “A 76‐Year‐Old Man with Fever, Dyspnea, Pulmonary Infiltrates, Pleural Effusions, and Confusion.” 9 Another interesting example drawn from the field of medicine concerns the extensive study now devoted to a small number of persons who seem resistant to the AIDS virus ( Buchbinder and Vittinghoff 1999 ; Haynes, Pantaleo, and Fauci 1996 ). Why are they resistant? What is different about these people? What can we learn about AIDS in other patients by observing people who have built‐in resistance to this disease?
Likewise, in psychology and sociology case studies may be comprised of deviant (in the social sense) persons or groups. In economics, case studies may consist of countries or businesses that overperform (e.g. Botswana; Microsoft) or underperform (e.g. Britain through most of the twentieth century; Sears in recent decades) relative to some set of expectations. In political science, case studies may focus on countries where the welfare state is more developed (e.g. Sweden) or less developed (e.g. the United States) than one would expect, given a set of general expectations about welfare state development. The deviant case is closely linked to the investigation of theoretical anomalies. Indeed, to say deviant is to imply “anomalous.” 10
Note that while extreme cases are judged relative to the mean of a single distribution (the distribution of values along a single variable), deviant cases are judged relative to some general model of causal relations. The deviant‐case method selects cases which, by reference to some (presumably) general relationship, demonstrate a surprising value. They are “deviant” in that they are poorly explained by the multivariate model. The important point is that deviant‐ness can only be assessed relative to the general (quantitative or qualitative) model. This means that the relative deviant‐ness of a case is likely to change whenever the general model is altered. For example, the United States is a deviant welfare state when this outcome is gauged relative to societal wealth. But it is less deviant—and perhaps not deviant at all—when certain additional (political and societal) factors are included in the model, as discussed in the epilogue. Deviance is model dependent. Thus, when discussing the concept of the deviant case it is helpful to ask the following question: Relative to what general model (or set of background factors) is Case A deviant?
Conceptually, we have said that the deviant case is the logical contrary of the typical case. This translates into a directly contrasting statistical measurement. While the typical case is one with a low residual (in some general model of causal relations), a deviant case is one with a high residual. This means, following our previous discussion, that the deviant case is likely to be an un representative case, and in this respect appears to violate the supposition that case‐study samples should seek to reproduce features of a larger population.
However, it must be borne in mind that the primary purpose of a deviant‐case analysis is to probe for new—but as yet unspecified—explanations. (If the purpose is to disprove an extant theory I shall refer to the study as crucial‐case, as discussed below.) The researcher hopes that causal processes identified within the deviant case will illustrate some causal factor that is applicable to other (more or less deviant) cases. This means that a deviant‐case study usually culminates in a general proposition, one that may be applied to other cases in the population. Once this general proposition has been introduced into the overall model, the expectation is that the chosen case will no longer be an outlier. Indeed, the hope is that it will now be typical , as judged by its small residual in the adjusted model. (The exception would be a circumstance in which a case's outcome is deemed to be “accidental,” and therefore inexplicable by any general model.)
This feature of the deviant‐case study should help to resolve questions about its representativeness. Even if it is not possible to measure the new causal factor (and thus to introduce it into a large‐ N cross‐case model), it may still be plausible to assert (based on general knowledge of the phenomenon) that the chosen case is representative of a broader population.
5 Influential Case
Sometimes, the choice of a case is motivated solely by the need to verify the assumptions behind a general model of causal relations. Here, the analyst attempts to provide a rationale for disregarding a problematic case or a set of problematic cases. That is to say, she attempts to show why apparent deviations from the norm are not really deviant, or do not challenge the core of the theory, once the circumstances of the special case or cases are fully understood. A cross‐case analysis may, after all, be marred by several classes of problems including measurement error, specification error, errors in establishing proper boundaries for the inference (the scope of the argument), and stochastic error (fluctuations in the phenomenon under study that are treated as random, given available theoretical resources). If poorly fitting cases can be explained away by reference to these kinds of problems, then the theory of interest is that much stronger. This sort of deviant‐case analysis answers the question, “What about Case A (or cases of type A)? How does that, seemingly disconfirming, case fit the model?”
Because its underlying purpose is different from the usual deviant‐case study, I offer a new term for this method. The influential case is a case that casts doubt upon a theory, and for that reason warrants close inspection. This investigation may reveal, after all, that the theory is validated—perhaps in some slightly altered form. In this guise, the influential case is the “case that proves the rule.” In other instances, the influential‐case analysis may contribute to disconfirming, or reconceptualizing, a theory. The key point is that the value of the case is judged relative to some extant cross‐case model.
A simple version of influential‐case analysis involves the confirmation of a key case's score on some critical dimension. This is essentially a question of measurement. Sometimes cases are poorly explained simply because they are poorly understood. A close examination of a particular context may reveal that an apparently falsifying case has been miscoded. If so, the initial challenge presented by that case to some general theory has been obviated.
However, the more usual employment of the influential‐case method culminates in a substantive reinterpretation of the case—perhaps even of the general model. It is not just a question of measurement. Consider Thomas Ertman's (1997) study of state building in Western Europe, as summarized by Gerardo Munck. This study argues
that the interaction of a) the type of local government during the first period of statebuilding, with b) the timing of increases in geopolitical competition, strongly influences the kind of regime and state that emerge. [Ertman] tests this hypothesis against the historical experience of Europe and finds that most countries fit his predictions. Denmark, however, is a major exception. In Denmark, sustained geopolitical competition began relatively late and local government at the beginning of the statebuilding period was generally participatory, which should have led the country to develop “patrimonial constitutionalism.” But in fact, it developed “bureaucratic absolutism.” Ertman carefully explores the process through which Denmark came to have a bureaucratic absolutist state and finds that Denmark had the early marks of a patrimonial constitutionalist state. However, the country was pushed off this developmental path by the influence of German knights, who entered Denmark and brought with them German institutions of local government. Ertman then traces the causal process through which these imported institutions pushed Denmark to develop bureaucratic absolutism, concluding that this development was caused by a factor well outside his explanatory framework. ( Munck 2004 , 118)
Ertman's overall framework is confirmed insofar as he has been able to show, by an in‐depth discussion of Denmark, that the causal processes stipulated by the general theory hold even in this apparently disconfirming case. Denmark is still deviant, but it is so because of “contingent historical circumstances” that are exogenous to the theory ( Ertman 1997 , 316).
Evidently, the influential‐case analysis is similar to the deviant‐case analysis. Both focus on outliers. However, as we shall see, they focus on different kinds of outliers. Moreover, the animating goals of these two research designs are quite different. The influential‐case study begins with the aim of confirming a general model, while the deviant‐case study has the aim of generating a new hypothesis that modifies an existing general model. The confusion stems from the fact that the same case study may fulfill both objectives—qualifying a general model and, at the same time, confirming its core hypothesis.
Thus, in their study of Roberto Michels's “iron law of oligarchy,” Lipset, Trow, and Coleman (1956) choose to focus on an organization—the International Typographical Union—that appears to violate the central presupposition. The ITU, as noted by one of the authors, has “a long‐term two‐party system with free elections and frequent turnover in office” and is thus anything but oligarchic ( Lipset 1959 , 70). As such, it calls into question Michels's grand generalization about organizational behavior. The authors explain this curious result by the extraordinarily high level of education among the members of this union. Michels's law is shown to be true for most organizations, but not all. It is true, with qualifications. Note that the respecification of the original model (in effect, Lipset, Trow, and Coleman introduce a new control variable or boundary condition) involves the exploration of a new hypothesis. In this instance, therefore, the use of an influential case to confirm an existing theory is quite similar to the use of a deviant case to explore a new theory.
In a quantitative idiom, influential cases are those that, if counterfactually assigned a different value on the dependent variable, would most substantially change the resulting estimates. They may or may not be outliers (high‐residual cases). Two quantitative measures of influence are commonly applied in regression diagnostics ( Belsey, Kuh, and Welsch 2004 ). The first, often referred to as the leverage of a case, derives from what is called the hat matrix . Based solely on each case's scores on the independent variables, the hat matrix tells us how much a change in (or a measurement error on) the dependent variable for that case would affect the overall regression line. The second is Cook's distance , a measure of the extent to which the estimates of all the parameters would change if a given case were omitted from the analysis. Cases with a large leverage or Cook's distance contribute quite a lot to the inferences drawn from a cross‐case analysis. In this sense, such cases are vital for maintaining analytic conclusions. Discovering a significant measurement error on the dependent variable or an important omitted variable for such a case may dramatically revise estimates of the overall relationships. Hence, it may be quite sensible to select influential cases for in‐depth study.
Note that the use of an influential‐case strategy of case selection is limited to instances in which a researcher has reason to be concerned that her results are being driven by one or a few cases. This is most likely to be true in small to moderate‐sized samples. Where N is very large—greater than 1,000, let us say—it is extremely unlikely that a small set of cases (much less an individual case) will play an “influential” role. Of course, there may be influential sets of cases, e.g. countries within a particular continent or cultural region, or persons of Irish extraction. Sets of influential observations are often problematic in a time‐series cross‐section data‐set where each unit (e.g. country) contains multiple observations (through time), and hence may have a strong influence on aggregate results. Still, the general rule is: the larger the sample, the less important individual cases are likely to be and, hence, the less likely a researcher is to use an influential‐case approach to case selection.
6 Crucial Case
Of all the extant methods of case selection perhaps the most storied—and certainly the most controversial—is the crucial‐case method, introduced to the social science world several decades ago by Harry Eckstein. In his seminal essay, Eckstein (1975 , 118) describes the crucial case as one “that must closely fit a theory if one is to have confidence in the theory's validity, or, conversely, must not fit equally well any rule contrary to that proposed.” A case is crucial in a somewhat weaker—but much more common—sense when it is most, or least, likely to fulfill a theoretical prediction. A “most‐likely” case is one that, on all dimensions except the dimension of theoretical interest, is predicted to achieve a certain outcome, and yet does not. It is therefore used to disconfirm a theory. A “least‐likely” case is one that, on all dimensions except the dimension of theoretical interest, is predicted not to achieve a certain outcome, and yet does so. It is therefore used to confirm a theory. In all formulations, the crucial‐case offers a most‐difficult test for an argument, and hence provides what is perhaps the strongest sort of evidence possible in a nonexperimental, single‐case setting.
Since the publication of Eckstein's influential essay, the crucial‐case approach has been claimed in a multitude of studies across several social science disciplines and has come to be recognized as a staple of the case‐study method. 11 Yet the idea of any single case playing a crucial (or “critical”) role is not widely accepted among most methodologists (e.g. Sekhon 2004 ). (Even its progenitor seems to have had doubts.)
Let us begin with the confirmatory (a.k.a. least‐likely) crucial case. The implicit logic of this research design may be summarized as follows. Given a set of facts, we are asked to contemplate the probability that a given theory is true. While the facts matter, to be sure, the effectiveness of this sort of research also rests upon the formal properties of the theory in question. Specifically, the degree to which a theory is amenable to confirmation is contingent upon how many predictions can be derived from the theory and on how “risky” each individual prediction is. In Popper's (1963 , 36) words, “Confirmations should count only if they are the result of risky predictions ; that is to say, if, unenlightened by the theory in question, we should have expected an event which was incompatible with the theory—and event which would have refuted the theory. Every ‘good’ scientific theory is a prohibition; it forbids certain things to happen. The more a theory forbids, the better it is” (see also Popper 1934/1968 ). A risky prediction is therefore one that is highly precise and determinate, and therefore unlikely to be achieved by the product of other causal factors (external to the theory of interest) or through stochastic processes. A theory produces many such predictions if it is fully elaborated, issuing predictions not only on the central outcome of interest but also on specific causal mechanisms, and if it is broad in purview. (The notion of riskiness may also be conceptualized within the Popperian lexicon as degrees of falsifiability .)
These points can also be articulated in Bayesian terms. Colin Howson and Peter Urbach explain: “The degree to which h [a hypothesis] is confirmed by e [a set of evidence] depends … on the extent to which P(eČh) exceeds P (e) , that is, on how much more probable e is relative to the hypothesis and background assumptions than it is relative just to background assumptions.” Again, “confirmation is correlated with how much more probable the evidence is if the hypothesis is true than if it is false” ( Howson and Urlbach 1989 , 86). Thus, the stranger the prediction offered by a theory—relative to what we would normally expect—the greater the degree of confirmation that will be afforded by the evidence. As an intuitive example, Howson and Urbach (1989 , 86) offer the following:
If a soothsayer predicts that you will meet a dark stranger sometime and you do in fact, your faith in his powers of precognition would not be much enhanced: you would probably continue to think his predictions were just the result of guesswork. However, if the prediction also gave the correct number of hairs on the head of that stranger, your previous scepticism would no doubt be severely shaken.
While these Popperian/Bayesian notions 12 are relevant to all empirical research designs, they are especially relevant to case‐study research designs, for in these settings a single case (or, at most, a small number of cases) is required to bear a heavy burden of proof. It should be no surprise, therefore, that Popper's idea of “riskiness” was to be appropriated by case‐study researchers like Harry Eckstein to validate the enterprise of single‐case analysis. (Although Eckstein does not cite Popper the intellectual lineage is clear.) Riskiness, here, is analogous to what is usually referred to as a “most‐ difficult” research design, which in a case‐study research design would be understood as a “least‐likely” case. Note also that the distinction between a “must‐fit” case and a least‐likely case—that, in the event, actually does fit the terms of a theory—is a matter of degree. Cases are more or less crucial for confirming theories. The point is that, in some circumstances, a paucity of empirical evidence may be compensated by the riskiness of the theory.
The crucial‐case research design is, perforce, a highly deductive enterprise; much depends on the quality of the theory under investigation. It follows that the theories most amenable to crucial‐case analysis are those which are lawlike in their precision, degree of elaboration, consistency, and scope. The more a theory attains the status of a causal law, the easier it will be to confirm, or to disconfirm, with a single case. Indeed, risky predictions are common in natural science fields such as physics, which in turn served as the template for the deductive‐nomological (“covering‐law”) model of science that influenced Eckstein and others in the postwar decades (e.g. Hempel 1942 ).
A frequently cited example is the first important empirical demonstration of the theory of relativity, which took the form of a single‐event prediction on the occasion of the May 29, 1919, solar eclipse ( Eckstein 1975 ; Popper 1963 ). Stephen Van Evera (1997 , 66–7) describes the impact of this prediction on the validation of Einstein's theory.
Einstein's theory predicted that gravity would bend the path of light toward a gravity source by a specific amount. Hence it predicted that during a solar eclipse stars near the sun would appear displaced—stars actually behind the sun would appear next to it, and stars lying next to the sun would appear farther from it—and it predicted the amount of apparent displacement. No other theory made these predictions. The passage of this one single‐case‐study test brought the theory wide acceptance because the tested predictions were unique—there was no plausible competing explanation for the predicted result—hence the passed test was very strong.
The strength of this test is the extraordinary fit between the theory and a set of facts found in a single case, and the corresponding lack of fit between all other theories and this set of facts. Einstein offered an explanation of a particular set of anomalous findings that no other existing theory could make sense of. Of course, one must assume that there was no—or limited—measurement error. And one must assume that the phenomenon of interest is largely invariant; light does not bend differently at different times and places (except in ways that can be understood through the theory of relativity). And one must assume, finally, that the theory itself makes sense on other grounds (other than the case of special interest); it is a plausible general theory. If one is willing to accept these a priori assumptions, then the 1919 “case study” provides a very strong confirmation of the theory. It is difficult to imagine a stronger proof of the theory from within an observational (nonexperimental) setting.
In social science settings, by contrast, one does not commonly find single‐case studies offering knockout evidence for a theory. This is, in my view, largely a product of the looseness (the underspecification) of most social science theories. George and Bennett point out that while the thesis of the democratic peace is as close to a “law” as social science has yet seen, it cannot be confirmed (or refuted) by looking at specific causal mechanisms because the causal pathways mandated by the theory are multiple and diverse. Under the circumstances, no single‐case test can offer strong confirmation of the theory ( George and Bennett 2005 , 209).
However, if one adopts a softer version of the crucial‐case method—the least‐likely (most difficult) case—then possibilities abound. Indeed, I suspect that, implicitly , most case‐study work that makes a positive argument focusing on a single case (without a corresponding cross‐case analysis) relies largely on the logic of the least‐ likely case. Rarely is this logic made explicit, except perhaps in a passing phrase or two. Yet the deductive logic of the “risky” prediction is central to the case‐study enterprise. Whether a case study is convincing or not often rests on the reader's evaluation of how strong the evidence for an argument might be, and this in turn—wherever cross‐ case evidence is limited and no manipulated treatment can be devised—rests upon an estimation of the degree of “fit” between a theory and the evidence at hand, as discussed.
Lily Tsai's (2007) investigation of governance at the village level in China employs several in‐depth case studies of villages which are chosen (in part) because of their least‐likely status relative to the theory of interest. Tsai's hypothesis is that villages with greater social solidarity (based on preexisting religious or familial networks) will develop a higher level of social trust and mutual obligation and, as a result, will experience better governance. Crucial cases, therefore, are villages that evidence a high level of social solidarity but which, along other dimensions, would be judged least likely to develop good governance, e.g. they are poor, isolated, and lack democratic institutions or accountability mechanisms from above. “Li Settlement,” in Fujian province, is such a case. The fact that this impoverished village nonetheless boasts an impressive set of infrastructural accomplishments such as paved roads with drainage ditches (a rarity in rural China) suggests that something rather unusual is going on here. Because her case is carefully chosen to eliminate rival explanations, Tsai's conclusions about the special role of social solidarity are difficult to gainsay. How else is one to explain this otherwise anomalous result? This is the strength of the least‐likely case, where all other plausible causal factors for an outcome have been minimized. 13
Jack Levy (2002 , 144) refers to this, evocatively, as a “Sinatra inference:” if it can make it here, it can make it anywhere (see also Khong 1992 , 49; Sagan 1995 , 49; Shafer 1988 , 14–6). Thus, if social solidarity has the hypothesized effect in Li Settlement it should have the same effect in more propitious settings (e.g. where there is greater economic surplus). The same implicit logic informs many case‐study analyses where the intent of the study is to confirm a hypothesis on the basis of a single case.
Another sort of crucial case is employed for the purpose of dis confirming a causal hypothesis. A central Popperian insight is that it is easier to disconfirm an inference than to confirm that same inference. (Indeed, Popper doubted that any inference could be fully confirmed, and for this reason preferred the term “corroborate.”) This is particularly true of case‐study research designs, where evidence is limited to one or several cases. The key proviso is that the theory under investigation must take a consistent (a.k.a. invariant, deterministic) form, even if its predictions are not terrifically precise, well elaborated, or broad.
As it happens, there are a fair number of invariant propositions floating around the social science disciplines (Goertz and Levy forthcoming; Goertz and Starr 2003 ). It used to be argued, for example, that political stability would occur only in countries that are relatively homogeneous, or where existing heterogeneities are mitigated by cross‐cutting cleavages ( Almond 1956 ; Bentley 1908/1967 ; Lipset 1960/1963 ; Truman 1951 ). Arend Lijphart's (1968) study of the Netherlands, a peaceful country with reinforcing social cleavages, is commonly viewed as refuting this theory on the basis of a single in‐depth case analysis. 14
Granted, it may be questioned whether presumed invariant theories are really invariant; perhaps they are better understood as probabilistic. Perhaps, that is, the theory of cross‐cutting cleavages is still true, probabilistically, despite the apparent Dutch exception. Or perhaps the theory is still true, deterministically, within a subset of cases that does not include the Netherlands. (This sort of claim seems unlikely in this particular instance, but it is quite plausible in many others.) Or perhaps the theory is in need of reframing; it is true, deterministically, but applies only to cross‐ cutting ethnic/racial cleavages, not to cleavages that are primarily religious. One can quibble over what it means to “disconfirm” a theory. The point is that the crucial case has, in all these circumstances, provided important updating of a theoretical prior.
Heretofore, I have treated causal factors as dichotomous. Countries have either reinforcing or cross‐cutting cleavages and they have regimes that are either peaceful or conflictual. Evidently, these sorts of parameters are often matters of degree. In this reading of the theory, cases are more or less crucial. Accordingly, the most useful—i.e. most crucial—case for Lijphart's purpose is one that has the most segregated social groups and the most peaceful and democratic track record. In these respects, the Netherlands was a very good choice. Indeed, the degree of disconfirmation offered by this case study is probably greater than the degree of disconfirmation that might have been provided by other cases such as India or Papua New Guinea—countries where social peace has not always been secure. The point is that where variables are continuous rather than dichotomous it is possible to evaluate potential cases in terms of their degree of crucialness .
Note that the crucial‐case method of case‐selection, whether employed in a confirmatory or disconfirmatory mode, cannot be employed in a large‐ N context. This is because an explicit cross‐case model would render the crucial‐case study redundant. Once one identifies the relevant parameters and the scores of all cases on those parameters, one has in effect constructed a cross‐case model that confirms or disconfirms the theory in question. The case study is thenceforth irrelevant, at least as a means of decisive confirmation or disconfirmation. 15 It remains highly relevant as a means of exploring causal mechanisms, of course. Yet, because this objective is quite different from that which is usually associated with the term, I enlist a new term for this technique.
7 Pathway Case
One of the most important functions of case‐study research is the elucidation of causal mechanisms. But which sort of case is most useful for this purpose? Although all case studies presumably shed light on causal mechanisms, not all cases are equally transparent. In situations where a causal hypothesis is clear and has already been confirmed by cross‐case analysis, researchers are well advised to focus on a case where the causal effect of X 1 on Y can be isolated from other potentially confounding factors ( X 2 ). I shall call this a pathway case to indicate its uniquely penetrating insight into causal mechanisms. In contrast to the crucial case, this sort of method is practicable only in circumstances where cross‐case covariational patterns are well studied and where the mechanism linking X 1 and Y remains dim. Because the pathway case builds on prior cross‐case analysis, the problem of case selection must be situated within that sample. There is no standalone pathway case.
The logic of the pathway case is clearest in situations of causal sufficiency—where a causal factor of interest, X 1 , is sufficient by itself (though perhaps not necessary) to account for Y 's value (0 or 1). The other causes of Y , about which we need make no assumptions, are designated as a vector, X 2 .
Note that wherever various causal factors are substitutable for one another, each factor is conceptualized (individually) as sufficient ( Braumoeller 2003 ). Thus, situations of causal equifinality presume causal sufficiency on the part of each factor or set of conjoint factors. An example is provided by the literature on democratization, which stipulates three main avenues of regime change: leadership‐initiated reform, a controlled opening to opposition, or the collapse of an authoritarian regime ( Colomer 1991 ). The case‐study format constrains us to analyze one at a time, so let us limit our scope to the first one—leadership‐initiated reform. So considered, a causal‐pathway case would be one with the following features: (a) democratization, (b) leadership‐initiated reform, (c) no controlled opening to the opposition, (d) no collapse of the previous authoritarian regime, and (e) no other extraneous factors that might affect the process of democratization. In a case of this type, the causal mechanisms by which leadership‐initiated reform may lead to democratization will be easiest to study. Note that it is not necessary to assume that leadership‐initiated reform always leads to democratization; it may or may not be a deterministic cause. But it is necessary to assume that leadership‐initiated reform can sometimes lead to democratization on its own (given certain background features).
Now let us move from these examples to a general‐purpose model. For heuristic purposes, let us presume that all variables in that model are dichotomous (coded as 0 or 1) and that the model is complete (all causes of Y are included). All causal relationships will be coded so as to be positive: X 1 and Y covary as do X 2 and Y . This allows us to visualize a range of possible combinations at a glance.
Recall that the pathway case is always focused, by definition, on a single causal factor, denoted X 1 . (The researcher's focus may shift to other causal factors, but may only focus on one causal factor at a time.) In this scenario, and regardless of how many additional causes of Y there might be (denoted X 2 , a vector of controls), there are only eight relevant case types, as illustrated in Table 28.2 . Identifying these case types is a relatively simple matter, and can be accomplished in a small‐ N sample by the construction of a truth‐table (modeled after Table 28.2 ) or in a large‐ N sample by the use of cross‐tabs.
Notes : X 1 = the variable of theoretical interest. X 2 = a vector of controls (a score of 0 indicates that all control variables have a score of 0, while a score of 1 indicates that all control variables have a score of 1). Y = the outcome of interest. A–H = case types (the N for each case type is indeterminate). G, H = possible pathway cases. Sample size = indeterminate.
Assumptions : (a) all variables can be coded dichotomously (a binary coding of the concept is valid); (b) all independent variables are positively correlated with Y in the general case; ( c ) X 1 is (at least sometimes) a sufficient cause of Y .
Note that the total number of combinations of values depends on the number of control variables, which we have represented with a single vector, X 2 . If this vector consists of a single variable then there are only eight case types. If this vector consists of two variables ( X 2a , X 2b ) then the total number of possible combinations increases from eight (2 3 ) to sixteen (2 4 ). And so forth. However, none of these combinations is relevant for present purposes except those where X 2a and X 2b have the same value (0 or 1). “Mixed” cases are not causal pathway cases, for reasons that should become clear.
The pathway case, following the logic of the crucial case, is one where the causal factor of interest, X 1 , correctly predicts Y while all other possible causes of Y (represented by the vector, X 2 ) make “wrong” predictions. If X 1 is—at least in some circumstances—a sufficient cause of Y , then it is these sorts of cases that should be most useful for tracing causal mechanisms. There are only two such cases in Ta b l e 28.2—G and H. In all other cases, the mechanism running from X 1 to Y would be difficult to discern either because X 1 and Y are not correlated in the usual way (constituting an unusual case, in the terms of our hypothesis) or because other confounding factors ( X 2 ) intrude. In case A, for example, the positive value on Y could be a product of X 1 or X 2 . An in‐depth examination of this case is not likely to be very revealing.
Keep in mind that because the researcher already knows from her cross‐case examination what the general causal relationships are, she knows (prior to the case‐ study investigation) what constitutes a correct or incorrect prediction. In the crucial‐ case method, by contrast, these expectations are deductive rather than empirical. This is what differentiates the two methods. And this is why the causal pathway case is useful principally for elucidating causal mechanisms rather than verifying or falsifying general propositions (which are already more or less apparent from the cross‐case evidence). Of course, we must leave open the possibility that the investigation of causal mechanisms would invalidate a general claim, if that claim is utterly contingent upon a specific set of causal mechanisms and the case study shows that no such mechanisms are present. However, this is rather unlikely in most social science settings. Usually, the result of such a finding will be a reformulation of the causal processes by which X 1 causes Y —or, alternatively, a realization that the case under investigation is aberrant (atypical of the general population of cases).
Sometimes, the research question is framed as a unidirectional cause: one is interested in why 0 becomes 1 (or vice versa) but not in why 1 becomes 0. In our previous example, we asked why democracies fail, not why countries become democratic or authoritarian. So framed, there can be only one type of causal‐pathway case. (Whether regime failure is coded as 0 or 1 is a matter of taste.) Where researchers are interested in bidirectional causality—a movement from 0 to 1 as well as from 1 to 0—there are two possible causal‐pathway cases, G and H. In practice, however, one of these case types is almost always more useful than the other. Thus, it seems reasonable to employ the term “pathway case” in the singular. In order to determine which of these two case types will be more useful for intensive analysis the researcher should look to see whether each case type exhibits desirable features such as: (a) a rare (unusual) value on X 1 or Y (designated “extreme” in our previous discussion), (b) observable temporal variation in X 1 , ( c ) an X 1 / Y relationship that is easier to study (it has more visible features; it is more transparent), or (d) a lower residual (thus indicating a more typical case, within the terms of the general model). Usually, the choice between G and H is intuitively obvious.
Now, let us consider a scenario in which all (or most) variables of concern to the model are continuous, rather than dichotomous. Here, the job of case selection is considerably more complex, for causal “sufficiency” (in the usual sense) cannot be invoked. It is no longer plausible to assume that a given cause can be entirely partitioned, i.e. rival factors eliminated. However, the search for a pathway case may still be viable. What we are looking for in this scenario is a case that satisfies two criteria: (1) it is not an outlier (or at least not an extreme outlier) in the general model and (2) its score on the outcome ( Y ) is strongly influenced by the theoretical variable of interest ( X 1 ), taking all other factors into account ( X 2 ). In this sort of case it should be easiest to “see” the causal mechanisms that lie between X 1 and Y .
Achieving the second desiderata requires a bit of manipulation. In order to determine which (nonoutlier) cases are most strongly affected by X 1 , given all the other parameters in the model, one must compare the size of the residuals for each case in a reduced form model, Y = Constant + X 2 + Res reduced , with the size of the residuals for each case in a full model, Y = Constant + X 2 + X 1 + Res full . The pathway case is that case, or set of cases, which shows the greatest difference between the residual for the reduced‐form model and the full model (ΔResidual). Thus,
Note that the residual for a case must be smaller in the full model than in the reduced‐ form model; otherwise, the addition of the variable of interest ( X 1 ) pulls the case away from the regression line. We want to find a case where the addition of X 1 pushes the case towards the regression line, i.e. it helps to “explain” that case.
As an example, let us suppose that we are interested in exploring the effect of mineral wealth on the prospects for democracy in a society. According to a good deal of work on this subject, countries with a bounty of natural resources—particularly oil—are less likely to democratize (or once having undergone a democratic transition, are more likely to revert to authoritarian rule) ( Barro 1999 ; Humphreys 2005 ; Ross 2001 ). The cross‐country evidence is robust. Yet as is often the case, the causal mechanisms remain rather obscure. In order to better understand this phenomenon it may be worthwhile to exploit the findings of cross‐country regression models in order to identify a country whose regime type (i.e. its democracy “score” on some general index) is strongly affected by its natural‐research wealth, all other things held constant. An analysis of this sort identifies two countries— the United Arab Emirates and Kuwait—with high Δ Residual values and modest residuals in the full model (signifying that these cases are not outliers). Researchers seeking to explore the effect of oil wealth on regime type might do well to focus on these two cases since their patterns of democracy cannot be well explained by other factors—e.g. economic development, religion, European influence, or ethnic fractionalization. The presence of oil wealth in these countries would appear to have a strong independent effect on the prospects for democratization in these cases, an effect that is well modeled by general theory and by the available cross‐case evidence.
To reiterate, the logic of causal “elimination” is much more compelling where variables are dichotomous and where causal sufficiency can be assumed ( X 1 is sufficient by itself, at least in some circumstances, to cause Y ). Where variables are continuous, the strategy of the pathway case is more dubious, for potentially confounding causal factors ( X 2 ) cannot be neatly partitioned. Even so, we have indicated why the selection of a pathway case may be a logical approach to case‐study analysis in many circumstances.
The exceptions may be briefly noted. Sometimes, where all variables in a model are dichotomous, there are no pathway cases, i.e. no cases of type G or H (in Table 28.2 ). This is known as the “empty cell” problem, or a problem of severe causal multicollinearity. The universe of observational data does not always oblige us with cases that allow us to independently test a given hypothesis. Where variables are continuous, the analogous problem is that of a causal variable of interest ( X 1 ) that has only minimal effects on the outcome of interest. That is, its role in the general model is quite minor. In these situations, the only cases that are strongly affected by X 1 —if there are any at all—may be extreme outliers, and these sorts of cases are not properly regarded as providing confirmatory evidence for a proposition, for reasons that are abundantly clear by now.
Finally, it should be clarified that the identification of a causal pathway case does not obviate the utility of exploring other cases. One might, for example, want to compare both sorts of potential pathway cases—G and H—with each other. Many other combinations suggest themselves. However, this sort of multi‐case investigation moves beyond the logic of the causal‐pathway case.
8 Most‐similar Cases
The most‐similar method employs a minimum of two cases. 16 In its purest form, the chosen pair of cases is similar in all respects except the variable(s) of interest. If the study is exploratory (i.e. hypothesis generating), the researcher looks for cases that differ on the outcome of theoretical interest but are similar on various factors that might have contributed to that outcome, as illustrated in Table 28.3 (A) . This is a common form of case selection at the initial stage of research. Often, fruitful analysis begins with an apparent anomaly: two cases are apparently quite similar, and yet demonstrate surprisingly different outcomes. The hope is that intensive study of these cases will reveal one—or at most several—factors that differ across these cases. These differing factors ( X 1 ) are looked upon as putative causes. At this stage, the research may be described by the second diagram in Table 28.3 (B) . Sometimes, a researcher begins with a strong hypothesis, in which case her research design is confirmatory (hypothesis testing) from the get‐go. That is, she strives to identify cases that exhibit different outcomes, different scores on the factor of interest, and similar scores on all other possible causal factors, as illustrated in the second (hypothesis‐testing) diagram in Table 28.3 (B) .
The point is that the purpose of a most‐similar research design, and hence its basic setup, often changes as a researcher moves from an exploratory to a confirmatory mode of analysis. However, regardless of where one begins, the results, when published, look like a hypothesis‐testing research design. Question marks have been removed: (A) becomes (B) in Table 28.3 .
As an example, let us consider Leon Epstein's classic study of party cohesion, which focuses on two “most‐similar” countries, the United States and Canada. Canada has highly disciplined parties whose members vote together on the floor of the House of Commons while the United States has weak, undisciplined parties, whose members often defect on floor votes in Congress. In explaining these divergent outcomes, persistent over many years, Epstein first discusses possible causal factors that are held more or less constant across the two cases. Both the United States and Canada inherited English political cultures, both have large territories and heterogeneous populations, both are federal, and both have fairly loose party structures with strong regional bases and a weak center. These are the “control” variables. Where they differ is in one constitutional feature: Canada is parliamentary while the United States is presidential. And it is this institutional difference that Epstein identifies as the crucial (differentiating) cause. (For further examples of the most‐similar method see Brenner 1976 ; Hamilton 1977 ; Lipset 1968 ; Miguel 2004 ; Moulder 1977 ; Posner 2004 .)
X 1 = the variable of theoretical interest. X 2 = a vector of controls. Y = the outcome of interest.
Several caveats apply to any most‐similar analysis (in addition to the usual set of assumptions applying to all case‐study analysis). First, each causal factor is understood as having an independent and additive effect on the outcome; there are no “interaction” effects. Second, one must code cases dichotomously (high/low, present/absent). This is straightforward if the underlying variables are also dichotomous (e.g. federal/unitary). However, it is often the case that variables of concern in the model are continuous (e.g. party cohesion). In this setting, the researcher must “dichotomize” the scoring of cases so as to simplify the two‐case analysis. (Some flexibility is admissible on the vector of controls ( X 2 ) that are “held constant” across the cases. Nonidentity is tolerable if the deviation runs counter to the predicted hypothesis. For example, Epstein describes both the United States and Canada as having strong regional bases of power, a factor that is probably more significant in recent Canadian history than in recent American history. However, because regional bases of power should lead to weaker parties, rather than stronger parties, this element of nonidentity does not challenge Epstein's conclusions. Indeed, it sets up a most‐difficult research scenario, as discussed above.)
In one respect the requirements for case control are not so stringent. Specifically, it is not usually necessary to measure control variables (at least not with a high degree of precision) in order to control for them. If two countries can be assumed to have similar cultural heritages one needn't worry about constructing variables to measure that heritage. One can simply assert that, whatever they are, they are more or less constant across the two cases. This is similar to the technique employed in a randomized experiment, where the researcher typically does not attempt to measure all the factors that might affect the causal relationship of interest. She assumes, rather, that these unknown factors have been neutralized across the treatment and control groups by randomization or by the choice of a sample that is internally homogeneous.
The most useful statistical tool for identifying cases for in‐depth analysis in a most‐ similar setting is probably some variety of matching strategy—e.g. exact matching, approximate matching, or propensity‐score matching. 17 The product of this procedure is a set of matched cases that can be compared in whatever way the researcher deems appropriate. These are the “most‐similar” cases. Rosenbaum and Silber (2001 , 223) summarize:
Unlike model‐based adjustments, where [individuals] vanish and are replaced by the coefficients of a model, in matching, ostensibly comparable patterns are compared directly, one by one. Modern matching methods involve statistical modeling and combinatorial algorithms, but the end result is a collection of pairs or sets of people who look comparable, at least on average. In matching, people retain their integrity as people, so they can be examined and their stories can be told individually.
Matching, conclude the authors, “facilitates, rather than inhibits, thick description” ( Rosenbaum and Silber 2001 , 223).
In principle, the same matching techniques that have been used successfully in observational studies of medical treatments might also be adapted to the study of nation states, political parties, cities, or indeed any traditional paired cases in the social sciences. Indeed, the current popularity of matching among statisticians—relative, that is, to garden‐variety regression models—rests upon what qualitative researchers would recognize as a “case‐based” approach to causal analysis. If Rosenbaum and Silber are correct, it may be perfectly reasonable to appropriate this large‐ N method of analysis for case‐study purposes.
As with other methods of case selection, the most‐similar method is prone to problems of nonrepresentativeness. If employed in a qualitative fashion (without a systematic cross‐case selection strategy), potential biases in the chosen case must be addressed in a speculative way. If the researcher employs a matching technique of case selection within a large‐ N sample, the problem of potential bias can be addressed by assuring the choice of cases that are not extreme outliers, as judged by their residuals in the full model. Most‐similar cases should also be “typical” cases, though some scope for deviance around the regression line may be acceptable for purposes of finding a good fit among cases.
X 1 = the variable of theoretical interest. X 2a–d = a vector of controls. Y = the outcome of interest.
9 Most‐different Cases
A final case‐selection method is the reverse image of the previous method. Here, variation on independent variables is prized, while variation on the outcome is eschewed. Rather than looking for cases that are most‐similar, one looks for cases that are most‐ different . Specifically, the researcher tries to identify cases where just one independent variable ( X 1 ), as well as the dependent variable ( Y ), covary, while all other plausible factors ( X 2a–d ) show different values. 18
The simplest form of this two‐case comparison is illustrated in Table 28.4 . Cases A and B are deemed “most different,” though they are similar in two essential respects— the causal variable of interest and the outcome.
As an example, I follow Marc Howard's (2003) recent work, which explores the enduring impact of Communism on civil society. 19 Cross‐national surveys show a strong correlation between former Communist regimes and low social capital, controlling for a variety of possible confounders. It is a strong result. Howard wonders why this relationship is so strong and why it persists, and perhaps even strengthens, in countries that are no longer socialist or authoritarian. In order to answer this question, he focuses on two most‐different cases, Russia and East Germany. These two countries were quite different—in all ways other than their Communist experience— prior to the Soviet era, during the Soviet era (since East Germany received substantial subsidies from West Germany), and in the post‐Soviet era, as East Germany was absorbed into West Germany. Yet, they both score near the bottom of various cross‐ national indices intended to measure the prevalence of civic engagement in the current era. Thus, Howard's (2003 , 6–9) case selection procedure meets the requirements of the most‐different research design: Variance is found on all (or most) dimensions aside from the key factor of interest (Communism) and the outcome (civic engagement).
What leverage is brought to the analysis from this approach? Howard's case studies combine evidence drawn from mass surveys and from in‐depth interviews of small, stratified samples of Russians and East Germans. (This is a good illustration, incidentally, of how quantitative and qualitative evidence can be fruitfully combined in the intensive study of several cases.) The product of this analysis is the identification of three causal pathways that, Howard (2003 , 122) claims, help to explain the laggard status of civil society in post‐Communist polities: “the mistrust of communist organizations, the persistence of friendship networks, and the disappointment with post‐communism.” Simply put, Howard (2003 , 145) concludes, “a great number of citizens in Russia and Eastern Germany feel a strong and lingering sense of distrust of any kind of public organization, a general satisfaction with their own personal networks (accompanied by a sense of deteriorating relations within society overall), and disappointment in the developments of post‐communism.”
The strength of this most‐different case analysis is that the results obtained in East Germany and Russia should also apply in other post‐Communist polities (e.g. Lithuania, Poland, Bulgaria, Albania). By choosing a heterogeneous sample, Howard solves the problem of representativeness in his restricted sample. However, this sample is demonstrably not representative across the population of the inference, which is intended to cover all countries of the world.
More problematic is the lack of variation on key causal factors of interest— Communism and its putative causal pathways. For this reason, it is difficult to reach conclusions about the causal status of these factors on the basis of the most‐different analysis alone. It is possible, that is, that the three causal pathways identified by Howard also operate within polities that never experienced Communist rule.
Nor does it seem possible to conclusively eliminate rival hypotheses on the basis of this most‐different analysis. Indeed, this is not Howard's intention. He wishes merely to show that whatever influence on civil society might be attributed to economic, cultural, and other factors does not exhaust this subject.
My considered judgment is that the most‐different research design provides minimal leverage into the problem of why Communist systems appear to suppress civic engagement, years after their disappearance. Fortunately, this is not the only research design employed by Howard in his admirable study. Indeed, the author employs two other small‐ N cross‐case methods, as well as a large‐ N cross‐country statistical analysis. These methods do most of the analytic work. East Germany may be regarded as a causal pathway case (see above). It has all the attributes normally assumed to foster civic engagement (e.g. a growing economy, multiparty competition, civil liberties, a free press, close association with Western European culture and politics), but nonetheless shows little or no improvement on this dimension during the post‐ transition era ( Howard 2003 , 8). It is plausible to attribute this lack of change to its Communist past, as Howard does, in which case East Germany should be a fruitful case for the investigation of causal mechanisms. The contrast between East and West Germany provides a most‐similar analysis since the two polities share virtually everything except a Communist past. This variation is also deftly exploited by Howard.
I do not wish to dismiss the most‐different research method entirely. Surely, Howard's findings are stronger with the intensive analysis of Russia than they would be without. Yet his book would not stand securely on the empirical foundation provided by most‐different analysis alone. If one strips away the pathway‐case (East Germany) and the most‐similar analysis (East/West Germany) there is little left upon which to base an analysis of causal relations (aside from the large‐ N cross‐national analysis). Indeed, most scholars who employ the most‐different method do so in conjunction with other methods. 20 It is rarely, if ever, a standalone method. 21
Generalizing from this discussion of Marc Howard's work, I offer the following summary remarks on the most‐different method of case analysis. (I leave aside issues faced by all case‐study analyses, issues that are explored in Gerring 2007 .)
Let us begin with a methodological obstacle that is faced by both Millean styles of analysis—the necessity of dichotomizing every variable in the analysis. Recall that, as with most‐similar analysis, differences across cases must generally be sizeable enough to be interpretable in an essentially dichotomous fashion (e.g. high/low, present/absent) and similarities must be close enough to be understood as essentially identical (e.g. high/high, present/present). Otherwise the results of a Millean style analysis are not interpretable. The problem of “degrees” is deadly if the variables under consideration are, by nature, continuous (e.g. GDP). This is a particular concern in Howard's analysis, where East Germany scores somewhat higher than Russia in civic engagement; they are both low, but Russia is quite a bit lower. Howard assumes that this divergence is minimal enough to be understood as a difference of degrees rather than of kinds, a judgment that might be questioned. In these respects, most‐different analysis is no more secure—but also no less—than most‐similar analysis.
In one respect, most‐different analysis is superior to most‐similar analysis. If the coding assumptions are sound, the most‐different research design may be quite useful for eliminating necessary causes . Causal factors that do not appear across the chosen cases—e.g. X 2a–d in Table 28.4 —are evidently unnecessary for the production of Y . However, it does not follow that the most‐different method is the best method for eliminating necessary causes. Note that the defining feature of this method is the shared element across cases— X 1 in Table 28.4 . This feature does not help one to eliminate necessary causes. Indeed, if one were focused solely on eliminating necessary causes one would presumably seek out cases that register the same outcomes and have maximum diversity on other attributes. In Table 28.4 , this would be a set of cases that satisfy conditions X 2a–d , but not X 1 . Thus, even the presumed strength of the most‐different analysis is not so strong.
Usually, case‐study analysis is focused on the identification (or clarification) of causal relations, not the elimination of possible causes. In this setting, the most‐ different technique is useful, but only if assumptions of causal uniqueness hold. By “causal uniqueness,” I mean a situation in which a given outcome is the product of only one cause: Y cannot occur except in the presence of X . X is necessary, and in some situations (given certain background conditions) sufficient, to cause Y . 22
Consider the following hypothetical example. Suppose that a new disease, about which little is known, has appeared in Country A. There are hundreds of infected persons across dozens of affected communities in that country. In Country B, located at the other end of the world, several new cases of the disease surface in a single community. In this setting, we can imagine two sorts of Millean analyses. The first examines two similar communities within Country A, one of which has developed the disease and the other of which has not. This is the most‐similar style of case comparison, and focuses accordingly on the identification of a difference between the two cases that might account for variation across the sample. A second approach focuses on communities where the disease has appeared across the two countries and searches for any similarities that might account for these similar outcomes. This is the most‐different research design.
Both are plausible approaches to this particular problem, and we can imagine epidemiologists employing them simultaneously. However, the most‐different design demands stronger assumptions about the underlying factors at work. It supposes that the disease arises from the same cause in any setting. This is often a reasonable operating assumption when one is dealing with natural phenomena, though there are certainly many exceptions. Death, for example, has many causes. For this reason, it would not occur to us to look for most‐different cases of high mortality around the world. In order for the most‐different research design to effectively identify a causal factor at work in a given outcome, the researcher must assume that X 1 —the factor held constant across the diverse cases—is the only possible cause of Y (see Table 28.4 ). This assumption rarely holds in social‐scientific settings. Most outcomes of interest to anthropologists, economists, political scientists, and sociologists have multiple causes. There are many ways to win an election, to build a welfare state, to get into a war, to overthrow a government, or—returning to Marc Howard's work—to build a strong civil society. And it is for this reason that most‐different analysis is rarely applied in social science work and, where applied, is rarely convincing.
If this seems a tad severe, there is a more charitable way of approaching the most‐different method. Arguably, this is not a pure “method” at all but merely a supplement, a way of incorporating diversity in the sub‐sample of cases that provide the unusual outcome of interest. If the unusual outcome is revolutions, one might wish to encompass a wide variety of revolutions in one's analysis. If the unusual outcome is post‐Communist civil society, it seems appropriate to include a diverse set of post‐Communist polities in one's sample of case studies, as Marc Howard does. From this perspective, the most‐different method (so‐called) might be better labeled a diverse‐case method, as explored above.
10 Conclusions
In order to be a case of something broader than itself, the chosen case must be representative (in some respects) of a larger population. Otherwise—if it is purely idiosyncratic (“unique”)—it is uninformative about anything lying outside the borders of the case itself. A study based on a nonrepresentative sample has no (or very little) external validity. To be sure, no phenomenon is purely idiosyncratic; the notion of a unique case is a matter that would be difficult to define. One is concerned, as always, with matters of degree. Cases are more or less representative of some broader phenomenon and, on that score, may be considered better or worse subjects for intensive analysis. (The one exception, as noted, is the influential case.)
Of all the problems besetting case‐study analysis, perhaps the most persistent— and the most persistently bemoaned—is the problem of sample bias ( Achen and Snidal 1989 ; Collier and Mahoney 1996 ; Geddes 1990 ; King, Keohane, and Verba 1994 ; Rohlfing 2004 ; Sekhon 2004 ). Lisa Martin (1992 , 5) finds that the overemphasis of international relations scholars on a few well‐known cases of economic sanctions— most of which failed to elicit any change in the sanctioned country—“has distorted analysts view of the dynamics and characteristics of economic sanctions.” Barbara Geddes (1990) charges that many analyses of industrial policy have focused exclusively on the most successful cases—primarily the East Asian NICs—leading to biased inferences. Anna Breman and Carolyn Shelton (2001) show that case‐study work on the question of structural adjustment is systematically biased insofar as researchers tend to focus on disaster cases—those where structural adjustment is associated with very poor health and human development outcomes. These cases, often located in sub‐Saharan Africa, are by no means representative of the entire population. Consequently, scholarship on the question of structural adjustment is highly skewed in a particular ideological direction (against neoliberalism) (see also Gerring, Thacker, and Moreno 2005) .
These examples might be multiplied many times. Indeed, for many topics the most‐studied cases are acknowledged to be less than representative. It is worth reflecting upon the fact that our knowledge of the world is heavily colored by a few “big” (populous, rich, powerful) countries, and that a good portion of the disciplines of economics, political science, and sociology are built upon scholars' familiarity with the economics, political science, and sociology of one country, the United States. 23 Case‐study work is particularly prone to problems of investigator bias since so much rides on the researcher's selection of one (or a few) cases. Even if the investigator is unbiased, her sample may still be biased simply by virtue of “random” error (which may be understood as measurement error, error in the data‐generation process, or as an underlying causal feature of the universe).
There are only two situations in which a case‐study researcher need not be concerned with the representativeness of her chosen case. The first is the influential case research design, where a case is chosen because of its possible influence on a cross‐case model, and hence is not expected to be representative of a larger sample. The second is the deviant‐case method, where the chosen case is employed to confirm a broader cross‐case argument to which the case stands as an apparent exception. Yet even here the chosen case is expected to be representative of a broader set of cases—those, in particular, that are poorly explained by the extant model.
In all other circumstances, cases must be representative of the population of interest in whatever ways might be relevant to the proposition in question. Note that where a researcher is attempting to disconfirm a deterministic proposition the question of representativeness is perhaps more appropriately understood as a question of classification: Is the chosen case appropriately classified as a member of the designated population? If so, then it is fodder for a disconfirming case study.
If the researcher is attempting to confirm a deterministic proposition, or to make probabilistic arguments about a causal relationship, then the problem of representativeness is of the more usual sort: Is case A unit‐homogeneous relative to other cases in the population? This is not an easy matter to test. However, in a large‐ N context the residual for that case (in whatever model the researcher has greatest confidence in) is a reasonable place to start. Of course, this test is only as good as the model at hand. Any incorrect specifications or incorrect modeling procedures will likely bias the results and give an incorrect assessment of each case's “typicality.” In addition, there is the possibility of stochastic error, errors that cannot be modeled in a general framework. Given the explanatory weight that individual cases are asked to bear in a case‐study analysis, it is wise to consider more than just the residual test of representativeness. Deductive logic and an in‐depth knowledge of the case in question are often more reliable tools than the results of a cross‐case model.
In any case, there is no dispensing with the question. Case studies (with the two exceptions already noted) rest upon an assumed synecdoche: The case should stand for a population. If this is not true, or if there is reason to doubt this assumption, then the utility of the case study is brought severely into question.
Fortunately, there is some safety in numbers. Insofar as case‐study evidence is combined with cross‐case evidence the issue of sample bias is mitigated. Indeed, the suspicion of case‐study work that one finds in the social sciences today is, in my view, a product of a too‐literal interpretation of the case‐study method. A case study tout court is thought to mean a case study tout seul . Insofar as case studies and cross‐case studies can be enlisted within the same investigation (either in the same study or by reference to other studies in the same subfield), problems of representativeness are less worrisome. This is the virtue of cross‐level work, a.k.a. “triangulation.”
11 Ambiguities
Before concluding, I wish to draw attention to two ambiguities in case‐selection strategies in case‐study research. The first concerns the admixture of several case‐ selection strategies. The second concerns the changing status of a case as a study proceeds.
Some case studies follow only one strategy of case selection. They are typical , diverse , extreme , deviant , influential , crucial , pathway , most‐similar , or most‐different research designs, as discussed. However, many case studies mix and match among these case‐selection strategies. Indeed, insofar as all case studies seek representative samples, they are always in search of “typical” cases. Thus, it is common for writers to declare that their case is, for example, both extreme and typical; it has an extreme value on X 1 or Y but is not, in other respects, idiosyncratic. There is not much that one can say about these combinations of strategies except that, where the cases allow for a variety of empirical strategies, there is no reason not to pursue them. And where the same cases can serve several functions at once (without further effort on the researcher's part), there is little cost to a multi‐pronged approach to case analysis.
The second issue that deserves emphasis is the changing status of a case during the course of a researcher's investigation—which may last for years, if not decades. The problem is acute wherever a researcher begins in an exploratory mode and proceeds to hypothesis‐testing (that is, she develops a specific X 1 / Y proposition) or where the operative hypothesis or key control variable changes (a new causal factor is discovered or another outcome becomes the focus of analysis). Things change. And it is the mark of a good researcher to keep her mind open to new evidence and new insights. Too often, methodological discussions give the misleading impression that hypotheses are clear and remain fixed over the course of a study's development. Nothing could be further from the truth. The unofficial transcripts of academia— accessible in informal settings, where researchers let their guards down (particularly if inebriated)—are filled with stories about dead‐ends, unexpected findings, and drastically revised theory chapters. It would be interesting, in this vein, to compare published work with dissertation prospectuses and fellowship applications. I doubt if the correlation between these two stages of research is particularly strong.
Research, after all, is about discovery, not simply the verification or falsification of static hypotheses. That said, it is also true that research on a particular topic should move from hypothesis generating to hypothesis‐testing. This marks the progress of a field, and of a scholar's own work. As a rule, research that begins with an open‐ended ( X ‐ or Y ‐centered) analysis should conclude with a determinate X 1 / Y hypothesis.
The problem is that research strategies that are ideal for exploration are not always ideal for confirmation. The extreme‐case method is inherently exploratory since there is no clear causal hypothesis; the researcher is concerned merely to explore variation on a single dimension ( X or Y ). Other methods can be employed in either an open‐ ended (exploratory) or a hypothesis‐testing (confirmatory/disconfirmatory) mode. The difficulty is that once the researcher has arrived at a determinate hypothesis the originally chosen research design may no longer appear to be so well designed.
This is unfortunate, but inevitable. One cannot construct the perfect research design until (a) one has a specific hypothesis and (b) one is reasonably certain about what one is going to find “out there” in the empirical world. This is particularly true of observational research designs, but it also applies to many experimental research designs: Usually, there is a “good” (informative) finding, and a finding that is less insightful. In short, the perfect case‐study research design is usually apparent only ex post facto .
There are three ways to handle this. One can explain, straightforwardly, that the initial research was undertaken in an exploratory fashion, and therefore not constructed to test the specific hypothesis that is—now—the primary argument. Alternatively, one can try to redesign the study after the new (or revised) hypothesis has been formulated. This may require additional field research or perhaps the integration of additional cases or variables that can be obtained through secondary sources or through consultation of experts. A final approach is to simply jettison, or de‐emphasize, the portion of research that no longer addresses the (revised) key hypothesis. A three‐case study may become a two‐case study, and so forth. Lost time and effort are the costs of this downsizing.
In the event, practical considerations will probably determine which of these three strategies, or combinations of strategies, is to be followed. (They are not mutually exclusive.) The point to remember is that revision of one's cross‐case research design is normal and perhaps to be expected. Not all twists and turns on the meandering trail of truth can be anticipated.
12 Are There Other Methods of Case Selection?
At the outset of this chapter I summarized the task of case selection as a matter of achieving two objectives: representativeness (typicality) and variation (causal leverage). Evidently, there are other objectives as well. For example, one wishes to identify cases that are independent of each other. If chosen cases are affected by each other (sometimes known as Galton's problem or a problem of diffusion), this problem must be corrected before analysis can take place. I have neglected this issue because it is usually apparent to the researcher and, in any case, there are no simple techniques that might be utilized to correct for such biases. (For further discussion of this and other factors impinging upon case selection see Gerring 2001 , 178–81.)
I have also disregarded pragmatic/logistical issues that might affect case selection. Evidently, case selection is often influenced by a researcher's familiarity with the language of a country, a personal entrée into that locale, special access to important data, or funding that covers one archive rather than another. Pragmatic considerations are often—and quite rightly—decisive in the case‐selection process.
A final consideration concerns the theoretical prominence of a particular case within the literature on a subject. Researchers are sometimes obliged to study cases that have received extensive attention in previous studies. These are sometimes referred to as “paradigmatic” cases or “exemplars” ( Flyvbjerg 2004 , 427).
However, neither pragmatic/logistical utility nor theoretical prominence qualifies as a methodological factor in case selection. That is, these features of a case have no bearing on the validity of the findings stemming from a study. As such, it is appropriate to grant these issues a peripheral status in this chapter.
One final caveat must be issued. While it is traditional to distinguish among the tasks of case selection and case analysis, a close look at these processes shows them to be indistinct and overlapping. One cannot choose a case without considering the sort of analysis that it might be subjected to, and vice versa. Thus, the reader should consider choosing cases by employing the nine techniques laid out in this chapter along with any considerations that might be introduced by virtue of a case's quasi‐experimental qualities, a topic taken up elsewhere ( Gerring 2007 , ch. 6 ).
Abadie, A. , Drukker, D. , Herr, J. L. , and Imbens, G. W. 2001 . Implementing matching estimators for average treatment effects in Stata. Stata Journal , 1: 1–18.
Google Scholar
Abbott, A. 2001 . Time Matters: On Theory and Method . Chicago: University of Chicago Press.
Google Preview
—— and Tsay, A. 2000 . Sequence analysis and optimal matching methods in sociology. Sociological Methods and Research , 29: 3–33. 10.1177/0049124100029001001
—— and Forrest, J. 1986 . Optimal matching methods for historical sequences. Journal of Interdisciplinary History , 16: 471–94. 10.2307/204500
Achen, C. H. , and Snidal, D. 1989 . Rational deterrence theory and comparative case studies. World Politics , 41: 143–69. 10.2307/2010405
Allen, W. S. 1965 . The Nazi Seizure of Power: The Experience of a Single German Town, 1930–1935 . New York: Watts.
Almond, G. A. 1956 . Comparative political systems. Journal of Politics , 18: 391–409.
Amenta, E. 1991 . Making the most of a case study: theories of the welfare state and the American experience. Pp. 172–94 in Issues and Alternatives in Comparative Social Research ed. C. C. Ragin . Leiden: E. J. Brill.
Barro, R. J. 1999 . Determinants of democracy. Journal of Political Economy , 107: 158–83. 10.1086/250107
Belsey, D. A. , Kuh, E. , and Welsch, R. E. 2004 . Regression Diagnostics: Identifying Influential Data and Sources of Collinearity . New York: Wiley.
Bennett, A. , Lepgold, J. , and Unger, D. 1994 . Burden‐sharing in the Persian Gulf War. International Organization , 48: 39–75. 10.1017/S0020818300000813
Bentley, A. 1908/ 1967 . The Process of Government . Cambridge, Mass.: Harvard University Press.
Brady, H. E. , and Collier, D. (eds.) 2004 . Rethinking Social Inquiry: Diverse Tools, Shared Standards . Lanham, Md.: Rowman and Littlefield.
Braumoeller, B. F. 2003 . Causal complexity and the study of politics. Political Analysis , 11: 209–33. 10.1093/pan/mpg012
Breman, A. , and Shelton, C. 2001. Structural adjustment and health: a literature review of the debate, its role‐players and presented empirical evidence. CMH Working Paper Series, Paper No. WG6: 6. WHO, Commission on Macroeconomics and Health.
Brenner, R. 1976 . Agrarian class structure and economic development in pre‐industrial Europe. Past and Present , 70: 30–75. 10.1093/past/70.1.30
Browne, A. 1987 . When Battered Women Kill . New York: Free Press.
Buchbinder, S. , and Vittinghoff, E. 1999 . HIV‐infected long‐term nonprogressors: epidemiology, mechanisms of delayed progression, and clinical and research implications. Microbes Infect , 1: 1113–20. 10.1016/S1286-4579(99)00204-X
Cohen, M. R. , and Nagel, E. 1934 . An Introduction to Logic and Scientific Method . New York: Harcourt, Brace and Company.
Collier, D. , and Mahoney, J. 1996 . Insights and pitfalls: selection bias in qualitative research. World Politics , 49: 56–91. 10.1353/wp.1996.0023
Collier, R. B. , and Collier, D. 1991/ 2002 . Shaping the Political Arena: Critical Junctures, the Labor Movement, and Regime Dynamics in Latin America . Notre Dame, Ind.: University of Notre Dame Press.
Colomer, J. M. 1991 . Transitions by agreement: modeling the Spanish way. American Political Science Review , 85: 1283–302. 10.2307/1963946
Converse, P. E. , and Dupeux, G. 1962 . Politicization of the electorate in France and the United States. Public Opinion Quarterly , 16: 1–23. 10.1086/267067
Coppedge, M. J. 2004. The conditional impact of the economy on democracy in Latin America. Presented at the conference “Democratic Advancements and Setbacks: What Have We Learnt?”, Uppsala University, June 11–13.
De Felice, E. G. 1986 . Causal inference and comparative methods. Comparative Political Studies , 19: 415–37. 10.1177/0010414086019003005
Desch, M. C. 2002 . Democracy and victory: why regime type hardly matters. International Security , 27: 5–47. 10.1162/016228802760987815
Deyo, F. (ed.) 1987 . The Political Economy of the New Asian Industrialism . Ithaca, NY: Cornell University Press.
Dion, D. 1998 . Evidence and inference in the comparative case study. Comparative Politics , 30: 127–45. 10.2307/422284
Eckstein, H. 1975 . Case studies and theory in political science. In Handbook of Political Science , vii: Political Science: Scope and Theory , ed. F. I. Greenstein and N. W. Polsby . Reading, Mass.: Addison‐Wesley.
Eggan, F. 1954 . Social anthropology and the method of controlled comparison. American Anthropologist , 56: 743–63. 10.1525/aa.1954.56.5.02a00020
Elman, C. 2003 . Lessons from Lakatos. In Progress in International Relations Theory: Appraising the Field , ed. C. Elman and M. F. Elman . Cambridge, Mass.: MIT Press.
—— 2005 . Explanatory typologies in qualitative studies of international politics. International Organization , 59: 293–326.
Emigh, R. 1997 . The power of negative thinking: the use of negative case methodology in the development of sociological theory. Theory and Society , 26: 649–84. 10.1023/A:1006896217647
Epstein, L. D. 1964 . A comparative study of Canadian parties. American Political Science Review , 58: 46–59. 10.2307/1952754
Ertman, T. 1997 . Birth of the Leviathan: Building States and Regimes in Medieval and Early Modern Europe . Cambridge: Cambridge University Press.
Esping‐Andersen, G. 1990 . The Three Worlds of Welfare Capitalism . Princeton, NJ: Princeton University Press.
Flyvbjerg, B. 2004 . Five misunderstandings about case‐study research. Pp. 420–34 in Qualitative Research Practice , ed. C. Seale , G. Gobo , J. F. Gubrium , and D. Silverman . London: Sage.
Geddes, B. 1990 . How the cases you choose affect the answers you get: selection bias in comparative politics. In Political Analysis , vol. ii, ed. J. A. Stimson . Ann Arbor: University of Michigan Press.
—— 2003 . Paradigms and Sand Castles: Theory Building and Research Design in Comparative Politics . Ann Arbor: University of Michigan Press.
George, A. L. , and Bennett, A. 2005 . Case Studies and Theory Development . Cambridge, Mass.: MIT Press.
—— and Smoke, R. 1974 . Deterrence in American Foreign Policy: Theory and Practice . New York: Columbia University Press.
Gerring, J. 2001 . Social Science Methodology: A Criterial Framework . Cambridge: Cambridge University Press.
—— 2007 . Case Study Research: Principles and Practices . Cambridge: Cambridge University Press.
—— Thacker, S. and Moreno, C. 2005. Do neoliberal policies save lives? Unpublished manuscript.
Goertz, G. and Starr, H. (eds.) 2003 . Necessary Conditions: Theory, Methodology and Applications . New York: Rowman and Littlefield.
—— and Levy, J. (eds.) forthcoming. Causal explanations, necessary conditions, and case studies: World War I and the end of the Cold War. Manuscript.
Goodin, R. E. and Smitsman, A. 2000 . Placing welfare states: the Netherlands as a crucial test case. Journal of Comparative Policy Analysis , 2: 39–64. 10.1080/13876980008412635
Gujarati, D. N. 2003 . Basic Econometrics , 4th edn. New York: McGraw‐Hill.
Hamilton, G. G. 1977 . Chinese consumption of foreign commodities: a comparative perspective. American Sociological Review , 42: 877–91. 10.2307/2094574
Haynes, B. F. Pantaleo, G. and Fauci, A. S. 1996 . Toward an understanding of the correlates of protective immunity to HIV infection. Science , 271: 324–8. 10.1126/science.271.5247.324
Hempel, C. G. 1942 . The function of general laws in history. Journal of Philosophy , 39: 35–48. 10.2307/2017635
Ho, D. E. Imai, K. King, G. and Stuart, E. A. 2004. Matching as nonparametric preprocessing for reducing model dependence in parametric causal inference. Manuscript.
Howard, M. M. 2003 . The Weakness of Civil Society in Post‐Communist Europe . Cambridge: Cambridge University Press.
Howson, C. and Urbach, P. 1989 . Scientific Reasoning: The Bayesian Approach . La Salle, Ill.: Open Court.
Humphreys, M. 2005 . Natural resources, conflict, and conflict resolution: uncovering the mechanisms. Journal of Conflict Resolution , 49: 508–37. 10.1177/0022002705277545
Jenicek, M. 2001 . Clinical Case Reporting in Evidence‐Based Medicine , 2nd edn. Oxford: Oxford University Press.
Karl, T. L. 1997 . The Paradox of Plenty: Oil Booms and Petro‐states . Berkeley: University of California Press.
Kazancigil, A. 1994 . The deviant case in comparative analysis: high stateness in comparative analysis. Pp. 213–38 in Comparing Nations: Concepts, Strategies, Substance , ed. M. Dogan and A. Kazancigil . Cambridge: Blackwell.
Kemp, K. A. 1986 . Race, ethnicity, class and urban spatial conflict: Chicago as a crucial case Urban Studies , 23: 197–208. 10.1080/00420988620080231
Kendall, P. L. and Wolf, K. M. 1949/ 1955 . The analysis of deviant cases in communications research. In Communications Research, 1948–1949 , ed. P. F. Lazarsfeld and F. N. Stanton. New York: Harper and Brothers. Reprinted as pp. 167–70 in The Language of Social Research , ed. P. F. Lazarsfeld and M. Rosenberg . New York: Free Press.
Kennedy, C. H. 2005 . Single‐case Designs for Educational Research . Boston: Allyn and Bacon.
Kennedy, P. 2003 . A Guide to Econometrics , 5th edn. Cambridge, Mass.: MIT Press.
Khong, Y. F. 1992 . Analogies at War: Korea, Munich, Dien Bien Phu, and the Vietnam Decisions of 1965 . Princeton, NJ: Princeton University Press.
King, G. Keohane, R. O. and Verba, S. 1994 . Designing Social Inquiry: Scientific Inference in Qualitative Research . Princeton, NJ: Princeton University Press.
Lakatos, I. 1978 . The Methodology of Scientific Research Programmes . Cambridge: Cambridge University Press.
Lazarsfeld, P. F. and Barton, A. H. 1951 . Qualitative measurement in the social sciences: classification, typologies, and indices. In The Policy Sciences , ed. D. Lerner and H. D. Lass‐ well. Stanford, Calif.: Stanford University Press.
Levy, J. S. 2002 . Qualitative methods in international relations. In Evaluating Methodology in International Studies , ed. F. P. Harvey and M. Brecher. Ann Arbor: University of Michigan Press.
Lijphart, A. 1968 . The Politics of Accommodation: Pluralism and Democracy in the Netherlands . Berkeley: University of California Press.
—— 1969 . Consociational democracy. World Politics , 21: 207–25. 10.2307/2009820
—— 1971 . Comparative politics and the comparative method. American Political Science Review , 65: 682–93.
—— 1975 . The comparable cases strategy in comparative research. Comparative Political Studies , 8: 158–77.
Lipset, S. M. 1959 . Some social requisites of democracy: economic development and political development. American Political Science Review , 53: 69–105. 10.2307/1951731
—— 1960/ 1963 . Political Man: The Social Bases of Politics . Garden City, NY: Anchor.
—— 1968 . Agrarian Socialism: The Cooperative Commonwealth Federation in Saskatchewan. A Study in Political Sociology . Garden City, NY: Doubleday.
—— Trow, M. A. and Coleman, J. S. 1956 . Union Democracy: The Internal Politics of the International Typographical Union . New York: Free Press.
Lynd, R. S. and Lynd, H. M. 1929/ 1956 . Middletown: A Study in American Culture . New York: Harcourt, Brace.
Mahoney, J. and Goertz, G. 2004 . The possibility principle: choosing negative cases in comparative research. American Political Science Review , 98: 653–69.
Martin, L. L. 1992 . Coercive Cooperation: Explaining Multilateral Economic Sanctions .Princeton, NJ: Princeton University Press.
Mayo, D. G. 1996 . Error and the Growth of Experimental Knowledge . Chicago: University of Chicago Press.
Meckstroth, T. 1975 . “Most different systems” and “most similar systems:” a study in the logic of comparative inquiry. Comparative Political Studies , 8: 133–77.
Miguel, E. 2004 . Tribe or nation: nation‐building and public goods in Kenya versus Tanzania. World Politics , 56: 327–62. 10.1353/wp.2004.0018
Mill, J. S. 1843/ 1872 . The System of Logic , 8th edn. London: Longmans, Green.
Monroe, K. R. 1996 . The Heart of Altruism: Perceptions of a Common Humanity . Princeton, NJ: Princeton University Press.
Moore, B., Jr. 1966 . Social Origins of Dictatorship and Democracy: Lord and Peasant in the Making of the Modern World . Boston: Beacon Press.
Morgan, S. L. and Harding, D. J. 2005. Matching estimators of causal effects: from stratification and weighting to practical data analysis routines. Manuscript.
Moulder, F. V. 1977 . Japan, China and the Modern World Economy: Toward a Reinterpretation of East Asian Development ca. 1600 to ca. 1918 . Cambridge: Cambridge University Press.
Munck, G. L. 2004 . Tools for qualitative research. Pp. 105–21 in Rethinking Social Inquiry: Diverse Tools, Shared Standards , ed. H. E. Brady and D. Collier . Lanham, Md. : Rowman and Littlefield.
Njolstad, O. 1990 . Learning from history? Case studies and the limits to theory‐building. Pp. 220–46 in Arms Races: Technological and Political Dynamics , ed. O. Njolstad . Thousand Oaks, Calif.: Sage.
Patton, M. Q. 2002 . Qualitative Evaluation and Research Methods . Newbury Park, Calif.: Sage.
Popper, K. 1934/ 1968 . The Logic of Scientific Discovery . New York: Harper and Row.
—— 1963 . Conjectures and Refutations . London: Routledge and Kegan Paul.
Posner, D. 2004 . The political salience of cultural difference: why Chewas and Tumbukas are allies in Zambia and adversaries in Malawi. American Political Science Review , 98: 529–46.
Przeworski, A. and Teune, H. 1970 . The Logic of Comparative Social Inquiry . New York: John Wiley.
Queen, S. 1928 . Round table on the case study in sociological research. Publications of the American Sociological Society, Papers and Proceedings , 22: 225–7.
Ragin, C. C. 2000 . Fuzzy‐set Social Science . Chicago: University of Chicago Press.
—— 2004 . Turning the tables. Pp. 123–38 in Rethinking Social Inquiry: Diverse Tools, Shared Standards , ed. H. E. Brady and D. Collier. Lanham, Md. : Rowman and Littlefield.
Reilly, B. 2000 –1. Democracy, ethnic fragmentation, and internal conflict: confused theories, faulty data, and the “crucial case” of Papua New Guinea. International Security , 25: 162–85. 10.1162/016228800560552
—— and Phillpot, R. 2003 . “Making democracy work” in Papua New Guinea: social capital and provincial development in an ethnically fragmented society. Asian Survey , 42: 906–27. 10.1525/as.2002.42.6.906
Rogowski, R. 1995 . The role of theory and anomaly in social‐scientific inference. American Political Science Review , 89: 467–70. 10.2307/2082443
Rohlfing, I. 2004. Have you chosen the right case? Uncertainty in case selection for single case studies. Working Paper, International University, Bremen.
Rosenbaum, P. R. 2004 . Matching in observational studies. In Applied Bayesian Modeling and Causal Inference from an Incomplete‐data Perspective , ed. A. Gelman and X.‐L. Meng . New York: John Wiley.
—— and Silber, J. H. 2001 . Matching and thick description in an observational study of mortality after surgery. Biostatistics , 2: 217–32. 10.1093/biostatistics/2.2.217
Ross, M. 2001 . Does oil hinder democracy? World Politics , 53: 325–61. 10.1353/wp.2001.0011
Sagan, S. D. 1995 . Limits of Safety: Organizations, Accidents, and Nuclear Weapons . Princeton, NJ: Princeton University Press.
Sekhon, J. S. 2004 . Quality meets quantity: case studies, conditional probability and counter‐ factuals. Perspectives in Politics , 2: 281–93.
Shafer, M. D. 1988 . Deadly Paradigms: The Failure of U.S. Counterinsurgency Policy . Princeton, NJ: Princeton University Press.
Skocpol, T. 1979 . States and Social Revolutions: A Comparative Analysis of France, Russia, and China . Cambridge: Cambridge University Press.
—— and Somers, M. 1980 . The uses of comparative history in macrosocial inquiry. Comparative Studies in Society and History , 22: 147–97.
Stinchcombe, A. L. 1968 . Constructing Social Theories . New York: Harcourt, Brace.
Swank, D. H. 2002 . Global Capital, Political Institutions, and Policy Change in Developed Welfare States . Cambridge: Cambridge University Press.
Tendler, J. 1997 . Good Government in the Tropics . Baltimore: Johns Hopkins University Press.
Truman, D. B. 1951 . The Governmental Process . New York: Alfred A. Knopf.
Tsai, L. 2007 . Accountability without Democracy: How Solidary Groups Provide Public Goods in Rural China . Cambridge: Cambridge University Press.
Van Evera, S. 1997 . Guide to Methods for Students of Political Science . Ithaca, NY: Cornell University Press.
Wahlke, J. C. 1979 . Pre‐behavioralism in political science. American Political Science Review , 73: 9–31. 10.2307/1954728
Yashar, D. J. 2005 . Contesting Citizenship in Latin America: The Rise of Indigenous Movements and the Postliberal Challenge . Cambridge: Cambridge University Press.
Yin, R. K. 2004 . Case Study Anthology . Thousand Oaks, Calif.: Sage.
Gujarati (2003) ; Kennedy (2003) . Interestingly, the potential of cross‐case statistics in helping to choose cases for in‐depth analysis is recognized in some of the earliest discussions of the case‐study method (e.g. Queen 1928 , 226).
This expands on Mill (1843/1872 , 253), who wrote of scientific enquiry as twofold: “either inquiries into the cause of a given effect or into the effects or properties of a given cause.”
This method has not received much attention on the part of qualitative methodologists; hence, the absence of a generally recognized name. It bears some resemblance to J. S. Mill's Joint Method of Agreement and Difference ( Mill 1843/1872 ), which is to say a mixture of most‐similar and most‐different analysis, as discussed below. Patton (2002 , 234) employs the concept of “maximum variation (heterogeneity) sampling.”
More precisely, George and Smoke (1974 , 534, 522–36, ch. 18 ; see also discussion in Collier and Mahoney 1996 , 78) set out to investigate causal pathways and discovered, through the course of their investigation of many cases, these three causal types. Yet, for our purposes what is important is that the final sample includes at least one representative of each “type.”
For further examples see Collier and Mahoney (1996) ; Geddes (1990) ; Tendler (1997) .
Traditionally, methodologists have conceptualized cases as having “positive” or “negative” values (e.g. Emigh 1997 ; Mahoney and Goertz 2004 ; Ragin 2000 , 60; 2004 , 126).
Geddes (1990) ; King, Keohane, and Verba (1994) . See also discussion in Brady and Collier (2004) ; Collier and Mahoney (1996) ; Rogowski (1995) .
The exception would be a circumstance in which the researcher intends to disprove a deterministic argument ( Dion 1998 ).
Geddes (2003 , 131). For other examples of casework from the annals of medicine see “Clinical reports” in the Lancet , “Case studies” in Canadian Medical Association Journal , and various issues of the Journal of Obstetrics and Gynecology , often devoted to clinical cases (discussed in Jenicek 2001 , 7). For examples from the subfield of comparative politics see Kazancigil (1994) .
For a discussion of the important role of anomalies in the development of scientific theorizing see Elman (2003) ; Lakatos (1978) . For examples of deviant‐case research designs in the social sciences see Amenta (1991) ; Coppedge (2004) ; Eckstein (1975) ; Emigh (1997) ; Kendall and Wolf (1949/1955) .
For examples of the crucial‐case method see Bennett, Lepgold, and Unger (1994) ; Desch (2002) ; Goodin and Smitsman (2000) ; Kemp (1986) ; Reilly and Phillpot (2003) . For general discussion see George and Bennett (2005) ; Levy (2002) ; Stinchcombe (1968 , 24–8).
A third position, which purports to be neither Popperian or Bayesian, has been articulated by Mayo (1996 , ch. 6 ). From this perspective, the same idea is articulated as a matter of “severe tests.”
It should be noted that Tsai's conclusions do not rest solely on this crucial case. Indeed, she employs a broad range of methodological tools, encompassing case‐study and cross‐case methods.
See also the discussion in Eckstein (1975) and Lijphart (1969) . For additional examples of case studies disconfirming general propositions of a deterministic nature see Allen (1965); Lipset, Trow, and Coleman (1956) ; Njolstad (1990) ; Reilly (2000–1) ; and discussion in Dion (1998) ; Rogowski (1995) .
Granted, insofar as case‐study analysis provides a window into causal mechanisms, and causal mechanisms are integral to a given theory, a single case may be enlisted to confirm or disconfirm a proposition. However, if the case study upholds a posited pattern of X/Y covariation, and finds fault only with the stipulated causal mechanism, it would be more accurate to say that the study forces the reformulation of a given theory, rather than its confirmation or disconfirmation. See further discussion in the following section.
Sometimes, the most‐similar method is known as the “method of difference,” after its inventor ( Mill 1843/1872 ). For later treatments see Cohen and Nagel (1934) ; Eggan (1954) ; Gerring (2001 , ch. 9 ); Lijphart (1971 ; 1975) ; Meckstroth (1975) ; Przeworski and Teune (1970) ; Skocpol and Somers (1980) .
For good introductions see Ho et al. (2004) ; Morgan and Harding (2005) ; Rosenbaum (2004) ; Rosenbaum and Silber (2001) . For a discussion of matching procedures in Stata see Abadie et al. (2001) .
The most‐different method is also sometimes referred to as the “method of agreement,” following its inventor, J. S. Mill (1843/1872) . See also De Felice (1986) ; Gerring (2001 , 212–14); Lijphart (1971 ; 1975) ; Meckstroth (1975) ; Przeworski and Teune (1970) ; Skocpol and Somers (1980) . For examples of this method see Collier and Collier (1991/2002) ; Converse and Dupeux (1962) ; Karl (1997) ; Moore (1966) ; Skocpol (1979) ; Yashar (2005 , 23). However, most of these studies are described as combining most‐similar and most‐different methods.
In the following discussion I treat the terms social capital, civil society, and civic engagement interchangeably.
E.g. Collier and Collier (1991/2002) ; Karl (1997) ; Moore (1966) ; Skocpol (1979) ; Yashar (2005 , 23). Karl (1997) , which affects to be a most‐different system analysis (20), is a particularly clear example of this. Her study, focused ostensibly on petro‐states (states with large oil reserves), makes two sorts of inferences. The first concerns the (usually) obstructive role of oil in political and economic development. The second sort of inference concerns variation within the population of petro‐states, showing that some countries (e.g. Norway, Indonesia) manage to avoid the pathologies brought on elsewhere by oil resources. When attempting to explain the constraining role of oil on petro‐states, Karl usually relies on contrasts between petro‐states and nonpetro‐states (e.g. ch. 10 ). Only when attempting to explain differences among petro‐states does she restrict her sample to petro‐states. In my opinion, very little use is made of the most‐different research design.
This was recognized, at least implicitly, by Mill (1843/1872 , 258–9). Skepticism has been echoed by methodologists in the intervening years (e.g. Cohen and Nagel 1934 , 251–6; Gerring 2001 ; Skocpol and Somers 1980 ). Indeed, explicit defenses of the most‐different method are rare (but see De Felice 1986 ).
Another way of stating this is to say that X is a “nontrivial necessary condition” of Y .
Wahlke (1979 , 13) writes of the failings of the “behavioralist” mode of political science analysis: “It rarely aims at generalization; research efforts have been confined essentially to case studies of single political systems, most of them dealing …with the American system.”
- About Oxford Academic
- Publish journals with us
- University press partners
- What we publish
- New features
- Open access
- Institutional account management
- Rights and permissions
- Get help with access
- Accessibility
- Advertising
- Media enquiries
- Oxford University Press
- Oxford Languages
- University of Oxford
Oxford University Press is a department of the University of Oxford. It furthers the University's objective of excellence in research, scholarship, and education by publishing worldwide
- Copyright © 2024 Oxford University Press
- Cookie settings
- Cookie policy
- Privacy policy
- Legal notice
This Feature Is Available To Subscribers Only
Sign In or Create an Account
This PDF is available to Subscribers Only
For full access to this pdf, sign in to an existing account, or purchase an annual subscription.
Case Selection Techniques in Case Study Research: A Menu of Qualitative and Quantitative Options
- Related Documents
Case Selection for Case‐Study Analysis: Qualitative and Quantitative Techniques
This article presents some guidance by cataloging nine different techniques for case selection: typical, diverse, extreme, deviant, influential, crucial, pathway, most similar, and most different. It also indicates that if the researcher is starting from a quantitative database, then methods for finding influential outliers can be used. In particular, the article clarifies the general principles that might guide the process of case selection in case-study research. Cases are more or less representative of some broader phenomenon and, on that score, may be considered better or worse subjects for intensive analysis. The article then draws attention to two ambiguities in case-selection strategies in case-study research. The first concerns the admixture of several case-selection strategies. The second concerns the changing status of a case as a study proceeds. Some case studies follow only one strategy of case selection.
Case Selection and Hypothesis Testing
This chapter discusses quantitative and qualitative practices of case-study selection when the goal of the analysis is to evaluate causal hypotheses. More specifically, it considers how the different causal models used in the qualitative and quantitative research cultures shape the kind of cases that provide the most leverage for hypothesis testing. The chapter examines whether one should select cases based on their value on the dependent variable. It also evaluates the kinds of cases that provide the most leverage for causal inference when conducting case-study research. It shows that differences in research goals between quantitative and qualitative scholars yield distinct ideas about best strategies of case selection. Qualitative research places emphasis on explaining particular cases; quantitative research does not.
Paying Attention to the Trees in the Forest, or a Call to Examine Agency-Specific Stories
Public administration scholarship needs to strike a better balance between large sample studies and in-depth case studies. The availability of large data sets has led us to engage in empirical research that is broad in scope but is frequently devoid of rich context. In-depth case studies can help to explain why we observe particular relationships and can help us to clarify gaps and inconsistencies in theory. Our argument for more case studies aims to encourage researchers to bridge insights from qualitative and quantitative research through triangulation. We describe the value of case study research, and qualitative and quantitative design options. We then propose opportunities for case study research in public personnel scholarship on patronage pressures, performance management, and diversity management.
Case Selection Techniques in Case Study Research
Blending the focused ethnographic method and case study research: implications regarding case selection and generalization of results.
In this article, we present the benefits of blending the methodological characteristics of the focused ethnographic method (FEM) and case study research (CSR) for a study on auxiliary work processes in a hospital in Barcelona, Spain. We argue that incorporating CSR logic and principles in the FEM produces a better form of inquiry, as this combination improves the quality of data and the ability to make comparisons in addition to enhancing the transferability of findings. These better outcomes are achieved because the characteristics of the case study methodological design reinforce many of the strengths of the FEM, such as its thematic concreteness and its targeted data collection. The role played by the theoretical framework of the study thus makes it easier to focus on the key variables of the initial theoretical model and to introduce a logic of multiple case comparison.
Qualitative Case Study Research Design
Qualitative case study research can be a valuable tool for answering complex, real-world questions. This method is often misunderstood or neglected due to a lack of understanding by researchers and reviewers. This tutorial defines the characteristics of qualitative case study research and its application to a broader understanding of stuttering that cannot be defined through other methodologies. This article will describe ways that data can be collected and analyzed.
Intersecting Mixed Methods and Case Study Research: Design Possibilities and Challenges
Robert k. yin. (2014). case study research design and methods (5th ed.). thousand oaks, ca: sage. 282 pages., cooperative banks & local government units - areas of collaboration (poland case study - research results), from the ‘frying-pan’ into the ‘fire’ antidotes to confirmatory bias in case-study research, export citation format, share document.
- Open access
- Published: 27 June 2011
The case study approach
- Sarah Crowe 1 ,
- Kathrin Cresswell 2 ,
- Ann Robertson 2 ,
- Guro Huby 3 ,
- Anthony Avery 1 &
- Aziz Sheikh 2
BMC Medical Research Methodology volume 11 , Article number: 100 ( 2011 ) Cite this article
779k Accesses
1040 Citations
37 Altmetric
Metrics details
The case study approach allows in-depth, multi-faceted explorations of complex issues in their real-life settings. The value of the case study approach is well recognised in the fields of business, law and policy, but somewhat less so in health services research. Based on our experiences of conducting several health-related case studies, we reflect on the different types of case study design, the specific research questions this approach can help answer, the data sources that tend to be used, and the particular advantages and disadvantages of employing this methodological approach. The paper concludes with key pointers to aid those designing and appraising proposals for conducting case study research, and a checklist to help readers assess the quality of case study reports.
Peer Review reports
Introduction
The case study approach is particularly useful to employ when there is a need to obtain an in-depth appreciation of an issue, event or phenomenon of interest, in its natural real-life context. Our aim in writing this piece is to provide insights into when to consider employing this approach and an overview of key methodological considerations in relation to the design, planning, analysis, interpretation and reporting of case studies.
The illustrative 'grand round', 'case report' and 'case series' have a long tradition in clinical practice and research. Presenting detailed critiques, typically of one or more patients, aims to provide insights into aspects of the clinical case and, in doing so, illustrate broader lessons that may be learnt. In research, the conceptually-related case study approach can be used, for example, to describe in detail a patient's episode of care, explore professional attitudes to and experiences of a new policy initiative or service development or more generally to 'investigate contemporary phenomena within its real-life context' [ 1 ]. Based on our experiences of conducting a range of case studies, we reflect on when to consider using this approach, discuss the key steps involved and illustrate, with examples, some of the practical challenges of attaining an in-depth understanding of a 'case' as an integrated whole. In keeping with previously published work, we acknowledge the importance of theory to underpin the design, selection, conduct and interpretation of case studies[ 2 ]. In so doing, we make passing reference to the different epistemological approaches used in case study research by key theoreticians and methodologists in this field of enquiry.
This paper is structured around the following main questions: What is a case study? What are case studies used for? How are case studies conducted? What are the potential pitfalls and how can these be avoided? We draw in particular on four of our own recently published examples of case studies (see Tables 1 , 2 , 3 and 4 ) and those of others to illustrate our discussion[ 3 – 7 ].
What is a case study?
A case study is a research approach that is used to generate an in-depth, multi-faceted understanding of a complex issue in its real-life context. It is an established research design that is used extensively in a wide variety of disciplines, particularly in the social sciences. A case study can be defined in a variety of ways (Table 5 ), the central tenet being the need to explore an event or phenomenon in depth and in its natural context. It is for this reason sometimes referred to as a "naturalistic" design; this is in contrast to an "experimental" design (such as a randomised controlled trial) in which the investigator seeks to exert control over and manipulate the variable(s) of interest.
Stake's work has been particularly influential in defining the case study approach to scientific enquiry. He has helpfully characterised three main types of case study: intrinsic , instrumental and collective [ 8 ]. An intrinsic case study is typically undertaken to learn about a unique phenomenon. The researcher should define the uniqueness of the phenomenon, which distinguishes it from all others. In contrast, the instrumental case study uses a particular case (some of which may be better than others) to gain a broader appreciation of an issue or phenomenon. The collective case study involves studying multiple cases simultaneously or sequentially in an attempt to generate a still broader appreciation of a particular issue.
These are however not necessarily mutually exclusive categories. In the first of our examples (Table 1 ), we undertook an intrinsic case study to investigate the issue of recruitment of minority ethnic people into the specific context of asthma research studies, but it developed into a instrumental case study through seeking to understand the issue of recruitment of these marginalised populations more generally, generating a number of the findings that are potentially transferable to other disease contexts[ 3 ]. In contrast, the other three examples (see Tables 2 , 3 and 4 ) employed collective case study designs to study the introduction of workforce reconfiguration in primary care, the implementation of electronic health records into hospitals, and to understand the ways in which healthcare students learn about patient safety considerations[ 4 – 6 ]. Although our study focusing on the introduction of General Practitioners with Specialist Interests (Table 2 ) was explicitly collective in design (four contrasting primary care organisations were studied), is was also instrumental in that this particular professional group was studied as an exemplar of the more general phenomenon of workforce redesign[ 4 ].
What are case studies used for?
According to Yin, case studies can be used to explain, describe or explore events or phenomena in the everyday contexts in which they occur[ 1 ]. These can, for example, help to understand and explain causal links and pathways resulting from a new policy initiative or service development (see Tables 2 and 3 , for example)[ 1 ]. In contrast to experimental designs, which seek to test a specific hypothesis through deliberately manipulating the environment (like, for example, in a randomised controlled trial giving a new drug to randomly selected individuals and then comparing outcomes with controls),[ 9 ] the case study approach lends itself well to capturing information on more explanatory ' how ', 'what' and ' why ' questions, such as ' how is the intervention being implemented and received on the ground?'. The case study approach can offer additional insights into what gaps exist in its delivery or why one implementation strategy might be chosen over another. This in turn can help develop or refine theory, as shown in our study of the teaching of patient safety in undergraduate curricula (Table 4 )[ 6 , 10 ]. Key questions to consider when selecting the most appropriate study design are whether it is desirable or indeed possible to undertake a formal experimental investigation in which individuals and/or organisations are allocated to an intervention or control arm? Or whether the wish is to obtain a more naturalistic understanding of an issue? The former is ideally studied using a controlled experimental design, whereas the latter is more appropriately studied using a case study design.
Case studies may be approached in different ways depending on the epistemological standpoint of the researcher, that is, whether they take a critical (questioning one's own and others' assumptions), interpretivist (trying to understand individual and shared social meanings) or positivist approach (orientating towards the criteria of natural sciences, such as focusing on generalisability considerations) (Table 6 ). Whilst such a schema can be conceptually helpful, it may be appropriate to draw on more than one approach in any case study, particularly in the context of conducting health services research. Doolin has, for example, noted that in the context of undertaking interpretative case studies, researchers can usefully draw on a critical, reflective perspective which seeks to take into account the wider social and political environment that has shaped the case[ 11 ].
How are case studies conducted?
Here, we focus on the main stages of research activity when planning and undertaking a case study; the crucial stages are: defining the case; selecting the case(s); collecting and analysing the data; interpreting data; and reporting the findings.
Defining the case
Carefully formulated research question(s), informed by the existing literature and a prior appreciation of the theoretical issues and setting(s), are all important in appropriately and succinctly defining the case[ 8 , 12 ]. Crucially, each case should have a pre-defined boundary which clarifies the nature and time period covered by the case study (i.e. its scope, beginning and end), the relevant social group, organisation or geographical area of interest to the investigator, the types of evidence to be collected, and the priorities for data collection and analysis (see Table 7 )[ 1 ]. A theory driven approach to defining the case may help generate knowledge that is potentially transferable to a range of clinical contexts and behaviours; using theory is also likely to result in a more informed appreciation of, for example, how and why interventions have succeeded or failed[ 13 ].
For example, in our evaluation of the introduction of electronic health records in English hospitals (Table 3 ), we defined our cases as the NHS Trusts that were receiving the new technology[ 5 ]. Our focus was on how the technology was being implemented. However, if the primary research interest had been on the social and organisational dimensions of implementation, we might have defined our case differently as a grouping of healthcare professionals (e.g. doctors and/or nurses). The precise beginning and end of the case may however prove difficult to define. Pursuing this same example, when does the process of implementation and adoption of an electronic health record system really begin or end? Such judgements will inevitably be influenced by a range of factors, including the research question, theory of interest, the scope and richness of the gathered data and the resources available to the research team.
Selecting the case(s)
The decision on how to select the case(s) to study is a very important one that merits some reflection. In an intrinsic case study, the case is selected on its own merits[ 8 ]. The case is selected not because it is representative of other cases, but because of its uniqueness, which is of genuine interest to the researchers. This was, for example, the case in our study of the recruitment of minority ethnic participants into asthma research (Table 1 ) as our earlier work had demonstrated the marginalisation of minority ethnic people with asthma, despite evidence of disproportionate asthma morbidity[ 14 , 15 ]. In another example of an intrinsic case study, Hellstrom et al.[ 16 ] studied an elderly married couple living with dementia to explore how dementia had impacted on their understanding of home, their everyday life and their relationships.
For an instrumental case study, selecting a "typical" case can work well[ 8 ]. In contrast to the intrinsic case study, the particular case which is chosen is of less importance than selecting a case that allows the researcher to investigate an issue or phenomenon. For example, in order to gain an understanding of doctors' responses to health policy initiatives, Som undertook an instrumental case study interviewing clinicians who had a range of responsibilities for clinical governance in one NHS acute hospital trust[ 17 ]. Sampling a "deviant" or "atypical" case may however prove even more informative, potentially enabling the researcher to identify causal processes, generate hypotheses and develop theory.
In collective or multiple case studies, a number of cases are carefully selected. This offers the advantage of allowing comparisons to be made across several cases and/or replication. Choosing a "typical" case may enable the findings to be generalised to theory (i.e. analytical generalisation) or to test theory by replicating the findings in a second or even a third case (i.e. replication logic)[ 1 ]. Yin suggests two or three literal replications (i.e. predicting similar results) if the theory is straightforward and five or more if the theory is more subtle. However, critics might argue that selecting 'cases' in this way is insufficiently reflexive and ill-suited to the complexities of contemporary healthcare organisations.
The selected case study site(s) should allow the research team access to the group of individuals, the organisation, the processes or whatever else constitutes the chosen unit of analysis for the study. Access is therefore a central consideration; the researcher needs to come to know the case study site(s) well and to work cooperatively with them. Selected cases need to be not only interesting but also hospitable to the inquiry [ 8 ] if they are to be informative and answer the research question(s). Case study sites may also be pre-selected for the researcher, with decisions being influenced by key stakeholders. For example, our selection of case study sites in the evaluation of the implementation and adoption of electronic health record systems (see Table 3 ) was heavily influenced by NHS Connecting for Health, the government agency that was responsible for overseeing the National Programme for Information Technology (NPfIT)[ 5 ]. This prominent stakeholder had already selected the NHS sites (through a competitive bidding process) to be early adopters of the electronic health record systems and had negotiated contracts that detailed the deployment timelines.
It is also important to consider in advance the likely burden and risks associated with participation for those who (or the site(s) which) comprise the case study. Of particular importance is the obligation for the researcher to think through the ethical implications of the study (e.g. the risk of inadvertently breaching anonymity or confidentiality) and to ensure that potential participants/participating sites are provided with sufficient information to make an informed choice about joining the study. The outcome of providing this information might be that the emotive burden associated with participation, or the organisational disruption associated with supporting the fieldwork, is considered so high that the individuals or sites decide against participation.
In our example of evaluating implementations of electronic health record systems, given the restricted number of early adopter sites available to us, we sought purposively to select a diverse range of implementation cases among those that were available[ 5 ]. We chose a mixture of teaching, non-teaching and Foundation Trust hospitals, and examples of each of the three electronic health record systems procured centrally by the NPfIT. At one recruited site, it quickly became apparent that access was problematic because of competing demands on that organisation. Recognising the importance of full access and co-operative working for generating rich data, the research team decided not to pursue work at that site and instead to focus on other recruited sites.
Collecting the data
In order to develop a thorough understanding of the case, the case study approach usually involves the collection of multiple sources of evidence, using a range of quantitative (e.g. questionnaires, audits and analysis of routinely collected healthcare data) and more commonly qualitative techniques (e.g. interviews, focus groups and observations). The use of multiple sources of data (data triangulation) has been advocated as a way of increasing the internal validity of a study (i.e. the extent to which the method is appropriate to answer the research question)[ 8 , 18 – 21 ]. An underlying assumption is that data collected in different ways should lead to similar conclusions, and approaching the same issue from different angles can help develop a holistic picture of the phenomenon (Table 2 )[ 4 ].
Brazier and colleagues used a mixed-methods case study approach to investigate the impact of a cancer care programme[ 22 ]. Here, quantitative measures were collected with questionnaires before, and five months after, the start of the intervention which did not yield any statistically significant results. Qualitative interviews with patients however helped provide an insight into potentially beneficial process-related aspects of the programme, such as greater, perceived patient involvement in care. The authors reported how this case study approach provided a number of contextual factors likely to influence the effectiveness of the intervention and which were not likely to have been obtained from quantitative methods alone.
In collective or multiple case studies, data collection needs to be flexible enough to allow a detailed description of each individual case to be developed (e.g. the nature of different cancer care programmes), before considering the emerging similarities and differences in cross-case comparisons (e.g. to explore why one programme is more effective than another). It is important that data sources from different cases are, where possible, broadly comparable for this purpose even though they may vary in nature and depth.
Analysing, interpreting and reporting case studies
Making sense and offering a coherent interpretation of the typically disparate sources of data (whether qualitative alone or together with quantitative) is far from straightforward. Repeated reviewing and sorting of the voluminous and detail-rich data are integral to the process of analysis. In collective case studies, it is helpful to analyse data relating to the individual component cases first, before making comparisons across cases. Attention needs to be paid to variations within each case and, where relevant, the relationship between different causes, effects and outcomes[ 23 ]. Data will need to be organised and coded to allow the key issues, both derived from the literature and emerging from the dataset, to be easily retrieved at a later stage. An initial coding frame can help capture these issues and can be applied systematically to the whole dataset with the aid of a qualitative data analysis software package.
The Framework approach is a practical approach, comprising of five stages (familiarisation; identifying a thematic framework; indexing; charting; mapping and interpretation) , to managing and analysing large datasets particularly if time is limited, as was the case in our study of recruitment of South Asians into asthma research (Table 1 )[ 3 , 24 ]. Theoretical frameworks may also play an important role in integrating different sources of data and examining emerging themes. For example, we drew on a socio-technical framework to help explain the connections between different elements - technology; people; and the organisational settings within which they worked - in our study of the introduction of electronic health record systems (Table 3 )[ 5 ]. Our study of patient safety in undergraduate curricula drew on an evaluation-based approach to design and analysis, which emphasised the importance of the academic, organisational and practice contexts through which students learn (Table 4 )[ 6 ].
Case study findings can have implications both for theory development and theory testing. They may establish, strengthen or weaken historical explanations of a case and, in certain circumstances, allow theoretical (as opposed to statistical) generalisation beyond the particular cases studied[ 12 ]. These theoretical lenses should not, however, constitute a strait-jacket and the cases should not be "forced to fit" the particular theoretical framework that is being employed.
When reporting findings, it is important to provide the reader with enough contextual information to understand the processes that were followed and how the conclusions were reached. In a collective case study, researchers may choose to present the findings from individual cases separately before amalgamating across cases. Care must be taken to ensure the anonymity of both case sites and individual participants (if agreed in advance) by allocating appropriate codes or withholding descriptors. In the example given in Table 3 , we decided against providing detailed information on the NHS sites and individual participants in order to avoid the risk of inadvertent disclosure of identities[ 5 , 25 ].
What are the potential pitfalls and how can these be avoided?
The case study approach is, as with all research, not without its limitations. When investigating the formal and informal ways undergraduate students learn about patient safety (Table 4 ), for example, we rapidly accumulated a large quantity of data. The volume of data, together with the time restrictions in place, impacted on the depth of analysis that was possible within the available resources. This highlights a more general point of the importance of avoiding the temptation to collect as much data as possible; adequate time also needs to be set aside for data analysis and interpretation of what are often highly complex datasets.
Case study research has sometimes been criticised for lacking scientific rigour and providing little basis for generalisation (i.e. producing findings that may be transferable to other settings)[ 1 ]. There are several ways to address these concerns, including: the use of theoretical sampling (i.e. drawing on a particular conceptual framework); respondent validation (i.e. participants checking emerging findings and the researcher's interpretation, and providing an opinion as to whether they feel these are accurate); and transparency throughout the research process (see Table 8 )[ 8 , 18 – 21 , 23 , 26 ]. Transparency can be achieved by describing in detail the steps involved in case selection, data collection, the reasons for the particular methods chosen, and the researcher's background and level of involvement (i.e. being explicit about how the researcher has influenced data collection and interpretation). Seeking potential, alternative explanations, and being explicit about how interpretations and conclusions were reached, help readers to judge the trustworthiness of the case study report. Stake provides a critique checklist for a case study report (Table 9 )[ 8 ].
Conclusions
The case study approach allows, amongst other things, critical events, interventions, policy developments and programme-based service reforms to be studied in detail in a real-life context. It should therefore be considered when an experimental design is either inappropriate to answer the research questions posed or impossible to undertake. Considering the frequency with which implementations of innovations are now taking place in healthcare settings and how well the case study approach lends itself to in-depth, complex health service research, we believe this approach should be more widely considered by researchers. Though inherently challenging, the research case study can, if carefully conceptualised and thoughtfully undertaken and reported, yield powerful insights into many important aspects of health and healthcare delivery.
Yin RK: Case study research, design and method. 2009, London: Sage Publications Ltd., 4
Google Scholar
Keen J, Packwood T: Qualitative research; case study evaluation. BMJ. 1995, 311: 444-446.
Article CAS PubMed PubMed Central Google Scholar
Sheikh A, Halani L, Bhopal R, Netuveli G, Partridge M, Car J, et al: Facilitating the Recruitment of Minority Ethnic People into Research: Qualitative Case Study of South Asians and Asthma. PLoS Med. 2009, 6 (10): 1-11.
Article Google Scholar
Pinnock H, Huby G, Powell A, Kielmann T, Price D, Williams S, et al: The process of planning, development and implementation of a General Practitioner with a Special Interest service in Primary Care Organisations in England and Wales: a comparative prospective case study. Report for the National Co-ordinating Centre for NHS Service Delivery and Organisation R&D (NCCSDO). 2008, [ http://www.sdo.nihr.ac.uk/files/project/99-final-report.pdf ]
Robertson A, Cresswell K, Takian A, Petrakaki D, Crowe S, Cornford T, et al: Prospective evaluation of the implementation and adoption of NHS Connecting for Health's national electronic health record in secondary care in England: interim findings. BMJ. 2010, 41: c4564-
Pearson P, Steven A, Howe A, Sheikh A, Ashcroft D, Smith P, the Patient Safety Education Study Group: Learning about patient safety: organisational context and culture in the education of healthcare professionals. J Health Serv Res Policy. 2010, 15: 4-10. 10.1258/jhsrp.2009.009052.
Article PubMed Google Scholar
van Harten WH, Casparie TF, Fisscher OA: The evaluation of the introduction of a quality management system: a process-oriented case study in a large rehabilitation hospital. Health Policy. 2002, 60 (1): 17-37. 10.1016/S0168-8510(01)00187-7.
Stake RE: The art of case study research. 1995, London: Sage Publications Ltd.
Sheikh A, Smeeth L, Ashcroft R: Randomised controlled trials in primary care: scope and application. Br J Gen Pract. 2002, 52 (482): 746-51.
PubMed PubMed Central Google Scholar
King G, Keohane R, Verba S: Designing Social Inquiry. 1996, Princeton: Princeton University Press
Doolin B: Information technology as disciplinary technology: being critical in interpretative research on information systems. Journal of Information Technology. 1998, 13: 301-311. 10.1057/jit.1998.8.
George AL, Bennett A: Case studies and theory development in the social sciences. 2005, Cambridge, MA: MIT Press
Eccles M, the Improved Clinical Effectiveness through Behavioural Research Group (ICEBeRG): Designing theoretically-informed implementation interventions. Implementation Science. 2006, 1: 1-8. 10.1186/1748-5908-1-1.
Article PubMed Central Google Scholar
Netuveli G, Hurwitz B, Levy M, Fletcher M, Barnes G, Durham SR, Sheikh A: Ethnic variations in UK asthma frequency, morbidity, and health-service use: a systematic review and meta-analysis. Lancet. 2005, 365 (9456): 312-7.
Sheikh A, Panesar SS, Lasserson T, Netuveli G: Recruitment of ethnic minorities to asthma studies. Thorax. 2004, 59 (7): 634-
CAS PubMed PubMed Central Google Scholar
Hellström I, Nolan M, Lundh U: 'We do things together': A case study of 'couplehood' in dementia. Dementia. 2005, 4: 7-22. 10.1177/1471301205049188.
Som CV: Nothing seems to have changed, nothing seems to be changing and perhaps nothing will change in the NHS: doctors' response to clinical governance. International Journal of Public Sector Management. 2005, 18: 463-477. 10.1108/09513550510608903.
Lincoln Y, Guba E: Naturalistic inquiry. 1985, Newbury Park: Sage Publications
Barbour RS: Checklists for improving rigour in qualitative research: a case of the tail wagging the dog?. BMJ. 2001, 322: 1115-1117. 10.1136/bmj.322.7294.1115.
Mays N, Pope C: Qualitative research in health care: Assessing quality in qualitative research. BMJ. 2000, 320: 50-52. 10.1136/bmj.320.7226.50.
Mason J: Qualitative researching. 2002, London: Sage
Brazier A, Cooke K, Moravan V: Using Mixed Methods for Evaluating an Integrative Approach to Cancer Care: A Case Study. Integr Cancer Ther. 2008, 7: 5-17. 10.1177/1534735407313395.
Miles MB, Huberman M: Qualitative data analysis: an expanded sourcebook. 1994, CA: Sage Publications Inc., 2
Pope C, Ziebland S, Mays N: Analysing qualitative data. Qualitative research in health care. BMJ. 2000, 320: 114-116. 10.1136/bmj.320.7227.114.
Cresswell KM, Worth A, Sheikh A: Actor-Network Theory and its role in understanding the implementation of information technology developments in healthcare. BMC Med Inform Decis Mak. 2010, 10 (1): 67-10.1186/1472-6947-10-67.
Article PubMed PubMed Central Google Scholar
Malterud K: Qualitative research: standards, challenges, and guidelines. Lancet. 2001, 358: 483-488. 10.1016/S0140-6736(01)05627-6.
Article CAS PubMed Google Scholar
Yin R: Case study research: design and methods. 1994, Thousand Oaks, CA: Sage Publishing, 2
Yin R: Enhancing the quality of case studies in health services research. Health Serv Res. 1999, 34: 1209-1224.
Green J, Thorogood N: Qualitative methods for health research. 2009, Los Angeles: Sage, 2
Howcroft D, Trauth E: Handbook of Critical Information Systems Research, Theory and Application. 2005, Cheltenham, UK: Northampton, MA, USA: Edward Elgar
Book Google Scholar
Blakie N: Approaches to Social Enquiry. 1993, Cambridge: Polity Press
Doolin B: Power and resistance in the implementation of a medical management information system. Info Systems J. 2004, 14: 343-362. 10.1111/j.1365-2575.2004.00176.x.
Bloomfield BP, Best A: Management consultants: systems development, power and the translation of problems. Sociological Review. 1992, 40: 533-560.
Shanks G, Parr A: Positivist, single case study research in information systems: A critical analysis. Proceedings of the European Conference on Information Systems. 2003, Naples
Pre-publication history
The pre-publication history for this paper can be accessed here: http://www.biomedcentral.com/1471-2288/11/100/prepub
Download references
Acknowledgements
We are grateful to the participants and colleagues who contributed to the individual case studies that we have drawn on. This work received no direct funding, but it has been informed by projects funded by Asthma UK, the NHS Service Delivery Organisation, NHS Connecting for Health Evaluation Programme, and Patient Safety Research Portfolio. We would also like to thank the expert reviewers for their insightful and constructive feedback. Our thanks are also due to Dr. Allison Worth who commented on an earlier draft of this manuscript.
Author information
Authors and affiliations.
Division of Primary Care, The University of Nottingham, Nottingham, UK
Sarah Crowe & Anthony Avery
Centre for Population Health Sciences, The University of Edinburgh, Edinburgh, UK
Kathrin Cresswell, Ann Robertson & Aziz Sheikh
School of Health in Social Science, The University of Edinburgh, Edinburgh, UK
You can also search for this author in PubMed Google Scholar
Corresponding author
Correspondence to Sarah Crowe .
Additional information
Competing interests.
The authors declare that they have no competing interests.
Authors' contributions
AS conceived this article. SC, KC and AR wrote this paper with GH, AA and AS all commenting on various drafts. SC and AS are guarantors.
Rights and permissions
This article is published under license to BioMed Central Ltd. This is an Open Access article distributed under the terms of the Creative Commons Attribution License ( http://creativecommons.org/licenses/by/2.0 ), which permits unrestricted use, distribution, and reproduction in any medium, provided the original work is properly cited.
Reprints and permissions
About this article
Cite this article.
Crowe, S., Cresswell, K., Robertson, A. et al. The case study approach. BMC Med Res Methodol 11 , 100 (2011). https://doi.org/10.1186/1471-2288-11-100
Download citation
Received : 29 November 2010
Accepted : 27 June 2011
Published : 27 June 2011
DOI : https://doi.org/10.1186/1471-2288-11-100
Share this article
Anyone you share the following link with will be able to read this content:
Sorry, a shareable link is not currently available for this article.
Provided by the Springer Nature SharedIt content-sharing initiative
- Case Study Approach
- Electronic Health Record System
- Case Study Design
- Case Study Site
- Case Study Report
BMC Medical Research Methodology
ISSN: 1471-2288
- General enquiries: [email protected]
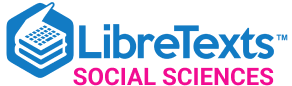
- school Campus Bookshelves
- menu_book Bookshelves
- perm_media Learning Objects
- login Login
- how_to_reg Request Instructor Account
- hub Instructor Commons
Margin Size
- Download Page (PDF)
- Download Full Book (PDF)
- Periodic Table
- Physics Constants
- Scientific Calculator
- Reference & Cite
- Tools expand_more
- Readability
selected template will load here
This action is not available.
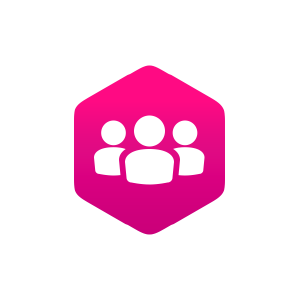
2.3: Case Selection (Or, How to Use Cases in Your Comparative Analysis)
- Last updated
- Save as PDF
- Page ID 135832
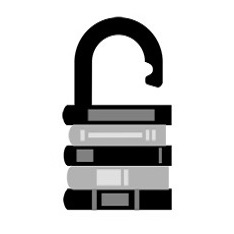
- Dino Bozonelos, Julia Wendt, Charlotte Lee, Jessica Scarffe, Masahiro Omae, Josh Franco, Byran Martin, & Stefan Veldhuis
- Victor Valley College, Berkeley City College, Allan Hancock College, San Diego City College, Cuyamaca College, Houston Community College, and Long Beach City College via ASCCC Open Educational Resources Initiative (OERI)
\( \newcommand{\vecs}[1]{\overset { \scriptstyle \rightharpoonup} {\mathbf{#1}} } \)
\( \newcommand{\vecd}[1]{\overset{-\!-\!\rightharpoonup}{\vphantom{a}\smash {#1}}} \)
\( \newcommand{\id}{\mathrm{id}}\) \( \newcommand{\Span}{\mathrm{span}}\)
( \newcommand{\kernel}{\mathrm{null}\,}\) \( \newcommand{\range}{\mathrm{range}\,}\)
\( \newcommand{\RealPart}{\mathrm{Re}}\) \( \newcommand{\ImaginaryPart}{\mathrm{Im}}\)
\( \newcommand{\Argument}{\mathrm{Arg}}\) \( \newcommand{\norm}[1]{\| #1 \|}\)
\( \newcommand{\inner}[2]{\langle #1, #2 \rangle}\)
\( \newcommand{\Span}{\mathrm{span}}\)
\( \newcommand{\id}{\mathrm{id}}\)
\( \newcommand{\kernel}{\mathrm{null}\,}\)
\( \newcommand{\range}{\mathrm{range}\,}\)
\( \newcommand{\RealPart}{\mathrm{Re}}\)
\( \newcommand{\ImaginaryPart}{\mathrm{Im}}\)
\( \newcommand{\Argument}{\mathrm{Arg}}\)
\( \newcommand{\norm}[1]{\| #1 \|}\)
\( \newcommand{\Span}{\mathrm{span}}\) \( \newcommand{\AA}{\unicode[.8,0]{x212B}}\)
\( \newcommand{\vectorA}[1]{\vec{#1}} % arrow\)
\( \newcommand{\vectorAt}[1]{\vec{\text{#1}}} % arrow\)
\( \newcommand{\vectorB}[1]{\overset { \scriptstyle \rightharpoonup} {\mathbf{#1}} } \)
\( \newcommand{\vectorC}[1]{\textbf{#1}} \)
\( \newcommand{\vectorD}[1]{\overrightarrow{#1}} \)
\( \newcommand{\vectorDt}[1]{\overrightarrow{\text{#1}}} \)
\( \newcommand{\vectE}[1]{\overset{-\!-\!\rightharpoonup}{\vphantom{a}\smash{\mathbf {#1}}}} \)
Learning Objectives
By the end of this section, you will be able to:
- Discuss the importance of case selection in case studies.
- Consider the implications of poor case selection.
Introduction
Case selection is an important part of any research design. Deciding how many cases, and which cases, to include, will clearly help determine the outcome of our results. If we decide to select a high number of cases, we often say that we are conducting large-N research. Large-N research is when the number of observations or cases is large enough where we would need mathematical, usually statistical, techniques to discover and interpret any correlations or causations. In order for a large-N analysis to yield any relevant findings, a number of conventions need to be observed. First, the sample needs to be representative of the studied population. Thus, if we wanted to understand the long-term effects of COVID, we would need to know the approximate details of those who contracted the virus. Once we know the parameters of the population, we can then determine a sample that represents the larger population. For example, women make up 55% of all long-term COVID survivors. Thus, any sample we generate needs to be at least 55% women.
Second, some kind of randomization technique needs to be involved in large-N research. So not only must your sample be representative, it must also randomly select people within that sample. In other words, we must have a large selection of people that fit within the population criteria, and then randomly select from those pools. Randomization would help to reduce bias in the study. Also, when cases (people with long-term COVID) are randomly chosen they tend to ensure a fairer representation of the studied population. Third, your sample needs to be large enough, hence the large-N designation for any conclusions to have any external validity. Generally speaking, the larger the number of observations/cases in the sample, the more validity we can have in the study. There is no magic number, but if using the above example, our sample of long-term COVID patients should be at least over 750 people, with an aim of around 1,200 to 1,500 people.
When it comes to comparative politics, we rarely ever reach the numbers typically used in large-N research. There are about 200 fully recognized countries, with about a dozen partially recognized countries, and even fewer areas or regions of study, such as Europe or Latin America. Given this, what is the strategy when one case, or a few cases, are being studied? What happens if we are only wanting to know the COVID-19 response in the United States, and not the rest of the world? How do we randomize this to ensure our results are not biased or are representative? These and other questions are legitimate issues that many comparativist scholars face when completing research. Does randomization work with case studies? Gerring suggests that it does not, as “any given sample may be widely representative” (pg. 87). Thus, random sampling is not a reliable approach when it comes to case studies. And even if the randomized sample is representative, there is no guarantee that the gathered evidence would be reliable.
One can make the argument that case selection may not be as important in large-N studies as they are in small-N studies. In large-N research, potential errors and/or biases may be ameliorated, especially if the sample is large enough. This is not always what happens, errors and biases most certainly can exist in large-N research. However, incorrect or biased inferences are less of a worry when we have 1,500 cases versus 15 cases. In small-N research, case selection simply matters much more.
This is why Blatter and Haverland (2012) write that, “case studies are ‘case-centered’, whereas large-N studies are ‘variable-centered’". In large-N studies we are more concerned with the conceptualization and operationalization of variables. Thus, we want to focus on which data to include in the analysis of long-term COVID patients. If we wanted to survey them, we would want to make sure we construct questions in appropriate ways. For almost all survey-based large-N research, the question responses themselves become the coded variables used in the statistical analysis.
Case selection can be driven by a number of factors in comparative politics, with the first two approaches being the more traditional. First, it can derive from the interests of the researcher(s). For example, if the researcher lives in Germany, they may want to research the spread of COVID-19 within the country, possibly using a subnational approach where the researcher may compare infection rates among German states. Second, case selection may be driven by area studies. This is still based on the interests of the researcher as generally speaking scholars pick areas of studies due to their personal interests. For example, the same researcher may research COVID-19 infection rates among European Union member-states. Finally, the selection of cases selected may be driven by the type of case study that is utilized. In this approach, cases are selected as they allow researchers to compare their similarities or their differences. Or, a case might be selected that is typical of most cases, or in contrast, a case or cases that deviate from the norm. We discuss types of case studies and their impact on case selection below.
Types of Case Studies: Descriptive vs. Causal
There are a number of different ways to categorize case studies. One of the most recent ways is through John Gerring. He wrote two editions on case study research (2017) where he posits that the central question posed by the researcher will dictate the aim of the case study. Is the study meant to be descriptive? If so, what is the researcher looking to describe? How many cases (countries, incidents, events) are there? Or is the study meant to be causal, where the researcher is looking for a cause and effect? Given this, Gerring categorizes case studies into two types: descriptive and causal.
Descriptive case studies are “not organized around a central, overarching causal hypothesis or theory” (pg. 56). Most case studies are descriptive in nature, where the researchers simply seek to describe what they observe. They are useful for transmitting information regarding the studied political phenomenon. For a descriptive case study, a scholar might choose a case that is considered typical of the population. An example could involve researching the effects of the pandemic on medium-sized cities in the US. This city would have to exhibit the tendencies of medium-sized cities throughout the entire country. First, we would have to conceptualize what we mean by ‘a medium-size city’. Second, we would then have to establish the characteristics of medium-sized US cities, so that our case selection is appropriate. Alternatively, cases could be chosen for their diversity . In keeping with our example, maybe we want to look at the effects of the pandemic on a range of US cities, from small, rural towns, to medium-sized suburban cities to large-sized urban areas.
Causal case studies are “organized around a central hypothesis about how X affects Y” (pg. 63). In causal case studies, the context around a specific political phenomenon or phenomena is important as it allows for researchers to identify the aspects that set up the conditions, the mechanisms, for that outcome to occur. Scholars refer to this as the causal mechanism , which is defined by Falleti & Lynch (2009) as “portable concepts that explain how and why a hypothesized cause, in a given context, contributes to a particular outcome”. Remember, causality is when a change in one variable verifiably causes an effect or change in another variable. For causal case studies that employ causal mechanisms, Gerring divides them into exploratory case-selection, estimating case-selection, and diagnostic case-selection. The differences revolve around how the central hypothesis is utilized in the study.
Exploratory case studies are used to identify a potential causal hypothesis. Researchers will single out the independent variables that seem to affect the outcome, or dependent variable, the most. The goal is to build up to what the causal mechanism might be by providing the context. This is also referred to as hypothesis generating as opposed to hypothesis testing. Case selection can vary widely depending on the goal of the researcher. For example, if the scholar is looking to develop an ‘ideal-type’, they might seek out an extreme case. An ideal-type is defined as a “conception or a standard of something in its highest perfection” (New Webster Dictionary). Thus, if we want to understand the ideal-type capitalist system, we want to investigate a country that practices a pure or ‘extreme’ form of the economic system.
Estimating case studies start with a hypothesis already in place. The goal is to test the hypothesis through collected data/evidence. Researchers seek to estimate the ‘causal effect’. This involves determining if the relationship between the independent and dependent variables is positive, negative, or ultimately if no relationship exists at all. Finally, diagnostic case studies are important as they help to “confirm, disconfirm, or refine a hypothesis” (Gerring 2017). Case selection can also vary in diagnostic case studies. For example, scholars can choose an least-likely case, or a case where the hypothesis is confirmed even though the context would suggest otherwise. A good example would be looking at Indian democracy, which has existed for over 70 years. India has a high level of ethnolinguistic diversity, is relatively underdeveloped economically, and a low level of modernization through large swaths of the country. All of these factors strongly suggest that India should not have democratized, or should have failed to stay a democracy in the long-term, or have disintegrated as a country.
Most Similar/Most Different Systems Approach
The discussion in the previous subsection tends to focus on case selection when it comes to a single case. Single case studies are valuable as they provide an opportunity for in-depth research on a topic that requires it. However, in comparative politics, our approach is to compare. Given this, we are required to select more than one case. This presents a different set of challenges. First, how many cases do we pick? This is a tricky question we addressed earlier. Second, how do we apply the previously mentioned case selection techniques, descriptive vs. causal? Do we pick two extreme cases if we used an exploratory approach, or two least-likely cases if choosing a diagnostic case approach?
Thankfully, an English scholar by the name of John Stuart Mill provided some insight on how we should proceed. He developed several approaches to comparison with the explicit goal of isolating a cause within a complex environment. Two of these methods, the 'method of agreement' and the 'method of difference' have influenced comparative politics. In the 'method of agreement' two or more cases are compared for their commonalities. The scholar looks to isolate the characteristic, or variable, they have in common, which is then established as the cause for their similarities. In the 'method of difference' two or more cases are compared for their differences. The scholar looks to isolate the characteristic, or variable, they do not have in common, which is then identified as the cause for their differences. From these two methods, comparativists have developed two approaches.
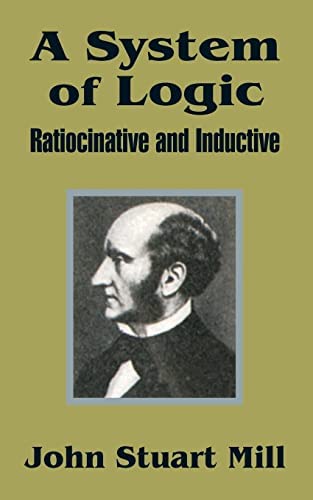
What Is the Most Similar Systems Design (MSSD)?
This approach is derived from Mill’s ‘method of difference’. In a Most Similar Systems Design Design, the cases selected for comparison are similar to each other, but the outcomes differ in result. In this approach we are interested in keeping as many of the variables the same across the elected cases, which for comparative politics often involves countries. Remember, the independent variable is the factor that doesn’t depend on changes in other variables. It is potentially the ‘cause’ in the cause and effect model. The dependent variable is the variable that is affected by, or dependent on, the presence of the independent variable. It is the ‘effect’. In a most similar systems approach the variables of interest should remain the same.
A good example involves the lack of a national healthcare system in the US. Other countries, such as New Zealand, Australia, Ireland, UK and Canada, all have robust, publicly accessible national health systems. However, the US does not. These countries all have similar systems: English heritage and language use, liberal market economies, strong democratic institutions, and high levels of wealth and education. Yet, despite these similarities, the end results vary. The US does not look like its peer countries. In other words, why do we have similar systems producing different outcomes?
What Is the Most Different Systems Design (MDSD)?
This approach is derived from Mill’s ‘method of agreement’. In a Most Different System Design, the cases selected are different from each other, but result in the same outcome. In this approach, we are interested in selecting cases that are quite different from one another, yet arrive at the same outcome. Thus, the dependent variable is the same. Different independent variables exist between the cases, such as democratic v. authoritarian regime, liberal market economy v. non-liberal market economy. Or it could include other variables such as societal homogeneity (uniformity) vs. societal heterogeneity (diversity), where a country may find itself unified ethnically/religiously/racially, or fragmented along those same lines.
A good example involves the countries that are classified as economically liberal. The Heritage Foundation lists countries such as Singapore, Taiwan, Estonia, Australia, New Zealand, as well as Switzerland, Chile and Malaysia as either free or mostly free. These countries differ greatly from one another. Singapore and Malaysia are considered flawed or illiberal democracies (see chapter 5 for more discussion), whereas Estonia is still classified as a developing country. Australia and New Zealand are wealthy, Malaysia is not. Chile and Taiwan became economically free countries under the authoritarian military regimes, which is not the case for Switzerland. In other words, why do we have different systems producing the same outcome?
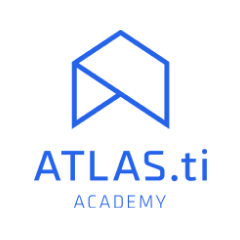
The Ultimate Guide to Qualitative Research - Part 1: The Basics
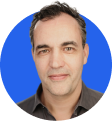
- Introduction and overview
- What is qualitative research?
- What is qualitative data?
- Examples of qualitative data
- Qualitative vs. quantitative research
- Mixed methods
- Qualitative research preparation
- Theoretical perspective
- Theoretical framework
- Literature reviews
Research question
- Conceptual framework
- Conceptual vs. theoretical framework
Data collection
- Qualitative research methods
- Focus groups
- Observational research
What is a case study?
Applications for case study research, what is a good case study, process of case study design, benefits and limitations of case studies.
- Ethnographical research
- Ethical considerations
- Confidentiality and privacy
- Power dynamics
- Reflexivity
Case studies
Case studies are essential to qualitative research , offering a lens through which researchers can investigate complex phenomena within their real-life contexts. This chapter explores the concept, purpose, applications, examples, and types of case studies and provides guidance on how to conduct case study research effectively.
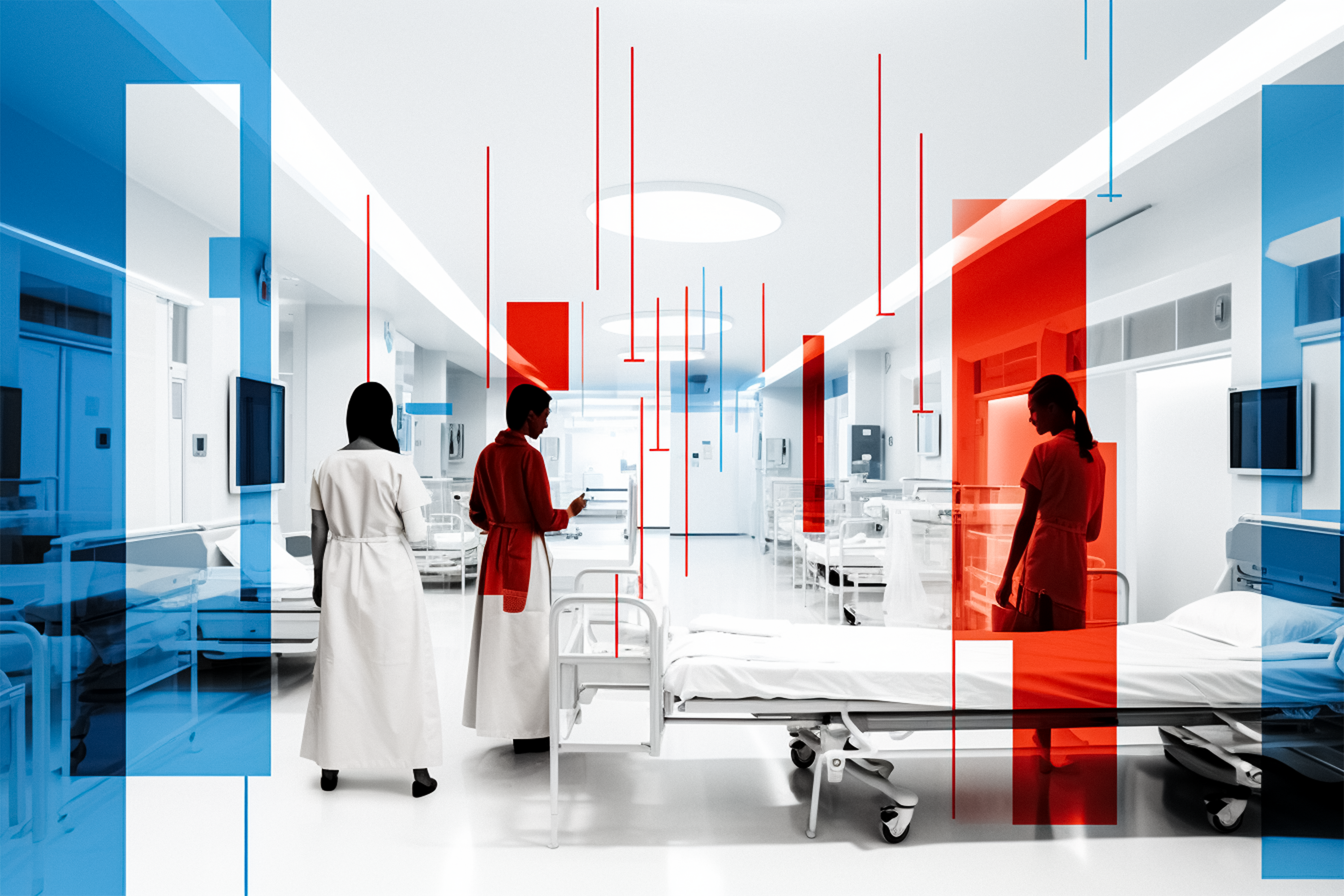
Whereas quantitative methods look at phenomena at scale, case study research looks at a concept or phenomenon in considerable detail. While analyzing a single case can help understand one perspective regarding the object of research inquiry, analyzing multiple cases can help obtain a more holistic sense of the topic or issue. Let's provide a basic definition of a case study, then explore its characteristics and role in the qualitative research process.
Definition of a case study
A case study in qualitative research is a strategy of inquiry that involves an in-depth investigation of a phenomenon within its real-world context. It provides researchers with the opportunity to acquire an in-depth understanding of intricate details that might not be as apparent or accessible through other methods of research. The specific case or cases being studied can be a single person, group, or organization – demarcating what constitutes a relevant case worth studying depends on the researcher and their research question .
Among qualitative research methods , a case study relies on multiple sources of evidence, such as documents, artifacts, interviews , or observations , to present a complete and nuanced understanding of the phenomenon under investigation. The objective is to illuminate the readers' understanding of the phenomenon beyond its abstract statistical or theoretical explanations.
Characteristics of case studies
Case studies typically possess a number of distinct characteristics that set them apart from other research methods. These characteristics include a focus on holistic description and explanation, flexibility in the design and data collection methods, reliance on multiple sources of evidence, and emphasis on the context in which the phenomenon occurs.
Furthermore, case studies can often involve a longitudinal examination of the case, meaning they study the case over a period of time. These characteristics allow case studies to yield comprehensive, in-depth, and richly contextualized insights about the phenomenon of interest.
The role of case studies in research
Case studies hold a unique position in the broader landscape of research methods aimed at theory development. They are instrumental when the primary research interest is to gain an intensive, detailed understanding of a phenomenon in its real-life context.
In addition, case studies can serve different purposes within research - they can be used for exploratory, descriptive, or explanatory purposes, depending on the research question and objectives. This flexibility and depth make case studies a valuable tool in the toolkit of qualitative researchers.
Remember, a well-conducted case study can offer a rich, insightful contribution to both academic and practical knowledge through theory development or theory verification, thus enhancing our understanding of complex phenomena in their real-world contexts.
What is the purpose of a case study?
Case study research aims for a more comprehensive understanding of phenomena, requiring various research methods to gather information for qualitative analysis . Ultimately, a case study can allow the researcher to gain insight into a particular object of inquiry and develop a theoretical framework relevant to the research inquiry.
Why use case studies in qualitative research?
Using case studies as a research strategy depends mainly on the nature of the research question and the researcher's access to the data.
Conducting case study research provides a level of detail and contextual richness that other research methods might not offer. They are beneficial when there's a need to understand complex social phenomena within their natural contexts.
The explanatory, exploratory, and descriptive roles of case studies
Case studies can take on various roles depending on the research objectives. They can be exploratory when the research aims to discover new phenomena or define new research questions; they are descriptive when the objective is to depict a phenomenon within its context in a detailed manner; and they can be explanatory if the goal is to understand specific relationships within the studied context. Thus, the versatility of case studies allows researchers to approach their topic from different angles, offering multiple ways to uncover and interpret the data .
The impact of case studies on knowledge development
Case studies play a significant role in knowledge development across various disciplines. Analysis of cases provides an avenue for researchers to explore phenomena within their context based on the collected data.
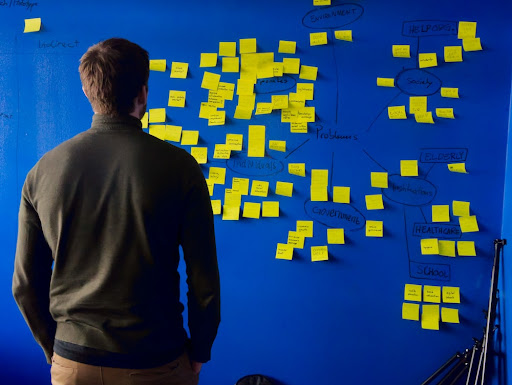
This can result in the production of rich, practical insights that can be instrumental in both theory-building and practice. Case studies allow researchers to delve into the intricacies and complexities of real-life situations, uncovering insights that might otherwise remain hidden.
Types of case studies
In qualitative research , a case study is not a one-size-fits-all approach. Depending on the nature of the research question and the specific objectives of the study, researchers might choose to use different types of case studies. These types differ in their focus, methodology, and the level of detail they provide about the phenomenon under investigation.
Understanding these types is crucial for selecting the most appropriate approach for your research project and effectively achieving your research goals. Let's briefly look at the main types of case studies.
Exploratory case studies
Exploratory case studies are typically conducted to develop a theory or framework around an understudied phenomenon. They can also serve as a precursor to a larger-scale research project. Exploratory case studies are useful when a researcher wants to identify the key issues or questions which can spur more extensive study or be used to develop propositions for further research. These case studies are characterized by flexibility, allowing researchers to explore various aspects of a phenomenon as they emerge, which can also form the foundation for subsequent studies.
Descriptive case studies
Descriptive case studies aim to provide a complete and accurate representation of a phenomenon or event within its context. These case studies are often based on an established theoretical framework, which guides how data is collected and analyzed. The researcher is concerned with describing the phenomenon in detail, as it occurs naturally, without trying to influence or manipulate it.
Explanatory case studies
Explanatory case studies are focused on explanation - they seek to clarify how or why certain phenomena occur. Often used in complex, real-life situations, they can be particularly valuable in clarifying causal relationships among concepts and understanding the interplay between different factors within a specific context.
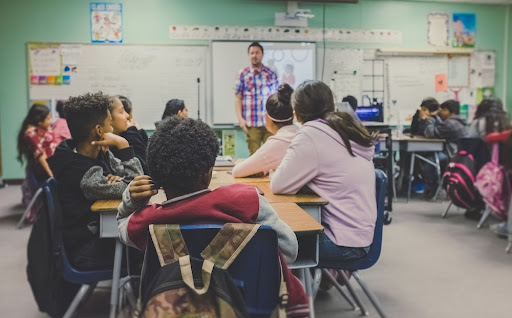
Intrinsic, instrumental, and collective case studies
These three categories of case studies focus on the nature and purpose of the study. An intrinsic case study is conducted when a researcher has an inherent interest in the case itself. Instrumental case studies are employed when the case is used to provide insight into a particular issue or phenomenon. A collective case study, on the other hand, involves studying multiple cases simultaneously to investigate some general phenomena.
Each type of case study serves a different purpose and has its own strengths and challenges. The selection of the type should be guided by the research question and objectives, as well as the context and constraints of the research.
The flexibility, depth, and contextual richness offered by case studies make this approach an excellent research method for various fields of study. They enable researchers to investigate real-world phenomena within their specific contexts, capturing nuances that other research methods might miss. Across numerous fields, case studies provide valuable insights into complex issues.
Critical information systems research
Case studies provide a detailed understanding of the role and impact of information systems in different contexts. They offer a platform to explore how information systems are designed, implemented, and used and how they interact with various social, economic, and political factors. Case studies in this field often focus on examining the intricate relationship between technology, organizational processes, and user behavior, helping to uncover insights that can inform better system design and implementation.
Health research
Health research is another field where case studies are highly valuable. They offer a way to explore patient experiences, healthcare delivery processes, and the impact of various interventions in a real-world context.
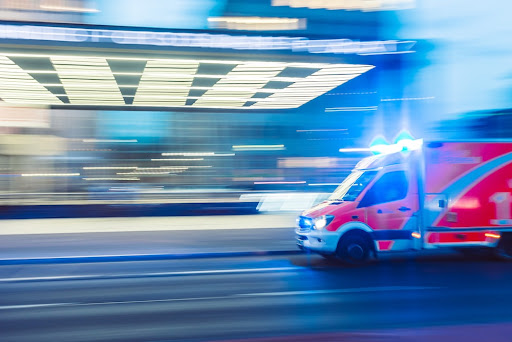
Case studies can provide a deep understanding of a patient's journey, giving insights into the intricacies of disease progression, treatment effects, and the psychosocial aspects of health and illness.
Asthma research studies
Specifically within medical research, studies on asthma often employ case studies to explore the individual and environmental factors that influence asthma development, management, and outcomes. A case study can provide rich, detailed data about individual patients' experiences, from the triggers and symptoms they experience to the effectiveness of various management strategies. This can be crucial for developing patient-centered asthma care approaches.
Other fields
Apart from the fields mentioned, case studies are also extensively used in business and management research, education research, and political sciences, among many others. They provide an opportunity to delve into the intricacies of real-world situations, allowing for a comprehensive understanding of various phenomena.
Case studies, with their depth and contextual focus, offer unique insights across these varied fields. They allow researchers to illuminate the complexities of real-life situations, contributing to both theory and practice.
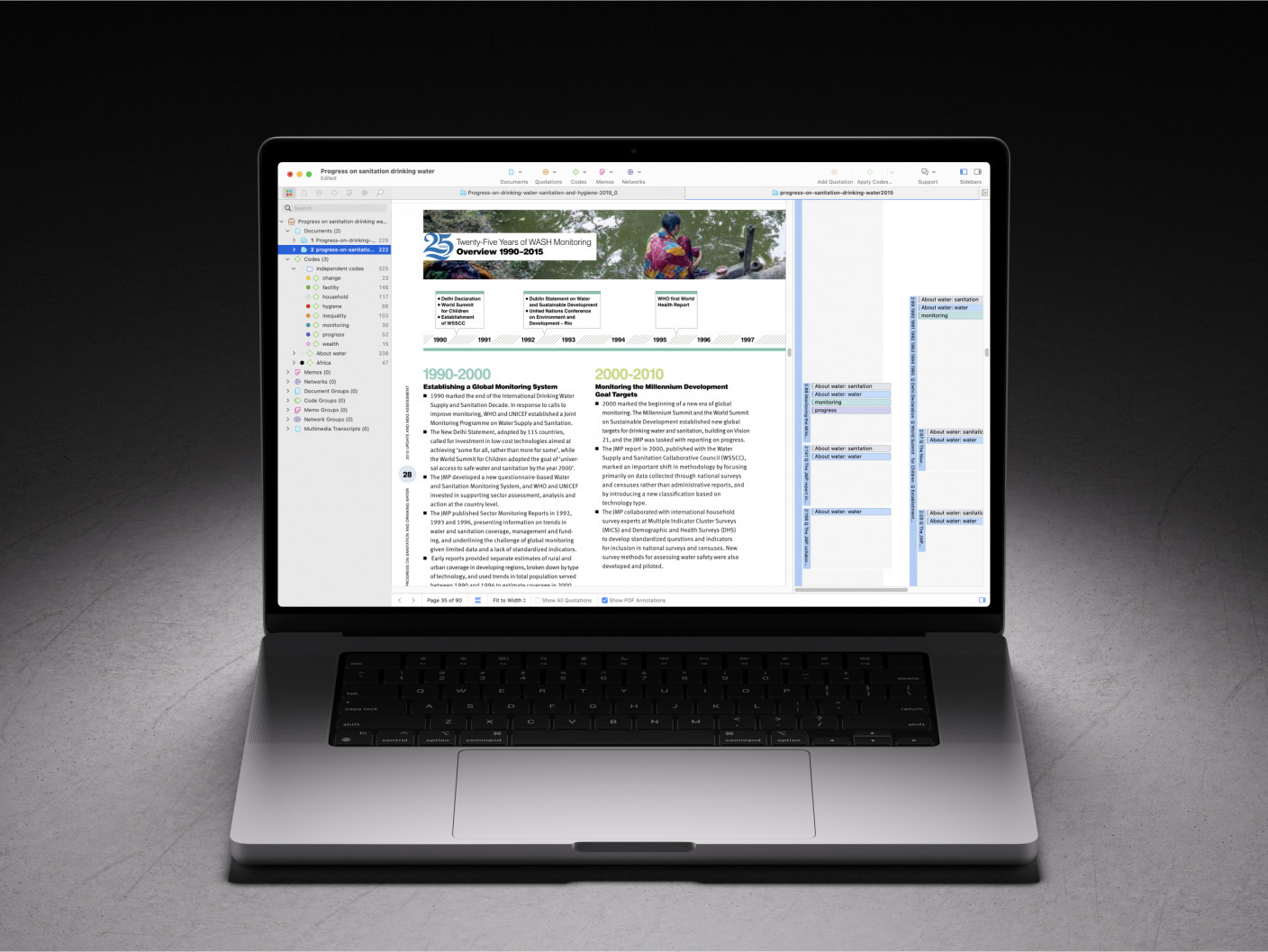
Whatever field you're in, ATLAS.ti puts your data to work for you
Download a free trial of ATLAS.ti to turn your data into insights.
Understanding the key elements of case study design is crucial for conducting rigorous and impactful case study research. A well-structured design guides the researcher through the process, ensuring that the study is methodologically sound and its findings are reliable and valid. The main elements of case study design include the research question , propositions, units of analysis, and the logic linking the data to the propositions.
The research question is the foundation of any research study. A good research question guides the direction of the study and informs the selection of the case, the methods of collecting data, and the analysis techniques. A well-formulated research question in case study research is typically clear, focused, and complex enough to merit further detailed examination of the relevant case(s).
Propositions
Propositions, though not necessary in every case study, provide a direction by stating what we might expect to find in the data collected. They guide how data is collected and analyzed by helping researchers focus on specific aspects of the case. They are particularly important in explanatory case studies, which seek to understand the relationships among concepts within the studied phenomenon.
Units of analysis
The unit of analysis refers to the case, or the main entity or entities that are being analyzed in the study. In case study research, the unit of analysis can be an individual, a group, an organization, a decision, an event, or even a time period. It's crucial to clearly define the unit of analysis, as it shapes the qualitative data analysis process by allowing the researcher to analyze a particular case and synthesize analysis across multiple case studies to draw conclusions.
Argumentation
This refers to the inferential model that allows researchers to draw conclusions from the data. The researcher needs to ensure that there is a clear link between the data, the propositions (if any), and the conclusions drawn. This argumentation is what enables the researcher to make valid and credible inferences about the phenomenon under study.
Understanding and carefully considering these elements in the design phase of a case study can significantly enhance the quality of the research. It can help ensure that the study is methodologically sound and its findings contribute meaningful insights about the case.
Ready to jumpstart your research with ATLAS.ti?
Conceptualize your research project with our intuitive data analysis interface. Download a free trial today.
Conducting a case study involves several steps, from defining the research question and selecting the case to collecting and analyzing data . This section outlines these key stages, providing a practical guide on how to conduct case study research.
Defining the research question
The first step in case study research is defining a clear, focused research question. This question should guide the entire research process, from case selection to analysis. It's crucial to ensure that the research question is suitable for a case study approach. Typically, such questions are exploratory or descriptive in nature and focus on understanding a phenomenon within its real-life context.
Selecting and defining the case
The selection of the case should be based on the research question and the objectives of the study. It involves choosing a unique example or a set of examples that provide rich, in-depth data about the phenomenon under investigation. After selecting the case, it's crucial to define it clearly, setting the boundaries of the case, including the time period and the specific context.
Previous research can help guide the case study design. When considering a case study, an example of a case could be taken from previous case study research and used to define cases in a new research inquiry. Considering recently published examples can help understand how to select and define cases effectively.
Developing a detailed case study protocol
A case study protocol outlines the procedures and general rules to be followed during the case study. This includes the data collection methods to be used, the sources of data, and the procedures for analysis. Having a detailed case study protocol ensures consistency and reliability in the study.
The protocol should also consider how to work with the people involved in the research context to grant the research team access to collecting data. As mentioned in previous sections of this guide, establishing rapport is an essential component of qualitative research as it shapes the overall potential for collecting and analyzing data.
Collecting data
Gathering data in case study research often involves multiple sources of evidence, including documents, archival records, interviews, observations, and physical artifacts. This allows for a comprehensive understanding of the case. The process for gathering data should be systematic and carefully documented to ensure the reliability and validity of the study.
Analyzing and interpreting data
The next step is analyzing the data. This involves organizing the data , categorizing it into themes or patterns , and interpreting these patterns to answer the research question. The analysis might also involve comparing the findings with prior research or theoretical propositions.
Writing the case study report
The final step is writing the case study report . This should provide a detailed description of the case, the data, the analysis process, and the findings. The report should be clear, organized, and carefully written to ensure that the reader can understand the case and the conclusions drawn from it.
Each of these steps is crucial in ensuring that the case study research is rigorous, reliable, and provides valuable insights about the case.
The type, depth, and quality of data in your study can significantly influence the validity and utility of the study. In case study research, data is usually collected from multiple sources to provide a comprehensive and nuanced understanding of the case. This section will outline the various methods of collecting data used in case study research and discuss considerations for ensuring the quality of the data.
Interviews are a common method of gathering data in case study research. They can provide rich, in-depth data about the perspectives, experiences, and interpretations of the individuals involved in the case. Interviews can be structured , semi-structured , or unstructured , depending on the research question and the degree of flexibility needed.
Observations
Observations involve the researcher observing the case in its natural setting, providing first-hand information about the case and its context. Observations can provide data that might not be revealed in interviews or documents, such as non-verbal cues or contextual information.
Documents and artifacts
Documents and archival records provide a valuable source of data in case study research. They can include reports, letters, memos, meeting minutes, email correspondence, and various public and private documents related to the case.
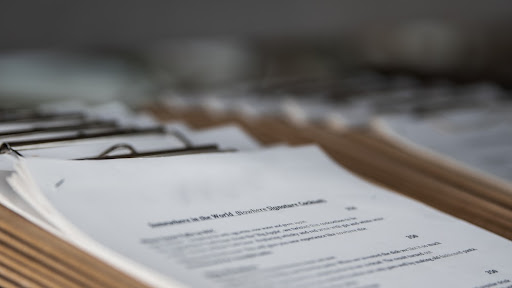
These records can provide historical context, corroborate evidence from other sources, and offer insights into the case that might not be apparent from interviews or observations.
Physical artifacts refer to any physical evidence related to the case, such as tools, products, or physical environments. These artifacts can provide tangible insights into the case, complementing the data gathered from other sources.
Ensuring the quality of data collection
Determining the quality of data in case study research requires careful planning and execution. It's crucial to ensure that the data is reliable, accurate, and relevant to the research question. This involves selecting appropriate methods of collecting data, properly training interviewers or observers, and systematically recording and storing the data. It also includes considering ethical issues related to collecting and handling data, such as obtaining informed consent and ensuring the privacy and confidentiality of the participants.
Data analysis
Analyzing case study research involves making sense of the rich, detailed data to answer the research question. This process can be challenging due to the volume and complexity of case study data. However, a systematic and rigorous approach to analysis can ensure that the findings are credible and meaningful. This section outlines the main steps and considerations in analyzing data in case study research.
Organizing the data
The first step in the analysis is organizing the data. This involves sorting the data into manageable sections, often according to the data source or the theme. This step can also involve transcribing interviews, digitizing physical artifacts, or organizing observational data.
Categorizing and coding the data
Once the data is organized, the next step is to categorize or code the data. This involves identifying common themes, patterns, or concepts in the data and assigning codes to relevant data segments. Coding can be done manually or with the help of software tools, and in either case, qualitative analysis software can greatly facilitate the entire coding process. Coding helps to reduce the data to a set of themes or categories that can be more easily analyzed.
Identifying patterns and themes
After coding the data, the researcher looks for patterns or themes in the coded data. This involves comparing and contrasting the codes and looking for relationships or patterns among them. The identified patterns and themes should help answer the research question.
Interpreting the data
Once patterns and themes have been identified, the next step is to interpret these findings. This involves explaining what the patterns or themes mean in the context of the research question and the case. This interpretation should be grounded in the data, but it can also involve drawing on theoretical concepts or prior research.
Verification of the data
The last step in the analysis is verification. This involves checking the accuracy and consistency of the analysis process and confirming that the findings are supported by the data. This can involve re-checking the original data, checking the consistency of codes, or seeking feedback from research participants or peers.
Like any research method , case study research has its strengths and limitations. Researchers must be aware of these, as they can influence the design, conduct, and interpretation of the study.
Understanding the strengths and limitations of case study research can also guide researchers in deciding whether this approach is suitable for their research question . This section outlines some of the key strengths and limitations of case study research.
Benefits include the following:
- Rich, detailed data: One of the main strengths of case study research is that it can generate rich, detailed data about the case. This can provide a deep understanding of the case and its context, which can be valuable in exploring complex phenomena.
- Flexibility: Case study research is flexible in terms of design , data collection , and analysis . A sufficient degree of flexibility allows the researcher to adapt the study according to the case and the emerging findings.
- Real-world context: Case study research involves studying the case in its real-world context, which can provide valuable insights into the interplay between the case and its context.
- Multiple sources of evidence: Case study research often involves collecting data from multiple sources , which can enhance the robustness and validity of the findings.
On the other hand, researchers should consider the following limitations:
- Generalizability: A common criticism of case study research is that its findings might not be generalizable to other cases due to the specificity and uniqueness of each case.
- Time and resource intensive: Case study research can be time and resource intensive due to the depth of the investigation and the amount of collected data.
- Complexity of analysis: The rich, detailed data generated in case study research can make analyzing the data challenging.
- Subjectivity: Given the nature of case study research, there may be a higher degree of subjectivity in interpreting the data , so researchers need to reflect on this and transparently convey to audiences how the research was conducted.
Being aware of these strengths and limitations can help researchers design and conduct case study research effectively and interpret and report the findings appropriately.
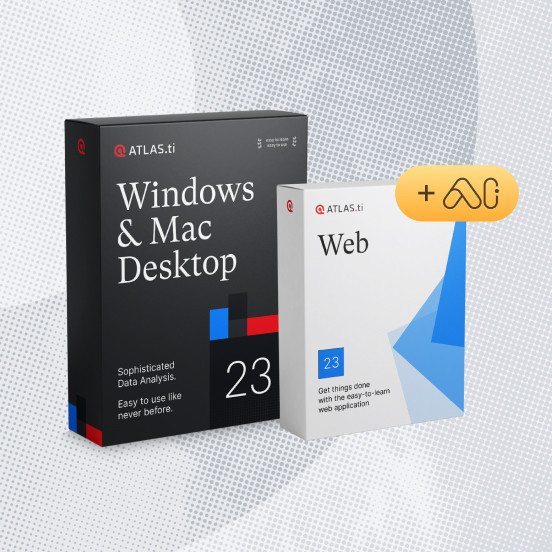
Ready to analyze your data with ATLAS.ti?
See how our intuitive software can draw key insights from your data with a free trial today.
How to select cases?
Social scientists try to make statements about a theoretical set of units. Sometimes we are able to study all units we are interested in. This means we study a census. In most cases, however, only a subset of cases is studied. Case selection is a crucial part of empirical research, and largely determines the extent to which you can generalise from your findings to the larger target population. Case selection is also called sampling , although this word is most frequently used when larger numbers of cases are selected. The main types of sampling are probability sampling and non-probability sampling. The first is mostly associated with large n studies. The second is associated with both large and smaller n studies. If the researcher does not have the intention to make an inference to a larger target population, other selection procedures are available.
Target population and sampling frame
The target population is the set of units we make statements about; companies, persons, newspaper articles, products. In many cases the target population is not easily accessed. A sampling frame is a set of units we can draw samples from. For example, if the target population is local businesses, a suitable sampling frame might be the listings in the business section of the telephone book. Preferably the sampling frame includes nearly all of the population, although this is not always possible. The sample frame is then used to select cases from.
Probability sampling
There are various types of probability sampling. All procedures use some reference to the known probability an element from the sampling frame is actually selected for study. Different procedures can be used to get a representative sample of units. A distinction is made between single stage sampling (for example, simple probability sampling, systematic sampling, stratified sampling and cluster sampling) and multi-stage sampling (in which different procedures are used sequentially (for example, first selecting municipalities and the random samples within each municipality).
Non-probability sampling
The set of non-probability sampling procedures is huge. Examples are self selection, snowball sampling and quota sampling. With all these procedures the danger is that the selection might be biased: i.e. over- or under- representing units with certain attributes. These procedures should therefore be avoided if possible.
If only a small number of cases is selected using probability sampling, the risk too is that certain attributes are over- or under-represented. To avoid this, cases must be selected on the basis of prior knowledge of their attributes (intentional selection). If the researcher tries to test a causal hypothesis het must at least select cases to ensure variation on the main independent and dependent variables. You cannot infer anything about the causes of the success of companies, for example, if you limit your selection to successful companies. Note, however, that if the number of cases is small, your conclusions can easily be the consequence of mere chance.
Selection of one case or only a few cases
If the researcher wants to explore a topic, or further develop an existing and well-tested theory several other case selection options are available. If the aim is exploration cases in single case studies are selected on the basis of the information they are expected to provide. Single cases can be selected because the case:
- is extreme (i.e. has an extremely low or high value on the central variable)
- is critical (i.e. ‘If it is valid for this case, it is valid for all (or many) cases’ or the converse, ‘If it is not valid for this case, then it is not valid for any (or only few) cases.’)
- is typical (i.e. an example of the phenomenon under investigation)
- is deviant (i.e. has a combination of characteristics different from most other units)
Note that all case selection procedures mentioned here assume the existence of a (preferably tested) theory and some general knowledge of the central variables in the units of analysis on the basis of which single cases can be selected.
Basic readings
- Babbie, Earl (2004). The Practice of Social Research (12 th edition) . Belmont: Wadsworth/Thomson. Chapter 7.
- Shadish, William R., Thomas D. Cook and Donald T. Cambell (2002). Experimental and Quasi-Experimental Designs for Generalized Causal Inference. Boston: Houghton Mifflin. Chapter 3.
- De Vaus, David (2001). Research Design in Social Research. London: Sage. Chapters 5, 8, 11, 14.
Additional readings
- King, Gary, Robert O. Keohane and Sidney Verba (1994). Designing Social Inquiry: scientific inference in qualitative research. Princeton: Princeton University Press.
- Yin, Robert K (1994). Case Study Research: Design and Methods (2 nd edition) . Thousand Oaks, California: Sage.
- Swanborn, P. G (1996). Case-study’s: Wat, wanneer en hoe? Amsterdam: Boom.
- Franzosi, Roberto P. (2004). Content Analysis , in: Melissa A. Hardy and Alan Bryman (eds). Handbook of Data Analysis. London, Sage
- Berg, Bruce (2007) Qualitative Research Methods for the Social Sciences . Boston, Pearson.
- Gerring, John (2001) Social Science Methodology: a criterial framework . Cambridge, Cambridge University Press. Chapter 8.
Strategies for model selection
Case studies:
A. SAT scores by state
B. Deeper dive into sex discrimination in base salary
Model selection
Best subsets
Stepwise methods
Case study A: State SAT scores
Predicting average 1982 SAT score by state.
Takers (percentage)
Income (parental income of SAT takers)
Years (years of education in key subjects)
Public (percent of takers in public high school)
Expend (state expenditure on high schools per student)
Rank (average percentile of students writing SAT
Case study B: Sex discrimination in employment
Same data as earlier, with additional features:
Age (years)
Educ (years)
Exper (months)
Senior (months)
Sal77 (salary in 1977 – used for a different analysis in the book)
Salary data
- In a given regression situation, there are often many choices to be made. Recall our usual setup (with intercept)
\[\begin{equation} Y_{n \times 1} = X_{n \times (p+1)} \beta_{(p+1) \times 1} + \epsilon_{n \times 1}. \end{equation}\]
- Any subset \(A \subset \{1, \dots, p\}\) yields a new regression model
\[\begin{equation} {\cal M}(A): Y_{n \times 1} = X[,A] \beta[A] + \epsilon_{n \times 1} \end{equation}\]
Why model selection?
Possible goals in the sat data.
States with low Takers typically have high Rank
Beyond this effect, what is important for understanding variability in SAT scores?
Maybe want a parsimonious model.
General problem & goals
When we have many predictors (with many possible interactions), it can be difficult to formulate a good model from outside considerations.
Which main effects do we include?
Which interactions do we include?
Model selection procedures try to simplify / automate this task.
General comments
This is generally an “unsolved” problem in statistics: there are no magic procedures to get you the “best model” or “correct model”.
Inference after selection is full of pitfalls!
General approach
To “implement” a model selection procedure, we first need a criterion / score / benchmark to compare two models.
Given a criterion, we also need a search strategy .
Search strategies
Exhaustive: With a limited number of predictors, it is possible to search all possible models.
Sequential: Select a model in a sequence of steps.
Exhaustive search ( leaps in R )
Candidate criteria.
\(R^2\) : not a good criterion. Always increase with model size \(\implies\) “optimum” is to take the biggest model.
Adjusted \(R^2\) : better. It “penalized” bigger models. Follows principle of parsimony / Occam’s razor.
Mallow’s \(C_p\) – attempts to estimate a model’s predictive power, i.e. the power to predict a new observation.
Setup for leaps
- leaps takes a design matrix as argument: throw away the intercept column or leaps will complain:
The problem with R^2
Plot of \(R^2\) of a model as a function of the model size.
The “best” model in terms of \(R^2\) does indeed include all variables.
Adjusted \(R^2\)
As we add more and more variables to the model – even random ones, \(R^2\) will increase to 1.
Recall that adjusted \(R^2\) tries to take this into account by replacing sums of squares by mean squares
\[R^2_a = 1 - \frac{SSE/(n-p-1)}{SST/(n-1)} = 1 - \frac{MSE}{MST}.\]
Plot of \(R^2_a\) of a model as a function of the model size.
The “best” model in terms of \(R^2_a\) is not the full model: has size 5 (4 variables plus intercept)
Mallow’s \(C_p\)
Definition:.
\[C_p({\cal M}) = \frac{SSE({\cal M})}{\widehat{\sigma}^2} + 2 \cdot p({\cal M}) - n.\]
\(\widehat{\sigma}^2=SSE(F)/df_F\) is the “best” estimate of \(\sigma^2\) we have (use the fullest model), i.e. in the SAT data it uses all 6 main effects.
\(SSE({\cal M})\) is the \(SSE\) of the model \({\cal M}\) .
\(p({\cal M})\) is the number of predictors in \({\cal M}\) .
This is an estimate of the expected mean-squared error of \(\widehat{Y}({\cal M})\) , it takes bias and variance into account.
Plot of \(C_p\) of a model as a function of the model size.
The “best” model in terms of \(C_p\) is not the full model: has size 5 (4 variables plus intercept)
Agrees with R^2_a .
Sequential search ( step in R )
- The step function uses a specific score for comparing models.
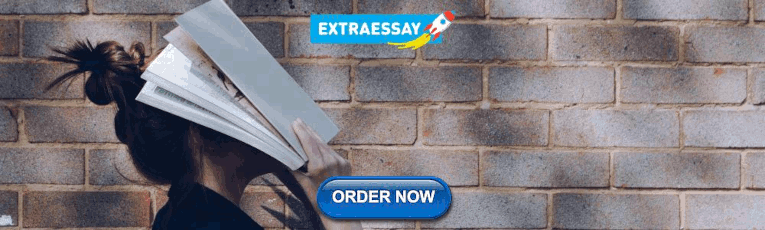
Akaike Information Criterion (AIC)
\[AIC({\cal M}) = - 2 \log L({\cal M}) + 2 \cdot p({\cal M})\]
- Above, \(L({\cal M})\) is the maximized likelihood of the model.
Bayesian Information Criterion (BIC)
\[BIC({\cal M}) = - 2 \log L({\cal M}) + \log n \cdot p({\cal M})\]
- Note: AIC/BIC be used for whenever we have a likelihood, so this generalizes to many statistical models.
AIC for regression
- In linear regression with unknown \(\sigma^2\)
\[-2 \log L({\cal M}) = n \log(2\pi \widehat{\sigma}^2_{MLE}) + n\]
- In linear regression with known \(\sigma^2\)
\[-2 \log L({\cal M}) = \frac{1}{\sigma^2} SSE({\cal M})\]
- AIC is very much like Mallow’s \(C_p\) with known variance.
Properties of AIC / BIC
BIC will typically choose a model as small or smaller than AIC (if using the same search direction).
As our sample size grows, under some assumptions, it can be shown that
- AIC will (asymptotically) always choose a model that contains the true model, i.e. it won’t leave any variables out.
- BIC will (asymptotically) choose exactly the right model.
SAT example
Let’s take a look at step in action. Probably the simplest strategy is forward stepwise which tries to add one variable at a time, as long as it can find a resulting model whose AIC is better than its current position.
When it can make no further additions, it terminates.
Interactions and hierarchy
Wildcard: . denotes any variable in SAT.df except SAT
.^2 denotes all 2-way interactions
- Note: when looking at trace=TRUE we see that step does not include an interaction unless both main effects are already in the model.
BIC example
The only difference between AIC and BIC is the price paid per variable. This is the argument k to step . By default k=2 and for BIC we set k=log(n) . If we set k=0 it will always add variables.
Compare to AIC
Backward selection.
Just for fun, let’s consider backwards stepwise. This starts at a full model and tries to delete variables.
There is also a direction="both" option.
Compare to forward
Summarizing results.
The model selected depends on the criterion used.
The selected model is random and depends on which method we use!
Pretty stable for this analysis.
Where we are so far
Many other “criteria” have been proposed: cross-validation (CV) a very popular criterion.
Some work well for some types of data, others for different data.
Check diagnostics!
These criteria (except cross-validation) are not “direct measures” of predictive power, though Mallow’s \(C_p\) is a step in this direction.
\(C_p\) measures the quality of a model based on both bias and variance of the model. Why is this important?
Bias-variance tradeoff is ubiquitous in statistics!
Case study B: sex discrimination
Possible goals in the salary data.
Interested in the effect of Sex but there could lots of other covariates we might adjust for…
Considering all second order effects (besides Sex ) in salary: \(2^{14}\) models!
Remove confounders, reduce variability of Sex effect.
Fit a model without Sex using a model selection technique.
Estimate the effect of Sex by adding it to this model.
How are we going to choose \(2^{14}\) possible choices!
Best second order model using \(C_p\) with leaps
Plot of \(c_p\) scores (fig 12.11 in book).
Plot of \(C_p\) score as a function of model size.
Two models are lower than the rest but quite close. These models used in subsequent analysis.
Estimating the effect of Sex
- On inspection, book drops one of these interactions:
What if we used step ?
Larger example.
As \(p\) grows, leaps will be too slow
Even step can get slow
HIV resistance
Resistance of \(n=633\) different HIV+ viruses to drug 3TC.
Features \(p=91\) are mutations in a part of the HIV virus, response is log fold change in vitro.

- Open access
- Published: 14 May 2024
Developing a survey to measure nursing students’ knowledge, attitudes and beliefs, influences, and willingness to be involved in Medical Assistance in Dying (MAiD): a mixed method modified e-Delphi study
- Jocelyn Schroeder 1 ,
- Barbara Pesut 1 , 2 ,
- Lise Olsen 2 ,
- Nelly D. Oelke 2 &
- Helen Sharp 2
BMC Nursing volume 23 , Article number: 326 ( 2024 ) Cite this article
31 Accesses
Metrics details
Medical Assistance in Dying (MAiD) was legalized in Canada in 2016. Canada’s legislation is the first to permit Nurse Practitioners (NP) to serve as independent MAiD assessors and providers. Registered Nurses’ (RN) also have important roles in MAiD that include MAiD care coordination; client and family teaching and support, MAiD procedural quality; healthcare provider and public education; and bereavement care for family. Nurses have a right under the law to conscientious objection to participating in MAiD. Therefore, it is essential to prepare nurses in their entry-level education for the practice implications and moral complexities inherent in this practice. Knowing what nursing students think about MAiD is a critical first step. Therefore, the purpose of this study was to develop a survey to measure nursing students’ knowledge, attitudes and beliefs, influences, and willingness to be involved in MAiD in the Canadian context.
The design was a mixed-method, modified e-Delphi method that entailed item generation from the literature, item refinement through a 2 round survey of an expert faculty panel, and item validation through a cognitive focus group interview with nursing students. The settings were a University located in an urban area and a College located in a rural area in Western Canada.
During phase 1, a 56-item survey was developed from existing literature that included demographic items and items designed to measure experience with death and dying (including MAiD), education and preparation, attitudes and beliefs, influences on those beliefs, and anticipated future involvement. During phase 2, an expert faculty panel reviewed, modified, and prioritized the items yielding 51 items. During phase 3, a sample of nursing students further evaluated and modified the language in the survey to aid readability and comprehension. The final survey consists of 45 items including 4 case studies.
Systematic evaluation of knowledge-to-date coupled with stakeholder perspectives supports robust survey design. This study yielded a survey to assess nursing students’ attitudes toward MAiD in a Canadian context.
The survey is appropriate for use in education and research to measure knowledge and attitudes about MAiD among nurse trainees and can be a helpful step in preparing nursing students for entry-level practice.
Peer Review reports
Medical Assistance in Dying (MAiD) is permitted under an amendment to Canada’s Criminal Code which was passed in 2016 [ 1 ]. MAiD is defined in the legislation as both self-administered and clinician-administered medication for the purpose of causing death. In the 2016 Bill C-14 legislation one of the eligibility criteria was that an applicant for MAiD must have a reasonably foreseeable natural death although this term was not defined. It was left to the clinical judgement of MAiD assessors and providers to determine the time frame that constitutes reasonably foreseeable [ 2 ]. However, in 2021 under Bill C-7, the eligibility criteria for MAiD were changed to allow individuals with irreversible medical conditions, declining health, and suffering, but whose natural death was not reasonably foreseeable, to receive MAiD [ 3 ]. This population of MAiD applicants are referred to as Track 2 MAiD (those whose natural death is foreseeable are referred to as Track 1). Track 2 applicants are subject to additional safeguards under the 2021 C-7 legislation.
Three additional proposed changes to the legislation have been extensively studied by Canadian Expert Panels (Council of Canadian Academics [CCA]) [ 4 , 5 , 6 ] First, under the legislation that defines Track 2, individuals with mental disease as their sole underlying medical condition may apply for MAiD, but implementation of this practice is embargoed until March 2027 [ 4 ]. Second, there is consideration of allowing MAiD to be implemented through advanced consent. This would make it possible for persons living with dementia to receive MAID after they have lost the capacity to consent to the procedure [ 5 ]. Third, there is consideration of extending MAiD to mature minors. A mature minor is defined as “a person under the age of majority…and who has the capacity to understand and appreciate the nature and consequences of a decision” ([ 6 ] p. 5). In summary, since the legalization of MAiD in 2016 the eligibility criteria and safeguards have evolved significantly with consequent implications for nurses and nursing care. Further, the number of Canadians who access MAiD shows steady increases since 2016 [ 7 ] and it is expected that these increases will continue in the foreseeable future.
Nurses have been integral to MAiD care in the Canadian context. While other countries such as Belgium and the Netherlands also permit euthanasia, Canada is the first country to allow Nurse Practitioners (Registered Nurses with additional preparation typically achieved at the graduate level) to act independently as assessors and providers of MAiD [ 1 ]. Although the role of Registered Nurses (RNs) in MAiD is not defined in federal legislation, it has been addressed at the provincial/territorial-level with variability in scope of practice by region [ 8 , 9 ]. For example, there are differences with respect to the obligation of the nurse to provide information to patients about MAiD, and to the degree that nurses are expected to ensure that patient eligibility criteria and safeguards are met prior to their participation [ 10 ]. Studies conducted in the Canadian context indicate that RNs perform essential roles in MAiD care coordination; client and family teaching and support; MAiD procedural quality; healthcare provider and public education; and bereavement care for family [ 9 , 11 ]. Nurse practitioners and RNs are integral to a robust MAiD care system in Canada and hence need to be well-prepared for their role [ 12 ].
Previous studies have found that end of life care, and MAiD specifically, raise complex moral and ethical issues for nurses [ 13 , 14 , 15 , 16 ]. The knowledge, attitudes, and beliefs of nurses are important across practice settings because nurses have consistent, ongoing, and direct contact with patients who experience chronic or life-limiting health conditions. Canadian studies exploring nurses’ moral and ethical decision-making in relation to MAiD reveal that although some nurses are clear in their support for, or opposition to, MAiD, others are unclear on what they believe to be good and right [ 14 ]. Empirical findings suggest that nurses go through a period of moral sense-making that is often informed by their family, peers, and initial experiences with MAID [ 17 , 18 ]. Canadian legislation and policy specifies that nurses are not required to participate in MAiD and may recuse themselves as conscientious objectors with appropriate steps to ensure ongoing and safe care of patients [ 1 , 19 ]. However, with so many nurses having to reflect on and make sense of their moral position, it is essential that they are given adequate time and preparation to make an informed and thoughtful decision before they participate in a MAID death [ 20 , 21 ].
It is well established that nursing students receive inconsistent exposure to end of life care issues [ 22 ] and little or no training related to MAiD [ 23 ]. Without such education and reflection time in pre-entry nursing preparation, nurses are at significant risk for moral harm. An important first step in providing this preparation is to be able to assess the knowledge, values, and beliefs of nursing students regarding MAID and end of life care. As demand for MAiD increases along with the complexities of MAiD, it is critical to understand the knowledge, attitudes, and likelihood of engagement with MAiD among nursing students as a baseline upon which to build curriculum and as a means to track these variables over time.
Aim, design, and setting
The aim of this study was to develop a survey to measure nursing students’ knowledge, attitudes and beliefs, influences, and willingness to be involved in MAiD in the Canadian context. We sought to explore both their willingness to be involved in the registered nursing role and in the nurse practitioner role should they chose to prepare themselves to that level of education. The design was a mixed-method, modified e-Delphi method that entailed item generation, item refinement through an expert faculty panel [ 24 , 25 , 26 ], and initial item validation through a cognitive focus group interview with nursing students [ 27 ]. The settings were a University located in an urban area and a College located in a rural area in Western Canada.
Participants
A panel of 10 faculty from the two nursing education programs were recruited for Phase 2 of the e-Delphi. To be included, faculty were required to have a minimum of three years of experience in nurse education, be employed as nursing faculty, and self-identify as having experience with MAiD. A convenience sample of 5 fourth-year nursing students were recruited to participate in Phase 3. Students had to be in good standing in the nursing program and be willing to share their experiences of the survey in an online group interview format.
The modified e-Delphi was conducted in 3 phases: Phase 1 entailed item generation through literature and existing survey review. Phase 2 entailed item refinement through a faculty expert panel review with focus on content validity, prioritization, and revision of item wording [ 25 ]. Phase 3 entailed an assessment of face validity through focus group-based cognitive interview with nursing students.
Phase I. Item generation through literature review
The goal of phase 1 was to develop a bank of survey items that would represent the variables of interest and which could be provided to expert faculty in Phase 2. Initial survey items were generated through a literature review of similar surveys designed to assess knowledge and attitudes toward MAiD/euthanasia in healthcare providers; Canadian empirical studies on nurses’ roles and/or experiences with MAiD; and legislative and expert panel documents that outlined proposed changes to the legislative eligibility criteria and safeguards. The literature review was conducted in three online databases: CINAHL, PsycINFO, and Medline. Key words for the search included nurses , nursing students , medical students , NPs, MAiD , euthanasia , assisted death , and end-of-life care . Only articles written in English were reviewed. The legalization and legislation of MAiD is new in many countries; therefore, studies that were greater than twenty years old were excluded, no further exclusion criteria set for country.
Items from surveys designed to measure similar variables in other health care providers and geographic contexts were placed in a table and similar items were collated and revised into a single item. Then key variables were identified from the empirical literature on nurses and MAiD in Canada and checked against the items derived from the surveys to ensure that each of the key variables were represented. For example, conscientious objection has figured prominently in the Canadian literature, but there were few items that assessed knowledge of conscientious objection in other surveys and so items were added [ 15 , 21 , 28 , 29 ]. Finally, four case studies were added to the survey to address the anticipated changes to the Canadian legislation. The case studies were based upon the inclusion of mature minors, advanced consent, and mental disorder as the sole underlying medical condition. The intention was to assess nurses’ beliefs and comfort with these potential legislative changes.
Phase 2. Item refinement through expert panel review
The goal of phase 2 was to refine and prioritize the proposed survey items identified in phase 1 using a modified e-Delphi approach to achieve consensus among an expert panel [ 26 ]. Items from phase 1 were presented to an expert faculty panel using a Qualtrics (Provo, UT) online survey. Panel members were asked to review each item to determine if it should be: included, excluded or adapted for the survey. When adapted was selected faculty experts were asked to provide rationale and suggestions for adaptation through the use of an open text box. Items that reached a level of 75% consensus for either inclusion or adaptation were retained [ 25 , 26 ]. New items were categorized and added, and a revised survey was presented to the panel of experts in round 2. Panel members were again asked to review items, including new items, to determine if it should be: included, excluded, or adapted for the survey. Round 2 of the modified e-Delphi approach also included an item prioritization activity, where participants were then asked to rate the importance of each item, based on a 5-point Likert scale (low to high importance), which De Vaus [ 30 ] states is helpful for increasing the reliability of responses. Items that reached a 75% consensus on inclusion were then considered in relation to the importance it was given by the expert panel. Quantitative data were managed using SPSS (IBM Corp).
Phase 3. Face validity through cognitive interviews with nursing students
The goal of phase 3 was to obtain initial face validity of the proposed survey using a sample of nursing student informants. More specifically, student participants were asked to discuss how items were interpreted, to identify confusing wording or other problematic construction of items, and to provide feedback about the survey as a whole including readability and organization [ 31 , 32 , 33 ]. The focus group was held online and audio recorded. A semi-structured interview guide was developed for this study that focused on clarity, meaning, order and wording of questions; emotions evoked by the questions; and overall survey cohesion and length was used to obtain data (see Supplementary Material 2 for the interview guide). A prompt to “think aloud” was used to limit interviewer-imposed bias and encourage participants to describe their thoughts and response to a given item as they reviewed survey items [ 27 ]. Where needed, verbal probes such as “could you expand on that” were used to encourage participants to expand on their responses [ 27 ]. Student participants’ feedback was collated verbatim and presented to the research team where potential survey modifications were negotiated and finalized among team members. Conventional content analysis [ 34 ] of focus group data was conducted to identify key themes that emerged through discussion with students. Themes were derived from the data by grouping common responses and then using those common responses to modify survey items.
Ten nursing faculty participated in the expert panel. Eight of the 10 faculty self-identified as female. No faculty panel members reported conscientious objector status and ninety percent reported general agreement with MAiD with one respondent who indicated their view as “unsure.” Six of the 10 faculty experts had 16 years of experience or more working as a nurse educator.
Five nursing students participated in the cognitive interview focus group. The duration of the focus group was 2.5 h. All participants identified that they were born in Canada, self-identified as female (one preferred not to say) and reported having received some instruction about MAiD as part of their nursing curriculum. See Tables 1 and 2 for the demographic descriptors of the study sample. Study results will be reported in accordance with the study phases. See Fig. 1 for an overview of the results from each phase.

Fig. 1 Overview of survey development findings
Phase 1: survey item generation
Review of the literature identified that no existing survey was available for use with nursing students in the Canadian context. However, an analysis of themes across qualitative and quantitative studies of physicians, medical students, nurses, and nursing students provided sufficient data to develop a preliminary set of items suitable for adaptation to a population of nursing students.
Four major themes and factors that influence knowledge, attitudes, and beliefs about MAiD were evident from the literature: (i) endogenous or individual factors such as age, gender, personally held values, religion, religiosity, and/or spirituality [ 35 , 36 , 37 , 38 , 39 , 40 , 41 , 42 ], (ii) experience with death and dying in personal and/or professional life [ 35 , 40 , 41 , 43 , 44 , 45 ], (iii) training including curricular instruction about clinical role, scope of practice, or the law [ 23 , 36 , 39 ], and (iv) exogenous or social factors such as the influence of key leaders, colleagues, friends and/or family, professional and licensure organizations, support within professional settings, and/or engagement in MAiD in an interdisciplinary team context [ 9 , 35 , 46 ].
Studies of nursing students also suggest overlap across these categories. For example, value for patient autonomy [ 23 ] and the moral complexity of decision-making [ 37 ] are important factors that contribute to attitudes about MAiD and may stem from a blend of personally held values coupled with curricular content, professional training and norms, and clinical exposure. For example, students report that participation in end of life care allows for personal growth, shifts in perception, and opportunities to build therapeutic relationships with their clients [ 44 , 47 , 48 ].
Preliminary items generated from the literature resulted in 56 questions from 11 published sources (See Table 3 ). These items were constructed across four main categories: (i) socio-demographic questions; (ii) end of life care questions; (iii) knowledge about MAiD; or (iv) comfort and willingness to participate in MAiD. Knowledge questions were refined to reflect current MAiD legislation, policies, and regulatory frameworks. Falconer [ 39 ] and Freeman [ 45 ] studies were foundational sources for item selection. Additionally, four case studies were written to reflect the most recent anticipated changes to MAiD legislation and all used the same open-ended core questions to address respondents’ perspectives about the patient’s right to make the decision, comfort in assisting a physician or NP to administer MAiD in that scenario, and hypothesized comfort about serving as a primary provider if qualified as an NP in future. Response options for the survey were also constructed during this stage and included: open text, categorical, yes/no , and Likert scales.
Phase 2: faculty expert panel review
Of the 56 items presented to the faculty panel, 54 questions reached 75% consensus. However, based upon the qualitative responses 9 items were removed largely because they were felt to be repetitive. Items that generated the most controversy were related to measuring religion and spirituality in the Canadian context, defining end of life care when there is no agreed upon time frames (e.g., last days, months, or years), and predicting willingness to be involved in a future events – thus predicting their future selves. Phase 2, round 1 resulted in an initial set of 47 items which were then presented back to the faculty panel in round 2.
Of the 47 initial questions presented to the panel in round 2, 45 reached a level of consensus of 75% or greater, and 34 of these questions reached a level of 100% consensus [ 27 ] of which all participants chose to include without any adaptations) For each question, level of importance was determined based on a 5-point Likert scale (1 = very unimportant, 2 = somewhat unimportant, 3 = neutral, 4 = somewhat important, and 5 = very important). Figure 2 provides an overview of the level of importance assigned to each item.
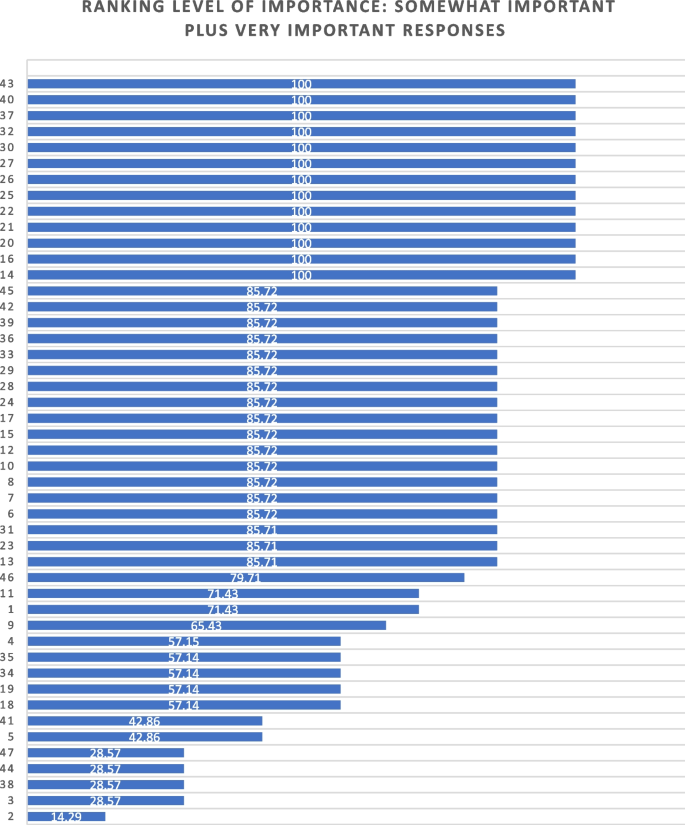
Ranking level of importance for survey items
After round 2, a careful analysis of participant comments and level of importance was completed by the research team. While the main method of survey item development came from participants’ response to the first round of Delphi consensus ratings, level of importance was used to assist in the decision of whether to keep or modify questions that created controversy, or that rated lower in the include/exclude/adapt portion of the Delphi. Survey items that rated low in level of importance included questions about future roles, sex and gender, and religion/spirituality. After deliberation by the research committee, these questions were retained in the survey based upon the importance of these variables in the scientific literature.
Of the 47 questions remaining from Phase 2, round 2, four were revised. In addition, the two questions that did not meet the 75% cut off level for consensus were reviewed by the research team. The first question reviewed was What is your comfort level with providing a MAiD death in the future if you were a qualified NP ? Based on a review of participant comments, it was decided to retain this question for the cognitive interviews with students in the final phase of testing. The second question asked about impacts on respondents’ views of MAiD and was changed from one item with 4 subcategories into 4 separate items, resulting in a final total of 51 items for phase 3. The revised survey was then brought forward to the cognitive interviews with student participants in Phase 3. (see Supplementary Material 1 for a complete description of item modification during round 2).
Phase 3. Outcomes of cognitive interview focus group
Of the 51 items reviewed by student participants, 29 were identified as clear with little or no discussion. Participant comments for the remaining 22 questions were noted and verified against the audio recording. Following content analysis of the comments, four key themes emerged through the student discussion: unclear or ambiguous wording; difficult to answer questions; need for additional response options; and emotional response evoked by questions. An example of unclear or ambiguous wording was a request for clarity in the use of the word “sufficient” in the context of assessing an item that read “My nursing education has provided sufficient content about the nursing role in MAiD.” “Sufficient” was viewed as subjective and “laden with…complexity that distracted me from the question.” The group recommended rewording the item to read “My nursing education has provided enough content for me to care for a patient considering or requesting MAiD.”
An example of having difficulty answering questions related to limited knowledge related to terms used in the legislation such as such as safeguards , mature minor , eligibility criteria , and conscientious objection. Students were unclear about what these words meant relative to the legislation and indicated that this lack of clarity would hamper appropriate responses to the survey. To ensure that respondents are able to answer relevant questions, student participants recommended that the final survey include explanation of key terms such as mature minor and conscientious objection and an overview of current legislation.
Response options were also a point of discussion. Participants noted a lack of distinction between response options of unsure and unable to say . Additionally, scaling of attitudes was noted as important since perspectives about MAiD are dynamic and not dichotomous “agree or disagree” responses. Although the faculty expert panel recommended the integration of the demographic variables of religious and/or spiritual remain as a single item, the student group stated a preference to have religion and spirituality appear as separate items. The student focus group also took issue with separate items for the variables of sex and gender, specifically that non-binary respondents might feel othered or “outed” particularly when asked to identify their sex. These variables had been created based upon best practices in health research but students did not feel they were appropriate in this context [ 49 ]. Finally, students agreed with the faculty expert panel in terms of the complexity of projecting their future involvement as a Nurse Practitioner. One participant stated: “I certainly had to like, whoa, whoa, whoa. Now let me finish this degree first, please.” Another stated, “I'm still imagining myself, my future career as an RN.”
Finally, student participants acknowledged the array of emotions that some of the items produced for them. For example, one student described positive feelings when interacting with the survey. “Brought me a little bit of feeling of joy. Like it reminded me that this is the last piece of independence that people grab on to.” Another participant, described the freedom that the idea of an advance request gave her. “The advance request gives the most comfort for me, just with early onset Alzheimer’s and knowing what it can do.” But other participants described less positive feelings. For example, the mature minor case study yielded a comment: “This whole scenario just made my heart hurt with the idea of a child requesting that.”
Based on the data gathered from the cognitive interview focus group of nursing students, revisions were made to 11 closed-ended questions (see Table 4 ) and 3 items were excluded. In the four case studies, the open-ended question related to a respondents’ hypothesized actions in a future role as NP were removed. The final survey consists of 45 items including 4 case studies (see Supplementary Material 3 ).
The aim of this study was to develop and validate a survey that can be used to track the growth of knowledge about MAiD among nursing students over time, inform training programs about curricular needs, and evaluate attitudes and willingness to participate in MAiD at time-points during training or across nursing programs over time.
The faculty expert panel and student participants in the cognitive interview focus group identified a need to establish core knowledge of the terminology and legislative rules related to MAiD. For example, within the cognitive interview group of student participants, several acknowledged lack of clear understanding of specific terms such as “conscientious objector” and “safeguards.” Participants acknowledged discomfort with the uncertainty of not knowing and their inclination to look up these terms to assist with answering the questions. This survey can be administered to nursing or pre-nursing students at any phase of their training within a program or across training programs. However, in doing so it is important to acknowledge that their baseline knowledge of MAiD will vary. A response option of “not sure” is important and provides a means for respondents to convey uncertainty. If this survey is used to inform curricular needs, respondents should be given explicit instructions not to conduct online searches to inform their responses, but rather to provide an honest appraisal of their current knowledge and these instructions are included in the survey (see Supplementary Material 3 ).
Some provincial regulatory bodies have established core competencies for entry-level nurses that include MAiD. For example, the BC College of Nurses and Midwives (BCCNM) requires “knowledge about ethical, legal, and regulatory implications of medical assistance in dying (MAiD) when providing nursing care.” (10 p. 6) However, across Canada curricular content and coverage related to end of life care and MAiD is variable [ 23 ]. Given the dynamic nature of the legislation that includes portions of the law that are embargoed until 2024, it is important to ensure that respondents are guided by current and accurate information. As the law changes, nursing curricula, and public attitudes continue to evolve, inclusion of core knowledge and content is essential and relevant for investigators to be able to interpret the portions of the survey focused on attitudes and beliefs about MAiD. Content knowledge portions of the survey may need to be modified over time as legislation and training change and to meet the specific purposes of the investigator.
Given the sensitive nature of the topic, it is strongly recommended that surveys be conducted anonymously and that students be provided with an opportunity to discuss their responses to the survey. A majority of feedback from both the expert panel of faculty and from student participants related to the wording and inclusion of demographic variables, in particular religion, religiosity, gender identity, and sex assigned at birth. These and other demographic variables have the potential to be highly identifying in small samples. In any instance in which the survey could be expected to yield demographic group sizes less than 5, users should eliminate the demographic variables from the survey. For example, the profession of nursing is highly dominated by females with over 90% of nurses who identify as female [ 50 ]. Thus, a survey within a single class of students or even across classes in a single institution is likely to yield a small number of male respondents and/or respondents who report a difference between sex assigned at birth and gender identity. When variables that serve to identify respondents are included, respondents are less likely to complete or submit the survey, to obscure their responses so as not to be identifiable, or to be influenced by social desirability bias in their responses rather than to convey their attitudes accurately [ 51 ]. Further, small samples do not allow for conclusive analyses or interpretation of apparent group differences. Although these variables are often included in surveys, such demographics should be included only when anonymity can be sustained. In small and/or known samples, highly identifying variables should be omitted.
There are several limitations associated with the development of this survey. The expert panel was comprised of faculty who teach nursing students and are knowledgeable about MAiD and curricular content, however none identified as a conscientious objector to MAiD. Ideally, our expert panel would have included one or more conscientious objectors to MAiD to provide a broader perspective. Review by practitioners who participate in MAiD, those who are neutral or undecided, and practitioners who are conscientious objectors would ensure broad applicability of the survey. This study included one student cognitive interview focus group with 5 self-selected participants. All student participants had held discussions about end of life care with at least one patient, 4 of 5 participants had worked with a patient who requested MAiD, and one had been present for a MAiD death. It is not clear that these participants are representative of nursing students demographically or by experience with end of life care. It is possible that the students who elected to participate hold perspectives and reflections on patient care and MAiD that differ from students with little or no exposure to end of life care and/or MAiD. However, previous studies find that most nursing students have been involved with end of life care including meaningful discussions about patients’ preferences and care needs during their education [ 40 , 44 , 47 , 48 , 52 ]. Data collection with additional student focus groups with students early in their training and drawn from other training contexts would contribute to further validation of survey items.
Future studies should incorporate pilot testing with small sample of nursing students followed by a larger cross-program sample to allow evaluation of the psychometric properties of specific items and further refinement of the survey tool. Consistent with literature about the importance of leadership in the context of MAiD [ 12 , 53 , 54 ], a study of faculty knowledge, beliefs, and attitudes toward MAiD would provide context for understanding student perspectives within and across programs. Additional research is also needed to understand the timing and content coverage of MAiD across Canadian nurse training programs’ curricula.
The implementation of MAiD is complex and requires understanding of the perspectives of multiple stakeholders. Within the field of nursing this includes clinical providers, educators, and students who will deliver clinical care. A survey to assess nursing students’ attitudes toward and willingness to participate in MAiD in the Canadian context is timely, due to the legislation enacted in 2016 and subsequent modifications to the law in 2021 with portions of the law to be enacted in 2027. Further development of this survey could be undertaken to allow for use in settings with practicing nurses or to allow longitudinal follow up with students as they enter practice. As the Canadian landscape changes, ongoing assessment of the perspectives and needs of health professionals and students in the health professions is needed to inform policy makers, leaders in practice, curricular needs, and to monitor changes in attitudes and practice patterns over time.
Availability of data and materials
The datasets used and/or analysed during the current study are not publicly available due to small sample sizes, but are available from the corresponding author on reasonable request.
Abbreviations
British Columbia College of Nurses and Midwives
Medical assistance in dying
Nurse practitioner
Registered nurse
University of British Columbia Okanagan
Nicol J, Tiedemann M. Legislative Summary: Bill C-14: An Act to amend the Criminal Code and to make related amendments to other Acts (medical assistance in dying). Available from: https://lop.parl.ca/staticfiles/PublicWebsite/Home/ResearchPublications/LegislativeSummaries/PDF/42-1/c14-e.pdf .
Downie J, Scallion K. Foreseeably unclear. The meaning of the “reasonably foreseeable” criterion for access to medical assistance in dying in Canada. Dalhousie Law J. 2018;41(1):23–57.
Nicol J, Tiedeman M. Legislative summary of Bill C-7: an act to amend the criminal code (medical assistance in dying). Ottawa: Government of Canada; 2021.
Google Scholar
Council of Canadian Academies. The state of knowledge on medical assistance in dying where a mental disorder is the sole underlying medical condition. Ottawa; 2018. Available from: https://cca-reports.ca/wp-content/uploads/2018/12/The-State-of-Knowledge-on-Medical-Assistance-in-Dying-Where-a-Mental-Disorder-is-the-Sole-Underlying-Medical-Condition.pdf .
Council of Canadian Academies. The state of knowledge on advance requests for medical assistance in dying. Ottawa; 2018. Available from: https://cca-reports.ca/wp-content/uploads/2019/02/The-State-of-Knowledge-on-Advance-Requests-for-Medical-Assistance-in-Dying.pdf .
Council of Canadian Academies. The state of knowledge on medical assistance in dying for mature minors. Ottawa; 2018. Available from: https://cca-reports.ca/wp-content/uploads/2018/12/The-State-of-Knowledge-on-Medical-Assistance-in-Dying-for-Mature-Minors.pdf .
Health Canada. Third annual report on medical assistance in dying in Canada 2021. Ottawa; 2022. [cited 2023 Oct 23]. Available from: https://www.canada.ca/en/health-canada/services/medical-assistance-dying/annual-report-2021.html .
Banner D, Schiller CJ, Freeman S. Medical assistance in dying: a political issue for nurses and nursing in Canada. Nurs Philos. 2019;20(4): e12281.
Article PubMed Google Scholar
Pesut B, Thorne S, Stager ML, Schiller CJ, Penney C, Hoffman C, et al. Medical assistance in dying: a review of Canadian nursing regulatory documents. Policy Polit Nurs Pract. 2019;20(3):113–30.
Article PubMed PubMed Central Google Scholar
College of Registered Nurses of British Columbia. Scope of practice for registered nurses [Internet]. Vancouver; 2018. Available from: https://www.bccnm.ca/Documents/standards_practice/rn/RN_ScopeofPractice.pdf .
Pesut B, Thorne S, Schiller C, Greig M, Roussel J, Tishelman C. Constructing good nursing practice for medical assistance in dying in Canada: an interpretive descriptive study. Global Qual Nurs Res. 2020;7:2333393620938686. https://doi.org/10.1177/2333393620938686 .
Article Google Scholar
Pesut B, Thorne S, Schiller CJ, Greig M, Roussel J. The rocks and hard places of MAiD: a qualitative study of nursing practice in the context of legislated assisted death. BMC Nurs. 2020;19:12. https://doi.org/10.1186/s12912-020-0404-5 .
Pesut B, Greig M, Thorne S, Burgess M, Storch JL, Tishelman C, et al. Nursing and euthanasia: a narrative review of the nursing ethics literature. Nurs Ethics. 2020;27(1):152–67.
Pesut B, Thorne S, Storch J, Chambaere K, Greig M, Burgess M. Riding an elephant: a qualitative study of nurses’ moral journeys in the context of Medical Assistance in Dying (MAiD). Journal Clin Nurs. 2020;29(19–20):3870–81.
Lamb C, Babenko-Mould Y, Evans M, Wong CA, Kirkwood KW. Conscientious objection and nurses: results of an interpretive phenomenological study. Nurs Ethics. 2018;26(5):1337–49.
Wright DK, Chan LS, Fishman JR, Macdonald ME. “Reflection and soul searching:” Negotiating nursing identity at the fault lines of palliative care and medical assistance in dying. Social Sci & Med. 2021;289: 114366.
Beuthin R, Bruce A, Scaia M. Medical assistance in dying (MAiD): Canadian nurses’ experiences. Nurs Forum. 2018;54(4):511–20.
Bruce A, Beuthin R. Medically assisted dying in Canada: "Beautiful Death" is transforming nurses' experiences of suffering. The Canadian J Nurs Res | Revue Canadienne de Recherche en Sci Infirmieres. 2020;52(4):268–77. https://doi.org/10.1177/0844562119856234 .
Canadian Nurses Association. Code of ethics for registered nurses. Ottawa; 2017. Available from: https://www.cna-aiic.ca/en/nursing/regulated-nursing-in-canada/nursing-ethics .
Canadian Nurses Association. National nursing framework on Medical Assistance in Dying in Canada. Ottawa: 2017. Available from: https://www.virtualhospice.ca/Assets/cna-national-nursing-framework-on-maidEng_20170216155827.pdf .
Pesut B, Thorne S, Greig M. Shades of gray: conscientious objection in medical assistance in dying. Nursing Inq. 2020;27(1): e12308.
Durojaiye A, Ryan R, Doody O. Student nurse education and preparation for palliative care: a scoping review. PLoS ONE. 2023. https://doi.org/10.1371/journal.pone.0286678 .
McMechan C, Bruce A, Beuthin R. Canadian nursing students’ experiences with medical assistance in dying | Les expériences d’étudiantes en sciences infirmières au regard de l’aide médicale à mourir. Qual Adv Nurs Educ - Avancées en Formation Infirmière. 2019;5(1). https://doi.org/10.17483/2368-6669.1179 .
Adler M, Ziglio E. Gazing into the oracle. The Delphi method and its application to social policy and public health. London: Jessica Kingsley Publishers; 1996
Keeney S, Hasson F, McKenna H. Consulting the oracle: ten lessons from using the Delphi technique in nursing research. J Adv Nurs. 2006;53(2):205–12.
Keeney S, Hasson F, McKenna H. The Delphi technique in nursing and health research. 1st ed. City: Wiley; 2011.
Willis GB. Cognitive interviewing: a tool for improving questionnaire design. 1st ed. Thousand Oaks, Calif: Sage; 2005. ISBN: 9780761928041
Lamb C, Evans M, Babenko-Mould Y, Wong CA, Kirkwood EW. Conscience, conscientious objection, and nursing: a concept analysis. Nurs Ethics. 2017;26(1):37–49.
Lamb C, Evans M, Babenko-Mould Y, Wong CA, Kirkwood K. Nurses’ use of conscientious objection and the implications of conscience. J Adv Nurs. 2018;75(3):594–602.
de Vaus D. Surveys in social research. 6th ed. Abingdon, Oxon: Routledge; 2014.
Boateng GO, Neilands TB, Frongillo EA, Melgar-Quiñonez HR, Young SL. Best practices for developing and validating scales for health, social, and behavioral research: A primer. Front Public Health. 2018;6:149. https://doi.org/10.3389/fpubh.2018.00149 .
Puchta C, Potter J. Focus group practice. 1st ed. London: Sage; 2004.
Book Google Scholar
Streiner DL, Norman GR, Cairney J. Health measurement scales: a practical guide to their development and use. 5th ed. Oxford: Oxford University Press; 2015.
Hsieh H-F, Shannon SE. Three approaches to qualitative content analysis. Qual Health Res. 2005;15(9):1277–88.
Adesina O, DeBellis A, Zannettino L. Third-year Australian nursing students’ attitudes, experiences, knowledge, and education concerning end-of-life care. Int J of Palliative Nurs. 2014;20(8):395–401.
Bator EX, Philpott B, Costa AP. This moral coil: a cross-sectional survey of Canadian medical student attitudes toward medical assistance in dying. BMC Med Ethics. 2017;18(1):58.
Beuthin R, Bruce A, Scaia M. Medical assistance in dying (MAiD): Canadian nurses’ experiences. Nurs Forum. 2018;53(4):511–20.
Brown J, Goodridge D, Thorpe L, Crizzle A. What is right for me, is not necessarily right for you: the endogenous factors influencing nonparticipation in medical assistance in dying. Qual Health Res. 2021;31(10):1786–1800.
Falconer J, Couture F, Demir KK, Lang M, Shefman Z, Woo M. Perceptions and intentions toward medical assistance in dying among Canadian medical students. BMC Med Ethics. 2019;20(1):22.
Green G, Reicher S, Herman M, Raspaolo A, Spero T, Blau A. Attitudes toward euthanasia—dual view: Nursing students and nurses. Death Stud. 2022;46(1):124–31.
Hosseinzadeh K, Rafiei H. Nursing student attitudes toward euthanasia: a cross-sectional study. Nurs Ethics. 2019;26(2):496–503.
Ozcelik H, Tekir O, Samancioglu S, Fadiloglu C, Ozkara E. Nursing students’ approaches toward euthanasia. Omega (Westport). 2014;69(1):93–103.
Canning SE, Drew C. Canadian nursing students’ understanding, and comfort levels related to medical assistance in dying. Qual Adv Nurs Educ - Avancées en Formation Infirmière. 2022;8(2). https://doi.org/10.17483/2368-6669.1326 .
Edo-Gual M, Tomás-Sábado J, Bardallo-Porras D, Monforte-Royo C. The impact of death and dying on nursing students: an explanatory model. J Clin Nurs. 2014;23(23–24):3501–12.
Freeman LA, Pfaff KA, Kopchek L, Liebman J. Investigating palliative care nurse attitudes towards medical assistance in dying: an exploratory cross-sectional study. J Adv Nurs. 2020;76(2):535–45.
Brown J, Goodridge D, Thorpe L, Crizzle A. “I am okay with it, but I am not going to do it:” the exogenous factors influencing non-participation in medical assistance in dying. Qual Health Res. 2021;31(12):2274–89.
Dimoula M, Kotronoulas G, Katsaragakis S, Christou M, Sgourou S, Patiraki E. Undergraduate nursing students’ knowledge about palliative care and attitudes towards end-of-life care: A three-cohort, cross-sectional survey. Nurs Educ Today. 2019;74:7–14.
Matchim Y, Raetong P. Thai nursing students’ experiences of caring for patients at the end of life: a phenomenological study. Int J Palliative Nurs. 2018;24(5):220–9.
Canadian Institute for Health Research. Sex and gender in health research [Internet]. Ottawa: CIHR; 2021 [cited 2023 Oct 23]. Available from: https://cihr-irsc.gc.ca/e/50833.html .
Canadian Nurses’ Association. Nursing statistics. Ottawa: CNA; 2023 [cited 2023 Oct 23]. Available from: https://www.cna-aiic.ca/en/nursing/regulated-nursing-in-canada/nursing-statistics .
Krumpal I. Determinants of social desirability bias in sensitive surveys: a literature review. Qual Quant. 2013;47(4):2025–47. https://doi.org/10.1007/s11135-011-9640-9 .
Ferri P, Di Lorenzo R, Stifani S, Morotti E, Vagnini M, Jiménez Herrera MF, et al. Nursing student attitudes toward dying patient care: a European multicenter cross-sectional study. Acta Bio Medica Atenei Parmensis. 2021;92(S2): e2021018.
PubMed PubMed Central Google Scholar
Beuthin R, Bruce A. Medical assistance in dying (MAiD): Ten things leaders need to know. Nurs Leadership. 2018;31(4):74–81.
Thiele T, Dunsford J. Nurse leaders’ role in medical assistance in dying: a relational ethics approach. Nurs Ethics. 2019;26(4):993–9.
Download references
Acknowledgements
We would like to acknowledge the faculty and students who generously contributed their time to this work.
JS received a student traineeship through the Principal Research Chairs program at the University of British Columbia Okanagan.
Author information
Authors and affiliations.
School of Health and Human Services, Selkirk College, Castlegar, BC, Canada
Jocelyn Schroeder & Barbara Pesut
School of Nursing, University of British Columbia Okanagan, Kelowna, BC, Canada
Barbara Pesut, Lise Olsen, Nelly D. Oelke & Helen Sharp
You can also search for this author in PubMed Google Scholar
Contributions
JS made substantial contributions to the conception of the work; data acquisition, analysis, and interpretation; and drafting and substantively revising the work. JS has approved the submitted version and agreed to be personally accountable for the author's own contributions and to ensure that questions related to the accuracy or integrity of any part of the work, even ones in which the author was not personally involved, are appropriately investigated, resolved, and the resolution documented in the literature. BP made substantial contributions to the conception of the work; data acquisition, analysis, and interpretation; and drafting and substantively revising the work. BP has approved the submitted version and agreed to be personally accountable for the author's own contributions and to ensure that questions related to the accuracy or integrity of any part of the work, even ones in which the author was not personally involved, are appropriately investigated, resolved, and the resolution documented in the literature. LO made substantial contributions to the conception of the work; data acquisition, analysis, and interpretation; and substantively revising the work. LO has approved the submitted version and agreed to be personally accountable for the author's own contributions and to ensure that questions related to the accuracy or integrity of any part of the work, even ones in which the author was not personally involved, are appropriately investigated, resolved, and the resolution documented in the literature. NDO made substantial contributions to the conception of the work; data acquisition, analysis, and interpretation; and substantively revising the work. NDO has approved the submitted version and agreed to be personally accountable for the author's own contributions and to ensure that questions related to the accuracy or integrity of any part of the work, even ones in which the author was not personally involved, are appropriately investigated, resolved, and the resolution documented in the literature. HS made substantial contributions to drafting and substantively revising the work. HS has approved the submitted version and agreed to be personally accountable for the author's own contributions and to ensure that questions related to the accuracy or integrity of any part of the work, even ones in which the author was not personally involved, are appropriately investigated, resolved, and the resolution documented in the literature.
Authors’ information
JS conducted this study as part of their graduate requirements in the School of Nursing, University of British Columbia Okanagan.
Corresponding author
Correspondence to Barbara Pesut .
Ethics declarations
Ethics approval and consent to participate.
The research was approved by the Selkirk College Research Ethics Board (REB) ID # 2021–011 and the University of British Columbia Behavioral Research Ethics Board ID # H21-01181.
All participants provided written and informed consent through approved consent processes. Research was conducted in accordance with the Declaration of Helsinki.
Consent for publication
Not applicable.
Competing interests
The authors declare they have no competing interests.
Additional information
Publisher’s note.
Springer Nature remains neutral with regard to jurisdictional claims in published maps and institutional affiliations.
Supplementary Information
Supplementary material 1., supplementary material 2., supplementary material 3., rights and permissions.
Open Access This article is licensed under a Creative Commons Attribution 4.0 International License, which permits use, sharing, adaptation, distribution and reproduction in any medium or format, as long as you give appropriate credit to the original author(s) and the source, provide a link to the Creative Commons licence, and indicate if changes were made. The images or other third party material in this article are included in the article's Creative Commons licence, unless indicated otherwise in a credit line to the material. If material is not included in the article's Creative Commons licence and your intended use is not permitted by statutory regulation or exceeds the permitted use, you will need to obtain permission directly from the copyright holder. To view a copy of this licence, visit http://creativecommons.org/licenses/by/4.0/ . The Creative Commons Public Domain Dedication waiver ( http://creativecommons.org/publicdomain/zero/1.0/ ) applies to the data made available in this article, unless otherwise stated in a credit line to the data.
Reprints and permissions
About this article
Cite this article.
Schroeder, J., Pesut, B., Olsen, L. et al. Developing a survey to measure nursing students’ knowledge, attitudes and beliefs, influences, and willingness to be involved in Medical Assistance in Dying (MAiD): a mixed method modified e-Delphi study. BMC Nurs 23 , 326 (2024). https://doi.org/10.1186/s12912-024-01984-z
Download citation
Received : 24 October 2023
Accepted : 28 April 2024
Published : 14 May 2024
DOI : https://doi.org/10.1186/s12912-024-01984-z
Share this article
Anyone you share the following link with will be able to read this content:
Sorry, a shareable link is not currently available for this article.
Provided by the Springer Nature SharedIt content-sharing initiative
- Medical assistance in dying (MAiD)
- End of life care
- Student nurses
- Nursing education
BMC Nursing
ISSN: 1472-6955
- General enquiries: [email protected]

A .gov website belongs to an official government organization in the United States.
A lock ( ) or https:// means you've safely connected to the .gov website. Share sensitive information only on official, secure websites.
- Join the National ALS Registry
- Research Publications
A new method for estimating under-recruitment of a patient registry: a case study with the Ohio Registry of Amyotrophic Lateral Sclerosis
Meifang Li [1], Xun Shi [1], Jiang Gui [2], Chao Song [3], Angeline S. Andrew [4], Erik P. Pioro [5], Elijah W. Stommel [4], Maeve Tischbein [4], and Walter G. Bradley [6]
- Department of Geography, Dartmouth College, Hanover, NH, USA.
- Department of Biomedical Data Science, Geisel School of Medicine at Dartmouth, Hanover, NH, USA.
- HEOA Group, West China School of Public Health and West China Fourth Hospital, Sichuan University, Chengdu City, Sichuan Province, China.
- Department of Neurology, Geisel School of Medicine, Dartmouth-Hitchcock Medical Center, Lebanon, NH, USA.
- Section of ALS and Related Disorders, Cleveland Clinic, Cleveland, OH, USA.
- Department of Neurology, Miller School of Medicine, University of Miami, Miami, FL, USA.
This paper proposes an alternative statistical method to capture-recapture in order to estimate under-ascertainment in the collection of ALS data for a disease registry in Ohio. The study utilized three statistical methods (z-score, straight section in a series, and Jenk’s natural breaks) to identify reference counties in Ohio with normal case-population relationships to build a model to estimate case counts in target counties with unrecruited cases. The researchers believe that this method has advantages over capture-recapture that allow it to be used to identify disease hotspots and associations between ALS and environment.
Link to Paper
Read the paper here !
National Amyotrophic Lateral Sclerosis (ALS) Registry
For everyone, public health.
- Open supplemental data
- Reference Manager
- Simple TEXT file
People also looked at
Original research article, metabolic dysfunction associated steatotic liver disease in patients with plaque psoriasis: a case–control study and serological comparison.
- 1 First Clinical Medical College of Zhejiang Chinese Medical University, Hangzhou, Zhejiang, China
- 2 The First Affiliated Hospital of Zhejiang Chinese Medical University, Hangzhou, Zhejiang, China
Background: The relationship between plaque psoriasis and both MASLD and lean MASLD has not been sufficiently explored in the current literature.
Method: This retrospective and observational study was carried out from January 2021 to January 2023 at The First Affiliated Hospital of Zhejiang Chinese Medical University. Patients diagnosed with plaque psoriasis and a control group consisting of individuals undergoing routine physical examinations were enrolled. The incidence of MASLD and lean MASLD among these groups was compared. Additionally, patients with plaque psoriasis were divided into those with MASLD, those with lean MASLD, and a control group with only psoriasis for a serological comparative analysis.
Results: The incidence of MASLD in the observation group and the control group was 43.67% (69/158) and 22.15% (35/158), respectively ( p < 0.01). Furthermore, the incidence of lean MASLD within the observation group and the control group was 10.76% (17/158) and 4.43% (7/158), respectively ( p < 0.01). After controlling for potential confounding variables, plaque psoriasis was identified as an independent risk factor for MASLD with an odds ratio of 1.88 (95% cl: 1.10–3.21). In terms of serological comparison, compared to the simple psoriasis group, we observed a significant elevation in the tumor marker CYFRA21-1 levels in both groups compared to the control group with simple psoriasis ( p < 0.01). Moreover, the MASLD group exhibited elevated levels of inflammatory markers and psoriasis score, whereas these effects were mitigated in the lean MASLD group.
Conclusion: The prevalence of MASLD and lean MASLD is higher among patients with psoriasis. Those suffering from psoriasis along with MASLD show increased psoriasis scores and inflammatory markers compared to those without metabolic disorders. MASLD likely worsens psoriasis conditions, indicating the necessity of targeted health education for affected individuals to reduce the risk of MASLD, this education should include guidelines on exercise and diet. In serological assessments, elevated levels of cytokeratin 19 fragment (CYFRA21-1) were noted in both MASLD and lean MASLD groups, implying a potential synergistic role between psoriasis and MASLD.
1 Introduction
Psoriasis is a chronic skin disease mediated by the immune system, affecting roughly 0.11% of the population in East Asia ( 1 ). Population-based research across six cities reveals a psoriasis prevalence of about 0.47% in China ( 2 ). The disease imposes a significant burden on patients, including economic and psychological strains such as increased absenteeism, high treatment costs, reduced sleep quality, and social stigma ( 3 – 5 ).
The pathogenesis of psoriasis involves genetic predispositions, environmental triggers, immune dysregulation, and other factors ( 6 ). Research into psoriasis has progressively clarified its pathogenesis. Studies have shown that psoriasis pathogenesis is controlled by a dynamic interplay between extracellular cytokine pathways and intracellular signaling molecules ( 7 ). The key role of the tumor necrosis factor-alpha (TNF-α)/interleukin-23 (IL-23)/interleukin-17 (IL-17) axis in psoriasis, especially plaque psoriasis, is well-documented ( 8 , 9 ). IL-23, produced by dendritic cells, activates intracellular signaling pathways when it binds to receptors on CD4+ helper T cells (Th17), leading to IL-17 secretion by these cells ( 10 ). IL-17 binding to keratinocyte receptors triggers the release of inflammatory mediators including TNF-α ( 11 ). TNF-α not only promotes the release of IL-23 from dendritic cells but also interacts with keratinocytes, creating an inflammatory feedback loop and promoting abnormal keratinocyte proliferation ( 12 , 13 ). Plaque psoriasis, the most common variant, features pruritus, xerosis, and scaly plaques. Other forms include erythrodermic psoriasis, pustular psoriasis, and psoriatic arthritis ( 14 ).
Furthermore, the metabolic complications associated with psoriasis have gained significant attention, increasingly viewed as an immunometabolic disorder ( 15 ). Key metabolic pathways such as glycolysis, the tricarboxylic acid cycle, lipid metabolism, and amino acid metabolism are vital in regulating keratinocytes and immune cells ( 16 , 17 ). The link between psoriasis and metabolic complications may stem from a common genetic basis, chronic inflammation, immune regulation, and oxidative stress ( 18 ). An expanding corpus of research strongly supports the relationship between psoriasis and metabolic disorders ( 19 – 21 ), making it crucial to further explore this connection to improve academic insight.
Nonalcoholic fatty liver disease (NAFLD) is characterized by triglyceride accumulation in the liver ( 22 ). The prevalence of NAFLD is rising, largely due to obesity and metabolic syndrome. Estimates suggest that up to 32% of adults worldwide are affected, highlighting significant global health concerns ( 23 ). The criteria for diagnosing NAFLD focus on liver steatosis while ruling out alcohol consumption and other hepatotoxic causes, thus providing a clear diagnosis ( 22 ). In 2023, the American, European, and Latin American Liver Societies implemented a modified Delphi process to update the terminology and definition of fatty liver disease. Consequently, NAFLD has been redefined as MASLD, which includes traditional hepatic steatosis and at least one of five common cardiometabolic risk factors ( 24 ).
Since its introduction, MASLD has shown several advantages over the traditional NAFLD terminology, including improved identification of the risk for hepatic and extrahepatic mortality, disease associations, and detection of high-risk individuals ( 25 ). The MASLD diagnostic criteria have gained broad acceptance across numerous prestigious medical societies for their precision, the simplified process by eliminating the need to exclude other liver diseases, and decreased stigma associated with the diagnosis ( 25 ). Despite the known link between MASLD and obesity, the emergence of lean MASLD has attracted significant attention ( 26 ). The criteria for diagnosing lean MASLD specify an individual with a normal Body Mass Index (BMI) diagnosed with MASLD. Previous research indicates a relationship between the onset and severity of psoriasis with metabolic syndrome and NAFLD ( 27 , 28 ). This study revisits these associations using the MASLD diagnostic criteria, marking it as the first to explore the connection with psoriasis under these new guidelines. Therefore, this case–control study aims to examine the link between plaque psoriasis and both MASLD and lean MASLD, seeking to identify potential serum-specific markers in patients with plaque psoriasis affected by MASLD or lean MASLD and clarify the potential mechanisms of their interaction.
2.1 Selection of patients
We carried out a retrospective, observational study on 158 patients diagnosed with plaque psoriasis at The First Affiliated Hospital of Zhejiang Chinese Medical University, China, from January 2021 to January 2023, referred to as the observation group. An equal number of individuals undergoing routine physical examinations at the Physical Examination Center of The First Affiliated Hospital of Zhejiang Chinese Medical University, China, formed the control group. The inclusion criteria were as follows: individuals aged over 18 years with a clinical diagnosis of plaque psoriasis; control group subjects matched with the observation group on age, gender, and BMI. The exclusion criteria included: individuals with significant alcohol consumption (more than 20 g/day) and other clinical conditions like viral hepatitis that could induce hepatic steatosis, known risk factors for steatotic liver disease (SLD) ( 29 ); those on systemic therapies such as methotrexate, cyclosporine, or biologics within the last month, which could confound liver disease or psoriatic symptoms ( 30 ); and those with conditions like hypothyroidism, tumors, or type 1 diabetes, which are associated with metabolic diseases ( 31 ); as well as pregnant, expecting, or lactating women, and individuals with severe infections, tuberculosis, or skin cancers. From the total of 158 patients, we recruited 37 with plaque psoriasis without metabolic disease (simple psoriasis group), 67 with plaque psoriasis and MASLD (MASLD group), and 16 with plaque psoriasis and lean MASLD (lean MASLD group) for serological analysis. Patients with incomplete data were excluded. The study protocol received approval from the Institutional Review Board, and the ethics committee waived the requirement for informed consent from participants, given the retrospective analysis of an existing database ( Figure 1 ).
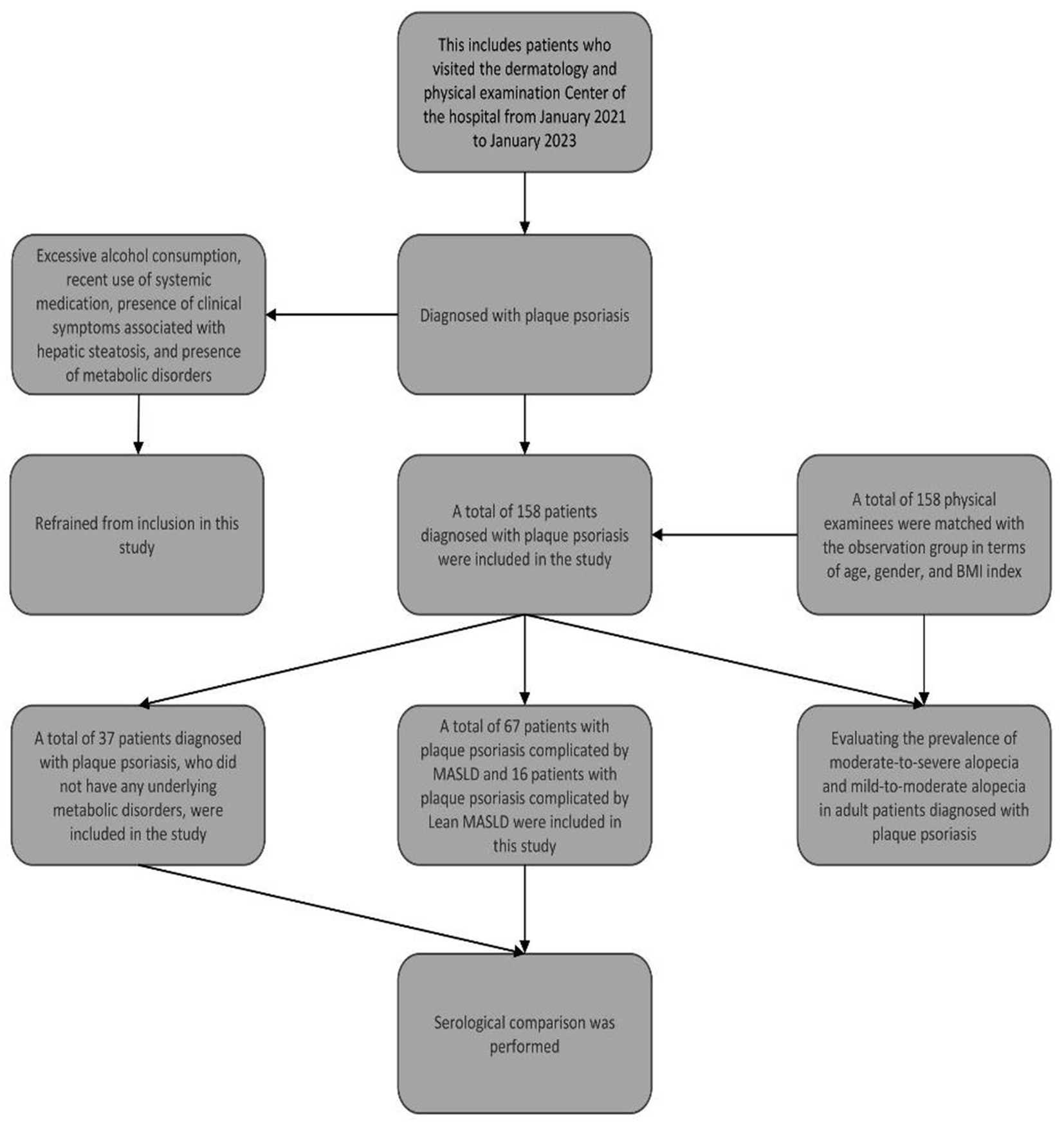
Figure 1 . Flow chart of research methods.
2.2 Research methodology
Clinical and laboratory parameters were recorded for all participants, including age, sex, weight, height, BMI, and medical history of hypertension, diabetes, and hyperlipidemia. Additionally, we recorded results from liver ultrasonography for SLD diagnosis, systolic and diastolic blood pressure, fasting blood glucose, blood triglycerides, total cholesterol, high-density lipoprotein cholesterol (HDL-C), and low-density lipoprotein cholesterol (LDL-C). We also calculated the incidence of MASLD and lean MASLD.
In parallel, laboratory serum data was collected for serological analysis. This included white blood cell count, absolute counts of neutrophils, lymphocytes, monocytes, eosinophils, and basophils, and hypersensitive C-reactive protein. Tumor markers such as alpha fetoprotein (AFP), carbohydrate antigen 19 (CA19-9), carbohydrate antigen 242 (CA242), carbohydrate antigen 50 (CA50), carbohydrate antigen 72-4 (CA72-4), CYFRA21-1 protein marker (CYFRA21-1); nerve-specific enolase marker (NSE) and squamous cell carcinoma-related antigen (SCC-Ag) were also evaluated. Biochemical markers measured comprised blood glucose levels, triglyceride levels, total cholesterol levels; high-density lipoprotein cholesterol levels (HDL-C); low-density lipoprotein cholesterol levels (LDL-C); total bilirubin, direct bilirubin, indirect bilirubin, glutamic oxalacetic transaminase (GOT), alanine aminotransferase (ALT), aspartate aminotransferase (AST), and AST: ALT ratios. Body surface area (BSA) and psoriasis area and severity index (PASI) were used to assess the severity of skin lesions in patients with plaque psoriasis. We collected these markers because routine blood tests cover most inflammatory markers, while biochemical routines encompass most metabolic factor markers. TNF-α exhibits diverse immunomodulatory effects and possesses the ability to recognize and eliminate tumor cells. Considering the key role of TNF-α in psoriasis pathogenesis ( 8 ) and its function in TNF-α-mediated apoptosis in MASLD ( 32 ), Previous studies have also elucidated the correlation between tumor biomarkers and psoriasis, such as squamous cell carcinoma-related antigens and psoriasis ( 33 ). Therefore, we hypothesized that psoriasis and MASLD could alter tumor marker profiles, prompting an investigation into their potential linkage. All serological parameters were collected after an overnight fast of at least 8 h from venous blood samples drawn from the antecubital vein, analyzed using standard procedures at our central laboratory.
The diagnosis of psoriasis is made by experienced dermatologists based on the 2023 edition of the guideline for the diagnosis and treatment of psoriasis in China ( 34 ), this process includes evaluating the characteristics of skin lesions and using auxiliary dermoscopy. The diagnosis of SLD is determined by experienced radiologists through ultrasound examination, following the guidelines for assessing and managing non-alcoholic fatty liver disease in the Asia-Pacific region ( 35 ). The diagnosis of MASLD and related metabolic diseases relies on results from the latest Delphi procedure ( 24 ). However, for classifying overweight, we use the obesity standard released by the Chinese Nutrition Society in June 2022, which better reflects the anthropometric characteristics of Chinese individuals ( 36 ) (Refer to Tables 1 , 2 for diagnostic criteria).
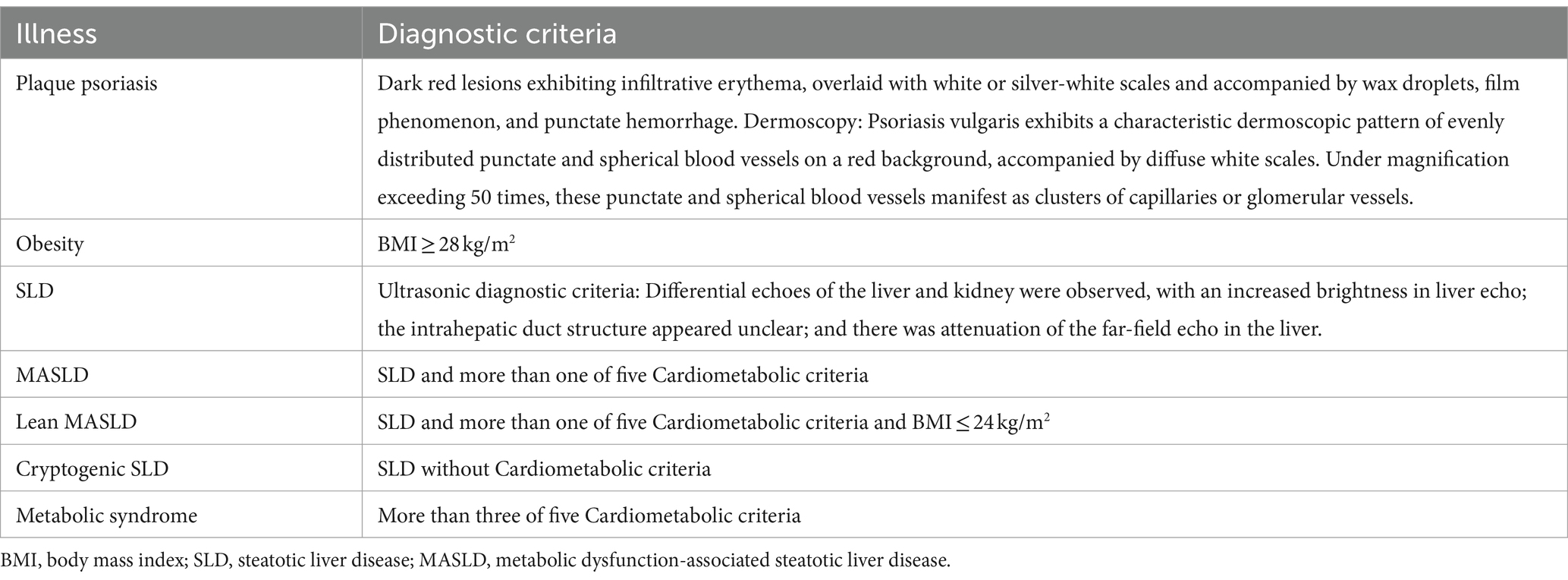
Table 1 . Diagnostic criteria for psoriasis and MASLD-related diseases by Chinese Society of Dermatology ( 34 ) and Asia-Pacific Working Party on NAFLD ( 35 ) and MASLD nomenclature consensus group ( 24 ).
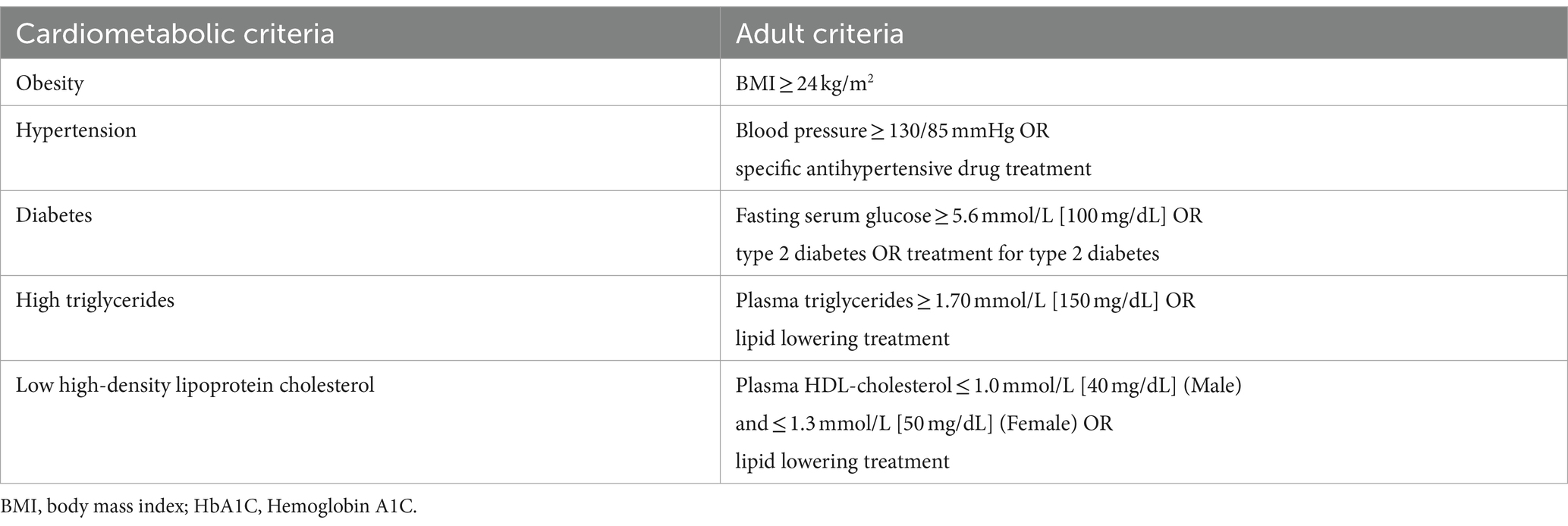
Table 2 . Adult criteria for five Cardiometabolic criteria by MASLD nomenclature consensus group ( 24 , 36 ).
2.3 Methodology for statistical analysis
Continuous data were presented as mean ± standard deviation, and categorical data were expressed as the number of patients (percentage). The normality of continuous data was verified using the Shapiro test and histograms, with p > 0.05 indicating a normal distribution. Differences between groups were analyzed using the t-test for normally distributed data, the Wilcoxon Mann–Whitney test for skewed data, and the Pearson chi-square test for nonparametric data; statistical significance was defined as p < 0.05. Logistic regression was utilized to explore the independent relationship between plaque psoriasis and MASLD, with MASLD as the dependent variable, adjusting for factors such as age, hypertension, diabetes, overweight status, low HDL-C, and high triglycerides. Data analysis was performed using SPSS 25 (SPSS Inc., Chicago, IL) and R version 4.3.1 (R Foundation for Statistical Computing).
3.1 Case control study
This study enrolled 158 patients with plaque psoriasis (observation group) and 158 gender-, age-, and BMI-matched healthy controls (control group) who visited The First Affiliated Hospital of Zhejiang Chinese Medical University between January 2021 and January 2023. The baseline characteristics of the study participants are presented in Table 3 , while the main clinical features are presented in Table 4 and visualized through a histogram ( Figure 2 ).
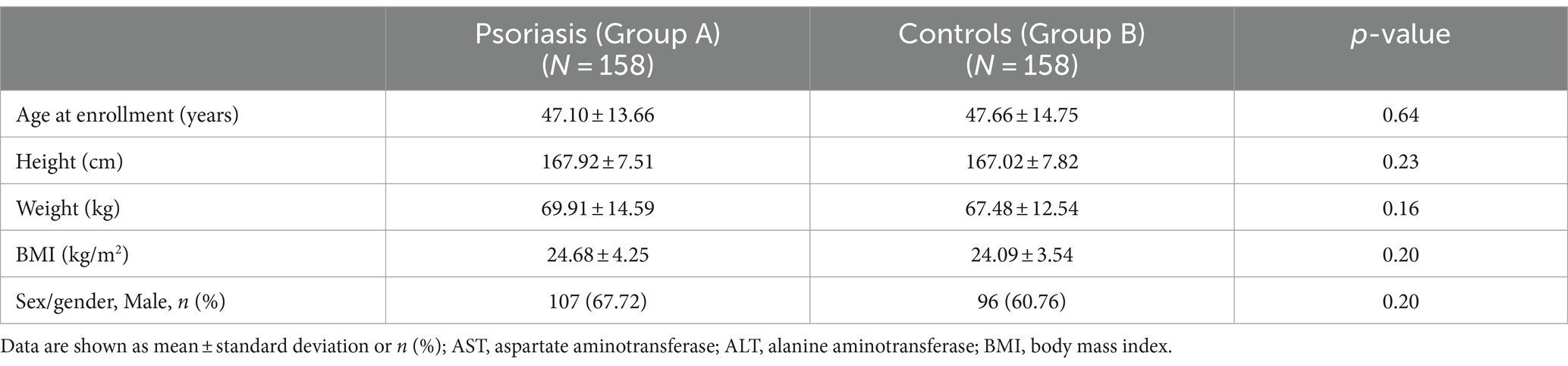
Table 3 . Relevant baseline characteristics of the study cohort.
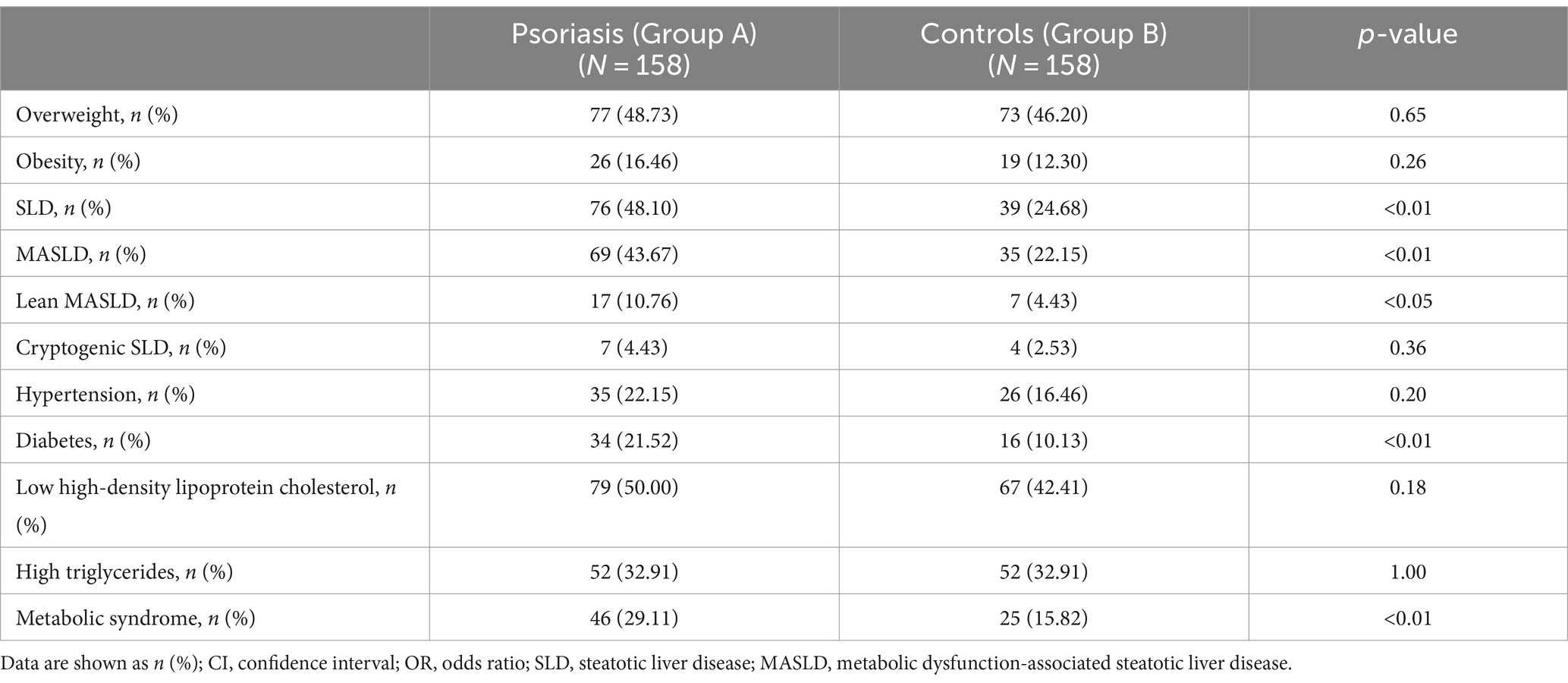
Table 4 . The main clinical characteristics of the study population.
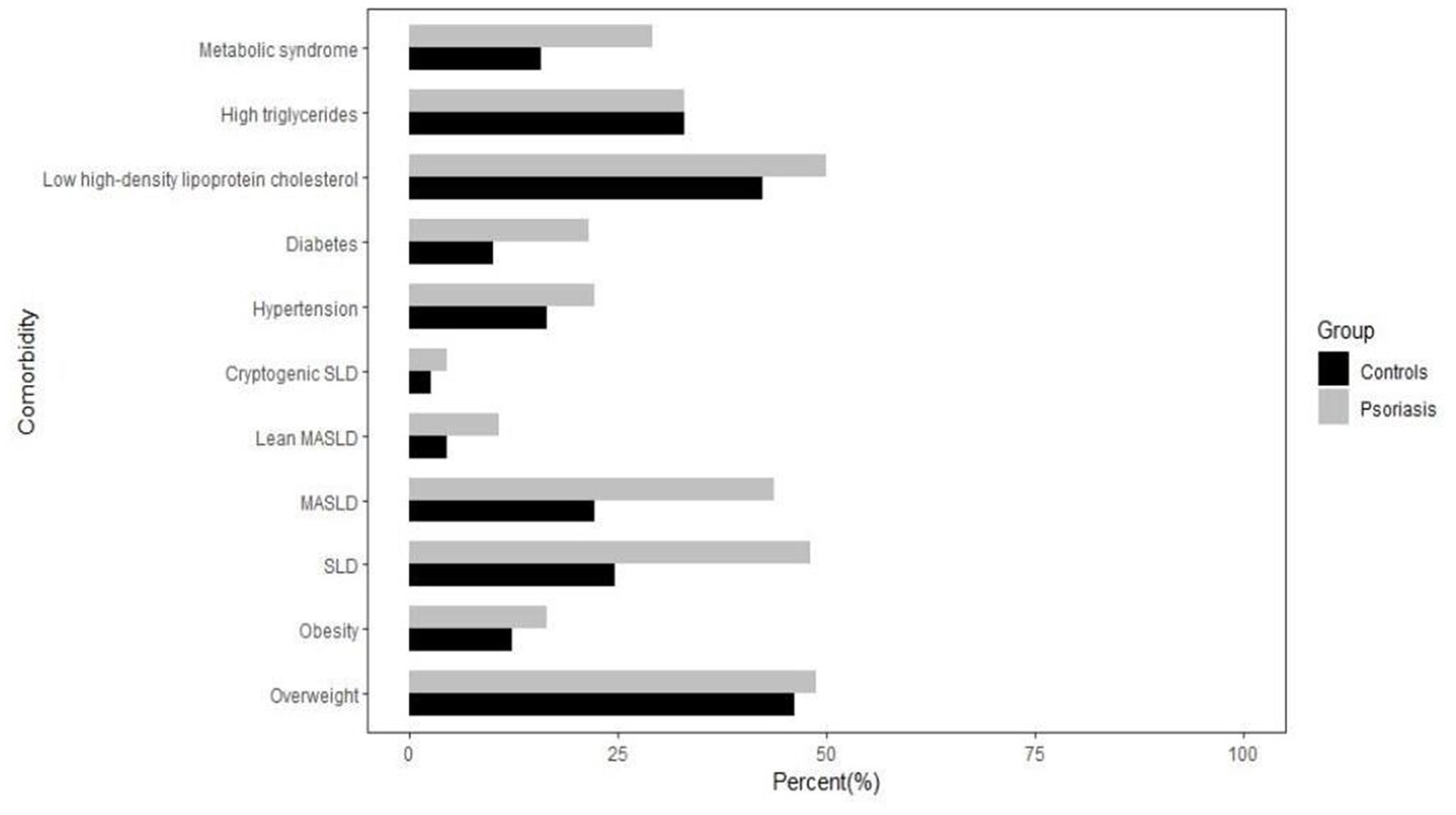
Figure 2 . The main clinical characteristics of the study population.
The age, height, weight, gender, and BMI did not exhibit any statistically significant differences between the two groups ( p > 0.05), indicating that the baseline characteristics of the observation group and control group were comparable and well-matched.
The incidence of MASLD in the observation group (43.67%, 69/158) was significantly higher than that in the control group (22.15%, 35/158) ( p < 0.01). The occurrence of lean MASLD in the observation group (10.76%, 17/158) was significantly higher compared to the control group (4.43%, 7/158) ( p < 0.05). Furthermore, compared to the control group, there was a significant increase in both diabetes and metabolic syndrome incidences within the observation group ( p < 0 0.05). However, no significant differences were found between groups concerning overweight, obesity, hypertension, low high-density lipoprotein cholesterol levels or hypertriglyceridemia incidences ( p > 0 0.05).
After adjusting for age, hypertension, diabetes, overweight, low HDL-C, and hypertriglyceridemia, logistic regression analysis was employed to examine the independent association between plaque psoriasis and MASLD (with MASLD as the dependent variable). The results are presented in Table 5 and visually represented using a forest plot ( Figure 3 ).
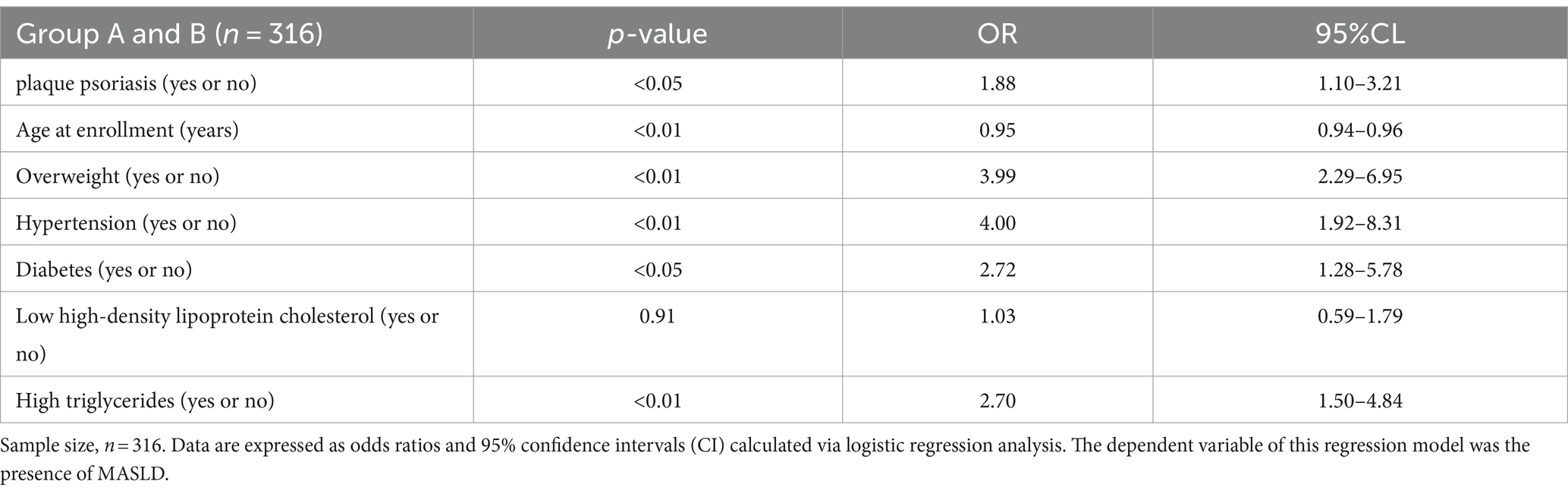
Table 5 . Independent predictors of MASLD.
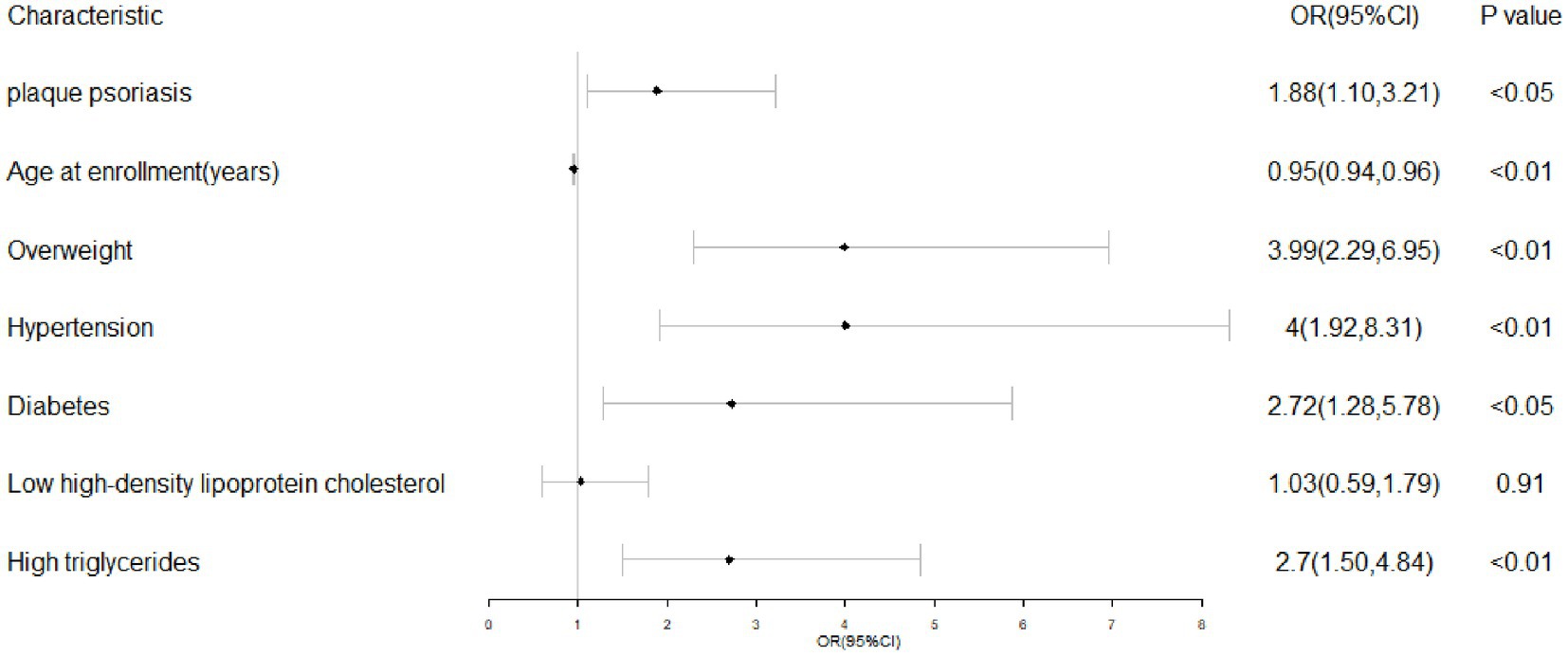
Figure 3 . The results of multivariate logistic regression analysis.
The association between plaque psoriasis and MASLD was examined in Table 5 (OR = 1.88, 95%CL: 1.10–3.21), after adjusting for potential confounders. Logistic regression analysis revealed significant associations of plaque psoriasis, overweight, hypertension, diabetes, and abnormal triglycerides with MASLD; however, no association was observed with abnormal HDL cholesterol.
3.2 Serological comparison
Serological comparisons were conducted among three subgroups: 37 patients with plaque psoriasis without metabolic disease (simple psoriasis group), 67 patients with plaque psoriasis accompanied by metabolic-associated fatty liver disease (MASLD group), and 16 patients with plaque psoriasis accompanied by lean MASLD group.
As presented in Table 6 , Simultaneously, we observed a significant elevation in the tumor marker CYFRA21-1 levels in both groups compared to the control group with simple psoriasis ( p < 0.01). Given the elevated levels of CYFRA21-1 observed in both MASLD and lean MASLD, we performed a correlation analysis between CYFRA21-1 and PASI, BSA, C-reactive protein (hs-CRP), and disease duration. However, the results indicated only weak positive correlations (cor < 0.3) with these indicators; hence they were not individually included in the table.
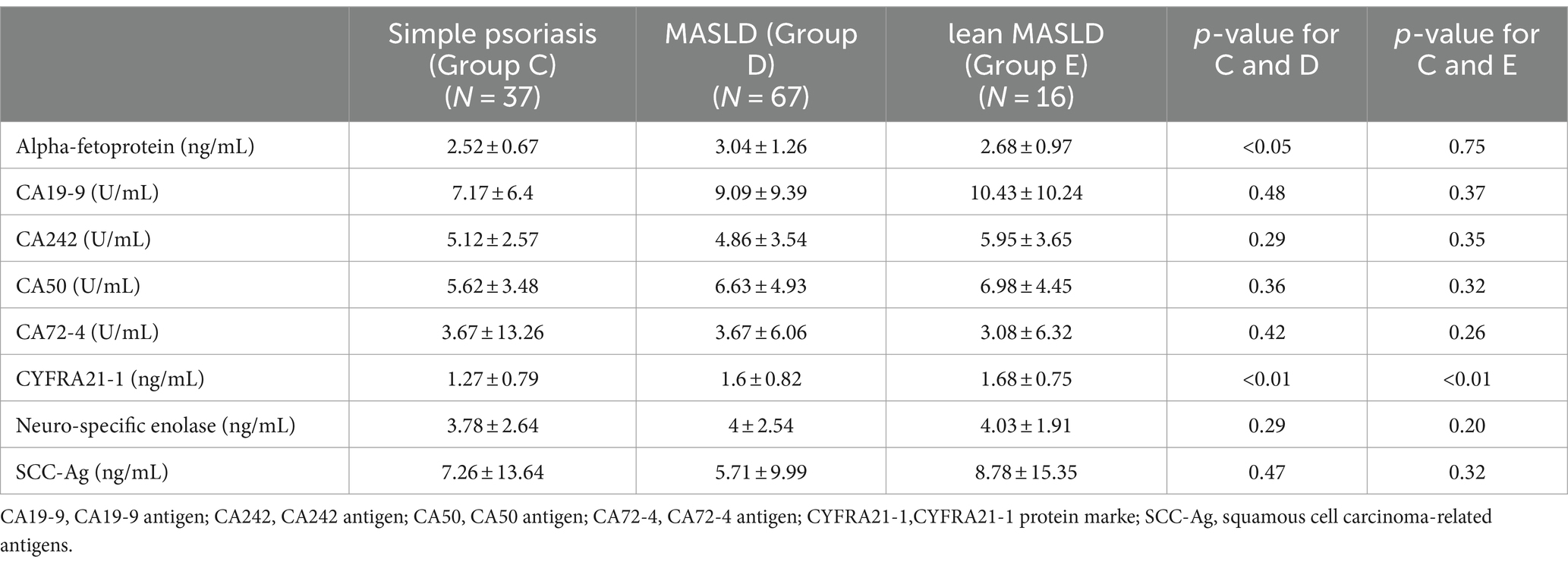
Table 6 . Tumor index of psoriasis with no comorbidities group and psoriasis with MASLD group and lean MASLD group.
As presented in Tables 7 – 9 , In terms of serological comparison, compared to the simple psoriasis group, the MASLD group exhibited higher PASI ( p < 0.05) and BSA ( p < 0.01); elevated white blood cell count ( p < 0.05), neutrophil count ( p < 0.01); increased high-sensitivity C-reactive protein levels ( p < 0.05); blood glucose ( p < 0.01), triglyceride ( p < 0.01), total cholesterol ( p < 0.01), and low-density lipoprotein cholesterol levels ( p < 0.01) were also elevate, while AST: ALT ratio was lower ( p < 0.01). There were no statistically significant differences observed in the remaining indicators ( p > 0.05).
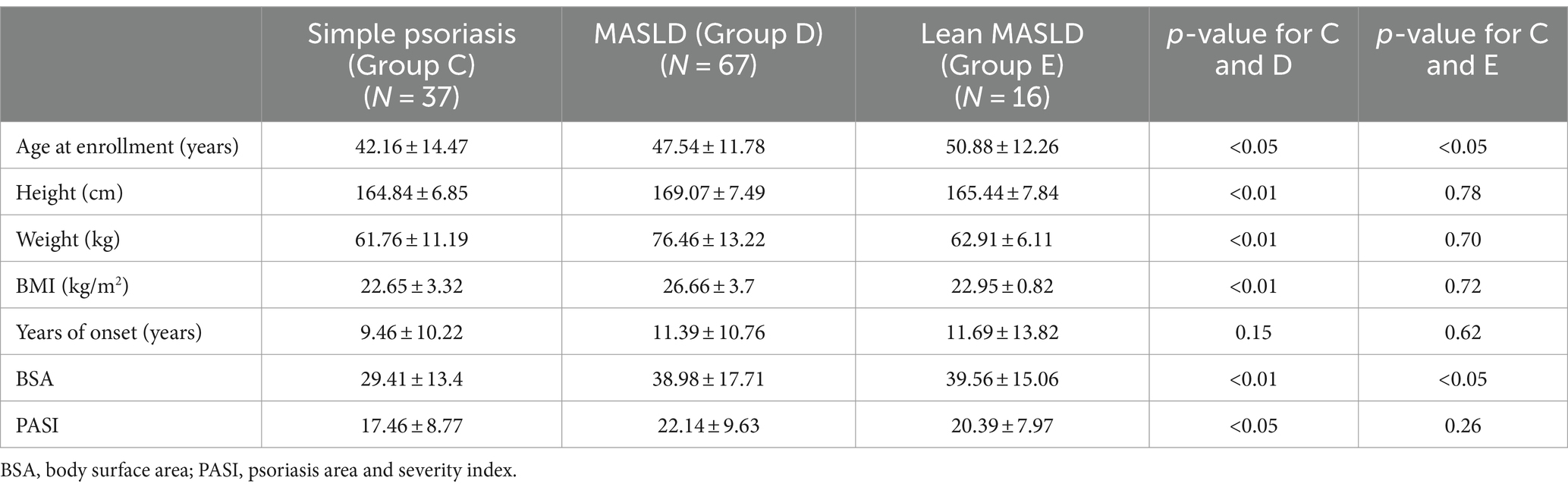
Table 7 . Baseline data and years of onset and psoriasis score of psoriasis with no comorbidities group and psoriasis with MASLD group and lean MASLD group.
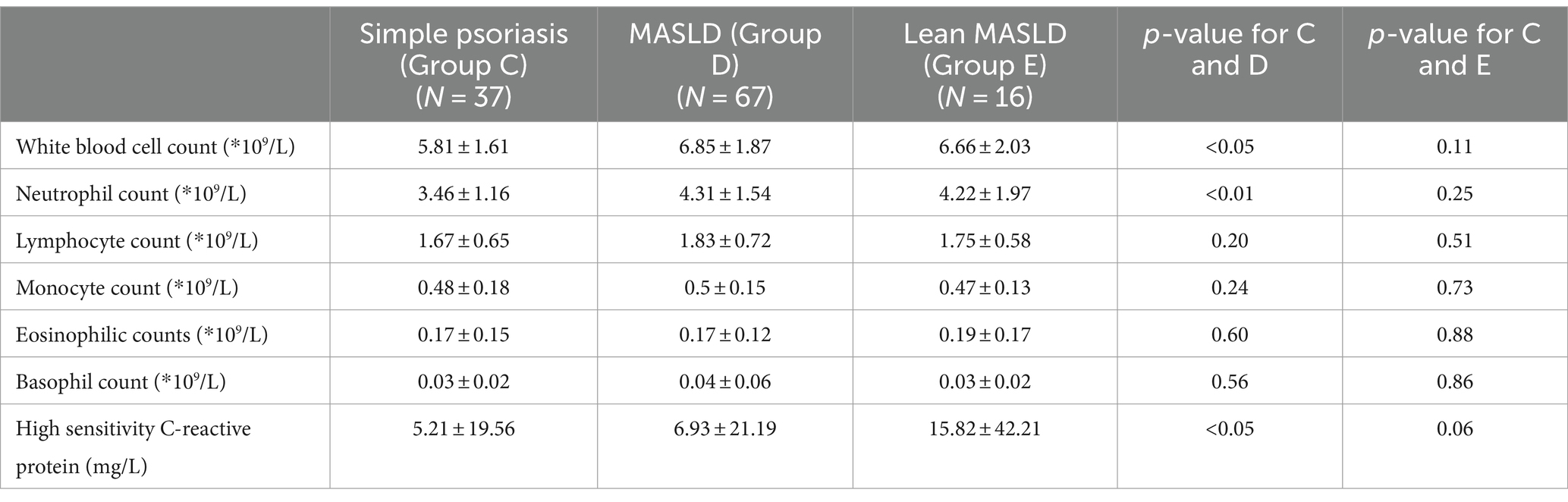
Table 8 . Blood routine examination of psoriasis with no comorbidities group and psoriasis with MASLD group and lean MASLD group.
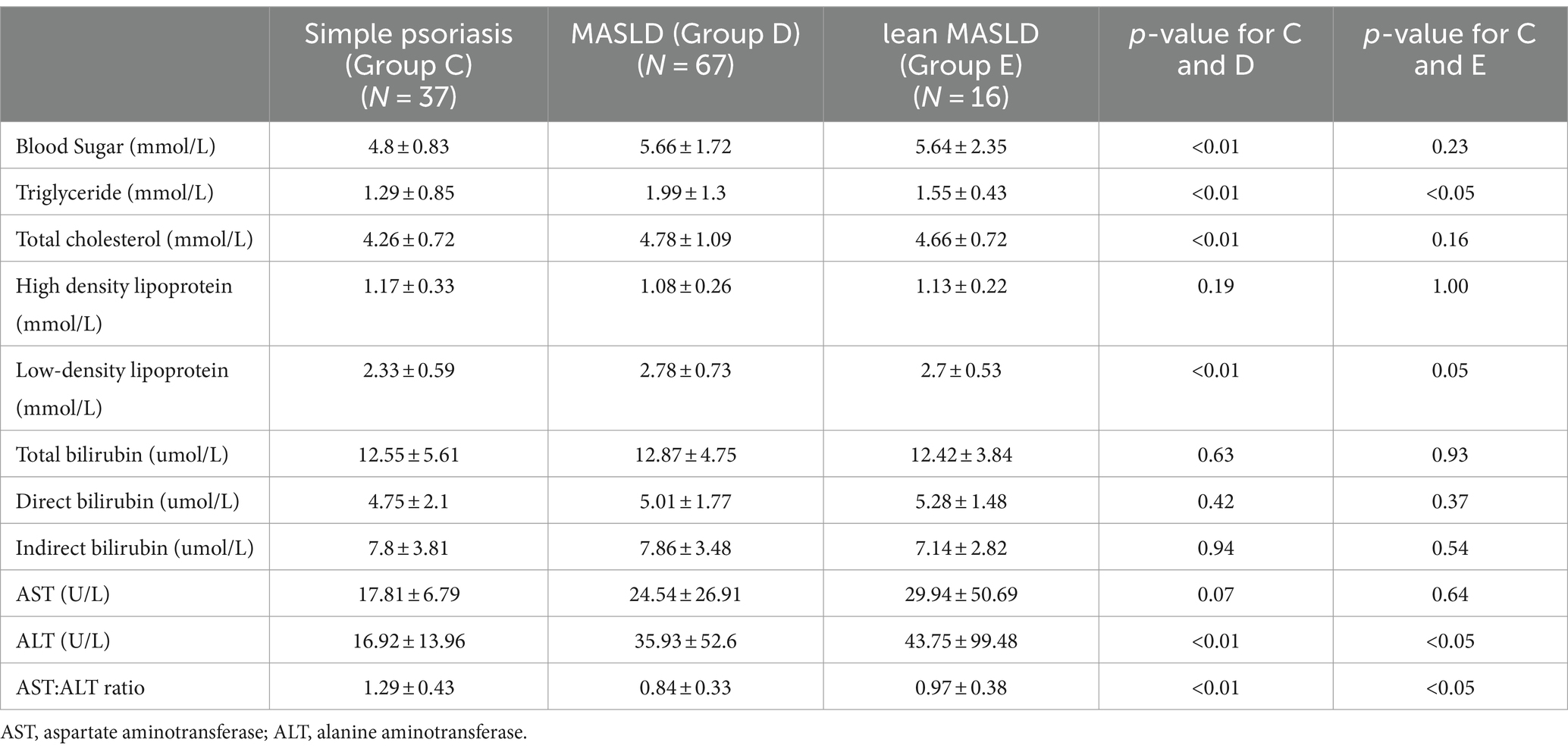
Table 9 . Biochemical index psoriasis with no comorbidities group and psoriasis with MASLD group and lean MASLD group.
Compared to the simple psoriasis group, the lean MASLD group exhibited a significantly higher BSA score ( p < 0.05), while no statistically significant difference was observed in PASI ( p = 0.26) and high-sensitivity C-reactive protein levels ( p = 0.06). Moreover, elevated platelet count were detected in blood routine analysis ( p < 0.05). Additionally, biochemical indexes indicated raised triglyceride levels and ALT activity ( p < 0.05), along with a decreased AST: ALT ratio ( p < 0.05). There were no statistically significant differences observed in the remaining indicators ( p > 0.05).
4 Discussion
4.1 discussion on case-control studies, focusing on lean masld.
To our knowledge, this is the first study to apply the MASLD diagnostic criteria to investigate its correlation with psoriasis following the recent update of MASLD’s definition. Similar to earlier studies linking psoriasis with NAFLD, 48.1% of our patients with plaque psoriasis were diagnosed with SLD ( 37 ), which can be attributed to our study’s rigorous exclusion of confounding factors such as alcohol intake and certain medications. Immunosuppressives like methotrexate and cyclosporine, often used in systemic psoriasis treatment, are known to significantly contribute to liver fibrosis ( 30 , 38 ). To avoid these confounding factors, we excluded participants who had used these medications in the month prior to enrollment. Patients were treated exclusively with topical agents and light therapy before entering the study, treatments which are generally considered to have minimal effects on hepatic function and metabolism ( 39 ). Assessments were conducted promptly upon admission, and since psoriasis treatment methods in this study showed limited impact on MASLD and other complications, their detailed discussion was not deemed crucial to the study’s scope. However, the potential hepatotoxicity of psoriasis medications highlights the importance of this investigation.
A novel finding from our study is the observed prevalence of MASLD among patients with plaque psoriasis at 43.67%, significantly higher than the 22.15% observed in the control group, aligning with previously reported MASLD prevalence ( 40 ). Our adjusted regression analysis further confirms a strong association between plaque psoriasis and MASLD prevalence. Notably, the prevalence of lean MASLD among psoriasis patients was significantly higher (10.76%) compared to that in healthy subjects (4.33%).
Several previous studies have consistently shown a higher prevalence of NAFLD among patients with psoriasis ( 41 ). Employing the new definition of MASLD, our findings are similar, confirming a strong association between MASLD and plaque psoriasis and highlighting the superior diagnostic coverage and accuracy of MASLD compared to NAFLD.
The link between MASLD and psoriasis could be due to the important role of insulin resistance in the metabolism of psoriatic and fatty liver conditions ( 42 , 43 ), and the dysfunction in psoriasis-associated immune cells, such as dendritic cells, which stems from abnormal lipid metabolism ( 44 , 45 ). Additionally, liver cell damage and the resulting cytokine secretion (e.g., FGF-7) may worsen psoriatic inflammation ( 46 , 47 ), while various prominent cytokines (including IL-17) might impact metabolism ( 48 ).
Research has indicated differences in susceptibility genes and pathogenetic mechanisms between lean MASLD and MASLD ( 26 ). Our data indicate that the prevalence of lean MASLD is also elevated in patients with plaque psoriasis, yet the specific overlaps and distinctions in the comorbidity mechanisms between lean MASLD and psoriasis, and those between MASLD and psoriasis, remain to be fully understood. Our findings could guide future research in this area.
4.2 Discussion of serologic comparisons, focusing on CYFRA21-1, and cytokeratin-19 (CK-19)
In our study, serological comparisons were made among groups with simple psoriasis, MASLD, and lean MASLD. We found that the MASLD group had a higher BMI than the simple psoriasis group, highlighting a strong link between overweight status and MASLD. Abnormal lipid metabolism and altered liver function indicators were consistent with the expected characteristics of MASLD. Importantly, novel observations indicated that the MASLD group had increased BSA and PASI, as well as elevated inflammatory markers such as hs-CRP, compared to the psoriasis vulgaris group. These findings suggest that MASLD exacerbates skin manifestations and inflammation of psoriatic lesions. Additionally, a Mendelian randomization study suggested that a rise in white blood cell count and neutrophils could be risk factors for the onset of psoriasis ( 49 ). Thus, the increased levels of these cells observed in the MASLD group might indicate an exacerbation of psoriasis when associated with MASLD.
We also examined serologic differences between the lean MASLD group and the simple psoriasis group, taking care to exclude potential confounding factors linked to overweight in the lean MASLD group. In the lean MASLD group, higher BSA scores were noted compared to the psoriasis-alone group; however, there were no significant differences in PASI and inflammatory markers such as C-reactive protein. These findings suggest that lean-type MASLD might cause less synergistic damage to plaque psoriasis than typical MASLD, pointing to weight management as a potential comprehensive strategy for treating patients with both plaque psoriasis and MASLD. A bicenter retrospective study published in 2023 also supports our perspective on the importance of weight management in psoriasis patients ( 50 ).
Surprisingly, our study identified an increase in CYFRA21-1 levels, a tumor marker typically associated with diagnostic value in lung non-small cell carcinoma ( 51 ). This elevation was noted when comparing serological differences between MASLD, lean MASLD, and psoriasis alone. Although CYFRA21-1 showed a weak correlation with skin lesion severity (PASI and BSA) and inflammation (hs-CRP) in chronic plaque psoriasis with MASLD, without reaching abnormal values, its significance for diagnosis and prognosis may be limited. However, this increase suggests a potential link between the mechanisms of MASLD and plaque psoriasis interaction. CYFRA21-1, the soluble fragment of CK-19 found in the bloodstream ( 52 ), could indicate increased tissue apoptosis, possibly due to increased TNF-α-mediated apoptosis in patients with psoriasis ( 53 ). This process may involve abnormal activity in keratinocytes ( 53 ) and hepatic stellate cells ( 54 ), potentially contributing to the coexistence of MASLD and psoriasis. It might also reflect a rise in intracellular CK-19 content. CK-19 is prevalent in epithelium-rich tissues such as skin and liver ( 55 , 56 ). Prior studies have shown that cytokeratin-6, cytokeratin-16, and cytokeratin-17, within the same family as CK-19, are upregulated in psoriasis, leading to excessive keratinocyte proliferation – supporting the involvement of the keratin system in this condition ( 57 ). Additionally, TGM2-dependent covalent CXCL12-Keratine 19 heterodimers can coat cancer cell surfaces, potentially interfering with T-cell-mediated immune responses, suggesting a role for CK-19 in immune regulation ( 58 ). Further, research has shown that the suppression of CK-19 gene expression prolongs the cell cycle in breast cancer cells, indicating that CK-19 is highly expressed in rapidly proliferating cells such as hepatocytes and keratin-forming cells ( 59 ). Confirmation of these findings would imply that targeting CK-19 expression to inhibit cell proliferation could be a promising therapeutic strategy for psoriasis and its related conditions. These findings align with a cellular experiment on keratin-17, corroborating our understanding of keratin properties and functions ( 60 ). Although these observations suggest a potential involvement of CK-19 in immune processes and cell proliferation/apoptosis related to psoriasis and MASLD, the pathogenesis of CK-19 in these conditions is yet to be thoroughly reported. Therefore, our conclusions require further validation through comprehensive cohort studies or biological experiments.
4.3 Summary
Our study has several limitations that merit consideration. Firstly, the study was conducted at a tertiary care center, where the majority of admitted patients presented with moderate to severe psoriasis, which may limit the generalizability of our findings. Secondly, the case–control design demonstrates a significant association but does not establish causality. Thirdly, while liver ultrasonography is sensitive and specific for diagnosing SLD, it is important to note that liver biopsy remains the gold standard for such diagnosis ( 35 ). Our study did not employ liver biopsy to assess liver fibrosis in psoriasis patients may affect the comprehensiveness of our findings. Simple steatosis is benign, but liver fibrosis is critical for the progression and prognosis of liver disease and the risk of hepatocellular carcinoma ( 61 ). We aim to address these limitations in future studies to increase the scope and depth of our research. Fourthly, the conclusions require further validation due to the small sample size of the lean MASLD group.
Despite these limitations, our study has notable strengths. It employs the novel diagnostic criteria for MASLD to explore its correlation with plaque psoriasis, representing a pioneering effort in this field as per our literature review. We specifically focused on the relationship between lean MASLD and psoriasis, laying groundwork for future investigations. Additionally, our study highlights CYFRA21-1 and CK-19 as potential markers that may help reveal the co-pathogenesis of MASLD and psoriasis. Lastly, the robust integrity of our comprehensive database, where all patients underwent detailed examinations (including liver ultrasonography) and blood samples were consistently collected at a single center.
In conclusion, our findings highlight the high prevalence of MASLD and lean MASLD among patients with plaque psoriasis. Consequently, this study advocates that treatment for patients with plaque psoriasis should extend beyond merely addressing skin lesions. It is crucial to include ultrasound screening and health education focusing on weight management. Additionally, when prescribing systemic therapy drugs such as methotrexate and cyclosporine, it is important to consider their impact on liver function. A careful assessment must be made regarding the suitability of these drugs for patients with psoriasis due to their potential liver burden. For patients with plaque psoriasis complicated by MASLD, biologics may be a preferable option, as current literature does not associate these with hepatic dysfunction. Collaboration between dermatologists and hepatologists could promote the evaluation of synergistic treatment options that benefit both conditions, ultimately improving patient outcomes. Moreover, this study opens up new avenues for research, such as exploring the potential overlap in pathogenesis between lean MASLD and psoriasis, and investigating the role of CK-19 in the development of both psoriasis and MASLD. Rigorous cohort studies and biological experiments are necessary to delve deeper into these topics.
Data availability statement
The original contributions presented in the study are included in the article/ Supplementary material , further inquiries can be directed to the corresponding author.
Ethics statement
The studies involving humans were approved by The First Affiliated Hospital of Zhejiang Chinese Medical University ethics review board (2024-KLS-139-01). The studies were conducted in accordance with the local legislation and institutional requirements. The ethics committee/institutional review board waived the requirement of written informed consent for participation from the participants or the participants’ legal guardians/next of kin because we conducted a retrospective analysis using an existing database containing relevant data.
Author contributions
ZL: Writing – review & editing, Writing – original draft, Software, Methodology, Formal analysis, Data curation, Conceptualization. Y-yS: Writing – review & editing, Validation, Data curation, Conceptualization. L-yY: Writing – review & editing, Investigation, Data curation. C-xM: Writing – review & editing, Investigation, Data curation. S-yP: Writing – review & editing, Validation, Conceptualization. YD: Writing – review & editing, Investigation, Data curation. Q-jZ: Writing – review & editing, Validation. YC: Writing – review & editing, Supervision, Resources, Project administration.
The author(s) declare that no financial support was received for the research, authorship, and/or publication of this article.
Conflict of interest
The authors declare that the research was conducted in the absence of any commercial or financial relationships that could be construed as a potential conflict of interest.
Publisher’s note
All claims expressed in this article are solely those of the authors and do not necessarily represent those of their affiliated organizations, or those of the publisher, the editors and the reviewers. Any product that may be evaluated in this article, or claim that may be made by its manufacturer, is not guaranteed or endorsed by the publisher.
Supplementary material
The Supplementary material for this article can be found online at: https://www.frontiersin.org/articles/10.3389/fmed.2024.1400741/full#supplementary-material
1. Parisi, R, Iskandar, IYK, Kontopantelis, E, Augustin, M, Griffiths, CEM, Ashcroft, DM, et al. National, regional, and worldwide epidemiology of psoriasis: systematic analysis and modelling study. BMJ . (2020) 369:m1590. doi: 10.1136/bmj.m1590
Crossref Full Text | Google Scholar
2. Ding, X, Wang, T, Shen, Y, Wang, X, Zhou, C, Tian, S, et al. Prevalence of psoriasis in China: a population-based study in six cities. Eur J Dermatol . (2012) 22:663–7. doi: 10.1684/ejd.2012.1802
PubMed Abstract | Crossref Full Text | Google Scholar
3. Villacorta, R, Teeple, A, Lee, S, Fakharzadeh, S, Lucas, J, and McElligott, S. A multinational assessment of work-related productivity loss and indirect costs from a survey of patients with psoriasis. Br J Dermatol . (2020) 183:548–58. doi: 10.1111/bjd.18798
4. Toledano, E, Hidalgo, C, Gomez-Lechon, L, Ibanez, M, Chacon, CC, Martin-Vallejo, J, et al. SLEEP quality in patients with psoriatic arthritis and its relationship with disease activity and comorbidities: a cross-sectional study. Sci Rep . (2023) 13:22927. doi: 10.1038/s41598-023-48723-z
5. Maspero, S, Ebert, C, Moser, S, Zink, A, Sichert, P, Schielein, M, et al. The potential of Instagram to reduce stigmatization of people with psoriasis: a randomized controlled pilot study. Acta Derm Venereol . (2023) 103:adv3513. doi: 10.2340/actadv.v103.3513
6. Hawkes, JE, Chan, TC, and Krueger, JG. Psoriasis pathogenesis and the development of novel targeted immune therapies. J Allergy Clin Immunol . (2017) 140:645–53. doi: 10.1016/j.jaci.2017.07.004
7. Vicic, M, Kastelan, M, Brajac, I, Sotosek, V, and Massari, LP. Current concepts of psoriasis Immunopathogenesis. Int J Mol Sci . (2021) 22:11574. doi: 10.3390/ijms222111574
8. Nair, RP, Duffin, KC, Helms, C, Ding, J, Stuart, PE, Goldgar, D, et al. Genome-wide scan reveals association of psoriasis with IL-23 and NF-kappa B pathways. Nat Genet . (2009) 41:199–204. doi: 10.1038/ng.311
9. Merola, JF, Landewe, R, McInnes, IB, Mease, PJ, Ritchlin, CT, Tanaka, Y, et al. Bimekizumab in patients with active psoriatic arthritis and previous inadequate response or intolerance to tumour necrosis factor-alpha inhibitors: a randomised, double-blind, placebo-controlled, phase 3 trial (BE COMPLETE). Lancet . (2023) 401:38–48. doi: 10.1016/S0140-6736(22)02303-0
10. Liu, T, Li, S, Ying, S, Tang, S, Ding, Y, Li, Y, et al. The IL-23/IL-17 pathway in inflammatory skin diseases: from bench to bedside. Front Immunol . (2020) 11:594735. doi: 10.3389/fimmu.2020.594735
11. Furue, M, Furue, K, Tsuji, G, and Nakahara, T. Interleukin-17A and keratinocytes in psoriasis. Int J Mol Sci . (2020) 21:275. doi: 10.3390/ijms21041275
12. Zaba, LC, Cardinale, I, Gilleaudeau, P, Sullivan-Whalen, M, Suarez-Farinas, M, Fuentes-Duculan, J, et al. Amelioration of epidermal hyperplasia by TNF inhibition is associated with reduced Th17 responses. J Exp Med . (2007) 204:3183–94. doi: 10.1084/jem.20071094
13. Chiricozzi, A, Guttman-Yassky, E, Suarez-Farinas, M, Nograles, KE, Tian, S, Cardinale, I, et al. Integrative responses to IL-17 and TNF-alpha in human keratinocytes account for key inflammatory pathogenic circuits in psoriasis. J Invest Dermatol . (2011) 131:677–87. doi: 10.1038/jid.2010.340
14. Rendon, A, and Schakel, K. Psoriasis pathogenesis and treatment. Int J Mol Sci . (2019) 20:1475. doi: 10.3390/ijms20061475
15. Armstrong, AW, and Read, C. Pathophysiology, clinical presentation, and treatment of psoriasis: a review. JAMA . (2020) 323:1945–60. doi: 10.1001/jama.2020.4006
16. Maurelli, M, Gisondi, P, and Girolomoni, G. Advanced glycation end products and psoriasis. Vaccines (Basel) . (2023) 11:617. doi: 10.3390/vaccines11030617
17. Matwiejuk, M, Mysliwiec, H, Chabowski, A, and Flisiak, I. An overview of growth factors as the potential link between psoriasis and metabolic syndrome. J Clin Med . (2023) 13:109. doi: 10.3390/jcm13010109
18. Greenberg, R, Goldsmith, T, Zeltser, D, Shapira, I, Berliner, S, Rogowski, O, et al. Comorbidities in patients with palmoplantar plaque psoriasis. J Am Acad Dermatol . (2021) 84:639–43. doi: 10.1016/j.jaad.2020.03.112
19. Arias-Santiago, S, Orgaz-Molina, J, Castellote-Caballero, L, Arrabal-Polo, MA, Garcia-Rodriguez, S, Perandres-Lopez, R, et al. Atheroma plaque, metabolic syndrome and inflammation in patients with psoriasis. Eur J Dermatol . (2012) 22:337–44. doi: 10.1684/ejd.2012.1714
20. Damevska, K, Neloska, L, Gocev, G, and Mihova, M. Metabolic syndrome in untreated patients with psoriasis: case-control study. J Dtsch Dermatol Ges . (2013) 11:1169–75. doi: 10.1111/ddg.12193
21. Gisondi, P, Tessari, G, Conti, A, Piaserico, S, Schianchi, S, Peserico, A, et al. Prevalence of metabolic syndrome in patients with psoriasis: a hospital-based case-control study. Br J Dermatol . (2007) 157:68–73. doi: 10.1111/j.1365-2133.2007.07986.x
22. Francque, SM, Marchesini, G, Kautz, A, Walmsley, M, Dorner, R, Lazarus, JV, et al. Non-alcoholic fatty liver disease: a patient guideline. JHEP Rep . (2021) 3:100322. doi: 10.1016/j.jhepr.2021.100322
23. Teng, ML, Ng, CH, Huang, DQ, Chan, KE, Tan, DJ, Lim, WH, et al. Global incidence and prevalence of nonalcoholic fatty liver disease. Clin Mol Hepatol . (2023) 29:S32–42. doi: 10.3350/cmh.2022.0365
24. Rinella, ME, Lazarus, JV, Ratziu, V, Francque, SM, Sanyal, AJ, Kanwal, F, et al. A multisociety Delphi consensus statement on new fatty liver disease nomenclature. J Hepatol . (2023) 79:1542–56. doi: 10.1016/j.jhep.2023.06.003
25. Gofton, C, Upendran, Y, Zheng, MH, and George, J. MAFLD: how is it different from NAFLD? Clin Mol Hepatol . (2023) 29:S17–31. doi: 10.3350/cmh.2022.0367
26. Danpanichkul, P, Suparan, K, Kim, D, and Wijarnpreecha, K. What is new in metabolic dysfunction-associated Steatotic liver disease in lean individuals: from bench to bedside. J Clin Med . (2024) 13:278. doi: 10.3390/jcm13010278
27. Balak, DMW, Piaserico, S, and Kasujee, I. Non-alcoholic fatty liver disease (NAFLD) in patients with psoriasis: a review of the hepatic effects of systemic therapies. Psoriasis (Auckl) . (2021) 11:151–68. doi: 10.2147/PTT.S342911
28. Singh, S, Young, P, and Armstrong, AW. An update on psoriasis and metabolic syndrome: a meta-analysis of observational studies. PLoS One . (2017) 12:e0181039. doi: 10.1371/journal.pone.0181039
29. Huang, SC, and Liu, CJ. Chronic hepatitis B with concurrent metabolic dysfunction-associated fatty liver disease: challenges and perspectives. Clin Mol Hepatol . (2023) 29:320–31. doi: 10.3350/cmh.2022.0422
30. Ruiz-Ponce, M, Cuesta-Lopez, L, Lopez-Montilla, MD, Perez-Sanchez, C, Ortiz-Buitrago, P, Barranco, A, et al. Decoding clinical and molecular pathways of liver dysfunction in psoriatic arthritis: impact of cumulative methotrexate doses. Biomed Pharmacother . (2023) 168:115779. doi: 10.1016/j.biopha.2023.115779
31. Mavromati, M, and Jornayvaz, FR. Hypothyroidism-associated dyslipidemia: potential molecular mechanisms leading to NAFLD. Int J Mol Sci . (2021) 22:2797. doi: 10.3390/ijms222312797
32. Tiegs, G, and Horst, AK. TNF in the liver: targeting a central player in inflammation. Semin Immunopathol . (2022) 44:445–59. doi: 10.1007/s00281-022-00910-2
33. Izuhara, K, Yamaguchi, Y, Ohta, S, Nunomura, S, Nanri, Y, Azuma, Y, et al. Squamous cell carcinoma antigen 2 (SCCA2, SERPINB4): an emerging biomarker for skin inflammatory diseases. Int J Mol Sci . (2018) 19:1102. doi: 10.3390/ijms19041102
34. Committee on Psoriasis CSoD. Guideline for the diagnosis and treatment of psoriasis in China (2023 edition). Chin J Dermatol . (2023) 56:573–625. doi: 10.35541/cjd.20220839
35. Farrell, GC, Chitturi, S, Lau, GK, and Sollano, JDAsia-Pacific Working Party on N. Guidelines for the assessment and management of non-alcoholic fatty liver disease in the Asia-Pacific region: executive summary. J Gastroenterol Hepatol . (2007) 22:775–7. doi: 10.1111/j.1440-1746.2007.05002.x
36. Control Society of Chinese Nutrition Society. Expert consensus on obesity prevention and treatment in China. Chin J Epidemiol . (2022) 43:619–31. doi: 10.3760/cma.j.cn112338-20220402-00253
37. Ortolan, A, Lorenzin, M, Tadiotto, G, Russo, FP, Oliviero, F, Felicetti, M, et al. Metabolic syndrome, non-alcoholic fatty liver disease and liver stiffness in psoriatic arthritis and psoriasis patients. Clin Rheumatol . (2019) 38:2843–50. doi: 10.1007/s10067-019-04646-7
38. Bichenapally, S, Khachatryan, V, Muazzam, A, Hamal, C, Velugoti, L, Tabowei, G, et al. Risk of liver fibrosis in methotrexate-treated patients: a systematic review. Cureus . (2022) 14:e30910. doi: 10.7759/cureus.30910
39. Stacey, SK, and McEleney, M. Topical corticosteroids: choice and application. Am Fam Physician . (2021) 103:337–43.
PubMed Abstract | Google Scholar
40. Chan, WK, Chuah, KH, Rajaram, RB, Lim, LL, Ratnasingam, J, and Vethakkan, SR. Metabolic dysfunction-associated Steatotic liver disease (MASLD): a state-of-the-art review. J Obes Metab Syndr . (2023) 32:197–213. doi: 10.7570/jomes23052
41. Mantovani, A, Gisondi, P, Lonardo, A, and Targher, G. Relationship between non-alcoholic fatty liver disease and psoriasis: a novel Hepato-dermal Axis? Int J Mol Sci . (2016) 17:217. doi: 10.3390/ijms17020217
42. Muzica, CM, Sfarti, C, Trifan, A, Zenovia, S, Cuciureanu, T, Nastasa, R, et al. Nonalcoholic fatty liver disease and type 2 diabetes mellitus: a bidirectional relationship. Can J Gastroenterol Hepatol . (2020) 2020:1–5. doi: 10.1155/2020/6638306
43. Nowowiejska, J, Baran, A, and Flisiak, I. Aberrations in lipid expression and metabolism in psoriasis. Int J Mol Sci . (2021) 22:6561. doi: 10.3390/ijms22126561
44. Zhang, X, Li, X, Wang, Y, Chen, Y, Hu, Y, Guo, C, et al. Abnormal lipid metabolism in epidermal Langerhans cells mediates psoriasis-like dermatitis. JCI Insight . (2022) 7:150223. doi: 10.1172/jci.insight.150223
45. Kanemaru, K, Matsuyuki, A, Nakamura, Y, and Fukami, K. Obesity exacerbates imiquimod-induced psoriasis-like epidermal hyperplasia and interleukin-17 and interleukin-22 production in mice. Exp Dermatol . (2015) 24:436–42. doi: 10.1111/exd.12691
46. Geervliet, E, Terstappen, L, and Bansal, R. Hepatocyte survival and proliferation by fibroblast growth factor 7 attenuates liver inflammation, and fibrogenesis during acute liver injury via paracrine mechanisms. Biomed Pharmacother . (2023) 167:115612. doi: 10.1016/j.biopha.2023.115612
47. Pu, J, Wang, R, Zhang, G, and Wang, J. FGF-7 facilitates the process of psoriasis by inducing TNF-alpha expression in HaCaT cells. Acta Biochim Biophys Sin Shanghai . (2019) 51:1056–63. doi: 10.1093/abbs/gmz095
48. Olveira, A, Augustin, S, Benlloch, S, Ampuero, J, Suarez-Perez, JA, Armesto, S, et al. The essential role of IL-17 as the Pathogenetic link between psoriasis and metabolic-associated fatty liver disease. Life (Basel) . (2023) 13:419. doi: 10.3390/life13020419
49. Zhou, G, Ren, X, Tang, Z, Li, W, Chen, W, He, Y, et al. Exploring the association and causal effect between white blood cells and psoriasis using large-scale population data. Front Immunol . (2023) 14:1043380. doi: 10.3389/fimmu.2023.1043380
50. Li, L, Liu, K, Duan, X, Xu, L, Yang, Q, and Liu, F. A comparison of clinical characteristics in overweight/obese and Normal weight patients with psoriasis vulgaris: a Bicentric retrospective observational study. Clin Cosmet Investig Dermatol . (2023) 16:1377–85. doi: 10.2147/CCID.S411636
51. Fu, L, Wang, R, Yin, L, Shang, X, Zhang, R, and Zhang, P. CYFRA21-1 tests in the diagnosis of non-small cell lung cancer: a meta-analysis. Int J Biol Markers . (2019) 34:251–61. doi: 10.1177/1724600819868234
52. Molyneaux, PL, Fahy, WA, Byrne, AJ, Braybrooke, R, Saunders, P, Toshner, R, et al. CYFRA 21-1 predicts progression in idiopathic pulmonary fibrosis: a prospective longitudinal analysis of the PROFILE cohort. Am J Respir Crit Care Med . (2022) 205:1440–8. doi: 10.1164/rccm.202107-1769OC
53. Kokolakis, G, Sabat, R, Kruger-Krasagakis, S, and Eberle, J. Ambivalent effects of tumor necrosis factor alpha on apoptosis of malignant and Normal human keratinocytes. Skin Pharmacol Physiol . (2021) 34:94–102. doi: 10.1159/000513725
54. Wang, WM, Xu, XS, and Miao, CM. Kupffer cell-derived TNF-alpha triggers the apoptosis of hepatic stellate cells through TNF-R1/caspase 8 due to ER stress. Biomed Res Int . (2020) 2020:8035671. doi: 10.1155/2020/8035671
55. Stasiak, PC, Purkis, PE, Leigh, IM, and Lane, EB. Keratin 19: predicted amino acid sequence and broad tissue distribution suggest it evolved from keratinocyte keratins. J Invest Dermatol . (1989) 92:707–16. doi: 10.1016/0022-202X(89)90186-3
56. Takano, M, Shimada, K, Fujii, T, Morita, K, Takeda, M, Nakajima, Y, et al. Keratin 19 as a key molecule in progression of human hepatocellular carcinomas through invasion and angiogenesis. BMC Cancer . (2016) 16:903. doi: 10.1186/s12885-016-2949-y
57. Zhang, X, Yin, M, and Zhang, LJ. Keratin 6, 16 and 17-critical barrier Alarmin molecules in skin wounds and psoriasis. Cells . (2019) 8:807. doi: 10.3390/cells8080807
58. Wang, Z, Moresco, P, Yan, R, Li, J, Gao, Y, Biasci, D, et al. Carcinomas assemble a filamentous CXCL12-keratin-19 coating that suppresses T cell-mediated immune attack. Proc Natl Acad Sci USA . (2022) 119:e2119463119. doi: 10.1073/pnas.2119463119
59. Sharma, P, Alsharif, S, Bursch, K, Parvathaneni, S, Anastasakis, DG, Chahine, J, et al. Keratin 19 regulates cell cycle pathway and sensitivity of breast cancer cells to CDK inhibitors. Sci Rep . (2019) 9:14650. doi: 10.1038/s41598-019-51195-9
60. Luo, Y, Pang, B, Hao, J, Li, Q, Qiao, P, Zhang, C, et al. Keratin 17 covalently binds to alpha-enolase and exacerbates proliferation of keratinocytes in psoriasis. Int J Biol Sci . (2023) 19:3395–411. doi: 10.7150/ijbs.83141
61. Roehlen, N, Crouchet, E, and Baumert, TF. Liver fibrosis: mechanistic concepts and therapeutic perspectives. Cells . (2020) 9:875. doi: 10.3390/cells9040875
Keywords: plaque psoriasis, MASLD, lean MASLD, CYFRA21-1, cytokeratin-19
Citation: Lin Z, Shi Y-y, Yu L-y, Ma C-x, Pan S-y, Dou Y, Zhou Q-j and Cao Y (2024) Metabolic dysfunction associated steatotic liver disease in patients with plaque psoriasis: a case–control study and serological comparison. Front. Med . 11:1400741. doi: 10.3389/fmed.2024.1400741
Received: 14 March 2024; Accepted: 29 April 2024; Published: 15 May 2024.
Reviewed by:
Copyright © 2024 Lin, Shi, Yu, Ma, Pan, Dou, Zhou and Cao. This is an open-access article distributed under the terms of the Creative Commons Attribution License (CC BY) . The use, distribution or reproduction in other forums is permitted, provided the original author(s) and the copyright owner(s) are credited and that the original publication in this journal is cited, in accordance with accepted academic practice. No use, distribution or reproduction is permitted which does not comply with these terms.
*Correspondence: Yi Cao, [email protected]
Evaluation of online job portals for HR recruitment selection using AHP in two wheeler automotive industry: a case study
- ORIGINAL ARTICLE
- Published: 12 May 2024
Cite this article
- S. M. Vadivel ORCID: orcid.org/0000-0002-5287-3693 1 &
- Rohan Sunny ORCID: orcid.org/0009-0002-2347-3081 2
38 Accesses
Explore all metrics
Automotive companies are booming worldwide in the economy. In order to sustain in the highly competitive world, every organization tries to create itself a trademark in the market. In our research, we looked at how two wheelers automotive company's selection enhances an organizational performance, which ensures the company's future growth. In today's fast-paced, globally integrated world, human resources are one of the most important production variables. It is critical to preserve and improve economic competitiveness by properly selecting and developing these resources. The main aim of this study is to identify the best online job portal website for recruitment at Two Wheeler Company and to suggest an HR strategy which resonates company’s values and culture. In this study, we have selected 6 criteria and 6 online popular job portals for recruitment with a sample of 15 candidates have been selected. Findings reveal that, AHP method has significant results on the selection of best employer, which helps HR Manager to finalize the decision making process/strategies. Towards the managerial implications section, the researcher aims to design an functional and effective HR strategy that can grasp, engage and retain the top talent in the organization.
This is a preview of subscription content, log in via an institution to check access.
Access this article
Price includes VAT (Russian Federation)
Instant access to the full article PDF.
Rent this article via DeepDyve
Institutional subscriptions
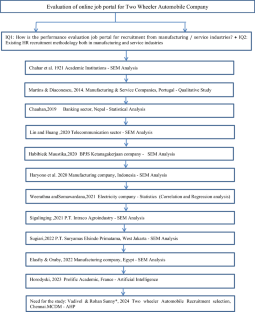
Similar content being viewed by others
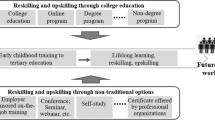
Reskilling and Upskilling the Future-ready Workforce for Industry 4.0 and Beyond
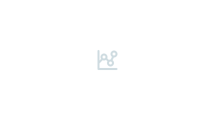
Exploring Human Resource Management Digital Transformation in the Digital Age
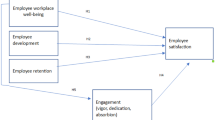
Work engagement and employee satisfaction in the practice of sustainable human resource management – based on the study of Polish employees
Availability of data and material.
'Not applicable' in this section.
Abbreviations
Analytic hierarchy process
Artificial intelligence
Analysis of variance
Chief Human Resources Officer
Consistency index
Curriculum vitae
Consistency ratio
Decision making
Faculty Development Programme
Hierarchical linear modelling
Human resources
Research and Development
Randomized index
Structural equation modelling
Search engine optimization
Triple bottom line
Technique for order preference by similarity
Maximum Eigen value
The normalized value of ith criterion for the jth alternative
The normalized value of jth criterion for the ith alternative
The number of alternatives for a certain MCDM problem
The number of criteria for a certain MCDM problem
Avinash Kapse S, Vishal Patil S, Nikhil PV (2021) E-Recruitment. Int J Eng Adv Technol (IJEAT) 1(4):82–86
Google Scholar
Chahar B, Jain SR, Hatwal V (2021) Mediating role of employee motivation for training, commitment, retention, and performance in higher education institutions. Probl Perspect Manag 19(3):95
Chauhan P (2019) Impact of training and development programs on motivation of employees in “A” graded commercial bank of Nepal. Int J Res Anal Rev 6(3):850–857
Elsafty A, Oraby M (2022) The impact of training on employee retention: an empirical research on the Private Sector in Egypt. Int J Bus Manage 17(5):58–74
Article Google Scholar
Habibie M, Mustika I (2020) The effect of training on work motivation and its impact on employee performance (Case Study at BPJS Ketenagakerjaan Headquarters). Int J Innovat Sci Res Technol 5(7):51–57
Haryono S, Supardi S, Udin U (2020) The effect of training and job promotion on work motivation and its implications on job performance: evidence from Indonesia. Manage Sci Lett 10(9):2107–2112
Horodyski P (2023a) Recruiter’s perception of artificial intelligence (AI)-based tools in recruitment. Comp Human Behav Reports 10:100298
Horodyski P (2023b) Applicants’ perception of artificial intelligence in the recruitment process. Comp Human Behav Reports 11:100303
Jack Walker H, Feild HS, Giles WF, Bernerth JB, Short JC (2011) ‘so what do you think of the organization? a contextual priming explanation for recruitment web site characteristics as antecedents of job seekers’ organizational image perceptions. Organ Behav Human Decis Process 2(2011):165–178
Khan N (2018) Does training & employee commitment predict employee retention. In: International Conference on Management and Information Systems (Vol. 21, pp. 120–124)
Lee I (2005) The evolution of E-Recruiting. A content analysis of Fortune 100 Career Websites. J Electronic Commerce Organ 3(3):57–68
Lievens F, Harris MM (2003) Research on Internet recruiting and testing: current status and future directions. Int Rev Ind Organ Psychol 16:131–165
Lin CY, Huang CK (2021) Employee turnover intentions and job performance from a planned change: the effects of an organizational learning culture and job satisfaction. Int J Manpow 42(3):409–423
Martins D, Diaconescu LM (2014) Expatriates recruitment and selection for long-term international assignments in Portuguese companies. Tékhne 12:48–57
RoyChowdhury T, Srimannarayana M (2013) Applicants’ perceptions on online recruitment procedures. Manage Labour Stud 38(3):185–199
Ryu G, Moon SG (2019) The effect of actual workplace learning on job satisfaction and organizational commitment: The moderating role of intrinsic learning motive. J Workplace Learn 31(8):481–497
Saaty TL (1990) How to make a decision: the analytic hierarchy process. Eur J Oper Res 48(1):9–26
Sengazhani Murugesan V, Sequeira AH, Shetty DS, Jauhar SK (2020) Enhancement of mail operational performance of India post facility layout using AHP. Int J Syst Assur Eng Manage 11(2):261–273
Sigalingging H, Pakpahan ME (2021) The Effect of training and work environment on employee performance with motivation as an intervening variable At PT Intraco Agroindustry. South East Asia Journal of Contemporary Business. Econ Law 24(6):130–139.
Sharawat K, Dubey SK (2018) An approach to vendor selection on usability basis by AHP and fuzzy topsis method. In: Soft computing: theories and applications: proceedings of SoCTA 2016, vol 2. Springer, Singapore, pp 595–604
Steil AV, de Cuffa D, Iwaya GH, Pacheco RCDS (2020) Perceived learning opportunities, behavioral intentions and employee retention in technology organizations. J Work Learn 32(2):147–159
Sugiarti E (2022) The influence of training, work environment and career development on work motivation that has an impact on employee performance at PT. Suryamas Elsindo Primatama In West Jakarta. Int J Artif Intell Res 6(1.2).
Sumrit D (2020) Supplier selection for vendor-managed inventory in healthcare using fuzzy multi-criteria decision-making approach. Decis Sci Lett 9(2):233–256
Thompson LF, Braddy PW, Wuensch KL (2008) E-recruitment and the benefits of organizational web appeal. Comp Human Behav 24(5):2384–2398
Turan FK, Scala NM, Sacre MB, Needy KL (2009) An Analytic Network Process (ANP) approach to the project portfolio management for Organizational Sustainability. In: Proceedings of the Industrial Engineering Research Conference. Institute of Industrial Engineers.
Wadhawan S, Sinha S (2018) Factors Influencing Young Job Seekers Perception towards Job Portals. AIMS Int J Manage 12(3).
Weerarathna RS, Somawardana WSD (2021) Impact on training and employee motivation in an electricity company. Future Work, 497.
Zusman R, Landis R (2002) Applicant preferences for Web-based versus traditional job postings. Comput Hum Behav 18(3):285–329
Download references
Acknowledgements
The authors would like to express their gratitude to two wheeler Automotive Industries in Chennai, Tamil Nadu, India, for their invaluable assistance and cooperation. We greatly acknowledge Ms. Ruchi Mishra, Research scholar from NIT Karnataka, for editing this manuscript in better form.
There is no funding provided in this research.
Author information
Authors and affiliations.
Operations Management Division, Vellore Institute of Technology Chennai, Vandalur-Kelambakkam Road, Chennai, 600127, India
S. M. Vadivel
Vellore Institute of Technology Chennai, Vandalur-Kelambakkam Road, Chennai, 600127, India
Rohan Sunny
You can also search for this author in PubMed Google Scholar
Contributions
S M Vadivel: Methodology, Writing—review & editing, Supervision. Rohan Sunny: Data Curation, Writing—original draft preparation.
Corresponding author
Correspondence to S. M. Vadivel .
Ethics declarations
Conflict of interests.
The authors declare that they have no competing interests.
Ethics approval and consent to participate
This manuscript has a research study involves human participants (Interview Candidates) for studying job portal evaluations in Indian two wheeler company running in Chennai, Tamil Nadu.
Consent for publication
‘ Not applicable’ in this section.
Additional information
Publisher's note.
Springer Nature remains neutral with regard to jurisdictional claims in published maps and institutional affiliations.
Rights and permissions
Springer Nature or its licensor (e.g. a society or other partner) holds exclusive rights to this article under a publishing agreement with the author(s) or other rightsholder(s); author self-archiving of the accepted manuscript version of this article is solely governed by the terms of such publishing agreement and applicable law.
Reprints and permissions
About this article
Vadivel, S.M., Sunny, R. Evaluation of online job portals for HR recruitment selection using AHP in two wheeler automotive industry: a case study. Int J Syst Assur Eng Manag (2024). https://doi.org/10.1007/s13198-024-02358-z
Download citation
Received : 04 December 2023
Revised : 07 April 2024
Accepted : 25 April 2024
Published : 12 May 2024
DOI : https://doi.org/10.1007/s13198-024-02358-z
Share this article
Anyone you share the following link with will be able to read this content:
Sorry, a shareable link is not currently available for this article.
Provided by the Springer Nature SharedIt content-sharing initiative
- Analytical hierarchy process (AHP)
- Online job portals
- Automobile two wheeler industries
- Artificial intelligence (AI)
- HR recruitment
- Find a journal
- Publish with us
- Track your research
Click through the PLOS taxonomy to find articles in your field.
For more information about PLOS Subject Areas, click here .
Loading metrics
Open Access
Peer-reviewed
Research Article
Adapting genetic algorithms for artificial evolution of visual patterns under selection from wild predators
Roles Formal analysis, Investigation, Methodology, Visualization, Writing – original draft, Writing – review & editing
* E-mail: [email protected]
Affiliation Faculty of Environment, Centre for Ecology and Conservation, Science and Economy, University of Exeter, Penryn, Cornwall, United Kingdom

Roles Conceptualization, Investigation, Methodology, Visualization, Writing – review & editing
Affiliations Faculty of Environment, Centre for Ecology and Conservation, Science and Economy, University of Exeter, Penryn, Cornwall, United Kingdom, Faculty of Biological and Environmental Sciences, University of Helsinki, Helsinki, Finland
Roles Conceptualization, Methodology, Supervision, Writing – review & editing
- Emmanuelle S. Briolat,
- George R. A. Hancock,
- Jolyon Troscianko
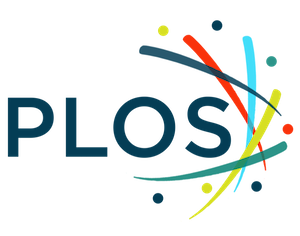
- Published: May 16, 2024
- https://doi.org/10.1371/journal.pone.0295106
- Peer Review
- Reader Comments
Camouflage is a widespread and well-studied anti-predator strategy, yet identifying which patterns provide optimal protection in any given scenario remains challenging. Besides the virtually limitless combinations of colours and patterns available to prey, selection for camouflage strategies will depend on complex interactions between prey appearance, background properties and predator traits, across repeated encounters between co-evolving predators and prey. Experiments in artificial evolution, pairing psychophysics detection tasks with genetic algorithms, offer a promising way to tackle this complexity, but sophisticated genetic algorithms have so far been restricted to screen-based experiments. Here, we present methods to test the evolution of colour patterns on physical prey items, under selection from wild predators in the field. Our techniques expand on a recently-developed open-access pattern generation and genetic algorithm framework, modified to operate alongside artificial predation experiments. In this system, predators freely interact with prey, and the order of attack determines the survival and reproduction of prey patterns into future generations. We demonstrate the feasibility of these methods with a case study, in which free-flying birds feed on artificial prey deployed in semi-natural conditions, against backgrounds differing in three-dimensional complexity. Wild predators reliably participated in this experiment, foraging for 11 to 16 generations of artificial prey and encountering a total of 1,296 evolved prey items. Changes in prey pattern across generations indicated improvements in several metrics of similarity to the background, and greater edge disruption, although effect sizes were relatively small. Computer-based replicates of these trials, with human volunteers, highlighted the importance of starting population parameters for subsequent evolution, a key consideration when applying these methods. Ultimately, these methods provide pathways for integrating complex genetic algorithms into more naturalistic predation trials. Customisable open-access tools should facilitate application of these tools to investigate a wide range of visual pattern types in more ecologically-relevant contexts.
Citation: Briolat ES, Hancock GRA, Troscianko J (2024) Adapting genetic algorithms for artificial evolution of visual patterns under selection from wild predators. PLoS ONE 19(5): e0295106. https://doi.org/10.1371/journal.pone.0295106
Editor: Matthew Shawkey, University of Akron, UNITED STATES
Received: November 14, 2023; Accepted: April 26, 2024; Published: May 16, 2024
Copyright: © 2024 Briolat et al. This is an open access article distributed under the terms of the Creative Commons Attribution License , which permits unrestricted use, distribution, and reproduction in any medium, provided the original author and source are credited.
Data Availability: Code for tools used in this paper can be found on GitHub ( https://github.com/GeorgeHancock471/CamoWild_Repository_CE.v1.2 ). The tools used for creating printed targets, including site randomisation, have been condensed and modified for greater ease of use in CamoEvo Version 2.0 and will be included in all future versions. Instructions on using these tools are provided in the CamoEvo 2.0 handbook on GitHub ( https://github.com/GeorgeHancock471/CamoEvo-v2.0-2022_Plugins/tree/main ). All other relevant data and analysis code are within the manuscript and its Supporting Information files.
Funding: GRAH was funded by a Natural Environment Research Council (NERC) GW4+ studentship (NE/S007504/1), JT & ESB by a NERC Independent Research Fellowship awarded to JT (NE/P018084/1). https://www.ukri.org/councils/nerc/
Competing interests: The authors have declared that no competing interests exist.
Introduction
Camouflage is a common anti-predator defence strategy, with a long history as a case study for evolution [ 1 ]. A vast body of work has investigated how visual patterns help conceal prey from predators, identifying a range of strategies from disruptive coloration and countershading to masquerade and iridescence [ 1 , 2 ]. Understanding which patterns produce the most effective protection is an important goal for camouflage research, particularly with respect to practical applications, yet determining which combinations of traits provide optimal camouflage is not straightforward. The typical approach of comparing the detectability of a set of phenotypes, varying in specific traits, has been hugely successful in determining relevant features for crypsis, but is poorly-suited to exploring camouflage optimisation: the vast phenotypic space available for colours and patterns, along with interacting effects of viewing conditions, backgrounds and receiver vision, make it unfeasible to test all possible combinations in this way [ 3 ]. Moreover, prey patterns are shaped by long-term interactions between communities of predators and prey, learning across repeated encounters and co-evolving over evolutionary time. In particular, search image formation, whereby predators learn to more efficiently detect common prey types, is thought to lead to apostatic selection, preventing the evolution of a stable optimum and favouring polymorphisms in prey patterns [ 4 , 5 ].
Inspired by the processes of natural selection, artificial evolution of visual patterns using genetic algorithms presents an alternative approach, allowing for exploration of a wide phenotypic space and accounting for the effects of repeated interactions between predators and prey. Originally developed as a general solution to optimisation problems with vast parameter spaces [ 6 ], genetic algorithms are an increasingly popular technique in biological research, ideally suited to exploring visual pattern space. So far, genetic algorithms have been used to investigate the evolution of several aspects of visual signalling, primarily camouflage patterns [ 4 , 7 – 12 ], but also aposematism [ 13 ], motion dazzle [ 14 ] and even nectar guides in flowers [ 15 ]. In brief, genetic algorithms set out both virtual genetic structures underpinning phenotypes and rules governing survival and reproduction into the next generation, based on the responses of an observer; across multiple encounters with the observer in successive generations, optimal solutions to the task should arise. When the observer is a foraging predator, prey items that are easiest to detect are selected against, and so lost from the population, while those that are most difficult to find will survive and breed, ultimately leading to the evolution of better-camouflaged patterns.
Following similar principles, early experiments in virtual ecology tracked populations of different-coloured pastry baits under selection from wild birds, with the proportion of different bait types in each generation determined by the ratio of survivors in the previous round [ 16 , 17 ]. Building on this simple paradigm, genetic algorithms incorporate processes of mutation and sexual reproduction, allowing for more complex variation in prey phenotype, and for novel traits to arise. In addition to the algorithms determining how patterns are retained, lost or modified between generations, the ways in which different pattern traits are encoded is also increasingly sophisticated. While no models claim to replicate the actual genetic architecture of a particular species, advances in our understanding of how animal patterns are genetically determined and physically produced during development [ 18 ] have inspired systems better able to produce a range of realistic natural patterns [ 12 ].
So far, these more complex genetic algorithms have generally been applied only in computer-based experiments, where they are most easily implemented, but which fail to capture the impacts of naturalistic viewing conditions and relevant receiver vision. Techniques such as projecting the search area on larger screens, using natural background images with occlusion layers, or simulating the visual system of dichromats can increase the realism of the search task [ 11 ], but still fall short of replicating the effects of real predator behaviour. To our knowledge, genetic algorithms have only been combined with selection by non-human predators in one scenario: seminal experiments on frequency-dependent selection in which blue jays ( Cyanocitta cristata ) searched for artificial moths with evolving patterns on screens [ 4 , 7 ]. Besides restricting prey patterns to greyscale targets, these experiments required highly-motivated captive predators—undergoing up to eight months of pre-training then 100 generations’ worth of trials [ 7 ]—so are difficult to expand to other species and contexts.
Here, we describe a more generalisable process that combines the benefits of field trials involving natural predators with state-of-the-art tools for artificial evolution, expanding on a recently-released genetic algorithm and pattern generation framework, the CamoEvo toolbox [ 12 ]. Prey patterns are generated by CamoEvo, with modifications facilitating implementation in the field, and deployed on physical prey items, concealing a food reward. Wild predators then interact with these items in semi-controlled arenas, and the order in which prey are attacked guides the evolution of subsequent generations of patterns. As a proof-of-principle, we present a case study in which we tested for effects of background complexity on the evolution of camouflage patterns, in artificial prey under selection from wild birds. We also replicated the field trials in computer-based experiments with human volunteers; this allowed us to compare the performance of the new field-based methods to well-established screen-based protocols, and explore how field experiments could be rapidly prototyped and parametrised in future applications.
Materials and methods
General principles.
We developed a methodology for generating and testing artificial prey in the field using genetic algorithms, by substantially adapting an existing screen-based implementation, the CamoEvo toolbox [ 12 ], released here as CamoPrint in CamoEvo V2.0 (see supporting code). The properties of the prey and predation scenarios can be customised, and multiple colour and pattern metrics can be used to analyse the outcomes of pattern evolution, providing flexibility to suit a range of research questions ( Fig 1 ). To begin with, an initial population of prey patterns is created by the CamoPrint software, following experimenter inputs for starting parameters, such as prey size, shape, population size and the colour space from which prey colours can be drawn (see [ 12 ] and supporting code). This first generation of patterns is applied to real physical objects and deployed where predators of interest can interact with them. Suitable prey items can take virtually any form required, so long as they can conceal a food reward accessible to predators, or otherwise provide a record of predation attempts. We describe a technique for transferring computer-generated patterns onto plasticine or 3D-printed shapes (see case study), but appropriate two- and three-dimensional targets can also be made more simply by printing on paper ( Fig 1 ). The setting, visual backgrounds and predators recruited will depend on the experimental question; these methods could be implemented in a wide range of scenarios, from relatively controlled predation trials with captive animals to more naturalistic experiments with multiple predators and prey scattered across a large area. As predators interact with prey, the timing or order of predation is recorded, whether through direct observation, filming, or regular checks, as in more traditional artificial predation experiments. Each population of prey can also be split into discrete groups, for example if prey are to be presented in a series of semi-controlled arenas (see case study). In that case, prey can be ranked according to the order of attack within each group; the size and number of groupings, and prey arrangement within arenas, can be customised as needed. Predation outcomes are then fed back into the genetic algorithm software, which uses this information to determine which patterns survive and reproduce into the next generation. To facilitate the integration of physical prey items into the existing CamoEvo workflow, we implemented several modifications to the pattern generation tools–to create the target shapes and shading used in the case study, assign prey to groups and positions in test arenas, and more easily input the results from predation trials–which can be adapted to suit each user’s needs (see Supporting Information (SI) for details, and supporting code). Repeating these processes of pattern generation, prey preparation, predation trials and data entry allows experimenters to follow selection by predators across multiple generations of prey.
- PPT PowerPoint slide
- PNG larger image
- TIFF original image
Users can customise a range of experimental parameters, and investigate multiple pattern traits when analysing results. Prey presented here include paper targets, two-dimensional with simple or more specific shapes (top), and three-dimensional rolled paper tubes, as well as 3D-printed shells (middle, as used in case study). Rightmost panels show example prey in situ .
https://doi.org/10.1371/journal.pone.0295106.g001
Finally, to explore the outcome of selection, experimenters can select a wide range of colour, luminance and pattern metrics to analyse changes in prey appearance across generations. CamoEvo can analyse the computer-generated prey patterns using a range of tools in the widely-used MICA and QCPA toolboxes in ImageJ [ 19 , 20 ], providing a fully open-access workflow, from experimental design to the assessment of pattern evolution. Alternatively, the physical prey items themselves can be photographed and processed using the same open-access tools, so that image analyses can account for the real 3D properties of prey and backgrounds, and relevant visual systems.
Case study—prey pattern evolution against backgrounds of varying three-dimensional complexity
As a proof-of-concept, we present an application of these methods, investigating the evolution of camouflage patterns in response to selection from wild birds. We deployed prey in arenas with specific visual backgrounds, to test the effect of differences in three-dimensional background complexity on the strength and direction of pattern evolution. Theoretical models [ 21 ] and experiments with both human and avian predators [ 22 – 24 ] suggest even relatively poorly-camouflaged targets are more difficult to detect against complex backgrounds, relaxing selection for increased similarity to the background [ 24 ]. In addition, three-dimensional backgrounds introduce variation in lighting conditions, between shadowed areas and those under direct lighting, which creates noise in both luminance and colour [ 25 , 26 ]. As a result, we expected to see improved camouflage in our targets across generations, but with a weaker signal of directional change in the population of prey displayed against backgrounds with greater three-dimensional complexity.
Field set-up.
Two sets of artificial predation experiments were run in different locations in the grounds of the University of Exeter’s Penryn campus (United Kingdom; 50° 10’ 12’’ N, 5° 7’ 22.8’’ W), from 17 th September to 21 st October and 10 th November to 11 th December 2021. Experiments were approved by the University of Exeter’s ethics committee (Application ID: eCORN003896 v3.1).
Our set-up was designed to encourage natural foraging behaviour by wild birds, while allowing us to control the backgrounds prey were viewed against, and accurately track predation events. Prey were placed in six wooden trays (53x53cm, 22cm above the ground), set out in areas where birds likely to interact with the set-up were regularly seen. Each tray was lined with a polypropylene ground cover membrane, allowing water to filter through, and a mix of gravel and construction sand, forming the visual background ( Fig 2 ). Many alternative backgrounds could be used here; this gravel mix was selected as a relatively natural and heterogeneous option, that was also stable enough to remain in place in poor weather conditions and could be sculpted to vary the complexity of the scene. To test for an effect of background complexity, each experimental run monitored the survival of two populations of prey, presented in trays in which the gravel was either smoothed flat or given 3D structure, with wavy furrows creating peaks and troughs in various orientations (henceforth, smooth and furrowed treatments; Fig 3 ).
Tray array for the first run (a), with the main predators involved, E . rubecula (b) and C . corone (c), and a side view of a tray and mechanical timing gate (d). Here, the first two items attacked were associated with the green and red marbles (leftmost prey).
https://doi.org/10.1371/journal.pone.0295106.g002
Inset: Diagram representing prey positioning in the trays, with strings connecting to the mechanical timing gate.
https://doi.org/10.1371/journal.pone.0295106.g003
Each population consisted of 24 prey, divided into six groups of four per tray. Every morning, half of each population was placed out into three trays with smooth or furrowed gravel respectively, so each generation of both populations was fully exposed to predators across two consecutive days ( Fig 3 ). The location of the smooth and furrowed backgrounds among the trays was alternated daily, and the prey positions in each tray were pseudo-randomised using a custom script in ImageJ [ 27 ], designed to reduce spatial clustering. In CamoPrint, custom additions to the CamoEvo toolbox automated the allocation of prey patterns to each tray and labelled them for easier identification (see SI and supporting code). The artificial prey items themselves consisted of 3D-printed oval shells (18x14 mm) which birds had to flip over to access a concealed food reward (dried mealworm and suet). Prey pattern was manipulated by covering the shells in a thin (~1mm) layer of white tack (UHU, Bühl, Germany), then applying custom patterns printed on waterslide transfer paper (Rolurious) with a laser printer (Colour Laser Jet Enterprise M553, HP, USA). Printing on waterslide paper is a practical way of printing on thin transparent film; after briefly soaking in water, the transparent film separates from its paper backing, and adheres strongly to the white tack surface. Printing targets in CIELAB space does not allow for manipulation of the UV component of colour patterns, which could be relevant to tetrachromatic avian vision; however, printing target patterns on paper with low UV reflectance is a widespread technique for artificial predation experiments testing camouflage strategies with avian predators (e.g. [ 22 ]). In this instance, both the targets and gravel backgrounds had low UV reflectance, and the printer could produce colours that matched patches of the background substrates even as perceived by tetrachromatic models of avian vision (ΔS < 1, see results , Fig 4 & S1 Data ).
Metrics of camouflage efficacy across generations, in targets from the first (a) and second run (b) of field trials, alongside expected trends for improved camouflage (c). All plots display targets shown against smooth backgrounds in the left panel, and furrowed backgrounds on the right. Trend lines and shaded ribbons represent final model predictions, with 95% confidence intervals. Generation was always initially fitted as a second-degree polynomial; non-linear trends are shown if the polynomial term was significant in final models.
https://doi.org/10.1371/journal.pone.0295106.g004
Prior to the experimental trials, wild birds were gradually attracted to the trays and trained to search for the artificial prey, starting with a single tray with visible baits, moving on to food in upturned prey shells, then concealed rewards, and finally increasing the number of trays and prey. During training, prey patterns consisted of checkerboards with colours sampled from the colour space of the experimental patterns. Trials began once visiting birds had consumed four prey in all six trays for at least three consecutive days. In the first run, the trays were visited by carrion crows ( Corvus corone ); in the second, a robin ( Erithacus rubecula ) was the main predator, with occasional visits from blackbirds ( Turdus merula ) and great tits ( Parus major ) ( Fig 2 ; S1 Fig in S1 File ; S1 Video ).
During experimental trials, the order in which prey items were attacked in each tray served as our measure of prey detectability. To automatically record this, the prey shells were tethered from the underside to coloured strings running under the trays, connecting them to a mechanical timing gate. The four prey items in each tray were always assigned string colours in the same order–red, green, blue and yellow from left to right–with marbles of matching colours in the timing gate; when an item was attacked, its string was pulled, releasing a marble of the corresponding colour to create a visible record of attack order (Figs 2 and 3 ). This system was not always sufficiently sensitive to record attacks from smaller birds, so as a fail-safe, each tray was also filmed continuously with a small spy camera (Coulax).
Prey were set out each morning (8–9am), and trays were checked every two to three hours; each trial ended once at least three items had been attacked per tray. If no prey were attacked over the course of the day, the trays were covered up overnight, and the same prey presented again the following day. Once each generation had been fully exposed to predation, the order of prey attacks in every tray was entered into the genetic algorithm software, and a new set of prey patterns was produced for the next generation. See Fig 3 for a full schematic of the experimental procedure.
Pattern generation and algorithm set-up.
Prey patterns were generated using the CamoEvo toolbox’s animal pattern generator, with a modified script for the 3D targets used in this experiment (see SI). Targets were oval, with colourations occupying a CIELAB space with ranges 0–100 in luminance, -50–50 in A (green-red) and -10–70 in B (blue-yellow). Every population began with an identical set of patterns, randomly-generated using these parameters with a uniform distribution of genes (Generation 0; S2 Fig in S1 File ). Details of the pattern generation and genetic algorithm framework can be found in [ 12 ].
CamoEvo is primarily designed for computer-based experiments, so we implemented several modifications to facilitate its application to a field experiment with physical prey items, where prey survival must be manually reported (described in full in SI). These modifications form the basis of the CamoPrint tools featured in CamoEvo V2.0. Once the trials for each generation were complete, prey were assigned a fitness ranking from 1 to 4 in every tray, based on the order in which they were attacked. The genetic algorithm then selected the last two patterns attacked in each tray (ranks 3 & 4) to survive and reproduce, creating the next generation of patterns. However, to reduce the likelihood of relatively well-camouflaged prey being eliminated by chance, we applied CamoEvo’s built-in rescue system [ 12 ]: if a prey pattern was ranked 1 or 2 in generation N, but ranked 4 (the highest possible rank) in generation N-1, the pattern also survived into the next generation (N+1). In the first run, trials stopped after generation 10, when crows stopped visiting the site; in the second, trials ended as planned after 15 generations. To account for the final set of predator decisions, we used CamoPrint to produce an additional generation of patterns (generation 11 or 16 respectively), included in subsequent analyses.
Prey camouflage was assessed with digital photography and image analysis, using well-established methods implemented in the Multispectral Image Calibration and Analysis (MICA) and QCPA plugins for ImageJ [ 19 , 20 ]. The following commonly-used metrics of camouflage efficacy were analysed, accounting for perception by relevant avian visual systems: chromatic (ΔS) and achromatic differences (ΔL) between mean colours in the Receptor Noise-Limited space [ 20 , 28 , 29 ], pattern difference based on granularity analyses [ 30 ], and target edge disruption, measured using the GabRat method [ 31 ]. To account for prey pattern, where component colours may better match the background than the mean prey colour, we also calculated a frequency-weighted average measure of colour difference between all colours in each prey pattern and the most similar colours in the background. Statistical analyses were carried out in R version 3.5.2 [ 32 ]. See SI for details.
Screen-based search tasks.
The outcomes of the field trials suggested our methods could be improved by refining the experimental parameters, to adjust task difficulty. To investigate this, we replicated the field trials with matching screen-based search tasks with human volunteers, allowing us to more rapidly test the impacts of different starting conditions; compared to a month-long run of field trials, each replicate of the screen-based task took roughly only half an hour for a participant to complete. These search tasks were also designed using the CamoEvo toolbox, and run using its built-in user interface for screen-based psychophysics experiments. All parameters were selected to mimic the field experiment as closely as possible, with the exception of starting colour space: target patterns occupied either the same colour space as prey in the field (henceforth the full colour space), or a narrower colour space, corresponding to 2 standard deviations around the mean colour of the background images. In the initial generation 0, targets from the full colour space were thus identical to generation 0 targets in the field, while those from the narrow colour space featured the same patterns but with a restricted range of colours (S5 Fig in S1 File ). Each participant viewed a single population of targets over 16 generations, shown on photographs of the same smooth or furrowed gravel backgrounds as in the field. Every image contained four targets to find, and participants were tasked with clicking on them as quickly as possible, within a maximum of 15 seconds per slide. Survival of targets into the next generation and pattern evolution were governed by the same rules as in the field, based on the order in which targets were clicked. Changes in the detectability of targets over successive generations were quantified using capture time, the time taken for participants to click on each target, as recorded by the CamoEvo toolbox. Measures of prey phenotype used to assess camouflage efficacy, and statistical analyses, were also selected to match those used for prey in the field trials as closely as possible (see SI for details of the experimental design and analyses). In addition, we used CamoEvo to produce 12 replicate populations, with identical starting conditions to the experimental populations, but in which prey patterns were selected at random to survive and reproduce into the next generations. These provide control trends, against which experimental populations can be compared to demonstrate the effect of selection by human volunteers, as opposed to neutral drift.
A total of 12 volunteers completed these screen-based tasks, between 23rd March and 13th April 2022 (three per combination of background type [smooth or furrowed] and colour space [full and narrow]). Experiments took place in a dark room, with images displayed on a 27” monitor (iiyama, Japan). Participants were recruited among staff and students at the University of Exeter, aged 18–70 with normal or corrected-to-normal vision and not knowingly colour-blind. The experiments complied with the declaration of Helsinki and received ethical approval from the University of Exeter’s CLES Cornwall Ethics committee (application ID: 512348, date approved: 22/03/22). Participants received an information sheet presenting the rationale and rules of the search task, and signed a consent form to approve the use of their anonymised data in this study.
Case study—pattern evolution in field trials
Prey populations showed evidence of improved camouflage across generations, though results varied between background types and experimental runs ( Fig 4 ). In the first run, colour and luminance differences between prey patterns and the background decreased, as expected, for the population shown on smooth gravel, but actually increased slightly for the population on furrowed gravel (linear model (lm), generation:background, F 2,570 = 7.625, F 2,570 = 17.425, p < 0.001, for colour difference between means and the weighted average colour difference respectively; F 2,570 = 18.997, p < 0.001 for luminance; Fig 4A(i-iii) ). Conversely, pattern differences decreased across generations in the more complex furrowed treatment, but increased in the smooth (lm, generation:background, F 1,572 = 16.282, p < 0.001; Fig 4A(iv) ), suggesting that the relative importance of pattern and colour matching varied between backgrounds. In the second run, luminance difference decreased in both populations, but most strongly on the furrowed backgrounds, (lm, generation:background, F 1,812 = 6.869, p = 0.009; Fig 4B (iii)). However, there was little improvement in colour-matching: differences between mean target and background colours declined across generations only on the furrowed background (lm, generation:background, F 2,810 = 10.761, p < 0.001; Fig 4B (i)) while the weighted average measure found little change in that population, and an increase in colour difference across generations on the smooth background (lm, generation:background, F 1,812 = 28.069, p < 0.001; Fig 4B (ii)). Unlike in the first run, there were no significant trends in pattern difference (lm, generation:background, F 2,810 = 1.328, p = 0.266; background, F 1,812 = 1.524, p = 0.217; generation, F 2,813 = 0.975, p = 0.378; Fig 4B (iv)). When improvements in background-matching were observed, effect sizes were small, and targets remained conspicuous, with colour and luminance differences well above accepted detection thresholds of 1 to 3 ΔS or ΔL. For example, in the smooth background population in the first run, colour differences between means declined from 10.28 ΔS in generation 0 to 9.32 ΔS in generation 11 ( Fig 4A(i) ). Luminance differences reached lower levels, suggesting that luminance matching was more critical for camouflage, but this could be due to lower starting values; for example, in the same population, mean luminance difference started at 7.07 ΔL in generation 0, reaching 3.47 ΔL by generation 11 ( Fig 4A(iii) ).
Most consistently, targets in all populations displayed increased edge disruption across generations ( Fig 4A, 4B(v) ); this improvement appeared to level off in the population shown on furrowed backgrounds in the first run (lm, generation:background type, F 2,570 = 4.754, p = 0.009), but not in the second (lm, generation:background, F 2,810 = 1.976, p = 0.139; background, F 1,812 = 0.531, p = 0.467; generation, F 1,814 = 72.172, p < 0.001).
Evolution in screen-based replicates
In the screen-based experiments, camouflage metrics reveal clear differences between populations of prey utilising the full and narrow colour spaces. For populations using the narrow colour space, patterns improved in every camouflage metric across generations ( Fig 5A ). There were significant decreases in luminance, colour and pattern differences between targets and the backgrounds (lme, for luminance: generation, χ 2 1 = 114.07, p < 0.001; for colour: generation, χ 2 2 = 191.31, p < 0.001; for pattern: generation, χ 2 1 = 8.466, p = 0.004), and an increase in edge disruption in target patterns (lme, for GabRat in L: generation, χ 2 1 = 8.043, p = 0.005), all with no significant differences between populations shown against smooth or furrowed backgrounds (see S1 Table in S1 File ). For the weighted average colour difference metric, there was a significant effect of the interaction between generation and background type, describing different patterns of overall decrease in colour differences (generation:background type, χ 2 2 = 7.434, p = 0.024; Fig 5A (iii)). These improvements in target camouflage made it harder for participants to find them, as suggested by a significant increase in the time taken to capture the first target on each slide over successive generations for the narrow colour space, regardless of background type (lme, generation: background, χ 2 2 = 4.762, p = 0.093, background: χ 2 1 = 3.826, p = 0.050, generation: χ 2 2 = 6.340, p = 0.012; Fig 5A (vi)).
Changes in camouflage metrics in the screen-based experiments, for prey populations using the narrow (a) and full (b) colour spaces. Plots show the mean and standard error of each metric per generation, along with predictions from the best statistical models. Shades of each colour represent different populations, with filled dots for those shown on smooth backgrounds, and open dots for furrowed backgrounds. Dotted lines represent population-level predictions, and thicker lines overall trends for fixed effects only.
https://doi.org/10.1371/journal.pone.0295106.g005
By contrast, for the full colour space, there was substantial variation in the trajectory of pattern evolution between replicate populations, making it difficult to see consistent evidence of selection for improved camouflage ( Fig 5B ). There were no significant trends in luminance or pattern difference between the targets and the background across generations and no evidence of increased edge disruption in prey patterns ( Fig 5B (i), (iv-v), S2 Table in S1 File ). There was however some evidence for improved colour-matching overall: average colour difference between targets and backgrounds decreased slightly across generations (lme, generation, χ 2 2 = 7.255, p = 0.027), and using the weighted average colour difference metric suggests that this improvement was greater against the smooth backgrounds (lme, generation:background, χ 2 2 = 6.965, p = 0.031, Fig 5B (ii-iii), S2 Table in S1 File ). Capture time was shaped by the interaction between generation and background type, but did not increase overall (lme, generation: background, χ 2 2 = 10.832, p = 0.004; Fig 5B (vi)).
Results from the control populations, simulating the effects of neutral drift, confirm that consistent selection for improved camouflage was only seen in experimental populations using the narrow colour space. Under those conditions, there were significant differences between the trajectories of experimental populations, selected by volunteers, and control replicates across generations: average colour and luminance differences between target patterns and their backgrounds decreased in the selected populations, while they increased slightly in the controls (lme, generation:scenario, for luminance χ 2 1 = 9.443, p = 0.002, for colour, χ 2 1 = 15.557, p < 0.001; S6 Fig in S1 File ). Similar trends for the weighted average colour difference metrics and edge disruption were not significant, suggesting that volunteers in our experiment were selecting primarily for improved colour and luminance match (see S6 Fig in S1 File , S3 Table in S1 File ). However, for the full colour space, analysing all replicates together revealed no significant interaction between scenarios (control versus experimental) and generation, for any metrics (see S6 Fig in S1 File , S4 Table in S1 File ).
This study presents methods to support investigations of camouflage evolution using genetic algorithms in the field, with prey items exposed to wild predators. Our detailed case study describes how to implement these tools, from modifications of the genetic algorithm software to techniques for making artificial prey and recording predation events, and demonstrates the feasibility of this approach. Free-flying birds reliably interacted with multiple generations of prey, and prey patterns improved in several metrics of camouflage efficacy, suggesting the potential for adaptive evolution.
However, this case study also highlights important factors to consider when designing this type of evolutionary experiment. Trends for improved camouflage in the field were relatively weak, and inconsistent among runs with different predators and background types. We tested whether these findings were linked to the starting colour space available for prey patterns, using screen-based search tasks with human volunteers, which closely replicated the field trials and were much quicker to run. These experiments revealed substantial variation in outcomes produced by individual participants, when target patterns utilised the same colour space as in the field: while camouflage improved across generations in some populations of prey, others became more conspicuous, such that few overall trends for improved camouflage were detected, and populations were not significantly different from control replicates, simulating neutral drift. These results were unexpected, as similar psychophysics experiments in artificial evolution, with starting parameters very close to the full colour space used here, typically find much clearer trends towards improved camouflage [ 12 ]. However, the number of prey and the way they are presented strongly affect learning in this type of experiment. Previous screen-based experiments with background-matching, disruptive or distractive camouflaged prey revealed that learning varied among human participants depending on whether targets were shown sequentially or simultaneously, and the number of prey shown together. For example, detection rates for high-contrast disruptive prey decreased faster than for background-matching prey when they were presented simultaneously, while there was no difference in learning over trials with sequential presentations of single prey [ 33 ]. Predator strategies, such as the formation of search images for specific prey types versus searching for more general features, will thus vary depending on the search scenario [ 33 ]. This will affect which prey will be detected first, shaping the evolutionary trajectories of prey patterns, so that outcomes are likely to be different in our multiple-prey paradigm compared to more traditional scenarios where prey are presented singly and sequentially. Here, the presence of multiple prey per image increases the influence of random factors, such as the position of prey relative to one another. Under these circumstances, participant learning outstripped target evolution, highlighting the unexplored power of predator learning in holding back the evolution of camouflage from a conspicuous evolutionary start point, a scenario that may be relevant to aposematic species, for example. Predator cognition, encompassing learning, neophobia, forgetting, recognition errors and adaptive decision-making, is a key consideration in the evolution and maintenance of aposematism as a signalling strategy [ 34 , 35 ]. Theoretical models suggest that elements of both predator psychology and prey ecology, such as prey density and aggregation, can contribute to the initial evolution of aposematism [ 36 ], and our set-up presents opportunities to investigate these effects, by varying the profitability and arrangement of prey in both the field and online systems. Predator behaviour and cognition are similarly important for the evolution of camouflage yet have received less attention in this context [ 37 ], so further experiments with real predators could also shed more light on the conditions required for crypsis to evolve, given different predator communities. In our experiments, the screen-based trials suggest the trajectory of evolution is essentially random when prey utilise the full colour space, so any specific results from the field case study should be interpreted with caution.
By contrast, the screen-based experiments showed that populations of prey utilising a narrower colour space, based on colours found in the backgrounds, were not only more cryptic from the start, but also consistently evolved to be better camouflaged over successive generations, and clearly behaved differently from control replicates. We therefore predict that field trials would similarly yield greater improvement in camouflage if the prey patterns started with a more restricted colour space, based on the colours of the background. In addition, very few metrics of camouflage efficacy revealed differences in prey pattern evolution between populations displayed against the smooth and furrowed backgrounds, even in the screen-based experiments with the narrow colour space. In the field runs, trends did vary between background types, but there was little consistency across metrics, or between repeats, and thus no clear sense that selection was stronger for populations on the simpler smooth background. This suggests that the variation in three-dimensional complexity between these backgrounds was also insufficient to select for different evolutionary trajectories in this scenario. As such, these experiments represent a proof-of-principle for virtual evolution in the wild, but future applications should carefully consider starting parameters, to better calibrate the difficulty of the task.
Researchers attempting similar work would benefit from testing their starting parameters in pilot experiments with human volunteers as “predators”, to establish conditions in which robust selection can be expected to take place before running more time-consuming experiments in the field. To that end, the methods described here present a significant advantage, as CamoEvo’s flexibility allows users to set up screen-based experiments that closely mirror their field setups, and rapidly collect data with human volunteers. Equally, the limitations of our field experiments highlight the difficulty of predicting the conditions under which prey patterns can be expected to evolve, which is in of itself an interesting area to explore. Using these field methods to investigate the scenarios that favour the evolution of camouflage in response to real predators would be extremely valuable, to better understand the role of predation as a selective force. In particular, the impact of the starting colour space available to prey has received little attention, yet is likely to be a significant constraint for the subsequent evolution of prey patterns. Beyond prey appearance, initial conditions are an important determinant of evolutionary trajectories more generally. For example, certain traits can promote or restrict the potential for adaptive radiation (eg. when competition between small individuals for a shared resource limits the emergence of larger individuals that could specialise on alternative resources [ 38 ]) while more evolutionarily labile traits can facilitate the spread of invasive species, or boost the resilience of native species they encounter [ 39 ]. So-called “historical difference experiments”, testing how populations that previously evolved under different conditions perform in a new situation, provide a means to explore the role of starting conditions and historical contingencies in evolution [ 40 ]. Our set-up provides opportunities to run this kind of experiment, potentially by combining screen and field experiments for efficiency, and approach these broad evolutionary questions through the study of pattern evolution.
The system we present here is designed to encourage future research on prey appearance, by providing open-access tools for every step of the process, from pattern generation and the genetic algorithm framework [ 12 ], to implementation in the field, and image analysis software for quantifying changes in prey properties [ 19 , 20 ]. Within CamoEvo and CamoPrint, parameters such as target shape, colour space and genetic algorithm rules are fully customisable, while the field set-up can be modified to meet individual needs, for different predators and backgrounds. Like all predation experiments relying on printed targets [eg. 22,41], matching colourations can be complicated, particularly if the observers can perceive a broader range of colours than humans (e.g. are sensitive to UV wavelengths). Moreover, the range of colours that can be reliably printed is somewhat limited; prey in these experiments could not, for example, evolve colours that were outside the printer’s colour gamut, or iridescence through structural coloration. As with all genetic algorithms, the parameters chosen for pattern generation and the operation of the genetic algorithm itself will define which traits can be subject to selection, so these should be carefully selected when designing experiments, and considered when interpreting results. However, acknowledging these limitations, the printing techniques shown here are relatively flexible, as they can be creatively applied to a range of materials, allowing for potential manipulation of UV reflectance if required. In addition, while CamoEvo’s built-in tools provide comparisons between target and background colours for human vision only, physical targets and backgrounds can be photographed post-hoc, and image analyses applied to estimate visual contrasts accounting for the full range of predator vision. These methods should therefore facilitate the application of virtual evolution to investigating a wide range of visual pattern types and functions. In particular, identifying optimal camouflage patterns remains a key question, with many practical applications. Recent studies tackling this problem have gained useful insights by defining optimal patterns based on the statistical properties of the background [ 41 ], or using deep learning to extrapolate from human responses to a limited set of phenotypes [ 3 ]. Yet genetic algorithms present an especially powerful tool for studying camouflage optimisation, particularly when paired with machine learning techniques [ 10 , 11 ]. Crucially, they account for the effects of repeated interactions between predators and prey, though implementation in screen-based experiments restricts their interpretation. Associating these tools with predation experiments in the field provides opportunities to investigate optimal camouflage while simultaneously considering a wider range of influential factors, from background properties and viewing conditions to predator vision, learning and prey population dynamics. Beyond camouflage, field-based evolutionary approaches supported by these tools could also provide valuable new insights into other forms of anti-predator colouration, such as aposematism or distant-dependent signalling.
Supporting information
https://doi.org/10.1371/journal.pone.0295106.s001
S1 File. SI1. Additional methodological information for case study field trials.
S1 Fig. Evidence of predation from a crow ( C . corone ) in run 1 (a), and a robin ( E . rubecula ), great tit ( P . major ) and blackbird ( T . merula ) in run 2 (b-d). Still frames extracted from footage captured by camera traps. SI 2. Additional information for screen-based search tasks. S4 Fig. Target image modification for screen-based replicate experiments. Unmodified target (left), and target as viewed by volunteers (right), after shadow and gloss layers have been applied to the target and a drop shadow has been applied to the background image. S5 Fig. Schematic of the screen-based search tasks, showing the targets in generation 0 and 15, for populations using the full and narrow colour spaces. Populations from both colour spaces were shown against either smooth or furrowed backgrounds, with three replicates per combination. Each replicate was completed by a single participant. For generation 15, each column of targets represents the six most successful targets (slowest to be found) for each replicate population. S6 Fig. Changes in camouflage metrics in the screen-based experimental populations and control replicates, using the narrow (a) and full (b) colour spaces. Plots show the mean and standard error of each metric per generation, coloured by population, along with predictions from the best statistical models. Coloured circles represent experimental populations, greyscale triangles control runs. Dotted lines represent population-level predictions; thicker lines show overall trends for fixed effects only. S1 Table. Model simplification tables for full models testing changes in metrics of camouflage efficacy in the screen-based experiment, for the narrow colour space. Models include population-level random slopes for generation, except for luminance and colour difference (a-b), edge disruption (e) and capture time (f). Models with a polynomial term for generation are fully simplified, then the usefulness of the 2 nd order polynomial term is tested with an ANOVA, comparing final models with and without the polynomial terms. Significant factors are highlighted in italics. S2 Table. Model simplification tables for full models testing changes in metrics of camouflage efficacy in the screen-based experiment, for the full colour space. Models include population-level random slopes for generation. Models with a polynomial term for generation are fully simplified, then the usefulness of the 2 nd order polynomial term is tested with an ANOVA, comparing final models with and without the polynomial terms. Significant factors are highlighted in italics. S3 Table. Model simplification tables for full models testing changes in metrics of camouflage efficacy between control and experimental populations of the screen experiment, for the narrow colour space. Models include population-level random slopes for generation, except for models of edge disruption. Models with a polynomial term for generation are fully simplified, then the usefulness of the 2 nd order polynomial term is tested with an ANOVA, comparing final models with and without the polynomial terms. Significant factors are highlighted in italics. S4 Table. Model simplification tables for full models testing changes in metrics of camouflage efficacy between control and experimental populations of the screen experiment, for the full colour space. Models include population-level random slopes for generation, except for models of edge disruption. Models with a polynomial term for generation are fully simplified, then the usefulness of the 2 nd order polynomial term is tested with an ANOVA, comparing final models with and without the polynomial terms. Significant factors are highlighted in italics.
https://doi.org/10.1371/journal.pone.0295106.s002
S1 Video. Example predations in field trials.
https://doi.org/10.1371/journal.pone.0295106.s003
Acknowledgments
We thank Juho Jolkkonen & Andrew Szopa-Comley for fieldwork assistance, all screen experiment volunteers, Will Allen for helpful comments on an earlier version, and two anonymous reviewers.
- View Article
- PubMed/NCBI
- Google Scholar
- 32. R Development Core Team. R: a language and environment for statistical computing. R Foundation for Statistical Computing. 2015. Available from: http://www.r-project.org

An official website of the United States government
The .gov means it’s official. Federal government websites often end in .gov or .mil. Before sharing sensitive information, make sure you’re on a federal government site.
The site is secure. The https:// ensures that you are connecting to the official website and that any information you provide is encrypted and transmitted securely.
- Publications
- Account settings
Preview improvements coming to the PMC website in October 2024. Learn More or Try it out now .
- Advanced Search
- Journal List
- BMC Med Res Methodol

The case study approach
Sarah crowe.
1 Division of Primary Care, The University of Nottingham, Nottingham, UK
Kathrin Cresswell
2 Centre for Population Health Sciences, The University of Edinburgh, Edinburgh, UK
Ann Robertson
3 School of Health in Social Science, The University of Edinburgh, Edinburgh, UK
Anthony Avery
Aziz sheikh.
The case study approach allows in-depth, multi-faceted explorations of complex issues in their real-life settings. The value of the case study approach is well recognised in the fields of business, law and policy, but somewhat less so in health services research. Based on our experiences of conducting several health-related case studies, we reflect on the different types of case study design, the specific research questions this approach can help answer, the data sources that tend to be used, and the particular advantages and disadvantages of employing this methodological approach. The paper concludes with key pointers to aid those designing and appraising proposals for conducting case study research, and a checklist to help readers assess the quality of case study reports.
Introduction
The case study approach is particularly useful to employ when there is a need to obtain an in-depth appreciation of an issue, event or phenomenon of interest, in its natural real-life context. Our aim in writing this piece is to provide insights into when to consider employing this approach and an overview of key methodological considerations in relation to the design, planning, analysis, interpretation and reporting of case studies.
The illustrative 'grand round', 'case report' and 'case series' have a long tradition in clinical practice and research. Presenting detailed critiques, typically of one or more patients, aims to provide insights into aspects of the clinical case and, in doing so, illustrate broader lessons that may be learnt. In research, the conceptually-related case study approach can be used, for example, to describe in detail a patient's episode of care, explore professional attitudes to and experiences of a new policy initiative or service development or more generally to 'investigate contemporary phenomena within its real-life context' [ 1 ]. Based on our experiences of conducting a range of case studies, we reflect on when to consider using this approach, discuss the key steps involved and illustrate, with examples, some of the practical challenges of attaining an in-depth understanding of a 'case' as an integrated whole. In keeping with previously published work, we acknowledge the importance of theory to underpin the design, selection, conduct and interpretation of case studies[ 2 ]. In so doing, we make passing reference to the different epistemological approaches used in case study research by key theoreticians and methodologists in this field of enquiry.
This paper is structured around the following main questions: What is a case study? What are case studies used for? How are case studies conducted? What are the potential pitfalls and how can these be avoided? We draw in particular on four of our own recently published examples of case studies (see Tables Tables1, 1 , ,2, 2 , ,3 3 and and4) 4 ) and those of others to illustrate our discussion[ 3 - 7 ].
Example of a case study investigating the reasons for differences in recruitment rates of minority ethnic people in asthma research[ 3 ]
Example of a case study investigating the process of planning and implementing a service in Primary Care Organisations[ 4 ]
Example of a case study investigating the introduction of the electronic health records[ 5 ]
Example of a case study investigating the formal and informal ways students learn about patient safety[ 6 ]
What is a case study?
A case study is a research approach that is used to generate an in-depth, multi-faceted understanding of a complex issue in its real-life context. It is an established research design that is used extensively in a wide variety of disciplines, particularly in the social sciences. A case study can be defined in a variety of ways (Table (Table5), 5 ), the central tenet being the need to explore an event or phenomenon in depth and in its natural context. It is for this reason sometimes referred to as a "naturalistic" design; this is in contrast to an "experimental" design (such as a randomised controlled trial) in which the investigator seeks to exert control over and manipulate the variable(s) of interest.
Definitions of a case study
Stake's work has been particularly influential in defining the case study approach to scientific enquiry. He has helpfully characterised three main types of case study: intrinsic , instrumental and collective [ 8 ]. An intrinsic case study is typically undertaken to learn about a unique phenomenon. The researcher should define the uniqueness of the phenomenon, which distinguishes it from all others. In contrast, the instrumental case study uses a particular case (some of which may be better than others) to gain a broader appreciation of an issue or phenomenon. The collective case study involves studying multiple cases simultaneously or sequentially in an attempt to generate a still broader appreciation of a particular issue.
These are however not necessarily mutually exclusive categories. In the first of our examples (Table (Table1), 1 ), we undertook an intrinsic case study to investigate the issue of recruitment of minority ethnic people into the specific context of asthma research studies, but it developed into a instrumental case study through seeking to understand the issue of recruitment of these marginalised populations more generally, generating a number of the findings that are potentially transferable to other disease contexts[ 3 ]. In contrast, the other three examples (see Tables Tables2, 2 , ,3 3 and and4) 4 ) employed collective case study designs to study the introduction of workforce reconfiguration in primary care, the implementation of electronic health records into hospitals, and to understand the ways in which healthcare students learn about patient safety considerations[ 4 - 6 ]. Although our study focusing on the introduction of General Practitioners with Specialist Interests (Table (Table2) 2 ) was explicitly collective in design (four contrasting primary care organisations were studied), is was also instrumental in that this particular professional group was studied as an exemplar of the more general phenomenon of workforce redesign[ 4 ].
What are case studies used for?
According to Yin, case studies can be used to explain, describe or explore events or phenomena in the everyday contexts in which they occur[ 1 ]. These can, for example, help to understand and explain causal links and pathways resulting from a new policy initiative or service development (see Tables Tables2 2 and and3, 3 , for example)[ 1 ]. In contrast to experimental designs, which seek to test a specific hypothesis through deliberately manipulating the environment (like, for example, in a randomised controlled trial giving a new drug to randomly selected individuals and then comparing outcomes with controls),[ 9 ] the case study approach lends itself well to capturing information on more explanatory ' how ', 'what' and ' why ' questions, such as ' how is the intervention being implemented and received on the ground?'. The case study approach can offer additional insights into what gaps exist in its delivery or why one implementation strategy might be chosen over another. This in turn can help develop or refine theory, as shown in our study of the teaching of patient safety in undergraduate curricula (Table (Table4 4 )[ 6 , 10 ]. Key questions to consider when selecting the most appropriate study design are whether it is desirable or indeed possible to undertake a formal experimental investigation in which individuals and/or organisations are allocated to an intervention or control arm? Or whether the wish is to obtain a more naturalistic understanding of an issue? The former is ideally studied using a controlled experimental design, whereas the latter is more appropriately studied using a case study design.
Case studies may be approached in different ways depending on the epistemological standpoint of the researcher, that is, whether they take a critical (questioning one's own and others' assumptions), interpretivist (trying to understand individual and shared social meanings) or positivist approach (orientating towards the criteria of natural sciences, such as focusing on generalisability considerations) (Table (Table6). 6 ). Whilst such a schema can be conceptually helpful, it may be appropriate to draw on more than one approach in any case study, particularly in the context of conducting health services research. Doolin has, for example, noted that in the context of undertaking interpretative case studies, researchers can usefully draw on a critical, reflective perspective which seeks to take into account the wider social and political environment that has shaped the case[ 11 ].
Example of epistemological approaches that may be used in case study research
How are case studies conducted?
Here, we focus on the main stages of research activity when planning and undertaking a case study; the crucial stages are: defining the case; selecting the case(s); collecting and analysing the data; interpreting data; and reporting the findings.
Defining the case
Carefully formulated research question(s), informed by the existing literature and a prior appreciation of the theoretical issues and setting(s), are all important in appropriately and succinctly defining the case[ 8 , 12 ]. Crucially, each case should have a pre-defined boundary which clarifies the nature and time period covered by the case study (i.e. its scope, beginning and end), the relevant social group, organisation or geographical area of interest to the investigator, the types of evidence to be collected, and the priorities for data collection and analysis (see Table Table7 7 )[ 1 ]. A theory driven approach to defining the case may help generate knowledge that is potentially transferable to a range of clinical contexts and behaviours; using theory is also likely to result in a more informed appreciation of, for example, how and why interventions have succeeded or failed[ 13 ].
Example of a checklist for rating a case study proposal[ 8 ]
For example, in our evaluation of the introduction of electronic health records in English hospitals (Table (Table3), 3 ), we defined our cases as the NHS Trusts that were receiving the new technology[ 5 ]. Our focus was on how the technology was being implemented. However, if the primary research interest had been on the social and organisational dimensions of implementation, we might have defined our case differently as a grouping of healthcare professionals (e.g. doctors and/or nurses). The precise beginning and end of the case may however prove difficult to define. Pursuing this same example, when does the process of implementation and adoption of an electronic health record system really begin or end? Such judgements will inevitably be influenced by a range of factors, including the research question, theory of interest, the scope and richness of the gathered data and the resources available to the research team.
Selecting the case(s)
The decision on how to select the case(s) to study is a very important one that merits some reflection. In an intrinsic case study, the case is selected on its own merits[ 8 ]. The case is selected not because it is representative of other cases, but because of its uniqueness, which is of genuine interest to the researchers. This was, for example, the case in our study of the recruitment of minority ethnic participants into asthma research (Table (Table1) 1 ) as our earlier work had demonstrated the marginalisation of minority ethnic people with asthma, despite evidence of disproportionate asthma morbidity[ 14 , 15 ]. In another example of an intrinsic case study, Hellstrom et al.[ 16 ] studied an elderly married couple living with dementia to explore how dementia had impacted on their understanding of home, their everyday life and their relationships.
For an instrumental case study, selecting a "typical" case can work well[ 8 ]. In contrast to the intrinsic case study, the particular case which is chosen is of less importance than selecting a case that allows the researcher to investigate an issue or phenomenon. For example, in order to gain an understanding of doctors' responses to health policy initiatives, Som undertook an instrumental case study interviewing clinicians who had a range of responsibilities for clinical governance in one NHS acute hospital trust[ 17 ]. Sampling a "deviant" or "atypical" case may however prove even more informative, potentially enabling the researcher to identify causal processes, generate hypotheses and develop theory.
In collective or multiple case studies, a number of cases are carefully selected. This offers the advantage of allowing comparisons to be made across several cases and/or replication. Choosing a "typical" case may enable the findings to be generalised to theory (i.e. analytical generalisation) or to test theory by replicating the findings in a second or even a third case (i.e. replication logic)[ 1 ]. Yin suggests two or three literal replications (i.e. predicting similar results) if the theory is straightforward and five or more if the theory is more subtle. However, critics might argue that selecting 'cases' in this way is insufficiently reflexive and ill-suited to the complexities of contemporary healthcare organisations.
The selected case study site(s) should allow the research team access to the group of individuals, the organisation, the processes or whatever else constitutes the chosen unit of analysis for the study. Access is therefore a central consideration; the researcher needs to come to know the case study site(s) well and to work cooperatively with them. Selected cases need to be not only interesting but also hospitable to the inquiry [ 8 ] if they are to be informative and answer the research question(s). Case study sites may also be pre-selected for the researcher, with decisions being influenced by key stakeholders. For example, our selection of case study sites in the evaluation of the implementation and adoption of electronic health record systems (see Table Table3) 3 ) was heavily influenced by NHS Connecting for Health, the government agency that was responsible for overseeing the National Programme for Information Technology (NPfIT)[ 5 ]. This prominent stakeholder had already selected the NHS sites (through a competitive bidding process) to be early adopters of the electronic health record systems and had negotiated contracts that detailed the deployment timelines.
It is also important to consider in advance the likely burden and risks associated with participation for those who (or the site(s) which) comprise the case study. Of particular importance is the obligation for the researcher to think through the ethical implications of the study (e.g. the risk of inadvertently breaching anonymity or confidentiality) and to ensure that potential participants/participating sites are provided with sufficient information to make an informed choice about joining the study. The outcome of providing this information might be that the emotive burden associated with participation, or the organisational disruption associated with supporting the fieldwork, is considered so high that the individuals or sites decide against participation.
In our example of evaluating implementations of electronic health record systems, given the restricted number of early adopter sites available to us, we sought purposively to select a diverse range of implementation cases among those that were available[ 5 ]. We chose a mixture of teaching, non-teaching and Foundation Trust hospitals, and examples of each of the three electronic health record systems procured centrally by the NPfIT. At one recruited site, it quickly became apparent that access was problematic because of competing demands on that organisation. Recognising the importance of full access and co-operative working for generating rich data, the research team decided not to pursue work at that site and instead to focus on other recruited sites.
Collecting the data
In order to develop a thorough understanding of the case, the case study approach usually involves the collection of multiple sources of evidence, using a range of quantitative (e.g. questionnaires, audits and analysis of routinely collected healthcare data) and more commonly qualitative techniques (e.g. interviews, focus groups and observations). The use of multiple sources of data (data triangulation) has been advocated as a way of increasing the internal validity of a study (i.e. the extent to which the method is appropriate to answer the research question)[ 8 , 18 - 21 ]. An underlying assumption is that data collected in different ways should lead to similar conclusions, and approaching the same issue from different angles can help develop a holistic picture of the phenomenon (Table (Table2 2 )[ 4 ].
Brazier and colleagues used a mixed-methods case study approach to investigate the impact of a cancer care programme[ 22 ]. Here, quantitative measures were collected with questionnaires before, and five months after, the start of the intervention which did not yield any statistically significant results. Qualitative interviews with patients however helped provide an insight into potentially beneficial process-related aspects of the programme, such as greater, perceived patient involvement in care. The authors reported how this case study approach provided a number of contextual factors likely to influence the effectiveness of the intervention and which were not likely to have been obtained from quantitative methods alone.
In collective or multiple case studies, data collection needs to be flexible enough to allow a detailed description of each individual case to be developed (e.g. the nature of different cancer care programmes), before considering the emerging similarities and differences in cross-case comparisons (e.g. to explore why one programme is more effective than another). It is important that data sources from different cases are, where possible, broadly comparable for this purpose even though they may vary in nature and depth.
Analysing, interpreting and reporting case studies
Making sense and offering a coherent interpretation of the typically disparate sources of data (whether qualitative alone or together with quantitative) is far from straightforward. Repeated reviewing and sorting of the voluminous and detail-rich data are integral to the process of analysis. In collective case studies, it is helpful to analyse data relating to the individual component cases first, before making comparisons across cases. Attention needs to be paid to variations within each case and, where relevant, the relationship between different causes, effects and outcomes[ 23 ]. Data will need to be organised and coded to allow the key issues, both derived from the literature and emerging from the dataset, to be easily retrieved at a later stage. An initial coding frame can help capture these issues and can be applied systematically to the whole dataset with the aid of a qualitative data analysis software package.
The Framework approach is a practical approach, comprising of five stages (familiarisation; identifying a thematic framework; indexing; charting; mapping and interpretation) , to managing and analysing large datasets particularly if time is limited, as was the case in our study of recruitment of South Asians into asthma research (Table (Table1 1 )[ 3 , 24 ]. Theoretical frameworks may also play an important role in integrating different sources of data and examining emerging themes. For example, we drew on a socio-technical framework to help explain the connections between different elements - technology; people; and the organisational settings within which they worked - in our study of the introduction of electronic health record systems (Table (Table3 3 )[ 5 ]. Our study of patient safety in undergraduate curricula drew on an evaluation-based approach to design and analysis, which emphasised the importance of the academic, organisational and practice contexts through which students learn (Table (Table4 4 )[ 6 ].
Case study findings can have implications both for theory development and theory testing. They may establish, strengthen or weaken historical explanations of a case and, in certain circumstances, allow theoretical (as opposed to statistical) generalisation beyond the particular cases studied[ 12 ]. These theoretical lenses should not, however, constitute a strait-jacket and the cases should not be "forced to fit" the particular theoretical framework that is being employed.
When reporting findings, it is important to provide the reader with enough contextual information to understand the processes that were followed and how the conclusions were reached. In a collective case study, researchers may choose to present the findings from individual cases separately before amalgamating across cases. Care must be taken to ensure the anonymity of both case sites and individual participants (if agreed in advance) by allocating appropriate codes or withholding descriptors. In the example given in Table Table3, 3 , we decided against providing detailed information on the NHS sites and individual participants in order to avoid the risk of inadvertent disclosure of identities[ 5 , 25 ].
What are the potential pitfalls and how can these be avoided?
The case study approach is, as with all research, not without its limitations. When investigating the formal and informal ways undergraduate students learn about patient safety (Table (Table4), 4 ), for example, we rapidly accumulated a large quantity of data. The volume of data, together with the time restrictions in place, impacted on the depth of analysis that was possible within the available resources. This highlights a more general point of the importance of avoiding the temptation to collect as much data as possible; adequate time also needs to be set aside for data analysis and interpretation of what are often highly complex datasets.
Case study research has sometimes been criticised for lacking scientific rigour and providing little basis for generalisation (i.e. producing findings that may be transferable to other settings)[ 1 ]. There are several ways to address these concerns, including: the use of theoretical sampling (i.e. drawing on a particular conceptual framework); respondent validation (i.e. participants checking emerging findings and the researcher's interpretation, and providing an opinion as to whether they feel these are accurate); and transparency throughout the research process (see Table Table8 8 )[ 8 , 18 - 21 , 23 , 26 ]. Transparency can be achieved by describing in detail the steps involved in case selection, data collection, the reasons for the particular methods chosen, and the researcher's background and level of involvement (i.e. being explicit about how the researcher has influenced data collection and interpretation). Seeking potential, alternative explanations, and being explicit about how interpretations and conclusions were reached, help readers to judge the trustworthiness of the case study report. Stake provides a critique checklist for a case study report (Table (Table9 9 )[ 8 ].
Potential pitfalls and mitigating actions when undertaking case study research
Stake's checklist for assessing the quality of a case study report[ 8 ]
Conclusions
The case study approach allows, amongst other things, critical events, interventions, policy developments and programme-based service reforms to be studied in detail in a real-life context. It should therefore be considered when an experimental design is either inappropriate to answer the research questions posed or impossible to undertake. Considering the frequency with which implementations of innovations are now taking place in healthcare settings and how well the case study approach lends itself to in-depth, complex health service research, we believe this approach should be more widely considered by researchers. Though inherently challenging, the research case study can, if carefully conceptualised and thoughtfully undertaken and reported, yield powerful insights into many important aspects of health and healthcare delivery.
Competing interests
The authors declare that they have no competing interests.
Authors' contributions
AS conceived this article. SC, KC and AR wrote this paper with GH, AA and AS all commenting on various drafts. SC and AS are guarantors.
Pre-publication history
The pre-publication history for this paper can be accessed here:
http://www.biomedcentral.com/1471-2288/11/100/prepub
Acknowledgements
We are grateful to the participants and colleagues who contributed to the individual case studies that we have drawn on. This work received no direct funding, but it has been informed by projects funded by Asthma UK, the NHS Service Delivery Organisation, NHS Connecting for Health Evaluation Programme, and Patient Safety Research Portfolio. We would also like to thank the expert reviewers for their insightful and constructive feedback. Our thanks are also due to Dr. Allison Worth who commented on an earlier draft of this manuscript.
- Yin RK. Case study research, design and method. 4. London: Sage Publications Ltd.; 2009. [ Google Scholar ]
- Keen J, Packwood T. Qualitative research; case study evaluation. BMJ. 1995; 311 :444–446. [ PMC free article ] [ PubMed ] [ Google Scholar ]
- Sheikh A, Halani L, Bhopal R, Netuveli G, Partridge M, Car J. et al. Facilitating the Recruitment of Minority Ethnic People into Research: Qualitative Case Study of South Asians and Asthma. PLoS Med. 2009; 6 (10):1–11. [ PMC free article ] [ PubMed ] [ Google Scholar ]
- Pinnock H, Huby G, Powell A, Kielmann T, Price D, Williams S, The process of planning, development and implementation of a General Practitioner with a Special Interest service in Primary Care Organisations in England and Wales: a comparative prospective case study. Report for the National Co-ordinating Centre for NHS Service Delivery and Organisation R&D (NCCSDO) 2008. http://www.sdo.nihr.ac.uk/files/project/99-final-report.pdf
- Robertson A, Cresswell K, Takian A, Petrakaki D, Crowe S, Cornford T. et al. Prospective evaluation of the implementation and adoption of NHS Connecting for Health's national electronic health record in secondary care in England: interim findings. BMJ. 2010; 41 :c4564. [ PMC free article ] [ PubMed ] [ Google Scholar ]
- Pearson P, Steven A, Howe A, Sheikh A, Ashcroft D, Smith P. the Patient Safety Education Study Group. Learning about patient safety: organisational context and culture in the education of healthcare professionals. J Health Serv Res Policy. 2010; 15 :4–10. doi: 10.1258/jhsrp.2009.009052. [ PubMed ] [ CrossRef ] [ Google Scholar ]
- van Harten WH, Casparie TF, Fisscher OA. The evaluation of the introduction of a quality management system: a process-oriented case study in a large rehabilitation hospital. Health Policy. 2002; 60 (1):17–37. doi: 10.1016/S0168-8510(01)00187-7. [ PubMed ] [ CrossRef ] [ Google Scholar ]
- Stake RE. The art of case study research. London: Sage Publications Ltd.; 1995. [ Google Scholar ]
- Sheikh A, Smeeth L, Ashcroft R. Randomised controlled trials in primary care: scope and application. Br J Gen Pract. 2002; 52 (482):746–51. [ PMC free article ] [ PubMed ] [ Google Scholar ]
- King G, Keohane R, Verba S. Designing Social Inquiry. Princeton: Princeton University Press; 1996. [ Google Scholar ]
- Doolin B. Information technology as disciplinary technology: being critical in interpretative research on information systems. Journal of Information Technology. 1998; 13 :301–311. doi: 10.1057/jit.1998.8. [ CrossRef ] [ Google Scholar ]
- George AL, Bennett A. Case studies and theory development in the social sciences. Cambridge, MA: MIT Press; 2005. [ Google Scholar ]
- Eccles M. the Improved Clinical Effectiveness through Behavioural Research Group (ICEBeRG) Designing theoretically-informed implementation interventions. Implementation Science. 2006; 1 :1–8. doi: 10.1186/1748-5908-1-1. [ PMC free article ] [ PubMed ] [ CrossRef ] [ Google Scholar ]
- Netuveli G, Hurwitz B, Levy M, Fletcher M, Barnes G, Durham SR, Sheikh A. Ethnic variations in UK asthma frequency, morbidity, and health-service use: a systematic review and meta-analysis. Lancet. 2005; 365 (9456):312–7. [ PubMed ] [ Google Scholar ]
- Sheikh A, Panesar SS, Lasserson T, Netuveli G. Recruitment of ethnic minorities to asthma studies. Thorax. 2004; 59 (7):634. [ PMC free article ] [ PubMed ] [ Google Scholar ]
- Hellström I, Nolan M, Lundh U. 'We do things together': A case study of 'couplehood' in dementia. Dementia. 2005; 4 :7–22. doi: 10.1177/1471301205049188. [ CrossRef ] [ Google Scholar ]
- Som CV. Nothing seems to have changed, nothing seems to be changing and perhaps nothing will change in the NHS: doctors' response to clinical governance. International Journal of Public Sector Management. 2005; 18 :463–477. doi: 10.1108/09513550510608903. [ CrossRef ] [ Google Scholar ]
- Lincoln Y, Guba E. Naturalistic inquiry. Newbury Park: Sage Publications; 1985. [ Google Scholar ]
- Barbour RS. Checklists for improving rigour in qualitative research: a case of the tail wagging the dog? BMJ. 2001; 322 :1115–1117. doi: 10.1136/bmj.322.7294.1115. [ PMC free article ] [ PubMed ] [ CrossRef ] [ Google Scholar ]
- Mays N, Pope C. Qualitative research in health care: Assessing quality in qualitative research. BMJ. 2000; 320 :50–52. doi: 10.1136/bmj.320.7226.50. [ PMC free article ] [ PubMed ] [ CrossRef ] [ Google Scholar ]
- Mason J. Qualitative researching. London: Sage; 2002. [ Google Scholar ]
- Brazier A, Cooke K, Moravan V. Using Mixed Methods for Evaluating an Integrative Approach to Cancer Care: A Case Study. Integr Cancer Ther. 2008; 7 :5–17. doi: 10.1177/1534735407313395. [ PubMed ] [ CrossRef ] [ Google Scholar ]
- Miles MB, Huberman M. Qualitative data analysis: an expanded sourcebook. 2. CA: Sage Publications Inc.; 1994. [ Google Scholar ]
- Pope C, Ziebland S, Mays N. Analysing qualitative data. Qualitative research in health care. BMJ. 2000; 320 :114–116. doi: 10.1136/bmj.320.7227.114. [ PMC free article ] [ PubMed ] [ CrossRef ] [ Google Scholar ]
- Cresswell KM, Worth A, Sheikh A. Actor-Network Theory and its role in understanding the implementation of information technology developments in healthcare. BMC Med Inform Decis Mak. 2010; 10 (1):67. doi: 10.1186/1472-6947-10-67. [ PMC free article ] [ PubMed ] [ CrossRef ] [ Google Scholar ]
- Malterud K. Qualitative research: standards, challenges, and guidelines. Lancet. 2001; 358 :483–488. doi: 10.1016/S0140-6736(01)05627-6. [ PubMed ] [ CrossRef ] [ Google Scholar ]
- Yin R. Case study research: design and methods. 2. Thousand Oaks, CA: Sage Publishing; 1994. [ Google Scholar ]
- Yin R. Enhancing the quality of case studies in health services research. Health Serv Res. 1999; 34 :1209–1224. [ PMC free article ] [ PubMed ] [ Google Scholar ]
- Green J, Thorogood N. Qualitative methods for health research. 2. Los Angeles: Sage; 2009. [ Google Scholar ]
- Howcroft D, Trauth E. Handbook of Critical Information Systems Research, Theory and Application. Cheltenham, UK: Northampton, MA, USA: Edward Elgar; 2005. [ Google Scholar ]
- Blakie N. Approaches to Social Enquiry. Cambridge: Polity Press; 1993. [ Google Scholar ]
- Doolin B. Power and resistance in the implementation of a medical management information system. Info Systems J. 2004; 14 :343–362. doi: 10.1111/j.1365-2575.2004.00176.x. [ CrossRef ] [ Google Scholar ]
- Bloomfield BP, Best A. Management consultants: systems development, power and the translation of problems. Sociological Review. 1992; 40 :533–560. [ Google Scholar ]
- Shanks G, Parr A. Proceedings of the European Conference on Information Systems. Naples; 2003. Positivist, single case study research in information systems: A critical analysis. [ Google Scholar ]
To read this content please select one of the options below:
Please note you do not have access to teaching notes, development of a dedicated process simulator for the digital twin in apparel manufacturing: a case study.
International Journal of Clothing Science and Technology
ISSN : 0955-6222
Article publication date: 16 May 2024
The purpose of this study is to introduce a dedicated simulator to automatically generate and simulate a balanced apparel assembly line, which is critical to the digital twin concept in apparel manufacturing. Given the low automation level in apparel manufacturing, this is a first step toward the implementation of a smart factory based on cyber-physical systems.
Design/methodology/approach
The mixed task assignment algorithm was implemented to automatically generate a module-based apparel assembly line in the developed simulator. To validate the developed simulator, a case study was conducted using process analysis data of technical jackets obtained from an apparel manufacturer. The case study included three scenarios: calculating the number of workers, selecting orders based on factory capacity and managing unexpected worker absences.
The developed simulator is approximately 97.2% accurate in assigning appropriate tasks to workstations using the mixed task assignment algorithm. The simulator was also found to be effective in supporting decision-making for production planning, order selection and apparel assembly line management. In addition, the module-based line generation algorithm made it easy to modify the assembly line.
Originality/value
This study contributes a novel approach to address the challenge of low automation levels in apparel manufacturing by introducing a dedicated simulator. This dedicated simulator improves the efficiency of virtual apparel assembly line generation and simulation, which distinguishes it from existing commercial simulation software.
- Task assignment algorithm
- Line balancing
- Apparel assembly line
- Automatic assembly line generation
- Apparel manufacturing
- Digital twin
Kim, M. and Kim, S. (2024), "Development of a dedicated process simulator for the digital twin in apparel manufacturing: a case study", International Journal of Clothing Science and Technology , Vol. ahead-of-print No. ahead-of-print. https://doi.org/10.1108/IJCST-01-2024-0017
Emerald Publishing Limited
Copyright © 2024, Emerald Publishing Limited
Related articles
We’re listening — tell us what you think, something didn’t work….
Report bugs here
All feedback is valuable
Please share your general feedback
Join us on our journey
Platform update page.
Visit emeraldpublishing.com/platformupdate to discover the latest news and updates
Questions & More Information
Answers to the most commonly asked questions here
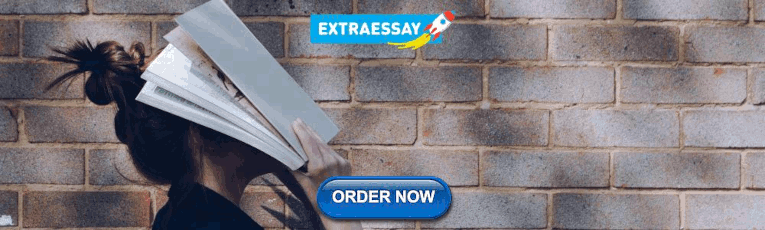
IMAGES
VIDEO
COMMENTS
Hence attention to purposive modes of sampling is needed. Yet, while the existing qualitative literature on case selection offers a wide range of suggestions for case selection, most techniques discussed require in-depth familiarity of each case. Seven case selection procedures are considered, each of which facilitates a different strategy for ...
While each of these techniques is normally practiced on one or several cases (the diverse, most‐similar, and most‐different methods require at least two), all may employ additional cases—with the proviso that, at some point, they will no longer offer an opportunity for in‐depth analysis and will thus no longer be "case studies" in the usual sense (Gerring 2007, ch. 2).
For case-study analysis, it is often the rareness of the value that makes a case valuable, not its positive or negative value (contrast Emigh 1997; Mahoney and Goertz 2004; Ragin 2000: 60; Ragin 2004: 126). Large-N Analysis. As we have said, extreme cases lie far from the mean of a variable. _.
This chapter discusses quantitative and qualitative practices of case-study selection when the goal of the analysis is to evaluate causal hypotheses. More specifically, it considers how the different causal models used in the qualitative and quantitative research cultures shape the kind of cases that provide the most leverage for hypothesis ...
Case-selection and qualitative comparisons. Methodological advice on the selection of cases in qualitative research stands in a long tradition. John Stuart Mill in his A System of Logic, first published in 1843, proposed five methods meant to enable researchers to make causal inferences: the method of agreement, the method of difference, the double method of agreement and difference, the ...
7.2.1 Case Selection Before the Processual Turn . Before "process tracing" entered the lexicon of social scientists, the dominant case selection strategy in case study research sought to maximize causal leverage via comparison, particularly via the "methods of agreement and difference" of John Stuart Reference Mill Mill (1843 [1974]: 388-391).
McMaster University, West Hamilton, Ontario, Canada. Qualitative case study methodology prov ides tools for researchers to study. complex phenomena within their contexts. When the approach is ...
motivates most similar case selection strategies and the process tracing anal-ysis that typically follows. I then give an overview of statistical matching methods. Because most existing matching algorithms are not ideally suited for qualitative case selection, I explain which methods can be most usefully adapted to the needs of case study analysts.
A case study is a detailed study of a specific subject, such as a person, group, place, event, organization, or phenomenon. Case studies are commonly used in social, educational, clinical, and business research. A case study research design usually involves qualitative methods, but quantitative methods are sometimes also used.
Design/methodology/approach This study used a case study of the Songkran festival in Chiang Mai to examine the dilemma between cultural rituals and hedonism for tourism, which brings lost or ...
A case study is a research approach that is used to generate an in-depth, multi-faceted understanding of a complex issue in its real-life context. It is an established research design that is used extensively in a wide variety of disciplines, particularly in the social sciences. A case study can be defined in a variety of ways (Table 5 ), the ...
An examination of case selection in a prominent study of rebel behaviour in civil war is then used to illustrate different applications of the Case Selector. ... Maoz, Z. (2002) 'Case Study Methodology in International Studies: From Storytelling to Hypothesis Testing', Evaluating Methodology in International Studies, ...
Finally, the selection of cases selected may be driven by the type of case study that is utilized. In this approach, cases are selected as they allow researchers to compare their similarities or their differences. Or, a case might be selected that is typical of most cases, or in contrast, a case or cases that deviate from the norm.
Current qualitative case study approaches are shaped by paradigm, study design, and selection of methods, and, as a result, case studies in the published literature vary. Differences between published case studies can make it difficult for researchers to define and understand case study as a methodology.
A good research question guides the direction of the study and informs the selection of the case, the methods of collecting data, and the analysis techniques. A well-formulated research question in case study research is typically clear, focused, and complex enough to merit further detailed examination of the relevant case(s). Propositions
Keywords comparative method; case selection; software; qualitative methods I n his seminal article on the compara-tive method, Arend Lijphart (1971) identifies and discusses four chal-lenges in the application of the compara-tive method to the study of politics. First, he critiques the discipline for limited methodological awareness. Second, he
Selection of one case or only a few cases. If the researcher wants to explore a topic, or further develop an existing and well-tested theory several other case selection options are available. If the aim is exploration cases in single case studies are selected on the basis of the information they are expected to provide. Single cases can be ...
Case studies: A. SAT scores by state. B. Deeper dive into sex discrimination in base salary. Model selection. Best subsets. Stepwise methods. Case study A: State SAT scores. Predicting average 1982 SAT score by state. Features: Takers (percentage) Income (parental income of SAT takers) Years (years of education in key subjects)
A system and method is provided for processing a whole slide image, WSI, of a biopsy such as a core needle biopsy or a vacuum assisted biopsy. A skeleton of the shape of the detected tissue is created and a skeleton path is determined. ... Value of Real-World Evidence for Treatment Selection: A Case Study in Colon Cancer. JCO Clin Cancer Inform ...
Falconer and Freeman studies were foundational sources for item selection. Additionally, four case studies were written to reflect the most recent anticipated changes to MAiD legislation and all used the same open-ended core questions to address respondents' perspectives about the patient's right to make the decision, comfort in assisting a ...
The study utilized three statistical methods (z-score, straight section in a series, and Jenk's natural breaks) to identify reference counties in Ohio with normal case-population relationships to build a model to estimate case counts in target counties with unrecruited cases. The researchers believe that this method has advantages over ...
Therefore, this case-control study aims to examine the link between plaque psoriasis and both MASLD and lean MASLD, seeking to identify potential serum-specific markers in patients with plaque psoriasis affected by MASLD or lean MASLD and clarify the potential mechanisms of their interaction. 2 Methods 2.1 Selection of patients
In this study, we have selected 6 criteria and 6 online popular job portals for recruitment with a sample of 15 candidates have been selected. Findings reveal that, AHP method has significant results on the selection of best employer, which helps HR Manager to finalize the decision making process/strategies.
Introduction. Camouflage is a common anti-predator defence strategy, with a long history as a case study for evolution [].A vast body of work has investigated how visual patterns help conceal prey from predators, identifying a range of strategies from disruptive coloration and countershading to masquerade and iridescence [1,2].Understanding which patterns produce the most effective protection ...
A case study is a research approach that is used to generate an in-depth, multi-faceted understanding of a complex issue in its real-life context. It is an established research design that is used extensively in a wide variety of disciplines, particularly in the social sciences. A case study can be defined in a variety of ways (Table.
Design/methodology/approach. The mixed task assignment algorithm was implemented to automatically generate a module-based apparel assembly line in the developed simulator. To validate the developed simulator, a case study was conducted using process analysis data of technical jackets obtained from an apparel manufacturer.